Notes
Article history
The research reported in this issue of the journal was funded by the EME programme as project number 11/30/11. The contractual start date was in April 2013. The final report began editorial review in April 2019 and was accepted for publication in November 2019. The authors have been wholly responsible for all data collection, analysis and interpretation, and for writing up their work. The EME editors and production house have tried to ensure the accuracy of the authors’ report and would like to thank the reviewers for their constructive comments on the final report document. However, they do not accept liability for damages or losses arising from material published in this report.
Declared competing interests of authors
Marie Braisher has received funding from the UK MS Society and the National Institute for Health Research (NIHR) Local Clinical Research Network. Ferran Prados receives a Guarantors of Brain fellowship. Sebastien Ourselin receives funding from the Engineering and Physical Sciences Research Council (EP/H046410/1, EP/J020990/1, EP/K005278), the Medical Research Council (MR/J01107X/1), the European Union’s Seventh Framework Programme for Research (FP7-ICT-2011-9-601055) and NIHR University College London Hospitals Biomedical Research Centre (BW.mn. BRC10269). Frederik Barkhof serves on the editorial boards of Brain, European Radiology, Journal of Neurology, Neurosurgery and Psychiatry, Neurology, Multiple Sclerosis and Neuroradiology, and serves as a consultant for Bayer Schering Pharma AG (Berlin, Germany), Sanofi-Aventis (Paris, France), Biogen-Idec Limited (Maidenhead, UK), TEVA Pharmaceutical (Petah Tikva, Israel), Genzyme (Cambridge, MA, USA), Merck Serono (Darmstadt, Germany), Novartis International AG (Basel, Switzerland), F. Hoffmann-La Roche Ltd (Basel, Switzerland), Synthon (Nijmegen, the Netherlands), Janssen Research (Beerse, Belgium) and H. Lundbeck A/S (Copenhagen, Denmark). Claudia AM Gandini Wheeler-Kingshott has received research grants (principal investigator and co-applicant) from Spinal Research (London, UK), Craig H. Neilsen Foundation (Encino, CA, USA), Engineering and Physical Sciences Research Council (Swindon, UK), Wings for Life-International (Salzburg, Austria), UK MS Society (London, UK), Horizon 2020, University College London Hospital and Biomedical Research Centre. Sharmilee Gnanapavan has received honoraria and meeting support from Biogen-Idec Limited, Novartis International AG, TEVA Pharmaceutical, Genzyme and research funds from Genzyme. Gavin Giovannoni is a steering committee member on the daclizumab trials for AbbVie, the BG12 and daclizumab trials for Biogen-Idec Limited, the fingolimod and siponimod trials for Novartis International AG, the laquinimod trials for TEVA Pharmaceutical and the ocrelizumab trials for F. Hoffmann-La Roche Ltd. He has also received consultancy fees for advisory board meetings for oral cladribine trials for Merck Serono, Sanofi Genzyme (Cambridge, MA, USA) and in relation to Data and Safety Monitoring Board activities for Synthon BV (Nijmegen, the Netherlands), as well as honoraria for speaking at the Physicians Summit and several medical education meetings. He is also the co-chief editor of Multiple Sclerosis and Related Disorders (Elsevier, Amsterdam, the Netherlands). In the last 3 years, Jeremy Chataway has received support from the Efficacy and Mechanism Evaluation (EME) programme, a Medical Research Council (MRC) and NIHR partnership, and the Health Technology Assessment (HTA) programme (NIHR), the UK MS Society, the US National MS Society and the Rosetrees Trust (London UK). He is supported in part by NIHR University College London Hospitals, Biomedical Research Centre, London, UK. He has been a local principal investigator for a trial in multiple sclerosis funded by the Canadian MS Society; he has been a local principal investigator for commercial trials funded by Actelion (Allschwil, Switzerland), Biogen-Idec Limited, Novartis International AG and F. Hoffmann-La Roche Ltd; he has received an investigator grant from Novartis International AG; and he has taken part in advisory boards/consultancy for Azadyne Ltd (Canterbury, UK), Biogen-Idec Limited, Celgene Corporation (Summit, NJ, USA), MedDay SA (Paris, France), Merck & Co. Inc. (Kenilworth, NJ, USA) and F. Hoffmann-La Roche Ltd.
Permissions
Copyright statement
© Queen’s Printer and Controller of HMSO 2020. This work was produced by De Angelis et al. under the terms of a commissioning contract issued by the Secretary of State for Health and Social Care. This issue may be freely reproduced for the purposes of private research and study and extracts (or indeed, the full report) may be included in professional journals provided that suitable acknowledgement is made and the reproduction is not associated with any form of advertising. Applications for commercial reproduction should be addressed to: NIHR Journals Library, National Institute for Health Research, Evaluation, Trials and Studies Coordinating Centre, Alpha House, University of Southampton Science Park, Southampton SO16 7NS, UK.
2020 Queen’s Printer and Controller of HMSO
Chapter 1 Introduction
Multiple sclerosis (MS) is an immune-mediated demyelinating disease of the central nervous system (CNS) with a reported prevalence of between 1 in 500 and 1 in 1500 in Europe, North America and Australasia, and represents the commonest non-traumatic cause of disability affecting young adults in temperate latitudes. 1 Approximately 120,000 people in the UK and 2.5 million people globally have MS. 2 MS generally starts with a relapsing–remitting clinical course, characterised by relapses, that is episodes of neurological dysfunction lasting at least 24 hours in the absence of infection, followed by varying degrees of recovery or remission. 3 After a mean of 10–15 years, most of the relapsing–remitting multiple sclerosis (RRMS) patients enter into a phase characterised by gradual progression of disability, called secondary progressive multiple sclerosis (SPMS). The underlying mechanisms of this secondary progression of MS are complex and still unclear; however, progressive neuroaxonal loss or neurodegeneration plays a major role in the accumulation of irreversible disability. 4–12 People with SPMS may experience severe limitations as a result of symptoms affecting walking, balance, vision, cognition, pain control, and bladder and bowel function. 7
The disease-modifying therapies (DMTs) available for RRMS have immunomodulatory and/or anti-inflammatory properties. They are effective in reducing relapse rate and the number of new lesions as detected by magnetic resonance imaging (MRI), and they can also delay the disability progression and the rate of conversion to SPMS. Unlike RRMS, for which an increasing number of DMTs have been being developed, and with the recent exception of siponimod (which has modest effectiveness13) there is a paucity of disease modification in SPMS. 14,15
Mechanistic processes underlying neurodegeneration in secondary progressive multiple sclerosis
In MS, the mechanisms of neurodegeneration may overlap with other conditions, and it is speculated that there are central neurodegenerative paths common to other neurodegenerative diseases, including Alzheimer’s disease, Parkinson’s disease and motor neuron disease. However, it should be highlighted that the pathology of SPMS has distinctive features: oligodendrocyte loss/demyelination, energy failure, glial (astrocyte/microglial)-mediated production of reactive oxygen/nitric oxide species, and excitotoxicity. 16
In demyelinated plaques, the pathological hallmark of MS, axons lack trophic support mediated by oligodendrocytes and become vulnerable to injury or physiological stress. Furthermore, demyelinated axons show a significant redistribution of sodium channels, which were previously restricted to the nodes of Ranvier. This adaptive response requires neurons to spend more energy to sustain electrical transmission, leading to a circumstance known as ‘energy failure’. 17–19 This is the result of an imbalance between energy demand and energy production in the demyelinated axons and results in an increased intracellular calcium influx, which is toxic to the axon. Axonal energy production is faulty as a result of mitochondrial damage mostly triggered by the production of reactive oxygen and nitric oxide species by activated microglia and macrophages. 20,21 In MS-derived tissue samples and in experimental models of MS, lactic acidosis due to energy failure leads to the activation of a class of acid-sensing ion channels (ASICs) on neurons and oligodendrocytes. 22,23 This represents an additional mechanism whereby intra-axonal cation excess leads to neuroaxonal damage. In addition, glutamate release by activated inflammatory cells, such as microglia, activates extra-synaptic N-methyl-D-aspartate (NMDA) receptors, which are cation channels permeable to calcium. The overstimulation of NMDA receptor-activated channels, known as excitotoxicity, is another mechanism implicated in the increased intracellular calcium concentration in neurons. 24,25
In summary, it is clear that the maladaptive ionic response to demyelination observed in MS sets in train a negative cycle of energy failure and linked processes including reactive oxygen species production by glia, mitochondrial inhibition and ASIC upregulation that converge around a common process of intra-axonal cation excess that initiates secondary calcium-mediated injury and, ultimately, death cascades including excitotoxicity.
Rationale for study and drug selection
Against the background of the neurodegenerative processes outlined above, we prospectively sought to identify existing putative neuroprotective drugs that target these pivotal neurodegeneration-causing pathways as the most biologically plausible approach to slowing disease progression in SPMS.
We followed a systematic approach to drug identification that includes drug rescue and repurposing, to shorten the time from target selection to regulatory approval. Drug rescue (drugs at an advanced stage of development but abandoned before approval) and repurposing (already approved drugs) exploit existing clinical efficacy, safety and regulatory data, and represent an interesting approach to reduce both cost and time in the process of drug licencing. 26,27 For this reason, we first undertook an MS-Clinical Trials Network commissioned systematic review of animal and human trials of putative neuroprotective drugs. 28,29 For the human analysis, given the existence of common pathways in neurodegeneration, we did not limit the research to MS, but also included amyotrophic lateral sclerosis, Huntington’s disease, Alzheimer’s disease and Parkinson’s disease. Searching PubMed, EMBASE, the Institute for Scientific Information (n = 27,000) and the Cochrane Group MS database (n = 2600) returned 120 drugs for which summaries were generated that included analysis of mechanism of action, scores for safety, study quality, efficacy and sample size. Two clinicians then independently reviewed the 120 drugs and excluded 68 as unsuitable using predefined criteria. A specially convened International MS Drug Selection meeting comprising expert representation from the Cochrane MS group, neuroscientists (including those from the US National Institutes of Health), neurologists, brain imaging experts, people with MS, trial methodologists and industry considered the remaining 52 drugs. Putting an emphasis on clinical efficacy, structured discussions over three rounds of detailed scrutiny identified seven drugs (ibudilast, riluzole, amiloride, pirfenidone, fluoxetine, oxcarbamazepine and polyunsaturated fatty acids class dietary supplements) that were further ranked and grouped against class of action/mechanistic plausibility noting that we were looking for drugs that target the pivotal neurodegenerative pathways discussed above. From those seven drugs, ibudilast, riluzole and amiloride were the three drugs originally chosen, but because of drug supply issues, fluoxetine replaced ibudilast. 28
MS-SMART tested the efficacy and mechanism of action of these three repurposed drugs (amiloride, fluoxetine and riluzole) in people with SPMS. MS-SMART was a Type B trial, as the investigational medicinal products were all in human use, had a good safety profile but were not used for this patient population. Critically for the purpose of MS-SMART, all three drugs had shown promise in early-phase human MS clinical trials and targeted one or more of the pivotal neurodegenerative-causing pathways implicated in SPMS. 29
Amiloride
Amiloride is a widely used diuretic and antagonises ASICs. This last mode of action is thought to be responsible for the myeloprotective and neuroprotective effects in both human and experimental models of progressive MS. Seventeen patients affected by primary progressive multiple sclerosis (PPMS) were enrolled in a Phase IIa trial, divided into a pre-treatment phase (1 year) and an amiloride treatment phase (1 year), at 5 mg bis in die (b.i.d.). During the amiloride treatment phase, patients showed a significant reduction in the whole-brain atrophy rate compared to pre treatment (p = 0.009). Also, corpus callosal radial diffusivity (RD) (myelin integrity) and thalamic mean diffusivity (MD) (structural integrity) showed a significant improvement. 30
Fluoxetine
Fluoxetine is a selective serotonin reuptake inhibitor (SSRI) that is widely used to treat depression. The main properties of this drug relevant for SPMS were the stimulation of glycogenolysis and the induction of production of brain-derived neurotrophic factor in rodent astrocyte cultures. A significantly improved cerebral white matter N-acetylaspartate (NAA)/creatine ratio was found on MRI, suggesting that the drug may improve axonal mitochondrial energy metabolism. Another possible mechanism of action of this drug is the suppression of the antigen-presenting capacity of glial cells. 31,32 Moreover, SSRIs have been demonstrated to ameliorate measures of dependence in patients with stroke. Two Phase IIa trials already tested fluoxetine in MS. In a cohort of 40 MS patients (10% SPMS) there was a significant reduction in relapse rate incidence to 0.54 (95% CI 0.29 to 0.98) with a trend towards reduction in new inflammatory lesions. 33 In the second trial, 42 patients with SPMS/PPMS (SPMS, n = 69%) were randomised in a 1 : 1 ratio to 40 mg or placebo over 2 years. 34 No statistical differences were seen in overall progression or in grey or white matter volume changes, but there were trends in favour of fluoxetine in relation to disability progression. This second trial was terminated early because of drug expiry issues and was considered to be underpowered.
Riluzole
Riluzole is a drug already licensed for motor neuron disease/amyotrophic lateral sclerosis and has two modes of action that may target neurodegeneration in SPMS: reducing glutamate release and antagonism of voltage-dependent sodium channels. 35 Sixteen patients with progressive MS were enrolled in a Phase IIa trial and studied for 1 year before treatment, followed by riluzole 50 mg b.i.d. for 1 year. 36 The primary outcome was the change in cervical spinal cord cross-sectional area, which showed a reduction from –2% (year 1) to –0.2% (year 2). Moreover, the increase in T1 hypointense lesion load was reduced from 15% in year 1 to 6% in year 2 and there was a reduction in brain parenchymal fraction of –1.0% (year 1) and –0.7% (year 2).
Paraclinical biomarkers of neurodegeneration
Magnetic resonance imaging
Magnetic resonance imaging is central to the diagnosis of MS as well as to guide and monitor treatment response. MRI-derived metrics have been extensively used as an interim outcome measure for randomised clinical trials in MS. In RRMS, quantification of new gadolinium-enhancing lesions or T2-weighted lesions from MRI is generally used as the primary outcome measure at phase 2 to test the efficacy of new DMTs in development. 37–39 In SPMS, although there may be some effect on new lesion count and lesion volume, the main MRI parameter for investigating neurodegeneration is the change (reduction) in brain volume (BV). Compared with age-matched healthy controls, there is a greater decrease in BV over time in SPMS, termed atrophy rate, which can be quantified by MRI. On average there is 0.5–1% loss of BV per year in SPMS. MRI studies have demonstrated a correlation between BV loss and disability in SPMS. A decrease in BV is seen at all stages of MS, and especially in SPMS. Neuroaxonal tissue constitutes a large proportion of BV and the increased rate of brain atrophy has been interpreted as evidence for neuroaxonal loss. Brain atrophy is correlated with disability and cognitive impairment in MS. 40–49
A large variety of advanced MRI techniques have been investigated in MS to both quantify and clarify neurodegeneration. In MS-SMART, other than BV and new and enlarging T2 lesion measures, several other MRI technologies were explored and are now described.
Proton magnetic resonance spectroscopy (MRS) was used to measure brain metabolites including glutamate, NAA and myoinositol. 50–52 High levels of glutamate, an excitatory neurotransmitter, are responsible for neuronal excitotoxicity, which was specifically targeted by riluzole as a proposed mechanism of action. NAA, a metabolite synthesised in mitochondria, is associated with axonal integrity and mitochondrial function and is used as a marker of neuroprotection and energy metabolism. Myoinositol is considered a marker of glial cell proliferation and activation (astrocytes and microglia), which are prominent features of CNS inflammation and contribute to neurodegeneration in SPMS.
Magnetisation transfer ratio (MTR) is a technique that strongly reflects the amount of myelin in the brain, but can also be influenced by inflammation and axonal density. There is an exchange of magnetisation between protons that are freely mobile and those that are bound to macromolecules. The extent of this exchange provides an indication of the amount of macromolecular structure in tissue and can be measured with the MTR. 53,54 Myelin has a major effect on MTR and in MS lower MTR is seen in demyelinated rather than remyelinated white matter lesions. The amount of signal decrease is thought to suggest damage to myelin or to the axonal membranes. The MTR measure provides an indication of the extent of axonal loss associated with new inflammatory-demyelinating white matter lesions. MTR has provided many insights regarding the evolution of demyelination and remyelination and was used in MS-SMART to measure the potential benefit on remyelination and putative neuroprotective therapy effects. 55
Diffusion tensor imaging (DTI) can quantitatively detect brain microstructural changes by calculating parameters, such as MD, which is determined by the overall water motion, and fractional anisotropy (FA), which reflects the uniformity of the direction of the diffusion of water molecules. DTI measures may be considered as markers of demyelination and axonal loss. 56 Strong diffusion-encoding gradients allow measurement of water diffusivity in the direction parallel to white matter fibres and perpendicular to this, and changes in these correlate with axonal damage and demyelination. FA derived from the diffusion tensor demonstrates the tendency of water molecules to diffuse in one direction and is a further marker of white matter integrity and myelination status. Abnormal DTI values have been demonstrated in both white matter lesions and normal-appearing white matter (NAWM) in MS, and further deterioration in the placebo arm is expected over the duration of the trial. Stabilisation of these values in treated arms of the study may indicate prevention of further tissue loss within white matter tracts.
In MS, both the brain and the spinal cord are affected. Indeed, up to 90% of patients with MS develop spinal cord focal lesions or diffuse abnormalities, which contribute to disability. Neuronal loss in the cord may occur for direct damage owing to white and grey matter (GM) demyelination or to secondary Wallerian degeneration. 57–61 These phenomena can be captured by quantification of spinal cord atrophy, an aspect that can be substantial in progressive MS. 62 The most common method for assessing in vivo spinal cord atrophy is to measure the cross-sectional area at specific anatomical levels, typically in the cervical region in the area from C2 to C5, using MRI. Image acquisition and segmentation of the upper cervical region are more efficient than in other parts of the cord from a technical perspective,63 and are thought to be more representative of the neurodegenerative process in the cord as lesions are more common in the cervical tract64 and this cord section may reflect destructive processes lower down in the cord via Wallerian degeneration. There is a strong clinical–radiological association between spinal cord atrophy and disability measures in MS, particularly in the progressive stages of the disease. 62,65–67 In a recent systematic review and meta-analysis (including 22 longitudinal studies and > 1000 patients), a mean upper cervical cord cross-sectional area of 73.07 mm2 in all MS patients and 68.55 mm2 in SPMS patients (vs. 80.87 mm2 in healthy controls68) was found, with a rate of spinal cord atrophy of 1.78% per year (95% CI 1.28% to 2.27% per year), potentially supporting the use of MRI-derived cervical cord area measurement as an outcome measure of neurodegeneration in MS trials.
Optical coherence tomography
The anterior visual pathway offers the possibility to study in vivo neuroaxonal loss in MS. Since the pioneering studies of Frisén and Hoyt,69 who described qualitative changes in the retinal nerve fibre layer (RNFL) in patients with MS, and Frohman,70 who found that about 50% of ganglion cells must be lost before focal RNFL defects are detectable, many attempts have been made to improve our ability to visualise the neuronal retinal layers. Optical coherence tomography (OCT) is an increasingly recognised, non-invasive imaging tool that allows investigation of the architecture of the neural retina, which had been virtually inaccessible until the advent of high-resolution spectral domain optical coherence tomography and has fundamentally changed our ability to qualitatively and quantitatively assess the eye. 71
Optical coherence tomography relies on interferometry of near-infrared light to construct very high-resolution images of the retinal layers. The most visible layer is the RNFL, comprising unmyelinated axons in a supportive connective tissue framework. The RNFL axons originate from retinal ganglion cell bodies, and continue through the optic nerve, chiasm and tract (where they are myelinated), to synapses in the lateral geniculate bodies. In acute optic neuritis, RNFL thickness increases because of optic nerve swelling. Subsequently, its thickness reduces, indicating significant axonal loss. 72 The optic nerve lesion leads to retrograde degeneration of the RNFL, a relatively pure compartment of unmyelinated axons whose thickness can be measured sensitively and non-invasively using OCT. Therefore, quantification of peripapillary retinal nerve fibre layer (pRNFL) thickness provides a plausible biomarker of axonal loss. 73 In addition, abnormalities of the retinal layers other than the pRNFL have been observed in post-mortem specimens from patients with MS, in which 79% of eyes exhibited ganglion cell loss and 40% showed amacrine and bipolar cell loss in the inner nuclear layer. These findings have been corroborated in vivo by OCT, demonstrating thinning of the ganglion cell layer (GCL) and inner plexiform layer (IPL), and are associated with reductions in visual function and vision-specific quality of life. 74
Many studies have reported an inverse correlation between pRNFL thickness and Expanded Disability Status Scale (EDSS) score in MS, and the strongest correlation was found for patients not affected by optic neuritis. 75 A recent meta-analysis examining studies using OCT in mixed cohorts of MS patients confirmed that, when compared with healthy controls, pRNFL and the complex GCL and IPL were decreased in both MS optic neuritis and non-optic neuritis eyes. 76 One study found that patients with MS and a pRNFL thickness of up to 87 µm (as measured with a Cirrus OCT machine; Carl Zeiss, Dublin, CA, USA) or 88 µm (as measured with a Spectralis OCT machine; Heidelberg Engineering, Heidelberg, Germany) had roughly twice the risk of disability worsening during follow-up compared with patients with thicker pRNFL. 77 This risk was independent of other factors known to be associated with disability worsening, including age, disease duration, baseline level of disability (EDSS) and the use of DMTs. It was suggested that the process of neurodegeneration within the retina could reflect similar processes occurring more diffusely in the brain and spinal cord. An extensive review of the literature confirmed that using OCT in MS can provide valid, reliable and reproducible data to track the process of neurodegeneration within the retina of patients with MS with optic neuropathy. pRNFL thickness and macular volume analyses could serve as a surrogate biomarker and primary outcome measure to confirm the neuroprotective effects of new drugs. 70
Optical coherence tomography has good analytical reproducibility, is cost-effective, correlates with clinical measures (loss of visual function, cognitive decline) and is predictive of a clinical outcome (poor visual recovery). 75 Finally, atrophy of the complex GCL and IPL appears to mirror whole-brain (and particularly GM) atrophy, especially in progressive MS, supporting the use of OCT in clinical trials. 78
Despite the evidence described above, and although serial OCT-measured pRNFL thickness has been proposed as a measure of neurodegeneration for clinical trials in MS, longitudinal observations are largely confined to RRMS, underscoring the need to develop the data set in progressive MS. 76,77
Cerebrospinal fluid
Cerebrospinal fluid (CSF) measures are potential outcome measures in SPMS trials.
Neurofilaments represent a component of the mature cytoskeleton of neurons,79 and are composed of neurofilament light (NfL), neurofilament medium (NfM) and neurofilament heavy (NfH) chain subunits. All the pathological processes that cause neuroaxonal damage release neurofilament proteins into the extracellular space, CSF and, depending on the extent of damage, the peripheral blood; therefore, CSF and blood neurofilament levels are considered as markers of neuroaxonal damage.
Disease-modifying therapies for RRMS, in particular the highly active therapies, have all demonstrated reduction in CSF NfL levels (fingolimod,80 natalizumab,81 ocrelizumab82), indicating a relationship between anti-inflammatory therapies and neuronal damage resolution. In SPMS, serum NfH and CSF NfH in a cohort of patients compliant with lamotrigine (a putative neuroprotectant), demonstrated lower levels than placebo. 83 This emphasises the mechanistic utility of sampling the CSF for such markers and exploring neurofilament and other measures in an established SPMS cohort.
Study objectives
The main aim of MS-SMART was to determine the efficacy of three likely neuroprotective agents, in a multiarm approach, to increase biological understanding of the disease process and to further our knowledge of interim markers of neurological damage. This was undertaken using MRI, disability measurements, OCT and targeted CSF analysis.
Primary objective
The primary objective of the MS-SMART study was to establish whether or not any of the three selected drugs (i.e. amiloride, fluoxetine and riluzole) were able to decrease the progression of BV loss in people with SPMS over 96 weeks, as assessed by MRI-derived percentage brain volume change (PBVC).
Secondary objectives
The secondary objectives were to:
-
establish that a multiarm trial strategy was an efficient way of screening drugs in SPMS and can become the template for future work
-
explore any anti-inflammatory drug activity (measured by counting the new and enlarging T2-weighted white matter lesions)
-
examine for evidence of pseudo-atrophy by MRI
-
examine the clinical effect of neuroprotection as measured by clinician- and patient-reported outcome measures (PROMs)
-
collect basic health-related quality-of-life data.
Exploratory objectives
The exploratory objectives were to:
-
assess neuroprotection in new lesions by estimating persistent new T1-weighted hypointense lesion (or ‘black holes’) count
-
assess cortical neuroprotection by evaluation of GM volume change
-
evaluate myelination using MTR
-
assess spinal cord neuroprotection using MRI
-
quantify neuronal mitochondrial dysfunction, prevention of glial cell inflammation and prevention of excitotoxicity as measured by MRS metabolites
-
quantify neuroprotection using DTI as an index of white matter integrity
-
evaluate neuroprotection using OCT measures
-
quantify neuroprotection using CSF neurofilaments
-
investigate treatment effect and predictive models of brain atrophy (by MRI) and clinical disability (by EDSS) at 96 weeks using baseline MRI and disability scores.
Patient and public involvement
The scientific approach in MS-SMART was recognised as being completely in tune with the wishes of people with SPMS.
Patient and public involvement (PPI) has been central to the genesis of this study. The UK MS Society has a long and active history of PPI. This was realised in the formation of the MS Society Clinical Trials Network. In the MS-SMART study this was evidenced by strong and repeated PPI sessions in the design and conduct of the study, specifically:
1) the Group emphasised the importance of working with early SPMS patients (EDSS scores below 6.5) prior to the ambulatory phase in light that any neuroprotective drug is likely to have most benefit; 2) a multi-arm design was preferable to standard single arm versus placebo to ensure that a maximum number of patients would have access to putative neuroprotective repurposed drugs compared to placebo; 3) they considered that the burden of advanced MRI protocol was acceptable to patients in view of the potential advancement that will come from the imaging analysis to the mechanistic elucidation.
All PPI recommendations were taken on board. One of the principal investigators (RB) was a person with progressive MS. The final study results were communicated in a specially developed video,84 in conjunction with the UK MS Society, to all trial participants as the trial results were announced. Participants were also told of their treatment allocation if they so wished.
Chapter 2 Methods
Trial design
MS-SMART was an investigator-led, multicentre, multiarm, double-blind, placebo-controlled, parallel-group, randomised Phase IIb trial that compared three putative neuroprotective therapies (i.e. amiloride, fluoxetine and riluzole) versus a shared placebo arm in people with SPMS. Participants were allocated 1 : 1 : 1 : 1 to active treatment with amiloride, fluoxetine or riluzole or a matching placebo. The duration of the trial for participants was 96 weeks.
Setting
MS-SMART took place at 13 UK clinical neuroscience centres in London, Edinburgh, Liverpool, Sheffield, Brighton, Truro, Oxford, Stoke-on-Trent, Plymouth, Newcastle, Leeds, Nottingham and Glasgow. The two main trial sites were University College London (UCL) Queen Square Institute of Neurology in London (hereafter called London) and the University of Edinburgh Anne Rowling Regenerative Neurology Clinic in Edinburgh (hereafter called Edinburgh).
Substudies
At the London and/or Edinburgh sites, the following optional substudies were in place:
-
the advanced MRI substudy (at both the London and Edinburgh sites, but with different imaging protocols)
-
CSF (only at the London site)
-
OCT (at both the London and the Edinburgh sites).
Study approvals
MS-SMART was approved by the Scotland A Research Ethics Committee (REC) on 13 January 2013 (REC reference: 13/SS/0007). The trial was assigned ClinicalTrials.gov identifier (NCT number) NCT01910259;2012-005394-3; and the International Standard Randomised Controlled Trial Number (ISRCTN) ISRCTN28440672.
Participants
Participants were aged 25–65 years, had a diagnosis of SPMS with evidence of disease progression independent of relapses in the previous 2 years and had an EDSS score of 4.0–6.5 at baseline.
Inclusion criteria
-
Confirmed diagnosis of SPMS. Steady progression rather than relapse must be the major cause of increasing disability in the preceding 2 years. Progression can be evident from either an increase of at least 1 point in EDSS score or clinical documentation of increasing disability in the patient’s notes.
-
EDSS score of 4.0–6.5.
-
Aged 25–65 years, inclusive.
-
Women and men with partners of childbearing potential had to use an appropriate method of contraception.
-
Women must have a negative pregnancy test within 7 days prior to the baseline.
-
Willing and able to comply with the trial protocol (e.g. can tolerate MRI and fulfils the requirements for MRI).
-
Give written informed consent.
Exclusion criteria
-
Pregnant or breastfeeding females.
-
Baseline MRI scan not of adequate quality for analysis.
-
Significant organ comorbidity.
-
Relapse within 3 months of baseline visit.
-
Intravenous or oral steroid treatment for a MS relapse/progression within 3 months of baseline visit.
-
Use of simvastatin at 80-mg dose within 3 months of baseline visit.
-
Commencement of fampridine within 6 months of baseline visit.
-
Use of immunosuppressants (e.g. azathioprine, methotrexate, ciclosporine) or first-generation disease-modifying therapies (β-interferons, glatiramer acetate) within 6 months of baseline visit.
-
Use of mitoxantrone, natalizumab, alemtuzumab, daclizumab, fingolimod, fumarate, teriflunomide, laquinomod or other experimental disease-modifying therapy within 12 months of baseline visit.
-
PPMS.
-
RRMS.
-
Known hypersensitivity to the active substances and their excipients to any of the active drugs for this trial.
-
Use of a SSRI within 6 months of the baseline visit.
-
Current use of tamoxifen.
-
Current use of herbal treatments containing St. John’s wort.
-
History of bleeding disorders or current use of anticoagulants.
-
Use of monoamine oxidase inhibitors, phenytoin, L-tryptophan and/or neuroleptic drugs, lithium, chlorpropamide, triamterene or spironolactone within 6 months of the baseline visit.
-
Current use of potassium supplements.
-
Significant signs of depression and a Beck Depression Inventory, version 2 (BDI-II), score of ≥ 19.
-
Bipolar disorder.
-
Epilepsy/seizures.
-
Receiving or previously received electroconvulsive therapy treatment.
-
Glaucoma.
-
Routine screening blood values: liver function tests > 3 × upper limit of normal of site reference ranges (aspartate aminotransferase, alanine aminotransferase, bilirubin, gamma-glutamyl transferase); potassium levels of < 2.8 mmol/l or > 5.5 mmol/l; sodium levels of < 125 mmol/l, creatinine levels of > 13 mmol/l; a white blood cell count of < 3 × 109/l; lymphocytes count of < 0.8 × 109/l; a neutrophil count of < 1.0 × 109/l; a platelet count of < 90 × 109/l; and haemoglobin levels of < 80 g/l.
Recruitment of participants
The identification of the potential MS-SMART participants was conducted through several routes:
-
in clinics run by principal investigators/neurologists, at participating hospital and clinic sites
-
using existing MS research and other neurological databases, such as the Scottish Health Research Register (SHARE) (URL: www.registerforshare.org), containing contact details of people who previously consented to be contacted about research
-
by primary care referrals
-
by participants’ self-referral through a dedicated MS-SMART website.
Pre-screening routes to check patients’ eligibility were allowed:
-
in-clinic briefing of potential participants about the study directly by a member of the clinical team
-
initial telephone contact from a member of the research team to explain the trial.
All the contacted potential participants were provided with a study patient information leaflet (PIL) and then at an interval no less than 24 hours after receiving the PIL they were re-contacted to determine if they wanted to proceed to face-to-face screening. If required, with permission, the participant’s general practitioner (GP)/neurologist was also contacted to provide written evidence that the patient met all the relevant eligibility criteria, before the screening visit in order to avoid unnecessary visits.
Following the pre-screening review at the London and Edinburgh sites, the potential participants were also provided with PILs for the optional relevant substudies.
The anonymity of all ineligible patients and all eligible patients who declined participation was maintained in every recruiting site. Anonymised information was collected including age, sex, date of screening, reason not eligible to participate (if applicable), reason for declining participation despite eligibility (if applicable) and any other reason for non-participation (if applicable).
Trial interventions
Investigational medicinal products
The intervention was a random allocation to amiloride (5 mg twice daily), or fluoxetine (20 mg twice daily), or riluzole (50 mg twice daily), or placebo (one capsule twice daily). For the first 4 weeks, participants were asked to take only one capsule daily (Figure 1). Details on dose modification and stopping rules are reported elsewhere. 29
FIGURE 1.
Drug titration scheme. AE, adverse event. Adapted from Connick et al. 29 This is an Open Access article distributed in accordance with the terms of the Creative Commons Attribution (CC BY 4.0) license, which permits others to distribute, remix, adapt and build upon this work, for commercial use, provided the original work is properly cited. See: http://creativecommons.org/licenses/by/4.0/.

The MS-SMART placebo comprised a size 00 capsule identical to the overencapsulated fluoxetine/amiloride/riluzole.
To evaluate the adherence to study drug, each participant was requested to bring back the unused study drug at each study visit and was asked about adherence. Participants had to record the number of capsules taken by using a diary card and to indicate any reason for non-adherence.
Screening visit
After the pre-screening phase, and at least 24 hours after receiving the PIL, patients underwent a formal face-to-face screening visit that involved documentation of written informed consent, review of medical history, concomitant medications, assessment of EDSS and BDI-II score, full physical clinical examination, recording of vital signs and collection of blood tests. After the screening phase, patients had a baseline brain MRI. In the majority of the recruitment sites, it was possible to perform the baseline MRI scan on the same day as the screening visit, decreasing travel costs and time commitment. A standard mandatory MRI protocol (defined as core MRI) was performed for all participants recruited in all sites. The London and Edinburgh sites performed optional MRI scan protocols (defined as advanced MRI), which included brain MTR, spectroscopy and DTI scans at the Edinburgh site, and brain MTR, spectroscopy and upper cervical cord at the UCL site. Each site subsequently transferred all scans to the Queen Square MS Centre Trial Office for central quality control and approval. Upon acceptance of the core MRI scans, the participants were then invited to take part to the baseline visit. At the London site, participants were also invited to take part in the optional OCT and CSF substudies. At the Edinburgh site, participants were invited to take part in the optional OCT substudy. Figure 2 shows the substudies carried out at the London and Edinburgh sites.
FIGURE 2.
Diagrammatic representation of the substudies.
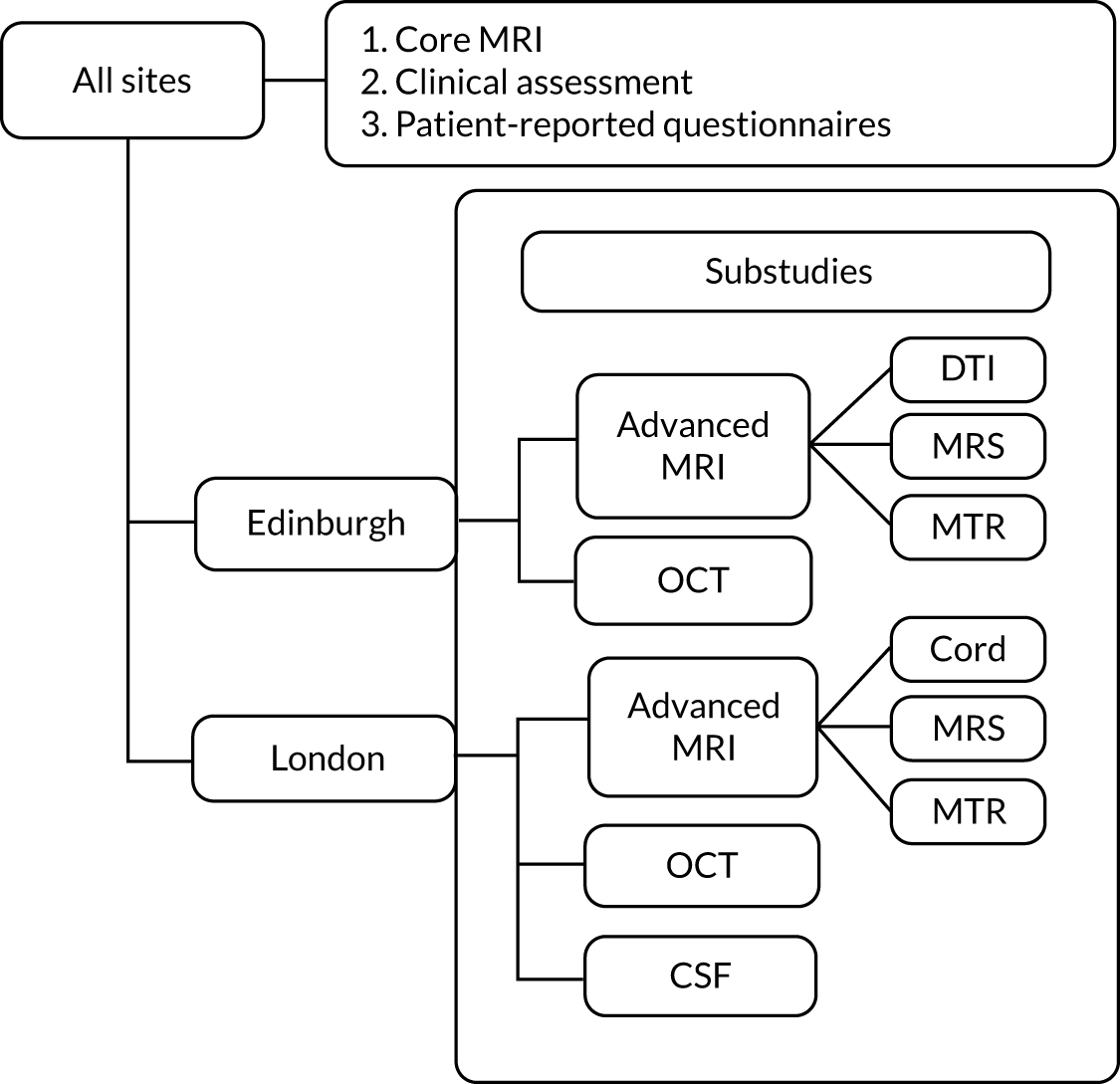
Baseline visit
The activities at the baseline visit included detailed neurological evaluation, randomisation and collection of participant self-reported questionnaires. Rejection of the advanced MRI protocol only did not impede the enrolment of patients and, whenever possible, the rejected advanced MRI scans were repeated at the baseline visit. After completion of the baseline visit and randomisation, the study drugs, emergency card and patient diary were given to the participant.
For patients who consented to the substudies, OCT and lumbar puncture were also performed.
Follow-up visits
After the baseline visit, participants were reviewed at weeks 4, 8, 12, 24, 36, 48, 72 and 96 (Figure 3). At the week 48 and week 96 visits, a full neurological assessment was repeated and patients completed self-reported questionnaires. The MRI scan, instead, was repeated at week 24 and week 96. Standard clinical laboratory blood tests (chemistry and haematology) were performed for safety monitoring at each visit. Finally, at week 100, a safety telephone call was performed.
FIGURE 3.
Patient visits. MSFC, multiple sclerosis functional composite; SDMT, Symbol Digit Modalities Test; SLCVA, Sloan Low Contrast Visual Acuity.

For patients taking part in the substudies, lumbar puncture was repeated at weeks 48 and 96, and OCT was repeated at week 96.
At each study site, there were treating physicians [responsible for dose adjustment and adverse event (AE) monitoring], research nurses and trained NeuroStatus-certified assessing physicians masked to the patients’ history, who performed baseline and yearly clinical outcome assessments. Follow-up assessments were undertaken preferably by the same assessing physician to improve consistency.
Outcomes
Primary end point
Percentage brain volume change measured at 96 weeks using the Structural Image Evaluation using Normalization of Atrophy (SIENA) technique was chosen as the primary outcome measure in this trial. SIENA is an automated method that registers the follow-up scan to the baseline scan and produces an integral of the edge motion existing in each voxel in both scans. It then directly calculates the PBVC from those values. 47,85 PBVC is considered as a marker of neurodegeneration. 48
Secondary end points
Secondary MRI end points were count of new and enlarging T2 lesions at 96 weeks and PBVC at 24 weeks (to estimate pseudoatrophy). Although new and enlarging T2 lesions appear to be less relevant than brain atrophy as a measure of neuroprotection in SPMS, they were included as a core outcome measure in order to detect an unanticipated immunomodulatory effect.
Clinical secondary end points were EDSS, Timed-25-Foot Walk (T25FW), 9-Hole Peg Test (9HPT), Paced Auditory Serial Addition Test (PASAT), Multiple Sclerosis Functional Composite (MSFC), Symbol Digit Modalities Test (SDMT), high-contrast visual acuity (HCVA) (100%), and Sloan Low Contrast Visual Acuity (SLCVA) (5%, 2.5%, 1.25%) obtained at baseline, 48 and 96 weeks. Time to first relapse was recorded. Patient-reported outcomes of the following were also measured at baseline and at 48 and 96 weeks: Multiple Sclerosis Impact Scale 29 items, version 2 (MSIS29v2), Multiple Sclerosis Walking Scale, version 2 (MSWSv2), Neurological Fatigue Index (NFI), Numeric Pain Rating Scale (NPRS), Brief Pain Inventory (BPI), Neuropathic Pain Scale (NPS) and health-related quality-of-life [EuroQol-5 Dimensions, five-level version (EQ-5D-5L)]. The MSFC z-score was normalised using participants’ baseline scores.
Exploratory end points
Exploratory MRI end points were as follows:
-
Proportion of new and enlarging T2 lesions at 24 weeks being persistently T1 hypointense at 96 weeks. Persistently T1 hypointense lesions exhibit greater axonal loss and could be a measure of neuroprotection. This end point was collected by all trial sites as part of the core MRI protocol.
-
Percentage GM volume change (to assess cortical neuroprotection) at 96 weeks. GM atrophy is abundant in SPMS and shows robust correlations with disability. GM atrophy was investigated as an additional measure of neuroprotection. This end point was collected by all trial sites as part of the core MRI protocol.
-
MR spectroscopy metabolite concentration including measures of NAA (reversal of neuroaxonal mitochondrial dysfunction), myoinositol (prevention of glial cell inflammation) and glutamate (prevention of excitotoxicity) at baseline and at 96 weeks. MR spectroscopy was part of the advanced MRI substudy and was performed at the London and Edinburgh sites.
-
MTR in the GM and in the new lesions to determine demyelination and remyelination and MTR of NAWM to investigate tissue integrity. It was measured at baseline and at 96 weeks. MTR was part of the optional advanced MRI substudy and was performed at the London and Edinburgh sites.
-
Upper cervical cord cross-sectional area (to investigate cord atrophy) measured on cervical cord three-dimensional (3D) phase-sensitive inversion recovery (PSIR) MRI scans acquired at baseline and 96 weeks. Spinal cord MRI was part of the optional advanced MRI substudy and was performed at the London site.
-
Four measures were derived from DTI acquisition at baseline and at 96 weeks: FA, axial diffusivity (AD), RD and MD. The peak width of skeletonised diffusivity (PWSD) was used as the outcome measure for each metric. This quantifies dispersion of values across all tracts within an individual subject and, therefore, offers improved responsiveness over measures of central tendency in a disease characterised by multifocal pathology. DTI was part of the optional advanced MRI substudy and was performed at the Edinburgh site.
Additional exploratory end points were:
-
Measurement of CSF neurofilament levels as part of the CSF substudy at the London site. The primary outcome of the CSF substudy was CSF NfL levels at 48 weeks. A secondary outcome was CSF NfL levels at 96 weeks and the change from 48 to 96 weeks. Additional CSF substudy outcomes included a panel of biomarkers: neural cell adhesion molecule (NCAM), NfH, glial fibrillary acidic protein (GFAP), ferritin, soluble cluster of differentiation 14 (CD14), matrix metalloproteinase 9 (MMP9) and neopterin.
-
Measurement of the peripapillary RNFL and retinal nerve GCL and IPL (GCL + IPL complex) with OCT to determine the extent of retinal layer thinning were obtained at baseline and at 96 weeks. OCT scans were acquired as part of the OCT substudy at the London and Edinburgh sites.
Magnetic resonance imaging protocol and analysis
Core magnetic resonance imaging
The following MRI sequences were obtained at all three MRI assessment visits for all participants:
-
sagittal localiser to identify the subcallosal line
-
axial dual echo fast/turbo spin echo proton density (PD)/T2 weighted from foramen magnum to vertex, in plane resolution 1 mm2, contiguous 3-mm slices
-
axial fluid attenuated inversion recovery from foramen magnum to vertex, in plane resolution 1 mm2, contiguous 3-mm slices
-
axial T1 from foramen magnum to vertex, in plane resolution 1 mm2, contiguous 3-mm slices
-
sagittal 3D T1 gradient echo with voxel resolution of 1 mm3.
Before the beginning of the trial, each site provided a ‘dummy scan’ (carried out on a healthy volunteer or a person with MS), which was sent the central MRI facility (Queen Square MS Centre Trial Office, London) for review and agreement on MRI parameters. Detailed MRI parameters according to scanner make and model are reported elsewhere. 29 Quality control feedback was generated by review at the central MRI facility soon after scan acquisition and was provided to the site.
The percentage brain volume change was calculated using the SIENA method. After the receipt of digital imaging and communications in medicine images to the central MRI facility and after quality control, the T2 lesions were outlined on PD scans by trained personnel blinded to clinical data using a semi-automatic method (Jim software, version 7.0, Xinapse Systems, Essex, UK). On further MS expert review, T2 lesion masks were used to lesion fill the 3D T1-weighed images using a patch-based method86 and, from these, brains were extracted and segmented into GM [i.e. cortical grey matter (CGM) plus deep grey matter (DGM)] and white matter volumes. 87 Finally, the SIENA method was applied to the 3D T1 images and the segmentations to calculate a percentage change in BV between baseline and week 96 scans.
Percentage change in GM volume was calculated by taking the difference in the GM volumes between 96 weeks and baseline divided by the baseline GM volume.
Advanced magnetic resonance imaging
An advanced MRI protocol was used at the London and Edinburgh sites.
At both the London and Edinburgh sites, the following scans were acquired as part of this advanced protocol:
-
brain MTR
-
brain MRS.
At the London site only, cervical cord 3D-PSIR scans were performed.
At the Edinburgh site only, brain DTI was performed.
Magnetic resonance spectroscopy
At the Edinburgh site, MRS was carried out at 3 T (Siemens Verio, Siemens Healthcare, Erlangen, Germany) using a standard 12-channel matrix head coil and a manufacturer-supplied prototype with semi-localisation by adiabatic selective refocusing (semi-LASER) excitation. Data were acquired from a single 10-mm-thick slice of spectroscopic voxels prescribed immediately above the lateral ventricles in the plane of the T2-weighted images. Spectroscopic data were analysed in Linear Combination of Model Spectra using a spectral basis set matching the semi-LASER sequence. Voxels were discarded if they had poor-quality spectra, were judged to be not completely inside the brain or the LCModel Cramér–Rao bounds (i.e. % standard deviations) of the fitted spectra exceeded 20% for any metabolite or 40% for myoinositol. 88
At the London site, the participating patients underwent a standardised MRS sequence on a Philips Achieva 3T MRI scanner (Philips Healthcare, Best, the Netherlands) using a 16-channel neurovascular coil. A 15-mm single-slice-thick volume of interest (VOI) was selected for MRS placed superior to the lateral ventricles. The MRS VOI was subdivided into a 21 × 16 grid, giving voxel size of 10 × 10 × 15 mm. Spectra were acquired using a 2D Point RESolved Spectroscopy (PRESS) sequence [short echo time (TE) 35 ms/repetition time (TR) 2000 ms]. Outer volume suppression using fat saturation was applied to limit artefacts, and the VOI was shimmed using the pencil beam-auto technique. CHEmical Shift Selective saturation (CHESS) pulses were used for water suppression. A reference scan with no water suppression was also collected with the same parameters in the same examination for quantification. Spectral post-processing analysis was completed using Linear Combination of Model Spectra (LCModel© version 6.3-1A). 89 Individual voxels were automatically rejected if the NAA, myoinositol and combined glutamate and glutamine (Glx) Cramér–Rao bounds were > 20%, full width half-maximum of the NAA peak was > 1.27 p.p.m. (parts per million) and signal–noise ratio was < 9. All voxels that passed the automated step were visually inspected by an experienced assessor to look for noisy baseline, non-random residuals or other artefacts that prevented the accurate measurement of neurometabolites.
At both sites, the total concentration of the following metabolites was finally measured: NAA, Glx and myoinositol. The NAA measure included N-acetylaspartylglutamate concentrations.
Magnetisation transfer ratio
Magnetisation transfer ratio data were acquired using a 3D slab-selective fast-field echo sequence with two echo times. Images acquired at the two TEs were averaged (thereby increasing the signal-to-noise ratio) for both the magnetisation transfer on (MTon) and off (MToff) data. The MTR sequences at the two sites (London and Edinburgh) were not identical, in terms of both imaging parameters and MT pulse characteristics (amplitude, pulse shape, duration, offset frequency). This was unavoidable as they were the sequences acquired on different scanners at the two sites. At the London site, MTR scans were acquired at 3 T using a Philips Achieva scanner with a 32-channel head coil and multitransmit technology. At the Edinburgh site, MTR scans were acquired at 3 T using a Siemens Verio (Siemens Healthcare, Erlangen, Germany) with a 12-channel head coil.
The MTon and MToff images were co-registered to a halfway point using NiftyReg90,91 and MTR maps (in percentage units) were calculated as [(MToff – MTon)/MToff) × 100]. The T1-weighted volume (in addition to lesion masks, and tissue segmentations for GM and NAWM) were coregistered to this same halfway point for further analysis. Whole-brain, GM, NAWM and T2 lesion masks were measured as part of the core MRI analysis.
Magnetisation transfer ratio maps were reviewed for artefacts, registration and segmentation quality.
Diffusion tensor imaging
Images were acquired at Edinburgh at 3 T (Magnetom Verio, Siemens AG, Healthcare Division GmbH, Erlangen, Germany) using a standard 12-channel head coil. The diffusion imaging protocol consisted of six T2-weighted [T2w (b = 0 smm–2)] and sets of diffusion-weighted (b = 1000 smm–2) whole-brain single-shot spin-echo echo-planar imaging volumes acquired with diffusion encoding gradients applied in 56 non-collinear directions. The acquisition parameters were field-of-view 240 × 240 mm; imaging matrix 96 × 96; 60 contiguous 2.5-mm-thick axial slices, giving 2.5 mm3 isotropic voxels. Repetition and ETs were 11,500 ms and 73.6 ms, respectively.
Diffusion tensor imaging images were corrected for eddy current-induced distortions and subject motion with the ‘eddy correct’ tool. 92 After brain tissue extraction using the Brain Extraction Tool, diffusion tensors and scalar diffusion parameters (FA, MD, AD and RD) were calculated using DTIFit (release 5.0.1. URL: https://fsl.fmrib.ox.ac.uk/fsl; accessed 18 June 2018; Analysis Group, FMRIB, Oxford, UK). 93,94
Twelve tracts of interest were identified from the diffusion MRI data using probabilistic neighbourhood tractography (PNT) as implemented in the TractoR package for fibre tracking analysis (release 3.1.0. URL: www.tractor-mri.org.uk; accessed 18 June 2018; Jon Clayden, UCL, London, UK). This technique optimises the choice of seed point for tractography by estimating the best matching tract to a reference tract derived from a white matter atlas, using a series of candidate seed points placed in a 7 × 7 × 7 voxel neighbourhood. Tracts assessed were the genu and splenium of the corpus callosum, and bilaterally the cingulum (divided into dorsal and ventral portions), corticospinal tracts, arcuate fasciculi and inferior longitudinal fasciculi. All generated tracts were visually assessed by an experienced observer and those that were deemed not to be anatomically acceptable representations of the fasciculi of interest were discarded from further analysis.
Four metrics were derived from DTI acquisition: FA, AD, RD and MD. The peak width of PWSD was used as the outcome measure for each metric defined as peak width of skeletonised fractional anisotropy (PWSFA), peak width of skeletonised axial diffusivity (PWSAD), peak width of skeletonised radial diffusivity (PWSRD), peak width of skeletonised mean diffusivity (PWSMD). PWSD quantifies dispersion of values across all tracts within an individual subject and, therefore, offers improved responsiveness over measures of central tendency in a disease characterised by multifocal pathology.
Cervical cord magnetic resonance imaging
The MRI of the cervical cord was carried out at the London site only. All the participants were scanned using the same scanner (3 T Philips Achieva system) using a 16-channel neurovascular coil to acquire spinal cord scans. The following scan was acquired: 3D-PSIR sequence (field of view = 256 × 256 mm2, matrix = 512 × 256, TR = 8 ms, TE = 3.7 ms, dual radiofrequency transmit, inversion time = 843.6 ms and number of averaged signals = 3; voxel dimensions 0.5 × 0.5 × 3 mm3 and duration 14 minutes). The cervical cord was imaged in the axial-oblique plane (i.e. slices perpendicular to the cord) containing 16 contiguous slices from C2–C4 with the centre of the imaging volume positioned at the level of C2–C3 intervertebral disc plane.
To calculate the mean upper cord cross-sectional area (MUCCA), an active surface model was used by means of Jim software (version 7.0). To achieve this, we manually marked the centre of five 3-mm-thick slices obtained from the 3D-PSIR image centred at C2–C3, then we ran the Jim software, which identified and contoured the cord edge equivalent to the cord area. Finally, we recorded and averaged the area of each of the five marked slices. 95 We repeated this procedure for all the baseline and week 96 cervical cord scans to obtain MUCCA for each patient at each single time point. The resulting contouring of the cord cross-sections was visually inspected and manually edited, if necessary.
Optical coherence tomography
Optical coherence tomography imaging was performed on a spectral domain OCT (software version 6.9.4.0 Spectralis, Heidelberg Engineering, Heidelberg, Germany). Room lighting was dimmed and no pharmacological pupil dilation was used. A circular scan [diameter 12°, 1536 A-scans, 1 B-scan, automatic real time (ART) 100] was manually centred on the optic nerve head to acquire a pRNFL image. In addition, to obtain macular images, we acquired volume scans of the macula (London site settings: 20° × 20° volume scan, 25 B-scans, 1024 A-scans per B-scan, vertical alignment, ART 9. Edinburgh site settings: 30° × 25° volume scans, 61 B-scans, 768 A-scans per B-scan, posterior pole alignment, ART 12) centred around the fovea. For the macular scan, we recorded the values using a thickness map on a 1-, 3- and 6-mm grid [Early Treatment Diabetic Retinopathy Study (ETDRS)]. 96 Retinal layer segmentation for quantification of GCL + IPL was obtained by automated segmentation software provided by the manufacturer (Spectralis, Heidelberg Engineering, Heidelberg, Germany).
For the GCL + IPL, we measured both the mean thickness from the ETDRS grid of the inner (i.e. 3-mm ring) and outer (i.e. 6-mm ring) quadrants together and of the inner quadrant alone, the latter being the more reliable measure and, hence, of primary interest. 97 For the measurement of the pRNFL thickness, the global mean of the entire pRNFL was used, but each single sector was also collected and reviewed for exploratory analyses.
Quality checks were performed in accordance with validated international consensus criteria. 98 Patients were excluded if they had high refractive error (more than +6 dioptres or less than –6 dioptres) or had concurrent ocular pathology not related to MS. Past history of optic neuritis was recorded. OCTs were performed by trained staff blinded to treatment allocation.
Cerebrospinal fluid
A lumbar puncture for assessment of baseline CSF NfL levels was performed at the baseline visit. A second and third lumbar puncture were then performed at weeks 48 and 96, respectively. Blood and urine samples were collected at baseline, and at weeks 12, 24, 48, 72 and 96. Lumbar punctures were performed according to standard or local practice, using atraumatic needles by trained clinical staff.
Neurofilament light was selected based on the unacceptable interlaboratory coefficient of variation for NfH, compared with the NfL assay99 and on the higher propensity of NfH to form aggregates. Finally, we used the commercial SimoaNF-light® assay (Quanterix®, UmanDiagnostics, Ballerica, MA, USA), a digital immunoassay for the quantitative determination of NfL in serum, plasma and CSF.
Sample size
The sample size was calculated based on the study reported by Altmann et al. 49 for measurement of PBVC using the SIENA method in SPMS, and based on further data obtained directly from the lead author (Dr Altmann). Ninety patients per arm would give > 90% power to detect a 40% reduction in PBVC on any active arm compared with placebo and 80% power to detect a 35% reduction, using Bonferroni adjustment for multiple comparisons of three 1.67% two-sided tests, giving 5% overall two-sided significance level. For a more exploratory analysis without adjusting for multiple comparisons, this sample size would give almost 90% power to detect a 35% reduction in atrophy. In addition, based on the experience from two UK Phase II trials,100,101 we expected 10% of the total cohort to drop out of the trial before week 48, and a further 10% of the total cohort to come for their week 96 visit, but to be off their medication. According to these figures, a total of 440 patients to be randomised equally (1 : 1 : 1 : 1) between the three active treatments and the placebo (i.e. 110 participants per treatment arm) would anticipate 90 participants per arm to complete the study.
Randomisation and blinding
Sequence generation
Randomisation was by a central, internet-based, secure password-protected randomisation database. The random allocation sequence was generated by the programmers at a UK Clinical Research Collaboration-registered trials unit [Edinburgh Clinical Trials Unit (ECTU)].
Type of randomisation
Patients were randomised to amiloride, fluoxetine, riluzole or placebo in a 1 : 1 : 1 : 1 ratio using a minimisation algorithm balanced according to sex, age (< 45 years or ≥ 45 years), baseline EDSS score (4.0–5.5; 6.0–6.5) and centre, with a random component to maintain unpredictability of treatment allocation.
Implementation
Eligible patients were randomised by study site personnel via a secure web-based randomisation service.
Blinding
Participants and all other personnel directly involved in the study were masked to treatment allocation. Amiloride, fluoxetine, riluzole and placebo capsules were overencapsulated to obtain an identical appearance to ensure that treatment allocation remained concealed to both staff and participants.
The MRI data were processed independently at a central reading site (Queen Square MS Centre Trial Office, UCL, London, UK) by staff unaware of trial group assignments.
Adherence
Adherence was defined as those participants taking, on average, > 90% of prescribed medication as reported in the patient diary in the 30 days prior to their clinic visit, across all clinic visits for which valid data on medication adherence were recorded.
Statistical analysis
Patient recruitment is reported by means of a Consolidated Standards of Reporting Trials (CONSORT) flow diagram. Baseline characteristics of participants are described using exploratory summary methods: continuous variables are summarised using summary statistics (mean, standard deviation, median, minimum and maximum) by treatment group and categorical variables are presented using frequency distributions by treatment group. Proportions of patients with missing 96-week MRI data in each treatment group are compared, as well as baseline data for patients with missing and non-missing 96-week follow-up data.
Unblinded safety data were monitored by a Data Monitoring Committee (DMC) to ensure the ongoing safety of patients in the study. No formal interim analyses were conducted.
A full statistical analysis plan was prepared that included details of methods for calculating derived variables, methods for handling missing data and withdrawals, any sensitivity analyses and approaches to testing the assumptions in the statistical analyses.
Primary outcome
The primary end point, the PBVC between baseline and 96 weeks, was calculated using a normal linear model to compare the three active treatment group arms with placebo adjusting for baseline normalised BV and the minimisation variables: age, sex, treatment centre (as a fixed effect) and baseline EDSS score. Baseline normalised BV was entered into the model as a continuous variable, as were the minimisation variables of age and EDSS score. Treatment centre was included as an explanatory factor variable with the UCL centre as the reference category. Brighton, Truro and Plymouth each had small numbers of patients (< 11 patients) and so these sites were combined in a ‘South Coast Other’ category. Similarly, the sites Liverpool, Stoke and Newcastle also had small numbers of patients (< 11 patients) and so were combined into a ‘Northern Other’ category. The efficacy measure for each active treatment was the mean difference in PBVC change versus placebo. All patients for whom baseline and 96-week BV data were available were included in the analysis according to the treatment group to which they were randomised, irrespective of which treatment(s) they might have received. Dunnett-adjusted 95% simultaneous confidence intervals (CIs) and p-values were calculated using a single-step Dunnett procedure in order to adjust for the multiple pairwise comparisons and maintain the overall family-wise error rate of a false-significant result < 5% for the primary outcome analysis. No formal comparison of the active treatments was undertaken.
The primary analysis was a complete-case analysis based on the intention-to-treat (ITT) population. This meant that all patients for whom baseline BV and PBVC at 96-week values were available were included in the analysis according to the treatment group to which they were randomised, regardless of the treatment they actually received. Patients who withdrew from the trial or who were non-compliant with medication were also included, provided baseline BV and PBVC data were recorded for them. In a secondary analysis, the same analysis was based on the per protocol population. The per protocol population included all randomised patients who were adherent to the protocol and compliant with the allocated treatment throughout the duration of follow-up. For this trial, patients were considered compliant with medication if they reported taking, on average, ≥ 90% of their prescribed medication (taking account of planned down-titrations and deferred up-titrations) in the 30 days preceding each clinic visit. Any patients who withdrew from the trial or who were not compliant with medication were excluded from the per protocol population. The per protocol population was formally agreed, prior to database lock, by the chief investigator who was blinded to treatment allocation.
In addition, based on the ITT population defined above, the effect of missing data on the primary outcome analysis was investigated by performing three separate sensitivity analyses.
The sensitivity analyses involved:
-
Missing 96-week PBVC observations were imputed using a pattern-mixture approach to multiple imputation under a missing-not-at-random (MNAR) assumption for missing values. 102–104 A regression approach was implemented utilising fully conditional specification and the imputation model contained the same variables as for the final analysis. Thirty imputation data sets were created. The imputed values for PBVC at 96 weeks were then adjusted to reflect the MNAR assumption by adding a constant value equal to the observed standard deviation in the primary outcome at 96 weeks. In the clinical context of MS-SMART, the MNAR concern was that at least some of the missing data at 96 weeks would be due to deteriorating patient outcomes and disability. We report how large an amount can be added to the imputed values without changing the clinical interpretation of the trial, which was suggested as a possible sensitivity analysis for clinical trials by White et al. 105 If there were any missing baseline BV values then these will be imputed using the same multiple imputation method but without the MNAR adjustment. In this case we made the missing-at-random (MAR) assumption,102 which means that given the other data observed in the trial and the statistical analysis model being used, the missingness of baseline BV data are assumed to be independent of baseline BV.
-
A standard multiple imputation analysis was performed assuming that any missing outcome values were MAR. We used the same multiple imputation method as in (1) except no adjustment of imputations with respect to a MNAR assumption was performed in this case.
-
A complete-case approach was used as for the primary analysis except that any outliers more than 4 standard deviations away from the mean were excluded for the PBVC outcome.
Secondary magnetic resonance imaging outcome
Pseudoatrophy
Using the same methods as for the primary analysis, the mean difference in PBVC from baseline to 24 weeks between the placebo group and each of the active treatment groups was also assessed. If the reduction in PBVC was significantly greater in any treatment group, a secondary analysis was performed to compare PBVC from week 24 to week 96 using normal linear modelling. As this was a secondary analysis, no formal adjustment for multiplicity was done.
Counts of new and enlarging T2 lesions
Each active treatment group was compared with placebo in terms of the number of new and enlarging T2 lesions between the baseline and 96-week MRI. Overdispersed Poisson regression models (see Agresti,106 pp. 149–150) were used to estimate the rate ratio (for each active treatment group compared with placebo) of new/enlarging T2 lesion count after adjusting for baseline T2 lesion volume (T2LV) and the minimisation variables: age, sex, treatment centre and baseline EDSS score.
Secondary clinical outcome
No adjustment for multiplicity was made when analysing the secondary and exploratory end points. The interpretation of secondary and exploratory outcome analyses will be suitably cautious to reflect the high number of outcomes considered.
When the change over time in discrete or continuous outcomes (i.e. EDSS, 9HPT, PASAT, MSFC, SDMT, SLCVA, MSIS29v2, MSWSv2, NFI, NPRS, BPI, NPS and EQ-5D-5L) were found to be reasonably normally distributed, these were compared between the active treatment and placebo groups using normal linear models. As for the primary outcome analysis, the linear models included trial arm as an explanatory factor variable (with placebo as the reference category), the baseline variable (corresponding to the outcome variable) and the minimisation variables: age, gender, treatment centre and baseline EDSS score. Treatment centre was included as an explanatory factor variable with the UCL centre as the reference category. Brighton, Truro and Plymouth each had small numbers of patients (< 11 patients) and so these sites were combined in a ‘South Coast Other’ category. Similarly, the sites of Liverpool, Stoke and Newcastle also had small numbers of patients (< 11 patients) and so were combined into a ‘Northern Other’ category. Normality could not be assumed for MSFC and so this outcome was transformed using the signed square root transformation prior to analysis, and results were checked using Mann–Whitney–Wilcoxon non-parametric comparison tests. For the EDSS outcome only, the 95% CIs were computed using a bootstrap method because of the ordinal nature of the outcome variable. Cox proportional hazard models (adjusting for the minimisation variables) were used for time to first relapse and T25FW, with the difference between each active treatment and placebo being expressed in terms of a hazard ratio. The variable ‘time to progress to a given EDSS score’ was not analysed using a Cox proportional hazard model as described in the protocol because it was only recorded at a maximum of three time points. Instead, the proportion of trial participants with an increase in EDSS score of at least 1.0 point at 96 weeks relative to baseline was analysed using a multiple logistic regression model adjusting for the minimisation variables.
For the NPS, the same linear regression analysis method was conducted as above, except that the analysis was applied to the individual questionnaire items rather than to any total score because the items are individually meaningful. For question 8 of the NPS, because this question has three categorical options and is in a different format to the other items, frequencies and percentages were calculated for each option at follow-up. Logistic regression was used to determine if there was a significant difference between the active treatment arms and placebo for each of the three binary options separately. These logistic regression models all adjusted for the minimisation variables to enable adjusted odds ratios to be computed.
Corresponding analyses were performed for clinical secondary outcomes measured at 48 weeks.
Exploratory outcomes
Statistical modelling assessed whether baseline MRI or CSF neurofilament levels or disability measures could be used to predict PBVC or EDSS score outcome at 96 weeks. Separate linear regression models were fitted for each potential baseline predictor of PBVC or EDSS score at 96 weeks. Trial arm was included as an explanatory factor variable in each model.
To investigate if treatment effect depends on baseline variables, the same analysis was performed as above, but with an interaction term included in the models representing the interaction between trial arm and the baseline variable. Specifically, we tested for interactions of treatment effect with the following baseline variables: Multiple Sclerosis Walking Scale (MSWSv2), MSIS total (MSIS29v2), MSIS physical, MSIS psychological, cross-sectional area of the upper cervical spinal cord, MSFC, EQ-5D-5L index, T25FW, 9HPT, SDMT score, PASAT, EDSS score at baseline, EDSS score at randomisation, HCVA, SLCVA 5%, SLCVA 2.5%, SLCVA 1.25%, CSF: NfH chain, CSF: NfL chain, T2LV, NAA and N-acetylaspartylglutamate concentration, deep grey matter volume (DGMV), total BV, cortical grey matter volume (CGMV), EQ-5D visual analogue scale (VAS), mean myoinositol concentration, mean Glx concentration, mean T2 hyperintense lesions MTR, mean whole-brain MTR, mean GM MTR, and mean NAWM MTR. Interactions found to be statistically significant suggest that the magnitude of the treatment effect differs according to the value of the baseline variable.
Regarding CSF neurofilament levels, we assessed the unique contribution of neurofilament levels (either NfL or NfH or both together) in predicting PBVC treatment response at 96 weeks over and above other baseline variables that were found to predict treatment response in the above analyses. This consisted of fitting a multiple linear regression model to PBVC at 96 weeks and comparing the model results with and without the neurofilament variables. The R2 coefficient and residual standard deviation were reported as indicators of model fit.
In addition, a meta-analytic approach was used to assess the value of using MRI or disability or neurofilament variables recorded at 24 or 48 weeks as potential surrogate end points;107 the rationale being that we aimed to predict the effect of treatment on PBVC/EDSS score at 96 weeks based on the observed treatment effect on a surrogate end point. Bivariate mixed-effects models were used to assess surrogacy separately for each investigational treatment arm versus placebo comparison, based on the estimated treatment effect measured across different centres. Each potential surrogate end point was analysed separately. Coefficients of determination at the centre level were reported for each treatment and each surrogate end point. The analysis was conducted using the package ‘Surrogate’ within R software [The R Foundation for Statistical Computing, Vienna, Austria; URL: www.r-project.org (accessed 3 February 2020)].
Magnetic resonance imaging
Proportion of new and enlarging T2 lesions at 24 weeks being persistently T1 hypointense at 96 weeks
This outcome was analysed using multiple linear regression adjusting for the minimisation variables.
Percentage grey matter volume change
The percentage of GM volume change was statistically compared between trial arms using a multiple linear regression method adjusting for baseline and the minimisation variables.
Magnetic resonance spectroscopy
Changes in the NAA, myoinositol, and Glx metabolite concentrations were statistically compared between trial arms using a multiple linear regression method adjusting for baseline and the minimisation variables.
Magnetic transfer ratio
Lesion, GM and NAWM MTR measures were statistically compared between trial arms using a multiple linear regression method adjusting for baseline and the minimisation variables.
Diffusion tensor imaging
Peak width of skeletonised diffusivity-FA, AD, RD and MD were statistically compared between trial arms using a multiple linear regression method adjusting for baseline and the minimisation variables.
Cervical cord magnetic resonance imaging
The MUCCA at week 96 was statistically compared between trial arms using a multiple linear regression method adjusting for baseline and the minimisation variables.
Optical coherence tomography
Left and right eye OCT parameters were analysed separately for the pRNFL (primary analysis) and the GCL + IPL. The thickness of the pRNFL was measured from the peripapillary circular OCT scan at baseline and 96 weeks for each eye separately. The primary analysis was the global average pRNFL thickness excluding eyes with optic neuritis. The analysis used a multiple linear regression method adjusting for baseline and the minimisation variables to calculate adjusted mean differences (AMDs) and 95% CIs for the individual pairwise comparisons between each active treatment and placebo. Specific pRNFL sectors of each eye were also analysed using the same approach described above.
Secondary analysis repeated the primary analysis but included all eyes regardless of optic neuritis status, adjusting for the presence of optic neuritis.
The same analyses as above were performed for the macular GCL + IPL thickness volume measured by OCT at 96 weeks.
Other variables from the peripapillary circular scan and the macula volume scan, such as the average macular thickness and volume, were analysed using similar regression methodology.
Cerebrospinal fluid
The primary analysis in the CSF substudy was to determine whether or not there was a reduction in CSF NfL levels at 48 weeks in each of the treatment arms versus placebo arm using multiple linear regression adjusted for baseline and the minimisation variables.
An analysis was also performed for:
-
NfL level at 96 weeks adjusting for baseline
-
change in NfL from 48 to 96 weeks adjusted for baseline.
Additional analyses (i.e. a panel of biomarkers including NCAM, NfH, GFAP, ferritin, soluble CD14, MMP9 and neopterin) are reported in Report Supplementary Material 1 (Tables 18 and 19).
All analyses listed above were undertaken as complete-case analyses on the ITT population, and were also separately analysed according to the per protocol population based on compliance. All other analyses in this section were undertaken only as complete-case analyses on the ITT population.
Spearman’s rank-order correlation coefficients were calculated to assess the bivariate correlation of neurofilament levels with PBVC and EDSS score at 96 weeks.
Safety reporting
All AEs were recorded in the source medical records based on the time a consent form was signed until the end of the study for that participant (this was week 100). Participants were asked whether any AEs or serious adverse events (SAEs) had occurred at each study visit. The data were also extrapolated from written information in the diary and from laboratory results. Symptoms felt to be due to the progression of MS were excluded. Relapses were collated and graded separately.
All SAEs were reported to the sponsor by site investigators within 24 hours of them becoming aware of the event. All suspected unexpected serious adverse reactions (SUSARs) were notified to the sponsor immediately (or at least within 24 hours). The sponsor notified the main REC and Medicines and Healthcare products Regulatory Agency of all SUSARs.
Safety results were reported for the safety analysis population, according to treatment received. The safety analysis population was defined as all patients who were randomised into MS-SMART and who received at least one prescription of study medication. Safety data were analysed according to which treatment was received, rather than the trial arm to which the patient was randomised.
The numbers of AEs, SAEs and SUSARs were reported, split by trial arm. The number and percentage of patients experiencing AEs were also reported. In addition, the AE, SAE and SUSAR tables were presented with the number and percentage of patients recording an event in each of the Medical Dictionary for Regulatory Activities (MedDRA) system organ class categories.
Data monitoring
An independent DMC was established and the terms of reference are reported in a charter that is held in the trial master file (TMF) at ECTU. Unblinded safety data were monitored by the DMC to ensure the ongoing safety of patients in the study. Stopping criteria were not prespecified to the DMC and no formal interim analyses were planned. A Trial Steering Committee (TSC) was established to supervise the conduct and progress of the trial. The terms of reference of the TSC were documented in a charter that was held in the TMF at ECTU.
Summary of protocol changes
There were five protocol amendments that are listed in Table 1, resulting in seven versions of the protocol. 29
Protocol update | Protocol version | Protocol date | Reason for amendment |
---|---|---|---|
NA | V1 | 1 January 2013 | NA |
Substantial amendment | V1 | 1 January 2013 | Addition of new sites |
Substantial amendment | V2,a V3 | 1 December 2013, 1 June 2014 | See note below. Replacement of the drug ibudilast with fluoxetine |
Substantial amendment | V4 | 25 May 2015 | Change to eligibility criteria to exclude patients on high-dose simvastatin. Clarification in patient information sheet about side effects of fluoxetine |
Substantial amendment | V5 | 1 November 2016 | Protocol updated to reflect changes to fluoxetine summary of product characteristics |
Non-substantial amendment | V6 | 5 October 2017 | To update new trials unit address and telephone numbers |
Substantial amendment | V6 | 5 October 2017 | To update change of principal investigator |
Non-substantial amendment | V7 | 4 June 2018 | To correct typing error of ClinicalTrials.gov number |
Chapter 3 Main results
Recruitment
A total of 547 participants were consented and screened between December 2014 and June 2016. The first patient was screened on 18 December 2014 and the first participant was randomised on 29 January 2015. The last patient was randomised on 22 June 2016 and the last patient last visit occurred on 4 July 2018.
Of the 547 patients consented and screened, 445 (81% of total screened) met all eligibility criteria and were consecutively randomised to one of the three active treatments or placebo. A few participants had already undergone the consent process and screening when the target 440th patient had been randomised; therefore, it was appropriate for them to continue in the study and be randomised if eligible. The rates of screening and randomisation are reported in Figure 4.
FIGURE 4.
Screening and randomisation: cumulative number of patients screened and randomised.

The proportion of patients randomised at each site and the distribution of treatment allocation across sites are reported in Figure 5.
FIGURE 5.
Distribution of patients and treatment across the sites.

Participant flow
A CONSORT flow diagram of recruitment is in Figure 6. The 445 eligible participants were randomised to receive amiloride (n = 111) or fluoxetine (n = 111) or riluzole (n = 111) or placebo (n = 112).
FIGURE 6.
Patient disposition. The figure reports the number of participants included in the primary outcome analysis. In total, there were 19 withdrawals from the trial post randomisation. Two withdrawals occurred after the 96-week primary end point and are not reported in this figure.

Two patients randomised to riluzole also received fluoxetine prescribed by their GP during follow-up towards the end of the trial. Only one patient (randomised to riluzole) was in the category of withdrawal by clinician; all other withdrawals were withdrawals by the patient. Two withdrawals (one each from the riluzole and placebo groups) happened shortly after the 96-week MRI scan and so these patients were still included in the primary outcome analysis.
Numbers analysed
Adherence to trial protocol
Only one protocol violation was recorded: eight patients at the Leeds site were randomised prior to baseline data collection. Eligibility was rechecked and all patients were confirmed to be eligible.
Protocol deviations (see Report Supplementary Material 1, Table 1) associated with randomised patients were recorded on 317 occasions, with similar frequencies across treatment groups. Most deviations (239; 75%) related to assessments occurring outside protocol-specified time windows. A further six deviations not linked to randomised patients were also recorded.
Adherence to trial medication
Overall, 337 participants were adherent to allocated trial medication. Adherence was similar across treatment groups: amiloride, 83 of 111 (75%); fluoxetine, 87 of 111 (78%); riluzole, 84 of 111 (76%); and placebo, 83 of 112 (74%). Eighty-five participants permanently discontinued their assigned treatment after randomisation [amiloride, 20 (18%); fluoxetine, 24 (22%); riluzole, 22 (20%); and placebo, 19 (17%)].
Adherence to remaining in the trial
Nineteen patients (4%) withdrew from the trial post randomisation (three deceased, one on instruction from their treating clinician and 15 at the request of the participant). Two withdrawals (both at the request of the participant) occurred after the 96-week primary end point. A further 13 patients (3%) could not be contacted and were recorded as lost to follow-up. Fifty-two patients (12%) did not attend the 96-week MRI follow-up: amiloride 12 (11%), riluzole 12 (11%), fluoxetine 15 (14%) and placebo 13 (12%).
Adherence to study blinding
The treatment allocation was unblinded six times during the course of the trial and the reasons were as follows: two deaths (one in the riluzole arm and one in the fluoxetine arm), three SAEs with hospitalisation (two patients were on riluzole, one was on placebo) and one for evidence of clinical worsening, which was suspected to be due to study drugs (the patient was on fluoxetine).
The success or otherwise of the study blinding was assessed via questionnaire for the participant or participant’s doctor. A total of 117 out of 386 participant respondents and 202 out of 389 clinician respondents did not venture a guess and selected the ‘Don’t know’ option. Out of those participants making a guess, we found that only 138 out of 269 participants (51%) correctly guessed their treatment allocation. The corresponding kappa statistic was calculated to be 0.039 (95% CI –0.060 to 0.139), which suggests very poor agreement between the participant’s guess and the true result. For clinicians, 111 out of 187 (59%) correctly guessed the participant’s treatment allocation, corresponding to a kappa statistic of 0.127 (95% CI –0.005 to 0.259). This again suggests poor agreement, albeit the observed agreement was slightly higher for the clinician than for the participant.
Baseline data
Demographic characteristics of the participants are shown in Table 2. The clinical characteristics, including EDSS, MSFC with its three subcomponents, SDMT, high and low visual acuity, MSIS29v2 with the two subcomponents and MSWSv2 are reported in Table 3.
Trial arm | Overall | ||||
---|---|---|---|---|---|
Amiloride | Fluoxetine | Riluzole | Placebo | ||
Age (years) | |||||
n | 111 | 111 | 111 | 112 | 445 |
Mean | 54.36 | 54.83 | 54.10 | 54.89 | 54.55 |
SD | 7.18 | 7.10 | 6.75 | 7.16 | 7.03 |
Gender, n (%) | |||||
Male | 36 (32.4) | 37 (33.3) | 37 (33.3) | 37 (33.0) | 147 (33.0) |
Female | 75 (67.6) | 74 (66.7) | 74 (66.7) | 75 (67.0) | 298 (67.0) |
Race, n (%) | |||||
White | 108 (97.3) | 105 (94.6) | 106 (95.5) | 108 (96.4) | 427 (96.0) |
Black | 2 (1.8) | 1 (0.9) | 1 (0.9) | 1 (0.9) | 5 (1.1) |
Asian | 1 (0.9) | 4 (3.6) | 3 (2.7) | 2 (1.8) | 10 (2.2) |
Other | 0 (0) | 1 (0.9) | 1 (0.9) | 1 (0.9) | 3 (0.7) |
MS disease duration (years) | |||||
n | 107 | 110 | 109 | 109 | 435 |
Mean | 21.40 | 22.47 | 21.94 | 20.73 | 21.64 |
SD | 9.92 | 9.59 | 9.58 | 9.66 | 9.68 |
SPMS disease duration (years) | |||||
n | 111 | 111 | 110 | 111 | 443 |
Mean | 7.79 | 7.44 | 7.86 | 7.05 | 7.54 |
SD | 5.51 | 5.74 | 5.71 | 5.66 | 5.65 |
Relapse count, number (pre-enrolment) | |||||
n | 110 | 111 | 111 | 112 | 444 |
Mean | 0.19 | 0.10 | 0.09 | 0.15 | 0.13 |
SD | 0.57 | 0.36 | 0.42 | 0.51 | 0.47 |
Median | 0 | 0 | 0 | 0 | 0 |
Minimum | 0 | 0 | 0 | 0 | 0 |
Maximum | 4 | 2 | 3 | 3 | 4 |
Trial arm | Overall | |||||
---|---|---|---|---|---|---|
Amiloride | Fluoxetine | Riluzole | Placebo | |||
EDSS, score | n | 111 | 111 | 111 | 112 | 445 |
Median | 6.0 | 6.0 | 6.0 | 6.0 | 6.0 | |
Minimum | 4.0 | 4.0 | 4.0 | 4.0 | 4.0 | |
Maximum | 6.5 | 6.5 | 6.5 | 6.5 | 6.5 | |
EDSS score band | ||||||
4.0–5.5 | n (%) | 29 (26.1) | 28 (25.2) | 28 (25.2) | 29 (25.9) | 114 (25.6) |
6.0–6.5 | n (%) | 82 (73.9) | 83 (74.8) | 83 (74.8) | 83 (74.1) | 331 (74.4) |
BDI-II | n | 111 | 111 | 111 | 112 | 445 |
Median | 6 | 6 | 7 | 7 | 6 | |
Minimum | 0 | 0 | 0 | 0 | 0 | |
Maximum | 18 | 18 | 18 | 18 | 18 | |
MSFC, z-score | n | 111 | 111 | 111 | 112 | 445 |
Mean | –0.19 | –0.02 | –0.09 | 0.00 | –0.07 | |
SD | 1.19 | 0.60 | 0.95 | 0.91 | 0.93 | |
PASAT, number of correct answers | n | 111 | 110 | 111 | 112 | 444 |
Mean | 39.02 | 36.60 | 36.91 | 41.46 | 38.51 | |
SD | 13.67 | 15.17 | 16.00 | 13.86 | 14.78 | |
T25FW, seconds | n | 111 | 109 | 111 | 112 | 443 |
Mean | 25.14 | 15.70 | 19.84 | 18.63 | 19.84 | |
SD | 38.84 | 12.38 | 28.46 | 28.53 | 28.82 | |
9HPT, sec-1 | n | 111 | 111 | 111 | 112 | 445 |
Mean | 0.03 | 0.03 | 0.03 | 0.03 | 0.03 | |
SD | 0.01 | 0.01 | 0.01 | 0.01 | 0.01 | |
SDMT, number of correct answers | n | 109 | 111 | 109 | 112 | 441 |
Median | 46 | 46 | 45 | 46.5 | 46 | |
Minimum | 17 | 17 | 18 | 13 | 13 | |
Maximum | 72 | 67 | 74 | 75 | 75 | |
HCVA OD, number of correct answers | n | 106 | 111 | 110 | 110 | 437 |
Mean | 48.82 | 52.72 | 48.78 | 49.92 | 50.08 | |
SD | 13.97 | 7.27 | 14.84 | 13.43 | 12.77 | |
Median | 53.50 | 53.00 | 54.00 | 54.00 | 54.00 | |
Minimum | 0 | 25 | 0 | 0 | 0 | |
Maximum | 60 | 60 | 60 | 60 | 60 | |
HCVA OS, number of correct answers | n | 107 | 111 | 110 | 111 | 439 |
Mean | 50.09 | 50.8 | 48.56 | 50.42 | 49.9 | |
SD | 11.21 | 10.8 | 14.8 | 12.71 | 12.57 | |
Median | 53 | 54 | 54 | 55 | 54 | |
Minimum | 0 | 4 | 0 | 0 | 0 | |
Maximum | 62 | 65 | 65 | 64 | 65 | |
SLCVA 5% OD, number of correct answers | n | 106 | 110 | 108 | 109 | 433 |
Mean | 31.66 | 35.15 | 30.71 | 34.15 | 32.94 | |
SD | 14.52 | 10.46 | 15.38 | 14.62 | 13.93 | |
Median | 35 | 35 | 32 | 38 | 35 | |
Minimum | 0 | 0 | 0 | 0 | 0 | |
Maximum | 60 | 57 | 56 | 60 | 60 | |
SLCVA 5% OS, number of correct answers | n | 107 | 110 | 108 | 110 | 435 |
Mean | 32.58 | 32.93 | 29.96 | 33.93 | 32.36 | |
SD | 13.40 | 12.82 | 16.13 | 14.57 | 14.31 | |
Median | 35.00 | 34.50 | 34.50 | 36.00 | 35.00 | |
Minimum | 0 | 0 | 0 | 0 | 0 | |
Maximum | 59 | 56 | 55 | 60 | 60 | |
SLCVA 2.5% OD, number of correct answers | n | 106 | 111 | 109 | 110 | 436 |
Mean | 19.90 | 19.86 | 19.12 | 20.74 | 19.91 | |
SD | 13.15 | 12.28 | 14.50 | 13.69 | 13.39 | |
Median | 20.50 | 19.00 | 18.00 | 22.00 | 19.00 | |
Minimum | 0 | 0 | 0 | 0 | 0 | |
Maximum | 48 | 50 | 55 | 58 | 58 | |
SLCVA 2.5% OS, number of correct answers | n | 107 | 111 | 109 | 111 | 438 |
Mean | 19.10 | 17.65 | 18.81 | 20.85 | 19.10 | |
SD | 12.71 | 12.36 | 14.16 | 13.98 | 13.33 | |
Median | 19.00 | 18.00 | 20.00 | 22.00 | 20.00 | |
Minimum | 0 | 0 | 0 | 0 | 0 | |
Maximum | 45 | 39 | 50 | 58 | 58 | |
SLCVA 1.25% OD, number of correct answers | n | 106 | 111 | 109 | 110 | 436 |
Mean | 9.07 | 8.94 | 7.69 | 9.84 | 8.88 | |
SD | 10.25 | 10.19 | 10.68 | 11.33 | 10.62 | |
Median | 5.50 | 5.00 | 1.00 | 5.00 | 4.00 | |
Minimum | 0 | 0 | 0 | 0 | 0 | |
Maximum | 42 | 40 | 43 | 42 | 43 | |
SLCVA 1.25% OS, number of correct answers | n | 107 | 111 | 109 | 111 | 438 |
Mean | 8.17 | 6.94 | 7.08 | 9.86 | 8.02 | |
SD | 10.67 | 9.59 | 10.66 | 11.88 | 10.76 | |
Median | 3.00 | 2.00 | 0.00 | 5.00 | 2.00 | |
Minimum | 0 | 0 | 0 | 0 | 0 | |
Maximum | 35 | 34 | 43 | 44 | 44 | |
MSIS29v2 total score | n | 111 | 111 | 111 | 112 | 445 |
Mean | 63.91 | 65 | 69.15 | 66.07 | 66.04 | |
SD | 13.37 | 13.83 | 15.01 | 14.39 | 14.25 | |
Median | 65.00 | 64.00 | 68.00 | 66.00 | 65.00 | |
Minimum | 33 | 32 | 31 | 35 | 31 | |
Maximum | 98 | 98 | 106 | 97 | 106 | |
MSIS29v2 psychological score | n | 111 | 111 | 111 | 112 | 445 |
Mean | 15.95 | 16.66 | 18.16 | 17.1 | 16.97 | |
SD | 4.511 | 4.76 | 5.43 | 4.98 | 4.98 | |
Median | 15.00 | 16.00 | 16.00 | 17.00 | 16.00 | |
Minimum | 9 | 9 | 9 | 9 | 9 | |
Maximum | 32 | 28 | 33 | 30 | 33 | |
MSIS29v2 physical score | n | 111 | 111 | 111 | 112 | 445 |
Mean | 47.97 | 48.34 | 50.99 | 48.97 | 49.07 | |
SD | 10.50 | 10.44 | 11.30 | 11.22 | 10.90 | |
Median | 48.00 | 49.00 | 51.00 | 48.50 | 49.00 | |
Minimum | 24 | 23 | 21 | 25 | 21 | |
Maximum | 70 | 74 | 77 | 74 | 77 | |
MSWSv2 total score | n | 111 | 111 | 110 | 112 | 444 |
Mean | 41.43 | 41.08 | 42.64 | 41.63 | 41.69 | |
SD | 9.18 | 9.77 | 9.32 | 9.93 | 9.54 | |
Median | 42.00 | 44.00 | 45.00 | 44.00 | 43.00 | |
Minimum | 14 | 14 | 14 | 18 | 14 | |
Maximum | 54 | 54 | 54 | 54 | 54 | |
EQ-5D-5L index | n | 111 | 111 | 110 | 111 | 443 |
Mean | 0.68 | 0.70 | 0.66 | 0.67 | 0.68 | |
SD | 0.17 | 0.16 | 0.17 | 0.18 | 0.17 | |
Median | 0.73 | 0.72 | 0.67 | 0.72 | 0.71 | |
EQ-5D-5L VAS: health state score | n | 111 | 111 | 111 | 112 | 445 |
Mean | 66.13 | 67.53 | 61.73 | 65.24 | 65.15 | |
SD | 16.90 | 19.48 | 21.01 | 20.33 | 19.55 | |
Median | 70.00 | 70.00 | 65.00 | 65.00 | 70.00 | |
NFI summary interval score | n | 111 | 110 | 109 | 108 | 438 |
Mean | 18.01 | 17.43 | 19.05 | 17.80 | 18.07 | |
SD | 4.20 | 3.89 | 4.80 | 3.86 | 4.23 | |
Median | 17.64 | 17.64 | 18.45 | 17.64 | 17.64 | |
NFI physical interval score | n | 111 | 111 | 109 | 110 | 441 |
Mean | 15.12 | 14.66 | 15.91 | 14.74 | 15.10 | |
SD | 3.78 | 3.87 | 4.34 | 3.65 | 3.93 | |
Median | 14.14 | 14.14 | 15.06 | 14.14 | 14.14 | |
NFI cognitive interval score | n | 111 | 110 | 109 | 109 | 439 |
Mean | 6.52 | 6.21 | 7.06 | 6.31 | 6.52 | |
SD | 2.21 | 2.24 | 2.33 | 2.38 | 2.31 | |
Median | 6.36 | 6.36 | 7.13 | 6.36 | 6.36 | |
NFI diurnal interval score | n | 111 | 111 | 110 | 110 | 442 |
Mean | 10.07 | 9.61 | 10.24 | 9.68 | 9.90 | |
SD | 2.78 | 2.93 | 3.10 | 2.50 | 2.84 | |
Median | 10.09 | 10.09 | 10.48 | 10.09 | 10.09 | |
NFI nocturnal interval score | n | 111 | 111 | 111 | 111 | 444 |
Mean | 7.75 | 7.60 | 8.24 | 7.96 | 7.89 | |
SD | 2.04 | 2.46 | 2.78 | 2.29 | 2.41 | |
Median | 7.83 | 7.83 | 8.52 | 7.83 | 7.83 |
Comorbidities were also recorded and are shown in Table 4. Seven conditions of interest were captured: asthma, depression, hypertension, hypothyroidism, hyperlipidaemia, osteoporosis and hysterectomy.
Comorbidity | Trial arm, n (%) | Overall, n (%) | |||
---|---|---|---|---|---|
Amiloride | Fluoxetine | Riluzole | Placebo | ||
Asthma | 9 (8.1) | 9 (8.1) | 5 (4.5) | 8 (7.1) | 31 (7.0) |
Depression | 4 (3.6) | 6 (5.4) | 11 (9.9) | 8 (7.1) | 29 (6.5) |
Hypertension | 15 (13.5) | 12 (10.8) | 17 (15.3) | 16 (14.3) | 60 (13.5) |
Hypothyroidism | 7 (6.3) | 9 (8.1) | 15 (13.5) | 10 (8.9) | 41 (9.2) |
Hyperlipidaemia | 11 (9.9) | 10 (9) | 11 (9.9) | 9 (8.0) | 41 (9.2) |
Osteoporosis | 6 (5.4) | 7 (6.3) | 2 (1.8) | 7 (6.3) | 22 (4.9) |
Hysterectomy | 6 (5.4) | 5 (4.5) | 6 (5.4) | 12 (10.7) | 29 (6.5) |
Other condition | 27 (24.3) | 25 (22.5) | 27 (24.3) | 27 (24.1) | 106 (23.8) |
Finally, MRI characteristics (including BV, T2LV, DGMV and CGMV) are reported in Table 5.
Trial arm | Overall (n = 445) | |||||
---|---|---|---|---|---|---|
Amiloride (n = 111) | Fluoxetine (n = 111) | Riluzole (n = 111) | Placebo (n = 112) | |||
BV (ml) | Mean | 1432.2 | 1413.1 | 1414.2 | 1431.0 | 1422.6 |
SD | 84.2 | 82.4 | 74.8 | 91.1 | 83.6 | |
T2LV (ml) | Mean | 13.0 | 14.1 | 13.0 | 13.6 | 13.4 |
SD | 12.4 | 12.7 | 12.3 | 12.5 | 12.4 | |
DGMV (ml) | Mean | 45.1 | 44.0 | 44.2 | 45.3 | 44.7 |
SD | 4.0 | 4.1 | 3.9 | 4.2 | 4.1 | |
Median | 45.4 | 44.0 | 44.3 | 44.9 | 44.7 | |
CGMV (ml) | Mean | 794.6 | 787.5 | 786.2 | 791.0 | 789.8 |
SD | 40.9 | 48.3 | 37.9 | 49.1 | 44.3 |
Overall, the baseline characteristics (including clinical measures, BV and T2LV) were similar across the four trial arms.
Outcomes and estimation
Primary outcome
There was no significant difference between any of the active arms and the placebo arm in the PBVC at week 96 (Table 6 and Figure 7). This finding was confirmed in sensitivity analysis (see Table 6).
Analysis | n a | AMD (%) (active treatment – placebo) | 95% CI | Raw p-value | Simultaneous CI | Dunnett-adjusted p-value |
---|---|---|---|---|---|---|
Amiloride | ||||||
Primary analysis (with Dunnett adjustment) | 393 | 0.036 | –0.320 to 0.391 | 0.843 | –0.391 to 0.462 | 0.995 |
Sensitivity analysis 1: multiple imputation based on a MNAR assumptiona | 445 | 0.064 | –0.310 to 0.437 | 0.738 | –0.376 to 0.503 | 0.776 |
Sensitivity analysis 2: multiple imputation based on a MAR assumption | 445 | 0.039 | –0.324 to 0.401 | 0.834 | –0.386 to 0.464 | 0.858 |
Sensitivity analysis 3: with outliers removed | 392 | 0.041 | –0.305 to 0.387 | 0.817 | –0.374 to 0.456 | 0.991 |
Secondary analysis: using per protocol population | 263 | 0.122 | –0.278 to 0.523 | 0.548 | –0.360 to 0.605 | 0.883 |
Fluoxetine | ||||||
Primary analysis (with Dunnett adjustment) | 393 | –0.116 | –0.474 to 0.243 | 0.527 | –0.546 to 0.315 | 0.863 |
Sensitivity analysis 1: multiple imputation based on a MNAR assumptionb | 445 | –0.137 | –0.508 to 0.235 | 0.471 | –0.575 to 0.302 | 0.541 |
Sensitivity analysis 2: multiple imputation based on a MAR assumption | 445 | –0.108 | –0.464 to 0.247 | 0.551 | –0.528 to 0.311 | 0.613 |
Sensitivity analysis 3: with outliers removed | 392 | –0.052 | –0.402 to 0.298 | 0.770 | –0.472 to 0.368 | 0.983 |
Secondary analysis: using per protocol population | 263 | –0.050 | –0.456 to 0.356 | 0.809 | –0.539 to 0.439 | 0.991 |
Riluzole | ||||||
Primary analysis (with Dunnett adjustment) | 393 | –0.143 | –0.499 to 0.214 | 0.432 | –0.570 to 0.285 | 0.771 |
Sensitivity analysis 1: multiple imputation based on a MNAR assumptionb | 445 | –0.120 | –0.496 to 0.256 | 0.531 | –0.562 to 0.322 | 0.594 |
Sensitivity analysis 2: multiple imputation based on a MAR assumption | 445 | –0.129 | –0.486 to 0.229 | 0.480 | –0.550 to 0.292 | 0.549 |
Sensitivity analysis 3: with outliers removed | 392 | –0.139 | –0.486 to 0.207 | 0.430 | –0.555 to 0.277 | 0.770 |
Secondary analysis: using per protocol population | 263 | 0.097 | –0.312 to 0.505 | 0.641 | –0.395 to 0.588 | 0.940 |
FIGURE 7.
Dot plot for PBVC at 96 weeks. Patients are stratified by trial arm. The vertical lines show the mean ± 1 standard deviation.

The percentage brain volume change at 96 weeks was –1.35% in the overall cohort (see Table 7), corresponding to a PBVC of around –0.7% per year. In the placebo arm, the PBVC was similar to the overall cohort (see Table 7).
Secondary outcomes
Pseudoatrophy
In Table 7, we report the summary of means and standard deviations of PBVC stratified by trial arm.
PBVC time frame | Trial arm | Overall | ||||
---|---|---|---|---|---|---|
Amiloride | Fluoxetine | Riluzole | Placebo | |||
PBVC 0–96 weeks | n | 99 | 96 | 99 | 99 | 393 |
Mean | –1.25 | –1.42 | –1.43 | –1.29 | –1.35 | |
SD | 1.03 | 1.45 | 1.48 | 1.10 | 1.28 | |
PBVC 0–24 weeks | n | 99 | 104 | 96 | 105 | 404 |
Mean | –0.28 | –0.59 | –0.47 | –0.27 | –0.40 | |
SD | 0.87 | 0.90 | 0.89 | 0.87 | 0.89 | |
PBVC 24–96 weeks | n | 89 | 89 | 89 | 93 | 360 |
Mean | –0.96 | –0.82 | –0.86 | –1.04 | –0.92 | |
SD | 1.02 | 1.42 | 1.16 | 1.17 | 1.20 |
Percentage brain volume change at 24 weeks was significantly lower in the fluoxetine arm than in the placebo arm (AMD –0.31, 95% CI –0.60 to –0.02; p = 0.032), but not for the other active treatment arms versus placebo (see Table 8). There was no difference between any of the active treatment arms and placebo for PBVC between 24 and 96 weeks.
Analysis | n | AMD (%) (active treatment – placebo) | 95% CI | Raw p-value | Simultaneous CI | Dunnett-adjusted p-value |
---|---|---|---|---|---|---|
Amiloride | ||||||
Secondary analysis: PBVC at 24 weeks | 404 | –0.006 | –0.248 to 0.236 | 0.960 | –0.297 to 0.285 | 1.000 |
Secondary analysis: PBVC between 24 and 96 weeks | 360 | 0.083 | –0.266 to 0.431 | 0.641 | –0.336 to 0.501 | 0.938 |
Fluoxetine | ||||||
Secondary analysis: PBVC at 24 weeks | 404 | –0.308 | –0.548 to –0.069 | 0.012 | –0.596 to –0.020 | 0.032 |
Secondary analysis: PBVC between 24 and 96 weeks | 360 | 0.221 | –0.129 to 0.571 | 0.215 | –0.199 to 0.641 | 0.461 |
Riluzole | ||||||
Secondary analysis: PBVC at 24 weeks | 404 | –0.179 | –0.424 to 0.066 | 0.151 | –0.473 to 0.115 | 0.342 |
Secondary analysis: PBVC between 24 and 96 weeks | 360 | 0.150 | –0.199 to 0.499 | 0.397 | –0.269 to 0.569 | 0.733 |
New and enlarging T2 lesions
The number of new and enlarging T2 lesions after 24 and 96 weeks is reported in Table 9.
New and enlarging T2 lesions | Trial arm | Overall | ||||
---|---|---|---|---|---|---|
Amiloride | Fluoxetine | Riluzole | Placebo | |||
Number of new and enlarging T2 lesions 0–24 weeks | n | 103 | 106 | 98 | 105 | 412 |
Mean | 1.11 | 0.57 | 0.76 | 0.85 | 0.82 | |
SD | 2.61 | 1.89 | 1.79 | 2.84 | 2.33 | |
Number of new and enlarging T2 lesions 0–96 weeks | n | 101 | 99 | 100 | 100 | 400 |
Mean | 3.74 | 1.77 | 2.83 | 2.95 | 2.83 | |
SD | 8.05 | 5.28 | 5.71 | 6.90 | 6.59 |
The adjusted rate ratio for the number of new and enlarging T2 lesions detected at the 96-week MRI scan is reported in Table 10. An adjusted rate ratio of < 1 indicates a lower rate of new and enlarging T2 lesions in the active treatment arm. The model was based on n = 400 patients. The scale parameter of the over-dispersed Poisson model was estimated to be 2.542.
Adjusted rate ratio for the number of new and enlarging T2 lesions at 96 weeks (active – placebo) and 95% CIa | p-valuea | |
---|---|---|
Amiloride | 1.24 (0.83 to 1.83) | 0.291 |
Fluoxetine | 0.54 (0.332 to 0.87) | 0.012 |
Riluzole | 0.95 (0.63 to 1.45) | 0.814 |
There was no significant difference in the number of new and enlarging T2 lesions detected at the 96-week MRI scan for amiloride, or for riluzole versus placebo (Table 10).
Clinical outcomes
Detailed summary statistics for the clinical secondary outcomes at 48 and 96 weeks, split by trial arm, are reported in Report Supplementary Material 1 (Table 2). Multiple regression analyses for the clinical secondary outcomes at 48 weeks are also reported in Report Supplementary Material 1 (Table 3).
Clinician-reported outcomes at 96 weeks
Adjusted mean differences for EDSS, MSFC, 9HPT, PASAT, SDMT, SLVCA, T25FW (95% CIs) and p-values for each of the treatment arms versus placebo are reported in Table 11.
Outcome variable | n | AMD (amiloride – placebo) | 95% confidence limitsa for AMD | p-value | AMD (fluoxetine – placebo) | 95% confidence limitsa for AMD | p-value | AMD (riluzole – placebo) | 95% confidence limitsa AMD | p-value |
---|---|---|---|---|---|---|---|---|---|---|
EDSS, score | 383 | 0.054 | –0.124 to 0.223 | 0.609 | –0.067 | –0.285 to 0.160 | 0.535 | 0.052 | –0.158 to 0.245 | 0.628 |
9HPT, sec-1 | 387 | 0.000765 | –0.000884 to 0.00241 | 0.362 | 0.000156 | –0.00152 to 0.00183 | 0.854 | 0.000933 | –0.000742 to 0.00261 | 0.274 |
MSFC,b z-score | 387 | 0.057 | –0.153 to 0.266 | 0.594 | –0.088 | –0.299 to 0.124 | 0.417 | 0.022 | –0.190 to 0.234 | 0.839 |
PASAT, number of correct answers | 385 | 0.938 | –1.859 to 3.735 | 0.510 | –1.053 | –3.898 to 1.792 | 0.467 | 0.452 | –2.404 to 3.309 | 0.756 |
SDMT, number of correct answers | 379 | –1.030 | –3.080 to 1.019 | 0.324 | –1.122 | –3.186 to 0.943 | 0.286 | –0.814 | –2.899 to 1.270 | 0.443 |
HCVA RE, number of correct answers | 376 | 0.901 | –1.444 to 3.245 | 0.450 | 0.161 | –2.190 to 2.513 | 0.893 | –0.116 | –2.485 to 2.254 | 0.924 |
HCVA LE, number of correct answers | 378 | 3.047 | 0.486 to 5.609 | 0.020 | 1.824 | –0.735 to 4.382 | 0.162 | 1.631 | –0.974 to 4.237 | 0.219 |
SLCVA 5% RE, number of correct answers | 371 | 2.007 | –0.970 to 4.985 | 0.186 | –0.465 | –3.443 to 2.512 | 0.759 | 0.351 | –2.664 to 3.367 | 0.819 |
SLCVA 5% LE, number of correct answers | 373 | 0.999 | –1.838 to 3.835 | 0.489 | 1.277 | –1.568 to 4.122 | 0.378 | 1.457 | –1.432 to 4.346 | 0.322 |
SLCVA 2.5% RE, number of correct answers | 375 | –0.764 | –3.447 to 1.920 | 0.576 | –1.984 | –4.663 to 0.695 | 0.146 | –0.542 | –3.265 to 2.181 | 0.696 |
SLCVA 2.5% LE, number of correct answers | 377 | 0.857 | –1.968 to 3.682 | 0.551 | 1.306 | –1.534 to 4.146 | 0.366 | 1.682 | –1.191 to 4.556 | 0.250 |
SLCVA 1.25% RE, number of correct answers | 375 | –0.978 | –3.126 to 1.170 | 0.371 | –1.925 | –4.072 to 0.221 | 0.079 | 0.007 | –2.177 to 2.190 | 0.995 |
SLCVA 1.25% LE, number of correct answers | 377 | –0.580 | –2.658 to 1.498 | 0.583 | –0.797 | –2.892 to 1.298 | 0.455 | 0.742 | –1.373 to 2.856 | 0.491 |
The multiple regression model for each outcome included trial arm as an explanatory factor variable (with placebo as the reference category), the baseline measurement and the minimisation variables: age, gender, treatment centre and EDSS score at randomisation. Positive AMDs are indicative of observed treatment benefit for all variables except EDSS score.
For the T25FW test, the results of the Cox proportional hazards regression analysis are shown in Table 12. There was no significant difference between any of the treatment arms and placebo.
n = 382 | Adjusted hazard ratio | 95% CI | p-value |
---|---|---|---|
Amiloride vs. placebo | 0.824 | 0.608 to 1.117 | 0.213 |
Fluoxetine vs. placebo | 0.807 | 0.595 to 1.096 | 0.170 |
Riluzole vs. placebo | 0.835 | 0.615 to 1.135 | 0.249 |
Patient-reported outcomes
Adjusted mean differences for MSIS29v2, MSWSv2, EQ-5D-5L and NFI (95% CIs) and p-values for each of the treatment arms versus placebo are reported in Table 13. AMDs for pain questionnaires (i.e. BPI, NPRS, NPS) are reported in Report Supplementary Material 1 (Table 4).
Outcome variable | n | AMD (amiloride – placebo) | 95% confidence limits for AMD | p-value | AMD (fluoxetine – placebo) | 95% confidence limits for AMD | p-value | AMD (riluzole – placebo) | 95% confidence limits for AMD | p-value |
---|---|---|---|---|---|---|---|---|---|---|
MSIS29v2 – total score | 390 | 3.697 | 0.233 to 7.161 | 0.037 | 0.468 | –3.035 to 3.972 | 0.793 | 0.941 | –2.582 to 4.464 | 0.600 |
MSIS29v2 – physical score | 392 | 2.187 | –0.333 to 4.707 | 0.089 | 0.745 | –1.799 to 3.289 | 0.565 | 0.573 | –1.991 to 3.136 | 0.661 |
MSIS29v2 – psychological score | 390 | 1.505 | 0.193 to 2.816 | 0.025 | –0.276 | –1.600 to 1.048 | 0.682 | 0.463 | –0.865 to 1.791 | 0.494 |
MSWSv2 score | 390 | 0.466 | –1.608 to 2.540 | 0.659 | 0.997 | –1.112 to 3.106 | 0.353 | 0.645 | –1.467 to 2.757 | 0.549 |
EQ-5D index score | 390 | –0.010 | –0.057 to 0.038 | 0.690 | –0.016 | –0.064 to 0.033 | 0.524 | –0.006 | –0.054 to 0.043 | 0.820 |
EQ-5D VAS score | 391 | –2.052 | –7.587 to 3.483 | 0.466 | –3.002 | –8.617 to 2.613 | 0.294 | –2.991 | –8.635 to 2.653 | 0.298 |
NFI summary interval score | 385 | 0.912 | –0.204 to 2.029 | 0.109 | 0.527 | –0.601 to 1.655 | 0.359 | 0.748 | –0.393 to 1.888 | 0.198 |
NFI physical interval score | 388 | 1.241 | 0.204 to 2.278 | 0.019 | 0.727 | –0.321 to 1.776 | 0.173 | 1.013 | –0.048 to 2.075 | 0.061 |
NFI cognitive score | 387 | 0.100 | –0.404 to 0.604 | 0.696 | 0.155 | –0.354 to 0.664 | 0.549 | 0.157 | –0.359 to 0.674 | 0.549 |
NFI diurnal interval score | 384 | –0.288 | –0.904 to 0.329 | 0.359 | –0.336 | –0.954 to 0.283 | 0.286 | –0.270 | –0.893 to 0.353 | 0.395 |
NFI nocturnal interval score | 390 | 0.636 | 0.134 to 1.138 | 0.013 | 0.398 | –0.109 to 0.905 | 0.124 | 0.116 | –0.392 to 0.624 | 0.653 |
The multiple regression model for each outcome included trial arm as an explanatory factor variable (with placebo as the reference category), the baseline measurement and the minimisation variables: age, gender, treatment centre and EDSS score at randomisation. Negative AMDs are indicative of observed treatment benefit for all variables except EQ-5D.
One-point increase in EDSS score at 96 weeks
For the calculation of the 1-point EDSS score increase, the fitted logistic regression model was unstable and had problems with convergence and so centre was recategorised into four categories when fitting the model: London, Edinburgh, Sheffield and Other, with ‘London’ as the reference category. Results from this analysis are shown in Table 14. There was no significant difference between the three treatment arms and placebo.
n = 383 | Adjusted odds ratio | 95% CI | p-value |
---|---|---|---|
Amiloride vs. placebo | 0.845 | 0.349 to 2.029 | 0.705 |
Fluoxetine vs. placebo | 0.999 | 0.422 to 2.369 | 0.999 |
Riluzole vs. placebo | 1.168 | 0.504 to 2.729 | 0.717 |
Relapses
Fifty-one patients (11%) experienced at least one relapse overall during follow-up. For each trial arm, patients experienced at least one relapse as follows: amiloride, n = 16 (14%); fluoxetine, n = 10 (9%); riluzole, n = 11 (10%); and placebo, n = 14 (12%).
Analysis from the Cox proportional hazards regression model showed no significant difference in the time to first relapse for any of the active arms compared with placebo (Table 15).
n = 445 | Adjusted hazard ratio | 95% CI | p-value |
---|---|---|---|
Amiloride vs. placebo | 1.145 | 0.557 to 2.354 | 0.713 |
Fluoxetine vs. placebo | 0.737 | 0.327 to 1.661 | 0.461 |
Riluzole vs. placebo | 0.784 | 0.355 to 1.731 | 0.547 |
The corresponding results for the clinical outcomes at 48 weeks are shown in Report Supplementary Material 1 (Table 3).
Safety
There were no emergent safety issues in the four trial arms. Table 16 shows the number of patients experiencing AEs, split by treatment received and MedDRA category.
MedDRA category | Treatment received | Total | ||||
---|---|---|---|---|---|---|
Amiloride | Fluoxetine | Riluzole | Riluzole + fluoxetinea | Placebo | ||
Total number of patients | 111 | 111 | 109 | 2 | 112 | 445 |
Blood and lymphatic system disorders, n (%) | 5 (5) | 3 (3) | 2 (2) | 0 | 3 (3) | 13 (3) |
Cardiac disorders, n (%) | 1 (1) | 3 (3) | 8 (7) | 0 | 2 (2) | 14 (3) |
Ear and labyrinth disorders, n (%) | 5 (5) | 3 (3) | 1 (1) | 0 | 5 (4) | 14 (3) |
Endocrine disorders, n (%) | 0 | 0 | 1 (1) | 0 | 0 | 1 (< 1) |
Eye disorders, n (%) | 13 (12) | 8 (7) | 9 (8) | 0 | 8 (7) | 38 (9) |
Gastrointestinal disorders, n (%) | 46 (41) | 62 (56) | 49 (45) | 0 | 36 (32) | 193 (43) |
General disorders and administration, n (%) | 26 (23) | 28 (25) | 27 (25) | 1 (50) | 32 (29) | 114 (26) |
Hepatobiliary disorders, n (%) | 2 (2) | 3 (3) | 0 | 0 | 1 (1) | 6 (1) |
Immune system disorders, n (%) | 1 (1) | 1 (1) | 3 (3) | 0 | 0 | 5 (1) |
Infections and infestations, n (%) | 68 (61) | 58 (52) | 62 (57) | 0 | 69 (62) | 257 (58) |
Injury, poisoning and procedural complications, n (%) | 26 (23) | 43 (39) | 29 (27) | 1 (50) | 28 (25) | 127 (29) |
Investigations, n (%) | 10 (9) | 20 (18) | 17 (16) | 0 | 8 (7) | 55 (12) |
Metabolism and nutrition disorders, n (%) | 2 (2) | 9 (8) | 7 (6) | 0 | 4 (4) | 22 (5) |
Musculoskeletal and connective tissue disorders, n (%) | 37 (33) | 26 (23) | 37 (34) | 1 (50) | 29 (26) | 130 (29) |
Neoplasms benign, malignant and unspecified, n (%) | 2 (2) | 1 (1) | 4 (4) | 0 | 2 (2) | 9 (2) |
Nervous system disorders, n (%) | 48 (43) | 46 (41) | 47 (43) | 0 | 44 (39) | 185 (42) |
Psychiatric disorders, n (%) | 21 (19) | 30 (27) | 22 (20) | 0 | 22 (20) | 95 (21) |
Renal and urinary disorders, n (%) | 9 (8) | 13 (12) | 10 (9) | 0 | 5 (4) | 37 (8) |
Reproductive system and breast disorders, n (%) | 4 (4) | 3 (3) | 2 (2) | 0 | 2 (2) | 11 (2) |
Respiratory disorders, n (%) | 15 (14) | 23 (21) | 13 (12) | 0 | 16 (14) | 67 (15) |
Skin and subcutaneous tissue disorders, n (%) | 16 (14) | 11 (10) | 13 (12) | 2 (100) | 17 (15) | 59 (13) |
Surgical and medical procedures, n (%) | 6 (5) | 3 (3) | 8 (7) | 0 | 7 (6) | 24 (5) |
Vascular disorders, n (%) | 4 (4) | 2 (2) | 3 (3) | 0 | 6 (5) | 15 (3) |
Table 17 shows the number of SAEs, split by treatment received and MedDRA category.
MedDRA category | Trial arm (n) | Total (n) | |||
---|---|---|---|---|---|
Amiloride | Fluoxetine | Riluzole | Placebo | ||
Cardiac disorders | 0 | 1 | 3 | 0 | 4 |
Gastrointestinal disorders | 2 | 0 | 0 | 1 | 3 |
General disorders and administration | 0 | 2 | 1 | 1 | 4 |
Hepatobiliary disorders | 2 | 1 | 0 | 3 | 6 |
Infections and infestations | 5 | 1 | 4 | 4 | 14 |
Injury, poisoning and procedural complications | 5 | 0 | 3 | 2 | 10 |
Investigations | 0 | 0 | 0 | 2 | 2 |
Musculoskeletal and connective tissue disorders | 0 | 1 | 0 | 0 | 1 |
Neoplasms benign, malignant and unspecified | 1 | 0 | 0 | 0 | 1 |
Nervous system disorders | 1 | 0 | 1 | 0 | 2 |
Psychiatric disorders | 0 | 1 | 1 | 1 | 3 |
Renal and urinary disorders | 1 | 1 | 0 | 0 | 2 |
Respiratory disorders | 1 | 0 | 1 | 0 | 2 |
Skin and subcutaneous tissue disorders | 0 | 0 | 0 | 1 | 1 |
Surgical and medical procedures | 1 | 0 | 1 | 1 | 3 |
Vascular disorders | 0 | 0 | 0 | 1 | 1 |
Total | 19 | 8 | 15 | 17 | 59 |
There was only one SUSAR; this was a hospitalisation due to suspected drug toxicity and the MedDRA category was ‘Injury, poisoning and procedural complications’. The patient was randomised to the riluzole treatment arm.
Three deaths occurred unrelated to the investigational agents; for two patients, death was unexpected and the cause of death remained unknown and one patient died of metastatic lung cancer.
Chapter 4 Substudy and exploratory outcome results
There were 244 patients originally consented to the advanced MRI substudy, 308 to the OCT substudy and 84 to the CSF substudy. There were 206 patients randomised to the advanced MRI substudy, 260 to the OCT substudy and 70 to the CSF substudy.
Magnetic resonance imaging
Summary statistics for the exploratory and advanced MRI substudy outcomes at baseline and 96 weeks are reported in Report Supplementary Material 1, Table 6. AMDs in the 96-week measures between each active treatment arm and placebo are shown in Table 18.
Outcome variable | n | AMD (amiloride – placebo) | 95% confidence limits for AMD | p-value | AMD (fluoxetine – placebo) | 95% confidence limits for AMD | p-value | AMD (riluzole – placebo) | 95% confidence limits for AMD | p-value |
---|---|---|---|---|---|---|---|---|---|---|
Percentage DGM volume change (%) | 380 | –0.371 | –0.815 to 0.074 | 0.102 | 0.085 | –0.364 to 0.535 | 0.709 | –0.304 | –0.752 to 0.144 | 0.183 |
Percentage CGM volume change (%) | 380 | –0.067 | –0.430 to 0.295 | 0.716 | –0.420 | –0.786 to –0.054 | 0.025 | –0.187 | –0.551 to 0.178 | 0.314 |
Mean NAA concentration (London) (millimolar units) | 121 | 0.060 | –0.278 to 0.398 | 0.725 | 0.124 | –0.233 to 0.481 | 0.492 | –0.180 | –0.521 to 0.161 | 0.297 |
Mean NAA concentration (Edinburgh) (millimolar units) | 36 | 219.980 | 14.452 to 425.507 | 0.037 | 110.551 | –70.491 to 291.593 | 0.221 | 110.623 | –86.264 to 307.510 | 0.259 |
Myoinositol concentration (London) (millimolar units) | 121 | –0.082 | –0.305 to 0.140 | 0.464 | –0.252 | –0.485 to –0.019 | 0.034 | 0.004 | –0.220 to 0.229 | 0.969 |
Myoinositol concentration (Edinburgh) (millimolar units) | 36 | –6.058 | –78.309 to 66.194 | 0.865 | 18.642 | –43.733 to 81.018 | 0.545 | 0.606 | –67.951 to 69.163 | 0.986 |
Glx concentration (London) (millimolar units) | 121 | –0.165 | –0.613 to 0.282 | 0.465 | –0.099 | –0.568 to 0.370 | 0.676 | –0.195 | –0.648 to 0.259 | 0.397 |
Glx concentration (Edinburgh) (millimolar units) | 36 | 38.947 | –119.41 to 197.304 | 0.618 | 2.508 | –138.545 to 143.561 | 0.971 | 39.327 | –112.318 to 190.972 | 0.599 |
Whole-brain MTR (mean) | 112 | –0.065 | –1.212 to 1.082 | 0.911 | –0.008 | –1.237 to 1.221 | 0.990 | 0.191 | –0.944 to 1.327 | 0.739 |
GM MTR (mean) | 112 | –0.175 | –1.375 to 1.026 | 0.774 | 0.156 | –1.131 to 1.442 | 0.811 | 0.086 | –1.104 to 1.276 | 0.887 |
NAWM MTR (mean) | 112 | –0.237 | –1.375 to 0.901 | 0.680 | 0.171 | –1.049 to 1.391 | 0.782 | 0.266 | –0.861 to 1.392 | 0.641 |
T2L MTR (mean) | 112 | –0.386 | –1.960 to 1.187 | 0.627 | 0.197 | –1.493 to 1.887 | 0.818 | 0.912 | –0.647 to 2.470 | 0.249 |
MUCCA (mm2) | 122 | –0.445 | –1.303 to 0.414 | 0.307 | –0.372 | –1.262 to 0.518 | 0.410 | –0.587 | –1.458 to 0.284 | 0.185 |
PWSAD (mm2/second) | 35 | –0.204 | –0.682 to 0.273 | 0.388 | –0.304 | –0.681 to 0.073 | 0.109 | –0.009 | –0.420 to 0.401 | 0.963 |
PWSFA (scaled between 0 and 1) | 35 | –0.00999 | –0.0283 to 0.00829 | 0.272 | –0.00845 | –0.0226 to 0.00573 | 0.232 | 0.000349 | –0.0149 to 0.0156 | 0.963 |
PWSMD (mm2/second) | 35 | –0.145 | –0.464 to 0.173 | 0.358 | –0.199 | –0.454 to 0.057 | 0.122 | 0.178 | –0.102 to 0.457 | 0.204 |
PWSRD (mm2/second) | 35 | –0.220 | –0.462 to 0.022 | 0.073 | –0.233 | –0.431 to –0.035 | 0.023 | 0.095 | –0.121 to 0.311 | 0.376 |
The MRI outcome of ‘Proportion of new and enlarging T2 lesions at 24 weeks being persistently T1 hypointense at 96 weeks’ was calculated as ‘Count of T1 hypointense lesions at week 96 which have a corresponding new or enlarging T2 hyperintense lesion at week 24’ divided by ‘Count of new and enlarging T2 hyperintense lesions since baseline at 24 weeks’. There was no significant difference in the proportion of new and enlarging T2 lesions at 24 weeks being persistently T1 hypointense at 96 weeks between the three active arms and placebo (Table 19).
Treatment effect | AMD (active treatment – placebo) | 95% confidence limits for AMD | p-value |
---|---|---|---|
Amiloride | 0.072 | –0.160 to 0.304 | 0.538 |
Fluoxetine | 0.065 | –0.191 to 0.321 | 0.616 |
Riluzole | –0.004 | –0.250 to 0.242 | 0.974 |
Optical coherence tomography
Summary statistics for the OCT left eye and right eye, pRNFL and GCL + IPL outcomes for all patients’ eyes and for patients’ eyes without previous history of optic neuritis at baseline and at 96 weeks are reported in Report Supplementary Material 1, Tables 7–14.
For pRNFL, left eye measures were obtained from 159 participants (119 participants had not suffered from previous optic neuritis) and right eye measures from 163 participants (113 participants had not suffered from previous optic neuritis).
For GCL + IPL, left eye measures were obtained from 161 participants (121 participants had not suffered from previous optic neuritis); and right eye measures from 165 participants (117 participants had not suffered from previous optic neuritis).
The AMDs between active drug and placebo for pRNFL thickness and complex GCL + IPL thickness are shown in Table 20.
Outcome variable | n | AMD (amiloride – placebo) | 95% confidence limits for AMD | p-value | AMD (fluoxetine – placebo) | 95% confidence limits for AMD | p-value | AMD (riluzole – placebo) | 95% confidence limits for AMD | p-value |
---|---|---|---|---|---|---|---|---|---|---|
Eyes with no optic neuritis only | ||||||||||
pRNFL thickness (µm) LE (primary OCT analysis) | 119 | –0.819 | –2.245 to 0.607 | 0.258 | 0.210 | –1.126 to 1.547 | 0.756 | –0.982 | –2.387 to 0.422 | 0.169 |
pRNFL thickness (µm) RE (primary OCT analysis) | 113 | –0.260 | –1.334 to 0.814 | 0.632 | 0.961 | –0.072 to 1.993 | 0.068 | –0.868 | –1.922 to 0.187 | 0.106 |
GCL + IPL thickness (µm) LE | 121 | 0.028 | –0.948 to 1.004 | 0.955 | 0.202 | –0.723 to 1.127 | 0.666 | –0.100 | –1.066 to 0.865 | 0.837 |
GCL + IPL thickness (µm) RE | 117 | –0.071 | –0.905 to 0.764 | 0.867 | 0.375 | –0.466 to 1.216 | 0.379 | 0.120 | –0.763 to 1.004 | 0.788 |
All eyes, adjusted for optic neuritis | ||||||||||
pRNFL thickness (µm) LE | 159 | –0.870 | –2.080 to 0.339 | 0.157 | 0.429 | –0.731 to 1.589 | 0.466 | –1.053 | –2.240 to 0.133 | 0.082 |
pRNFL thickness (µm) RE | 163 | –0.037 | –0.901 to 0.827 | 0.933 | 1.033 | 0.236 to 1.829 | 0.011 | –0.408 | –1.221 to 0.404 | 0.323 |
GCL + IPL thickness (µm) LE | 161 | 0.248 | –0.630 to 1.127 | 0.577 | 0.235 | –0.614 to 1.084 | 0.585 | 0.097 | –0.762 to 0.956 | 0.824 |
GCL + IPL thickness (µm) RE | 165 | 0.568 | –0.340 to 1.475 | 0.218 | 1.015 | 0.117 to 1.913 | 0.027 | 0.829 | –0.078 to 1.736 | 0.073 |
Secondary analyses including individual pRNFL sector thickness, as well as analyses of the global (inner plus outer) macular thickness from the ETDRS grid, are shown in Report Supplementary Material 1, Table 15.
Cerebrospinal fluid
Summary statistics of the CSF substudy at week 48 and week 96 are reported in the supplementary material (see Report Supplementary Material 1, Tables 16 and 17). The results of the panel of additional biomarkers (i.e. NCAM, NfH, GFAP, ferritin, soluble CD14, MMP9 and neopterin) are also described in the supplementary material (see Report Supplementary Material 1, Tables 18–20).
A total of 39 participants (fluoxetine, n = 11; riluzole, n = 9; amiloride, n = 10; placebo, n = 9) completed the CSF add-on study. The baseline NfL and NfH values were on average similar across the groups: NfL mean range 829–1079 pg/ml and NfH mean range 452–479 pg/ml (Table 21).
Outcome variable | n | AMD (amiloride – placebo) | 95% confidence limits for AMD | p-value | AMD (fluoxetine – placebo) | 95% confidence limits for AMD | p-value | AMD (riluzole – placebo) | 95% confidence limits for AMD | p-value |
---|---|---|---|---|---|---|---|---|---|---|
NfL at 48 weeks, pg/ml | 39 | 75.22 | –173.72 to 324.15 | 0.542 | 149.30 | –92.75 to 391.36 | 0.218 | 93.21 | –162.06 to 348.47 | 0.462 |
NfH at 48 weeks, pg/ml | 39 | –20.34 | –91.10 to 50.42 | 0.562 | 52.22 | –16.82 to 121.26 | 0.133 | 41.74 | –30.57 to 114.04 | 0.248 |
NfL at 96 weeks, pg/ml | 35 | –415.85 | –1118.12 to 286.42 | 0.235 | –186.00 | –897.99 to 525.99 | 0.596 | –371.05 | –1113.65 to 371.55 | 0.314 |
NfH at 96 weeks, pg/ml | 35 | –232.83 | –558.09 to 92.43 | 0.153 | –190.60 | –523.54 to 142.35 | 0.250 | –205.35 | –562.45 to 151.75 | 0.248 |
NfL change from 48 to 96 weeks, pg/ml | 32 | –685.92 | –1305.60 to –66.24 | 0.031 | –515.86 | –1136.49 to 104.78 | 0.099 | –538.27 | –1200.42 to 123.88 | 0.106 |
NfH change from 48 to 96 weeks, pg/ml | 32 | –296.13 | –639.75 to 47.49 | 0.088 | –314.46 | –661.71 to 32.80 | 0.074 | –334.01 | –711.92 to 43.90 | 0.081 |
PPP: NfL at 48 weeks, pg/ml | 23 | 71.45 | –334.35 to 477.25 | 0.713 | 241.70 | –235.29 to 718.69 | 0.297 | 68.90 | –335.24 to 473.05 | 0.721 |
PPP: NfH at 48 weeks, pg/ml | 23 | –15.49 | –106.08 to 75.10 | 0.721 | 59.54 | –45.90 to 164.99 | 0.247 | 63.45 | –27.80 to 154.69 | 0.159 |
PPP: NfL at 96 weeks, pg/ml | 22 | 168.47 | –275.83 to 612.77 | 0.430 | 469.27 | –39.78 to 978.32 | 0.068 | 339.48 | –120.71 to 799.66 | 0.136 |
PPP: NfH at 96 weeks, pg/ml | 22 | 101.73 | –23.61 to 227.06 | 0.104 | 149.06 | 3.23 to 294.89 | 0.046 | 88.48 | –44.47 to 221.42 | 0.175 |
PPP: NfL change from 48 to 96 weeks, pg/ml | 21 | –92.63 | –517.51 to 332.25 | 0.645 | 66.22 | –415.68 to 548.13 | 0.77 | –60.23 | –481.22 to 360.76 | 0.762 |
PPP: NfH change from 48 to 96 weeks, pg/ml | 21 | 123.95 | 20.19 to 227.72 | 0.023 | 96.15 | –20.71 to 213.00 | 0.10 | 4.12 | –102.70 to 110.94 | 0.935 |
Predictive modelling of the primary and Expanded Disability Status Scale outcomes according to baseline magnetic resonance imaging/disability scores
As part of the exploratory analyses, additional statistical modelling scores explored whether or not baseline MRI/disability could be used to predict temporal evolution of SPMS.
Separate linear regression models were fitted for each potential baseline predictor of PBVC at 96 weeks and EDSS score at 96 weeks and the results are shown in Tables 22 and 23, respectively. Trial arm was included as an explanatory factor variable in each model. Tables 22 and 23 are ordered according to the magnitude of the standardised coefficient (largest to smallest).
Baseline variable | n | Coefficient | 95% CI | p-value | Standardised coefficient | R 2 |
---|---|---|---|---|---|---|
NfH (pg/ml) | 49 | –0.0033 | –0.0057 to –0.0009 | 0.0076 | –0.382 | 0.184 |
NfL (pg/ml) | 49 | –0.0009 | –0.0017 to –0.0001 | 0.0200 | –0.347 | 0.151 |
SLCVA 2.5% RE (number of correct answers) | 385 | 0.0191 | 0.0095 to 0.0288 | 0.0001 | 0.196 | 0.043 |
SLCVA 5% RE (number of correct answers) | 382 | 0.0166 | 0.0072 to 0.0259 | 0.0006 | 0.177 | 0.036 |
SDMT (number of correct answers) | 389 | 0.0170 | 0.0069 to 0.0271 | 0.0010 | 0.167 | 0.031 |
Mean myoinositol (London) (millimolar units) | 133 | –0.3714 | –0.7560 to 0.0132 | 0.0583 | –0.164 | 0.066 |
HCVA RE (number of correct answers) | 386 | 0.0163 | 0.0059 to 0.0266 | 0.0022 | 0.157 | 0.028 |
SLCVA 2.5% LE (number of correct answers) | 387 | 0.0150 | 0.0054 to 0.0247 | 0.0024 | 0.155 | 0.028 |
SLCVA 1.25% REa (number of correct answers) | 385 | 0.0181 | 0.0061 to 0.0301 | 0.0032 | 0.151 | 0.027 |
HCVA LE (number of correct answers) | 388 | 0.0150 | 0.0048 to 0.0252 | 0.0040 | 0.147 | 0.025 |
T2L MTR (mean) | 156 | 0.0307 | –0.0034 to 0.0649 | 0.0772 | 0.142 | 0.040 |
SLCVA 5% LE (number of correct answers) | 384 | 0.0124 | 0.0033 to 0.0215 | 0.0074 | 0.137 | 0.023 |
SLCVA 1.25% LE (number of correct answers) | 387 | 0.0160 | 0.0042 to 0.0278 | 0.0079 | 0.136 | 0.022 |
T2LV (× 10,000 mm3) | 393 | –0.1399 | –0.2425 to –0.0372 | 0.0077 | –0.135 | 0.022 |
PASAT | 393 | 0.0107 | 0.0021 to 0.0193 | 0.0147 | 0.124 | 0.019 |
Whole-brain MTR (mean) | 156 | 0.0319 | –0.0101 to 0.0738 | 0.1352 | 0.121 | 0.034 |
Mean NAA concentration (London) (millimolar units) | 133 | 0.2062 | –0.0867 to 0.4992 | 0.1660 | 0.121 | 0.054 |
MUCCA (mm2) | 128 | 0.0197 | –0.0091 to 0.0486 | 0.1778 | 0.120 | 0.045 |
NAWM MTR (mean) | 156 | 0.0269 | –0.0141 to 0.0679 | 0.1965 | 0.104 | 0.030 |
MSFCa (z-score) | 393 | 0.1358 | –0.0011 to 0.2727 | 0.0518 | 0.099 | 0.014 |
GM MTR (mean) | 156 | 0.0263 | –0.0163 to 0.0689 | 0.2250 | 0.098 | 0.029 |
9HPT (sec-1) | 393 | 9.5974 | –2.9570 to 22.1518 | 0.1336 | 0.076 | 0.010 |
BV (× 10,000 mm3) | 393 | 0.0113 | –0.0042 to 0.0268 | 0.1519 | 0.073 | 0.009 |
DGMV (× 10,000 mm3) | 393 | 0.2061 | –0.1078 to 0.5200 | 0.1974 | 0.066 | 0.008 |
EDSS score at randomisation | 393 | –0.1035 | –0.2729 to 0.0659 | 0.2305 | –0.061 | 0.008 |
EDSS score at baseline | 393 | –0.1021 | –0.2716 to 0.0675 | 0.2373 | –0.060 | 0.008 |
MSWSv2 score | 392 | –0.0077 | –0.0209 to 0.0055 | 0.2515 | –0.058 | 0.007 |
MSIS20v2 physical score | 393 | –0.0067 | –0.0183 to 0.0049 | 0.2550 | –0.058 | 0.007 |
Mean Glx concentration (London) (mM) | 133 | –0.0848 | –0.3824 to 0.2127 | 0.5736 | –0.049 | 0.042 |
MSIS29v2 total score | 393 | –0.0041 | –0.0130 to 0.0048 | 0.3607 | –0.047 | 0.006 |
CGMV (× 10,000 mm3) | 393 | 0.0132 | –0.0158 to 0.0423 | 0.3712 | 0.045 | 0.006 |
EQ-5D-5L – VAS score | 393 | –0.0019 | –0.0085 to 0.0047 | 0.5685 | –0.029 | 0.005 |
EQ-5D-5L – index score | 391 | –0.1687 | –0.9002 to 0.5628 | 0.6505 | –0.023 | 0.005 |
T25FW (seconds) | 391 | –0.0006 | –0.0052 to 0.0039 | 0.7783 | –0.014 | 0.004 |
MSIS29v2 psychological score | 393 | –0.0016 | –0.0272 to 0.0240 | 0.9038 | –0.006 | 0.004 |
Baseline variable | n | Coefficient | 95% CI | p-value | Standardised coefficient | R 2 |
---|---|---|---|---|---|---|
EDSS score at baseline | 383 | 1.01745 | 0.92075 to 1.11414 | < 0.0001 | 0.729 | 0.532 |
EDSS score at randomisation | 383 | 0.99912 | 0.90058 to 1.09767 | < 0.0001 | 0.716 | 0.513 |
MSWSv2 | 383 | 0.06392 | 0.05489 to 0.07296 | < 0.0001 | 0.582 | 0.340 |
MSIS physical score | 383 | 0.0431 | 0.03424 to 0.05195 | < 0.0001 | 0.443 | 0.196 |
MUCCA (mm2) | 131 | –0.0411 | –0.0596 to –0.0227 | < 0.0001 | –0.366 | 0.139 |
MSIS29v2 total score | 383 | 0.0262 | 0.01906 to 0.03333 | < 0.0001 | 0.351 | 0.122 |
MSFC,a z-score | 383 | –0.3977 | –0.3494 to –0.5060 | < 0.0001 | –0.349 | 0.122 |
EQ-5D-5L index score | 381 | –2.0406 | –2.6361 to –1.4451 | < 0.0001 | –0.330 | 0.109 |
T25FW (seconds) | 381 | 0.01145 | 0.00786 to 0.01503 | < 0.0001 | 0.310 | 0.096 |
9HPT (sec-1) | 383 | –30.548 | –40.607 to –20.489 | < 0.0001 | –0.294 | 0.087 |
NfH (pg/ml) | 51 | 0.00201 | 1.5E-05 to 0.00401 | 0.0484 | 0.285 | 0.094 |
SDMT (number of correct answers) | 379 | –0.0248 | –0.0333 to –0.0163 | < 0.0001 | –0.284 | 0.081 |
NfL (pg/ml) | 51 | 0.00046 | –0.0002 to 0.00108 | 0.1416 | 0.221 | 0.059 |
T2LV (× 10,000 mm3) | 383 | 0.16266 | 0.06886 to 0.25646 | 0.0007 | 0.173 | 0.031 |
PASAT (number of correct answers) | 383 | –0.0112 | –0.0186 to –0.0039 | 0.0030 | –0.153 | 0.024 |
Mean NAA concentration (London) | 136 | 0.15369 | –0.0421 to 0.34943 | 0.1228 | 0.135 | 0.022 |
DGMV (× 10,000 mm3) | 383 | –0.33831 | –0.59672 to –0.07990 | 0.0104 | –0.132 | 0.018 |
BV (× 10,000 mm3) | 383 | –0.01552 | –0.02843 to –0.00262 | 0.0185 | –0.121 | 0.016 |
CGMV (× 10,000 mm3) | 383 | –0.02845 | –0.05261 to –0.00430 | 0.0211 | –0.118 | 0.015 |
EQ-5D-5L VAS | 383 | –0.0056 | –0.011 to –0.0001 | 0.0447 | –0.104 | 0.012 |
Mean myoinositol concentration (London) (millimolar units) | 136 | 0.15008 | –0.107 to 0.4072 | 0.2503 | 0.101 | 0.014 |
SLCVA 2.5% LE (number of correct answers) | 377 | –0.0074 | –0.0157 to 0.00079 | 0.0762 | –0.092 | 0.009 |
SLCVA 5% RE (number of correct answers) | 373 | –0.0071 | –0.0151 to 0.00091 | 0.0823 | –0.091 | 0.009 |
SLCVA 5% LE (number of correct answers) | 375 | –0.0069 | –0.0146 to 0.00089 | 0.0827 | –0.090 | 0.009 |
SLCVA 2.5% RE (number of correct answers) | 375 | –0.0068 | –0.0151 to 0.00143 | 0.1045 | –0.084 | 0.008 |
Mean Glx concentration (London) (millimolar units) | 136 | –0.07 | –0.2698 to 0.12974 | 0.4893 | –0.061 | 0.008 |
HCVA RE (number of correct answers) | 376 | –0.004 | –0.0129 to 0.00479 | 0.3694 | –0.047 | 0.003 |
T2L MTR (mean) | 156 | –0.0084 | –0.0368 to 0.02003 | 0.5610 | –0.047 | 0.032 |
BV MTR (mean) | 156 | –0.0061 | –0.041 to 0.02877 | 0.7300 | –0.028 | 0.030 |
HCVA LE (number of correct answers) | 378 | –0.002 | –0.011 to 0.00696 | 0.6617 | –0.023 | 0.002 |
MSIS psychological score | 383 | 0.00472 | –0.0174 to 0.02679 | 0.6744 | 0.022 | 0.002 |
SLCVA 1.25% LE (number of correct answers) | 377 | –0.0014 | –0.0116 to 0.0088 | 0.7891 | –0.014 | 0.001 |
GM MTR (mean) (millimolar units) | 156 | –0.0029 | –0.0384 to 0.03259 | 0.8721 | –0.013 | 0.030 |
SLCVA 1.25% RE (number of correct answers) | 375 | –0.0013 | –0.0118 to 0.00929 | 0.8152 | –0.012 | 0.001 |
NAWM MTR (mean) | 156 | –0.0019 | –0.0362 to 0.03236 | 0.9130 | –0.009 | 0.029 |
A high number of variables were statistically significant and/or had a high standardised coefficient as shown in Tables 22 and 23. In further analysis, we investigated the effect of multiple baseline variables at once on PBVC at 96 weeks.
In model 1 we included (1) all baseline variables that were statistically significant with p < 0.05, (2) any variables with standardised coefficient greater than the lowest standardised coefficient among the baseline variables with p < 0.05 (PASAT) and (3) MSFC, which appeared predictive of outcome after including interaction terms. The model, therefore, included 14 variables: SLCVA 2.5% right eyes, SLCVA 5% right eyes, SDMT, mean myoinositol concentration (London), HCVA right eyes, SLCVA 2.5% left eyes, SLCVA 1.25% right eyes, HCVA left eyes, mean T2 lesion MTR, SLCVA 5% left eyes, SLCVA 1.25% left eyes, T2LV, PASAT and MSFC. PBVC at 96 weeks was the outcome variable. The fitted model (n = 117) produced an R2 value of 0.220, adjusted R2 value of 0.086 and residual standard deviation of 1.416.
The advanced MRI variables were found to have a substantially lower sample size than other variables, and so in order to maximise the available sample size we repeated the analysis after removing the advanced MRI variables with a sample size below 380. To further simplify the model, we also excluded SLCVA 5% right eyes, SLCVA 5% left eyes and SLCVA 1.25% right eyes because they were strongly correlated with at least one other variable with a higher standardised coefficient (correlation greater than 0.75). Therefore, in model 2 we included nine variables in total: SLCVA 2.5% right eyes, SDMT, HCVA right eyes, SLCVA 2.5% left eyes, HCVA left eyes, SLCVA 1.25% left eyes, T2LV, PASAT and MSFC. Again, PBVC at 96 weeks was the outcome variable. This model (n = 383) produced an R2 value of 0.060, adjusted R2 value of 0.029 and residual standard deviation of 1.254.
In Report Supplementary Material 1, Table 21, we show the improvement in the model fit when one or both of the baseline CSF neurofilament variables are included in model 1 or 2 (in addition to all the other variables in the models).
The results in Report Supplementary Material 1, Table 21, suggest that there is a substantial improvement in both R2 and adjusted-R2 after including CSF NfH, even in models with as many as 14 baseline variables. We found that CSF NfH significantly predicted PBVC at 96 weeks (see Report Supplementary Material 1, Table 22) in models 1 and 2 even after adjusting for many other variables that were predictive of outcome [standardised coefficients –0.427 (p = 0.04) and –0.362 (p = 0.03) for models 1 and 2, respectively]; therefore, baseline CSF NfH may have utility in prediction of PBVC at 96 weeks.
Modelling of treatment effect according to baseline magnetic resonance imaging/disability scores
When fitting models including interaction terms, only two baseline variable analyses had a significant interaction term at the 5% level (MSFC and SLCVA 1.25% right eyes) (see Report Supplementary Material 1, Tables 23 and 24). No interaction terms were significant at the 1% level.
Surrogate end-point analysis
In the absence of any treatment effect, the results of this modelling are not reported in the monograph.
Chapter 5 Discussion
In this multiarm, double-blind, placebo-controlled Phase IIb trial of three potential neuroprotective agents, we have found that amiloride, fluoxetine and riluzole did not significantly reduce the rate of brain atrophy over 96 weeks in the randomised cohort of 445 patients with SPMS.
From a clinical perspective, none of the measures, including EDSS, MSFC, T25FW, 9HPT, SLCVA, SDMT and PASAT, showed statistically significant or clinically meaningful changes versus placebo. We saw significant differences in some of the PROMs (e.g. MSIS29v2 total and psychological score or NFI nocturnal interval); however, these findings were inconsistent across the trial arms, and we did not feel that they had clinical relevance and were probably due to multiple testing.
The drugs were safe and well adhered to.
A multiarm approach with the simultaneous evaluation of three compounds was effective and efficient and able to be performed in the UK health-care environment with MRI as the primary outcome. The MRI atrophy measure performed as expected with PBVC of –0.65 to –0.70% per year. It effectively allowed three Phase IIb studies to be performed over the trial period – essentially compressing 15 years of work into 5 years. There was excellent buy-in from both the patient community and the neurologists and investigators trying to find a disease-modifying therapy in progressive MS.
Our assessment of the study blinding suggests that blinding was highly successful in this trial.
MS-SMART allowed us to investigate the pathobiological substrate in SPMS. Despite the lack of treatment effect on BV, there may have been focal/multifocal preservation of neural tissue as evidenced by DTI PWSD for RD. In addition, there may have been sparing of neuronal functional health as demonstrated by reduced myoinositol concentration on MR spectroscopy. Notably, these were both seen in the fluoxetine arm. Further analysis of metabolites in tissue-specific volumes is also planned. Moreover, there was a significant decrease in both PBVC at 24 weeks and the number of new/enlarging T2 lesions at 96 weeks from this arm. This is intriguing. However, there was no effect on the primary outcome, and the recently reported results of the FLUOX-PMS trial108 involving 137 progressive patients treated with fluoxetine/placebo have been negative. Therefore, in the round, and with multiple testing, we do not feel there is a therapeutic effect with fluoxetine.
No treatment effect was seen from the OCT study as a whole.
Limitations to the study would stem from the inherent difficulties in fully understanding the neuropathological processes underlying SPMS. It is rare to obtain brain tissue in life to study and the animal models have well-described drawbacks. One central question is whether or not patients with SPMS should be treated with anti-inflammatory DMTs. When the study was set up and indeed finished, this was not the case in UK standard-of-care practice. Recent Phase III trial data have persuaded the regulatory authorities (Food and Drug Administration and European Medicines Agency) that siponimod should be used in SPMS. We would anticipate that this would be the standard of care (after NICE consideration) in the next 1–2 years and future trials would add on potential neuroprotective agents. That being said, the underlying ‘inflammatory’ activity in our cohort was low, with the median number of new T2 lesions being zero and about 10% of participants having an ’on-study’ relapse. Of course, the study was not set up to measure other inflammatory parameters such as microglial activity. In retrospect, to determine the proportion with gadolinium enhancement at baseline would have been instructive. Finally, the question of how to trial patients who are not suitable for DMT (e.g. those who fail the NICE criteria) will remain.
There were some missing data on the primary outcome, but this seems to be robust to the sensitivity analyses performed.
In addition, the CSF cohort was small (n = 39) and this limits interpretation. Going forward it would seem unlikely that the UK population will participate in large numbers in CSF studies in SPMS and we would favour the development of blood markers. This looks to be achievable with new SIMOA® (Quanterix Corporation, Billerica, MA, USA) based technology.
Chapter 6 Conclusions
The MS-SMART was ground-breaking in its design and its approach to an intractable neurodegenerative disease. It demonstrated that this type of highly efficient trial can be performed in progressive MS, and supports its application in further trials in progressive MS and potentially other neurodegenerative environments. This will enable the research community to accelerate the testing of drugs in these very demanding situations, which have large health-care costs and burdens associated with them.
The trial was well powered, blinding was robust, adherence was high and retention was high. In addition, the primary outcome progressed as expected in the placebo arm, in a similar way to what has been observed in other recent trials in SPMS. 13,101,109 Valuable information was obtained across the board for all secondary and exploratory measures, which will help to decide their place in future trial design as indicative and mechanistic measures.
Drug selection was performed through a rigorous and systematic process. Proof of concept that this process conveyed translational predictive value is evidenced by the fact that two of the shortlisted compounds have subsequently been demonstrated to exhibit neuroprotective effects in progressive MS at phase 2. 109,110
In summary, MS-SMART has laid down the template for future phase 2 drug testing in neurodegenerative disease.
Acknowledgements
MS-SMART is an investigator-led project sponsored by UCL. This independent research is awarded by the Efficacy and Mechanism Evaluation (EME) programme and funded by the Medical Research Council (MRC), the UK MS Society and the US National Multiple Sclerosis Society (NMSS), and is managed by the National Institute for Health Research (NIHR) on behalf of the MRC–NIHR partnership.
Additional support comes from the University of Edinburgh; the NIHR UCL Hospitals (NIHR-UCLH) Biomedical Research Centre (BRC) and UCL; NIHR Leeds Clinical Research Facility (Dental Translational and Clinical Research Unit). CJW and RAP were supported in this work by NHS Lothian via the ECTU. JC is supported in part by the NIHR, University College London Hospitals, Biomedical Research Centre, London, UK.
We thank David Miller, Lorraine Smith, Marios Yiannakas, Marcello Moccia, Almudena Garcia, Alberto Calvi, Tim Friede, Michael Hutchinson, Stanley Hawkins, Robert Swingler, John Thorpe, John O’Brien and Allan Walker; we acknowledge the support of Alan Thompson; we thank all the MS-SMART investigators; and we thank all those people with MS, who volunteered to take part in this trial and made it possible, as well as their families and friends, who provided invaluable encouragement and help.
MS-SMART Investigators
Jeremy Chataway, Claudia AM Gandini Wheeler-Kingshott, Floriana De Angelis, Domenico Plantone, Anisha Doshi, Nevin John, Thomas Williams, Jonathan Stutters, Ferran Prados Carrasco, David MacManus, Frederik Barkhof, Sebastien Ourselin, Marie Braisher, Tiggy Beyene, Vanessa Bassan, Alvin Zapata (Queen Square Multiple Sclerosis Centre, University College London and University College London Hospitals NHS Foundation Trust, London, UK); Siddharthan Chandran, Peter Connick, Dawn Lyle, James Cameron, Daisy Mollison, Shuna Colville, Baljean Dhillon (Anne Rowling Regenerative Neurology Clinic, The University of Edinburgh, Royal Infirmary of Edinburgh, NHS Lothian, Edinburgh, UK); Christopher J Weir, Richard A Parker, Moira Ross, Gina Cranswick, Allan Walker, Lorraine Smith (Edinburgh Clinical Trials Unit, Usher Institute, University of Edinburgh, Edinburgh, UK); Gavin Giovannoni, Sharmilee Gnanapavan (Blizard Institute, Barts and The London School of Medicine and Dentistry, Queen Mary University, Barts Health NHS Trust, London, UK); Richard Nicholas (Imperial College Healthcare NHS Trust, London, UK); Waqar Rashid, Julia Aram (Brighton and Sussex University Hospitals NHS Trust, Brighton, UK); Helen Ford (Leeds General Infirmary, Leeds Teaching Hospitals NHS Trust, Leeds, UK); Sue H Pavitt (Dental Translational and Clinical Research Unit, University of Leeds, Leeds, UK); James Overell (The Queen Elizabeth University Hospital Glasgow, NHS Greater Glasgow and Clyde, Glasgow, UK); Carolyn Young, Heinke Arndt (The Walton Centre NHS Foundation Trust, Liverpool, UK); Martin Duddy, Joe Guadagno (Royal Victoria Infirmary, The Newcastle upon Tyne Hospital NHS Foundation Trust, Newcastle, UK); Nikolaos Evangelou (Queens Medical Centre, Nottingham University Hospital NHS Trust, Nottingham, UK); Matthew Craner, Jacqueline Palace (John Radcliffe Hospital, Oxford University Hospitals NHS Foundation Trust, Oxford, UK); Jeremy Hobart (Derriford Hospital, University Hospitals Plymouth NHS Trust, Plymouth, UK); Basil Sharrack, David Paling (Royal Hallamshire Hospital, Sheffield Teaching Hospitals NHS Foundation Trust, Sheffield, UK); Clive Hawkins, Seema Kalra (Royal Stoke University Hospital, University Hospitals of North Midlands NHS Trust, Stoke-on-Trent, UK); Brendan McLean (Royal Cornwall Hospitals NHS Trust, Truro, UK); Nigel Stallard (Statistics and Epidemiology, Division of Health Sciences, Warwick Medical School, University of Warwick, Coventry, UK); and Roger Bastow (patient representative).
Contributions of authors
Floriana De Angelis (https://orcid.org/0000-0002-9833-1435) (Clinical Research Associate) contributed to the study conduct, patient enrolment, MRI and OCT analysis, interpretation of data and the writing of the report.
Peter Connick (https://orcid.org/0000-0002-3892-8037) (Senior Clinical Research Fellow) contributed to the study conduct, patient enrolment, DTI analysis, interpretation of data and the writing of the report.
Richard A Parker (https://orcid.org/0000-0002-2658-5022) (Senior Statistician) contributed to the analysis and interpretation of data and the writing of the report.
Domenico Plantone (https://orcid.org/0000-0001-6666-7244) (Clinical Research Associate) contributed to the patient enrolment and critical revision of the report.
Anisha Doshi (https://orcid.org/0000-0001-6699-8668) (Clinical Research Associate) contributed to the study conduct, patient enrolment, MRI analysis and critical revision of the report.
Nevin John (https://orcid.org/0000-0002-9834-4498) (Clinical Research Associate) contributed to the study conduct, MRS analysis, writing and critical revision of the report.
Jonathan Stutters (https://orcid.org/0000-0002-9151-0844) (Data Manager) contributed to the MRI data analysis and critical revision of the report.
David MacManus (https://orcid.org/0000-0002-0902-977X) (Principal Research Associate) contributed to the supervision of MRI data collection, quality assurance and analysis and critical revision of the report.
Ferran Prados (https://orcid.org/0000-0002-7872-0142) (Senior Research Fellow) contributed to the supervision of MRI data analysis and critical revision of the report.
Ian Marshall (https://orcid.org/0000-0003-4445-1551) (Professor of Magnetic Resonance Physics) contributed to the supervision of MRS data analysis, writing and critical revision of the report.
Bhavana Solanky (https://orcid.org/0000-0002-5946-7604) (Senior Research Associate) contributed to the supervision of MRS data analysis and critical revision of the report.
Rebecca S Samson (https://orcid.org/0000-0002-0197-702X) (Senior Research Associate) contributed to the supervision of MTR data analysis, writing and critical revision of the report.
Frederik Barkhof (https://orcid.org/0000-0003-3543-3706) (Professor of Radiology) contributed to the supervision of MRI analysis, interpretation of the data and critical revision of the report.
Sebastien Ourselin (https://orcid.org/0000-0002-5694-5340) (Professor of Physics) contributed to the supervision of MRI analysis and critical revision of the report.
Marie Braisher (https://orcid.org/0000-0003-0634-8350) (Research Manager) contributed to the co-ordination of the study at the London site and critical revision of the report.
Moira Ross (https://orcid.org/0000-0003-2998-136X) (Principal Trial Manager) contributed to the main co-ordination of the trial and critical revision of the report.
Gina Cranswick (https://orcid.org/0000-0002-4479-6034) (Trial Manager) contributed to the co-ordination of the study and critical revision of the report.
Sue H Pavitt (https://orcid.org/0000-0001-7447-440X) (Professor Translational and Applied Health Research) contributed to the study concept, design and conduct, and critical revision of the report.
Sharmilee Gnanapavan (https://orcid.org/0000-0003-2817-9922) (Consultant Neurologist and Honorary Senior Clinical Lecturer) contributed to the supervision and analysis of the CSF substudy, and the writing and critical revision of the report.
Gavin Giovannoni (https://orcid.org/0000-0001-9995-1700) (Professor of Neurology) contributed to the CSF substudy concept and design, and critical revision of the report.
Claudia AM Gandini Wheeler-Kingshott (https://orcid.org/0000-0002-4832-1300) (Professor of Magnetic Resonance Physics) contributed to the concept and design of the advanced MRI substudy, supervision of the MRI analysis and critical revision of the report.
Clive Hawkins (https://orcid.org/0000-0002-5114-232X) (Professor of Clinical Neurology) contributed to the study concept and critical revision of the report.
Basil Sharrack (https://orcid.org/0000-0003-2406-6365) (Professor of Clinical Neurology) contributed to the study concept and critical revision of the report.
Roger Bastow (https://orcid.org/0000-0001-6139-1306) (UK MS Society representative patient) contributed to the study concept and critical revision of the report.
Christopher J Weir (https://orcid.org/0000-0002-6494-4903) (Professor of Statistics) contributed to the study design, analysis and interpretation of the data, and the writing of the report.
Nigel Stallard (https://orcid.org/0000-0001-7781-1512) (Professor of Medical Statistics) contributed to the study concept and critical revision of the report.
Siddharthan Chandran (https://orcid.org/0000-0001-6827-1593) (Professor of Neurology) contributed to the study design, conduct, data interpretation and the writing of the report.
Jeremy Chataway (https://orcid.org/0000-0001-7286-6901) (Professor of Neurology) contributed to the study concept, design and conduct, patient enrolment, data interpretation and the writing of the report.
All authors have approved the final version of the report to be published.
Publications
Journal articles
Chataway J, De Angelis F, Connick P, Parker RA, Plantone D, Doshi A, John N, et al. Efficacy of three neuroprotective drugs in secondary progressive multiple sclerosis (MS-SMART): a phase 2b, multiarm, double-blind, randomised placebo-controlled trial [published online ahead of print January 22 2020]. Lancet Neurol 2020.
Connick P, De Angelis F, Parker RA, Plantone D, Doshi A, John N, et al. Multiple Sclerosis-Secondary Progressive Multi-Arm Randomisation Trial (MS-SMART): a multiarm phase Iib randomised, double-blind, placebo-controlled clinical trial comparing the efficacy of three neuroprotective drugs in secondary progressive multiple sclerosis. BMJ Open 2018;8:e021944.
Marshall I, Thrippleton MJ, Bastin ME, Mollison D, Dickie DA, Chappell FM, et al. Characterisation of tissue-type metabolic content in secondary progressive multiple sclerosis: a magnetic resonance spectroscopic imaging study. Neurol 2018;265:1795–1802.
Conference abstracts
Chataway J, Chandran S, Miller D, Connick P, Giovannoni G, Pavitt S, et al. (2016). Recruitment in Multiple Sclerosis Trials: The MS-SMART Experience. Presented at the Annual Meeting of the Association of British Neurologists (ABN), Brighton, UK, 17–19 May, 2016.
Connick P, Miller D, Pavitt S, Giovannoni G, Wheeler-Kingshott C, Weir C, et al. MS-SMART Trial Design and Recruitment Status: A Multi-arm Phase IIB Randomised Double Blind Placebo-controlled Clinical Trial Comparing the Efficacy of Three Repositioned Neuroprotective Drugs in Secondary Progressive Multiple Sclerosis. Presented at the European Committee for Treatment and Research in Multiple Sclerosis (ECTRIMS), London, 14–17 September 2016.
De Angelis F, Cameron J, Connick P, Miller DH, Pavitt S, Giovannoni G, et al. Optical Coherence Tomography in Secondary Progressive Multiple Sclerosis: A Baseline Data Report from the MS-SMART Trial. Presented at the European Committee for Treatment and Research in Multiple Sclerosis (ECTRIMS), London, 14–17 September 2016.
Doshi A, Plantone D, De Angelis F, Wheeler-Kingshott C, Ciccarelli O, Chataway J. Exploring Cognition In Secondary Progressive Multiple Sclerosis (SPMS). Presented at the Annual Meeting of the Association of British Neurologists (ABN), Brighton, UK, 17–19 May 2016.
Plantone D, Solanky B, Pavitt S, Giovannoni G, Wheeler-Kingshott C, De Angelis F, et al. Initial Cross-sectional MR Spectroscopy Analysis of a Cohort of Secondary Progressive MS Patients Enrolled in the MS-SMART Trial. Presented at the European Committee for Treatment and Research in Multiple Sclerosis (ECTRIMS), London, 14–17 September 2016.
De Angelis F, Gomez AG, Parker R, Plantone D, Doshi A, Barkhof F, et al. Examining Cross-sectional Relationships of Optical Coherence Tomography, Cervical Cord MRI and Disability in Secondary Progressive MS. Presented at the 7th Joint European Committee for Treatment and Research in Multiple Sclerosis (ECTRIMS)/Americas Committee for Treatment and Research in Multiple Sclerosis (ACTRIMS), Paris, 25–28 October 2017.
Calvi A, Prados F, Tur C, De Angelis F, John N, Doshi A, et al. Characterizing the Slowly Evolving Lesions (SELs) in a Cohort of Secondary Progressive Multiple Sclerosis Patients. Presented at the 4th Congress of the European Academy of Neurology (EAN), Lisbon, 16–19 June 2018.
Chataway J, De Angelis F, Connick P, Parker R, Plantone D, Doshi A, et al. MS-SMART Trial: A Multi-arm Phase 2b Randomised, Double Blind, Parallel Group, Placebo-controlled Clinical Trial Comparing the Efficacy of Three Neuroprotective Drugs in Secondary Progressive Multiple Sclerosis [NCT01910259]. Presented at the 34th Congress of the European Committee for Treatment and Research in Multiple Sclerosis (ECTRIMS), Berlin, 10–12 October 2018.
De Angelis F, Garcia A, Stutters J, Eshaghi A, Parker R, Plantone D, et al. Retinal Nerve Fibre Layer Thickness in the Temporal Quadrant Predicts Clinical Disability and Cervical Cord Cross-sectional Area in Secondary Progressive Multiple Sclerosis. Presented at the 34th Congress of the European Committee for Treatment and Research in Multiple Sclerosis (ECTRIMS), Berlin, 10–12 October 2018.
Doshi A, De Angelis F, Muhlert N, Prados F, Stutters J, Macmanus D, et al. Cognition in Secondary Progressive Multiple Sclerosis (SPMS). Presented at the 34th Congress of the European Committee for Treatment and Research in Multiple Sclerosis (ECTRIMS), Berlin, 10–12 October 2018.
Doshi A, De Angelis F, Muhlert N, Stutters J, Eshaghi A, Prados F, et al. Multiple Sclerosis Impact Scale and Brain Volume are Independent Predictors of Cognitive Impairment in Secondary Progressive Multiple Sclerosis. Presented at the 4th Congress of the European Academy of Neurology (EAN), Lisbon, 16–19 June 2018.
Monteverdi A, Solanky B, De Angelis F, Plantone D, Stutters J, John N, et al. Exploring Metabolite Profiling of Patients with Secondary Progressive Multiple Sclerosis. Presented at International Society for Magnetic Resonance in Medicine (ISMRM), Paris, 16–21 June 2018.
Ammoscato F, De Angelis F, Plantone D, Parker RA, Wheeler-Kingshott CAMG, Pavitt S, et al. CSF Neurofilament Heavy Chain Release (NfH) is Important in Secondary Progressive MS (SPMS) – Results from the MS-SMART Trial. Presented at the 35th Congress of the European Committee for Treatment and Research in Multiple Sclerosis (ECTRIMS)/24th Annual Conference of Rehabilitation in MS (RIMS), Stockholm, 11–13 September 2019.
John NA, Solanky B, De Angelis F, Stutters J, Prados F, Plantone D, et al. A Neurometabolic Profile of Secondary Progressive Multiple Sclerosis: The Relationship between Brain Metabolites and Clinical Disability. Presented at the Americas Committee for Treatment and Research in Multiple Sclerosis (ACTRIMS) Dallas, TX, 28 February–2 March 2019.
Data-sharing statement
All data requests should be submitted to the corresponding author for consideration. Access to available anonymised data may be granted following review.
Patient data
This work uses data provided by patients and collected by the NHS as part of their care and support. Using patient data is vital to improve health and care for everyone. There is huge potential to make better use of information from people’s patient records, to understand more about disease, develop new treatments, monitor safety, and plan NHS services. Patient data should be kept safe and secure, to protect everyone’s privacy, and it’s important that there are safeguards to make sure that it is stored and used responsibly. Everyone should be able to find out about how patient data are used. #datasaveslives You can find out more about the background to this citation here: https://understandingpatientdata.org.uk/data-citation.
Disclaimers
This report presents independent research. The views and opinions expressed by authors in this publication are those of the authors and do not necessarily reflect those of the NHS, the NIHR, the MRC, NETSCC, the EME programme or the Department of Health and Social Care. If there are verbatim quotations included in this publication the views and opinions expressed by the interviewees are those of the interviewees and do not necessarily reflect those of the authors, those of the NHS, the NIHR, NETSCC, the EME programme or the Department of Health and Social Care.
References
- Karussis D. The diagnosis of multiple sclerosis and the various related demyelinating syndromes: a critical review. J Autoimmun 2014;48–9:134-42. https://doi.org/10.1016/j.jaut.2014.01.022.
- Multiple Sclerosis International Federation (MSIF) . Atlas of MS 2013 n.d. www.msif.org (accessed 12 November 2018).
- Thompson AJ, Banwell BL, Barkhof F, Carroll WM, Coetzee T, Comi G, et al. Diagnosis of multiple sclerosis: 2017 revisions of the McDonald criteria. Lancet Neurol 2017;17:162-73. https://doi.org/10.1016/S1474-4422(17)30470-2.
- Bjartmar C, Kinkel RP, Kidd G, Rudick RA, Trapp BD. Axonal loss in normal-appearing white matter in a patient with acute MS. Neurology 2001;57:1248-52. https://doi.org/10.1212/wnl.57.7.1248.
- Kutzelnigg A, Lucchinetti CF, Stadelmann C, Brück W, Rauschka H, Bergmann M, et al. Cortical demyelination and diffuse white matter injury in multiple sclerosis. Brain 2005;128:2705-12. https://doi.org/10.1093/brain/awh641.
- Mandolesi G, Gentile A, Musella A, Fresegna D, De Vito F, Bullitta S, et al. Synaptopathy connects inflammation and neurodegeneration in multiple sclerosis. Nat Rev Neurol 2015;11:711-24. https://doi.org/10.1038/nrneurol.2015.222.
- Compston A, Coles A. Multiple sclerosis. Lancet 2008;372:1502-17. https://doi.org/10.1016/S0140-6736(08)61620-7.
- Trapp BD, Nave KA. Multiple sclerosis: an immune or neurodegenerative disorder?. Annu Rev Neurosci 2008;31:247-69. https://doi.org/10.1146/annurev.neuro.30.051606.094313.
- Hauser SL, Chan JR, Oksenberg JR. Multiple sclerosis: Prospects and promise. Ann Neurol 2013;74:317-27. https://doi.org/10.1002/ana.24009.
- Kapoor R. Neuroprotection in multiple sclerosis: therapeutic strategies and clinical trial design. Curr Opin Neurol 2006;19:255-9. https://doi.org/10.1097/01.wco.0000227034.85576.16.
- Lucchinetti CF, Popescu BF, Bunyan RF, Moll NM, Roemer SF, Lassmann H, et al. Inflammatory cortical demyelination in early multiple sclerosis. N Engl J Med 2011;365:2188-97. https://doi.org/10.1056/NEJMoa1100648.
- Popescu BF, Lucchinetti CF. Pathology of demyelinating diseases. Annu Rev Pathol 2012;7:185-217. https://doi.org/10.1146/annurev-pathol-011811-132443.
- Kappos L, Bar-Or A, Cree BAC, Fox RJ, Giovannoni G, Gold R, et al. Siponimod versus placebo in secondary progressive multiple sclerosis (EXPAND): a double-blind, randomised, phase 3 study. Lancet 2018;391:1263-73. https://doi.org/10.1016/S0140-6736(18)30475-6.
- Ontaneda D, Fox RJ, Chataway J. Clinical trials in progressive multiple sclerosis: lessons learned and future perspectives. Lancet Neurol 2015;14:208-23. https://doi.org/10.1016/S1474-4422(14)70264-9.
- De Angelis F, Plantone D, Chataway J. Pharmacotherapy in secondary progressive multiple sclerosis: an overview. CNS Drugs 2018;32:499-526. https://doi.org/10.1007/s40263-018-0538-0.
- Frischer JM, Bramow S, Dal-Bianco A, Lucchinetti CF, Rauschka H, Schmidbauer M, et al. The relation between inflammation and neurodegeneration in multiple sclerosis brains. Brain 2009;132:1175-89. https://doi.org/10.1093/brain/awp070.
- Lappe-Siefke C, Goebbels S, Gravel M, Nicksch E, Lee J, Braun PE, et al. Disruption of Cnp1 uncouples oligodendroglial functions in axonal support and myelination. Nat Genet 2003;33:366-74. https://doi.org/10.1038/ng1095.
- Waxman SG. Membranes, myelin, and the pathophysiology of multiple sclerosis. N Engl J Med 1982;306:1529-33. https://doi.org/10.1056/NEJM198206243062505.
- Nave KA, Trapp BD. Axon-glial signaling and the glial support of axon function. Annu Rev Neurosci 2008;31:535-61. https://doi.org/10.1146/annurev.neuro.30.051606.094309.
- Su KG, Banker G, Bourdette D, Forte M. Axonal degeneration in multiple sclerosis: the mitochondrial hypothesis. Curr Neurol Neurosci Rep 2009;9:411-17. https://doi.org/10.1007/s11910-009-0060-3.
- Dutta R, McDonough J, Yin X, Peterson J, Chang A, Torres T, et al. Mitochondrial dysfunction as a cause of axonal degeneration in multiple sclerosis patients. Ann Neurol 2006;59:478-89. https://doi.org/10.1002/ana.20736.
- Friese MA, Craner MJ, Etzensperger R, Vergo S, Wemmie JA, Welsh MJ, et al. Acid-sensing ion channel-1 contributes to axonal degeneration in autoimmune inflammation of the central nervous system. Nat Med 2007;13:1483-9. https://doi.org/10.1038/nm1668.
- Vergo S, Craner MJ, Etzensperger R, Attfield K, Friese MA, Newcombe J, et al. Acid-sensing ion channel 1 is involved in both axonal injury and demyelination in multiple sclerosis and its animal model. Brain 2011;134:571-84. https://doi.org/10.1093/brain/awq337.
- Macrez R, Stys PK, Vivien D, Lipton SA, Docagne F. Mechanisms of glutamate toxicity in multiple sclerosis: biomarker and therapeutic opportunities. Lancet Neurol 2016;15:1089-102. https://doi.org/10.1016/S1474-4422(16)30165-X.
- Pitt D, Werner P, Raine CS. Glutamate excitotoxicity in a model of multiple sclerosis. Nat Med 2000;6:67-70. https://doi.org/10.1038/71555.
- Ashburn TT, Thor KB. Drug repositioning: identifying and developing new uses for existing drugs. Nat Rev Drug Discov 2004;3:673-83. https://doi.org/10.1038/nrd1468.
- Pushpakom S, Iorio F, Eyers PA, Escott KJ, Hopper S, Wells A, et al. Drug repurposing: progress, challenges and recommendations. Nat Rev Drug Discov 2019;18:41-58. https://doi.org/10.1038/nrd.2018.168.
- Vesterinen HM, Connick P, Irvine CM, Sena ES, Egan KJ, Carmichael GG, et al. Drug repurposing: a systematic approach to evaluate candidate oral neuroprotective interventions for secondary progressive multiple sclerosis. PLOS ONE 2015;10. https://doi.org/10.1371/journal.pone.0117705.
- Connick P, De Angelis F, Parker RA, Plantone D, Doshi A, John N, et al. Multiple Sclerosis-Secondary Progressive Multi-Arm Randomisation Trial (MS-SMART): a multiarm phase IIb randomised, double-blind, placebo-controlled clinical trial comparing the efficacy of three neuroprotective drugs in secondary progressive multiple sclerosis. BMJ Open 2018;8. https://doi.org/10.1136/bmjopen-2018-021944.
- Arun T, Tomassini V, Sbardella E, de Ruiter MB, Matthews L, Leite MI, et al. Targeting ASIC1 in primary progressive multiple sclerosis: evidence of neuroprotection with amiloride. Brain 2013;136:106-15. https://doi.org/10.1093/brain/aws325.
- Kong EK, Peng L, Chen Y, Yu AC, Hertz L. Up-regulation of 5-HT2B receptor density and receptor-mediated glycogenolysis in mouse astrocytes by long-term fluoxetine administration. Neurochem Res 2002;27:113-20. https://doi.org/10.1023/a:1014862808126.
- Allaman I, Fiumelli H, Magistretti PJ, Martin JL. Fluoxetine regulates the expression of neurotrophic/growth factors and glucose metabolism in astrocytes. Psychopharmacology 2011;216:75-84. https://doi.org/10.1007/s00213-011-2190-y.
- Mostert JP, Admiraal-Behloul F, Hoogduin JM, Luyendijk J, Heersema DJ, van Buchem MA, et al. Effects of fluoxetine on disease activity in relapsing multiple sclerosis: a double-blind, placebo-controlled, exploratory study. J Neurol Neurosurg Psychiatry 2008;79:1027-31. https://doi.org/10.1136/jnnp.2007.139345.
- Mostert J, Heersema T, Mahajan M, Van Der Grond J, Van Buchem MA, De Keyser J. The effect of fluoxetine on progression in progressive multiple sclerosis: a double-blind, randomized, placebo-controlled trial. ISRN Neurol 2013;2013. https://doi.org/10.1155/2013/370943.
- Bellingham MC. A review of the neural mechanisms of action and clinical efficiency of riluzole in treating amyotrophic lateral sclerosis: what have we learned in the last decade?. CNS Neurosci Ther 2011;17:4-31. https://doi.org/10.1111/j.1755-5949.2009.00116.x.
- Killestein J, Kalkers NF, Polman CH. Glutamate inhibition in MS: the neuroprotective properties of riluzole. J Neurol Sci 2005;233:113-15. https://doi.org/10.1016/j.jns.2005.03.011.
- Miller DH, Khan OA, Sheremata WA, Blumhardt LD, Rice GP, Libonati MA, et al. A controlled trial of natalizumab for relapsing multiple sclerosis. N Engl J Med 2003;348:15-23. https://doi.org/10.1056/NEJMoa020696.
- Kappos L, Antel J, Comi G, Montalban X, O’Connor P, Polman CH, et al. Oral fingolimod (FTY720) for relapsing multiple sclerosis. N Engl J Med 2006;355:1124-40. https://doi.org/10.1056/NEJMoa052643.
- Kappos L, Radue EW, O’Connor P, Polman C, Hohlfeld R, Calabresi P, et al. A placebo-controlled trial of oral fingolimod in relapsing multiple sclerosis. N Engl J Med 2010;362:387-401. https://doi.org/10.1056/NEJMoa0909494.
- Losseff NA, Wang L, Lai HM, Yoo DS, Gawne-Cain ML, McDonald WI, et al. Progressive cerebral atrophy in multiple sclerosis. A serial MRI study. Brain 1996;119:2009-19. https://doi.org/10.1093/brain/119.6.2009.
- Kalkers NF, Bergers E, Castelijns JA, van Walderveen MA, Bot JC, Adèr HJ, et al. Optimizing the association between disability and biological markers in MS. Neurology 2001;57:1253-8. https://doi.org/10.1212/wnl.57.7.1253.
- Fisher E, Lee JC, Nakamura K, Rudick RA. Gray matter atrophy in multiple sclerosis: a longitudinal study. Ann Neurol 2008;64:255-65. https://doi.org/10.1002/ana.21436.
- De Stefano N, Giorgio A, Battaglini M, Rovaris M, Sormani MP, Barkhof F, et al. Assessing brain atrophy rates in a large population of untreated multiple sclerosis subtypes. Neurology 2010;74:1868-76. https://doi.org/10.1212/WNL.0b013e3181e24136.
- Miller DH, Barkhof F, Frank JA, Parker GJM, Thompson AJ. Measurement of atrophy in multiple sclerosis: pathological basis, methodological aspects and clinical relevance. Brain 2002;125:1676-95. https://doi.org/10.1093/brain/awf177.
- Panitch H, Miller A, Paty D, Weinshenker B. North American Study Group on Interferon beta-1b in Secondary Progressive MS . Interferon beta-1b in secondary progressive MS: results from a 3-year controlled study. Neurology 2004;63:1788-95. https://doi.org/10.1212/01.WNL.0000146958.77317.3E.
- Molyneux PD, Kappos L, Polman C, Pozzilli C, Barkhof F, Filippi M, et al. The effect of interferon beta-1b treatment on MRI measures of cerebral atrophy in secondary progressive multiple sclerosis. European Study Group on Interferon beta-1b in secondary progressive multiple sclerosis. Brain 2000;123:2256-63. https://doi.org/10.1093/brain/123.11.2256.
- Smith SM, Zhang Y, Jenkinson M, Chen J, Matthews PM, Federico A, et al. Accurate, robust, and automated longitudinal and cross-sectional brain change analysis. Neuroimage 2002;17:479-89. https://doi.org/10.1006/nimg.2002.1040.
- Bermel RA, Bakshi R. The measurement and clinical relevance of brain atrophy in multiple sclerosis. Lancet Neurol 2006;5:158-70. https://doi.org/10.1016/S1474-4422(06)70349-0.
- Altmann DR, Jasperse B, Barkhof F, Beckmann K, Filippi M, Kappos LD, et al. Sample sizes for brain atrophy outcomes in trials for secondary progressive multiple sclerosis. Neurology 2009;72:595-601. https://doi.org/10.1212/01.wnl.0000335765.55346.fc.
- Srinivasan R, Sailasuta N, Hurd R, Nelson S, Pelletier D. Evidence of elevated glutamate in multiple sclerosis using magnetic resonance spectroscopy at 3T. Brain 2005;128:1016-25. https://doi.org/10.1093/brain/awh467.
- De Stefano N, Filippi M. MR spectroscopy in multiple sclerosis. J Neuroimaging 2007;17:31-5. https://doi.org/10.1111/j.1552-6569.2007.00134.x.
- Kapeller P, Brex PA, Chard D, Dalton C, Griffin CM, Mclean MA, et al. Quantitative 1H MRS imaging 14 years after presenting with a clinically isolated syndrome suggestive of multiple sclerosis. Mult Scler J n.d.;8:207-10. https://doi.org/10.1191/1352458502ms822oa.
- Schmierer K, Scaravilli F, Altmann DR, Barker GJ, Miller DH. Magnetization transfer ratio and myelin in postmortem multiple sclerosis brain. Ann Neurol 2004;56:407-15. https://doi.org/10.1002/ana.20202.
- Hayton T, Furby J, Smith KJ, Altmann DR, Brenner R, Chataway J, et al. Longitudinal changes in magnetisation transfer ratio in secondary progressive multiple sclerosis: data from a randomised placebo controlled trial of lamotrigine. J Neurol 2012;259:505-14. https://doi.org/10.1007/s00415-011-6212-9.
- Fox RJ, Beall E, Bhattacharyya P, Chen JT, Sakaie K. Advanced MRI in multiple sclerosis: current status and future challenges. Neurol Clin 2011;29:357-80. https://doi.org/10.1016/j.ncl.2010.12.011.
- Sbardella E, Tona F, Petsas N, Pantano P. DTI measurements in multiple sclerosis: evaluation of brain damage and clinical implications. Mult Scler Int 2013;2013. https://doi.org/10.1155/2013/671730.
- Evangelou N, Konz D, Esiri MM, Smith S, Palace J, Matthews PM. Regional axonal loss in the corpus callosum correlates with cerebral white matter lesion volume and distribution in multiple sclerosis. Brain 2000;123:1845-9. https://doi.org/10.1093/brain/123.9.1845.
- Evangelou N, DeLuca GC, Owens T, Esiri MM. Pathological study of spinal cord atrophy in multiple sclerosis suggests limited role of local lesions. Brain 2005;128:29-34. https://doi.org/10.1093/brain/awh323.
- Rashid W, Davies GR, Chard DT, Griffin CM, Altmann DR, Gordon R, et al. Upper cervical cord area in early relapsing-remitting multiple sclerosis: cross-sectional study of factors influencing cord size. J Magn Reson Imaging 2006;23:473-6. https://doi.org/10.1002/jmri.20545.
- Mann RS, Constantinescu CS, Tench CR. Upper cervical spinal cord cross-sectional area in relapsing remitting multiple sclerosis: application of a new technique for measuring cross-sectional area on magnetic resonance images. J Magn Reson Imaging 2007;26:61-5. https://doi.org/10.1002/jmri.20959.
- Bergers E, Bot JCJ, De Groot CJA, Polman CH, Lycklama à Nijeholt GJ, Castelijns JA, et al. Axonal damage in the spinal cord of MS patients occurs largely independent of T2 MRI lesions. Neurology 2002;59:1766-71. https://doi.org/10.1212/01.WNL.0000036566.00866.26.
- Kearney H, Miller DH, Ciccarelli O. Spinal cord MRI in multiple sclerosis – diagnostic, prognostic and clinical value. Nat Rev Neurol 2015;11:327-38. https://doi.org/10.1038/nrneurol.2015.80.
- Wheeler-Kingshott CA, Stroman PW, Schwab JM, Bacon M, Bosma R, Brooks J, et al. The current state-of-the-art of spinal cord imaging: applications. Neuroimage 2014;84:1082-93. https://doi.org/10.1016/j.neuroimage.2013.07.014.
- Stankiewicz JM, Neema M, Alsop DC, Healy BC, Arora A, Buckle GJ, et al. Spinal cord lesions and clinical status in multiple sclerosis: a 1.5T and 3T MRI study. J Neurol Sci 2009;279:99-105. https://doi.org/10.1016/j.jns.2008.11.009.
- Rocca MA, Horsfield MA, Sala S, Copetti M, Valsasina P, Mesaros S, et al. A multicenter assessment of cervical cord atrophy among MS clinical phenotypes. Neurology 2011;76:2096-102. https://doi.org/10.1212/WNL.0b013e31821f46b8.
- Losseff NA, Webb SL, O’Riordan JI, Page R, Wang L, Barker GJ, et al. Spinal cord atrophy and disability in multiple sclerosis. A new reproducible and sensitive MRI method with potential to monitor disease progression. Brain 1996;119:701-8. https://doi.org/10.1093/brain/119.3.701.
- Stevenson VL, Leary SM, Losseff NA, Parker GJ, Barker GJ, Husmani Y, et al. Spinal cord atrophy and disability in MS: a longitudinal study. Neurology 1998;51:234-8. https://doi.org/10.1212/wnl.51.1.234.
- Casserly C, Seyman EE, Alcaide-Leon P, Guenette M, Lyons C, Sankar S, et al. Spinal cord atrophy in multiple sclerosis: a systematic review and meta-analysis. J Neuroimaging 2018;28:556-86. https://doi.org/10.1111/jon.12553.
- Frisén L, Hoyt WF. Insidious atrophy of retinal nerve fibers in multiple sclerosis. Fundoscopic identification in patients with and without visual complaints. Arch Ophthalmol 1974;92:91-7. https://doi.org/10.1001/archopht.1974.01010010097001.
- Frohman E, Costello F, Zivadinov R, Stuve O, Conger A, Winslow H, et al. Optical coherence tomography in multiple sclerosis. Lancet 2006;5:853-63. https://doi.org/10.1016/S1474-4422(06)70573-7.
- Calabresi PA, Balcer LJ, Frohman EM. Optical Coherence Tomography in Neurologic Diseases. New York, NY: Cambridge University Press; 2015.
- Toosy AT, Mason DF, Miller DH. Optic neuritis. Lancet Neurol 2014;13:83-99. https://doi.org/10.1016/S1474-4422(13)70259-X.
- Raftopoulos R, Hickman SJ, Toosy A, Sharrack B, Mallik S, Paling D, et al. Phenytoin for neuroprotection in patients with acute optic neuritis: a randomised, placebo-controlled, phase 2 trial. Lancet Neurol 2016;15:259-69. https://doi.org/10.1016/S1474-4422(16)00004-1.
- Balcer LJ, Miller DH, Reingold SC, Cohen JA. Vision and vision-related outcome measures in multiple sclerosis. Brain 2015;138:11-27. https://doi.org/10.1093/brain/awu335.
- Petzold A, de Boer JF, Schippling S, Vermersch P, Kardon R, Green A, et al. Optical coherence tomography in multiple sclerosis: a systematic review and meta-analysis. Lancet Neurol 2010;9:921-32. https://doi.org/10.1016/S1474-4422(10)70168-X.
- Petzold A, Balcer LJ, Calabresi PA, Costello F, Frohman TC, Frohman EM, et al. Retinal layer segmentation in multiple sclerosis: a systematic review and meta-analysis. Lancet Neurol 2017;16:797-812. https://doi.org/10.1016/S1474-4422(17)30278-8.
- Martinez-Lapiscina EH, Arnow S, Wilson JA, Saidha S, Preiningerova JL, Oberwahrenbrock T, et al. Retinal thickness measured with optical coherence tomography and risk of disability worsening in multiple sclerosis: a cohort study. Lancet Neurol 2016;15:574-84. https://doi.org/10.1016/S1474-4422(16)00068-5.
- Saidha S, Al-Louzi O, Ratchford JN, Bhargava P, Oh J, Newsome SD, et al. Optical coherence tomography reflects brain atrophy in multiple sclerosis: a four-year study. Ann Neurol 2015;78:801-13. https://doi.org/10.1002/ana.24487.
- Wang H, Wu M, Zhan C, Ma E, Yang M, Yang X, et al. Neurofilament proteins in axonal regeneration and neurodegenerative diseases. Neural Regen Res 2012;7:620-6. https://doi.org/10.3969/j.issn.1673-5374.2012.08.010.
- Kuhle J, Disanto G, Lorscheider J, Stites T, Chen Y, Dahlke F, et al. Fingolimod and CSF neurofilament light chain levels in relapsing-remitting multiple sclerosis. Neurology 2015;84:1639-43. https://doi.org/10.1212/WNL.0000000000001491.
- Gunnarsson M, Malmeström C, Axelsson M, Sundström P, Dahle C, Vrethem M, et al. Axonal damage in relapsing multiple sclerosis is markedly reduced by natalizumab. Ann Neurol 2011;69:83-9. https://doi.org/10.1002/ana.22247.
- Bar-Or A, Gelfand J, Fiore D, Harp C, Ma X, Herman A, et al. Interim analysis of the OBOE (Ocrelizumab Biomarker Outcome Evaluation) study in multiple sclerosis (MS) (S24.002). Neurology 2018;90.
- Gnanapavan S, Grant D, Morant S, Furby J, Hayton T, Teunissen CE, et al. Biomarker report from the phase II lamotrigine trial in secondary progressive MS – neurofilament as a surrogate of disease progression. PLOS ONE 2013;8. https://doi.org/10.1371/journal.pone.0070019.
- Multiple Sclerosis Society UK . MS-SMART Results n.d. www.mssociety.org.uk/research/explore-our-research/research-we-fund/search-our-research-projects/can-three-existing-drugs-protect-nerves-from-damage-in-ms/ms-smart-results (accessed 11 April 2019).
- Smith SM, Jenkinson M, Woolrich MW, Beckmann CF, Behrens TE, Johansen-Berg H, et al. Advances in functional and structural MR image analysis and implementation as FSL. Neuroimage 2004;23:208-19. https://doi.org/10.1016/j.neuroimage.2004.07.051.
- Prados F, Cardoso MJ, Kanber B, Ciccarelli O, Kapoor R, Gandini Wheeler-Kingshott CAM, et al. A multi-time-point modality-agnostic patch-based method for lesion filling in multiple sclerosis. Neuroimage 2016;139:376-84. https://doi.org/10.1016/j.neuroimage.2016.06.053.
- Cardoso MJ, Modat M, Wolz R, Melbourne A, Cash D, Rueckert D, et al. Geodesic information flows: spatially-variant graphs and their application to segmentation and fusion. IEEE Trans Med Imaging 2015;34:1976-88. https://doi.org/10.1109/TMI.2015.2418298.
- Marshall I, Thrippleton MJ, Bastin ME, Mollison D, Dickie DA, Chappell FM, et al. Characterisation of tissue-type metabolic content in secondary progressive multiple sclerosis: a magnetic resonance spectroscopic imaging study. J Neurol 2018;265:1795-802. https://doi.org/10.1007/s00415-018-8903-y.
- Provencher SW. Estimation of metabolite concentrations from localized in vivo proton NMR spectra. Magn Reson Med 1993;30:672-9. https://doi.org/10.1002/mrm.1910300604.
- Modat M, Cash DM, Daga P, Winston GP, Duncan JS, Ourselin S. Global image registration using a symmetric block-matching approach. J Med Imaging 2014;1. https://doi.org/10.1117/1.JMI.1.2.024003.
- Modat M, Ridgway GR, Taylor ZA, Lehmann M, Barnes J, Hawkes DJ, et al. Fast free-form deformation using graphics processing units. Comput Methods Programs Biomed 2010;98:278-84. https://doi.org/10.1016/j.cmpb.2009.09.002.
- Andersson JLR, Sotiropoulos SN. An integrated approach to correction for off-resonance effects and subject movement in diffusion MR imaging. Neuroimage 2016;125:1063-78. https://doi.org/10.1016/j.neuroimage.2015.10.019.
- Behrens TEJ, Berg HJ, Jbabdi S, Rushworth MFS, Woolrich MW. Probabilistic diffusion tractography with multiple fibre orientations: what can we gain?. Neuroimage 2007;34:144-55. https://doi.org/10.1016/j.neuroimage.2006.09.018.
- Behrens TE, Woolrich MW, Jenkinson M, Johansen-Berg H, Nunes RG, Clare S, et al. Characterization and propagation of uncertainty in diffusion-weighted MR imaging. Magn Reson Med 2003;50:1077-88. https://doi.org/10.1002/mrm.10609.
- Kearney H, Yiannakas MC, Abdel-Aziz K, Wheeler-Kingshott CA, Altmann DR, Ciccarelli O, et al. Improved MRI quantification of spinal cord atrophy in multiple sclerosis. J Magn Reson Imaging 2014;39:617-23. https://doi.org/10.1002/jmri.24194.
- Early Treatment Diabetic Retinopathy Study research group . Photocoagulation for diabetic macular edema. Early Treatment Diabetic Retinopathy Study report number 1. Arch Ophthalmol 1985;103:1796-806. https://doi.org/10.1001/archopht.1985.01050120030015.
- Balk LJ, Twisk JWR, Steenwijk MD, Daams M, Tewarie P, Killestein J, et al. A dam for retrograde axonal degeneration in multiple sclerosis?. J Neurol Neurosurg Psychiatry 2014;85:782-9. https://doi.org/10.1136/jnnp-2013-306902.
- Schippling S, Balk L, Costello F, Albrecht P, Balcer L, Calabresi P, et al. Quality control for retinal OCT in multiple sclerosis: validation of the OSCAR-IB criteria. Mult 2015;21:163-70. https://doi.org/10.1177/1352458514538110.
- Petzold A, Altintas A, Andreoni L, Bartos A, Berthele A, Blankenstein MA, et al. Neurofilament ELISA validation. J Immunol Methods 2010;352:23-31. https://doi.org/10.1016/j.jim.2009.09.014.
- Kapoor R, Furby J, Hayton T, Smith KJ, Altmann DR, Brenner R, et al. Lamotrigine for neuroprotection in secondary progressive multiple sclerosis: a randomised, double-blind, placebo-controlled, parallel-group trial. Lancet Neurol 2010;9:681-8. https://doi.org/10.1016/S1474-4422(10)70131-9.
- Chataway J, Schuerer N, Alsanousi A, Chan D, MacManus D, Hunter K, et al. Effect of high-dose simvastatin on brain atrophy and disability in secondary progressive multiple sclerosis (MS-STAT): a randomised, placebo-controlled, phase 2 trial. Lancet 2014;383:2213-21. https://doi.org/10.1016/S0140-6736(13)62242-4.
- Little RJA, Rubin DB. Statistical Analysis with Missing Data. Hoboken, NJ: John Wiley & Sons, Inc.; 2002.
- Rubin DB. Multiple Imputation for Nonresponse in Surveys. Hoboken, NJ: John Wiley & Sons, Inc.; 1987.
- Little RJA. Pattern-mixture models for multivariate incomplete data. J Am Stat Assoc 1993;88. https://doi.org/10.2307/2290705.
- White IR, Horton NJ, Carpenter J, Pocock SJ. Strategy for intention to treat analysis in randomised trials with missing outcome data. BMJ 2011;342. https://doi.org/10.1136/bmj.d40.
- Agresti A. Categorical Data Analysis. Hoboken, NJ: John Wiley & Sons Inc.; 2013.
- Burzykowski T, Buyse M. Surrogate threshold effect: an alternative measure for meta-analytic surrogate endpoint validation. Pharm Stat 2006;5:173-86. https://doi.org/10.1002/pst.207.
- Cambron M, Mostert J, D’Hooghe M, Nagels G, Willekens B, Debruyne J, et al. Fluoxetine in progressive multiple sclerosis: the FLUOX-PMS trial. Mult Scler 2019;25:1728-35. https://doi.org/10.1177/1352458519843051.
- Spain R, Powers K, Murchison C, Heriza E, Winges K, Yadav V, et al. Lipoic acid in secondary progressive MS: a randomized controlled pilot trial. Neurol Neuroimmunol Neuroinflamm 2017;4. https://doi.org/10.1212/NXI.0000000000000374.
- Fox RJ, Coffey CS, Conwit R, Cudkowicz ME, Gleason T, Goodman A, et al. Phase 2 trial of ibudilast in progressive multiple sclerosis. N Engl J Med 2018;379:846-55. https://doi.org/10.1056/NEJMoa1803583.
List of abbreviations
- 3D
- three-dimensional
- 9HPT
- 9-Hole Peg Test
- AD
- axial diffusivity
- AE
- adverse event
- AMD
- adjusted mean difference
- ART
- automatic real time
- ASIC
- acid-sensing ion channel
- BDI-II
- Beck Depression Inventory, version 2
- b.i.d.
- bis in die (twice a day)
- BPI
- Brief Pain Inventory
- BV
- brain volume
- CD14
- cluster of differentiation 14
- CGM
- cortical grey matter
- CGMV
- cortical grey matter volume
- CI
- confidence interval
- CNS
- central nervous system
- CONSORT
- Consolidated Standards of Reporting Trials
- CSF
- cerebrospinal fluid
- DGM
- deep grey matter
- DGMV
- deep grey matter volume
- DMC
- Data Monitoring Committee
- DMT
- disease-modifying therapy
- DTI
- diffusion tensor imaging
- ECTU
- Edinburgh Clinical Trials Unit
- EDSS
- Expanded Disability Status Scale
- EQ-5D-5L
- EuroQol-5 Dimensions, five-level version
- ETDRS
- Early Treatment Diabetic Retinopathy Study
- FA
- fractional anisotropy
- GCL
- ganglion cell layer
- GFAP
- glial fibrillary acidic protein
- Glx
- combined glutamate and glutamine
- GM
- grey matter
- GP
- general practitioner
- HCVA
- high-contrast visual acuity
- IPL
- inner plexiform layer
- ITT
- intention to treat
- MAR
- missing at random
- MD
- mean diffusivity
- MedDRA
- Medical Dictionary for Regulatory Activities
- MMP9
- matrix metalloproteinase 9
- MNAR
- missing not at random
- MRC
- Medical Research Council
- MRI
- magnetic resonance imaging
- MRS
- magnetic resonance spectroscopy
- MS
- multiple sclerosis
- MSFC
- Multiple Sclerosis Functional Composite
- MSIS29v2
- Multiple Sclerosis Impact Scale 29 items, version 2
- MS-SMART
- Multiple Sclerosis-Secondary Progressive Multi-Arm Randomisation Trial
- MSWSv2
- Multiple Sclerosis Walking Scale, version 2
- MToff
- magnetisation transfer off
- MTon
- magnetisation transfer on
- MTR
- magnetisation transfer ratio
- MUCCA
- mean upper cord cross-sectional area
- NAA
- N-acetylaspartate
- NAWM
- normal-appearing white matter
- NCAM
- neural cell adhesion molecule
- NfH
- neurofilament heavy
- NFI
- Neurological Fatigue Index
- NfL
- neurofilament light
- NIHR
- National Institute for Health Research
- NMDA
- N-methyl-D-aspartate
- NPRS
- Numeric Pain Rating Scale
- NPS
- Neuropathic Pain Scale
- OCT
- optical coherence tomography
- PASAT
- Paced Auditory Serial Addition Test
- PBVC
- percentage brain volume change
- PD
- proton density
- PIL
- patient information leaflet
- PPI
- patient and public involvement
- PPMS
- primary progressive multiple sclerosis
- pRNFL
- peripapillary retinal nerve fibre layer
- PROM
- patient-reported outcome measure
- PSIR
- phase-sensitive inversion recovery
- PWSAD
- peak width of skeletonised axial diffusivity
- PWSD
- peak width of skeletonised diffusivity
- PWSFA
- peak width of skeletonised fractional anisotropy
- PWSMD
- peak width of skeletonised mean diffusivity
- PWSRD
- peak width of skeletonised radial diffusivity
- RD
- radial diffusivity
- REC
- Research Ethics Committee
- RNFL
- retinal nerve fibre layer
- RRMS
- relapsing–remitting multiple sclerosis
- SAE
- serious adverse event
- SDMT
- Symbol Digit Modalities Test
- Semi-LASER
- semi-localisation by adiabatic selective refocusing
- SIENA
- Structural Image Evaluation using Normalization of Atrophy
- SLCVA
- Sloan Low Contrast Visual Acuity
- SPMS
- secondary progressive multiple sclerosis
- SSRI
- selective serotonin reuptake inhibitor
- SUSAR
- suspected unexpected serious adverse reaction
- T25FW
- Timed-25-Foot Walk
- T2LV
- T2 lesion volume
- TE
- echo time
- TMF
- trial master file
- TR
- repetition time
- TSC
- Trial Steering Committee
- UCL
- University College London
- VAS
- visual analogue scale
- VOI
- volume of interest
Notes
Supplementary material can be found on the NIHR Journals Library report page (https://doi.org/10.3310/eme07030).
Supplementary material has been provided by the authors to support the report and any files provided at submission will have been seen by peer reviewers, but not extensively reviewed. Any supplementary material provided at a later stage in the process may not have been peer reviewed.