Notes
Article history
The research reported in this issue of the journal was funded by the EME programme as project number 13/122/02. The contractual start date was in February 2016. The final report began editorial review in February 2020 and was accepted for publication in December 2020. The authors have been wholly responsible for all data collection, analysis and interpretation, and for writing up their work. The EME editors and production house have tried to ensure the accuracy of the authors’ report and would like to thank the reviewers for their constructive comments on the final report document. However, they do not accept liability for damages or losses arising from material published in this report.
Permissions
Copyright statement
Copyright © 2021 Embleton et al. This work was produced by Embleton et al. under the terms of a commissioning contract issued by the Secretary of State for Health and Social Care. This is an Open Access publication distributed under the terms of the Creative Commons Attribution CC BY 4.0 licence, which permits unrestricted use, distribution, reproduction and adaption in any medium and for any purpose provided that it is properly attributed. See: https://creativecommons.org/licenses/by/4.0/. For attribution the title, original author(s), the publication source – NIHR Journals Library, and the DOI of the publication must be cited.
2021 Embleton et al.
Chapter 1 The MAGPIE study main report
Introduction
Preterm birth accounts for 10% of all births and is associated with significant short- and long-term adverse outcomes for the infant, along with significant impacts on the family and the NHS. Over the last 20 years, there has been an increasing interest in the role of nutrition in improving a range of outcomes in preterm infants and collaborative trials have successfully been completed in the UK. Understanding both the actions of interventions in reducing disease and the disease mechanisms in a high-risk and vulnerable group, such as premature infants, presents many challenges, yet is best conducted in the setting of randomised controlled trials (RCTs). The aim of the Mechanisms Affecting the Gut of Preterm Infants (MAGPIE) study was to explore gut mechanisms using interventions tested within RCTs and mechanisms leading to disease. The MAGPIE study was planned to be nested within two RCTs [the Speed of Increasing milk Feeds Trial (SIFT)1 and the Enteral LactoFerrin In Neonates (ELFIN) trial2] conducted in the UK; however, owing to changing timelines for the original RCTs, the infants were recruited from within only one RCT: the ELFIN trial. 2 Infants recruited to the MAGPIE study had stool and urine samples collected to enable determination of the impact of enteral lactoferrin on gut bacterial patterns and metabolic function, as well as to explore the mechanisms leading to disease onset. The two diseases that we focused on were late-onset sepsis (LOS) and necrotising enterocolitis (NEC), which, combined, affect around 30% of very preterm infants and are associated with significant morbidity and mortality. This report explains the context for the MAGPIE study, the different methods employed to explore biological mechanisms (gut bacterial community and metabolic function analyses) and sets the results within the context of the main overarching RCT.
Preterm birth
Globally, 1 in 10 infants is born preterm and, in the UK, more than 8000 infants each year are born > 8 weeks early, a number that continues to rise year on year. 3 Costs to society and families from preterm birth are high in both financial and emotional terms. 4 Preterm birth requires significant NHS resource both for acute care for preterm infants, with intensive care costing around £2000 per day, and in managing the long-term consequences of early birth, which affects developmental outcomes. The length of stay in intensive care is primarily determined by gestational age at birth, as are the complications of prematurity. These complications include the need for respiratory support and feeding/nutrition support, as well as health-care-associated infections and other complications of intensive care. Over the last 20 years, the rate of death from respiratory disease in infants born at < 32 weeks’ gestation has fallen, but the rate of death from infections and a specific preterm bowel condition, NEC, has risen. 5 These significant individual and health-care costs, along with an increasing recognition that nutritional interventions affect rates of sepsis and NEC,6 were key drivers in the development of two UK Health Technology Assessment programme-funded trials exploring (1) the effect of milk feeds, SIFT,1 and (2) the impact of a supplemental milk protein with anti-infective properties, the ELFIN trial. 2
Late-onset sepsis and necrotising enterocolitis
Late-onset sepsis and NEC are common complications of birth at < 32 weeks’ gestation. Around 20–25% of these infants will experience infection beginning > 72 hours after birth (LOS), with an associated mortality of around 10%, and the incidence and mortality is highest in the most preterm infants. 7 NEC almost exclusively affects preterm infants, with around 10% of those born at < 32 weeks’ gestation having at least one episode. 8 The aetiology of NEC and LOS is poorly understood, making prevention difficult; however, receipt of mother’s own breast milk reduces the risk of both. 9 In addition, patterns of gut bacteria are different in infants who do and infants who do not develop LOS and NEC, suggesting that there is a link to the gut microbiome and feeding. 10 Distinguishing LOS from NEC can be complex: there are currently no predictive markers for LOS or NEC and there is no single diagnostic test for NEC in clinical use. 11 In addition, LOS and NEC can co-exist. For some infants with LOS, a causative organism is identified in blood culture; however, many infants appear clinically infected and have a negative blood culture (so-called culture-negative LOS). Common organisms in LOS in preterm infants are those colonising the gut, with the causative organisms for LOS often identified as the dominant gut organism 24–48 hours before the onset of LOS, suggesting that gut translocation may contribute to LOS. Central venous line care ‘bundles’ (packages of care) and NEC bundles that are designed to lower the rates of LOS and NEC have failed to consistently and sustainably reduce LOS and NEC, and both diseases remain key challenges in the UK.
Gut bacterial community patterns in preterm infants
The gut is host to the largest bacterial community in the body and is the largest immune organ; in the newborn period, it is the main site experiencing rapid and dramatic interactions between host and microbes. In infants born full term, this process generally proceeds without difficulty and a bacterial community, termed the microbiota, is established over the first weeks and months. Microbiota refers to the bacteria present, whereas microbiome refers to the organisms and their genes; however, in practice, these terms are often used interchangeably. Over the next few months and up to early childhood, this progresses in a predictable way towards an adult-type pattern, influenced primarily by feed type, environmental exposures and genetics, resulting in a pattern of gut microbiota that is well tolerated by the host immune system and is relatively stable. 12
In preterm infants, establishment of a stable gut bacterial community is more challenging and variable, with large day-to-day variation in microbes in individuals. 13 Substantial variation exists between individuals without clear explanation, and many infants develop unusual microbial patterns. Collectively, the lack of bacterial richness or diversity, the lack of key bacteria associated with health in full-term infants (e.g. Bifidobacterium) and the dominance by bacteria more often associated with disease (e.g. Klebsiella and Enterobacter) is described as dysbiosis, although no single definition exists. In part, dysbiosis occurs because of to the exposures associated with neonatal intensive care unit (NICU) practices and environments, including limited maternal skin-to-skin contact; a reduced intake of milk, especially mothers’ own breast milk; breast milk pasteurisation, which reduces maternal bacterial transfer; frequent antibiotic use; and exposure to microbes that colonise the NICU environment. Immaturity of the preterm immune system, with a dominant innate system, also affects the development of the microbiome. These frequent changes in bacterial community are then less well tolerated by the immune system, with a propensity for an exaggerated inflammatory response that results in damage to intestinal cells, with resultant translocation of bacteria (LOS) or bowel inflammation (NEC).
Observational data suggest that the gut bacteria that are present, typically measured by 16S ribosomal ribonucleic acid (rRNA)-based techniques, differ between healthy preterm infants and infants who develop NEC or LOS, but there are important challenges in data interpretation. 14 These studies typically utilise longitudinal stool sampling and compare community structures in health and disease states. Attempts to allow for other influential factors on the microbiota are made by matching cases and controls for gestational age at birth, postnatal age of sample and delivery mode, where possible, but such studies are not able to control for other important variables. The differences observed in diseased infants are collectively termed ‘dysbiosis’, but this is problematic given that there is no agreement on what constitutes a healthy pattern of microbiota (eubiosis) and the fact that these terms lack precise definitions and understanding in preterm neonatal settings. Importantly, although individual studies see a difference between healthy and diseased infants, the specific taxa that differ between studies in different NICUs potentially reflect the importance of the NICU site as a determinant in the changes seen in disease states. 15 Morrow et al. 16 identified two bacterial profiles associated with NEC, one Enterococcus dominant and one Escherichia dominant, whereas Sim et al. 17 identified a clostridia-associated NEC group and a Klebsiella-dominant NEC group, with both patterns also occurring in healthy infants. Warner et al. 18 identified an increase in the relative abundance of Gammaproteobacteria in NEC; however, this reached significance after day 30 only, whereas the average onset of NEC was on day 24. 18 Meta-analysis of microbiome analyses has been attempted, but only 61 cases of NEC were included. 19 Changes were discernible at the phyla/class level, with a reduction in Firmicutes and Bacteroidetes and a relative increase in Proteobacteria before NEC. Stewart et al. 13 demonstrated greater stability and fewer changes between so-called preterm gut community types in health as opposed to disease. The focus to date has been on whole-community next-generation sequencing (NGS) comparison, and blooms in key taxa or changes in overall diversity; however, more precision and sensitivity may be required to identify the key drivers of processes (keystone species) and greater focus on gut bacterial metabolic function is likely to be important. Overall, the bacterial metabolic capacity of the gut may be more functionally relevant in maintaining host health than simply the pattern of bacteria, and specific metabolomic signatures have been observed in small numbers of infants with NEC/LOS to date. 13,20–24
Interventions that directly or indirectly mediate milk (or milk constituents) exposure, gut bacteria, gut immune function or a combination of these can be expected to affect rates of LOS, rates of NEC, neurodevelopmental outcome and survival, or combinations of these outcomes. These interventions include the use of supplemental ‘probiotic’ bacteria (examined in > 30 interventional trials25), the rate of milk advancement (assessed in SIFT), the addition of bioactive milk constituents (including lactoferrin), the addition of prebiotics, antibiotic use and the use of donor milk. Some of these interventions can be explored in randomised trial settings, whereas others, such as exposure to fresh maternal milk, cannot be studied in this way. Likewise, some interventions come with high perceived associated risks, for example oral antibiotics, which are shown historically to reduce NEC,26 but further clinical trials of which have not been conducted for fear of promoting antimicrobial resistance.
Collaborative neonatal trials in the UK
The UK neonatal community, in collaboration with clinical trials units and, importantly, the National Perinatal Epidemiology Unit (NPEU), has a long track history of delivering and continues to demonstrate the capacity to deliver large multicentre neonatal RCTs in several areas, including nutrition,27 oxygen,28 probiotics8 and drugs. The multicentre approach, and utilisation of the benefits of the National Institute for Health Research (NIHR) portfolio system, has enabled studies of several thousand very preterm infants to be delivered within a reasonably short recruitment time frame (2–3 years). This means that the intervention is being tested in a time frame in which other elements of treatment may have remained relatively stable, which is important in neonatal medicine as it is a technical and rapidly evolving specialty. Large trials mean that relatively rare events, such as NEC, which has an incidence of < 10%, can be studied in clinically relevant time frames. Standardised reporting systems, outcome definitions (LOS and NEC), data collection forms and experienced research staff enable high-quality data, high follow-up rates and high data completion rates, thereby reducing bias and increasing the generalisability of study outcomes.
Consequently, the UK neonatal community has delivered large RCTs of, for example, oxygen saturation targets [Benefits Of Oxygen Saturation Targeting (BOOST II) UK]28 and iodine supplementation,27 with successful completion to time and target. However, these studies included only relatively modest, if any, embedded mechanistic elements. For pragmatic trials with a ‘positive’ end point, such as BOOST II UK (for which mortality was lower in one trial arm), this may not matter given that clinical practice will change based on clinical differences between trial arms. However, for trials that do not reject the null hypothesis (and, potentially, also for all RCTs), opportunities for better understanding of disease processes, aetiology, outcome modulators and future hypothesis generation are more limited without a mechanistic study. The MAGPIE study aimed to embed the exploration of the gut-mediated mechanisms that might lead to LOS and NEC and how these are modulated in preterm infants within the context of a RCT.
The potential benefits of embedding even limited mechanistic work were shown by a recent NIHR study, the Probiotics in Preterm Infants Trial. 8 This trial addressed the pragmatic question of whether or not receipt of Bifidobacterium breve BBG-001 would reduce LOS compared with placebo. The primary outcome showed no difference between placebo and intervention arms for NEC (9% vs. 10%), LOS (11% vs. 12%) or mortality (8% vs. 9%). However, gut bacteria analysis showed that 21% of the placebo arm were colonised with the probiotic at age 2 weeks and almost half (49%) at 36 weeks’ postmenstrual age. 29 This strongly suggests cross-contamination of infants in the placebo arm with the probiotic acquired within the NICU environment. This was the first probiotic RCT to assess colonisation across all NICU sites, which demonstrated that this was universally present. Analysis by colonisation state showed a trend towards reduced NEC, LOS and mortality compared with the null finding in the primary outcome. 29 This shows that mechanistic work may reveal important aspects within large pragmatic clinical trials that affect study interpretation, change clinical perspectives and alter future study design.
Probiotics and lactoferrin in interventional RCTs have demonstrated impacts on LOS and NEC. 25,30 Probiotics are an extensively studied, enterally delivered intervention that shares potential similarities with lactoferrin in mechanisms of action: both impact on gut microbiota community structure and the metabolic function of the bacterial community may change, and both may have primary effects on host immune function or may modulate this through changes in the microbiota. Mechanistic work on probiotics in RCTs is also limited, but includes studies demonstrating altered faecal cytokines and calprotectin, decreased inflammatory cytokines [interleukin (IL) 6, IL-8, IL-17] and increased anti-inflammatory cytokines (IL-10), and the production of beneficial metabolites, such as short-chain fatty acids. 31–33
Research challenges in preterm infants
The understanding of mechanisms of disease processes in general is extremely limited in preterm infants, and few mechanistic studies take place. This includes all steps of disease development, with poor understanding of pathogenesis, pathways of disease evolution and prognosis. In part, this is because of the difficulties in accessing any biological samples for scientific study given the small size and fragility of the preterm infant, who may weigh < 500 g and have a circulating blood volume of < 40 ml, thus restricting deliberate sampling for research purposes. 34 Disease occurrence in preterm infants is also sporadic, individually rare within NICUs and sudden in onset, making discussion regarding research sampling at the time of illness difficult. Historically, there has been reluctance to ask the parents of sick, fragile infants to give permission for such studies; however, this is changing and data increasingly show that parents wish to participate in such studies. In addition, data show that multiple trial participation is not problematic for parents. 35,36 Preterm infants are also disadvantaged by experiencing some diseases that are (virtually) unique to them (such as NEC), making learning from an older child or adult counterpart less possible. Likewise, although animal models exist for NEC and LOS, given the key roles of human milk constituents and human gut bacteria in disease processes, these models have currently not resulted in data that have translated into a change in clinical practice for preterm infants. Given these difficulties, we established a ‘sample salvage’ system that, with parental consent, takes stool, urine, residual breast milk, residual blood from necessary tests and gut tissue where it has been resected as part of a necessary operation. This allows translational research work to be undertaken with a view to understanding these complex diseases in preterm infants. Having piloted this methodology successfully, we used this approach in units participating in the ELFIN trial to obtain urine, stool and tissue for mechanistic work embedded in a RCT.
Lactoferrin: mechanisms of action
Lactoferrin is the third most abundant protein in human milk and is present at a particularly high concentration in colostrum. In vitro, it has direct antifungal, antiviral and antibacterial properties and it has been shown to have direct gastrointestinal effects on enterocyte growth and tight junctions in animal models. 37 In addition, lactoferrin has prebiotic effects that promote the growth of ‘beneficial bacteria’, the presence of which is associated with less NEC, such as Bifidobacterium,38–40 and a reduction in the growth of potentially pathogenic bacteria, such as Enterobacter species. In turn, this may lead to more stable microbial communities, with less dysbiosis. Altered microbial community members, greater community stability or enhanced tight junctions could result in lower rates of LOS or NEC in preterm infants; however, none has previously been explored in the context of large, pragmatic randomised trials of lactoferrin in very preterm infants. The MAGPIE study aimed to explore these putative mechanisms of benefit from bovine lactoferrin given enterally to preterm infants.
The iron-binding properties were originally thought to be key to the antibacterial function of lactoferrin. 41 Given that some bacterial species are dependent on free iron to replicate, the iron-binding capacity of lactoferrin was thought to be its main protective mechanism. The holo form is saturated and the apo form is unsaturated. In breast milk, human lactoferrin is a folded polypeptide chain that is normally < 20% saturated with iron, with the unsaturated (apo) form capable of binding and holding iron that would otherwise be free, such that bacterial siderophores cannot access iron for bacterial replication. 42 However, protection against bacterial infection by lactoferrin is also configured by other mechanisms, including the disruption of cell walls leading to cell death. In Gram-negative bacteria, this is through the binding of lactoferrin to the bacterial porins, which causes the cell to release lipopolysaccharide but creates a disrupted cell wall membrane at that point, leading to cell death. The released combination of lipopolysaccharide and lactoferrin acts on CD14+ immune cells, blocking MYD88 activation of the inflammatory response and promoting an anti-inflammatory or tolerant profile. The high affinity of lactoferrin for lipopolysaccharide has led to some commercial preparations being contaminated with lipopolysaccharide, affecting in vitro studies of function. 43
Lactoferrin is also known to be cleaved by pepsin to release the lactoferricin domain, a beta-pleated sheet from the N1 domain. 44 In vitro, lactoferrin has been shown to be capable of biofilm disruption, specifically those biofilms associated with Staphylococcus epidermidis, and the beta-pleated sheet is thought to give better adherence for disruption of the film. Given that the most common organisms grown in preterm blood cultures are coagulase-negative staphylococci (CoNS),7 including but not exclusively S. epidermidis, and these infants often have venous access lines (long lines) that may harbour biofilms, this may be a relevant mode of action for lactoferrin to reduce preterm LOS if lactoferrin (or a functional metabolite) was able to reach the infected site. The ELFIN trial delivered lactoferrin enterally, and it is uncertain how much lactoferrin and its associated peptides may cross into the bloodstream (if any) to exert a direct effect on cannula or long-line biofilm at a ‘distant’ site. Impacts on such infections may be mediated through reductions in gut microbiota CoNS, leading to less surface (skin) CoNS and lower associated line contamination.
Lactoferrin exerts antibacterial effects not simply by reducing the numbers of bacteria present, but by neutralising secreted proteins that are part of the adherence/invasion apparatus of bacteria, thereby reducing their virulence. 45 This has been demonstrated for Escherichia coli in vitro and in animal models, in which enteral lactoferrin has been shown to reduce invasive E. coli infection in guinea pigs and gut-associated infection rates. 43 For Gram-positive bacteria, including Staphylococcus aureus and group A streptococci, lactoferrin has been shown in vitro to bind to both bacterial adhesins and host cells to prevent intracellular invasion. Binding within the gut may similarly prevent invasion of species, reducing LOS with gut-derived organisms.
In the gut, the antiviral and antifungal properties of lactoferrin are also important, given that evolutionary niches for bacteria may be liberated by viral or fungal cell death, and changes in the bacterial microbiome have been shown to be associated with fluconazole use. 46 Lactoferrin has been shown to be fungicidal to six common Candida species, including Candida albicans, Candida parapsilosis and Candida glabrata, responsible for most neonatal candidaemia. Candidaemia in association with NEC may also be reduced by exogenously delivered lactoferrin in the gut.
In animal models, piglets fed transgenic lactoferrin-enhanced milk had better weight gain and more Bifidobacterium in the ileum and more Lactobacillus in the colon than those fed standard milk. Similarly, obese mice fed lactoferrin had lower levels of pro-inflammatory cytokines (TNFα and IL1β) in association with lower systemic lipopolysaccharide levels and enhanced tight junction protein production. Gut microbiota analysis revealed higher levels of Bifidobacterium and lower levels of Bacteroidetes. Bifidobacterium spp. in stool were also enhanced in full-term infants who received lactoferrin-supplemented formula by age 3 months, but not sooner, suggesting that gut or immune maturity may have an impact on the effects of enteral lactoferrin supplementation.
The results of giving piglets either bovine lactoferrin or recombinant human lactoferrin in transgenic bovine milk show that orally administered exogenous lactoferrin is biologically active in the neonatal piglet intestine. Piglets that received supplemental lactoferrin have longer crypt depth, larger crypt area and higher enterocyte proliferation than piglets who did not receive supplemental lactoferrin. 43 Threefold-higher expression of messenger ribonucleic acid of beta-catenin by enterocytes was found in association with this, suggesting that exogenous lactoferrin can affect gene expression of the host.
Lactoferrin also has direct effects on the intestinal cells,42 promoting proliferation, but the effects of iron-bound and -unbound lactoferrin differ: a potentially important factor for exogenously administered ‘therapeutic’ lactoferrin. These cellular actions are mediated through binding of the lactoferrin receptor, which is located in the brush border of intestinal cell membranes in humans and pigs and expressed in fetuses, and, therefore, probably in preterm infants also. Bovine lactoferrin has also been shown to bind to the intestinal lactoferrin receptor in non-bovine species. Intracellular lactoferrin then promotes signalling pathways that mediate cell proliferation, with apo lactoferrin promoting this preferentially. Dose-dependent effects are of important consideration for the preterm infant, for whom total protein delivery is limited and other essential amino acids are also needed, that is there may be a limit to how much lactoferrin can be given without resulting in an abnormal delivery of amino acids for a rapidly growing infant.
Lactoferrin may also modulate the host immune response through direct contact with the gut-associated lymphoid tissue and the mucosa-associated innate T cells, and the subsequent cytokine modulation. 47 Peyer’s patches are larger in mice and piglets receiving supplemental lactoferrin and immunoglobulin A (IgA) secretion is higher. IgA may be important in NEC development, with recent data suggesting that IgA-unbound bacteria are on the causal pathway for NEC. 48
Lactoferrin: impact on key gut bacterial species
Bifidobacteria are key constituents of the healthy microbiota in infancy, dominating in breastfed full-term infants and promoted by the presence of human milk oligosaccharides that are digestible by bifidobacteria only and not by the host. In preterm infants, health has been shown to be associated with higher bifidobacterial relative abundancy, with less NEC/LOS in infants with stable bifidobacterial-dominated microbiota. 13 In vitro, lactoferrin has been shown to promote bifidobacterial growth under certain conditions; these conditions are dependent on the holo or apo forms and iron availability, as well as the strain of Bifidobacterium. 49 Lactoferrin from both bovine species and humans has been shown to be bifidogenic, with bifidogenic activity increasing with increasing iron saturation: bovine lactoferrin being bifidogenic to B. breve and Bifidobacterium infantis, but human lactoferrin being bifidogenic to Bifidobacterium bifidum only. 40 It has also been demonstrated in vitro that bifidobacteria can utilise the sugars bound to lactoferrin to provide them with additional substrate for growth. 50 Bifidogenic peptides derived from the N-terminal cationic region of the N-lobe or C-lobe have also been demonstrated in human and bovine lactoferrin, providing a further mechanism for enhanced bifidobacteria in gut communities in association with supplemental enteral lactoferrin. 38,40 In coculture models, the complexities of these interactions are demonstrated by the fact that bovine lactoferrin inhibits E. coli growth but not the growth of B. infantis. This means that rapidly growing pathogens, such as E. coli, that require a good supply of iron may be prevented from doing so if the iron is sequestered by the lactoferrin.
The level of both Salmonella and E. coli in stool was reduced by supplemental oral lactoferrin in piglets receiving high-dose lactoferrin, demonstrating the ability of exogenous oral lactoferrin to affect the stool microbial pathogenic community. Lactobacillus is another constituent of the healthy full-term infant microbiota, and observational data from breastfed infants revealed a correlation between lactoferrin levels in the milk and lactobacilli levels in the stool in full-term infants. 39
Lactoferrin: impacts on immune function
Oral lactoferrin affects the host gut bacteria community and host immune function. In rats, the immune effect appears to require an intact microbiome. Lactoferrin-driven immunomodulatory profiles were studied in dysbiotic rats which had or had not been given antibiotics. Lactoferrin alone produced a T helper type 1 (Th1)-type response, with elevated IFNγ and lower IgA levels. Lactoferrin with antibiotics in rats with an ablated microbiome did not result in the same change in host immune function, nor did antibiotics alone, suggesting that the mechanism of action is lactoferrin via the microbiota. Differences in the stool microbiome were explained by changes in the phyla Firmicutes, Bacteroidetes and Proteobacteria on day 5 of exposure, but by day 33, only the Firmicutes and Bacteroidetes were persistently different. By day 33, clostridia, Lactobacillus and Oscillibacter were also different between groups. Oscillibacter is regarded as anti-inflammatory and suppresses T helper type 17 cell (Th17) polarisation, promoting anti-inflammatory regulatory T (Treg)/type 1 regulatory T (Tr1) cells in the gut.
Similarly, in mice, recombinant oral lactoferrin treatment was seen to decrease intestinal Th17 inflammatory cells and increase Treg cells, with a microbiota mirroring this. Anti-inflammatory and short-chain fatty acid-producing Firmicutes were increased in lactoferrin recipients, and Th17-promoting segmented filamentous bacteria were decreased. Lactoferrin has also been shown in vitro to enhance dendritic cell function, in turn promoting Th1 differentiation from naive T helper type 0 (Th0) neonatal cells.
Macrophages in the gut may contribute to breaches in barrier integrity, leading to LOS and a pro-inflammatory state contributing to NEC through toll-like receptor (TLR) signalling. Full-term infants’ cord blood monocyte-derived macrophages were exposed to lactoferrin to enable an understanding of whether or not macrophage behaviour was modulated by lactoferrin. Lactoferrin down-regulated TLR4 receptors, decreased TNFα production after stimulation with lipopolysaccharide and reduced TLR signalling. Mechanistically, this appeared to be because of lower phosphorylation of the signalling proteins NF-Kβ and ERK1/2. These data suggest that modulation of macrophage function by lactoferrin could be important mechanistically in protection against preterm LOS.
Clinical trials of lactoferrin in preterm infants
When the ELFIN trial was originally planned (in 2009), there was just a single RCT of supplementation of enteral lactoferrin in very preterm infants. 51 This trial was undertaken in Italian units and the placebo that was used was dextrose solution. The intervention was 100 mg of lactoferrin per day for 30 days (or 45 days) and the primary outcome was the first culture-positive infection in blood, cerebrospinal fluid or peritoneum. A total of 472 infants were recruited: 153 received supplemental lactoferrin alone, 168 received placebo, and the remainder received the probiotic Lactobacillus rhamnosus GG® (LGG) (Chr Hansen Holding A/S, Hørsholm, Denmark) and lactoferrin. Comparing infants who received lactoferrin with those who received placebo, there were fewer cases of LOS in the lactoferrin group [5.9% vs. 17.3%, risk ratio (RR) 0.34, 95% confidence interval (CI) 0.17 to 0.7]. However, most of the clinical benefit was because of a reduction in fungal infections, which fell from 5.4% in the placebo group to 0% in the lactoferrin group (p = 0.04), with bacterial infections alone not being significantly reduced across the groups (5.3% vs. 11%).
In UK NICUs, fungal infection is rare (< 1%);7 therefore, it is important that much of the overall benefit in the Italian trial51 was from reductions in fungal infection. Despite the apparent reduction in LOS, the Italian trial51 did not show a reduction in overall mortality as might be expected given the usually high mortality from fungal infection. The ELFIN trial took place in a UK setting with low fungal infection rates and determined whether or not supplemental enteral bovine lactoferrin would reduce LOS. The ELFIN trial determined blood-culture positive and carefully defined culture-negative infections as the primary outcome (all cases of LOS), and analysed culture-positive infections alone as a prespecified secondary outcome. In addition, the ELFIN trial gave a per-kg dose of lactoferrin based on the finding in the Italian trial51 that there was a significant reduction in LOS in the subgroup of infants weighing < 1000 g (and, therefore, receiving a higher per-kg dose) only. The ELFIN trial also gave the trial investigational medicinal product (IMP) until 34 weeks’ postmenstrual age to attempt to provide the intervention for the whole period that preterm infants remain at risk of LOS.
Full-term infants receiving bovine lactoferrin-enhanced formula (1 g/l) for 14 days had increased levels of bifidobacteria in their stool compared with those receiving standard formula, unless they already had bifidobacteria levels above a certain limit, in which case lactoferrin did not increase this further. This suggests that an ecological niche exists in the neonatal gut for bifidobacteria and that bifidobacteria can be helped to occupy this by providing lactoferrin up to a certain level that then cannot be exceeded. In fact, very high supplementation may suppress certain species of Bifidobacterium, such as B. breve. 52
Since the ELFIN trial was planned, and after the publication of the Italian trial,51 the impact of lactoferrin in preterm infants has been further studied and reviewed in a meta-analysis. 30 Individual studies remain small and mechanistic work is minimal. 53,54 In total, 190 infants who had a birthweight of < 2500 g in Peru were randomised to receive 200 mg/kg/day of lactoferrin or placebo (maltodextrin) for 4 weeks, with a reduction in the risk of sepsis from 22.1% to 12.6% in the lactoferrin group. Although the hazard ratio did not reach significance, in the smallest infants, that is those weighing < 1500 g, the impact appeared greater, with risks of sepsis of 20% and 37.5%, respectively; an analysis of the time of receipt of lactoferrin was also suggestive of an effect. 55
Additional infants were recruited by the Italian trial,51 the results of which were published as a further paper in 2014 with NEC as the focus,56 showing (in a total population of 743 infants) a reduction in NEC of stage ≥ 2 to 2% (5/247 infants) in those receiving lactoferrin, compared with 5.4% (14/258) of control infants (RR 0.37, 95% CI 0.136 to 1.005; p = 0.055). This was associated with a reduction in the combined incidence of death and/or NEC, which was 4.0% in the lactoferrin group and 10% in the control group (RR 0.39, 95% CI 0.19 to 0.80; p = 0.008).
A small (n = 50) Turkish study53 of infants born at < 32 weeks’ gestation who were given 200 mg/kg of bovine lactoferrin or placebo (saline) until discharge did not find evidence of any effect of lactoferrin on the rates of LOS or NEC (19% vs. 32%, RR 0.57, 95% CI 0.2 to 1.63; 0% vs. 20%, RR 0.1, 95% CI 0.01 to 1.76, respectively); however, this study was underpowered for these clinical outcomes. Sherman et al. 54 used recombinant human lactoferrin (talactoferrin) in a RCT of 120 infants, giving doses of lactoferrin higher than most other studies, at 300 mg/day for 28 days, to infants weighing between 750 and 1500 g at birth. 54 Bloodstream infections occurred in 33% of the control group and in 14% of the lactoferrin group, with a reduction in CoNS in the lactoferrin group being primarily responsible for the findings.
In Canada, 170 infants who were born at < 31 weeks’ gestation were randomised to receive either 100 mg/day of bovine lactoferrin or placebo until 36 weeks’ postmenstrual age, which did not result in any identified significant change in LOS (17.5% vs. 25%) or NEC (2.5% vs. 5%). 57 A study enrolling infants with birthweights of < 2000 g in India, and randomising them to either bovine lactoferrin or placebo for 28 days recruited 130 infants and showed no statistical difference between the groups. Two infants in the lactoferrin group and nine in the control group had LOS, with a RR of 0.24 (95% CI 0.05 to 1.05).
Six clinical studies, with a total of 886 infants, were included in a Cochrane meta-analysis. 30 LOS occurred less frequently in infants receiving lactoferrin, with a RR of 0.59 (95% CI 0.40 to 0.87) and an associated number needed to treat for a beneficial outcome of 17 infants. NEC was reported in four trials with 750 infants and occurred less frequently in infants receiving lactoferrin, with a RR of 0.40 (95% CI 0.18 to 0.86) and a number needed to treat for a beneficial outcome of 25 infants. No effect was seen on all-cause mortality. Owing to concerns around methods of blinding, randomisation, allocation concealment, outcome assessment and moderate to severe heterogeneity of studies, the data quality was graded as low and further well-designed and -delivered studies were recommended.
The only mechanistic work undertaken within the context of a RCT of neonatal lactoferrin was embedded in the studies of recombinant lactoferrin (tal-lactoferrin) by Sherman et al. 54 and bovine lactoferrin by Akin et al. 53 Sherman et al. performed a microbiome analysis of the stool of 21 infants (placebo, n = 11; lactoferrin, n = 10), who participated in the RCT of recombinant human lactoferrin in two NICUs. Stool samples collected on one occasion (on day 21) were analysed, with receipt of the IMP taking place between day 1 and day 28. Importantly, antibiotic use and duration of parenteral nutrition were different between NICUs; therefore, NICU site significantly influenced the microbiota. No data are provided on infections in these 21 infants nor information regarding the timing of antibiotic administration in relation to stool sampling. In the original study, there were significantly more Gram-negative infections in the placebo group, and an associated reduction in the proportion of staphylococcal operational taxonomic units (OTUs) in the stool microbiota of the 10 studied infants receiving lactoferrin. A reduction in Enterobacteriaceae was also associated with lactoferrin receipt; however, this was highly influenced by NICU site. Enterobacteriaceae have been associated with NEC development in some studies. In tal-lactoferrin-receiving infants, the accompanying proportional increase in OTUs was seen in Citrobacter.
Akin et al. 53 included assessment of the circulating lymphocyte profile, with a focus on Treg cells in cord blood and at discharge. Although no differences were seen between the lactoferrin and placebo groups overall, at either birth or discharge, the increase in numbers of Treg cells between birth and discharge was larger in the lactoferrin group, with differential FOXP3 expression. The authors hypothesise that this could be part of a protective mechanism of lactoferrin in the gut, given that FOXP3 Treg cells prevent colitis in an animal induction model. 58,59
Microbiomic and metabolomic study: strengths and limitations
Changes in the gut microbial communities (dysbiosis) may or may not be associated with alterations in metabolic function, and both are potentially important in modulating disease mechanisms and maintaining health in preterm infants. Determining changes in metabolic function of bacteria is complex and it is not possible to easily study the functional effect of a single bacterial species in vivo. In addition, it is important to note that the data set generated by gut bacterial and metabolomic analysis is vast and not amenable to analysis by standard statistical techniques. For example, several taxa of bacteria may be present in larger or smaller proportions when two samples are compared, and techniques such as principal co-ordinate analysis are necessary. This type of approach allows the analysis to determine differences or changes without determining which specific taxa increase or decrease.
In addition to the vastness of microbiome data sets, it is also important to consider whether increases or decreases in the proportion of specific bacteria actually impact on the metabolites that they produce. Ideally, studies would assess bacterial community members and function of the gut microbiome, and immunological and metabolic impacts on the host, which also modulate disease and health. Currently, most mechanistic studies of neonatal gut interventions focus on bacterial presence, not bacterial function, and few assess the impact on the host metabolism or immune system. In the last 10 years, there has been a move away from purely traditional microbiological techniques that identified bacteria by culture and promoted traditional classification by physical characteristics and phylogeny into taxonomic ranks: phylum, class, order, family, genus and species. The most recent studies now use genetic sequencing-based technologies that rely on similarity in deoxyribonucleic acid (DNA) sequences to determine organisms’ phylogenetic relatedness to other organisms, so-called NGS. Supplementation of these methods with more traditional microbiology techniques or whole-genome sequencing (WGS) is increasingly recognised as necessary, with differences at the strain level potentially explaining the mechanisms of action of interventions. However, costs would probably prevent the routine use of WGS in large data sets and, therefore, 16S rRNA enables many more samples to be analysed.
Sequencing methods use the identification of DNA code sequences through the whole genome (metagenomics) or using specific marker genes, commonly the 16S rRNA gene. Sequences with more than a prespecified level of similarity are classified as belonging to the same OTU. This is then cross-referenced with databases to identify bacterial genus (or higher level if genus is unavailable). The length read of the 16S rRNA gene sequencing (≈ 250 base pairs) prevents classification of OTUs to the species level, which needs metagenomic sequencing to computationally assemble DNA to the species level. These methods allow high-throughput untargeted detection of the microbial community, allowing rapid generation of large data sets on large numbers of samples. However, data can be biased by the specifics of DNA extraction, primer selection in polymerase chain reaction (PCR), sequencing technology and bioinformatic processing. Individual studies frequently present results equivalent to different taxonomic levels, for example family compared with genus, making comparisons across studies difficult.
In the MAGPIE study, we sought to test the hypotheses that receipt of supplemental enteral bovine lactoferrin would affect overall gut microbiota communities using NGS as assessed by increases in the diversity of bacteria measured by the Shannon diversity index; increases in the presence of bacteria associated with health (e.g. Bifidobacterium species); decreases in the presence of potentially pathogenic bacteria (e.g. Enterobacter species); increases in the proportion of key taxa prior to the onset of LOS or NEC in preterm infants, such as Staphylococcus or Enterobacter species; and increases in the amounts of metabolites produced by gut bacteria and or by the host using gas chromatography–mass spectrometry (GCMS) and liquid chromatography–mass spectrometry (LCMS). 33
Aim and objectives
The aim of the MAGPIE study was to explore the actions of feeding interventions on gut bacteria and metabolites tested in RCTs in preterm infants. The initial aim was to study interventions that were tested in two large NIHR-sponsored RCTs: (1) SIFT and (2) ELFIN. Initially, these studies were planned and expected to be open to recruitment at the same time in participating hospitals. However, ultimately, SIFT recruited infants between June 2013 and June 2015, and the ELFIN trial recruited between May 2014 and September 2017. The MAGPIE study took much longer to set up than anticipated and did not commence recruitment until June 2016. Therefore, all infants were recruited to the ELFIN trial, and the MAGPIE study explored the actions of supplemental enteral bovine lactoferrin. The study aimed to determine the effects of lactoferrin on gut microbiota and metabolites in stool and urine, and any increases or decreases in the proportion of likely pathogens or potentially healthy bacteria that may occur prior to the onset of NEC or LOS. We aimed to determine effects in both the stool and the urinary metabolome because these may reflect changes in bacterial or host metabolism, or both. We aimed to determine changes in the bacterial community and overall metabolome profiles using both LCMS and GCMS. Our specific objectives were to test the following hypotheses:
-
Lactoferrin will result in detectable increases or decreases in the proportion of key bacterial taxa and these will be paralleled by changes in metabolites in the stool or urine.
-
There will be increases in the proportions of likely pathogens or pathogens directly causing neonatal sepsis, and decreases in potentially healthy bacteria, such as bifidobacteria, and these will be paralleled by changes in metabolites in infants developing NEC or LOS in their stool and/or urine that occur in the 7-day period prior to disease onset compared with control infants.
-
There will be upregulation in key immune pathways in samples of tissue affected by NEC compared with controls in tissue resected at operation required for clinical reasons.
We planned to achieve these objectives by determining the following outcomes:
-
We will use 16S NGS of bacterial DNA extracted from stool samples on days 1–3, 7, 10–14 and 21 (± 1) of life to determine the presence and proportions of key bacterial taxa.
-
We will use GCMS and/or LCMS of stool samples to determine effects on the metabolome and how increases or decreases in the proportion of key metabolites are associated with gut bacterial patterns.
-
We will analyse samples up to 1 week before disease onset and compare them with samples from healthy matched controls to determine increases or decreases in the proportions of key gut microbiota and/or metabolites during this period.
-
We will use any gut tissue that has been surgically resected and use immunohistochemistry to compare samples affected by disease (NEC) with control tissue. Control tissues will be samples of non-affected tissue from the same infant that is remote from any localised disease or from an infant who required surgery but did not have NEC, for example owing to spontaneous (focal) intestinal perforation.
Chapter 2 Methods
Participant eligibility and exclusions
Preterm infants at any of the 12 participating NHS hospital trusts (13 separate NICUs) were eligible for the trial if they met the enrolment criteria for the ELFIN trial, which included preterm infants of < 32 weeks’ gestation and < 72 hours’ postnatal age. Infants with a severe congenital anomaly, or who were not expected to survive or who were likely not to be enterally fed for > 14 days were ineligible. Infants enrolled in the ELFIN trial were randomised to receive supplemental enteral bovine lactoferrin (150 mg/kg/day) or blinded placebo (sucrose) from as soon as enteral milk feeds were tolerated until 34 weeks’ postmenstrual age. 60
Potential infants meeting the eligibility criteria for the MAGPIE study were identified by the local health-care team. Responsibility for enrolment was typically delegated to good clinical practice-trained research nurses or other individuals by the site principal investigator. We considered that parents whose first language was not English would be eligible if the local team deemed them eligible for recruitment to the ELFIN trial. Parents were approached for written informed consent after they had received a verbal and written explanation of the MAGPIE study. This could occur at any time after enrolment in the ELFIN trial, but was usually within the first 3 days and frequently occurred at the same time as consent was given for participation in the ELFIN trial. This meant that the first stool sample collected for the MAGPIE study may not have been the first meconium sample passed, but, in some cases, the first stool collected will also have been the first stool passed given that initial stool passage may occur at only a few days of age in sick preterm infants. Written parent information sheets explicitly stated the intention to share and use data collected for the purposes of the ELFIN trial, collect urine and stool samples, and retrieve any residual resected gut tissue available in NHS pathology departments if surgery had been required for clinical reasons (i.e. NEC or intestinal perforation). We obtained research ethics approval to collect stool and urine samples and to store them in the NICU for up to 1 week prior to signed consent for the MAGPIE study, but were to destroy any such samples if consent was not subsequently obtained. Parents were given the option of consenting to all aspects of the study or declining longer-term sample storage in an approved biobank and/or the research use of retrieved gut tissue. Examples of MAGPIE study documentation, such as parent information sheets, consent forms, sample collection logs and courier transfer logs, are available on the journal website (https://fundingawards.nihr.ac.uk/award/13/122/02).
Case definitions of necrotising enterocolitis and late-onset sepsis
The SIFT and ELFIN trial used internationally accepted definitions of NEC and LOS that were confirmed at Blinded End-point Review Committees (BERCs) conducted by at least two senior clinician investigators. 60 Briefly, confirmed LOS required microbiological culture of potentially pathogenic bacteria or fungi from fluid aseptically sampled > 72 hours after birth from blood or cerebrospinal fluid, or clinically suspected sepsis (meeting three objective clinical criteria), and the intention for antibiotic treatment for ≥ 5 days. NEC was diagnosed at surgery, post mortem or clinically where at least one clinical sign was present (bilious gastric aspirate or emesis, abdominal distension, or occult or gross blood in stool) and at least one radiological feature was present (pneumatosis intestinalis, hepatobiliary gas or pneumoperitoneum). Infants who satisfied the definition of NEC but at surgery or post mortem had a ‘focal gastrointestinal perforation’ were not coded as having confirmed NEC. The BERCs ensured consistency in diagnosis of these diseases, but we acknowledge that methods of coding disease, especially NEC, differ between many of the published studies.
Sample selection for analysis
Although the primary aim of the study was to determine the gut microbiomic mechanisms of action of supplemental enteral lactoferrin rather than simply the mechanisms leading to NEC or LOS, we were limited in the number of samples that we would be able to analyse owing to funding and resource issues. Given the devastating nature and impacts of NEC and LOS, we considered it important to analyse samples from all infants who developed disease, where adequate samples existed. We, therefore, selected the samples from all cases of NEC or LOS in the 7 days prior to disease onset. We expected to have between 70 and 100 infants who developed disease (LOS and NEC combined) and would, therefore, need to select around 100 healthy infants to act as controls. Having selected samples from diseased infants and their matched controls (see Matching of healthy controls for each case of disease), we then selected further samples from healthy infants to explore the impact of lactoferrin during the NICU stay.
Matching of healthy controls for each case of disease
For each case of NEC or LOS, we identified one healthy control infant who had samples available at a similar postnatal age, on a week-by-week basis, from the same NICU. This is because data show that NICU site (hospital) is a key driver of gut bacterial communities, and a control matched tightly on gestation from another hospital may be a less good match than an infant from the same NICU. We defined a healthy infant as an infant who did not have a confirmed or suspected episode of NEC or a confirmed episode of LOS. We aimed to select a healthy control who was within 1 week of the same corrected weeks of gestation at birth, choosing the younger infant if there were two equally well-sampled infants. Because antibiotics are widely used and likely to affect the gut microbiota, yet the majority of infants on the NICU receive at least one course of antibiotics, we aimed to use samples from healthy infants in whom antibiotics had not been administered in the 3 days prior to selected samples, and ideally for as long as 7 days prior. We did use a small number of control infants who on one occasion were coded as having suspected LOS, but we used only those infants who did not have prolonged antibiotic exposure (> 7 days) and whose enteral feeds were not stopped as part of that episode for > 48 hours (i.e. did not have any clinical features of medical NEC). Some of the infants initially selected as healthy matched controls were also twins or triplets. In this case, we used the first infant meeting the criteria as the control, but we endeavoured to then use the co-twin/triplet in the analysis of the impact of lactoferrin on gut microbiota over the NICU stay (see Impact of lactoferrin in healthy infants).
We also identified and collected stool samples for analysis from a small number of infants who died but did not have a confirmed diagnosis of NEC or LOS. We expected that a number of these infants may indeed have had NEC or LOS given that these diseases are the most common cause of death in a NICU, and we were keen to use the opportunity to conduct microbiome profiling in infants for whom we did not have permission to store stool or urine samples in a biobank longer term. We did not have permission to access the certified cause of death and recognise that there are often multiple aetiological factors in preterm infants who die after the first few days. Many of these infants were placed ‘nil-by-mouth’ enterally and indeed may have had NEC or LOS that preceded (or caused) death, even if this was not coded by the local research team.
Impact of lactoferrin in healthy infants
The main aim of the MAGPIE study was to explore the impact of supplemental lactoferrin on gut bacteria and metabolites. These healthy infants were either (1) infants who had already been identified as controls for disease cases (as described above) or (2) infants who were healthy and could be matched to another infant from the same NICU. Twins and triplets who are healthy are well matched to their siblings because they receive care in the same NICU, share genetic factors and will be exposed to the same microbes on the mother’s skin or from breast milk. This meant that we often selected a healthy twin to act as control for the other. The gut microbiota of the preterm infant is substantially different from that in full-term infants, shows frequent shifts in taxa proportions and is affected by a range of maternal (e.g. diet, lifestyle and genetic factors) and pregnancy-related factors (e.g. reason for preterm delivery, delivery mode, medications and antibiotics). In addition, one of the largest impacts on gut microbiota is nutritional and feeding-related practices, including the volume and type of milk feed (i.e. days of enteral milk feeds, breast vs. formula milk, etc.). It is not possible to identify matches on all of these factors; however, using twins (or higher-order multiples) from the same pregnancy who remain healthy, and are probably tolerating similar volumes of milk, provided us with an opportunity to ‘control’ for a substantial number of the effects caused by maternal, pregnancy- and delivery-related factors. Around half of all twins recruited to the MAGPIE study would, by random allocation, receive the same IMP (i.e. both receive lactoferrin) or placebo (we termed this ‘concordant’ for trial IMP), while the other half would receive different IMP (i.e. one infant receives lactoferrin while the other receives placebo) (‘discordant’ for trial IMP). We selected twins or triplets only if none of the infants had developed NEC or LOS, and we avoided sampling at time points following recent antibiotics, ideally not using samples from the subsequent 5–7 days. However, in cases where one infant developed disease and the other remained healthy, we selected the healthy infant as a control for the infant who developed disease.
Our original aim was to identify a sample collected at the following postnatal ages: 0–3, 7, 10–14 and 21 days. In addition, we also identified samples after the first few weeks, noting that several cases of NEC and LOS presented after the first month, so we selected additional samples up to 34 weeks’ corrected age. It is common for preterm infants not to pass stool in the first few days of life, and many infants may pass stool erratically thereafter. It is also possible that on any specific day the bedside nurse may have been too busy or not collected a sample. Where a sample was not available on the chosen day, we looked for samples collected 1–2 days earlier or later. Infants who developed disease in the first week of life may have been enrolled to the MAGPIE study for only a few days, during which time some infants may not have passed any stool samples.
Clinical data collection
We used data collected as part of the ELFIN trial by electronic encrypted data transfer from the clinical trials unit (NPEU). Relevant data used for the MAGPIE study included:
-
hospital site, anonymised ELFIN trial and MAGPIE study number
-
maternal age, use of antenatal steroids, absence/reversed end-diastolic umbilical arterial blood flow, duration of membrane rupture and delivery mode
-
gestation, birthweight and twin/triplet status
-
daily use of parenteral nutrition
-
milk type received on each postnatal day (breast, formula or mixed)
-
receipt of the ELFIN trial IMP and ELFIN trial group.
We remained blinded to the ELFIN trial group until analysis was complete. We supplemented this with additional items collected on bedside samples logs for the MAGPIE study, which included daily information on antibiotic type and use of prophylactic antifungal agents (either nystatin or fluconazole) or probiotics; all NICUs that used probiotics used the same commercially available product that includes three species – B. infantis, B. bifidum and Lactobacillus acidophilus. Bedside logs also detailed the actual date of stool and urine collection, and each sample was given a unique code before storage and transport.
Standard operating procedures: sample collection, storage and transport
Samples were collected, anonymised and analysed in accordance with standard operating procedures (SOPs) developed by the project team. Stool and urine collection pots, spoons and vials were provided by the research team and were the same for each NICU. Stool samples were collected at routine clinical nursing care times from the nappy using a clean disposable plastic spoon and placed in a glass pot with a lid. The use of a glass pot for stool collection enabled metabolomic profiling using GCMS. Urine samples were collected in accordance with standard NICU procedure, which involved collecting urine passed spontaneously onto a sterile cotton wool ball, squeezing it out using a sterile syringe and aliquoting into two 2-ml cryovials. Samples were labelled and placed in a –20 °C freezer located in the NICU as soon as possible, although we did not record the time between stool passage and placement into the freezer. Samples were transferred frozen, in batches of approximately 500 samples, from the local NICU to Northumbria University using Human Tissue Act-compliant61 NHS-approved couriers, where they were then stored at –80 °C prior to analysis. Courier transport of samples took place every 2–4 months.
Methods: gut tissue identification, collection and analysis
Knowledge of the molecular aetiology of NEC is extremely limited. Given that the most at-risk population are preterm infants, biological tissue access for research is ethically and practically challenging. In response to this, the Newcastle SERVIS (Supporting Research in Vulnerable Infants) study biorepositories successfully established the research infrastructure for obtaining consent from parents of preterm infants to access NHS-collected surgical tissue specimens that are surplus to diagnostic requirements. Given that many modern/emerging cutting-edge molecular techniques, including transcriptomics, immunohistochemistry and immunofluorescence, require only a single or small number of 4-µm slices of formalin-fixed, paraffin-embedded (FFPE) tissue, this material provides substantial opportunity for immune research discovery and validation.
We aimed to retrieve gut tissue resected during surgery for either NEC or other conditions, for example spontaneous intestinal perforation (SIP) from FFPE blocks located in NHS pathology archives after all clinical tests have been complete. We anticipated that only around 10–12 infants recruited to the ELFIN trial would undergo surgery for NEC and have residual tissue available. Therefore, we planned, if necessary, to use additional tissue samples from preterm infants, available from the lead hospital site (Newcastle Hospitals NHS Foundation Trust), that had been enrolled in SIFT or other local studies, which had full research ethics permission to access those samples. Because lactoferrin may have a range of immunomodulatory and anti-inflammatory actions in the preterm infant gut, we planned tissue-based analyses to explore the aberrant innate and adaptive immune mechanisms, and validate immune pathways or biomarkers that were identified by microbiomic or metabolomic profiling. At the outset, we recognised that we would be able to access very small numbers of tissue samples only and, therefore, the study would be underpowered to explore the impact of lactoferrin on histological features.
When we commenced the MAGPIE study, it was unclear which immune cell populations might be the key drivers of inflammation in NEC. Accordingly, as preparatory work supported by separate funds from the Newcastle University MRC Confidence in Concept Fund, a hypothesis-free analysis of the gene expression profile of tissue from surgical resections was carried out for NEC to identify key immune pathways, which were then validated through a protein expression analysis using immunohistochemistry. FFPE small bowel (ileal) tissue samples were identified along with control (non-NEC) infants, and histologically defined microanatomical zones within ileal tissue were identified from each infant: ‘healthy resection margin’; ‘transitional’ tissue, showing architectural distortion and inflammation without overt necrosis; and ‘necrosis’.
Methods: bacterial deoxyribonucleic acid analyses of stool samples using 16S ribosomal ribonucleic acid
Stool sample collection and preparation
Nurses at each of the 13 NICUs (12 hospital sites) collected samples from enrolment to ≥ 34 weeks’ corrected gestation, aiming to collect a daily stool and urine sample. Stool samples were collected in accordance with SOPs (as described earlier). Many stool samples were identified as requiring multiple analyses, and these samples were removed from –80 °C storage in randomised batches of 188 samples and stored on ice. In turn, each sample was transferred to a class II laminar flow hood and split into 0.1-g aliquots using a sterile scalpel. Each aliquot was transferred to a sterile glass pot, which was immediately sealed. A sample-splitting negative control, consisting of an empty sterile glass pot sealed at the same time as the samples, accompanied each batch of samples split for both volatile organic compound (VOC) and LCMS analysis. A 0.1-g aliquot of each stool sample was simultaneously transferred to an individual well of the DNeasy PowerSoil® HTP 96 Kit (Qiagen, Hilden, Germany) and stored at –80 °C overnight prior to isolation of bacterial DNA.
Isolation of genomic deoxyribonucleic acid from stool samples
Stool samples, split for 16S rRNA-based bacterial community analysis, were defrosted at ambient temperature in individual wells of a DNeasy PowerSoil HTP 96 Kit PowerBead DNA plate (Qiagen). Extraction was carried out as per the manufacturer’s instructions, utilising two 10-minute bead beating steps at 20 Hz on a TissueLyser II (Qiagen), but with altered centrifugation at 2250 × g rather than 4500 × g. For this reason, centrifugation times were extended accordingly. Further modifications included a 15-minute incubation at ambient temperature following addition of the elution buffer prior to eluting DNA from the silicon filter to maximise yields.
The DNA extractions were carried out in batches of 188 samples, split between two HTP 96-well plates (Qiagen). Each plate contained an extraction kit negative (an empty well processed as per the kit extraction protocol) and one completely blank well, left blank for inclusion of sequencing negative during library preparation protocol. Following the isolation of sample DNA, all samples were sealed and stored at –80 °C until sequencing library preparation.
Sample deoxyribonucleic acid library preparation for sequencing of bacterial 16S ribosomal ribonucleic acid gene
Sequencing libraries were prepared using the protocol described by Kozich et al. ,62 using primers targeting the V4 region of the 16S rRNA gene (515f: 5′-GTG-CCA-GCM-GCC-GCG-GTA-A-3′; 806r: 5′-GGA-CTA-CHV-GGG-TWT-CTA-AT-3′). 63 A zero template PCR negative was included at this stage that formed a sequencing negative for each batch.
The PCR amplicons were normalised and cleaned of excess deoxynucleoside triphosphates (dNTPs) and oligonucleotides with the SequalPrep normalisation kit (Thermo Fisher, Waltham, MA, USA) before pooling barcoded amplicons in multiplex libraries of 24 samples. Multiplex libraries were further normalised to a concentration of 2 nmol, before being combined into sequencing libraries containing 96 samples. Each sequencing library contained a kit negative and sequencing negative, and was sequenced on the Illumina MiSeq platform (Illumina, San Diego, CA, USA) using V2 2 × 250 chemistry.
Sequence data processing and microbial community analysis
Sequences were downloaded in FASTQ format from BaseSpace (Illumina) and processed using Mothur v.1.42.364 in accordance with the MiSeq SOP (Illumina). 62 Briefly, forward- and reverse-paired end reads were merged, quality filtered, aligned to known bacterial 16S rRNA gene sequences, screened for chimeras, clustered into OTUs and assigned consensus taxonomies based on OTU representative sequence similarity to the SILVA 16S rRNA gene sequence database (release 132, Quast et al. 65). Outputs of FASTQ sequence processing in Mothur consisted of feature tables of OTUs per sample and consensus taxonomic classification tables in which each OTU was classified to the genus level. These outputs were imported into RStudio (RStudio, Boston, MA, USA) for further analysis using the phyloseq package. 66 Owing to the limitations on taxonomic resolution inherently associated with sequencing 16S rRNA gene regions, all OTUs were merged at the genus level for analysis unless otherwise stated. Where necessary, species-level classification of single OTUs was inferred by performing a blastN (Basic Local Alginment Search Tool – Nucleotide) search on representative OTU sequences extracted from Mothur. OTUs contributing fewer than 10 reads across all samples were culled from the analysis.
Microbial compositions of sequencing and kit negative controls were compared with samples by analysis of similarities (ANOSIM), utilising 999 permutations. The vegan package in R (The R Foundation for Statistical Computing, Vienna, Austria) (Oksanen et al. 67) was used to calculate the alpha- and beta-diversity of microbial communities. Alpha-diversity was calculated as rarefied Shannon diversity. To ensure independence of sampling, depth rarefaction was performed prior to analysis, in which any samples with a library size of < 1000 reads were culled. Beta-diversity was calculated as Bray–Curtis dissimilarity to account for abundance rather than simply presence/absence of OTU features within the sample. Fisher’s exact test was utilised to identify significant differences between counts of discrete variables, while the Kruskal–Wallis rank-sum test was employed to identify significant differences between means of continuous variables. The pairwise Mann–Whitney Wilcoxon test was used when multiple means of continuous variables were compared and corrected for multiple hypothesis testing with false discovery rate. Adonis permutational multivariate analysis of variance (PERMANOVA) was used to identify variables affecting the dissimilarity between samples and was corrected for multiple hypothesis testing with Bonferroni correction. To account for repeated measures, where necessary, only one sample per infant was considered for analysis, such as when comparing the effect of lactoferrin supplementation or longitudinal effects on the microbiota. When multiple samples were available for a single infant, the sample closest to lactoferrin exposure was selected for analysis.
Methods: gas chromatography–mass spectrometry analyses of stool samples
The analysis of faecal samples was based on our robust protocols and procedures developed over several years. 68–74
Gas chromatography–mass spectrometry sample preparation
Faecal samples were stored at –80 °C at Northumbria University, Newcastle upon Tyne, before being shipped to the Liverpool laboratory on dry ice and being stored at –20 °C. Prior to analysis, samples were weighed and aliquots were transferred to 10-ml glass headspace vials with magnetic septum caps (Crawford Scientific, Lanarkshire, UK) in a hood: a mean of 80.6 mg [standard deviation (SD) 12.3 mg] of stool was used for the analysis. During aliquoting, an empty vial remained unsealed in the hood to collect circulating air, which was then sealed before being removed from the hood and stored with the prepared samples. These air blanks were analysed alongside the samples to determine whether or not there were contaminants in the air when the samples were aliquoted.
Gas chromatography–mass spectrometry methods
Volatile organic compound analysis was performed using GCMS on a PerkinElmer Clarus 500 GCMS quadrupole benchtop system (PerkinElmer, Beaconsfield, UK) and Combi PAL auto-sampler (CTC Analytics, Zwingen, Switzerland). VOCs were extracted using solid-phase micro-extraction with a divinylbenzene-carboxen-polydimethylsiloxane (DVB-CAR-PDMS)-coated fibre (Sigma-Aldrich, Dorset, UK); otherwise, the protocol and GCMS conditions were the same as those published by Reade et al. 70 The fibre was preconditioned before use in accordance with the manufacturer’s manual. Samples were heated to 60 °C for 30 minutes prior to fibre exposure. The fibre was exposed to the headspace gases at 60 °C for 20 minutes, and then VOCs were thermally desorbed for 5 minutes at 220 °C.
The gas chromatography (GC) column used was a 60-m Zebron ZB-624 (inner diameter 0.25 mm, length 60 m, film thickness 1.4 µm) (Phenomenex, Macclesfield, UK). The carrier gas was 99.996% pure helium (BOC Ltd, Sheffield, UK) that was passed through a helium purification system, Excelasorb™ (Supelco, Inc., Belllefonte, PA, USA), at 1 ml per minute. The initial temperature of the GC oven was set at 40 °C and was held for 2 minutes, and then the temperature was increased to 220 °C at a rate of 5 °C per minute and held for 4 minutes, giving a total run time of 41 minutes. The mass spectrometry (MS) was operated in electron impact ionisation EI+ mode, scanning ion mass fragments from 10 m/z to 300 m/z, with an interscan delay of 0.1 seconds and a resolution of 1000 at full width at half-maximum (FWHM). The sensitivity of the instrument was determined with 2-pentanone only and will vary for other compounds. The limit of detection was three times the signal-to-noise ratio: for the method for 2-pentanone with DVB-CAR-PDMS, this is 16 ppm.
Volatile organic compound data and statistical analysis
The VOC data were processed using the Automated Mass Spectral Deconvolution System (version 2.71, 2012, URL: www.amdis.net) coupled to the National Institute of Standards and Technology mass spectral library (version 2.3, 2017) to putatively identify VOCs. The R package metab was used to align data. VOC data were analysed using R (version 3.4.2). To start, the VOC table was adjusted as follows: only those compounds observed in ≥ 25% of samples were kept, normalisation by natural log-transformation was performed using the log1p() function and missing values were adjusted to 1. PERMANOVA was performed using the adonis2() function of the vegan package. 67 Bray–Curtis distance was used and samples were stratified for patient identification (ID). Principal component analysis was performed with the prpcomp() function. Generalised linear mixed effects, the glmer() function of the lme4 package, were used to assess whether or not there was correlation between the number of VOCs and infant age (days). Finally, linear mixed-effects model analysis was performed with the lmer() function of the lme4 package. 75 Patient ID was used as a random factor, while infant age (days), GCMS run batch and trial group were fixed factors. The ggplot2 package was used to produce the charts. 76
Methods: liquid chromatography–mass spectrometry analyses of stool and urine samples
The LCMS methods were based on robust protocols developed by the team at Birmingham and other published bioinformatic techniques. 77–81 In total, 253 urine and 196 stool samples collected longitudinally were shipped to the Phenome Centre Birmingham, University of Birmingham, for ultra high-performance LCMS analysis. This analysis represents the untargeted metabolomic profiling element of the project and aimed to detect small molecules that represent the functional products of cellular processes.
Preparation of urine samples for ultra high-performance liquid chromatography–mass spectrometry analysis
The specific gravity of each urine sample was measured using a Reichert TS 400 Total Solids Refractometer (Fisher Scientific, Loughborough, UK). Urine samples were thawed on ice, vortex mixed (10 seconds) and centrifuged (5000 × g, 5 minutes, 4 °C). A total of 100 µl of supernatant was loaded onto the refractometer and the urine-specific gravity (USG) measurement was taken. A USG linear standard curve was created by taking the urine sample with the highest USG and carrying out a serial dilution of the urine with water [neat, 80%, 60%, 40%, 20%, 10%, 5% and blank (with LCMS-grade water, VWR, Leicestershire, UK)]. The equation of the line from the standard curve was used to calculate the dilution factor of each urine sample based on its USG measurement. Each sample was diluted with water (LCMS grade, VWR) to make the samples equivalent in concentration according to the USG reading.
A total of 50 µl of the diluted urine sample was mixed with 150 µl of ice-cold, 50 : 50 methanol–water (LCMS grade, VWR). Samples were vortex mixed (15 seconds), centrifuged (13,000 × g, 20 minutes, 4 °C) and 100 µl of the clear supernatant was transferred to a glass LC autosampler vial [VI-04–12–02RVG 300-µl plastic vial (Chromatography Direct, UK)] for immediate ultra high-performance LCMS analysis. A single pooled quality control (QC) sample was prepared by combining 25-µl aliquots from each diluted urine sample and vortex mixing (2 minutes). Aliquots (50 µl) of the pooled QC sample were prepared as defined above for the urine samples. Extraction blank samples were prepared by adding 100 µl of water to a blank urine collection tube and vortex mixing (1 minute). A total of 50 µl of this solution was removed and prepared as defined above for the samples.
Preparation of faeces samples for ultra high-performance liquid chromatography–mass spectrometry analysis
Frozen faeces samples were weighed (mass range 50–124 mg) and 80 : 20 methanol–water (LCMS grade, VWR) was added to each sample; the volume of added solvent was normalised to faeces mass (15 µl of solvent was added per mg of mass). Samples were homogenised in a bead-based homogeniser [Precellys 24 system (Bertin Instruments, Montigny-le-Bretonneux, France) and 2-ml tubes containing CK14 ceramic beads (Stretton Scientific, URL: www.strettonscientific.co.uk/)] at 6400 rpm (revolutions per minute) for two bursts of 10 seconds separated by a 10-second break. The homogenate was transferred to an Eppendorf tube and centrifuged (13,000 × g, 20 minutes, 4 °C). A total of 650 µl of supernatant (equivalent to 43 mg of biomass) was removed, placed in a clean tube and dried in a SpeedVac concentrator (Thermo Fisher Scientific). To create QC samples, an additional 100 µl of supernatant was taken from each sample, pooled, vortex mixed (2 minutes), aliquoted into 650-µl aliquots and dried in a SpeedVac concentrator. Dried extracts were resuspended in 430 µl of 1.5 : 2.5 methanol–water, vortexed (30 seconds) and centrifuged (13,000 × g, 20 minutes, 4 °C). A total of 100 µl of the clear supernatant was transferred to a glass LC autosampler vial (VI-04-12-02RVG 300-µl plastic vial) for immediate ultra high-performance LCMS analysis. Several extraction blanks were prepared. The 50- to 100-mg pieces of nappy, sampling equipment (plastic spoons) or 100 µl of water were extracted in the same way as for the samples.
Ultra high-performance liquid chromatography–mass spectrometry
The samples were maintained at 4 °C and were analysed by applying an ultra high-performance LCMS method using a Dionex UltiMate™ 3000 Rapid Separation LC system (Thermo Fisher Scientific) coupled with a heated electrospray Q Exactive Focus mass spectrometer (Thermo Fisher Scientific). Polar extracts were analysed on a Hypersil GOLD™ C18 aqueous column (100 mm × 2.1 mm × 2.6 µm) (Thermo Fisher Scientific). Mobile phase A consisted of water with 0.1% formic acid (LCMS grade, Sigma Aldrich) and mobile phase B consisted of methanol with 0.1% formic acid. For both positive and negative ion mode, the initial flow rate was set to 0.30 ml/minute, rising to 0.40 ml/minute between 11 and 14.9 minutes. The gradient was as follows (where t = time in minutes and B = mobile phase B): t = 0.0, 1% B; t = 0.5, 1% B; t = 2.0, 50% B; t = 10.5, 99% B; t = 11.0, 99% B; t = 11.5, 1% B; t = 15, 1% B. All changes were linear with curve = 5 except for two instances: 10.5 minutes (curve = 8) and 11.5–15 minutes (curve = 7). The precise methods used for column temperature, injection volume, data acquisition and ion source parameters are provided in more detail in a recent publication. 82 Data-dependent MS2 in discovery mode was used for the MS/MS spectra acquisition using the following settings: resolution 17,500 (FWHM at m/z 200); isolation width 3.0 m/z; stepped collision energies 20, 40, 100 (positive ion mode)/40, 60, 130 (negative ion mode). MS/MS mass spectra were acquired in five different precursor m/z ranges: 100–260, 250–360, 350–510, 500–700 and 690–1500. Two blank samples were analysed, the first as the sixth injection and the second at the end of each batch.
Mass spectrometry raw data processing
Raw data acquired in each analytical batch were converted from the instrument-specific format to the mzML file format, applying the open access ProteoWizard (version 3.0.11417) (ProteoWizard, Palo Alto, CA, USA) msconvert tool. 77 During this procedure, peak picking and centroiding were achieved using vendor algorithms. Isotopologue parameter optimisation (version 1.0.0) using XCMS™ (version 1.46.0)79 (The Scripps Research Institute, La Jolla, CA, USA) was used to perform automatic optimisation of XCMS80 peak-picking parameters. For the centWave peak-picking algorithm, the following parameters and ranges were used: min_peakwidth (from 2 to 10), max_peakwidth (from 20 to 60), parts per million (ppm) (from 5 to 15), mzdiff (–0.001 to 0.01), snthresh (10), noise (10,000), prefilter (3), value_of_prefilter (100), mzCenterFun (wMean), integrate (1), fitgauss (FALSE) and verbose.columns (FALSE). Optimised XCMS parameters for raw data files deconvolution were applied and a data matrix of metabolite features (m/z-retention time pairs) versus samples was constructed with peak areas provided where the metabolite feature was detected for each sample.
Peak matrix processing
The data for pooled QC samples were applied to perform QC filtering. The first five QCs for each batch were used to equilibrate the analytical system and, therefore, were subsequently removed from the data before the data were processed and analysed. The data from the pooled QC samples were used to apply QC filtering. For each metabolite feature detected, QC samples 1–8 were removed (i.e. leaving a blank and two QCs at the start of each batch) and the relative SD and percentage detection rate were calculated using the remaining QC samples. Blank samples at the start and end of a run were used to remove features from non-biological origins. Any feature with an average QC intensity < 20 times the average intensity of the blanks was removed. Any samples with > 50% missing values were excluded from further analysis. Metabolite features with a relative standard deviation of > 30% present in < 90% of the QC samples were deleted from the data set. Features with a < 50% detection rate over all samples were also removed.
Metabolite annotation
Putative annotation of metabolites or metabolite groups was performed by applying the Birmingham mEtabolite Annotation for Mass Spectrometry (BEAMS) workflow, which is maintained by Phenome Centre Birmingham and available on GitHub (https://github.com/computational-metabolomics/beams; accessed 1 October 2020), where further details of the technique used are available. It is important to note that different metabolites will be detected with the same m/z (e.g. isomers with the same molecular formula) and it is also possible that a single metabolite could be detected as multiple molecules, depending on the specific type of ion, for example if the ions are protonated. All molecules were annotated in accordance with guidelines for reporting chemical analysis results, specifically to the 2007 Metabolomics Standards Initiative level 2. 83
Univariate statistics
For univariate statistics, the probabilistic quotient-normalised data were used to avoid including imputed values in the calculations. For comparisons with two groups (study 1), Mann–Whitney U-tests were applied. For comparisons with more than two groups (study 2), one-way analysis of variance (ANOVA) was applied to determine features showing a significant difference between groups (p < 0.05). For features found to be significant by ANOVA, Tukey’s honest significant difference was applied to determine between which groups there was a significant difference (p < 0.05). A false discovery rate correction (Benjamini–Hochberg) was applied to adjust for multiple testing and control the number of false positives (q < 0.05) for ANOVA and Fisher’s exact test. Pathway enrichment analysis was performed by applying the software MetaboAnalyst (Xia Lab, McGill University, Sainte-Anne-de-Bellevue, QC, Canada). 78
Methods: urinary analyses of intestinal fatty acid-binding protein
To supplement the metabolomic data, we analysed urine samples for intestinal fatty acid-binding protein (iFABP) to provide additional data. iFABP is a water-soluble protein that is expressed by enterocytes and may be released when the gut epithelium is inflamed or damaged; a small number of studies have explored its potential use as a marker or predictor of incipient NEC. 84–88 Its half-life in plasma is around 11 minutes in adults, but may be longer in preterm infants, before it passes into the urinary compartment. Levels of iFABP in urine may, therefore, reflect average plasma levels over the preceding few hours (or longer) and act as a non-invasive marker of gut damage. In sick preterm infants, hydration status and renal function can change very rapidly, so, to adjust for differences in urine concentration, the results can be normalised for creatinine concentration.
Urinary samples from informative cases were selected when available in the period leading to disease onset, and samples from healthy infants were used as controls. We used samples from infants who had also undergone 16S rRNA analyses, but in some infants we did not have any residual or available urine. Urine samples were prepared by first centrifuging samples at 1500 × g to separate any debris, and 15 µl of urine was added to a given well of a 96-well plate. To this well, 285 µl of sample dilution buffer for iFABP was added to the urine sample to create a 20× dilution. Some of the dilution factors were modified as their absorbances were outside the standard curve. A series of seven standards were created by serially diluting 225 µl of reconstituted iFABP standard in an equal volume of sample dilution buffer, giving a series of 3000, 1500, 750, 375, 187, 94 and 47 pg/ml. Standards were fitted with a four-parameter logistic curve, as recommended by the ELISA (enzyme-linked immunosorbent assay) kit manufacturers, and unknowns were calculated from the standard curve. Samples were analysed in duplicate and poor replicates were repeated until acceptable. Creatinine standards were made and diluted to 5, 2.5, 1 and 0.5 mmol, with a blank of distilled water as 0 mmol. The kit contains two reagents labelled 1 µl and 2.5 µl of standard or sample is added to a 96-well plate in duplicate followed by 225 µl of reagent 1. Undiluted urine was used to determine the creatinine content in each sample. The plate was read at 555 nm.
Sample size, randomisation and blinding
Sample size estimates for each element of the MAGPIE study were considered separately and, where possible, based on published data. However, the types of data collected and analysed using microbiomic and metabolomic techniques are generally not suitable for traditional statistical power analysis. Planned sample size was also in part determined by certain practical and logistical aspects. Overall, we based sample size on the following:
-
The anticipated start date and recruitment rate of the main ELFIN trial, which did not receive the full go-ahead until June 2015, following successful completion of the internal pilot.
-
The efficacy of lactoferrin in reducing the incidence of NEC and LOS. We estimated that recruiting 480 infants to the MAGPIE study would identify between 70 and 100 cases of LOS and/or NEC, and 10–20 cases requiring gut surgery in whom tissue might be available. It would also provide a large enough number of well-sampled healthy infants with a range of gestations, feed and antibiotic exposures.
-
The complexities of microbiomic data analysed using 16S rRNA sequencing mean that the sample size necessary to evaluate the actions of different interventions and the incidence of disease is dependent on effect size, the number of interacting factors and their correlation. For a power of 80% to detect a 50% difference in community profile patterns arising from a categorical descriptor of microbial community variation, using a two-sided test at a significance level of 5%, the study needs approximately 200 samples.
-
GCMS VOC analysis identifies several individual compounds; data from our research group showed that, on average, 31.3 ± 10.5 (mean ± SD) VOCs were identified per sample. 70 At a power of 80% and two-sided significance at the 5% level, we estimated that we would need 50 infants per ELFIN trial group to show an increase in five VOCs.
-
Using immunohistochemical data from our research group, assuming that any differences between disease and control will be greater than those in healthy individuals, and using a two-sample t-test, a sample size of 20 would give 80% power to detect a difference of 0.66 (66%) in cells/crypt cross-section at a significance level of 5% and an approximated SD of 0.5 (50%).
The ELFIN trial was a randomised controlled Clinical Trial of an Investigational Medicinal Product (CTIMP) and blinding was maintained until clinical data were fully analysed. In the MAGPIE study, sample selection, laboratory analysis and statistical analysis were also completed while all research staff remained blinded to trial group allocation.
Interim ‘futility’ analysis
We planned a ‘futility’ analysis after samples were available from ≥ 150 infants, prior to commencing further microbiomic and metabolomic analyses in the entire cohort to demonstrate feasibility of study design. We planned to select around 120 infants, anticipating that this would give us ≥ 50 infants from each ELFIN trial group, and to analyse 16S rRNA to determine gut bacterial composition. We chose day 10 because we expected that most infants would have received trial intervention for around 1 week, given that most infants commence increasing feeds around day 3–4. We planned to analyse a single stool sample using MAGPIE study sample logs in which the infant appeared well and did not appear to have had confirmed NEC or LOS, that is avoiding cases in which the infant had received prolonged antibiotics and/or any antibiotics in the preceding 2–3 days. The aim was to determine if there were significant differences in bacterial taxa between trial groups, but not to undertake more detailed analyses, and to remain blinded to trial group allocation. The samples analysed in this analysis were used in the final analysis.
Changes to the MAGPIE study protocol after commencement
There were no major changes to the study plans and procedures during the MAGPIE study, although we set up more NICU sites than initially planned. This was because Health Research Authority procedures took longer to complete and overall recruitment to the ELFIN trial progressed more quickly than anticipated, so we needed a larger number of recruiting sites to reach our target. As previously explained, the MAGPIE study included infants recruited to the ELFIN trial only, given that SIFT had completed recruitment.
Transportation and courier
We had planned to transport samples from individual NICUs to our central freezers every 6–8 weeks, but the high costs of Human Tissue Act-approved61 couriers and the number of recruiting sites meant that we decided to transport samples in batches every 2–4 months only. We do not feel that this impacted on the outcomes of the study.
Ongoing sample analyses
We initially hoped to confirm some cases of NEC or LOS that developed during the phase of sample collection and identify suitable controls for these cases as the study progressed. This would have allowed us to process samples, to biobank or store residual samples and to destroy any samples that we did not need, to minimise –80 °C freezer requirements. As the study progressed, it became clear that BERC confirmation of disease often took place several months (or longer) after reporting and we, therefore, needed to store all samples until laboratory analyses could be undertaken. In addition, some reported cases of disease were not confirmed following BERC, meaning that waiting provided us with more reliable data.
Metabolomic analysis technique
Our initial protocol planned to undertake metabolomic analysis using LCMS at Northumbria University. One of the major challenges for metabolomic study of stool and urine specimens is precise metabolite identification, which requires considerable experience and a large database library. Recognising the unique potential of the MAGPIE study and because of savings we had made from our consumable budget, we established an additional collaboration at one of our co-applicant institutions (the University of Birmingham) to provide high-quality data on paired stool/urine samples. This was approved by the MAGPIE Study Steering Committee.
Chapter 3 Results
The MAGPIE study timelines
The ELFIN trial completed an internal pilot in 2015, opened to recruitment in a staged manner across all sites in July 2015 and closed to recruitment in September 2017. The MAGPIE study only opened to recruitment in the lead site in June 2016. However, we were not able to open any of the other sites to recruitment until November 2016 and the final site was not opened until May 2017. The main delay was receiving HRA and individual site approvals. The last infant was recruited to the MAGPIE study in September 2017, when recruitment to the ELFIN trial ceased. Sample collection continued until December 2017, when the last infant reached the postmenstrual age of 34 weeks. Database cleaning and locking from the ELFIN trial was not completed until May 2018, following which we were able to reliably identify disease and control cases, and select samples.
Demographic and other baseline characteristics
We recruited 479 preterm infants of < 32 weeks’ gestation who had been enrolled in the ELFIN trial from 13 separate NICUs (12 NHS hospital trusts): 239 were allocated to receive lactoferrin and 240 to receive placebo. Box 1 shows the recruiting hospital sites and Table 1 shows the numbers consenting to different aspects of the study. NICUs recruited a mean of 37 infants each (range 12–78 infants per site). Recruitment to the MAGPIE study typically occurred at the same time as recruitment to the ELFIN trial, although it may have occurred later in some cases. In total, 224 infants (46.8%) were female and 255 were male, and 145 (30.2%) were from multiple pregnancies (twins, triplets or quads) and 334 were singletons. A total of 270 infants (56.3%) were born by caesarean section and 113 (23.6%) had prolonged rupture of the membranes for over 24 hours prior to delivery. Sixty infants (12.5%) had absent or reversed end-diastolic flow in the umbilical artery and 35 (7.3%) did not receive any antenatal steroids. The mean (± SD) birthweight was 1120 g (± 358 g), with a range of 445–2110 g, and the mean gestation (± SD) was 28.4 weeks (± 2.3 weeks), with a range of 22.7–31.86 weeks. These demographic variables suggest that the MAGPIE study infants were a similar population to the entire ELFIN trial cohort.
Jessop Wing, Sheffield Teaching Hospital, Sheffield.
James Cook University Hospital, Middlesbrough.
Nottingham City Hospital, Nottingham.
Queen’s Medical Centre, Nottingham.
Birmingham Women’s Hospital, Birmingham.
Sunderland Royal Hospital, Sunderland.
Royal Victoria Infirmary, Newcastle upon Tyne.
University Hospital of North Tees, Stockton-on-Tees.
Bradford Royal Infirmary, Bradford.
Leeds General Infirmary, Leeds.
Leicester Royal Infirmary, Leicester.
Princess Anne Hospital, Southampton.
Queen Alexandra Hospital, Portsmouth.
Site | Number recruited (n) | Confirmed NEC cases (n) | Confirmed LOS cases (n) | Declined biobank (n) | Declined gut tissue (n) | Declined gut and biobank (n) |
---|---|---|---|---|---|---|
A | 78 | 7 | 11 | 1 | 0 | 0 |
B | 25 | 0 | 3 | 0 | 0 | 0 |
C | 12 | 0 | 5 | 0 | 0 | 0 |
D | 44 | 2 | 6 | 0 | 0 | 0 |
E | 71 | 4 | 13 | 1 | 2 | 0 |
F | 43 | 3 | 4 | 0 | 0 | 0 |
G | 18 | 3 | 5 | 0 | 1 | 1 |
H | 10 | 3 | 3 | 0 | 0 | 0 |
I | 31 | 3 | 9 | 0 | 2 | 2 |
J | 33 | 1 | 6 | 0 | 1 | 1 |
K | 33 | 1 | 5 | 0 | 1 | 1 |
P | 59 | 3 | 7 | 2 | 32 | 11 |
S | 22 | 1 | 4 | 0 | 0 | 0 |
Total | 479 | 31 | 81 | 4 | 39 | 16 |
Results of the interim ‘futility’ analysis
We conducted a ‘futility’-type analysis after samples were available from ≥ 150 infants to demonstrate feasibility and test the hypothesis that one of the mechanisms of action of enteral lactoferrin would have an effect on gut bacterial composition. In December 2017, we selected 120 infants from whom we had collected and archived stool specimens at around day 10 of life. We remained blinded to the ELFIN trial group allocation at this stage and anticipated that this would give us ≥ 50 infants from each ELFIN trial group. We chose day 10 because we expected that most infants would have received trial intervention for around 1 week, given that most infants commence increasing feeds around day 3–4. We identified a single stool sample using the MAGPIE study sample logs at approximately 10 days of age for which the infant appeared well and did not appear to have had confirmed NEC or LOS, thereby avoiding infants who had received prolonged antibiotics and/or any antibiotics in the preceding 2–3 days. This resulted in samples from 119 infants that could be used for gut bacterial analyses using 16S rRNA. The clinical trials unit at NPEU confirmed that these 119 cases included ≥ 50 cases from each ELFIN trial group. After 16S analysis was completed, NPEU provided group allocation as A or B, that is the project team remained blinded as to whether the infant was allocated to receive placebo or the intervention. Two of the 119 infants who appeared to have had disease and/or were receiving prolonged antibiotics at the time that the sample was collected were subsequently excluded when further data became available. This analysis confirmed significant differences in bacterial taxa between the two ELFIN trial groups. We did not undertake more detailed analysis at that stage, but results from these 119 samples were subsequently included in the full analyses.
Incidence of necrotising enterocolitis and late-onset sepsis
Eighty-one infants (16.9%) had at least one confirmed episode of LOS (of whom 13 had two or more episodes) and 67 (14.0%) additional infants had at least one episode of suspected LOS, meaning that, overall, 31% of infants (148/479) had at least one episode of sepsis. Table 2 shows details of the organisms causing confirmed LOS. The first episode of LOS occurred at a median age of 12 days (range 3–52 days). Sixteen infants with confirmed LOS also had a confirmed episode of NEC. In total, 30 infants (6.3%) had at least one confirmed episode of NEC, of whom three had a second episode and a further 15 were coded as having suspected NEC but did not meet the criteria for confirmed NEC (i.e. modified Bell’s stage II or III). NEC was diagnosed at a median age of 20 days (range 2–69 days). The number of cases varied between NICU sites, but this may simply reflect case mix, that is whether the unit was a fetal medicine or neonatal surgical referral unit.
Organism | Episode (n) | Total episodes (n) | ||
---|---|---|---|---|
First | Second | Third | ||
Klebsiella spp. | 3 | 3 | ||
Enterobacter spp. | 3 | 3 | ||
E. coli | 12 | 2 | 1 | 15 |
Roseomonas gilardii | 1 | 1 | ||
Other Gram-negative organisms | 1 | 1 | ||
Group B streptococci | 3 | 3 | ||
Enterococcus spp. | 2 | 1 | 3 | |
Enterococcus faecalis | 3 | 2 | 5 | |
S. aureus | 4 | 1 | 5 | |
S. epidermidis | 6 | 1 | 1 | 8 |
Staphylococcus capitis | 4 | 4 | ||
Other CoNS | 34 | 6 | 1 | 41 |
Bifidobacterium spp. | 2 | 2 | ||
Gram-positive cocci | 3 | 3 | ||
Total | 81 | 13 | 3 | 97 |
Case selection for microbiomic and metabolomic analyses
Disease occurred in the first 7 days of life in 7 out of 30 cases of NEC and 18 out of 81 cases of LOS (overall ≈ 22% of disease cases), meaning that very few or no stool samples were available in such cases prior to disease onset that we could analyse. However, cases occurring in the first few days of life will have occurred before the infant was exposed to more than a small level of lactoferrin. In addition, some cases of disease presented in infants who were older than 7 days of age but had no stool samples collected in the 7 days prior to disease onset or who were not in a MAGPIE study recruiting centre at disease onset (i.e. prior to transfer to a surgical unit with NEC), or presented following back-transfer to their local regional hospital. We performed 16S rRNA analysis on at least one stool sample from 201 infants with disease and healthy control infants who, overall, were broadly similar to the entire MAGPIE study cohort but were, on average, of slightly lower birthweight than the entire MAGPIE study cohort. Overall, the median (range) birthweight and gestational age of infants who provided samples for microbial analysis were 965 g (500–2000 g) and 27 weeks (22–31 weeks). As described, we first selected all infants with stool samples who had confirmed NEC or LOS and identified one healthy infant control match for each case. We also selected healthy infants who were either twins or triplets, some of whom will have been allocated to the same (concordant) trial IMP (i.e. either lactoferrin or placebo) and some of whom will have received a different IMP (discordant). We also analysed stool samples from six infants who died but were not recorded as having NEC or LOS, and stool samples from six other infants who acted as healthy controls but had one episode of suspected (not confirmed) sepsis that was remote from the period when stool samples were selected. This resulted in the following types of infants:
-
Confirmed NEC – infants with stage II or III NEC with at least one stool sample pre disease. In the 20 cases with confirmed NEC, the median day of disease onset was 22 days (range 3–69 days).
-
Confirmed LOS – all infants with a positive blood culture and at least one stool sample pre disease (see Table 3).
-
Died – infants who died but in whom there was no confirmed episode of NEC or LOS.
-
Healthy infants who were twins or triplets – including sets of healthy infant twin/triplets without NEC or LOS who received the same ELFIN trial IMP (ELFIN trial concordant) or who received a different ELFIN trial IMP (ELFIN trial discordant).
-
Healthy control infants who were singletons – the next recruited infant from the same NICU who provided the closest gestational week match for an index case of disease with either NEC or LOS, and who had samples available at a similar postnatal age.
-
Other – one infant with SIP who did not have NEC or LOS, and five infants in whom there was a single episode of suspected LOS according to case definitions, but who were not exposed to antibiotics or disease for at least 3–7 days before stool samples were obtained, were used as ‘controls’.
Table 3 provides demographic information of the infants who provided samples for 16S analyses, and Table 4 shows the organisms confirmed as causing sepsis in the cases analysed.
Infant description | Number of infants (n) | Birthweight (g), mean ± SD | Gestation (weeks), mean ± SD |
---|---|---|---|
Confirmed NEC | 20 | 905 ± 207 | 26.5 ± 2.0 |
Confirmed LOS | 51 | 862 ± 248 | 26.1 ± 2.1 |
Died (not NEC or LOS) | 6 | 741 ± 211 | 25.5 ± 2.1 |
Healthy control infants | 41 | 899 ± 305 | 26.0 ± 2.1 |
ELFIN trial concordant twins/triplets | 24 | 1270 ± 318 | 29.2 ± 1.5 |
ELFIN trial discordant twins/triplet/quads | 53 | 1228 ± 299 | 28.7 ± 1.8 |
Other | 6 | 825 ± 180 | 26.0 ± 1.8 |
Total | 201 | 1015 ± 331 | 27.1 ± 2.4 |
As expected, infants who developed NEC or LOS tended to be smaller and more premature than the entire cohort recruited to the MAGPIE study, but were well matched to infants selected as healthy controls based on gestation. In addition, as expected, infants who were healthy and did not develop disease were larger and more mature. Some of the healthy infants from multiple pregnancies were also used as controls for disease cases if they were the most suitable infant from that NICU. In this situation, their data were used in the case–control analysis of disease, as well as the longitudinal study determining the impact of lactoferrin in healthy infants. Nine out of the 20 NEC cases also had a confirmed episode of LOS. Seven out of the nine cases of LOS plus NEC (five cases of CoNS and one each of S. aureus, E. coli and Enterobacter spp.) occurred within 24 hours of the diagnosis of NEC and were not considered a separate disease episode. The two remaining cases of LOS occurred 2 and 4 weeks following NEC onset, and were both because of CoNS.
LOS-confirmed bacteria | Number of cases (n) |
---|---|
Coagulase-negative staphylococcia | 28 |
S. aureus | 2 |
Enterococcus spp. | 4 |
Group B streptococci | 2 |
Bifidobacterium spp. | 1 |
E. coli | 8 |
Enterobacter spp. | 2 |
Other Gram-negative bacteria | 1 |
Klebsiella spp. | 3 |
Total | 51 |
Overall, among the 201 infants analysed, the median age at starting feeds was 5 days (range 0–37 days), and the median age at commencing the IMP was 5 days (range 0–46 days). The median age at the first stool sample collection was 6 days (range 0–73 days). The types of milk given on the day that feeds were started were breast milk (n = 165), formula (n = 12) and a mixture of breast milk and formula (‘mixed’) (n = 23). One infant was never started on milk and was also the only infant not to receive at least one dose of IMP. A total of 110 out of 165 infants received only breast milk for the duration of stool collection, but the data collected did not distinguish between mother’s own breast milk and donor breast milk.
Description of infants selected for gas and liquid chromatography–mass spectrometry metabolomic analyses
We aimed to select samples for GCMS or LCMS analyses; however, owing to the available funding, we were limited in the number of samples that we could analyse and we conducted metabolomic analyses only in those infants in whom we also conducted analyses of 16S bacterial RNA. We, therefore, focused GCMS analyses by aiming to measure VOCs in all disease cases pre disease onset along with matched control samples. Next, we identified healthy twin/triplet pairs to determine the impact of lactoferrin on gut VOCs over time. This resulted in 98 infants who had stool samples analysed using GCMS, of whom 60 were healthy controls (49/60 had a healthy twin sibling and 11/60 were singletons), 25 had samples taken before confirmed LOS and 13 had samples taken before confirmed NEC.
For LCMS analyses, we also focused sampling on infants from whom samples were taken before disease onset, along with their matched controls, but, where possible, we aimed to select and analyse cases for whom we also had paired stool and urine samples. Most of the LCMS analyses in healthy infants were carried out using twin/triplet controls who were discordant for the ELFIN trial IMP to measure the impact of lactoferrin in infants who would be otherwise well matched for feeding exposures (i.e. the same mother and breast milk or milk formula choices) and other demographic variables (gestation, pregnancy complications, reasons for labour and delivery mode). This resulted in 99 infants who had at least one stool or urine sample analysed using LCMS, of whom 39 were healthy twins or triplets discordant for the ELFIN trial IMP, two were twins concordant for the ELFIN trial IMP, 16 were healthy singleton controls, 21 developed LOS, 18 developed NEC and three died without a confirmed diagnosis of NEC or LOS.
Results of 16S gut bacterial microbiota analyses
A total of 33,331 stool and urine samples of sufficient volume that matched labelling and containment criteria were collected from 467 infants across the 13 satellite NICUs and were transferred to the central laboratory at Northumbria University. Of the total samples, 10,990 were stool samples and 22,341 were urine samples. The sampling across the individual recruiting NICUs is shown in Figure 1.
FIGURE 1.
Stool and urine samples collected per NICU site.

Microbial community analysis
A total of 1304 samples, including 30 kit and sequencing negatives, that were selected from 201 infants as previously described were sequenced for microbial community analysis. Of the 1274 samples that were analysed, we removed 25 further samples at the rarefaction/clean-up stage in accordance with our described methods, making a total of 1249 samples for the full analysis. Of the 201 infants from whom samples were sequenced, 97 were from the lactoferrin group and 104 were from the placebo group, with infants from each ELFIN trial group contributing to the analyses from every NICU site other than site C (see Figure 1). The overall cohort of infants selected for 16S rRNA gene-based microbial community analysis included infants who subsequently developed NEC (11% of the cohort) and infants who developed LOS (31% of the cohort) (see Appendix 1).
Although sample collection continued until ≥ 34 weeks’ corrected age, the samples chosen for the analyses were focused on the first 6 weeks of life, with the largest number of infants being selected from sites A (n = 34), P (n = 30) and E (n = 27). In sites with lower recruitment rates and/or low incidence of disease (NEC or LOS), fewer infants were selected: site H (n = 7), site S (n = 6) and site C (n = 3). The temporal distribution of sampling within each infant across each NICU site is illustrated in Appendix 2. Bacterial 16S rRNA gene sequencing yielded 6.0 × 107 sequence reads across all 1304 samples, including kit and sequencing negatives. Sequences were clustered into OTUs using a threshold of 97% sequence similarity. This yielded a total of 21,372 bacterial OTUs. All OTUs with fewer than 10 sequence reads (< 1.0 × 10–5%) across the entire data set were removed from the analysis, resulting in a total of 4.2 × 104 reads being culled from the analysis and a reduction in the number of OTUs to 4595. The community composition of kit and sequencing negatives was significantly different from that of stool samples (ANOSIM: R2 = 0.15; p = 0.001). In addition, significantly fewer sequence reads were observed in kit [Mann–Whitney–Wilcoxon (MWW): p(adj) = 0.005] and sequencing negatives [MWW: p(adj) < 0.001] than in stool samples (Figure 2). Owing to the significant dissimilarity in composition and library size between samples and controls, all remaining taxa were included in the downstream analysis.
Kit and sequencing controls were removed from the data set, leaving a total of 5.9 × 107 sequence reads spread across 1249 samples. The median number of reads per sample was 4.0 × 104 ± 2.0 × 104. Owing to the limited taxonomic resolution of 16S rRNA sequencing experiments, all OTUs were agglomerated at the genus level, yielding a total of 1163 bacterial genera.
FIGURE 2.
Library sizes of positive community controls, kit and sequencing negative controls and stool samples analysed in this study. Library size is expressed on a log-10 scale. Each box describes the range between the upper and lower quartiles, with the middle line depicting the median. Whiskers extend to the full range of data, excluding outliers.

Preterm stool communities are dominated by similar bacterial taxa, regardless of neonatal intensive care unit site
Dominant bacterial taxa identified in these data sets match closely with those seen in our previous studies from one of the participating NICUs,20,89 with samples predominantly containing Enterobacteriaceae, Staphylococcus spp., Escherichia spp., Veillonella spp., Enterococcus spp. and Bifidobacterium spp. (Figure 3). These patterns were consistent across all NICUs. The higher relative abundance of Staphylococcus observed in site C may be because of the small number of infants enrolled from that site.
FIGURE 3.
The average abundance of the 10 most abundant bacterial taxa across each NICU site from which infants were sampled for this study. Each bar represents the average relative abundance observed for each bacterial genus or family (colour coded as per legend).
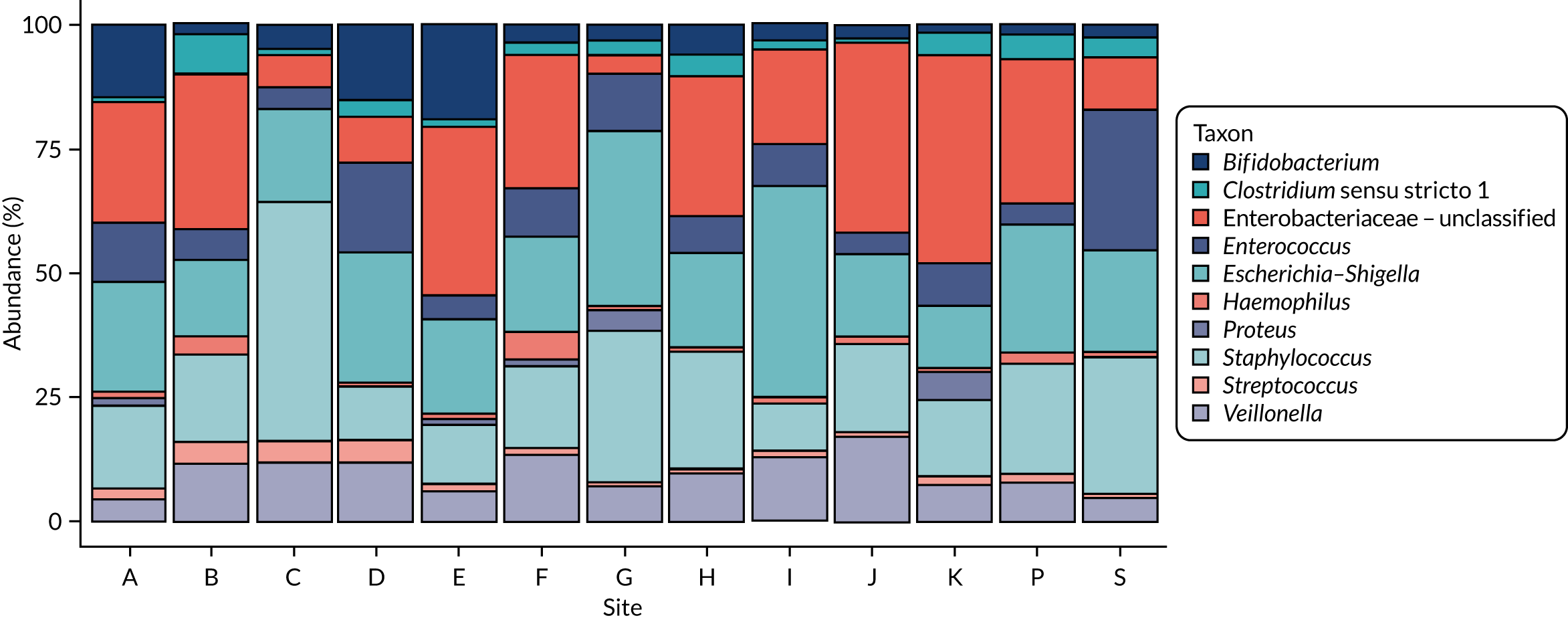
Bifidobacterium spp. were observed in greater abundance in sites that used routine supplemental probiotics (i.e. sites A, D and E) [median 8.16%, interquartile range (IQR) 0.21–24.79%] than in those not using routine supplemental probiotics (median 0.02%, IQR 0.00–0.03%). A Kruskal–Wallis rank-sum test found no significant difference in Bifidobacterium abundance between NICUs using probiotics and NICUs not using probiotics; however, this may be because of scant bifidobacterial abundance in very early samples (Figure 4).
FIGURE 4.
Relative abundance of Bifidobacterium spp. in samples from infants admitted to NICUs regularly administering supplemental probiotics. Each point represents an individual sample. Boxes extend to the upper and lower quartiles, with the middle line representing the median. Whiskers extend to the full range of data, excluding outliers.

Individual infant and age explain most of the dissimilarity between communities
An initial analysis was carried out to determine the clinical variables explaining the greatest variance in community composition. The bacterial diversity of samples was calculated as Shannon diversity in samples once rarefied to 1000 reads. Briefly, any sample with a library size of < 1000 reads was excluded from the analysis, reducing the number of samples from 1249 to 1220 (2% reduction in sample numbers). Alpha-diversity values were significantly lower in samples from infants prior to a diagnosis of NEC than in samples from control infants (Kruskal–Wallis p < 0.01) (Figure 5a). This comparison includes a small number of post-diagnosis samples; therefore, the reduced diversity may be an artefact of clinical intervention or disease progression. Samples also showed increasing diversity over time, with a significant but weak positive correlation between infant age at sampling and alpha-diversity (R2 = 0.03; p < 0.001) (see Figure 5b).
FIGURE 5.
Differences in (a) alpha-diversity between NEC and control infants, (b) alpha-diversity over time and (c) beta-diversity between samples analysed. Alpha-diversity is calculated as rarefied Shannon diversity, computed following rarefaction of samples to 1000 reads. Significantly greater alpha-diversity (Kruskal–Wallis: p = 0.008) was observed in control infants than in those who developed NEC (a). Furthermore, a significant increase in diversity occurred with increasing age at sampling (R2 = 0.03; p < 0.001) (b). Each point in alpha-diversity plots represents an individual sample colour coded by (a) NEC status or (b) infant age at sampling. The linear regression line is included (b), with standard error of the model illustrated by the shaded area. ** denotes significant difference between alpha diversity of control and NEC infant stools. Beta-diversity was calculated as weighted Bray–Curtis dissimilarity index. All measured clinical variables had a significant relationship (p < 0.05) with community structure, other than NEC status. The greatest variance in community compositions was explained by individual infant [R2 = 0.34; p(adj) = 0.001].



The community structure of stool samples was assessed by beta-diversity, calculated as weighted Bray–Curtis dissimilarity. Pairwise PERMANOVA identified the strongest correlations between community structure and clinical variables, including individual infant [R2 = 0.34; p(adj) = 0.001], infant age in days at sampling [R2 = 0.13; p(adj) = 0.001] and NICU site [R2 = 0.08; p(adj) = 0.001]. Further variables exhibiting significant correlations with community structure included LOS [R2 = 0.01; p(adj) = 0.001] and lactoferrin exposure [R2 = 0.002; p(adj) = 0.01], although these were much weaker associations than both individual infant and infant age at sampling (see Figure 5c). Notably, the residual variance, unexplained by the clinical measures included in the model, exceeded that explained by any single clinical measure (R2 = 0.43), indicating that several other factors influence community composition.
Owing to the high impact of infant age on microbiota composition (13% total variance), further analyses aimed to determine which specific bacterial genera explained the largest changes in relative abundance over time.
To do this, the cohort was divided into strata based on days of life and sampling periods specified in the protocol: 0–6, 7–9, 10–14, 20–27 and 30–60 days. Only single samples taken from each infant at each time point were included in analysis to avoid repeated measures. The number of samples included in each sampling time point is displayed in Figure 6a.
FIGURE 6.
Longitudinal change in the relative abundance of the 10 most abundant bacterial taxa over time in exclusively healthy infants. (a) Total numbers of samples (one per infant) per time point included in the analysis; (b) mean relative abundance per genus per time point. Coloured lines represent each genus or family while the shaded area represents the standard error.
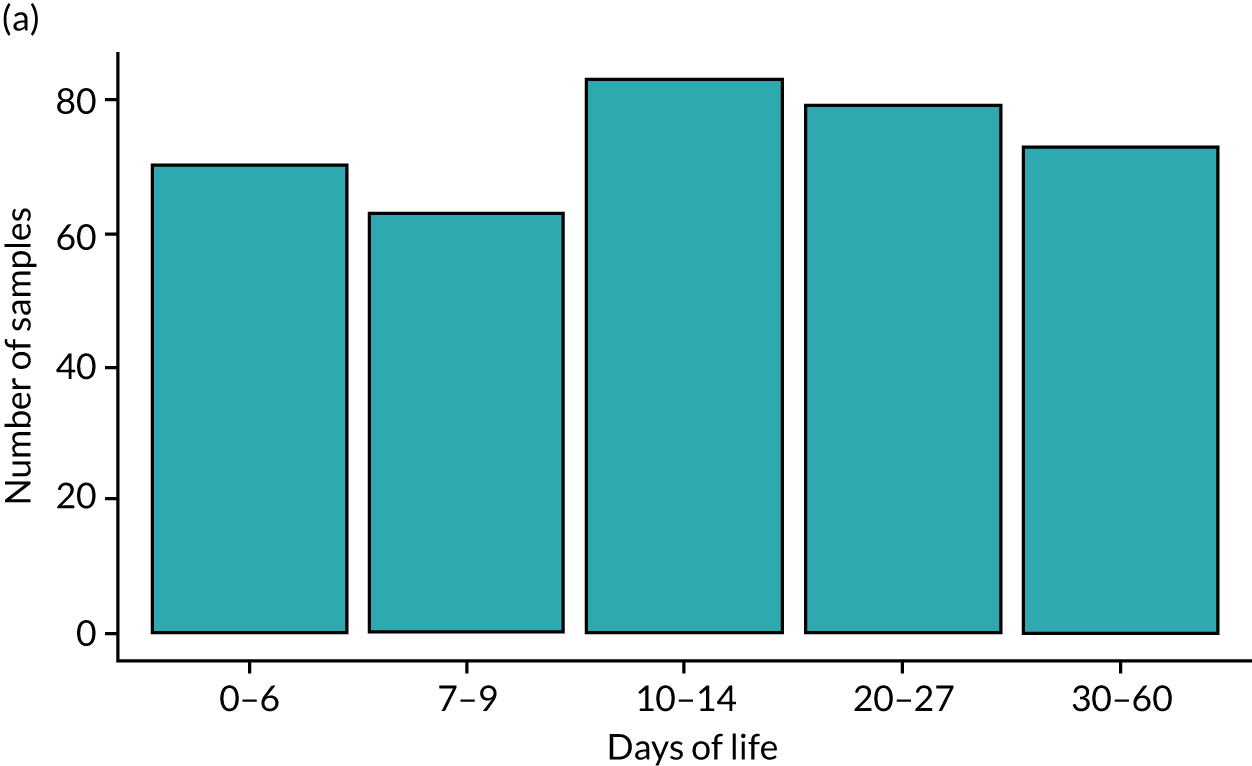

The greatest change in relative abundance over time was observed in Staphylococcus, which decreased from 42% at 7–9 days of life to only 2% at 30–60 days of life (p < 0.001). This reduction in relative abundance of Staphylococcus was mirrored by an increase in relative abundance of Veillonella (from 4% to 12%; p < 0.001) and Enterobacteriaceae (from 13% to 32%; p = 0.002). Other genera for which significant differences in relative abundance were observed between time points included Finegoldia, which increased in relative abundance over time (p < 0.001), and Streptococcus, which decreased in relative abundance over time (p = 0.001) (Table 5; see Figure 6). Both Staphylococcus and Streptococcus are skin commensals, suggesting that the longitudinal reduction in these two genera may be linked to niche specialisation in the gut environment.
Taxon | Mean abundance per time point (days of life) | p(adj)-value | ||||
---|---|---|---|---|---|---|
0–6 | 7–9 | 10–14 | 20–27 | 30–60 | ||
Staphylococcus | 24.50 | 41.78 | 20.95 | 5.47 | 2.03 | 9.98 × 10–13 |
Veillonella | 4.51 | 2.68 | 7.26 | 12.27 | 12.57 | 1.31 × 10–6 |
Finegoldia | 0.009 | 0.003 | 0.11 | 0.114 | 0.21 | 3.24 × 10–4 |
Streptococcus | 4.65 | 0.67 | 1.97 | 1.91 | 1.58 | 1.28 × 10–3 |
Enterobacteriaceae – unclassified | 13.03 | 16.59 | 24.18 | 26.813 | 32.27 | 1.59 × 10–3 |
Escherichia–Shigella | 28.02 | 15.50 | 15.21 | 25.77 | 20.94 | 3.70 × 10–3 |
Enterococcus | 6.73 | 8.88 | 7.72 | 7.51 | 5.48 | 0.053 |
Corynebacterium 1 | 0.27 | 0.24 | 0.56 | 0.11 | 0.22 | 0.075 |
Bifidobacterium | 3.62 | 8.44 | 13.27 | 8.60 | 9.25 | 0.140 |
Lactobacillus | 0.20 | 0.953 | 1.16 | 0.63 | 0.65 | 0.157 |
Lactoferrin exposure has a limited impact on microbiota composition
Identifying clinical variables with the greatest impact on community composition enabled analyses of the impact of lactoferrin on the gut microbiota to be controlled accordingly, and we included only those samples from infants who were never diagnosed with either NEC or sepsis to avoid the confounding effects of disease or treatment (e.g. prolonged antibiotic exposures). Samples were stratified according to predefined time points (days of life 0–6, 7–9, 10–14, 20–27 and 30–60) and a single sample from each infant per time point was used, which provided 368 samples for analysis (lactoferrin group, n = 182; placebo group, n = 186).
Small but significant differences in community composition were observed between samples in each ELFIN trial group by comparing weighted Bray–Curtis dissimilarity (Adonis PERMANOVA: R2 = 0.005; p = 0.04). These differences in community composition are illustrated in the ordination plot in Figure 7a.
FIGURE 7.
Impact of lactoferrin exposure on gut microbiota. Single samples per infant at predefined sampling time points are included in the analysis. Each point across all panels represents an individual sample. (a) Differences in community structure (beta-diversity calculated as weighted Bray–Curtis dissimilarity) between samples from infants within each treatment group are illustrated by sPLS-DA ordination. (b) Alpha-diversity calculated as the Shannon index and changes over time; significant differences between diversity across time points are highlighted by asterisks. (c) The relative abundance of the most discriminant taxa making up ≥ 1% of the total community data set. Significant differences in mean relative abundance between exposure groups were observed in all genera to the left of the dotted line. PLS-DA, partial least squares – discriminant analysis; sPLS-DA, sparce partial least squares – discriminant analysis.


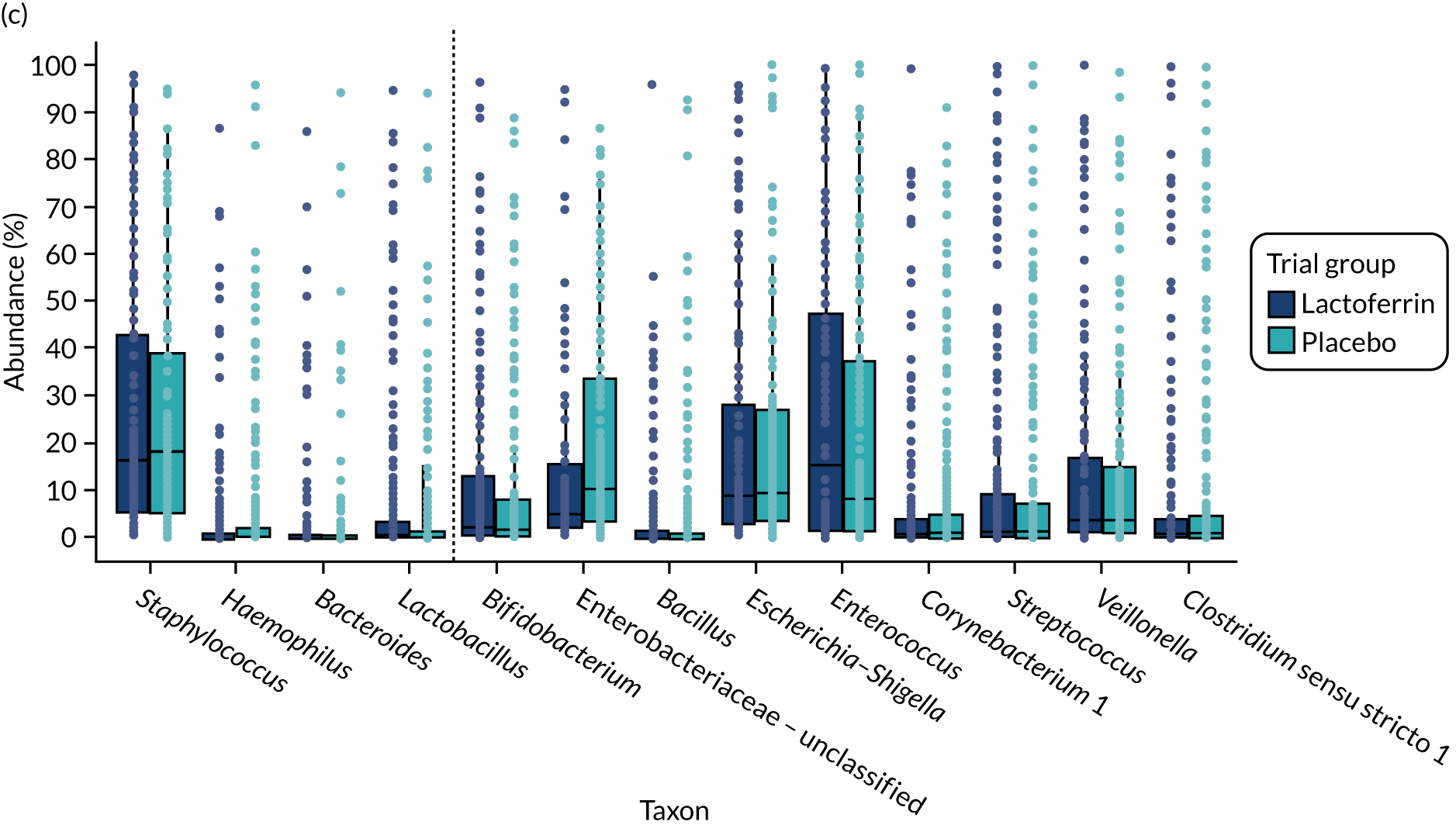
Shannon diversity was compared across the sampling time points (see Figure 6b). Following an initial reduction in diversity between 0–6 and 7–9 days, upwards trends in alpha-diversity were observed longitudinally in both trial groups. Despite this, a significant correlation between alpha-diversity and days of life was observed in the placebo group only (R2 = 0.04; p < 0.01). No significant differences were observed in alpha-diversity between trial groups at any of the predefined time points, although the lactoferrin group did have an impact on temporal change in alpha-diversity. Overall, the Bonferroni-corrected pairwise Mann–Whitney test demonstrated few significant differences; however, alpha-diversity of placebo group infants demonstrated a difference between 7–9 days of life and 30–60 days of life [p(adj) = 0.016] that was not observed in lactoferrin group infants (see Figure 7b).
Bacterial taxa contributing to these shifts in community structure were identified by comparing mean relative abundance across the two lactoferrin exposure groups. The results of the pairwise Kruskal–Wallis test with false discovery rate correction identified three genera with greater mean relative abundance in the placebo group than in the lactoferrin group: Staphylococcus (p < 0.01), Haemophilus (p < 0.01) and Lactobacillus (p = 0.01). Conversely, Bacteroides (p < 0.01) was the only genus observed in greater mean relative abundance in the lactoferrin group than in the placebo group (see Figure 7c).
Determining the impact of lactoferrin exposure on bacterial community structure in healthy infants
The large number of twin pairs included in this study (n = 36) enabled a well-matched comparison of community dissimilarity between infants who received the same lactoferrin exposure and infants who did not receive the same lactoferrin exposure. In this approach, selecting twins meant that the confounding effect of other exposures (e.g. maternal microbiota and genetics, breast milk exposure and NICU site) would be minimised and enable the impact of lactoferrin to be determined more precisely. A total of 22 twin pairs (44 infants) were randomly assigned to different lactoferrin exposure groups (termed ELFIN trial discordant). In addition, 14 twin pairs (28 infants) were randomly assigned to the same lactoferrin exposure group; in these twin pairs, any difference in bacterial community structure could not be because of the trial intervention (i.e. lactoferrin or placebo). Twin pairs were included in comparisons of the impact of lactoferrin on inter-twin and intratwin pair microbial dissimilarity as long as a single sample was available per twin, per pair, for each time point, which resulted in 17 discordant twin pairs and 11 concordant twin pairs.
Weighted Bray–Curtis dissimilarity was used as the measure of beta-diversity; again, twin pair and time point represented the most community dissimilarity in both concordant and discordant twins, when compared by Adonis PERMANOVA (Figure 8a). To compare inter-twin and intratwin pair community dissimilarity, an sparce partial least squares – discriminant analysis (sPLS-DA) model was constructed, from which the distance of each point per twin pair to the centroid of the ordinated points per twin pair was calculated, along with the distance of each point per time point to the centroid of the same ordinated points per time point. The mean centroid distances per infant were calculated as the intratwin pair dissimilarity for both concordant and discordant twin pairs, while mean centroid distances per time point represented the interinfant dissimilarity.
FIGURE 8.
Inter-twin and intratwin pair community dissimilarity in twin pairs. Analyses use weighted Bray–Curtis dissimilarity and identified measured variables with the greatest impact on community beta-diversity in both (a) discordant and (b) concordant twin pairs. (c) The mean distance of sPLS-DA ordinated points to the centroid (mean sample variance) for both intratwin (infant) and inter-twin (time point) twin pair groups. Boxes extend to the upper and lower quartile ranges, with the solid line representing the average.



No significant differences were observed in either the inter-twin pair or the intratwin pair dissimilarity between concordant and discordant twin pairs, indicating no greater homogeneity between microbial communities in twin pairs who were concordant for lactoferrin exposure and twin pairs who were discordant for lactoferrin exposure (see Figure 8b). This agrees with the results of the Adonis PERMANOVA, which suggested that twin pairs had a greater impact on community composition than lactoferrin exposure.
Results of volatile organic compound gas chromatography–mass spectrometry analysis
In total, 317 faecal samples from 117 infants were analysed; these included twin pairs, most of whom were discordant for the study treatment. Twenty-eight infants with NEC (n = 7), LOS (n = 15) or both (n = 6) were studied. The median number of VOCs from faecal samples was 21 (IQR 14–24) VOCs. A total of 36 VOCs were found in ≥ 25% of samples and 11 VOCs were found in > 60% of all samples (Table 6).
Compound name | Occurrence in all samples (%) |
---|---|
Hexanal | 91.2 |
Acetic acid | 89.3 |
Nonanal | 83.0 |
3-Methylbutanal | 79.8 |
Heptanal | 77.3 |
Heptan-4-one | 73.2 |
Butane-2,3-dione | 72.9 |
Octanal | 71.9 |
2-Methylbutanal | 69.1 |
2-Pentylfuran | 68.8 |
Oct-1-en-3-ol | 63.1 |
3-Methylbutanoic acid | 61.2 |
2-Ethylhexan-1-ol | 60.9 |
6-Methylhept-5-en-2-one | 60.6 |
We investigated factors that might influence the VOCs: a PERMANOVA was performed that considered the patient, age, duration of gestation, feeding regime (within 48 hours of sampling), NICU, treatment (lactoferrin/placebo) and GCMS batch. The data are reported as R2 and p-values: the R2 is the percentage of the variance that is explained by a factor. In total, 43% of the variance of the VOCs is determined by the individual infant (p < 0.001); the second most important variable is the NICU (9.6% of variance; p = 0.02) in which that infant was managed; and the third most important is the age of the infant when the sample was obtained (6.7%; p = 0.005) (Table 7). Neither trial group nor feeding regimen was a significant factor.
Factor | R 2 | p-value |
---|---|---|
Patient | 0.43 | 0.001 |
Hospital | 0.096 | 0.02 |
Infant age (days) | 0.067 | 0.001 |
Batch (GCMS run) | 0.016 | 0.005 |
Gestational age (weeks) | 0.014 | 0.032 |
Feed type (48 hours prior to sample) | 0.012 | NS |
ELFIN trial group | 0.004 | NS |
These data can be visualised using principal component analysis (PCA). The first PCA shows the influence of age on faecal VOCs [see Figure 10a, in which there appears to be a cluster of samples from the first 5 days of life in the left lower quadrant (navy dots)]. The median number of VOCs per sample was 14 VOCs in the first 10 days of life, which plateaued at 21–24 VOCs for the following 25 days (Figure 9). A generalised linear mixed-effects analysis showed a modest increase in the number of VOCs with age (slope 0.004; p = 0.0617).
FIGURE 9.
Number of VOCs at different infant ages. Scatterplot with histograms showing the number of VOCs in relation to the age of the infant. Each dot represents a sample. Reproduced with permission from Frau et al. 90 This is an Open Access article distributed in accordance with the terms of the Creative Commons Attribution (CC BY 4.0) license, which permits others to distribute, remix, adapt and build upon this work, for commercial use, provided the original work is properly cited. See: https://creativecommons.org/licenses/by/4.0/. The figure includes minor additions and formatting changes to the original figure.
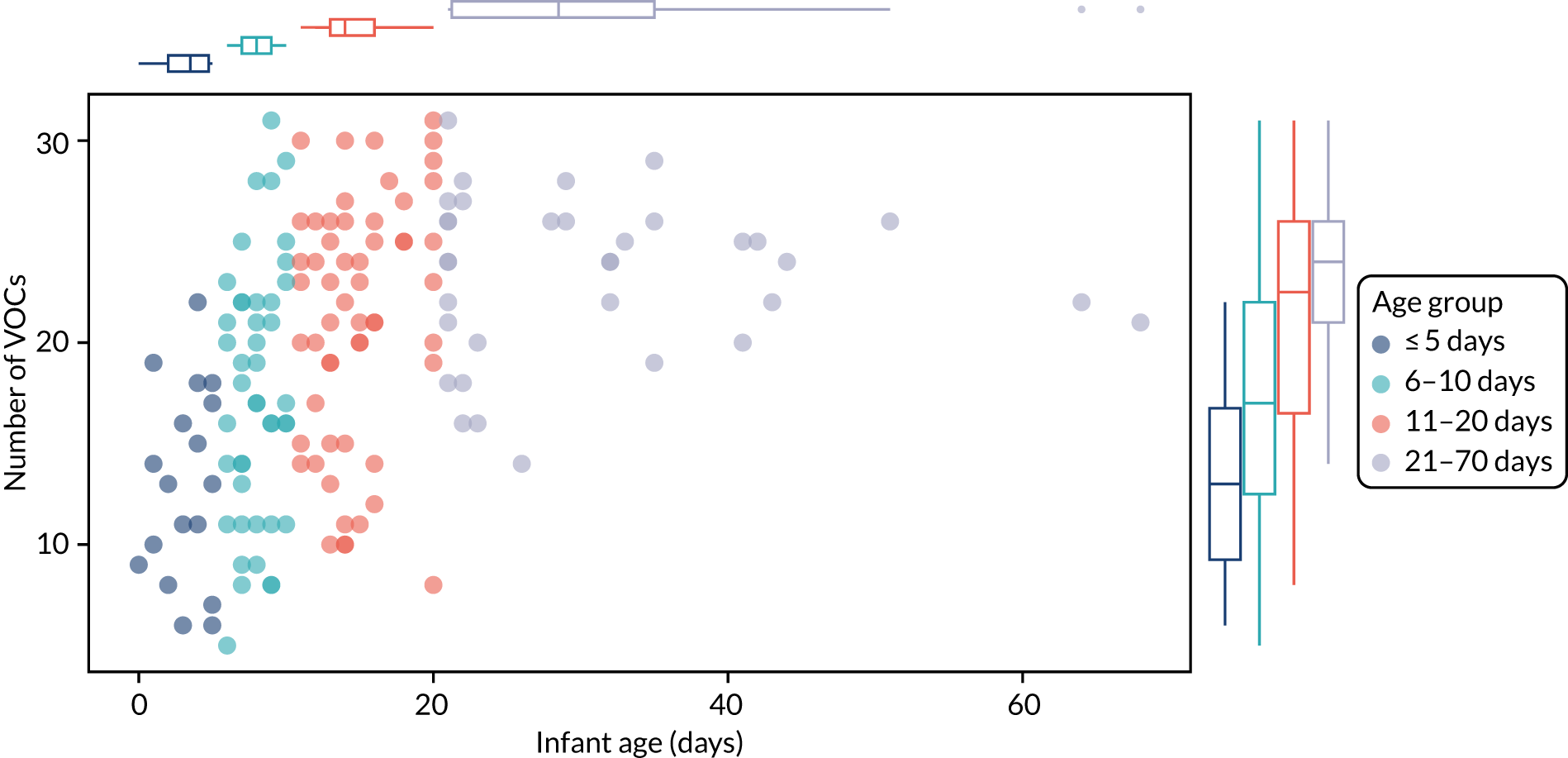
FIGURE 10.
Influence of age on the VOC profiles. A PCA plot of samples grouped by age: (a) all samples were used or (b) the first 5 days were compared with samples from infants aged 11–20 days. PC1, principal component 1; PC2, principal component 2.

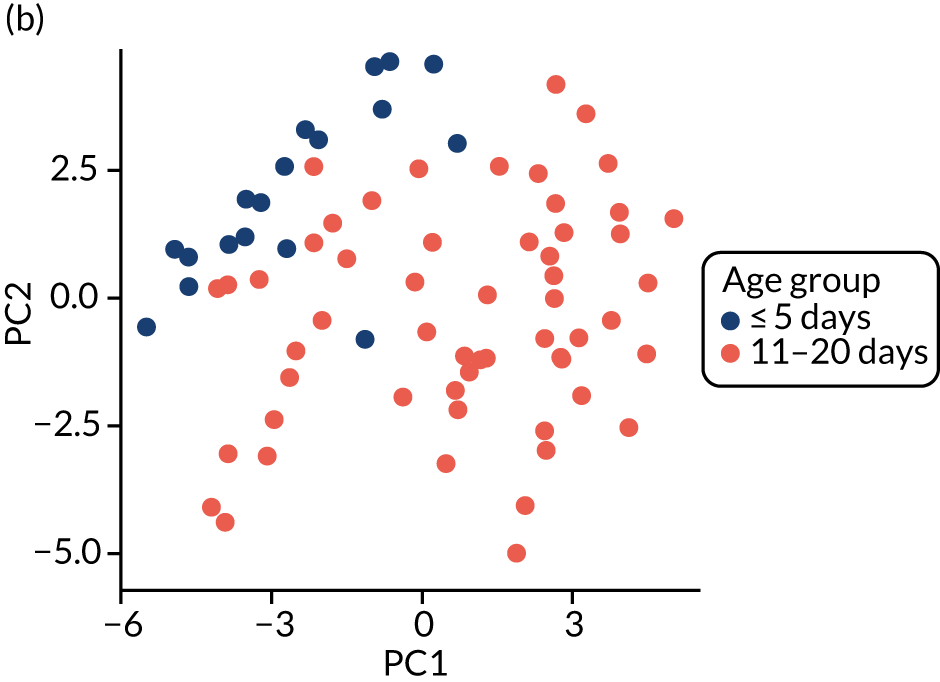
Subsequently, we removed groups of samples that were obtained from days 6–10 and 21–70; the PCA plot in Figure 10b shows that samples from the first 5 days of life are different from later samples. There was a change in the abundance of about one-third of the VOCs (Table 8). We investigated a subset of these and found that, in general, they were absent in the first 5 days of life, but rapidly rose and plateaued shortly afterwards. Of these VOCs, the esters tended to appear after day 10 and the short-chain fatty acids after day 7; however, what was striking was the appearance of two branched-chain fatty acids (2-Methylbutanoic acid and 3-Methylbutanoic acid) that were evident from days 3 to 5.
Acids | Aldehydes |
---|---|
|
|
Alcohols | |
|
Ketones
|
Furans | |
|
An effect of the feed regime was hard to detect (Figure 11a). This is probably because most infants were fed expressed breast milk, which meant that the study was underpowered to detect an effect of feed type. By contrast, the trial groups were well balanced, and the PCA shows that there was no difference between the two groups (Figure 11b). Gestational age at birth also had little measurable impact on the metabolome (Figure 12).
FIGURE 11.
Impact of milk feed type and treatment group on the metabolome. PCA plots showing (a) milk type and (b) effect of trial group, either lactoferrin or placebo. PC1, principal component 1; PC2, principal component 2.


FIGURE 12.
Influence of gestational age at birth on the metabolome. PCA plot with data presented in gestational age groups. PC1, principal component 1; PC2, principal component 2.

Results of liquid chromatography mass–spectrometry metabolomic analysis
Mann–Whitney U-tests were performed to compare placebo treatment with lactoferrin treatment in healthy infants only. In the urine samples, no metabolite peaks were statistically significant after correction for multiple testing. Two metabolite peaks were statistically significant (after correction for multiple testing) when the comparison was performed in faeces (Table 9). In total, > 50 metabolite peaks were statistically significant when the comparison was performed both on urine and in faeces, with no correction for multiple testing. However, to ensure robust biological interpretation, it is recommended that data corrected for multiple testing are applied.
Metabolite annotation(s) | Fold change (lactoferrin/placebo) | p-value (after correction for multiple testing) |
---|---|---|
Sedoheptulose | 0.50 | 0.008 |
Alpha-D-mannoheptulopyranose | ||
Glycero-D-manno-heptose | ||
Galacto-heptulose | ||
Manno-heptulose | ||
Erythro-galacto-nonulose | ||
Trimethyluric acid | ||
6-Hydroxyl-1,6-dihydropurine ribonucleoside | ||
Aspartyl-histidine | ||
Lysophosphatidylglycerol | 3.86 | 0.040 |
Impact of necrotising enterocolitis or late-onset sepsis on the stool and urinary metabolome
One-way ANOVA was carried out to compare healthy infants with NEC and LOS infants, independent of placebo and lactoferrin treatment. In total, 394 metabolite peaks were statistically significant in urine and 75 metabolite peaks were statistically significant in faeces, following correction for multiple testing. The urinary metabolites that were the most statistically different in NEC and LOS infants compared with control infants included tryptophan, 12-oxo-20-trihydroxy-leukotriene B4, N-[(5-hydroxy-2-pyridinyl)methyl]adenosine, 5,9,11-trihydroxyprosta-6E and 14Z-dien-1-oate (all putatively identified). In comparison, the stool metabolites that were the most statistically different in NEC and LOS infants compared with control infants included hexadecatrienoic acid, methyl 3-carbazolecarboxylate, C17 sphingosine 1-phosphate and nor-linolenic acid (all putatively identified). Four metabolite peaks were statistically significant in both faeces and urine following correction for multiple testing (Table 10).
Sample type | ANOVA (FDR), p-value | Post hoc analysis, p-value | Putative metabolite annotation | ||
---|---|---|---|---|---|
Disease | LOS – NEC | LOS – control | NEC – control | ||
Urine | 0.021 | 1.00 | 0.02 | 1.00 |
|
Faeces | 0.007 | 0.86 | 0.00 | 1.00 | |
Urine | 0.019 | 1.00 | 0.02 | 1.00 |
|
Faeces | 0.001 | 1.00 | 0.00 | 1.00 | |
Urine | 0.018 | 1.00 | 0.02 | 1.00 | Unidentified A |
Faeces | 0.001 | 1.00 | 0.00 | 1.00 | |
Urine | 0.019 | 1.00 | 0.03 | 1.00 | Unidentified B |
Faeces | 0.007 | 1.00 | 0.00 | 1.00 |
Next, we moved beyond looking at individual metabolite peaks and explored differences at the pathway level. This pathway enrichment analysis accounts for the fact that several metabolites will be integral to a given pathway, and biologically important pathways underlying disease processes will have several significant metabolites at consistently higher/lower levels across a pathway between NEC and LOS in comparison with healthy controls.
In urine, five metabolic pathways appeared to be enriched following pathway enrichment analysis in MetaboAnalyst; however, no pathway was statistically significant after accounting for the multiple testing required because > 300 metabolites were included in the analysis. The five pathways were aminoacyl-tRNA (transfer RNA) biosynthesis, tyrosine metabolism, tryptophan metabolism, arginine and proline metabolism, and purine metabolism. Because MetaboAnalyst does not include all metabolite classes and pathways, further manual interpretation was carried out. Carbohydrate metabolism, bile acids, peptides, sphingosine 1-phosphate, fatty acids, oxidised fatty acids and lyso-glycerophospholipids were identified as being potentially enriched metabolite classes. Notably, the most statistically significant metabolite peaks in NEC infants were different from those observed in healthy infants and LOS infants.
In faeces, no metabolic pathway was statistically significantly enriched and no pathway had more than four hits following pathway enrichment analysis in MetaboAnalyst. Following further manual interpretation, oxidised fatty acids (five metabolites) and peptides (seven metabolites) were identified as being potentially enriched metabolite classes. As with the urine pathway analysis, the most statistically significant metabolite peaks in NEC infants were different from those observed in healthy infants and LOS infants.
Results of intestinal fatty acid-binding protein in urine
We selected between one and six urine samples per infant from 65 infants, depending on availability, and focused on samples from the 7-day period prior to disease (LOS or NEC) onset to determine if any sequential trends existed. We then selected samples from infants of a similar gestation at equivalent postnatal ages, as well as a small number of samples from infants who died or had SIP. In total, we analysed 286 urine samples from 27 infants with LOS (mean gestation 26 weeks; mean birthweight 886 g), 11 infants with NEC (mean gestation 27 weeks; mean birthweight 911 g), three infants who died, one infant who had SIP and 23 healthy controls (mean gestation 26 weeks; mean birthweight 861 g). Urinary iFABP levels were adjusted for creatinine concentration and analysed as a ratio of pmol iFABP to nmol creatinine. Overall, urinary iFABP ratios showed considerable variation in both cases and controls, with no significant differences between healthy and diseased infants (Figure 13). There were also no apparent differences due to gestation or postnatal age at sample collection (data not shown).
FIGURE 13.
The iFABP results for all samples analysed (n = 286 urine samples from 65 patients).

A sample collected on any specific day represents just a single point in a 24-hour period, and it is also possible that the short plasma half-life results in rapid clearance of the peptide, perhaps even before clinical disease is suspected. Therefore, to compare whether infants with LOS or NEC might have had a significantly raised iFABP concentration immediately prior to disease onset, we chose the sample that had the highest ratio at any time in the 3-day period prior to recorded disease onset (recognising that the ‘true’ day of disease onset may easily differ from when it is recognised clinically) and compared this with the highest value measured at any point in time in a control infant (i.e. just one sample per infant). The postnatal age of the samples was 21 days (15 days since starting feeds) in healthy infants, 25 days (18 days after starting feeds; one infant had not received any enteral feed) in infants with NEC and 15 days (9 days after starting feeds; five infants had not received any enteral feeds) in infants with LOS. The median iFABP concentration ratio (pmol iFABP to nmol creatinine) was 17.2 (range 0.52–107.53) in LOS cases, 35.57 (range 0.7–240.12) in NEC cases and 29.1 (range 4.04–174.9) in healthy controls, and there were no significant differences between groups (Figure 14).
FIGURE 14.
Highest relevant iFABP concentration comparing NEC, LOS and control (one sample per infant).

Results of the gut tissue analysis
Tissue samples for molecular immune phenotyping
An initial analysis was performed using ileal (distal small bowel) FFPE tissue samples that were identified from 10 preterm infants of < 32 weeks’ gestation, of whom four were recruited to the ELFIN trial or SIFT and six were other preterm infants for whom tissue was available in accordance with Research Ethics Committee approvals. Infants had undergone surgery for ileal NEC, and an age- and gestation-matched disease control group with limited intestinal inflammation was established from three preterm infants who had undergone surgical resection for SIP (i.e. 13 infants in total). For each infant, three histologically defined microanatomical zones within the resection specimen were identified: ‘healthy resection margin’; ‘transition zone’, showing architectural distortion and inflammation without overt necrosis; and ‘necrosis’. Representative haematoxylin and eosin (H&E)-stained sections from these anatomical zones are shown in Appendix 4. The figures and the data on whole-genome expression analysis (see Whole-genome expression analysis of FFPE tissue) are from these 13 infants, and were used to develop the analyses. In addition to the four infants who were recruited to the ELFIN trial or SIFT, we identified FFPE tissue from an additional eight infants recruited to the ELFIN trial and three infants recruited to SIFT, which will be included in future analyses depending on the initial results (Table 11).
Infant ID | Gestation (weeks) | Trial | Disease | Site | Age at resection (days) |
---|---|---|---|---|---|
303a | 25 | SIFT | NEC | Small bowel | 5 |
565 | 24 | ELFIN | NEC | Small bowel | 54 |
592 | 26 | ELFIN | NEC | Small bowel | 19 |
501 | 25 | ELFIN | NEC | Small bowel | 38 |
529 | 23 | ELFIN | NEC | Small bowel | 26 |
573 | 27 | ELFIN | NEC | Small bowel | 4 |
308 | 24 | SIFT | NEC | Small bowel | 55 |
313 | 28 | SIFT | NEC | Large bowel | 22 |
337 | 25 | SIFT | NEC | Small bowel | 65 |
410 | 23 | ELFIN | NEC | Large bowel | 18 |
441 | 26 | ELFIN | NEC | Small bowel | 9 |
462 | 24 | ELFIN | NEC | Small bowel | 12 |
312a | 26 | SIFT | NEC | Small bowel | 4 |
315a | 24 | SIFT | NEC | Small bowel | 14 |
385a | 25 | SIFT and ELFIN | NEC | Small bowel | 10 |
Whole-genome expression analysis of formalin-fixed, paraffin-embedded tissue
Tissue preparation was conducted in collaboration with the Medical Research Council (MRC) Newcastle Molecular Pathology Node. All equipment was cleaned with ribonuclease (RNase) decontamination solution prior to tissue microtomy or dissection. Multiple serial 4-µm sections of FFPE tissue were removed from each tissue block to discard potentially oxidised tissue, then multiple sections from each block were stored at 4 °C to allow later molecular analysis by immunohistochemistry. Where each tissue zone was embedded in a single block with no other type of tissue zone, 4 × 20-µm curl sections were collected by microtomy to conserve the block and transferred to 1.5-ml deoxyribonuclease (DNase)-/RNase-free microcentrifuge tubes. Where several tissue zones existed in a single FFPE block, 5-mm full-tissue-thickness cores were taken out of each histologically defined zone of tissue and transferred to 1.5-ml DNase-/RNase-free microcentrifuge tubes. Samples were shipped on dry ice to Hologic Ltd (Manchester, UK) for RNA extraction and genome-wide expression quantification using the GeneChip® Human Transcriptome Array 2.0 (Affymetrix, Santa Clara, CA, USA) using the WT Pico Reagent kit (Affymetrix) to prepare hybridisation-ready targets. All samples were processed in a single batch. Bioinformatic analysis was conducted in collaboration with the Bioinformatic Support Unit at Newcastle University using the Limma (Linear Models for Microarray Data) package in R. Data quality control was conducted using Affymetrix expression console software (Affymetrix). p-values were adjusted for multiple comparisons and an adjusted value of < 0.05 was deemed significant.
Immunohistochemistry
Immunohistochemistry was carried out on 4-µm FFPE tissue sections from all 13 infants (SIP, n = 3; NEC, n = 10) in the MRC Newcastle Molecular Pathology Node using a Ventana Discovery Ultra Autostainer (Ventana, Oro Valley, AZ, USA). Each antibody was optimised for intestinal tissue (Table 12). All slides were digitised using an iScan HT microscope scanner (Ventana) and images were captured using Aperio ImageScope software (Leica, Wetzlar, Germany).
Antibody | Host | Clone | Antigen retrieval | Detection kit | Manufacturer | Dilution |
---|---|---|---|---|---|---|
COX-2 | Rabbit | SP21 | SCC1 (64 minutes in EDTA) | Ventana Ultraview DAB | Ventana | Pre dilute |
NP57 | Rabbit | Polyclonal | None | Ventana Ultraview DAB | Dako (Santa Clara, CA, USA) | 1/100 |
CD68P | Mouse | PGM1 | SCC1 (64 minutes in EDTA) | Ventana Ultraview DAB | Dako | 1/200 |
CD44 | Mouse | VFF-7 | MCC1 (32 minutes in EDTA) | Ventana Ultraview DAB | Leica | 1/400 |
ICAM | Rabbit | EPR4776 | SCC1 (64 minutes in EDTA) | Ventana Ultraview DAB | Abcam (Cambridge, MA, USA) | 1/400 |
IL1R1 | Rabbit | Polyclonal | SCC1 (64 minutes in EDTA) | Discovery Chromomap DAB OmniMap anti-Rb | Sigma-Aldrich | 1/200 |
MMP-1 | Rabbit | Polyclonal | SCC1 (64 minutes in EDTA) | Discovery Chromomap DAB OmniMap anti-Rb | Sigma-Aldrich | 1/400 |
Quality control of formalin-fixed, paraffin-embedded tissue for transcriptome data
The analysis first determined if transcriptomic array data from each infant passed quality control (QC). Using Affymetrix Expression Console Software (Affymetrix), samples from all three infants with SIP passed quality control (total of three arrays). For each of the 10 infants with NEC, we had transcriptomic data from the healthy resection margin, transition zone and necrosis (total of 30 arrays). In one array from each of four infants (two necrotic and two transition zones, four arrays in total), QC was outside bounds. These four infants were, therefore, entirely excluded from subsequent analysis, which left matched transcriptomic data from all three anatomical zones in six infants with NEC.
Comparison of healthy resection margins in necrotising enterocolitis and spontaneous intestinal perforation demonstrated no differential gene expression
Given that no entirely healthy ileal tissue would ever be resected from a preterm infant, we used SIP as the non-disease control tissue for comparison with the healthy resection margin from infants with NEC. Consistent with this being an appropriate control, no differential gene expression between these tissue types was seen. This suggested that the healthy resection margin was a suitably matched comparator group to necrotic and transitional tissue in NEC.
Whole-genome expression identifies multiple innate immunity pathways associated as upregulated during necrotising enterocolitis
In the six infants with NEC, comparison of transitional zone tissue with healthy margins demonstrated no differentially expressed genes. Comparison of transitional zone tissue with necrosis revealed three transcripts that were relatively increased in necrosis. Two were transcripts for PLIN2, which encodes perilipin-2, and one transcript was for SLC2A3, which encodes the glucose transporter 3 (GLUT3) protein. Both proteins have roles in metabolic cellular activity, including pro-inflammatory monocyte/macrophage function (unique PubMed identifiers 30402637 and 22876317).
Comparison of matched necrotic tissue with healthy resection margins within the six NEC infants revealed 82 significantly differentially expressed genes with a fold change of > 2 (Figure 15 and Table 13). This identified multiple innate immune proteins or pathways, including granulocyte/neutrophil chemotaxis (CXCL8/IL8, CXCL5 and S100A9), neutrophil activation (CXCL5) and macrophage activation (CXCL8). Multiple genes in the IL1 family were upregulated, including IL1α, IL1β, IRAK3 and IL1R2. Coupled with this, the increased expression of genes encoding IκBα (NFKBIA), TNFα-induced protein 3 (TNFαIP3) and matrix metalloproteinase 1 (MMP-1) implicates a dominate innate myeloid mechanism driving inflammation during NEC. Consistent with this, a gene expression analysis did not identify adaptive T-cell responses as associated with necrosis.
FIGURE 15.
Volcano plot comparing matched gene expression data between necrosis and healthy resection margins in six infants with NEC.

Gene | Fold change | Adjusted p-value |
---|---|---|
CXCL8 | 187.42 | 0.0255 |
CXCL8 | 34.52 | 0.0107 |
CXCL5 | 19.66 | 0.0270 |
PTGS2 | 12.66 | 0.0093 |
HBB | 12.32 | 0.0446 |
AQP9 | 10.51 | 0.0131 |
KCNJ15 | 8.85 | 0.0199 |
IL1β | 7.42 | 0.0179 |
NAMPT | 6.13 | 0.0107 |
ICAM1 | 6.02 | 0.0204 |
SOD2 | 5.15 | 0.0253 |
MMP-1 | 5.07 | 0.0285 |
C5AR1 | 4.72 | 0.0197 |
MMP-1 | 4.52 | 0.0217 |
IRAK3 | 4.46 | 0.0396 |
IRAK3 | 4.43 | 0.0107 |
SAMSN1 | 4.33 | 0.0158 |
TREM1 | 4.15 | 0.0093 |
SLC2A3 | 4.06 | 0.0059 |
SERPINE1 | 4.05 | 0.0279 |
MMP-1 | 4.05 | 0.0426 |
IL1R2 | 3.96 | 0.0158 |
FCER1G | 3.91 | 0.0199 |
TNFαIP6 | 3.91 | 0.0460 |
HIF1A-AS2 | 3.86 | 0.0453 |
SERPINE1 | 3.81 | 0.0098 |
SLC2A3 | 3.79 | 0.0032 |
SRGN | 3.72 | 0.0239 |
TNC | 3.69 | 0.0309 |
THBS1 | 3.62 | 0.0163 |
CD44 | 3.61 | 0.0279 |
IFITM2 | 3.59 | 0.0218 |
ALOX5AP | 3.35 | 0.0096 |
CD44 | 3.25 | 0.0096 |
HIF1A | 3.23 | 0.0157 |
STC1 | 3.18 | 0.0093 |
PLAUR | 3.17 | 0.0107 |
ST3GAL1 | 3.03 | 0.0115 |
ACSL1 | 2.97 | 0.0258 |
SRGN | 2.93 | 0.0107 |
CXCL5 | 2.87 | 0.0375 |
TIMP1 | 2.83 | 0.0197 |
PLEK | 2.83 | 0.0093 |
CD53 | 2.81 | 0.0218 |
DDX21 | 2.79 | 0.0273 |
TNFαIP3 | 2.63 | 0.0475 |
MNDA | 2.61 | 0.0290 |
BCL2A1 | 2.59 | 0.0158 |
FPR1 | 2.48 | 0.0206 |
CD55 | 2.47 | 0.0158 |
FAM43A | 2.46 | 0.0285 |
IFITM2 | 2.43 | 0.0453 |
CTSL | 2.33 | 0.0299 |
ARRDC3 | 2.31 | 0.0255 |
CCL3L3 | 2.26 | 0.0290 |
CCL3L3 | 2.25 | 0.0270 |
FCGR2A | 2.19 | 0.0092 |
RNU7-76P | 2.19 | 0.0257 |
S100A8 | 2.18 | 0.0344 |
ZFAND5 | 2.14 | 0.0446 |
PLIN2 | 2.13 | 0.0104 |
CD93 | 2.11 | 0.0427 |
SLC25A37 | 2.10 | 0.0107 |
LINC01272 | 2.09 | 0.0235 |
IL1α | 2.08 | 0.0491 |
NFKBIA | 2.07 | 0.0299 |
ATP13A3 | 2.04 | 0.0093 |
PLIN2 | 2.02 | 0.0096 |
INHBA | 2.08 | 0.0158 |
RIPK2 | 2.00 | 0.0477 |
PCSK5 | 0.49 | 0.0393 |
POF1B | 0.49 | 0.0341 |
CREB3L3 | 0.48 | 0.0341 |
CXCL14 | 0.48 | 0.0163 |
GCG | 0.46 | 0.0158 |
GUCY2C | 0.46 | 0.0446 |
PLEKHS1 | 0.44 | 0.0300 |
KCNJ13 | 0.43 | 0.0423 |
CYP3A4 | 0.41 | 0.0341 |
ERBB3 | 0.33 | 0.0205 |
ADGRG7 | 0.33 | 0.0393 |
CYP2C19 | 0.33 | 0.0375 |
SULT1E1 | 0.32 | 0.0128 |
UGT2A3 | 0.19 | 0.0459 |
SI | 0.17 | 0.0428 |
To validate the importance of aberrant innate immunity during NEC and, in particular, myeloid recruitment and activation highlighted by gene expression analysis, we optimised several immunohistochemistry antibodies in GI tissue. These include the pro-inflammatory mediator COX-2, encoded by prostaglandin–endoperoxide synthase (PTGS2); neutrophil elastase (NP57); the tissue phagocyte/macrophage marker CD68 (CD68P); the cell adhesion molecule CD44; the vascular adhesion molecule, that is intercellular adhesion molecule (ICAM) that is responsible for extravasation of leucocytes to into the gut during inflammation; and the innate immune mediators MMP-1 and the IL-1 receptor (IL1R1). Further analyses of the protein expression profiles will be undertaken. Representative images of immunohistochemical staining are shown in Figure 16.
FIGURE 16.
Representative immunohistochemical images for (a) PTGS2/COX-2 (necrosis); (b) IL1R (transitional tissue); (c) CD68 (healthy margin); and (d) ICAM1 (healthy margin).
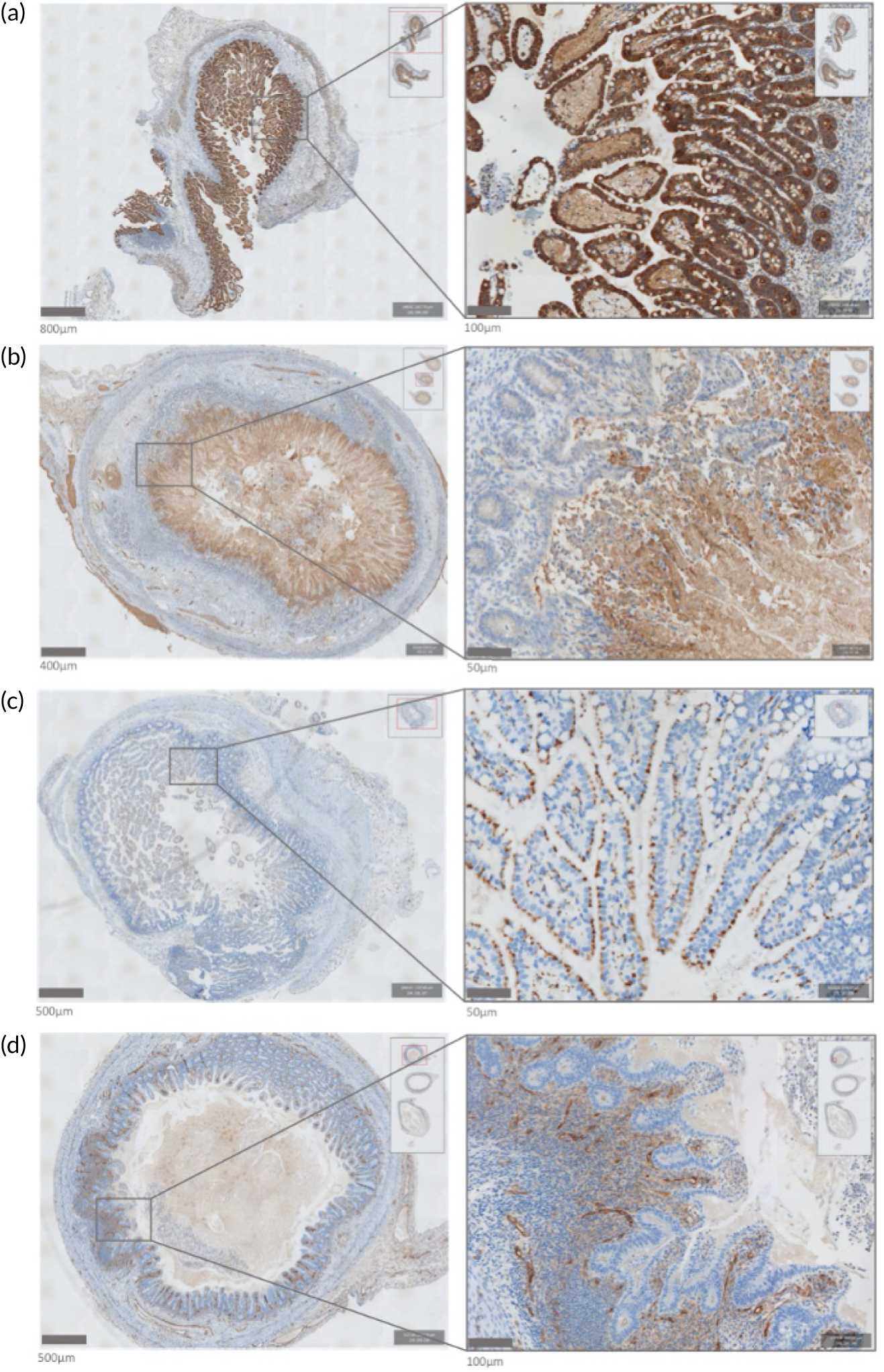
Adverse events
The MAGPIE study was an observational study nested in a RCT of an IMP, and sample collection for the MAGPIE study did not require infants to undergo any invasive procedures. The study team were notified of a single adverse event, which was a thumb laceration suffered by a parent who was placing the lid on a glass vial, which contained a stool sample, when it broke. The MAGPIE study procedures had clearly documented that samples should be obtained only by the research nurse or nurse caring for the infant. The study team was not made aware of any other untoward events. Two NICUs reported an occasion when the freezer had been unplugged or disconnected for several hours. In these cases, most samples still appeared frozen or partially frozen and were, therefore, transported to central freezers as they represented only ≈ 1–2% of all available samples.
Chapter 4 Discussion
The MAGPIE study successfully recruited 479 very preterm infants of < 32 weeks’ gestation, from 13 separate NICUs, who were representative of the entire ELFIN trial cohort in terms of maternal/pregnancy characteristics, birthweight, gestation and rates of NEC (6%) and LOS (31%), and who were evenly split between the two ELFIN trial IMPs: lactoferrin or placebo. We successfully obtained and transferred over 33,000 stool and urine samples of sufficient volume for analysis from 467 infants from every NICU, of which 10,990 were stool samples and 22,341 were urine samples. We analysed over 1300 samples to determine the gut bacterial community structure of 201 infants (lactoferrin, n = 97; placebo, n = 104), of whom 11% had NEC and 31% had LOS; the remaining infants were largely free of confirmed disease. The samples that were analysed were focused on the first 6 weeks of age, when most disease (NEC or LOS) occurs. Our carefully designed study enabled access to a large number of samples with extensive clinical information, allowed us to identify carefully matched controls for each case of disease and allowed us to identify a cohort of healthy infants to determine gut effects of lactoferrin over time on the NICU. We have shown that it is feasible to conduct robust mechanistic analyses of large numbers of biological samples from a large cohort, within a RCT design, in a vulnerable high-risk patient group, across multiple hospital sites, and use state-of-the-art technologies in four separate laboratories (Newcastle University, Northumbria University, the University of Liverpool and the University of Birmingham). Our study provides considerable added value to the overarching RCT and made use of existing clinical information.
Patterns of gut bacteria in health and disease and the impact of lactoferrin
We found that the dominant bacterial taxa were similar across all NICU sites and stool samples, predominantly Enterobacteriaceae, Staphylococcus, Escherichia, Veillonella and Enterococcus. Apart from Veillonella, most of these are organisms that have been identified as key pathogens causing LOS or have been associated with NEC in preterm infants. Bifidobacteria were noted in all NICUs but, unlike full-term infants, were not the dominant flora even after the first weeks in healthy infants who appeared to be exclusively breastfed. NICUs using supplemental probiotics appeared to have higher abundances of bifidobacteria; however, this finding was not significant on multiple testing, perhaps because early samples were from infants yet to be started on probiotics and some were from infants who were unwell and not tolerating many/any milk feeds. Samples showed increasing bacterial diversity over time, but there was lower diversity in samples from infants immediately preceding onset of NEC.
The bacterial community structure (beta-diversity) was most strongly correlated with the individual infant (R2 = 0.34), postnatal age (R2 = 0.13) and NICU site (R2 = 0.08), whereas lactoferrin had relatively minimal effect (R2 = 0.002), although this was still significant [p(adj) = 0.01]. Importantly, the residual variance, which was unexplained by clinical variables in the model, exceeded that explained by any single measure (R2 = 0.43). This emphasises the complexity and apparently random nature of gut bacterial communities, and exemplifies the challenges that researchers and clinicians experience when attempting to determine risk factors for disease, test the effects of interventions exerting an effect in the gut likely to affect disease (e.g. probiotics) and plan the best medical care that reduces the risk of disease (e.g. antibiotic use).
To explore the dynamic nature of gut microbial communities and, therefore, to understand the context in which supplemental lactoferrin may exert any effect, we used a single sample from each healthy infant at five specific time points up to day 60. This showed that the greatest change in relative abundance was observed in Staphylococcus, which decreased from 42% at age 7–9 days to only 2% at age 30–60 days. This is important because cases of confirmed LOS occurred at a median age of 12 days and were predominantly because of CoNS (i.e. staphylococci infections). Importantly, our analyses showed that lactoferrin exerted only a very small effect on alpha-diversity over time. Staphylococcus (along with Haemophilus and Lactobacillus) showed lower relative abundance in infants allocated to the lactoferrin groups than in those allocated to placebo groups, whereas Bacteroidetes was the only taxon to show higher abundance in infants allocated to receive lactoferrin.
Our primary finding, therefore, is that lactoferrin does have a measurable impact on gut bacteria; however, the effect is small and much less important than other clinical variables, including NICU site. Although lactoferrin does have a slight impact on reducing staphylococcal relative abundance, there was no impact on the rates of LOS according to the main ELFIN trial. Importantly, we also noted that the median age at commencing lactoferrin was around day 4–5 and the median age at onset of LOS was day 12 (i.e. only 1 week later). In addition, given that the ELFIN trial was a pragmatic trial that used an intention-to-treat analysis, some infants who developed LOS will have been sicker infants, some of whom will have commenced the IMP after day 5 and some who may not have received any IMP at all. Although lactoferrin may exert an effect through multiple pathways (e.g. microbiomic, metabolic and cell signalling), it seems unlikely that supplemental enteral lactoferrin administered for less than 5–7 days will reduce the incidence of LOS caused by CoNS. This is likely to be especially true where sepsis may have been secondary to skin colonisation with CoNS, resulting in infection at the site of a venous catheter (the predominant type of LOS observed in preterm infants).
Gut bacteria metabolic function
Our study was carefully designed to utilise multiple methods of exploring any gut-mediated effects of lactoferrin. Our analyses of gut bacterial patterns were supported using state-of-the-art metabolomic methods using both GCMS and LCMS, each of which will provide different targeted and untargeted data. Given the complexity and cost of these techniques, we were not able to analyse as many samples as we did for 16S, but we did analyse over 750 samples from infants with extremely detailed clinical information.
The key determinant of the distribution of VOCs in faeces samples was the individual infant, which was a greater contributor than age, milk type or the NICU hospital in which the infant's care took place. The use of lactoferrin or placebo had no measurable overall impact on the metabolome, as assessed using GCMS. In addition, there was no measurable impact from the milk type, but this might simply reflect the fact that most infants received breast milk. There was also no measurable or lasting impact from the gestational age at delivery. We had expected that a very immature intestine may produce different metabolites in response to microbiota. Again, these data may reflect sample selection: the most premature infants have the highest risk of developing NEC or sepsis and are, therefore, less well represented in the longitudinal analysis.
The influence of age was unexpected. We had expected that faecal VOCs would become more diverse as infants mature. In fact, the number of VOCs increased in the first 2 weeks of life then plateaued. It was striking that two branched-chain fatty acids, 3-Methylbutanoic acid and 2-Methylbutanoic acid, increased significantly in the first week, followed by acids and then esters. These fatty acids are derived from leucine and isoleucine, and may be produced by bifidobacteria. 91 These data suggest that they may be metabolites from the protein-rich amniotic fluid. Lactobacilli and bifidobacteria are a source of butanoic acid. Acids and alcohols start to appear as infants receive milk feeds, and these are followed by esters, which are synthesised from acids and alcohols.
We are confident that these data confirm that lactoferrin was exerting minimal, if any, impact on gut bacterial metabolic function. The reliability of our data is further strengthened by the blinded nature of all microbiota and metabolomic analyses. All investigators remained blinded to the ELFIN trial group throughout the analytical process.
Although lactoferrin had no measurable metabolic impact, the current study and data analysis have highlighted the potential of untargeted ultra high-performance LCMS metabolomic assays to identify metabolic changes, such as NEC and LOS. The results indicate that hundreds of individual metabolites and several metabolic pathways/classes were perturbed in urine and stool samples, with urine showing the strongest signals. When applying a robust statistical analysis with correction for multiple testing, we found that NEC infants were different from LOS infants and healthy controls, whereas the difference between LOS infants and control infants was less distinct.
Our methods also meant that the same sample could be analysed using more than one technique, affording the opportunity to conduct future work to explore links between relevant clinical ‘exposures’ (e.g. milk type, disease and demographics) and specific patterns of bacterial colonisation, and combine these with metabolic correlates. Our data are relatively unique in that we collected stool and urine samples at the same time, and single stool samples were split, rather than using two samples collected a few hours apart. This identified multiple differences in urinary metabolites pre disease that deserve further explanation. Given the challenges of obtaining regular stool samples, especially in sick infants, urinary metabolomic examination urgently deserves further study. It is possible that urine might be a more useful biological fluid/sample than stool, especially because it may require less preparation than stool samples and might be amenable to point-of-care testing in the future.
Urinary intestinal fatty acid-binding protein analysis
We explored the utility of using a single-spot measure of iFABP as a means of confirming a diagnosis of NEC or LOS that might have been because of translocation of a gut-derived organism. We found that wide variations in iFABP existed, even when normalised to urinary concentration using creatinine, both in healthy and in diseased infants. We noted relatively low levels of iFABP preceding a diagnosis of NEC in several cases, but also very high levels in infants who remained completely healthy, that is where there was no evidence that feeds were stopped or antibiotics started, even without a confirmed diagnosis of NEC or LOS. It is possible that there are several confounders, although there was no clear impact of gestational or postnatal age. We did not collect any information on other variables that might alter fluid or feed status, such as recent blood transfusions, presence of a patent arterial duct, blood transfusions, fluid volume intake and use of diuretics. We do not have any additional measures of gut motility or gastric emptying, but these are not routinely measured in clinical practice. With such a short plasma half-life, use of urine may provide a better ‘cumulative’ measure than plasma, although urine can still be rapidly voided in preterm infants. Because of the small number of cases, we did not separately analyse the samples based on severity of NEC diagnosis. It is possible that infants not undergoing surgery have extensive inflammatory disease (producing more iFABP) and that some infants who undergo laparotomy have relatively smaller areas of intestine affected. Furthermore, in the absence of gut tissue (at operation or post mortem) a diagnosis of NEC may be difficult to confirm and some cases of LOS may have been misclassified as NEC (or vice versa). Even if an unequivocal diagnosis of NEC is made, the precise day of onset can be difficult to define, meaning that the ‘peak’ release of iFABP may have passed or have yet to occur.
Further analysis of these cases or additional samples might allow more accurate determination of potential confounders; however, it appears that (1) iFABP may not be a reliable predictor of NEC or gut-derived sepsis, (2) high iFABP levels may erroneously suggest that a preterm infant is unwell, when in fact they are healthy, and (3) iFABP is not useful in confirming NEC in preterm infants. However, as previously described, a more extensive exploration of urinary peptides or metabolites using ‘omic’ technology may identify other useful markers.
Gut tissue analysis
We successfully demonstrated that NHS FFPE tissue that is surplus to diagnostic requirements can be used as a powerful research resource in the devastating condition of NEC. Where RNA sequencing (RNAseq) requires fresh tissue to extract high-quality RNA, we have demonstrated that FFPE tissue, which is unsuitable for RNAseq, can be successfully used for array-based transcriptomic analysis. In this study, in which matched tissue from three anatomical areas of the ileum was required for analysis, failure of QC in four arrays led to the exclusion of four patients. Despite this, we noted that nearly 90% of samples passed QC, which confirms the utility of FFPE tissue for whole-genome-expression studies in NEC and other conditions for which available fresh tissue for research is limited.
During the study, we identified three histological microanatomical ‘zones’ within the intestine during NEC: healthy margins, an inflamed transition zone and necrosis. Transcriptomic data from this study have strongly implicated myeloid, in particular monocyte/macrophage and neutrophil, recruitment and activation as an important mediator of inflammation in NEC. The IL-1 signalling pathway appears to have a dominant role, which is evidenced by upregulation of multiple genes, providing proof of concept that targeting these immune cells or pathways may be beneficial in avoidance or treatment of NEC.
Our study is unique in obtaining gut tissue from preterm infants in the context of a RCT. Tissue analysis provided novel data on mechanisms associated with NEC, demonstrating differential gene expression between healthy and diseased tissue. We now plan to explore differential protein expression between necrosis and healthy resection margins, and we will explore the capacity of tissue resection specimens to yield information regarding mucosa-associated microbes in NEC by combining immunofluorescence for human leucocyte and epithelial proteins, as well as probing for bacterial 16S rRNA by in situ hybridisation.
Population characteristics and generalisability
Our results are likely to be generalisable to all preterm infants across the UK, as well as further afield, and our methods could be replicated in future studies. We recruited large numbers of infants from multiple sites representative of NICU care in the UK, and collected large numbers of biological samples and data without exposing the infants to any risk or discomfort (i.e. samples were all non-invasive). Details of recruitment per hospital site and over the course of the study are available in Appendix 5. Our large sample size and the nature of premature delivery meant that we recruited large numbers of twins, and our precise clinical data meant that we could identify twin pairs who remained healthy and could, therefore, act as well-matched controls for each other. Differences in gut bacteria between healthy twin pairs who received the same IMP are unlikely to be due to maternal or pregnancy characteristics or differences in nutritional exposures. They may have received slightly different exposures to antibiotics (although antibiotic use in infants without confirmed disease was generally low) and they may, of course, have genetic or epigenetic differences given that most will not be monochorionic twins, and some will have endured different in utero conditions if selective growth restriction or placental function occurred. Nevertheless, our unique approach allowed us to control for many more factors than would be the case if only singleton infants were used.
Limitations and challenges of the MAGPIE study
Infant recruitment, sample logistics and analysis
Despite the multiple strengths and novel nature of our mechanistic study, we encountered many challenges in conducting the study and collecting and analysing the samples; interpretation of our findings needs to be made in the context of several important factors. Although we recruited 479 preterm infants, we were able to obtain samples of usable volume from only 467 infants. In addition, disease onset occurred in the first 7 days of life in 7 out of 30 cases of NEC and 18 out of 81 cases of LOS, representing ≈ 22% of disease cases overall. NEC occurring in the first week of life is likely to represent the end result of a different set of risk factors to that of NEC disease occurring after the age of 4–6 weeks. Most older infants with NEC are likely to have tolerated full milk feeds for a prolonged period and will have a very different gut microbiota composition to infants in the first 10 days of life.
Stool samples were collected from the nappy, but we could not record how long it had been since the stool had been passed, potentially affecting the occurrence and certain types of metabolites, especially some VOCs that may rapidly decrease in concentration in the residual stool. There may also have been a delay between collection into a sample pot (stool and urine) and transfer into the NICU freezer, and there will always have been a delay of a few weeks when the sample was stored at –20 °C before transport to the central –80 °C freezers. It is also likely that stool samples were not always collected or available on informative days owing to work pressures in the NICU. This is likely to have been more challenging in the sickest infants, as they pass stool less frequently and bedside nurses may have had more important clinical priorities when caring for the infant than collecting samples for research. Our methods, therefore, represent a compromise for a large clinical study in a busy NICU environment involving hundreds of bedside nurses in obtaining thousands of samples. Oversampling was necessary, and many of the samples might not be used in analysis because it is not possible to predict which infant will develop disease, and when.
Samples were transferred on dry ice from the NICU to the central storage using Human Tissue Act-approved61 couriers and, although all samples arrived frozen, we did not record the temperature during courier transport. There were also considerable logistical challenges to overcome in co-ordinating courier transports from multiple sites that met the Human Tissue Act standards,61 sometimes pressured because of limited freezer space in the NICU. Many NICUs struggled to locate more than a relatively small –20 °C freezer within the NICU footprint. Two NICUs reported that the freezers containing batches of samples had been inadvertently turned off and, thus, partial defrosting may have occurred. The large number of samples collected created considerable logistical challenges, and we required more –80 °C storage than originally anticipated. This was exacerbated by the rapid recruitment rates to the ELFIN trial from more NICUs than planned, over a much shorter time period, as changes to the HRA process of approvals delayed site set-up by several months and considerably reduced our window for recruitment.
Originally, we planned to conduct analyses as samples accrued, but this would have required precise determination of disease presence or absence at the time that the cases presented. However, the ELFIN trial design meant that all reported cases of NEC or LOS underwent BERC review. Typically, this took place several months after data collection for that case had been completed, as BERC review could be carried out by experienced clinicians only. Combined with the rapid recruitment rate over a small time period, this meant that most microbiomic analyses started towards the end of the recruitment period. After robust identification of disease, it was also important that control cases were confirmed as not having developed disease, and finding a ‘good’ match for each case based on gestational and postnatal age from the same NICU meant that we used controls who may have been born a few months after the index case.
We used data collected for the ELFIN trial that had already been independently verified, and supplemented these data with additional bedside data collection. This was necessary so that we could accurately determine postnatal age and the relationship to antibiotic exposure. Although most daily logs appeared complete, there were cases of infants coded as having LOS in whom recorded antibiotic use did not appear to be accurate, that is daily doses were not recorded for ≥ 5 days (which was an essential criterion for a diagnosis in the ELFIN trial). In a minority of cases, infants with clearly confirmed LOS were recorded as having not received any antibiotics, so it is possible that other short-duration antibiotic exposures occurred without suspected or confirmed LOS that were similarly not accurately recorded.
In such a large study, it was also not possible to validate sample time and collection, that is it is possible that some samples were attributed to the previous or following day when research nurses anonymised samples. In a very small number of cases, samples that were recorded as being sent via courier could not be identified on arrival. A small number of samples arrived without logs or clear labelling and then had to be destroyed. Use of supplemental probiotics and fungal prophylaxis appeared to be well recorded, but again there were occasions when it seems that these were not recorded accurately. We also identified a small number of infants with either prolonged periods of receiving antibiotics or periods of not being fed who are likely to have had either suspected LOS or suspected NEC, but for whom there was no accompanying case report form. This may have been the case for infants who died without a confirmed diagnosis of NEC or LOS. There were also a small number of infants who would have received parenteral nutrition based on their size and gestation, but this had not been recorded on case report forms. We are confident that key demographic variables (e.g. gestation and birthweight) and major outcomes (e.g. LOS and NEC) are correctly recorded, but this study emphasises that using large databases that include multiple clinical variables on a daily basis can be extremely challenging, especially in a busy intensive care environment.
Analytical and technical challenges
Using 16S rRNA technology has the advantage of being relatively robust and affordable; however, it will not provide data at the species level for most bacteria. In the important genus of Staphylococcus, 16S rRNA does not distinguish between the key pathogens S. aureus and Staphylococcus epidermidis, which may have different aetiologies, presentations and consequences when causing invasive LOS on a NICU. Future work using WGS or other methods may help resolve this. In addition, it is important to note that 16S rRNA provides data on relative (not absolute) abundance and that stool collection from the nappy reflects gut bacterial patterns throughout the gut, whereas in most cases of NEC it is possible that bacterial community patterns in the small intestine are the most predictive or causative for NEC. Using 16S rRNA, we cannot distinguish between B. bifidum that may have been administered with probiotic supplements and Bifidobacterium infantis that may have been promoted using maternal breast milk. Furthermore, routine data recording did not distinguish between use of mothers’ own breast milk and use of donated human breast milk in those NICUs that use it. Future analysis of the MAGPIE study samples for WGS will provide additional data.
We identified numerous metabolites indicating multiple potential metabolic pathways in both stool and urine. However, stool metabolites might reflect bacterial metabolism that on its own may have relatively little functional impact on the infant. We have previously shown that rapid changes in the microbiome might still be associated with relatively metabolomic stability. 92 Although both GCMS and LCMS control for the volume of stool used, normalising the concentration of any metabolite in stool is challenging owing to other stool constituents that may be present in variable amounts, for example undigested oligosaccharides; urine analysis may be less problematic. Nevertheless, absolute concentrations in urine will reflect hydration status and intravascular fluid volume, which are known to be significantly affected during acute illness. Urinary metabolites might better reflect host metabolism, but the compounds identified are hugely varied and might be bacterial metabolites, host metabolites or metabolites from the breakdown of acute-phase proteins, such as C-reactive protein. In addition, some metabolites might be small peptides released from damaged gut tissue. Distinguishing these different metabolites might provide important insights into disease mechanisms and/or such metabolites could act as biomarkers in future studies, especially for a disease like NEC that lacks a gold standard diagnosis.
If further research and additional analysis of our urine samples enables us to precisely identify compounds in urine that reflect damage to deeper gut layers, such as the lamina propria or gut muscularis layer, these compounds could be used to confirm diagnosis and may alter clinical decisions in the future, such as the decision to perform a laparotomy. Currently used biomarkers, such as serum C-reactive protein, urinary iFABP or stool calprotectin, are non-specific and insensitive measures of the clinical syndrome that clinicians term NEC. We also found that absolute urinary iFABP does not appear to be a sensitive or specific marker of NEC. However, our ability to precisely identify the metabolites relies on their inclusion in an existing library of compounds. Even if an identified compound is present in the library, there could still be a lack of precision for distinguishing between very similar compounds. For example, an identified compound, such as the amino acid leucine, may be misclassified if the library does not also contain isoleucine, which is a very similar chemical compound. The results of our novel study can be retested in the future, as libraries are rapidly developing. Indeed, it is likely that most of the compounds that we identified in this study could not have been precisely identified when we planned the study just 5 years ago.
Challenges associated with gut tissue retrieval and co-ordination of multiple recruiting and analytical sites
As previously mentioned, access to and analysis of gut tissue resected for clinical indications was a novel aspect of our study, but this presented additional challenges. In two tertiary-level NICUs, surgery was performed on a separate site, and in one case this was part of a different hospital NHS trust, and 5 out of the 13 NICUs did not perform neonatal surgery. On average, there were only one to three NEC cases per recruiting site, and identifying the availability of FFPE tissue specimens was challenging or not possible in several cases. It is, therefore, likely that some suitable specimens were not identified or could not be retrieved. However, we have demonstrated that collecting suitable specimens is feasible and provides considerable added value. We plan to explore the possibility of analysing such tissue that was resected from sick infants during other RCTs such as BOOST II UK, in which differences in mortality associated with the trial intervention (i.e. different levels of oxygen saturations) appear to be in part due to a higher incidence of NEC.
Mechanistic insights relevant to the ELFIN (lactoferrin) trial
The MAGPIE study shows that, although lactoferrin does affect gut microbiota diversity and reduces the relative abundance of certain key bacteria, the effect of this is that relatively small, and important common pathogens, such as staphylococci, are not substantially reduced. Furthermore, the impact of lactoferrin is much smaller than that of other clinical variables. The lack of a clear metabolic signal in urine also suggests that lactoferrin or one of its metabolites, such as lactoferricin or other peptides, may not cross the gut epithelium in any significant concentration, perhaps making the chance of an ‘anti-infective’ effect on a site remote from the gut, such as a peripherally sited cannula, quite small. If there was to be any such effect, it might also be expected to be related to the duration of lactoferrin exposure. Given that half of all cases of LOS occurred by the end of day 12 of life, it is perhaps not surprising that lactoferrin did not reduce LOS in a large pragmatic RCT, even if it does have some other clinical benefit on gut health. Furthermore, in the ELFIN trial, the primary outcome was LOS, which we defined as sepsis occurring at any time after aged 72 hours. It would, therefore, have been possible to meet the primary outcome of LOS before any lactoferrin exposure had occurred.
Lactoferrin is an evolutionarily highly conserved mammalian protein that is found in milks and is present in especially high levels in maternal colostrum. The ELFIN trial was designed to provide preterm infants with an amount roughly equivalent to that of a healthy breastfed infant in a small volume as soon as enteral feeds were tolerated. Given the very limited impact on the microbiome or metabolome, it seems unlikely that a larger dose would have made a difference. Using a much larger dose could be problematic because the amino acids are likely to be nutritionally available and lactoferrin administration might then need to be considered within the overall dietary supply of protein.
We used bovine lactoferrin as the only widely available source and were restricted in the number of commercial suppliers able to meet quality and good manufacturing practice standards. There are no data to suggest, and we have no reason to suspect, that the ‘quality’ of bioactivity of the product used in the ELFIN trial was inferior to that of other products available; however, in vitro studies have documented clear differences between different lactoferrin preparations. 93 Omic analysis of similar nutritional supplements, such as milk fat globule membrane, also shows significant variations between commercially available sources, suggesting that there may be important differences in functional activity of dietary supplements such as lactoferrin. 94 Our study was designed to explore the gut impact of lactoferrin, but we were able to explore microbiomic and metabolomic aspects only, and it is possible (but unlikely) that there were other sites of action that may be of functional relevance. For example, the brain is known to express lactoferrin receptors, suggesting that other effects may be important. It is also important to appreciate that bovine lactoferrin, although similar in structure to human lactoferrin, has a different protein structure and amino acid composition, and there may be further differences in protein function owing to the presence of specific glycans. 95,96
Nutrients are not pharmaceutical agents and should not be expected to have similar dynamics, similar kinetics or as rapid effects as other compounds such as antibiotics or antifungal agents, which are absorbed rapidly from the gut and reach bactericidal or fungicidal levels in tissue very quickly. Human evolution provided breast milk lactoferrin directly to the gut, where it is likely to have the greatest impact. The benefits of breast milk in reducing gut-derived and other infections, as well as diarrhoea (especially in resource-poor settings), last throughout the duration of breastfeeding, which may be several months or years. By contrast, the ELFIN trial focused on a time period of just a few weeks, which may have been too short for beneficial effects to become apparent, given that most cases of NEC and sepsis present before age 3 weeks.
Chapter 5 Conclusions
The MAGPIE study shows that while supplemental lactoferrin has a statistically significant impact on the gut microbiome in reducing the relative proportion of staphylococci, the impact is relatively small and is not associated with any measurable metabolic change. Routine supplemental lactoferrin, is not currently recommended in routine clinical settings, as demonstrated in the ELFIN trial. We have shown that it is feasible to embed detailed mechanistic studies within large RCTs utilising a range of state-of-the-art technology and using non-invasive biological samples that would otherwise be discarded, and we have also demonstrated the utility of using gut tissue stored in NHS pathology archives. In addition, we have found high consent rates, both to sample collection and to longer-term biobanking, in a high-risk population that will inform future study designs and act as a resource for further research. Our data also suggest that urinary analysis can provide significant mechanistic insights and might provide biomarkers of disease for early disease confirmation in a clinical context, provide validation in research settings and/or enable risk stratification for treatments enabling novel interventions to be tested in those at the highest risk. All of these benefits may lead to a more individualised medicine approach to treatment and research in preterm infants.
Preterm delivery is associated with significant health-care costs (≈ £3B per year in the UK) that are considerably higher in those infants who develop disease. The lifetime costs of caring for a child who developed NEC as a preterm infant and then suffered from developmental delay or cerebral palsy will be in excess of £5M. Although there is evidence that rates of LOS have decreased in some NICUs, it remains an important disease, and NEC and LOS together are responsible for more childhood deaths than all childhood cancers combined. Mechanistic study of LOS and NEC in the UK in preterm infants is extremely limited. Without a better understanding of the underlying mechanisms, clinicians and researchers will not be able to develop optimal trial designs to compare clinical effectiveness of currently used treatments or test novel interventions.
Recommendations for future research
The MAGPIE study and ELFIN trial have generated a vast data set. Although we do not have the resources to biobank every sample collected, we have contributed thousands of samples of stool, urine and extracted bacterial DNA to an existing biorepository of samples, the Great North Neonatal Biobank (Newcastle). The Great North Neonatal Biobank now contains samples and clinical data on more than 1000 very preterm infants. The creation of biobanks to better understand NEC has been strongly supported worldwide by patient advocacy organisations. 97 We will use existing data and sample analysis results, and analyse further samples stored in the biobank, to undertake further research.
Future work to improve the use of metabolomics in research and practice is as follows:
-
Better understand the interactions between the microbiome and the metabolome using advanced techniques (‘mixomics’) that allow us to integrate the data. This will improve knowledge of which specific bacterial taxa have the greatest metabolic effects and potential benefits, for example species that might be incorporated into probiotics.
-
Determine the metabolomic profile of infants receiving complex diets and treatments. This will help to identify and subsequently control for key metabolites in sick infants so that we can identify biomarkers and mechanisms as a result of disease in contrast to the treatments associated with those diseases, for example parenteral nutrition, antibiotics and inotropes.
Future work to better understand the pattern of bacteria in health infants is as follows:
-
Explore the clinical factors associated with microbiomic features that are potentially associated with better health outcomes, for example determine why some infants develop bifidobacterial colonisation but others do not.
-
Explore the microbiomic impact of exposure to breast milk compared with formula milk. This will help to determine microbial taxa that might be preferentially supported by formula milk as an antecedent risk factor for diseases such as NEC.
-
Undertake WGS to determine the species of staphylococci associated with sepsis or the species of Bifidobacterium associated with breast milk exposure.
-
Use WGS or other techniques to look for the presence of antimicrobial resistance patterns and relate these to clinical variables, such as antibiotic exposures, or differences between NICUs that may reflect infection control and other procedures.
Future work to understand gut cell and mucosal pathology in NEC is as follows:
-
Integrate 16S sequence data from stool with molecular assays from gut tissue to derive a better understanding of which microbes are the most important for host immune–gut microbe cross-talk.
-
Further explore the capacity of gut tissue resection specimens to yield information regarding mucosa-associated microbes in NEC, by combining immunofluorescence for human leucocytes and epithelial proteins, as well as probing for bacterial 16S rRNA by in situ hybridisation.
-
Examine pharmacological and non-pharmacological means to manipulate the molecular pathways identified by this study. These may include myeloid trafficking to the gut, neutrophil or macrophage activation, IL-1 signalling and lipid metabolism.
Acknowledgements
We gratefully acknowledge the following, without whom the MAGPIE study would not have been possible:
-
the parents who contributed to the design of the study, and those who consented for their infants to be enrolled in the MAGPIE study and ELFIN trial
-
the local principal investigators and research nurses at each of the collaborating NICUs; and the clinical staff, especially bedside nurses, who performed sample collection
-
Professor Warwick Dunn and the staff at the Phenome Centre Birmingham who undertook analysis of LCMS specimens
-
Professor Simon Eaton and the staff at University College London, London, who performed the iFABP assays
-
the research team and research and development department at Newcastle Hospitals NHS Trust, especially Mr Christopher Price, Mrs Wendy Mitson, Mrs Julie Groombridge, Dr Tom Skeath and Dr Stefan Zalewski
-
all members of the NPEU team, Oxford and everyone who contributed to the ELFIN trial and SIFT
-
members of the Study Steering Committee, including Professor Ben Stenson (chairperson), Professor Jonathon Wyllie, Dr Anne Dale and Mrs Sharon McLeod (parent representative).
Preparatory work was supported by separate funds from the Newcastle University MRC Confidence in Concept fund.
Patient and public involvement
The MAGPIE study was designed following the experience gained in the lead and other NICUs that had been involved in stool and urine collection. We developed the parent information leaflet by sharing it with local parents. A parent of a preterm infant joined our study steering committee. We plan to work with parent advocacy groups, including BLISS (London, UK) and NEC UK (Nottingham, UK), in disseminating the results.
Contributions of authors
Professor Nicholas Embleton (https://orcid.org/0000-0003-3750-5566) (Consultant Neonatologist) designed the clinical aspects of the study, including sample and data collection; liaised with individual hospital sites, undertook site set up and acted as a clinical lead during the study; took responsibility for study governance, co-ordination of the Study Steering Committee and liaison with the overarching SIFT and ELFIN trial; organised the logistical aspects of transport storage, transport and archiving; and selected samples for analysis and determined which residual samples to biobank.
Dr Janet Berrington (https://orcid.org/0000-0002-6185-2843) (Consultant Neonatologist) designed the clinical aspects of the study, including sample and data collection; liaised with individual hospital sites, undertook site set up and acted as a clinical lead during the study; took responsibility for study governance, co-ordination of the Study Steering Committee and liaison with the overarching SIFT and ELFIN trial; organised the logistical aspects of transport storage, transport and archiving; and selected samples for analysis and determined which residual samples to biobank.
Professor Stephen Cummings (https://orcid.org/0000-0001-5298-2075) (Dean) supported the 16S rRNA microbiomic analyses and planned the initial modelling analysis of the microbiomic data.
Professor Jon Dorling (https://orcid.org/0000-0002-1691-3221) (Professor of Pediatrics) designed the clinical aspects of the study, including sample and data collection, and led the overarching SIFT and ELFIN trial.
Professor Andrew Ewer (https://orcid.org/0000-0002-3825-4781) (Consultant Neonatologist) designed the clinical aspects of the study, including sample and data collection.
Dr Alessandra Frau (https://orcid.org/0000-0002-6885-1042) (Research Associate) led the GCMS analysis at the University of Liverpool.
Professor Edmund Juszczak (https://orcid.org/0000-0001-5500-2247) (Head of Trials) took responsibility for study governance, co-ordination of the Study Steering Committee and liaison with the overarching SIFT and ELFIN trial.
Professor John Kirby (https://orcid.org/0000-0003-2543-4131) (Dean) planned the tissue analysis.
Dr Christopher Lamb (https://orcid.org/0000-0002-7271-4956) (Consultant Gastroenterologist) planned, conducted and analysed the tissue analysis.
Dr Clare Lanyon (https://orcid.org/0000-0002-5200-9336) (Senior Lecturer) supported the 16S rRNA microbiomic analyses and planned the initial modelling analysis of the microbiomic data.
Dr Lauren Lett (https://orcid.org/0000-0002-3623-0321) (Research Associate) led the GCMS analysis at the University of Liverpool.
Professor William McGuire (https://orcid.org/0000-0001-8572-3467) (Professor of Child Health) designed the clinical aspects of the study, including sample and data collection, and led the overarching SIFT and ELFIN trial.
Professor Christopher Probert (https://orcid.org/0000-0003-4550-0239) (Consultant Gastroenterologist) led the GCMS analysis at the University of Liverpool.
Professor Stephen Rushton (https://orcid.org/0000-0001-8443-5228) (Professor of Biological Modelling) planned the initial modelling analysis of the microbiomic data.
Dr Mark Shirley (https://orcid.org/0000-0002-6220-2578) (Research Associate) planned the initial modelling analysis of the microbiomic data.
Dr Christopher Stewart (https://orcid.org/0000-0002-6033-338X) (Senior Research Fellow) organised the logistical aspects of transport storage, transport and archiving; selected samples for analysis and determined which residual samples to biobank; led the 16S rRNA microbiomic analyses; co-ordinated the LCMS analysis, supported by the team at the University of Birmingham; planned and conducted the initial modelling analysis of the microbiomic data; and planned the tissue analysis.
Dr Gregory R Young (https://orcid.org/0000-0001-5342-1421) (Senior Research Fellow) organised the logistical aspects of transport storage, transport and archiving; selected samples for analysis and determined which residual samples to biobank; led the 16S rRNA microbiomic analyses; organised urine samples for analysis undertaken by the team at University College London; and conducted the initial modelling analysis of the microbiomic data.
All authors made substantial contributions to the conception or design of the work; the acquisition, analysis or interpretation of data for the work; drafting the work or revising it critically for important intellectual content; and final approval of the version to be published.
Data-sharing statement
We shall make data available to the scientific community with as few restrictions as feasible. Anonymised data will be deposited and made available to encourage wider use. Requests for access to other data will be considered and should be addressed to the corresponding author. Access to samples in the biobank should be discussed with the corresponding author in the first place, who will provide further information as needed. The Great North Neonatal Biobank operates under a Human Tissue Act61 license to Newcastle University and requests will be considered by an access committee that includes public representation.
Patient data
This work uses data provided by patients and collected by the NHS as part of their care and support. Using patient data is vital to improve health and care for everyone. There is huge potential to make better use of information from people’s patient records, to understand more about disease, develop new treatments, monitor safety, and plan NHS services. Patient data should be kept safe and secure, to protect everyone’s privacy, and it’s important that there are safeguards to make sure that it is stored and used responsibly. Everyone should be able to find out about how patient data are used. #datasaveslives You can find out more about the background to this citation here: https://understandingpatientdata.org.uk/data-citation.
Disclaimers
This report presents independent research. The views and opinions expressed by authors in this publication are those of the authors and do not necessarily reflect those of the NHS, the NIHR, the MRC, NETSCC, the EME programme or the Department of Health and Social Care. If there are verbatim quotations included in this publication the views and opinions expressed by the interviewees are those of the interviewees and do not necessarily reflect those of the authors, those of the NHS, the NIHR, NETSCC, the EME programme or the Department of Health and Social Care.
References
- Abbott J, Berrington J, Bowler U, Boyle E, Dorling J, Embleton N, et al. The Speed of Increasing milk Feeds: a randomised controlled trial. BMC Pediatr 2017;17. https://doi.org/10.1186/s12887-017-0794-z.
- The ELFIN trial investigators group . Enteral lactoferrin supplementation for very preterm infants: a randomised placebo-controlled trial. Lancet 2019;393:423-33. https://doi.org/10.1016/S0140-6736(18)32221-9.
- March of Dimes, The Partnership for Maternal, Newborn and Child Health, Save the Children, World Health Organization (WHO) . Born Too Soon: The Global Action Report on Preterm Birth 2012.
- Mangham LJ, Petrou S, Doyle LW, Draper ES, Marlow N. The cost of preterm birth throughout childhood in England and Wales. Pediatrics 2009;123:e312-27. https://doi.org/10.1542/peds.2008-1827.
- Berrington JE, Hearn RI, Bythell M, Wright C, Embleton ND. Deaths in preterm infants: changing pathology over 2 decades. J Pediatr 2012;160:49-53.e1. https://doi.org/10.1016/j.jpeds.2011.06.046.
- Cleminson JS, Zalewski SP, Embleton ND. Nutrition in the preterm infant: what’s new?. Curr Opin Clin Nutr Metab Care 2016;19:220-5. https://doi.org/10.1097/MCO.0000000000000270.
- Vergnano S, Menson E, Kennea N, Embleton N, Russell AB, Watts T, et al. Neonatal infections in England: the NeonIN surveillance network. Arch Dis Child Fetal Neonatal Ed 2011;96:F9-14. https://doi.org/10.1136/adc.2009.178798.
- Costeloe K, Hardy P, Juszczak E, Wilks M, Millar MR. Bifidobacterium breve BBG-001 in very preterm infants: a randomised controlled phase 3 trial. Lancet 2015;6736:1-12. https://doi.org/10.1016/S0140-6736(15)01027-2.
- Quigley M, Embleton ND, Mcguire W. Formula versus donor breast milk for feeding preterm or low birth weight infants. Cochrane Database Syst Rev 2018;6. https://doi.org/10.1002/14651858.CD002971.pub4.
- Berrington JE, Stewart CJ, Embleton ND, Cummings SP. Gut microbiota in preterm infants: assessment and relevance to health and disease. Arch Dis Child Fetal Neonatal Ed 2013;98:F286-90. https://doi.org/10.1136/archdischild-2012-302134.
- Garg BD, Sharma D, Bansal A. Biomarkers of necrotizing enterocolitis: a review of literature. J Matern Fetal Neonatal Med 2018;31:3051-64. https://doi.org/10.1080/14767058.2017.1361925.
- Stewart CJ, Ajami NJ, O’Brien JL, Hutchinson DS, Smith DP, Wong MC, et al. Temporal development of the gut microbiome in early childhood from the TEDDY study. Nature 2018;562:583-8. https://doi.org/10.1038/s41586-018-0617-x.
- Stewart CJ, Embleton ND, Marrs ECL, Smith DP, Fofanova T, Nelson A, et al. Longitudinal development of the gut microbiome and metabolome in preterm neonates with late onset sepsis and healthy controls. Microbiome 2017;5. https://doi.org/10.1186/s40168-017-0295-1.
- Zalewski S, Stewart CJ, Embleton ND, Berrington JE. Brief guide to the analysis, interpretation and presentation of microbiota data. Arch Dis Child Educ Pract Ed 2018;103:327-30. https://doi.org/10.1136/archdischild-2017-313838.
- Taft DH, Ambalavanan N, Schibler KR, Yu Z, Newburg DS, Ward DV, et al. Intestinal microbiota of preterm infants differ over time and between hospitals. Microbiome 2014;2. https://doi.org/10.1186/2049-2618-2-36.
- Morrow AL, Lagomarcino AJ, Schibler KR, Taft DH, Yu Z, Wang B, et al. Early microbial and metabolomic signatures predict later onset of necrotizing enterocolitis in preterm infants. Microbiome 2013;1. https://doi.org/10.1186/2049-2618-1-13.
- Sim K, Shaw AG, Randell P, Cox MJ, McClure ZE, Li M-S, et al. Dysbiosis anticipating necrotizing enterocolitis in very premature infants. Clin Infect Dis 2015;60:389-97. https://doi.org/10.1093/cid/ciu822.
- Warner BB, Deych E, Zhou Y, Hall-Moore C, Weinstock GM, Sodergren E, et al. Gut bacteria dysbiosis and necrotising enterocolitis in very low birthweight infants: a prospective case-control study. Lancet 2016;387:1928-36. https://doi.org/10.1016/S0140-6736(16)00081-7.
- Pammi M, Cope J, Tarr PI, Warner BB, Morrow AL, Mai V, et al. Intestinal dysbiosis in preterm infants preceding necrotizing enterocolitis: a systematic review and meta-analysis. Microbiome 2017;5. https://doi.org/10.1186/s40168-017-0248-8.
- Stewart CJ, Nelson A, Treumann A, Skeath T, Cummings SP, Embleton ND, et al. Metabolomic and proteomic analysis of serum from preterm infants with necrotising enterocolitis and late-onset sepsis. Pediatr Res 2016;79:425-31. https://doi.org/10.1038/pr.2015.235.
- Wilson K, Hawken S, Ducharme R, Potter BK, Little J, Thébaud B, et al. Metabolomics of prematurity: analysis of patterns of amino acids, enzymes, and endocrine markers by categories of gestational age. Pediatr Res 2014;75:367-73. https://doi.org/10.1038/pr.2013.212.
- Wu GD. The gut microbiome, its metabolome, and their relationship to health and disease. Nestle Nutr Inst Workshop Ser 2016;84:103-10. https://doi.org/10.1159/000436993.
- Wandro S, Osborne S, Enriquez C, Bixby C, Arrieta A, Whiteson K. The microbiome and metabolome of preterm infant stool are personalized and not driven by health outcomes, including necrotizing enterocolitis and late-onset sepsis. MSphere 2018;3:e00104-18. https://doi.org/10.1128/mSphere.00104-18.
- Hill CJ, Lynch DB, Murphy K, Ulaszewska M, Jeffery IB, O’Shea CA, et al. Evolution of gut microbiota composition from birth to 24 weeks in the INFANTMET Cohort. Microbiome 2017;5. https://doi.org/10.1186/s40168-016-0213-y.
- Van Den Akker CHP, van Goudoever JB, Szajewska H, Embleton ND, Hojask I, Reid D, et al. Probiotics for preterm infants: a strain specific systematic review and network meta-analysis. J Pediatr Gastroenterol Nutr 2018;67:103-22. https://doi.org/10.1097/MPG.0000000000001897.
- Bury RG, Tudehope D. Enteral antibiotics for preventing necrotizing enterocolitis in low birthweight or preterm infants. Cochrane Database Syst Rev 2001;1. https://doi.org/10.1002/14651858.CD000405.
- Williams FLR, Ogston S, Hume R, Watson J, Stanbury K, Willatts P, et al. Supplemental iodide for preterm infants and developmental outcomes at 2 years: an RCT. Pediatrics 2017;139. https://doi.org/10.1542/peds.2016-3703.
- Stenson BJ, Tarnow-Mordi WO, Darlow BA, Simes J, Juszczak E, Askie L, et al. Oxygen saturation and outcomes in preterm infants. N Engl J Med 2013;368:2094-104. https://doi.org/10.1056/NEJMoa1302298.
- Millar M, Seale J, Greenland M, Hardy P, Juszczak E, Wilks M, et al. The microbiome of infants recruited to a randomised placebo-controlled probiotic trial (PiPS Trial). EBioMedicine 2017;20:255-62. https://doi.org/10.1016/j.ebiom.2017.05.019.
- Pammi M, Suresh G. Enteral lactoferrin supplementation for prevention of sepsis and necrotizing enterocolitis in preterm infants. Cochrane Database Syst Rev 2017;6. https://doi.org/10.1002/14651858.CD007137.pub5.
- Indrio F, Riezzo G, Tafuri S, Ficarella M, Carlucci B, Bisceglia M, et al. Probiotic supplementation in preterm: feeding intolerance and hospital cost. Nutrients 2017;9. https://doi.org/10.3390/nu9090965.
- Underwood MA, Salzman NH, Bennett SH, Barman M, Mills DA, Marcobal A, et al. A randomized placebo-controlled comparison of 2 prebiotic/probiotic combinations in preterm infants: impact on weight gain, intestinal microbiota, and fecal short-chain fatty acids. J Pediatr Gastroenterol Nutr 2009;48:216-25. https://doi.org/10.1097/MPG.0b013e31818de195.
- Embleton ND, Berrington JE, Dorling J, Ewer AK, Juszczak E, Kirby JA, et al. Mechanisms affecting the gut of preterm infants in enteral feeding trials. Front Nutr 2017;4. https://doi.org/10.3389/fnut.2017.00014.
- Embleton ND, Turnbull E, Turner S, Berrington JE. Successful blood salvaging from preterm infants: maximizing opportunities, minimizing interventions. Acta Paediatr 2013;102:e527-9. https://doi.org/10.1111/apa.12373.
- Davis JM, Baer GR, Portman R, Nelson R, Storari L, Aranda JV, et al. Enrollment of neonates in more than one clinical trial. Clin Ther 2017;39:1959-69. https://doi.org/10.1016/j.clinthera.2017.09.006.
- Richards J, Rankin J, Juszczak E, Dorling J, McGuire W, Embleton ND. Parental experiences of being approached to join multiple neonatal clinical trials: qualitative study (PARENT). Arch Dis Child Fetal Neonatal Ed 2021;106:84-7. https://doi.org/10.1136/archdischild-2020-319031.
- Embleton ND, Berrington JE, McGuire W, Stewart CJ, Cummings SP. Lactoferrin: antimicrobial activity and therapeutic potential. Semin Fetal Neonatal Med 2013;18:143-9. https://doi.org/10.1016/j.siny.2013.02.001.
- Rahman MM, Kim WS, Ito T, Kumura H, Shimazaki K. Growth promotion and cell binding ability of bovine lactoferrin to Bifidobacterium longum. Anaerobe 2009;15:133-7. https://doi.org/10.1016/j.anaerobe.2009.01.003.
- Mastromarino P, Capobianco D, Campagna G, Laforgia N, Drimaco P, Dileone A, et al. Correlation between lactoferrin and beneficial microbiota in breast milk and infant’s feces. Biometals 2014;27:1077-86. https://doi.org/10.1007/s10534-014-9762-3.
- Kim WS, Ohashi M, Tanaka T, Kumura H, Kim GY, Kwon IK, et al. Growth-promoting effects of lactoferrin on L. acidophilus and Bifidobacterium spp. Biometals 2004;17:279-83. https://doi.org/10.1023/b:biom.0000027705.57430.f1.
- Aguila A, Herrera AG, Morrison D, Cosgrove B, Perojo A, Montesinos I, et al. Bacteriostatic activity of human lactoferrin against Staphylococcus aureus is a function of its iron-binding properties and is not influenced by antibiotic resistance. FEMS Immunol Med Microbiol 2001;31:145-52. https://doi.org/10.1111/j.1574-695X.2001.tb00511.x.
- Liao Y, Jiang R, Lönnerdal B. Biochemical and molecular impacts of lactoferrin on small intestinal growth and development during early life. Biochem Cell Biol 2012;90:476-84. https://doi.org/10.1139/o11-075.
- Reznikov EA, Comstock SS, Yi C, Contractor N, Donovan SM. Dietary bovine lactoferrin alters mucosal and systemic immune cell responses in neonatal piglets. J Nutr 2014;144:525-32. https://doi.org/10.3945/jn.113.190264.
- Farnaud S, Evans RW. Lactoferrin – a multifunctional protein with antimicrobial properties. Mol Immunol 2003;40:395-40. https://doi.org/10.1016/S0161-5890(03)00152-4.
- Wada Y, Lönnerdal B. Bioactive peptides derived from human milk proteins – mechanisms of action. J Nutr Biochem 2014;25:503-14. https://doi.org/10.1016/j.jnutbio.2013.10.012.
- Stewart CJ, Nelson A, Scribbins D, Marrs EC, Lanyon C, Perry JD, et al. Bacterial and fungal viability in the preterm gut: NEC and sepsis. Arch Dis Child Fetal Neonatal Ed 2013;98:F298-303. https://doi.org/10.1136/archdischild-2012-302119.
- Liu KY, Comstock SS, Shunk JM, Monaco MH, Donovan SM. Natural killer cell populations and cytotoxic activity in pigs fed mother’s milk, formula, or formula supplemented with bovine lactoferrin. Pediatr Res 2013;74:402-7. https://doi.org/10.1038/pr.2013.125.
- Gopalakrishna KP, Macadangdang BR, Rogers MB, Tometich JT, Firek BA, Baker R, et al. Maternal IgA protects against the development of necrotizing enterocolitis in preterm infants. Nat Med 2019;25:1110-15. https://doi.org/10.1038/s41591-019-0480-9.
- Rahman SF, Olm MR, Morowitz MJ, Banfield JF. Functional potential of bacterial strains in the premature infant gut microbiome is associated with gestational age. BioRxiv 2019. https://doi.org/10.1101/530139.
- Davis JC, Totten SM, Huang JO, Nagshbandi S, Kirmiz N, Garrido DA, et al. Identification of oligosaccharides in feces of breast-fed infants and their correlation with the gut microbial community. Mol Cell Proteomics 2016;15:2987-3002. https://doi.org/10.1074/mcp.M116.060665.
- Manzoni P, Rinaldi M, Cattani S, Pugni L, Romeo MG, Messner H, et al. Bovine lactoferrin supplementation for prevention of late-onset sepsis in very low-birth-weight neonates: a randomized trial. JAMA 2009;302:1421-8. https://doi.org/10.1001/jama.2009.1403.
- Woodman T, Strunk T, Patole S, Hartmann B, Simmer K, Currie A. Effects of lactoferrin on neonatal pathogens and Bifidobacterium breve in human breast milk. PLOS ONE 2018;13. https://doi.org/10.1371/journal.pone.0201819.
- Akin IM, Atasay B, Dogu F, Okulu E, Arsan S, Karatas HD, et al. Oral lactoferrin to prevent nosocomial sepsis and necrotizing enterocolitis of premature neonates and effect on T-regulatory cells. Am J Perinatol 2014;31:1111-20. https://doi.org/10.1055/s-0034-1371704.
- Sherman MP, Sherman J, Arcinue R, Niklas V. Randomized control trial of human recombinant lactoferrin: a substudy reveals effects on the fecal microbiome of very low birth weight infants. J Pediatr 2016;173:S37-42. https://doi.org/10.1016/j.jpeds.2016.02.074.
- Ochoa TJ, Zegarra J, Cam L, Llanos R, Pezo A, Cruz K, et al. Randomized controlled trial of lactoferrin for prevention of sepsis in Peruvian neonates less than 2500 g. Pediatr Infect Dis J 2015;34:571-6. https://doi.org/10.1097/INF.0000000000000593.
- Manzoni P, Meyer M, Stolfi I, Rinaldi M, Cattani S, Pugni L, et al. Bovine lactoferrin supplementation for prevention of necrotizing enterocolitis in very-low-birth-weight neonates: a randomized clinical trial. Early Hum Dev 2014;90:60-5. https://doi.org/10.1016/S0378-3782(14)70020-9.
- Asztalos EV, Barrington K, Lodha A, Tarnow-Mordi W, Martin A. Lactoferrin infant feeding trial_Canada (LIFT_Canada): protocol for a randomized trial of adding lactoferrin to feeds of very-low-birth-weight preterm infants. BMC Pediatr 2020;20. https://doi.org/10.1186/s12887-020-1938-0.
- Furusawa Y, Obata Y, Fukuda S, Endo TA, Nakato G, Takahashi D, et al. Commensal microbe-derived butyrate induces the differentiation of colonic regulatory T cells. Nature 2013;504:446-50. https://doi.org/10.1038/nature12721.
- Burger-van Paassen N, van der Sluis M, Bouma J, Korteland-van Male AM, Lu P, Van Seuningen I, et al. Colitis development during the suckling-weaning transition in mucin Muc2-deficient mice. Am J Physiol Gastrointest Liver Physiol 2011;301:G667-78. https://doi.org/10.1152/ajpgi.00199.2010.
- Griffiths J, Jenkins P, Vargova M, Bowler U, Juszczak E, King A, et al. Enteral lactoferrin supplementation for very preterm infants: a randomised placebo-controlled trial. Lancet 2019;393:P423-33.
- Great Britain . Human Tissue Act 2004 2004.
- Kozich JJ, Westcott SL, Baxter NT, Highlander SK, Schloss PD. Development of a dual-index sequencing strategy and curation pipeline for analyzing amplicon sequence data on the MiSeq Illumina sequencing platform. Appl Environ Microbiol 2013;79:5112-20. https://doi.org/10.1128/AEM.01043-13.
- Caporaso JG, Lauber CL, Walters WA, Berg-Lyons D, Lozupone CA, Turnbaugh PJ, et al. Global patterns of 16S rRNA diversity at a depth of millions of sequences per sample. Proc Natl Acad Sci U.S.A 2011;108:4516-22. https://doi.org/10.1073/pnas.1000080107.
- Schloss PD, Westcott SL, Ryabin T, Hall JR, Hartmann M, Hollister EB, et al. Introducing mothur: open-source, platform-independent, community-supported software for describing and comparing microbial communities. Appl Environ Microbiol 2009;75:7537-41. https://doi.org/10.1128/AEM.01541-09.
- Quast C, Pruesse E, Yilmaz P, Gerken J, Schweer T, Yarza P, et al. The SILVA ribosomal RNA gene database project: improved data processing and web-based tools. Nucleic Acids Res 2013;41:D590-6. https://doi.org/10.1093/nar/gks1219.
- McMurdie PJ, Holmes S. phyloseq: an R package for reproducible interactive analysis and graphics of microbiome census data. PLOS ONE 2013;8. https://doi.org/10.1371/journal.pone.0061217.
- Oksanen J, Blanchet FG, Kindt R, Legendre P, Minchin PR, O’Hara B, et al. Vegan: Community Ecology Package n.d. https://CRAN.R-project.org/package=vegan (accessed 16 June 2021).
- Ahmed I, Greenwood R, Costello B, Ratcliffe N, Probert CS. Investigation of faecal volatile organic metabolites as novel diagnostic biomarkers in inflammatory bowel disease. Aliment Pharmacol Ther 2016;43:596-611. https://doi.org/10.1111/apt.13522.
- Probert C, Greenwood R, Mayor A, Highes D, Aggio R, Jackson RE, et al. Faecal volatile organic compounds in preterm babies at risk of necrotising enterocolitis: the DOVE study. Arch Dis Child Fetal Neonatal Ed 2019:6-11. https://doi.org/10.1136/fetalneonatal-2019-318221.
- Reade S, Mayor A, Aggio R, Khalid T, Pritchard DM, Ewer AK, et al. Optimisation of sample preparation for direct SPME-GC-MS analysis of murine and human faecal volatile organic compounds for metabolomic studies. J Anal Bioanal Tech 2014;5. https://doi.org/10.4172/2155-9872.1000184.
- Garner CE, Ewer AK, Elasouad K, Power F, Greenwood R, Ratcliffe NM, et al. Analysis of faecal volatile organic compounds in preterm infants who develop necrotising enterocolitis: a pilot study. J Pediatr Gastroenterol Nutr 2009;49:559-65. https://doi.org/10.1097/MPG.0b013e3181a3bfbc.
- Rossi M, Aggio R, Staudacher HM, Lomer MC, Lindsay JO, Irving P, et al. Volatile organic compounds in feces associate with response to dietary intervention in patients with irritable bowel syndrome. Clin Gastroenterol Hepatol 2018;16:385-91.e1. https://doi.org/10.1016/j.cgh.2017.09.055.
- Garner CE, Smith S, de Lacy Costello B, White P, Spencer R, Probert CS, et al. Volatile organic compounds from feces and their potential for diagnosis of gastrointestinal disease. FASEB J 2007;21:1675-88. https://doi.org/10.1096/fj.06-6927com.
- Aggio R, Villas-Bôas SG, Ruggiero K. Metab: an R package for high-throughput analysis of metabolomics data generated by GC-MS. Bioinformatics 2011;27:2316-18. https://doi.org/10.1093/bioinformatics/btr379.
- Bates D, Mächler M, Bolker B, Walker S. Fitting linear mixed-effects models using lme4. J Stat Softw 2015;67:1-48. https://doi.org/10.18637/jss.v067.i01.
- Wickham H. Ggplot2: Elegant Graphics for Data Analysis. New York, NY: Springer Publishing; 2016.
- Chambers MC, Maclean B, Burke R, Amodei D, Ruderman DL, Neumann S, et al. A cross-platform toolkit for mass spectrometry and proteomics. Nat Biotechnol 2012;30:918-20. https://doi.org/10.1038/nbt.2377.
- Chong J, Soufan O, Li C, Caraus I, Li S, Bourque G, et al. MetaboAnalyst 4.0: towards more transparent and integrative metabolomics analysis. Nucleic Acids Res 2018;46:W486-94. https://doi.org/10.1093/nar/gky310.
- Libiseller G, Dvorzak M, Kleb U, Gander E, Eisenberg T, Madeo F, et al. IPO: a tool for automated optimization of XCMS parameters. BMC Bioinformatics 2015;16. https://doi.org/10.1186/s12859-015-0562-8.
- Smith CA, Want EJ, O’Maille G, Abagyan R, Siuzdak G. XCMS: processing mass spectrometry data for metabolite profiling using nonlinear peak alignment, matching, and identification. Anal Chem 2006;78:779-87. https://doi.org/10.1021/ac051437y.
- Dieterle F, Ross A, Schlotterbeck G, Senn H. Probabilistic quotient normalization as robust method to account for dilution of complex biological mixtures. Application in 1H NMR metabonomics. Anal Chem 2006;78:4281-90. https://doi.org/10.1021/ac051632c.
- D’Elia RV, Goodchild SA, Winder CL, Southam AD, Weber RJM, Stahl FM, et al. Multiple metabolic pathways are predictive of ricin intoxication in a rat model. Metabolomics 2019;15. https://doi.org/10.1007/s11306-019-1547-9.
- Sumner LW, Amber A, Barrett D, Beale MH, Beger R, Daykin CA, et al. Proposed minimum reporting standards for chemical analysis: Chemical Analysis Working Group (CAWG) Metabolomics Standards Initiative (MSI). Metabolomics 2007;3:211-21. https://doi.org/10.1007/s11306-007-0082-2.
- Thuijls G, Derikx JP, van Wijck K, Zimmermann LJ, Degraeuwe PL, Mulder TL, et al. Non-invasive markers for early diagnosis and determination of the severity of necrotizing enterocolitis. Ann Surg 2010;251:1174-80. https://doi.org/10.1097/SLA.0b013e3181d778c4.
- Robinson JR, Rellinger EJ, Hatch LD, Weitkamp J-H, Speck KE, Danko M, et al. Surgical necrotizing enterocolitis. Semin Perinatol 2017;41:70-9. https://doi.org/10.1053/j.semperi.2016.09.020.
- Reisinger KW, Derikx JP, Thuijls G, van der Zee DC, Brouwers HA, van Bijnen AA, et al. Noninvasive measurement of intestinal epithelial damage at time of refeeding can predict clinical outcome after necrotizing enterocolitis. Pediatr Res 2013;73:209-13. https://doi.org/10.1038/pr.2012.160.
- Gregory KE, Winston AB, Yamamoto HS, Dawood HY, Fashemi T, Fichorova RN, et al. Urinary intestinal fatty acid binding protein predicts necrotizing enterocolitis. J Pediatr 2014;164:1486-8. https://doi.org/10.1016/j.jpeds.2014.01.057.
- Zamora IJ, Stoll B, Ethun CG, Sheikh F, Yu L, Burrin DG, et al. Low abdominal NIRS values and elevated plasma intestinal fatty acid-binding protein in a premature piglet model of necrotizing enterocolitis. PLOS ONE 2015;10. https://doi.org/10.1371/journal.pone.0125437.
- Young GR, Smith DL, Embleton ND, Berrington JE, Schwalbe EC, Cummings SP, et al. Reducing viability bias in analysis of gut microbiota in preterm infants at risk of NEC and sepsis. Front Cell Infect Microbiol 2017;7. https://doi.org/10.3389/fcimb.2017.00237.
- Frau A, Lett L, Slater R, Young GR, Stewart CJ, Berrington J, et al. The stool volatile metabolome of pre-term babies. Molecules 2021;26. https://doi.org/10.3390/molecules26113341.
- LeBlanc JG, Chain F, Martín R, Bermúdez-Humarán LG, Courau S, Langella P. Beneficial effects on host energy metabolism of short-chain fatty acids and vitamins produced by commensal and probiotic bacteria. Microb Cell Fact 2017;16. https://doi.org/10.1186/s12934-017-0691-z.
- Stewart CJ, Embleton ND, Marrs EC, Smith DP, Nelson A, Abdulkadir B, et al. Temporal bacterial and metabolic development of the preterm gut reveals specific signatures in health and disease. Microbiome 2016;4. https://doi.org/10.1186/s40168-016-0216-8.
- Lönnerdal B, Du X, Jiang R. Biological activities of commercial bovine lactoferrin sources [published online ahead of print July 24 2020]. Biochem Cell Biol 2020. https://doi.org/10.1139/bcb-2020-0182.
- Brink LR, Herren AW, McMillen S, Fraser K, Agnew M, Roy N, et al. Omics analysis reveals variations among commercial sources of bovine milk fat globule membrane. J Dairy Sci 2020;103:3002-16. https://doi.org/10.3168/jds.2019-17179.
- Parc AL, Karav S, Rouquié C, Maga EA, Bunyatratchata A, Barile D. Characterization of recombinant human lactoferrin N-glycans expressed in the milk of transgenic cows. PLOS ONE 2017;12. https://doi.org/10.1371/journal.pone.0171477.
- Newburg DS, Morelli L. Human milk and infant intestinal mucosal glycans guide succession of the neonatal intestinal microbiota. Pediatr Res 2015;77:115-20. https://doi.org/10.1038/pr.2014.178.
- Chaaban H, Markel TA, Canvasser J, Good M. Biobanking for necrotizing enterocolitis: needs and standards. J Pediatr Surg 2020;55:1276-9. https://doi.org/10.1016/j.jpedsurg.2019.05.002.
Appendix 1 Number of infants undergoing 16S ribosomal ribonucleic acid analyses split by site
FIGURE 17.
Number of infants who underwent analyses of microbial communities. (a) Total number of infants, (b) infants who developed NEC and (c) infants who developed LOS. Each panel shows the total number of infants in each group on the left, with the spread of infants across the MAGPIE study consortium NICUs on the right.



Appendix 2 Longitudinal samples and ages per infant stratified by site
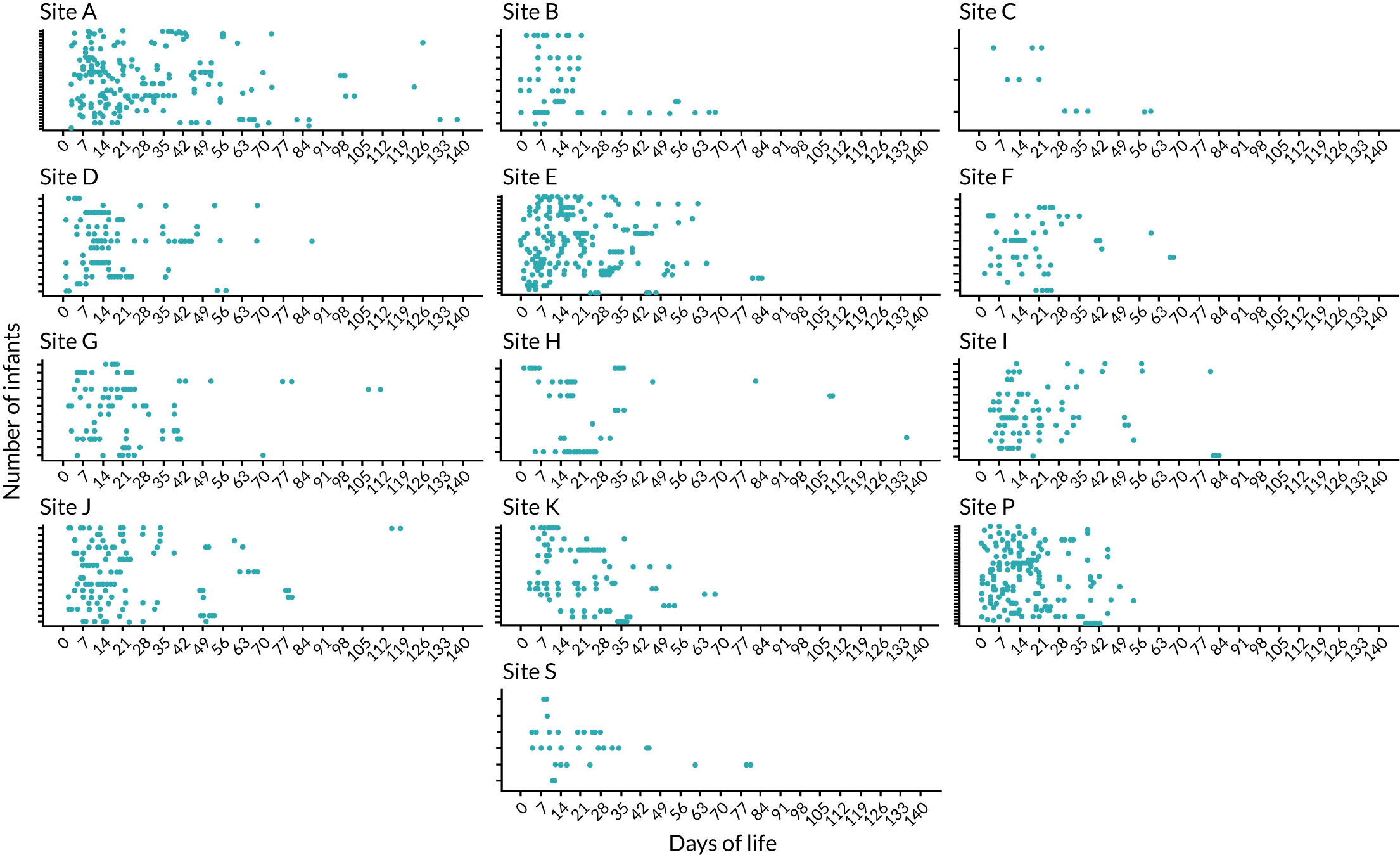
Appendix 3 Box plots of six volatile organic compounds significantly influenced by postnatal age

Appendix 4 Representative haematoxylin and eosin images of controls and necrotising enterocolitis
FIGURE 18.
Representative images of H&E-stained sections from (a) the three infants with SIP. Representative H&E-stained sections of resection specimens from three infants with NEC are shown in (b), (c) and (d). For each NEC infant, left panel = healthy resection margin, centre panel = transition zone and right panel = necrosis. All scale bars represent 50 µm.
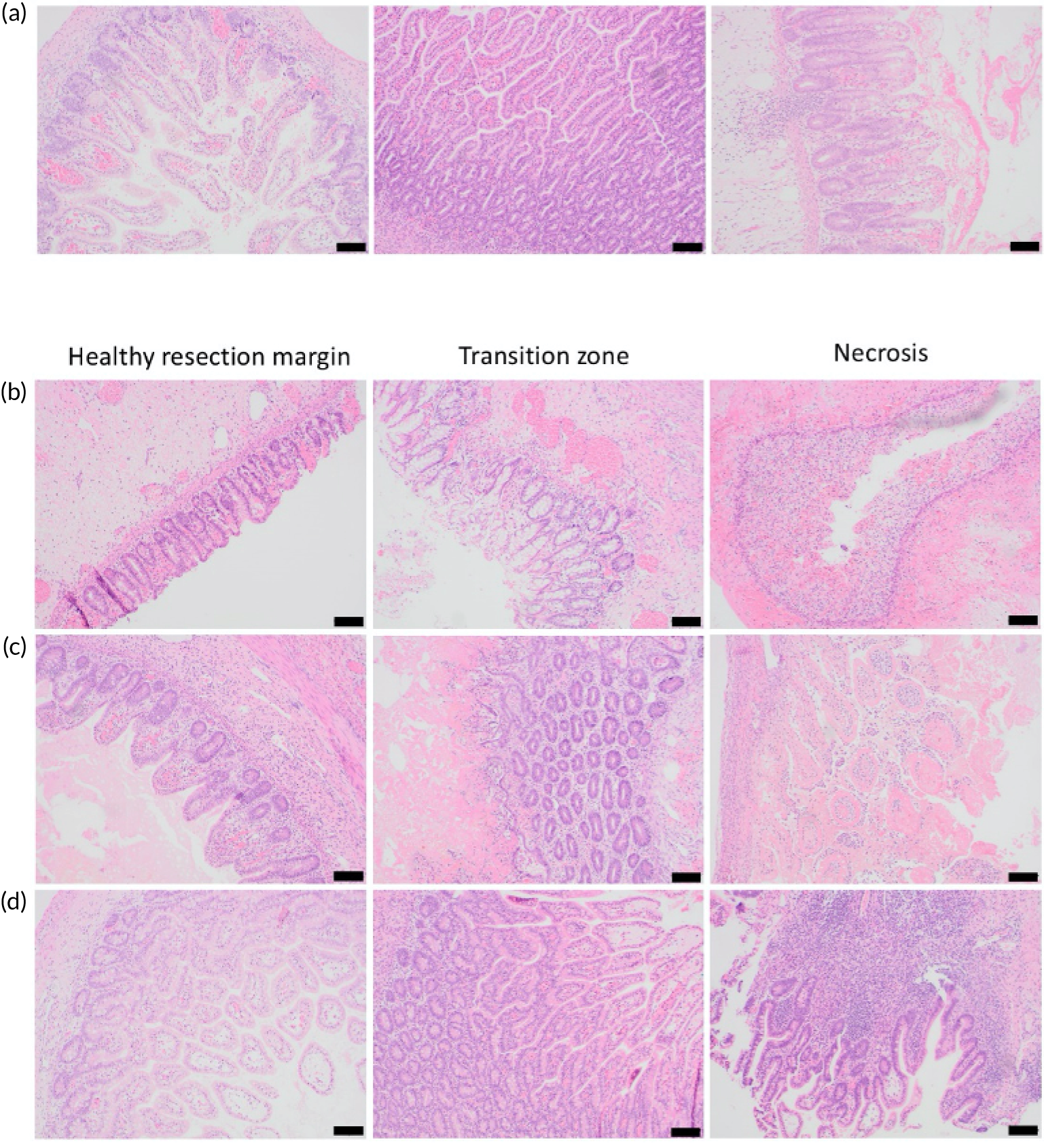
Appendix 5 Recruitment graphs: monthly recruits and per-site breakdown
FIGURE 19.
Anticipated and actual recruitment rates, by time point and site. The dashed line represents the recruitment rate according to protocol, and the solid line represents the actual recruitment rate.

Recruitment
Please note that, originally, 481 infants in total were reported from recruiting centres. We subsequently identified one infant who had been inadvertently recruited when they were transferred to a second NICU (transferred for NEC). The two records were merged. In addition, one further infant was allocated a study number incorrectly (i.e. was not recruited), leaving a total of 479 infants.
Monthly recruitment
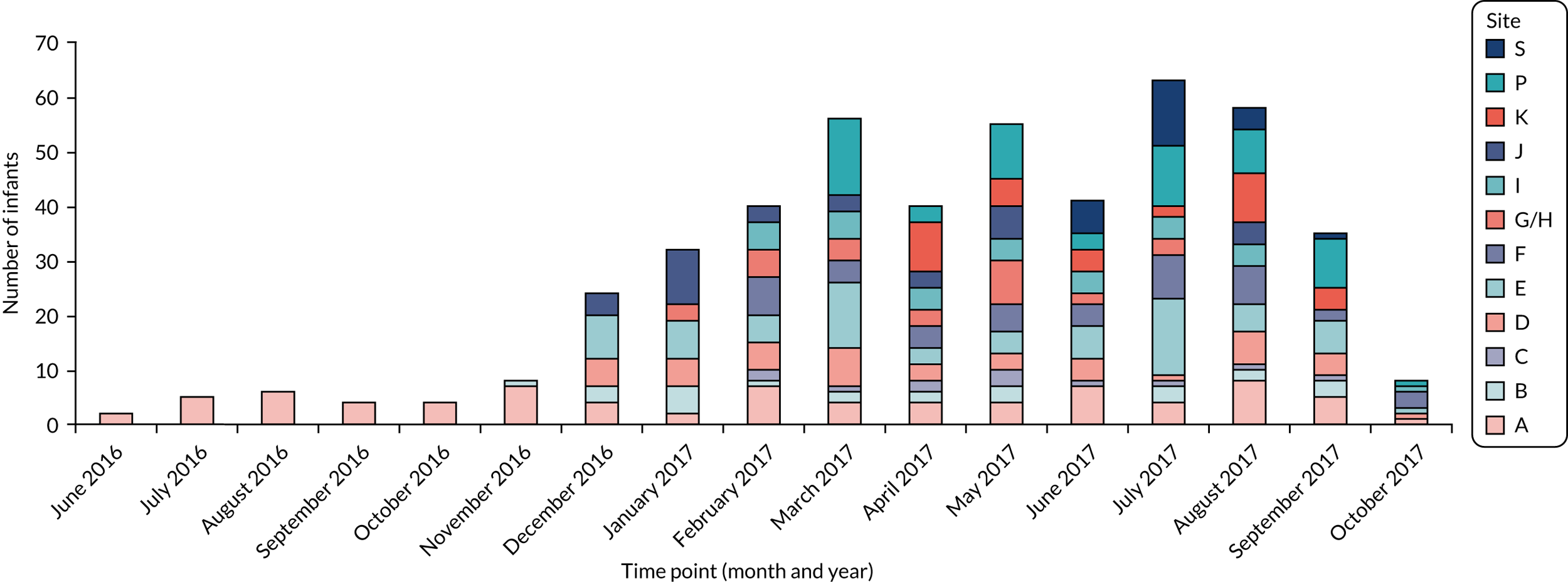
Per-site breakdown
Site code | 2016 (n) | 2017 (n) | Total (N) | |||||||||||||||
---|---|---|---|---|---|---|---|---|---|---|---|---|---|---|---|---|---|---|
June | July | August | September | October | November | December | January | February | March | April | May | June | July | August | September | October | ||
A | 2 | 5 | 6 | 4 | 4 | 7 | 4 | 2 | 7 | 4 | 4 | 4 | 7 | 4 | 8 | 5 | 1 | 78 |
B | 0 | 0 | 0 | 0 | 0 | 1 | 3 | 5 | 1 | 2 | 2 | 3 | 0 | 3 | 2 | 3 | 0 | 25 |
C | 0 | 0 | 0 | 0 | 0 | 0 | 0 | 0 | 2 | 1 | 2 | 3 | 1 | 1 | 1 | 1 | 0 | 12 |
D | 0 | 0 | 0 | 0 | 0 | 0 | 5 | 5 | 5 | 7 | 3 | 3 | 4 | 1 | 6 | 4 | 1 | 44 |
E | 0 | 0 | 0 | 0 | 0 | 0 | 8 | 7 | 5 | 12 | 3 | 4 | 6 | 14 | 5 | 6 | 1 | 71 |
F | 0 | 0 | 0 | 0 | 0 | 0 | 0 | 0 | 7 | 4 | 4 | 5 | 4 | 8 | 7 | 2 | 3 | 44 |
G/H | 0 | 0 | 0 | 0 | 0 | 0 | 0 | 3 | 5 | 4 | 3 | 8 | 2 | 3 | 0 | 0 | 0 | 28 |
I | 0 | 0 | 0 | 0 | 0 | 0 | 0 | 0 | 5 | 5 | 4 | 4 | 4 | 4 | 4 | 0 | 1 | 31 |
J | 0 | 0 | 0 | 0 | 0 | 0 | 4 | 10 | 3 | 3 | 3 | 6 | 0 | 0 | 4 | 0 | 0 | 33 |
K | 0 | 0 | 0 | 0 | 0 | 0 | 0 | 0 | 0 | 0 | 9 | 5 | 4 | 2 | 9 | 4 | 0 | 33 |
P | 0 | 0 | 0 | 0 | 0 | 0 | 0 | 0 | 0 | 14 | 3 | 10 | 3 | 11 | 8 | 9 | 1 | 59 |
S | 0 | 0 | 0 | 0 | 0 | 0 | 0 | 0 | 0 | 0 | 0 | 0 | 6 | 12 | 4 | 1 | 0 | 23 |
Total (monthly) | 2 | 5 | 6 | 4 | 4 | 8 | 24 | 32 | 40 | 56 | 40 | 55 | 41 | 63 | 58 | 35 | 8 | 481 |
Total (cumulative) | 2 | 7 | 13 | 17 | 21 | 29 | 53 | 85 | 125 | 181 | 221 | 276 | 317 | 380 | 438 | 473 | 481 |
List of abbreviations
- ANOSIM
- analysis of similarities
- ANOVA
- analysis of variance
- BERC
- Blinded End-point Review Committee
- BOOST II
- Benefits Of Oxygen Saturation Targeting
- CI
- confidence interval
- CoNS
- coagulase-negative staphylococci
- DNA
- deoxyribonucleic acid
- DNase
- deoxyribonuclease
- DVB-CAR-PDMS
- divinylbenzene-carboxen-polydimethylsiloxane
- ELFIN
- Enteral LactoFerrin In Neonates
- FFPE
- formalin fixed, paraffin embedded
- FWHM
- full width at half maximum
- GC
- gas chromatography
- GCMS
- gas chromatography–mass spectrometry
- H&E
- haematoxylin and eosin
- HRA
- Health Research Authority
- ID
- identification
- iFABP
- intestinal fatty acid-binding protein
- IgA
- immunoglobulin A
- IL
- interleukin
- IMP
- investigational medicinal product
- IQR
- interquartile range
- LCMS
- liquid chromatography–mass spectrometry
- LOS
- late-onset sepsis
- MAGPIE
- Mechanisms Affecting the Gut of Preterm Infants
- MRC
- Medical Research Council
- MS
- mass spectrometry
- MWW
- Mann–Whitney–Wilcoxon
- NEC
- necrotising enterocolitis
- NGS
- next-generation sequencing
- NICU
- neonatal intensive care unit
- NIHR
- National Institute for Health Research
- NPEU
- National Perinatal Epidemiology Unit
- OTU
- operational taxonomic unit
- PCA
- principal component analysis
- PCR
- polymerase chain reaction
- PERMANOVA
- permutational multivariate analysis of variance
- ppm
- parts per million
- QC
- quality control
- RCT
- randomised controlled trial
- RNA
- ribonucleic acid
- RNase
- ribonuclease
- RNAseq
- RNA sequencing
- RR
- risk ratio
- rRNA
- ribosomal ribonucleic acid
- SD
- standard deviation
- SIFT
- Speed of Increasing milk Feeds Trial
- SIP
- spontaneous intestinal perforation
- SOP
- standard operating procedure
- Th1
- T helper type 1 cell
- Th17
- T helper type 17 cell
- TLR
- toll-like receptor
- TNF
- tumour necrosis factor
- TNFα
- tumour necrosis factor alpha
- TNFαIP3
- tumour necrosis factor alpha-induced protein 3
- Tr1
- type 1 regulatory T cell
- Treg
- regulatory T cell
- USG
- urine-specific gravity
- VOC
- volatile organic compound
- WGS
- whole-genome sequencing