Notes
Article history
The research reported in this issue of the journal was funded by the EME programme as project number 11/133/07. The contractual start date was in June 2014. The final report began editorial review in December 2019 and was accepted for publication in December 2020. The authors have been wholly responsible for all data collection, analysis and interpretation, and for writing up their work. The EME editors and production house have tried to ensure the accuracy of the authors’ report and would like to thank the reviewers for their constructive comments on the final report document. However, they do not accept liability for damages or losses arising from material published in this report.
Permissions
Copyright statement
© Queen’s Printer and Controller of HMSO 2021. This work was produced by Beardsall et al. under the terms of a commissioning contract issued by the Secretary of State for Health and Social Care. This issue may be freely reproduced for the purposes of private research and study and extracts (or indeed, the full report) may be included in professional journals provided that suitable acknowledgement is made and the reproduction is not associated with any form of advertising. Applications for commercial reproduction should be addressed to: NIHR Journals Library, National Institute for Health Research, Evaluation, Trials and Studies Coordinating Centre, Alpha House, University of Southampton Science Park, Southampton SO16 7NS, UK.
2021 Queen’s Printer and Controller of HMSO
Chapter 1 Introduction
Parts of this report have been reproduced with permission from Thompson et al. 1 and Beardsall et al. 2–4 in accordance with the terms of the Creative Commons Attribution (CC BY 4.0) license, which permits others to distribute, remix, adapt and build upon this work, for commercial use, provided the original work is properly cited. See: http://creativecommons.org/licenses/by/4.0/. The text includes minor additions and formatting changes to the original text.
Increasing numbers of infants are being born preterm. These infants require intensive care and have a high risk of early mortality and short-term morbidity. 5 Among surviving infants, the incidence of long-term health problems, including learning difficulties, is high, with significant long-term costs to the NHS and society. 6 Treatable neonatal causes of long-term health problems have been difficult to establish. National priorities for research include investigation of the management of infants who are born too early or too small, and evaluation of the reasons for variations in outcome of ‘high-risk’ neonates. Early postnatal glucose control may be an important and modifiable risk factor for clinical outcomes. In utero, glucose levels are normally maintained between 4 and 6 mmol/l,7 but infants born preterm are at risk of both hyperglycaemia (20–86%, depending on how it is defined) and hypoglycaemia (17%; blood glucose level < 2.6 mmol/l). 8
Existing research
Hyperglycaemia and hypoglycaemia in the preterm infant
Hyperglycaemia and hypoglycaemia have both been associated with increased mortality and morbidity for preterm infants. 9,10 Hyperglycaemia can lead to acute problems of persistent osmotic diuresis and metabolic acidosis, which can be difficult to control, and has been associated with increased risk of intraventricular haemorrhage. 11 Hyperglycaemia has also been associated with increased long-term morbidity, including increased risk of retinopathy of prematurity (ROP). 9,12,13 Pivotal single-centre studies in adult intensive care have demonstrated that tight glycaemic control can reduce both mortality and morbidity. 14 However, these results have been difficult to replicate because of the risk of hypoglycaemia. 15 A trial in paediatric intensive care, targeted to reduce hyperglycaemia, demonstrated a reduction in length of intensive care admission and mortality, but a significant increase in hypoglycaemia. 16 This is of particular concern as attempts to reduce the prevalence of hyperglycaemia may increase the risk of hypoglycaemia in preterm infants because of their variable sensitivity to insulin. In addition, the developing brain appears to be particularly vulnerable to both hyperglycaemic17 and hypoglycaemic insults. A Cochrane review9 has highlighted the need for further studies into the impact of interventions to improve glucose control in these infants.
Current glucose monitoring in neonatal intensive care
Although glucose monitoring is implemented in all very preterm infants, it is currently limited to intermittent blood sampling, with long periods when glucose levels are unknown. The reason for the intermittent nature of glucose monitoring is that current methodologies for measurement of glucose levels are dependent on blood sampling either from a central arterial line or by heel prick. The very small circulating volumes of blood in preterm infants mean that it is also important to minimise the number of blood samples and volume of blood sampled in this way. Furthermore, current practice in neonatal intensive care units (NICUs) aims to reduce the frequency of handling of infants, as this has been shown to improve outcomes. 18 By contrast, other physiological parameters, such as oxygen saturation, blood pressure and heart rate, are all monitored continuously to prevent wide fluctuations. It is increasingly thought that fluctuations in glucose levels may also have a significant impact on long-term outcomes. 19
If clinical interventions to optimise glucose control are to be safe and effective in the intensive care setting, robust methods of monitoring glucose levels in real time need to be in place. Furthermore, to fully understand the clinical significance of hyperglycaemia and hypoglycaemia, it is imperative that robust data are available regarding their true prevalence throughout the period of intensive care. Current follow-up studies are dependent on infrequent measurements of blood glucose, typically taken for clinical reasons and with the inherent associated bias. Continuous glucose monitoring (CGM) therefore has the potential to both safely guide acute clinical management to optimise control and provide robust data on which to assess the long-term impact.
Continuous glucose monitoring
A range of technological methods have been tested for their abilities to support CGM. Currently, the only CGM method used in clinical practice for the management of patients with diabetes involves measurement of interstitial glucose levels, which are calibrated against blood glucose measurements. CGM devices comprise a disposable subcutaneous oxidase-based platinum electrode that catalyses interstitial glucose, generating an electrical signal that is transmitted to a monitor for recording or display. Three companies have devices on the market for CGM: Dexcom Inc. (San Diego, CA, USA), Abbott Laboratories UK (Berkshire, UK) and Medtronic plc (Watford, UK). However, because of the size of extremely preterm infants and issues of insertion and attachment to these small infants, only the Medtronic plc devices are currently suitable for use in the extremely preterm infant.
Although this is a rapidly changing field, early CGM devices were used to collect data in real time, but these data could be downloaded only retrospectively to be reviewed and used to guide future clinical treatment. This is useful (1) in the setting of research, (2) to provide advice on long-term behavioural or medical interventions and (3) in the case of patients with stable diabetes, to review patterns of glucose control over time. These devices have also been used in preterm infants without side effects and identified significant periods of both hyperglycaemia and hypoglycaemia that were undetected clinically. 20–22 However, they are not suitable for providing data that is useful for acute clinical management. Newer CGM devices allow the data to be viewed in real time, providing information on glucose trends, with the potential to identify episodes of hyperglycaemia and hypoglycaemia, thus allowing the possibility of earlier intervention and prevention.
Development of continuous glucose monitors
The development of CGM devices provides the opportunity for data on glucose levels to be recorded every 5 minutes and viewed continuously. A number of different devices are available for clinical use and they are increasingly found to help reduce glycated haemoglobin and glycaemic variability in patients with type 1 diabetes without increasing the risk of hypoglycaemia. These devices allow patients to monitor their glucose levels by displaying absolute glucose concentrations as well as trends. Some systems depend on intravenous access, some use microdialysis and others measure interstitial glucose levels. Their use has been trialled in adults in intensive care and in patients requiring cardiac surgery. 23 Although these studies have been limited, they have shown that the devices are accurate and safe in cardiac surgical patients and can reduce the risk of hypoglycaemia in adults in intensive care. 24 Some have raised concerns about the lack of absolute sensitivity of individual readings at glucose thresholds, emphasising the potential importance of CGM as an early-warning system for both hyperglycaemia and hypoglycaemia. 25
These studies have highlighted the need for methodologies that can provide real-time data and allow adjustment of clinical management in the setting of a rapidly changing clinical picture of intensive care. 26,27 These benefits have been seen in the adult intensive care setting, where blood glucose levels are routinely measured much more frequently than in NICUs. Therefore, the potential for benefits in the NICU setting, where the frequency of blood glucose measurements is much lower, is likely to be more clinically significant. Key recent developments in these devices include extended sensor life, such that sensors can now remain in situ for 6 days (previously 72 hours), and changes to the sensor construction and the calibration algorithms, which have led to improved overall accuracy. 28 This is particularly important in terms of sensitivities to detect threshold levels of hypoglycaemia and hyperglycaemia.
Previous use of continuous glucose monitors in neonates
There is limited use of CGM in NICUs. We have used blinded CGM devices as part of an international multicentre trial in preterm infants and assessed the accuracy of these devices compared with current clinical practice. 29,30 These studies suggested that the value of CGM could be in providing early warning of fluctuations in glucose levels to guide the need for blood glucose assessment. In addition, by providing a continuous readout, episodes of hyperglycaemia and hypoglycaemia could be anticipated and therefore avoided. We have previously collected ‘blinded’ CGM data as part of the Neonatal Insulin Therapy [Nirture (Neonatal Insulin Replacement Therapy in Europe Study)] trial using a CGM (Medtronic plc)21 and in a small feasibility study using the iPro™2 (Medtronic plc). In the small feasibility study of 10 infants with a birthweight ≤ 1200 g monitored from birth for up to 7 days, infants had a median gestational age at birth of 28.4 weeks and a median birthweight of 840 g. The median time in the target glucose range of 2.6–10 mmol/l was 58% and the median time in the target glucose range of 4–8 mmol/l was 40%.
The REACT project
The purpose of the REACT project was to evaluate the role of CGMs in the detection and management of hyperglycaemia and hypoglycaemia in NICUs. The project was funded by the National Institute for Health Research Efficacy and Mechanism Evaluation programme, with additional support of CGM equipment provided by Medtronic plc and sponsorship from the University of Cambridge Addenbrookes Hospital NHS Trust (Cambridge, UK). There were three stages:
-
to undertake a single-centre feasibility study to inform the design of a multicentre randomised controlled trial (RCT)
-
to evaluate the clinical role of CGMs, in terms of efficacy, safety, utility and cost-effectiveness, through a multicentre RCT
-
to pilot the potential use of a closed-loop system for glucose control using CGMs.
Chapter 2 Feasibility study
Aim
The aim of the study was to carry out preliminary evaluation of feasibility of real-time CGM to inform the design of a larger RCT in the preterm population.
Objectives
The objectives of the feasibility study were to determine provisional data regarding:
-
recruitment potential for a RCT
-
accuracy and safety of the devices for use in the preterm population
-
user acceptability, including the impact on clinical care and frequency of blood glucose testing
-
prevalence of the anticipated primary outcome measure (i.e. time in glucose target range of 2.6–10 mmol/l).
Methods
Study design
This was a single-centre study, with infants recruited from the NICU at Addenbrooke’s Hospital, Cambridge, UK. Ethics and trust research and development approval were obtained prior to the start of study recruitment, and informed parental consent was obtained prior to any study procedures. Inclusion criteria were infants with a birthweight ≤ 1200 g and aged ≤ 48 hours for whom informed parental consent had been received. Infants were excluded if they had a major congenital malformation or an underlying metabolic disorder, or if the mothers had a pregnancy complicated with diabetes. The intervention lasted for up to 7 days. Data regarding glucose levels, clinical condition and nutritional intake, as well as insulin use, were collected prospectively.
Continuous glucose monitoring and management
Real-time CGM was performed using the Paradigm® Veo™ system (Medtronic plc) (Figure 1). Enlite™ sensors (Medtronic plc) were linked to the Paradigm® Veo™. The sensor glucose data could be viewed in real time and were used in conjunction with the guideline to support clinical management. The guideline provided simple guidance, was not a rigid guideline and had not undergone formal in silico testing. The nurses recorded the sensory glucose value, alongside standard hourly clinical observation, using it to guide the need for blood glucose testing. The guideline prompted review and intervention based on both absolute glucose levels and change. The CGM devices were calibrated at least twice daily using a blood glucose measured on the point-of-care (POC) StatStrip® meter (Nova Biomedical, Waltham, MA, USA). The StatStrip meter was chosen because it has been validated for accuracy in the newborn and intensive care settings.
FIGURE 1.
Continuous glucose monitoring system. (a) Enlite sensor; (b) Paradigm Veo and MiniLink® REAL-Time Transmitter (Medtronic plc) attached to a sensor; and (c) preterm infant with device in situ. Reproduced with permission from Thomson et al. 1 This is an Open Access article distributed in accordance with the terms of the Creative Commons Attribution (CC BY 4.0) license, which permits others to distribute, remix, adapt and build upon this work, for commercial use, provided the original work is properly cited. See https://creativecommons.org/licenses/by/4.0/. The figure includes minor additions and formatting changes to the original figure.
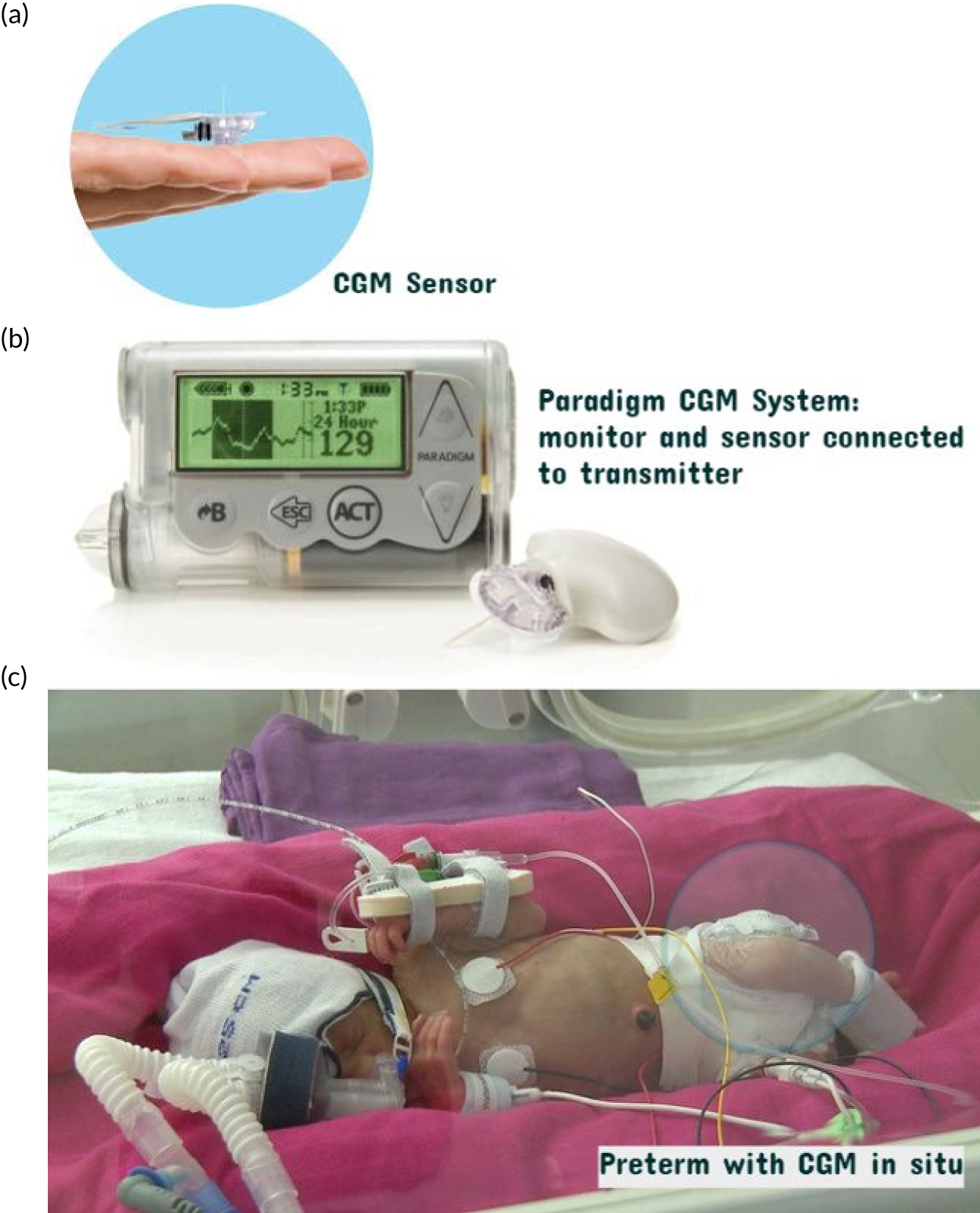
Blood glucose monitoring
Blood glucose levels were measured using a combination of arterial, venous and capillary samples, and were tested on the blood gas analyser (cobas b 221; Roche Diagnostics, Welwyn Garden City, UK) and the Nova StatStrip.
Analyses
Accuracy of the continuous glucose monitor
Prespecified comparative analyses were based on any glucose levels that were recorded within 5 minutes of each other. The median relative difference was calculated as the percentage difference between the two measures. Absolute differences were determined at each time point in terms of compliance with International Organization for Standardization (ISO) 2003 and 2013 standards. 31,32 Bland–Altman analysis was used for assessment of error between glucose measurements and error grid plots were used to explore potential clinical impact.
Safety of the continuous glucose monitor
Safety outcomes were defined as prevalence of hypoglycaemia (percentage of time with sensor glucose level < 2.6 mmol/l) and any single blood glucose reading of < 2.6 mmol/l, and/or more than six sensor glucose readings of < 2.6 mmol/l (i.e. > 30 minutes).
Utility
The clinical care team were invited to complete a comments sheet daily for immediate feedback, as well as an anonymised questionnaire for summative review. The questionnaires explored initial expectations and experiences of using the CGM. These questionnaires were developed in collaboration with nurses on the unit to ensure that questions were easy to understand and relevant to the individuals concerned.
Efficacy outcome
Predefined efficacy outcomes included time in target range (i.e. percentage of time with a sensor glucose level 2.6–10 mmol/l or 4–8 mmol/l), prevalence of hyperglycaemia (i.e. percentage of time with a sensor glucose level > 10 mmol/l) and prevalence of severe hyperglycaemia (i.e. percentage of time with a sensor glucose level > 15 mmol/l).
Results are expressed as mean [standard deviation (SD)], median [interquartile range (IQR)] or frequencies (percentages), as appropriate.
Results
Recruitment
Twenty-one infants were successfully recruited to the feasibility study. One infant was withdrawn because of failure of sensor insertion. The baseline demographic details of the remaining 20 infants with CGM data are shown in Table 1. The recruitment rate fluctuated widely from month to month and was delayed by two factors: (1) availability of eligible infants to approach and (2) availability of appropriately trained staff to undertake the study procedures. During the recruitment period, the NICU underwent a period of reduced admissions associated with the introduction of a new electronic medical records system, resulting in fewer infants being admitted and available for recruitment. In addition, sensor insertion was initially limited to key study staff to facilitate a core of experience within the team. However, the unpredictability of preterm deliveries, combined with the need for many mothers to be transferred from other hospitals, resulted in a very small window for recruitment and meant that staff with the key skills for sensor insertion were not always available.
Baseline demographic data | Feasibility study (N = 20) |
---|---|
Gestational age at birth (weeks), mean (SD) | 26.14 (1.9) |
Birthweight (g), mean (SD) | 809 (156) |
Sex (male-to-female) | 10 : 10 |
Antenatal variable, n (%) | |
Antenatal steroids | 19 (95) |
Maternal smoking | 5 (25) |
Chorioamnionitis | 1 (5) |
PROM | 6 (30) |
Hypertension | 1 (5) |
Accuracy of continuous glucose monitors
Comparative data providing more than two glucose measurements (blood glucose or sensor glucose) taken within 5 minutes of each other were available at 247 time points. Bland–Altman analyses provided a mean bias between POC and CGM of –0.27 [95% confidence interval (CI) –0.35 to –0.19] (Figure 2). Error grid analyses (comparing sensor glucose with either blood glucose methodology) demonstrated that 98% of values lay within area A or B (see Figure 2). Performance in relation to the ISO 200331 and ISO 201332 standards is provided in Table 2.
FIGURE 2.
Feasibility study: Bland–Altman and error grid plots. 33 (a) Bland–Altman comparison of CGM sensor glucose with StatStrip meter; (b) error grid plot comparing CGM sensor glucose with StatStrip meter or blood gas (cobas b 221) glucose values; (c) Bland–Altman comparison of POC StatStrip meter with blood gas (cobas b 221) glucose values; (d) error grid plot comparing POC StatStrip meter with blood gas (cobas b 221) glucose values; (e) Bland–Altman comparison of CGM sensor glucose with blood gas (cobas b 221) glucose values; and (f) error grid plot comparing CGM sensor glucose with blood gas (cobas b 221) glucose values. BG, blood glucose; SG, sensor glucose. Orange dots represent outliers whose results were reviewed and were deemed to be secondary to pre-analytical error in blood glucose sampling. The reference lines show the estimate of the mean difference, 95% CIs around the mean estimate and the predictive interval, the region in which a new observation would be expected, with 95% confidence, to be observed. Reproduced with permission from Thomson et al. 1 This is an open access article distributed in accordance with the Creative Commons Attribution (CC BY 4.0) license, which permits others to copy, redistribute, remix, transform and build upon this work for any purpose, provided the original work is properly cited, a link to the licence is given, and indication of whether changes were made. See: https://creativecommons.org/licenses/by/4.0/. The figure includes minor additions and formatting changes to the original figure.



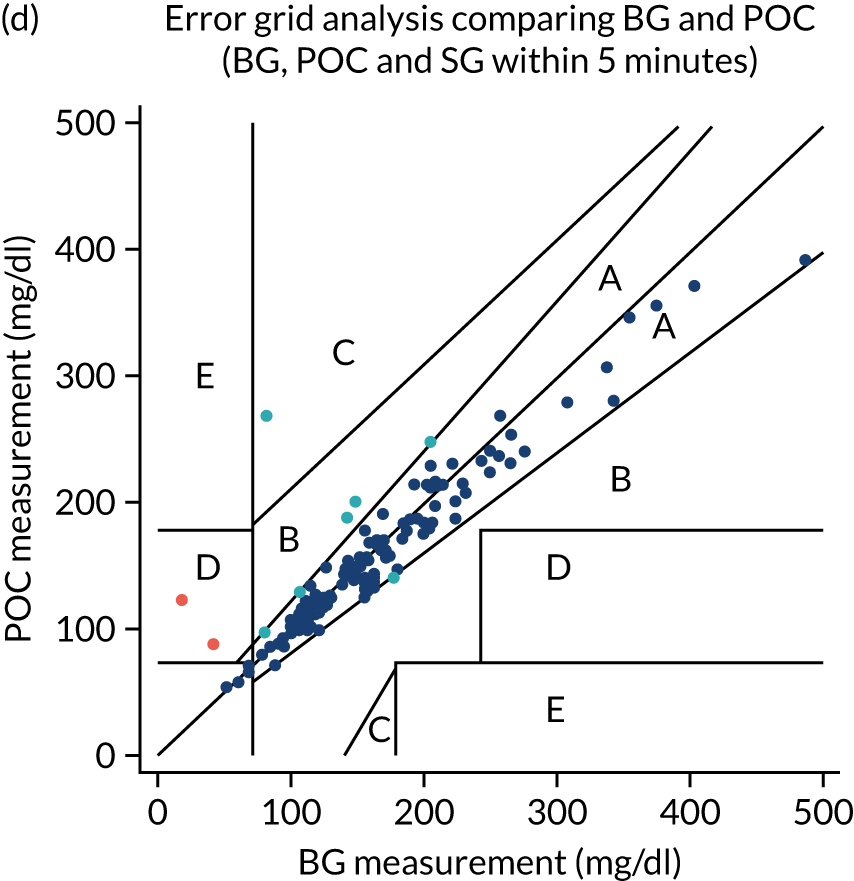
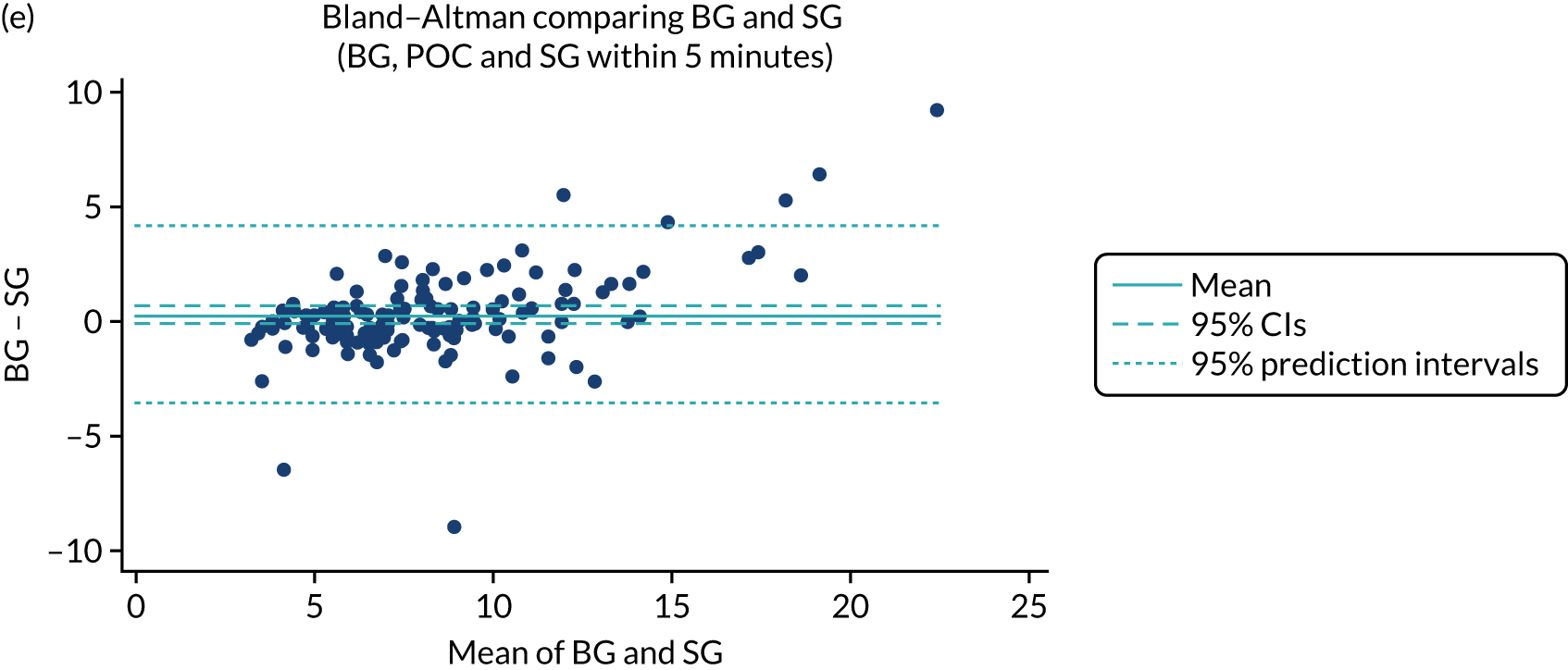

Standard | Criterion | Blood glucose (%) | POC (%) |
---|---|---|---|
MARD | Per cent difference between sensor glucose and reference (sensor glucose – reference)/reference × 100 | 11 | 10 |
ISO 200331 | Per cent of sensor glucose values within ± 20% of the blood glucose value | 84 | 90 |
ISO 201332 | Per cent of sensor glucose values within ± 0.83 mmol/l of blood glucose value | 73 | 78 |
Safety
There were no serious adverse device effects (SADEs). One infant died prior to discharge from hospital, but after the intervention period. Death was due to prematurity and felt to be unrelated to the study intervention. One infant was withdrawn from the study early as the mother became acutely unwell and, in the light of the mother’s mental health, it was felt appropriate to withdraw the infant from the study. One infant was withdrawn because of failure of sensor insertion. This was the second infant recruited to the study. The Enlite sensor failed to insert on two attempts and remained within the needle guard. There were no concerns about infection or trauma at the site of sensor insertion and the CGM device did not interfere with clinical care.
There were three infants who had clinically documented episodes of hypoglycaemia, defined as any blood glucose measurement < 2.6 mmol/l. In each of these infants, the falling sensor glucose level had prompted action to be taken to check the blood glucose level. There were no concerns about the infants during these episodes and without the falling sensor glucose level the blood glucose level would not have been checked. After confirming a blood glucose level < 2.6 mmol/l, these infants were treated with intravenous dextrose. In two cases, the falling sensor glucose level also revealed failure of central access. In two of these episodes, sensor glucose values were also < 2.6 mmol/l (recorded on the CGM devices as episodes lasting 40 and 25 minutes, respectively). In the other case, the lowest sensor glucose value was 2.7 mmol/l. There was one further episode when the sensor glucose fell to < 2.6 mmol/l for 70 minutes, but this was not documented clinically because of a protocol deviation, as blood glucose was not measured at this time. There were no concerns about any of the infants during any of these episodes and the infants were not receiving any insulin.
Utility
The overall assessment of the CGM devices by research and clinical staff was that they were easy to use in terms of insertion and calibration. There were occasions when there was loss of connectivity between the sensor and the monitor (e.g. if an infant was moved out of an incubator for kangaroo care or someone stood between the infant and the monitor). On such occasions, it was evident that the transmitter range was limited if transmission was blocked by objects between the sensor and the monitor. This could be resolved by moving the monitor closer to the infant. Staff found the use of both predictive and threshold alarms challenging, considering them an unnecessary addition to recording sensor glucose levels hourly. As a result of frequent requests to silence these alarms, they were subsequently turned off in the early stages of the study to ensure continued staff engagement.
With a need for twice-daily calibrations, the median number of blood samples taken for glucose measurement per 24-hour period did not appear to be excessive or significantly different from the number of samples taken in other infants of similar gestational and postnatal age on the unit. The median of the mean number of samples per day per infant was 4.1 (range 3.3–4.3).
Staff expectations varied greatly, with some nurses excited about the potential to limit the need for frequent blood glucose sampling and avoiding ‘hurting the baby’; however, others had concerns about increased workload, sensor insertion, risk of ‘tissue damage’ or difficulties in positioning an infant following sensor insertion. Comments varied from ‘exciting’ to ‘how much extra time will it take up?’. After caring for an infant using CGM, there was an over-riding view that the intervention improved the quality of care (Figure 3). Comments included ‘found the monitor to be useful when the infant was on insulin’, ‘the chart . . . is really useful’, ‘I think this is the best treatment’ and ‘it is not extra workload’. Nursing staff reported positively about the impact on care in terms of feeling more empowered to manage the infant’s glucose control. They did not report any significant negative effects for the infant or the clinical/nursing team caring for the infant.
FIGURE 3.
Feasibility study: staff assessment of the use of continuous glucose monitoring. 1 (a) Do you think the baby is distressed?; (b) How did you find the monitor to read and calibrate?; (c) Do you think the device has interfered with the nursing care?; (d) Did having the baby on the monitor significantly affect your workload?; and (e) Did you think that being able to monitor glucose continuously had an impact on clinical care? Data are presented as a per cent of the responses received. Reproduced with permission from Thomson et al. 1 This is an open access article distributed in accordance with the Creative Commons Attribution (CC BY 4.0) license, which permits others to copy, redistribute, remix, transform and build upon this work for any purpose, provided the original work is properly cited, a link to the licence is given, and indication of whether changes were made. See: https://creativecommons.org/licenses/by/4.0/. The figure includes minor additions and formatting changes to the original figure.




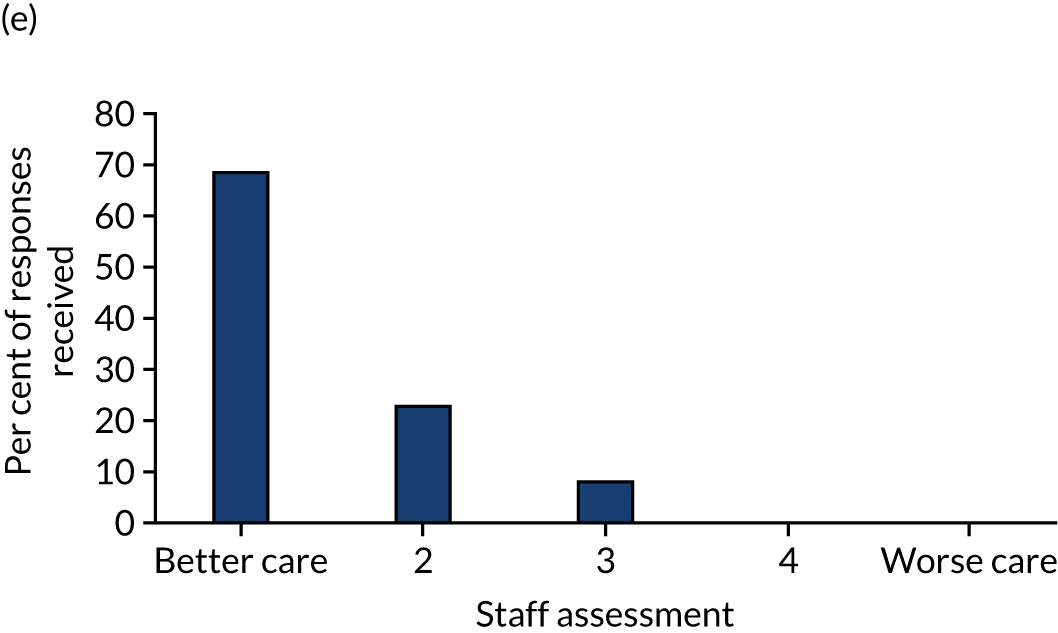
Efficacy
A total of 2626 hours of CGM data were collected from 20 infants, with a median for individual infants of 144 (range 7–166) hours. There was wide variability in glucose control in this population. Data demonstrating the percentage of time within different target thresholds are provided in Table 3. The median percentage of time in the target range of 2.6–10 mmol/l was 78% (IQR 59–94%), with values > 10 mmol/l recorded 22% of the time.
Outcome | Value (n = 20) |
---|---|
Percentage of time sensor glucose in range | |
2.6–10.0 mmol/la | 78 (59–94) |
4.0–8.0 mmol/l | 46 (35–66) |
> 10.0 mmol/l | 22 (6–41) |
< 2.6 mmol/l | 0 (0–0) |
Mean sensor glucose (mmol/l) | 8.3 (7.4–9.4) |
SD of sensor glucose (mmol/l) | 2.39 (1.77–3.67) |
Hypoglycaemia (sensor data) | |
Number of infants with more than one episode of hypoglycaemia | 2 |
Number of episodes of hypoglycaemia | 2 |
Total length of episodes (minutes) | 40–70 |
Blood glucose (< 2.6 mmol/l) | |
Number of episodes of hypoglycaemia | 3b |
Discussion
This study was the first to explore the potential role of ‘real-time’ CGM to support targeting of glucose control, with guidance on insulin and glucose delivery in preterm infants. The feasibility study highlighted the challenges of recruiting to a complex interventional study when recruitment has to occur at unpredictable times and within a very short time frame. The devices were found to be sufficiently accurate to guide clinical management and the sensors were well tolerated, despite the infants’ low birthweight, limited subcutaneous tissue and potential risk of infection. Furthermore, the staff felt that the intervention led to improved care.
Recruitment within the feasibility study was noted to be onerous to the research team. This was related to the unpredictability of infants being delivered, combined with the tight time frame. The feasibility study also showed that the burden of recruitment, combined with a complex intervention, was likely to present considerable challenges to the delivery of a multicentre trial. In most units, key staff are usually trained to undertake consent for trials, but interventions such as a change in feeding practice or even investigational medicinal product delivery can be undertaken by core clinical staff. As a device study, identifying the key skill sets needed to undertake the study and the optimal number of staff needed to support recruitment was seen as critical. There was a need for staff with the key skills for sensor insertion and who were able to guide the clinical team regarding the protocol to be available 24 hours a day, 7 days a week, for optimal recruitment. This informed the development of training packages for unit staff, including a video guide to sensor insertion and packages of example cases for the use of the guideline for glucose control and further informed the plans for the subsequent RCT.
The data showed the CGM devices to be of comparable accuracy to the POC devices that are currently used in clinical practice. The median absolute relative difference was similar to that reported in other paediatric and intensive care studies. 34,35 There were episodes when only one of sensor glucose or blood glucose level reached the threshold for hypoglycaemia, but the trend in falling sensor glucose level had prompted the measurement of blood glucose. The blood glucose level would not have been checked if the sensor had not triggered a clinical concern and the episode of hypoglycaemia would have been clinically undetected. Differences may, in part, be related to the physiological differences between blood and interstitial glucose levels, particularly with rapidly changing blood glucose levels. 36
There was no increase in risk of hypoglycaemia with a more proactive approach to management of hyperglycaemia, that is when using the guideline alongside the real-time monitoring. This is in contrast to previous attempts to target glucose control with insulin in adult or neonatal intensive care, which have resulted in significant increases in the prevalence of hypoglycaemia. 15 In this study, use of CGM devices provided an opportunity to track changes in glucose control in real time. This was important in providing guidance to clinical staff about glucose management in a population, that is infants in the NICU, in whom drug infusions (both types of drugs and dosing), as well as insulin sensitivity and secretion, change both frequently and rapidly, leading to a risk of hypoglycaemia. This was highlighted by the fact that two infants experienced hypoglycaemic episodes (related to loss of central access) that first became apparent because of the falling sensor glucose value.
The aim was not for CGM to replace blood glucose sampling, but to augment it, and the number of blood glucose samples taken in these infants was comparable to the number of samples taken from other infants of a similar gestational and postnatal age within the unit. This is in keeping with the advice given to the clinical nurses during the study, which was to check blood glucose values before modifying any aspect of the management of glucose control. For example, changes in insulin dosing were undertaken only in response to a persistent rising glucose level on the CGM device and supported by a confirmatory blood glucose measurement. During the study, however, nursing staff became more confident in the accuracy of the sensor glucose values and, with the guideline, they began to change dosing based on sensor glucose values alone. They began to use blood glucose values in a more proactive way to confirm observed trends in the CGM. Increasing confidence in the accuracy of CGM devices and the desire to limit handling of preterm infants may lead to reduced blood glucose sampling in the future.
One infant (the second infant recruited) was withdrawn from the study because of failure on two occasions to successfully place a sensor. For this infant, on both occasions, the sensor remained within the needle guard and did not insert into the thigh. This event had been previously reported in paediatric and adult patients, but had not been experienced by the research team with previous Softsense sensors (Medtronic plc). On reviewing the potential causes, the advice on insertion technique was modified to focus on ensuring that the needle and sensor remained at 90° to the hub until after the introducer needle was removed. There were no further occurrences during the feasibility study. The sensor appeared to be safe, with no reports of infection or debridement of skin at the sensor site in this preterm population.
The lack of benefit from predictive trend alarms was disappointing, but it may be that with more experience the alarms could be used. However, the system was reported by the nursing staff to be beneficial to clinical care. The clinical team reported feeling that the combination of the CGM device and guideline was useful in caring for the infant.
We chose a strategy that aimed to optimise nutritional intake, using the intervention to inform decisions on insulin and glucose therapy in the event of hyperglycaemia or hypoglycaemia. Other strategies can also be used to target glucose control in preterm infants, each with different risks and benefits. One such alternative strategy, that of reducing parenteral intake, risks compromised nutritional delivery, as found in a recent study in preterm infants using CGM and a computer algorithm to modify glucose intake in response to hyperglycaemia. 37 Our study is unique in combining the use of a CGM with a guideline for the use of insulin and glucose therapy. This design is easy to adopt in clinical practice as it does not involve the need for frequent changes to parenteral nutrition, which may affect nutritional and electrolyte delivery, as well as staff workload and cost. Moreover, we have shown in this feasibility study that this strategy does not increase the risk of hypoglycaemia.
The efficacy outcome of percentage of time with glucose level in the target range (i.e. 2.6–10 mmol/l) was reviewed by the trial statistician to inform power calculations for the planned multicentre RCT of real-time CGM compared with standard care. The data were used to confirm sample size considerations based on a final analysis comparing the percentage of time in the target range (2.6–10 mmol/l), that is a continuous variable, in terms of the mean values between the treatment arms. Based on these data, the SD of this end point was conservatively estimated at 22%; hence, a sample size of 200 infants would provide 90% power to detect a difference of 10% at the 5% significance level. Consensus was reached among expert opinion at the Trial Steering Committee (TSC) and Data Monitoring Ethics Committee (DMEC) meetings that a 10% difference would be clinically meaningful. The comparison between the mean values in Table 3 provides support to an effect size of 10%, or more being plausible.
Summary
The feasibility study confirmed reasonable accuracy and utility of the combination of CGM plus the guideline for blood glucose testing and subsequent intervention within a clinical trial. It further informed the need to innovate to maximise recruitment potential and staff engagement, while providing data to substantiate the power calculations for the primary outcomes within such a trial.
Chapter 3 REACT trial design
Introduction
Data from the feasibility study helped to design this RCT. Those involved in recruitment pointed out that the short time period from birth to study entry was a challenge and, therefore, an online training video was developed to enable training of a larger number of staff in recruiting sites to support recruitment and delivery of the intervention. There were no safety flags in terms of the CGM device itself. In the context of using CGM as an adjunct to current methods of blood glucose monitoring, CGM was confirmed to be sufficiently accurate to safely support glucose management. Provisional data on the combination of the CGM and guideline to guide targeting of glucose control did not give rise to any safety concerns in terms of risks of hypoglycaemia. Data on the prevalence of the anticipated primary outcome measure of time in glucose target range (i.e. 2.6–10 mmol/l) confirmed an estimated SD of 22%, which was in keeping with the planned sample size of 200 infants. Furthermore, the staff questionnaire demonstrated that the clinical team caring for infants felt that CGM has the potential to improve clinical care.
Aim
To evaluate the clinical role of CGM in the preterm infant, in terms of efficacy, safety, utility and cost-effectiveness, through a multicentre RCT.
Hypothesis
We hypothesised that the use of real-time CGM could increase the amount of time that an infant’s glucose levels (measured using CGM) are within the target range of 2.6–10 mmol/l (i.e. the widely accepted clinical target for glucose control) compared with standard clinical practice (with blinded CGM data collection).
Trial objectives
The primary efficacy outcome was percentage of time for which glucose levels were within the glucose target range of 2.6–10 mmol/l over 6 days, following recruitment within 24 hours of birth.
Secondary outcomes related to:
-
efficacy (i.e. mean sensor glucose level, percentage of time sensor glucose levels are in the target range of 4–8 mmol/l, sensor glucose level variability, percentage of time sensor glucose levels are > 15 mmol/l)
-
safety, as measured by both device effects and incidence of hypoglycaemia
-
utility assessed by staff and parent questionnaires
-
cost-effectiveness
-
exploratory analyses.
Methods
Study design
This study was an international open-label parallel-group RCT, with infants recruited between July 2016 and January 2019 from 13 NICUs in the UK, Spain and the Netherlands. The protocol has been published. 2
Eligibility criteria
Infants were eligible if they were within 24 hours of birth, weighed ≤ 1200 g and were born at ≤ 36+6 weeks gestation, and if written parental consent had been obtained. Exclusion criteria included any lethal congenital malformations and any congenital metabolic disorder known at trial entry. Infants were also ineligible if, in the opinion of the treating clinical consultant, they had no realistic prospect of survival.
Recruitment procedure
All infants were recruited within 24 hours of birth at one of the NICUs that had been approved for study participation as a level 3 unit with capacity and capability to recruit. Owing to the short time frame from birth to recruitment, potentially eligible infants were identified in a number of ways: (1) liaison with the obstetric team to highlight mothers at risk of preterm delivery, (2) liaison with the neonatal transport team to identify infants who had been born in local units and being transferred to a study centre and (3) liaison with the NICU clinical team of study centres. Screening of eligible infants was undertaken in collaboration with the clinical team and families were approached for consent only if they were considered eligible. Screening logs were reviewed regularly by the co-ordinating centre to identify any issues around recruitment. The investigator or a suitably qualified person designated by the principal investigator was able to receive written informed consent from the infant’s parent/legally acceptable representative before any trial-specific activity was performed.
Randomisation and blinding
Randomisation to the standard-care and CGM arms of the study in a 1 : 1 ratio was undertaken centrally using a web randomisation system, Trans European Network ALEA software (TENALEA; ALEA Clinical, Abcoude, The Netherlands). This used block randomisation, with blocks of random size (i.e. 4, 6, 8) stratifying by recruiting centre and gestation (< 26 or ≥ 26 weeks’ gestation). The management of glucose control and use of CGM from recruitment at aged ≤ 24 hours until day 7 were predetermined in the protocol for each study arm. 2 Clinical outcome data were collected until 36 weeks’ corrected gestational age. No other aspects of concomitant care were prohibited during the trial.
Intervention
All infants were fitted with a sensor (i.e. the Enlite glucose sensor) that was linked to a MiniMed™ 640G System (Medtronic plc). Sensors were inserted subcutaneously (into the thigh) by hand and not using the standard insertion device, thereby ensuring that the sensor was inserted into the subcutaneous tissue. The sensors are soft and flexible, approximately 8.75 mm in length, and are mounted inside a hollow needle to allow for subcutaneous insertion. Once the sensor was inserted, the introducer needle was withdrawn and the sensor attached to a small Guardian™ 2 Link transmitter [Medtronic plc; Conformité Européenne (CE) Mark 2013; certificate number 8858] for data transfer to the MiniMed 640G System for data viewing. The sensors were secured with a clear occlusive dressing so that the insertion site could be inspected daily. A blood sample was required every 12 hours for calibration. For consistency across sites, all units were provided with Nova StatStrip meters for calibration of the CGM.
Intervention: real-time continuous glucose monitoring with guideline
The CGM data were available to view by the clinical team during the first week of life. Clinical staff were advised to read and record the sensor glucose data hourly as part of standard clinical care. They were provided with a specifically designed guideline to guide the management of glucose control and the use of insulin. The guideline was developed during the REACT feasibility study. 1
Control: standard care with blinded continuous glucose monitor data collection
Blinding of the study intervention between study groups was not feasible. To ensure that CGM data were not available to the clinical team caring for infants in the standard-care arm, the monitors were secured in an opaque bag with a tamper-proof seal. The bags were opened for calibration every 12 hours and a time log was kept of when the tamper tag was broken/resealed to document compliance.
Infants’ glucose control was monitored and managed in accordance with standard clinical practice based on intermittently sampled blood glucose levels. Nutritional requirement and insulin delivery were prescribed in accordance with standard clinical guidelines within each unit. The CGM device collected glucose data continuously, but the clinical team were masked to the data, as described above.
Medical devices: MiniMed 640G System
The MiniMed 640G System is indicated for glucose monitoring for the management of diabetes (Figure 4). The system being used comprised the Enlite sensor linked using the Guardian™ 2 Link transmitter to the MiniMed 640G. The Enlite sensor (CE certificate number 21024) has been described previously and comprises a disposable subcutaneous oxidase-based platinum electrode that catalyses interstitial glucose, generating an electrical current every 10 seconds that is transmitted to a monitor. The data were recorded as an averaged value every 5 minutes, giving a total of 288 readings per day. Glucose values outside the range of 2.2–24 mmol/l (40–430 mg/dl) are reported as < 2.2 mmol/l (40 mg/dl) or > 24 mmol/l (430 mg/dl).
FIGURE 4.
The MiniMed 640G System (courtesy of Medtronic plc). (a) The Enlite glucose sensor; (b) the Enlite glucose sensor with the Guardian 2 Link transmitter attached; and (c) the MiniMed 640G.



Data management and schedule of assessments
Data collection was undertaken from birth to 36 weeks’ corrected gestational age, and comprised the collection of clinical data recorded on a paper case report form (CRF) and electronic transfer of CGM data. Key time points for clinical data collection were recruitment, daily for the first week, day 14 followed by end of study at 36 weeks’ corrected gestational age and, if appropriate, at the point of withdrawal from the study. Parent and staff questionnaires were completed in the CGM group at study days 3 and 7. CGM data were transferred electronically after download from the CGM device on study day 7 or the day of sensor removal. Safety data were collected and reported in accordance with regulatory guidance. If an infant was discharged from their recruiting NICU, the research team used local and national databases, local contacts and links with parents to ensure that complete follow-up data were obtained. All CRF data were sent to the co-ordinating centre in Cambridge to be entered onto a macro-database. All CGM files were sent to the co-ordinating centre in Cambridge for validation on format and consistency before processing and analysis. All data were collected, transferred (using a study identifier), cleaned and stored to comply with good clinical practice and data protection legislation.
Efficacy
Efficacy was assessed by comparing the data collected from the CGM in the CGM and standard-care arms of the study.
Safety
Safety was assessed in three areas: (1) incidence of hypoglycaemia, measured as part of clinical care (blood glucose levels) and after review of sensor glucose data; (2) device safety through adverse device effect (ADE) reporting; and (3) acute mortality and morbidity outcomes as part of the CRF.
Utility
Nursing staff and parents caring for the infants in the CGM arm of the study were asked to complete a study-specific questionnaire.
Health economic evaluation
Data were collected on the health service resources used in the treatment of infants during the period between randomisation and 36 weeks’ gestational age, and based on the British Association of Perinatal Medicine standard criteria for level of care,38 as well as neonatal complications. Current UK unit costs were applied to each resource item and a per diem cost for each level of neonatal care was based on Department of Health and Social Care reference costs calculated on a full absorption costing basis. 39
Outcomes
Primary outcome
The primary outcome was defined as the percentage of time that the sensor glucose level was in the target range of 2.6–10 mmol/l. This was selected as the internationally most widely accepted clinical target range for glucose control in this population.
Secondary outcomes
Efficacy
-
Percentage of time sensor glucose level is in the target range of 4–8 mmol/l.
-
Mean sensor glucose level during the first 6 days.
-
Sensor glucose level variability within infants (as assessed by within-infant SD).
-
Percentage of time that glucose levels are in hyperglycaemic range (i.e. a sensor glucose level > 15 mmol/l).
Safety
-
Incidence of moderate hypoglycaemia, defined as any episode of blood glucose > 2.2 mmol/l and < 2.6 mmol/l.
-
Incidence of severe hypoglycaemia, defined as any episode of blood glucose ≤ 2.2 mmol/l.
-
Incidence of continuous hypoglycaemia, defined as a continuous episode of sensor glucose level < 2.6 mmol/l for > 1 hour.
Utility
-
The questionnaire comprised key questions that were scored using a Likert scale.
Health economic outcomes
Cost-effectiveness was expressed in terms of incremental cost per additional case of adequate glucose control between 2.6 and 10 mmol/l.
Exploratory outcomes
Exploratory outcomes included mortality before 36 weeks’ corrected gestational age, maximum severity of ROP, bronchopulmonary dysplasia (BPD) (i.e. the need for supplemental oxygen or respiratory support at 36 weeks’ corrected gestational age), microbiologically confirmed or clinically suspected late-onset invasive infection, necrotising enterocolitis (NEC), patent ductus arteriosus (PDA) requiring medical or surgical treatment, maximum grade of intracranial haemorrhage (Papile and Burstein grading), growth (weight, length and head circumference at the end of week 1 and at 36 weeks’ corrected gestational age), nutritional intake during the first week of life (carbohydrate, protein and lipid), and use of insulin during the first and second week of life.
Sample size
The sample size was based on data from the REACT feasibility study and historical control data, which conservatively assumed that the SD of the primary end point (i.e. time in sensor glucose target range of 2.6–10 mmol/l over a 6-day period) would be 22%. A sample size of 200 participants would enable a treatment effect of a 10% increase in the mean value of the primary end point to be detected with 90% power using a two-sided 5% significance test in the primary analysis. Based on a consensus of expert opinion, a difference of 10% was believed to be of clinical relevance. It was expected that a small number of infants would be withdrawn from the study because of transfer to local units, withdrawal of parental consent or death.
At an interim analysis, a revised blinded estimate of the SD for the primary end point suggested that the original sample size would provide 92.5% power. After review of recruitment rates and undertaking a revised power calculation using the revised blinded estimate of the SD for the primary end point, a decision was made by the independent DMEC, TSC and sponsor to stop recruitment when 182 infants had been recruited. At this time, it was considered that concluding the study with 182 infants rather than the planned 200 would reduce the conservative estimate of power from 92.5% to 90%, which was felt to be an acceptable compromise to be able to report trial results within the desired timelines.
Statistical analyses
Efficacy analyses
Analyses were predetermined to be undertaken based on ‘full analysis’ (primary outcome) and ‘per-protocol’ populations to compare the CGM group with the standard-care group and were prespecified in the statistical analysis plan. Linear regression was used to estimate the absolute difference in the percentage of time that the sensor glucose level was in the target range of 2.6–10 mmol/l, adjusting for baseline variables (i.e. centre and gestation). Estimates of treatment effect, with 95% CIs and p-values, were calculated. Secondary end points that were continuous variables were analysed in a similar fashion and binary/ordinal variables were analysed using logistic/ordinal logistic regression.
To control the study-wide significance level to 5%, a Benjamini–Hochberg multiple testing procedure was applied to three end points of equal importance (i.e. the percentage of time that the sensor glucose level was in the target range of 2.6–10 mmol/l, the percentage of time that the sensor glucose level was in the target range of 4–8 mmol/l and mean sensor glucose level), followed by a gatekeeping sequence applied in the following order: sensor glucose variability, as assessed by within-infant SD; and percentage of time that glucose levels were in the hyperglycaemic range (i.e. sensor glucose level > 15 mmol/l). Nominal p-values and CIs are presented, unless otherwise stated.
Predefined subgroup analyses were undertaken by estimating, using the regression framework in an exploratory, non-confirmatory manner, treatment interaction effects with the following baseline variables: centre, sex, corrected gestational age, birthweight standard deviation score (SDS), use of antenatal steroids, maternal chorioamnionitis, maternal diabetes and first sensor glucose level.
Utility analyses
Acceptability of the intervention was assessed by a specifically designed staff questionnaire, which was completed anonymously by staff caring for an infant in the CGM arm on days 3 and 7, and by a similar parent questionnaire (completed on day 7). Compliance in the CGM group was assessed as a percentage of time that sensor glucose readings were recorded on the CRF and when the insulin rate of infusion was recorded if the sensor glucose > 8 mmol/l. In the standard-care group, non-compliance was defined as 12 or more CGM readings available during the time between the tamper tag being broken and resealed on at least one occasion.
Cost-effectiveness analyses
An incremental cost-effectiveness analysis was performed, details of which are presented in Chapter 5.
Study management
The study was co-ordinated and monitored by the Cambridge Clinical Trials Unit (Cambridge, UK) in accordance with international guidelines. The study was adopted onto the National Institute for Health Research Clinical Research Network portfolio of studies.
Regulatory requirements
Ethics and regulatory approvals were obtained and the protocol is in the public domain (ISRCTN12793535),2 with a list of protocol amendments provided in Appendix 1. A DMEC and TSC were appointed and reviewed the data in accordance with their formal charters (see Appendices 2 and 3). Research teams at each site were required to have up-to-date good clinical practice training and have undertaken training in study procedures, including the use of the CGMs and Nova Biomedical POC devices. Paper and online resources, as well as a call line, were available to support the research teams.
Clinical trial authorisation was granted by the Medicines and Healthcare products Regulatory Agency (MHRA) (reference CI/2016/0011). Written approvals were received from individual hospital sites prior to recruitment. Approvals were also obtained from the Medical Ethics Review Committee (Vrije Universiteit University Medical Centre, Amsterdam, the Netherlands) and Heath Care Inspectorate (Heerlen, the Netherlands) (reference 2017-1 398434/VlO 14949), the Research Ethics Committee, Sant Joan de Déu Research Foundation (Barcelona, Spain), and the Ministry of Health Social Services and Equality (reference 591/16/EC) (Madrid, Spain). The trial was conducted in accordance with the principles of the Declaration of Helsinki40 and in conformity with the European Union medical devices regulations and any relevant amendments. 41
Monitoring
Data returns were continually monitored by the central team for the completeness and timeliness of all data returned. Compliance with intervention strategy in each study arm was also reviewed to ensure that there was no ‘crossover’ between study arms. A monitoring plan was in place, determining frequency and scope of site monitoring based on continuing risk review. Face-to-face monitoring visits were initially undertaken within the first 6 months; the interval between visits was then adjusted following assessment of recruitment rate and the number of data queries and serious adverse event (SAE) reports. The study sites were provided with direct access to all trial-related source data and reports for the purpose of monitoring and auditing by the central study team, sponsor and regulatory authorities, as required.
Data and safety monitoring
The DMEC chairperson was responsible for safeguarding the interests of the trial participants and making recommendations to the TSC. The REACT DMEC roles and responsibilities and operating procedures are defined in the REACT DMEC Charter (see Appendices 2 and 3). The DMEC was composed of three independent multidisciplinary experts who were not involved in the conduct of the trial in any way. They met prior to the initiation of enrolment and determined a plan to review the protocol, compliance, safety and adverse events (AEs), and outcome data after a prespecified number of infants had been recruited. The TSC was composed of six independent members, whose roles and responsibilities were defined by a charter. The TSC provided advice, through its chairperson, to the chief investigator and reported to the trial sponsor and trial funder.
Safety was assessed continuously during each infant’s stay in NICU. The frequency of AEs and SAEs that would normally require reporting within a clinical trial, as defined by the International Council for Harmonisation of Technical Requirements for Pharmaceuticals for Human Use,42 was anticipated to be high in the population being studied, despite the low risk of the study intervention. Following discussions with the MHRA and in accordance with regulatory guidance that allows for exceptions in such circumstances, a modified reporting plan was agreed.
Any ADE was recorded and reported to the co-ordinating centre. All device deficiencies that might have led to a SADE if suitable action had not been taken, the intervention had not been made or if circumstances had been less fortunate were reported to the sponsor, as for SAEs and SADEs. AEs were recorded in the notes and captured as exploratory outcomes as part of the CRF. SAEs are known to be common in this population and, therefore, the MHRA requested the following expectations for safety reporting.
During the intervention period of the study (study days 1–7)
The following expected SAEs were required to be recorded in the CRF (safety log) and reported, using the CRF, to the sponsor within 24 hours of awareness of the event: (1) death, (2) culture-positive infection, (3) severe hypoglycaemia (glucose level < 2.6 mmol/l), (4) seizures and (5) any other related SAE.
Post intervention period (study day 8 until end of study)
It was agreed with the MHRA that important medical outcomes, including those normally considered as a SAE for a clinical trial, would be captured in the CRF at the 36 weeks’ corrected gestational age assessment (i.e. SAEs were viewed to be anticipated events for this study population and, therefore, they were not required to be recorded or reported separately as a SAE, if judged by the clinical team to be unrelated to the study).
Patient and public involvement
Members of the local parent support group were consulted to help inform trial design. Parents were asked to provide feedback on study involvement as part of the protocol, through both formal review of the protocol/patient information sheet and informal discussions at parent groups within the local NICU. The TSC included a lay person. We sent newsletters to parents to update them on study progress, and an end-of-study update for families.
Dissemination
The findings of the trial have been presented at national meetings, published in international journals and communicated back to the families involved.
Chapter 4 REACT trial results
Recruitment
A Consolidated Standards of Reporting Trials (CONSORT) flow diagram is presented in Figure 5. A total of 1350 families were screened based on an anticipated birthweight ≤ 1200 g, with 918 families not approached because of the short recruiting window of 24 hours. Of the remaining 360 families who were approached, 182 consented, resulting in a consent rate of 51%. Two infants were excluded prior to randomisation, as they were > 24 hours of age. One infant who was found to be ineligible was excluded post randomisation. There were 11 withdrawals from the trial and eight deaths (control, n = 6; intervention, n = 2).
FIGURE 5.
A Consolidated Standards of Reporting Trials (CONSORT) flow diagram. Reproduced with permission from Beardsall et al. 4 in accordance with the terms of the Creative Commons Attribution (CC BY 4.0) license, which permits others to distribute, remix, adapt and build upon this work, for commercial use, provided the original work is properly cited. See: http://creativecommons.org/licenses/by/4.0/. The figure includes minor additions and formatting changes to the original figure.

Demographic details
Demographic details of all recruited infants are provided in Table 4. These data show no apparent differences between study arms at baseline in terms of premorbidity and the results are in keeping with what would be anticipated for this preterm population.
Variable | Statistic | Standard-care arm | CGM arm |
---|---|---|---|
Gestational age (weeks) | n | 95 | 84 |
Mean (SD) | 27.4 (1.9) | 27.7 (2.1) | |
Sex, % (n/N) | Male | 47 (45/95) | 54 (45/84) |
Ethnicity, % (n/N) | Asian | 12 (11/95) | 8 (7/84) |
Black | 8 (8/95) | 8 (7/84) | |
Mixed | 5 (5/95) | 7 (6/84) | |
Other | 5 (5/95) | 4 (3/84) | |
White | 69 (66/95) | 73 (61/84) | |
Number of infants delivered, % (n/N) | Singleton | 74 (70/95) | 77 (65/84) |
Multiple | 26 (25/95) | 23 (19/84) | |
CRIB II score | n | 91 | 80 |
Mean (SD) | 10 (2.9) | 10 (2.9) | |
Median | 10 | 10 | |
Minimum, maximum | 4, 19 | 4, 19 | |
Birthweight (g) | n | 95 | 84 |
Mean (SD) | 880 (180) | 910 (160) | |
Median | 920 | 930 | |
Minimum, maximum | 470, 1170 | 390, 1190 | |
Birthweight SDS | n | 95 | 84 |
Mean (SD) | –0.8 (0.92) | –0.82 (0.98) | |
Median | –0.66 | –0.76 | |
Minimum, maximum | –2.6, 1.32 | –2.97, 1.2 | |
Use of antenatal steroids > 24 hours prior to delivery, % (n/N) | Yes | 73 (69/95) | 82 (69/84) |
Use of antibiotics in 24 hours prior to delivery, % (n/N) | Yes | 34 (32/94) | 30 (25/84) |
Maternal diabetes, % (n/N) | Yes | 8 (8/95) | 6 (5/84) |
Maternal chorioamnionitis, % (n/N) | Yes | 22 (21/95) | 23 (19/84) |
Delivery mode, % (n/N) | Spontaneous vaginal delivery | 33 (31/95) | 35 (29/84) |
Caesarean section pre onset of labour | 42 (40/95) | 49 (41/84) | |
Caesarean section post onset of labour | 23 (22/95) | 1 (13/84) | |
Assisted vaginal delivery | 2 (2/95) | 1 (1/84) |
Compliance with intervention and control procedures
The number of CGM data points in the CGM and standard-care arms was a comparable mean of 1538 (SD 341) and 1412 (SD 424), respectively (Figure 6).
FIGURE 6.
Randomised controlled trial: CGM data recorded by treatment group.
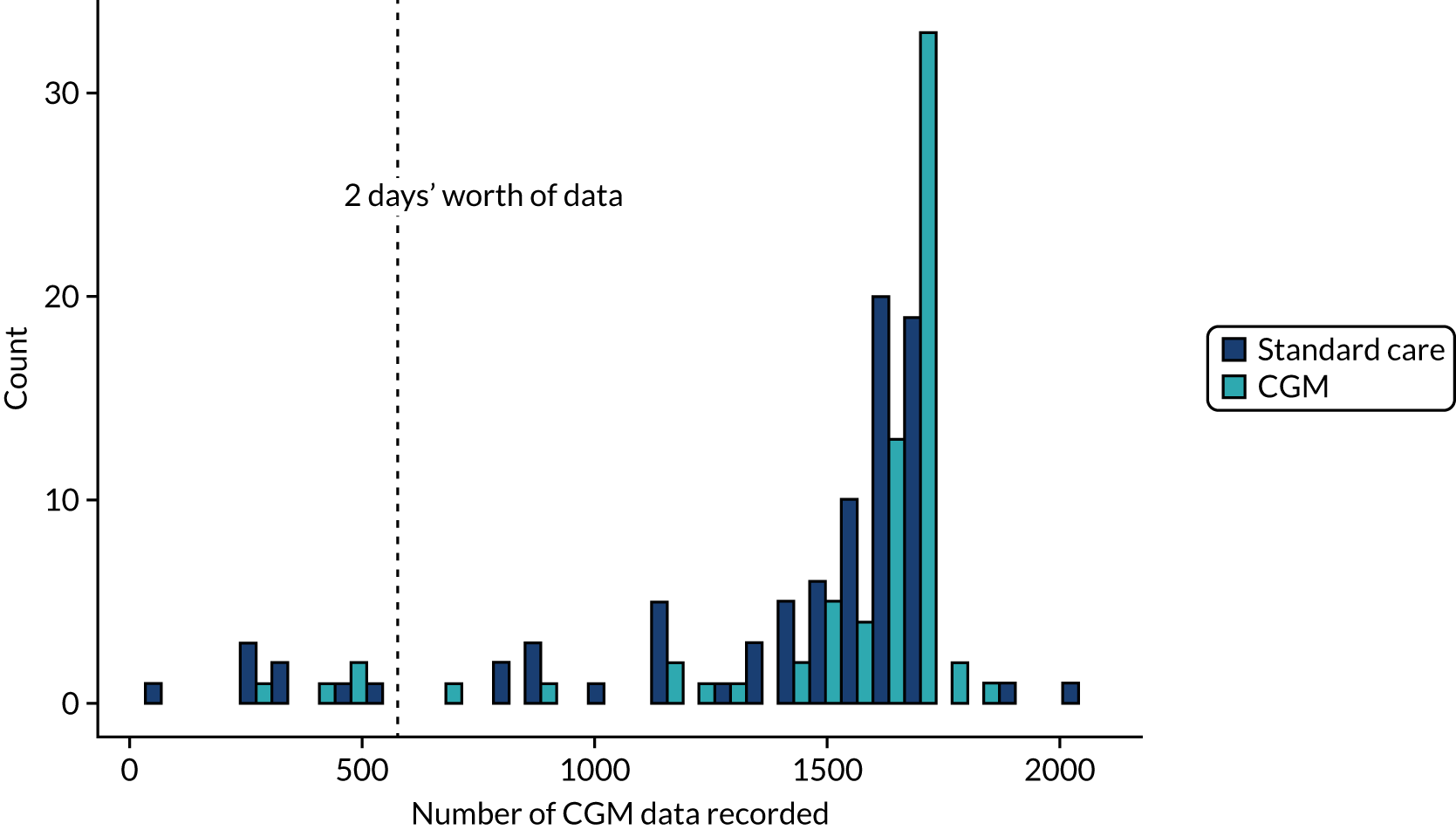
In the CGM group, the median number of times sensor glucose was recorded per hour on the CRF was 0.98 (range 0.56–1.45) based on hours’ worth of data (number of CGM readings/12) and 0.90 (range 0.29–1.28) based on total sensor duration (hours). The mean percentage of time that the insulin rate of infusion was recorded when the sensor glucose was > 8 mmol/l was 54% (SD 38%). Assessment of compliance with blinding in the control study arm was recorded using a log of time between the tamper tag being opened and resealed. Failure to comply with blinding [prespecified as 12 or more CGM readings available (equivalent to 60 minutes of time) between the tag being opened and resealed] occurred in 15% (14/95) of infants. The median length of time the tags were open for was 0.167 (range 0–23.9) hours, with a mean of 0.377 (SD 1.34) hours. These figures are represented graphically in Figure 7.
FIGURE 7.
Randomised controlled trial: clinically recorded glucose profiles and use of insulin in the CGM intervention study arm. SG, sensor glucose.

Primary outcome measure
The primary results for the full analysis population demonstrated that the time during which sensor glucose was in the target range of 2.6–10 mmol/l was 9% higher in the CGM arm than in the standard-care arm (95% CI 3% to 14%; p = 0.002) (Table 5). Full model-fitting results exploring the effect of centre and gestation ≥ 26 weeks (Table 6), and a range of sensitivity analyses (see Appendix 4, Tables 24 and 25), all gave similar treatment effects, suggesting that the results are robust. In per-protocol analyses, CGM, compared with standard control, resulted in a 10 percentage-point increase in the time that sensor glucose was in the target range of 2.6–10 mmol/l (95% CI 4% to 17%; p = 0.002). Model-fitting results for per-protocol analyses are provided in Appendix 4, Table 25.
Variable | Statistic | Standard-care arm | CGM arm | Linear regression model | |
---|---|---|---|---|---|
Adjusted mean differencea (95% CI) | p-value | ||||
Percentage of time sensor glucose is in target range of 2.6–10 mmol/l | n | 85 | 70 | 8.9 (3.4 to 14.4) | 0.002 |
Mean (SD) | 84 (22) | 94 (11) | |||
Median | 97 | 99 | |||
Minimum, maximum | 14, 100 | 51, 100 | |||
Mean sensor glucose level (mmol/l) | n | 85 | 70 | –0.46 (–1 to 0.09) | 0.99 |
Mean (SD) | 7 (2.1) | 6.5 (1.2) | |||
Median | 6.5 | 6.4 | |||
Minimum, maximum | 3.1, 12.2 | 3, 9.9 | |||
Percentage of time sensor glucose is in target range of 4–8 mmol/l | n | 85 | 70 | 11.5 (3.7 to 19.2) | 0.004 |
Mean (SD) | 62 (29) | 74 (20) | |||
Median | 65 | 78 | |||
Minimum, maximum | 0, 100 | 16, 100 | |||
Sensor glucose variability (mmol/l) | n | 85 | 70 | –0.01 (–0.15 to 0.13) | 0.9 |
Mean (SD) | 3.6 (4.3) | 2.7 (3) | |||
Median | 2.1 | 1.9 | |||
Minimum, maximum | 0.07, 22 | 0.6, 23.1 | |||
Percentage of time in hyperglycaemic range (i.e. > 15 mmol/l) | n | 85 | 70 | –0.92 (–2.03 to 0.19) | 0.1 |
Mean (SD) | 1.3 (4.2) | 0.3 (2.1) | |||
Median | 0 | 0 | |||
Minimum, maximum | 0, 24 | 0, 16 |
Outcome | Covariate | Estimate (SE) | 95% CI | p-value |
---|---|---|---|---|
Percentage of time sensor glucose is in the target range 2.6–10 mmol/l | Intercept | 80.6 (3.7) | 73.4 to 87.9 | < 0.001 |
CGM vs. standard care | 8.9 (2.8) | 3.4 to 14.4 | 0.002 | |
N36 (reference N01) | –7.9 (12.3) | –32.2 to 16.5 | 0.5 | |
N42 | 7.6 (5.9) | –4.1 to 19.3 | 0.2 | |
N43 | 0.2 (4.2) | –8.2 to 8.6 | 0.96 | |
N68 | 0.2 (4.2) | –8.1 to 8.5 | 0.97 | |
N73 | 0.3 (5.5) | –10.6 to 11.2 | 0.95 | |
N74 | 10 (12) | –14 to 34 | 0.4 | |
N85 | 8.2 (6.9) | –5.4 to 21.8 | 0.2 | |
N86 | –16 (8.9) | –33.7 to 1.6 | 0.08 | |
N87 | –12.2 (12.4) | –36.6 to 12.2 | 0.3 | |
N88 | –29 (8) | –44.8 to –13.2 | < 0.001 | |
ND3 | 6.9 (6.9) | –6.7 to 20.5 | 0.3 | |
SP3 | 9.6 (8.9) | –8 to 27.2 | 0.3 | |
Gestation ≥ 26 weeks | 4.9 (3.6) | –2.1 to 12 | 0.2 | |
RSD = 17.02 |
Significant subgroup effects included sex (compared with standard care, CGM resulted in a 15 percentage-point increase in the time that sensor glucose level was in the target range in male infants and a 2 percentage-point increase in female infants; see Appendix 4, Table 26).
Secondary outcomes
Efficacy
Secondary outcome analyses showed that time in the target range of 4–8 mmol/ was 12 percentage-points higher in the CGM arm than in the standard-care arm. The mean sensor glucose level, sensor glucose variability and percentage of time sensor glucose level was > 15 mmol/l were all lower in the CGM arm than in the standard-care arm, but none of these differences reached statistical significance. Results are provided with multiple testing procedure data (see Appendix 4, Table 27).
Safety
Continuous glucose monitor data showed that more infants in the standard-care arm (15%) than in the CGM arm (6%) were exposed to at least one episode of sensor glucose < 2.6 mmol/l for > 1 hour. Safety outcome data are provided in Table 7. AEs and ADEs are reported in Tables 8 and 9, respectively. The tables show that episodes of blood glucose levels ≤ 2.2 mmol/l and 2.2–2.6 mmol/l occurred in 7% and 12%, respectively, of the standard-care group, and in 13% and 15%, respectively, of the CGM group. Collection of the blood glucose data was biased by the result of a low sensor glucose level in the CGM arm, prompting blood glucose to be checked, and is reflected in the higher percentage of infants in the standard-care arm who were exposed to at least one episode of hypoglycaemia lasting > 1 hour. Owing to the small numbers and interinfant variability, none of these differences was statistically significant.
Variable | Statistic | Standard-care arm | CGM arm | Logistic regression | |
---|---|---|---|---|---|
Adjusted ORa (95% CI) | p-value | ||||
Blood glucose level range 2.2–2.6 mmol/l | 12% (11/93) | 15% (11/75) | 1.2 (0.48 to 3.2) | 0.7 | |
Blood glucose level ≤ 2.2 mmol/l | 7% (6/93) | 13% (10/75) | 2.2 (0.7 to 7.1) | 0.2 | |
Continuous episode of sensor glucose < 2.6 mmol/l for > 1 hour | 15% (13/85) | 6% (4/70) | 0.361 (0.0919 to 1.2) | 0.1 | |
Length of time sensor glucose level is < 2.6 mmol/l (hours) | n | 85 | 70 | ||
Mean (SD) | 1.0 (3.2) | 0.5 (1.7) | |||
Median | 0 | 0 | |||
Minimum, maximum | 0, 22 | 0, 11.2 | |||
Percentage of time that the sensor glucose level is < 2.6 mmol/l | n | 85 | 70 | ||
Mean (SD) | 1.1 (3.2) | 1 (5.3) | |||
Median | 0 | 0 | |||
Minimum, maximum | 0, 17 | 0, 41 |
Variable | Statistic | Standard-care arm, % (n/N) | CGM arm, % (n/N) | Total, % (n/N) |
---|---|---|---|---|
AE description | Culture-positive infection | 0 (0/95) | 1 (1/84) | 1 (1/179) |
Moderate hypoglycaemia (sensor): sensor glucose 2.2–2.6 mmol/l for > 1 hour | 0 (0/95) | 6 (5/84) | 3 (5/179) | |
Moderate hypoglycaemia: blood glucose level range 2.2–2.6 mmol/l | 9 (9/95) | 6 (5/84) | 8 (14/179) | |
Persistent pulmonary hypertension | 1 (1/95) | 0 (0/84) | 1 (1/179) | |
Pulmonary haemorrhage | 1 (1/95) | 0 (0/84) | 1 (1/179) | |
Seizures | 1 (1/95) | 0 (0/84) | 1 (1/179) | |
Severe hypoglycaemia: blood glucose level ≤ 2.2 mmol/l | 5 (5/95) | 10 (8/84) | 7 (13/179) | |
Severe prematurity | 2 (2/95) | 0 (0/84) | 1 (2/179) | |
Transient: sensor glucose level < 2.6 mmol/l for < 1 hour | 0 (0/95) | 2 (2/84) | 1 (2/179) |
Variable | Statistic | Standard-care arm, % (n/N) | CGM arm, % (n/N) | Total, % (n/N) |
---|---|---|---|---|
ADE description | Bruising at sensor site | 3 (3/95) | 4 (3/84) | 3 (6/179) |
Clinically significant discrepancy between sensor glucose and blood glucose levels | 0 (0/95) | 8 (7/84) | 4 (7/179) | |
Excoriated skin at sensor site | 1 (1/95) | 0 (0/84) | 1 (1/179) | |
Failure to re-establish connectivity during intervention | 4 (4/95) | 2 (2/84) | 3 (6/179) | |
Failure to transmit data to the MiniMed 640G because of loss of connectivity | 7 (7/95) | 6 (5/84) | 7 (12/179) | |
Pressure mark on skin | 2 (2/95) | 0 (0/84) | 1 (2/179) | |
Sensor displaced | 1 (1/95) | 2 (2/84) | 2 (3/179) | |
Sensor insertion failure | 22 (21/95) | 13 (11/84) | 18 (32/179) | |
Transient: sensor glucose level < 2.6 mmol/l for < 1 hour | 0 (0/95) | 2 (2/84) | 1 (2/179) | |
Unable to calibrate | 23 (22/95) | 20 (17/84) | 22 (39/179) | |
User error | 1 (1/95) | 0 (0/84) | 1 (1/179) |
The mean percentage of time for which sensor glucose level was < 2.6 mmol/l was 1% (SD 3.2%) in the standard-care group and 1% (SD 5.3%) in the CGM group. The percentage of time that sensor glucose level was < 2.6 mmol/l depended on the number of CGM data available, which explains why it is similar in both groups, despite the total length of time that sensor glucose level was < 2.6 mmol/l being greater in the standard-care arm. Further details of SAEs and SADEs are provided in Tables 8 and 9, and details of withdrawals from the intervention or study, and deaths are shown in Appendix 4, Tables 28–30.
Utility
Table 10 shows summary statistics for the number of sensors used, the individual sensor duration and the number of CGM data collected (hours). We received CGM data from 155 infants, with loss of data due to reported failure of sensor insertion, calibration failure and lack of data at download. Insertion of the first sensor failed in 18% of infants and, therefore, the average number of sensors needed per infant was 1.2. Details of withdrawals are provided in Appendix 4, Tables 28 and 29. The success of sensor insertion varied markedly between centres. Difficulties in calibrating the sensor with the blood glucose level were reported in 22% of infants and episodes where data could not be linked between the transmitter and monitor occurred in 7% of infants. ADEs reported included bruising at the sensor site in 3% of infants, and significant discrepancy between sensor glucose and blood glucose in seven (4%) infants in the CGM study arm. In three infants, the sensor became displaced from under the skin (fell out).
Variable | Statistic | Standard-care arm | CGM arm | Total |
---|---|---|---|---|
Sensor duration (hours) | n | 92 | 81 | 173 |
Mean (SD) | 128 (47) | 127 (48) | 127 (48) | |
Median | 145 | 145 | 145 | |
Minimum, maximum | 4, 192 | 2, 191 | 2, 192 | |
Number of CGM data (hours) | n | 85 | 70 | 155 |
Mean (SD) | 118 (35) | 128 (28) | 122 (33) | |
Median | 133 | 140 | 136 | |
Minimum, maximum | 6, 170 | 24, 155 | 6, 170 | |
Number of sensors used | n | 95 | 84 | 179 |
Mean (SD) | 1.25 (0.48) | 1.13 (0.43) | 1.20 (0.46) | |
Median | 1 | 1 | 1 | |
Minimum, maximum | 0, 3 | 0, 2 | 0, 3 |
The staff and parent questionnaire results are presented in Figures 8 and 9, respectively. Most staff found the devices easy to calibrate (86%) and did not think that the CGM device interfered with the infant’s nursing care (87%) or caused any distress (94%). Seventy per cent of staff felt that the use of CGM improved clinical care. The majority of parents reported that the CGM did not interfere with the infant’s nursing care (96%) or cause any distress (89%), and 80% felt that the use of CGM improved clinical care.
FIGURE 8.
Randomised controlled trial: staff questionnaires of utility. Stacked bar chart showing proportion of response for each Likert question. Reproduced with permission from Beardsall et al. 4 in accordance with the terms of the Creative Commons Attribution (CC BY 4.0) license, which permits others to distribute, remix, adapt and build upon this work, for commercial use, provided the original work is properly cited. See: http://creativecommons.org/licenses/by/4.0/. The figure includes minor additions and formatting changes to the original figure.

FIGURE 9.
Randomised controlled trial: parent questionnaire of acceptability. Stacked bar chart showing proportion of response for each Likert question. Reproduced with permission from Beardsall et al. 4 in accordance with the terms of the Creative Commons Attribution (CC BY 4.0) license, which permits others to distribute, remix, adapt and build upon this work, for commercial use, provided the original work is properly cited. See: http://creativecommons.org/licenses/by/4.0/. The figure includes minor additions and formatting changes to the original figure.

Concomitant medications
Details of complementary medical treatment received during the intervention period in both study arms are provided in Table 11. These data are in keeping with what would be considered standard clinical care for this population and there were no apparent differences between study arms.
Variable | Statistic | Standard-care arm | CGM arm | Total |
---|---|---|---|---|
Inotropes given (days) | n | 94 | 80 | 174 |
Mean (SD) | 0.8 (1.6) | 0.7 (1.5) | 0.7 (1.5) | |
Median | 0 | 0 | 0 | |
Minimum, maximum | 0, 7 | 0, 7 | 0, 7 | |
Antibiotics given (days) | n | 94 | 80 | 174 |
Mean (SD) | 4.3 (2.3) | 4.5 (2.4) | 4.4 (2.4) | |
Median | 4 | 5 | 5 | |
Minimum, maximum | 0, 7 | 0, 7 | 0, 7 | |
Caffeine given (days) | n | 94 | 80 | 174 |
Mean (SD) | 6.4 (1.6) | 6.6 (1.4) | 6.5 (1.5) | |
Median | 7 | 7 | 7 | |
Minimum, maximum | 1, 7 | 1, 7 | 1, 7 | |
Morphine given (days) | n | 94 | 80 | 174 |
Mean (SD) | 1.7 (2.5) | 1.8 (2.6) | 1.7 (2.5) | |
Median | 0 | 0 | 0 | |
Minimum, maximum | 0, 7 | 0, 7 | 0, 7 | |
Corticosteroids given (days) | n | 94 | 80 | 174 |
Mean (SD) | 0.12 (0.53) | 0.09 (0.51) | 0.1 (0.52) | |
Median | 0 | 0 | 0 | |
Minimum, maximum | 0, 4 | 0, 4 | 0, 4 | |
Insulin given (days) | n | 94 | 80 | 174 |
Mean (SD) | 1.6 (2.4) | 2.6 (2.5) | 2.1 (2.5) | |
Median | 0 | 2.5 | 0 | |
Minimum, maximum | 0, 7 | 0, 7 | 0, 7 | |
Insulin units, days 1–7 (per kg per day) | n | 94 | 80 | 174 |
Mean (SD) | 0.6 (2.1) | 0.6 (0.94) | 0.6 (1.7) | |
Median | 0 | 0.19 | 0 | |
Minimum, maximum | 0, 19.8 | 0, 5.39 | 0, 19.8 | |
Insulin units, days 8–14 (per kg per day) | n | 88 | 75 | 163 |
Mean (SD) | 0.15 (0.34) | 0.16 (0.43) | 0.16 (0.38) | |
Median | 0 | 0 | 0 | |
Minimum, maximum | 0, 1.72 | 0, 2.83 | 0, 2.83 | |
Glucose (g/kg/day) | n | 95 | 84 | 179 |
Mean (SD) | 9.1 (2.6) | 9.5 (3.1) | 9.3 (2.9) | |
Median | 9.3 | 10.1 | 9.9 | |
Minimum, maximum | 0, 14.9 | 0, 17.2 | 0, 17.2 | |
Glucose (ml/kg/day) | n | 95 | 84 | 179 |
Mean (SD) | 84 (24) | 85 (28) | 84 (26) | |
Median | 86 | 92 | 88 | |
Minimum, maximum | 0, 143 | 0, 185 | 0, 185 | |
Lipids (g/kg/day) | n | 92 | 80 | 172 |
Mean (SD) | 1.95 (0.78) | 1.89 (0.77) | 1.92 (0.78) | |
Median | 2.1 | 1.95 | 2 | |
Minimum, maximum | 0.04, 3.9 | 0.04, 4.7 | 0.04, 4.7 | |
Lipids (ml/kg/day) | n | 92 | 80 | 172 |
Mean (SD) | 9.9 (3.7) | 9.7 (3.7) | 9.8 (3.7) | |
Median | 10.6 | 9.9 | 10.4 | |
Minimum, maximum | 1.8, 19.5 | 1.9, 23.7 | 1.8, 23.7 | |
Amino acids (g/kg/day) | n | 94 | 81 | 175 |
Mean (SD) | 2.2 (1.3) | 2.2 (1.2) | 2.2 (1.3) | |
Median | 2.4 | 2.5 | 2.5 | |
Minimum, maximum | 0, 7.2 | 0, 6.1 | 0, 7.2 | |
Oral nutrition (ml/kg/day) | n | 94 | 81 | 175 |
Mean (SD) | 18 (23) | 20 (21) | 19 (22) | |
Median | 11 | 14 | 12 | |
Minimum, maximum | 0, 108 | 0, 94 | 0, 108 |
Exploratory outcome measures
Further data on glucose profiles in the CGM and standard-care arms are provided in Figures 10 and 11, respectively.
FIGURE 10.
Randomised controlled trial: profile plots of CGM data – CGM arm. SG, sensor glucose.

FIGURE 11.
Randomised controlled trial: profile plots of CGM data – standard-care arm. SG, sensor glucose.
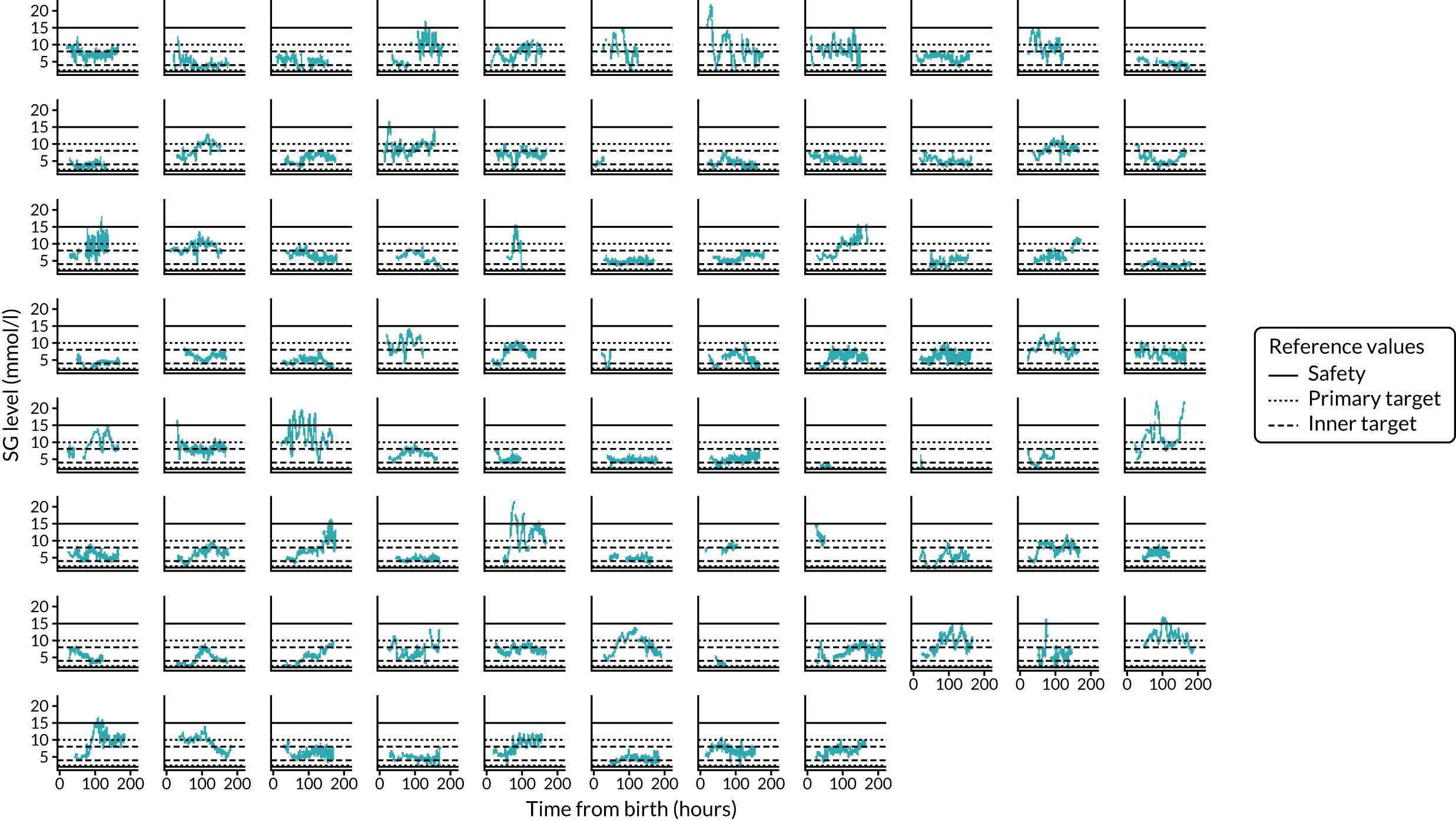
Exploratory analyses of the use of insulin can be found in Figure 12. Figure 12 shows that variability in the use of insulin during study days 5–7 was greater in the standard-care arm than in the CGM arm. There were no differences in any of the growth parameters between study arms at any study time points.
FIGURE 12.
Randomised controlled trial: mean (95% CI) insulin and sensor glucose against study day. (a) Sensor glucose; and (b) insulin. SG, sensor glucose.

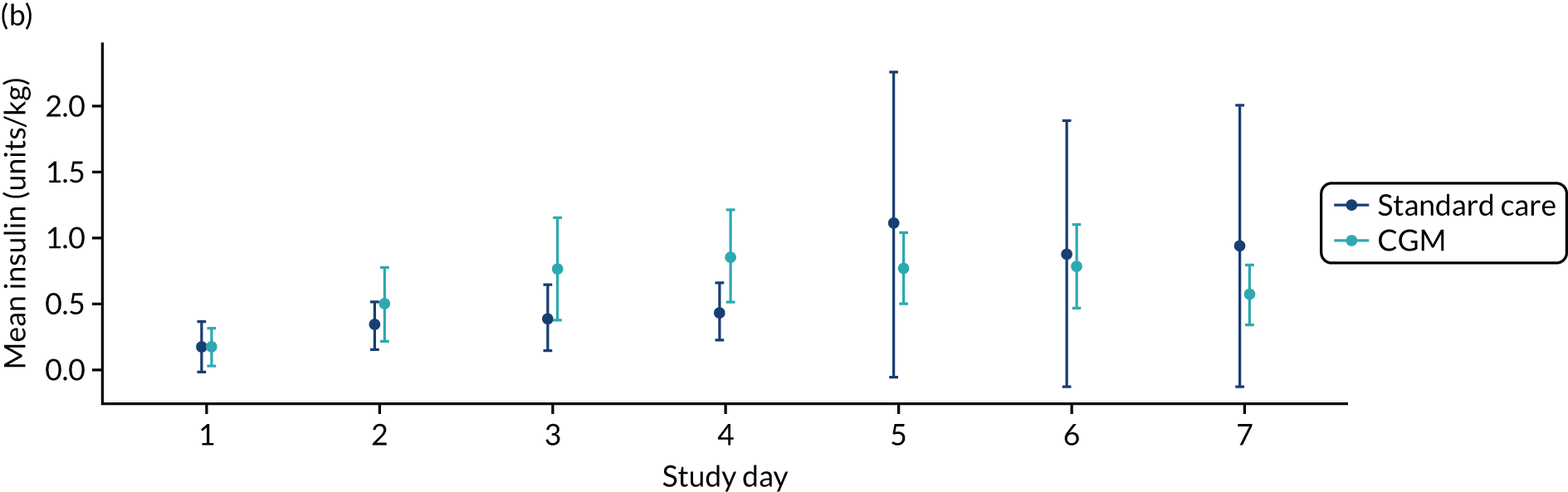
Details of causes of death are provided in Appendix 4, Table 30. Details of growth parameters are provided in Appendix 4 for at birth (see Appendix 4, Table 31), day 7 (see Appendix 4, Table 32), day 14 (see Appendix 4, Table 33) and at 36 weeks’ corrected gestational age (see Appendix 4 Table 34). Changes in growth SDS are provided from birth to 36 weeks’ corrected gestational age for weight (Figure 13), body length (Figure 14) and head circumference (Figure 15).
FIGURE 13.
Randomised controlled trial: change in mean weight SDS over time. Error bars represent standard error of the mean.

FIGURE 14.
Randomised controlled trial: mean body length SDS over time. Error bars represent standard error of the mean.
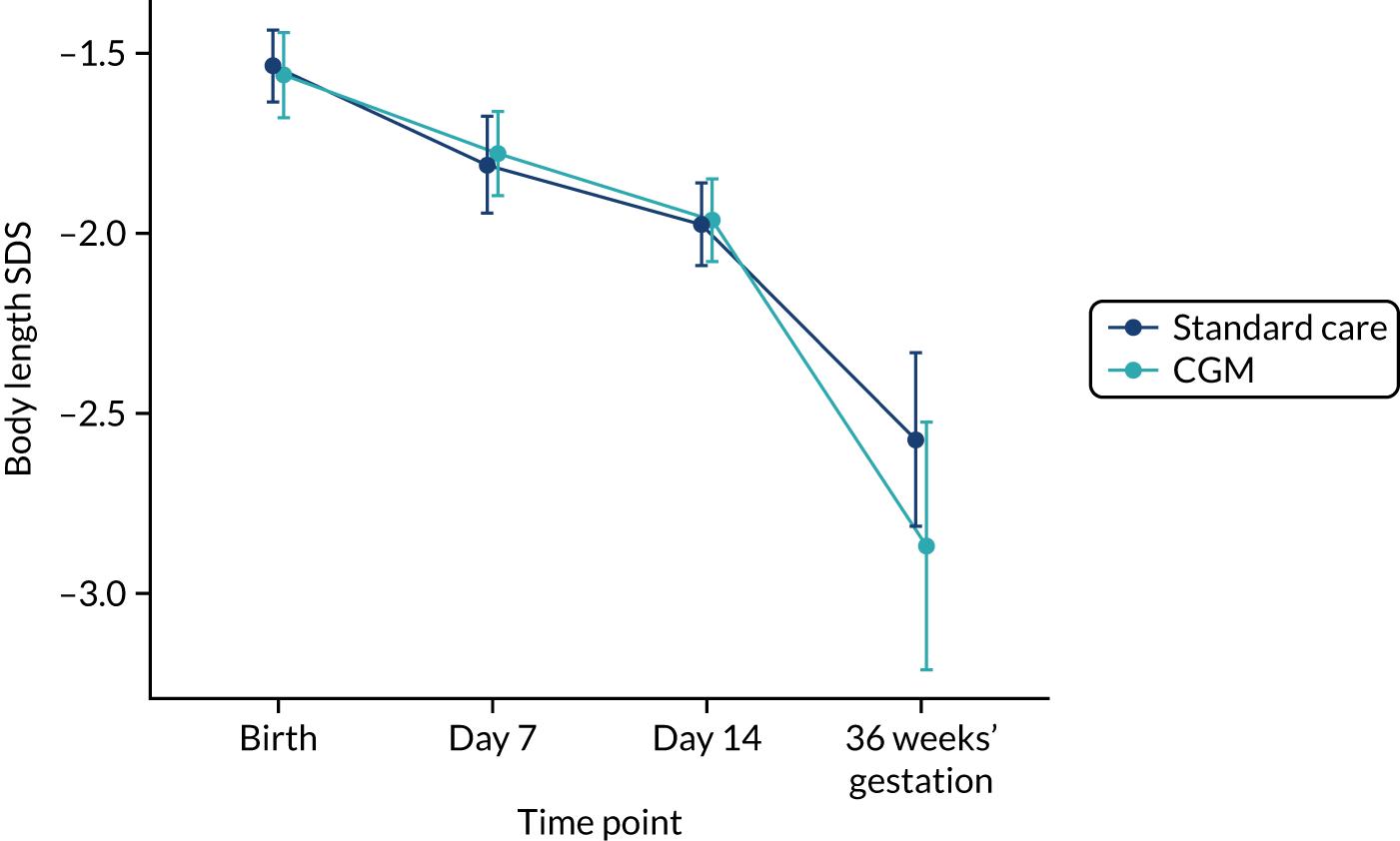
FIGURE 15.
Randomised controlled trial: change in mean head circumference SDS over time. Error bars represent standard error of the mean.

Summary statistics of the exploratory clinical outcomes analyses and model fitting are provided in Tables 12 and 13, respectively. More infants in the standard-care arm (12%) than in the CGM arm (5%) required surgery for PDA, but the difference did not reach statistical significance. CGM was associated with a significant reduction in the exploratory outcome of NEC as 28% of infants in the standard-care arm were diagnosed with NEC, compared with 13% of infants in the CGM arm [odds ratio (OR) 0.33, 95% CI 0.13 to 0.78; p = 0.01].
Variable | Statistic | Standard-care arm | CGM arm | Total |
---|---|---|---|---|
Mortality, % (n/N) | Yes | 6 (6/95) | 2 (2/84) | 4 (8/179) |
Maximum severity of ROP | n | 78 | 68 | 146 |
Mean (SD) | 1.5 (3.6) | 1.1 (3.8) | 1.3 (3.7) | |
Median | 0 | 0 | 0 | |
Minimum, maximum | 0, 22 | 0, 22 | 0, 22 | |
Maximum severity of ROP (categorical), % (n/N) | < 3 | 88 (69/78) | 96 (65/68) | 92 (134/146) |
≥ 3 | 12 (9/78) | 4 (3/68) | 8 (12/146) | |
BPD, % (n/N) | Yes | 66 (56/85) | 60 (45/75) | 63 (101/160) |
BPD severity, % (n/N) | Mild | 32 (18/56) | 22 (10/45) | 28 (28/101) |
Moderate | 46 (26/56) | 64 (29/45) | 54 (55/101) | |
Severe | 21 (12/56) | 13 (6/45) | 18 (18/101) | |
Infection, % (n/N) | Yes | 62 (53/85) | 68 (50/74) | 65 (103/159) |
NEC requiring medical or surgical treatment, % (n/N) | Yes | 28 (24/85) | 13 (10/75) | 21 (34/160) |
NEC treatment, % (n/N) | Medically managed | 83 (20/24) | 80 (8/10) | 82 (28/34) |
Surgically managed | 17 (4/24) | 20 (2/10) | 18 (6/34) | |
PDA requiring medical or surgical treatment, % (n/N) | Yes | 31 (26/85) | 26 (19/74) | 28 (45/159) |
PDA treatment, % (n/N) | Medically managed | 88 (23/26) | 95 (18/19) | 91 (41/45) |
Surgically managed | 12 (3/26) | 5 (1/19) | 9 (4/45) | |
Intracerebral pathology, % (n/N) | Yes | 32 (27/84) | 33 (25/75) | 33 (52/159) |
Intracerebral pathology type, % (n/N) | Germinal layer haemorrhage | 44 (12/27) | 40 (10/25) | 42 (22/52) |
IVH without ventricular dilatation | 33 (9/27) | 44 (11/25) | 38 (20/52) | |
IVH with ventricular dilatation | 26 (7/27) | 32 (8/25) | 29 (15/52) | |
Parenchymal involvement | 22 (6/27) | 12 (3/25) | 17 (9/52) |
Model | Outcome | Covariate | Estimate (SE)a | 95% CI | p-value |
---|---|---|---|---|---|
Linear regression | Maximum ROP | Intercept | 2.53 (0.78) | 0.98 to 4.08 | 0.002 |
CGM vs. standard care | –0.26 (0.56) | –1.37 to 0.85 | 0.6 | ||
Weight SDS, day 7 | Intercept | –0.88 (0.16) | –1.19 to –0.57 | < 0.001 | |
CGM vs. standard care | 0.05 (0.12) | –0.19 to 0.28 | 0.7 | ||
Weight SDS, 36 weeks’ gestational age | Intercept | –1.17 (0.21) | –1.59 to –0.76 | < 0.001 | |
CGM vs. standard care | –0.04 (0.15) | –0.34 to 0.25 | 0.8 | ||
Body length SDS, day 7 | Intercept | –1.72 (0.22) | –2.15 to –1.28 | < 0.001 | |
CGM vs. standard care | –0.02 (0.17) | –0.36 to 0.31 | 0.9 | ||
Body length SDS, 36 weeks’ gestational age | Intercept | –4.05 (0.67) | –5.4 to –2.7 | < 0.001 | |
CGM vs. standard care | –0.38 (0.4) | –1.19 to 0.42 | 0.3 | ||
Head circumference, day 7 | Intercept | –1.59 (0.17) | –1.93 to –1.25 | < 0.001 | |
CGM vs. standard care | 0.07 (0.14) | –0.2 to 0.35 | 0.6 | ||
Head circumference, 36 weeks’ gestational age | Intercept | –2.14 (0.4) | –2.94 to –1.35 | < 0.001 | |
CGM vs. standard care | 0.13 (0.29) | –0.44 to 0.7 | 0.6 | ||
Daily glucose (g/kg) | Intercept | 9.53 (0.55) | 8.44 to 10.6 | < 0.001 | |
CGM vs. standard care | 0.42 (0.41) | –0.39 to 1.22 | 0.3 | ||
Daily amino acids (g/kg) | Intercept | 2.59 (0.16) | 2.29 to 2.9 | < 0.001 | |
CGM vs. standard care | 0.17 (0.12) | –0.06 to 0.4 | 0.2 | ||
Daily lipids (g/kg) | Intercept | 1.67 (0.12) | 1.44 to 1.9 | < 0.001 | |
CGM vs. standard | –0.04 (0.09) | –0.21 to 0.13 | 0.6 | ||
Daily oral nutrition (ml/kg/d) | Intercept | 5.9 (3.8) | –1.6 to 13.4 | 0.1 | |
CGM vs. standard care | 2 (2.8) | –3.6 to 7.6 | 0.5 | ||
Total insulin, week 1 (units/kg) | Intercept | 8 (2.3) | 3.4 to 12.5 | < 0.001 | |
CGM vs. standard care | 0.08 (1.7) | –3.3 to 3.5 | 0.96 | ||
Total insulin, week 2 (units/kg) | Intercept | 2 (0.58) | 0.89 to 3.2 | < 0.001 | |
CGM vs. standard care | 0.1 (0.44) | –0.76 to 0.96 | 0.8 | ||
Logistic regression | Mortality | CGM vs. standard care | 0.263 [0.23] | 0.0353 to 1.3 | 0.1 |
Infection | CGM vs. standard care | 1 [0.4] | 0.46 to 2.2 | 0.99 | |
NEC | CGM vs. standard care | 0.328 [0.15] | 0.129 to 0.78 | 0.01 | |
PDA | CGM vs. standard care | 0.52 [0.243] | 0.199 to 1.3 | 0.2 | |
Intracerebral pathology | CGM vs. standard care | 1.02 [0.365] | 0.51 to 2.1 | 0.9 | |
Ordinal logistic regression | BPD | CGM vs. standard care | 1.2 [3.2] | 0.52 to 2.8 | 0.7 |
Chapter 5 REACT randomised controlled trial health economic analyses
Parts of this text have been reprinted from Seminars in Perinatology, vol. 45, Petrou S, Kim S, Bond S, Allison A, Beardsall K, the REACT collaborative, Cost-effectiveness of real time continuous glucose monitoring to target glucose control in preterm infants, pp. 151392, Copyright (2021),43 with permission from Elsevier.
Introduction
The main goal of the health economic evaluation for the REACT RCT was to evaluate the comparative cost-effectiveness of the two interventions (CGM and standard care) in preterm infants weighing ≤ 1200 g and aged ≤ 24 hours. The economic analysis adopted a NHS and Personal Social Services (PSS) perspective in accordance with the National Institute for Health and Care Excellence (NICE) methodological recommendations. 44 For the purposes of the economic evaluation, cost-effectiveness was primarily presented in terms of incremental cost per additional case of adequate glucose control during the first 6 days of life for the trial participants. However, clinical data were prospectively collected until 36 weeks’ corrected gestational age. As a result, the costs incurred until 36 weeks and secondary outcomes were also reflected in the cost-effectiveness analysis. Study participants were hospitalised in a NICU and the majority of participants were in hospital at the 36 weeks’ corrected gestational age assessment. The time horizon covered the period from randomisation to the end of follow-up at the 36 weeks’ corrected gestational age assessment. Costs and outcomes were therefore not discounted because of the short time horizon of the economic evaluation, extending to either 7 days’ or 36 weeks’ corrected gestational age.
Methods
Measurement of resource use and costs
Health economic data were collected on:
-
resource use and costs associated with CGM (i.e. direct intervention costs)
-
broader health service use during the 36-week follow-up period.
All costs were presented in Great British pounds and valued in 2016–17 prices. If necessary, costs were inflated or deflated to 2016–17 prices using the Hospital and Community Health Services Pay and Price Inflation Index. 45
Direct intervention costs
Direct intervention costs encompassed the costs associated with the application of the comparator intervention (i.e. CGM with guideline) over and above the cost of standard care. Infants in the CGM arm were fitted with Enlite glucose sensors, which were linked to a MiniMed 640G System and calibrated with POC blood glucose levels. As a result, the costs of the intervention included the cost of the Enlite sensor and MiniMed 640G System, the costs associated with fitting the device to infants (hospital staff time) and the costs associated with the removal of sensors. The lifetime cost of the Medtronic plc system was annuitised and then further adjusted to reflect the duration of use of the device over the trial period. However, to reflect clinical practice outside the confines of a RCT, the resource inputs associated with masked CGM and the use of associated devices were not costed in the standard-care arm, although the relevant resource inputs were prospectively measured. The resource use associated with the direct intervention costs was collected prospectively. Table 14 provides an outline of the types of direct intervention-related resource inputs that were measured and the sources of the unit costs of each resource input.
Resource type | Resource use | Unit cost source |
---|---|---|
Medtronic plc MiniMed 640G System and Enlite sensors | Cost of MiniMed 640G System and Enlite sensors | Manufacturers |
Enlite sensor insertion and set-up of MiniMed 640G System | Staff time | NHS Agenda for Change46 and clinician’s advice |
Sensor glucose monitoring | Staff time | NHS Agenda for Change46 and clinician’s advice |
Removal of sensor | Staff time | NHS Agenda for Change46 and clinician’s advice |
POC (StatStrip) | Cost of Nova Biomedical devices | Manufacturers |
Cost estimates for staff inputs associated with key clinical events, such as time associated with sensor insertion and removal, were based on expert clinical advice about the duration of each event, and the unit cost values for staff inputs were obtained from the NHS Agenda for Change 2016/17 pay scales. 46
The total direct intervention cost for each participant was estimated by estimating the compound of the associated resource use input values multiplied by their unit cost values.
Measuring broader resource use
The different types of resource categories for broader health-care costs and the sources of their unit costs are presented in Table 15.
Resource type | Resource use | Unit cost source |
---|---|---|
Length of stay by level of care (e.g. intensive care, special care) | Staff salaries, on-costs, equipment, consumables, revenue and capital overheads | NHS reference costs47 |
Medications | Cost of medications | BNF48 |
Investigations | Staff time to deliver the investigations, associated costs | NHS reference costs47 |
Medical (surgical) treatment | Staff time to deliver treatment, associated costs | NHS reference costs47 |
Blood transfusion products | Cost of blood transfusion products | NHS Blood and Transplant Price List 2017 49 |
Surgical procedures | Staff time to deliver the interventions, associated costs | NHS reference costs;47 NHS Agenda for Change46 |
Mode of discharge | Mode and distance of transfer or discharge to home | UK government50 |
Broader resource use data were collected for each infant using data extraction sheets that were completed by research nurses in each clinical centre. The nurses extracted the relevant data directly from routine hospital systems and patient records following the final follow-up assessments at 36 weeks’ corrected gestational age. The CRFs recorded details of length of inpatient stays by intensity of care, investigations performed [e.g. ultrasound scan, magnetic resonance imaging (MRI), electrocardiography (ECG), computed tomography (CT) scan, X-ray, cranial ultrasound scan and electroencephalography (EEG)], medications and procedures performed (e.g. lumbar punctures, ventricular taps, long lines, surgical procedures and interventions for ROP treatment). The CRFs also captured information surrounding transfers between hospitals, and the modes of transport used for hospital transfers and at final hospital discharge. Distances associated with transfers between hospitals were calculated using Google Maps (Google Inc., Mountain View, CA, USA).
Valuation of resource use
Unit cost data were mainly based on NHS reference costs. 47
Costs for sensor insertion, sensor monitoring and sensor removal were calculated by multiplying the duration of each activity, based on clinical advice, by the unit cost extracted from the NHS Agenda for Change pay scales (2016/17). 46 The costs of medications for infants were estimated based on the doses and frequencies reported on the CRF, with medication costs obtained from the British National Formulary. 48 The costs of blood transfusion products, such as platelets, red blood cells and frozen plasma, were obtained from NHS Blood and Transplant Price List 201749 and multiplied by the volume of each product used.
When infants were transferred to another hospital, the distance (miles) between the study site and transferred location was calculated using Google Maps and fuel costs were calculated using the advisory fuel rates obtained from the UK government. 50 The fuel costs applied to the distances of hospital transfers in clinical sites located outside the UK were estimated in the same manner.
Measurement of outcomes
The primary clinical outcome measure for this study was the percentage of time sensor glucose readings were within the target range of 2.6–10 mmol/l during the first 6 days of life. Cost-effectiveness was primarily expressed in terms of incremental cost per additional case of adequate glucose control during the first 6 days of life. For the primary cost-effectiveness analysis, adequate glucose control was defined as at least 80% of readings falling within the target range. However, to assess the robustness of the cost-effectiveness results, a number of sensitivity analyses were also performed that varied the threshold for number of readings falling within the target range of 2.6–10 mmol/l. Therefore, adequate glucose control was re-estimated at alternative thresholds of 60%, 70% and 90% of readings falling within the target range. Given the nature of the outcome measure used in this project and the methodological constraints surrounding utility measurement in newborn infants, cost–utility analysis using a preference-based measure, such as quality-adjusted life-years, was not performed.
The secondary clinical outcome measures selected for secondary expressions of cost-effectiveness were NEC and BPD. Cost-effectiveness analyses using these secondary clinical outcome measures were expressed using the incremental cost-effectiveness ratio (ICER), and expressed in terms of incremental cost per case of NEC averted and incremental cost per case of BPD averted, using imputed costs for the entire period extending to 36 weeks’ corrected gestation.
Cost-effectiveness analysis methods
Missing data
Missing data may be a particular issue for costs and the health outcome measures of interest; therefore, it is important to deal with them in a standardised way. RCTs have been criticised in the health economic literature for failing to use appropriate methods to address missing data. 51
Multiple imputation was adopted to impute missing data and avoid biases associated with complete-case analysis. Multiple imputation was conducted based on Rubin’s rule. 52 Multiple imputation was carried out on the main and secondary clinical outcome measures, using Markov chain Monte Carlo and predicted mean matching. 53 Predicted mean matching is a semiparametric imputation approach and generally performs better than linear regression, despite the similarities in method. 54
It is recommended that potential predictors are incorporated into analytical models for multiple imputation. 55 Inclusion of explanatory variables enables the analyst to use multivariate imputation through chained equations. In chained equations, missing values in variable X are replaced by draws from the posterior predictive distribution of X and imputation is repeatedly performed using the values of other independent variables. 55 Consequently, the multiple imputation models used baseline covariates (i.e. gestational age, sex, site and randomisation arm), as well as health outcomes and cost components (i.e. glucose level, and costs associated with inpatient days, investigation, medical treatment and surgical procedures).
For the CGM outcome, before implementing multiple imputation, linear interpolation using the known values of CGM, before and after any missing values, was conducted first, given that the data followed a time series format. 56 Interpolation requires that the dependent variable be a function of independent variables, so glucose level was also interpolated for values of the time that the glucose level was checked. 56,57 Then, likewise, the interpolated values were imputed using a chained equation with baseline covariates and costs. Five imputed data sets were generated, as this has been considered to be sufficient to obtain valid results. 53
Regression analysis
Generalised linear modelling (GLM) was used to estimate total costs and effectiveness. GLM is an estimation strategy well suited to modelling skewed data. 58 Exponentiation of the mean of the logs generates the geometric mean of the skewed dependent variable (in this case, costs), which is a downwards-biased estimate of the arithmetic mean. 58 In contrast, GLM predicts the mean of the log without using a smearing factor and, therefore, tends to yield more consistent results. After conducting statistical tests, such as the Pregibon link test and Pearson correlation test, on cost data, the gamma family and log-link function were chosen as an appropriate generalised linear model for these trial data.
By specifying the treatment group as an indicator within the GLM, the incremental costs and outcomes attributable to CGM were estimated, while controlling for baseline covariates (i.e. treatment arm, study site, gestational age, sex, weight at birth, whether or not the mother received antenatal steroids > 24 hours prior to delivery, and whether or not the mother had diabetes during pregnancy).
Presentation of cost-effectiveness results
Summary statistics were estimated for resource use variables by treatment allocation and duration, covering either days 1–7 or the 36-week follow-up period. Between-group differences in resource use and costs were compared using the two-sample t-test. Standard errors (SEs) are reported for CGM and standard-care group means. Mean differences are reported with 95% CIs. When there was evidence of non-normality in the continuous outcome measure, non-parametric bootstrapping with 1000 samples was used to estimate the effect of the intervention and bootstrapped 95% CIs for mean differences are reported. Cost-effectiveness was estimated using a bootstrap method to minimise sampling uncertainty. Non-parametric bootstrap methods generate multiple replications of the statistic of interest by sampling replications from the original data. 58,59 A total of 1000 bootstrap samples were drawn and incremental effectiveness (with associated 95% CIs) was calculated. Estimates from each imputed data set were used to run regressions using Rubin’s rule. 52
To express uncertainty around ICERs and to display results across a range of cost-effectiveness thresholds, cost-effectiveness acceptability curves (CEACs) were generated on the basis of bootstrapped sample data. 60,61 These curves show the probability that CGM is cost-effective at different levels of the threshold based on modelled variation in observed patient outcomes. 62
To determine cost-effectiveness, the use of CGMs was compared with standard care on the basis of the cost per additional case of adequate glucose control (ICER). An alternative that is less effective and more costly, on average, than one or a linear combination of other alternatives is considered ‘dominated’ in health economic terms and, therefore, it is not considered when estimating ICERs. An alternative that is more effective (i.e. provides the greatest additional adequate glucose control) and offers an ICER less than or equal to a benchmark value (the cost-effectiveness threshold) can be deemed ‘cost-effective’ compared with other alternatives. 62 However, there are currently no published cost-effectiveness thresholds for the primary and secondary clinical outcomes of the REACT trial. We searched the stated and revealed preference literature to identify any external evidence with respect to population preferences for health changes associated with adequate glucose control and for health changes associated with the secondary clinical outcomes, and no such preference values were revealed. Therefore, ranges of hypothetical cost-effectiveness threshold values have been selected for our analyses. As a result, the net monetary benefit (NMB) of CGM compared with standard care was calculated across three predetermined cost-effectiveness thresholds, namely £1000, £5000 and £10,000 per additional case of adequate glucose control. 63 Additionally, alternative cost-effectiveness threshold values were introduced for the secondary outcome analyses (£10,000, £30,000 and £50,000 cost-effectiveness thresholds). A positive incremental NMB indicates that the CGM is cost-effective compared with standard care at the given cost-effectiveness threshold.
Sensitivity and secondary outcomes analyses
Several sensitivity analyses were carried out to assess the robustness of the cost-effectiveness estimates. These were conducted under the following scenarios: (1) restricting the analyses to complete cases (i.e. those with complete cost and outcome data for the 7 days or up to 36 weeks’ corrected gestation); and (2) estimating the incremental cost-effectiveness using different thresholds of 60%, 70% and 90% for adequate glucose control. The same sensitivity analyses described above were repeated over alternative follow-up periods of the first 7 days of life or extending to 36 weeks’ corrected gestational age.
Two sets of prespecified subgroup analyses were conducted to explore the heterogeneity in the cost-effectiveness results. Subgroup analysis was performed for each study site and for two gestational age groups: extremely preterm (i.e. < 28 weeks’ gestation) and very preterm (i.e. 28–32 weeks’ gestation). A post hoc subgroup analysis was subsequently carried out using sex as an additional subgroup category. All subgroup analyses were based on cases with imputed cost and outcomes data at 7 days or 36 weeks’ corrected gestational age.
Results of economic analysis
Table 35 in Appendix 4 shows the completeness of health economic data by treatment allocation. The table shows that there were no missing data for resource use. Likewise, there were no missing data for the items such as use of the MiniMed 640G 3.0-ml insulin pump device, Guardian 2 Link and the Generation Enlite single sensor. Cases with data that were not available or not applicable are also reported if trial participants were withdrawn from the intervention and/or trial.
Health-care resource use
Table 16 shows resource use values for participants by trial group allocation and resource use category over the 7-day intervention period and the entire follow-up period for complete cases. The resource values are presented for subcategories of resource use, including hospital inpatient medications, investigation, medical treatment and surgical procedures. Use of Enlite sensors and glucose monitoring was reported for the 7-day intervention period only, as infants used the devices for this period only. Clinical recording of sensor glucose values was performed, on average, 121 times in infants in the CGM arm, but was not performed in infants in the standard-care arm, as the clinical team was masked to the sensor data.
Intervention period | Item | CGM arm (n = 71), mean (SE) | Standard-care arm (n = 83), mean (SE) | Total (n = 154), mean (SE) |
---|---|---|---|---|
Days 1–7 | ||||
Enlite sensor (MiniMed system) (number of items) | MiniMed 640G 3.0-ml insulin pump | 1.00 (0.00) | n/a | |
Guardian 2 Link | 1.00 (0.00) | n/a | ||
Generation Enlite single sensor: MMT-7008B | 1.17 (0.04) | n/a | ||
Glucose monitoring (number of activities) | Glucose sensor insertion | 1.17 (0.04) | n/a | |
Glucose sensor monitoring | 121.14 (4.56) | n/a | ||
Glucose sensor removal | 1.17 (0.04) | n/a | ||
POC testing: used to calibrate the MiniMed 640G System (number of strips) | Nova Biomedical StatStrip meter | 20.28 (1.05) | 15.59 (0.91) | 17.75 (0.71) |
Length of stay (days) | Intensive care | 6.01 (0.23) | 5.95 (0.23) | 5.98 (0.16) |
High-dependency care | 0.96 (0.23) | 0.87 (0.21) | 0.91 (0.15) | |
Special care | 0.01 (0.01) | 0.08 (0.08) | 0.05 (0.05) | |
Transitional care | 0.00 (0.00) | 0.00 (0.00) | 0.00 (0.00) | |
Medications (days of use during intervention period) | Inotropes | 0.59 (0.17) | 0.69 (0.17) | 0.64 (0.12) |
Antibiotics | 4.61 (0.29) | 4.48 (0.24) | 4.54 (0.18) | |
Caffeine | 6.73 (0.13) | 6.69 (0.11) | 6.71 (0.09) | |
Morphine | 1.77 (0.31) | 1.67 (0.27) | 1.72 (0.20) | |
Corticosteroids | 0.04 (0.03) | 0.06 (0.04) | 0.05 (0.02) | |
Insulin (ml/kg) | Insulin | 0.02 (0.00) | 0.01 (0.00) | 0.01 (0.00) |
Investigations (number of items) | Cranial ultrasound | 2.25 (0.12) | 2.27 (0.12) | 2.26 (0.08) |
Other ultrasound | 0.01 (0.01) | 0.04 (0.02) | 0.03 (0.01) | |
CT | 0.00 (0.00) | 0.00 (0.00) | 0.00 (0.00) | |
X-ray | 3.79 (0.27) | 4.34 (0.29) | 4.08 (0.20) | |
EEG | 0.00 (0.00) | 0.00 (0.00) | 0.00 (0.00) | |
MRI | 0.00 (0.00) | 0.00 (0.00) | 0.00 (0.00) | |
Echocardiography | 0.58 (0.12) | 0.63 (0.09) | 0.60 (0.07) | |
ECG | 0.00 (0.00) | 0.00 (0.00) | 0.00 (0.00) | |
Blood transfusion products (number of products) | Platelets | 0.15 (0.06) | 0.14 (0.05) | 0.15 (0.04) |
Red blood cells | 0.63 (0.12) | 0.76 (0.13) | 0.70 (0.09) | |
Fresh-frozen plasma | 0.08 (0.04) | 0.07 (0.03) | 0.08 (0.02) | |
Medical treatment (number of treatments) | Lumbar punctures | 0.11 (0.04) | 0.11 (0.04) | 0.11 (0.03) |
Ventricular taps | 0.00 (0.00) | 0.00 (0.00) | 0.00 (0.00) | |
Long lines | 0.93 (0.07) | 0.90 (0.09) | 0.92 (0.06) | |
Surgical procedures (number of procedures) | Ventriculoperitoneal shunts | 0.01 (0.01) | 0.00 (0.00) | 0.01 (0.01) |
Surgical procedures for central access | 0.01 (0.01) | 0.01 (0.01) | 0.01 (0.01) | |
Interventions for ROP treatment | 0.01 (0.01) | 0.00 (0.00) | 0.01 (0.01) | |
Other procedures | 0.00 (0.00) | 0.06 (0.03) | 0.03 (0.02) | |
n = 71 | n = 82 | n = 153 | ||
Day 8 to 36 weeks’ corrected gestation | ||||
Length of stay (days) | Intensive care | 13.15 (1.64) | 16.71 (1.83) | 15.06 (1.24) |
High-dependency care | 25.06 (2.00) | 24.8 (1.51) | 24.92 (1.23) | |
Special care | 16.55 (1.68) | 15 (1.51) | 15.72 (1.12) | |
Transitional care | 0.44 (0.25) | 0.22 (0.11) | 0.32 (0.13) | |
Medication (ml) | Insulin (ml/g) | 0.004 (0.00) | 0.003 (0.00) | 0.00 (0.00) |
Investigations (number of items) | Cranial ultrasound | 2.87 (0.29) | 3.02 (0.20) | 2.95 (0.17) |
Other ultrasound | 0.17 (0.08) | 0.38 (0.20) | 0.28 (0.11) | |
CT | 0 (0.00) | 0.01 (0.01) | 0.01 (0.01) | |
X-ray | 3.87 (0.58) | 4.93 (0.65) | 4.44 (0.44) | |
EEG | 0 (0.00) | 0.01 (0.01) | 0.01 (0.01) | |
MRI | 0.01 (0.01) | 0.05 (0.02) | 0.03 (0.01) | |
Echocardiography | 0.96 (0.17) | 1.18 (0.17) | 1.08 (0.12) | |
ECG | 0.04 (0.02) | 0 (0.00) | 0.02 (0.01) | |
Blood transfusion products (number of products) | Platelets | 0.07 (0.03) | 0.04 (0.02) | 0.05 (0.02) |
Red blood cells | 1.75 (0.25) | 2.23 (0.30) | 2.01 (0.20) | |
Fresh-frozen plasma | 0.11 (0.05) | 0.11 (0.05) | 0.11 (0.04) | |
Medical treatment (number of treatments) | Lumbar punctures | 0.1 (0.04) | 0.23 (0.06) | 0.17 (0.04) |
Ventricular taps | 0.27 (0.07) | 0.5 (0.13) | 0.39 (0.08) | |
Long lines | 0 (0.00) | 0.04 (0.03) | 0.02 (0.01) | |
Surgical procedures (number of procedures) | Ventriculoperitoneal shunts | 0.03 (0.02) | 0.05 (0.02) | 0.04 (0.02) |
Surgical procedures for central access | 0 (0.00) | 0.02 (0.02) | 0.01 (0.01) | |
Interventions for ROP treatment | 0 (0.00) | 0.01 (0.01) | 0.01 (0.01) | |
Other procedures | 0.01 (0.01) | 0.07 (0.03) | 0.05 (0.02) | |
Transfers to other hospital (number by mode) | Air ambulance transfers | 0 (0.00) | 0.02 (0.02) | 0.01 (0.01) |
Road transfers | 0.49 (0.06) | 0.43 (0.05) | 0.46 (0.04) |
Economic costs
Table 17 summarises the total NHS and PSS costs associated with resource use during days 1–7, day 8 to 36 weeks’ corrected gestational age and the entire study period for complete cases. Table 18 breaks these costs down by subcategory. The mean direct intervention costs over the first 7 days were £11,198 in the CGM arm and £9934 for the standard-care arm; the mean difference was not statistically significant at the 5% level. The cost of using the Enlite sensor and glucose monitoring was not included in the standard-care arm, as it is not part of standard care. The total cost during the period covering day 8 to 36 weeks’ corrected gestational age was higher in the standard-care arm (£61,065 vs. £65,464), but the difference was not statistically significant at the 5% level. The main driver for the higher cost in the standard-care arm during the follow-up period was the higher cost of neonatal care. Likewise, the mean total NHS and PSS cost throughout the entire follow-up period was higher in the standard-care group (£75,348) than in the CGM group (£71,909), but the mean between-group cost difference of –£3439 was not statistically significant at the 5% level.
Cost | CGM arm (n = 71), mean (SE) | Standard-care arm (n = 83), mean (SE) | Total (n = 154), mean (SE) | Mean difference | Parametric 95% CI | p-value | Bootstrapped 95% CI |
---|---|---|---|---|---|---|---|
Days 1–7 | |||||||
Equipment | 87 (2) | n/a | n/a | n/a | n/a | n/a | n/a |
Monitoring | 165 (6) | n/a | n/a | n/a | n/a | n/a | n/a |
POC testing | 6 (0.3) | 5 (0.3) | 5 (0.2) | 1.4 | 0.6 to 2.2 | 0.001 | 0.6 to 2.2 |
Length of stay | 8656 (97) | 8534 (132) | 8590 (84) | 121 | –213 to 455 | 0.5 | –172 to 482 |
Medications | 79 (2) | 77 (1) | 78 (1) | –2.8 | –2.8 to 5.3 | 0.5 | –3.3 to 4.9 |
Investigation | 571 (31) | 630 (33) | 603 (23) | –59 | –148 to 31 | 0.2 | –148 to 32 |
Blood transfusion products | 2 (2) | 35.7 (34) | 20 (19) | –14 | –63 to 35 | 0.4 | –68 to 35 |
Medical treatment | 92 (17) | 106 (18) | 100 (12) | 17 | –153 to 186 | 0.6 | –139 to 196 |
Surgical procedures | 544 (57) | 527 (62) | 535 (43) | –34 | –108 to 39 | 0.8 | –132 to 2 |
Other procedures | 2 (2) | 36 (34) | 20 (19) | –34 | –108 to 39 | 0.4 | –144 to 3 |
Total | 11,199 (976) | 9934 (184) | 10,517 (462) | 1265 | 68 to 4168 | 0.2 | –560 to 3090 |
Cost | CGM arm (n = 71), mean (SE) | Standard-care arm (n = 82), mean (SE) | Total (n = 153), mean (SE) | Mean difference | Parametric 95% CI | p-value | Bootstrapped 95% CI |
Day 8 to 36 weeks’ corrected gestational age | |||||||
Length of stay | 59,901 (2296) | 62,807 (2059) | 61,458 (1533) | –2906 | –8983 to 3170 | 0.3 | –9020 to 2400 |
Medication: insulin only | 0.3 (0.1) | 0.2 (0.1) | 0.2 (0.1) | 0.1 | –0.1 to 0.4 | 0.2 | –0.1 to 0.4 |
Investigation | 677 (76) | 841 (97) | 765 (63) | –165 | –414 to 84 | 0.2 | –443 to 53 |
Blood transfusion products | 222 (34) | 279 (39) | 252 (26) | –57 | –161 to 47 | 0.3 | –157 to 52 |
Medical treatment | 244 (64) | 531 (105) | 398 (65) | –287 | –540 to –35 | 0.03 | –535 to –65 |
Surgical procedures | 20 (14) | 909 (837) | 496 (449) | –889 | –2667 to 889 | 0.3 | –3289 to –21 |
Other procedures | 0 (0) | 94 (62) | 50 (33) | –94 | –225 to 38 | 0.2 | –244 to –5 |
Mode of transfers | 2.8 (0.4) | 3.6 (1) | 3.2 (0.6) | –0.8 | –3.1 to 1.4 | 0.5 | –3.3 to 0.8 |
Total | 61,065 (2376) | 65,464 (2381) | 63,423 (1690) | –4399 | –11,080 to 2283 | 0.2 | –10,713 to 1773 |
Cost | CGM arm (n = 69), mean (SE) | Standard-care arm (n = 81), mean (SE) | Total (n = 150), mean (SE) | Mean difference | Parametric 95% CI | p-value | Bootstrapped 95% CI |
Entire study period | |||||||
Equipment | 87 (2) | n/a | n/a | n/a | n/a | n/a | n/a |
Monitoring | 165 (6) | n/a | n/a | n/a | n/a | n/a | n/a |
POC testing | 5.8 (0.3) | 4.5 (0.3) | 5.1 (0.2) | 1.3 | 0.5 to 2.1 | 0.002 | 0.6 to 2.2 |
Length of stay | 68,144 (2563) | 71,273 (2101) | 69,833 (1636) | –3129 | –9616 to 3358 | 0.3 | –9577 to 3043 |
Medications | 79 (2) | 78 (1) | 78 (1) | –2.7 | –2.7 to 5.5 | 0.5 | –3 to 5.5 |
Investigation | 1260 (94) | 1471 (115) | 1374 (76) | –212 | –513 to 89 | 0.2 | –505 to 88 |
Blood transfusion products | 320 (49) | 387 (53) | 356 (36) | –67 | –211 to 78 | 0.4 | –203 to 71 |
Medical treatment | 798 (99) | 1062 (131) | 941 (85) | –264 | –598 to 70 | 0.1 | –587 to 45 |
Surgical procedures | 1047 (994) | 939 (848) | 989 (645) | 109 | –2457 to 2674 | 0.9 | –2313 to 3007 |
Other procedures | 2 (2) | 131 (72) | 72 (39) | –130 | –284 to 25 | 0.1 | –294 to –7 |
Mode of transfers | 2.8 (0.4) | 3.6 (1) | 3.3 (0.6) | –0.8 | –3.1 to 1.5 | 0.5 | –3.2 to 1 |
Total | 71,910 (2779) | 75,348 (2476) | 73,767 (1849) | –3439 | –10,645 to 2939 | 0.4 | –10,774 to 3896 |
Cost | Item | CGM arm (n = 71), mean (SE) | Standard-care arm (n = 83), mean (SE) | Total (n = 154), mean (SE) | Mean difference | Parametric 95% CI | p-value | Bootstrapped 95% CI |
---|---|---|---|---|---|---|---|---|
Days 1–7 | ||||||||
Enlite sensor (MiniMed System) (item) | MiniMed 640G | 14 (0) | n/a | n/a | n/a | n/a | n/a | n/a |
Guardian 2 Link | 9 (0) | n/a | n/a | n/a | n/a | n/a | n/a | |
Generation Enlite single sensor: MMT-7008B | 64 (2.4) | n/a | n/a | n/a | n/a | n/a | n/a | |
Glucose monitoring | Enlite sensor insertion | 6 (0.2) | n/a | n/a | n/a | n/a | n/a | n/a |
Enlite sensor monitoring | 157 (6) | n/a | n/a | n/a | n/a | n/a | n/a | |
Enlite sensor removal | 2.5 (0.1) | n/a | n/a | n/a | n/a | n/a | n/a | |
POC testing | Nova Biomedical StatStrip meter for calibration of 640G device | 5.9 (0.3) | 4.5 (0.3) | 5.1 (0.2) | 1.4 | 0.6 to 2.2 | 0.001 | 0.6 to 2.1 |
Length of stay | Intensive care | 7788 (304) | 7708 (297) | 7745 (212) | 81 | –762 to 923 | 0.9 | –720 to 810 |
High-dependency care | 859 (205) | 778 (188) | 816 (138) | 81 | –467 to 629 | 0.8 | –416 to 648 | |
Special care | 8 (8) | 49 (49) | 30 (27) | –41 | –146 to 65 | 0.4 | –167 to 17 | |
Transitional care | 0 (0) | 0 (0) | 0 (0) | 0 | ||||
Medications | Inotropes | 0.1 (0.0) | 0.1 (0.0) | 0.1 (0.0) | 0 | –0.1 to 0 | 0.7 | –0.1 to 0.1 |
Antibiotics | 3.5 (0.2) | 3.4 (0.2) | 3.4 (0.1) | 0.1 | –0.5 to 0.6 | 0.7 | –0.5 to 0.6 | |
Caffeine | 74 (1.5) | 73 (1.2) | 74 (0.9) | 0.5 | –3.3 to 4.3 | 0.8 | –3.4 to 4.1 | |
Morphine | 0 (0) | 0 (0) | 0 (0) | 0 | 0 to 0 | 0.8 | 0 to 0.0 | |
Corticosteroids | 0 (0) | 0 (0) | 0 (0) | 0 | 0 to 0 | 0.7 | 0 to 0.0 | |
Insulin | 1.1 (0.2) | 0.5 (0.1) | 0.8 (0.1) | 0.6 | 0.2 to 1.2 | 0.007 | 0.2 to 1.1 | |
Investigations | Cranial ultrasound | 159 (9) | 160 (8) | 160 (6) | –0.8 | –25 to 23 | 0.9 | –27 to 23 |
Other ultrasound | 1 (1) | 2.6 (1.5) | 1.8 (0.9) | –1.6 | –5 to 2 | 0.4 | –4.9 to 2.1 | |
CT | 0 (0) | 0 (0) | 0 (0) | 0 | ||||
X-rays | 360 (25) | 412 (27) | 388 (19) | –52 | –127 to 22 | 0.2 | –121 to 25 | |
EEG | 0 (0) | 0 (0) | 0 (0) | 0 | ||||
MRI | 0 (0) | 0 (0) | 0 (0) | 0 | ||||
Echocardiography | 51 (10) | 55 (8) | 53 (6) | –4 | –30 to 21 | 0.7 | –30 to 22 | |
ECG | 0 (0) | 0 (0) | 0 (0) | 0 | ||||
Blood transfusion products | Platelets | 14 (5) | 13 (4) | 14 (3) | 0.9 | –13 to 14 | 0.9 | –12 to 14 |
Red blood cells | 76 (14) | 91 (15) | 84 (10) | –15 | –56 to 26 | 0.5 | –52 to 28 | |
Fresh-frozen plasma | 2.4 (1.1) | 2.1 (0.8) | 2.2 (0.7) | 0.3 | –2.3 to 3 | 0.8 | –2 to 3.4 | |
Medical (surgical) treatments | Lumbar puncture | 148 (50) | 143 (51) | 145 (36) | 6 | –136 to 147 | 0.9 | –137 to 149 |
Ventricular tap | 0 (0) | 0 (0) | 0 (0) | 0 | ||||
Long line | 396 (30) | 385 (40) | 390 (25) | 11 | –89 to 111 | 0.8 | –93 to 92 | |
Surgical procedures | Interventions for ROP treatment | 10 (10) | 0 (0) | 4.5 (4.5) | 10 | –8 to 28 | 0.3 | 0 to 36 |
Surgical procedures for central access | 22 (22) | 19 (19) | 20 (14) | 3 | –53 to 59 | 0.9 | –51 to 65 | |
Ventriculoperitoneal shunts | 967 (967) | 0 (0) | 446 (446) | 967 | –799 to 2732 | 0.3 | 0 to 3550 | |
Other procedures | 1.5 (1.5) | 36 (34) | 20 (19) | –34 | –108 to 39 | 0.4 | –144 to 2.6 | |
Total costs | 11,199 (976) | 9934 (184) | 10,517 (462) | 1265 | 68 to 4168 | 0.2 | –560 to 3090 | |
Cost | Item | CGM arm (n = 71), mean (SE) | Standard-care arm (n = 82), mean (SE) | Total (n = 153), mean (SE) | Mean difference | Parametric 95% CI | p-value | Bootstrapped 95% CI |
Day 8 to 36 weeks’ corrected gestational age | ||||||||
Length of stay | Intensive care | 17,036 (2119) | 21,636 (2368) | 19,501 (1611) | –4600 | –10,962 to 1762 | 0.2 | –10,885 to 1774 |
High-dependency care | 22,476 (1791) | 22,250 (1352) | 22,355 (1099) | 226 | –4143 to 4594 | 0.9 | –3824 to 4645 | |
Special care | 9549 (970) | 8655 (874) | 9070 (648) | 894 | –1679 to 3467 | 0.5 | –1649 to 3617 | |
Transitional care | 10,840 (1160) | 10,266 (1037) | 10,533 (772) | 575 | –2491 to 3640 | 0.7 | –2274 to 3952 | |
Medications | Insulin | 0.3 (0.1) | 0.2 (0.1) | 0.2 (0.1) | 0.1 | –0.1 to 0.4 | 0.2 | –0.1 to 0.4 |
Investigations | Cranial ultrasound | 203 (21) | 214 (14) | 209 (12) | –11 | –59 to 38 | 0.7 | –58 to 38 |
Other ultrasound | 12 (6) | 27 (14) | 20 (8) | –15 | –46 to 17 | 0.4 | –48 to 12 | |
CT | 0 (0) | 1.4 (1.4) | 0.8 (0.8) | –1.4 | –4.4 to 1.6 | 0.4 | –6.1 to 0 | |
X-ray | 368 (56) | 468 (61) | 422 (42) | –100 | –266 to 66 | 0.2 | –256 to 66 | |
EEG | 0 (0) | 15 (15) | 8.2 (8.2) | –15 | –48 to 17 | 0.4 | –58 to 0 | |
MRI | 3.3 (3.3) | 11 (6) | 7.6 (3.4) | –8 | –21 to 5.2 | 0.2 | –22 to 3.9 | |
Echocardiography | 85 (15) | 104 (15) | 95 (11) | –20 | –62 to 23 | 0.4 | –60 to 24 | |
ECG | 5.6 (3.2) | 0 (0) | 2.6 (1.5) | 5.6 | –0.3 to 12 | 0.06 | 0 to 14 | |
Blood transfusion products | Platelets | 10 (4.6) | 9.9 (4.7) | 10 (3.3) | 0.3 | –13 to 13 | 1.0 | –14 to 13 |
Red blood cells | 210 (31) | 268 (36) | 241 (24) | –58 | –154 to 37 | 0.2 | –164 to 31 | |
Fresh-frozen plasma | 2 (0.9) | 1 (0.6) | 1.5 (0.5) | 1 | –1.1 to 3 | 0.4 | –1 to 3.3 | |
Medical (surgical) treatments | Lumbar puncture | 130 (47) | 305 (80) | 224 (49) | –175 | –366 to 16 | 0.07 | –380 to –11 |
Ventricular tap | 114 (32) | 213 (55) | 167 (33) | –99 | –230 to 33 | 0.1 | –246 to 3.8 | |
Long line | 0 (0) | 13 (9.7) | 7 (5.2) | –13 | –34 to 7.6 | 0.2 | –38 to 0 | |
Surgical procedures | Interventions for ROP treatment | 20 (14) | 34 (17) | 27 (11) | –14 | –58 to 29 | 0.5 | –60 to 28 |
Surgical procedures for central access | 0 (0) | 38 (26) | 20 (14) | –38 | –93 to 19 | 0.2 | –106 to 0 | |
Ventriculoperitoneal shunts | 0 (0) | 837 (837) | 449 (449) | –837 | –2615 to 941 | 0.4 | –3566 to 0 | |
Other procedures | 0 (0) | 93.7 (62) | 50 (33) | –94 | –225 to 38 | 0.2 | –241 to –5.3 | |
Mode of transfers | Air ambulance | 0 (0) | 0.9 (0.6) | 0.5 (0.3) | –0.9 | –2.5 to 0 | 0.2 | –2.2 to 0.5 |
Road | 2.8 (0.4) | 2.7 (0.5) | 2.7 (0.3) | 0.1 | –1.3 to 1.5 | 0.9 | –1.2 to 1.5 | |
Total | 61,065 (2376) | 65,464 (2381) | 63,423 (1690) | –4399 | –11,080 to 2283 | 0.2 | –10,972 to 2187 | |
Cost | Item | CGM arm (n = 69), mean (SE) | Standard-care arm (n = 81), mean (SE) | Total (n = 150), mean (SE) | Mean difference | Parametric 95% CI | p-value | Bootstrapped 95% CI |
Entire study period | ||||||||
Enlite sensor (MiniMed System) | MiniMed 640G System | 14 (0) | n/a | n/a | n/a | n/a | n/a | n/a |
Guardian 2 Link | 9 (0) | n/a | n/a | n/a | n/a | n/a | n/a | |
Generation Enlite single sensor MMT-7008B | 64 (2.4) | n/a | n/a | n/a | n/a | n/a | n/a | |
Glucose monitoring | Enlite sensor insertion | 6.1 (0.2) | n/a | n/a | n/a | n/a | n/a | n/a |
Enlite sensor monitoring | 157 (6) | n/a | n/a | n/a | n/a | n/a | n/a | |
Enlite sensor removal | 2.5 (0.1) | n/a | n/a | n/a | n/a | n/a | n/a | |
POC testing | Nova Biomedical StatStrip meter for calibration of 640G device | 5.8 (0.3) | 4.5 (0.3) | 5.1 (0.2) | 1.3 | 0.5 to 2.1 | 0.002 | 0.5 to 2 |
Length of stay (days) | Intensive care | 25,149 (2273) | 29,433 (2480) | 27,463 (1702) | –4284 | –11,021 to 2452 | 0.2 | –11,182 to 1828 |
High-dependency care | 23,439 (1782) | 22,946 (1339) | 23,173 (1089) | 494 | –3839 to 4826 | 0.8 | –3381 to 4696 | |
Special care | 8973 (998) | 8669 (890) | 8809 (662) | 304 | –2331 to 2938 | 0.8 | –2138 to 2963 | |
Transitional care | 10,583 (1177) | 10,225 (1049) | 10,389 (781) | 358 | –2749 to 3465 | 0.8 | –2679 to 3392 | |
Medications | Inotropes | 0.1 (0) | 0.1 (0) | 0.1 (0) | 0 | –0.1 to 0.1 | 0.7 | –0.1 to 0.1 |
Antibiotics | 3.5 (0.2) | 3.4 (0.2) | 3.4 (0.1) | 0.1 | –0.5 to 0.6 | 0.8 | –0.5 to 0.7 | |
Caffeine | 74 (1.5) | 73 (1.3) | 74 (1) | 0.5 | –3.4 to 4.4 | 0.8 | –4.2 to 4 | |
Morphine | 0 (0) | 0 (0) | 0 (0) | 0 | 0 to 0 | 0.9 | 0 to 0 | |
Corticosteroids | 0 (0) | 0 (0) | 0 (0) | 0 | 0 to 0 | 0.7 | 0 to 0 | |
Insulin | 1.5 (0.3) | 0.6 (0.1) | 1 (0.2) | 0.8 | 0.2 to 1.6 | 0.01 | 0.2 to 1.5 | |
Investigations | Cranial ultrasound | 365 (24) | 375 (19) | 370 (15) | –9.7 | –70 to 50 | 0.8 | –65 to 58 |
Other ultrasound | 13 (6.2) | 30 (15) | 22 (8.5) | –16 | –50 to 17 | 0.3 | –57 to 8.3 | |
CT | 0 (0) | 1.4 (1.4) | 0.8 (0.8) | –1.4 | –4.5 to 1.6 | 0.4 | –5 to 0 | |
X-ray | 734 (67) | 877 (79) | 811 (53) | –143 | –352 to 65 | 0.2 | –336 to 55 | |
EEG | 0 (0) | 16 (16) | 8.4 (8.4) | –16 | –49 to 18 | 0.4 | –53 to 0 | |
MRI | 3.4 (3.4) | 12 (5.6) | 7.7 (3.4) | –8.1 | –22 to 5.4 | 0.2 | –21 to 3.7 | |
Echocardiography | 138 (21) | 161 (20) | 151 (15) | –23 | –81 to 35 | 0.4 | –81 to 33 | |
ECG | 5.8 (3.3) | 0 (0) | 2.7 (1.5) | 5.8 | –0.2 to 12 | 0.06 | 1.8 to 14 | |
Blood transfusion products | Platelets | 258 (8.7) | 23 (7.9) | 24 (5.8) | 1.5 | –22 to 25 | 0.9 | –20 to 25 |
Red blood cells | 290 (42) | 360 (48) | 328 (32) | –70 | –198 to 58 | 0.3 | –203 to 47 | |
Fresh-frozen plasma | 4.5 (1.4) | 3.2 (1) | 3.8 (0.8) | 1.4 | –1.9 to 4.7 | 0.4 | –1.6 to 5.4 | |
Medical (surgical) treatments | Lumbar puncture | 286 (76) | 455 (104) | 377 (66) | –169 | –431 to 93 | 0.2 | –432 to 74 |
Long line | 512 (50) | 594 (72) | 556 (45) | –82 | –262 to 98 | 0.4 | –266 to 64 | |
Ventricular tap | 0 (0) | 13 (9.9) | 7.2 (5.3) | –13 | –34 to 7.8 | 0.2 | –38 to 0 | |
Surgical procedures | Interventions for ROP treatment | 30 (17) | 35 (17) | 33 (12) | –4 | –52 to 44 | 0.9 | –54 to 42 |
Surgical procedures for central access | 22 (22) | 57 (42) | 41 (25) | –35 | –134 to 64 | 0.5 | –143 to 47 | |
Ventriculoperitoneal shunts | 995 (995) | 847 (847) | 915 (645) | 147 | –2418 to 2713 | 0.9 | –1716 to 3269 | |
Other procedures | 1.5 (1.5) | 131 (72) | 72 (39) | –130 | –284 to 25 | 0.10 | –319 to –8.4 | |
Mode of transfers | Air ambulance | 0 (0) | 0.9 (0.6) | 0.5 (0.3) | –0.9 | –2.2 to 0.4 | 0.2 | –2.6 to 0 |
Road | 2.8 (0.4) | 2.8 (0.5) | 2.8 (0.3) | 0.1 | –1.3 to 1.5 | 0.9 | –1.5 to 1.4 | |
Total costs | 71,910 (2779) | 75,348 (2476) | 73,766.5 (1849) | –3439 | –10,645 to 2939 | 0.4 | –10,774 to 3896 |
Cost-effectiveness results
The cost-effectiveness results are presented in Tables 19 and 20 for days 1–7 and the entire study period, respectively. The associated CEACs and cost-effectiveness scatterplots are graphically represented in Figures 16–19.
Scenario | Trial arm, mean (SE) cost (£) | Incremental cost (£) (95% CI) | Trial arm, mean (SE) cost (£) | Incremental effectiveness (95% CI) | ICER | Probability of cost-effectiveness at threshold | NMB (£) at threshold | ||||||
---|---|---|---|---|---|---|---|---|---|---|---|---|---|
CGM | Standard care | CGM | Standard care | £1000 | £5000 | £10,000 | £1000 (95% CI) | £5000 (95% CI) | £10,000 (95% CI) | ||||
Base-case analysis | |||||||||||||
Imputed costs and cases of adequate glucose control (80% threshold), covariate adjusted | 10,354 (597) | 9749 (282) | 605 (–379 to 1589) | 0.92 (0.05) | 0.68 (0.05) | 0.23 (0.07 to 0.4) | 2583 | 0.31 | 0.86 | 0.97 | –276 (–308 to –245) | 718 (677 to 759) | 1961 (1899 to 2023) |
Sensitivity analyses | |||||||||||||
Complete-case attributable costs and cases of adequate glucose control (80% threshold) | 11,117 (743) | 10,013 (370) | 1104 (–39 to 2247) | 0.93 (0.05) | 0.7 (0.06) | 0.22 (0.05 to 0.4) | 4923 | 0.08 | 0.56 | 0.89 | –827 (–864 to –790) | 138 (90 to 185) | 1344 (1276 to 1413) |
Imputed costs and cases of adequate glucose control (90% threshold), covariate adjusted | 10,354 (597) | 9749 (282) | 605 (–379 to 1589) | 0.80 (0.1) | 0.58 (0.06) | 0.23 (–0.03 to 0.48) | 2670 | 0.33 | 0.81 | 0.93 | –266 (–376 to –157) | 752 (581 to 923) | 2025 (1740 to 2309) |
Imputed costs and cases of adequate glucose control (70% threshold), covariate adjusted | 10,354 (597) | 9749 (282) | 605 (–379 to 1589) | 0.97 (0.04) | 0.75 (0.05) | 0.21 (0.06 to 0.36) | 2870 | 0.27 | 0.85 | 0.97 | –292 (–396 to –189) | 621 (499 to 742) | 1762 (1590 to 1935) |
Imputed costs and cases of adequate glucose control (60% threshold), covariate adjusted | 10,354 (597) | 9749 (282) | 605 (–379 to 1589) | 0.97 (0.03) | 0.83 (0.04) | 0.14 (0.04 to 0.25) | 4223 | 0.26 | 0.65 | 0.90 | –370 (–475 to –265) | 233 (114 to 352) | 987 (835 to 1139) |
Subgroup analysisa | |||||||||||||
Stratification by centre | |||||||||||||
Cambridge N01 | 12,542 (2275) | 9873 (357) | 2670 (–1819 to 7158) | 0.88 (0.06) | 0.63 (0.1) | 0.26 (0.03 to 0.48) | 10,469 | 0.17 | 0.19 | 0.27 | –2444 (–3015 to –1874) | –2251 (–2821 to –1681) | –2009 (–2582 to –1437) |
Norwich N42 | 7770 (1163) | 10,297 (251) | –2527 (–4871 to –182) | 1 | 0.86 (0.12) | 0.14 (–0.09 to 0.37) | –17,686 | 0.96 | 0.98 | 0.99 | 3666 (3345 to 3987) | 4087 (3753 to 4420) | 4612 (4251 to 4973) |
Luton and Dunstable N43 | 10,622 (182) | 9309 (686) | 1312 (–88 to 2714) | 0.91 (0.08) | 0.69 (0.13) | 0.22 (–0.06 to 0.49) | 6056 | 0.19 | 0.28 | 0.49 | –982 (–1196 to –768) | –495 (–704 to –285) | 114 (–108 to 336) |
Wolverhampton N68 | 9592 (383) | 9470 (788) | 122 (–1517 to 1760) | 0.82 (0.12) | 0.69 (0.15) | 0.13 (–0.26 to 0.51) | 968 | 0.36 | 0.53 | 0.62 | –317 (–498 to –136) | 21 (–192 to 234) | 444 (179 to 708) |
Southampton N73 | 9181 (889) | 8112 (546) | 1069 (–1169 to 3307) | 1 | 0.83 (0.1) | 0.17 (–0.04 to 0.37) | 6413 | 0.63 | 0.71 | 0.87 | 824 (291 to 1357) | 1582 (1128 to 2036) | 2529 (2100 to 2958) |
Amsterdam ND3 | 10,282 (535) | 9290 (853) | 992 (–848 to 2832) | 1 (0) | 0.75 (0.17) | 0.25 (–0.08 to 0.58) | 3967 | 0.40 | 0.56 | 0.72 | –758 (–859 to –658) | –462 (–583 to –341) | –92 (–302 to 118) |
Stratification by gestational age at birth | |||||||||||||
Extremely preterm (< 28 weeks’ gestation) | 10,035 (292) | 10,018 (254) | 17 (–820 to 854) | 0.88 (0.06) | 0.64 (0.06) | 0.24 (0.08 to 0.4) | 71 | 0.69 | 0.99 | 1 | 221 (133 to 310) | 1191 (1067 to 1315) | 2403 (2212 to 2594) |
Very preterm (28–32 weeks’ gestation) | 11,531 (1724) | 8937 (370) | 2594 (–785 to 5972) | 0.93 (0.05) | 0.79 (0.08) | 0.14 (–0.05 to 0.33) | 18,501 | 0.03 | 0.16 | 0.29 | –2541 (–2886 to –2196) | –1947 (–2305 to –1589) | –1204 (–1601 to –807) |
Stratification by sex | |||||||||||||
Male | 9539 (331) | 9735 (299) | –196 (–1041 to 650) | 0.91 (0.05) | 0.60 (0.08) | 0.31 (0.12 to 0.5) | –632 | 0.88 | 1 | 1 | 498 (410 to 585) | 1734 (1608 to 1859) | 3278 (3074 to 3483) |
Female | 11,666 (1595) | 9517 (269) | 2150 (–1037 to 5336) | 0.88 (0.06) | 0.78 (0.07) | 0.10 (–0.07 to 0.27) | 21,496 | 0.08 | 0.22 | 0.27 | –2077 (–2400 to –1753) | –1678 (–2010 to –1346) | –1179 (–1540 to –819) |
£10,000 | £30,000 | £50,000 | £10,000 (95% CI) | £30,000 (95% CI) | £50,000 (95% CI) | ||||||||
Secondary analysis | |||||||||||||
Imputed costs and cases of BPD averted, covariate adjusted | 10,354 (597) | 9749 (282) | 605 (–379 to 1589) | 0.66 (0.17) | 0.52 (0.12) | 0.14 (–0.28 to 0.56) | 4286 | 0.65 | 0.73 | 0.74 | 962 (819 to 1105) | 3936 (3531 to 4342) | 6910 (6240 to 7580) |
Imputed costs and cases of NEC averted, covariate adjusted | 10,354 (597) | 9749 (282) | 605 (–379 to 1589) | 0.87 (0.05) | 0.71 (0.06) | 0.16 (0 to 0.33) | 3697 | 0.82 | 0.95 | 0.97 | 1085 (885 to 1285) | 4296 (3781 to 4812) | 7508 (6662 to 8354) |
Scenario | Trial arm, mean (SE) cost (£) | Incremental cost (£) (95% CI) | Trial arm, mean (SE) cost (£) | Incremental effectiveness (95% CI) | ICER | Probability of cost-effectiveness at threshold | NMB (£) at threshold | ||||||
---|---|---|---|---|---|---|---|---|---|---|---|---|---|
CGM | Standard care | CGM | Standard care | £1000 | £5000 | £10,000 | £1000 (95% CI) | £5000 (95% CI) | £10,000 (95% CI) | ||||
Base-case analysis | |||||||||||||
Imputed costs and cases of adequate glucose control (80% threshold), covariate adjusted | 73,505 (2758) | 76,382 (2258) | –2877 (–10,026 to 4272) | 0.92 (0.05) | 0.68 (0.05) | 0.23 (0.07 to 0.4) | –12,273 | 0.8 | 0.86 | 0.92 | 3227 (3000 to 3454) | 4221 (3990 to 4452) | 5464 (5225 to 5703) |
Sensitivity analyses | |||||||||||||
Complete-case attributable costs and cases of adequate glucose control (80% threshold) | 73,200 (2480) | 74,773 (2237) | –1574 (–6557 to 3410) | 0.93 (0.05) | 0.7 (0.06) | 0.22 (0.05 to 0.4) | –7016 | 0.07 | 0.62 | 0.91 | 1802 (1643 to 1961) | 2768 (2602 to 2933) | 3974 (3797 to 4151) |
Imputed costs and cases of adequate glucose control (90% threshold), covariate adjusted | 73,505 (2758) | 76,382 (2258) | –2877 (–10,026 to 4272) | 0.8 (0.1) | 0.58 (0.06) | 0.23 (–0.03 to 0.48) | –12,687 | 0.81 | 0.86 | 0.91 | 3375 (2593 to 4156) | 4393 (3576 to 5210) | 5666 (4789 to 6542) |
Imputed costs and cases of adequate glucose control (70% threshold), covariate adjusted | 73,505 (2758) | 76,382 (2258) | –2877 (–10,026 to 4272) | 0.97 (0.04) | 0.75 (0.05) | 0.21 (0.06 to 0.36) | –13,637 | 0.81 | 0.85 | 0.9 | 3348 (2572 to 4124) | 4262 (3476 to 5047) | 5403 (4599 to 6207) |
Imputed costs and cases of adequate glucose control (60% threshold), covariate adjusted | 73,505 (2758) | 76,382 (2258) | –2877 (–10,026 to 4272) | 0.97 (0.03) | 0.83 (0.04) | 0.14 (0.04 to 0.25) | –20,066 | 0.81 | 0.83 | 0.89 | 3271 (2494 to 4048) | 3874 (3085 to 4663) | 4628 (3820 to 5436) |
Subgroup analysisa | |||||||||||||
Stratification by centre | |||||||||||||
Cambridge N01 | 82,336 (2895) | 79,688 (4287) | 2648 (–7706 to 13,002) | 0.88 (0.06) | 0.63 (0.1) | 0.26 (0.03 to 0.48) | 10,385 | 0.3 | 0.38 | 0.56 | –1997 (–3043 to –951) | –963 (–2006 to 81) | 330 (–722 to 1381) |
Norwich N42 | 63,545 (19515) | 73,956 (4990) | –10,411 (–48,985 to 28,162) | 1 | 0.86 (0.12) | 0.14 (–0.09 to 0.37) | –72,879 | 0.79 | 0.79 | 0.82 | 12,753 (8126 to 17,379) | 13,695 (9059 to 18,331) | 14,874 (10,222 to 19,525) |
Luton and Dunstable N43 | 70,091 (6103) | 74,115 (4544) | –4024 (–18,633 to 10,586) | 0.91 (0.08) | 0.69 (0.13) | 0.22 (–0.06 to 0.49) | –18,561 | 0.74 | 0.77 | 0.8 | 4385 (2910 to 5860) | 5208 (3714 to 6701) | 6236 (4708 to 7764) |
Wolverhampton N68 | 79,446 (6431) | 88,220 (5321) | –8774 (–25,199 to 7651) | 0.82 (0.12) | 0.69 (0.15) | 0.13 (–0.26 to 0.51) | –69,708 | 0.79 | 0.8 | 0.81 | 7539 (5856 to 9222) | 7954 (6170 to 9737) | 8473 (6553 to 10,392) |
Southampton N73 | 56,614 (12,085) | 61,969 (7603) | –5355 (–32,051 to 21,341) | 1 | 0.83 (0.1) | 0.17 (–0.04 to 0.37) | –32,129 | 0.81 | 0.81 | 0.82 | 5994 (2283 to 9705) | 6904 (3220 to 10,589) | 8043 (4386 to 11,700) |
Amsterdam ND3 | 72,631 (16,207) | 75,867 (8455) | –3236 (–38,650 to 32,177) | 1 (0) | 0.75 (0.17) | 0.25 (–0.08 to 0.58) | –12,945 | 0.67 | 0.68 | 0.71 | 4415 (162 to 8669) | 5810 (1521 to 10,099) | 7553 (3212 to 11,893) |
Stratification by gestational age at birth | |||||||||||||
Extremely preterm (< 28 weeks’ gestation) | 84,532 (2548) | 87,024 (2423) | –2492 (–8872 to 3888) | 0.88 (0.06) | 0.64 (0.06) | 0.24 (0.08 to 0.4) | –10,443 | 0.81 | 0.88 | 0.91 | 2524 (1874 to 3174) | 3493 (2824 to 4162) | 4705 (4004 to 5406) |
Very preterm (28–32 weeks’ gestation) | 59,983 (3187) | 57,829 (2146) | 2154 (–5541 to 9849) | 0.93 (0.05) | 0.79 (0.08) | 0.14 (–0.05 to 0.33) | 15,365 | 0.37 | 0.4 | 0.45 | –1506 (–2304 to –707) | –911 (–1720 to –102) | –169 (–1001 to 664) |
Stratification by sex | |||||||||||||
Male | 74,004 (3800) | 81,783 (3860) | –7779 (–17,801 to 2243) | 0.91 (0.05) | 0.6 (0.08) | 0.31 (0.12 to 0.5) | –25,123 | 0.93 | 0.96 | 0.99 | 7580 (6559 to 8602) | 8816 (7763 to 9870) | 10,361 (9262 to 11,460) |
Female | 72,839 (3641) | 71,301 (2778) | 1538 (–6991 to 10,067) | 0.88 (0.06) | 0.78 (0.07) | 0.1 (–0.07 to 0.27) | 15,381 | 0.33 | 0.35 | 0.37 | –1732 (–2592 to –872) | –1333 (–2200 to –466) | –835 (–1717 to 48) |
£10,000 | £30,000 | £50,000 | £10,000 (95% CI) | £30,000 (95% CI) | £50,000 (95% CI) | ||||||||
Secondary analysis | |||||||||||||
Imputed costs and cases of BPD averted, covariate adjusted | 73,505 (2758) | 76,382 (2258) | –2877 (–10,026 to 4272) | 0.66 (0.17) | 0.52 (0.12) | 0.14 (–0.28 to 0.56) | –20,368 | 0.82 | 0.82 | 0.81 | 4466 (4167 to 4764) | 7440 (6920 to 7959) | 10,414 (9645 to 11,183) |
Imputed costs and cases of NEC averted, covariate adjusted | 73,505 (2758) | 76,382 (2258) | –2877 (–10,026 to 4272) | 0.87 (0.05) | 0.71 (0.06) | 0.16 (0 to 0.33) | –17,568 | 0.88 | 0.94 | 0.97 | 4726 (3923 to 5529) | 7937 (6986 to 8888) | 11,149 (9971 to 12,327) |
FIGURE 16.
Randomised controlled trial: (a) CEAC and (b) scatterplot at intervention period for 80% threshold of adequate glucose control, imputed and covariate adjusted.


FIGURE 17.
Randomised controlled trial (a) CEAC and (b) scatterplot at intervention period for 80% threshold of adequate glucose control, complete case and covariate adjusted.

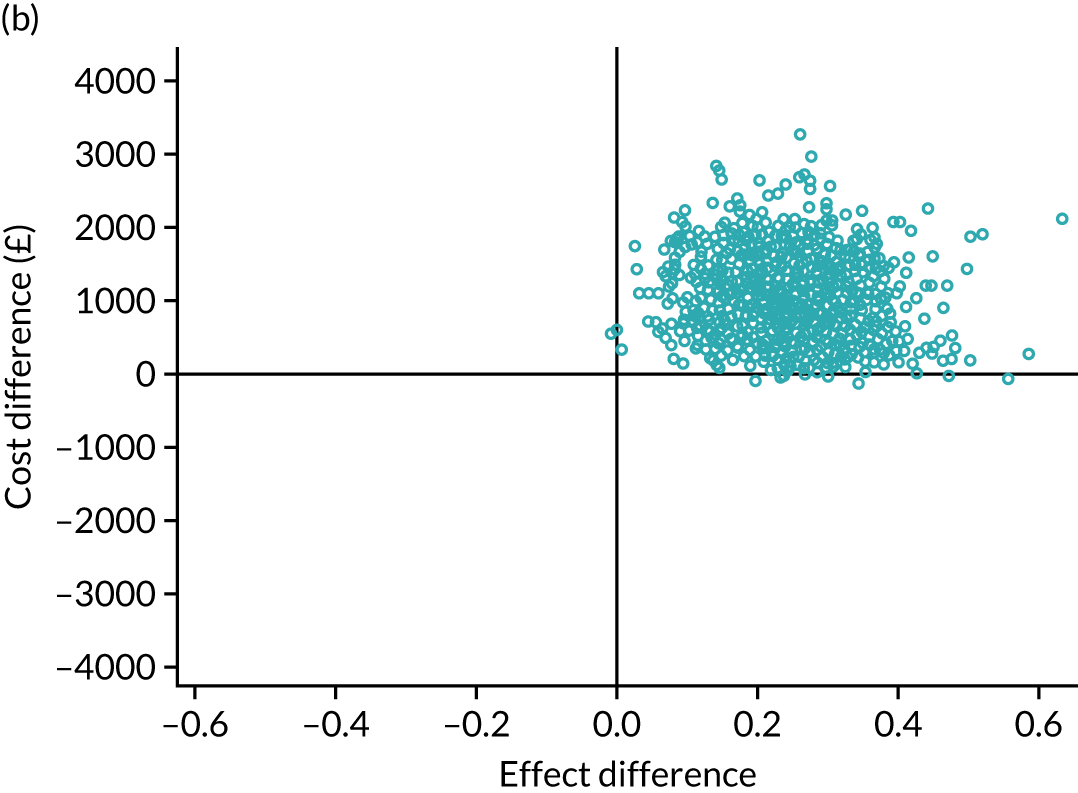
FIGURE 18.
Randomised controlled trial: (a) CEAC and (b) scatterplot over entire follow-up period for 80% threshold of adequate glucose control, imputed and covariate adjusted.


FIGURE 19.
Randomised controlled trial: (a) CEAC and (b) scatterplot at entire follow-up period over 80% threshold of adequate glucose control, complete case and covariate adjusted.


Base-case analysis
Two base-case analyses were performed. The first was imputed 7-day intervention costs and cases of adequate glucose control (see Table 19), with an 80% threshold selected for definition of adequate glucose control. The second was imputed costs and cases of adequate glucose control (80% threshold), but with costs extending to 36 weeks’ corrected gestational age and outcomes restricted to the intervention period (see Table 20). For the first base-case analysis, trial participants in the CGM arm experienced a statistically significant increase in cases of adequate glucose control based on 1000 bootstrap simulations (i.e. a 23 percentage-point increase in the rate of adequate glucose control) during the first 7 days. The mean NHS and PSS costs (see Table 19) were also lower in the standard-care group (mean cost difference £605). The ICER for the base-case analysis in Table 19 indicates that using CGM is, on average, more costly and more effective than standard care. Assuming cost-effectiveness thresholds of £1000, £5000 and £10,000 per additional case of adequate glucose control, the probability of cost-effectiveness for CGM reaches 90% at approximately £6000 (see Figure 16), whereas the NMB associated with use of a CGM became positive at a cost-effectiveness threshold of £5000 (see Table 19).
For the second base-case analysis (see Table 20), the trial participants in the CGM arm experienced lower costs (mean cost difference –£2877) and a 23 percentage-point increase in cases of adequate glucose control, as before. CGM was not only less costly, but also the more effective strategy in this second base-case analysis and, therefore, dominant in health economic terms. To summarise, the base-case analyses reported in Table 20 show that CGM is the dominant strategy in health economics terms.
Sensitivity analyses
It was found that the cost-effectiveness results from the sensitivity analyses using different analytical scenarios (i.e. complete case and imputed costs with 60%, 70% and 90% thresholds selected for definitions of additional adequate glucose control) generally supported the base-case findings. In the complete-case analysis (see Table 19), mean costs were higher in the CGM arm (mean cost difference £1104, 95% CI –£38.56 to £2247.11) than in the standard-care arm. The effectiveness results followed the same pattern as that of the base-case analysis and showed that participants in the CGM arm experienced a statistically significant increase in the proportion of glucose readings within the target range over the 7-day intervention period (0.22, 95% CI 0.05 to 0.4). For the four sets of sensitivity analyses (see Table 19), the probabilities of cost-effectiveness of using CGM reach approximately 90% at cost-effectiveness thresholds of £10,000. Adopting 60%, 70% and 90% thresholds for proportions of glucose readings within the target ranges, the costs for each group remained similar, with £10,354 for the CGM group and £9749 for the standard-care group, but effectiveness varied. However, the pattern of increased effectiveness of CGM remained consistent across the scenarios when using different levels of adequate glucose thresholds. The results presented in Table 20 support this finding. The notable difference is that CGM becomes the dominant strategy in health economic terms in the sensitivity analyses (see Table 20) because of the lower costs of CGM compared with standard care. In brief, the sensitivity analyses generally support the findings of the base-case analyses.
Subgroup analyses
Subgroup analyses were performed using the variables of site, gestational age and sex. A few study sites were dropped because the number of observations was insufficient. In all study sites presented in Table 19, effectiveness was greater in the CGM arm than in the standard-care arm and costs were also higher [except in site N42 (Norwich)] than in the standard-care group. Subgroup analyses based on gestational age at birth and sex demonstrated the same pattern, that is that costs and effectiveness were generally higher in the CGM arm than in the standard-care arm. Another notable finding was that costs were lower for male infants than female infants in the subgroup analyses.
Secondary outcome analyses
Secondary outcome analyses were performed using the two outcomes of NEC and BPD. For these outcomes, increased effectiveness can be interpreted as reduced cases of NEC and BPD and, hence, the denominator of the ICER has been inverted. The secondary outcome analyses revealed that, when the analysis is restricted to the 7-day intervention period, CGM averts more cases of BPD and NEC, but with higher costs, than standard care. Different cost-effectiveness threshold values were adopted for the secondary outcome analyses, namely £10,000, £30,000 and £50,000. The secondary outcomes displayed in Table 20 show that the probability of cost-effectiveness of CGM reached 80% at a £10,000 cost-effectiveness threshold. In the secondary analyses reported in Table 19, mean costs were higher in the CGM arm (mean cost difference £605, 95% CI –£379 to £1589) than the standard-care arm. When valued over a time horizon extending to 36 weeks’ corrected gestational age (see Table 20), the effectiveness followed the same pattern as the base-case analysis, but the costs were lower for CGM (mean cost difference –£2877, 95% CI –£10,026 to £4272) than standard care, making CGM dominant in health economic terms.
Chapter 6 REACT trial discussion
Discussion
This study demonstrates that CGM can be used to guide the use of insulin in preterm infants. The use of CGM leads to a reduction in exposure to hyperglycaemia and is also associated with a lower incidence of prolonged periods of hypoglycaemia. Previous studies found that the use of insulin in preterm infants led to an increased risk of hypoglycaemia, which was often clinically silent. 21,64 In contrast, this study showed that CGM allowed the safe titration of insulin, as well as highlighting clinically silent episodes of hypoglycaemia, in the standard-care group.
The strength of the study is that our findings on glucose exposure are not biased by the frequency of blood glucose sampling, as continuous data were available in both study arms. The accuracy of CGM at low glucose thresholds and the potential impact of glucose levels used for calibration remain controversial. However, CGM provides more detailed data on both glucose variability and the duration of exposure to any given target level, and it is increasingly apparent that these two parameters are more important than any prespecified threshold. Further analyses will be undertaken to explore the relationship between sensor and blood glucose levels in the trial.
The study highlights the wide variation in patterns of glucose control between infants and the significant difficultly for clinical staff in managing these infants with infrequent intermittent blood glucose monitoring. Furthermore, it demonstrates the challenges for staff in designing simple clinical guidelines for management of glucose control when the responses to interventions can be so varied. Comparison of mean daily sensor glucose and insulin infusion rates suggests that CGM led to earlier use of insulin, which prevented the early rise in glucose levels. Those in the standard-care arm received similar total levels of insulin during the intervention period but insulin was infused in the second half of the week and was associated with higher glucose levels. The significant interaction effects between treatment and both sex and first sensor glucose value are in keeping with expectations from clinical practice. This suggests that the most significant benefits are achieved in male infants and those with higher early glucose levels. The underlying pathophysiology driving this warrants further investigation.
The effect size is small, but similar to the clinically important difference of 10% that was prespecified in the sample size calculation. The effect size may have been limited by a number of factors, including compliance with the guideline for standard care, number of CGM data and improvements in standard care over time. In this context, in the CGM group, the sensor glucose level was recorded on the CRF > 90% of the time; however, the percentage of time during which the rate of insulin infusion was recorded when the glucose was > 8 mmol/l was only 54%. This may be because the guideline was designed to support clinical management, not to be a strict protocol. It may reflect the clinical team choosing to change rates of dextrose infusion rather than starting insulin. Alternatively, in some units that recruited only a few infants, proactive use of the CGM data and guideline was limited by the nature of intensive care (i.e. where the study outcomes are dependent on a large number of clinical staff being confident to deliver the study intervention over a 24-hour period). Increased use in these units may have led to further increases in time in target range, with increasing confidence in the accuracy of the sensor glucose level and the potential benefits of a more proactive approach allowing even better targeting of glucose control.
However, an increase from this level is remarkable, given the higher than predicted percentage of time within the target range of 84% (from 58% in initial masked data and 78% in the feasibility trial). This increase in time in the target range in the standard-care arm may be the result of changes in clinical practice either unrelated or related to the study. An increased emphasis placed on the importance of glucose control while undertaking the trial may well have had an impact on the care of infants in the standard-care arm, a common phenomenon in the setting of a RCT.
Although not powered for clinical outcomes, a number of exploratory outcomes were explored. There were more deaths in the standard-care arm than in the CGM arm (6% vs. 2%), but the difference did not reach statistical significance. It was anticipated that the use of CGM might lead to increased insulin treatment and nutritional intake, and therefore have an impact on growth. However, there were no differences between study arms in total insulin infusion rates or nutritional intakes over the first week of life, and there were no differences in growth parameters. More infants in the standard-care arm (12%) than in the CGM arm (5%) required surgery for PDA, but the difference did not reach statistical significance and might be related to differences in fluid management. In the case of the exploratory outcome of incidence of NEC, CGM was associated with significantly reduced risk (OR 0.33, 95% CI 0.13 to 0.78; p = 0.01), with 28% of infants in the standard-care arm being diagnosed with NEC, compared with 13% of infants in the CGM arm. There is evidence to link hyperglycaemia with increased risk of microbial gut translocation. 65 However, larger studies would be needed to confirm such a link.
Compared with the feasibility study, more difficulties with initial insertion and sensor calibration were experienced in this trial. 1 This was initially thought to be because of the lack of experience of the procedure in the sites. Although site training was undertaken, and staff were provided with an online training video and a 24-hour helpline, it was challenging to support staff remotely. This was particularly the case given that this new procedure needed to be undertaken within a short, time-critical window after birth. The MiniMed 640G System and Enlite sensor are not specifically designed for neonatal use and this presents challenges to their use in this setting. The inserter provided cannot be used because of the small amount of subcutaneous tissue in preterm infants as the normal insertion angle of 90° would result in the needle passing through the subcutaneous tissue into muscle and other tissues below. The rate of successful insertion varied between centres. It was difficult for some individuals to gain significant experience and confidence within a study setting when undertaking a procedure infrequently. In discussion with Medtronic plc, it was suggested that the introducer itself plays a role in optimising the condition of the sensor after insertion by ensuring a rapid and smooth insertion technique.
Furthermore, because of the display of error codes on the MiniMed 640G System, it was difficult to always be clear as to the primary reason for ‘sensor failure’ and whether this was failure of insertion or a calibration error. There were substantially more calibration errors with the MiniMed 640G System than with the Paradigm Veo System (used in the feasibility study). This was discussed and reviewed in collaboration with Medtronic plc. A number of changes to the MiniMed 640G System algorithm were made to improve safety and increase accuracy at low threshold. These included (1) the use of artefact detection, such that signal dips that were deemed to not reflect true physiological glucose levels were filtered by the algorithm; (2) changes to signal threshold limits, which is the allowable range of interstitial signal that the algorithm can use; and (3) reduction in the calibration ratio limit (i.e. the difference between blood glucose and the sensor glucose). These factors, combined with the introduction of a lockout following two consecutive error codes during calibration, led to increased reports of failure to calibrate and loss of sensor data.
Lessons learned included the fact that the increased calibration error rate seen in some infants (particularly in the first 24 hours after birth) may have been because of high glucose variability and not failed sensor insertion. In some infants, the sensor was demonstrated to benefit from additional ‘wetting time’ to be able to generate sufficient signal for a reading. In both situations, allowing additional time for the sensor to settle in, either a few hours or, in the study setting, overnight, led to successful calibration and further monitoring. This is similar to the situation in lipodystrophy patients, in whom more time may be required prior to the first calibration to allow for ‘wetting’ of the sensor. In addition, staff were advised to avoid calibration when glucose levels were changing rapidly or immediately after one calibration error. This was counterintuitive in an intensive care setting, but prevented sensor lockout.
There were more deaths in the standard-care arm than in the CGM arm (six vs. two), but the difference did not reach statistical significance. Importantly, there were no SAEs related to the use of the devices in this extremely vulnerable population or related to episodes of infection at the sensor site. Despite the challenges for staff of undertaking the paperwork required for such a trial, both staff and parents reported that they felt that CGM improved clinical care. The inclusion of a number of centres in both the UK and Europe make these results generalisable to a range of neonatal units that care for extremely preterm infants.
Limitations of the study include potential bias to study recruitment resulting from a short recruitment window of 24 hours, meaning that many infants’ parents were not approached. This may have biased the study towards infants born in the local recruiting centre. We were unable to blind staff to the study intervention, but compliance with blinding of the CGM data in the standard-care arm was good. The lack of data for a number of infants could also lead to bias, but there was no significant difference in the outcomes based on full analysis and per-protocol populations, suggesting that the findings are robust. The study was limited by its dependence on a large number of clinical staff being confident to deliver the study intervention throughout a 24-hour period. Rapid developments in sensor and Bluetooth® technology (Bluetooth Special Interest Group, Kirkland, WA, USA), newer generations of sensors that do not require calibration and centralised platforms for closed-loop insulin delivery may further support the use of CGM in these infants.
Interpretation of the results of the economic evaluation was constrained by an absence of external evidence, either from external guidelines, or from stated or revealed preference studies, on economic values associated with health changes resulting from adequate glucose control or for health changes associated with the secondary clinical outcomes. Consequently, our assessments of cost-effectiveness rely on hypothetical cost-effectiveness threshold values for improvements in the relevant clinical outcomes. Furthermore, the economic evaluation was limited to the time horizon of the trial, extending to 36 weeks’ corrected gestational age. The effects of CGM for targeting glucose control in neonates on longer-term economic costs and health consequences, including its potential to prevent the sequelae of NEC and BPD, remain a topic for future research.
In conclusion, this study demonstrates that CGM can safely guide the use of insulin and lead to a reduction in exposure to hyperglycaemia in extremely preterm infants. In addition, CGM is associated with a high probability of cost-effectiveness. Despite challenges, both staff and parents reported that they felt that CGM improved the clinical care of these infants. Further studies will be important to evaluate the longer-term impact of targeting glucose control on clinical outcomes.
The trial results in context
This study is in keeping with previous studies that have used CGM in the newborn population to monitor glucose levels and has highlighted clinically silent episodes of both hyperglycaemia and hypoglycaemia. 37,66 However, previous studies of CGM in infants have been much smaller, single centre and included a very small number of extremely preterm infants. These infants are those known to be most at risk of glucose dysregulation, in whom glucose control is most challenging and who have the highest risk of mortality and morbidity. It is these infants in whom any intervention has the potential to have the most clinical impact (on both morbidity and mortality). In this context, the higher rate of NEC in the standard-care group than in the CGM group, alongside evidence to link hyperglycaemia with increased risk of microbial gut translocation, warrants further investigation,65 with larger studies needed to confirm such a link.
This study demonstrates that CGM can safely guide the use of insulin and lead to a reduction in exposure to hyperglycaemia without increasing the risk of hypoglycaemia in extremely preterm infants. The international multicentre design of this study suggests that the intervention can be safely applied across a range of practices considered as standard neonatal care and outside centres specialising in using CGM. Despite challenges, both staff and parents reported that they felt that the use of CGMs improved the clinical care of these infants. Further studies will be important to evaluate the longer-term impact of targeting glucose control on clinical outcomes.
Chapter 7 Closed-loop study
Introduction
The wide variation in individual insulin sensitivity and limited staff resources within intensive care make it challenging for the full potential of CGMs to be realised. 1 Adaptive computerised algorithms utilising blood glucose measurements have been evaluated in adults67–69 and neonates undergoing intensive care. 37,70 The addition of frequent glucose levels obtained by CGM allows the development of closed-loop systems, as recently investigated in adult intensive care patients. 71,72 This study hypothesised that closed-loop insulin delivery, based on subcutaneous CGM, could be more effective in targeting glucose control in extremely preterm infants than CGM with insulin therapy and a guideline.
Aim
To pilot the use of a closed-loop system for glucose control using CGM.
Methods
Study design
This was a single-centre feasibility study in infants recruited from the NICU at the Cambridge University Hospitals NHS Foundation Trust. All parents of eligible infants admitted to the unit within 48 hours of birth were approached (if a member of the research team was available to do so). The study applied a randomised open-label parallel design, with infants randomised to CGM alone supported by a guideline or to CGM with an additional intervention period of closed-loop CGM. Research Ethics Committee approval and informed written parental consent were obtained prior to study procedures. Eligibility criteria included birthweight ≤ 1200 g and aged ≤ 48 hours. Infants were excluded if they had a major congenital malformation or an underlying metabolic disorder, or if the mother’s pregnancy had been complicated by diabetes. Criteria were chosen based on previous data that identified these infants as at most risk of glucose dysregulation, while avoiding potential bias. 21
A real-time CGM device was inserted in all infants and remained in situ for up to 7 days. The guideline advised on the use of insulin or additional dextrose support. For a prespecified period of 24 hours, between 48 and 72 hours post birth, a closed-loop system controlled glucose in infants during the closed-loop intervention, whereas infants in the control group continued to receive CGM alongside the guideline. This window for closed-loop intervention was preselected to be a standard time from birth in all infants to allow time for informed parental consent to be obtained and because previous data (from masked CGMs) had shown this to be the time when hyperglycaemia is most prevalent. 21 Randomisation applied the minimisation methods using the Minim randomisation software (version 1, London School of Hygiene and Tropical Medicine, London, UK), with stratification according to gestational age and birthweight.
Common study procedures
With the exception of glucose control over the prespecified period, all other aspects of care were identical between treatment groups. Blood glucose monitoring was undertaken on the POC glucose StatStrip meter. Actrapid® insulin (Novo Nordisk, Bagsværd, Denmark) at a concentration of 25 units/kg in 50 ml of 0.9% saline was used in both treatment groups. For CGM, an Enlite sensor was inserted by hand into the lateral thigh of each infant and linked to the Paradigm Veo for display of the sensor glucose concentration. Outside the intervention period (48–72 hours), CGM data were used in combination with the guideline by the clinical team to guide glucose control in all infants. Nurses calibrated the CGM device at least once every 12 hours, with a blood glucose measurement taken on the POC glucose StatStrip meter.
Paper guideline
The guideline for insulin delivery has previously been described. 1 It provided guidance based on the absolute sensor glucose value, as well as glucose trends. If sensor glucose levels are outside the target range, the guideline recommends review of clinical care and the dextrose infusion rate, and that modification of insulin delivery be considered, which could be instigated by the nurse at cotside. The insulin and dextrose were delivered by Alaris™ pumps (CareFusion, San Diego, CA, USA).
Closed-loop glucose control between 48 and 72 hours
The closed-loop system comprised (1) an Enlite sensor, (2) a laptop computer running a model predictive control algorithm and (3) two Alaris syringe pumps. We used a control algorithm based on the model predictive control approach,71,72 optimised and tuned in silico using a computer simulation environment validated for glucose control in the critically ill,73 aiming to keep sensor glucose levels between 4 and 8 mmol/l. The algorithm calculated insulin requirements or, at low glucose values, 20% dextrose infusion rates based on real-time sensor glucose values. A study nurse entered sensor glucose values into the laptop and modified insulin and dextrose pumps, as directed by the control algorithm, every 15 minutes. During the closed-loop intervention, Actrapid insulin in a concentration of 25 units/kg in 50 ml of 0.9% saline was used to allow for finer-dose titration in accordance with the algorithm. The algorithm calculations utilised a compartment model of glucose kinetics,74 describing the effect of insulin on sensor glucose excursions. The algorithm was initialised using an infant’s weight and adapted itself to a particular infant by updating two model parameters: (1) a rapidly changing glucose flux, correcting for errors in model-based predictions; and (2) a slowly changing estimate of an insulin rate to maintain normoglycaemia. The individualised model forecasted plasma glucose excursions over a 1.5-hour prediction horizon when calculating the insulin rate and a 30- to 40-minute horizon when calculating the dextrose rate. Information about enteral or parenteral nutrition was not provided to the algorithm. A reference blood glucose value was used every 6 hours for calibration of the glucose sensor. If sensor readings were not available because of sensor failure or loss of data capture, then hourly blood glucose levels were used to inform the algorithm for up to 4 hours. At this time, the algorithm continued to provide advice every 15 minutes.
Assessments and data collection
Demographic and clinical characteristics were collected with the following antenatal variables based on clinical diagnoses recorded in the maternal medical file: antenatal steroids (having received at least one dose prior to delivery), prolonged rupture of membranes (a rupture > 24 hours prior to delivery), hypertension and chorioamnionitis. All blood glucose measurements, insulin administration, type and volume of enteral and parenteral nutrition, and additional intravenous glucose administration were recorded from the time of randomisation to the end of CGM.
Statistical analysis
The outcome measures and the statistical analysis plan were agreed in advance. The primary outcome was a comparison of the time that sensor glucose was in the glucose target range of 4–8 mmol/l between the study arms. Secondary outcomes were time spent with sensor glucose levels between 2.6 and 10 mmol/l, prevalence of hyperglycaemia (percentage of time sensor glucose is > 10 mmol/l) and mean (and SD) sensor glucose level. Safety end points included frequency of hypoglycaemia (any blood glucose levels < 2.6 mmol/l) and other AEs. Sample size was selected to reflect this study’s status as a pilot to ensure that we had comparable control data, while minimising patient exposure. Formal power calculations were not performed and all analyses were based on intention to treat. An independent t-test was used to compare normally distributed variables. Non-normally distributed variables were compared using a Mann–Whitney U-test. Calculations were carried out using IBM SPSS Statistics version 23 (IBM Corporation, Armonk, NY, USA). Values are given as mean (SD) or median (IQR). p-values < 0.05 were considered statistically significant.
Results
Twenty-one infants were randomly assigned to the study arms, but one infant in the control group died within 48 hours of birth and so was excluded from the analyses. The baseline characteristics of the two groups (n = 20) were similar (Table 21). There were no significant problems with sensor insertion or removal, with only one infant (in the control group) having to have a sensor replaced, as there was no connection to the monitor. All 20 infants remained in the study throughout the intervention period from 48 to 72 hours, with similar numbers of sensor glucose data available for analysis in each group [for both study groups: median 24 (IQR 23.8–24) hours]. Control algorithm-directed insulin therapy was followed, at all times, during the prespecified 48- to 72-hour period. The maximum period of sensor signal loss during the closed loop was 3.5 hours, during which hourly blood glucose values were used by the control algorithm. There was minimal loss of data for the remainder of the study. One infant in the control arm was transferred out of the unit on study day 5 and one infant in the closed-loop arm died on study day 6, and so no further data could be collected for either infant. Therefore, the mean duration of collection of glucose data was 137 (SD 16.4) hours in the control arm and 136 (SD 8.7) hours in the closed-loop arm.
Demographic data | Closed-loop arm (N = 10) | Control arm (N = 10) |
---|---|---|
Gestational age at birth (weeks), mean (SD) | 27.0 (2.4) | 27.5 (2.8) |
Birthweight (g), mean (SD) | 962 (164) | 823 (282) |
Sex (male-to-female) | 5 : 5 | 5 : 5 |
Antenatal variable, n (%) | ||
Antenatal steroids | 10 (100) | 9 (90) |
Maternal smoking | 1 (10) | 2 (20) |
Chorioamnionitis | 2 (20) | 3 (30) |
PROMa | 4 (40) | 4 (40) |
Hypertension | 1 (10) | 1 (10) |
Glucose control and insulin and dextrose administration between 48 and 72 hours
There was no difference between the study groups in the baseline (48 hours) mean sensor glucose level (Table 22). During the intervention period, the median time spent in the target range of sensor glucose level (i.e. 4–8 mmol/l) was significantly higher in the closed-loop arm (91%, IQR 78–99%) than in the control arm (26%, IQR 6–64%) (p < 0.001). This was equivalent to 22 (IQR 19–24) hours in the closed-loop group, compared with 6 (IQR 1–15) hours in the control group. Similarly, the time spent in the wider target range of 2.6–10 mmol/l was higher in the closed-loop group (median 100% vs. median 84%). This was because the median percentage of time with sensor glucose values > 10 mmol/l was lower in the control arm than in the closed-loop arm (16% compared with 0%). There was no difference in the time spent with sensor glucose levels < 2.6 mmol/l. The median sensor glucose level was lower in the closed-loop arm (6.2 mmol/l, IQR 6.1–7.1 mmol/l) than in the control arm (8.6 mmol/l, IQR 7.4–11.1 mmol/l) (p = 0.002). Glucose variability, as measured by the SD of sensor glucose, was similar (p = 0.6).
Variable | Closed loop arm (n = 10) | Control arm (n = 10) | p-value |
---|---|---|---|
Time spent with sensor glucose level (%) | |||
4–8 mmol/la | 91 (78–99) | 26 (6–64) | < 0.001 |
2.6–10 mmol/l | 100 (94–100) | 84 (46–98) | 0.1 |
> 10 mmol/l | 0 (0–6) | 16 (2–54) | 0.1 |
< 2.6 mmol/l | 0.0 (0.0–0.0) | 0.0 (0.0–0.0) | 0.7 |
Baseline sensor glucose level (mmol/l) | 7.9 (6.9–11.5) | 8.2 (7.0–12.4) | 0.2 |
Mean sensor glucose level (mmol/l) | 6.2 (6.1–7.1) | 8.6 (7.4–11.1) | 0.002 |
SD of sensor glucose level (mmol/l) | 1.0 (0.8–1.9) | 1.3 (0.9–2.5) | 0.6 |
Episodes of blood glucose < 2.6 mmol/lb | 1 | 0 | 1 |
Nutritional intake | |||
Insulin (units/kg/hour) | 0.04 (0.03–0.07) | 0.02 (0.00–0.11) | 0.4 |
Dextrose (mg/kg/minute) | 8.4 (7.2–10.3) | 8.5 (4.2–10.6) | 0.6 |
Protein (g/kg/day) | 3.2 (2.5–4.1) | 3.5 (1.6–4.1) | 1 |
Lipid (g/kg/day) | 1.8 (1.0–1.8) | 1.4 (0.9–2.2) | 0.9 |
Trophic feeds | 4 | 4 | 1 |
Summative glucose profiles and insulin delivery are provided in Figure 20. Nine out of the 10 infants in the closed-loop group had received insulin prior to the 24-hour intervention, with the one remaining infant being started on insulin during the 24-hour closed-loop period. For comparison, four infants in the control arm received insulin prior to the intervention period and eight infants received insulin during the intervention period. Four infants in the closed-loop arm received additional 20% dextrose for short periods during the intervention period (up to 3.5 hours). The mean infusion rate in these infants ranged from 0.13 to 0.53 ml/kg/hour, with the highest rate being infused in an infant who was hypoglycaemic prior to the start of the closed-loop intervention. There was no statistical difference in the total amount of insulin infused or nutritional intake between the study groups during the 24-hour intervention period.
FIGURE 20.
Closed-loop study: glucose control and insulin delivery. 3 Median (IQR) sensor glucose level and insulin infusion rate in infants randomised to closed-loop management or CGM with paper guideline (control). The closed-loop intervention period is denoted by the vertical lines and the glucose target range of 4–8 mmol/l is denoted by horizontal lines. The shaded area represents the IQR of sensor glucose values and insulin infusion rates, respectively. Reproduced with permission from Beardsall et al. 3 This is an open access article distributed in accordance with the Creative Commons Attribution (CC BY 4.0) license, which permits others to copy, redistribute, remix, transform and build upon this work for any purpose, provided the original work is properly cited, a link to the licence is given, and indication of whether changes were made. See: https://creativecommons.org/licenses/by/4.0/. The figure includes minor additions and formatting changes to the original figure.

Glucose control and insulin and dextrose administration between 72 and 160 hours
In the post-intervention period, after 72 hours, there was a trend of increased time in both glucose target ranges (i.e. 4–8 mmol/l and 2.6–10 mmol/l) in the closed-loop arm compared with the control arm (Table 23), but these differences did not reach statistical significance.
Variable | Closed loop arm (n = 10) | Control arm (n = 10) | p-value |
---|---|---|---|
Time spent with sensor glucose level (%) | |||
4–8 mmol/l | 64 (39–90) | 42 (30–55) | 0.05 |
2.6–10 mmol/l | 95 (79–97) | 78 (61–97) | 0.2 |
> 10 mmol/l | 3 (1–21) | 22 (3–36) | 0.2 |
< 2.6 mmol/l | 0.0 (0.0–0.8) | 0.0 (0.0–0.2) | 0.7 |
Mean sensor glucose level (mmol/l) | 7.0 (6.8–8.5) | 8.3 (7.3–9.2) | 0.2 |
SD of sensor glucose level (mmol/l) | 1.7 (1.5–2.1) | 1.7 (1.3–2.8) | 0.8 |
Episodes of blood glucose < 2.6 mmol/l | 1 | 0 | 1 |
Nutritional intake | |||
Insulin (units/kg/hour) | 0.02 (0.00–0.04) | 0.03 (0.00–0.05) | 0.4 |
Dextrose (mg/kg/minute) | 9.4 (7.0–10.6) | 8.7 (5.4–10.9) | 0.5 |
Protein (g/kg/day) | 3.7 (2.7–4.3) | 3.8 (2.9–4.4) | 1 |
Lipid (g/kg/day) | 2.0 (1.5–2.9) | 1.8 (1.2–2.8) | 0.6 |
Oral milk intake (ml/kg/day) | 4.4 (3.5–11.5) | 5.0 (0.5–13.0) | 0.7 |
Nutrition and clinical care
All infants received parenteral and enteral nutrition in accordance with the standard local neonatal unit protocol. During the closed-loop intervention period, between 48 and 72 hours, four infants in each study group were receiving minimal amounts of trophic feeds. In the post-intervention period, after 72 hours, there was no difference between the two study groups in the volume of milk received (see Table 23). None of the infants received hydrocortisone, but seven infants in the control arm and two infants in the closed-loop arm received inotropes during the first week. During the intervention period, the median number of blood glucose values taken per day was 5.5 (IQR 4.8–6.6) in the control arm and 6.3 (IQR 5.1–6.5) in the closed-loop arm. Over the whole study period, the median number of blood glucose values taken per day was 5.1 (IQR 4.3–5.4) in the control arm and 5.3 (IQR 4.6–5.8) in the closed-loop arm.
Safety
No reported concerns about the sensor site, in terms of inflammation or infection, were reported either during the study or after removal of the sensor. Two infants in the closed-loop arm experienced documented episodes of hypoglycaemia, with a blood glucose level < 2.6 mmol/l. One episode occurred when checking the baseline blood glucose prior to the start of the closed-loop intervention. At that time, maintenance fluids were being changed, but no insulin was being infused. The second episode was on day 6 and, again, this was associated with a change of maintenance fluids. A further two infants experienced periods (after the 72-hour closed loop) when sensor glucose levels fell below 2.6 mmol/l, but the blood glucose level at that time was documented to be above 2.6 mmol/l. In the control arm, no infants had a documented blood glucose value of < 2.6 mmol/l. One infant in the control arm experienced an episode lasting 205 minutes when the sensor glucose fell to < 2.6 mmol/l (blood glucose level was not checked at this time). None of the infants was on insulin and there was no clinical evidence of hypoglycaemia in these infants during any of these episodes.
Discussion
To the best of our knowledge, this study is the first to show that a closed-loop system utilising subcutaneous glucose measurements to guide insulin delivery may improve glucose control in extremely preterm infants. This new approach could represent a step change in care, providing greater safety and tighter control, while minimising staff time at bedside and changes in fluid/insulin treatment. Compared with CGM alone, the closed-loop intervention increased threefold the percentage of time when sensor glucose was in the target range of between 4 and 8 mmol/l. In the high-intensity and high-cost setting of NICUs, these preliminary data support further development of closed-loop systems with real-time glucose-responsive insulin and dextrose delivery to support the care of these infants.
Reflecting the current controversy regarding optimal targets for glucose control in NICUs, we adopted a moderate glucose target range of between 4 and 8 mmol/l, rather than the tight glycaemic regimen of KU Leuven and Normoglycemia in Intensive Care Evaluation–Survival Using Glucose Algorithm Regulation (NICE-SUGAR) studies. 16,75,76 These moderate target ranges represent physiological in utero levels,77 and the upper threshold reflects a postnatal glucose level that has been associated with increased mortality and morbidity in preterm infants. 10 However, the optimal target range for glucose levels in these infants is still to be determined and this study does not resolve this long-standing debate in the field. Instead, it shows how an automated system can be used to achieve control to a given target range.
Different strategies are currently utilised to target glucose control in the preterm infant, each with different risks and benefits. A reduction in dextrose load risks compromised nutritional intake, whereas insulin therapy can lead to hypoglycaemia. The level of insulin use in this study is likely to reflect both the extreme prematurity of the infants recruited and the current practice in the NICU, which aims to ‘optimise’ nutritional delivery. In this study, there were no episodes of hypoglycaemia related to advice from the closed-loop algorithm, but CGM highlighted clinically silent episodes of hypoglycaemia in both study arms, independent of insulin use. The prevalence of hypoglycaemia was comparable to that reported by others in this population (27%)64 and to that reported using masked CGM, which highlights prolonged periods of clinically silent hypoglycaemia. 21
The CGM devices were calibrated using the StatStrip meter, which is validated for use in neonates and has US Food and Drug Administration approval for use in intensive care. 78 These meters are the standard of care for clinical management in the neonatal unit. Although there remains controversy regarding the clinical significance of clinically silent episodes of hypoglycaemia detected by CGM, there is recent evidence of an association between these episodes and substantially increased risk of impaired executive function and visual motor difficulty at 4.5 years. 79,80
The frequency of blood glucose sampling in preterm infants is typically much lower than in adults and children in intensive care, and therefore CGM has the potential to have an even greater impact. Differences in frequency of blood glucose monitoring may have had an impact on the level of glucose control. However, among infants in the control arm, more than four blood glucose samples were taken every 24 hours in the first 3 days of life. Notably, the insulin administered in the intervention arm was more diluted than that used in the control group to allow for more frequent changes in dose titration. Despite randomisation, more infants in the control arm received inotropes during the first week, and inotropic drugs will reduce insulin secretion and insulin sensitivity. However, given the pattern of improved glucose control during the intervention period, we do not think that these differences can account for the differences in glucose control that were observed.
Previous studies have explored the potential for CGM to guide changes in dextrose delivery,37 although intervention still depended on staff responding to trends in sensor glucose or to alarms. 37 In contrast, in the present study, targeting of glucose control was proactively driven by the closed-loop algorithm, which responded to frequently sampled sensor glucose data. This study is unique in exploring a control approach belonging to the family of model predictive control algorithms and optimised on a validated computer simulation environment73 prior to study commencement to ensure a favourable outcome.
The strengths of our study are the randomised controlled study design, and the comparability of the study groups and nutritional intakes. The study limitations include a small sample size, which means that, despite randomisation, baseline differences could remain between the study groups. Although the study groups appeared comparable, more infants in the control group than in the closed-loop group were on inotropes, and more infants in the intervention arm were on insulin at the start of study intervention. With such a design, it was not possible to blind the clinicians to the intervention. The study duration was short and, therefore, as it is known that insulin sensitivity varies over time from birth, it is important to explore how the algorithm works over longer periods.
In addition, this was a single-centre study and, as nutritional policies and practices vary between centres, it will be important to test the algorithm in different units with different nutritional strategies to test its generalisability. The algorithm was not designed to alter nutritional delivery, as this was predetermined by the clinical team, with the intervention designed as a simple adjunct to support targeting glucose control, alongside the clinical plan for nutritional support. Alternative designs might consider a combined approach to guide nutritional intake alongside insulin treatment. Further studies are required to explore the impact of a fully automated system with infusion pumps providing insulin and 20% dextrose under fully automated computer control.
To the best of our knowledge, this is the first randomised study to evaluate the feasibility of a closed-loop control based on CGM in preterm infants to guide insulin delivery to support glucose control. This closed-loop system appears to be a potential adjunct for targeting glucose control in preterm infants requiring intensive care and warrants further study.
Chapter 8 Conclusions
Implications for future health
These studies have demonstrated that CGM can support the targeting of glucose control in the extremely preterm infant without increasing the risk of hypoglycaemia. Staff felt that CGM improved the care of infants. The economic evaluation demonstrated that, over the first 7 days, CGM is, on average, more costly and more effective than standard care. Assuming cost-effectiveness thresholds of £1000, £5000 and £10,000 per additional case of adequate glucose control, respectively, the probability of cost-effectiveness of CGM reached 90% at approximately £6000, while the NMB associated with CGM became positive at a cost-effectiveness threshold of £5000. Over a time horizon extending to 36 weeks’ corrected gestational age, CGM was less costly and more effective, on average, than standard care and was therefore dominant in health economic terms.
The international nature of the study means that the results are generalisable across a range of NICU settings. The findings highlight the need for specific developments in the technology to improve the utility of CGM devices for use in this unique population. They further demonstrate the potential of a ‘closed-loop’ system to support targeting of glucose control, which could help to reduce staff workload and therefore cost.
The feasibility study highlighted the potential of CGM to identify infants at risk of incipient hypoglycaemia, which may be silent in this population. This study was unusual in undertaking a robust assessment of a new medical device in NICU patients before its introduction into clinical practice. The importance of designing devices to address the unique physiological and pathological challenges presented by these infants needs to be emphasised. More robust pathways are needed to encourage the development and validation of devices for use in such vulnerable populations. It is also important to ensure that these devices can be introduced into clinical practice in a robust manner, which requires adequate support and training for staff so that the devices are used effectively and safely in this population. Further developments in technology involving ‘closed-loop systems’ would be important in NICUs to limit blood sampling and provide a more personalised approach to care.
Specific post-study reflections
Continuous glucose monitoring versus point-of-care blood glucose
Considerable controversy remains as to the best way to manage neonatal glucose control, primarily because of a lack of good evidence on which to base clinical practice. 81,82 Measurement of glucose levels has evolved greatly over the last 40 years, with CGM being introduced in the last 20 years. The increasingly rapid innovations in technology could open up the opportunity for better understanding and management of the many infants who present with disordered glucose levels.
Current clinical care depends on a spectrum of medical devices and a range of clinical practices regarding the frequency of glucose sampling. Standard care includes capillary, venous or arterial blood sampling, and POC devices that have the advantage of rapid results and small samples compared with the ‘gold standard’ of laboratory analyses, which can result in a significant delay before results are available. 83 CGM could provide consistency in terms of interstitial levels measured continuously in real time. Over the course of our studies, the regulatory landscape for CGM models has changed during the time of these studies from an adjunct to care (requiring confirmatory blood glucose levels) to one where some CGM models are approved as being accurate enough to be a standalone guide to insulin dosing in patients with diabetes. 84
The fact that CGM measures not blood but interstitial glucose levels has given rise to some concern about its ‘validity’. Even when CGM devices are calibrated to blood glucose levels, there can be differences, particularly in certain circumstances. 85 However, it can be argued that interstitial glucose levels provide much more useful physiological and, therefore, clinical information, as cellular glucose levels may be particularly relevant to neuroglycopenia and the developing brain. 85
The acknowledged lag of up to 15 minutes in sensor glucose level compared with blood glucose levels needs to be placed in a clinical context. Although there may be a short lag of 15 minutes using CGM, the system still provides a continuous trend in real time. In contrast, the current standard of care using intermittent blood sampling can result in a delay of many hours between levels being checked. CGM can therefore reveal significant periods of clinically silent hypoglycaemia. 86,87 This was highlighted in our studies, in which falling sensor values highlighted the need for blood glucose levels to be taken. 1 However, there were no SAEs related to a ‘falsely’ reassuring sensor glucose level during an episode of hypoglycaemia. The detection of clinically silent hypoglycaemic episodes may be the most useful impact of CGM, highlighted by the association of such episodes with deficits in attention and visual acuity at 4.5 years. 79 Although, theoretically, this could lead to overmedicalisation, the potential to prevent harm should not be missed.
The unbiased nature of the CGM data also has the potential to help us better understand the physiology and pathology of changes in glucose levels. However, CGM devices are not currently licensed for use in the neonate and are not designed for ease of use with these infants. The next steps are to engage with the industry to address issues specific to this population.
The use of paper and computerised algorithms
This programme of studies involved the use of CGM technology combined with a variety of algorithms, both paper and computer based. The interventions should therefore be considered ‘combined interventions’.
We purposefully designed the paper algorithm as a guideline and not as a protocol. Although a ‘protocolised’ approach to ensure compliance may be considered optimal within a clinical trial, it was clear that the complexity needed for such a paper protocol would have increased risk. This was based on our previous experience, which highlighted wide variation in drug infusions, clinical complications and interventions across multiple sites. During the feasibility study, the algorithm evolved, as early iterations that aimed to avoid hypoglycaemia resulted in infusions being stopped and restarted more often than was deemed optimal. The advice was simplified for use by nursing staff, without requiring formal changes in prescriptions (similar to following a sliding scale). This was important as it provided a safe framework for the nursing staff to be able to titrate insulin infusion rates based on sensor glucose trends over short periods of time and nursing staff took ownership of decision-making, with a more comprehensive approach to care.
The ‘closed-loop’ study was entirely exploratory, but dramatically demonstrated the potential impact of combing the wealth of data from CGM with a computer-based algorithm. It provided a more personalised approach to care. A fully automated system has the potential to optimise clinical care by targeting glucose control and reducing workload. However, there are significant challenges to realisation, including CGM technology designed for the preterm infant, manufacturing engagement with open access to sensor and pump data, and regulatory hurdles. However, this route clearly could have significant impact for infants and staff.
Device trials and future regulations
Large multicentre device trials are uncommon compared with drug trials, and very limited clinical data are required for devices to obtain CE marking. This is particularly relevant for the neonatal population, in whom the unique risks and benefits may not have been fully assessed.
This trial highlighted some of the challenges of undertaking device trials, highlighting both practical clinical complexities and the importance of working closely with the company, with complete transparency about developments in the technology during the study.
Prior to starting the feasibility study, the sensor technology had been redesigned from the Medtronic plc Sof-Sensor® to the Medtronic plc Enlite, with a change in the angle of insertion. This was an advance for the adult market, but made sensor insertion more challenging in our preterm infants, who have limited subcutaneous tissue. It was necessary to modify procedures and study guidance. Further modifications were made to the Enlite sensor (from Enlite to Enlite plus) during the studies to improve the accuracy of the sensor technology.
On completion of the feasibility study, a new monitor was due to be released to supersede the Paradigm Veo. The advantages included better accuracy at low glucose levels and a clearer user interface. Therefore, after consideration and discussion with the TSC about risk and benefits, a decision was made to use the latest model. However, it became apparent that changes to the algorithm were having an impact on the calibration frequency and lockout of sensors which then needed to be replaced. Discussions with the company revealed that changes made to the algorithm to provide better accuracy and safety led to increased error readings within our population. There were further modifications to the algorithm to address some of these issues during the study. These potentially subtle changes from a manufacturing perspective had a significant impact on our sensor failure rate at the beginning of the study, but could not have been anticipated. This shows that the rapid advances in the technology present clinical challenges and it is imperative that manufacturers and clinicians work together with complete transparency.
Clinical aspects
Training the large number of staff within a busy intensive care unit to use devices as part of a clinical trial requires significant resources, both financial and time. In our studies, the short recruitment window increased this challenge for recruiting sites, and therefore the provision of on-site training and an online training video, as well as a 24-hour helpline, was important. It was clear that the level of support required for an interventional device trial was greater than that for an investigational medicinal product study.
Recommendation for further research
The findings of the REACT project raise the following important questions that need to be addressed in follow-on studies:
-
What is the impact of ‘optimising’ glucose control on long-term health outcomes?
-
What is the optimal glucose target range for preterm infants?
-
What is the impact of silent hypoglycaemia in these preterm infants?
-
Can we design a CGM that is better suited to the unique physiology and pathology of the newborn?
-
What is the physiological basis for the differences found between sexes?
Acknowledgements
We would like to thank all of the parents who enabled their children to join the REACT studies.
Trial Steering Committee
S Coaker, K Costeloe (chairperson), T Cole, D Dunger, A Ewer and P Watkinson.
Trial Management Group
A Allison, K Beardsall, S Bond, C Guy, B Pantaleo and L Thomson.
Cambridge Clinical Trials Unit
A Allison, S Bond, B Pantaleo and I Wilkinson.
Economic evaluation
S Petrou and SW Kim.
Data Monitoring and Ethics Committee
D Elbourne, D Field (chairperson) and J Hawdon.
Medtronic plc
L Cunningham, C Sargent and J Da Silva.
Nova Biomedical
L McGough.
Recruiting centres: principal investigators and their team
[Principal investigator(s) and lead research nurse(s) are listed first and italicised.]
Addenbrooke’s Hospital
A Ogilvy-Stuart and L Thomson.
Hospital Sant Joan de Déu (Barcelona)
I Iglesias.
John Radcliffe Hospital
Z Molnar, S Barlow and S Baugh.
Leeds General Infirmary
K Johnson, L Uryn and C Spencer.
Leicester Royal Infirmary
M Hubbard, J Hamilton, TC Kwok and S Mittal.
Luton and Dunstable University Hospital
S Somisetty, O Adesiyan, J Kapadia and Y Millar.
New Cross Hospital
K Gurusamy, L Bibb and K Jones.
Norfolk and Norwich University Hospital
P Muthukumar, A Nichols and all of the nurses and the medical team at the Norfolk and Norwich University Hospital NICU.
Princess Anne Hospital
M Johnson, J Pond, P Crowley, C Mellish and H Dewar.
The Royal London Hospital
D Shah, P Vincent, A Iringan, M Abraham, S Anilkumar, B Aninakwa and RA Dalangin-Chalmers.
St Michael’s Hospital
A Collins.
St Peter’s Hospital
P Reynolds, N Holland and K Wells.
Vrije Universiteit University Medical Center: Amsterdam University Medical Center
M van Weissenbruch and A de Lange.
National Institute for Health Research Evaluation, Trials and Studies Coordinating Centre
R Bloom, C Dukes and S Hammerson.
UK Clinical Research Network
Patient and public involvement
In each of the studies, families on the neonatal unit were asked individually and during informal family meetings to review and advise on the language of the patient information sheets and consent forms. There were two parents on the TSC throughout the multicentre trial who provided helpful insight into optimising consent, including increasing engagement with midwifery colleagues. These parents also provided advice on the content of parental newsletters prior to distribution. We have drafted a newsletter in collaboration with the lay member of the TSC and will circulate this once we have confirmation of publication, initially to a small group of families and then to all families who participated in the trial.
Contributions of authors
Kathryn Beardsall (https://orcid.org/0000-0003-3582-183X) (Consultant in Neonatal Intensive Care/Clinical Lecturer) conceived the idea and original design of the study and was the chief investigator. She was involved in designing the final design and conduct of research, and was a member of the Trial Management Group (TMG) and the TSC. She was also involved in the development of the trial protocol, the conduct of research interpretation and the reporting of results, and she revised the manuscript for important intellectual content.
Lynn Thomson (https://orcid.org/0000-0002-6629-0237) (Research Nurse) was the lead nurse for the trial and was involved from the conception of the trial design, and was involved in the set up, conduct and acquisition of these research data.
Catherine Guy (https://orcid.org/0000-0002-9686-7623) (Study Manager) was the trial manger and was involved in the set-up, running and management of the trial.
Simon Bond (https://orcid.org/0000-0003-2528-1040) (Senior Trial Statistician) was a member of the TMG and TSC. He provided leadership of the statistical aspects of the trial.
Annabel Allison (https://orcid.org/0000-0002-9122-6341) (Trial Statistician) was a member of the trial management group. She undertook complex data analysis and produced relevant statistical reports.
Beatrice Pantaleo (https://orcid.org/0000-0003-4860-3743) (Data Manager) was a member of the TMG. She led on the design of the CRFs and MACRO database, and developed Pepepuz software to assist with validating and analysing the CGM data. She was also responsible for management of the trial data.
Stavros Petrou (https://orcid.org/0000-0003-3121-6050) (Investigator) was involved in the trial design and led on the health economic data analysis.
Sungwook Kim (https://orcid.org/0000-0002-1254-5038) (Health Economist) was involved in the health economic data analysis.
David Dunger (https://orcid.org/0000-0002-2566-9304) (Investigator) was a member of the TSC and was involved in the trial design.
Roman Hovorka (https://orcid.org/0000-0003-2901-461X) (Investigator) was involved in the trial design.
Publications
Li T, Thomson L, Ogilvy Stuart A, Beardsall K. Glucose monitoring and management in the NICU – how are we doing? Infant 2017;13:182–6.
Saha P, Beardsall K. Perioperative continuous glucose monitoring in a preterm infant. BMJ Case Rep 2018;2018:bcr2018224728.
Beardsall K, Thomson L, Guy C, van Weissenbruch MM, Iglesias I, Muthukumar P, et al. Protocol of a randomised controlled trial of real-time continuous glucose monitoring in neonatal intensive care ‘REACT’. BMJ Open 2018;8:e020816.
Beardsall K. Real time continuous glucose monitoring in neonatal intensive care. Early Hum Dev 2019;138:104844.
Stewart ZA, Thomson L, Murphy HR, Beardsall K. A feasibility study of paired continuous glucose monitoring intrapartum and in the newborn in pregnancies complicated by type 1 diabetes. Diabetes Technol Ther 2019;21:20–7.
Thomson L, Elleri D, Bond S, Howlett J, Dunger DB, Beardsall K. Targeting glucose control in preterm infants: pilot studies of continuous glucose monitoring. Arch Dis Child Fetal Neonatal Ed 2019;104:F353–9.
Beardsall K, Thomson L, Elleri D, Dunger DB, Hovorka R. Feasibility of automated insulin delivery guided by continuous glucose monitoring in preterm infants. Arch Dis Child Fetal Neonatal Ed 2020;105:279–84.
Beardsall K, Thomson L, Guy C, Iglesias-Platas I, van Weissenbruch MM, Bond S, et al. Real-time continuous glucose monitoring in preterm infants (REACT): an international, open-label, randomised controlled trial. Lancet Child Adolesc Health 2021;5:265–73.
Petrou S, Kim S, Bond S, Allison A, Beardsall K, the REACT collaborative. Cost-effectiveness of real time continuous glucose monitoring to target glucose control in preterm infants. Semin Perinatol 2021;45:151392.
Data-sharing statement
All data requests should be submitted to the corresponding author for consideration. Access to anonymised data may be granted following review.
Patient data
This work uses data provided by patients and collected by the NHS as part of their care and support. Using patient data is vital to improve health and care for everyone. There is huge potential to make better use of information from people’s patient records, to understand more about disease, develop new treatments, monitor safety, and plan NHS services. Patient data should be kept safe and secure, to protect everyone’s privacy, and it’s important that there are safeguards to make sure that it is stored and used responsibly. Everyone should be able to find out about how patient data are used. #datasaveslives You can find out more about the background to this citation here: https://understandingpatientdata.org.uk/data-citation.
Disclaimers
This report presents independent research. The views and opinions expressed by authors in this publication are those of the authors and do not necessarily reflect those of the NHS, the NIHR, the MRC, NETSCC, the EME programme or the Department of Health and Social Care. If there are verbatim quotations included in this publication the views and opinions expressed by the interviewees are those of the interviewees and do not necessarily reflect those of the authors, those of the NHS, the NIHR, NETSCC, the EME programme or the Department of Health and Social Care.
References
- Thomson L, Elleri D, Bond S, Howlett J, Dunger DB, Beardsall K. Targeting glucose control in preterm infants: pilot studies of continuous glucose monitoring. Arch Dis Child Fetal Neonatal Ed 2019;104:F353-9. https://doi.org/10.1136/archdischild-2018-314814.
- Beardsall K, Thomson L, Guy C, van Weissenbruch MM, Iglesias I, Muthukumar P, et al. Protocol of a randomised controlled trial of real-time continuous glucose monitoring in neonatal intensive care ‘REACT’. BMJ Open 2018;8. https://doi.org/10.1136/bmjopen-2017-020816.
- Beardsall K, Thomson L, Elleri D, Dunger DB, Hovorka R. Feasibility of automated insulin delivery guided by continuous glucose monitoring in preterm infants. Arch Dis Child Fetal Neonatal Ed 2020;105:279-84. https://doi.org/10.1136/archdischild-2019-316871.
- Beardsall K, Thomson L, Guy C, Iglesias-Platas I, van Weissenbruch MM, Bond S, et al. Real-time continuous glucose monitoring in preterm infants (REACT): an international, open-label, randomised controlled trial. Lancet Child Adolesc Health 2021;5:265-73. https://doi.org/10.1016/S2352-4642(20)30367-9.
- Costeloe K, Hennessy E, Gibson AT, Marlow N, Wilkinson AR. The EPICure study: outcomes to discharge from hospital for infants born at the threshold of viability. Pediatrics 2000;106:659-71. https://doi.org/10.1542/peds.106.4.659.
- Petrou S. The economic consequences of preterm birth during the first 10 years of life. BJOG 2005;112:10-5. https://doi.org/10.1111/j.1471-0528.2005.00577.x.
- Economides DL, Proudler A, Nicolaides KH. Plasma insulin in appropriate and small for gestational age fetuses. Am J Obstet Gynecol 1989;160:1091-4. https://doi.org/10.1016/0002-9378(89)90167-1.
- Beardsall K, Vanhaesebrouck S, Ogilvy-Stuart AL, Vanhole C, Palmer CR, Ong K, et al. Prevalence and determinants of hyperglycemia in very low birth weight infants: cohort analyses of the NIRTURE study. J Pediatr 2010;157:715-19.e1. https://doi.org/10.1016/j.jpeds.2010.04.032.
- Bottino M, Cowett RM, Sinclair JC. Interventions for treatment of neonatal hyperglycemia in very low birth weight infants. Cochrane Database Syst Rev 2009;1. https://doi.org/10.1002/14651858.CD007453.pub2.
- Hays SP, Smith EO, Sunehag AL. Hyperglycemia is a risk factor for early death and morbidity in extremely low birth-weight infants. Pediatrics 2006;118:1811-18. https://doi.org/10.1542/peds.2006-0628.
- Finberg L. Dangers to infants caused by changes in osmolal concentration. Pediatrics 1967;40:1031-4.
- Garg R, Agthe AG, Donohue PK, Lehmann CU. Hyperglycemia and retinopathy of prematurity in very low birth weight infants. J Perinatol 2003;23:186-94. https://doi.org/10.1038/sj.jp.7210879.
- Kao LS, Morris BH, Lally KP, Stewart CD, Huseby V, Kennedy KA. Hyperglycemia and morbidity and mortality in extremely low birth weight infants. J Perinatol 2006;26:730-6. https://doi.org/10.1038/sj.jp.7211593.
- van den Berghe G, Wouters P, Weekers F, Verwaest C, Bruyninckx F, Schetz M, et al. Intensive insulin therapy in critically ill patients. N Engl J Med 2001;345:1359-67. https://doi.org/10.1056/NEJMoa011300.
- Cryer PE. Hypoglycaemia: the limiting factor in the glycaemic management of the critically ill?. Diabetologia 2006;49:1722-5. https://doi.org/10.1007/s00125-006-0306-4.
- Vlasselaers D, Milants I, Desmet L, Wouters PJ, Vanhorebeek I, van den Heuvel I, et al. Intensive insulin therapy for patients in paediatric intensive care: a prospective, randomised controlled study. Lancet 2009;373:547-56. https://doi.org/10.1016/S0140-6736(09)60044-1.
- Alexandrou G, Skiöld B, Karlén J, Tessma MK, Norman M, Adén U, et al. Early hyperglycemia is a risk factor for death and white matter reduction in preterm infants. Pediatrics 2010;125:e584-91. https://doi.org/10.1542/peds.2009-0449.
- Peters KL, Rosychuk RJ, Hendson L, Coté JJ, McPherson C, Tyebkhan JM. Improvement of short- and long-term outcomes for very low birth weight infants: Edmonton NIDCAP trial. Pediatrics 2009;124:1009-20. https://doi.org/10.1542/peds.2008-3808.
- Hirshberg E, Larsen G, Van Duker H. Alterations in glucose homeostasis in the pediatric intensive care unit: hyperglycemia and glucose variability are associated with increased mortality and morbidity. Pediatr Crit Care Med 2008;9:361-6. https://doi.org/10.1097/PCC.0b013e318172d401.
- Beardsall K, Ogilvy-Stuart AL, Ahluwalia J, Thompson M, Dunger DB. The continuous glucose monitoring sensor in neonatal intensive care. Arch Dis Child Fetal Neonatal Ed 2005;90:F307-10. https://doi.org/10.1136/adc.2004.051979.
- Beardsall K, Vanhaesebrouck S, Ogilvy-Stuart AL, Vanhole C, Palmer CR, van Weissenbruch M, et al. Early insulin therapy in very-low-birth-weight infants. N Engl J Med 2008;359:1873-84. https://doi.org/10.1056/NEJMoa0803725.
- Harris DL, Battin MR, Weston PJ, Harding JE. Continuous glucose monitoring in newborn babies at risk of hypoglycemia. J Pediatr 2010;157:198-202.e1. https://doi.org/10.1016/j.jpeds.2010.02.003.
- Schierenbeck F, Franco-Cereceda A, Liska J. Accuracy of 2 different continuous glucose monitoring systems in patients undergoing cardiac surgery. J Diabetes Sci Technol 2017;11:108-16. https://doi.org/10.1177/1932296816651632.
- Holzinger U, Warszawska J, Kitzberger R, Wewalka M, Miehsler W, Herkner H, et al. Real-time continuous glucose monitoring in critically ill patients: a prospective randomized trial. Diabetes Care 2010;33:467-72. https://doi.org/10.2337/dc09-1352.
- Price GC, Stevenson K, Walsh TS. Evaluation of a continuous glucose monitor in an unselected general intensive care population. Crit Care Resusc 2008;10:209-16.
- De Block C, Manuel-y-Keenoy B, Rogiers P, Jorens P, Van Gaal L. Glucose control and use of continuous glucose monitoring in the intensive care unit: a critical review. Curr Diabetes Rev 2008;4:234-44. https://doi.org/10.2174/157339908785294460.
- Preiser JC, Chase JG, Hovorka R, Joseph JI, Krinsley JS, De Block C, et al. Glucose control in the ICU: a continuing story. J Diabetes Sci Technol 2016;10:1372-81. https://doi.org/10.1177/1932296816648713.
- Keenan DB, Cartaya R, Mastrototaro JJ. Accuracy of a new real-time continuous glucose monitoring algorithm. J Diabetes Sci Technol 2010;4:111-18. https://doi.org/10.1177/193229681000400114.
- Beardsall K, Dunger D. Insulin therapy in preterm newborns. Early Hum Dev 2008;84:839-42. https://doi.org/10.1016/j.earlhumdev.2008.09.013.
- Beardsall K, Vanhaesebrouck S, Ogilvy-Stuart AL, Vanhole C, VanWeissenbruch M, Midgley P, et al. Validation of the continuous glucose monitoring sensor in preterm infants. Arch Dis Child Fetal Neonatal Ed 2013;98:F136-40. https://doi.org/10.1136/archdischild-2012-301661.
- International Organization for Standardization . ISO 13485:2003. Medical Devices – Quality Management Systems – Requirements for Regulatory Purposes n.d. www.iso.org/standard/36786.html (accessed 12 February 2021).
- International Organization for Standardization . ISO/IEC/27001:2013./Information/Technology/–/Security/Techniques/–/Information/Security/Management/Systems/–/Requirements n.d. www.iso.org/standard/54534.html (accessed 12 February 2021).
- Bland JM, Altman DG. Statistical methods for assessing agreement between two methods of clinical measurement. Lancet 1986;1:307-10.
- Edge J, Acerini C, Campbell F, Hamilton-Shield J, Moudiotis C, Rahman S, et al. An alternative sensor-based method for glucose monitoring in children and young people with diabetes. Arch Dis Child 2017;102:543-9. https://doi.org/10.1136/archdischild-2016-311530.
- Raizman JE, Shea J, Daly CH, Karbasy K, Ariadne P, Chen Y, et al. Clinical impact of improved point-of-care glucose monitoring in neonatal intensive care using Nova StatStrip: evidence for improved accuracy, better sensitivity, and reduced test utilization. Clin Biochem 2016;49:879-84. https://doi.org/10.1016/j.clinbiochem.2016.05.002.
- Schmelzeisen-Redeker G, Schoemaker M, Kirchsteiger H, Freckmann G, Heinemann L, Del Re L. Time delay of CGM sensors: relevance, causes, and countermeasures. J Diabetes Sci Technol 2015;9:1006-15. https://doi.org/10.1177/1932296815590154.
- Galderisi A, Facchinetti A, Steil GM, Ortiz-Rubio P, Cavallin F, Tamborlane WV, et al. Continuous glucose monitoring in very preterm infants: a randomized controlled trial. Pediatrics 2017;140. https://doi.org/10.1542/peds.2017-1162.
- British Association of Perinatal Medicine . Categories of Care 2011 n.d. https://hubble-live-assets.s3.amazonaws.com/bapm/attachment/file/43/CatsofcarereportAug11.pdf (accessed July 2021).
- Department of Health and Social Care . Reference Costs 2013–14 n.d. https://assets.publishing.service.gov.uk/government/uploads/system/uploads/attachment_data/file/380322/01_Final_2013-14_Reference_Costs_publication_v2.pdf (accessed July 2021).
- World Medical Association . WMA Declaration of Helsinki – Ethical Principles For Medical Research Involving Human Subjects n.d. www.wma.net/policies-post/wma-declaration-of-helsinki-ethical-principles-for-medical-research-involving-human-subjects (accessed July 2021).
- European Commission . Overview n.d. https://ec.europa.eu/health/md_sector/overview_en#new_regulations (accessed July 2021).
- European Medicines Agency . International Council on Harmonisation of Technical Requirements for Registration of Pharmaceuticals for Human Use (ICH) n.d. www.ema.europa.eu/en/partners-networks/international-activities/multilateral-coalitions-initiatives/international-council-harmonisation-technical-requirements-registration-pharmaceuticals-human-use (accessed July 2021).
- Petrou S, Kim S, Bond S, Allison A, Beardsall K. the REACT collaborative . Cost-effectiveness of real time continuous glucose monitoring to target glucose control in preterm infants. Semin Perinato 2021;45. https://doi.org/10.1016/j.semperi.2021.151392.
- National Institute for Health and Care Excellence (NICE) . Guide to the Methods of Technology Appraisal 2013 2013.
- Curtis LA, Burns A. Unit Costs of Health and Social Care 2017 n.d. www.pssru.ac.uk/project-pages/unit-costs/unit-costs-2017/ (accessed 1 August 2019).
- NHS Employers . NHS Terms and Conditions (AfC) Pay Scales – Annual n.d. www.nhsemployers.org/pay-pensions-and-reward/agenda-for-change/pay-scales/annual (accessed 1 December 2018).
- OpenDataNI . Health Trust Reference Costs 2016–17 n.d. https://data.gov.uk/dataset/489e83d2-7b05-41e1-b8f3-c06c1a8a9de4/health-trust-reference-costs-2016-17 (accessed 1 December 2018).
- British National Formulary. London: BMJ Group and Pharmaceutical Press; 2018.
- NHS . NHS Blood and Transplant Price List 2017 n.d. http://hospital.blood.co.uk/media/28230/component-price-list-2016-2017.pdf (accessed 15 August 2018).
- GOV.UK . Advisory Fuel Rates n.d. www.gov.uk/government/publications/advisory-fuel-rates/how-advisory-fuel-rates-are-calculated (accessed 1 August 2019).
- Gomes M, Ng ES, Grieve R, Nixon R, Carpenter J, Thompson SG. Developing appropriate methods for cost-effectiveness analysis of cluster randomized trials. Med Decis Making 2012;32:350-61. https://doi.org/10.1177/0272989X11418372.
- Rubin DB. Multiple Imputation for Nonresponse in Surveys. Chichester: John Wiley & Sons; 2004.
- van Buuren S, Groothuis-Oudshoorn K. mice: Multivariate Imputation by Chained Equations in R. J Stat Softw 2011;45:1-68. https://doi.org/10.18637/jss.v045.i03.
- Horton NJ, Lipsitz SR. Multiple imputation in practice: comparison of software packages for regression models with missing variables. Am Stat 2001;55:244-54. https://doi.org/10.1198/000313001317098266.
- White IR, Royston P, Wood AM. Multiple imputation using chained equations: issues and guidance for practice. Stat Med 2011;30:377-99. https://doi.org/10.1002/sim.4067.
- Cox NJ. Speaking Stata: a set of utilities for managing missing values. Stata J 2015;15:1174-85. https://doi.org/10.1177/1536867X1501500413.
- Moler C. Numerical Computing with Matlab. Philadelphia, PA: Society for Industrial and Applied Mathematics; 2004.
- Glick H, Doshi JA, Sonnad SS, Polsky D. Economic Evaluation in Clinical Trials. Oxford: Oxford University Press; 2014.
- Efron B, Tibshirani R. An Introduction to the Bootstrap. New York, NY: Chapman & Hall; 1993.
- Briggs AH, Claxton K, Sculpher MJ. Decision Modelling for Health Economic Evaluation. Oxford: Oxford University Press; 2006.
- Fenwick E, O’Brien BJ, Briggs A. Cost-effectiveness acceptability curves – facts, fallacies and frequently asked questions. Health Econ 2004;13:405-15. https://doi.org/10.1002/hec.903.
- Drummond MF, Sculpher MJ, Claxton K, Stoddart GL, Torrance GW. Methods for the Economic Evaluation of Health Care Programmes. Oxford: Oxford University Press; 2015.
- Claxton K, Martin S, Soares M, Rice N, Spackman E, Hinde S, et al. Methods for the estimation of the National Institute for Health and Care Excellence cost-effectiveness threshold. Health Technol Assess 2015;19. https://doi.org/10.3310/hta19140.
- Alsweiler JM, Harding JE, Bloomfield FH. Tight glycemic control with insulin in hyperglycemic preterm babies: a randomized controlled trial. Pediatrics 2012;129:639-47. https://doi.org/10.1542/peds.2011-2470.
- Thaiss CA, Levy M, Grosheva I, Zheng D, Soffer E, Blacher E, et al. Hyperglycemia drives intestinal barrier dysfunction and risk for enteric infection. Science 2018;359:1376-83. https://doi.org/10.1126/science.aar3318.
- Nally LM, Bondy N, Doiev J, Buckingham BA, Wilson DM. A feasibility study to detect neonatal hypoglycemia in infants of diabetic mothers using real-time continuous glucose monitoring. Diabetes Technol Ther 2019;21:170-6. https://doi.org/10.1089/dia.2018.0337.
- Cordingley JJ, Vlasselaers D, Dormand NC, Wouters PJ, Squire SD, Chassin LD, et al. Intensive insulin therapy: enhanced model predictive control algorithm versus standard care. Intensive Care Med 2009;35:123-8. https://doi.org/10.1007/s00134-008-1236-z.
- Preiser JC, Devos P, Ruiz-Santana S, Mélot C, Annane D, Groeneveld J, et al. A prospective randomised multi-centre controlled trial on tight glucose control by intensive insulin therapy in adult intensive care units: the Glucontrol study. Intensive Care Med 2009;35:1738-48. https://doi.org/10.1007/s00134-009-1585-2.
- Pachler C, Plank J, Weinhandl H, Chassin LJ, Wilinska ME, Kulnik R, et al. Tight glycaemic control by an automated algorithm with time-variant sampling in medical ICU patients. Intensive Care Med 2008;34:1224-30. https://doi.org/10.1007/s00134-008-1033-8.
- Le Compte A, Chase JG, Lynn A, Hann C, Shaw G, Wong XW, et al. Blood glucose controller for neonatal intensive care: virtual trials development and first clinical trials. J Diabetes Sci Technol 2009;3:1066-81. https://doi.org/10.1177/193229680900300510.
- Leelarathna L, English SW, Thabit H, Caldwell K, Allen JM, Kumareswaran K, et al. Feasibility of fully automated closed-loop glucose control using continuous subcutaneous glucose measurements in critical illness: a randomized controlled trial. Crit Care 2013;17. https://doi.org/10.1186/cc12838.
- Leelarathna L, English SW, Thabit H, Caldwell K, Allen JM, Kumareswaran K, et al. Accuracy of subcutaneous continuous glucose monitoring in critically ill adults: improved sensor performance with enhanced calibrations. Diabetes Technol Ther 2014;16:97-101. https://doi.org/10.1089/dia.2013.0221.
- Wilinska ME, Blaha J, Chassin LJ, Cordingley JJ, Dormand NC, Ellmerer M, et al. Evaluating glycemic control algorithms by computer simulations. Diabetes Technol Ther 2011;13:713-22. https://doi.org/10.1089/dia.2011.0016.
- Hovorka R, Shojaee-Moradie F, Carroll PV, Chassin LJ, Gowrie IJ, Jackson NC, et al. Partitioning glucose distribution/transport, disposal, and endogenous production during IVGTT. Am J Physiol Endocrinol Metab 2002;282:E992-1007. https://doi.org/10.1152/ajpendo.00304.2001.
- Finfer S, Chittock DR, Su SY, Blair D, Foster D, Dhingra V, et al. Intensive versus conventional glucose control in critically ill patients. N Engl J Med 2009;360:1283-97. https://doi.org/10.1056/NEJMoa0810625.
- Finfer S, Heritier S. NICE Study Management Committee and SUGAR Study Executive Committee. The NICE-SUGAR (Normoglycaemia in Intensive Care Evaluation and Survival Using Glucose Algorithm Regulation) Study: statistical analysis plan. Crit Care Resusc 2009;11:46-57.
- Economides DL, Nicolaides KH, Campbell S. Metabolic and endocrine findings in appropriate and small for gestational age fetuses. J Perinat Med 1991;19:97-105. https://doi.org/10.1515/jpme.1991.19.1-2.97.
- U.S. Food & Drug Administration . FDA Recommendations for All Hospital Patients Including ITU n.d. www.fda.gov/food/resources-you-food/healthcare-professionals (accessed July 2021).
- McKinlay CJD, Alsweiler JM, Anstice NS, Burakevych N, Chakraborty A, Chase JG, et al. Association of neonatal glycemia with neurodevelopmental outcomes at 4.5 Years. JAMA Pediatr 2017;171:972-83. https://doi.org/10.1001/jamapediatrics.2017.1579.
- McKinlay CJD, Chase JG, Dickson J, Harris DL, Alsweiler JM, Harding JE. Continuous glucose monitoring in neonates: a review. Matern Health Neonatol Perinatol 2017;3. https://doi.org/10.1186/s40748-017-0055-z.
- Ogilvy-Stuart AL, Harding JE, Beardsall K. Thresholds for hypoglycaemic screening-a cause for concern?. Arch Dis Child Educ Pract Ed 2019;104:33-4. https://doi.org/10.1136/archdischild-2017-314135.
- Cornblath M, Ichord R. Hypoglycemia in the neonate. Semin Perinatol 2000;24:136-49. https://doi.org/10.1053/sp.2000.6364.
- Beardsall K. Measurement of glucose levels in the newborn. Early Hum Dev 2010;86:263-7. https://doi.org/10.1016/j.earlhumdev.2010.05.005.
- Dexcom . Dexcom G6® CGM System Receives CE Mark n.d. https://dexcom.gcs-web.com/news-releases/news-release-details/dexcom-g6r-cgm-system-receives-ce-mark (accessed 26 May 2021).
- Cengiz E, Tamborlane WV. A tale of two compartments: interstitial versus blood glucose monitoring. Diabetes Technol Ther 2009;11:11-6. https://doi.org/10.1089/dia.2009.0002.
- Wackernagel D, Dube M, Blennow M, Tindberg Y. Continuous subcutaneous glucose monitoring is accurate in term and near-term infants at risk of hypoglycaemia. Acta Paediatr 2016;105:917-23. https://doi.org/10.1111/apa.13479.
- Uettwiller F, Chemin A, Bonnemaison E, Favrais G, Saliba E, Labarthe F. Real-time continuous glucose monitoring reduces the duration of hypoglycemia episodes: a randomized trial in very low birth weight neonates. PLOS ONE 2015;10. https://doi.org/10.1371/journal.pone.0116255.
Appendix 1 Summary of protocol amendments
Amendment number | Date of submission | Details |
---|---|---|
1 | 17 December 2015 | Protocol amendment (v2, dated 17 December 2015):
|
2 | 4 August 2016 | Protocol amendment (v3, dated 4 August 2015):
|
3 | 4 August 2016 | Addition of one site:
|
4 | 2 December 2016 | Protocol amendment (v4, dated 7 November 2016):
|
5 | 16 December 2016 | Addition of two sites:
|
6 | 9 March 2017 | Addition of one site:
|
7 | 25 May 2017 | Change of principal investigator at John Radcliffe Hospital, Oxford |
8 | 28 July 2017 | Request to extend study until 30 November 2018 |
9 | 14 September 2017 | Addition of six sites:
|
10 | 29 June 2018 | Minor amendments, including:
|
11 | 7 August 2018 | GDPR information sheet |
12 | 21 September 2018 | Request to extend study until 31 July 2019 |
13 | 6 December 2018 | Minor amendments, including:
|
Appendix 2 Trial Steering Committee terms of reference
Appendix 3 Data Monitoring Committee Charter
Appendix 4 Randomised controlled trial: additional analyses
Model | Covariate | Estimate (SE) | 95% CI | p-value |
---|---|---|---|---|
Linear regression weighted by number of sensor glucose observations | Intercept | 80 (3.4) | 73.3 to 86.7 | < 0.001 |
CGM vs. standard | 8.5 (2.6) | 3.4 to 13.6 | 0.001 | |
N36 (reference N01) | –5 (15) | –34 to 25 | 0.8 | |
N42 | 7.0 (5.5) | –3.9 to 17.9 | 0.2 | |
N43 | –0.04 (3.8) | –7.6 to 7.5 | 0.99 | |
N68 | 1.7 (4.0) | –6.1 to 9.5 | 0.7 | |
N73 | 3.6 (5.4) | –7.1 to 14.2 | 0.5 | |
N74 | 10 (12) | –15 to 35 | 0.4 | |
N85 | 8.3 (6.4) | –4.4 to 21 | 0.2 | |
N86 | –21 (8.7) | –38.1 to –3.9 | 0.02 | |
N87 | –17 (12) | –40 to 7 | 0.2 | |
N88 | –26.5 (7.2) | –40.8 to –12.2 | < 0.001 | |
ND3 | 6.3 (6.2) | –6 to 18.6 | 0.3 | |
SP3 | 9.2 (7.6) | –5.9 to 24.3 | 0.2 | |
Gestation ≥ 26 weeks | 6.1 (3.3) | –0.4 to 12.6 | 0.07 | |
RSD = 15.8 | ||||
Linear regression adjusted for start time of first sensor glucose and first sensor glucose | Intercept | 82.8 (4.7) | 73.6 to 92 | < 0.001 |
CGM vs. standard | 9.1 (2.6) | 3.9 to 14.3 | < 0.001 | |
Time from birth (hours) to first sensor glucose measurement | 0.04 (0.13) | –0.23 to 0.3 | 0.8 | |
First sensor glucose measurement (mmol/l) | –2.3 (0.55) | –3.4 to –1.21 | < 0.001 | |
N36 (reference N01) | –12.8 (12.3) | –37.1 to 11.6 | 0.3 | |
N42 | 2.8 (6) | –9.1 to 14.6 | 0.6 | |
N43 | 0.005 (4) | –8 to 8 | 0.999 | |
N68 | –2.8 (4.2) | –11 to 5.5 | 0.5 | |
N73 | –0.4 (5.3) | –10.9 to 10 | 0.9 | |
N74 | 3.8 (12.2) | –20.2 to 27.9 | 0.8 | |
N85 | 4.9 (6.6) | –8.1 to 18 | 0.5 | |
N86 | –14.6 (8.5) | –31.4 to 2.3 | 0.09 | |
N87 | –16.4 (11.9) | –39.9 to 7.2 | 0.2 | |
N88 | –26.9 (7.7) | –42.2 to –11.6 | < 0.001 | |
ND3 | 2.7 (6.7) | –10.6 to 16 | 0.7 | |
SP3 | 7.13 (8.5) | –9.6 to 23.9 | 0.4 | |
Gestation ≥ 26 weeks | 1.3 (3.5) | –5.7 to 8.2 | 0.7 | |
RSD = 16.1 | ||||
Linear regression with interaction between time to first sensor glucose and treatment | Intercept | 82.6 (5.7) | 71.3 to 93.9 | < 0.001 |
CGM vs. standard | 9.5 (6.8) | –4 to 23 | 0.2 | |
Time from birth (hours) to first sensor glucose measurement | 0.04 (0.18) | –0.32 to 0.41 | 0.8 | |
First sensor glucose measurement (mmol/l) | –2.3 (0.56) | –3.4 to –1.2 | < 0.001 | |
N36 (reference N01) | –12.8 (12.4) | –37.4 to 11.7 | 0.3 | |
N42 | 2.7 (6.1) | –9.4 to 14.8 | 0.7 | |
N43 | –0.007 (4.1) | –8 to 8 | 0.999 | |
N68 | –2.8 (4.2) | –11 to 5.5 | 0.5 | |
N73 | –0.5 (5.3) | –11 to 10.1 | 0.9 | |
N74 | 4.0 (12.4) | –20.5 to 28.5 | 0.7 | |
N85 | 4.9 (6.6) | –8.2 to 18 | 0.5 | |
N86 | –14.6 (8.6) | –31.7 to 2.4 | 0.09 | |
N87 | –17 (12) | –40 to 7 | 0.2 | |
N88 | –27 (8) | –42.7 to –11.2 | < 0.001 | |
ND3 | 2.7 (6.8) | –10.6 to 16 | 0.7 | |
SP3 | 7.1 (8.5) | –9.7 to 23.9 | 0.4 | |
Gestation ≥ 26 weeks | 1.3 (3.5) | –5.7 to 8.2 | 0.7 | |
Treatment effect modification [CGM – Standard : time from birth (hours) to first sensor glucose measurement] | –0.02 (0.24) | –0.49 to 0.47 | 0.95 | |
RSD = 16.2 | ||||
Linear regression with interaction between first sensor glucose measurement and treatment | Intercept | 80 (4.6) | 70.8 to 89.1 | < 0.001 |
CGM vs. standard | 10.3 (2.6) | 5.2 to 15.5 | < 0.001 | |
First sensor glucose measurement (mmol/l) | –3.59 (0.67) | –4.82 to –2.16 | < 0.001 | |
Time from birth (hours) to first sensor glucose measurement | 0.06 (0.13) | –0.20 to 0.31 | 0.6 | |
N36 (reference N01) | –12 (12) | –35 to 12 | 0.3 | |
N42 | 1.8 (5.9) | –9.8 to 13.4 | 0.8 | |
N43 | 0.8 (3.9) | –7 to 8.6 | 0.8 | |
N68 | –2.6 (4.1) | –10.6 to 5.5 | 0.5 | |
N73 | –0.2 (5.2) | –10.4 to 10 | 0.97 | |
N74 | 2.7 (11.9) | –20.8 to 26.1 | 0.8 | |
N85 | 4.8 (6.4) | –7.97 to 17.5 | 0.5 | |
N86 | –19.1 (8.5) | –35.9 to –2.4 | 0.03 | |
N87 | –16.8 (11.6) | –39.7 to 6.1 | 0.1 | |
N88 | –27.1 (7.5) | –42 to –12.2 | < 0.001 | |
ND3 | 3.1 (6.5) | –9.8 to 16.1 | 0.6 | |
SP3 | 5.6 (8.3) | –10.7 to 21.9 | 0.5 | |
Gestation ≥ 26 weeks | 3.4 (3.5) | –3.5 to 10.3 | 0.3 | |
Treatment effect modification [CGM – Standard : first sensor glucose measurement (mmol/l)] | 3.1 (1) | 1 to 5.1 | 0.004 | |
RSD = 15.7 | ||||
Generalised least squares regression allowing for heteroscedastic variances between treatment groups | Intercept | 81.9 (3.4) | 75.2 to 88.7 | < 0.001 |
CGM vs. standard | 8.9 (2.7) | 3.6 to 14.2 | 0.001 | |
N36 (reference N01) | 2.9 (9.4) | –15.5 to 21.3 | 0.8 | |
N42 | 8.3 (5.2) | –1.8 to 18.4 | 0.1 | |
N43 | 3.9 (3.3) | –2.6 to 10.3 | 0.2 | |
N68 | –0.08 (3.2) | –6.4 to 6.2 | 0.98 | |
N73 | 4.7 (4.2) | –3.6 to 13 | 0.3 | |
N74 | 9.2 (9.4) | –9.2 to 27.6 | 0.3 | |
N85 | 8.5 (5.5) | –2.3 to 19.2 | 0.1 | |
N86 | –23.2 (8.2) | –39.2 to –7.3 | 0.005 | |
N87 | –11.8 (15.7) | –42.5 to 18.9 | 0.5 | |
N88 | –12.2 (6.5) | –25 to 0.5 | 0.06 | |
ND3 | 7.3 (5.5) | –3.5 to 18.1 | 0.2 | |
SP3 | 9 (6.8) | –4.3 to 22.3 | 0.2 | |
Gestation ≥ 26 weeks | 1.5 (2.9) | –4.2 to 7.3 | 0.6 | |
RSD = 9.6 | ||||
Random intercept for site | Intercept | 79.5 (4.1) | 71.3 to 87.7 | < 0.001 |
CGM vs. standard | 9.3 (2.8) | 3.8 to 14.8 | 0.001 | |
Gestation ≥ 26 weeks | 5.2 (3.6) | –1.8 to 12.2 | 0.1 | |
RSD = 17.2 | ||||
Linear regression adjusting for gestation only | Intercept | 80.1 (3.5) | 73.3 to 86.9 | < 0.001 |
CGM vs. standard | 9.4 (2.9) | 3.7 to 15.1 | 0.001 | |
Gestation ≥ 26 weeks | 5.3 (3.6) | –1.9 to 12.4 | 0.1 | |
RSD = 17.8 |
Outcome | Covariate | Estimate (SE) | 95% CI | p-value |
---|---|---|---|---|
Percentage of time sensor glucose is in the target range 2.6–10 mmol/l | Intercept | 79.3 (4) | 71.3 to 87.2 | < 0.001 |
CGM vs. standard | 10.1 (3.3) | 3.7 to 16.6 | 0.002 | |
N36 (reference N01) | –19 (18) | –54 to 15 | 0.3 | |
N42 | 9.4 (6.4) | –3.3 to 22 | 0.1 | |
N43 | 0.7 (4.7) | –8.6 to 9.9 | 0.9 | |
N68 | 5.47 (4.54) | –3.53 to 14.5 | 0.2 | |
N73 | –3.4 (7.1) | –17.5 to 10.6 | 0.6 | |
N74 | 11 (13) | –14 to 36 | 0.4 | |
N85 | 8.5 (9.1) | –9.6 to 26.6 | 0.4 | |
N86 | –23 (10) | –44 to –3 | 0.03 | |
N87 | –11 (13) | –35 to 14 | 0.4 | |
N88 | –27.7 (8.2) | –43.9 to –11.5 | < 0.001 | |
ND3 | 8 (9.2) | –10.1 to 26.2 | 0.4 | |
SP3 | 16 (13) | –9 to 41 | 0.2 | |
Gestation ≥ 26 weeks | 4.3 (3.9) | –3.5 to 12.2 | 0.3 | |
RSD = 17.2 |
Subgroup | Covariate | Estimate (SE) | 95% CI | p-value |
---|---|---|---|---|
Site | Intercept | 82.7 (4.2) | 74.3 to 91 | < 0.001 |
CGM vs. standard | 5.8 (4.7) | –3.6 to 15.1 | 0.2 | |
N36 (reference N01) | –23 (17) | –57 to 11 | 0.2 | |
N42 | 7 (7.2) | –7.3 to 21.1 | 0.3 | |
N43 | –4.2 (5.7) | –15.6 to 7.1 | 0.5 | |
N68 | 1.3 (5.7) | –10 to 12.7 | 0.8 | |
N73 | –4.8 (7.6) | –19.9 to 10.3 | 0.5 | |
N74 | 13 (17) | –21 to 47 | 0.4 | |
N85 | 8.7 (9.1) | –9.3 to 26.6 | 0.3 | |
N86 | –10.8 (10.3) | –31.3 to 9.6 | 0.3 | |
N87 | –14 (12) | –38 to 11 | 0.3 | |
N88 | –47 (10) | –68 to –27 | < 0.001 | |
ND3 | 7.2 (9.1) | –10.7 to 25.1 | 0.4 | |
SP3 | 12 (12) | –12 to 37 | 0.3 | |
Gestation ≥ 26 weeks | 4.2 (3.6) | –2.9 to 11.3 | 0.2 | |
[CGM – treatment : N36 (reference N01) – site] | 30 (24) | –18 to 78 | 0.2 | |
[CGM – treatment : N42 – site] | 0.4 (13) | –25 to 25 | 0.97 | |
[CGM – treatment : N43 – site] | 9.6 (8.4) | –6.9 to 26.2 | 0.3 | |
[CGM – treatment : N68 – site] | –2.2 (8.2) | –18.5 to 14.1 | 0.8 | |
[CGM – treatment : N73 – site] | 10.6 (10.8) | –10.8 to 32.1 | 0.3 | |
[CGM – treatment : N74 – site] | –6 (24) | –54 to 42 | 0.8 | |
[CGM – treatment : N85 – site] | –1 (14) | –29 to 26 | 0.9 | |
[CGM – treatment : N86 – site] | –23 (20) | –62 to 17 | 0.3 | |
[CGM – treatment : N88 – site] | 46 (16) | 14 to 77 | 0.006 | |
[CGM – treatment : ND3 – site] | –0.9 (14) | –28 to 26 | 0.95 | |
[CGM – treatment : SP3 – site] | –5 (18) | –40 to 30 | 0.8 | |
[CGM – treatment : N87 – site] | n/a to n/a | |||
RSD = 16.9 | ||||
Sex | Intercept | 86.8 (4.1) | 78.6 to 94.9 | < 0.001 |
CGM vs. standard | 2.3 (3.9) | –5.4 to 10 | 0.6 | |
Male vs. female | –11.3 (3.7) | –18.7 to –3.9 | 0.003 | |
N36 (reference N01) | –11 (12) | –35 to 13 | 0.4 | |
N42 | 5.7 (5.8) | –5.8 to 17.2 | 0.3 | |
N43 | –0.7 (4.1) | –8.9 to 7.5 | 0.9 | |
N68 | 1.1 (4.1) | –7 to 9.2 | 0.8 | |
N73 | –0.1 (5.4) | –10.9 to 10.6 | 0.98 | |
N74 | 12 (12) | –12 to 36 | 0.3 | |
N85 | 9.6 (6.7) | –3.7 to 22.9 | 0.2 | |
N86 | –17.5 (8.7) | –34.8 to –0.3 | 0.05 | |
N87 | –13 (12) | –36 to 11 | 0.3 | |
N88 | –28.2 (7.8) | –43.6 to –12.8 | < 0.001 | |
ND3 | 7.9 (6.8) | –5.5 to 21.4 | 0.2 | |
SP3 | 6.9 (8.8) | –10.5 to 24.2 | 0.4 | |
Gestation ≥ 26 weeks | 4.8 (3.5) | –2.1 to 11.7 | 0.2 | |
[CGM – treatment : Male – sex] | 12.6 (5.5) | 1.8 to 23.5 | 0.02 | |
RSD = 16.6 | ||||
Sexa | Intercept | 86.8 (4.1) | 78.6 to 94.9 | < 0.001 |
Male vs. female | –11.3 (3.7) | –18.7 to –3.9 | 0.003 | |
N36 (reference N01) | –11 (12) | –35 to 13 | 0.4 | |
N42 | 5.7 (5.8) | –5.8 to 17.2 | 0.3 | |
N43 | –0.7 (4.1) | –8.9 to 7.5 | 0.9 | |
N68 | 1.1 (4.1) | –7 to 9.2 | 0.8 | |
N73 | –0.1 (5.4) | –10.9 to 10.6 | 0.98 | |
N74 | 12 (12) | –12 to 36 | 0.3 | |
N85 | 9.6 (6.7) | –3.7 to 22.9 | 0.2 | |
N86 | –17.5 (8.7) | –34.8 to –0.3 | 0.05 | |
N87 | –13 (12) | –36 to 11 | 0.3 | |
N88 | –28.2 (7.8) | –43.6 to –12.8 | < 0.001 | |
ND3 | 7.9 (6.8) | –5.5 to 21.4 | 0.2 | |
SP3 | 6.9 (8.8) | –10.5 to 24.2 | 0.4 | |
Gestation ≥ 26 weeks | 4.8 (3.5) | –2.1 to 11.7 | 0.2 | |
[Female – sex : CGM – treatment] | 2.3 (3.9) | –5.4 to 10 | 0.6 | |
[Male – sex : CGM – treatment] | 14.9 (3.8) | 7.4 to 22.5 | < 0.001 | |
RSD = 16.6 | ||||
Sex (interaction with first sensor glucose measurement and treatment) | Intercept | 89.2 (4) | 81.3 to 97.2 | < 0.001 |
CGM vs. standard | 2.9 (3.6) | –4.2 to 10 | 0.4 | |
Male vs. female | –11.7 (3.4) | –18.4 to –4.9 | < 0.001 | |
First sensor glucose measurement (mmol/l) | –1.23 (0.98) | –3.16 to 0.7 | 0.2 | |
N36 (reference N01) | –13 (11) | –35 to 9 | 0.2 | |
N42 | 1.9 (5.3) | –8.7 to 12.4 | 0.7 | |
N43 | –0.6 (3.8) | –8.1 to 6.8 | 0.9 | |
N68 | –2.1 (3.8) | –9.6 to 5.4 | 0.6 | |
N73 | 0.2 (5) | –9.6 to 10 | 0.97 | |
N74 | 5 (11) | –18 to 25 | 0.8 | |
N85 | 4.1 (6.2) | –8.2 to 16.3 | 0.5 | |
N86 | –19 (8.1) | –35.1 to –3 | 0.02 | |
N87 | –17 (11) | –38 to 5 | 0.1 | |
N88 | –28.5 (7.2) | –42.6 to –14.3 | < 0.001 | |
ND3 | 3.6 (6.3) | –8.9 to 16.1 | 0.6 | |
SP3 | 5.1 (8) | –10.6 to 20.9 | 0.5 | |
Gestation ≥ 26 weeks | 2.2 (3.4) | –4.5 to 8.9 | 0.5 | |
[CGM – treatment : Male – sex] | 13.2 (5) | 3.2 to 23.1 | 0.01 | |
[CGM – treatment : First sensor glucose measurement] | 0.6 (1.4) | –2.2 to 3.4 | 0.7 | |
[Male – sex : First sensor glucose measurement] | –3.8 (1.3) | –6.3 to –1.2 | 0.004 | |
[CGM – treatment : Male – sex : First sensor glucose measurement] | 3.8 (2) | –0.07 to 7.7 | 0.05 | |
RSD = 15 | ||||
Corrected gestational age | Intercept | 111 (13) | 85 to 137 | < 0.001 |
CGM vs. standard | –9 (19) | –46 to 28 | 0.6 | |
Corrected age | 109 (52) | 6 to 211 | 0.04 | |
N36 (reference N01) | –9 (12) | –32 to 16 | 0.5 | |
N42 | 6.9 (6) | –5.1 to 18.8 | 0.3 | |
N43 | 0.6 (4.2) | –7.7 to 8.9 | 0.9 | |
N68 | 0.6 (4.2) | –7.6 to 8.9 | 0.9 | |
N73 | –1.4 (5.6) | –12.5 to 9.7 | 0.8 | |
N74 | 9.4 (12.3) | –14.9 to 33.6 | 0.4 | |
N85 | 7.7 (6.9) | –6 to 21.3 | 0.3 | |
N86 | –17.7 (8.9) | –35.3 to –0.01 | 0.05 | |
N87 | –12 (12) | –36 to 13 | 0.4 | |
N88 | –30.8 (8.1) | –46.8 to –14.8 | < 0.001 | |
ND3 | 6 (6.9) | –7.6 to 19.6 | 0.4 | |
SP3 | 8.9 (8.9) | –8.7 to 26.4 | 0.3 | |
[CGM – treatment : corrected age] | –72 (76) | –223 to 79 | 0.4 | |
RSD = 16.9 | ||||
Birthweight SDS | Intercept | 83.6 (3.9) | 75.8 to 91.3 | < 0.001 |
CGM vs. standard | 5.4 (3.7) | –1.9 to 12.6 | 0.1 | |
Weight SDS | 5.3 (2.1) | 1 to 9.5 | 0.02 | |
N36 (reference N01) | –4 (12) | –28 to 20 | 0.8 | |
N42 | 6.3 (5.9) | –5.4 to 18.1 | 0.3 | |
N43 | –0.6 (4.2) | –9 to 7.6 | 0.9 | |
N68 | –0.4 (4.1) | –8.6 to 7.8 | 0.9 | |
N73 | 2 (5.5) | –8.8 to 12.8 | 0.7 | |
N74 | 8 (12) | –16 to 32 | 0.5 | |
N85 | 8.9 (6.8) | –4.5 to 22.3 | 0.2 | |
N86 | –13.9 (8.8) | –31.4 to 3.5 | 0.1 | |
N87 | –20 (13) | –44 to 5 | 0.1 | |
N88 | –29.3 (8.1) | –45.3 to –13.3 | < 0.001 | |
ND3 | 6.7 (6.8) | –6.7 to 20.1 | 0.3 | |
SP3 | 9.5 (8.9) | –8.1 to 27.1 | 0.3 | |
Gestation ≥ 26 weeks | 6.6 (3.6) | –0.5 to 13.7 | 0.07 | |
[CGM – treatment : weight SDS] | –4.2 (3.1) | –10.4 to 2 | 0.2 | |
RSD = 16.8 | ||||
Antenatal steroids | Intercept | 81.4 (3.9) | 73.7 to 89 | < 0.001 |
CGM vs. standard | 7.5 (3.2) | 1.2 to 13.9 | 0.02 | |
Antenatal steroids (no vs. yes) | –2.8 (4.7) | –12 to 6.4 | 0.5 | |
N36 (reference N01) | –6 (13) | –31 to 19 | 0.6 | |
N42 | 8.3 (6.2) | –4 to 20.5 | 0.2 | |
N43 | 0.4 (4.6) | –8.6 to 9.5 | 0.9 | |
N68 | 0.5 (4.2) | –7.9 to 8.9 | 0.9 | |
N73 | –0.08 (5.6) | –11.1 to 11 | 0.99 | |
N74 | 12 (13) | –13 to 37 | 0.4 | |
N85 | 9.4 (7.2) | –4.8 to 23.6 | 0.2 | |
N86 | –15.5 (9) | –33.3 to 2.3 | 0.09 | |
N87 | –13 (12) | –37 to 12 | 0.3 | |
N88 | –28.5 (8.1) | –44.4 to –12.6 | < 0.001 | |
ND3 | 7.3 (7.1) | –6.7 to 21.2 | 0.3 | |
SP3 | 9 (9) | –8.9 to 26.8 | 0.3 | |
Gestation ≥ 26 weeks | 4.7 (3.7) | –2.5 to 11.9 | 0.2 | |
[CGM – treatment : No – antenatal steroids] | 6 (7) | –8 to 19.9 | 0.4 | |
RSD = 17.1 | ||||
Maternal chorioamnionitis | Intercept | 80.6 (4.9) | 70.9 to 90.3 | < 0.001 |
CGM vs. standard | 10.8 (5.7) | –0.5 to 22.1 | 0.06 | |
Maternal chorioamnionitis (no vs. yes) | –0.4 (4.6) | –9.6 to 8.8 | 0.9 | |
Maternal chorioamnionitis (unknown vs. yes) | 15 (13) | –11 to 40 | 0.3 | |
N36 (reference N01) | –7 (12) | –32 to 18 | 0.6 | |
N42 | 8 (6) | –3.8 to 19.8 | 0.2 | |
N43 | 0.6 (4.3) | –8 to 9.1 | 0.9 | |
N68 | 0.4 (4.3) | –8.2 to 8.9 | 0.9 | |
N73 | 0.6 (5.6) | –10.5 to 11.7 | 0.9 | |
N74 | 11 (12) | –14 to 36 | 0.4 | |
N85 | 8.3 (7) | –5.6 to 22.1 | 0.2 | |
N86 | –15.2 (9.1) | –33.1 to 2.7 | 0.1 | |
N87 | –12 (13) | –37 to 13 | 0.3 | |
N88 | –28.4 (8.2) | –44.6 to –12.3 | < 0.001 | |
ND3 | 7.3 (7) | –6.5 to 21.1 | 0.3 | |
SP3 | 9.7 (9) | –8.1 to 27.6 | 0.3 | |
Gestation ≥ 26 weeks | 4.5 (3.7) | –2.7 to 11.8 | 0.2 | |
[CGM – treatment : No – maternal chorioamnionitis] | –2.1 (6.7) | –15.3 to 11.1 | 0.8 | |
[CGM – treatment : Unknown – maternal chorioamnionitis] | –19 (18) | –55 to 18 | 0.3 | |
RSD = 17.2 | ||||
Maternal diabetes | Intercept | 78.7 (7.4) | 64 to 93.4 | < 0.001 |
CGM vs. standard | 13 (11) | –8 to 34 | 0.2 | |
Maternal diabetes (no vs. yes) | 2.1 (6.8) | –11.2 to 15.5 | 0.8 | |
N36 (reference N01) | –8 (12) | –33 to 17 | 0.5 | |
N42 | 7.7 (6) | –4.1 to 19.4 | 0.2 | |
N43 | 0.2 (4.3) | –8.3 to 8.6 | 0.97 | |
N68 | –0.1 (4.3) | –8.6 to 8.3 | 0.98 | |
N73 | 0.2 (5.6) | –10.8 to 11.2 | 0.97 | |
N74 | 10 (12) | –15 to 34 | 0.4 | |
N85 | 8.3 (7.2) | –5.8 to 22.5 | 0.2 | |
N86 | –16.2 (9) | –34 to 1.6 | 0.08 | |
N87 | –12 (13) | –37 to 12 | 0.3 | |
N88 | –28.7 (8.1) | –44.7 to –12.7 | < 0.001 | |
ND3 | 6.8 (7) | –7 to 20.5 | 0.3 | |
SP3 | 9.5 (9) | –8.3 to 27.2 | 0.3 | |
Gestation ≥ 26 weeks | 5 (3.7) | –2.2 to 12.2 | 0.2 | |
[CGM – treatment : No – maternal diabetes] | –5 (11) | –27 to 17 | 0.7 | |
RSD = 17.2 |
Test | Outcome | Nominal p-value | Rank | Significant |
---|---|---|---|---|
Hochberg | Percentage of time sensor glucose is in the target range 2.6–10 mmol/l | 0.002 | 1 | Yes |
Hochberg | Percentage of time sensor glucose is in the target range 4–8 mmol/l | 0.004 | 2 | Yes |
Hochberg | Mean sensor glucose | 0.099 | 3 | No |
Gate-keeping | Sensor glucose variability (unconditional) | 0.9 | 4 | No |
Gate-keeping | Percentage of time sensor glucose is in the hyperglycaemic range (> 15 mmol/l) | 0.1 | 5 | No |
Subject ID | Group | Date of randomisation | Date of withdrawal | Reason | Description | Number of intervention days completed | Later withdrawn from trial? |
---|---|---|---|---|---|---|---|
N43-1032 | Standard | 26 April 2017 | 1 May 2017 | Clinical decision | Yes | ||
N43-1034 | Standard | 4 May 2017 | 9 May 2017 | Clinical decision | No | ||
N68-1091 | Standard | 1 March 2018 | 2 March 2018 | Clinical decision | 2 | No | |
N74-1092 | Standard | 1 March 2018 | 3 March 2018 | Clinical decision | 2 | No | |
N86-1131 | Standard | 13 July 2018 | 16 July 2018 | Clinical decision | 3 | No | |
N87-1168 | Standard | 21 November 2018 | 28 November 2018 | Clinical decision | 6 | No | |
N01-1083 | Standard | 5 February 2018 | 6 February 2018 | Other | Clinical decision. Third sensor failed and inappropriate to attempt fourth sensor | 2 | No |
N36-1021 | Standard | 25 January 2017 | 26 January 2017 | Other | Sensor would not work | No | |
N36-1066 | Standard | 19 December 2017 | 20 December 2017 | Other | Unable to get sensor to calibrate | 1 | No |
N36-1102 | Standard | 28 March 2018 | 29 March 2018 | Other | Unable to get sensor to calibrate | 1 | No |
N42-1049 | Standard | 21 August 2017 | 27 August 2017 | Other | Sensor stopped working | 6 | No |
N68-1068 | Standard | 22 December 2017 | 25 December 2017 | Other | Device-related issue | 3 | No |
N68-1082 | Standard | 4 February 2018 | 6 February 2018 | Other | Infant transferred to another hospital and so sensor was removed | 2 | No |
N68-1107 | Standard | 10 April 2018 | 13 April 2018 | Other | Transferred to another hospital for intervention | 4 | No |
N73-1090 | Standard | 27 February 2018 | 4 March 2018 | Other | Interstitial signal not recording and therefore sensor removed | 5 | No |
N73-1164 | Standard | 13 November 2018 | 17 November 2018 | Other | Infant transferred to Salisbury | 5 | No |
N88-1151 | Standard | 20 September 2018 | 21 September 2018 | Other | Joint agreement. Equipment malfunction | 1 | No |
N01-1048 | CGM | 21 August 2017 | 22 August 2017 | Clinical decision | 1 | Yes | |
N36-1022 | CGM | 25 January 2017 | 26 January 2017 | Clinical decision | No | ||
N36-6022 | CGM | 1 November 2017 | 3 November 2017 | Clinical decision | 2 | No | |
N43-1027 | CGM | 30 March 2017 | 31 March 2017 | Clinical decision | No | ||
N43-1028 | CGM | 30 March 2017 | 2 April 2017 | Clinical decision | No | ||
N68-1138 | CGM | 7 August 2018 | 10 August 2018 | Clinical decision | 3 | No | |
N85-1175 | CGM | 15 January 2019 | 17 January 2019 | Clinical decision | 2 | No | |
N01-1059 | CGM | 9 November 2017 | 11 November 2017 | Other | Failed sensor. Parents did not want a replacement sensor inserted | 3 | No |
N68-1080 | CGM | 1 February 2018 | 1 February 2018 | Other | Could not get a sensor into the infant | 0 | No |
N73-1165 | CGM | 14 November 2018 | 17 November 2018 | Other | Sensor readings lost | 4 | No |
N74-1128 | CGM | 23 June 2018 | 29 June 2018 | Other | Advice from trial team because the infant was 9 days old (not 7 days) because of the delay in inserting the sensor | 5 | No |
N88-1173 | CGM | 18 December 2018 | 19 December 2018 | Other | Equipment malfunctioning. Could not get transmitters to work despite good sensor placement | 2 | No |
SP3-1085 | CGM | 14 February 2018 | 15 February 2018 | Other | Monitor not working | 0 | No |
Subject ID | Group | Date of randomisation | Date of withdrawal | Study timing | Reason | Description |
---|---|---|---|---|---|---|
N01-1052 | Standard | 13 October 2017 | 13 October 2017 | Days 1–7 | Parental decision | |
N36-1117 | Standard | 22 May 2018 | 23 May 2018 | Days 1–7 | Parental decision | |
ND3-1062 | Standard | 30 November 2017 | 30 November 2017 | Days 1–7 | Other | No sensor readings because infant was small. Inserted new sensor; still no readings |
N87-1148 | Standard | 14 September 2018 | 15 September 2018 | Days 8–14 | Other | No interstitial signal or BMG reading on MiniMed |
N01-1144 | CGM | 1 September 2018 | 1 September 2018 | Days 1–7 | Parental decision | |
N01-1006 | CGM | 15 September 2016 | 18 September 2016 | Days 8–14 | Clinical decision | |
N36-1033 | CGM | 3 May 2017 | 4 May 2017 | Days 8–14 | Clinical decision | |
N73-1141 | CGM | 21 August 2018 | 22 August 2018 | Days 8–14 | Clinical decision | |
N42-1016 | CGM | 8 December 2016 | 12 December 2016 | Days 8–14 | Parental decision | |
N87-1142 | CGM | 23 August 2018 | 25 August 2018 | Days 8–14 | Parental decision | |
N87-1172 | CGM | 16 December 2018 | 17 December 2018 | Days 8–14 | Other | Large IVH for reorientation of care. Death 18 December 2018 |
Subject ID | Group | Date of randomisation | Date of death | Study timing |
---|---|---|---|---|
N43-1038 | Standard | 29 May 2017 | 30 May 2017 | Days 1–7 |
N73-1095 | Standard | 14 March 2018 | 15 March 2018 | Days 1–7 |
N01-1147 | Standard | 10 September 2018 | 23 September 2018 | Days 8–14 |
N36-1018 | Standard | 5 January 2017 | 2 February 2017 | Post day 14 |
N43-1032 | Standard | 26 April 2017 | 13 May 2017 | Post day 14 |
N68-1139 | Standard | 11 August 2018 | 26 August 2018 | Post day 14 |
N01-1048 | CGM | 21 August 2017 | 7 October 2017 | Post day 14 |
N01-1087 | CGM | 20 February 2018 | 10 March 2018 | Post day 14 |
Variable | Statistic | Standard-care arm | CGM arm | Total |
---|---|---|---|---|
Birthweight (g) | n | 95 | 84 | 179 |
Mean (SD) | 880 (180) | 910 (160) | 890 (170) | |
Median | 920 | 930 | 930 | |
Minimum, maximum | 470, 1170 | 390, 1190 | 390, 1190 | |
Birthweight SDS | n | 95 | 84 | 179 |
Mean (SD) | –0.8 (0.92) | –0.82 (0.98) | –0.81 (0.95) | |
Median | –0.66 | –0.76 | –0.74 | |
Minimum, maximum | –2.6, 1.32 | –2.97, 1.2 | –2.97, 1.32 | |
Body length (cm) | n | 64 | 55 | 119 |
Mean (SD) | 33 (2.5) | 33.2 (2.8) | 33 (2.6) | |
Median | 33 | 33 | 33 | |
Minimum, maximum | 26, 38 | 24.5, 38 | 24.5, 38 | |
Body length SDS | n | 64 | 55 | 119 |
Mean (SD) | –1.54 (0.81) | –1.56 (0.89) | –1.55 (0.84) | |
Median | –1.45 | –1.41 | –1.41 | |
Minimum, maximum | –3.87, –0.41 | –3.73, 0.24 | –3.87, 0.24 | |
Head circumference (cm) | n | 87 | 75 | 162 |
Mean (SD) | 24.1 (1.9) | 24.5 (1.8) | 24.3 (1.9) | |
Median | 24.5 | 24.8 | 24.5 | |
Minimum, maximum | 19, 28 | 19, 28 | 19, 28 | |
Head circumference SDS | n | 87 | 75 | 162 |
Mean (SD) | –1.16 (0.86) | –1.12 (0.99) | –1.14 (0.92) | |
Median | –1.14 | –1.04 | –1.1 | |
Minimum, maximum | –3.03, 0.82 | –3.5, 1.11 | –3.5, 1.11 |
Variable | Statistic | Standard-care arm | CGM arm | Total |
---|---|---|---|---|
Weight (g) | n | 87 | 76 | 163 |
Mean (SD) | 890 (180) | 930 (160) | 910 (170) | |
Median | 920 | 960 | 930 | |
Minimum, maximum | 480, 1220 | 450, 1220 | 450, 1220 | |
Weight SDS | n | 87 | 76 | 163 |
Mean (SD) | –1.3 (0.75) | –1.26 (0.79) | –1.28 (0.77) | |
Median | –1.14 | –1.19 | –1.16 | |
Minimum, maximum | –3.11, 0.16 | –3.2, 0.17 | –3.2, 0.17 | |
Body length (cm) | n | 63 | 56 | 119 |
Mean (SD) | 33.7 (2.7) | 33.8 (2.7) | 33.7 (2.7) | |
Median | 34 | 34 | 34 | |
Minimum, maximum | 24, 39.5 | 24.5, 39.3 | 24, 39.5 | |
Body length SDS | n | 63 | 56 | 119 |
Mean (SD) | –1.81 (1.07) | –1.78 (0.87) | –1.8 (0.97) | |
Median | –1.59 | –1.67 | –1.6 | |
Minimum, maximum | –7.01, 0.16 | –4.19, –0.28 | –7.01, 0.16 | |
Head circumference (cm) | n | 72 | 60 | 132 |
Mean (SD) | 24.1 (1.8) | 24.4 (1.6) | 24.2 (1.7) | |
Median | 24 | 24.5 | 24.3 | |
Minimum, maximum | 19.7, 28 | 20, 27.7 | 19.7, 28 | |
Head circumference SDS | n | 72 | 60 | 132 |
Mean (SD) | –1.84 (0.77) | –1.76 (0.83) | –1.81 (0.8) | |
Median | –1.85 | –1.56 | –1.73 | |
Minimum, maximum | –3.6, 0.03 | –3.91, 0.03 | –3.91, 0.03 |
Variable | Statistic | Standard-care arm | CGM arm | Total |
---|---|---|---|---|
Weight (g) | n | 89 | 76 | 165 |
Mean (SD) | 980 (200) | 1020 (200) | 1000 (200) | |
Median | 980 | 1040 | 1000 | |
Minimum, maximum | 480, 1390 | 530, 1380 | 480, 1390 | |
Weight SDS | n | 89 | 76 | 165 |
Mean (SD) | –1.38 (0.76) | –1.39 (0.77) | –1.38 (0.76) | |
Median | –1.35 | –1.35 | –1.35 | |
Minimum, maximum | –3.06, 0.21 | –3.06, 0.31 | –3.06, 0.31 | |
Body length (cm) | n | 59 | 51 | 110 |
Mean (SD) | 34.5 (2.6) | 34.6 (2.7) | 34.6 (2.6) | |
Median | 35 | 35 | 35 | |
Minimum, maximum | 26.5, 40 | 26, 38.5 | 26, 40 | |
Body length SDS | n | 59 | 51 | 110 |
Mean (SD) | –1.98 (0.88) | –1.96 (0.83) | –1.97 (0.85) | |
Median | –1.93 | –1.94 | –1.93 | |
Minimum, maximum | –4.71, –0.11 | –3.89, –0.56 | –4.71, –0.11 | |
Head circumference (cm) | n | 74 | 66 | 140 |
Mean (SD) | 25 (2.3) | 25.4 (2.4) | 25.2 (2.4) | |
Median | 25 | 25.5 | 25.2 | |
Minimum, maximum | 19.2, 35.6 | 20.5, 36.5 | 19.2, 36.5 | |
Head circumference SDS | n | 74 | 66 | 140 |
Mean (SD) | –1.9 (1.1) | –1.8 (1.2) | –1.9 (1.2) | |
Median | –2.1 | –1.9 | –1.9 | |
Minimum, maximum | –4.1, 3.5 | –4.5, 4 | –4.5, 4 |
Variable | Statistic | Standard-care arm | CGM arm | Total |
---|---|---|---|---|
Weight (g) | n | 85 | 75 | 160 |
Mean (SD) | 2030 (390) | 2040 (360) | 2030 (380) | |
Median | 2050 | 2020 | 2050 | |
Minimum, maximum | 1130, 2820 | 1160, 2980 | 1130, 2980 | |
Weight SDS | n | 85 | 75 | 160 |
Mean (SD) | –1.54 (0.94) | –1.56 (0.93) | –1.55 (0.93) | |
Median | –1.47 | –1.57 | –1.5 | |
Minimum, maximum | –3.83, 0.45 | –3.92, 0.65 | –3.92, 0.65 | |
Body length (cm) | n | 41 | 27 | 68 |
Mean (SD) | 41.3 (3.4) | 40.7 (3.9) | 41.1 (3.6) | |
Median | 42 | 41 | 41 | |
Minimum, maximum | 34, 47 | 29.6, 47 | 29.6, 47 | |
Body length SDS | n | 41 | 27 | 68 |
Mean (SD) | –2.6 (1.6) | –2.9 (1.8) | –2.7 (1.6) | |
Median | –2.3 | –2.8 | –2.6 | |
Minimum, maximum | –6, 0.09 | –7.8, 0.03 | –7.8, 0.09 | |
Head circumference (cm) | n | 71 | 59 | 130 |
Mean (SD) | 30.9 (2.1) | 31.2 (2.2) | 31 (2.1) | |
Median | 30.8 | 31 | 30.9 | |
Minimum, maximum | 25.6, 36 | 26.5, 39.7 | 25.6, 39.7 | |
Head circumference SDS | n | 71 | 59 | 130 |
Mean (SD) | –1.3 (1.6) | –1.1 (1.7) | –1.2 (1.6) | |
Median | –1.4 | –1.3 | –1.3 | |
Minimum, maximum | –5.5, 2.8 | –4.8, 5.6 | –5.5, 5.6 |
Data | Item | CGM arm (N = 84), n (%) | Standard-care arm (N = 95), n (%) | Total (N = 179), n (%) | |||||||||
---|---|---|---|---|---|---|---|---|---|---|---|---|---|
Completed | CRF not available | CRF not applicable | Data on variable missing | Completed | CRF not available | CRF not applicable | Data on variable missing | Completed | CRF not available | CRF not applicable | Data on variable missing | ||
Enlite sensor (MiniMed system) | MiniMed 640G 3.0-ml insulin pump | 81 (96) | 0 (0) | 3 (4) | 0 (0) | 0 (0) | 0 (0) | 95 (100) | 0 (0) | 81 (45) | 0 (0) | 98 (55) | 0 (0) |
Guardian 2 Link | 81 (96) | 0 (0) | 3 (4) | 0 (0) | 0 (0) | 0 (0) | 95 (100) | 0 (0) | 91 (45) | 0 (0) | 98 (55) | 0 (0) | |
Generation Enlite single sensor: MMT-7008B | 81 (96) | 0 (0) | 3 (4) | 0 (0) | 0 (0) | 0 (0) | 95 (100) | 0 (0) | 91 (45) | 0 (0) | 98 (55) | 0 (0) | |
Glucose monitoring | Glucose sensor insertion | 81 (96) | 0 (0) | 3 (4) | 0 (0) | 0 (0) | 0 (0) | 95 (100) | 0 (0) | 81 (45) | 0 (0) | 98 (55) | 0 (0) |
Glucose sensor monitoring | 75 (89) | 6 (7) | 3 (4) | 0 (0) | 0 (0) | 0 (0) | 95 (100) | 0 (0) | 75 (42) | 6 (3) | 98 (55) | 0 (0) | |
Glucose sensor removal | 81 (96) | 0 (0) | 3 (4) | 0 (0) | 0 (0) | 0 (0) | 95 (100) | 0 (0) | 81 (45) | 0 (0) | 98 (55) | 0 (0) | |
POC testing used to calibrate MiniMed 640G | Nova StatStrip meter | 75 (89) | 0 (0) | 9 (11) | 0 (0) | 93 (98) | 0 (0) | 2 (2) | 0 (0) | 168 (94) | 0 (0) | 11 (6) | 0 (0) |
Length of stay | Total: days 1–7 | 83 (99) | 1 (1) | 0 (0) | 0 (0) | 94 (99) | 1 (1) | 0 (0) | 0 (0) | 177 (99) | 2 (1) | 0 (0) | 0 (0) |
Total: randomisation to 36 weeks | 73 (87) | 11 (13) | 0 (0) | 0 (0) | 83 (87) | 12 (13) | 0 (0) | 0 (0) | 156 (87) | 23 (13) | 0 (0) | 0 (0) | |
Medication | Any medication use: days 1–7 | 81 (96) | 3 (4) | 0 (0) | 0 (0) | 94 (99) | 1 (1) | 0 (0) | 0 (0) | 175 (98) | 4 (2) | 0 (0) | 0 (0) |
Inotrope use | 81 (96) | 3 (4) | 0 (0) | 0 (0) | 94 (99) | 1 (1) | 0 (0) | 0 (0) | 175 (98) | 4 (2) | 0 (0) | 0 (0) | |
Antibiotic use | 81 (96) | 3 (4) | 0 (0) | 0 (0) | 94 (99) | 1 (1) | 0 (0) | 0 (0) | 175 (98) | 4 (2) | 0 (0) | 0 (0) | |
Caffeine use | 81 (96) | 3 (4) | 0 (0) | 0 (0) | 94 (99) | 1 (1) | 0 (0) | 0 (0) | 175 (98) | 4 (2) | 0 (0) | 0 (0) | |
Morphine use | 81 (96) | 3 (4) | 0 (0) | 0 (0) | 94 (99) | 1 (1) | 0 (0) | 0 (0) | 175 (98) | 4 (2) | 0 (0) | 0 (0) | |
Corticosteroid use | 81 (96) | 3 (4) | 0 (0) | 0 (0) | 94 (99) | 1 (1) | 0 (0) | 0 (0) | 175 (98) | 4 (2) | 0 (0) | 0 (0) | |
Insulin use | 81 (96) | 3 (4) | 0 (0) | 0 (0) | 94 (99) | 1 (1) | 0 (0) | 0 (0) | 175 (98) | 4 (2) | 0 (0) | 0 (0) | |
Insulin use over post-intervention period | 77 (92) | 7 (8) | 0 (0) | 0 (0) | 89 (94) | 6 (6) | 0 (0) | 0 (0) | 166 (93) | 13 (7) | 0 (0) | 0 (0) | |
Investigations | Any investigation use | 74 (88) | 10 (12) | 0 (0) | 0 (0) | 85 (89) | 10 (11) | 0 (0) | 0 (0) | 159 (89) | 20 (11) | 0 (0) | 0 (0) |
Cranial ultrasound | 74 (88) | 10 (12) | 0 (0) | 0 (0) | 85 (89) | 10 (11) | 0 (0) | 0 (0) | 159 (89) | 20 (11) | 0 (0) | 0 (0) | |
Other ultrasound | 74 (88) | 10 (12) | 0 (0) | 0 (0) | 85 (89) | 10 (11) | 0 (0) | 0 (0) | 159 (89) | 20 (11) | 0 (0) | 0 (0) | |
CT | 74 (88) | 10 (12) | 0 (0) | 0 (0) | 85 (89) | 10 (11) | 0 (0) | 0 (0) | 159 (89) | 20 (11) | 0 (0) | 0 (0) | |
X-ray | 74 (88) | 10 (12) | 0 (0) | 0 (0) | 85 (89) | 10 (11) | 0 (0) | 0 (0) | 159 (89) | 20 (11) | 0 (0) | 0 (0) | |
EEG | 74 (88) | 10 (12) | 0 (0) | 0 (0) | 85 (89) | 10 (11) | 0 (0) | 0 (0) | 159 (89) | 20 (11) | 0 (0) | 0 (0) | |
MRI | 74 (88) | 10 (12) | 0 (0) | 0 (0) | 85 (89) | 10 (11) | 0 (0) | 0 (0) | 159 (89) | 20 (11) | 0 (0) | 0 (0) | |
Echocardiography | 74 (88) | 10 (12) | 0 (0) | 0 (0) | 85 (89) | 10 (11) | 0 (0) | 0 (0) | 159 (89) | 20 (11) | 0 (0) | 0 (0) | |
ECG | 74 (88) | 10 (12) | 0 (0) | 0 (0) | 85 (89) | 10 (11) | 0 (0) | 0 (0) | 159 (89) | 20 (11) | 0 (0) | 0 (0) | |
Blood transfusion products | Any product use | 74 (88) | 10 (12) | 0 (0) | 0 (0) | 85 (89) | 10 (11) | 0 (0) | 0 (0) | 159 (89) | 20 (11) | 0 (0) | 0 (0) |
Fresh-frozen plasma | 74 (88) | 10 (12) | 0 (0) | 0 (0) | 85 (89) | 10 (11) | 0 (0) | 0 (0) | 159 (89) | 20 (11) | 0 (0) | 0 (0) | |
Red blood cells | 74 (88) | 10 (12) | 0 (0) | 0 (0) | 85 (89) | 10 (11) | 0 (0) | 0 (0) | 159 (89) | 20 (11) | 0 (0) | 0 (0) | |
Platelets | 74 (88) | 10 (12) | 0 (0) | 0 (0) | 85 (89) | 10 (11) | 0 (0) | 0 (0) | 159 (89) | 20 (11) | 0 (0) | 0 (0) | |
Medical treatment | Any medical treatment use | 74 (88) | 10 (12) | 0 (0) | 0 (0) | 85 (89) | 10 (11) | 0 (0) | 0 (0) | 159 (89) | 20 (11) | 0 (0) | 0 (0) |
Lumbar puncture | 74 (88) | 10 (12) | 0 (0) | 0 (0) | 85 (89) | 10 (11) | 0 (0) | 0 (0) | 159 (89) | 20 (11) | 0 (0) | 0 (0) | |
Ventricular tap | 74 (88) | 10 (12) | 0 (0) | 0 (0) | 85 (89) | 10 (11) | 0 (0) | 0 (0) | 159 (89) | 20 (11) | 0 (0) | 0 (0) | |
Long line | 74 (88) | 10 (12) | 0 (0) | 0 (0) | 85 (89) | 10 (11) | 0 (0) | 0 (0) | 159 (89) | 20 (11) | 0 (0) | 0 (0) | |
Surgical procedures | Any surgical procedure use | 74 (88) | 10 (12) | 0 (0) | 0 (0) | 85 (89) | 10 (11) | 0 (0) | 0 (0) | 159 (89) | 20 (11) | 0 (0) | 0 (0) |
Ventriculoperitoneal shunts | 74 (88) | 10 (12) | 0 (0) | 0 (0) | 85 (89) | 10 (11) | 0 (0) | 0 (0) | 159 (89) | 20 (11) | 0 (0) | 0 (0) | |
Surgical procedures for central access | 74 (88) | 10 (12) | 0 (0) | 0 (0) | 85 (89) | 10 (11) | 0 (0) | 0 (0) | 159 (89) | 20 (11) | 0 (0) | 0 (0) | |
Interventions for ROP treatment | 74 (88) | 10 (12) | 0 (0) | 0 (0) | 85 (89) | 10 (11) | 0 (0) | 0 (0) | 159 (89) | 20 (11) | 0 (0) | 0 (0) | |
Mode of discharge if infant is transferred to another hospital (n = 75)a | 38 (100) | 0 (0) | 0 (0) | 0 (0) | 37 (100) | 0 (0) | 0 (0) | 0 (0) | 75 (100) | 0 (0) | 0 (0) | 0 (0) |
List of abbreviations
- ADE
- adverse device effect
- AE
- adverse event
- BPD
- bronchopulmonary dysplasia
- CE
- Conformité Européenne
- CEAC
- cost-effectiveness acceptability curve
- CGM
- continuous glucose monitoring
- CI
- confidence interval
- CONSORT
- Consolidated Standards of Reporting Trials
- CRF
- case report form
- CT
- computed tomography
- DMEC
- Data Monitoring Ethics Committee
- ECG
- electrocardiography
- EEG
- electroencephalography
- GLM
- generalised linear modelling
- ICER
- incremental cost-effectiveness ratio
- IQR
- interquartile range
- ISO
- International Organization for Standardization
- MHRA
- Medicines and Healthcare products Regulatory Agency
- MRI
- magnetic resonance imaging
- NEC
- necrotising enterocolitis
- NICU
- neonatal intensive care unit
- NMB
- net monetary benefit
- OR
- odds ratio
- PDA
- patent ductus arteriosus
- POC
- point of care
- PSS
- Personal Social Services
- RCT
- randomised controlled trial
- REACT
- REAl-time Continuous glucose moniToring in neonatal intensive care
- ROP
- retinopathy of prematurity
- SADE
- serious adverse device effect
- SAE
- serious adverse event
- SD
- standard deviation
- SDS
- standard deviation score
- SE
- standard error
- TMG
- Trial Management Group
- TSC
- Trial Steering Committee