Notes
Article history
The research reported here is the product of an HSDR Rapid Service Evaluation Team, contracted to undertake real time evaluations of innovations and development in health and care services, which will generate evidence of national relevance. Other evaluations by the HSDR Rapid Service Evaluation Teams are available in the HSDR journal.
The research reported in this issue of the journal was funded by the HSDR programme or one of its preceding programmes as project number NIHR132703. The contractual start date was in August 2020. The final report began editorial review in March 2022 and was accepted for publication in July 2022. The authors have been wholly responsible for all data collection, analysis and interpretation, and for writing up their work. The HSDR editors and production house have tried to ensure the accuracy of the authors’ report and would like to thank the reviewers for their constructive comments on the final report document. However, they do not accept liability for damages or losses arising from material published in this report.
Permissions
Copyright statement
Copyright © 2023 Fulop et al. This work was produced by Fulop et al. under the terms of a commissioning contract issued by the Secretary of State for Health and Social Care. This is an Open Access publication distributed under the terms of the Creative Commons Attribution CC BY 4.0 licence, which permits unrestricted use, distribution, reproduction and adaption in any medium and for any purpose provided that it is properly attributed. See: https://creativecommons.org/licenses/by/4.0/. For attribution the title, original author(s), the publication source – NIHR Journals Library, and the DOI of the publication must be cited.
2023 Fulop et al.
Chapter 1 Context
Background
In December 2019, a new form of coronavirus, severe acute respiratory syndrome coronavirus 2 (SARS-CoV-2), was identified in Wuhan in China. 1 The SARS-CoV-2 virus causes an infectious disease, which is referred to as ‘COVID-19’. 2 In January 2020, the World Health Organization (WHO) declared the outbreak to be a ‘public health emergency of international concern’. 3 COVID-19 has been responsible for millions of deaths and hospitalisations worldwide. 4 The WHO has reported that, globally, there have been 761,071,826 confirmed cases and 6, 879,677 deaths (as of 22 March 2023). 5 COVID-19 cases have continued to fluctuate since January 2020. For example, since January 2020, England alone has experienced three waves of the COVID-19 pandemic (wave 1: March to May 2020, wave 2: October 2020 to February 2021, wave 3: began in July 2021 but has been complex to track and is therefore currently difficult to estimate when the end date was). 6
COVID-19 is characterised by some common symptoms including a fever, a continuous cough, tiredness and loss of taste and/or smell. 2 In wave 1, there was a lack of knowledge of COVID-19 and treatment options compared with later waves. During the COVID-19 pandemic, restrictions on travel, social interaction and access to public spaces, known as a ‘lockdown’, were introduced worldwide. For example, within the UK, individuals were asked to stay at home (with the exception of critical workers, e.g. health-care professionals, food retail workers) and to socially distance from others. 7 To minimise spread of the disease and increase capacity of health-care services, many routine health-care appointments were cancelled across the NHS or delivered remotely,8–11 and parts of the workforce were redeployed.
COVID-19 is an acute disease with differential effects on the population. Diagnosis of COVID-19 can have many health, mental health, economic and social impacts on individuals. While many individuals develop mild to moderate illness, for some the disease can be life-threatening. 2 COVID-19 has been responsible for thousands of deaths and hospitalisations in the UK. 6 As at 2 March 2023, the UK had reported 209,396 COVID-19 deaths. 5 In particular, some groups have been shown to have worse mortality and outcomes from COVID-19 – for example, older adults, those with significant medical comorbidities, males, those living in more deprived areas, black and Asian ethnic groups, and those working in certain occupations (such as taxi/bus/coach drivers, retail assistants, construction workers, social care workers and nurses). 12
Patients with COVID-19 may present with ‘silent hypoxia’ (very low oxygen saturations, often without breathlessness). 13,14 Patients may not be aware that they have low blood oxygen saturations, which may lead to delays in patients being escalated and admitted to hospital, presenting with more serious advanced symptoms of the disease. This has resulted in some patients needing invasive treatments and/or being admitted to intensive care units (ICUs), with a higher likelihood of mortality. 15,16
To deliver better and more personalised care to people in their own homes, services which enable patients to self-manage their health and care at home (e.g. remote home monitoring) have been identified as a priority within the NHS @home programme17 and the NHS Long Term Plan. 18 Prior to the pandemic, remote home monitoring models have been used to monitor and provide care for patients with chronic health conditions [e.g. heart failure, chronic obstructive pulmonary disease (COPD), diabetes, kidney disease, cancer]. 19–21 However, the COVID-19 pandemic enhanced and accelerated the need for health-care services to use technology in care delivery. 22
To reduce pressure on hospitals and infection transmission, and to ensure that patients with COVID-19 receive appropriate care in the right place and are appropriately escalated as early as possible, COVID-19 remote home monitoring models using pulse oximetry were developed and implemented in many different countries during the pandemic,23–32 and are now recommended by the WHO. 33 The type of remote monitoring varies, in relation to the frequency of monitoring, mode of monitoring and recording (analogue or technology enabled), referral criteria and the inclusion of pulse oximetry. 23–32
Within remote monitoring models, patients take readings at home and submit these to a health-care professional in another location for review. 34 Pulse oximeters are small devices that can be placed on a person’s finger and used to measure blood oxygen levels. Pulse oximeters were used as previous research indicates that oxygen saturation may predict outcomes such as mortality and admissions to ICUs. 35 Patients, or their carer, measure blood oxygen saturations with a pulse oximeter (together with other measurements, e.g. temperature) at home. They record these readings in one of two ways: (1) recording readings on a paper diary and providing readings via telephone (analogue), or (2) recording and submitting readings using digital technology such as smartphone applications (apps), automated telephone or text (technology enabled). Once patients have submitted readings, a health-care provider reviews these readings elsewhere, escalating care when necessary. 32,36
In England, during the first wave of the pandemic, COVID-19 remote home monitoring models (pre-hospital and early discharge models) using pulse oximeters were implemented in a number of areas. 36 During 2020, NHS England and NHS Improvement (NHSEI) purchased and distributed them to clinical commissioning groups (CCGs) throughout England. NHSEI purchased 706,000 oximeters across waves 1 and 2 (Zofja Zolna, NHS England, 2020 personal communication). These pulse oximeters were European Conformity (CE) marked, as recommended by Medicines and Healthcare products Regulatory Agency (MHRA) guidance,37 and were available to local sites within three days through an ordering system set out in the standard operating procedure (SOP). In November 2020, NHSEI launched a national roll-out of COVID Oximetry @home (CO@h) services. 38 Patients were referred within the community to the CO@h service (e.g. via general practices (GPs), COVID-19-specific community clinics, called ‘hot hubs’, and emergency departments). In addition, in January 2021, NHSEI launched a national roll-out of ‘COVID virtual ward’ (CVW) models, whereby patients were referred on to services upon being discharged from hospital early. 39 CVW models aimed to reduce pressures on hospitals by enabling early discharge and continuation of recovery at home. The national model of COVID-19 remote home monitoring, advocated by NHSEI, was for self-monitoring and self-escalation (with reference to clinical judgement), based on their learning from wave 1. 38,39 Self-monitoring and self-escalation refer to patients (or carers, where appropriate) monitoring their own readings and escalating their own care as necessary, with an option for prompts or check-in calls to take readings on certain days. This differs from remote home monitoring models whereby patients (or carers) submit readings to providers who monitor these readings remotely. NHSEI supported the national roll-out and local implementation of COVID-19 remote home monitoring services, including providing financial support for primary care to establish and implement COVID-19 remote home monitoring services,40 resourcing NHS Digital to supply data to COVID-19 remote home monitoring providers to identify people who may benefit from these services,41 and the commissioning and funding of Academic Health Science Network (AHSN) patient safety collaboratives. This study included both CO@h and CVW models. Collectively, throughout this report, we refer to these services as remote home monitoring for COVID-19 patients (Figure 1).
FIGURE 1.
A summary of remote home monitoring for COVID-19 services.
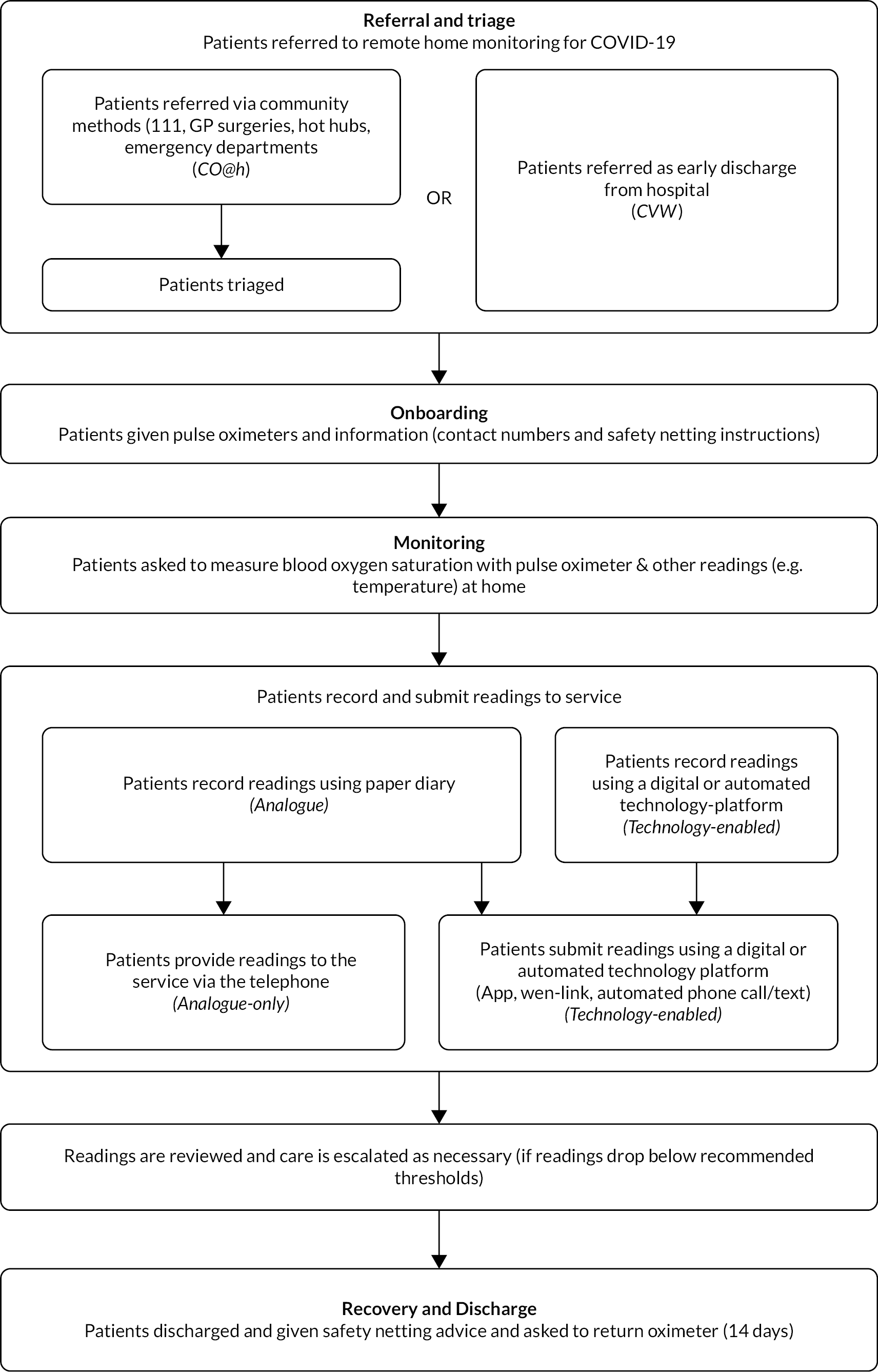
In addition, NHS user experience (NHSX) supported the implementation of COVID-19 remote home monitoring services through facilitation of the use of technology-enabled platforms. In the initial stages of roll-out (during wave 1), NHSX helped to pilot sites to use technology-enabled platforms as part of their CO@h and CVW services by signposting services to technology companies (however, it was up to local sites to decide whether the technology offered by these companies met their needs). In wave 2, NHSX funded and supported the implementation and evaluation of technology-enabled solutions for COVID-19 remote home monitoring services.
Despite previous research on the use of remote home monitoring models for other chronic health conditions,19–21 there is a lack of studies exploring models of care developed to implement remote home monitoring across different health-care contexts during the COVID-19 pandemic. It is important to explore the implementation of remote home monitoring models within the context of a pandemic as it is likely that this context differs in relation to health-care pressures, patient needs and uncertainties. There is also a lack of studies evaluating the effectiveness and implementation of remote home monitoring models for patients with COVID-19, including in-depth analyses of patients’ and staff’s experiences of receiving and delivering care. This mixed-methods evaluation of remote home monitoring models in England aimed to address this gap by exploring the impact of the implementation of COVID-19 remote home monitoring models [on length of stay (LOS) in hospital, re-admission and health outcomes], the costs of implementing these models, the experiences of patients with remote home monitoring, staff involvement and experiences of delivering care and the processes used to implement these models at national and local levels. The study had a particular focus on inclusivity of these services and potential impact on inequalities.
Study aims
Phase 1
The phase 1 study comprised:
-
an international rapid systematic review of remote home monitoring services for patients with COVID-1932
-
a rapid study of implementation of remote home monitoring services for patients with COVID-19 during the first wave of the coronavirus pandemic in England (mixed methods). 36
The rapid systematic review aimed to analyse the implementation and impact of remote home monitoring models for patients with COVID-19.
The empirical implementation study aimed to develop a conceptual map of remote home monitoring models, to explore the experiences of staff implementing these models during the COVID-19 pandemic, to document impact of services, to understand the use of data for monitoring progress against outcomes, and document variability in staffing and resource allocation.
Phase 2
Building on phase 1, we conducted a multisite, mixed methods national evaluation involving 28 purposively selected COVID-19 remote home monitoring services (October 2020 to November 2021).
This evaluation aimed to explore effectiveness, costs, implementation and experience (staff/patients) of COVID-19 remote home monitoring, and comprised four workstreams:
-
effectiveness
-
cost analysis
-
national survey of implementation and staff and patient experience
-
in-depth case studies of implementation and staff and patient experience.
Research questions
Phase 1
Systematic review:
-
What are the aims of remote home monitoring models?
-
What are the main components of these models?
-
What are the patient populations considered appropriate for remote monitoring?
-
How is patient deterioration determined and flagged?
-
What are the expected outcomes of implementing remote home monitoring?
-
How have these models been evaluated?
-
What are the benefits and limitations of implementing these models?
Implementation study:
-
What were the conceptual models guiding the implementation of remote home monitoring models during the COVID-19 pandemic?
-
What were the processes that acted as barriers and facilitators in the design and implementation of pilots of these models during wave 1 of the pandemic?
-
What were the expected outcomes of the virtual wards implemented during wave 1 of the pandemic?
-
What data were collected by pilot sites and how has it helped them monitor progress against their expected outcomes?
-
What quantitative evidence did the sites use from national and international experiences of these models to help inform clinical management decisions?
-
How were resources allocated (including staffing models) to implement the remote home monitoring pilots during wave 1 of the pandemic?
-
What are the lessons learned from implementing remote home monitoring models during wave 1 of the pandemic? Can some of these lessons be used for planning care delivery for winter 2020–21?
Phase 2
-
What was the impact of remote home monitoring for patients with COVID-19 on mortality and use of hospital services? (workstream 1)
-
What were the costs of setting up and running CO@h and CVW models? (workstream 2)
-
What were the factors influencing delivery and implementation of remote home monitoring for patients with COVID-19? (workstream 3)
-
What were the experiences and behaviours (i.e. engagement, use of other services) of patients receiving remote home monitoring for patients with COVID-19? (workstream 3)
-
Were there potential impacts of remote home monitoring for patients with COVID-19 on existing health inequalities? (workstream 3)
-
What were the experiences of staff delivering remote home monitoring for patients with COVID-19? (workstream 3)
See Chapter 2 for protocol deviations.
Structure of this report
-
Chapter 1 (context) outlines the rationale for this evaluation
-
Chapter 2 (methods) presents the design and methods used (phases 1 and 2). Detailed methods are presented within each findings chapter.
-
Chapter 3–11 (findings) outline the findings.
-
Chapter 3 – phase 1
-
Chapter 4 – implementation study (workstream 3 and 4).
-
Chapters 5 and 6 – effectiveness study (workstream 1).
-
Chapter 7 – cost analysis (workstream 2).
-
Chapters 8 to 11– patient and staff experience findings (workstreams 3 and 4).
-
-
Chapter 12 (discussion and conclusion) – key findings, strengths and limitations, implications/lessons learned and future research.
Other related evaluations
Two other evaluations of remote home monitoring models for COVID-19 were commissioned alongside this evaluation, conducted by: (1) Institute of Global Health Innovation, National Institute for Health and Care Research (NIHR) Patient Safety Translational Research Centre, Imperial College London and, (2) the Improvement Analytics Unit (IAU; a partnership between the Health Foundation and NHSEI). Both evaluations investigated the impact of the CO@h service on mortality and hospital service use. 42–45 Imperial College London also examined inequalities in the enrolment of eligible patients on to the CO@h service and conducted quantitative evaluations of differences between technology-enabled and analogue pathways. 46
The Birmingham, RAND and Cambridge Evaluation Centre (BRACE) and Rapid Service Evaluation Team (RSET) also investigated the use of pulse oximetry in care homes across England and completed a mixed-methods rapid evaluation using an online national survey sent to all care homes in England and qualitative interviews with staff from six care homes acting as case study sites (see Sidhu et al. 47).
Working with stakeholders
Throughout the evaluation, the team worked with a range of stakeholders at national and local levels (phase 1: community of practice, phase 2: NHSEI, NHS Digital, NHSX, community of practice, national learning network and Clinical advisory group).
Chapter 2 An overview of the methods used in the COVID-19 remote home monitoring evaluation
This chapter outlines the methods used in phase 1 and phase 2 of the evaluation. Individual chapters provide further details.
Phase 1
This phase was developed as a result of a 4-week scoping exercise including initial scoping of the literature, discussions with a small number of sites, documentary analysis and discussions with colleagues at Public Health England (PHE) and NHSEI.
Methods
Setting
In England, within health-care organisations (trusts/CCGs) who delivered remote home monitoring services for COVID-19 patients (including CO@h and CVW models). The evaluation took place between July and September 2020.
Procedure
Systematic review
A rapid systematic review of virtual ward models (led by primary and secondary care) was conducted. The systematic review followed the method proposed by Tricco et al. 48 (see Chapter 3).
Implementation study
A rapid multisite implementation study which combined qualitative and quantitative approaches. Interviews were conducted with staff across eight sites in England. Additionally, we collected data on impact, costs, and staffing models (see Chapter 3).
Ethical approval
This phase received ethical approval from the University of Birmingham humanities and social sciences ethics committee (ERN_13-1085AP37) and was categorised as a service evaluation by the Health Research Authority (HRA) decision tool and University College London/University College London Hospitals (UCL/UCLH) Joint Research Office.
Phase 2
The protocol was informed by phase 1 and developed with input from our clinical advisory group (colleagues at PHE, NHSEI, NHS Digital and NHSX), and with other research teams working in this area.
Methods
Setting
In England, within health-care organisations (trusts/CCGs) that delivered remote home monitoring services for patients with COVID-19 (including CO@h and CVW models). The evaluation took place between October 2020 and November 2021 (data collection took place between February and June 2021, but the patient survey retrospectively included January 2021).
Design
A rapid multisite study combining qualitative and quantitative approaches to analyse the implementation and impact of remote home monitoring services for patients with COVID-19. The design of phase 2 built on phase 1 methods and findings and consultation with our clinical advisory patient and public involvement (PPI) groups and the 70@70 nurses. Specifically, findings from phase 1 informed focus on outcomes and patient experience, and the sampling approach.
Ethical approval
The study protocol was divided into two separate protocols:
-
Covered workstreams 1 and 2 and the staff elements of workstreams 3 and 4, received ethical approval from the University of Birmingham humanities and social sciences ethics committee (ERN_13-1085AP39) and was categorised as a service evaluation by the HRA decision tool and UCL/UCLH Joint Research Office (January 2021).
-
The patient experience study (survey and case study interviews – workstreams 3 and 4) was reviewed and given favourable opinion by the London-Bloomsbury Research Ethics Committee (REC reference: 21/HRA/0155: Feb 2021).
The study team was aware of the sensitive nature of this research for organisations and individuals, and has experience in conducting research on similar sensitive topics. The team maintained the independence of the research, followed an informed consent process, and maintained the anonymity of participants and organisations.
The patient experience study was categorised as an urgent public health study. The primary and local clinical research networks supported the team to get local approvals and participant identification centre agreement sign off at each site.
Theoretical lens
We analysed remote home monitoring models using different theoretical lenses. We explored remote home monitoring models within the social and political context in which they are designed and implemented (including the clinic and home), the multiple realities, assumptions and values that play a role in their implementation, the organisational structures that shape experiences of receiving and delivering care and the sociopolitical issues that frame development, diffusion and use of technology. 49 This lens went beyond an analysis of remote home monitoring solely as a technological innovation to consider dimensions such as: self-management, accountability and clinical responsibility, ‘personalised care’, inequalities in access to care and ‘caring at a distance’. 50–52
Furthermore, we used a framework with a sociotechnical lens that incorporates non-adoption, abandonment and challenges to the scale-up, spread, and sustainability of technologies for health and social care (see Chapter 11). This includes expected and necessary changes/adaptation to staff working practices and the context for widespread use of the technology. This framework (informed by theory and evidence) describes the barriers to successful uptake of innovations and provides a guide to the type of issues that should be considered by evaluators. 53
Procedure
The evaluation consisted of four workstreams (see Figure 2).
FIGURE 2.
Overview of the methods for each workstream.

Workstream 1: the clinical effectiveness of COVID-19 remote home monitoring services
Workstream 1 aimed to use combinations of aggregate population-level data and hospital administrative data to both describe and estimate the impact of the roll-out of remote home monitoring models for CO@h and CVW services.
The study of the impact of CO@h on mortality and admissions was designed as an area-level analysis combining aggregated data from different sources. The aim was to use these data as time series to enable us to investigate ‘dose–response’ relationships between the evolving rates of enrolment to the services within each area and their outcomes, ‘enrolment rates’ being defined as the ratio of people enrolled on to services to the number of new cases each fortnight. For the in-hospital outcomes, we used an observational design relating in-hospital mortality and lengths of stay at an individual patient level to the rate of enrolment to CO@h services within the area at the time of admission.
For the analysis of impact of virtual ward services, we designed an admission-level multivariate regression analyses for patients with COVID-19 being discharged from hospital. The aim was to examine the association between various factors – including the availability of a virtual ward – with the length of COVID-19 inpatient spells, and likelihood of subsequent COVID-19 readmissions.
We evaluated the CO@h service across the CCG areas in England (N = 37) where we judged there was complete data on numbers of people enrolled on to services (onboarded) between 2 November 2020 and 21 February 2021. The study population included anyone aged 65 years or over with a laboratory-confirmed positive test for COVID-19 and any hospital admission within that age group for COVID-19 or suspected COVID-19.
For the virtual ward study, we extracted information from inpatient Hospital Episode Statistics (HES) data on all individuals discharged alive from any of 123 English hospital trusts where there had been a confirmed or suspected COVID-19 International Statistical Classification of Diseases and Related Health Problems, 10th revision (ICD-10) primary diagnosis code recorded during the stay. We included all patients discharged between 17 August 2020 and 28 February 2021, a period covering the beginning, the peak, and the start of the decline of England’s second COVID wave. Where a patient had two or more relevant inpatient stays, all stays were included in our analysis.
For our analysis we used data from a number of sources (see Report Supplementary Material 1).
For CO@h we analysed four main outcomes: mortality from COVID-19, hospital admissions for people with confirmed or suspected COVID-19, in-hospital mortality for these admissions and their lengths of stay. Because we were using population-level data, we first calculated enrolment rates for CO@h over time and then used multivariate regression models to investigate relationships between levels of enrolment and outcomes.
To analyse outcomes for patients admitted to hospital, we used individual-level HES. Again, we used multivariate regression models relating enrolment rates to outcomes, adjusting for individual patient characteristics (see Report Supplementary Material 1).
For the CVW analysis we developed multivariate regression models to examine the impact of the availability of CVW on two outcomes: (1) the LOS of the COVID-19 inpatient stay, and (2) on subsequent readmissions for COVID-19. Our models adjusted for the following person, inpatient spell, and trust characteristics: age at admission, gender, ethnic group, Charlson Comorbidity Index category,54 whether the stay was the person’s first COVID-19 hospital stay, whether the inpatient stay was an emergency admission, Index of Multiple Deprivation (IMD) decile, the time period of the discharge date, hospital trust, and a trust-and-week-specific measure giving the proportion of all acute beds occupied by patients with COVID-19.
Further details are provided in Report Supplementary Material 1.
Workstream 2: economic analysis
The aim of workstream 2 was to conduct a cost analysis of remote home monitoring that comprised an analysis of setting up and running remote home monitoring services for patients with COVID-19. As described in the study protocol, we originally planned to undertake a cost–utility analysis of remote home monitoring services for patients with COVID-19. 55 However, in the absence of any impacts on mortality or hospitalisation being demonstrated by the effectiveness analysis (workstream 1), a cost–utility was considered uninformative and our analysis focused only on analysing the costs of setting up CO@h and CVW services and mean running cost per patient monitored.
Remote home monitoring models were costed using data on staff (clinical and non-clinical) and non-staff resource use during the second wave. Contacts at each of the 28 sites (see workstream 3/4 for further details) were asked to complete a form in Microsoft Word (Microsoft Corporation, Redmond, WA, USA) (see Project website documentation – Study instruments) and 26 sites returned it electronically to the researchers. The data collection process was conducted between April and June 2021. Information was collected on staff (including the number of staff, their function/seniority and the number of hours worked) and non-staff resources used for setting up and running the sites (including information on the type and costs of digital monitoring platform used, oximeters, other medical equipment, etc.). We also collected information regarding the number of patients involved (triaged, monitored, deteriorated, escalated and deaths). The cost analysis was conducted separately for CO@h and CVW services.
Costs of setting up remote home monitoring services for patients with COVID-19 We calculated the costs incurred for setting up and running the CO@h and CVW models, also examining the variation by whether data submission was undertaken using technology-enabled with analogue modes versus analogue-only modes. The set-up costs were reported separately as mean costs per site. Note that the lifetime of the sites extends beyond the period observed in the study (e.g. some of the equipment, such as digital platforms and thermometers, can be used for longer than the study time) and therefore we did not calculate the mean set-up costs per patient or per unit of time. The running costs were calculated as mean costs per patient monitored and included the costs of staff (clinical and non-clinical) who were involved in running the services, and non-staff costs including costs of pulse oximeters (based on the number of patients monitored and assuming that 70% of pulse oximeters would be returned and reused) and other monthly expenditures related to maintaining the digital platforms. We adjusted the resources used and costs incurred per patient by applying weights based on the number of patients monitored by each service (CO@h and CVW) and the total numbers of patients monitored at each site. To investigate the effect of the type of service, data submission mode, seniority of full-time equivalent (FTE) staff and the total number of patients monitored factors on the mean running cost, we have used an ordinary least square regression model.
Technology-enabled versus analogue-only modes To explore the differences in staff, resources, types of patients and patient outcomes further between tech-enabled and analogue versus analogue-only data submission modes, additional data were collected from four of the 28 sites. All of these four sites used both tech-enabled and analogue data submission modes. We conducted an in-depth analysis of the time spent per patient for specific activities of the programme and calculated the cost per patient for all the activities. The mean cost for each activity per patient was calculated based on the time spent for each activity and staff working on each activity.
Workstreams 3 and 4: implementation and patient and staff experiences
The aim of these workstreams was to (1) understand the development of national COVID-19 remote home monitoring services and, (2) analyse the implementation of COVID-19 remote home monitoring services, and patient and staff experiences of care.
These workstreams included data from: staff surveys and interviews, patient/carer surveys and interviews, interviews with national leaders and documentary analysis of relevant national operating procedures (see Report Supplementary Material 1 for detailed methods).
Selection of sites Twenty-eight services were included in our national evaluation. To obtain maximum variation, we sampled services based on a range of criteria, including the setting (primary care or secondary care), type of model (pre-hospital, early discharge, both), mechanism for patient monitoring (paper-based, app, both), geographic location (across different areas of the country), timing of implementation (implemented since wave 1 of the pandemic or recently implemented) and involvement in the evaluation with the other evaluation partners (Imperial and IAU).
To conduct an in-depth analysis of implementation, staff and patient experiences, 17 of these sites were selected as in-depth case study sites using the aforementioned criteria. In four of these sites, we also conducted in-depth comparisons of technology-enabled versus analogue models (see Economic analysis above). These sites were identified by NHSX to ensure inclusion of different digital platforms.
Sites were recruited through an expression of interest process whereby we presented our study at local and national meetings and asked sites to express interest in participating. Clinical Research Networks facilitated the set-up of sites and local governance approvals. Some sites were identified through our phase 1 evaluation.
Staff (survey and interviews) We conducted a survey of staff involved in delivering COVID-19 remote home monitoring services in 28 services (including clinical leads, delivery staff and data staff). The study coordinator at participating sites distributed links to the online surveys to staff involved in delivering/leading services via e-mail.
For staff interviews, we purposively sampled staff involved in delivering services, leading services and data processes at each of the 17 sites. Potential participants were approached by staff at participating sites and were introduced to the researcher or asked to contact the researcher if they would like to take part. The researcher sent potential participants an information sheet. If they agreed to participate, they were asked to sign a consent form.
Patients and carers (survey and interviews) To take part in our evaluation, participants needed to be
-
18 or over
-
proficient in English (or one of Polish, Bengali, Urdu, Punjabi, French and Portuguese)
-
eligible to receive remote home monitoring services for COVID-19, and must also have been offered and received COVID-19 remote home monitoring services.
We were flexible within our sampling to consider both national and local eligibility criteria.
A total of 25 of the 28 sites agreed to run the patient survey. NHS staff from participating services sent the patient survey to patients and carers either via post, text or e-mail. Patients/carers completed the surveys and returned these to the researchers either electronically or via post.
For patient interviews, we aimed to purposively sample four to six patients or carers who received services or declined/disengaged from services at each of the 17 case study sites. We sampled using a range of criteria including age, gender, ethnicity, employment status, mechanism for onboarding, type of monitoring and outcomes. Potential participants were approached by staff at participating sites and were asked if they would be interested in taking part. Participants were then sent an information sheet by the researcher. If the patient/carer agreed to take part in the study, they were asked to sign consent forms and send them to the researcher ahead of the interview.
National lead interviews and documentary analysis To understand development of the national service and capture changes in design and implementation over time, we conducted interviews with national leaders who were purposively selected from different roles across organisations. We also analysed key documents (e.g. SOPs).
For the national lead interviews, national leads were contacted via e-mail and asked if they would like to take part. If happy to take part, they were asked to give their consent in advance of the interview. Researchers then arranged a convenient time for the interview.
Staff (survey and interviews) The staff survey was developed for this study. We developed different questions for different groups (service leads and staff delivering the service). The staff survey included questions about experiences delivering remote home monitoring services for patients with COVID-19. The questions were reviewed and piloted prior to use (see Project website documentation – Study instruments for survey questions).
We developed a topic guide for staff interviews. Different questions were developed for different groups: (1) service leads, (2) staff delivering the service, (3) data. The topic guide included questions about the service, experiences of delivering services and views on patient engagement (see Project website documentation – Study instruments for topic guide). Interviews at four of the 17 sites included think-aloud questions about using the technology.
Patients/carers (survey and interviews) The patient/carer survey was developed for this study. The survey included questions about experiences receiving remote home monitoring services for patients with COVID-19. The questions were reviewed and piloted prior to use (see Project website documentation – Study instruments for survey questions).
We developed a topic guide for patient/carer interviews. Different questions were developed for those who received the service, and those who declined or disengaged. The topic guide included questions on their experiences of receiving services and engagement (see Project website documentation – Study instruments for topic guide).
Survey and interview questions were informed by relevant service documentation,38,39 theoretical frameworks relating to social, political and technical contexts49–53 and behaviour,56 and previous literature on engagement. 57,58 Questions on demographic characteristics were informed by previous literature. 59–64
National leads (interviews) We developed a topic guide for national lead interviews. This included questions about the service, leadership and governance, data and implementation (see Project website documentation – Study instruments for topic guide).
Staff (survey and interviews) Staff were asked to follow an online link to complete the survey. The survey began with an information sheet and consent form. Survey sites were asked to keep a record of the number of surveys sent to determine response rates.
Interviews were conducted by one of six postdoctoral researchers (MS, CV, HW, LH, IL, JB); each site had a different lead researcher. Interviews were carried out via telephone or an online platform as preferred by the participant.
Patients/carers (survey and interviews) Patients were asked to complete the survey in one of two ways: (1) if monitored through use of a tech-enabled mode, patients/carers were e-mailed or texted the link to the online survey, or (2) if the patient was monitored using analogue methods, they received the survey in the post, together with a pre-paid envelope. Surveys were distributed at onboarding to the remote home monitoring service or discharge from the remote home monitoring service, depending on service procedures. The survey included an information sheet and consent form.
Interviews were conducted by one of six postdoctoral researchers (MS, CV, HW, LH, IL, JB); each site had a different lead researcher. Interviews were carried out via telephone or an online platform as preferred by the participant.
National leads (interviews) National lead interviews were conducted by four researchers (MS, CV, HW, NJF). Interviews were carried out via telephone or an online platform such as Zoom (Zoom Video Communications, San Jose, CA, USA) or Microsoft Teams (MS Teams) (Microsoft Corporation, Redmond, WA, USA) as preferred by the participant.
Data management Surveys (patients and staff) Electronic surveys were transferred directly into Research Electronic Data Capture (REDCap, IBM Corporation, Armonk, NY). Surveys received via post were returned to the Nuffield Trust or UCL offices, stored securely in locked filing cabinets and inputted into REDCap by members of the research team. Data were securely stored on UCL’s Data Safe Haven portal.
Interviews (national lead, patients/carers and staff) All interviews were audio-recorded (with consent), transcribed verbatim, anonymised and stored securely.
Site characteristics Sites were characterised with respect to their population size,65 the proportion in urban versus rural areas,66 the proportion in the most and least deprived areas (with respect to national quintiles),67 and ethnicity. 68 For sites based on CCG areas we calculated these characteristics using publicly available data at lower-layer super output area (LSOA) level mapped to CCGs,69 while for trust-based sites we used data derived from inpatient HES admissions during the financial year 2019–20, in addition to web searches for the trust catchment populations.
Surveys (patients/carers and staff) Survey data were analysed using descriptive statistics, multivariate and univariate analyses on Statistical Package for the Social Sciences (SPSS, version 25). Participating sites were provided with a report on the patient survey responses from their site compared with overall findings.
Interviews (national lead, patients/carers and staff) Data collection and analysis was facilitated through the use of rapid assessment procedure (RAP) sheets. 70 RAP sheets were developed per site to facilitate cross-case comparisons and per population (to make comparisons between subgroups). The categories used in the RAP sheets were based on the questions included in the interview topic guide, maintaining flexibility to add categories as the study progressed. RAP sheets were used to develop coding frameworks to explore themes and subthemes in more depth and identify example quotes. Further details on analysis methods are reported within each chapter (see Chapters 4 and 8–11).
Although our study was rapid, many steps were taken to ensure the transparency, rigour and robustness of our qualitative research methods. For example, our study used purposive sampling to recruit a large number of participants with a range of characteristics. In terms of analysis, our study used both rapid analysis methods (e.g. RAP),70 together with traditional qualitative analysis methods (e.g. in-depth coding of transcripts and development of themes and subthemes). Our study involved a range of experienced qualitative researchers, and our analysis was strengthened by continuous team discussions about the interpretation of our data. We also discussed findings with those providing the service and our clinical advisory group to sense check our interpretation. Additionally, our findings were triangulated with quantitative, economic and other qualitative analyses. Our methodological approaches are consistent with previous recommendations on conducting trustworthy, robust and rigorous qualitative research. 71–74
Integration
Data from all four workstreams were integrated to answer our research questions and to facilitate processes of triangulation. The study team met weekly throughout the study. We included findings from each workstream within our RAP sheet. We produced RAP sheets at site level and also at population level (including for subgroups of staff and patients). For example, findings on local barriers and facilitators to implementation and patient and staff experiences helped the interpretation of findings on outcomes, service use and costs. Quantitative data on resource allocation were understood in relation to qualitative data on staff experiences of planning and delivering services. Data from the case studies were used to explain the survey findings (representing experiences and trends at a national scale). In addition, emerging findings from this study were discussed in relation to those from the two other evaluation partners (Imperial and IAU).
Deviations from protocol
We planned to undertake a cost–utility analysis of the COVID-19 remote home monitoring services but in the absence of any impacts on mortality or hospitalisation, as demonstrated by the effectiveness analysis (see Chapters 5 and 6), the economic analysis only included the costs of setting up and running services (see Chapter 7).
We intended to conduct ‘think aloud’ sessions with patients who had used tech-enabled data submission from the four sites selected for in-depth analysis of tech-enabled platforms. However, patients did not have access to the platforms after discharge and recall of the use of these platforms during their illness was poor. Consequently, the ‘think aloud’ methodology was discontinued for patient interviews.
Patient and public involvement
Members of the study team met with service user and public members of the BRACE PPI group and health and care panel and patient representatives from RSET in a series of workshops. These workshops informed study design, data collection tools, data interpretation and to dissemination for phase 2. For example, patient-facing documents, such as consent forms, topic guides, patient survey and patient information sheets were reviewed by this group. Additionally, PPI members helped to pilot patient surveys and interview guides with the research team. We also asked some members of the public to pilot the patient survey.
Dissemination and formative feedback
Throughout the project, we regularly shared feedback with stakeholders on emerging findings and lessons learned. The study will inform clinical practice for COVID-19 but will also inform the use of remote monitoring models in other conditions and areas of the NHS. Dissemination has been facilitated through various existing and new networks. Findings have been and will be published in peer-reviewed journals.
Chapter 3 Capturing and informing early stages of design and implementation of COVID-19 remote home monitoring services (phase 1)
Overview
This chapter draws on two papers by Vindrola-Padros et al.,32,36 published in Lancet eClinicalMedicine: https://doi.org/10.1016/j.eclinm.2021.100965 and https://doi.org/10.1016/j.eclinm.2021.100799. These are open access articles under the term of the Creative Commons Attribution 4.0 International License (CC BY 4.0), which permits use, distribution and reproduction in any medium, provided that the original work is properly cited (see https://creativecommons.org/licenses/by/4.0/).
What was already known
-
Previous research has explored the use of remote home monitoring models for non-COVID-19 conditions.
-
COVID-19 remote home monitoring services were implemented rapidly around the world, but implementation processes were rarely documented.
What this chapter adds
-
The COVID-19 remote home monitoring services varied in relation to the health-care settings and mechanisms used for patient triage, monitoring and escalation.
-
Good communication within clinical teams, culturally appropriate information for patients/carers and the combination of multiple approaches for patient monitoring (app and paper-based) were considered facilitators in implementation.
-
We could not reach substantive conclusions regarding patient safety and the identification of early deterioration due to lack of standardised reporting and missing data.
Aims of the phase 1 evaluation
In July 2020, we began a rapid mixed-methods evaluation to identify the key characteristics of COVID-19 remote home monitoring models operating during the first wave of the COVID-19 pandemic, explore the experiences of staff implementing these models, understand the use of data for monitoring progress against outcomes, document variability in staffing and resource allocation, document patient numbers and impact, draw out lessons learned for the development of models for the 2020–21 winter period and identify areas for further research. To achieve these aims, phase 1 was divided in two main workstreams: (1) a rapid review of the literature, and (2) a rapid mixed-methods evaluation to document implementation and identify the lessons learned during wave 1 of the pandemic. Findings from both these phases have been published,32,36 so we provide a summary here.
A rapid systematic review of the evidence
Remote home monitoring models have been implemented in the United States, Australia, Canada, the Netherlands, Ireland, China and UK, with some variation in the frequency of patient monitoring, modality (a combination of telephone or video calls and use of applications or online portals), patient admission criteria, staffing used for patient monitoring and level of clinical oversight and use of pulse oximetry. However, there is limited published literature on the models of care developed to implement remote home monitoring across different health-care contexts during the COVID-19 pandemic, the experiences of staff implementing these models and patients receiving care, the use of data for monitoring progress, resources required, as well as the impact of these models on clinical, process and economic outcomes. The aim of this review was to analyse the implementation and impact of remote home monitoring models for confirmed or suspected COVID-19 patients, identifying their main components, processes of implementation, target patient populations, impact on outcomes, costs and lessons learned.
Methods
Design
We followed an established rapid review method. 48 This method uses an adapted systematic review approach, with the aim to reduce time taken to carry out the review (e.g. using a large multidisciplinary team to review abstracts/full texts and extract data, having a percentage of excluded articles reviewed by a second reviewer, and using software for data extraction). 48
We used the Preferred Reporting Items for Systematic Reviews and Meta-Analysis (PRISMA) statement. 75 The protocol was registered with PROSPERO (CRD42020202888, registered 6 August 2020).
Search strategy
We used a phased search approach. 48 This involved gradually adding search terms based on the keywords used in the identified literature (see Vindrola-Padros et al. 32 for search terms). We used the following databases: MEDLINE, CINAHL PLUS, EMBASE, TRIP, medRxiv and Web of Science (searches carried out by CV on 9 July 2020 and updated on 21 August 2020, 21 September 2020 and 5 February 2021). To identify additional publications, we manually screened reference lists of included articles. We removed duplicates.
Study selection, inclusion and exclusion criteria
One researcher (CVP) screened titles, with researchers cross-checking exclusions in abstract (KS) and full-text phases (KS/MS). Disagreements were discussed until consensus was reached. To be included, studies needed to: (1) focus on monitoring of confirmed or suspected patients with COVID-19, (2) focus on pre-hospital monitoring, monitoring after emergency department presentation and step-down wards for early discharge, (3) focus on monitoring at home (excluding monitoring done while the patient is in health-care facilities), and (4) be published in English.
Data extraction and management
We analysed articles using a data extraction form developed in REDCap. We extracted data on design, populations, process, clinical outcomes and economic impact. The form was independently piloted by two researchers (CV/KS) and finalised. Data extraction was checked by three researchers (TG/CSJ/ST).
Data synthesis
The information entered in free text boxes was exported from REDCap and analysed using framework analysis. 76 We did not assess quality of studies.
Results
We present a summary of findings below (see Vindrola-Padros et al. for further information). 32
Search results
The initial search yielded 902 articles. After screening, 155 articles remained for full-text review. After full-text review (n = 11), identifying additional references from reference lists (n = 3) and updated searches (n = 13), 27 articles met the inclusion criteria (see Report Supplementary Material 332 for PRISMA flow chart).
Characteristics of included remote home monitoring models
Remote home monitoring models were implemented in different countries, including the United States (n = 11), UK (n = 9), Canada (n = 2), Netherlands (n = 2), China (n = 1), Ireland (n = 1), Brazil (n = 1), Australia (n = 1). Different study designs were used: descriptive studies (n = 12), evaluations (n = 6), observational studies (n = 7), feasibility study (n = 1), news feature (n = 1). See Vindrola-Padros et al. 32 for further details.
Aims and designs of remote home monitoring models
The primary aim of remote home monitoring models was to enable early identification of deterioration for patients self-managing COVID-19 symptoms at home (including those who had not been admitted to hospital as well as those who had been discharged).
A secondary aim of the models was to reduce the rate of hospital infection and demand for beds in the acute care sector (including preventing admitting those suitable for home management and discharging those admitted to hospital earlier with care from a remote team).
Most of the remote home monitoring models were led by teams in secondary care (n = 23), with some led by primary care (n = 3) and some led by both primary and secondary care (n = 2). Thirteen of the models functioned as pre-admission wards (preventing admission of patients to hospital) and five were designed for patients admitted to hospital who could be discharged and monitored at home (step down wards), and 10 were both (with separate pathways).
Patient populations appropriate for remote monitoring
Most of the models established broad criteria for patient eligibility, defining the patient group as adult (over 18 years) patients with COVID-19 symptoms (suspected and confirmed cases). Some models limited referrals to confirmed cases. Some models excluded certain groups (e.g. patients over 65 years with significant comorbidities, or pregnant women). The size of patient cohorts varied considerably (ranging from 12 patients to 6853; see Vindrola-Padros et al. 32 for further details).
Stages of remote home monitoring
The articles described five main stages in remote home monitoring for COVID-19: (1) referral and triage to determine eligibility (including recording patient demographics, clinical variables, health data for risk assessment and vital signs data, and in some cases a risk assessment); (2) onboarding of patient to remote home monitoring service (provision of information to patient and/or carer on monitoring process, mechanisms for escalation and self-care); (3) monitoring [including recording of observations, communication of the information via a paper-based system and telephone or digital technologies such as apps or online forms, inclusion of pulse oximetry (in some cases), assessment of the information by the medical team]; (4) escalation (if required according to pre-established thresholds); and (5) discharge from the pathway (once their symptoms improved or patient opted out of the pathway).
Expected outcomes
Outcomes of remote home monitoring models were grouped in three main categories: (1) process outcomes related to the remote home monitoring pathway (e.g. time from swab to assessment, time to escalation, ambulance attendance/emergency activation), (2) process outcomes related to secondary care (e.g. LOS) and (3) patient outcomes, including clinical outcomes such as emergency department attendance/reattendance, hospital admission, ICU admission, readmission, mortality, ventilation needs and experience (e.g. patient satisfaction).
Impact on outcomes
It was difficult to carry out an analysis of the impact of remote home monitoring across all examples because not all articles reported data on the same outcomes. In terms of clinical outcomes, mortality rates were low, admission or readmission rates ranged from 0 to 29%, and emergency department attendance or reattendance ranged from 4% to 36%. Six of the models reported data on patient feedback and indicated high satisfaction rates.
Information about remote home monitoring process outcomes was limited (n = 6 articles), but time, from swab to assessment ranged from 2 to 3.7 days and virtual LOS from 3.5 days to 13 days (see Vindrola-Padros et al.). 32
Economic impact
Very few of the selected studies for this rapid review provided a descriptive form of economic analysis. The amount spent per patient on remote monitoring varied by country and type of costs included in the analysis.
Strengths and limitations of review
The last search was carried on 5 February 2021, so any articles published after this date were not included. However, the inclusion of preprints helped to mitigate this issue. Although we employed multiple broad search terms, it is possible that we missed articles that did not use these terms. Owing to the variability in study designs and the descriptive nature of the articles we did not assess these articles for quality using standardised tools for assessment. However, we feel it is important to note that we found several cases of missing data and inconsistencies in the reporting of evaluations that would lead to low quality ratings.
Future research areas identified
The review highlighted that further research should focus on exploring patient experience, implementation, outcome evaluation and cost-effectiveness.
A rapid mixed-methods evaluation of the early implementation of remote home monitoring services
A number of remote home monitoring models were set-up during the first wave of the COVID-19 pandemic in England with a high degree of variability in relation to the mechanisms implemented for patient assessment and triage, monitoring and escalation.
Despite previous research on the use of remote home monitoring models for other conditions and their widespread use during the COVID-19 pandemic, studies on their implementation and impact are rare. This study aimed to identify the key characteristics of these services, explore staff experiences, understand the use of data for monitoring progress against outcomes, and document variability in staffing/resource allocation.
Methods
Design
A multisite mixed-methods study that combined qualitative and quantitative approaches to analyse the implementation and impact of remote home monitoring models during the first wave of the COVID-19 pandemic (July to September 2020) in England (see Vindrola-Padros et al.). 36
Sample and recruitment
We recruited staff with experience delivering these models across eight pilot sites in England. We purposively recruited sites to represent a range of characteristics including the type of remote home monitoring service, different population sizes, areas of the country and monitoring approaches (see Vindrola-Padros et al.). 36
Data collection
We conducted interviews with 22 staff. Most of these interviews were carried out by pairs of researchers. Interviews were conducted using a topic guide, were audio-recorded and lasted 30–60 minutes. We also collected data on staffing models and resource allocation (number of staff, their function/seniority, the number of hours worked, all other resources used in setting up and running pilots and associated costs).
Data analysis
Detailed notes from interviews were added to RAP sheets. 70 Multiple researchers cross-checked data to ensure consistency. The team met weekly to discuss emerging findings and develop themes. Set up costs were reported as mean cost per site, and for running costs, mean costs of patient were calculated for the pilot period (see Vindrola-Padros et al.). 36
Findings
We present a summary of findings below (see Vindrola-Padros et al. 36).
Models implemented
The models varied in relation to the health-care settings and mechanisms used for patient triage, monitoring and escalation. The main components of models were triage, admission to service (including provision of pulse oximeter), patient provides readings through phone calls or app, medical team monitor observations and escalate cases, and discharge at 14 days or when symptoms have improved.
Barriers and facilitators to implementation
Staff described high levels of patient engagement. Implementation was embedded in existing staff workloads and budgets. Implementation facilitators included: good communication within clinical teams, culturally appropriate information for patients/carers and the combination of multiple approaches for patient monitoring (app and paper-based).
Barriers to implementation included: unclear referral criteria and processes, difficulties carrying out non-verbal assessments over telephone, lack of culturally appropriate patient information, lack of administrative support and workforce availability.
Cost
The mean cost per monitored patient varied from £400 to £553, depending on the model. Mean costs were higher in pre-hospital than early discharge from hospital model.
Strengths and limitations
The study was limited in the sense that we could not determine the effectiveness of these models due to lack of comparator data.
Future research areas identified
We identified the sustainability of the models and patient experience (considering the extent to which some of the models exacerbate existing inequalities in access to care) as future areas of research.
Dissemination and use of findings
The findings from the rapid review and the rapid mixed-methods evaluation on the implementation of COVID-19 remote home monitoring models across eight sites in England were disseminated widely and used to inform decisions in relation to the roll-out of services during autumn/winter 2020–21. The main channels for the dissemination of findings included journal articles,13,32,36 blogs,77–79 a slide set80 and presentations (see Project website documentation – Dissemination).
Impact
A senior director at NHSEI provided the following feedback on how findings from phase 1 of our evaluation influenced the national roll-out of COVID-19 remote home monitoring services in England:
-
Setting very simple entry criteria [65 and over OR clinically extremely vulnerable (CEV)].
-
Ensured that we did not go down a tech-only or tech-first route.
-
Produced translations of all the patient facing guidance and an easy-read version at the outset. It is possible that we may have missed the importance of this point.
-
National SOP was focused on patient self-escalation and our workforce calculations were informed by this work.
-
Created a minimum standard for out-of-hours to ensure that the links were clear.
-
We required services to be established within two months and had confidence that we could encourage all CCGs to set up by 31 December.
-
Ensured that we were able to get pulse oximeters out to CCGs within three working days.
-
Provide CCGs with a feed of patients that have tested positive and identify people within the cohort (65 years and over or CEV).
Informing phase 2 of the evaluation
The findings from phase 1 of the evaluation were also used to inform the design of phase 2, including the selection of outcome measures, cost analysis and identification of key areas of focus such as: the sustainability of these models during the second and third waves of the pandemic, patient and carer experiences with the services and how these varied according to the type of model (including analogue vs. digital models), factors that could have created or exacerbated inequalities in health and access to care and the costs involved in delivering these services.
Chapter 4 The development and implementation of COVID-19 remote home monitoring services (phase 2)
Overview
What was already known
-
COVID-19 remote home monitoring services were implemented internationally during wave 1 of the pandemic.
-
During our phase 1 evaluation (wave 1 of the pandemic), some facilitators and barriers to COVID-19 remote home monitoring services were identified.
-
It is important to explore local variation to fully understand implementation and to identify factors that might explain variation in effectiveness and cost outcomes across service models.
What this chapter adds
-
Rapid development of national remote home monitoring services took place in three phases: (1) local development, (2) national development and roll-out, and (3) local implementation.
-
Patient enrolment to COVID-19 remote home monitoring services across England was lower than expected.
-
There was large variability in the models implemented for COVID-19 remote home monitoring services.
-
Variation was influenced by patient, workforce, organisational and resource factors, and the rapid and evolving COVID-19 situation.
-
These factors might help to explain variation in outcomes/effectiveness of different service models.
Introduction
It is important to understand the impact of local factors on adoption when implementing national policy initiatives81–83 as evidence suggests local factors affect service design,84–86 ability to meet service aims,87 sustainability of the service and can also hinder the accurate assessment of efficacy and cost-effectiveness. 57,81,83,84,88
To increase our understanding of how local conditions affect rapid implementation of national policy during a public health crisis89–92 and support future development and implementation of services,93–96 it is necessary to explore the development, enrolment rates and implementation of COVID-19 remote home monitoring services (see Research question 6, Chapter 1).
This chapter provides:
-
an overview of the development of COVID-19 remote home monitoring services including the national roll-out and the enrolment rates of the services
-
a description of how services have been implemented and used locally
-
an analysis of the local factors that help explain why the services varied across sites
-
a backdrop for findings presented in Chapters 5–11.
Methods
A summary of the methods is provided below; further details can be found in Chapter 2, Report Supplementary Material 1.
Sample and recruitment
Twenty-eight sites were recruited to our study. Sites were included from all regions across England, represented CCGs and trusts, varied in urban/rural, deprivation scores, ethnicity, and size (see Chapter 2, Report Supplementary Material 2).
Data collection
To identify when new services started and to estimate the proportion of new diagnosed cases who were onboarded (enrolment rates), we drew on a selection of data sources (see Report Supplementary Material 1), including Kent, Surrey and Sussex AHSN (KSS AHSN) data (see Chapter 6), bespoke onboarding data and data on diagnoses of COVID-19 provided by PHE (now the UK Health Security Agency).
To describe the development and roll-out of services we conducted interviews with national leads (see Chapter 2). To explore implementation, we conducted a documentary analysis of service documentation, surveys with patients and staff and interviews with patients, carers and staff, and a cost survey (see Chapter 2).
Data analysis
Survey and interview data
Inductive thematic analysis was used to analyse interview data inputted into RAP sheets. 70 Survey data were analysed using descriptive statistics (using SPSS, version 25). To allow for comparison, quantitative and qualitative data sets were mapped against the SOP components (referral, triage, onboarding, monitoring, discharge).
National data on enrolment
For the CO@h programme, we defined rates of enrolment as the proportion of new cases diagnosed who were onboarded. For each CCG, we estimated these rates by dividing the numbers onboarded each fortnight by the number of new cases of COVID-19 diagnosed in the same fortnight. We could only do this for CCGs where the onboarding data were judged to be complete, and to make this judgement we used a combination of management information provided by the programme and data collected by our own costing survey (see Report Supplementary Material 1).
For the CVW programme, data quality issues left us unable to use NHS situation reports (SITREPs) data on the number of patients with COVID-19 discharged from hospital to virtual wards. We were able, however, to carry out a limited analysis of enrolment rates for seven hospital trust-based sites by comparing the number of patients monitored on a post-discharge pathway (as reported in our costs survey, see Chapter 7) to the number of patients with COVID-19 discharged alive, according to national HES Admitted Patient Care (APC) data.
Findings
Within this section, the following findings are presented: (1) a summary of interview and survey data (n = 28 sites), (2) an overview of the development of COVID-19 remote home monitoring services (including (a) development of these services, and (b) coverage of these services nationally and rates of enrolment), (3) a description of the different typologies and implementation of services between sites, and (4) factors influencing the implementation of these services.
Participant characteristics
We received 292 staff surveys (39% response rate) across 28 sites and 1069 patient and carer surveys (18% response rate) across 25 sites (see Report Supplementary Material 2 for demographic characteristics). Interviews were conducted with national leads (n = 5), staff (n = 58 across 17 sites), and patients or carers (n = 62 across 17 sites). See Report Supplementary Material 2 for demographic characteristics.
An overview of the development of COVID-19 remote home monitoring services including the national roll-out and the uptake/coverage of the resultant service
Within this section, we first present an overview of the three phases of development and implementation of these services. Secondly, we present the uptake/coverage of the final service.
Development of COVID-19 remote home monitoring services
The development of COVID-19 remote home monitoring services occurred rapidly, in three stages: (1) local development and implementation, (2) national development and roll-out, and (3) local implementation (see Figure 4 for timeline and key groups involved in each phase).
FIGURE 4.
Development of COVID-19 remote home monitoring services.
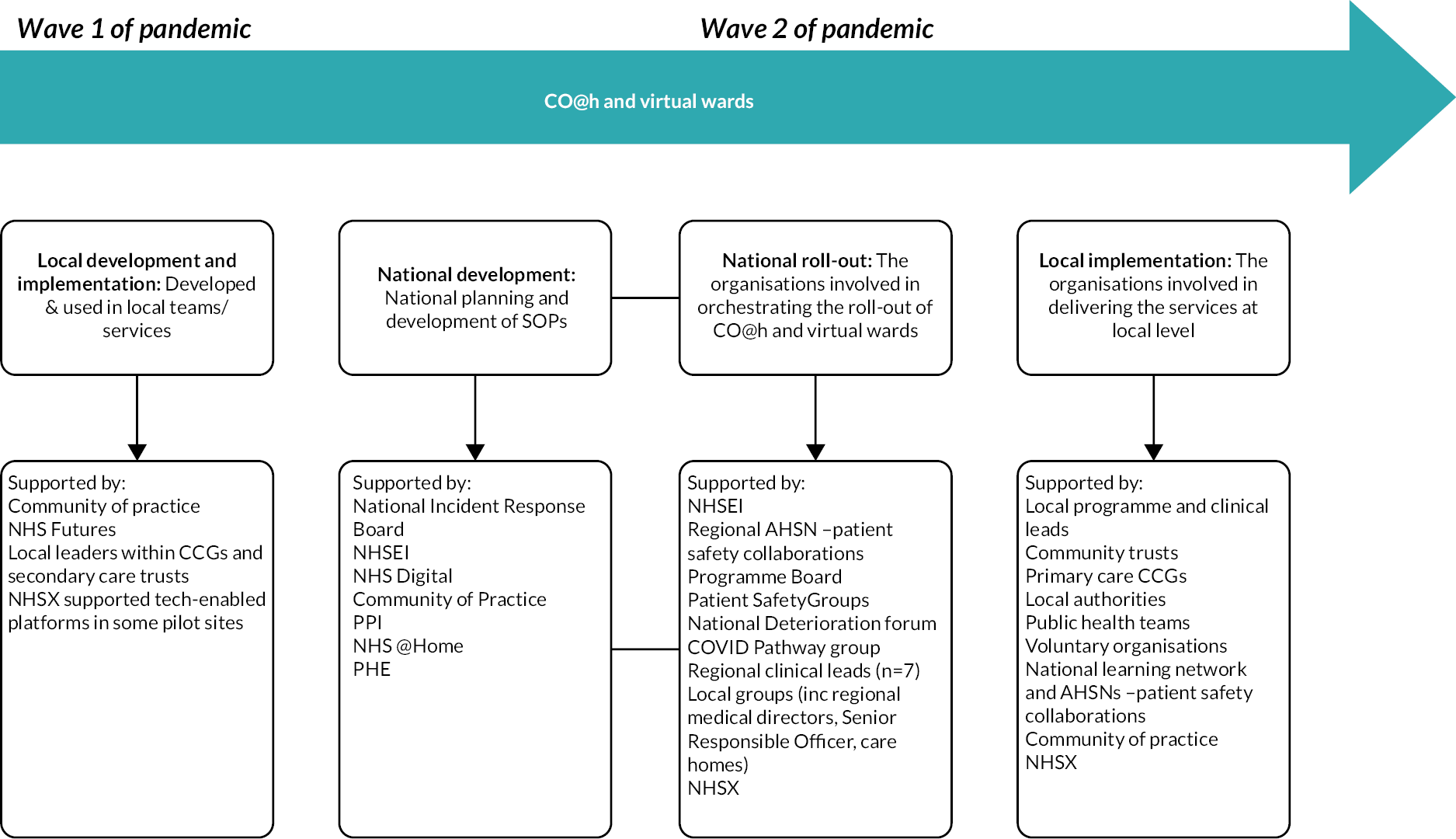
Local development and implementation – wave 1 and the first use of pulse oximetry in patients’ homes
The use of oximetry to monitor patients with COVID-19 in their home began in wave 1 as several, initially disconnected, local services were established, motivated by attempts to mitigate silent hypoxia (very low oxygen saturations, often without breathlessness). Pulse oximetry was initially used to monitor patients in the community. 36 During phase 1, local clinical leaders within CCGs and secondary care trusts had identified a need for this service and were integral in facilitating the initial development, set-up and implementation of services, and instigated the development of an informal community of practice. NHSX supported a few of the pilot sites to use tech-enabled models by putting them in touch with tech companies. The local development and implementation of services gained interest from national stakeholders.
An evaluation of phase 1 was commissioned and conducted by researchers from NIHR RSET and NIHR BRACE (see Chapter 3). The purpose of this evaluation was to provide lessons for the ongoing development/roll-out of the services.
Two learning communities were set up prior to the national roll-out, to support local implementation: a community of practice and a national learning network.
The community of practice was an informal group, led by key national clinical and policy leads and attended by national and local clinical leaders for local services adopting these pulse oximetry services. The community of practice held regular online meetings (every couple of weeks) to share best practice quickly from national leads to local teams, and between local teams, offering mutual support and leadership. This community of practice grew rapidly.
A national learning network (the National Deterioration and COVID-19 Forum) was set up by the AHSN patient safety collaboratives to support clinical leads and provide resources to facilitate implementation of these services. The set-up of the national learning network was prompted through NHS @home17 (via the national team and regional medical directors). Additionally, regular webinars were held to share learning. 97 The learning network was hosted within the Future NHS collaboration platform (an online platform designed to help services to co-develop services, share materials and work together). 98
The conversations and shared learning from both these networks played a key role in shaping the basic pathway underpinning these services. These communities were set up to support local clinical leads and share experiences.
National development and roll-out
Between wave 1 and 2, there was a period of gathering evidence (research and clinical consensus), discussions around how services should be developed and development of safety netting guidance. This evidence included our evaluation findings from phase 1 (see Chapter 3).
During the summer and autumn of 2020, NHSEI began to develop SOPs for CO@h, with leadership and governance from the NHS @home team. The National Incident Response Board approved operationalisation and national roll-out of the CO@h service as the appropriate next step.
The SOP for CO@h38 (see Appendix 1, Table 24) that emerged was informed by the learning gained from previous services which provided care at home, experience gained from managing wave 1 in the community including the various pilots and case studies. It was shared through the NHS Futures website98 and the pre-existing evidence base. 32,36 It was intended that services would be inclusive by design and enabled by, but not dependent on, technology (to remain accessible to the most vulnerable patient groups).
In January 2021, a SOP for CVW (early discharge from hospital) was published and these services were rolled out. 39 Virtual ward models were designed to be run by acute trusts, with responsibility for services being with secondary care services39 (see Appendix 1, Table 24).
Following service development, the AHSNs were commissioned through the AHSN’s patient safety collaboratives to facilitate regional roll-out. 97 A programme board with all key stakeholders was established and met weekly to discuss a number of workstreams including data management, workforce/training, patient safety, operations, pathways, evaluation and oximeter distribution. The programme was monitored via comprehensive data collection for CO@h which aimed to track how the programme was developing/performing with a focus on using the data as shared learning. As part of this process any safety incidents were recorded, using patient safety groups where appropriate.
National roll-out of CO@h and virtual ward services was supported by 20 highly attended regional launch events, which included question and answer sessions. To support national roll-out, national clinical leads and seven regional clinical leads were funded to support local implementation. NHSEI agreed to purchase pulse oximeters. NHSEI purchased 706,000 oximeters across waves 1 and 2. These pulse oximeters were CE marked (as recommended by MHRA guidance)37 and were available to local sites within three days through an ordering system set out in the SOP. NHS Digital supported data management. 99 NHSX were a partner in the roll-out and implementation of tech-enabled platforms within COVID-19 remote home monitoring services. NHSX committed to supporting (funding) up to 50 organisations to use a tech-enabled model for CO@h and/or virtual ward models during wave 2 (sites bid for this funding and then chose and procured the platform they wanted to use). CO@h and virtual ward services were rolled out between November 2020 and February 2021.
Local implementation
NHSEI were integral in supporting the local implementation of COVID-19 remote home monitoring services, including providing financial support for primary care to establish and implement these services,40 resourcing NHS Digital to supply data to service providers to identify people who may benefit from these services41 and the commissioning and funding of AHSN support.
National leads described the importance of local leads assuming responsibility and accountability for their services, guided by the SOPs for CO@h and CVW.
While there was some variation in responsibility and accountability, local CCGs were primarily responsible and accountable for delivery of CO@h and secondary care trusts were responsible and accountable for delivery of CVW. Each local service had its own local governance and management structure, which were expected to minimise national input and allow local services to be more responsive to and reflective of the needs of local populations. This local governance was overseen and supported by the AHSNs. NHSX managed data reporting on tech-enabled deployment.
With local services responsible for the day-to-day running of CO@h and CVW, services allocated resources, designed staffing models, and distributed equipment and educational materials. The management teams particularly of integrated services (i.e. where CO@h was combined with CVW) consisted of colleagues drawn from across multiple settings including primary and secondary care, but also the ambulance service, mental health trusts, care homes, and patient safety collaboratives. The precise mix depended upon the scope of services within the local environment. Regional ‘clinics’ were established by the local AHSNs to facilitate regular engagement and support the initial set-up. Two-way relationships were developed between policymakers and strategic management and front-line NHS workers as a way of sense-checking the work completed. Significant support and buy-in from senior management within acute trusts and across CCGs helped to set up COVID-19 remote home monitoring services. There was, however, a need to have supportive local authority and public health teams to integrate CO@h and CVW services with existing ways of data sharing.
Throughout the national roll-out, local services were supported by the community of practice and the national learning network (as described earlier).
National coverage of COVID-19 remote home monitoring services and enrolment
COVID Oximetry @home
We judged that the national onboarding data for CO@h were complete for 37 of 135 CCGs (see Report Supplementary Material 1), so our analysis of coverage and enrolment was restricted to these areas.
The dates sites within each of the 37 CCGs started onboarding patients are plotted in Figure 5. Three of these CCGs were onboarding patients in October and all 37 were onboarding patients in the fortnight beginning 11 January 2021.
FIGURE 5.
Number of CCGs with sites operating the CO@h programme by fortnight (data for the 37 CCGs where the onboarding data were assumed to be complete).
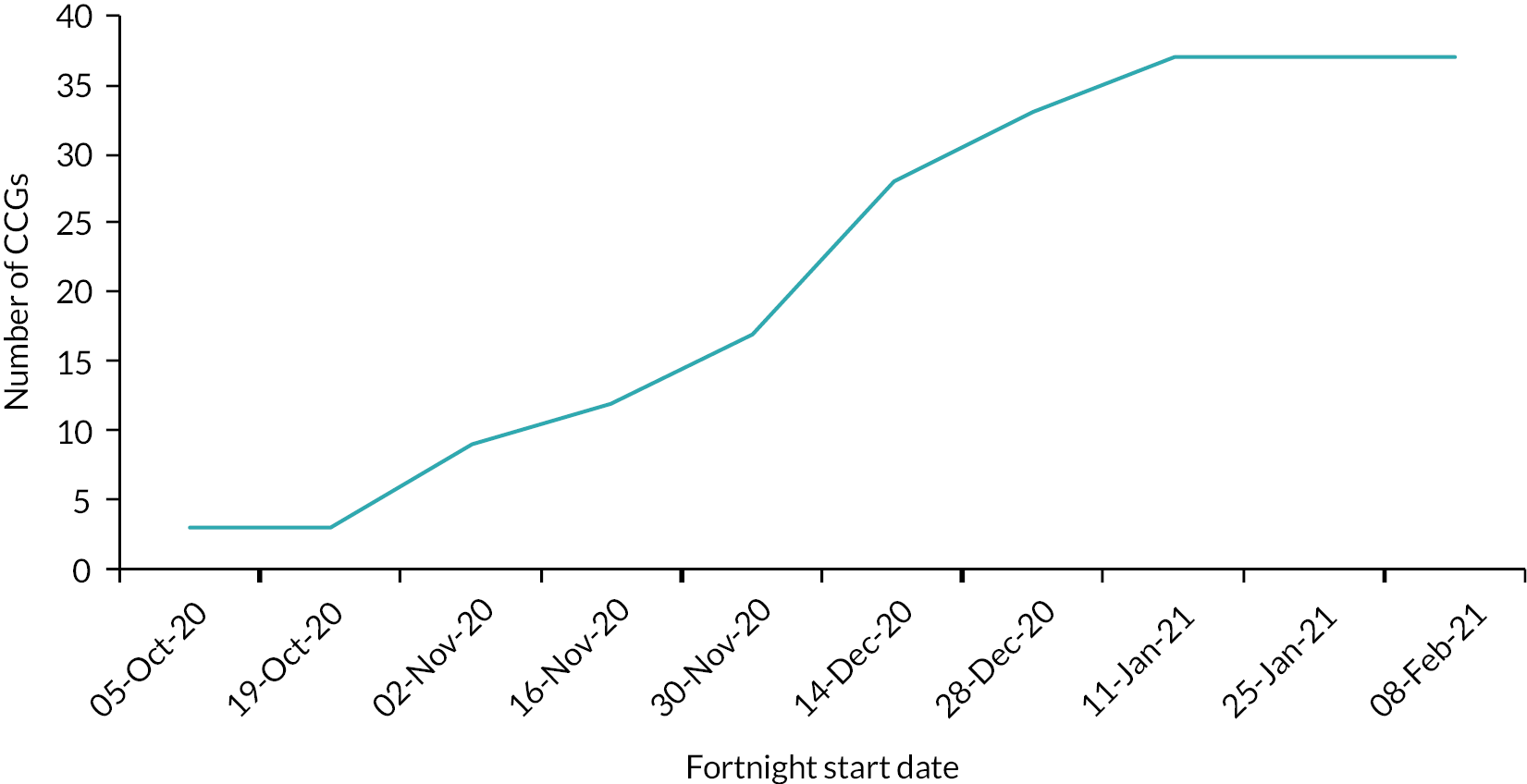
Once services started operating and up until 4 April 2021, enrolment across the 37 CCGs among people aged 65 years or over is shown in Figure 6. The CCG with the highest enrolment rates had values that were more than twice the next highest; 22 CCGs (59%) enrolled fewer than 10%. The overall enrolment rate over the period for this age group across all 37 CCGs was 8.7%.
FIGURE 6.
Enrolment of CO@h services among people aged 65 years or over by CCG after implementation started up until 4 April 2021 (enrolment is defined as the ratio of numbers onboarded to new cases over the period and calculated from 19 October 2020 for any services in operation before then).

Virtual wards
Dates acute trusts opened their virtual wards are plotted in Figure 7. A majority of services (62%) launched in January and February 2021. For seven trust-based sites included in our costs survey (see Chapter 7), we were able to estimate an approximate enrolment proportion: that is, the proportion of patients with COVID-19 discharged who were monitored on a virtual ward. Two sites had fewer than 10% of patients monitored, one site had 20% and four had more than 50% (but below 65%) of patients monitored. Otherwise, with national SITREP data not useable because of data quality issues, we had no further information on virtual ward enrolment.
FIGURE 7.
Start dates of CVW services in England’s non-specialist acute hospital trusts (n = 123 trusts); 14 trusts had no CVW by 22 February 2021.

A description of the different typologies and implementation of services between sites
We created a typology (classification system) and categorised sites according to seven domains (see Table 1): (1) type of model (CO@h/CVW/Integrated); (2) sector leading services (primary care/secondary care/both); (3) type of monitoring (analogue-only (paper and telephone)/tech-enabled and analogue); (4) admission criteria (age and clinical vulnerability); (5) workforce (number and type of staff); (6) date service started; and (7) enrolment rates within the CCGs where the sites were located. As above, enrolment rates were defined as the ratio of patients onboarded to new cases among people aged 65 or over by CCG after implementation started up until 4 April 2021. It was calculated from 19 October 2020 for any services in operation before then.
All models (n = 28) | CO@h models (n = 13/28) | CVW models (n = 4/28) | Integrated models (n = 11/28) | ||
---|---|---|---|---|---|
1. Type of model | CO@h (referral from community providers, e.g. GP, hot hub, emergency department) | 13 | 13 | ||
CVW (referral through early discharge from hospital) | 4 | 4 | |||
Integrated model (community and early discharge referrals) | 11 | 11 | |||
2. Sector leading the service | Primary/community care | 15 | 11 | 1 | 3 |
Secondary care | 5 | 0 | 3 | 2 | |
Both | 6 | 1 | 0 | 5 | |
Not specified | 2 | 1 | 0 | 1 | |
3. Method of monitoring | Analogue only (paper and telephone) | 7 | 1 | 2 | 4 |
Tech-enabled and analogue | 21 | 12 | 2 | 7 | |
4. Admission criteria a. Agea |
18+ years old | 13 | 8 | 2 | 3 |
50+ years old | 11 | 4 | 0 | 7 | |
65+ years old | 1 | 1 | 0 | 0 | |
Did not use age as a criterion | 3 | 0 | 2 | 1 | |
b. Risk factors | Yes | 25 | 13 | 2 | 10 |
None specified | 3 | 0 | 2 | 1 | |
5. Workforce a. Number of staff |
Setting up (range across 26 sites)b | 2–20+ staff members | 2–20 staff members | 2–20 staff members | 2–20+ staff members |
Running (range across 26 sites)c | 2–70+ staff members | 2–70+ | 2–10 | 2–60 | |
b. Type of staff involved in monitoring | Clinical staff only | 15 | 5 | 4 | 6 |
Clinical and administrative staff | 10 | 7 | 0 | 3 | |
Not known | 3 | 1 | 0 | 2 | |
6. Month started | Before November 2020 | 11 | 6 | 1 | 4 |
In November 2020 | 12 | 5 | 2 | 5 | |
After November 2020 | 5 | 2 | 1 | 2 | |
7. Enrolment rates within CCGs where sites were located (population age 65 years or over – CO@h models only) | > 30% | 1 | 0 | 1 | |
20–30% | 1 | 1 | |||
10–20% | 4 | 3 | 1 | ||
<10% | 6 | 3 | 3 | ||
Incompleted | 11 | 5 | 6 |
Different models of COVID-19 remote home monitoring services were implemented across the 28 local sites. Below, we describe variability in relation to the stages of services outlined in the national SOPs (see Report Supplementary Material 4). 38,39
Entry criteria
In terms of entry criteria, most services used age criteria of either 18 years or over, or 50 years or over, rather than the age criteria recommended within the SOP of 65 years or over. Many sites adapted and reduced their enrolment age throughout the period. Most sites used risk factors alongside age to enrol patients, but each site varied in the risk factors used.
Workforce
As shown in Table 1, there were large differences across sites with regard to the number of staff involved in setting up (n = 2–20+) and running (n = 2–70+) the service, and with regard to the type of staff involved in monitoring. Most sites used clinical staff only to support monitoring.
Triage
As recommended by national guidance, most sites had processes in place for patient triage (n = 24/25). However, interview findings indicate that triage processes varied across different models (e.g. in terms of who checks against admission criteria, and the processes used). See Chapter 11 for a description of models categorised by risk-stratification.
Onboarding
In line with national guidance, all of the sites provided patients with oximeters. However, their means of distribution varied, including delivery (n = 27 sites), given to patients at GP/hospital (n = 9 sites), patients/family members collecting oximeters (n = 7 sites). Some patients described difficulties in collecting oximeters due to their poor health and isolation.
In line with national guidance, most sites provided patients with information (n = 22/25). Most sites provided patients with written information or provided information over the telephone.
Monitoring
Sites differed in the type of options they offered for patients submitting data (e.g. tech-enabled and/or analogue) and the level of interaction with staff (see Appendix 2, Table 25). Most services were tech-enabled with telephone options offered when tech-enabled modes of submission were not possible. Members of staff were involved in taking and reporting readings at all sites; none of the services solely asked all their patients to self-monitor and self-escalate care (see Appendix 2, Table 25). However, interview findings indicated that two sites offered some of their patients (exact number not known) a self-monitoring option. Self-monitoring is defined as patients or carers (where appropriate) monitoring their readings and escalating their own care as necessary (see Report Supplementary Material 4).
Escalation
Escalation processes varied, with some tech-enabled solutions identifying patients for escalation (with the addition of phone or face-to-face assessment in some cases) and some escalation processes being manually initiated (with the addition of phone or face-to-face assessment in some cases) (see Report Supplementary Material 4).
Discharge
In line with national guidance, many but not all sites had explicit processes in place for patient discharge (n = 19/25). Our findings on the flexibility of discharge were in line with the SOP, in that most sites reported patients were discharged after 14 days, but that patients could be discharged earlier or kept on if needed. However, only 73.4% (n = 769) of patients were aware of discharge processes (range across sites: 33.3–90.9%). Oximeter return processes were also not apparent at every site with only 69% of patients being asked to return the oximeter (range across sites: 21.5–100%).
Factors influencing implementation of COVID-19 remote home monitoring services
We observed differences in the design and delivery of services between local sites and between the national guidance and local implementation. Implementation may have been influenced by a number of site-specific factors relating to patients, staff, the organisation delivering the programme, and the resources at their disposal (see Report Supplementary Material 4).
Patient-related factors
Patient factors relate to demographic characteristics, disease prevalence, digital access, and patient engagement. Sites were charged with responding to the needs of local populations and the degree to which they did so was shaped by the specific needs of the local population, influenced by factors such as socioeconomic status, ethnic and cultural background, prevalence of comorbidities, the ability of patients to access or utilise digital technologies and their level of engagement with the programme (see Box 1). However, sites varied in the time and resources dedicated to attempt to engage with the vulnerable and disadvantaged groups disproportionately affected by COVID-19.
Although all of our sites used telephones to collect readings, some sites would also use these calls to offer comfort and reassurance to isolating patients or otherwise signpost them to other services or community groups. Some sites reported that staff would visit patients in-person if particularly concerned about their wellbeing or if the patient was visually impaired. This additional support was at the discretion of sites and not prescribed by the national guidance.
In site O, they developed materials in a range of languages. They also used community-based partnerships and voluntary organisations to help onboard and monitor patients. Patients were drawn from a range of vulnerable populations leading them to successfully target minority ethnic groups, those who were morbidly obese and members from the local homeless population.
Staff factors
A number of staff-related factors influenced the way in which services varied between sites. These related to their level of training and experience, whether they worked remotely or in a shared workspace with more direct clinical oversight, and the overall workload they experienced (Box 2). For example, those that were redeployed to services tended to be nurses working in secondary care, whereas those asked to take on the responsibility of delivering these services alongside their current roles tended to be drawn from primary care.
The knowledge, skills and confidence of the staff charged with delivering services varied between sites, and these differences in confidence and knowledge were particularly evident when services relied on non-clinical staff. Across many sites, the routine availability of senior clinical oversight was deemed important.
In site M, where staff worked remotely from other staff, they described how their training was minimal and this gave rise to a number of concerns. These included their ability to use the software through which they managed their caseload, but also more fundamental issues around their lack of experience of teleconsultations, remote monitoring of respiratory conditions, and how this left them uncertain about whether they were offering the correct advice to patients or their non-clinical colleagues with patient-facing roles.
Organisational factors
A range of organisational factors impacted on the design of individual services including staffing models, the culture and work practices that supported the learning of individuals and/or the organisation,100 and the engagement of senior staff in changing practice and supporting innovative delivery. 101 The timing of the introduction of remote home monitoring services in each local area may have influenced variation in services observed between sites. The SOP produced in November 2020 contained the caveat that those that had already established a service would not be expected to adhere to the national guidance.
A key factor in shaping services was the level of successful collaboration between the organisations involved at each site (Box 3). The importance of robust communication pathways between senior managers at local sites was recognised, as was the value of collaborating with other teams and specialities, particularly in refining and improving services and for supporting patients with additional needs (e.g. those with existing health conditions or requiring mental health support).
At site K, there was close collaboration between different sectors. Their service was run by primary care and secondary care organisations together with each organisation/sector operating within clearly defined roles (identification of patients, running hot hubs/assessments, monitoring). Staff within this service reported a history of integrated working relationships between secondary, primary care and voluntary/community sectors. These long-standing relationships were felt to better facilitate the implementation.
Resource factors
Resource factors related to the availability of reliable pulse oximeters and the availability and functionality of the software used to support the service. One major investment in these services was the provision of pulse oximeters by NHSEI. However, the perceived quality of these oximeters reportedly varied and not all sites had the links with the voluntary sector that many used to deliver the instruments (in workable order) to patients in the community.
The tech-enabled platforms selected by local systems varied across regions and local systems, with each possessing various levels of functionality and interoperability. The more readily service data could be integrated with existing databases the more effective the programme could operate. In its absence, valuable information on patients would be unavailable, for example, current medications, prior history of respiratory illness, or susceptibility to anxiety. Several sites reported that technology platforms were not always ready for use at the onset (due to the speed at which services were developed and implemented). This meant switching mid-pandemic without the necessary time for training or familiarisation (Box 4).
At site J, the site originally used (platform 1) but this did not meet their requirements to automatically send three reminders to patients per day. Therefore, mid-way through the second wave the service switched over to (platform 2). However, this presented its own challenges of moving from one system to another while the service was under enormous pressure and they had to temporarily return to using (platform 1) due to fears that some patients were being omitted.
Discussion
Key findings
The rapid development of COVID-19 remote home monitoring services in England took place in three phases: (1) local development and implementation (in wave 1 and 2), (2) national development and roll-out (in wave 2), and (3) local implementation. The proportion of new diagnosed cases who were onboarded on to these services was lower than expected. The implementation of services varied between sites and from national guidance. A range of service typologies emerged across our evaluation including noticeable variation in relation to enrolment rates, the nature of onboarding, referral criteria, monitoring processes, and discharge and pulse oximeter return procedures. Patient factors (e.g. needs of the local population), staff factors (e.g. workload and work environment), organisational factors (e.g. staffing models and collaboration) and resource factors (e.g. software) influenced implementation and enrolment.
How findings relate to previous research
The implementation of COVID-19 remote home monitoring services in England can be seen as an example of major system change or ‘large system transformations’, defined as ‘coordinated, systemwide change affecting multiple organisations and care providers, with the goal of significant improvements in the efficiency of health-care delivery, the quality of patient care, and population-level patient outcomes’ (Best et al.,102 p. 422). A conceptual framework proposes five main stages of major system change: (1) decision to change influenced by drivers to change, governance and leadership of decision-making, (2) decision on which model to implement, (3) the implementation approach, (4) implementation outcomes, and (5) intervention outcomes. 103 There are different ways of implementing major system change (top-down and bottom-up approaches). Previous research suggests that a combination of both top-down and bottom-up approaches may be beneficial. 104 Our findings indicate that rapid implementation processes may follow a bottom-up (local services make decision to change and which model to implement), top-down (national service makes decision to change and provides guidance on which model to implement), then bottom-up process (local services interpret guidance from national service and decide which model to implement) (as described in Chapter 4). Therefore, rapid implementation may vary from major system change in non-rapid contexts (e.g. Fulop et al.). 103 However, our findings support previous research which indicates that this combination of approaches may impact on consistency of implementation approach and uptake. 105
Prior to this evaluation, there was limited evidence regarding how these services have been implemented. Our findings indicated that most remote home monitoring services studied had low rates of enrolment and that there was considerable variation in which model was implemented and how, according to local context. These findings support previous research demonstrating likelihood of variation when implementing national services generally81–83,89–92 and in response to the COVID-19 pandemic. 106–108 Previous research has highlighted differences in the models implemented and the ways in which services are implemented must be considered when interpreting effectiveness and cost-effectiveness of services. 57,81,83,84,88
Strengths and limitations
This study used mixed-methods data from a wide range of sources, including national quantitative data, cost data, national surveys and case study interviews. Additionally, this evaluation is a large rapid scale evaluation, including 28 health-care services throughout England. Therefore, the findings provide a comprehensive picture of the development, coverage and implementation of COVID-19 remote home monitoring services through phase 2 of the pandemic.
Given the rapid implementation of services, we are unable to say whether the degree of variation we witnessed is a result of the rapid roll-out or whether it would have been observed if lengthier implementation processes had been followed.
Different data sources were triangulated to analyse the findings presented in this chapter. Sometimes, different data sources or respondents disagreed and consensus needed to be reached. For example, across several sites, more than one clinical lead/service manager completed the staff survey and there were several instances of responses being inconsistent (to overcome this problem, we sought further information from services as necessary and selected one clinical lead per site using relevance of job title).
Our assessment of service enrolment within CCGs relied on their onboarding data being complete. To judge completeness, we relied on assessments made by individual CCGs, although as they were not necessarily the service providers, and therefore removed from the data entry process, we were not sure about the accuracy of this information in all cases. We were able to cross-check against the data provided by the 28 study sites themselves and found a reasonable match for most but not all, and we were unable to verify the data from the CCGs that were not study sites in the same way.
Implications
Our findings have implications for interpretation of quantitative outcomes of the evaluation. Our findings on low rates of enrolment and variation in implementation can be used to help interpret findings relating to effectiveness and cost of the services (as we discuss in Chapters 5–7). However, we were not able to look at how specific variation linked with particular service outcomes or enrolment more generally.
Several valuable lessons for service development have emerged from our work. Findings indicate that when developing and rapidly implementing national roll-out of services, service planners must consider the context and priorities of local organisations (e.g. their patient population characteristics and resources). It may be helpful to consult with patient groups to ensure that strategies to ensure reach are appropriate and feasible, and that services meet the needs of the local population. Flexibility of workforce is needed for those delivering the service.
Future research
Future research should explore how similar nationally implemented programmes vary from their intended design when not implemented at such speed and the degree to which local variation acts favourably in sustaining the service. Further evidence is needed on self-monitoring models of remote home monitoring for COVID-19, including the groups of patients that this might be appropriate for and whether its use would enable services to onboard more patients. Further research is needed to explore why enrolment was lower than expected, and how to improve enrolment to these services in future.
Conclusions
Local and national leadership were integral in the development and implementation of COVID-19 remote home monitoring services. Enrolment to these services was lower than expected. There was large variability in the models implemented and that variation may have been influenced by patient, workforce, organisational and resource factors. Together, low enrolment and variation in implementation indicate that it is difficult to evaluate the true extent of effectiveness of COVID-19 remote home monitoring services.
Chapter 5 The impact of COVID-19 Oximetry @home on mortality and the use of hospital inpatient services
Overview
This chapter draws on a manuscript by Sherlaw-Johnson et al. 109 published in Lancet eClinicalMedicine (2022): https://doi.org/10.1016/j.eclinm.2022.101318. This is an open access article under the term of the Creative Commons Attribution 4.0 International License (CC BY 4.0), which permits use, distribution and reproduction in any medium, provided the original work is properly cited (see https://creativecommons.org/licenses/by/4.0/).
What was already known
-
Previous quantitative studies have mostly assessed remote oximetry monitoring services for patients with COVID-19 at individual sites and focused on their safety.
-
There is some existing evidence that remote monitoring of patients with COVID-19 can be locally effective, particularly among higher-risk patient groups. However, effectiveness of national programmes has been little studied.
What this chapter adds
-
We have found no evidence that national implementation of pre-admission home monitoring of older people diagnosed with COVID-19 impacts on mortality, hospital admission rates, in-hospital mortality or length of hospital stay.
-
Missing data and lower than expected enrolment of patients to services may have influenced our results, and the effectiveness of some local programmes could have been lost among the analysis of national data.
-
Future implementation requires better data collection strategies and effective learning from areas that have achieved higher patient enrolment.
Background
Among the aims of remote monitoring of the CO@h service was the reduction in mortality through earlier identification of deterioration. Furthermore, it was hypothesised that if fewer patients with COVID-19 were admitted to hospital with advanced disease and critically low oxygen levels, there may be a reduction in the use of critical care facilities, fewer deaths within hospital and shorter lengths of stay. The anticipated impact on numbers of hospital admissions was less certain since the aim of the programme was not to reduce admissions, but to make sure people who needed to be in hospital were admitted sooner. However, any consequence on the number and mix of patients admitted with COVID-19 would be useful to understand as remote monitoring may have different impacts on different types of individuals.
Earlier studies of the use of oximetry for remote monitoring within England during the country’s first wave focused on aspects of safety and implementation, but were unable to establish reliable comparators for measuring impact. 32,110
This chapter presents findings from the clinical effectiveness workstream of the study addressing the specific research questions:
-
What was the impact of CO@h on mortality?
-
What was the impact of CO@h on the incidence of hospital admission for COVID-19 or suspected COVID-19 and on the characteristics of those admitted?
-
For these admissions, what was the impact on in-hospital mortality and LOS?
Our evaluation was requested by NHSEI alongside two other evaluations of clinical effectiveness which were intended to complement each other. 43,45,55 Our approach used combinations of unlinked, aggregated population-level data and hospital administrative data. We were able to undertake a rapid analysis that worked alongside the other evaluations and provided valuable insight in the future evaluation of similar programmes implemented at scale.
Methods
Our methods are described in Chapter 2 and Report Supplementary Material 1.
Results
Data completeness and patient enrolment
As we mentioned in Chapter 2, because of the design of our study we were only able to evaluate services within 37 CCGs where we judged there was complete data on numbers of people enrolled on to services (onboarded) between 2 November 2020 and 21 February 2021. The study population included anyone aged 65 years or over with a laboratory-confirmed positive test for COVID-19 and any hospital admission within that age group for COVID-19 or suspected COVID-19.
The included CCGs had no notable differences in mean age, proportions of non-white population or proportions resident in most deprived areas when compared with the remaining 98 that were not included, although included CCGs had a lower incidence of positive test results (Table 2). There were also regional differences: no CCGs from the East NHS region were included, and only one from the North East and Yorkshire (see Figure 8). The South West, North West and Midlands were the best represented regions.
Included CCGs (n = 37) | Excluded CCGs (n = 98) | p-value for difference between groupsa | |||
---|---|---|---|---|---|
Mean | (Standard error) | Mean | (Standard error) | ||
Mean proportion aged 65 years or more | 18.1% | (0.7%) | 17.9% | (0.5%) | 0.77 |
Mean proportion aged 80 years or more | 4.8% | (0.2%) | 4.8% | (0.1%) | 0.91 |
Mean proportion in non-white ethnic groups | 17.3% | (2.7%) | 17.7% | (1.8%) | 0.97 |
Mean CCG-level IMD | 22.7 | (1.3) | 22.6 | (0.8) | 0.96 |
Incidence of laboratory-confirmed COVID-19 per 1000 people | |||||
All ages | 41.9 | (1.7) | 46.8 | (1.4) | 0.03 |
Age 65 years or over | 30.4 | (1.3) | 35.0 | (1.2) | 0.01 |
FIGURE 8.
Number of CCGs with complete data by NHS region (% complete shown in each bar).

Fortnightly patient enrolment to CO@h among people aged 65 years or over within the 37 CCGs is shown in Figure 9. Rates were particularly low earlier in the period because many sites had not commenced implementation. The numbers of CCGs where there were sites onboarding patients within each fortnight is shown along the horizontal axis. Sites within nine (24%) CCGs were operating services in the first fortnight, which had risen to 33 (89%) within the fortnight beginning 28 December 2020. Services were operating within all CCGs during the fortnight beginning 11 January 2021. The median enrolment rate only exceeded 10% in the final fortnight, although, from the end of November 2020, the maximum was consistently above 30%, with one or two CCGs each fortnight achieving much higher rates than the rest. The overall enrolment rate over the period across all 37 CCGs was 5.9%. If we exclude fortnights during which services were either not operating or operating for only part of the fortnight, the overall enrolment rate was 8.7% with only one CCG averaging a rate of more than 30%.
FIGURE 9.
Variation in enrolment rates of CO@h among people aged 65 years or over with laboratory-confirmed COVID-19 across the 37 CCGs included in the analysis.
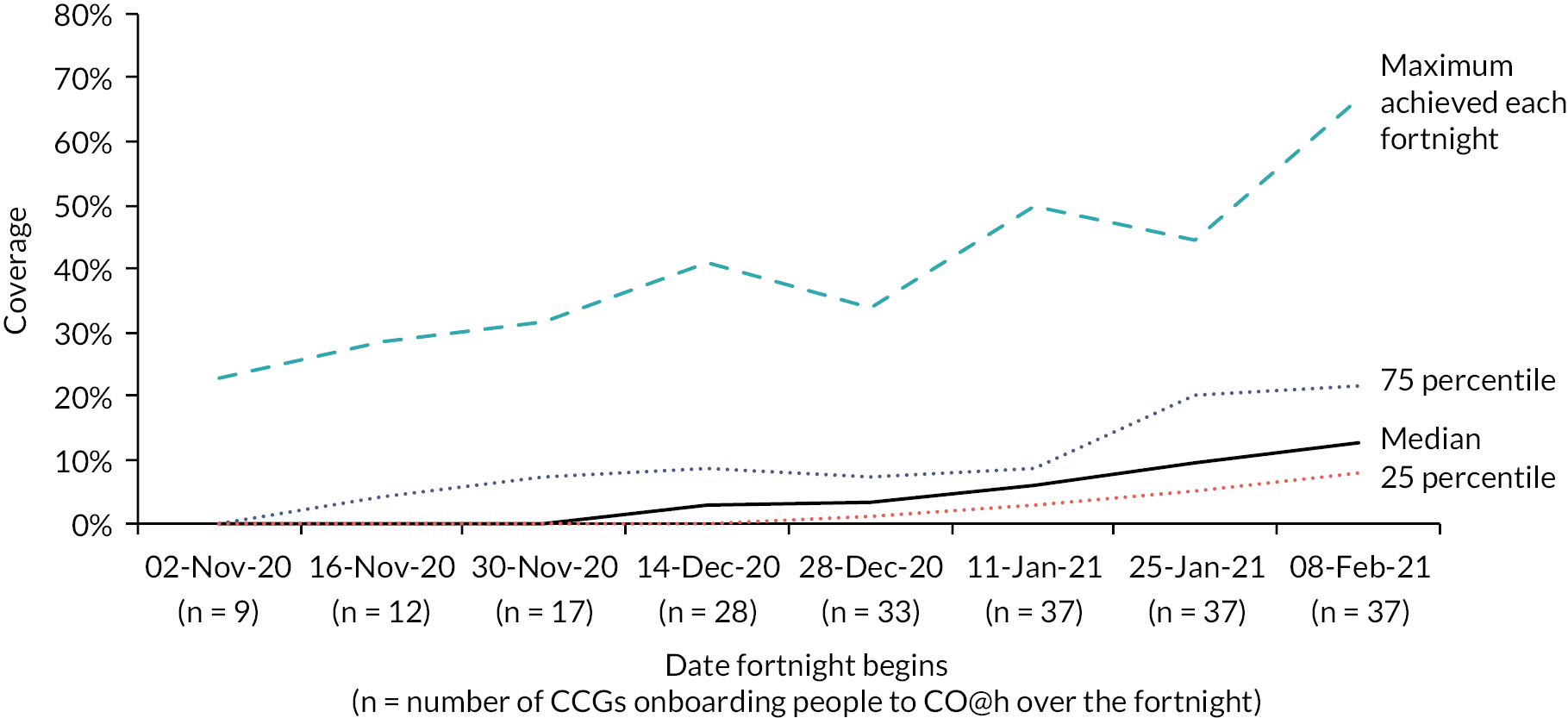
Summary data and outcomes
Summary data and outcomes for the period from 2 November 2020 to 21 February 2021 for the 37 CCGs by age band are shown in Table 3. Although there were many more positive tests recorded among the under 65s, there were approximately half as many deaths and equivalent numbers of hospital admissions. Enrolment rates after implementation was low across both age groups and highest among those aged 65–79 years.
Age band | Both age groups | ||
---|---|---|---|
65–79 | 80+ | ||
Positive tests and onboarding over the whole period: | |||
New positive diagnoses (n) | 41,437 | 27,944 | 69,381 |
Patients onboarded to CO@h (n)a | 2734 | 1382 | 4116 |
Enrolment rate (%) | 6.60 | 4.95 | 5.93 |
Positive tests and onboarding since implementationb | |||
New positive diagnoses (n) | 26,507 | 18,636 | 45,143 |
Patients onboarded to CO@h (n)a | 2610 | 1320 | 3930 |
Enrolment rate (%) | 9.85 | 7.08 | 8.71 |
Mortality: | |||
Deaths (n) | 4269 | 8699 | 12,968 |
New diagnoses over the period (%)c | 10.30 | 31.13 | 18.69 |
Hospital admissions: | |||
Admissions (n) | 12,351 | 12,128 | 24,479 |
New diagnoses over the period (%)c | 29.81 | 43.40 | 35.28 |
Hospital admissions (excluding readmissions within 28 days) (n) | 10,895 | 10,683 | 21,578 |
New diagnoses over the period (%)c | 26.29 | 38.23 | 31.10 |
Within-hospital outcomes: | |||
In-hospital deaths (n) | 2748 | 3948 | 6696 |
In-hospital deaths per admission (%) | 22.25 | 32.55 | 27.35 |
Median LOS in days (IQR) | 8 (3–15) | 9 (4–17) | 8 (4–16) |
Patients staying 2 weeks or more (n) | 3489 | 4134 | 7623 |
Proportion staying 2 weeks or more (%) | 28.25 | 34.09 | 31.14 |
Time series plots of numbers of newly diagnosed cases and numbers onboarded to the programme within the selected 37 CCGs over the study period (2 November 2020 and 21 February 2021) are shown in Figures 10 and 11. New diagnoses peaked in the fortnight straddling the new year, while onboarding peaked in the following fortnight. Profiles of all COVID-19 deaths and admissions for confirmed or suspected COVID-19 are shown in Figures 12 and 13.
FIGURE 10.
New diagnoses of COVID-19 by fortnight; data for the 37 CCGs included within the analysis.

FIGURE 11.
People onboarded to the CO@h programme by fortnight; data for the 37 CCGs included within the analysis.
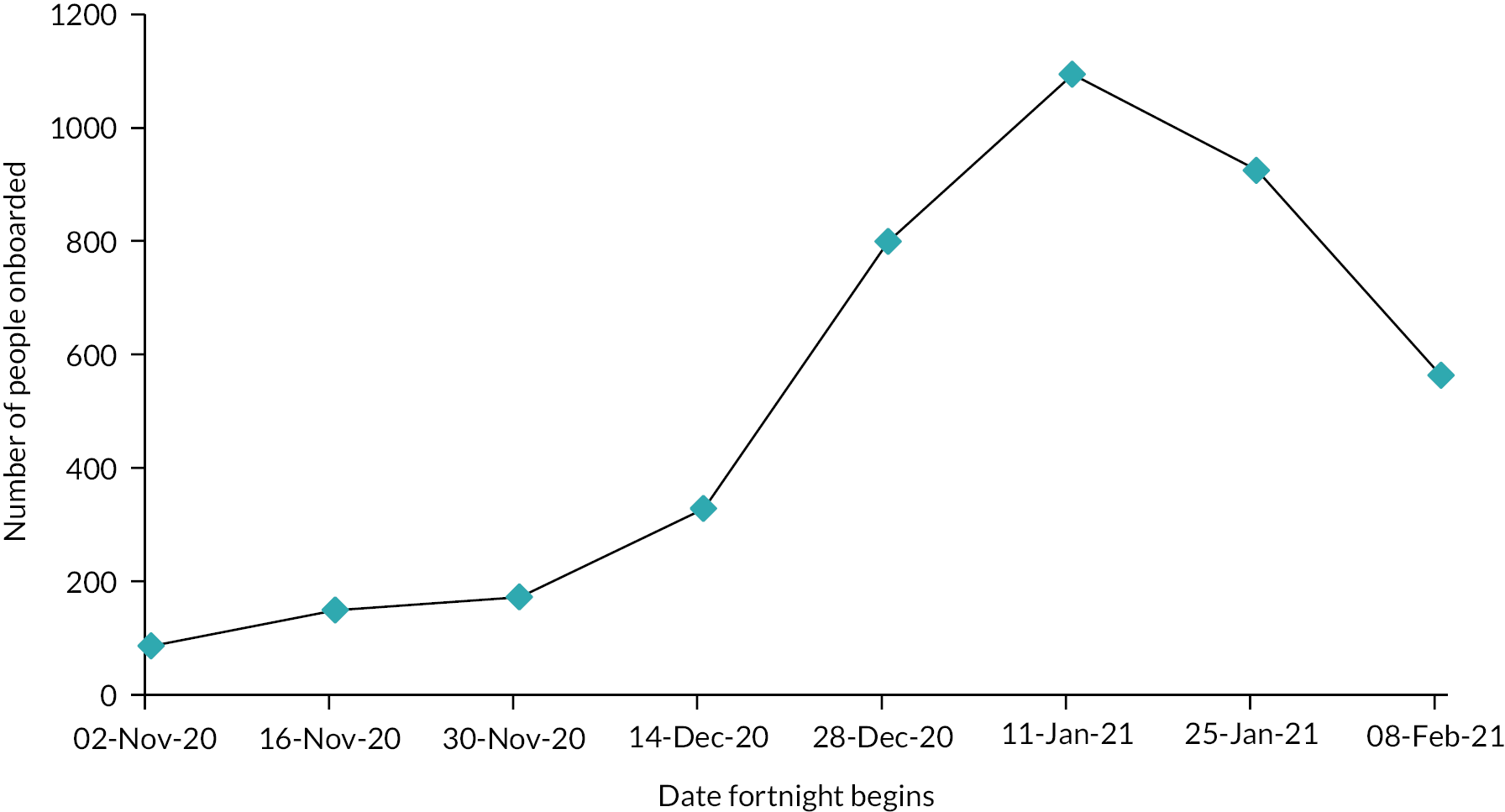
FIGURE 12.
All deaths from COVID-19 by fortnight within the 37 CCGs included in the analysis.
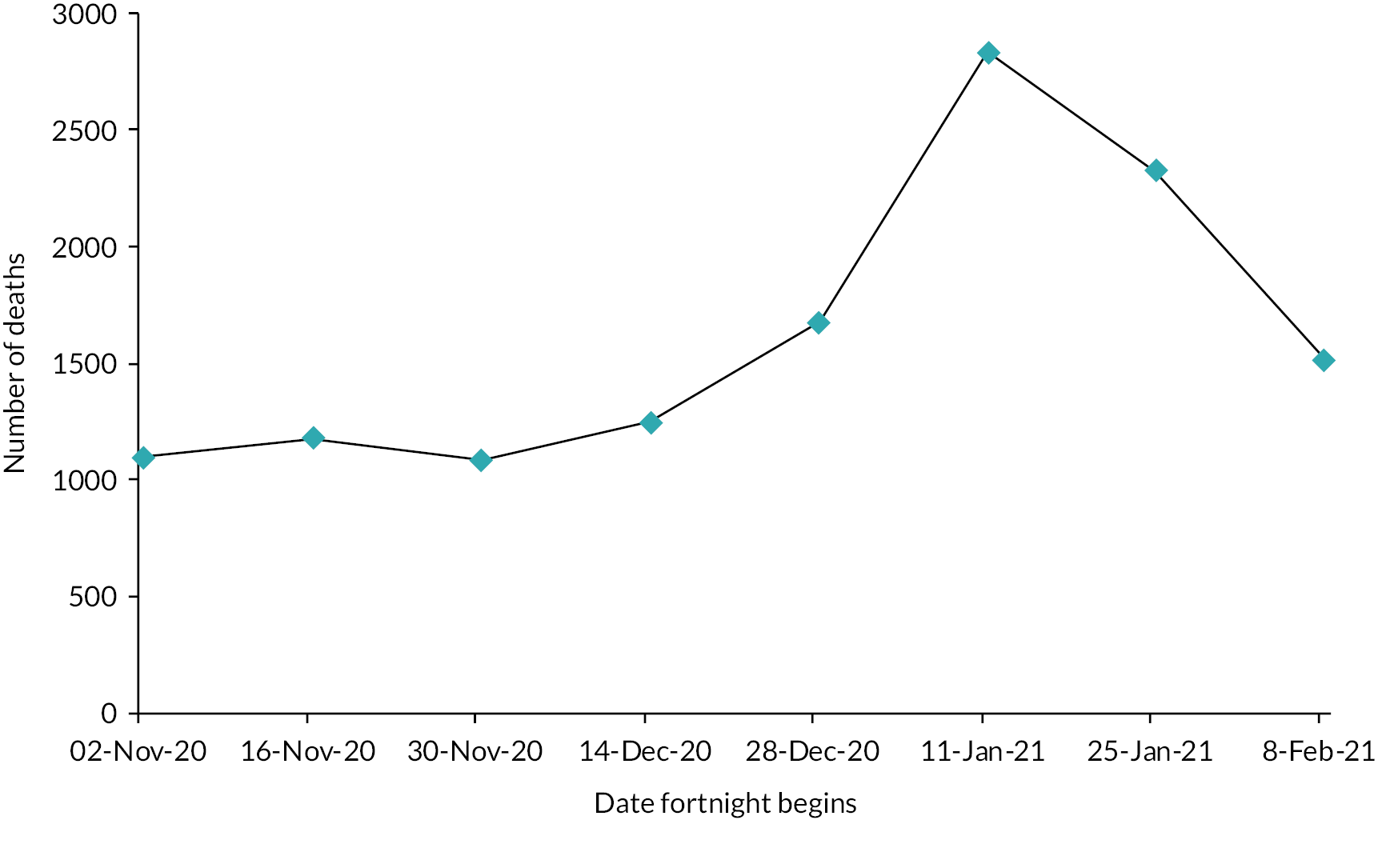
FIGURE 13.
Hospital admissions for confirmed or suspected COVID-19 by fortnight within the 37 CCGs included in the analysis.

Rates of in-hospital mortality and median lengths of stay for patients admitted to hospital with confirmed or suspected COVID-19 are shown in Figures 14 and 15. Mortality rates peak at approximately 30% over the new year and decline thereafter to around 21%. Median lengths of stay decline from the end of November 2020 while admission numbers increase.
FIGURE 14.
In-hospital mortality by fortnight for patients resident within the 37 CCGs and admitted with confirmed or suspected COVID-19.
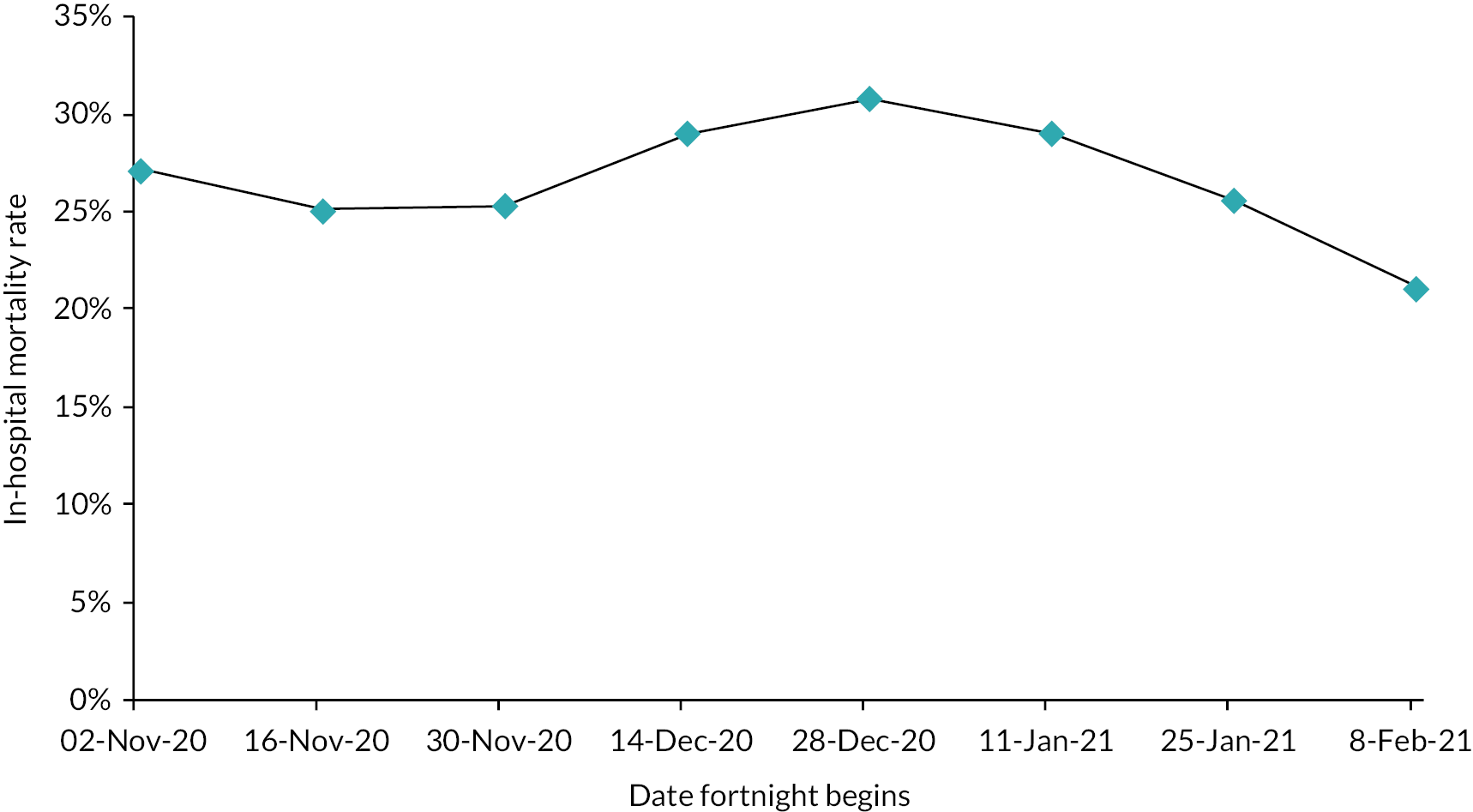
FIGURE 15.
Median LOS by fortnight of admission for patients resident within the 37 CCGs and admitted with confirmed or suspected COVID-19.
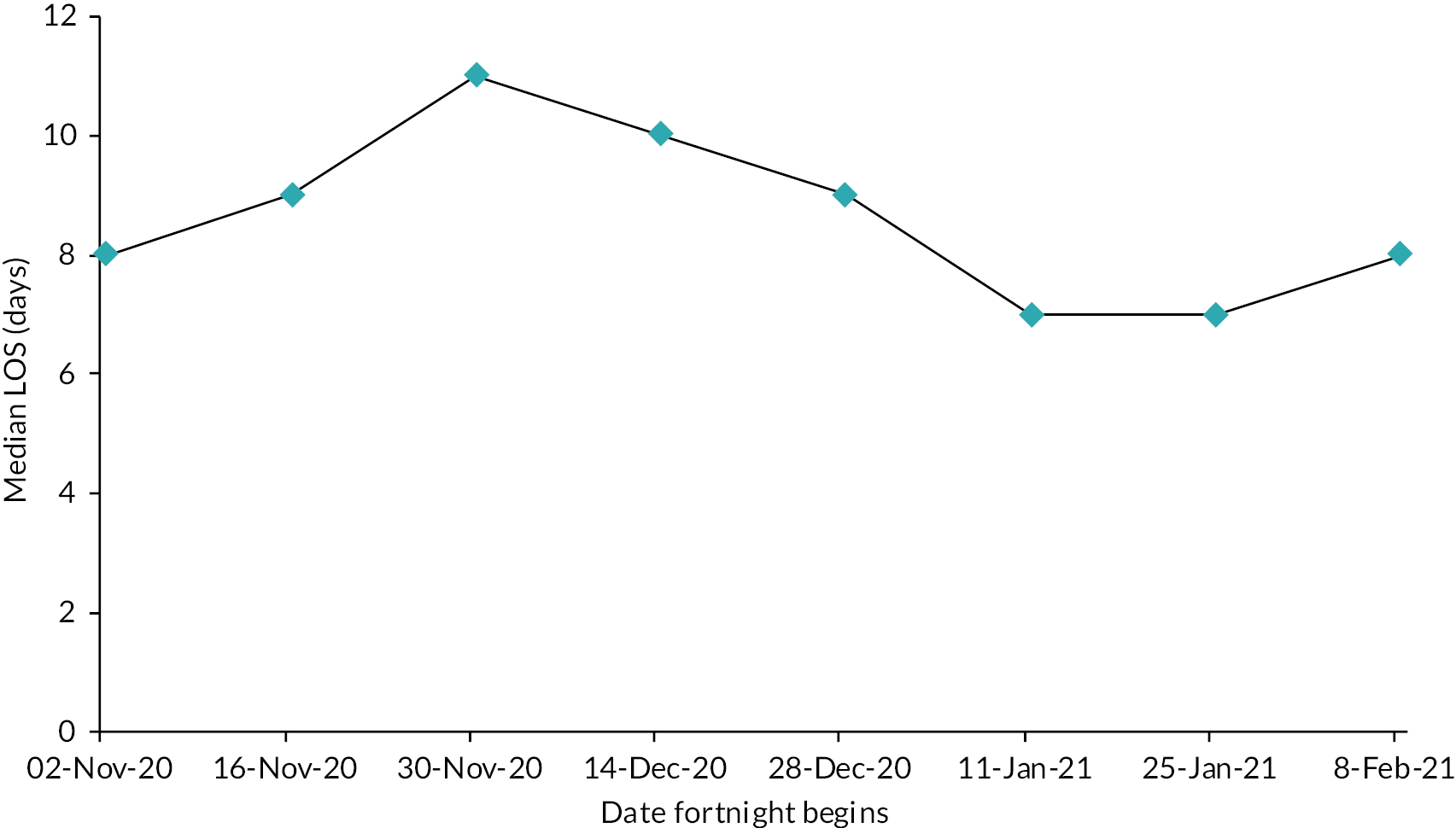
The characteristics of patients admitted with confirmed or suspected COVID-19 over the study period are shown in Table 4.
Explanatory variable | Value | Patients | |
---|---|---|---|
(n) | (%) | ||
Ethnicity | White | 19,811 | 88.99 |
Non-white | 2450 | 11.01 | |
Charlson Index | ≤5 | 19,442 | 79.42 |
>5 | 5037 | 20.58 | |
Sex | Male | 12,807 | 52.32 |
Female | 11,672 | 47.68 | |
Deprivation | Most deprived quintile | 5295 | 21.75 |
Excluding most deprived quintile | 19,053 | 78.25 | |
Age (Years) | 65–79 | 12,351 | 50.46 |
80+ | 12,128 | 49.54 | |
Virtual ward active on admission | Yes | 9537 | 27.98 |
No | 13,562 | 28.94 | |
COVID-19 bed occupancy on admission | <15% | 5932 | 24.23 |
15–22% | 6131 | 25.05 | |
22–29% | 5944 | 24.28 | |
>29% | 6472 | 26.44 | |
Weighted enrolment | <5% | 15,914 | 65.01 |
5–10% | 4182 | 17.08 | |
> 10% | 4383 | 17.91 |
Mortality and hospital admissions
Results from our models for mortality and hospital admissions are shown in Table 5, Figures 16 and 17. For every 10% increase in enrolment, mortality fell by 2% [relative risk = 0.98, 95% confidence interval (CI) 0.96 to 1.01] and admissions increased by 3% (relative risk = 1.03, 95% CI 0.99 to 1.07), but neither result is statistically significant. There is, however, a significantly higher risk of mortality and admission among the older age group (p < 0.001) and higher risk of mortality in the months following November 2020 (p < 0.001 for each month in comparison with November).
Variable | Relative risk of death (95% CI) | Relative risk of hospital admission (95% CI) | |||
---|---|---|---|---|---|
Enrolment | Every 10% increase in enrolment | 0.98 | (0.96 to 1.01) | 1.03 | (0.99 to 1.07) |
Age (years) | 80+ vs. 65 to 79 | 3.06 | (2.94 to 3.17) | 1.55 | (1.48 to 1.63) |
Month | December 2020 vs. November 2020 | 1.25 | (1.17 to 1.34) | 1.06 | (1.01 to 1.11) |
January 2021 vs. November 2020 | 1.24 | (1.14 to 1.34) | 1.03 | (0.97 to 1.11) | |
February 2021 vs. November 2020 | 1.40 | (1.27 to 1.55) | 0.97 | (0.88 to 1.07) |
FIGURE 16.
Impact of each variable used in the multivariate model on the risk of death (mortality rate ratios). Error bars denote 95% CIs.

FIGURE 17.
Impact of each variable used in the multivariate model on the risk of hospital admission (admission rate ratios). Error bars denote 95% CIs.
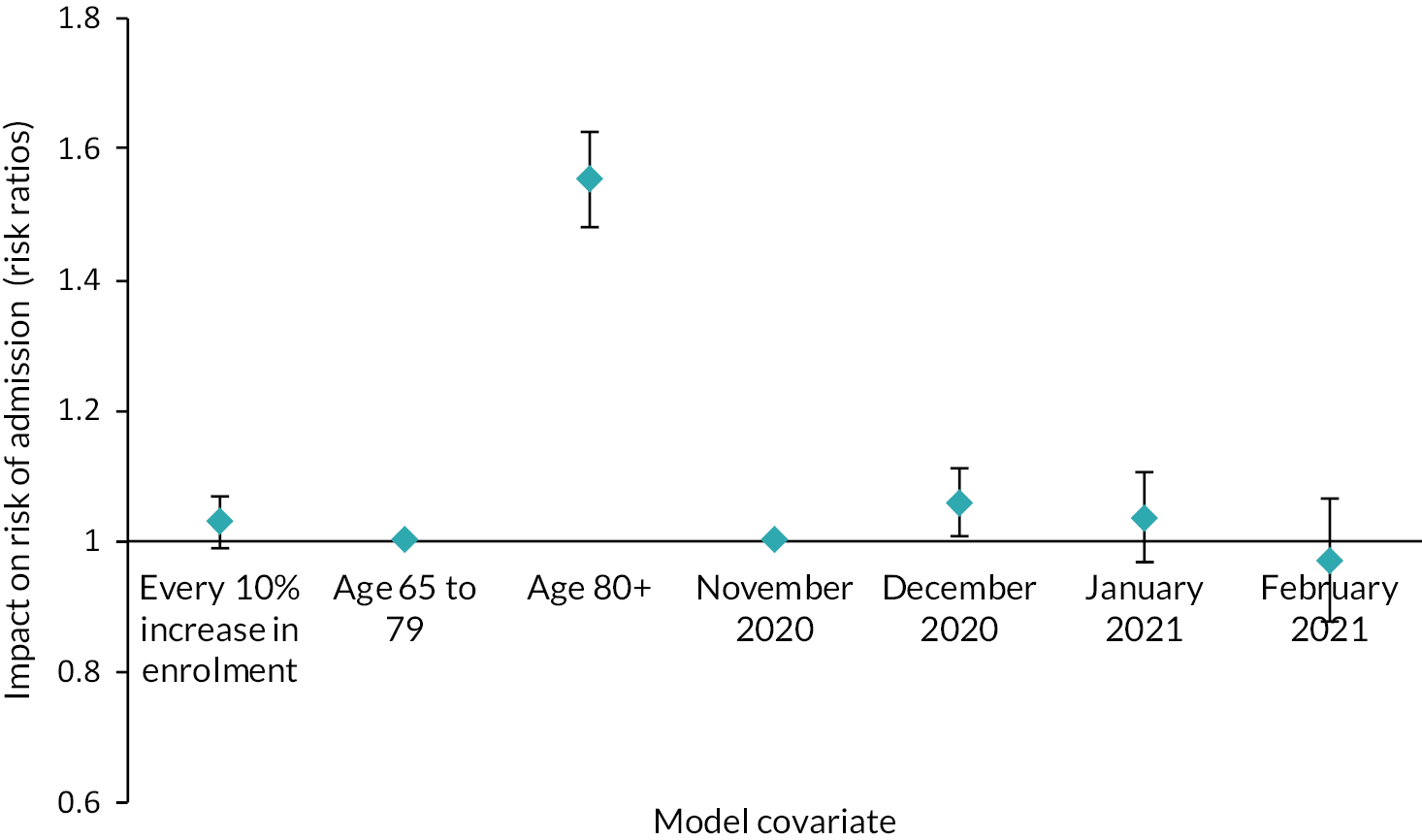
We also found no significant relationship between rates of enrolment to CO@h and the ethnicity, sex, deprivation or health status of patients admitted to hospital with COVID-19 or suspected COVID-19 (Table 6). The impact on age is of borderline statistical significance with each 10% increase in enrolment associated with a reduction of nearly four months (95% CI −7.9 months to 0.2 months, p = 0.07).
Characteristic | Percentage change with each 10% increase in enrolment (95% CI) | |
---|---|---|
Proportion non-white ethnicity | 0.43 | (−8.99 to 10.82) |
Proportion female | 0.07 | (−1.9 to 2.11) |
Proportion resident in most deprived areas (by quintile) | 0.30 | (−7.19 to 8.41) |
Proportion with Charlson scores > 5 | −2.75 | (−6.37 to 1.00) |
Change in mean age with each 10% increase in enrolment (95% CI) | ||
Mean age | −3.9 months | (−7.9 to 0.2) |
In-hospital outcomes
The results of our analysis of in-hospital outcomes are shown in Table 7, Figure 18 and Figure 19. For every 10% increase in patient enrolment, in-hospital mortality fell by 3% (relative risk = 0.97, 95% CI 0.92 to 1.03) and LOS increased by 1.8% (95% CI −1.2% to 4.9%). Again, neither result is statistically significant. Non-white ethnicity and existing COVID-19 bed occupancy on admission were both associated with shorter lengths of stay.
Variable | OR associated with in-hospital mortality (95% CI) | Relative change in LOS (95% CI) | |||
---|---|---|---|---|---|
Enrolment | Every 10% increase in enrolment | 0.97 | (0.92 to 1.03) | 1.8 | (−1.2 to 4.9) |
Age (years) | 80 + vs. 65 to 79 | 1.73 | (1.63 to 1.84) | 6.5 | (3.1 to 9.7) |
Month | December 2020 vs. November 2020 | 1.27 | (1.14 to 1.42) | −4.2 | (−9.5 to 1.5) |
January 2021 vs. November 2020 | 1.12 | (1.00 to 1.26) | −11.5 | (−14.9 to −8.1) | |
February 2021 vs. November 2020 | 0.88 | (0.76 to 1.03) | −19.7 | (−27.4 to −11.1) | |
Sex | Female | 0.71 | (0.66 to 0.76) | 2.5 | (0.0 to 5.0) |
Charlson score | >5 | 2.14 | (1.96 to 2.33) | 1.1 | (−2.2 to 4.6) |
Ethnicity | Non-white ethnicity | 1.24 | (1.07 to 1.42) | −12.0 | (−16.2 to −7.6) |
Deprivation | Lowest IMD quintile | 0.98 | (0.89 to 1.07) | 0.0 | (−3.0 to 3.1) |
Has a virtual ward | 0.96 | (0.88 to 1.04) | 1.0 | (−6.5 to 9.1) | |
COVID-19 bed occupancy in the trust on admission | Every 10% increase in occupancy | 1.04 | (0.97 to 1.11) | −5.2 | (−7.6 to −2.7) |
FIGURE 18.
Impact of each variable used in the multivariate model on the risk of in-hospital mortality for people admitted with confirmed or suspected COVID-19 (OR). Error bars denote 95% CIs.
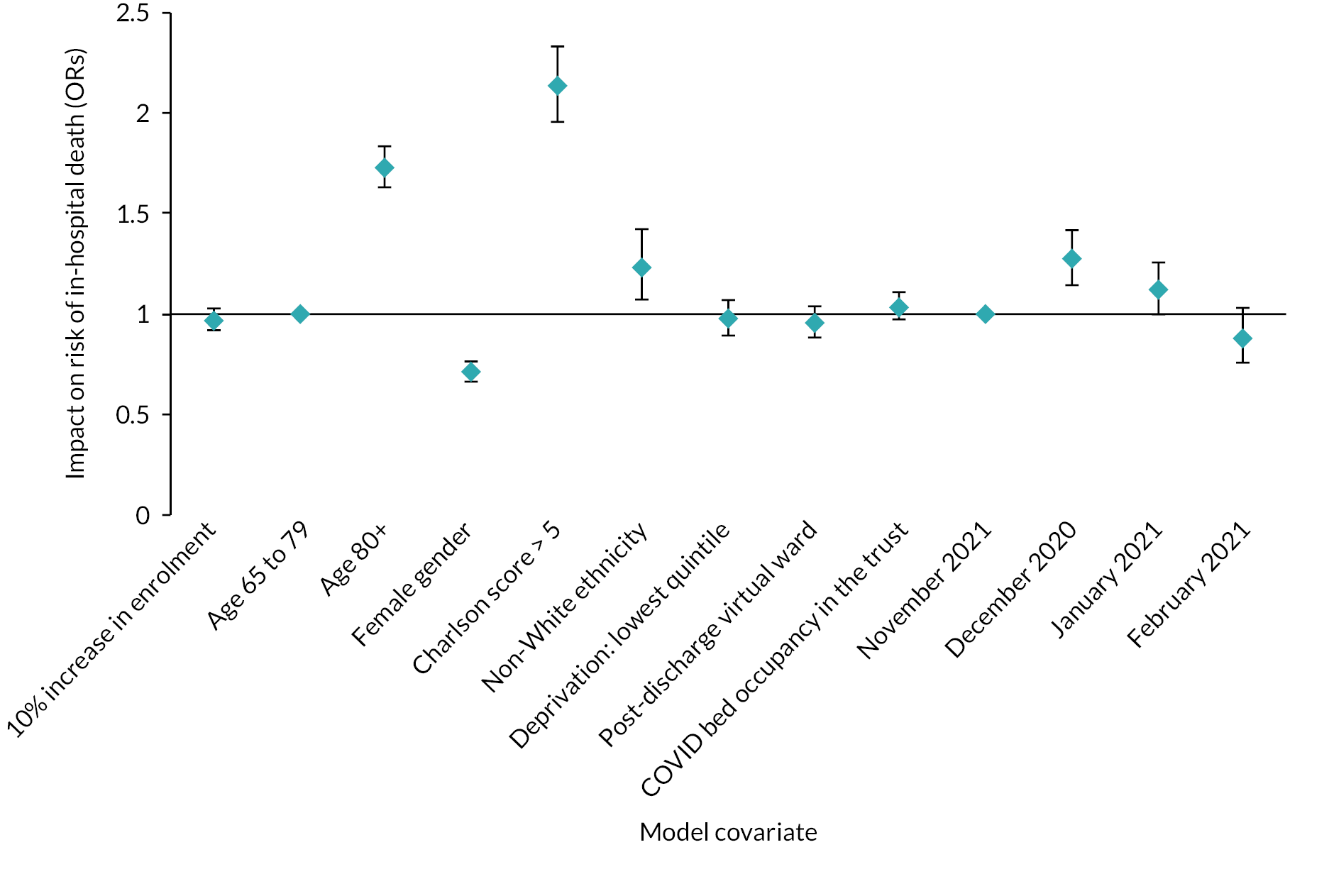
FIGURE 19.
Impact of each variable used in the multivariate model on LOS for people admitted with confirmed or suspected COVID-19 (% change). Error bars denote 95% CIs.

Sensitivity analysis
Results from sensitivity analyses are shown in Report Supplementary Material 1. None of the changes we made in our assumptions affected our findings with respect to the association between coverage of remote oximetry and outcomes.
Discussion
Summary of findings
In this study, we have found no significant association between rates of patient enrolment to the CO@h programme, and COVID-19-associated mortality or COVID-19-associated admission to hospital. For such hospital admissions, we found no significant relationship to in-hospital mortality or LOS. Also, for COVID-19-associated admissions, there appears to have been no associated change in the patient characteristics we have tested.
Interpretation
There are several possible explanations for these results, and it would be premature to conclude that the CO@h programme has had no impact. Firstly, limitations in data completeness meant that we were only able to analyse onboarding data for one-quarter of CCGs across England. This, combined with lower than expected patient enrolment within these CCGs, meant that our ability to detect any possible impact was smaller than anticipated. This also meant that some areas of the country were under-represented. Secondly, individual-level associations may not have been seen at the aggregate level. Thirdly, qualitative findings from our wider study revealed that CO@h was implemented in a variety of ways by different services within CCGs, some of which may have had more impact on outcomes than others (see Chapter 4). The possible impact of the intervention on hospital admissions was always uncertain, since the aim of the programme was to ensure people who needed hospital treatment were admitted at the right time. Also, once in hospital, the determinants of LOS are complex and multifactorial, and may have varied during the time course of the second wave of COVID-19 infection in England.
Strengths and limitations
The anticipated value of our approach with aggregated data was that it would complement the other two simultaneous quantitative evaluations of CO@h,43,45 could be carried out more rapidly and, if COVID-19 continues to stretch national health services, it could be more readily repeated as new data become available, provided the right information is routinely collected at source.
We were able to handle small number suppression and the rounding of aggregate data by multiple random sampling throughout the range of possible values and it was encouraging to discover that this uncertainty did not have a large impact on results.
Using aggregated data has some limitations because it does not enable us to trace direct links between the onboarding of an individual and their outcomes. There may also be an ecological fallacy, where individual-level effects are not observable in the aggregated data. 111 However, obtaining linked individual-level data is a complex and potentially long process that may not always be feasible when there is a need to provide rapid feedback to a developing programme and where resources are stretched. Unfortunately, however, our ability to provide rapid feedback was compromised by delays in obtaining onboarding data which proved an understandable challenge for local services in the midst of a pandemic.
The wider mixed-methods study has added insight into some of our findings and provided locally collected bespoke data against which we could verify information we received centrally about patient enrolment and data completeness. However, these checks could only be made against the 28 study sites and we were not able to verify the data from the other CCGs in the same way. Findings from surveys and interviews have helped interpret what we have found and, conversely, our data analyses have helped provide a context against which to understand the relative importance of the qualitative findings (see Chapters 4 and 8–11).
The qualitative study has also shed light on the different ways that the services had been implemented. However, with just 12 of the 28 study sites being among the 37 included in our analysis, in combination with low enrolment, it was not possible to undertake a quantitative study of the relative performance of the different types of services implemented.
We anticipated that finding a suitable comparator group during the national implementation of a programme was likely to be problematic, and we therefore avoided this problem by treating the relationship between rates of enrolment and outcome as a dose–response. However, the power to detect any impact in such an analysis depends on the level of enrolment which, in practice, was lower than we hoped. This approach also assumes any selection bias with those being enrolled is negligible.
During the period of our analysis the vaccination programme was starting and by the end of our study period 88% of people aged 65 years or over had had at least one dose. 112 Although this study investigates outcomes of people after being diagnosed with COVID-19, there is evidence that vaccination changes the subsequent risks of mortality and hospital admission113 which could have had a confounding effect on our analysis.
In the context of fortnightly data, we assumed a minimal lag between the diagnosis of COVID-19 and onboarding to CO@h. However, there was evidence from sites that they sometimes encountered delays in identifying positive cases,114 although the overall impact on this assumption is uncertain.
Comparison with other studies
Prior to this study, very little was known about the quantitative impact of the use of pulse oximeters for COVID-19 remote home monitoring. One of the other evaluations of the CO@h programme in England also found no significant impact on mortality or health service utilisation. 43 However, the study did find reductions in mortality and increases in hospital attendance (yet with lower use of critical care) among people enrolled on to the programme after attending the emergency department. 42 A study of 4384 high-risk patients receiving home monitoring of vital signs, including pulse oximetry, in one region of Galicia, Spain, found lower admissions, lengths of hospital stays and in-hospital mortality when compared with other local regions. 115 A retrospective cohort study from South Africa evaluated the use of pulse oximetry for home monitoring of people diagnosed with COVID-19 and, as with the Spanish study, deemed to be of high clinical risk. 116 They found a significant improvement in mortality but no impact on admissions to hospital; the reduction in mortality being explained by earlier admission. A recent study of CO@h carried out at one site in England demonstrated reductions in 30-day mortality and lengths of stay among people admitted to hospital over the second wave. 117 The control group in this study were patients admitted with COVID-19 who were not on the CO@h programme and it is not clear whether that would be an inherently higher risk group. In another study implemented in the UK during the first wave, patients with suspected COVID-19 attending the emergency department were discharged home with an oximeter. They observed a reattendance rate of 4.7% compared with 22.7% among a retrospective control group. 118 However, this was a younger cohort (median age 41 years) and the absolute numbers of reattendance were small (nine in all). Other studies have reported on the safety of similar programmes, but have lacked comparators. 28,43,110,119–121
Implications and further research
At the start of this study, we anticipated that services would have higher rates of patient enrolment and that complete data would be available from more CCGs. Although the use of aggregated population-level data can enable more rapid evaluation of a new service, these two elements had an influence on the power of the analysis to detect an impact. The resulting shortfall in expected data reflects the challenges of trying to centrally manage a bespoke data collection while services are already stretched. However, sufficient quantities of data are vital to determining whether a service is effective, so it is important to understand how this can be improved, for example, by concentrating data collection in a few sites and using routinely collected data wherever possible.
Furthermore, low patient enrolment raises questions about capacity of both staff and resources amid high infection rates and how it is possible to secure the best value from such a service under the circumstances. The fact that at least one CCG managed to achieve reasonably good rates of enrolment indicates the possibility for learning from others.
Other studies, as described above, have provided evidence of positive impacts on high-risk cohorts, and similar findings may follow from focusing evaluation of the CO@h programme in the same way.
Conclusions
This study provides an evaluation of the national implementation of remote home monitoring of pulse oximetry for people diagnosed with COVID-19 across the English NHS. Although we detected no significant impact on outcomes, there are potential explanations for this finding that are unrelated to the effectiveness of the programme. Taking due account of populations that may respond less well to oximetry, there is no evidence that future implementation of similar programmes would be unsafe. However, the challenges of providing sufficient data so that effectiveness can be adequately measured need to be overcome.
Chapter 6 Impact of COVID virtual wards on hospital activity
Overview
This chapter draws on a manuscript by Georghiou et al. 122 published in Lancet eClinicalMedicine (2022): https://doi.org/10.1016/j.eclinm.2022.101441. This is an open access article under the term of the Creative Commons Attribution 4.0 International License (CC BY 4.0), which permits use, distribution and reproduction in any medium, provided the original work is properly cited (see https://creativecommons.org/licenses/by/4.0/).
What was already known
-
Post-hospital discharge virtual wards have been found to have a positive impact on patient outcomes when focused on patients with specific diseases, for example those with heart disease. There has been less evidence of impact for more heterogenous groups of patients.
-
There has been little evidence thus far that post-hospital discharge virtual wards (using pulse oximetry monitoring) have helped to reduce the LOS of patients hospitalised with COVID-19 or to change rates of subsequent readmissions for COVID-19.
What this chapter adds
-
This national-scale study has found no evidence that the roll-out of post-hospital discharge virtual ward services for patients with COVID-19 in England reduced lengths of stay in hospital, or impacted rates of readmission.
-
While there is currently an absence of evidence of positive impacts for patients with COVID-19 discharged to a virtual ward, our study emphasises the need for quality data to be collected as part of future service implementation.
Introduction
There has been a long-standing ambition in the NHS in England to develop remote monitoring services to better support people with long-term conditions out of hospital. 18 Virtual ward models have been proposed as one way of easing the transition of patients being discharged home, and there is some evidence that such models (distinguished from less intensive telemonitoring) may help to reduce mortality and readmissions, especially where the intervention is focused on patients with a specific disease, for example heart failure. 123
In facilitating the national roll-out of CVW services in January 2021, NHSEI stated that ‘CVWs have been proven to reduce admissions/bed occupancy and improve LOS’, and so could act to mitigate the pressure on hospital beds, which was severe at the time. 6 However, evidence of the impact of such services on hospital activity has been lacking. A US study found a reduction in rates of emergency department presentation or readmission for a group of 225 patients enrolled on to a post-discharge monitoring programme,124 and two studies (from the Netherlands, and a case study from England) estimated reduced lengths of hospital stays, but with small patient cohorts and uncontrolled methods. 125,126 Elsewhere, where outcomes have been reported, they have tended to focus on rates of patient escalation (including readmissions) in the absence of a comparator. Occasionally, outcomes are considered against a perceived optimal level (e.g. a 10% conversion rate to admissions for ambulatory care pathways),31 or the reported performance of services elsewhere. 25,31,36,121,127–129
Data on which patients were enrolled to CVWs in England were not collected, but information was assembled on launch dates of CVWs at each hospital trust. By late February 2021, the large majority of acute hospital trusts in England had a service available for use. The analysis described in this chapter aimed to use patient-level admissions data to provide quantitative evidence of the impact of the service, to contribute to future development of post-discharge home monitoring programmes during the remainder of the COVID-19 pandemic, and beyond.
The primary aim of the analysis described in this chapter was to address the following research question:
What was the impact of CVWs on the length of COVID-19 hospitalisations and rates of subsequent readmission for COVID-19?
A secondary aim, in the context of COVID-19s widely reported differential effects on outcomes for groups of patients (e.g. by gender, age and ethnicity),12,130 was to reflect on associations found between patient characteristics and hospital outcomes.
Methods
A summary of the methods is provided below; further details can be found in Chapter 2 and Report Supplementary Material 1.
We used HES APC data to extract information on patients with a confirmed or suspected primary diagnosis of COVID-19 (ICD-10 codes U071 or U072) who were discharged alive between 17 August 2020 and 28 February 2021 (during England’s second COVID-19 wave). We limited our analysis to 123 acute hospital trusts for which there was a known CVW service start date (from a data set collected by KSS AHSN).
Using patient-level (pseudonymised) data, we developed multivariate models to examine the impact of the availability of CVW (the primary independent variable of interest) on two outcomes: the length of inpatient stay for COVID-19, and on subsequent readmissions for COVID-19. Factors included in our models are shown in Table 8.
Modelling factors | Categories assigned to each COVID-19 patient discharge | Source |
---|---|---|
Age at admission (years) | 0–17, 18–49, 50–64, 65–79, 80s+ | HES APC |
Gender | Male, female | HES APC |
Ethnic group | Asian, black, white, mixed, other, unknown | HES APC |
Charlson comorbidity index category54 | 0, 1, 2, 3, 4, 5, 6+ | HES APC (activity during 2 years prior to discharge) |
Whether the stay was the person’s first COVID-19 hospital stay | Yes, no | HES APC |
Whether the inpatient stay was an emergency admission | Yes, no (elective) | HES APC |
Deprivation quintile IMD (2019) | 1 – most deprived to 5 – least deprived, with a sixth category for unknown area | UK Ministry of Housing, Communities and Local Government, linked via LSOA in HES APC |
Period of the discharge date | 14 categories, each covering a 14-day period starting from 17 August 2020 | HES APC |
Proportion of all acute beds occupied by patients with COVID-19 | A trust- and week-specific continuous measure (mapped to week of discharge) | HES APC |
Availability of CVW | Yes, No | KSS AHSN bespoke data collection |
For our main analyses negative binomial regression was used to examine the relationship between independent variables and LOS, and logistic regression was similarly used for occurrence of any readmission. To account for clustering at the level of the hospital trust, generalised estimating equation approaches were used. Descriptive statistics and a number of sensitivity analyses were also carried out.
Results
Participants
We found 139,619 discharges between 17 August 2020 and 28 February 2021 where the patient had a primary diagnosis of COVID-19 recorded during their inpatient stay, and had not died in hospital. These discharges belonged to 129,461 individuals. Table 9 shows characteristics of these COVID-19 patient discharges.
Characteristic | CVW availability | p value of differencea | |||||
---|---|---|---|---|---|---|---|
All COVID-19 discharges | Discharges with no CVW available | Discharges with CVW available | |||||
N = 139,619 (100 %) | N = 81,174 (58.1%) | N = 58,445 (41.9%) | |||||
(N) | (%) | (N) | (%) | (N) | (%) | ||
Gender | 0.127 | ||||||
Male | 75,429 | (54.0) | 43,714 | (53.9) | 31,715 | (54.3) | |
Female | 64,190 | (46.0) | 37,460 | (46.1) | 26,730 | (45.7) | |
Age band | < 0.0001 | ||||||
0–17 | 2041 | (1.5) | 1242 | (1.5) | 799 | (1.4) | |
18–49 | 30,076 | (21.5) | 17,647 | (21.7) | 12,429 | (21.3) | |
50–64 | 39,776 | (28.5) | 22,620 | (27.9) | 17,156 | (29.4) | |
65–79 | 38,140 | (27.3) | 22,384 | (27.6) | 15,756 | (27.0) | |
80+ | 29,586 | (21.2) | 17,281 | (21.3) | 12,305 | (21.1) | |
Ethnic group | < 0.0001 | ||||||
Asian | 16,360 | (11.7) | 9089 | (11.2) | 7271 | (12.4) | |
Black | 6002 | (4.3) | 3172 | (3.9) | 2830 | (4.8) | |
Other | 4969 | (3.6) | 2501 | (3.1) | 2468 | (4.2) | |
White | 93,688 | (67.1) | 56,357 | (69.4) | 37,331 | (63.9) | |
Mixed | 1394 | (1.0) | 779 | (1.0) | 615 | (1.1) | |
Unknown | 17,206 | (12.3) | 9276 | (11.4) | 7930 | (13.6) | |
IMD 2019 quintile of residence | < 0.0001 | ||||||
1 (most deprived) | 38,247 | (27.4) | 23,848 | (29.4) | 14,399 | (24.6) | |
2 | 31,794 | (22.8) | 18,413 | (22.7) | 13,381 | (22.9) | |
3 | 26,391 | (18.9) | 14,975 | (18.4) | 11,416 | (19.5) | |
4 | 22,697 | (16.3) | 12,859 | (15.8) | 9838 | (16.8) | |
5 (least deprived) | 19,354 | (13.9) | 10,367 | (12.8) | 8987 | (15.4) | |
Unknown | 1136 | (0.8) | 712 | (0.9) | 424 | (0.7) | |
Charlson comorbidity score | 0.402 | ||||||
0 | 47,904 | (34.3) | 27,858 | (34.3) | 20,046 | (34.3) | |
1 | 15,012 | (10.8) | 8667 | (10.7) | 6345 | (10.9) | |
2 | 23,403 | (16.8) | 13,658 | (16.8) | 9745 | (16.7) | |
3 | 14,677 | (10.5) | 8528 | (10.5) | 6149 | (10.5) | |
4 | 9538 | (6.8) | 5587 | (6.9) | 3951 | (6.8) | |
5 | 8585 | (6.1) | 5056 | (6.2) | 3529 | (6.0) | |
6+ | 20,500 | (14.7) | 11,820 | (14.6) | 8680 | (14.9) | |
First COVID-19 admission? | < 0.0001 | ||||||
Yes | 129,041 | (92.4) | 75,948 | (93.6) | 53,093 | (90.8) | |
No | 10,578 | (7.6) | 5226 | (6.4) | 5352 | (9.2) | |
COVID-19 admission – emergency? | < 0.0001 | ||||||
Yes | 136,643 | (97.9) | 79,642 | (98.1) | 57,001 | (97.5) | |
No | 2976 | (2.1) | 1532 | (1.9) | 1,444 | (2.5) | |
COVID-19 stay discharge date (from date) | < 0.0001 | ||||||
17/08/2020 | 541 | (0.4) | 488 | (0.6) | 53 | (0.1) | |
31/08/2020 | 619 | (0.4) | 512 | (0.6) | 107 | (0.2) | |
14/09/2020 | 1561 | (1.1) | 1354 | (1.7) | 207 | (0.4) | |
28/09/2020 | 2952 | (2.1) | 2625 | (3.2) | 327 | (0.6) | |
12/10/2020 | 4767 | (3.4) | 4188 | (5.2) | 579 | (1.0) | |
26/10/2020 | 7872 | (5.6) | 6661 | (8.2) | 1211 | (2.1) | |
09/11/2020 | 10,014 | (7.2) | 8246 | (10.2) | 1768 | (3.0) | |
23/11/2020 | 9135 | (6.5) | 7119 | (8.8) | 2016 | (3.4) | |
07/12/2020 | 8696 | (6.2) | 6452 | (7.9) | 2244 | (3.8) | |
21/12/2020 | 14,214 | (10.2) | 10,274 | (12.7) | 3940 | (6.7) | |
04/01/2021 | 24,578 | (17.6) | 15,410 | (19.0) | 9168 | (15.7) | |
18/01/2021 | 25,632 | (18.4) | 11,637 | (14.3) | 13,995 | (23.9) | |
01/02/2021 | 18,193 | (13.0) | 4600 | (5.7) | 13,593 | (23.3) | |
15/02/2021 | 10,845 | (7.8) | 1608 | (2.0) | 9237 | (15.8) | |
Proportion of beds occupied by patients with COVID-19 at time of discharge | < 0.0001 | ||||||
0−9.99% | 17,370 | (12.4) | 13,439 | (16.6) | 3931 | (6.7) | |
10−19.99% | 34,052 | (24.4) | 18,865 | (23.2) | 15,187 | (26.0) | |
20−29.99% | 35,514 | (25.4) | 20,173 | (24.9) | 15,341 | (26.2) | |
30−39.99% | 24,841 | (17.8) | 13,999 | (17.2) | 10,842 | (18.6) | |
40−49.99% | 16,757 | (12.0) | 8476 | (10.4) | 8281 | (14.2) | |
50−59.99% | 10,035 | (7.2) | 5550 | (6.8) | 4485 | (7.7) | |
60−69.99% | 938 | (0.7) | 560 | (0.7) | 378 | (0.6) | |
70−79.99% | 112 | (0.1) | 112 | (0.1) | 0 | (0.0) |
The number of patients with COVID-19 discharged in this time across England (not limited to the 123 analysis trusts) was 142,216. Thus, our analysis includes 98.2% of recorded inpatient stays of patients in England with a primary COVID-19 diagnosis, discharged alive.
Table 9 also shows the characteristics of the patients split into two key analysis groups: patients discharged where no CVW was available (N = 81,174, 58.1%), and those discharged where a CVW was available (N = 58,445, 41.9%). These two sets of patients were well matched in terms of gender and comorbidities. Patients discharged where a CVW was available were more likely to be in the age range 50 to 64 and members of non-white ethnic groups, and less likely to be from the most deprived areas. As a consequence of the accelerated set-up of CVW services from early January 2021 (Figure 20), discharge dates of patients where a CVW was available tended to take place towards the end of the analysis period, with nearly four in five occurring on or after 4 January 2021 (compared with two in five of the discharges where a CVW was not available). Discharges of patients taking place where a CVW was available were more likely to be occurring in the context of higher levels of beds occupied by patients with COVID-19.
FIGURE 20.
Cumulative start dates of CVW services in England’s non-specialist acute hospital trusts (n = 123 trusts); 14 trusts had no CVW by 22 February 2021. The grey box represents the main period of this analysis.

COVID-19 length of stay
The mean LOS for patients discharged where a CVW was available was 10.1 days [standard deviation (SD) 11.3 days], compared with 8.9 days (standard deviation 10.3 days) for patients where a CVW was not available (Figure 21). This was an unadjusted incidence rate ratio (IRR) of 1.13, or 13% longer for discharges taking place from hospital trusts with a CVW. Distributions of LOS for COVID-19 for patients discharged with and without an available CVW are provided in Report Supplementary Material 5.
FIGURE 21.
Mean (unadjusted) length of COVID-19 hospitalisations and adjusted IRRs, with respect to categories of factors in multivariate model.
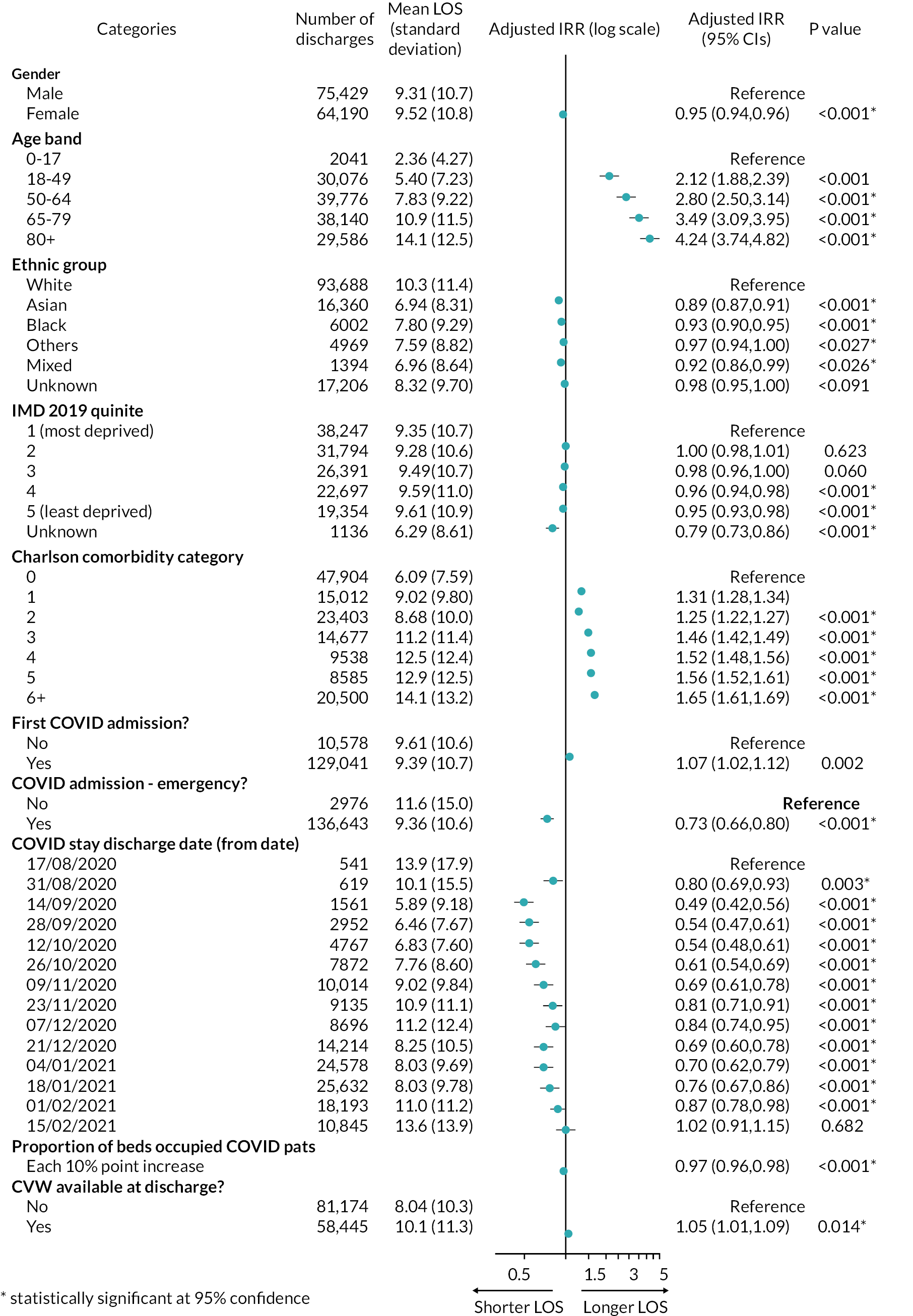
Fully adjusting for all covariates, we found an adjusted IRR of 1.05 (95% CI 1.01 to 1.09) for discharges where a CVW was available compared with those where a CVW was not available, indicating that the LOS was 5% longer for relevant COVID-19 inpatient stays where a CVW had been available.
We additionally found strong, generally positive gradients of LOS for increasing age and comorbidity (note that these relationships relate to all 139,619 discharges and not just those with a CVW available). Females had shorter lengths of stay than males (adjusted IRR 0.95, 95% CI 0.94 to 0.96), as did all non-white ethnic groupings, compared with the white group (e.g. Asian patients had an adjusted IRR of 0.89, 95% CI 0.87 to 0.91, and black patients an adjusted IRR of 0.93, 95% CI 0.90 to 0.95). Patients resident in the most affluent population quintiles tended to have slightly shorter lengths of stay than those in the most deprived quintile. First COVID-19 inpatient stays were longer than subsequent stays (adjusted IRR 1.07, 95% CI 1.02 to 1.12). The LOS shortened significantly for all time periods after 31 August 2020, until mid-February 2021. As the proportion of acute beds occupied by patients with COVID-19 increased, LOS tended to fall: for every ten-percentage-point increase in this proportion, the adjusted IRR was 0.97 (CI 0.96 to 0.98).
Model variants carried out as sensitivity analyses marginally altered the resulting adjusted IRR of the CVW-available discharges (see Report Supplementary Material 5). Shortening the time period variable to seven days reduced the IRR slightly such that the difference was no longer significant at 95% statistical confidence level (IRR 1.04, 95% CI 0.997 to 1.08), while increasing the period to 28 days increased the adjusted IRR to 1.08 (95% CI 1.04 to 1.13). The addition of earlier (wave 1) data made the two groups effectively indistinguishable from one another (IRR 1.01, 95% CI 0.97 to 1.05). The two alternative LOS outcomes tested had little impact on the adjusted IRRs compared with the base model.
COVID-19 readmission within 28 days
Of the patients discharged from trusts with a CVW available, 13.0% were readmitted with COVID-19 within 28 days, compared with 13.2% from trusts where no CVW was available (Figure 22). Adjusting for all variables, we found a non-significant difference in COVID-19 readmissions within 28 days for discharges where a CVW was available compared to those where a CVW was not available (adjusted OR of 0.97, 95% CI 0.91 to 1.03). Our sensitivity analyses demonstrated the robustness of these findings (see Report Supplementary Material 5).
FIGURE 22.
Numbers (%) of patients readmitted for COVID-19 within 28 days and adjusted OR with respect to factors in multivariate model.

As with LOS, we found generally positive gradients of relative odds of readmission with increasing age and comorbidity. Females had significantly lower odds of readmission than males (adjusted OR 0.78, 95% CI 0.75 to 0.81). Black patients had lower odds of readmission compared with white patients (adjusted OR 0.91, 95% CI 0.84 to 0.99), while no other ethnic categories (for patients with known ethnicity) showed similarly significant differences. There were no apparent differences in the odds of COVID-19 readmission for patients resident in less deprived areas compared with those in the most deprived quintile areas.
For both LOS and readmissions models, adding variables one by one to our multivariate models (an example is shown in Report Supplementary Material 5) revealed that the addition of the 14-day time period categories, and the clustering by hospital trust had large impacts on the estimated adjusted ratios, but all additional variables (age, comorbidity index, and so on) had relatively marginal additional impacts.
Discussion
Key findings
In this analysis of patients with COVID-19 discharged from hospital during the second wave of the COVID-19 pandemic in England, we found no evidence of a relationship between the availability of hospital-based CVWs and subsequent rates of readmission for COVID-19. We did, however, find that the LOS for patients hospitalised with COVID-19 was 5% longer where a CVW was available.
Comparisons with other studies
There are few studies available against which we can compare our results. Our finding of no difference with respect to readmissions appears to contrast with that of an analysis of a post-discharge remote monitoring model implemented in five Boston (MA) hospitals. This study found that enrolled patients had a reduced odds (OR 0.54, p-value 0.04) of attending emergency department or being readmitted within 30 days of discharge, compared to those not enrolled. 124 However, given the relatively large CIs in their estimate, emergency department attendances being much more common than readmissions for the enrolled patient cohort, and those readmissions being for any cause, our findings may not be inconsistent.
Two other analyses have claimed reductions in length of hospitalisation associated with post-discharge models, on a scale of 30–40%. One, related to implementation in a Netherlands hospital, estimated a reduction in the LOS of 5.0 days (with a resulting mean LOS of 10.6 days),125 and a second, in an English hospital trust, reported a reduced LOS of 10 days, against an average of 17 days prior to implementation. 126 Both analyses, however, were based on small patient cohorts with no formal statistical testing. In the first analysis it was not clear how estimates of reductions were made, and in the second there was no attempt made to control for differences between the compared groups.
More generally, there is evidence of non-COVID-19-related post-discharge virtual wards having a positive impact on readmissions when employed as a disease-specific intervention (at least in the case of heart failure), but there is not similar evidence of impact for more mixed groups of patients at high-risk of chronic disease. 123 Our findings may be consistent with this picture, considering the heterogeneity of patients with COVID-19, and the multiorgan effects of COVID-19 infection. 131–133
Strengths and limitations
With respect to the analysis of post-discharge COVID monitoring services, our study is currently unique in its scope. We have made use of a national administrative hospital data set, and have analysed almost 140,000 COVID-19 admissions in an effort to detect an impact of a national-scale roll-out of post-discharge remote monitoring services. We have been pragmatic in our use of the available data and have controlled for characteristics available to us.
Nevertheless, there are several limitations, and our results should be interpreted with caution. We did not know which patients were enrolled on to a CVW, and so treated all patients as potentially having received the intervention where one was available at a trust. We had no information about important clinical factors (e.g. ICU admission, clinical readings, including oxygen saturation levels, and specific treatments received). The location to which patients were discharged (home, care home, etc.) was not known, and we also had no information about out-of-hospital deaths (and this during a period in which rates of out-of-hospital deaths had been persistently above long-term norms). 134 We extracted data only on patients discharged alive, so the impact (if any) of patients with COVID-19 who died during their hospital stay has not been accounted for. Our analyses have assumed that these factors, which are likely to have been associated to a greater or lesser degree with eligibility for referral, and/or with risks of readmission and long stays, were well balanced between the two key groupings of patients after having adjusted for model factors, but the extent to which this is the case is not known. However, in terms of at least one of these missing factors, an analysis of a cohort of 419 patients with COVID-19 managed on a post-discharge pathway suggests that out-of-hospital deaths may have been relatively rare (<0.1%). 120
As outlined in Chapter 4, for seven hospital-based sites we were able to estimate a range of 4–65% of discharged patients with COVID-19 may have been monitored on a CVW. But nationally, we did not know the scale of each trust’s implementation and our analysis wasn’t able to examine potential dose–response relationships.
We also know that services were implemented with significant differences, depending on the locally adopted models of care. Our analysis treated the intervention as being homogeneous, and was not able to estimate differential impacts by hospital trust, although these impacts may have existed.
Interpretation
We found longer COVID-19 inpatient stays associated with implementation of CVWs; this was not expected for a service that aimed to support the early discharge of patients. One explanation might be that CVW eligibility criteria influenced hospitals’ discharge decisions such that patients were kept in a bed for longer than they otherwise might have been. It is not clear whether local pre-hospital (CO@h) services starting at similar times might have affected admissions in such a way that patients were admitted sooner and stayed for longer. The finding might, in part, also be a consequence of many CVW services launching during the falling (improving) edge of England’s second COVID-19 wave, with longer lengths of stay being a consequence of improving bed capacity.
We did attempt to control for the latter effect in our addition of trust-specific weekly occupied beds data, and with our inclusion of a time period variable in our multivariate analyses. In one sensitivity analysis, when we shortened the time period to seven days (from 14), we no longer found a statistically significant difference in LOS at the 95% level (adjusted IRR 1.04, 95% CI 1.00 to 1.08, p-value 0.07). The time factor used in our fully adjusted models was somewhat arbitrary, and it is not clear that 14 days was better used above seven days. The finding of a longer LOS associated with CVW therefore needs to be considered with some care.
The expected impact of such services on readmissions following discharge is not obvious. These services aimed to detect early deterioration for more rapid escalation to potentially improve ultimate outcomes. It may be the case therefore, that readmissions might have been expected to increase. Unfortunately, with limitations in the available data described above, we were not able to assess any changes in the severity of patients on readmission.
As a result of the limitations in the available data outlined above, there is a strong possibility that any positive impacts – at a local hospital trust level, or for specific versions of the pathway – were not ultimately able to be detected. This limits the extent to which this analysis can contribute to the further development of these, or similar services, and highlights the importance of making routine data collection – appropriate for use in evaluation – an intrinsic part of services’ operations.
Briefly, we reflect on observed relationships between patient characteristics and COVID-19 lengths of stay, and readmissions. Positive relationships between age and comorbidities and these outcomes have been observed elsewhere. 135–138 Meanwhile, differences with respect to gender (in this study’s case both outcomes were lower for females vs. males) tend to have been absent. 131,136,138,139 We found shorter lengths of stay and lower rates of readmissions for patients belonging to specific ethnic groups with respect to white patients (this was the case for patients belonging to black ethnic groups for both outcomes, and also Asian, mixed and ‘other’ ethnic groups for LOS). Evidence from elsewhere is mixed, but at least one US study found shorter lengths of stay for hospitalised non-white patient groups. 138 Ultimately, however, care is needed when interpreting findings for specific characteristics separate from the context of the other model variables. It is possible, for example, that non-white ethnic groups (to give one example) may be acting as fine-scale markers of urban or more deprived areas (in addition to the model deprivation categories) and as markers of younger patients (within modelled age bands).
Conclusion
Our analysis has not shown any evidence of early discharges or changes to rates of readmissions associated with the roll-out of CVWs in England. While this may reflect the true impact of the service, it may be, in part, a consequence of the lack of certain data: on which patients were enrolled (or even how many), what COVID-19 treatments were received while in hospital, and their clinical observations. It is possible that CVW services had a range of impacts that we have ultimately not been able to reveal.
Chapter 7 Cost evaluation of remote home monitoring services for COVID-19 patients in England during wave 2 from October 2020 to April 2021
Overview
What was already known
-
Previous economic analyses of remote home monitoring for patients with COVID-19 have reported the resources used and the amount spent per patient monitored.
What this chapter adds
-
The mean running cost per patient monitored under CO@h services was slightly lower compared with CVW services (£527.5 vs. £599.1).
-
For both CO@h and CVW services the mean cost per patient monitored at home was lower in sites using both tech-enabled and analogue modes of data submission compared with the sites using analogue-only modes.
-
Mortality rates were slightly higher for the patients under CO@h services in comparison with patients under CVW services (0.9% vs. 0.7% respectively).
-
The majority of the staff involved in running CO@h and CVW services were clinical staff. Over 50% of staff (clinical and non-clinical staff combined) were employed at band 5 or below in the CO@h service, whereas in CVW services there were slightly more staff at band 6 or above.
Background
While cost analyses have been carried out for remote home monitoring of some conditions,140 there is a gap in the literature regarding effectiveness, costs, resources and workforce associated with remote monitoring for COVID-19. 36 The aim of this study was to evaluate the costs of implementing remote home monitoring for patients with COVID-19 during wave 2 of the pandemic (October 2020–April 2021). Costs related to setting up and running the CO@h and CVW services were identified and measured and stratified by the mode of technology used for patients’ data submission, whether tech-enabled and analogue modes were used, or analogue-only.
The research questions that this analysis addressed were:
-
What were the numbers of patients involved (triaged, monitored, deteriorated, escalated, and number of deaths) in the CO@h and CVW services for the period October 2020 to April 2021?
-
What was the composition of the workforce involved in providing these services, and did this vary between tech-enabled and analogue and analogue-only data submission modes?
-
What were the costs of setting up the CO@h and CVW services and how do these costs vary between tech-enabled and analogue and analogue-only data submission modes?
-
What were the mean costs per patient monitored when running the CO@h and CVW services, and how do these costs vary between tech-enabled and analogue and analogue-only data submission modes?
-
How much time did sites spend on activities required to run the CO@h and CVW services and how did these times vary between tech-enabled and analogue and analogue-only data submission modes?
Methods
Data collection
We gathered retrospective information using a data collection form provided to the 28 sites that was also used for the analyses in Chapters 4–6. Designated site leads and data leads at each research site were contacted and asked to complete the form in Microsoft Word, and return it electronically to a member of the research team. During May to June 2021, we collected data for the period 1 October 2020 to 30 April 2021 (see Chapter 2 for further information). We collected information regarding number of patients involved (triaged, monitored, deteriorated, escalated, and number of deaths), and the number of staff and resources used for setting up and running the sites (see Project website documentation – Study instruments).
To explore the differences in staff, resources, patients involved and patient outcomes further between tech-enabled and analogue versus analogue-only data submission modes, additional data were collected from four of the 28 sites. All of these four sites were using both tech-enabled and analogue data submission modes. We conducted an in-depth analysis of the time spent per patient for specific activities of the programme and calculated the cost per patient for all the activities. The data collection form for these four sites included additional questions regarding the total number of patients monitored using each data submission mode, staffing (role/band, time spent per patient on every activity) and the frequency of contacts made with patients (see Project website documentation – Study instruments).
Data analysis
Patient and staff numbers
Sites were asked to report the number of patients onboarded on to both services (CO@h and CVW), the number of patients whose health deteriorated and were escalated to different pathways, as well as the number of deaths reported among monitored patients (see Chapter 2 for more detailed information). For sites running both CO@h and CVW services we have summarised the number of patients in each of these services.
We examined the numbers of staff by grade (converted into FTE numbers of staff) during the running of the programme. Staff were grouped into clinical and non-clinical groups. Clinical staff were from a range of backgrounds including medicine and nursing, and non-clinical staff had either an administrative role [e.g. any managerial role, information technology (IT) assistant, etc.] or other support (e.g. drivers, technical instructors). Clinical staff were divided into three subgroups (i.e. bands 5 and below, bands 6–7 and bands 8 and above) and non-clinical staff into two subgroups (bands 5 and below, bands 6 and above). In the workforce analysis, sites were stratified into four groups based on the numbers of patients onboarded: very small (200 patients or fewer), small (201–600 patients), medium (601–1000 patients) and large (1001+ patients). Owing to data limitations, we assumed that the services were running for the same time period in all sites.
The number of FTE staff involved per site could be affected by other factors such as the type of service, data submission mode and the size of the site. We checked for all these factors and reported on the results of the multivariate regression analysis (we regressed the total number of FTE staff, and FTE staff in different groups, against all these factors jointly in an ordinary least squares regression analysis).
Costs
We calculated the costs incurred for setting up and running the CO@h and CVW services, also examining the variation by data submission mode (tech-enabled and analogue vs. analogue-only). The costs of setting up the sites were reported as mean costs per site. Running costs were calculated as mean costs per patient for the period (October 2020 to April 2021) and included: (1) staff that were involved in running the service, (2) pulse oximeters, and (3) other monthly expenditures related to the maintaining of the tech-enabled platforms. Staff running costs were based on the information about staff hours and band. 141 We assumed that the unit cost for staff employed at Band 3 and below was equal to that of band 4 staff. 141 The number of pulse oximeters was based on the number of patients monitored. It was assumed that 70% of pulse oximeters would be returned and reused. This decision was based on the results from the patient and carer survey (n = 1069) that showed that 69% of patients or carers had been asked to return the pulse oximeter (range: 21.5–100% by site). The oximeters were provided by NHSEI, with the unit cost per pulse oximeter varying between £20 and £25. However, 15 of the 26 sites purchased all pulse oximeters from alternative sources while 2 of the 26 sites purchased part of them.
The mean running cost per patient could be affected by factors like the type of service, data submission mode, seniority of FTE staff and total number of patients monitored. As above, to investigate the effect of all these factors on the mean running cost, we used an ordinary least square regression model.
Additional detailed information provided by the four selected sites was used to investigate the time spent on different activities per patient associated with each patient data submission mode (see Project website documentation – Study instruments).
We adjusted the resources used and costs incurred per patient by applying weights based on the number of patients monitored by each service (CO@h and CVW) and the total number at each site.
Measuring cost-effectiveness
In the study protocol we originally planned to undertake a cost–utility analysis of remote home monitoring for patients with COVID-19. 55 In the absence of any impact on mortality or hospitalisation being demonstrated by the primary quantitative analyses (see Chapters 5 and 6), a cost–utility analysis was considered uninformative. We therefore focused on the programme costs alone.
Results
A total of 26 of the 28 sites returned the data collection form, of which 13 provided CO@h services, 4 provided CVW services and nine both CO@h and CVW (integrated) services.
Patients onboarded and the impact on outcomes
The number of patients triaged for both CO@h and CVW services (26,126 and 1,761 respectively) was higher compared with the number of patients monitored (17,424 and 1,711). The percentage of the monitored patients over total triaged was much higher for CO@h (149.9%) if compared with CVW services (103.3%). This may be because hospitals had a less heterogenous pool of people qualifying for services and had much more prior information about them. The proportion of the patients escalated and deaths over those monitored was higher for the CO@h services compared to the CVW services (16.6% and 10.8% respectively for escalated patients and 0.9% and 0.7%, respectively, for the number of deaths; Table 10).
Throughput and outcome | CO@h service | CVW service | ||
---|---|---|---|---|
Patients (n) | Monitored patients (%) | Patients (n) | Monitored patients (%) | |
Patients triaged | 26,126 | 149.9 | 1761 | 103.0 |
Average number of patients triaged per site | 1188 | 135 | ||
Patients monitored | 17,424 | 100.0 | 1711 | 100.0 |
Average number of patients monitored per site | 792 | 132 | ||
Patients deteriorated and escalated | 2898 | 16.6 | 185 | 10.8 |
Deaths | 160 | 0.9 | 12 | 0.7 |
Patients deteriorated and escalated | Patients ( n ) | Deteriorated and escalated patients (%) | Patients ( n ) | Deteriorated and escalated patients (%) |
Emergency department | 2240 | 77.3 | 135 | 73.0 |
Admitted to the hospital | 1257 | 43.4 | 161 | 87.2 |
ICU | 403 | 13.9 | 7 | 3.5 |
Primary care | 1806 | 62.3 | 73 | 39.4 |
Of the 22 sites running the CO@h service, 16 used tech-enabled and analogue modes, and 6 used the analogue-only mode (see Report Supplementary Material 2). Of the 13 sites running a CVW service, 6 used the tech-enabled and analogue data submission modes and 7 the analogue-only mode. Our results show that from the total number of patients onboarded in the CO@h services 76.8% were followed up using the tech-enabled and analogue data submission mode while in the CVW services 60.8% of patients onboarded were followed up using analogue-only data submission mode.
The structure of the workforce involved in providing CO@h and CVW services
The total number of FTE staff involved in running the CO@h service was on average 7.4 per site [range: 1.0–39.0] and for the CVW service this was 2.1 per site [range: 0.1–4.8]. Staff involved were a mix of medical consultants, emergency department staff, general practitioners, nurses, advanced nurse practitioners (ANPS) and medical students. Our analysis of the staff employed in running the services showed that there were no clear patterns in the differences in terms of clinical/non-clinical staff or seniority of the staff involved between CO@h and CVW services.
Table 11 provides the mean number of FTE staff by role and band, stratified by type of service and size of site (see Report Supplementary Material 2 for the total number of FTE staff involved). For both CO@h and CVW services, most of the staff involved in monitoring the patients were clinical (68.4% for CO@h service and 86.7% for CVW service).
Type of site (by no. patients)a | Sitesa | Staff FTE (mean n) | Non-clinical staff (FTE equivalent) | Clinical staff (FTE equivalent) | |||||
---|---|---|---|---|---|---|---|---|---|
Non-clinical staff n (%) | Band 6 + n (as % over total) | Band ≤5 n (as % over total) | Clinical staff mean n (%) | Band 8 + n (as % over total) | Band 6–7 n (as % over total) | Band ≤5 n (as % over total) | |||
CO@h service | |||||||||
The total sample | |||||||||
Very small sites (0–200 patients) | 3 | 3.7 | 1.1 (28.7) | 0.6 (16.6) | 0.4 (12.0) | 2.6 (71.3) | 0.1 (2.5) | 0.9 (24.9) | 1.6 (43.9) |
Small sites (201–600 patients) | 6 | 4.2 | 1.3 (31.6) | 0.2 (4.3) | 1.1 (27.3) | 2.8 (68.4) | 1.3 (6.4) | 1.1 (26.3) | 0.4 (10.2) |
Medium sites (601–1000 patients) | 5 | 9.7 | 3.8 (39.1) | 0.6 (5.7) | 3.2 (33.4) | 5.9 (60.9) | 0.6 (5.9) | 2.9 (29.3) | 2.5 (25.7) |
Large sites (1001 + patients) | 8 | 9.8 | 2.7 (27.4) | 0.2 (2.2) | 2.5 (25.2) | 7.1 (72.6) | 1.1 (22.7) | 3.3 (33.3) | 2.8 (28.6) |
Total sample | 22 | 7.4 | 2.3 (31.6) | 0.3 (4.5) | 2.0 (27.1) | 5.1 (68.4) | 0.9 (11.3) | 2.3 (30.5) | 1.9 (25.9) |
Tech-enabled and analogue | |||||||||
Very small sites (0–200 patients) | 3 | 3.7 | 1.1 (28.7) | 0.6 (16.6) | 0.4 (12.0) | 2.6 (71.3) | 0.1 (2.5) | 0.9 (24.9) | 1.6 (43.9) |
Small sites (201–600 patients) | 2 | 4.0 | 2.8 (69.3) | 0.1 (2.2) | 2.7 (67.1) | 1.2 (30.7) | 0.3 (6.4) | 1.0 (24.3) | 0.0 (0.0) |
Medium sites (601–1000 patients) | 5 | 9.7 | 3.8 (39.1) | 0.6 (5.7) | 3.2 (33.4) | 5.9 (60.9) | 0.6 (5.9) | 2.9 (29.3) | 2.5 (25.7) |
Large sites (1001 + patients) | 6 | 5.9 | 2.0 (34.7) | 0.2 (3.2) | 1.9 (31.5) | 3.9 (65.3) | 1.3 (22.7) | 2.2 (37.4) | 0.3 (5.3) |
Total tech-enabled and analogue | 16 | 6.4 | 2.5 (38.8) | 0.4 (5.7) | 2.1 (33.1) | 3.9 (61.2) | 0.7 (11.3) | 2.0 (31.2) | 1.2 (18.6) |
Analogue-only | |||||||||
Small sites (201–600 patients) | 4 | 4.2 | 0.6 (13.8) | 0.2 (5.3) | 0.4 (8.5) | 3.7 (86.2) | 1.9 (43.9) | 1.2 (27.2) | 0.6 (15.1) |
Large sites (1001 + patients) | 2 | 21.6 | 4.6 (21.4) | 0.3 (1.4) | 4.3 (20.1) | 17.0 (78.6) | 0.2 (1.0) | 6.5 (30.0) | 10.3 (47.7) |
Total analogue-only | 6 | 10.0 | 1.9 (19.3) | 0.2 (2.5) | 1.7 (16.8) | 8.1 (80.7) | 1.3 (13.1) | 2.9 (29.2) | 3.9 (38.5) |
CVW service | |||||||||
The total sample | |||||||||
Very small sites (0–200 patients) | 11 | 1.8 | 0.2 (11.5) | 0.02 (1.3) | 0.2 (10.2) | 1.6 (88.5) | 0.2 (10.4) | 0.5 (28.4) | 0.9 (49.7) |
Small sites (201–600 patients) | 2 | 3.7 | 0.7 (18.1) | 0.0 (0.0) | 0.7 (18.1) | 3.0 (81.9) | 0.1 (2.9) | 2.9 (79.0) | 0.0 (0.0) |
Total sample | 13 | 2.1 | 0.3 (13.3) | 0.02 (0.9) | 0.3 (12.3) | 1.8 (86.7) | 0.2 (8.4) | 0.9 (42.0) | 0.8 (36.3) |
Tech-enabled and analogue | |||||||||
Very small sites (0–200 patients) | 5 | 1.9 | 0.2 (12.7) | 0.04 (2.0) | 0.2 (10.7) | 1.6 (87.3) | 0.1 (4.5) | 0.6 (34.0) | 0.9 (48.8) |
Small sites (201–600 patients) | 1 | 4.0 | 1.3 (33.2) | 0.0 (0.0) | 1.3 (33.2) | 2.7 (66.8) | 0.2 (5.3) | 2.5 (61.5) | 0.0 (0.0) |
Total tech-enabled and analogue | 6 | 2.2 | 0.4 (18.9) | 0.03 (1.4) | 0.4 (17.5) | 1.8 (81.1) | 0.1 (4.7) | 0.9 (42.3) | 0.8 (34.1) |
Analogue-only | |||||||||
Very small sites (0–200 patients) | 6 | 1.8 | 0.2 (10.4) | 0.01 (0.6) | 0.2 (9.8) | 1.6 (89.6) | 0.3 (15.5) | 0.4 (23.5) | 0.9 (50.6) |
Small sites (201–600 patients) | 1 | 3.4 | 0.0 (0.0) | 0.0 (0.0) | 0.0 (0.0) | 3.4 (100.0) | 0.0 (0.0) | 3.4 (100.0) | 0.0 (0.0) |
Total analogue-only | 7 | 2.0 | 0.2 (7.9) | 0.01 (0.5) | 0.1 (7.4) | 1.9 (92.1) | 0.2 (11.8) | 0.8 (41.8) | 0.8x (38.5) |
Table 11 also shows that for all sites running CO@h services, 53.0% of staff were employed at band 5 or below (27.1% non-clinical and 25.9% clinical staff). Sites using tech-enabled and analogue modes had a higher proportion of non-clinical staff at band 5 or below (33.1% non-clinical and 18.6% clinical staff) compared to analogue-only mode (16.8% non-clinical and 38.5% clinical staff).
Across all sites running the CVW services, 51.3% of all staff (50.4% clinical and 0.9% non-clinical) involved were employed at band 6 and above. A similar trend was observed for the sites using analogue-only mode where 54.1% of all staff involved (53.6% clinical and 0.5% non-clinical) were employed at band 6 and above whereas for the tech-enabled and analogue mode most staff involved (51.6%; 34.1% clinical and 17.5% non-clinical) were at band 5 and below.
Our analysis of the effect of other factors (i.e. type of services, data submission mode and size of the site) on the number of FTE staff involved revealed that, as expected, a key determinant was the size of sites. Note that these findings should be considered with caution due to the small sample size (N = 35 sites) (see Report Supplementary Material 6).
The costs of setting-up and running the sites
Table 12 presents detailed information on the mean running cost per patient triaged and monitored over the study period for both CO@h and CVW services (see Report Supplementary Material 6). These data show that most funds during the setting-up phase were spent on non-staff items (e.g. the technology platform, medical and other equipment). Our analysis of running costs showed that the mean cost per patient monitored using the CO@h service was lower compared with the CVW service (£527.5 vs. £599.1). The mean cost per patient monitored was lower for sites using tech-enabled and analogue compared with the sites using analogue-only data submission mode for both CO@h (£515 vs. £561) and CVW (£584 vs. £612) services.
Cost per patient | Total Covid-19 Care at Home | |||||
---|---|---|---|---|---|---|
CO@h service | CVW service | |||||
Mean cost per patient | 10th percentile | 90th percentile | Mean cost per patient | 10th percentile | 90th percentile | |
Triaged | £351.80 (£73.70) |
£67.60 | £512.20 | £581.80 (£139.90) |
£74.40 | £1366.80 |
Monitored | £527.50 (£110.50) |
£101.40 | £768.00 | £599.10 (£144.00) |
£76.60 | £1407.40 |
Tech-enabled and analogue mode | ||||||
Triaged | £343.40 (£83.80) |
£78.80 | £464.10 | £567.00 (£208.10) |
£129.00 | £1152.50 |
Monitored | £514.90 (£125.60) |
£118.20 | £695.80 | £583.90 (£214.30) |
£132.80 | £1186.80 |
Analogue-only mode | ||||||
Triaged | £374.10 (£165.20) |
£68.90 | £821.10 | £594.50 (£204.10) |
£130.10 | £1220.90 |
Monitored | £560.90 (£247.80) |
£103.20 | £1231.10 | £612.10 (£210.20) |
£134.00 | £1257.10 |
The regression analysis of the effect of factors (i.e. type of services, data submission mode, seniority of FTE staff and the number of the patients monitored) on the mean running cost per patient revealed that, as expected, a key determinant was the number of patients monitored. Another significant factor was the type of service (i.e. CO@h or CVW). Even though the type of the data submission mode and the FTE difference between the senior and junior staff involved were not statistically significant predictors of mean running costs per patient, the direction of their effect was consistent with the descriptive findings. Note that these findings should be considered with caution because of the small sample size (N = 35 sites) (see Report Supplementary Material 6).
We investigated whether the mean running costs per patient at each site were associated with the total number of patients monitored. Figures 23 and 24 show a positive relationship (slope coefficient for the CO@h service 0.54 (95% CI 0.19 to 0.89), and for CVW service 2.10 (95% CI 0.16 to 4.03)) between the number of patients monitored and the mean running cost per patient for the CO@h and CVW services, respectively. This suggests that running costs increase as the number of patients monitored increases. However, this finding should be treated with caution for several reasons. First, the number of sites involved in this analysis is small. Second, as evidenced by the two figures, there are outlying sites in terms of mean costs and number of patients monitored. Third, the figures are based on running costs only; if set-up costs were included, these costs would fall as the number of patients monitored increased. Fourth, we do not have data on the maximum patient capacity of sites on which total running costs would be based.
FIGURE 23.
The mean cost per patient for CO@h services. Source: Based on data provided by each site; 22 sites used CO@h services.

FIGURE 24.
The mean cost per patient for CVW services. Source: Based on data provided by each site; 13 sites used CVW services.
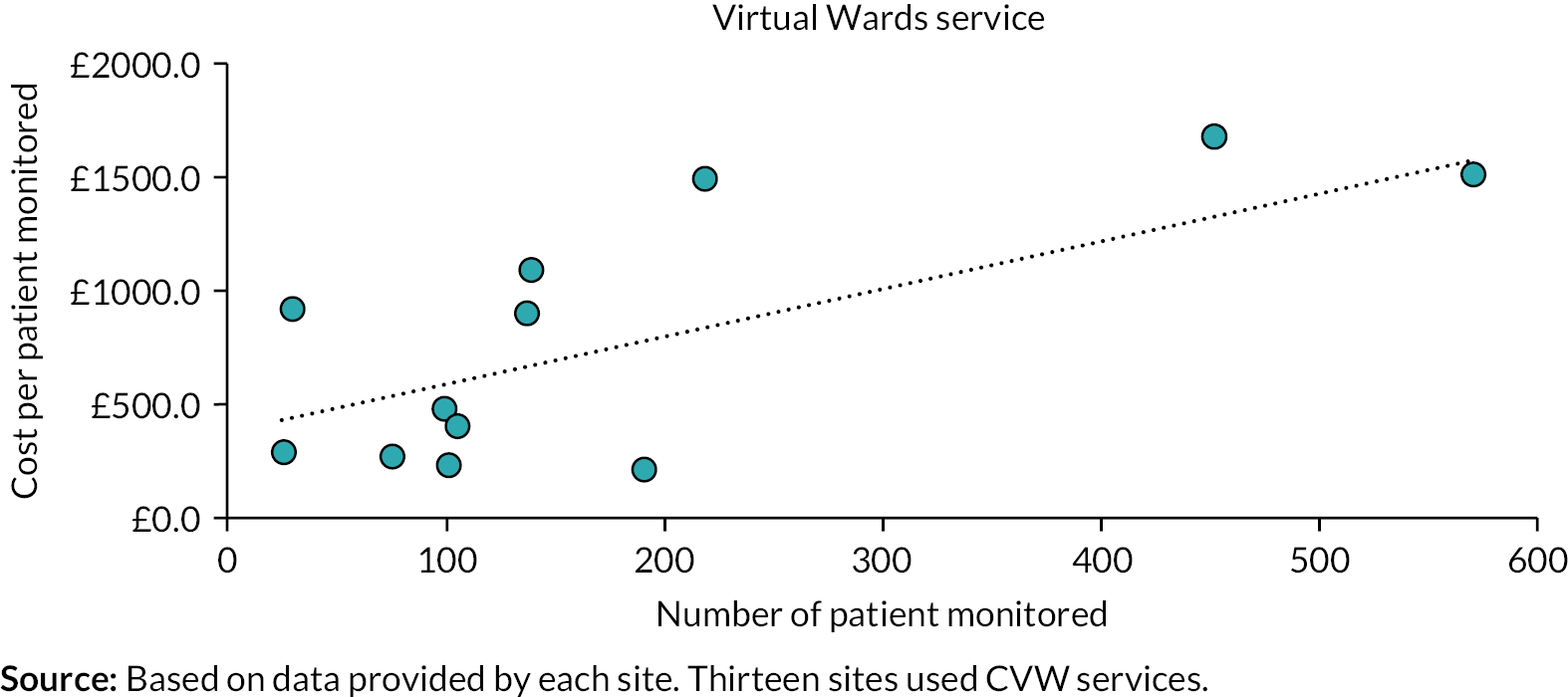
Time spent on monitoring activities
This part of the analysis used data that were available only from the four sites where we undertook a more detailed investigation of the monitoring activities. All four sites used tech-enabled and analogue and analogue-only data submission modes. The total number of patients monitored at these four sites was 3366, of whom 2824 were monitored using tech-enabled and analogue mode and 542 were monitored using analogue-only mode (see Report Supplementary Material 2).
The most time-consuming activity was patient’s monitoring, and on average less staff time was spent on this under tech-enabled and analogue mode compared with analogue-only (68.0 minutes vs. 72.5 minutes respectively; note that this activity by staff might be removed in the case of self-monitoring by patients). Based on time spent and staff working on each activity (see Report Supplementary Material 6), we calculated the average cost of each activity per patient, for the tech-enabled and analogue and analogue-only modes (£115.1 vs. £98.3, respectively; if the patient monitoring costs were removed, as might be the case in a self-monitoring service with no monitoring by staff, and assuming all other staff inputs/costs remained constant, these costs would fall to £80.0 and £60.8, respectively; see Report Supplementary Material 6). Please note that because of the amount of missing data, these findings should be viewed with caution.
These four sites reported on average 8.7 contacts per patient using tech-enabled and analogue mode throughout the time they were in the CO@h service, and 15.5 contacts per patient using the analogue-only option.
Discussion
Key findings
A total of 26 of the 28 sites contacted provided information for this analysis, of which 13 provided the CO@h service, 4 provided the CVW service and 9 provided both services. In total, 17 sites used tech-enabled and analogue and 9 analogue-only data submission modes. The mean running cost per patient monitored under the CO@h service was lower compared with CVW service. The main driver of the cost per patient was the number of FTE staff and the grade of staff involved, and this was regardless of the type of service adopted. A comparison with findings from wave 1 (March to August 2020)36 showed that the mean cost per patient monitored remained fairly constant from wave 1 to wave 2 for the CO@h service (£553 vs. £527) and increased from wave 1 to wave 2 for the CVW service (£400 vs. £599).
The mean costs per patient in the sites using tech-enabled and analogue were lower compared with analogue-only sites, mainly due to shorter time needed to collect patient medical information. However, as other studies have shown, the potential for technology to save costs must be treated with caution unless the digital assessments are shorter in time and they are proven to decrease follow-up and deterioration of symptoms, thus leading to lower commitment of staff time for overall service delivery. 142,143
Comparison with other studies
Previous studies have found that remote patient monitoring for conditions other than COVID-19 was associated with improved clinical outcomes and medication compliance and reduced the number of emergency room visits, hospital admissions and patient travel. 144–146 A comparison with data from wave 1 (March to August 2020)36 showed that escalated patient rates for the period October 2020 to April 2021 was higher for the CO@h service (16.6% vs. 10.0%). Whereas for the CVW service the escalated patients’ rates were slightly higher for wave 2 in comparison with March to August 2020 period (10.8% vs. 12.2%). The mortality rates for the period October 2020 to April 2021 were 0.9% for the CO@h and 0.7% for the CVW, slightly lower than the rates previously reported for the period March to August 2020 (1.1% and 0.9%, respectively). 36
A previous systematic review found that there was no evidence showing that telemedicine and telecare interventions can be cost-effective compared with conventional health care. 147 However, it has been also highlighted that cost-effectiveness analysis of remote monitoring of patients for different diseases is often integrated into other home health-care services making it challenging to isolate and allocate its cost appropriately. 147 The evidence on resources used and costs of remote home monitoring for patients with COVID-19 specifically is also very limited. Our previous literature review on similar interventions revealed that very few studies have conducted an economic analysis and in most cases this is limited to describing costs incurred and resources used. 32 In general, previous studies have stated that similar interventions may be cost-saving by reducing downstream costs (in terms of avoiding hospital admissions and bed-days). 31 Our findings are consistent with a recent Australian study where staffing costs were the main contributors to the total costs of monitoring. 148 In other health-care contexts, the use of telehealth for the provision of health care has previously shown to be associated with cost savings, especially in reaching remote areas. 149 However, any potential cost saving of technology relies on the assumption that digital assessment is shorter in time and decreases follow-up contacts and deterioration of symptoms, which may result in new admissions to hospital. Caution is warranted as such assumptions may not always hold. 142
Strengths and weaknesses
The main strength of this analysis is that we achieved good geographical coverage of sites across England (see Chapter 4), obtained primary data on CO@h and CVW services use and distinguished between the tech-enabled and analogue versus analogue-only data submission modes. Additionally, this study fills a literature gap regarding COVID-related remote monitoring services, since it is the first to provide this level of detail about costs, workforce and activities.
There are several weaknesses with this study. First, we were not able to perform a full economic analysis (i.e. cost–utility analysis) as the effectiveness analysis found no evidence of the CO@h service on mortality or downstream hospital utilisation (see Chapters 5 and 6). Second, the study design did not allow the use of patient-level data, which means we were unable to examine patient-level variation in service costs. Third, cost- and resource-use data provided by some sites were incomplete. For this reason, the in-depth analysis using the four sites was very limited and the results should be treated with caution. Fourth, the selection of the four sites used for the in-depth analysis was based on purposive and convenience sampling, and this may insert selection bias. Fifth, there was wide variation in service models across sites and much variation in how services were designed and delivered (see Chapter 4). Sixth, the generalisation of our findings to all patients with COVID-19 is difficult as pre-existing conditions and level of severity were not taken into consideration. Seventh, the limited number of sites which have been used for this analysis does not allow further grouping in terms of their size, considering both the number of patients and the length of time establishing this programme. Finally, any interpretation of results should be taken with caution as we do not have the information on the maximum capacity of the sites and can only observe the current flow of patients. For sites with a higher planned capacity the underutilisation of resources will mean that the cost per patient may go down with the increase in number of patients.
Implications
Our analysis showed a lower cost of remote monitoring per patient with COVID-19 during wave 2 of the pandemic in the UK. Our results indicated that the tech-enabled and analogue monitoring was less costly, in terms of mean cost per patient, than analogue-only monitoring. It offers a model for future research in the way it covers a range in the size of sites and raises questions about differential practices within the overall envelope of remote monitoring – differences that are necessarily present in any geographically diverse and large-scale health system.
Future interventions on remote monitoring for other health conditions need to consider their impact on primary and secondary care. Such services may differ in costs, band of the staff involved and worked hours based on the health sector of primary impact. Consideration of the differences between the CO@h and CVW services should also be considered given the different pool of patients they serve and the level of information the staff have on them. Future studies should also gather information on total capacity and how this relates to costs; given the fixed costs in delivering services, this may provide a better view on actual costs and may also possibly help future planning. Future research, including more sites, may provide additional evidence and shed light on the areas where the availability of this study’s data was poor. Availability of routine data on health outcomes (instead of having to use site questionnaires) would also facilitate future research. Overall, there is pressing scope for seeing remote monitoring within a more holistic view of patients’ and staff experiences.
Chapter 8 Staff experiences of delivering COVID-19 remote home monitoring services
Overview
This chapter draws on a manuscript by Sidhu et al. 150 published in Journal of Health Services Research and Policy (2023): https://doi.org/10.1177/13558196231172586. This is an open access article under the term of the Creative Commons Attribution 4.0 International License (CC BY 4.0), which permits use, distribution and reproduction in any medium, provided the original work is properly cited (see https://creativecommons.org/licenses/by/4.0/).
What was already known
-
Remote home monitoring may have some benefits for patients, health-care systems and staff (e.g. flexibility/independence/fast access to clinicians).
-
Some challenges and concerns with remote home monitoring have been highlighted (e.g. lack of supportive infrastructure within NHS organisations to facilitate successful implementation and delivery, staff training, treating patients living with a range of long-term conditions which may include physical and/or cognitive impairment).
-
However, there is a paucity of evidence on staff experiences and the factors influencing delivery of COVID-19 remote home monitoring services.
What this chapter adds
-
Delivery of remote home monitoring services for COVID-19 involved delivery of either technology-enabled and analogue services, or analogue-only services. Staff were involved in a range of tasks including monitoring, escalation, and service evaluation.
-
Staff generally reported positive experiences of delivering the service, felt that it was easy to deliver and valued support, but staff would have benefited from further training.
-
Factors influencing delivery of remote home monitoring services for COVID-19 included: support for delivering the service, NHS resources and capacity on staff workload, multidisciplinary team dynamics, and patient (dis)engagement.
Introduction
The impact of remote home monitoring services on NHS staff or staff experiences is not yet known. 36,151–154 Delivering remote home monitoring services may affect job satisfaction for staff155,156 – that is, if staff are reluctant to deliver remote services full-time, due to valuing face-to-face interactions with patients11,156,157 and concerns over ‘call-centre medicine’. 157 Previous research has indicated that many staff acknowledge benefits of remote home monitoring, including flexibility and independence for staff, fast access to clinicians for patients living with chronic (e.g. respiratory and heart failure) and acute (e.g. COVID-19) conditions,158,159 and improved continuity of care and communication of risks between staff and patients. 160 Despite these putative benefits, clinicians have expressed concerns that some patient characteristics, including physical and cognitive impairments, may present challenges for remote home monitoring services. 160 Additionally, staff report concerns about lack of training and the exclusion of some service user groups if alternatives to remote care are not provided. 161,162
Despite previous research on remote home monitoring models for other conditions, there is a paucity of evidence on staff experiences of delivering care as part of remote home monitoring services. A range of individual, organisational and environmental factors have been previously proposed to influence health-care professional behaviours,163 but the factors (barriers and facilitators) influencing delivery of COVID-19 remote home monitoring services are not yet known.
This study explored staff experience of delivering COVID-19 remote home monitoring services. The study reported in this chapter aimed to answer the following questions:
-
What was the nature of ‘work’ that staff undertook to care for patients with COVID-19?
-
How were staff supported to deliver remote home monitoring services?
-
What factors (barriers and facilitators) influenced delivery of COVID-19 remote monitoring services?
Methods
A summary of the methods is provided below; further details can be found in Chapter 2 and Report Supplementary Material 1.
Design
This chapter draws on data from workstream 3 (staff survey data) and workstream 4 (staff interviews). Data collection for this study took place between February and June 2021.
Sample
Staff who led and/or delivered COVID-19 remote home monitoring services (see Chapter 2 and Chapter 4 for site and staff sample details).
Measures
We iteratively developed the survey and semistructured topic guides specifically for this study (see Chapter 2, Project website documentation – Study instruments).
Procedure
We conducted a survey with staff from 28 sites, and interviews with staff from 17 sites (see Chapter 2 for details).
Analysis
Inductive thematic analysis was used to analyse interview data inputted into RAP sheets. 70 Additionally, a subset of transcripts (n = 17) were independently thematically coded to validate interpretations from the analysis of RAP sheets.
Survey data were analysed on a complete case basis (excluding participants with missing data) using descriptive statistics (using SPSS, version 25). Open text responses were coded using inductive thematic analysis.
Interview and survey findings were triangulated to compare consistency of findings relating to research questions. 71,72
Results
Participant characteristics
Surveys were received from 292 NHS staff (70 clinical leads/service managers, 222 delivery staff) and 58 interviews were conducted with staff (23 clinical leads, 28 delivery staff, 7 data staff. See Chapter 4 and Report Supplementary Material 2 for demographic characteristics.
Findings
The nature of ‘work’ that staff undertook to care for patients with COVID-19 remotely
Staff were involved in remote monitoring services delivered in one of two ways: technology-enabled with analogue services (21/28 sites), and analogue-only services (7/28 sites) (see Chapter 4 for further details).
Daily, staff would assess the nature of referrals made to the service, monitor data uploaded by patients regarding oxygen saturation, temperature, or breathlessness (as a minimum), and if necessary, determine whether data warranted escalation in patient care (phone call to the patient, sending paramedics to the patient, directing patients to seek emergency acute secondary care).
Staff survey responders reported a range of work-related tasks they had been involved with when delivering their respective services (see Table 13). The more frequently cited tasks included monitoring activities (80%) and escalation processes (70%).
Service managers/clinical leads (n = 70) | Delivery staff (n = 222) | Total (n = 292) | ||
---|---|---|---|---|
Aspect of the service involved with n (%) | Development and/or piloting of the service | 50 (71) | 40 (18) | 90 (31) |
Set-up and design | 53 (76) | 22 (10) | 75 (26) | |
Service management | 54 (77) | 32 (14) | 86 (30) | |
Referring patients | 30 (43) | 58 (26) | 88 (30) | |
Triaging patients | 29 (41) | 112 (51) | 141 (48) | |
Monitoring | 35 (50) | 199 (90) | 234 (80) | |
Escalation | 43 (61) | 162 (73) | 205 (70) | |
Discharge | 34 (49) | 156 (70) | 190 (65) | |
Monitoring/evaluating the service | 50 (71) | 61 (28) | 111 (38) | |
Other tasks (e.g. delivering equipment) | 3 (4) | 15 (7) | 18 (6) |
The majority of clinical leads and delivery staff felt services they provided had a positive or very positive impact (47%, n = 127/266) or neutral impact (32%, n = 85/266) on their workload. However, delivery staff and service leads also reported that services increased their workload (21%, n = 54/266).
Support and training provided to staff to deliver remote home monitoring services
Service leads reported being supported in many ways by NHSEI and other organisations; for example, obtaining pulse oximeters (54%) and receiving guidance for implementation (43%). Delivery staff (88%) reported that they were adequately supported in their role (see Table 14).
Findings from staff survey | Responses | n (%) | |
---|---|---|---|
Support in delivering the service | Staff adequately supported in their role (n = 221)a | Yes | 195 (88) |
No | 9 (4) | ||
Not sure | 17 (8) | ||
Support provided by NHSEI (or other organisations) (n = 70)b | Obtaining pulse oximeters | 38 (54) | |
Guidance for implementation | 30 (43) | ||
Setting up and using systems for recording data | 18 (26) | ||
Training for staff | 11 (16) | ||
Distributing pulse oximeters | 10 (14) | ||
Training for patients | 5 (7) | ||
Other | 2 (3) | ||
Staff reported experience of service | Confidence in carrying out daily responsibilities (n = 222)a | Very confident or confident | 204 (92) |
Neutral | 15 (7) | ||
Not very confident | 3 (1) | ||
Staff clear about the requirements of their role (n = 221)a | Yes | 202 (91) | |
No | 11 (5) | ||
Not sure | 8 (4) | ||
Staff reported experience of tasksa | Remote monitoring of patients (n = 201) | Very easy or easy | 146 (73) |
Neutral | 47 (23) | ||
Difficult or very difficult | 8 (5) | ||
Escalation processes (n = 202) | Very easy or easy | 144 (71) | |
Neutral | 42 (21) | ||
Difficult or very difficult | 16 (8) | ||
Using IT systems (n = 217) | Very easy or easy | 144 (66) | |
Neutral | 56 (26) | ||
Difficult or very difficult | 17 (8) | ||
Working with other COVID-related services (n = 179) | Very easy or easy | 97 (54) | |
Neutral | 73 (41) | ||
Difficult or very difficult | 9 (6) | ||
Triage processes (n = 181) | Very easy or easy | 114 (63) | |
Neutral | 57 (32) | ||
Difficult or very difficult | 10 (6) | ||
Impact of service on workload, job satisfaction and work-related stress | Impact on workload (n = 266) | Very positive or positive | 127 (47) |
Neutral | 85 (32) | ||
Negative or very negative | 54 (21) | ||
Impact on job satisfaction (n = 288) | Very positive or positive | 216 (75) | |
Neutral | 63 (22) | ||
Negative or very negative | 9 (3) | ||
Impact on work-related stress | Very positive or positive | 60 (22) | |
Neutral | 152 (57) | ||
Negative or very negative | 55 (20) |
In terms of training, 68% (n = 200/292) of staff survey respondents (managers/leads and delivery staff) reported having received training or training resources as part of their role. However, the amount and nature of training received was difficult to determine. Delivery staff lacked knowledge of and familiarity with the NHS CO@h competency framework (which sets guidelines for staff training to deliver both services and undertake patient assessment); only 9.5% (n = 21/220) reported having completed the framework and 35.5% (n = 78/220) were unsure. See Report Supplementary Material 7 for a training and support summary.
Forty-one per cent (n = 28/69) of clinical leads/service managers and 12% (n = 26/222) of delivery staff reported that they had further training or support needs (beyond that already received) (Table 15).
Additional training need | Service managers or clinical leads, n (%) | Delivery staff, n (%) | ||
---|---|---|---|---|
Clinical role (n = 19) | Non-clinical role (n = 12) | Clinical role (n = 21) | Non-clinical role (n = 5) | |
Clinical pathways | 17 (89.5) | 11 (91.6) | 15 (71.4) | 3 (60) |
Processes for triage | 12 (63.2) | 7 (58.3) | 7 (33.3) | 0 (0) |
Processes to monitor patients | 13 (68.4) | 10 (83.3) | 4 (19) | 0 (0) |
Processes to escalate patients | 15 (78.9) | 9 (75) | 8 (38) | 1 (20) |
The IT systems being used | 15 (78.9) | 9 (75) | 7 (33.3) | 0 (0) |
Training on conflict resolution | – | – | 4 (19) | 0 (0) |
Other | 2 (10.5) | 0 (0) | 2 (9.5) All mentioned ongoing support |
1 (20) |
Interview findings highlighted that clinical delivery staff, particularly nurses, raised concerns that training led by senior clinicians largely took the form of shadowing and/or receiving brief overviews on each part of the service. As a result, initially many nurses felt a lack of confidence, especially when communicating risks associated with COVID-19, and remained dependent on senior clinicians. This dependency subsided as their experience grew. During this period, nurses welcomed those senior clinicians who were readily accessible:
[. . .] you don’t need to know everything. You’re not a doctor. You just need to get all the information and you can always get a doctor to give them a call. [ . . . ]. So, don’t panic. Just, yes, reassure them and if you’re unsure, just get a doctor to give them a call.
(Site L, interviewee 1 – health-care professional delivering the service
Factors influencing delivery of COVID-19 remote home monitoring services
Staff knowledge and confidence
Delivery staff reported feeling confident or very confident in their ability to carry out their responsibilities (92%, n = 204/222) and were clear about the requirements of their role (91%, n = 202/221). Delivery staff reported few difficulties completing tasks as part of delivering services and most staff reported these tasks to be easy or very easy (range between 54% and 73% across tasks). The most challenging tasks seemed to be using IT systems and working with other COVID-related services – with lower numbers of staff reporting these tasks as easy (see Table 14).
Although the survey data indicated that staff felt satisfied with the support and training received as well as confident delivering the service, not all felt this way. Staff reported that the need to develop new knowledge, skills and confidence about engaging with online monitoring platforms and undertaking clinical assessments via telephone, early on in the implementation of these services was a barrier to delivery, as training was not always embedded in a timely way during service implementation. A significant minority of nursing staff felt unsupported and distressed at having to work on a remote home monitoring service. At one site, staff initially ‘hated [the service] with a passion’ with some staff finding the accountability of assessing patient risk remotely and then determining appropriate treatment too stressful and threatened to leave their roles unless changes were made to their training (e.g. increasing the number of hours of training, who was providing training, and greater shadowing when staff first deliver the service). Nursing staff, at times felt ‘at risk’ of potentially making mistakes in relation to monitoring and decisions about potential escalation in the rapid scale-up of services. Often staff were relying on their previous experiences of working with SOPs (in GP and secondary care) and were unfamiliar with newly established practices when delivering care remotely and hence transitioning to a new remote workplace culture with risk assessment, interpretation, and potential escalation of patient care at its centre was difficult. However, there were a number of exceptions:
Working in A and E I can see some really acutely unwell patients and we use kind of an A to E approach where it’s kind of a system that we look at looking at airway, breathing, circulation, etc. And then, say, when I’m speaking to a person on the phone, what we are concerned about is the breathing and things, so you’re kind of able to transfer that knowledge.
(Site K, interviewee 3 – health-care professional delivering the service)
Influences on staff workload
Members of staff felt their workload was increased more than initially expected (Table 15) as they were often dealing with wider determinants of ill health that were having a substantial impact on patients’ well-being. For example, given the nature of COVID-19, staff were supporting some patients who had lost employment, were taking care of others diagnosed with COVID-19, or who also had mental health problems.
While the majority of clinical leads/service managers felt there were enough staff and sufficient capacity to deliver services as intended, delivery staff reported having to balance their role within these services with other roles (Table 16). Redeployment of staff facilitated capacity to deliver services and scale-up when needed, as these staff were often working solely on remote home monitoring services.
Service managers/clinical leads n (%) (n = 70) | Delivery staff (n = 222) n (%) | Total n (%) (n = 292) | ||
---|---|---|---|---|
Sharing COVID-19 remote monitoring role with any other role(s) | Yes | 52 (74) | 137 (62) | 189 (65) |
No | 13 (19) | 78 (35) | 91 (31) | |
Not applicable | 5 (7) | 7 (3) | 12 (4) |
Both clinical leads and delivery staff expressed concern that redeployed staff members would not be available during future surges in patient cases.
We delivered on existing staffing. So it was mainly done via bank. We did have bank admin and bank drivers for deliveries and collections. [. . .] But it was done on existing staffing. So the staff that we already had stepped up, took on extra shifts, it was all done on bank and goodwill.
(Site F, interviewee 4, health-care professional delivering the service)
Staff reported having discussions within their team regarding which technology-enabled platforms should be used (N = 4) and could be easily integrated with existing patient record systems. For example, staff welcomed apps and/or online platforms that could be integrated with existing familiar primary care patient record systems. Technology which could not be integrated meant greater time taken by staff to upload patient data on to multiple databases, which was resource intensive, cumbersome, and increased the risk for potential mistakes. In other sites (N = 3), staff did not feel included in decisions on which apps and/or online platforms would be deployed for remote monitoring. The use of these platforms felt imposed and limited early engagement with the technology:
And my honest personal view was at the time I felt like we had a clever little app that we were trying to fit a service for, that was my personal view. And so, I took a little bit of convincing . . .
(Site I, interviewee 2, service lead)
Multidisciplinary team dynamics
Where sites adopted a multidisciplinary team model of working, there were at times tensions between professional groups, especially where there was historically a lack of co-working between the groups. This remained problematic until services were more established and roles became more distinguished (e.g. GPs understanding that remote home monitoring services were not replacing their duty to continue doing home visits during the pandemic).
A key facilitator was determining the correct mix of staff that needed to be involved in the delivery of the service. Of the staff survey respondents, 71% (n = 206/292) reported their role within services to be clinical, 29% (n = 86/292) non-clinical, and 2% (n = 5/292) were students/volunteers. In a small number of sites, only clinical staff were involved in monitoring patients, whereas most sites involved a mix of both clinical and administrative staff. Volunteers were also used at two sites to support the administrative duties associated with setting up services.
Implementation was facilitated by good communication between members of the clinical team. Service leads tried to identify staff with the necessary communication skills to undertake remote monitoring consultations via telephone, such as active listening, and picking up cues regarding a patient’s current condition without physical examination. Delivery team members, in turn, wanted to make service managers aware that they needed flexibility regarding how each call was made as staff wanted to be able to provide patients with emotional support as well, which, at times meant longer phone calls.
You spend a lot of your time actually reassuring patients that they’re doing all right. They’re frightened. They’re very frightened. And they always know somebody that’s been in hospital and has had a really bad experience.
(Site I, interviewee 4, health-care professional delivering the service)
Staff experiences of patent engagement or disengagement
All staff felt that delivery of remote home monitoring services was facilitated by patients engaging with services and having confidence as well as sufficient digital literacy to send their measurements and readings. Survey staff reported high levels of patient engagement with their service (96%, n = 209/219). Delivery staff generally reported that service users experienced few difficulties in using the oximeter to take readings (91% well/very well: n = 193/212) and providing readings over the phone (91% well/very well: n = 186/205). However, providing readings via an app was reported to have more difficulties (78% well/very well: n = 134/172). Staff felt that those patients who were living with a long-term respiratory condition (e.g. COPD) were more comfortable dealing with episodes of breathlessness and therefore were less in need of the advice and exercises provided by nurses for managing COVID symptoms.
Staff also identified specific groups which they felt required more support and found it more difficult to engage (e.g. those who were older, had health difficulties or lacked sufficient digital literacy), and other groups who were more likely to disengage with services (e.g. younger patients, those who had returned to work following self-isolation, or those with few acute symptoms associated with COVID-19). Often, asking next of kin or family members to provide readings helped to maintain engagement which also facilitated effective delivery of the service. In addition, delivery staff felt that some patients preferred to provide oximetry readings with the support of a member of the remote home monitoring team over the telephone. There were a number of barriers to delivering remote home monitoring services relating to patient engagement (e.g. having to chase patients who did not submit readings, patients not answering the phone, delays in provision of oximeters or other equipment and patients receiving too little written or oral information).
Discussion
Key findings
Staff were involved in flexibly delivering a range of technology-enabled and analogue remote monitoring models for COVID-19. Staff generally reported positive experiences of delivering the service, felt that it was easy to deliver and valued support. A range of training and support opportunities were provided but staff would have benefited from further training especially when undertaking clinical assessments remotely, determining how best to escalate care of patients whose condition may be deteriorating from COVID-19, and how best to provide follow-up care. Findings also indicated different factors which influenced delivery of remote home monitoring models, including appropriate support and training, staff knowledge and confidence, capacity and workload, multidisciplinary team dynamics, and patient engagement or disengagement.
How findings relate to previous research
Given the rapid transition to using remote home monitoring models and technology to support people living with COVID-19 and long-term health conditions, our findings extend literature on the training needs and experiences of staff asked to use such methods within a wider context of workforce challenges across primary and secondary care. 154 Staff were generally comfortable pivoting away from their usual front-line services during the pandemic; however, the impact on how staff managed this transition varied with some feeling an additional burden while others welcomed greater responsibility and the opportunity to support the NHS’s response to the pandemic. For some delivery staff, remote home monitoring brought new challenges especially when completing consultations over the telephone compared to face-to-face. In some cases, such a change led staff to reconsider their professional role, beliefs, as well as capabilities when treating patients. Similar learning has been found in previous reviews, citing nursing staff in particular as being concerned about the impact of digitally enhanced modes of working on the staff-patient relationship. 164 However, patient experience findings indicated that the staff-patient relationship was not jeopardised by remote monitoring (see Chapter 9 or Walton et al. 165). Staff experiences on the modality of COVID-19 remote home monitoring and impact of staff are explored elsewhere (see Chapter 11).
Staff received a range of training and support to deliver remote monitoring models as described in our evaluation. Unfamiliarity with nationally designed competency frameworks and a desire for bespoke training to reflect local organisational working meant that some staff may have been better equipped to work more independently than others. Front-line delivery staff, in particular, were asked to rapidly transition from usual methods of providing face-to-face treatment to remote ways of working with limited available evidence of the effectiveness, benefits/challenges and staff experiences of remote home monitoring services. 153 Given the speed at which remote home monitoring services were implemented, this left a significant gap between staff skills, competency and confidence.
The nature and quality of training delivered to front-line delivery staff was dependent on those providing senior clinical insight. Consequently, some delivery staff experienced substantial levels of uncertainty about using their own clinical judgement to independently assess and communicate risk. This was, to some extent, later addressed by the development of SOPs and other working materials. This uncertainty may have impacted on the ability of delivery staff to communicate risks effectively with patients, especially those living with multimorbidities as well as COVID-19 which may have led to patients to revaluate self-management practices. Remote home monitoring may not always remove the need for in-person examinations. 166
Our findings demonstrated workload differences for those balancing their workload with other commitments versus those who were redeployed. This supports findings from the first wave of the pandemic, in which staff were able to focus on delivery of remote home monitoring services due to cancellation of elective care/other activities, and the redeployment of staff. 36 Delivery staff, particularly nurses, remained committed to taking a patient-centred approach to providing care that took account of the emotional and physical impact of COVID-19 on patients. As with previous research,152 our findings highlighted that staff were often not directly involved or lacked a level of autonomy with respect to wider organisational change, despite wanting to share views on how models should be implemented locally.
Given the range of skills required to deliver remote home monitoring models, a multidisciplinary approach is needed for COVID-19 and chronic disease management in general. 167 Previous research from the United States showed that team-based care (physicians, nurses, administrative staff) led to more effective implementation of COVID-19 remote home monitoring services and higher patient satisfaction than single practitioner-led models. 129 Similar findings were found in another study with chronic heart failure patients, who expressed a greater desire to speak with a range of health professionals when being digitally monitored using a range of technology enabled devices. 168
Strengths and limitations
One strength is that we used mixed methods to study staff experiences of COVID-19 remote home monitoring services, and our study included a large sample of participants working across a range of job roles in primary and secondary care. However, variation between case study sites (see Chapter 4) made it difficult to synthesise findings, which may limit transferability of findings.
Our findings may not be representative of all staff views and experiences. For example, the response rate for our staff survey was 39%. Therefore, it is possible that staff who were less engaged with services may have been less likely to complete the survey.
Implications for policy and practice
Current levels of training may not be adequate to support both clinical leads and delivery staff to undertake a wide range of ‘work’ when managing patients with COVID-19. Staff need the opportunity to be better informed for delivering the service; e-resources should be available to staff, that can be accessed as and when required, and staff should receive bespoke training relevant to their service and organisation. Service leads should consider whether they need to introduce formal sign-off mechanisms to ensure staff have been trained adequately and to a required standard. Staff largely felt confident in their ability to deliver remote home monitoring services but found apps and online platforms difficult to navigate as part of monitoring. It would be valuable, where possible, to involve staff when deciding which apps and/or online platforms to adopt during service development and implementation. Notably, delivery staff lacked confidence when communicating the risks associated with COVID-19 to patients. As a result, organisations implementing remote home monitoring services should promote multidisciplinary approaches where delivery staff have ready access to clinical oversight, especially when a patient has deteriorated to a stage where escalation is required.
Primary and secondary care organisations need to consider staff capacity for delivering home monitoring services, given redeployed staff will no longer be available as routine health-care services recommence.
For staff to take a patient-centred approach, staff should be given flexibility within services to discuss wider contextual issues that can contribute to ill health and to provide emotional support to patients experiencing anxiety and other difficulties.
Future research
Further research is needed to explore the following topics: staff experiences of delivering care for COVID-19 and other health conditions using remote monitoring models, the competency and skill set required to deliver remote monitoring services, how training should be designed, and how best to embed training during the set-up and implementation of services across primary and secondary care sectors.
Conclusions
Remote monitoring models can play a crucial role in managing a large number of patients for a range of health conditions; however, the success of delivery is dependent on the right workplace infrastructure for delivering these models of care. While staff in our study show high levels of acceptability for managing patients using remote models, issues remain around confidence when making clinically informed decisions and a dependency on clinical oversight. Delivery of remote home monitoring services is also influenced by patient engagement or disengagement with these services.
Chapter 9 Patients’ and carers’ experiences of, and engagement with, COVID-19 remote home monitoring services
Overview
This chapter draws on a manuscript by Walton et al. 165 published in Health Expectations (2022): https://doi.org/10.1111/hex.13548. This is an open access article under the term of the Creative Commons Attribution 4.0 International License (CC BY 4.0), which permits use, distribution and reproduction in any medium, provided the original work is properly cited (see https://creativecommons.org/licenses/by/4.0).
What was already known
-
Remote home monitoring services for patients with COVID-19 have been implemented throughout England.
-
These services may place more responsibility on to patients/families.
-
It is not known how patients and carers experience these services, or how they found engaging with the activities.
What this chapter adds
-
Patients and carers found the human contact with staff reassuring.
-
Patients with COVID-19 (an acute condition) can engage with remote monitoring services but may require support from staff and family/friends to do so.
-
Engagement is conditional on a range of factors including patient factors, support and resources, and service characteristics.
-
Burden of treatment may be experienced by patients and families with acute conditions.
Introduction
The shift in health-care delivery from traditional face-to-face care models52 towards remote home monitoring models22,169 is consistent with recent moves towards self-management and patient activation within health care, whereby accountability for care has changed. 170–173 Patients are becoming more involved in self-management, for example, learning how to detect and manage their symptoms, and treatments, and escalation of care associated with their condition,51,174–178 and health-care tasks (e.g. managing medication, organising care appointments, taking measurements). 179 While some patients may welcome this move,180 there have been concerns that self-management and models such as remote home monitoring shift burden (including responsibility, commitment and workload) on to patients and families, rather than facilitating shared care. 171,180 This may not be suitable for everyone,181 as some people may be unable to meet expectations and may experience negative impacts from treatment burden171 – for example, health consequences faced by patients due to not adhering to treatment and professional, social, emotional and financial consequences. 179 Different individuals may tolerate different levels of treatment burden, and needs should be assessed regularly as tolerance changes over time. 171,182 As many situational, personal, and structural factors can worsen treatment burden,179 formal and informal support networks are needed to support patients. 50,51
Treatment burden may negatively impact on patient experience and levels of engagement. This is problematic given that patient engagement with remote home monitoring is crucial. Patient engagement has been defined as patients understanding the information they are given (‘receipt’) and being able to perform the required activities (‘enactment’). 57,58 For this service, engagement refers to the behaviours that patients must undertake as part of the service – for example, reading/listening to and understanding the information given to them by the service, using the oximeter to take readings, recording and submitting readings and self-escalating care as required. If patients do not engage with these services, they may be at risk of negative outcomes, such as silent hypoxia (very low oxygen saturations, often without breathlessness)13 and/or delayed admission to hospital. 15,16 Additionally, limited engagement makes it difficult to evaluate whether or not these services influence key outcome measures such as any changes in mortality or hospital use.
While previous research indicates factors which may influence patient engagement with treatment models more generally,50,51,171,179 there is a lack of research on patient experience and engagement with COVID-19 remote home monitoring services (see Chapter 3 or Vindrola-Padros et al. 32 and Vindrola-Padros et al. 36). This chapter addresses this gap by evaluating patient experience of, and engagement with COVID-19 remote home monitoring services.
This study aimed to explore what formal and informal support patients received as part of COVID-19 remote home monitoring services, and patient experience of, and engagement with these services. This chapter addressed the following questions:
-
What types of formal and informal support did patients receive as part of COVID-19 remote home monitoring services? What was the burden of treatment on patients and carers in informal support roles?
-
What were patients’ and carers’ experiences of engaging with COVID-19 remote home monitoring services?
-
What were the factors influencing burden of treatment and ability to engage with COVID-19 remote home monitoring services?
Methods
A summary of the methods is provided below; further details can be found in Chapter 2 (or Walton et al. 165).
Design
This chapter draws on data from workstream 3 (patient and carer survey data) and workstream 4 (patient and carer interviews). Data collection took place between February and June 2021 (patient surveys retrospectively included January).
Sample
Patients and carers who had received COVID-19 remote home monitoring services (see Chapter 2 and Chapter 4 for site and patient sample details).
Measures
We developed the survey and semistructured topic guides specifically for this study (see Chapter 2, Project website documentation – Study instruments).
Data collection
We conducted a survey with patients and carers from 25 sites and interviews with patients and carers from 17 sites (see Chapter 2 for details).
Analysis
Survey data were analysed using descriptive statistics (using SPSS, version 25). All cases were analysed (whether carer, patient or unknown). Where data were missing for specific questions, cases were excluded from the analysis and the denominator reported. Open text responses for three questions (434 responses for anything else to say about the service, 61 responses for how carers supported friend/family member while they had COVID-19 and 200 responses for recommendations to improve service).
Inductive thematic analysis was used to analyse interview data inputted into RAP sheets. 70 We then developed a framework based on these themes and subthemes and one researcher used this framework to extract quotes from all original transcripts.
Survey and interview findings were triangulated.
Results
Participant characteristics
Surveys were received from patients (n = 936) and carers (n = 48), and 62 interviews were conducted with patients (n = 59) and carers (n = 3). See Chapter 4 and Report Supplementary Material 2 for demographics.
Most patients were referred to services via community methods (Table 17). Patients and carers used a range of methods to record and report their readings to the service, including analogue (paper and phone) and tech-enabled methods (Table 17).
Survey participants (N = 1069), n (%) | Interview participants (N = 62), n (%) | ||
---|---|---|---|
Pathway | CO@h (referred pre-hospital via community methods) | 749 (70) | 44 (71) |
CVW (referred via early discharge from hospital) | 168 (16) | 13 (21) | |
Both | N/A | 2 (3) | |
Unknown | 152 (14) | 2 (3) | |
Not applicable | N/A | 1 (1) | |
Method used to record and report readings | Analogue (paper and phone) | 522 (49) | 19 (31) |
Tech-enabled (such as text, app, weblink or automated phone) | 547 (51) | 27 (44) | |
Combination of tech-enabled and analogue | N/A | 14 (23) | |
Not known | N/A | 1 (2) | |
Not applicable | N/A | 1 (2) |
What type of formal and informal support did patients receive as part of COVID-19 remote home monitoring services?
Formal support from staff
The ‘care’ on offer differed across sites and patients, with variation in the type and frequency of monitoring offered by services (see Chapter 4).
The frequency with which patients had contact with a member of staff ranged from several times a day to not at all. Most patients and carers had contact either once a day, or several times a week. A few patients and carers reported not speaking to staff at all (Table 18). Findings indicate that patients were supported by staff throughout different stages of the service, including providing information, monitoring, escalating care, signposting and comfort and reassurance.
Frequency of contact with staff member | Percentage of survey participants, n (%) | Range across sites (%) |
---|---|---|
Several times a day (n = 1060) | 169 (16) | 0–45 |
Once a day (n = 1060) | 276 (26) | 7–91 |
Several times a week (n = 1060) | 270 (25) | 0–62 |
Once a week (n = 1060) | 139 (13) | 0–31 |
Less than once a week (n = 1060) | 142 (13) | 0–27 |
Not at all (n = 1060) | 64 (6) | 0–27 |
Burden of treatment on patients and carers in informal support roles
Survey findings indicated that almost all patients used an oximeter to record readings when receiving the service. Many patients reported completing a diary and providing readings over the phone or using technology-enabled methods. Escalation-related behaviours (seeking further help, checking readings) were reported less frequently by patients (Table 19).
Remote home monitoring activities that patients reported doing | Percentage of survey participants, n (%) | Range across sites (%) |
---|---|---|
Using the oximeter (n = 1069) | 1014 (95) | 81–100 |
Completing a diary (n = 1069) | 555 (52) | 11–89 |
Providing readings over the phone (n = 1069) | 498 (47) | 19–93 |
Providing readings via text (n = 1069) | 309 (29) | 0–74 |
Recording readings in a digital app (n = 1069) | 264 (25) | 0–89 |
Providing readings via e-mail (n = 1069) | 15 (1) | 0–5 |
Seeking further help due to readings being lower than the recommended threshold (n = 1069) | 344 (32) | 18–71 |
Checking over readings for issues (n = 1069) | 215 (20) | 7–41 |
Many patients were supported by family and friends to engage with the service. One-quarter of survey respondents needed help to use equipment (25%, range 11–50% across sites), and more than half of the interview participants were supported by family members.
Most patients and carers reported having informal support to help them use the oximeter and support with taking and recording readings (Table 20). Qualitative findings highlighted that family and friends provided support with many activities, including support submitting readings, monitoring and collecting the oximeter. However, not all patients had support with using the oximeter (16%) or taking/recording readings (18%). Some patients did not have support due to their family members having COVID-19.
Support for remote home monitoring activities | Survey participants, n (%) | Range across sites (%) | |||
---|---|---|---|---|---|
Having someone to help use the oximeter when needed (n = 1058) | Yes | 782 (74) | 50–100 | ||
No | 169 (16) | 0–44 | |||
Not applicable | 107 (10) | 0–22 | |||
Support taking and recording readings if needed (n = 1057) | Yes | 666 (63) | 43.8–90 | ||
No | 190 (18) | 7–33 | |||
Not applicable | 201 (19) | 0–37.5 |
What are patients’ and carers’ experiences of engaging with COVID-19 remote home monitoring services?
Patients mostly had positive views of the services; 93% (n = 970/1045) of survey respondents rated services as excellent or good, 90% (n = 923/1028) of respondents found the services helpful and 91% (n = 944/1037) would recommend the services to their family and friends.
Findings indicated that most patients and carers found the services reassuring and supportive (91% (n = 946/1040) of survey respondents). Qualitative findings indicated that patients and carers valued the human contact with staff and found it reassuring due to having someone watching over them, particularly for those who were living alone, had no support nearby or had existing conditions.
Because it’s obviously keeping an eye on you, isn’t it really? And I was getting the phone calls every day. How are you feeling? [. . .]. But someone who was on their own, who had no- who was living on their own, you know, it’s a bit of a lifesaver isn’t it?
(Site A, interviewee 4)
A minority of patients and carers felt that there were gaps in the service, and it was not holistic. Some felt that services were narrowly focused on managing known symptoms of COVID-19 which did not always suit those with other symptoms, health conditions or who required wider support. A few patients reported feeling that services were isolating and unsupportive (e.g. they only received a call about the oximeter drop-off/return, but not for monitoring).
Most patients and carers felt the care provided was appropriate and preferred to be at home instead of being in hospital (given the pandemic context). Reasons for preferring home over hospital included freeing up space for others in need, fears of going to hospital during a pandemic, and communication barriers. However, some patients and carers spoke about preferring to be in the hospital rather than at home, to feel more secure, feeling scared and wanting to be seen face-to-face.
And I didn’t feel too embarrassed that I was using up valuable resources because I thought, Well I’m sitting here at home, there’s no reason for me to go in Hospital, bother anyone and waste people’s time.
(Site N, interviewee 3)
A minority of patients and carers spoke about how these services were the only available care and that they would have liked to have received care from other health-care professionals such as their GP in parallel. Many patients and carers were not aware of these services prior to referral.
Some patients and carers also spoke about how these services helped them to monitor their own improvement and that it potentially improved their outcomes.
Patients and carers reported very positive views of the workforce and that they were helpful and put patients at ease and were professional and potentially even life saving. Continuity of staff was thought to be important.
A few patients had negative experiences with individual staff members (e.g. that they were dismissive, did not recognise that they needed help, were not interested or lacked clinical expertise to support patients or answer their queries).
What are the factors influencing burden of treatment and ability to engage with COVID-19 remote home monitoring services?
Findings indicated that patients and carers generally found it easy or very easy to engage with these services and the resulting activities, including understanding information, monitoring using the oximeter, recording readings and providing readings and escalating care (Table 21). Most survey respondents indicated that they did not experience problems with services (72%, n = 771/1069) and did not report barriers to engagement with services (80%, n = 858/1069).
Experience engaging with service activities | Survey participants who reported easy/very easy, n (%) |
---|---|
Understanding the information they were given (n = 1040) | 970 (93) |
Monitoring using the oximeter (n = 1049) | 1022 (97) |
Recording readings (n = 949) | 913 (96) |
Providing readings to the remote home monitoring team (n = 1010) | 979 (97) |
Seeking further help (if applicable) (n = 857) | 738 (86) |
Engagement with service activities was not without challenges, with some patients and carers reporting issues with the information provided or needing further information. Some patients found monitoring difficult due to other health conditions, or that monitoring made them feel worried. Some patients and carers wanted more support or found recording burdensome. Finally, some issues related to escalating care were identified in the interviews. The uncertainty of COVID-19, perceptions of hospital as a frightening place and uncertainty around interpretation of readings and thresholds, meant that some patients and carers were hesitant to self-escalate their care, waited for a member of staff to advise them to escalate their care or reported not wanting to go to hospital or seek further support, even when advised to by staff members.
really I should have probably rung when the readings were that bad but I didn’t. [. . .]And when I did send them through they said, ‘No, get to the doctors now’.
(Site C, interviewee 6)
The most frequent challenges reported within the survey were returning the oximeter, contacting health-care professionals when needed and seeking further help (Table 22). While many survey respondents discussed problems with the team (35%, n = 87/249) or had their problems resolved (33%, n = 76/232), over half of these participants said problems had not been resolved (54%, n = 126/232).
Survey participants, n (%) | ||
---|---|---|
Challenges experienced with service activities | Using the oximeter (n = 1069) | 34 (3) |
Recording readings in an app or diary (n = 1069) | 27 (3) | |
Contacting health-care professionals when needed (n = 1069) | 56 (5) | |
Seeking further help (n = 1069) | 57 (5) | |
Returning the oximeter (n = 1069) | 136 (13) | |
Other (n = 1069) | 42 (4) | |
Discussion and resolution of problems | Discussed problems with remote home monitoring team (n = 249) | 87 (35) |
Had problems resolved (n = 232) | 76 (33) | |
Did not have problems resolved (n = 232) | 126 (54) |
Findings from the surveys and interviews indicated three overarching themes that influenced burden of treatment and patient’s ability to engage, with COVID-19 remote home monitoring services: (1) patient factors; (2) wider support and resources; and (3) service factors (see Report Supplementary Material 8 for example quotes).
Patient factors
Knowledge, memory, physical health, attitudes towards services and having time to complete the required tasks influenced engagement (see Report Supplementary Material 8). Interview findings indicated that patients in poorer health (e.g. due to COVID-19, other health conditions) found it harder to engage with the service. Patients and carers who felt they had sufficient knowledge about what they needed to do found it easier to engage [e.g. 55% (n = 583/1069) of survey respondents felt that knowing what to do helped them to engage with the service]. On the other hand, a lack of knowledge on how to complete the activities limited engagement.
Wider support and resources
Support from staff/service, support from family members/friends, accessibility and availability of materials, equipment and technology influenced engagement (see Report Supplementary Material 8). For example, support from staff members (e.g. 46% (n = 488/1069) of survey respondents) and family/friends [e.g. 25% (n = 266/1069) of survey respondents] was crucial in helping many patients to use the service.
Service factors
Monitoring characteristics, service characteristics, scope of service and availability of treatment influenced engagement (see Report Supplementary Material 8). For example, some participants felt that the inconsistent timing of calls was a barrier, and some felt that calls were too frequent, whereas others felt they were not frequent enough. Additionally, some patients and carers felt that service scope was a barrier to engagement, in that it did not cover wider symptoms of COVID-19 and was not holistic.
Discussion
Key findings
Patients can engage with remote home monitoring services, even when experiencing acute illnesses (e.g. COVID-19). However, many patients required formal input from staff and informal support from family and friends to complete the necessary tasks. Patients and carers had positive experiences receiving remote home monitoring. The human contact from staff provided patients and carers with reassurance and patients reported services were mostly easy to engage with. However, patients’ ability to engage with services was conditional on a range of factors, including having support from family/friends and staff, being in good health and receiving clear instructions on what they needed to do and how to do it, and the level of commitment from patients while on the service.
How findings relate to previous research
Previous research highlighted that little was known regarding patient experiences of COVID-19 remote home monitoring services in the context of pressured health services and concerned patients (see Chapter 3 or Vindrola-Padros et al.)32,36 These findings extend earlier findings by highlighting patients’ and carers’ positive views of the service, challenges and concerns relating to engagement with remote home monitoring for acute conditions, and tangible recommendations on how to improve remote home monitoring services (see Appendix 3, Table 26), many of which support wider themes reported in patient experience literature (e.g. the importance of information provision). 183,184
Previous research outlines concerns relating to remote care and telemedicine and the loss of interpersonal dimensions involved in caring relationships. 170 Findings extend the evidence base by showing that care does not need to take place face-to-face for patients to feel reassured and supported. Patients largely felt that care provided at a distance was appropriate and that they were being monitored. This is consistent with previous research indicating that technology may support closer contact with professionals. 170 However, findings may have been affected by the pandemic context in that data were collected during the height of wave 2 of the pandemic, so patients may have been more likely to accept remotely delivered services to help minimise risk to themselves, family members and staff. Patients and carers may feel differently about remote home monitoring and care delivered at a distance in non-pandemic contexts.
New models of health care such as COVID-19 remote home monitoring services sought to change the traditional model of in-person care. Instead, within these models, staff engage with patients to share the care burden while equipping patients and carers to self-monitor and manage care in the absence of staff members. Our findings demonstrate that concepts of treatment burden and difficulties engaging with health-care demands171,179,182 also apply to remote monitoring models for COVID-19. Some patients reported problems engaging with remote home monitoring services for a range of reasons, including feeling too poorly, not having enough knowledge on what to do, and lack of support from staff and/or family/friends. Others reported the necessity of support from their family/friends when engaging with the service. This extends knowledge by showing that social networks may undertake self-monitoring tasks on behalf of (potentially very poorly) patients in addition to helping patients cope with burden of treatment171 and self-management of conditions. 51,185 Those who feel more poorly (either due to COVID-19 or existing conditions) may require more formal or informal support to manage care. Yet, this increases the caring burden for family/friends. This finding also raises concerns regarding appropriateness of care for those who do not have informal support networks in place.
This chapter builds on earlier research by providing a nuanced interpretation of the factors that influenced engagement with remote home monitoring services for acute conditions such as COVID-19. Our finding that many non-health related factors influence engagement is consistent with other studies in a range of other conditions and interventions. 51,179,186–189 Our findings add to prior knowledge by demonstrating that many factors were exacerbated due to the acute nature of COVID-19 and policy factors surrounding COVID. For example, physical health factors limiting engagement may be worsened by the acute nature of COVID-19 and the severity of symptoms that some patients faced, thus affecting a patient’s ability to engage. Furthermore, policy regulations and lockdown restrictions imposed within the UK may have meant that physical social support available to patients may have been limited by members of their support networks living elsewhere. As COVID-19 is easily transmissible, social distancing recommendations were in place, therefore, many patients were distancing from family members living in the same space. Findings demonstrate that despite difficulties imposed by COVID-19, social networks were crucial for many patients in facilitating engagement with services and ensuring that care needs were met. This highlights the need for alternative support where necessary (particularly for those living alone or those who are socially isolated). The reliance on informal support networks has implications for burden of treatment and may not be appropriate for all individuals. These findings support previous theoretical frameworks indicating that social, political and technical contexts influence engagement. 49–52
Previous research has explored concepts of self-management,50,51,186 engagement187 and treatment burden179 in chronic conditions, but little research had been conducted on remote home monitoring and self-management in acute conditions such as COVID-19 in which care needs to be urgently escalated in an efficient and time-sensitive manner. Findings extend earlier work by demonstrating challenges of remote home monitoring models for acute conditions. For example, due to uncertainty of COVID-19, perceptions of hospital being a frightening place and uncertainty around readings and thresholds, some patients were hesitant to self-escalate care and in many cases, patients waited until advised by staff to escalate care. This finding indicates that in situations where there is a need for timely escalation, but concerns around infection transmission from going to hospital, it may be suitable to have formal support from staff members. Together, staff and patients can collaboratively decide when to seek further help, rather than placing responsibility on to patients. This finding contrasts with recommendations within the national SOP for COVID-19 remote home monitoring services,38,39 which indicate that pathways should encourage patients to self-escalate care.
Some patients felt that they were able to engage with services because they had manageable symptoms and felt comfortable with tasks. This indicates that different levels of remote monitoring support are needed for different individuals. This finding supports previous research indicating that the success of telehealth services including remote home monitoring may rely on the fit between individuals’ needs and services. 50,170
Strengths and limitations
Integration of mixed-methods data helped to provide in-depth perspectives on patient and carer experiences of, and engagement with COVID-19 remote home monitoring services.
Findings may not be representative of all patient and carer groups and experiences, for the following reasons: (1) our patient sample was under-representative of some groups (e.g. older patients and ethnic minorities) and over-representative of other groups, when compared with patient onboarding data,114 (2) survey response rate was only 17.5%, and (3) we were unable to recruit patients who had declined or dropped out of the service, or who did not want to take part in the research.
The focus of our research was on patients’ experiences of remote home monitoring services. Therefore, it is possible that we have not captured carers’ experiences in detail. However, some carers shared their own experiences during the interviews and in responding to the survey.
Implications
Burden of treatment may not only affect those with multimorbidity or chronic conditions, but can also affect those with acute conditions. Findings indicate that remote monitoring may increase treatment burden for some patients and families.
COVID-19 remote home monitoring services aimed to target patient groups at higher risk from COVID-19, yet many of these groups appear more likely to report difficulties in engagement with these services (e.g. older patients and those with health problems). Remote monitoring may not be appropriate for everyone (e.g. those without support). Services need to gauge a person’s support network and any concerns surrounding remote home monitoring when assessing eligibility for these services. Services must then tailor the health-care offer to enable patients to engage (e.g. providing further support for those from at-risk groups or who do not have informal support, or linking patients with care networks if needed). All patients should be given contact details to contact services should problems arise. Face-to-face support (e.g. for monitoring) from staff and families has implications for infection transmission.
These findings may have implications for remote home monitoring services more generally. Service developers should consider the type of condition when designing pathways. For example, services for acute conditions may require support from staff to ensure that patients are escalated for further care as necessary. Services must plan logistics for delivery and collection of equipment, ensure sufficient information provision and that patients know what they need to do and that they feel able to engage with the service. Some patients felt that services offered were too narrow and do not consider wider social, emotional or condition-related needs. Service adaptations may be necessary for those receiving remote home monitoring for acute conditions in addition to care for other chronic conditions.
Future research
Further research is needed to explore: (1) experiences of those who decline or disengage from these services; (2) burden of treatment for acute conditions versus chronic conditions; (3) which groups are able to engage with and tolerate burden from remote home monitoring services; and (4) the impact of treatment burden from informal caring responsibilities on families.
Conclusions
COVID-19 remote home monitoring services place a large responsibility on patients and carers in relation to monitoring and escalating care. While patients and carers found services reassuring and a positive experience, many factors influenced their ability to engage. This indicates that services may be conditional on a range of factors relating to the patient (e.g. knowledge and memory), their support and resources (e.g. support from family, friends and staff) and service factors (e.g. service scope and frequency of monitoring).
Chapter 10 Examining disparities relating to service reach and patient engagement with COVID-19 remote home monitoring services
Overview
This chapter draws on a manuscript by Crellin et al. 190 uploaded as a preprint to medRxiv. This is an open access article under the term of the Creative Commons Attribution 4.0 International License (CC BY 4.0), which permits use, distribution and reproduction in any medium, provided the original work is properly cited (see https://creativecommons.org/licenses/by/4.0).
What was already known
-
Evidence shows that COVID-19 has a disproportionate impact on certain population groups, such as ethnic minority groups, older adults and those with comorbidities.
-
Literature exploring the effectiveness of strategies to reduce health disparities for remote monitoring services and the impact of remote monitoring services on health disparities is scarce.
-
The rapid adoption and spread of remote home monitoring services in England must be accompanied by evaluations at a local level to monitor the impact on health disparities.
What this chapter adds
-
Increased understanding of how remote home monitoring services can be designed and delivered to address local population needs to increase accessibility and facilitate engagement with the service.
-
We identified a number of local service adaptations, including prioritising vulnerable groups, creating referral pathways, offering translation services, offering non-digital options, and providing face-to-face assessments, and we discuss their potential impact on health disparities.
-
We found that despite efforts to adapt services, disparities across patient groups in their experience of, and engagement with, the service were reported (related to age, health status, ethnicity, level of education, employment status and gender).
Introduction
The COVID-19 pandemic has shone a spotlight on existing health disparities in the UK. The pandemic has disproportionately affected the most marginalised communities, particularly people living in more socioeconomically deprived areas, those from minority ethnic communities, older adults, people with a learning disability and those residing in care homes, and people in key worker occupations. 12,130,191
The pandemic has also led to changes to the way that health services are delivered at an unprecedented pace,192,193 with an acceleration of the move towards remote monitoring models of care. Yet, little is known as to whether and how this shift might affect existing health disparities. 194–196
The benefits brought about by remote methods of delivering health care are not experienced by all: for tech-enabled remote methods, digital exclusion may negatively impact access to or quality of care. 197–199 Other factors may exacerbate disparities in access, engagement or quality of care. Some patient groups are more likely to experience inequities (e.g. older adults, people with coexisting conditions, those with difficulties communicating, and those isolated or experiencing language barriers, those with lower health literacy or understanding of how health systems work) and may need additional support to engage with services. 200
Inequities can be introduced at all stages of the planning and delivery of health interventions, including access, diagnostic accuracy, patient uptake and engagement. 201 Given the recent rapid shift towards remote models of care, it is important to explore whether disparities exist in how patients access, engage with and experience services, and to understand whether and how remote services can be designed and delivered to promote inclusion. This study addressed the following questions:
-
Were COVID-19 remote home monitoring services adapted at a local level to increase service reach and patient engagement for different groups?
-
Were there disparities in patients’ reports of their ability to engage with the service, and were these moderated by the modality of the service? What were the potential impacts of service adaptations on health disparities according to patients?
-
Were there disparities in patients’ reports of their experience of the service?
Methods
A summary of the methods is provided below; further details can be found in Chapter 2 or Report Supplementary Material 1.
Design
This chapter draws on staff and patient/carer surveys and staff and patient/carer interviews. Data collection took place between February and June 2021 (patient surveys retrospectively included January).
Sample
Patients/carers who received these services and staff leading and delivering services (see Chapter 2 and Chapter 4 for sample details).
Measures
We developed the survey and semistructured topic guides specifically for this study (see Chapter 2 and Project website documentation – Study instruments).
Data collection
We conducted a survey with patients/carers from 25 sites and with staff from 28 sites. We conducted interviews with staff, patients and carers from 17 sites (see Chapter 2 for details).
Analysis
Descriptive statistics were calculated to explore staff survey responses relating to perceived patient groups facing barriers. Open text responses relating to local service adaptations and patient groups facing barriers were analysed; responses were triangulated with service lead interview data, coded and grouped into themes.
For patient survey data, non-parametric univariate analyses (i.e. Mann–Whitney U-tests and Kruskal–Wallis tests) were conducted to explore patient engagement with services and experience of services across patient characteristics. Where statistically significance differences were detected (at the p < 0.01 level) across more than two groups, post hoc analyses were conducted. For both patient and staff survey responses, where data were missing for specific questions, available case analysis was performed and the denominator reported.
We conducted logistic regression modelling to examine whether modality of the service, age, education, health status and ethnicity were associated with likelihood of patients reporting at least one problem with the service. Patient open text survey responses providing feedback about services and any suggested changes or improvements were triangulated with patient interview data, coded and grouped into themes.
Service lead and patient interviews
To understand whether and how local services were adapted to address health disparities and their potential impacts (RQ1), a thematic analysis was carried out on primary data from service lead interviews. 202,203 Interview transcripts were coded inductively and organised into higher-order themes.
To examine the potential impacts of adaptations from patients’ perspectives, RAP sheets70 were analysed. Data was deductively coded using the themes from the service lead interviews.
Results
Participant characteristics
Surveys were received from 292 NHS staff and 1069 surveys were received from patients and carers. We conducted 23 interviews with service delivery leads and 62 interviews with patients/carers (see Chapter 4 and Report Supplementary Material 2 for demographic characteristics).
Comparison of the characteristics of patient survey respondents with those of more than 26,000 patients onboarded to CO@h services114 indicate the survey sample to be relatively representative of patients engaging with the service. However, several differences should be noted. The survey sample is under-representative of patients over 80 years (5% vs. 10%) and under 50 years of age (21% vs. 33%), and over-representative of patients aged 50–80 years (74% vs. 57%). The survey sample comprises a higher proportion of patients from white ethnic groups (91%) compared to those onboarded to services (76%). Finally, our sample is over-representative of patients in the least deprived deciles of the IMD (18% vs. 13%) and under-representative of patients in the most deprived deciles (24% vs. 28%).
Service adaptations to increase service reach and patient engagement
Staff reported their views of patient groups facing barriers to engaging with the service. Some 39% (n = 113/292) reported patients for whom English was not their first language, 33% (n = 97/292) patients with a visual or hearing impairment, 31% (n = 91/292) cognitive impairment, 22% (n = 63/292) patients with a learning disability, 21% (n = 61/292) those who are digitally excluded, 13% (n = 37/292) older adults, 7% (n = 20/292) ethnic minority groups, and 5% (n = 16/292) unpaid carers. Other patient groups that staff reported in surveys or interviews included those living alone without support, patients unable to collect the oximeter, people with mental health problems, asthmatics, pregnant patients, patients whose GP had not engaged with the service, those with difficulty communicating via phone, and patients without a fixed abode.
Two-thirds of service leads (16/24 sites) reported that their service had been adapted at a local level to accommodate specific service user needs or requirements. Examples included providing information in different languages, offering translation services, offering non-digital monitoring options, face-to-face assessments, and flexibility of monitoring. Service-lead interviews and survey data indicated there was considerable local variation in the number of strategies adopted by sites. For example, several services made considerable efforts to standardise the coverage of the service, invest resources into setting up special pathways targeting hard-to-reach, vulnerable or at-risk groups, and monitor uptake. National strategies and local service adaptations reported by staff are set out in Appendix 4, Table 27.
Disparities in patients’ reports of their ability to engage with the service
In this section, we present findings from patient-reported engagement with these services in terms of the accessibility of information, the achievability of tasks, and problems reported via the survey (drawn from the survey analysis). See Report Supplementary Material 8 for further details. We also use findings from patient interviews and open-text survey responses (referred to as ‘qualitative data’) relating to patient experiences of the impact of service adaptations aimed at addressing inequalities.
Accessing the service
In interviews, patients reported being referred to services by many different organisations and pathways. However, sites were not always consistent in the admission criteria applied to access the service and there was sometimes a lack of clarity in how patients were referred to the service; some patients reported inconsistencies across local areas and several patients from sites that used active case finding reported they did not know how they were referred. Collaboration between services was perceived to have facilitated more rapid access to care; several patients highlighted that they were supported quickly because of close liaison between services and their GP practice, hospital or ambulance services. Once enrolled into the service, there were challenges relating to obtaining the appropriate equipment; some patients reported it was difficult to collect the oximeter when they were unwell and relied on family members or friends.
Accessibility of the information about the service
Interviews and survey responses indicated variation across and between sites in the information available to patients and how it was provided. We found evidence of a difference in understanding information provided by services with patient age (p = 0.005) and ethnicity (p = 0.001). There were also some marginally non-significant differences with health status (p = 0.014).
Patients aged 80 years and over reported less ease in understanding information; a lower proportion of patients reported understanding the information to be ‘very easy’ (40%) compared with all other age categories (60–67%). However, the number of patients aged 80 years and over was small and when ‘very easy’ and ‘easy’ responses were combined there was little difference across age categories. Patient ratings of helpfulness of the information was also statistically significantly lower for older patients (p = 0.005), with similar patterns of responses to understanding the information. Patients from minority ethnic groups reported less ease in understanding information provided; 81% of patients from minority ethnic groups reported understanding the information to be ‘easy’ or ‘very easy’ compared to 96% of patients from white ethnic groups.
Interviews identified several other factors affecting the accessibility of patient information. Several patients noted it was particularly difficult to take on the information when they were unwell, while those with visual impairments would have welcomed written information in a larger font, and several patients would have liked additional information about the service, their condition and recovery (e.g. how to interpret oxygen readings, how to manage their recovery, and where to seek help).
Patients’ ability to complete tasks as part of the service
We found evidence of differences in patient-reported ease of completing monitoring activities (i.e. using an oximeter, recording and providing readings) and escalation activities (i.e. seeking further help) with age, gender, health status, ethnicity, and level of education (see Report Supplementary Material 8 for further details).
Monitoring activities
Overall, our analysis found that most patients reported monitoring activities easy to engage with. The proportion of patients reporting difficulties was low; however, some patient groups might require additional support to engage with the service. This is consistent with qualitative data; some patients reported receiving additional support beyond the monitoring protocol or national guidance, such as receiving face-to-face visits.
Survey analysis found evidence of a difference in patient-reported ease of recording readings with health status (p = 0.004). Fewer patients with a health problem or disability rated recording readings as ‘very easy’ (70%) compared with those without a health problem (79%), however when this response option was combined with ‘easy’ there was little difference between groups. A similar trend was found for health status and providing readings to services, however, was marginally non-significant (p = 0.017).
There was evidence of differences between ethnic groups for recording readings (p < 0.001). Fewer patients from minority ethnic groups reported recording readings (91%) to be ‘easy’ or ‘very easy’ compared with white groups (98%). A similar pattern was found for providing readings to services however was marginally non-significant (p = 0.013). While translated patient materials were available to services, the extent that these were used by services is unclear.
Staff interviews indicated translation services were often needed and not always available for patients for whom English was not their first language. Services often relied on liaising with friends or family to support communication (when translation services were not available) and this was not always possible.
Although not statistically significant, there was some evidence of differences in ease of recording (p = 0.022) and providing readings (p = 0.016) with age. There was little difference in the proportion of patients reporting tasks as ‘easy’ or ‘very easy’ across age categories, but fewer patients aged over 80 years reported recording readings (55%) and providing readings (57%) to be ‘very easy’ compared with those under 50 years (80%, 79%) and in the 50–64 years age category (77%, 78%). Older patients reported support from friends, family and health-care professionals was more important for their engagement with services: 26% (n = 11/43) of patients over 80 years reported they had support from family and friends to use equipment and 49% (n = 21/43) reported support from health-care professionals helped them to engage with the service. This compares with 21% (n = 40/195) of patients under 50 years of age reporting support from family and friends, and 43% (n = 83/195) reporting support from health-care professionals.
A statistically significant difference in providing readings to services (p = 0.001) was also identified for gender: fewer male patients reported providing readings (70%) to be ‘very easy’ compared with female patients (80%). A higher proportion of males (25%, n = 97/385) also reported the importance of friends and family in supporting their engagement with services compared with females (19%, n = 102/531).
There were no statistically significant differences (at the p < 0.01 level) in engagement with monitoring activities with employment or living situation. In interviews several patients in paid employment reported that they would prefer to reduce the number of daily readings or that they preferred to receive calls at a specific time each day. Patients living with others reported greater reliance on support networks, friends and family; 10% (n = 14/137) of patients living alone reported support from family or friends helped them to engage with the service compared with 23% (n = 175/754) of those living with others. Many patients reported the support from family members, friends or carers to take and provide readings, and liaise with the remote monitoring team was crucial. This was particularly important for patients who were tired or more acutely unwell.
Escalation processes
As for escalation processes (i.e. seeking further help when needed), we found evidence of a difference with employment status (p = 0.007). Fewer patients who were not in employment reported seeking further help to be ‘easy’ or ‘very easy’ (84%) compared with those who were employed (89%). There was also some evidence of differences in ease of engagement with escalation processes across ethnic groups (p = 0.015) and with level of education (p = 0.015), however these were marginally non-significant (at the p < 0.01 level). Patients with higher education attainment and those from minority ethnic groups were less likely to report seeking further help to be ‘easy’ or ‘very easy’.
Disparities in patient engagement by the mode of services
Overall, 25% (n = 228/936) of patients reported at least one problem completing tasks that were part of services (e.g. using the oximeter, providing or recording readings, or seeking further help). Logistic regression analysis found mode of monitoring was not related to whether patients reported a problem, nor were health status or ethnicity (see Report Supplementary Material 8). Increasing age and a higher level of educational attainment were associated with an increased likelihood of reporting a problem. The odds of reporting at least one problem with services were approximately 7.6 times higher for older adults (80 years and over) than younger adults (under 50 years of age), for patients aged 65–79 years the odds were 2.9 times higher and for patients aged 50–64 years the odds were 2.3 times higher compared with patients under 50 years. The odds of patients who were educated to A level or equivalent reporting a problem were three times higher than patients who had received no formal qualification.
Disparities in patients’ reports of their experience of the service
We found evidence of differences in patient reported experience and helpfulness of services with age and employment status, however reassurance provided did not differ markedly across patient groups.
Older patients reported services to be less helpful (p = 0.004); fewer patients aged 80 years and over reported services to be ‘helpful’ or ‘very helpful’ (88%) compared with those in the under 50 years (92%) and 50–64 years age categories (92%). Older patients also reported a less positive experience of services (p = 0.034), although this was not statistically significant (at the p < 0.01 level). The less positive experiences of patients aged 80 years and over might be explained by differences in understanding information about services and ease of completing monitoring tasks; however, they might also be due to the smaller sample size of the 80 years and over age category.
Patients in employment rated services more positively (p = 0.001) and found services more helpful (p = 0.003); 96% rated it ‘good’ or ‘excellent’ and 93% ‘helpful’ or ‘very helpful’ compared with 92% and 87%, respectively, among patients not in employment. The differences in patient experiences based on employment status might be related to other factors, such as health status, level of education or age, with older patients more likely to be retired.
Living situation was not identified in the survey data to be a significant factor in patient reported experience, or reassurance provided. However patient interview data indicated living situation and strength of social network as important factors; provided by services was reported to be particularly important for those socially isolated or living alone.
Discussion
Key findings
At a local level many sites designed and adapted their service to be more inclusive to the needs of their local population and to expand their reach. Strategies included: providing information in different languages or formats, offering translation services, offering analogue and tech-enabled options for relaying readings, face-to-face assessments, delivering oximeters, providing additional training or support, flexible monitoring processes, and liaising with family or friends. There was considerable local variation in the adaptations employed by services. The adoption of such strategies was often dependent on local leaders as to how patient groups were approached and supported,90 and on the resources available to deliver the service.
Despite efforts to adapt services to meet local needs, there were disparities across patient groups in their experience of, and engagement with, services including age, employment status, ethnicity, level of education, health status and gender.
How findings relate to previous research
Despite previous evaluations investigating models of remote home monitoring and implementation of such services for COVID-19 patients,36,109 to our knowledge, this is the first research that focused on strategies adopted by services to address disparities. Our findings are consistent with previous research of strategies that can be used to reduce disparities in access to and use of health services more broadly,204–207 for example, expanding admission criteria and referral routes, and providing information in a range of formats (although support was provided at a national level, not all services provided information in formats accessible to all patients). Our findings extend research by outlining some strategies not previously identified; for example, staff providing additional support beyond monitoring (linking with social support, or more contact time), amending protocols to patient need and liaising with family/carers. Some possible areas for improvement included the need to routinely collect and analyse data on relevant populations, co-developing platforms with patients and staff (there was limited opportunity due to the rapid implementation) and working with communities to promote the service.
Critiques of remote models of care often focus on possible harms arising from digital exclusion. Having analogue options available as part of COVID-19 remote home monitoring services is, therefore, crucial to prevent increasing health disparities. 208 This is particularly important given the overlap between patient groups at greater risk of severe illness from COVID-19 (e.g. older adults) and those who are at greater risk of digital exclusion. Our finding that mode of service did not impact on patient engagement or experiences might be explained by patient satisfaction or the fact that all sites offered analogue modes (see Chapter 11).
Implications
Our evaluation generates a range of recommendations for the design and delivery of remote monitoring services for patients with COVID-19, and other health conditions. Services must consider the needs of their local population and be adapted accordingly, monitor the impact of services on population groups at risk of health disparities and work with existing local systems (e.g. community groups) to engage harder to reach groups.
Given that many of the groups of patients (e.g. clinically vulnerable, older adults, those without support) that services aimed to reach found it more difficult to engage with the service, patients’ needs and circumstances should be assessed upon referral in a standardised way and services must be tailored to provide appropriate levels of support for patients and carers (see Chapters 9 and 10 results and Miles and Huberman,203 Cooper et al. 209 and Centre for Ageing Better210). Examples of appropriate tailoring could include patient information in a range of formats,211 additional information, education or support in navigating services,212 and flexible monitoring processes.).
When implementing remote models of care, adequate resources and infrastructure should be provided to allow patients to be involved in the design and implementation. Co-design can help to ensure that services are better suited to patient needs and can facilitate adherence and engagement. 213 Holistic health care and collaboration or linking between services have previously shown to be important for reducing inequalities, particularly for those who are unwell or have comorbidities. 209,213
Strengths and limitations
The analysis of experience and engagement across patient groups could have been subject to false positives due to the number of comparisons made, although a more stringent p-value was used in reporting significant results (p < 0.01). When making comparisons between patient groups, the sample size of several groups was relatively small (e.g. patients aged over 80 years), limiting generalisability. The planned multivariate regressions investigating whether mode of monitoring and patient characteristics were associated with patient experience of the service were not possible due to the skew within the dependent variable (and therefore insufficient power to detect any differences). The interviews with patients focused on their experiences of services more broadly and not necessarily the identification of inequalities in access or quality of care, so much of our analysis of the latter draws on staff perspectives or patient survey data.
Future research
There is little published literature on the implementation of remote monitoring and health disparities. There are other populations at risk of health disparities that were beyond the scope of this evaluation and should be considered in any future evaluations (e.g. the homeless community, people with disabilities and unpaid carers).
The scope of our evaluation also did not allow us to examine the impact of specific strategies used to increase accessibility or patient engagement, or the impact of specific adaptations made by local services. We are therefore not able to make any conclusions regarding the effectiveness of strategies in relation to health outcomes for particular patient groups. This is an area for future research.
Conclusions
When health services undergo such a rapid transformation, as we have seen with the shift towards remote models of care, evaluations of the effectiveness of such services must include an impact assessment to ensure services are accessible and available to all patients, and to monitor the impact on health disparities. Addressing health disparities must therefore be a key focus in the planning, design and delivery of remote monitoring models for COVID-19 and other conditions, both nationally and locally, so that remote models of care can be of value to all population groups. Staff and patients from groups typically experiencing disparities in their health care must play an active role in service design to ensure their needs, experiences and expectations are accounted for.
Chapter 11 Technology-enabled and analogue models of remote home monitoring care for COVID-19: a rapid mixed-method evaluation of patient and staff experiences
This chapter draws on a manuscript by Herlitz et al. 214 uploaded as a preprint to MedRXiv. This is an open access article under the term of the Creative Commons Attribution 4.0 International License (CC BY 4.0), which permits use, distribution and reproduction in any medium, provided the original work is properly cited (see https://creativecommons.org/licenses/by/4.0/).
What was already known
-
Studies have shown that patients and staff like remote home monitoring, but there are some barriers to delivery and engagement, and it may not be appropriate for all patient groups.
-
The availability of dependable, high-quality, and clinically-useful technology systems that patients and staff can engage with influences implementation of services.
-
Few studies have explored patient and staff experience of COVID-19 remote home monitoring services.
What this chapter adds
-
Older patients, patients with a lower level of educational attainment and ethnic minorities were more likely to relay symptoms through phone calls with the service.
-
Staff considered tech-enabled and analogue models better equipped to manage large patient numbers; but improvements were needed to improve functionality of technology systems to better fit clinical and operational needs.
-
Tech-enabled and analogue models were not a substitute for human contact, as phone calls were used in both tech-enabled and analogue services and analogue-only services.
Background
COVID-19 remote home monitoring services have been implemented using a range of technology-enabled and analogue modes (see Vindrola-Padros et al. 32 and Vindrola-Padros et al. 36 and Chapters 1, 3 and 4).
For patients to engage with remote home monitoring services, previous research has indicated that patients need access to private space, carer support, the necessary digital infrastructures required to engage with remote home monitoring services,208 a choice of modality and access to technical support. 215
For staff to deliver remote home monitoring services, previous research has highlighted the importance of the accessibility and functionality of technology for meeting the needs of work practices, interoperability with existing data systems and sufficient staffing for monitoring and responding to data. 164,216–218 Furthermore, the beneficial impacts of technology on work activity can be reduced by technical problems and a lack of technological infrastructure. 219
Research indicates that patients and staff have emphasised the need to maintain human contact in the clinical relationship to preserve high quality care. 216,220–222
While previous research provides an indication of the types of factors that may enable staff and patient use of remote home monitoring services, the experience of patients and staff when using different modes of COVID-19 remote home monitoring have not yet been fully explored. Emerging evidence indicates that digital apps may facilitate the follow-up of a higher number of patients with COVID-19 compared with telephone calls,32 but might also limit the engagement of older patients.
Within this chapter, we report on patient and staff experiences of engaging with rapidly implemented tech-enabled and analogue models of remote home monitoring of patients with COVID-19. We hypothesise that differences in how patients relay readings in the models may impact on how patients and staff engage with and experience the service. We aimed to answer the following questions:
-
Which models of tech-enabled and analogue COVID-19 remote home monitoring were adopted in the UK?
-
Were there differences in the characteristics of patients using these models?
-
What were patients’ experiences of relaying symptom data using tech-enabled and analogue models?
-
What were staff experiences of processing symptom data for large numbers of patients using tech-enabled and analogue models?
-
How did the use of tech-enabled and analogue models affect patients’ and staff’s experience of the patient–clinician relationship?
Methods
A summary of the methods is provided below; further details can be found in Chapter 2 or Report Supplementary Material 1.
We used the technologies (dependability, functionality and familiarity) and clinical relationship (patient–clinician interaction, knowledge of patient and trust) domains of the Planning and Evaluating Remote Consultation Services (PERCS) conceptual framework to examine the newly established services. 208
Design
This chapter draws on staff and patient/carer surveys and staff and patient/carer interviews. Data collection took place between February and June 2021 (patient surveys retrospectively included January).
Sample
Patients/carers who received these services and staff leading and delivering services or involved in service data collection/analysis (see Chapter 2 and Chapter 4 for sample details).
Measures
We developed the survey and semistructured topic guides specifically for this study (see Chapter 2 and Project website documentation – Study instruments). Interviews with delivery staff included a ‘think aloud’ section where staff narrated the process of using the platform.
Data collection
We conducted a survey with patients/carers from 25 sites and with staff from 28 sites. We conducted interviews with staff, patients and carers from 17 sites (see Chapter 2 for details).
Data analysis
Inductive thematic analysis was used to analyse interview data (relating to patient views/experiences of relaying symptom data, staff processing data and interactions between patients and clinicians), inputted into RAP sheets. 70 The PERCS domains of technologies and the clinical relationship were used as a sensitising lens. 208 Transcripts were reviewed to gain further in-depth data on reasons for adoption of models and knowledge gained from models. Each code’s data were checked for consistency of interpretation and re-coded as necessary. The data were organised into lower- and higher-order themes. We constructed the typology of the models based on two key characteristics: the modality of the technology and human contact between patients and clinicians. Data relating to modality and frequency of human contact were coded deductively.
Survey data were analysed using SPSS (version 25). The following patient data were analysed: sociodemographic information, patient-reported ease of completing activities as part of their care, frequency and quality of contact with the remote monitoring team, and whether they had received any support from family or friends. Data related to experience of relaying data and the clinical relationship were also coded deductively from two open-ended survey questions. Survey responses were compared with patients who had reported they had relayed readings via tech-enabled or analogue modes. We analysed staff survey respondent characteristics. Staff reported on their experience of delivering the service, capacity/resources to deliver the service, the impact of the role on their workload and their views of patient engagement with the service. Data related to processing and responding to symptom data and the clinical relationship were coded deductively from one open-ended survey question. To make comparisons across models, staff were categorised as delivering a tech-enabled and analogue model if sites adopted a mixed approach, or an analogue-only model if a tech-enabled platform was not used.
For patient and staff survey data, we used descriptive statistics including frequencies and percentages, and univariate analyses were performed. Where data were missing for specific questions, available case analysis was performed and the denominator reported. We conducted Pearson’s chi-square tests and logistic regression modelling to compare the characteristics of patients using tech-enabled and analogue-only models and to determine the association between patient characteristics and mode of submission. We used non-parametric Mann–Whitney U-tests to compare patient-rated experiences of receiving services and staff experiences of delivering services for tech-enabled and analogue-only models.
Results
Participant characteristics
Surveys were received from 292 NHS staff and 1069 patients and carers. We conducted 58 interviews with staff and 62 interviews with patients/carers (see Chapter 4 and Report Supplementary Material 2 for demographic characteristics).
The majority of staff reported delivering tech-enabled and analogue services (81% interview participants, 77% survey participants) compared with analogue-only models (19% interview participants, 23% survey participants).
More patients reported using a tech-enabled mode for data submission (66% of interview participants and 51% of survey participants) than an analogue-only mode (31% of interview participants and 49% of survey participants). Of the patients surveyed: 29% reported providing readings via text, 25% via digital app, and 1% via e-mail.
A comparison of participant characteristics for survey participants who used tech-enabled compared with survey participants who used analogue methods of submission is shown in Report Supplementary Material 8. The logistic regression analysis found statistical evidence of differences in patient characteristics between survey participants who used tech-enabled versus analogue-only models, for age (p = 0.005), level of education (p = 0.011) and ethnicity (p = 0.043) (see Report Supplementary Material 8).
Models of COVID-19 remote home monitoring adopted
Services were categorised into four models (Figure 25). Services within model 1 (10/17 services) and model 2 (4/17 services) offered tech-enabled and analogue services submission modes. Services within model 3 (1/17 service) and model 4 (2/17 services) offered analogue-only modes of submission. Models 1 and 3 outline ‘universal’ services which offered the same submission options and frequency of human contact to all participants, whereas models 2 and 4 outline ‘risk-stratified’ services which adapted the submission method and/or frequency of contact depending on patients’ needs. For example, two services within model 2 offered self-monitoring (analogue-only options to low-risk patients) and some services within model 4 offered more frequent phone calls or face-to-face visits for high-risk patients. See Report Supplementary Material 4 for further details.
FIGURE 25.
Models of COVID-19 remote home monitoring.

Patients’ experiences of using tech-enabled and analogue methods to relay symptoms
We constructed two key themes related to patients’ experiences of relaying symptom data using tech-enabled and analogue, and analogue-only modes: (1) allocation of mode; and (2) ease of relaying readings and the functionality of tech-enabled systems.
Allocation of mode
Most patient interviewees did not recall being given a choice about the mode in which they could submit readings; however, most patients were happy with the mode they were given. Several patients mentioned that it had been unproblematic to switch modes during their time with the service. Staff interviewees across many sites reported that they were aware that older patients, in particular, might lack digital skills/infrastructure or experience cognitive/physical impairments that might make engaging with tech-enabled systems challenging. These patients were offered phone calls as appropriate. Given the differences between patient groups using tech-enabled and analogue-only modes, staff may have been attuned to which option might best suit patients or may have assumed that certain patients (e.g. older patients) would not be able to manage a tech-enabled approach.
Ease of relaying readings and the functionality of tech-enabled systems
Most patient interviewees across sites reported that it was ‘easy’, ‘simple’ or ‘straightforward’ to relay readings whether by tech-enabled or analogue modes. A number of patients thought that the simplicity of the tech-enabled systems was its strength:
I’m really un-techy, but . . . the text message reminder came through, you clicked on it, you put in . . . your name and date of birth, it took you straight through it . . . you could be as thick as you like and you would manage to do that. It was really [laughs] . . . no technical prowess required.
(Site M, patient 1)
Similarly, survey analysis found patients rated both tech-enabled and analogue-only modes for submitting data positively. Patients using a tech-enabled mode reported that recording readings (p = 0.009) and providing readings (p = 0.001) to services was easier than patients using analogue-only methods (see Report Supplementary Material 8). However, differences might reflect differing characteristics of patient groups.
The main barriers to submitting readings (for both tech-enabled and analogue, and analogue-only models) reported by patient and staff interviewees were feeling too poorly or tired to engage or forgetting to submit readings. Many patients and staff highlighted that family members or carers had assisted patients in relaying readings, particularly for patients using a tech-enabled modality, who lacked confidence in their technical skills:
In the beginning I found it [entering the information] difficult because I was poorly but then I had a member of my family to help me and then it was just easy to do and I’m not good at things like that. I found it easier to do after a while.
(Site C, patient 6)
The functionality of the technologies was not discussed in detail by patients, however several patients and staff reported issues particularly related to set-up because they required downloading and remembering log-in details. Two sites offered face-to-face support to patients at onboarding for this.
Staff experiences of using the models to process symptom data for large numbers of patients
Two themes emerged as key to staff experiences of processing symptom data: the scalability of the models, and the ease-of-use and functionality of tech-enabled systems.
Scalability of the models
Tech-enabled and analogue models were considered by staff to be more scalable than analogue-only models. Service leads from three sites reported in interviews that they had adopted tech-enabled platforms to ensure services could cope with a high volume of patients, and staff from six tech-enabled sites highlighted that there were efficiency gains from data being entered by patients in real time:
At the peak we were on I think hundred and ninety-six on the service . . . and each of them were submitting three or four points of data, three times a day . . . . that’s well over two thousand bits of data coming in a day. There’s just no way manually we would have been able to do that.
(Site G, interviewee 1 – service lead)
Staff interviewees from two analogue-only sites noted that phone calls were labour intensive and necessitated a larger workforce (relative to patient numbers), and manual data entry, was required for each patient so that services could keep abreast of patients using the service. Staff survey findings indicated that mixed tech-enabled and analogue models had more positive impact (p = 0.034) on workload compared with analogue-only services: 52% of staff using tech-enabled models reported a positive impact while only 35% of staff using analogue-only did so (see Report Supplementary Material 8). However, service lead/manager perceptions of the capacity/resources needed to deliver services (as reported in the survey) were not statistically significantly different (p = 0.554) across models (although the smaller sample size for this question should be noted, see Report Supplementary Material 8).
Ease-of-use and functionality of tech-enabled systems
Staff reported monitoring patients as statistically significantly easier (p = 0.005) for tech-enabled and analogue service models (77% of staff reported monitoring as easy/very easy) compared with analogue-only models (56% of staff reported monitoring as easy/very easy; see Report Supplementary Material 8). However, staff interviewees reported an extensive number of ways that tech-enabled platforms could be enhanced to support their clinical and operational needs (see Appendix 5, Table 28), and staff across several sites reported that they were frustrated by the length of time it took for tech providers to make changes to platforms.
Patients’ and staff’s experiences of the clinical relationship
Three themes emerged relating to patients’ and staff’s experiences of the patient-clinician relationship embodied in the remote home monitoring models: staff knowledge of patients’ condition, reassurance and providing appropriate care, and concordance in the patient-clinician relationship.
Staff knowledge of patients’ condition
Phone calls were an essential element of both models, enabling staff to gain comprehensive knowledge of their patients’ condition. Staff using tech-enabled and analogue models used the data they received to get an overview of patients’ health status and called patients if there were signs of deterioration to conduct a more thorough assessment (e.g. assessing breathlessness, retaking readings, measuring oxygen saturation after exertion, and assessing patients’ coherence and anxiety). Phone calls were also useful for gaining information about patients’ social circumstances and mental health, sometimes prompting referrals to other services:
Many of the patients when we come across . . . live on their own. So, it’s not just their health it’s the . . . wellbeing of the patients as well . . . There are many times where I have done the referrals . . . and provided more support for them, for food and other medications and things like that.
(Site D, interviewee 4 – health-care professional delivering the service)
Some staff members (across both tech-enabled and analogue and analogue-only models) highlighted that on the phone (or in person) patients were more likely to mention other medical symptoms in passing, which might require attention. Staff from two analogue-only sites reported that the depth of knowledge gained from phone calls was one of the key reasons for not adopting a tech-enabled model of care.
Reassurance and providing appropriate care
Patient and staff interviewees across all sites reported that patients found services very reassuring at a time when they were feeling very unwell, isolated or anxious about the disease. There was no evidence of a difference (p = 0.559) between models in how patients rated contact with the service team (see Report Supplementary Material 8). Staff across all sites reported that the opportunity for patients to discuss symptoms with a clinician reassured them and prevented patients from unnecessarily contacting emergency services or encouraged deteriorating patients to go into hospital when they were afraid to:
So a lot of [patients], when you speak to them, and you say, ‘Actually look, your levels were 92. I’ve exerted you for a minute they’ve dropped down to 89, can you hear yourself gasping on the phone?’ They say, ‘Yes.’ And I say, ‘Well that’s really serious you do need to call an ambulance.’ And then they will come around to it.
(Site A, interviewee 4 – health-care professional delivering the service)
Regardless of the mode (p = 0.158), patient survey respondents reported that contacting a health professional was relatively easy. However, seeking further help if they had concerns about their health was statistically significantly easier (p = 0.006) for patients using tech-enabled modes (see Report Supplementary Material 8). Several patients using tech-enabled models reported that despite receiving an automatic prompt to escalate their care when readings were low they preferred to first speak to the service for a more personalised/thorough assessment.
Managing the patient-clinician relationship
Patient interviewees reported that being contacted by staff if they had missed readings gave them a sense of security and reassurance. However, for staff, chasing and managing non-submitters could be time-consuming, frustrating and worrying, consuming valuable resources. Services tried to encourage patients to relay data by setting out service requirements at onboarding, and emphasising the importance of relaying readings:
What we found initially was that [expectation of submitting twice a day] wasn’t explained fully and then we were having to chase them a lot for readings. So that is really explicit at the start now that . . . almost like as daft as it sounds like a verbal contract. You know, ‘We will do all of this however, what we expect of you is you know readings twice a day to be compliant’.
(Site I, interviewee 3 – staff member involved in data)
Several staff delivering tech-enabled and analogue models highlighted the importance of patients understanding that while readings were reviewed regularly, communication was not synchronous and patients still needed to call emergency services if oxygen saturation levels were low. However, some patients reported preferring to speak to services before escalating their care.
Discussion
Key findings
Tech-enabled care was not a substitute for human contact in COVID-19 remote monitoring services; phone calls and human relationships were a feature of all models of remote monitoring and patients found services reassuring (across models). This indicates that technology cannot completely replace phone calls. Patients using tech-enabled methods tended to be younger, well-educated and more likely to identify as white British compared with those using analogue methods and most patients did not recall being given a choice about the mode offered. Staff considered tech-enabled and analogue services to be more scalable and able to deal with larger volumes of data and patients than analogue-only services.
How findings relate to previous research
Consistent with previous studies,164,216,218 staff noted that better functioning systems that fit with their work practices would have greatly improved efficiency, highlighting the need to involve clinicians in the design and development of technical platforms. Patient involvement was also missing from their development; patient participation groups and patient experience work were considered non-essential early on in the pandemic. 223 As a number of different tech-enabled models have now been established, there is an opportunity for service leads to trial different systems and incorporate patient feedback/involvement.
Patients in all models were contacted by telephone if their symptoms deteriorated or they did not submit readings, with the exception of two sites that offered a self-monitoring service. Staff reported that pursuing non-submitting patients could unnecessarily consume resources. Consistent with previous research showing staff concerns about patient safety in chronic conditions, the acute nature of COVID-19 caused staff anxiety about rapid deterioration. 164 There is a question of whether services’ resources could have been better optimised for patients’ needs, by addressing concerns about risk in staff supervision and training, by offering more lower-risk patients self-monitoring, by adapting tech-enabled platforms to offer varying levels of automated contact, and/or following more stringent protocols for managing non-submitters (e.g. discharging patients back to the care of their GP if they miss three data submission points).
Previous studies have shown that both patients and staff have responded positively to remote home monitoring for chronic health conditions,216,224 and a small number of studies have been conducted on patients’ experiences of COVID-19 remote home monitoring. 211 This is the first study to examine in-depth patient and staff experiences of using tech-enabled and analogue modalities to respectively relay and process COVID-19 symptom data and compare experiences of the patient-clinician relationship. It builds on our work looking more broadly at patients’ experiences of COVID-19 remote home monitoring services (see Chapter 9).
This study supports prior work that has shown that some patients may need additional support to engage with technology or access to analogue options,208,220 and the importance of maintaining human contact. 221,222 Older patients, less well-educated patients and patients identifying as non-white were more likely to relay symptoms through phone calls with the service, with staff primarily determining the allocation of mode. Co-designing digital health services with patients, improving digital health literacy in the population, training and supporting the workforce to build digital skills and confidence, and gathering data on patients’ access to technology and digital skills could widen patient participation in digital health care. 206,215
Staff engagement has been explored in previous studies,164,216 but this study extends previous research by considering the scalability of models, with staff considering tech-enabled and analogue models better equipped for managing large patient numbers without jeopardising clinical relationships. However, future models of remote monitoring should tailor their frequency of contact to patients’ level of risk and include systems which create a dialogue between patients and staff as to their preferences.
Strengths and limitations
There was a high level of variation between different models (see Chapter 4). Few sites adopted analogue-only modes of data submission, and no sites used tech-enabled-only modes, making it difficult to assess whether differences in patient and staff experiences between tech-enabled and analogue models were due to the engagement with the technology, or related to other service factors. This also meant that it was not possible to use multivariate analyses to look at staff experiences (based on survey responses) across models, due to the limited sample size in analogue-only sites. The univariate analyses of staff and patient experiences could therefore have been subject to false positives due to the number of comparisons made. However, in-depth data collection from staff on the functionality of tech-enabled models at four sites enabled us to draw out specific tech-related findings.
We had originally planned to use ‘think aloud’ methodology with patients but in practice, these did not work because patients no longer had access to the tech-enabled platforms they had used and their recall of their use and functionality during ill health was poor.
The language and terminology describing technology was unfamiliar to some staff and patients, for example, understanding the difference between a web-link and a digital app.
Conclusions
Organisations implementing tech-enabled remote home monitoring at scale should consider providing flexible models which can tailor processes and resources to accommodate patients with different needs and requirements. These include risk levels, abilities to engage with and access technology, support available at home from family or carers, and levels of desire for support versus self-management. Services routinely collecting and monitoring data on patients’ access to technological infrastructure and skills could inform digital inclusion strategies for remote home monitoring.
Chapter 12 Discussions and conclusions
Overview
This study was a mixed-methods evaluation of COVID-19 remote home monitoring services in England.
Phase 1 of the evaluation (rapid systematic review, empirical mixed-methods implementation study) aimed to analyse implementation and impact of COVID-19 remote home monitoring services, develop a conceptual map of these services, explore implementation and staff experiences and understand data use, staffing and resource allocation (see Chapter 1 and Vindrola-Padros et al. 32 and Vindrola-Padros et al. 36). We also shared findings in a timely way so these could inform the national roll-out of COVID-19 remote home monitoring services implemented throughout England.
Phase 2 aimed to explore effectiveness, cost, implementation, and staff and patient experiences of COVID-19 remote home monitoring services (see Chapter 1). It comprised four workstreams. Workstream 1 explored effectiveness of COVID-19 remote home monitoring services (see Chapters 5 and 6). Workstream 2 included an analysis of the composition of the workforce involved in providing COVID-19 remote home monitoring services and a cost analysis of these services (see Chapter 7). Workstreams 3 and 4 included a national survey and in-depth case studies of implementation, staff and patient experience, including a focus on health disparities and mode of data submission offered by services, whether technology-enabled and analogue or analogue-only (see Chapters 4 and 8–11).
Within this chapter, we provide a summary of key findings with a focus on our research questions (see Chapter 1). We then discuss the implications and lessons learned, the strengths and limitations of our evaluation, the potential impact of our findings, future research areas and conclusions.
Summary of key findings
Below, we describe key findings from phases 1 and 2 of our evaluation (Figure 26 for a summary).
FIGURE 26.
Summary of key findings.

Key findings from phase 1 (see Chapter 3)
Systematic review of COVID-19 remote home monitoring services32
-
Remote home monitoring services have been implemented internationally for COVID-19, an acute condition, during the pandemic.
-
Models of remote home monitoring for COVID-19 varied (online platforms, paper-based systems with telephone calls or wearable sensors), but models using phone calls were considered more inclusive.
-
This review was unable to reach substantive conclusions regarding patient safety and cost, due to study design, missing data and the lack of standardised reporting.
-
Gaps in evidence for effectiveness, cost-effectiveness and patient experience of COVID-19 remote monitoring services.
Implementation of services during wave 136
-
COVID-19 remote home monitoring services implemented throughout England varied in relation to the health-care settings and mechanisms used for patient triage, monitoring and escalation.
-
Many factors facilitated implementation, including good communication within clinical teams, culturally appropriate information for patients and carers, and the combination of multiple approaches for patient monitoring (app and paper-based).
-
The mean cost per monitored patient varied from £400 to £553, depending on the service.
Key findings from phase 2
Development and implementation of services during wave 2 (see Chapter 4)
-
The rapid development of national remote home monitoring services took place in three phases: local development, national development and roll-out and local implementation.
-
Development and implementation were facilitated by learning from wave 1, a range of national and local stakeholders and organisations, and a range of initiatives (community of practice, national learning network, AHSN patient safety collaborations). 225
-
Despite national roll-out, patient enrolment to COVID-19 remote home monitoring services was lower than expected.
-
There was large variability in the models of remote home monitoring services that were implemented.
-
Variation was influenced by patient, workforce, organisational and resource factors.
Outcomes (see Chapters 5 and 6)
-
We found no association between rates of patient enrolment to CO@h services and COVID-19-associated mortality or admission to hospital. Additionally, for hospital admissions, no relationships were found between enrolment rates and in-hospital mortality or LOS (see Chapter 5).
-
We found no evidence that the roll-out of CVW services reduced LOS in hospital or rates of readmission for patients hospitalised with COVID-19 (see Chapter 6).
-
Low rates of patient enrolment and incomplete data may have affected the chances of detecting any possible impact.
Cost (see Chapter 7)
-
We were unable to conduct a cost utility analysis due to the absence of impact of the services on mortality or hospitalisation.
-
For CO@h services, the mean cost per patient triaged was £351.80 and per patient monitored was £527.50. For CVW services, the mean cost per patient triaged was £581.80 and per patient monitored was £599.10.
-
The mean cost per patient monitored was lower for tech-enabled and analogue sites than analogue-only sites (CO@h and CVW services).
-
Most staff involved in running COVID-19 remote home monitoring services were clinical staff. Examples of clinical roles included consultants, emergency department staff, GPs, nurses, ANPs and medical students.
Workforce experience and delivery (see Chapter 8)
-
Staff generally reported positive experiences of delivery and felt that services were easy to deliver.
-
Staff valued support provided nationally and locally. But some staff would have benefited from further training.
-
Support for delivering the service, NHS resources, capacity and staff workload, multidisciplinary team dynamics, and patient (dis)engagement influenced delivery.
Patient experience and engagement (see Chapter 9)
-
Many patients and carers reported positive experiences and felt that services and human contact received reassured them and were mostly easy to engage with.
-
Engagement was conditional on a range of factors including patient factors (e.g. knowledge and health), support and resources, and service characteristics.
-
Findings indicate that burden of treatment may be experienced by patients and families when implementing remote monitoring for COVID-19.
Disparities (see Chapter 10)
-
Many sites designed their service in an attempt to be inclusive of the needs of local populations and to ensure a broad reach.
-
Despite local adaptations to services, disparities were reported across patient groups (e.g. with age, health status, ethnicity and level of education) in their experience of, and engagement with services in terms of accessibility, achievability of tasks, and problems experienced.
-
Certain groups may therefore require additional support to engage with COVID-19 remote home monitoring services.
Modality of service (see Chapter 11)
-
Most services offered both analogue and tech-enabled submission options.
-
The patient cohort using tech-enabled modes of relaying symptom data differed from patients using analogue methods (i.e. by age, level of education and ethnicity).
-
The choice of mode used by patients was primarily made by staff, but most patients reported being satisfied with the mode allocated.
-
Staff considered the tech-enabled models better equipped to manage large patient numbers.
-
Many improvements were suggested to improve functionality of technology systems to better fit staff and patient needs.
-
Tech-enabled engagement was not a substitute for human contact.
How findings relate to previous research
In this section we present findings in relation to previous research.
Implementation
The use of remote home monitoring
In recent years, remote home monitoring services have become a priority for policy-makers and the NHS. 18,158 Previous research demonstrated that remote home monitoring models have been widely implemented for a range of chronic conditions. 19–21 This evaluation extends the evidence base by showing that remote home monitoring models have also been widely used internationally for patients with COVID-19 (see Chapters 3 and 4). We provide evidence for the range of services implemented for COVID-19 and the types and frequencies of remote home monitoring used. Additionally, our review and implementation study provide insights into the components and processes involved in these services.
Major system change
Our evaluation provides nuanced understanding of major system change in a rapid emergency context (i.e. a global pandemic). There are different ways of implementing major system change (top-down and bottom-up approaches), but a combination of approaches may be beneficial. 104 Findings from COVID-19 remote home monitoring services indicate that rapid implementation processes may follow a bottom-up, top-down then bottom-up process (as described in Chapter 4). Therefore, rapid implementation may vary from major system change in non-rapid contexts such as demonstrated by Fulop et al. 103
Previous research outlined five conditions for major system change: involving stakeholders from all levels, establishing feedback loops, attending to history, engaging health-care providers, and engaging patients and families. 102,104 Our findings offer support for the involvement of professionals and patients and families in service change as staff and patient engagement was integral in implementing COVID-19 remote home monitoring services. While engagement from staff and patients/carers is critical when implementing services rapidly, it is also potentially more challenging due to time constraints. Additionally, we highlight the importance of national and local clinical leaders and their pivotal roles in developing and shaping services, and the facilitating impact of collaboration between organisations.
Factors influencing implementation and variation
Prior to this evaluation, there was limited evidence regarding how COVID-19 remote home monitoring services have been implemented. This evaluation (see Chapters 3 and 4) found that most remote home monitoring services studied had low patient enrolment and that there was considerable variation in which model was implemented and how according to local context. These findings support previous research demonstrating likelihood of variation when implementing national services generally81–83,89–92 and in response to the COVID-19 pandemic. 106–108 Previous research has highlighted differences in the models implemented and the ways in which services are implemented must be considered when interpreting effectiveness and cost-effectiveness of services. 57,81,83,84,88
The context of rapidly developing COVID-19 remote home monitoring services during a global pandemic may explain some of the variation identified between national guidance and local implementation. Findings demonstrated that service models were initially developed locally, then further developed and rolled out nationally, before being implemented locally (see Chapter 4). It is likely that the rapid process of development of services may have culminated in variation (between national guidance and local services, and between different local services), as sites were often running prior to national guidance. Given the low enrolment rates and variation demonstrated in our evaluation, further consideration is needed to identify the ‘right’ balance between adhering to national SOPs (e.g. NHS)38,39 and flexibility needed within local services. This balance may be challenging to achieve for services like COVID-19 remote home monitoring, which have been rapidly developed and implemented during a pandemic, when time is limited. This limits the ability for national and local stakeholders to give, receive and implement feedback and also to understand how different approaches to implementation may relate to effectiveness.
Additionally, our evaluation offers support for previous research which has highlighted the role that local factors have on implementation. 81–87 We demonstrate that local factors (patient, staff, organisational and resource factors) are of importance when implementing national services for COVID-19 (see Chapter 4). Our Phase 1 study also demonstrated facilitators (e.g. clear communication channels, positive engagement from patients, dedicated clinical leaders and support from senior management) and barriers (e.g. unclear referral processes, challenges monitoring patients remotely, lack of administrative support and resources, workforce capacity and lack of data systems) (see Chapter 3 and Vindrola-Padros et al.). 36
Evaluating effectiveness and cost of services
Effectiveness of COVID-19 remote home monitoring services
Prior to this evaluation, there was little robust evidence for the effectiveness and cost of COVID-19 remote home monitoring services. Our findings on the national CO@h programme are consistent with other evaluations of the same programme, which found no impact on mortality or health service utilisation. 43 However, our findings (for both CO@h and CVW) contrast with potentially promising effects of remote home monitoring services for patients with COVID-19 found by others,115–118,124–126 and individuals with other health and care needs. 226,227 Our findings differ from previous COVID-19 research findings possibly due to differences in study design and limitations associated with these (including with respect to the intervention cohorts, outcomes, and comparator groups). In addition, these other studies typically reported on single-site or single-area implementation (see Chapters 5 and 6 for discussion of these studies). The low rates of patient enrolment and lack of complete, or linkable data may have affected the ability of our studies to detect any true impact that may have existed.
Despite some evidence that remote monitoring of patients with COVID-19 can be locally effective we have not been able to replicate such findings at a wider level. Because of the influence of missing data, lower enrolment to the CO@h service than expected and the lack of linkable data for the virtual wards, the more immediate concerns are for better data collection processes and enrolment rather than any judgement as to whether the services are worthwhile. Indeed, the effectiveness of some local programmes could have been lost among the analysis of national data.
Cost of COVID-19 remote home monitoring services
Prior to this evaluation, there was limited evidence of cost analyses for remote home monitoring services (see Chapter 3 and Vindrola-Padros et al.)32 Previous studies have stated that similar interventions may be cost saving by reducing downstream costs (in terms of avoiding hospital admissions and bed-days). 31 Findings from our cost analysis of COVID-19 remote home monitoring services were consistent with a recent Australian study where staffing costs were the main contributors to the total costs of monitoring. 148
Staff and patient experiences
Staff-patient relationships
Previous research has highlighted concerns over the staff-patient relationship being jeopardised by remote delivery of health-care services. 164,170 However, learning from our staff and patient studies indicated that this was not the case. For example, our analysis of staff experience delivering tech-enabled and analogue modes of remote home monitoring indicated that staff valued remote home monitoring services as they were able to support patients and appropriately identify the right place of care for them, allowing a therapeutic relationship to develop (see Chapter 11). Additionally, the patient study indicated that patients felt reassured by the contact provided by staff remotely and that the care provided at a distance was appropriate (see Chapter 9). Remote home monitoring services do not necessarily jeopardise staff-patient relationships usually built during face-to-face appointments. That said, our findings indicate that some form of human contact is imperative within these services, supporting previous research. 221,222
Staff experiences delivering COVID-19 remote home monitoring services
Previous research indicated that there were several potential benefits and challenges of remote home monitoring services for staff. 153,155,157,158 This evaluation extends previous research by demonstrating that staff generally had positive experiences of delivering remote home monitoring services in this context, but that there are specific barriers that need to be overcome, for example relating to training and provision of oversight by senior clinicians. However, it must be acknowledged that some staff felt that there was additional burden and workload associated with delivering remote home monitoring services and, more generally, it is clear that delivery relies heavily on staff.
Patient engagement
Previous research has explored patient involvement in health-care delivery (e.g. self-management, engagement and treatment burden) for many chronic conditions,50,51,179,186,187 but little research had been conducted into patient experience and involvement in remote home monitoring services (using tech-enabled and analogue modes) for acute conditions such as COVID-19. Our evaluation offers considerable evidence to indicate that patients were able to engage with remote home monitoring services when managing acute conditions such as COVID-19 (see Chapters 9 and 11), but that this engagement was conditional on a range of patient, staff, organisational and resource factors and heavily relied on support from family and friends (see Chapter 9).
Staff and patient qualitative and survey findings indicated that there are groups of individuals for whom remote home monitoring services may be less appropriate due to enhanced difficulties engaging with services (see Chapters 8–11). This finding is consistent with previous research. 197–200,208,215,228,229 Additionally, we highlight further challenges that must be considered for COVID-19, including infection transmission for family members providing support, and uncertainty and fear around seeking help in hospital settings.
Co-production
This evaluation extends previous research by outlining the importance of co-production of services with staff involved in delivering these services and patients receiving these services. Previous research has highlighted that it is necessary to involve patients and staff in development and implementation to ensure that services meet their needs230 and that service components, such as digital solutions, are fit for purpose. 164,216,218,223 Our results indicated that efficiency, utility and value functionality of tech-enabled solutions could be improved by involving staff in co-design and offering them opportunities to observe and test different platforms. While findings indicated services did try to develop and implement strategies to meet the needs of their population (see Chapter 10), patient involvement was not necessarily consistently incorporated throughout service development and implementation (see Chapter 4). The rapid nature of development and set-up of services appeared to limit scope for involving staff and patients in decision-making. This meant that staff and patient experience was at times hindered by decisions made without involvement (see Chapter 11). There remain gaps on if and how conventional methods of engagement can work within rapid timescales; it is possible that engagement approaches for coproduction may need to be reconsidered within rapid contexts.
Implications
In this section we outline overarching implications arising from these findings which relate to implementation, evaluating remote home monitoring services, and staff and patient experience (see Table 23 for implications). These implications apply to COVID-19 but may also apply to remote home monitoring services for patients with other conditions.
Topic | Implication |
---|---|
Implementation | |
The use of remote home monitoring |
|
Implementation of COVID-19 remote home monitoring services |
|
Factors influencing implementation and variation |
|
|
|
|
|
Evaluating remote home monitoring services | |
Effectiveness |
|
Service reach |
|
Cost |
|
|
|
|
|
Staff and patient experiences | |
Staff–patient relationships |
|
Staff experiences |
|
Patient engagement |
|
|
|
Co-production |
|
Strengths and limitations
Strengths
Our evaluation was a large-scale mixed methods evaluation which included analysis of national data sets, and primary data collection from 28 sites that were representative of a range of regions, levels of deprivation, ethnicity, size of population, and a range of urban versus rural sites. However, it is possible that our study may not be representative of all sites. Using mixed methods (consisting of a systematic review, qualitative data collection and analysis, quantitative data collection and analysis and economic data collection and analysis), enabled us to explore a range of topics and triangulate data from multiple perspectives and sources. For example, we were able to explore possible reasons for low patient enrolment demonstrated by the quantitative national data sets within the qualitative case-study findings.
This evaluation was a rapid evaluation completed over two time periods. Our phase 1 study was completed within one months, and our phase 2 mixed-methods study was completed within one year, with data collection taking less than six months. Our rapid approach to this evaluation meant that learnings from the evaluation were able to be used in real time to inform future service development and evaluation (see Project website documentation – Dissemination). Additionally, conducting our evaluation over two time periods (coinciding with waves 1 and 2 of the pandemic) enabled us to capture learnings and constants/changes over time.
Throughout our evaluation, a number of actions were taken to ensure robustness of qualitative findings (see Chapter 2).
Our evaluation team (a multidisciplinary research team) worked collaboratively with, yet independently from two other evaluation teams and key stakeholders from NHSEI and other organisations. This meant that evaluation teams were able to share findings to evaluate services from multiple perspectives, thus providing findings with more credibility. Additionally, our evaluation team worked closely with our PPI group and the 70@70 cohort throughout the study.
Limitations
Data completeness was a limitation of the quantitative and cost elements of this study. The evaluation of effectiveness for CO@h could only be carried out for CCGs where the onboarding data was believed to be complete, which was only one quarter of all CCGs. For CVWs, we were able to undertake a national analysis, but we had no knowledge about which patients were referred to a virtual ward, or even how many. Additionally, cost and resource use data provided by some sites were incomplete.
Our CO@h effectiveness study (see Chapter 5) and the cost study (see Chapter 7) used aggregated data rather than individual patient-level data for key analyses. This meant that patient-level variation in outcomes and programme costs could not be examined. However, patient-level data on outcomes were examined by the other evaluation teams. 42–45
It was not possible to conduct all aspects of the evaluation as planned in the protocol. For example, a cost–utility analysis was not undertaken, given there was no evidence found of an effect of COVID-19 remote home monitoring services on mortality or downstream hospital utilisation. Additionally, for the qualitative study, we were unable to fully embed ‘think aloud’ interviews within our tech-enabled four case study sites as this was not feasible in practice.
Compared with patient onboarding data,114 the sample in the patient experience study was under-representative of some groups (e.g. older patients, ethnic minorities and most deprived) and over-representative of other groups. Additionally, the response rate for the patient and carer survey was fairly low (17.5%). For patient experience surveys and interviews, participants who had declined services could not be recruited. Additionally, our study would not have included the views of staff or patients who were unable or did not want to take part in surveys and interviews. Therefore, our study may not be representative of all patient and staff groups and experiences.
These services were rapidly developed to respond to the global pandemic. Additionally, our evaluation was rapidly conducted within a pandemic. Therefore, our conclusions may lack generalisability to other acute conditions, as staff and patient expectations and attitudes may be different during a pandemic (e.g. perhaps staff and patients may be more appreciative of the service, given the wider context).
Future research
While this evaluation has contributed to growing evidence on COVID-19 remote home monitoring services, there are still some further avenues of future research which would benefit from being explored.
First, further research, including longitudinal evidence, is needed to explore further the effectiveness and cost-effectiveness of COVID-19 remote home monitoring services. However, for this to happen, high quality complete data need to be collected for the uptake and use of these services that can be linked to routine data sets.
Second, further research is needed on the most appropriate type of model for remote home monitoring for different groups of patients. For example, further research could compare the effectiveness, cost-effectiveness, implementation and staff/patient experiences of remote home monitoring services which are supported by staff, versus those which are purely self-monitoring models (patients monitor and escalate their own care). Additionally, research could explore whether there is an optimum frequency of contact for patients with varying needs and risks; which models of remote home monitoring services are most appropriate for which groups of patients; how ‘standard’ models can be adapted to be more accessible and appropriate for diverse groups; and whether different models impact on number of patients onboarded to these services.
Finally, further research is needed to explore other experiences which may not have been captured within this evaluation. For example, sites who were less research active, groups of staff whose views may not have been included or were limited in this evaluation (i.e. ambulance staff and volunteers) and patients who we were unable to reach in this evaluation (e.g. those who declined or disengaged from the service). Additionally, future research needs to explore carers’ perspectives in more detail and any treatment burden associated with informal care provided as part of these services.
Equality, diversity and inclusion
Participant representation
Within this evaluation, we aimed to ensure that participants were representative of different locations within England and different backgrounds.
Site characteristics
We recruited 28 sites to our national staff and patient surveys and 17 sites for staff and patient interviews. The 28 sites included services from all regions across England, represented CCGs and trusts, varied in urban/rural characteristics, deprivation scores, ethnicity and size (see Chapter 4 and Report Supplementary Material 2).
Strategies to improve representation
Within the study we undertook a range of strategies to try and ensure that our study was inclusive. For example, we offered a choice of paper or electronic surveys for patients, and attempted to make it easy for participants to return surveys by providing free post envelopes. We also provided patient and carer study materials (information sheets, surveys) in English, Polish, Bengali, Urdu, Punjabi, French and Portuguese and offered translation services for interviews if needed. While sites offered potential participants translated materials, uptake of these was low and the research team received no completed translated surveys. We also aimed to purposively sample using a range of criteria (e.g. age, gender, ethnicity, onboarding mechanism, type of monitoring and outcome), to ensure that our sample was as inclusive and representative as possible. We offered different modes of interview (telephone, MS Teams or Zoom) and different times depending on participants’ preferences and to ensure accessibility for as many people as possible. We offered the option for a family member to answer on behalf of patients for both the survey and interview if appropriate.
Further efforts are needed to ensure that studies are as representative as they can be in future (e.g. raising awareness of translated materials and opportunities for interpretation services). There were limited opportunities in our study to reach out further due to services being gatekeepers to potential participants and the rapid pragmatic timescales of our study.
Participant characteristics
Our patient and carer surveys and interviews included a range of participants of different ages, living circumstances, educational qualifications, work situations, disability, and deprivation score. However, our patient and carer survey and interview participants were mostly white, straight and had English as a first language (see Chapter 4 and Report Supplementary Material 2). We also compared our participant demographics with service patient onboarding data,114 and found that our survey and interview sample was under-representative of some groups (e.g. older patients and ethnic minorities) and over-representative of other groups (e.g. participants from white ethnic groups and patients in the least deprived deciles of the IMD). Additionally, patient and carer response rates for the survey was only 18%. We were unable to interview or survey anyone who had declined the service or disengaged.
Staff included in our surveys and interviews varied in their role, whether they were redeployed, length of time in position, and the location they were based (see Chapter 4 and Report Supplementary Material 2). Additionally, the staff response rate for the survey was only 39%. We did not collect data relating to staff personal demographics (e.g. ethnicity) as we focused on professional characteristics.
Research topics relating to equality, diversity and inclusion
Our study had a clear focus on inequalities of COVID-19 remote home monitoring services, including an analysis of disparities relating to service reach and patient engagement with these services (see Chapter 10) and an analysis of patient and staff experiences of different modes of submission (see Chapter 11).
Reflections on research team and wider involvement
Research team
The research team consisted of researchers from two rapid evaluation teams: RSET and BRACE. Overall, our team included researchers from academic university settings and think tanks. The research team was a large, mixed-methods multidisciplinary team from many different disciplines (statistics, sociology, applied health research, health psychology, health economics and project management) and team members differed in seniority from professors to postdoctoral researchers and research fellows. The research team comprised a mix of backgrounds in relation to gender and ethnicity. Team members who took maternity/paternity leave during the course of the study were supported to remain engaged with the study and to ensure their contributions were recognised.
The team held weekly project meetings and weekly data collection and analysis meetings throughout the project to ensure that all members of the team were well supported. All members of the team have been involved in all aspects of the project, from conception, to data collection and analysis and dissemination of findings – both written (papers for publication and chapters) and oral (see Contributions of Authors). All researchers were supported by the principal investigator and senior members of the team.
Wider involvement
In addition to the research team, our evaluation also included input from our Clinical Advisory Group and RSET and BRACE PPI groups, who were actively involved throughout the project (including project planning, analysis and dissemination). Our PPI panel, involved throughout the project, included six members (representing a range of ages, gender and ethnicity). All our PPI panel members have experience with engaging with PPI activity across research and evaluation prior to their involvement in this study. Our PPI panel members have experience with a range of long-term conditions and all have experience of navigating and/or working within the health-care system. Through online meetings, workshops and e-mail, PPI members were involved throughout all stages of the research, including shaping the research questions, focus and methods, developing data collection tools for staff and patients, interpreting findings, and dissemination (e.g. all PPI members had the opportunity to comment on all chapters/papers if they wanted to and based on their interests, prior to their submission).
We attempted to work with services to seek specific PPI input from patients who had received COVID-19 remote home monitoring services, in relation to survey development and topic guide development; though this proved difficult to achieve due to rapid timescales. We also could have developed a specific study PPI group, but again this would have been difficult within the urgent rapid time frame.
Conclusions
To conclude, our evaluation was unable to provide evidence regarding the effectiveness of COVID-19 remote home monitoring services, but this may have been influenced by incomplete data and lower patient enrolment to services than expected. However, this evaluation offers insight into the cost, implementation, staff and patient experience of these services, and lessons learned. There was large variability in the models implemented in relation to the design and intensity of monitoring, workforce, uptake levels and enrolment criteria. Implementation was influenced locally by a range of patient, staff, organisational and resource factors. Tech-enabled monitoring was widely used and all sites were offered an analogue monitoring option. Services proactively used a range of strategies to ensure that their services were inclusive. Staff and patients generally viewed COVID-19 remote home monitoring services positively and felt that they provided reassurance for patients. However, these services were not without limitations, with staff and patients identifying some challenges to delivery and engagement. Findings indicate that for some patients and carers, remote home monitoring services may be burdensome, particularly for some groups. Future remote home monitoring services for COVID-19 and other conditions should operate alongside effective data collection processes, ensure that staff are well supported and have capacity to deliver these services and that patients have appropriate support, ability, and understanding to engage with them.
Acknowledgements
We are indebted to all of the services that participated in this study and to all of the patients, carers and staff who participated in our surveys and interviews. Thank you to the following: Dr Jennifer Bousfield for supporting with study design and data collection, Simon Barnes for supporting with data entry; Jon Sussex for advice given throughout the project; our NIHR BRACE and NIHR RSET PPI members (Raj Mehta, Tina Coldham, June Sadd, Richard Allen, Trevor Fernandes and Fola Tayo) for providing feedback throughout the study and for commenting on drafts of chapters; the NIHR 70@70 Senior Nurse Research Leaders for providing feedback on the development of our study; Russell Mannion for peer-reviewing our study protocol; the NIHR Clinical Research Networks for supporting study set-up and data collection; PHE and the Institute of Global Health Innovation, NIHR Patient Safety Translational Research Centre, Imperial College London, NHSEI and Kent, Surrey and Sussex Academic Health Sciences Network for providing data; Cono Ariti (University of Cardiff) for statistical advice; and Eilís Keeble (Nuffield Trust) for her approach to classifying COVID-19 spells in HES. We thank the NHS Digital CO@h Evaluation Workstream Group chaired by Professor Jonathan Benger and NHSX (in particular Breid O’Brien and Chris Richmond) for facilitating and supporting the evaluation, and to the other two evaluation teams for their collaboration throughout this evaluation: (1) Institute of Global Health Innovation, NIHR Patient Safety Translational Research Centre, Imperial College London; and (2) the IAU (partnership between the Health Foundation and NHSEI). Many thanks to our clinical advisory group for providing insights and feedback throughout the project: Dr Karen Kirkham (whose previous role was the Integrated Care System Clinical Lead, NHSE/I Senior Medical Advisor Primary Care Transformation, Senior Medical Advisor to the Primary Care Provider Transformation team), Dr Matt Inada-Kim (Clinical Lead Deterioration and National Specialist Advisor Sepsis, National Clinical Lead – Deterioration and Specialist Advisor Deterioration, NHS England & Improvement) and Dr Allison Streetly (Senior Public Health Advisor, Deputy National Lead, Healthcare Public Health, Medical Directorate NHS England).
Contributions of authors
Naomi J Fulop (https://orcid.org/0000-0001-5306-6140) (Professor of Health Care Organisation and Management) was the principal investigator and led the overall evaluation. She led on, and contributed to conception, design, analysis and write-up of all aspects of the study, and is lead author of this report.
Holly Walton (https://orcid.org/0000-0002-8746-059X) (Research Fellow) contributed to design and data collection of workstreams 3 and 4 (phase 2), co-led the analysis for the implementation and patient experience chapters, and supported analysis of the workforce chapter. She contributed to the design and interpretation of all other aspects of the study (phase 2). She was involved in contributing to the drafting of the final report (including drafting Chapters 1, 2 and 12, leading/co-leading Chapters 4 and 9, and supporting Chapter 8).
Nadia Crellin (https://orcid.org/0000-0002-9497-5874) (Fellow) contributed to design and data collection of workstreams 3 and 4 (phase 2), co-led the analysis for the implementation and disparities chapters, and supported analysis of the workforce and modality chapter. She contributed to the design and interpretation of all other aspects of the study (phase 2). She was involved in contributing to the drafting of the final report (including leading/co-leading Chapters 4 and 10 and supporting Chapters 2, 8 and 11).
Theo Georghiou (https://orcid.org/0000-0001-9532-876X) (Senior Fellow) contributed to design and data collection of the Phase 1 study and workstream 1 (Phase 2), led on the effectiveness of virtual wards chapter and supported on the effectiveness of CO@h chapter. He contributed to the design and interpretation of all other aspects of the study. He was involved in contributing to the drafting of the final report (including leading Chapter 6 and supporting on Chapters 2 and 5).
Lauren Herlitz (https://orcid.org/0000-0003-2497-9041) (Research Fellow) contributed to design and data collection of workstreams 3 and 4 (phase 2), led the analysis for the modality chapter, and supported analysis of the disparities chapter. She contributed to the design and interpretation of all other aspects of the study (phase 2). She was involved in contributing to the drafting of the final report (including leading Chapter 11 and supporting Chapters 2 and 10).
Ian Litchfield (https://orcid.org/0000-0002-1169-5392) (Research Fellow) contributed to design and data collection of workstreams 3 and 4 (phase 2) and lead/co-lead the analysis for the implementation chapter. He contributed to the design and interpretation of all other aspects of the study (Phase 2). He was involved in contributing to the drafting of the final report (including co-leading Chapter 4 and supporting Chapter 2).
Efthalia Massou (https://orcid.org/0000-0003-0488-482X) (Research Associate) contributed to design and data collection of workstream 2 (phase 2), and co-lead on the cost analysis chapter. She contributed to the design and interpretation of all other aspects of the study (phase 2). She was involved in contributing to the drafting of the final report (including co-leading Chapter 7 and supporting Chapter 2).
Chris Sherlaw-Johnson (https://orcid.org/0000-0002-4851-6060) (Senior fellow) contributed to design and data collection of the Phase 1 study and workstream 1 (phase 2). He led on the effectiveness of CO@h chapter and supported on the effectiveness of virtual wards chapter. He contributed to the design and interpretation of all other aspects of the study (phases 1 and 2). He was involved in contributing to the drafting of the final report (including leading Chapter 5 and supporting on Chapters 2 and 6).
Manbinder Sidhu (https://orcid.org/0000-0001-5663-107X) (Research Fellow) contributed to design and data collection of the phase 1 study and workstreams 3 and 4 (phase 2). He led the analysis for the workforce chapter, and supported analysis of the disparities chapter. He contributed to the design and interpretation of all other aspects of the study (phases 1 and 2). He was involved in contributing to the drafting of the final report (including leading Chapter 8 and supporting Chapters 2 and 11).
Sonila M Tomini (https://orcid.org/0000-0002-4241-2121) (Research Fellow) contributed to design and data collection of the phase 1 study and workstream 2 (phase 2), and co-lead on the cost analysis chapter. She contributed to the design and interpretation of all other aspects of the study (phases 1 and 2). She was involved in contributing to the drafting of the final report (including co-leading Chapter 7 and supporting Chapter 2).
Cecilia Vindrola-Padros (https://orcid.org/0000-0001-7859-1646) (Senior Research Fellow) contributed to design and data collection of the phase 1 study, and workstreams 3 and 4 (phase 2). Cecilia led the analysis for phase 1, and supported analysis of the patient experience and modality chapters. She contributed to the design and interpretation of all other aspects of the study (phases 1 and 2). She was involved in contributing to the drafting of the final report (including leading Chapter 3 and supporting Chapters 2 and 9).
Jo Ellins (https://orcid.org/0000-0001-8138-9385) (Senior Fellow) contributed to the design and analysis of all aspects of the evaluation, in particular supporting with analysis and interpretation of findings relating to workstreams 3 and 4, and supported with drafting of the final report.
Stephen Morris (https://orcid.org/0000-0002-5828-3563) (Professor of Health Services Research) contributed to the design and analysis of all aspects of the evaluation, led and oversaw workstream 2, and supported with drafting the final report.
Pei Li Ng (https://orcid.org/0000-0001-8411-220X) (Project Manager) led on the project management of the evaluation and supported with drafting of the final report.
All authors contributed to integrating the findings of the study. All authors made critical revisions to the report content and approved the final manuscript. All authors agree to be accountable for all aspects of this work in ensuring that questions related to the accuracy or integrity of any part of the final report are appropriately investigated and resolved.
Publications
Published:
Georghiou T, Sherlaw-Johnson C, Massou E, Morris S, Crellin NE, Herlitz L, et al. The impact of post-hospital remote monitoring of COVID-19 patients using pulse oximetry: a national observational study using hospital activity data. eClinicalMedicine 2022;48:101441. doi: https://doi.org/10.1016/j.eclinm.2022.101441
Greenhalgh T, Knight M, Inda-Kim M, Fulop NJ, Leach J, Vindrola-Padros C. Remote management of covid-19 using home pulse oximetry and virtual ward support. BMJ 2021;372:n677. https://doi.org/10.1136/bmj.n677
Sherlaw-Johnson C, Georghiou T, Morris S, Crellin N, Litchfield I, Massou E, et al. The impact of remote home monitoring of people with COVID-19 using pulse oximetry: a national population and observational study. EClinicalMedicine 2022;45:101318. https://doi.org/10.1016/j.eclinm.2022.101318
Sidhu MS, Ford GA, Fulop NJ, Roberts CM. Learning networks in the pandemic: mobilising evidence for improvement. BMJ 2022;379:e070215. https://doi.org/10.1136/bmj-2022-070215
Sidhu M, Walton H, Crellin N, Ellins J, Herlitz L, Litchfield I, et al. Staff experiences of training and delivery of remote home monitoring services for patients diagnosed with COVID-19: a mixed-methods study. J Health Serv Res Policy 2023;0(0). https://doi.org/10.1177/13558196231172586
Vindrola C, Fulop N, Greenhalgh T. Virtual wards: caring for COVID-19 patients at home could save lives. The Conversation. 2020. URL: https://theconversation.com/virtual-wards-caring-for-covid-19-patients-at-home-could-save-lives-149292 (accessed 18 July 2023).
Vindrola-Padros C, Sidhu MS, Georghiou T, Sherlaw-Johnson C, Singh KE, Tomini SM, et al. The implementation of remote home monitoring models during the COVID-19 pandemic in England. EClinicalMedicine 2021;34:100799. https://doi.org/10.1016/j.eclinm.2021.100799
Vindrola-Padros C, Singh KE, Sidhu MS, Georghiou T, Sherlaw-Johnson C, Tomini SM, et al. Remote home monitoring (virtual wards) for confirmed or suspected COVID-19 patients: a rapid systematic review. EClinicalMedicine 2021;37:100965. https://doi.org/10.1016/j.eclinm.2021.100965
Walton H, Crellin N, Sidhu MS, Sherlaw-Johnson C, Herlitz L, Litchfield I, et al. Undertaking rapid evaluations during the COVID-19 pandemic: Lessons from evaluating COVID-19 remote home monitoring services in England. Front Sociol 2023;8:982946. https://doi.org/10.3389/fsoc.2023.982946
Walton H, Fulop NJ. Virtual wards and Covid-19: An explainer. Nuffield Trust. 2022. URL: www.nuffieldtrust.org.uk/resource/virtual-wards-and-covid-19-an-explainer (accessed 18 July 2023).
Walton H, Vindrola-Padros C, Crellin N, Sidhu MS, Herlitz L, Litchfield I, et al. Patients’ and carers’ experiences of, and engagement with remote home monitoring services for COVID-19 patients: a rapid mixed-methods study. Health Expectations 2022:25:2386–404. https://doi:10.1111/hex.13548.
Preprints/submitted for review
Crellin N, Herlitz L, Sidhu MS, Ellins J, Georghiou T, Litchfield I, et al. Examining disparities relating to service reach and patient engagement with COVID-19 remote home monitoring services in England: a mixed-methods rapid evaluation. medRxiv 2021. https://doi.org/10.1101/2022.02.21.22270793
Herlitz L, Crellin N, Vindrola-Padros C, Ellins J, Georghiou T, Litchfield I, et al. Technology-enabled and analogue models of remote home monitoring care for COVID-19: a rapid mixed-method evaluation of patient and staff experiences. Submitted for publication June 2023.
Ethical approval
Phase 1 received ethical approval from the University of Birmingham humanities and social sciences ethics committee (ERNE_13-1085AP37) and was categorised as a service evaluation by the Health Research Authority (HRA) decision tool and University College London/University College London Hospitals (UCL/UCLH) Joint Research Office. The study protocol was divided into two separate protocols: (1) covered workstreams 1 and 2 and the staff elements of workstreams 3 and 4, received ethical approval from the University of Birmingham Humanities and Social Sciences ethics committee (ERN_13-1085AP39) and was categorised as a service evaluation by the HRA decision tool and UCL/UCLH Joint Research Office (January 2021); (2) the patient experience study (survey and case study interviews – workstreams 3 and 4) was reviewed and given favourable opinion by the London-Bloomsbury Research Ethics Committee (REC reference: 21/HRA/0155 (February 2021).
Data-sharing statement
Individual patient-level data and data supplied under specific data-sharing agreements cannot be made available by the study team. Sources for data that are already publicly available are supplied either in the text or the references. Aggregate survey data collected by the study team are presented within this final report. The anonymised qualitative data that support the findings of this study are available on request from the corresponding authors. To protect anonymity of interviewees, the data are not publicly available. Further information can be obtained from the corresponding authors.
Funding
This project was funded by the National Institute for Health and Care Research (NIHR) Health and Social Care Delivery Research programme [(RSET: 16/138/17; BRACE: 16/138/31) and NHSEI] and will be published in full in Health and Social Care Delivery Research; Vol. 11, No. 13. See the NIHR Journals Library website for further project information. The views expressed in this publication are those of the authors and not necessarily those of the National Institute for Health and Care Research or the Department of Health and Social Care.
Disclaimers
This report presents independent research funded by the National Institute for Health and Care Research (NIHR). The views and opinions expressed by authors in this publication are those of the authors and do not necessarily reflect those of the NHS, the NIHR, the HSDR programme or the Department of Health and Social Care. If there are verbatim quotations included in this publication the views and opinions expressed by the interviewees are those of the interviewees and do not necessarily reflect those of the authors, those of the NHS, the NIHR, the HSDR programme or the Department of Health and Social Care.
References
Appendix 1 A summary of the SOPs for CO@h38 and CVW39
CO@ha | ||||||
---|---|---|---|---|---|---|
Characteristics of service | Patient journey | |||||
Entry criteria | Staffing and oversight | Referral (stage 1) | Triage (stage 2) | Onboarding (stage 3) | Monitoring (stage 4) | Recovery and discharge (stage 5) |
(1) Diagnosed with COVID-19 (clinically or positive test result); and (2) symptomatic and either ≥ 65 years or <65 years and CEV to COVID-19a | Models primarily implemented in GP (including hot hubs, community teams); responsibility lies with CCGs | Timely referral that meets entry requirements from all relevant providers (NHS 111, COVID-19 clinical assessment service, test and trace and hospital emergency departments) | Patients should receive standard assessment (with potential for face-to-face clinical assessment if necessary); same day as referral; hot sites (face-to-face assessment); phone/video (virtual assessment using standard questionnaire and baseline oximetry reading either at hot site or home visit) | Patients should be given pulse oximeter and supporting information (including paper diary in variety of languages, app/regular call mechanism), contact details to report readings/symptoms and safety netting instructions: immediately if patient seen face-to-face; within 12 hours if assessment remotely. Instruct patients to record oximetry readings daily (usually 3× day). Also give option of prompts at days 2, 5, 7, 10, 12 (text message, e-mail, or non-clinician phone call). Instruct patients on how to access help if needed and provide 24/7 access to support. Agree how patients will return oximeter | Patients should be sent a text message or e-mailed or called as agreed; check-in calls should check patient is using oximeter and diary correctly and that readings are 95% or above; frequency of calls can be reviewed as appropriate | Discharge patients and give leaving information, safety netting and safe advice on how to return oximeter (hot site, NHS volunteers) within 14 days; patients can be discharged earlier if negative result or if determined at clinical review; patients still symptomatic at 14 days: further assessments |
CVW b | ||||||
Entry criteria | Staffing and oversight | Hospital discharge assessment (stage 1) | Onboarding (stage 2) | Monitoring (stage 3) | Recovery and discharge (stage 4) | |
(1) Adults in hospital with diagnosis of COVID-19 who have an improving clinical trajectory and no fever for 48 hours (2) Patients seen in secondary care or by ambulance clinicians who do not require emergency admission but do require oximetry follow-up |
Models implemented by one or more acute trust | Completion of satisfactory exercise test: saturations 95–100% and low NEWS2 and improving clinical trajectories can be discharged to CVW; saturations 93–94% with improving clinical trajectories can be considered for CVW (if clinically appropriate); saturations 92% or lower or experiencing moderate/severe shortness of breath are unsuitable for early supported discharge unless patient is stable; clinical judgement necessary | Agree a discharge/escalation plan which includes monitoring arrangements; patients given a patient information leaflet, oximeter, instructions and diary; patients given hospital number to call for advice or support between 8 a.m. and 8 p.m., 7 days a week; onboarding should include provision of a patient held escalation plan | Patient takes three readings each day and enters into a diary; patient asked to call hospital immediately if reading <92% If not possible attend emergency department within an hour or call 999; service contact the patient by phone every day | Discharged at 14 days (or before if appropriate); GP should be informed; if still symptomatic, receive a further clinical assessment; friend/family or NHS volunteer collects and returns oximeter for decontamination and reuse | |
Responsibility lies with hospitals | ||||||
Clinical, governance and administrative responsibilities provided by appropriately trained staff (including shielding staff); staff should be supervised by a clinically registered professional | ||||||
CVW led by a named consultant or ST3 + doctor with relevant COVID-19 experience | ||||||
Requires staffing for at least 12 hours a day (8 a.m. to 8 p.m.) 7 days a week plus out of hours cover |
Appendix 2 Summary of monitoring approach and frequency of monitoring calls (based on staff interviews and clinical lead surveys)
Summary of monitoring approacha | Frequency of calls (for submission of readings) | Sites (n) | Example |
---|---|---|---|
Self-monitoring only | No scheduled telephone calls from members of staff for all patients (tech-enabled or calls patient-initiated) | 0 | N/A |
Tech-enabled and analogue self-monitoring option if tech-enabled not possible | Regular contact from member of staff regardless of whether using tech-enabled or analogue mode of submitting readings (welcome call, courtesy call mid-way and discharge call). Patients on analogue option asked to ring if any problems with monitoring (self-monitoring) | 1 | At site D, three options of monitoring are offered: (1) Analogue option: patients record using paper diaries and are advised to record readings three times a day and ring the service if they need help (self-monitoring) (2) Tech-enabled option: text message readings once a day (encouraged to take readings more than once a day) (3) Tech-enabled option for those with chronic conditions: submit readings once a day via an app |
Tech-enabled and analogue if tech-enabled not possible (non-automated telephone calls to submit readings) | Once a day or less (with some exceptions)b | 10 | At site B, three options of monitoring are offered: (1) Tech-enabled option: patients submit readings by text 3 times a day. (2) Tech-enabled option: patients submit readings by automated calls 3 times a day (3) Analogue option: patients record readings using paper diary and patients are called by service once a day to give their readings |
Tech-enabled and analogue if tech-enabled not possible (non-automated telephone calls to submit readings) | More than once a day | 9 | At site Q, two options of monitoring are offered: (1) Analogue option: patients telephoned three times a day to provide readings (or less if stable or less contact preferred) (2) Tech-enabled option: patients submit readings via app three times a day |
Tech-enabled monitoring and analogue (non-automated telephone calls to submit readings) if tech-enabled not possible | Regular contact from member of staff regardless of whether using tech-enabled or analogue mode of submitting readings (days 2, 5, 6, 12, 14); telephone calls to submit readings (for analogue patients): more than once a day | 1 | At site N, three options of monitoring are offered: (1) Tech-enabled option: patients are texted to submit readings two or three times day (2) Tech enabled option: patients receive automated calls to submit readings (3) Analogue option: patients are phoned three times a day to submit readings |
Analogue-only; regular telephone calls from member of staff to submit readings | Varying frequencies (once a day or less to more than once a day depending on needs)c | 5 | At site R, one option of monitoring is offered: (1) Analogue option: patients telephoned once a day to provide readings (or more frequently if concerns) |
At site V, three analogue options are offered: (1) Analogue option: patients telephoned daily to provide readings (red category) (2) Analogue option: patients telephoned every other day (amber category) (3) Analogue option: patients are telephoned weekly to provide readings (green category) |
|||
Analogue-only; regular telephone calls from member of staff to submit readings and face-to-face option (for monitoring) if needed | Varying frequencies (once a day or less to more than once a day depending on needs) | 2 | At site F, two analogue options are offered: (1) Analogue option: patients receive daily, twice daily or on alterative days depending on patient preferences and ability (2) Analogue option: if patients are struggling with oximeters and readings, are older or have certain conditions, or are too poorly, patients receive face-to-face appointments every day |
Appendix 3 Patient/carer recommendations to improve the service
Category | Recommendation |
---|---|
Patient awareness | Need more publicity about the service |
Need to know about the service sooner or earlier referral | |
Need to improve link to NHS Track and Trace | |
Need national standardised approach or automatic referral | |
Patient enablement | Need more information provision about the service at referral, about escalation and about discharge |
Need a dedicated contact number for patients to contact service if needed | |
Some patients require some face-to-face visits | |
Need reminders to submit readings | |
Workforce | More continuity of staff and continuity of information |
More contact with doctors desired by some patients | |
Individual differences | More personalised approach |
Flexibility of methods and patient choice | |
Equipment | Provision of thermometers |
Support with using technology (oximeter and digital platforms) | |
Logistics of service | Consider timing of monitoring calls and having a specific time slot |
Need to receive calls when promised | |
Need to ensure practical and efficient arrangement of oximeter delivery and return | |
Signposting | Following patients up after discharge |
A point of contact after discharge to ask questions to | |
Signposting to places that can support them while on the service | |
Community drives to ease concerns over hospitals |
Appendix 4 Local service adaptations to increase reach and facilitate patient engagement
Adaptations to service | Patient group (to benefit from adaptation) | |
---|---|---|
Adaptations to increase inclusivity/reach | ||
Broadening the service entry criteria |
|
At-risk or vulnerable groups prioritised by services, for example, patients with a learning disability, severe mental illness, ethnic minority groups, and pregnant women |
|
||
|
||
Active case finding |
|
Hard-to-reach patients (i.e. those less likely to seek help from their GP), could include those with limited digital, numerical or written literacy, frail or elderly patients, or patients with high levels of anxiety |
|
||
Design of referral pathways |
|
Referral pathways can target specific patient groups (e.g. carers, people living in sheltered or supported accommodation, people residing in secure units) |
|
||
|
||
Monitoring service uptake by different at-risk groups |
|
At-risk or vulnerable groups prioritised by services |
Adaptations to increase patient engagement | ||
Providing additional support for patients beyond monitoring protocol or national guidance |
|
Vulnerable or particularly unwell patients may need more assistance, for example, patients living alone/socially isolated, patients who are carers, patients that are very anxious or patients at risk of being digitally excluded |
|
||
|
||
Collaborating with other teams or specialist services |
|
Patients requiring additional support such as those with a learning disability or severe mental illness, or patients with long COVID-19 or specific health needs (e.g. pre-existing or associated with their COVID-19 diagnosis). |
|
||
Delivery of oximeters |
|
Particular value for patients living alone, without a support network, or where several family members isolating at the same time |
|
||
|
||
Adapting patient information |
|
Patients with a visual impairment, learning disability, cognitive or hearing impairment or difficulty communicating, and patients without digital access |
|
||
|
||
|
||
|
||
Translation services |
|
Patients for whom English was not their first language |
|
||
|
||
|
||
|
||
Amending the monitoring processes/ protocols |
|
To better suit the needs of specific patient groups, such as those with a visual or hearing impairment, cognitive impairment, patients living alone, anxious or working patients, those with low digital literacy or patients who were particularly unwell |
|
||
|
||
|
||
Mode of monitoring |
|
|
|
||
Providing additional equipment |
|
Patients without access to appropriate technology |
Face-to-face assessments or home visits and/or video consultations |
|
|
|
|
|
Liaising with family, friends or carer |
|
If patients live alone or do not feel supported by their household, services need to be mindful that they may require additional support to engage with the service |
Appendix 5 Functions of COVID-19 remote home monitoring platforms that staff valued or felt needed improvements
Valuable functions | Staff views |
---|---|
Interoperability with other data systems | Staff valued tech-enabled platforms that integrated with existing patient health systems, enabling them to view patients GP records, automate referrals from GP practices, update GP practices on patients’ care |
Plasticity | Staff liked features that enabled them to change what they viewed according to their needs and add or change patient readings or notes; for example, being able to: |
|
|
|
|
|
|
|
|
Filtering, ordering and sorting information | Staff valued platforms that enabled them to filter, order and sort patients according to their clinical needs or the service’s operational needs, for example, by red/amber/green rating, oxygen saturation levels, by staff allocation, by patients yet to be reviewed |
Task management | Task management functions (i.e. automated messages from the system relating to allocated tasks) were valued if they fit the service’s needs. In some sites, automated tasks to call patients could facilitate work allocation. In others, inappropriately automated tasks became an additional message to remove because oversensitive alerts were raised for patients |
Exact readings | Staff wanted exact readings for patients’ measurements (rather than ranges); for example, oxygen saturation levels (essential when patients were weaned off oxygen) and temperature. In one site, inexact readings for patients using oxygen led to staff calling patients for readings they had already provided through the app. In one site, temperature readings were provided in Fahrenheit rather than Celsius and had to be continually converted by staff |
Automated discharge report | One platform produced automated discharge reports that could be shared with patients’ GPs, which was valued by staff. A staff member from another site highlighted the potential efficiency gains from automating the discharge process through the platform so that the tech provider and GP could both be notified of a patients’ discharge from the service (and the need for equipment to be collected) |
Reporting | For most tech-enabled platforms, reports on patients using the service were provided on a daily or weekly basis by the tech provider. However, built-in reporting function would enable staff to view and interrogate the data themselves |
Data dashboard | Staff reported the utility of a data dashboard to have an overview of all patient records, including referral, onboarding, review and discharge dates |
Visibility of information | Staff valued being able to see patients’ readings visually, for example, in tables or charts that could be viewed clearly on one page. Including data points for missing data from non-submission was also useful |
List of abbreviations
- AHSN
- Academic Health Science Network
- ANPs
- advanced nurse practitioners
- BRACE
- The Birmingham, RAND and Cambridge Evaluation Centre
- CCGs
- clinical commissioning groups
- CE
- European Conformity
- CEV
- clinically extremely vulnerable
- CI
- confidence interval
- CO@h
- COVID Oximetry @home
- COPD
- chronic obstructive pulmonary disease
- CVW
- COVID virtual wards
- FTE
- full-time equivalent
- GPs
- general practices
- HES
- Hospital Episode Statistics
- HES APC
- Hospital Episode Statistics Admitted Patient Care
- HRA
- Health Research Authority
- IAU
- Improvement Analytics Unit
- ICD-10
- International Statistical Classification of Diseases and Related Health Problems, Tenth Revision
- ICU
- intensive care unit
- IMD
- Index of Multiple Deprivation
- IRR
- incidence rate ratios
- IT
- information technology
- KSS AHSN
- Kent, Surrey and Sussex Academic Health Science Network
- LOS
- length of stay
- LSOA
- lower-layer super output area
- MHRA
- Medicines and Healthcare Products Regulatory Agency
- MS Teams
- Microsoft Teams
- NHSEI
- NHS England and NHS Improvement
- NHSX
- NHS user experience
- NIHR
- National Institute for Health and Care Research
- OR
- odds ratio
- PERCS
- Planning and Evaluating Remote Consultation Services
- PHE
- Public Health England
- PPI
- Patient and Public Involvement
- RAP sheets
- Rapid Assessment Procedure sheets
- REDCap
- Research Electronic Data Capture
- RSET
- Rapid Service Evaluation Team
- SARS-CoV-2
- severe acute respiratory syndrome coronavirus
- SITREPs
- Situation Reports
- SOP
- standard operating procedure
- SPSS
- Statistical Package for the Social Sciences
- UCL
- University College London
- UCLH
- University College London Hospitals
- WHO
- World Health Organization
Notes
Supplementary material can be found on the NIHR Journals Library report page (https://doi.org/10.3310/FVQW4410).
Supplementary material has been provided by the authors to support the report and any files provided at submission will have been seen by peer reviewers, but not extensively reviewed. Any supplementary material provided at a later stage in the process may not have been peer reviewed.