Notes
Article history
The research reported in this issue of the journal was funded by the HS&DR programme or one of its proceeding programmes as project number 10/1011/70. The contractual start date was in March 2012. The final report began editorial review in September 2013 and was accepted for publication in July 2014. The authors have been wholly responsible for all data collection, analysis and interpretation, and for writing up their work. The HS&DR editors and production house have tried to ensure the accuracy of the authors’ report and would like to thank the reviewers for their constructive comments on the final report document. However, they do not accept liability for damages or losses arising from material published in this report.
Declared competing interests of authors
none
Permissions
Copyright statement
© Queen’s Printer and Controller of HMSO 2014. This work was produced by Weich et al. under the terms of a commissioning contract issued by the Secretary of State for Health. This issue may be freely reproduced for the purposes of private research and study and extracts (or indeed, the full report) may be included in professional journals provided that suitable acknowledgement is made and the reproduction is not associated with any form of advertising. Applications for commercial reproduction should be addressed to: NIHR Journals Library, National Institute for Health Research, Evaluation, Trials and Studies Coordinating Centre, Alpha House, University of Southampton Science Park, Southampton SO16 7NS, UK.
Chapter 1 Background
Introduction
Rates of compulsory admissions to psychiatric inpatient beds have been rising in recent decades in many Western European countries,1,2 including England. 3–5 This trend appears to have accelerated since the introduction of the Mental Health Act (2007) (MHA):1,5–8 compulsory admissions to hospital in England increased by 14% in the first five years after the introduction of the MHA (2007). 9 Taking into account the widespread use of Community Treatment Orders (CTOs),10 introduced in 2008, the numbers of people subject to the MHA since 2007/8 has increased by about 5% per annum. 9 The total number of detentions in England exceeded 50,000 for the first time ever in 2012/1311 (not counting short-term assessment orders), and this is now a matter of significant public, government and regulatory concern in England. 12–14 The reasons for this remain unclear, particularly since this trend has continued despite the development of a range of community-based services such as Community Mental Health Teams, Assertive Outreach, Crisis Resolution Home Treatment (CRHT) and Early Intervention services. 15
Different explanations for increasing compulsory admissions have been proposed, including secular changes in social support networks,16,17 bed shortages18 and ‘local system failures in facilitation of timely and appropriate access to care’,13 including difficulties accessing effective crisis care in the community, accident and emergency (A&E) departments and appropriate Place of Safety settings,14 and bed shortages. 13,18 Recent evidence that rates of detention are also increasing among patients initially admitted voluntarily suggests that consent to treatment is now being withdrawn more often after patients have been exposed to inpatient environments. 13 Although illicit drugs and alcohol use is often considered to be a cause of mental disorder, suicide and violence,3 recent evidence of falling rates of misuse argue against this as a primary cause of rising compulsory admission rates. 19 Increases in compulsory admission rates in England have coincided with an equally dramatic reduction in the number of mental illness beds. 20 Fewer beds may lead to higher occupancy levels, delays in admission,21 increased illness severity thresholds for admission, inpatient units that are more disturbed and frightening,22–24 and early discharge resulting in relapse and readmission. 3 Analysis for England shows that the number of NHS compulsory admissions increased by over 80% between 1988 and 2008, while the number of mental illness beds fell by around 60% during the same period. 5,20 While closure of mental illness beds has been part of deinstitutionalisation of care for the mentally ill in high-income countries,25 the UK has taken this further than most other developed economies,26 despite having higher levels of funding for mental health services overall. 27
Clues to understanding increasing compulsory admission rates may lie in variation in use of the MHA between different types of individual, and between different places and health-care providers. People from black and minority ethnic (BME) groups have very different experiences of mental health services from the rest of population in the UK. 28–33 These groups experience significantly greater rates of admission, including compulsory detention. 31,34 Black mental health service users experience pathways to acute psychiatric care that are more coercive and more likely to involve referral for MHA assessment35 and the police and criminal justice system. 36,37 These inequalities have persisted despite major policy initiatives,38–40 additional investment, workforce developments and the general improvements in the coverage and quality of mental health services over the last decade. 39,41 There are also significant geographical differences in use of the MHA; a twofold difference in the rate of compulsory admission between strategic health authorities (SHAs) was noted in 2011/12. 9 This may reflect variation in investment in mental health services by commissioners. In 2007, per-adult mental health spend by primary care trusts (PCTs) in England varied by a factor of three after adjusting for age, population need and overall PCT spending. 42 The gains associated with improved community services may not be experienced equally, and compulsory admission rates may be increasing disproportionately in places where social networks are weakest, demands on services are greatest and community services are hardest to deliver, particularly urban areas as well as remote rural communities.
Increasing compulsory admission rates are unpopular with service users and carers (even when viewed in retrospect as necessary)43,44 and sit uneasily with professionals, who have spent decades attempting to improve engagement with care and reduce fear and stigma. 22–24,31,45 Observational studies show that people who were admitted compulsorily had worse social functioning and higher suicide rates [but not more (re)admissions or greater lengths of stay] than those admitted voluntarily, although the former also expressed lower levels of satisfaction with treatment. 46 Despite services being increasingly patient- and carer-centred, responsive to need, recovery-focused, timely and popular,22,47 recent developments in community mental health services have not reduced involuntary admissions. 15,48–51 Despite early promise,52 subsequent rigorous, high-quality randomised controlled trials of individual-level interventions, most notably joint crisis plans,2 advance directives53 and CTOs10 have all failed to demonstrate significant reductions in compulsory admissions. Although it is possible that enhanced, intensive interventions for those most at risk of compulsory admission may prove more effective,8 indications to date are not promising.
This is also a source of concern to commissioners and service providers, given the high costs of inpatient care. The increasing rate of involuntary treatment represents a major financial obstacle to investment in community services and preventative and public health initiatives, particularly at times of austerity. Understanding the determinants of this trend is imperative if it is to be arrested or reversed. 15
Research aims and objectives
This study seeks to identify factors that contribute to the increasing rate of compulsory admission in England. This will be achieved by means of the following aims and objectives.
Research aim 1
To establish if, and to what extent, compulsory admission rates vary across different places and between providers and commissioners of (mental) health care in England, and, if so, where this variance occurs.
Research objective 1
The geography of mental health care is complex and comprises overlapping, partially nested spatial clusters that include individuals, general practices, commissioners (previously PCTs), providers (NHS trusts or independent providers) and regional health authorities (SHAs). We will use multilevel statistical models to measure and compare variance in the rate of compulsory admission across England at each of these levels.
Research aim 2
To explore and quantify the extent to which variance in the rate of compulsory admission can be explained by:
-
the individual characteristics of people using services (e.g. age, sex and ethnicity)
-
the local area (i.e. contextual) socioeconomic and sociodemographic factors (e.g. small area socioeconomic deprivation, urbanicity and ethnic density)
-
the features of local mental health services [e.g. bed availability, number of admissions, length of stay (LOS) in hospital and investment in mental health services].
Research objective 2
There are many factors that may influence (i.e. increase or decrease) the risk of a patient being compulsorily admitted to hospital. We will undertake substantial data linkage using multiple routine (secondary) data sources in order to create models that incorporate information about people, places and health-care organisations. We will use multilevel statistical models to examine the impact of specific risk factors at both the individual (patient) level and at each of the spatial levels in the Mental Health Minimum Data Set (MHMDS) for England in 2010/11, simultaneously, on variance in the rate of compulsory admission across England.
Finally, we estimated associations between risk factors operating at different spatial levels and compulsory admission, after adjusting for other model covariates.
These research aims were addressed through secondary analyses of routinely collected national mental health-care data, described in detail in the next chapter.
Chapter 2 Methods
Study overview and design
This study is a cross-sectional, multilevel secondary analysis of annual, routinely collected data, from providers of secondary mental health-care services in England during 2010/11, augmented by linkage to external data sets across a range of different spatial levels.
Our initial intention was to estimate longitudinal changes in the variance of compulsory admission rates at different spatial levels (see Appendix 1). Data quality (and completeness) problems in MHMDS data before 2010/11, combined with far richer data linkages than expected, caused us to change our study design at the outset of the project. This was reported to the funders in both of our interim progress reports, and was documented in an approved amendment to the study protocol (see Appendix 2).
Ethics
Ethical approval for this study was obtained from Warwick Medical School Biomedical Research Ethics Committee on 12 January 2012 (REF 166-01-2012).
The Mental Health Minimum Data Set
The primary data source for this study is the 2010/11 MHMDS. 54 The MHMDS is a national, person-based, individual-level data set describing mental health service users and the care they receive. MHMDS includes data on all adults aged 18 years or older (with no upper age limit) who have received specialist adult mental health-care services in a secondary care setting. A small number of individuals younger than 18 years of age who are receiving treatment within a specialist secondary adult mental health service are also included in the provider trust returns. 55 In 2011 (at the time this project began), the MHMDS was collated and analysed by the NHS Information Centre (NHSIC). In 2013, the NHSIC was re-organised to be the NHS Health and Social Care Information Centre, or the HSCIC (www.hscic.gov.uk). For consistency, this report will refer to the HSCIC throughout. The MHMDS contains confidential patient-level data and our research team were granted access to the anonymised data for this project by the NHS Data Access and Advisory Group.
Brief overview of the Mental Health Minimum Data Set
The MHMDS was initially developed to support monitoring of the mental health element of the then government’s ‘Health of the Nation’ strategy (1992–7). 56 Since 2003 the MHMDS has become a mandatory quarterly data return for all providers of NHS-funded specialist mental health services for adults, and for some independent sector providers since 2011. 54,57 The MHMDS provides data on a wide range of mental health services, including those provided in hospital, in outpatient clinics and in the community (where the majority of people in contact with these services are treated). 54 The MHMDS is an approved NHS Information Standard that aims to deliver robust, comprehensive, nationally consistent and comparable person-based information on patients in contact with specialist secondary mental health services. It captures key information from the mental health-care pathway. 58 The MHMDS supports a variety of secondary use functions such as commissioning, clinical audit, research, service planning, inspection and regulation, monitoring key government policies and legislation including the National Service Framework for Mental Health and MHA, performance management and benchmarking at both local and national levels, and national reporting and analysis. 58
Systems for MHMDS data collection, collation and curatorship have improved dramatically since the data set was first created, and this is reflected in increasing reliance on MHMDS for the national governance of NHS mental health services. Nevertheless, significant data quality issues have been a constant feature, and pose challenges to its use. It became clear in the course of our research that, while the 2010/11 MHMDS was of sufficient completeness to support the cross-sectional examination of variance in compulsory admission rates, the data from previous years were much less reliable and of far inferior quality.
The 2010/11 MHMDS was designed to support the legal requirement of the NHS to administer and apply the 2007 MHA. 55 Providers make quarterly data returns which are then assembled to create an annual database, covering a reporting period from 1 April 2010 to 31 March 2011. During this 2010/11 reporting period, a total of 72 providers of mental health services submitted data to the MHMDS: three were independent sector providers, 10 were also NHS commissioners and 59 were (solely) NHS mental health providers.
The MHMDS is a large and complex data set. In 2010/11, a total of 1,287,730 individuals had contact with specialist mental health services in England. Each record in the MHMDS represents the complete, continuous spell of care for an individual, from initial referral to final discharge (referred to as a mental health-care spell – or care spell for short). This could cover any type of treatment from a short community or outpatient episode to an extended care spell over many years and covering all aspects of mental health services. 59 The care spell is assembled from separate records of care episodes, patient activity and contacts with mental health professionals. The vast majority of patients (89%) have only one care spell and therefore one record in the 2010/11 MHMDS; the remaining patients have more than one care spell and more than one record. Although multiple records are likely to arise for a variety of reasons (e.g. transfer of care from one mental health provider to another), the data set does not include this level of detail. This means that there are more records in the 2010/11 MHMDS (n = 1,443,858) than there are patients (n = 1,287,730). For the purposes of this study, each patient was counted once only using specific selection rules, which are outlined in detail later in this report. This is common practice in national analyses of the same data by the HSCIC. 54
Defining the study outcome
Defining compulsory admission
The main study outcome is compulsory admission, and this terminology will be used throughout the report. Those who were admitted but not subject to the MHA (2007) are referred to as voluntary inpatients. We have not used the terms ‘informal’ and ‘formal’ (or ‘involuntary’) in relation to inpatient admissions, although these are sometimes used to denote voluntary and compulsory admissions, respectively.
Compulsory admission was defined as time spent in an inpatient mental illness bed subject to the MHA (2007) during the 2010/11 reporting period. We did not include patients who were detained only under sections of the MHA (2007) that apply only to conveyance to, and assessment in, a Place of Safety (S135 and S136), or for the purposes of assessment only [e.g. Sections 4 and 5(2)] where detention for longer than 72 hours does not occur. Likewise (because it does not relate to inpatient admission per se) we did not include sections of the Act relating only to guardianship or the supervision of community treatment. Patients who were detained under these short-term assessment sections and subsequently admitted under Sections 2 or 3 were included as cases of compulsory admission; those discharged from Sections 135 or 5(2), or admitted to hospital voluntarily, were not. The data did not allow us to distinguish between patients who were detained under the MHA (2007) at the time of admission and those admitted voluntarily and subsequently detained.
No single variable in the MHMDS data set described the study outcome. It was therefore necessary to derive this outcome using a number of other variables, starting with those concerned with inpatient admission.
The MHMDS contains several treatment and care variables which indicate if the patient was admitted to hospital during the reporting period, including number of days spent as an inpatient, number of admissions to hospital and number of discharges from hospital. There are also a number of variables relating to the nature of the admission to hospital (e.g. whether or not the patient was subject to the MHA), some of which apply to the reporting period (a year) and others to the entire course of the care spell (no definitive time period). These variables will be described in detail in the next sections.
Treatment in the community
Most patients in the MHMDS received all of their mental health care in the community, and were not admitted to hospital (either voluntarily or compulsorily). MHMDS collects information relating to what type of treatment the patient received in the community, for example the number of contacts a patient had with a community psychiatric nurse or the number of times a patient attended outpatient appointments. Combining these data with information from the number of days spent in hospital (bed-days) and number of admissions/discharges enabled us to identify patients who received only community care during the study year, with a high degree of accuracy.
Admission to hospital
A number of variables in the MHMDS provide information on admissions to hospital. The variable ‘bed-days’ is the number of days a patient spent as a mental health inpatient, including any days spent in a secure mental health unit. The number of admissions and discharges occurring during the reporting period are also recorded. However, the HSCIC discovered that there were inconsistencies and missing data, such that information on bed-days and on admissions/discharges does not concur for every patient. This is noted in the MHMDS Data Quality and Methodology Manual:
A very small number of trusts are failing to record consistent information about Inpatient spells in the Hospital Provider Spell (IPEP) and Ward Stay within Hospital Provider Spell (WARDSTAYS) tables in the input data and this affects the accuracy of the trust level results for these organisations . . . discrepancies in the source data result in discrepancies between the reported admissions, occupied bed days and patients categorised as ’admitted’. It also means that some trusts that certainly have beds have no patients in the ‘admitted’ category.
p. 959
However, IPEP and WARDSTAYS variables are generated by the HSCIC data assembler when the provider trust makes a submission but are not available to external users of the MHMDS. We were therefore unable to conduct any missing data analysis on these variables and had to conduct our own exploratory analyses to ascertain if a patient spent time as an inpatient subject to the MHA during the 2010/11 reporting period. We were not able to distinguish the type of mental health service providing inpatient treatment, and therefore these data will include people receiving specialist treatment in old age, learning disability (LD) and forensic mental health services.
Information from all relevant variables was used to optimise certainty about whether or not a patient spent time as an inpatient during the reporting period. Patients were therefore classified as follows:
-
‘admitted’ if there was any evidence that the individual spent time in hospital as an inpatient during 2010/11
-
‘only non-admitted care’ if there was evidence that the individual received treatment in the community but there is no evidence that the individual spent time as an inpatient during 2010/11
-
‘no care’ if there was no information about care provided during 2010/11.
A small proportion of patients (6.7%) had no care information in their 2010/11 record. This group may include patients recently referred to mental health services but not yet seen. It is more likely, however, that data-uploading errors were responsible. We assumed that these patients received some care in the community but no hospital admissions.
Table 1 provides a breakdown of the number of patients in each of the defined care status categories for the 2010/11 reporting period.
Care status category | Description (for 2010/11) | Number of patients (n = 1,287,730) |
---|---|---|
Admitted | Evidence of time in hospital as an inpatient | 106,719 |
Only non-admitted care | Evidence that patient received treatment in the community only but no evidence of specific record of being an inpatient in 2010/11 admission | 1,094,138 |
No care | No evidence of direct care being provided in 2010/11 | 86,873 |
These estimates match those reported in the annual MHMDS Bulletin,59 providing evidence of the validity of our methodology.
In Table 1, the ‘admitted’ category includes all patients who were admitted to hospital during 2010/11, regardless of whether they were subject to the MHA or not. This ‘admitted’ category required further classification according to legal status (voluntary versus compulsory admission) using additional information relating to the patient’s MHA legal status classification.
Specifying the reference group
The reference group for this study comprised all patients receiving any type of care other than compulsory admission to hospital under the MHA (including informal admission and community care only). Patients were only counted once; for patients with more than one care spell during the 2010/11 reporting period, the care spell with the most restrictive level of care was then selected.
Legal status and nature of admission to hospital
Legal Status Classification
The MHMDS contains a number of variables which provide information on the patient’s legal status under the MHA (2007) during 2010/11. Legal Status Classification (LSC) specifies whether or not, on the last day of the reporting period (i.e. 31 March 2011), the patient was subject to any section of the MHA, including sections which allow for assessment or the removal of a person to a place of safety. If a patient was not formally detained, the LSC provides information on whether or not the patient was being treated in hospital on a voluntary basis (i.e. informally admitted). If the patient was not an inpatient on a voluntary or compulsory basis on the last day of the reporting period, LSC is not applicable.
Legal Status Restrictiveness
The original stated intention of the MHMDS was to collect information on the most restrictive legal status (referred to as LSR) during the reporting period. 55 In a major data incident, the HSCIC discovered that data-uploading instructions contained an error that resulted in all provider trusts recoding the most restrictive legal status at any point during the care spell rather than during the reporting period. 59 This meant that the LSR code could refer to any point during the care spell (which has no definitive time period), rather than in the annual period of interest (1 April 2010 to 31 March 2011). This posed a major and unexpected challenge to the study.
The HSCIC estimated that ‘around 11% (4,650) of patients categorised as being formally detained (in 2010/11) probably experienced detention in an early reporting period’ (p. 13). 59 This estimate was obtained using linked data (at patient level) from the 2009/10 and 2010/11 MHMDS. Although there is a detailed description (and syntax) provided in the MHMDS Data Quality and Methodology Manual,59 key variables that are necessary to replicate this estimation are not routinely provided to general external users of the MHMDS. Our request for access to these data was declined. Of the 55,852 patients who had a LSR of Section 2 or Section 3 in their current care spell, 19,631 (35%) had no recorded inpatient bed-days during the study year.
We therefore had to establish a means of ascertaining which inpatients in 2010/11 had been compulsory inpatients during this time.
Identifying patients who were admitted compulsorily in 2010/11
Further to establishing if patients had received treatment as an inpatient in 2010/11 reporting period, we had to ascertain if this was subject to the MHA (2007).
Providers are required to specify start and end dates for each care spell. The mental health-care spell began during the 2010/11 reporting period for around one-half of patients (54%) in the 2010/11 MHMDS. This means that the LSR referred to the reporting period under investigation (i.e. 1 April 2010 to 31 March 2011).
We accessed data from the 2009/10 MHMDS to assist in ascertaining LSR in 2010/11 for patients whose care spells began before this reporting period. We used unique MHMDS identification numbers to link care spell records from 2009/10 and 2010/11. We used all variables relating to admission and detention, including LSR and LSC (as described above) discharge, bed-days, revocation of CTOs, Section 17 leave and the start and end dates for care spells to determine whether or not patients were admitted compulsorily in the 2010/11 reporting period. Two main complications were evident: (1) there was often inconsistency between the 2009/10 and 2010/11 MHMDS databases in terms of information relating to care spell start and end dates; and (2) missing data in one or both of the reporting periods made it difficult to get a clear picture of the patient’s admission history.
Information relating to the patient’s ‘care status’ during 2010/11 (see Table 1) was used together with the corresponding LSR and LSC codes to ascertain which inpatients spent time in hospital during 2010/11 compulsorily, i.e. subject to the MHA (2007). The main classification rules were as follows:
-
Anyone with a qualifying 2009/10 LCS code (i.e. for a compulsory admission other than for an assessment section or guardianship) on 31 March 2010 was assumed to have still been compulsorily admitted on 1 April 2010 (i.e. the first day of the 2010/11 reporting period).
-
Anyone who was an inpatient during 2010/11 and had a qualifying LSR code in the 2010/11 MHMDS was coded as having had a compulsory admission during 2010/11.
-
Anyone who was an inpatient during 2010/11 and had a LSR code of a compulsory admission to hospital in the 2010/11 MHMDS, but only for an assessment section or guardianship, was coded as a voluntary admission in 2010/11.
-
Anyone who was an inpatient during 2010/11 but who was not subject to the MHA on the last day of the 2009/10 or 2010/11, and whose LSR for 2010/11 was the same as the LSR for 2009/10, was coded as a voluntary admission in 2010/11. This decision was based on the assumption that the 2010/11 LSR code relates to a time before the 2010/11 reporting period.
This exercise was critically important for the main aims of this study but it also served as a quality assurance task for the project. As will be discussed in greater detail in Chapter 3, Results and discussion, the number of patients deemed to be compulsorily admitted to hospital during the 2010/11 reporting period (n = 44,442) was consistent with the HSCIC estimates54 for compulsory admissions during the same reporting period (n = 43,941).
Geographical and spatial identifiers
The MHMDS records include information about where patients live, the identity of their general practitioner (GP) practice and where they received mental health care. There are several geographical or spatial levels in the MHMDS at which variance in rates of compulsory admissions might occur, and which can be used as points of linkage to other data sets. These are detailed in the following sections.
Patient
Each patient record (n = 1,287,730) in the MHMDS contains information about their contact with mental health services during the care spell. This clinical record also includes fields for diagnosis and Health of the Nation Outcome Scale (HoNOS), a global clinician-rated measure of clinical status. The MHMDS record includes fields for a number of demographic variables including patient age, sex, ethnicity, marital status, housing and employment status.
Lower layer super output area
Lower layer super output areas (LSOAs) are a set of stable geographical areas developed to facilitate estimation and dissemination of neighbourhood statistics and are also used for the reporting of Census data. On average, LSOAs typically include 672 households and 1614 residents. In 2001, there were 34,378 LSOAs across England (n = 32,482) and Wales (n = 1896) (www.ons.gov.uk/ons/guide-method/geography/beginner-s-guide/census/super-output-areas--soas-/index.html). The 2010/11 MHMDS used the 2001 LSOA boundaries. LSOA data were missing for 12,557 patients (1.0% of the sample). Almost all (98.1%) of the LSOAs in England were represented in the study sample. A small number of patients (n = 1014) (0.08% of the sample) received treatment from an English provider trust but had a LSOA in Wales; these patients were retained in the analysis. The mean number of patients per LSOA (i.e. in contact with an English mental health-care provider) was much higher (39.2) in England than in Wales (1.8).
General practice
A total of 9492 of 10,203 GP practices in England in 2011 (93.0%) were represented in the data set.
Primary care trust (commissioner)
In 2010/11, there were 152 PCTs in England; 146 (95.4%) of these were represented in the MHMDS. At the time the data were collected, PCTs were responsible for commissioning health care, including specialist mental health services but not forensic services. Several variables were present in the MHMDS regarding PCTs: Code of Commissioner, Code of PCT of GP practice and Code of PCT of residence were available and in agreement for ∼1.1 million records (86% of patients). Code of GP practice, an indirect PCT identifier, was also available for these patients. The Code of Commissioner was therefore used to specify PCT for these patients. Where one or more of these variables were missing or not in agreement with the others, we prioritised Code of Commissioner and Code of PCT of GP practice over Code of PCT of residence, since we were primarily interested in the effects of investment in mental health services and judged that these were more likely to indicate the organisations that commissioned care. Data on all PCT variables (including Code of GP practice) were missing for just 3128 patients (0.2% of the sample). No other information in the MHMDS could be used to identify PCTs for these patients and therefore these patient care spells were excluded from the analysis when PCT was included in multilevel models.
Provider trust
The Code of provider trust variable was present for all patients, since it is automatically derived at the time of annual data submission. This meant that there was 100% certainty about where the patient received their mental health care during the care spell. Patients receive mental health care from providers, mainly NHS trusts but also a small number of independent sector providers. In total, there are 72 mental health service providers (both NHS and independent sector) in the 2010/11 MHMDS. Independent sector providers are units registered with the Healthcare Commission to accept patients detained under the MHA 198360 (as amended by the MHA 2007), as specified under Section 2(3)(b) of the Care Standards Act 2000. 61 These independent hospitals that are registered to receive detained patients are considered to be hospitals for the purposes of the MHA (2007). In contrast to NHS provider trusts, these independent sector providers were coded by the service provider company in the MHMDS and not by individual units or according to geographical location.
Strategic Health Authority
The SHA was derived from Code of provider trust, and was likewise present for all patients. There were 10 SHAs in England in 2010/11. SHAs were responsible for improving health services in their local area and ensuring these were of a high quality and performing well.
Data quality
Following preliminary data exploration, data from eight provider trusts were removed from subsequent analysis. The three independent sector provider groups were excluded, since these were coded only by their corporate name and not by location, and because some of these units provided only secure, specialist inpatient treatment on transfer from other providers (i.e. the decision to admit the patient compulsorily was made elsewhere and could not have been influenced by factors operating locally). Tavistock and Portman NHS Foundation Trust was excluded because it had neither inpatient beds nor compulsory admissions. Four additional providers (South West Yorkshire Partnership Trust, Gateshead Health NHS Foundation Trust, Derbyshire County PCT and Oxford Health NHS Foundation Trust) were excluded because they had been discovered by HSCIC to have failed to return any data on patients’ legal status. 59 Excluding these providers resulted in a 3.8% reduction in the size of the study from 1,287,730 to 1,238,188.
In addition to missing and inconsistent PCT data, a number of other spatial identifiers were also subject to data-coding errors and missing data. LSOA was missing (i.e. not recorded by the provider when making the submission) for 11,479 patients in the study sample and Code of GP practice was missing for 22,334 patients.
Given different levels of missing data across the spatial levels, the study sample size changed slightly depending on the combination of spatial levels used for each statistical model. Specific details of the sample size and model used to address each research question will be outlined in Chapter 3.
Explanatory variables and data linkage
Individual level variables
The MHMDS contained reasonably complete data on a limited number of (individual-level) patient characteristics, namely age, sex and ethnicity. Levels of missing data across these variables varied considerably, however: age was missing for 84 patients (< 0.01%); sex was missing for 454 patients (0.03%); and ethnicity was missing for 119,392 patients (9.6%).
Several other individual-level variables were not included in our analyses because there were high levels of missing data. For example, marital status was missing for almost 15% of patients and psychiatric diagnosis was missing for around 81% of patients. 59 Accommodation status was missing for 920,288 (64%) and employment status was missing for 1,099,311 (75%) of all care spells. Recent clinical status (as measured by the HoNOS) was recorded for only 25% of all patient care spells. Other information of potential salience to our research questions included medication history (e.g. use of antipsychotics), drug and alcohol use, family and household composition (including if a patient lives alone, or has children) and social support were not recorded in the 2010/11 MHMDS. Although we were able to ascertain reliably whether or not a patient had been admitted during the reporting period, we did not have information about the number of admissions per patient. The same consideration applied to ascertaining the number of compulsory admissions during the reporting period, or if a person was admitted to hospital voluntarily and subsequently detained under the MHA.
The following section describes the external data sources that were linked to the MHMDS at different spatial levels to facilitate the study of factors that influence variation in compulsory admission across England.
Linkage at lower layer super output area level
Population density
We used Office for National Statistics (ONS) mid-2010 population estimates (derived from 2001 Census data) for linkage at LSOA level for two reasons: first, because MHMDS LSOA identifiers were based on the 2001 Census classification; and, second, because the study began before the release of 2011 Census data, and relevant linkages and modelling had been completed by this time. To assess if this was likely to have affected our findings, we plotted mid-2010 population estimates against 2011 Census results (for all ages and ethnic groups) for 33,519 LSOAs and found a correlation of 0.898 (results available from authors). Population density data were set as missing for the 11,479 patients for whom LSOA information was missing (0.9% of study sample). Population density estimates for LSOAs were converted into categories based on quintiles, with the lowest-density quintile serving as the reference category, as follows, in number of people per hectare: 0.0–6.8, 6.9–24.0, 24.1–41.2, 41.3–61.4 and ≥ 61.5.
Urbanicity
Urbanicity was defined using the ONS classification (www.ons.gov.uk/ons/guide-method/geography/products/area-classifications/rural-urban-definition-and-la/rural-urban-definition--england-and-wales-/index.html), based on LSOA of patient’s residence.
Area-level deprivation
The English Indices of Deprivation 201062 are 38 separate indicators recorded at LSOA level and used to calculate the Index of Multiple Deprivation (IMD). These indicators reflect seven domains of deprivation, namely health and disability; living environment; income; employment; crime; education, skills and training; and barriers to housing and services. Only the overall summary IMD score was used. Those patients in the study sample with missing data on their LSOA or those living in a LSOA in Wales (n = 12,437; 1.0% of study sample) were assigned a missing value for the IMD total score. A categorical variable for the IMD score for each LSOA was then created by dividing the score into quintiles, with the least deprived quintile serving as the reference category.
Ethnic density
We took the decision to delay the study analyses slightly to wait for 2011 Census ethnic population estimates, given the large shift in the demographic composition of England between 2001 and 2011. 63 Whereas mid-year estimates of LSOA (total) population sizes were highly correlated with the actual LSOA population sizes in the 2011 Census, there were no reliable mid-year estimates of LSOA ethnic composition. The 2011 Census contains estimates for the ethnic composition of each LSOA in England and Wales under several broad ethnic groups: white, black, Asian, mixed and other (www.ons.gov.uk/ons/guide-method/census/2011/index.html). There are minor differences between the LSOAs used in the 2001 Census (and used in the MHMDS to identify the patient’s area of residence) and the 2011 Census. 64 The overall number of LSOAs in England and Wales increased from 34,378 in 2001 (32,482 in England and 1896 in Wales) to 34,753 in 2011 (32,844 in England and 1909 in Wales). This meant that linkage of ethnic density data from the 2011 Census to the MHMDS resulted in some missing data because of changes in boundaries and sizes of the LSOAs. In total, ethnic density was missing for 50,938 individuals (4.0% of the sample). For remaining individuals, a single continuous variable representing the proportion of adults reporting white ethnicity at the LSOA level served as the ethnic density indicator. This variable was chosen in the light of evidence that white groups have the lowest risk of being compulsorily admitted to hospital. 28–30 These data were negatively skewed (with some extreme outliers); overall, the majority of LSOAs across the country had a high proportion of residents who classified themselves as white British [mean percentage of white British residents across LSOAs was 79.26% (standard deviation 22.9%), range 1–100%]. These data were therefore subsequently transformed into a five-category variable to represent the distribution of the data (representing LSOAs with very low to very high percentages of residents of white British origin).
Linkage at primary care trust level
Investment in mental health services
The Department of Health sponsored the annual National Survey of Investment in Adult Mental Health Services, an annual finance mapping exercise regarding spending on mental health services in England (www.gov.uk/government/publications/2010-11-working-age-adult-and-older-adult-national-survey-of-investment-in-mental-health-services). Data from 2010 provided details of investment in mental health services for working age adults (aged 18–64 years) in England, by PCT (commissioner).
Using data on the population density of PCTs in conjunction with the investment survey data, we calculated the level of investment (spend in pounds) per working member of the adult PCT population aged 16–64 years, including spending on:
-
all mental health services
-
accommodation services
-
continuing care services
-
personality disorder services
-
psychological therapies services [both Improving Access to Psychological Therapies (IAPT) and non-IAPT]
-
secure and high-dependency provision
-
assertive outreach team
-
community mental health team
-
CRHT team
-
early intervention in psychosis services.
Full investment survey data were available for the all PCTs in the MHMDS; however, as previously outlined, PCT of residence was missing for 63,402 patients (and was therefore also missing for the investment survey data).
Linkage at provider trust level
Mental illness bed availability and occupancy
The numbers of available and occupied NHS mental illness and LD beds are available from the Department of Health [beds open overnight (BOO)], and are derived from aggregated quarterly KH03 returns (a quarterly collection from all NHS organisations that operate beds; www.england.nhs.uk/statistics/statistical-work-areas/bed-availability-and-occupancy). This return includes beds in units managed by the provider, not beds commissioned from other providers. In 2010, provider trusts submitted quarterly bed data returns (http://transparency.dh.gov.uk/2012/07/05/bed-availability-and-occupancy-overnight/) and these were combined and averaged to derive annual estimates of:
-
total number of available mental illness and LD beds
-
total number of occupied mental illness and LD beds.
For the purposes of our analyses, we disaggregated data for mental illness and LD beds, since it was possible that well-publicised pressures on mental illness beds18 might be offset by reduced LD bed occupancy.
It is important to note how the occupancy of mental illness beds is recorded in BOO.
An occupied bed is defined as one which is occupied at midnight on a given day. A bed allocated to a patient on home leave is recorded as unavailable and therefore not occupied. If this same bed is subsequently made available for another patient, it will be then be recorded as available and occupied (once another patient occupies it). In this way, the official bed occupancy in BOO never exceeds 100%,65 despite reports that true occupancy (including beds occupied by those technically on leave) is often higher than this.
Unlike other spatial levels (e.g. LSOA, PCT), there are no publicly available population density estimates for provider trusts (HSCIC, March 2013, personal communication). Since it was not possible to determine the size of the adult population that each provider trust served, we were unable to estimate the provision of mental illness and LD beds relative to the local population size. Instead, we estimated bed occupancy as the annual average percentage of available mental illness and LD beds which were occupied. Preliminary descriptive analysis of these data revealed that the annual mean mental illness (but not LD) bed occupancy at provider trust level was 86.1% (standard deviation 6.1%), range 69.3–97.3%. This means that, based on official bed data (and the method of counting occupancy), all of the provider trusts included in our sample were operating below full capacity. Complete BOO data at trust level were available (i.e. linkage resulted in no missing data).
Hospital Episode Statistics
Hospital Episode Statistics (HES) is a data warehouse containing details of all admissions (including compulsory admissions), outpatient appointments and A&E attendances in England. It is a records-based system that covers all NHS trusts in England, including acute hospitals, PCTs and mental health trusts. HES data are collected monthly and managed by the HSCIC. We obtained a subset of 2010/11 HES data at provider trust level, namely:
-
number of admission episodes by mental illness and LD specialities, specifically LD (700), adult mental illness (710), child and adolescent psychiatry (711), forensic psychiatry (712), psychotherapy (713) and old age psychiatry (715)
-
LOS in hospital.
Full HES data at trust level were available (i.e. linkage resulted in no missing data).
Patient Environment and Action Team data
The Patient Environment and Action Team (PEAT) is an annual self-assessment of all inpatient health-care sites in England with more than 10 beds. Assessments are conducted by trusts and they are free to choose which staff members conduct the assessment. The National Patient Safety Agency (NPSA) recommends involving patients as well as staff from estates and facilities, domestic, catering, nursing and dietetic departments. Some trusts also involve patient forum representatives. Results are fed back to the NPSA and shared with the NHS, Care Quality Commission (CQC), Monitor and the Department of Health. PEAT assesses non-clinical aspects of patient care including environment, food, privacy and dignity. Scores demonstrate how well individual health-care providers believe they are performing in key areas including food, cleanliness, infection control and patient environment (including bathroom areas, décor, lighting, floors and patient areas). Scores range from 0 to 5, with higher scores indicating better performance for each trust in that domain. Data are managed by the HSCIC and were available for 2010 (www.nrls.npsa.nhs.uk/patient-safety-data/peat/). PEAT scores were not available for one trust, resulting in missing data for 3146 patients (0.3% of sample total).
Inpatient Service Users Survey
The Mental Health Acute Inpatient Service Users Survey (2009) was conducted by the Healthcare Commission. 66 This postal survey sought to find out about the inpatient experiences of people treated at 64 NHS trusts providing mental health inpatient services.
Patients were eligible to participate in the survey if they were aged 16–65 years, had stayed on an acute ward or a psychiatric intensive care unit for at least 48 hours between 1 July 2008 and 31 December 2008 and were not current inpatients at the time of the survey. Trusts with fewer than 1000 discharges in this period sampled all patients. Trusts with more than 1000 patients took a consecutive sample of the 1000 most recently discharged service users within the sampling period 1 July 2008 to 31 December 2008. Fieldwork took place between April and June 2009. A total of 7527 people took part in the survey, amounting to a 28% response rate. There were 47 questions covering 7 areas and 12 domains:
-
introduction to the ward (one domain)
-
about the ward (one domain)
-
hospital staff (two domains)
-
your care and treatment (five domains)
-
your rights (one domain)
-
leaving hospital (one domain)
-
overall rating of inpatient experience (one domain).
Individual patient responses were combined at trust level on a scale of 0–100 (where 100 represents the best possible response) and standardised for the age and sex of respondents, to ensure comparability across trusts. These benchmarked data allowed trusts to be compared on each of the above domains, for which they were rated as better, worse or about the same as other trusts. 67 In the absence of specific hypotheses about associations between individual domain scores and compulsory admission, we used the ‘Overall’ domain as an indicator of trust performance in our multilevel models. Data were unavailable for five trusts, resulting in missing data for 37,327 patients (2.9% of the sample).
Community Mental Health Survey
The NHS Community Mental Health Service User Survey 2011 was conducted by the CQC. 68 The survey involved 65 NHS trusts in England (including combined mental health and social care trusts, foundation trusts and PCTs that provide mental health services). The survey aimed to find out about the experiences of people using mental health services in the community. Service users aged 16 years and over were eligible if they were receiving specialist care for a mental health condition and had been seen by the trust between 1 July 2010 and 30 September 2010. Each trust compiled a list of all patients who attended the service as community patients during this time period. A simple random sample of 900 patients was selected from this list and the questionnaire was posted to these patients. Over 17,000 service users responded to the survey, a response rate of 33%. 69 The questionnaire contained 45 questions covering nine domains:
-
health and social care workers
-
medications
-
talking therapies
-
care co-ordinator
-
care plan
-
your care review
-
crisis care
-
day-to-day living
-
overall rating of care in the last 12 months.
Individual patient responses were combined at trust level on a scale of 0–100 (where 100 represents the best possible response) and standardised for the age and sex of respondents, to ensure comparability across trusts. These benchmarked data allowed trusts to be compared on each of the above domains, and rated as better, worse or about the same as other trusts. 50 In the absence of specific hypotheses about associations between individual domain scores and compulsory admission, we used the ‘Overall’ domain as an indicator of trust performance in our multilevel models. Data were not available for three trusts in total, resulting in missing data for 18,736 patients (1.5% of the study sample).
Trust location
Using information from NHS organisational look-up tables, all provider trusts were categorised according to whether their location was in London or outside London. We chose to include this variable on the grounds that London is different from all other areas of England in terms of scale, extremes of affluence and deprivation, and ethnic composition.
NHS staff survey
The 2010 NHS staff survey is the eighth annual survey of NHS staff (www.cqc.org.uk/content/2010-nhs-staff-survey). The results of this survey help trusts to review and improve the experiences of their staff, enabling them to provide better care to patients. The survey covers a wide range of work related domains including workload, staff health and well-being, staff satisfaction with job, job performance and communication among staff within the NHS. The staff survey response rates across provider trusts varied from 44–70%; at a summary level, however, full data were available for each trust. Given the very large number of survey items, the items in the list below were chosen a priori as salient to the research questions:
-
per cent of staff feeling satisfied with the quality of work and patient care they deliver
-
per cent of staff agreeing that their role makes a difference to patients
-
per cent of staff suffering work-related stress in last 12 months
-
per cent of staff experiencing physical violence from patients/relatives/members of the public in last 12 months
-
per cent of staff reporting good communication between senior management and staff
-
per cent of staff able to contribute towards improvements at work
-
staff job satisfaction (score)
-
staff intention to leave jobs (score).
Strategies for analysis
The overarching aim of the project was to estimate variance in the prevalence of compulsory admission in England at different spatial levels and to evaluate the extent to which this is explained by the characteristics of people (e.g. age, gender or ethnicity), places (e.g. deprivation) and health-care organisations (e.g. mental illness bed numbers and occupancy).
An important objective of this project, however, was to undertake linkage to external data sources to provide additional contextual information for each of the spatial levels in the MHMDS. For example, the MHMDS provides information on the LSOA (geographical area) in which the patient lives. This information can be used to determine what region of the country that person lives in but no additional information about that geographical area is contained in the MHMDS. The ONS, however, collects and records a wide variety of information for each LSOA including population size (from which population density can be derived) and ethnic composition (and, by derivation, ethnic density). By linking this type of information to the MHMDS, we aimed to establish the conditions for estimating the effect of area-level characteristics (for example) on the risk of being involuntarily admitted to hospital. As explained in detail earlier, we undertook linkage to several external data sources.
Multilevel models
As outlined earlier, there are six discrete and identifiable spatial levels in the MHMDS at which variance in rates of involuntary admissions might occur. Multilevel modelling is a widely used method for analysing nested (or clustered) data because it mirrors the structure of the data to be analysed and allows explanation to be correctly assigned to the various ‘levels’ within the data set (e.g. individual, LSOA, provider trust). The structure of the MHMDS is complex, and the various spatial levels do not nest or cluster neatly within each other. For example, approximately two-thirds of LSOAs were not nested completely within (i.e. served exclusively by) a single provider trust. This is known as a cross-classified data structure and is illustrated schematically in Figure 1. This illustrates a three-level model with patients (level 1) nested within LSOA (level 2) and a non-nesting hierarchy between LSOA and provider trust (level 3). ‘Non-nesting’ is because LSOAs straddled provider trust boundaries and therefore more than one provider trust delivered care to people living in some LSOAs. These level 2 and 3 units can be conceptualised as ‘classifications’: combinations of particular LSOAs and provider trusts. This classification structure is recorded for each individual within the data set. Ignoring cross-classification complexity would result in incorrect estimates of area-level variance and biased estimations of statistical validity. We refer to individuals, and the geographical or health-care areas with which they are associated, as ‘levels’ but it is important to acknowledge that variation across these levels takes into account this non-nested, cross-classified data structure. A fuller description of cross-classified models can be found elsewhere. 70
FIGURE 1.
An example of a cross-classified data structure.
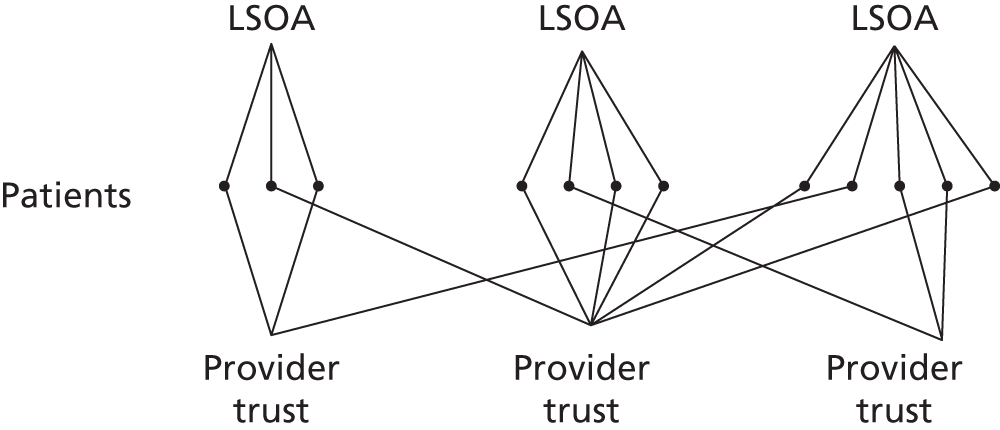
The specification of multilevel models (MLMs) depends on the research question or hypothesis being tested; details of these models are provided in Chapter 3, Results.
Despite the pre-eminence of MLwiN (MLwiN 2.27, Centre for Multilevel Modelling, Bristol, UK) as a multilevel modelling program, it has finite limitations in computational power (when the sample size is very large) and model stability (when estimating more than three levels). Given the size of the database (∼1.2 million patients), we were aware that estimating cross-classified models with more than three spatial levels would be difficult because of these program limitations.
Null models
The most basic MLM is the null model, which estimates the total variance in compulsory admission in the study sample, without the inclusion of any explanatory or potentially confounding variables. This variance is equal to the sum of the variances at the different spatial levels. Further models can then be developed which attempt to reduce and explain this initial geographical variability in the rates of involuntary admission by including explanatory variables relating to the characteristics of individuals or the higher-level geographies (e.g. LSOA, provider trust).
We ran all permutations of four-level models (using combinations of individuals, LSOAs, GP practices, provider trusts and PCTs) to identify consistent cross-model patterns and compare goodness of fit. However, where model stability allowed, we included a fifth level (i.e. SHA) to ensure that we had derived the most parsimonious model for the data set. This stage provided an estimate of the variance in compulsory admission to hospital at these various different spatial levels, before characteristics of the given levels were taken into account. It also provided a clear rationale for building more complicated four-level models with explanatory variables, ensuring that only spatial levels with significant proportions of variance were considered for inclusion.
Markov chain Monte Carlo (MCMC) estimation methods were used to estimate the variance of our outcome variable across these geographic classifications (i.e. LSOA, provider trust, PCT, etc.). Analyses of the cross-classified multilevel data structure were undertaken using a logit link function to model the binary response of compulsory admission (denoted by a ‘1’) or not (denoted by a ‘0’). 71 Furthermore, we assumed that our binary observations were derived from an underlying continuous latent variable so that (in this case) compulsory admission to hospital under the MHA during 2010/11 (denoted by ‘1’) is assumed to occur after a certain threshold has been exceeded in the severity of illness. Level 1 variance on this latent variable was the standardised logistic variance of π2/3 = 3.29. 71
The number of iterations used for each model depended on the number and arrangement of levels in the null model, but this ranged from 500 to 100,000 iterations and was checked using the Raftery–Lewis diagnostic. 72 The mean variance estimate was recorded for each higher level along with the 95% credible interval. The total variation in each model was calculated by adding up the estimated variance at each level, and the relative percentages occurring at the various spatial levels were calculated. A commonly used statistic to compare the fit of alternative MLMs is the Bayesian deviance information criterion (DIC) goodness-of-fit statistic. 73 The DIC compares alternative models based on a trade-off between the fit of the data to the model and the corresponding complexity of the model. 74 It is considered analogous to the Bayesian information criterion (BIC)75 or the Akaike information criterion (AIC)76 fit statistics for models estimated using MCMC. Models with lower DIC values are preferred to those with larger values and, as a general rule of thumb, a difference of 10 or more is considered to be substantial. The main limitation is that the DIC value is comparable only across models with exactly the same observed data (nesting of models, however, is not required). 77 DIC statistics were therefore estimated using only cases that had complete data for all of the model variables, to ensure identical samples.
Modelling and mapping residuals
After selecting the optimal four-level null model (research aim 1), we controlled for basic demographic characteristics of the patient population served by the provider trust (i.e. individual age and sex) and examined the provider trust-level residuals produced by the model. A residual at provider trust level is the difference between the actual (observed) risk of compulsory admission and the risk predicted by the (age- and sex-adjusted) model. Positive residuals indicate that the model is underpredicting the risk of admission and negative residuals indicate that the model is overpredicting risk (compared with the actual risk). Residual results were reported graphically in two ways: using ‘caterpillar’ plots [showing the raw residual for each trust in rank order, as a point estimate with 95% confidence interval (CI) error bars] and by mapping the normal score of the residual (i.e. the normal deviates of each residual). Upper and lower CIs were obtained by multiplying the standard error of each residual by 1.96 and adding and subtracting this value from each estimate, respectively. Normal scores were used to allow comparison of residual size across different models. To produce a digital map of the residual normal scores, we derived the postcode of the provider trust headquarters and, using standard lookup tables available from The UK Data Service,62 we derived the associated National Grid co-ordinate for the postcode centroid. The normalised residual value and its associated co-ordinate were then ‘attached’ to a digital boundary of England and Wales (available from Edina Digimap Ordnance Survey Service) and mapped using ArcMap version 10.1 (Redlands, CA, USA). 63 This exercise was repeated for the fully adjusted, final model (research aim 2) as described next.
This was essentially an exploratory diagnostic tool designed to help us identify ‘missing’ explanatory variables, i.e. hypothetical variables whose existence might be posited to explain observed variance in the study outcome. If negative residuals are clustered together and positive residuals clustered together (i.e. model residuals are positively spatially auto-correlated), this would suggest a missing spatially clustered explanatory variable. If residuals are not spatially auto-correlated, then we would expect to find a random arrangement of positive residuals and negative residuals and there will be no clustering. Such a finding would direct us away from a search for a ‘missing’ explanatory spatial variable.
Modelling explanatory variables
We extended null MLM to include explanatory variables at different spatial levels. Our aim was to estimate the effect of doing so on the variance in compulsory admission rates identified in our null models (including the residuals at provider trust level).
Extending the MLM in this way increased the complexity of the model and placed additional demands on the computing capacity of the statistical software MLwiN. Given the sample size and the potential number of linked variables (from external data sources), multivariate models with more than three (cross-classified) levels proved unstable. For this reason, we had to choose the most important spatial levels for inclusion in these more complex models, and we did so this on the basis of our null model results.
Adding explanatory variables across one or more spatial levels allowed us to quantify the proportion of variance explained by these risk factors for compulsory admission. This involved modelling the predicted value of the (assumed) underlying continuous latent variable by using an equation with both fixed [intercept plus regression coefficient(s) for explanatory variable(s)] and random parts. 78 The proportion of variance explained at, for example, level 1 was estimated as the proportion of total variance attributable to the fixed part of a model that included individual-level explanatory variables, divided by the total variance in the underlying latent variable. The total variance is equal to the sum of the variances of the fixed part of the model and the (unexplained) variances at each of the higher levels. Variance terms were derived from the MLwiN output.
Handling missing data
The issue of missing data in statistical modelling is both complex and important. This is because missing data can be missing for a variety of reasons – often difficult to establish – which may or may not affect inferences drawn from the model. There are three main types of missing data: missing completely at random (MCAR), missing at random (MAR) and missing not at random (MNAR). 79 MCAR data are the simplest to understand, as missingness occurs by chance alone. MAR data occur when data are missing because of a particular factor, but not because of the missing value itself. If the missingness is dependent on the value of the missing value itself (such as a missing patient outcome due to the patient being too ill to attend the clinic), the mechanism is MNAR. Principled methods to account for missing values exist but are dependent on the mechanism driving the missingness, and unfortunately no statistical tests exist to determine the mechanism of missingness.
Levels of missing data varied substantially between variables in the study. At the individual level, for example, patient age and sex data were almost fully complete, with less than 0.0004% missing data, whereas patient ethnicity data was missing for 9.6% of patients. It was unclear from the MHMDS specification documents why there was such a high level of missing data for patient ethnicity (although, as previously outlined, even higher levels of missing data were common among many important patient-level variables such as diagnosis).
Among higher-level spatial identifiers, there were considerably lower levels of missing data at LSOA level (mainly because of missing data on the patient’s LSOA identifier in the MHMDS, rather than missing data in the external data sources) and largely complete data at provider trust level. The patient surveys were a notable exception: five provider trusts had no data for the 2009 Inpatient Service Users Survey and three provider trusts had no data for the 2011 Community Mental Health Survey.
We considered two approaches to missing data: (1) restricting analyses to cases (patients) with complete data and (2) modelling the missing data as a separate category of exposure. A listwise deletion approach to missing data involves dropping any patient who has missing data on either the spatial-level identifiers (e.g. LSOA, GP practice, PCT or provider trust) or any explanatory variable (patient ethnicity, etc.), to remove the case from the analysis. This approach, known as complete case analysis, ensures that only those patients with complete and full data across all variables to be included in the MLM analyses are included in the model. This is a simple approach and is best used when the mechanism for missing data is MCAR. However, our data were not likely to be suitable for complete case analysis, as some variables may be MNAR or MAR. Ethnicity is a good example. Although it is possible that patients from particular ethnic groups (e.g. those of non-white ethnicity) did not declare their ethnicity (for example) because they believed that it would affect their treatment (MNAR), a more likely explanation is that trusts with little ethnic diversity among its patient population are less rigorous in identifying and recording this variable, since almost everyone is white (MAR).
Our approach involved retaining all cases and modelling missing data for each variable as a separate category. This is a commonly used practice in social science research when we suspect that the missing cases are not occurring in a random fashion (MNAR). 80 This allowed us to determine how the missing group are behaving, but we are also able to use the non-missing characteristics of those observations elsewhere in our model, optimising statistical power. The risk is that this approach may result in biased estimates of the model parameters.
Given the size of the data set, a simple sensitivity analysis was undertaken for ethnicity data by incorporating missing values into each of the other categories in turn (results available from authors). This allowed us to investigate whether or not the model was robust to the most extreme perturbations possible by seeing what happened when we assumed that those with missing values belonged to one ethnic group (by pooling the missing data with each of these groups in turn). The odds ratios (ORs) for compulsory admission (compared with the reference group) were reduced across all model permutations. While the OR for the white group had the smallest change overall, this was not unexpected, as it was also the largest group (over 80% of the sample). The OR for compulsory admission for the missing group was very small (0.2), i.e. indicating a rate of compulsory admission in this group that was five times lower than that of the white reference group. This further implies that these patients have different experiences from the other ethnic groups. Hence, these results reinforced the need to retain these cases in our models rather than exclude them.
Chapter 3 Results
Description of the study sample
As outlined in Chapter 2, Methods, the main outcome variable was compulsory admission to hospital under the MHA during the 2010/11 reporting period, compared with all other types of care (including informal hospital admission and community-only care). Table 2 shows the demographic characteristics for the study sample by admission and treatment status.
Characteristic | Treatment and admission status in 2010/11 (n = 1,238,188) | ||
---|---|---|---|
Community care only 1,133,541 (91.5%) | Voluntary admission only 61,655 (5.0%) | Compulsory admission 42,915 (3.5%) | |
Sex | |||
Male | 495,945 (43.7%) | 31,739 (51.4%) | 22,920 (53.4%) |
Female | 637,177 (56.2%) | 29,972 (48.6%) | 19,983 (46.6%) |
Missing | 419 (< 0.01%) | 21 (< 0.01%) | 12 (< 0.01%) |
Age | |||
< 18 years | 20,861 (1.8%) | 620 (1.0%) | 402 (0.9%) |
18–35 years | 296,268 (26.1%) | 16,219 (27.5%) | 13,874 (32.3%) |
36–64 years | 455,252 (40.2%) | 26,401 (42.7%) | 20,006 (46.6%) |
≥ 65 years | 361,078 (31.9%) | 17,733 (28.8%) | 8633 (20.2%) |
Missing | 82 (< 0.01%) | 759 (0.01%) | 0 |
Ethnicity | |||
White | 911,292 (80.4%) | 53,747 (87.1%) | 32,130 (74.9%) |
Mixed | 11,965 (1.1%) | 789 (1.3%) | 1027 (2.4%) |
Asian | 41,780 (3.7%) | 1993 (3.2%) | 2771 (6.5%) |
Black | 32,116 (2.8%) | 2168 (3.5%) | 4965 (11.6%) |
Other | 19,992 (1.8%) | 1009 (1.6%) | 1052 (2.5%) |
Missing | 116,396 (10.2%) | 2026 (3.3%) | 970 (2.1%) |
Marital status | |||
Single | 415,022 (36.6%) | 26,579 (43.1%) | 23,811 (55.5%) |
Married | 325,675 (28.7%) | 16,219 (26.3%) | 8541 (19.9%) |
Divorced | 66,619 (5.9%) | 5024 (8.1%) | 3529 (8.2%) |
Widowed | 122,866 (10.8%) | 6102 (9.9%) | 2984 (7.0%) |
Separated | 27,949 (2.5%) | 2201 (3.6%) | 1322 (3.1%) |
Missing | 175,410 (15.5%) | 5607 (9.0%) | 2728 (6.3%) |
Regiona | |||
North East | 71,703 (6.4%) | 3915 (6.5%) | 2681 (6.4%) |
North West | 163,738 (14.6%) | 9408 (15.6%) | 6343 (15.2%) |
Yorks and Humber | 101,946 (9.1%) | 5026 (8.3%) | 3391 (8.1%) |
East Midlands | 107,096 (9.5%) | 5183 (8.6%) | 3296 (7.9%) |
West Midlands | 128,463 (11.4%) | 6366 (10.6%) | 3974 (9.5%) |
East of England | 107,533 (9.6%) | 7757 (12.9%) | 3439 (8.2%) |
London | 177,293 (15.8%) | 9445 (15.7%) | 11,264 (27.0%) |
South East | 157,329 (14.0%) | 7715 (12.8%) | 3948 (9.5%) |
South West | 108,921 (9.7%) | 5343 (8.9%) | 3235 (7.8%) |
Wales | 694 (< 0.01%) | 148 (< 0.01%) | 116 (< 0.01%) |
Missing | 8825 (< 0.01%) | 1349 (2.2%) | 1228 (2.9%) |
Urbanicitya | |||
Urban (> 10,000) | 958,282 (85.2%) | 52,165 (86.5%) | 37,600 (90.2%) |
Town and fringe | 91,800 (8.2%) | 4608 (7.6%) | 2204 (5.3%) |
Village and hamlet | 74,634 (6.6%) | 3533 (5.9%) | 1883 (4.5%) |
Missing | 8825 (0.01%) | 1426 (2.2%) | 1228 (2.9%) |
Approximately 3.5% of all patients in the MHMDS spent time in hospital during 2010/11 as compulsory inpatients. A further 5.0% of patients spent time in hospital on a voluntary basis, that is they did not meet the study criteria for compulsory admission. The vast majority of patients were treated in a community setting and did not require hospitalisation.
Null models (research aim 1)
The first research aim was to estimate and compare variance in the rate of compulsory admission across England during 2010/11 between people, places and health-care organisations. As described previously, cross-classified models with more than four levels proved unstable, and we therefore sought to explore all permutations of four-level models. SHA was omitted from these models, as exploratory analyses suggested limited variance at this level that failed to reach statistical significance (details available from authors).
Table 3 shows permutations and sample sizes (excluding missing data) for the remaining four-level cross-classified null models that included individual (patient) as the first level.
Models | Sample size (n) | |
---|---|---|
Model 1 | ||
Level 1 | Individual | 1,149,541 |
Level 2 | LSOA | |
Level 3 | GP practice | |
Level 4 | PCT | |
Model 2 | ||
Level 1 | Individual | 1,207,916 |
Level 2 | LSOA | |
Level 3 | GP practice | |
Level 4 | Provider trust | |
Model 3 | ||
Level 1 | Individual | 1,167,316 |
Level 2 | LSOA | |
Level 3 | PCT | |
Level 4 | Provider trust | |
Model 4 | ||
Level 1 | Individual | 1,157,010 |
Level 2 | GP practice | |
Level 3 | PCT | |
Level 4 | Provider trust |
Table 4 shows the estimates of the variances for the risk of compulsory admission for individuals alongside the variance estimates for different permutations of four-level null models.
Model and levels | Variance (standard error) | p-value | % unexplained variance (95% CI) | DIC statistica |
---|---|---|---|---|
Model 1 | ||||
Individual | 3.29 | 84.5 | 322,505.7 | |
LSOA | 0.248 (0.008) | < 0.001 | 6.4 (6.0 to 6.8) | |
GP | 0.075 (0.004) | < 0.001 | 1.9 (1.7 to 2.1) | |
PCT | 0.280 (0.036) | < 0.001 | 7.2 (5.5 to 8.9) | |
Model 2 | ||||
Individual | 3.29 | 83.7 | 321,749.2 | |
LSOA | 0.264 (0.010) | < 0.001 | 6.7 (6.2 to 7.2) | |
GP | 0.106 (0.005) | < 0.001 | 2.7 (2.4 to 2.9) | |
Provider trust | 0.271 (0.053) | < 0.001 | 6.9 (4.3 to 9.5) | |
Model 3 | ||||
Individual | 3.29 | 75.1 | 321,392.94 | |
LSOA | 0.280 (0.008) | < 0.001 | 6.4 (6.1 to 6.7) | |
PCT | 0.271 (0.037) | < 0.001 | 6.2 (4.5 to 7.8) | |
Provider trust | 0.539 (0.104) | < 0.001 | 12.3 (7.7 to 17.0) | |
Model 4 | ||||
Individual | 3.29 | 79.8 | 324,247.78 | |
GP | 0.108(0.004) | < 0.001 | 2.6 (2.4 to 2.8) | |
PCT | 0.230 (0.032) | < 0.001 | 5.6 (4.1 to 7.1) | |
Provider trust | 0.496 (0.096) | < 0.001 | 12.0 (7.5 to 16.6) |
Overall, between 75.1% and 84.5% of the unexplained variance in this outcome was observed between individuals; variance at LSOA level was estimated to be between 6.4% and 6.7%; unexplained variance at PCT level ranged from 5.6% to 7.2%; GP-level variance was between 1.9% and 2.7% of the total; and the unexplained variance between provider trusts was estimated to be between 6.9% and 12.3%. The variance at each level was statistically significant (< 0.001). The biggest differences in these estimates were observed between models 1 and 2, on the one hand, and models 3 and 4, on the other. When both PCT and provider trust were included, there appeared to be a reduction in individual-level variance and an increase in variance between provider trusts from about 7% to about 12%.
Spatial clustering of primary care trusts and provider trusts
Reasons for the differences in variance estimates when PCT and provider trust levels were both included were unclear. To investigate this further, we explored the distribution of PCTs and provider trusts. Although most provider trusts were associated with three or four local PCTs (commissioners) at most, some appeared to be treating (a few) patients from many different PCTs around the country. This was probably because of specialised tertiary care services, as well as movement of patients around the country for personal reasons.
In one-third of provider trusts, over 80% patients originated from a single PCT. Indeed, examination of the data matrix mapping patient counts for all provider trusts against all PCTs revealed that about one-quarter of the cells had counts of 0 or 1. This could account for the decrease of variance at the individual level in models 3 and 4, as there may be possible conflation between individual and higher-level variances when the cell counts contain just one observation – a problem that is also exacerbated by the cross-classification occurring in the data structure. 81,82 We therefore considered the estimates of higher-level variance in models 1 and 2 to be more reliable than those of models 3 and 4.
Comparing model fit using deviance information criterion statistic
As noted in Chapter 2, Methods, DIC statistics can be compared only when the sample used in each model is exactly the same. The four null models were therefore re-estimated using complete cases only (i.e. by including only patient records that had complete data across LSOA, GP practice, PCT and provider trust; n = 1,149,541). This reduced the sample size but ensured that the DIC statistic could be used to compare model fit (see Table 3). Results in Table 4 revealed that models 2 and 3 were superior to models 1 and 4, with model 3 having a slightly better fit than model 2.
Given the greater reliability of estimates of higher level variances in models 1 and 2 (i.e. those models that contained either PCT or provider trust but not both together), we selected model 2 as the preferred four-level model. This choice was strengthened by the a priori importance of provider trusts to the study of compulsory admission rates. Provider trusts deliver mental health care and are ultimately the locus at which decisions about whether or not an individual patient should be admitted to hospital compulsorily are made. In the same vein, future interventions to reduce compulsory admission rates will almost certainly have to involve provider trusts.
Estimating the effect of including Strategic Health Authority as a fifth level in the null model
We re-estimated the four-level model of choice (model 2) by including SHA as a fifth spatial level (model 2a) to test whether or not this improved model fit using data from the same number of patients. This meant that it was appropriate to use the DIC statistic to compare the fit of the models and to statistically test whether or not including SHA as a spatial level enhances the model fit. The DIC statistic for model 2 (336,537.7) was smaller than that of model 2a (336,538.2). The proportion of unexplained variance at SHA level was not statistically significant (2.4%, 95% CI 0.0% to 5.9%; χ2 = 2.04; p = 0.15). SHA was therefore not considered for inclusion in further models.
Examining model residuals at provider trust level
We examined the model residuals at provider trust level using model 2 and adjusting (at the level of individuals) for patients’ age and sex. A residual (at provider trust level) is the difference between the actual risk of compulsory admission and the risk predicted by the model given the age and sex composition of each trust. Positive residuals indicate that the model is underpredicting the risk of compulsory admission, and negative residuals indicate that the model is over-predicting risk (compared with the actual risk).
Model residuals are portrayed graphically using a caterpillar plot (Figure 2), which shows the provider trust residuals in rank order (with error bars representing 95% CIs). The horizontal line represents the average risk of compulsory admission across all provider trusts in England during 2010/11. Residuals are regarded as not differing from the average to a statistically significant extent if the error bar crosses zero (represented by the horizontal line). We can see from Figure 2 that 21 (out of 64) trusts had rates significantly higher than expected and 24 had rates significantly lower than the average, after accounting for the age and sex profiles of patients attending the provider trusts. Overall, therefore, the observed rate of compulsory admission differed significantly from that predicted by the null model for 45 (70.3%) trusts, reflecting the limitation of a model that did not include any explanatory variables at higher spatial levels (i.e. adjusting only for age and sex at the individual level).
FIGURE 2.
Residual plots (with 95% CI error bars) for provider trust level variance (four-level cross-classified model of individuals within LSOAs within GP practices within provider trusts, MCMC estimation adjusting for age and sex). Provider trust residuals are shown in rank order.
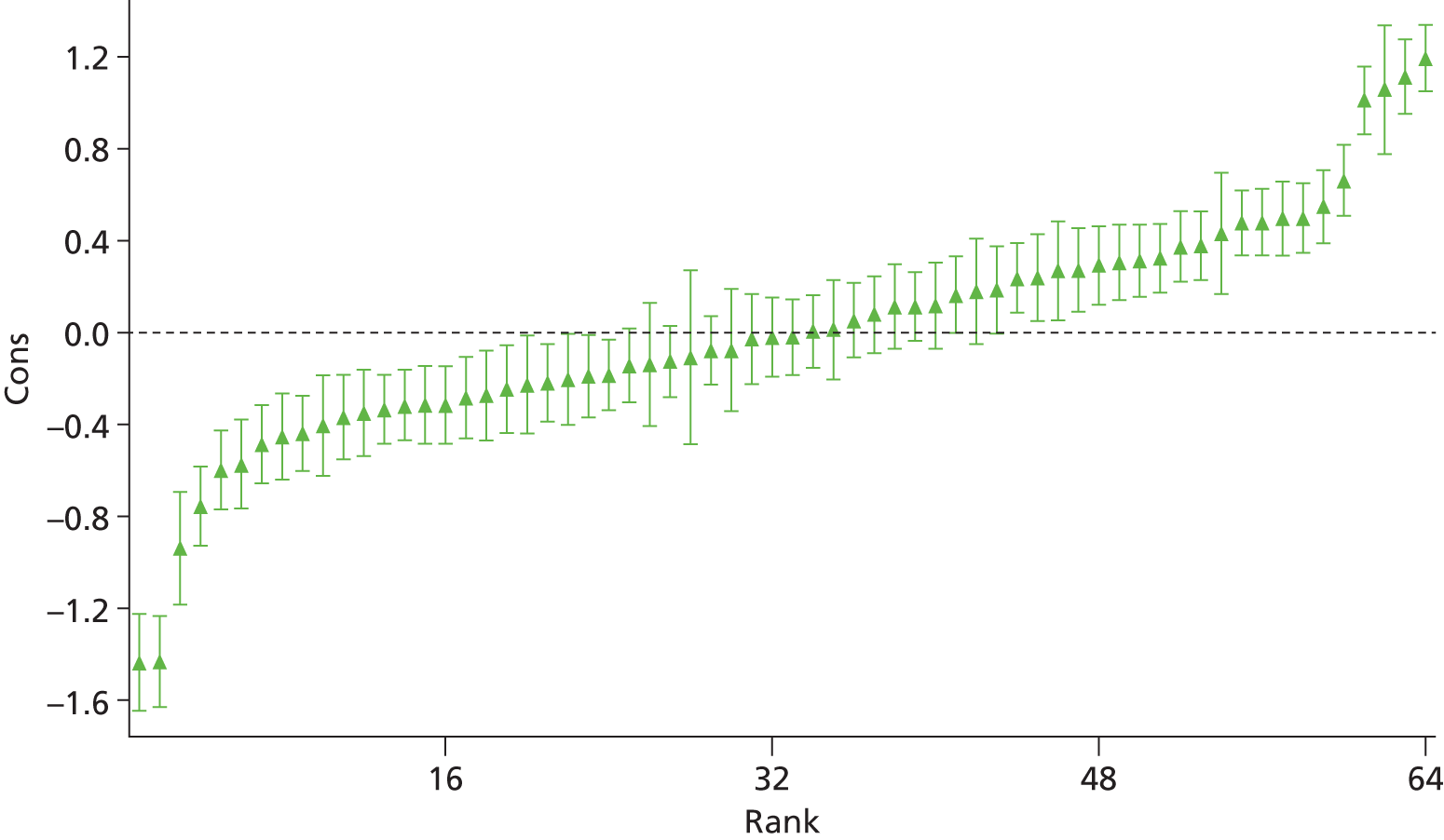
Figure 2 shows the full distribution of rates of compulsory admission at provider trust level, and the difference between each of these values and the overall model average. Model residuals at provider trust level were also plotted on a map of England to try to ascertain if there was spatial clustering of negative or positive residual values (Figure 3). The circles on this map represent provider trust-level residuals; the size of the circle reflects the size of the residual and the colour indicates whether the provider trust is located in an area that has a risk of admitting patients to hospital under the MHA similar to the national average (black), statistically significantly lower than the national average (blue shades) or statistically significantly higher than the national average (green shades).
FIGURE 3.
Provider trust standardised residual values (adjusted for patient age and sex) mapped geographically.
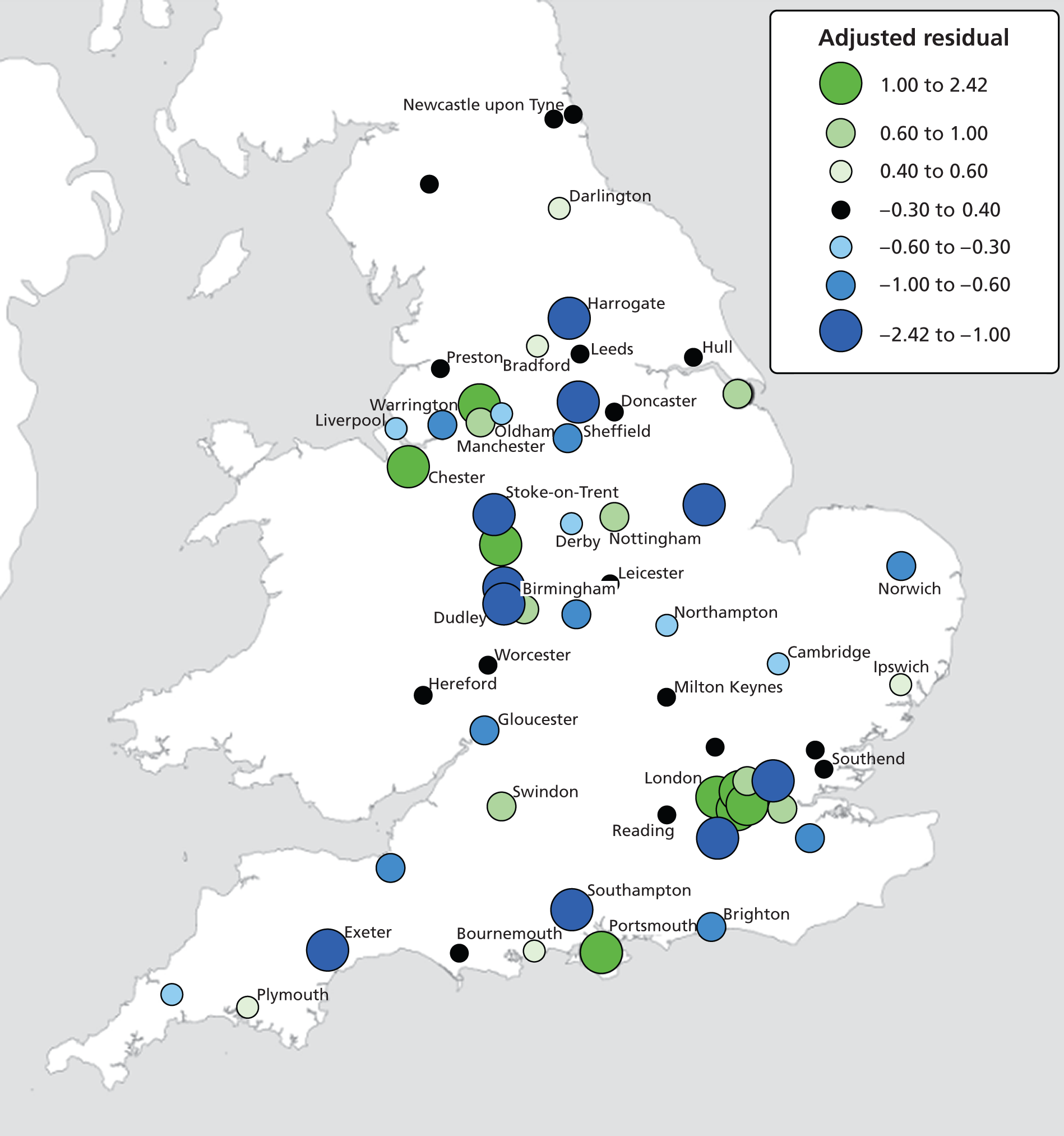
The map in Figure 3 suggests that, in general, there is no strong spatial auto-correlation across the residuals (i.e. clustering of same sign residuals). For example, there is certainly no evidence of a north–south divide in model residuals or a strong suggestion of an urban–rural divide. There is, however, a tendency for larger positive residuals to be found across London and parts of the North West (e.g. Chester and Manchester). This indicates that trusts in these areas have rates of compulsory admission which are significantly higher than the national average (given their age and sex composition). Larger residuals are also present around the urban areas of the West Midlands but the residuals here are both positive and negative, suggesting that the urban penalty is not consistently evidenced in this region. A similar pattern is shown around the urban areas of the South West, where Southampton appears to have rates lower than expected whereas rates are higher than expected around Portsmouth. Furthermore, urban areas are not always associated with significantly larger residuals; rates around Newcastle and Leeds, for example, are similar to the overall national average rate of compulsory admission.
Modelling explanatory variables (research aim 2)
The second research question advances upon the first by exploring the extent to which variance in rates of compulsory detention rates are explained by:
-
the individual characteristics of people using services (e.g. age, sex and ethnicity)
-
the local area (i.e. contextual) socioeconomic and sociodemographic factors (e.g. small area socioeconomic deprivation, urbanicity and ethnic density)
-
the features of local mental health services (e.g. bed availability, number of admissions, LOS in hospital).
As noted previously, multivariate models with more than three spatial (cross-classified) levels proved unstable. We therefore selected the most important spatial levels for inclusion in these more complex models. We did this on the basis of our null models, and following the a priori view that individual and provider trust were essential to any model: the former because this was the level at which most variance occurred, and the latter because it represented the (most distal) locus at which compulsory admissions decisions were made. We opted to include LSOA because this offered the greatest scope for modelling the effects of area-level effects, including population and ethnic density as well as socioeconomic deprivation. These levels had the further advantage of having a large amount of externally linked data. Details of the explanatory variables included at each spatial level are provided next. The choice of explanatory variables was constrained by model stability and the need to include a reasonable number and breadth of variables at each level.
Individual-level variables:
-
patient sex – binary coded with women as reference group
-
current age, in four-categories – under 18 years (reference group), 18–35 years, 36–64 years and 65 years or older
-
ethnicity – white (reference group), black, Asian, mixed, other.
Lower layer super output area-level variables:
-
population density
-
ethnic density
-
area deprivation.
Provider trust-level variables:
-
mental illness bed occupancy
-
number of finished consultant admission episodes
-
overall performance of community mental health services
-
overall performance of inpatient mental health services
-
percentage of staff feeling satisfied with the quality of work and the patient care that they are able to deliver
-
PEAT privacy and dignity score
-
median LOS in hospital (days) across consultant specialities (700–715)
-
trust location (in London or outside London).
We began by estimating the main three-level null model comprising individuals, LSOAs and provider trusts (model 5). The model was then extended by including:
-
individual-level patient characteristics (model 6)
-
individual- and LSOA-level characteristics (model 7)
-
individual-, LSOA- and provider trust-level characteristics (model 8).
The null model (Table 5) results indicate that 8.3% of the variance in compulsory detentions occurred at the LSOA level and 7.0% occurred at provider trust level; the remaining variance occurred at the individual level (model 5). In building the fully adjusted models, we estimated that the individual-level risk factors included in this study explained 8.0% of the risk of being compulsorily admitted, whereas LSOA- and provider trust-level variables each explained 1.1% of the total variance in the compulsory admission rate. Our results also indicate that adjusting for explanatory variables at these three spatial levels had little impact on the relative distribution of unexplained variance. Overall, the inclusion of explanatory variables increased the proportion of unexplained variance occurring at the individual level (from 84.7% in the null model to 87.6% in the fully adjusted model), with a commensurate reduction in unexplained variance at the provider trust level (from 7.0% to 5.2%). The unexplained variance at LSOA level fell slightly, from 8.3% to 7.5%.
Model and levels | Variance (standard error) | p-value | % unexplained variance (95% CI) | % variance explained | Sample size (% study sample) |
---|---|---|---|---|---|
Model 5 (null) | |||||
Individual | 3.29 | 84.7 | N/A | 1,226,709 (99.1) | |
LSOA | 0.323 (0.009) | < 0.001 | 8.3 (7.9 to 8.8) | ||
Trust | 0.271 (0.051) | < 0.001 | 7.0 (4.4 to 9.6) | ||
Model 6 (+ individual-level variables) | |||||
Individuala | 3.29 | 86.9 | 8.0 | 1,226,252 (99.0) | |
LSOA | 0.284 (0.007) | < 0.001 | 7.5 (7.1 to 7.9) | ||
Trust | 0.213 (0.039) | < 0.001 | 5.6 (3.6 to 7.6) | ||
Model 7 (+ individual- and LSOA-level variables) | |||||
Individuala | 3.29 | 87.2 | 9.1 | 1,185,912 (95.8) | |
LSOAb | 0.280 (0.009) | < 0.001 | 7.4 (7.0 to 7.9) | ||
Trust | 203 (0.039) | < 0.001 | 5.2 (3.3 to 6.9) | ||
Model 8 (+ individual-, LSOA- and provider trust-level variables) | |||||
Individuala | 3.29 | 87.6 | 10.2 | 1,182,847 (95.5) | |
LSOAb | 0.283 (0.008) | < 0.001 | 7.5 (7.1 to 7.9) | ||
Trustc | 0.195 (0.045) | < 0.001 | 5.2 (2.8 to 7.5) |
Associations between explanatory variables and compulsory admission
We extended our analyses by estimating associations between the explanatory variables used in our adjusted models and the risk of compulsory admission. Since running each model required several thousand iterations, these were computationally demanding and often took several hours to run. It was not possible, therefore, to estimate unadjusted associations for each of the study variable. We did just this, however, for two variables – the number of mental illness beds in each provider trust, and mental illness bed occupancy – given previous evidence of, and concerns about, the association between the availability of mental illness beds and rates of compulsory admission. 5 We estimated associations with compulsory admission by re-estimating the three-level model, controlling just for patient age and sex and for each of these trust-level variables in turn. We found statistically significant associations with each of these variables, although that for mental illness beds (OR 1.0009, 95% CI 1.0006 to 1.0012; p < 0.001) was smaller than that found between bed occupancy and compulsory admission (OR 1.03, 95% CI 1.02 to 1.04; p < 0.0001). We therefore used only the latter in our fully adjusted models.
Table 6 shows the odds ratios for the fully adjusted associations between each of the explanatory variables and compulsory admission in the fully adjusted three-level cross-classified model (model 8). These findings show that men have a higher probability of being compulsorily admitted to hospital than women (OR 1.29, 95% CI 1.27 to 1.31). Patients who were 18 years old or younger were the least likely to be admitted compulsorily; compared with this group, the risk of compulsory admission was greatest among those aged 18–35 years (OR 1.92, 95% CI 1.82 to 2.02) and fell with age, but remained statistically significant even in the oldest age group (65 years and older) (OR 1.12, 95% CI 1.02 to 1.22). Adult black patients had the highest rate of compulsory admission (OR 2.94, 95% CI 2.90 to 2.98). Patients of Asian and mixed ethnicity were significantly more likely to have been admitted compulsorily than the white reference group. Compared with white patients, adults whose ethnicity data were not available in the MHMDS (i.e. coded as ‘missing’) had a significantly lower probability – around four times lower – of being admitted compulsorily.
Level | Explanatory variables | OR (95% CI) | p-value | |
---|---|---|---|---|
Level 1 (individual) | Sex | Male | 1.29 (1.27 to 1.31) | < 0.0001 |
Age (vs. ≤ 18 years) | 18–35 years | 1.92 (1.82 to 2.02) | < 0.0001 | |
36–64 years | 1.79 (1.68 to 1.89) | < 0.0001 | ||
65 years and older | 1.12 (1.02 to 1.22) | 0.03 | ||
Ethnicity (vs. white) | Missing | 0.25 (0.18 to 0.32) | < 0.0001 | |
Mixed | 1.81 (1.74 to 1.88) | < 0.0001 | ||
Asian | 1.41 (1.37 to 1.46) | < 0.0001 | ||
Black | 2.94 (2.90 to 2.98) | < 0.0001 | ||
Other | 1.00 (0.93 to 1.07) | 0.96 | ||
Level 2 (LSOA) | Deprivation | First quintile (least deprived) | 1.00 | |
Second quintile | 1.14 (1.09 to 1.18) | < 0.0001 | ||
Third quintile | 1.20 (1.16 to 1.24) | < 0.0001 | ||
Fourth quintile | 1.21 (1.17 to 1.26) | < 0.0001 | ||
Fifth quintile (most deprived) | 1.22 (1.18 to 1.27) | < 0.0001 | ||
Population density (people/hectare) | First quintile (0–6.8) | 1.00 | ||
Second quintile (6.9–24.0) | 1.02 (0.97 to 1.07) | 0.52 | ||
Third quintile (24.1–41.2) | 1.04 (0.99 to 1.09) | 0.11 | ||
Fourth quintile (41.3–61.4) | 1.01 (0.96 to 1.06) | 0.78 | ||
Fifth quintile (≥ 61.5) | 1.01 (0.95 to 1.07) | 0.76 | ||
Ethnic density (% white British) | 95–100 | 1.00 | ||
81–94 | 1.14 (1.10 to 1.18) | < 0.0001 | ||
68–80 | 1.28 (1.23 to 1.34) | < 0.0001 | ||
22–67 | 1.40 (1.34 to 1.46) | < 0.0001 | ||
1–21 | 1.51 (1.43 to 1.59) | < 0.0001 | ||
Level 3 (provider trust) | Bed occupancy | % of occupied mental illness beds | 1.01 (0.99 to 1.03) | 0.14 |
LOS | Median LOS (days) | 0.99 (0.98 to 1.01) | 0.13 | |
Community mental health services survey | Worse than other trusts | 1.00 | ||
Same as/better than other trusts | 1.93 (1.39 to 2.48) | 0.02 | ||
Missing | 1.12 (0.21 to 2.03) | 0.34 | ||
Inpatient survey | Worse than other trusts | 1.00 | ||
Same as/better than other trusts | 1.01 (0.56 to 1.45) | 0.97 | ||
Missing | 1.45 (0.66 to 2.24) | 0.35 | ||
PEAT survey | Privacy and dignity score | 1.18 (0.67 to 1.69) | 0.53 | |
Staff survey | % satisfied with quality of care | 0.99 (0.97 to 1.02) | 0.50 | |
Admissions | Hospital admissions (cat 700–715) | 1.00 (1.00 to 1.00) | 0.65 | |
Trust location | In London | 1.00 | ||
Outside London | 0.74 (0.32 to 1.17) | 0.17 |
After adjusting for all of the other variables in Table 6, compulsory admission was associated with area-level deprivation in a manner which strongly suggested a dose–response effect. ORs for compulsory admission rose steadily, with deprivation score quintiles from 1.14 (95% CI 1.09 to 1.18) for those living in areas in the second-lowest quintile, to 1.22 (95% CI 1.18 to 1.27) for those in areas with deprivation scores in the top quintile (most deprived). We did not find a statistically significant association between compulsory admission and population density. However, the association between compulsory admission and ethnic density was statistically significant and appeared to show a dose–response effect, with patients living in LSOAs composed of higher proportions of white British residents having lower risks of being admitted compulsorily.
After adjusting for the other study variables, no statistically significant associations were found between compulsory admission and bed capacity (average bed occupancy), median LOS, annual number of admissions or PEAT privacy and dignity scores. Similarly, there was no statistically significant difference in the rate of compulsory admission in London trusts from those located outside the capital. Only one of the provider trust-level covariates included in the model was a statistically significant predictor of compulsory admission after adjusting for all of the study variables. Patients receiving care from a provider trust whose community mental health services were rated as ‘same as other trusts’ in the national survey of community mental health services were almost twice as likely to be admitted compulsorily as patients receiving care from providers rated as ‘worse than other trusts’ (OR 1.93, 95% CI 1.39 to 2.48).
Residuals at provider trust level in the fully adjusted model
The final stage in our analyses involved examining provider trust residuals for the fully adjusted three-level model (model 8). One provider trust was excluded from model 8 because its data on the PEAT privacy and dignity score were completely missing. The residual plot therefore compared 63 provider trusts in terms of their rate of compulsory admission compared with the national average. When controlled for all of the explanatory variables in this model, 10 provider trusts had rates of compulsory admission to hospital that were lower than the national average to a statistically significant extent, and 10 provider trusts had rates that were significantly higher (Figure 4). This compares with 24 provider trusts that had statistically significantly lower detention rates than the mean detention and 21 that had significantly higher rates, when only patient age and sex were accounted for (see Figure 2).
FIGURE 4.
Residual plots (with 95% CI error bars) for provider trust-level variance (three-level cross-classified model of individuals within LSOAs within provider trusts, MCMC estimation adjusting for explanatory variables in model 8). Provider trust residuals are shown in rank order.
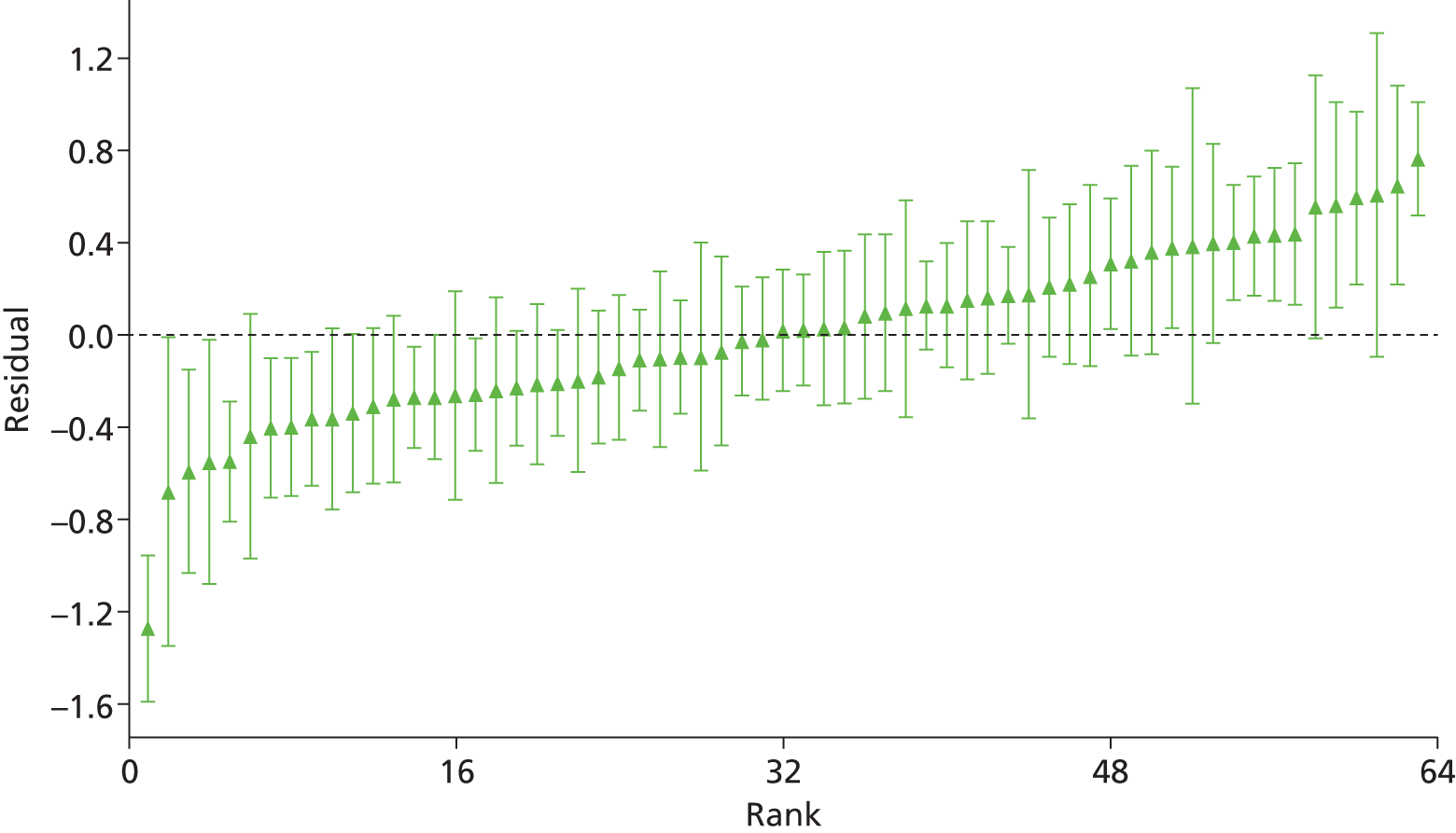
Fully adjusted, standardised model residuals were mapped to explore spatial clustering of negative or positive values (Figure 5), and to compare this with the map based on the null model (see Figure 3). Again there appeared to be no evidence of spatial auto-correlation amongst the residuals derived from the fully adjusted model. As before, there was no strong suggestion of an urban effect. Large positive and negative residuals were found in the urban cores, and some urban areas had non-significant residuals.
FIGURE 5.
Provider trust residual values (adjusted for all explanatory variables in model 8) mapped geographically.
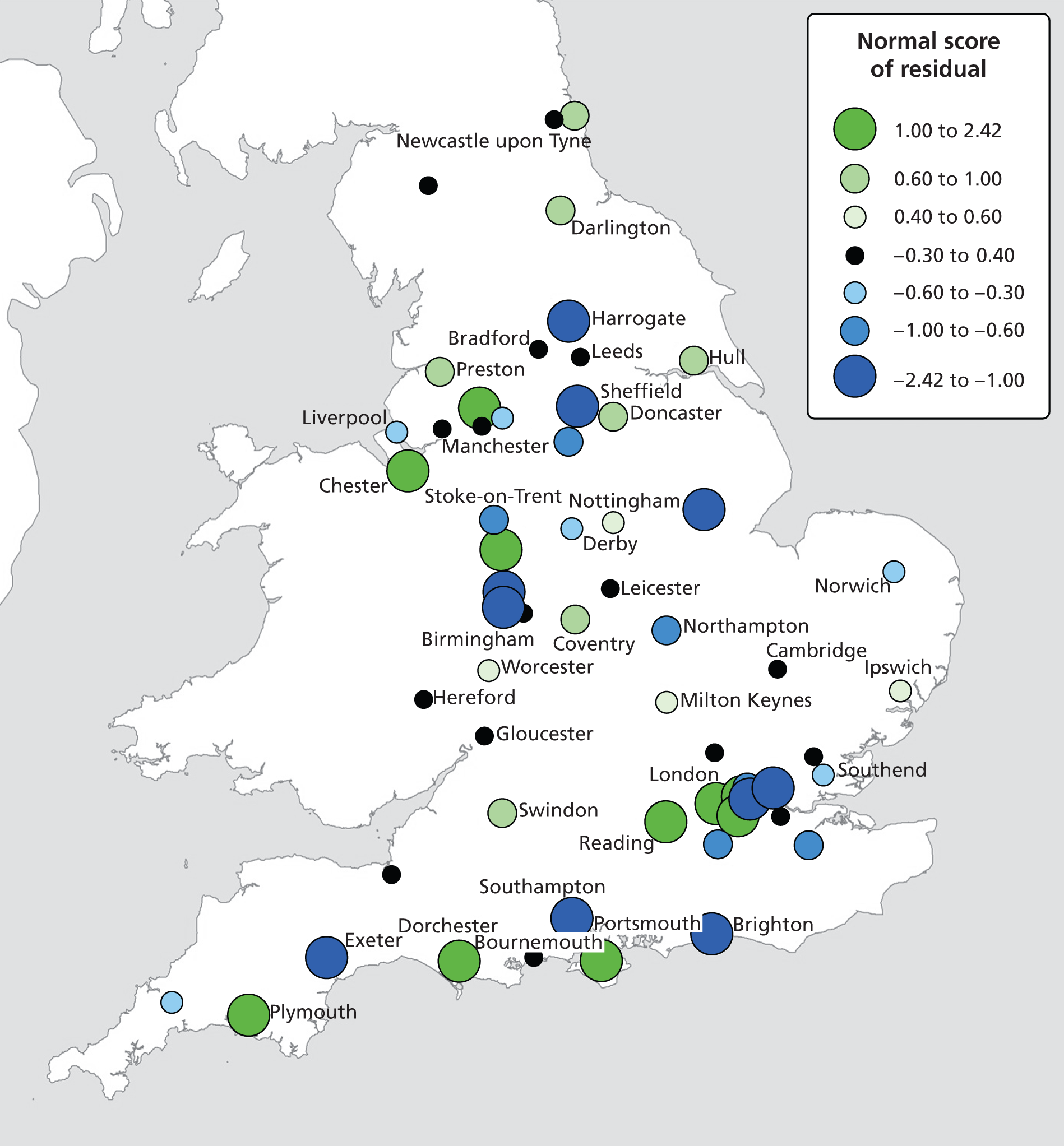
Association between primary care trust investment and compulsory admission
As previously outlined in Chapter 3, the four-level cross-classified null model results showed that a significant percentage of the variance in compulsory admission, of the order of 7%, occurred at PCT level. As noted previously, model results and further examination of the data structure suggested that models that included both PCT and provider trust may have been prone to conflation of individual and higher-level variances, and that the latter may not have been reliable. However, we felt that it was desirable to assess the association between investment in mental health services by commissioners (PCTs) and the rate of compulsory admission. As outlined in Chapter 2, Methods, we established linkage to data on investment in adult mental health services by PCTs in England in 2010. We selected the following variables, as spend in pounds per adult of working age (16–65 years):
-
total reported investment in all mental health services
-
total spend on assertive outreach teams
-
total spend on community mental health teams
-
total spend on CRHTs.
Since investment in specific services (e.g. CRHT) was included in the overall total reported investment in all mental health services, it was necessary to estimate two separate models, one with the overall adjusted spend on all mental health services and the other with the three specialised services of interest (CRHT, community teams and assertive outreach). These services were chosen on the grounds that they target patients at high risk of admission, and might therefore be viewed as providing alternatives to admission.
Given the potential instability of multivariate models with more than three spatial levels, we reverted to a three-level model. For the purposes of these analyses we retained individual and LSOA levels, and specified PCT as the highest spatial level. The explanatory variables of interest at individual level and LSOA level were the same as those specified previously, namely patient sex, age and ethnicity (at individual level) and population density, deprivation and ethnic density (at LSOA level). PCT explanatory variables were as specified above (Table 7).
Model and levels | Variance (standard error) | p-value | % unexplained variance (95% CI) | % variance explained (vs. model 9) | DIC statistic |
---|---|---|---|---|---|
Model 9 (null model, no explanatory variables) | |||||
Individual | 3.29 | N/A | |||
LSOA | 0.287 (0.008) | < 0.001 | 7.3 (6.8 to 7.7) | ||
PCT | 0.288 (0.036) | < 0.001 | 6.9 (5.0 to 8.7) | ||
Model 10 (individual + LSOA variables) | |||||
Individual | 3.29 | 9.2 | 311,504.3 | ||
LSOA | 0.256 (0.008) | < 0.001 | 6.8 (6.4 to 7.2) | ||
PCT | 0.211 (0.028) | < 0.001 | 5.9 (4.4 to 7.2) | ||
Model 11 (individual + LSOA variables + total PCT investment) | |||||
Individual | 3.29 | 9.3 | 311,513.1 | ||
LSOA | 0.258 (0.008) | < 0.001 | 6.9 (6.4 to 7.3) | ||
PCT | 0.212 (0.027) | < 0.001 | 5.6 (4.2 to 7.0) | ||
Model 12 (individual + LSOA variables + PCT investment by service) | |||||
Individual | 3.29 | 9.3 | 311,500.9 | ||
LSOA | 0.259 (0.007) | < 0.001 | 6.7 (6.4 to 7.1) | ||
PCT | 0.211 (0.028) | < 0.001 | 5.9 (4.2 to 7.6) |
In the three-level (individual, LSOA and PCT) null model (model 9), 7.3% of the (unexplained) variance in compulsory admission occurred at LSOA level and 6.9% occurred at PCT level. The percentage of variance explained in the rate of compulsory admission to hospital by these models was around 9.0%, and nearly all of this was the result of including individual- and LSOA-level explanatory variables. Almost no additional variance was explained when PCT investment variables were included in the model. DIC statistics (based on identical samples) indicate that there were no substantial differences between the fit of models 10, 11 and 12. These results confirm that, compared with model 10, the inclusion of PCT investment variables (models 11 and 12) did not increase model fit. We note that there was a small difference between models 11 and 12 (DIC value difference 12.2), although neither represented an improvement on model 10.
We found only limited evidence of statistically significant associations between PCT investment in mental health services and compulsory admission. In model 11, total investment in mental health services was not significantly associated with compulsory admission (OR 1.0009, 95% CI 0.999 to 1.0025; p = 0.32). In model 12, the association with investment in community mental health teams reached statistical significance, although the effect size was small (OR 1.01, 95% CI 1.00 to 1.02; p = 0.03). Investments in assertive outreach teams (OR 0.97, 95% CI 0.93 to 1.02; p = 0.39) and CRHTs (OR 0.99, 95% CI 0.97 to 1.01; p = 0.55) were not statistically significant.
Chapter 4 User involvement
Two groups of users were involved and consulted in the course of the study: users of mental health services and those with experience of caring for people with serious mental illness (through partnership with the Mental Health Foundation); and potential users of the evidence arising from the research, in the form of senior NHS mental health service managers and clinicians (via the Mental Health Network of the NHS Confederation).
Service user and carer involvement
Service user and carer involvement was undertaken in partnership with the Mental Health Foundation, and was led by DC-K.
Mental Health Foundation
The Mental Health Foundation (MHF) (www.mentalhealth.org.uk) is the UK’s leading mental health research, policy and service improvement charity. Service user involvement was coordinated by DC-K, Head of Empowerment and Social Inclusion at the MHF (and co-applicant on this project). The main aim of this partnership was to ensure meaningful patient and public involvement (PPI) such that the service user perspective shaped the research questions, influenced analysis and interpretation, and assisted in disseminating the findings.
Background
This aspect of the project comprised three meetings with people with direct personal experience of compulsory admission, or of caring for someone who had been admitted compulsorily. These meetings were held at key points during the research process and focused on relevant issues at each stage of the project.
Aims
The aim of the first meeting was to seek service user input into the analysis by encouraging service users to suggest reasons for different rates of compulsory admission. The intention was to use the content of these discussions to guide the researchers, particularly in selecting explanatory variables through data linkage.
The second meeting was used to reflect on interim findings and help guide their interpretation by exploring experiences of compulsory admission, including those that occur before, during and after the inpatient spell, upon discharge. At the third meeting, people examined how the study findings might be used to reduce rates and variability of compulsory admission, and improve service user experiences in future.
Methods
Recruitment
The aim was to recruit an expert group of at least five or six people who had personal experience of compulsory admission and/or of caring for someone who had been detained. The study was advertised though the MHF mental health policy network and the National Survivor User Network (NSUN). Being mindful of the importance of the issue of ethnic variation in compulsory admissions, it was a priority to ensure the PPI groups were ethnically diverse. We were also very determined to ensure heterogeneity in terms of geography, gender and age. A number of recruitment strategies were therefore employed, and these evolved in the light of the experience of the first two groups.
The first meeting focused particularly on recruiting people from BME groups by targeting specialist BME groups. This meeting was held in London in August 2012. Given the preponderance of participants from London and the South East at the first meeting, we sought greater geographical and demographic diversity at the second meeting. We recruited via national networks and held the meeting at Warwick University (near Coventry) in March 2013. The third meeting consisted of participants from the first and second meetings and was also held at Warwick University in July 2013.
Participants
Twenty people were recruited and participated in one or more meetings: 17 participants had been admitted compulsorily under the MHA (two of whom also had experiences of caring for someone who had been admitted compulsorily) and one person had carer experience only. Most participants were women (Table 8). There was a reasonable spread of ages and participants derived from a diverse ethnic and geographical background. Participants had experience of being detained under various sections of the MHA.
Demographic characteristic | n | |
---|---|---|
Sex | Male | 5 |
Female | 10 | |
Not disclosed | 5 | |
Age group (years) | 26–35 | 5 |
36–45 | 4 | |
46–55 | 5 | |
56–65 | 1 | |
66–75 | 2 | |
Not disclosed | 3 | |
Ethnicity | White | 10 |
Mixed | 1 | |
Asian | 2 | |
Black | 3 | |
Not specified | 4 | |
Geographical location | London | 10 |
Yorkshire | 2 | |
Cheshire | 1 | |
Manchester | 1 | |
Birmingham | 2 | |
Cambridgeshire | 1 | |
Oxfordshire | 1 | |
Hampshire | 2 | |
Detention related to section of MHA | Section 2 | 4 |
Section 3 | 2 | |
Section 4 | 1 | |
Section 17 | 2 | |
Section 52 | 1 | |
Section 136 | 3 | |
Not disclosed | 4 |
Results
Meeting 1: exploring factors that might affect rates of detention
At the start of the research process, the priority for PPI was helping to guide the research team in identifying factors that may explain different rates of detention. Questions such as ‘Are some people more likely to be detained than others?’ and ‘What factors should the researchers explore in this project?’ aided the discussion.
The following personal characteristics were suggested by the groups as issues that may have an impact on rates of compulsory admission:
-
ethnicity
-
age
-
sex
-
being unemployed
-
poverty
-
being homeless
-
drug and alcohol misuse
-
culture
-
previous detention.
When asked why ‘rates of detention might be different in different areas’, participants suggested that it was important to consider the following factors in the research project, if possible:
-
local police attitudes and experience
-
urban settings – may be more stressful
-
the accessibility of crisis services or alternatives to detention
-
people living on their own – more bedsits?
-
drug/drink culture
-
poorer estates.
Service users were also asked their opinions and experiences of what events might be important in leading up to (precipitating) compulsory admission. The following circumstances or events were suggested as being associated with detention:
-
family breakdown
-
bereavement
-
loss of children (custody)
-
domestic violence
-
bullying
-
drug abuse
-
loss of job
-
court proceedings.
Discussions were wide ranging and participants expressed great interest in the study. It was noted that not all of the suggested lines of enquiry could be examined by means of analysis of the MHMDS and externally linked data sources, since they specified variables that were not available to the researchers; however, participants thought that there may be a number of proxies that would enable investigation. It was also thought useful to log questions that could not currently be explored as an avenue for future research.
A number of people highlighted the importance of discrimination, stigma and public attitudes as a contributory factor in compulsory admission. Although this clearly falls outside the remit of the English National Study of Compulsory Admissions (ENSCA) project, it remains an issue worth noting.
Meeting 2: experiences around detention
The PPI priority for this stage of the research was to understand the experience of compulsory admission and subsequent detention. Much of the second meeting was spent in exploring people’s stories (which were often highly moving) of the causes, process and impact of compulsory detention, and, in so doing, thinking about modifiable factors on the causal pathway.
Experience leading to detention
Seeking help
A number of people spoke of actively seeking help before compulsory detention, either from primary care or from their existing community teams. In some cases this request was not, in their view, taken seriously. A number of people felt that compulsory admission could have been avoided if their requests for help, including in one case a voluntary admission, had been met. People felt that they had a great deal of experience and insight into when they needed help, what triggered deterioration and what the key signs were. They felt that this should be given greater weight by professionals.
Avoiding help
Conversely, some people spoke of actively avoiding help when their mental health deteriorated. Two distinct explanations were offered for this and they would require different approaches to reduce their impact on compulsory admission. The first explanation was prior experience of services. Some participants talked about their reluctance to seek help based on previous bad experiences, for example having had a request for help declined in the past. To address this, people needed acknowledgement from services that things had gone wrong in the past and ways of making improvements needed to be identified.
The second explanation was that their distress prevented them from seeking help. Some participants recalled experiences when they did not recognise that they needed help, or when the distress they were experiencing at that time made seeking help very difficult. Some people were very aware that they sometimes lose the ability to assess their own state of mental health and that they are not always aware when they need help. However, often in these cases they have people around them who are more aware (e.g. family, friends or peers from support groups). There appear to be times when the views of family and friends need to be taken into account. This clearly raises ethical issues, particularly if there is direct disagreement or conflict between the needs and/or wishes of someone in distress and those of their family or friends; however, participants felt that it would be possible to address at least some of these issues through advanced directives, crisis planning or a trusted advocate.
Where people recognised the need for help but were unable to seek it, two different difficulties emerged: being able to articulate the need for help; and actually getting in contact with the appropriate service. This was considered to be an issue of access. Services might be able to address this issue by having longer opening hours, named contacts, fast access telephone numbers for people identified as likely to need rapid support, self-referral for those who have used a service previously and quicker routes from primary to secondary care.
Life events
People also identified a number of life events that had preceded their detention, including:
-
moving away from a familiar area
-
loss of employment
-
change of family situation (e.g. bereavement or separation)
-
other family difficulties and financial problems.
While events are not avoidable or even always predictable, they are known to significantly increase the risk of deteriorating mental health and therefore could be identified as potential triggers as well as flags for more rapid access to support.
Experience of compulsory admission
Detention itself was distressing. Although it is an intrinsically disempowering process, people felt that it could, even when it is necessary, be done in a more empowering way. Some people raised concerns about the involvement of police or highly visible professionals involved in the compulsory admission process, and about the effect of this on how they were viewed within their communities. This was seen as frustrating and sometimes humiliating, particularly by those who had sought help and not received it. It was also associated by some with a sense of failure and significantly reduced people’s self-confidence.
Some agreed that detention was necessary (and, in at least one case, life-saving). However, even these individuals expressed concern that neither the expected outcomes of detention nor the proposed treatments were adequately explained at the time. These people felt that they would have been more inclined to accept being in hospital if they had understood that there was a good reason for their admission.
The feeling was expressed that there was a strong focus on treatment and risk avoidance but little or no consideration given to understanding the trigger events that may have caused the distress in the first place.
One participant noted:
I sometimes wonder whether senior managers or clinician don’t section someone, merely to avoid the possibility of something happening out there in the community and therefore bad press, law suits and all the rest.
Most people took the view that the deterioration in their mental health before admission had some cause beyond a simple ‘chemical imbalance’. These were sometimes social or practical problems that had pushed people beyond their ability to cope (as previously mentioned). Many participants felt that little was done during their admission to address these problems and so people still had to deal with them upon discharge as well as adjusting to life outside the hospital. These stressors were seen as potentially leading to further time in hospital.
Participants subsequently tended to speak about their experiences of admission and subsequent discharge, rather than the process of compulsory admission itself.
Some people felt that the detention had damaged their relationships in the community. This included family relationships, social relationships and even relationships with community-based mental health staff. It was, however, also possible for detention to have a positive effect on family relationships where the hospital admission provided a break in an unhealthy or strained relationship.
The problems caused to relationships with community professionals took two forms: a breakdown in trust where the professional had been part of the compulsory admission process (though this was sometimes reversible if people felt, in retrospect, that the detention was appropriate); and a breakdown in the therapeutic relationship where clinical responsibility switched to a different team, or where the community team did not have regular contact with the patient when they were in hospital. This was felt to be particularly important in the weeks before discharge from hospital.
Experiences of discharge
In addition to re-establishing community relationships, there were two further issues highlighted about the time just before leaving hospital: little preparation for leaving hospital; and issues that were causing distress not addressed during admission.
The experience as a detained patient was described as controlled and people were not expected (or able) to do much for themselves. This meant that, when they came to leave hospital, they did not feel prepared to cope effectively. This experience was worse for those people who experienced longer LOS. Thoughts about how to address this included more use of extended leave and better contact with community teams before discharge.
Most people described some difficulties adjusting to life after (compulsory) admission. As well as having to deal with the consequences of being away from their homes, family and friends, sometimes for extended periods, some people had suffered interruptions to employment or education and training. This increased the risk of isolation and the feelings of failure and inadequacy that people expressed as part of the detention experience. Some people thought that this might increase the risk of subsequent mental health problems and reduce the likelihood that they would seek help in future. These potential consequences highlighted a need for hospitals to help people maintain links with employers or colleges/universities and for services to help people to return to work or education as quickly as was reasonably possible.
One participant commented:
It’s just about integrating all the different services to deliver around the care plan or lifestyle plan of the individual.
The final frustration that a number of people spoke of at the end of any particular spell in hospital was the absence of a process that helped everyone involved to learn from the experience. Some people had clearly built up a great deal of experience from admissions over the years but were unable either to escape from particular cycles of behaviour or to articulate ways that others could help to do so. They felt that an opportunity to talk through the whole experience and constructively consider how everyone involved should respond, were similar circumstances to arise in future, could achieve a number of useful ends; it would allow people to take something positive from an otherwise almost wholly negative experience, increase the chances of avoiding detention in future and allow people to positively contribute to their future care and treatment even if they become unable to articulate their needs.
This final point reinforces both people’s desire to be more actively involved in their care and treatment before, during and immediately after a crisis of the severity that warrants admission, and that services remain poor at finding mechanisms to allow this to happen.
Meeting 3: what could be done to reduce rates of detention
The third and final meeting was used to reflect on the main study findings, and to discuss how these might be applied to improve the experience of service users and carers to reduce rates of compulsory detention in the future. This discussion began with a brief outline of the headline findings by SW.
Getting the environment right
Two problems were identified with conventional psychiatric settings: the stigma attached to mental ill-health extended to psychiatric institutions and people did not want to be seen in a psychiatric environment; and mental health services maintained a focus on psychiatric treatment and compliance rather than understanding the broader problems people faced in their daily lives.
The issue of stigma clearly reduces people’s desire to seek help when their mental health is deteriorating. In addition, because crises were often precipitated by life events, people felt that these events needed addressing as part of the service response. If these issues were not addressed, then people thought readmission was more likely.
Cultures and conditions within mental health services were also thought to have an impact on how likely people would be to seek help in future. The following characteristics were identified as important:
-
ward design
-
staff behaviour and attitudes
-
cultural sensitivity
-
good-quality information about treatments, their side effects and alternatives
-
choice of services and staff
-
ability to see the same staff over time, to build rapport and reduce repetitive questioning
-
continuity of care and staff between community and hospital
-
therapeutic environment
-
decision-making and intelligent risk-taking encouraged.
One participant noted:
My experience is that ward staff huddle in the nursing station and patients are on the ward and there’s no interaction or association between them.
Another commented:
Being given a choice about medication, so for example, I said no I don’t want this and it was listened to and heard.
One service user offered:
She had kids and the one (psychiatric drug) that made her sleepy made her lose her kids and her kids got taken off her because she couldn’t stay awake enough to care for them, so she as a service user her response was she needed to be able to make that choice of which medication to have.
Learning from experience
Where people had experienced repeated admissions, they felt there was an opportunity to learn from this more constructively by identifying triggers and early warning signs, paying more attention to carers’ understanding and use of advocates and tools such as crisis cards to help people articulate their needs at times when mental ill-health made it difficult for people to do this for themselves.
Some people thought that you could learn positive lessons from recovering from crisis that could make future recovery quicker and less distressing. People identified a clear role for self-management, peer support and advanced planning for crises.
Crisis prevention
People thought more effort was needed in terms of avoiding crises as opposed to responding to them. In particular, the following approaches were offered as having the potential to reduce the need for admission:
-
more attention paid to social, economic and physical health issues, especially housing and employment
-
easier and quicker access to services
-
people being given self-management skills.
Alternatives to admission
A theme that ran through many of the discussions that took place was the absence or disappearance of a viable alternative to hospital admission.
One participant said:
Therapeutic communities, crisis houses, having alternatives, for me it would have been about understanding the spiritual nature of my situation.
A number of people mentioned having previously received good support from services that have since closed. Several people had received good support from voluntary sector services but others reported finding it difficult to find services, particularly at short notice or outside normal working hours or at weekends. Self-help or peer support was thought to be useful both before crisis and after leaving hospital, but not necessarily appropriate for periods of crisis.
One of the reported advantages of self-help or peer support was that people felt they were contributing towards supporting others as well as being supported. This was one of the few approaches that seemed to help increase people’s self-confidence.
Service user and carer involvement summary
The service user and carer involvement component of this study brought members of the research team together with people who had direct personal or caring experience of compulsory admission, significantly adding to the diversity of experience available to the research team. During the early part of the study, this partnership provided an opportunity for the research team to explore lines of enquiry relevant to the lives of the people most directly affected by compulsory detention. The service users and carers drew on their own experience to ask a range of questions that they thought could be answered by the study.
As the study developed, the service user and carer perspective offered a qualitative perspective to sit alongside the quantitative analysis. In particular, descriptions of events and service use before admission, the experience of admission itself, and the care received during detention and following discharge helped to indicate potential explanations for variations in compulsory admission rates across different service providers. Towards the end of the study the service user and carer involvement was used to highlight some of the practical applications of the emerging findings.
Service user and carer involvement in the dissemination of the findings will ensure a much wider reach and consequent impact than could otherwise have been achieved. Compulsory admissions have always been a very important issue to service users and carers, as deprivation of liberty is one of the most serious interventions that our law allows. Involvement in the study has led to a high level of interest amongst the service users and carers and their broader networks.
The MHF plans to produce a briefing about the context and findings of the study and publicise it via MHF networks, which include an e-newsletter (reaching 45,000 people), local and national groups, service users and carers, as well as contacts within large number of service providers and commissioners. We shall also publicise the findings at a service user event organised in collaboration with the NSUN.
Mental Health Network (NHS Confederation)
The NHS Confederation represents more than 90% of the organisations that make up the NHS. Its members include the majority of NHS acute trusts, ambulance trusts, foundation trusts, mental health trusts, PCTs, special health authorities and SHAs in England; provider trusts and local health boards in Wales; and health and social service trusts and boards in Northern Ireland. We aimed to recruit senior NHS mental health service managers to participate in a consultation event regarding the project’s main research aims and findings.
The consultation event was convened by members of the ENSCA project team in December 2012. Participants were provided with a background to the study, including the main aims and objectives and a brief overview of the main headline findings at that stage in the project.
The group confirmed that the headline estimates of the proportions of patients detained under the MHA, those admitted voluntarily and those treated in the community only were correct. There was also a consensus that the research was important, and that the current levels of compulsory detention were worryingly high. The ensuing discussion was wide-ranging but has been captured under a series of headings for the sake of providing an accurate summary of the different views expressed.
Underlying trends
The participants concurred with the report of historic trends (1988–2008), and felt certain that rates of detention were likely to have increased in the meantime; one participant noted that these rates had increased by 6% in the last year alone. It was also noted, and agreed generally, that the majority of inpatients across the country are now detained patients.
Professionals’ views about reasons for increased rates of detention
This was one of the major areas of discussion, and produced a comprehensive view of possible factors to be investigated.
Risk aversion (and fear) among mental health professionals
This was a trend that many identified, with roots in the serious untoward events and negative publicity of the 1990s and subsequent proposals for the proactive detention of people with so-called Dangerous and Severe Personality Disorders. The overall effect had been political and societal pressures to detain more people with serious mental illness. The last 20 years were judged to have seen greater emphasis on risk assessment and risk management in the delivery of mental health care. The decision to admit a patient to hospital is made on the grounds not of accessing treatment or therapy, but of avoiding untoward outcomes outside hospital.
Introduction of crisis resolution and home treatment services
Crisis resolution and home treatment teams have had great success in providing alternatives to admission (through home treatment) for many people in crisis. The downside is that admission is not always avoidable, and some people may be kept out of hospital and admitted only when severely unwell. A view was expressed that those who could be kept out of hospital were being kept out, but that there was a core of patients who were too ill to benefit from this kind of care. These individuals would never be suitable for home treatment during acute episodes of illness. Since the introduction of CRHTs, the average LOS in hospital has fallen to around 35 days (figure suggested by participants). Shorter LOS was seen as a possible factor in (early) readmission. The benefits of CRHTs are not realised uniformly or universally. Rural areas were discussed, and it was noted that it is almost impossible to deliver crisis resolution services in remote places. Rural poverty and social isolation are causes of mental illness, and access to firearms heightens risk. People who are unwell can stand out in small communities. Lack of public transport and other services often means that admission is necessary.
Increasing use of Section 136 by the police
This was being addressed currently through the opening of place of safety suites in mental health provider units, and the move away from looking after people who are detained in public places in police stations. It was noted that there had been a 36% increase in Section 136 detentions in the past year.
Introduction of electronic patient records
Although electronic patient records should allow more accurate management decisions to be made out of hours, the reality is often that even they are incomplete or unavailable. It was also suggested that this information might include more information about risk and therefore a greater chance of detention, particularly where assessment was undertaken by professionals without prior knowledge of the patient.
Greater preparedness to manage challenging behaviours assertively
This was seen as including out-of-area placement in independent-sector units. This has also seen the expansion of NHS low-security forensic beds, and reflects a general tendency towards the assertive management of risk through admission.
The demise of psychiatric rehabilitation as a specialty
Psychiatric rehabilitation was a specialty that previously cared for patients most at risk of admission. This change in service delivery went with the reduction in long-stay beds.
Lack of effective and acceptable interventions to reduce cycles of readmission
This was highlighted in a consultation event involving users of mental health services, where social interventions were valued in particular. ‘Lack of purposeful life opportunities’ was mentioned in this respect. This was echoed later in a discussion about the lack of alternatives to admission (other than CRHTs) in many parts of the country, and even the ‘best’ CRHTs can visit only two or three times per day at most.
Poverty and benefits cuts (including housing benefits exclusions)
One factor leading to great pressure on inpatient service was the greater geographical mobility as patients are displaced to areas with cheaper housing costs. Employment and social care may be less available, and those affected are likely to experience greater isolation and more difficulty accessing services. This will result in weaker therapeutic relationships, and the likelihood that professionals will encounter a patient for the first time when in crisis.
Discontinuities in care
This was a major theme, and was seen as arising from a widely adopted ‘functional’ model involving highly specialised clinical teams and numerous boundaries and care interfaces. Clinicians who know a patient well may be less likely to detain them in a crisis, and perhaps more likely to negotiate a voluntary admission. As one participant put it ‘stories don’t move with patients any more’. Electronic records are no replacement for relationships. The view was expressed that care is becoming depersonalised as a result of functionalisation.
Loss of ‘specialist’ status and skills among inpatient services
Inpatient services are often viewed as the hardest, least desirable and least prestigious places to work for professionals, and where patients go when other treatments have failed. High levels of staff turnover, absenteeism and use of bank and agency staff were identified as major problems, and factors associated with higher rates of compulsory admission. Permanent staff tend to be relatively inexperienced, and therefore perhaps more likely to detain patients than more experienced clinicians.
The view was expressed that pressures on inpatient services to minimise LOS mean that less time is spent on assessment at the time of admission. Lack of knowledge and understanding of the causes and consequences of illness probably increased the risk that patients would be undertreated and remediable risk factors for relapse overlooked. Longer-term care planning (including help to reduce relapses) was less likely to be undertaken now than in the past, and was thought to be directly related to shorter LOS and busier, more disturbed wards.
As wards become more disturbed they also become more frightening and aversive, and less therapeutic. This was a vicious cycle, and it was not unknown in some places for patients to agree to a voluntary admission but refuse to stay when they saw the ward. This was considered a cause of avoidable detention.
Influence of reduced budgets and greater pressure on services
Demoralisation and destabilisation of services at a time of austerity and service cuts were seen as contributing to increased bed pressures, and greater rates of compulsory admission. Cutbacks and use of locum staff result in fewer professionals with previous knowledge of patients who present in crisis. It was suggested that this might affect compulsory admission rates by reduced investment in staff training and development. There was also support for the notion that reduced bed numbers could contribute to increased rates of detention. It was noted that in some parts of the country being placed on a section of the MHA is necessary to gain access to a bed, but can involve waiting for up to two weeks after a successful application (after which the application expires and the patient has to be reassessed, which itself is not unknown). Bed occupancy was typically at or above 100% in most places, according to participants. This places greater pressure on teams to discharge patients, at the expense of time spent on relapse prevention. It is no longer possible for patients to be admitted to hospital for short periods of respite, to prevent imminent crises. The relationship between LOS in hospital and detention rates is likely to be important.
Greater attention to the care of people with personality disorders
The National Institute for Health and Care Excellence guidelines make it clear that mental health services have a duty of care, and such patients often present in crisis and expressing ideas of self-harm. Such patients can be difficult to keep out of hospital, and are often difficult to engage in therapeutic relationships. A significant proportion of the current pressure on beds is thought to reflect the increasing prevalence of personality disorders among inpatient populations.
Changing role of mental health social workers
The role of mental health social workers has altered dramatically in the past 10–15 years, and this group of professionals has gone from multidisciplinary mental health team members to Approved Mental Health Professionals concentrating mainly on MHA assessments. Reduction in social care budgets (as part of local authority financial challenges) is fast resulting in the breakdown of joint working across health and social care.
Increasing prevalence of substance misuse and therefore dual diagnoses
It was suggested that in some units, especially in London, almost 100% of inpatients meet criteria for dual diagnosis.
Introduction of Community Treatment Orders
Community Treatment Orders were introduced to optimise adherence to treatment in those at highest risk of relapse, and this may be happening. However, they are probably not reducing admissions, since they require greater supervision of patients and therefore more awareness of discontinuation of treatment and relapse. They are very good for some patients (especially those on depot medication), but probably not for everyone.
Useful and desirable outcomes of the research
Following this lengthy discussion, the participants were aware of the main aims and objectives of the ENSCA project. They were then asked to give their opinions about what specific evidence they would like to see being derived from the project; in other words, how they felt the evidence from this research study might be useful for professionals. The following points were raised:
-
What is the association between LOS in hospital and the compulsory admission rate?
-
What implication does the increasing compulsory admission rate have on the availability of resources in other areas of care (e.g. services to help prevent relapse)?
-
What evidence is there to support the ‘reinvention’ of inpatient services and ‘upskilling’ of ward staff in an attempt to reduce the compulsory admission rate?
-
What impact does the commissioning of CRHT services have on the rate of compulsory admission to hospital?
As outlined in the MHF PPI section, it was not be feasible within the current project (given the limitations of the MHMDS and external data sources) to address all of these proposed avenues for analyses raised in this consultation event. The content of the discussion, however, did inform the selection of explanatory variables as described in Chapter 2, Methods. These recommendations were also considered as opportunities for future research studies, as covered in Chapter 5, Discussion and conclusions.
Chapter 5 Discussion and conclusions
The study had two main aims: to estimate variance in rates of compulsory admission between people, places and healthcare organisations (research aim 1); and to evaluate the extent to which this variance could be explained by characteristics of these different spatial levels (research aim 2). A subsidiary aim was to evaluate associations between compulsory admission and specific risk factors (explanatory variables) that were derived from data linkages across all spatial levels within the study data, including bed occupancy and investment in mental health services.
We set out to achieve these aims through (secondary) multilevel statistical analysis of the MHMDS, which comprises the routine data returns on all specialist mental health treatment activity in England. We encountered significant MHMDS data quality issues; these were offset by richer than expected data linkages but meant that we were able to undertake cross-sectional analysis of only one year of MHMDS data (2010/11). Our outcome was compulsory admission, defined as inpatient treatment in a mental illness bed while subject to section(s) of the MHA (2007) other than those concerned only with conveyance to a place of safety and/or short-term (maximum 72-hour) detention for the purposes of assessment or guardianship.
We identified six spatial levels in the MHMDS, namely individual, LSOA (the primary census unit of local geography), GP practice, PCT (as commissioner of services), provider trust and SHA. We made full use of the facility that this data structure offered for linking to external data sets, and these were the source of most explanatory variables used in our analyses.
Summary of main findings
The first aim of the study was to model and assess variance in rates of compulsory admission in England across a comprehensive range of spatial levels. We set out to identify the most parsimonious four-level, cross-classified model, and to model the residuals across provider trusts after adjusting for age and sex.
Patterns of variance in the null model
The choice of an optimal four-level, cross-classified null model was complicated, and involved careful comparison of competing models (including model fit statistics) and further examination of the data structure in respect of PCTs and provider trusts. We began with the a priori assumptions that individual (patient) and provider trust should remain in all models, since the former is the level at which outcome is classified (and where most variance occurred) and the latter represents the locus at which decisions about admission and detention are made, and therefore represents an important target and/or location for future interventions.
The relatively small number of PCTs (commissioners) per provider trust meant that higher-level variances were likely to be unreliable in models that included both PCT and provider trust. We therefore selected the four-level cross-classified null model comprising individuals, LSOA (based on Census area geography), GP practice and provider trust. Although around 84% of the (unexplained) variance in compulsory admission in this model reflected differences between individuals, substantial and statistically significant variance (∼7% in both instances) was found between areas (LSOA) and between provider trusts. The variance at GP level was smaller (2.7%) but statistically significant. The model fit was not improved by including SHA, and there was no evidence of statistically significant variance at this (higher) level.
Examining and mapping null model residuals
The limitations of even the best-fitting null model were evidenced by examining the provider trust-level residuals. These differed from the modelled average to a statistically significant degree for 45 out of 64 (70%) provider trusts included in the study sample even after adjusting for patients’ age and sex.
We mapped these residuals to explore their geographical distribution, and particularly the spatial distribution of positive and negative values. This was an exploratory diagnostic tool designed to help identify potentially ‘missing’ explanatory variables. Missing variables would be considered more likely if negative residuals were clustered together and positive residuals clustered (i.e. if there were evidence of spatial auto-correlation among residuals). Although there are no formal statistical tests with which to assess this, inspection of the map based on null model residuals did not suggest clustering of this type, with the possible exception of London, where (notwithstanding the limitations of the map itself) there appeared to be a cluster of large positive model residuals.
The next stage (research aim 2) involved attempting to explain this variance by introducing variables that characterised the different spatial levels within the data set. To maintain model stability while accommodating explanatory variables, we were forced to reduce the model from four to three spatial levels, and retained individual, LSOA and provider trust. The distribution of variance in this three-level null model was broadly in keeping with that of the null four-level model described above. In the fully adjusted model we estimated that individual-level risk factors explained 8.0% of the risk of being compulsorily admitted, while LSOA- and provider trust-level variables each explained 1.1% of the total variance in the study outcome.
On examining the fully adjusted model residuals we found that the number of provider trusts whose observed compulsory admission rate differed from the model average to a statistically significant extent had fallen from 45, in the null model, to 20. Mapping of these residuals did not reveal any striking evidence of spatial auto-correlation.
In our adjusted models, we found significantly higher rates of compulsory admission for men, and for patients of black, Asian and mixed ethnicity (compared with white patients). The finding that patients of black ethnicity were almost three times more likely to be admitted compulsorily than white patients is consistent with evidence from elsewhere. 28–30 The rate of compulsory admission was highest in patients aged 18–35 years, and fell with age thereafter. The lowest rate was observed in those aged under 18 years.
We found statistically significant associations with area-level deprivation (which appeared to show a dose–response effect) and ethnic density, but not with population density. The risk of compulsory admission was inversely associated with the proportion of white British LSOA residents. This finding highlights, and raises further questions about, ethnic inequalities in the experience of mental health care.
We found very little evidence of significant associations between compulsory admission and provider trust-level factors. Only one such covariate was a statistically significant predictor of compulsory admission: patients receiving care in provider trusts with community mental health services rated as average (‘same as other trusts’) had a significantly higher risk of being admitted compulsorily than in those rated as poor (‘worse than other trusts’) on the survey of patient experience.
One important finding concerns bed occupancy and rates of compulsory admission. Before adjusting for other covariates (and potential confounders), we found a statistically significant association between (higher) average annual trust-level mental illness bed occupancy and compulsory admission. However, this association no longer reached statistical significance after adjusting for the other covariates in the model. Although there was a trend towards lower rates of compulsory admission in trusts outside than in London, this association did not reach statistical significance in the fully adjusted model.
For the reasons described we did not include PCT (commissioner) in our null or adjusted models. Instead we carried out separate analyses in which we examined associations between PCT investment in mental health services and rates of compulsory admission. Our results indicated that inclusion of these variables explained almost no additional variance in the rate of compulsory admission after adjusting for individual- and LSOA-level variables. No statistically significant association was found between total investment in mental health services and compulsory admission in adjusted models. A small but statistically significant association was, however, found for investment in community mental health teams, but not with investment in assertive outreach or crisis resolution teams.
Strengths of the study
There are no directly comparable studies, and none with similar geographical and spatial coverage, or statistical power. The size and representativeness of the sample used in this study were unprecedented. The MHMDS includes the treatment records on every person – over 1.2 million individuals – who received secondary care mental health services in England in 2010/11. The sample size ensured a high degree of precision in our estimates of variances and residuals. The complexity of the data set, which reflects the real world settings in which mental health services are commissioned, delivered and received, means that our results are highly generalisable to England as a whole. Arguably the most important of these complexities was the cross-classified nature of the data themselves, with multiple overlapping and intersecting geographies which defied simple description or representation.
Our first aim was to model and assess variance in rates of compulsory admission in England across a comprehensive range of spatial levels. We set out to identify the most parsimonious four-level, cross-classified model, and to model the residuals across provider trusts. This was done as a precursor to identifying factors that might account for this variance, and to model potential impacts of future interventions. Ours was the first study to model these variances simultaneously.
The use of multilevel statistical modelling (and MLwiN software) was a particular strength, since this was capable of handling cross-classified data and therefore of estimating variances across a highly complex geography of places and health-care commissioners and providers. This was the first study of which we are aware that modelled variances in compulsory admission rates across several spatial levels simultaneously.
One important strength of the study data set was its large number of spatial identifiers, which enabled the many data linkages employed in our efforts to address research aim 2. In fact the search for data and variables that characterised the different spatial levels in our data set proved highly productive, and exceeded expectations. The number and quality of these linked variables contributed significantly to our ability to achieve the study aims.
Limitations
There were a number of study limitations that need to be taken into account when interpreting the findings. The first of these was the secondary nature of the study design, based on use of routine, retrospective data. Secondary analysis is always reliant on variables that have been collected for other purposes and on the quality and completeness of the data. Such research is always limited by an inability to specify variables of primary interest (including the study outcome itself).
The data quality issues (which we have highlighted throughout this report) prevented us from pursuing all of the research questions in our original protocol, most notably the longitudinal analysis of trends in compulsory admission over time. It is important to note that we were the first researchers ever to undertake this type of analysis of the MHMDS, and the HSCIC had little or no experience of supporting use of the data for research purposes. We were therefore forging a path for others to follow. Unbeknown to us, the data for years before 2010/11 were simply too incomplete to enable longitudinal analysis or modelling, and after careful reflection we decided to concentrate on undertaking a high-quality cross-sectional study of the 2010/11 data. This decision was supported by the discovery of far richer (cross-sectional) data linkages than expected.
The use of secondary analysis also constrained the questions we could address and the hypotheses we could test. This was highlighted by the many salient suggestions of potential explanatory factors proposed by participants in both of our user groups. For instance, we were unable to study factors such as ‘continuity of care’, ‘inpatient environment’ or ‘carer involvement’. Through a combination of data specification and completion difficulties, we were unable to accurately establish the number of admissions, LOS (bed-days) or number of discrete compulsory admissions for individual patients in the data set. This had one major consequence: we were unable to study patterns of admission and readmission. As a result we cannot comment on the extent to which compulsory admission rates are influenced by the ‘revolving door’ effect whereby a small number of patients account for a large number of (compulsory) admissions. Likewise, the data did not allow us to distinguish between patients who were detained under the MHA (2007) at the time of admission to hospital and those admitted voluntarily and subsequently detained.
We were unable to distinguish between the type of inpatient units and mental illness beds involved, and those admitted compulsorily will have included those treated in specialist old age, LD and forensic mental health services. However, the numbers treated in each of these services is very small in relation to general adult services. 20
We were also constrained by the way in which certain variables were specified. The best example of this was bed occupancy: according to the coding rules of BOO, beds allocated to patients on home leave are recorded as unavailable and therefore not occupied. Consequently, official bed occupancy can never exceed 100%, despite reports that true occupancy is often higher than this.
As described in this report, the data set itself is large, complex and beset by data quality and coding challenges, as well as high levels of missing data on many potential variables of interest, particularly at the individual level (such as diagnosis or other clinical outcome measures). While our null models would not have been affected, it is likely that we underestimated the proportion of variance that would have been explained had we been able to control for diagnosis, previous treatment experiences, length of illness, engagement with care and socioeconomic status in particular. Data on variables that are likely to determine thresholds for admission (e.g. suicidality, agitation/aggression, substance use and homelessness) would arguably have led to significant insights into variation in rates of compulsory admission. Furthermore, this unmeasured confounding could have resulted in overestimates of the association between compulsory admission and individual- (e.g. ethnicity) and LSOA-level factors such as deprivation and ethnic density. Likewise, we had no information about alternatives to inpatient treatment, such as crisis houses or respite facilities. 83 Although previous research has suggested that such facilities are less suitable for those who are severely unwell,84 their presence may reduce the need for compulsory admission in some cases.
Perhaps the single greatest obstacle that we encountered was the MHMDS specification oversight which resulted in admission and legal status data being recorded for the care spell rather than the reporting period. We succeeded in overcoming this problem, and many others, through painstaking workarounds. We have described in detail some of the unforeseen difficulties that we encountered, many of which were concerned with data quality. It should be acknowledged that the HSCIC is primarily resourced to support the reporting of data for healthcare decision-making, rather than research. While it is very assiduous in the curatorship of data, it was rarely able to offer solutions to problems that it had only belatedly become aware of. The HSCIC was particularly reluctant to share the primary data used to derive complex care spell and reporting period variables, and we were compelled to find alternative ways of deriving study outcomes. By linking a small number of data from the 2009/10 MHMDS data set, we were able to establish (and validate) a method for ascertaining if patients had been admitted to inpatient mental health beds and subject to compulsory detention under the MHA (2007) during the study year. This delayed the project somewhat but resulted in estimates of compulsory admission for 2010/11 that were very close to those produced by the HSCIC itself.
Despite the evident strengths of multilevel modelling and MLwiN software, the complexity and size of the models meant that these often took several hours to run, and many proved unstable. We therefore had to be selective and focused in our analysis strategy and we were forced to make a priori judgements about which of the (very large number of) explanatory variables to include at each level. Similarly we were not able, for instance, to undertake univariate tests of association with each of the variables included in the study.
The cross-sectional nature of the study was a further limitation. Although a significant advance on previous ecological research,5 this design makes it difficult to distinguish between reverse causality, (residual) confounding and causation as explanations for the observed associations. Nevertheless, we were able to model variance in the proportion of patients known to mental health services who experienced at least one compulsory admission in 2010/11 using patient-level data, and to estimate associations between this outcome and explanatory variables operating at a range of spatial levels. After linking two annual MHMDS databases (to access important information in relation to a patient’s mental health-care spell in the 2009/10 and 2010/11 reporting periods), we noted important reporting inconsistencies across these periods. We came to the conclusion that the quality of the MHMDS data before 2010/11 was insufficient to permit longitudinal analyses, although this had been part of our original project plan. However, the cross-sectional analyses that we were able to undertake were richer and more complex than expected, by virtue of more extensive data linkage than was originally planned. This enabled us to obtain a clear understanding of the factors influencing the rates of involuntary admissions at different spatial levels.
We did not study use of Section 136 (place of safety) orders or CTOs. Ours was a study of compulsory admission, defined as time spent in an inpatient mental illness bed subject to the MHA (2007). We did not include patients who were detained only under sections of the MHA (2007) that apply only to conveyance to, and assessment in, a place of safety (Sections 135 and 136), or for the purposes of assessment only [e.g. Sections 4 and 5(2)] where detention for longer than 72 hours does not occur. We did not include sections of the Act relating only to guardianship or orders relating to supervision in the community. The reasons for this were twofold: first, because we were interested in compulsory admission and its relationship to aspects of service provision (such as mental illness beds) rather than use of the MHA (2007) per se; and, second, because the MHMDS for 2010/11 would not permit this. The method of recording and classifying compulsory assessment and treatment comprised noting the most restrictive legal status for the reporting period and/or care spell. For this reason, the only people for whom this would be coded as Section 136 (for example) are those seen for assessment and either discharged or admitted voluntarily. There was no way to identify those brought to a place of safety under Section 136 and subsequently admitted under the MHA, since the later section (e.g. Section 2) would have been recorded against LSC and LSR. Although MHMDS did include data on CTOs and their revocation, we did not judge these data to be sufficiently complete or accurate to enable analysis, which was in any event outside the scope of our study aims.
User involvement
User involvement in this project, and especially the contribution of service users and carers through the MHF, was a significant success. This took the form of consultation events rather than qualitative interviews or focus groups, and provided not only great encouragement to the research team but also a context to our work. The very thoughtful comments of both sets of users (potential users of the evidence and users of services) provided nuanced and insightful reflections on wider contextual factors that might explain rising rates of compulsory admission in England. While most of these comments focused on individual- and service-level factors, the (unprompted) reflections of service users on local and contextual (area-level) factors resonated with our finding that a large and significant amount of variance in compulsory admission rates occurs between LSOAs.
The user consultation meetings highlighted a number of themes for further investigation, specifically the effects of what some perceived to be a declining inpatient ‘culture’ (including not just the physical environment but also the difficulty of seeing these as therapeutic spaces in which the object was to discharge patients as soon as possible, leading to higher staff turnover and greater levels of disturbance). Similarly, several professional participants spoke of the primacy of avoiding risk to the decision to admit (and detain), rather than the prospect of effective treatment. Many people (both professional and service users) felt that this made it more likely that someone would be admitted compulsorily, a risk that was further increased by the lack of continuity of care. Most participants agreed that it was very common (and possibly the norm) for MHA assessments to be undertaken by professionals who had never met the patient before. These meetings also highlighted the complexity of the experience of compulsory admission; while few had relished the experience or wanted to experience it again, a number of participants noted that it had been an important (and even life-saving) intervention. The overwhelming feeling, however, was that compulsory admission might not have been necessary had help been provided sooner and/or in a different way. Participants also spoke of avoiding treatment on occasion, often as a result of stigma and sometimes because of earlier adverse experiences of services.
In considering the finding that services with ‘better’ community mental health teams have higher rates of compulsory admission than those where services are rated as poor (‘worse than others’), it is possible that the alternative to high rates of compulsory admission (in some places) is undertreatment, greater individual risk and even more adverse outcomes.
Clinical implications
The increasing (and accelerating) number of compulsory admissions is a major concern. The recent annual review of use of the MHA by the CQC highlighted nationally occurring difficulties in accessing care during a mental health crisis,13 including difficulties accessing effective crisis care in the community, A&E departments and appropriate place of safety settings,14 and bed shortages. 13,18 Fewer beds means delays in admission21 and increased illness severity thresholds for admission. 13 Increasing detention among patients initially admitted voluntarily is worrying, as it suggests that consent is being withdrawn after exposure to inpatient environments. 13
Our findings suggest that bed numbers are not the whole explanation. Although most of the variation in compulsory admission occurred between individuals, we report significant variance (of the order of 12–14% of total variance) between places – including between local areas and between service providers (NHS trusts). 85,86 Relatively little of the variance in rates of compulsory admission were explained by differences in local (commissioner) investment in mental health services. 42 One interpretation of these findings is that gains arising from the development of community mental health services may not be experienced equally. In other words, it is possible that compulsory admission rates may be occurring disproportionately in places where it is more difficult to deliver community treatments, including more deprived, highly urban and/or very remote places. 35
Understanding the effects of local contexts on mental health-care outcomes may be the key to explaining why, despite being increasingly patient-centred, responsive to need, recovery-focused, timely and popular,22,47 transformative developments in community mental health services over the past decade (and longer) have not succeeded in reducing national involuntary admission rates. 10,48–51 It may also account, in part, for persistent ethnic inequalities in the experience of mental health services, and explain why people from BME groups (who tend to reside in socioeconomically deprived urban areas) continue to experience more coercive care pathways, including higher rates of compulsory admission. 13,30,36,37,39,87 These phenomena are mirrored in the results of clinical trials of interventions targeted at individual patients, including joint crisis plans,2,88,89 advance directives53 and CTOs,10,90 which failed to demonstrate significant reductions in compulsory admissions (or indeed time spent in hospital in the case of the Oxford Community Treatment Order Evaluation Trial, OCTET)10,90 despite very promising pilot trial results in one locality. 91 Increasing involuntary treatment has significant cost implications for service providers and commissioners and has been highlighted by the CQC as unacceptable from a regulatory and care standards point of view. 13
Finding ways to reverse this trend is imperative. As well as noting the role of recovery-based care in reducing relapse and crises, the recent Mental Health Crisis Care Concordat highlighted local variation in access to timely crisis resolution services. 14 That report stressed the importance of interagency working, and directed a number of ways in which commissioners (NHS England and clinical commissioning groups), local authorities, police, mental health trusts and voluntary sector agencies should work together to improve outcomes for people with mental health crises. In keeping with this systems-level approach, we suggest that reducing compulsory admissions may be achieved best (and perhaps only) through local partnerships in which those responsible for commissioning and delivering services come together to prioritise and then implement interventions by tailoring these to local circumstances.
Conclusions
Using complete data for England, we found that 3.5% of people using specialist secondary care mental health services experienced at least one compulsory admission in 2010/11, defined as time spent in an inpatient mental illness bed under the MHA (2007). Cross-classified multilevel statistical models revealed that 84.5% of the variance in compulsory admission rates occurred between individuals, 6.7% between local areas (LSOAs), 2.7% between GP practices and 6.9% between provider trusts. All of these estimates of variance were statistically significant.
Although we adjusted for a large number of individual-, area- and provider-level variables (including individual age, sex and ethnicity, area-level deprivation and mental illness bed occupancy), these explained only around 10% of the total variance in the study outcome. People of black ethnicity experienced compulsory admission rates almost three times greater than white patients, and compulsory admission rates increased with area-level deprivation and the proportion of non-white residents in local areas. There was a small but statistically significant association between (higher) bed occupancy and compulsory admission, but this was confounded by adjusting for other study covariates. Despite evidence of a small but significant association between compulsory admission and (greater) investment in community mental health teams, levels of PCT (commissioner) investment in mental health services (whether in total or by specific community) did not add to the overall model fit after adjusting for individual- and area-level characteristics. Thus, although we were able to determine where variance was occurring (mostly between individuals but also between places and service providers), the exact causes of differences in rates of compulsory admission remain largely unexplained.
This was the largest and most complete study of its kind, and the representativeness of the data set was a major strength. Despite the enormous value of the MHMDS as a research resource, there were inevitably limitations in the nature, quality and completeness of the (routine) data that were available to us. Data coding practices made it difficult to establish when periods of admission began and ended, and so we were unable to identify readmissions or to study LOS. The dearth of data regarding the characteristics of individual patients (including diagnosis, ethnicity and socioeconomic status) meant that we were unable to exclude the confounding some of the associations we identified (e.g. with ethnicity and LSOA-level ethnic density). Although these data limitations could not have altered the results of our null models (or estimates of the variance in compulsory admission at the different levels within our analyses), they may explain why we were unable to account for more of this variance in our adjusted models. Finally, we were unable to consider many of the very salient hypotheses suggested to us by the service users and mental health professionals we consulted as part of the project, which have influenced our research recommendations.
Recommendations for future research
We have demonstrated that there is statistically significant (and largely unexplained) variance in compulsory admission between places (areas) and mental health provider trusts. Extending these findings requires mixed methods approaches to (1) undertake longitudinal analysis and (2) generate and test hypotheses about the causes of the observed variance. There is therefore a need for in-depth qualitative research to explore factors that might explain local variation in compulsory admission, for which there are no existing data.
We have confirmed – for the first time – that the MHMDS can be used to address critical research questions about the outcomes of mental health care in England. The development of this data set over the past 18 months (while this study was under way) is likely to have resulted in this excellent resource becoming more user- and researcher-friendly in the meantime. There is cause to believe that within the next 12 months usable longitudinal MHMDS data may be available for the first time, enabling research to be undertaken on chronological trends in use of the MHA. Better and more detailed recording of activity should also help to overcome the problems we faced in identifying distinct treatment episodes (including the number of admissions and compulsory treatment episodes in any given reporting period). This will be undoubtedly be matched by greater complexity of data management, and this will need to be considered when commissioning and resourcing further studies. Nevertheless, MHMDS remains a unique and greatly valued research resource, with huge potential to support future studies.
Specific research questions which should be addressed, using a range of methodologies, include:
-
What are the rates and chronological patterns of repeat compulsory admission, and what proportion of these are accounted for by the same individuals?
-
How do the characteristics of individual patients, especially diagnosis, previous treatment experiences, length of illness, engagement with care and socioeconomic status affect the likelihood of compulsory admission?
-
Given that most of the variance in compulsory admission rates remains unexplained, what effect do other local service characteristics, including the physical, social and therapeutic environments on inpatient units, and continuity of care, have on compulsory admission rates?
-
To what extent do longitudinal trends in compulsory admission rates vary between places? Are there areas and health-care providers where the rates of increase has been halted or reversed, and are there places where rates are accelerating? Can these differences be explained?
-
What effect do CTOs have on compulsory admission rates over the long term, and can longitudinal analysis reveal any evidence that these result in fewer patients being readmitted under the MHA (2007)?
-
Around 15% of the variance in compulsory admission occurs between places, mental health-care providers, GP practices and commissioners. In addition, some of the individual-level variance may reflect personal experience of local services. Is it feasible to develop and deliver interventions at the level of local health-care systems that could reduce compulsory admissions, and would this prove effective?
There is also an imperative to move towards developing and evaluating interventions to reduce compulsory admission rates, but this will depend in part on further mixed-methods research designed to further our understanding of the reasons why these rates remain so high.
Acknowledgements
This report presents independent research commissioned by the National Institute for Health Research (NIHR). The views and opinions expressed by authors in this publication are those of the authors and do not necessarily reflect those of the NHS, NIHR, NIHR Evaluation, Trials and Studies Coordinating Centre (NETSCC), Health Services and Delivery Research (HS&DR) programme or Department of Health. The views and opinions expressed by the PPI participants in this publication are those of the interviewees and do not necessarily reflect those of the authors or those of the NHS, NIHR, NETSCC, HS&DR programme or Department of Health. This project was funded by the NIHR HS&DR programme (project number 10/1011/70). We are grateful to Paddy Cooney from the Mental Health Network (NHS Confederation) for organising and cofacilitating the professionals’ consultation meeting.
Contribution of authors
Scott Weich was the Chief Investigator and guarantor of the research. He had overall responsibility for the design, delivery and interpretation of the study findings, as well as research governance and data security. He chaired project team meetings and attended (and co-led) user consultation events. He was responsible for the final version of this report.
Orla McBride undertook all of the study analyses, drafted interim and final study reports and contributed fully to the interpretation of the study findings and their reporting.
Liz Twigg provided health geography and multilevel statistical modelling expertise, and advised OM on data linkages and analyses. She contributed to the management of the study, interpretation and analysis of results, and the drafting of the study reports.
Patrick Keown, Kamaldeep Bhui and Jan Scott contributed to the design of the study, attended project team meetings, commented on and contributed to the interpretation of results throughout the project, and commented in detail on study reports.
Helen Parsons was the study statistician and advised on data analysis. She attended project meetings and the service user consultation meetings, and contributed to interpretation and reporting of study findings.
David Crepaz-Keay was responsible for coordinating and leading the mental health service user consultation throughout the project. Together with Eva Cyhlarova he attended all project team meetings, drafted the report of the service user involvement work and contributed to the interpretation and reporting of the study findings.
Disclaimers
This report presents independent research funded by the National Institute for Health Research (NIHR). The views and opinions expressed by authors in this publication are those of the authors and do not necessarily reflect those of the NHS, the NIHR, NETSCC, the HS&DR programme or the Department of Health. If there are verbatim quotations included in this publication the views and opinions expressed by the interviewees are those of the interviewees and do not necessarily reflect those of the authors, those of the NHS, the NIHR, NETSCC, the HS&DR programme or the Department of Health.
References
- Priebe S, Badesconyi A, Fioritti A, Hansson L, Kilian R, Torrez-Gonzales F, et al. Reinstitutionalisation in mental health care: comparison of data on service provision from six European countries. BMJ 2005;330:123-6. http://dx.doi.org/10.1136/bmj.38296.611215.AE.
- Thornicroft G, Farrelly S, Szmukler G, Birchwood M, Waheed W, Flach C, et al. Clinical outcomes of Joint Crisis Plans to reduce compulsory treatment for people with psychosis: a randomised controlled trial. Lancet 2013;381:1634-41. http://dx.doi.org/10.1016/S0140-6736(13)60105-1.
- Wall S, Hotopf M, Wessely S, Churchill R. Trends in the use of the Mental Health Act: England, 1984–96. BMJ 1999;318:1520-1. http://dx.doi.org/10.1136/bmj.318.7197.1520.
- Hotopf M, Wall S, Buchanan A, Wessely S, Churchill R. Changing patterns in the use of the Mental Health Act 1983 in England, 1984–1996. Br J Psychiatry 2000;176:479-84. http://dx.doi.org/10.1192/bjp.176.5.479.
- Keown P, Weich S, Bhui KS, Scott J. Association between provision of mental illness beds and rate of involuntary admissions in the NHS in England 1988–2008: ecological study. BMJ 2011;343. http://dx.doi.org/10.1136/bmj.d3736.
- Salize JH, Dressing H. Epidemiology of involuntary placement of mentally ill people across the European Union. Br J Psychiatry 2004;184:163-8. http://dx.doi.org/10.1192/bjp.184.2.163.
- Lay B, Salize H, Dressing H, Rusch N, Schonenberger T, Buhlmann M, et al. Preventing compulsory admission to psychiatric inpatient care through psycho-education and crisis focused monitoring. BMC Psychiatry 2012;12. http://dx.doi.org/10.1186/1471-244X-12-136.
- Mental Health Act 2007. London: The Stationery Office; 2007.
- In-Patients Formally Detained in Hospitals under the Mental Health Act 1983 and Patients Subject to Supervised Community Treatment, Annual Figures, England 2009/10. Leeds: Health and Social Care Information Centre; 2010.
- Burns T, Rugkåsa J, Molodynski A, Dawson J, Yeeles K, Vazquez-Montes M, et al. Community treatment orders for patients with psychosis (OCTET): a randomised controlled trial. Lancet 2013;381:1627-33. http://dx.doi.org/10.1016/S0140-6736(13)60107-5.
- Inpatients Formally Detained in Hospitals under the Mental Health Act 1983, and Patients Subject to Supervised Community Treatment: Annual Report, England, 2013. London: Government Statistical Service; 2013.
- Post-Legislative Scrutiny of the Mental Health Act 2007: First Report of Session 2013–14. London: House of Commons; 2013.
- Monitoring the Mental Health Act in 2012/13. Newcastle upon Tyne: Care Quality Commission; 2014.
- Improving Outcomes for People Experiencing Mental Health Crisis. London: HM Government; 2014.
- Johnson S. Can we reverse the rising tide of compulsory admissions?. Lancet 2013;381:1603-4. http://dx.doi.org/10.1016/S0140-6736(13)60442-0.
- van der Post LFM, Peen J, Dekker JJM. A prediction model for the incidence of civil detention for crisis patients with psychiatric illnesses; the Amsterdam study of acute psychiatry VII. Soc Psychiatry Psychiatr Epidemiol 2013;49:283-90.
- van der Post LFM, Cornelis L, Mulder CL, Peen J, Visch I, Dekker J, et al. Social support and risk of compulsory admission: part IV of the Amsterdam Study of Acute Psychiatry. Psychiatr Serv 2012;63:577-83. http://dx.doi.org/10.1176/appi.ps.201100080.
- Davidge M, Elias S, Jayes B, Wood K, Yates J. Survey of English Mental Illness Hospitals. Birmingham: Health Service Management Centre; 1993.
- Falling Drug Use: The Impact of Treatment. London: National Health Service; 2013.
- Keown P, Mercer G, Scott J. A retrospective analysis of hospital episode statistics, involuntary admissions under the Mental Health Act 1983, and the number of psychiatric beds in England 1996–2006. BMJ 2008;337.
- Bindman J, Tighe J, Thornicroft G, Leese M. Poverty, poor services, and compulsory psychiatric admission in England. Soc Psychiatry Psychiatr Epidemiol 2002;37:341-5. http://dx.doi.org/10.1007/s00127-002-0558-3.
- Weich S, Griffith L, Commander M, Bradby H, Sashidharan SP, Pemberton S, et al. Experiences of acute mental health care in an ethnically diverse inner city: qualitative interview study. Soc Psychiatry Psychiatr Epidemiol 2012;47:119-28. http://dx.doi.org/10.1007/s00127-010-0314-z.
- The Pathway to Recovery: A Review of NHS Acute Inpatient Mental Health Services. London: Healthcare Commission; 2008.
- Future Perfect? Outlining an Alternative to the Pain of Psychiatric In-Patient Care. Kingston upon Thames: Rethink Severe Mental Illness; 2005.
- Saxena S, Thornicroft G, Knapp M, Whiteford H. Resources for mental health: scarcity, inequity, and inefficiency. Lancet 2007;370:878-89. http://dx.doi.org/10.1016/S0140-6736(07)61239-2.
- Mental Health Atlas. Geneva: WHO; 2005.
- 2009/10 National Survey of Investment in Adult Mental Health Services. London: Department of Health; 2010.
- Count Me In: Results of a National Census of Inpatients in Mental Health Hospitals and Facilities in England and Wales. London: Commission for Healthcare Audit and Inspection; 2005.
- Count Me In 2007: Results of the 2007 National Census of Inpatients in Mental Health and Learning Disability Services in England and Wales. London: Commission for Healthcare Audit and Inspection; 2007.
- Count Me In 2008: Results of the 2008 National Census of Inpatients in Mental Health and Learning Disability Services in England and Wales. London: Commission for Healthcare Audit and Inspection; 2008.
- Bhui K, Stansfeld S, Hull S, Priebe S, Mole F, Feder G. Ethnic variations in pathways to and use of specialist mental health services in the UK. Br J Psychiatry 2003;182:105-16. http://dx.doi.org/10.1192/bjp.182.2.105.
- Breaking the Circles of Fear: A Review of the Relationship between Mental Health Services and the African and Caribbean Communities. London: SCMH; 2002.
- Morgan C, Mallett R, Hutchinson G, Leff J. Negative pathways to psychiatric care and ethnicity: the bridge between social science and psychiatry. Soc Sci Med 2004;58:739-52. http://dx.doi.org/10.1016/S0277-9536(03)00233-8.
- Commander MJ, Sashidharan SP, Odell SM, Surtees PG. Access to mental health services in an inner city health district. II: associations with demographic factors. Br J Psychiatry 1997;170:317-20. http://dx.doi.org/10.1192/bjp.170.4.317.
- Singh SP, Burns T, Tyrer P, Islam Z, Parsons H, Crawford MJ. Ethnicity as a predictor of detention under the Mental Health Act. Psychol Med 2014;44:997-1004. http://dx.doi.org/10.1017/S003329171300086X.
- Morgan C, Mallet R, Hutchinson G, Bagalkote H, Morgan K, Fearon P, et al. Pathways to care and ethnicity. I: sample characteristics and compulsory admission. Br J Psychiatry 2005;186:281-9. http://dx.doi.org/10.1192/bjp.186.4.281.
- Commander MJ, Cochrane R, Sashidharan SP, Akilu F, Wildsmith E. Mental health care for Asian, black and white patients with non-affective psychoses: pathways to psychiatric hospital, in-patient and aftercare. Soc Psychiatry Psychiatr Epidemiol 1999;34:484-91. http://dx.doi.org/10.1007/s001270050224.
- Delivering Race Equality in Mental Health Services: An Action Plan for Reform Inside and Outside Services. London: : Department of Health; 2005.
- Appleby L. Services for ethnic minorities: a question of trust. Psychiatr Bull 2008;32:401-2. http://dx.doi.org/10.1192/pb.bp.107.018895.
- Inside Outside: Improving Mental Health Services for Black and Minority Ethnic Groups in England. Leeds: Department of Health; 2003.
- Appleby L. The National Service Framework for Mental Health: Five Years On. London: Department of Health; 2004.
- Appleby J, Gregory S. NHS Spending. Local Variations in Priorities: An Update. London: The King’s Fund; 2008.
- Katsakou C, Rose D, Amos T, Bowers L, McCabe R, Oliver D, et al. Psychiatric patients’ views on why their involuntary hospitalisation was right or wrong: a qualitative study. Soc Psychiatry Psychiatr Epidemiol 2012;47:1169-79. http://dx.doi.org/10.1007/s00127-011-0427-z.
- Jankovic J, Yeeles K, Katsakou C, Amos T, Morriss R, Rose D, et al. Family caregivers’ experiences of involuntary psychiatric hospital admissions of their relatives: a qualitative study. PLOS ONE 2011;6. http://dx.doi.org/10.1371/journal.pone.0025425.
- Weich S. Availability of inpatient beds for psychiatric admissions in the NHS. BMJ 2008;337:970-1. http://dx.doi.org/10.1136/bmj.a1561.
- Kallert TW, Glockner M, Schutzwohl M. Involuntary vs. voluntary hospital admission: a systematic literature review on outcome diversity. Eur Arch Psychiatry Clin Neurosci 2008;258:195-209. http://dx.doi.org/10.1007/s00406-007-0777-4.
- Holloway F. ‘Gentlemen, we have no money therefore we must think’: mental health services in hard times. Psychiatrist 2011;35:81-3. http://dx.doi.org/10.1192/pb.bp.110.030627.
- Dean C, Phillips J, Gadd E, Joseph M, England S. Comparison of a community based service with a hospital based service for people with acute, severe psychiatric illness. BMJ 1993;307:642-6. http://dx.doi.org/10.1136/bmj.307.6902.473.
- Glover G, Arts G, Babu KS. Crisis resolution/home treatment teams and psychiatric admission rates in England. Br J Psychiatry 2006;189:441-5. http://dx.doi.org/10.1192/bjp.bp.105.020362.
- Craig TKJ, Garety P, Power P, Rahaman N, Colbert S, Fornells-Ambrojo M, et al. The Lambeth Early Onset (LEO) Team: randomised controlled trial of the effectiveness of specialised care for early psychosis. BMJ 2004;329:1067-71. http://dx.doi.org/10.1136/bmj.38246.594873.7C.
- Johnson S, Nolan F, Pilling S, Sandor A, Hoult J, McKenzie N, et al. Randomised controlled trial of acute mental health care by a crisis resolution team: the north Islington crisis study. BMJ 2005;331:586-90. http://dx.doi.org/10.1136/bmj.38519.678148.8F.
- Flood C, Byford S, Henderson C, Leese M, Thornicroft G, Sutherby K, et al. Joint crisis plans for people with psychosis: economic evaluation of a randomised controlled trial. BMJ 2006;333. http://dx.doi.org/10.1136/bmj.38929.653704.55.
- Campbell LA, Kisely SR. Advance treatment directives for people with severe mental illness. Cochrane Database Syst Rev 2009;1. http://dx.doi.org/10.1002/14651858.CD005963.pub2.
- Mental Health Bulletin: Fifth Report from Mental Health Minimum Dataset (MHMDS) Annual Returns, 2011. Leeds: Health and Social Care Information Centre; 2011.
- National Dataset Services: Draft Mental Health Minimum Dataset (MHMDS) (MHMDS) v3.0 Specification and Guidance. Leeds: NHS Information Centre for Health and Social Care; 2008.
- The Health of the Nation: A Strategy for Health in England. London: HMSO; 1992.
- Glover G. Adult mental health care in England. Eur Arch Psychiatry Clin Neurosci 2007;257:71-82. http://dx.doi.org/10.1007/s00406-006-0694-y.
- The Mental Health Minimum Dataset v4.1. Leeds: Information Standards Board for Health and Social Care; 2012.
- MHMDS Statistics: Data Quality and Methodology. Leeds: Health and Social Care Information Centre; 2011.
- Mental Health Act 1983. London: The Stationery Office; 1983.
- Care Standard Act 2000. London: The Stationery Office; 2000.
- The English Indices of Deprivation 2010: Neighbourhoods, Statistical Release. London: Department for Communities and Local Government; 2011.
- Office for National Statistics . 2011 Census: Population and Household Estimates for England and Wales, March 2011 2012. www.ons.gov.uk/ons/rel/census/2011-census/population-and-household-estimates-for-england-and-wales/stb-e-w.html (accessed 28 October 2014).
- Office for National Statistics . Super Output Areas 2011 n.d. www.ons.gov.uk/ons/guide-method/geography/beginner-s-guide/census/super-output-areas--soas-/index.html (accessed 28 October 2014).
- Department of Health . KH03 Quarterly Bed Availability and Occupancy UNIFY2 Non-DCT Provider Collection: Data Definitions 2010. www.england.nhs.uk/statistics/wp-content/uploads/. /dh_124212-5.doc (accessed 28 October 2014).
- Mental Health Acute Inpatient Service Users Survey, 2009. Colchester: UK Data Archive; 2010.
- Care Quality Commission . 2009 Mental Health Inpatient Survey: ‘Experience of Patients’ Performance Indicator Calculation 2009 n.d. www.nhssurveys.org/surveys/520 (accessed 28 October 2014).
- Mental Health Trusts: Community Mental Health Service User Survey, 2010. Colchester: UK Data Archive; 2010.
- Care Quality Commission . Mental Health Trusts: Community Mental Health Service User Survey, 2010: NHS National Patient Survey Programme: Data Weighting Issues 2011 n.d. www.nhssurveys.org/surveys/606 (accessed 28 October 2014).
- Fielding A, Goldstein H. Cross-Classified and Multiple Membership Structures in Models: An Introduction and Review. Research Report RR791 for DfES. London: Department for Education and Skills; 2006.
- Goldstein H, Rasbash J. Improved approximations for multilevel models with binary responses. J R Stat Soc Ser A 1996;159:505-13. http://dx.doi.org/10.2307/2983328.
- Raftery AE, Lewis SM. One long run with diagnostics: implementation strategies for Markov chain Monte Carlo. Stat Sci 1992;7:493-7. http://dx.doi.org/10.1214/ss/1177011143.
- Spiegelhalter D, Best N, Carlin B, van der Linde A. Bayesian measures of model complexity and fit. J R Stat Soc Ser B 2002;64:583-639. http://dx.doi.org/10.1111/1467-9868.00353.
- An Introduction to MCMC Methods and Bayesian Statistics. Bristol: University of Bristol; 2012.
- Schwartz G. Estimating the dimension of a model. Ann Stat 1978;6:461-4. http://dx.doi.org/10.1214/aos/1176344136.
- Akaike H. Factor analysis and the AIC. Psychometrika 1987;52:317-32. http://dx.doi.org/10.1007/BF02294359.
- Software BUGS. Advanced Use of BUGS Language 2009 n.d. www://openbugs.net/w/FrontPage (accessed 28 October 2014).
- Snijders TA, Bosker RJ. Introduction to Multilevel Analysis. London: Sage; 1999.
- Little RJA, Rubin DB. Statistical Analysis with Missing Data. New York, NY: Wiley; 1987.
- Cohen J, Cohen P. Applied Multiple Regression and Correlation Analysis for the Behavioral Sciences. Hillsdale, NJ: Erlbaum; 1985.
- Clarke P. When can group level clustering be ignored? Multilevel models versus single-level models with sparse data. J Epidemiol Community Health 2008;62:752-8. http://dx.doi.org/10.1136/jech.2007.060798.
- Rasbash J, Steele F, Browne WJ, Goldstein H. A User’s Guide to MLwiN, v2.26. Centre for Multilevel Modelling: University of Bristol; 2012.
- Johnson S, Gilburt H, Lloyd-Evans B, Osborn DPJ, Boardman J, Leese M, et al. In-patient and residential alternatives to standard acute psychiatric wards in England. Br J Psychiatry 2009;194:456-63. http://dx.doi.org/10.1192/bjp.bp.108.051698.
- Morant N, Lloyd-Evans B, Gilburt H, Slade M, Osborn D, Johnson S. Implementing successful residential alternatives to acute in-patient psychiatric services: lessons from a multi-centre study of alternatives in England. Epidemiol Psychiatr Sci 2012;21:175-85. http://dx.doi.org/10.1017/S204579601100076X.
- Wierdsma AI, Mulder CL. Does mental health service integration affect compulsory admissions?. Int J Integr Care 2009;9.
- In-Patients Formally Detained in Hospitals under the Mental Health Act, 1983, and Patients Subject to Supervised Community Treatment, Annual Figures, England 2010/11. Leeds: Health and Social Care Information Centre; 2011.
- Raleigh VS, Irons R, Hawe E, Scobie S, Cook A, Reeves R, et al. Ethnic variations in the experience of mental health service users in England. Br J Psychiatry 2007;191:304-12. http://dx.doi.org/10.1192/bjp.bp.106.032417.
- Thornicroft G, Farrelly S, Birchwood M, Marshall M, Szmukler G, Waheed W, et al. CRIMSON [CRisis plan IMpact: Subjective and Objective coercion and eNgagement] protocol: a randomised controlled trial of joint crisis plans to reduce compulsory treatment of people with psychosis. Trials 2010;11. http://dx.doi.org/10.1186/1745-6215-11-102.
- Barrett B, Waheed W, Farrelly S, Birchwood M, Dunn G, Flach C, et al. Randomised controlled trial of joint crisis plans to reduce compulsory treatment for people with psychosis: economic outcomes. PLOS ONE 2013;8. http://dx.doi.org/10.1371/journal.pone.0074210.
- Burns T, Molodynski A. Community treatment orders: background and implications of the OCTET trial. Psychiatrist 2014;38:3-5. http://dx.doi.org/10.1192/pb.bp.113.044628.
- Sutherby K, Szmukler GI, Halpern A, Alexander M, Thornicroft G, Johnson C, et al. A study of ‘crisis cards’ in a community psychiatric service. Acta Psychiatr Scand 1999;100:56-61. http://dx.doi.org/10.1111/j.1600-0447.1999.tb10914.x.
- Dyer C. Psychiatrists are concerned by rise in patients subject to compulsory mental health treatment. BMJ 2010;341. http://dx.doi.org/10.1136/bmj.c5596.
- Gould M. Mental health patients complain of ‘zombification’. The Guardian 2011.
- Snijders TAB, Bosker RJ. Multilevel Analysis: an Introduction to Basic and Advanced Multilevel Modeling. London: Sage; 1999.
- Fourth Report from Mental Health Minimum Dataset (MHMDS) Annual Returns, 2010. London: Health and Social Care Information Centre; 2011.
- Goldstein H. Multilevel Statistical Models. London: Edward Arnold; 1995.
- Browne WJ. MCMC Estimation in MLwiN v2.1. Centre for Multilevel Modelling: University of Bristol; 2009.
Appendix 1 Original study protocol
Full title of project
Understanding the Increasing Rate of Involuntary Admissions in NHS Mental Health Care.
Aims and objectives
Involuntary admissions under the Mental Health Act in England have increased dramatically in the last 20 years, and this coincides with an equally stark reduction in mental health bed numbers. It is also recognised that people from black and minority ethnic communities are consistently over-represented among those detained in psychiatric hospitals and in secure settings. Bed reductions may disproportionately affect their detention rates as well as the detention rates of other groups that share the same mediating characteristics, for example residing in urban and/or deprived areas.
Our aims will be to explore variation between places in England in rates of involuntary admission and to identify factors that might explain this, including socio-economic context (e.g. area deprivation), features of local services (including level of investment by commissioners), and characteristics of service users (especially socio-economic status and ethnicity). Interpretation of the study findings and their translation into actionable conclusions for the NHS will be aided by consultation with mental health service users and senior NHS managers.
Research questions
-
How variable are rates of involuntary admission across different places, namely Strategic Health Authorities (SHAs), NHS Trusts and independent sector hospitals, and Primary Care Trusts (PCTs) in England?
-
To what extent is this variance explained by local factors, such as small area socio-economic deprivation, urbanicity, ethnic density, PCT investment in mental health services, and by characteristics of local mental health services including the availability and occupancy of NHS mental illness beds?
-
To what extent is variance in rates of involuntary admission explained by local factors, such as small area socio-economic deprivation, urbanicity, ethnic density, PCT investment in mental health services, and by features of local mental health services and the experiences of those who use them?
-
To what extent is variance in rates of involuntary admission explained by the characteristics of people using services, including socio-economic status and ethnicity?
-
Is there evidence of geographical variation in the use of Community Treatment Orders (CTOs), and if so, how is this associated with bed numbers and occupancy, length of stay, area-level factors (like deprivation and PCT investment), experience and quality of local services, and rates of involuntary admission?
-
Using the model of best fit based on local services and settings and including historical data on trends in bed numbers, can we predict the future need for involuntary treatment with sufficient accuracy to support commissioners and NHS managers in determining optimal local bed numbers?
Background
See Final Study Protocol.
How the proposed research will add to the body of knowledge with reference to current NHS policy and practice
See Final Study Protocol.
Importance of the proposed research
See Final Study Protocol.
Research methods
Design
Secondary analysis of routinely collected data. Data will be organised to permit analysis as cross-sectional survey, cohort and (nested) case control studies.
Data sources
Most of the data for the proposed study will be obtained from the NHS Information Centre (http://www.ic.nhs.uk), which is responsible for collecting and collating national health and social care data in the UK. The Information Centre also provides support and advice to end users through its Secondary Uses Service (SUS) and Medical Research Information Service (http://www.connectingforhealth.nhs.uk/systemsandservices/sus and http://www.ic.nhs.uk/services/medical-research-information-service), in partnership with NHS Connecting for Health. The NHS Information Centre ensures appropriate governance checks for the use of these data, to protect the confidentiality of those on whom data is collected, through the National information Governance Board for Health and Social Care (NIGB). The NHS Information Centre also collates geographical data, including deprivation and other neighbourhood statistics, and provides support in ensuring appropriate linkage to other data sets (http://www.ic.nhs.uk/services/population-geography-information/geographical-information).
The data archive at the NHS Information Centre includes historic information on geographic boundaries and organisation changes in the NHS, which will enable us to track this over time and to make adjustments for changes over time in the configuration of boundaries relating to the regulation, commissioning and delivery of NHS services. One example of this is the PCT mapping tool (http://www.ic.nhs.uk/statistics-and-data-collections/population-and-geography/pct-mapping-tool), which enables older service use data to be correctly mapped onto current PCT boundaries (http://www.statistics.gov.uk/geography/england_health.asp).
The significant investment on the part of the NHS in software resources and staffing to support secondary users through the NHS Information Centre represents a major strength of the proposed research. Although the proposed data linkages will be methodologically challenging, we are confident that the study aims are achievable.
The data sets which we propose to access and link are set out below in Table 9, followed by more detailed descriptions about each of these. Outcomes will be ascertained using the first two of these data sets (Mental Health Minimum Dataset and Hospital Episode Statistics); the remainder will be used to provide exposure (risk factor) information via data linkage. The data on In-Patients Formally Detained in Hospital will be used to validate data on involuntary admissions in MHMDS and HES, and to generate study weights if necessary.
Dataset | Key variables | Smallest data level | Level of proposed linkage | Available from |
---|---|---|---|---|
Mental Health Minimum Dataset (MHMDS) | Patients admitted involuntarily; patient characteristics inc ethnicity and SES; HoNOS scores | Individual | PCT | 2007/8 |
Hospital Episode Statistics (HES) | All admissions, inc involuntary admissions; diagnosis, length of stay; patient characteristics inc ethnicity and SES | Individual | PCT | 1998/99 |
In-Patients Formally Detained in Hospital (IPFDH) | All in-patient episodes involving MHA; type of section; whether admitted voluntarily then detained; CTOs (from 2008/9). To be used to validate outcomes from MHMDS and HES | Provider (Trust or independent hospital) | Provider | 1998/99 |
Beds Open Overnight (BOO) previously Hospital Activity Statistics | Bed numbers inc availability and occupancy | Provider (Trust or independent hospital) | Provider | 2000/1 |
Community Mental Health Activities (CMHA) | Contacts with community services, esp. crisis teams, assertive outreach and early intervention services | NHS Trust | Provider (NHS Trust) | 2003/4 |
National Patient Survey (NPS) | Patient experiences of and satisfaction with care; patient choice | Provider (NHS Trust) | Provider (NHS Trust) | 2004/5 |
Investment in Mental Health Services (IMHS) | Spend per person on mental health care for adults of working age | PCT | PCT | 2003/4 |
Census & other geographical data | Area-level features (e.g. urbanicity, socio-economic deprivation) and population composition | Census output area, electoral ward, local authority district or PCT | PCT | 2001 (inc. mid-year estimates) |
1. Mental Health Minimum Dataset (MHMDS)
The Mental Health Minimum Dataset will be our primary data source for the main cross-sectional analyses in this study, by virtue of the detail recorded at the individual patient level. Although (limited) MHMDS data are available from 2003, comprehensive data on service use are available from 2007/8 to present. This includes anonymised record-level (i.e. individual patient-level) data about adults and older people using secondary mental health services. The Mental Health Minimum Data Set describes Adult Mental Health Care Spells, and is based on quarterly returns from NHS Trusts. MHMDS includes records of voluntary and involuntary in-patient admission, out-patient attendances, day treatment and all other episodes of care. The MHMDS data is reported quarterly and describes complete spells of care for an individual, from initial referral to final discharge.
Rather than counting uses of the Mental Health Act, MHMDS counts people subject to the Act and provides opportunity for analysis by age and ethnic group. Such information is not part of the collection that provides the source data for the National Statistics (known as KP90), which is reported in In-Patients Formally Detained in Hospital, IPFDH (see below). Involuntary admissions are denoted by recording legal status at end of reporting period, coded using the most restrictive legal status (i.e. whether detained or treated informally) that applied during the (quarterly) reporting period. Community Treatment Orders and their revocation and recall to hospital are also recorded in MHMDS, and we will validate this data against IPFDH. A unique patient identifier (the MHMDS ID) is attached to each record making it possible to link multiple spells of care for an individual patient across different reporting periods and different providers. This process will also ensure that an involuntary admission spanning two or more reporting periods is not double counted when data are aggregated for the whole year. MHMDS also includes geographic identifiers (Trust or hospital, PCT and electoral ward of residence) and patient-level data on socio-economic status (employment, accommodation status), ethnicity, diagnosis and Health of the National Outcome Scale (HoNOS) ratings (a validated measure of clinical status).
We will use MHMDS data for 4 years (2007/8 through 2010/11). We will seek NHS Research Ethics approval for our research, and will apply to the NHS Information Centre through its Security and Confidentiality Advisory Group for access to anonymised patient-level MHMDS data. Data from independent sector providers will be available for the first time with the 2010/11 MHMDS release in January 2012. The advantage of MHMDS over other data sets, and especially Hospital Episode Statistics (HES), is that it comprises all episodes of mental health treatment regardless of setting. HES is restricted to in-patient admissions and hospital out-patient attendances but does not include use of community mental health services.
2. Hospital Episode Statistics (HES)
HES contains details of all admissions (including those under the Mental Health Act) to NHS hospitals and independent hospitals in England funded by the NHS. HES also contains details of all NHS outpatient appointments in England and is available from 1998 to the present.
HES comprises patient-level records and contains data on diagnosis, length of stay, age, gender and ethnicity. It also includes information about where treatment was delivered and place of residence. We will seek NHS Research Ethics approval for our research, and will apply to the NHS Information Centre through its Security and Confidentiality Advisory Group for access to anonymised, patient-level HES data. We shall also seek access to hospital admission data aggregated at the level of Census Outputs Areas as well as PCTs. This will enable linkage to other data sources, particularly the Mental Health Minimum Dataset (MHMDS). One major advantage of HES data is the length of time over which these have been collected. This will enable us to model trajectories of admissions and detentions by place over time, and to explore the extent to which these patterns are explained by individual- and area-level characteristics.
3. In-Patients Formally Detained in Hospital (IPFDH)
This data set includes information about all admissions to hospital under the Mental Health Act (MHA) and detentions after admission. This includes admission to in-patient units managed by NHS Trusts (including medium secure forensic units), the three high security ‘special’ psychiatric hospitals (Broadmoor, Rampton and Ashworth), and independent hospitals as defined by the Care Standards Act 2000. IPFDH is based on KP90 returns from providers and includes data on Supervised Community Treatment Orders (CTOs), which were introduced in November 2008 following changes to the Mental Health Act in 2007. Data is available by type of section, reason for detention (mental illness versus mental impairment) and by change of status (i.e. informal admission followed by detention). Data in IPFDH (e.g. total number of detentions per annum) are aggregated at the level of mental health service provider: http://www.ic.nhs.uk/pubs/inpatientdetmha0910.
Since they are based on official returns from all providers, these data are probably the most accurate in the recording of involuntary admissions. They will therefore be used to validate the data found in HES and MHMDS. The latter may prove the least accurate, since legal status is recorded only once for each reporting period, possibly resulting in an under-estimate of the total number of involuntary admissions. We will explore using findings from IPFDH to weight data obtained from HES and MHMDS, should differences in reported rates of involuntary admission be identified between these data sets.
4. Beds Open Overnight (BOO)
The number of available and occupied NHS mental illness and learning disability beds are available via the Department of Health, and comprise aggregated quarterly KH03 returns by each provider. These data are available from 2000, and were previously known as Hospital Activity Statistics: http://www.dh.gov.uk/en/Publicationsandstatistics/Statistics/Performancedataandstatistics/Beds/index.htm.
5. Community Mental Health Activities (CMHA)
In 2003 the Department of Health began collecting information regarding community mental health teams via UNIFY2. The scope of this data set was later altered (in 2009) to concentrate on the activity of specialist community mental health services, specifically Crisis Resolution Home Treatment, Early Intervention and Assertive Outreach teams. The quarterly CMHA data set also includes information about follow-up of patients admitted to hospital under the Care Programme Approach (CPA). These data are aggregated at provider level but also include information about patient sex and ethnicity. This data set will provide an invaluable barometer of the activity of those community services that are viewed as critical to the acute care pathway in adult mental health services: http://www.ic.nhs.uk/services/omnibus-survey/using-the-service/data-collections/community-mental-health-activities.
6. National Patient Survey (NPS)
The NHS Patient Surveys are coordinated by Picker Institute Europe on behalf of the Care Quality Commission (http://www.nhssurveys.org) and the data are available via the UK Data Archive (http://www.data-archive.ac.uk). Surveys of community mental health service users were undertaken from 2004 to 2008, and again in 2010 and 2011. A survey of users of in-patient mental health services was undertaken in 2009. Data were collected by questionnaire from between 17,000 and 27,000 users of community mental health services at each survey, and from around 7,500 users of in-patient services. The latter highlighted service users’ concerns about a lack of safety on some wards. Both community and in-patient surveys include data on experiences of care, involvement in decision-making, perceived quality of relationships with professionals, treatments offered and satisfaction with care. Recent surveys have collected data on service users’ ethnicity. These data are aggregated at provider level, enabling linkage with other data sets.
7. Investment in Mental Health Services (IMHS)
The Department of Health sponsors the annual National Survey of Investment in Adult Mental Health Services, an annual finance mapping exercise regarding spending on mental health services in England. Data are available for each year since 2003, and provide details of investment in mental health services for working age adults (aged 18-64) in England, by PCT (commissioner). Previous years’ investment have been adjusted by the GDP Deflator Figures for 31 March 2010 published by HM Treasury to reflect past investment at today’s prices: http://www.dh.gov.uk/en/Publicationsandstatistics/Publications/PublicationsPolicyAndGuidance/DH_117488.
8. Census and other geographical data
We will use area-level deprivation indices to characterise the places in which services are delivered. We will use population statistics (mostly derived from the UK census) to estimate ethnic composition) and population density for different areas, and across different spatial levels as determined by the other data sets to which we will establish linkages. The Office for National Statistics provides details on urbanicity via the England and Wales Rural/Urban definition (http://www.ons.gov.uk/about-statistics/geography/products/area-classifications/rural-urban-definition-and-la-classification/rural-urban-definition/index.html.
Participants
See Final Study Protocol.
Setting
See Final Study Protocol.
Primary outcome
See Final Study Protocol.
Data analysis
We will undertake both cross-sectional and longitudinal analyses, and both will require significant data linkage between multiple data sets. This linkage will take place at the smallest possible spatial scale: as indicated above, in our description of the data sets to be used, the most common linkages will be by provider Trust and (commissioning) PCT. The primary statistical technique employed will be multilevel modelling, which mirrors the structure of the data to be analysed and allows explanation to be correctly assigned to the various ‘levels’ within the data set (i.e. individual or area) and is therefore a widely used method for analysing nested (or clustered) data.
Strategy of analysis
1. Cross-sectional multilevel analysis using patient-level data at the lowest level. MHMDS will be used for this (rather than Hospital Episode Statistics) since the data comprise information about all those using mental health services rather than just those admitted to hospital. The reference group will therefore be those receiving mental health care but not admitted to hospital involuntarily. This will have the advantage of adjusting for the overall prevalence of mental illness in particular areas, and for the accessibility of mental health services generally (36). This is not the case for HES data, where the reference population would be those using hospital services (in-patients admitted voluntarily and those seen in hospital out-patient clinics) but not including those accessing community mental health services. We will explore the possibility of pooling two years’ worth of MHMDS data to optimise the sample size.
Data from other sources will be linked at the appropriate level, e.g. by PCT and/or Trust. This will enable us (for example) to explore the effects of age, sex, ethnicity (at the individual level), bed numbers (and types of available beds), overall bed occupancy rates, average length of in-patient stay (at Trust level), investment in services (at PCT level) and socio-economic deprivation, urbanicity and ethnic composition (at area level) on the likelihood that a user of mental health services will be admitted to hospital involuntarily. As well as assessing the main effects of these risk factors, we will explore variance at different spatial scales and test for cross-level interactions, ie the extent to which associations vary between places.
2. Cross-sectional multi-level analysis using aggregated involuntary admissions by PCT. The data set of choice for this analysis will be Hospital Episode Statistics (HES) for 2009/10, validated against data for the same year from In-Patients Formally Detained in Hospital (IPFDH) (and weighted if necessary). IPFDH data are obtained from providers in statutory returns. HES is preferred to IPFDH for these analyses because data can be aggregated at PCT (and even Census Output Area) level rather than provider (Trust); and HES is preferred to MHMDS because of its more detailed recording of involuntary in-patient spells, and because it contains information about numbers of in-patient episodes and length of stay. Unlike MHMDS, HES distinguishes between admissions under the Mental Health Act (from the outset) and those where admission was initially voluntary and where the person was later detained. Both HES and IPFDH include data on admissions to independent sector facilities that are funded by the NHS; these data are not yet available in MHMDS. We will explore variance at different spatial scales, assess the extent to which this is accounted for by a range of explanatory variables and test for cross-level interactions, i.e. the extent to which associations vary between places.
3. Longitudinal analyses. The primary aim of this research is to understand the determinants of changing rates of involuntary admission. Therefore the preferred data set for our longitudinal analyses will Hospital Episode Statistics (HES), which are available from 1998 to the present. As for our cross-sectional analyses, we will undertake a validation exercise comparing HES with data from IFDH, and where necessary we will derive appropriate weights.
Longitudinal analysis of HES data will explore and assess the extent to which increases in rates of involuntary admission over time vary between PCTs and between different areas of England (including comparing urban versus other types of area), or whether these increases are similar across the country. We will also test hypotheses about changes in rates of involuntary admission and prior (time lagged) changes in bed numbers, bed occupancy, and/or in the experiences of, and investment in, mental health services. Data linkage and the number of years for which data are available will enable us to explore and model the effects of variables at different spatial levels, and over different intervals. These analyses will also allow us to explore the effects of changes to the Mental Health Act in 2007, and the introduction of Community Treatment Orders in 2008. We will also model the growth in involuntary admissions in both secure (forensic) units and the independent sector, to estimate their contribution to overall increases in the use of detention and to see if changes in these rates differ from those pertaining to adult mental illness beds in NHS general psychiatric settings.
4. Predictive modelling. Finally, we will use longitudinal models to predict future rates of involuntary admission based on multivariate, multilevel models. In particular, we will model the effects of reducing bed numbers, increasing bed occupancy and rising numbers of Community Treatment Orders to predict future changes in involuntary admissions. We will do so using multilevel synthetic estimation, a methodology developed by one of the applicants (37,38) and validated independently by the Department of Health (39).
Multilevel modelling
Datasets will be described using standard statistical methods including frequency tables and chi-squared tests. Further analysis, and testing of the study hypotheses, will be undertaken using multilevel modelling (MLM) (40). MLM enables variances at different spatial levels to be modelled simultaneously and accurately, and in a way that reflects the structure of the data (i.e. ensuring that standard errors are adjusted to reflect spatial clustering within the hierarchical data). We will estimate how much of the observed variation is explained by individual and/or ‘compositional’ factors (such as the age, gender and ethnic composition of the local population), and how much is due to area characteristics, otherwise known as ‘contextual’ factors such as area deprivation or service investment. Multilevel approaches will also allow us to test for independent cross-level interactions, ie the extent to which individual level associations with involuntary admission vary between types of places (i.e. in terms of service investment, quality, socio-economic deprivation etc.).
Multilevel models will be developed using MLwiN software (41). In each instance a null, random effects model will be derived that estimates variance at each level: for example, individuals nested in hospitals (and/or Trusts), with Trusts nested in PCTs and PCTs nested in SHAs (or regions). These null models will serve to indicate the degree to which variance in rates of involuntary admission vary within and between these different levels, before the introduction of any (potential) explanatory variables. Subsequent modelling will be used to explore the extent to which this variance is explained by factors that include individual characteristics (like age, sex, diagnosis and ethnicity, where these are available) and area-level exposures such as urbanicity, socio-economic deprivation and the size of minority ethnic populations. Each of these variables will be allocated (and introduced to the model) at the appropriate level. Likewise, modelling will explore associations between rates of involuntary admission and the availability (and occupancy) of different types of inpatient beds (at provider level). A major advantage of multi-level modelling is the opportunity to measure cross-level interactions (e.g. between bed numbers and PCT mental health spend, for instance).
Our primary outcome (involuntary admission) will be analysed in two ways: (i) as an individual-level outcome, coded as the number of involuntary admissions per year; and (ii) as the rate of involuntary admission by PCT (for example). The latter can be treated as a continuous outcome across PCTs and analysed using a normal iterative generalised least squares hierarchical regression. The former outcome is measured at an individual level, whereby the individual risk or likelihood of being detained over a given year is treated as a dichotomous variable (i.e. detained or not). This type of outcome will lend itself to multilevel logistic regression where analyses will be undertaken using a logit link function and assumed non-constant, between-individual variance based on a Bernoulli distribution (42). In such models, the constant term will be the logit (loge of the odds) of a person in the base (reference) category experiencing an involuntary admission in a given year. The proportion of each involuntary admission will therefore be estimated from the constant term in the null model, which is equal to ln(p/1+p). Although unlikely, it is possible that some people may be detained more than once in a year and if this is found to be sufficiently common to model, then it is possible to develop multinomial multilevel models (i.e. modelling the risk of being detained once, twice, three times etc.).
Sample size
It is not possible to derive precise sample size estimates for our analyses, and nor are these needed in the design of our research since no new data are to be collected. The data sets at our disposal are extremely large, and are arguably the most comprehensive population-based sources of data on the use of mental health services anywhere in the world. This reflects both the nature of nationalised health care provision in the UK, and high levels of investment in the collection and collation of routine activity data.
Our analyses will cover all of England, and will include information from NHS and independent sector providers of in-patient mental health care. In 2009/10 this comprised 10 Strategic Health Authorities (signifying region), 151 Primary Care Trusts (as commissioners), 58 NHS Mental Health Trusts (including Care Trusts and Mental Health Foundation Trusts) and 211 registered independent mental hospitals. In 2009/10 more than 1.25m people accessed mental health services in England (2.7% of the population). (36) In the same year, 107,765 people were in-patients during the year and 42,479 were detained in hospital under the MHA (39.4% of all admissions). Of these, 2717 (4.8% of all detentions) were in independent mental hospitals and 760 (1.8% of all detentions) were under court or prison disposal orders.
Whilst sample size is unlikely to be a problem for the multilevel rates analyses, the individual logistic analyses may have relatively few observations nested within small areas. To overcome this problem, parameters will be estimated using second-order Taylor expansion with predictive quasi likelihood (PQL). This estimation procedure is considered superior to first or second order marginal quasi-likelihood (MQL) when observations within clusters, such as individuals within small areas are small (42). Markov chain Monte Carlo (MCMC) methods will further improve the accuracy of such estimates (43).
Dissemination and projected outputs
See Final Study Protocol.
Plan of investigation Timetable
See Final Study Protocol.
Project management
See Final Study Protocol.
Approval by ethics committees
See Final Study Protocol.
Service users/public involvement
See Final Study Protocol.
Expertise and justification of support required
See Final Study Protocol.
Justification of support requested
See Final Study Protocol.
References
See Final Study Protocol.
Appendix 2 Final study protocol
Full title of project
Understanding the Increasing Rate of Involuntary Admissions in NHS Mental Health Care.
Aims and objectives
Involuntary admissions under the Mental Health Act in England have increased dramatically in the last 20 years, and coincide with an equally stark reduction in mental health bed numbers. It is also recognised that people from black and minority ethnic communities are consistently over-represented among those detained in psychiatric hospitals and in secure settings. Bed reductions may disproportionately affect their detention rates as well as the detention rates of other groups that share the same mediating characteristics, for example residing in urban and/or deprived areas.
Our aims will be to explore variation between places in England in rates of involuntary admission and to identify factors that might explain this, including socio-economic context (e.g. area deprivation), features of local services (including level of investment by commissioners), and characteristics of service users (especially socio-economic status and ethnicity). Interpretation of the study findings and their translation into actionable conclusions for the NHS will be aided by consultation with mental health service users and senior NHS managers.
Research questions
-
To what extent are national rates of involuntary admission applicable to lower level health administrative areas? In other words, how variable are rates of involuntary admission across different places, namely Strategic Health Authorities (SHAs), NHS Trusts and independent sector hospitals, and Primary Care Trusts (PCTs) in England?
-
What proportion of the total variance in rates of involuntary admissions occurs between SHAs, trusts/hospitals and PCTs, respectively?
-
To what extent is variance in rates of involuntary admission explained by local factors, such as small area socio-economic deprivation, urbanicity, ethnic density, PCT investment in mental health services, and by features of local mental health services and the experiences of those who use them?
-
To what extent is variance in rates of involuntary admission explained by the characteristics of people using services, including socio-economic status and ethnicity?
-
After taking into account characteristics of areas, services, commissioner (PCT) investment and individual service users, to what extent can the variance in involuntary admissions be attributed to the availability and occupancy of NHS mental illness beds?
Our original proposal included two additional research questions:
-
Is there evidence of geographical variation in the use of Community Treatment Orders (CTOs), and if so, how is this associated with bed numbers and occupancy, length of stay, area-level factors (like deprivation and PCT investment), experience and quality of local services, and rates of involuntary admission?
-
Using the model of best fit based on local services and settings and including historical data on trends in bed numbers, can we predict the future need for involuntary treatment with sufficient accuracy to support commissioners and NHS managers in determining optimal local bed numbers?
Given our knowledge and experience with the MHMDS at this stage in the project, it is not feasible to explore these two research questions due to data quality issues. The complexities of the MHMDS structure and issues with data quality will be outlined throughout this revised protocol and have already been provided in detail in our interim progress reports submitted in September 2012 and February 2013.
Background
Increasing rates of involuntary psychiatric in-patient admission and falling bed numbers
Rates of involuntary admission to psychiatric in-patient beds have been rising in many Western European countries,1 including in England since the introduction of the Mental Health Act (MHA) in 1983. 3,4 The reasons for this remain unclear, particularly since this trend has continued despite the development of a range of community-based services such as Community Mental Health Teams, Assertive Outreach, Crisis Resolution Home Treatment and Early Intervention service. Despite being patient- and carer-centred, responsive to need, recovery-focused, timely and popular,22,47 none of the developments in community mental health services has been shown to reliably reduce involuntary admissions. 48–51
The upward trend in rates of detention appears to have accelerated since the introduction of the Mental Health Act (2007). Formal admissions alone (i.e. patients admitted to hospital under the Act as opposed to those detained after removal to a place of safety, or subsequent to informal admission) increased by 7.3% from 2008/9 to 2009/10, the largest increase in three years. 92 This increase was due solely to increased rates of formal admission to NHS facilities and concealed a decline in numbers of formal admissions to private sector units. 86 Formal admissions to NHS facilities under Section 2 of the Act (the most commonly used section, which allows for assessment rather than treatment) increased by almost 15% in 2009/10. By comparison, this number fell by 10% in the private sector over the same period.
The increasing rate of compulsory admission in England has coincided with an equally dramatic reduction in the number of mental illness beds. 20 Analysis for England shows that the number of NHS involuntary admissions increased by over 80% between 1988 and 2008, while the number of mental illness beds fell by around 60% during the same period (Keown et al. , personal communication based on submitted manuscript). 5 While closure of mental illness beds has been part of the deinstitutionalisation of care for the mentally ill for decades in high income countries,25 the United Kingdom has taken this further than its neighbours and most other developed economies. There are substantially fewer psychiatric in-patient beds in the UK (5.8 per 10,000 population in 2005) than the median for Europe (8.0 per 10,000 population) or high income countries as a whole (7.5 per 10,000 population). 26 These figures should be seen in the context of high overall levels of funding for mental health services in the UK, which currently account for 10% of the total health budget. 27 The United Kingdom has greater numbers of psychiatrists (11 per 100,000 population) and psychiatric nurses (104 per 100,000 population) than the medians for Europe (9.8 and 24.8, respectively) and high income countries as a whole (10.5 and 33.0, respectively).
People from black and minority ethnic groups experience poorer mental health services compared to the rest of population in the UK. 28–32,34,36,87 Disparities in rates of admission, including compulsory detention31,34 and more coercive pathways to acute psychiatric care, involving the police and criminal justice system disadvantage black service users in particular. 30,36,37 These inequalities have persisted despite major policy initiatives,38–40 additional investment, workforce developments and the general improvements in the coverage and quality of mental health services over the last decade. 30,39,41
Supervised Community Treatment (SCT) in the form of Community Treatment Orders (CTOs) was introduced as part of the new Mental Act in 2007. Since November 2008 it has been possible for patients detained under Section 3 of the Act to be discharged from hospital by means of a CTO, subject to the possibility of recall to hospital if necessary. This order requires the patient to adhere to specific conditions, most often including taking their medication. In the first full year of operation, 4103 such orders were made – significantly greater numbers than anticipated – while only 1789 were revoked, suggesting that some people are being kept on these orders for long periods of time. 86 SCT remains controversial, and its effect on and associations with bed numbers, occupancy, detention rates and therapeutic outcomes remain unknown. It has been suggested that SCT is both a means to ensure early discharge where beds are scarce (particularly where therapeutic to medication are restricted or unavailable), and a defensive and alienating response to managing risk. 93
The increase in the use of compulsory detention sits uneasily with most professionals, who have spent two decades attempting to improve engagement with care and to reduce the fear and stigma associated with mental health services. 22–24,31,45 It is also a source of concern to commissioners and service providers, given the high costs of in-patient care. The increasing rate of involuntary treatment represents a major financial obstacle to investment in community services, particularly at times of austerity. The acute care pathway in adult mental health services (the most expensive of all service areas) is particularly vulnerable to budgetary pressures. The growing strain on these services may explain recent acceleration detention rates. Understanding the determinants of this trend – and how to identify and therefore prevent potentially catastrophic disinvestment – is therefore imperative.
Different explanations for the increase in involuntary admissions have been proposed, including secular increases in the use of illicit drugs and alcohol. 3 But empirical evidence is extremely limited. An alternative hypothesis is that the rise in involuntary admissions is due to bed shortages arising from reductions in mental health bed numbers (and especially long stay or ‘rehabilitation’ beds) dating back to the 1950s in England. 18 It has been suggested that fewer beds results in greater pressure to discharge patients from hospital, leading to relapse and readmission. 3
An ecological study across eight hospital trusts in England provided some support for this view, finding that the rate of involuntary admission was associated with delays in accessing in-patient beds (as well as with socio-economic deprivation and the size of minority ethnic populations). 21 More recently, our own research based on time-series analysis of national data on mental illness beds and detentions for England between 1988 and 2008 found the strongest association between these variables was observed when a time lag of one year was introduced, with bed reductions preceding increases in involuntary admissions. This association increased in magnitude when analyses were restricted to civil (non-forensic) involuntary admissions and non-secure beds. Our findings support the view that that the annual reduction in mental illness bed numbers is directly associated with the number of involuntary admissions over the short to medium term. We have estimated that for every two mental illness beds that are closed there will be one additional involuntary admission in the following year (Keown et al. , personal communication based on submitted manuscript). 5 This research has also highlighted differential rates of bed closure by type of bed: while long stay bed numbers have fallen dramatically, there has been a small but steady increase in secure beds (i.e. beds in specialist forensic mental health units, sometimes referred to as ‘medium secure units’).
Despite these important findings, there are limitations to this type of ecological analysis based on national-level data. Not only can this overlook informative local variations occurring over smaller areas, but correlation at a national level may be confounded by controlling for other associations at the level of region, trust or even hospital. The most obvious potential confounder is (area-level) socio-economic deprivation. This has never been fully explored.
Studying the geography of involuntary admission
There is an urgent need to understand the determinants of the persistent and seemingly irrevocable trend towards ever higher rates of involuntary psychiatric admission in the UK. This is even more imperative in the light of evidence that this trend may have accelerated following changes to the Mental Health Act in 2007. 86,92 Studying the geography of involuntary admission offers the opportunity to explore variations in this trend at local level and therefore to identify factors associated with higher and lower rates of involuntary admission. It therefore represents the logical next step towards achieving the aim of understanding the causes of the upward national trend.
Preliminary investigation reveals marked variation in rates of involuntary admission between regions of England. In 2009/10, there was a two-fold difference between regions with the highest (London SHA, 146 detentions per 100,000 population) and lowest (South Central SHA, 70 per 100,000) rates of formal detention, after excluding Place of Safety orders. 86
There is substantial variation in investment by PCTs on mental health services per head of population. It was estimated that the crude ratio between the PCTs with the highest and lowest mental health spend was 4.6 in 2007, falling slightly to 2.9 after weighting for age, population need and overall PCT spending allocations. 42 It is not yet known whether and how this variation is associated with differences in mental health bed numbers, occupancy, length of stay, detention rates, and access to services or patient experiences of care.
We suggest that urbanicity (i.e. population density and settlement size), ethnic density, socio-economic deprivation and the availability (or otherwise) of in-patient beds are likely to be the most important local factors that contribute to needs for involuntary treatment. While there is now limited evidence of a direct association with (fewer) mental health beds, we propose to test the hypothesis that detention under the Mental Health Act is also related to the availability and quality of community mental health services, as well as the lack of barriers to using these (as indicated by area-level deprivation). One key hypothesis is that the gains associated with improved community services have not been enjoyed equally by all, and that rates of involuntary admission may be growing fastest in those places where social networks are weakest and community services are the hardest to deliver, namely the deprived inner cities with large minority ethnic populations.
We will also explore the extent to which urbanicity and ethnic composition interact (as opposed to acting independently of one another) in their associations with rates of involuntary admission. The rate of involuntary admission may be higher in areas with diverse minority populations than in places with greater ethnic density (i.e. concentrations of people of the same ethnicity), where social networks might be stronger. By establishing linkages to Census data, we will assess the independent and interactive effects of ethnic diversity and density, and urbanicity on rates of involuntary admission.
How the proposed research will add to the body of knowledge with reference to current NHS policy and practice
In the present economic climate, further closure of in-patient beds appears the most likely strategy for funding improvements in community-based mental health services. While this is in keeping with providing care in the least restrictive setting and near to home, there is little evidence to guide the relative and absolute provision of short stay, long stay and secure (forensic) mental illness beds in the NHS. The current imperative for efficiency savings is also leading commissioners and service providers to consider disinvesting in a number of specialist community-based services. This could have a counter-productive effect by increasing the pressure on beds and the cost base on which services are delivered, particularly as there is evidence that fewer beds (and greater occupancy) delays admission and makes involuntary admission more likely. In this scenario there will be ever-diminishing scope for new investment in community-based services, with consequences for the quality and experience of care. The recent addition of Community Treatment Orders (CTOs), driven by a desire to reduce risk albeit at the risk of increased coercion, may represent a recipe for accelerating involuntary admission rates.
This project will add to knowledge in this area and help in future decisions about the provision and balance of inpatient and community psychiatric services in England. An important aim of this research will be to derive comprehensive and sophisticated, multivariate predictive models (based on a validated methodology pioneered by one of the applicants) that will allow individual NHS Trusts (and their commissioners) to estimate the numbers of involuntary admissions they might expect over time, and what impact further bed closures would have on this number.
Importance of the proposed research
Patients are clear about their dislike for in-patient treatment, which is frightening and undermining of autonomy and choice. 22–24 Involuntary admission to hospital is even more distressing for patients and their carers, and typically results in longer lengths of stay in hospital. Involuntary admissions are more common in some groups (especially among black and minority ethnic groups), and in some places. Though often necessary to prevent immediate harm, involuntary admission impairs longer term therapeutic relationships, increases stigma and reduces confidence in and subsequent engagement with services.
Despite a decade or more of investment in more patient-centred, community-based treatments the rate of involuntary admission continues to rise and have coincided with reductions in mental illness bed numbers. There is also evidence that this may have increased further since the introduction of Supervised Community Treatment in 2008. But other, as yet unstudied factors may also be important, including ethnicity, urbanicity, and socio-economic status and diagnosis (patients with psychosis are more likely to be detained). Although it was an original aim of the project to look at the association between diagnosis and involuntary admission rates, the MHMDS only has reliable diagnostic information for ∼19% of all patients. This level of missing data is extremely high and was unexpected; we are not able to include psychiatric diagnosis in our analytical plan for this project. The rate of involuntary admission is of interest to many groups and is likely to remain highly relevant for a number of reasons (clinical effectiveness, ethical, and health economic reasons).
At a time of austerity, tough decisions are being made about the shape of mental health care for the next decade. One important decision concerns the optimal balance between inpatient and community services. Understanding the relationship between bed numbers and involuntary admission is an important part of this. But including measures of the configuration, quality and experience of community service at the same time as analysing the association between bed numbers (and occupancy) and involuntary admission means that the proposed research will result in usable evidence about how to improve acute care pathways in all settings across England. Identifying and quantifying the wider societal and service-level determinants of the rise in involuntary admission, and modelling the impact of further bed closures are all vitally important for the commissioning and delivery of services.
By working with service users (through the mental Health Foundation) and senior NHS managers (with the support of the NHS Confederation), we will ensure that our scientifically robust findings are translated into actionable conclusions for the NHS. These will allow NHS Trusts to anticipate the impact of bed closures on the rate of involuntary admissions to those beds and the consequent impact on psychiatric admission wards. The results of this study will have a major bearing on the commissioning of mental health services, which in the current economic climate are highly dependent on the release of resource from (very costly) in-patient services to support further development of community-based treatments.
Research methods
Design
Secondary analysis of routinely collected data. Data will be organised to permit analysis as cross-sectional survey, cohort and (nested) case control studies.
Data sources
As the time this protocol was submitted, the main data sources were to be obtained from the NHS Information Centre. In 2013, the NHSIC was re-organised to be the NHS Health and Social Care Information Centre, or the HSCIC (http://www.hscic.gov.uk/). As a result, many of the services (and corresponding webpage links) we outlined in our original protocol are no longer available and we have updated this section of the protocol accordingly.
The HSCIC is responsible for collecting and collating national health and social care data in the UK. The HSCIC ensures appropriate governance checks for the use of these data, to protect the confidentiality of those on whom data is collected, through the National information Governance Board for Health and Social Care (NIGB). It also collates geographical data, including deprivation and other neighbourhood statistics, and provides support in ensuring appropriate linkage to other data sets. The significant investment on the part of the NHS in software resources and staffing to support secondary users through the HSCIC represents a major strength of the proposed research. Although the proposed data linkages will be methodologically challenging, we are confident that the study aims are achievable.
The data sets which we propose to access and link are set out below in Table 10, followed by more detailed descriptions about each of these. Since the original protocol was submitted, our research team has become very familiar with the structure, content, and capabilities of the data sets we proposed to analyse to achieve the aims of this project. In light of this, a number of amendments and additions have been made to the data sources we aim to work with in this project. Specifically, we will focus on the Mental Health Minimum Dataset (MHMDS) as our main data source to derive the outcome variable for the study, and link in information from the Hospital Episode Statistics (HES) data as well as other data sources to provide additional contextual information relevant to the study of psychiatric admission rates. Table 10 below, which was included in the original protocol, has been modified to reflect these changes. As outlined previously (and in our interim reports submitted in September 2012 and February 2013), the quality and reliability of the MHMDS is of a relatively high quality from 2010/11 onwards but poor from 2007–2009. Given that the project will focus on comprehensive cross-sectional analyses of the most comprehensive data available, additional data sources relevant to this recent annual period (2010/11) and to the study of psychiatric admissions have been sourced for linkage to the MHMDS (Table 10).
Data set | Key variables | Smallest data level | Level of proposed linkage | Time frame | |
---|---|---|---|---|---|
1 | Mental Health Minimum Dataset (MHMDS) | Patients admitted involuntarily; patient characteristics inc ethnicity, age, and gender. In our original protocol, we had hoped to include other individual-level characteristics such SES, HoNOS, and diagnosis; due to high levels of missing data and inconsistencies in recording data by the Providers submitting to the MHMDS, this is not now possible | Individual | PCT | 2010–11 |
2 | Hospital Episode Statistics (HES) | All admissions, inc involuntary admissions; diagnosis, length of stay; patient characteristics inc ethnicity and SES | Individual | PCT | 2010/11 |
3 | Census & other geographical data | Area-level features (e.g. urbanicity, socio-economic deprivation) and population composition | Lower-layer super output area level | Lower-layer super output area; PCT | 2009–2011 (inc mid-year estimates) |
4 | Beds Open Overnight (BOO) – previously Hospital Activity Statistics | Bed numbers inc availability and occupancy | Provider (Trust or independent hospital) | Provider | 2010/11 |
5 | Patient Environment and Action Team (PEAT) | Summary scores for performance in patient safety in the following areas: environment, food, and dignity and respect | Provider | Provider | 2011 |
6 | Community Mental Health Services Survey | Provider performance on a variety of domains including health and social care workers, medication, helpfulness of talking therapies, care plan management, crisis care, and day-to-day living | Provider | Provider | 2011 |
7 | Inpatient Service User Survey | Provider performance on a variety of domains including ward accommodation, staff communication, medications and treatment, and patient rights | Provider | Provider | 2009 |
8 | NHS Staff Survey | Workplace related indicators including workloads, training, staff communication, health and wellbeing etc. | Provider | Provider | 2010/11 |
9 | Investment in Adult Mental Health Services (IMHS) | Spend per person on mental health care for adults of working age | PCT | PCT | 2010/11 |
1. Mental Health Minimum Dataset (MHMDS)
As previously mentioned, the Mental Health Minimum Dataset will be our primary data source for the analyses in this study, by virtue of the detail recorded at the individual patient level. The MHMDS comprises anonymised record-level (i.e. individual patient-level) data about adults and older people using secondary mental health services. Each record in the MHMDS represents the complete, continuous spell of care for an individual, from initial referral to final discharge (referred to as a mental health care spell – or ‘care spell’ for short). Each care spell contains information relating to voluntary and involuntary in-patient admissions, out-patient attendances, day treatment and all other episodes of care. The MHMDS data is reported quarterly and describes complete spells of care for an individual, from initial referral to final discharge. Quarterly data are aggregated into an annual database which is released by the NHS Health and Social Care Information centre to registered users of the data.
Although (limited) MHMDS data are available from 2003, the data quality has been quite poor (i.e. high levels of missing data and inconsistent recording of patient care by Provider Trusts) until very recently. Our project team have gained access to data from 2007/08 and are confident that the most comprehensive data on service use are available from 2010/11. Details of these data exploration tasks have been documented in detail in our interim reports (submitted September 2012 and February 2013). This means that only data from the 2010/11 annual reporting period will be used to investigate this study’s research questions. Whilst longitudinal analysis will be feasible as time progress (i.e. from 2010/11 onwards), earlier years of MHMDS are simply too poor to be able to confidently link patient records across years for the purposes of longitudinal analyses. No other more recent data (i.e. 2011/12) is available at the present time. Thus, we will focus on the most recent and comprehensive data available to use (i.e. 2010/11 MHMDS) but we also make use of 2009/10 MHMDS database for quality assurance purposes. We will seek NHS Research Ethics approval for our research, and will apply to the NHS Information Centre through its Security and Confidentiality Advisory Group for access to anonymised, patient-level MHMDS data. Data from independent sector providers will be available for the first time with the 2010/11 MHMDS release in January 2012. The advantage of MHMDS over other data sets, and especially Hospital Episode Statistics (HES), is that it comprises all episodes of mental health treatment regardless of setting. By contrast, HES is restricted to in-patient admissions. Therefore the reference group for analyses based on these data are people using local mental health services but who have not been admitted to hospital involuntarily in a specified period. The findings will therefore be generalizable to people who use mental health services.
Rather than counting uses of the Mental Health Act, MHMDS counts people subject to the Act and provides opportunity for analysis by age and ethnic group. Involuntary admissions are denoted by recording legal status at end of reporting period, coded using the most restrictive legal status (i.e. whether detained or treated informally) that applied during the mental health care spell. Community Treatment Orders (CTOs) and their revocation and recall to hospital are also recorded in MHMDS. As mentioned earlier in this revised protocol, however, the CTOs data are not currently sufficient to permit a thorough exploration of these data in our current study. After much interrogation of the MHMDS over the course of this project, our team now has a clear understanding of what is capable in terms of determining the number of patients who were admitted to hospital under the MHA during the time frame under study (2010/11). This has not been without difficulties, and we have documented these stages in our interim progress reports. Nevertheless, we have been successful in navigating the MHMDS to derive the outcome variable for the study and our estimates of the number of patients detained are very consistent with those published by the HSCIC.
Having derived our outcome variable for the study, the next task involved understanding the different levels within the MHMDS that could be used to explore what regions of the country have different rates of psychiatric detentions and what factors contribute to this variation. In addition to recording detailed information on the patient’s medical history, the MHMDS records contain information about where the patient lives, where their GP is located, and where they receive their mental health care. This type of information is very useful for trying to build a picture of the type of environment in which patients live, which may give clues as to the reasons for variation in involuntary admission rates in different areas across England. There are numerous discrete and identifiable spatial levels in the MHMDS at which variance in rates of involuntary admissions might occur:
-
Patient-level: each patient record (n = 1,287,730) in the MHMDS contains detailed information about the patient’s contact with mental health services.
-
Lower layer super output area-level (LSOA): The LSOA is a geographical area comprising of a minimum of 1000 people. There are approximately 33,000 LSOAs in England. Knowing the LSOA in which a person lives can provide a lot of information about the type of area they inhabit (e.g. population density, ethnic density, and socio-economic deprivation). Importantly, it allows us to link in other pertinent data sets such as the UK Census and the Index of Multiple Deprivation.
-
GP Practice-level: GP Practices, of which there are approximately 8000 in England, deliver primary care services. This will include delivering some mental health services, but also covering referrals to more specialist secondary care mental health services.
-
Primary Care Trust-level (PCTs): PCTs were in charge of primary care and had a major role around commissioning secondary care, providing community care services.
-
Provider Trust-level: Patients receive mental health care from Providers, mainly NHS Trusts but also some Independent sector Providers. There are 58 specialist NHS Mental Health Trusts in England. These Trusts deliver specialist mental health care services. In total, there are 72 Providers (both NHS and Independent sector) in the 2010/11 MHMDS.
-
Strategic Health Authority-level (SHA): There are currently 10 SHAs in England. SHAs are responsible for, amongst other things, improving health services in their local area and ensuring local health services are of a high quality and are performing well.
2. Hospital Episode Statistics (HES)
HES contains details of all admissions (including those under the Mental Health Act) to NHS hospitals and independent hospitals in England funded by the NHS. HES also contains details of all NHS outpatient appointments in England and is available from 1998 to the present. HES comprises patient-level records and contains data on diagnosis, length of stay, age, gender and ethnicity. It also includes information about where treatment was delivered and place of residence. We will seek NHS Research Ethics approval for our research, and will apply to the HSCIC through its Security and Confidentiality Advisory Group for access to hospital admission data for linkage to the MHMDS. We are particularly interested in accessing admission-related data from HES at Provider Trust and PCT level, for example length of hospital stay and number of finished consultant episodes, to create a more comprehensive picture of the Trusts who are delivering mental health services (and PCTs who are commissioning these services) to patients in the MHMDS.
3. Census and other geographical data
We will use area-level deprivation indices to characterise the places in which services are delivered. In the original protocol, we envisaged that it would be necessary to make do with area-level population estimates from the 2001 Census data, supplemented with more recent mid-year estimates, due to lack of availability of more recent data. We are pleased to report that we have been able to access the 2011 Census population statistics to determine ethnic composition and population density for different areas, and across different spatial levels (supplemented by mid-2009/2010 estimates where necessary when this is the most recent available data). We will also obtain details on urbanicity classification for England and Wales from the Office for National Statistics (http://www.ons.gov.uk/ons/guide-method/geography/products/area-classifications/rural-urban-definition-and-la/index.html).
4. Beds Open Overnight (BOO) – previously Hospital Activity Statistics
The number of available and occupied NHS mental illness and learning disability beds are available via the Department of Health, and comprise aggregated quarterly KH03 returns by each provider. Data are available for 2010 (http://transparency.dh.gov.uk/2012/07/05/bed-availability-and-occupancy-overnight/). We aim to source detailed information on the average annual number of available and occupied beds for each consultant speciality treatment patients with mental illness.
5. Patient Environment and Action Team (PEAT)
PEAT is an annual assessment of inpatient healthcare sites in England that have more than 10 beds. It is a benchmarking tool to ensure improvements are made in the non-clinical aspects of patient care including environment, food, privacy and dignity. The scores demonstrate how well individual healthcare providers believe they are performing in key areas including food, cleanliness, infection control and patient environment (including bathroom areas, décor, lighting, floors and patient areas). Data are managed by the NHS Health and Social Care Information Centre and are available for 2010 (http://www.nrls.npsa.nhs.uk/patient-safety-data/peat/).
6. Community Mental Health Services Survey
The Community Mental Health Services Survey is conducted by the Care Quality Commission (http://www.nhssurveys.org/surveys/515). The 2010 survey comprises a sample of service users aged 16 and over who had been in contact with NHS mental health services in the three month period 1 July 2009 to 30 September 2009 and who were receiving specialist-help for a mental health condition. The survey aimed to find out about the experiences of people using mental health services in the community. These services provide care and treatment to people who have been referred to a psychiatric outpatient clinic, local community mental health team, or other community-based mental health services. The survey compares the performance of Trusts on a variety of issues, including care plan reviews, use of care coordinators, medications, talking therapies, etc. Data will be linked to the MHMDS at Trust level.
7. Inpatient Service User Survey
The Inpatient Service User Survey is conducted by the Care Quality Commission (http://www.nhssurveys.org/survey/1016). Over 69,000 adult patients from 162 acute and specialist NHS trusts in England responded to the survey, a response rate of 52%. The survey was carried out between September 2009 and January 2010 and gathered the views of patients who were discharged from hospital before the end of August 2009. The survey aimed to find out about the experiences of people using inpatient mental health services and covered a wide range of topics including, ward accommodation, medication, staff communication etc.
8. NHS Staff Survey
The 2010 NHS Staff Survey is the eighth annual survey of NHS staff. The results of this survey help Trusts to review and improve the experiences of their staff, enabling them to provide better care to patients. The survey covers a wide range of work related domains including work load, staff health and wellbeing, staff satisfaction with job, job performance and communication among staff within the NHS. Data will be linked at Trust level.
9. Investment in Mental Health Services (IMHS)
The Department of health sponsors the annual National Survey of Investment in Adult Mental Health Services, an annual finance mapping exercise regarding spending on mental health services in England (https://www.gov.uk/government/publications/2010-11-working-age-adult-and-older-adult-national-survey-of-investment-in-mental-health-services). Data from 2010 are relevant to this project’s timeframe and provide details of investment in mental health services for working age adults (aged 18–64) in England, by PCT (commissioner).
Participants
Although there will be no primary data collection (field work), our subjects will be people who have used NHS and independent sector mental health services in England, about whom data is collected routinely during episodes of care. Other analyses will be undertaken at the level of service provider (NHS Trusts and independent sector providers) and commissioning Primary Care Trusts (PCTs).
Setting
The proposed research will be conducted in England, and will include analysis of data covering all Strategic Health Authorities (SHAs), Primary Care Trusts (PCTs) and all NHS and independent sector providers of in-patient mental health care.
‘Independent sector providers’ are those units that are registered with the Healthcare Commission to accept patients detained under the Mental Health Act 1983 (as amended by the Mental Health Act 2007), as specified under S. 2(3) (b) of the Care Standards Act 2000. These independent Hospitals that are registered to receive detained patients are considered to be ‘hospitals’ for the purposes of the Mental Health Act.
Data preparation
Primary outcome
As previously described, a great deal of time and effort has been spent on deriving the main outcome for the study, that is, categorising patients in terms of their admission to hospital under the Mental Health Act 2007 (MHA) during the 2010/11 reporting period. Details of this process have been documented in our interim progress reports. Briefly, the outcome variable will be dichotomous: patients who were admitted to hospital under the MHA during 2010/11 versus all other patients who received any other type of care (including voluntary admission to hospital, community treatment only, or no care). This categorisation will also permit us to determine the rate of involuntary compulsory admissions to hospital at, for example, Provider Trust and PCT level (as outlined in our original protocol).
Data linkage
The next main task will be to link the MHMDS to a wide variety of relevant external data sources. The purpose of data linkage is to create a data set that will specify multiple distinct levels at which variance in detention rates might be quantified and explained, including geography (e.g. physical environment and demographic composition), service provider (most commonly NHS Trust), commissioner (PCT) and General Practice. Creating such linkages is complex and involves finding suitable data sources and appropriate methods for linkage to the MHMDS. This linkage will take place at the smallest possible spatial scale: as indicated above in Table 10, the most common linkages will be by provider Trust and (commissioning) PCT.
Data analysis
The primary statistical technique employed will be multilevel modelling, which mirrors the structure of the data to be analysed and allows explanation to be correctly assigned to the various ‘levels’ within the data set (i.e. individual or area) and is therefore a widely used method for analysing nested (or clustered) data.
Strategy of analysis
This section was included in the original protocol but has been redrafted to provide greater clarity and to describe the refocused emphasis on conducting details and robust cross-sectional analysis of the best data sources available to our team: the 2010/11 MHMDS linked to contextually-rich external data sources. Linking the MHMDS to external data sources will enable us (for example) to explore the effects of age, sex, ethnicity (at the individual level), bed numbers (and types of available beds), overall bed occupancy rates, average length of in-patient stay (at Trust level), investment in services (at PCT level) and socio-economic deprivation, urbanicity and ethnic composition (at area level) on the likelihood that a user of mental health services will be admitted to hospital involuntarily. As well as assessing the main effects of these risk factors, we will explore variance at different spatial scales and test for cross-level interactions, i.e. the extent to which associations vary between places.
Multilevel modelling (MLM)
Testing of the study hypotheses will be undertaken using multilevel modelling (MLM). 94 Multilevel models will be developed using MLwiN software. MLM enables variances at different spatial levels to be modelled simultaneously and accurately, and in a way that reflects the structure of the data (i.e. ensuring that standard errors are adjusted to reflect spatial clustering within the hierarchical data). We will estimate how much of the observed variation is explained by individual and/or ‘compositional’ factors (such as the age, gender and ethnic composition of the local population), and how much is due to area characteristics, otherwise known as ‘contextual’ factors such as area deprivation or service investment. Multilevel approaches will also allow us to test for independent cross-level interactions, i.e. the extent to which individual level associations with involuntary admission vary between types of places (i.e. in terms of service investment, quality, socio-economic deprivation etc.).
In a multilevel model, it is important to structure the data correctly. In a classic MLM, the various spatial levels nest neatly within one another and, in this sense, we can create a nested hierarchy – i.e. individuals (level 1) nested within LSOAs (level 2) within Provider Trusts (level 3). The structure of the MHMDS is quite complex and the data are not nested neatly. If we were to run a MLM that assumed the data were nested neatly then the resultant estimates of variance across the different geographies would not be correct. Therefore, rather than regard the geographical areas as ‘levels’ within a hierarchy, they are instead regarded as ‘classifications’ and for each individual within the data set, the classification structure is recorded, allowing for a non-nested, cross-classified data structure. Markov chain Monte Carlo (MCMC) estimation methods are available in MLwiN to estimate the variance of our outcome variable across these geographic classifications (i.e. LSOA, Commissioner, Provider Trust etc.), within these types of multilevel models.
Our first aim was is to estimate how much of the observed variation in involuntary admission rates across England is explained by individual factors such as gender and age and how much is due to factors operating at these different spatial levels. The most basic MLM is the null model, which estimates the total unexplained variance in involuntary detentions in the study sample (this is equal to the sum of the unexplained variances at the different spatial levels).
Given the size of the database (∼1.2 million patients), estimating models with more than three spatial levels would be difficult due to problems with model stability and computing power. A series of three-level models will be estimated, varying the structure of the spatial levels in an attempt to identify where the largest variance in involuntary admissions might be occurring, and in order to guide us in populating more definitive models. Subsequent modelling will be used to explore the extent to which this variance is explained by factors such that include individual characteristics (like age, sex, and ethnicity, where these are available) and area-level exposures such as urbanicity, socio-economic deprivation and the size of minority ethnic populations. Each of these variables will be allocated (and introduced to the model) at the appropriate level. Likewise, modelling will explore associations between rates of involuntary admission and the availability (and occupancy) of different types of inpatient beds (at provider level). A major advantage of multi-level modelling is the opportunity to measure cross-level interactions (e.g. between bed numbers and PCT mental health spend, for instance).
Sample size
It is not possible to derive precise sample size estimates for our analyses, and nor are these needed in the design of our research since no new data are to be collected. The data sets at our disposal are extremely large, and are arguably the most comprehensive population-based sources of data on the use of mental health services anywhere in the world. This reflects both the nature of nationalised health care provision in the UK, and high levels of investment in the collection and collation of routine activity data.
Our analyses will cover all of England, and will include information from NHS and independent sector providers of in-patient mental health care (as previously, these independent sector providers are coded by the service provider (company) and not by individual units or according to geographical location). In 2009/10 this comprised 10 Strategic Health Authorities (signifying region), 151 Primary Care Trusts (as commissioners), 58 NHS Mental Health Trusts (including Care Trusts and Mental Health Foundation Trusts) and 211 registered independent mental hospitals. In 2009/10 more than 1.25 M people accessed mental health services in England (2.7% of the population). 95 In the same year, 107,765 people were in-patients during the year and 42,479 were detained in hospital under the MHA (39.4% of all admissions). Of these, 2717 (4.8% of all detentions) were in independent mental hospitals and 760 (1.8% of all detentions) were under court or prison disposal orders.
Whilst sample size is unlikely to be a problem for the multilevel rates analyses, the individual logistic analyses may have relatively few observations nested within small areas. To overcome this problem, parameters will be estimated using second-order Taylor expansion with predictive quasi likelihood (PQL). This estimation procedure is considered superior to first or second order marginal quasi-likelihood (MQL) when observations within clusters, such as individuals within small areas, are small. 96 Markov chain Monte Carlo (MCMC) methods will further improve the accuracy of such estimates. 97
Dissemination and projected outputs
Our strategy for dissemination is built around two key partnerships. In undertaking this project, we shall be working closely with the Mental Health Foundation and the Mental Health Network (which is part of the NHS Confederation) from the outset. These partnerships will help ensure that our analyses focus on the outcomes and associations of greatest importance to service users, NHS managers and commissioners, and that the findings are translated into actionable conclusions. Both partners have well established and effective communications strategies which we shall utilise so that our results reach the widest possible audience. This will involve Briefing Papers (available on line and in hard copy), hyperlinks to our reports via MHF and NHS Confederation web pages and press releases. We will explore opportunities for other dissemination events, including hosting stakeholder workshops.
We will also pursue more traditional modes of academic dissemination by submitting our findings to high impact journals, and by presenting these at national and international scientific conferences. Finally, we also propose to hold workshop for key stakeholders in each of the three regions from which the clinical academic applicants are drawn: London, the Midlands and the North East.
Plan of investigation
Timetable
This project was granted a no-cost time extension to extend the project end date from 31/05/2013 to 31/08/2013. The reasons for this extension were outlined in our interim reports (September 2012 and February 2013) and were largely due to administrative delays in hiring the Research Fellow to the project on a full-time basis. This section has been amended accordingly to reflect this extension.
Months –3 to 0: Applications for (a) research ethics and NIGB approval and (b) permission for access to anonymised patient-level data from the NHS Information Centre’s Security and Confidentiality Advisory Group; further data and software acquisition. Research Fellow will begin on-line training in multilevel modelling through the Centre for Multilevel Modelling (University of Bristol). First meeting of full study team.
Months 1 to 3: Induction of Research Fellow and completion of on-line multilevel modelling training; secure NIGB and NRES permissions and finalise acquisition of all study data; liaison with NHS Information Centre Secondary Uses Service to support data linkage. Plan & execute data management. Further meeting of the full study team.
Months 4 to 15: Data analysis. Regular study team meetings; regular supervision of Research Fellow (Warwick and Portsmouth). Initial meetings with service users (via Mental Health Foundation, MHF), and with NHS mental health service clinicians and managers (via Mental Health Network, MHN).
Months 16 to 18: Interpretation of findings, final meetings of full study team. Further consultation event with service users (facilitated by Mental Health Foundation) NHS mental health service Chief Executives (facilitated by Mental Health Network); report writing and dissemination (including formal briefing reports to be published by MHF and the NHS Confederation (governing body of the MHN).
Project management
Management of the project is of the utmost importance, given that the research team will be spread out across five sites within the UK. As we describe below, this project has arisen from strong research partnerships and collaborations that span the past decade. We have an established track record of working together very productively, and this is further evidenced by the significant research outputs that have accrued between the time of the outline submission and the present. We have published numerous papers together in high impact scientific journals, including several notable publications in the last year alone. We are a tried and tested collaborative of like-minded and committed academics who know one another very well, and we have established an effective modus operandi over many years.
SW, who is based at the University of Warwick, will be the Chief Investigator and Project Manager, and will have day to day responsibility for all aspects of the project. His time is budgeted at 10%. SW has considerable management experience, and is also Clinical Director of West Midlands (South) CLRN, where he is responsible for an annual budget of £6.7 m. He is also chair of the Psychological and Community Therapies Panel at the NIHR Health Technology Assessment programme.
PK (in Newcastle) will be Deputy Project Manager, and his time has also been costed at 10%. He has a wealth of experience of using a number of the study data sets, and of liaising with the NHS Information Centre.
There will frequent communication between members of the research team, and we have scheduled regular face-to-face meetings every two months at rotating venues. We will hold a teleconference (assisted where appropriate by Skype) between these meetings, to ensure that the full study team discuss progress at least once a month. These meetings will be minuted and will be used to monitor progress and to identify any necessary action points.
The proposed Research Fellow, Dr McBride, has a proven record of academic achievement and a well-deserved reputation for being able to work independently. Her CV (which we attach) testifies to her drive and ambition, as well as her expertise. She has undertaken very productive visiting fellowships overseas, where she has increased her knowledge and learning but has also produced significant research outputs. She has worked and published previously with SW, and the physical separation is unlikely to be a hindrance. We have budgeted for her to travel regularly to England where she will be supervised both by SW but also by Dr Twigg in Portsmouth, by Liz Twigg. Dr Twigg has worked extensively with SW and has been recruited to the project for her experience as a senior health geographer and her considerable expertise in multilevel statistical modelling.
We have also set out our proposals for working with our two partners, the Mental Health Foundation and the Mental Health Network below. The former will be enhanced by the David Crepaz-Keay’s membership of the project steering group.
Approval by ethics committees
Although this is secondary research and we will not be collecting any new data, we will require research ethics approval for specific aspects of the proposed research.
We will seek NHS Research Ethics approval for our research, and will apply to the NHS Information Centre through its Security and Confidentiality Advisory Group for access to anonymised, patient-level HES data. The Information Centre and its associated Secondary Uses Service and Medical Research Information Service have been very helpful to date in signposting processes for securing the necessary permissions and approvals, and we will follow their direction in these matters.
Most of the other data that we will be using will be aggregated at the PCT or Trust level, and is in the public domain. Nevertheless, our research ethics application will address the issue of data linkage, since in theory it might be possible to identify someone by triangulated the multiple area-level indicators in a linked data set.
Service users/public involvement
We propose to engage two sets of ‘users’: users of mental health services, and senior NHS mental health service managers and clinicians.
We will involve mental health service users through our partnership with the Mental Health Foundation (MHF) (http://www.mentalhealth.org.uk), the UK’s leading mental health research, policy and service improvement charity. Service user involvement will be coordinated by David Crepaz-Keay, Head of Empowerment and Social Inclusion at the MHF and a co-application on this proposal. The main aim of this partnership is to ensure that the service user perspective shapes the research questions, influences analysis and interpretation and assists in disseminating the findings.
Working through and with the MHF, we propose to circulate a summary of the study aims and methods to a network of mental health service users for comment. We will also seek feedback from service users on our preliminary findings.
We have also established a partnership with representatives of another key ‘user’ group, namely senior NHS mental health service managers. We will work with the Mental Health Network (part of the NHS Confederation) to recruit participants to two consultation events. The NHS Confederation represents more than 90% of the organisations that make up the NHS. Its members include the majority of NHS acute trusts, ambulance trusts, foundation trusts, mental health trusts, primary care trusts, special health authorities and strategic health authorities in England; trusts and local health boards in Wales; and health and social service trusts and boards in Northern Ireland.
Both mental health service users and NHS mental health managers (and chief executives) will be asked to comment on our research aims, hypotheses at the start of the project, and their views on how best to interpret our findings will be solicited at the end, but before formal dissemination. This will ensure that the research questions are framed to address key concerns of those responsible for delivering mental health care in the UK as well as those on the receiving end. Feedback from both sets of consultations will enable us to present our findings in the form of actionable conclusions.
Expertise and justification of support required
Project team
The project team is based at Warwick, Newcastle, London and Portsmouth. All members of the group have worked together on several prior studies over 10 years (SW and LT), demonstrating effective collaboration and numerous research outputs. SW, PK, JS and KB are all Consultant Psychiatrists with long experience of developing, leading and delivering adult mental health services in the UK. SW and PK will assume responsibility for delivering the project, and together with LT will supervise the Research Fellow. LT will provide specialist statistical expertise. All applicants will contribute to the formulation of study aims, monitoring progress, interpreting findings and supporting dissemination.
Scott Weich is a psychiatric epidemiologist with extensive experience of secondary analysis using complex data sets in the study of social, geographical, ethnic and gender inequalities in rates of mental disorder. He has published widely on these, including work with Liz Twigg using multilevel modelling (MLM). He and Kam Bhui have worked together on studies of ethnic inequality in the experience of mental health care. He has also worked with Orla McBride (Research Fellow) on two recent publications. He will have overall responsibility for project management.
Patrick Keown has detailed knowledge of the relevant data sets and has experience in evaluating the impact of service innovations at a local level as well as changes across England. He was the lead author on the 2008 BMJ paper documenting increased detention rates, and is first author on the time series analysis (using HES data) that is being considered by the BMJ.
Jan Scott has a track record in clinical service developments (e.g. Longbenton community service, cited as an example of good practice in the Key Areas Handbook) and was previously an Honorary SMO at the Department of Health working on mental health policy. She has expertise in the analysis of large and complex data sets such as process and outcome research in psychotherapy. She chaired a workshop on the European Mental Health Programme at the EU in Brussels in 2009 and with John Bowis (EuroMP) led a similar initiative in 2009 at the European Health Conference in Gastein. These meetings compared cross-national resource utilisation and voluntary and involuntary admissions rates. She is currently involved in a multi-national project on barriers to service use and engagement in young adults with severe mental disorders, including how stigma delays help-seeking.
Kam Bhui is an epidemiologist and cultural psychiatrist and has experience of working with large data sets to assess inequalities. He has worked extensively on different aspects of the national race equality programme using complex qualitative and quantitative data. Together with Scott Weich, he undertook a national evaluation of the Focused Implementation Site programme, the flagship for Delivering Race Equality.
Liz Twigg is a senior health geographer who specialises in the analysis of large and complex spatially referenced data sets using multilevel modelling methods. She has used MLM techniques to look at geographical variation in a number of health outcomes (including common mental disorders) and health related behaviours and has much experience of using the results of such models to produce small area estimates of health outcomes.
David Crepaz-Keay is Head of Empowerment and Social Inclusion at the Mental Health Foundation. He has over 20 years’ experience in service user involvement, and was previously chief executive of Mental Health Media, former board member and vice-chair for the Commission for Patient and Public Involvement in Health, founding member of National Survivor User Network (NSUN), and former chair and treasurer of Survivors Speak Out. I am also a member of the All Wales Mental Health Promotion Board and an advisor to World Health Organisation (WHO) Europe on empowerment. He has personal experience of using mental health services and of involuntary admission.
Justification of support requested
We note that the project budget has increased since the outline submissions. The main reasons for this were to (i) ensure appointment of a Research Fellow capable of undertaking the work; (ii) include expertise in health geography and multilevel modelling; (iii) increase the time commitment of the applicants to ensure successful delivery of the study; (iv) secure effective patient and public involvement; and (v) ensure that the study findings are translated into actionable conclusions for NHS managers and commissioners.
We are seeking support for a 15-month project. The main challenge of this project will be collating, merging and managing a complex data set, and therefore it is imperative that the project researcher is capable of undertaking this. Past experience shows that recruiting someone with the necessary skill set, and who is also a capable statistical analyst, is difficult. At the behest of the Reviewers we have budgeted for a Research Fellow rather than a Research Associate. The largest cost associated with this project is the salary of an experienced Research Fellow (Dr Orla McBride, CV attached). Dr McBride is shortly to take up a post at the University of Ulster, from where (if our application is successful) we shall second her to this project. She has collaborated previously with the Chief Investigator, and has extensive experience of analysing complex data sets used a range of advanced statistical methods. She has a background in psychology and clinical experience in mental health settings. An honorary contract will also be sought for Dr McBride at Warwick University (for which there are no cost implications). We are seeking a limited training budget to support Dr McBride in developing her skills in multi-level modelling. We note that the centre for Multilevel Modelling at Bristol University, the leading centre of its kind in the UK, now delivers its renowned training on-line: http://www.bristol.ac.uk/cmm/learning/course.html.
In response to the Reviewers’ comments, we have expanded the project team in three ways: (i) to ensure robust PPI involvement; (ii) to engage another key set of ‘users’, namely senior NHS managers; and finally (iii) to secure expertise in health geography multilevel statistical modelling (Dr Liz Twigg). We are delighted to report that both the Mental Health Foundation (MHF) and the Mental Health Network of the NHS Confederation have agreed to join us as partners, and to help us facilitate consultation events with service users and NHS managers and clinicians, respectively. David Crepaz-Keay, the Head of Empowerment and Social Inclusion at the MHF, has agreed to become an applicant on this proposal. We have also noted the Reviewers’ concerns about the collective time to be spent by the applicants on the project and have increased this so that the project lead (SW), deputy lead (PK) and LT will all spend 10% of their time on this, and JS and KB 5% each. We have requested support for the involvement of DC-K (2 days per month for the duration of the project) and a small amount of time for the MHF’s Director of Research, Dr Eva Cyhlarova.
The budget includes requests to support consultation events (two each) hosted by the Mental Health Foundation (mental health service users) and the Mental Health Network (senior NHS managers and clinicians). Each of these meetings will comprise 8–10 participants plus members of the research team.
We have applied for travel funds to enable regular meetings of the project team, and for Dr McBride to travel to England (Portsmouth and Warwick) for supervision. We have allowed a modest amount in the budget for software, data acquisition, consumables and dissemination.
List of abbreviations
- A&E
- accident and emergency
- BME
- black and minority ethnic
- BOO
- beds open overnight
- CI
- confidence interval
- CQC
- Care Quality Commission
- CRHT
- Crisis Resolution Home Treatment
- CTO
- Community Treatment Order
- DIC
- deviance information criterion
- ENSCA
- English National Study of Compulsory Admissions
- GP
- general practitioner
- HES
- Hospital Episode Statistics
- HoNOS
- Health of the Nation Outcome Scale
- HSCIC
- Health and Social Care Information Centre
- LD
- learning disability
- LOS
- length of stay
- LSC
- Legal Status Classification
- LSOA
- lower layer super output area
- LSR
- Most Restrictive Legal Status Classification Code
- MCMC
- Markov chain Monte Carlo
- MHA
- Mental Health Act
- MHF
- Mental Health Foundation
- MHMDS
- Mental Health Minimum Data Set
- MLM
- multilevel model
- NHSIC
- National Health Service Information Centre
- NSUN
- National Survivor User Network
- ONS
- Office for National Statistics
- OR
- odds ratio
- PCT
- primary care trust
- PEAT
- Patient Environment and Action Team
- PPI
- patient and public involvement
- SHA
- strategic health authority