Notes
Article history
The research reported in this issue of the journal was funded by the HS&DR programme or one of its preceding programmes as project number 10/2002/06. The contractual start date was in July 2012. The final report began editorial review in January 2014 and was accepted for publication in August 2014. The authors have been wholly responsible for all data collection, analysis and interpretation, and for writing up their work. The HS&DR editors and production house have tried to ensure the accuracy of the authors’ report and would like to thank the reviewers for their constructive comments on the final report document. However, they do not accept liability for damages or losses arising from material published in this report.
Declared competing interests of authors
none
Permissions
Copyright statement
© Queen’s Printer and Controller of HMSO 2015. This work was produced by Hardcastle et al. under the terms of a commissioning contract issued by the Secretary of State for Health. This issue may be freely reproduced for the purposes of private research and study and extracts (or indeed, the full report) may be included in professional journals provided that suitable acknowledgement is made and the reproduction is not associated with any form of advertising. Applications for commercial reproduction should be addressed to: NIHR Journals Library, National Institute for Health Research, Evaluation, Trials and Studies Coordinating Centre, Alpha House, University of Southampton Science Park, Southampton SO16 7NS, UK.
Chapter 1 Background and aims
Introduction
Life expectancy at birth in high-income countries has increased by about 8 years since 1950, and approximately half of this can be attributed to health care. 1 Improvements in health care offer many opportunities to increase the quality and length of life. For example, the population of England experiences approximately 60,000 potentially avoidable deaths annually, which is higher than in comparable countries. 2 Deficits in quality of care have been reported from high-income countries over many years,3–6 and we have previously reported substantial variations in quality standards achieved in England for older people with chronic conditions. 7,8
A wealth of international evidence has identified care processes that are suitable quality indicators of health care for many conditions. Quality indicators describe the minimum levels of acceptable health care, based on evidence of effectiveness in increasing patient health and reducing the risk of morbidity. The quality indicators used in this study were originally developed by researchers at RAND Corporation, who combined the best available evidence with expert opinion to assess appropriateness of treatment, and they were then adapted for use in the UK. 9
The UK has a long-established ‘free at the point of use’ health-care system, with relatively strong primary care services. Since 2004 there has been a major drive to improve receipt of indicated care through the Quality and Outcomes Framework (QOF), a scheme to pay general practices according to their performance with respect to quality indicators. Despite concentrated efforts to improve the quality of care, there is only weak evidence of improvement in quality of care since the introduction of the QOF. 10 Many geriatric conditions commonly affecting older people, such as osteoarthritis, are not included in this scheme. There is evidence that quality of care has not improved for some excluded conditions, such as falls. 8,11,12 QOF data are only available at the level of practices, precluding a detailed analysis of how individual patient characteristics (such as age, sex or degree of deprivation) might influence receipt of care.
The English Longitudinal Study of Ageing (ELSA) is a national survey following a cohort of people aged 50 years or older every 2 years since 2002. Questions assessing receipt of quality of care using quality indicators have been included since 2004. The baseline results derived from the 2004 data7 show that the amount of care that people were eligible for, and the amount they subsequently received, was highest for ischaemic heart disease (IHD) (83%) but lower for hypertension (72%), diabetes (74%), depression (64%) and osteoarthritis (29%). It is not known if receipt of this indicated care has improved since 2004 or whether or not patient characteristics are associated with variability in quality of care. ELSA has a broad range of participant demographics, and using these data it is possible to extract much more information on each participant than is available at a practice level. Gaining a clearer picture of who is at most risk of receiving poor quality of care is needed in order to inform the design of targeted interventions to improve quality of care, and to improve access for previously underserved groups.
Aims
Our objective was to analyse data from four waves from ELSA in order to identify opportunities for improving health care in older adults in England.
We aimed to assess changes over time in the receipt of effective health-care interventions for people aged ≥ 50 years in England with cardiovascular disease, depression, diabetes or osteoarthritis; to identify how quality varied with participant characteristics; and to compare the distribution of illness burden in the population with the distributions of diagnosis and treatment.
We had three research questions:
-
Research question 1: how does the prevalence of good quality of health care vary over time?
-
Research question 2: what characteristics are shared by people aged ≥ 50 years who received or did not receive specific items of recommended NHS or non-NHS care for cardiovascular disease, depression, diabetes and osteoarthritis in a national population-based study followed up for 6 years?
-
Research question 3: are factors such as wealth, patient education, physical activity, obesity and coexisting other chronic conditions, or practical factors such as good eyesight or marital status, independently associated with the quality of health care received, from burden of illness to diagnosis and, finally, to treatment?
Ethical approval
The English Longitudinal Study of Ageing received ethical approval from the National Research Ethics Service (reference number 09/H0505/124). Participants gave written informed consent before taking part.
Background
Cardiovascular disease
Cardiovascular disease is an umbrella term for a number of associated conditions. ELSA gathered information on achievement of quality indicators for IHD, hypertension and cerebrovascular disease (CVD) (stroke). A systematic review of 17 quality-of-care papers reporting on the RAND Corporation’s Assessing Care of Vulnerable Elders (ACOVE) quality indicators13 revealed that achievement rates are often high in primary care settings for indicated care for hypertension (72%) and stroke (69%).
Data from all 8409 general practices in the UK between 2005 and 2006, collected for the QOF, showed that quality indicator achievement for cardiovascular prescribing was generally high, with a mean achievement for all 26 indicators of 79.0% (standard deviation 4.4%). 14 This included antiplatelet therapy (89.75%), hypertension (74.83%) and beta-blockers (50.94%). An audit of 254 general practitioners (GPs) in the USA between 2007 and 2009, using quality indicators from the National Quality Forum and RAND Corporation, found that eligible patients with coronary artery disease were prescribed lipid-lowering medication on 76% of occasions and that beta-blockers were prescribed post myocardial infarction (MI) on 84% of occasions, though achievement for a quality indicator assessing prescriptions of antiplatelet therapy was lower at 48%. 15
Fürthauer et al. 16 assessed adherence to nine quality indicators by 58 GPs covering a range of cardiovascular conditions and interviewed patients and practitioners about the reasons for deviations from guideline recommendations. Approximately 70% of non-adherence was accounted for by physicians’ lack of knowledge and patients’ lack of awareness of the guidelines. Ludt et al. 17 sought to identify patient and practice characteristics that predicted good quality of care for prevention of cardiovascular disease using data from the clinical records of 7846 patients across primary care settings in 10 European countries. They did not find that practice factors predicted outcomes, but good risk factor control was more likely in those with higher health-related quality of life and lower for those whose marital status was single or who had achieved lower educational levels.
Diabetes
In 2009–10 the national audit on diabetes care18 reported that 1.8 million people were diagnosed with type 1 or type 2 diabetes and registered with an English general practice (5.24% adult prevalence). Despite 95.4% of patients with type 2 diabetes having consulted their GP at least once in the last year, the proportion receiving all processes included in a bundle of nine diabetes quality indicators19 was low (52.9%). Some processes [e.g. weight measurement 90.5% and glycosylated haemoglobin (HbA1c) measurement 92.6%] were more frequently measured than others (e.g. retinopathy screening 78.9%, foot risk assessment 85.2% and renal risk assessment 54.4%). Similarly, De Wet et al. 20 reported compliance with 10 individual diabetes quality indicators and a ‘care bundle’ – a composite measure of the individual quality indicators – in nine Scottish general practices (56,948 patients). Achievement of individual quality indicators was high (ranging from 85.9% to 97.8%), but all-or-nothing receipt of the care bundle was lower (58.4%).
There is considerable variation in the delivery of core diabetes care between providers. 18 For example, practices located in more deprived areas perform less well relative to their wealthier counterparts. 21,22 Less is known about patient factors, even though we need to better understand why such variation arises in order to improve receipt of basic care processes. 20 The national diabetes audit18 reported that social deprivation, sex and time since diagnosis did not affect the likelihood of receiving a complete care bundle, although patient age (< 55 years or ≥ 85 years vs. 55–84 years) and ethnicity (‘non-white’ vs. ‘white’ patients) were associated. Kontopantelis et al. 22 analysed longitudinal data (2000–1 to 2006–7) from 23,920 patients with diabetes who were registered with one of 148 practices. Older patients (≥ 65 years vs. 17–39 years), those with comorbidities (three or more vs. none), and number of years living with diabetes (1–4 years vs. newly diagnosed) were more likely to receive a complete package, although no sex effect was observed.
Osteoarthritis
Osteoarthritis is the most prevalent chronic joint disease globally. 23 Key clinical practice guidelines for osteoarthritis include non-pharmacological interventions (e.g. exercise and weight optimisation), provision of information regarding the disease and treatments, and joint replacement for severe disease. 23
Previous work assessing quality of care for osteoarthritis7 found that indicated care was received on only 29% of occasions. A meta-analysis of 17 studies using ACOVE quality indicators found that achievement rates for quality indicators for osteoarthritis had an interquartile range of 29–41%. 13 A recent review of chronic disease management found similarly poor quality of care for osteoarthritis across the quality domains of effectiveness, efficiency, safety, timeliness and appropriateness and patient-centred care, despite the availability of several evidence-based clinical practice guidelines. 23 A longitudinal study following 268 patients newly referred to an orthopaedic specialist in the UK over 12 months found that information was provided regarding their condition, pain management and exercise on 42%, 35% and 43% of occasions, respectively. 24 There is a need for research to assess quality of care for osteoarthritis over a longer time period. Using basic patient descriptors taken from medical records, Li et al. 25 found that receipt of advice on exercise and weight optimisation was not provided equitably across sex, age, disability and levels of formal education in 1349 patients with osteoarthritis of the knee and/or hip.
Depression
Quality indicators of health-care processes for depression focus on the provision of pharmacological and psychotherapy treatments. A recent meta-analysis13 of studies using quality indicators developed for the ACOVE project found low achievement of quality indicators for depression in both primary care settings (31%) and in nursing homes (16%). Duhoux et al. 26 assessed adherence rates to 27 quality indicators for depression in 915 adults who consulted a GP, regardless of the reason for the consultation, who also met established criteria for a major depressive episode. Adherence to guidelines was high (> 75%) for around one-third of assessed quality indicators, but less impressive for over half of quality indicators (< 60% achievement). Only 52.2% of the sample received at least one minimally acceptable treatment for depression (i.e. pharmacotherapy or psychotherapy). Patient characteristics that were associated with receipt of indicated care included old age (≥ 65 years), having a comorbid anxiety disorder, having a family physician, having supplementary insurance cover and the increasing severity of the depressive episode.
Chapter 2 Methods
The English Longitudinal Study of Ageing
The English Longitudinal Study of Ageing is a survey of adults aged ≥ 50 years and their partners living in private households in England. It began in 2002 and the respondents have been followed up with 2-yearly interviews, or waves. There have been five waves of data released to date – wave 1 2002–3, wave 2 2004–5, wave 3 2006–7, wave 4 2008–9 and wave 5 2010–11 – with a sixth wave due for release in early 2014. The original sample (2002–3) was drawn from households that had previously responded to the Health Survey for England (HSE) in 1998, 1999 and 2001. These years were chosen from the HSE because they were recent and could provide a sufficiently large sample size to make the survey representative of all adults living in England. The HSE is an annual cross-sectional household survey that gathers a broad range of biometric and health data. It was intended that the original sample of ELSA in 2002–3 would be representative of older people living independently in England. Further information about ELSA, including all questionnaires, is available from the ELSA website. 27 ELSA data are available from the UK Data Service. 28
Scope of the English Longitudinal Study of Ageing
The aim of ELSA is ‘to explore what it means to grow older in the 21st century and to understand which factors account for the variety of observed patterns’. 29 There is, therefore, an emphasis in the interviews on measuring change over time, with some measures being repeated exactly at each wave and others asking about change or giving respondents the opportunity to amend or update previous responses. A core set of modules on specific domains was included in all waves, with certain topics additionally covered at various waves. ELSA aimed to cover a broad array of salient domains, using standardised measures where possible. The modules common to all waves are listed in Table 1.
Module | Description |
---|---|
Household demographics | Answered by one person on behalf of the household. Collects basic demographic characteristics of everyone in the household. Uses feed forward data from HSE |
Individual demographics | Answered by all. Included questions on marital status, family members and childhood circumstances |
Health | Answered by all. Included self-reported general health; long-standing illness or disability; eyesight and hearing; specific diagnoses, symptoms and care processes received; pain; difficulties with activities and instruments of daily living; health behaviours; and quality of care. Those aged ≥ 60 years were asked about falls and fractures |
Social participation | Answered by all. Covered frequency of participation in social activities, limitations to these activities, their care giving and usage of public transport |
Work and pensions | Answered by all. Included current work activities; and current or past pensions, including amounts if currently receiving them |
Income and assets | If a couple’s finances are kept together one of them answers. If separate, both answer. Asked about all income and assets |
Housing | Answered by one person on behalf of the household. Included: current housing situation (e.g. size, quality); housing-related expenses; ownership of durables and cars; expenditure on food. House owners were asked about value of property, mortgage details, etc. |
Cognitive function | Answered by all at start of ‘private modules’ block. Measured cognitive function including memory, speed, mental flexibility and numeracy |
Expectations | Answered privately by all. Measured expectations regarding financial decision-making and certainty of future events |
Psychosocial health | Answered privately by all. Measured respondents’ views on life including a depression scale and attitudes towards ageing |
Walking speed | Completed by those aged ≥ 60 years when safe to do so. Measured time taken to walk 2.4 m (taken twice) |
Self-completion | Completed either during other members’ private blocks or after and returned by post |
Data collection methods
Face-to-face interviews were conducted using computer-assisted personal interviews (CAPIs) and took place in respondents’ homes. A self-completion questionnaire was also given to respondents for them to complete and return at their convenience. If there were several respondents for a given household, interviews could be conducted at the same time using concurrent interview techniques, with modules requiring private answers still performed individually. Both the CAPI and self-completion instruments were piloted for each wave approximately 1 year before the main data collection, using a separate sample of households.
Additionally, a nurse visited the respondents in waves 2 (2004) and 4 (2008), and collected anthropometric data and blood samples. The blood samples were analysed for HbA1c and many other biomarkers.
The CAPI instrument used in ELSA adopted the technique of ‘feeding forward’ data. That is, certain responses that individuals made in previous waves were used to improve consistency of their responses across waves, or to aid recall. For example, a respondent who had previously reported a certain diagnosis would be asked at subsequent waves to confirm that they previously had that diagnosis and whether or not they still had it. Previous responses were also used in the routing of the computerised interviews.
Sample design
Three cohorts of participants make up the ELSA sample. Recruits were sourced from HSE samples, as these were nationally representative. Households were eligible for ELSA if they contained at least one individual from one of the respective core HSE samples who was still living at a private residential address in England at the wave of ELSA at which that cohort was introduced. They also had to be age-eligible and had given permission to be recontacted in the future (though they were not specifically informed of ELSA at HSE). ‘Cohort 1′ was introduced at wave 1 (2002) and was selected from HSE samples in 1998, 1999 and 2001, with people being age-eligible if they were born on or before 29 February 1952. The HSE in 1999 originally included a ‘boost’ sample that represented ethnic minorities, but funding constraints meant that this sample were not included in ELSA. ‘Cohort 3′ was introduced at wave 3 (2006) to replenish the ageing sample with people aged 50–52 years and was taken from HSE in 2001–4. ‘Cohort 4′ was introduced at wave 4 (2008) to both replenish the youngest age range, as in cohort 3, and bolster the sample of people aged 52–74 years that had lost numbers due to participant attrition over the waves. It was sourced from people born between 1 March 1933 and 29 February 1958 who participated in HSE in 2006, which was chosen because it included a nurse visit with measures taken that could be compared with those obtained in the wave 4 nurse visits.
Recruits selected for one of these cohorts were termed ‘core members’ of their respective cohort and remained eligible to take part in ELSA at subsequent waves as long as they had not moved out of Britain or had died. At each wave, partners of core members who lived in the household could also be interviewed. From wave 2 onwards, core members who had moved out of the private sector (e.g. into a nursing home) since the previous wave were approached for an ‘institution interview’. Proxy interviews were attempted if cognitive impairment, physical or mental ill health prevented someone from participating in a face-to-face interview. The person who answered on behalf of the respondent was aged ≥ 16 years and knew enough about the individual’s circumstances to provide the required information. Not all of the core modules were included in the proxy interviews, and we excluded proxies for the purposes of all our analyses. Box 1 summarises the different types of sample member eligible for ELSA. Further details of the sampling design and fieldwork procedures can be found in the ELSA technical reports. 31–34
Core members were participants who were living within the household that participated in HSE (not all were personally interviewed for HSE). They were age-eligible for ELSA (≥ 50 years of age) and had their first ELSA interview at a private residential address in England.
Core partners were participants who, like core members, were living within the household at the time of the HSE interview and were age-eligible for inclusion in ELSA. They differ from core members in that they were not interviewed when first approached. Therefore, they were only approached by virtue of their being the partner of a core member.
Younger partners were the cohabiting younger spouses or partners of core members, who had been living within the household at the time of HSE and the first ELSA interview, but who were not age-eligible.
Older partners (for cohorts 3 and 4 only) were the cohabiting spouses or partners of age-eligible sample members selected for ELSA, who had been living within the household at the time of the HSE or ELSA interview, but were themselves over the ages eligible for these cohorts.
New partners were the cohabiting spouses or partners (of any age) of core members at the time of the ELSA interview who had joined the household since the HSE interview.
Participant response rates
The technical reports for the first four waves of ELSA provide a variety of in-depth response rates. 31–34 Here, we report the ‘individual response rates’ from initial HSE samples to the entry wave of ELSA for each of the three cohorts, the total number of core members at each wave and the ‘study response rate’ of cohort 1 core members (the longitudinal sample) across the five waves (Table 2). 67% of individuals determined to be eligible for ELSA from HSE in 1998, 1999 and 2001 took part in ELSA wave 1. 34 Of individuals from HSE in 2001–4 who were eligible for ELSA as part of cohort 3, 60% were interviewed at wave 3. 32 A response rate of 65% was achieved for cohort 4 core members sourced from HSE in 2006. 31 Of the 11,392 core members from cohort 1 interviewed at wave 1, only 5315 (47%) completed all subsequent waves up to and including wave 5. The replenishment samples from cohorts 3 and 4 meant that numbers of all core members ranged from 8780 in wave 2 to 9886 in wave 4.
Wave of ELSA | Total interviews (all samples types), n | Total core members (all available cohorts), n | Longitudinal sample size (cohort 1), n | Longitudinal sample retained in next wave, % |
---|---|---|---|---|
Wave 1: 2002–3 | 12,100 | 11,392 | 11,392 | 100.0 |
Wave 2: 2004–5 | 9432 | 8780 | 8780 | 77.1 |
Wave 3: 2006–7 | 9771 | 8810 | 7197 | 63.2 |
Wave 4: 2008–9 | 11,050 | 9886 | 6018 | 52.8 |
Wave 5: 2010–11 | 10,274 | 9090 | 5315 | 46.7 |
Creating the data sets for analysis
The core ELSA data (see Table 1 for included modules) for each wave were contained in separate data sets, which needed to be merged into a single data set for analyses. Additionally, there were data sets containing financial derived variables for waves 1–4 and data sets for nurse data collected at waves 2 and 4. Table 3 lists the data sets that were merged.
Data set | Filename as retrieved from UK Data Archive | Description |
---|---|---|
Wave 1 core data | Wave_1_Core_Data_v2.1 | Data from core ELSA modules for wave 1 |
Wave 1 financial derived variables | Wave_1_Financial_Derived_Variables_v2 | Financial variables derived by the Institute of Fiscal Studies for wave 1 |
Wave 2 core data | Wave_2_Core_Data_v2 | Data from core ELSA modules for wave 2 |
Wave 2 financial derived variables | Wave_2_Financial_Derived_Variables | Financial variables derived by the Institute of Fiscal Studies for wave 2 |
Wave 2 nurse data | Wave_2_Nurse_Data_v2 | Data obtained from the nurse visit in wave 2 |
Wave 3 core data | Wave_3_Core_Data_Phase_2_v2 | Data from core ELSA modules for wave 3 |
Wave 3 financial derived variables | Wave_3_Financial_Derived_Variables | Financial variables derived by the Institute of Fiscal Studies for wave 3 |
Wave 4 core data | Wave_4_Core_Data_Phase_2 | Data from core ELSA modules for wave 4 |
Wave 4 financial derived variables | Wave_4_Financial_Derived_Variables | Financial variables derived by the Institute of Fiscal Studies for wave 4 |
Wave 4 nurse data | Wave_4_Nurse_Data | Data obtained from the nurse visit in wave 4 |
Wave 5 core data | elsa_wave_5_with_dvs_ | Data from core ELSA modules for wave 5 |
Before a data set was merged, the variables it contained were given a prefix that identified the wave to which it belonged. Participants’ data were linked across data sets by a unique individual identification number. For each merge, no participant was excluded, even if they had no data in the new data set or if they had not been present in previous data sets. This allowed our final data set to include participants who had missed a number of waves, or had joined the ELSA sample as part of cohorts 3 and 4. Table 4 displays the number of participants merged at each step. There were 16,778 ELSA responders in the final data set. New participants were added to the sample from the core data set at each wave owing to partners being interviewed, or owing to the additions of cohorts 3 and 4.
Data set merged | Total sample size, N | Number of participants matched on merger | Number of participants not matched on merger | |
---|---|---|---|---|
Not in added data set | New in added data set | |||
Wave 1 core data (start point) | 12,100 | N/A | N/A | N/A |
Wave 1 financial derived variables | 12,100 | 12,099 | 1 | 0 |
Wave 2 core data | 12,208 | 9324 | 2776 | 108 |
Wave 2 financial derived variables | 12,208 | 9432 | 2776 | 0 |
Wave 2 nurse data | 12,208 | 7666 | 4542 | 0 |
Wave 3 core data | 13,944 | 8043 | 4165 | 1736 |
Wave 3 financial derived variables | 13,948 | 9767 | 4177 | 4 |
Wave 4 core data | 16,636 | 8362 | 5586 | 2688 |
Wave 4 financial derived variables | 16,636 | 11,050 | 5586 | 0 |
Wave 4 nurse data | 16,636 | 8643 | 7993 | 0 |
Wave 5 core data | 16,778 | 10,132 | 6504 | 142 |
Longitudinal and cross-sectional samples
To answer our research questions, cross-sectional samples from each wave of ELSA and a longitudinal sample of those who were interviewed at all waves were required. Proxy interviews were excluded, as we required accurate reporting of diagnoses and received care, as were interviews held in institutions, because these would not be representative of those living in private residences. The 5114 ELSA cohort 1 core members who were interviewed at all four waves, excluding proxies and those in institutions, formed our longitudinal sample (Table 5). This represents 45.5% of the original sample of 11,233 who responded at wave 1. The additions of cohorts 3 and 4 kept the cross-sectional samples above 8500 core members each. For analysis of the diabetes care bundle, we used a cross-sectional sample of all core members in 2010–11, who had also been non-proxy participants in 2008–9 (n = 8222).
Sample | n |
---|---|
Wave 1 | 11,322 |
Wave 2 | 8688 |
Wave 3 | 8628 |
Wave 4 | 9578 |
Wave 5 | 8729 |
Longitudinal (all waves) | 5114 |
Quality indicators
The health module of ELSA included questions on the receipt of health care, as described in quality indicators. The quality indicators were originally developed by RAND Corporation for the ACOVE project35 and adapted for use in patient interview surveys in England9 to assess care for a range of common medical conditions affecting older people, as described in more detail in Quality indicators in the English Longitudinal Study of Ageing, below. Studies using these indicators have found a substantial shortfall in quality of care in both the USA in 19996 and the ELSA. 7
Composite measures of quality
It is possible to combine individual quality indicators into composites that summarise quality of care at a higher level, such as for a condition or even for all health care in a system. There are both advantages and disadvantages of this approach. Summary measures are useful for conveying a complex range of information to policy-makers and the public, but important variations in individual health-care processes can be masked if combined without care. 36 Composites should, therefore, avoid overgeneralisation and have a specific focus and aim, such as formative quality improvement for specific conditions. 37
‘Care bundles’ are composites that comprise processes of care which should all be received by the eligible population for certain conditions, such as annual monitoring checks for patients with diabetes. The ‘gap’ in performance between individual indicators and the care bundle may be a more valid reflection of variation in expected care delivery. 20
There are a number of different methods for deriving composite measures, with different strengths and weaknesses. Below, we describe the main methods.
Indicator-level achievement
Indicator-level achievement is the number of a group of indicators achieved, divided by the number of that group of indicators triggered. This is the most straightforward measure to calculate, and the most transparent in that it is clear where the underlying data come from. We use this measure to describe quality indicator achievement for each of the four conditions in this study. Its weakness is that the most prevalent conditions will drive the final composite score, and the scores for rare (but perhaps important) conditions can be lost in the greater numbers.
Average indicator-level achievement
The mean of the set of indicators is calculated, representing the average rate at which the indicated care was received. For example, if a condition had three quality indicators and their achievements at a given wave were 75%, 80% and 85%, then the indicator-level achievement for that condition would be (75 + 80 + 85)/3 = 80%. This method is suitable for comparing heterogeneous samples, as it is not greatly affected by the number of components included. Rare processes (those for which a comparatively small sample are eligible) are given the same importance as common processes of health care. 38
Patient-level achievement
This approach represents the average percentage of triggered aspects of care that were successfully met for each participant per condition. It is calculated by dividing the number of quality indicators each participant passed by the number they were eligible for per condition, taking the mean of the results and expressing them as a percentage. For example, if a participant is eligible for all five diabetes quality indicators at a given wave and passed three of them, their score would be 3/5 = 0.6, or 60%. The mean of all participants’ scores would be the average for that condition.
This method is best at assessing quality of care delivered to each patient. It can provide scores for individual patients and can therefore be used in a wide range of statistical analyses. 38
All-or-nothing achievement
In this method, the care for a patient is achieved only if all quality indicators for which they were eligible for that condition were achieved. This approach is helpful when considering sets of quality indicators for a condition that easily form ‘care bundles’, such as the three annual monitoring checks for diabetes. This is a stringent criterion and in our data set we were able to apply the care bundle approach only to diabetes, as the quality indicators available all map onto those required for annual diabetes checks. The patient-level achievement is calculated as above for the set of indicators that make a care bundle. Those whose percentage of received care was 100% were scored 0 and those who did not meet this criterion were scored 1. This is used to predict who did not receive all the bundled care items, so the proportion of eligible participants who were scored 1 is calculated.
The derived scores are intrinsically affected by the number of components included, as the more care processes involved, the harder it is to achieve all of them,20 and so only a few carefully selected quality indicators should be used with this method. Weighting can be hard to interpret with this approach and common care processes (those for which a comparatively large sample is eligible) can dominate the scoring. 38 However, this method is amenable to a broad range of statistical analyses38 and may be more clinically meaningful, as receipt of all indicated health care should be the ideal.
Quality indicators in the English Longitudinal Study of Ageing
In total, 32 quality of care indicators were used in ELSA. These quality indicators were adapted from a set of 236 quality indicators originally developed in the USA for the ACOVE project39 for conditions chosen for their prevalence, impact, effectiveness of treatment or prevention, importance in older people, feasibility of measurement and potential for quality improvement. They were based on systematic reviews of evidence of improved outcomes and focus on processes of care rather than health outcomes, as processes are under the control of the health-care system and are not subject to the array of other factors that influence health outcomes. Approximately half of the ACOVE indicators could be used in an interview survey without the use of clinical records, and these were rated for validity by an expert panel of clinicians for validity in patient interview surveys in England, using an adapted form of the RAND Corporation/University of California in Los Angeles appropriateness method for combining evidence with expert opinion. 9,40 The clinical panel reviewed the evidence for 119 quality indicators and scored them on the extent to which they reflected good practice in England. Two rounds of anonymous rating were used, with face-to-face discussion between the rounds. Indicators were excluded if the panel disagreed on their validity. The quality indicators were designed to reflect the minimum acceptable standard of care rather than the optimum, and the panel were informed that they were to be used in a national longitudinal panel survey.
The panel identified 17 of the 119 indicators that were not suitable for use in the UK. Forty-three of the remaining 102 quality indicators were not piloted in ELSA owing to difficulties in implementing them, or because they did not relate to conditions found to be a common cause of morbidity in HSE 2000. A further 27 were not used in ELSA owing to problems found with their implementation in the pilots or main survey. 7 The remaining 32 indicators were incorporated in wave 2 of ELSA, with many also repeated at subsequent waves. These covered 13 conditions: CVD (stroke), depression, diabetes mellitus, falls, hearing problems, hypertension, IHD, osteoarthritis, osteoporosis, pain management, smoking cessation, urinary incontinence and cataracts.
Generation of quality indicators
From wave 2 onwards, the health module contained questions that enabled generation of the quality indicators developed by ACOVE and adapted for use in England that were implemented in ELSA. In total, there are five quality indicators for diabetes, three for depression, one for CVD (stroke), one for hypertension, five for IHD and four for osteoarthritis. There were also seven questions about patient-centred care. Quality indicators were generated at every wave for which they were available (see Availability of quality indicators across waves). A non-software specific syntax is given in Appendix 2.
Description of quality indicators and their generation from English Longitudinal Study of Ageing questions
The quality indicators for each condition are described below. Each quality indicator starts with an ‘if’ clause that determines the eligible population, followed by a ‘then’ clause that states the indicated care. The quality indicators specify that they are for those with the relevant condition who are aged ≥ 50 years or over. Some of the quality indicators specify that treatment should be offered ‘at least once’ or relate to treatments that are usually continuous in practice. For these quality indicators, participants who were eligible at the current wave and had reported receiving the indicated treatment at a previous wave were deemed to have also passed the indicator at the current wave. The derivation of each quality indicator follows its description. Appendix 1 shows the translation of each quality indicator and patient-centred question into its corresponding ELSA questions.
Cerebrovascular disease (stroke)
Stroke antihypertensives description
IF a person aged ≥ 50 years has had a previous stroke, THEN the patient should be offered antihypertensive medication.
Stroke antihypertensives generation
At each wave, participants were asked ‘Has a doctor ever told you that you have (or have had) any of the conditions on this card [includes ‘stroke (cerebral vascular disease)’]?’ Participants who reported a diagnosed stroke at that wave, or who confirmed a previous report of diagnosed stroke from an earlier wave, formed the eligible sample if they had also given a yes/no response to the question ‘Did a doctor or nurse ever suggest you take any medication to lower your blood pressure?’ at any wave up to and including the current wave. Respondents from this sample passed the quality indicator if they answered ‘yes’ to this last question at least once.
Hypertension
Hypertension treatment description
IF a person aged ≥ 50 years remains hypertensive after non-pharmacological intervention, THEN pharmacological antihypertensive treatment should be initiated.
Hypertension treatment generation
At each wave, participants were asked ‘Has a doctor ever told you that you have (or have had) any of the conditions on this card (includes ‘high blood pressure or hypertension’)?’ Participants who reported diagnosed hypertension at the current wave, or confirmed that they still had hypertension reported at an earlier wave, formed the eligible sample if they had also given a yes/no response to the question ‘Did a doctor or nurse ever suggest you take any medication to lower your blood pressure?’ at any wave up to and including the current wave. Respondents from this sample passed the quality indicator if they answered ‘yes’ to this last question at least once.
Ischaemic heart disease
Four of the five quality indicators for IHD specify that they are for those with established coronary heart disease (CHD). We defined CHD as prevalent angina and/or MI. At each wave, participants were asked ‘Has a doctor ever told you that you have (or have had) any of the conditions on this card (includes ‘angina’ and ‘heart attack’)?’ We determined participants to have established CHD if they reported angina or MI at the current wave, or confirmed that they still had angina and/or a previous report of MI.
Ischaemic heart disease cholesterol description
IF a person aged ≥ 50 years has established CHD and low-density lipoprotein (LDL) cholesterol > 3 mmol/l, THEN he or she should be offered an intervention to lower cholesterol.
Ischaemic heart disease cholesterol generation
Participants whom we determined to have established CHD at the current wave and who gave a yes/no response to the question ‘Has any doctor talked to you about how to lower your cholesterol? This would include changing your diet, losing weight, getting more exercise or taking medication’ formed the eligible sample. Those who answered ‘yes’ to this question passed the indicator.
Ischaemic heart disease antiplatelets description
IF a person aged ≥ 50 years has established CHD and is not on warfarin, THEN he or she should be offered antiplatelet therapy.
Ischaemic heart disease antiplatelets generation
Participants whom we determined to have established CHD at the current wave, who also answered ‘no’ to the question ‘Are you taking warfarin?’ and who gave a yes/no response to the question ‘Did a doctor suggest that you take medication to thin your blood such as warfarin or aspirin, Plavix, Ticlid, or other blood thinning medication?’ formed the eligible sample. Participants passed the indicator if they answered ‘yes’ to this last question.
Ischaemic heart disease smoking description
IF a person aged ≥ 50 years with established CHD smokes, THEN he or she should be offered counseling for smoking cessation.
Ischaemic heart disease smoking generation
Participants were eligible for this quality indicator if we determined them to have established CHD at the current wave, if they answered ‘yes’ to the question ‘Do you smoke cigarettes at all nowadays?’ at the current wave and if they gave a yes/no response to the question ‘Has a doctor or nurse ever advised you to stop smoking?’ at any wave up to and including the current wave. Participants passed the indicator if they answered ‘yes’ to this last question at least once.
Myocardial infarction beta-blocker description
IF a person aged ≥ 50 years has had a recent MI, THEN he or she should be offered a beta-blocker.
Myocardial infarction beta-blocker generation
Unlike most other quality indicators, this quality indicator requires incident cases and not prevalent cases. We determined participants to have incident MI if they reported a MI in response to the question ‘Has a doctor ever told you that you have (or have had) any of the conditions on this card (includes ‘heart attack’)?’ at the current wave, but not at the previous wave. Of these cases, those who gave a yes/no response to the question ‘Did any doctor ever tell you that you should take a medication called a beta-blocker?’ or indicated whether or not they were taking a beta-blocker in response to the question ‘Please could you show me the medications or the repeat prescription list for any medications you have taken over the last week (checked against card F beta-blockers)?’ formed the eligible sample. Participants from this sample passed the indicator if they either answered ‘yes’ to the former question, or indicated that they were taking beta-blockers at the latter question.
International normalised ratio test description
IF a person aged ≥ 50 years is prescribed warfarin, THEN an international normalised ratio (INR) should be determined at least every 12 weeks.
International normalised ratio test generation
Participants who reported CHD at the current wave were filtered to the question ‘Are you taking warfarin?’ Those who answered ‘yes’ were then filtered to the question ‘Many doctors ask patients on warfarin to get their blood tested regularly. The test checks how thin their blood is, and is sometimes called an INR test. Do you get your blood checked for this?’ Participants who answered ‘yes’ to this question were then filtered to the question ‘Have you had this blood test in the past 12 weeks?’ Those who gave a yes/no response to this question formed the eligible population and passed the indicator if their answer was ‘yes’.
Diabetes mellitus
Diabetes glycosylated haemoglobin description
IF a person aged ≥ 50 years has diabetes, THEN his or her HbA1c or fructosamine level should be measured at least annually.
Diabetes glycosylated haemoglobin generation
At each wave from wave 2 onwards, participants were asked ‘Has a doctor ever told you that you have diabetes?’ Those who responded ‘yes’ at any wave up to and including the current wave were filtered to the question ‘Have you ever had a special blood test to see how well your blood sugar was controlled? This test is called a glycosylated haemoglobin, or haemoglobin A1c, or fructosamine. This is a blood test taken at a doctor’s surgery or health centre or laboratory’. Participants who gave a yes/no response to this question at the current wave formed the eligible sample. Those who responded ‘yes’ to this question were filtered to a further question that asked ‘Have you had this test (glycosylated haemoglobin or fructosamine) performed in the past 12 months?’ Eligible participants who responded ‘yes’ to this final question passed the quality indicator.
Diabetes proteinuria description
IF a diabetic person aged ≥ 50 years does not have established renal disease and is not receiving an angiotensin-converting enzyme (ACE) inhibitor or angiotensin II receptor blocker, THEN he or she should receive an annual test for proteinuria.
Diabetes proteinuria generation
Participants who responded ‘yes’ to the question ‘Has a doctor ever told you that you have diabetes?’ at any wave up to and including the current wave were filtered to the question ‘I would like to check whether any of the medications you are taking are on this list of ACE inhibitor or A2 receptor blockers (show card G ACE inhibitors)?’ Those who answered ‘no’ to this question formed the eligible sample if they also answered ‘no’ to the question ‘Has a doctor ever told you that your diabetes has caused trouble with your kidneys?’ and gave a yes/no response to the question ‘Have you had a urine test for protein in the last 12 months?’ Participants who answered ‘yes’ to this final question passed the indicator.
Diabetes cholesterol description
IF a diabetic person aged ≥ 50 years has a fasting total cholesterol level of ≥ 5 mmol/l, THEN he or she should be offered an intervention to lower cholesterol.
Diabetes cholesterol generation
Participants who responded ‘yes’ to the question ‘Has a doctor ever told you that you have diabetes?’ at any wave up to and including the current wave, and who also reported high cholesterol at the current wave in response to the question ‘Has a doctor ever told you that you have (or have had) any of the conditions on this card (includes ‘high cholesterol’)?’, were filtered to the question ‘Has any doctor talked to you about how to lower your cholesterol? This would include changing your diet, losing weight, getting more exercise or taking medication’. Those who answered this question formed the eligible sample. Participants who answered ‘yes’ passed the indicator.
Diabetes ACE inhibitor description
IF a diabetic person aged ≥ 50 years has one additional cardiac risk factor (i.e. smoker, hypertension, hypercholesterolemia or renal insufficiency/microalbuminuria), THEN he/she should be offered an ACE inhibitor or receptor blocker.
Diabetes ACE inhibitor generation
Participants who responded ‘yes’ to the question ‘Has a doctor ever told you that you have diabetes?’ at any wave up to and including the current wave were filtered to the questions ‘Has a doctor discussed with you whether you should take a medication called an ACE inhibitor or A2 receptor blocker?’ and ‘I would like to check whether any of the medications you are taking are on this list of ACE inhibitor or A2 receptor blockers (show card G ACE inhibitors)?’ Those who gave a yes/no response to either of these questions formed the eligible sample if they also met at least one of the following criteria:
-
Answered ‘yes’ to the question ‘Do you smoke cigarettes at all nowadays?’
-
Answered ‘yes’ to the question ‘Has a doctor ever told you that your diabetes has caused trouble with your kidneys?’
-
Answered ‘yes’ to the question ‘Has a doctor ever told you that you have protein in your urine?’
-
Reported high cholesterol when asked ‘Has a doctor ever told you that you have (or have had) any of the conditions on this card (includes ‘high cholesterol’)?’ at the current wave, or confirmed they still have high cholesterol reported at a previous wave.
-
Reported hypertension when asked ‘Has a doctor ever told you that you have (or have had) any of the conditions on this card (includes ‘high blood pressure or hypertension’)?’ at the current wave, or confirmed they still have hypertension reported at a previous wave.
Waves 2 and 4 only (nurse data): their mean systolic blood pressure was ≥ 140 mmHg or their mean diastolic blood pressure was ≥ 90 mmHg.
Eligible participants who answered ‘yes’ to either being offered or taking an ACE inhibitor/A2 receptor blocker passed the indicator.
Diabetes foot check description
ALL diabetic persons aged ≥ 50 years should have an annual examination of his or her feet.
Diabetes foot check generation
Participants who responded ‘yes’ to the question ‘Has a doctor ever told you that you have diabetes?’ at any wave up to and including the current wave were filtered to the question ‘Some doctors suggest that some patients with diabetes have a regular foot examination. In the past year, has any doctor or nurse examined your bare feet?’ Those who gave a yes/no response to this question formed the eligible population. Participants who answered ‘yes’ passed the indicator.
Osteoarthritis
At each wave, participants were asked ‘Has a doctor ever told you that you have (or have had) any of the conditions on this card [includes ‘arthritis (including osteoarthritis, or rheumatism)’]?’ Those who reported arthritis were filtered to the question ‘Which type or types of arthritis do you have (includes ‘osteoarthritis’)?’ Participants who reported arthritis at a previous wave were also asked to confirm if they still had the condition. Below, we refer to participants as having current osteoarthritis if they reported osteoarthritis at the current wave, or confirmed that they still have arthritis previously reported, if the type of arthritis was also osteoarthritis.
Osteoarthritis exercise description
IF an ambulatory person aged ≥ 50 years has had a diagnosis of symptomatic osteoarthritis of the knee for longer than 3 months and has no contraindications to exercise and is physically and mentally able to exercise, THEN a directed or supervised strengthening or aerobic exercise programme should have been prescribed at least once.
Osteoarthritis exercise generation
All participants were asked ‘Are you often troubled with pain?’ All who responded ‘yes’ were filtered to the question ‘How would you rate your pain if you were walking on a flat surface? Please rate your pain from 0–10 for each of the following where 0 is no pain and 10 is severe or excruciating pain, as bad as you can imagine’. Unless respondents stated that they could not walk or never walk, they were then filtered to a set of questions that rated pain in different body parts, including their knees. Participants who rated the pain in their knees from 1–10 (i.e. at least some pain), and who reported current osteoarthritis, were filtered to the question ‘How long has the pain in your knee been bothering you?’ Respondents who reported that the pain had been bothering them for over 3 months were then asked ‘Has a doctor or nurse suggested that you receive physiotherapy or attend a supervised exercise program for your knee pain?’ Participants were eligible for this quality indicator if they gave a yes/no response to this final question at any wave up to and including the current wave and passed the indicator if their answer was ‘yes’ at least once.
Osteoarthritis education description
IF an ambulatory person aged ≥ 50 years has a diagnosis of symptomatic osteoarthritis, THEN education regarding the natural history, treatment and self-management of the disease should be offered at least once.
Osteoarthritis education generation
Participants with current osteoarthritis were filtered to the three questions ‘Has any doctor or nurse ever talked to you about . . . what your arthritis or joint pain will be like as time goes on?’, ‘. . . how to keep your arthritis or joint pain from getting worse?’ and ‘. . . how your arthritis or joint pain will be treated?’ Those with osteoarthritis at the current wave were eligible for this quality indicator if they gave a yes/no response to all three questions at any wave up to and including the current wave. Participants passed the quality indicator if they answered ‘yes’ to all three questions at least once.
Osteoarthritis paracetamol description
IF oral pharmacological therapy is initiated to treat osteoarthritis among people aged ≥ 50 years, THEN paracetamol should be the first drug used, unless there is a contraindication to use.
Osteoarthritis paracetamol generation
Participants with current osteoarthritis were filtered to the question ‘Some doctors suggest trying paracetamol as the first medication for arthritis or joint pain. Did any doctor or nurse recommend you try paracetamol before other medicines for your join pain?’ Those who gave a yes/no response to this question at any wave up to and including the current wave formed the eligible sample. Participants passed the indicator of they answered ‘yes’ at least once.
Osteoarthritis referral description
IF a person aged ≥ 50 years with severe symptomatic osteoarthritis of the knee or hip has failed to respond to non-pharmacological and pharmacological therapy, THEN the patient should be offered referral to an orthopaedic surgeon to be evaluated for total joint replacement within 6 months unless surgery is contraindicated.
Osteoarthritis referral generation
All participants were asked ‘Are you often troubled with pain?’ All who responded ‘yes’ were filtered to the question ‘How would you rate your pain if you were walking on a flat surface? Please rate your pain from 0–10 for each of the following where 0 is no pain and 10 is severe or excruciating pain, as bad as you can imagine’. Unless respondents stated that they could not walk or never walk, they were then filtered to a set of questions that rated pain in different body parts, including their knees and hips. Participants who rated the pain in their knee or hip from 6–10, and who reported current osteoarthritis, were filtered to the question ‘Has your knee or hip pain been bothering you for more than 6 months?’ Those who responded ‘yes’ were then asked ‘Do exercises and medicines control the pain in your knee or hip?’ If participants responded ‘no’, they were asked ‘Did any doctor recommend that you should have surgery or joint replacement?’ All who gave a yes/no response to this final question were eligible for this quality indicator and passed the indicator if their answer was ‘yes’.
Depression
All of the quality indicators for depression used incident cases only. At each wave, participants were asked ‘Has a doctor ever told you that you have (or have had) any of the conditions on this card (includes ‘any emotional, nervous or psychiatric problems’)?’ Those reporting emotional, nervous or psychiatric problems were filtered to a question that asked ‘What type of emotional, nervous or psychiatric problems do you have (includes ‘depression’)?’ Participants who reported such problems at a previous wave were also asked to confirm if they still had the condition. Below, we define incident depression as reports of depression at the current wave, excluding those who reported depression at the previous wave.
Depression suicide description
IF a person aged ≥ 50 years receives a diagnosis of a new depression episode, THEN the diagnosing physician should ask on the day of diagnosis if the person aged ≥ 50 years had any thoughts about suicide.
Depression suicide generation
Participants reporting psychiatric problems were filtered to the question ‘When you talked about these feelings with a doctor or nurse, did they ask you on that day if you had thoughts about suicide?’ Participants were eligible for this quality indicator if they reported incident depression and gave a yes/no response to the question. Those who answered ‘yes’ passed the indicator.
Depression treatment description
IF a person aged ≥ 50 years is diagnosed with clinical depression, THEN antidepressant treatment, talking treatment or electroconvulsive therapy should be offered within 2 weeks after diagnosis unless within that period the patient has improved, or unless the patient has substance abuse or dependence, in which case treatment may wait until 8 weeks after the patient is in a drug- or alcohol-free state.
Depression treatment generation
Participants reporting depression were filtered to the question ‘I have some questions about any treatment you may have had for your depression. Did a doctor or nurse suggest that you take medication, or see a mental health professional for counselling?’ Those with incident depression were eligible for this quality indicator if they responded to this question. Participants passed the indicator if the indicated that they had been offered a form of treatment and answered ‘yes’ to the question ‘Did you start medication or counselling within 2 weeks of being offered this treatment?’
Depression review description
IF a person aged ≥ 50 years has no meaningful symptom response after 6 weeks of treatment, THEN one of the following treatment options should be initiated by the eighth week of treatment: medication dose should be optimised (if initial treatment was medication), or medication should be initiated (if initial treatment was psychotherapy alone), or referral to a psychiatrist should be offered.
Depression review generation
Participants reporting depression were filtered to the question ‘I have some questions about any treatment you may have had for your depression. Did a doctor or nurse suggest that you take medication, or see a mental health professional for counselling?’ Those who indicated that they were on a treatment for depression were then asked ‘Did you start medication or counselling within 2 weeks of being offered this treatment?’ Participants who answered ‘yes’ to this question were filtered to a question that asked ‘Did you feel better within 6 weeks after starting to take medication, or seeing a mental health professional for counselling?’ Those who answered ‘no’ were eligible for this quality indicator if they had incident depression and gave a yes/no response to the question ‘Did any doctor or nurse start you on medication, change the dose of the medication that you were taking or, change the medications to help you feel better?’ Participants passed the indicator if they answered ‘yes’ to this final question.
Patient-centred care
Diabetes training description
Some people with diabetes receive training to help them manage their diabetes themselves. Have you ever participated in a course or class about diabetes, or received special training on how you can live with your diabetes from day to day?
Diabetes training generation
Participants who responded ‘yes’ to the question ‘Has a doctor ever told you that you have diabetes?’ at any wave up to and including the current wave were filtered to the question ‘Some people with diabetes receive training to help manage their diabetes themselves. Have you ever participated in a course or class about diabetes, or received special training on how you can live with your diabetes from day to day?’ Participants formed the eligible sample if they gave a yes/no response to this question at any wave and passed the indicator if they ever answered ‘yes’.
Diabetes knowledge description
How much do you think you know about managing your diabetes?
Diabetes knowledge generation
Participants who responded ‘yes’ to the question ‘Has a doctor ever told you that you have diabetes?’ at any wave up to and including the current wave were filtered to the question ‘How much do you think you know about managing your diabetes?’ Possible responses were ‘just about everything you need to know’, ‘most of what you need to know’, ‘some of what you need to know’, ‘a little of what you need to know’ and ‘almost none of what you need to know’. Participants formed the eligible sample if they gave a response and passed the indicator if they answered ‘just about everything you need to know’ or ‘most of what you need to know’.
Cholesterol explanation description
Has a doctor or nurse explained high cholesterol in a way you could understand?
Cholesterol explanation generation
Participants who reported high cholesterol in response to the question ‘Has a doctor ever told you that you have (or have had) any of the conditions on this card (includes ‘high cholesterol’)?’ were filtered to the question ‘Has a doctor or nurse explained high cholesterol in a way you could understand?’ Participants formed the eligible sample if they gave a yes/no response to this question and passed the indicator if their response was ‘yes’.
Cholesterol preferences description
Have doctors or nurses taken your preferences into account when making treatment decisions about your high cholesterol?
Cholesterol preferences generation
Participants who reported high cholesterol in response to the question ‘Has a doctor ever told you that you have (or have had) any of the conditions on this card (includes ‘high cholesterol’)?’ were filtered to the question ‘Have doctors or nurses taken your preferences into account when making treatment decisions about your high cholesterol?’ Participants formed the eligible sample if they gave a yes/no response to this question and passed the indicator if their response was ‘yes’.
Blood pressure explanation description
Has a doctor or nurse explained high blood pressure in a way you could understand at any time since you were first told you had high blood pressure?
Blood pressure explanation generation
Participants who reported hypertension in response to the question ‘Has a doctor ever told you that you have (or have had) any of the conditions on this card (includes ‘high blood pressure or hypertension’)?’ at the current wave, or who confirmed that they still had hypertension reported at an earlier wave, were filtered to the question ‘Has a doctor or nurse explained high blood pressure in a way you could understand at any time since you were first told you had high blood pressure?’ Participants formed the eligible sample if they gave a yes/no response to this question at any wave and passed the indicator if their response was ‘yes’ at any wave.
Blood pressure choice description
In general, have doctors or nurses given you any choice about how to treat your high blood pressure?
Blood pressure choice generation
Participants who reported hypertension in response to the question ‘Has a doctor ever told you that you have (or have had) any of the conditions on this card (includes ‘high blood pressure or hypertension’)?’ at the current wave, or who confirmed they still had hypertension reported at an earlier wave, were filtered to the question ‘In general, have doctors or nurses given you any choice about how to treat your high blood pressure?’ Participants formed the eligible sample if they gave a yes/no response to this question at any wave and passed the indicator if their response was ‘yes’ at any wave.
Osteoarthritis treatment purpose description
Has any doctor or nurse ever talked to you about what the specific purpose of the treatment for your arthritis or joint pain is?
Osteoarthritis treatment purpose generation
Participants with current osteoarthritis were filtered to the question ‘Has any doctor or nurse ever talked to you about how your arthritis or joint pain will be treated?’ Those who answered ‘yes’ were then filtered to the question ‘Has any doctor or nurse ever talked to you about what the specific purpose of the treatment for your arthritis or joint pain is?’ Participants formed the eligible sample if they gave a yes/no response to this last question at any wave and passed the indicator if their response was ‘yes’ at any wave.
Availability of quality indicators across waves
There were changes in the questions included in ELSA between the waves. As a result, not all quality indicators could be generated at all waves. Table 6 displays the availability of the quality indicators. All quality indicators were generated at wave 2. Wave 5 was the next most complete wave, where all quality indicators except those for depression could be generated. Apart from wave 2, depression quality indicators were available only at wave 4, and then only two out of the three could be derived. For diabetes, CVD and osteoarthritis, there were at least two waves at which quality of care could be compared using all quality indicators.
Quality indicator | Wave 2 | Wave 3 | Wave 4 | Wave 5 |
---|---|---|---|---|
Diabetes mellitus | ||||
Diabetes HbA1c | ✓ | ✓ | ✓ | ✓ |
Diabetes proteinuria | ✓ | ✓ | ✓ | ✓ |
Diabetes cholesterol | ✓ | ✓ | ||
Diabetes ACE inhibitor | ✓ | ✓ | ✓ | ✓ |
Diabetes foot check | ✓ | ✓ | ✓ | ✓ |
Cardiovascular disease | ||||
Stroke antihypertensives | ✓ | ✓ | ✓ | ✓ |
Hypertension treatment | ✓ | ✓ | ✓ | ✓ |
IHD cholesterol | ✓ | ✓ | ||
IHD antiplatelets | ✓ | ✓ | ||
IHD smoking | ✓ | ✓ | ||
MI beta-blocker | ✓ | ✓ | ||
INR test | ✓ | ✓ | ||
OA | ||||
OA exercise | ✓ | ✓ | ✓ | ✓ |
OA education | ✓ | ✓ | ✓ | ✓ |
OA paracetamol | ✓ | ✓ | ✓ | ✓ |
OA referral | ✓ | ✓ | ✓ | ✓ |
Depression | ||||
Depression suicide | ✓ | ✓ | ||
Depression treatment | ✓ | ✓ | ||
Depression review | ✓ | |||
Patient-centred care | ||||
Diabetes training | ✓ | ✓ | ✓ | |
Diabetes knowledge | ✓ | ✓ | ✓ | |
Cholesterol explanation | ✓ | |||
Cholesterol preferences | ✓ | |||
Blood pressure explanation | ✓ | |||
Blood pressure choice | ✓ | |||
OA treatment purpose | ✓ | ✓ | ✓ |
Variables
Potential covariates
Possible covariates were selected by a panel consisting of academics, clinicians, researchers and Patient and Public Patient Involvement in Research (PPIRes) members of the team. The choices were based on clinical relevance, feasibility to extract the data from ELSA and previous work. Covariates were grouped into five domains: demographics, health and disability, health behaviours, physical measures, and psychological and social measures. Table 7 lists all covariates that were used in any of the analyses in this work. Any relevant descriptors, explanations and groups are also shown.
Covariate | Description | Groups |
---|---|---|
Demographics | ||
Age groups | Age. Participants ≥ 90 years old have their age recorded as ‘over 90 years’ to help maintain anonymity | 50–64 years, 65–74 years, ≥ 75 years |
Sex | Women, men | |
Marital status | Cohabiting/not cohabiting | |
NS-SEC | Three-class version used | Managerial/professional, intermediate, routine/manual |
Education | Highest educational qualification achieved | No qualifications, intermediate or degree or higher education below a degree |
Age-specific total wealth quintile | Net financial wealth and physical wealth, private and state pension, and primary housing value less housing debt | Quintiles defined within the three age bands |
Weighting (see Weighting) | Wave specific cross-sectional or longitudinal | |
Health and disability | ||
General health | Self-reported general health | Excellent/very good/good/fair/poor |
Eyesight | Self-reported eyesight | Excellent/very good/good/fair/poor/blind |
Hearing | Self-reported hearing | Excellent/very good/good/fair/poor |
Chronic pain | Self-reported severity of chronic pain | None/mild/moderate/severe |
Long-standing illness | Whether or not reports long-standing illness, and whether or not this illness limits activities | None/long-standing illness/limiting long-standing illness |
Basic activities of daily living | Number of self-reported difficulties with basic activities of daily living of dressing, walking across a room, bathing, eating, getting in/out of bed and using the toilet | None/one, two or more |
Instrumental activities of daily living | Number of self-reported difficulties with instrumental activities of daily living of orientation, preparing meals, shopping, using the telephone, taking medications, housekeeping and money management | None/one, two or more |
Health behaviours | ||
Health literacy | Number of correct responses to four questions on health literacy. This was not available at 2008–9 so was taken from 2010–11 | No errors (high), one or more errors (low) |
Alcohol consumption | Self-reported frequency of alcohol consumption | 1–2 days/week or less, 3–4 days/week or more |
Smoking history | Smoking status | Never smoked, smoked in past, currently smokes |
Physical activity level | Classified using the reported level of work activity and frequency of low, moderate and vigorous leisure-time activity. Definition in ELSA wave 5 documentation (Banks et al.29) | Moderate/high, sedentary/low |
Diet | Whether eats five portions of fruit and/or vegetables per day | Eats ‘5 a day’, does not eat ‘5 a day’ |
Psychological and social measures | ||
Access to GP | The answer to ‘how easy or difficult is it to get to your GP using your usual form of transport?’ | Very easy/quite easy/quite difficult/very difficult/unable to go/do not wish to go |
Social detachment | Social detachment is a multidimensional construct. Four domains are derived: civic participation, leisure activities, cultural engagement and social networks. Those classified as detached on three or more of these domains were classified as socially detached. See ELSA wave 5 documentation for derivation27 | Not socially detached, socially detached |
Quality of life | The CASP-19 scale41 transformed into tertiles | High, medium, low |
Cognitive impairment | Composite score of prospective memory, attention, processing speed, verbal fluency, orientation, immediate word recall, delayed word recall and numeracy. Component scores were standardised and summed to form a cognitive performance scale. This scale was then standardised and the bottom 10% of scores were classified as cognitively impaired42 | Not cognitively impaired, cognitively impaired |
Lives alone | Whether or not respondent lives alone (reports no cohabitors) | No, yes |
LOC | Respondents rated the extent to which they agreed with the statement ‘what happens in life is often determined by factors beyond [his/her] control’ on a six-point Likert scale. Those who strongly/slightly agreed were classified as having ‘external’ LOC and those who strongly/slightly disagreed were classified as having ‘internal’ LOC | Internal, external |
Physical measures | ||
BMI category | Height and weight assessed in a nurse visit. BMI calculated from weight/(height)2 and defined from WHO criteria | Underweight/normal weight (≤ 25 kg/m2), overweight (≥ 25, < 30 kg/m2), obese (≥ 30 kg/m2) |
HbA1c levels | Used to define symptomatic diabetes, samples taken in waves 2 and 4 for analysis of HbA1c. Symptomatic diabetes defined as a fasting HbA1c level of ≥ 7.5% (DCCT) | Has symptomatic diabetes/do not have symptomatic diabetes |
Total cholesterol | Blood total cholesterol levels in mmol/l. QOF cut-off of > 5 mmol/l indicates high cholesterol | High cholesterol/acceptable cholesterol |
MAP in mmHg | Defined as the average arterial pressure during a single cardiac cycle. MAP = diastolic BP + 1/3 (systolic BP – diastolic BP) | High ≥ 110 mmHg/regular < 110 mmHg |
Disease-specific variables | ||
Comorbidities | Number of comorbid conditions measured in ELSA | ≥ 2/none or one |
Previous care (diabetes) | Whether or not the indicated care was achieved at the previous wave for the care bundle of annual monitoring checks (all-or-nothing achievement) and diabetes ACE inhibitor. New cases since the last wave were added as a separate category | Achieved, not achieved, new case |
Knows mostly everything about managing diabetes | Responses to the question ‘How much do you think you know about managing your diabetes?’ | Just about everything/most of what they need to know (yes), some/a little/almost none of what you need to know (no) |
Time since diagnosis for all conditions | Measured in waves of ELSA (2-year increments) since the diagnosis was reported. IHD split into time since heart attack and time since angina. Hypertension given in wave first diagnosed | Range of 0 (new case in 2010–11) to 3 (reported in 2004–5). Entered as ordinal variable |
CES-D depression classification | Self-reported depressive symptoms (eight items) using the CES-D scale43 | Not depressed, depressed (≥ 4 symptoms) |
Rose Angina Scale | Defined as grade 2 on the Rose Angina Scale44 | Has symptomatic angina/does not have symptomatic angina |
Joint replacement payment (used in OA) | Was your joint replacement paid for by the NHS or privately or a mixture of both? | NHS/private and mixed NHS private payment |
Cataract extraction payment (used in cataract) | Was your cataract extraction paid for by the NHS or privately or a mixture of both? | NHS/private and mixed NHS private payment |
Symptomatic OA by pain and disability45 | Often troubled by pain in knee or hip, and this pain was rated 5 or more out of 10 when walking on a flat surface. Also had some or much difficulty or were unable to walk a quarter of a mile | Has symptomatic OA/does not have symptomatic OA |
Standardised scales
Standardised scales available to use as covariates are described below.
Rose Angina Scale
The Rose Angina Scale is an epidemiological measure of angina pectoris. 45 It defines angina according to standard criteria as chest pain or discomfort that fulfils all of the following criteria:
-
It is brought on by exertion.
-
It is situated in the central or left anterior chest or left arm or sternum.
-
It forces the subject to slow down or stop.
-
It is relieved if the subject does slow down or stop.
-
It is relieved within 10 minutes.
This is further subcategorised into:
-
A: severe (grade II) if exertional pain came on while walking on level ground.
-
B: not severe (grade I) if chest pain only came on when hurrying or walking uphill.
Centre for Epidemiological Studies – Depression scale
A short form of the Centre for Epidemiological Studies – Depression Scale (CES-D), consisting of eight items, was included in the psychosocial health module at each wave from wave 2 onwards. Participants were asked to say whether or not eight statements relating to symptoms of depression were true of them in the past week, giving yes/no responses. The established criterion of likely depression is the presence of four or more symptoms. 43
Control, Autonomy, Self-realisation and Pleasure-19 quality of life scale
This scale is designed for use with older people and assesses quality of life over four domains, from which its name is taken: Control, Autonomy, Self-realisation and Pleasure (CASP-19). 41 It consists of 19 items, which were asked of participants in the self-completion module. Responses to these items are summed, after reverse scoring where necessary, to produce an overall measure of quality of life.
Activities of daily living
Markers of physical function available in ELSA included grip strength, walking speed, use of stick or mobility aids, leg raise, tandem or side stand, and the ability to walk a quarter of a mile without special equipment. We used the activities of daily living (ADL) scale in this study, as it is a well-known and widely used measure of physical function. 46,47
Definition of comorbidities
In our regression analyses we used a measure of comorbid conditions and these were defined as having two or more comorbid conditions as opposed to one or none. This is a commonly used definition of multimorbidity. 48 This variable was created by counting the number of comorbid conditions that every participant had out of a possible 16 conditions, and excluding those conditions closely related to the specific condition. For example, those with osteoarthritis often also had the more general diagnosis of ‘arthritis’, and this was not counted as a condition comorbid to osteoarthritis. For diabetes, hypertension, congestive heart failure, diabetes and high cholesterol were not considered comorbid conditions; for depression, Alzheimer’s disease and dementia were not considered comorbidities. For IHD, hypertension, prevalent angina, prevalent heart attack, heart murmur, arrhythmia, CVD and high cholesterol were not considered comorbid conditions.
Excluded variables
It was agreed by the clinical screening panel that ethnicity should be tested for association with health care; however, owing to < 2% of the sample being non-white this could not be used in the regression analyses. Other covariates that were not included in analyses were country of birth (not specific enough as recoded as UK or non-UK), geography (not available), internet use (missing data), employment status (majority not employed as they were past retirement age) and fasting glucose measurements (not used in QOF as a measure of diabetes). Previous work on ELSA accounted for the clustering and stratification of the participants in the HSE. On advice from the ELSA data manager at the National Centre for Social Research (NatCen), we did not include these variables as the ELSA sample is now effectively unclustered owing to non-response and people moving.
Additional variables excluded from the diabetes care bundle analysis
A number of the selected covariates measure very similar concepts. To reduce colinearity, the clinical screening panel selected one covariate to assess a domain where there were several options. Thus, National Statistics Socio-Economic Classification (NS-SEC)49 was used to represent socioeconomic status, and highest educational qualification and age-stratified wealth were excluded. Reports of long-standing illness, including limiting long-standing illness, were used as a measure of overall health, and participants’ reports of general health and comorbidities were excluded. Variables for whether or not respondents exceeded QOF criteria for high HbA1c and cholesterol were also excluded, as they were highly associated with whether or not participants had previously received indicated care. Ratings of the accessibility of participants’ GPs were excluded as < 10% of respondents’ ratings were anything other than ‘very easy’.
Data quality
Many covariates had missing data for some participants. In most cases, the frequency of missing data was small (< 2%). However, the frequency of missing data was much larger for some covariates, particularly those taken from the self-completion module or nurse visits, which had lower response rates or covariates that were derived scales and required responses to all scale components. To maximise the samples available for regression modelling, missing data were coded as an extra category for each covariate if the frequency was > 2% (continuous covariates were first transformed into tertiles). This criterion was chosen as a balance between including as many respondents as possible in the models and not causing multicolinearity issues in models due to low category frequencies. The frequencies of all covariates’ categories were checked for the samples to be used in statistical models. Where there were < 10% of responses in a category, we combined categories or, if combining categories was not suitable, excluded the covariate on the basis of poor data quality.
Weighting
The English Longitudinal Study of Ageing is designed to be analysed either cross-sectionally or longitudinally. Cross-sectional analysis uses data collected at a single wave, whereas longitudinal data analysis uses data collected in more than one wave. Owing to non-response, the refreshment samples in waves 3 and 4, and the original clustered recruitment from the HSE, sampling weights have been calculated for use in analyses to allow for these factors.
Longitudinal weights were designed to adjust for historical non-response as well as to response since the last wave; therefore, at wave 5 the longitudinal weights adjust for non-response to HSE as well as for non-response to each wave of ELSA. Cross-sectional weights were designed to adjust the sample to be representative of the English population ≥ 50 years old.
In our analyses we used the weighting where appropriate, depending on the wave used, and whether we were performing cross-sectional or longitudinal analyses. The precise methods of calculating the weights are given in the ELSA user guides and technical reports, available from the Economic and Social Data Service.
Statistical methods
All analyses described below were conducted using Stata SE version 12.1 (StataCorp LP, College Station, Texas, USA).
Quality indicator achievement
The entire ELSA sample for this study consisted of all participants who had been interviewed at any of the four waves (n = 16,773). The primary outcome was achievement of each quality indicator, which was the number of times the indicator was achieved divided by the number of times it was triggered, expressed as a percentage, with possible values between 0% and 100%. The quality indicator achievement at the level of each condition (cardiovascular disease, depression, diabetes or osteoarthritis) was the number of times any indicator for that condition was achieved divided by the number of times any indicator for that condition was triggered, expressed as a percentage, with possible values between 0% and 100% (see Table 9).
The percentage change in achievement was calculated between wave 2 and the most recent year of follow-up, and the statistical significance of changes over time were tested with Pearson’s chi-squared test for comparing proportions (see Table 10). Some data points were paired within individuals between wave 2 and the most recent year of follow-up, and some were not, as different individuals were eligible for indicators at different waves. For this reason we did not use McNemar’s test for paired data, although McNemar’s test would have had greater power and precision had it been appropriate to use. We excluded ‘don’t know’ responses from both the numerator and the denominator when calculating quality scores.
We used correlation analysis to investigate whether or not quality of care for one condition was associated with quality of care in another.
Transitions in receipt of care across time
We explored the extent to which participants with diabetes, cardiovascular disease, osteoarthritis and depression transitioned from non-receipt of indicated care to subsequently receiving it, and vice versa, across the available waves of ELSA. To achieve this, we used cross-tabulations of whether or not an indicator was passed at one wave with whether or not it was subsequently passed at the wave at which it was next available. Some quality indicators were only available at one wave other than the baseline (wave 2), either wave 4 or wave 5 (see Availability of quality indicators across waves). As a result, owing to participant attrition and changes in individuals’ health status, sample sizes for these quality indicators were too small for comparisons across waves. Additionally, sample sizes were too small for osteoarthritis referral and osteoarthritis paracetamol was not suitable, as if paracetamol for pain relief was not the first prescribed drug, this could never be rectified. We therefore report the proportions of individuals who successfully transition from non-receipt of care to receiving their care, who transition from receipt of care to subsequent non-receipt of care, and those who do not transition, for stroke antihypertensives, hypertension treatment, diabetes HbA1c, diabetes proteinuria, diabetes ACE inhibitor, diabetes foot check, all-or-nothing receipt of the care bundle for diabetes, osteoarthritis exercise and osteoarthritis education.
Modelling for longitudinal quality of care
For the modelling we used a longitudinal data set comprising only those participants who had completed interviews at all four waves (n = 5114) (see Table 13). We calculated quality indicator achievement for these 5114 participants in exactly the same way as we calculated it for the entire data set, described in Quality indicator achievement – that is, the quality indicator achievement at the level of each condition was the number of times any indicator for that condition was achieved divided by the number of times any indicator for that condition was triggered, expressed as a percentage, with possible values between 0% and 100% (see Table 15).
For the regression, modelling we needed to calculate quality indicator eligibility by participant rather than by condition, as the covariates were linked to participants rather than to conditions (cardiovascular disease, depression, diabetes or osteoarthritis). Each participant might have been eligible for more than one indicator within each condition, and so we reshaped the data into ‘long’ format in Stata statistical software, with each participant having a separate record for each indicator. These person-indicator level records of quality achievement were the unit of analysis for the modelling, using ‘svy’ commands in Stata ‘long’ format. This was done for each condition separately, and the outcome was expressed as the odds of achievement of any quality indicator that a participant was eligible for, within the relevant condition, with adjustment for participant characteristics. Intraperson correlation of outcomes was accounted for using robust adjustment with Stata, with each participant’s unique identifier included in the regression equation as a cluster variable. All data were weighted for historical non-response and non-response since the last wave (see Table 15).
Age group (50–64, 65–74 and ≥ 75 years) and sex were included in all regression models, and the following were examined as potential explanatory variables, and included in final models if they were significantly associated with the outcome before adjustment for other variables: wealth, marital status, education, socioeconomic status, NHS or independent sector delivery of joint surgery, general health, eyesight, hearing, chronic pain, limiting illnesses, instrumental activities of daily living (IADL) (scale of 1–7), ADL (scale of 1–6), GP access, the Rose Angina Scale,44 cognitive impairment, comorbidities, smoking, eating five portions of fruit and vegetables a day, alcohol consumption, physical activity levels, body mass index (BMI), blood pressure, blood glucose (HbA1c) levels, self-perceived social status, CASP-19, social detachment, and depression (CES-D).
We categorised BMI according to the World Health Organization criteria,21 blood glucose [HbA1c ≥ 7.5% (Diabetes Control and Complications Trial: DCCT) or 59 mmol/mol (International Federation of Clinical Chemistry and Laboratory Medicine: IFCC)] and cholesterol (≤ 5 mmol/l or less) according to the QOF criteria,8 and depression according to the CES-D (a score of 4 or more was classified as depression). 22
A single fasting plasma glucose was used to estimate the prevalence of diabetes, rather than the two separate measurements recommended by the World Health Organization. This approach is not recommended for clinical care, but is acceptable at population level and has been used in other studies. 50 It may overestimate diabetes51 but will also not identify the small number of people whose diabetes can be detected only on an oral glucose tolerance test. 52
All characteristics that were significantly associated with quality of care at the 5% level for the conditions using univariate regression analyses were used in the final models.
Predicting non-receipt of indicated care for diabetes care bundles
We used the care bundle approach as an alternative method to model quality of care for diabetes, to explore which characteristics were predictive of not receiving indicated care for diabetes 2 years later. For the diabetes care bundle analyses we used a data set comprising ELSA core members at wave 5 who also responded at wave 4 (n = 8222) (see Table 17). The latest outcomes were deemed to be the most clinically relevant to model, so we used wave 5 outcomes and covariates from wave 4. A covariate of particular interest was whether or not participants had previously received the indicated care.
We therefore had two outcomes: all-or-nothing receipt of the care bundle and whether or not the diabetes ACE inhibitor quality indicator was passed. Separate logistic regression models were developed for each of the two outcomes using the following process: short-listed covariates were first entered into univariate regressions, adjusted for age and sex, and the models were weighted for non-response using the wave 5 cross-sectional sample weights (see Weighting). All characteristics that were significantly associated with quality of care at the 5% level for either outcome were then entered into a forced entry logistic regression, adjusted and weighted as above, for both outcomes (see Table 17).
Patient characteristics associated with the illness burden, diagnosis and treatment pathway
To examine whether or not factors such as wealth are associated with quality of health care received through the care pathway, we defined burden of illness, diagnosis and treatment for four conditions from data generated from the four waves of ELSA. We used the sample of ELSA core members who had been interviewed at any of the four waves (n = 16,773).
Variable definitions
The illness burden for angina was defined as grade 2 on the Rose Angina Scale [pain or discomfort in chest when walking at an ordinary pace on the level on most occasions or more often, which makes subject stop or slow down if occurs while walking, and which then goes away within 10 minutes, and which includes either sternum (any level), or left arm and left anterior chest]. Illness burden for diabetes was defined as a fasting HbA1c level of > 7.5%. Illness burden for depression was defined as a score of ≥ 3 on the eight-item CES-D. The application of these standardised scales in ELSA has been described previously. Illness burden for osteoarthritis was defined as self-reported pain in the hip or knee of ≥ 5 on a scale of 0 to 10.
A medical diagnosis was considered to exist if a participant answered ‘yes’ when asked if a doctor had ever told them they had the condition of interest. For arthritis, a follow-up question asked whether they had been told that they had osteoarthritis, rheumatoid or other arthritis.
Treatment for depression and diabetes was defined by reported achievement of quality of care indicators, derived through a robust process of literature reviews, expert panel assessment and piloting. For depression, the quality indicator was about receipt of treatment since the previous wave: ‘if a person is diagnosed with clinical depression, then antidepressive treatment, talking treatment or electroconvulsive treatment should be offered within 2 weeks after diagnosis unless within that period the patient has improved, or unless the patient has substance abuse or dependence, in which case treatment may wait until 8 weeks after the patient is in a drug-or alcohol-free state’. For diabetes, treatment was measurement of HbA1c or fructosamine levels in the preceding 12 months. Treatment for angina was defined as ever being offered or currently taking beta-blockers (ELSA variables hebeta or hebetb). Treatment for osteoarthritis was defined as reporting ever having had surgery for the condition; this excluded those with hips or knees replaced due to fracture. Data on hip and knee replacements were available only for respondents aged 60 and over, and so respondents aged < 60 years (n = 3186) were excluded from the analysis of osteoarthritis.
Wealth was defined as the sum of financial, physical and housing wealth plus state and private pension income. Age was categorised into three groups: 50–59 years, 60–74 years and ≥ 75 years (see Table 20).
Analysis
We used two approaches to analysis: a main analysis using serial cross-sectional data and then a subsidiary analysis using longitudinal data. Multivariable logistic regression analysis was used, with the outcome variables defined as one of illness burden, medical diagnosis or treatment for each of the four conditions in each cross-sectional wave (Stata statistical software version 12.1). This regression analysis was repeated for each of the four waves of ELSA from 2004 to 2011 separately and then ‘overall’ for all four waves combined. For the ‘overall’ analysis, the data were reshaped into ‘long’ format in Stata statistical software, with each participant having a separate record for each indicator and wave, as explained earlier (see Statistical methods and Modelling for longitudinal quality of care). Intraperson correlation of outcomes was accounted for using robust adjustment with Stata, with each participant’s unique identifier included in the regression equation as a cluster variable. Missing data were excluded from the analyses.
The independent variables were age group, sex and slope order of inequality. We used the slope order of inequality as an independent variable to estimate the relationship between the outcome measures and the categorised measure of wealth. The slope order of inequality consisted of wealth quintiles with values of 0.1, 0.3, 0.5, 0.7 and 0.9, that is the mid-points of each quintile on a scale of zero (least wealthy) to one (most wealthy). The slope order of inequality was modelled as a continuous variable, and its odds ratio (OR) represents the ratio of the least wealthy compared with the wealthiest participant. This OR is also known as a relative index of inequality. Advantages of this method of quantifying inequality are that it includes all participants, instead of just comparing the highest and lowest quintiles, it accounts for the number of participants in each category and it provides a single overall measure of inequality.
We included all participants in the main cross-sectional analysis in order to compare the distribution of illness burden in the whole population with the distributions of diagnoses and treatments in the whole population. This meant that diagnosis was assessed even in those who did not meet the criteria for ‘illness burden’, and treatment was assessed even in those with no diagnosis. For the subsidiary analysis using longitudinal data, we estimated the OR of receiving a diagnosis by a subsequent wave only for those who had met the criteria for ‘illness burden’ in a previous wave, and then the likelihood of receiving treatment only for those who had received a diagnosis in a previous wave. This was a subsidiary analysis as the numbers of participants who could be followed over time in this manner was small, particularly for treatment in angina and depression.
Others factors, such as health literacy, obesity, access to GP, comorbidities, education and health behaviours, were used to investigate whether or not they had an additional impact on the care pathway as well as wealth. This was performed not at each wave but as an overall measure, where burden of illness, diagnosis and treatment were defined as having the burden of disease, diagnosis or receiving treatment at any wave, respectively. The sensitivity analyses adjusted for age group, sex and slope index of inequality at wave 5 as well as one other confounder at a time.
Chapter 3 Results
The results are presented in this chapter in the following four sections: Demographics for all waves of the English Longitudinal Study of Ageing, Achievement of quality indicators over time (this section also gives the results for the diabetes care bundle and for transitions in quality over time), Regression modelling for quality of care and Population distribution of illness burden, diagnosis and treatment.
Demographics for all waves of the English Longitudinal Study of Ageing
At any of the four waves 16,773 participants were interviewed, of whom 3810 had at least one condition at wave 2 and 5404 had at least one condition of interest at wave 5 (Table 8). The mean age of the participants at baseline was 65.8 years (range 52 to > 90 years), and just under half were women. This sample was used to address research question 1: how does the prevalence of good quality of health care vary over time?
Characteristics | 2004–5: wave 2 | 2006–7: wave 3 | 2008–9: wave 4 | 2010–11: wave 5 |
---|---|---|---|---|
Total number at wave | 9432 | 9771 | 1150 | 10,274 |
Age (years), mean | 65.8 | 64.6 | 65.3 | 66.8 |
Rangea | 52 to > 90 | 54 to > 90 | 56 to > 90 | 58 to > 90 |
Sex | ||||
Female, n (%) | 4125 (47.3) | 4295 (44.0) | 4925 (44.6) | 4569 (44.5) |
Male, n (%) | 5307 (56.3) | 5476 (56.0) | 6125 (55.4) | 5705 (55.5) |
Ethnicity | ||||
White, n (%), wave 2 | 9432 (97.5) | |||
Non-white, n (%) | 229 (2.4) | |||
Education (highest qualification), n (%) | ||||
Degree or equivalent | 2721 (16.2) | |||
Intermediate | 4335 (25.9) | |||
No qualifications | 5008 (29.9) | |||
Missing | 4709 (28.1) | |||
Total net household financial wealth, £ | ||||
Median | 188,851 | 207,631 | 217,098 | 231,049 |
Range | –111,700 to 9,297,227 | –1,578,980 to 2.08 × 107 | –255,580 to 3.93 × 107 | –10,500 to 1.06 × 107 |
One or more conditions, n (%) | 3868 (41) | 4754 (49) | 5404 (49) | 3810 (37) |
Number of conditions for which participants were eligible | ||||
Mean | 1 | 1 | 1 | 1 |
Range | 0 to 6 | 0 to 4 | 0 to 4 | 0 to 3 |
Number of indicators for which participants were eligible | ||||
Mean | 1 | 1 | 1 | 1 |
Range | 0 to 13 | 0 to 8 | 0 to 12 | 0 to 12 |
Clinical incidence over 2 years, n (%) | ||||
CVD | 110 (1.2) | 168 (1.6) | 136 (1.4) | |
Depression | 125 (1.3) | 288 (2.7) | ||
IHD | 298 (3.1) | |||
Osteoarthritis | 877 (10.7) | 1105 (11.8) | 877 (10.7) | |
Hypertension | 817 (12.8) | 1599 (18.9) | 933 (13.2) | |
Clinical prevalence, n (%) | ||||
Diabetes mellitus | 739 (7.8) | 1000 (10.1) | 1330 (11.7) | 1566 (14.6) |
Achievement of quality indicators over time
Achievement of quality indicators
The eligibility and achievement rates of individual quality indicators for each wave of data for every participant (n = 16,773) show that quality indicator pass rates varied substantially within conditions, with the depression suicide indicator being achieved by 46.5% in wave 2 and treatment by 78.9% at the same wave (Table 9). There was also variation between pass rates at different waves; the greatest percentage increase in quality indicator achievement over the waves was seen in osteoarthritis exercise, where there was a 20% improvement in passing the quality indicator, while the worst performing indicator was depression treatment, with a 17% drop between the two waves where it was measured.
Quality indicator (n = 32) or patient-centred question (n = 7) | 2004–5, wave 2: % pass rate (95% CI); n pass/n eligible | 2006–7, wave 3: % pass rate (95% CI); n pass/n eligible | 2008–9, wave 4: % pass rate (95% CI); n pass/n eligible | 2010–11, wave 5: % pass rate (95% CI); n pass/n eligible | % change wave 2 to most recent follow-up wave |
---|---|---|---|---|---|
Stroke antihypertensives | 77.3 (71.0 to 83.5); 136/176 | 74.9 (70.7 to 79.2); 305/407 | 82.1 (78.5 to 85.7); 354/431 | 84.8 (81.4 to 88.2); 375/442 | + 7.5 |
Depression suicide | 46.5 (37.8 to 55.2); 60/129 | N/A | 57.8 (52.0 to 63.7); 162/280 | N/A | + 11.3 |
Depression treatment | 78.9 (71.9 to 86.0); 105/133 | N/A | 61.8 (56.0 to 67.4); 176/285 | N/A | – 17.1 |
Depression review | 75.8 (59.3 to 92.4); 22/29 | N/A | N/A | N/A | N/A |
Diabetes HbA1c | 83.0 (80.2 to 85.8); 562/677 | 83.5 (80.9 to 86.1); 648/776 | 80.4 (77.8 to 83.0); 723/899 | 82.0 (76.0 to 84.4); 815/994 | – 1.0 |
Diabetes proteinuria | 83.2 (79.1 to 87.4); 263/316 | 80.9 (76.6 to 85.2); 263/325 | 78.4 (74.1 to 82.7); 279/356 | 79.5 (75.6 to 83.4); 326/410 | – 3.7 |
Diabetes cholesterol | 85.6 (79.9 to 91.4); 125/146 | N/A | N/A | 88.7 (82.2 to 95.0); 86/97 | + 3.1 |
Diabetes ACE inhibitor | 48.1 (44.3 to 52.0); 311/646 | 53.1 (49.2 to 56.9); 345/650 | 56.6 (53.2 to 59.9); 489/864 | 56.9 (53.7 to 60.1); 511/898 | + 8.8 |
Diabetes foot check | 83.7 (81.0 to 86.3); 606/724 | 82.3 (79.7 to 84.9); 671/815 | 81.8 (79.3 to 84.2); 781/955 | 82.8 (80.5 to 85.1); 863/1042 | – 0.9 |
Hypertension treatment (prevalent hypertension) | 71.6 (68.0 to 75.1); 451/630 | 67.8 (64.8 to 70.9); 614/905 | 80.3 (78.0 to 82.6); 915/1139 | 74.6 (71.1 to 78.1); 453/607 | + 3.0 |
Hypertension treatment (incident hypertension) | 84.6 (82.9 to 86.3); 1423/1682 | 87.1 (85.9 to 88.3); 2736/3130 | 90.7 (89.7 to 91.6); 3277/3615 | 92.7 (91.8 to 93.5); 3299/3560 | + 8.1 |
IHD cholesterol | 85.2 (80.0 to 90.3); 161/189 | N/A | N/A | 86.2 (78.8 to 93.6); 75/87 | + 1.0 |
IHD antiplatelets | 82.6 (78.8 to 86.5); 309/374 | N/A | N/A | 82.4 (79.8 to 84.9); 719/873 | – 0.2 |
IHD smoking | 89.7 (84.8 to 94.7); 132/147 | N/A | N/A | 90.7 (86.4 to 94.9); 165/182 | + 3.0 |
MI beta-blocker (incident MI) | 64.0 (53.9 to 74.2); 57/89 | N/A | N/A | 75.6 (66.3 to 84.8); 65/86 | + 11.6 |
MI beta-blocker (prevalent MI) | 69.1 (60.8 to 77.4); 85/123 | N/A | N/A | 72.8 (65.6 to 80.0); 110/151 | + 3.7 |
INR test | 100 (100 to 100); 44/44 | N/A | N/A | 93.9 (89.8 to 98.1); 124/132 | – 6.1 |
OA exercise | 26.1 (21.4 to 30.8); 88/337 | 34.6 (30.3 to 38.9); 161/465 | 41.6 (38.7 to 44.5); 459/1103 | 46.2 (43.5 to 48.9); 613/1326 | + 20.6 |
OA education | 21.6 (17.7 to 25.3); 99/459 | 20.0 (18.3 to 21.8); 404/2019 | 25.6 (23.9 to 27.4); 606/2366 | 32.1 (30.3 to 33.9); 824/2569 | + 10.5 |
OA paracetamol | 45.2 (40.6 to 49.8); 206/456 | 42.6 (38.7 to 46.4); 273/641 | 44.8 (41.9 to 47.7); 497/1109 | 46.0 (43.1 to 48.8); 552/1201 | + 0.8 |
OA referral | 35.8 (28.3 to 43.3); 57/159 | 39.2 (31.0 to 47.3); 56/143 | 39.1 (29.8 to 48.4); 43/110 | 33.6 (24.8 to 42.5); 38/113 | – 2.2 |
Diabetes training | 25.4 (22.2 to 28.6); 184/724 | 29.8 (27.0 to 32.7); 290/972 | 33.2 (30.6 to 35.7); 426/1284 | N/A | + 7.8 |
Diabetes knowledge | 78.0 (74.9 to 81.0); 564/723 | 78.2 (75.3 to 81.0); 634/811 | 81.3 (78.9 to 83.8); 772/949 | N/A | + 3.3 |
Cholesterol explanation | 78.6 (76.7 to 80.5); 1356/1725 | N/A | N/A | N/A | N/A |
Cholesterol preferences | 49.1 (46.7 to 51.4); 839/1708 | N/A | N/A | N/A | N/A |
Blood pressure explanation | 68.7 (66.4 to 70.9); 1151/1676 | N/A | N/A | N/A | N/A |
Blood pressure choice | 42.7 (40.3 to 45.1); 704/1648 | N/A | N/A | N/A | N/A |
OA treatment purpose | 77.9 (72.2 to 83.6); 162/208 | 79.1 (76.3 to 81.8); 654/827 | 81.9 (80.0 to 84.1); 974/1189 | N/A | + 4.0 |
We used quality indicator achievement at condition level to see whether care for specific conditions had increased or decreased over the 6 years of the study (Table 10). A statistically significant difference between wave 2 (2004–5) and wave 5 (2010–11) was seen only for osteoarthritis quality indicators. Although the achievement rates for osteoarthritis show a small increase, they are still very low (36% in 2010–11). We used the chi-squared test for associations, as the number of people with conditions at both time points was small (n = 185 for cardiovascular disease, n = 6 for depression, n = 349 for diabetes and n = 366 for osteoarthritis).
Condition | 2004–5, wave 2: % achievement (95% CI); n pass/n eligible | 2006–7, wave 3: % achievement (95% CI); n pass/n eligible | 2008–9, wave 4: % achievement (95% CI); n pass/n eligible | 2010–11, wave 5: % achievement (95% CI); n pass/n eligible | aχ2 |
---|---|---|---|---|---|
Cardiovascular disease | 82.7 (79.9 to 85.5); 703/843 | N/A | N/A | 84.2 (82.1 to 86.2); 1148/1360 | 0.53 |
Depression | 63.3 (57.6 to 69.0); 187/291 | N/A | 59.8 (52.4 to 64.3); 338/565 | N/A | 0.21 |
Diabetes | 76.0 (74.1 to 77.8); 1867/2509 | 76.6 (74.8 to 78.5); 1927/2566 | 75.3 (73.5 to 77.1); 2272/3074 | 76.5 (74.8 to 78.1); 2661/3441 | 0.67 |
Osteoarthritis | 31.2 (28.5 to 33.8); 450/1411 | 23.9 (22.4 to 25.4); 894/3268 | 31.2 (29.8 to 32.7); 1605/4688 | 35.6 (34.2 to 37.1); 2027/5209 | < 0.001 |
Those who reported ‘don’t know’ were not included in the analyses. The numbers reporting this were extremely low and in wave 2 (total n at wave 2 = 9432) only four did not know if they had been asked about osteoarthritis and paracetamol use. This dropped to two at wave 5 (total n at wave 5 = 10,274). The offer of antihypertensive medication after a stroke was not known by 19 people at wave 2 and by two at wave 5.
Associations of care between conditions
We assessed whether or not quality of care for one condition was associated with quality of care for another condition at each wave that data were available (see Chapter 2, Availability of quality indicators across waves, and Table 6). The only statistically significant association was at wave 2, with quality of care for diabetes and cardiovascular disease correlated (r = 0.13, p = 0.035). No associations between quality of care for other diseases were found at wave 2 (osteoarthritis and diabetes, r = 0.16, p = 0.18; osteoarthritis and cardiovascular disease r = –0.044, p = 0.72; depression and osteoarthritis r = –0.17, p = 0.48; depression and cardiovascular disease r = 0.20, p = 0.41; and depression and diabetes r = 0.43, p = 0.28). At wave 3 quality of care for osteoarthritis and diabetes were not associated (r = 0.078, p = 0.27). At wave 4, depression and osteoarthritis and depression and diabetes care showed no associations (r = 0.18, p = 0.11 and r = 0.037, p = 0.85, respectively). Likewise, there was no relationship between quality of care at wave 4 for osteoarthritis and diabetes (r = 0.075, p = 0.23). At wave 5, similarly, there were no associations found between quality of care in the different diseases (cardiovascular disease and diabetes r = 0.14, p = 0.074; cardiovascular disease and osteoarthritis r = 0.057, p = 0.3; and osteoarthritis and diabetes r = 0.039, p = 0.47)
Achievement of diabetes care bundle quality indicators
This analysis used participants who were core members at wave 5 and had also responded at wave 4 (n = 8222). Eligibility and non-achievements rates of the individual indicators that made up the diabetes care bundle and the one indicator (diabetes ACE inhibitor) were calculated. At least one of the three monitoring checks included in the care bundle was not received in 288 out of 907 cases in 2010–11 [weighted rate = 32.2%, 95% confidence interval (CI) 29.1% to 35.5%]. This is equivalent to achievement of 67.8% for all three quality indicators in the bundle. The HbA1c test, proteinuria test and foot check quality indicators had similar, low rates of non-achievement (weighted 2010–11 range = 17.4–22.8%), although the ACE inhibitor quality indicator had much higher non-achievement (weighted 2010–11 score = 44.5%). The non-achievements of the individual quality indicators and the care bundle varied little between waves in the study (Table 11).
Quality indicator | 2008–9 | 2010–11 | ||
---|---|---|---|---|
Failed/eligible | % (95% CI) | Failed/eligible | % (95% CI) | |
Diabetes HbA1c | 130/692 | 20.0 (16.8 to 23.3) | 154/861 | 17.4 (14.8 to 20.1) |
Diabetes proteinuria | 58/273 | 22.7 (17.3 to 28.0) | 76/349 | 22.8 (18.0 to 27.6) |
Diabetes foot check | 129/735 | 17.8 (14.8 to 20.8) | 150/905 | 17.5 (14.8 to 20.2) |
Care bundlea | 235/736 | 32.8 (29.2 to 36.4) | 288/907 | 32.2 (29.1 to 35.5) |
Diabetes ACE inhibitor | 289/665 | 44.6 (40.6 to 48.6) | 325/759 | 44.5 (40.7 to 48.3) |
Transitions in quality of care across time
Transitions from receiving indicated care in one wave to not receiving care in a subsequent wave, and also from not receiving care to subsequently receiving care, were calculated for wave 2 to wave 3, wave 3 to wave 4, and wave 4 to wave 5 for the quality indicators available at every wave (see Chapter 2, Availability of quality indicators across waves) (Table 12). Different patterns were seen for different conditions. Two quality indicators for cardiovascular disease could be derived at every wave: the quality indicator for stroke antihypertensives and the quality indicator for hypertension treatment. The percentage of eligible participants who did not receive the indicated care for stroke antihypertensives in either the earlier or subsequent wave of each wave pairing ranged from 65.4% in waves 2–3 to 73.1% in waves 4–5. Only 26.9–34.6% transitioned to receiving their care at the subsequent wave. For hypertension treatment the percentage of those who were not offered an antihypertensive who then transitioned to subsequently passing the quality indicator was much higher (59.6–69.0%), though 31.0–40.4% continued to not receive the indicated care. In contrast, only 1.5–2.8% of those who were previously offered antihypertensive medication transitioned to not receiving this care at the subsequent wave.
Quality indicator | W2 to W3 | W3 to W4 | W4 to W5 | |||||||||
---|---|---|---|---|---|---|---|---|---|---|---|---|
No → no, n (% of participants who did not receive this care at W2) | No → yes, n (% of participants who did not receive this care at W2) | Yes → no, n (% of participants who received this care at W2) | Yes → yes, n (% of participants who received this care at W2) | No → no, n (% of participants who did not receive this care at W3) | No → yes, n (% of participants who did not receive this care at W3) | Yes → no, n (% of participants who received this care at W3) | Yes → yes, n (% of participants who received this care at W3) | No → no, n (% of participants who did not receive this care at W4) | No → yes, n (% of participants who did not receive this care at W4) | Yes → no, n (% of participants who received this care at W4) | Yes → yes, n (% of participants who received this care at W4) | |
Stroke antihypertensa | 17 (65.4) | 9 (34.6) | N/A | 92 (100) | 46 (73.0) | 17 (27.0) | N/A | 201 (100) | 38 (73.1) | 14 (26.9) | N/A | 239 (100) |
Hypertension treatment | 26 (31.0) | 58 (69.0) | 25 (2.8) | 862 (97.2) | 57 (40.4) | 84 (59.6) | 29 (1.6) | 1821 (98.4) | 44 (36.4) | 77 (63.6) | 36 (1.5) | 2350 (98.5) |
Diabetes HbA1c | 32 (40.5) | 47 (59.5) | 47 (11.3) | 368 (88.7) | 30 (36.6) | 52 (63.4) | 72 (15.9) | 382 (84.1) | 34 (28.3) | 86 (71.7) | 76 (14.1) | 462 (85.9) |
Diabetes proteinuria | 6 (26.1) | 17 (73.9) | 25 (21.6) | 91 (78.5) | 12 (42.9) | 16 (57.1) | 17 (14.3) | 102 (85.7) | 12 (35.3) | 22 (64.7) | 17 (13.0) | 114 (87.0) |
Diabetes ACE inhibitor | 132 (64.1) | 74 (35.9) | 46 (22.6) | 158 (77.5) | 127 (63.8) | 72 (36.2) | 42 (17.0) | 205 (83.0) | 162 (66.4) | 82 (33.6) | 68 (19.8) | 275 (80.2) |
Diabetes foot check | 42 (46.2) | 49 (53.9) | 42 (9.1) | 422 (91.0) | 40 (38.8) | 63 (61.2) | 47 (9.6) | 444 (90.4) | 48 (38.1) | 78 (61.9) | 52 (8.6) | 550 (91.4) |
Diabetes bundleb | 75 (47.2) | 84 (52.8) | 84 (21.2) | 313 (78.8) | 81 (45.5) | 97 (54.5) | 101 (24.2) | 317 (75.8) | 112 (48.3) | 120 (51.7) | 97 (19.4) | 402 (80.6) |
Osteoarthritis exercisea | 147 (91.3) | 14 (8.7) | N/A | 64 (100) | 186 (92.1) | 16 (7.9) | N/A | 106 (100) | 397 (83.8) | 77 (16.2) | N/A | 321 (100) |
Osteoarthritis educationa | 170 (85.0) | 30 (15.0) | N/A | 65 (100) | 861 (87.7) | 121 (12.3) | N/A | 251 (100) | 1026 (86.3) | 163 (13.7) | N/A | 414 (100) |
A similar pattern to that seen with hypertension was observed in diabetes HbA1c, proteinuria and foot check and the care bundle of these three indicators. Of eligible participants who did not receive all indicated care in the bundle at the earlier wave, 51.7–54.5% did transition to receiving the care at the subsequent wave, while 45.5–48.3% continued to not receive the full bundle. Of those who received all three annual monitoring checks at the earlier wave, only 19.4–24.2% subsequently transitioned to not receiving the complete bundle, as 75.8–80.6 received all the indicated care at both waves across the wave pairings. The diabetes quality indicator for ACE inhibitors showed a slightly different pattern. Achievement of this quality indicator was lower (see Table 9), and a greater proportion (63.8–66.4%) of eligible participants who were not offered the indicated medication at the earlier wave continued to not receive the care at the subsequent wave. In comparison, only 17.0–22.6% of those who were offered the indicated medication at the earlier wave subsequently transitioned to not pass the indicator.
As described above (see Achievement of quality indicators), achievements of the osteoarthritis quality indicators were low. Most of the eligible participants who did not receive their indicated care continued to subsequently not receive their care (osteoarthritis exercise 83.8–92.1%; osteoarthritis education 85.0–87.7%). Only a small minority transitioned to passing the quality indicators at the subsequent wave (osteoarthritis exercise 7.9–16.2%; osteoarthritis education 12.3–15.0%).
Regression modelling for quality of care
Demographics for the longitudinal data set
In all four waves 5114 participants were interviewed, and the number with at least one condition of interest in any wave ranged from 1998 to 2961 (Table 13). The mean age of participants was 65 years (range 52 to > 90 years) in 2004–5 and just over half were women. This sample was used to address research question 2: what characteristics are shared by people aged ≥ 50 years who received or did not receive specific items of recommended NHS or non-NHS care for cardiovascular disease, depression, diabetes and osteoarthritis in a national population-based study followed up for 6 years? A detailed breakdown of the whole ELSA sample by covariate and wave is given in Appendix 5.
Characteristics | 2004–5 | 2006–7 | 2008–9 | 2010–11 |
---|---|---|---|---|
Age (years), mean | 64.8 | 66.6 | 68.7 | 70.9 |
Sex | ||||
Female, n (%) | 2882 (56.4) | 2882 (56.4) | 2882 (56.4) | 2882 (56.4) |
Male, n (%) | 2232 (43.6) | 2232 (43.6) | 2232 (43.6) | 2232 (43.6) |
One or more conditions, n (%) | 1998 (39) | 2738 (54) | 2961 (58) | 2290 (44.8) |
Number of conditions for which participants were eligible | ||||
Mean | 1 | 1 | 1 | 1 |
Range | 0–6 | 0–4 | 0–4 | 0–3 |
Number of indicators for which participants were eligible | ||||
Mean | 1 | 1 | 1 | 1 |
Range | 0–13 | 0–7 | 0–8 | 0–8 |
Clinical incidence over 2 years, n (%)a | ||||
CVD | 32 (0.7) | 53 (1.1) | 60 (1.2) | |
Depression | 65 (1.3) | 53 (1.1) | ||
IHD | 183 (3.7) | |||
Osteoarthritis | 415 (11.1) | 372 (9.7) | 415 (11.1) | |
Hypertension | 253 (8.5) | 453 (13.5) | 334 (10.7) | |
Clinical prevalence, n (%) | ||||
Diabetes mellitus | 351 (6.9) | 447 (8.7) | 542 (10.6) | 634 (12.4) |
Time varying covariates
Some covariates were expected to change over time, but in fact the changes we found in the data were generally small. The greatest change in the number of participants reporting potentially time-varying covariates was seen in cognitive impairment, which was reported by over twice as many participants in wave 5 as in wave 2 (Table 14). This was explored further in the regression models, which found that the only condition in which cognitive impairment was associated with quality was diabetes (see Associations between quality of care and patient characteristics and Table 16). Only 5.8% more participants with diabetes also had cognitive impairment in wave 5 than did those in wave 2.
Covariate | Wave 2 (n unless % stated) | Wave 5 (n unless % stated) | Change (n unless % stated) |
---|---|---|---|
Cognitively impaired | 256 | 557 | + 301 |
Cognitively impaired and diabetes | 29 | 89 | + 60 |
Cognitively impaired as percentage of participants with diabetes | 8.2% | 14.0% | 5.8% |
Mean number of ADLs | |||
1 | 472 | 558 | + 86 |
2 | 186 | 237 | + 51 |
3 | 95 | 111 | + 16 |
4 | 56 | 67 | + 11 |
5 | 34 | 50 | + 24 |
6 | 11 | 12 | + 1 |
Mean number of IADLs | |||
1 | 537 | 518 | – 19 |
2 | 218 | 189 | – 29 |
3 | 90 | 68 | – 22 |
4 | 38 | 32 | – 6 |
5 | 17 | 24 | + 7 |
6 | 4 | 5 | + 1 |
7 | 4 | 6 | + 2 |
MAP > 110 mmHg | 458 | 372 | – 86a |
HbA1c ≥ 7.5% DCCT values | 89 | 132 | + 43a |
BMI | |||
Normal/underweight | 1231 | 1159 | – 72a |
Overweight | 2033 | 1931 | – 102a |
Obese | 1328 | 1405 | + 77a |
Quality indicator achievement in the entire data set of 16,773 participants is reported earlier in this results chapter (see Tables 10 and 11). Here, we report quality indicator achievement in the smaller longitudinal data set of 5114 participants who were used for regression modelling, before going on to give the regression results. Quality achievement for this data set was very similar to that for the entire data set. Receipt of indicated care in 2010–11 varied substantially and ranged from 89% (95% CI 85% to 92%) for CVD to 34% (95% CI 32% to 36%) for osteoarthritis (Table 15). As a further check that the data sets were similar, we compared the overall quality indicators achievement in both data sets. Overall, quality indicator achievement dropped slightly from 59% (95% CI 57% to 61%) in 2004–5 to 54% (95% CI 53% to 55%) in 2010–11 (p < 0.001), giving a drop of 3.4% for the core longitudinal sample of 5114 participants. This compared with an overall 3.7% drop in achievement of quality indicators using the 16,773 participants who had been interviewed at any wave of ELSA.
Condition | Wave 2 | Wave 3 | Wave 4 | Wave 5 | ||||
---|---|---|---|---|---|---|---|---|
n pass/n eligible | % (95% CI) | n pass/n eligible | % (95% CI) | n pass/n eligible | % (95% CI) | n pass/n eligible | % (95% CI) | |
CVD | 61/73 | 83.6 (74.9 to 92.2) | 160/205 | 78.0 (72.3 to 83.8) | 208/248 | 83.9 (79.3 to 88.5) | 264/298 | 88.6 (85.0 to 92.2) |
Depression | 105/157 | 67.5 (60.4 to 74.6) | 73/118 | 61.9 (52.7 to 71.0) | ||||
Diabetes | 935/1245 | 76.3 (73.7 to 79.0) | 1081/1428 | 77.5 (75.0 to 80.0) | 1273/1732 | 75.2 (72.9 to 77.6) | 1541/2048 | 76.6 (74.6 to 78.7) |
Hypertension | 222/320 | 69.4 (64.3 to 74.5) | 219/324 | 67.6 (62.5 to 72.7) | 169/247 | 68.4 (62.6 to 74.3) | 208/268 | 77.6 (72.6 to 82.6) |
IHD | 321/375 | 77.7 (72.2 to 83.1) | 701/820 | 81.4 (78.4 to 84.4) | ||||
Osteoarthritis | 229/780 | 29.4 (25.8 to 32.9) | 512/1989 | 22.4 (20.4 to 24.3) | 788/2508 | 28.3 (26.3 to 30.2) | 1091/2986 | 33.6 (31.7 to 35.5) |
Associations between quality of care and patient characteristics
We found few statistically significant associations between quality of care and most participant characteristics (Table 16 and Figure 1). This analysis was conducted as described in Chapter 2 (see Statistical methods and Modelling for longitudinal quality of care). For each condition, univariate models tested each of the covariates listed in Table 7 for association with quality indicator achievement at the 5% level of statistical significance. For all covariates that were associated at the 5% level for each condition, we ran a multivariate model that also included age and sex (see Table 16).
Conditiona | Characteristic | % quality indicator achievement | OR (95% CI) |
---|---|---|---|
CVD | Wealth | ||
Quintile 1 (poorest) | 92 | 1.0 | |
Quintile 2 | 84 | 0.4 (0.1 to 1.1) | |
Quintile 3 | 84 | 0.4 (0.1 to 1.0) | |
Quintile 4 | 69 | 0.2 (0.1 to 0.5) | |
Quintile 5 (richest) | 87 | 0.5 (0.2 to 1.3) | |
Diabetes | Cognitive impairment | ||
No | 77 | 1.0 | |
Yes | 63 | 0.5 (0.4 to 0.7) | |
Cohabiting | |||
Lives with partner | 69 | 1.0 | |
Lives alone | 79 | 1.7 (1.3 to 2.0) | |
Hypertension | Age (years) | ||
50–64 | 61 | 1.0 | |
65–74 | 79 | 2.2 (1.6 to 3.1) | |
75+ | 86 | 3.2 (2.0 to 5.3) | |
IHD | Age (years) | ||
50–64 | 89 | 1.0 | |
65–74 | 87 | 1.0 (0.6 to 1.6) | |
75+ | 79 | 0.5 (0.3 to 0.8) | |
Cohabiting | |||
Lives with partner | 81 | 1.0 | |
Lives alone | 88 | 1.8 (1.3 to 2.5) | |
Osteoarthritis | Pain | ||
Mild | 30 | 1.0 | |
Moderate | 30 | 1.0 (0.8 to 1.2) | |
Severe | 42 | 1.8 (1.4 to 2.2) | |
Limiting Illness | |||
No | 26 | 1.0 | |
Yes | 37 | 1.8 (1.5 to 2.1) | |
BMI | |||
Under-/normal weight (< 25 kg/m2) | 26 | 1.0 | |
Overweight (25 to 30 kg/m2) | 29 | 1.2 (0.9 to 1.5) | |
Obese (> 30 kg/m2) | 37 | 1.6 (1.2 to 2.0) |
FIGURE 1.
Direction and size of associations between quality of care and participant characteristics by condition and logistic regression ORs. OA, osteoarthritis; Q, quintile.
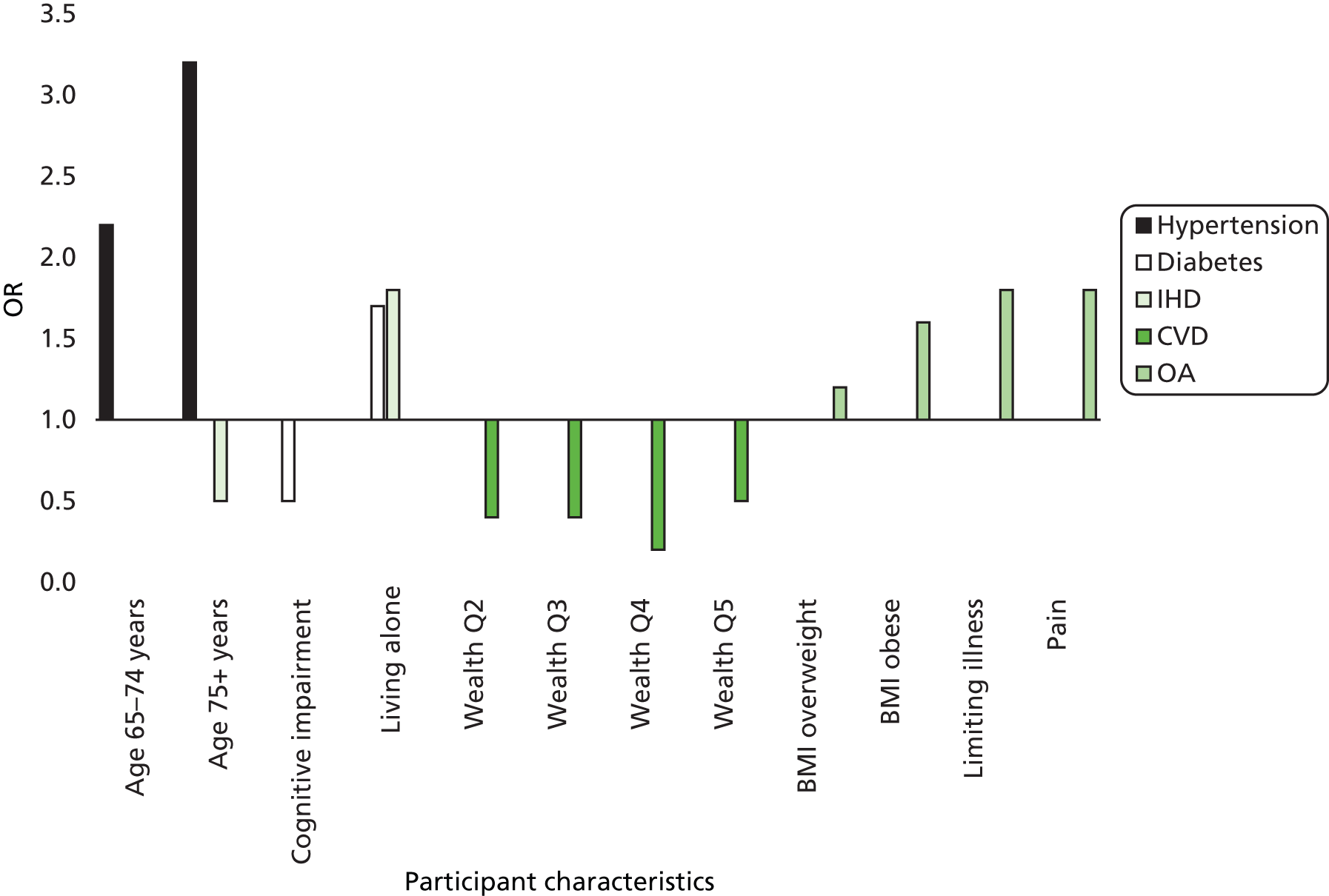
We found univariate associations between CVD and wealth, BMI and CASP-19. In the multivariate model only increased wealth was associated with poorer care for CVD. Hypertension care was associated in the univariate analyses with age, BMI and mean arterial blood pressure. In the final multivariate model only increasing age was associated with better care for hypertension. IHD care showed univariate associations with age, cognitive impairment, physical activity level, mean arterial blood pressure and cohabiting status, and in the final multivariate regression model, only cohabiting status and increased age were significantly associated with quality of care.
Diabetes care was associated with socioeconomic classification, wealth, cognitive impairment, physical activity and cohabiting status in the univariate analyses. In the multivariate model, only being cognitively impaired and living alone were associated with quality of care for diabetes.
Osteoarthritis care was associated with age, pain, BMI, limiting illness, ADL, IADL, frequency of alcohol consumption, physical activity level, CASP and cohabiting status at the univariate 5% level, but only obesity, limiting illness and pain remained significantly associated with better quality of care for osteoarthritis in the multivariate model.
We did not model depression as the relatively small number of participants with depression in the longitudinal data set (see Table 15) led to very small numbers of participants with depression and specific covariates. Instead, we included depression as a covariate in the regression models for the other condition, and, notably, depression was not associated with worse quality of care. The full results from the multivariate regression models, including statistically non-significant results, are reported in Appendix 6.
Demographics for the diabetes care bundle
The sample for modelling associations between participant characteristics and achievement of the diabetes care bundle consisted of ELSA core members at wave 5 who had also responded at wave 4 (n = 8222). At wave 5, 907 (11.0%) of this sample had diabetes and were eligible for at least one quality indicator in the diabetes care bundle and 759 (9.2%) had diabetes with additional cardiac risk factors and were eligible for the diabetes ACE inhibitor quality indicator. Participants in these two groups tended to comprise more males than the whole sample, had more respondents in the 75-and-older age group and fewer in the youngest group, were more likely to be from the lowest socioeconomic classification and had a greater proportion with no educational qualification (Table 17).
Covariate | Whole sample (n = 8222), n (%) | Care bundle (n = 907), n (%) | Diabetes ACE inhibitor (n = 759), n (%) |
---|---|---|---|
Sex | |||
Female | 4561 (55.5) | 425 (46.8) | 363 (47.8) |
Male | 3661 (44.5) | 483 (53.2) | 396 (52.2) |
Age (years) | |||
50–64 | 4250 (51.7) | 369 (40.7) | 306 (40.3) |
65–74 | 2533 (30.8) | 316 (34.8) | 274 (36.10) |
75 + | 1439 (17.5) | 222 (24.5) | 179 (23.58) |
NS-SEC | |||
Managerial/professional | 2827 (34.4) | 249 (27.5) | 207 (27.3) |
Intermediate | 2085 (25.4) | 208 (22.9) | 173 (22.8) |
Routine | 3193 (38.8) | 432 (47.6) | 364 (48.0) |
Missing | 117 (1.4) | 18 (2.0) | 15 (2.0) |
Previous carea | |||
Achieved | – | 496 (54.7) | 342 (45.1) |
Not achieved | – | 225 (24.8) | 240 (31.6) |
New case | – | 175 (19.3) | 144 (19.0) |
Missing | – | 11 (1.2) | 33 (4.4) |
Eyesight | |||
Good to excellent | 7227 (87.9) | 726 (80.0) | 602 (79.3) |
Fair to blind | 995 (12.1) | 181 (20.0) | 157 (20.7) |
Hearing | |||
Good to excellent | 6610 (80.4) | 673 (74.2) | 563 (74.2) |
Fair to poor | 1612 (19.6) | 234 (25.8) | 196 (25.8) |
Chronic pain | |||
None/mild | 5893 (71.7) | 545 (60.1) | 438 (57.7) |
Moderate/severe | 2278 (27.7) | 354 (39.0) | 315 (41.5) |
Has long-standing illness | |||
No | 3761 (45.7) | 121 (13.4) | 100 (13.2) |
Yes | 1725 (21.0) | 318 (35.0) | 253 (33.3) |
Yes – limiting | 2735 (33.3) | 468 (51.5) | 406 (53.5) |
Difficulties with basic ADL | |||
None/one | 7588 (92.3) | 783 (86.3) | 651 (85.8) |
Two or more | 634 (7.7) | 124 (13.7) | 108 (14.2) |
ADL | |||
None/one | 7560 (92.0) | 773 (85.2) | 649 (85.5) |
Two or more | 662 (8.1) | 134 (14.8) | 110 (14.5) |
Cognitive impairment | |||
No | 6757 (82.2) | 699 (77.0) | 593 (78.1) |
Yes | 634 (7.7) | 115 (12.7) | 96 (12.7) |
Missing | 831 (10.1) | 93 (10.4) | 70 (9.2) |
Health literacy | |||
High | 5798 (70.5) | 548 (60.4) | 470 (61.9) |
Low | 2069 (25.2) | 278 (30.7) | 227 (29.9) |
Missing | 355 (4.3) | 81 (8.9) | 62 (8.2) |
Time since diagnosisb | |||
Wave 5 (new case) | – | 165 (18.2) | 134 (17.7) |
Wave 4 | – | 216 (23.8) | 176 (23.2) |
Wave 3 | – | 154 (17.0) | 126 (16.6) |
Wave 2 | – | 371 (40.9) | 371 (40.9) |
Alcohol consumption | |||
1–2 times/week or less | 4538 (55.2) | 579 (63.8) | 498 (65.6) |
3–4 times/week or more | 2670 (32.5) | 178 (19.6) | 148 (19.5) |
Missing | 1014 (12.3) | 150 (16.5) | 113 (14.9) |
Physical activity level | |||
Moderate/high | 5907 (71.8) | 499 (55.0) | 406 (53.5) |
Sedentary/low | 2314 (28.1) | 407 (44.8) | 353 (46.5) |
Smoking status | |||
Never | 3258 (39.6) | 304 (33.5) | 251 (33.1) |
In past | 3828 (46.6) | 478 (52.7) | 394 (51.9) |
Current smoker | 1108 (13.5) | 123 (13.6) | 113 (14.9) |
Diet | |||
Eats ‘5-a-day’ | 3113 (37.9) | 320 (35.3) | 267 (35.2) |
Does not eat ‘5-a-day’ | 2393 (29.1) | 259 (28.6) | 229 (30.2) |
Missing | 2716 (33.0) | 328 (36.2) | 263 (34.7) |
Knows mostly everything about managing diabetes | |||
Yes | – | 604 (66.6) | 505 (66.5) |
No | – | 123 (13.6) | 109 (14.4) |
Missing | – | 180 (19.9) | 145 (19.1) |
Married/civil partnership | |||
Yes | 5456 (66.4) | 558 (61.5) | 460 (60.6) |
No | 2765 (33.6) | 348 (38.4) | 299 (39.4) |
Social detachment | |||
Not detached | 5631 (68.5) | 523 (57.7) | 432 (56.9) |
Detached | 961 (11.7) | 142 (15.7) | 125 (16.5) |
Missing | 1630 (19.8) | 242 (26.7) | 202 (26.6) |
Quality of life (CASP-19) | |||
High | 2116 (25.7) | 150 (16.5) | 109 (14.4) |
Mid | 2534 (30.8) | 252 (27.8) | 216 (28.5) |
Low | 2351 (28.6) | 328 (36.2) | 295 (38.9) |
Missing | 1221 (14.9) | 177 (19.5) | 139 (18.3) |
Locus of control | |||
Internal | 1659 (20.2) | 134 (14.8) | 108 (14.2) |
External | 5545 (67.4) | 618 (68.1) | 532 (70.1) |
Missing | 1018 (12.4) | 155 (17.1) | 119 (15.7) |
CES-D depression classification | |||
Not depressed | 6970 (84.8) | 708 (78.1) | 579 (76.3) |
Depressed | 1132 (13.8) | 179 (19.7) | 163 (21.5) |
Missing | 120 (1.5) | 20 (2.2) | 17 (2.2) |
Lives alone | |||
No | 2773 (33.7) | 252 (27.8) | 215 (28.3) |
Yes | 5140 (62.5) | 618 (68.1) | 512 (67.5) |
Missing | 309 (3.8) | 37 (4.1) | 32 (4.2) |
Logistic regression models for the diabetes care bundle
Participant characteristics were modelled on subsequent non-receipt of care two years later. The key predictor of non-receipt of care for the diabetes care bundle was non-receipt 2 years earlier, which more than trebled the odds of subsequent non-receipt of the care bundle (OR 3.26, 95% CI 2.24 to 4.74; p < 0.001) and increased the odds nearly sevenfold for the diabetes ACE inhibitor quality indicator for diabetes with other cardiac risk factors (OR 6.94, 95% CI 4.54 to 10.60; p < 0.001) (Table 18). Besides previous care, the two models diverged in their significant predictors. Respondents classified as cognitively impaired had 78% increased odds of non-receipt of the care bundle (OR 1.78, 95% CI 1.09 to 2.89; p = 0.021). Those who did not feel they knew at least most of what they needed to know about managing their diabetes had double the odds of not receiving all three of the annual checks included in the bundle (OR 2.03, 95% CI 1.28 to 3.22; p = 0.003), but knowledge of diabetes management was not significantly associated with not being offered an ACE inhibitor (OR 1.32, 95% CI 0.77 to 2.26; p = 0.31).
Covariate | Care bundle, OR (95% CI) | Diabetes ACE inhibitor, OR (95% CI) |
---|---|---|
Sex | ||
Female | 1.0 (–) | 1.0 (–) |
Male | 1.14 (0.76 to 1.70) | 1.11 (0.73 to 1.70) |
Age group, years (trend)a | 1.19 (0.95 to 1.49) | 0.77 (0.60 to 1.00)b |
50–64 | 1.0 (–) | 1.0 (–) |
65–74 | 1.26 (0.86 to 1.86) | 0.91 (0.61 to 1.37) |
75 + | 1.40 (0.89 to 2.22) | 0.57 (0.34 to 0.97)b |
Previous carec | ||
Achieved | 1.0 (–) | 1.0 (–) |
Not achieved | 3.26 (2.24 to 4.74)d | 6.94 (4.54 to 10.60)d |
New case | 1.16 (0.35 to 3.81) | 2.23 (0.66 to 7.49) |
Missing | – | 2.17 (0.92 to 5.11) |
NS–SEC (trend)a | 1.24 (1.01 to 1.51)b | 1.17 (0.94 to 1.45) |
Managerial/professional | 1.0 (–) | 1.0 (–) |
Intermediate | 0.88 (0.55 to 1.41) | 1.14 (0.68 to 1.91) |
Routine/manual | 1.41 (0.95 to 2.10) | 1.36 (0.89 to 2.10) |
Missing | 1.41 (0.45 to 4.47) | 1.53 (0.47 to 5.06) |
Eyesight | ||
Excellent/good | 1.0 (–) | 1.0 (–) |
Fair/blind | 0.96 (0.63 to 1.46) | 1.03 (0.65 to 1.63) |
Has long-standing illness (trend)a | 0.96 (0.76 to 1.21) | 1.04 (0.80 to 1.36) |
No | 1.0 (–) | 1.0 (–) |
Yes | 0.96 (0.56 to 1.63) | 0.96 (0.53 to 1.74) |
Yes to limiting | 0.91 (0.55 to 1.51) | 1.06 (0.61 to 1.84) |
Cognitively impaired | ||
No | 1.0 (–) | 1.0 (–) |
Yes | 1.78 (1.09 to 2.89)b | 1.63 (0.90 to 2.97) |
Missing | 1.59 (0.93 to 2.73) | 1.04 (0.52 to 2.08) |
Health literacy | ||
High | 1.0 (–) | 1.0 (–) |
Low | 1.10 (0.77 to 1.57) | 1.51 (1.01 to 2.26)b |
Missing | 0.93 (0.50 to 1.72) | 2.46 (1.22 to 4.99)b |
Time since diagnosis (waves) | 1.04 (0.84 to 1.30) | 0.99 (0.79 to 1.25) |
Knows everything about managing diabetes | ||
Yes | 1.0 (–) | 1.0 (–) |
No | 2.03 (1.28 to 3.22)e | 1.32 (0.77 to 2.26) |
Missing | 3.40 (1.16 to 9.94)b | 2.46 (0.79 to 7.66) |
BMI category (trend)a | 0.97 (0.75 to 1.25) | 0.70 (0.53 to 0.92)b |
Under-/normal weight | 1.0 (–) | 1.0 (–) |
Overweight | 1.06 (0.59 to 1.91) | 0.71 (0.37 to 1.37) |
Obese | 0.98 (0.55 to 1.73) | 0.49 (0.26 to 0.90)b |
Missing | 0.88 (0.45 to 1.72) | 0.64 (0.31 to 1.33) |
Married/in civil partnership | ||
Yes | 1.0 (–) | 1.0 (–) |
No | 1.09 (0.73 to 1.63) | 2.17 (1.40 to 3.38)d |
CES–D depression classification | ||
No | 1.0 (–) | 1.0 (–) |
Yes | 0.92 (0.61 to 1.40) | 0.98 (0.62 to 1.55) |
Missing | 0.68 (0.24 to 1.93) | 2.96 (0.84 to 10.47) |
Lives alone | ||
No | 1.0 (–) | 1.0 (–) |
Yes | 0.75 (0.48 to 1.19) | 0.96 (0.59 to 1.55) |
Missing | 0.66 (0.28 to 1.55) | 0.78 (0.29 to 2.07) |
Log-likelihood | –519.22 | –424.94 |
Wald χ2 | 86.54 | 134.17 |
p-value | 0.000 | 0.000 |
Pseudo R2 | 0.103 | 0.198 |
n | 903 | 757 |
On the other hand, patients with low health literacy had 50% higher odds for non-receipt of ACE inhibitor (OR 1.51, 95% CI 1.01 to 2.26; p = 0.043) than those with high health literacy, with no significant difference apparent for the care bundle. The odds of not being offered an ACE inhibitor were decreased in the oldest age group (OR 0.57, 95% CI 0.34 to 0.97; p = 0.038) and in those categorised as obese (OR 0.49, 95% CI 0.26 to 0.90; p = 0.021), but were increased over twofold for those not married or in a civil partnership (OR 2.17, 95% CI 1.40 to 3.38; p < 0.001).
Sensitivity analyses for missing data
Sensitivity analyses were conducted to determine whether or not using multiple imputation of missing data would alter the final results for non-receipt of the diabetes care bundle and the ACE inhibitor/receptor blocker quality indicator (Table 19). The results from the model using multiple imputation were remarkably similar to the original models for both the care bundle and the ACE inhibitor quality indicator. A small number of differences were evident. For the model assessing non-receipt of the ACE inhibitor quality indicator, the difference found between those aged ≥ 75 years and those aged 50–64 years was no longer significant, though the ORs and CIs were very similar. Cognitive impairment became significant (p < 0.05) in this model when using multiple imputation. The biggest difference between the two methods was apparent for the ‘new case’ category in the ‘previous care’ covariate, that is those reporting a diagnosis of diabetes only at the latest wave (2010–11). These participants were found to be at greatly increased odds of not receiving the care bundle and/or the ACE inhibitor quality indicator in the models using multiple imputation. However, it should be noted that, as we cannot tell at what point between the two waves these participants were told they had diabetes, it is inappropriate to draw conclusions on their likelihood of not receiving care. It may be the case that they have not had the diagnosis for sufficiently long to have the opportunity to receive a complete care cycle. Thus, we feel that it is sensible to treat the ‘new case’ category as if it were a special kind of ‘missing data’ in its own right.
Covariate | Missing data as a category, OR (95% CI) | Multiple imputation of missing data, OR (95% CI) |
---|---|---|
Sex | ||
Female | 1.0 (–) | 1.0 (–) |
Male | 1.14 (0.76 to 1.70) | 1.16 (0.78 to 1.72) |
Age (years) | ||
50–64 | 1.0 (–) | 1.0 (–) |
65–74 | 1.26 (0.86 to 1.86) | 1.21 (0.83 to 1.78) |
75 + | 1.40 (0.89 to 2.22) | 1.37 (0.86 to 2.17) |
Previous care | ||
Complete | 1.0 (–) | 1.0 (–) |
Incomplete | 3.26 (2.24 to 4.74)a | 3.16 (2.17 to 4.61)a |
New case | 1.16 (0.35 to 3.81) | 2.80 (1.47 to 5.31)b |
NS-SEC | ||
Managerial/professional | 1.0 (–) | 1.0 (–) |
Intermediate | 0.88 (0.55 to 1.41) | 0.87 (0.53 to 1.43) |
Routine/manual | 1.41 (0.95 to 2.10) | 1.37 (0.90 to 2.08) |
Missing | 1.41 (0.45 to 4.47) | – |
Eyesight | ||
Excellent/good | 1.0 (–) | 1.0 (–) |
Fair/blind | 0.96 (0.63 to 1.46) | 0.95 (0.63 to 1.43) |
Has long-standing illness | ||
No | 1.0 (–) | 1.0 (–) |
Yes | 0.96 (0.56 to 1.63) | 0.93 (0.55 to 1.57) |
Yes to limiting | 0.91 (0.55 to 1.51) | 0.90 (0.54 to 1.48) |
Cognitively impaired | ||
No | 1.0 (–) | 1.0 (–) |
Yes | 1.78 (1.09 to 2.89)c | 1.78 (1.12 to 2.88)c |
Missing | 1.59 (0.93 to 2.73) | – |
Health literacy | ||
High | 1.0 (–) | 1.0 (–) |
Low | 1.10 (0.77 to 1.57) | 1.11 (0.77 to 1.62) |
Missing | 0.93 (0.50 to 1.72) | – |
Time since diagnosis (waves) | 1.04 (0.84 to 1.30) | 1.01 (0.81 to 1.25) |
Knows everything about managing diabetes | ||
Yes | 1.0 (–) | 1.0 (–) |
No | 2.03 (1.28 to 3.22)b | 1.98 (1.25 to 3.13)c |
Missing | 3.40 (1.16 to 9.94)c | – |
BMI category | ||
Under-/normal weight | 1.0 (–) | 1.0 (–) |
Overweight | 1.06 (0.59 to 1.91) | 1.00 (0.55 to 1.82) |
Obese | 0.98 (0.55 to 1.73) | 0.93 (0.52 to 1.65) |
Missing | 0.88 (0.45 to 1.72) | – |
Married/in civil partnership | ||
Yes | 1.0 (–) | 1.0 (–) |
No | 1.09 (0.73 to 1.63) | 1.06 (0.72 to 1.58) |
CES–D depression classification | ||
No | 1.0 (–) | 1.0 (–) |
Yes | 0.92 (0.61 to 1.40) | 0.90 (0.59 to 1.36) |
Missing | 0.68 (0.24 to 1.93) | – |
Lives alone | ||
No | 1.0 (–) | 1.0 (–) |
Yes | 0.75 (0.48 to 1.19) | 0.73 (0.46 to 1.16) |
Missing | 0.66 (0.28 to 1.55) | – |
Population distribution of illness burden, diagnosis and treatment
Demographics
The sample of participants aged 50 years or over who had responded to at least one wave of ELSA from wave 2 in 2004–5 until wave 5 in 2010–11 and were core members was used to address research question 3: are factors such as wealth, or patient education, physical activity, obesity, coexisting other chronic conditions or practical factors such as good eyesight or marital status independently associated with the quality of health care received, from symptoms to diagnosis and, finally, to treatment?
There were 12,765 core members in the sample, and 8729 in wave 5, of whom slightly over half were women, and slightly over half were aged ≥ 60 years (Table 20). Diagnosed diabetes, angina and osteoarthritis were more prevalent with increasing age, but the opposite trend was seen with depression, with 10% of the youngest age group reporting a diagnosis compared with only 3% in the oldest age group. The highest prevalence of all conditions was seen in the poorest quintile. For example, the prevalence of depression was 4% in the wealthiest quintile and 11% in the least wealthy.
Characteristics | n | Angina, % | Depression, % | Diabetes, % | Osteoarthritis, % |
---|---|---|---|---|---|
Sex | |||||
Male | 3886 | 8.2 | 5.4 | 13.3 | 19.8 |
Female | 4843 | 6.3 | 7.8 | 9.4 | 32.9 |
Age (years) | |||||
50–59 | 1906 | 2.2 | 10.1 | 7.2 | 17.1 |
60–74 | 4766 | 5.8 | 7.0 | 11.0 | 28.1 |
75 + | 2057 | 15.0 | 2.9 | 15.0 | 34.1 |
Wealth quintile | |||||
1 (wealthiest) | 1716 | 3.4 | 4.1 | 6.0 | 21.5 |
2 | 1714 | 4.9 | 5.9 | 8.0 | 24.2 |
3 | 1723 | 6.6 | 5.7 | 11.3 | 25.7 |
4 | 1716 | 8.2 | 6.7 | 13.6 | 31.6 |
5 (poorest) | 1715 | 12.9 | 11.5 | 16.7 | 33.1 |
Missing | 145 | 5.5 | 4.8 | 9.7 | 20.0 |
Total | 8729 | 7.2 | 6.7 | 11.1 | 27.1 |
There was very little variation in the prevalence of illness burden, diagnosis and treatment between the waves for each of the four conditions (Table 21).
Wave | Angina, n (%) | Depression, n (%) | Diabetes, n (%) | Osteoarthritis, n (%) |
---|---|---|---|---|
Illness burden | ||||
Wave 2 (2004–5) | 397 (4.6) | 2037 (23.4) | 160 (1.8) | 1106 (12.7) |
Wave 3 (2006–7) | 300 (3.6) | 1929 (23.3) | N/A | 917 (11.1) |
Wave 4 (2008–9) | 300 (3.1) | 2049 (21.4) | 220 (2.3) | 1088 (11.4) |
Wave 5 (2010–11) | 254 (2.9) | 1956 (22.4) | N/A | 1046 (12.0) |
Medical diagnosis | ||||
Wave 2 | 668 (7.6) | 402 (4.6) | 715 (8.2) | 1861 (21.4) |
Wave 3 | 591 (7.1) | 490 (5.9) | 935 (11.3) | 1952 (23.6) |
Wave 4 | 645 (6.7) | 601 (6.3) | 1215 (12.7) | 2262 (23.6) |
Wave 5 | 655 (7.5) | 602 (6.9) | 1413 (16.2) | 2416 (27.7) |
Treatment | ||||
Wave 2 | 85 (1.0) | 98 (1.1) | 552 (6.4) | 202 (2.3) |
Wave 3 | N/A | N/A | 618 (7.5) | 141 (1.7) |
Wave 4 | N/A | 155 (1.6) | 671 (7.0) | 226 (2.4) |
Wave 5 | 88 (1.0) | N/A | 748 (8.6) | 208 (2.4) |
Modelling with illness burden, diagnosis and treatment
The hypothetically poorest participant had higher odds of illness burden than the hypothetically richest for each of the four conditions at all four time points (ORs ranging from 3.9 to 16.0) (Table 22). The odds were generally smaller for diagnosis (ORs 0.6 to 5.3) and appropriate treatment (ORs 0.2 to 3.3). The substantially higher illness burden in less wealthy participants was not matched by higher levels of diagnosis and treatment (Figure 2).
Wave 2 (2004–5), adjusted ORa (95% CI) | Wave 3 (2006–7), adjusted OR (95% CI) | Wave 4 (2008–9), adjusted OR (95% CI) | Wave 5 (2010–11), adjusted OR (95% CI) | Overall, adjusted OR (95% CI) | |||||||||||
---|---|---|---|---|---|---|---|---|---|---|---|---|---|---|---|
Illness burden | Diagnosis | Treatment | Illness burden | Diagnosis | Treatment | Illness burden | Diagnosis | Treatment | Illness burden | Diagnosis | Treatment | Illness burden | Diagnosis | Treatment | |
Angina | 5.6 (3.8 to 8.3) | 2.9 (2.2 to 3.9) | 2.6 (1.2 to 5.7) | 8.7 (5.5 to 13.8) | 4.9 (3.6 to 6.8) | 6.7 (4.2 to 10.5) | 4.3 (3.2 to 5.9) | 8.4 (5.1 to 13.7) | 5.3 (3.9 to 7.3) | 3.3 (1.5 to 7.3) | 7.6 (5.4 to 10.8) | 4.5 (3.3 to 6.0) | 3.2 (1.7 to 6.0) | ||
Depression | 5.1 (4.3 to 6.2) | 4.8 (3.3 to 7.0) | 0.6 (0.1 to 2.9) | 6.9 (5.7 to 8.5) | 0.7 (0.4 to 1.4) | 5.9 (4.9 to 7.1) | 0.7 (0.4 to 1.2) | 2.4 (1.0 to 5.9) | 5.9 (4.8 to 7.1) | 1.7 (1.0 to 2.8) | 6.4 (5.5 to 7.5) | 3.3 (2.4 to 4.5) | 2.6 (1.1 to 6.1) | ||
Diabetes | 4.4 (2.5 to 8.0) | 3.1 (2.3 to 4.2) | 0.7 (0.3 to 1.5) | 3.4 (2.6 to 4.4) | 1.2 (0.6 to 2.4) | 3.9 (2.4 to 6.4) | 3.9 (2.4 to 6.4) | 0.2 (0.1 to 0.6) | 4.3 (3.4 to 5.4) | 0.8 (0.4 to 1.6) | 4.2 (2.6 to 6.8) | 4.0 (3.1 to 5.2) | 0.9 (0.5 to 1.4) | ||
OA | 11.0 (8.1 to 14.9) | 1.6 (1.3 to 2.0) | 1.1 (0.7 to 1.9) | 12.7 (9.1 to 17.8) | 0.6 (0.4 to 0.8) | 1.0 (0.6 to 1.9) | 14.0 (10.3 to 19.1) | 0.6 (0.4 to 0.8) | 1.0 (0.6 to 1.6) | 16.0 (11.7 to 21.8) | 0.6 (0.4 to 0.8) | 1.2 (0.7 to 2.0) | 15.1 (11.4 to 20.0) | 1.1 (0.9 to 1.3) | 1.2 (0.8 to 1.6) |
FIGURE 2.
Illness burden, medical diagnosis and treatment of angina, depression, diabetes and osteoarthritis, comparing the least wealthy with the most wealthy: overall ORs (adjusted for age and sex) and 95% confidence bars (logistic regression).

For angina, the overall OR for meeting the criteria for illness burden was 7.6, indicating that the hypothetically least wealthy individual was almost eight times more likely to have angina symptoms (defined by the Rose Angina Scale) than the wealthiest. The OR for reported medical diagnosis was 4.5, suggesting that some less wealthy people with angina symptoms had not received a diagnosis of angina, as the expected OR for equitably distributed diagnosis would be 7.6. The OR for treatment was 3.2, and again the expected OR for equitably distributed treatment would have been around 8.
For depression, the overall OR for illness burden was 6.4, while for medical diagnosis it was 3.3 and for treatment it was 2.6, again suggesting that some poorer people with illness burden of depression were less likely to have received a diagnosis or indicated health care.
For diabetes, the overall ORs for illness burden were 4.2 and 4.0 for diagnosis, suggesting that diabetes diagnosis was distributed equitably. However, the OR for treatment was 0.9 and not statistically different from 1, again suggesting that some less wealthy people with medically diagnosed diabetes had not received treatment, as the expected OR for equitably distributed treatment would have been around 4.
We also calculated the ORs of receiving a diagnosis in a subsequent wave, only for those who were estimated to have had the relevant long-term condition in a previous wave, and then the likelihood of receiving treatment, only for those who had received a medical diagnosis in a previous wave. This is a secondary analysis as the numbers of participants that could be followed over time in this manner was small, particularly for treatment in angina and depression, and so these results should be interpreted with great caution. The substantial inequalities in the estimated prevalence of conditions by wealth are similar to Table 23, as expected, and subsequently the numbers are too small for the results to be statistically significant. The general pattern, however, is consistent with less medical diagnosis and treatment in less wealthy participants. Taking osteoarthritis as an example, ORs were 12.7 (95% CI 9.1 to 17.8) for estimated prevalence in wave 3, 0.7 (95% CI 0.3 to 1.8) for diagnosis in wave 4 and 0.7 (95% CI 0.3 to 1.6) for treatment in wave 5 (Table 23).
Condition | ORa (95% CI) | n receiving treatmentb | ||
---|---|---|---|---|
Illness burden (wave 3) | Medical diagnosis (wave 4) | Treatment (wave 5) | ||
Angina | 8.7 (5.5 to 13.8) | 1.4 (0.5 to 4.0) | 1.6 (0.3 to 9.0) | 11 |
Osteoarthritis | 12.7 (9.1 to 17.8) | 0.7 (0.3 to 1.8) | 0.7 (0.3 to 1.6) | 30 |
Illness burden (wave 2) | Medical diagnosis (wave 3) | Treatment (wave 4) | ||
Depression | 5.1 (4.3 to 6.2) | 0.4 (0.1 to 1.4) | 17.3 (0.5 to 604) | 12 |
Diabetes | 4.4 (2.5 to 8.0) | 0.1 (0.0 to 3.6) | 0.5 (0.2 to 1.1) | 99 |
Modelling with illness burden, diagnosis and treatment: additional covariates
The regression models immediately above for comparing the distribution by wealth of illness burden, diagnosis and treatment, respectively (see previous section), were adjusted for age, sex and slope index of inequality only. This section reports the results when additional covariates were added to the models above and the results when one more covariate at a time was added to the models, which are presented separately for illness burden (see Table 24), diagnosis (see Table 25) and treatment (see Table 26).
Illness burden
Table 24 presents the results for illness burden.
Covariate added to the age, sex and slope index of inequality model | Angina, OR (95% CI) | Depression, OR (95% CI) | Diabetes, OR (95% CI) | Osteoarthritis, OR (95% CI) |
---|---|---|---|---|
Highest educational qualification vs. none | ||||
Degree or higher education | 0.6 (0.5 to 0.8) | 0.7 (0.7 to 0.8) | 0.7 (0.5 to 1.0) | 0.6 (0.5 to 0.7) |
Physical activity level vs. sedentary | ||||
High | 0.3 (0.2 to 0.5) | 0.2 (0.2 to 0.3) | 0.3 (0.2 to 0.5) | 0.07 (0.05 to 0.09) |
Comorbidities vs. none or one | ||||
Two or more | 7.5 (6.0 to 9.4) | 2.0 (1.8 to 2.2) | 2.0 (1.5 to 2.6) | 3.1 (2.7 to 3.5) |
Eyesight vs. excellent | ||||
Fair/poor/blind | 2.8 (2.1 to 4.0) | 3.3 (2.8 to 4.0) | 2.5 (1.5 to 4.1) | 3.9 (3.0 to 5.0) |
Cohabiting | ||||
vs. living alone | 0.9 (0.8 to 1.1) | 0.6 (0.5 to 0.6) | 0.9 (0.7 to 1.2) | 1.0 (0.9 to 1.2) |
Access to GP | ||||
Difficult/impossible vs. very easy | 2.4 (1.8 to 3.2) | 4.3 (3.5 to 5.4) | 1.5 (0.9 to 2.4) | 6.9 (5.5 to 8.9) |
Health literacy vs. literate | ||||
Health illiterate | 0.8 (0.7 to 1.0) | 0.7 (0.6 to 0.8) | 0.8 (0.6 to 1.1) | 0.7 (0.6 to 0.9) |
BMI vs. normal/underweight | ||||
Overweight | 1.5 (1.2 to 2.0) | 0.9 (0.8 to 1.0) | 1.7 (1.1 to 2.7) | 1.4 (1.2 to 1.8) |
Obese | 2.3 (1.8 to 3.0) | 1.2 (1.1 to 1.4) | 4.0 (2.6 to 6.1) | 3.7 (3.0 to 4.6) |
Having two or more comorbidities is associated with having increased odds of having angina illness burden [OR 7.5 (95% CI 6.0 to 9.4)]; there is a smaller, but still significant effect for the other diseases. Having poorer eyesight was also associated with increasing odds of having illness burden of all four diseases, with ORs ranging from 2.5 (95% CI 1.5 to 4.1) for diabetes to 3.9 (95% CI 3.0 to 5.0) for osteoarthritis. Difficulty in accessing a GP was also associated with increasing odds for having illness burden for all four diseases [osteoarthritis OR 6.9 (95% CI 5.5 to 8.9)]. Looking at BMI, there was an increment in odds of having illness burden the more the BMI increased for all the diseases, though the effect was lowest in depression [overweight 0.9 (95% CI 0.8 to 1.0) and obese 1.2 (95% CI 1.1 to 1.4)].
Diagnosis
Very similar findings were seen in the adjusted diagnosis model, where the odds of having diagnosis of angina were 11-fold if you had two or more comorbidities. There was still a gradient seen in increasing BMI and increasing odds of being diagnosed for all four conditions (Table 25).
Covariate added to the age, sex and slope index of inequality model | Angina, adjusted OR (95% CI) | Depression, adjusted OR (95% CI) | Diabetes, adjusted OR (95% CI) | Osteoarthritis, adjusted OR (95% CI) |
---|---|---|---|---|
Highest educational qualification vs. none | ||||
Degree or higher education | 0.7 (0.6 to 0.9) | 1.2 (0.9 to 1.4) | 0.7 (0.6 to 0.9) | 0.9 (0.8 to 1.1) |
Physical activity level vs. sedentary | ||||
High | 0.4 (0.3 to 0.5) | 0.5 (0.3 to 0.6) | 0.3 (0.2 to 0.4) | 0.5 (0.4 to 0.6) |
Comorbidities vs. none or one | ||||
Two or more | 11.0 (8.8 to 13.8) | 1.3 (1.1 to 1.5) | 1.9 (1.7 to 2.2) | 1.7 (1.5 to 1.9) |
Eyesight vs. excellent | ||||
Fair/poor/blind | 2.5 (1.9 to 3.3) | 1.8 (1.4 to 2.5) | 1.7 (1.3 to 2.2) | 1.6 (1.3 to 2.0) |
Cohabiting | ||||
vs. living alone | 0.9 (0.8 to 1.1) | 0.7 (0.6 to 0.8) | 1.0 (0.9 to 1.2) | 1.0 (0.9 to 1.2) |
Access to GP | ||||
Difficult/impossible vs. very easy | 1.8 (1.4 to 2.3) | 1.9 (1.4 to 2.6) | 2.0 (1.6 to 2.6) | 2.0 (1.0 to 2.5) |
Health literacy vs. literate | ||||
Health illiterate | 1.3 (1.0 to 1.6) | 1.1 (0.9 to 1.3) | 0.9 (0.8 to 1.0) | 1.0 (0.9 to 1.1) |
BMI vs. normal/underweight | ||||
Overweight | 1.2 (1.0 to 1.6) | 0.9 (0.8 to 1.2) | 1.7 (1.3 to 2.1) | 1.3 (1.1 to 1.5) |
Obese | 1.6 (1.3 to 2.0) | 1.6 (1.3 to 2.0) | 4.0 (3.1 to 5.0) | 1.8 (1.5 to 2.1) |
Treatment
For the treatment model, having comorbidities was less associated with angina [OR 0.9 (95% CI 0.3 to 2.7)] than in the diagnosis or illness burden models. Being more physically active was associated with increased odds of being treated for angina [OR medium physical activity 3.3 (95% CI 1.2 to 8.6); OR high physical activity 6.5 (95% CI 1.1 to 37.1)]. Depression and osteoarthritis treatment was associated with being overweight or obese, and the strongest predictor for diabetes treatment was high physical activity and not living alone [OR 1.5 (95% CI 0.5 to 4.2) and OR 1.5 (95% CI 0.9 to 2.5)], respectively (Table 26).
Covariate added to the age, sex and slope index of inequality model | Angina, OR (95% CI) after adjusting for age, sex, slope index | Depression, OR (95% CI) after adjusting for age, sex, slope index | Diabetes, OR (95% CI) after adjusting for age, sex, slope index | Osteoarthritis, OR (95% CI) after adjusting for age, sex, slope index |
---|---|---|---|---|
Highest educational qualification vs. none | ||||
Intermediate | 2.6 (1.1 to 6.0) | 1.1 (0.6 to 2.0) | 0.9 (0.5 to 1.5) | 0.9 (0.7 to 1.1) |
Degree or higher education | 2.1 (1.1 to 6.0) | 0.9 (0.5 to 1.7) | 1.2 (0.6 to 2.3) | 0.9 (0.7 to 1.2) |
Physical activity level vs. sedentary | ||||
Medium | 3.3 (1.2 to 8.6) | 0.6 (0.2 to 1.7) | 1.2 (0.6 to 2.5) | 0.7 (0.5 to 0.9) |
High | 6.5 (1.1 to 37.1) | 0.5 (0.1 to 1.4) | 1.5 (0.5 to 4.2) | 0.5 (0.4 to 0.8) |
Comorbidities vs. two or more | ||||
None or one | 0.9 (0.3 to 2.7) | 1.2 (0.7 to 2.0) | 1.1 (0.7 to 1.7) | 1.3 (1.1 to 1.6) |
Eyesight vs. excellent | ||||
Fair/poor/blind | 1.5 (0.4 to 5.3) | 1.1 (0.5 to 2.7) | 2.2 (0.8 to 5.8) | 1.1 (0.8 to 1.6) |
Cohabiting | ||||
vs. living alone | 1.4 (0.7 to 3.0) | 1.2 (0.7 to 2.0) | 1.5 (0.9 to 2.5) | 1.0 (0.8 to 1.3) |
Access to GP | ||||
Easy vs. very easy | 1.7 (0.6 to 4.3) | 1.2 (0.7 to 2.0) | 0.9 (0.5 to 1.5) | 1.2 (1.0 to 1.5) |
Difficult/impossible vs. very easy | 2.4 (0.8 to 7.2) | 1.5 (0.5 to 4.4) | 0.9 (0.4 to 2.1) | 2.2 (1.6 to 3.0) |
Health literacy | ||||
Health illiterate vs. literate | 1.7 (0.8 to 2.1) | 0.7 (0.4 to 1.4) | 1.0 (0.6 to 1.6) | 0.8 (0.7 to 1.0) |
BMI | ||||
Overweight vs. normal/underweight | 0.7 (0.2 to 2.1) | 1.8 (0.9 to 3.5) | 0.8 (0.3 to 1.9) | 2.0 (1.4 to 2.7) |
Obese vs. normal/underweight | 0.4 (0.2 to 1.3) | 1.8 (0.9 to 3.7) | 1.0 (0.4 to 2.3) | 3.8 (2.7 to 5.2) |
Joint replacement payment type | ||||
Private vs. NHS | 1.7 (0.8 to 3.6) |
Chapter 4 Discussion
These analyses of quality of care and participant characteristics from the ELSA have shown that, despite relatively high levels of quality achievement in cardiovascular disease and individual diabetes care processes, important shortfalls in quality of care have persisted over 6 years. Shortfalls were greater for the diabetes care bundle, for depression and particularly for osteoarthritis, with less than half of indicated health care achieved for osteoarthritis compared with the other three conditions. Osteoarthritis was, however, the only condition where quality had improved over time, perhaps partly because of the low starting point.
Variations in quality by participant characteristics were generally small, with the few statistically significant associations including worse care for diabetes in participants with cognitive impairment, and better care in those living alone. Hypertension care was better for those aged > 74 years, and osteoarthritis care was better for those with greater pain, limiting illness and obesity. Previous non-achievement of the diabetes care bundle was the biggest predictor of non-achievement 2 years later. The surprising lack of variation in quality of care by participant characteristics suggests that future quality improvement interventions may be more effective when directed at health-care delivery systems rather than at individuals.
The comparison of the distribution by wealth of illness burden with the distribution of diagnoses and treatments showed that the relatively high prevalence of specific illness burden in poorer participants was not matched by an equally high prevalence of diagnosis or treatment, suggesting that barriers to equity may exist at the stage at diagnosis.
Comparison of main results with previous research
Quality indicator achievement
Our findings are broadly consistent with the findings of a systematic review by Askari et al. 13 of 17 papers reporting on achievement of the ACOVE quality indicators, which showed large variation between conditions, and notably low rates for osteoarthritis quality indicators. This review only found a few conditions with a stable pass rate range over multiple studies, and noted that caution is required in making direct comparisons of quality indicator achievement between studies. Most indicators were reported in only a small number of studies and were drawn from different countries and diverse populations (e.g. nursing home residents or primary care settings).
For the cardiovascular disease indicators reported here, the proportion of eligible patients who reported receiving care ranged between 64% for MI beta-blocker and 100% for INR test in wave 2. By wave 5 these figures ranged between 73% for MI beta-blocker and 94% for INR test. Improvements were observed in the achievement rates for some indicators between waves 2 and 5 (stroke antihypertensives +7%, hypertension treatment +8%, MI beta-blocker +12%). However, achievement rates of the remaining indicators stayed constant across time with the exception of the INR test, which declined by 6% from the 100% attainment noted at wave 2. Askari et al. 13 reported comparable achievement rates in primary care settings for hypertension (72% vs. 75% prevalent hypertension treatment at wave 5) and stroke (69% vs. 85% achievement in ELSA) indicators.
For the diabetes indicators, achievement rates in wave 2 ranged between 48% for ACE inhibitor and 83% for diabetes HbA1c testing. By wave 5 these figures ranged between 57% for ACE inhibitor and 89% for diabetes cholesterol test. Most diabetes indicators remained largely unchanged across time, with the exception of the ACE inhibitor one, which increased by 9% between waves 2 and 5. Our findings are broadly consistent with figures reported for 2009–10 in the recent National Diabetes Audit18 which examined all available clinical records in the UK. This audit found achievement rates of 93%, 74% and 85% for HbA1c checks, renal risk checks and foot checks, respectively. This compares with wave 5 data, where 82% patients reported having HbA1c levels checked, 79% had a proteinuria check and 83% received a foot check.
Receipt of care for depression at wave 2 was 46% (suicide), 79% (treatment) and 76% (review), and at wave 4 was 58% (suicide) and 62% (treatment). Although depression is a condition included in the QOF for UK general practice, the QOF indicators do not map perfectly to the criteria used in ELSA and no direct comparisons can be made.
Achievement of the osteoarthritis indicators was lower than for any cardiovascular disease, depression or diabetes indicators, ranging from 26% (education) to 45% (paracetamol) at wave 2, and from 32% (education) to 46% (exercise) at wave 5. Although a prevalent condition in later life, osteoarthritis is not included in the QOF. For both depression and osteoarthritis we found no national audit data that could be compared with our sample. The review by Askari et al. 13 found very similar rates of achievement of osteoarthritis indicators (interquartile range 29% to 41%), which they described as ‘notably low’.
Transitions in quality indicator achievement over time
We explored transitions in quality indicator achievement across time by tabulating whether or not patients received their care at a given wave with subsequent receipt of that care by the next wave. This allowed us to investigate the rates of transitions from poor-quality care to good-quality care and vice versa, as well as the rates of continued receipt of poor- or good-quality care. Not all quality indicators were suitable for this analysis as some indicators (e.g. depression) referred to the management of incident disease within specific time scales.
For other quality indicators it was not possible to transition from receiving care at one wave to subsequently not receiving it, as the indicated care either only needed to be provided once (osteoarthritis exercise and education), or referred to the provision of long-term treatment (stroke antihypertensives). However, there were relatively low rates of transitions from not receiving the care to subsequently receiving it, especially for the two osteoarthritis quality indicators. The majority of eligible people who did not receive indicated care continued to receive poor quality of care subsequently.
A different pattern emerged from the follow-up for the hypertension quality indicator, the three quality indicators for annual monitoring checks for diabetes (HbA1c test, proteinuria and foot check) that compose the diabetes care bundle, and the quality indicator assessing the prescription of ACE inhibitors to those with diabetes and additional cardiac risk factors. For these quality indicators, more participants who did not receive their care at a given wave transitioned to subsequently receiving it than to continuing to not receive it. There were comparatively low rates of patients transitioning from receiving good quality of care to not receiving their care (e.g. 2% to 3% for hypertension and 19% to 24% for the diabetes care bundle) in relation to those who continued to receive poor-quality care (e.g. 31% to 40% for hypertension and 45% to 48% for the diabetes care bundle). Overall, our results suggest that those participants who received poor care tended to persistently receive poor care, and that time alone did not rectify deficits in care.
Participant characteristics associated with receipt of indicated health care
The results of multivariate logistic regression modelling for individual conditions found that very few of the wide range of personal characteristics that we measured were significantly and independently associated with eligible individuals achieving indicated care. At condition level, some participant characteristics were associated with achievement of care. For example, achievement of diabetes care was higher for patients without cognitive impairment, or for patients who lived alone than those cohabiting. In contrast, care achievement for patients with osteoarthritis was associated with participants reporting severe pain or having a limiting illness, or being obese compared with their healthier counterparts.
Modelling achievement of the care bundle for diabetes
This analysis included 8222 ELSA participants at wave 5 who also responded at wave 4, of whom 11% had diabetes. Despite low non-achievement for the individual diabetes quality indicators (17% to 23%) in wave 5, we found that at least one of the three bundled annual monitoring checks was not achieved in one-third of eligible patients in 2010–11 (33%). Our figure is somewhat lower than data from the National Diabetes Audit in 2009–10,18 which found that 47% of patients did not receive all nine National Institute for Health and Care Excellence quality indicated diabetes care processes.
Only a limited number of variables were found to be independently and significantly associated with receipt of incomplete care. Previous (2008–9) non-achievement of the care bundle was the best predictor of subsequent non-achievement 2 years later, increasing the odds of this by over threefold. Patients who had previously reported in 2008–9 that they did not know ‘everything about managing their diabetes’ or who were cognitively impaired were more likely to subsequently report not having a complete diabetes care bundle. Individuals who were single or had low health literacy were more likely to report not having been offered an ACE inhibitor. In contrast, people in the oldest age group, or classified as obese according to their BMI category, were more likely to have been offered an ACE inhibitor.
The National Diabetes Audit modelled, in a cross-sectional sample, the impact of patient characteristics (available from routine records) on the likelihood of receiving a complete bundle of care. 18 Common to both studies is the finding that only a limited number of patient characteristics explained variability in the receipt of incomplete care. Patient social deprivation, sex and duration of diabetes were not found to affect the likelihood of receiving complete care, but age and ethnicity were. Significant geographical variation was observed across the 151 primary care trusts that contributed data.
The variations in indicator achievement by condition were much larger than those by participant characteristics. This, together with the findings from the diabetes care bundle analysis, suggests that the major causes of variations in health-care quality may lie elsewhere than with the patient, although ELSA could not account for all patient factors which contribute to variation and are difficult to capture in a survey, such as patient behaviour. We were unable to model health service performance data as they are not in ELSA, although participants were asked to consent to linkage of ELSA data to NHS data (e.g. Hospital Episode Statistics), which opens the possibility for future research on linked data sets.
Distribution of illness burden, diagnosis and treatment
This analysis suggested that barriers to equitable receipt of health care according to need may exist predominantly at the stage at diagnosis rather than treatment. For angina, depression, diabetes and osteoarthritis, the hypothetically poorest participant always had higher odds of illness burden than the hypothetically richest. Poorer participants usually had no higher odds for subsequent receipt of diagnosis or treatment. This serial cross-sectional analysis of four waves of ELSA included all eligible participants in each wave in order to maximise the sample size. This approach means that some participants with a diagnosed condition would no longer have had illness burden, if they were being successfully treated. Examples would be diabetic participants whose blood sugar levels were being successfully controlled by treatment, and participants with successfully treated depression. We checked our main results with the secondary (longitudinal) analysis, which assessed subsequent diagnosis in those with illness burden, and subsequent treatment in those with illness burden, and found a similar picture of inequalities in illness burden not being fully addressed by appropriately greater levels of diagnosis and treatment for poorer participants.
Care-seeking behaviour and patient preferences may differ with wealth. Given the same information, patients may be less likely to seek treatment than their doctors53,54 and pessimism about availability of treatment may make older people reluctant to seek help. 55 People from poorer backgrounds may view living with illness burden (such as pain or emotional problems) as a normal part of ageing rather than a disease. 56 It is possible to imagine that the response of the primary care physician may also vary according to the wealth of the patient, however subconsciously. For example, a physician might initially consider symptoms such as aches and pains to be part of the tapestry of life, or the natural ageing process, when they occur in a patient from a deprived background. The same symptoms in a wealthier, well-educated and articulate patient might arguably be considered more unusual and, therefore, more likely to lead to a diagnosis. Our results fit with research showing that while people from deprived groups were more likely to have Rose angina, they were not more likely to receive a GP diagnosis of CHD. 57 Comorbidity is more common in deprived populations, and may make diagnosis of all conditions harder for doctors within the constraints of a short consultation. 58
Strengths and limitations
This project is the first to investigate a wide range of clinical, behavioural, psychosocial and sociodemographic patient characteristics as predictors of subsequent receipt of care in older people in England, encompassing four common health conditions. Its major strengths include that this is the first national cohort study to measure quality of care for a range of common chronic conditions, with individual-level data on a wide range of participant characteristics. We were able to pair reports of receipt of care indicated by evidence- and consensus-based standards developed specifically for older people35 with in-depth, sophisticated patient characteristics assessed using established measures, whereas previous work has usually been limited to basic patient descriptors available from routine records (e.g. age or sex). The quality indicators for all four conditions were developed using the same robust methods, and were based on similar strengths of evidence of effectiveness.
Limitations include the difficulty, common to all longitudinal studies (particularly of ageing), of maintaining a sample that is representative of the national population. The ELSA cohorts were selected from HSE to be representative of people aged ≥ 50 years living in private accommodation in England. However, as Table 2 shows, there was inevitably attrition of the sample over time. ELSA added replenishment samples at waves 3 and 4 to bolster the younger age bands and correct for attrition to some extent. Therefore, cross-sectional analyses, or comparisons of wave pairs, are less affected by this limitation. All analyses were weighted for differential non-response, using longitudinal or cross-sectional sample weights as appropriate, to mitigate any potential lack of representativeness. Ethnic groups other than white British represented approximately 2% of the sample, which is reasonably representative of the population at that age in the UK, and too small a sample to allow separate analysis of quality indicator achievement. It could be argued that people who respond to ELSA over several years are also more likely to use and benefit from health services. For example, their GP would have been notified of any abnormal results identified at the nurse visit, and so we expect that the ELSA quality indicator achievement rates presented in this paper are likely to be slightly higher than in the general population.
Minor technical problems with the computer-assisted questionnaire that filtered ELSA participants to different parts of the ELSA questionnaire temporarily affected the sample sizes of those eligible for diabetes cholesterol and IHD cholesterol quality indicators, as is explained in the ELSA wave 2 documentation. 33 This did not affect the quality of data from those quality indicators.
The ELSA questionnaire, including the quality indicators, was based on self-reports about processes of care that participants might have received up to 2 years previously. The indicators used in ELSA were highly selected on the basis of suitability for use with self-report in an interview survey, as described in Chapter 2 (see Quality indicators in the English Longitudinal Study of Ageing). The ACOVE researchers compared interview data and medical notes where feasible and found that the ACOVE interview data were comparable with notes data, and for some indicators respondents remembered higher rates of achievement than had been recorded in the medical notes. Approximately half of the 236 ACOVE indicators could be used in an interview survey without the use of clinical records, and after conducting literature reviews, expert clinical panel assessment for validity in England, and piloting in ELSA, only 32 indicators were taken forward for implementation in ELSA on the basis that they could be answered meaningfully in a survey. For certain areas of care, recorded data are more likely to reflect actual clinical practice, but for some chronic conditions and process of care measures, including prescription of medication, self-reported data appear to be at least as accurate as clinical records. Clinical records do not contain in-depth information on patient characteristics, and for some of the ELSA questions, such as the presence of pain, it could be argued that the patient’s report is more likely to be accurate than the medical record, and so finding a perfect data source is difficult. Self-reports tend to score the same as, or higher than, medical records. ELSA did not include potential participants if they had severe cognitive issues, and we excluded proxy respondents from our selected samples. Our findings are comparable with other work as discussed above, including an audit of care for diabetes using all clinical records in the UK. 59,60
The statistical significance of the changes in achievement between wave 2 and the most recent year of follow-up was tested with Pearson’s chi-squared test for comparing proportions (see Table 10). This test was chosen because some data points were paired within individuals between wave 2 and the most recent year of follow-up, and some were not, as different individuals were eligible for indicators at different waves. For this reason we did not use McNemar’s test for paired data, although McNemar’s test would have had greater power and precision had it been appropriate to use.
If key covariates change over time, and the analysis only considers baseline values, then this can be a source of bias. In this study, changes in potentially time-varying covariates were generally small. The greatest change in the number of participants reporting potentially time-varying covariates was seen in cognitive impairment, which was reported by over twice as many participants in wave 5 as in wave 2 (see Chapter 3, Regression modelling for quality of care, Demographics for the diabetes care bundle, and Table 14). The only condition in which cognitive impairment was associated with quality was diabetes, and only 5.8% more participants with diabetes also had cognitive impairment in wave 5 than in wave 2. Because the change over time was only 5.8%, any impact on the overall results was thought to be minimal. To check this we also repeated the final regression model for diabetes in Table 19 with both the baseline values for cognitive impairment and the value at wave 5. The result was that cognitive impairment was no longer significant in wave 2 or wave 5, supporting the idea that there was no additional explanatory effect from cognitive impairment at wave 5 that had not been captured by cognitive impairment at wave 2.
If missing data are not properly accounted for, they can bias the results of a study. We considered the alternate approaches of multiple imputation, and including missing data as a ‘missing’ category in the analyses. We recognise that multiple imputation is a powerful and flexible approach for handling missing data in epidemiological studies. However, as with all procedures for handling missing data, multiple imputation has advantages and disadvantages. For example, although the chained equations approach often works well in practice, it does not necessarily have a strong theoretical basis. Other multiple imputation approaches typically require distributional assumptions (e.g. multivariate normality). Based on our experience of other studies, we decided to adopt an alternative strategy for handling missing data: use of a missing category. This approach, although ad hoc, is easily communicated to non-methodologists and often gives results that are consistent with more formal model-based procedures (including multiple imputation). We conducted sensitivity analyses to determine whether or not using multiple imputation of missing data would alter the final results for the diabetes care bundle and the ACE inhibitor/receptor blocker quality indicator (see Chapter 3, Regression modelling for quality of care, Demographics for the diabetes care bundle, and Table 19). The results from the model using multiple imputation were generally very similar to the original models for both the care bundle and the ACE inhibitor quality indicator.
One specific potential limitation of the analysis of distribution of illness burden, diagnoses and treatment is that standardised measures were used to assess illness burden of depression, angina and diabetes, but not illness burden of osteoarthritis and cataract. This means that the illness burden we attributed to osteoarthritis and cataract may not be 100% specific for those conditions, as indeed they are not in clinical situations. However, this lack of specificity in unlikely to vary with wealth, and so should not be a source of bias. Self-reported data may be a source of bias if self-report varies by factors other than objective health status, such as wealth or social experience. This is a recognised problem with some self-reported morbidity data, but is less of a problem with measures of pain, where self-report is the best means of assessment. 61
We did not adjust in the main analysis for related factors that are also more prevalent in poorer populations, such as smoking, obesity and comorbidity, because none of these is a reason for not making a diagnosis. Comorbid conditions are more common in those with lower socioeconomic status, but there is no evidence that comorbidities make diagnosis less likely. On the contrary, a higher number of comorbid conditions in older people may be associated with higher quality of care. 62 We adjusted for the effects of adding individual additional covariates in additional analyses found different patterns in different conditions, which fits with other research showing that wealth acts differently in different conditions. 63
Generalisability
The results are likely to be generalisable to the population of England aged ≥ 50 years, bearing in mind the limitations discussed above, because the sample was large and selected to be nationally representative, the results were weighted for non-response, and achievement rates were comparable with QOF. They may also be generalisable with caution to other countries with similar population demographics, but are likely to vary according to health system and availability of care. Previous US studies have used similar methods and found similar quality scores.
Chapter 5 Conclusions
This project is the first to investigate a wide range of clinical, behavioural, psychosocial and sociodemographic participant characteristics as predictors of subsequent receipt of care in older people in England, encompassing four common health conditions. The results show that there were persistent shortfalls in quality for all conditions, and particularly for osteoarthritis, and that these changed little over 6 years of follow-up, although quality for osteoarthritis did improve slightly. The analysis of transitions shows that the strongest predictor of future poor care was past poor care, which means that it was often the same people who did not receive indicated care year after year. We found that quality varied little by participant characteristics, despite the wide range of characteristics (e.g. patient education, socioeconomic status, health and health behaviours, and cognition) available for analysis. There were some participant characteristics that were significantly associated with achievement of quality indicators, but these were generally different for the different conditions. For example, we found that participants with diabetes, and who had cognitive impairment or who were cohabiting (as opposed to living alone), were more likely to report poor-quality care. The good news is that, for those with one of the four diagnosed health conditions studied, receipt of indicated care is generally distributed equitably across the population.
The implications for policy are that shortfalls in quality of care for these four common and important long-term conditions have persisted over 6 years, despite much policy attention and major initiatives such as payment for performance in primary care through the QOF. The variations in quality within and between conditions are not, for the most part, explained by participant characteristics, so a different source or sources of variation must be driving these. Health system characteristics are known to be strongly associated with quality of care in other countries, and are a possible explanation for the shortfalls in quality of care that are not explained by patient characteristics. Fisher et al. 64 showed that health care is often driven by the availability of specialist services rather than by the health needs of the population, with no detectable difference in health outcomes.
Health system characteristics affect the quality of health care received by patients at micro (individual clinician), meso (general practice or hospital) and macro (regional and national) levels. The shortfall in quality was particularly marked for osteoarthritis. This research has found that the QOF seems to have had little effect on the quality of care received by ELSA participants for the four conditions studied. Common features of successful models of care for older people are strong generalist or primary care, the use of care plans, and electronic medical records with continuity of care. 65,66
Participant characteristics may not explain access to treatment, but they, especially wealth, do explain large variations in the distribution of illness burden for the four conditions studied. The analysis of inequalities in access to diagnosis and treatment presented here is preliminary and limited by the availability of data, but suggests that less-wealthy people may be less likely to be diagnosed. This matters as, after diagnosis, the results show that, for the most part, treatment is remarkably equitable. Diagnosis is a key step in equitable access to treatment for those with long-term conditions.
Recommendations for further research
Further research could compare the results with measurements from directly observed clinical care and from medical records. As this research has shown that the substantial variations in quality of care are generally not explained by participant characteristics, further research is needed into the association between quality and health system characteristics at micro (individual clinician), meso (general practice or hospital) and macro (regional and national) levels. Future research could use linked data sets (such as the GP patient survey, and Hospital Episode Statistics) to examine the effect of health service characteristics on the quality of care received at the individual patient level. For the few areas where specific participant characteristics were associated with quality of care, further qualitative research could explore how these characteristics affect treatment quality.
Further research could explore why quality is so much lower in osteoarthritis than for other conditions studied, and this may include investigating GPs’ knowledge about treatment options, and availability of further care such as physiotherapy. The preliminary finding that there may be system level inequalities in the receipt of diagnosis that make it harder for disadvantaged people with specific illness burden to receive an appropriate diagnosis could be investigated further. What are the barriers that may differentially hinder access to an appropriate diagnosis? A mixed qualitative and quantitative approach could explore the delivery of care in different systems, and link care to patient health outcomes.
Acknowledgements
Dave Stott and Amander Wellings, representatives of PPIRes, brought a helpful lay perspective to this research, including specific comments on earlier versions of this report.
Antonia Hardcastle was supported between grants by Research Capability Funding from NHS South Norfolk Clinical Commissioning Group.
Contributions of authors
Dr Antonia C Hardcastle, Senior Research Associate, Primary Care, wrote the first and subsequent drafts of the report and conducted analyses.
Dr Luke TA Mounce, Associate Research Fellow, Primary Care, drafted the report and conducted analyses.
Dr Suzanne H Richards, Senior Lecturer, Primary Care, drafted and revised the report.
Professor Max O Bachmann, Professor of Health Services Research, Academic Public Health, contributed to study design, statistical advice and revising the report.
Dr Allan Clark, senior lecturer in medical statistics, contributed statistical advice and revised the report.
Professor William E Henley, Professor of Medical Statistics, contributed statistical advice and revised the report.
Professor John L Campbell, Professor of General Practice, Health Services Research, contributed to the study design and revised the report.
Professor David Melzer, Professor of Epidemiology, Primary Care, contributed to the study design.
Dr Nicholas Steel, Clinical Reader in Primary Care, was principal investigator and contributed conception, design and analysis, and drafted and revised the report.
This article presents independent research commissioned by the National Institute for Health Research National Institute for Health Research under the Health Services and Delivery Research programme. The views expressed in this publication are those of the authors and not necessarily those of the NHS, the National Institute for Health Research or the Department of Health.
Publications
Steel N, Hardcastle AC, Clark A, Mounce LTA, Bachmann MO, Richards SH, et al. Self-reported quality of care for older adults from 2004 to 2011: a cohort study. Age Ageing 2014;43:716–20.
Steel N, Hardcastle AC, Bachmann MO, Richards SH, Mounce LTA, Clark A, et al. Economic inequalities in burden of illness, diagnosis and treatment of five long-term conditions in England: panel study. BMJ Open 2014;4:e005530.
Mounce LTA, Steel N, Hardcastle AC, Henley WE, Bachmann MO, Campbell JL, et al. Patient characteristics predicting failure to receive indicated care for type 2 diabetes. Diabetes Res Clin Pract 2015;107:247–58.
Disclaimers
This report presents independent research funded by the National Institute for Health Research (NIHR). The views and opinions expressed by authors in this publication are those of the authors and do not necessarily reflect those of the NHS, the NIHR, NETSCC, the HS&DR programme or the Department of Health. If there are verbatim quotations included in this publication the views and opinions expressed by the interviewees are those of the interviewees and do not necessarily reflect those of the authors, those of the NHS, the NIHR, NETSCC, the HS&DR programme or the Department of Health.
References
- Bunker JP. The role of medical care in contributing to health improvements within societies. Int J Epidemiol 2001;30:1260-3. http://dx.doi.org/10.1093/ije/30.6.1260.
- Leatherman S, Sutherland K. The Quest for Quality in the NHS. A Chartbook on Quality of Care in the UK. Oxford: Radcliffe; 2005.
- Grol R. Successes and failures in the implementation of evidence-based guidelines for clinical practice. Med Care 2001;39:II46-54. http://dx.doi.org/10.1097/00005650-200108002-00003.
- McGlynn EA, Asch SM, Adams J, Keesey J, Hicks J, DeCristofaro A, et al. The quality of health care delivered to adults in the United States. N Engl J Med 2003;348:2635-45. http://dx.doi.org/10.1056/NEJMsa022615.
- Seddon ME, Marshall MN, Campbell SM, Roland MO. Systematic review of studies of quality of clinical care in general practice in the UK, Australia and New Zealand. Qual Health Care 2001;10:152-8. http://dx.doi.org/10.1136/qhc.0100152.
- Wenger NS, Solomon DH, Roth CP, MacLean CH, Saliba D, Kamberg CJ, et al. The quality of medical care provided to vulnerable community-dwelling older patients. Ann Intern Med 2003;139:740-7. http://dx.doi.org/10.7326/0003-4819-139-9-200311040-00008.
- Steel N, Bachmann M, Maisey S, Shekelle P, Breeze E, Marmot M, et al. Self reported receipt of care consistent with 32 quality indicators: national population survey of adults aged 50 or more in England. BMJ 2008;337. http://dx.doi.org/10.1136/bmj.a957.
- Steel N, Maisey S, Clark A, Fleetcroft R, Howe A. Quality of clinical primary care and targeted incentive payments: an observational study. Br J Gen Pract 2007;57:449-54.
- Steel N, Melzer D, Shekelle PG, Wenger NS, Forsyth D, McWilliams BC. Developing quality indicators for older adults: transfer from the USA to the UK is feasible. Qual Saf Health Care 2004;13:260-4. http://dx.doi.org/10.1136/qshc.2004.010280.
- Steel N, Willems S. Research learning from the UK Quality and Outcomes Framework: a review of existing research. Qual Prim Care 2010;18:117-25.
- Gillam S, Siriwardena A, Steel N. Pay-for-Performance in the United Kingdom: impact of the Quality and Outcomes Framework – a systematic review. Ann Fam Med 2012;10:461-8. http://dx.doi.org/10.1370/afm.1377.
- Campbell S, Reeves D, Kontopantelis E, Sibbald B, Roland M. Effects of Pay for Performance on the quality of primary care in England. N Engl J Med 2009;361:368-78. http://dx.doi.org/10.1056/NEJMsa0807651.
- Askari M, Wierenga PC, Eslami S, Medlock S, de Rooij SE, Abu-Hanna A. Assessing quality of care of elderly patients using the ACOVE quality indicator set: a systematic review. PLOS ONE 2011;6. http://dx.doi.org/10.1371/journal.pone.0028631.
- Fleetcroft R, Cookson R, Steel N, Howe A. Correlation between prescribing quality and pharmaceutical costs in English primary care: national cross-sectional analysis. Br J Gen Pract 2011;61:e556-64. http://dx.doi.org/10.3399/bjgp11X593839.
- Holmboe E, Weng W, Arnold G, Kaplan S, Normand S, Greenfield S. The comprehensive care project: measuring physician performance in ambulatory practice. Health Serv Res 2010;45:1912-33. http://dx.doi.org/10.1111/j.1475-6773.2010.01160.x.
- Fürthauer J, Flamm M, Sonnichsen A. Patient and physician related factors of adherence to evidence based guidelines in diabetes mellitus type 2, cardiovascular disease and prevention: a cross sectional study. BMC Fam Pract 2013;14. http://dx.doi.org/10.1186/1471-2296-14-47.
- Ludt S, Wensing M, Campbell SM, Ose D, van Lieshout J, Rochon J, et al. The challenge of cardiovascular prevention in primary care: implications of a European observational study in 8928 patients at different risk levels. Eur J Prev Cardiol 2014;21:203-13. http://dx.doi.org/10.1177/2047487312462798.
- The National Diabetes Audit 2009–10. Leeds: NHS Information Centre; 2011.
- Diabetes in Adults Quality Standard. London: National Institute for Health and Care Excellence; 2011.
- de Wet C, McKay J, Bowie P. Combining QOF data with the care bundle approach may provide a more meaningful measure of quality in general practice. BMC Health Serv Res 2012;12. http://dx.doi.org/10.1186/1472-6963-12-351.
- Doran T, Fullwood C, Kontopantelis E, Reeves D. Effect of financial incentives on inequalities in the delivery of primary clinical care in England: analysis of clinical activity indicators for the quality and outcomes framework. Lancet 2008;372:728-36. http://dx.doi.org/10.1016/S0140-6736(08)61123-X.
- Kontopantelis E, Reeves D, Valderas JM, Campbell S, Doran T. Recorded quality of primary care for patients with diabetes in England before and after the introduction of a financial incentive scheme: a longitudinal observational study. BMJ Qual Saf 2013;22:53-64. http://dx.doi.org/10.1136/bmjqs-2012-001033.
- Brand CA, Ackerman IN, Bohensky MA, Bennell KL. Chronic disease management: a review of current performance across quality of care domains and opportunities for improving osteoarthritis care. Rheum Dis Clin North Am 2013;39:123-43. http://dx.doi.org/10.1016/j.rdc.2012.10.005.
- McHugh GA, Campbell M, Luker KA. Quality of care for individuals with osteoarthritis: a longitudinal study. J Eval Clin Pract 2012;18:534-41. http://dx.doi.org/10.1111/j.1365-2753.2010.01616.x.
- Li LC, Sayre EC, Kopec JA, Esdaile JM, Bar S, Cibere J. Quality of nonpharmacological care in the community for people with knee and hip osteoarthritis. J Rheumatol 2011;38:2230-7. http://dx.doi.org/10.3899/jrheum.110264.
- Duhoux A, Fournier L, Gauvin L, Roberge P. Quality of care for major depression and its determinants: a multilevel analysis. BMC Psychiatry 2012;12. http://dx.doi.org/10.1186/1471-244X-12-142.
- English Longitudinal Study of Ageing. London: Institute for Fiscal Studies; 2011.
- English Longitudinal Study of Ageing. Colchester: UK Data Service; n.d.
- Banks J, Nazroo J, Steptoe A. The Dynamics of Ageing; Evidence from the English Longitudinal Study of Ageing 2002–2010 (Wave 5). London: Institute for Fiscal Studies; 2012.
- Banks J, Breeze E, Lessof C, Nazroo J. Retirement, Health and Relationships of the Older Population in England: the 2004 English Longitudinal Study of Ageing (Wave 2). London: Institute for Fiscal Studies; 2006.
- Cheshire H, Hussey D, Medina J, Pickering K, Wood N, Ward K, et al. Financial Circumstances, Health and Well-Being of the Older Population in England: The 2008 English Longitudinal Study of Ageing: Technical Report. London: Institute for Fiscal Studies; 2012.
- Scholes S, Medina J, Cheshire H, Cox K, Hacker E, Lessof C. Living in the 21st Century: Older People in England. The 2006 English Longitudinal Study of Ageing: Technical Report. London: Institute for Fiscal Studies; 2009.
- Scholes S, Taylor R, Cheshire H, Cox K, Lessof C. Retirement, Health and Relationships of the Older Population in England: The 2004 English Longitudinal Study of Ageing: Technical Report. London: Institute for Fiscal Studies; 2008.
- Taylor R, Conway L, Calderwood L, Lessof C, Cheshire H, Cox K, et al. Health, Wealth and Lifestyles of the Older Population in England: The 2002 English Longitudinal Study of Ageing: Technical Report. London: Institute for Fiscal Studies; 2007.
- Wenger N, Shekelle P, Davidoff F, Mulrow C. Quality indicators for assessing care of vulnerable elders. Ann Intern Med 2001;135:641-758.
- Guthrie B. Measuring the quality of healthcare systems using composites. BMJ 2008;337. http://dx.doi.org/10.1136/bmj.a639.
- van Doorn-Klomberg A, Braspenning J, Feskens R, Bouma M, Campbell S, Reeves D. Precision of individual and composite performance scores: the ideal number of indicators in an indicator set. Med Care 2013;51:115-21. http://dx.doi.org/10.1097/MLR.0b013e3182726bf1.
- Reeves D, Campbell SM, Adams J, Shekelle PG, Kontopantelis E, Roland MO. Combining multiple indicators of clinical quality: an evaluation of different analytic approaches. Med Care 2007;45:489-96. http://dx.doi.org/10.1097/MLR.0b013e31803bb479.
- Wenger NS, Shekelle PG. Assessing Care of Vulnerable Elders: ACOVE project overview. Ann Intern Med 2001;135:642-6. http://dx.doi.org/10.7326/0003-4819-135-8_Part_2-200110161-00002.
- Brook RH, Chassin MR, Fink A, Solomon DH, Kosecoff J, Park RE. A method for the detailed assessment of the appropriateness of medical technologies. Int J Technol Assess Health Care 1986;2:53-6. http://dx.doi.org/10.1017/S0266462300002774.
- Hyde M, Wiggins RD, Higgs P, Blane DB. A measure of quality of life in early old age: the theory, development and properties of a needs satisfaction model (CASP-19). Aging Ment Health 2003;7:186-94. http://dx.doi.org/10.1080/1360786031000101157.
- Cajipe MD, Chu D, Bakaeen FG, Casal RF, LeMaire SA, Coselli JS, et al. Video-assisted thoracoscopic lobectomy is associated with better perioperative outcomes than open lobectomy in a veteran population. Am J Surg 2012;204:607-12. http://dx.doi.org/10.1016/j.amjsurg.2012.07.022.
- Radloff LS. The CES-D Scale: a self-report depression scale for research in the general population. Appl Psychol Meas 1977;1:385-401. http://dx.doi.org/10.1177/014662167700100306.
- Rose GA. The diagnosis of ischaemic heart pain and intermittent claudication in field surveys. Bull World Health Organ 1962;27:645-58.
- Steel N, Melzer D, Gardener E, McWilliams B. Need for and receipt of hip and knee replacement – a national population survey. Rheumatology 2006;45:1437-41. http://dx.doi.org/10.1093/rheumatology/kel131.
- Katz S, Akpom CA. 12. Index of ADL. Med Care 1976;14:116-18. http://dx.doi.org/10.1097/00005650-197605001-00018.
- Pearson VI, Kane RL, Kane RA. Assessing Older Persons: Measures, Meaning and Practical Applications. Oxford: Oxford University Press; 2000.
- Mercer SW, Smith SM, Wyke S, O’Dowd T, Watt GC. Multimorbidity in primary care: developing the research agenda. Fam Pract 2009;26:79-80. http://dx.doi.org/10.1093/fampra/cmp020.
- SOC2010 Volume 3: The National Statistics Socio-Economic Classification (NS-SEC Rebased on the SOC2010). London: ONS; 2010.
- Pierce MB, Zaninotto P, Steel N, Mindell J. Undiagnosed diabetes-data from the English longitudinal study of ageing. Diabet Med 2009;26:679-85. http://dx.doi.org/10.1111/j.1464-5491.2009.02755.x.
- Thomas MC, Walker MK, Emberson JR, Thomson AG, Lawlor DA, Ebrahim S, et al. Prevalence of undiagnosed type 2 diabetes and impaired fasting glucose in older British men and women. Diabet Med 2005;22:789-93. http://dx.doi.org/10.1111/j.1464-5491.2005.01516.x.
- Decode Study Group . Age- and sex-specific prevalences of diabetes and impaired glucose regulation in 13 European cohorts. Diabetes Care 2003;26:61-9. http://dx.doi.org/10.2337/diacare.26.1.61.
- Montgomery AA, Fahey T. How do patients’ treatment preferences compare with those of clinicians?. Qual Health Care 2001;10:i39-43. http://dx.doi.org/10.1136/qhc.0100039.
- Steel N. Thresholds for taking antihypertensive drugs in different professional and lay groups: questionnaire survey. BMJ 2000;320:1446-7. http://dx.doi.org/10.1136/bmj.320.7247.1446.
- Sanders C, Donovan JL, Dieppe PA. Unmet need for joint replacement: a qualitative investigation of barriers to treatment among individuals with severe pain and disability of the hip and knee. Rheumatology 2004;43:353-7. http://dx.doi.org/10.1093/rheumatology/keh044.
- Murray J, Banerjee S, Byng R, Tylee A, Bhugra D, Macdonald A. Primary care professionals’ perceptions of depression in older people: a qualitative study. Soc Sci Med 2006;63:1363-73. http://dx.doi.org/10.1016/j.socscimed.2006.03.037.
- Richards H, McConnachie A, Morrison C, Murray K, Watt G. Social and gender variation in the prevalence, presentation and general practitioner provisional diagnosis of chest pain. J Epidemiol Community Health 2000;54:714-18. http://dx.doi.org/10.1136/jech.54.9.714.
- Mercer SW, Guthrie B, Furler J, Watt GC, Hart JT. Multimorbidity and the inverse care law in primary care. BMJ 2012;344. http://dx.doi.org/10.1136/bmj.e4152.
- Chang JT, MacLean CH, Roth CP, Wenger NS. A comparison of the quality of medical care measured by interview and medical record. J Gen Intern Med 2004;19:109-10.
- Tisnado DM, Adams JL, Liu H, Damberg CL, Chen WP, Hu FA, et al. What is the concordance between the medical record and patient self-report as data sources for ambulatory care?. Med Care 2006;44:132-40. http://dx.doi.org/10.1097/01.mlr.0000196952.15921.bf.
- Sen A. Health: perception versus observation. BMJ 2002;324:860-1. http://dx.doi.org/10.1136/bmj.324.7342.860.
- Min LC, Reuben DB, MacLean CH, Shekelle PG, Solomon DH, Higashi T, et al. Predictors of overall quality of care provided to vulnerable older people. J Am Geriatr Soc 2005;53:1705-11. http://dx.doi.org/10.1111/j.1532-5415.2005.53520.x.
- McBride D, Hardoon S, Walters K, Gilmour S, Raine R. Explaining variation in referral from primary to secondary care: cohort study. BMJ 2010;341. http://dx.doi.org/10.1136/bmj.c6267.
- Fisher ES, Wennberg DE, Stukel TA, Gottlieb DJ, Lucas FL, Pinder EL. The implications of regional variations in Medicare spending. Part 1: the content, quality, and accessibility of care. Ann Intern Med 2003;138:273-87. http://dx.doi.org/10.7326/0003-4819-138-4-200302180-00006.
- Boult C, Green AF, Boult LB, Pacala JT, Snyder C, Leff B. Successful models of comprehensive care for older adults with chronic conditions: evidence for the Institute of Medicine’s ‘retooling for an ageing America’ report. J Am Geriatr Soc 2009;57:2328-37. http://dx.doi.org/10.1111/j.1532-5415.2009.02571.x.
- Cornwell J, Sonola L, Levenson R, Poteliakhoff E. Continuity of Care for Older Hospital Patients: a Call for Action. London: The King’s Fund; 2012.
Appendix 1 Translating quality indicators to the English Longitudinal Study of Ageing
Condition, quality indicator abbreviated title and quality indicator/question text | Denominatora | Numeratora | Corresponding ELSA variable(s)a and question(s) |
---|---|---|---|
Diabetes mellitus | |||
Diabetes HbA1c. IF a person aged ≥ 50 years has diabetes, THEN his or her glycosylated haemoglobin or fructosamine level should be measured at least annually | Age ≥ 50 years. All diabetes cases up to current wave (heacd) | Hesuga = ‘yes’ | Heacd: Has a doctor ever told you that you have diabetes? Hesug: Have you ever had a special blood test to see how well your blood sugar was controlled? This test is called a glycosylated haemoglobin, or haemoglobin A1c, or fructosamine. This is a blood test taken at a doctor’s surgery or health centre or laboratory Hesuga: Have you had this test (glycosylated haemoglobin or fructosamine) performed in the past 12 months? |
Diabetes proteinuria. IF a diabetic person aged ≥ 50 years does not have established renal disease and is not receiving an ACE inhibitor or angiotensin II receptor blocker, THEN he or she should receive an annual test for proteinuria | Age ≥ 50 years. All diabetes cases up to current wave, and hekidn = ‘no’, and heacea = ‘not taking ACE inhibitor or A2 receptor blocker’ | Hewee = ‘yes’ | Hekidn: Has a doctor ever told you that your diabetes has caused trouble with your kidneys? Heacea: I would like to check whether any of the medications you are taking are on this list of ACE inhibitor or A2 receptor blockers (show card G ACE inhibitors)? Hewee: Have you had a urine test for protein in the last 12 months? |
Diabetes cholesterol. IF a diabetic person aged ≥ 50 years has a fasting total cholesterol level of ≥ 5 mmol/l, THEN he or she should be offered an intervention to lower cholesterol | Age ≥ 50 years. All diabetes cases up to current wave, and ‘high cholesterol’ at current wave, but not at previous wave | Hechol = ‘yes’ | Hediaa: Has a doctor ever told you that you have (or have had) any of the conditions on this card (includes ‘high cholesterol’)? Hechol: Has any doctor talked to you about how to lower your cholesterol? This would include changing your diet, losing weight, getting more exercise or taking medication (asked if has diabetes and high cholesterol) |
Diabetes ACE inhibitor. IF a diabetic person aged ≥ 50 years has one additional cardiac risk factor (i.e. smoker, hypertension, hypercholesterolemia, or renal insufficiency/microalbuminuria), THEN he/she should be offered an ACE inhibitor or receptor blocker | Age ≥ 50 years. All diabetes cases up to current wave, and at current wave [heska = ‘yes’, or systolic blood pressure (BP) ≥ 140 or diastolic BP ≥ 90], or hekidn = ‘yes’, or heweea = ‘yes’, or reported diagnosed ‘high cholesterol’ or ‘high blood pressure or hypertension’, or prevalent hypertension | Heace = ‘yes’ or Heacea = ‘yes’ | Hediaa: Has a doctor ever told you that you have (or have had) any of the conditions on this card (includes ‘high blood pressure or hypertension’ and ‘high cholesterol’)? Hewee: Some doctors check to see if patients with diabetes have protein in their urine. Have you had a urine test for protein in the past 12 months? (This test may also be called a microalbumin test, and involves a first morning urine sample or 24-hour urine collection) Heweea: Has a doctor ever told you that you have protein in your urine? Hekidn: Has a doctor ever told you that your diabetes has caused trouble with your kidneys? Heska: Do you smoke cigarettes at all nowadays? Sysval: valid mean systolic BP (measured) Diaval: valid mean diastolic BP (measured) Heace: Has a doctor discussed with you whether you should take a medication called an ACE inhibitor or A2 receptor blocker? Heacea: I would like to check whether any of the medications you are taking are on this list of ACE inhibitor or A2 receptor blockers (show card G ACE inhibitors)? |
Diabetes foot check. ALL diabetic persons aged ≥ 50 years should have an annual examination of his or her feet | Age ≥ 50 years. All diabetes cases up to current wave | Heftchk = ‘yes’ | Heftchk: Some doctors suggest that some patients with diabetes have a regular foot examination. In the past year, has any doctor or nurse examined your bare feet? |
CVD | |||
Stroke antihypertensives. IF a person aged ≥ 50 years has had a previous stroke, THEN the patient should be offered antihypertensive medication | Age ≥ 50 years. Newly reported stroke (hediaa), or confirmed stroke previously reported (hediac) | Hehibpb1 = ‘yes’ | Hediaa: Has a doctor ever told you that you have (or have had) any of the conditions on this card [includes ‘stroke (cerebral vascular disease)’]? Hediac: Whether confirms stroke diagnosis Hehibpb1: Did a doctor or nurse ever suggest you take any medication to lower your blood pressure? |
Hypertension | |||
Hypertension treatment. IF a person aged ≥ 50 years remains hypertensive after non-pharmacological intervention, THEN pharmacological antihypertensive treatment should be initiated | Age ≥ 50 years. Newly reported hypertension (hediaa), or still has hypertension previously reported (hedias) | Hehibpb = ‘yes’ | Hedias: Do you still have (hypertension)? Hehibpb: Some doctors suggest that some patients take medication to lower their blood pressure. Did a doctor or nurse ever suggest that you take medication to lower your blood pressure? |
IHD | |||
IHD cholesterol. IF a person aged ≥ 50 years has established CHD and LDL cholesterol > 3 mmol/l, THEN he or she should be offered an intervention to lower cholesterol | Age ≥ 50 years. Diagnosed high cholesterol at current wave (hediaa) and diagnosed angina or heart attack (including MI or coronary thrombosis) at current wave (hediaa) or still has angina (hedias) or confirms heart attack previously reported (hediac) | Hechol = ‘yes’ | Hechol: Has any doctor talked to you about how to lower your cholesterol? This would include changing your diet, losing weight, getting more exercise or taking medication |
IHD antiplatelets. IF a person aged ≥ 50 years has established CHD and is not on warfarin, THEN he or she should be offered antiplatelet therapy | Age ≥ 50 years. Diagnosed angina or heart attack (including MI or coronary thrombosis) at current wave (hediaa) or still has angina (hedias) or confirms heart attack previously reported (hediac), and Hehrtc = ‘no’ | Hehrta = ‘yes’ | Hehrtc: Are you taking warfarin? Hehrta: Did a doctor suggest that you take medication to thin your blood such as warfarin or aspirin, Plavix, Ticlid, or other blood-thinning medication? |
IHD smoking. IF a person aged ≥ 50 years with established CHD smokes, THEN he or she should be offered counselling for smoking cessation | Age ≥ 50 years. Diagnosed angina or heart attack (including MI or coronary thrombosis) at current wave (hediaa) or still has angina (hedias) or confirms heart attack previously reported (hediac), and heska = ‘yes’ | Hecgstp = ‘yes’ | Heska: Do you smoke cigarettes at all nowadays? Hecgstp: Has a doctor or nurse ever advised you to stop smoking? |
MI beta-blocker. IF a person aged ≥ 50 years has had a recent MI, THEN he or she should be offered a beta-blocker | Age ≥ 50 years. Diagnosed heart attack (including MI or coronary thrombosis) at current wave (hediaa) and not at previous wave | Hebeta = ‘yes’ or Hebetb = currently taking beta-blockers | Hebeta: Did any doctor ever tell you that you should take a medication called a beta-blocker? Hebetb: Please could you show me the medications or the repeat prescription list for any medications you have taken over the last week (checked against card F beta-blockers)? |
INR test. IF a person aged ≥ 50 years is prescribed warfarin, THEN an INR should be determined at least every 12 weeks | Age ≥ 50 years. Diagnosed angina or heart attack (including MI or coronary thrombosis) at current wave (hediaa) or still has angina (hedias) or confirms heart attack previously reported (hediac), and Hehrtc = ‘yes’ | Hehrte = ‘yes’ | Hehrtc: Are you taking warfarin? Hehrtd: Many doctors ask patients on warfarin to get their blood tested regularly. The test checks how thin their blood is, and is sometimes called an INR test. Do you get your blood checked for this? Hehrte: Have you had this blood test in the past 12 weeks? |
OA | |||
OA exercise. IF an ambulatory person aged ≥ 50 years has had a diagnosis of symptomatic osteoarthritis of the knee for longer than 3 months and has no contraindications to exercise and is physically and mentally able to exercise, THEN a directed or supervised strengthening or aerobic exercise programme should have been prescribed at least once | Age ≥ 50 years. Diagnosed osteoarthritis at current wave (heart) or still has osteoarthritis (hedids + heart), and knee pain (hekne) for more than 3 months (heknea) | Hekneb = ‘yes’ | Heart: Which type or types of arthritis do you have (includes ‘osteoarthritis’)? Hedids: Do you still have (arthritis)? Hekne: How would you rate the pain in your knees? (‘Where 0 is no pain and 10 is severe or excruciating pain, as bad as you can imagine.’ Range: 0–10) Heknea: Has your knee pain been bothering you for more than 3 months? Hekneb: Has a doctor or nurse suggested that you receive physiotherapy or attend a supervised exercise program for your knee pain? |
OA education. IF an ambulatory person aged ≥ 50 years has a diagnosis of symptomatic osteoarthritis, THEN education regarding the natural history, treatment and self-management of the disease should be offered at least once | Age ≥ 50 years. Diagnosed osteoarthritis at current wave (heart) or still has osteoarthritis (hedids + heart) | Hearta = ‘yes’ and Heartb = ‘yes’ and Heartc = ‘yes’ | Has any doctor or nurse ever talked to you about: Hearta: What your arthritis or joint pain will be like as time goes on? Heartb: How to keep your arthritis or joint pain from getting worse? Heartc: How your arthritis or joint pain will be treated? |
OA paracetamol. IF oral pharmacological therapy is initiated to treat osteoarthritis among people aged ≥ 50 years, THEN paracetamol should be the first drug used, unless there is a contraindication to use | Age ≥ 50 years. Diagnosed osteoarthritis at current wave (heart) or still has osteoarthritis (hedids + heart) | Hearte = ‘yes’ | Hearte: Did any doctor or nurse recommend you to try paracetamol before other medicines for your joint pain? |
OA referral. IF a person aged ≥ 50 years with severe symptomatic osteoarthritis of the knee or hip has failed to respond to non-pharmacological and pharmacological therapy, THEN the patient should be offered referral to an orthopaedic surgeon to be evaluated for total joint replacement within 6 months unless surgery is contraindicated | Age ≥ 50 years. All diagnosed cases of osteoarthritis up to current wave (heartoa), and knee or hip pain for more than 6 months (hekne and hehip), both rated 6–10, and not controlled by treatment (hepae = ‘no’) | Hepaf = ‘yes’ | Hehip: How would you rate the pain in your hips? (‘Where 0 is no pain and 10 is severe or excruciating pain, as bad as you can imagine.’ Range: 0–10) Hepac: Has your knee or hip pain been bothering you for more than 6 months? Hepad/hepmed:b Are you taking or have you taken any medication or exercises to control the pain in your knee or hip? Hepae/heknefb and hehipc:b Do exercises and medicines control the pain in your knee or hip? Hepaf/hypesur:b Did any doctor recommend that you should have surgery or joint replacement? |
Depression | |||
Depression suicide. IF a person aged ≥ 50 years receives a diagnosis of a new depression episode, THEN the diagnosing physician should ask on the day of diagnosis whether or not the person aged ≥ 50 years had any thoughts about suicide | Age ≥ 50 years. Diagnosed depression at current wave, but not at previous wave (hediab) | Hepsye = ‘yes’ | Hediab: Has a doctor ever told you that you have (or have had) any of the conditions on this card (includes ‘any emotional, nervous or psychiatric problems’)? Hepsy: What type of emotional, nervous or psychiatric problems do you have (includes ‘depression’)? Hepsye: When you talked about these feelings with a doctor or nurse, did they ask you on that day if you had thoughts about suicide? |
Depression treatment. IF a person aged ≥ 50 years is diagnosed with clinical depression, THEN antidepressant treatment, talking treatment, or electroconvulsive therapy should be offered within 2 weeks after diagnosis unless within that period the patient has improved, or unless the patient has substance abuse or dependence, in which case treatment may wait until 8 weeks after the patient is in a drug- or alcohol-free state | Age ≥ 50 years. Diagnosed depression at current wave, but not at previous wave (hediab), and hepsya ≠ ‘none’ | Hepsyb = ‘yes’ | Hepsya: I have some questions about any treatment you may have had for your depression. Did a doctor or nurse suggest that you take medication, or see a mental health professional for counselling? Hepsyb: Did you start medication or counselling within 2 weeks of being offered this treatment? |
Depression review. IF a person aged ≥ 50 years has no meaningful symptom response after 6 weeks of treatment, THEN one of the following treatment options should be initiated by the 8th week of treatment: medication dose should be optimised (if initial treatment was medication), or medication should be initiated (if initial treatment was psychotherapy alone), or referral to a psychiatrist should be offered | Age ≥ 50 years. Diagnosed depression at current wave, but not at previous wave (hediab), and Hepsyc = ‘no’ | Hepsyd = ‘yes’ | Hepsyc: Did you feel better within 6 weeks after starting to take medication, or seeing a mental health professional for counselling? Hepsyd: Did any doctor or nurse start you on medication, change the dose of the medication that you were taking or, change the medications to help you feel better? |
Patient-centred care | |||
Diabetes training. Some people with diabetes receive training to help manage their diabetes themselves. Have you ever participated in a course or class about diabetes, or received special training on how you can live with your diabetes from day to day? | Age ≥ 50 years. All diabetes cases up to current wave (heacd) | Heslfcr = ‘yes’ at any wave | Heslfcr: Some people with diabetes receive training to help manage their diabetes themselves. Have you ever participated in a course or class about diabetes, or received special training on how you can live with your diabetes from day to day? |
Diabetes knowledge. How much do you think you know about managing your diabetes (‘just about everything you need to know’ or ‘most of what you need to know’)? | Age ≥ 50 years. All diabetes cases up to current wave (heacd) | Heslfcb = 1 (‘just about everything . . .’) or 2 (‘most . . .’) | Heslfcb: How much do you think you know about managing your diabetes? Do you know . . . 1 just about everything you need to know; 2 most of what you need to know; 3 some of what you need to know; 4 a little of what you need to know; or 5 almost none of what you need to know? |
Cholesterol explanation. Has a doctor or nurse explained high cholesterol in a way you could understand? | Age ≥ 50 years. Diagnosed high cholesterol at current wave (hediaa), or confirms still has high cholesterol previously reported (hedias) | Hecholb = ‘yes’ at any wave | Hedias: Do you still have (high cholesterol)? Hecholb: Has a doctor or nurse explained high cholesterol in a way you could understand? |
Cholesterol preferences. Have doctors or nurses taken your preferences into account when making treatment decisions about your high cholesterol? | Age ≥ 50 years. Diagnosed high cholesterol at current wave (hediaa), or confirms still has high cholesterol previously reported (hedias) | Hecholc = ‘yes’ at any wave | Hecholc: Have doctors or nurses taken your preferences into account when making treatment decisions about your high cholesterol? |
Blood pressure explanation. Has a doctor or nurse explained high blood pressure in a way you could understand at any time since you were first told you had high blood pressure? | Age ≥ 50 years. Newly reported hypertension (hediaa), or still has hypertension previously reported (hedias) | Hehibp = ‘yes’ at any wave | Hehibp: Has a doctor or nurse explained high blood pressure in a way you could understand at any time since you were first told you had high blood pressure? |
Blood pressure choice. In general, have doctors or nurses given you any choice about how to treat your high blood pressure? | Age ≥ 50 years. Newly reported hypertension (hediaa), or still has hypertension previously reported (hedias) | Hehibpa = ‘yes’ at any wave | Hehibpa: In general, have doctors or nurses given you any choice about how to treat your high blood pressure? |
OA treatment purpose. Has any doctor or nurse ever talked to you about what the specific purpose of the treatment for your arthritis or joint pain is? | Age ≥ 50 years. Diagnosed osteoarthritis at current wave (heart) or still has osteoarthritis (hedids + heart), and doctor has discussed treatment (Heartc = ‘yes’) | Heartd = ‘yes’ | Heartc: Has any doctor or nurse ever talked to you about how your arthritis or joint pain will be treated? Heartd: Has any doctor or nurse ever talked to you about what the specific purpose of the treatment for your arthritis or joint pain is? |
Appendix 2 Syntax to generate quality of care variables
Recode multiresponse items to generate diagnostic variables
Recoding: waves 1 and 2 flashcard questions need recoding to gather up responses into one variable. These variables are then used to calculate the prevalence rates for quality of care work; not needed after wave 3 as replaced by feed forward variables.
Wave 1
Coded 0 if they mention having no problem (i.e. refusals and don’t knows are excluded)
make w1_dim01_d = 0 if w1_hedim01=96
label variable w1_dim01_d ‘Hypertension’
make w1_dim02_d = 0 if w1_hedim01=96
label variable w1_dim02_d ‘Angina’
make w1_dim03_d = 0 if w1_hedim01=96
label variable w1_dim03_d ‘Heart attack’
make w1_dim04_d = 0 if w1_hedim01=96
label variable w1_dim04_d ‘Congestive heart failure’
make w1_dim05_d = 0 if w1_hedim01=96
label variable w1_dim05_d ‘Heart murmur’
make w1_dim06_d = 0 if w1_hedim01=96
label variable w1_dim06_d ‘Abnormal heart rhythm’
make w1_dim07_d = 0 if w1_hedim01=96
label variable w1_dim07_d ‘diabetes or high blood sugar’
make w1_dim08_d = 0 if w1_hedim01=96
label variable w1_dim08_d ‘Stroke’
replace w1_dim01_d = 1 if (w1_hedim01=1 or w1_hedim02=1 or w1_hedim03=1 or w1_hedim04=1 or w1_hedim05=1 or w1_hedim06=1 or w1_hedim07=1)
replace w1_dim02_d = 1 if (w1_hedim01=2 or w1_hedim02=2 or w1_hedim03=2 or w1_hedim04=2 or w1_hedim05=2 or w1_hedim06=2 or w1_hedim07=2)
replace w1_dim03_d = 1 if (w1_hedim01=3 or w1_hedim02=3 or w1_hedim03=3 or w1_hedim04=3 or w1_hedim05=3 or w1_hedim06=3 or w1_hedim07=3)
replace w1_dim04_d = 1 if (w1_hedim01=4 or w1_hedim02=4 or w1_hedim03=4 or w1_hedim04=4 or w1_hedim05=4 or w1_hedim06=4 or w1_hedim07=4)
replace w1_dim05_d = 1 if (w1_hedim01=5 or w1_hedim02=5 or w1_hedim03=5 or w1_hedim04=5 or w1_hedim05=5 or w1_hedim06=5 or w1_hedim07=5)
replace w1_dim06_d = 1 if (w1_hedim01=6 or w1_hedim02=6 or w1_hedim03=6 or w1_hedim04=6 or w1_hedim05=6 or w1_hedim06=6 or w1_hedim07=6)
replace w1_dim07_d = 1 if (w1_hedim01=7 or w1_hedim02=7 or w1_hedim03=7 or w1_hedim04=7 or w1_hedim05=7 or w1_hedim06=7 or w1_hedim07=7)
replace w1_dim08_d = 1 if (w1_hedim01=8 or w1_hedim02=8 or w1_hedim03=8 or w1_hedim04=8 or w1_hedim05=8 or w1_hedim06=8 or w1_hedim07=8)
Recode eye diseases wave 1
Coded 0 if they mention having no problem (i.e. refusals and don’t knows are excluded)
make w1_opt1_d=0 if w1_heopt1=96
label variable w1_opt1_d ‘glaucoma’
make w1_opt2_d=0 if w1_heopt1=96
label variable w1_opt2_d ‘diabetic eye disease’
make w1_opt3_d=0 if w1_heopt1=96
label variable w1_opt3_d ‘macular degeneration’
make w1_opt4_d=0 if w1_heopt1=96
label variable w1_opt4_d ‘cataract’
make w1_opt96_d=0 if w1_heopt1=96
label variable w1_opt96_d ‘no eye disease’
replace w1_opt1_d=1 if (w1_heopt1=1 or w1_heopt2=1 or w1_heopt3=1 or w1_heopt4=1)
replace w1_opt2_d=1 if (w1_heopt1=2 or w1_heopt2=2 or w1_heopt3=2 or w1_heopt4=2)
replace w1_opt3_d=1 if (w1_heopt1=3 or w1_heopt2=3 or w1_heopt3=3 or w1_heopt4=3)
replace w1_opt4_d=1 if (w1_heopt1=4 or w1_heopt2=4 or w1_heopt3=4 or w1_heopt4=4)
replace w1_opt96_d=1 if (w1_heopt1=96)
Recode hedia which is the cardiovascular flashcard question merged with hedix (any other heart conditions)
Coded 0 if they mention having no problem (i.e. refusals and don’t knows are excluded)
Recode dia and dib variables to make those who do not have a cardio problem = 96
make w1_dia01_d=0 if w1_hedia01=96
label variable w1_dia01_d ‘hypertension’
make w1_dia02_d=0 if w1_hedia01=96
label variable w1_dia02_d ‘Angina’
make w1_dia03_d=0 if w1_hedia01=96
label variable w1_dia03_d ‘MI’
make w1_dia04_d=0 if w1_hedia01=96
label variable w1_dia04_d ‘CHF’
make w1_dia05_d=0 if w1_hedia01=96
label variable w1_dia05_d ‘murmur’
label values w1_dia05_d recode
make w1_dia06_d=0 if w1_hedia01=96
label variable w1_dia06_d ‘abnormal rhythm’
make w1_dia07_d=0 if w1_hedia01=96
label variable w1_dia07_d ‘diabetes’
make w1_dia08_d=0 if w1_hedia01=96
label variable w1_dia08_d ‘stroke’
make w1_dia95_d=0 if w1_hedia01=96
label variable w1_dia95_d ‘other heart trouble’
make w1_dia96_d=0 if w1_hedia01=96
label variable w1_dia96_d ‘no diagnosed heart trouble’
replace w1_dia01_d=1 if (w1_hedia01=1 or w1_hedia02=1 or w1_hedia03=1 or w1_hedia04=1 or w1_hedia05=1 or w1_hedia06=1 or w1_hedia07=1 or w1_hedia08=1 or w1_hedia09=1)
replace w1_dia02_d=1 if (w1_hedia01=2 or w1_hedia02=2 or w1_hedia03=2 or w1_hedia04=2 or w1_hedia05=2 or w1_hedia06=2 or w1_hedia07=2 or w1_hedia08=2 or w1_hedia09=2)
replace w1_dia03_d=1 if (w1_hedia01=3 or w1_hedia02=3 or w1_hedia03=3 or w1_hedia04=3 or w1_hedia05=3 or w1_hedia06=3 or w1_hedia07=3 or w1_hedia08=3 or w1_hedia09=3)
replace w1_dia04_d=1 if (w1_hedia01=4 or w1_hedia02=4 or w1_hedia03=4 or w1_hedia04=4 or w1_hedia05=4 or w1_hedia06=4 or w1_hedia07=4 or w1_hedia08=4 or w1_hedia09=4)
replace w1_dia05_d=1 if (w1_hedia01=5 or w1_hedia02=5 or w1_hedia03=5 or w1_hedia04=5 or w1_hedia05=5 or w1_hedia06=5 or w1_hedia07=5 or w1_hedia08=5 or w1_hedia09=5)
replace w1_dia06_d=1 if (w1_hedia01=6 or w1_hedia02=6 or w1_hedia03=6 or w1_hedia04=6 or w1_hedia05=6 or w1_hedia06=6 or w1_hedia07=6 or w1_hedia08=6 or w1_hedia09=6)
replace w1_dia07_d=1 if (w1_hedia01=7 or w1_hedia02=7 or w1_hedia03=7 or w1_hedia04=7 or w1_hedia05=7 or w1_hedia06=7 or w1_hedia07=7 or w1_hedia08=7 or w1_hedia09=7)
replace w1_dia08_d=1 if (w1_hedia01=8 or w1_hedia02=8 or w1_hedia03=8 or w1_hedia04=8 or w1_hedia05=8 or w1_hedia06=8 or w1_hedia07=8 or w1_hedia08=8 or w1_hedia09=8)
replace w1_dia95_d=1 if (w1_hedia01=95 or w1_hedia02=95 or w1_hedia03=95 or w1_hedia04=95 or w1_hedia05=95 or w1_hedia06=95 or w1_hedia07=95or w1_hedia08=95 or w1_hedia09=95)
replace w1_dia96_d=1 if (w1_hedia01=96)
Recode hedib: non-cardio disease flashcard question
Coded 0 if they mention having no problem (i.e. refusals and don’t knows are excluded)
make w1_dib01_d=0 if w1_hedib01=96
label variable w1_dib01_d ‘chronic bronchitis/emphysema’
make w1_dib02_d=0 if w1_hedib01=96
label variable w1_dib02_d ‘asthma’
make w1_dib03_d=0 if w1_hedib01=96
label variable w1_dib03_d ‘arthritis’
make w1_dib04_d=0 if w1_hedib01=96
label variable w1_dib04_d ‘osteoporosis’
make w1_dib05_d=0 if w1_hedib01=96
label variable w1_dib05_d ‘cancer’
make w1_dib06_d=0 if w1_hedib01=96
label variable w1_dib06_d ‘Parkinson’s’
make w1_dib07_d=0 if w1_hedib01=96
label variable w1_dib07_d ‘psychiatric problems’
make w1_dib08_d=0 if w1_hedib01=96
label variable w1_dib08_d ‘Alzheimer’s’
make w1_dib09_d=0 if w1_hedib01=96
label variable w1_dib09_d ‘dementia’
make w1_dib96_d=0 if w1_hedib01=96
label variable w1_dib96_d ‘none of these’
replace w1_dib01_d=1 if (w1_hedib01=1 or w1_hedib02=1 or w1_hedib03=1 or w1_hedib04=1 or w1_hedib05=1 or w1_hedib06=1)
replace w1_dib02_d=1 if (w1_hedib01=2 or w1_hedib02=2 or w1_hedib03=2 or w1_hedib04=2 or w1_hedib05=2 or w1_hedib06=2)
replace w1_dib03_d=1 if (w1_hedib01=3 or w1_hedib02=3 or w1_hedib03=3 or w1_hedib04=3 or w1_hedib05=3 or w1_hedib06=3)
replace w1_dib04_d=1 if (w1_hedib01=4 or w1_hedib02=4 or w1_hedib03=4 or w1_hedib04=4 or w1_hedib05=4 or w1_hedib06=4)
replace w1_dib05_d=1 if (w1_hedib01=5 or w1_hedib02=5 or w1_hedib03=5 or w1_hedib04=5 or w1_hedib05=5 or w1_hedib06=5)
replace w1_dib06_d=1 if (w1_hedib01=6 or w1_hedib02=6 or w1_hedib03=6 or w1_hedib04=6 or w1_hedib05=6 or w1_hedib06=6)
replace w1_dib07_d=1 if (w1_hedib01=7 or w1_hedib02=7 or w1_hedib03=7 or w1_hedib04=7 or w1_hedib05=7 or w1_hedib06=7)
replace w1_dib08_d=1 if (w1_hedib01=8 or w1_hedib02=8 or w1_hedib03=8 or w1_hedib04=8 or w1_hedib05=8 or w1_hedib06=8)
replace w1_dib09_d=1 if (w1_hedib01=9 or w1_hedib02=9 or w1_hedib03=9 or w1_hedib04=9 or w1_hedib05=9 or w1_hedib06=9)
replace w1_dib96_d=1 if (w1_hedib01=96)
Recode heart: arthritis
Coded 0 if mentioned arthritis, 1 if the form of arthritis is dealt with by that variable
make w1_art1_d=0 if w1_heart10 & not missing(w1_heart1)
label variable w1_art1_d ‘osteoarthritis’
make w1_art2_d=0 if w1_heart10 & not missing(w1_heart1)
label variable w1_art2_d ‘rheumatoid arthritis’
make w1_art3_d=0 if w1_heart10 & not missing(w1_heart1)
label variable w1_art3_d ‘other arthritis’
make w1_art8_d=0 if w1_heart10 & not missing(w1_heart1)
label variable w1_art8_d ‘unknown arthritis type’
replace w1_art1_d=1 if (w1_heart1=1 or w1_heart2=1 or w1_heart3=1)
replace w1_art2_d=1 if (w1_heart1=2 or w1_heart2=2 or w1_heart3=2)
replace w1_art3_d=1 if (w1_heart1=3 or w1_heart2=3 or w1_heart3=3)
replace w1_art8_d=1 if (w1_heart1=-8 or w1_heart2=-8 or w1_heart3=-8)
Recode hepsy
Coded 0 if mentioned psych difficulty, 1 if the form of psychiatric difficulty dealt with by that variable
make w1_psy1_d=0 if w1_hepsy10 & not missing(w1_hepsy1)
label variable w1_psy1_d ‘hallucinations’
make w1_psy2_d=0 if w1_hepsy10 & not missing(w1_hepsy1)
label variable w1_psy2_d ‘anxiety’
make w1_psy3_d=0 if w1_hepsy10 & not missing(w1_hepsy1)
label variable w1_psy3_d ‘depression’
make w1_psy4_d=0 if w1_hepsy10 & not missing(w1_hepsy1)
label variable w1_psy4_d ‘emotional problems’
make w1_psy5_d=0 if w1_hepsy10 & not missing(w1_hepsy1)
label variable w1_psy5_d ‘schizophrenia’
make w1_psy6_d=0 if w1_hepsy10 & not missing(w1_hepsy1)
label variable w1_psy6_d ‘psychosis’
make w1_psy7_d=0 if w1_hepsy10 & not missing(w1_hepsy1)
label variable w1_psy7_d ‘mood swings’
make w1_psy8_d=0 if w1_hepsy10 & not missing(w1_hepsy1)
label variable w1_psy8_d ‘manic depression’
make w1_psy95_d=0 if w1_hepsy10 & not missing(w1_hepsy1)
label variable w1_psy95_d ‘other psych problem’
replace w1_psy1_d=1 if (w1_hepsy1=1 or w1_hepsy2=1 or w1_hepsy3=1 or w1_hepsy4=1 or w1_hepsy5=1 or w1_hepsy6=1 or w1_hepsy7=1)
replace w1_psy2_d=1 if (w1_hepsy1=2 or w1_hepsy2=2 or w1_hepsy3=2 or w1_hepsy4=2 or w1_hepsy5=2 or w1_hepsy6=2 or w1_hepsy7=2)
replace w1_psy3_d=1 if (w1_hepsy1=3 or w1_hepsy2=3 or w1_hepsy3=3 or w1_hepsy4=3 or w1_hepsy5=3 or w1_hepsy6=3 or w1_hepsy7=3)
replace w1_psy4_d=1 if (w1_hepsy1=4 or w1_hepsy2=4 or w1_hepsy3=4 or w1_hepsy4=4 or w1_hepsy5=4 or w1_hepsy6=4 or w1_hepsy7=4)
replace w1_psy5_d=1 if (w1_hepsy1=5 or w1_hepsy2=5 or w1_hepsy3=5 or w1_hepsy4=5 or w1_hepsy5=5 or w1_hepsy6=5 or w1_hepsy7=5)
replace w1_psy6_d=1 if (w1_hepsy1=6 or w1_hepsy2=6 or w1_hepsy3=6 or w1_hepsy4=6 or w1_hepsy5=6 or w1_hepsy6=6 or w1_hepsy7=6)
replace w1_psy7_d=1 if (w1_hepsy1=7 or w1_hepsy2=7 or w1_hepsy3=7 or w1_hepsy4=7 or w1_hepsy5=7 or w1_hepsy6=7 or w1_hepsy7=7)
replace w1_psy8_d=1 if (w1_hepsy1=8 or w1_hepsy2=8 or w1_hepsy3=8 or w1_hepsy4=8 or w1_hepsy5=8 or w1_hepsy6=8 or w1_hepsy7=8)
replace w1_psy95_d=1 if (w1_hepsy1=95 or w1_hepsy2=95 or w1_hepsy3=95 or w1_hepsy4=95 or w1_hepsy5=95 or w1_hepsy6=95 or w1_hepsy7=95)
Wave 2
For dim variable use the same coding as wave 1
For dia variable use the same coding as wave 1
For dib variable use the same coding as wave 1
For opt variable use:
make w2_opt1_d=0 if w2_heopt1=96
label variable w2_opt1_d ‘w2 glaucoma’
make w2_opt2_d=0 if w2_heopt1=96
label variable w2_opt2_d ‘w2 diabetic eye disease’
make w2_opt3_d=0 if w2_heopt1=96
label variable w2_opt3_d ‘w2 macular degeneration’
make w2_opt4_d=0 if w2_heopt1=96
label variable w2_opt4_d ‘w2 cataract’
make w2_opt96_d=0 if w2_heopt1=96
label variable w2_opt96_d ‘w2 no eye dis’
replace w2_opt1_d=1 if (w2_heopt1=1 or w2_heopt2=1)
replace w2_opt2_d=1 if (w2_heopt1=2 or w2_heopt2=2)
replace w2_opt3_d=1 if (w2_heopt1=3 or w2_heopt2=3)
replace w2_opt4_d=1 if (w2_heopt1=4 or w2_heopt2=4)
replace w2_opt96_d=1 if (w2_heopt1=96)
For arthritis variable use
make w2_art1_d=0 if w2_heart10 & not missing(w2_heart1)
label variable w2_art1_d ‘w2 osteoarthritis’
make w2_art2_d=0 if w2_heart10 & not missing(w2_heart1)
label variable w2_art2_d ‘w2 rheumatoid arthritis’
make w2_art3_d=0 if w2_heart10 & not missing(w2_heart1)
label variable w2_art3_d ‘w2 other arthritis’
make w2_art8_d=0 if w2_heart10 & not missing(w2_heart1)
label variable w2_art8_d ‘w2 unknown arthritis type’
replace w2_art1_d=1 if (w2_heart1=1 or w2_heart2=1)
replace w2_art2_d=1 if (w2_heart1=2 or w2_heart2=2)
replace w2_art3_d=1 if (w2_heart1=3 or w2_heart2=3)
replace w2_art8_d=1 if (w2_heart1=-8 or w2_heart2=-8)
For psy variable use
make w2_psy1_d=0 if w2_hepsy10 & not missing(w2_hepsy1)
label variable w2_psy1_d ‘w2 hallucinations’
make w2_psy2_d=0 if w2_hepsy10 & not missing(w2_hepsy1)
label variable w2_psy2_d ‘w2 anxiety’
make w2_psy3_d=0 if w2_hepsy10 & not missing(w2_hepsy1)
label variable w2_psy3_d ‘w2 depression’
make w2_psy4_d=0 if w2_hepsy10 & not missing(w2_hepsy1)
label variable w2_psy4_d ‘w2 emotional problems’
make w2_psy5_d=0 if w2_hepsy10 & not missing(w2_hepsy1)
label variable w2_psy5_d ‘w2 schizophrenia’
make w2_psy6_d=0 if w2_hepsy10 & not missing(w2_hepsy1)
label variable w2_psy6_d ‘w2 psychosis’
make w2_psy7_d=0 if w2_hepsy10 & not missing(w2_hepsy1)
label variable w2_psy7_d ‘w2 mood swings’
make w2_psy8_d=0 if w2_hepsy10 & not missing(w2_hepsy1)
label variable w2_psy8_d ‘w2 manic depression’
make w2_psy95_d=0 if w2_hepsy10 & not missing(w2_hepsy1)
label variable w2_psy95_d ‘w2 other psych problem’
replace w2_psy1_d=1 if (w2_hepsy1=1 or w2_hepsy2=1 or w2_hepsy3=1 or w2_hepsy4=1 or w2_hepsy5=1 or w2_hepsy6=1)
replace w2_psy2_d=1 if (w2_hepsy1=2 or w2_hepsy2=2 or w2_hepsy3=2 or w2_hepsy4=2 or w2_hepsy5=2 or w2_hepsy6=2)
replace w2_psy3_d=1 if (w2_hepsy1=3 or w2_hepsy2=3 or w2_hepsy3=3 or w2_hepsy4=3 or w2_hepsy5=3 or w2_hepsy6=3)
replace w2_psy4_d=1 if (w2_hepsy1=4 or w2_hepsy2=4 or w2_hepsy3=4 or w2_hepsy4=4 or w2_hepsy5=4 or w2_hepsy6=4)
eplace w2_psy5_d=1 if (w2_hepsy1=5 or w2_hepsy2=5 or w2_hepsy3=5 or w2_hepsy4=5 or w2_hepsy5=5 or w2_hepsy6=5)
replace w2_psy6_d=1 if (w2_hepsy1=6 or w2_hepsy2=6 or w2_hepsy3=6 or w2_hepsy4=6 or w2_hepsy5=6 or w2_hepsy6=6)
replace w2_psy7_d=1 if (w2_hepsy1=7 or w2_hepsy2=7 or w2_hepsy3=7 or w2_hepsy4=7 or w2_hepsy5=7 or w2_hepsy6=7)
replace w2_psy8_d=1 if (w2_hepsy1=8 or w2_hepsy2=8 or w2_hepsy3=8 or w2_hepsy4=8 or w2_hepsy5=8 or w2_hepsy6=8)
replace w2_psy95_d=1 if (w2_hepsy1=95 or w2_hepsy2=95 or w2_hepsy3=95 or w2_hepsy4=95 or w2_hepsy5=95 or w2_hepsy6=95)
Generate incidence and prevalence for diagnosed conditions
Wave 1
Eye disease
make w1_eye_d=0 if w1_heopt1=96
replace w1_eye_d=1 if w1_heopt10 & w1_heopt1<96
Coronary heart disease
make w1_chd_d=0 if not missing(w1_finstat)
replace w1_chd_d=1 if w1_dim02_d=1orw1_dim03_d=1
Wave 2
NOTE: there was an error at w2 whereby not everyone with a cardiovascular disease at w1 was fed forward to w2. This makes ascertaining prevalence rates for w2 CVD conditions tricky. We decided that we would use report of the condition at w1 or w2 for prevalence of the typically chronic CVD conditions: hypertension, heart attack, heart murmur, heart failure, diabetes and stroke. For angina and abnormal heart rhythm, we will need to use the feed forward variable ‘confirms diagnosis from previous wave’ (hediac) and ‘still has condition from previous wave’ (hedias) for the conditions respectively, because many reporting the condition at the previous wave may not still have it at w2.
Incident depression
make depres_d=0 if member of the sample at that wave
replace depres_d=1 if psy3_d=1 & w1_psy3_d!=1
Prevalent hypertension
make hibp_d=0 if member of the sample at that wave
replace hibp_d=1 if w1_dim01_d=1ordim01_d=1
Prevalent angina
make angina_d=0 if member of the sample at that wave
replace angina_d=1 if hediac2=1ordim02_d=1
Prevalent heart attack
make hrtattack_d=0 if member of the sample at that wave
replace hrtattack_d=1 if w1_dim03_d=1ordim03_d=1
Coronary heart disease/myocardial infarction
make chd_d=0 if member of the sample at that wave
replace chd_d=1 if angina_d=1orhrtattack_d=1
Prevalent congestive heart failure
make hrtfail_d=0 if member of the sample at that wave
replace hrtfail_d=1 if w1_dim04_d=1ordim04_d=1
Prevalent heart murmur
make murmur_d=0 if member of the sample at that wave
replace murmur_d=1 if w1_dim05_d=1ordim05_d=1
Prevalent abnormal heart rhythm
make rhythm_d=0 if member of the sample at that wave
replace rhythm_d=1 if hedias6=1ordim06_d=1
Prevalent diabetes
make diab_d=0 if member of the sample at that wave
replace diab_d=1 if heacd=1
Prevalent stroke
make stroke_d=0 if member of the sample at that wave
replace stroke_d=1 if w1_dim08_d=1ordim08_d=1
Prevalent high cholesterol
make hichol_d=0 if member of the sample at that wave
replace hichol_d=1 if dia09_d=1
Prevalent lung disease
make lungdis_d=0 if member of the sample at that wave
replace lungdis_d=1 if hedids1=1ordib01_d=1
Prevalent asthma
make asthma_d=0 if member of the sample at that wave
replace asthma_d=1 if hedids2=1ordib02_d=1
Prevalent arthritis
make arthritis_d=0 if member of the sample at that wave
replace arthritis_d=1 if hedids3=1ordib03_d=1
Prevalent osteoporosis
make osteop_d=0 if member of the sample at that wave
replace osteop_d=1 if hedids4=1ordib04_d=1
Prevalent cancer
make cancer_d=0 if member of the sample at that wave
replace cancer_d=1 if hedids5=1ordib05_d=1
Prevalent Parkinson’s disease
make parkins_d=0 if member of the sample at that wave
replace parkins_d=1 if hedids6=1ordib06_d=1
Prevalent psychiatric difficulties
make psychdiff_d=0 if member of the sample at that wave
replace psychdiff_d=1 if hedids7=1ordib07_d=1
Prevalent Alzheimer’s disease
make alzheimers_d=0 if member of the sample at that wave
replace alzheimers_d=1 if hedids8=1ordib08_d=1
Prevalent dementia
make dementia_d=0 if member of the sample at that wave
replace dementia_d=1 if hedids9=1ordib09_d=1
Prevalent eye condition
make eye_d=0 if heopt1=96
replace eye_d=1 if heops=1or(heopt10 & heopt1<96)
Wave 3 onwards
Prevalent stroke
make stroke_d=0 if member of the sample at that wave
replace stroke_d=1 if hedimst=1 or hedacst=1
Incident depression
make depres_d=0 if member of the sample at that wave
replace depres_d=1 if hepsyde=1 & hedibps=1
Prevalent diabetes
make diab_d=0 if member of the sample at that wave
replace diab_d=1 if heacd=1at any previous wave
Prevalent hypertension
make hibp_d=0 if member of the sample at that wave
replace hibp_d=1 if hedimbp=1 or hedasbp=1
Prevalent angina
make angina_d=0 if member of the sample at that wave
replace angina_d=1 if hediman=1 or hedasan=1
Prevalent heart attack
make hrtattack_d=0 if member of the sample at that wave
replace hrtattack_d=1 if hedimmi=1 or hedacmi=1
Coronary heart disease/myocardial infarction
make chd_d=0 if member of the sample at that wave
replace chd_d=1 if angina_d=1orhrtattack_d=1
Prevalent high cholesterol
make hichol_d=0 if member of the sample at that wave
replace hichol_d=1 if hedimch=1 or hedasch=1
Prevalent heart murmur
make murmur_d=0 if member of the sample at that wave
replace murmur_d=1 if hedimhm=1 or hedashm=1
Prevalent abnormal heart rhythm
make rhythm_d=0 if member of the sample at that wave
replace rhythm_d=1 if hedimar=1 or hedasar=1
Prevalent congestive heart failure
make hrtfail_d=0 if member of the sample at that wave
replace hrtfail_d=1 if hedimhf=1 or hedashf=1
Prevalent lung disease
make lungdis_d=0 if member of the sample at that wave
replace lungdis_d=1 if hediblu=1 or hedbslu=1
Prevalent asthma
make asthma_d=0 if member of the sample at that wave
replace asthma_d=1 if hedibas=1 or hedbsas=1
Prevalent arthritis
make arthritis_d=0 if member of the sample at that wave
replace arthritis_d=1 if hedibar=1 or hedbsar=1
Prevalent osteoporosis
make osteop_d=0 if member of the sample at that wave
replace osteop_d=1 if hedibos=1 or hedbsos=1
Prevalent cancer
make cancer_d=0 if member of the sample at that wave
replace cancer_d=1 if hedibca=1 or hedbsca=1
Prevalent Parkinson’s disease
make parkins_d=0 if member of the sample at that wave
replace parkins_d=1 if hedibpd=1 or hedbspd=1
Prevalent psychiatric difficulties
make psychdiff_d=0 if member of the sample at that wave
replace psychdiff_d=1 if hedibps=1 or hedbdps=1
Prevalent Alzheimer’s disease
make alzheimers_d=0 if member of the sample at that wave
replace alzheimers_d=1 if hedibad=1 or hedbdad=1
Prevalent dementia
make dementia_d=0 if member of the sample at that wave
replace dementia_d=1 if hedibde=1 or hedbsde=1
Prevalent eye condition
make eye_d=0 if member of the sample at that wave
replace eye_d=1 if (heopsgl=1orheopsdi=1orheopsmd=1orheopsca=1) or (heoptgl=1orheoptdi=1orheoptmd=1orheoptca=1)
Generate quality indicators
Wave 2 followed by subsequent waves.
Wave 2
dk=don’t knows
ref=refusals
Indicator stroke antihypertensives
IF a person aged 50 or older has had a previous stroke, THEN the patient should be offered antihypertensive medication.
make w2_bpmed=w2_hehbpb
replace w2_bpmed=w2_hehbpb1 if (w2_hehbpb=-1)
make w2_basecvd4=0 if not missing(w2_finstat)
replace w2_basecvd4=1 if w2_stroke_d=1 & w2_bpmed0 & not missing(w2_bpmed)
make w2_dkcvd4=0 if not missing(w2_finstat)
replace w2_dkcvd4=1 if w2_stroke_d=1 & w2_bpmed=-8
make w2_refcvd4=0 if not missing(w2_finstat)
replace w2_refcvd4=1 if w2_stroke_d=1 & w2_bpmed=-9
make w2_passcvd4=0 if w2_basecvd4=1
replace w2_passcvd4=1 if w2_basecvd4=1 & w2_bpmed=1
Indicator depression suicide
IF a person aged 50 or older receives a diagnosis of a new depression episode, THEN the diagnosing physician should ask on the day of diagnosis whether the person aged 65 or older had any thoughts about suicide.
make w2_basedep1=0 if not missing(w2_finstat)
replace w2_basedep1=1 if w2_depres_d=1 & w2_hepsye=0
make w2_dkdep1=0 if not missing(w2_finstat)
replace w2_dkdep1=1 if w2_depres_d & w2_hepsye=-8
make w2_refdep1=0 if not missing(w2_finstat)
replace w2_refdep1=1 if w2_depres_d & w2_hepsye=-9
make w2_passdep1=0 if w2_basedep1=1
replace w2_passdep1=1 if w2_basedep1=1 & w2_hepsye=1
Indicator depression treatment (first iteration, diagnosed depression)
IF a person aged 50 or older is diagnosed with clinical depression, THEN antidepressant treatment, talking treatment, or electroconvulsive therapy should be offered within 2 weeks after diagnosis unless within that period the patient has improved, or unless the patient has substance abuse or dependence, in which case treatment may wait until 8 weeks after the patient is in a drug- or alcohol-free state.
make w2_basedep2=0 if not missing(w2_finstat)
replace w2_basedep2=1 if w2_depres_d & w2_hepsya=0 & not missing(w2_hepsya)
make w2_dkdep2=0 if not missing(w2_finstat)
replace w2_dkdep2=1 if w2_depres_d & w2_hepsya=-8
make w2_dkrdep2=0 if not missing(w2_finstat)
replace w2_dkrdep2=1 if w2_depres_d & w2_hepsya<-7
make w2_passdep2=0 if w2_basedep2=1
replace w2_passdep2=1 if w2_basedep2=1 & w2_hepsyb=1
Indicator depression review
IF a person aged 50 or older has no meaningful symptom response after 6 weeks of treatment, THEN one of the following treatment options should be initiated by the 8th week of treatment: medication dose should be optimised (if initial treatment was medication), or medication should be initiated (if initial treatment was psychotherapy alone), or referral to a psychiatrist should be offered.
make w2_basedep3=0 if not missing(w2_finstat)
replace w2_basedep3=1 if w2_depres_d & w2_hepsyc=2
make w2_passdep3=0 if w2_basedep3=1
replace w2_passdep3=1 if w2_basedep3=1 & w2_hepsyd=1
Indicator diabetes HbA1c
IF a person aged 50 or older has diabetes, THEN his or her glycosylated haemoglobin or fructosamine level should be measured at least annually.
make w2_basediab1=0 if not missing(w2_finstat)
replace w2_basediab1=1 if w2_hesug0 & w2_hesuga-8 & not missing(w2_hesug)
make w2_passdiab1=0 if w2_basediab1=1
replace w2_passdiab1=1 if w2_basediab1=1 & w2_hesuga=1
Indicator diabetes proteinuria
IF a diabetic person aged 50 or older does not have established renal disease and is not receiving an ACE inhibitor or angiotensin II receptor blocker, THEN he or she should receive an annual test for proteinuria.
make w2_basediab2=0 if not missing(w2_finstat)
replace w2_basediab2=1 if w2_hekidn!=1 & w2_heacea=2 & w2_hewee=0 & not missing(w2_hewee)
make w2_dkdiab2=0 if not missing(w2_finstat)
replace w2_dkdiab2=1 if w2_hekidn!=1 & w2_heacea=2 & w2_hewee=-8
make w2_dkrdiab2=0 if not missing(w2_finstat)
replace w2_dkrdiab2=1 if w2_hekidn!=1 & w2_heacea=2 & w2_hewee<-7
make w2_passdiab2=0 if w2_basediab2=1
replace w2_passdiab2=1 if w2_basediab2=1 & w2_hewee=1
Indicator diabetes cholesterol
IF a diabetic person aged 50 or older has a fasting total cholesterol level of 5 mmol/l or greater, THEN he or she should be offered an intervention to lower cholesterol.
make w2_basediab6=0 if not missing(w2_finstat)
replace w2_basediab6=1 if w2_heacd=1 & w2_hechol=0 & not missing(w2_hechol)
make w2_passdiab6=0 if w2_basediab6=1
replace w2_passdiab6=1 if w2_basediab6=1 & w2_hechol=1
Indicator diabetes ACE inhibitor
IF a diabetic person aged 50 or older has one additional cardiac risk factor (ie, smoker, hypertension, hypercholesterolemia, or renal insufficiency/microalbuminuria), THEN he/she should be offered an ACE inhibitor or receptor blocker.
make w2_highbp2=0 if not missing(w2_finstat)
replace w2_highbp2 = 1 if (w2n_sysval=140 & not missing(w2n_sysval)) or (w2n_diaval=90 & not missing(w2n_diaval))
make w2_basediab8=0 if not missing(w2_finstat)
replace w2_basediab8 = 1 if w2_heacd=1 &(w2_heska=1orw2_highbp2=1orw2_hekidn=1orw2_heweea=1orw2_hichol_d=1orw2_hibp_d=1)& ((w2_heace0 & not missing(w2_heace))or(w2_heacea=0 & not missing(w2_heacea)))
make w2_dkdiab8=0 if not missing(w2_finstat)
replace w2_dkdiab8=1 if w2_heacd=1 &(w2_heska=1orw2_highbp2=1orw2_hekidn=1orw2_heweea=1orw2_hichol_d=1orw2_hibp_d=1)& (w2_heace=-8 & w2_heacea=-8)
make w2_passdiab8=0 if w2_basediab8=1
replace w2_passdiab8 = 1 if w2_basediab8=1 & (w2_heace=1orw2_heacea=1)
Indicator diabetes foot check
ALL diabetic persons aged 50 or older should have an annual examination of his/her feet.
make w2_basediab9=0 if not missing(w2_finstat)
replace w2_basediab9=1 if w2_heacd=1 & not missing(w2_heftchk) & w2_heftchk=0
make w2_passdiab9=0 if w2_basediab9=1
replace w2_passdiab9=1 if w2_basediab9=1 & w2_heftchk=1
Indicator hypertension treatment: incident hypertension
IF a person aged 50 or older remains hypertensive after non-pharmacological intervention, THEN pharmacological antihypertensive treatment should be initiated.
make w2_basehyp2=0 if not missing(w2_finstat)
replace w2_basehyp2=1 if w2_dim01_d=1 & w1_dim01_d!=1 & w2_hehbpb=0 & not missing(w2_hehbpb)
make w2_passhyp2=0 if w2_basehyp2=1
replace w2_passhyp2=1 if w2_basehyp2=1 & w2_hehbpb=1
Indicator hypertension treatment: prevalent hypertension
IF a person aged 50 or older remains hypertensive after non-pharmacological intervention, THEN pharmacological antihypertensive treatment should be initiated.
make w2_basehyp2a=0 if not missing(w2_finstat)
replace w2_basehyp2a=1 if w2_hibp_d=1 & w2_hehbpb=0 & not missing(w2_hehbpb)
make w2_passhyp2a=0 if w2_basehyp2a=1
replace w2_passhyp2a=1 if w2_basehyp2a=1 & w2_hehbpb=1
Indicator ischaemic heart disease cholesterol (cholesterol first iteration) reported IDH and reported high cholesterol (previous variable: w2_basechol1a & w2_passchol1a)
IF a person aged 50 or older has established CHD and LDL cholesterol 3 mmol/l, THEN he or she should be offered an intervention to lower cholesterol.
make w2_baseihd1=0 if not missing(w2_finstat)
replace w2_baseihd1=1 if w2_hichol_d=1 & (w2_angina_d=1orw2_hrtattack_d=1) & w2_hechol=0 & not missing(w2_hechol)
make w2_passihd1=0 if w2_baseihd1=1
replace w2_passihd1=1 if w2_baseihd1=1 & w2_hechol=1
Indicator ischaemic heart disease antiplatelets
IF a person aged 50 or older has established CHD and is not on warfarin, THEN he or she should be offered antiplatelet therapy.
make w2_baseihd2=0 if not missing(w2_finstat)
replace w2_baseihd2=1 if (w2_angina_d=1orw2_hrtattack_d=1) & (w2_hehrta=0 & not missing(w2_hehrta)) & w2_hehrtc!=1
make w2_dkihd2=0 if not missing(w2_finstat)
replace w2_dkihd2=1 if (w2_angina_d=1orw2_hrtattack_d=1) & w2_hehrta=-8 & w2_hehrtc!=1
make w2_passihd2=0 if w2_baseihd2=1
replace w2_passihd2=1 if w2_baseihd2=1 & w2_hehrta=1
Indicator ischaemic heart disease smoking
IF a person aged 50 or older with established CHD smokes, THEN he or she should be offered counselling for smoking cessation.
make w2_baseihd3=0 if not missing(w2_finstat)
replace w2_baseihd3=1 if (w2_angina_d=1orw2_hrtattack_d=1) & not missing(w2_hecgstp) & w2_hecgstp=0
make w2_dkihd3=0 if not missing(w2_finstat)
replace w2_dkihd3=1 if (w2_angina_d=1orw2_hrtattack_d=1) & w2_hecgstp=-8
make w2_passihd3=0 if w2_baseihd3=1
replace w2_passihd3=1 if w2_baseihd3=1 & w2_hecgstp=1
Indicator myocardial infarction beta-blocker: incident myocardial infarction
IF a person aged 50 or older has had a recent MI, THEN he or she should be offered a beta-blocker.
make w2_betab=0 if not missing(w2_finstat)
replace w2_betab=1 if w2_hebeta=1orw2_hebetb=1
replace w2_betab= if (mi(w2_hebeta)orw2_hebeta<0) & (mi(w2_hebetb)orw2_hebetb<0)
make w2_baseihd5=0 if not missing(w2_finstat)
replace w2_baseihd5=1 if w2_dia03_d=1 & w1_dim03_d!=1 & not missing(w2_betab)
make w2_dkihd5=0 if not missing(w2_finstat)
replace w2_dkihd5=1 if w2_dia03_d=1 & w1_dim03_d!=1 & (w2_hebeta=-8 & w2_hebetb=-8)
make w2_passihd5=0 if w2_baseihd5=1
replace w2_passihd5=1 if w2_baseihd5=1 & w2_betab=1
Indicator myocardial infarction beta-blocker: prevalent myocardial infarction
IF a person aged 50 or older has had a recent MI, THEN he or she should be offered a beta-blocker.
make w2_baseihd5a=0 if not missing(w2_finstat)
replace w2_baseihd5a=1 if w2_hrtattack_d=1 & not missing(w2_betab)
make w2_dkihd5a=0 if not missing(w2_finstat)
replace w2_dkihd5a=1 if w2_hrtattack_d=1 & (w2_hebeta=-8 & w2_hebetb=-8)
make w2_passihd5a=0 if w2_baseihd5a=1
replace w2_passihd5a=1 if w2_baseihd5a=1 & w2_betab=1
Indicator international normalised ratio test
(IHD extra indicator – previously med3) limited to IHD (MI and angina) by questionnaire so aggregate as IHD variable.
IF a person aged 50 or older is prescribed warfarin, THEN an INR should be determined at least every 12 weeks.
make w2_baseihd6=0 if not missing(w2_finstat)
replace w2_baseihd6=1 if w2_hehrtc=1 & (w2_hehrte=0 & not missing(w2_hehrte))
make w2_dkihd6=0 if not missing(w2_finstat)
replace w2_dkihd6=1 if w2_hehrtc=1 & w2_hehrtd=-8
make w2_passihd6=0 if w2_baseihd6=1
replace w2_passihd6=1 if w2_baseihd6=1 & w2_hehrte=1
Indicator osteoarthritis exercise
IF an ambulatory person aged 50 or older has had a diagnosis of symptomatic osteoarthritis of the knee for longer than 3 months and has no contraindications to exercise and is physically and mentally able to exercise, THEN a directed or supervised strengthening or aerobic exercise programme should have been prescribed at least once.
make w2_baseoa2=0 if not missing(w2_finstat)
replace w2_baseoa2=1 if w2_hekneb=0 & not missing(w2_hekneb)
make w2_passoa2=0 if w2_baseoa2=1
replace w2_passoa2=1 if w2_baseoa2=1 & w2_hekneb=1
Indicator osteoarthritis education
IF an ambulatory person aged 50 or older has a diagnosis of symptomatic osteoarthritis, THEN education regarding the natural history, treatment and self-management of the disease should be offered at least once.
make w2_alleduc=0 if not missing(w2_finstat)
replace w2_alleduc=1 if w2_hearta=1 & w2_heartb=1 & w2_heartc=1
replace w2_alleduc= if w2_hearta<0 or w2_heartb<0 or w2_heartc<0 or mi(w2_hearta, w2_heartb, w2_heartc)
make w2_baseoa3=0 if not missing(w2_finstat)
replace w2_baseoa3=1 if w2_heartoa=1 & not missing(w2_alleduc)
make w2_dkoa3=0 if not missing(w2_finstat)
replace w2_dkoa3=1 if w2_heartoa=1 & (w2_hearta=-8 or w2_heartb=-8 or w2_heartc=-8)
make w2_passoa3=0 if w2_baseoa3=1
replace w2_passoa3=1 if w2_baseoa3=1 & w2_alleduc=1
Indicator osteoarthritis paracetamol
IF oral pharmacological therapy is initiated to treat osteoarthritis among people aged 50 or older, THEN paracetamol should be the first drug used, unless there is a contraindication to use.
make w2_baseoa4=0 if not missing(w2_finstat)
replace w2_baseoa4=1 if w2_heartoa=1 & w2_hearte=0 & not missing(w2_hearte)
make w2_dkoa4=0 if not missing(w2_finstat)
replace w2_dkoa4=1 if w2_heartoa=1 & w2_hearte=-8
make w2_passoa4=0 if w2_baseoa4=1
replace w2_passoa4=1 if w2_baseoa4=1 & w2_hearte=1
Indicator osteoarthritis referral
IF a person aged 50 or older with severe symptomatic osteoarthritis of the knee or hip has failed to respond to non-pharmacological and pharmacological therapy, THEN the patient should be offered referral to an orthopaedic surgeon to be evaluated for total joint replacement within 6 months unless surgery is contraindicated.
make w2_baseoa6=0 if not missing(w2_finstat)
replace w2_baseoa6=1 if w2_hepae=2 & w2_hepaf=0 & not missing(w2_hepaf)
make w2_dkoa6=0 if not missing(w2_finstat)
replace w2_dkoa6=1 if w2_hepae=2 & w2_hepaf=-8
make w2_passoa6=0 if w2_baseoa6=1
replace w2_passoa6=1 if w2_baseoa6=1 & w2_hepaf=1
Patient-centered care
Indicator diabetes training
Some people with diabetes receive training to help manage their diabetes themselves. Have you ever participated in a course or class about diabetes, or received special training on how you can live with your diabetes from day to day?
make w2_basepat1=0 if not missing(w2_finstat)
replace w2_basepat1=1 if w2_heacd=1 & not missing(w2_heslfcr) & w2_heslfcr=0
make w2_passpat1=0 if w2_basepat1=1)
replace w2_passpat1=1 if w2_basepat1=1 & w2_heslfcr=1
Indicator diabetes knowledge
How much do you think you know about managing your diabetes? (‘just about everything you need to know’ or ‘most of what you need to know’).
make w2_basepat2=0 if not missing(w2_finstat)
replace w2_basepat2=1 if w2_heacd=1 & not missing(w2_heslfcb) & w2_heslfcb=0
make w2_dkpat2=0 if not missing(w2_finstat)
replace w2_dkpat2=1 if w2_heacd=1 & w2_heslfcb=-8
make w2_passpat2=0 if w2_basepat2=1
replace w2_passpat2=1 if w2_basepat2=1 & (w2_heslfcb=1 or w2_heslfcb=2)
Indicator cholesterol explanation
Has a doctor or nurse explained high cholesterol in a way you could understand?
replace w2_basepat3=1 if w2_hecholb=1 or w2_hecholb=2
make w2_passpat3=0 if w2_basepat3=1
replace w2_passpat3=1 if w2_basepat3=1 & w2_hecholb=1
Indicator cholesterol preferences
Have doctors or nurses taken your preferences into account when making treatment decisions about your high cholesterol?
make w2_basepat4=0 if not missing(w2_finstat)
replace w2_basepat4=1 if w2_hecholc=1 or w2_hecholc=2
make w2_passpat4=0 if w2_basepat4=1
replace w2_passpat4=1 if w2_basepat4=1 & w2_hecholc=1
Indicator blood pressure explanation
Has a doctor or nurse explained high blood pressure in a way you could understand at any time since you were first told you had high blood pressure?
make w2_basepat5=0 if not missing(w2_finstat)
replace w2_basepat5=1 if w2_hehbp=1 or w2_hehbp=2
make w2_passpat5=0 if w2_basepat5=1
replace w2_passpat5=1 if w2_basepat5=1 & w2_hehbp=1
Indicator blood pressure choice
In general, have doctors or nurses given you any choice about how to treat your high blood pressure?
make w2_basepat6=0 if not missing(w2_finstat)
replace w2_basepat6=1 if w2_hehbpa=1 or w2_hehbpa=2
make w2_passpat6=0 if w2_basepat6=1
replace w2_passpat6=1 if w2_basepat6=1 & w2_hehbpa=1
Indicator osteoarthritis treatment purpose
Has any doctor or nurse ever talked to you about what the specific purpose of the treatment for your arthritis or joint pain is?
make w2_basepat7=0 if not missing(w2_finstat)
replace w2_basepat7=1 if w2_heartoa=1 & (w2_heartd=1 or w2_heartd=2)
make w2_passpat7=0 if w2_basepat7=1
replace w2_passpat7=1 if w2_basepat7=1 & w2_heartd=1
Subsequent waves
Wave number is named X, one wave before is X-1, 2 waves before is X-2 and three waves before is X-3.
NB: not all indicators can be generated at every wave.
Indicator stroke antihypertensives
make wX_bpmed=wX_hehbpb
replace wX_bpmed=wX_hehbpb1 if (wX_hehbpb=-1)
make wX_basecvd4=0 if not missing(wX_finstat)
replace wX_basecvd4=1 if wX_stroke_d=1 & ((wX-3_bpmed>0 & not missing(wX-3_bpmed)) or (wX-2_bpmed>0 & not missing(wX-2_bpmed)) or (wX-1_bpmed>0 & not missing(wX-1_bpmed)) or(wX_bpmed>0 & not missing(wX_bpmed)))
make wX_passcvd4=0 if wX_basecvd4=1
replace wX_passcvd4=1 if wX_basecvd4=1 & (wX-3_bpmed=1orwX-2_bpmed=1orwX-1_bpmed=1orwX_bpmed=1)
Indicator depression suicide
make wX_basedep1=0 if not missing(wX_finstat)
replace wX_basedep1=1 if wX_depres_d=1 & wX_hepsye>=0 & not missing(wX_hepsye)
make wX_passdep1=0 if wX_basedep1=1
replace wX_passdep1=1 if wX_basedep1=1 & wX_hepsye=1
Indicator depression treatment
make wX_basedep2=0 if not missing(wX_finstat)
replace wX_basedep2=1 if wX_depres_d=1 & wX_hepsya>=0 & not missing(wX_hepsya)
make wX_passdep2=0 if wX_basedep2=1
replace wX_passdep2=1 if wX_basedep2=1 & wX_hepsyb=1
Indicator diabetes HbA1c
make wX_basediab1=0 if not missing(wX_finstat)
replace wX_basediab1=1 if wX_hesug>=0 & wX_hesuga>-8 & not missing(wX_hesug, wX_hesuga)
make wX_passdiab1=0 if wX_basediab1=1
replace wX_passdiab1=1 if wX_basediab1=1 & wX_hesuga=1
Indicator diabetes proteinuria
make wX_basediab2=0 if not missing(wX_finstat)
replace wX_basediab2=1 if wX_hekidn!=1 & wX_heacea=2 & wX_hewee>=0 & not missing(wX_hewee)
make wX_passdiab2=0 if wX_basediab2=1
replace wX_passdiab2=1 if wX_basediab2=1 & wX_hewee=1
Indicator diabetes cholesterol
make wX_basediab6=0 if not missing(wX_finstat)
replace wX_basediab6=1 if wX_diab_d=1 & wX_hechol>=0 & not missing(wX_hechol)
make wX_passdiab6=0 if wX_basediab6=1
replace wX_passdiab6=1 if wX_basediab6=1 & wX_hechol=1
Indicator diabetes ACE inhibitor
make wX_basediab8=0 if not missing(wX_finstat)
replace wX_basediab8=1 if wX_diab_d=1 &
(wX_heska=1orwX_hekidn=1orwX_heweea=1orwX_hichol_d=1orwX_hibp_d=1) &
( (wX_heace>0 & not missing(wX_heace)) or (wX_heacea>=0 & not missing(wX_heacea)) )
make wX_passdiab8=0 if wX_basediab8=1
replace wX_passdiab8=1 if wX_basediab8=1 & (wX_heace=1orwX_heacea=1)
Indicator diabetes foot check
make wX_basediab9=0 if not missing(wX_finstat)
replace wX_basediab9=1 if wX_heftchk>=0 & not missing(wX_heftchk)
make wX_passdiab9=0 if wX_basediab9=1
replace wX_passdiab9=1 if wX_basediab9=1 & wX_heftchk=1
Indicator hypertension treatment: incident hypertension
make wX_basehyp2=0 if not missing(wX_finstat)
replace wX_basehyp2=1 if (wX_hedimbp=1 & wX_hedasbp!=1) & wX_hehbpb>=0 & not missing(wX_hehbpb)
make wX_passhyp2=0 if wX_basehyp2=1
replace wX_passhyp2=1 if wX_basehyp2=1 & wX_hehbpb=1
Indicator hypertension treatment: prevalent hypertension
make wX_basehyp2a=0 if not missing(wX_finstat)
replace wX_basehyp2a=1 if wX_hibp_d=1 & wX_hehbpb>=0 & not missing(wX_hehbpb)
make wX_passhyp2a=0 if wX_basehyp2a=1
replace wX_passhyp2a=1 if wX_basehyp2a=1 & wX_hehbpb=1
Indicator ischaemic heart disease cholesterol
make wX_baseihd1=0 if not missing(wX_finstat)
replace wX_baseihd1=1 if wX_chd_d=1 & wX_hechol>=0 & not missing(wX_hechol)
make wX_passihd1=0 if wX_baseihd1=1
Indicator ischaemic heart disease antiplatelets
make wX_baseihd2=0 if not missing(wX_finstat)
replace wX_baseihd2=1 if wX_chd_d=1 & (wX_hehrta>=0 & not missing(wX_hehrta)) & wX_hehrtc!=1
make wX_passihd2=0 if wX_baseihd2=1
replace wX_passihd2=1 if wX_baseihd2=1 & wX_hehrta=1
Indicator ischaemic heart disease smoking
make wX_baseihd3=0 if not missing(wX_finstat)
replace wX_baseihd3=1 if wX_chd_d=1 & ((wX-3_hecgstp>0 & not missing(wX-3_hecgstp)) or (wX_Hecgstp>0 & not missing(wX_Hecgstp)) )
make wX_passihd3=0 if wX_baseihd3=1
replace wX_passihd3=1 if wX_baseihd3=1 & (wX-3_hecgstp=1orwX_Hecgstp=1)
Indicator myocardial infarction beta-blocker: incident myocardial infarction
make wX_betab=0 if wX_hebeta=2orwX_hebetb=2
replace wX_betab=1 if wX_hebeta=1orwX_hebetb=1
make wX_baseihd5=0 if not missing(wX_finstat)
replace wX_baseihd5=1 if wX_hedimmi=1 & wX-1_hedimmi!=1 & not missing(wX_betab)
make wX_passihd5=0 if wX_baseihd5=1
replace wX_passihd5=1 if wX_baseihd5=1 & wX_betab=1
Indicator myocardial infarction beta-blocker: prevalent myocardial infarction
make wX_baseihd5a=0 if not missing(wX_finstat)
replace wX_baseihd5a=1 if wX_hrtattack_d=1 & not missing(wX_betab)
make wX_passihd5a=0 if wX_baseihd5a=1
replace wX_passihd5a=1 if wX_baseihd5a=1 & wX_betab=1
Indicator ischaemic heart disease international normalised ratio test
make wX_baseihd6=0 if not missing(wX_finstat)
replace wX_baseihd6=1 if wX_hehrtc=1 & (wX_hehrte>=0 & not missing(wX_hehrte))
make wX_passihd6=0 if wX_baseihd6=1
replace wX_passihd6=1 if wX_baseihd6=1 & wX_hehrte=1
Indicator osteoarthritis exercise
make wX_baseoa2=0 if not missing(wX_finstat)
replace wX_baseoa2=1 if wX_heartoa=1 & ( (wX-3_hekneb>0 & not missing(wX-3_hekneb)) or(wX-2_hekneb>0 & not missing(wX-2_hekneb)) or (wX-1_hekneb>0 & not missing(wX-1_hekneb)) or (wX_hekneb>0 & not missing(wX_hekneb)) )
make wX_passoa2=0 if wX_baseoa2=1
replace wX_passoa2=1 if wX_baseoa2=1 & (wX-3_hekneb=1or wX-2_hekneb=1or wX-1_hekneb=1or wX_hekneb=1)
Indicator osteoarthritis education
make wX_alleduc=0 if wX_hearta>0 & wX_heartb>0 & wX_heartc>0 & not missing(wX_hearta, wX_heartb, wX_heartc)
replace wX_alleduc=1 if wX_hearta=1 & wX_heartb=1 & wX_heartc=1
make wX_baseoa3=0 if not missing(wX_finstat)
replace wX_baseoa3=1 if wX_heartoa=1 &
(not missing(wX-3_alleduc)or not missing(wX-2_alleduc)or not missing(wX-1_alleduc)or not missing(wX_alleduc))
make wX_passoa3=0 if wX_baseoa3=1
replace wX_passoa3=1 if wX_baseoa3=1 &
(wX-3_alleduc=1orwX-2_alleduc=1orwX-1_alleduc=1or wX_alleduc=1)
Indicator osteoarthritis paracetamol
make wX_baseoa4=0 if not missing(wX_finstat)
replace wX_baseoa4=1 if wX_heartoa=1 & ((wX-3_hearte>0 & not missing(wX-3_hearte)) or
(wX-2_hearte>0 & not missing (wX-2_hearte)) or (wX-1_hearte>0 & not missing (wX-1_hearte)) or(wX_hearte>0 & not missing(wX_hearte)))
make wX_passoa4=0 if wX_baseoa4=1
replace wX_passoa4=1 if wX_baseoa4=1 & (wX-3_hearte=1or wX-2_hearte=1or wX-1_hearte=1or wX_hearte=1)
Indicator osteoarthritis referral
make wX_baseoa6=0 if not missing(wX_finstat)
replace wX_baseoa6=1 if wX_heartoa=1 & wX_hehipc=2 & wX_hepsur>=0 & not missing(wX_hepsur)
make wX_passoa6=0 if wX_baseoa6=1
replace wX_passoa6=1 if wX_baseoa6=1 & wX_hepsur=1
Indicator diabetes training
make wX_basepat1=0 if not missing(wX_finstat)
replace wX_basepat1=1 if wX_diab_d=1 & ((w2_heslfcr>0 & not missing(w2_heslfcr)) or(w3_heslfcr>0 & not missing(w3_heslfcr)) or (wX_heslfcr>0 & not missing(wX_heslfcr)) )
make wX_passpat1=0 if wX_basepat1=1
replace wX_passpat1=1 if wX_basepat1=1 & (wX-2_heslfcr=1or wX-1_heslfcr=1or wX_heslfcr=1)
Indicator diabetes knowledge
make wX_basepat2=0 if not missing(wX_finstat)
replace wX_basepat2=1 if wX_diab_d=1 & not missing(wX_heslfcb) & wX_heslfcb>=0
make wX_passpat2=0 if wX_basepat2=1
replace wX_passpat2=1 if wX_basepat2=1 & (wX_heslfcb=1 or wX_heslfcb=2)
Indicator osteoarthritis treatment purpose
make wX_basepat7=0 if not missing(wX_finstat)
replace wX_basepat7=1 if wX_heartoa=1 & ((wX-2_heartd>0 & not missing(wX-2_heartd)) or(wX-1_heartd>0 & not missing(wX-1_heartd)) or(wX_heartd>0 & not missing(wX_heartd)) )
make wX_passpat7=0 if wX_basepat7=1
replace wX_passpat7=1 if wX_basepat7=1 & (wX-2_heartd=1orwX-1_heartd=1orwX_heartd=1)
Appendix 3 Protocol: key changes
The dynamics of quality: a national panel study of evidence-based standards
Primary outcome measures: owing to differences in the way questions were asked to the respondents, quality indicators are not available for all conditions at all four waves; the following table shows data availability.
Quality indicators for the four diseases | Availability of data |
---|---|
Cardiovascular disease | |
Hypertension treatment | All |
IHD cholesterol | 2 and 5 |
IHD antiplatelets | 2 and 5 |
INR test | 2 and 5 |
IHD smoking | 2 and 5 |
MI beta-blocker | 2 and 5 |
OA | |
OA exercise | All |
OA education | All |
OA paracetamol | All |
OA referral | All |
Receipt of hip or knee joint replacement if indicated, and whether received through NHS or non-NHS health care | All for receipt of joint replacement; 4 and 5 for payment |
Diabetes | |
Diabetes HbA1c | All |
Diabetes proteinuria | All |
Diabetes cholesterol | 2 and 5 |
Diabetes ACE inhibitor | 2, 4 and 5 |
Diabetes foot check | All |
Depression | |
Depression suicide | 2 and 4 |
Depression treatment | 2 and 4 |
Depression review | 2 |
In addition to those indicators listed above, we can generate stroke antihypertensive use at all four waves.
Patient-centred care
-
Diabetes training: waves 2 and 4.
-
Diabetes knowledge: waves 2 and 4.
-
Cholesterol explanation: wave 2 only.
-
Cholesterol preferences: wave 2 only.
-
Blood pressure explanation: wave 2 only.
-
Blood pressure choice: wave 2 only.
-
Osteoarthritis treatment purpose: waves 2 and 4.
Effects of loss of quality indicator data
Cardiovascular disease, osteoarthritis and diabetes have sufficient measurements for all the indicators for longitudinal analyses. Depression has two quality indicators available in waves 2 and 4 for longitudinal analyses. Unfortunately, one quality indicator was only asked at wave 2, so comparisons over time cannot be drawn for that indicator.
Appendix 4 Public and Patient Involvement in Research report
The PPIRes project in Norfolk and Suffolk is a local initiative to enable and encourage volunteer members of the public to collaborate with researchers in local trusts and universities. Our study had two PPIRes steering committee members, Mrs Amander Wellings and Mr Dave Stott. They joined the study steering group meetings and usefully contributed to the research team discussions about factors that might be associated with quality of care. It is worth noting that it was difficult achieving meaningful patient involvement in this study which largely evaluated professional practice.
After the first meeting there was discussion about whether or not ELSA could be used to explore information regarding patient perceptions of their interactions with their clinician. There were also discussions regarding what biographical factors should be used to predict quality of care. With regard to patient perceptions, we used the patient-centred quality indicators. These are not ideal measures as they were primarily designed to measure the performance of the health-care system and so focus on the offer of effective care. The idea was to be able to give clinicians credit for offering/discussing good care, without penalty if their patients made an informed decision not to take a particular treatment. The biographical data discussion was based around availability and ethical considerations of using these data. Age, sex, ethnicity and sexual orientation were mentioned, and barring sexual orientation (which is being asked in wave 6 onwards and hence the data are not available for this work), these factors were included in the modelling process. Geography was discussed; however, this is not routinely released to protect patient confidentiality, and was not used in our analyses.
When we were amalgamating factors that might be associated with quality of care, a ‘long-list’ was sent to the PPIRes representatives as a base on which they could build further ideas for us to model. The following points were raised as ideas to follow up if possible:
-
The proportion of patients: making use of telephone consultations or maybe using repeat prescriptions or non-attending pre-booked appointments or using home visits or using emergency after hours/weekend locum call-outs or having referrals to hospital consultants or other non-practice-based specialist services?
-
The proportion of patients: seeing multiple clinicians within a practice for the same or maybe different ailments.
-
Some other biographical factors might include patients’ mental health history (more than just the CES-D depression scale) or pattern of illegal drug or alcohol use – more than the ‘days since having had an alcoholic drink’ question. More ‘left field’, we wondered whether ELSA had any information on patients’ religion.
-
Geographical distance of patients’ homes to GP surgery, or even mode of transport from home to surgery. In part this might tap in to the ‘access’ issue that remains a key strand of the national health-care agenda. It might also link with the availability/mobility of carers or other helpful family/neighbours?
-
The proportion of patients who attend appointments accompanied by a carer, friend, family member or other more formal ‘advocate’ or representative? Probably not captured in patient records or ELSA but nonetheless interesting. Again quite easy to understand the additional pressures/encouragement for a clinician to do more or appear to do more/better when there is a third – though arguably relatively high status – independent presence in the consultation room.
-
We also thought about the extent to which equity of health care might relate to the presentation of multiple ailments at the same consultation? One can easily imagine patients – ourselves included – saving up symptoms into a bundle that makes a surgery visit worthwhile/economical. Though similar, we guess, to the ‘one more thing doctor before I leave’ scenario, we do not feel it is entirely the same phenomena. It would be quite easy to see how some patients simultaneously presenting with say three or four problems might inadvertently downplay or forget the most critical one – possibly due to consultation stress or issues of ‘cognition’. This could result in some GPs understandably focusing more upon on the first or least problematic of presented symptoms. (We are, however, well aware that GPs normally ask patients to focus on one problem per visit or invite them produce a hierarchy of problems but in reality. . .) Empathising somewhat with the plight of clinicians the phrase ‘hard to see the wood for the trees’ came to mind.
-
One other variant of this phenomena could be patients with multiple problems, i.e. patients with a pre-existing problem, for which they are already being treated but who subsequently develop symptoms of other problems but about which they delay telling their GP, sometimes until its very or too late. Why? We are both aware of some patients who feel they have used up their NHS ‘entitlement’ to health care by having just one health problem and therefore defer going to their GP for fear of developing a practice reputation as a worrier or hypochondriac. How and when these patients alert their GP to these other/secondary issues is interesting but probably not for this project?
-
We know that some clinicians, previously inclined so to do, no longer mark or otherwise signal on patients files their surgery reputation as being awkward, difficult, truculent, badly behaved – though anecdotally are aware locally of things like NfN (Normal for Norfolk). That said we could fully understand the temptation for clinicians not to be as thorough, sympathetic or caring towards certain types of patient – even down to ushering them out of the consultation door as quickly as possible. We cannot help but feel patient behaviour/reputation might well have a role in patients receipt of quality health care though can think of no way of getting access to or testing this, well at least not as part of this study. Whilst on this theme we wondered in that same ‘left field’ way, and howsoever broached whether criminal conviction or even a propensity among some patients for ‘crying wolf’ might be worth exploring.
-
Finally we had an even wilder thought about whether a synergy between an individual patient’s profile with that of his/her GP might be an interesting avenue to explore. We are not really expecting a response to this one but believe we have read somewhere about evidence drawn from wider therapeutic encounters to suggest the greater the ‘fit’ – biographical/social/political/personal, etc. – between clinician and patient the more likely the patient is to feel they have received a better quality of care. We even had some momentary some fun with the notion that ELSA might have enquired about patients’ political affiliations!
-
Patients being scared about going to their clinician – the ‘white coat syndrome’, e.g. how this syndrome could affect some patients say with obesity/BMI. The GP says ‘lose weight‘ but the patient is not emotionally ready to take this advice on board at that point resulting in them not going back to the GP or at least not doing so for some considerable time or at a crisis, or looking for a different GP. Also possibly elongating the time between the onset of a health condition and the patient actually presenting themselves for a diagnosis and treatment. So link BMI with length of time from onset to presentation. Also possibly link with alcohol consumption/smoking and presentation.
-
The potentially important role of patients’ spouses in their quality of health care.
-
Comparing/contrasting the optimists and pessimists, i.e. feeling satisfied how life has turned out and feeling what has happened is out of their control. If one feels life is out of control it assumes cannot do anything about improving my own health.
-
Carer support – (a) Importance of cognitive status. This could usefully link to the availability and importance to a patient having the support of a ‘carer’. With reference to ‘cognition’ there is an important ‘time’ variable between a patient gaining their educational qualifications – possibly many years ago – and their present cognitive state and therefore inability to actually use their education/knowledge/skills ‘now’ to help them more effectively problem solve and ask for or gain the quality of healthcare they so need. (b) Eyesight/hearing and linked it with the availability or otherwise and level of carer support. (c) You rightly identified ‘access to care’ as a big issue in primary care. In this context you began to explore the notion of ‘home visits and also a patient’s ability to walk to the surgery. Again the role of a carer could be key in such patients accessing high quality GP care. Look at last wave to see if cutbacks to care plays a part? Also could look at financial status e.g. total wealth amount of care from the 1st wave to the last. Is this carer a paid carer in the questionnaire?
These comments were mapped to the information that we could extract from the ELSA questionnaire, and the following table shows the result of this mapping.
Mapping PPIRes ideas to ELSA data | |
---|---|
Question/translation | Use ability? |
Telephone consults, repeat prescriptions, non-attendance, home visits, locum call outs, number of hospital referrals, use of non-practice based services | Not available, may be examined if linkage with GPPS if it asks those questions |
Multiple clinicians within a practice being seen by patient | N/A |
Mental health history – more than CES-D Pattern of illegal drug use Religion |
N/A N/A General question in self-completion asks if member of organisations/clubs/societies including church. Used in social detachment measure (scorg) |
Distance from house to surgery Transport from house to surgery (access issues) |
How easy is it for you to get to GP? In self-completion module, scale very easy to don’t want to go |
Attendance of carer/friend at GP visits | N/A |
Presentation of multiple ailments at one visit – ‘There’s one more thing before I go doctor. . .’ | Comorbidities measured no information on whether these are all identified at one visit or several. Do have time since diagnosis, perhaps could link together to see if diagnosed with several conditions very quickly? |
Multiple problems i.e. if being treated for one condition and develop and second/third why do they not seek the doctor’s advice earlier | Have a measure of comorbidities and also time since diagnosis for the 4 main diseases of interest. Can’t measure why they do not seek advice earlier |
‘Normal for Norfolk’ (NfN)/crying wolf/patient behaviour | If patient is ‘badly behaved’ or perceived as NfN do they get worse health care? Can’t measure this |
Synergy between GP and patient Political affiliations |
General question in self-completion asks if member of organisations/clubs/societies including church. Used in social detachment measure (scorg) |
Time since diagnosis and BMI/alcohol/smoking – tests for white coat syndrome – if I go to my GP she/he will tell me to lose weight, stop drinking/smoking therefore I won’t go about my disease/illness | Can look at, time since diagnosis going into main regression model anyway. Also BMI/smoking/alcohol intakes |
Role of spouses in quality of health care | Have marital status, carer availability and whether the care is useful or not |
Sense of control and optimism/pessimism | Sense of control in model, can’t really address the differences between optimists/pessimists |
Carer support part 1 | |
Present cognition/knowledge i.e. there is a length of time between when a person gets their qualifications and present cognitive state. I take this to mean that some old people feel a lack of control over their environment e.g. using a computer, electronics etc. as this is very different from what they learnt about | Could use sense of control CASP-19 this is already in model Time between qualification to present day cannot do |
Carer support part 2 | |
Eyesight/hearing. Do you have sufficient support from your carer due to these difficulties? | Sight and hearing in model. Also carer availability, see above |
Carer support part 3 | |
Access to care Have there been cutbacks to care? Role of carer in access to GP |
Question asked in self-completion module N/A |
Comments on the role of Public and Patient Involvement from Mrs Wellings
In my view the role of the public and patient involvement (PPI) member of the team often seemed vague especially due to the statistical nature of this piece of research and the reliance on analysis of secondary data. Much of what I thought as a lay member of the team ended up not being relevant within the confines of the data. Much of the statistical debate went on without me really understanding what was being discussed. There were none of the parts of research where PPI members can play an active role as this research was not recruiting patients. I felt like the team tried to include me but it was often difficult. I thought the study would be interesting as its policy and practical interventions seemed very good and I could see where it could make a real difference to patient outcomes. ‘A clearer picture of who is at most risk from poor quality care would inform the design of targeted interventions to improve quality of care, and/or improve access for previously under-served groups. It would inform the further development and refinement of national quality of care surveys: with co-applicant JC there is a real opportunity to follow up results with targeted questions in the General Practice Patient Survey or similar surveys, to improve primary care quality. This proposal is limited to four conditions, and if successful this methodology can be extended to other common chronic conditions in primary care’ (quote from the protocol). My interest being, how the health service seems to work better for some than others and why barriers exist and how we can remove them. As a life-long carer of people with mental health problems, learning disabilities, autism and stroke heart failure and head injury to name but a few of the conditions I have experience of, it is important for me to make a visit to the GP as easy as possible. I would be interested in future work in this area, but not a very large statistical study as I feel my strengths did not really reflect what was needed in this team.
Appendix 5 Detailed breakdown of the whole English Longitudinal Study of Ageing sample
Detailed breakdown of the whole ELSA Sample by wave and covaraite
Covariate | Wave 2, n (%) | Wave 3, n (%) | Wave 4, n (%) | Wave 5, n (%) |
---|---|---|---|---|
Sex | ||||
Female | 5307 (56) | 5476 (56) | 6125 (56) | 5705 (56) |
Male | 4125 (44) | 4295 (44) | 4925 (44) | 4569 (44) |
Age (years) | ||||
50–64 | 4424 (48) | 4919 (53) | 5571 (52) | 4718 (47) |
65–74 | 2643 (29) | 2371 (25) | 3047 (28) | 3061 (30) |
75 + | 2104 (23) | 2053 (22) | 2131 (20) | 2316 (23) |
NS-SEC | ||||
Managerial/professional | 2557 (28) | N/A | 2769 (28) | 3428 (35) |
Intermediate | 3612 (39) | N/A | 3919 (39) | 2543 (26) |
Routine | 2993 (33) | N/A | 3258 (33) | 3938 (40) |
Highest educational qualification | ||||
None | 5008 (30) | N/A | N/A | 2665 (16) |
Intermediate | 4335 (36) | N/A | N/A | 3967 (24) |
Degree or higher education below a degree | 2721 (16) | N/A | N/A | 3563 (21) |
Eyesight | ||||
Excellent/very good | 8089 (86) | 8301 (85) | 9577 (87) | 8881 (86) |
Good/fair, poor, blind | 1337 (14) | 1463 (15) | 1465 (13) | 1381 (14) |
General health | ||||
Excellent/good | 6711 (72) | 8868 (93) | 7819 (74) | 7176 (74) |
Fair/poor | 2583 (28) | 666 (7) | 2775 (26) | 2549 (26) |
Hearing | ||||
Good to excellent | 7436 (79) | 7689 (79) | 8808 (80) | 8121 (79) |
Fair to poor | 1992 (21) | 2077 (21) | 2233 (20) | 2142 (21) |
Chronic pain | ||||
None | 5786 (62) | 5942 (62) | 6413 (61) | 5861 (60) |
Mild | 968 (10) | 1091 (11) | 1252 (12) | 1176 (12) |
Moderate | 1822 (20) | 1733 (19) | 2102 (20) | 1888 (19) |
Severe | 715 (8) | 761 (8) | 819 (8) | 797 (8) |
Has long-standing illness | ||||
No | 4103 (44) | 4473 (46) | 5057 (46) | 4645 (45) |
Yes | 1982 (21) | 2001 (20) | 2208 (20) | 2007 (20) |
Yes: limiting | 3341 (35) | 3290 (34) | 3776 (34) | 3608 (35) |
ADL | ||||
None/one | 8586 (90) | 8838 (90) | 10,036 (91) | 9309 (91) |
Two or more | 946 (10) | 933 (10) | 1014 (9) | 965 (9) |
IADL | ||||
None/one | 8368 (89) | 8717 (89) | 9945 (90) | 9562 (93) |
Two or more | 1064 (11) | 1054 (11) | 1105 (10) | 712 (7) |
Cognitive impairment | ||||
No | 8063 (48) | 8103 (48) | 8495 (51) | 8511 (51) |
Yes | 896 (5) | 901 (5) | 944 (6) | 950 (6) |
Health literacy | ||||
Low | 1108 (7) | N/A | N/A | 915 (5) |
Medium | 1805 (11) | N/A | N/A | 1506 (9) |
High | 5989 (36) | N/A | N/A | 6868 (41) |
Alcohol consumption | ||||
1–2 times/week or less | 5256 (31) | 5099 (30) | 5757 (34) | 5725 (34) |
3–4 times/week or more | 2888 (17) | 2818 (17) | 3346 (20) | 3137 (19) |
Physical activity level | ||||
Sedentary | 556 (6) | 686 (7) | 835 (8) | 801 (8) |
Low | 2309 (25) | 2263 (23) | 2562 (23) | 2452 (24) |
Medium | 4688 (50) | 4884 (50) | 5388 (49) | 4996 (49) |
High | 1744 (19) | 1972 (20) | 2254 (20) | 2014 (20) |
Smoking status | ||||
Never | 3472 (37) | 3770 (39) | 4336 (40) | 3827 (38) |
In past | 4482 (48) | 3485 (46) | 5001 (46) | 5000 (49) |
Current smoker | 1475 (16) | 1510 (15) | 1545 (14) | 1312 (13) |
Diet | ||||
Eats ‘5-a-day’ | N/A | 2592 (15) | 3869 (23) | 4972 (30) |
Does not eat ‘5-a-day’ | N/A | 2142 (13) | 3089 (18) | 3791 (23) |
Access to GP | ||||
Very/quite easy | 7663 (46) | N/A | 8625 (51) | 8258 (49) |
Difficult/unable to attend | 378 (2) | N/A | 515 (3) | 518 (3) |
Social detachment | ||||
Not detached | 5956 (36) | 5954 (35) | 7024 (42) | 6704 (40) |
Detached | 1089 (6) | 996 (6) | 1265 (19) | 1260 (8) |
Locus of control | ||||
Internal | 1874 (11) | 1811 (11) | 2100 (13) | 2154 (13) |
External | 6264 (37) | 6287 (37) | 6995 (42) | 6658 (40) |
Lives alone | ||||
No | 3452 (21) | 3584 (21) | 4062 (24) | 3754 (22) |
Yes | 5740 (34) | 5855 (35) | 6584 (39) | 6038 (58) |
Married/civil partnership | ||||
No | 3162 (34) | 3232 (33) | N/A | 3341 (33) |
Yes | 6269 (66) | 6538 (67) | N/A | 6927 (67) |
Appendix 6 Complete breakdown of longitudinal quality of care modelling results
Diabetes
Characteristic | OR | 95% CI |
---|---|---|
Age (years) | ||
50–64 | 1.0 | – |
65–74 | 1.1 | 0.8 to 1.4 |
75 + | 0.9 | 0.7 to 1.2 |
Sex | ||
Female | 1.0 | – |
Male | 1.0 | 0.8 to 1.1 |
NS-SEC | ||
Routine | 1.0 | |
Intermediate/managerial and professional | 1.2 | 0.9 to 1.5 |
Education | ||
None | 1.0 | – |
Up to degree level | 1.2 | 0.9 to 1.5 |
Wealth | ||
Quartile 1 | 1.0 | – |
Quartile 2 | 1.2 | 0.9 to 1.7 |
Quartile 3 | 1.3 | 0.9 to 1.8 |
Quartile 4 | 1.1 | 0.8 to 1.6 |
Quartile 5 | 1.5 | 1.0 to 2.2 |
Eyesight | ||
Less than excellent | 1.0 | – |
Excellent | 1.0 | 0.8 to 1.4 |
Hearing | ||
Less than excellent | 1.0 | – |
Excellent | 1.2 | 0.8 to 1.6 |
Chronic pain | ||
None | 1.0 | – |
Mild/moderate | 0.8 | 0.5 to 1.2 |
Severe | 1.2 | 0.8 to 1.6 |
General health | ||
Less than excellent | 1.0 | – |
Excellent | 1.0 | 0.6 to 1.5 |
Limiting illness | ||
None | 1.0 | – |
Has limiting long-standing illness | 1.0 | 0.8 to 1.3 |
ADL | ||
None/one | 1.0 | – |
Two or more | 1.0 | 0.9 to 1.1 |
IADL | ||
None/one | 1.0 | – |
Two or more | 0.9 | 0.8 to 1.0 |
Access to GP | ||
Very easy | 1.0 | – |
Quite easy | 0.9 | 0.7 to 1.2 |
Difficult/can’t go | 1.1 | 0.6 to 1.8 |
Cognitive impairment | ||
No | 1.0 | – |
Yes | 0.5 | 0.4 to 0.7 |
BMI (kg/m2) | ||
Under-/normal weight | 1.0 | – |
Overweight | 1.1 | 0.7 to 1.7 |
Obese | 1.3 | 0.8 to 2.0 |
Alcohol | ||
Infrequently | 1.0 | – |
Daily | 0.9 | 0.7 to 1.3 |
Marital status | ||
Lives with partner | 1.0 | – |
Lives alone | 1.7 | 1.3 to 2.0 |
HbA1c | ||
< 7.5% DCCT | 1.0 | – |
≥ 7.5% DCCT | 1.1 | 0.8 to 1.4 |
Smoking | ||
Never | 1.0 | – |
Past or present | 0.9 | 0.7 to 1.1 |
Physical activity | ||
Sedentary | 1.0 | – |
Low | 1.6 | 0.9 to 2.6 |
Medium | 1.4 | 0.9 to 2.2 |
High | 1.8 | 1.0 to 3.3 |
CASP-19 | ||
Low | 1.0 | – |
Medium | 1.1 | 0.8 to 1.5 |
High | 1.2 | 0.9 to 1.6 |
Rose angina | ||
No | 1.0 | – |
Yes | 1.1 | 0.7 to 1.7 |
MAP | ||
< 110 mmHg | 1.0 | – |
≥ 110 mmHg | 0.9 | 0.6 to 1.3 |
CES-D depression | ||
Not depressed | 1.0 | – |
Depressed | 0.8 | 0.6 to 1.1 |
Social detachment | ||
Not detached | 1.0 | – |
Detached | 1.3 | 0.9 to 2.0 |
Cerebrovascular disease
Characteristic | OR | 95% CI | |
---|---|---|---|
Age (years) | |||
50–64 | 1.0 | – | |
65–74 | 1.0 | 0.5 to 2.1 | |
75 + | 0.9 | 0.4 to 1.9 | |
Sex | |||
Female | 1.0 | – | |
Male | 0.8 | 0.4 to 1.5 | |
NS-SEC | |||
Routine | 1.0 | – | |
Intermediate/managerial and professional | 0.9 | 0.5 to 1.6 | |
Education | |||
None | 1.0 | – | |
Up to degree level | 0.9 | 0.5 to 1.7 | |
Wealth | |||
Quartile 1 | 1.0 | – | |
Quartile 2 | 0.4 | 0.1 to 1.1 | |
Quartile 3 | 0.4 | 0.1 to 1.0 | |
Quartile 4 | 0.2 | 0.1 to 0.5 | |
Quartile 5 | 0.5 | 0.2 to 1.3 | |
Eyesight | |||
Less than excellent | 1.0 | – | |
Excellent | 0.9 | 0.3 to 2.5 | |
Hearing | |||
Less than excellent | 1.0 | – | |
Excellent | 0.6 | 0.3 to 1.2 | |
Chronic pain | |||
None | 1.0 | – | |
Mild/moderate | 0.6 | 0.2 to 1.4 | |
Severe | 0.9 | 0.4 to 2.1 | |
General health | |||
Less than excellent | 1.0 | – | |
Excellent | 0.5 | 0.2 to 1.6 | |
Limiting illness | |||
None | 1.0 | – | |
Has limiting long-standing illness | 1.2 | 0.6 to 2.1 | |
ADL | |||
None/one | 1.0 | – | |
Two or more | 1.3 | 1.0 to 1.7 | |
IADL | |||
None/one | 1.0 | – | |
Two or more | 1.1 | 0.8 to 1.3 | |
Access to GP | |||
Very easy | 1.0 | – | |
Quite easy | 1.3 | 0.6 to 2.7 | |
Difficult/can’t go | 1.2 | 0.3 to 5.1 | |
Cognitive impairment | |||
No | 1.0 | – | |
Yes | 1.0 | 0.4 to 2.6 | |
BMI (kg/m2) | |||
Under-/normal weight | 1.0 | – | |
Overweight | 1.4 | 0.6 to 3.5 | |
Obese | 1.9 | 0.6 to 6.3 | |
Alcohol | |||
Infrequently | 1.0 | – | |
Daily | 0.6 | 0.3 to 1.1 | |
Marital status | |||
Lives with partner | 1.0 | – | |
Lives alone | 1.3 | 0.7 to 2.6 | |
HbA1c | |||
< 7.5% DCCT | 1.0 | – | |
≥ 7.5% DCCT | 0.8 | 0.3 to 2.1 | |
Smoking | |||
Never | 1.0 | – | |
Past or present | 0.6 | 0.3 to 1.1 | |
Physical activity | |||
Sedentary | 1.0 | – | |
Low | 2.8 | 0.7 to 12.1 | |
Medium | 1.6 | 0.4 to 6.2 | |
High | 1.4 | 0.3 to 7.2 | |
CASP-19 | |||
Low | 1.0 | – | |
Medium | 0.3 | 0.1 to 0.8 | |
High | 0.3 | 0.1 to 0.9 | |
Rose angina | |||
No | 1.0 | – | |
Yes | 1.3 | 0.4 to 4.1 | |
MAP | |||
< 110 mmHg | 1.0 | – | |
≥ 110 mmHg | 2.0 | 0.4 to 11.1 | |
CES-D depression | |||
Not depressed | 1.0 | – | |
Depressed | 1.6 | 0.7 to 3.6 | |
Social detachment | |||
Not detached | 1.0 | – | |
Detached | 2.5 | 0.7 to 8.3 |
Hypertension
Characteristic | OR | 95% CI |
---|---|---|
Age (years) | ||
50–64 | 1.0 | – |
65–74 | 2.2 | 1.6 to 3.1 |
75+ | 3.2 | 2.0 to 5.3 |
Sex | ||
Female | 1.0 | – |
Male | 0.9 | 0.6 to 1.1 |
NS-SEC | ||
Routine | 1.0 | – |
Intermediate/managerial and professional | 0.9 | 0.6 to 1.2 |
Education | ||
None | 1.0 | – |
Up to degree level | 0.9 | 0.7 to 1.2 |
Wealth | ||
Quartile 1 | 1.0 | – |
Quartile 2 | 0.9 | 0.6 to 1.5 |
Quartile 3 | 1.2 | 0.8 to 1.9 |
Quartile 4 | 0.7 | 0.5 to 1.1 |
Quartile 5 | 1.0 | 0.6 to 1.5 |
Eyesight | ||
Less than excellent | 1.0 | – |
Excellent | 1.4 | 0.9 to 2.2 |
Hearing | ||
Less than excellent | 1.0 | – |
Excellent | 1.1 | 0.7 to 1.5 |
Chronic pain | ||
None | 1.0 | – |
Mild/moderate | 0.9 | 0.6 to 1.3 |
Severe | 0.8 | 0.5 to 1.5 |
General health | ||
Less than excellent | 1.0 | – |
Excellent | 0.9 | 0.6 to 1.4 |
Limiting illness | ||
None | 1.0 | – |
Has limiting long-standing illness | 1.1 | 0.8 to 1.5 |
ADL | ||
None/one | 1.0 | – |
Two or more | 1.0 | 0.9 to 1.2 |
IADL | ||
None/one | 1.0 | – |
Two or more | 1.0 | 0.8 to 1.2 |
Access to GP | ||
Very easy | 1.0 | – |
Quite easy | 1.0 | 0.7 to 1.4 |
Difficult/cannot go | 1.6 | 0.6 to 4.1 |
Cognitive impairment | ||
No | 1.0 | – |
Yes | 1.1 | 0.5 to 2.4 |
BMI (kg/m2) | ||
Under-/normal weight | 1.0 | – |
Overweight | 1.3 | 0.8 to 1.9 |
Obese | 1.0 | 0.7 to 1.6 |
Alcohol | ||
Infrequently | 1.0 | – |
Daily | 0.9 | 0.7 to 1.2 |
Marital status | ||
Lives with partner | 1.0 | – |
Lives alone | 1.0 | 0.7 to 1.4 |
HbA1c | ||
< 7.5% DCCT | 1.0 | – |
≥ 7.5% DCCT | 2.6 | 0.5 to 12.7 |
Smoking | ||
Never | 1.0 | – |
Past or present | 0.9 | 0.7 to 1.2 |
Physical activity | ||
Sedentary | 1.0 | – |
Low | 1.1 | 0.5 to 2.5 |
Medium | 0.9 | 0.4 to 1.9 |
High | 0.7 | 0.3 to 1.6 |
CASP-19 | ||
Low | 1.0 | – |
Medium | 1.0 | 0.7 to 1.4 |
High | 0.9 | 0.6 to 1.4 |
Rose angina | ||
No | 1.0 | – |
Yes | 1.3 | 0.6 to 2.9 |
MAP | ||
< 110 mmHg | 1.0 | – |
≥ 110 mmHg | 1.4 | 0.9 to 2.1 |
CES-D depression | ||
Not depressed | 1.0 | – |
Depressed | 0.8 | 0.5 to 1.2 |
Social detachment | ||
Not detached | 1.0 | – |
Detached | 1.3 | 0.8 to 2.1 |
Ischaemic heart disease
Characteristic | OR | 95% CI |
---|---|---|
Age (years) | ||
50–64 | 1.0 | – |
65–74 | 1.0 | 0.6 to 1.6 |
75 + | 0.5 | 0.3 to 0.8 |
Sex | ||
Female | 1.0 | – |
Male | 1.0 | 0.7 to 1.3 |
NS-SEC | ||
Routine | 1.0 | – |
Intermediate/managerial and professional | 1.0 | 0.7 to 1.6 |
Education | ||
None | 1.0 | – |
Up to degree level | 1.3 | 0.9 to 1.9 |
Wealth | ||
Quartile 1 | 1.0 | – |
Quartile 2 | 0.8 | 0.5 to 1.4 |
Quartile 3 | 0.7 | 0.4 to 1.2 |
Quartile 4 | 0.9 | 0.5 to 1.6 |
Quartile 5 | 0.8 | 0.5 to 1.5 |
Eyesight | ||
Less than excellent | 1.0 | – |
Excellent | 0.8 | 0.4 to 1.3 |
Hearing | ||
Less than excellent | 1.0 | – |
Excellent | 0.9 | 0.5 to 1.5 |
Chronic pain | ||
None | 1.0 | – |
Mild/moderate | 1.6 | 0.7 to 3.6 |
Severe | 0.8 | 0.5 to 1.2 |
General health | ||
Less than excellent | 1.0 | – |
Excellent | 2.3 | 0.5 to 9.7 |
Limiting illness | ||
None | 1.0 | – |
Has limiting long-standing illness | 0.8 | 0.5 to 1.1 |
ADL | ||
None/one | 1.0 | – |
Two or more | 1.0 | 0.8 to 1.1 |
IADL | ||
None/one | 1.0 | – |
Two or more | 1.0 | 0.8 to 1.1 |
Access to GP | ||
Very easy | 1.0 | – |
Quite easy | 0.8 | 0.5 to 1.2 |
Difficult/can’t go | 0.8 | 0.4 to 1.6 |
Cognitive impairment | ||
No | 1.0 | – |
Yes | 1.1 | 0.5 to 2.4 |
BMI (kg/m2) | ||
Under-/normal weight | 1.0 | – |
Overweight | 1.1 | 0.7 to 1.9 |
Obese | 0.9 | 0.5 to 1.5 |
Alcohol | ||
Infrequently | 1.0 | – |
Daily | 1.1 | 0.7 to 1.7 |
Marital status | ||
Lives with partner | 1.0 | – |
Lives alone | 1.6 | 1.1 to 2.5 |
HbA1c | ||
< 7.5% DCCT | 1.0 | – |
≥ 7.5% DCCT | 0.6 | 0.3 to 1.2 |
Smoking | ||
Never | 1.0 | – |
Past or present | 1.2 | 0.8 to 1.7 |
Physical activity | ||
Sedentary | 1.0 | – |
Low | 1.8 | 1.0 to 3.4 |
Medium | 1.6 | 0.8 to 2.9 |
High | 2.0 | 0.9 to 4.5 |
CASP-19 | ||
Low | 1.0 | – |
Medium | 1.3 | 0.9 to 2.1 |
High | 1.5 | 0.9 to 2.4 |
Rose angina | ||
No | 1.0 | – |
Yes | 1.3 | 0.8 to 2.1 |
MAP | ||
< 110 mmHg | 1.0 | – |
≥ 110 mmHg | 1.1 | 0.5 to 2.4 |
CES-D depression | ||
Not depressed | 1.0 | – |
Depressed | 1.1 | 0.7 to 1.7 |
Social detachment | ||
Not detached | 1.0 | – |
Detached | 1.0 | 0.6 to 1.3 |
Osteoarthritis
Characteristic | OR | 95% CI |
---|---|---|
Age (years) | ||
50–64 | 1.0 | – |
65–74 | 0.8 | 0.6 to 1.0 |
75 + | 1.1 | 0.8 to 1.4 |
Sex | ||
Female | 1.0 | – |
Male | 0.9 | 0.8 to 1.1 |
NS-SEC | ||
Routine | 1.0 | – |
Intermediate/managerial and professional | 1.0 | 0.9 to 1.2 |
Education | ||
None | 1.0 | – |
Up to degree level | 1.0 | 0.8 to 1.2 |
Wealth | ||
Quartile 1 | 1.0 | – |
Quartile 2 | 0.8 | 0.6 to 1.0 |
Quartile 3 | 0.8 | 0.6 to 1.0 |
Quartile 4 | 0.9 | 0.7 to 1.2 |
Quartile 5 | 0.8 | 0.6 to 1.0 |
Eyesight | ||
Less than excellent | 1.0 | – |
Excellent | 1.0 | 0.8 to 1.3 |
Hearing | ||
Less than excellent | 1.0 | – |
Excellent | 1.0 | 0.8 to 1.3 |
Chronic pain | ||
None | 1.0 | – |
Mild/moderate | 1.0 | 0.8 to 1.2 |
Severe | 1.8 | 1.4 to 2.2 |
General health | ||
Less than excellent | 1.0 | – |
Excellent | 0.8 | 0.5 to 1.0 |
Limiting illness | ||
None | 1.0 | – |
Has limiting long-standing illness | 1.8 | 1.5 to 2.1 |
ADL | ||
None/one | 1.0 | – |
Two or more | 1.0 | 0.9 to 1.2 |
IADL | ||
None/one | 1.0 | – |
Two or more | 1.0 | 0.9 to 1.2 |
Access to GP | ||
Very easy | 1.0 | – |
Quite easy | 1.2 | 1.0 to 1.4 |
Difficult/cannot go | 1.0 | 0.8 to 1.5 |
Cognitive impairment | ||
No | 1.0 | – |
Yes | 1.2 | 0.8 to 1.8 |
BMI (kg/m2) | ||
Under-/normal weight | 1.0 | – |
Overweight | 1.2 | 0.9 to 1.5 |
Obese | 1.6 | 1.2 to 2.0 |
Alcohol | ||
Infrequently | 1.0 | – |
Daily | 1.0 | 0.8 to 1.3 |
Marital status | ||
Lives with partner | 1.0 | – |
Lives alone | 1.2 | 0.9 to 1.4 |
HbA1c | ||
< 7.5% DCCT | 1.0 | – |
≥ 7.5% DCCT | 0.9 | 0.5 to 1.6 |
Smoking | ||
Never | 1.0 | – |
Past or present | 1.0 | 0.9 to 1.2 |
Physical activity | ||
Sedentary | 1.0 | – |
Low | 0.9 | 0.6 to 1.3 |
Medium | 1.0 | 0.7 to 1.6 |
High | 0.9 | 0.6 to 1.5 |
CASP-19 | ||
Low | 1.0 | – |
Medium | 1.1 | 0.9 to 1.4 |
High | 1.0 | 0.8 to 1.3 |
MAP | ||
< 110 mmHg | 1.0 | – |
≥ 110 mmHg | 1.0 | 0.8 to 1.4 |
CES-D depression | ||
Not depressed | 1.0 | – |
Depressed | 1.1 | 0.9 to 1.4 |
Social detachment | ||
Not detached | 1.0 | – |
Detached | 1.1 | 0.9 to 1.5 |
List of abbreviations
- ACE
- angiotensin-converting enzyme
- ACOVE
- Assessing Care of Vulnerable Elders
- ADL
- activities of daily living
- BMI
- body mass index
- CAPI
- computer-assisted personal interview
- CASP-19
- Control, Autonomy, Self-realisation and Pleasure-19 quality of life scale
- CES-D
- Centre for Epidemiological Studies – Depression Scale
- CHD
- coronary heart disease
- CI
- confidence interval
- CVD
- cerebrovascular disease
- DCCT
- Diabetes Control and Complications Trial
- ELSA
- English Longitudinal Study of Ageing
- GP
- general practitioner
- HbA1c
- glycosylated haemoglobin
- HSE
- Health Survey for England
- IADL
- instrumental activities of daily living
- IHD
- ischaemic heart disease
- INR
- international normalised ratio
- LDL
- low-density lipoprotein
- MI
- myocardial infarction
- NS-SEC
- National Statistics Socio-Economic Classification
- OR
- odds ratio
- PPIRes
- Patient and Public Involvement in Research
- QOF
- Quality and Outcomes Framework