Notes
Article history
The research reported in this issue of the journal was funded by the HS&DR programme or one of its preceding programmes as project number 11/2004/28. The contractual start date was in December 2012. The final report began editorial review in October 2014 and was accepted for publication in June 2015. The authors have been wholly responsible for all data collection, analysis and interpretation, and for writing up their work. The HS&DR editors and production house have tried to ensure the accuracy of the authors’ report and would like to thank the reviewers for their constructive comments on the final report document. However, they do not accept liability for damages or losses arising from material published in this report.
Declared competing interests of authors
none
Permissions
Copyright statement
© Queen’s Printer and Controller of HMSO 2016. This work was produced by Bedford et al. under the terms of a commissioning contract issued by the Secretary of State for Health. This issue may be freely reproduced for the purposes of private research and study and extracts (or indeed, the full report) may be included in professional journals provided that suitable acknowledgement is made and the reproduction is not associated with any form of advertising. Applications for commercial reproduction should be addressed to: NIHR Journals Library, National Institute for Health Research, Evaluation, Trials and Studies Coordinating Centre, Alpha House, University of Southampton Science Park, Southampton SO16 7NS, UK.
Chapter 1 Introduction
What is acute kidney injury?
Acute kidney injury (AKI), previously known as acute renal failure, is an all too common clinical problem characterised by an acute decline in renal function, the results of which range from minimal alteration in serum creatinine to anuric renal failure necessitating renal replacement therapy (RRT). This abrupt rise in serum creatinine results from an insult or injury that causes a functional or structural change in the kidney. The aetiologies and risk factors for AKI are numerous, but now well defined. 1–4 Even without the need for RRT, AKI may impact on a patient’s clinical course with complications such as fluid overload, acidosis and hyperkalaemia, all of which may lead to an increase in morbidity, length of stay and, ultimately, mortality in both the long and short term. Renal outcomes include full renal recovery, no renal recovery, development of chronic kidney disease (CKD) and progression of pre-existing CKD. 5–9
Acute kidney injury is increasingly well recognised as a public health issue and is both a consequence and a predictor of CKD. 10–12 Its prevalence (4.9% among hospitalised patients in the USA) is increasing,13 owing to an ageing population and the growing burden of the same risk factors contributing to the rise in CKD (including obesity, diabetes and hypertension), and also to better recognition.
Conceptual model
The conceptual model of CKD is well established, and continues to inform clinical medicine, research and public health. 14–16 In contrast, the concept of acute kidney diseases and disorders (AKD) is relatively new and attempts to map itself onto the widely accepted CKD concept. The definition of AKI, in contradistinction to CKD, describes an abrupt, time-limited reduction of function which has at least the potential to recover.
Both AKI and CKD describe decreased function which can lead to complications, including end-stage renal disease (ESRD) and mortality. Risk factors for AKI and CKD are similar, and there is a conceptual overlap and interplay between the two. AKI and CKD are both risk factors for each other, and also worsen the prognosis of each other.
Underlying biology
If we now consider the situation at a biological level, in the elderly CKD population several factors could lead to increased susceptibility to AKI. Changes in the renal vasculature occur with age, just as in other vascular beds, often owing to comorbidity, but also in the absence of comorbidity. 17 It is suggested that these changes eventually cause cortical glomerulosclerosis, interstitial fibrosis and tubular atrophy, and compensatory hypertrophy and hyperfiltration of glomeruli in the medulla, contributing to the development of CKD. 18 With increasing age and CKD, function in both proximal and distal tubules is compromised, hampering the ability to control fluid and electrolyte balance and affecting tubuloglomerular feedback. 17,19 These changes, related to age and CKD, may exacerbate clinical events such as dehydration and drug toxicity, which carry a high risk of AKI. 18
Contrary to the idea that the diseased kidney is at increased risk of AKI is the ‘intact nephron’ hypothesis. 20,21 In surviving nephrons of a kidney with CKD there remains homogeneity of function and regulatory capacity. The kidney responds in a predictable and organised manner to maintain homeostasis in the face of a number of challenges. There may be fewer functional nephrons available, and a reduced reserve, but available nephrons are functionally intact. This is evident until the late stages of the disease and should, therefore, not produce an increased risk of AKI; however, it may impart an increased severity when it develops, which is therefore more likely to be clinically evident. There is also the concept of priming or conditioning, where the ischaemic or diseased kidney in CKD is more ‘used to’ insults and can, therefore, maintain function.
Given that people with CKD have an increased burden of vascular disease, it may be that less of a vascular insult is required to provoke AKI. There are supportive data from animal models of AKI which suggest that AKI is a form of ‘vasomotor nephropathy’. 22,23 People with CKD and a greater burden of vascular disease may have increased severity of AKI when it develops, which is more likely to be clinically apparent and require hospitalisation and, thus, be captured in epidemiological studies. Patients without CKD, and with less vascular disease, may have less severe AKI, which manifests as ‘silent and discrete’ episodes in the community and may not be captured in existing epidemiological studies, thereby indicating an increased incidence of AKI in CKD.
Further prospective studies are required to assess the true incidence of AKI in patients with CKD and correct more accurately for comorbidity and hospitalisation.
But what happens following AKI? Renal tissue has the ability to recover from sublethal or lethal cellular damage. 24–27 However, function may not be fully restored, which results in the development of CKD. 26 It is suggested that kidney function can be directly related to a cycle of cell injury and recovery following AKI (Figure 1a). 28 This involves renal tubular epithelial cells, damage to which is thought to be extended by renal vascular endothelial injury and dysfunction. It is believed that endothelial repair is important to overall renal recovery and may impact on long-term function. 29 This model, however, considers acute tubular necrosis (ATN) as the cause of AKI. What happens most frequently is limited to the very early part of this process. In patients developing CKD (Figure 1b), the initiating insult leading to damage, inflammation and repair (initiation) may result in fibrosis (extension) and then further damage in a self-perpetuating cycle of progression (maintenance) to ESRD. Early intervention at the stages of initiation and extension may prevent CKD and ESRD, while later intervention during the maintenance stage may only delay progression, with the extent of delay determined by the success or otherwise of intervention. Patients with AKI may or may not have pre-existing CKD (Figure 1c). Okusa et al. 30 (pathophysiological concepts from Sutton et al. 28) suggest that, following AKI, there are four possible outcomes: (1) full recovery; (2) incomplete recovery resulting in CKD; (3) exacerbation of pre-existing CKD accelerating progression to ESRD; and (4) non-recovery of function leading to ESRD. Recovery from AKI may also be incomplete, leading to step-down in glomerular filtration rate (GFR) falling short of CKD. The fact that patients experiencing AKI are likely to also have risk factors for CKD could suggest that patients without a known background CKD who develop AKI already have unrecognised renal disease and reduced functional reserve, which has not yet manifested as CKD. These patients are programmed to develop future CKD, and the AKI episode simply speeds up the development of overt CKD. In this respect, renal outcomes of AKI and CKD are the same, which is further evidence that they are part of the same pathophysiological pathway.
FIGURE 1.
Conceptual model of GFR and cellular pathology over time in AKI, CKD, and AKI and CKD. (a) Model of AKI over time: the cellular phases of AKI leading to repair, highlighting the possibility of initiating a self-perpetuating cycle of inflammation producing fibrosis leading to CKD; (b) model of CKD over time: the phases of cellular injury in CKD. Following an initial insult there is initiation of the inflammatory response with repair. This may then lead to the extension phase with added fibrosis. Past a point of no return, the disease process embarks upon a self-perpetuating cycle of cellular damage and fibrosis (maintenance phase) leading to deterioration in GFR, and progression to ESRD. The figure also shows the effect of intervention on the disease process; and (c) AKI and CKD: the effect of episodes of AKI on the progression of CKD, with three possible outcomes: complete recovery, stepwise progression and inexorable decline. D, damage; F, fibrosis; GFR, glomerular filtration rate; I, inflammation, R, repair.
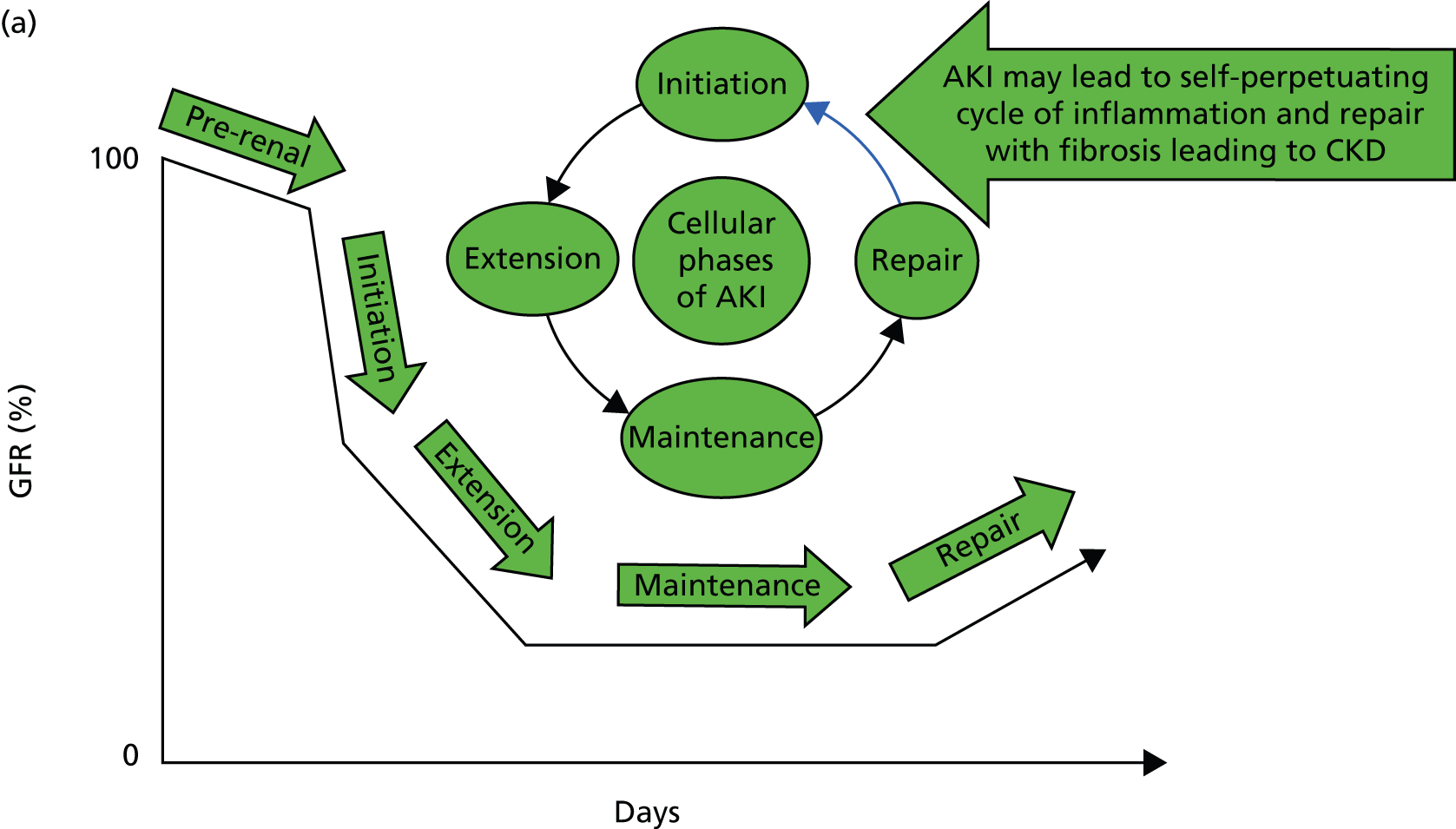

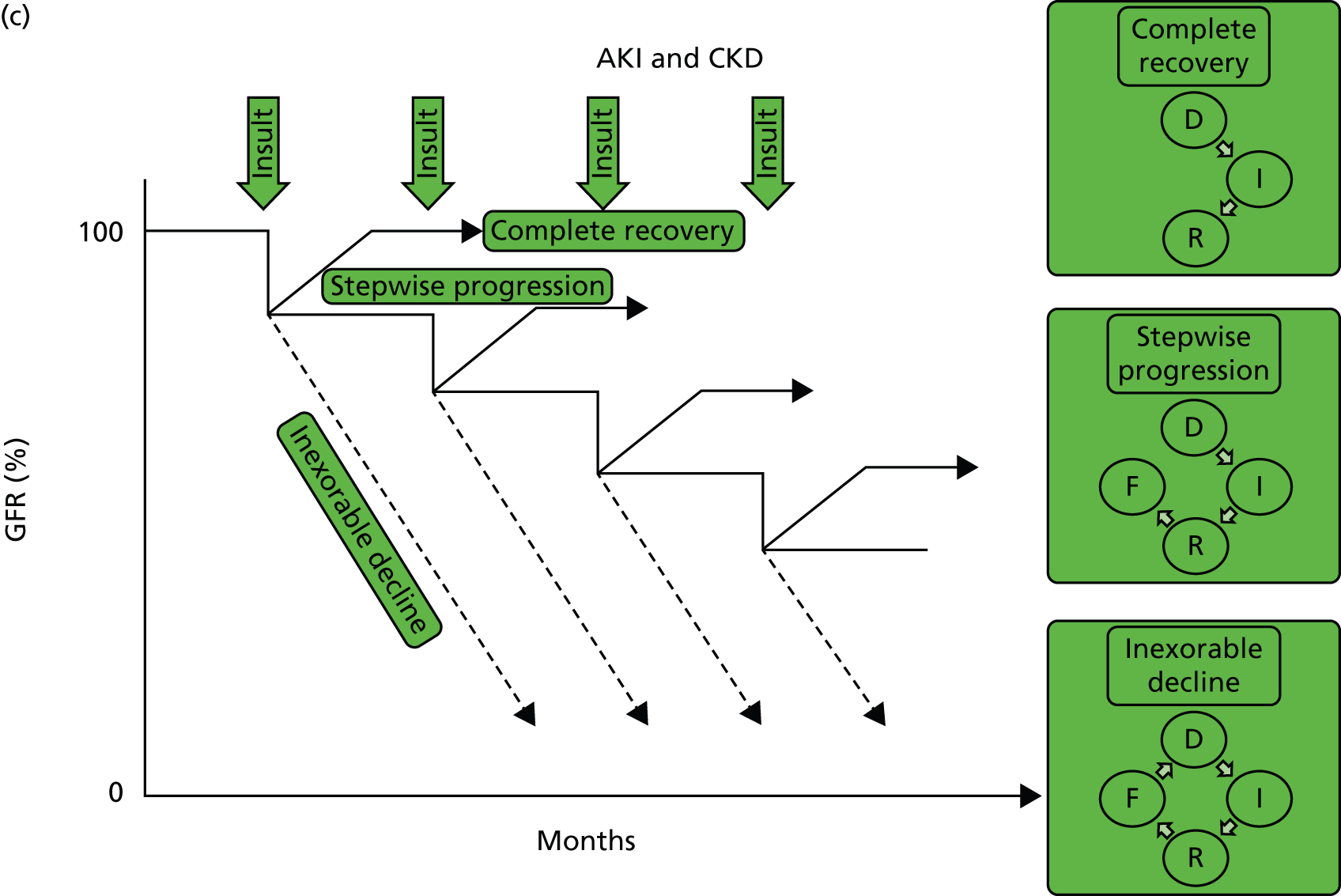
A key question is whether the ‘I’ in AKI truly stands for injury or actually stands for impairment and/or injury. Is it underpinned by histopathological damage and, if so, when does this become relevant in terms of future CKD or CKD progression? Do undetected episodes of AKI in the community lead to CKD? When patients present with CKD without an obvious cause, is the pathophysiology related to multiple undetected AKI events in the community?
In an ischaemia–reperfusion injury model of AKI in rats, Basile et al. 29 found permanent alterations in renal structure and function associated with the development of features indicative of CKD. They suggest that permanent changes in renal blood flow occur following AKI, resulting in tubulointerstitial fibrosis and altered medullary tonicity (causing impairment of urinary concentrating ability). 29 They also suggest a loss of microvasculature, resulting in a build-up of extracellular matrix, contributing to the development of interstitial fibrosis,29 which in turn leads to development of CKD. They hypothesise that, as long as there is adequate functional reserve, the single-nephron GFR of surviving nephrons increases to maintain a constant total GFR. 29 This suggests that, even in patients in whom creatinine levels and GFR return to baseline, there may be underlying permanent damage masked by compensatory mechanisms. These patients may subsequently have an increased risk of CKD and AKI, owing to underlying ‘subclinical’ damage.
These results are borne out further by studies of renal transplant patients, which demonstrate that delayed graft function (most commonly ATN31) is an independent risk factor for graft survival. 31–33 The kidney has the ability to restore structure and function following AKI, but there are some changes and damage which are permanent. This may lead to development of CKD (or progression of existing CKD) if there is not sufficient functional reserve to compensate. In cases where compensation maintains baseline GFR, there may then be increased risk of future development of CKD. This ‘subclinical’ damage will be important in the management of these patients following AKI, for preventing progression or development of CKD.
Are AKI and CKD biologically part of the same pathway, with eventual glomerulosclerosis and interstitial fibrosis? The discrete episode of AKI leads to fibrosis by setting up the cycle of inflammation and cell repair.
Incidence
How many people does AKI affect? It is estimated that in the USA 17 million admissions per year are complicated by AKI. 34 In developing countries AKI is a disease of the young35–37 and children;38,39 in developed countries it is a disease of the elderly. 40,41 With overall decreases in birth rate and mortality, life expectancy is increasing and is now longer than 80 years in most developed countries. This ageing population, with age-related changes in the kidney, systemic vasculature and immunological system, along with the burden of comorbidity and exposure to iatrogenic insults such as medications, radiocontrast agents and surgery, will lead to a continued rise in the incidence of AKI. It has been suggested that incidence will nearly double in the next decade. 40,42
The incidence of AKI has been reported as 486–630 per million people per year in data from the past 10 years. 43–45 In 2003, Ali et al. ,46 from a population of 523,390 in the Grampian region of Scotland, conducted a population-based study of AKI, reporting an incidence of 1811 cases of AKI and 336 of acute-on-chronic kidney failure per million people. This study reported a much higher incidence than previous work and may be closer to the true incidence of AKI.
Outcomes of acute kidney injury
What are the outcomes for patients with AKI? There is clear evidence that outcomes for AKI are poor. According to local data, only 56% of patients who experienced severe AKI in hospital survived to discharge; only 28% survived to 3 years post discharge. 43 Most studies of AKI in the intensive care unit (ICU) report a mortality exceeding 50%. It is clear that ICU and in-hospital mortality increases alongside severity of AKI. 1,4,46–56 Despite the trend of lower mortality in recent years, AKI still remains an important negative prognostic factor, particularly in critically ill patients. Even small isolated increases in serum creatinine levels have an associated increase in short-term morbidity and mortality and in longer-term outcomes, including 1-year mortality;38,48,57–62 this is even more the case when RRT is required. 5,6,13,34,63 A study from the Medicare Sample Beneficiary Analytical File from 1992 to 2001 found that patients with AKI who required RRT had an in-hospital mortality rate of 32.9%, compared with 27.5% in patients with AKI not requiring RRT, and 4.6% in patients without AKI. Importantly, it found that the mortality rate was 32.6% in patients with AKI coded as a secondary diagnosis, and 15.2% in patients with AKI coded as a primary diagnosis. 40 This confirms what is already known: that isolated AKI without other organ involvement has a better prognosis than AKI in the context of multiple organ failure.
Chronic kidney disease is an independent predictor of morbidity and mortality. 64,65 Hence we would assume that AKI in CKD has a summative effect on outcome, giving rise to the question whether or not the outcome of AKI differs with the presence of background CKD. Studies actually demonstrate lower in-hospital mortality rates in patients with AKI with a background of CKD than in patients without a background of CKD. 41,53,57,66–69 This would seem counterintuitive. One explanation might be that patients with CKD require less of an insult to manifest clinically apparent AKI, and thus the severity of the AKI episode is less in these patients, resulting in better outcomes. In addition, patients experiencing ‘silent and discrete’ AKI, which remains unrecognised and does not lead to admission, will not be captured by epidemiological studies. Only the more seriously ill patients with clinically apparent and recognised AKI will be included, leading to a bias in mortality statistics. Conversely, those with CKD may have more resilience to acute insults secondary to conditioning or priming, and tolerate AKI better. It is also possible that those with CKD receive better/different care from their non-CKD counterparts when AKI is identified, thus impacting outcomes. Finally, it been suggested that results may be confounded by malnutrition (lower serum creatinine values from low muscle mass). 57
Even small isolated increases in serum creatinine levels have an associated increase in short-term morbidity and mortality and in longer-term outcomes, including 1-year mortality. 1,2,4,47,58,59,70,71 ‘Silent and discrete’ episodes of AKI in the community, therefore, require further research directed at recognition and early indentification, as intervention in this group may have a significant effect on outcomes.
Another outcome of paramount interest is renal recovery. According to the annual report of the US Renal Disease Survey 2006,60 approximately 6% of patients with AKI progressed to ESRD within 2 years, and two-thirds of hospitalised patients who had AKI and progressed to ESRD had background CKD. CKD or comorbid conditions leading to CKD are risk factors that predict dialysis dependence following AKI. 61,62
Wald et al. 72 looked at outcomes of chronic dialysis and death in AKI patients requiring in-hospital dialysis who survived free of dialysis for at least 30 days after discharge, from a 10-year cohort of all adult patients in Ontario, Canada. Patients with AKI were three times more likely than those without AKI to require chronic dialysis. 72 Individuals with pre-existing CKD who had AKI requiring dialysis had a twofold higher risk of chronic dialysis than patients with CKD without AKI requiring dialysis. Patients with AKI requiring dialysis without pre-existing CKD had a 15-fold higher risk of chronic dialysis than patients with CKD without AKI. 72
Other observational and database studies demonstrate that AKI, with a background of CKD, leads to ESRD at a higher frequency than does AKI alone. 12,40,46 Ishani et al. 12 assessed, based on Medicare claims, a random cohort of 233,803 hospitalised patients aged ≥ 67 years on discharge and without previous ESRD or AKI. Patients with concomitant AKI and CKD were far more likely to develop ESRD, indicating a strong multiplicative effect of the interaction on ESRD development. 12 A population-based study by Ali et al. 46 compared patients with ACKD and those with AKI alone. Patients with ACKD were older, with less chance of renal recovery.
Importantly, these studies all depend on the definitions of both CKD and AKI, which may not be accurate or comparable. For example, the definition of CKD is based on diagnostic coding or pre-operative GFR taken as a baseline function. These can introduce bias in AKI and CKD detection. Singh et al. 73 suggested that differences could also reflect greater specificity of administrative codes for AKI among patients without CKD. This underlines the need for consensus on the definition of baseline function. The debate surrounding definition will be discussed in more detail later.
There is mounting evidence that AKI contributes significantly to CKD and CKD progression, leading to ESRD. Okusa et al. 30 (pathophysiological concepts from Sutton et al. 28) suggest that, following AKI, there are four possible outcomes: (1) full recovery; (2) incomplete recovery resulting in CKD; (3) exacerbation of pre-existing CKD accelerating progression to ESRD; and (4) non-recovery of function leading to ESRD.We suggest a fifth outcome: AKI does not necessarily have to be associated with progressive CKD; instead, incomplete recovery may occur, leading to step-down in GFR, which subsequently remains stable.
There is no doubt that mortality from AKI is high. In those who survive, there may be decline in function, in some cases leading to ESRD, either at the time of AKI or in the future. 51,74–77 Even in patients with complete recovery there is still reduced survival and increased incidence of CKD in the years following AKI. 12,78 Patients experiencing AKI are likely to also have risk factors for CKD. It may be that patients without known background CKD who develop AKI already have unrecognised renal disease and reduced functional reserve, not yet manifested as CKD. These patients are programmed to develop future CKD, and the AKI episode simply speeds up the development of overt CKD. Ishani et al. ,12 based on Medicare claims, reported that of patients with AKI and no background of CKD, 72.1% had CKD documented within 2 years of AKI. Triverio et al. 79 demonstrated that AKI progressed to CKD within 3 years in 50% of patients without background CKD. Hsu et al. 80 suggested that the growth of ESRD incidence (in the USA) could not be accounted for solely by the rise in CKD incidence. Growth in ESRD incidence may partly be attributable to AKI.
There are further studies suggesting that development of CKD and dialysis dependency follow AKI. 1,2,7,9,67,81 Amdur et al. 82 tested the hypothesis that AKI, and specifically ATN, causes CKD. A total of 5404 out of 113,272 patients (US Department of Veterans Affairs database, 1999–2005) had diagnostic codes indicating AKI or ATN without background CKD. A diagnosis of ATN without background CKD was associated with a similar time to development of stage 4 CKD to that of a patient with early CKD. Twenty per cent of survivors of ATN rapidly progressed to CKD stage 4. Diagnostic codes of AKI and ATN were associated with a significant decline in function over time after hospital discharge. Survivors of AKI were more likely than controls to progress to late-stage CKD. The authors concluded that AKI might be an important cause of CKD. 82
If AKI is a cause of CKD, it seems logical that AKI may exacerbate pre-existing CKD. There are, however, difficulties in testing this hypothesis. A large number of risk factors for AKI are the same as those for CKD. AKI also occurs more frequently in an older population with greater burden of comorbidity, in which there may be greater risk of CKD progression anyway. Many studies looking at outcome following AKI concentrate on survival and subsequent ESRD development; however, resultant CKD and CKD progression are less well reported. These studies again depend on the definitions used.
Could minor episodes of AKI in the community, which are not acknowledged to have occurred because renal function is either not tested or not properly assimilated, be contributing to development and/or progression of CKD? The effect of ‘silent and discrete’ episodes of AKI in the community on CKD progression is presently unknown. There is growing evidence that ‘multiple hits’ may well contribute to progression in susceptible individuals.
Following an episode of AKI, Kidney Disease: Improving Global Outcomes (KDIGO) guidelines83 suggest that we should evaluate patients within 3 months for resolution of AKI, and at 3 months or after for new onset or worsening of pre-existing CKD. They also suggest that if patients do not have CKD they should be considered at increased risk, on the assumption that one AKI episode demonstrates ‘susceptibility’ and qualifies a high-risk population. Further research is warranted to inform the optimal follow-up period and to facilitate better understanding of the clinical consequences of AKI in patients with and without underlying CKD. 83
Why is acute kidney injury under the spotlight?
The concept of AKI, or, as it was previously, acute renal failure, is not new. There was renewed interest in acute renal failure dating from the now classical description of tubular degeneration and tubular pigmented casts, together with intact glomeruli, in patients crushed by fallen masonry during the London Blitz. 84 However, in the past 5–10 years there has been a growing impetus and focus on AKI both within the academic community and politically within government and the NHS. Within the Department of Health, NHS Kidney Care has brought together experts in AKI from across the country to form the AKI Delivery Group. They have increased awareness of AKI and actively promoted better clinical management. We also have the development of guidelines, for example the KDIGO AKI guidelines,83 the recent National Institute for Health and Care Excellence (NICE) guidance on AKI85 and the updated Renal Association Guidelines. 86 AKI has also now been incorporated as a clinical pathway in the Enhancing Quality Initiative.
So, why is AKI, which is not a new entity, now under the spotlight?
Definition
The first debate surrounding AKI concerned the definition. Over the years, one inherent problem in both diagnosing AKI clinically and reviewing and comparing studies published in the literature has been the numerous definitions used for AKI. 87 The use of these differing definitions in different locations with different populations has only worsened the problem. This also precluded the appreciation of the true problem of AKI in terms of incidence and outcomes.
Risk, Injury, Failure, Loss of function, and End-stage renal failure
In 2003, the Acute Dialysis Quality Initiative group published guidelines to define AKI as a 1.5-fold increase in serum creatinine levels, a decrease in estimated glomerular filtration rate (eGFR) of > 25% or a reduction in urine output to < 0.5ml/kg/hour over 6 hours. 58 They developed the RIFLE (Risk, Injury, Failure, Loss, and End-stage renal failure) classification to define patients by changes in serum creatinine level or urine output criteria. 58 Risk was defined as a 1.5- to 2.0-fold increase, injury as a 2.0- to 3.0-fold increase and failure as a > 3.0-fold increase in serum creatinine level. 58 Loss was defined as a complete loss of kidney function requiring RRT for > 4 weeks and end-stage renal failure as complete loss of kidney function for > 3 months. 58
The Acute Kidney Injury Network
In 2007, the Acute Kidney Injury Network (AKIN) modified the RIFLE criteria, defining three stages of AKI (Table 1): AKI stage 1, equating to the ‘R’ of the RIFLE criteria, with the inclusion of a 1.5-fold or 26.4 µmol/l (0.3 mg/dl) rise in serum creatinine levels; AKI stage 2, representing the ‘I’ of the RIFLE criteria; and AKI stage 3 representing the ‘F’ of the RIFLE criteria. 88 The ‘L’ and ‘E’ (end-stage renal failure) were redefined as outcomes.
Stage | Serum creatinine levels | Urine output |
---|---|---|
1 | ≥ 1.5–1.9 times baseline OR 0.3 mg/dl (26.4 µmol/l) increase |
< 0.5 ml/kg/hour for 6–12 hours |
2 | ≥ 2.0–2.9 times baseline | < 0.5 ml/kg/hour for ≥ 12 hours |
3 | ≥ 3.0 times baseline OR Increase in creatinine to ≥ 4 mg/dl (354 µmol/l) OR RRT OR In patients aged < 18 years, a decrease of eGFR to < 35 ml/minute/1.73 m2 |
< 0.3 ml/kg/hour for ≥ 24 hours OR Anuria for ≥ 12 hours |
The addition of a rise in 26.4 µmol/l to define AKI stage 1 was based on two large studies which demonstrated an independent association between an increase in serum creatinine of 26.4 µmol/l and in-hospital mortality. 34,63 The RIFLE and AKIN are consensus definitions that have now been validated and correlate well with patient outcomes. 89,90
Kidney Disease: Improving Global Outcomes
In the recent KDIGO AKI guideline, AKI is defined as a syndrome, including direct injury to the kidney as well as acute impairment of function. 83
The guideline defines AKI as:
-
increase in serum creatinine level of > 0.3 mg/dl within 48 hours, or
-
increase in serum creatinine level of > 1.5-fold above the baseline which is known or presumed to have occurred within 7 days, or
-
urine volume < 0.5 ml/kg/hour for 6 hours.
The importance of staging AKI (see Table 1) is stressed, as adverse outcomes worsen with increasing stage. 46–49,83,91–93
The definitions of both AKI and CKD are time dependent. For AKI there must be an increase in serum creatinine levels over a period of 2 (AKIN) to 7 (RIFLE) days. For CKD, GFR must be reduced for at least 3 months. These definitions may not capture all cases of AKI and CKD. Certain causes of AKI and CKD may lead to changes in serum creatinine levels and GFR over a time period outside those currently specified, precluding definition. These cases should not be neglected, as intervention may be required. For this reason, the KDIGO AKI Work Group proposed an operational definition for AKD to provide an integrated clinical approach to patients with abnormalities of kidney function and structure, and provide a diagnostic algorithm for defining AKD, AKI and CKD (Table 2). 83
Type of disease | Functional criteria | Structural criteria |
---|---|---|
AKI | Increase in serum creatinine level by 50% within 7 days OR Increase in serum creatinine level by 0.3 mg/dl within 2 days OR Oliguria |
No criteria |
CKD | GFR < 60 ml/minute/1.73m2 for > 3 months | Damage for > 3 months |
AKD | AKI OR GFR < 60 ml/minute/1.73 m2 for < 3 months OR Decrease in GFR by ≥ 35% or increase in serum creatinine level by > 50% for < 3 months |
Kidney damage for < 3 months |
NKD | GFR ≥ 60 ml/minute/1.73 m2 Stable serum creatinine |
No damage |
Baseline
Although we now have an internationally agreed on and validated definition of AKI, the time constraints of these definitions raise the big question and primary focus of the present debate in AKI, which concerns how we define the baseline kidney function of a patient. The absolute and relative rises in serum creatinine levels to define AKI are now used widely in clinical practice and research studies, allowing better comparison of data sets. However, what baseline kidney function are these rises from? The AKIN criteria suggest a rise in serum creatinine levels over a period of 2 days, whereas the RIFLE criteria suggest a rise over a period of 7 days. However, a large number of patients presenting acutely to hospital will not have had blood tests in the preceding 2, or in fact 7, days. The question and debate is then twofold: how far back do we look for a baseline kidney function and what value over this time period do we take?
A retrospective cohort study by Lafrance and Miller94 assessed 1,126,636 veterans (US Department of Veterans Affairs healthcare system) who were hospitalised at least once between 2000 and 2005. The highest serum creatinine level during hospitalisation was compared with the lowest using four different baseline periods (in-hospital only, 3, 6 or 12 months before admission). AKI was defined as a rise in serum creatinine level ≥ 1.5 times or an increase of 0.3–0.5 mg/dl over the baseline. 94 The cumulative incidence of AKI ranged from 12.5% (in-hospital baseline) to 18.3% (baseline up to 12 months before admission). By extending the baseline period to at least 3 months, the authors found that the discriminative power increased slightly (the c-statistic increased from 0.846 to 0.855; p = 0.001). They suggested the need for consensus regarding how baseline serum creatinine levels should be determined in database studies.
A clinician’s definition of the baseline kidney function is often achieved through the visualisation of serum creatinine level results graphically represented (Figure 2). The problem with using the lowest serum creatinine levels in the preceding 12 months (which is done to allow a computer algorithm to define AKI from creatinine level results in a structured database) is that a spuriously low result recorded on the pathology database, either from an error or, more often, from an event such as fluid loading (and hence dilution) during a previous hospital admission, will be taken as the baseline kidney function (see Figure 2a). This could, in fact, be significantly lower than the patient’s true baseline kidney function, leading to an incorrect trigger of a diagnosis of AKI on a new blood test.
FIGURE 2.
Errors in defining acute kidney injury as the lowest creatinine in the 12 months prior to the present test. (a) Here, the kidney function (creatinine) can be seen to be stable; however, two spuriously low results, which may be a result of error or more likely fluid loading and dilution may result in an incorrect definition of AKI; (b) here, there has been a stepwise deterioration in kidney function around 4 months previously (likely to be an AKI at that point in time), and since then the kidney function has been stable. However, using a 12-month baseline will continue define this as an acute event; (c) here, a progressive decline in CKD over the course of 12 months. Using the lowest creatinine in 12 months as the baseline will trigger a definition of AKI.

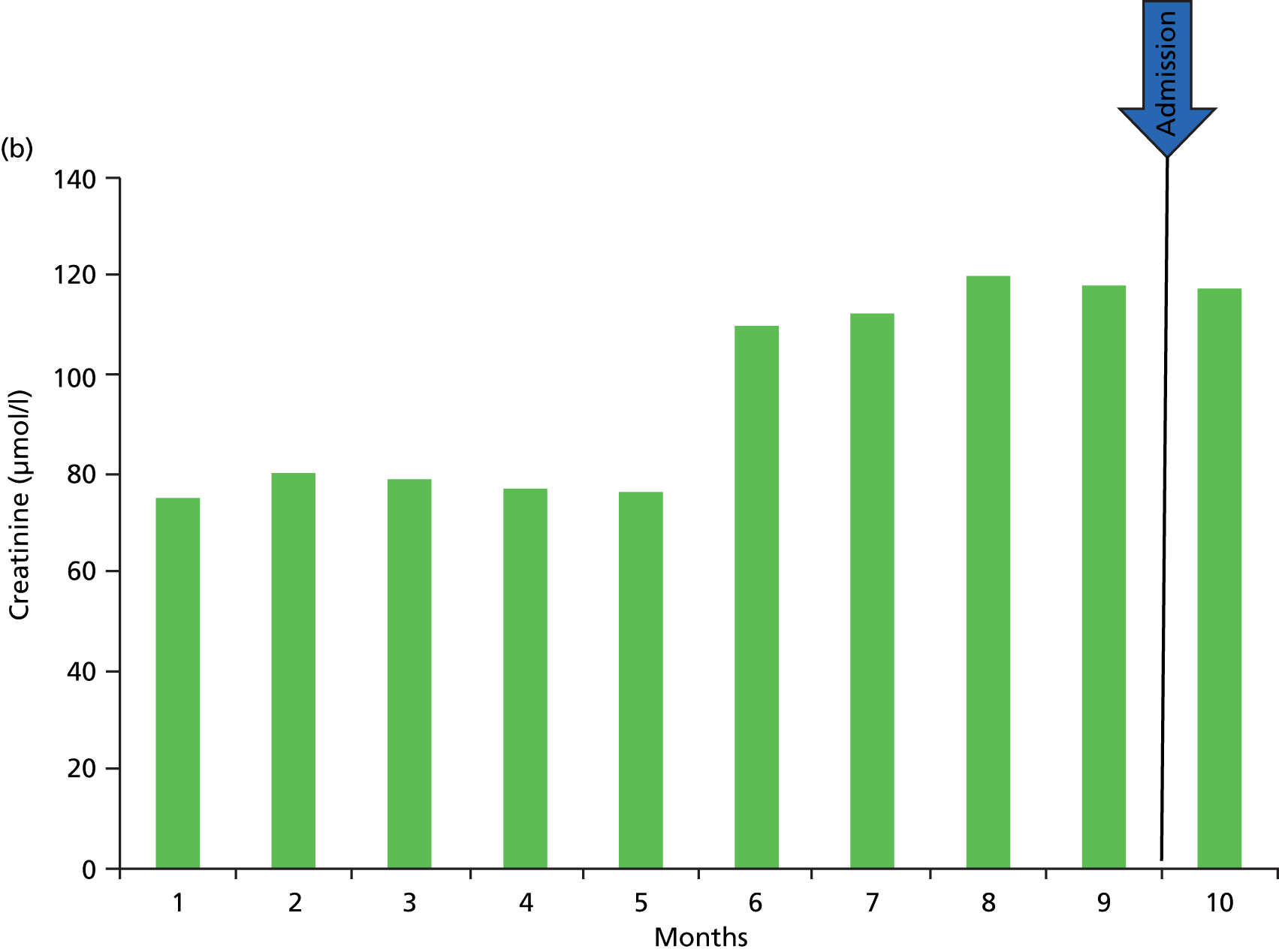

There is also the possibility that a patient with progressive CKD may trigger a diagnosis of AKI based on a baseline defined as the lowest serum creatinine level in the preceding 12 months, when actually the kidney function has slowly deteriorated over the 12-month period, but comparison of the present creatinine level with that of 12 months prior triggers a diagnosis of AKI (see Figure 2c). In the same way, within a 12-month period a patient may have a stepwise reduction in kidney function (probably owing to an AKI) and hence an increase in creatinine that then remains stable. However, a new serum creatinine test, although at the same level as the previous number of months, may be higher than the stepwise increase in creatinine in the past 12 months (see Figure 2b).
In an attempt to tackle some of these issues, other strategies have been suggested: taking the average of values between 7 and 365 days prior to admission;95 back-calculating reference serum creatinine for missing values from an assumed modification of diet in renal disease (MDRD) GFR of 75 ml/minute/1.73 m2,96 and (most recently) a method employing multiple imputation using known comorbidity strengthened by factoring in the lowest admission serum creatinine level. 97
If there are no serum creatinine results in the preceding 12 months, the KDIGO AKI guideline suggests that an estimated creatinine level can be used, provided there is no evidence of CKD. 83 However, there remain cases of CKD in the community that have not been previously appreciated; therefore, estimating baseline creatinine level may lead to diagnosis of AKI in patients with previously unrecognised CKD. These problems with definition make the assessment of AKI and CKD, and their complex interplay, more problematic.
One other point to note is the fact that serum creatinine is a poor biomarker of kidney injury, requiring 48 hours for levels to rise following insult. This emphasises the need for new biomarkers and point-of-care devices to facilitate early identification of patients, which would aid early intervention, as well as more accurate risk assessment to identify patients who may go on to develop AKI.
Although the debate of baseline kidney function in the definition of AKI continues, the now-accepted staging of AKI leads to the following question: when using these accepted definitions, what are the true impacts of AKI?
NHS England patient safety alert
NHS England released a patient safety alert, stage three directive on 9 June 2014, to ensure both that all acute NHS hospital trusts in England are alerting to AKI (by 9 March 2015) and that there is standardisation in the identification of AKI using a single algorithm (www.england.nhs.uk/aki-algorithm). Although similar, our study does not use the same algorithm because it was designed and the analysis was completed before the publication of this directive. In our work following this study we have been using the NHS England algorithm to validate our models so that when we implement the models in clinical practice in the next phase of our work we can ensure standardisation and generalisability.
National Confidential Enquiry into Patient Outcome and Death
From what we already know about AKI, it is apparent that early recognition and effective management of AKI is essential, a concept highlighted in the Renal National Service Framework. However, the 2009 National Confidential Enquiry into Patient Outcome and Death (NCEPOD) in the setting of AKI highlighted systematic failings in identification and subsequent management. 98
The aim of the NCEPOD study was to assess the care of patients who died in hospital with AKI in order to highlight deficiencies in care and provide recommendations for improving clinical management in the future. An advisory group, comprising nephrologists, anaesthetists, intensivists and general physicians, was brought together to review the care of these patients, with a focus on seven main themes:
-
diagnosis and recognition of AKI
-
recognition of risk factors associated with AKI
-
prevention of AKI
-
assessment of patients recognised as having AKI
-
management of established AKI
-
recognition and management of complications of AKI
-
organisational factors relevant to the treatment of AKI.
All NHS hospitals in England, Wales and Northern Ireland were expected to participate. Hospitals in the independent sector and public hospitals in the Isle of Man, Guernsey and Jersey also participated. The inclusion criteria were set as any patient aged 16 years or older who died in hospital between 1 January 2007 and 31 March 2007, inclusive, and who had a coded diagnosis of AKI. Patients were excluded if they were already receiving renal replacement therapy or if their admission was for palliative care from the outset. At each hospital, the NCEPOD local reporter, who acted as a liaison between the NCEPOD and hospital staff, facilitated the identification of these cases within the inclusion criteria, and then facilitated the dissemination of questionnaires and data collation for these patients. For each patient identified, a clinical questionnaire was sent to the clinician responsible for the patient’s care at the time of death and an organisational questionnaire was sent to each hospital. Photocopies of the patient’s case notes were also made and sent to the NCEPOD. The case notes and questionnaires were anonymised before the advisory group reviewed each case.
In total, 1518 patients from 215 hospitals met the inclusion criteria. Of these, 473 were subsequently excluded either for not being indicative of AKI or because the admission was for palliative care at the outset. In a further 69 cases, the case notes were reported as being lost or the consultant in charge of the patient at the time of their death had left the trust. This left 976 patients. A clinical questionnaire and/or case notes were received for 700 patients (72%).
Of the 700 patients, half were from the specialties of general medicine and elderly care medicine. In 14% of patients, it was the clinician’s opinion that the AKI was avoidable. In an overall assessment of care, only 50% of patients were assessed to have received a ‘good’ standard of care and, importantly, in the majority of cases in which the care was considered less than good, there was judged to be room for improvement in the clinical care rather than at an organisational level. This suggests inadequacies in the clinicians’ recognition of AKI and of its subsequent management.
Complications of AKI were missed in 13% of patients and, importantly, in 17% of patients the advisors concluded that the complications of AKI were avoidable. In 22%, the complications were managed badly. In relation to the management and assessment of AKI, the advisors found that there was an adequacy of investigation of AKI in only 67% of patients. The advisors also concluded that in one in six cases there was a failure to recognise the severity of the illness.
In patients who developed AKI post admission, one-fifth were deemed to have been predictable and avoidable, and in 43% of cases there was judged to have been an unacceptable delay in recognising AKI.
Although the NCEPOD report does have its limitations, notably the dependency on clinical coding and its inherent inaccuracies to define AKI, and a patient population in which the outcome in each case was death, the conclusions are very clear: there are currently significant deficiencies in the recognition and clinical management of patients with AKI. The NCEPOD report recommends risk assessment for AKI in all emergency admissions to hospital, and suggests that predictable and avoidable AKI should never occur. 98
The NCEPOD report, with its clear conclusions, has been a key factor in the growing impetus of and focus on AKI and the fact that AKI is now under the spotlight. This also leads to the question of how we can improve the clinical management of AKI. Although strategies can be put in place to alert clinicians to the presence of AKI and allow early intervention to improve clinical outcomes, as with any disease process, our ultimate aim should be prevention.
Risk prediction
In order to prevent AKI we must have a clear understanding of what factors convey a risk of AKI to the patient, and in what situations. This also then highlights time points in the clinical pathway at which risk assessment should be carried out and clinical intervention informed/directed.
As described, the 2009 NCEPOD report,98 in the setting of AKI, highlighted systematic failings in identification and subsequent management of AKI. 98 One of the report’s key recommendations was that risk assessment for AKI should be carried out for all emergency admissions. Following on from this report, the 2010 NCEPOD report, ‘An Age Old Problem: a review of the care received by elderly patients undergoing surgery’,99 suggested that when risk assessing patients prior to surgery the risk factors for acute kidney injury should also be taken into account and also suggested a need for post graduate education of physicians, surgeons and anaesthetists to include education of the assessment of risk factors for the development of AKI in elderly surgical patients’. 99
However, a number of patients present to hospital who already have AKI. The risk of AKI in these patients can be assessed as they enter the emergency department to ensure that patients at high risk have their kidney function checked, to confirm their underlying AKI. In patients who have established AKI, the risk assessment should be extended to determine which patients are at high risk of experiencing worsening AKI and hence are likely to require specialist or critical care intervention.
Once the most important of the large number of factors involved in the development of AKI, or of worsening AKI, have been identified, these can then be employed in risk models and risk scores to determine the individual risk of a patient and thereby more accurately guide clinical management. To date, the development of risk models in AKI has mainly focused on the clinical areas of cardiac surgery and radiology contrast scans.
In the disease process of AKI there are two points (Figure 3) at which we can intervene. When the patient experiences an event which carries a risk of AKI we can intervene in order to prevent the development of AKI, and when the patient already has AKI we can intervene in order to reduce the resultant sequelae of AKI and prevent worsening AKI. At these points of clinical intervention we can then provide an assessment of risk factors in order to guide clinical intervention in high-risk patients.
FIGURE 3.
Points of clinical intervention in the disease process of AKI.
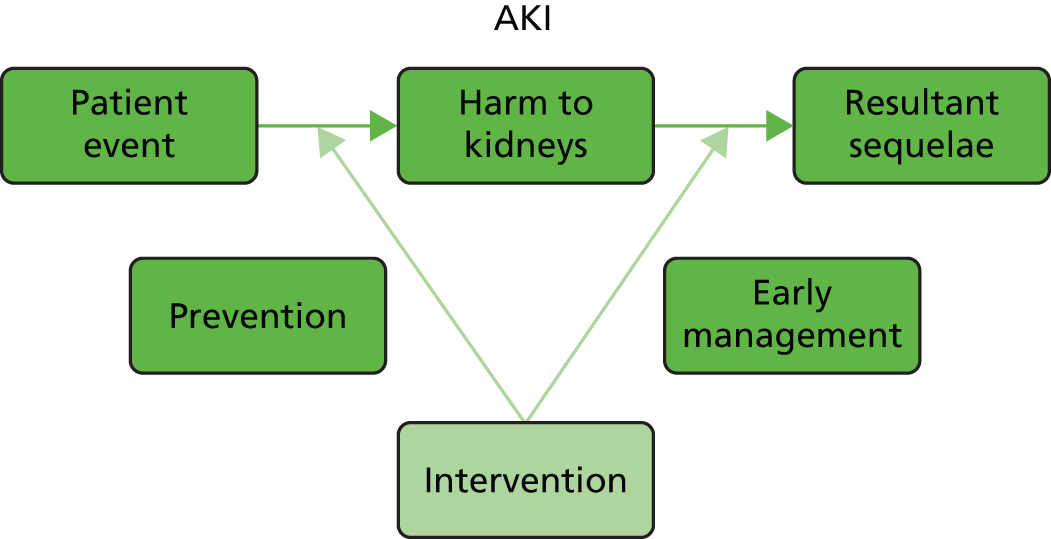
The KDIGO clinical practice guidelines for AKI published in March 201283 suggests that risk assessment should be carried out at four points:
-
before exposure to an insult
-
after exposure to an insult
-
on development of AKI
-
after recovery from AKI.
Before exposure to an insult
In clinical medicine there are certain procedures or treatments that a patient may experience as part of their clinical management that are essential in their care, but carry an inherent risk of precipitating AKI. Some insults may not be avoidable and in these cases the patient’s care can be optimised to reduce the risk of AKI. In some cases, when the risk of AKI is fully appreciated it may outweigh the benefit of the procedure or treatment. In other cases, although the benefit of a procedure or treatment may outweigh the risk, modifications in dosing or exposure may reduce the risk of AKI.
Risk assessing the patient in the context of the expected exposure to the insult can allow appropriate decisions to be made regarding the risk benefit of the procedure or treatment, modifications in dosing and exposure, and clinical optimisation prior to the procedure.
After exposure to an insult
After a patient is exposed to an insult, whether this is iatrogenic or the presenting disease of the patient, as it takes up to 48 hours for the creatinine level to rise in AKI, it may not be immediately apparent. In such cases we can predict which patients are at risk of developing AKI from the insult they have sustained. In these patients, we can provide clinical intervention in terms of both making management changes (Box 1) to aid prevention of AKI following the insult and ensuring that repeat kidney function checks are carried out to monitor for AKI.
Regularly assess fluid status.
Reverse hypovolaemia/hypotension.
Stop and avoid nephrotoxic agents (angiotensin-converting enzyme inhibitors, angiotensin receptor blockers, non-steroidal anti-inflammatory drugs).
Assess drug dosages with respect to level of kidney function.
Look for and treat infection early.
Recognise and treat hypoxia.
Check for acidosis.
Urinalysis.
Renal tract ultrasound.
Daily kidney function.
On development of acute kidney injury
When we know a patient has AKI, as shown by blood testing, this is not the end of the story. At this point we have the opportunity to intervene to make management changes [often the same as prior to the development of AKI (see Box 1)] to effectively manage the AKI and prevent both the worsening of AKI and the development of sequelae including morbidity and mortality. By risk assessing patients at this point and identifying patients at high risk of worsening AKI or resultant morbidity and mortality, we can focus clinical and specialist care on these patients.
After recovery from acute kidney injury
Following an episode of AKI, a patient may have complete recovery of their kidney function; however, as described Outcomes of acute kidney injury, in some patients this recovery may not be complete, resulting in new CKD or the progression of pre-existing CKD. Patients who have experienced an episode of AKI may also be at increased risk of morbidity, including cardiovascular disease, and mortality in the future.
In this case, risk modelling may be important to highlight patients who require follow-up to diagnose or manage resultant CKD, and other resultant morbidity, following an episode of AKI.
Defining risk in clinical practice
If each patient’s risk of AKI, or worsening AKI if already present, can be defined, then clinicians can be alerted to these patients and management changes and interventions can be put in place in order to prevent, or at least reduce, the risk of the patient developing AKI or worsening AKI.
In any disease process the ultimate treatment is prevention. In the disease process of AKI, risk factors include pre-existing comorbid disease, the presenting illness and also the treatment given for this illness. From these risk factors we can attempt to define a patient’s risk.
In the literature, the majority of the reports focus on the need for RRT after cardiac surgery. One of the first of these was by Chertow et al. ,100 who produced a risk model for predicting AKI after cardiac surgery based on a population of 40,000 patients who underwent cardiac bypass or valvular surgery in 43 Veterans Administration Hospitals in Virginia, USA. A risk stratification algorithm was formulated on the basis of interactions between potential risk factors. There were inherent flaws in the study cohort, specifically a lack of femaled and African American patients. Thakar et al. 101 produced a clinical risk score to predict post-cardiac surgery AKI requiring RRT, based on 33,217 patients who underwent cardiac surgery at the Cleveland Clinic between 1993 and 2002. The scoring system was derived based on 13 preoperative factors, which were weighted; the sum of the scores, ranging from 0 to 17, allowed for stratification of postoperative risk of AKI from low to high. The lowest-risk group (score of 0–2) had a risk for AKI requiring RRT of 0.4%, in contrast to those in the high-risk stratum (score of 9–13), who had a RRT risk of 21.5%. Table 3 demonstrates the risk factors.
Risk factor | Points |
---|---|
Female gender | 1 |
Congestive heart failure | 1 |
Left ventricular ejection fraction < 35% | 1 |
Preoperative use of IABP | 2 |
COPD | 1 |
Insulin-requiring diabetes | 1 |
Previous cardiac surgery | 1 |
Emergency surgery | 2 |
Valve surgery only (reference to CABG) | 1 |
CABG and valve (reference to CABG) | 2 |
Other cardiac surgeries | 2 |
Preoperative creatinine level 1.2 to < 2.1 mg/dl (reference to 1.2)a | 2 |
Preoperative creatinine level ≥ 2.1 (reference to 1.2) | 5 |
There is, however, a paucity in the literature of studies regarding the risk and risk prediction of AKI in unselected emergency admissions to a district general hospital. Finlay et al. 102 published a recent study of risk factors (Table 4) associated with AKI in patients admitted to acute medical units in a study conducted over two separate 24-hour periods in a total of 10 acute medical units. Forni et al. 103 developed a model for predicting AKI in a subset of medical patients admitted to a UK hospital, which included some physiological markers. Alongside this there have been reports in the literature of the development of AKI alert systems, but none presently demonstrate significant clinical benefit. 104,105
Risk factor | Description/explanation |
---|---|
Age | > 75 years |
Hypotension | SBP < 100 mmHg or decrease of ≥ 40 mmHg from usual baseline |
Sepsis | Two or more criteria for SIRS owing to suspected infection |
Hypovolaemia | Suggested by history or on clinical examination |
CKD | Stage 3–5 (eGFR < 60 ml/minute/1.73 m2) |
Vascular disease | History of atherosclerotic vascular disease |
Congestive cardiac failure | History of congestive cardiac failure or current presentation consistent with acute cardiac failure |
Diabetes mellitus | |
Jaundice | Clinical or biochemical jaundice |
Nephrotoxic medication | Nephrotoxic medication used in the week prior to admission |
Aims
The aims of this study were as follows:
-
Identification of AKI – accurately identify and report patients with AKI.
-
Predictive model – based on factors identified in primary and secondary care records and the admission characteristics of each patient, develop a predictive model to stratify the risk of (1) AKI on arrival in hospital, (2) developing AKI during the admission and (3) worsening AKI if it is already present.
-
Clinical algorithm – use the predictive model to develop an algorithm for all patients admitted to hospital to stratify them according to risk of developing AKI.
-
Integration into clinical practice – define the most effective way to incorporate the risk model into a clinical decision support system (CDSS) that can be integrated into everyday clinical practice. This will inform the follow-on study from this project.
The objectives were to define risk of AKI at three time points (Figure 4):
-
the point of admission to hospital – to predict patients who are already likely to have AKI and hence should have kidney function testing
-
following initial admission blood tests in the first 24 hours – to predict patients who will develop AKI, or worsening AKI if already present, within the first 72 hours of admission
-
at 72 hours – to predict patients who will develop AKI, or worsening AKI if already present, during the rest of the hospital admission.
FIGURE 4.
Acute kidney injury points of risk modelling.

Chapter 2 Methods
Quantitative methods
Design and theoretical/conceptual framework
This project involved both quantitative and qualitative methodology. Quantitative methodology was used to (1) formulate the predictive risk model and (2) validate the risk model in the East Kent population, and a second population and a NHS trust (Medway NHS Foundation Trust). Qualitative methodology was employed to plan the CDSS development and facilitate effective integration of the CDSS into everyday clinical care.
Setting/context
For risk model development and validation in the first population the study population comprised all patients presenting to the three acute hospitals of East Kent Hospitals University NHS Foundation Trust (EKHUFT; Kent and Canterbury Hospital in Canterbury, William Harvey Hospital in Ashford, and Queen Elizabeth the Queen Mother Hospital in Margate) in the calendar year of 2011, excluding maternity admissions and elective admissions. The renal tertiary referral centre is based at Kent and Canterbury Hospital. The secondary validation population included all patients presenting to Medway NHS Foundation Trust over the same time period and with the same exclusions.
Research governance
Ethics
The project received full ethical approval from the Kent Research Ethics Committee (reference number 10/H1101/89) on 20 December 2010. This approval is for patients in East Kent. We subsequently submitted a substantial amendment on 16 June 2011 to the Research Ethics Committee to include validation of the risk model in the Medway (Medway NHS Foundation Trust) population, and this was formally approved on 13 July 2011. The project was also reviewed and had agreement of methods from the National Information Governance Board. The ethical considerations in this project were:
-
Consent – the risk model development involved retrospective assessment of patient data of all admissions to hospital over a 12-month period. For a retrospective analysis of this type, it would not have been appropriate or practical to seek consent from all patients previously admitted to hospital. We had the support and endorsement of our medical director and chief executive of the trust/primary care trust and our Patient Safety Board to run this study.
-
Confidentiality – patient information was extracted and stored anonymously under a unique identification number on a secure server. We had confirmation from our Caldicott Guardian that the project satisfied the information governance issues of confidentiality and data security.
There were no potential risks or burdens for research participants as the risk model development used retrospective data.
Public and patient involvement
We had public and patient involvement in the study design process; however, as this study was a mathematical analysis of retrospective data there was no public and patient involvement during the study.
Data collection
Prior to study commencement and funding applications, an assessment had been made of the data set available for the study in terms of database access and variables available. Following commencement of the study, interfaces were developed with the following clinical systems/databases to enable data extraction.
Hospital episode data
The hospital data warehouse holds data from coded episodes of care on all admissions and outpatient activity in East Kent. From these data, the Hospital Episode Statistics and the Secondary Uses Service data that were collected nationally were derived. A modified Charlson Comorbidity Index score was calculated using a modified version of the Charlson score developed by Dr Foster Intelligence™. 106 The variables available from this database are demonstrated in Appendix 1.
Pathology
The pathology database holds records of blood tests in East Kent requested in primary and secondary care in both the outpatient and inpatient setting. All NHS blood-testing results in East Kent are recorded on this database. The variables available, and thought to be of clinical importance, from this database are demonstrated in Appendix 1.
Electronic discharge notification
At EKHUFT all discharges from hospital are recorded on an electronic discharge notification, which includes a summary of the admission and the medications the patient is taking on discharge. This summary is sent to the general practitioner in primary care at discharge. The variables available from this database are demonstrated in Appendix 1.
Operation data
Every operation performed in theatres at EKHUFT is recorded in an electronic operation database. The system records the date and time of procedure, duration of procedure, duration of anaesthetic, surgeon and anaesthetist, name of procedure, International Classification of Diseases, Tenth Edition (ICD-10) and Read code for the procedure and American Society of Anaesthesiologists physical status classification score (see Appendix 1). Each operation was coded with a severity score ranging from 1 (least severe) to 5 (most severe) (see Appendix 1).
Data analysis
The main aim of this study was the development of predictive models for identifying and stratifying the risk of AKI at the point of, and during, hospital admission. These models included a large set of potential risk factors identified from secondary care records, as well as admission characteristics of each patient. Both traditional and Bayesian modelling techniques were assessed in order to develop these models. We will describe these methods separately.
Traditional methods
Traditional methods were employed using Stata (version 12.1; StataCorp LP, College Station, TX, USA) to develop the following models:
-
risk model 1 – the point of admission to hospital
-
risk model 2 – predicting new AKI at 72 hours
-
risk model 3 – predicting worsening AKI at 72 hours.
Traditional model development
Risk model 1: the point of admission to hospital
The data analysis considered emergency admissions for patients without pre-admission AKI. Patients with pre-admission AKI were omitted from the analysis. However, patients whose pre-admission AKI status was unknown were kept in the data analysis. Non-emergency admissions were also omitted, as were admissions associated with childbirth and pregnancy. Patients with no information on AKI at admission were also omitted from the analysis.
After omissions, the full data set was split into a ‘development’ data set for constructing the risk model and a ‘validation’ data set on which to evaluate the performance of the model. To allow a larger number of data on which to construct the model, a 3 : 1 ratio was employed, with the development data set being the larger of the two. Selection of admissions to one of the two data sets was done at random.
The outcome variable was AKI stage, which was considered as an ordinal measure, the categories being:
-
no AKI
-
AKI stage 1
-
AKI stage 2
-
AKI stage 3.
To allow for the ordinal nature of the outcome, all analysis was performed using ordinal logistic regression. There were multiple admissions for some patients; to allow for this in the data analysis, robust standard errors were used.
Initially, the individual association between each factor and AKI stage was examined individually in a series of univariable analyses (see Chapter 3, Risk model 1: the point of admission to hospital).
There were no missing data for the key demographics (e.g. age, sex). In some cases the primary diagnosis was missing, and these patients would have been excluded from the final model. For the blood test variables missingness was deemed to be informative (i.e. data missing not at random) and hence missing values were given their own category.
Subsequently, the joint association between the factors and AKI stage was examined in a multivariable analysis. Variance inflation factors were used to assess collinearity between the predictor variables. Where collinearity was found, action was taken to allow for this. This included either excluding variables from this stage of the analysis or combining variables together. A backwards selection procedure was used to retain only the statistically significant variables in the final model.
Risk model 2: predicting new acute kidney injury at 72 hours
A specific patient group was selected for risk model 2, to predict AKI at 72 hours based on data available up to the end of the first 24 hours after hospital admission. The following patients were excluded from the data set:
-
non-emergency admissions
-
patients with pre-admission AKI
-
patients with AKI at admission
-
childbirth/pregnancy admissions
-
patients with no information on AKI at 72 hours.
After omissions, the full data set was split into a ‘development’ data set for constructing the risk model and a ‘validation’ data set on which to evaluate the performance of the model. To allow a larger number of data on which to construct the model, a 3 : 1 ratio was employed, with the development data set being the larger of the two. Selection of admissions to one of the two data sets was done at random using pseudo-random numbers.
The outcome variable was AKI stage at 72 hours, which was considered as an ordinal measure, the categories being:
-
no AKI
-
AKI stage 1
-
AKI stage 2
-
AKI stage 3.
To allow for the ordinal nature of the outcome, all analysis was performed using ordinal logistic regression. There were multiple admissions for some patients; to allow for this in the data analysis, robust standard errors were used.
Initially, the individual association between each factor and AKI stage was examined individually in a series of univariable analyses.
Subsequently, the joint association between the factors and AKI stage was examined in a multivariable analysis. Variance inflation factors were used to assess collinearity between the predictor variables. Where collinearity was found, action was taken to allow for this. This included either excluding variables from this stage of the analysis or combining variables together. A backwards selection procedure was used to retain only the statistically significant variables in the final model.
Risk model 3: predicting worsening acute kidney injury at 72 hours
The third risk model is designed to predict worsening AKI by 72 hours in patients with AKI stage 1 or AKI stage 2 at admission. Note that AKI stage 3 patients could not get any worse and so were not included in the analysis.
A specific patient group was selected for the 72-hour increase in AKI risk model. The following patients were excluded from the data set:
-
non-emergency admissions
-
patients with pre-admission AKI
-
patients with no AKI at admission
-
patients with AKI stage 3 at admission
-
childbirth/pregnancy admissions
-
patients with no information on AKI at 72 hours.
After omissions, the full data set was split into a ‘development’ data set for constructing the risk model and a ‘validation’ data set on which to evaluate the performance of the model. To allow more data on which to construct the model, a 3 : 1 ratio was employed, with the development data set being the larger of the two. Selection of admissions to one of the two data sets was done at random using pseudo-random numbers.
The outcome was defined as a worsening in AKI, considered as a binary variable. A worsening in this case was regarded as a higher AKI stage. In other words, worsening was defined as a change to AKI stage 2 or AKI stage 3 for patients with AKI stage 1 on admission, and a change to AKI stage 3 for patients with AKI stage 2 on admission.
As there were repeated admissions for some patients, the analysis was performed using multilevel statistical methods. Two-level models were used, with admissions nested within patients. To allow for the binary nature of the outcome, all analysis was performed using multilevel logistic regression.
Initially, the individual association between each factor and AKI stage was examined individually in a series of univariable analyses. Subsequently, the joint association between the factors and AKI stage was examined in a multivariable analysis. Variance inflation factors were used to assess collinearity between the predictor variables.
Traditional model validation
Validation in this population
The risk models for AKI were developed using three-quarters of the original patient group meeting the eligibility criteria. The model was, therefore, validated on the remaining one-quarter of the data.
The validation concentrated on two aspects of the model, the ability to discriminate between the cases with a high and low risk of AKI, and the calibration of the model, whether or not the risk of AKI from the fitted model matches that in the observed data.
The model was fitted considering AKI on a 4-point scale: no AKI, AKI stage 1, AKI stage 2 and AKI stage 3. Thus, the model can be used to obtain predicted probabilities of being in each of the four AKI categories. Although this is useful, it is harder to validate the model with a 4-point outcome scale. Thus, for the purposes of validation, two different cut-off points were used. First, the probabilities were combined to give the probability of AKI (AKI stage 1, 2 or 3), which was compared with the occurrence of AKI in the data. A second set of analyses split the data into no AKI and AKI stage 1 versus AKI stage 2 and AKI stage 3, and compared this with the occurrence of AKI stage 2 and AKI stage 3 in the data.
The first approach used was to split the validation data set into risk groups based on the predicted probabilities. For each analysis, four different risk categories were considered. Within each risk category, the actual occurrence of AKI was assessed and compared with the predictions; this assesses both the discrimination and the calibration of the model.
Second, the discrimination between high- and low-risk cases was assessed by calculating the area under the receiver operating characteristic (ROC) curve. The area under the ROC (AUROC) curve was calculated and interpreted. One suggested interpretation of the area under the curve (AUC) values is:
-
0.5–0.6: no discrimination
-
0.6–0.7: poor discrimination
-
0.7–0.8: fair discrimination
-
0.8–0.9: good discrimination
-
0.9–1.0: excellent discrimination.
Note that the AUROC value is equivalent to the c-statistic, sometimes used for model assessment.
A final set of analyses examined the difference between the observed outcome and that predicted by the model using the Hosmer–Lemeshow test. This divided the admissions into the same four categories described earlier and compares the observed number and the predicted number in each category. A non-significant result would imply little difference between observed and expected numbers, and thus a good fit of the model to the data.
Validation in a second population
The population demographic in East Kent is older and has fewer members of ethnic minorities than the general population of England. It was, therefore, important that we validated our models in a second population in order to assess the generalisability of the models across the NHS. For our second population we chose Medway NHS Foundation Trust, which constitutes both a different demographic population and a different NHS trust from which to extract data.
The method of validation is equivalent to that used in the East Kent data set. The model assessed differs slightly from that detailed in the East Kent data set, as it excludes the number of drugs given, which was not measured in this data set.
Bayesian methods
Bayesian methods were employed, using MATLAB 2014 (MathWorks, Cambridge, UK) statistical software, to develop the following risk models:
-
risk model 1 – the point of admission to hospital
-
risk model 2 – predicting new AKI at 72 hours.
The response variable in the case of risk model 1 was AKI on admission, and for risk model 2 it was AKI at 72 hours. AKI was treated as a binary variable. All of the models considered were logistic regression models. The usual assumptions applied, and these included:
-
The link function of the true conditional probabilities and the independent variables was the logit function.
-
All predictors that were considered important were included.
-
Collinear variables were avoided.
-
There were no errors in the measurement of all possible predictors.
-
The episodes included in the database were independent.
-
All possible predictors are not linear combinations of each other.
Bayesian model development
As the primary variable consisted of a nominal variable with 19 categories, it was transformed into 19 dummy variables, one for each category/type of primary diagnosis. This allowed us to independently access the effect of each diagnosis on the response variable.
We followed the usual process of all other Bayesian analyses; that is, we worked out the likelihood function of the data, placed a prior distribution over all of the unknown parameters and used the Bayes theorem to calculate the posterior distribution over all parameters. The Bayesian framework should more naturally allow for modelling biases and systematic errors, and does not suffer from the large sample constraint (i.e. we do not need to worry about how large our sample should be in order to be able to carry out inference on the parameters). The results of a Bayesian analysis are direct statements about the quantities of interest: in our case, the probability of developing AKI.
The likelihood used here matches that of the classical approach; therefore, the likelihood contribution from the fifth episode is:
where Y is the binary response variable, X1, X2, . . . Xp are the predictor variables, and b0, b1, . . ., bp are the unknown predictor coefficients (the unknown parameters). As the episodes are assumed to be independent of each other, the likelihood function over the whole data set will then be the nth product of the above equation.
At the inception of the project we wanted to place an informative prior on the unknown coefficients. However, the study of predicting AKI is in its infancy, particularly with respect to Bayesian methods, and a strong opinion as to what characteristics the prior distribution should have could not be agreed; the approach for variable selection problems is to employ a normal prior. We therefore selected a normal distribution prior for the unknown coefficients and within that incorporated the stochastic search variable selection (SSVS) approach described in George and McCullogh. 107 The SVSS approach specifies a hierarchical mixture prior that in turn is informed by the data to assign a larger posterior probability to the subset of predictors that are more likely to have an effect on the response. The most promising subsets of predictors can be identified as those with higher posterior probability. The Gibbs sampler was then used to indirectly sample from this posterior distribution on the set of possible subset choices. The most promising predictors can be identified by their more frequent appearance in the Gibbs sample. This means that the major advantage of SSVS is the fact that it allows for model averaging while avoiding the problem of calculating probabilities for all 2p subsets (p is the number of predictors). The complete set-up of our Bayesian logistic regression SSVS approach is as follows:
The latent variable is gj and defines if the jth variable is included in the model. A criticism of this approach is that it is necessary to choose the value of s2. If s2 is too large then we are faced with the danger of overfitting. As we have standardised the continuous variables, we chose s2 = 1. We have assumed a priori that five variables should be in the model, adhering to the beliefs of the clinical team. This then implied that p = 5. We feel that this is a reasonable choice as we want to avoid hj being too large.
Bayesian model validation
To assess the predictive accuracy of the models, we randomly divided the data sets in half: half was used for in-sample estimation/development and the other half used for out-of-sample prediction. The out-of-sample prediction was made in terms of sensitivity and specificity; that is, we calculated the probability that the model accurately predicts episodes with AKI and the probability that the model accurately predicts episodes without AKI.
Although there seem to be large numbers of missing data in the analysis, this appears to be attributable to failure to conduct a specific test rather than to data being missing in the usual respect. In order to explore the impact of these data and the fact that they were not missing at random, we created dummy variables indicating the absence or presence of a particular test result and explored the impact of this on the outcomes as a sensitivity analysis.
Qualitative methods
The exploratory arm of the study employed both focus group and individual interview designs. The purpose of this was to inform and plan the follow-up study, adopting a user involvement approach. The aim of the qualitative methodology was to identify perceptions of the AKI alert system presently in place at the EKHUFT and explore the best communication and information pathways that would permit an alert system to both alert and provide actionable recommendations to clinicians for decision-making. This will allow accurate planning of the new alerting system, which will include AKI and AKI risk alerting following this study, to ensure effective integration into everyday clinical practice.
Sample
The sample consisted of six renal consultants for the individual interviews and six outreach nurses who attended the focus group. All of the consultants worked across the three hospitals within the trust, and there was representation from all hospitals from the outreach nurses; however, the nurses did not work across all three sites. The consultants used the alert system to identify patients with the more serious AKI stage 3 and alert medical teams to offer advice and review if required, and the outreach nurses identified AKI stage 2 patients and provided clinical review of the patients, whose condition was more amenable to preventative action, on the ward.
In terms of the accessibility of the consultant and nurse groups, it was established early on that different qualitative approaches would need to be used on account of their individual availability and potential to meet together. The consultants had to cover three sites and group meetings were difficult to convene; therefore, interviews were the method of choice. Conversely, the outreach nurses were difficult to capture individually but attended a training session as a group once per month, which provided an opportunity for a focus group. Tuning different methods to the requirements of the population group, in order to maximise attendance and enrich data, is considered to be good practice. 108
Instrumentation and data collection
For the focus group, a schedule was developed that explored perceptions of the impact on clinical practice, aiming to identify the best methods for delivering the alerts and recommendations. This covered aspects such as accessibility of information, hardware, whom the recipient should be (junior doctor or consultant), what form the alert should take (additional e-mail or text), how to avoid alert fatigue and alerts being ignored. The focus group lasted 1 hour and was facilitated by an experienced researcher from a university external to the clinical team. Interviews took place at a convenient time and location, and were carried out by the same experienced researcher.
The template and coding framework for both the focus group and the interviews has been included in Appendix 3.
Data analysis
Data consisted of six 30-minute interviews and a 1-hour focus group. The data were transcribed verbatim and subjected to a thematic analysis using a predetermined theme, derived from the interview schedule, as an initial template for analysis. Clinical team members were not involved at all in the data collection or analysis of the data; this was undertaken by a researcher from a university external to the clinical team. Regarding the interview and focus group schedules, sections 1, 2 and 4 are identical. Section 3 has been altered to account for the differing clinical roles in the project and communication experiences (i.e. with different people at different times) regarding exposure to the project. There are, however, similarities, as both sections focus on communication with teams, changes to clinical practice and impacts. Although it is accepted that data obtained from focus groups are influenced by group dynamics and consensus, data sources from different qualitative methodological approaches can be blended and contrasted, provided that they are at first analysed thematically in a separate manner. An overarching, thematic pattern matching can be used to determine an explanation of the data, which is the method used here. 109
The analytical approach taken was Flick’s content analysis,110 whereby themes and subthemes were categorised within a pre-existing template (within the instrumentation). With this approach, however, care must be taken not to artificially represent data within the template but to introduce new themes when identified. This approach required peer review to ensure analytical trustworthiness, which was conducted within the research team. The analysis from the focus group concentrated on the identification of best methods for delivering the alerts and recommendations in order to inform the AKI and AKI risk alert system development and implementation in the follow-up study.
We would aim to have two further focus group waves in our next clinical intervention study to deliver AKI and AKI-risk alerting to clinicians at the point of care. The qualitative analysis described here is, therefore, part of a larger longitudinal qualitative research design. Longitudinal qualitative research involves repeat interviews or observations of, ideally, the same research subjects over time. 111 In recent years, longitudinal qualitative research has been used in a number of health-related areas to generate rich data and a deeper understanding of people’s perspectives and experiences and how and why these may change over time in order to improve practice. 112–115 Rather than comparing findings at a number of distinct moments, longitudinal qualitative research is concerned with the comparison of different, continuous processes of change.
The benefits of the longitudinal design will be that it will permit the same participants to be involved in identifying practice challenges and solutions, in developing methods for how alerts and recommendations can be best delivered for action, and for examining and reflecting on the effects with regard to practice change as well as system evaluation and improvement. The qualitative aspect of both this and the follow-on study will strengthen the production of potentially transferable practice guidelines and system accessibility across the NHS.
The focus group method is a favoured approach in the health-care setting not only for expanding ideas, but also for gaining consensus on views and promoting good practice. 108 This particular research study promotes new and innovative ideas that may benefit from being explored within a group, particularly with a longitudinal approach. Variations in perception and experience will encourage deeper discussion and illuminate impacts, as well as reveal the nature and cause of practice changes in relation to the intervention.
Chapter 3 Quantitative results
Clinical practice algorithm development
Points of decision-making
The study was designed to develop and validate risk models to define risk of AKI or of worsening AKI during hospital admission. We determined three time points during the period of admission when significant clinical decision-making takes place at which the use of risk models would have the greatest impact on clinical care and patient management. These time points are described below.
The point of admission to hospital
The model applied in this case (referred to as risk model 1) uses all electronic data up until the point of admission (and the reason for admission to hospital) to determine risk of patients already having AKI on admission. In this way, the model is guiding the testing of kidney function to ensure that patients who are likely to have AKI have their kidney function tested to unmask the condition and allow efficient appropriate clinical intervention to treat the AKI.
After 24 hours of admission
These models use all electronic data both prior to admission and up to 24 hours after admission to determine the risk of developing AKI (model 2) or of worsening AKI (model 3) in the first 72 hours after admission. At the 24-hour point, patients will be admitted to the ward and are likely to then be reviewed by the admitting clinical team on the post-take ward round, or by the ward team on their ward round of new patients. The models guides clinical management at this point to define patients at risk of AKI who require:
-
Management changes to include the stopping of nephrotoxic medication, fluid assessment and ensuring fluid replete, monitoring of blood pressure and ensuring adequate blood pressure and appropriate use of antihypertensive drugs.
-
Daily renal function testing to observe for development of AKI. If there is consideration at this point of discharge from hospital, the models at this point may inform the discharge decision or, if discharge is still intended, guide follow-up in primary care to observe for AKI (and also management of medications that may have been stopped temporarily while the risk of AKI exists).
After 72 hours of admission
The purpose of this model was to predict patients who will develop AKI, or worsening AKI if already present, during the rest of the hospital admission. During the progression of this study, the clinical experts on the board (informed by the results of the risk modelling) determined that this point of risk assessment would not add clinical benefit for a number of reasons:
-
The risk models at this point were not sufficiently accurate to determine risk and guide clinical management, which are also related to and a consequence of the other reasons.
-
Most patients remain in hospital for < 3 days (72 hours). For those who do stay in hospital over 72 hours, the period of admission may range from 3 days up to as high as 90–365 days. It is very difficult to determine risk of developing AKI in a widely varying time period, most importantly because these patients will develop new conditions and changes in blood results (variables in the risk models) after 72 hours, which change their risk and cannot be accounted for.
Clinical alerting
The purpose of the risk models is to guide clinical management. This will be achieved through alerting clinicians at the point of care. These systems have been developed as part of this project, but are awaiting formal assessment in a trial setting. The clinical alerting system will not solely alert to risk of AKI; it will also alert to patients with established AKI and provide clinical guidance for improving the management of these patients and reducing both the progression of AKI and the development of resultant sequelae. It is therefore important that both alerting to AKI and alerting to AKI risk are brought together in a clinical practice algorithm to guide both the alerting and the clinical management pathway following the alert.
Alerting at the point of admission
Initial risk model development for the point of admission included pre-admission AKI as a variable in the models. As would be expected, if a patient has AKI just prior to admission then there is a high risk that they will still have AKI at the point of admission. The initial models developed confirmed that the variable ‘pre-admission AKI’ was by far the strongest predictor of AKI on admission and diluted other variables in the model. As part of the clinical practice algorithm, the decision was made by the clinical experts on the project team that all patients with pre-admission AKI should be alerted by the alerting system as ‘pre-admission AKI’, irrespective of risk assessment. In the cases of these patients, renal function testing should always occur on admission and the management changes above should be implemented. Patients with pre-admission AKI were, therefore, removed from the admission models for predicting AKI, and hence the population of assessment for the point of admission models included only patients without pre-admission AKI or patients who did not have pre-admission AKI status determined because they did not undergo renal function testing pre admission.
Alerting after 24 hours of admission
For patients who have AKI on admission, the clinical alerting system will suggest appropriate management interventions as above listed (see Points of decision-making), including daily renal function testing. Again, as part of the clinical practice algorithm, patients with AKI on admission were removed from the risk models at 24 hours to predict AKI at 72 hours, as these patients have already been highlighted by the system. Moreover, including AKI on admission as a variable in a model to predict AKI at 72 hours would be highly predictive, dilute the other variables and reduce the clinical utility of the system, especially as these patients will already be flagged up by the system anyway.
This led to the development of the clinical practice algorithm (Figure 5) to guide:
-
AKI alerting
-
patients to be assessed by the risk models at the point of admission and at 24 hours after admission
-
appropriate renal function testing
-
appropriate management interventions.
FIGURE 5.
Acute kidney injury clinical practice algorithm. A&E, accident and emergency.
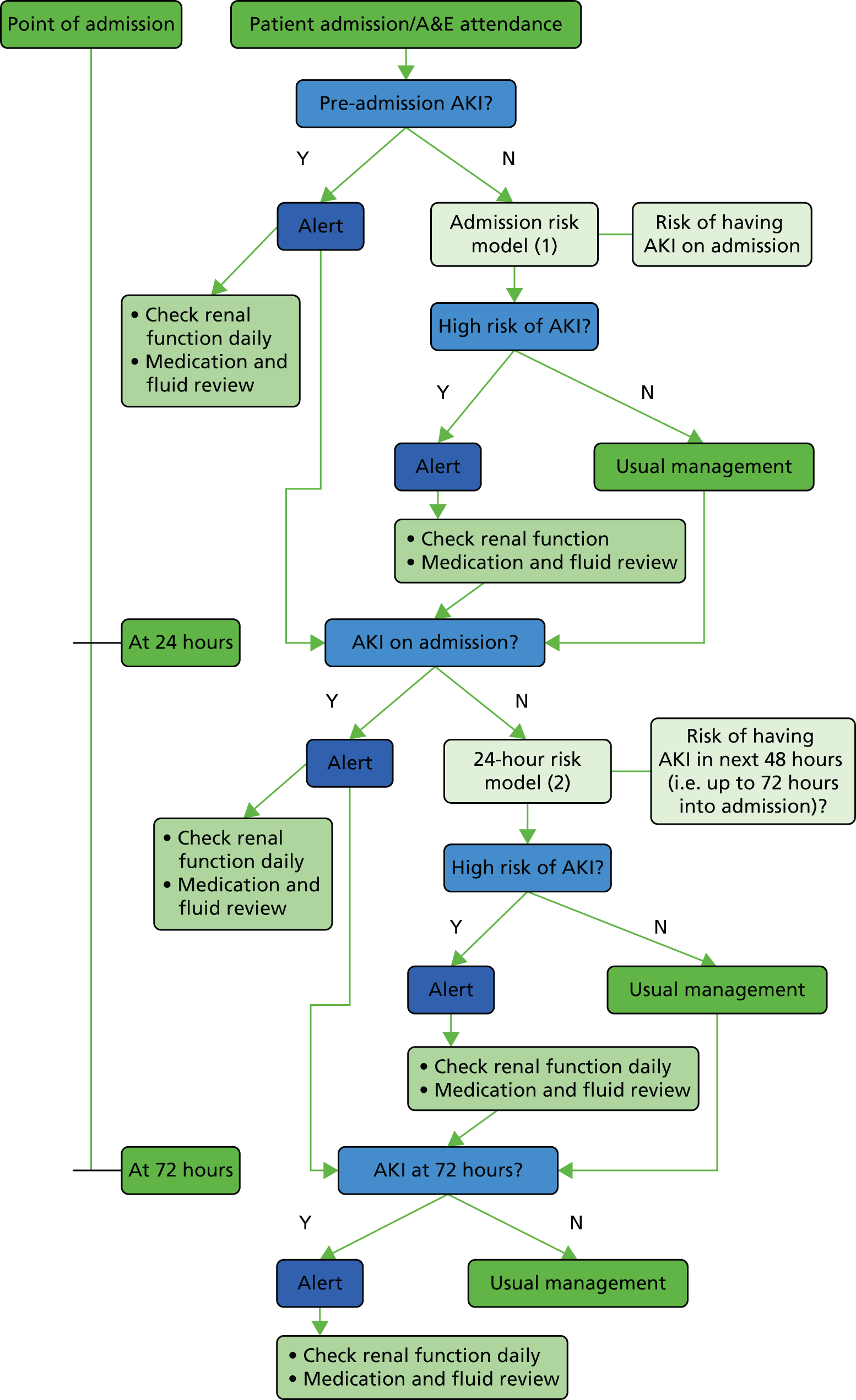
Relationships
The first stage of the risk modelling work was to determine relationships between risk factors and AKI. This was carried out following the definition and extraction of appropriate variables for the model. Of key importance was assessing the relationship between pathology results and AKI. Once we had established a normal range, variables were converted from continuous to categorical. Rather than simply using the laboratory normal range, the point at which each variable became associated with AKI was used; in the majority of cases this assessment agreed with laboratory normal ranges. For each of the pathology blood tests we performed a univariate analysis to create a plot of blood-test value on the x-axis and risk of AKI on the y-axis. The clinical experts in the project group then assessed this in order to define the normal ranges for the tests.
In the risk models, the options for a given pathology variable were therefore:
-
not tested
-
normal
-
abnormal.
The assessment of the pathology tests in this way is reported in Appendix 2.
Traditional risk modelling analysis
The purpose of the first risk model is to both guide renal function testing and highlight patients most likely to benefit from detailed review with respect to AKI at the point of admission. This model uses electronic data from prior to admission, as well as the reason for hospital admission, to predict AKI already being present at the point of admission. In line with the clinical practice algorithm already discussed, patients who have confirmed AKI pre admission (in the 2 weeks prior to admission to hospital) will automatically be highlighted by the AKI alert and CDSS as having pre-admission AKI and the need for urgent retesting and clinical review. Therefore, the population assessed by this first risk model at the point of admission excludes patients with pre-admission AKI, but includes patients with no testing of renal function pre admission.
The variables used in this first model are included in Appendix 3, Table 32. We used both traditional and Bayesian methods of risk model development to determine the most effective method of providing risk assessment in this setting. We will present the results of these models separately.
Risk model 1: the point of admission to hospital
Development
After exclusions from the full database (as described in Chapter 2), three-quarters of the data set was randomly selected for the development database. This consisted of 27,532 admissions with available AKI-on-admission data. These admissions occurred in 20,330 different patients.
A series of separate univariable ordinal logistic regression analyses were performed to examine factors associated with AKI on admission. A summary of the analysis results is given in Appendix 3, Table 32. The sizes of effects are reported in the form of odds ratios. The odds ratio is calculated as the exponential of the parameter estimates (beta). For categorical variables, these give the odds of being in the next highest outcome category (e.g. AKI stage 1 rather than no AKI, AKI stage 3 rather than AKI stage 2) for each category relative to a baseline category. For the continuous predictor variables, the odds ratios represent the relative change in the odds of being in the next highest category for a given increase in that predictor. The p-value indicating the overall significance of each variable is also reported.
The results in Appendix 3, Table 32, are interpretable for the categorical predictor variables. The results for the continuous variables are less interpretable, and the results for these variables are best showed graphically.
The graph in Figure 6 shows the relationship between age and the probability of AKI. For graphical purposes, the occurrence of any AKI is plotted.
FIGURE 6.
Risk model 1: relationship between age and probability of AKI in the univariable analysis.

A similar graph for baseline eGFR is shown in Figure 7.
FIGURE 7.
Risk model 1: relationship between baseline eGFR and probability of AKI in the univariable analysis.

The next stage in the analyses examined the joint association between the variables and AKI stage in a multivariable analysis.
The collinearity between the different predictors was examined. The examinations suggested collinearity between platelet count (PLT), white blood cell count (WBC) and haemoglobin (Hb) levels. Additionally, collinearity was found between potassium (K) and sodium (Na). The nature of the collinearity was that these tests were performed on the same patients, and so the ‘not measured’ category was almost equivalent where collinearity was found.
Before the main multivariable analysis was performed, an additional analysis was performed, examining the association between PLT, WBC and Hb levels on AKI stage on the subgroup of patients with all tests performed. The analyses suggested that only Hb was statistically significant, with WBC and platelets not significant after adjusting for Hb. As a result, only Hb was considered for the multivariable analysis. A similar analysis was performed for K and Na in the subgroup where these variables were measured. This analysis suggested that both were independently associated with AKI stage. As a result, a combined variable was derived, consisting of the following categories: one/both tests not measured, both tests normal, Na only abnormal, K only abnormal and both tests abnormal.
A backwards selection procedure was used to retain the statistically significant variables in the final model. This procedure omitted the following variables (in order of removal):
-
wound swab/fluid aspirate
-
combined Na/K
-
calcium
-
gender
-
faeces
-
sputum
-
number of outpatient appointments.
The final model is summarised in Table 5.
Variable | Category/term | Odds ratio (95% CI) | p-value |
---|---|---|---|
Agea | Linear term | 3.35 (1.82 to 6.16) | < 0.001 |
Quadratic term | 0.89 (0.81 to 0.98) | ||
Cubic term | 1.003 (0.998 to 1.009) | ||
Admissions (last 30 days) | No | 1 | < 0.001 |
Yes | 0.82 (0.74 to 0.91) | ||
Admissions (last 2–12 months) | 0 | 1 | < 0.001 |
1–2 | 1.28 (1.16 to 1.42) | ||
3–5 | 1.54 (1.36 to 1.75) | ||
6+ | 2.01 (1.70 to 3.80) | ||
6+ | 1.67 (1.52 to 1.84) | ||
Primary diagnosis | Neoplasms | 1 | < 0.001 |
Infectious diseases | 1.37 (1.05 to 1.80) | ||
Blood diseases | 0.50 (0.35 to 0.72) | ||
Circulatory system | 0.66 (0.55 to 0.80) | ||
Digestive system | 0.83 (0.68 to 1.02) | ||
Diseases of head/neck | 0.30 (0.15 to 0.63) | ||
Genitourinary system | 1.76 (1.43 to 2.15) | ||
Musculoskeletal | 0.39 (0.30 to 0.49) | ||
Nervous system | 0.53 (0.39 to 0.72) | ||
Respiratory system | 0.90 (0.75 to 1.09) | ||
Skin | 0.87 (0.68 to 1.16) | ||
Endocrine/metabolic | 1.64 (1.26 to 2.13) | ||
Injury/poisoning | 0.55 (0.45 to 0.67) | ||
Mental disorders | 0.79 (0.55 to 1.12) | ||
Symptoms/signs | 0.53 (0.44 to 0.64) | ||
Other | 0.36 (0.16 to 0.79) | ||
CRP | Not measured | 1 | 0.009 |
Normal (≤ 10 mg/l) | 0.99 (0.86 to 1.14) | ||
Abnormal | 1.19 (1.04 to 1.35) | ||
Hb | Not measured | 1 | < 0.001 |
Normal (female: 11–15 g/dl)b | 0.79 (0.70 to 0.89) | ||
Abnormal | 1.10 (0.98 to 1.24) | ||
HbA1c (12-month average) | Not measured | 1 | 0.008 |
Normal (≤ 7.5%) | 1.06 (0.96 to 1.17) | ||
Abnormal | 1.24 (1.08 to 1.43) | ||
Troponin | 0 | 1 | < 0.001 |
1 | 1.27 (1.11 to 1.45) | ||
2+ | 1.46 (1.15 to 1.85) | ||
Blood culture | Not taken | 1 | < 0.001 |
Not significant | 1.49 (1.29 to 1.73) | ||
Significant | 3.12 (2.36 to 4.12) | ||
Charlson score | ≤ 0 | 1 | < 0.001 |
1–10 | 1.08 (0.97 to 1.21) | ||
11+ | 1.37 (1.23 to 1.53) | ||
Not recorded | 1.11 (0.96 to 1.29) | ||
Baseline eGFRc | Linear term | 0.93 (0.60 to 1.43) | < 0.001 |
Quadratic term | 0.83 (0.74 to 0.93) | ||
Cubic term | 1.03 (1.02 to 1.04) | ||
Drugs takend | 0 | 1 | < 0.001 |
1 | 1.15 (1.04 to 1.27) | ||
2 or 3 | 1.38 (1.20 to 1.58) | ||
Not recorded | 0.86 (0.77 to 0.95) | ||
MSU or CSU | Not taken | 1 | < 0.001 |
Not significant | 1.05 (0.80 to 1.36) | ||
Significant | 1.37 (1.19 to 1.59) | ||
Proteinuria (worst result) | Not done | 1 | < 0.001 |
1 | 1.07 (0.96 to 1.19) | ||
2 or 3 | 1.38 (1.23 to 1.54) |
The results suggested that most factors had a smaller effect after adjustment for the other factors in the model. This is to be expected, as there will be correlations between many of the risk factors. However, the results for the majority of predictors showed a similar effect to the results from the univariable analyses.
The exception was for an admission in the last 30 days. In the univariable analyses patients with an admission in this time period had a higher AKI stage; however, after adjustments for the other factors these patients had a lower status.
The results for the continuous variables (age and baseline GFR) are again difficult to visualise from the odds ratios alone. The adjusted predicted probabilities for age are shown in Figure 8 (assuming ‘average’ values for all other variables). This graph shows a broadly similar relationship for age to that seen in the univariable analyses, although the increased risk peaks around the age of 75 years.
FIGURE 8.
Risk model 1: relationship between age and probability of AKI in the multivariable analysis.
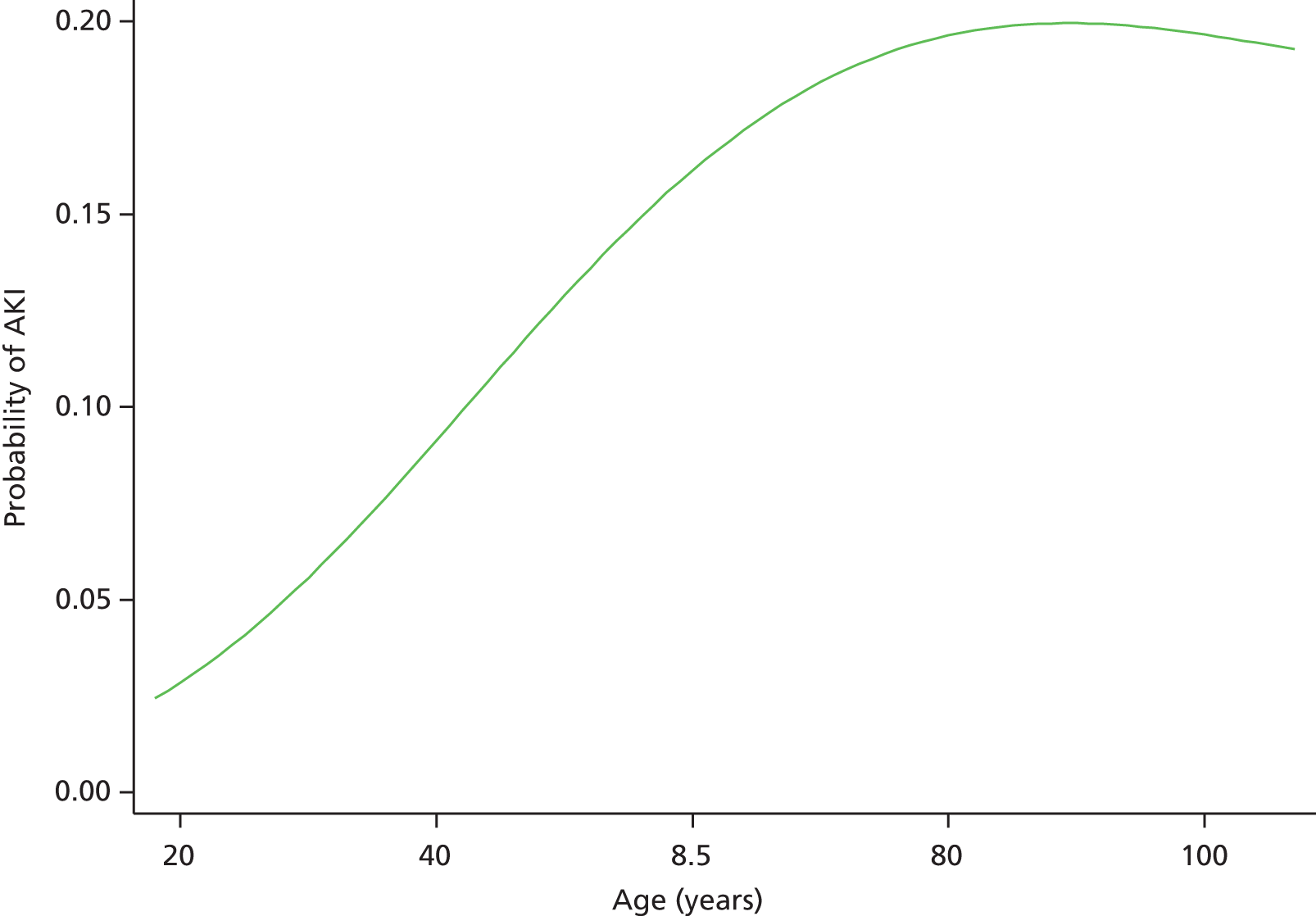
The adjusted relationship for eGFR is shown in Figure 9. After adjusting for the other variables, it seems that those with the highest values have a lower risk, around the same risk as those with the lowest eGFR values. The peak risk is around 100.
FIGURE 9.
Risk model 1: relationship between baseline eGFR and probability of AKI in the multivariable analysis.

Validation
After exclusions from the full database (as described earlier), one-quarter of the data were randomly selected for the validation database. This consisted of 9177 admissions. However, owing to a small number of missing values, predicted probabilities were obtained for 9157 admissions.
First, the development and validation data sets were compared, with the finding that the two data sets were very matched in terms of the model variables. This was not surprising given the selection of the two data sets at random.
The first set of analyses split the patients into risk groups based on the predicted probability of AKI. The ‘expected’ risk of AKI in each category based on the predicted probabilities was calculated, along with the observed occurrence of AKI in each category. Analyses were performed based on the probability of any AKI, and then again based on the predicted probability of either AKI stage 2 or AKI stage 3. The results are summarised in Table 6.
Categorisation | Risk category (%) | n | Mean expected (%) | Observed (%) |
---|---|---|---|---|
Any AKI | ≤ 10 | 3325 | 6.2 | 5.0 |
> 10–20 | 3057 | 14.3 | 14.8 | |
> 20–40 | 2178 | 27.5 | 28.4 | |
> 40 | 597 | 51.7 | 51.6 | |
AKI stage 2/3 | ≤ 2 | 3962 | 1.2 | 0.8 |
> 2–5 | 3358 | 3.2 | 3.8 | |
> 5–10 | 1294 | 6.9 | 7.2 | |
> 10 | 543 | 15.8 | 11.4 |
The results suggested reasonably good discrimination between the risk groups for both categorisations. For both models the observed results increased with increased risk, with the categories correctly ordered.
The calibration of the models was also good, with the observed percentages falling within the risk boundaries for both categorisations. In addition, there was a fairly good agreement between the predicted percentages and those observed by the data. The slight exception was for the highest risk subjects for the AKI stage 2/3 categorisation, in whom the observed percentage of AKI stage 2/3 cases was lower than that predicted by the model.
The next set of analyses further examined the discrimination of the two categorisations using ROC curves based on the predicted probabilities. A summary of the AUROC values is given in Table 7.
Categorisation | AUROC (95% CI) | Interpretation |
---|---|---|
Any AKI | 0.75 (0.74 to 0.77) | Fair |
AKI stage 2/3 | 0.75 (0.73 to 0.78) | Fair |
The ROC curve analyses suggested that the model had some discriminatory power, although the AUC values were only ‘fair’.
The ROC curve for any AKI is shown in Figure 10.
FIGURE 10.
Risk model 1: ROC curve for the prediction of any AKI.

Figure 11 shows the ROC curve for the prediction of AKI stages 2 and 3.
FIGURE 11.
Risk model 1: ROC curve for the prediction of AKI stages 2 and 3.
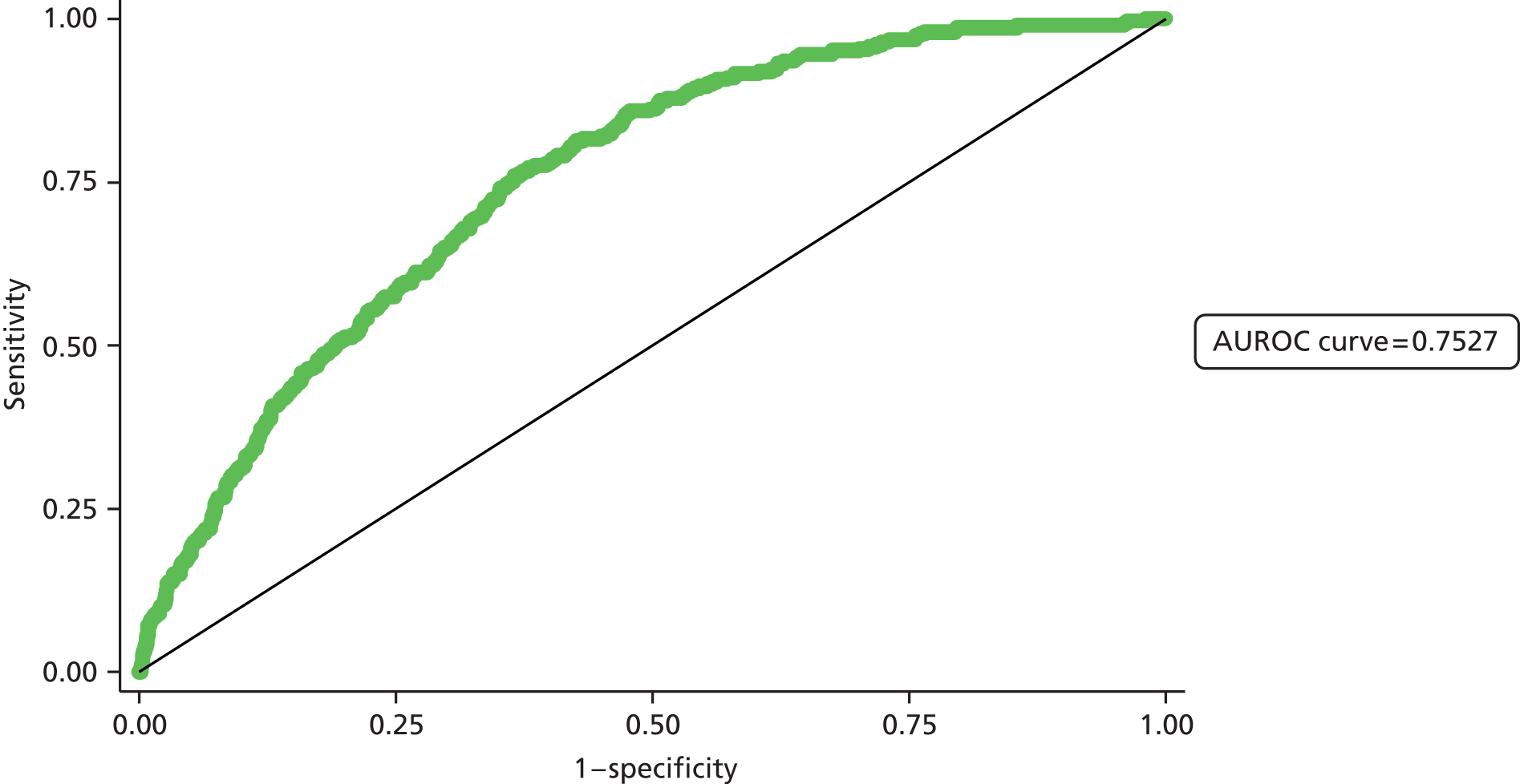
The final analyses compared the observed numbers of patients with AKI in each AKI group and the numbers predicted by the model using the Hosmer–Lemeshow test. A summary of the results is given in Table 8.
Categorisation | Risk category (%) | No AKIa (n) | AKIa (n) | χ2b | p-value |
---|---|---|---|---|---|
Observed (expected) | Observed (expected) | ||||
Any AKI | ≤ 10 | 3158 (3119) | 167 (206) | 9.4 | 0.009 |
> 10–20 | 2606 (2619) | 451 (438) | |||
> 20–40 | 1559 (1580) | 619 (598) | |||
> 40 | 289 (289) | 308 (308) | |||
AKI stage 2/3 | ≤ 2 | 3931 (3916) | 31 (46) | 16.4 | 0.0003 |
2–5 | 3232 (3250) | 126 (108) | |||
5–10 | 1201 (1205) | 93 (89) | |||
> 10 | 481 (457) | 62 (86) |
The results suggested that for both models there was a significant difference between the observed numbers and those expected by the model. This suggests some lack of fit of the model to the data. The fit of the model for any AKI is slightly better than that for AKI stage 2/3.
For any AKI, the model overpredicts the number of cases of AKI in the lowest-risk group, but provides a good prediction in the upper risk group. For the AKI stage 2/3 predictions, the model overpredicts the number of AKI stages 2 and 3 cases in the upper risk group, as seen in Table 6.
Despite the test suggesting a lack of fit to the data, this test is known to be fairly sensitive to slight deviations between observed and expected frequencies, and so a statistically significant difference often occurs.
Validation in a second population
The same exclusions were applied to the Medway data set as to those applied to the East Kent data for the admission AKI model (risk model 1). After excluding certain episodes, the data set consisted of 4726 admissions. Predicted probabilities of AKI were obtained for all admissions.
Initially, the East Kent data set (the development data set only) and Medway data sets were compared for the admissions suitable for inclusion in the admission model. This revealed some differences between the two sets of data, as AKI was more common in the Medway data set, occurring in approximately 23% of patients, compared with 17% in East Kent. There were also differences in a number of the patient characteristics that were included in the model.
The first set of analyses looked at the occurrence of AKI in each category. Analyses were performed on patients split into risk groups based on the predicted probability of AKI. The ‘expected’ risk of AKI in each category was calculated based on the predicted probabilities, along with the observed probability of any AKI, and then again based on the predicted probability of either AKI stage 2 or AKI stage 3. The results are summarised in Table 9.
Categorisation | Risk category (%) | n | Mean expected (%) | Observed (%) |
---|---|---|---|---|
Any AKI | ≤ 10 | 1961 | 6.1 | 10.7 |
> 10–20 | 1597 | 14.1 | 23.0 | |
> 20–40 | 960 | 27.1 | 39.8 | |
> 40 | 208 | 51.1 | 63.0 | |
AKI stage 2/3 | ≤ 2 | 2329 | 1.1 | 2.1 |
> 2–5 | 1657 | 3.2 | 5.9 | |
> 5–10 | 552 | 6.8 | 9.6 | |
> 10 | 188 | 15.3 | 18.6 |
The results suggested reasonably good discrimination between the risk groups for both categorisations. For both models the observed results increased with increased risk, with the categories correctly ordered.
The calibration of the models was less good, with the observed outcomes in each category higher than those ‘expected’ by the model. In some instances the observed percentage in each category was outside the range of the risk category. For example, for all AKI, in those patients with a predicted risk of 10–20% the observed percentage with AKI was 23.0%, which was higher than the upper boundary of the prediction.
The next set of analyses further examined the discrimination of the two categorisations using ROC curves based on the predicted probabilities. A summary of the AUROC values is given in Table 10.
Categorisation | AUROC (95% CI) | Interpretation |
---|---|---|
Any AKI | 0.72 (0.71 to 0.74) | Fair |
AKI stage 2/3 | 0.71 (0.68 to 0.75) | Fair |
The ROC curve analyses suggested that the model had some discriminatory power, although the AUC values were only ‘fair’. These values compare with values of 0.75 (for both categorisations) that were obtained from the internal validation with the East Kent data.
The ROC curve for any AKI is shown in Figure 12.
FIGURE 12.
Risk model 1: ROC curve for the prediction of any AKI in the second population.

Figure 13 shows the ROC curve for the prediction of AKI stages 2 and 3.
FIGURE 13.
Risk model 1: ROC curve for the prediction of AKI stages 2 and 3 in the second population.
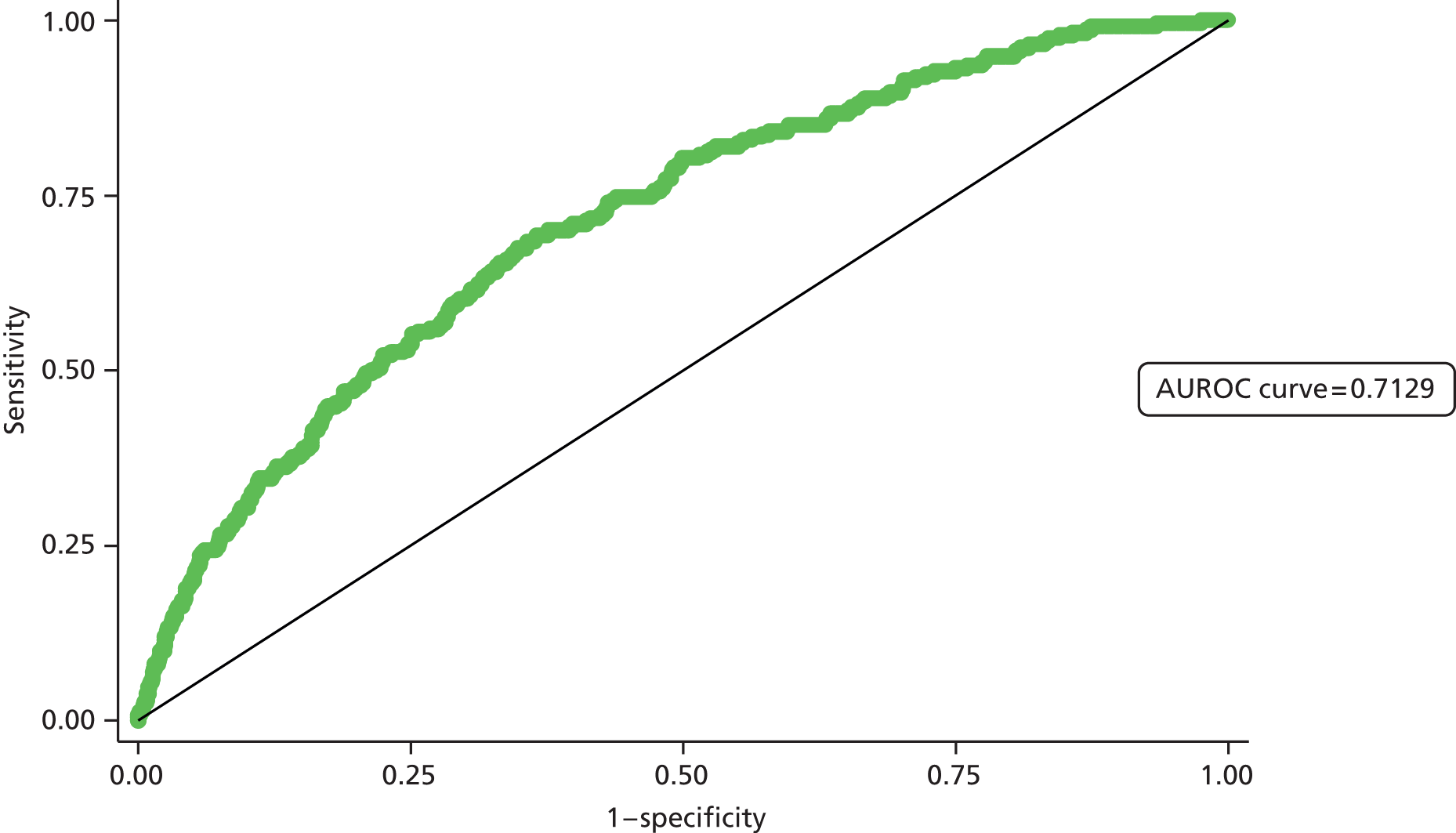
The final analyses compared the observed numbers of patients with AKI in each AKI group and the numbers predicted by the model using the Hosmer–Lemeshow test. A summary of the results is given in Table 11.
Categorisation | Risk category (%) | No AKIa (n) | AKIa (n) | χ2b | p-value |
---|---|---|---|---|---|
Observed (expected) | Observed (expected) | ||||
Any AKI | ≤ 10 | 1752 (1842) | 209 (119) | 269.6 | < 0.0001 |
> 10–20 | 1229 (1372) | 368 (225) | |||
> 20–40 | 578 (700) | 382 (260) | |||
> 40 | 77 (119) | 131 (106) | |||
AKI stage 2/3 | ≤ 2 | 2280 (2302) | 49 (27) | 66.9 | < 0.0001 |
> 2–5 | 1560 (1604) | 97 (52) | |||
> 5–10 | 499 (515) | 53 (37) | |||
> 10 | 153 (159) | 35 (29) |
The results suggested that for both analyses there was a strong overall lack of fit for the models to the validation data set. In both instances the number of cases of AKI and AKI stage 2/3 was higher than that which might be expected by the model. This mirrors the results observed earlier.
Summary
The results suggest that the model is fairly well calibrated, with the predictions of AKI in quite close agreement with the observed occurrence of AKI. This is perhaps unsurprising, as the characteristics of the development data set and the occurrence of AKI were very similar to those of the validation data set.
The model was found to have some predictive powers, and generally was found to be able to distinguish between low- and high-risk cases. However, the model was by no means perfect in terms of producing correct predictions for all patients.
The results of the validation in the second population indicate that, using the Medway data, the East Kent risk model underpredicts the risk both of any AKI and of the occurrence of AKI stage 2/3. This could potentially be partly attributable to the higher occurrence of both of these outcomes in the Medway data than in the East Kent data.
However, the discrimination of the model is still reasonable on this external data set, suggesting that it is able to separate out the high-risk from the low-risk cases. In terms of AUROC curve, the result was only slightly worse than that obtained when performing the internal validation.
Risk model 2: predicting new acute kidney injury at 72 hours
Development
After exclusions from the full database (as described in Chapter 2), the data consisted of 10,075 admissions. Of these, three-quarters were randomly selected for the development database, resulting in 7556 admissions for the development of the model. These admissions occurred in 6626 different patients.
A series of separate univariable ordinal logistic regression analyses were performed to examine factors associated with AKI at 72 hours. A summary of the analysis results is given in Appendix 3, Table 33. The size of effects is reported in the form of odds ratios. For categorical variables, these give the odds of being in the next highest outcome category (e.g. AKI stage 1 rather than no AKI, AKI stage 3 rather than AKI stage 2) for each category relative to a baseline category. For the continuous predictor variables, the odds ratios represent the relative change in the odds of being in the next highest category for a given increase in that predictor. The p-value indicating the overall significance of each variable is also reported.
The results in the above table are interpretable for the categorical predictor variables.
A linear term only was found to be sufficient for age. A 10-year increase in age was associated with a 28% increase in the odds of AKI being in the next highest category. This result is also shown in Figure 14, which plots age and the probability of AKI. For graphical purposes the occurrence of any AKI is plotted.
FIGURE 14.
Risk model 2: relationship between age and probability of AKI in the univariable analysis.

A quadratic (squared) term was necessary for baseline eGFR, and thus the odds ratios are difficult to interpret and the relationship is best viewed graphically. A graph, similar to that for age, is shown in Figure 15 for baseline eGFR.
FIGURE 15.
Risk model 2: relationship between baseline eGFR and probability of AKI in the univariable analysis.
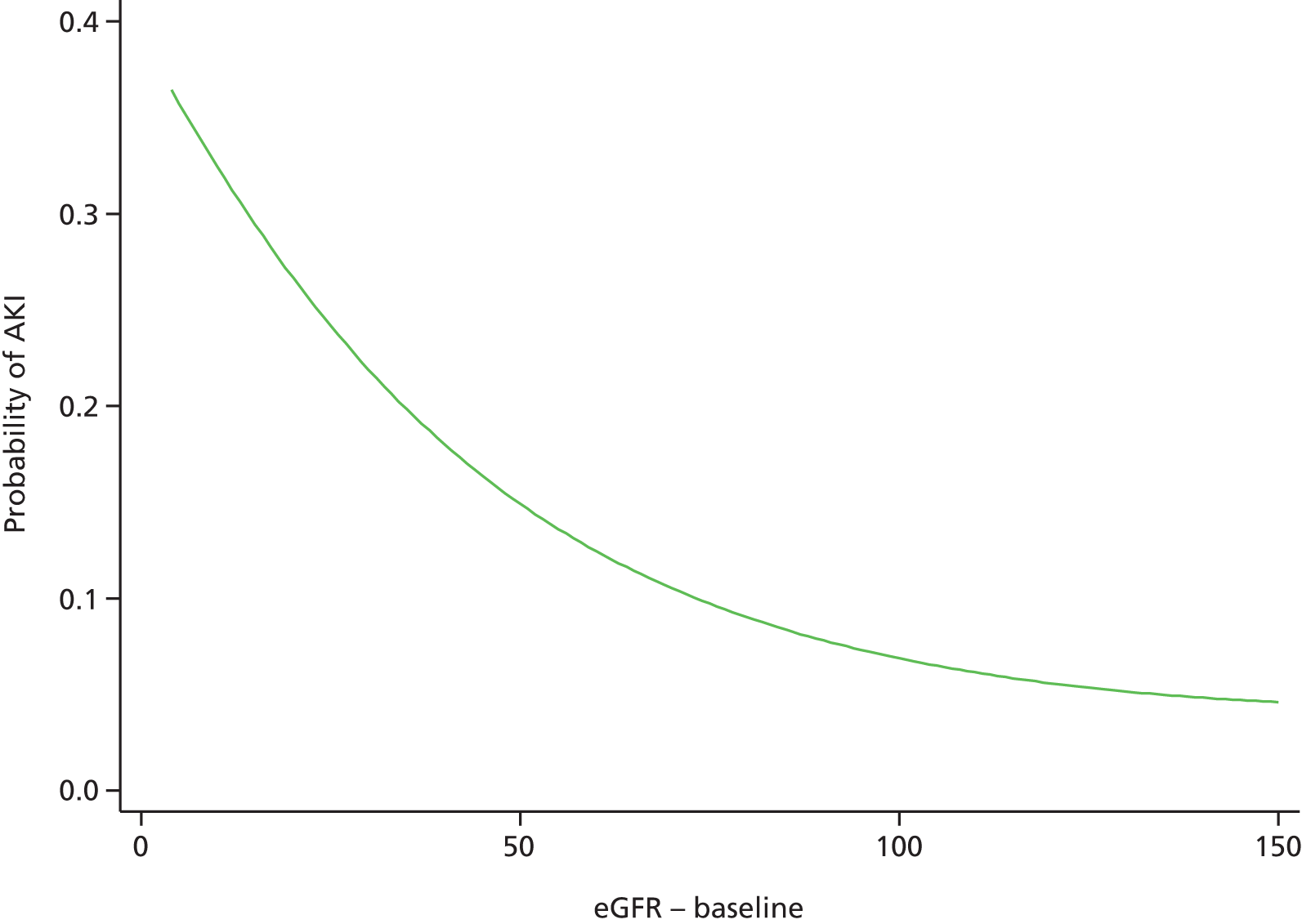
The next stage in the analyses examined the joint association between the variables and AKI stage in a multivariable analysis.
The collinearity between the different predictors was examined. The examinations indicated no strong evidence of collinearity between any of the predictors; therefore, all predictors were considered for the multivariable analyses.
The univariable analyses suggested that patients with normal and abnormal glycated haemoglobin (HbA1c) (from the 12-month average) had almost equivalent risks of AKI. As a result, for this second stage in the analysis, this variable was recategorised as ‘not measured’ and ‘measured’.
A backwards selection procedure was used to retain the statistically significant variables in the final model. This procedure omitted the following variables (in order of removal):
-
calcium
-
gender
-
B-type natriuretic peptide (BNP) (12-month average)
-
creatinine kinase (CK)
-
number of outpatients’ appointments in 12 months
-
sputum pre admission
-
number of contrast radiology scans
-
wound swab/fluid aspirate pre admission
-
wound swab/fluid aspirate at admission
-
Na
-
alanine transaminase (ALT)
-
mid-stream specimen urine (MSU) or catheter specimen urine (CSU) pre admission
-
faeces at admission
-
Hb
-
sputum at admission
-
MSU or CSU at admission
-
faeces pre admission
-
operation score at 12 hours
-
PLT
-
admissions within 30 days of current admission
-
blood culture
-
number of drugs
-
amylase (AMY).
The final model is summarised in Table 12.
Variable | Category/term | Odds ratio (95% CI) | p-value |
---|---|---|---|
Agea | Linear term | 1.78 (1.22 to 2.60) | < 0.001 |
Quadratic term | 0.97 (0.95 to 1.00) | ||
Admissions (last 2–12 months) | 0 | 1 | < 0.001 |
1–2 | 1.41 (1.14 to 1.75) | ||
3–5 | 1.58 (1.20 to 2.09) | ||
6+ | 2.24 (1.57 to 3.19) | ||
Primary diagnosis | Neoplasms | 1 | < 0.001 |
Infectious diseases | 1.53 (0.90 to 2.61) | ||
Blood diseases | 0.58 (0.25 to 1.36) | ||
Circulatory system | 0.77 (0.50 to 1.20) | ||
Digestive system | 0.84 (0.55 to 1.30) | ||
Genitourinary system | 1.52 (0.95 to 2.43) | ||
Musculoskeletal | 0.79 (0.39 to 1.58) | ||
Nervous system | 0.57 (0.23 to 1.38) | ||
Respiratory system | 0.59 (0.38 to 0.91) | ||
Skin | 0.88 (0.46 to 1.72) | ||
Endocrine/metabolic | 0.71 (0.34 to 1.46) | ||
Injury/poisoning | 1.04 (0.67 to 1.60) | ||
Mental disorders | 0.18 (0.02 to 1.32) | ||
Symptoms/signs | 0.78 (0.49 to 1.26) | ||
Other | 1.33 (0.36 to 4.85) | ||
CRP | Not measured | 1 | 0.05 |
Normal (≤ 10 mg/l) | 0.89 (0.65 to 1.22) | ||
Abnormal | 1.15 (0.85 to 1.55) | ||
HbA1c (12-month average) | Not measured | 1 | 0.03 |
Measured | 1.26 (1.03 to 1.56) | ||
K | Not measured | 1 | 0.02 |
Normal (3.5–5.3 mmol/l) | 0.69 (0.50 to 0.94) | ||
Abnormal | 0.86 (0.58 to 1.26) | ||
Magnesium | Not measured | 1 | 0.02 |
Normal (0.7–1.0 mmol/l) | 0.88 (0.65 to 1.17) | ||
Abnormal | 1.69 (1.14 to 2.50) | ||
Troponin | 0 | 1 | < 0.001 |
1 | 1.42 (1.15 to 1.77) | ||
2+ | 2.19 (1.67 to 2.87) | ||
WBC | Not measured/normal | 1 | 0.03 |
Abnormal | 1.18 (1.00 to 1.40) | ||
Charlson score | ≤ 0 | 1 | 0.05 |
1–10 | 1.14 (0.87 to 1.49) | ||
11+ | 1.35 (1.05 to 1.75) | ||
Not recorded | 1.41 (1.02 to 1.95) | ||
Baseline eGFRb | Linear term | 0.40 (0.28 to 0.57) | < 0.001 |
Quadratic term | 1.11 (1.06 to 1.16) | ||
Proteinuria (worst result) | Not done | 1 | 0.001 |
1 | 0.95 (0.73 to 1.22) | ||
2 or 3 | 1.52 (1.19 to 1.95) |
The final model suggested that a combination of 12 variables could help to predict AKI. A number of these variables [C-reactive protein (CRP) and Charlson score] were only of borderline statistical significance, but were retained in the final model.
The univariable results suggested that only a linear term for age was required. However, after adjusting for the other factors, a quadratic term was found to improve the fit of the model and was, therefore, included. As a result of the inclusion of this term, the results for age are difficult to visualise from the odds ratios alone. The adjusted predicted probabilities for age are shown in Figure 16 (assuming ‘average’ values for all other variables). This graph shows a broadly similar relationship for age to that seen in the univariable analyses, although the increased risk with age tails off for subjects over 80 years.
FIGURE 16.
Risk model 2: relationship between age and probability of AKI in the multivariable analysis.

The adjusted relationship for eGFR is also best viewed graphically, and this relationship is shown in Figure 17. After adjusting for the other variables, the graph suggests that patients with a baseline eGFR of around 90 ml/minute/1.73m2 have the lowest risk, with a slight rise in risk for those with higher baseline eGFR values.
FIGURE 17.
Risk model 2: relationship between baseline eGFR and probability of AKI in the multivariable analysis.
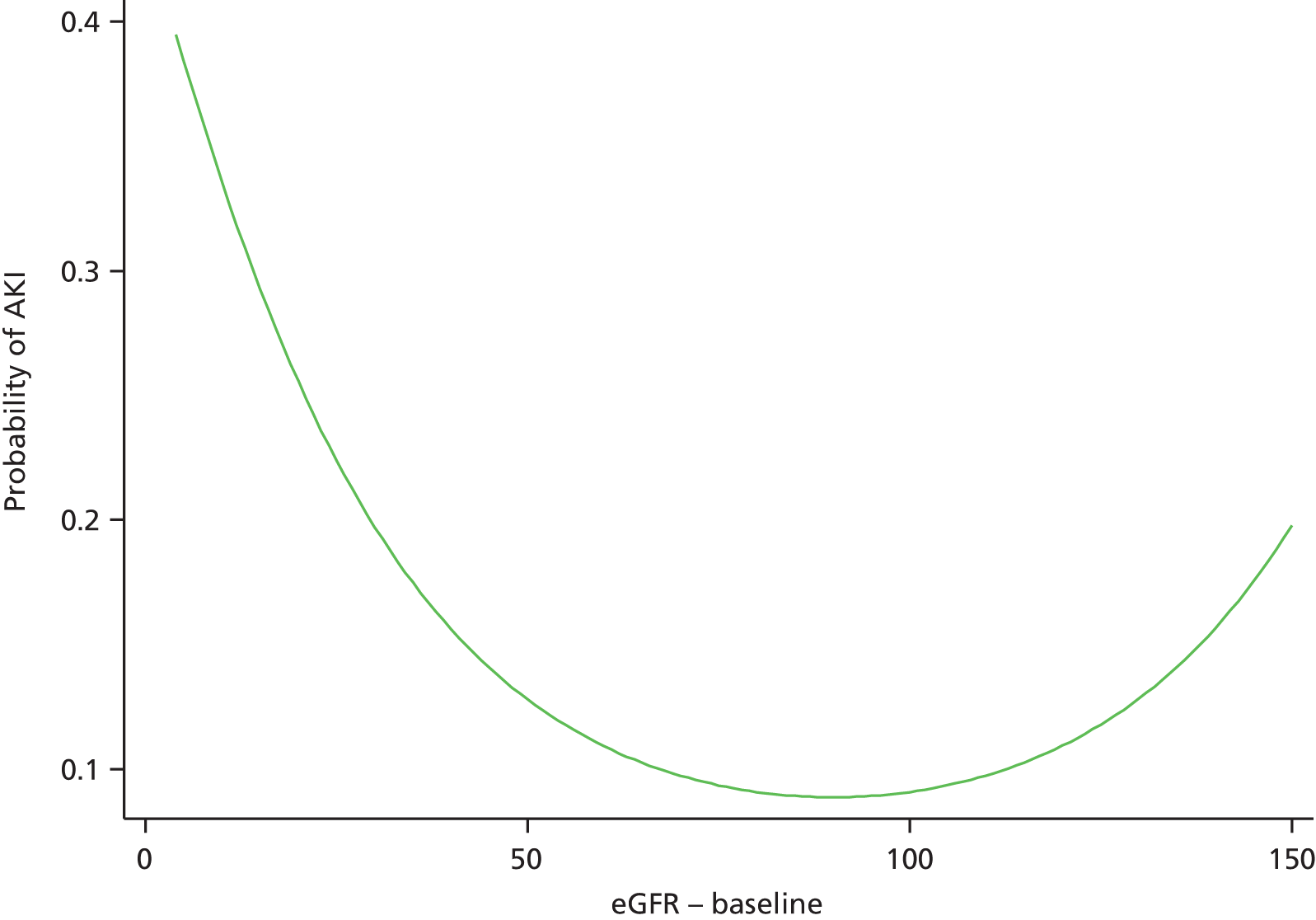
Validation
After exclusions from the full database (as described in Chapter 2), one-quarter of the data were randomly selected for the validation database. These consisted of 2519 admissions. However, owing to a small number of missing values, predicted probabilities were obtained for 2514 admissions.
Initially, the development and validation data sets were compared, with the finding that the two data sets were very matched in terms of the model variables. This is not surprising given that the selection of the two data sets was at random.
The first set of analyses split the patients into risk groups based on the predicted probability of AKI. The ‘expected’ risk of AKI in each category based on the predicted probabilities was calculated, along with the observed occurrence of AKI in each category. Analyses were performed based on the probability of any AKI, and then again based on the predicted probability of either AKI stage 2 or 3. The results are summarised in Table 13.
Categorisation | Risk category (%) | n | Mean expected (%) | Observed (%) |
---|---|---|---|---|
Any AKI | ≤ 5 | 709 | 3.3 | 4.5 |
> 5–10 | 1023 | 7.3 | 7.7 | |
> 10–20 | 633 | 13.7 | 15.3 | |
> 20 | 149 | 26.9 | 22.1 | |
AKI stage 2/3 | ≤ 1 | 1441 | 0.6 | 0.8 |
> 1–2 | 737 | 1.4 | 2.6 | |
> 2–3 | 210 | 2.4 | 3.3 | |
> 3 | 126 | 4.3 | 2.4 |
The results suggested reasonably good discrimination between the risk groups for any AKI. For the prediction of this outcome, the observed results increased with increased risk, with the categories correctly ordered.
The discrimination for the prediction of AKI stage 2 or AKI stage 3 was less impressive. AKI stage 2/3 was less common in the lowest-risk group. However, there was a similar occurrence of AKI stage 2/3 in the three higher-risk groups, with this outcome occurring slightly more frequently in the second highest-risk group than in the highest-risk group. However, it should be noted that AKI stage 2/3 was relatively rare in this patient group and the group sizes were relatively small for the higher-risk groups.
The calibration of the model for the prediction of AKI was good, with the observed percentages falling within the risk boundaries. In addition, there was a fairly good agreement between the predicted percentages and those observed by the data.
The calibration for the prediction of AKI stage 2/3 was less good, with an observed occurrence of AKI stage 2/3 in the 1–2% and 2–3% categories above the risk category boundary. Additionally, the observed occurrence of AKI stage 2/3 for the > 3% category was below the category boundary; however, it is noted that the risk boundaries were relatively tight for the prediction of this outcome.
The next set of analyses further examined the discrimination of the two categorisations using ROC curves based on the predicted probabilities. A summary of the AUROC values is given in Table 14.
Categorisation | AUROC (95% CI) | Interpretation |
---|---|---|
Any AKI | 0.67 (0.64 to 0.71) | Poor |
AKI stage 2/3 | 0.68 (0.61 to 0.76) | Poor |
The ROC curve analyses suggested that the model had there was some discriminatory power, with the lower bounds for the confidence intervals (CIs) higher than 0.5 for both categorisations. Despite the model having some predictive ability, the AUC values were classed as ‘poor’ (although approaching ‘fair’).
The ROC curve for any AKI is shown in Figure 18.
FIGURE 18.
Risk model 2: ROC curve for the prediction of any AKI.

Figure 19 shows the ROC curve for the prediction of AKI stages 2 and 3.
FIGURE 19.
Risk model 2: ROC curve for the prediction of AKI stages 2 and 3.
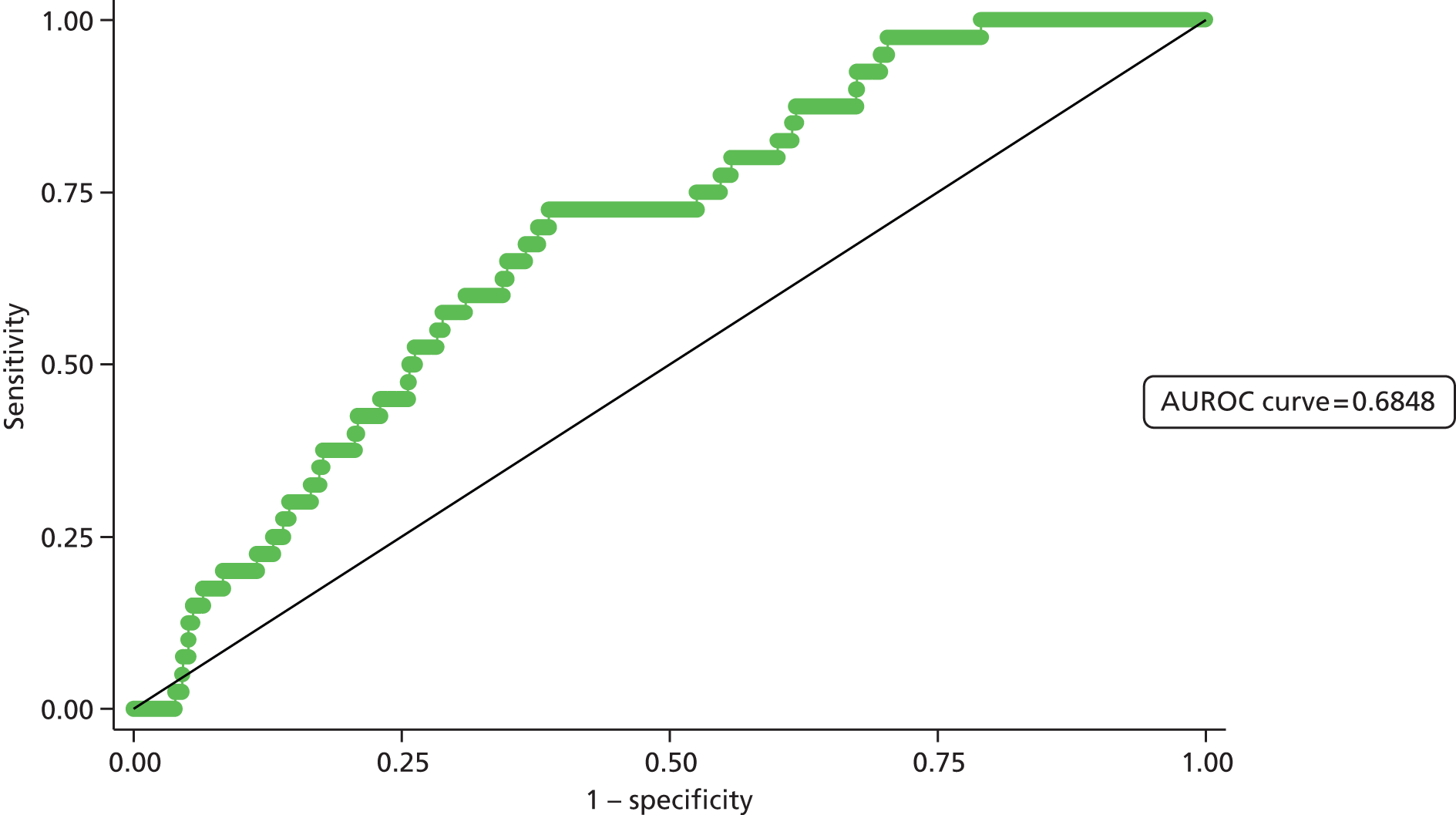
The final analyses compared the observed numbers of patients with AKI in each AKI group and the numbers predicted by the model using the Hosmer–Lemeshow test. A summary of the results is given in Table 15.
Categorisation | Risk category (%) | No AKIa (n) | AKIa (n) | χ2b | p-value |
---|---|---|---|---|---|
Observed (expected) | Observed (expected) | ||||
Any AKI | ≤ 5 | 677 (685) | 32 (24) | 6.4 | 0.04 |
> 5–10 | 944 (949) | 79 (74) | |||
> 10–20 | 536 (546) | 97 (87) | |||
> 20 | 116 (109) | 33 (40) | |||
AKI stage 2/3 | ≤ 1 | 1430 (1432) | 11 (9) | 10.4 | 0.005 |
> 1–2 | 718 (727) | 19 (10) | |||
> 2–3 | 203 (205) | 7 (5) | |||
> 3% | 123 (120) | 3 (5) |
The results indicate that for both models there was a significant difference between the observed numbers and those expected by the model. This suggests some lack of fit of the model to the data. The fit of the model for any AKI was better than that for AKI stage 2/3, with the lack of fit only just statistically significant.
For any AKI, the model underpredicts the number of cases of AKI in the three lowest-risk groups, but overpredicts in the upper risk group. The results for the AKI stage 2/3 predictions are similar.
Despite the test suggesting a lack of fit to the data, this test is known to be fairly sensitive to slight deviations between observed and expected frequencies, and so a statistically significant difference often occurred.
Validation in a second population
The same exclusions were applied to the Medway data set as those applied to the East Kent data for the 72-hour AKI model (as described in Risk model 2: predicting new acute kidney injury at 72 hours). After excluding certain episodes, the data set consisted of 1585 admissions. Predicted probabilities of AKI were obtained for all admissions.
Initially, the East Kent data set (the development data set only) and Medway data sets were compared for the admissions suitable for inclusion in the 72-hour model.
This suggests some differences between the two sets of data. However, results for AKI by 72 hours were relatively similar in the two data sets. Any AKI occurred in 8.9% of the East Kent patients, compared with 7.6% of the Medway patients. AKI stage 2/3 was rare in both data sets, but the occurrence was similar in the two groups.
The first set of analyses looked at the occurrence of AKI in each category. Analyses were performed on patients split into risk groups based on the predicted probability of AKI. The ‘expected’ risk of AKI in each category based on the predicted probabilities was calculated, along with the observed probability of any AKI, and then again based on the predicted probability of either AKI stage 2 or AKI stage 3. The results are summarised in Table 16.
Categorisation | Risk category (%) | n | Mean expected (%) | Observed (%) |
---|---|---|---|---|
Any AKI | ≤ 5 | 760 | 3.0 | 3.7 |
> 5–10 | 563 | 6.8 | 8.5 | |
> 10–20 | 214 | 13.4 | 15.0 | |
> 20 | 48 | 26.5 | 25.0 | |
AKI stage 2/3 | ≤ 1 | 1218 | 0.5 | 0.7 |
> 1–2 | 262 | 1.4 | 0.8 | |
> 2–3 | 69 | 2.4 | 1.4 | |
> 3 | 36 | 4.3 | 0.0 |
The results suggested reasonably good discrimination between the risk groups for any AKI. For this categorisation the observed results increased with increased risk, with the categories correctly ordered. The discrimination was less good for the prediction of AKI stage 2/3. The first three categories are correctly ordered, although there is little difference in the observed occurrence of AKI stage 2/3 in the lowest two groups. The highest-risk group actually has the lowest occurrence of AKI stage 2/3 (no observed cases); however, it is noted that the group is small in number.
The calibration of the models was good for any AKI. The observed outcomes in each category are broadly similar to those ‘expected’ by the model. For the first three risk categories the model underpredicted slightly. In all instances, the observed percentage in each category was within the range of the risk category. The calibration of the AKI stage 2/3 model was less good, with the model overpredicting the occurrence of AKI stage 2/3 in all risk categories.
The next set of analyses further examined the discrimination of the two categorisations using ROC curves based on the predicted probabilities. A summary of the AUROC values is given in Table 17.
Categorisation | AUROC (95% CI) | Interpretation |
---|---|---|
Any AKI | 0.71 (0.67 to 0.76) | Fair |
AKI stage 2/3 | 0.63 (0.52 to 0.75) | Poor |
The ROC curve analyses suggested that there was some discriminatory power for the prediction of any AKI, although the AUC values were only ‘fair’. This is comparable with the value of 0.68 obtained from the internal validation with the East Kent data; therefore, this is a slightly better performance than that of the internal data.
The AUROC was poorer for the prediction of AKI stage 2/3, with a value of 0.63, which is a fairly poor value. This is a lower value than that obtained from the East Kent internal validation.
The ROC curve for any AKI is shown in Figure 20. Figure 21 shows the ROC curve for the prediction of AKI stages 2 and 3.
FIGURE 20.
Risk model 2: ROC curve for the prediction of any AKI in the second population.
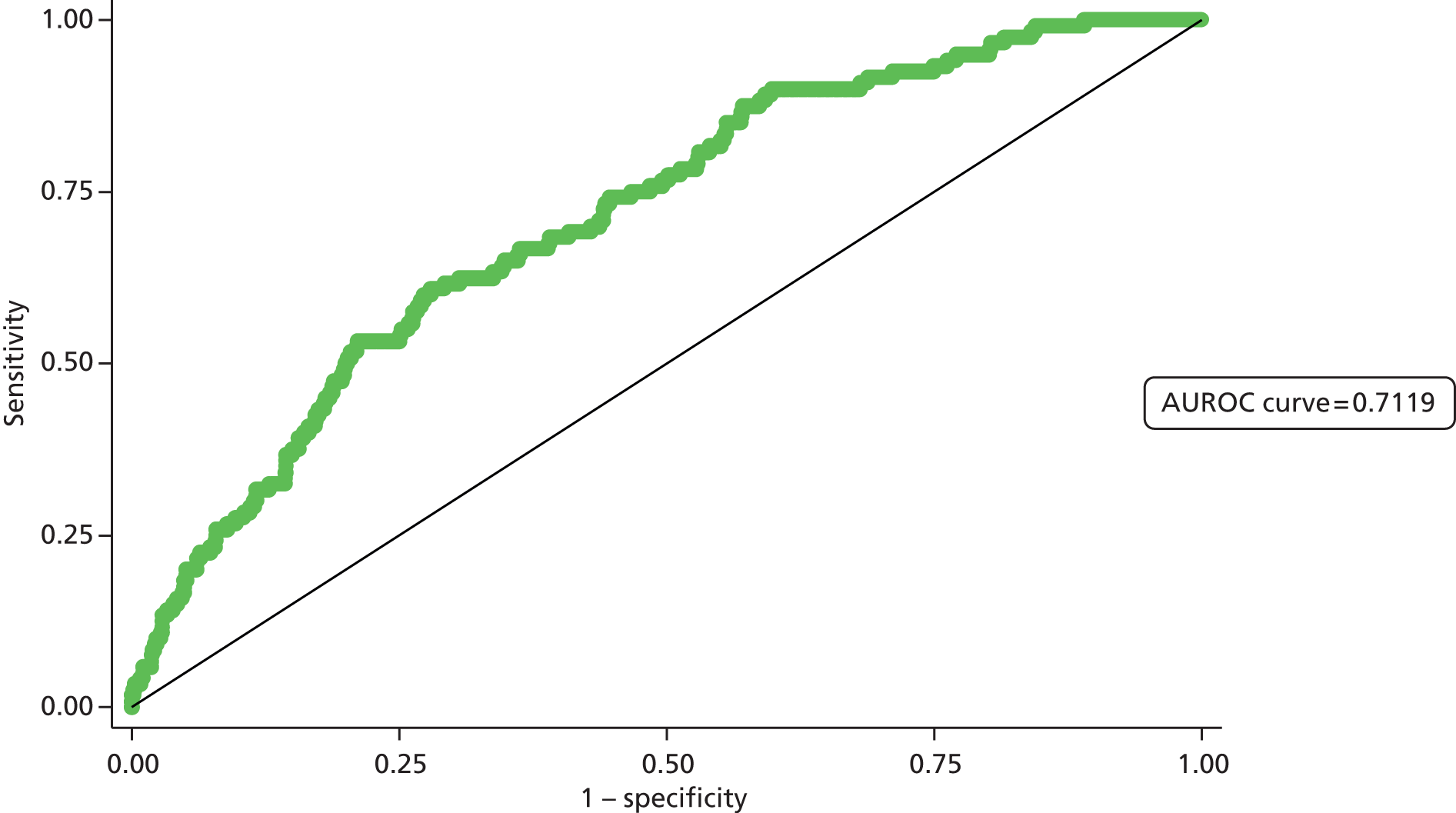
FIGURE 21.
Risk model 2: ROC curve for the prediction of AKI stages 2 and 3 in the second population.

The final analyses compared the observed numbers of patients with AKI in each AKI group and the numbers predicted by the model using the Hosmer–Lemeshow test. A summary of the results is given in Table 18.
Categorisation | Risk category (%) | No AKIa | AKIa | χ2b | p-value |
---|---|---|---|---|---|
Observed (expected) | Observed (expected) | ||||
Any AKI | ≤ 5 | 732 (737) | 28 (23) | 4.3 | 0.12 |
> 5–10 | 515 (525) | 48 (38) | |||
> 10–20 | 182 (185) | 32 (29) | |||
> 20 | 36 (35) | 12 (13) | |||
AKI stage 2/3 | ≤ 1 | 1209 (1211) | 9 (6.2) | 4.0 | 0.14 |
> 1–2 | 260 (258) | 2 (3.6) | |||
> 2–3 | 68 (67) | 1 (1.7) | |||
> 3 | 36 (34) | 0 (1.6) |
The results suggested that for both models there was no statistically significant difference between the observed numbers and those expected by the model. This typically suggests a fairly good fit of the model to the data.
Summary
The results suggest that the model performs better for the prediction of any AKI than for the prediction of AKI stage 2/3. However, it is noted that AKI stage 2/3 is relatively rare in this cohort of patients. The model for the prediction of AKI is relatively well calibrated, although its discriminatory ability is poorer than for the model predicting AKI on hospital admission.
The results of validation in a second population indicate that, using the Medway data, the East Kent risk model has a fairly good calibration for the prediction of any AKI. The discrimination of the model is as good as, if not slightly better than, observed when validating on the East Kent data.
Both the calibration and the discrimination of the prediction of AKI stage 2/3 were poorer when tested on this Medway data set. The performance of the model for the prediction of this outcome is probably not sufficiently good for use in practice. However, it is noted that AKI stages 2/3 in this patient group is rare, making it more difficult to predict the outcome.
Risk model 3: predicting worsening acute kidney injury at 72 hours
Development
After exclusions from the full database (as described in Chapter 2), the data consisted of 3111 admissions. Of these, three-quarters were randomly selected for the development database, resulting in 2333 admissions for the development of the model. These admissions occurred in 2159 different patients.
A series of separate univariable logistic regression analyses were performed to examine factors associated with worsening AKI at 72 hours. A summary of the analysis results is given in Appendix 3, Table 34. The sizes of effects are reported in the form of odds ratios. For categorical variables, these give the odds of worsening AKI for each category relative to a baseline category. For the continuous predictor variables, the odds ratios represent the relative change in the odds of worsening AKI for a given increase in that predictor. The p-value indicating the overall significance of each variable is also reported.
The next stage in the analyses examined the joint association between the variables and AKI stage in a multivariable analysis.
The collinearity between the different predictors was examined. The examinations indicated no strong evidence of collinearity between any of the predictors. Therefore, all predictors were considered for the multivariable analyses.
A backwards selection procedure was used to retain the statistically significant variables in the final model. This procedure omitted the following variables (in order of removal):
-
admissions within the last 12 months
-
faeces at admission
-
troponin
-
number of radiology scans
-
K
-
HbA1c
-
gender
-
AMY
-
BNP
-
MSU or CSU pre admission
-
wound swab/fluid aspirate pre admission
-
Na
-
admissions within 30 days
-
calcium
-
outpatient appointments within the last 12 months
-
sputum pre admission
-
WBC
-
faeces on admission
-
MSU or CSU on admission
-
blood culture
-
sputum on admission
-
Charlson Comorbidity Index score
-
number of drugs
-
age
-
proteinuria
-
CRP
-
ALT.
The final model is summarised in Table 19.
Variable | Category/term | Odds ratio (95% CI) | p-value |
---|---|---|---|
Primary diagnosis | Neoplasms | 1 | 0.01 |
Infectious diseases | 7.91 (2.06 to 30.3) | ||
Circulatory system | 2.20 (0.67 to 7.23) | ||
Digestive system | 1.30 (0.38 to 4.44) | ||
Genitourinary system | 3.54 (1.04 to 12.0) | ||
Musculoskeletal | 1.48 (0.20 to 10.8) | ||
Nervous system | 0.81 (0.07 to 9.45) | ||
Respiratory system | 1.92 (0.58 to 6.38) | ||
Skin | 1.27 (0.27 to 6.12) | ||
Endocrine/metabolic | 1.03 (0.25 to 4.32) | ||
Injury/poisoning | 2.64 (0.74 to 9.43) | ||
Symptoms/signs | 1.18 (0.31 to 4.55) | ||
Other | 0.41 (0.04 to 4.37) | ||
Magnesium | Not measured | 1 | 0.03 |
Normal (0.7–1.0 mmol/l) | 1.10 (0.64 to 1.90) | ||
Abnormal | 2.25 (1.23 to 4.13) | ||
PLT | Not measured/normal | 1 | 0.06 |
Abnormal | 1.48 (0.99 to 2.12) | ||
CK | Not measured | 1 | 0.01 |
Normal (≤ 1000 U/l) | 1.54 (0.70 to 3.39) | ||
Abnormal | 5.44 (1.72 to 17.2) | ||
Baseline eGFRa | Linear term | 1.19 (1.04 to 1.37) | 0.01 |
Operation score at 12 hours | 0 | 1 | 0.003 |
Score 1–2 | 1.16 (0.44 to 3.03) | ||
Score 3–4 | 4.68 (1.91 to 11.5) | ||
Wound swab/fluid aspirate | Not taken | 1 | 0.001 |
Admission | Taken | 2.63 (1.49 to 4.64) |
The final model suggested that a combination of seven variables could help to predict worsening in AKI. Of these variables, one (PLT) was only of borderline statistical significance but was retained in the final model.
Validation
After exclusions from the full database (as described above), one-quarter of the data were randomly selected for the validation database. These consisted of 778 admissions. However, owing to a small number of missing values, predicted probabilities were obtained for 775 admissions.
First, the development and validation data sets were compared. This suggested that the two data sets were closely matched in terms of the model variables. This is not surprising given that the selection of the two data sets was at random.
The first set of analyses split the patients into risk groups based on the predicted probability of worsening AKI. Three risk categories were used (as opposed to four in previous risk models) because of the smaller sample size for this data set and the low occurrence of worsening AKI.
The ‘expected’ risk of worsening AKI in each category based on the predicted probabilities was calculated, along with the observed occurrence of worsening AKI in each category. The results are summarised in Table 20.
Risk category (%) | n | Mean expected (%) | Observed (%) |
---|---|---|---|
≤ 4 | 445 | 2.6 | 7.4 |
> 4–10 | 244 | 5.9 | 7.0 |
> 10 | 86 | 19.7 | 11.6 |
The results indicated fairly poor discrimination between the risk groups for worsening AKI. The first two risk categories had little difference in the observed percentage of patients with worsening AKI, with the occurrence in the highest-risk category being not significantly higher.
The calibration of the model was also not particularly good. There was an overprediction of the risk of worsening AKI for the lowest-risk category.
The next set of analyses further examined the discrimination of the model using ROC curves based on the predicted probabilities of worsening AKI. The AUC value was found to be 0.53 (95% CI 0.45 to 0.61). This suggests that the model has little or no discriminatory ability. The ROC curve is shown in Figure 22.
FIGURE 22.
Risk model 3: ROC curve for the prediction of worsening AKI.
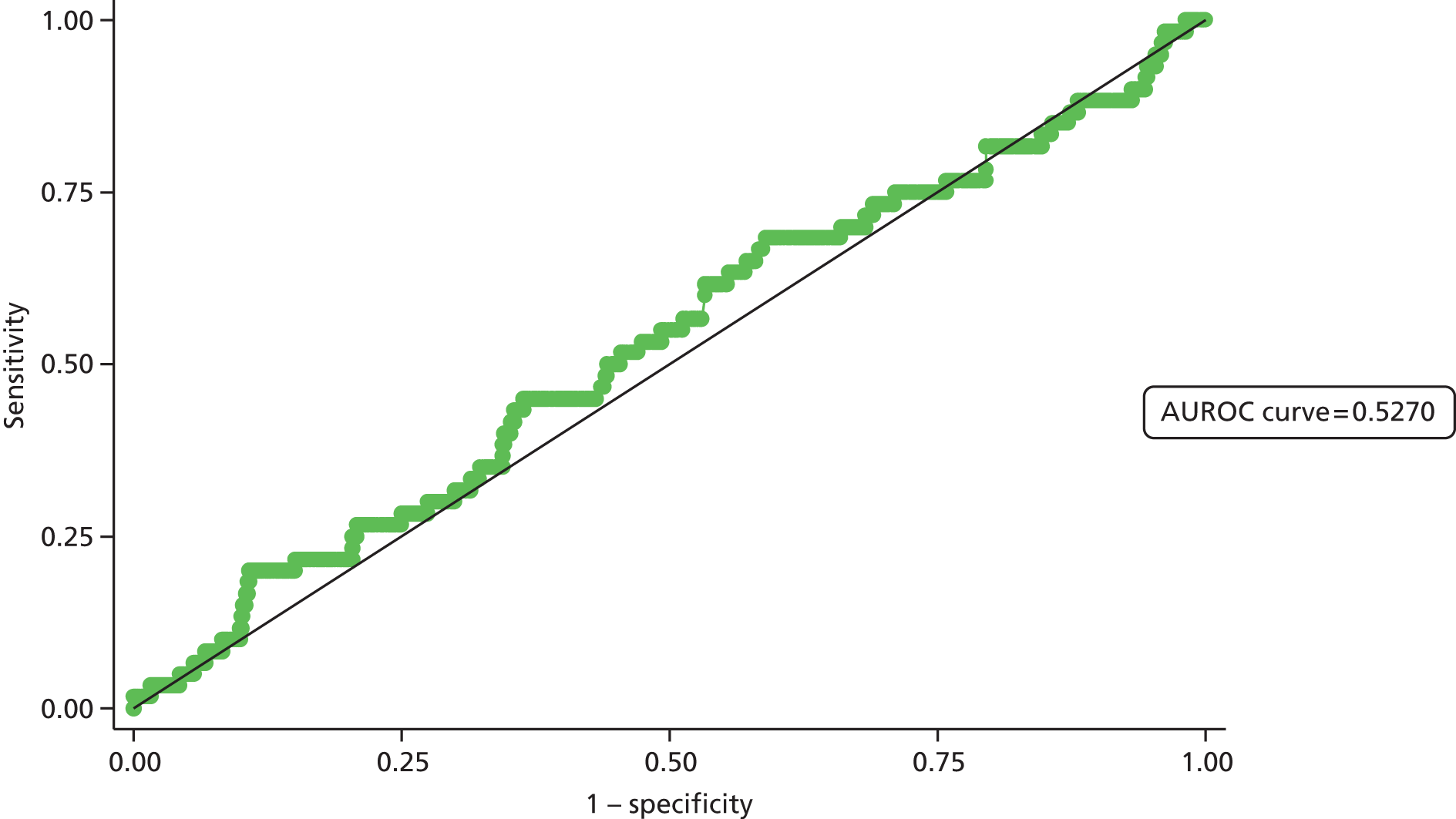
The final analyses compared the observed numbers of patients with worsening AKI in each AKI group against the numbers predicted (expected) by the model using the Hosmer–Lemeshow test. A summary of the results is given in Table 21.
Risk category (%) | No worsening (n) | Worsening (n) | χ2a | p-value |
---|---|---|---|---|
Observed (expected) | Observed (expected) | |||
≤ 4 | 412 (433) | 33 (40) | 44.8 | < 0.001 |
> 4–10 | 227 (229) | 17 (15) | ||
> 10 | 76 (69) | 10 (17) |
The results indicated that there was a highly significant difference between the observed numbers and those expected by the model. This suggests a lack of fit of the model to the data.
The model overpredicts the number of cases of worsening AKI in the lowest-risk group, but underpredicts the number in the upper risk group.
Summary
The results suggest a poor performance of the model in all validation assessments. As a result, this model is unlikely to be useful in clinical practice in predicting which patients are more likely to have worsening AKI. The poor performance of the model may be because there are considerably fewer patients in this group, because key variables have not been included in the model (e.g. physiological variables) or, finally, because development of AKI in this setting is subject to a random effect.
Bayesian risk modelling analysis
Risk model 1: the point of admission to hospital
Development
After exclusions from the full database (as described in Chapter 2), half of the data were randomly selected for the development database. Table 22 displays the variables that were selected via the SSVS method for the Bayesian logistic regression model.
Variable | Median | 95% credible interval | Mean |
---|---|---|---|
Intercept | –1.1835 | –1.2318 to –1.1375 | –1.1839 |
Variables with probability of inclusion > 0.5 | |||
Episode | 0.1352 | 0.0800 to 0.1920 | 0.1353 |
Primary diagnosis: diseases of the genitourinary system | 0.0988 | 0.0530 to 0.1398 | 0.0980 |
Charlson Comorbidity Index score | 0.1480 | 0.0966 to 0.1975 | 0.1478 |
Baseline eGFR | –0.2255 | –0.2812 to –0.1693 | –0.2255 |
Proteinuria 12-month test count | 0.0683 | 0 to 0.2045 | 0.0047 |
HbA1c recent result provided | –0.0839 | –0.3052 to 0 | –0.0760 |
Variables with probability of inclusion > 0.25 | |||
Primary diagnosis: diseases of the respiratory system | 0.0009 | 0 to 0.1069 | 0.0290 |
MSU or CSU in previous 2 weeks | 0.0007 | 0 to 0.1045 | 0.0335 |
Platelet result available | –0.0005 | –0.1364 to 0 | –0.0246 |
This model considers only the main effects. SSVS calculates the probability of inclusion of variables in the model. A high inclusion probability implies that the variable is important. A good cut-off point for this probability is 0.5. Based on this, the model should include six variables: spell type, primary diagnosis – diseases of the genitourinary system, Charlson Comorbidity Index score, baseline eGFR, proteinuria 12-month test count and whether or not the HbA1c recent result has been provided. Based on this model we can make the following statements, which are illustrated in Figure 23:
-
The odds of developing AKI are 1.15 times greater for non-elective admission than for elective.
-
The odds of developing AKI are 1.1 times greater for an episode with primary diagnosis = 12; that is, a diagnosis of a disease of the genitourinary system.
-
The odds of developing AKI are 1.16 times greater for an episode with Charlson Comorbidity Index score = 10 than for an episode with Charlson Comorbidity Index score = 9.
-
The odds of developing AKI decrease by a factor of 0.80 when the baseline eGFR increases by 1 unit.
-
The odds of developing AKI increase by a factor of 1.07 when the proteinuria 12-month test count increases by 1 unit.
-
The odds of developing AKI decrease by a factor of 0.92 when the recent HbA1c blood result is provided.
FIGURE 23.
Graphical representation of variables indicating the probability of their inclusion in the final model.

The effects of a diagnosis of a disease of the respiratory system, of MSU or CSU taken 2 weeks earlier and of the provision of recent PLT are very small, which means that the odds are very close to 1, suggesting that the risk of AKI is the same.
Validation
Validation of the developed Bayesian model was performed as described in the methods. Half of the data set had been randomly selected for use in model development, and half had been selected for model validation.
For this Bayesian model, the in-sample sensitivity was 35% and specificity was 98%, and out-of sample sensitivity was 32% and specificity was 97%.
The specificity, which is the probability that the model accurately predicts the episodes when AKI will not develop or, better, who will not develop AKI, is high. The sensitivity is poor.
Summary
The results of the Bayesian modelling demonstrate excellent specificity and hence this model is good at defining patients at very low risk of having AKI; however, the sensitivity is poor and so the model cannot accurately determine which patients are at high risk. This model would, therefore, be good for defining the population of patients who do not need renal function testing to assess for AKI; by inference it can, therefore, determine the population for testing. However, a large number of the population for testing will be false positives.
Risk model 2: predicting new acute kidney injury at 72 hours
Development
After exclusions from the full database (as described in Chapter 2), half of the data were randomly selected for the development database. Table 23 displays the variables that were selected via the SSVS method for the Bayesian logistic regression model.
Variable | Median | 95% credible interval |
---|---|---|
Intercept | –1.3377 | –1.5866 to –1.2238 |
Variables with probability of inclusion > 0.5 | ||
Charlson Comorbidity Index score | 0.1744 | 0 to 0.2714 |
Variables with probability of inclusion > 0.25 | ||
Outpatient attendances in the last 12 months | 0.0010 | –1.6156 to 0 |
This model considers only the main effects. SSVS calculates the probability of inclusion of variables in the model. A high inclusion probability implies that the variable is important. A good cut-off point for this probability is 0.5. Based on this cut-off the model should include only one variable, the Charlson Comorbidity Index score. Based on a cut-off of 0.25 only one other variable is included, the outpatient attendances in the last 12 months. The second and third columns of Table 23 display the median effect and 95% credible interval (for the effect) of the selected variables. Based on this model we can make the following statements, which are illustrated in Figure 24:
-
The odds of developing AKI are 1.20 times greater for an episode with Charlson Comorbidity Index score = 10 than for an episode with Charlson Comorbidity Index score = 9.
-
The odds of developing AKI decrease by a factor of 1 when the outpatient attendance in the last 12 months increases by one additional attendance. This suggests that this variable has a very insignificant impact on the risk of developing AKI.
FIGURE 24.
Overall plot of variables indicating the probability of their inclusion in the final model.
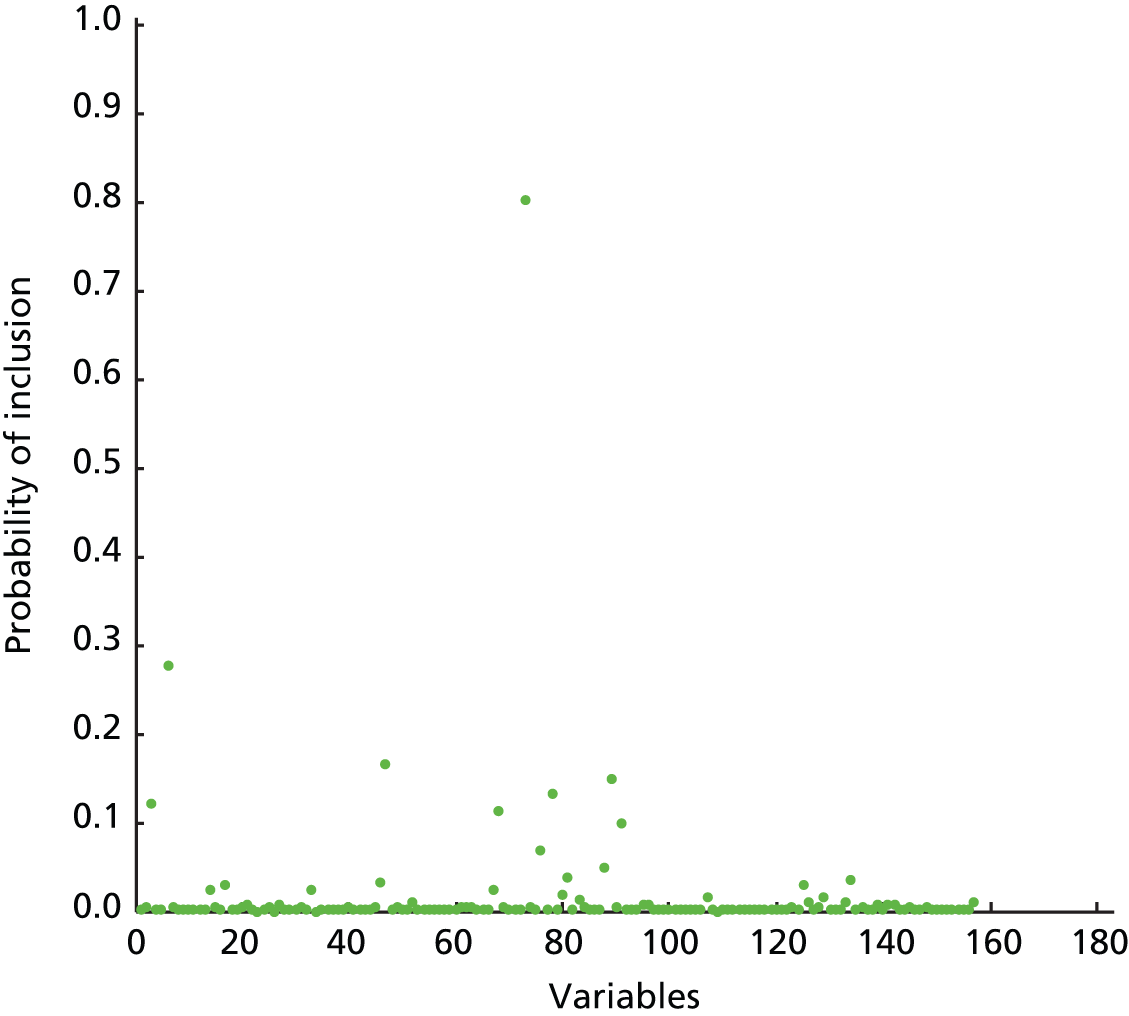
Validation
Validation of the developed Bayesian model was performed as described in the methods. Half of the data set had been randomly selected for use in model development and half had been selected for model validation.
For this Bayesian model the in-sample sensitivity was 33% and specificity was 98%, and out-of sample sensitivity was 32% and specificity was 97%.
The specificity, which is the probability that the model predicts accurately the episodes without AKI or, better, who not develop AKI, is high. The sensitivity is poor.
Summary
The results of the Bayesian modelling demonstrate an excellent specificity and hence this model is good at defining patients at very low risk of having AKI; however, the sensitivity is poor and so cannot accurately determine those patients at high risk. This model would, therefore, be good for defining the population of patients who do not need renal function testing to assess for AKI; by inference it can, therefore, determine the population for testing. However, a large number of the population for testing will be false positives.
Chapter 4 Qualitative analysis
Analysis of qualitative data
This chapter reports on the findings of the individual interviews with renal consultants and the focus group with outreach nurses from critical care conducted approximately 18 months following the introduction of the alert system. The purpose of this part of the study was to gather information on perceptions of the system, levels of interaction with the technology, communication with medical teams, impacts on patients and clinical practice, and recommendations for improvements.
Table 24 lists the main themes and subthemes emanating from the data. Quotes from the respondents are used in the analysis to justify interpretation, with ‘C’ referring to a consultant response and ‘ON’ referring to an outreach nurse response. Further numerical coding refers to the interviewee code for the respondents and the page number of the transcript from which the quote was derived. For example, C3:4 = consultant 3, transcript page 4.
Themes | Subthemes |
---|---|
1 Introducing the alert system | 1.1 Getting to know the alert system 1.2 First reactions to the system |
2 Using the technology | 2.1 General comments 2.2 Issues with user friendliness 2.3 Timing and workload 2.4 Accessing data and making decisions |
3 Interacting with medical teams | 3.1 Finding the right doctor 3.2 Discussing the cases 3.3 Awareness of the alert system |
4 Monitoring cases | 4.1 To follow up or not to follow up? 4.2 Acting on advice |
5 Impacts on patient care and clinical practice | 5.1 Making a difference to patient care 5.2 Impacts on clinical practice 5.3 Perceptions of cost-effectiveness |
6 Recommendations for improvements | 6.1 Improving trust-wide knowledge of the alert system 6.2 Improving the technology |
At the time it was assessed, the AKI alert system at EKHUFT was delivered through QlikView, a browser-based reporting tool; references to ‘QlikView’ in quotes are, therefore, referring to the AKI alert system.
Each section of the analysis is followed by a short summary highlighting the main points raised.
Introducing the alert system
This first theme focuses on how respondents first heard of the alert system and were instructed how to use it, and their initial reactions to becoming part of the system.
Getting to know the alert system
Some respondents had already used a similar system and so found the transition to using it for the research straightforward:
I’ve used QlikView already so I’m familiar with it . . . [researchers] took me through the system and I basically got on with it.
C6:1
. . . I’d used QlikView previously, some of the trust data’s on QlikView itself . . . I think [researcher] probably discussed it at one of our senior doctor meetings and then it was introduced, and we’d had a chat about using it . . . that the on-call consultant would use it every day to identify patients.
C4:1
I basically was using it in a previous job role . . . to access A&E [accident and emergency] data so we was [verbatim] kind of familiar with it from there. And then . . . I’ve just adapted the bits of it that I’m using really.
ON2:1
The nature of the first introduction appeared to be informal and brief; some participants had difficulty remembering it:
I think it was at a meeting that [researcher] briefly mentioned but it wasn’t a formal you know, unveiling of the system . . .
C1:2
I don’t think there was any formal training that I remember, just opened it and started using it.
C3:1
I can’t remember actually . . . I suppose [researchers] must have shown us, shown me how to use it but I honestly can’t remember.
C2:1
The outreach nurses appeared to have had more formal training, which they rated highly in terms of meeting their needs, and they particularly valued the accessibility of the researcher:
. . . when [researcher] trained us, I certainly felt that it was very adequate. We had several sessions with him. And I feel very much that if I needed anything I could always get what I wanted from [researcher] so it wasn’t . . . it wasn’t just an informal thing for us.
ON1:4
I mean [researcher] is very adaptable and approachable in terms of giving us what we need, I think, as a whole team across the sites in terms of education and updates and stuff.
ON3:4
Some of the consultants had been involved in the project’s conception so they were already knowledgeable about it, as explained here:
I was aware of its development and you know [researcher] would talk about it whilst it was being developed and ask for ideas and suchlike . . .
C5:2
Most respondents felt that the system was relatively straightforward and that they were able to learn experientially, without the need for more formal training:
. . . a lot of it was sort of learning as you tried to use the system, you try . . . and you learned how to access this bit of it or that bit of it . . .
C1:1
. . . it’s not very complicated, but yes we were given passwords for access to QlikView and the AKI system and then I think [researcher] took a quick whizz through and that was it.
C5:1
. . . you learn the tricks as you go, so I think expectations of how you’re taught how a system works are probably too high . . . the practicalities of doing it – actually that experience is quite a useful tool.
C4:3
First reactions to the system
All respondents appeared to be keen to support colleagues and welcomed the alert system. There was also an acknowledgement of its importance and potential contribution to innovation and change in renal medicine:
. . . well I thought it was a good idea because we I mean we have been doing this with chronic kidney disease and advising GP [general practitioner] practices so I thought with acute kidney injury . . . it was quite a good idea and I was keen to continue with it and help out the research process.
C1:1
I think it’s great that there’s a system in place to pick people up.
ON4:26
It’s important work, not only for the trust but it fits with the recommendations of the NCEPOD report for AKI . . . and the general Department of Health Research and Innovation Agenda . . . yeah, so good for patients and practice.
C6:1
In addition to recognising how the system could improve patient care and impact on practice, other respondents discussed the proactive nature of the alert system in bringing this about:
. . . people can be going ‘off’ without anybody realising, so having an alert system that actually picked it up and prompted the doctors looking after them to actually have a think about what might be going on with this patient seemed like a very good idea.
C5:1
. . . it’s our only means of identifying some of the sick patients . . . and quite often some of these groups of patients we will only pick up on because they’ve been identified through the alert system . . . Had we not had that access to that we probably would never even know they’re in hospital.
ON2:5
. . . well I think it’s a very good idea . . . I think is very important and using the benefits of the expertise that we have in the trust for building these platforms I think makes a lot of sense, I’m enthusiastic.
C2:1
One respondent, however, did feel a sense of imposition, suggesting that there was little choice in terms of participation:
. . . we were told that we had to start using it, we were told that it was part of a research and that they were trying to sort of incorporate it into our normal daily activities and practice.
C1:1
The outreach nurses also had some collective reservations about the added workload and goodness of fit with their role, an aspect elaborated on later:
I’m not sure we were totally warm to it because it was just something else to add to our job . . . And we’ve got one of those umbrella jobs where we . . . ‘Outreach will do it anyway’ . . . And then it was like oh. But . . . it is quite appropriate to see the patients very often because they’re sick and they have renal failure . . . But we weren’t exactly, ‘Yay!’
ON1:4/5
I . . . I sort of personally queried why this wasn’t being taken up by the renal satellite unit . . . and I did feel a little bit well I’m not quite sure that that’s appropriate for us.
ON3:5
Summary
Initial responses to the alert system appeared to be encouraging. There was strength of opinion regarding the desire to support colleagues and the research endeavour, and an understanding of its potential contribution to renal medicine. Given that the alert system has been in operation for nearly 2 years, the sentiments expressed by most respondents appeared to be enduring, evidenced by their use of the present tense when describing their views. Alongside this, however, there was a tendency for the outreach nurses to greet the system with a degree of uncertainty regarding their roles. Outreach nurses had more formal training, which they appreciated, and, although seemingly brief and informal for the consultants, the induction process appeared to be sufficient for engaging colleagues given that the technology was not complex.
Using the technology
The second theme explores opinions and use of the system technology and includes a general overview of accessibility and functionality, followed by a deeper analysis of issues concerning user friendliness, timing and workload, and factors relating to accessing data and making decisions.
General comments
General comments about the technology are summarised by the quotes below and include opinions about accessibility of information and the population group:
I think the accessibility is good, the fact that it’s web based so you don’t have to have software installed on a specific computer and the fact that I can access it from my iPad® [Apple Inc., Cupertino, CA, USA] at home . . . I don’t have a problem with it, it’s quite intuitive, visual impact you know that’s fine again.
C2:3
I use it really just to identify the patients with AKI 3 and those who have not had intervention . . . I only deal with the ones who have not had any comments, so the new ones.
C4:5
With respect to its overall functionality, a frequent comment related to how respondents restricted their use to certain features deemed most relevant:
. . . there are a lot of buttons at the top but I never use most of them . . . most of the information is, the important things, that they’ve got AKI 3, where they are and what team they’re under . . .
C4:8
I appreciate that there are a lot of tabs along the top that I just haven’t explored really through lack of time more than anything else but in terms of the core functionality it’s very easy to use.
C2:1
To be honest I only access the first page and then I go into other systems because they provide for more information.
C5:4
Issues with user friendliness
More detailed exploration with respondents highlighted a number of issues regarding the ease or difficulty of navigating the system and accessing data. The following quotes describe the main difficulties experienced. First, the speed and responsiveness of the system appeared problematic:
. . . sometimes the pages wouldn’t load and if you were trying to filter out things it wouldn’t, it would take a while to . . . get to the filter page which you wanted to look at so sometimes a lot of clicking and logging out of the system and logging back onto it . . .
C1:2
The system is very variable in terms of it does seem to not want to upload or refresh and stuff quite regularly.
ON2:20
. . . there’s a way you can add comments so that your colleagues for the following day know that you’ve already sort of dealt with that patient and that can be a little bit sluggish to upload . . .
C6:4
One respondent also commented on the ‘modernity’ of the system:
. . . it’s not very pretty . . . because actually we’re used to doing most stuff on webpages then you go to Amazon or whatever else you know that’s what we’re used to, and it’s a very different system and I don’t think it’s knowledge user friendly.
C4:9
Issue were also raised about the mechanisms through which identifiable patient data could be retrieved and information added:
. . . sometimes just when you start, when you log in you have to clear all the selections and then start again otherwise you don’t get all the patients quite right . . . you might miss patients.
C4:4
. . . if you were reporting on a certain patient you have to manually enter sort of details, for example the NHS number or whatever and that was a bit time-consuming . . .
C1:2
. . . it’s been hard to be able to print . . . off the lists and then when I’ve gone back at the end of the day to try and put something in I get locked out again or not being able to log in.
ON4:20
if you’re documenting in notes, on a handover sheet . . . then try and do it on the QlikView as well and you think . . . It’s an endless process.
ON2:20
Given that the system was in development, the research team appeared responsive to some of the difficulties respondents were experiencing:
Things that [researcher] has changed which I like is that now it actually updates more quickly when you actually fill in a form and that information goes in there much more quickly now.
C5:2
Timing and workload issues
Although, overall, respondents appeared keen to participate, a further theme that came to light concerned the amount of time it took to use the alert system. The following quotes, from a consultant and an outreach nurse, respectively, summarise the different patterns of system use and associated activity:
. . . an average month about three times, and then when you’re on the wards, which is 1 month every fourth month you’re on the ward, then actually on top of that three you’ll do two weekends so you’ll access it another six times that month.
C5:6
. . . [we access it] Every day. And then we look at the people that are scoring twos and maybe threes. We, you know, we don’t exclude the threes because they might not have been enquired about at the point that we go.
ON3:7
Consultants were particularly keen to highlight the relationship with work plans. Each time they are on call, they encounter between one and eight new cases of AKI stage 3. This respondent appeared reconciled to accommodating the work:
You just incorporate it into your work plan . . . yes, other things get pushed down the list but it’s swings and roundabouts.
C6:4
However, most respondents found the integration of this additional work troublesome in that it was not officially incorporated into their work plans:
. . . there’s been no time put in my job plan to do it . . . it depends on the day but often it’s quite a burden . . .
C5:7
. . . you need a good one and a half to two hours of just you and the computer and the phone . . . I think that it needs to be accounted for . . . I think because it’s a big chunk of work that we have to do . . .
C1:5
A further issue that came to light concerned difficulties prioritising the work, particularly as there was a significant time commitment; reasons for this are explored more fully in a later section (see Interacting with medical teams):
. . . trying to fit it in there was very difficult and it didn’t always happen to be perfectly honest and I used to slightly resent it then but there you go, just one of those things.
C2:4
. . . a lot of time is wasted especially when you’re on call and you have to have other things you know, if you have got a ward round that day, clinic that day, so it takes time.
C3:3
. . . we’ve all got lots of other things going on and OK it’s only once every 10 days so it’s not that often but it’s just a bit irritating having to do it . . .
C4:8
Accessing data and making decisions
An issue of concern related to the fact that the data on the alert system provided information that was up to 24 hours old; this posed problems for locating patients and created uncertainty about their medical condition and concerns about wasted time:
. . . sometimes the ward has changed . . . you have to go on some other system to find where exactly the patient is and sometimes you have to, when we ring a ward they say, well, they’ve moved to the other one.
C3:4
. . . a lot of the time the results will be from A&E and they’ll flag up on QlikView from A&E so you think I’ll go into the computer system and track a patient. Oh where are they now on the site you know. You’ll trawl all the way over there to find out they‘ve just had their bloods done and their result’s better so you’ve wasted like half an hour.
ON2:18
. . . you are in a way acting on yesterday’s data so you’re coming in up to 24 hours later when a lot more things would have happened to that patient in the time period, which is a small problem I think.
C1:3
There was a view among some respondents that this time lag had its advantages:
I’m not sure true real time would be a necessary advantage actually . . . because I think that 24 hours of hindsight allows certain things to sort themselves out . . . the patient that’s admitted clearly dying . . . and me phoning up the team looking after them and saying this patient’s got acute kidney injury . . . would probably not serve any useful benefit to either the patient or the team.
C2:3
So there’s somebody who’s flagged up as [AKI stage] three, but the team have already started treating and if you can wait till the results are back you might see that their results are already improving, so . . . it’s sort of a good thing . . .
C4:4
Respondents described in more detail how patients with AKI were identified and how decisions were made to formalise the alert and contact relevant teams. Given the time lag, it was clear that other data sets were needed to support the decision-making process, as the alert system provided a first-level identification of patients that only included such details as NHS number, AKI stage and the ward:
. . . you would have to log on to other systems . . . the iSOFT system, to sometimes look at the past clinical history from clinic letters and you’d also have to log onto the X-ray system to look at the current images and the historical images.
C1:3
. . . I use all the other trust IT systems . . . so PAS [patient administration system (iSOER)] to identify where they are . . . and you can get in and use the blood results system either on that or DART [pathology database] to see what the latest result is and also the new VitalPAC system I’ll look up their observations and things . . . you know you have to go and find it, yeah you have to open it up . . . there’s no link directly onto QlikView.
C4:5
. . . we can also look on the clinical functions at previous documents where they might have been to clinics so you can get a bit of a background but not all patients have those updated.
ON3:22
For some this was frustrating, and one outreach nurse summed up the general feeling among the nurses:
None of these systems interact with each other and it’s just a nightmare really.
ON3:20
It was considered preferable to have all the data in one system; however, the absence of this was not perceived to be too much of an imposition:
I suspect it would be quite complicated to put interfaces with other systems so I think it, the other information is easily accessible on the net so it’s not too complicated to access it . . . I would say it probably takes me 5 or 10 minutes to look at all the systems and formulate an opinion as to what might be going on with the patient before I try to ring them . . .
C5:5/7
. . . the system identifies the patients, that’s the crucial bit yeah I can access the other information, oh it’s on another, I have to click another box and put in another password or whatever, but yes it’s not the end of the world . . .
C6:6
Summary
Most respondents restricted their use of the technology to the few alert system functions that were necessary for identifying the AKI patients and initiating the alert to medical teams. Observations were made about lack of user friendliness with regard to the sluggishness of navigating the system and inability to access data, and consultants raised the issue of increased workloads that were not built into work plans. Although some felt able to assimilate this extra work, a number found this to be quite onerous. Much discussion focused on the need to access data from other sources to facilitate decision-making about whether or not to alert the medical team, and the pros and cons of having a system that provided data that were 24 hours out of date.
Interacting with medical teams
A prominent issue for all respondents was accessing and interacting with medical teams to action the alert. This third theme describes how respondents tracked down the appropriate doctor, the differing experiences with communicating and discussing the cases, and the extent to which respondents felt that medical teams were aware of the alert system.
Finding the right doctor
Consultants in particular described this as a ‘chasing game’ that was frustrating, took up valuable time and tried their patience. The following quotes elaborate on typical communication attempts in detail, focusing on difficulties tracking junior doctors and the ‘tyranny’ of bleep identification:
. . . the main problem . . . is mapping junior doctor or junior doctor team to an individual patient and I think that work wasn’t sufficiently developed before the system was rolled out . . . a patient is flagged up as having AKI you then have to . . . go through switchboard to identify which junior doctor is looking after the patient, you may phone the ward where . . . you get given one bleep number, it turns out not to be the right bleep number . . . and then that person doesn’t answer that bleep and so you try a different member of the team and you know it can take half an hour to get hold of the right junior doctor and that’s not an exaggeration . . . that actually gets very tedious when you’ve got lots of other stuff to do to be bleeping about five different people before you get a response and I think that’s a fairly common issue with it.
C2:2
And we have this daft system in this trust where the [junior doctors] look after the wards at a weekend but because they’re not actually on call . . . the switchboards don’t carry their bleep number . . . they haven’t got any number for them so you have to ring up the on-call team to actually ask them which [junior doctor] is on for the weekend and what bleep are they carrying.
C5:11
. . . it’s teams, and it’s partly because teams are so unstable because the registrar’s on holiday, the SHO [senior house officer]’s just done nights so it’s only the house officer and actually they’re in the middle of a ward round for a different team . . . that is the big problem.
C4:12
For all respondents, the frustration of trying to find the team responsible for the patient was clear, and the alert system did not appear to help in this regard:
I mean you can’t get hold of the right person, the person you eventually get hold of does not know the patient . . . you know the conversation doesn’t take very long, it’s just getting hold of people and whether they know about it or not.
C3:3
You deal with it generally out of hours by contacting a doctor who’s not really responsible for that speciality of patient who is already generally busy and probably overworked so it’s not an ideal system.
ON2:12
. . . that’s the real downside of this, I don’t actually mind any of the other bits but I get so frustrated about trying to actually pin down somebody who actually knows something about this person who’s willing to take responsibility and take it forward . . .
C5:8
Very difficult. Very difficult. I think there was a thing, a page where you could look at the contact details of the teams and sometimes I cannot access that information from the actual QlikView dashboards.
C1:5
There were added difficulties when the patient was admitted through accident and emergency (A&E) and when trying to contact certain specialties:
. . . trying to identify which team you’re meant to be talking to when it’s an A&E patient, so somebody comes in with AKI 3 into A&E, it’ll flag up as A&E and then you have to track them down which is, I mean it’s possible using the other IT systems but it’s just a bit frustrating . . .
C4:5/6
. . . surgery – particularly orthopaedics they, you can ring around quite a number of F2s [junior doctors] before you actually find someone who’ll take responsibility or go and see the patient.
C5:7
. . . what you need to do is get the best person that’s going to give the best treatment to that patient and generally unfortunately that’s not always the orthopaedic doctor.
ON2:13
One respondent felt that the European directive on working time contributed towards these difficulties:
. . . what I’ve noticed is, you know, numbers of junior teams who don’t know their patients because they weren’t on call or they weren’t, you know, or they were on a different ward round . . . it’s European working time that’s mucked the whole thing up.
C4:6/7
One respondent felt that more trust-wide communication at the beginning of the project would have been better:
I think it probably wasn’t fully sorted out and like anything you introduce a system and actually you have to anticipate there’s going to be problems and there will be a grumpy clinician somewhere who gets a bit silly about it all . . .
C4:2
Respondents had adapted to these difficulties by developing a range of strategies to improve their chances of accessing the right doctors:
. . . usually I get hold of the right person straight away by actually phoning the ward . . . I guess then that reflects the fact that the nurses just have the local knowledge on the ground on the day.
C2:2
I’m not sure how reliable those bleep numbers are but . . . I don’t even look at them now I still go back to ringing switchboard and trying to find out.
C3:6
. . . it’s very difficult to locate an orthopaedic doctor so I guess that our first port of call isn’t actually the orthopaedic team, it would be the on-call medical team or ITU [intensive therapy unit].
ON3:12
. . . we’re just communicating. We just talk to people a lot of the time.
ON3:19
Discussing the cases
Once the appropriate doctors had been located to formally alert them to an AKI patient in their care, there were differences between consultants’ and nurses’ experiences because of the differing intervention pathways; however, there were also similarities. First, these respondents provide a general overview of their approaches. A consultant describes their general intervention pathway:
I phone them up and I give them advice and make sure that they are repeating the bloods and doing all the appropriate things I think they need to do and it may be a case of just saying ‘are you aware’ and they say ‘yes’ and I say ‘fine you’re doing all the right things’ or it may be much more detailed advice depending on the situation . . .
C2:5
For outreach nurses, there were some pathway differences between the sites because of the professional judgements and discretion of the outreach team members. This did have the potential, however, to cause confusion among doctors who worked across three sites:
. . . we look to see where the patients are . . . and then we go out to the wards to see the patients and speak to the teams so we’ll put a sticker in the notes alerting the team to the patient . . . and we’ll find out . . . what’s happening with the patient . . . then we’ll speak directly with the teams about that. And then if we feel that they need continued monitoring then we’ll retain them on our list. If not, that will be our only contact with them.
ON3:7
Other people on that initial visit, even if it’s basic, might still go on to do a complete full assessment . . . And I think that’s when then you get a differing expectation maybe from the medical teams.
ON2:10
However, respondents found that in most cases action had already been taken:
. . . by and large I think that by the time I get round to speaking to the team they’re usually aware that the patient has AKI. I cannot really recall any instance, maybe one or two instances in the last year or however long since we rolled it out, that I phoned a team and they were genuinely unaware.
C2:9
. . . the doctors that I’ve spoken to will say ‘oh yes we know about the patient, we’ve done A, B and C and we’re awaiting X, Y and Z’, so most of the times they are already on the case and our advice isn’t needed.
C1:5
Although nurses had similar experiences, they felt that the stickers were instrumental in bringing about awareness:
Quite honestly, I think the stickers have been quite successful – generally [the teams have] already identified that they’ve got acute kidney injury, they’ve already started all the processes on the advice sticker and we stick the sticker on the notes anyway, even though it’s all happening.
ON3:7
When it came to reactions from doctors to these interventions, respondents had mixed experiences. This respondent, for example, felt that consultant colleagues were supportive overall:
I have spoken to other consultants, medical consultants in other hospitals and they say it’s a good thing that someone else is keeping an eye and providing that extra layer of advice to their teams.
C1:4
For outreach nurses, despite the sticker often being applied after the event, these respondents were keen to point out that this did not seem to interfere with interpersonal relationships within the teams:
. . . but they’ve identified it, they’ve done it all and then we’ve come along with our sticker! But I think there is sort of quite a . . . a good rapport with them . . . And we just go, ‘Ha, ha, ha. I’m afraid it’s got to go in there,’ because it’s like an audit trail, isn’t it? . . . but I’ve never felt anybody was really cross about it . . .
ON1:9
Not ever had a negative response from anybody.
ON2:14
. . . we’re quite good at . . . at rapport . . . There’s sometimes a bit of a competitive spirit as well. They go, ‘I’ve done it already!’
ON3:15
For other respondents, the general experience of doctors’ reactions varied, with some respondents perceiving some indifference because action had already been taken:
. . . it’s a mixture of positive and indifferent but not negative. I’ve not had anyone getting irritated, cross, abusive or obviously lacking in gratitude. Indifferences maybe one-third and people actually sort of sounding quite positive’s probably two-thirds and that is irrespective of the sort of level if you like, of the person I’m speaking to . . .
C2:5
. . . they’ve got you know other priorities they are busy . . . they just give you the information and if you say something to them they will just note it down but I don’t think they’re hugely interested.
C3:5
Another respondent highlighted that there could sometimes be an issue with understanding the urgency of the situation for the patient:
. . . sometimes you’ll get a registrar who’ll say ‘well I’m in clinic I’ll deal with it later’ and you say ‘no you can’t’ . . . and yeah, so occasionally perhaps there’s a perception issue as to the significance of the problem, it’s how you handle that . . .
C4:10
As intimated previously, some of the respondents did notice a difference between the teams in the trust in terms of how the patients were dealt with:
. . . medical teams usually have it under control, it’s the surgical teams that have the challenges . . .
C6:5
. . . often I find that the ward manager knows far more about what’s going on with Mrs Bloggs than the surgical junior doctors . . .
C5:10
. . . you are dependent . . . on one on-call doctor at a junior level who specialises in people with broken or damaged bones who then have – this is a slightly sweeping statement that I’m going to make, – the inability to do anything about anything that’s not to do with an injured bone! It’s just like, ‘That’s not my job.’
ON3:12
This respondent had a sympathetic opinion regarding these differences:
. . . I am aware that in many instances . . . the surgeons are less aware but they’re surgeons . . . physicians should be looking after these patients . . . surgeons should get on with the job they’re best at . . . they’re not particularly concerned with post-op . . .
C6:3
It was of interest, though, that many of the consultants recognised the potentially intrusive nature of the alert system and were empathetic about how the telephone calls could be received:
I feel very guilty about troubling them . . . the post-take ward round is probably the most stressful point in the week when your workload is at its highest. And to then have somebody bugging you about a patient you already know has got AKI to tell you they’ve got AKI, I feel slightly guilty about to be perfectly honest . . . they are remarkably restrained I think.
C2:7
If I had a patient coming in under my care and a doctor phoned me up asking me basic questions, I would probably get a bit upset but that’s me, every doctor is different.
C1:6
. . . it’s sort of stepping on professional toes a little bit . . . there is perhaps sometimes a worry that we almost take a bit of a holier-than-thou attitude . . . you know, somebody coming in and, not even invited . . . and saying ‘well you’ve got this wrong and this needs to happen’, it could be perceived as you know a little bit arrogant or a bit rude. Not the intention at all.
C4:2
Awareness of the alert system
One of the factors that influenced the response by the teams was the extent to which they were aware of the alert system. Some felt that this was an enduring problem caused by working patterns, especially among junior doctors:
The juniors . . . haven’t had a clue about them at all but once you get to registrar level and above they’re very receptive to it . . .
ON4:14
. . . even now I think a lot of them are basically not certain why we’re ringing them . . . it happens, because there are so many clinicians all over and surgeons, and physicians and nurses, junior doctors. I don’t think everybody knows about it.
C3:2
. . . teams are changing all the time. Junior doctors change every year . . . you just get one group used to the system then a new group arrives with no knowledge, no training in dealing with patients with AKI . . . so it’s a challenge.
C6:11
This respondent highlighted the importance of communication skills:
Sometimes they’ll go ‘ugh, no!’ and don’t know about the system . . . they’re not fully aware that they might get a phone call, so how you introduce yourself in the first instance I think sets a tone of the rest of your discussion . . .
C4:10
For others, there had been more problems at the beginning of the project:
I think in the early days people were surprised that someone else was aware of that clinical issue . . . sometimes if you rang you’d end up speaking to the ward . . . and then they would say ‘sorry, who are you? And what system is this?’ . . . So it’s almost like sometimes I felt I was a bogus caller . . . then eventually, eventually now it’s old hat they generally are aware.
C1:4
Well, we did some work early on in getting round to tell people about the system . . . getting buy-in . . .
C6:3
Summary
There were similarities between how consultants and nurses approached ‘alerting’ and discussing cases with the relevant medical teams; however, for outreach nurses, the extent of professional involvement in this varied between the hospital sites, which could cause confusion among doctors. The difficulties respondents had in tracking down the appropriate junior doctor manifested themselves as a major problem, and it was clearly frustrating and costly in time. Respondents highlighted the many intricate instances of how communication can fail to connect them to the right person in a timely manner. This included failures with how bleeps are managed, the complexity of junior doctors’ working patterns, how their responsibilities are organised, the instability of medical teams, and how the movement of patients is monitored. All respondents had developed strategies to cope with this frustration, which mainly entailed telephoning the nursing staff on the wards, who they felt had more immediate knowledge of who to contact, and maintaining good channels of communication.
In the face of these difficulties with contact, the general experience of the respondents was that once the appropriate doctor was located and cases were discussed, most found that teams were already aware and were dealing with the situation. Outreach nurses noted the success of the stickers in bringing about awareness. Some respondents felt a degree of discomfort with ringing colleagues uninvited for little justification, noting that responses sometimes implied disinterest. On the whole, however, responses were not negative and there was recognition of the importance of interpersonal communication skills. It appeared that there were differences between specialties; not only did some respondents seem to have greater difficulties accessing surgical and orthopaedic teams than physicians, but surgical teams were, by and large, less aware of their AKI patient and how to action the alert.
When it came to colleagues’ awareness of the alert system, respondents were mixed in their responses. Some felt uncertain about whether or not there was full understanding of why they were being contacted. The shifting nature of junior doctors’ employment explained some of this. Others felt that there were problems at the outset but that there was now generally a good understanding.
Monitoring cases
Although the function of the alert system is to ensure that AKI patients are identified, that accountable clinicians are informed and that appropriate interventions have been or will be actioned, respondents were asked whether they undertook any monitoring or follow-up of cases. The two sections here focus on differing views of whether or not respondents felt that they should follow up, and the extent to which they felt that advice was acted on.
To follow up or not to follow up?
The responses were varied; these quotes from consultants, for example, describe experiences in which respondents were particularly concerned about inappropriate management and took action:
. . . we had one last week where I felt very strongly that the patient had been inappropriately managed . . . my colleague went and saw the patient, you know, absolutely agreed that this was an avoidable situation, that really they should have been more aware of the risks and the fact that the patient already was developing AKI . . .
C2:6/7
. . . there are a couple of cases where I’ve been really, really worried about somebody . . . then I will actually follow it up and look at it the next day and the next day to make sure that actually they’re getting better . . .
C5:10
. . . probably once every 6 or 9 months then you know I feel actually this hasn’t been appropriately managed and there needs to be a bit of follow-through for this.
C2:6
As intimated in earlier sections, outreach nurses’ involvement varied across sites according to the condition of the patient and professional judgement:
. . . in my workload . . . they either get a sticker and we’d never ever promise to do anything more than one visit, or they are a documented outreach patient, so they’ll have a full assessment.
ON1:10
. . . if they have already identified AKI then . . . then that’s it and we don’t really necessarily go back.
ON3:11
. . . you’ll find people that are more complex, that have got a lot of other ongoing problems, that have got maybe multiple potential causes of their AKI that are very sick . . . you keep on the outreach list because they’re sick, not necessarily just because they’ve got an AKI.
ON2:9/10
These consultants, however, felt that the teams should be accountable following the alert communication:
I really don’t see the need for further monitoring, once it’s handed over then it’s the responsibility of that team . . .
C6:5
I think really the ball should be in their court, whose patient it is, that they should be just chasing up and let us know if there is progress or lack or it rather than, you know, we chasing them all the time which is happening at the moment . . .
C3:5
Whether or not they followed up cases, all respondents made efforts to ensure that a communication was recorded to alert the teams to their involvement, or that a mechanism was in place should more advice be needed:
. . . following up, no . . . I try to remember . . . put that as a final comment you know, registrar or SHO [senior house officer] informed, discussed blah blah blah, team aware to contact renal if they have any further concerns or issues.
C4:8
. . . if I was going to carry on monitoring or seeing a patient from a trigger . . . I would write something at the end of the notes along the lines of ‘we will continue to monitor this patient’s progress’ and something along those lines.
ON2:10
. . . obviously the team can contact you but sometimes the team will contact the on-call doctor as well or would have already done that so there is a way of, for the team to follow up those cases.
C1:3
Many of the patients eventually came under the care of the renal team:
. . . a lot of them do end up on dialysis and they eventually come to our ward anyway so there is that follow-up. I think out of all the cases I’ve done there was one that I was particularly interested in and kept contacting the team and . . . then eventually they came over to our ward . . .
C1:3
Acting on advice
There were distinct differences between the consultants and the outreach nurses on this subject. For consultants, the extent to which advice was acted on was not generally known because of work patterns. One respondent stated:
. . . you generally aren’t really aware of whether it’s been carried out or not – your recommendation – because the next day you’re not on call again . . . On one occasion I did ring to speak to the doctors and I advised them to call me back, and they never did. And for whatever reason they probably felt that they’d finished the case effectively and they didn’t need my advice, I’m assuming.
C1:4
A further concern related to this was the failure of teams to make a written note of the discussions with respondents, which impacted on the audit trail and clinical decision-making process. This respondent summarises consultant experiences:
What I hadn’t done until recently was actually ask them to document the discussion that had been had with them . . . There’s no documentation of these discussions happening, and I suspect that’s because often the discussions happen when the doctor’s not in front of the notes because we’re ringing them and . . . catching them wherever they are.
C5:8
The outreach nurses found that there were clear comparisons between different medical teams, and significant concerns were raised again regarding the lack of appropriate trauma and orthopaedic team responses and accountability. The following responses highlight the extremes to which the nurses feel they need to go to ensure that patients are appropriately managed:
. . . the medics are really very good at dealing with the acute kidney injury. The area that we have a huge problem with . . . is the trauma and orthopaedic areas . . . you know, they . . . they will watch their patient’s renal function deteriorate and then the nurses will call the outreach team to say, ‘This patient’s got a really big problem,’ and then we’ll tip up and start the whole ball rolling really . . . in extreme cases they’ve already contacted the renal team and they completely ignore the advice. And that’s really an area that needs a lot of work . . .
ON3:11
We’ve started using words like ‘life-threatening’ ‘this patient will die’ and then . . . and then the next thing we do is we say, ‘Shall we phone [the renal team] for you? Here you are; they’re on the phone’.
ON2:15
. . . we’ve highlighted it, shown the doctors the stickers in the notes and said to them, ‘This is actually quite serious now and this patient looks like they have a potential to deteriorate. We feel that you need to take this further,’ and the doctors have walked past us and carried on talking to other nurses and . . . and we’ve had to wait and wait our turn to then come back to them and say, ‘Did you quite understand that this is serious? Can you please listen to us?’
ON4:15
Summary
There were differences in consultants’ and outreach nurses’ experiences regarding monitoring patients and acting on advice given. For consultants, monitoring or follow-up of cases was conducted on few occasions and only when patients gave cause for concern and confidence in managing the patient was not high. Some felt quite strongly that their involvement should end following the alert, as teams should take over responsibility. Ongoing monitoring by outreach nurses varied between the three sites and was dependent on professional judgement and the condition of the patient.
Most consultants were not aware whether or not advice had been acted on, and there were some concerns regarding the documenting of the advice given. However, all respondents, including nurses, agreed that they would ensure the availability of a contact for further advice if needed. Outreach nurses focused on challenges with trauma and orthopaedic teams and highlighted the difficulties with passing on the management responsibility of AKI patients to them.
Impacts on patients and clinical practice
The final theme is concerned with establishing the extent to which respondents felt that their involvement in the alert system had any effects, beneficial or otherwise, on patient care and clinical practice, as well as cost-effectiveness.
Making a difference to patient care
Some respondents could point to circumstances in which they might have had some positive impact on patient outcomes, and outreach nurses tended to have a firm conviction that they were essentially contributing to patient benefit:
. . . for the patients. I know . . . I mean I don’t know how many we’ve seen . . . but I know that there are some that we’ve changed things for.
ON1:24
. . . how can it not be a benefit? It has to be, doesn’t it? It has to be.
ON2:25
. . . most AKI is . . . secondary to sepsis or dehydration, or excess diuresis, you know, so actually if you can alert the teams to it and get them to intervene early, then actually you will significantly improve outcomes.
C5:12
I think sometimes . . . when we pitch up a lot of the relatives and the patients are, ‘Oh thank goodness somebody’s going to come and do something,’ because they’ve got . . . they’ve noticed that there’s something wrong and it looks as if somebody’s coming to sort it out so that’s a patient benefit, isn’t it, from their perception.
ON3:26
But consultants were largely sceptical about the benefits. This was mostly because of the observation that either teams were already aware or full-scale intervention was not clinically appropriate:
I question whether phoning a junior doctor team or consultant . . . about a patient admitted the day before with acute kidney injury and who, they must be aware if they’ve looked at the blood results, has AKI, I wonder whether that actually provides any added value at all.
C2:7
. . . I’d say three-quarters of the patients you ring up . . . they’re either dying and there is nothing you can do or the team’s got it in hand and they know exactly what they’re doing and they’re doing fine. So you know it does feel sometimes like quite a lot of work for very little benefit.
C5:11
One respondent indicated that the lack of perceived benefits stemmed from advice not being followed:
I think that generally speaking it doesn’t improve patient care . . . there was one case only where I did give advice which was the correct advice and then we subsequently found that the advice wasn’t followed and that patient was eventually transferred here for dialysis therapy so I feel that it doesn’t really add to patient management . . .
C1:5
Comments were also made that suggested a need for proper comparative research and larger samples to better estimate benefits:
. . . once you’ve, you know, given the information and instructions, what happens it’s not really followed up and whether it would have made any difference you would have to compare it with somebody who has not had that, you know, data so you have to compare it with something to know whether there is a difference or not.
C3:5
I think there are too few numbers of patients to really point to whether it has a benefit or not, but it’s brought AKI to people’s attention . . . we just need to have the means to track patients . . .
C6:10
The following respondent indicated that more benefits could potentially be achieved through the outreach team, who focus on patients with the earlier stages of AKI:
I think the outreach team have been looking at going out to see people with acute kidney injury stage 2 and they’ve actually physically seen patients and put information in the notes . . . I suspect that probably the biggest benefits will have come from that intervention, getting in there a little bit earlier and actually steering people in the right direction.
C5:12
Impacts on clinical practice
Most respondents saw both positive and negative impacts on clinical practice. The following statements refer to cases in which intervention can be positive and serve an educational purpose:
I think that there are certain groups in the hospital that are very poor at managing the sick patients and particularly patients with AKI . . . so I think that actually, I think that’s where I see that we have the benefit . . . when I ring up some, one of the surgeons to say did you realise your patient has deteriorating renal function . . . they usually haven’t got a clue . . . so it does prompt them to go and actually look and get their medical review.
C5:9/10
. . . sometimes it’s not direct intervention, it’s almost preventing intervention . . . you know, facilitating the team making a clinical judgement on what is or isn’t appropriate for the demented ninety seven year old with multiple other illnesses . . . So some of them are sort of much more soft interventions about sort of management strategy rather than medical treatment . . . some of it’s educational as well.
C4:13
The outreach nurses specifically identified the positive and proactive contribution of the stickers, as well as the ability of their involvement to reduce unnecessary work:
And they’re actually now approaching us when we go onto the unit and say, ‘Can we have a sticker?’ and they will have people that haven’t even been identified on the database as acute kidney injury and they want stickers for those notes.
ON3:7
. . . whoever’s on the nightshift will go round in the early hours of the morning, we’d go into QlikView, get the list out, go and identify these patients and their notes so that when they’re being post-take in the morning it’s identified to the teams . . . Which is . . . certainly cutting out one ward round and potentially 1 day’s worth of medical input.
ON2:17
In addition, outreach nurses in particular saw the educational opportunities for themselves:
I think for me it’s been positive because I’ve . . . it’s done a lot for my knowledge and maybe . . . maybe I see patients in a different way now. Sharing skills which is part of our job, people show interest in the stickers and you’re able to tell them what you’re looking for and why so they might pick things up about the drugs that you’re looking at and so on.
ON1:24
The following comment from a consultant indicates the benefits of an AKI database, again in terms of enhancing educational opportunities in the trust:
. . . the system does mean that we’ve now got a very good database of actually what sort of AKI we have in the trust and where it is . . . which groupings is it happening under and maybe an idea as to you know, who’s doing well and who’s not doing so well and to allow us to focus some education on those areas that aren’t doing well.
C5:11
Conversely, other comments related to the negative personal impact on practice brought about by the alert system:
. . . I think it adds to my busy day already when I’ve put you know, I can actually do other things . . .
C1:6
. . . I’m not sure it’s the best use of my time to be perfectly honest . . . a lot of it’s an administrative task which is pretty tedious.
C2:9
However, all respondents highlighted concerns regarding clinical responsibility for the patient and the impact of the alert system. This comment describes what this means in practice for consultants:
I have quite a lot of concerns about ownership of the patients. So for example if a patient was admitted on my take, I would assume a hundred per cent responsibility for . . . acting and looking into each problem and dealing with it. And when you include a system like this I feel that number one ownership could be eroded and doctors . . . could become more nonchalant and lethargic and say ‘oh there is this computer system that could pick this patient up and because they have renal failure it’s not my problem any more’ and I feel strongly about that.
C1:6
As has been noted, outreach nurses had particular concerns about where their involvement began and ended, and how some doctors interpreted this:
I think ownership of the . . . ownership of the alert system from my feeling is . . . becomes an issue. My feeling is that once you document in the notes ‘Outreach’, you then become the first point of contact for anything that ever goes wrong or problems . . . you then become embroiled in the rest of the unfolding of the events.
ON2:6/7
. . . some doctors will see the sticker and your signature and think that every day you’re going to make sure their bloods are done and you’re going to check the results. Other teams won’t. Other teams will just manage what they do but it’s very varied.
ON1:9
Even from the medical teams; ‘Mrs so-and-so – you’ve seen this person?’ ‘I’ve kind of not really; I just stuck an . . . alert sticker in the notes and signed it.’
ON3:6
This respondent made particular reference to the establishment of a specialist orthogeriatric system that compounded the problem of patient accountability within certain surgical teams:
. . . part of the problem with trauma and orthopaedics is now that there is this orthogeriatric system in place, it’s almost exacerbated the problem of them now completely ignoring anything . . . there’s almost a mentality now that; out of hours – oh that can wait, the orthogeries will be on in the morning.
ON2:15
There were also concerns that the alert system had generally impacted on their professional roles in a negative way, reducing it to an unsatisfactory screening process as a result of being provided with by ‘lists’:
I think the problem for us is that we have so many parts of our role and we’ve . . . we’ve become very much a list and screening culture, so we . . . we start off every morning with a list from the alert system, we have a list from the VitalPAC, we have our list of patients and it feels very much as though we’re trawling around the hospital to keep a lot of lists . . . you obviously have to go through that process to get to people that you’re going to have effective interactions with but it’s beginning to feel like we are part of this list culture . . . that’s not very nice really.
ON3:17
Continuing with outreach nurse perceptions of professional issues, respondents were aware that there were numerical differences in patient interactions brought about by the alert system between the three hospital sites. This was explained by an administrative problem with recording on two of the sites (the numbers recorded did not in fact reflect activity in reality) and that there were fewer identified patients on the third site:
. . . we go and see these patients but we don’t always at the end of the day sit down and put them on the system . . . Now, unless we keep all of that documentation we can’t go back retrospectively and put it on the system so we . . . we miss that time slot. So there are a lot of interactions we do on this site that we don’t put on the system.
ON3:19
Probably at [site] our patient numbers are lower . . . And it is doable within our working hours.
ON1:19
Perceptions of cost-effectiveness
It was generally difficult for respondents to express the extent to which they felt that the alert system saved money, but the following comments demonstrate that it is certainly an aspiration:
Well it costs £150 to dialyse a patient, and that’s around £30,000 per patient per year . . . so it would be nice to think it was making some impact on that . . .
C6:10
If you’re highlighting early people that have got an acute kidney injury that’s then being treated and managed it’s got to save money in terms of length of stay and ongoing treatment or higher levels of treatment.
ON2:26
. . . so do we save money? Don’t know . . . do we improve patient outcomes? One would hope to think so and actually you could then say well improving outcomes is going to be a cost saving in terms of if you can prevent somebody needing to go onto dialysis or needing to go up to ITU for haemofiltration, yes you will save money, you will shorten their patient inpatient stay.
C4:14
This respondent, however, was more sceptical:
. . . if somebody’s already in ITU and being managed and they know about AKI I don’t think that will save money just to tell them that he has AKI.
C3:7
Other, more comparative views focused on the cost of respondent time:
I don’t know how much QlikView costs, I’m assuming it’s part of the great IT budget of the hospital so in terms of everything else it’s probably a small cost, but in terms of time at the moment it’s quite costly on time.
C1:7
. . . well it’s highly cost-effective at the moment because it’s not included within any of our job plans to my knowledge, so essentially the trust is getting this additional work for – for nothing!
C2:8
Summary
Despite some respondents, largely outreach nurses, perceiving positive proactive, preventative and educational benefits for patient outcomes and clinical practice, other respondents were not wholly convinced of the value of the system in terms of inducing these benefits, nor of being cost-effective. This scepticism resulted from the observation that most clinical teams already had the situation in hand, or that intervention was not indicated. In addition, the small number of patients made impacts difficult to assess. An issue of concern centred on the potential of the alert system to divert perceived clinical responsibility of the patient away from the clinician who was actually accountable, and outreach nurses in particular felt that this aspect impacted on their professional roles. It was also revealed that discrepancies in outreach nurse system activity between the sites were largely caused by an administrative recording issue.
Recommendations for improvement
Improving trust-wide knowledge of the alert system
Knowledge of the alert system could be improved by the following means: inclusion of a user manual; an initial comprehensive and wide exposure of clinicians and nurses to the new system; an ongoing programme of updates; a focus on junior doctors’ education; and greater visibility of the stickers.
maybe a user’s guide . . . you know, an idiot’s guide how to quickly get to the reporting page . . .
C1:2
. . . perhaps if they would have maybe sat down with everybody at the beginning and explained how the system works and what to do and how to go through the system.
C1:1
I think that it would have probably been advisable to have made it more obvious, more visible to other staff in the trust . . . you’d have to kind of redo that every time you induced a new load of junior doctors across multiple sites, I’m not sure that would be practical so maybe some, you know some pre rollout announcements and so on . . .
C2:2
I think [junior doctors] need to be made aware of that but that will have to be a very extensive education programme, because it’s across three sites and it includes all specialities surgical, medical and the juniors change as well.
C6:4
I have to say the stickers are good. I do think the stickers need to be a bit more in your face, though, because I sometimes put them in the notes and think mmmm, do you know what; I’d just like something a bit more out there.
ON2:23
Improving the technology
Aligning the data systems and technology
The following methods should be employed to improve alignment: improving the accessibility of clinical data sources within the alert system and its speed; providing up-to-date clinical information; improving how NHS numbers are accessed; developing a link directly to the clinician responsible; and having an accessible electronic form to improve reporting.
. . . well it’d be really nice if it was all in one place, that would be amazing, but you know, it’s a new project and I think . . . you might want the Ferrari but having the Ford Fiesta is a start!
C4:5
. . . if it’s incorporated into the VitalPAC system where you know you are highlighting patients with acute kidney injury and you can just with the click of a button look at their observations and then the historic blood results and then what has been ordered and what is awaited and it’s live data, that would be . . . helpful.
C1:6
. . . if the system was actually – represented what, you know was real time, then . . . it would save you quite a lot of time . . .
C5:6
. . . there should be facility to copy and paste for example the NHS number because that’s how it identifies it on the monitoring form but you can’t do that so you have to write it down first and then put it in there.
C3:3
. . . in an ideal world there should . . .some sort of link system whereby the alerts can be sent . . . directly to the teams looking after that patient that says, ‘Your patient, Mr Smith, is an AKI 2. Deal with it please.’ . . . if we need to be contacted for our input then we’re there, however it’s kind of cutting out the middle man in a sense.
ON2:17
. . . doctors don’t record the discussion in the notes, so there’s no evidence that it existed or how it was acted on . . . so it would be good to have an electronic form that could be universally accessed . . .
C6:5
From a reporting point of view, we wanted to know last year how many patients we’d seen on the system . . . so I guess for us it would be nice if we could have an easy to pull reporting system.
ON3:23
Establishing clinical ownership of the patient and responsiveness
Clinical ownership should involve greater organisational accountability for tracking the whereabouts of junior doctors; developing a ‘response’ alert; improving pathways to clinical responsibility for patient management; and creating a clearer understanding of the role of outreach nurses, as well as reassessing their function in the alert system.
I suspect the nursing staff on the ground probably know amongst themselves who the right person will be but that knowledge has to be translated up the corporate structure so that actually, the corporation, the organisation knows exactly how individual patients are mapped onto the junior teams.
C2:3
. . . to have an alert that links to advice and then to have an escalation system whereby . . . we can see where alerts hadn’t been responded to . . . I think that would be you know a huge advantage.
C2:9
doctors . . . could become more nonchalant and lethargic and say ‘oh there is this computer system that could pick this patient up and because they have renal failure it’s not my problem any more’.
C1:6
. . . the difficulties are that we’re . . . we’re acting as screening people . . . I’m not sure that that couldn’t be screened by somebody else and then we could be alerted to the fact that they need us.
ON3:6
Chapter 5 Discussion and conclusions
Discussion
The overall aim of this study was to develop risk models for the development of AKI in patients admitted to hospital and, using qualitative analysis, to provide personal insight into and explanation of the processes involved in using an alert system through an experiential narrative gleaned via semistructured interviews.
In this study we developed and assessed both Bayesian and traditional methods of providing risk models for the prediction of new or worsening AKI in patients presenting to hospital. The results of the Bayesian modelling demonstrated an excellent specificity, indicating that the models were good at defining patients at very low risk of having AKI; however, as the sensitivity was poor, they would not be able to accurately determine those patients at high risk. These models would therefore be good at defining the population of patients who do not need renal function testing to assess for AKI, and could determine a population to test by inference. However, a large proportion of the population tested would be false positives. It became apparent during the modelling iterations that the traditional methods were superior to the Bayesian in terms of providing risk models that could be used in clinical practice to provide clinical benefit. We therefore progressed the traditional modelling further and will carry this forward in our future work. We will, however, look into assessing the use of more specific priors that may provide greater benefit in the future with a more defined data set.
From the traditional risk modelling presented here, we have developed a risk model which is designed to predict patients who will have AKI at admission to hospital, and to guide renal function testing alongside the clinical practice algorithm. The ROC curve analyses suggest that the model has discriminatory power and, with an AUC value of 0.75, it is acceptable for use in clinical practice. This has been validated in a second population. We have also developed a risk model designed to predict patients who will develop new AKI in the first 72 hours of admission to hospital. The ROC curve analyses suggest that this second model has discriminatory power but that it is not as effective as the admission model, with an AUC value of 0.68. This has again been validated in a second population. The third model for predicting worsening AKI in the first 72 hours of hospital admission did not prove to have any discriminatory power and would not provide clinical benefit. This was therefore not validated further. The results suggest that we have not included key variables in the third model or that the development of AKI in hospital is subject to a random effect which is not measured. The model for predicting new AKI in the first 72 hours may be refined further once physiological data becomes more commonly available electronically across the NHS.
The methodology used has its limitations, and further analysis and testing, including continuous modelling, non-linear modelling and interaction exploration, may refine the model further. This study provides valuable evidence of the relationships between AKI and key variables available from hospital electronic records. This work also gives other insights as to which variables may not be associated with AKI, something that has been lacking in the literature.
Risk models in the setting of acute kidney injury
In the literature, the majority of the reports focus on the need for RRT after cardiac surgery. One of the first of these was by Chertow et al. ,34 who produced a risk model for predicting AKI after cardiac surgery, based on a population of 40,000 patients who underwent cardiac bypass or valvular surgery in 43 Veterans Administration Hospitals in Virginia, USA. A risk stratification algorithm was formulated on the basis of interactions between potential risk factors. There were inherent flaws in the study cohort, specifically a lack of female patients and African-American patients. Thakar et al. 101 produced a clinical risk score to predict post–cardiac surgery AKI requiring RRT, based on 33,217 patients who underwent cardiac surgery at the Cleveland Clinic between 1993 and 2002. The scoring system was derived based on 13 preoperative factors which were weighted; the sum of the scores, ranging from 0 to 17, allowed for stratification of postoperative risk of AKI from low to high. The lowest-risk group (score of 0–2) had a risk of AKI requiring RRT of 0.4%, in contrast to the high-risk stratum (score of 9–13) who had a RRT risk of 21.5%. However, there is a paucity in the literature of studies about the risk and risk prediction of AKI in unselected emergency admissions to a district general hospital. Finlay et al. 102 published a recent study of AKI risk factors associated with AKI in patients admitted to acute medical units in a study conducted over two separate 24-hour periods at a total of 10 acute medical units. Forni et al. 103 have developed a model for predicting AKI in a subset of medical patients admitted to a UK hospital. Their model included some physiological markers.
NHS England initiatives in acute kidney injury
NHS England has recently made considerable progress in improving patient safety with respect to AKI. A national algorithm has been developed to standardise the definition of AKI. A patient safety alert was issued on 9 June 2014 requiring all hospital trusts to embed the algorithm in routine pathology reporting. Although this will go a long way towards the identification of established AKI, it does not address the issue of prevention of harm in the first place, hence the requirement for the development of risk assessment tools. In addition, the reporting of AKI will not require a response by the attending medical team; in other words, there is currently no requirement for a standardised response to alerts to established AKI. Although this work goes along way towards highlighting established AKI, it does not address the issue of assessing the risk of AKI. This work and a future study examining the impact of an automated alert will inform further national strategy with respect to AKI prevention.
Our study and further studies examining the complexities of implementation of auditable clinical alerts have informed these work streams and in the future will provide more clarity in a complex area.
Intervention studies
To date there have been no intervention studies in comparable unselected populations that have shown a reduction in episodes of AKI. A formal health economic analysis of the impact and costs of such intervention needs to be performed, along with a technology appraisal to maximise the benefit from electronic alerting.
Generalisability
Quantitative analysis
Using a number of combined hospital databases, we have identified a number of key variables that predict AKI; this methodology can therefore be used to highlight patients at risk of AKI. We have validated our findings in second data sets from the same hospitals and then a further data set from a second hospital trust with very different population characteristics. The key variables used in these models are available in most UK hospitals and the majority of them are not susceptible to coding bias; however, the potential for such bias is always a concern. The use of physiological parameters to predict AKI for inpatients may pose a problem for some hospitals across the NHS because of the lack of electronic recording. Many hospitals are adopting electronic recording of physiological parameters as part of their patient-safety programmes, so these data are likely to become more ubiquitous in the future; the application of these models across the NHS is therefore a feasible proposition. As discussed previously, the model would benefit from further refinement and analysis.
Qualitative analysis
The aim of the qualitative arm of this project was to provide personal insight into, and explanation of, the processes involved in using the alert system through an experiential narrative gleaned via semistructured interviews. A total of 12 members of staff participated and, given this low number, generalisability cannot be applied to measure rigour nor to infer fittingness of the findings to the wider population; this is not the intention of qualitative research. Instead, the study has conformed to two leading qualitative principles to ensure the collection of rich experiences and to achieve credibility. First, there was a high level of adequacy regarding representation within the sample; the majority of consultant and outreach nurse users across the trust participated, thereby ensuring a full complement of views concerning the alert system. Second, the data collected through the use of semistructured interviews were, on the one hand, rich and diverse, but, on the other, clearly revealed consensus of opinion through thematic representation. Agreement of the strengths and weaknesses of the system, alongside contextual issues that helped or hindered, was reached in a number of core areas; thus it can be implied that data saturation was achieved. The high level of user representation alongside consensus of opinion suggests that this arm of the project has added a credible explanatory component to the wider study in terms of taking the system forward to the next stage.
Delivery of alerts
During the course of this project we have explored a number of methods of alert delivery. NHS England has implemented alerts to the presence of AKI across the NHS; however, there are no plans to alert to risk of AKI. Desktop alerting systems can easily be made available across the NHS with little adaption of existing information technology (IT) systems. The use of ‘push notification’ to mobile devices may require infrastructure changes in some NHS hospitals; however, there has been a significant increase in the availability of wireless communication and mobile devices in recent years.
Strengths and weaknesses of the study
Weaknesses of this study include the fact that this was a retrospective cohort study using hospital databases to find predictors of AKI. It was carried out in a small geographical area of England (Kent) and covered a 1-year period. Neither of the hospitals studied have cardiothoracic services. The combination of such data sets is labour intensive and, as such, would not provide generalisable results unless an easily accessible subset of the data was found to contain useful predictors. Our work has provided a list of candidate variables for the prediction of AKI at admission and 72 hours. Importantly, we have also provided a comprehensive analysis of variables that do not appear to influence the risk of AKI. We have been unable to produce a predictive model for worsening AKI during admission. This may be because key candidate variables have not been included or because of a random effect.
Further analysis and testing, including continuous modelling, non-linear modelling and interaction exploration, may refine the models further.
Certain groups of patients were excluded from the analysis (e.g. those with established AKI) requiring the use of a clinical practice algorithm as well as multiple predictive models for use in different scenarios. Finally, the delivery of alert systems to doctors and allied health professionals at the point of care will require modification for different hospitals with differing IT infrastructure.
However, this study used a very large unselected data set, which represents the kind of populations presenting to the majority of UK hospitals. While there was no cardiothoracic surgery performed at either hospital, AKI in this setting has been extensively studied and represents a very small and unrepresentative group of people with AKI. The study was carried out using robust methodology and the initial data set used to develop the models was randomly selected from all hospital admissions over a year on a 3 : 1 ratio. The model was validated in the remainder of the population and then subsequently tested in a second population from a different hospital trust, demonstrating that ethnic and social differences had little effect on the model, nor did the potential difference in coding practices between organisations. We have therefore been able to demonstrate that we can transfer our model to one other hospital trust; however, these models may only provide a platform for further analysis and refinement before they can be employed more widely.
Although the qualitative analysis has provided accurate guidance as to the best approach to further implementation of this technology to highlight patients at risk of AKI in our hospitals, it was a small-scale analysis in one centre and, as such, may not be generalisable. Finally, we have tested a number of the methods of delivery of such alerts and have developed a reliable and user-friendly approach, maximising the chances of successful implementation and assessment in a wider health-care setting.
What our study adds
Our study adds the following to the literature:
-
We have developed clear clinical practice algorithm to guide clinical alerting and risk modelling for AKI in emergency hospital admissions.
-
We have identified the key variables that are associated with the risk of AKI at the point of admission and during admission to hospital. Importantly, this study used an unselected population, unlike many in the literature.
-
Our study also provides a comprehensive list of variables that do not appear to be associated with the risk of AKI at the time points discussed.
-
Relationships between key variables available from hospital electronic records and AKI were identified, which will aid other groups in the development and validation of risk models in this and other settings, for example primary care.
-
Risk models were validated in two populations to predict AKI on admission to hospital, and the development of new AKI in the first 72 hours of admission to hospital.
-
Risk models to predict worsening AKI during admission were not successful, suggesting that this requires further study or that there is a random effect.
-
A qualitative analysis provides guidance as to the best approach to the implementation of clinical alerting designed to highlight patients at risk of AKI in hospitals.
Conclusion
In our studies we have been able to demonstrate that routinely available data can be used to highlight patients at risk of AKI both at the point of admission to hospital and following admission. However, the methodology used has its limitations, and further analysis and testing, including continuous modelling, non-linear modelling and interaction exploration, may refine the model further. This study provides valuable evidence of the relationships between AKI and key variables available from hospital electronic records. Some of the models may be refined further once physiological data become more commonly available across the NHS. This study has also highlighted the complexity of the implementation and delivery of alerting systems to the clinical front line.
Recommendations for future work
The next stage of this work is to test these risk models in terms of their clinical, logistic and economic impact in routine clinical practice in a clinical intervention pilot study. Alongside this there should be further analysis and testing, including continuous modelling, non-linear modelling and interaction exploration, which may refine the model further. There should also be further work to investigate the development of clinical risk models in different settings (e.g. elective surgery or radiocontrast investigations) within clinical practice, as we believe there a number of different risk models that we need to employ across the different settings within the secondary care environment.
There should also be work to investigate the development of risk models to predict the presence of AKI in patients presenting to their general practitioner in primary care, to guide testing in this setting.
The qualitative analysis reported here has provided guidance as to the best approach in terms of delivering AKI and AKI-risk alerting to the point of care in our hospitals; however, this was a small-scale analysis in one centre and, as such, may not be generalisable. A further multicentre qualitative analysis is required to inform delivery across the NHS.
The qualitative analysis has suggested that different methods of delivery of clinical alerting to the point of care may be beneficial. This requires further investigation in terms of technical appraisal and usability.
Acknowledgements
Dr Sanjay Suman (Consultant Physician and AKI Clinical Lead for Medway NHS Foundation Trust) acted as the liaison with Medway to facilitate data extraction for the second population. Mr Brian Hughes (Business Intelligence Manager at Medway) provided the data extraction for the second population at Medway.
Contributions of authors
Michael Bedford was responsible for study design, clinical expertise, variable selection, data analysis and the preparation of the manuscript.
Paul Stevens was responsible for study design, clinical expertise and the review of the manuscript.
Simon Coulton was responsible for study design, methodological support and the preparation of the manuscript.
Jenny Billings was responsible for study design, qualitative analysis and the preparation of the manuscript.
Marc Farr was responsible for data access and assurance, and the review of the manuscript.
Toby Wheeler was responsible for data extraction, and cleaning and validation.
Maria Kalli was responsible for study design, quantitative analysis and the preparation of the manuscript.
Tim Mottishaw was responsible for project management.
Chris Farmer was responsible for study design, clinical expertise, variable selection, data analysis and the preparation of the manuscript.
Data sharing statement
The corresponding author will provide the detailed results of the statistical analysis on request. When possible, anonymous data will also be provided.
Disclaimers
This report presents independent research funded by the National Institute for Health Research (NIHR). The views and opinions expressed by authors in this publication are those of the authors and do not necessarily reflect those of the NHS, the NIHR, NETSCC, the HS&DR programme or the Department of Health. If there are verbatim quotations included in this publication the views and opinions expressed by the interviewees are those of the interviewees and do not necessarily reflect those of the authors, those of the NHS, the NIHR, NETSCC, the HS&DR programme or the Department of Health.
References
- Mehta RL, Pascual MT, Soroko S, Savage BR, Himmelfarb J, Ikizler TA, et al. Spectrum of acute renal failure in the intensive care unit: the PICARD experience. Kidney Int 2004;66:1613-21. http://dx.doi.org/10.1111/j.1523-1755.2004.00927.x.
- Metnitz PG, Krenn CG, Steltzer H, Lang T, Ploder J, Lenz K, et al. Effect of acute renal failure requiring renal replacement therapy on outcome in critically ill patients. Crit Care Med 2002;30:2051-8. http://dx.doi.org/10.1097/00003246-200209000-00016.
- Liangos O, Wald R, O’Bell JW, Price L, Pereira BJ, Jaber BL. Epidemiology and outcomes of acute renal failure in hospitalized patients: a national survey. Clin J Am Soc Nephrol 2006;1:43-51. http://dx.doi.org/10.2215/CJN.00220605.
- Uchino S, Kellum JA, Bellomo R, Doig GS, Morimatsu H, Morgera S, et al. Acute renal failure in critically ill patients: a multinational, multicenter study. JAMA 2005;294:813-18. http://dx.doi.org/10.1001/jama.294.7.813.
- Schrier RW. Early intervention in acute kidney injury. Nat Rev Nephrol 2010;6:56-9. http://dx.doi.org/10.1038/nrneph.2009.170.
- Gruberg L, Mintz GS, Mehran R, Gangas G, Lansky AJ, Kent KM, et al. The prognostic implications of further renal function deterioration within 48 h of interventional coronary procedures in patients with pre-existent chronic renal insufficiency. J Am Coll Cardiol 2000;36:1542-8. http://dx.doi.org/10.1016/S0735-1097(00)00917-7.
- Clermont G, Acker CG, Angus DC, Sirio CA, Pinsky MR, Johnson JP. Renal failure in the ICU: comparison of the impact of acute renal failure and end-stage renal disease on ICU outcomes. Kidney Int 2002;62:986-96. http://dx.doi.org/10.1046/j.1523-1755.2002.00509.x.
- Thakar CV, Worley S, Arrigain S, Yared JP, Paganini EP. Influence of renal dysfunction on mortality after cardiac surgery: modifying effect of preoperative renal function. Kidney Int 2005;67:1112-19. http://dx.doi.org/10.1111/j.1523-1755.2005.00177.x.
- Druml W. Long term prognosis of patients with acute renal failure: is intensive care worth it?. Intensive Care Med 2005;31:1145-7. http://dx.doi.org/10.1007/s00134-005-2682-5.
- Waikar SS, Liu KD, Chertow GM. The incidence and prognostic significance of acute kidney injury. Curr Opin Nephrol Hypertens 2007;16:227-36. http://dx.doi.org/10.1097/MNH.0b013e3280dd8c35.
- Hoste EA, Lameire NH, Vanholder RC, Benoit DD, Decruyenaere JM, Colardyn FA. Acute renal failure in patients with sepsis in a surgical ICU: predictive factors, incidence, comorbidity, and outcome. J Am Soc Nephrol 2003;14:1022-30. http://dx.doi.org/10.1097/01.ASN.0000059863.48590.E9.
- Ishani A, Xue JL, Himmelfarb J, Eggers PW, Kimmel PL, Molitoris BA, et al. Acute kidney injury increases risk of ESRD among elderly. J Am Soc Nephrol 2009;20:223-8. http://dx.doi.org/10.1681/ASN.2007080837.
- DeFrances CJ, Lucas CA, Buie VC, Golosinsking A. National Hospital Discharge Survey. National Health Statistics Report: No. 5. Hyattsville, MD: National Centre for Health Staistics; 2008.
- National Kidney Foundation . K/DOQI Clinical practice guidelines for chronic kidney disease: evaluation, classification and stratification. Am J Kidney Dis 2002;39:S1-266.
- Levey AS, Eckardt KU, Tsukamoto Y, Levin A, Coresh J, Rossert J, et al. Definition and classification of chronic kidney disease: a position statement from Kidney Disease: Improving Global Outcomes (KDIGO). Kidney Int 2005;67:2089-100. http://dx.doi.org/10.1111/j.1523-1755.2005.00365.x.
- Levey AS, Deo A, Jaber BL. Filtration markers in acute kidney injury. Am J Kidney Dis 2010;56:619-22. http://dx.doi.org/10.1053/j.ajkd.2010.08.001.
- Martin JE, Sheaff MT. Renal ageing. J Pathol 2007;211:198-205. http://dx.doi.org/10.1002/path.2111.
- Chronopoulos A, Cruz DN, Ronco C. Hospital-acquired acute kidney injury in the elderly. Nat Rev Nephrol 2010;6:141-9. http://dx.doi.org/10.1038/nrneph.2009.234.
- Fliser D. Ren sanus in corpore sano: the myth of the inexorable decline of renal function with senescence. Nephrol Dial Transplant 2005;20:482-5. http://dx.doi.org/10.1093/ndt/gfh710.
- Bricker NS. On the meaning of the intact nephron hypothesis. Am J Med 1969;46:1-11. http://dx.doi.org/10.1016/0002-9343(69)90053-9.
- Bricker NS, Morrin PA, Kime SW. The pathologic physiology of chronic Bright’s disease. An exposition of the ‘intact nephron hypothesis’. J Am Soc Nephrol 1997;8:1470-6.
- Bonventre JV, Weinberg JM. Recent advances in the pathophysiology of ischemic acute renal failure. J Am Soc Nephrol 2003;14:2199-210. http://dx.doi.org/10.1097/01.ASN.0000079785.13922.F6.
- Oken DE. Hemodynamic basis for human acute renal failure (vasomotor nephropathy). Am J Med 1984;76:702-10. http://dx.doi.org/10.1016/0002-9343(84)90299-7.
- Bonventre JV. Mechanisms of ischemic acute renal failure. Kidney Int 1993;43:1160-78. http://dx.doi.org/10.1038/ki.1993.163.
- Finn WF, Lazarus JM, Brenner BM. Acute Renal Failure. New York, NY: Churchill Livingstone; 1993.
- Humes HD, Lake EW, Liu S. Renal tubule cell repair following acute renal injury. Miner Electrolyte Metab 1995;21:353-65.
- Humes HD, Liu S. Cellular and molecular basis of renal repair in acute renal failure. J Lab Clin Med 1994;124:749-54.
- Sutton TA, Fisher CJ, Molitoris BA. Microvascular endothelial injury and dysfunction during ischemic acute renal failure. Kidney Int 2002;62:1539-49. http://dx.doi.org/10.1046/j.1523-1755.2002.00631.x.
- Basile DP, Donohoe D, Roethe K, Osborn JL. Renal ischemic injury results in permanent damage to peritubular capillaries and influences long-term function. Am J Physiol Renal Physiol 2001;281:F887-99. http://dx.doi.org/10.1152/ajprenal.0050.2001.
- Okusa MD, Chertow GM, Portilla D. Acute Kidney Injury Advisory Group of the American Society of Nephrology. The nexus of acute kidney injury, chronic kidney disease, and World Kidney Day 2009. Clin J Am Soc Nephrol 2009;4:520-2. http://dx.doi.org/10.2215/CJN.06711208.
- McKay D, Milford E, Tolkoff-Rubin N, Brenner BM. The Kidney. Philadelphia, PA: Saunders; 2000.
- Perez Fontan M, Rodriguez-Carmona A, Bouza P, Valdes F. The prognostic significance of acute renal failure after renal transplantation in patients treated with cyclosporin. QJM 1998;91:27-40. http://dx.doi.org/10.1093/qjmed/91.1.27.
- Ojo AO, Wolfe RA, Held PJ, Port FK, Schmouder RL. Delayed graft function: risk factors and implications for renal allograft survival. Transplantation 1997;63:968-74. http://dx.doi.org/10.1097/00007890-199704150-00011.
- Chertow GM, Burdick E, Honour M, Bonventre JV, Bates DW. Acute kidney injury, mortality, length of stay, and costs in hospitalized patients. J Am Soc Nephrol 2005;16:3365-70. http://dx.doi.org/10.1681/ASN.2004090740.
- Kohli HS, Bhat A, Jairam A, Aravindan AN, Sud K, Jha V, et al. Predictors of mortality in acute renal failure in a developing country: a prospective study. Ren Fail 2007;29:463-9. http://dx.doi.org/10.1080/08860220701260651.
- Arije A, Kadiri S, Akinkugbe OO. The viability of hemodialysis as a treatment option for renal failure in a developing economy. Afr J Med Med Sci 2000;29:311-4.
- Chugh KS. Renal disease in India. Am J Kidney Dis 1998;3:Ivii-ix.
- Vachvanichsanong P, Dissaneewate P, Lim A, McNeil E. Childhood acute renal failure: 22-year experience in a university hospital in southern Thailand. Pediatrics 2006;118:e786-91. http://dx.doi.org/10.1542/peds.2006-0557.
- Askenazi DJ, Feig DI, Graham NM, Hui-Stickle S, Goldstein SL. 3–5 Year longitudinal follow-up of pediatric patients after acute renal failure. Kidney Int 2006;69:184-9. http://dx.doi.org/10.1038/sj.ki.5000032.
- Xue JL, Daniels F, Star RA, Kimmel PL, Eggers PW, Molitoris BA, et al. Incidence and mortality of acute renal failure in Medicare beneficiaries, 1992 to 2001. J Am Soc Nephrol 2006;17:1135-42. http://dx.doi.org/10.1681/ASN.2005060668.
- Waikar SS, Curhan GC, Wald R, McCarthy EP, Chertow GM. Declining mortality in patients with acute renal failure, 1988 to 2002. J Am Soc Nephrol 2006;17:1143-50. http://dx.doi.org/10.1681/ASN.2005091017.
- Hsu CY, McCulloch CE, Fan D, Ordonez JD, Chertow GM, Go AS. Community-based incidence of acute renal failure. Kidney Int 2007;72:208-12. http://dx.doi.org/10.1038/sj.ki.5002297.
- Stevens PE, Tamimi NA, Al-Hasani MK, Mikhail AI, Kearney E, Lapworth R, et al. Non-specialist management of acute renal failure. QJM 2001;94:533-40. http://dx.doi.org/10.1093/qjmed/94.10.533.
- Metcalfe W, Simpson M, Khan IH, Prescott GJ, Simpson K, Smith WC, et al. Acute renal failure requiring renal replacement therapy: incidence and outcome. QJM 2002;95:579-83. http://dx.doi.org/10.1093/qjmed/95.9.579.
- Hegarty J, Middleton RJ, Krebs M, Hussain H, Cheung C, Ledson T, et al. Severe acute renal failure in adults: place of care, incidence and outcomes. QJM 2005;98:661-6. http://dx.doi.org/10.1093/qjmed/hci109.
- Ali T, Khan I, Simpson W, Prescott G, Townend J, Smith W, et al. Incidence and outcomes in acute kidney injury: a comprehensive population-based study. J Am Soc Nephrol 2007;18:1292-8. http://dx.doi.org/10.1681/ASN.2006070756.
- Hoste EA, Clermont G, Kersten A, Venkataraman R, Angus DC, De Bacquer D, et al. RIFLE criteria for acute kidney injury are associated with hospital mortality in critically ill patients: a cohort analysis. Crit Care 2006;10. http://dx.doi.org/10.1186/cc4915.
- Uchino S, Bellomo R, Goldsmith D, Bates S, Ronco C. An assessment of the RIFLE criteria for acute renal failure in hospitalized patients. Crit Care Med 2006;34:1913-17. http://dx.doi.org/10.1097/01.CCM.0000224227.70642.4F.
- Ostermann M, Chang RW. Acute kidney injury in the intensive care unit according to RIFLE. Crit Care Med 2007;35:1837-43.
- Nash K, Hafeez A, Hou S. Hospital-acquired renal insufficiency. Am J Kidney Dis 2002;39:930-6. http://dx.doi.org/10.1053/ajkd.2002.32766.
- Morgera S, Kraft AK, Siebert G, Luft FC, Neumayer HH. Long-term outcomes in acute renal failure patients treated with continuous renal replacement therapies. Am J Kidney Dis 2002;40:275-9. http://dx.doi.org/10.1053/ajkd.2002.34505.
- Cosentino F, Chaff C, Piedmonte M. Risk factors influencing survival in ICU acute renal failure. Nephrol Dial Transplant 1994;9:179-82.
- Chertow GM, Christiansen CL, Cleary PD, Munro C, Lazarus JM. Prognostic stratification in critically ill patients with acute renal failure requiring dialysis. Arch Intern Med 1995;155:1505-11. http://dx.doi.org/10.1001/archinte.1995.00430140075007.
- Bernieh B, Al Hakim M, Boobes Y, Siemkovics E, El Jack H. Outcome and predictive factors of acute renal failure in the intensive care unit. Transplant Proc 2004;36:1784-7. http://dx.doi.org/10.1016/j.transproceed.2004.07.016.
- Levy EM, Viscoli CM, Horwitz RI. The effect of acute renal failure on mortality. A cohort analysis. JAMA 1996;275:1489-94. http://dx.doi.org/10.1001/jama.1996.03530430033035.
- Abosaif NY, Tolba YA, Heap M, Russell J, El Nahas AM. The outcome of acute renal failure in the intensive care unit according to RIFLE: model application, sensitivity, and predictability. Am J Kidney Dis 2005;46:1038-48. http://dx.doi.org/10.1053/j.ajkd.2005.08.033.
- Waikar SS, Liu KD, Chertow GM. Diagnosis, epidemiology and outcomes of acute kidney injury. Clin J Am Soc Nephrol 2008;3:844-61. http://dx.doi.org/10.2215/CJN.05191107.
- Bellomo R, Ronco C, Kellum JA, Mehta RL, Palevsky P. Acute Dialysis Quality Initiative workgroup. Acute renal failure – definition, outcome measures, animal models, fluid therapy and information technology needs: the Second International Consensus Conference of the Acute Dialysis Quality Initiative (ADQI) Group. Crit Care 2004;8:R204-12. http://dx.doi.org/10.1186/cc2872.
- McCullough PA, Soman SS. Contrast-induced nephropathy. Crit Care Clin 2005;21:261-80. http://dx.doi.org/10.1016/j.ccc.2004.12.003.
- United States Renal Data System Annual Data Report, 2006. Minneapolis, MN: USRDS Coordinating Center; 2006.
- Bagshaw SM, Laupland KB, Doig CJ, Mortis G, Fick GH, Mucenski M, et al. Prognosis for long-term survival and renal recovery in critically ill patients with severe acute renal failure: a population-based study. Crit Care 2005;9:R700-9. http://dx.doi.org/10.1186/cc3879.
- Korkeila M, Ruokonen E, Takala J. Costs of care, long-term prognosis and quality of life in patients requiring renal replacement therapy during intensive care. Intensive Care Med 2000;26:1824-31. http://dx.doi.org/10.1007/s001340000726.
- Lassnigg A, Schmidlin D, Mouhieddine M, Bachmann LM, Druml W, Bauer P, et al. Minimal changes of serum creatinine predict prognosis in patients after cardiothoracic surgery: a prospective cohort study. J Am Soc Nephrol 2004;15:1597-605. http://dx.doi.org/10.1097/01.ASN.0000130340.93930.DD.
- Go AS, Chertow GM, Fan D, McCulloch CE, Hsu CY. Chronic kidney disease and the risks of death, cardiovascular events, and hospitalization. N Engl J Med 2004;351:1296-305. http://dx.doi.org/10.1056/NEJMoa041031.
- Anavekar NS, Gans DJ, Berl T, Rohde RD, Cooper W, Bhaumik A, et al. Predictors of cardiovascular events in patients with type 2 diabetic nephropathy and hypertension: a case for albuminuria. Kidney Int Suppl 2004;92:S50-5. http://dx.doi.org/10.1111/j.1523-1755.2004.09213.x.
- Chertow GM, Soroko SH, Paganini EP, Cho KC, Himmelfarb J, Ikizler TA, et al. Mortality after acute renal failure: models for prognostic stratification and risk adjustment. Kidney Int 2006;70:1120-6. http://dx.doi.org/10.1038/sj.ki.5001579.
- Mehta RL, Pascual MT, Soroko S, Chertow GM. PICARD Study Group . Diuretics, mortality, and nonrecovery of renal function in acute renal failure. JAMA 2002;288:2547-53. http://dx.doi.org/10.1001/jama.288.20.2547.
- Paganini EP, Larive B, Kanagasundaram NS. Severity scores and outcomes with acute renal failure in the ICU setting. Contrib Nephrol 2001;132:181-95. http://dx.doi.org/10.1159/000060091.
- Uchino S, Bellomo R, Morimatsu H, Morgera S, Schetz M, Tan I, et al. External validation of severity scoring systems for acute renal failure using a multinational database. Crit Care Med 2005;33:1961-7. http://dx.doi.org/10.1097/01.CCM.0000172279.66229.07.
- Levy MM, Macias WL, Vincent JL, Russell JA, Silva E, Trzaskoma B, et al. Early changes in organ function predict eventual survival in severe sepsis. Crit Care Med 2005;33:2194-201. http://dx.doi.org/10.1097/01.CCM.0000182798.39709.84.
- Praught ML, Shlipak MG. Are small changes in serum creatinine an important risk factor?. Curr Opin Nephrol Hypertens 2005;14:265-70. http://dx.doi.org/10.1097/01.mnh.0000165894.90748.72.
- Wald R, Quinn RR, Luo J, Li P, Scales DC, Mamdani MM, et al. Chronic dialysis and death among survivors of acute kidney injury requiring dialysis. JAMA 2009;302:1179-85. http://dx.doi.org/10.1001/jama.2009.1322.
- Singh P, Rifkin DE, Blantz RC. Chronic kidney disease: an inherent risk factor for acute kidney injury?. Clin J Am Soc Nephrol 2010;5:1690-5. http://dx.doi.org/10.2215/CJN.00830110.
- Briggs JD, Kennedy AC, Young LN, Luke RG, Gray M. Renal function after acute tubular necrosis. Br Med J 1967;3:513-16. http://dx.doi.org/10.1136/bmj.3.5564.513.
- Schiffl H. Renal recovery from acute tubular necrosis requiring renal replacement therapy: a prospective study in critically ill patients. Nephrol Dial Transplant 2006;21:1248-52. http://dx.doi.org/10.1093/ndt/gfk069.
- Finkenstaedt JT, Merrill JP. Renal function after recovery from acute renal failure. N Engl J Med 1956;254:1023-6. http://dx.doi.org/10.1056/NEJM195605312542203.
- Lowe KG. The late prognosis in acute tubular necrosis; an interim follow-up report on 14 patients. Lancet 1952;1:1086-8. http://dx.doi.org/10.1016/S0140-6736(52)90744-7.
- Hobson CE, Yavas S, Segal MS, Schold JD, Tribble CG, Layon AJ, et al. Acute kidney injury is associated with increased long-term mortality after cardiothoracic surgery. Circulation 2009;119:2444-53. http://dx.doi.org/10.1161/CIRCULATIONAHA.108.800011.
- Triverio PA, Martin PY, Romand J, Pugin J, Perneger T, Saudan P. Long-term prognosis after acute kidney injury requiring renal replacement therapy. Nephrol Dial Transplant 2009;24:2186-9. http://dx.doi.org/10.1093/ndt/gfp072.
- Hsu CY, Vittinghoff E, Lin F, Shlipak MG. The incidence of end-stage renal disease is increasing faster than the prevalence of chronic renal insufficiency. Ann Intern Med 2004;141:95-101. http://dx.doi.org/10.7326/0003-4819-141-2-200407200-00007.
- Liano F, Junco E, Pascual J, Madero R, Verde E. The spectrum of acute renal failure in the intensive care unit compared with that seen in other settings. The Madrid Acute Renal Failure Study Group. Kidney Int Suppl 1998;66:S16-24.
- Amdur RL, Chawla LS, Amodeo S, Kimmel PL, Palant CE. Outcomes following diagnosis of acute renal failure in U.S. veterans: focus on acute tubular necrosis. Kidney Int 2009;76:1089-97. http://dx.doi.org/10.1038/ki.2009.332.
- Kidney Disease: Improving Global Outcomes (KDIGO) Acute Kidney Injury Work Group . KDIGO Clinical Practice Guideline for Acute Kidney Injury. Kidney Int 2012;2:1-138.
- Bywaters EG, Beall D. Crush injuries with impairment of renal function. Br Med J 1941;1:427-32. http://dx.doi.org/10.1136/bmj.1.4185.427.
- Acute Kidney Injury: Prevention, Detection and Management of Acute Kidney Injury up to the Point of Renal Replacement Therapy. London: NICE; 2013.
- Lewington A, Kanagasundaram S. Acute Kidney Injury Clinical Practice Guideline. Hamsphire: The Renal Association; 2011.
- Mehta RL, Chertow GM. Acute renal failure definitions and classification: time for change?. J Am Soc Nephrol 2003;14:2178-87. http://dx.doi.org/10.1097/01.ASN.0000079042.13465.1A.
- Mehta RL, Kellum JA, Shah SV, Molitoris BA, Ronco C, Warnock DG, et al. Acute Kidney Injury Network: report of an initiative to improve outcomes in acute kidney injury. Crit Care 2007;11. http://dx.doi.org/10.1186/cc5713.
- Ricci Z, Cruz D, Ronco C. The RIFLE criteria and mortality in acute kidney injury: a systematic review. Kidney Int 2008;73:538-46. http://dx.doi.org/10.1038/sj.ki.5002743.
- Kellum JA. Acute kidney injury. Crit Care Med 2008;36:S141-5. http://dx.doi.org/10.1097/CCM.0b013e318168c4a4.
- Bagshaw SM, George C, Dinu I, Bellomo R. A multi-centre evaluation of the RIFLE criteria for early acute kidney injury in critically ill patients. Nephrol Dial Transplant 2008;23:1203-10. http://dx.doi.org/10.1093/ndt/gfm744.
- Joannidis M, Metnitz B, Bauer P, Schusterschitz N, Moreno R, Druml W, et al. Acute kidney injury in critically ill patients classified by AKIN versus RIFLE using the SAPS 3 database. Intensive Care Med 2009;35:1692-702. http://dx.doi.org/10.1007/s00134-009-1530-4.
- Thakar CV, Christianson A, Freyberg R, Almenoff P, Render ML. Incidence and outcomes of acute kidney injury in intensive care units: a Veterans Administration study. Crit Care Med 2009;37:2552-8. http://dx.doi.org/10.1097/CCM.0b013e3181a5906f.
- Lafrance JP, Miller DR. Defining acute kidney injury in database studies: the effects of varying the baseline kidney function assessment period and considering CKD status. Am J Kidney Dis 2010;56:651-60. http://dx.doi.org/10.1053/j.ajkd.2010.05.011.
- Siew ED, Ikizler TA, Matheny ME, Shi Y, Schildcrout JS, Danciu I, et al. Estimating baseline kidney function in hospitalized patients with impaired kidney function. Clin J Am Soc Nephrol 2012;7:712-9. http://dx.doi.org/10.2215/CJN.10821011.
- Siew ED, Matheny ME, Ikizler TA, Lewis JB, Miller RA, Waitman LR, et al. Commonly used surrogates for baseline renal function affect the classification and prognosis of acute kidney injury. Kidney Int 2010;77:536-42. http://dx.doi.org/10.1038/ki.2009.479.
- Siew ED, Peterson JF, Eden SK, Moons KG, Ikizler TA, Matheny ME. Use of multiple imputation method to improve estimation of missing baseline serum creatinine in acute kidney injury research. Clin J Am Soc Nephrol 2013;8:10-8. http://dx.doi.org/10.2215/CJN.00200112.
- Stewart J, Findlay G, Smith N, Kelly K, Mason M. Adding Insult to Injury: A Review of the Care of Patients Who Died in Hospital with a Primary Diagnosis of Acute Kidney Injury (Acute Renal Failure). London: NCEPOD; 2009.
- An Age Old Problem. A Review of the Care Received by Elderly Patients Undergoing Surgery. London: NCEPOD; 2010.
- Chertow GM, Lazarus JM, Christiansen CL, Cook EF, Hammermeister KE, Grover F, et al. Preoperative renal risk stratification. Circulation 1997;95:878-84.
- Thakar CV, Arrigain S, Worley S, Yared JP, Paganini EP. A clinical score to predict acute renal failure after cardiac surgery. J Am Soc Nephrol 2005;16:162-8.
- Finlay S, Bray B, Lewington AJ, Hunter-Rowe CT, Banerjee A, Atkinson JM, et al. Identification of risk factors associated with acute kidney injury in patients admitted to acute medical units. Clin Med 2013;13:233-8.
- Forni LG, Dawes T, Sinclair H, Cheek E, Bewick V, Dennis M, et al. Identifying the patient at risk of acute kidney injury: a predicitve scoring system for the development of acute kidney injury in acute medical patients. Nephron Clin Pract 2013;123:143-50.
- Porter CJ, Juurlink I, Bisset LH, Bavakunji R, Mehta RL, Devonald MA. A real-time electronic alert to improve detection of acute kidney injury in a large teaching hospital. Nephrol Dial Transplant 2014;29:1888-93.
- Thomas M, Sitch A, Dowswell G. The initial development and assessment of an automatic alert warning of acute kidney injury. Nephrol Dial Transplant 2011;26:2161-8.
- Charlson ME, Pompei P, Ales KL, MacKenzie CR. A new method of classifying prognostic comorbidity in longitudinal studies: development and validation. J Chronic Dis 1987;40:373-83.
- George CI, McCulloch RE. Variables selection via Gibbs sampling. J Am Stat Assoc 1993;88:881-9.
- Pope C, Mays N. Qualitative Research in Health Care. London: BMJ Books; 2006.
- Yin RK. Case Study Research: Design and Methods. Thousand Oaks, CA: Sage Publications; 2003.
- Flick U. Introduction to Qualitative Research. Thousand Oaks, CA: Sage Publications; 1998.
- Smith N. Cross-sectional profiling and longitudinal analysis: Research notes on analysis in the longitudinal qualitative study, ‘Negotiating transitions to citizenship’. Int J Social Res Methodol 2003;6:273-7.
- Boyd KJ, Worth A, Kendall M, Pratt R, Hockly J, Denvir M, et al. Making sure services deliver for people with advanced heart failure: a longitudinal qualitative study of patients, family carers, and health professionals. Palliat Med 2009;23:767-76.
- Eilertsen G, Kirkevold M, Bjork IT. Recovering from a stroke: a longitudinal, qualitative study of older norwegian women. J Clin Nurs 2010;19:2004-13.
- Lawton J, Rankin D, Peel E, Douglas M. Patients’ perceptions and experiences of transitions in diabetes care: a longitudinal qualitative study. Health Expect 2009;12:138-48.
- Taylor C, Richardson A, Cowley S. Restoring embodied control following surgical treatment for colorectal cancer: a longitudinal qualitative study. Int J Nurs Stud 2010;47:94-56.
- The Diabetes Control and Complications Trial Research Group . The effect of intensive treatment of diabetes on the development and progression of long-term complications in insulin-dependent diabetes mellitus. N Engl J Med 1992;329:977-86.
Appendix 1 Variable definitions
Data entry | Calculated variable | Variable description |
---|---|---|
NHS number | Not used in analysis | Identifier used to link patient data removed following data linkage |
Trust unique episode number | Not used in analysis | Identifier used following anonymisation |
Gender | Gender | Gender of the patient. Coded as: 1 = male 2 = female |
Age on admission | Age on admission | Age of the patient on admission to hospital |
Spell type | Spell type | Type of patient admission. Coded as: DC = day case EL = elective NEL = non-elective |
Admission date and time | Admissions (last 12 months) | Using admission date, the number of admissions in the last 12 months for this patient is calculated |
Admission date and time | Admissions (last 30 days) | Using admission date, the number of admissions in the last 30 days for this patient is calculated |
Length of stay | Length of stay | Number of days the patient remained in hospital as an inpatient |
Died in hospital | Died in hospital | Whether or not the patient died in this admission to hospital |
Admission source | Increase in care on discharge | By comparison of admission source and discharge destination an assessment of increase in care (from home to residential or nursing care) is made |
Discharge destination | Increase in care on discharge | By comparison of admission source and discharge destination an assessment of increase in care (from home to residential or nursing care) is made |
Primary diagnosis | Primary diagnosis group | Individual primary diagnosis for this admission coded by ICD-10 is recoded into ICD-10 group |
Secondary diagnoses | Individual comorbidity:
|
Up to 12 secondary diagnoses are coded per hospital episode. All previous episode (i.e. not this present episode of care) secondary diagnoses are used to define the Charlson individual comorbidities using validated coding algorithms |
Secondary diagnoses | Modified Charlson Comorbidity Index score | Up to 12 secondary diagnoses are coded per hospital episode. All previous episode (i.e. not this present episode of care) secondary diagnoses are used to define the modified Charlson Comorbidity Index score using a validated algorithm |
Outpatient appointment date | Outpatient attendances (last 12 months) | Using outpatient appointment date and the outcome field (coded as ATT = attended) the number of outpatient attendances in the last 12 months for this patient is calculated |
Renal modality | Not used in analysis | This data variable was linked from the renal system at the EKHUFT, using NHS number, in order to remove patients on RRT from the analysis |
Data entry | Calculated variable | Variable description |
---|---|---|
ALT | 12-month average | Average of all ALT results in the 12 months prior to hospital admission |
Most recent result | Most recent ALT result within the last 30 days prior to hospital admission | |
Admission result | Peak ALT result within 12 hours prior to 12 hours post admission time (taken from ‘Admission Date and Time’ data entry in Table 25) | |
72-hour peak | Peak ALT result within 12 hours post admission to 72 hours post admission time (taken from ‘Admission Date and Time’ data entry in Table 25) | |
AMY | 12-month average | Average of all AMY results in the 12 months prior to hospital admission |
Most recent result | Most recent AMY result within the last 30 days prior to hospital admission | |
Admission result | Peak AMY result within 12 hours prior to 12 hours post admission time (taken from ‘Admission Date and Time’ data entry in Table 25) | |
72-hour peak | Peak AMY result within 12 hours post admission to 72 hours post admission time (taken from ‘Admission Date and Time’ data entry in Table 25) | |
BNP | 12-month average | Average of all BNP results in the 12 months prior to hospital admission |
Most recent result | Most recent BNP result within the last 30 days prior to hospital admission | |
Ca | 12-month average | Average of all calcium results in the 12 months prior to hospital admission |
Most recent result | Most recent calcium result within the last 30 days prior to hospital admission | |
Admission result | Peak calcium result within 12 hours prior to 12 hours post admission time (taken from ‘Admission Date and Time’ data entry in Table 25) | |
72-hour peak | Peak calcium result within 12 hours post admission to 72 hours post admission time (taken from ‘Admission Date and Time’ data entry in Table 25) | |
CRP | 12-month average | Average of all CRP results in the 12 months prior to hospital admission |
Most recent result | Most recent CRP result within the last 30 days prior to hospital admission | |
Admission result | Peak CRP result within 12 hours prior to 12 hours post admission time (taken from ‘Admission Date and Time’ data entry in Table 25) | |
72-hour peak | Peak CRP result within 12 hours post admission to 72 hours post admission time (taken from ‘Admission Date and Time’ data entry in Table 25) | |
Hb | 12-month average | Average of all Hb results in the 12 months prior to hospital admission |
Most recent result | Most recent Hb result within the last 30 days prior to hospital admission | |
Admission result | Peak Hb result within 12 hours prior to 12 hours post admission time (taken from ‘Admission Date and Time’ data entry in Table 25) | |
72-hour peak | Peak Hb result within 12 hours post admission to 72 hours post admission time (taken from ‘Admission Date and Time’ data entry in Table 25) | |
HbA1c | 12-month average | Average of all HbA1c results in the 12 months prior to hospital admission |
Most recent result | Most recent HbA1c result within the last 30 days prior to hospital admission | |
K | 12-month average | Average of all K results in the 12 months prior to hospital admission |
Most recent result | Most recent K result within the last 30 days prior to hospital admission | |
Admission result | Peak K result within 12 hours prior to 12 hours post admission time (taken from ‘Admission Date and Time’ data entry in Table 25) | |
72-hour peak | Peak K result within 12 hours post admission to 72 hours post admission time (taken from ‘Admission Date and Time’ data entry in Table 25) | |
Mg | 12-month average | Average of all Mg results in the 12 months prior to hospital admission |
Most recent result | Most recent Mg result within the last 30 days prior to hospital admission | |
Admission result | Peak Mg result within 12 hours prior to 12 hours post admission time (taken from ‘Admission Date and Time’ data entry in Table 25) | |
72-hour peak | Peak Mg result within 12 hours post admission to 72 hours post admission time (taken from ‘Admission Date and Time’ data entry in Table 25) | |
Na | 12-month average | Average of all Na results in the 12 months prior to hospital admission |
Most recent result | Most recent Na result within the last 30 days prior to hospital admission | |
Admission result | Peak Na result within 12 hours prior to 12 hours post admission time (taken from ‘Admission Date and Time’ data entry in Table 25) | |
72-hour peak | Peak Na result within 12 hours post admission to 72 hours post admission time (taken from ‘Admission Date and Time’ data entry in Table 25) | |
PLT | 12-month average | Average of all PLT results in the 12 months prior to hospital admission |
Most recent result | Most recent PLT result within the last 30 days prior to hospital admission | |
Admission result | Peak PLT result within 12 hours pre to 12 hours post admission time (taken from ‘Admission Date and Time’ data entry in Table 25) | |
72-hour peak | Peak PLT result within 12 hours post admission to 72 hours post admission time (taken from ‘Admission Date and Time’ data entry in Table 25) | |
WBC | 12-month average | Average of all WBC results in the 12 months prior to hospital admission |
Most recent result | Most recent WBC result within the last 30 days prior to hospital admission | |
Admission result | Peak WBC result within 12 hours pre to 12 hours post admission time (taken from ‘Admission Date and Time’ data entry in Table 25) | |
72-hour peak | Peak WBC result within 12 hours post admission to 72 hours post admission time (taken from ‘Admission Date and Time’ data entry in Table 25) | |
CK | Most recent result | Most recent CK result within the last 30 days prior to hospital admission |
Admission result | Peak CK result within 12 hours pre to 12 hours post admission time (taken from ‘Admission Date and Time’ data entry in Table 25) | |
72-hour peak | Peak CK result within 12 hours post admission to 72 hours post admission time (taken from ‘Admission Date and Time’ data entry in Table 25) | |
BC | Taken within 2 weeks | Binary blood culture taken (Y/N) within the 2 weeks prior to hospital admission |
2 weeks significant growth | Binary blood culture reported with significant growth (Y/N) within the 2 weeks prior to hospital admission | |
Taken within 24 hours | Binary blood culture taken (Y/N) within 12 hours pre to 12 hours post admission time (taken from ‘Admission Date and Time’ data entry in Table 25) | |
Taken within 72 hours | Binary blood culture taken (Y/N) within 12 hours pre to 72 hours post admission time (taken from ‘Admission Date and Time’ data entry in Table 25) | |
72 hours significant growth | Binary blood culture reported with significant growth (Y/N) within 12 hours pre to 72 hours post admission time (taken from ‘Admission Date and Time’ data entry in Table 25) | |
FAE | Taken within 2 weeks | Binary faeces culture taken (Y/N) within the 2 weeks prior to hospital admission |
2 weeks significant growth | Binary faeces culture reported with significant growth (Y/N) within the 2 weeks prior to hospital admission | |
Taken within 24 hours | Binary faeces culture taken (Y/N) within 12 hours pre to 12 hours post admission time (taken from ‘Admission Date and Time’ data entry in Table 1) | |
Taken within 72 hours | Binary faeces culture taken (Y/N) within 12 hours pre to 72 hours post admission time (taken from ‘Admission Date and Time’ data entry in Table 25) | |
72 hours significant growth | Binary faeces culture reported with significant growth (Y/N) within 12 hours pre to 72 hours post admission time (taken from ‘Admission Date and Time’ data entry in Table 25) | |
MSU or CSU | Taken within 2 weeks | Binary MSU OR CSU taken (Y/N) within the 2 weeks prior to hospital admission |
2 weeks significant growth | Binary MSU OR CSU reported with significant growth (Y/N) within the 2 weeks prior to hospital admission | |
Taken within 24 hours | Binary MSU OR CSU taken (Y/N) within 12 hours pre to 12 hours post admission time (taken from ‘Admission Date and Time’ data entry in Table 25) | |
Taken within 72 hours | Binary MSU OR CSU taken (Y/N) within 12 hours pre to 72 hours post admission time (taken from ‘Admission Date and Time’ data entry in Table 25) | |
72 hours significant growth | Binary MSU OR CSU reported with significant growth (Y/N) within 12 hours pre to 72 hours post admission time (taken from ‘Admission Date and Time’ data entry in Table 25) | |
SPU | Taken within 2 weeks | Binary sputum culture taken (Y/N) within the 2 weeks prior to hospital admission |
2 weeks significant growth | Binary sputum culture reported with significant growth (Y/N) within the 2 weeks prior to hospital admission | |
Taken within 24 hours | Binary sputum culture taken (Y/N) within 12 hours pre to 12 hours post admission time (taken from ‘Admission Date and Time’ data entry in Table 25) | |
Taken within 72 hours | Binary sputum culture taken (Y/N) within 12 hours pre to 72 hours post admission time (taken from ‘Admission Date and Time’ data entry in Table 25) | |
72 hours significant growth | Binary sputum culture reported with significant growth (Y/N) within 12 hours pre to 72 hours post admission time (taken from ‘Admission Date and Time’ data entry in Table 25) | |
SAP | Taken within 2 weeks | Binary SAP taken (Y/N) within the 2 weeks prior to hospital admission |
2 weeks significant growth | Binary SAP reported with significant growth (Y/N) within the 2 weeks prior to hospital admission | |
Taken within 24 hours | Binary SAP taken (Y/N) within 12 hours pre to 12 hours post admission time (taken from ‘Admission Date and Time’ data entry in Table 25) | |
Taken within 72 hours | Binary SAP taken (Y/N) within 12 hours pre to 72 hours post admission time (taken from ‘Admission Date and Time’ data entry in Table 25) | |
72 hours significant growth | Binary SAP reported with significant growth (Y/N) within 12 hours pre to 72 hours post admission time (taken from ‘Admission Date and Time’ data entry in Table 25) | |
Cr | Baseline creatinine level | Lowest creatinine level in the 12 months prior to hospital admission (not including the 2 weeks prior to admission) |
Baseline eGFR | eGFR calculated using the MDRD equation, based on the baseline Cr | |
Baseline CKD stage | CKD stage based on the baseline eGFR | |
Pre-admission creatinine level | Peak creatinine level in the 2 weeks prior to hospital admission [from 12 hours pre admission up to 2 weeks (taken from ‘Admission Date and Time’ data entry in Table 25)] | |
Pre-admission AKI stage | AKI stage based on the pre-admission creatinine level | |
Admission creatinine level | Peak creatinine level within 12 hours pre to 12 hours post admission time (taken from ‘Admission Date and Time’ data entry in Table 25) | |
Admission AKI stage | AKI stage based on the admission creatinine level | |
72-hour peak creatinine level | Peak creatinine level within 12 hours post admission to 72 hours post admission time (taken from ‘Admission Date and Time’ data entry in Table 25) | |
72-hour AKI stage | AKI stage based on the 72-hour peak creatinine level | |
Trop | 12-month test count | Number of troponin tests performed in the 12 months prior to hospital admission |
12-month positive count | Number of troponin tests classed as positive for myocardial infarction in the 12 months prior to hospital admission | |
Admission result | Peak trop result within 12 hours pre to 12 hours post admission time (taken from ‘Admission Date and Time’ data entry in Table 25) | |
Admission result tested | Binary troponin tested (Y/N) within 12 hours pre to 12 hours post admission time (taken from ‘Admission Date and Time’ data entry in Table 25) | |
Admission result positive | Binary troponin result positive for myocardial infarction (Y/N) within 12 hours pre to 12 hours post admission time (taken from ‘Admission Date and Time’ data entry in Table 25) | |
72-hour peak | Peak troponin result within 12 hours post admission to 72 hours post admission time (taken from ‘Admission Date and Time’ data entry in Table 25) | |
72-hour result tested | Binary troponin tested (Y/N) within 12 hours post admission to 72 hours post admission time (taken from ‘Admission Date and Time’ data entry in Table 25) | |
72-hour peak result positive | Binary troponin result positive for myocardial infarction (Y/N) within 12 hours post admission to 72 hours post admission time (taken from ‘Admission Date and Time’ data entry in Table 25) | |
ACR and PCR | Proteinuria – 12-month test count | Number of proteinuria tests performed in the 12 months prior to hospital admission (taken from ‘Admission Date and Time’ data entry in Table 25) |
Proteinuria – 12-month worst stage | Worst proteinuria stage [either ACR or PCR, as defined by KDIGO (see Table 29)] in the 12 months prior to hospital admission (taken from ‘Admission Date and Time’ data entry in Table 25) |
Data entry | Calculated variable | Variable description |
---|---|---|
Medication name | ACEi/ARB count | From medication name field of last discharge summary for that patient and cross reference with lookup table of medication names and parent groups, calculation of number of this class of medication |
Medication name | Non-steroidal anti-inflammatory count | From medication name field of last discharge summary for that patient and cross reference with lookup table of medication names and parent groups, calculation of number of this class of medication |
Medication name | Diuretic count | From medication name field of last discharge summary for that patient and cross reference with lookup table of medication names and parent groups, calculation of number of this class of medication |
Medication name | Total number of medications | Total number of entries in the medication name field |
Data entry | Calculated variable | Variable description |
---|---|---|
Operation date and time | 24-hour operation performed | Binary (Y/N) operation performed in the first 24 hours of admission (taken from ‘Admission Date and Time’ data entry in Table 25) |
Operation score | 24-hour maximum operation score [1 (least severe) to 5 (most severe)] |
Maximum operation score in the first 24 hours of admission (taken from ‘Admission Date and Time’ data entry in Table 25) |
Measure | Categories | ||
---|---|---|---|
Normal to mildly increased (1) | Moderately increased (2) | Severely increased (3) | |
AER (mg/24 hours) | < 30 | 30–300 | > 300 |
PER (mg/24 hours) | < 150 | 150–500 | > 500 |
ACR | |||
mg/mmol | < 3 | 3–30 | > 30 |
mg/g | < 30 | 30–300 | > 300 |
PCR | |||
mg/mmol | < 15 | 15–50 | > 50 |
mg/g | < 150 | 150–500 | > 500 |
Protein reagent strip | Negative to trace | Trace to + | + or greater |
OPCS subsection code | OPCS subsection description | Operative Severity Score |
---|---|---|
# | Blank or unknown code | |
A32 | Other decompression of cranial nerve | 2 |
A33 | Neurostimulation of cranial nerve | 1 |
A36 | Other operations on cranial nerve | 2 |
A39 | Repair of dura | 4 |
A40 | Drainage of extradural space | 4 |
A41 | Drainage of subdural space | 4 |
A47 | Other destruction of spinal cord | 4 |
A48 | Other operations on spinal cord | 4 |
A52 | Therapeutic epidural injection | 1 |
A53 | Drainage of spinal canal | 1 |
A54 | Therapeutic spinal puncture | 1 |
A55 | Diagnostic spinal puncture | 1 |
A57 | Operations on spinal nerve root | 3 |
A59 | Excision of peripheral nerve | 1 |
A60 | Destruction of peripheral nerve | 1 |
A61 | Extirpation of lesion of peripheral nerve | 1 |
A62 | Microsurgical repair of peripheral nerve | 1 |
A64 | Other repair of peripheral nerve | 1 |
A65 | Release of entrapment of peripheral nerve at wrist | 1 |
A66 | Release of entrapment of peripheral nerve at ankle | 1 |
A67 | Release of entrapment of peripheral nerve at other site | 1 |
A68 | Other release of peripheral nerve | 1 |
A69 | Revision of release of peripheral nerve | 1 |
A70 | Neurostimulation of peripheral nerve | 1 |
A73 | Other operations on peripheral nerve | 1 |
A75 | Excision of sympathetic nerve | 2 |
A76 | Chemical destruction of sympathetic nerve | 1 |
A77 | Cryotherapy to sympathetic nerve | 1 |
A78 | Radiofrequency controlled thermal destruction of sympathetic nerve | 1 |
A79 | Other destruction of sympathetic nerve | 2 |
A81 | Other operations on sympathetic nerve | 2 |
A83 | Electroconvulsive therapy | 2 |
A84 | Neurophysiological operations | 2 |
B08 | Excision of thyroid gland | 3 |
B09 | Operations on aberrant thyroid tissue | 2 |
B10 | Operations on thyroglossal tissue | 2 |
B12 | Other operations on thyroid gland | 2 |
B14 | Excision of parathyroid gland | 2 |
B16 | Other operations on parathyroid gland | 2 |
B22 | Excision of adrenal gland | 4 |
B27 | Total excision of breast | 3 |
B28 | Other excision of breast | 2 |
B29 | Reconstruction of breast | 2 |
B30 | Prosthesis for breast | 2 |
B32 | Biopsy of breast | 1 |
B33 | Incision of breast | 1 |
B34 | Operations on duct of breast | 1 |
B35 | Operations on nipple | 1 |
B36 | Reconstruction of nipple and areola | 1 |
B37 | Other operations on breast | 2 |
C01 | Excision of eye | 3 |
C02 | Extirpation of lesion of orbit | 2 |
C03 | Insertion of prosthesis of eye | 2 |
C04 | Attention to prosthesis of eye | 1 |
C05 | Plastic repair of orbit | 2 |
C06 | Incision of orbit | 2 |
C08 | Other operations on orbit | 2 |
C09 | Replacement of canthal tendon | 2 |
C10 | Operations on eyebrow | 1 |
C11 | Operations on canthus | 1 |
C12 | Extirpation of lesion of eyelid | 1 |
C13 | Excision of redundant skin of eyelid | 1 |
C14 | Reconstruction of eyelid | 1 |
C15 | Correction of deformity of eyelid | 1 |
C16 | Other plastic repair of eyelid | 1 |
C17 | Other repair of eyelid | 1 |
C18 | Correction of ptosis of eyelid | 1 |
C19 | Incision of eyelid | 1 |
C20 | Protective suture of eyelid | 1 |
C22 | Other operations on eyelid | 1 |
C24 | Operations on lacrimal gland | 1 |
C25 | Connection between lacrimal apparatus and nose | 1 |
C26 | Other operations on lacrimal sac | 1 |
C27 | Operations on nasolacrimal duct | 1 |
C29 | Other operations on lacrimal apparatus | 1 |
C31 | Combined operations on muscles of eye | 2 |
C32 | Recession of muscle of eye | 2 |
C33 | Resection of muscle of eye | 2 |
C35 | Other adjustment to muscle of eye | 2 |
C37 | Other operations on muscle of eye | 2 |
C39 | Extirpation of lesion of conjunctiva | 1 |
C40 | Repair of conjunctiva | 1 |
C43 | Other operations on conjunctiva | 1 |
C44 | Other plastic operations on cornea | 1 |
C45 | Extirpation of lesion of cornea | 1 |
C46 | Plastic operations on cornea | 1 |
C47 | Closure of cornea | 1 |
C48 | Removal of foreign body from cornea | 1 |
C49 | Incision of cornea | 1 |
C51 | Other operations on cornea | 2 |
C53 | Extirpation of lesion of sclera | 1 |
C54 | Buckling operations for attachment of retina | 2 |
C55 | Incision of sclera | 2 |
C57 | Other operations on sclera | 2 |
C59 | Excision of iris | 2 |
C60 | Filtering operations on iris | 1 |
C61 | Other operations on trabecular meshwork of eye | 1 |
C62 | Incision of iris | 1 |
C64 | Other operations on iris | 1 |
C65 | Operations following glaucoma surgery | 2 |
C66 | Extirpation of ciliary body | 2 |
C69 | Other operations on anterior chamber of eye | 2 |
C71 | Extracapsular extraction of lens | 2 |
C72 | Intracapsular extraction of lens | 2 |
C73 | Incision of capsule of lens | 2 |
C74 | Other extraction of lens | 2 |
C75 | Prosthesis of lens | 2 |
C77 | Other operations on lens | 2 |
C79 | Operations on vitreous body | 2 |
C80 | Operations on retinal membrane | 2 |
C81 | Photocoagulation of retina for detachment | 1 |
C82 | Destruction of lesion of retina | 1 |
C84 | Other operations on retina | 2 |
C85 | Fixation of retina | 2 |
C86 | Other operations on eye | 2 |
C89 | Operations on posterior segment of eye | 2 |
C90 | Local anaesthetics for ophthalmology procedures | 1 |
D01 | Excision of external ear | 1 |
D02 | Extirpation of lesion of external ear | 1 |
D03 | Plastic operations on external ear | 1 |
D04 | Drainage of external ear | 1 |
D06 | Other operations on external ear | 1 |
D07 | Clearance of external auditory canal | 1 |
D08 | Other operations on external auditory canal | 1 |
D10 | Exenteration of mastoid air cells | 2 |
D12 | Other operations on mastoid | 2 |
D13 | Attachment of bone anchored hearing prosthesis | 2 |
D14 | Repair of eardrum | 2 |
D15 | Drainage of middle ear | 1 |
D16 | Reconstruction of ossicular chain | 2 |
D17 | Other operations on ossicle of ear | 2 |
D19 | Extirpation of lesion of middle ear | 2 |
D20 | Other operations on middle ear | 2 |
D23 | Operations on inner ear | 2 |
D26 | Operations on vestibular apparatus | 2 |
D28 | Other operations on ear | 2 |
E01 | Excision of nose | 2 |
E02 | Plastic operations on nose | 1 |
E03 | Operations on septum of nose | 1 |
E04 | Operations on turbinate of nose | 1 |
E05 | Surgical arrest of bleeding from internal nose | 1 |
E06 | Packing of cavity of nose | 1 |
E07 | Other plastic operations on nose | 1 |
E08 | Other operations on internal nose | 1 |
E09 | Operations on external nose | 1 |
E10 | Other operations on nose | 1 |
E12 | Operations on maxillary antrum using sublabial approach | 3 |
E13 | Other operations on maxillary antrum | 2 |
E14 | Operations on frontal sinus | 2 |
E15 | Operations on sphenoid sinus | 2 |
E16 | Other operations on frontal sinus | 2 |
E17 | Operations on unspecified nasal sinus | 2 |
E19 | Excision of pharynx | 4 |
E20 | Operations on adenoid | 2 |
E21 | Repair of pharynx | 3 |
E23 | Other open operations on pharynx | 3 |
E24 | Therapeutic endoscopic operations on pharynx | 2 |
E25 | Diagnostic endoscopic examination of pharynx | 1 |
E27 | Other operations on pharynx | 3 |
E28 | Operations on cricopharyngeus muscle | 2 |
E29 | Excision of larynx | 4 |
E30 | Open extirpation of lesion of larynx | 3 |
E31 | Reconstruction of larynx | 3 |
E33 | Other open operations on larynx | 3 |
E34 | Microtherapeutic endoscopic operations on larynx | 2 |
E35 | Other therapeutic endoscopic operations on larynx | 2 |
E36 | Diagnostic endoscopic examination of larynx | 1 |
E38 | Other operations on larynx | 3 |
E39 | Partial excision of trachea | 3 |
E40 | Plastic operations on trachea | 3 |
E41 | Open placement of prosthesis in trachea | 3 |
E42 | Exteriorisation of trachea | 3 |
E43 | Other open operations on trachea | 3 |
E48 | Therapeutic fibre-optic endoscopic operations on lower respiratory tract | 1 |
E49 | Diagnostic fibre-optic endoscopic examination of lower respiratory tract | 1 |
E50 | Therapeutic endoscopic operations on lower respiratory tract using rigid bronchoscope | 1 |
E51 | Diagnostic endoscopic examination of lower respiratory tract using rigid bronchoscope | 1 |
E52 | Other operations on bronchus | 4 |
E59 | Other operations on lung | 4 |
E85 | Ventilation support | 3 |
E95 | Tuberculosis support | 1 |
F01 | Partial excision of lip | 1 |
F02 | Extirpation of lesion of lip | 1 |
F03 | Correction of deformity of lip | 1 |
F04 | Other reconstruction of lip | 1 |
F05 | Other repair of lip | 1 |
F06 | Other operations on lip | 1 |
F08 | Implantation of tooth | 1 |
F09 | Surgical removal of tooth | 2 |
F10 | Simple extraction of tooth | 1 |
F12 | Surgery on apex of tooth | 1 |
F13 | Restoration of tooth | 1 |
F14 | Orthodontic operations | 1 |
F15 | Other orthodontic operations | 1 |
F16 | Other operations on tooth | 1 |
F17 | Operations on teeth using dental crown or bridge | 1 |
F18 | Excision of dental lesion of jaw | 2 |
F20 | Operations on gingiva | 2 |
F22 | Excision of tongue | 4 |
F23 | Extirpation of lesion of tongue | 2 |
F24 | Incision of tongue | 2 |
F26 | Other operations on tongue | 3 |
F28 | Extirpation of lesion of palate | 2 |
F29 | Correction of deformity of palate | 3 |
F30 | Other repair of palate | 2 |
F32 | Other operations on palate | 2 |
F34 | Excision of tonsil | 2 |
F36 | Other operations on tonsil | 2 |
F38 | Extirpation of lesion of other part of mouth | 2 |
F39 | Reconstruction of other part of mouth | 3 |
F40 | Other repair of other part of mouth | 3 |
F42 | Other operations on mouth | 2 |
F43 | Other examinations of mouth | 1 |
F44 | Excision of salivary gland | 2 |
F45 | Extirpation of lesion of salivary gland | 1 |
F46 | Incision of salivary gland | 1 |
F48 | Other operations on salivary gland | 1 |
F51 | Open extraction of calculus from salivary duct | 1 |
F53 | Other open operations on salivary duct | 1 |
F56 | Manipulative removal of calculus from salivary duct | 1 |
F58 | Other operations on salivary duct | 1 |
F63 | Insertion of dental prosthesis | 1 |
G02 | Total excision of oesophagus | 4 |
G07 | Repair of oesophagus | 4 |
G09 | Incision of oesophagus | 3 |
G10 | Open operations on varices of oesophagus | 4 |
G13 | Other open operations on oesophagus | 4 |
G14 | Fibre-optic endoscopic extirpation of lesion of oesophagus | 2 |
G15 | Other therapeutic fibre-optic endoscopic operations on oesophagus | 2 |
G16 | Diagnostic fibre-optic endoscopic examination of oesophagus | 1 |
G17 | Endoscopic extirpation of lesion of oesophagus using rigid oesophagoscope | 2 |
G18 | Other therapeutic endoscopic operations on oesophagus using rigid oesophagoscope | 2 |
G19 | Diagnostic endoscopic examination of oesophagus using rigid oesophagoscope | 2 |
G21 | Other operations on oesophagus | 3 |
G23 | Repair of diaphragmatic hernia | 3 |
G24 | Antireflux operations | 3 |
G25 | Revision of antireflux operations | 3 |
G27 | Total excision of stomach | 4 |
G28 | Partial excision of stomach | 4 |
G29 | Open extirpation of lesion of stomach | 3 |
G31 | Connection of stomach to duodenum | 3 |
G32 | Connection of stomach to transposed jejunum | 4 |
G33 | Other connection of stomach to jejunum | 3 |
G34 | Artificial opening into stomach | 2 |
G35 | Operations on ulcer of stomach | 3 |
G36 | Other repair of stomach | 3 |
G38 | Other open operations on stomach | 3 |
G40 | Incision of pylorus | 3 |
G42 | Other fibre-optic endoscopic extirpation of lesion of upper gastrointestinal tract | 2 |
G43 | Fibre-optic endoscopic extirpation of lesion of upper gastrointestinal tract | 2 |
G44 | Other therapeutic fibre-optic endoscopic operations on upper gastrointestinal tract | 2 |
G45 | Diagnostic fibre-optic endoscopic examination of upper gastrointestinal tract | 1 |
G46 | Therapeutic fibre-optic endoscopic operations on upper gastrointestinal tract | 2 |
G47 | Intubation of stomach | 2 |
G49 | Excision of duodenum | 4 |
G51 | Bypass of duodenum | 3 |
G52 | Operations on ulcer of duodenum | 3 |
G53 | Other open operations on duodenum | 3 |
G55 | Diagnostic endoscopic examination of duodenum | 1 |
G57 | Other operations on duodenum | 3 |
G58 | Excision of jejunum | 4 |
G59 | Extirpation of lesion of jejunum | 3 |
G60 | Artificial opening into jejunum | 2 |
G61 | Bypass of jejunum | 3 |
G63 | Other open operations on jejunum | 3 |
G64 | Therapeutic endoscopic operations on jejunum | 2 |
G67 | Other operations on jejunum | 3 |
G69 | Excision of ileum | 4 |
G71 | Bypass of ileum | 3 |
G72 | Other connection of ileum | 3 |
G73 | Attention to connection of ileum | 3 |
G74 | Creation of artificial opening into ileum | 3 |
G75 | Attention to artificial opening into ileum | 2 |
G76 | Intra-abdominal manipulation of ileum | 3 |
G78 | Other open operations on ileum | 3 |
G80 | Diagnostic endoscopic examination of ileum | 1 |
G82 | Other operations on ileum | 3 |
H01 | Emergency excision of appendix | 2 |
H02 | Other excision of appendix | 2 |
H03 | Other operations on appendix | 2 |
H04 | Total excision of colon and rectum | 4 |
H05 | Total excision of colon | 4 |
H06 | Extended excision of right hemicolon | 4 |
H07 | Other excision of right hemicolon | 4 |
H08 | Excision of transverse colon | 4 |
H09 | Excision of left hemicolon | 4 |
H10 | Excision of sigmoid colon | 4 |
H11 | Other excision of colon | 4 |
H12 | Extirpation of lesion of colon | 3 |
H13 | Bypass of colon | 3 |
H14 | Exteriorisation of caecum | 3 |
H15 | Other exteriorisation of colon | 3 |
H16 | Incision of colon | 3 |
H17 | Intra-abdominal manipulation of colon | 3 |
H18 | Open endoscopic operations on colon | 2 |
H19 | Other open operations on colon | 3 |
H20 | Endoscopic extirpation of lesion of colon | 2 |
H21 | Other therapeutic endoscopic operations on colon | 2 |
H22 | Diagnostic endoscopic examination of colon | 1 |
H24 | Other therapeutic endoscopic operations on lower bowel using fibre-optic sigmoidoscope | 2 |
H25 | Diagnostic endoscopic examination of lower bowel using fibre-optic sigmoidoscope | 1 |
H26 | Endoscopic extirpation of lesion of sigmoid colon using rigid sigmoidoscope | 2 |
H27 | Other therapeutic endoscopic operations on sigmoid colon using rigid sigmoidoscope | 2 |
H28 | Diagnostic endoscopic examination of sigmoid colon using rigid sigmoidoscope | 1 |
H29 | Subtotal excision of colon | 4 |
H30 | Other operations on colon | 3 |
H33 | Excision of rectum | 4 |
H34 | Open extirpation of lesion of rectum | 3 |
H35 | Fixation of rectum for prolapse | 3 |
H36 | Other abdominal operations for prolapse of rectum | 3 |
H40 | Operations on rectum through anal sphincter | 2 |
H41 | Other operations on rectum through anus | 2 |
H42 | Perineal operations for prolapse of rectum | 2 |
H44 | Manipulation of rectum | 2 |
H46 | Other operations on rectum | 3 |
H47 | Excision of anus | 4 |
H48 | Excision of lesion of anus | 3 |
H49 | Destruction of lesion of anus | 3 |
H50 | Repair of anus | 2 |
H51 | Excision of haemorrhoid | 2 |
H52 | Destruction of haemorrhoid | 2 |
H53 | Other operations on haemorrhoid | 2 |
H54 | Dilatation of anal sphincter | 2 |
H55 | Other operations on perianal region | 2 |
H56 | Other operations on anus | 2 |
H57 | Other operations on the anal sphincter to control continence | 2 |
H58 | Drainage through perineal region | 2 |
H59 | Excision of pilonidal sinus | 2 |
H60 | Other operations on pilonidal sinus | 2 |
H62 | Other operations on bowel | 3 |
H68 | Diagnostic endoscopic examination of enteric pouch using colonoscope | 1 |
H69 | Diagnostic endoscopic examination of enteric pouch using fibre-optic sigmoidoscope | 1 |
H70 | Diagnostic endoscopic examination of enteric pouch using rigid sigmoidoscope | 1 |
J02 | Partial excision of liver | 4 |
J04 | Repair of liver | 4 |
J08 | Therapeutic endoscopic operations on liver using laparoscope | 3 |
J09 | Diagnostic endoscopic examination of liver using laparoscope | 2 |
J10 | Transluminal operations on blood vessel of liver | 2 |
J12 | Other therapeutic percutaneous operations on liver | 2 |
J13 | Diagnostic percutaneous operations on liver | 2 |
J14 | Other puncture of liver | 2 |
J15 | Transluminal insertion of prosthesis into blood vessel of liver | 2 |
J18 | Excision of gall bladder | 3 |
J20 | Repair of gall bladder | 3 |
J21 | Incision of gall bladder | 2 |
J23 | Other open operations on gall bladder | 3 |
J24 | Therapeutic percutaneous operations on gall bladder | 2 |
J32 | Repair of bile duct | 3 |
J33 | Incision of bile duct | 3 |
J34 | Plastic repair of sphincter of Oddi using duodenal approach | 3 |
J37 | Other open operations on bile duct | 3 |
J40 | Endoscopic retrograde placement of prosthesis in bile duct | 2 |
J41 | Other therapeutic endoscopic retrograde operations on bile duct | 2 |
J42 | Therapeutic endoscopic retrograde operations on pancreatic duct | 2 |
J43 | Diagnostic endoscopic retrograde examination of bile duct and pancreatic duct | 2 |
J47 | Therapeutic percutaneous insertion of prosthesis into bile duct | 2 |
J48 | Other therapeutic percutaneous operations on bile duct | 2 |
J50 | Percutaneous examination of bile duct | 2 |
J52 | Other operations on bile duct | 2 |
J57 | Other partial excision of pancreas | 4 |
J60 | Other open operations on pancreatic duct | 3 |
J67 | Diagnostic percutaneous operations on pancreas | 2 |
J68 | Other operations on pancreas | 3 |
J69 | Total excision of spleen | 4 |
J72 | Other operations on spleen | 4 |
J76 | Therapeutic percutaneous operations on bile duct | 2 |
K14 | Other open operations on septum of heart | 4 |
K47 | Repair of coronary artery | 4 |
K49 | Transluminal balloon angioplasty of coronary artery | 2 |
K60 | Cardiac pacemaker system introduced through vein | 2 |
K61 | Other cardiac pacemaker system | 2 |
K62 | Therapeutic transluminal operations on heart | 2 |
L12 | Other open operations on pulmonary artery | 4 |
L13 | Transluminal operations on pulmonary artery | 3 |
L16 | Extra-anatomic bypass of aorta | 4 |
L18 | Emergency replacement of aneurysmal segment of aorta | 4 |
L19 | Other replacement of aneurysmal segment of aorta | 4 |
L20 | Other emergency bypass of segment of aorta | 4 |
L21 | Other bypass of segment of aorta | 4 |
L22 | Attention to prosthesis of aorta | 4 |
L25 | Other open operations on aorta | 4 |
L26 | Transluminal operations on aorta | 3 |
L27 | Transluminal insertion of stent graft for aneurysmal segment of aorta | 3 |
L28 | Transluminal operations on aneurysmal segment of aorta | 3 |
L29 | Reconstruction of carotid artery | 3 |
L30 | Other open operations on carotid artery | 3 |
L31 | Transluminal operations on carotid artery | 3 |
L37 | Reconstruction of subclavian artery | 4 |
L38 | Other open operations on subclavian artery | 4 |
L39 | Transluminal operations on subclavian artery | 3 |
L41 | Reconstruction of renal artery | 4 |
L42 | Other open operations on renal artery | 4 |
L43 | Transluminal operations on renal artery | 2 |
L45 | Reconstruction of other visceral branch of abdominal aorta | 4 |
L47 | Transluminal operations on other visceral branch of abdominal aorta | 2 |
L48 | Emergency replacement of aneurysmal iliac artery | 4 |
L49 | Other replacement of aneurysmal iliac artery | 4 |
L50 | Other emergency bypass of iliac artery | 4 |
L51 | Other bypass of iliac artery | 4 |
L52 | Reconstruction of iliac artery | 4 |
L53 | Other open operations on iliac artery | 4 |
L54 | Transluminal operations on iliac artery | 2 |
L56 | Emergency replacement of aneurysmal femoral artery | 4 |
L57 | Other replacement of aneurysmal femoral artery | 4 |
L58 | Other emergency bypass of femoral artery | 4 |
L59 | Other bypass of femoral artery | 4 |
L60 | Reconstruction of femoral artery | 4 |
L62 | Other open operations on femoral artery | 4 |
L63 | Transluminal operations on femoral artery | 2 |
L65 | Revision of reconstruction of artery | 4 |
L66 | Other therapeutic transluminal operations on artery | 3 |
L67 | Excision of other artery | 4 |
L68 | Repair of other artery | 4 |
L70 | Other open operations on other artery | 4 |
L71 | Therapeutic transluminal operations on other artery | 3 |
L72 | Diagnostic transluminal operations on other artery | 3 |
L74 | Arteriovenous shunt | 2 |
L75 | Other arteriovenous operations | 3 |
L76 | Endovascular placement of stent | 3 |
L79 | Other operations on vena cava | 4 |
L84 | Combined operations on varicose vein of leg | 2 |
L85 | Ligation of varicose vein of leg | 2 |
L86 | Injection into varicose vein of leg | 2 |
L87 | Other operations on varicose vein of leg | 2 |
L88 | Transluminal operations on varicose vein of leg | 2 |
L89 | Other endovascular placement of stent | 2 |
L90 | Open removal of thrombus from vein | 2 |
L91 | Other vein related operations | 2 |
L92 | Unblocking of access catheter | 1 |
L93 | Other open operations on vein | 2 |
L94 | Therapeutic transluminal operations on vein | 2 |
L95 | Diagnostic transluminal operations on vein | 2 |
L96 | Percutaneous removal of thrombus from vein | 2 |
L97 | Other operations on blood vessel | 3 |
L98 | Operations on microvascular vessel | 2 |
L99 | Other therapeutic transluminal operations on vein | 2 |
M02 | Total excision of kidney | 4 |
M03 | Partial excision of kidney | 4 |
M04 | Open extirpation of lesion of kidney | 3 |
M05 | Open repair of kidney | 3 |
M06 | Incision of kidney | 3 |
M09 | Therapeutic endoscopic operations on calculus of kidney | 2 |
M10 | Other therapeutic endoscopic operations on kidney | 2 |
M11 | Diagnostic endoscopic examination of kidney | 2 |
M13 | Percutaneous puncture of kidney | 2 |
M14 | Extracorporeal fragmentation of calculus of kidney | 2 |
M15 | Operations on kidney along nephrostomy tube track | 2 |
M16 | Other operations on kidney | 3 |
M18 | Excision of ureter | 4 |
M19 | Urinary diversion | 3 |
M20 | Replantation of ureter | 3 |
M21 | Other connection of ureter | 3 |
M22 | Repair of ureter | 3 |
M23 | Incision of ureter | 3 |
M25 | Other open operations on ureter | 3 |
M26 | Therapeutic nephroscopic operations on ureter | 2 |
M27 | Therapeutic ureteroscopic operations on ureter | 2 |
M28 | Other endoscopic removal of calculus from ureter | 2 |
M29 | Other therapeutic endoscopic operations on ureter | 2 |
M30 | Diagnostic endoscopic examination of ureter | 2 |
M31 | Extracorporeal fragmentation of calculus of ureter | 2 |
M32 | Operations on ureteric orifice | 3 |
M33 | Percutaneous ureteric stent procedures | 2 |
M34 | Total excision of bladder | 3 |
M35 | Partial excision of bladder | 3 |
M37 | Other repair of bladder | 3 |
M38 | Open drainage of bladder | 3 |
M39 | Other open operations on contents of bladder | 3 |
M41 | Other open operations on bladder | 3 |
M42 | Endoscopic extirpation of lesion of bladder | 2 |
M43 | Endoscopic operations to increase capacity of bladder | 2 |
M44 | Other therapeutic endoscopic operations on bladder | 2 |
M45 | Diagnostic endoscopic examination of bladder | 1 |
M47 | Urethral catheterisation of bladder | 1 |
M48 | Operations on bladder | 3 |
M49 | Other operations on bladder | 3 |
M52 | Abdominal operations to support outlet of female bladder | 3 |
M53 | Vaginal operations to support outlet of female bladder | 3 |
M56 | Therapeutic endoscopic operations on outlet of female bladder | 3 |
M58 | Other operations on outlet of female bladder | 3 |
M61 | Open excision of prostate | 3 |
M65 | Endoscopic resection of outlet of male bladder | 3 |
M66 | Other therapeutic endoscopic operations on outlet of male bladder | 3 |
M67 | Other therapeutic endoscopic operations on prostate | 3 |
M68 | Endoscopic insertion of prosthesis into prostate | 2 |
M70 | Other operations on outlet of male bladder | 3 |
M71 | Other operations on prostate | 3 |
M72 | Excision of urethra | 3 |
M73 | Repair of urethra | 3 |
M75 | Other open operations on urethra | 3 |
M76 | Therapeutic endoscopic operations on urethra | 2 |
M77 | Diagnostic endoscopic examination of urethra | 1 |
M79 | Other operations on urethra | 2 |
M81 | Operations on urethral orifice | 2 |
M83 | Other operations on urinary tract | 3 |
M85 | Diagnostic endoscopic examination of urinary diversion | 1 |
N01 | Extirpation of scrotum | 2 |
N03 | Other operations on scrotum | 2 |
N05 | Bilateral excision of testes | 2 |
N06 | Other excision of testis | 2 |
N07 | Extirpation of lesion of testis | 2 |
N08 | Bilateral placement of testes in scrotum | 2 |
N09 | Other placement of testis in scrotum | 2 |
N10 | Prosthesis of testis | 2 |
N11 | Operations on hydrocele sac | 1 |
N13 | Other operations on testis | 2 |
N15 | Operations on epididymis | 1 |
N17 | Excision of vas deferens | 1 |
N19 | Operations on varicocele | 1 |
N20 | Other operations on spermatic cord | 1 |
N22 | Operations on seminal vesicle | 1 |
N24 | Operations on male perineum | 2 |
N26 | Amputation of penis | 2 |
N27 | Extirpation of lesion of penis | 2 |
N28 | Plastic operations on penis | 2 |
N30 | Operations on prepuce | 1 |
N32 | Other operations on penis | 2 |
O02 | Transluminal balloon assisted coil embolisation of aneurysm of artery | 2 |
O03 | Transluminal stent assisted coil embolisation of aneurysm of artery | 2 |
O05 | Operations on dural arteriovenous fistula | 4 |
O06 | Hybrid prosthetic replacement of shoulder joint using cemented humeral component | 3 |
O07 | Hybrid prosthetic replacement of shoulder joint using cemented glenoid component | 3 |
O08 | Hybrid prosthetic replacement of shoulder joint using cement | 3 |
O11 | Other upper digestive tract | 2 |
O12 | Branch of external carotid artery | 3 |
O14 | Other lymph node | 2 |
O15 | Operations on blood vessel | 2 |
O17 | Secondary closed reduction of fracture of bone and internal fixation | 3 |
O18 | Hybrid prosthetic replacement of knee joint using cement | 4 |
O19 | Other therapeutic endoscopic operations on other joint structure | 2 |
O20 | Endovascular placement of stent graft | 3 |
O21 | Total prosthetic replacement of elbow joint using cement | 4 |
O22 | Total prosthetic replacement of elbow joint not using cement | 4 |
O23 | Total prosthetic replacement of elbow joint | 4 |
O24 | Prosthetic replacement of head of radius using cement | 3 |
O25 | Prosthetic replacement of head of radius not using cement | 3 |
O26 | Other prosthetic replacement of head of radius | 3 |
O27 | Other stabilising operations on joint | 3 |
O29 | Excision of bone | 3 |
P01 | Operations on clitoris | 1 |
P03 | Operations on Bartholin gland | 1 |
P05 | Excision of vulva | 3 |
P06 | Extirpation of lesion of vulva | 2 |
P09 | Other operations on vulva | 2 |
P11 | Extirpation of lesion of female perineum | 2 |
P13 | Other operations on female perineum | 2 |
P14 | Incision of introitus of vagina | 2 |
P15 | Other operations on introitus of vagina | 2 |
P17 | Excision of vagina | 3 |
P18 | Other obliteration of vagina | 3 |
P19 | Excision of band of vagina | 2 |
P20 | Extirpation of lesion of vagina | 2 |
P21 | Plastic operations on vagina | 2 |
P22 | Repair of prolapse of vagina and amputation of cervix uteri | 3 |
P23 | Other repair of prolapse of vagina | 3 |
P24 | Repair of vault of vagina | 3 |
P25 | Other repair of vagina | 3 |
P26 | Introduction of supporting pessary into vagina | 1 |
P27 | Exploration of vagina | 1 |
P29 | Other operations on vagina | 2 |
P31 | Operations on pouch of Douglas | 3 |
P32 | Other plastic operations on vagina | 2 |
Q01 | Excision of cervix uteri | 2 |
Q02 | Destruction of lesion of cervix uteri | 2 |
Q03 | Biopsy of cervix uteri | 2 |
Q05 | Other operations on cervix uteri | 2 |
Q07 | Abdominal excision of uterus | 3 |
Q08 | Vaginal excision of uterus | 3 |
Q09 | Other open operations on uterus | 3 |
Q10 | Curettage of uterus | 2 |
Q11 | Other evacuation of contents of uterus | 2 |
Q12 | Intrauterine contraceptive device | 1 |
Q14 | Introduction of abortifacient into uterine cavity | 2 |
Q15 | Introduction of other substance into uterine cavity | 2 |
Q16 | Other vaginal operations on uterus | 2 |
Q17 | Therapeutic endoscopic operations on uterus | 2 |
Q18 | Diagnostic endoscopic examination of uterus | 2 |
Q20 | Other operations on uterus | 2 |
Q22 | Bilateral excision of adnexa of uterus | 3 |
Q23 | Unilateral excision of adnexa of uterus | 3 |
Q24 | Other excision of adnexa of uterus | 3 |
Q25 | Partial excision of fallopian tube | 3 |
Q27 | Open bilateral occlusion of fallopian tubes | 2 |
Q28 | Other open occlusion of fallopian tube | 2 |
Q29 | Open reversal of female sterilisation | 3 |
Q30 | Other repair of fallopian tube | 3 |
Q31 | Incision of fallopian tube | 2 |
Q32 | Operations on fimbria | 2 |
Q34 | Other open operations on fallopian tube | 3 |
Q35 | Endoscopic bilateral occlusion of fallopian tubes | 2 |
Q38 | Other therapeutic endoscopic operations on fallopian tube | 2 |
Q39 | Diagnostic endoscopic examination of fallopian tube | 2 |
Q41 | Other operations on fallopian tube | 2 |
Q43 | Partial excision of ovary | 3 |
Q45 | Repair of ovary | 3 |
Q47 | Other open operations on ovary | 3 |
Q48 | Oocyte recovery | 2 |
Q49 | Therapeutic endoscopic operations on ovary | 2 |
Q50 | Diagnostic endoscopic examination of ovary | 2 |
Q51 | Other operations on ovary | 3 |
Q55 | Other examination of female genital tract | 1 |
R01 | Therapeutic endoscopic operations on fetus | 2 |
R05 | Diagnostic percutaneous examination of fetus | 2 |
R10 | Other operations on amniotic cavity | 2 |
R12 | Operations on gravid uterus | 2 |
R14 | Surgical induction of labour | 2 |
R17 | Elective caesarean delivery | 3 |
R18 | Other caesarean delivery | 3 |
R19 | Breech extraction delivery | 2 |
R20 | Other breech delivery | 2 |
R21 | Forceps cephalic delivery | 2 |
R22 | Vacuum delivery | 2 |
R23 | Cephalic vaginal delivery with abnormal presentation of head at delivery without instrument | 2 |
R24 | Normal delivery | 2 |
R27 | Other operations to facilitate delivery | 2 |
R28 | Instrumental removal of products of conception from delivered uterus | 2 |
R29 | Manual removal of products of conception from delivered uterus | 2 |
R30 | Other operations on delivered uterus | 2 |
R32 | Repair of obstetric laceration | 2 |
R37 | Non-routine obstetric scan for fetal observations | 1 |
R43 | Ultrasound monitoring | 1 |
S01 | Plastic excision of skin of head or neck | 1 |
S02 | Plastic excision of skin of abdominal wall | 1 |
S03 | Plastic excision of skin of other site | 1 |
S04 | Other excision of skin | 1 |
S05 | Microscopically controlled excision of lesion of skin | 1 |
S06 | Other excision of lesion of skin | 1 |
S08 | Curettage of lesion of skin | 1 |
S09 | Photodestruction of lesion of skin | 1 |
S10 | Other destruction of lesion of skin of head or neck | 1 |
S11 | Other destruction of lesion of skin of other site | 1 |
S13 | Punch biopsy of skin | 1 |
S14 | Shave biopsy of skin | 1 |
S15 | Other biopsy of skin | 1 |
S17 | Distant flap of skin and muscle | 3 |
S18 | Distant flap of skin and fascia | 3 |
S19 | Distant pedicle flap of skin | 3 |
S20 | Other distant flap of skin | 3 |
S21 | Hair-bearing flap of skin | 3 |
S23 | Flap operations to relax contracture of skin | 3 |
S24 | Local flap of skin and muscle | 3 |
S25 | Local flap of skin and fascia | 3 |
S26 | Local subcutaneous pedicle flap of skin | 3 |
S27 | Other local flap of skin | 3 |
S30 | Other operations on flap of skin to head or neck | 3 |
S31 | Other operations on flap of skin to other site | 3 |
S33 | Hair-bearing graft of skin to scalp | 2 |
S35 | Split autograft of skin | 2 |
S36 | Other autograft of skin | 2 |
S37 | Other graft of skin | 2 |
S38 | Graft of mucosa | 2 |
S39 | Graft of other tissue to skin | 2 |
S40 | Other closure of skin | 1 |
S41 | Suture of skin of head or neck | 1 |
S42 | Suture of skin of other site | 1 |
S43 | Removal of repair material from skin | 1 |
S44 | Removal of other inorganic substance from skin | 1 |
S45 | Removal of other substance from skin | 1 |
S47 | Opening of skin | 1 |
S48 | Insertion of skin expander into subcutaneous tissue | 1 |
S49 | Attention to skin expander in subcutaneous tissue | 1 |
S50 | Introduction of other inert substance into subcutaneous tissue | 1 |
S52 | Introduction of therapeutic substance into subcutaneous tissue | 1 |
S53 | Introduction of substance into skin | 1 |
S54 | Exploration of burnt skin of head or neck | 1 |
S55 | Exploration of burnt skin of other site | 1 |
S56 | Exploration of other skin of head or neck | 1 |
S57 | Exploration of other skin of other site | 1 |
S58 | Larvae therapy of skin | 1 |
S60 | Other operations on skin | 1 |
S62 | Other operations on subcutaneous tissue | 1 |
S64 | Extirpation of nail bed | 1 |
S66 | Other operations on nail bed | 1 |
S68 | Excision of nail | 1 |
S70 | Other operations on nail | 1 |
T01 | Partial excision of chest wall | 4 |
T03 | Opening of chest | 4 |
T05 | Other operations on chest wall | 4 |
T07 | Open excision of pleura | 4 |
T08 | Open drainage of pleural cavity | 3 |
T12 | Puncture of pleura | 2 |
T16 | Other repair of diaphragm | 4 |
T19 | Simple excision of inguinal hernial sac | 2 |
T20 | Primary repair of inguinal hernia | 2 |
T21 | Repair of recurrent inguinal hernia | 2 |
T22 | Primary repair of femoral hernia | 2 |
T23 | Repair of recurrent femoral hernia | 2 |
T24 | Primary repair of umbilical hernia | 2 |
T25 | Primary repair of incisional hernia | 2 |
T26 | Repair of recurrent incisional hernia | 2 |
T27 | Repair of other hernia of abdominal wall | 2 |
T28 | Other repair of anterior abdominal wall | 2 |
T29 | Operations on umbilicus | 2 |
T30 | Opening of abdomen | 3 |
T31 | Other operations on anterior abdominal wall | 2 |
T34 | Open drainage of peritoneum | 3 |
T36 | Operations on omentum | 3 |
T37 | Operations on mesentery of small intestine | 3 |
T38 | Operations on mesentery of colon | 3 |
T39 | Operations on posterior peritoneum | 3 |
T41 | Other open operations on peritoneum | 3 |
T42 | Therapeutic endoscopic operations on peritoneum | 2 |
T43 | Diagnostic endoscopic examination of peritoneum | 2 |
T45 | Image-controlled operations on abdominal cavity | 2 |
T46 | Other drainage of peritoneal cavity | 3 |
T48 | Other operations on peritoneum | 3 |
T50 | Transplantation of fascia | 2 |
T51 | Excision of fascia of abdomen | 2 |
T52 | Excision of other fascia | 2 |
T55 | Release of fascia | 2 |
T56 | Other excision of other fascia | 2 |
T57 | Other operations on fascia | 2 |
T59 | Excision of ganglion | 2 |
T60 | Re-excision of ganglion | 2 |
T62 | Operations on bursa | 2 |
T64 | Transposition of tendon | 2 |
T65 | Excision of tendon | 2 |
T67 | Primary repair of tendon | 2 |
T68 | Secondary repair of tendon | 2 |
T69 | Freeing of tendon | 2 |
T70 | Adjustment to length of tendon | 2 |
T71 | Excision of sheath of tendon | 2 |
T72 | Other operations on sheath of tendon | 2 |
T74 | Other operations on tendon | 2 |
T76 | Transplantation of muscle | 3 |
T77 | Excision of muscle | 2 |
T79 | Repair of muscle | 2 |
T80 | Release of contracture of muscle | 2 |
T81 | Biopsy of muscle | 2 |
T83 | Other operations on muscle | 2 |
T85 | Block dissection of lymph nodes | 2 |
T86 | Sampling of lymph nodes | 1 |
T87 | Excision or biopsy of lymph node | 1 |
T88 | Drainage of lesion of lymph node | 1 |
T90 | Contrast radiology of lymphatic tissue | 1 |
T91 | Operations on sentinel lymph node | 2 |
T94 | Operations on branchial cleft | 2 |
T96 | Other operations on soft tissue | 2 |
T97 | Repair of recurrent umbilical hernia | 2 |
U07 | Diagnostic imaging of chest | 1 |
U08 | Diagnostic imaging of abdomen | 1 |
U09 | Diagnostic imaging of pelvis | 1 |
U11 | Diagnostic imaging of vascular system | 1 |
U16 | Diagnostic imaging of hepatobiliary system | 1 |
U20 | Diagnostic echocardiography | 1 |
U21 | Diagnostic imaging procedures | 1 |
U24 | Diagnostic audiology | 1 |
V05 | Other operations on cranium | 4 |
V06 | Excision of maxilla | 3 |
V07 | Excision of bone of face | 3 |
V08 | Reduction of fracture of maxilla | 2 |
V09 | Reduction of fracture of other bone of face | 2 |
V10 | Division of bone of face | 2 |
V11 | Fixation of bone of face | 2 |
V13 | Other operations on bone of face | 2 |
V14 | Excision of mandible | 3 |
V15 | Reduction of fracture of mandible | 2 |
V16 | Division of mandible | 2 |
V17 | Fixation of mandible | 2 |
V19 | Other operations on mandible | 2 |
V21 | Other operations on temporomandibular joint | 2 |
V22 | Primary decompression operations on cervical spine | 3 |
V24 | Decompression operations on thoracic spine | 3 |
V25 | Primary decompression operations on lumbar spine | 3 |
V26 | Revisional decompression operations on lumbar spine | 3 |
V27 | Decompression operations on unspecified spine | 3 |
V28 | Insertion of lumbar interspinous process spacer | 3 |
V29 | Primary excision of cervical intervertebral disc | 3 |
V33 | Primary excision of lumbar intervertebral disc | 3 |
V34 | Revisional excision of lumbar intervertebral disc | 3 |
V35 | Excision of unspecified intervertebral disc | 3 |
V37 | Primary fusion of joint of cervical spine | 3 |
V38 | Primary fusion of other joint of spine | 3 |
V39 | Revisional fusion of joint of spine | 3 |
V40 | Stabilisation of spine | 3 |
V41 | Instrumental correction of deformity of spine | 3 |
V44 | Decompression of fracture of spine | 3 |
V46 | Fixation of fracture of spine | 3 |
V47 | Biopsy of spine | 1 |
V48 | Denervation of spinal facet joint of vertebra | 2 |
V49 | Exploration of spine | 2 |
V50 | Manipulation of spine | 2 |
V52 | Other operations on intervertebral disc | 3 |
V54 | Other operations on spine | 3 |
V55 | Levels of spine | 1 |
V60 | Primary percutaneous decompression using coblation to intervertebral disc | 2 |
V67 | Other primary decompression operations on lumbar spine | 2 |
V68 | Other revisional decompression operations on lumbar spine | 2 |
W01 | Complex reconstruction of thumb | 2 |
W02 | Other complex reconstruction of hand | 2 |
W03 | Complex reconstruction of forefoot | 3 |
W04 | Complex reconstruction of hindfoot | 3 |
W05 | Prosthetic replacement of bone | 4 |
W06 | Total excision of bone | 3 |
W07 | Excision of ectopic bone | 3 |
W08 | Other excision of bone | 3 |
W09 | Extirpation of lesion of bone | 3 |
W10 | Open surgical fracture of bone | 3 |
W12 | Angulation periarticular division of bone | 2 |
W13 | Other periarticular division of bone | 2 |
W14 | Diaphyseal division of bone | 2 |
W15 | Division of bone of foot | 2 |
W16 | Other division of bone | 2 |
W17 | Other reconstruction of bone | 3 |
W18 | Drainage of bone | 2 |
W19 | Primary open reduction of fracture of bone and intramedullary fixation | 2 |
W20 | Primary open reduction of fracture of bone and extramedullary fixation | 2 |
W21 | Primary open reduction of intra-articular fracture of bone | 2 |
W22 | Other primary open reduction of fracture of bone | 2 |
W23 | Secondary open reduction of fracture of bone | 2 |
W24 | Closed reduction of fracture of bone and internal fixation | 2 |
W25 | Closed reduction of fracture of bone and external fixation | 2 |
W26 | Other closed reduction of fracture of bone | 2 |
W27 | Fixation of epiphysis | 2 |
W28 | Other internal fixation of bone | 2 |
W29 | Skeletal traction of bone | 2 |
W30 | Other external fixation of bone | 2 |
W31 | Other autograft of bone | 3 |
W32 | Other graft of bone | 3 |
W33 | Other open operations on bone | 3 |
W35 | Therapeutic puncture of bone | 2 |
W36 | Diagnostic puncture of bone | 2 |
W37 | Total prosthetic replacement of hip joint using cement | 4 |
W38 | Total prosthetic replacement of hip joint not using cement | 4 |
W39 | Other total prosthetic replacement of hip joint | 4 |
W40 | Total prosthetic replacement of knee joint using cement | 4 |
W41 | Total prosthetic replacement of knee joint not using cement | 4 |
W42 | Other total prosthetic replacement of knee joint | 4 |
W43 | Total prosthetic replacement of other joint using cement | 4 |
W44 | Total prosthetic replacement of other joint not using cement | 4 |
W45 | Other total prosthetic replacement of other joint | 4 |
W46 | Prosthetic replacement of head of femur using cement | 3 |
W47 | Prosthetic replacement of head of femur not using cement | 3 |
W48 | Other prosthetic replacement of head of femur | 3 |
W49 | Prosthetic replacement of head of humerus using cement | 3 |
W50 | Prosthetic replacement of head of humerus not using cement | 3 |
W51 | Other prosthetic replacement of head of humerus | 3 |
W52 | Prosthetic replacement of articulation of other bone using cement | 3 |
W53 | Prosthetic replacement of articulation of other bone not using cement | 3 |
W54 | Other prosthetic replacement of articulation of other bone | 3 |
W55 | Prosthetic interposition reconstruction of joint | 3 |
W56 | Other interposition reconstruction of joint | 3 |
W57 | Excision reconstruction of joint | 3 |
W58 | Other reconstruction of joint | 3 |
W59 | Fusion of joint of toe | 3 |
W60 | Fusion of other joint and extra-articular bone graft | 3 |
W61 | Fusion of other joint and other articular bone graft | 3 |
W62 | Other primary fusion of other joint | 3 |
W63 | Revisional fusion of other joint | 3 |
W64 | Conversion to fusion of other joint | 3 |
W65 | Primary open reduction of traumatic dislocation of joint | 2 |
W66 | Primary closed reduction of traumatic dislocation of joint | 2 |
W67 | Secondary reduction of traumatic dislocation of joint | 2 |
W68 | Primary reduction of injury to growth plate | 2 |
W69 | Open operations on synovial membrane of joint | 2 |
W71 | Other open operations on intra-articular structure | 2 |
W72 | Prosthetic replacement of ligament | 2 |
W74 | Other reconstruction of ligament | 2 |
W75 | Other open repair of ligament | 2 |
W76 | Other operations on ligament | 2 |
W77 | Stabilising operations on joint | 2 |
W78 | Release of contracture of joint | 2 |
W79 | Soft tissue operations on joint of toe | 2 |
W80 | Debridement and irrigation of joint | 2 |
W81 | Other open operations on joint | 2 |
W82 | Therapeutic endoscopic operations on semilunar cartilage | 2 |
W83 | Therapeutic endoscopic operations on other articular cartilage | 2 |
W84 | Therapeutic endoscopic operations on other joint structure | 2 |
W85 | Therapeutic endoscopic operations on cavity of knee joint | 2 |
W86 | Therapeutic endoscopic operations on cavity of other joint | 2 |
W87 | Diagnostic endoscopic examination of knee joint | 2 |
W88 | Diagnostic endoscopic examination of other joint | 2 |
W89 | Other therapeutic endoscopic operations on other articular cartilage | 2 |
W90 | Puncture of joint | 2 |
W91 | Other manipulation of joint | 2 |
W92 | Other operations on joint | 2 |
W93 | Hybrid prosthetic replacement of hip joint using cemented acetabular component | 4 |
W94 | Hybrid prosthetic replacement of hip joint using cemented femoral component | 4 |
W95 | Hybrid prosthetic replacement of hip joint using cement | 4 |
W96 | Total prosthetic replacement of shoulder joint using cement | 4 |
W97 | Total prosthetic replacement of shoulder joint not using cement | 4 |
W98 | Total prosthetic replacement of shoulder joint | 4 |
X07 | Amputation of arm | 3 |
X08 | Amputation of hand | 3 |
X09 | Amputation of leg | 3 |
X10 | Amputation of foot | 3 |
X11 | Amputation of toe | 3 |
X12 | Operations on amputation stump | 3 |
X14 | Clearance of pelvis | 4 |
X20 | Correction of congenital deformity of forearm | 3 |
X21 | Correction of congenital deformity of hand | 3 |
X22 | Correction of congenital deformity of hip | 3 |
X23 | Correction of congenital deformity of leg | 3 |
X24 | Primary correction of congenital deformity of foot | 3 |
X25 | Other correction of congenital deformity of foot | 3 |
X27 | Correction of minor congenital deformity of foot | 3 |
X28 | Intermittent infusion of therapeutic substance | 1 |
X29 | Continuous Infusion of therapeutic substance | 1 |
X30 | Injection of therapeutic substance | 1 |
X32 | Exchange blood transfusion | 1 |
X33 | Other blood transfusion | 1 |
X35 | Other intravenous injection | 1 |
X36 | Blood withdrawal | 1 |
X37 | Intramuscular injection | 1 |
X38 | Subcutaneous injection | 1 |
X40 | Compensation for renal failure | 2 |
X41 | Placement of ambulatory apparatus for compensation for renal failure | 2 |
X42 | Placement of other apparatus for compensation for renal failure | 2 |
X45 | Donation of organ | 4 |
X46 | Donation of other tissue | 3 |
X48 | Immobilisation using plaster cast | 1 |
X49 | Other external support of limb | 1 |
X50 | External resuscitation | 4 |
X53 | Extirpation of unspecified organ | 4 |
X55 | Other operations on unspecified organ | 3 |
X56 | Intubation of trachea | 1 |
X59 | Anaesthetic without surgery | 1 |
X62 | Assessment | 1 |
Y02 | Placement of prosthesis in organ NOC | 2 |
Y03 | Attention to prosthesis in organ NOC | 2 |
Y05 | Excision of organ NOC | 4 |
Y06 | Excision of lesion of organ NOC | 3 |
Y07 | Obliteration of cavity of organ NOC | 3 |
Y08 | Laser therapy to organ NOC | 2 |
Y09 | Chemical destruction of organ NOC | 3 |
Y11 | Other destruction of organ NOC | 3 |
Y13 | Other destruction of lesion of organ NOC | 3 |
Y14 | Placement of stent in organ NOC | 2 |
Y15 | Attention to stent in organ NOC | 2 |
Y16 | Connection of organ NOC | 3 |
Y18 | Release of organ NOC | 2 |
Y20 | Biopsy of organ NOC | 2 |
Y22 | Drainage of organ NOC | 2 |
Y25 | Suture of organ NOC | 2 |
Y26 | Other repair of organ NOC | 3 |
Y27 | Graft to organ NOC | 3 |
Y29 | Removal of foreign body from organ NOC | 2 |
Y30 | Incision of organ NOC | 2 |
Y31 | Exploration of organ NOC | 2 |
Y32 | Re-exploration of organ NOC | 2 |
Y33 | Puncture of organ NOC | 2 |
Y35 | Introduction of removable radioactive material into organ NOC | 2 |
Y38 | Injection of therapeutic substance into organ NOC | 2 |
Y39 | Injection of other substance into organ NOC | 2 |
Y40 | Dilatation of organ NOC | 2 |
Y41 | Examination of organ NOC | 2 |
Y42 | Manipulation of organ NOC | 2 |
Y44 | Other methods of operation on organ NOC | 2 |
Y48 | Approach to spine through back | 2 |
Y49 | Approach through thoracic cavity | 4 |
Y50 | Approach through abdominal cavity | 3 |
Y51 | Approach to organ through artificial opening into gastrointestinal tract | 3 |
Y52 | Approach to organ through other opening | 2 |
Y53 | Approach to organ under image control | 2 |
Y58 | Harvest of skin for graft | 2 |
Y59 | Harvest of flap of skin and fascia | 3 |
Y60 | Other harvest of fascia | 2 |
Y61 | Harvest of flap of skin and muscle of trunk | 3 |
Y63 | Harvest of flap of muscle of trunk | 3 |
Y65 | Harvest of tendon | 2 |
Y66 | Harvest of bone | 3 |
Y67 | Harvest of other multiple tissue | 3 |
Y69 | Harvest of other tissue | 2 |
Y70 | Early operations NOC | 1 |
Y71 | Late operations NOC | 1 |
Y74 | Minimal access to thoracic cavity | 3 |
Y75 | Minimal access to abdominal cavity | 2 |
Y76 | Minimal access to other body cavity | 2 |
Y78 | Arteriotomy approach to organ under image control | 2 |
Y80 | General anaesthetic | 2 |
Y81 | Spinal anaesthetic | 1 |
Y82 | Local anaesthetic | 1 |
Y96 | In vitro fertilisation | 1 |
Y99 | Donor status | 1 |
Appendix 2 Variable relationships with acute kidney injury
Alanine transaminase
Alanine transaminase is a liver enzyme. It is often measured clinically to determine liver function. In the event of acute hepatocellular injury the ALT level rises. In cases of chronic liver damage and cirrhosis there may, however, be a reduced level of ALT as production of the enzyme is decreased. The relationship between ALT and AKI in this data set (Figure 25), suggest that only a low ALT level is associated with an increased risk of AKI.
FIGURE 25.
Relationship between ALT level and AKI.
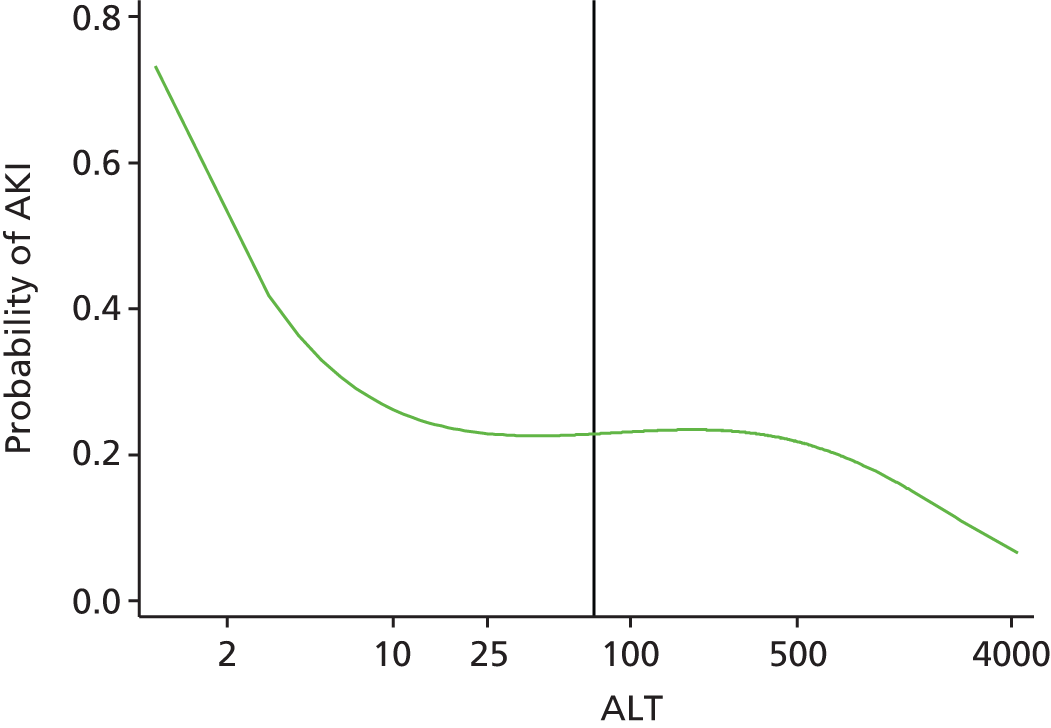
Normal range (laboratory) = 0–70 U/l.
Range determined as normal for risk modelling = ≤ 50 U/l.
Amylase
Amylase is an enzyme produced in the pancreas that aids the digestion of carbohydrates. A raised level of AMY can signify the presence of pancreatitis, which we believe may carry a risk of AKI. However, as shown in Figure 26, the relationship between AMY level and AKI in this data set suggests that only a low AMY level is associated with an increased risk of AKI.
FIGURE 26.
Relationship between AMY level and AKI.

Normal range (laboratory) = 0–125 U/l.
Range determined as normal for risk modelling = ≤ 125 U/l.
Brain natriuretic peptide
Brain natriuretic peptide is an amino acid produced by the cardiac myocytes when they are under strain, and, in this way, BNP is associated with heart failure. Heart failure may result in reduced perfusion of the kidneys and thus carry a risk of AKI. The relationship between BNP level and AKI in this data set (Figure 27) suggests that, above a BNP of 25 ng/l, with a rising BNP value there is an increasing risk of AKI.
FIGURE 27.
Relationship between BNP level and AKI.

Normal range (laboratory) = 0–99 ng/l.
Range determined as normal for risk modelling = ≤ 25 ng/l.
Corrected calcium
Corrected calcium is the calcium, a mineral in the blood, corrected for the albumin level in the blood. Raised calcium levels can lead to dehydration, which can result in AKI. Low calcium levels can signify acute disease, which may have a risk of AKI. The relationship between calcium level and AKI in this data set (Figure 28) suggests that both low calcium and high calcium levels signify an increased risk of AKI, and that the risk increases the further away the value is from the normal range.
FIGURE 28.
Relationship between calcium and AKI.
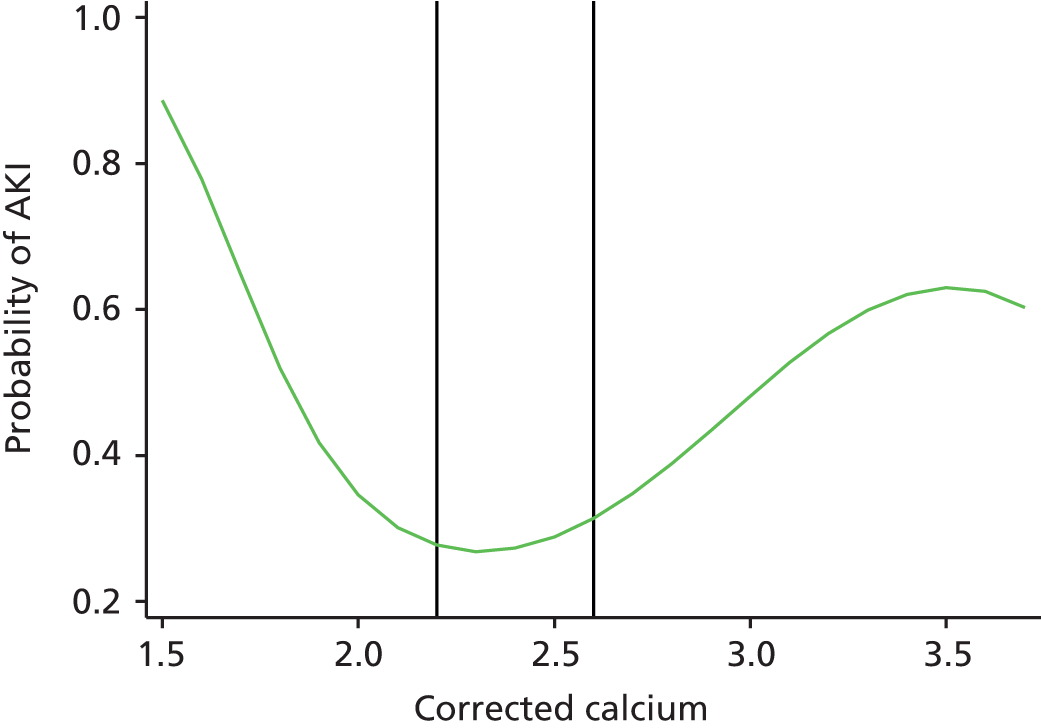
Normal range (laboratory) = 2.2–2.6 mmol/l.
Range determined as normal for risk modelling = 2.1–2.6 mmol/l inclusive.
C-reactive protein
C-reactive protein is a marker of infection or inflammation. Infection and, importantly, sepsis carry a significant risk of AKI. The higher the CRP level the greater the severity of the infection and, it would be expected, the higher the risk of AKI. The relationship between CRP level and AKI in this data set (Figure 29) suggests that the risk of AKI increases with rising CRP levels.
FIGURE 29.
Relationship between CRP level and AKI.

Normal range (laboratory) = ≤ 10 mg/l.
Range determined as normal for risk modelling = ≤ 10 mg/l.
Haemoglobin
Haemoglobin is the iron-containing oxygen transport metalloprotein in red blood cells. A low Hb level is a marker of acute or chronic disease, and we would therefore expect it to be associated with AKI. The relationship between Hb level and AKI in this data set (Figure 30) suggests that both low and high Hb levels signify an increased risk of AKI and that risk increases the further away the value is from the normal range.
FIGURE 30.
Relationship between Hb and AKI.
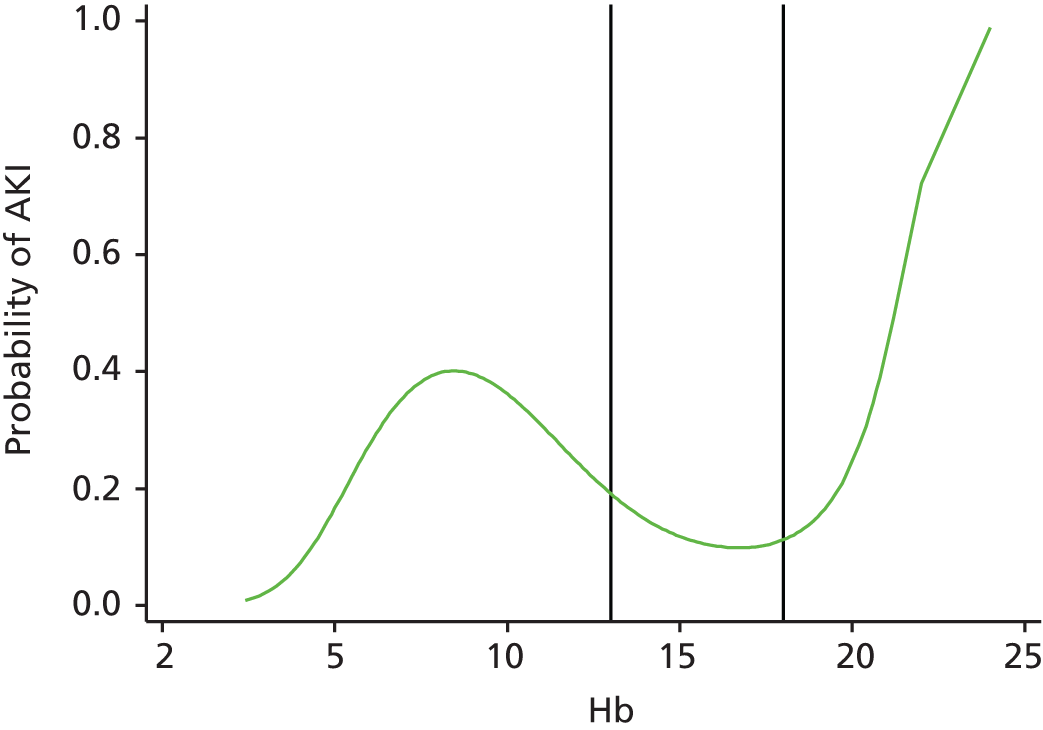
Normal range (laboratory) = 11–15 g/l (women); 13–18 g/l (men).
Range determined as normal for risk modelling = 11–15 g/l inclusive if female, 13–18 g/l inclusive if male.
Glycated haemoglobin
Glycated haemoglobin gives an average of blood sugar readings over the last 120 days. A value of > 6.5% indicates a patient with diabetes. Patients with diabetes have an increased risk of AKI. A value of > 7.5% indicates that the diabetes is not well controlled; it would be expected that the higher the value, the worse the diabetic control and, therefore, the higher the risk of AKI. The relationship between HbA1c and AKI in this data set (Figure 31) suggests that having HbA1c tested (as probably defining diabetes) indicates an increased risk of AKI. This risk increases with rising HbA1c up to a value of approximately 12%, at which point the risk starts to decrease.
FIGURE 31.
Relationship between HbA1c and AKI.
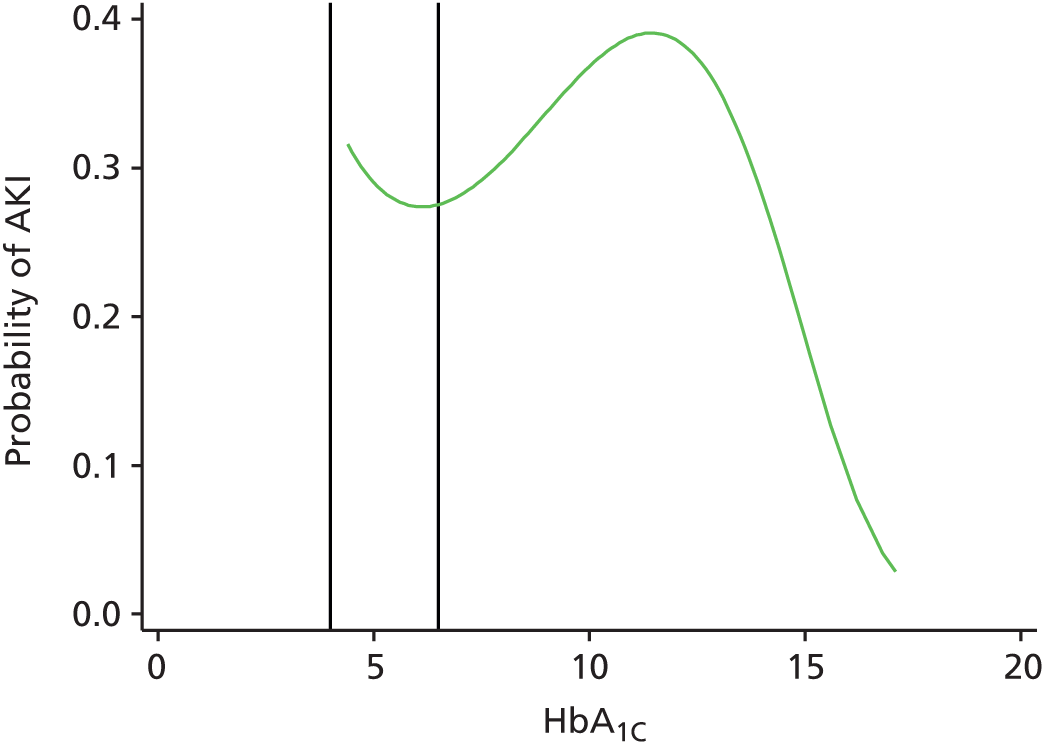
Normal range (laboratory) = 4–6.5% (Diabetes Control and Complications Trial).*
Range determined as normal for risk modelling = ≤ 7.5% (Diabetes Control and Complications Trial116).*
*The International Federation of Clinical Chemistry (www.ifcc.org) recommended standardisation of HbA1c following extraction of this data set.
Potassium
Potassium is an electrolyte that is essential for the normal functioning of cells, importantly cardiac cells. Maintaining the gradient across the cellular membrane is essential, and changes in this can lead to cardiac arrhythmias. K level itself in blood would not be expected to have a causal relationship with the development of AKI, but may be a reflection of acute illness and changes in electrolyte and fluid balance. The relationship between K level and AKI in this data set (Figure 32), suggests that both a low and a high K level indicates an increased risk of AKI and that risk increases the further away the value is from the normal range.
FIGURE 32.
Relationship between K and AKI.
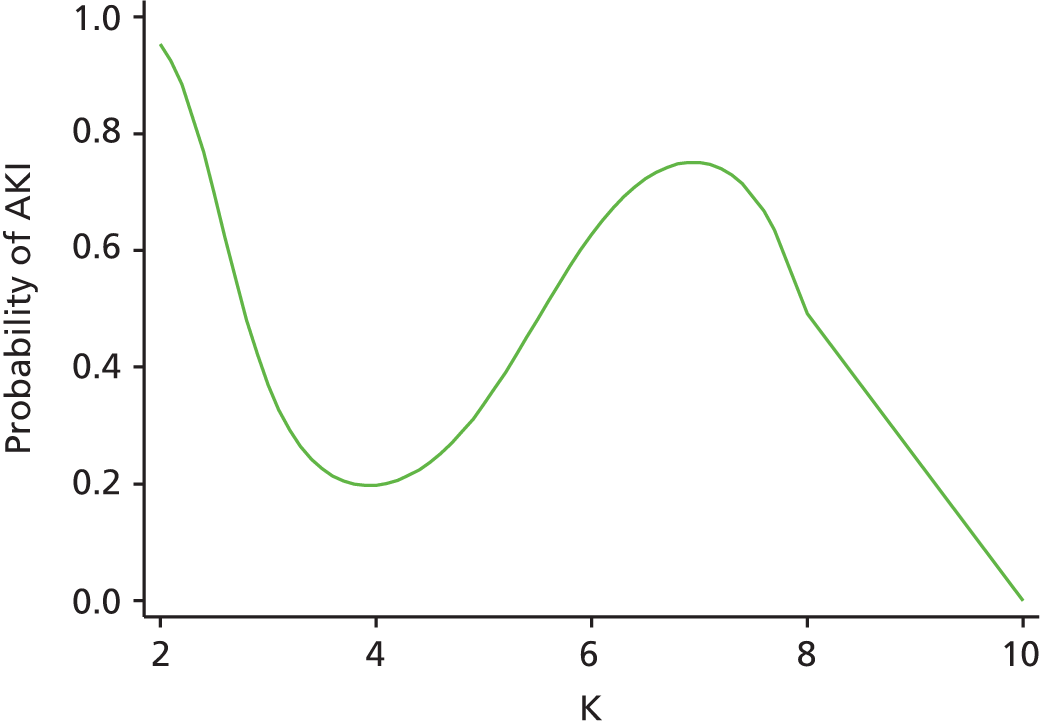
Normal range (laboratory) = 3.5–5.3 mmol/l.
Range determined as normal for risk modelling = 3.5–5.3 mmol/l, inclusive.
Magnesium
Magnesium is an electrolyte that is essential for the normal functioning of cells. The magnesium (Mg) level itself in blood would not be expected to have a causal relationship with the development of AKI, but may be a reflection of acute illness and changes in electrolyte and fluid balance. The relationship between Mg level and AKI in this data set (Figure 33), suggests that both a low and a high Mg level indicates an increased risk of AKI and that risk increases the further away the value is from the normal range.
FIGURE 33.
Relationship between Mg and AKI.

Normal range (laboratory) = 0.70–1.05 mmol/l.
Range determined as normal for risk modelling = 0.7–1.0 mmol/l, inclusive.
Sodium
Sodium is an electrolyte that is essential for the normal functioning of cells. The Na level itself in blood would not be expected to have a causal relationship with the development of AKI, but may be a reflection of acute illness and changes in electrolyte and fluid balance. A low Na level may also signify diuretic medication use. The relationship between Na and AKI in this data set (Figure 34) suggests that both low and high Na levels indicate an increased risk of AKI, and that risk increases the further away the value is from the normal range.
FIGURE 34.
Relationship between NA and AKI.
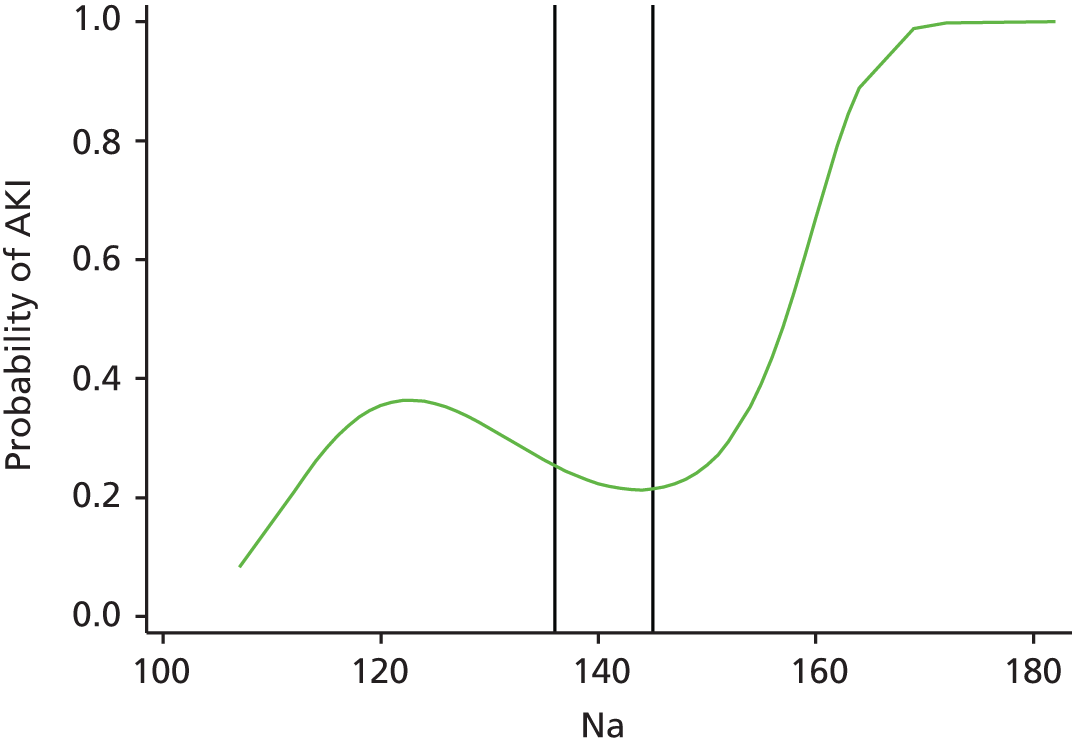
Normal range (laboratory) = 136–145 mmol/l.
Range determined as normal for risk modelling = 136–145 mmol/l, inclusive.
Platelet count
Platelet count is a measure of the number of platelets in the blood. Platelets are essential for the clotting of the blood. The platelet level itself in blood would not be thought to have a causal relationship with the development of AKI. However, a low PLT may be related to haematological disease or acute illness, and a high PLT may also signify acute illness, specifically inflammation/infection. The relationship between PLT and AKI in this data set (Figure 35) suggests that both a low and a high PCT indicates an increased risk of AKI, and that risk increases the further away the value is from the normal range.
FIGURE 35.
Relationship between PLT and AKI.

Normal range (laboratory) = 150–400 × 109/l.
Range determined as normal for risk modelling = 150– 400 × 109/l, inclusive.
White blood cell count
White blood cell count is a direct marker of infection. A low (< 4 × 109/l) or high (> 11 × 109/l) WBC can signify infection, and an infection brings a risk of AKI. The relationship between WBC and AKI in this data set (Figure 36) suggests that both a low and a high WBC indicates an increased risk of AKI, and that risk increases the further away the value is from the normal range.
FIGURE 36.
Relationship between WBC and AKI.

Normal range (laboratory) = 4–11 × 109/l.
Range determined as normal for risk modelling = 1–11 × 109/l, inclusive.
Creatinine kinase
Creatinine kinase is a product of muscle breakdown. When excess muscle breakdown occurs, high levels of CK can cause damage to the kidneys and result in AKI. It would therefore be expected that the greater the CK the greater the risk of AKI. However, the relationship between creatinine kinase level and AKI in this data set (Figure 37) suggests that the fact that CK has been tested defines a patient with a higher risk of AKI; however, lower levels of CK seem to be related to higher risk of AKI.
FIGURE 37.
Relationship between CK and AKI.

Normal range (laboratory) = 0–142 U/l.
Range determined as normal for risk modelling = ≤ 1000 U/l.
Appendix 3 Quantitative univariable analysis results
Variable | Category/term | Odds ratio (95% CI) | p-value |
---|---|---|---|
Agea | Linear term | 0.85 (0.51 to 1.41) | < 0.001 |
Quadratic term | 1.08 (0.99 to 1.18) | ||
Cubic term | 0.996 (0.991 to 1.000) | ||
Sex | Male | 1 | < 0.001 |
Female | 0.78 (0.73 to 0.84) | ||
Admissions (last 30 days) | No | 1 | 0.002 |
Yes | 1.13 (1.05 to 1.21) | ||
Admissions (last 2–12 months) | 0 | 1 | < 0.001 |
1–2 | 1.64 (1.52 to 1.77) | ||
3–5 | 2.24 (2.03 to 2.46) | ||
6+ | 2.80 (2.43 to 3.22) | ||
Outpatients appointments (last 12 months) | 0 | 1 | < 0.001 |
1–2 | 1.10 (1.01 to 1.20) | ||
3–5 | 1.29 (1.17 to 1.41) | ||
6+ | 1.67 (1.52 to 1.84) | ||
Primary diagnosis | Neoplasms | 1 | < 0.001 |
Infectious diseases | 1.47 (1.13 to 1.90) | ||
Blood diseases | 0.62 (0.43 to 0.87) | ||
Circulatory system | 0.71 (0.59 to 0.86) | ||
Digestive system | 0.66 (0.55 to 0.80) | ||
Diseases of head/neck | 0.19 (0.09 to 0.40) | ||
Genitourinary system | 1.57 (1.30 to 1.91) | ||
Musculoskeletal | 0.33 (0.26 to 0.41) | ||
Nervous system | 0.44 (0.33 to 0.60) | ||
Respiratory system | 1.02 (0.85 to 1.23) | ||
Skin | 0.84 (0.65 to 1.08) | ||
Endocrine/metabolic | 1.74 (1.47 to 2.23) | ||
Injury/poisoning | 0.47 (0.38 to 0.57) | ||
Mental disorders | 0.59 (0.42 to 0.83) | ||
Symptoms/signs | 0.44 (0.37 to 0.53) | ||
Other | 0.35 (0.16 to 0.73) | ||
Calcium | Not measured | 1 | < 0.001 |
Normal (2.2–2.6 mmol/l) | 1.24 (1.13 to 1.36) | ||
Abnormal | 1.89 (1.34 to 2.66) | ||
CRP | Not measured | 1 | < 0.001 |
Normal (≤ 10 mg/l) | 0.86 (0.78 to 0.96) | ||
Abnormal | 1.49 (1.36 to 1.62) | ||
Hb | Not measured | 1 | < 0.001 |
Normal (female: 11–15 g/dl)b | 0.77 (0.71 to 0.84) | ||
Abnormal | 1.76 (1.62 to 1.91) | ||
HbA1c (12-month average) | Not measured | 1 | < 0.001 |
Normal (≤ 7.5%) | 1.43 (1.31 to 1.56) | ||
Abnormal | 1.93 (1.71 to 2.17) | ||
K | Not measured | 1 | < 0.001 |
Normal (3.5–5.3 mmol/l) | 1.17 (1.09 to 1.25) | ||
Abnormal | 1.79 (1.52 to 2.10) | ||
Na | Not measured | 1 | < 0.001 |
Normal (136–145) | 1.11 (1.04 to 1.20) | ||
Abnormal | 1.50 (1.36 to 1.65) | ||
K/Na combined | Not measured | 1 | < 0.001 |
Both normal | 1.07 (1.00 to 1.16) | ||
Na only abnormal | 1.44 (1.31 to 1.60) | ||
K only abnormal | 1.66 (1.35 to 2.05) | ||
Both abnormal | 1.96 (1.50 to 2.55) | ||
PLT | Not measured | 1 | < 0.001 |
Normal (150–400 × 109/l) | 1.08 (1.01 to 1.16) | ||
Abnormal | 1.44 (1.28 to 1.62) | ||
Troponin | 0 | 1 | < 0.001 |
1 | 2.33 (2.07 to 2.62) | ||
2+ | 3.38 (2.72 to 4.19) | ||
WBC count | Not measured | 1 | < 0.001 |
Normal (1–11 × 109/l) | 1.13 (1.05 to 1.21) | ||
Abnormal | 1.26 (1.12 to 1.41) | ||
Blood culture | Not taken | 1 | < 0.001 |
Not significant | 1.95 (1.70 to 2.22) | ||
Significant | 4.67 (3.61 to 6.04) | ||
Charlson score | ≤ 0 | 1 | < 0.001 |
1–10 | 1.55 (1.40 to 1.72) | ||
11+ | 3.11 (2.84 to 3.41) | ||
Not recorded | 1.04 (0.91 to 1.19) | ||
Baseline eGFRc | Linear term | 0.63 (0.43 to 0.94) | < 0.001 |
Quadratic term | 0.93 (0.84 to 1.03) | ||
Cubic term | 1.01 (1.00 to 1.02) | ||
Drugs takend | 0 | 1 | < 0.001 |
1 | 1.30 (1.18 to 1.43) | ||
2 or 3 | 1.93 (1.71 to 2.18) | ||
Not recorded | 0.62 (0.57 to 0.67) | ||
Faeces | Not taken | 1 | < 0.001 |
Taken | 1.74 (1.32 to 2.30) | ||
MSU or CSU | Not taken | 1 | < 0.001 |
Not significant | 1.20 (0.95 to 1.52) | ||
Significant | 1.75 (1.54 to 2.00) | ||
Proteinuria (worst result) | Not done | 1 | < 0.001 |
1 | 1.39 (1.27 to 1.53) | ||
2 or 3 | 2.66 (2.42 to 2.91) | ||
Sputum | Not taken | 1 | 0.90 |
Taken | 1.02 (0.71 to 1.48) | ||
Wound swab/fluid aspirate | Not taken | 1 | 0.07 |
Not significant | 0.62 (0.39 to 0.99) | ||
Significant | 1.14 (0.91 to 1.44) |
Variable | Category/term | Odds ratio (95% CI) | p-value |
---|---|---|---|
Agea | Linear term | 1.28 (1.21 to 1.35) | < 0.001 |
Sex | Male | 1 | 0.27 |
Female | 0.91 (0.78 to 1.07) | ||
Admissions (last 30 days) | No | 1 | 0.56 |
Yes | 0.95 (0.79 to 1.14) | ||
Admissions (last 2–12 months) | 0 | 1 | < 0.001 |
1–2 | 1.31 (1.09 to 1.58) | ||
3–5 | 1.55 (1.22 to 1.97) | ||
6+ | 1.88 (1.37 to 2.58) | ||
Outpatients appointment (last 12 months) | 0 | 1 | 0.07 |
1–2 | 1.03 (0.84 to 1.28) | ||
3–5 | 1.25 (1.00 to 1.55) | ||
6+ | 1.28 (1.02 to 1.62) | ||
Primary diagnosis | Neoplasms | 1 | < 0.001 |
Infectious diseases | 1.80 (1.08 to 3.00) | ||
Blood diseases | 0.63 (0.28 to 1.43) | ||
Circulatory system | 1.09 (0.73 to 1.62) | ||
Digestive system | 0.71 (0.47 to 1.08) | ||
Genitourinary system | 1.54 (0.98 to 2.42) | ||
Musculoskeletal | 0.74 (0.37 to 1.47) | ||
Nervous system | 0.53 (0.23 to 1.22) | ||
Respiratory system | 0.78 (0.52 to 1.19) | ||
Skin | 0.89 (0.47 to 1.69) | ||
Endocrine/metabolic | 0.70 (0.34 to 1.43) | ||
Injury/poisoning | 0.97 (0.64 to 1.48) | ||
Mental disorders | 0.14 (0.02 to 1.04) | ||
Symptoms/signs | 0.72 (0.46 to 1.14) | ||
Other | 1.02 (0.29 to 3.65) | ||
ALT | Not measured | 1 | 0.84 |
Normal (≤ 50 U/l) | 1.06 (0.86 to 1.32) | ||
Abnormal | 1.08 (0.79 to 1.48) | ||
AMY | Not measured | 1 | 0.55 |
Normal (≤ 125 U/l) | 0.90 (0.73 to 1.10) | ||
Abnormal | 1.05 (0.62 to 1.79) | ||
BNP (12-month average) | Not measured | 1 | 0.49 |
Measured | 1.19 (0.73 to 1.96) | ||
Calcium | Not measured | 1 | 0.45 |
Normal (2.2–2.6 mmol/l) | 1.09 (0.92 to1.30) | ||
Abnormal | 1.24 (0.75 to 2.03) | ||
CRP | Not measured | 1 | < 0.001 |
Normal (≤ 10 mg/l) | 0.81 (0.59 to 1.10) | ||
Abnormal | 1.16 (0.87 to 1.53) | ||
Hb | Not measured | 1 | < 0.001 |
Normal (female: 11–15 g/dl)b | 0.65 (0.41 to 1.01) | ||
Abnormal | 0.94 (0.60 to 1.47) | ||
HbA1c (12-month average) | Not measured | 1 | < 0.001 |
Normal (≤ 7.5%) | 1.51 (1.24 to 1.85) | ||
Abnormal | 1.52 (1.13 to 2.02) | ||
K | Not measured | 1 | 0.003 |
Normal (3.5–5.3 mmol/l) | 0.67 (0.50 to 0.91) | ||
Abnormal | 0.94 (0.65 to 1.37) | ||
Mg | Not measured | 1 | 0.008 |
Normal (0.7–1.0 mmol/l) | 0.87 (0.66 to 1.15) | ||
Abnormal | 1.72 (1.19 to 2.48) | ||
Na | Not measured/normal | 1 | 0.46 |
Abnormal | 1.06 (0.90 to 1.25) | ||
PLT | Not measured/normal | 1 | 0.10 |
Abnormal | 1.17 (0.97 to 1.41) | ||
Troponin | 0 | 1 | < 0.001 |
1 | 1.35 (1.10 to 1.66) | ||
2+ | 2.33 (1.86 to 2.93) | ||
WBC | Not measured/normal | 1 | 0.03 |
Abnormal | 1.20 (1.02 to 1.40) | ||
CK | Not measured | 1 | 0.67 |
Normal (≤ 1000 U/l) | 0.96 (0.63 to 1.47) | ||
Abnormal | 1.35 (0.68 to 2.67) | ||
Blood culture | Not taken | 1 | 0.02 |
Taken | 1.28 (1.03 to 1.59) | ||
Charlson score | ≤ 0 | 1 | < 0.001 |
1–10 | 1.45 (1.12 to 1.87) | ||
11+ | 2.33 (1.86 to 2.93) | ||
Not recorded | 1.39 (1.03 to 1.88) | ||
Number of contrast radiology scans | 0 | 1 | 0.82 |
1+ | 1.03 (0.78 to 1.37) | ||
Baseline eGFRc | Linear term | 0.54 (0.40 to 0.74) | < 0.001 |
Quadratic term | 1.03 (1.00 to 1.08) | ||
Drugs takend | 0 | 1 | < 0.001 |
1 | 1.25 (1.00 to 1.58) | ||
2 or 3 | 1.92 (1.42 to 2.59) | ||
Not recorded | 0.85 (0.71 to 1.03) | ||
Faeces (pre admission) | Not taken | 1 | 0.30 |
Taken | 1.36 (0.76 to 2.43) | ||
Faeces (admission) | Not taken | 1 | 0.42 |
Taken | 0.78 (0.43 to 1.42) | ||
MSU or CSU (pre admission) | Not taken | 1 | 0.07 |
Not significant | 0.63 (0.29 to 1.36) | ||
Significant | 1.40 (1.00 to 1.96) | ||
MSU or CSU (admission) | Not taken | 1 | 0.001 |
Taken | 1.43 (1.15 to 1.78) | ||
Operation score at 12 hours | 0 | 1 | 0.13 |
Score 1–2 | 0.99 (0.68 to 1.44) | ||
Score 3–4 | 1.47 (1.01 to 2.13) | ||
Proteinuria (worst result) | Not done | 1 | < 0.001 |
1 | 1.27 (1.01 to 1.60) | ||
2 or 3 | 2.56 (2.08 to 3.15) | ||
Sputum (pre admission) | Not taken | 1 | 0.73 |
Taken | 1.14 (0.54 to 2.42) | ||
Sputum (admission) | Not taken | 1 | 0.12 |
Taken | 0.54 (0.24 to 1.17) | ||
Wound swab/fluid aspirate (pre admission) | Not taken | 1 | 0.42 |
Taken | 0.81 (0.48 to 1.36) | ||
Wound swab/fluid aspirate (admission) | Not taken | 1 | 0.55 |
Taken | 1.11 (0.80 to 1.53) |
Variable | Category/term | Odds ratio (95% CI) | p-value |
---|---|---|---|
Agea | Linear term | 0.94 (0.86 to 1.04) | 0.26 |
Sex | Male | 1 | 0.32 |
Female | 1.18 (0.86 to 1.61) | ||
Admissions (last 30 days) | No | 1 | 0.53 |
Yes | 0.89 (0.61 to 1.129) | ||
Admissions (last 2–12 months) | 0 | 1 | 0.92 |
1–2 | 0.89 (0.61 to 1.29) | ||
3–5 | 0.88 (0.56 to 1.38) | ||
6+ | 0.98 (0.54 to 1.78) | ||
Outpatients appointment (last 12 months) | 0 | 1 | 0.42 |
1–2 | 0.86 (0.57 to 1.33) | ||
3–5 | 0.69 (0.42 to 1.11) | ||
6+ | 1.00 (0.66 to 1.52) | ||
Primary diagnosis | Neoplasms | 1 | < 0.001 |
Infectious diseases | 6.39 (1.88 to 21.7) | ||
Circulatory system | 1.90 (0.63 to 5.69) | ||
Digestive system | 1.46 (0.47 to 4.57) | ||
Genitourinary system | 2.60 (0.85 to 7.94) | ||
Musculoskeletal | 1.67 (0.27 to 10.3) | ||
Nervous system | 0.82 (0.08 to 8.08) | ||
Respiratory system | 1.46 (0.48 to 4.42) | ||
Skin | 1.15 (0.27 to 4.99) | ||
Endocrine/metabolic | 1.06 (0.28 to 4.02) | ||
Injury/poisoning | 2.70 (0.84 to 8.66) | ||
Symptoms/signs | 0.92 (0.26 to 3.29) | ||
Other | 0.43 (0.04 to 4.12) | ||
ALT | Not measured | 1 | 0.07 |
Normal (≤ 50 U/l) | 1.05 (0.69 to 1.63) | ||
Abnormal | 1.73 (0.98 to 3.05) | ||
AMY | Not measured | 1 | 0.24 |
Measured | 1.25 (0.86 to 1.82) | ||
BNP (12-month average) | Not measured | 1 | 0.86 |
Measured | 1.09 (0.45 to 2.62) | ||
Calcium | Not measured | 1 | 0.92 |
Normal (2.2–2.6 mmol/l) | 0.93 (0.66 to 1.32) | ||
Abnormal | 1.01 (0.47 to 2.19) | ||
CRP | Not measured | 1 | 0.05 |
Normal (≤ 10 mg/l) | 0.80 (0.32 to 1.96) | ||
Abnormal | 1.40 (0.62 to 3.17) | ||
Hb | Not measured | 1 | 0.73 |
Normal (female: 11–15 g/dl)b | 1.02 (0.38 to 2.70) | ||
Abnormal | 0.89 (0.34 to 2.37) | ||
HbA1c (12-month average) | Not measured | 1 | 0.85 |
Normal (≤ 7.5%) | 1.06 (0.72 to 1.58) | ||
Abnormal | 1.14 (0.71 to 1.83) | ||
K | Not measured | 1 | 0.76 |
Normal (3.5–5.3 mmol/l) | 0.89 (0.43 to 1.86) | ||
Abnormal | 1.03 (0.47 to 2.27) | ||
Mg | Not measured | 1 | < 0.001 |
Normal (0.7–1.0 mmol/l) | 1.29 (0.80 to 2.11) | ||
Abnormal | 2.92 (1.71 to 4.98) | ||
Na | Not measured/normal | 1 | 0.55 |
Abnormal | 1.10 (0.80 to 1.52) | ||
PLT | Not measured/normal | 1 | 0.05 |
Abnormal | 1.43 (1.00 to 2.05) | ||
Troponin | 0 | 1 | 0.66 |
1 | 0.90 (0.58 to 1.39) | ||
2+ | 1.16 (0.75 to 1.78) | ||
WBC | Not measured/Normal | 1 | 0.02 |
Abnormal | 1.51 (1.08 to 2.11) | ||
CK | Not measured | 1 | 0.008 |
Normal (≤ 1000 U/l) | 1.49 (0.73 to 3.04) | ||
Abnormal | 4.90 (1.73 to 13.9) | ||
Blood culture | Not taken | 1 | 0.04 |
Taken | 1.47 (1.03 to 2.12) | ||
Charlson score | ≤ 0 | 1 | 0.44 |
1–10 | 1.40 (0.82 to 2.39) | ||
11+ | 1.10 (0.68 to 1.78) | ||
Not recorded | 1.45 (0.76 to 2.79) | ||
Number of contrast radiology scans | 0 | 1 | 0.05 |
1+ | 1.77 (1.01 to 3.09) | ||
Baseline eGFRc | Linear term | 1.18 (1.040 to 1.32) | 0.007 |
Drugs takend | 0 | 1 | 0.23 |
1 | 1.09 (0.69 to 1.72) | ||
2 or 3 | 1.53 (0.90 to 2.61) | ||
Not recorded | 1.41 (0.96 to 2.07) | ||
Faeces (pre admission) | Not taken | 1 | 0.69 |
Taken | 1.24 (0.42 to 3.66) | ||
Faeces (admission) | Not taken | 1 | 0.22 |
Taken | 1.57 (0.78 to 3.20) | ||
MSU or CSU (pre admission) | Not taken | 1 | 0.50 |
Taken | 1.20 (0.71 to 2.04) | ||
MSU or CSU (admission) | Not taken | 1 | 0.20 |
Taken | 1.28 (0.88 to 1.85) | ||
Operation score at 12 hours | 0 | 1 | < 0.001 |
Score 1–2 | 1.55 (0.68 to 3.52) | ||
Score 3–4 | 4.65 (2.24 to 9.67) | ||
Proteinuria (worst result) | Not done | 1 | 0.84 |
1 | 1.00 (0.65 to 1.55) | ||
2 or 3 | 1.12 (0.76 to 1.65) | ||
Sputum (pre admission) | Not taken | 1 | 0.68 |
Taken | 0.65 (0.08 to 5.17) | ||
Sputum (admission) | Not taken | 1 | 0.02 |
Taken | 2.95 (1.17 to 7.42) | ||
Wound swab/fluid aspirate (pre admission) | Not taken | 1 | 0.10 |
Taken | 1.95 (0.87 to 4.34) | ||
Wound swab/fluid aspirate (admission) | Not taken | 1 | < 0.001 |
Taken | 3.16 (1.94 to 5.13) |
Appendix 4 Qualitative analysis documentation
Data analysis framework
Introducing the AKI alert system | |
---|---|
Analytical construct | Quote and code |
Coming to know about the AKI alert system | |
Introduction to the system, how this happened | |
Preparation for use, training | |
Length of time system used | |
How introduction could be improved | |
Other | |
Using the technology | |
General experiences of using the technology | |
Accessibility | |
Ease of navigation | |
Visual impact | |
Opinions of information on the system | |
Ease of identifying cohort | |
Relevance of information | |
Accuracy | |
Sufficiently up to date | |
How information is used | |
Accessing information | |
Communicating information | |
Monitoring behaviour | |
Influencing treatments/interventions | |
Communicating to clinician looking after the patient | |
Strengths of technology | |
Weaknesses of technology | |
Improvements to technology | |
Other | |
Impacts on clinical practice and patients: consultants | |
Changes to clinical practice from the AKI alert system | |
Positive changes | |
Negative changes | |
Communicating to teams | |
Team’s understanding of AKI | |
Difference to patient care | |
Positive differences | |
Negative differences | |
Added value, cost-effectiveness | |
Other | |
Impacts on clinical practice and patients: nurses | |
Changes to clinical practice from the AKI alert system | |
Positive changes | |
Negative changes | |
Assessment behaviour | |
Explanation of disparities between areas | |
Working with medical teams | |
Communication issues | |
Reactions to requests | |
Response to referrals | |
Other | |
Comments/suggestions for improvement/other | |
Appendix 5 Consultant interview schedule
Appendix 6 Outreach nurse focus group schedule
List of abbreviations
- A&E
- accident and emergency
- ACKD
- acute-on-chronic kidney disease
- AKD
- acute kidney diseases and disorders
- AKI
- acute kidney injury
- AKIN
- Acute Kidney Injury Network
- ALT
- alanine transaminase
- AMY
- amylase
- ATN
- acute tubular necrosis
- AUC
- area under the curve
- AUROC
- area under the receiver operating characteristic
- BNP
- B-type natriuretic peptide
- CDSS
- clinical decision support system
- CI
- confidence interval
- CK
- creatinine kinase
- CKD
- chronic kidney disease
- CRP
- C-reactive protein
- CSU
- catheter specimen urine
- eGFR
- estimated glomerular filtration rate
- EKHUFT
- East Kent Hospitals University NHS Foundation Trust
- ESRD
- end-stage renal disease
- GFR
- glomerular filtration rate
- Hb
- haemoglobin
- HbA1c
- glycated haemoglobin
- ICD-10
- International Classification of Diseases, Tenth Edition
- ICU
- intensive care unit
- IT
- information technology
- ITU
- intensive therapy unit
- KDIGO
- Kidney Disease: Improving Global Outcomes
- MDRD
- modification of diet in renal disease
- MSU
- mid-stream specimen urine
- NCEPOD
- National Confidential Enquiry into Patient Outcome and Death
- NICE
- National Institute for Health and Care Excellence
- PLT
- platelet count
- RIFLE
- Risk, Injury, Failure, Loss, and End-stage renal failure classification
- ROC
- receiver operating characteristic
- RRT
- renal replacement therapy
- SSVS
- stochastic search variable selection
- WBC
- white blood cell count