Notes
Article history
The research reported in this issue of the journal was funded by the HS&DR programme or one of its preceding programmes as project number 09/1801/1054. The contractual start date was in September 2010. The final report began editorial review in March 2016 and was accepted for publication in March 2017. The authors have been wholly responsible for all data collection, analysis and interpretation, and for writing up their work. The HS&DR editors and production house have tried to ensure the accuracy of the authors’ report and would like to thank the reviewers for their constructive comments on the final report document. However, they do not accept liability for damages or losses arising from material published in this report.
Declared competing interests of authors
Helen Snooks is a member of the Health Technology Assessment Editorial Board and scientific advisor to the Health Services and Delivery Research Programme Board.
Permissions
Copyright statement
© Queen’s Printer and Controller of HMSO 2018. This work was produced by Snooks et al. under the terms of a commissioning contract issued by the Secretary of State for Health. This issue may be freely reproduced for the purposes of private research and study and extracts (or indeed, the full report) may be included in professional journals provided that suitable acknowledgement is made and the reproduction is not associated with any form of advertising. Applications for commercial reproduction should be addressed to: NIHR Journals Library, National Institute for Health Research, Evaluation, Trials and Studies Coordinating Centre, Alpha House, University of Southampton Science Park, Southampton SO16 7NS, UK.
2018 Queen’s Printer and Controller of HMSO
Chapter 1 Introduction
Background
An increasingly older population and rising incidence of chronic conditions places a significant and unprecedented demand on health-care services. 1,2 In 2012–13, in England alone, there were 5.3 million emergency admissions to hospitals, costing approximately £12.5B. 3 Around half of these admissions can be attributed to just 5% of the population – typically older people with comorbidities. 4,5 Patients with chronic conditions are more likely to experience emergency hospital admissions for potentially avoidable causes, resulting in a ‘triple fail event’ of suboptimal health outcomes, significant health costs and poor patient experience. 6 An emergency admission to hospital is disruptive and unsettling, exposing patients to clinical and psychological risks and increasing their dependency. 7
Primary- and community-based care offers the opportunity to deliver efficient, co-ordinated and individualised care that can prevent emergency admissions, improve care quality and reduce costs. 8,9
A recent Health Foundation and Nuffield Trust report estimates that up to one in five emergency admissions are avoidable,10 especially where they relate to ambulatory care-sensitive conditions – conditions amenable to community prevention. 11 These include instances in which problems develop over longer periods and the risk of a crisis leading to emergency hospital admission can be reduced by timely and effective self-care, primary and community care or outpatient care. 12 Admissions related to common chronic conditions such as asthma, chronic obstructive pulmonary disease (COPD), diabetes mellitus and hypertension are included in most ambulatory care-sensitive condition lists. Recent analysis in England suggests that better management of ambulatory care could achieve yearly savings of > £1.4B,13 as well as improving patient health and service quality. In England and Wales, > 16 million people have a long-term (or chronic) condition – and their care accounts for 70% of expenditure on health and social care. 4
Recognising such challenges and opportunities, England in 2005,5 and Wales in 2007,14 developed ‘evidence-based’ frameworks for the care of patients with long-term conditions. They shared key features of early identification and response to patient needs, joined-up care, and holistic support centred on the person rather than on specific conditions.
Both models championed risk profiling as a means of identifying patients at risk (case finding) who may benefit from proactive management. The English model included systematic risk profiling as one of three primary drivers for the model, alongside integrated locality teams and systematised support for patients to manage themselves. 5
The underlying theoretical model for introduction of emergency admission predictive risk models is that they are expected to improve patient outcomes and experience and provide better value for money by identifying those at high risk of admission and enabling the targeting of proactive management to prevent health deteriorations requiring emergency care. 15,16 This proactive targeting of services at people at defined risk has retained prominence in UK Government policy ever since, notably within efforts to introduce integrated care. 17
Given the challenges of rising emergency admissions, their association with long-term conditions, and the financial and political impetus, there has been considerable international investment in the systematic risk profiling of emergency admissions. The approach is based on proactive targeting and support for those at risk, with the aim of preventing health deterioration and emergency admissions to hospital. To be cost-effective, however, preventative interventions must use case-finding techniques that target those at risk. 3,15,18
Identifying those at risk
A number of approaches to identifying patients at risk have been explored. One approach is to ask clinicians to select at-risk patients based on their knowledge and experience, but a study by Allaudeen et al. 19 found that the predictions made by junior doctors, senior doctors, nurses and case managers were statistically no different from chance. An alternative is to use a criteria-based approach, whereby individuals meeting certain conditions are selected for intervention. For example, in the UK Evercare pilots, in nine English primary care trusts (PCTs), patients aged ≥ 65 years with two or more emergency admissions in the previous year were eligible for case management by community matrons. The intervention did not show an effect, however, with the accuracy of the approach to identifying patients at risk criticised. 20 Such criteria-based approaches systematically exclude those who may be at high risk, but who do not meet what are typically a narrow set of criteria (such as younger people and those who have not had a recent emergency admission). 21 Furthermore, such an approach is susceptible to the statistical phenomenon of regression to the mean. 16 Offering hospital avoidance interventions to patients identified merely from history of emergency admissions can therefore be inefficient. 22
An alternative approach to identifying patients at risk that has gained widespread support is the use of a range of demographic and clinical data (such as age, gender, body mass index and medical history to calculate disease risk), or to predict an undesirable outcome (such as an emergency admission). The resulting clinical prediction models are intended to help clinicians make better decisions by providing more objective estimates of probability as a supplement to other clinical information. 23,24 Building on the successful implementation of risk models predicting diabetes mellitus (e.g. QDiabetes®) and cardiovascular disease (Framingham Risk Score25), emergency admission risk prediction (EARP) models have been widely developed – Table 1 provides examples. In calculating individualised risk for a given population, the models use data from up to three sources: self-reported data from patients; routinely collected administrative data; and data from the clinical record or other primary data source. 26
Model | Developer | Outcome |
---|---|---|
PRISM (Predictive RIsk Stratification Model) | Informing Healthcare and Health Dialog for NHS Wales | Risk of emergency admission in 1 year |
Combined model | The King’s Fund for the Department of Health | Risk of emergency admission in 1 year |
QAdmissions | ClinRisk Ltd, UK | Risk of emergency admission in 1 and 2 years |
PEONY (Predicting Emergency Admissions Over the Next Year) | NHS Tayside, UK | Risk of emergency admission in 1 year |
PARR (Patients At Risk of Re-hospitalisation) | The King’s Fund for Department of Health | Risk of hospital re-admission |
ACGs | Johns Hopkins University, Baltimore, MD, USA | Range of outcomes, including risk of hospitalisation (planned and unplanned) |
HARP (Hospital Admission Risk Programme) | Health Quality Ontario, Toronto, ON, Canada and the Canadian Institute for Health Information, Canada | Risk of emergency admission in 30 days and 15 months |
A 2014 systematic review of the technical performance of EARP models identified 27 tools, 18 using routine or primary data, with nine using self-reported data. 26 The majority of models (18 of 27) were developed to predict emergency hospital admission at 12-month follow-up. Those models that performed best (in terms of predictive accuracy, as measured by c-statistics of > 0.8), featured ‘prior health care utilization variables, multimorbidity or polypharmacy measures, and named medical diagnoses or named prescribed medications variables’. These better-performing models all used routinely collected clinical patient data rather than self-reported patient data, and it is recognised that models reliant on self-reported (questionnaire) data are limited by response rates, recall issues and respondent burden. 27
Implementing risk prediction models
Although the EARP models are widely advocated in international policy and practice, notably in the UK, Europe and the USA,5,14,28,29 evidence regarding their implementation and effects is limited. A 2015 NHS England paper on the Next Steps for Risk Stratification in the NHS recognised the need for robust evidence, and a pressing need for further research and evaluation, using high-quality study designs. 15 We present a systematic review of the existing published evidence in Chapter 2. The review confirms that the most common intervention used in emergency admission avoidance are various forms of case management. Although definitions of case management vary, Hutt et al. described it as ‘the process of planning, co-ordinating and reviewing the care of an individual’ (as cited in Ross et al. 22). Case management often covers a range of activities, but it is recognised that these can vary widely between programmes. 22
Evidence for case management is mixed. A 2013 review found good evidence that many common aspects of case management are effective, including continuity of care with a general practitioner (GP), structured discharge planning and advanced care planning (as cited in Lewis15). However, a recent systematic review by Stokes et al. concludes that there is little evidence that case management is effective in reducing secondary care use or costs, but does show a positive impact on patient satisfaction. 30 Of the 36 studies included, only four used routine data risk prediction tools to identify patients for intervention, with most relying on referral criteria or identification by clinicians unaided by a risk model. The results of the subgroup of four remaining studies using routine data generated risk models to predict emergency admission were favourable, although none was from the UK. 30
Contractual incentives to adopt risk models
Despite the lack of evidence concerning effectiveness, over the past few years in the UK, a range of contracts has promoted and financially incentivised the use of emergency admission risk models in general practice. For example, NHS England’s Enhanced Service Specification. Avoiding Unplanned Admissions: Proactive Case Finding and Patient Review for Vulnerable People enhanced service committed funds of £480M over 3 years (2014–17). 18 Through ‘more personalised care’, the initiative aimed to improve the quality and co-ordination of care for high-risk patients to improve their health and well-being and reduce hospital admissions and emergency department (ED) attendances. 18 It funded practices to create a register of patients at high risk of avoidable unplanned admissions, for proactive case management.
Such initiatives have prompted further development of risk tools and widespread take-up in the UK. It is estimated that over 7500 English GP practices have participated in the enhanced service initiative, which generally relies on predictive risk scores to identify patients for selection for case management – over 95% of all practices (NHS Digital, 2015, personal communication).
Wales also introduced a variation to the general practice contract in 2013/14, encouraging the use of EARP tools to support hospital avoidance. 31 This work was funded through quality and productivity indicators within the Quality and Outcomes Framework (QOF) contract, and aligned with an ‘increased focus on integration of services’, and a vision of additional services within primary and community care. 31 Box 1 lists the relevant quality and productivity indicators (QPIs) for the 2013/14 financial year. Practices were encouraged to participate in this work, but it was not mandatory.
The practice produces a list of 5% of patients in the practice who are predicted to be at significant risk of unscheduled admission to secondary care or community-based alternatives (10 points).
QP101WThe practice identifies a minimum of 10% (with a maximum of 0.5% of the practice list) of those patients from the list produced in indicator QP100W who would most benefit from review and ensures there is an active management plan is in place for each patient. The active management plan must identify the lead clinician or care co-ordinator and an appropriate review date. The frequency of each patient’s review should be determined in light of their clinical and care needs. The practice will be responsible for ensuring that an appropriate system is in place for monitoring and review of the patients identified (10 points).
QP102WThe practice has at least four meetings during the year to review the delivery of care for the patients identified in QP16. These meetings should be open to all relevant professionals engaged in the delivery of care to this group. The meetings should be used to review the clarity of care plans and the effectiveness of service delivery. Patient and carer feedback should play a key role in informing this assessment. Participants should seek to identify opportunities to improve systems of care and to enact changes where possible (22.5 points).
Introducing the Predictive RIsk Stratification Model
This study relates to an evaluation of the Predictive RIsk Stratification Model (PRISM), an EARP tool introduced in Wales. PRISM is a web-based emergency admission predictive risk tool commissioned by the Welsh Assembly (now Welsh Government), with development led by the NHS Wales Informatics Service (NWIS – formerly Informing Healthcare). PRISM was closely aligned to the chronic conditions management model and framework,14 and built on a similar model in England (the combined predictive model). 32
PRISM provides individual risk scores for patients and stratifies general practice population into four levels based on the individual patient risk of an emergency admission to hospital in the following 12 months. The tool generates a predicted risk (out of 100%) of emergency admission for each patient on a practice list. It also stratifies patients into four risk groups according to the relative risk within the practice as a whole. So, for example, using the default stratification, the 0.5% of patients with the highest practice risk will appear at level 4; those in the top 0.5 –5% in level 3 (moderate risk); those between 5% and 20% in level 2 (low risk); and the remainder in level 1 (very low risk). 33 PRISM was developed and tested (validated) for accuracy of prediction on hospital and GP records of 300,000 people in Wales (10% of the Welsh population), from which 37 variables with the highest predictive power were selected. The variables used to develop PRISM were drawn from routinely available data on inpatient, outpatient and primary care episodes and from the Welsh Index of Multiple Deprivation (WIMD), which includes data on employment, income, housing, environment, education and health.
Following initial testing in 25 practices, PRISM distribution to all general practices in Wales was planned for April 2010. In anticipation, our original research proposal involved a study across three areas of Wales. However, this distribution did not proceed. We revised our study plan following discussions with Abertawe Bro Morgannwg University Health Board (ABM UHB), which remained keen for PRISM to be introduced in its area. Following an offer of support from NWIS to help with the technical support for PRISM, we were able to propose a revised cluster randomised study in a single site. Following approval from the National Institute for Health Research (NIHR) Health Services and Delivery Research (HSDR) programme and a study extension, we carried out this study according to our published protocol,34 rather than our original project description as submitted to the NIHR HSDR (then NHS Service Delivery and Organisation) programme.
The following chapters include a systematic review of the implementation of EARP models, followed by a summary of methods and findings reported over four chapters: clinical effectiveness, cost-effectiveness, findings from stakeholder consultation and a brief section on service user involvement. There follows a discussion featuring research recommendations and conclusions. Appendices include documents associated with data collection, training and supplementary information.
Chapter 2 Systematic review of the costs, effects and implementation of emergency admission risk prediction models in primary care
Introduction
There is limited evidence on what works in reducing avoidable emergency admissions. There are many local initiatives to prevent avoidable emergency admissions including risk prediction tools, case management, hospital alternatives and telemedicine, but limited evidence on what works. 3
We carried out a systematic review of studies reporting effects, costs and implementation of using risk prediction models in primary care to identify patients with chronic conditions at risk of future emergency admissions.
Methods
We registered the systematic review with PROSPERO – the International Prospective Register Of Systematic Reviews – on 14 April 2015 (reference number CRD42015016874), and outlined our methods in a protocol publication. 35 We carried out the review in accordance with Preferred Reporting Items for Systematic Reviews and Meta-Analyses guidelines. 36
Search strategy
We searched the following electronic databases: Applied Social Sciences Index and Abstracts via ProQuest, Cumulative Index to Nursing and Allied Health Literature via EBSCOhost, The Cochrane Library and Cochrane Central Register of Controlled Trials and Economic Evaluations, Health Management Information Consortium via Ovid, ISI Web of Science, MEDLINE via EBSCOhost and Scopus. We also hand-searched BMC Family Practice, the British Journal of General Practice and the International Journal of Integrated Care, all known to have published related work. We searched references and citations of included articles, undertook a further search using the names or other identifiers of risk models identified in included studies, and consulted experts in EARP. Our systematic review protocol35 provides a detailed listing of the search terms.
We selected 2005 as the earliest publication date, to precede relevant policy initiatives that prompted the development of the risk tool,5,14,37,38 and to ensure relevance to contemporary primary and community care. Searches were completed to the end of May 2015.
Study selection
We included studies reporting use of risk prediction models within primary care. Our inclusion criteria are shown in Table 2.
Criterion | Description |
---|---|
Population | Patients registered with general practices, or consulting primary or community care practitioners |
Intervention |
Models in primary care using routine data to predict risk of hospital admission for patients with, or at risk of, chronic conditions Exclusion: models that rely on patient-reported (questionnaire or interview) data |
Comparators | External (e.g. trial) or internal (e.g. cohort). None for qualitative studies |
Outcomes | Clinical effectiveness or cost-effectiveness, views of patients or health professionals on EARP models, or implementation of model |
Study design |
Studies that report empirical data Published 2005–15; no language restriction Exclusion: commentaries or editorials |
Two reviewers (MRK and KN) independently assessed initial eligibility of identified studies by screening titles, abstracts and keywords. Two reviewers (MRK and BAE) independently assessed potentially eligible full texts for inclusion. Disagreements were resolved through discussion.
Data extraction
Mark Kingston and Hayley Hutchings extracted data independently and in duplicate from all eligible studies. Disagreements were managed by consensus. A standardised data extraction form, building on guidance from the NHS Centre for Reviews and Dissemination39 was developed, tested and subsequently used following minor adjustments.
We sought to extract data based on a primary outcome of the number of emergency hospital admissions, and:
-
other health-care resource use, implementation costs, reported facilitators and barriers, and clinicians’ and patients’ views, notably satisfaction
-
study characteristics (setting, objectives, design and methods)
-
study population, notably selection criteria
-
nature and purpose of risk prediction model
-
implementation of risk prediction model – who used it and how?
Additional data were sought from authors when necessary.
Quality assessment
Two reviewers (MRK and HH) independently assessed general study quality as strong, moderate or weak, resolving any differences through discussion with a third reviewer (BAE). To account for a range of study designs we used two bias assessment tools. Thomas et al. ’s 2004 tool is suitable for quantitative study designs. 40 This tool assesses study design, sample selection, identification and analysis of confounders, blinding of outcome assessors and participants, validity and reliability of data collection methods, and nature and extent of withdrawals. We also used the Walsh and Downe framework to appraise the qualitative studies according to their scope and purpose, design, sampling, analysis, interpretation, reflexivity, ethical dimensions and relevance. 41
Data synthesis
We grouped and tabulated included studies according to their characteristics, including narrative summaries of risk prediction models used, how they were implemented and reported effects. Owing to the range of study design and limited overlap in study outcomes, it was not appropriate to undertake meta-analysis or to undertake statistical tests for heterogeneity. 42
We used narrative synthesis to review qualitative data on model implementation, and staff and patients views, informed by the framework developed by Popay et al. 43
Results
Search results
Our initial electronic database search identified 10,244 papers, with a further 11 identified through other sources. Following the removal of duplicates, 6621 papers were screened by title and abstract, with 215 reviewed in full-text format. Thirteen papers, from 11 studies, met all criteria and underwent full data extraction (Figure 1). 7,44–55
FIGURE 1.
Search results PRISMA flow diagram.

Quality assessment
An overview of the results of the quality assessment can be found in Tables 3 and 4. Most studies were rated as being of low quality.
Author (year of publication) | Selection bias | Design | Confounders | Blinding | Data collection methods | Withdrawals and dropouts | Overall quality rating |
---|---|---|---|---|---|---|---|
Baker et al. (2012)44 | Weak | Moderate | Weak | Weak | Strong | Moderate | Weak |
Dhalla et al. (2014)45 | Strong | Strong | Moderate | Weak | Strong | Strong | Strong |
Freund et al. (2011)46 | Weak | Weak | Weak | Weak | Weak | Moderate | Weak |
Levine et al. (2012)47 | Weak | Strong | Moderate | Moderate | Moderate | Moderate | Moderate |
Reilly et al. (2011)48 | Moderate | Moderate | Weak | Weak | Moderate | Weak | Weak |
Roland et al. (2012)49 | Moderate | Moderate | Strong | Weak | Moderate | Weak | Weak |
Takahashi et al. (2012)50 | Weak | Strong | Moderate | Weak | Moderate | Moderate | Weak |
Upatising et al. (2013)51 | Weak | Strong | Moderate | Weak | Moderate | Moderate | Weak |
Author (year of publication) | Scope and purpose | Design | Sampling strategy | Analysis | Interpretation | Reflexivity | Ethical dimensions | Relevance and transferability | Overall quality rating |
---|---|---|---|---|---|---|---|---|---|
Abell et al. (2010)52 | B | B | A | B | C | C | A | B | |
Sauto Arce et al. (2014)53 | B | B | B | C | C | C | B | B | C |
Freund et al. (2012)27 | B | B | A | B | B | C | B | B | B |
Hall et al. (2011)54 | B | B | A | B | B | C | A | A | B |
McEvoy et al. (2011)55 | B | B | A | B | C | C | B | B | B |
Settings
Of the 11 studies, eight were European, consisting of four studies (and five papers) from England,48,49,52,54,55 two (related studies) in Germany,27,46 one in Scotland44 and one in Spain. 53 Three studies were undertaken in North America, one in Canada45 and two in the USA (yielding three papers). 47,50,51 A summary of studies is presented in Table 5.
Author (year of publication) | Country | Aim | Design and methods | Description of interventions | Data collection/coverage |
---|---|---|---|---|---|
Abell et al. (2010)52 | Manchester, UK | To explore processes that influence patient selection for case management and to explore the factors within the service that promote a differential service | Uncontrolled prospective cohort study
|
Various methods including predictive risk tools used to identify patients at risk of unplanned hospital admissions, eligible for case management. Case management through a range of primary care staff (e.g. community matrons, district nurses, community nurses) across 10 PCTs. No control group | 2007–8 |
Sauto Arce et al. (2014)53 | Basque area, Spain | To explore implementation of a risk tool | Qualitative. Three focus groups with users (12 GPs and 11 nurses) | Predictive Risk Stratification tool to identify target populations for services such as secondary prevention activities by primary care practice staff for patients with hypercholesterolaemia, high blood pressure, or type 2 diabetes mellitus, not suffering heart failure or ischaemic heart disease | December 2011 |
Baker et al. (2012)44 | Nairn, UK | To describe results ACP aimed at reducing unplanned hospitalisations of older patients who are frail | Before-and-after comparison. Comparative analysis of ACP vs. usual care – cases identified by use of predictive risk tool | Patients identified through use of predictive risk tool (Nairn Case Finder). Case management (ACP) featuring care plans for older patients who are frail. Supported by case managers (1.2 WTE), care workers (2 WTE), physiotherapists (1 WTE) and OTs (0.5 WTE) | April 2007 to November 2008 |
Dhalla et al. (2014)45 | Toronto, ON, Canada | To explore whether or not a virtual ward could improve health outcomes and reduce re-admissions after hospital discharge in a high-risk population | Parallel-group randomised trial with patients randomised at hospital discharge in a 1 : 1 ratio to either the virtual ward model of care or usual care. Eligibility for trial determined by use of predictive model for risk of readmission (LACE). No comparative process or outcome data related to predictive risk stratification available – all patients identified for virtual ward management using predictive risk tool | Virtual ward, admitted on day of discharge from hospital, with care co-ordinator (similar to case manager), p/t pharmacist, p/t nurse or nurse practitioner, f/t physician, clerical assistant. Regular MDT discussion, care plans, liaison with primary care physician, home visit by co-ordinator. Further follow-up in home, in hospital clinic, or elsewhere (e.g. family physicians office). Average discussion 6.3 times in MDT team meetings, 2.8 home visits | 29 June 2010 to 2 June 2014 |
Freund et al. (2011)46 | South-west Germany | To compare patient selection for care management interventions by physicians and by predictive modelling | Observational study comparing patients selected by predictive model with those selected by physicians | Study relates to testing performance of predictive risk tool, as part of intervention development process | |
Freund et al. (2012)27 | South-west Germany | To explore how physicians select patients for care management and how risk prediction may complement their case finding | Qualitative: 12 interviews with physicians from 10 primary care practices | Study relates to views about use of predictive risk tool to identify patients for case management, as part of intervention development process | Not reported |
Hall et al. (2011)54 | London, UK | To evaluate how community matrons select their case load | Qualitative service evaluation. Interviews with seven community matrons and two GPs | Case selection, potentially for case management via community matrons | Not reported |
Levine et al. (2012)47 | Three Los Angeles county regions, CA, USA | To assess the efficacy of a home care programme designed to improve access to medical care for older adults with multiple chronic conditions at risk of hospitalisation | RCT in which participants were assigned to the home care intervention (Choices for Healthy Ageing programme) or usual care. Routine data, costs and telephone patient satisfaction questionnaires. Eligibility for trial determined by use of predictive model for risk of readmission (SCAN). No comparative process or outcome data related to predictive risk stratification available – all patients identified for home care programme management using predictive risk tool | Home care intervention programme – early identification and treatment of exacerbation of the illness; patient-specific health education; self-management or caregiver management of the disease; advance care planning and other psychosocial issues. Care delivered via interdisciplinary team, with core team members consisting of physician, nurse practitioner, nurse care manager and a social worker | January to July 2008 |
McEvoy et al. (2011)55 | Northern England, UK | The evaluation aimed:
|
Formative evaluation self-completion questionnaire and semistructured interview. Predictive risk tool (PARR) used to identify patients for case management. No comparative process or outcome data available | Case management for high-intensity service users via three community matrons who acted as both advanced clinical practitioners and case managers, six case managers and an administrator | February 2007 to August 2008 |
Reilly et al. (2011)48 | Manchester, UK | Evaluate the implementation and impact of case management for long-term conditions in 10 PCTs | Cross-sectional postal survey, interviews and retrospective cohort analysis. Various case finding methods used including predictive risk stratification and direct referral, handsearching | Predictive risk stratification not part of intervention. Case management through a range of primary care staff (e.g. community matrons, district nurses, community nurses) across 10 PCTs | July 2007 interviews; 1 July 2005 to 1 October 2006 cohort analysis |
Roland et al. (2012)49 | UK | Evaluation of English integrated care pilots | Questionnaire to staff and patients. Analysis of secondary care utilisation using HES data on 3646 patients and 17,311 matched controls. Various case finding methods used including predictive risk tools. Before/after comparison of outcomes for case management | Predictive risk tool not necessarily part of intervention. Virtual wards, with nurse-led case management. Plus focus on delivery system redesign and improved clinical information systems | Staff questionnaires : summer 2010 and spring 2011. Patient questionnaires: autumn 2009 and autumn 2010 |
Takahashi et al. (2012)50 | Rochester, MN, USA | To determine the difference in hospitalisations and emergency room visits in older adults using telemonitoring vs. standard care | RCT. Eligibility for trial determined by use of predictive model for risk of readmission (ERA). All patients identified for telemonitoring intervention using predictive risk tool. Comparative analysis of outcomes of telemonitoring | The telemonitoring intervention included usual medical care and telemonitoring case management. Using telemonitoring the participant’s blood pressure, pulse, oxygen saturation, blood glucose level and weight were measured as per an individualised protocol, based on their medical condition. Usual care included various types of face-to-face visits, telephone services and home health care available to all primary care patients. Home health care includes provision of home health nursing and/or physical and occupational therapist visits | November 2009 to July 2012 |
Upatising et al. (2013)51 | Rochester, MN, USA | To evaluate the effectiveness of home telemonitoring in reducing the decline of worsening frailty states in older adults aged ≥ 60 years with comorbid health problems | Secondary analysis of RCT data.50 Eligibility for trial determined by use of predictive model for risk of readmission (ERA). All patients identified for telemonitoring intervention using predictive risk tool. Comparative analysis of outcomes of telemonitoring | The telemonitoring intervention included usual medical care and telemonitoring case management. Using telemonitoring the participant’s blood pressure, pulse, oxygen saturation, blood glucose level, and weight were measured as per an individualised protocol, based on their medical condition. Usual care included various types of face-to-face visits, telephone services and home health care available to all primary care patients. Home health care includes provision of home health nursing and/or physical and occupational therapist visits | November 2009 to July 2012 |
All studies related to interventions in primary and community care, including one study that randomised patients to community-based care on discharge from hospital. 45
All papers were published from 2010 onwards with reported data collection or coverage between 2005 and 2014.
Study designs
Study designs comprised three randomised controlled trials,45,47,50 three cohort studies,44,48,56 one cross-sectional study55 and one further observational study. 46 Three wholly qualitative studies were included,27,53,54 with qualitative methods also featuring in two others. 52,55
Risk tools identified
The majority of studies reported the use of a single tool predicting emergency admissions to hospital. Three studies reported findings relating to the use of multiple risk tools, as applied in different PCT areas,48,52,56 although none provided a disaggregation of results by area or tool used. The Patients at Risk of Re-hospitalisation (PARR) tool was used in five studies. 48,49,52,54,55 PARR uses data on prior hospitalisations to predict risk of rehospitalisation and, hence, calculates risk only for those patients with a previous admission. The combined predictive model was reported in three studies,48,49,52 with single references to the Elder Risk Assessment (ERA),50 Case Smart Suite Germany,46 Length of stay, Acuity, Comorbidities, ED visits in previous 6 months (LACE),45 Nairn Case Finder,44 High Impact User Manager52 and Adjusted Clinical Groups (ACGs). 53 An unnamed tool developed by the Senior Care Action Network Health Plan was used for the Levine et al. study. 47
All except two tools were reported as predicting the likelihood of an emergency hospital admission (or re-admission in the case of the PARR tool), within 12 months. The LACE tool predicts risk within 30 days45 and the ERA tool51 predicts risk within 24 months. The time span for the ACG tool used in Sauto Arce et al. ’s qualitative study53 was not provided.
Only one paper explicitly addressed risk tool technical performance – Baker et al. 44 – reporting an area under the receiver operating characteristic (ROC) curve of 0.794 for the Nairn Case Finder. A number of papers did include a reference45,48,50–55 to resources where details of the development and validation of the respective risk tools could be found. No technical performance details or references were included in three papers. 46,47,56
Risk tools users
Identification of who used the risk tools was included in all studies, except three (Table 6). 47,48,55
Author (year of publication) | Risk prediction model | Outcome and time span of risk model | Validation | Population risk model applied to | Users of risk model | Case selection method |
---|---|---|---|---|---|---|
Abell et al. (2010)52 | PARR; combined predictive model |
PARR: likelihood of rehospitalisation within the next 12 months Combined model: risk of emergency admission within the next 12 months |
Not discussed, but reference supplied for PARR tool development and validation paper (see Billings et al.57) | Unspecified number of patients across 10 PCTs providing case management services | Case managers including community matrons | Risk models (10/10 PCTs); referrals from professionals (10/10); criteria (threshold) (9/10); and, assessment framework (4/10), disease registry (3/10) and other (3/10) |
Sauto Arce et al. (2014)53 | ACGs | Risk score for health costs, risk score for risk of admission to hospital. Time span not reported | Not discussed. Link to ACG website provided | 2.2 million people in Basque Health Service area | GPs and primary care nurses | Risk score and presence of diabetes mellitus, high blood pressure or hypercholesterolaemia |
Baker et al. (2012)44 | Nairn Case Finder | Risk of admission to hospital within next 12 months | Reports area under the ROC curve of 0.794. Refers to appendix for further information | General practice with 10,860 patients | Practice staff | From each monthly list, the 1% of patients with the highest risk of admission were defined as the ACP cohort, along with all care home patients |
Dhalla et al. (2014)45 | LACE | Predict the risk of death or non-elective re-admission within 30 days after discharge | Not discussed, but reference provided to development and validation paper (see van Walraven et al.58) | 30,143 patients discharged from four Toronto hospitals | A research co-ordinator assessed patient eligibility at each hospital | Risk model only |
Freund et al. (2011)46 | Case Smart Suite Germany | Likelihood of hospitalisation within the next 12 months | No details provided | 6026 beneficiaries of a statutory health insurance programme in Germany | Primary care physicians | Mix (study explored case selection by physician and by risk model) |
Freund et al. (2012)27 | Case Smart Suite Germany | Likelihood of hospitalisation within the next 12 months | No details provided | 6026 beneficiaries of a statutory health insurance programme in Germany | Primary care physicians | Mix (study explored case selection by physician and by risk model) |
Hall et al. (2011)54 | PARR | PARR: likelihood of rehospitalisation within the next 12 months | Not discussed but reference supplied for PARR tool development and validation paper (see Billings et al.57) | No details provided | Community matrons (data supplied by e-mail by PCT) | Mix (PARR) referrals and identification by community matrons |
Levine et al. (2012)47 | Unnamed tool developed by SCAN Health Plan | At risk of hospitalisation. Time span not provided | No details provided | 1362 patients eligible from three Los Angeles county regions | Not specified | Risk model only (intervention and control) |
McEvoy et al. (2011)55 | PARR | Risk of re-admission within 12 months | Not discussed but reference to development and validation paper provided (see Billings et al.57) | High-risk patients in northern England | Not specified (likely to be community matrons/GPs) | Not specified though all had a PARR score of > 40, indicating that there was a high risk they would require emergency re-admission to hospital |
Reilly et al. (2011)48 | PARR; combined predictive model; High Impact User Manager (Dr Foster) |
PARR and High Impact User Manager: risk of re-admission within 12 months Combined predictive model: risk of emergency admission with 12 months |
Not discussed, but reference supplied for PARR tool development and validation paper (see Billings et al.57) | Unspecified number of patients across 10 PCTs providing case management services | Case managers including community matrons | Risk model and clinician referral (referrals in all 10 sites) |
Roland et al. (2012)49 | PARR; combined predictive model |
PARR: risk of re-admission within 12 months Combined predictive model: risk of emergency admission with 12 months |
No details provided | Six integrated care pilot sites in England | Varies across six sites, but includes GPs and case managers | Mix of risk profiling tools, medical history and clinical judgement to identify older people at risk |
Takahsahi et al. (2012)50 | ERA | Risk of admission to hospital within 2 years | Reference provided to development of ERA tool (see Crane et al.59) | Residents of four Mayo Clinic sites in Minnesota | Not specified | Risk model (and aged ≥ 60 years) |
Upatising et al. (2013)51 | ERA | Risk of admission to hospital within 2 years | As above | Residents of four Mayo Clinic sites in Minnesota | Not specified | Risk model (and aged over ≥ 60 years) |
Interventions
Predictive risk stratification was generally used as a tool for identifying patients suitable for a further intervention (e.g. virtual ward), rather than as a formal part of that intervention. In some cases, a predictive risk tool was used as one of several methods of case-finding. No studies reported comparative data about processes or outcomes related to predictive risk stratification. In each of the RCTs, predictive risk tools were used to identify patients eligible for the trial – and were therefore used in both trial arms.
With regard to the follow-on (secondary) interventions, eight of the studies focused on case (or care) management of patients at high risk of emergency admission to hospital. 44–48,52,54,55 Two studies featured the use of telemedicine46,50 as part of an overall package of care. A range of primary care and community staff delivered, or were proposed to deliver, these secondary interventions. This included the use of community matrons48,52,54,55 – senior nurses with a care co-ordination function,54 who were introduced following Department of Health funding in support of the care of patients with long-term conditions. 60 The intended use of multidisciplinary teams (MDTs) was noted in five studies,44,45,47,49,54 and virtual wards featured in three. 45,54,56 The virtual ward model is based on the use of predictive models to identify those at risk of emergency admission, and the provision of a period of intensive, multidisciplinary case management at home using the processes and staff associated with hospital wards. 56 One study related to gathering evidence to inform the development of an intervention. 46
Main quantitative results
There were no comparative data available related to effects of predictive risk stratification on processes or outcomes of care.
Main qualitative results
Organisational issues, staff attitudes towards risk prediction models, together with staff skills and access to computer equipment affected use within primary and community care (Table 7).
Barriers | Facilitators |
---|---|
Not an organisational priority55 | Supportive organisational processes for risk prediction models53 |
Difficult to fit use of model into reactive way of working53,54 | Individual and organisational support for a population management approach to primary care delivery53 |
Low interest in using risk prediction models and new ways of working54,55 | Training for staff53,54 |
Priority placed on personal and clinical knowledge over risk model information27 | Interest in new approaches to primary care delivery54 |
Questions over accuracy and timeliness of risk model data27,53 | Confidence and skills in IT54 |
Inadequate access to IT equipment54 |
Primary and community care context
Political, organisational and cultural issues were reported to affect how risk prediction models were introduced and used. Lack of organisational leadership and guidance meant that staff took individual decisions about if and how to use the model. 55 GPs and nurses were more likely to accept and use risk prediction models if they were involved in developing the implementation process, could see how it fitted into their practice and had confidence in the organisation promoting its use. 53 According to GPs, the structure of general practice and the dependencies of patients forced them into delivering reactive care. Against a backdrop of multiple new initiatives, they said limited time and heavy workload prevented them working differently. 53
Staff attitudes
Existing knowledge of risk prediction processes and an understanding of its principles were reported to affect willingness to use risk prediction models in included studies. Support for new ways of working was also required. 27,53–55 GPs and nurses who perceived risk prediction as a population health management approach were more likely to use a risk model to identify patients at higher risk, especially those they did not already know, in order to prevent patients becoming ill or more ill. 53 Hall et al. 54 reported that community matrons also welcomed a system which could identify patients before they presented to hospital and those high users of services who were not known to their GP. However, the community matrons said effective multidisciplinary working was necessary in order to deliver selected care to high-risk patients and this depended on a common understanding and outlook between staff from different disciplines.
Health-care staff recognised that risk prediction tools could successfully identify high-risk patients,53,54 although concerns about the reliability of tools limited their use. Data could be out of date, inaccurate or not provide new information. 53,54 Confidence in risk prediction data was affected by which data were included in the algorithm. Those tools with the widest data sources (ideally health and social data from a whole population) were reported to be most popular because they were perceived to be most equitable and highlighted the most appropriate patients for treatment. 53 However, staff who lacked confidence in the model data or who prioritised clinical experience and knowledge were less likely to use risk models. 27 Studies reported that staff used risk prediction alongside other information sources to get the fullest picture of a patient’s needs. 52–55
Information technology skills and access to equipment
Limited access to the risk prediction model was a common issue for staff who worked in the community. Hall et al. 54 reported that community matrons wanted access to a patient’s data on a computer system and to understand the systems they were using in order to make best use of risk prediction data. McEvoy et al. 55 reported that staff in one site could use systems of colleagues in social services, community services and secondary care, but many staff were denied access to all data in another site. Although McEvoy et al. 55 and Abell et al. 52 did not describe how case managers accessed risk prediction data, both reported that collaborative working was difficult for staff and delayed use of the risk prediction tool until pragmatic solutions were agreed to meet information governance requirements. Lack of information technology (IT) skills also inhibited staff from using risk prediction models. 53,54
Discussion
To the best of our knowledge, this is the first review of studies into costs, effects and implementation of EARP tools. Our review highlights the shortage of high-quality evidence in this area. Given the small number of heterogeneous studies and the predominant use of predictive risk tools to identify patients, rather than as part of an intervention, our review did not provide strong evidence for or against the use of EARP models. A search for unpublished articles was beyond the scope of this review, although we consider it unlikely that our findings would have been greatly affected.
The studies included demonstrate heterogeneity in interventions. It is important to note that emergency admission predictive risk tools can form part of a complex intervention, which may vary according to the nature of the target population and delivery – although most featured case management based on identified high-risk patients. Case selection approaches varied, with some interventions including patients referred by health-care professionals rather than identified by risk tool, but not differentiating outcomes in relation to the different recruitment approaches. In some cases, the use of mixed methods of case selection illustrated a lack of intervention fidelity. It is not possible to separate out effects of the predictive risk tool from those of the associated (secondary) intervention in the published studies, as none reported comparative data about processes or outcomes related to predictive risk stratification.
A handful of studies included staff feedback on the use of risk prediction tools. These data indicated that, although there was support for the use of the tools, there were concerns over the accuracy of models and access to data. The review revealed a deficit of evidence regarding patient perspectives.
There was poor quality of reporting in relation to the technical performance of the tools.
This review supports the need for further studies that address important gaps in our understanding of the effectiveness of EARP tools. The Predictive Risk Stratification: A Trial in Chronic Conditions Management (PRISMATIC) study aims to address this gap.
Chapter 3 Methods
Study aim
To evaluate the introduction of PRISM in primary care.
Objectives
The objectives of the study were to:
-
measure the effects on service usage, particularly emergency admissions to hospital
-
assess the effects of PRISM on quality of life and satisfaction
-
assess the technical performance of PRISM
-
estimate the costs of PRISM implementation and its effects
-
describe the processes of change associated with PRISM.
Design
We carried out a mixed-methods, progressive, cluster randomised trial with a quantitative evaluation and health economic analysis sited within a health board in south-west Wales and qualitative fieldwork across the whole of Wales. The trial site, ABM UHB, is the second largest of seven health boards in Wales, serving > 500,000 people. The 77 general practices are arranged in three localities (Swansea, Neath Port Talbot and Bridgend) and 11 community networks (local groupings of practices, health, social care, and voluntary sector professionals) (Table 8). We invited all of these practices to participate and included those that volunteered. Based on the distribution of practices willing to participate we created 11 study clusters (Table 9), based as closely as possible on these community networks. The practices were grouped to ensure as limited contamination across practices within the intervention and control phases as possible.
Locality | Network | Practices (n) | Population (n) |
---|---|---|---|
Neath Port Talbot | Neath | 8 | 56,640 |
Afan | 10 | 50,603 | |
Upper Valleys | 5 | 30,615 | |
Subtotal | 23 | 137,858 | |
Bridgend | Bridgend North | 9 | 51,251 |
Bridgend East | 6 | 67,394 | |
Bridgend West | 4 | 34,099 | |
Subtotal | 19 | 152,744 | |
Swansea | Llwchwr | 6 | 45,233 |
Penderi | 6 | 37,715 | |
Cwmtawe | 5 | 42,392 | |
CityHealth | 10 | 55,437 | |
BayHealth | 8 | 68,047 | |
Subtotal | 35 | 248,824 | |
Total | 77 | 539,426 |
Locality | Cluster | Practices (n) | Population (n) |
---|---|---|---|
Neath Port Talbot | Afan B | 3 | 15,033 |
Neath A | 4 | 25,332 | |
Neath B | 4 | 31,179 | |
Upper Valleys | 3 | 9856 | |
Afan A | 3 | 16,807 | |
Bridgend | Bridgend East | 2 | 25,696 |
Bridgend North | 4 | 22,544 | |
Swansea | Bayhealth | 2 | 28,332 |
Cwmtawe | 2 | 17,691 | |
Llwchwr | 2 | 17,155 | |
Penderi/City | 3 | 19,804 | |
Total | 32 | 229,429 |
The study fulfilled the last of the three major steps (that of evaluating clinical performance), in researching multivariable prognostic models identified by the recent series in the British Medical Journal. 23
To ensure that all participating practices had the opportunity to implement and use the intervention during the study period, we used a progressive cluster randomised trial design (also known as randomised multiple interrupted time series or stepped-wedge design). 61–63 As the trial progressed, the number of intervention practices increased and the number of control practices fell (Figure 2). This design protects against some sources of bias, including inherent differences and contamination between practices, as well as the ‘resentful demoralisation’ of controls deprived of the intervention.
FIGURE 2.
Randomised multiple interrupted time series study design overview. Reproduced from Hutchings HA, Evans BA, Fitzsimmons D, Harrison J, Heaven M, Huxley P, et al. Predictive risk stratification model: a progressive cluster randomised trial in chronic conditions management (PRISMATIC) research protocol. Trials 2013;14:301. 34 This article is published under license to BioMed Central Ltd. This is an Open Access article distributed under the terms of the Creative Commons Attribution License (http://creativecommons.org/licenses/by/2.0), which permits unrestricted use, distribution, and reproduction in any medium, provided the original work is properly cited.

All participating practices began as control practices without the trial intervention, received the intervention (PRISM package and training) and, thereafter, were able to use PRISM with clinical and technical support. Swansea Trials Unit (STU) used random number tables to choose the order in which practice clusters received the intervention, stratified by locality. We concealed when this would happen from practices until 6 weeks before the planned start of the intervention, when we notified them by telephone and e-mail. We arranged training before the start of the intervention.
Intervention
We describe the intervention according to the Template for Intervention Description and Replication checklist, as summarised below and in Table 10:
-
Name PRISM intervention.
-
Why? Identification of patients at risk of emergency admission can support preventative allocation of resources and reduce that risk.
-
What (materials)? PRISM software and user-friendly PRISM handbook.
-
What (procedures)? Practice-based training, clinical support through two locally appointed ‘GP champions’, and telephone and e-mail ‘help desk’.
-
Who provided? Primary care team.
-
How? We installed PRISM software on practice PCs. Whenever possible, one of the GP champions and the PRISMATIC project manager delivered the training. Thereafter the GP champions provided clinical support, and the primary care service desk at NWIS provided technical support.
-
Where? We initiated PRISM intervention in each of 32 participating practices within ABM UHB.
-
When and how much? All practices received one training session on PRISM. We provided extra training on request. Clinical support from GP champions was also available on request throughout the intervention phase. Each practice nominated a PRISMATIC GP lead, responsible for co-ordinating their practice’s participation in the research and intervention, to include engagement with other clinical and practice staff as required.
-
Tailoring Individual practices were advised that they could alter the default risk settings on PRISM tool and were given flexibility with which to use the tool within their practice.
-
Modifications None.
-
How well? We delivered the intervention as planned in all 32 practices.
Component | Description |
---|---|
PRISM software | Installed on computers in each practice and activated when the practice begins the intervention |
Practice-based training | 1-hour session delivered in the practice by a GP champion to PRISM lead GP, the PM and any other interested staff |
GP champions | Two local GPs employed for two sessions per month to support practices in clinical use of PRISM |
Technical help desk | Telephone and e-mail support provided in office hours by NWIS to address enquiries about technical aspects of using PRISM |
PRISM handbook | 25-page, user-friendly handbook explaining how to set up and access PRISM, demonstrating the range of functions available in PRISM, and suggesting how to use it within the practice |
Outcomes
We compared between intervention and control phases.
Primary outcome
-
Emergency hospital admissions.
Secondary outcomes
-
ED attendances.
-
Primary care events.
-
Outpatient attendances.
-
Emergency admission bed-days.
-
Health-related quality of life (Short Form questionnaire-12 items; SF-12).
-
Patient satisfaction.
-
NHS implementation costs.
-
NHS recurrent costs.
Although not an effectiveness outcome per se, we compared deaths between groups to check for unexpected effects.
We also explored in detail:
-
technical performance of the PRISM tool – predicted compared with actual emergency admissions to hospital
-
practitioner, commissioner and policy-maker views about PRISM implementation, adoption and effects.
Methods
To address our study objectives, we used anonymised linked routine data on processes of health care for all patients registered at the participating practices. We sent postal questionnaires to a sample of patients. We were aware that the highest-risk patients were likely to be older and have more chronic conditions and, as a result, be less likely to return the questionnaire. In order to ensure representation from this highest risk group, we weighted the sampling frame to include proportionally more patients at higher levels of risk. We also conducted focus groups and one-to-one interviews with service providers, commissioners and policy-makers. Figure 2 shows the progress of the trial and the points at which we collected data.
Data collection and sources
Table 11 outlines how we addressed our study objectives, the data sources we used and the times when we collected those data.
Objective | Data source | Sample | Collection time |
---|---|---|---|
1. Measure effects on service usage, particularly emergency admissions to hospital | Anonymised routine linked data (including PRISM data) | All patients from participating practices | Baseline |
6 months | |||
18 months | |||
Questionnaire data: CSRI | Random sample of patients from participating practices (n = 800 at each time point) | Baseline | |
6 months | |||
18 months | |||
2. Assess the effect of PRISM on quality of life and patient satisfaction | Questionnaire data: SF-12, QCM | Random sample of patients from participating practices (n = 800 at each time point) | Baseline |
6 months | |||
18 months | |||
3. Assess the technical performance of PRISM | PRISM data | PRISM risk data for patients at participating practices | Baseline |
6 months | |||
18 months | |||
Anonymised routine linked data | Routine health data | Baseline | |
6 months | |||
18 months | |||
4. Estimate costs of PRISM implementation and its effects | Anonymised routine linked data (including PRISM data) | All patients from participating practices | Baseline |
6 months | |||
18 months | |||
Questionnaire data: SF-12 was used to derive SF-6D score | Random sample of patients from participating practices (n = 800 at each time point) | Baseline | |
6 months | |||
18 months | |||
Structured interviews | PRISM users from all participating practices (n = up to 40) | 18 months | |
5. Describe processes of change associated with PRISM | Focus groups | GPs, practice nurses and managers from participating practices (n = 4); local health services managers and community staff managers (n = 1) | Baseline |
Interviews | GPs from participating practices who were unable to attend focus groups (n = 12) | ||
Health board managers from sites not participating in main study (n = 6); policy-makers and NHS managers (n = 5) | |||
Interviews | PRISM users from half of all participating practices, purposively sampled (n = 16 practices) | After 3 months following PRISM going live (mid-trial point) and at the end of the intervention (end of the trial) | |
Questionnaire | PRISM users from remaining half of all participating practices (n = 16 practices) | ||
Focus group | Local health services managers and community staff managers (n = 1) | 18 months | |
Interviews | ABM UHB health service managers (n = 3) |
Clinical effectiveness outcomes
Anonymised linked data
We used routine data from the Secure Anonymised Information Linkage (SAIL) databank64 to compare services (emergency, acute and primary care) delivered to patients across the spectrum of risk – between intervention and control phases. The SAIL databank includes routine Welsh hospital data on emergency admissions (ED data set), secondary care (Patient Episode Database for Wales) and general practice data. We derived PRISM risk scores from GP data and linked these to data on health service use for all study patients who did not dissent from the postal survey.
Postal questionnaires
We sent postal questionnaires to sampled patients at three points: at baseline, and at 6 and 18 months after initiating PRISM in the first study practice. The questionnaire comprised three validated tools: the adapted Client Service Receipt Inventory65 to estimate individual health service use; the Quality of Care Monitor (QCM66) to measure patient satisfaction; and the SF-1267 to measure patient-reported outcomes. We aimed to recruit samples of 800 patients stratified by risk level to complete the questionnaires at each time point (Table 12). This equated to around 70 patients per practice for screening at each time point.
PRISM risk level (default score range) | Proportion of sample (%) | Sample (number of patients) for screening in each practice |
---|---|---|
Level 4 (50–100) | 20 | 15 |
Level 3 (20–50) | 50 | 35 |
Level 2 (10–20) | 15 | 10 |
Level 1 (0–10) | 15 | 10 |
Total sample | 100 | 70 |
To ensure representation from the very small risk groups 3 and 4 (4.5% and 0.5% of patients), we sampled proportionately more from these groups. We excluded patients who were aged < 18 years or > 100 years, or had recently left a participating practice. NWIS undertook this sampling from their anonymised PRISM data set so that selected patients were identifiable only by their practices. Those practices then checked their lists of sampled patients to assess their suitability to receive questionnaires. They excluded patients who lacked the capacity, support or resilience to complete questionnaires. No additional patients were selected at this stage if patients were excluded. Participating practices then sent questionnaires packs (letter from GP, information sheet, consent form, questionnaire and business reply envelope), direct to approved patients. The letter asked patients to return completed questionnaires and consent forms direct to the study team. Completed consent forms gave us access to patients’ demographic information. Practices sent a second pack to patients who had not responded to the first after 2 weeks.
The two subsequent surveys followed the same approach. Practices checked that the original sampled patients were still alive and suitable to participate, and sent them follow-up questionnaires. NWIS then replaced each patient lost to follow-up by another patient of the same age, sex and risk level.
Clinical effectiveness analysis
We complied with STU’s standard operating procedure (SOP) on statistics. We undertook primary analysis by treatment allocated. Our primary outcome was the number of emergency admissions per patient, analysed as binary, count and rate variables and, hence, modelled using an appropriate generalised linear model in which we were able to take account of explanatory factors (including whether or not the participant’s practice had yet adopted PRISM) and covariates, including baseline observations, time-varying covariates and days at risk.
Outcomes were analysed using an appropriate generalised linear mixed model; specifically, we used linear models for measurement outcomes (assuming normality, also assessed via residual diagnostics), in which study practice was considered as a random factor; logistic regression for binary (yes/no) outcomes; negative binomial regression models for counts; we also analysed SF-12 and QCM scores for participants returning questionnaires using a repeated measures linear model. Analyses, which always included a PRISM effect, considered the following covariates and factors: gender, age (in years) at study day 1, an overall WIMD score and, separately, its health component (both taken from 2011), an initial PRISM score (dated around study day 1), seasonality, trend and ‘days at risk’ in the control and intervention phases.
Models also included a participant-level random factor to account for paired ‘control’ and ‘intervention’ observations from participants. Modelling progressed by eliminating, in turn and starting with the least significant, all covariates and factors found to be not statistically significant (that is, with a coefficient with a p-value > 0.05), and concluded when all remaining covariates and factors were statistically significant.
Raw and adjusted comparisons between groups were made, with some indication of the extent of the intracluster correlation coefficient (ICC) in variables between participants at the same study practice, and details of statistically significant factors and covariates. The adjusted comparisons reflected the nature of the variable under consideration: we present an odds ratio (OR) for logistic regression models for binary variables; an incidence or event ratio, Λ from negative binomial models for count variables, and an additive group effect (Δ, in the same units as the dependent variable) for linear models for continuous variables. To test the effect of the positive skewness on the statistical results, data were log-transformed and the generalised linear model re-run as described above.
Predictive risk stratification model risk groups
Each participant was assigned to one of four PRISM risk groups, based on the PRISM score at study day 1 and its relative position within the PRISM scores for the participant’s practice. Within each study practice, PRISM risk group 1 comprises those participants with the lowest 80% of scores in that practice. PRISM risk group 2 comprises those participants with the next 15% of scores, and PRISM risk group 3 comprises those participants with the next 4.5% of ranked scores, so that PRISM risk group 4 comprises those participants whose PRISM score is in the top 0.5% of scores for that practice (i.e. those who are predicted to be at the highest risk of emergency hospital admission). PRISM risk groups for the complete sample are formed by combining the practice-level groups.
Technical performance
To investigate the technical performance of the PRISM tool, we focused on data available prior to the introduction of PRISM (to avoid potential contamination between its predictive ability and its use) and, consistent with the interpretation that the PRISM risk score is a probability of an emergency admission in 12 months, sought to analyse data on PRISM scores and emergency admissions in a 1-year period.
Health economics
Health economic analysis
We undertook an evaluation of the costs from the perspective of the UK NHS. Costs were assigned to the resources utilised by each patient in the 32 trial practices. These consisted of the PRISM implementation costs, primary care costs and secondary care costs (including ED attendances, emergency admissions, outpatient visits, and elective and emergency inpatient stays). Unit costs (pounds sterling, cost year 2014) were derived from published information. 68 In view of the time scale of each phase (control and intervention) of the project not exceeding 1 year, no discounting was applied to the costs or benefits. Cost differences between the two study phases were determined and used in conjunction with differences in the primary outcome (emergency admissions) to undertake a cost-effectiveness analysis. The Short Form questionnaire-6 Dimensions (SF-6D) scores were used to undertake a cost–utility analysis. Other secondary outcomes were included in a cost–consequences analysis.
Resource use and costs
Predictive risk stratification model implementation costs
We estimated the cost of PRISM implementation, based on the roll-out of the PRISM software across 32 general practices in the ABM UHB area, using information and data obtained within the trial. The base-case costs were derived from the general practices in the trial. The figures do not include the PRISM software development costs.
Implementation of the PRISM software in general practices required several individual steps, which were considered and costed separately as detailed below.
The pre-activation phase consisted of distributing information sharing agreements (ISAs), which was co-ordinated by the trial team members. The ISAs were completed and returned to NWIS by the general practice’s Caldicott Guardian [i.e. the GP or practice manager (PM) responsible for protecting the confidentiality of patient and service user information and enabling appropriate information sharing]. This process was evaluated as GP/PM opportunity costs [for GPs, General and Personal Medical Services (GMS) activity, excluding direct care staff costs and without qualification], from the NHS perspective using published unit costs. 68 Following receipt of completed ISAs, NWIS sent the PRISM activation details (a set of four passwords) to the practice Caldicott Guardian via e-mail.
Three potential cost drivers were identified during the PRISM activation and set-up phase, comprising NWIS IT support, GP training and capital investment (i.e. equipment costs).
The activation or set-up phase of the trial involved the distribution and download of the PRISM software onto general practice computer hardware. This also reflected real practice for general practices to gain access to PRISM. Using the passwords e-mailed to the Caldicott Guardian, PRISM was activated within the British Medical Journal Informatica’s Audit+ clinical system, which runs on all general practice computers in NHS Wales. Activation instructions were provided by the trial team in a two-page Microsoft Word document (Microsoft Corporation, Redmond, WA, USA), and usually the PM proceeded with activation. Any general practice requiring help during the downloading and activation of the software could telephone the NWIS IT help desk for assistance. When telephone assistance did not solve the issue, an IT information officer provided on-site assistance during a visit to the general practice. The formal electronic application for access to the PRISM software and NWIS verification (by the NWIS governance team) was not assessed and costed as it was expected to require very little time or resources.
The second component assessed for cost was the monitoring of the activation status of the PRISM software as practice staff went through the process of downloading software (a cost incurred by NWIS). An information officer (NHS band 4) monitored the status of PRISM software activation and reported back to each practice. This involved the first data transfer of files and confirmation that data had been uploaded. NWIS support staff costs were taken from the Agenda for Change NHS 2014 pay scale. 69
The second resource-intensive component of the set-up phase was GP training. Two GPs were employed during the trial to deliver training on using the PRISM software to designated GP staff in all participating general practices. These GPs were paid £62.50 per hour as part of the trial. The GP trainers were trained by the trial team and then provided the training to GPs in each trial practice. The practice GP opportunity costs were also assessed for those GPs in practice spending time being trained on the use of PRISM. GP opportunity costs (GMS activity, excluding direct care staff costs and without qualification) were estimated using published unit costs. 70
There was a requirement for NWIS to download relatively large numbers of data from the participating general practices onto their own servers to activate the scoring process. During the trial no new servers were acquired as NWIS used current capacity and as such no costs were allocated for this resource. No equipment costs were therefore considered.
Predictive risk stratification model running costs
Running and maintenance costs of the PRISM tool for NWIS were expected to be minimal. An IT staff member at NWIS was expected to oversee the monthly data uploads from GP surgeries, activate PRISM scoring and confirm updates to general practices. NWIS also provided ongoing IT help desk support. This support was primarily set up as an e-mail support system. NWIS support staff costs were taken from the Agenda for Change NHS 2014 pay scale. 69
An evaluation tool was developed which was made up of five questions to capture practice staff activity with regard to using the software and data generated by PRISM in reviewing patient care. Practice staff were asked to provide information on frequency and duration of use as well as the job title of the member of staff who would usually work with PRISM. This evaluation took place at 3–9 months after implementation and at the end of the trial. User login data were also provided by NWIS to establish the activity within general practices. General practice staff time spent using the PRISM software was considered as GP or PM opportunity costs (for GPs, GMS activity, excluding direct care staff costs and without qualification), and costed using published unit costs. 70
Primary care resource use and costs
Primary care resource use was derived from SAIL, which delivers data on the number of GP event-days on a patient level. Owing to the fact that SAIL does not provide specification of the nature of the GP event, GP events were costed following the publication of Trends in Consultation Rates in General Practice 1995/96 to 2008/09: Analysis of the QResearch® Database in 2009 by the NHS Information Centre and the Department of Health. 71 It was assumed in the base case that 82% of GP events are surgery visits of 11.7 minutes’ duration, 15% are telephone consultations (of 7.1 minutes’ duration) and 3% are home visits (30 minutes’ duration assumed, including travel time). 70 Of these, 62% would be undertaken by a GP and 38% by a practice nurse resulting in a unit cost per GP event of £32.10. In the sensitivity analysis, the impact of lowest available cost (all appointments done by nurses) of £10.20 and highest available cost (all appointments done by GP) of £45.40 on the results were investigated.
Secondary care resource use and unit costs
The number of ED attendances (admitted and discharged), emergency admission-related inpatient stays, outpatient visits and elective inpatient stays were derived on a patient level through SAIL. Unit costs were taken from NHS Reference Costs 2014/1568 and weighted for specialties and activity. Because the target population of PRISM is mostly older patients suffering from chronic conditions, we excluded paediatric and obstetric codes from the analysis. Emergency admission-related inpatient stays were costed according to actual length of stay for each individual patient as observed in the study. However, owing to unavailability of length of stay data, elective inpatient stays were costed, assuming a length of stay of 5.4 days, according to the national average weighted by specialties and activity. 68 Table 13 summarises the unit costs used in the base-case analysis and ranges used for the sensitivity analysis.
Parameter | Base-case unit cost (£) (lower, upper for sensitivity analysis) |
---|---|
ED attendance (discharged) | 113.60 (94.30, 128.50) |
ED attendance (admitted) | 191.60 (152.00, 220.80) |
Emergency admission-related bed-day (short stay) | 541.30 (369.00, 631.00) |
Emergency admission-related bed-day (long stay) | 458.20 (326.70, 540.10) |
Outpatient visit | 110.60 (67.60, 127.80) |
Inpatient stay | 3605.50 (2624.30, 4299.10) |
Cost-effectiveness analysis
We calculated the incremental cost per emergency admission avoided. Owing to the difference in duration of the control and intervention phases, as a result of the stepped-wedge trial design, the control and intervention phase data of the primary and secondary health-care costs were adjusted for loss to follow-up (e.g. patients who died in the control phase and no data were available for the intervention phase) and annualised. Difference in total cost data between the control and intervention phase were then modelled using a generalised linear model incorporating an appropriate discrete distribution; consistent with the statistical analyses employed. Analyses always included a PRISM effect and considered gender, age (in years) at study day 1, an overall WIMD score and, separately, its health component (both taken from 2011), an initial PRISM score (dated around study day 1), season and trends as covariates and factors. Models also included a participant-level random factor to account for paired ‘control’ and ‘intervention’ observations from participants. Modelling progressed by eliminating all covariates and factors found to be not statistically significant (that is, with a coefficient with a p-value of > 0.05) starting with the least significant, and concluded when all remaining covariates and factors were statistically significant. To test the effect of the positive skewness inherent to cost data on the statistical results, total cost data were log-transformed and the generalised linear model rerun as described above.
The incremental cost of the intervention was calculated as the difference between the cost in the intervention phase (implementation cost of PRISM plus the primary and secondary care costs, as observed during the study intervention period) and the control phase (primary and secondary care costs, as observed during the study control period). This was then compared with the adjusted number of emergency admissions for both trial phases to generate an incremental cost-effectiveness ratio (ICER). Generally, the results of cost-effectiveness analyses are expressed as ICERs calculated by dividing the cost difference between the two alternatives being compared by the difference in the effect/benefit.
Cost–utility analysis
Differences in total health-care cost and SF-6D scores derived from the SF-12 questionnaires completed by a subset of participants were used to calculate the incremental cost per quality-adjusted life-year (QALY) gained. In cost–utility analysis, the effect is expressed in QALYs, which incorporates quantity of life (additional life-years) and quality of life into one measure. Thus, by dividing the difference in costs by the difference in QALYs, cost per QALY can be calculated for each comparison.
Generally, the National Institute for Health and Care Excellence (NICE) considers an intervention cost-effective if one of the following applies:
-
The intervention is less costly and more clinically effective than all other relevant alternatives. In this case, no ICER is calculated as the strategy in question dominates the alternatives.
-
The intervention has an ICER of < £20,000 per QALY compared with the next best alternative. This means that an investment of up to £20,000 in order to achieve an additional QALY is considered cost-effective.
The ICER is generally compared with the willingness-to-pay threshold of £20,000 per QALY gained as ‘accepted’ by NICE. A cost-effectiveness acceptability curve is produced to illustrate the probability of the intervention being cost-effective at different thresholds.
If the intervention is less effective and more costly than the comparator, the intervention is considered dominated. In this case, no ICER or cost-effectiveness acceptability curve is produced.
Cost–consequence analysis
We presented a tabular representation of costs versus changes in primary and secondary outcomes in a cost–consequence analysis. The cost–consequence approach presents all relevant outcome measures alongside the costs (without combining them into an ICER), to leave decision-makers the option to form their own view of relative importance.
Health economics: sensitivity analysis
Deterministic (univariate) sensitivity analyses investigated the robustness of the results to changes in estimated costs and outcomes. All ICERs were recalculated after changing the value of a range of parameters individually to estimate the robustness of the ICER (Table 14).
Parameter | Change from base case |
---|---|
PRISM pre-activation | Minimum (all done by PM) and maximum (all done by GP) cost |
PRISM activation support | Minimum (no site visits required) and maximum (all surgeries need site visit to assist with set-up) cost |
PRISM opportunity time for GP surgeries | Minimum and maximum time spent during trial period |
Number of emergency admissions | 95% CI |
Primary care costs | Minimum (all done by nurse) and maximum (all done by GP) cost |
Secondary care costs | Lower and upper quartile costs for all secondary cost components, as reported in NHS Reference Costs 2014/1568 |
Probabilistic sensitivity analysis with changes to the values of all chosen parameters [usually within the 95% confidence intervals (CIs) or a reasonable, defined range], used bootstrap resampling to determine the probability that the intervention was cost-effective when all uncertainty associated with the individual parameters was considered. The results of the probabilistic sensitivity analysis were expressed as percentage probability that the intervention was cost-effective.
Budget impact analysis
The budgetary impact of the adoption of the PRISM scoring tool in primary care was estimated from a NHS perspective based on the differences between the cost of emergency admissions and total cost, as obtained as part of the trial. We calculated the total budget impact per 100,000 patients registered in the trial GP surgeries, as observed over the trial period. For transparency, unadjusted and adjusted analyses are presented.
Deterministic sensitivity analysis used the lower and upper quartile unit costs – weighted across Healthcare Resource Groups and for activity – to estimate worst- and best-case scenarios of PRISM budget impact.
Stakeholder views
Focus groups, interviews and questionnaire
We collected qualitative data from GPs and practice staff, at baseline and after launching the PRISM intervention, to explore current practice in chronic conditions management and changes initiated by PRISM (see Table 11 for details regarding numbers of focus groups planned). Questions explored attitudes, expectations and experience relating to predictive risk stratification, specifically the PRISM tool, including barriers to and facilitators of use.
Before activating PRISM, we conducted four focus groups with staff from participating practices, two in the largest locality within the health board, and one in each of the other two localities. We interviewed GPs who could not take part in a focus group, by telephone or face to face. We also conducted a focus group with senior managers and community-based practitioners. Focus groups were used to explore different views and experiences by encouraging group interaction. 72
We also interviewed GPs and practice staff after activating PRISM to gain information in more depth about the adoption, use and perceived effectiveness of PRISM. We purposively sampled half the participating practices and sampled a PRISM user in each for interviews face to face between 3 and 6 months after PRISM was activated in their practice, and again at the end of the intervention phase, approximately 18 months after activation in the first practices. This enabled us to describe changes over time. We asked the other participating practices to complete online questionnaires at the same time points. We used responses from early adopters to inform later interviews. This enabled us to explore variations between participating practices.
In addition, at 18 months, we interviewed a senior manager within ABM UHB to explore area-wide issues in patient management and the effects of PRISM in general practices. Two other managers declined because they had moved to other roles. To gain historical, managerial and political insights into the development and scope of PRISM, we also interviewed six managers (health services commissioners and policy-makers) with all-Wales perspectives at baseline, either face to face or by telephone. We also conducted six interviews with seven respondents (one interview had two respondents) from non-participating health boards across Wales to examine their experience of PRISM and their views of its role and potential.
We contacted all respondents by e-mail. Three experienced researchers from the study team (BAE, AP and MRK) conducted all focus groups and interviews, lasting between 30 and 90 minutes. We recorded and transcribed these, with participants’ consent, after giving a participant information sheet explaining their purpose. We made field notes after each focus group or interview.
Qualitative analysis
We recorded and transcribed the focus groups and interviews and analysed them thematically. We chose this approach as it is a systematic and transparent method of analysis that generates themes from the explicit and implicit ideas contained in the original accounts of participants. Four team members (researchers BAE, MRK, AP and service user SW) read the transcripts and developed a coding framework. One researcher then led the analysis with the others independently supporting key stages of coding, generating themes and interpretation, thus encouraging a critical stance to test and confirm findings. 73–75
We used normalisation process theory (NPT)76,77 to inform our understanding of how the PRISM innovation came into practice. NPT is a conceptual framework to explain implementation of innovations in health care. 78 NPT offers four constructs to describe how innovation comes into normal practice:
-
How people understand the innovation and its purpose (coherence).
-
What decisions people take about using it, based on perceived advantages (cognitive participation).
-
What people do to bring the innovation into everyday use (collective action).
-
How the innovation is reviewed, modified or abandoned (reflexive monitoring). 77
Normalisation process theory suggests that each of these tasks is shaped by factors that promote or inhibit the extent to which participants look on a new practice as meaningful.
Sample size and power
We derived data on the number of emergency admissions per patient, the primary outcome for this study, from routine data, in principle, for all non-dissenting patients. We expected SAIL to yield data on about 250,000 people. We excluded people not registered with a study practice on day 1, and defined their final date in the study as the earliest of the date of death, the date of registration with another practice (including another study practice) or the end of the study. The Consolidated Standards of Reporting Trials (CONSORT) flow chart (see Figure 3) recorded the numbers dissenting or withdrawing from the study, or otherwise leaving before its end.
In considering sample sizes for postal questionnaires measuring secondary outcomes, we identified few general practice studies with ICCs of > 0.01. This led us to seek 800 respondents in each of the three questionnaire phases to detect differences in resource use between current intervention and control practices across the spectrum of risk. This would allow us to have 80% power when using a 5% significance level to detect a difference of 15% in the proportion of patients at defined risk levels receiving specified resources.
Missing data
Following the STU’s SOP on statistics, we adopted a consistent approach to missing data relating to effectiveness or cost-effectiveness except where individual outcome measures required variation in that approach. Although SAIL yielded data on our primary outcome for non-dissenting participants, some individual data were missing. We summarised the frequency of missing data for each variable, which directly influenced sample sizes, and hence the statistical power of analyses.
Project management
We organised the review, approval and adoption of the trial by STU. In undertaking the trial, we adhered to all relevant STU SOPs in the conduct, management and monitoring of the study. We set up a Research Management Group (RMG), which was responsible for the strategic management of the trial. This RMG met quarterly and comprised the chief investigator, all co-applicants, all research staff, two service users and two local participating GPs. We managed operational issues through a monthly research team meeting which was made up of the researchers, clerical support, the principal investigator and one of the co-applicants. We set up a data management task and finish group to oversee all data management and analysis issues. We used the STU SOP on data management to develop a data management plan, outlining details of data entry, coding, security and storage, including any related processes to promote data quality. We set up an independent Trial Steering Committee (TSC) that provided overall oversight and ensured the rigorous conduct of the trial. In addition, we organised an independent Data Monitoring and Ethics Committee (DMEC) which had oversight of the data management and analysis issues and which fed information into the TSC. We organised TSC meetings every 6 months, with the DMEC being held just prior to these meetings. The TSC was made up of an independent chairperson with an interest in emergency care, an academic in primary care, a consultant in public health, a statistician and two service users (with no previous involvement in the trial). The DMEC was chaired by the statistician with experience in trials and was also attended by the consultant in public health, the academic GP and the two service users. We adopted the principles outlined in STU’s SOPs on quality assurance and we carried out independent trial monitoring through the STU.
Service user involvement
In accordance with STU’s SOP for service user inclusion,79 we recruited two service users who acted throughout the study as collaborators in our research partnership. 80,81 As members of the RMG, they attended the quarterly meetings responsible for strategic and operational decisions about the study. They contributed, as equal team members, in all meetings to ensure we considered patients’ perspectives at all stages of the study.
We recruited these service users through Service Users with Chronic Conditions Encouraging Sensible Solutions (SUCCESS), a group of patients and carers engaged in research linked to the chronic conditions management policy in Wales (URL: www.invo.org.uk/posttypeconference/an-alternative-success-model/). The two service users linked the study to the wider SUCCESS group by reporting back on the study and seeking feedback from the SUCCESS group to inform their contributions.
We recruited two service users to the TSC through Involving People (URL: www.wales.nhs.uk/sites3/page.cfm?orgid=1023&pid=59261) to ensure their independence. We followed best practice by ensuring all users received honoraria, expenses, training and support, a named contact, information and networking opportunities. 81 We used the notes of meetings and other documents, including attendance records and draft papers, to describe their contributions to research structures and processes.
Ethics
We obtained full ethics approval for the main protocol and all subsequent amendments from the Multicentre Research Ethics Committee for Wales (reference number 10/MRE09/25). We received research and development permissions to conduct the trial across Wales, and Information Governance Review Panel permission to use the SAIL databank.
We complied with the CONSORT guidelines82 for reporting randomised trials and completed the CONSORT checklist when presenting findings from the trial. We also completed the CONSORT extension checklists for cluster trials, patient-reported outcomes, abstracts and harms.
Changes to the published protocol
The follow-up qualitative interviews/questionnaires were carried out at the end of the intervention phases (between October 2014 and January 2015). They were originally planned for 9 months after implementation of the intervention within each network.
We originally intended to compare time to first emergency admission but revised this plan without carrying out this analysis – on consideration, we concluded that emergency admissions per patient per year at risk would be the most appropriate analysis for this highly skewed data.
We did not include mortality as an outcome in our original protocol. We think it is important to document this as part of the study and have included it within Chapter 4.
Chapter 4 Clinical effectiveness
Participant flow
Figure 3 covers participant flow over the study period, extending from 1 February 2013 until 30 September 2014, based on specific dates within that period. Figure 3 gives (for each interval between such dates) the numbers of participant histories ending as a result of deregistration at a study practice (categorised as due to death, or for any other reason), and partitioned by study practice status (based on a ‘treatment allocated’ date) at the date of deregistration, and participant transfers from the control to intervention phase.
FIGURE 3.
Patient flow for primary outcome.
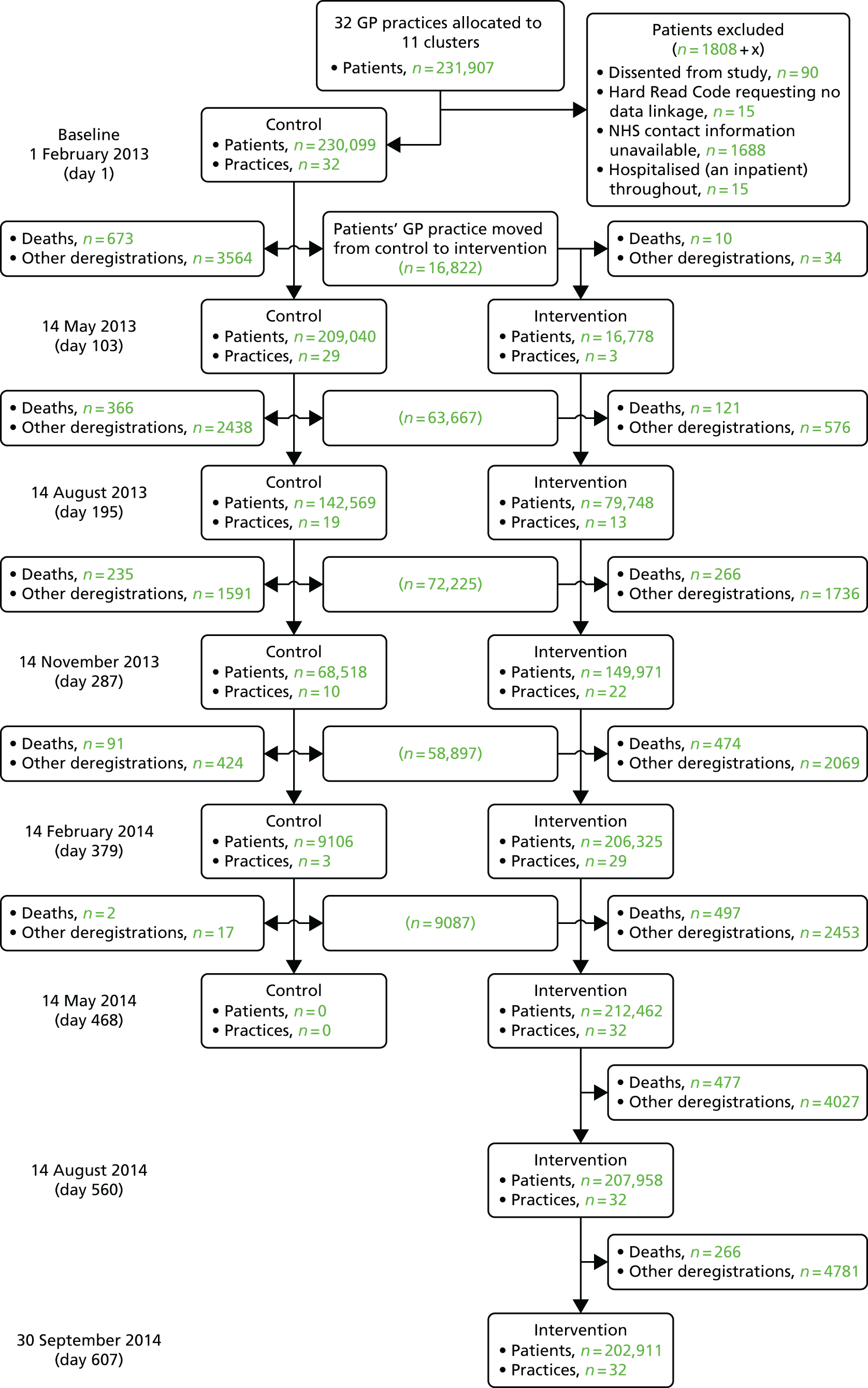
Figure 4 reprises the ‘stepped-wedge’ study design, and provides the transfer of first GP champion practices, then other study practices (grouped into 11 clusters each with a common, randomly allocated intervention date), from control to intervention phase. Some practices were unable to facilitate PRISM receipt and training in their allocated month, and hence their receipt dates occurred later in the intervention phase in Figure 4.
FIGURE 4.
Intervention allocation months and actual receipt dates.

Clinical effectiveness results
Data analysed and baseline characteristics
We have history of NHS contacts over the study period [from 1 February 2013 (study day 1) until 30 September 2014] on 230,114 participants, 15 of whom spent the whole study period in hospital. We were therefore able to include outcomes from routine NHS records in control and/or intervention phases on 230,099 participants. Table 15 summarises various characteristics of these participants, both overall and by PRISM risk group.
Variable | Proportion | % | |
---|---|---|---|
Gender | |||
Group | |||
All | |||
Female | 115,251/230,098 | 50.1 | |
Male | 114,847/230,098 | 49.9 | |
PRISM risk group 1 | |||
Female | 89,610/182,954 | 49.0 | |
Male | 93,344/182,954 | 51.0 | |
PRISM risk group 2 | |||
Female | 18,534/34,311 | 54.0 | |
Male | 15,777/34,311 | 46.0 | |
PRISM risk group 3 | |||
Female | 5820/10,292 | 56.5 | |
Male | 4472/10,292 | 43.5 | |
PRISM risk group 4 | |||
Female | 598/1129 | 53.0 | |
Male | 531/1129 | 47.0 | |
Mean | SD | n | |
Age at study day 1 (years) | |||
Group | |||
All | 41.2 | 23.4 | 230,099 |
PRISM risk group 1 | 36.6 | 20.5 | 182,955 |
PRISM risk group 2 | 56.7 | 25.2 | 34,311 |
PRISM risk group 3 | 69.8 | 21.4 | 10,292 |
PRISM risk group 4 | 70.2 | 22.1 | 1129 |
WIMD score (2011) | |||
Group | |||
All | 24.03 | 16.26 | 226,298 |
PRISM risk group 1 | 23.32 | 15.96 | 180,085 |
PRISM risk group 2 | 26.66 | 17.23 | 33,754 |
PRISM risk group 3 | 27.31 | 17.15 | 10,026 |
PRISM risk group 4 | 27.58 | 17.05 | 1093 |
WIMD health component (2011) | |||
Group | |||
All | 27.08 | 22.09 | 226,298 |
PRISM risk group 1 | 26.26 | 21.80 | 180,085 |
PRISM risk group 2 | 29.99 | 23.02 | 33,754 |
PRISM risk group 3 | 30.97 | 22.88 | 10,026 |
PRISM risk group 4 | 31.57 | 22.53 | 1093 |
PRISM score | |||
Group | |||
All | 6.53 | 6.89 | 228,687 |
PRISM risk group 1 | 4.16 | 1.61 | 182,955 |
PRISM risk group 2 | 11.49 | 3.18 | 34,311 |
PRISM risk group 3 | 26.15 | 7.97 | 10,292 |
PRISM risk group 4 | 60.87 | 13.63 | 1129 |
Durations in the control and intervention phases
As Figure 4 illustrates, the study design includes a relatively short initial period in which all participants are in the control phase, and a longer final period in which all participants still registered at a study practice are in the intervention phase; between these periods, participants transfer from one phase to another as the PRISM tool is made available at their practices. This design means that the mean length of time spent by a participant in the intervention phase is longer than in the control phase. Table 16 summarises these durations based on the ‘treatment allocated’ date at which the PRISM risk tool becomes available in study practices.
Variable | Mean | SD | n |
---|---|---|---|
Days in the control phase | |||
Group | |||
All | 226.3 | 91.3 | 230,099 |
PRISM risk group 1 | 226.9 | 91.0 | 182,955 |
PRISM risk group 2 | 226.7 | 91.2 | 34,311 |
PRISM risk group 3 | 219.2 | 93.8 | 10,292 |
PRISM risk group 4 | 202.3 | 99.1 | 1129 |
Days in the intervention phase | |||
Group | |||
All | 364.0 | 101.3 | 220,698 |
PRISM risk group 1 | 365.3 | 100.0 | 176,217 |
PRISM risk group 2 | 362.5 | 102.1 | 32,936 |
PRISM risk group 3 | 349.2 | 113.4 | 9356 |
PRISM risk group 4 | 318.8 | 128.1 | 898 |
Outcomes from anonymised routine linked NHS data
Tables 17–22 present the results of primary and secondary outcomes derived from anonymised routine linked NHS data sets. We provide raw and adjusted comparisons between groups, ICC in variables between participants at the same study practice, and details of statistically significant factors and covariates. The adjusted comparison reflects the nature of the variable under consideration: we present an OR for logistic regression models for binary variables; an incident or event rate ratio Λ from negative binomial models for count variables, and an additive group effect (Δ, in the same units as the dependent variable) for linear models for continuous variables.
Table 17 covers the primary outcome, emergency hospital admissions, in which we summarise separate analyses of a binary variable (whether or not a participant had any emergency hospital admission in each phase); a count variable (the number of such admissions); and a measurement variable (an annualised rate of such admissions, based on the ‘days at risk’ of such an admission, which excludes days in which participants were known to be hospitalised). Thus, ‘days at risk’ features explicitly in the analysis of rates of admissions, and is included as a potential covariate in the analysis of binary and count variables. Table 18 and so on follow broadly the same format for selected secondary outcomes; Table 21, on inpatient visits, considers only the days per year each participant is hospitalised in each phase.
Outcome | Group | Phase (uncorrected for phase duration) | Adjusted comparison | 95% CI | ICC | ||
---|---|---|---|---|---|---|---|
Intervention | Control | Estimate | 95% CI | ||||
Proportion of participants with one or more emergency hospital admission: proportion (%) | Alla | 15,743/220,683 (7.1) | 10,870/230,087 (4.7) | OR = 1.444 (p < 0.001) | 1.395 to 1.495 | 0.0009 | 0.0005 to 0.0015 |
Risk group 1b | 7746/176,214 (4.4) | 5029/182 to 952 (2.7) | OR = 1.199 (p < 0.001) | 1.144 to 1.257 | |||
Risk group 2c | 4521/32,929 (13.7) | 3031/34 to 307 (8.8) | OR = 1.665 (p < 0.001) | 1.561 to 1.776 | |||
Risk group 3d | 2881/9352 (30.8) | 2230/10 to 288 (21.7) | OR = 1.941 (p < 0.001) | 1.784 to 2.112 | |||
Risk group 4e | 497/897 (55.4) | 504/1128 (44.7) | OR = 1.707 (p < 0.001) | 1.400 to 2.081 | |||
Number of emergency hospital admissions per participant: mean (SD) [n] | Allf | 0.1045 (0.5323) [220,683] | 0.0635 (0.3429) [230,087] | Λ = 1.429 (p < 0.001) | 1.385 to 1.474 | 0.0007 | 0.0004 to 0.0011 |
Risk group 1g | 0.0557 (0.3107) [176,214] | 0.0337 (0.2247) [182,952] | Λ = 1.226 (p < 0.001) | 1.171 to 1.284 | |||
Risk group 2h | 0.2095 (0.8218) [32,929] | 0.1188 (0.4805) [34,307] | Λ = 1.630 (p < 0.001) | 1.538 to 1.728 | |||
Risk group 3i | 0.5362 (1.2799) [9352] | 0.3193 (0.7471) [10,288] | Λ = 1.716 (p < 0.001) | 1.623 to 1.814 | |||
Risk group 4j | 1.3088 (2.0484) [897] | 0.8688 (1.4037) [1128] | Λ = 1.389 (p < 0.001) | 1.225 to 1.575 | |||
Numbers of emergency hospital admissions per participant per year at risk: mean (SD) [n] | Allk | 0.1666 (2.0794) [220,683] | 0.1607 (2.1634) [230,087] | Δ = 0.005 (p = 0.425) | –0.007 to 0.016 | 0.0001 | 0 to 0.0002 |
Risk group 1l | 0.0663 (0.7455) [176,214] | 0.0636 (0.6987) [182,952] | Δ = 0.002 (p = 0.351) | –0.002 to 0.006 | |||
Risk group 2m | 0.3663 (3.6970) [32,929] | 0.2943 (2.3583) [34,307] | Δ = 0.053 (p = 0.019) | 0.009 to 0.097 | |||
Risk group 3n | 1.0509 (5.4392) [9352] | 1.0655 (6.9002) [10,288] | Δ = –0.035 (p = 0.684) | –0.204 to 0.134 | |||
Risk group 4o | 3.3003 (10.9239) [897] | 3.4817 (15.3970) [1128] | Δ = –0.063 (p = 0.915) | –1.216 to 1.090 | |||
Log-transformed numbers of emergency hospital admissions per participant per year at risk: mean (SD) [n] | Allp | 0.0664 (0.2762) [220,683] | 0.0566 (0.2819) [230,087] | ΔL = 0.011 (p < 0.001) | 0.010 to 0.013 | 0.0007 | 0.0004 to 0.0011 |
Risk group 1q | 0.0355 (0.1811) [176,214] | 0.0294 (0.1879) [182,952] | ΔL = 0.0061 (p < 0.001) | 0.005 to 0.007 | |||
Risk group 2r | 0.1326 (0.3888) [32,929] | 0.1065 (0.3794) [34,307] | ΔL = 0.0260 (p < 0.001) | 0.021 to 0.031 | |||
Risk group 3s | 0.3454 (0.6267) [9352] | 0.2987 (0.6484) [10,288] | ΔL = 0.0609 (p < 0.001) | 0.045 to 0.076 | |||
Risk group 4t | 0.7978 (0.9315) [897] | 0.7344 (0.9761) [1128] | ΔL = 0.1097 (p = 0.002) | 0.040 to 0.179 |
Table 17 shows, following adjustment for length of time in each phase and all other significant covariates, an increase in the proportion of participants who experienced an emergency admission to hospital in the intervention phase compared with the control phase. The number of emergency admissions per participant was also higher in the intervention phase. These data are highly skewed, with most participants (> 90%) not experiencing any admissions, but a few experiencing multiple admissions. Analysis using log-transformed data is therefore appropriate, and shows an increase of 1% in emergency admissions per participant per year at risk in the intervention phase. These effects were consistent across risk groups, and increased with predicted risk level.
Table 18 shows, following adjustment for length of time in each phase and all other significant covariates, an increase in the proportion of participants who attended the ED in the intervention phase compared with the control phase. The number of ED attendances per participant was also higher in the intervention phase. These data are also highly skewed, with most participants (> 80%) not experiencing any attendances, but a few attending on multiple occasions. Analysis using log-transformed data is therefore appropriate, and shows an increase of 3% in emergency admissions per participant per year at risk in the intervention phase. These effects were consistent across risk groups, and increased with predicted risk level.
Outcome | Group | Phase (uncorrected for phase duration) | Adjusted comparison | 95% CI | ICC | ||
---|---|---|---|---|---|---|---|
Intervention | Control | Estimate | 95% CI | ||||
Proportion of participants with one or more ED attendance: proportion (%) | Alla | 43,991/220,683 (19.9) | 30,350/230,087 (13.2) | OR = 1.116 (p < 0.001) | 1.092 to 1.141 | 0.0038 | 0.0023 to 0.0057 |
Risk group 1b | 31,075/176,214 (17.6) | 20,688/182,952 (11.3) | OR = 1.047 (p = 0.001) | 1.019 to 1.074 | |||
Risk group 2c | 8471/32,929 (25.7) | 6017/34,307 (17.5) | OR = 1.221 (p < 0.001) | 1.161 to 1.283 | |||
Risk group 3d | 3684/9352 (39.4) | 2925/10,288 (28.4) | OR = 1.495 (p < 0.001) | 1.384 to 1.614 | |||
Risk group 4e | 510/897 (56.9) | 535/1128 (47.4) | OR = 1.257 (p = 0.033) | 1.018 to 1.552 | |||
Number of ED attendances per participant: mean (SD) [n] | Allf | 0.3200 (0.8863) [220,683] | 0.1894 (0.6139) [230,087] | Λ = 1.115 (p < 0.001) | 1.095 to 1.136 | 0.0037 | 0.0022 to 0.0056 |
Risk group 1g | 0.2649 (0.7164) [176,214] | 0.1543 (0.5143) [182,952] | Λ = 1.045 (p < 0.001) | 1.022 to 1.068 | |||
Risk group 2h | 0.4464 (1.1010) [32,929] | 0.2589 (0.7155) [34,307] | Λ = 1.226 (p < 0.001) | 1.177 to 1.277 | |||
Risk group 3i | 0.7951 (1.7995) [9352] | 0.4820 (1.0669) [10,288] | Λ = 1.380 (p < 0.001) | 1.306 to 1.459 | |||
Risk group 4j | 1.5385 (2.82864) [897] | 1.1135 (2.3650) [1128] | Λ = 1.173 (p = 0.012) | 1.036 to 1.327 | |||
Numbers of ED attendances per participant per year at risk: mean (SD) [n] | Allk | 0.3613 (1.7877) [220,683] | 0.3593 (2.1014) [230,087] | Δ = 0.006 (p = 0.299) | –0.005 to 0.016 | 0.0013 | 0.0007 to 0.0020 |
Risk group 1l | 0.2705 (1.0942) [176,214] | 0.2658 (1.0969) [182,952] | Δ = 0.005 (p = 0.148) | –0.002 to 0.011 | |||
Risk group 2m | 0.5453 (2.7529) [32,929] | 0.4941 (1.9043) [34,307] | Δ = 0.044 (p = 0.010) | 0.011 to 0.077 | |||
Risk group 3n | 1.1663 (4.1921) [9352] | 1.2432 (6.2355) [10,288] | Δ = –0.066 (p = 0.340) | –0.122 to 0.146 | |||
Risk group 4o | 3.0370 (8.3959) [897] | 3.235 (14.4377) [1128] | Δ = –0.134 (p = 0.793) | –1.093 to 0.908 | |||
Log-transformed numbers of ED attendances per participant per year at risk: mean (SD) [n] | Allp | 0.1802 (0.4035) [220,683] | 0.1528 (0.4259) [230,087] | ΔL = 0.030 (p < 0.001) | 0.028 to 0.032 | 0.0034 | 0.0021 to 0.0052 |
Risk group 1q | 0.1506 (0.3542) [176,214] | 0.1253 (0.3755) [182,952] | ΔL = 0.026 (p < 0.001) | 0.024 to 0.029 | |||
Risk group 2r | 0.2477 (0.4793) [32,929] | 0.2084 (0.4928) [34,307] | ΔL = 0.040 (p < 0.001) | 0.034 to 0.047 | |||
Risk group 3s | 0.4390 (0.6540) [9352] | 0.3887 (0.7000) [10,288] | ΔL = 0.058 (p < 0.001) | 0.042 to 0.075 | |||
Risk group 4t | 0.8096 (0.9094) [897] | 0.7649 (0.9543) [1128] | ΔL = 0.074 (p = 0.035) | 0.005 to 0.143 |
Table 19 shows a more mixed picture. Following adjustment for length of time in each phase and all other significant covariates, we found a decrease in the proportion of participants with GP event-days recorded in the intervention phase compared with the control phase, an effect that was consistent across risk groups. However, the number of days when GP activity was recorded per participant per year at risk was higher in the intervention phase. Although these data are less skewed, with most participants (> 80%) experiencing event-days, the rate of events is still heavily weighted to the smaller numbers at highest predicted risk of emergency admission to hospital. Analysis using log-transformed data is, again, therefore, appropriate, and shows an increase of 1% in days on which GP activity was recorded per participant per year at risk in the intervention phase. This effect was reversed among the two highest risk groups.
Outcome | Group | Phase (uncorrected for phase duration) | Adjusted comparison | 95% CI | ICC | ||
---|---|---|---|---|---|---|---|
Intervention | Control | Estimate | 95% CI | ||||
Proportion of participants with one or more GP event-days: proportion (%) | Alla | 185,814/220,683 (84.2) | 185,372/230,087 (80.6) | OR = 0.697 (p < 0.001) | 0.683 to 0.712 | 0.1603 | 0.1047 to 0.2215 |
Risk group 1b | 143,792/176,214 (81.6) | 140,582/182,952 (76.8) | OR = 0.733 (p < 0.001) | 0.717 to 0.750 | |||
Risk group 2c | 31,249/32,929 (94.9) | 32,774/34,307 (95.5) | OR = 0.2718 (p < 0.001) | 0.245 to 0.302 | |||
Risk group 3d | 8942/9352 (95.6) | 9892/10,288 (96.2) | OR = 0.384 (p < 0.001) | 0.322 to 0.459 | |||
Risk group 4e | 856/897 (95.4) | 1079/1128 (95.7) | OR = 0.495 (p < 0.001) | 0.307 to 0.796 | |||
Number of GP event-days per participant: mean (SD) [n] | Allf | 13.275 (16.268) [220,683] | 8.389 (11.020) [230,087] | Λ = 0.960 (p < 0.001) | 0.957 to 0.964 | 0.0372 | 0.0230 to 0.0544 |
Risk group 1g | 9.330 (11.374) [176,214] | 5.738 (7.385) [182,952] | Λ = 0.966 (p < 0.001) | 0.961 to 0.971 | |||
Risk group 2h | 26.393 (19.607) [32,929] | 16.792 (13.446) [34,307] | Λ = 0.961 (p < 0.001) | 0.955 to 0.967 | |||
Risk group 3i | 38.538 (25.942) [9352] | 25.108 (18.923) [10,288] | Λ = 0.966 (p < 0.001) | 0.955 to 0.977 | |||
Risk group 4j | 46.641 (32.218) [897] | 32.072 (25.518) [1128] | Λ = 0.912 (p < 0.001) | 0.879 to 0.946 | |||
Numbers of GP event-days per participant per year at risk: mean (SD) [n] | Allk | 14.075 (32.593) [220,683] | 14.100 (23.124) [230,087] | Δ = 0.139 (p = 0.011) | 0.032 to 0.246 | 0.0165 | 0.0101 to 0.0244 |
Risk group 1l | 9.418 (13.673) [176,214] | 9.319 (12.548) [182,952] | Δ = 0.044 (p = 0.113) | –0.010 to 0.098 | |||
Risk group 2m | 29.239 (43.279) [32,929] | 27.784 (24.957) [34,307] | Δ = 0.301 (p = 0.196) | –0.155 to 0.756 | |||
Risk group 3n | 47.186 (109.44) [9352] | 46.590 (53.813) [10,288] | Δ = 1.973 (p = 0.002) | 0.741 to 3.204 | |||
Risk group 4o | 67.297 (68.665) [897] | 78.721 (120.98) [1128] | Δ = –8.383 (p = 0.035) | –16.186 to 0.581 | |||
Log-transformed numbers of GP event-days per participant per year at risk: mean (SD) [n] | Allp | 2.027 (1.260) [230,087] | 2.015 (1.292) [230,087] | Δ = 0.011 (p < 0.001) | 0.007 to 0.014 | 0.1118 | 0.0716 to 0.1580 |
Risk group 1q | 1.755 (1.157) [176,214] | 1.723 (1.193) [182,952] |
ΔL = 0.023 (p < 0.001) |
0.018 to 0.027 | |||
Risk group 2r | 3.019 (0.999) [32,929] | 3.052 (0.935) [34,307] |
ΔL = 0.042 (p < 0.001) |
–0.049 to 0.035 | |||
Risk group 3s | 3.512 (0.993) [9352] | 3.545 (0.947) [10,288] |
ΔL = –0.031 (p < 0.001) |
–0.044 to 0.018 | |||
Risk group 4t | 3.860 (1.069) [897] | 3.955 (1.076) [1128] |
ΔL = –0.090 (p < 0.001) |
–0.139 to 0.041 |
Table 20 also shows a mixed picture. Following adjustment for length of time in each phase and all other significant covariates, we found overall no difference in the proportion of participants with outpatient visits in the intervention phase compared with the control phase, with varying effects across the risk groups. Analysis using log-transformed data shows an increase of 5% in outpatient attendances per participant per year at risk in the intervention phase, an effect related to an increase in the two lowest risk groups.
Outcome | Group | Phase | Adjusted comparison | 95% CI | ICC | ||
---|---|---|---|---|---|---|---|
Intervention | Control | Estimate | 95% CI | ||||
Proportion of participants with one or more outpatient visits: proportion (%) | Alla | 81,619/220,683 (37.0) | 67,324/230,087 (29.3) | OR = 1.015 (p = 0.177) | 0.993 to 1.038 | 0.0039 | 0.0023 to 0.0059 |
Risk group 1b | 52,954/176,214 (30.1) | 40,532/182,952 (22.2) | OR = 1.082 (p < 0.001) | 1.055 to 1.110 | |||
Risk group 2c | 20,495/32,929 (62.2) | 18,459/34,307 (53.8) | OR = 0.880 (p < 0.001) | 0.835 to 0.927 | |||
Risk group 3d | 7055/9352 (75.4) | 7153/10,288 (69.5) | OR = 0.785 (p < 0.001) | 0.708 to 0.870 | |||
Risk group 4e | 740/897 (82.5) | 862/1128 (76.4) | OR = 0.788 (p = 0.200) | 0.547 to 1.135 | |||
Number of outpatient visits per participant: mean (SD) [n] | Allf | 1.5446 (3.4375) [220,683] | 0.9439 (2.3067) [230,087] | Λ = 1.002 (p = 0.717) | 0.990 to 1.014 | 0.0022 | 0.0012 to 0.0033 |
Risk group 1g | 1.0590 (2.6408) [176,214] | 0.6089 (1.7004) [182,952] | Λ = 1.050 (p < 0.001) | 1.033 to 1.067 | |||
Risk group 2h | 3.0792 (4.6168) [32,929] | 1.9572 (3.2040) [34,307] | Λ = 0.956 (p < 0.001) | 0.937 to 0.975 | |||
Risk group 3i | 4.8505 (6.1833) [9352] | 3.1632 (4.2297) [10,288] | Λ = 0.955 (p = 0.002) | 0.927 to 0.983 | |||
Risk group 4j | 6.7592 (8.5298) [897] | 4.5142 (5.3923) [1128] | Λ = 0.936 (p = 0.073) | 0.870 to 1.006 | |||
Numbers of outpatient visits per participant per year at risk: mean (SD) [n] | Allk | 1.717 (9.746) [220,683] | 1.704 (9.984) [230,087] | Δ = 0.035 (p = 0.158) | –0.014 to 0.085 | 0.0004 | 0.0002 to 0.000 |
Risk group 1l | 1.086 (5.630) [176,214] | 1.022 (4.543) [182,952] | Δ = 0.058 (p < 0.001) | 0.028 to 0.088 | |||
Risk group 2m | 3.417 (11.207) [32,929] | 3.460 (13.720) [34,307] | Δ = –0.015 (p = 0.846) | –0.172 to 0.141 | |||
Risk group 3n | 6.547 (27.087) [9352] | 6.693 (27.846) [10,288] | Δ = –0.006 (p = 0.984) | –0.618 to 0.606 | |||
Risk group 4o | 13.503 (65.558) [897] | 13.833 (59.869) [1128] | Δ = –0.147 (p = 0.955) | –5.246 to 4.952 | |||
Log-transformed numbers of outpatient visits per participant per year at risk: mean (SD) [n] | Allp | 0.5266 (0.7975) [220,683] | 0.4760 (0.8213) [230,087] | ΔL = 0.055 (p < 0.001) | 0.051 to 0.058 | 0.0034 | 0.0020 to 0.005 |
Risk group 1q | 0.3919 (0.6812) [176,214] | 0.3344 (0.6859) [182,952] | ΔL = 0.056 (p < 0.001) | 0.053 to 0.060 | |||
Risk group 2r | 0.9752 (0.9311) [32,929] | 0.9280 (0.9881) [34,307] | ΔL = 0.050 (p < 0.001) | 0.040 to 0.060 | |||
Risk group 3s | 1.3791 (1.0361) [9352] | 1.3605 (1.0996) [10,288] | ΔL = 0.025 (p = 0.016) | 0.005 to 0.046 | |||
Risk group 4t | 1.7931 (1.1689) [897] | 1.7711 (1.2411) [1128] | ΔL = –0.007 (p = 0.851) | –0.076 to 0.063 |
Table 21 shows, following adjustment for length of time in each phase and all other significant covariates, no effect in mean bed-days per patient per year at risk. However, these data are highly skewed, with most participants (> 90%) not experiencing any hospital stays, but a few attending on multiple occasions and some with very long lengths of stay. Analysis using log-transformed data is therefore appropriate, and shows an increase of 3% in mean bed-days per participant per year at risk in the intervention phase. This effect was consistent across risk groups, and increased with predicted risk level.
Outcome | Group | Phase, mean (SD) [n] | Adjusted comparison | 95% CI | ICC | ||
---|---|---|---|---|---|---|---|
Intervention | Control | Estimate | 95% CI | ||||
Days per year hospitalised per participant | Alla | 0.728 (5.886) [220,683] | 0.792 (9.934) [230,087] | Δ = –0.010 (p = 0.628) | –0.051 to 0.031 | 0 | n/a |
Risk group 1b | 0.263 (3.316) [176,214] | 0.276 (7.577) [182,952] | Δ = 0.006 (p = 0.660) | –0.020 to 0.032 | |||
Risk group 2c | 1.620 (8.711) [32,929] | 1.631 (12.165) [34,307] | Δ = 0.002 (p = 0.975) | –0.147 to 0.151 | |||
Risk group 3d | 5.157 (15.314) [9352] | 5.613 (22.571) [10,288] | Δ = –0.148 (p = 0.549) | –0.633 to 0.336 | |||
Risk group 4e | 13.384 (25.907) [897] | 15.148 (33.928) [1128] | Δ = –1.121 (p = 0.360) | –3.522 to 1.280 | |||
Log-transformed days per year hospitalised per participant | Allf | 0.1251 (0.5271) [220,683] | 0.0996 (0.5070) [230,087] | ΔL = 0.029 (p < 0.001) | 0.026 to 0.031 | 0.0007 | 0.0004 to 0.0011 |
Risk group 1g | 0.0621 (0.3374) [176,214] | 0.04707 (0.3170) [182,952] | ΔL = 0.015 (p < 0.001) | 0.013 to 0.017 | |||
Risk group 2h | 0.2635 (0.7631) [32,929] | 0.1976 (0.7157) [34,307] | ΔL = 0.066 (p < 0.001) | 0.056 to 0.076 | |||
Risk group 3i | 0.6993 (1.2060) [9352] | 0.5711 (1.2056) [10,288] | ΔL = 0.150 (p < 0.001) | 0.120 to 0.180 | |||
Risk group 4j | 1.4642 (1.5466) [897] | 1.3158 (1.6480) [1128] | ΔL = 0.197 (p < 0.001) | 0.073 to 0.320 |
Table 22 shows mortality by practice cluster. We have not carried out full, adjusted analyses for this variable as this was not a formally set outcome; however, we present this as a background check of safety and have found no clear effect associated with trial phase.
Cluster | Treatment allocated date | Phase | |||
---|---|---|---|---|---|
Intervention | Control | ||||
Proportion of deaths | Deaths per 1000 patients per year | Proportion of deaths | Deaths per 1000 patients per year | ||
1 | 30 April 2013 | 229/16,822 | 9.57 | 43/17,061 | 10.45 |
2 | 31 May 2013 | 305/24,645 | 9.26 | 97/25,166 | 11.82 |
3 | 30 June 2013 | 259/21,879 | 9.43 | 82/22,442 | 8.95 |
4 | 31 July 2013 | 169/17,143 | 8.43 | 82/17,754 | 9.37 |
5 | 31 August 2013 | 322/30,295 | 9.80 | 170/31,193 | 9.43 |
6 | 30 September 2013 | 231/27,349 | 8.42 | 166/28,624 | 8.78 |
7 | 31 October 2013 | 174/14,581 | 13.00 | 143/15,246 | 12.59 |
8 | 30 November 2013 | 125/18,067 | 8.28 | 130/19,596 | 8.01 |
9 | 31 December 2013 | 134/24,470 | 7.29 | 205/25,849 | 8.69 |
10 | 31 January 2014 | 105/16,360 | 9.64 | 154/17,440 | 8.85 |
11 | 28 February 2014 | 58/9087 | 10.84 | 95/9728 | 9.09 |
Weighted average (based on cluster size) | 9.25 | 9.58 |
Table 23 illustrates that there was not a large variation in the profile of PRISM scores between clusters of practices (mean range between 5.76 to 7.44 out of a maximum of 100).
Cluster | PRISM risk scores | ||||
---|---|---|---|---|---|
Mean (SD) [n: ≈missing] | Minimum, maximum | ||||
Risk group 1 | Risk group 2 | Risk group 3 | Risk group 4 | ||
1 | 7.31 (7.37) [17,017: ≈44] | 1.68, 9.49 | 7.44, 21.31 | 16.64, 50.70 | 46.72, 99.55 |
2 | 6.63 (7.02) [25,120: ≈46] | 1.72, 9.04 | 6.93, 20.72 | 14.90, 54.21 | 44.82, 96.49 |
3 | 6.52 (7.00) [21,788: ≈654] | 1.78, 9.41 | 4.07, 20.39 | 6.76, 53.81 | 19.40, 98.81 |
4 | 6.56 (6.84) [17,718: ≈36] | 1.72, 8.39 | 8.01, 19.25 | 17.52, 49.12 | 42.14, 97.05 |
5 | 6.61 (6.66) [31,167: ≈26] | 1.72, 8.85 | 7.44, 19.89 | 15.98, 48.39 | 37.21, 91.13 |
6 | 5.76 (6.18) [28,408: ≈216] | 1.69, 7.02 | 6.79, 16.21 | 15.78, 41.84 | 41.98, 96.35 |
7 | 7.44 (7.46) [15,231: ≈15] | 1.74, 11.57 | 8.62, 23.11 | 19.05, 54.66 | 47.28, 99.84 |
8 | 6.97 (7.36) [19,547: ≈49] | 1.72, 9.65 | 8.12, 21.95 | 17.59, 60.48 | 48.91, 99.64 |
9 | 5.84 (6.45) [25,764: ≈85] | 1.68, 7.06 | 6.77, 16.55 | 15.59, 46.02 | 44.24, 98.66 |
10 | 6.28 (6.89) [17,217: ≈223] | 1.72, 7.639 | 7.41, 17.44 | 16.93, 51.20 | 48.45, 97.85 |
11 | 6.85 (7.03) [9710: ≈18] | 1.84, 8.84 | 6.76, 20.18 | 12.49, 49.44 | 35.72, 89.91 |
All clusters | 6.53 (6.89) [228,687: ≈1412] | 1.68, 11.57 | 4.07, 23.11 | 6.76, 60.48 | 19.40, 99.84 |
Self-reported outcomes
We analysed data from 2362 questionnaire responses from 1403 distinct participants; these responses yield SF-12 Mental Health Component and Physical Health Component scores data, and hence SF-6D values, and a quality of care measure. As questionnaires were sent to a sample deliberately skewed towards those with higher PRISM scores, we separately summarise characteristics of respondents in Table 24.
Variable | Proportion | % | |
---|---|---|---|
Gender | |||
Group | |||
All | |||
Female | 736/1403 | 52.5 | |
Male | 667/1403 | 47.5 | |
PRISM risk group 1 | |||
Female | 119/217 | 54.8 | |
Male | 98/217 | 45.2 | |
PRISM risk group 2 | |||
Female | 201/374 | 53.7 | |
Male | 173/374 | 46.3 | |
PRISM risk group 3 | |||
Female | 346/670 | 51.6 | |
Male | 324/670 | 48.4 | |
PRISM risk group 4 | |||
Female | 68/140 | 48.6 | |
Male | 72/140 | 51.4 | |
Mean | SD | n | |
Age at study day 1 (years) | |||
Group | |||
All | 68.2 | 16.4 | 1403 |
PRISM risk group 1 | 50.1 | 15.5 | 217 |
PRISM risk group 2 | 67.8 | 14.6 | 374 |
PRISM risk group 3 | 73.6 | 13.5 | 670 |
PRISM risk group 4 | 71.3 | 15.0 | 140 |
WIMD (2011) | |||
Group | |||
All | 27.87 | 16.67 | 1379 |
PRISM risk group 1 | 23.06 | 14.57 | 217 |
PRISM risk group 2 | 28.51 | 16.70 | 369 |
PRISM risk group 3 | 28.98 | 17.00 | 654 |
PRISM risk group 4 | 28.68 | 16.95 | 137 |
WIMD (2011) health component | |||
Group | |||
All | 31.58 | 22.58 | 1379 |
PRISM risk group 1 | 26.74 | 20.73 | 217 |
PRISM risk group 2 | 32.76 | 22.54 | 369 |
PRISM risk group 3 | 32.46 | 23.40 | 654 |
PRISM risk group 4 | 31.92 | 20.80 | 137 |
PRISM score | |||
Group | |||
All | 23.14 | 17.33 | 1401 |
PRISM risk group 1 | 4.26 | 1.86 | 217 |
PRISM risk group 2 | 12.77 | 3.98 | 374 |
PRISM risk group 3 | 27.09 | 9.15 | 670 |
PRISM risk group 4 | 61.19 | 14.00 | 140 |
Table 25 shows no difference in Mental Health Component scores but higher (improved) Physical Health Component scores in respondents in the intervention phase, with a trend towards greater improvement in those in the higher-risk groups. However, no differences were evident when questionnaire responses were summarised by an overall SF-6D. Satisfaction scores were slightly lower overall in the intervention phase, although there was no clear pattern across risk groups.
Outcome | Group | Phase, mean (SD) [n] | Adjusted comparison | 95% CI | ICC | ||
---|---|---|---|---|---|---|---|
Intervention | Control | Estimate | 95% CI | ||||
SF-12 Mental Health Component | Alla | 43.77 (9.67) [1410] | 44.62 (9.47) [662] | Δ = –0.720 (p = 0.060) | –1.469 to 0.030 | 0.0164 | 0.0063 to 0.0320 |
Risk group 1b | 49.44 (8.34) [242] | 49.03 (8.07) [83] | Δ = 1.736 (p = 0.037) | 0.106 to 3.366 | |||
Risk group 2c | 43.72 (8.97) [381] | 45.18 (8.85) [163] | Δ = –0.802 (p = 0.268) | –2.222 to 0.618 | |||
Risk group 3d | 42.54 (9.53) [659] | 44.13 (9.80) [322] | Δ = –1.187 (p = 0.040) | –2.317 to 0.056 | |||
Risk group 4e | 39.61 (10.35) [127] | 41.45 (9.10) [94] | Δ = –1.911 (p = 0.084) | –4.079 to 0.258 | |||
SF-12 Physical Health Component | Allf | 41.72 (9.20) [1410] | 40.07 (7.30) [662] | Δ = 1.465 (p < 0.001) | 0.774 to 2.157 | 0.0091 | 0.0024 to 0.0210 |
Risk group 1g | 34.41 (9.09) [242] | 36.77 (7.62) [83] | Δ = –4.385 (p < 0.001) | –6.106 to 2.664 | |||
Risk group 2h | 41.90 (8.18) [381] | 40.65 (7.12) [163] | Δ = 0.882 (p = 0.159) | –0.345 to 2.108 | |||
Risk group 3i | 43.54 (8.45) [659] | 40.18 (7.15) [322] | Δ = 3.205 (p < 0.001) | 2.218 to 4.191 | |||
Risk group 4j | 45.56 (8.76) [127] | 41.61 (7.11) [94] | Δ = 4.103 (p < 0.001) | 2.230 to 5.977 | |||
SF-6D score | Allk | 0.6380 (0.0682) [1467] | 0.6382 (0.0719) [692] | Δ = –0.002 (p = 0.584) | –0.008 to 0.004 | 0.0097 | 0.0026 to 0.0222 |
Risk group 1l | 0.6293 (0.0687 [247] | 0.6417 (0.0773) [83] | Δ = –0.019 (p < 0.001) | –0.034 to 0.003 | |||
Risk group 2m | 0.6387 (0.0716) [399] | 0.6408 (0.0752) [166] | Δ = –0.003 (p = 0.649) | –0.015 to 0.010 | |||
Risk group 3n | 0.6414 (0.0673) [690] | 0.6375 (0.0700) [341] | Δ = 0.002 (p = 0.628) | –0.006 to 0.010 | |||
Risk group 4o | 0.6347 (0.0598) [130] | 0.6333 (0.0687) [102] | Δ = 0.002 (p = 0.787) | –0.013 to 0.017 | |||
Quality of care | Allp | 4.196 (0.737) [1408] | 4.268 (0.695) [660] | Δ = –0.074 (p = 0.014) | –0.133 to 0.015 | 0.0392 | 0.0198 to 0.0651 |
Risk group 1q | 4.188 (0.720) [189] | 3.957 (0.860) [62] | Δ = 0.169 (p = 0.095) | –0.030 to 0.368 | |||
Risk group 2r | 4.244 (0.694) [391] | 4.275 (0.716) [162] | Δ = –0.080 (p = 0.156) | –0.191 to 0.031 | |||
Risk group 3s | 4.200 (0.736) [697] | 4.360 (0.607) [340] | Δ = –0.107 (p = 0.008) | –0.186 to 0.029 | |||
Risk group 4t | 4.040 (0.867) [130] | 4.130 (0.762) [96] | Δ = –0.100 (p = 0.291) | –0.286 to 0.086 |
Technical performance
Data analysed
As Figure 3 shows, relatively few participants (based on ‘treatment allocation’ dates) were still in the control phase on 31 January 2014. To mitigate this reduction, we therefore included in this part of the analysis data from the ‘lead-in’ period from 1 August 2012 to 31 January 2013 and used this in conjunction with the corresponding data from study day 1 onwards. The earliest PRISM score within this extended window is dated 2 September 2012 and is available for 96,314 out of the 230,999 participants. Just over half (n = 51,570) of these 96,314 participants were still in the control phase on 31 July 2013 and this subsample of 51,570 participants is the basis of the analysis in this section. Table 26 summarises the characteristics of these participants.
Variable | Proportion | % | |
---|---|---|---|
Gender | |||
Group | |||
All | |||
Female | 25,454/51,570 | 49.4 | |
Male | 26,116/51,570 | 50.6 | |
PRISM risk group 1 | |||
Female | 19,763/41,119 | 48.1 | |
Male | 21,356/41,119 | 51.9 | |
PRISM risk group 2 | |||
Female | 4172/7834 | 53.3 | |
Male | 3662/7834 | 46.7 | |
PRISM risk group 3 | |||
Female | 1385/2367 | 58.5 | |
Male | 982/2367 | 41.5 | |
PRISM risk group 4 | |||
Female | 134/250 | 53.6 | |
Male | 116/250 | 47.4 | |
Mean | SD | n | |
Age at study day 1 (years) | |||
Group | |||
All | 42.2 | 23.2 | 51,570 |
PRISM risk group 1 | 37.4 | 20.2 | 41,119 |
PRISM risk group 2 | 58.1 | 24.7 | 7834 |
PRISM risk group 3 | 71.1 | 20.9 | 2367 |
PRISM risk group 4 | 70.9 | 19.9 | 250 |
WIMD (2011) | |||
Group | |||
All | 23.79 | 19.24 | 50,256 |
PRISM risk group 1 | 22.92 | 20.68 | 40,090 |
PRISM risk group 2 | 26.94 | 20.59 | 7644 |
PRISM risk group 3 | 28.09 | 21.44 | 2281 |
PRISM risk group 4 | 28.72 | 19.24 | 241 |
WIMD (2011) health component | |||
Group | |||
All | 26.55 | 24.09 | 50,256 |
PRISM risk group 1 | 25.69 | 23.64 | 40,090 |
PRISM risk group 2 | 29.56 | 25.53 | 7644 |
PRISM risk group 3 | 31.09 | 25.36 | 2281 |
PRISM risk group 4 | 30.81 | 25.46 | 241 |
Study PRISM score | |||
Group | |||
All | 6.42 | 6.71 | 51,570 |
PRISM risk group 1 | 4.08 | 1.61 | 41,119 |
PRISM risk group 2 | 11.25 | 3.07 | 7834 |
PRISM risk group 3 | 25.44 | 7.49 | 2367 |
PRISM risk group 4 | 59.48 | 13.13 | 250 |
PRISM score at 2 September 2012a | |||
Group | |||
All | 6.08 | 6.31 | 51,570 |
PRISM risk group 1 | 4.04 | 1.76 | 41,119 |
PRISM risk group 2 | 10.39 | 4.13 | 7834 |
PRISM risk group 3 | 22.77 | 10.47 | 2367 |
PRISM risk group 4 | 48.42 | 21.02 | 250 |
Analysis
The data are summarised, first, as a ROC curve (Figure 5) and, second, again consistent with interpreting a PRISM score, as a probability of an emergency hospital admission in 12 months, by comparing observed and expected numbers of emergency hospital admissions between 1 August 2012 and 31 July 2013, for both the overall subsample and the PRISM risk group (Table 27).
FIGURE 5.
Receiver operating characteristic curve based on PRISM scores in September 2012 and emergency hospital admission between 1 August 2012 and 31 July 2013. Diagonal segments are produced by ties.
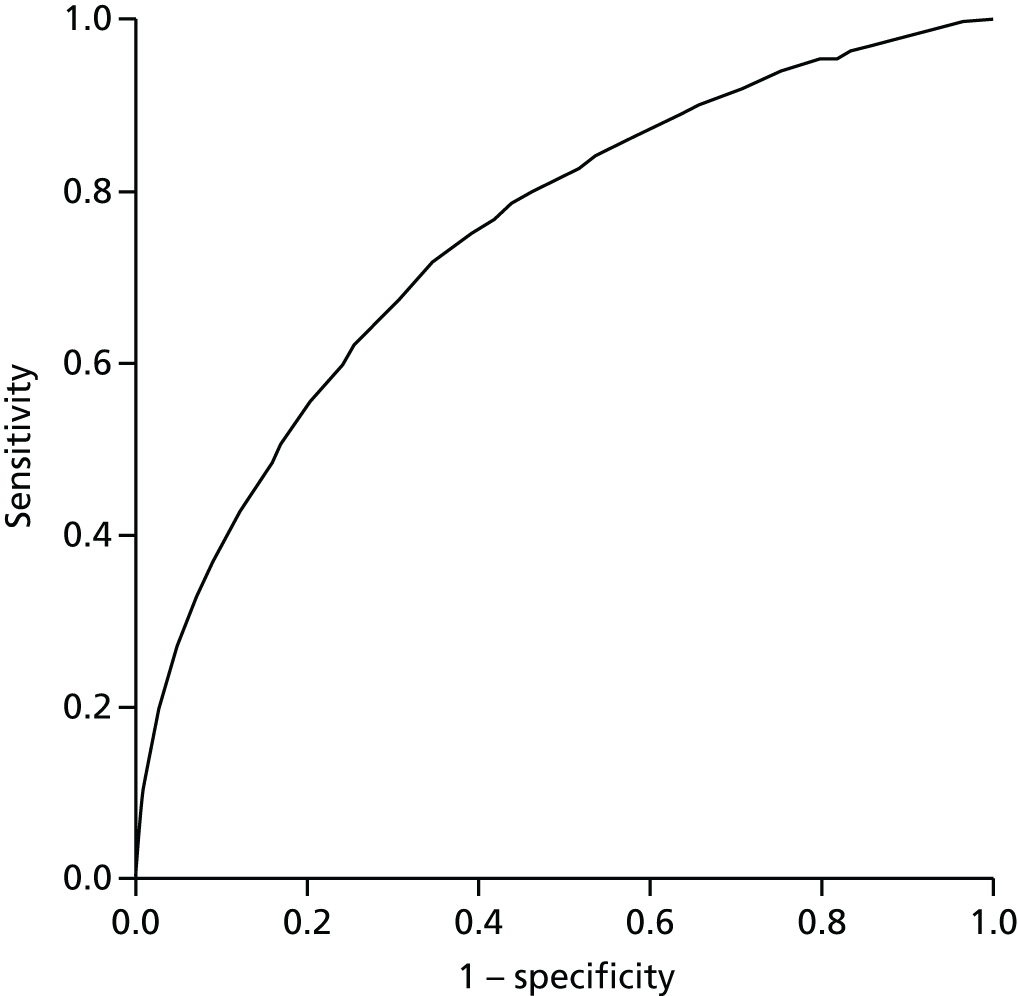
Group | Average PRISM score | n | Number of emergency hospital admissions from 1 August 2012 to 31 July 2013 | |
---|---|---|---|---|
Observed | Expected | |||
All | 6.08 | 51,570 | 3213 | 3133.8 |
PRISM risk group 1 | 4.04 | 41,119 | 1141 | 1659.8 |
PRISM risk group 2 | 10.39 | 7834 | 1078 | 814.0 |
PRISM risk group 3 | 22.77 | 2367 | 808 | 538.9 |
PRISM risk group 4 | 48.42 | 250 | 186 | 121.1 |
The area under the ROC curve is 0.749, indicating fair agreement. Table 27 shows that overall PRISM performed well, with some variation – fewer than expected admissions at risk level 1, but more admissions than expected at risk levels 2–4.
Chapter 5 Health economics results
Predictive risk stratification model implementation costs
Pre-activation and activation costs
Table 28 summarises the resource use and costs associated with the PRISM pre-activation phase, PRISM activation and set-up, and NWIS activity, during activation of the software in general practices. Processing of the ISAs was estimated to take 20 minutes from discussion with the research team. The different downloading and activation scenarios are shown with associated costs. These were based on support requirements in the actual trial general practices.
Cost component/resources required | Staff involved | Cost (£) | |
---|---|---|---|
Unit | Overall | ||
Pre-activation phase | |||
General practice staff opportunity costs: processing ISAs approximately 20 minutes per practice (the calculations are based on 32 practices) | GP; PM | 109.00/hour,a 36.33 per practice; 13.95/hour,a 4.65 per practice | 1162.56; 148.80 |
It was assumed for the base case that in 50% of cases a GP would do the ISA | 655.68 | ||
Activation phase | |||
Distribution of PRISM software: intended to be an internet-based system of downloading required software with IT support via telephone help desk or IT tech visit if required | |||
Scenario 1 | |||
Successful download of PRISM software via the internet by practice staff (PM): average time taken was 20 minutes per practice (calculations based on 32 practices either successfully activating PRISM software without assistance or failing to initially activate software and requiring further assistance) | PM | 13.95/hour,a 4.65 per practice | 148.80 |
Scenario 2 | |||
Help required from the IT help desk via telephone for guidance to download the PRISM software:
|
IT help desk: band 4 information officer, 19 calls × 15 minutes | 10.58/hour,b 2.65 per practice | 50.26 |
Help required from the IT help desk via telephone for guidance to download the PRISM software:
|
PM opportunity cost: 19 calls × 15 minutes | 13.95/hour,a 3.49 per practice | 66.26 |
Scenario 3 | |||
Site visit required by NWIS IT technician to download the PRISM software:
|
IT technician; band 4 information officer, 11 practices × approximately 1 hour 40 minutes of IT technician time + travel | £10.58/hourb (£0.18/minute), 40 minutes = £7.05; 1-hour travel, £10.58; £27.00, travel expenses; £44.63 per practice | 490.93 |
NWIS backend costs during the set-up phase | |||
|
IT technician; band 4 information officer | £10.58/hourb | £10.58 |
The total cost of PRISM activation was £1423.00 equating to £44.00 per practice. This cost of setting up PRISM represents the costs incurred during the trial period. Resource use and cost of GP trainers are shown in Table 29. The costs include GP trainer training, GP trainer time during training, as well as GP opportunity cost.
Cost component/resource used | Staff involved | Cost (£) | |
---|---|---|---|
Unit | Overall | ||
Training costs of GP PRISM trainer who carried out training in trial practices on behalf of NWIS | |||
Training of GP champions to deliver PRISM training (2.5 hours of GP time at a rate agreed during trial) GPs participating in the trial were paid £62.50 per hour. Trial team members travelled to the GPs to deliver training |
Two trainer GPs × 2.5 hours | 62.50/hour | 312.50 |
Training session in general practice on how to use PRISM software (it was assumed that GP trainers would deliver training in 1-hour sessions) It was estimated that a GP trainer from each region would be available to deliver PRISM training with an average travel distance of 30 miles = 1 hour of travel time (travel to practice and travel back) Trial travel costs were based on £0.45 per mile |
Trainer GP × 1-hour training and 1-hour travel cost (plus fuel costs) | 62.50/hour; 125.00 per practice; plus 27.00 fuel costs | 4000.00 plus 864.00 = 4864.00 |
Additional PRISM training (four practices requested extra training during trial) | Trainer GP × 2 hours (training plus travel) | 62.50/hour; 125.00 × 4; 27.00 fuel | 500.00 plus 108.00 = 608.00 |
Trainee GP opportunity costs: 1 hour of training on PRISM software for each participating general practice plus extra training (one GP trainee per practice assumed) | Trainee GP × 36 | 109.00/hour | 3924.00 |
Training of trainers and practice GPs cost £9709.00 in total and £303.00 per practice, which means that setting up PRISM in general practices (pre-activation and activation cost, NWIS activation support and GP training), during the trial period cost £11,131.00 in total and £348.00 per practice.
Predictive risk stratification model running and maintenance costs
The tasks required and costs of PRISM on a regular basis are summarised in Table 30.
Cost component/resource used | Staff involved | Cost (£) | |
---|---|---|---|
Unit | Overall | ||
Running monthly data uploads and providing e-mail support for general practices: the IT support tasks carried out by NWIS to maintain the running of the PRISM software are listed below:
|
IT technician; band 4 information officer | 10.58/hour; 7.5 hours | 79.35 per month |
Maintenance/updates of PRISM software: NWIS estimate that PRISM software would be further developed and updated every 2 years. This would take approximately 15 days of a band 7 IT technician’s time Investment in new servers in the longer term: servers would be expected to be upgraded every 3–4 years It is estimated that it would take 30 days to migrate and update systems No new servers were required for the trial use of PRISM and no costs were allocated |
Band 7 IT technician | 18.22/hour; 136.65/day | 2048.25 every 2 years |
The annual running costs were calculated using the unit cost of each component of operating and maintaining the PRISM software system and deriving an annual cost from the unit cost. Running monthly data uploads were calculated at £79 per month, which equates to £952 per year for all practices. It was estimated that maintenance of the PRISM software would be required every 2 years and calculated to cost £2048, which equates to £1024 per year.
The staff cost (opportunity cost) of participating general practices was calculated as part of the implementation costs for the PRISM scoring tool. Two general practices (numbers 13 and 30) were excluded from this analysis. Practice 13 was the GP champion practice working alongside the trial research team and hence logged into the PRISM software far more frequently than would be expected from routine use (29 times). Practice 30 was also initially a GP champion practice, but dropped out after approximately 1 month. The high frequency of logins by GPs during this early phase was also considered non-routine use.
Frequency of use was determined using login data obtained from the NWIS, which collected activity by:
-
the number of practice staff users per practice
-
the number of logins per practice
-
which staff members logged on and how many times each logged on.
However, the login statistics from NWIS did not provide information on how much time was spent using the PRISM software. Average length of time spent using PRISM software/data was therefore derived from the interviews held with general practice staff and the corresponding online questionnaire. However, there are several limitations associated with the following data.
-
The 9-month questionnaire data were incomplete; only 12 out of 31 practices analysed provided data (10 practices did not respond to the online questionnaire and nine practices indicted that there was no regular pattern of use).
-
Using the 12 available responses regarding the ‘time spent using PRISM’, a mean time of 60 minutes was calculated and imputed for practices that did not respond to the online survey and practices that responded that there was ‘no regular pattern’ of using PRISM software.
-
The available data did not specify which member of staff used PRISM for the length of time indicated if there was more than one user per practice; therefore, it has been assumed that the time spent using PRISM is an indication of time spent by the practice staff member who was the main PRISM user in that practice but is also applied to any other users in the practice, costs are weighted by the number of logins per PRISM user to get an approximate practice cost of using the PRISM software.
-
The ‘time spent using PRISM’ is a graded response which has been interpreted as follows:
-
approximately 1 hour = 60 minutes
-
1–2 hours = 90 minutes
-
> 2 hours = 120 minutes
-
up to 15 minutes = 15 minutes
-
up to 30 minutes = 30 minutes
-
up to 45 minutes = 45 minutes.
-
Table 31 shows the available data on frequency and duration of PRISM use by different users within the participating practices.
Practice ID | Number of PRISM users per practice | Number of logins | Average time (minutes) spent using prism | Staff using PRISM | Total cost (£) per practice | |||||||||
---|---|---|---|---|---|---|---|---|---|---|---|---|---|---|
GP | PM | Practice nurse | Other administration (band 3 IT administrator) | |||||||||||
Number of logins | Cost (£) by time used (£109.00/hour) | Total GP cost | Number of logins | Cost (£) by time used (£13.95/hour) | Total PM cost (£) | Number of logins and cost (£) per hour (£41.00) | Total cost (£) | Number of logins and cost (£) per hour (£9.22) | Total cost (£) | |||||
1 | 1 | 2 | 60 | N/A | 2 | 13.95 | 27.90 | 27.90 | ||||||
2 | 2 | 11 | 90 | 6 | 163.50 | 981.00 | 5 | 20.93 | 104.65 | 1085.65 | ||||
3 | 4 | 12 | 45 | 3 | 81.75 | 245.25 | N/A | 7 × 6.92 | 48.44 | 293.69 | ||||
4 | 2 | 6 | 90 | 3 | 163.50 | 490.50 | N/A | 490.50 | ||||||
5 | 2 | 9 | 60 | 3 | 109.00 | 327.00 | 6 | 13.95 | 83.70 | 410.70 | ||||
6 | 1 | 8 | 60 | 8 | 109.00 | 872.00 | N/A | 872.00 | ||||||
7 | 4 | 9 | 60 | 1 | 109.00 | 109.00 | 1 | 13.95 | 13.95 | 2 × 41 | 82 | 5 × 9.22 | 46.10 | 251.05 |
8 | 2 | 9 | 90 | 7 | 163.50 | 1144.50 | 2 | 20.93 | 41.86 | 1186.36 | ||||
9 | 2 | 10 | 60 | 1 | 109.00 | 109.00 | 9 | 13.95 | 125.55 | 234.55 | ||||
10 | 2 | 7 | 15 | 3 | 27.25 | 81.75 | 4 | 3.49 | 13.96 | 95.71 | ||||
11 | 1 | 6 | 60 | N/A | 6 | 13.95 | 83.70 | 83.70 | ||||||
12 | 1 | 9 | 60 | N/A | 9 | 13.95 | 125.55 | 125.55 | ||||||
13 | 3 | 29 | 60 | N/A | 0.00 | 0.00 | ||||||||
14 | 2 | 11 | 60 | 3 | 109.00 | 327.00 | 8 | 13.95 | 111.60 | 438.60 | ||||
15 | 1 | 9 | 30 | N/A | 9 | 6.98 | 62.82 | 62.82 | ||||||
16 | 1 | 5 | 60 | N/A | 5 | 13.95 | 69.75 | 69.75 | ||||||
17 | 1 | 3 | 60 | N/A | 3 | 13.95 | 41.85 | 41.85 | ||||||
18 | 1 | 4 | 60 | N/A | 4 | 13.95 | 55.80 | 55.80 | ||||||
19 | 2 | 8 | 60 | 1 | 109.00 | 109.00 | 7 | 13.95 | 97.65 | 206.65 | ||||
20 | 6 | 15 | 60 | 8 | 109.00 | 872.00 | 2 | 13.95 | 27.90 | 899.90 | ||||
21 | 1 | 7 | 60 | N/A | 7 | 13.95 | 97.65 | 97.65 | ||||||
22 | 2 | 9 | 60 | N/A | 9 | 13.95 | 125.55 | 125.55 | ||||||
23 | 2 | 6 | 120 | 4 | 163.50 | 654.00 | 2 | 20.93 | 41.86 | 695.86 | ||||
24 | 1 | 5 | 60 | N/A | 5 | 13.95 | 69.75 | 69.75 | ||||||
25 | 1 | 6 | 30 | N/A | 6 | 6.98 | 41.88 | 41.88 | ||||||
26 | 2 | 4 | 60 | 1 | 109.00 | 109.00 | 4 | 13.95 | 55.80 | 164.80 | ||||
27 | 1 | 7 | 60 | N/A | 7 | 13.95 | 97.65 | 97.65 | ||||||
28 | 2 | 7 | 60 | 7 | 109.00 | 763.00 | N/A | 763.00 | ||||||
29 | 2 | 7 | 60 | N/A | 5 | 13.95 | 69.75 | 2 × 9.22 | 18.44 | 88.19 | ||||
30 | 1 | 11 | 60 | 11 | 109.00 | 1199.00 | N/A | 1199.00 | ||||||
31 | 1 | 3 | 30 | N/A | 3 | 6.98 | 20.94 | 20.94 | ||||||
32 | 1 | 6 | 60 | N/A | 6 | 13.95 | 83.70 | 83.70 |
The overall staff cost in the first 9 months post implementation for all 30 trial practices included in the analysis was £9182.00. This equates to a mean cost of £296.00 per practice. The minimum staff cost for a practice was £21.00 where a PM logged into PRISM three times for approximately 30 minutes per time over a 9-month period. The maximum cost per practice was £1186.00, where there were seven GP logins and two PM logins for approximately 90 minutes per login, over a 9-month period.
Overall costs of setting up and operating Predictive RIsk Stratification Model software
The overall cost of PRISM was calculated over 1 year based on the trial intervention phase duration. Costs associated with general practice staff using the PRISM software were calculated by extrapolating the 9-month cost of £9182 to 12 months resulting in a yearly staff opportunity cost of £12,242, which equates to £408 per practice. The total annual cost of operating and maintaining the PRISM software tool across the general practices of the trial area are therefore estimated to be £14,218.60 and £473.95 per practice. This means that use of the PRISM software might be estimated to cost £25,350.00 and thus £822.00 per general practice in year 1 (includes activation and training), and would be expected to cost £474.00 per practice in every subsequent year (this figure does not include discounting). These figures are based on trial figures, include staff opportunity cost but exclude any server or other hardware requirements.
The 32 practices included in the study had 230,099 patients registered at the beginning of the study, of which 220,683 were included in the intervention phase analysis, equating to a PRISM implementation cost of £0.12 per patient.
Cost analysis of primary and secondary health care
The mean annualised costs of primary and secondary care per patient, as observed in the control and intervention phases, are summarised in Table 32.
Health-care provision | Phase, mean cost (SD) | Difference in cost (£) | |
---|---|---|---|
Intervention | Control | ||
ED attendances (discharged) | 30.10 (97.70) | 29.90 (120.60) | 0.19 |
ED attendances (admitted) | 13.30 (107.40) | 13.30 (126.50) | –0.05 |
Outpatient visits | 173.10 (407.60) | 172.60 (427.30) | 0.43 |
Elective inpatient stays | 549.30 (3978.50) | 545.00 (4436.50) | 4.31 |
Emergency-related inpatient stays | 418.50 (4052.40) | 406.10 (5131.30) | 12.34 |
GP events | 364.57 (446.56) | 367.69 (446.32) | –3.12 |
Total cost | 1548.26 (6226.39) | 1534.54 (7260.49) | 13.72 |
Table 33 summarises the differences in total health-care costs after adjustment for covariates for the entire patient cohort and individual risk groups.
Group | Phase, mean cost (SD) [n] | Adjusted comparison | ||
---|---|---|---|---|
Intervention | Control | Estimated (p-value) | 95% CI | |
Alla | 1548.26 (6226.34) [220,683] | 1534.54 (7260.49) [230,087] | Δ = 75.91 (p < 0.001) | 45.83 to 105.99 |
Risk group 1b | 867.60 (3895.53) [176,214] | 808.59 (4784.65) [182,952] | Δ = 60.85 (p < 0.001) | 37.98 to 83.72 |
Risk group 2c | 3266.72 (9119.38) [32,929] | 3182.73 (9687.06) [34,307] | Δ = 136.62 (p = 0.020) | 21.58 to 251.67 |
Risk group 3d | 7027.45 (14,538.30) [9352] | 7428.90 (17,412.04) [10,288] | Δ = 133.50 (p = 0.446) | –209.78 to 476.78 |
Risk group 4e | 15,570.46 (23,732.79) [897] | 15,879.08 (26,475.56) [1128] | Δ = 907.56 (p = 0.300) | –809.96 to 2625.07 |
The difference between the total cost in the control phase and intervention phase was found to be statistically significant (p < 0.001) in the overall cohort and for risk groups 1 and 2. Differences were no longer statistically significant in risk groups 3 and 4. Statistical significance (p < 0.001) remained after log-transformation.
Health-care costs per patient in the intervention phase were generally slightly higher, but £3 lower in primary care and £0.05 lower for ED attendances followed by admission. The main cost drivers were found to be inpatient stays (elective and emergency) and GP visits. These differences were based on raw data (i.e. unadjusted for covariate effects).
Including all primary and secondary care costs and after covariate adjustment, the total cost difference between the control and intervention phase shows an increase of £75.91 (total health-care costs only) and £76.03 (total health-care costs and PRISM implementation costs) per patient, respectively, in the intervention phase.
Cost-effectiveness analysis
All costs were annualised to account for the difference in the duration of the control and intervention phases. Base-case analysis showed a small difference in total health-care costs between the control and intervention phases (see Table 25). This was mainly attributable to the relatively small implementation cost of PRISM per patient and the marginal cost increases per patient during the intervention phase. The clinical effectiveness analysis found an increase in the number of emergency admissions from 0.1607 [standard deviation (SD) 2.1634] per patient in the control phase to 0.1666 (SD 2.0794) per patient during the intervention phase. Therefore, no emergency admissions were avoided while an incremental cost of £0.12 per patient per year was spent to implement PRISM in general practice. The intervention is therefore less effective and more costly than the control and can hence be concluded to be dominated by the control. As a consequence, no ICERs were calculated as the PRISM tool cannot be considered cost-effective.
Sensitivity analysis
The parameter changes applied in the one-way sensitivity analysis are outlined in Table 34.
Parameter | Base case | Sensitivity analysis | Change from base case |
---|---|---|---|
PRISM pre-activation (£) | 655.68 | 148.80–1162.56 | Use minimum (all done by PM) and maximum (all done by GP) cost |
PRISM activation support (£) | 490.93 | 0.00–1428.16 | Assume minimum (no site visits required) and maximum (all surgeries need site visit to assist with set-up) cost |
PRISM opportunity cost for GP surgeries (for 12 months, £) | 394.91 | 27.92–1581.81 | Use minimum and maximum time spent during trial period |
Difference in number of emergency admissions per patient per year at risk | 0.016 | 0.004–0.027 | Use 95% CI to recalculate ICER |
Primary care costs (£) | 32.05 | 10.21–45.43 | Minimum (all done nurse) and maximum (all done by GP) cost |
ED attendance (discharged) cost (£) | 113.60 | 94.30–128.50 | Lower and upper quartile costs reported NHS Reference Costs 2014/1568 |
ED attendance (admitted) cost (£) | 191.6 | 152.00–220.80 | Lower and upper quartile costs reported in NHS Reference Costs 2014/1568 |
Outpatient visits cost (£) | 110.6 | 67.60–127.80 | Lower and upper quartile costs reported in NHS Reference Costs 2014/1546 |
Elective inpatient stays (£) | 3605.50 | 2624.30–4299.10 | Lower and upper quartile costs reported in NHS Reference Costs 2014/1568 |
Emergency admission-related inpatient stay per bed-day (short stay, £) | 541.30 | 369.00–631.00 | Lower and upper quartile costs reported in NHS Reference Costs 2014/1568 |
Emergency admission-related inpatient stay per bed-day (long stay, £) | 458.20 | 326.70–540.10 | Lower and upper quartile costs reported in NHS Reference Costs 2014/1568 |
The results appeared robust in the univariate sensitivity analysis and did not change significantly from base case. Based on the difference in number of emergency admissions between control and intervention phase, the intervention remained dominated by the control (routine practice) in all analyses.
Total health-care costs ranged from £965.00 (SD £5165.00) to £1995.00 (SD £8667.90) in the control phase and from £976.00 (SD £4415.50) to £2010 (SD £7459.50) in the intervention phase. Covariate-adjusted incremental costs per patient incurred in the intervention phase (including PRISM implementation), ranged from £45.83 to £105.99. Considering the pooled uncertainty of all parameters, probabilistic sensitivity analysis showed a probability of the intervention being cost-effective of 21% if a willingness-to-pay threshold of £3666.00 (average cost of an emergency admission-related inpatient stay68) was assumed.
Cost–utility analysis
Utility scores (QALYs) were slightly lower in the intervention phase (0.6382) than in the control phase (0.638; see Table 36). After adjustment for covariate effects, the difference of –0.002 was not statistically significant (p = 0.584). Considering the higher cost of the PRISM scoring tool and generally higher health-care cost in the intervention phase, the intervention is dominated by the control. No ICER was therefore generated for the cost per QALY gained. Probabilistic sensitivity analysis showed that the probability that the intervention is cost-effective at a willingness-to-pay threshold of £20,000 is 46% when all parameter uncertainty is considered.
Cost–consequence analysis
Table 35 summarises the results of the cost–consequence analysis.
Cost/health impact | Phase | Difference | p-value | |
---|---|---|---|---|
Intervention | Control | |||
Annual costs (£) impact | ||||
Implementation cost: total | 25,349.61 | 0.00 | 25,349.61 | |
Implementation cost per GP practice | 821.79 | 0.00 | 821.79 | |
Implementation cost per patient | 0.12 | 0.00 | 0.12 | |
Primary care cost per patient | 364.57 (SD 446.56) | 367.69 (SD 446.32) | –3.12 | |
ED attendances (discharged) | 30.10 (SD 97.70) | 29.90 (SD 120.60) | 0.19 | |
ED attendances (admitted) | 13.30 (SD 107.40) | 13.30 (SD 126.50) | –0.05 | |
Outpatient visits | 173.10 (SD 407.60) | 172.60 (SD 427.30) | 0.43 | |
Elective inpatient stays | 549.30 (SD 3978.50) | 545.00 (SD 4436.50) | 4.31 | |
Emergency-related inpatient stays | 418.50 (SD 4052.40) | 406.10 (SD 5131.30) | 12.34 | |
Total health-care cost per patient per year | 1548.26 (SD 6226.39) | 1534.54 (SD 7260.49) | 75.91 (adjusted: 45.83 to 105.99) | < 0.001 |
Health impact | ||||
Number of emergency admissions per patient per year at risk | 0.1666 (SD 2.0794) | 0.1607 (SD 2.1634) | 0.005 (adjusted: –0.0074 to 0.016) | 0.425 |
Number of A&E attendances per patient per year at risk | 0.3613 (SD 1.7877) | 0.3593 (SD 2.1014) | 0.006 (adjusted: –0.005 to 0.016) | 0.299 |
Number of GP event-days per patient per year at risk | 14.075 (SD 32.593) | 14.100 (SD 23.124) | 0.139 (adjusted: 0.032 to 0.246) | 0.011 |
Number of outpatient visits per patient per year at risk | 1.717 (SD 9.746) | 1.704 (SD 9.984) | 0.035 (adjusted: –0.014 to 0.085) | 0.158 |
SF-12 mental health score | 43.77 (SD 9.67) [n = 1410] | 44.62 (SD 9.47) [n = 662] | –0.720 (adjusted: –1.469 to 0.030) | 0.060 |
SF-12 physical health score | 41.72 (SD 9.20) [n = 1410] | 40.07 (SD 7.30) [n = 662] | 1.465 (adjusted: 0.774 to 2.157) | < 0.001 |
SF-6D utility scores | 0.6380 (SD 0.0682) [n = 1467] | 0.6382 (SD 0.0719) [n = 692] | –0.002 (adjusted: –0.008 to 0.004) | 0.584 |
Budget impact of the Predictive RIsk Stratification Model in the study area
The annualised budget impact of PRISM per 100,000 population in the trial area during the intervention phase is detailed in Table 36.
Budget impact (£) | Phase | Difference | |
---|---|---|---|
Intervention | Control | ||
Total health-care cost | 154,821,008.00 | 153,450, 874.00 | 1,370,134.00 |
PRISM implementation cost | 11,486.89 | 0.00 | 11,486.89 |
Total budget impact | 154,832,495.00 | 153,450,874.00 | 1,381,621.00 |
During the 1-year duration of the intervention phase, the total cost of primary and secondary care was £1.370M higher than the annualised health-care costs in the control phase. Taking into consideration the implementation costs of PRISM of £11,487 per 100,000 population, this results in an increased cost of £1.382M per 100,000 patients per year.
The results of the deterministic sensitivity analysis show that, using the lower and upper quartile unit costs for primary and secondary care, the estimated budget impact of PRISM in the trial area over 1 year and 100,000 population in the intervention phase can be expected to have been between £1102M and £1.477M and £11.02 and £14.77 per patient, respectively. Furthermore, after adjustment for covariate effects, the rise in health-care costs that coincided with the PRISM scoring tool in the trial area and 1-year intervention phase gave an estimated increase in budgetary impact to a mean £7.591M (95% CI £4.583M to £10.599M) per 100,000 population.
Limitations of the health economic analysis
There are several limitations associated with the economic analysis.
The implementation cost for the PRISM scoring tool was costed according to the procedure and use as recorded in the trial. Any changes in routine use will result in costs to differ from the ones reported here.
Hospital admissions in the SAIL database are coded as elective, emergency and other. As there was no indication about the nature of ‘other hospitalisation’, these were excluded from the economic analysis, which might introduce bias by underestimating actual hospitalisation costs.
No length of stay data were available for this study for elective inpatient stays. They were therefore costed according to the national average as reported in the NHS Reference Costs 2014/15,68 which will introduce bias. However, this was addressed in the sensitivity analysis.
Any hospitalisations that started in the control phase and lasted while the patient’s GP surgery entered the intervention phase were counted entirely as a control phase hospitalisation. However, hospitalisation length of stay was censored at the end of the study. Hence, the analysis will underestimate the true hospitalisation costs during the intervention phase. Although this will introduce bias, considering that the intervention phase was already found to be more costly, this will not change the direction of the results.
Secure anonymised information linkage records GP event-days. However, the nature of the interaction is unknown. We have therefore costed GP event-days according to consultation trends in general practice, which might not be entirely accurate as the trend data only includes the period between 1998 and 2008. Effects of this were addressed in the sensitivity analysis.
Ideally, the economic evaluation would have been undertaken from a societal perspective. However, the SAIL database does not capture patients’ out-of-pocket expenses or data on social and community care usage. We planned to use questionnaire data (Client Service Receipt Inventory) to estimate the cost of social care but because of the complexity of the data linkage with the SAIL database, social care costs could not be included in the analysis, which will cause an underestimation of the true costs in both the control and intervention phases.
Although the cost difference between the intervention and control phase is statistically significant, this result has to be interpreted with caution because of the large sample size included in the analysis and the skewness of the data. Although generalised linear models and log-transformation are not ideal to address the common problems with cost data (i.e. positive skewness, excess zeros, multimodality and heavy tails), the sample size used in the analysis should be large enough for a near-mean distribution to be assumed and so the number of large costs is sufficient for the answers not to be unduly influenced by a few, very large, outlying costs.
The budget impact analysis only considered the trial population rather than an all-Wales or other per country-based population. Further examination (e.g. to take into account differing demographic structures across regions) would be needed to provide robust estimations of the impact of implementing PRISM over a 5-year period. However, the trial-based analysis provides an illustration of the likely budgetary demands (based on a 100,000 population) of the PRISM scoring tool on the NHS.
Chapter 6 Stakeholder views: the Predictive RIsk Stratification Model implementation and use
Introduction
The qualitative component of the PRISMATIC study was designed to describe how the PRISM tool was understood, communicated, adopted and used by practitioners, managers, local commissioners and policy-makers. In particular, it aimed to identify the processes of change associated with introducing and implementing the PRISM tool within primary care services.
This chapter is structured roughly chronologically. We start by presenting the views of policy-makers involved with development and roll-out of PRISM, and staff from Welsh health boards who were invited to pilot PRISM within their chronic conditions management programmes. We then explore local aspects of the context for the PRISMATIC study, by reporting on the expectations of and views on PRISM from community health staff and health board staff in the ABM UHB areas at baseline of the study. We then examine the process of adoption of PRISM in general practice within the PRISMATIC trial, through reporting the views and experiences of staff from the 32 general practices at three time points: baseline, mid-trial and end of trial. This analysis is informed by the NPT described in Chapter 3. The four components or tasks associated with implementing innovation in normal health-care practice are summarised in Box 2. We conclude the presentation of qualitative data with reflections from an AMB UHB manager at the end point of the PRISMATIC trial intervention on the potential and use of the PRISM tool in their area.
-
How people understand the innovation and its purpose (coherence).
-
What decisions people take about using it, based on perceived advantages (cognitive participation).
-
What people do to bring the innovation into everyday use (collective action).
-
How the innovation is reviewed, modified or abandoned (reflexive monitoring). 77
This chapter also contains information on the implementation and use of PRISM from the surveys administered to participating practices, to complement the interviews and focus groups.
Normalisation process theory suggests that each of these tasks is shaped by factors that promote or inhibit the extent to which participants look on a new practice as meaningful.
Respondents
Table 37 summarises the stages in which each of the three main staff groups participated in the qualitative data collection, as well as the number of staff involved.
Staff group | Time point | ||
---|---|---|---|
Baseline: pre PRISM activation | Mid-trial: 3–6 months post PRISM activation | End of trial: 18 months post PRISM activation | |
All-Wales policy-makers and health board staff | 12 interviews | Not interviewed | Not interviewed |
Local health board and community staff | 1 focus group (n = 7) | Not interviewed | 1 interview |
General practice staff |
4 focus groups (GPs, n = 21; PMs, n = 10; nurses, n = 2) 11 interviews (GPs, n = 10; PMs, n = 1) |
22 interviews (GPs, n = 18; PMs, n = 4) 9 questionnaires (GPs, n = 7; PMs, n = 2) |
19 interviews (GPs, n = 17; PMs, n = 2) 15 questionnaires (GPs, n = 14; PMs, n = 1) |
Health services policy-makers, managers and community health staff
During 2013 we conducted face-to-face interviews with policy and health board managers (n = 12) to explore the story of developing the PRISM tool. Six respondents had responsibility for supporting and implementing chronic conditions management policy (including developing the PRISM tool) at an all-Wales level and worked for the Welsh Government or an agency which advised the Welsh Government on this matter. The other six respondents had regional responsibility for planning and delivering chronic conditions management services in Welsh health boards.
At baseline (before PRISM was introduced to GP practices), we conducted a focus group with seven ABM UHB staff with a responsibility for the management, redesign and/or delivery of primary and/or community care services. Two of them were practising nurses involved in delivering community care, and one was a GP working in a management role at the time of the focus group. One respondent had a role which spanned the whole of ABM UHB, whereas the remaining six respondents worked in a particular locality or network. Six of the seven respondents were female. Part-way through the focus group, we presented a handout explaining PRISM and giving examples of screenshots.
At the end of the trial, we interviewed one ABM UHB manager. Two other ABM UHB locality managers declined to participate, saying they had changed roles and had no knowledge of the PRISM implementation. We also circulated a short questionnaire about the PRISM implementation to members of the baseline ABM UHB focus group, but none was returned completed.
Staff from general practitioner practices trialling the Predictive RIsk Stratification Model tool
At baseline, we invited each GP who had been nominated as lead for PRISM in a participating practice to attend one of four focus groups, along with other staff members (such as PM or nurse), if desired. Thirty-three respondents attended focus groups: group A (GPs, n = 5; PMs, n = 4; nurses, n = 1), group B (GPs, n = 4; PMs, n = 3; nurses, n = 1), group C (GPs, n = 7; PMs, n = 1), group D (GPs, n = 5; PMs, n = 2). We also interviewed 10 GPs who were unable to attend a focus group, by telephone (n = 7) or in person (n = 3); in one of the face-to-face interviews, the GP was joined by their PM. This gave a total of 44 participants from across all 32 practices. Part-way through the focus group or interview, we presented a handout explaining PRISM and giving examples of screenshots.
At two time points after PRISM was introduced (at the mid-point and at the end of the trial), we carried out follow-up data collection with respondents from the practices. For half of the participating practices (n = 16), we interviewed the PRISM lead GP in order to understand how the tool was introduced and used over the study period. The PM or practice nurse also contributed to a small number of these interviews. The mid-trial interviews took place at the time when practices were preparing reports required for QOF: interviews with practices who gained access to the tool early in the PRISMATIC trial occurred up to 6 months before the QOF deadline, whereas later implementing practices were interviewed during the few months or weeks before they submitted their reports. By the time of the interviews at the end of the trial, the QOF payment for focusing on patients at high risk of emergency admissions had ended.
At the same two time points (at the mid-point and at the end of the trial), we circulated a short questionnaire based on the interview schedule to the other half of the lead GPs (n = 16) to identify any experiences that diverged from those reported in interviews.
Characteristics of practices and respondents are described in Appendix 10.
Figure 6 illustrates the timing of mid-trial interviews with participating GPs in relation to the QOF reporting deadline.
FIGURE 6.
The timing of mid-trial interviews with participating GP practices in relation to the QOF reporting deadline.

In the presentation of results in this chapter, quotations are selected to be illustrative and typical of respondents’ comments unless otherwise stated. Where a respondent emphasised a word or phrase, that emphasis is indicated by bold type. Quotations are identified by respondent role (GP, PM, practice nurse), practice-unique identifier, time point (baseline, mid-trial, end of trial). For example, ‘GP01base’ would identify a quotation from the baseline interview with the GP from practice number 1, ‘PM14end’ would identify a quotation from a PM in the interview at the end of the trial at practice number 14. Quotations for policy and health board managers are identified by PHB and the unique number (e.g. PHB01).
Views of health service managers at strategic level: pre implementation
How the Predictive RIsk Stratification Model risk tool was planned and developed across Wales
This section presents results of interviews with policy-makers and health services managers (n = 12) responsible for chronic condition management in Hywel Dda, Betsi Cadwaladr, Powys, Cardiff and Vale, Cwm Taf, and Aneurin Bevan Health Boards.
Interview respondents reported broad support for the introduction of a risk prediction tool in Wales. One commented that, at a Wales-wide meeting, ‘there was a complete consensus’ (PHB06) regarding the tool’s development, driven in part by a focus on reducing emergency hospital admissions:
We’re demonstrating at the moment about 8–10 per cent increases in emergency admissions year on year. So, it’s completely unsustainable, but it’s massive pressure on hospitals and we have to do far more to stop people coming in.
PHB01
We knew that we had to look at these patients who were multiple admissions to hospital, and that there was a keenness to stop people going into hospital, and to keep them in the community, and keep them at home.
IPHB09
I think it’s critical. You should be able to plan, as far as you can, and try and understand where your demands for health care are going to come from.
PHB07
Several respondents recalled that PRISM had been anticipated for some time. They indicated that there were high levels of awareness and enthusiasm across different staff groups with a strategic interest in chronic conditions management:
. . . there’s strong support amongst the audience, primarily the local health board, chronic condition [staff?] and managers, planning implementation manager, they like the tool, want it, and were . . . quite keen to say ‘well – but when are we going to get it?’
PHB 02
The work that we did on chronic conditions management when the national framework came out was very much sort of looking at an overview of services overall . . . Within that we’ve always been waiting for PRISM . . . because we always talked about ‘when PRISM comes we’ll do that’.
PHB10
Most respondents were aware that PRISM had initially been proposed as a tool to support the planning of services. A minority of respondents expressed the opinion that this original vision was preferable to the subsequent emphasis on use within GP practices:
. . . the original concept was based . . . on population, managing the needs and allocating the services and support more appropriately to meet those needs . . . [. . .] the original concept was that it was a locality tool that we could use to kind of do a population-based planning . . . and we got sucked down into the primary care individual level.
PHB03
This scepticism about the role of the tool in case finding of at-risk patients at practice level could be linked to the attitudes that respondents reported observing in some GPs at the time of the tool’s development and piloting. Though some interest and openness to the tool was reported, respondents also identified many aspects of caution and reluctance among GPs and practice colleagues. These included comments that:
-
PRISM was a threat to professional autonomy:PMB06
. . . this way of working is not one that many people are comfortable with, because it takes the initial responsibility of identifying people away from clinicians. And I think that that’s quite difficult for many people to come to terms with.
-
there was a lack of evidence to justify it:PMB02
Some of them would say, ‘I will use it when I know more about it, when it’s more developed, when it’s more understood’. . .
-
it would not provide new or timely information on patients:PMB09
The first thing that practices were coming up with was, ‘Well, we know who our top patients are, we know who these patients in the top of the pyramid are, why would we need a piece of software to do that?’ And it was actually trying to explain to them the concept of not just looking at those patients who you knew were very ill, and were likely to be experiencing admissions, but it was those people who were further down the pyramid, but starting to fall over into a higher need bracket.
-
it would generate additional work.
Constraints on implementation pre PRISMATIC
Several respondents reflected on the constraints on implementation of PRISM shortly after its development. They reported their perception of the reasons at national level for the delay in Wales-wide implementation. These reasons related to concerns about the accuracy and value of the tool; concerns about confidentiality and patient security expressed by professional body representatives, including the British Medical Association (BMA); a lack of central ownership and top-down drive to continue to develop and promote PRISM; and a lack of GP champion to provide peer support and encouragement:
I think public health [representatives] didn’t have enough confidence that it [PRISM] was accurate; they have their own parameters. GPs and the Welsh BMA, they had concerns about: was the supporting infrastructure around confidentiality and patient security enough?
PMB02
It was the BMA in the end who . . . had enough reservations that prevented us from making it more widely available.
PMB03
There was no new development work, there was nobody really we could say was a PRISM person. [. . .] which is a shame, because we invested a lot of effort initially to it and then suddenly it just died.
PMB05
There’s no other way GPs will engage, they will engage more with their own colleagues initially.
PMB08
Views of health service managers and community health providers at local level: pre implementation
As we were interested in how PRISM would fit in with a wider picture of community-based provision, we talked to other relevant members of the local health community in the ABM UHB area, by holding a focus group with staff with responsibility for management, redesign and/or delivery of primary and/or community care services. This focus group took place at baseline, before implementation of the tool through the PRISMATIC study.
Awareness and understanding of the Predictive RIsk Stratification Model
Awareness of the PRISM tool among community health staff and managers at baseline was generally high, largely because respondents had already come across it, and in some cases worked directly with it. It had been an intrinsic component of a pilot virtual ward development in part of the ABM UHB area, when piloted prior to the PRISMATIC study. Three respondents who had worked directly with it in their care role reported responding to it favourably – ‘another weapon in the armoury’, as one said (respondent 6, focus group E). However, they were also frustrated by technical hitches and delays, and the fact that, because of the short-term nature of the pilot, use of PRISM became a ‘fizzled out firework’ (respondent 7, focus group E).
Many of the respondents indicated a generally high level of understanding of the details of PRISM in terms of its technical functioning, though there were still some uncertainties about, for example, the differences between relative and absolute risk. A minority of respondents, despite feeling generally positive about the potential benefits of PRISM, reported some specific concerns. Some of these concerns were around the accuracy of the data and the fact that scores may not take full account of patients’ level of anxiety or competence to self-manage. Respondents also identified a potential problem with the PRISM approach of identifying relative risk, practice by practice – and as practice lists vary widely, this could produce inequity as patients in the highest category of risk in one practice might actually be at much less risk than patients in another practice, who were further down in the stratification:
Well, how do we make – how do we manage need across a whole population, not just at a practice level?
Respondent 5, focus group E
Respondents described their understanding of how PRISM could be used as a resource by practitioners in order to support case finding of patients who might benefit from case management. This understanding of the role of PRISM in case finding extended to take in the concept of ‘impactability’, and the selection from a list of patients at high risk of being those for whom interventions would be offered:
Some of the concerns of GPs have been, they’re going to have this whole, you know, great tranche of people. But, actually, you can keep select – you can select however many you want. You don’t have to manage them all, you can select out those that are most at risk.
Respondent 1, focus group E
One nurse saw value in using PRISM for case finding, but was uncertain about how to manage that because she also had to accept referrals from GPs – who may, themselves, not have been using PRISM. Another community nurse, working across four different practices, was concerned about another practical challenge. As PRISM rolled out, she would have to visit each practice to access PRISM separately, and would need to enter four different access codes.
In terms of delivering a model of proactive care, risk scores might be used as a basis of deciding not just when a patient goes onto the nurse’s caseload, but also when they might be discharged:
I’d hate to be the bean counter, but actually that’s part of what I have to do. How do we make sure we target our resources to the most effective area, and how do we ensure that people come out of service as well as come in?
Respondent 5, focus group E
Discharge decisions are not necessarily straightforward, though, as patients’ needs and risk level can fluctuate over time:
I have what I call a backburner, where I put – park people if you like, who are not technically on my caseload, but I know they’re probably going to come back on.
Respondent 5, focus group E
Perceived opportunities and limitations of the Predictive RIsk Stratification Model
Respondents were generally aware of the wider debate which had taken place prior to PRISM’s introduction, including the concerns expressed by the BMA and the local medical committee – about risks to confidentiality, liability, and the possibility of being misled by regression to the mean – though respondents did not generally endorse these concerns. One respondent suggested that, for those GP practices that had not signed up to the PRISMATIC study, there was a list of anxieties that were still current:
. . . having spoken to some of the GPs, [comments I have received have been] ‘We don’t believe it will add value’; ‘we already know [our high risk patients]’; and ‘. . . fear of all this additional work that we will now have to do to manage people we didn’t know were risky before’.
Respondent 5, focus group E
In addition to its role in case finding, PRISM’s potential to support service development and planning was extensively discussed by respondents in a number of different ways, particularly around remodelling of care. As one health service manager with responsibility for service redesign said:
Clearly the PRISMATIC tool is of great interest to me in terms of how that can support redesign in the community . . . if you start to understand what it is that determines risk, you then see where you’re going to have the biggest impact, and where you need to direct your resources.
Respondent 1, focus group E
A number of respondents talked about the potential role of PRISM in supporting patients’ self-care and self-management, and ‘explaining to them how that [risk score] can shift’ (respondent 1, focus group E). Self-management was seen as something which would in itself need support services:
It’s about – it’s more than just managing the condition as well. It’s getting them to take ownership. It’s about promoting their health and their self-preservation skills and that kind of thing. And it’s developing that into the services and the teams that we plan.
Respondent 3, focus group E
Respondents identified scope for PRISM to support strategic service development and management in further ways. The first way was to measure the effectiveness of service change – instead of waiting to see impact on admissions, see what change there is in risk scores. A second way would be to support workforce modelling as part of service redesign. Third, respondents talked about the potential for using PRISM as a way of benchmarking within a network:
[If] you can start to see maybe some networks, maybe some practices, have got these much higher risks then – and then you try and work out why. Why is it they’re different? You can then target the education to them, and so it sort of helps with that, as well as resources per se – it’s about education too.
Respondent 1, focus group E
There was an acknowledgement, though, that if the tool were seen as part of a performance management regime, this could create resistance to its adoption in practices. In terms of the work of putting PRISM into practice, respondents felt that practices were likely to vary greatly in their enthusiasm, and that peer-to-peer influence would be helpful, particularly through the practice networks.
Views of general practitioners and practice staff on the Predictive RIsk Stratification Model risk prediction tool
We now present the views of GPs and practice staff from data collected both before they had access to the PRISM tool and while it was available for use in their practices (mid-trial and end-of-trial fieldwork). To understand how these potential users perceived the technology and implemented it within their health-care practice, we present findings in line with the theoretical framework offered by NPT. Table 38 presents a summary of key findings in relation to the four components of NPT, which we then expand on in the text which follows.
NPT component | Questions to consider within the NPT framework | Findings from interviews with GPs using PRISMATIC |
---|---|---|
Coherence (i.e. meaning and sense-making by participants) | Is the intervention easy to describe? | GPs found the PRISM tool easy to understand because they recognised the principles of risk prediction |
Is it clearly distinct from other interventions? | PRISM predicted risk resulting from health and social factors in contrast to condition-specific risk tools | |
Does it have a clear purpose for all relevant participants? | GPs and managerial staff recognised the potential value of a formal, systematic process for assessing patients’ risks alongside clinical expertise | |
What benefits will the intervention bring and to whom? | PRISM could complement current clinical practice and intuitive risk assessments and identify patients for case management | |
Are these benefits likely to be valued by potential participants? | It could be a useful systematic contribution to case-finding and identify patients at high risk of emergency admissions, in line with policy imperatives | |
Will it fit with the overall goals and activity of the organisation? | The PRISM tool was perceived to fit within local and national priorities to implement case management, reduce unplanned health demands and encourage self-management by patients | |
Cognitive participation (i.e. commitment and engagement by participants) | Are target user groups likely to think it is relevant and usable? | GPs found that PRISM was very relevant in helping complete QOF requirements |
Will they see the point of the intervention easily? | PRISM could add to clinical judgement, was in line with the emphasis on proactive care and generally seemed to provide accurate data | |
What affects their willingness to invest time, energy and work in it? | Protected time and financial incentives encouraged GPs to use PRISM within routine practice. Competing demands on time and resources and technical problems limited their willingness to use PRISM | |
Collective action (i.e. the work participants do to make the intervention function) | Will staff require extensive training before they can use it? | Training provided practical information on using PRISM. Ideas about how to use the data in patient care came from focus groups, their own aims or QOF |
How will the intervention affect the work of user groups? | GPs felt that PRISM helped them complete the tasks required by QOF. Other use was infrequent and inconsistent across GPs | |
Will it promote or impede their work? | PRISM was easy to use, but technical problems limited quick and regular access | |
How will use of the intervention be divided among users? | The lead GP in each practice was the main PRISM user and provided information for discussion at multidisciplinary meetings | |
What effect will it have on responding to patients’ needs? | GPs described four types of actions to respond to patient needs:
|
|
Reflexive monitoring (i.e. participants reflect on or appraise the intervention) | Will it be clear what effects the intervention has had? | GPs were unsure of effects on emergency admissions |
Is it likely to be perceived as advantageous for patients or staff? | PRISM raised GPs awareness of high-risk patients. It was difficult to fit use of PRISM into the reactive structure of practices | |
How are users likely to perceive the intervention once it has been in use for a while? | PRISM was not used in practices after the completion of the QOF process | |
Can users/staff contribute feedback about the intervention once it is in use? | Lack of time and treatment options for high-risk patients limited use of PRISM | |
Can the intervention be adapted or improved on the basis of experience? | Respondents identified technical changes to make PRISM more usable |
Understandings and expectations of the Predictive RIsk Stratification Model tool
In this section, we describe how respondents discussed what they understood PRISM to be before they started to use it, and how it offered something distinct from existing practice. These views relate to the first component of the NPT framework – coherence or making sense of the intervention and are drawn from the interviews and focus groups conducted at baseline.
The PRISM tool was not seen as something entirely new. Many respondents made a comparison between the principles and process of PRISM and condition-specific risk prediction tools with which they were already familiar, such as the Wells’ Criteria (estimating the probability of deep-vein thrombosis or pulmonary embolism) and Framingham Risk Score (cardiovascular risk). Views varied on whether or not the PRISM technology would be more accurate than the GPs’ own judgement. Although they strongly defended their clinical expertise and patient knowledge, some respondents recognised that a formal and systematic process could complement current clinical practice, including the ‘intuitive risk assessments’ (GP30base, focus group D) they routinely carried out. Most agreed that risk scores needed to be interpreted by GPs, informed by their knowledge of individual patient situations. It was also observed that the tool could provide a technological rationalisation or justification for clinical decision-making:
We can always refer back to that tool that ‘this is the scoring system, that was the reason I admitted this patient or they did not need admission’.
GP25base, focus group B
The dominant view was that PRISM was a potentially useful technological contribution to case-finding as part of the current trend in general practice towards identifying individuals for case management, through a more proactive, rather than reactive, model of care:
We want a systematic way rather than just seeing people opportunistically because they are ill, we want to get there before they’re ill and on the point of going into hospital. We want to do it in a more orderly manner.
GP30base, focus group D
Many respondents described the potential of PRISM in supporting policy imperatives to prevent admissions – ‘something that we’re very much being pressured to do’ (GP16base, interview) – whereas at the same time enabling practices to meet contractual and reporting responsibilities. PRISM was seen as being able to help with the identification of patients at high risk of emergency admission, so that home-based nursing care could be provided through a community support team:
That’s how I would go about it, you see, identifying the patients that may have a problem, put in place, or mobilise forces that might be able to go in, and help a little bit – you know, if somebody has a chest infection and they’re having difficulty in breathing, they’re in need of – intravenous antibiotics, the [community support] team can do that at home – she doesn’t have to go in.
GP09base, interview
However, a range of reservations was expressed by many of the respondents. One was that, although the tool might be able to identify patients at risk, its purpose would be limited in that it would not necessarily be telling practice staff anything they did not already know:
I suppose, one of the overwhelming senses, was that if somebody were to give us a list of our top 100 patients, we’d say, ‘Well, yeah, you know, we already know about them.’ . . . We felt that the chance of this churning out unexpected patients was probably pretty low.
GP15base, interview
The second reservation was that, though patients may be identified as high risk, it was not necessarily possible to do anything to mitigate that risk:
The idea is that you can predict who they are and you can do something about it, so they don’t need to be going into hospital. And that sort of assumes lots of things, really. I mean, it assumes first of all that you can predict it, but secondly it presumes that some of these things are modifiable.
GP15base, interview
There were also concerns that resources might not be available to meet any identified needs. If these were not available, then the scope for PRISM to make a difference would be limited:
We have to have a system in place, where there is an alternative . . . if there’s no alternative, then all of our GPs, all we’re gonna do is just say ‘admit, admit, admit’.
GP11base, interview
Respondents described how being unable to respond to identified needs would be likely to create frustration among clinicians and disappointment among patients. There were also, among a minority of respondents, anxieties about implications in terms of performance management, if practices were to identify needs but then fail to respond to them:
So, if your patient has a certain level of risk, in the top 5% . . . and you haven’t [been able] to get them lower . . . that’s my worry, because it’ll be like a stick to beat you.
GP12base, focus group C
Though the function of PRISM was generally identified as being individual case finding, a minority of respondents recognised a potential role in service planning. They saw PRISM as an opportunity to demonstrate how to improve community services by highlighting patients’ unmet needs:
If this helps us define and gives evidence to what we need, that’s where I see it coming from.
GP13base, focus group C
In interviews and focus groups, most respondents reflected on PRISM from an individual perspective, rather than reporting any collective viewpoint in their practice. However, a minority of respondents did report on discussions with colleagues about PRISM, with GPs saying that they had persuaded partners, and some PMs that they had convinced GPs, to take the opportunity to try the technology and they described plans for working together with other practice staff to bring PRISM into use:
We’ll involve the whole practice. We’ll involve the nurses – the clinical side, isn’t it? And we’ll have a look at it in clinical meetings, which we have in any case, and we’ll go from there, I think.
GP01base, interview
It wouldn’t be everybody using it, but I think as long as we’ve got a doctor on-board . . . I’m there, and then it’s the nurse – a small group . . . Sometimes if you get too many people involved, nothing gets done.
PM07base, focus group A
However, more often there was a sense that there was no coherent interest in PRISM across the practice, with some individuals being more inclined to use it than others. There was an acknowledgement that it might not be easy to persuade colleagues to use PRISM, because it could be seen as bringing additional work, in a context where clinicians already felt fully stretched:
We struggle at the moment anyway – everybody’s the same with time, with clinics, scripts, mail – everything. After all of this there’s not much time to put in.
PM04base, focus group C
As well as concerns about the work involved in implementing PRISM (learning how to use it, logging on, running searches), there were some concerns about the additional work which might come from needing to actively case manage any patients identified.
General practitioners and practice staff showed a willingness and open-mindedness about trying the PRISM risk prediction tool as a way to move away from current practices that were seen as unsustainable, a case of ‘trying to keep our head above water’ (GP18base, focus group B). However, there was consensus among respondents that PRISM’s value was not as a tool in isolation, but as a way of identifying people for referral to community services or resources.
Commitment and engagement with the Predictive RIsk Stratification Model by general practice staff
In this section, we explore respondents’ initial reactions to PRISM, formed as they started to use the tool. These relate to how context affected the process of introducing it in practices, the perceived relevance of PRISM and the willingness of GP practice staff to use the tool. This links to the cognitive participation component of the NPT framework (see Table 31). The data are drawn from the interviews and focus groups conducted at the mid-point of the trial and the end of the trial period.
During the early period of the PRISMATIC trial, GPs were offered incentive payments through the QOF for 2013–14, which required them to identify 0.5% of patients at risk of emergency admissions and prepare individual case management plans. 31 This provided participating GPs with a particular structure and purpose for using PRISM.
Perceived relevance and value of the Predictive RIsk Stratification Model to general practices
General practitioners said they understood the relevance of PRISM to delivering proactive care tailored to reduce patients’ risks. As practices entered the QOF-reporting period, when they were required to review patients at high risk of emergency admissions, the PRISM tool appeared to gain a new relevance related to the QOF task. The wider potential of emergency admissions risk stratification tended to be overshadowed as GPs and PMs focused on the immediate requirements of QOF and how PRISM helped to meet these:
I think that’s the main negative I would have for you, is that QOF has interfered, I would say, with the study, because it made us use it for QOF and we’ve, essentially, not done anything with it otherwise.
GP03mid
In interviews at the end of the study, some respondents reflected that they realised the relevance of PRISM to general practice because QOF had given them an opportunity to see the tool in action. Ideas for how to use PRISM included reviewing different patient groups, such as those with lower-risk scores who could improve self-management, or to identify patients who missed routine health checks. However, respondents said that the annual rhythm of contractual requirements meant they had prioritised tasks that were linked to financial payments and then moved onto ‘the next hoop they’re making you jump through’ (GP17end). As a result of a changed context, they had not ‘capitalised’ (GP19end) on PRISM or used it within regular practice since the QOF contract ended, for which several respondents apologised.
In general, respondents said that PRISM appeared to provide accurate data and, overall, GPs had confidence in it. However, some respondents questioned its value as, they believed, it identified patients whom they already knew to be high risk, based on their own clinical judgement, whereas others reported that it could be some months out of date or appear to give illogical scores for some patients:
When we were doing the QOF stuff, we did use the tool and people felt it identified people that we knew already, who were already had every input we could think of.
GP17end
. . . there were some patients in those higher risk categories that weren’t obvious and were surprising. But then when you look back at the actual data that generates the risk profile, you think: well that’s spurious . . . and then it depends on the accuracy of the coding from the episode in hospital which, obviously, isn’t accurate.
GP14mid
Though many high-risk patients were already known, most respondents also said the tool highlighted some patients they would not normally consider high risk. These included people with addictions, homeless people, housebound patients not regularly attended by practice staff, and children with life-threatening illnesses, who could not easily be treated in primary care. They also agreed that PRISM could usefully alert them to lower-risk patients who were not routinely seen and who were potentially amenable to interventions to prevent their health deteriorating:
Those at high risk, they’d be known to us, those in the middle bracket were the kind of patients that you were possibly able to help more.
PM02end
In this way, they said, it could add value to GPs’ clinical expertise by replacing some guesswork with insight:
It gave you more of the structured and focused sort of target of what you could try to do to improve things with some sort of feeling like there was evidence of that, it was an area that might be beneficial rather than it being a bit more than guesswork.
GP13end
PRISM has shown that you can reasonably intelligently interpret and allocate points for ailments and rank patients . . . I have never seen that done in anything else except the GP’s mind.
GP10end
Willingness to engage with the Predictive RIsk Stratification Model
The willingness and speed with which respondents used PRISM once it was introduced in the practice varied according to their personal motivations and awareness of QOF. Proximity of the QOF deadline was likely to prompt immediate use. Those practices that received PRISM early in the roll-out were not under immediate pressure to fulfil the QOF requirements, and some respondents reported taking an exploratory approach to their first use. In contrast, practices that gained access to PRISM nearer the deadline for completing QOF tasks took a more focused and less exploratory approach to using PRISM:
. . . within the first couple of weeks of having it, and we all sat down and we went through the ones who were on the top – sort of the top 30 so to speak, and just went through what we thought about it and what interventions we thought might work. So it was more of a – without any plan, we just discussed it.
GP06mid
We did it straight away, because the QOF timetable really meant that we had to have the reviews done by the April, and ready for QOF . . . And so we thought: We’d better crack on and deal with it . . . And that’s the main use we’ve had from [PRISM], actually, is identifying the people for the QOF part of the risk profiling.
GP03mid
I think it’s kind of been hijacked really by the QOF requiring us to do certain things and so we’ve tended to sort of focus on what the QOF requirements were, really, you know, for the first couple of months, particularly, as it actually had to be done.
GP17mid
Even those who had hoped to use the tool more broadly ended up taking a narrow approach as the QOF requirements took priority over other practice activity:
What we hoped to do is not what actually happened. So, the idea was that we would do the review and it would be with practice nurses right the way through to, possibly, even the district nurses . . . what we did in the end was, me and the other three partners went through the patients and argued the toss, basically, about it. We tried to discuss a few of them with the district nurses at the end of a palliative care meeting . . . But it wasn’t within that original meeting, it was done over the new few weeks.
GP06end
The winter months were described as being a particularly difficult time to implement a new system because of workload.
Making the Predictive RIsk Stratification Model work: how general practice staff used the Predictive RIsk Stratification Model
In this section, we explore how respondents used PRISM in every day practice. In terms of NPT, this section considers the collective action required by participants to make PRISM function within general practice. We draw on data from the interviews and focus groups conducted mid-trial and at the end.
Training staff to use the Predictive RIsk Stratification Model
Predictive RIsk Stratification Model was introduced to each practice in a training session for the GPs and PMs who would be using the tool. Respondents reported that the training session had been useful. The tool was straightforward to use and worked as they expected:
I suppose I’m fairly computer literate anyway, so I do pick up on the computer very- fairly easily, and it’s . . . just a logical programme, if you ask me.
GP03mid
I mean at the end of the day, I think the website gives me what I was told to expect, really, was that it would give me risk scores for people and stratify them and show them on pages, and that’s sort of what it did.
GP15mid
The training focused on the practical aspects of accessing the PRISM data. Respondents said their ideas about how to put risk score data to use came from the focus groups that some participants had attended early in the study, from participants’ own aims or the QOF requirements:
. . . we only really wanted to know what we needed to know, to do the piece of work that we were gonna get judged on.
GP32mid
Usability of the Predictive RIsk Stratification Model
Respondents said they had found the PRISM tool easy and intuitive to use. They said it was ‘straightforward’ (GP02end), ‘user-friendly’ (GP06end), ‘made sense’ (GP08end), ‘very organised, very matter of fact’ (GP10end) and the filtering options were ‘very good’ (GP02end). However, several GPs had experienced technical problems with the system crashing or locking, or passwords not working; in some cases, slow local broadband speeds and big practice lists prevented them quickly accessing and interrogating data. One GP’s effort to access the technical help desk was ‘a nightmare’ (GP18end). With only small periods of available time to use PRISM between other responsibilities, respondents said that it was vital to have guaranteed quick access. One GP explained his frustration when he tried to obtain some risk scores:
So here you are, flying out with the speed of light and thinking, well, okay, I’ll just get those . . . and download it. Right, where’s my log-in number? And I found it and logged on and – it froze. And it froze one time with [PM] as well so what she did was take a screen print . . . the truth is that cannot happen. That is anathema to general practice. You have no time to breathe, so if it’s going to work, this has got to be so robust.
GP05end
General practitioners who looked at data frequently reported that the system could be out of date. Many were also frustrated that the risk scores and patient data were not interlinked with the clinical information on practice information systems so they had to toggle between screens and programs or print data and make manual comparisons.
How the Predictive RIsk Stratification Model affected the work of general practitioners
All respondents reported that they used PRISM to identify patients at high risk of emergency admissions in order to fulfil the QOF requirements. They focused on the top PRISM tier using the tool to generate a list of patients from which they selected a percentage for review:
It was fantastic because we were able to pick out the patients that the local health board had highlighted, for the QOF thing.
GP11end
During the QOF work, we did use PRISM to help inform out meetings, to understand what happened to the 52 patients that we looked at, used it as a base for discussions with other team members that attended meetings. . . . and we did do these with care planning around those individuals, mostly by house visits from the relevant GPs, so spread the work between us.
GP08end
We used it to pull off all the data that was necessary for the QOF.
GP18mid
In most cases, the lead GP or (less often) the PM looked at the PRISM data on screen, saved information in an electronic spreadsheet or printed off lists, logging on very few times in order to acquire the information, and did not filter patients in the top tier. However, others reported that they had used PRISM more frequently and creatively. A small number who had more time before completing the QOF report, explained how they explored patient data within the PRISM tool, to understand why some patients had high scores or to review certain patient groups. One GP said that he had used PRISM on about 10 occasions during the first 3 months to gather information to inform his practice:
I’ve gone back in to look at some of the patients . . . and why they’re risky. So, I sort of go back into it, and sort of say, ‘well, I’ve got this patient to see,’ and I’ll sometime go back in and sort of look at what PRISM’s identifying the risk to be so that when I go and do a review I can put that in context.
GP08mid
Another GP filtered high-risk patients to identify those with COPD in the top tier and COPD patients with rising risk scores in the next tier down, plus elderly people likely to have social needs in those two tiers:
We changed tack a little bit. And we tried to focus in on specifics and we also tried to focus in on those that were climbing. So, we identified anyone with an arrow meaning their risk had gone up since last time and we tried to pin those down and do a chronic disease review on them.
GP06end
Some GPs expressed disappointment at the information that PRISM provided and were uncertain about how they could use it:
What I wanted, for instance was to download my 53 patients and download the information that would allow me to work out why they’re on that list, in terms of what chronic diseases did they have, and how many hospital admissions had they had, and how many drugs were they on, and so on . . . And it really disappointed me that a lot of that I had to do manually.
GP15mid
Respondents reported that involvement with PRISM usually fell to a single individual within the practice, who would then disseminate the information to others at practice or multidisciplinary meetings. Respondents reported that they shared the PRISM information (patient lists with risk scores) within the practice through routine practice meetings. Some respondents, particularly from practices that received PRISM closer to the QOF deadline, said that they divided the list of high-risk patients among the partners and worked individually:
We had another meeting then, to just discuss the patients who we were going to see. Some of us, I think, well, sort of chose patients that perhaps we knew already, which perhaps wasn’t the ideal thing to do, really, you know . . . it was more trying to meet the requirements of the QOF, rather than get a meaningful outcome from it, I think.
GP17mid
I allocated people to different clinicians . . . I said ‘right you can have diabetes you can have COPD, you can have asthma, or whatever’ and then I looked at the rest of them and there were something like 12 or 14, you know, who didn’t fit into any of these, who were odd ones really, and we just sort of divvied these out . . . I mean, you can’t review 53 patients collectively.
GP15mid
In most practices, few staff other than those taking the lead on PRISM were reported as making direct use of PRISM. Lack of interest, on top of demands on GPs’ time and difficulties incorporating the PRISM information into practice routine, meant that respondents were not able to make widespread changes to the way practices functioned. Several of the practices were short of GPs because of illness, retirement or maternity leave. Some were planning to close or merge. Respondents were concerned that other practice staff did not have the capacity or skills to take on more patients and also reported cuts in community services and outpatient clinics. Many respondents reported in the end of study interviews that they had not accessed the PRISM data after practices completed and submitted their QOF reports. A small number of respondents said they had planned to keep using PRISM, but technical problems or lack of time meant they were no longer using it. Only two practices reported that they were still using PRISM at the time of the end-of-trial interviews.
How the Predictive RIsk Stratification Model affected the way general practitioners responded to patient needs
General practitioners said that the QOF process encouraged them to intervene with identified high-risk patients. They made an effort to respond with different or additional activities for the small percentage of at-risk patients they selected from the top PRISM tier.
Respondents described types of actions that they took in response to a patient’s high-risk score. All respondents reported carrying out an extra level of review and care to fine-tune the existing treatments that these high-risk patients already received. They contacted many patients, paying a house call, inviting them into surgery for a check-up or telephoning to see if anything was needed for ‘optimisation in their treatment’ (GP31end), for example updating blood tests, bringing forward annual check-ups or making a referral to outpatient clinics or nursing teams. However, they were concerned that teams did not have the resources to respond to more activity and questioned whether or not patients were actually followed up:
I think QOF work highlighted our lack of support in managing these individuals. So, although they did have medical case review, that didn’t really generate much extra activity, particularly almost no response from district nursing. So, consequently, the work had a fairly negative impact.
GP08end
In other instances, GPs felt that patients were already optimally managed and they used the contact to provide reassurance:
Some of them we were doing everything we could and it was just telling them that we were as well.
GP03end
Some practices used the opportunity to inform patients about ways to better self-manage. Two practices (10 and 11) identified frequent ED attenders and discussed, with patients and family members, other ways of managing crises. Practice 19 asked the nurse to talk with patients about issues such as identifying infections, weight management and spotting early warning signs:
We got these patients and tried to (take) more time in educating them . . . you teach them what to do, how to identify a source of infection . . . with heart failure, exactly how to understand, keep weighing themselves if they’re getting weight, more short of breath.
GP19end
Some GPs focused on non-medical needs which could reduce the chances of a patient deteriorating, by referring patients to other care agencies and encouraging families to self-refer. However, they admitted that it was difficult to engage other services, especially if the care was preventative rather than reactive:
. . . we would sit down, me, the PM, the district nurses, the midwives if necessary and the health worker if necessary and we’d discuss the more difficult patients . . . highlight the problems that were there or the potential problems . . . so that they were aware if we started running into problems and we’d phone one of them.
GP11end
I’ve tried to involve social services or the CIIS [Community Integrated Intermediate Care Service] team or packages of care and things to be put in place sooner rather than waiting for the crisis . . . start the ball rolling earlier rather than leaving it 6 months down the line and them needing urgent respite or something.
GP13end
Practice 3 changed arrangements to allow high-risk patients to always see the GP of their choice and flagged this arrangement on their notes and for receptionists to see when arranging appointments, so patients received continuity of care. Practice 6 made up emergency drug, steroid and antibiotic packs for identified patients to use at weekends and bank holidays if they felt their health was deteriorating. Practice 2 was able to modify a patient’s treatment following discussion at a multidisciplinary meeting:
It wasn’t until we discussed it with everybody in the room . . . so you had nurses saying ‘Oh yes, she’s terrible, she doesn’t take any of her medications after 10 o’clock in the morning’. And the GPs went, ‘well, nobody’s told us’. So we can structure that and do it, so rather than twice daily medication, give her once daily medication and solve the problem.
GP02mid
Just one GP, from practice 5, said that he did nothing above normal care and described the process as ‘an exercise in form filling’ (GP05end).
Reflections on the Predictive RIsk Stratification Model by general practice staff
Respondents were invited to reflect on the PRISM tool and their experiences of using it, including how it affected patients and their practices.
Perceived effects on emergency admissions and emergency department attendances
General practitioners thought it unlikely that using PRISM had any effect on emergency admissions and ED attendances. There was a widespread feeling that admissions initiated by GPs were already low and could therefore not be much reduced.
There are odd occasions where you were able to proactively help somebody or put a plan in place to stop them being admitted to hospital. I think it’s a fallacy to think that you could reduce emergency admissions from primary care because the primary care admissions are so small. The vast majority of admissions to hospital go through A&E . . . as a practice, our admission rate is 0.8% . . . one case a week, if that.
GP02end
I have seen cases where you try and get involved and actually you’re referring more, treating more and more medication and then ending up using more resources, maybe appropriately, maybe not. But the evidence isn’t there as yet, that I’ve seen, that doing a lot of things early on, whether that makes much of a difference long term or not.
GP31end
Media coverage already made GPs very aware that they should not use emergency services inappropriately. Although they acknowledged that primary care ‘was set up to be reactive’ (GP08end), they seemed very concerned to use resources appropriately and carefully:
You think about it all the time, even if you’re in an out-of-hours setting and the budget isn’t yours, and when you’re prescribing a drug, that the thing that’s at the back of the mind is the money, how much of the resources are you using?
GP11end
However, some respondents could identify instances where an emergency admission may have been avoided and the two GPs who each targeted a patient with frequent ED attendance reported that those patients’ use of 999 services had fallen. Only one GP suggested that the PRISM reviewing may have been associated with increased hospital admissions:
We did bring in a certain number of people and do care plans with them and then we ended up admitting them because we’d seen them and they looked unwell.
GP31end
Effects on general practitioners and the way they worked
The majority of respondents described how PRISM changed their awareness of high-risk patients and increased their contact with some of this group while they were undertaking QOF tasks in order to reassure themselves that everything was being done to prevent a possible crisis:
It [a high risk score] does have an effect of making you sit up and think ‘bloomin’ heck, what’s he doing up?
GP05end
We’d sit down and discuss the odd patient or so that were highlighted and see whether we could improve things for them . . . see whether we could do something about it.
GP11end
General practitioners reported that because the activity was tied to their contract, they were incentivised to spend time on this work. They carried out a number of house calls, telephone contacts or extra appointments themselves. They also coordinated care to involve other practitioners or clinicians:
Made some clinicians aware of them, initiated home visits, changes to medications, getting other people involved in their care.
PM02end
Reviewing high-risk patients also resulted in additional work because the process meant they identified unmet need that they then had to address:
You’re trying to be focused but actually what you’re actually doing is picking up people for other reasons.
GP06end
You spent some time looking at their records, and it was then communicating to them what changes if any were needed to be made and double checking then that everything was as we thought it was and monitoring their blood pressure and things like that . . . I think it probably was more than we needed to do.
GP03end
It did throw up quite a few surprises, some additional potential work that we weren’t addressing . . . some of those are the people that would admit themselves and bypass us.
GP08end
They also understood that their actions, by giving patients extra attention, could increase a patient’s risk score:
The very fact that we were seeing these patients . . . resulted in a flurry of referrals on their record that put their risk higher . . . it will initially look like higher levels of risk by doing whatever interventions we’ve done, put these patients at more risk really (is) how it would appear.
GP03end
Some respondents were more proactive with patients, and this raised their confidence and pride in their work. The GP from practice 17 said that she encouraged her patients to engage with her and the available services in order to prevent health crises:
More awareness of the benefits of getting people to engage with the chronic disease clinics and more awareness of being proactive with managing people’s ongoing conditions in the consultation . . . I think it makes me feel like I’m a better doctor for doing it.
GP17end
The GP from practice 6 said that using PRISM had broadened his perspective when treating patients and described how he would review a patient whose risk score had risen:
You can’t do anything about an admission that’s already happened but it gives you an idea of where your efforts are best suited . . . you can’t change that management with that patient. Everything you’re doing is what you’re already doing, but you can see if somebody else has an intervention . . . we might need to optimise their COPD care or their diabetic care, that’s what’s going to make the difference with that patient.
GP06end
Some GPs were concerned that they were focusing on a small number of patients, usually known to the practice, when other patients may also need the same attention.
I thought I’d be using PRISM for the people who aren’t coming in, who have got those risks and we’re not seeing them . . . so far we haven’t done that. Because, as I say, time meant we had to do this particular 100 so we’ve not done anybody else yet.
GP03mid
Everyone knows their sickest patients, not everyone knows their third or fourth sickest patients and I think that’s probably where it [PRISM] will become useful. I’ll be honest, we haven’t tackled that yet, probably because it seems a bit complicated.
GPmid06
Most respondents agreed that it would be most useful to study and intervene with patients from lower-risk tiers because it was possible to prevent health deterioration. However, the QOF requirements had not included this and no respondents reported that they intervened with any of these patients. They also felt that taking this approach would require too much time:
Those in the middle bracket were the kind of patients that you were possibly able to help more than those . . . in the higher echelons that were already having all the input that was available. But it was the ones in the next cohort down which might have been more useful because you can actually put in things that will stop them from going up the pyramid.
GP02end
It’s the second tier down where we might be able to make a difference. The way that we were required to do the QOF data meant that we had to use the highest risk group so I don’t think we got the best out of it that we could’ve done because that top risk group we knew all the names . . . and were already doing all we could for them. Unfortunately we haven’t had the time to look at the people in the groups further down.
GP03end
Effects on general practitioner interaction with patients
General practitioners reflected on the effect that PRISM had on their interaction with patients, particularly those in the highest risk group, even when no new interventions were available. Several respondents thought that the increased GP–patient interaction could have been beneficial, regardless of the medical interventions delivered:
I think the experience from the patient, when we actually popped in to see them, they actually liked that, that we were actively going to see them . . . I can see there is a patient need around this. It’s just whether it’s deliverable at the moment.
GP08end
Was it just that we were being particularly caring this year?
GP32end
Although GPs did not generally tell patients about PRISM or share the risk score, they used opportunities to tailor self-management advice. However, they were careful not to alarm and precipitate self-referrals:
It [risk score] wasn’t directly volunteered. It was just a ‘we think that this would perhaps stop you needing to go in this year, we’re hoping that this will stop you needing to go to hospital’ . . . they don’t understand the difference between high risk of admission and high risk of being ill. They think that’s the same thing. So their use of the health service right the way from just telephone advice through to rocking up at out-of-hours or A&E would go up.
GP06end
Several respondents questioned how far their actions did, or could, benefit patients when the process was constrained by the QOF framework and limited by availability of resources:
There will have been people who were reviewed or assessed who otherwise wouldn’t have been, but whether this translates into change in outcomes is a separate issue.
GP14end
Facilitators and barriers affecting use of the Predictive RIsk Stratification Model
Respondents said that lack of time was the single most important limitation on use of PRISM. Respondents said they felt ‘overloaded . . . and burnt out’ (GP02end), ‘running so fast (we) can’t take a step off’ (GP32end) and ‘crisis-driven’ (GP18end):
We’re fire fighting and trying to get that switch, between fire fighting and more proactive care is challenging because we’ve got too many fires to fight.
GP08end
They welcomed the principle of risk prediction using PRISM, but felt that it was unrealistic to work this way without extra resources to support changed practice. They had been able to use PRISM through the QOF system because it provided funding for more GP hours, which released them from the imperative of the appointment schedule:
Having the money enabled us to spend the time that was needed to meet together and discuss everything and go through the notes individually, contact the patients, do a management plan . . .
GP17end
However, this meant that most respondents could not see any way of using PRISM other than how it had been used to complete the QOF tasks. Some recognised that potential benefits had not been properly explored and considered and that ‘it was sad because it wasn’t done very well’ (GP05end). However, all respondents came to the conclusion that continuing to use the PRISM risk prediction tool was not a realistic way for them to practise:
You know, if it makes my day easier and its safer and improves patients’ health, then I’m happy to use it. But I haven’t the time to go looking in depth without, you know, having some protected time.
GP10mid
In a perfect world, PRISM would be great. At the moment we are like a swan, frantically trying to keep afloat.
GP02end
Many respondents also highlighted the very limited range of available treatment options to support high-risk patients. They were disappointed that the intervention did not include additional services or skilled staff to support care and enhance treatment for patients. Consequently, they felt deterred from using the tool because it had little value unless they could access extra services to support identified patients. GPs said that nurses were already working at capacity, and other community services provided reactive not preventative care: all that GPs had currently was ‘access in a crisis’ (GP08end). They identified that they needed a network of community health and social services to make the system work:
[PRISM] is a tool we’re going to use but there’s nothing that we can actually then do with what we find out, other than us. So no other service linked to it. No add-on when things are so stretched already. And I can remember it taking me a little while to [understand] because I thought, I really thought I was missing something.
GP13end
You think ‘right, OK, I need to get the CRT team into that one’, you ring them up and you get ‘I’m awfully sorry we’re booked up for 2 days’. And that’s an issue because (a) it’s a waste of my time and that took a precious 10 minutes to get through, and to find the right person, and to have the conversation, and then to be told it’s 2 days, is useless to me. So then I’ve got to ring the nursing home back and say ‘they’ve got to go in’, because they’re not drinking now, you know. So the resource that will prevent the admission, which is what I’m assuming PRISMATIC’s all about, is not there.
GP05mid
Several respondents stated that they were reluctant to invest much of their time in using PRISM while it was still being trialled:
If there were examples where the amount of effort produced a magnified return, then I would be quite interested to read that topic. And if you can prove that sitting down with your district nurses to look at your top – say, let’s filter everybody over the age of 70, who are the top ten over 70, and that proves, has got proof of reducing admissions, then why should I do that? Why should I spend my time doing that it if it doesn’t stop me doing something else, if it doesn’t save my time, or if it doesn’t earn income.
GP10mid
Suggestions for ways to improve the Predictive RIsk Stratification Model tool
Respondents proposed technical changes to enable integration of PRISM into their practice routines. They wanted a single document with all of a patient’s data, to avoid needing to look at different sets of records for clinical information, correspondence and PRISM, in order to properly interpret a patient’s risk score and treatment history. The GP from practice 8 wished he could:
Jump backwards and forwards between patients . . . if it was all real-time as opposed to closer to real time, I think you’d probably be more aware of what good you were doing and the impact it would have. And things like secondary calls and sending messages and letters to coordinate care and things would be much more easy if it was connected to the system, i.e. giving a print out of the reports to the district nurses and things. It would be a lot easier if it was more seamless between the two.
GP08end
Integrating PRISM with the clinical software was cited as something that would not only make using the tool easier, but might encourage GPs to use it more frequently:
It has to be that easily accessed, and that might just trigger: oh my goodness, you know, they’ve fallen and I didn’t realise they had, or whatever other trigger they have on that list.
GP05mid
Views of a health service manager on the Predictive RIsk Stratification Model risk tool: at the end point of the trial
At the end of the implementation period, we interviewed a senior manager in ABM UHB with a strategic role for governance and operational issues for the professional side of primary care, who also had oversight of the research and development projects. Although she had high-level responsibility for PRISM within the health board, she had little capacity for engaging with practices to support its implementation, but did have close contact with the research team, including the GP champions, and with clinical directors working at local level.
The manager reflected on the implementation and impact of PRISM during the PRISMATIC study. Her perception was that PRISM had a limited impact on practice, though it had helped some GPs to identify hitherto unknown people at risk, particularly younger people with alcohol problems. She saw the adoption of PRISM into general practice as being inhibited by the fact that it was seen as additional work, and not as something which would be of immediate help to them. She also believed that the tool had proved ‘clunky’ in use, with the disadvantage that:
. . . it wasn’t embedded in the GP system . . . so, if they were in the middle of a busy clinic, a surgery, and a patient came in and they wanted to know what risks they were at . . . they would’ve had to have closed down their current system and then opened PRISM . . . So, they weren’t gonna do that.
She also suggested that PRISM had not been used within ABM UHB to support service planning.
The manager identified a number of contextual issues which developed during the course of the study period – some at local level, some at national level – which may have influenced implementation. At local level, the old management structure of three localities was abolished and new clusters of practices were set up, with GPs:
. . . being expected, within the contract, to look at their population health within their areas, and to really try to re-design and influence it.
At the same time, there was a loss of GPs and practice nurses from primary care in the local area, many leaving practice as they reached retirement age, with newly arriving clinicians less inclined to enter into partnerships, and more likely to opt to be part-time employees. Smaller practices, in particular, were seen to be struggling to be viable, and there were a number of practice closures. Combined with the increasing pressures of demand from an ageing population and a health system stretched in both primary and secondary care, this led to a situation described by the manager as:
. . . an absolute crisis out there. And it’s probably difficult for people who aren’t involved to know just what a crisis point we’re at . . . we need solutions. So, I mean, this cannot go on as it is.
The Welsh Government set up an Intermediate Care Fund, which supported health boards to develop community resource teams to provide multidisciplinary support for people at risk of admission. Three of these teams were established in the ABM UHB area. Though they were seen by the manager as valuable, the team’s focus was very much on providing interventions to those patients ‘at the tipping point’, that is, at immediate risk of admission, rather than on working in a longer-term, proactive way.
The introduction and then disappearance of the QOF points associated with identifying high-risk patients was seen by the manager as encouraging use of the PRISM tool, but only in a very limited way:
I think it did encourage and promote looking at the top 5%, because that’s all they would be paid for . . . People will just do what they’re paid for . . . and they will not do the extra . . . the criticism of QOF is that it’s become tick-box, and that GPs just do things to tick a box and to get points, and money, and that that detracts from a more holistic approach.
The manager was very much aware of the challenges of trying to implement an intervention as part of a research study, as a strong evidence base was not yet available to justify the intervention, and more particularly the time needed to support and promote it and to educate people in its use.
I do think that’s partly because we didn’t . . . want to be promoting something we didn’t really – we weren’t really sure worked . . . if people are expending a lot of energy on something, you want to be sure it’s evidence-based . . . And it’s a bit of a catch 22.
Summary of practice staff questionnaires
These findings summarise questionnaire data from general practice staff at intervention practices. Two rounds of questionnaires were conducted: mid-trial (3–6 months post intervention) for a sample of invited practices and at the end of the trial (18 months post intervention) for all practices in the study. Participation in the first round was 9 out of 16 practices, and in the second round 25 out of 32 practices. In the summary of results that follow, ‘3m’ refers to the mid-trial questionnaire point, and ‘18m’ to the end of the trial. Text comments include the same numerical practice codes used elsewhere, and confirmation of whether the comment was received at mid-trial or at the end. Questionnaires were completed by PRISMATIC GP leads or their delegates (fellow GP or PM).
Training and induction
At the mid-trial point we asked respondents to indicate the usefulness of the training they received on using PRISM. Seven out of eight respondents to this question found the training useful (six ‘very useful’ and one ‘moderately’). Two-thirds of respondents questioned at the mid-trial point first used PRISM within 1 week of their training.
Reported use of the Predictive RIsk Stratification Model
We asked respondents at the mid-trial point to estimate how many times they had logged into PRISM over the preceding 3 months (Figure 7). The number of logins reported ranged from zero to 11+, with most reporting between one and six logins. At the end of the trial we asked respondents to estimate how many times they had logged into PRISM during the preceding 9 months; once again, most respondents reported one to six logins (see Figure 7).
FIGURE 7.
Please estimate how many times you have logged on to PRISM in the past 3 months (3m, n = 9) and 9 months (9m, n = 25).

We asked respondents at the end of the trial to indicate when they last logged on to the PRISM website. For over half of respondents, this was over 4 months previously, with 4 of the 14 respondents indicating that they last used PRISM over 6 months previously. When asked at the end of the trial whether PRISM has been used more or less over the past 6 months than in the first few months, 9 of 13 (69%) of respondents reported less use.
The user-reported data are consistent with patterns of logins as recorded by NWIS (see Table 31 and Figure 8). PRISM logins by registered users at PRISMATIC study practices (NWIS, 2016, personal communication) below.
FIGURE 8.
Predictive risk stratification model logins by registered users at PRISMATIC study practices (source: NWIS).

Targeting patient groups
We asked respondents to indicate which groups of patients they had reviewed using PRISM (Figure 9). At both the trial mid-point and at the end, patients in the highest risk groups (3 and 4) were reported as the most frequent groups of patients reviewed; at the end of the trial, a wider range of patient groups was reported as being reviewed.
FIGURE 9.
When you use PRISM, which groups of patients do you review? Please select all that apply (3m, n = 9; 18m, n = 13).

When asked to indicate which one group was most frequently reviewed, respondents at mid-point were most likely (5/9) to say it was those at level 3 (high risk); at end-point, however, the most commonly cited group (5/13) was those patients at level 4 (the highest risk group).
Who uses the Predictive RIsk Stratification Model, and how?
We asked respondents at both time points to indicate who in their practice had been using PRISM, and how (Table 39). Respondents indicated that use is largely by GPs (particularly those leading on PRISM) and PMs. The most common way for clinicians and PMs to make use of PRISM data was by discussing these in meetings, including MDT meetings within the practice.
Practice staff | Logged in to see PRISM on screen | Seen PRISM data on a paper print-out | Discussed in meetings (including MDT) | Discussed in informal meetings | ||||
---|---|---|---|---|---|---|---|---|
3m | 18m | 3m | 18m | 3m | 18m | 3m | 18m | |
GP leading on PRISM | 5 | 7 | 4 | 6 | 9 | 12 | 4 | 3 |
Other GPs in your practice | 0 | 3 | 1 | 5 | 8 | 12 | 1 | 2 |
Practice nurse | 0 | 0 | 0 | 1 | 4 | 5 | 0 | 1 |
PM | 5 | 6 | 3 | 6 | 9 | 14 | 2 | 4 |
Other – e.g. district nurse | 0 | 1 | 0 | 0 | 7 | 6 | 0 | 3 |
Actions taken after using the Predictive RIsk Stratification Model
We asked respondents what actions were taken in terms of patient care after reviewing the PRISM data; their responses are shown in Table 40.
Action | Time point | |
---|---|---|
3m (n = 9) | 18m (n = 25) | |
GP consultation in person | 6 | 14 |
GP consultation by telephone | 5 | 13 |
GP consultation by home visit | 4 | 12 |
Practice nurse appointment | 2 | 8 |
Chronic conditions clinic in GP practice (e.g. diabetes mellitus, asthma) | 3 | 12 |
Prepare active management plan | 4 | 15 |
Communicate with or refer to the district nurse | 3 | 7 |
Communicate with or refer to the health visitor | 2 | 1 |
Refer to community nurse or case manager (e.g. respiratory, mental health, chronic conditions) | 3 | 4 |
Communicate with or refer to mental health services | 1 | 3 |
Refer to therapist (e.g. physiotherapist, occupational therapist, speech therapist, rehabilitation therapist) | 3 | 3 |
Refer to social services | 3 | 4 |
Refer to an alternative medicine provider | 0 | 2 |
Refer to voluntary sector (please identify who below) | 2 | 3 |
Other (please identify who below) | 3 | 2 |
Not used PRISM | 1 | Not asked |
Have used PRISM, but not yet taken any actions | 0 | 3 |
At the mid-trial point, respondents reported that GP consultations (including face to face, telephone, home visit) were the most frequent course of action. At the end of the trial, although GP contacts and referrals to community providers were still widely reported, the single most frequently reported action for patients identified through PRISM was the preparation of an active management plan:
What has been beneficial is the concept of the active management plan. I think this concept has come partly from using the PRISM data and one that we still use. We are hoping to train a nurse to advance nurse practitioner status to act as a chronic condition practitioner which would massively change the way we would be able to respond and use PRISM.
22, 18m
Two respondents provided particular detail on how PRISM supported care for patients with COPD who were identified as high risk. The first described using PRISM as a way to initiative contact with specialists – though challenges remained to finding ways to implement change:
Involved local consultant respiratory physician, as there was a pattern of patients with respiratory problems appearing in patients on level 4. Sharing of information, plan to possibly meet again to discuss patients . . . with COPD to try and develop a more collaborative approach to care. Useful meeting but was not followed up on due to general busyness on both sides.
9, 3m
Patients did seem to value and appreciate the applications of PRISM, e.g. we targeted those with COPD and implemented self-management plans which included having ‘just in case’ antibiotics at home.
28, 18m
Issues affecting Predictive RIsk Stratification Model use
We asked respondents at both time points to describe what issues had affected their use of PRISM (Table 41).
Factor affecting PRISM use | 3m (n = 9) | 18m (n = 25) |
---|---|---|
Lack of clarity about PRISM | 1 | 3 |
Speed of the website | 3 | 8 |
Issues with logging in | 4 | 7 |
Concerns about the accuracy of the PRISM data | 0 | 2 |
Concerns about data sharing | 0 | 0 |
Demands on time to access and understand the PRISM data | 2 | 12 |
Demands on time to respond to the data | 3 | 14 |
Anxieties about liability | 0 | 0 |
Concerns that PRISM does not provide new information | 0 | 4 |
Lack of resources to respond to identified needs | 1 | 15 |
Staff trained to use PRISM had left practice | Not asked | 1 |
Other (please specify below) | 3 | 3 |
At the mid-trial point, the emphasis from respondents was on technological barriers, such as speed of the PRISM website, and difficulties with logging in. Although these remained issues in the end-of-trial findings, demands on time and lack of resources emerged as the most cited issues:
The issue is having dedicated time to analyse the PRISM data and implement the resulting workload. At present it feels that as a GP we are running to stand still. PRISM may well be helpful, but when the resources and time are available to implement it within this practice.
22, 18m
Among the ‘other’ issues identified was the technical one of PRISM not being integrated into existing practice computer systems.
The role of the 2012/13 General Medical Services contract
We asked respondents at the end of the trial to describe how, if at all, the 2012/13 GMS contract, which included QPIs encouraging the use of a risk prediction tool, influenced the use of PRISM in their practice, and 13 respondents gave free-text responses to this question. Most emphasised the importance of the contract in encouraging the use of PRISM, with a small number going on to emphasise the value of PRISM in supporting delivery of this QPI:
A very welcome helpful tool to identify in a systematic way the patients required for this QPI. I am not sure how easy it would have been to do without the tool.
30, 18m
However, there was also an awareness among some that the contract had been the sole driver of PRISM use:
I don’t think we would have used PRISM if it was not for the contract.
22, 18m
We used it almost exclusively because of QOF.
15, 18m
Opinions on the Predictive RIsk Stratification Model
We asked respondents at the mid-trial point to indicate how strongly they agreed with a number of statements relating to how PRISM has changed the way they work (Figure 10).
FIGURE 10.
Looking back over the past 3 months, what difference has PRISM made to the way you work? (3m, n = 9).
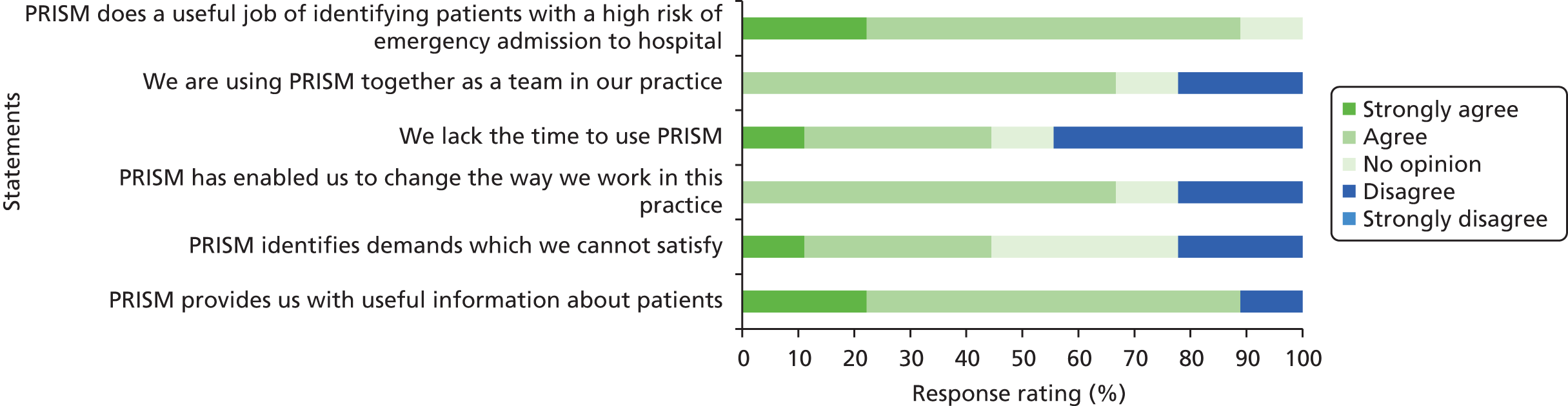
Almost 90% agreed or strongly agreed that PRISM did a useful job at identifying patients at high risk of emergency admission, and over 60% agreed that PRISM had enabled respondents to make a change to the way they worked within the practice.
We asked the same questions at the end of the trial time point (Figure 11).
FIGURE 11.
Looking back over the past 9 months, what difference has PRISM made to the way you work? (18m, n = 13).

Agreement that PRISM did a useful job at identifying patients at risk was somewhat lower, at 72%. The proportion of respondents agreeing or strongly agreeing that PRISM had enabled them to change they worked had fallen to < 30%. Lack of time to use PRISM was an issue, and few practices agreed that they were working together as a team to use it.
Future Predictive RIsk Stratification Model use
At the end of the trial, we asked respondents how they expected to use PRISM over the following 6 months; 13 out of 31 responded with free-text answers. There was a range of responses, from those who did not expect to use it at all, to those who had definite plans:
We will continue to use PRISM to identify patients at risk and patients who should be included in our palliative register.
23, 18m
We are planning on looking at the data with our chronic conditions nurse to identify patients that need input.
25, 18m
There was a fairly consistent theme running through responses that future use of PRISM would depend on time capacity within the practice, and, to a lesser extent, on being able to access resources or support services to meet identified needs.
Summary of stakeholder views on implementation and use of the Predictive RIsk Stratification Model
The qualitative component of the study revealed some consistent themes in terms of attitudes to PRISM across all stakeholder groups. All stakeholder groups seemed to be aware of a lack of certainty about the intended role and function of PRISM, with its original purpose being identified as supporting service planning, while later implementation focused on individual case-finding.
General practitioners and practice staff showed a willingness and open-mindedness about trying the PRISM risk prediction tool as a way to move away from current reactive practice, which was seen as unsustainable. However, there were concerns expressed – both before implementation and after – by all stakeholder groups about its ability to support change in patient care without associated investment in new community services or resources.
Almost all practices that responded to the end-of-trial survey reported that they made some use of PRISM, although the total number of logins was not high and became less frequent during the course of the intervention period, with only two practices reporting that they were still using it at the end.
The extent to which PRISM was used varied greatly across practices. A range of ways of using the PRISM information was reported, with some practices printing off PRISM data (lists) for later discussion. Generally, patients were discussed in practice meetings (often initiated by QOF requirements), some of which were attended by staff from other disciplines and organisations. Respondents reported four types of interventions taken in response to a patient’s high-risk score: (1) fine-tuning existing treatment, (2) improving self-management skills, (3) trying to address non-medical needs and (4) making changes to the way that treatment and care was provided.
The introduction of PRISM to general practices coincided with contractual requirements (QOF) to select 0.5% of their practice patients at high risk of emergency hospital admission and prepare case management plans. The QOF requirement appears to have been a major driver of PRISM use, and also to have shaped the exact way in which practices used the tool – focusing on those patients in the highest risk group. After the QOF reporting period ended, use of PRISM appears to have fallen away.
General practitioners cited as barriers to using PRISM: the lack of time to work prospectively; inadequate referral services; limited internet access; out-of-date data; and the PRISM data not being integrated with practice records. They said that they needed financial incentives alongside additional community-based services for identified patients in order to regularly use the tool.
Reflecting on PRISM, GPs reported that they were unsure whether or not using it had any effect on emergency admissions and ED attendances, but two GPs who each targeted a patient with frequent ED attendance reported that those patients’ use of 999 services had fallen. Respondents felt that PRISM changed their awareness of patients and focused them on targeting at-risk patients to reassure themselves all steps were taken to prevent a possible crisis. All had concentrated on high-risk patients, despite feeling these were least suitable for proactive management, yet they believed that they had provided more attention and treatment, which was reassuring to patients.
Strengths and limitations of qualitative strand of study
Strengths
The multiple data collection methods (interviews, focus groups, survey, login information) allowed us to triangulate findings, which helped interpretation of quantitative findings. Collection of data at different time points allowed us to track how attitudes to and use of PRISM changed over time.
Using the NPT framework to interpret interview data enabled us to fully consider the stages and actions associated with implementing an intervention in a health-care setting to increase our understanding of the ways they responded in this study.
Limitations
Interviews with policy and health board staff about the story of PRISM took place some years after PRISM was developed, potentially affecting their memory of events because of the time period and role changes.
In most cases, we talked to only one respondent from participating general practices. This was usually the GP responsible for leading use of PRISM, and their knowledge and use of PRISM was not always shared by other staff. In a minority of practices, the PRISM lead GP changed between the three data collection points, because they retired or moved practice.
We were unable to gather the views of community health staff after the tool was implemented, as most staff declined to attend a follow-up focus group because their roles had changed. Only one health services manager agreed to be interviewed at the end of the study.
General practitioners taking part in the PRISMATIC trial volunteered for the study. In interviews, several identified themselves as interested in, or supportive of, research and wanted to contribute to knowledge generation by participating. They were therefore likely to be atypical of many GPs and their response to PRISM may not be that of other members of their profession.
Chapter 7 Service user involvement
In line with good practice, service user involvement in the PRISMATIC trial was intended from the outset and throughout. A summary of service user involvement can be found in Table 42.
Type of involvement activity | Role | Process | Comments |
---|---|---|---|
Supporting service user involvement | Service users were actively involved across all activities associated with delivering the PRISMATIC trial | Information, guidance, honoraria, expenses and briefing sessions were provided to facilitate active involvement |
Named individual (BAE) supported the trial manager (MRK) to ensure active involvement. In addition, provided single contact for service users Service users recruited through the SUCCESS group: membership of this group gave access to mutual support and a wider service user perspective Mid-study meeting held to review involvement and further support needed Long time scales enabled strong relationships across all research partners |
RMG meetings | Two service users were invited to be members of the RMG, and contributed to all decisions about managing and undertaking the study |
24 meetings scheduled (2010–16) Two service users were at almost all meetings; just one meeting took place without a service user member present |
One individual remained involved throughout the study; the second place was taken by four different individuals Individuals received induction before starting role and were offered pre-meeting briefings Service user perspectives were sought at all stages, especially when developing patient consent process, preparing patient information and questionnaires, holding a prize draw for questionnaire respondents, interpreting final results |
TSC meetings | Two service users were invited to be members of the six-person TSC, and provided independent study scrutiny and oversight |
Five meetings held (2013–15) Three out of the five meetings attended by at least one service user |
Service users received training before joining the TSC which covered the role of a TSC and provided background about the PRISMATIC trial Opportunity provided for briefing before each TSC meeting |
Qualitative analysis and write-up of results | One service user was invited to the qualitative subcommittee to develop coding framework and review drafts of qualitative results |
Two meetings held (2013–14) Both meetings were attended by one service user and three researchers. All contributed to e-mail discussions following meetings and reviewed drafts of the results |
|
Writing events | To contribute service user perspective to all discussions about communications and dissemination |
Six meetings held (2012–15) Two service users were invited to each meeting: at least one attended each meeting |
Workshop sessions held at two meetings to consider potential study outputs for service user audience Discussions held about how to describe the PRISMATIC trial service user involvement in final report Service users contributed to all discussions as equal team members and agreed publications plan |
Publicity and dissemination | Service users contributed to publicity and dissemination materials about PRISMATIC | Service users reviewed and contributed to seven PRISMATIC newsletters, aimed at participating GP practices and health services managers |
One service user contributed to edition 6, writing about her experience of being involved in research One service user contributed to edition 7, explaining how risk prediction tools can help patients, especially those with early-stage chronic illness who can expect their condition to deteriorate Two newsletters contained articles by GP champions linked to the research team The SUCCESS group members advised on, and reviewed, the patient information pages of the PRISMATIC trial website |
Service users involved in publicising PRISMATIC to patients |
Service users suggested production and distribution of a poster about the PRISMATIC trial, for display in GP practices, to inform patients about the study One service user and one GP champion were interviewed by a TV crew about the study. The package was screened on the BBC Wales evening news |
||
Service users were co-authors on all study outputs |
Service users co-authored conference presentations Service users co-authored journal publication reporting baseline qualitative findings (Porter et al. )84 |
||
Further bid development | Service users collaborated in developing further research about risk prediction | Service users contributed through research development groups and reviewing draft applications |
Seven service users attended a research discussion group to consider risk prediction research priorities: outcome informed future bid development At least one service user was named as a co-applicant on all large-scale research proposals linked to use of risk prediction tools and proactive care |
Recruiting and supporting service users to be involved in the PRISMATIC trial
We recruited two service users to the RMG. As people changed, five individuals were involved over 5 years. They were all members of the SUCCESS group. All were diagnosed with chronic conditions and some also cared for family members. One of these individuals was actively involved throughout the whole period of the PRISMATIC trial. Poor health meant three other individuals had to give up their role. To reduce the burden on individuals, we recruited a reserve service user so there were potentially three to attend meetings and less need for anyone with family responsibilities or feeling unwell. Despite receiving briefings and project information, the reserve person reported that it was difficult to retain continuity when not regularly involved and there were long periods between meetings. When his health limited his ability to drive, the study team agreed not to recruit another person and resumed working with two service users.
Halfway through the trial (December 2013), we held a meeting for service users and core research staff (BAE, MRK and HH) to review their experience and identify ways to enable them to sustain and increase their involvement.
Study meetings
At least one, often two, service users attended almost all RMG meetings (23/24). We recruited two different service users to the TSC; they attended three of five meetings.
We also involved service users in task-related meetings such as writing days, meetings to undertake qualitative analysis and research development groups discussing further research linked to the topic of risk prediction.
Research activities
Throughout the period of the PRISMATIC trial, service users were involved in a range of research activities including:
-
RMG meetings – all aspects in an equal role with other RMG members
-
reviewing research information
-
format of patient questionnaires
-
patient letters and information sheets
-
interview schedules
-
abstracts and posters presented at conferences
-
-
qualitative data analysis
-
commenting on strategies to increase questionnaire response rates (prize draw)
-
deciding to have a patient page on the PRISMATIC trial website (RMG decision)
-
developing and reviewing the patient page of the PRISMATIC trial website (at SUCCESS group meetings)
-
commenting on consent issues relating to the use of anonymised data
-
publicity (British Broadcasting Corporation, newsletters); focus of British Broadcasting Corporation publicity was on one of the service users
-
TSC – two members
-
developing and piloting service user terms of reference (at SUCCESS group meetings and RMG)
-
informal dissemination about the PRISMATIC trial to patient networks
-
co-applicant on further research bids, including a systematic review of risk prediction models and developing an intervention to communicate risk scores with patients.
Reflections from one service user member of the PRISMATIC study
I considered the PRISMATIC research to be advantageous to service users and I was pleased to be part of its development. I was able to pose questions and ask for explanations as part of the PRISMATIC team but felt there were some aspects of the research which could have taken a wider view.
As a service user with chronic conditions I am aware of the problems with access to health services especially primary services, for example, GP surgeries. The research did not address the initial presentation at a GP surgery. GP receptionists were not able to identify the patients on the top of the PRISM pyramid (this was a problem when these patients were requesting an appointment). The remit for PRISMATIC did not include this and was considered to be the responsibility of the surgeries and I accept this.
I do not have any issues to raise about involving service users as it has got to be beneficial in a research study. Service users, to be an asset to research and researchers, need to have some training and understanding of working within this environment. They must also understand the remit but never be afraid to put forward issues that they consider will benefit patients. That should be why we are taking part in research and we must not lose sight of this.
I do think it was good enough involvement. What could be done better is explaining the remit more. There have been times when I could not see how a particular research project can work without some other basic changes made to make the research work in practice. When I have posed these questions I can tell the information is not compatible with the research criteria.
Being part of a research project like PRISMATIC works for me. But what really worked for me was when I asked [name] to explain in plain English where we were at a particular time and the newsletter he produced after the event.
My reflections on the results and report are ’didn’t we do well’.
Chapter 8 Discussion and conclusions
Summary of key findings
Systematic review
-
We found that previous research related to implementation of PRISMs for the management of emergency admissions to hospital has shown minimal effects overall, but has been largely observational in nature, suffered from operational issues in implementation, tended to focus on processes of care rather than health outcomes, and that studies were heterogeneous in terms of intervention, populations and study design.
Primary outcome
-
The rate, and proportion, of people with emergency admissions increased overall in the intervention phase of the trial, effects that were consistent across predicted risk levels.
Secondary outcomes
-
Attendances at the ED were higher in the intervention phase than in the control phase; GP event-days were slightly lower; there were no clear effects on outpatient visits; bed-days overall were higher; mental health quality-of-life scores were similar between phases, but physical health scores were higher in the intervention phase; satisfaction scores were slightly lower in the intervention phase.
-
Mortality was similar across intervention and control periods.
-
Technical performance of the tool was good. Evidence of underprediction of risk was apparent at higher-risk levels, balanced by overprediction of risk at the lowest risk level.
Costs
-
The implementation cost of PRISM in the first year was estimated to be £822 per practice, £0.12 per patient.
-
Total health-care costs after the implementation of the PRISM scoring tool in primary care practices were £76 per patient higher than in the control phase.
-
Considering the increased number of emergency admissions in the intervention phase, the PRISM tool can be considered dominated by the control (routine practice).
Processes of change associated with the Predictive RIsk Stratification Model
Usage of PRISM appeared to be low and declined over time. Usage was strongly driven by the QOF requirements in the GP contract, focusing on a small proportion (0.5%) identified as being at the highest risk of emergency admission to hospital. GPs were generally open to trying PRISM, but extreme pressures on their role limited their time and capacity for using it to its full potential. All stakeholders were aware of the limited potential of PRISM to support improvements to patient care without additional resources being put into community-based care services. GPs reported that PRISM changed their awareness of patients and focused them on targeting the highest-risk patients, though these may have been least suitable for proactive management. They agreed that PRISM was potentially very useful to manage patients from lower-risk tiers.
Strengths and limitations of study
Our stepped-wedge study design, with randomised allocation of clusters of GP practices to receive the PRISM tool over a 1-year period and using linked data follow-up, allowed us to carry out a rigorous evaluation of this population-level intervention that included primary outcomes for > 250,000 people. We were able to anonymously link self-reported questionnaires for a sample of patients to our routine data outcomes, giving us a picture of effects on health service use as well as quality of life and satisfaction. Using linked data allowed us to include almost the whole population for those general practices that participated in the study. Inclusion of outcomes for such a high number of participants means that even small differences are detected and are statistically significant. In this case, effects were small but consistent, and across such high numbers of participants, resulted in large cost differences between phases.
Our mixed-methods approach allowed us to explore implementation and reported usage as well as perceived challenges and benefits. The incorporation of qualitative methods, health economic analyses, as well as the investigation of technical performance, has ensured that a comprehensive evaluation has been undertaken to inform health-care decision-making of the value (from clinical, service, patient and economic outcomes) of PRISM.
This is the first evaluation of the effects of the introduction of a PRISM in a real-life setting, although the tools have now been widely introduced across the UK as part of a comprehensive policy for the care of people with chronic conditions, with higher rates of management of patients outside hospital, through primary- or community-based services or self-care. However, within the constraints of a funded evaluation, we were only able to include outcomes up to 18 months from implementation of PRISM at the first practices. We do not know what the longer-term effects would be.
Self-reported health-related quality-of-life and satisfaction findings are based on a sample which was weighted to favour patients at higher levels of risk. These scores therefore need further analysis to account for non-responders and for this weighting, so that findings are representative of the whole population.
There were a number of practical and analytical challenges associated with using anonymised linked routine data for the assessment of cost-effectiveness. With respect to the cost-effectiveness analyses, there is little literature available on the conduct of health economic analyses alongside trial designs of this nature. We demonstrated that appropriate methods can be applied; a particular strength of our analyses is that we undertook cost, cost-effectiveness, cost–consequences and cost–utility analyses, and trial-based budget impact to provide as full a picture as possible of the economic impact of PRISM. However, several challenges were unexpected. The economic analysis (other than the implementation costs associated with PRISM) could not be done until the SAIL data were made available for final analysis; and logistical aspects such as accessing the data proved problematic throughout the analysis period. Another essential lesson learnt was the need to work closely with the statistical analysis (e.g. a fully integrated statistical and economic analysis plan should be produced) to ensure standardisation and harmonisation of outcomes given their relative importance to reporting both clinical effectiveness and cost-effectiveness.
Further limitations relate to the dynamic context, with changing policy and practice environment before, during and after recruitment. We believe our study design is well suited to this context, frequently encountered by evaluative studies in health care. Against this background, beyond the PRISM tool it was challenging to define the wider intervention that was designed to reduce emergency admissions, and we were unable to disentangle effects of PRISM from the introduction of QOF targets for the care of those at the highest level of risk of emergency admissions to hospital.
Implications for policy and practice
This research addresses a major policy concern for the NHS – how best to manage patients in the community to avoid unnecessary, disruptive and costly emergency admissions. In 2012–13 there were 5.3 million emergency admissions to hospitals costing approximately £12.5B. 3 Over 2 million unplanned admissions per year are for those aged ≥ 65 years – accounting for 68% of hospital bed-days – and the use of more than 51,000 acute beds at any one time. 85 In 2009/10 the average length of stay was approximately 3 days for patients aged < 65 years, but 9 days for patients aged ≥ 65 years. 7 Approximately half of all bed-days are attributable to just 5% of the population – typically older people with multimorbidity. 3,86 An emergency admission to hospital is a disruptive and unsettling experience which can have a negative impact on a patient’s life as they are exposed to clinical and psychological risks and likely to have increased their dependency on discharge. 7 It is widely recognised that there are opportunities to improve management of care for older patients with multimorbidity in primary and community settings – and that doing so is necessary to reduce avoidable emergency admissions. 3
The use of EARP tools is widely advocated16 to support the identification of vulnerable patients for proactive targeted care aimed at preventing emergency hospitalisation – a ‘triple fail’ event that is harmful, costly and results in poor patient satisfaction. 6 ‘It is now well recognised in the NHS that predictive risk tools are essential to use if high quality care is to be offered’ (Jennifer Dixon, Chief Executive of the Health Foundation). 87 EARP tools can support the allocation of scarce resources through the identification of patients in need who can be proactively targeted with support appropriate to their needs. The use of EARP tools is widely advocated in academic, policy and clinical literature and is, for example, a core component of both the English and Welsh chronic/long-term conditions models. 5,14
Provisional indications from a UK-wide survey led by one of the co-applicants are that > 70% of UK practices now have access to an EARP tool. The development and validity of the tools has been widely researched,26 but little research has been undertaken into their effectiveness. 15,26 The 2015 NHS England paper Next Steps for Risk Stratification in the NHS notes a ‘pressing need for further research’ about the effectiveness of hospital-avoidance interventions. 15
There is some debate about how best to use EARP tools – and whether or not focus should be on those at the highest level of risk. Lewis,15 for instance, suggests that at this level there is little scope for improvement, whereas Wallace et al. suggest that numbers at this level are so small that overall impact is limited when following this approach. 88 However, UK health policy is clearly focused on the management of patients at the highest level of risk. GP contracts have incentivised EARP use for case management of patients at high risk of hospitalisation, with over £480M allocated for the Avoiding Unplanned Admissions Enhanced Service in England between 2014 and 2017. 18 Participation in the Enhanced Services (ES) is widespread with around 7500 practices taking part in the ES – over 95% of all practices (NHS Digital, 2015, personal communication). Their responsibilities are to use a risk prediction model or alternative to identify vulnerable older people, high-risk patients and patients needing end-of-life care who are at risk of unplanned admission, and create a register of at least 2% of patients aged > 18 years. 18
For those patients on the register, the practice is expected to offer care co-ordination and active care planning and review, involving patients as partners in the management of their health. The ES is aligned with NHS policy guidance for patient-centred care and supporting self-management, with GPs encouraged to involve patients in their care planning through shared decision-making. 18,89 The King’s Fund’s 2013 list of priorities for sustainable health services begins with active support for self-management and primary prevention, including the targeting of high-risk groups. 85
The reach and scale of ES and the potential impact on patients and health and care resources is considerable, with over 800,000 patients across the whole of England on the case management register in each of the intervention years (2014/15 and 2015/16).
Incentivisation of the targeting of those at the very highest levels of risk has become routine practice in Wales through QOF measures and in England through the funding of an enhanced service, although originally predictive risk stratification tools were seen as a way of managing care across the spectrum of risk. In 2013, Geraint Lewis, Chief Data Officer, NHS England, drew attention to this:
It is important to remember that patients at very high-predicted risk of hospitalisation only account for a modest proportion of all unplanned admissions. Therefore, to have a meaningful impact on admission rates at the population level, it will be important to consider less-intensive, lower-cost interventions for patients at moderately high-predicted risk. Indeed, there is a danger that by focusing exclusively on the integration of care for very high-risk patients, virtual wards may be diverting attention away from the integration of care for lower risk patients.
There has been an assumption that, if EARP tools predict those at high risk of emergency admission to hospital with reasonable accuracy, patients will benefit from targeted care to improve their health and reduce unplanned episodes of care. However, this is based on assumptions – with little empirical evidence to date – about how risk prediction tools and incentivisation of care work in practice. Our findings have shown effects that are opposite to those intended. Although GPs reported that the use of the PRISM tool overall was low, they also reported carrying out reviews of the PRISM-generated lists of people predicted to be at the highest level of risk of emergency admission to hospital. They reported that they felt very stretched without additional resources to allocate specifically to the care of these patients. We have found an increase in emergency admissions and ED attendances at all risk levels, associated with introduction of the PRISM tool, and once we had adjusted for all significant covariates, including age, season and trend over time. This is challenging to explain, and is an effect that would not have been detected in a traditional evaluation that focused on relatively small numbers of those at the highest level of risk. Our use of anonymised linked routine data has allowed us to detect effects across the whole population. We refer to recently published results showing a similar unexpected effect on emergency episodes related to introduction of an air pollution alert system. 91 We can hypothesise that PRISM, alongside QOF targets to target care at those at highest risk of emergency hospital admission, both sensitised GPs and practice staff to need at this level, while shifting the focus from others at lower risk, thereby missing opportunities to avoid emergency admission to hospital in those seen as less frail.
Conclusions
We have carried out the first large study of what happens when you introduce EARP in the real world. Use of anonymised data linkage has allowed us to carry out an experimental study with a randomised design at population level, with a very high rate of inclusion of primary and secondary routine outcomes, as well as self-reported outcomes for a sample of patients and health economic analysis.
Our results are surprising and alarming – increases in activity and costs across the board. We do not fully understand how or why this is happening; possible reasons include sensitisation of GPs and identification of unmet need; lack of resources to respond to need apart from hospitalisation; and concentration on those at highest predicted risk may mean that attention slips from those with lower predicted risk scores.
Despite low reported use of PRISM, we found clinically and operationally important effects of the introduction of the new risk stratification tool alongside contractual (QOF) incentives to target those at the highest risk of emergency admission to hospital. These effects were unexpected and in the opposite direction to those intended. We are unable to disentangle the effects of the introduction of the PRISM tool from those of the QOF targets, but this reflects practice across the UK, where emergency admission predictive risk stratification tools have been introduced alongside an incentivised enhanced service. We see no reason to expect that findings would be different from those found across this mixed urban and semi-urban population in south Wales.
Recommendations for research in order of priority
-
Evaluation of targeting of different services to different levels of risk, rather than the strong current focus on the very highest level of risk (QOF and enhanced service). In the PRISMATIC trial our evaluation of PRISM became an evaluation of PRISM alongside incentivisation of targeted care for those at highest risk through QOF targets. The PRISM tool itself can only be part of a wider intervention. PRISM was not designed to be used with such a focus on the highest level of risk, but UK health policy has focused on these patients and PRISM was used to identify patients to meet these targets. We do not know what effects would be found if efforts – and resources – were to be targeted at those at a slightly lower-risk level, and whether or not the generation of a risk score per se can have the effects that we found. We recommend further exploration of this aspect, as risk tools are not going away – we cannot ‘unknow’ what we know – and we need to understand mechanisms and effects much better if they are to be used to improve care and outcomes for patients. This will require a new study based on an experimental design – preferably a randomised trial clustered by practice to exclude biases caused by changing policy and practice within the study time frame. This study must be underpinned by theory and a logic model, and include a strong process evaluation to understand mechanisms of change. We recommend also that this study should be carried out in sites across England and Wales and that longer-term outcomes are included.
-
Investigation of effects on vulnerable populations, health inequalities and by health condition type; in the PRISMATIC trial we have detected effects at aggregate and risk group levels, but have not delved into differential effects within the general practice population. This could partially be achieved by secondary analysis of the PRISMATIC trial data set, but some new investigation would also be required.
-
Acceptability of predictive risk stratification and communication of scores to patients and practitioners is an important topic to explore, but it is more important at this stage to establish effects, costs and mechanisms of change.
Acknowledgements
This study was only possible thanks to the expertise and commitment of a wide range of people and organisations. Although we specifically acknowledge the following parties, we would like to extend our thanks to all of those who supported this work.
-
All the participating general practices and their staff.
-
All patients and stakeholders who participated in the trial.
-
NWIS, which developed the PRISM tool, and provided considerable support and expertise throughout the trial. We would particularly like to thank the following staff members: Ken Leake, Dave Price, Mick Kelly, Cecilia Jones, David Leach, Tracey Taylor, Claire John and Simon Scourfield.
-
The research support staff at the National Institute for Social Care and Health Research (now rebranded Health and Care Research Wales). In particular, for their support with practice engagement and research tasks, we thank Kathy Malinovszky, Carol Thomas, Zoe Abbott and Rachel McGrath.
-
Our GP champions: Deborah Burge-Jones, Sarah Smallcombe and Kerry Bailey-Jones.
-
NIHR HSDR (previously the Service Delivery and Organisation programme) for funding and then supporting this research throughout, and especially in light of the changes from the original funded proposal.
-
Geraint Lewis for specialist advisory support.
-
Sherry Jenkins, Anne Surman, Claire Evans, Moira Morgan, Rhiannon Griffiths, Kayleigh Nelson and Ashra Khanom for administrative support.
-
Clare Boucher (Swansea University Medical School subject librarian).
-
Members of our TSC and DMEC: Sarah Purdy, Gareth Davies, Adrian Edwards, Ben Carter and Chris Jones.
-
Our service user representatives: Jan Davies, Shirley Whitman, Jackie McCarthy and Rosita Wilkins.
-
Christine Foley for transcription services, and Opinion Research Services for assisting with the processing of anonymous questionnaires.
-
The SAIL DATABANK team at Swansea University for their help with our data linkage. In particular, we would like to thank Cynthia McNerney, Caroline Brooks, Dan Thayer and Ronan Lyons and members of the Information Governance Review Panel.
Contributions of authors
Helen Snooks (Professor of Health Services Research), chief investigator, led the development of the research question, study design, and was responsible for trial delivery and conduct.
Kerry Bailey-Jones (GP) and Deborah Burge-Jones (GP) acted as study GP champions, offering general practice insight and experience throughout.
Jeremy Dale (Professor of Primary Care), co-applicant, provided expertise in primary and emergency care research.
Jan Davies (Service User Representative), RMG member and service user advisor.
Bridie Evans (Research Officer), service user involvement lead and qualitative support.
Angela Farr (Researcher in Swansea Centre for Health Economics) helped prepare implementation costs section.
Deborah Fitzsimmons (Professor of Health Outcomes Research) supported the management of the health economic analysis and contributed to the draft of the cost-effectiveness chapter.
Jane Harrison (Public Health Wales, previously ABM UHB Assistant Medical Director for Primary Care) led the introduction of PRISM in ABM UHB.
Martin Heaven (Senior analyst at FARR Institute @ CIPHER) provided expertise and support for data linkage.
Helen Howson (Welsh Government) advised on policy concerning chronic conditions management throughout the study.
Hayley Hutchings (Professor of Health Services Research and Deputy Director of STU), research manager, supervised staff, and led the writing of the study protocol and methods chapter.
Gareth John (Information Manager at NWIS) supported implementation of PRISM, advised and facilitated data linkage, and was key liaison for NWIS throughout the study.
Mark Kingston (Research Officer), project and data manager, co-ordinated the day-to-day delivery of the trial, including site liaison, and wrote first drafts of the introduction and systematic review.
Leo Lewis (Senior Fellow, International Foundation for Integrated Care) advised on predictive risk stratification implementation throughout the study.
Ceri Phillips (Professor of Health Economics), co-applicant, helped develop the original study and support health economics components.
Alison Porter (Associate Professor), qualitative lead.
Bernadette Sewell (Health Economist) wrote the analysis plan for the health economic evaluation, analysed health economics data and led draft of cost-effectiveness chapter.
Daniel Warm (Service Transformation Programme Manager, Hywel Dda UHB) provided advice on information systems management.
Alan Watkins (Associate Professor), senior statistician, developed analysis plan and analysed data. Led draft of clinical effectiveness chapter.
Shirley Whitman (Service User Representative), RMG member and service user advisor.
Victoria Williams (Research Officer) supported the qualitative data analysis and chapter draft.
Ian T Russell (Emeritus Professor of Clinical Trials), co-applicant, provided methodological support, including statistical expertise.
All authors contributed to the writing of the report and approved the final version.
Publications
Hutchings HA, Evans BA, Fitzsimmons D, Harrison J, Heaven M, Huxley P, et al. Predictive risk stratification model: a progressive cluster-randomised trial in chronic conditions management (PRISMATIC) research protocol. Trials 2013;14:301.
Kingston MR, Evans BA, Nelson K, Hutchings HA, Russell IT, Snooks HA. Costs, effects and implementation of emergency admission risk prediction models in primary care for patients with, or at risk of, chronic conditions: a systematic review protocol. BMJ Open 2016;6:e009653.
Porter A, Kingston MR, Evans BA, Hutchings H, Whitman S, Snooks H. It could be a ‘Golden Goose’: a qualitative study of views in primary care on an emergency admission risk prediction tool prior to implementation. BMC Fam Pract 2016;17:14.
Data sharing statement
Data are stored within the SAIL databank at the Health Information Research Unit at Swansea University. All proposals to use SAIL data sets must comply with Health Information Research Unit’s information governance policy.
Disclaimers
This report presents independent research funded by the National Institute for Health Research (NIHR). The views and opinions expressed by authors in this publication are those of the authors and do not necessarily reflect those of the NHS, the NIHR, NETSCC, the HS&DR programme or the Department of Health. If there are verbatim quotations included in this publication the views and opinions expressed by the interviewees are those of the interviewees and do not necessarily reflect those of the authors, those of the NHS, the NIHR, NETSCC, the HS&DR programme or the Department of Health.
References
- Noncommunicable Diseases Country Profiles 2011. Geneva: World Health Organization; 2011.
- Abegunde DO, Mathers CD, Adam T, Ortegon M, Strong K. The burden and costs of chronic diseases in low-income and middle-income countries. Lancet 2007;370:1929-38.
- Emergency Admissions to Hospital: Managing the Demand. London: National Audit Office; 2013.
- Improving Quality of Life for People with Long Term Conditions. London: Department of Health; 2013.
- The National Service Framework for Long-term Conditions. London: Department of Health; 2005.
- Lewis G, Kirkham H, Duncan I, Vaithianathan R. How health systems could avert ‘triple fail’ events that are harmful, are costly, and result in poor patient satisfaction. Health Aff 2013;32:669-76. https://doi.org/10.1377/hlthaff.2012.1350.
- Older People and Emergency Bed Use: Exploring Variation. London: The King’s Fund; 2012.
- Freund T, Wensing M, Mahler C, Gensichen J, Erler A, Beyer M, et al. Development of a primary care-based complex care management intervention for chronically ill patients at high risk for hospitalization: a study protocol. Implement Sci 2010;5. https://doi.org/10.1186/1748–5908–5-70.
- Integrated Care and Support: Our Shared Commitment. London: Department of Health; 2013.
- Focus on Preventable Admissions: Trends in Emergency Admissions for Ambulatory Care Sensitive Conditions, 2001 to 2013. London: The Health Foundation and Nuffield Trust; 2013.
- Purdy S. Avoiding Hospital Admissions. What Does the Research Evidence Say?. London: The King’s Fund; 2010.
- Sanderson C, Dixon J. Conditions for which onset or hospital admission is potentially preventable by timely and effective ambulatory care. J Health Serv Res Policy 2000;5:222-30.
- Tian Y, Dixon A, Gao H. Data Briefing: Emergency Hospital Admissions for Ambulatory Care-Sensitive Conditions. London: The King’s Fund; 2012.
- Designed to Improve the Health and Management of Chronic Conditions in Wales. An Integrated Model and Framework. Cardiff: Welsh Assembly Government; 2007.
- Lewis G. Next Steps for Risk Stratification in the NHS. London: NHS England; 2015.
- Choosing a Predictive Risk Model: A Guide for Commissioners in England. London: Nuffield Trust; 2011.
- Stokes J, Checkland K, Kristensen SR. Integrated care: theory to practice. J Health Serv Res Policy 2016;21:282-5. https://doi.org/10.1177/1355819616660581.
- Enhanced Service Specification. Avoiding Unplanned Admissions: Proactive Case Finding and Patient Review for Vulnerable People. London: NHS England; 2014.
- Allaudeen N, Schnipper JL, Orav EJ, Wachter RM, Vidyarthi AR. Inability of providers to predict unplanned readmissions. J Gen Intern Med 2011;26:771-6. https://doi.org/10.1007/s11606–011–1663–3.
- The King’s Fund . Evercare Needs Evaluation of Cost Effectiveness Before It Is Rolled Out Further, Says The King’s Fund 2005. www.kingsfund.org.uk/press/press-releases/evercare-needs-evaluation-cost-effectiveness-it-rolled-out-further-says-kings (accessed 16 September 2015).
- Donnan PT, Dorward DT, Mutch B, Morris AD. Development and validation of a model for predicting emergency admissions over the next year (PEONY): a UK historical cohort study. Arch Intern Med 2008;168:1416-22. http://dx.doi.org/10.1001/archinte.168.13.1416.
- Ross P, Curry C, Goodwin N. Case Management. What it is and How it Can Best be Implemented?. London: The King’s Fund; 2011.
- Moons KG, Royston P, Vergouwe Y, Grobbee DE, Altman DG. Prognosis and prognostic research: what, why, and how?. BMJ 2009;338.
- Meehl P, Waller NG, Yonce LJ, Grove WM, Faust D, Lezenweger MF. A Paul Meehl Reader: Essays on the Practice of Scientific Psychology. Mahwah, NJ: Lawrence Erlbaum Associates; 2006.
- Kivimäki M, Batty GD, Singh-Manoux A, Ferrie JE, Tabak AG, Jokela M, et al. Validating the Framingham Hypertension Risk Score: results from the Whitehall II study. Hypertension 2009;54:496-501. https://doi.org/10.1161/HYPERTENSIONAHA.109.132373.
- Wallace E, Stuart E, Vaughan N, Bennett K, Fahey T, Smith SM. Risk prediction models to predict emergency hospital admission in community-dwelling adults: a systematic review. Med Care 2014;52:751-65. http://dx.doi.org/10.1097/mlr.0000000000000171.
- Freund T, Wensing M, Geissler S, Peters-Klimm F, Mahler C, Boyd CM, et al. Primary care physicians’ experiences with case finding for practice-based care management. Am J Manag Care 2012;18:e155-61.
- Haas LR, Takahashi PY, Shah ND, Stroebel RJ, Bernard ME, Finnie DM, et al. Risk-stratification methods for identifying patients for care coordination. Am J Manag Care 2013;19:725-32.
- European Innovation Partnership on Active and Healthy Ageing. A compilation of Good Practices: Replicating and Tutoring Integrated Care for Chronic Diseases, Including Remote Monitoring at Regional Level. Brussels: European Commission; 2013.
- Stokes J, Panagioti M, Alam R, Checkland K, Cheraghi-Sohi S, Bower P. Effectiveness of case management for ‘at risk’ patients in primary care: a systematic review and meta-analysis. PLOS ONE 2015;10. https://doi.org/10.1371/journal.pone.0132340.
- Quality and Outcomes Framework Guidance for the GMS Contract Wales 2013/14. Cardiff: BMA Cymru Wales; 2013.
- Wennberg D, Siegel M, Darin B, Filipova N, Russell R, Kenney L, et al. Combined Predictive Model Final Report 2006. www.kingsfund.org.uk/sites/files/kf/field/field_document/PARR-combined-predictive-model-final-report-dec06.pdf (accessed 27 May 2015).
- Kingston MR. Initial Uses of the PRISM Risk Stratification Tool in CCM Demonstrator Sites: a Qualitative Study. Llantrisant: National Leadership and Innovation Agency for Healthcare; 2010.
- Hutchings HA, Evans BA, Fitzsimmons D, Harrison J, Heaven M, Huxley P, et al. Predictive risk stratification model: a progressive cluster-randomised trial in chronic conditions management (PRISMATIC) research protocol. Trials 2013;14. http://dx.doi.org/10.1186/1745-6215-14-301.
- Kingston MR, Evans BA, Nelson K, Hutchings H, Russell I, Snooks H. Costs, effects and implementation of routine data emergency admission risk prediction models in primary care for patients with, or at risk of, chronic conditions: a systematic review protocol. BMJ Open 2016;6. http://dx.doi.org/10.1136/bmjopen-2015-009653.
- Moher D, Liberati A, Tetzlaff J, Altman DG. PRISMA Group . Reprint – preferred reporting items for systematic reviews and meta-analyses: the PRISMA statement. Phys Ther 2009;89:873-80.
- The NHS Improvement Plan: Putting People at the Heart of Public Services. London: HMSO; 2004.
- Kocher RP, Adashi EY. Hospital readmissions and the Affordable Care Act: paying for coordinated quality care. JAMA 2011;306:1794-5. https://doi.org/10.1001/jama.2011.1561.
- Systematic Reviews: CRD’s Guidance for Undertaking Reviews in Health Care. York: Centre for Reviews and Dissemination, University of York; 2009.
- Thomas BH, Ciliska D, Dobbins M, Micucci S. A process for systematically reviewing the literature: providing the research evidence for public health nursing interventions. Worldviews Evid Based Nurs 2004;1:176-84. http://dx.doi.org/10.1111/j.1524–475X.2004.04006.x.
- Walsh D, Downe S. Appraising the quality of qualitative research. Midwifery 2006;22:108-19.
- Higgins J, Green S. Cochrane Handbook for Systematic Reviews of Interventions 2011. http://handbook.cochrane.org.
- Popay J, Roberts H, Sowden A, Petticrew M, Arai L, Rodgers M, et al. Guidance on the Conduct of Narrative Synthesis in Systematic Reviews. Lancaster: Institute of Health Research; 2006.
- Baker A, Leak P, Ritchie LD, Lee AJ, Fielding S. Anticipatory care planning and integration: a primary care pilot study aimed at reducing unplanned hospitalisation. Br J Gen Pract 2012;62:e113-20. https://doi.org/10.3399/bjgp12X625175.
- Dhalla IA, O’Brien T, Morra D, Thorpe KE, Wong BM, Mehta R, et al. Effect of a postdischarge virtual ward on readmission or death for high-risk patients: a randomized clinical trial. JAMA 2014;312:1305-12. https://doi.org/10.1001/jama.2014.11492.
- Freund T, Mahler C, Erler A, Gensichen J, Ose D, Szecsenyi J, et al. Identification of patients likely to benefit from care management programs. Am J Manag Care 2011;17:345-52.
- Levine S, Steinman BA, Attaway K, Jung T, Enguidanos S. Home care program for patients at high risk of hospitalization. Am J Manag Care 2012;18:e269-76.
- Reilly S, Abell J, Brand C, Hughes J, Berzins K, Challis D. Case management for people with long-term conditions: impact upon emergency admissions and associated length of stay. Prim Health Care Res Dev 2011;12:223-36. http://dx.doi.org/10.1017/s1463423611000028.
- Roland M, Lewis R, Steventon A, Abel G, Adams J, Bardsley M, et al. Case management for at-risk elderly patients in the English integrated care pilots: observational study of staff and patient experience and secondary care utilisation. Int J Integ Care 2012;12.
- Takahashi PY, Pecina JL, Upatising B, Chaudhry R, Shah ND, Van Houten H, et al. A randomized controlled trial of telemonitoring in older adults with multiple health issues to prevent hospitalizations and emergency department visits. Arch Intern Med 2012;172:773-9. http://dx.doi.org/10.1001/archinternmed.2012.256.
- Upatising B, Hanson GJ, Kim YL, Cha SS, Yih Y, Takahashi PY. Effects of home telemonitoring on transitions between frailty states and death for older adults: a randomized controlled trial. Int J Gen Med 2013;6:145-51. http://dx.doi.org/10.2147/IJGM.S40576.
- Abell J, Hughes J, Reilly S, Berzins K, Challis D. Case management for long-term conditions: developing targeting processes. Care Manag J 2010;11:11-8.
- Sauto Arce R, De Ormijana AS, Orueta JF, Gagnon MP, Nuño-Solinís R. A qualitative study on clinicians’ perceptions about the implementation of a population risk stratification tool in primary care practice of the Basque health service. BMC Fam Pract 2014;15. https://doi.org/10.1186/1471-2296-15-150.
- Hall S, Kulendran M, Sadek A, Green S, de Lusignan S. Variability in selecting patients to manage in the community: a service evaluation of community matron’s case-finding strategies. Fam Pract 2011;28:414-21. http://dx.doi.org/10.1093/fampra/cmq095.
- McEvoy P, Escott D, Bee P. Case management for high-intensity service users: towards a relational approach to care co-ordination. Health Soc Care Community 2011;19:60-9. https://doi.org/10.1111/j.1365–2524.2010.00949.x.
- Roland M, Abel G. Reducing emergency admissions: are we on the right track?. BMJ 2012;345. https://doi.org/10.1136/bmj.e6017.
- Billings J, Dixon J, Mijanovich T, Wennberg D. Case finding for patients at risk of readmission to hospital: development of algorithm to identify high risk patients. BMJ 2006;333.
- van Walraven C, Dhalla IA, Bell C, Etchells E, Stiell IG, Zarnke K, et al. Derivation and validation of an index to predict early death or unplanned readmission after discharge from hospital to the community. CMAJ 2010;182:551-7. http://dx.doi.org/10.1503/cmaj.091117.
- Crane SJ, Tung EE, Hanson GJ, Cha S, Chaudhry R, Takahashi PY. Use of an electronic administrative database to identify older community dwelling adults at high-risk for hospitalization or emergency department visits: the elders risk assessment index. BMC Health Serv Res 2010;10:1-7. http://dx.doi.org/10.1186/1472-6963-10–338.
- Supporting People with Long Term Conditions: Commissioning Personalised Care Planning a Guide for Commissioners. London: HMSO; 2009.
- Bojke C, Philips Z, Sculpher M, Campion P, Chrystyn H, Coulton S, et al. Cost-effectiveness of shared pharmaceutical care for older patients: RESPECT Trial findings. Br J Gen Pract 2010;60:e20-7. http://dx.doi.org/10.3399/bjgp09X482312.
- Richmond S, Morton V, Chi Kei Wong I, Russell I, Philips Z, Miles J, et al. Effectiveness of shared pharmaceutical care for older patients: RESPECT trial findings. Br J Gen Pract 2010;59:14-20.
- Brown CA, Lilford RJ. The stepped wedge trial design: a systematic review. BMC Med Res Methodol 2006;6.
- Ford DV, Jones KH, Verplancke JP, Lyons RA, John G, Brown G, et al. The SAIL Databank: building a national architecture for e-health research and evaluation. BMC Health Serv Res 2009;9. https://doi.org/10.1186/1472-6963-9-157.
- Chisholm D, Knapp MR, Knudsen HC, Amaddeo F, Gaite L, van Wijngaarden B. Client socio-demographic and service receipt inventory – European version: development of an instrument for international research. EPSILON Study 5. European Psychiatric Services: Inputs Linked to Outcome Domains and Needs. Br J Psychiatry Suppl 2000:s28-33.
- Carey RG, Seibert JH. A patient survey system to measure quality improvement: questionnaire reliability and validity. Med Care 1993;31:834-45.
- SF-36.org . The SF-12®. An Even Shorter Health Survey n.d. www.sf-36.org/tools/sf12.shtml (accessed 16 January 2013).
- NHS Reference Costs 2014/15. London: Department of Health; 2015.
- NHS Careers . Pay for NHS Health Informatics Staff 2014. www.nhscareers.nhs.uk/working-in-the-nhs/pay-and-benefits/agenda-for-change-pay-rates/ (accessed 5 October 2015).
- Curtis L. Unit Costs of Health and Social Care. Canterbury: Personal Social Services Research Unit, University of Kent; 2010.
- Trends in Consultation Rates in General Practice 1995/96 to 2008/09: Analysis of the QResearch® Database. Nottingham: University of Nottingham; 2009.
- Bryman A. Social Research Methods. Oxford: Oxford University Press; 2012.
- Marshall C, Rossman GB. Designing Qualitative Research. Los Angeles, CA: Sage; 2011.
- Ziebland S, McPherson A. Making sense of qualitative data analysis: an introduction with illustrations from DIPEx (personal experiences of health and illness). Med Educ 2006;40:405-14.
- Miles MB, Huberman AM. Qualitative Data Analysis: An Expanded Sourcebook. London: Sage; 1994.
- May C, Finch T, Mair F, Ballini L, Dowrick C, Eccles M, et al. Understanding the implementation of complex interventions in health care: the normalization process model. BMC Health Serv Res 2007;7.
- Murray E, Treweek S, Pope C, MacFarlane A, Ballini L, Dowrick C, et al. Normalisation process theory: a framework for developing, evaluating and implementing complex interventions. BMC Med 2010;8. https://doi.org/10.1186/1741-7015-8-63.
- McEvoy R, Ballini L, Maltoni S, O’Donnell CA, Mair FS, MacFarlane A. A qualitative systematic review of studies using the normalization process theory to research implementation processes. Implement Sci 2014;9. http://dx.doi.org/10.1186/1748-5908-9-2.
- Evans BA, Bedson E, Bell P, Hutchings H, Lowes L, Rea D, et al. Involving service users in trials: developing a standard operating procedure. Trials 2013;14. https://doi.org/10.1186/1745–6215–14–219.
- Walter I, Davies H, Nutley S. Increasing research impact through partnerships: evidence from outside health care. J Health Serv Res Policy 2003;8:58-61. https://doi.org/10.1258/135581903322405180.
- INVOLVE Briefing Notes for Researchers: Involving the Public in NHS, Public Health and Social Care Research. Eastleigh: INVOLVE; 2012.
- Schulz KF, Altman DG, Moher D. CONSORT 2010 statement: updated guidelines for reporting parallel group randomised trials. BMC Med 2010;8.
- Curtis L. Unit Costs of Health and Social Care 2014. Canterbury: Personal Social Services Research Unit, University of Kent; 2014.
- Porter A, Kingston MR, Evans BA, Hutchings H, Whitman S, Snooks H. It could be a ‘Golden Goose’: a qualitative study of views in primary care on an emergency admission risk prediction tool prior to implementation. BMC Fam Pract 2016;17.
- Transforming our Health Care System: Ten Priorities for Commissioners. London: The King’s Fund; 2013.
- Raising the Profile of Long Term Conditions Care: A Compendium of Information. London: Department of Health; 2008.
- Nuffield Trust . The Future of Predictive Risk Tools for the NHS 2011. www.nuffieldtrust.org.uk/media-centre/press-releases/future-predictive-risk-tools-nhs (accessed 23 March 2015).
- Wallace E, Smith SM, Fahey T, Roland M. Reducing emergency admissions through community based interventions. BMJ 2016;352. https://doi.org/10.1136/bmj.h6817.
- Liberating the NHS: No Decision Without Me, About Me. London: NHS; 2012.
- Lewis G, Vaithianathan R, Wright L, Brice MR, Lovell P, Rankin S, et al. Integrating care for high-risk patients in England using the virtual ward model: lessons in the process of care integration from three case sites. Int J Integr Care 2013;13.
- Lyons RA, Rodgers SE, Thomas S, Bailey R, Brunt H, Thayer D, et al. Effects of an air pollution personal alert system on health service usage in a high-risk general population: a quasi-experimental study using linked data. J Epidemiol Community Health 2016;70:1184-90. http://dx.doi.org/10.1136/jech-2016–207222.
Appendix 1 Baseline focus group guide for general practice staff
-
What knowledge did you previously have of predictive risk stratification tools?
-
What risk tools have you used?
-
Generic versus disease specific.
-
-
Views of risk stratification in general?
-
-
Does your practice seek to identify people at high risk of hospitalisation at present?
-
How does it do this?
-
How do you use that information?
-
Do not identify high-risk patients?
-
-
How does your practice currently deal with people considered at high risk of hospitalisation?
-
Proactively, responsively, not at all, other?
-
How does your practice know which patients are in hospital or have been discharged, at any one time?
-
-
How does your practice feel about using PRISM?
-
Do you have a clear understanding of what the tool is for?
-
Who will use it/some more than others?
-
What motivated your practice to sign up for PRISM?
-
Who/what influenced your practice in the decision?
-
-
When did you/your practice first become aware of PRISM?
-
What did you know of the origin and developments of PRISM?
-
-
-
Do you have any concerns about PRISM or how it will be implemented?
-
Concerns about what it’s for/overall purpose?
-
Practical/technical difficulties with using it?
-
Concerns about accuracy – frequency of update, measures feeding into it?
-
Concerns about data handling and sharing?
-
Demands on time to input/update?
-
Use as performance monitoring tool – compliance, liability?
-
-
How do you expect to use PRISM?
-
Do not expect to use.
-
Focus for discussion.
-
New approach to dealing with patients?
-
Expected strengths/weaknesses?
-
-
Do you expect PRISM to change the way you work?
-
Which staff do you expect to use PRISM?
-
Appendix 2 Baseline focus group guide for community/health staff
-
Can you briefly describe your role? (Ask all these prompts for everyone.)
-
Job title.
-
What it involves (clinical/non-clinical, managerial)?
-
Where you are based?
-
What geographic area are you responsible for?
-
What input do you have into the design of health and social services in your locality/network/community?
-
-
What knowledge did you have of predictive risk tools before the PRISMATIC study?
-
Generic (i.e. emergency admissions) versus disease specific.
-
Have you used any predictive risk tools?
-
Do you currently use any predictive risk tools?
-
How do you use them/what do you do with the information?
-
Views of risk tools in general?
-
-
Do you currently seek to identify people at high risk of hospitalisation?
-
How do you do this?
-
How do you use that information?
-
Proactively, reactively, not at all, other.
-
Any variation between localities?
-
-
-
Do you/your organisation know which patients are in hospital or have been recently discharged at any time?
-
What do you see as advantages of identifying people at high risk of hospitalisation?
-
At practice level.
-
At locality level.
-
At health board level.
-
-
When did you first become aware of PRISM?
-
What did you know of the origin and developments of PRISM?
-
(Present PRISM summary including summary of origin and purpose of tool. Explain justification for predicting EAs. Explain regression to the mean.)
-
How do you feel about PRISM?
-
Do you have a clear understanding of what the tool is for?
-
How do you think it could be used/will be used?
-
Neath Port Talbot/Bridgend/Swansea variation?
-
-
What will influence GPs’ use of PRISM?
-
Why do you think some GP practices have declined PRISM/the PRISMATIC study?
-
-
How do you expect PRISM will influence your work?
-
No influence.
-
Focus for discussion.
-
New approach to delivering care.
-
Expected strengths/weaknesses.
-
Individual changes or organisational changes?
-
Neath Port Talbot/Bridgend/Swansea variation?
-
-
What scope do you have in your role to respond to PRISM?
-
-
Do you expect PRISM to change the way that people with chronic conditions are managed in Abertawe Bro Morgannwg?
-
How?
-
Which staff do you expect to be affected?
-
Individual or organisational changes?
-
Neath Port Talbot/Bridgend/Swansea variation?
-
-
-
Do you have any concerns about PRISM?
-
Implementation?
-
Concerns about what it’s for/overall purpose?
-
Concerns about feasibility to respond?
-
Demands on time to respond?
-
Practical/technical difficulties with GP practices using it?
-
Concerns about accuracy – frequency of update, measures feeding into it?
-
Concerns about data handling and sharing?
-
Use as performance monitoring tool – compliance, liability?
-
-
Bearing everything in mind that we have talked about today, what impact overall do you think PRISM will have in Abertawe Bro Morgannwg?
Appendix 3 Interview guide for chronic conditions management leads across Wales
Please describe your role in this health board and how it relates to PRISM.
-
Other than PRISM, what risk prediction tools are you aware of?
-
What risk tools are being used in your health board?
-
Generic versus disease specific.
-
Any tools stratifying whole population?
-
-
-
What are your views on risk prediction/stratification tools in general?
(Probe: individual views vs. colleagues/organisation.)
-
When was PRISM first considered as a tool for use in your health board?
-
When (timing/policy–political context)?
-
Who proposed it (Welsh Assembly Government/local health board)?
-
Why was it proposed (i.e. what was PRISM aiming to achieve)?
-
What was the role of the chronic conditions management demonstrator in testing PRISM?
-
Extent of support (from you, from health board colleagues, from GPs, from other health practitioners).
-
Extent of knowledge and understanding (from you, from health board colleagues, from GPs, from other health practitioners).
-
-
How was PRISM expected to be used?
-
By whom? (Official view vs. your view.)
-
To do what? (Official view vs. your view.)
-
Anticipated effect on planning and managing chronic conditions care?
-
Anticipated effect on individuals delivering chronic conditions care?
-
-
Vision for PRISM? (Official view vs. your view.)
-
How was PRISM to be funded/supported/made to happen? (Official view vs. your view?)
-
Strengths/weaknesses of PRISM? (Official view vs. your view?)
-
-
To what extent has PRISM been implemented in your health board?
-
What has helped in the process of implementing PRISM?
-
Political/financial context.
-
Organisational restructuring (health boards, National Leadership and Innovation Agency for Healthcare/Informing Healthcare).
-
Views of GPs and health professionals.
-
Health boards/managers.
-
-
What has hindered the process of implementing PRISM?
-
Political/financial context.
-
Organisational restructuring (health boards, National Leadership and Innovation Agency for Healthcare/Informing Healthcare).
-
Views of professional bodies: BMA/General Practitioners Committee Wales.
-
Views of GPs and health professionals.
-
Health boards/managers.
-
-
-
How much of your role was it to communicate information about PRISM to your colleagues?
-
How did you do this?
-
How did people respond?
-
Did you have to change your method or your message?
-
-
How do you predict the story of PRISM will unfold from now?
-
What is needed to implement PRISM?
-
Will that happen?
-
Are you considering using another risk stratification tool instead of PRISM?
-
-
-
The latest QOF contract is likely to include the use of risk stratification tools to help target patients at risk of hospitalisation. What impact will that have on your health board?
-
If a chronic conditions management demonstrator site, how has being a demonstrator site, and testing PRISM, affected views about PRISM?
-
Your views.
-
Other managers/commissioners.
-
GPs’ views.
-
Other health practitioners.
-
Appendix 4 Interview guide for policy leads across Wales
When was risk stratification first considered as a tool to help provide health services in Wales?
-
Who proposed it (individual/department)?
-
When (timing/policy–political context)?
-
Why?
-
Extent of support?
Why was PRISM developed rather than use an existing risk stratification tool?
-
Who made the decision?
-
Vision for PRISM? (Official view vs. your view.)
-
Extent of support?
-
Strengths/weaknesses of PRISM?
How was PRISM expected to be used?
-
By whom? (Official view vs. your view.)
-
To do what? (Official view vs. your view.)
-
Anticipated effect on organisations planning and managing chronic conditions care?
-
Anticipated effect on individuals delivering CC care?
-
-
How was PRISM to be funded/supported/made to happen? (Official view vs. your view.)
Can you describe how far you think Wales has got with implementing PRISM?
-
How much variation has there been across Wales?
-
Demonstrator versus non-demonstrator site.
-
-
What has helped in the process of implementing PRISM?
-
Political/financial context.
-
Organisational restructuring (health boards, National Leadership and Innovation Agency for Healthcare/Informing Healthcare).
-
Views of GPs and health professionals.
-
Health boards/managers.
-
-
What has hindered the process of implementing PRISM?
-
Political/financial context.
-
Organisational restructuring (health boards, National Leadership and Innovation Agency for Healthcare/Informing Healthcare).
-
Views of GPs and health professionals.
-
Health boards/managers.
-
How do you predict the story of PRISM will unfold from now?
-
What is needed to implement PRISM?
-
Do you feel that will that happen?
Appendix 5 Interview guide for general practice staff: mid-trial
-
Your practice received training to use PRISM about 3 months ago. What happened next?
-
Used immediately/delayed start.
-
What decisions were made with colleagues – at PRISM training or after?
-
Who used it initially?
-
Not used.
-
How easy/how difficult was it to start using PRISM?
-
-
-
What influence did the training have on your planning for and use of PRISM?
-
Views on training session.
-
Views on handbook.
-
-
Over the past 3 months, how has your practice been using PRISM?
-
How frequently?
-
How long do you usually spend each time you review PRISM data?
-
Who has been involved?
-
Single user.
-
Routine practice meetings.
-
Special meetings to discuss PRISM.
-
Who with: MDT, GPs, community network staff?
-
-
Informal meetings.
-
Clarify how long other people spend reviewing PRISM data.
-
-
How do you use the information provided by PRISM?
-
Do you review individual or grouped data?
-
Do you inform the patient that you are using PRISM?
-
-
Please think back to the most recent occasion you used PRISM. Can you talk me through what you did?
-
Used at screen/reviewed printed lists?
-
Alone/in meetings?
-
Which groups of patients (risk levels, conditions, other categories)?
-
What actions have you taken as a result of the information provided by PRISM?
-
GP consultation in person.
-
GP consultation by telephone.
-
GP consultation by home visit.
-
Practice nurse appointment.
-
Clinic in GP practice (e.g. diabetes mellitus, asthma).
-
Prepare active management plan.
-
Communication with/refer to Community Resource Team.
-
Communication with/refer to district nurse.
-
Communication with/refer to health visitor.
-
Refer to community nurse/case manager (e.g. respiratory, mental health, chronic conditions).
-
Communication with/refer to mental health services (identify which).
-
Communication with/refer to therapist (e.g. physiotherapist, occupational therapist, speech therapist, rehabilitation) – identify which.
-
Communication with/refer to social services.
-
Refer to alternative medicine provider.
-
Refer to voluntary sector service (say what).
-
Other (please give details).
-
-
Have you needed to identify additional resources when taking action as a result of information provided by PRISM? Probe what.
-
-
How has the way you use PRISM changed during the past 3 months?
-
Used by different people.
-
Started then stopped using PRISM.
-
Only recently started using PRISM.
-
Change in patients being reviewed through PRISM.
-
Changed frequency of use – how?
-
-
Is there anything that has limited your use of PRISM?
-
Concerns about what it’s for/overall purpose.
-
Practical/technical difficulties with using it.
-
Issues with web access/password logins.
-
-
Concerns about accuracy – frequency of update, measures feeding into it.
-
Concerns about data handling and sharing.
-
Demands on time to access and understand.
-
Use as performance monitoring tool – compliance, liability.
-
Does not provide new information.
-
Unable to respond to identified needs.
-
-
How do you feel about using PRISM at the moment?
-
Do you have a clear understanding of what the tool is for?
-
Does it give you new/useful information?
-
Value for money?
-
What has influenced your/your colleagues’ use of PRISM?
-
How does your experience match your expectations?
-
Impact of QOF in deciding how you use PRISM.
-
-
What difference has PRISM made to the way you work and patient care in the past 3 months?
-
Why?
-
Individual changes or organisational changes?
-
Extent of your role in communicating/using PRISM in the practice.
-
Do you think this is making a difference to emergency admissions?
-
-
How do you expect to use PRISM over the next 6 months?
-
Do not expect to use.
-
Expect to use differently from first 3 months.
-
Focus for discussion.
-
New approach to dealing with patients.
-
Expected strengths/weaknesses.
-
Extent of your role in communicating/using PRISM in the practice.
-
Impact of QOF.
-
-
Thank you for telling me what you think about PRISM. If I talked to your colleagues, would they share your views or do they feel differently?
-
Their experience of using it.
-
Their experience of impact on their work.
-
Their expectations for next 6 months.
-
Other GPs, practice nurse, PM, etc.
-
Appendix 6 Interview guide for general practice staff: end trial
If interviewing a different person from the 3 month respondent . . .
Last time we visited your practice, we talked to Dr . . . Could you explain why this interview is taking place with you?
-
Change of staff (doctors left and arrived).
-
Change of person leading PRISM in the practice and why.
-
When did this change take place?
-
Any other staff changes in past 6 months?
-
Last time we talked to you/your practice, you said you mainly used PRISM for . . . (check back to 3-month interview content).
Over the past 6 months, how has your practice been using PRISM?
-
How frequently?
-
How long do you usually spend each time you review PRISM data?
-
Who has been involved?
-
Single user.
-
Routine practice meetings.
-
Special meetings to discuss PRISM.
-
Who with – MDT, GPs, community network staff?
-
-
Informal meetings.
-
Clarify how long other people spend reviewing PRISM data.
-
-
How do you use the information provided by PRISM?
-
Do you review individual or grouped data?
-
Do you inform the patient that you are using PRISM?
-
Have you discussed individual emergency admissions risk scores with patients?
-
-
What has influenced the use of PRISM in your practice in the past 6 months?
-
Received guidance from:
-
ABM UHB
-
locality teams
-
Deb Burge-Jones.
-
-
Change of QOF/QPI requirements to identify high-risk patients.
-
Identifying what works best in this practice.
-
Anything which has supported your use of PRISM?
-
Time of year (winter vs. summer).
-
Other influences on how you use PRISM?
-
-
How has the way you use PRISM changed during the past 6 months?
-
Used by different people.
-
Started then stopped using PRISM.
-
Only recently started using PRISM.
-
Change in patients being reviewed through PRISM.
-
Changed frequency of use – how?
-
-
Please think back to the most recent occasion you used PRISM. Can you talk me through what you did?
-
Used at screen/reviewed printed lists?
-
Alone/in meetings?
-
Which groups of patients (risk levels, conditions, other categories)?
-
Used in individual patient consultation?
-
What actions have you taken as a result of the information provided by PRISM?
-
GP consultation in person.
-
GP consultation by telephone.
-
GP consultation by home visit.
-
Practice nurse appointment.
-
Clinic in GP practice (e.g. diabetes mellitus, asthma).
-
Prepare active management plan.
-
Communication with/refer to Community Resource Team.
-
Communication with/refer to district nurse.
-
Communication with/refer to health visitor.
-
Refer to community nurse/case manager (e.g. respiratory, mental health, chronic conditions).
-
Refer to secondary care.
-
Communication with/refer to mental health services (identify which).
-
Communication with/refer to therapist (e.g. physiotherapist, occupational therapist, speech therapist, rehabilitation) – identify which.
-
Communication with/refer to social services.
-
Refer to alternative medicine provider.
-
Refer to voluntary sector service (say what).
-
Other (please give details).
-
-
Have you needed to identify additional resources when taking action as a result of information provided by PRISM? Probe what.
-
Is this action typical of your normal use – what else have you done?
-
-
Is there anything that has limited your use of PRISM?
-
Concerns about what it’s for/overall purpose.
-
Practical/technical difficulties with using it.
-
Issues with web access/password logins.
-
Lack of integration with practice systems.
-
-
Concerns about accuracy – frequency of update, measures feeding into it.
-
Concerns about data handling and sharing.
-
Demands on time to access and understand.
-
Use as performance monitoring tool – compliance, liability.
-
Does not provide new information.
-
Unable to respond to identified needs.
Last time we talked to you, you said . . . limited your use of PRISM. How has that changed?
-
-
How do you feel about using PRISM at the moment?
-
Do you have a clear understanding of what the tool is for?
-
Does it give you new/useful information?
-
Value for money?
-
What has influenced your/your colleagues’ use of PRISM?
-
How does your experience match your expectations?
-
Impact of QOF in deciding how you use PRISM? (To be reworded when we know whether the 2014/15 QOF requirements are relevant.)
-
-
What difference has PRISM made to the way you work and patient care in the past 6 months?
-
Compared with 3 months before?
-
Compared with pre PRISM?
-
Why?
-
Individual changes or organisational changes?
-
Extent of your role in communicating/using PRISM in the practice?
-
Do you think this is making a difference to emergency admissions?
-
Do you think this is making a difference to any other outcomes?
When we talked to you 6 months ago, you said PRISM made . . . difference. Why do you think this has changed? (If there is a change.)
-
-
How do you expect to use PRISM over the next 6 months?
-
Do not expect to use.
-
Expect to use differently from past 6 months.
-
Focus for discussion.
-
New approach to dealing with patients.
-
Expected strengths/weaknesses.
-
Extent of your role in communicating/using PRISM in the practice.
-
Impact of QOF (depending on 2014/15 QOF requirements).
-
Becoming a routine part of practice?
-
-
Thank you for telling me again what you think about PRISM. If I talked to your colleagues, would they share your views or do they feel differently?
-
Their experience of using it.
-
Their experience of impact on their work.
-
Their expectations for next 6 months.
-
Other GPs, practice nurse, PM, etc.
-
Appendix 7 Predictive risk stratification model use questionnaire for practices post intervention
Appendix 8 Patient questionnaire pack
Appendix 9 Predictive risk stratification model training plan
Appendix 10 Profile of practice staff participation in qualitative data collection
Practice | Role | Years in role | FT/PT | Age (years) | Gender | Data collection method | |||
---|---|---|---|---|---|---|---|---|---|
ID | Size | Baseline | Mid-study | End of study | |||||
1 | M | GP | 13 | FT | 45–54 | Female | I | Q | Q |
1 | PM | 5 | FT | 35–44 | Female | I | – | – | |
1 | PN | NS | NS | NS | Female | FG | – | – | |
2 | L | GP | 12 | FT | 35–34 | Male | FG | I | I |
2 | PM | 9 | FT | 35–44 | Male | FG | I | I | |
3 | L | GP | 10 | FT | 35–44 | Female | TI | I | I |
4 | M | GP | 6 | FT | 35–44 | Male | FG | Q | Q |
4 | PM | 4 | FT | 25–34 | Female | FG | – | – | |
5 | M | GP | 28 | FT | 55–64 | Female | FG | I | I |
6 | M | GP | 9 | FT | 25–34 | Male | FG | I | I |
7 | L | GP | 6 | FT | 35–44 | Male | FG | Q | Q |
7 | PM | 16 | FT | 45–54 | Male | FG | – | – | |
8 | M | GP | 18 | FT | 45–54 | Male | FG | I | I |
9 | M | GP | 33 | FT | 55–64 | Male | TI | Q | Q |
10 | S | GP | 23 | FT | 45–54 | Male | FG | I | I |
10 | PM | 6 | FT | 35–44 | Female | FG | – | – | |
11 | S | GP | 25 | FT | 55–64 | Male | TI | I | I |
12 | L | GP | 19 | FT | 45–54 | Male | FG | Q | Q |
13 | M | GP | 20 | FT | 45–54 | Female | FG | – | – |
13 | GP | 15 | FT | 35–44 | Female | – | I | I | |
14 | S | GP | 20 | FT | 45–54 | Male | TI | I | I |
14 | PM | 20 | FT | 45–54 | Female | – | I | – | |
15 | L | GP | 25 | FT | 45–54 | Male | I | I | I |
16 | M | GP | 13 | PT | 35–44 | Female | TI | Q | Q |
17 | L | GP | 14 | FT | 45–54 | Female | FG | I | I |
18 | L | GP | 25 | FT | 45–54 | Male | – | I | – |
18 | GP | 16 | FT | 35–44 | Female | FG | I | I | |
18 | PM | 10 | PT | 55–64 | Female | FG | – | – | |
19 | S | GP | 10 | FT | 35–44 | Female | FG | I | I |
19 | PM | 9 | FT | 55–64 | Female | FG | – | – | |
20 | M | GP | 10 | FT | 35–44 | Male | FG | Q | Q |
21 | M | GP | 18 | FT | 45–54 | Male | FG | – | – |
21 | GP | 28 | FT | 45–54 | Male | – | I | I | |
22 | S | GP | 10 | FT | 25–34 | Male | – | Q | Q |
22 | PM | 7 | FT | 35–44 | Female | FG | – | – | |
22 | PM | 2.5 | FT | 35–44 | Female | FG | – | – | |
23 | S | GP | 20 | FT | 55–64 | Female | TI | Q | Q |
24 | S | GP | 6 | FT | 35–44 | Male | FG | Q | Q |
24 | PM | 13 | FT | 55–64 | Female | FG | Q | Q | |
25 | M | GP | 8 | FT | 45–54 | Male | FG | Q | Q |
26 | M | GP | 28 | FT | 45–54 | Male | I | Q | Q |
27 | M | GP | 11 | FT | 35–44 | Female | TI | I | I |
28 | M | GP | 15 | FT | 35–44 | Male | FG | Q | Q |
28 | PM | NS | NS | NS | Female | FG | – | – | |
29 | NS | PM | 7 | FT | 35–44 | Female | FG | – | – |
30 | M | GP | 10 | FT | 35–44 | Female | FG | Q | Q |
31 | S | GP | 3 | FT | 25–34 | Male | – | I | I |
31 | PM | 19 | FT | 45–54 | Female | FG | – | – | |
31 | PN | 7 | FT | 35–44 | Female | FG | – | – | |
32 | L | GP | 17 | FT | 45–54 | Male | FG | I | I |
32 | PM | NS | NS | NS | Male | – | I | – |
List of abbreviations
- ABM UHB
- Abertawe Bro Morgannwg University Health Board
- ACG
- Adjusted Clinical Group
- BMA
- British Medical Association
- CI
- confidence interval
- CONSORT
- Consolidated Standards of Reporting Trials
- COPD
- chronic obstructive pulmonary disease
- DMEC
- Data Monitoring and Ethics Committee
- EARP
- emergency admission risk prediction
- ED
- emergency department
- ERA
- Elder Risk Assessment
- ES
- Enhanced Services
- GMS
- General and Personal Medical Services
- GP
- general practitioner
- HSDR
- Health Services and Delivery Research
- ICC
- intracluster correlation coefficient
- ICER
- incremental cost-effectiveness ratio
- ISA
- information sharing agreement
- IT
- information technology
- LACE
- Length of stay, Acuity, Comorbidities, ED visits in previous 6 months
- MDT
- multidisciplinary team
- NICE
- National Institute for Health and Care Excellence
- NIHR
- National Institute for Health Research
- NPT
- normalisation process theory
- NWIS
- NHS Wales Informatics Service
- OR
- odds ratio
- PARR
- Patients at Risk of Re-hospitalisation
- PCT
- primary care trust
- PM
- practice manager
- PRISM
- Predictive RIsk Stratification Model
- PRISMATIC
- Predictive Risk Stratification: A Trial in Chronic Conditions Management
- QALY
- quality-adjusted life-year
- QCM
- Quality of Care Monitor
- QOF
- Quality and Outcomes Framework
- QPI
- quality and productivity indicator
- RMG
- Research Management Group
- ROC
- receiver operating characteristic
- SAIL
- Secure Anonymised Information Linkage
- SD
- standard deviation
- SF-6D
- Short Form questionnaire-6 Dimensions
- SF-12
- Short Form questionnaire-12 items
- SOP
- standard operating procedure
- STU
- Swansea Trials Unit
- SUCCESS
- Service Users with Chronic Conditions Encouraging Sensible Solutions
- TSC
- Trial Steering Committee
- WIMD
- Welsh Index of Multiple Deprivation