Notes
Article history
The research reported in this issue of the journal was funded by the HS&DR programme or one of its preceding programmes as project number 14/156/15. The contractual start date was in November 2015. The final report began editorial review in July 2017 and was accepted for publication in December 2017. The authors have been wholly responsible for all data collection, analysis and interpretation, and for writing up their work. The HS&DR editors and production house have tried to ensure the accuracy of the authors’ report and would like to thank the reviewers for their constructive comments on the final report document. However, they do not accept liability for damages or losses arising from material published in this report.
Declared competing interests of authors
none
Permissions
Copyright statement
© Queen’s Printer and Controller of HMSO 2019. This work was produced by Rivas et al. under the terms of a commissioning contract issued by the Secretary of State for Health and Social Care. This issue may be freely reproduced for the purposes of private research and study and extracts (or indeed, the full report) may be included in professional journals provided that suitable acknowledgement is made and the reproduction is not associated with any form of advertising. Applications for commercial reproduction should be addressed to: NIHR Journals Library, National Institute for Health Research, Evaluation, Trials and Studies Coordinating Centre, Alpha House, University of Southampton Science Park, Southampton SO16 7NS, UK.
2019 Queen’s Printer and Controller of HMSO
Chapter 1 Background and introduction
Patient experience surveys
Increasing attention is being paid by health-care providers to the patient-reported experience, which is at the core of all that the NHS does. 1 Patient feedback on their experiences has the potential to drive care-quality improvements, highlight system failures and improve safety, reduce patient harm and increase satisfaction with health-care provision. 2–4
Patient experience is often determined formally through surveys. These are considered to be important indicators of the quality of health service provision and service improvement priorities5–9 because they involve patients’ – or sometimes their carers’ – own evaluations. The NHS has led the way internationally in mandating a national patient survey programme in England since 2001. 10 There are currently hundreds of such surveys administered at various levels – locally, nationally and internationally – across a range of institutional settings and patient groups. 10 The Cancer Patient Experience Survey (CPES), the survey at the core of this study, is an example of a condition-specific patient experience survey (PES). There are many informal sources of patient experience that further add to the knowledge base on NHS websites and other websites dedicated to patient feedback, such as PatientOpinion (www.careopinion.org.uk), iWantGreatCare (www.iwantgreatcare.org), NHS Choices (www.nhs.uk/pages/home.aspx), blogs, social media and online fora.
It is important for patient feedback data to be properly used, and this requires appropriate tools for the collection, analysis, presentation, engagement with and understanding of the data if they are to improve health services effectively. Surveys mostly comprise closed questions, that is, questions with a fixed set of possible answers. This includes yes/no and multiple-choice responses, Likert scales, visual analogue scales and rating questions; the defining factor is that all of these are measures that can be easily quantified for meaning-making. Methods of analysing these have been well developed. 11 Using these, the CPES, which began in 2010, is widely acknowledged as the most successful national PES in enabling and embedding service improvement. 12 This has been achieved by providing trusts with tailored (trust-specific) statistical feedback of responses to the CPES closed questions, which benchmarks their performance against that of all other trusts. 13 More generally, there is evidence that PESs are also effective when their findings are linked to government-led campaigns, targets and incentives,6 such as Care Quality Commission (CQC) assessments.
Patient experience survey free-text comments
To add context to closed-question responses, it is common practice for open-ended questions to be provided within PESs for respondents to leave free-text comments. 7 CPES respondents, for example, are offered three such questions; one in three patients writes comments. 9,12 The ≥ 70,000 free-text comments produced by the CPES each year are anonymised by Quality Health, then selectively provided to NHS trusts, but in unstructured raw data form (unlike the closed-question responses), and so cannot be easily linked to the quantitative analysis or used in any systematic way nationally. The National Institute for Health Research (NIHR) commissioning brief [Health Services and Delivery Research (HSDR) programme 14/156] for this study noted that few organisations have sufficient analytical capacity to interpret large numbers of such complex data. The brief further noted that there was uncertainty as to how to present such data in a meaningful and granular way to stimulate local action, and this is where the study focus was placed.
Current limitations in the usefulness of free-text comments
Critically, there is currently no system to efficiently and usefully analyse and report free-text responses in PESs. 7 The conventional approach is manual thematic analysis (i.e. researchers reading through all of the text and assigning topic or theme codes to each comment). Such work requires considerable staff resource and can take months; thus, the number and costs of analysing these data each year using qualitative methods currently precludes its systematic analysis or reporting. 7 The usefulness of the data thus depends on individual willingness and capacity. 7 Even when analyses are undertaken, as with the 2013 London CPES free-text comments, there is a significant time lag; the London data were released in June 2013, the analysis was completed in December 2014 and was then published in June 2015. 14 There are several problems with such delays. First, the services the comments relate to may have changed considerably and most comments may no longer be relevant. Second, staff could become demoralised if they received misplaced negative feedback on services that they have improved in the meantime. Third, patients themselves worry that their data are not effectively used. Fourth, because the manual approach is resource-heavy in human labour, financial cost and time, it cannot practically be reapplied to new waves of data.
Overall then, because these data are not presented to trusts in a structured and easily accessed and assimilated form, they are unlikely to have much of an impact on service change and commissioning decisions. However, free-text comments potentially provide rich insights into patient experiences that underpin, illustrate and complement the closed-question responses. 7,12,15 The HSDR programme brief reflects the current need for better use of such data and highlights this as being critical in informing service provision in a challenged NHS. 16,17
Alternative approaches to the analysis of free-text comments
Despite the limitations of manual thematic analysis, there have been few previous attempts to analyse large-survey, unstructured, free-text responses in health care, including cancer services, to inform clinical and health-care practice. 7,12,15,18,19 There are notable exceptions,14,18–34 but these all represent one-off analyses (see Chapter 2).
NVivo version 9 (QSR International, Warrington, UK) and other qualitative data analysis (QDA) packages, including dedicated text-analysis software, such as QDA Miner (Provalis Research, Montreal, QC, Canada), are often used to organise manual thematic analyses. This might be considered particularly helpful with large data sets. However, in the experience of the study team, NVivo is unstable when handling a large amount of text; the software creates considerable metadata from relatively small text inputs, resulting in file sizes of several gigabytes. These restrictions became problematic when there were around 4600 comments in previous work. 19 Rapid automated QDA outputs that might be thought useful include automated coding, word clouds, concordance and word-frequency tables and associated statistics (against a comparator dictionary or data set). However, each of these uses basic word-search technology and ignores semantics and syntactics. Automated codes augment but cannot replace even basic manual coding. Word-cloud software generates ‘pictures’ of words from word-frequency lists, but only includes the more frequent words and does not take account of synonyms or closely related words. Depending on the ‘cloud’ shape chosen by the user, the words selected will vary considerably. Word-frequency tables enable the most used words and significant differences between data sets to be determined, but can be hard to interpret. Concordance software enables the user to click on a word to access its use in context, so that a more detailed understanding can be obtained. All of these approaches enable the big picture to be quickly obtained, but present an overwhelming number of irrelevant as well as relevant words, and more so the larger the sample size. Many of the words will be filler words (devoid of semantic content, but used in natural talk and text for flow or to hold attention) and many will be meaningless out of context. Text lists and word clouds will also contain many words with similar meanings that will appear to be of lower frequency because they have not been combined, when they may together represent something very significant. 35 If survey text responses are fairly simple – lists of symptoms, for instance – statistics-based solutions such as these may be useful. With even slightly more complex text responses, as with PESs, both specificity and sensitivity will be compromised.
A possible solution to the problem is to develop analyses of the data using more advanced computational approaches, which some people refer to as artificial intelligence, although the definition of this term is discipline dependent. 36 Text mining has been previously tried with the 2013 Wales CPES (WCPES) free-text comments. 19 The so-called template-based (otherwise known as memory- or instance-based) approach, a form of machine learning, was used; this has often been used for comparable classification tasks. 37 This approach might be expected to be an improvement over manual methods and basic QDA approaches. The work was certainly useful in showing the challenges to quality of life that are often faced by cancer survivors, and associated gaps in health care. 19 This led to a service model showing links between factors that negatively affected patient quality of life and potentially mediating factors.
However, a manual thematic analysis of 80% of the data was still needed to be undertaken using NVivo, meaning that the analysis process still took many months and, therefore, was only slightly faster than a fully manual approach. This is because memory- or instance-based learning approaches such as this classify new data by comparison against stored, ready-labelled instances. The version that was used, from R statistics software (The R Foundation for Statistical Computing, Vienna, Austria), relied on the k-nearest neighbour (KNN) algorithm, which is based on regression analysis and is one of the simplest machine learning approaches. In general, supervised learning methods take pairs of data points (X, Y) as input, in which X are the predictor variables (features) and Y is the target variable (label). The supervised learning method then uses these pairs as training sets and learns a model F, where F(X) is as close to Y as possible. This model F is then used to predict Ys for new data points X that are input into the system, using a statistical similarity measure such as Euclidean distance or cosine similarity. The KNN algorithm works out the distances between the new data point and all stored data points, determines from these which stored points are closest (the KNNs) and then labels the new data point using either a majority or a weighted vote based on this calculation. In other words, the closer the new data point is in its aggregate distance score to one that is already stored, the more likely it is to receive the same label as the stored data point. As this approach uses stored examples for these calculations (i.e. as templates to ‘learn’ or work out where new data will be classified), it cannot analyse data accurately if (1) the new data do not match these templates well or (2) the distances between the templates are too great. This means that for good sensitivity and specificity, this approach requires approximately two-thirds of the data to be manually analysed and labelled as templates,38 as was previously found. 32 The remaining one-third of the data will then be matched accurately.
Although the previous study32 ultimately achieved a sensitivity of 78%, a precision of 83.5% and an overall F-score of 80% (see Chapter 8 for a further explanation of these scores), this approach is of limited use for repeated survey analyses. First, as explained above, it is not sufficiently automated to be useful for repeated waves of data and still does not possess the benefits of immediacy. Second, the algorithms that were used were pre-set by the R software developer and could not be modified. The ‘black-box’ approach was hard to evaluate,39 domain non-specific (i.e. not developed for health-care data per se) and insensitive to unexpected data. Each time new data need analysing, such algorithms need more training with new terms and words that may have arisen in the interim, such as new treatment names, because the algorithms are limited to the ‘controlled vocabulary’ of the templates. 39
Information retrieval
Given these limitations, a memory-based template approach to text mining is not appropriate for repeated waves of data, such as those generated by the annual CPES. The solution is to customise a rule-based IR or knowledge-extraction approach. This approach has been used worldwide in biomedicine. Herland et al. 40 provided a detailed description of some of the recent uses in health care, whereas Ordenes et al. 41 explored the widespread use of this approach to analyse comments made about commercial products and provide customer feedback.
Despite the significant use of rule-based IR over many years, at the time that funding was applied for, it had not been tried for PES free-text comment data. Indeed, it is still believed that this is the first such application; however, since funding was obtained, some teams and commercial organisations have developed analyses for the Friends and Family Test on patient satisfaction (a different concept from experience) and social media and website patient feedback. 42,43
Instead of using templates, rule-based IR methods involve a stage of lexicosyntactic preprocessing and development of gazetteers (sets of lists containing words and phrases for specific entities or concepts). This is followed by cascades of pattern-matching syntactic (grammar and other language structure) applications designed to find the targets for IR – in the case of the study, comments that match particular themes – in combination with rules that look up terms in the gazetteers.
The approach was based on the GATE open-source system, the developers of which have labelled their process as text or knowledge engineering,44 whereas the term rule-based IR is used in this report to encompass the whole analysis process; the study’s ‘text engineers’ have added further applications and lookup rules or search queries to the basic GATE pipeline (for an explanation of these terms, see Chapter 4). To develop the gazetteers and rules, theme labels and data have been used from previous manual (human hand-coded) and template-based machine learning projects that the study team has been involved in.
The aim in relation to IR of text has been to explore how a highly transferable rule-based approach might perform; however, this performance could be further improved by using the rule-based outputs as templates for template machine learning.
Benefits of the approach
This approach has many benefits. Lookup rules can tag or annotate comments as belonging to quite nuanced literal themes, by combining gazetteers using Boolean logic. The functions of the syntactic applications include part-of-speech (POS) tagging, in which an individual word (X in the X–Y annotation used above) is tagged on the basis of the POS it represents (e.g. whether it is a noun, verb or adjective). This can, for example, distinguish between the different uses of the test in ‘the test came back negative’ and ‘I will test the new treatment’. Importantly, words (X) are not independent of each other, because if the previous word was an adjective, it is far more likely that the next word will be a noun than a verb. This enables new words to be more likely to be categorised correctly than not, given these various points of information. Such features of IR have been used to develop an analytical process that can cope with the fragmented phrases and sentences that typify survey free text. The approach also makes use of semantic (meaning-making) information, such as co-location. Thus, for example, if a comment says ‘The nurse was present. She did not speak’, the system will know that ‘She’ refers to the nurse because that is the relevant POS in the nearest sentence. Sentiment analysis has also been used, in which the label Y is used to reveal the sentiment (positive or negative) of each comment. These and other advantages of rule-based IR over template-based machine learning, and the rationale for choosing it, can be summarised as follows:
-
External knowledge repositories [such as WordNet®, version 3.1 (Princeton University, Princeton, NJ, USA)], can be used in categorisation, so that the system can cope with novel data linked in these repositories to existing labels.
-
New words can be added to gazetteers in the time that it takes to type them.
-
By writing rules that are not dependent on specific words, but that take into account the arrangements of words within syntactic and semantic contexts, the software can handle new data with good sensitivity and specificity.
-
It is not required to annotate a large number of data to start the process, in contrast to memory-based machine learning. Thus, once the rules are written, the system can be used in virtual real time for future iterations of PESs with no modification to minimal modification (a matter of hours rather than months). This gives it potential speed advantages in future years.
-
The system can be made domain specific, increasing the relevance of its outputs,45 as the rules are written by specific individuals.
-
New rules could be written very quickly and added to the system in the future and, hence, the approach makes for a potentially more accurate, more adaptable and more future-proofed system than template-based machine learning.
-
The system could be easily modified by others adapting the system in the future; transferability to other health-care surveys was an important element in the HSDR programme brief.
-
Rules could be specifically written to tackle some specific issues with free-text comments (see Chapter 4).
-
The approach is amenable to real-time data processing.
-
It does not require training examples (using resource-consuming manual data categorisation) or redevelopment of the whole system each time novel data are added.
Previous research has shown that linguistics-based IR models such as this can outperform manual (human) categorisation of customer feedback reviews,46 an area in which IR is particularly used. Therefore, it shows promise in the analysis of patient experience feedback.
Engagement with the data: making it meaningful for all
The health sciences lag behind other disciplines in the use of computational approaches, such as IR. 47 It has been suggested that this owes much to the chasm between person-centred care and the depersonalised approach of computing. 47 In other words, it is unclear how it can be ensured that computational analyses benefit individual patients. There has been little work to bridge the chasm and use the outputs of computational approaches to directly improve health care for patients. Accordingly, when study design commenced, the pre-funding patient and public involvement (PPI) input included the concern that the results would be mechanistic and reductionist, with the survey respondents and the people and health-care processes they wrote about in their free-text comments being reduced to mere numbers and data points. 48 There was concern that automation, generalisations and breadth would be favoured over the insights the comment boxes were meant to provide.
The study design therefore prioritises PPI (see Chapter 10) and includes a number of co-design-type substudies with patients, carers and health-care professionals that feed into the main analysis and the design of a digital toolkit with a dashboard or visual summary of the data, as well as the presentation of the newly structured (i.e. thematically grouped) free-text comments themselves. This is what Halford and Savage49 call a symphonic approach, with small qualitative and quantitative studies being used in a complementary fashion with large data set (‘big data’) computational approaches. For this reason, the outputs should lead to improvements in the health care of people with cancer in ways that are meaningful to them and incorporate and represent their perspectives and language. 50,51
Aims and objectives
The primary aim was therefore to improve the use and usefulness of PES free-text comments in order to drive improvements in the patient experience, and to do so, it was considered important to use a mixture of rule-based IR and complementary smaller studies.
Therefore, it was intended to develop and validate a novel use of rule-based IR to provide rapid automated thematic analysis of large amounts of survey free text (using the CPES as the first case), and to develop and validate a linked ‘dashboard’ (which later became a toolkit) to display the results in a summary format that can be drilled down to the original free text and used by patients and staff alike.
The toolkit, and the use of co-design with stakeholders, gives the approach added value over a simple thematic analysis, in addition to the speed and automaticity that rule-based IR enables. The display is intended to illuminate service gaps and areas in which the patient experience can be practically improved at the team, NHS trust and national levels.
As one of the secondary aims, the transferability of the approach was explored. Manuals ensured transferability to free text in other health surveys more generally. The rule-based IR process (i.e. the topic-oriented automated free-text analysis) and the toolkit were designed to be quickly reproducible and easily modifiable across health-care topics.
The objectives were to integrate co-design and implementation science into the approach, output thematic analyses into a digital display, produce recommendations on toolkit design, validate the approach and ensure transferability. The work primarily asked:
-
Is the novel approach a valid, accurate way to analyse large-volume CPES free-text responses?
-
Can the approach be transferred to similar surveys on other health-care topics?
-
Is co-design (as defined here) with mixed UK stakeholders (patients, their partners/carers, NHS managers and clinicians) feasible and effective for the approach?
-
Is Normalisation Process Theory (NPT) useful for the approach?
Overview of the study
The study comprises three main stages with subcomponents in each, which are summarised in Figure 1. Parts of this figure are repeated throughout the report to orient the reader.
FIGURE 1.
Overview of the study. The flow back from stage 3 to stage 2 represents the final refinements that were made to outputs as a result of stage 3 work.

Stage 1: preliminary (scoping) work
Review: see Chapter 2
A scoping review determined the key health-care dashboard design principles.
Dashboard-scoping survey and term and theme mindmapping survey: see Chapter 3
Patients, carers and health-care professionals completed an online survey to input into the text-analytics work that asked them to mindmap relevant terms and themes. In addition, they completed a separate survey on dashboard design to find out what potential end-users considered to be the key features for a good dashboard.
Rapid review of themes: see Chapter 3
Through a rapid review of the literature and incorporation of themes from previous relevant research, a draft taxonomy of themes was constructed, which was further developed in stage 2.
Stage 2: main development phase
Rule-based information retrieval work: see Chapter 4
The rule-based IR work involved modifications to GATE and further programming to solve issues with the analysis of PES data.
Prototype toolkit development: see Chapter 5
Prototypes were developed from stage 1 findings and refined through stage 2 as new data were collected and analysed.
Group workshops and interviews: see Chapter 6
The workshops incorporated group consensus concept-mapping techniques for naming and validating themes, and design discussions around dashboard prototypes, as a result of which the single-screen dashboard developed into a fuller toolkit. The interviews explored the issues in more depth.
Stage 3: validation and evaluation phase
Discrete choice experiment: see Chapter 7
A discrete choice experiment (DCE) was used to objectively validate a set of core features required of the toolkit for it to be taken up within health care, and it included a cost–benefit analysis based on the findings.
Validating the rule-based information retrieval: see Chapter 8
The sensitivity, specificity and accuracy of the approach was tested, as well as its transferability.
Toolkit evaluation through structured walk-through techniques: see Chapter 9
Techniques were used that are standard in technology evaluations to consider the usability and usefulness of the analysis and the toolkit and, combined with NPT, the work that these would do within health-care settings.
Theory
Normalisation Process Theory was used throughout the study. NPT is used to understand actions related to the implementation, embedding and integration of new technology or complex interventions. 52,53 It comprises four core ideas or constructs: (1) coherence, (2) cognitive participation, (3) collective action and (4) reflexive monitoring. Each of these constructs is subdivided into four and, thus, there are 16 constructs in total. The NPT toolkit website [www.normalizationprocess.org/npt-toolkit.aspx (accessed 9 October 2018)] contains example questions that can be used to explore these, and represents the results graphically in radar plots.
Chapter 2 Scoping review of clinical digital toolkit design

Introduction
To ensure that the dashboard, as a core element in the study, was designed to meet standards of best practice, a scoping review was undertaken of the existing evidence on digital toolkit design for use within health or social care or for the dissemination of health or social care data. Scoping reviews are typically used when an area is comparatively poorly understood. 54 Good dashboard design principles have been developed by web designers and programmers,55–61 but there was a dearth of evidence concerning health care specifically. Informal conversations with relevant web developers revealed that they tended to design sites using their technology-focused explicit and tacit knowledge, from a brief developed with or by commissioners of the website, who tend not to be the ultimate users. Consultation with patients and the public was not routine, although was sometimes undertaken, particularly for research studies and – in the last few years – some health smartphone applications. 62,63
In 2009, the NHS Connecting for Health initiative began the Clinical Dashboards Programme. This was incorporated within what was then the Health and Social Care Information Centre [http://webarchive.nationalarchives.gov.uk/20130502102046/http://www.hscic.gov.uk/about-us (accessed 9 October 2018)] and is now called NHS Digital. This provided toolkits for local groups to develop tailored dashboards from local quantitative data,64 an attempt to make dashboard development more accessible to health-care professionals as end-users. However, its recommendations do not appear to have been based on domain-specific considerations that incorporated patient views or were informed by empirical work on health-care dashboard usability and design considerations. Moreover, this programme was so flexible that it led to very divergent design approaches that often breached gestalt design principles. 65 Such flexibility was considered to be useful by the professionals concerned. However, gestalt design principles were developed from basic cognitive processes (see Chapter 10), and good dashboards should tailor their designs to specific end-user requirements without breaking these principles. In other words, end-users need to work with, rather than instead of, designers. In a pre-study exercise, at a PRESENT study launch workshop, three mixed groups of health-care professionals and patients at a PRESENT study launch workshop were formed to rate clinical dashboard programme designs (the Bolton series66) for the information they imparted and for attractiveness. Participants criticised each one as being unsatisfactory. Moreover, the three groups diverged considerably when asked to rank the examples in order of preference. It was clear, therefore, that the field would benefit from some clear direction on the core principles in health-care dashboard design.
As La Grouw61 states, dashboard design ‘starts with understanding the business. Anyone can design a dashboard; however designing an effective dashboard is a combination of brain science and business process knowledge’ [p. 4 (our emphasis)]. This domain-specific scoping review was therefore important in filling an evidence gap in the ‘business process’, as well as being useful in developing the evidence-based dashboard.
Method
A scoping review methodology67–69 with six stages was used: (1) identifying the research question; (2) identifying relevant studies; (3) selecting studies; (4) charting the data; (5) collating, summarising and reporting the results; and (6) consulting knowledge users. The first five steps of the framework are detailed below; for step 6, see Chapter 6.
Identifying the research question
The research question was shaped by the larger study and was ‘How does existing empirical evidence suggest that a digital health-care dashboard or toolkit should be designed to optimise its usefulness and usability?’.
Definitions and scope
The PICOT (participants, intervention, comparison, outcomes, types of study) framework70 was followed to determine inclusion and exclusion criteria and develop the search strategy. Each PICOT element is described in the following sections.
Participants
The focus for the larger study was on digital dashboards or toolkits used to improve health care, but the scope was broadened for the review to include any digital dashboard or toolkit study in which the dashboard or toolkit is intended for use by patients and carers, or by health-care professionals, health service managers, social and community workers or other professionals involved in health services or public health. Data that may be transferable to health-care improvement dashboards were not excluded. The different groups were not defined more precisely than this in order to ensure that sufficient useful information was obtained. Thus, information relevant to the digital dashboard or toolkit design for any health-care or health-care-related service, condition or topic was included.
Intervention
There are many dashboard definitions in common use, but the one provided by La Grouw61 was taken as the working definition: ‘a means to provide “at a glance” visibility into key performance using simple visual elements displayed within a single digital screen’. The following text was added to extend the definition: ‘and that is dynamic, that is, it can be mined down to see the original data’.
From this, a health service or social care dashboard as a digital display of data was defined as:
-
giving health-care or social care providers and stakeholders relevant information on health service performance or social or patient care or satisfaction, or, as in this study (although the review’s scope was broader), the patient experience at a glance; and
-
including some element of data transparency or granularity, so that higher-level summaries can be mined down at areas of interest. 61
The development of dashboards with higher-level summaries and lower-level detail was a key recommendation for the NHS in Lord Darzi’s High Quality Care for All: NHS Next Stage Review Final Report,16 as well as in The Health Informatics Review: Report. 71
Dashboards often form a part of digital toolkits and digital health-care interventions, so the study aimed to be inclusive in the search terms to capture a comprehensive collection of evidence. Therefore, any dashboard or toolkit was considered, or any other form of predominantly graphic communication or information provision intended for digital – usually web-based – use. This was especially important because the terminology in the area is constantly shifting. 72 It proved to be useful when it was decided, on the basis of participant feedback, to expand the dashboard that was being developed into a toolkit (see Chapter 6). A toolkit, as defined by the study team, is a type of internet portal that provides a range of resources, such as advice, information and networking targeted at a specific community.
The main exclusions were non-digital interfaces and digital or non-digital infographics, as they follow different design principles. Infographics are designed to tell a story to disseminate knowledge rather than to result in actionable events. However, static dashboards were not excluded from the search, despite the fact that the working definition included dynamism. This is because the greatest importance should be attached to the summary data page, as many users may not go beyond this (see Chapter 9); thus, analyses of static pages could contribute useful knowledge. Studies of dashboards that did not provide metrics, measures or indicators, or other numeric data, qualitative data or health-care information on a single screen intended to summarise data for future action were excluded.
Comparison
Studies did not need to include comparators.
Outcomes
A broad, and inclusive, approach to outcomes was undertaken within the areas of usability and design, display, communication and engagement features, such as the type and number of infographics, use of colour and font size. Health-related outcomes or effectiveness were not considered.
Types of studies
Any study design was considered and no restrictions were imposed on date. The search was restricted to English-language studies.
Study inclusion and exclusion criteria
Table 1 shows the study inclusion and exclusion criteria, as a summary of the previous sections.
Criteria | |
---|---|
Inclusion | Exclusion |
Studies in which the intervention being evaluated was some form of digital visual representation of information designed to inform future action | Studies in which the evaluation of the design or usability digital visual intervention was not an objective (e.g. which considered only clinical outcomes resulting from use) |
Studies in which the intervention was designed for use by any stakeholder within health or social care or related domains | Not used for health or social care data or related domains |
Strategic, tactical, operational, local or public purpose of the intervention | |
Any date | Non-English-language papers |
All peer-reviewed empirical primary study designs | Narrative and review papers, and grey literature |
Data for display could be qualitative or quantitative, although the dashboard is being designed to summarise qualitative data, but the intervention had to include a summary data page |
Search strategy
To develop the search strategy, environmental scans were undertaken using the iterative process usually used tacitly in systematic reviews. The process began with the first 100 results in each database; these display results by relevance using a link-analysis system or algorithms. Specificity was considered (identifying keywords of the first 100 articles in each database that may be associated with their relevance or lack of relevance) and this was balanced against the total number of articles retrieved (sensitivity). This approach led to the refinement of the search several times, including or excluding particular terms or words. See Appendix 1 for the final search terms for PubMed, which combined synonyms and metonyms for (1) dashboard, health informatics or related terms; (2) words relating to the design or use of a dashboard or similar technology; or (3) the terms health or health care, and variants thereof.
The scoping review was conducted by a team of five researchers. After the search terms were selected and refined by the whole team, four of the researchers extracted the search results from the databases into EndNote X7.4 [Clarivate Analytics (formerly Thomson Reuters), Philadelphia, PA, USA] for group sharing. The databases were PubMed, Cumulative Index to Nursing and Allied Health Literature (CINAHL), PsycINFO, Web of Knowledge, the Association for Computing Machinery Digital Library, the Journal of Medical Internet Research and EMBASE. The final search was undertaken in March 2017.
Two researchers (Daria Tkacz, Esther Irving/Chun Borodzicz/Johanna Nayoan) independently screened the search results by title, then abstract, then full text, depending on the level of detail needed to reach a decision for inclusion or exclusion. If the reviewers disagreed, a third reviewer (Carol Rivas) would have arbitrated, but this was not necessary. The reviewers assessed all results for relevance using the inclusion criteria in Table 1. In total, 13,823 articles were excluded after reading the titles or abstracts. The remaining 418 articles were subjected to full-text checks. Further articles were identified by backward and forward citation tracking of included studies. Forty-three articles were identified as fitting the criteria and included in the final review. The Preferred Reporting Items for Systematic Reviews and Meta-Analyses (PRISMA) flow diagram detailing the assessment process is shown in Appendix 2 (PRISMA is an evidence-based minimum set of items for reporting in systematic reviews and meta-analyses).
Assessment of methodological quality
A formal quality assessment of the included studies was not undertaken, as the main interest was in using the results to develop the health-care dashboard.
Charting the data and collating, summarising and reporting the results
A data extraction form was developed to ensure consistency in the review process across researchers. This included details of each study’s design, as well as the key constructs and other information needed to answer the research question. The focus was particularly on the format and audience of the dashboard, and results of the evaluation of its features. After two researchers extracted the data from all included studies (with cross-checks for quality control), one researcher (Daria Tkacz) undertook a narrative synthesis of the results. This was checked back against the original documents by Daria Tkacz and Carol Rivas for consistency and face validity. Results were also summarised in tabular form and discussed by the research team, steering committee and user advisory group (AG) members in relation to the PRESENT dashboard design. The DCE and structured walk-through results were mapped onto this table to inform both the final design and the general recommendations for health-care digital dashboard/toolkit design.
Results
Forty-three studies were included in the review. Study settings and intervention types included general practice,73 quality-of-life information systems,74,75 primary care,76,77 emergency departments,78,79 personal health dashboards,80 hospital care79,81–85 and sexual health. 86
The evaluation methods used in studies varied from focus groups and interviews74,87–89 to walk-throughs,74,76,78,82,90,91 eye-tracking82 and questionnaires. 79,82,91
The study findings covered access, flexibility and individualisation, usability, use of images and videos, chart types, data interrogation, print and export, community features, security and privacy and offering recommendations and solutions, which are considered in turn in the following sections.
Access
Access was considered by 9 of the 43 studies. Dashboards and toolkits were reportedly more effective if provided as part of a workflow, integrated into an already existing system92 or otherwise easy or effortless to access or constantly in sight. 76,93–95 Stand-alone systems requiring the user to initiate access via a separate login were less likely to be used regularly. Users may be reluctant to register for access, particularly if the process requires effort. 89,90,94 Any registration process should therefore be clear and short, preferably limited to one screen. 96 The value of the system and its benefits for the user need to be clearly defined before registration, for instance through an engaging homepage. 96
The URL should be simple, memorable and clearly associated with the focus of the dashboard. 89
Having real-time access to a dashboard during clinics with patients was seen as a valuable feature for clinicians. 77
Flexibility and individualisation
The theme of flexibility and individualisation was reported in 11 articles. Users valued the ability to tailor a system to individual and organisational needs. 94,97–100 This functionality could include personalised alerts and reminders,77,79,93,100–102 the option to set default filters75,87 and the ability to pin particular information to the screen to appear at all times, enabling comparisons. 77 Benefits were also reported from being able to dynamically add, remove and adjust elements of the system to fit the user’s focus and workflow. 74,82,103
The appropriate style to use when presenting the data is highly related to the nature of the data set and its audience, and, hence, it was recommended in four studies that the user is presented with a choice of graphs, dials or tables that can be adapted to individual preferences. 74,82,102–104
Usability and navigation
Usability was considered in 24 studies (as reported in 26 papers). Study authors considered a simple-to-follow, clear layout that anticipates the user’s workflow as key to usability. 75,87,90,102,103,105 Faster interpretation of the data is enabled by applying colour to emphasise differentiations and by adopting ‘most common’ lists. 79 Scrolling should be avoided or limited according to study authors,89,96,106 and information should always be accessible in as few mouse clicks as possible. 79,100
Using bright, distinct and highly contrasting colours for the interface is recommended. 84,86,96,104,107,108 Warm colours have been found to be inviting. 109 Black fonts on contrasting, light-coloured backgrounds are favoured. 109 The font must be large and clear in style to ensure accessibility. 76,89,100,106,108
To ensure comfortable navigation, the browsing options should always be clearly visible on all pages. 76,96,110 The navigation buttons should be large and clearly labelled. 90,108 A left-to-right menu has been found to be more successful than a top-down menu. 95 It is also important to consider different screen sizes and browsers to ensure that the layout resizes well and looks suitable on different devices;73,76,111 in other words, the system should be responsive.
Stakeholder preferences for layouts and ways of presenting the data depended on their role. Simple, static dashboard screens were reported to be helpful for ‘at-a-glance’ overviews during busy clinics, whereas interactive views were described as being more helpful for in-depth analytics. 74,103 Correspondingly, simple layouts were found to be appropriate for most users, whereas more complex, highly interactive dashboards were more suitable for ‘power users’ with analytical responsibilities. 74,82,103 Overall, to support the effective interpretation of information on the dashboard, it was recommended that the pages are not cluttered or overburdened with information. 90,96,104,106,109 The information at the bottom of the page tends not to be found easily, thus key points should appear at the top. 82,87 Limiting the immediately visible textual information and giving users an option to view more detail has been found to be effective. 111
The language must be kept simple and abbreviations and jargon should be avoided. 76,89,90,93,104,109,111 One study suggested that the complexity should be kept below a seventh grade (in the USA – equivalent to year 8 in England) reading level, and first-person narrative should be used in the text for a more engaging style. 106 Coyne et al. ,104 who designed and evaluated a dashboard for young people, emphasised that the language should be empowering, not patronising. 104 To avoid misinterpretation, it is important to provide definitions for any dashboard elements that may not be immediately obvious. 74,100,103,112
Use of images and videos
Although images are considered to be effective at fostering engagement with dashboards,87,105 one study emphasised the need to ensure that their purpose was informative, not solely decorative. 109 The choice of pictures should reflect users’ realities regarding factors such as age, gender or ethnicity. 105,106 Videos were found useful both for providing instructions on how to use the dashboard and for providing content, for instance, in the form of patient stories. 88,106,107
Chart types
Four studies reported that line and bar charts were the clearest and most appropriate charts for providers. 76,84,87,103 Line graphs were considered to be particularly suitable for comparisons over time, and they can be used with tool tips. 76 By contrast, one study found that speed dials were more effective than bar charts or radar plots. 77 This might have been linked to the nature of the displayed data, which focused on lifestyle. A sixth study compared the effectiveness of graphs and tables and found that the former were more appropriate for tasks that required analysing relationships and the latter were more appropriate for extracting specific values. 101 This study also showed that graphical formats were suitable for providers with a lower level of analytical skills, whereas tabular formats were more accurate for complex tasks.
Decision-making users with highly developed analytical skills may benefit from innovative graphs showing different data simultaneously82,84 (e.g. with multiple graphs on one screen). 80 Users often stated a desire for historical comparisons. 76,83,84,87,93,97,98,112 When reference data points, such as national averages, are included, interpretation is facilitated.
Regardless of the type, graphs need to be clearly labelled. 74,76,91 Displaying proportions and sample sizes is important for informed judgement74 and using highly contrasting colours to differentiate data points on the graphs helps to avoid misinterpretation. 82,84 The red/amber/green (RAG) traffic-light colour system has been found to be universally recognisable across health care. 76,78,79,81,83,95,112
Data interrogation
The ability to filter the data in real time and to sort these by any level and quality indicator was found to be valuable. 73,76,112 Importantly, the filter parameters need to be practical, clearly defined and aligned with the user’s work. 73,76,80,112 The design needs to make it clear that the page has changed after filtering. 76
Search boxes were also found to be useful for interrogating the data, and a drop-down list or dictionary of suggested search terms was recommended to meet the needs of users who may not be sure what to look for. 90
Hartzler et al. 99 considered the use of pictographs on a dashboard designed for patients and found that weather pictographs were more effective at illustrating patient-reported outcomes than the overly simplistic ones based on smiley and sad faces or charged batteries.
Print and export functions
A feature highlighted in four studies,74,98,104,109 as desired by users, was the option to print or download information and data, for patients to use or share with friends and family, and for professionals to use or share with colleagues.
Community features
A number of studies found that fora, chat rooms or similar community features were highly desired by users, to encourage an exchange of experience and information-swapping and to establish communication between patients and other patients or clinicians. 74,86,89,97,99,101,107,108,111
Discussion and impact on the project
The scoping review provided us with useful recommendations for the development and delivery of the dashboard (see Appendix 3). The evidence suggests that a clear definition of value gains on the homepage and a simple, short registration process are essential for initial user engagement. Ensuring convenience of access and integration with a user’s workflow are important for continued engagement. This can be achieved by offering users the ability to tailor the dashboard to their needs and preferences, making information available quickly and in a clear format, as well as offering a range of filters to enable quick analysis. Thus, the over-riding finding from studies, and the focus of any health-care dashboard designer, should be on ensuring minimum user effort and making role-specific tasks simple and quick to achieve.
Strengths and limitations
This was a scoping review, and for this reason, although it was rigorous and systematic, it had a broad research question. Most studies considered the health-care professional user, and few evaluated dashboards that were designed for patients. Many of the features elucidated are no different to more generalised good dashboard design features (see Chapter 10). For example, many common recommendations from the review studies accord with La Grouw’s61 assertion that a good dashboard requires appropriate high-level summary data, display features that are appropriate for the message and design features that are customised to the requirements of the specific users. Nonetheless, sufficient papers were located to provide a clear picture of health-care dashboards as needing to be easily integrated within existing workflows and simple and effortless to use, but flexible. It was clear that health-care dashboard users were pressed for time.
Implications for research and health care
This scoping review provides a valuable summary of evaluation-based recent evidence on desirable design features for health-care dashboards. The findings have been used to develop a list of recommendations for evidence-based health-care dashboard design, combined with other work. This list of recommendations can be checked by dashboard researchers, commissioners and developers within health care when developing new dashboards, for their optimisation.
How this informed later stages of the study
The findings of this scoping review were fed into a report shared among research team members. The immediate implications for the design of the dashboard were discussed with the programmer. Many of the suggestions raised in the articles discussed in this chapter were considered in the topic guide for the workshops and follow-up interviews, when the opinions were sought of the stakeholders to whom the dashboard is addressed. These were then cumulatively discussed by the research team and considered in relation to the scope of the project. This informed the design decisions for the beta version of the prototype, which was subsequently tested in the proof-of-concept stage.
The scoping review was also essential for designing the DCE. The key design features identified in this chapter informed the list of attributes for the DCE (see Chapter 7). The cove research team met with the economist working on the project, Emmanouil Mentzakis, on three separate occasions, during which the list and variations of the attributes were further discussed and adapted. The final list of attributes was shared with the AG for comments before the DCE survey went live.
Chapter 3 Scoping studies

In this chapter, consideration is given to the data and information that were collected and used to develop the initial plans are considered for the rule-based IR and dashboard/toolkit development, and the involvement of various stakeholders (patients, carers and health-care professionals). The results from the different data sources are synthesised towards the end of the chapter, with findings from the scoping review of the literature (see Chapter 2) also included. The chapter is concluded by considering the limitations of the approach, the implications of the findings for research and health care, and the impact on the project, including recommendations to inform the rule-based IR and toolkit design.
Introduction
The scoping stage enabled us to build on existing research and incorporate the stated needs of the different stakeholders in health care. The stage comprised the following elements:
-
development of preliminary themes from prior research on the patient experience, in particular, the prior machine learning work and a rapid review of thematic analysis of similar PES data (with themes summarised in Table 2)
-
two online surveys, important in giving the different stakeholders (patients, carers and health-care professionals) a voice in the shaping of the study from the start; although it was originally planned to use crowdsourcing for these, it was advised when applying for ethics approval that a more focused approach would need to be undertaken.
These elements are now considered in more detail.
Rapid review of prior research
A rapid review of the literature on patient experience themes was undertaken, using a focused search strategy that was not intended to be exhaustive, but rather to help us to develop the taxonomy of themes. Studies were included across health-care domains that might be relevant to the transferability of the approach. Searches were undertaken in May 2016 and updated on 31 May 2017.
The inclusion criteria were:
-
the study grouped into themes the free-text comments completed by patients as part of a patient health-care experience survey
-
in mixed-methods analyses, free-text themes had to be distinguishable from other themes
-
studies published since 2006
-
English-language studies
-
peer-reviewed studies.
The exclusion criteria were:
-
not satisfying the inclusion criteria
-
patient satisfaction surveys (such as the Friends and Family Test, which probes for a value judgement on whether or not expectations were met, rather than encouraging patients to be specific about how points of care were experienced)
-
patient experience surveys that focused on aspects of care that were not generalisable
-
statistical analyses.
The search was limited to the last 10 years to obtain an up-to-date perspective in the context of modern health care. Only peer-reviewed articles in English were considered because this was a rapid review and in order to avoid unpublished reports that may have been biased by survey or health-care provider interests, and three main databases were searched: Web of Science including PsycINFO, CINAHL and PubMed. See Appendix 1 for the search strategy. This combined synonyms and related terms for digital dashboards with potential outcome measures, such as usability, and with terms for health or health care.
Altogether, 148 potentially relevant articles were identified from these three sources. Once articles were rejected that did not fit the inclusion criteria on the basis of their titles and abstracts, 25 full-text articles were considered. Survey analyses of intervention uptake (n = 1) or service evaluation (n = 2) were then excluded. Studies that analysed only the free text for symptoms (n = 2), text analytics that provided word lists rather than themes (n = 2), very focused non-generalisable questions (such as those on the lesbian, gay, bisexual and transgendered experience) (n = 2), and methodological comparisons of survey comments and interviews (n = 1) were excluded. This left 15 articles for inclusion (see Appendix 4 for the PRISMA flow diagram).
The 36 themes identified in this review are summarised in Table 2. This table shows that transport, hospital environment and food were the three most common structural themes across studies. Considering health-care systems, access was commonly mentioned and no mention was made of broader system constraints, such as NHS funding. Communication and information was the most common theme in the individual needs category and overall; other themes in this category were broken down into quite granular levels. Waiting times, co-ordinated care, and aftercare were the most commonly reported process themes. Studies by team members and an independent analysis of the Scottish CPES contributed the most themes, highlighting the importance of the study and the richness of CPES as a data source.
Theme: presence or absence of | Study | Tippens et al.31 | McKinnon et al.34 | York et al.33 | Poole et al.30 | Lian et al.28 | Henrich et al.26 | Fradgley et al.24 | Cunningham et al.22,23 | Number of studies | Suggestions from the survey | |||||||
---|---|---|---|---|---|---|---|---|---|---|---|---|---|---|---|---|---|---|
CQC standards | Bracher et al.21 | Wiseman et al.14 | Wagland et al.32 | Iversen et al.27 | McLemore et al.29 | Hazzard et al.25 | Attanasio et al.20 | |||||||||||
Transport- or travel-related issues | ✓ | ✓ | ✓ | ✓ | ✓ | 5 | ||||||||||||
Hospital environment and equipment | ✓ | ✓ | ✓ | ✓ | ✓ | ✓ | ✓ | 7 | ✓ | |||||||||
Resources | ✓ | 1 | ||||||||||||||||
Financial concerns | ✓ | 1 | ||||||||||||||||
Safety | ✓ | ✓ | 2 | |||||||||||||||
Staff knowledge, skills and abilities | ✓ | ✓ | ✓ | ✓ | 4 | |||||||||||||
Consent around treatment | ✓ | ✓ | 2 | |||||||||||||||
Food | ✓ | ✓ | ✓ | ✓ | 4 | |||||||||||||
Staffing levels | ✓ | ✓ | ✓ | ✓ | 4 | ✓ | ||||||||||||
Out-of-hours and weekend care | ✓ | 1 | ||||||||||||||||
Signposting to external sources of support, such as charities | ✓ | ✓ | 2 | |||||||||||||||
Privacy | ✓ | 1 | ||||||||||||||||
System | ||||||||||||||||||
Accessing the care system | ✓ | ✓ | ✓ | ✓ | ✓ | ✓ | 7 | ✓ | ||||||||||
Feeling that the system had caused their health to worsen | ✓ | 1 | ||||||||||||||||
Trust in staff or system/confidence in the system | ✓ | ✓ | 2 | ✓ | ||||||||||||||
Individual needs | ||||||||||||||||||
Clear information/communication between patients and staff/candour | ✓ | ✓ | ✓ | ✓ | . ✓ | ✓ | ✓ | ✓ | ✓ | ✓ | ✓ | 12 | ✓ | |||||
Involvement in decision-making | ✓ | ✓ | ✓ | 3 | ✓ | |||||||||||||
Emotional, social and psychological support | ✓ | ✓ | ✓ | ✓ | 4 | ✓ | ||||||||||||
Empowerment | ✓ | 1 | ||||||||||||||||
Empathy and compassion | ✓ | ✓ | 3 | ✓ | ||||||||||||||
Person-centred care, dignity and respect | ✓ | ✓ | ✓ | 3 | ✓ | |||||||||||||
Involving the patient’s family | ✓ | ✓ | ✓ | 4 | ||||||||||||||
Good support | ✓ | 1 | ||||||||||||||||
Preparation for side effects | ✓ | ✓ | ✓ | ✓ | 4 | |||||||||||||
Managing expectations | ✓ | 1 | ||||||||||||||||
Good clinical care | ✓ | ✓ | 2 | |||||||||||||||
Processes | ||||||||||||||||||
Speedy and efficient processes | ✓ | 1 | ||||||||||||||||
Administration problems | ✓ | 1 | ||||||||||||||||
Long waits and delays/waiting for appointments/waiting on the day | ✓ | ✓ | ✓ | ✓ | ✓ | ✓ | ✓ | ✓ | 9 | ✓ | ||||||||
Co-ordinated vs. fragmented care/communication between staff and staff, staff and institutions and institutions and institutions | ✓ | ✓ | ✓ | ✓ | ✓ | ✓ | 6 | |||||||||||
Organisation of services | ✓ | 1 | ||||||||||||||||
Diagnosis and referral | ✓ | ✓ | ✓ | 3 | ||||||||||||||
Discharge | ✓ | ✓ | ✓ | 3 | ||||||||||||||
Continuity of care | ✓ | ✓ | 2 | |||||||||||||||
Follow-up and aftercare | ✓ | ✓ | ✓ | ✓ | ✓ | 5 | ||||||||||||
Good clinical care | ✓ | ✓ | ✓ | ✓ | 4 | |||||||||||||
Total = 36 themes | 11 | 13 | 8 | 9 | 3 | 8 | 6 | 2 | 4 | 4 | 4 | 2 | 4 | 6 | 26 |
To these themes, CQC fundamentals of care were added, as suggested by the AG.
Themes from prior research by the team in more detail
Prior research by the wider PRESENT team, and included in the rapid review, is considered in more detail in this report for two reasons. First because this shows the team’s natural progression in the thinking about text analysis and second, it includes a study commissioned by Macmillan Cancer Support,21 which involved the thematic analysis of free text from the 2013 WCPES. The same data set was used independently of this analysis to develop the prototype system.
The WCPES analysis, reported by Bracher et al. ,21 was manual and took several months, using a four-stage process of coding and analysis. This involved literal coding (i.e. concrete coding based on respondent’s own words113) for areas of cancer patient experience (i.e. concrete coding based on respondents’ own words;113 literal coding for specific categories within different areas of cancer patient experience; identification of more abstract themes; and comparisons between closed questions and free-text responses). This research highlighted the need for a more automated approach to analysis for these data. The results from the original analysis by Bracher et al. 21 were compared with the PRESENT text-analytics process outputs in the study’s sensitivity and specificity analysis (see Chapter 8).
The main themes from the study by Bracher et al. 21 were:
-
communication between patients and staff
-
waiting for appointments
-
waiting to be seen on the day
-
communication between staff and/or institutions
-
concerns about staffing levels
-
out-of-hours and weekend care
-
investigations and diagnostic services
-
aftercare
-
emotional, social and psychological support
-
hospital environments
-
travel-related issues
-
food and catering
-
financial concerns.
Wiseman et al. 14 undertook a framework analysis of free-text data from the 2012–13 National CPES subsample for two London Integrated Cancer Systems, covering 27 trusts. This comprised 15,403 comments from > 6500 patients. The dominant themes were poor care, poor communication and waiting times in outpatient departments, consistent with the analysis by Bracher et al. 21
Team members had also previously undertaken a memory-based machine learning analysis of the 1688 free-text comments in a population-based survey of colorectal cancer survivors. This necessitated the manual analysis of a large proportion of the data, to train the machine learning algorithms. The main themes from this study were similar to those for the WCPES analysis, relating to:
-
emotional support
-
diagnosis and referral
-
preparation for treatment side effects
-
signposting
-
aftercare
-
co-ordinated care.
New surveys
In spring 2016, patients, carers and health-care professionals were invited to complete an online survey, using the SoGo (Herndon, VA, USA) survey platform (www.sogosurvey.com), which prompted them to mindmap terms and phrases that they might use when writing about their experiences as a patient or potential patient. This is a modification of an approach used by the GATE developers; 15 respondents are considered to provide sufficient information. 114 Respondents were asked to mindmap words in four different categories, relating to their condition, treatments, health-care settings and health-care staff. Respondents were also asked to suggest topics or themes for inclusion in the PRESENT dashboard.
A second ‘dashboard-scoping’ survey was sent out at the same time, which asked a similar range of stakeholders to tell us what they thought a good health-care dashboard should be like functionally and visually, and what good existing examples they could recommend to us. The later DCE data (see Chapter 7) make for an interesting comparison with this study.
As inclusion criteria for the surveys, participants:
-
had to be NHS staff, patients and carers
-
should have a confirmed diagnosis of the cancer or other condition that had led to their being sent the survey, or care for such a person informally (e.g. partner) or professionally (e.g. nurse); non-cancer patients were included to make the approach robust and potentially transferable
-
must be aged ≥ 18 years
-
should have acknowledged that they read the information form (two consent boxes in the questionnaire must be ticked before the participant can proceed).
The surveys were piloted with six health-care professionals and two patients. The aim was to recruit up to 100 participants within 3 months, which was considerably more than the minimum needed, to ensure that diverse voices were heard. Participants were not contacted directly, but invited to fill in the surveys via the relevant networks of study collaborators, for a broad, UK-wide, but targeted, approach. This included internet-based and non-internet-based platforms.
Responses, in the form of ideas, concepts, words and phrases, were collated by Daria Tkacz and analysed by hand by Daria Tkacz and Peter West. The findings were shared with the rest of the team to inform both text analysis and dashboard design.
For the terms and themes mindmapping survey, there were 77 respondents, 70% of whom were female and 83% of whom identified as being of white ethnicity. Of these, the majority who specified their professional role self-identified as allied health professionals or nurses. Health-care professionals provided more names, phrases and figures of speech for treatments than for cancer or the patient experience, whereas patients provided a more even spread of terms (Figure 2). Carers also specified names for settings, but as there were only two carers, their data are not discussed further here. No participants specified alternative names for staff.
FIGURE 2.
Proportion (%) of total words suggested for each category by staff, patients and carers in the terms and themes mindmapping survey. Note that there were only two carers. HCP, health-care professional.
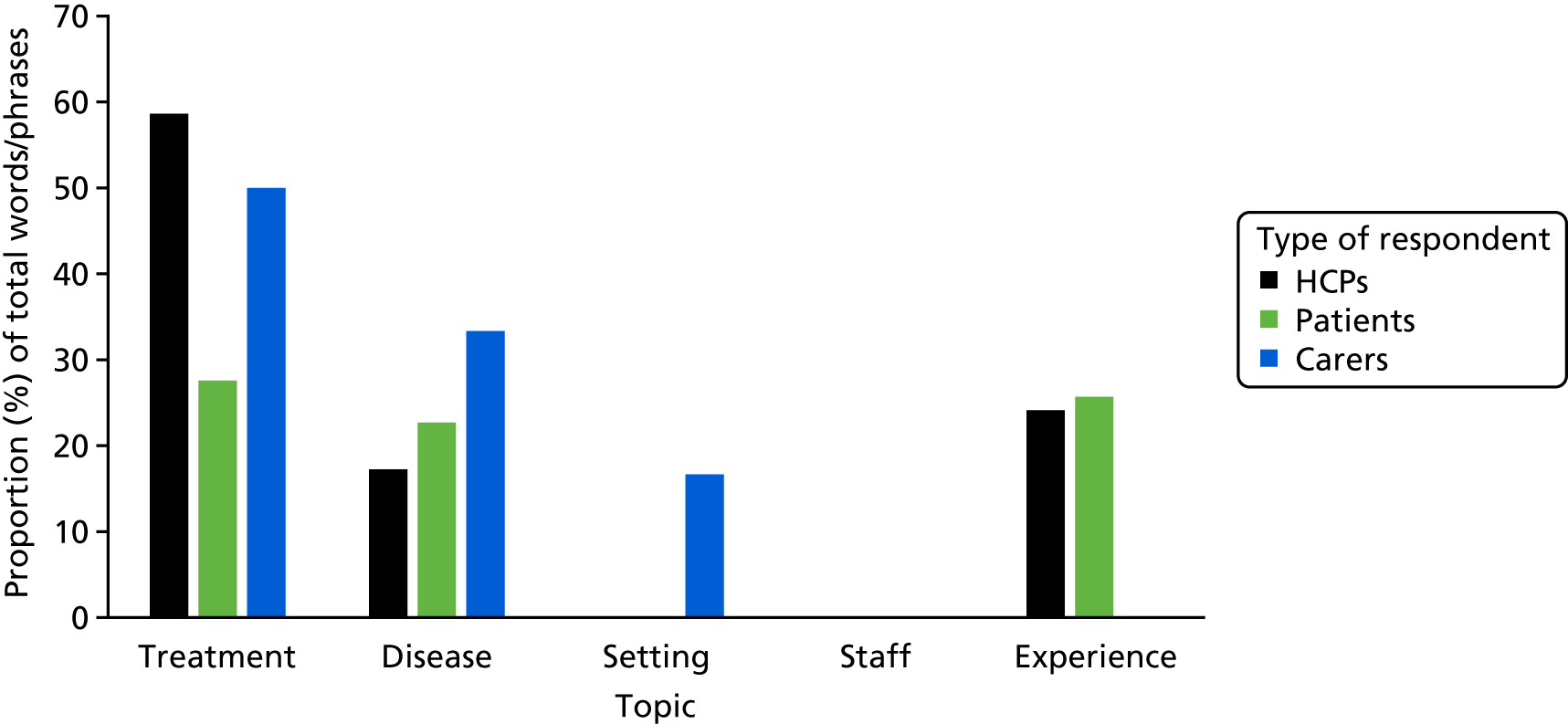
When health-care professionals provided treatment names, they were more likely than patients to use euphemisms (e.g. ‘servicing the cancer’), indicating that they considered that the topic might be sensitive to patients. When patients used euphemisms, they were more likely to convey emotion or dynamism (e.g. ‘whipping the prostate out’). Health-care professionals also tended to use acronyms or abbreviations, whereas patients tended to use synecdoche as abbreviations. Synecdoche is the naming of a part of something to refer to the whole, such as in the expression ‘the knife’, which stands for ‘the surgical knife’, or ‘the line’ instead of ‘the catheter line’.
When talking about the focal illness or condition, both patients and staff often used euphemisms, indicating the delicate nature of illness talk. Patients also tended to express emotions, use metaphors for expressiveness (e.g. ‘waterworks problems’) or use understatement, which may be a way of coping. 115 Health-care professionals tended to use abbreviations and metonymy, both being concise and efficient ways of referring to something rather than emotive terms. Metonymy refers to terms that are associated in meaning rather than being part of the original, such as saying ‘the Crown’ instead of ‘the Queen’.
When referring to the illness experience, both patients and staff used metaphors to suggest a fight or a journey (such as ‘I’m going down the tubes’ said by a patient). Patients also expressed their emotions and the uncertainties of their illness with phrases such as ‘living a game of snakes and ladders’, ‘living under a shadow’ and ‘going through hell’. Staff used idioms that focused on the work the patients did to fight their disease and conceal their emotions, such as ‘bottling it up’ and ‘the slog’.
Overall, patients and staff showed the greatest similarity in their talk of treatments and the greatest difference in their terms for the illness experience. Patients emphasised their emotional and uncertain journey, whereas staff believed that patients concealed their emotions. For all the categories of words, staff words and phrases tended to have a more efficient and practical orientation, although it was noted that the number of patients was relatively low, meaning that this research needs to be extended.
Responses were received from 35 respondents for the dashboard-scoping survey, 33 of whom were health-care professionals, but only 70% of whom used dashboards routinely. Respondents rated the quality of the data presented as the most important feature of dashboards, with relevant, focused, meaningful and structured displays. The second most important feature was the clarity and simplicity in the dashboard design, with an intuitive, easy-to-navigate interface. Users wanted visualisations that conveyed the meaning of the data through format choices, such as colour and graphic style. As a third most important feature, they felt that the dashboard should be designed to be used by executive board members as well as clinical staff, so that it had a real impact on the quality of care. Anonymity, flexibility, regular updates and visual appeal all received fewer votes from staff, although they were also considered to be important.
As special features and tools, staff suggested that the dashboard should include comparison features, the raw data, a ‘predictive intelligence capability’ to inform and help plan capacity and the power to differentiate between different areas of care and staff groups in the comments.
The suggested themes were as follows:
-
staff caring
-
involvement in decision-making
-
trust in staff
-
cleanliness
-
being asked about fears/concerns/feeling safe
-
waiting times
-
availability of specialist staff
-
patient satisfaction with access to information
-
availability of hospital staff
-
ease of access to services
-
time to listen to concerns
-
waiting times to be seen in clinic and day care.
These were compared with the themes determined from previous research (see Table 2) and it was found that they incorporated the most common themes and others that were moderately common within the literature. This is encouraging, given that the surveys were predominantly completed by health-care professionals and the themes reported in Table 2 were, with the exception of CQC themes, based on patient feedback.
Impact of the scoping stage on the initial prototype
A list of PES themes was collected from the different data sources to create a tentative taxonomy of themes, which was built on in further stages of the study. The final taxonomy has the potential for use in other research. This initial theme list was important in informing both the text-analytics rule-writing and the prototype dashboard display. Suggestions for priority themes influenced what was shown in the first prototype. Mindmapped terms and phrases were incorporated into the text-analytics gazetteers to enhance sensitivity. The differences were considered in perspective between staff and patients/carers were considered when facilitating stage 2 concept-mapping workshops (see Chapter 6) and these will be reported in the dashboard toolkit.
An attempt was made to accommodate all of the suggested features in the first prototype dashboard design. Theme icons were developed to be clicked on to drill down to more detail, including original comments, enabling the rationale for higher-level directives to be understood at the clinical ‘coalface’ through detailed example. High-level overviews were developed to highlight problem areas and benchmark regional and national performance on one computer screen. As well as providing text analyses, the facility was provided for users to be able to upload and incorporate quantitative data, so that their link to the rule-based IR-generated data could be explored. Importantly, for the health of patients and the NHS, this would maximise the usefulness of all of the data sets, not just the free text. A simple predictive tool and alert flags to indicate data reliability were also incorporated. It should be noted that as the study progressed, as a result of further research and input, some of these features were modified or disenabled. These early examples could, however, be quickly replaced.
The strengths of this phase of the work are that it draws on several different types of data and thus it is likely to have captured the most significant themes. It enables the different stakeholders in health care to contribute in a cheap, simple and efficient way. Thus, the approach could be adopted in complex interventions research as a way to engage the different stakeholders in early parts of a study. The limitations are that the rapid review of themes was undertaken by one researcher and was limited, the sample sizes for the surveys were small and there was considerable sampling bias.
As this stage relies partly on surveys, there was concern that this would immediately exclude some people from commenting. However, this research was intended only to inform the development of the digital dashboard; therefore, this was not the limitation that it might appear to be at first. The need for computer literacy and access to computers in order to be able to see such information in the age of big data is, however, something that needs to be considered within larger debates on the accessibility of big data as is also the need for opportunities to contribute to such data or its analysis and representation.
Chapter 4 Information extraction (rule-based information retrieval)

Having undertaken the groundwork for developing the free-text analysis and display approach, in this chapter the processes involved in rule-based IR are considered in more detail. This is followed by a detailed explanation of the solutions to problems with the analysis of survey free-text comments. In addition, the chapter describes problems that became apparent a posteriori; for example, it was realised that real-name redaction by the data suppliers [QualityWatch; URL: www.qualitywatch.org.uk (accessed 9 October 2018)] was incomplete, and a new secondary aim was to provide a solution to this.
Introduction
GATE (a General Architecture for Text Engineering) is a software framework and collection of resources that can be used for various natural language processing (NLP) tasks. 44,116 NLP is a field of computer science that involves getting computers to process and interpret human language. This covers a range of possibilities, such as:
-
parsing (i.e. sequentially ‘reading’ or analysing) natural language and ‘annotating’ it for grammatical features and other syntactic elements
-
extracting information from segments of natural language
-
actually ‘understanding’ natural language.
Each of these possibilities usually begins by ‘tokenising’ text, that is, breaking it up into words, punctuation marks, numbers and other discrete features. This is a very basic level of analysis, but provides a lot of information and packages the text into units for further processing. The next stage is usually to classify words into their grammatical categories or POSs and label them accordingly, which is known as POS tagging or tagging. Each token is associated with a POS. Tokens and tags are forms of linguistic annotation of the text.
One branch of NLP that is relevant and of interest to us is best described as information extraction (IE) from the text. 117 IE is concerned with the extraction of structured data from unstructured or semistructured data. Free-text answers on a survey are an example of unstructured text data. An example of semistructured text data is the ingredient lines in recipes for cooking food. Data that are grouped into themes provide an example of structured data.
Rules-based NLP approaches, such as the one used in the study, are based on an expert system of rules hand-coded by humans (see Alternative approaches to the analysis of free-text comments and Information retrieval for the rationale for choosing this approach). As the system becomes more complex, the interactions of these rules can also become more and more complex. Other non-rule-based approaches include statistical NLP approaches: NLP using statistics, probabilities, machine learning and similar techniques. Machine learning can be supervised (annotated corpora as learning sets), unsupervised or semisupervised. Different approaches can be combined.
GATE is being used in PRESENT for a gazetteer lookup and rule-based approach to IE from the free-text responses to survey questions. The rule-based approach is an approach that is suitable for the type of IE the study is interested in (i.e. theme extraction), whereas more complex information understanding would require more complex approaches. Tweaking gazetteers and rules to work with new data is far less time-consuming for a small team than annotating large numbers of new training data to create a sufficiently varied training set for every new set of survey questions for the template-based machine learning approach that members of the current team have used previously. This was a key factor in the choice of GATE for the study.
Individual GATE resources perform different elements of the work of parsing a piece of text and annotating it to allow extraction of information from it. These resources are assembled together into pipelines (series of resources), allowing the results of one resource’s processing to be used by other resources further along the pipeline. The result is a set of annotations to the original text.
The gazetteer component of the approach allows us to make use of resources, such as WordNet, and lists of words and phrases specifically related to the health-care domain. The use of gazetteers allows us to improve the ability of the system to specifically recognise the topics of interest for a given survey and tweak what is being looked for to suit differing questions in new surveys.
The rules portion consists of sets of rules written in Java Annotation Patterns Engine (JAPE). This is the core of the rule-based approach within GATE: a set of rules for matching patterns in the text and performing actions on the text. It provides regular expression-based pattern-matching; this simply means that it can ‘recognise’ or match elements of grammar, such as POSs, and the text annotations created by prior JAPE rules, gazetteer lookup or any of the other prior steps in the processing flow. These JAPE rules can add new annotations when a positive match is made. The rules can also undertake complex processing once potential patterns have been matched, to further determine if a new annotation should be made, and better disambiguate which type to make an annotation in cases in which a possible match can be made for more than one annotation type or entity type (i.e. a single unique object, such as a person, object or organisation).
Challenges in analysing survey free-text comments and key foci
Free-text comments raise a number of challenges for NLP programming:
-
‘messy’ language that does not neatly follow formal language rules: unusual punctuation, typos, capitalisation, spelling, use of slang, regional variations and figures of speech, sarcasm, garden path phrases (e.g. ‘the horse jumped over the gate fell’)
-
terse structure with messy syntax (e.g. short partial sentences, abrupt topic switches and odd lists of unrelated items, such as ‘the food, bed and staff’
-
lack of context, giving rise to ambiguities (e.g. comment boxes containing only the words ‘excellent’ or ‘nothing’, which might be responses to the original survey questions – ‘Was there anything particularly good about your NHS cancer care?’, ‘Was there anything that could have been improved?’, ‘Any other comments?’)
-
word use – emotive word use (e.g. ‘soooo not good’), words with multiple meanings (e.g. ‘may’), new words or acronyms [e.g. TBH (to be honest)]
-
co-reference – for example, using people’s names (e.g. ‘Bob’) to refer to the condition or body part affected and then starting the next sentence with ‘He’ might lead the process to annotate ‘He’ as if it were Bob (see Chapter 3).
These features of free-text comments are a challenge to language identification tools and can lead to linguistic pre-processing failure that may decrease the performance of standard IE tools considerably. Errors early in the pipeline can have a knock-on effect. For example, the GATE developers at the University of Sheffield report a decrease from ≈90% to ≈40% accuracy when the standard GATE program is run on tweets rather than on news articles. 118 A similar reduced accuracy was found when WCPES free-text comments were run through the standard GATE program.
The initial key foci, or research problems, were therefore to:
-
determine figures of speech that might confuse the system [through survey consultation and experimentation (see Chapter 3)]
-
cope with the syntactic irregularities and terse style of the texts (through programming)
-
develop gazetteers that could cope with word-use issues (including programming)
-
associate answers with the questions to reduce ambiguity (through programming).
A sentiment analysis was also incorporated that could work with the peculiarities of these data (through programming). Comments for a theme could be positive or negative, and this was indicated rather than separating comments into positive and negative themes per se.
As data were worked on, further problems were determined, which are described with their solutions in the section Additional issues realised during the study and their possible solutions.
Data used to develop and test the approach
A list of potential target themes from previous work (see Chapter 3) was developed to enable domain-specific gazetteers and possible rules to be developed, which mapped to existing themes, concepts and structures referred to within the NHS. The study team was required to go through a rigorous NHS permissions and advanced data management training process before it was possible to obtain the most current national England CPES data. Hence, half of the data from the 2013 WCPES were used to determine the challenges that the team was required to meet and to test the solutions and debug them [i.e. identify and remove coding errors; the other 50% was used in the validation stage (see Chapter 8)]. (The study team had permission to use WCES data as a result of approvals gained during the earlier work, although not to disseminate them via the new system.) As is usual in text analytics, this was an iterative process, with sensitivity and specificity improved through refinement of the gazetteers and rules. Less commonly in text analytics, the original target themes were modified through the co-design work (see Chapter 6), meaning that gazetteers and rules were added to at an advanced stage of the process, and further debugging was needed.
The main task: coping with the syntactic irregularities and terse style
The nature of survey free-text comment data compared with more formal text meant that some difficulties needed to be overcome to provide an accurate automatic thematic analysis. The sentences are highly complex and often diverge greatly from standard syntactic structure, as described in the section Challenges in analysing survey free-text comments and key foci. This rules out ‘Word_A is within n words of Word_B’ rules and means that standard computational syntax cascades cannot be applied without further modules to pre-process the data (notably this also means that the training set approach would require more templates than for more formal text – further reducing its usefulness compared with the solution that has been developed – and without necessarily effectively resolving conflicting or ambiguous annotations).
Approach rationale
The way the target themes were named meant that rules could be written that did not require the subject, verb and object of the comment to be determined, although the code that Laurence Antao wrote is easily adaptable to do so. Nonetheless, syntax was still important for accurate rule-writing and for sentiment analysis. The study team wished to identify subclauses to break the comments down into meaning units, but with standard GATE parsing this was often inaccurate. Laurence Antao determined that using noun phrases, verb phrases (including object noun phrases) and adjectival phrases, and also prepositional phrases along with subclauses, made it possible to develop an effective rule-based approach to create syntactically viable lists of topics using domain-specific gazetteers.
Syntactic patterns
Apache OpenNLP, version 1.8.4 (The Apache Software Foundation, Forest Hill, MD, USA), a GATE parser plugin (i.e. a software component that adds to an existing computer program), is good at determining noun phrases and verb phrases, and annotates them hierarchically. This has two important (non-mutually exclusive) features that are of great use. First, noun phrases may contain multiple noun phrases within them (likewise, verb phrases may contain multiple verb phrases – as in the garden path example in Challenges in analysing survey free-text comments and key foci). Second, verb phrases may contain noun phrases. Whatever the structure of a sentence, a clause will always have reference to a verb phrase and a subject phrase, whether that be within the clause itself, in another clause within the sentence or in another sentence completely (although this is not true of single-word sentences or very short sentence fragments that stand alone, this study is considering surveys, in which there is always a question being asked). By pairing up verb phrases to noun phrases, with phrases that are able to be paired multiple times, syntactically correct semantic meaning can be drawn from highly complex sentences and non-sentences (Figure 3). The full meaning of a sentence can then be gleaned by using rules to interpret conjunctions and verb tenses (e.g. a conditional tense implies the negative of that action in the present).
FIGURE 3.
How a complex sentence can be broken down into its constituent noun phrases and verb phrases (prepositional phrases were also noted). These can be parsed in turn by the system so that it picks up each and all variations. NP, noun phrase; PP, prepositional phrase; VP, verb phrase.

The parser that was used outputs subclause category SyntaxTreeNodes (tree representations of the abstract syntactic structure of the programming source code), which means that rules can be created to differentiate between noun and verb phrases that are next to each other but not necessarily directly connected.
GATE pipeline
In Figure 4, the ‘processing resources’ (PRs) highlighted in yellow are those that interpret the clause structures and produce the syntactically feasible lists of topics contained in the verb phrase and noun phrase ‘pairs’. The PRs take, as input, the keywords produced by the blue elements, some of which contain a polarity (‘negative’ Boolean) and ‘degree’ (0 = average, 1 = very, 2 = extremely). The ‘themes’ JAPE transducer PR (highlighted in red), then assigns the different ‘pairs’ to their corresponding themes. The green highlighted PR merely annotates hospital names or trust names with their corresponding trust. The non-highlighted PRs are the default PRs of GATE known as ANNIE (‘A Nearly-New IE’ system) PRs (although the sentence splitter has been swapped with a more efficient one). The JAPE transducer works out whether or not the phrase is negative, taking into account modal verbs and multiple negatives, as well as ‘if’ statements; there is not a necessity to factor in negative conjunctions. For convenience (at the theme stage), if a keyword is determined to be negative, its majorTypes or minorTypes list entry will be preceded by ‘not’.
FIGURE 4.
The PRESENT GATE pipeline.
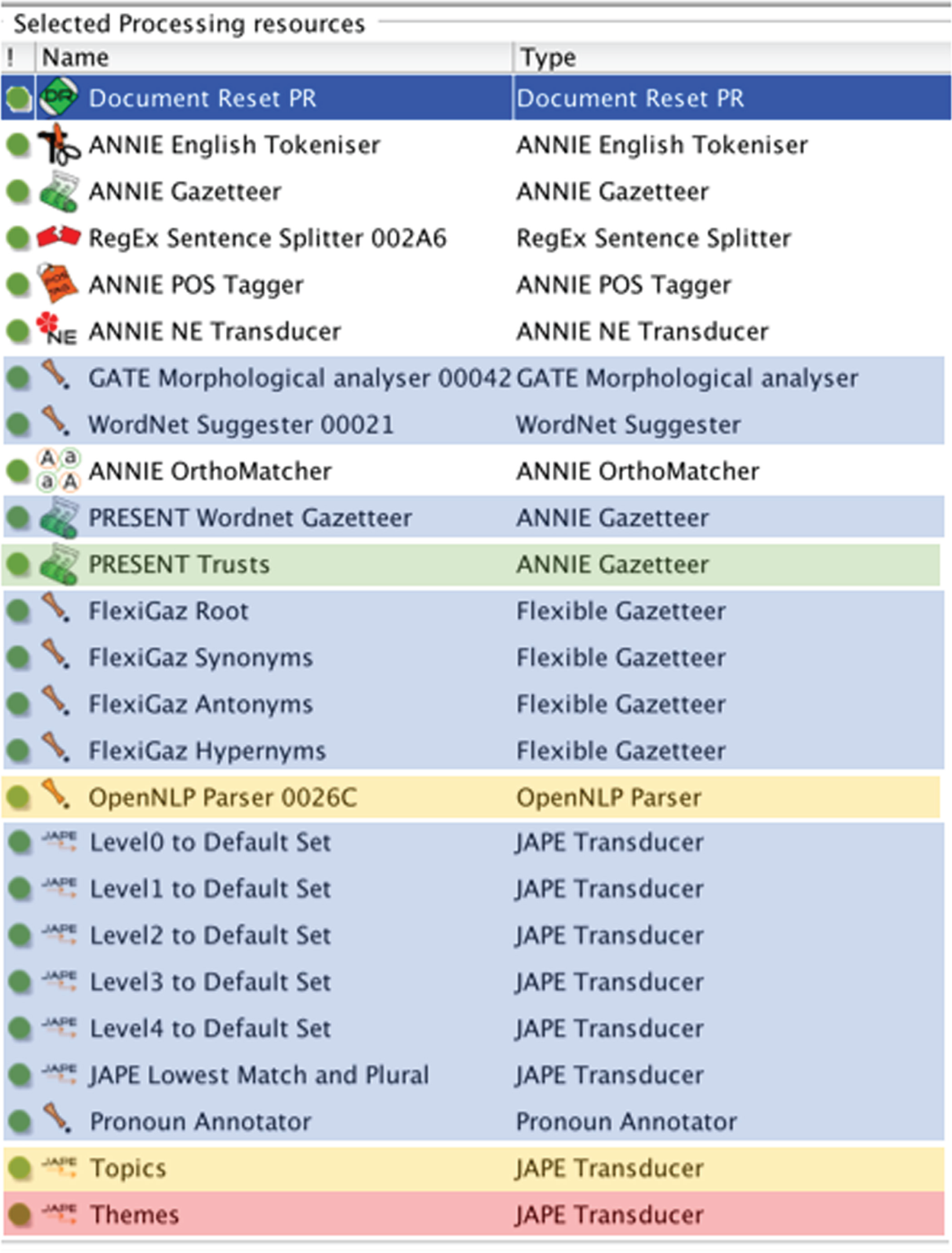
The GATE sentiment analysis JAPE was used and extended by adding relevant domain-specific entities to the JAPE so that the sentiment analysis can recognise specific entities from the domain-specific work to apply sentiment to.
Rule logic
Rules depended on a hierarchical approach upstream and an ‘if–then’ format and Boolean logic downstream and for gazetteer lookup.
An example of a rule that draws on gazetteers using Boolean logic is given below, where | means ‘or’. Rules can be easily tweaked by adapting or changing the gazetteer chosen.

Word use issues and WordNet
Although a rule-based system is potentially better able to cope with the unfamiliar than memory-based systems, it is not foolproof. The system has been built to take this into account and accommodate a wider possibility of previously unseen input words in a parsimonious way, to allow for the interpretation of unexpected vocabulary and reduce the reliance on human prediction. This has been achieved using the WordNet dictionary to tag words with synonyms and hypernyms and then annotating the most specific gazetteer entry with the flexible gazetteer PR. A flexible gazetteer was used rather than JAPE rules to make for easier thematic decisions, as it essentially presents the features to be added to the terms in a spreadsheet. This should be better in terms of legacy and accessibility, and should also make adding terms quicker.
One drawback of using WordNet is that it could lead to words being included in lookup that are inappropriate for the task and, therefore, lead to inaccuracies. Thus, it might become useful to put in delimiters. Users will need to balance the sensitivity and the specificity.
Output granularity
Through the use of metadata annotations, theme data were generated:
-
at the individual, local and national levels
-
considering demographics (ethnicity, gender and age of respondents)
-
considering tumour type, year or region
-
by sentiment.
The text and tags that were produced by the GATE pipeline were used to populate the dashboard at these different levels. Programming code was used to enable a user to run the GATE application via GATE Developer and then update and use the dashboard directly from a computer.
Additional issues realised during the study and their possible solutions
Issues of providing full comments publicly
One issue is that patients did not consent to have their comments publicly displayed when they completed the CPES. Therefore, the full comments cannot be shown on an open site.
Trust disambiguation
Respondents to a survey may be referred from the trust to which their survey is attributed to a centre in another trust for some aspects of their care. Previous work by some of the research team suggests that this occurs in 9% of cases and has the potential to cause significant problems. 14,21
Rules can be created that compare the trust name in the metadata for each comment with the name of organisations mentioned in the comment to see whether or not they correspond. The system includes such a rule, and also, in case the trust files need to be updated or edited for specificity of trust (names have several shortened forms), there is a Python program (version 3.6.5; The Python Software Foundation, Wilmington, DE, USA) to automatically create the gazetteer files from the Extensible Markup Language (XML) data file available on the NHS website containing trust names.
Redactions
Personal names were identified for redaction to ensure the privacy of patients and other individuals and confidentiality of patient information. This required disambiguation between the names of persons and of hospitals that happen to contain personal names, such as Jimmy’s for St James’s. Real names were replaced with [health-care professional name], [patient name], [hospital name] or any relevant alternative.
HealthWatch redactions, such as [xxxx] or [name removed], were replaced by the use of programming to indicate the entity type, such as [hospital].
Words with multiple meanings
Rules were developed to identify words that might be confused with a person’s name, such as X-ray or hospital ward.
Data volume
Loading large batches of data into the GATE graphical user interface (GUI), to play with visually, may cause problems for the GATE GUI. The developers of GATE advocate the use of their cloud system as an alternative. ‘GATE Embedded’ (a version of GATE usually used by developers) can handle much bigger data sets than the GUI can, but the GUI is more useful for looking at things when developing new rules. This means that refinements in development should be made on subsets of any data, but for the final outputs, large batch-loading is not a problem. Therefore, the option of choosing to load into a corpus up to 500 or 1000 rows only was included.
Further possible refinements
The development of systems such as this is always iterative, and other adaptations could be made to it; this is currently being considered. A couple of such possible new features are specified here.
Themes extracted
The themes for the process were determined through co-design work with the different stakeholders in health care, but this work was started with a finite pool of themes determined from previous research (see Chapter 3), an initial survey (see Chapter 3) and the literature (see Chapter 2). This is a deductive approach. Using Python, a latent Dirichlet allocation (LDA) option was developed. This is an unsupervised clustering technique that can be used to group texts by discovering apparent topics that they share through looking at words that occur together in some of the texts but not all (words that occur in all of the texts are expected to be common words rather than words indicating a topic). LDA can be used as an inductive topic-modelling/topic-discovery approach; the PyLDAVis library (The Python Software Foundation, Wilmington, DE, USA) (a Python library for interactive topic model visualisation) was used to better visualise the results of the LDA. This is potentially useful to:
-
see how close results come to what humans with domain knowledge have worked out the topics of interest are
-
see if the LDA finds topics that the humans had not thought of, but that are of interest.
For future data sets, topic discovery could make a useful addition to the process of humans mindmapping which topics are of interest. This option is particularly useful for free text for which the topics have not been predetermined or when there is insufficient prior information for a useful list to be drawn up deductively. It could also be used for discovery within the existing data set.
Latent Dirichlet allocation/topic-modelling does have drawbacks that need to be considered with the data:119
-
Topic discovery is a black-box approach and is prone to overfitting.
-
Efficiency decreases when a very large number of topics are used to fit the LDA.
-
The LDA performs better when the underlying topics are well separated (in the sense of Euclidean distance).
-
‘Topics’ discovered in an unsupervised way may not match the true topics in the data.
-
It is theoretically impossible to guarantee the identification of topics from a small number of documents/comments.
-
Short documents, such as free-text comments, may result in poor performance of the LDA.
In addition to LDA, the system could incorporate further unsupervised machine learning techniques in similar supporting roles to improve the automation of the overall system.
Case sensitivity
Existing PRESENT gazetteer entries in the pipeline are case insensitive (case sensitive = false). The assumption was made that words would not mean something else entirely if they were uppercase versus lowercase. This eliminates the need to make a duplicate of every lowercase word that might be at the start of a sentence within the gazetteers. It also helps to prevent errors arising when the case is used wrongly within a comment. This means that if there are any words that need to be case sensitive in the future, a second case sensitive = true gazetteer needs to be added to the pipeline for these words. This might be required, for instance, if acronyms are used that would otherwise be taken as normal words.
Transferability to other surveys and data sets
There are two key aspects to transferability of a domain-specific system to other surveys and health-care data sets. First, and most importantly, a system needs to be easily adapted. The power of the PRESENT system is derived from its modular nature, which also allows it to be quickly adapted and refined. Modification need only occur in:
-
The lower-level rules and gazetteers, hence it is relatively uncomplicated to make modifications.
-
Relating the responses to the questions, which are likely to differ across surveys. This may be incorporated into the automatic analysis.
Second, the system needs to be able to use alternative sources of data. For PRESENT, an approach was developed to batch-load text from a comma-separated values (CSV) file that could be varied in accordance with the number of header rows in the data file and the column of the spreadsheet in which the data occurred, as well as an identifier tag for the comment.
General maintenance needs
The system could run as is for a few years on the CPES, but is likely to become increasingly in need of maintenance because of:
-
changes in technology and software
-
modifications to the survey
-
changes in topic needs.
Implications for research and health care
Using the approach, a structure can be systematically applied to PES free text to make it more accessible to health-care providers and more quickly summarised than by existing approaches. The approach produces literal themes in a deductive process that researchers could use as the first stage in more conceptual analyses.
For the sensitivity, specificity and transferability tests, see Chapter 8.
Summary
Rule-based parsing was applied to patient answers to the free-text questions in the CPES via gazetteers and custom JAPE rules, using the GATE framework augmented with Python programming. Responses were categorised for their use in improving feedback to cancer care trusts on the patient experience. Rule-writing has sought to solve the following, in addition to producing a basic thematic analysis:
-
Accurate interpretation of disparate and syntactically errant free-text comments. The modular hierarchical nature of the rules allows this to be done by operating the lower-level (e.g. verb phrases, noun phrases) ‘best-match’ tags from the SUPPLE parser (University of Sheffield Prolog Parser for Language Engineering; University of Sheffield, Sheffield, UK). Thus, as with word recognition, interpretation is not reliant on the development corpus, and disparately structured entries will be interpretable.
-
Incorporating a WordNet feature. This means that the study’s gazetteers do not have to be exhaustive, but rather they need to have a sufficient range of words for WordNet to augment with synonyms and antonyms to provide a vastly expanded lookup vocabulary.
-
Relating answers to the survey question so that responses that would otherwise have been incomprehensible, such as ‘good’ or ‘nothing’, can be contextually analysed.
-
Reducing the ‘misattribution’ problem to accommodate situations in which a patient associated with one trust/site mentions receiving treatment at a different site. Names of hospitals and trusts were identified and disambiguated to enable feedback to be directed to the correct institution.
-
Sentiment analysis of the responses was performed by adapting a GATE tool that was originally designed for use on Twitter (Twitter, Inc., San Francisco, CA, USA; www.twitter.com) to the health-care domain. This was made even more sensitive by using WordNet to identify metonyms and programming to assign one of five levels of sentiment (‘very good’, ‘good’, ‘neutral’, ‘needs improvement’ and ‘needs a lot of improvement’).
-
A system was developed for batch-loading new data sets.
-
Rules were developed to identify and mark people’s names for redaction, which takes account of hospital names containing personal names. The study team also aimed to replace the redactions throughout with entity types.
-
Rules were written to identify words that might be confused with a person’s name, such as X-ray or hospital ward.
Transferability and maintenance were explored, and key issues were dealt with. The modular nature of the system and the need to alter only lower-level rules and gazetteers make this relatively unproblematic. This included the potential deployment of LDA to identify or discover possible topics of interest in the corpus of patient responses.
Through the IE work, theme data can be:
-
generated at the individual, local and national levels
-
generated considering demographics (ethnicity, gender and age of respondents)
-
generated considering tumour type, year or region
-
scored using sentiment analysis to measure the polarity and strength of mood expressed in a comment.
Chapter 5 Dashboard development
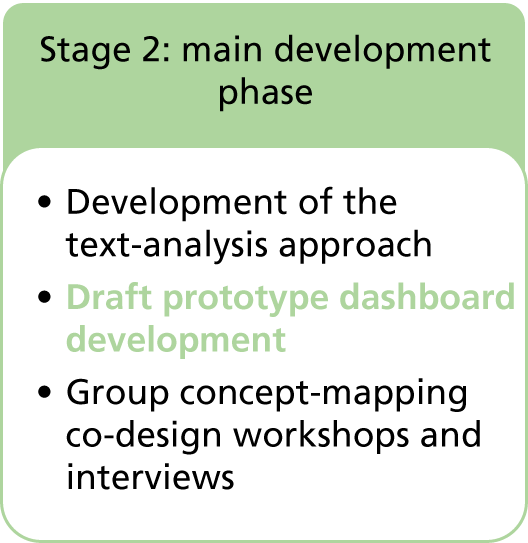
This chapter will explore the iterative process that was used to develop the dashboard. It shows how the study stage 1 findings were used to guide the design of the early prototypes and contributed to decisions on the basic infrastructure of the dashboard. The ways in which subsequent fieldwork (described in subsequent chapters) led to refinements and further decision-making is then considered.
Introduction
Using data from stage 1 (see Chapter 3) and the scoping review of the literature (see Chapter 2), a list of criteria was constructed to aid us in developing the dashboard prototype. The dashboard differs from those formulated as part of the Clinical Dashboards Programme64 in that it displays a thematic grouping of text comments rather than numerical outcomes and performance data. In the original proposal, it was intended to include quantitative data, but NHS England asked the study instead to link to the sites it was developing for this. Users of the system who would wish to adapt it could still easily include feeds from other data in a minor revision.
Currently, the way that patient experience free-text comments are used may be considered as strategic, that is, for future goal-setting. 61 The intention was to develop a dashboard/toolkit that could be used across La Grouw’s61 five levels (p. 130), although the launch workshop suggested that this might be difficult to achieve (see Chapter 1). Thus, it was intended that the dashboard/toolkit would be used:
-
strategically by managers and executives to review operational and strategic information; this requires the inclusion of targets, trends, comparisons and results
-
tactically by higher-level managers to consider whether or not improvement initiatives should be used or if they are working, with one eye on strategic objectives
-
operationally by units, such as wards, to consider how the unit is performing and how this relates to NHS performance requirements
-
locally by teams and individuals working at the ‘coalface’ to manage single elements of performance and improve the patient experience
-
publicly, so that patients could learn from other patients’ experiences.
The first priority, as per the protocol, was level 4 in the above list and the second priority was level 5. Thus, the aim was to include detail that was fine-grained enough for local staff to use in small health-care improvement initiatives, to make immediate decisions and adjustments in response to current data.
Benefits
Linked to these five levels, the main benefits of the dashboard/toolkit, as the study team perceived them at the start and aimed to uphold, were that it enabled:
-
the routine systematic use of patient experience free-text comment data (at all levels, including the public level)
-
enhanced decision-making in health-care improvements (strategic and operational use)
-
facilitation of immediate targeted decisions to improve patient care (local use)
-
improved quality-of-care benchmarking against other sites (operational use)
-
reduced time and effort to assimilate information on the patient experience (all levels, including public use)
-
increased staff satisfaction (and hence performance), as teams feel ownership of the local level of care quality delivered (local use)
-
improved data quality as a result of information being displayed locally in a tested approach (local use)
-
improved standard of care and experience for patients by aiding service planning and decision-making (all levels, including public use).
It should, however, be noted that it was always intended that its use would be qualified, given inherent limitations in the data (see Chapter 10), which was factored in from the start of the design and development process.
Given the dashboard/toolkit’s purpose in disseminating information from free-text comments on the patient experience that can be used to help to drive health-care improvements, it was determined that some of the key features the version should have are:
-
easy access to the data in a visual and usable format
-
data provided in a way that can be engaged with by members of the public and by professionals
-
summary data that can be mined down
-
locally relevant information displayed alongside relevant regional and national data
-
information provision that is relevant across all levels of use and with multidisciplinary teams, enabling organisational working –
-
front-line staff delivering care at the ‘coalface’ may benefit from the fine detail and will also benefit from seeing change through the years
-
the toolkit should facilitate discussion between commissioners, the clinical team and others to improve care quality, potentially acting as leverage to drive change
-
-
information provision in ways that can meaningfully inform service improvement within the contexts of local care
-
a full account of the limitations of the data and its appropriate use
-
information provision in ‘real time’, when the data are available for this, or (as is currently the situation with annual large-batch CPES free-text data) analysis and display within hours to days after the data become available.
Building dashboard prototypes
The remit of the dashboard was considered first, extending the considerations beyond those discussed above. The purpose of the PRESENT project has been to develop techniques for automatically making sense of free-text responses in PESs and, from this, to present meaningful visualisations and insights.
The potential users of these data are health-care providers and professionals, who may use the information to decide where resources should be placed to improve services at a local or broader level, and patients, who may use the information to help to decide what they should expect from their health care. The clear differences in the requirements of these stakeholders led the dashboard programmers to consider two separate dashboards: one for clinicians (slightly modified for commissioners) and one for patients. Initial requirements were drawn from stage 1 data and expanded on in the group concept-mapping workshops and associated interviews.
The expectation was that patients would be more interested in high-level patient feedback about particular trusts and topics, whereas clinicians (and commissioners) would want to be able to make statistical comparisons between trusts. This led the team to focus on a faceted dashboard [inspired by TripAdvisor (TripAdvisor, Inc., Needham, MA, USA)] for navigating comments in the clinical dashboard, and a more traditional hierarchical website (inspired by police.uk) for the patient dashboard. Furthermore, because of the risk of personal information being present in free-text responses, discussions with NHS England and governance considerations meant that, at present, it would be unlikely that members of the public would be allowed to see individual responses, so only the clinicians’ dashboard was set up to allow displays of data at this level of granularity.
The dashboards were implemented separately; each of two programmers focused on one. The implementations were complementary in terms of programming language [JavaScript, v8 (Google Chrome, Joyent Inc., San Francisco, CA, USA)] and tools [node.js, d3 (Google Chrome, Joyent Inc., San Francisco, CA, USA)] used.
Liaison with Insight NHS England and its cancer dashboard
NHS England was an important stakeholder in the project as the custodian of the CPES data. This led to constraints in how the data were used that were appreciated from a governance point of view, but that were frustrating for the other stakeholders. An all-parliamentary research group has considered the issues, which are true for big data in general (see Chapter 10).
NHS England was developing its cancer dashboard at the time the study began [beta version available at www.cancerdata.nhs.uk/dashboard#?tab=Overview (accessed 29 January 2019)]. The study team therefore collaborated with NHS England in face-to-face meetings with team members and by e-mail, to explore the links between the works and to ensure that the two dashboards did not duplicate the same information. The Insight NHS England executive team and web developers suggested that the study team signpost users of the dashboard to theirs, and that once ours was live they would reciprocate. They also suggested that responses to six of the quantitative questions in the CPES could be included to provide a stronger link. These were included in an early draft of the prototype, but there was consensus from users that their presence was confusing. These may be reinstated once the system becomes live and further work is undertaken on this with NHS England.
The presence of the names of health-care staff in the data was discussed with NHS England. It appeared that QualityHealth did not redact these when positive comments were made – at least that was the understanding – and this was not believed to be appropriate. At the same time, there were accounts in the media of accidental leaks of non-related NHS data, and NHS England withdrew all CPES data from its archives, while all remaining personally identifiable data were removed. In some ways, this might be seen as an example of PRESENT’s impact, but it also meant that the system could not be developed using 2015 data as planned. Hence, the study team members used 2013 WCPES data that they already had an agreement to use in research but not to share in disseminations outside the research process. This has limited the visible final outputs, but did not otherwise negatively impinge on the study itself. In addition, more alternative data sets were used than in the protocol [i.e. including patientopinion.org data and free-text data from three questions rather than one from the Life After Prostate Cancer Diagnosis (LAPCD) study] to test the system transferability more thoroughly, as a pragmatic decision to maximise the use of the system.
The iterative process
The PRESENT project followed a participatory design process, which involved stakeholders (namely patients and health-care providers, professionals and managers) in the design of the dashboards. This meant having an iterative refinement process that was reactive to participants’ feedback. The feedback was often unexpected, demonstrating the importance of involving stakeholders in the design process.
The main aim of the dashboard, as specified in the protocol, is to lead to health-care improvement that improves the patient experience – where this is indicated as being necessary. The target should be the embedding of good-quality care in health-care services, rather than simply ensuring adequate care that does not fall below a critical threshold.
To enable this, what the dashboard shows should be meaningful to both the staff who may need to act on it and the patients reporting their care experiences, the achievement of which has been attempted through the different stages of the study. The following sections include a consideration of the results from these stages and how they have informed the iterative development of the dashboard/toolkit.
Requirements elicitation
In the workshops (see Chapter 6), participants were asked how they would use the CPES free-text data in a dashboard. It was thought to be likely by patients and carers, who dominated the early groups, that there would be differences between what health-care professionals would want compared with what patients would want. Patient and carer use suggestions were:
-
making hospitals/the government accountable for common problems for people with particular conditions – participants mentioned that the publication of data can lead to change (and social media was suggested as a way to drive that)
-
informing medical students on the patient experience to help their education (some participants said that this might help doctors to support patients)
-
links to external sources of support (groups, etc.) – this was also considered by patients as diplomatic, so as to not give an impression of trying to put too much pressure on health-care professionals
-
the desire to see comments made by ‘patients like me’ to compare experiences
-
audit trail of comments by patients so that they can check that their comment has not been misconstrued.
In the structured walk-throughs (see Chapter 9), similar questions were asked of health-care professionals. Participants identified that the primary aim is to see where improvements need to be made in a timely and easy-to-understand manner (instead of the years that qualitative analyses can take) and to be able to use this evidence to make a justification for funding. Many also wanted to be able to use the dashboard to find out what is going well, to let patients know and to boost the morale of staff. A few said that this could also provide useful evidence for appraisals. Some mentioned that they would like to know that patients see that their comments are being used constructively.
Dashboard/toolkit features development
Data were accumulated on the desired features in three ways (see Chapters 2 and 3). Here, the summary of these and the prioritisation table are not reported, but it is demonstrated how this was an iterative process. See Appendix 5 for a few examples of the feedback received and the actions subsequently taken in the early stages. In subsequent sections (see Chapter 9), the final decisions are discussed in more detail.
In the first workshops, two different dashboard designs were presented for comparison, labelling these the patient one and the professional one on the basis of what had been learned so far during the project. However, it became clear that professionals also preferred most features of the dashboard labelled as the ‘patient’ dashboard, so that over time the two versions morphed into one.
There was the feeling among patients that they should have access to the same data as professionals, as this was ultimately their data. As per the protocol, a version of the dashboard suited to health-care professional use was completed and tested. The study team believes that this should also be the template for the patient dashboard, as a result of study findings, with some changes (for a discussion, see Chapter 9).
The feedback received from the early workshops mainly pertained to patients. This was a limiting factor at that time. Another limitation was that in using WCPES data, only data that had been vetted for an absence of personally identifiable information and a lack of specificity in the patient experience could be shown. Therefore, the system was tested in the group concept-mapping workshops using only 35 comments. Participants noticed that many of the data visualisations showed very few data points (in some cases pointing out that this may make such comparisons unreliable). This was also picked up during an AG meeting. As a result, it was difficult to demonstrate the value of the data visualisations with this data set, so that feedback pertained mostly to the dashboard navigation and design, and not the data visualisations. Unfortunately, this meant that data representations did not see much refinement, and their suitability for clinicians and patients is less known. On the other hand, the issues of structuring unstructured big data into too-small groupings became an unexpected topic of general debate.
Participants in the first two workshops and the user AG (UAG) suggested an Amazon (Amazon.com, Inc., Bellevue, WA, USA)-style chart (Figure 5), as a way of showing the distribution of sentiment and, therefore, the subjectivity inherent in the data. This was tested in the subsequent workshops, but once implemented, there was unanimity that this was confusing and appropriate only for the commercial environment in which it was used by Amazon, rather than for something like health-care services.
FIGURE 5.
Example of an Amazon-style chart for the responses for one hospital.

The study team also experimented with another Amazon-style graphic: stars to show the level of sentiment (Figure 6).
FIGURE 6.
Comment with star ratings. Star ratings can be seen below the comments (when the sentiment is unknown, there will be no star rating).
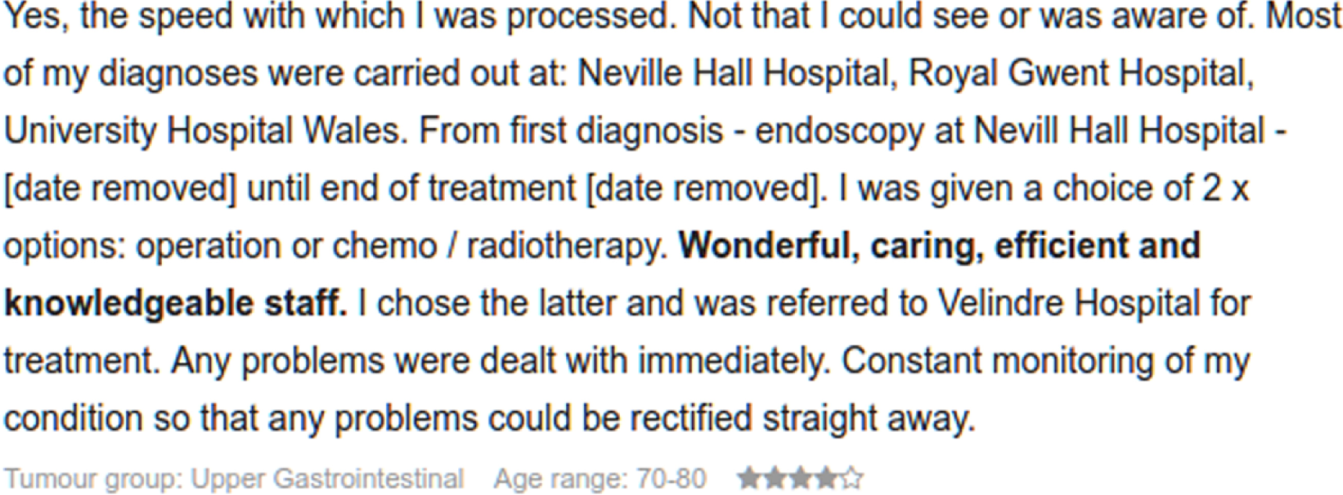
Coalescing dashboard efforts
As the dashboards were being developed, the programmers realised the potential for generalising certain aspects of the source code so that they were not both solving the same problems separately. They decided that there were two core areas of generalisability: a data application programming interface (API), which would be used to interrogate survey response data, and a visualisation API for generating charts. A core advantage of generalising these APIs is that components of the dashboards can be reused to easily develop new dashboard designs based on future feedback; this may also be critical when modifying the dashboard for alternative PES data or CPES modifications.
The display decisions
This section reports on the most significant display decisions that had to be made.
Difference between indicators and metrics
A metric is a numerical measure of a specified attribute, such as prostate-specific antigen level or the miles per hour being driven shown on a car dashboard speedometer, and needs to be precisely reported. An indicator, as the name suggests, indicates something about a metric – usually quality or safe levels. Thus, a car dashboard speed gauge shows whether or not the speed is within the legal limits. Indicators are often developed from rates or proportions. The co-design work showed that participants believed that the free-text comments should not be transformed into pseudometrics but should always be shown as relative frequencies or indicators.
Data presentation for indicators
Comparative displays used in NHS dashboards typically include funnel plots, bullet or spine charts, or bar charts. 61 As explained previously (see Chapter 3), non-NHS stakeholder participants in the study often liked pie charts (Figure 7) despite their inherent inaccuracies, whereas NHS staff preferred bar charts.
FIGURE 7.
Examples of pie charts from an early prototype.

Another option, commonly used in dashboards to show changes over time, is a run chart of the score of something (such as the number of negative comments) plotted over time (hence shown as a rate or indicator). This needs at least seven data points to reliably demonstrate a change, and so was not possible with the current dashboard, which would need data from 7 years, although it could be incorporated as needed. At the moment, the bar chart approach would be able to show comparisons, but would become overcrowded within 3–6 years.
Spine charts for indicators are created within NHS dashboards using Spiegelhalter statistical process control (SPC). SPC charts are generally recommended by the NHS because they enable both a focus on the median or mean position and graphical control limits. Thus, one small image conveys a lot of important indicator information – the essence of a dashboard. A value that falls outside 2 standard deviations (SDs) is typically considered as an ‘alert’, and if it falls outside 3 SDs it is conventionally considered as an ‘alarm’ within NHS dashboards. This is usually shown using RAG colours. SPC charts tend to help to reduce service variability, as teams aim to keep their services within the limits. This form of presentation was not thought of as helpful for the data by stakeholders, as the original data were not quantitative.
Overall, the visualisation choices that were made depended considerably on the reliability of the data (e.g. the acceptability of displaying small numbers of results on a graph).
Comparisons
Frequency in the dashboard/toolkit uses three comparators: national, within site (i.e. within the user’s hospital year on year) and regional. Although comparisons between individual sites could be used, following talks with NHS England, the study team is currently not doing so. It was felt that the data could be too open to misinterpretation. For example, when comparing organisations, it is important to compare ‘like’ with ‘like’ (such as hospital size, rural location) and the collection and recording of the necessary data was outside the scope of the study and the permissions obtained from NHS England. Broad comparisons, such as by region and nationally, reduce the risks to an acceptable level. Nonetheless, national or regional values do not always provide the best benchmarks, as they combine good- and poor-quality sites. It may be possible in future years to show comparisons by hospital, as the NHS changes the consent process it uses for the collection of CPES data.
Data are shown by theme, using charts based on the relative frequency of comments compared with the national level. Qualitative overviews are enabled, as the user can generate a list of the comments in a theme. This can be filtered, as can the chart data. The text of each comment is displayed, along with the sentiment score. This ensures that the richness of the CPES data is not lost.
Navigation
The way the toolkit can be navigated has depended on how the data were structured. For example, the themes were written hierarchically (i.e. with subthemes), but were not shown in this way. An option to show ‘tags’ (Figure 8) enables the user to get to the lower levels of the hierarchies, whereas the listed themes represent generally higher levels.
FIGURE 8.
The three different ways in which users can display data: by ticking a box next to an icon or choosing a lower-level ‘tag’, by using tag names or by text input.

Sentiment analysis
A decision was needed as to whether sentiment was represented on a three-, four- or five-level scale. It was decided that neutral values should not be displayed, as they confused users and reduced the utility of the displays. The team thus ended up with a four-level scale for sentiment (very positive, positive, negative or very negative) plus a separate ‘unclassified comments’ group, which would have shown up as neutral on the scales and weighted this group had these not been kept separate. After stage 3, it was decided that sentiment should be displayed as simply positive and negative plus unclassified.
Back-end decisions
As well as considering the interface presented to users, which was developed with the stakeholders (see Chapter 6), some decisions about the structure behind this interface were also needed.
Channels or platforms
The main access point was expected to be via a web browser. It had to be assumed that some displays would be old and with poor resolution. The system was also made to be reactive, so that it would adjust to different resolutions, screen sizes and smartphone displays.
Extract, transform and load facilities
The basic functions that the system had to do were as follows:
-
Extract data from source systems into a defined format. The source data are generally supplied in CSV format, although any data that GATE accepts could potentially be used. CSV is the de facto interchange format for this type of data, and tools such as Microsoft Excel® (Microsoft Corporation, Redmond, WA, USA) and SPSS [(Statistical Product and Service Solutions) SPSS Inc., Chicago, IL, USA] will readily export to this format. The first rows may contain header information.
-
Split the data from the CSV file into chunks. The data are processed in chunks within the GATE application so that the rule-based IR system can work on each chunk separately to keep random-access memory (RAM) usage to a sensible level. When processing > 3000 responses (each with three free-text responses), it was found that standard workstations were unable to cope with the RAM requirement.
-
Generate the data file needed by the toolkit. The output file contains the theme classifications from the rule-based IR process and also a reference to the respondent. The data also include basic demographic information on the respondents to drive the toolkit filters that relate to those demographics.
-
Loading of the data into the toolkit display. Two approaches to this were undertaken: (1) a Ruby script (Ruby 2.3; Yukihiro Matsumoto, Japan) that loads data stored in GATE’s native XML format and generates the file needed for the toolkit; and (2) use of the GATE’s JavaScript Object Notation (JSON) support to generate the toolkit file directory, negating the need for a separate script.
-
Toolkit presentation. The toolkit shows a thematic overview of the data at the site (hospital) level. The overview level uses infographics to provide a general view, and when a user selects a particular theme to investigate, the individual text comments are shown for that theme.
-
Reporting. Functionality that allowed for the creation of specialised reports summarising the data in filtered specified ways had been planned. This could not be achieved with current NHS data-sharing constraints.
-
Manual data entry. This is a functionality in which a health service professional would be able to upload their own CSV file for presentation in the toolkit. This functionality was present in earlier versions of the toolkit, but restrictions on how the NHS data could eventually be used meant that the information needed to relate it to the user-provided data could not be shown.
-
Modification. The toolkit is packaged as Hypertext Markup Language (HTML) files for easy transferability and modification. The toolkit website itself does not have an active server component – the files are plain HTML, image files, Cascading Style Sheets (CSS) (World Wide Web Consortium, Massachusetts Institute of Technology, Cambridge, MA, USA) and JavaScript (Google Chrome, Joyent Inc., San Francisco, CA, USA). This approach was chosen to limit the attack surface as much as possible, and to reduce the cost of server maintenance, as the files can be moved to a new webserver without having to port/upgrade an active web server application.
Infrastructure and security
-
Data storage: provision of secure data storage will be via the University of Southampton secure data management services or NHS secure sites, depending on the agreements reached.
-
Servers: provided by the University of Southampton.
-
Information security: managed by a University of Southampton-dedicated site.
-
Directory services: a University of Southampton site dedicated to the sharing of tools such as ours will be able to provide support documentation.
Conceptual information flow
In summary, the data are extracted using common data interchange formats (XML, CSV, etc.) through the modified GATE pipeline, in which they are transformed for display in the presentation layer (dashboard). Screen displays can be printed using the usual browser print facility.
Software
Toolkit interactivity and transferability
The website is built using HTML, JavaScript and CSS. These files are available in a Git repository and the website files are built using a Ruby script (build.rb). Each specific page (located in the source folder) has unique content, and the script adds the website structure menus and the header/footer areas based on a template (stored in the template folder).
The script requires the commodity software package ‘Ruby’ to be installed. This is installed by default on Mac OS X (Apple, Inc., Cupertino, CA, USA) and is available as free software for Microsoft Windows® (Microsoft Corporation, Redmond, WA, USA) from www.ruby-lang.org/en/ (accessed 19 July 2017). To build the website, simply run the Ruby script as a command:

After the script, the website files are available in the www folder. These files can be transferred to a webserver as static files to drive the toolkit. The commodity webserver package Apache HyperText Transfer Protocol daemon (HTTPD) (version 2.1; Apache Software Foundation, Forest Hill, MD, USA) was used to serve the files, but any webserver system, including WordPress (WordPress Foundation, USA), could be used to drive the toolkit website.
Toolkit navigation structure
An excerpt from the menu structure is defined in the build.rb file as follows:
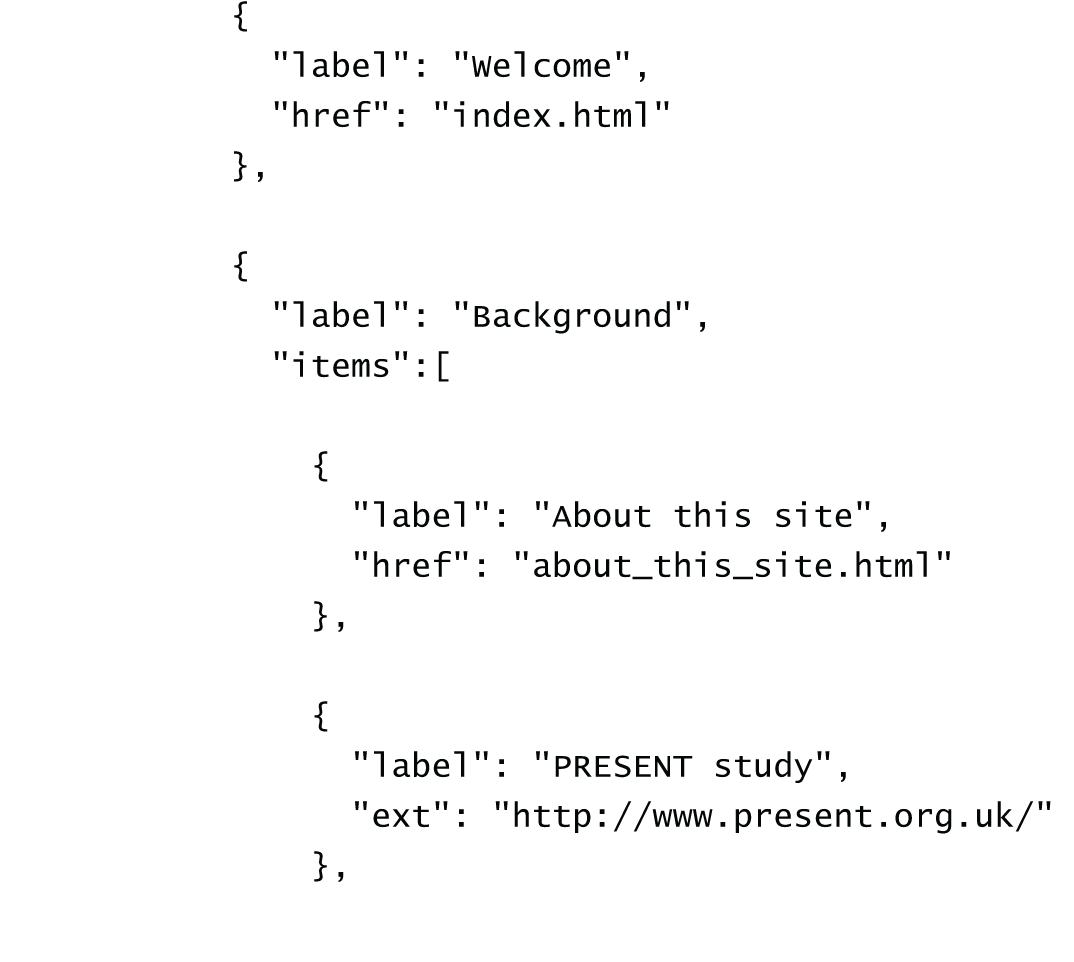
These are the first four entries for the PRESENT toolkit website. Each menu item has a label, which is the text used for the visual appearance of the menu item. Each top-level item may have subentries using the items entry.
Each item may have either (1) a href entry with the relative URL of a page local to the toolkit; (2) an ext entry with the absolute URL of an external web page resource; or (3) neither a href nor an ext entry, which means that it is not a link and is shown only as a placeholder.
The excerpt above was used to create the portion of the website navigation shown in Figure 9.
FIGURE 9.
Navigation tab for the toolkit.

Feeding data from GATE to toolkit
GATE uses an XML file format (known as GATE XML), which the user can export to using the GATE Developer interface. This is the file format that contains all of the information that is generated by GATE, including intermediate annotations made on the data during the rule-based IR process. There is a GATE JSON format, but when an attempt was made to use this format, it was discovered that it did not contain all of the data of the XML format.
JSON is the preferred data format for websites. Graphing libraries import data from JSON (and often CSV) with ease, unlike XML, in which there are often too many different ways to define the data and manual intervention is often necessary.
Non-toolkit view of the data
For the manual validation of the rule-based IR process, a script was written that takes a collection of GATE XML files and produces a CSV file suitable for a person to see a matrix of comments and each possible theme that the analyser can produce. The script is called generate_review_file.rb and is located in the present_dashboard Git repository.
Each ‘cell’ of this matrix is actually three cells in Microsoft Excel
-
The output from the rule-based IR process. A ‘1’ indicates a match and an empty cell indicates no match.
-
The response from the reviewer in the same format. A ‘1’ indicates a match and an empty cell indicates no match.
-
A correlation field to be added after the review process that simply compares the first two cells.
To use the Ruby script, supply the XML files as command-line arguments, for example:

Once complete, the script will generate an output file with the name output.csv. This file can then be reformatted, as necessary, for the review process. The review process spreadsheet, as shown in the screenshot (Figure 10), was prepared by the usual Microsoft Excel functions for formatting and presentation. The cells were locked except for the second column for each tag, so that the reviewer did not accidentally modify content areas other than the intended areas. The greyed-out areas represent the comments, redacted for this report for governance reasons.
FIGURE 10.
Microsoft Excel spreadsheet for reviewing GATE outputs without using the toolkit.

The review process has three columns for every comment/tag combination:
-
the output from GATE
-
the assessment by the human reviewer
-
the correlation of the first two (as a Microsoft Excel formula comparing the first two cells).
Discussion
Technologies are usually developed in an iterative fashion, but for this project, the iterative process was more protracted than is usual, and reactivity to user feedback began at the start of the design process. This differed from brief-taking, because stakeholders’ feedback continued through the development process, meaning that the study team was accountable to them throughout for all of the design decisions. This led to some surprises and challenges, but also means that the toolkit, having been co-designed with its ultimate users, should be a better match to their needs and have greater usability.
Nonetheless, ‘executive decisions’ were required on which stakeholder feedback points to incorporate within the toolkit. Sometimes there was a lack of consensus, in which case, what was technically appropriate and what was, in the opinion of the study authors, appropriate for the data was chosen. At other times, it was considered that points were useful but not practical for implementation, and these points have therefore been included in the list of recommendations for the future use of the toolkit (see Tables 12–16). Overall, however, user feedback contributed significantly to the overall design, as is clear from the difference between the original and final drafts of the toolkit.
Chapter 6 Group concept-mapping workshops and interviews
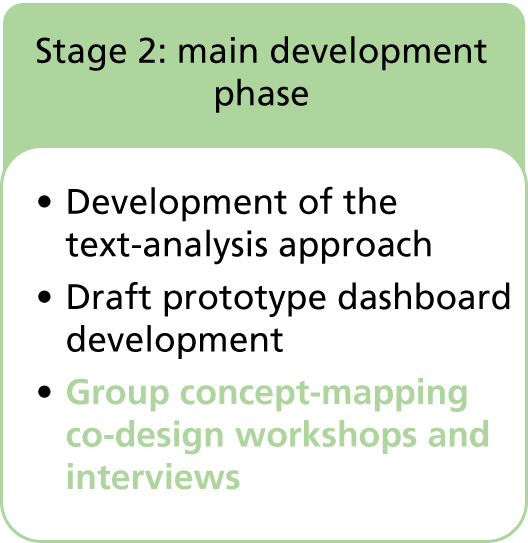
Introduction to concept mapping and the novel variation of this
In this chapter, the novel use of group concept mapping120 in the co-design work is described. Group concept mapping is considered to be effective in capturing and summarising the complexity found in social phenomena,121 as a ‘participatory mixed methods approach to social and behavioural research’120 ( p. 1). This made it a natural choice for our co-design work. It is a structured consensus-building approach, but unlike the often-used Delphi method,122 there is only one round of data structuring. During this round, participants work independently of each other to sort text statements (‘items’), label them with code names and rate them. This individual work serves ‘to limit the possibility of “groupthink” or peer pressure’ at this early stage123 (p. 245). This means that a range of different stakeholders, or ‘representatives of the target population’124 (p. 7), may be involved together without the voices of the less-dominant participants being suppressed. This satisfies a key requirement in co-design work, without which it is usually difficult to reconcile the differing viewpoints (e.g. clinical vs. patient),50,51 and this was the main reason for selecting this method. It was also important in the study that the approach enabled participants rather than researchers to rapidly code and label the data.
Group concept mapping is considered to be a mixed-methods approach because the qualitative sorting, labelling and rating of task outputs are aggregated and transformed into quantitative data as an integral part of the process, usually by specialist software. This transformation is achieved by rapid multivariate statistical methods of multidimensional scaling (MDS) and hierarchical cluster analysis, with the computation of average ratings for each item and cluster of items. 120 The effect of this is that ‘consensus is not forced, but emerges organically from the data’123 (p. 245). Coding schemas and ratings are represented visually ‘in the language of the participants’123 (p. 1), that is, using their labels, through concept (cluster) maps. These maps are x–y matrices of the aggregated individual task results, and simultaneously enable individual coded pieces of text, clusters and ratings, and their inter-relationships, to be displayed and explored.
As part of this exploration, cluster boundaries may be shifted through negotiation between group members until consensus is reached. Each person is anonymised on the visual display, which facilitates discussions on difference and tighter consensus-forming. It is for this reason in particular that Rosas124 describes the approach as participatory – the final result is effectively co-created by all participants.
Usually, the whole process is undertaken remotely (in 85.3% of cases, according to Rosas’ overview). 121 The group negotiation typically involves individuals being fed back the overall group results online, with the opportunity to modify their own sorting, labelling and rating; after two or three rounds, consensus is usually achieved. Sometimes, negotiation may include webinar group discussions involving the original participants, and at other times, different participants may gather for a face-to-face consensus-forming discussion, as in the PRESENT study, but (unlike the PRESENT study) having completed other stages online.
Difference between group concept-mapping workshop discussions and traditional focus groups
In the PRESENT protocol, the choice was made to call the consensus-mapping workshops ‘large focus groups’ to emphasise the discursive elements. This does not mean that they resemble the small focus groups typically used in qualitative research. 125 Concept-mapping groups discuss divergences and similarities to develop group consensus. 120 Small focus groups are not intended to reach consensus, but to reveal group norms, a different concept. 125 Although small focus groups require homogeneity for successful interactions that converge to the group norm, large focus groups (group concept-mapping workshops) work best with heterogeneity. 120 This is important, as revealed by the stage 1 results in which the different perspectives of health-care professionals and patients became clear (see Chapter 3, Impact of the scoping stage on the initial prototype). For example, sexual dysfunction resulting from prostate cancer treatment may be variously highlighted as a side effect of treatment, a relationship issue or a body change with age, depending on perspective. In order for the themes-based toolkit to work effectively, that is, for it to be understood by health-care professionals while having meaning for patients, such differences in perspective needed to be reconciled through negotiated consensus. The study team was committed to making the toolkit intuitive to use and useful in driving health-care improvement and, therefore, more likely to be implemented within regular practice, with sustained use.
Methods
Aims and research questions for this part of the overall study
The main aim of this part of the overall study was to determine the labels and definitions to use for themes in the text-analytics rules and the six themes to be used for the default view in the toolkit.
The secondary aim, in this part of the overall study, in a linked qualitative discussion (see Study design), was to explore the perceptions and preferences for different toolkit design and functionality features.
Using concept mapping and follow-on interviews, the study team therefore aimed to answer the following questions:
-
According to the different stakeholders in the patient experience, how should priority areas for feedback be labelled and why is this?
-
What is the difference, if any, between what these stakeholders consider to be most feasible and most important?
-
Do patients/carers and other stakeholders perceive these labels and rankings differently?
-
Do stakeholders in the patient experience of cancer and other chronic conditions perceive these labels and rankings differently to stakeholders in multiple sclerosis (MS)?
Study design
A decision was made to diverge from the normal approach in several ways, in addition to the decision to use face-to-face group work throughout:
-
The whole process was to be undertaken in one workshop session (see Figure 11).
-
As the study team already had a set of > 8000 free-text comments (from the WCPES 2013) that it had begun to use in the developmental work, the usual first phase of the concept-mapping process – in which the statements or items are produced from a mindmapping session – was excluded and a selection of existing comments were used as the items to sort and rate.
-
To ensure that no potential participants were excluded because they lacked computer literacy, the individual sorting, labelling and rating task was undertaken by hand, with researchers then inputting results into the software for automated MDS and clustering.
-
The study team could have discussed the results as they were input, for example, by asking people for confirmation of particular label names. However, a decision was made to use the time to hold a workshop discussion around early prototypes of the toolkit.
The plan for the last phase of the process did not deviate from the usual concept-mapping process,120 that is, an in-depth discussion of the concept maps was held, thus aiming to form a consensus by the end of the day. Semistructured qualitative interviews with some participants in the weeks following the workshops were used to further explore areas of contention in which reaching a consensus had been tricky or impossible.
In a mentoring event held in Sweden in March 2016 for users of the software to meet the developers and discuss their projects, it was discussed whether the process should be used to validate the themes developed from the text-analytics process or to gather data to inform the process. The latter was preferred by the PRESENT team, and this was also the opinion of the method mentors, although it was pointed out that both approaches made innovative use of concept mapping.
Choosing statements
A total of 100 ‘statements’ (mostly single sentences) were initially chosen from > 8000 comments in the WCPES 2013. These were selected to represent the working set of themes decided on from the stage 1 work to enable us to build up the JAPE programming rules. The two key criteria that had to be met in preparing the statements for the concept-mapping sorting task were:
-
1. Each statement had to contain only one concept, as it could be sorted into only one theme or pile by each participant.
-
2. Each theme had to be represented by at least two statements (selected on the basis of the previous qualitative analysis of the data),21 as the sorting task requires each pile to consist of more than one item.
In addition, the study team aimed to include:
-
3. Statements that were considered to be unambiguous. This was to steer the sorting to some extent towards the predetermined theme and avoid the generation of new themes entirely unrelated to the original analysis.
-
4. Statements that were relatively ambiguous – thus, in the original WCPES 2013 analysis, a statement that was grouped under communication might also have been grouped under resources.
The third criterion in particular produced an inherent, strong bias in the task, but was justified, as the focus was on the development of a functional toolkit rather than the generation of higher-order conceptualisations. A more conceptually focused analysis had already been undertaken and had informed theme selection. 21 The fourth criterion was intended to balance this bias and to generate discussion around the ‘fuzzy edges’ of existing themes to tease out differences between the groups when decisions were less clear cut.
It quickly became clear that to satisfy all four selection criteria using 100 statements, not all themes could be included. The themes that were the most common and the most transferable across conditions were therefore chosen.
To select individual statements, a random list of five statements per theme from the manual WCPES analysis was generated, and this random generation was repeated until sufficient statements were found to fulfil the criteria.
Two researchers independently went through the statements and made their selections, with 76% agreement; differences were resolved by discussion to form the final selection.
To ensure that each statement would be considered independently, each one was given a random number (it was also ensured that cards were shuffled before being given to participants on the day).
Piloting the day and final choice of statements to include
Two pilots were undertaken. At the first pilot, the team worked out efficient ways of entering the data and practised this to determine their data entry rate, using one set of statements sorted into themes by an independent volunteer from within the Faculty of Health Sciences at the University of Southampton. The second pilot involved four volunteers from the faculty, naive to this type of task, and three research team members (CR, DT and Mike Bracher) undertaking the sorting, labelling and rating tasks at their convenience and timing how long this took them. The study team then entered sorted piles, taking care to ensure that a team member’s own pile was given to a different team member to enter. From the second pilot, participant and data entry capacities were able to be confidently calculated. During the first pilot, the number of comments was reduced from the planned 100 to 76. After the second pilot, the number of comments was further reduced to 60. This involved the same two-researcher process as in the initial statement selection process. This decision took into account volunteer feedback that the sorting task took 45–60 minutes, which gave team members a maximum of 3 hours to enter all of the data from up to 15 participants, depending on the workshop schedule. Data entry rates were 30 minutes per 76-statement pile, consistently across the team; thus, each team member could manage to enter data from six participants in the 3 hours, and the study team wished to ensure that should any one team member need to miss the workshop, it would still be manageable with 15 participants.
Topic guide development for discussion part of the day
The topic guide for the workshop was informed by the scoping review of the dashboard design (see Chapter 2) and the two developers working on the dashboard.
Screenshots from the first prototype dashboards and interactive exploration of the live toolkit were used to support the discussion.
Participant inclusion and exclusion criteria
The inclusion criteria were that participants had to:
-
be a cancer or, depending on the workshop, non-cancer patient/carer or relevant health-care professional
-
have a confirmed diagnosis to ensure that they had sufficient experience of the relevant services.
Using convenience samples, the study team aimed for a sampling balance across professional roles (service providers, charities, policy-makers, budget holders and commissioners). The aim was to sample patients/carers by demographics, health and health-care-related features (gender, age, cancer stage and type and treatment type). Each of the five workshops was intended to contain up to 15 such stakeholders in health care, mixed for heterogeneity.
Participants were excluded if they:
-
were aged < 18 years
-
could not travel to the group location easily and with same-day return
-
could not provide full informed consent.
Participants were invited from across the nation, but the venues were in three UK cities only: London, Southampton and Leeds.
Recruitment procedure for the workshops
The study team approached the AG, study steering committee (SSC) and UAG for support. The UAG group members disseminated the ‘call of interest’ among their patient networks, including other groups with which they were associated. The AG and SCC advised us on particular professionals to invite. Macmillan Cancer Support, a collaborator on the study with representation on the AG, disseminated the call via its professional and user networks. The study team approached the local networks at the University of Southampton and made clinical contacts within Wessex. Clinical Commissioning Groups (CCGs) in London, Wessex and Leeds were e-mailed; the Leeds co-applicant (Adam Glaser) and the study chief investigator (CR) both drew on their clinical and commissioning networks for further participants.
The ‘call of interest’ leaflet and participant information sheet included an Eventbrite (Eventbrite, San Francisco, CA, USA) link for registration. This enabled the study team to better prepare for the day by monitoring the number and type of participants and ensuring that no single group was oversubscribed. Reminders were sent 48 hours before the meeting, with an electronic copy of the consent form and information sheet to give participants the time to familiarise themselves with these documents and make a note of any questions they may have wanted to ask about the study.
Transferability
Although the first four groups focused on cancer, the study team invited people with MS or people involved in their care to the last session to explore the transferability of the system to a very different long-term condition. The patients who attended this workshop were members of a well-established user group that regularly convened at the Royal London Hospital.
Format of the day, including workshop discussions
The workshops lasted up to 5 hours, usually between 10.30 and 15.30, to take patient accessibility issues and travel times into account. Refreshments were available throughout the day and a relaxed informal approach was used. Each session began with team and participant introductions, and an outline of the context and objectives of the study and the workshops. Participants had the opportunity to ask questions. Each participant received a hard copy of the information sheet and two copies of the consent form and was asked to sign the forms and return one to the team if they were happy with all of the tasks involved. All of the participants who attended gave their written consent. A team member ascertained and highlighted housekeeping, comfort and safety rules, assistance needs and confidentiality rules, and explained the plans for the day.
Participants were asked to read through and consider the 60 free-text comment ‘statements’, sort them into thematic piles in accordance with the sorting rules and name each pile. The rules were that they had to create more than one pile, no pile could contain only one statement, no statement could be included in more than one pile and no pile could be labelled ‘miscellaneous’. Participants were encouraged to name their piles in ways that were meaningful to them and were supported when they felt unsure about the process or lacking in confidence. The study team pointed out that the approach required no prior expertise.
Participants were then asked to rate each statement on the basis of how important they felt the issue to be, relative to other issues and assuming that all were feasible, using a scale from 1 (lowest) to 5 (highest). Relativity was important to ensure a good spread of rating values. A second rating focused on how feasible the participants felt it was to address the issue, given the current state of the NHS. This was used to determine theme ranking and, hence, default themes for the toolkit.
Both tasks were completed individually with support from the team, with 45–60 minutes allocated to this part of the day (Figure 11). Two or three team members (depending on the number of participants attending) collected the sorted and rated statements and entered them onto the concept-mapping software.
FIGURE 11.
A summary of the process that was used. All but the first stage occurred within the same workshop, and each took approximately 45 minutes.

While they did so, another team member facilitated discussion on the design and usability of the toolkit prototypes. Drafts of both the patient toolkit and the professional toolkit were reviewed; a third toolkit, for commissioners specifically, was considered in the first workshop as per protocol, but was subsequently discarded as it was not considered to be helpful. Each version was discussed for 45 minutes with a half-hour lunch break.
In the final part of the day, several iterations of cluster repartitioning were discussed in the group, until consensus was reached. The software default representation of clusters is one-fifth of the number of statements, which in the case of the study was 12. However, through simple instruction, the software can repeatedly recalculate the cluster boundaries as the group actively negotiates consensus. The fewer the clusters, the bigger and more spread out they are. Outliers may be closer to proximal clusters than to central points in the same cluster, meaning that clusters have too much conceptual overlap, or else clusters may be seen within clusters. Conversely, having too many clusters may lead to overfragmentation with weak conceptualisations. The facilitator sought to achieve a balance in cluster size; a rule of thumb is to have not more than four more clusters than the largest number of sort piles produced by a participant.
As part of the process, bridging values were examined; these show how often a statement was sorted with other statements in the cluster, with lower values indicating a closer conceptual relationship between statements. Labels that people had given to the cluster were also examined; the programme shows those given to the piles closest to the representational clustering. Importance ratings for clusters and for individual points were considered to help the group to make decisions. Finally, the study team discussed ‘regions’ in the map on the basis that clusters closer together might form a higher-order conceptualisation and that clusters at the centre tend to be higher-order concepts linking several other clusters.
The data were cumulatively aggregated in each of the five groups, ensuring that a consensus was reached across groups. The data from individual groups were also stored for later analysis of the similarities and differences between the groups.
Toolkit design discussions
After the first concept-mapping tasks, while team members entered participants’ data into the software, one researcher facilitated a discussion on the design and usability of the toolkit. An interactive, online version of the prototypes was shared on a computer display, driven by the data from the WCPES 2013, and screenshot printouts were provided. The professional and patient (public) versions of the toolkit were discussed separately and cross-comparisons were made. The discussion focused on:
-
what the participants would like from the system and what they would use it for
-
how participants would browse and navigate the toolkit and how the prototypes could be improved
-
what the preferred ways of displaying the data would be (e.g. type of graphs, sentiment analysis)
-
what features should be included (such as the ability to personalise what is displayed)
-
analytics (e.g. filtering, search boxes, comparators).
Interviews
Immediately after each workshop, two researchers scanned the transcripts to identify areas of disagreement in the group discussion. This informed an interview topic guide. Semistructured qualitative interviews126 were then undertaken to gain a more in-depth understanding of the different arguments underpinning the disagreements. Thus, participants were selected from the groups for a follow-up interview if it was felt that they might be illuminating in this regard. The interviews also gave us a chance to invite participants to explore points that they may have been reluctant to talk about in the workshops.
Initially, potential participants were contacted by e-mail, and received up to two reminders by telephone or e-mail; in the second reminder, they were told that the study team appreciated their involvement in the workshops and would not contact them further regarding a possible interview, although they could contact the team.
It was intended to invite participants for interview within 2 weeks of the concept-mapping group to aid in their recall of the discussion. However, delays earlier in the project meant that the groups were held mostly in November and, therefore, many participants were unavailable for interview in the run-up to Christmas. As a result, some interviews were undertaken in January 2017. Therefore, the interval between group discussion and interview was a mean of 48 days (range 16–82 days).
Analysis of importance and feasibility ratings
The software ladder graphs were considered and Pearson’s r statistic for mean cluster ratings was calculated. Differences of interest between these in the comparisons were analysed using Welch’s t-test, a two-sample unpooled adaptation of Student’s t-test for unequal variances. 127
Reliability of the data
The study team considered the ‘stress’ value, a goodness-of-fit statistic routinely used in MDS analyses, which summarises the degree to which the cluster point map is a reliable representation of the conceptual relationships generated by participant sorting. The lower the stress value, the better the fit, the more reliable the results and the closer they may be considered to be to a representation of a larger population. 128
The stress values for each group were calculated, as well as combined values, to better understand group inconsistencies, as the groups were very heterogeneous.
Qualitative analysis
The discussions leading to consensus and the follow-up interviews were transcribed and analysed by two researchers using NVivo software to manage the data. An initial coding framework was developed using deductive coding based on stage 1 of the study and inductive coding from the first two workshop transcripts. The researchers discussed any ambiguities and disagreements, and modified the wording as needed to create an operationalised set of codes. The remaining workshop and follow-up interview transcripts were then divided between the researchers for analysis with further coding changes developed through discussion as needed. Given that the analysis was intended to inform the toolkit design rather than conceptualisations, a kappa statistic was not calculated.
Results
Concept-mapping workshop participants
Overall, 34 participants took part in the concept-mapping workshops (4–9 participants per group), 26 completed the feasibility ratings and 25 completed the importance ratings. Tables 5–9 show their breakdown by role, health condition most relevant to them or their work and demographics. Most were patients with no one condition dominating, but many were not represented; cancer was not the only condition represented in any one group (condition homogeneity was not required, but complicates comparisons with the MS group). Participants were typically female, in their 50s and self-reported as being from a cross-section of ethnic groups (see Appendix 6).
Recruitment patterns
The sessions were predominantly attended by patients; recruitment of health-care professionals was challenging because the sessions involved a whole day. Some health-care professionals cancelled at the last minute because of changes in clinic times and other commitments. The number of caregivers was also lower than anticipated. However, many patients identified themselves as carers too, and were able to share their experiences from both perspectives. Recruitment issues were partially caused by the relatively short time from invitation to the workshops (approximately 1 month), which was itself attributable to delays and uncertainties in the finalisation of the prototype dashboards. The turnout was 70–90% of those who booked. However, the original goal of 15–25 participants per face-to-face session could have compromised the quality of discussions; this was confirmed by one participant who said that the group size was perfect.
Interview participants
As most workshop participants were patients, the study team was able to recruit patient interviewees from both the cancer and non-cancer groups. One academic and three professionals were also recruited. The study team failed to recruit carers per se, although two patients discussed both perspectives. As the population was limited to those who took part in the workshops, the study team considered inviting more patients, but reached saturation of themes at 12 (hence not needing to recruit the per protocol 15–20 participants).
Cluster labels and stress values
The five workshops led to 224 unique labels for sorted statement piles. Labels suggested by more than one participant were facilities, complaints, travel, transport, aftercare, resources, funding, communication and administration. Several other labels were similar to these. The final seven-cluster map with consensus labels is shown in Figure 12. This provided the most conceptually meaningful and internally consistent solution, as determined through the consensus-forming process among participants. Clusters were explored with participants, resulting in six themes chosen for the dashboard default:
-
referral from primary care and initial diagnosis
-
travel (e.g. buses, no parking)
-
staff attitude and role (e.g. empathy from nurses who are expected to be caring – referred to as ‘clinical positive’ in the diagrams)
-
information for patients (e.g. on treatments, health issues, external sources of support)
-
patient’s finances (e.g. claiming benefits, affording parking)
-
hospital resources (e.g. broken equipment, mixed wards, lack of beds).
FIGURE 12.
Final negotiated consensus cluster map for all participants and all workshops. Numbers in bold are cluster numbers; other numbers refer to the statements.
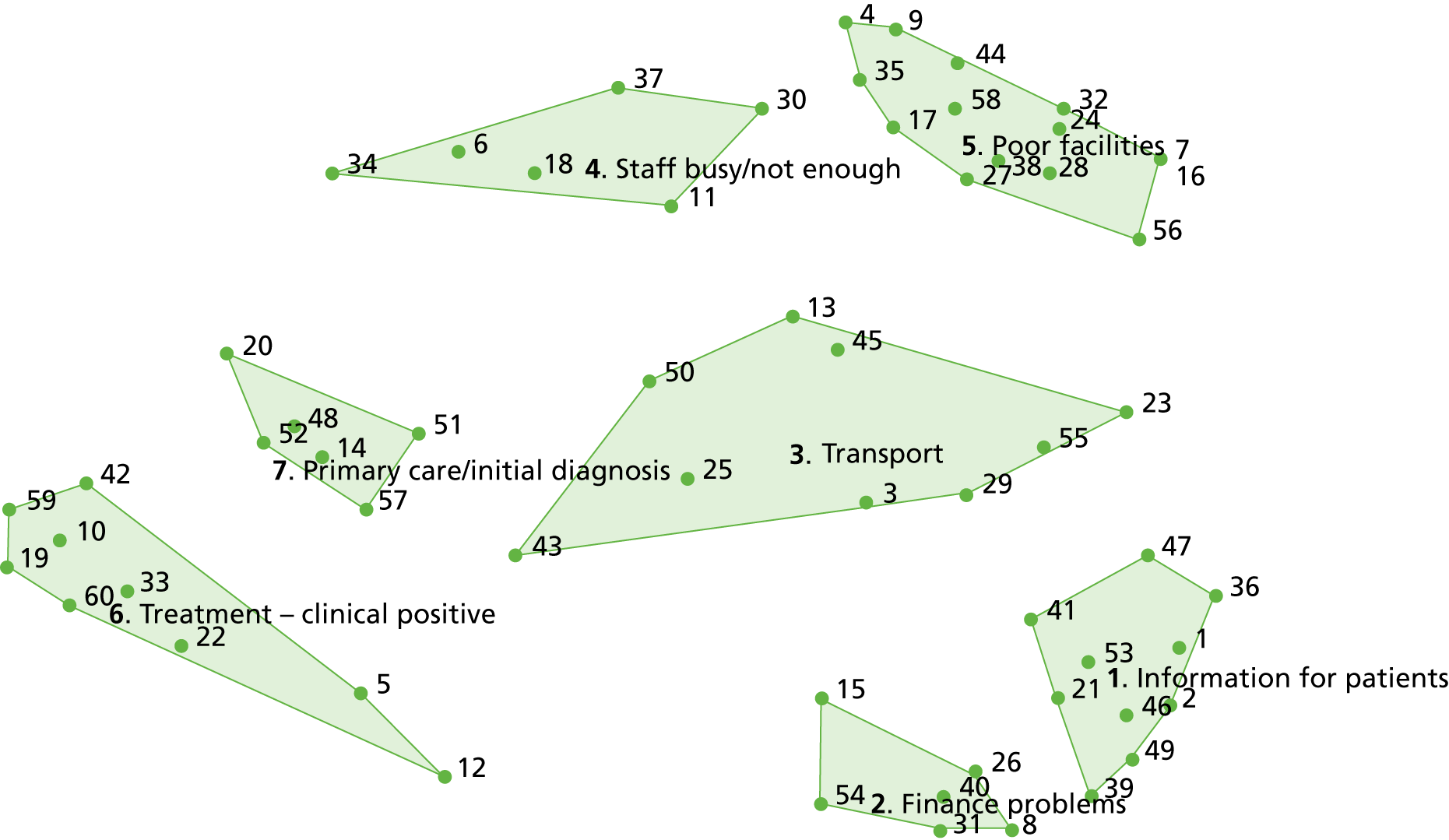
The final maps by role (i.e. for patients and carers, health-care professionals and others separately, not provided) showed us how boundaries and theme names were similar but not identical across these.
Individual statements (items), their clusters (themes) and bridging values were calculated for the three different groups by role. Among the health-care professionals (pile count range 4–14), contact and hygiene were the most tightly bound according to their bridging values, with stress values of 0.25 and 0.09, respectively, which means that these are the most conceptually distinct; other clusters in this group were less well defined. Among the academics and others (pile count range 6–11), attitude, communications, transport and facilities were the most tightly bound, with stress values of 0.24, 0.22, 0.04 and 0.20, respectively. Among the patients and carers (pile count range 6–12), waiting times and NHS issues were the most tightly bound, with stress values of 0.23 and 0.09, respectively. Clusters in the patient and carer group that were not conceptually distinct were administration and patient experience. The MS participants had more distinct clusters than the cancer participants; the latter focused on treatment and treatment consent issues, neither of which appeared in the MS group data. The differences between the MS group and the cancer groups were considered by a t-test of the four cancer groups versus all five groups, to ensure the same number of themes in each comparison group.
Sturrock and Rocha129 determined that for two-dimensional MDS solutions in which 100 objects have been scaled, stress values of < 0.39 indicate a < 1% probability that the MDS arrangement is random; in other words, this indicates good internal validity or a match between the participant-structured input and the final mathematically generated output. Stress values in all three groups were 0.27–0.29, thus groupings were unlikely to be random; as the stress values were all similar, this shows that all groups performed the task to a similar level. The relatively high stress values reflect the heterogeneity within each role group.
Ranking by priority and feasibility
The importance of themes in the final consensus map is shown by the depth of the cluster polygons (Figure 13), with the following all ranking highest: referral from primary care and initial diagnosis; travel; staff attitude and role; and information for patients.
FIGURE 13.
Bridging values for the final consensus map.

Feasibility ratings were similar, suggesting that participants were unable to bracket this off when considering importance ratings, with a Pearson’s correlation coefficient of 0.95 (Figure 14).
FIGURE 14.
Ladder diagram for participants, with importance ratings presented on the x-axis and feasibility on the y-axis.

For the cancer-focused workshops, it was found that the top themes by importance for patients and carers were legal and safety issues; staff attitudes; teamwork; diagnosis and primary care; funding and resources; and facilities and environment of the hospital.
For health-care professionals, the top themes by importance were legal and safety issues; staff training and skills; facilities and environment of the hospital; staff attitudes; teamwork/communication; and including patients’ family members in treatment and decision-making.
For the MS-focused workshops, patient and carer ratings for top themes by importance were hospital cleanliness; staff expertise and attitudes; patients’ consent and decision-making; family support; treatment choices; and hospital resources.
For health-care professionals in the MS group, the top themes by importance were staff expertise and attitudes; treatment choices; diagnosis; availability of support; human contact and empathy; and hospital cleanliness.
This heterogeneity shows the importance of including theme choices in the toolkit.
In terms of feasibility, the patients and carers in the cancer-focused workshops decided that the six key themes were legal and safety issues; staff attitudes; service and clinical quality; diagnosis and primary care; facilities and environment of the hospital; and teamwork/communication.
For health-care professionals, the key themes by feasibility were facilities and environment of the hospital; including patients’ family in treatment and decision-making; legal and safety issues; quality of care; staff attitudes; and teamwork/communication.
For the MS-focused workshop, the key themes by feasibility for patients and carers were treatment choices; cleanliness; staff approach, knowledge and empathy; information for patients; general practitioner (GP) experience; and staff expertise and attitude.
For the MS professionals, the key themes by feasibility were treatment choices; hospital cleanliness; practical support following diagnosis; staff approach, knowledge and empathy; family support; and communication and teamwork.
Dashboard design discussion
The findings from the workshop dashboard design discussions focused on four main areas: (1) outcomes and uses of the system; (2) functions; (3) presentation and output of data; and (4) data integrity, security and interpretation. These were further explored in the interviews.
The findings are summarised in Table 3.
Feature category | Subcategory | Details | How this affected the development work |
---|---|---|---|
Outcomes and uses | Health-care professionals |
|
|
Patients |
|
|
|
Functions | Exploring and interrogating the data |
|
|
Navigation |
|
|
|
Search |
|
|
|
Filters |
|
|
|
Registration |
|
|
|
User input (comments, etc.) |
|
|
|
Navigation |
|
|
|
Numerical representation |
|
|
|
Presentation and output of data | Graphical representations of output |
|
|
Print and export |
|
|
|
Textual–semantic content (summaries, etc.) |
|
|
|
Data integrity, security and interpretation | Anonymity and security |
|
|
Integrity |
|
|
Disagreements in facilitated discussion
The key areas of disagreement related to:
-
the potential uses of the toolkit and whether or not patients would benefit from access to it
-
functions available on the toolkit, such as enabling user input through a community forum or the facility to add comments to the page; choice of filters; what labels should be used to indicate the sentiment of patient feedback; whether or not access should be restricted by user registration
-
issues of data presentation, including whether patients’ comments should appear in full or be summarised; the type of graphs perceived to represent trends most effectively; how to represent the data numerically; and how to best support an intuitive navigation through the toolkit based on users’ experiences with other websites
-
concerns regarding the representativeness, anonymity and security of the data.
Example comments were:
I think as what I might call an ordinary patient I think probably not [would not use the dashboard]. I’m not sure that they would go onto it. I think it’s more of interest to patients like me who are representatives of groups, and taking a greater interest in perhaps seeking improvement in the way that the hospitals or whatever respond. And I think that the ordinary member of the public, who’s a patient, I think they’re just concerned with getting their own health back to normal. I think as far as other patients are concerned it’s going to be those patients who are what I would call campaigning.
Patient 7, group 3
Because I’m just wondering what the media could do with it, and so I’m just thinking about that pie chart. That actually media’s interpretation of the work that we do is usually incorrect. And it just made me . . . it did make me think that actually if we are saying four of these patients have experiences and one of them has experiences, if the media want to they could just take it and turn it into a percentage.
Patient 2, group 2
Discussion
Group concept mapping proved to be suited to exploration of patient experience free-text comments by mixed stakeholder groups, and transformation of the comments into themes that could be interpreted by health-care professionals to drive service improvements but preserve the patient voice. Results were used to create a taxonomy of themes and their relative importance for different stakeholder groups, and to inform the labelling of clusters produced by the rule-based IR process. The rating guided us in selecting the key issues to be represented on the toolkit. The feedback from the discussions and interviews focused on desired outcomes and uses of the system; its functions; the presentation and output of data; and issues of data integrity, security and interpretation.
The study has also been able to show where different stakeholders diverge in their conceptualisations of the patient experience and their importance and feasibility rankings of topics for health-care change, both within and across health conditions. There was only one group dominated by non-cancer participants, so the study can report only tentative differences relating to condition, but participants in cancer-dominant groups focused on treatment and treatment consent issues, whereas patients with MS focused more on support. The labels also differed for the cancer and MS groups, showing the need to amend theme names and possibly rules if the system is used for other conditions.
Concept mapping is usually used for the evaluation of interventions in health and social care and research,130 although it has also been used to develop learning outcomes for an interdisciplinary module in medicine and engineering. 124 The study’s approach is innovative, but some parts have their precedence in work by Jackson and Trochim. 131 These authors have previously used concept mapping to group free-text survey responses into themes. They argue that the benefits compared with a purely manual approach are the inclusion of statistical analysis and results based on respondents’ judgements, rather than this being researcher driven. In an overview of 69 individual concept-mapping studies conducted over 10 years, Rosas and Kane130 determined that 14.5% of the total were carried out face to face, with 20–649 participants in the 69 studies, fewest in face-to-face approaches (mean number of face-to-face approaches 62.10, SD 49.14). Rosas and Kane130 also showed that the ideal number of sorters is 20–30, with limited gains in the stress values, and hence consistency of fit, with higher numbers. The recruited numbers are therefore consistent with other work and fall within the ideal range. The recommended minimum number of sorters is 15,131 which was comfortably exceeded. Overall, the average number of raters was 13.94 in the Rosas and Kane overview;130 given that sorting and rating were undertaken in the same session in the PRESENT study approach, the PRESENT study did not experience the attrition that many studies have. The 69 studies Rosas and Kane reviewed used a mean of 96.32 statements, with a range of 45–132 statements, and these were sorted into a median of 9.93 (SD 2.22) piles. The number of statements in the largest clusters was 18.634 (SD 4.94 statements, range 9–32 statements) and in the smallest clusters was 4.9 (SD 1.94 statements, range 1–10 statements). Overall then, not only was the approach informative in terms of the process design, but it was well within the specification ranges recommended by Rosas and Kane. 130 Importantly, its usefulness was confirmed, and the changes that were made to the emergent work as a result are now described.
Amendments to themes and rules
At the time the workshops were held, some tentative rules and 36 theme names for the rule-based IR process had been developed. As a result of the group concept-mapping work, several of these were changed, resulting in a smaller taxonomy of 19 themes. The six default themes for the toolkit were those considered to be most important by the participants overall.
Although the study team was mainly guided by the overall consensus map, participants sorted only 60 statements, and the themes were not exactly the same in their scope as those used for the text-analysis rules, so at times new labels had to be added to the themes uncovered in the workshops or the suggested ones had to be adapted. Whenever the team members did so, they tried to draw on the discussion transcripts and to use the participants’ words.
Amendments to the toolkit design
The team met to review results and make final decisions on changes to the prototype for stage 3. Considering larger decisions, as a result of the workshops and interviews, it was decided that the patient and public version of the toolkit would need to be different from the health-care professional version and that each dashboard would be expanded into a toolkit. This was determined from comments about registration, data security, graph preferences and aims of using the toolkit.
Patients were more likely to use the toolkit on a one-off basis to learn about treatment side effects and experiences of others suffering from the same condition, and to gain information on a site where they were to receive treatment. In contrast, health-care professionals declared that they would use it on a more regular basis and would therefore benefit from more tailoring options to adapt the settings to their workflow. The theme selection needed to be relevant to their work environment, making it easy to identify which teams are able to address issues highlighted by the patients.
Strengths and limitations
The concept mapping was undertaken to enable mixed stakeholder co-design of the theme names and scope, as well as the dashboard (which they subsequently expanded into a toolkit). These goals were achieved. However, as a wider piece of work exploring the conceptualisations of different stakeholders in cancer care, the work has several limitations. The study team considered only a small selection of statements, smaller than the number usually used (≥ 100) when the process is undertaken online. Participants worked with 60 statements, yet the data set contained several thousands of comments that inevitably included a wider selection of themes.
Nonetheless, these represented 26 of the 36 themes (72%) that had been determined deductively before the workshops or that became evident through further discussion within the workshops, and participants did not consider there to be major gaps in the themes used. The data had good construct validity, as statements were taken from a large data set and because the original comments from which they were derived were relatively simple compared with longer texts. 131
The comments were selected by the study team from the WCPES 2013 free-text comments to cover a range of themes in a variety of ways (positive, negative, ambiguous and explicit) for maximal variation of topics, while ensuring that no topic was represented by only one comment. This process necessarily involved a top-down filtering approach – comments were chosen in accordance with themes identified in a manual analysis of the same WCPES data,21 then piloted and the selection was refined to ensure that effective sorting was achievable. More inductive theme development might have better external validity. Nonetheless, the final list of themes had satisfactory external validity, as the stakeholders themselves determined theme names and rankings.
Even a sophisticated computerised text-analytics approach, such as the one that the study team developed, cannot make judgements about the underlying relationships between two different actions or events, such that these still need to be based on the judgements of the researchers and programmers who decide on the rules, exceptions and cut-off points. 132 Thus, the more people contribute to these judgements, the greater the external validity. Nonetheless, the published meta-analysis has shown that 20–30 participants are sufficient for this in group concept mapping120 and this number was met. Data were collected from 34 participants in total; the original aim was 60 participants, but it was realised on piloting the approach that the size of each group (and hence the overall number of participants) had to be restricted owing to the time it took the team to input the manually sorted data. Such a small sample size inevitably limits generalisability. However, the study team never aimed for generalisability, but was rather adapting the process to enable co-design work. Moreover, the mean cluster rating for any given cluster is the average of all mean factor ratings within that cluster that reduces the variance within clusters, enabling statistically significant findings from small mean differences and from the small sample size.
The participants were mainly patients; the team intends to collect further data online from health-care professionals, but this will not include the face-to-face negotiations that were an important feature of the work.
This study has also shown the feasibility of adapting the software-based approach to enable full participation that can include people who are not computer literate. The team has demonstrated how a simple technique could be used to obtain information to modify the rule-based IR toolkit system for different conditions, and how concept mapping can be used in iterative intervention development work more generally. Outputs were composite summaries grounded in what participants decided and determined through a combination of statistical analysis, participants’ personal judgement and researcher expertise. However, it is also advised that developers exercise caution; ultimately, the products of this approach might be used as guidance but not as a definitive list, even when combined with discussions. The results can be tested in the future in further research.
Chapter 7 Elicitation of individual preferences
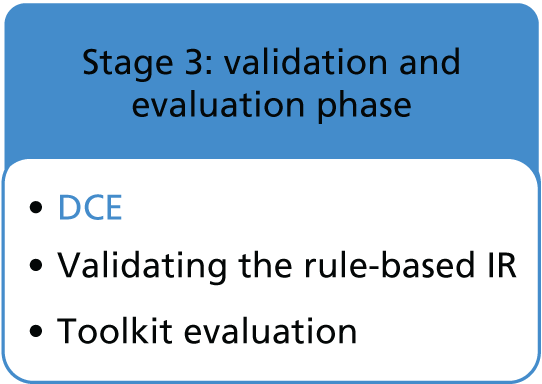
Introduction
This chapter presents and discusses the applicability of stated preference techniques in identifying and eliciting individuals’ preferences for the feature-packed digital toolkit, the prototype for which was developed in stage 2. The chapter discusses the development of the experiment and the analysis of the data to prioritise and elicit the trade-offs individuals observe with, as well as the relative importance of, the individual features of the online toolkit. Preferences are translated into monetary values that individuals indicate they are willing to pay for the various features and the toolkit as a whole. Using these monetary value as guide prices, a basic cost–benefit analysis for the development and deployment of the online toolkit is presented. This part of the study was added after funding was obtained, with NIHR approval, and an ethics amendment was granted on 19 January 2017.
Background: stated preference techniques in context
Constrained budgets within health-care systems and the need to efficiently allocate resources often necessitate the valuation of health-care interventions and services. For economists, markets provide the main mechanism through which the true value of a good is revealed. When someone chooses to purchase a good or service, this implies a preference, whereby the utility (or satisfaction) the person derives from their purchase is at least as big as the cost/price they had to pay. In other words, the price they are willing to pay to obtain the good is an appropriate market price. However, what happens when preference is considered for a good or service for which there is no current market?
Traditionally, methods such as the opportunity cost or the market replacement cost are employed. Both focus on the valuation of the time component of an activity. For instance, for informal carers, the opportunity cost value would be the carer’s benefits that are forgone as a result of the time spent caring, often assumed to be the carer’s wage. Correspondingly, the market replacement value would be the wage of a professional carer who could be hired to provide help instead of the informal carer.
When considering a good without a market, two further dimensions need consideration:
-
A market will most probably eventually exist, but often the developer requires some prior insight of the market structure, so as to place the product correctly.
-
A product could have an impossibly large number of different specifications, and choosing which to supply, that is, which features are the most sought after and important for eventual users, requires prior intelligence during the development stage.
A tool that satisfies all such requirements and allows the elicitation of monetary values not only of the product but of each individual feature is the DCE, a type of stated preference methodology. Stated preference techniques are based on survey/primary data. They are preference and value elicitation techniques, with respondents stating their intentions in relation to a hypothetical question presented to them. They provide researchers with the ability to place individuals in controlled environments (situations) and investigate issues in isolation without potential confounding biases.
Discrete choice experiments are founded on the consumer behaviour and preferences theory of Lancaster133 and Rosen. 134 They postulate that utility is not derived from the consumption of a good per se, but from the characteristics that this good possesses. Thus, the characteristics of a good determine its utility value, and hence differences in the characteristics result in different degrees of desirability for the individual. In essence, the researcher describes a good in terms of its features, with various combinations of features available as if they were multiple different hypothetical products. Potential users are then asked which product version they would purchase if they were given the chance.
The underlying intuition is that the user will consider the features of the different products presented to them and will trade off among them, as well as against the specific price for each product. They will then choose to purchase (or rather state their intention to purchase) the one that they deem to be the most attractive or desirable given the price.
The above context is directly applicable to the development of the online/software toolkit that this project has set out to achieve. The development work and the evaluation process undertaken here were somewhat distinct (i.e. limited feedback into the development process), because of delays earlier in the project that led to the very late development of the list of attributes needed for the experiment. However, the wealth of intelligence that the experimental methodology provides is used to tweak the customisation of the product, as well as being useful for its evaluation and market placement. Specifically, the analysis of the DCE will be able to inform us of how much individuals are willing to pay for each of the features of the online toolkit and how likely they are to purchase or not purchase a toolkit on the basis of its features.
Discrete choice experiment design methodology
The identification of specific features that are clearly distinct, separable and visible is paramount for utility identification in DCEs. Researchers administer surveys to participants to elicit their preferences. In conducting a DCE, a number of steps need attention so as to maximise the quality of responses and results:
-
The features (called attributes) of the service in question need to be selected. Commonly, these attributes are based on expert knowledge and discussion, focus groups, literature reviews and often intuition. A price attribute may be included for willingness-to-pay (WTP) calculations, in other words, to determine the price one is willing to pay for a specific feature.
-
For each of those attributes, the number of levels and the levels themselves have to be chosen. They should be realistic and cover the range of potential values that individuals’ preferences would fall within. For attributes of a discrete nature, the identification of levels is usually straightforward (e.g. colours for cars, availability or not of a search word function in a text document). However, for continuous attributes, the task is slightly more complicated, and it is advisable that levels that support the extreme values of the attribute are used. 135
-
Statistical theory is employed to combine one level of each attribute for all attributes to create hypothetical alternative specifications of the product/service. These alternatives are placed in groups of two or three to create the scenarios (or choice sets) that are presented to respondents and that form the basis of the survey.
-
The survey is then administered to respondents, who are asked to indicate their preferred alternative within each choice set.
-
Following data collection, appropriate discrete choice econometric models are used to estimate the relative importance of the various levels and features of the product and their respective WTP values.
Statistical theory for the design of discrete choice experiments in more detail
Common experimental designs use the full factorial (i.e. the total number of combinations of attributes and levels is used). For example, with five attributes of two levels each, the number of combinations would be 25 = 32 or LA, where L is the number of levels and A is the number of attributes. The main advantage of full factorial designs is that they possess enough information to estimate the main effects of all of the attributes, as well as all their interactions. However, the number of combinations grows very large as the number of levels and attributes increases. A first attempt to control the size of the design is to redefine or exclude certain characteristics or reduce levels. With failure to reduce the full factorial to a manageable size, the analyst can resort to what is called a fractional factorial design. By using a fraction of the possible combinations, they attain a manageable size, but at the cost of lost information and estimation power. However, looking at linear models, Dawes and Corrigan136 found that 70–90% of the variance may be explained by the main effects and 5–15% by two-way interactions. Therefore, depending on the research question of the study, designs for main-effects estimation in most cases are sufficient to elicit the information required for the problem at hand.
The efficiency of the fractional factorial design depends on certain statistical properties, orthogonality, level balance, minimal overlap and utility balance. 137,138 Orthogonality requires that all attributes are independent of each other (zero correlations). Level balance requires that the levels of each attribute appear with equal frequency. Minimal overlap implies that the alternatives within each choice set do not have overlapping attribute levels. Finally, utility balance requires that the utility of each alternative within a choice set is the same. This final principle ensures that there are no ‘dominant’ alternatives within a choice set (i.e. alternatives that are ‘better’ at one attribute and at least as good on the rest of the attributes, which would force the rational individual to choose them without making any trade-offs between the alternatives’ characteristics). These four properties are combined to ensure maximum D-efficiency (a statistic based on the determinant of the covariance matrix), which itself is a relative measure compared with the efficiency of a fully identified orthogonal design.
Methods
Identifying attributes and levels
In the development of the PRESENT toolkit, 21 potential attributes, excluding cost, were initially identified from the rapid review of the literature (see Chapter 2) that were also relevant to the prototype toolkit design that had been developed. The cost-attribute values were determined through cognitive interviews and piloting of the survey. The study team elicited from potential users the maximum that they would be willing to pay for some of the combinations they liked most, and also whether or not this should be costed as an individual or team purchase.
The initial design used 12 choices per respondent. Each of these choices involved three hypothetical toolkits. Within each choice, the three toolkits varied in only 5 out of the 12 attributes. Two versions were developed, one for patients and one for health-care professionals. Some attributes were generic across these and some were suited to only one or the other; the final list contained 11 attributes per version and, therefore, one attribute had to be lost from each version, as the design requires even numbers. Following initial feedback on the DCE design, it was felt that it might be too difficult for patients to understand because they were not used to the type of toolkit being explored, and the study therefore continued with only the health-care professional version.
The study team ensured inclusion of some attributes that were deterministic, in other words, that all participants would be expected to either prefer or disprefer. For example, it was known with enough certainty that respondents prefer lower prices for a given product, and hence the sign of the price attribute would be negative. Similarly, it seems likely that whether or not users could save data would have a positive sign (i.e. for a given product, users would prefer to have the option of saving rather than not). This prior assumption about the influence of an attribute helped Emmanouil Mentzakis to create a more informative design for the task at hand; it was important to optimise the survey, as the study team expected to recruit only low numbers of respondents.
The final list of attributes and levels is given in Box 1.
-
1. Option to search for data on a specific hospital OTHER THAN YOUR OWN:
-
yes: choose a hospital from a drop-down list
-
yes: keyword search (type in part of the word and suggestions appear)
-
yes: map and postcode (choose from a map or type in postcode to see the list of hospitals in the area)
-
no: only regional data visible.
-
-
2. Graphs:
-
fixed graphs or pie charts
-
user can choose and change graphs.
-
-
3. Data resolution:
-
show graphs and pie charts only if more than six responses, but show all comments
-
show graphs and pie charts as well as comments, even if there is only one comment for the chosen topic.
-
-
4. Language:
-
technical, with a dictionary of terms
-
lay, that is, with no jargon.
-
-
5. Indicators displayed:
-
six fixed indicators of patient experience shown at the same time
-
user can choose up to 6 out of 12 indicators of patient experience.
-
-
6. Filter:
-
filter data by gender AND age AND ethnicity AND condition/illness
-
filter only by condition or illness (e.g. type of cancer).
-
-
7. Staff role:
-
filter comments by staff role
-
do not filter by staff role.
-
-
8. Upload your own data:
-
yes
-
no.
-
-
9. Predictive intelligence (see how lack of given resources may influence particular areas of health care):
-
predictive intelligence capability to inform and help to plan capacity
-
no predictive intelligence capability.
-
-
10. Annual team membership cost:
-
£250
-
£500
-
£1000
-
£1500.
-
Piloting
The attributes and levels were piloted online on a small sample of three health-care professionals who fitted the profile of potential users to gauge clarity and understanding of the survey and the experiment. Formal feedback was sought via a comment window incorporated in the survey, and a number of issues were raised:
Interesting survey and well explained but there are some complex instructions/ideas to get your head around which may put people off.
It’s interesting and very well explained but it is quite difficult, not something you can just dash off in 5 minutes! As long as people know that, I think they will be happy to work through it. Also, if your target health professionals are interested in using the dashboard, that will focus their minds.
It will be important to emphasise that this survey will take a while to complete.
At the start saying you will do 10 choices could be the difference between people giving up or not, if you have done six and know there are 10 you might be able to push yourself to finish it.
At first I was confused about the additional question that pops up at the bottom of a page once you have made your selection – it asks ‘Suppose you were given the option to opt out of subscribing to this service . . .’ but I did see why it was being asked once I’d gone through one choice set.
Very nice survey design.
As a result of the pilot user suggestions, the main change was to make the text on the welcome page and instructions page shorter, and including bold and underline to highlight the most significant information.
Further feedback was sought immediately after these modifications via cognitive interviews with two stakeholders. This was fewer than intended; the aim was to interview five stakeholders, but the survey took time, there was no compensation for helping us and the team was very short of time. However, further changes were made:
-
The text on the welcome page and the instructions page was further shortened, broken into smaller paragraphs and the font size was increased.
-
A graphic was added to the home page to make it more visually appealing.
-
A link to a prototype toolkit, which had been included in the survey to enable respondents to get a feel for the product, was dropped and replaced with a screenshot. The interviewed participants had not seen any relation between the toolkit prototype and the survey, and were confused as to why they were asked to engage with it.
-
A table explaining the different toolkit features was made more comprehensive and definitions of the features were made clearer. The link to this table, which was previously displayed vertically next to each choice set, was changed to display horizontally under the choice set.
-
A clearer definition of the cost attribute was provided and the subscription prices were increased to correspond more closely to those currently found on the market.
-
A conditional question about the pay bands/grades was added for the NHS staff respondents.
As many of these changes were made because of feedback that the survey was complicated to complete, the study team discussed whether or not training sets should be used. However, given that this would add to the cognitive burden, the team decided against this. The team also discussed a visit–revisit option. However, it was considered that this would reduce validity, as participants might forget the theme, topic and context.
Experiment design
Following the identification of attributes and their levels, a statistical theory was used to create a DCE with eight attributes of two levels and two attributes of four levels. To obtain enough information to identify the main effects of this size, each respondent would have been required to respond to a minimum of 15 choice sets of two alternatives each (i.e. 15 pairwise sets of two alternative hypothetical toolkits). However, with 10 attributes (potentially with different levels) in each of the hypothetical alterative toolkits, the cognitive burden placed on respondents to process this information and indicate their preferred alternative in each of these pairwise choice sets was deemed to be too large.
For this reason, a number of steps were taken to reduce such cognitive burden and render the DCE more manageable for the respondents. First, the number of hypothetical alternative toolkits within each choice set was increased to three (from two). This automatically reduced the number of choice sets required per respondent to eight. Second, given that all 10 attributes could vary simultaneously among the three alternative toolkits, implying that comprehending and evaluating the possible trade-offs among all attributes across all three alternatives for each choice set was impossible, an alternative type of design was used, namely partial profile design. Such designs vary only a limited number of attributes among the three alternatives in each choice set, but with different attributes across different choice sets. For this project, it was deemed that 4 out of the 10 attributes would vary in any given choice set (i.e. a different group of four attributes across different choice sets). This convenience and discount in cognitive burden, however, comes with the cost of a larger number of choice sets that each respondent must see. Finally, using one of the features of the experimental design software, three different versions of the exact same design were created (each respondent receiving only one version) that allowed more variation in the combination of attributes and levels presented to individuals.
To improve the power of the experiment, it was originally intended that the three versions would be distributed randomly to participants, with a balance of respondents across versions. However, the study team discussed the problems that a computer-driven random allocation would cause if people started but did not complete the survey, and decided against the randomisation.
Forcing individuals to indicate their preferred alternative out of the three in each choice set results in what is commonly called a ‘forced-choice’ experiment. Individuals are forced to trade off between the attributes of the alternatives without the possibility of choosing to decline the purchase. However, in such cases, it is impossible for the researcher to elicit true purchasing behaviour, given that the corresponding real-world choice would be first whether or not to purchase a toolkit service at all and, subsequently, which version one would like. 139 For this reason, a two-stage elicitation strategy was implemented, whereby individuals were first asked which of the three alternatives they prefer and, subsequently, whether they would rather keep their preferred alternative or opt out altogether if they were given the chance.
Construction of experimental designs from first principles was not necessary; JMP software, version 11 (SAS Institute, Marlow, UK), was used, which contains preprogrammed routines that are able to create tailor-made experimental designs.
Survey administration
The survey was platformed on the iSurvey toolkit (University of Southampton, Southampton, UK). Over 200 stakeholders were invited to participate through the existing professional networks and Macmillan Cancer Support and its digital team. The study team contacted all of the CCGs in England, as well as a number of cancer charities. The professionals who had participated in other stages of the study, had previously shown interest in the study or had attended the launch event were invited and encouraged to share the link with colleagues. The survey was also disseminated via members of the AG and steering committee, and shared within the Faculty of Health Sciences at the University of Southampton. The survey was open for 9 weeks, and there was one reminder.
Analysis
Participant descriptive statistics
The mean age of the 32 completer responders was 49 years, with 81% being female and 38% working for the NHS (Table 4). Among the 38% of responders working for the NHS, the most common band was band 7 (50%), and management was the most common professional area (58%). This was the target group for the DCE, as the responders were likely to be able to influence the use of the toolkit and make use of it. Individuals with academic roles constituted 53% of the respondents; many were academic clinicians (incomplete reporting means that a final figure cannot be attributed to these respondents).
Characteristic | Descriptive statistics | |
---|---|---|
Mean | Number of responders (min., max.) | |
Age (years) | 48.5 | 32.0 (30.0, 77.0) |
Frequency | Percentage | |
Sex | ||
Male | 6.0 | 18.8 |
Female | 26.0 | 81.3 |
NHS staff | ||
No | 20.0 | 62.5 |
Yes | 12.0 | 37.5 |
Band/grade | ||
Band 7 | 6.0 | 50.0 |
Band 8a | 2.0 | 16.7 |
Band 8b | 1.0 | 8.3 |
Band 8d | 2.0 | 16.7 |
Other | 1.0 | 8.3 |
Professional area | ||
Clinical psychology | 1.0 | 8.3 |
Management | 7.0 | 58.3 |
Medicine/surgery | 1.0 | 8.3 |
Nursing | 1.0 | 8.3 |
Other | 1.0 | 8.3 |
Physiotherapy/occupational therapy | 1.0 | 8.3 |
Area of specialty | ||
Commissioning | 1.0 | 9.1 |
Communications and engagement | 1.0 | 9.1 |
Complaints, patient experience and risk management | 1.0 | 9.1 |
Diabetes mellitus and obesity | 1.0 | 9.1 |
Innovation evaluation and implementation | 1.0 | 9.1 |
Musculoskeletal | 1.0 | 9.1 |
Obstetrics and gynaecology | 1.0 | 9.1 |
Operational/commissioning | 1.0 | 9.1 |
Service development manager | 1.0 | 9.1 |
Urology | 1.0 | 9.1 |
Communications and patient experience | 1.0 | 9.1 |
Role | ||
Academic | 9.0 | 52.9 |
Charity | 1.0 | 5.9 |
Health researcher | 1.0 | 5.9 |
HealthWatch | 1.0 | 5.9 |
Professional | 1.0 | 5.9 |
Community engagement | 1.0 | 5.9 |
Local authority officer | 1.0 | 5.9 |
Manager of charity | 1.0 | 5.9 |
Patient | 1.0 | 5.9 |
Discrete choice experiment data
The analysis of the DCE data was based on random utility theory140 and discrete choice econometric models. 141 Random utility theory assumes, as in neoclassical economic theory, that individuals have perfect information on their preferences and perfect discrimination capabilities. 140 On the other hand, the researcher has incomplete information and does not observe the full decision process of the individual. Hence, a component of uncertainty (with various potential sources) has to be included in modelling utility. The utility of the ith alternative for the qth individual is:
where:
is the deterministic part of the utility (with k attributes) and εiq is the error term capturing the uncertainty and the unobserved (by the researcher) part of individual heterogeneity. βk are the utility parameters, independent of q (i.e. homogeneous across the population). Assuming that the error term is independent and identically distributed, extreme value type 1 gives rise to the McFadden’s conditional logit (CL),142 in which the alternative i is chosen out of j alternatives with probability:
The probability in the CL depends on differences among alternatives and, therefore, attributes that do not vary by alternative will not influence the probabilities. Hence, demographic characteristics cannot directly influence the choice within each choice set. Similarly, given the use of a partial profile design, any attributes that do not vary among the three alternatives in any given choice set (i.e. 6 out of the 10 attributes) also cannot influence the choice in the choice set (i.e. any variation in choices comes from differences in the preference over the attributes that vary within a choice set).
Taking the CL further, models have been developed that relax some of its restrictive assumptions, mainly with more flexible structures in the covariance matrix of the model. A common specification is the nested logit (NL) model, in which the alternatives are grouped into nests (i.e. purchase or not purchase), under the assumption that the independence of irrelevant alternatives property holds within each nest but not across nests. 143 The probability of the individual q choosing alternative i from nest g is the product of the probability of the individual choosing an alternative from nest g (i.e. choosing nest g) and of the probability of choosing alternative i conditional on nest g:
which is the product of two simple logits. Assuming that there are indirect utilities with both of these decisions and rewriting:
From above, the probability of the NL is:
where:
is the inclusive value, which links the two levels of the nested model and is used to establish the extent of dependence or independence between linked choices. 134 λg is the scale parameter to be estimated in the range 0–1. Every level in the NL has its own scale parameter, and for identification reasons, one of them has to be normalised to 1. Table 5 shows the DCE estimation results.
Decision | Conditional logit | Nested logit | |
---|---|---|---|
Forced choice | Opt out | ||
(1) Attribute estimates | |||
Search: yes, choose a hospital from a drop-down list | 3.792*** (1.796) | 2.763*** (1.040) | 6.321*** (4.241) |
Search: yes, keyword search | 2.962** (1.386) | 2.539*** (0.915) | 4.730** (2.939) |
Search: yes, map and postcode | 2.948** (1.423) | 2.318*** (0.742) | 3.007 (2.059) |
Graphs: user can choose and change graphs | 1.627* (0.407) | 1.666** (0.361) | 1.848** (0.508) |
Data resolution: show graphs and pie charts only if more than six responses, but show all comments | 1.232 (0.302) | 1.331 (0.299) | 1.317 (0.434) |
Language: lay, that is, no jargon | 1.895** (0.561) | 1.125 (0.232) | 1.714 (0.569) |
Indicators displayed: user can choose up to 6 out of 12 indicators of patient experience | 1.424* (0.305) | 1.221 (0.226) | 1.576 (0.437) |
Filter: filter data by gender AND age AND ethnicity AND condition/illness | 3.703*** (0.907) | 3.193*** (0.699) | 5.023*** (1.647) |
Staff role: filter comments by staff role | 1.798** (0.454) | 1.568*** (0.247) | 2.033** (0.618) |
Upload own data: yes | 2.596*** (0.723) | 1.742*** (0.355) | 2.823*** (1.119) |
Predictive intelligence: predictive intelligence capability to inform and help plan capacity | 1.144 (0.261) | 1.144 (0.276) | 1.144 (0.356) |
Fee | 0.999*** (0.0002) | 0.999*** (0.0002) | 0.999*** (0.0002) |
Decline the service (opt out) | 8.823*** (4.574) | ||
(2) Probability to decline the service | |||
Age | 0.994 (0.029) | ||
Sex (1 if female) | 29.35*** (29.29) | ||
NHS staff | 1.322 (0.891) | ||
Constant | 0.0506 (0.092) | ||
Number of respondents | 33 |
Willingness-to-pay values
Following the estimation of models and coefficients for each attribute level, WTP values can be calculated. Coefficients in DCE models depict part-worth utility (i.e. the contribution of that attribute level to the utility enjoyed from the alternative), and for this reason present the relative importance of attribute levels. By taking the ratios of coefficients, one can calculate the marginal rates of substitution between them [i.e. how much of one attribute level one is willing to lose to gain more of another (Table 6)].
Decision | Conditional logit | Nested logit | |
---|---|---|---|
Forced choice | Opt out | ||
Search: yes, choose a hospital from a drop-down list | 167,376 (2.19)** | 1836.33 (2.34)** | 2446.80 (2.37)** |
Search: yes, keyword search | 1363.52 (2.06)** | 1683.55 (2.33)** | 2062.10 (2.42)** |
Search: yes, map and postcode | 1357.49 (2.01)** | 1518.86 (2.41)** | 1460.96 (1.67)* |
Graphs: user can choose and change graphs | 611.41 (1.57) | 922.53 (1.57) | 815.25 (1.61) |
Data resolution: show graphs and pie charts only if more than six responses, but show all comments | 261.76 (0.78) | 516.10 (1.11) | 365.61 (0.77) |
Language: lay, that is, no jargon | 802.76 (1.89) | 212.45 (0.61) | 715.41 (1.56) |
Indicators displayed: user can choose up to 6 out of 12 indicators of patient experience | 443.44 (1.63) | 360.70 (1.24) | 603.35 (1.76)* |
Filter: filter data by gender AND age AND ethnicity AND condition/illness | 1643.74 (2.85)*** | 2097.37 (2.65)*** | 2141.81 (2.55)** |
Staff role: filter comments by staff role | 736.55 (2.13)** | 812.63 (2.06)** | 941.73 (2.03)** |
Upload own data: yes | 1197.61 (2.29)** | 1002.80 (2.01)** | 1376.96 (2.04)** |
Predictive intelligence: predictive intelligence capability to inform and help plan capacity | 168.78 (0.54) | 243.31 (0.51) | 178.27 (0.40) |
Incorporating a price component (as a numeraire) into the attributes list allows one to translate the trade-offs and marginal rates of substitution between attributes into their monetary representations. These WTP values represent the amount of money an average individual is willing to pay to obtain a service/product that has a specific feature attribute level. For the CL, the WTP calculation would be:
Predicted probabilities
Further inference on the results can be drawn from calculating predicted probabilities. These probabilities represent the likelihood that an individual will or will not purchase a given product, or the change in the likelihood of purchasing or not purchasing a product when its features change. Specifically, three types of predicted probabilities are presented.
The first one is used to provide some better insight into the magnitude of the effect of each attribute level (i.e. how much it contributes to the attractiveness of the product). For this, the study team initially defined a baseline product with the features shown in Box 2.
No, only regional data visible.
GraphsFixed graphs or pie charts.
Data resolutionShow graphs and pie charts as well as comments even if there is only one comment for the chosen topic.
LanguageTechnical, with a dictionary of terms.
Indicators displayedFixed indicators of patient experience shown at the same time.
FilterFilter only by condition or illness (e.g. type of cancer).
Staff roleDo not filter by staff role.
Upload own dataNo.
Predictive intelligenceNo predictive intelligence capability.
Annual subscription fee£250.
Subsequently, the team created alternative toolkits that are identical to the baseline with the exception of one attribute level. For instance, such an alternative toolkit would be one that was identical to the baseline but with the capability to upload own data (i.e. attribute: upload own data – yes). The possible configurations (i.e. the number of possible alternative products) are numerous, so, without any loss of generality, the team members restricted themselves to changing only one attribute level at a time (see above), which resulted in a total of 12 alternative toolkits. The probability of purchase for each of these alternative toolkits was then calculated and compared with the baseline. This difference indicates the change in the predicted probability of purchase when one attribute level changes with respect to the baseline.
Given that the study team intentionally chose the baseline toolkit to contain the least desired level of each feature, with the exception of price when it was the cheapest, probability differences were expected to be positive, implying that a higher number of desired features increased the probability of selecting the toolkit.
The second type of predicted probability followed a similar logic, but gave an assessment of the importance of each attribute level in preventing an average individual from opting out of purchasing the toolkit. In this case, the probability of opting out for the baseline, as well as for all other alternative toolkits, was calculated and, again, compared. More desirable features were expected to reduce the probability of opting out.
Given that, in these calculations, the presence of the opt-out as an option was necessary, this was possible for only the second stage of the DCE choice. Recall that a two-stage elicitation strategy was implemented, whereby individuals were first asked which of three alternative toolkits they preferred and were subsequently offered the option to keep their preferred alternative or opt out altogether.
The third type calculated the unconditional predicted probability of purchase and of opt-out (i.e. decline purchase) for three hypothetical toolkits (i.e. the baseline, a fully featured low-price alternative and a fully featured high-price alternative). Such figures give a sense of the overall tendency to purchase from the sample of respondents and can aid in building market scenarios that can guide market placement and revenue streams.
Estimation results and product valuation
Out of 152 individuals starting the survey, 33 respondents completed it (i.e. completion/retention rate of about 22%). Although the identification of coefficients does not rest on sample size (i.e. the experimental design ensures that enough information is available to estimate parameters), the generalisability or robustness of the findings would therefore need to be verified.
Estimation results
Table 7 presents the estimation result from three models. The CL can be used to estimate the first stage of the DCE question (i.e. forced choice without an opt-out option) and the first and second stage of the DCE questions combined (i.e. three alternative toolkits plus an opt-out option to choose from). This latter combination (i.e. first and second stage combined) is also analysed using a NL. Results for all three are given in odds ratios, which indicate how much more likely one is to choose an alternative that features an attribute level compared with the baseline.
Feature | Ranking |
---|---|
Search: yes, choose a hospital from a drop-down list | 1 |
Search: yes, keyword search | 3 |
Search: yes, map and postcode | 4 |
User can choose and change graphs | 9 |
Data resolution: show graphs and pie charts only if more than six responses, but show all comments | 11 |
Language: lay, that is, no jargon | 7 |
Indicators displayed: user can choose up to 6 out of 12 indicators of patient experience | 10 |
Filter data by gender AND age AND ethnicity AND condition/illness | 2 |
Filter comments by staff role | 8 |
Upload own data | 5 |
Predictive intelligence capability to inform and help plan capacity | 12 |
Fee: £1500 | 6 |
Common patterns appear across models (see Table 9). Being able to filter data (by gender AND age AND ethnicity AND condition/illness) seems to be the most influential feature, followed by the ability to search for a hospital from a drop-down list. All three search features are highly desirable for respondents, although the ability to upload one’s own data is almost equally important. Furthermore, filtering comments (by staff roles) and customisation of graphs also appear to be important, whereas the use of lay language and indicators display achieved limited significance. Interestingly, data resolution and predictive intelligence were not identified as important in any of the models. Finally, the cost of the toolkit was highly significant, suggesting that respondents were sensitive to price changes as predicted by economic theory. Overall, on average, individuals were very likely to decline to purchase the service (i.e. almost nine times more likely to opt out than to purchase it) at the highest price compared with the lowest price, and females were much more likely to do so, as indicated by the NL model.
Willingness-to-pay monetary valuations
Table 8 presents WTP calculations. Given that WTP calculations are essentially non-linear combinations of the estimated coefficients, statistical significance and relative size closely follow the coefficient results. All three models point to an identical list of important attribute levels, albeit there is some variation in the magnitude of the elicited WTP. This is largely explained by the different model assumptions and other model features and for the vast majority, WTP calculations were not statistically different across the three models.
Feature | Conditional logit | Nested logit | |||
---|---|---|---|---|---|
Forced choice | Opt out | ||||
Difference in predicted probability from baseline | Difference in predicted probability from baseline | Change in predicted probability of opt-out | Difference in predicted probability from baseline | Change in predicted probability of opt-out | |
Search: yes, choose a hospital from a drop-down list | 32.2 | 10.9 | –9.1 | 27.9 | –4.2 |
Search: yes, keyword search | 26.4 | 9.7 | –8.1 | 23.8 | –3.4 |
Search: yes, map and postcode | 26.3 | 8.4 | –7.0 | 16.8 | –2.1 |
Graphs: user can choose and change graphs | 11.6 | 4.5 | –3.7 | 9.0 | –1.0 |
Data resolution: show graphs and pie charts only if more than six responses, but show all comments | 4.8 | 2.3 | –1.9 | 3.9 | –0.4 |
Language: lay, that is, no jargon | 15.4 | 0.9 | –0.7 | 7.8 | –0.9 |
Indicators displayed: user can choose up to 6 out of 12 indicators of patient experience | 8.3 | 1.5 | –1.3 | 6.5 | –0.7 |
Filter: filter data by gender AND age AND ethnicity AND condition/illness | 31.6 | 13.2 | –11.0 | 24.7 | –3.5 |
Staff role: filter comments by staff role | 14.0 | 3.8 | –3.2 | 10.5 | –1.2 |
Upload own data: yes | 23.2 | 4.9 | –4.1 | 15.8 | –2.0 |
Predictive intelligence: predictive intelligence capability to inform and help plan capacity | 3.1 | 1.0 | –0.8 | 1.8 | –0.2 |
Fee: £1500 | –17.7 | –3.6 | 3.1 | –10.0 | 1.0 |
Overall, respondents were willing to pay around £1674–2447 for their most preferred search feature (i.e. a drop-down list of hospitals), followed by the filter (by age, gender and condition), which was valued at £1644–2142. The other two search options, keyword and map/postcode, were valued at £1364–2062 and £1357–1461, respectively. The capability to upload one’s own data was valued at £1198–1377, and finally, filtering by staff role was valued at £736–941.
It should be noted that although the rest of the attribute levels do not appear to be as significant, this does not imply that they are not desired in the toolkit. As discussed previously, in Willingness-to-pay values, coefficients convey a relative piece of information, namely, how much more a feature is desired compared with the baseline or the baseline level. For this reason, statistically insignificant WTP values imply that respondents did not systematically value the toolkit with these attribute levels more than they valued a toolkit with the respective baseline attribute level. For instance, in the case of graphs [with two levels: (1) fixed graphs or pie charts and (2) user can choose and change graphs], an insignificant WTP suggests that respondents were not willing to pay more for a toolkit with the user-specified graphs than they were for a toolkit with fixed graphs. This is vastly different from the notion that graphs are altogether not important to the toolkit users.
Predicted probabilities calculations
The results from the predicted probabilities calculations are given in Table 9. Recall that the values in the table are changes in predicted probabilities and, for this reason, are interpreted as percentage-point changes. Positive values imply an increase in the chances of purchasing an alternative toolkit (i.e. identical to the baseline toolkit in all aspects, apart from the specific attribute indicated) compared with the baseline toolkit. Correspondingly, negative values suggest that the attribute level evaluated is less desired than the respective baseline attribute level and, for this reason, the chances of purchasing the alternative toolkit are lower than those of the baseline toolkit.
Feature | Toolkit | ||
---|---|---|---|
Baseline | Fully featured, low price | Fully featured, high price | |
Search | No, only regional data visible | Yes, choose a hospital from a drop-down list | Yes, choose a hospital from a drop-down list |
Graphs | Fixed graphs or pie charts | User can choose and change graphs | User can choose and change graphs |
Data resolution | Show graphs and pie charts as well as comments, even if there is only one comment for the chosen topic | Show graphs and pie charts only if more than six responses, but show all comments | Show graphs and pie charts only if more than six responses, but show all comments |
Language | Technical, with a dictionary of terms | Lay, that is no jargon | Lay, that is no jargon |
Indicators displayed | Fixed indicators of patient experience shown at the same time | Users can choose up to 6 out of 12 indicators of patient experience | User can choose up to 6 out of 12 indicators of patient experience |
Filter | Filter only by condition or illness (e.g. type of cancer) | Filter data by gender AND age AND ethnicity AND condition/illness | Filter data by gender AND age AND ethnicity AND condition/illness |
Staff role | Do not filter by staff role | Filter comments by staff role | Filter comments by staff role |
Upload own data | No | Yes | Yes |
Predictive intelligence | No predictive intelligence capability | Predictive intelligence capability to inform and help plan capacity | Predictive intelligence capability to inform and help plan capacity |
Fee | £250 | £250 | £1500 |
Probability to accept purchase | 9% | 89% | 80.5% |
Probability to decline purchase | 91% | 11% | 19.5% |
All three models point to a similar ranking across attribute levels, with the search feature of a drop-down hospital list increasing the chances of an alternative being selected by 32 percentage points in the forced-choice model and 28 percentage points in the NL model. Equally large changes in likelihood were obtained by the filter (by age, gender and condition) feature, increasing the chances by 32 percentage points and 25 percentage points, respectively. The remaining search levels and data-uploading capabilities follow. Looking at the price effect, an increase in the annual fee from £250 to £1500 drops the probability of purchase by around 18 percentage points in the forced-choice model and 10 percentage points in the NL model.
Moving on to the second type of predicted probabilities through which the change in the predicted probability of opting out was calculated, negative values imply that an attribute level will result in a lower probability to opt out (i.e. a desired feature), whereas positive values suggest an increased chance of opting out and not purchasing (i.e. an unattractive feature). For both the CL and NL models, it can be seen that the changes in the opt-out probabilities were relatively small, with around a 13-percentage points and 3.5-percentage points drop in the CL and NL, respectively, for filtering (by age, gender and condition). Search features follow, with values between 7 percentage points and 9 percentage points for the CL and 4.2 percentage points and 2 percentage points for the NL model, suggesting that all features are desirable and reducing the chances of opt-out.
It is noted that such drops in the opt-out probability potentially appear to be small. However, this is to be expected, given that there is a large tendency of respondents to opt out of purchasing the baseline toolkit, which, in essence, is the least desirable toolkit that can be configured, given the attributes specified. To gain insight into the overall attractiveness of the digital toolkit and the potential market demand, the third type of predicted probabilities is presented in Table 9. Individuals in the vast majority of cases (i.e. 91%) declined purchase for the baseline toolkit, but would choose to purchase when a fully featured alternative is offered, with 89% for the lower priced options and 80.5% for the higher priced options. From this, the effect of price on a highly desirable toolkit can also be seen, whereby an increase of £1250 (i.e. from £250 to £1500) reduces the probability of purchase by around 8.5 percentage points.
Basic cost–benefit evaluation of the online toolkit
Given the results of the DCE, a basic cost–benefit simulation exercise was performed to evaluate the profitability of a digital toolkit venture. This may also be useful when considering transferability. An evaluation for the three toolkits from Table 9 was performed (i.e. the baseline, a fully featured low-price alternative and a fully featured high-price alternative). Furthermore, it was assumed that the toolkit was offered to 100, 500 and 1000 potentially interested clients and the product uptake purchasing behaviour was calculated from Table 9. For the calculation of costs, only the initial development cost was taken into account (i.e. the value of the grant: £412,242) and a varying annual maintenance cost was assumed, depending on the number of clients (i.e. £50,000 for 100 potential clients, £100,000 for 500 potential clients, £150,000 for 1000 potential clients). Finally, a product life of 5 years and an interest rate of 3% were assumed.
From Table 10, it is apparent that the baseline toolkit is not a viable option for any number of potential clients, largely because of its very low chances of uptake (i.e. even for 1000 potential clients, only 90 purchases are predicted). Moving onto the full-featured option with the low-pricing strategy, the product becomes profitable over a 5-year period only for 1000 potential clients (i.e. 890 purchases per year). Finally, for the full-featured option in the high-pricing strategy, the product becomes highly profitable for 500 potential clients (i.e. 403 purchases per year) and 1000 potential clients (i.e. 805 purchases per year).
Feature | Toolkit | ||
---|---|---|---|
Baseline | Fully featured, low price | Fully featured, high price | |
Revenues | |||
Probability of purchase (out of potential clients) (%) | 9.0 | 89.0 | 80.5 |
Product price (£) | 250 | 250 | 1500 |
Number of clients/users per annum | 100 | 500 | 1000 |
Annual revenue (£) | |||
For 100 potential clients | 2250 | 22,250 | 120,760 |
For 500 potential clients | 11,250 | 111,250 | 603,760 |
For 1000 potential clients | 22,500 | 222,500 | 1,207,500 |
Revenues (£) | |||
Present value of total revenue (over a period of 5 years with an interest rate of 3%) | |||
For 100 potential clients | 10,304 | 101,898 | 553,000 |
For 500 potential clients | 51,522 | 509,492 | 2,764,998 |
For 1000 potential clients | 103,043 | 1,018,985 | 5,529,996 |
Costs (£) | |||
Development cost | 412,242.00 | ||
For potential clients | For 100 potential clients | For 500 potential clients | For 1000 potential clients |
Annual maintenance cost | 50,000 | 100,000 | 150,000 |
Present value of total costs (over a period of 5 years with an interest rate of 3%) | 641,227 | 870,213 | 1,099,198 |
Discussion
This chapter has presented the development of the online survey DCE to elicit individual preferences for features of an online toolkit and explored the results. It should be noted that the study team was not interested in the exact content of the toolkit, but in the availability of features and customisation options and how they affect individuals’ purchasing behaviours. In other words, the purchasing behaviour elicited in this context assumes that individuals are already aware of the importance of the information offered by an online toolkit, and for this reason, the study team assessed how the various features of such a toolkit influence the probability of purchasing the product. In this way, the team can determine which features are the most important. At the same time, the team allowed individuals to opt out of purchasing, which affords the opportunity to elicit a demand function for the product. Individuals could opt out either because they disliked the product or because they disliked its features, and it cannot be determined which is the reason.
The final design that was used in the DCE had 10 choice sets of three alternatives each and an opt-out in the second stage. Within each choice set, only four attributes were allowed to vary across the three alternatives. Three versions of the design were created, with each respondent assigned to one of the three. For an assumed sample of 50 respondents, the design had a D-efficiency of around 85%.
Discrete choice experiments are a very useful tool in the development and evaluation of this type of intervention, as they render feasible an assessment of the intervention’s dimensions prior to its release in the market. Findings suggest that certain features are highly desirable, namely the search function, filtering and uploading one’s own data being the top choices. Furthermore, a range of WTP values is observed, mostly towards the upper end of the distribution that was specified in the price attribute, again suggesting that certain features were highly valued and would contribute significant added value in a potential toolkit. Finally, in terms of the market demand for three representative toolkits, it was found that purchasing behaviour is very much dependent on the toolkit features, going from a 10% to a 90% probability of purchase when the team moved from using a baseline toolkit to using a fully featured one.
However, extrapolating actual market demand from the experimental findings should be treated with caution for various reasons. First, although a response rate cannot be calculated in online surveys, the study had a moderate retention rate of approximately 20%. It is unknown whether those who logged into the survey but did not complete it did not do so because they were not interested in the product, not interested in participating or not able to devote the required time, or for some other reason. For this reason, it is not clear what the uptake would be among the overall stakeholder population. Second, although the study team made every effort to appear realistic in the way in which the toolkit and its features were presented to participants, the DCE itself and the purchasing behaviour is of a hypothetical nature, whereby individuals express their intentions. There is some literature to suggest that discrepancies between stated and actual behaviours are not uncommon,144 and DCE results are to be verified and further replicated before generalisations can be made. Third, in general, individuals are assumed to be perfect information processors, although this is not always the case. 145,146 Moreover, the problems of the potential inconsistency of the respondents’ answers to the hypothetical situations increase with the size of the experiment and the difficulty of the tasks. 147,148 Often, individuals find answering the necessary questions increasingly difficult, while fatigue also sets in. 149 These problems could inhibit individuals in using compensatory behaviour (trading off one attribute for another), and instead they may either answer at random or use other types of non-compensatory techniques, which could result in a lack of robustness in the results. 145,150
Overall, with these concerns in mind, and with a few further assumptions, a basic evaluation exercise suggests that the development of the online toolkit and its roll-out in the market would result in a positive net benefit and suggests which features are the most important to develop in such a toolkit. It should be highlighted that this cost–benefit analysis offers a lower-bound estimate of the net benefit, as it does not acknowledge or incorporate any of the non-monetary benefits that would result from the use of the online toolkit and from which the main benefits are expected to arise (i.e. improved health-care services, improved health outcomes, enhanced data availability and research, etc.). However, given that such evaluation is outside the scope of this project, this would have to be explored in future research.
Chapter 8 Evaluation of the rule-based information retrieval
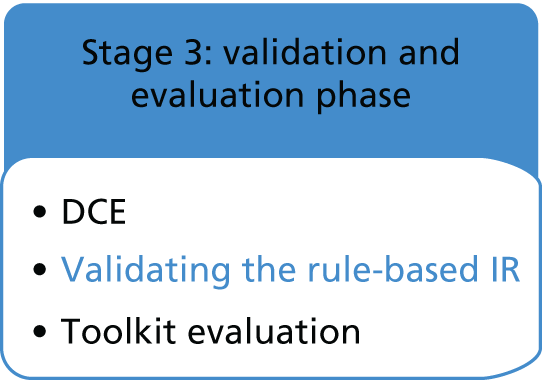
This short chapter considers the validity and reliability of the text analytics using statistical methods. The WCPES data and transferability performance are considered.
Methods
Statistics
A standard contingency table was used to classify performance (Table 11). For a binary classification problem, the table has two rows and two columns. Across the top are the observed class labels and down the side are the predicted class labels. Each cell contains the number of predictions made by the classifier that fall into that cell. Using this table, standard calculations were made for accuracy, precision, sensitivity and the F-score.
Predicted | True (observed) | |
---|---|---|
Positive | Negative | |
Positive | True positive | False positive |
Negative | False negative | True negative |
Classification accuracy is a common performance statistic. It is the number of correct predictions made divided by the total number of predictions made, expressed as a percentage. However, accuracy statistics alone should not be relied on, because, for example, it may be desirable to compromise accuracy to achieve greater predictive power. This is known as the ‘accuracy paradox’:
Precision is the number of TPs divided by the number of TPs and FPs. It is also called the positive predictive value. Low precision (< 1) can mean that the system throws up a large number of FTPs:
Sensitivity is the number of TPs divided by the number of TPs and the number of FNs. This is also known as the recall or TP rate:
The F-score shows the balance between precision and sensitivity. Thus:
When calculating these statistics for the WCPES data, the study team used the half data set that had been reserved for this purpose (having built the analytics from the other half).
Adapting the approach for the analysis of Life After Prostate Cancer Diagnosis study data
The LAPCD study is a UK-wide patient-reported outcomes study that has generated information to improve the health and well-being of men with prostate cancer. 151 Prostate cancer survivors (18–42 months post diagnosis) in all four UK countries (≈70,000) were identified through cancer registration systems and were sent postal surveys. A survey instrument was developed, covering a range of generic and cancer-specific patient-reported outcome measures, with additional items covering treatments received, sociodemographic characteristics and the patient perspective on their disease, treatment and experiences. The survey was structured into sections covering specific issues facing prostate cancer survivors [e.g. treatment decision-making (TDM) and decision regret; emotional well-being; and coping and self-management]. In addition to the closed-response items, eight free-text response questions were included at the end of each survey section for respondents to add further detail or to capture other relevant issues not covered in the section.
The adapted GATE software was used to identify themes within the responses to two free-text questions. First, at the end of section 3 of the questionnaire, which elicited 10,358 comments, a free-text question asks respondents the following:
-
Please add anything else that you would like to tell us about your diagnosis, treatment and the decision-making process.
Second, a final ‘catch-all’ free-text question, to which 6682 respondents provided comments, asks the following:
-
Is there anything else you would like to tell us about what life has been like for you following your prostate cancer?
This catch-all question was analysed as a direct test of transferability.
With regard to the first question on TDM, the LAPCD data research team involved four members of the study UAG to help inform the development of a gazetteer. Following a teleconference between the research team and the UAG on 30 March 2017, a random sample of 400 free-text responses to the TDM question were equally divided between the UAG members. The UAG members then read the comments and identified words and phrases that indicated comments describing the TDM process experienced; the treatment options with which they were presented; the level of involvement they had in the decision-making process; the amount of information and preparation they received concerning potential side effects; and their subsequent satisfaction with the treatment received. UAG members returned these TDM lists of words/phrases to the research team members, who then collated them and categorised them under subheadings.
Immediately preceding the TDM free-text question in the LAPCD data questionnaire is a closed question asking respondents to indicate their level of involvement in the decision-making process that determined their treatment (Box 3).
9a. Do you think your views were taken into account when the team of doctors and nurses caring for you were discussing which treatment you should have? Please tick one of the following boxes:
□ Yes, definitely
□ Yes, to some extent
□ No, my views were not taken into account
□ I didn’t know my treatment was being discussed by a team of doctors/nurses
□ Not sure/can’t remember
Comments provided by respondents were categorised on the basis of the level of involvement that patients indicated they had in the TDM process. This was done to identify common themes within the comments pertaining to levels of TDM involvement.
This information was used to develop gazetteers and rules to test for transferability.
patientopinion.org data
The study team obtained 10,000 comments from patientopinion.org (now known as Care Opinion) to test the approach on a different form of patient feedback free-text comments with often lengthy narratives.
Results and discussion
With the current approach, the following statistics for the WCPES data were calculated:
-
accuracy = 86%
-
precision = 88%
-
sensitivity = 96%
-
F-score = 92%.
The system was able to handle comments such as:
I am convinced [word unreadable] helped me. The special [word unreadable] drinks/desserts could be available post-surgery also.
It annotated this as belonging to hospital resources, drinks, food and helpful.
WordNet led to some overcategorisation through its use of metonyms, but also meant that the system was surprisingly accurate in ambiguous cases. For example, it was able to code patient-centred care well, even though this might be seen as a more conceptual code.
Some FPs were subjective. For example:
The level of support available and the quality of care has been high.
The above was classified as errors and safety, which was deemed to be incorrect, but which may be considered a positive example (care quality).
Some FNs were hard to explain. For example, the following was not categorised under waiting times, even though the word ‘waiting’ is in the comment:
The care given to me and the time I spent waiting for my operation was very good, fast turnaround. In fact, having the mammogram and quick diagnosis saved my life.
This may be a function of using NLP, which has inherent problems as well as advantages:
-
NLP has problems processing noisy data, reducing the overall accuracy.
-
Choices need to be made at each stage of the pipeline, which increases flexibility, but also the potential for problems; therefore, rules sometimes lead to unexpected results.
As the study team also found, NLP is computationally resource heavy, slowing the process and meaning that computers with large memories are needed.
Overall, the approach is reliable, performs better than the previous R algorithms19 and similar systems in development, and is likely to pick up most comments, although results will also contain some comments that are not correctly themed. This is better than missing a lot of comments but always placing those it catches correctly, as it is harder to check for what is missed than what is placed wrongly. However, users of the system may perceive it to be performing less well if they see the errors, so this is something that needs to be explained in the toolkit user guide.
Statistics for the LAPCD study data are less favourable. Thus:
-
accuracy = 47%
-
precision = 68%
-
sensitivity = 47%
-
F-score = 56%.
This is because the data contain much information about daily living rather than health care. Thus, a statement by a patient to say that they do not go out much anymore because they need to go to the toilet all the time as a result of their prostate cancer was categorised by the system as a negative comment about facilities.
At the time of writing this report, the study team has not yet run calculations on patientopinion.org data, which was not part of the original remit. This will be an interesting test of the system, as the comments are much longer.
Conclusions
The approach performs well on CPES data and, although transferability statistics seem disappointing, the study team was exploring the ‘worst-case scenario’, in which no adaptations were made. In fact, the system can be easily modified to increase the accuracy of different data sets. It is because of the ease with which rules can be tweaked that rule-based IR was chosen over template-based machine learning.
It should also be noted that the study team was conservative in scoring for the LAPCD data and this raises the point that such calculations depend on the research question. Although it was felt that the toilet example was wrongly coded, at least one senior LAPCD data researcher did not, as their research question was not so much ‘How can health care be improved?’ but ‘What is this patient’s overall experience of cancer?’.
The study team will also need to explore whether or not modifications for transferability reduce the accuracy of the system for CPES data; the more complex rule-based approaches become, the more unstable they may be. Thus, it may be important to keep different uses packaged separately.
It should also be noted here that any system (computational and manual), including that of the study team, has limitations, such as problems with some comments. No result from any form of IR should be taken at face value. It is essential that results are checked and confirmed, and this involves manually delving into the text under study.
Problems for the system included:
-
redacted comments, such as ‘Was not referred to a lymphedema clinic until I attended a mobile [word unreadable] unit workshop’ (although often the sytem got these right)
-
some comments with bizarre punctuation (e.g. ‘I chose . . . ’) or only blank spaces.
The error rates that these cause are data set specific. For the WCPES, these problems accounted for 0.5% of the data. This should not affect the practical overall accuracy of the system, as these comments are very unlikely to contain useful information anyway. Therefore, none of the calculations includes these comments. This means that the calculations are based on cleaned data. This approach was chosen because the study team was using the statistics to determine the areas in which the rules and gazetteers needed refinement.
The ability of the system to handle the more conceptual code of patient-centred care needs further exploration.
Chapter 9 Evaluation of the toolkit

This chapter explores the usability and goal-setting value of the toolkit using feedback from potential health-care professional users. It describes how the study team used findings to make final refinements to the toolkit output.
Introduction to the toolkit evaluation
New information technologies often fail, not only as a consequence of poor technical design, but also because there is a mismatch between the technologies and both the user needs and the organisational contexts. Moreover, users often interpret and use technologies in ways that were not anticipated by the designers, or abandon them as being inappropriate to their needs and goals. 152 The purpose of the toolkit evaluation described in this chapter was to minimise these risks by identifying any emergent or residual problems that might act as a barrier to use and effectiveness. The previous stages had sought to understand user needs, organisational contexts and user interpretations during development of the prototype. However, there is often a mismatch between what people say they want and what works in practice,144 and so in the final part of the study, the study team wished to consider how people actually used a working prototype that they could explore in front of the team members.
The major goals of technology evaluative processes should be to limit defensiveness on the part of system designers and to provide users (participants) with the freedom to express what they think. To help to ensure this, the study team organised the evaluation as a formal structured process – a structured walk-through. This evaluated the quality of the user interface and system behaviour, not the actions of participants. The participants ‘walked through’ the toolkit, while the researcher ensured that the walk-through agenda was followed, and encouraged and facilitated full participation.
The walk-through had three components:
-
heuristic evaluation
-
goal-oriented evaluation
-
implementation evaluation.
These are described in more detail in the following section.
Methods
Recruitment
The study team chose participants on the basis of geographic and sociodemographic variation, and poorer and better performance in the CPES 2014. Participants were selected from cancer services, and also other health areas, to enable the study to consider the transferability of the intervention to other specialties.
Inclusion criteria
NHS staff belonging to any of the following groups:
-
lead members of multidisciplinary treatment teams
-
ward managers
-
associate directors, medical clinician leads, nursing and/or general managers
-
members of the trust board
-
lead commissioners in CCGs/Health and Wellbeing Board members
-
general practitioner practice leads.
Exclusion criteria
-
Not fitting any of the inclusion criteria.
-
Private (non-NHS)-only staff.
-
Unable to undertake full informed consent.
-
Having taken part in stage 1 or 2 (as they would be ‘contaminated’ by previous exposure to earlier stages of the toolkit).
-
Unable to use a digital interface.
Invitations were sent through collaborator newsletters, networks and announcements, including both patients and professionals, and to people in their own networks. The study team initially invited staff from three UK NHS trusts (Wessex, London and Leeds), but to get variation in professional roles, recruitment was extended outside the three original areas, while ensuring that the team maintained variation in the trust performance indicators. Selected participants were e-mailed the participant information sheet and consent form. The signed consent form was either returned securely digitally or collected at the start of the session.
Setting
Walk-throughs took place at participants’ workplaces, and lasted an average of 2 hours per participant. An iPad (Apple Inc., Cupertino, CA, USA) was used to video-record the session. The iPad was set up on a flexible arm attached to the participant’s desk, with their hands and computer screen in view. An audio-recorder was placed on the desk, the participant was asked a final time if they consented to proceed (participants were reminded that they could stop at any time), then recording and the evaluative process began. Typically a scribe attends walk-throughs with the moderator (facilitator), to take notes and produce a list of required changes for the developers. After the first three sessions, the study team realised that, as team members were video-recording the session, the scribe was not needed. Therefore, the remainder of the walk-throughs were continued with just one researcher present who facilitated, scribed and recorded.
Heuristic evaluation (standard usability principles)
The starting point was to rely on the classic principles of (1) usability engineering and (2) the support of psychological flow in information systems, and to define these as benchmarks to be evaluated by users. 152,153 The first stage of the evaluation was therefore a heuristic evaluation. 154 This helps to identify usability problems in a user interface through potential users (i.e. evaluators) who examine the interface and judge its compliance with recognised guidelines and usability principles (the heuristics). This evaluation method takes less time and uses fewer resources than a full systemic evaluation, but is widely seen as being successful in uncovering usability problems during the design phase. For this reason, it is widely used in the industry in the design of user interfaces and especially in the design of new media. The guidelines that were selected to ask participants questions about were identified by Jakob Neilsen155 and Jill Gerhardt-Powals. 156
Participants were shown the website with the toolkit for the first time (naive) and asked to use the toolkit on their own without any prompts. They were then asked to read themselves the following questions, answering in an instinctive ‘think-aloud’ manner (facilitated by the researcher, with questions and responses spoken aloud and audio- and video-recorded):
-
Does the toolkit keep me informed about what is going on through appropriate feedback within a reasonable time?
-
Does the toolkit speak my language using words, phrases and concepts familiar to me?
-
Do I find that I make errors when using this toolkit?
-
If I commit an error, is the help that the toolkit provides clear?
-
How do I correct something if I make a mistake?
-
Do I have to remember a lot of information, or are prompts, etc., visible and accessible?
-
As I become more familiar with the toolkit, can I take shortcuts and achieve the same result?
-
Is the visual effect of the toolkit confusing?
Severity ratings
Severity ratings are useful in making clear to designers the magnitude of usability problems, and to allocate resources to address those problems. It is not advisable to release a system that has severe usability problems that are persistent, but one might decide to go ahead with the release of a system if the problems are cosmetic. 157
Participants were asked for information that enabled the research team to rate the usability problems encountered by participants:
-
The frequency of the issue: is it common or rare?
-
The impact of the issue: how easy is it to overcome it?
-
The persistence or recurrence of the issue: will users overcome the problem after they encounter it once, or will it be a continuing problem?
Participants were also asked to rate the severity of the usability issues that they encountered:
-
0 = I don’t agree that this is a usability problem at all
-
1 = cosmetic problem only – does not need to be fixed unless extra time is available on the project
-
2 = minor usability problem – fixing this should be given low priority
-
3 = major usability problem – important to fix, so should be given high priority
-
4 = usability catastrophe – imperative to fix this before the product can be released.
Goal-oriented evaluation
Following the heuristic evaluation, a goal-oriented evaluation was conducted. Task- or goal-oriented evaluations focus on the extent to which a system supports the goals or tasks set by users. This is a logical extension of a heuristic evaluation, but although a heuristic evaluation evaluates the usability from a user-interface perspective, a task-oriented evaluation examines whether or not the system actually helps users to achieve their goals or complete their tasks. 158
Participants were asked to examine the toolkit and ask themselves the following questions (facilitated by the researcher, with questions and responses spoken aloud and audio- and video-recorded):
-
What is my goal of using this toolkit?
-
What actions do I need to take to achieve this goal?
-
Is it obvious to me how to take this action?
-
What feedback do I need from the toolkit to achieve my goal?
-
Am I getting appropriate feedback?
To help the participants with this and other tasks in the session, the team members suggested that they imagine real goals that they might try to use the toolkit to achieve.
Implementation (Normalisation Process Theory) and diffusion of innovation
After the walk-through, the study team determined:
-
the relevant knowledge and skill set of the evaluators
-
the evaluators’ roles and reasons for potentially using the process
-
the evaluators’ ability to support others to do so.
For this, the team developed walk-through topic guide extensions to the main questions in the various walk-through components, including questions drawing on diffusion of innovations theory and network contagion theory, as well as NPT.
Normalisation Process Theory
The NPT provided a conceptual framework for understanding and evaluating the processes by which new health technologies are integrated and embedded (see Chapter 1, Theory). NPT has an additional practical value in that it can help us to understand what barriers there might be to new ways of thinking, acting and organising – if these can be overcome, there may be an increased likelihood of a new system becoming embedded in the health-care systems. 52,53
Drawing on NPT, participants were asked to imagine using the toolkit in practice and to think about its possible use within their teams when answering questions.
These questions were intended to explore the four main constructs in NPT:
-
coherence – sense-making work (meaning-making)
-
cognitive participation (commitment/buy-in)
-
collective action – enacting work (action)
-
reflexive monitoring – appraisal (formal and informal evaluations).
After piloting a questionnaire, and after discussion with Carl May (one of the originators of NPT and a study co-applicant), the study researcher modified the topic guide to make the questions more comprehensible for participants. This followed suggestions from May et al. 159
Participants were also shown the following:
-
the NPT toolkit [www.normalizationprocess.org/npt-toolkit/ (accessed 29 January 2019)] – 16 sliding-scale questions, responses to which are displayed on radar plots to show where additional work is needed to improve the implementation of a new intervention/way of working
-
the e-HIT (e-Health Implementation Toolkit) [www.ucl.ac.uk/iehc/research/primary-care-and-population-health/research/ehealth/resources/tools-accordian/e-hit (accessed 29 January 2019)] – 21 sliding-scale questions adapted from NPT for e-health and other interventions.
Walk-through participants were asked if these would be useful within the toolkit for staff to understand and reflect on how ensembles of social practices – such as interventions developed as a result of considering the free-text comments – can become routinely embedded and made workable in dynamic settings.
Diffusion of innovations theory
Diffusion of innovations theory seeks to explain technology spread. Everett Rogers160 proposed that four main elements influence the spread of a new idea: (1) the innovation itself, (2) communication channels, (3) time and (4) a social system. This process relies heavily on human capital. The innovation must be widely adopted in order to self-sustain. 161 People may be considered as innovators, early adopters, early majority (i.e. being among the first to adopt something once it has become mainstream), late majority and laggards. 160 Diffusion manifests itself in different ways and is highly subject to the type of adopters within the potential innovation pathway and the innovation decision process. Therefore, an individual self-assessment of participants’ adopter categories was included in this phase of the study.
Three types of innovation decisions have been identified:160
-
optional innovation decision – made by an individual who is in some way distinguished from others
-
collective innovation decision – made collectively by all participants
-
authority innovation decision – made for the entire social system by individuals in positions of influence or power.
Health-care professional participants were asked to assess what kinds of decision-making organisations they work in (see Figure 15). This helped us to consider the implementation and sustainability potential for the toolkit. It also provided context for the different responses in the walk-throughs.
Analysis
The data were analysed with respect to the different features of the toolkit, which provided the key ‘themes’. The purpose was to understand the usability and implementation of, and response to, the toolkit,162,163 rather than to develop or test theories164 or higher-order concepts. The study team thus charted the data; that is, features of the toolkit and implementation issues were the row labels of a table, whereas the columns of the table represented more granular themes. The table is not shown in this report as it was a working document.
Results
Participants
The plan was to recruit 15 participants, but because of time pressures, the study team stopped at 13 participants, hence not following up the six who had agreed to participate and were booked, but then cancelled. All participants were nurse directors and managers, and they came from across Wessex, London and Leeds. Table 12 contains information on those recruited.
Professional roles that the study team planned to recruit from | Locations | Gender | Total completed |
---|---|---|---|
Lead GPs | London, Leeds and Southampton | One male, two female | 3 |
Ward managers, nurse directors | London | One female, one male | 2 |
Associate directors, lead clinicians, trust board members | Southampton and Leeds | Two male | 2 |
Lead commissioners in CCGs | Southampton | One male | 1 |
Lead members of MDTs | Southampton, London and Leeds | Two female, one male | 3 |
Other – data analysts in NHS Improvement (A&E), patient experience leads | Southampton and London | One male, one female | 2 |
Total | 13 | 13 |
Heuristic evaluation of the toolkit
A summary and solutions implemented in the study-end toolkit prototype are shown in Table 13. In terms of usability, all participants found the toolkit simple and ‘easy on the eye’, and liked the visual summary, with the further ability to see actual comments sorted into themes. But most participants found the infographic dial in the prototype uninterpretable. This is being changed in the final prototype to bar charts. Although visual simplicity was considered an asset, all participants wanted more navigation tools (e.g. select all, back, clear all, keyword search and help/prompts available on the dashboard, not on a separate page).
Questions | Responses | What the study team did |
---|---|---|
Does the toolkit keep me informed about what is going on through appropriate feedback within a reasonable time? |
Modal response: yes Participants commented that the toolkit is fast, loads immediately, no timer symbol needed |
No change needed |
Does the toolkit speak my language using words, phrases and concepts that are familiar to me? |
Modal response: qualified yes Severity: 2. Minor usability problem: fixing this should be given low priority Most agree that it does in terms of filter group and theme names; however, some pointed out that filter choices could be refined Specific examples: |
Although low priority, theme definitions had already been written, which were added Filter names could be easily changed within the HTML, but should be considered only once the system goes live, as they need to correspond to the current questions in the survey Referral and diagnosis were combined by participants in the group consensus-mapping workshops and have been left combined |
Do I find that I make errors when using this toolkit? |
Modal response: qualified yes Severity: 2. Minor usability problem: fixing this should be given low priority Most concur that it is quite intuitive, easy to navigate and select/unselect filters, but wanted more navigation tools such as going back, selecting all, clear all |
Back buttons are not generally used with modern browsers; although they could be added, the study team chose not to. More intuitive select-all and clear-all functions were added |
If I commit an error, is the help that the toolkit provides clear? |
Modal response: no Severity: 2. Minor usability problem: fixing this should be given low priority The majority said that they could not tell if they had made an error or if it was even possible to make an error Several commented that the ‘Help’ page was too wordy and not in the right place, as the last page on the website: help, FAQs and instruction prompts need to come earlier (e.g. on the ‘Welcome’ page or as hover-over options actually in the toolkit) |
Although low priority, this was easy to change, so the study team did |
How do I correct something if I make a mistake? |
Modal response: no Severity: 2. Most could not see that mistakes are possible, but suggested more navigation functions to address this scenario: all, back and clear selections |
Amended as above |
Do I have to remember a lot of information or are prompts, etc., visible and accessible? |
Modal response: qualified no Severity: 2. Minor usability problem: fixing this should be given low priority Participants agreed that it is intuitive; however, a few commented on the lack of prompts: ‘There are no prompts at all. That’s one thing that is needed’ |
The study team believes that the ‘video tour’ will help to address this and other comments |
As I become more familiar with the toolkit, can I take shortcuts and achieve the same result? |
Modal response: no Severity: 3. Major usability problem: important to fix, so should be given high priority Most participants were not able to find shortcuts (e.g. being able to access a comment within a theme by clicking on one of the dials from the Overview screen. One participant suggested that it would be good to be able to find certain comments again at a later date – might require identifiers – this would be important when UK-wide data were entered) |
Amended shortcuts Identifiers are possible |
Are the visual effects of the toolkit clear? |
Modal response: no Severity: 4. Usability catastrophe: imperative to fix this before product can be released Although there was unanimous agreement that the site is clear, clean and ‘very easy on the eye’, there was near unanimous agreement that the ‘dial’ infographic was neither interpretable nor useful. Most wanted a clear visual summary, such as ‘RAG’ coding for negative, neither/unclassified (not included in the graphic) and positive; and proportional representation of denominator and respondent numbers (actual and percentage) fitting rating groups. Many said that they prefer traditional graphs, such as pie charts or bar charts, over dials, simply because these do not require extra time to work out how to interpret them, therefore rapid assessments can be made ‘at a glance’. There were also negative comments about the images and wording in pages before and after the toolkit (too wordy, inappropriate photos, logos not in the right positions, management tools not needed, links that could be added) |
Option to choose graphics is being implemented |
Two senior clinicians and a Macmillan Cancer Support lead observed that it might be hard to reconcile the differences between performance targets and patient experience – being good at one does not necessarily equal good feedback in the other. They commented that a manager can be ‘sacked’ for poor performance but not necessarily for poor patient experience, and that major improvements may require long-term, whole-culture changes.
When participants were asked to rate the severity of problematic issues they found during the heuristic evaluation, most agreed that the prototype was not market ready; infographic (dial) re-design was needed for simple interpretation and the navigation tools needed improvement; otherwise, the system was highly desired. Some of these issues were subsequently addressed.
Wider website design comments were also made. Most participants suggested the following: change the images on the ‘Welcome’ page; reduce the wordiness of the ‘About’ page; the ‘Background’ page could include a summary of data collected from the CPES that was used to populate the toolkit and key findings; instructions on how to use the toolkit are needed; further links could be to the CQC, CCGs, National Institute for Health and Care Excellence (NICE) and other key organisations, along with CPES qualitative summaries, Cancer Research UK (CRUK), NHS and other key cancer resources that may be useful to service improvement. Many of these had already been identified by the concept-mapping workshop participants (see Chapter 6), and most were implemented in the final prototype.
Goal-directed enquiry: can the toolkit fulfil the working needs of health-care professionals?
All participants considered that the toolkit had the potential to help them achieve goals in service improvement, justify requests for funding/designing new initiatives, gather data to show successes (and boost morale), support appraisals and support the reporting of service data (although an export/report output function is needed). Once these were addressed, all participants agreed that the system would ‘revolutionise’ the way in which patient free-text comments could be used in service improvement. Results and solutions are summarised in Table 14.
Questions | Responses | What the study team did |
---|---|---|
What is my main goal in using this toolkit? | Participants identified that the primary aim is to identify areas in which improvements are needed in a timely and easy-to-understand manner, and to use this evidence to make business cases for funding. Many would also want to use the toolkit to find out what is going well, to let patients know and boost staff morale. A few said that this could also provide useful information for appraisals. Some mentioned that they would like to know that patients see that their comments are being used constructively | N/A |
What actions do I need to take to achieve this goal? | Participants want to see an overview ‘at a glance’ and be able to ‘drill down’ into the specific comments. Some participants (mostly female) were not so interested in the infographic as they were in the comments themselves, whereas others (mostly male) said that they would first focus on the infographics (because of time pressures). The majority would need some export or reporting function to use the results that they find (for meetings and other documents), as well as theme definitions, expansion of filters, better navigation and help/prompts. Some pointed out that it would be useful to be able to identify comments so that they can be found easily again |
Theme definitions were added as always planned, filters were expanded, navigation and help prompts were added; see the second row in Table 13 Export or report functions were included in the first prototype, but are currently removed as a result of GCP issues with resharing the data in ways in which the patients who completed the CPES had not agreed to When large numbers of data are analysed and a user wants to keep a note of comments to use in service improvement, the study team agrees that identifiers would be useful. These have not yet been implemented. Customised reports would be helpful |
Is it obvious to me how to take this action? | Most said ‘yes’ to ‘at-a-glance view and more exploration of comments’ (when improved), but ‘no’ to export/report or print-friendly options | The help pages tell users how to print using the web browser |
What feedback do I need from the toolkit to achieve my goal? | Some suggested that it would be good to be able to see comments at a more specific ward, practice or practitioner level to focus remedial efforts. Some said that it was hard to see how comments currently related to themes, as the whole comment was included, not just the sentence related to the assigned theme. However, others said that this was important for context (see Chapter 6). Several expressed the need for the system to be able to process patient comments from other sources (the Friends and Family Test, etc.) to be able to access summaries of all patient feedback in one place |
Providing data at these levels may be easily added if NHS England agrees and the data are provided at this level of granularity. This option is not currently provided An upload feature for other free-text survey comments was included in an early prototype; this would be easy to read as needed |
Am I getting appropriate feedback? | Most participants said that the themes, filters, actual comments and clarity of view gave most participants exactly what they need to be able to work within service improvement – as long as there are not long delays following CPES data collection. A couple mentioned the tension between patient experience and hospital performance targets – how do they connect? | The process takes from hours to minutes to do, depending on the size of the data set and the local computing capacities. Thus, it is appropriate for real-time use. However, the CPES is collected annually and QualityHealth takes months to enter the data from hard-copy surveys and clean it, redacting personally identifying information. The study team has no control over this delay |
Implementation (based on Normalisation Process Theory toolkit questions)
The majority of participants do not currently have an efficient or reliable method for using patient experience free-text comments and would support the implementation of the present toolkit. Equally, the majority believe that their teams/organisation would also support the use of such a system, and would see the purpose and value of it. Better use of patient-derived free-text experience needs to be made, and the toolkit could enable this. Most participants could imagine, and had examples of, service-improvement initiatives as a result of qualitative data from patients and were aware of the methods for assessing the impact of such initiatives. Many participants are overwhelmed by the many local systems to use patient experience and would like to see one system that could process all free text from several different sources.
The implementation data are summarised in Table 15. There was some overlap in concept between the coherence construct and goal-directed questions summarised in Table 14, in which several more explicit actionable points are listed. The main implementation barriers and issues identified were time, absence of run charts, being overwhelmed already with online systems, password protection if used, data misuse problems, the possibility that the system would lead to ‘easy wins’ and neglect more deeply rooted problems, and a lack of funding for the system. Enablers were mentioned less frequently, but included the use of local champions, the timely use of data and the possibility of comparing data year on year. The study team did not explore deeper implementation issues that might be important to ensure uptake and sustainability, as participants were not presented with an implementation plan to discuss; this was the result of governance issues mentioned earlier, which made this premature. As a result, the study team was not able to maximise the potential of the questions here.
Questions | Responses | What the study team did |
---|---|---|
What do you currently do to analyse survey free-text comments (if anything) and is the toolkit very different? | The majority of participants said that current practice is the manual collation of hand-written patient satisfaction comments. A few said that they do nothing at all, and a few used other systems; those mentioned were ‘I want great care’, the Friends and Family Test, Real Time Patient Feedback System (available at only four London hospitals) and NHS Choices. All participants agreed that the PRESENT toolkit system is very different | N/A |
What do you see as the purpose of the toolkit – how worthwhile is it and does it have any value to you? | All participants can see that the purpose of the toolkit is to use the patient experience in service improvement | N/A |
Do you think that the toolkit should be used as part of your work? | There was unanimity that the patient free-text experience data should be used, and this system could ‘revolutionise’ ways of organising data that are collected but not currently well used. It could also be useful for sharing learning from other hospitals (e.g. best-practice visits) | N/A |
What are your personal barriers to using it? | Every participant said ‘time’; however, this system offers the promise of saving time. Some said that the infographic dial took too long to interpret. If text sensitivity and labelling is incorrect, this would impede use. Similarly, patients need to express themselves clearly, otherwise feedback on the toolkit may be difficult to interpret. One participant asked who will fund/host this after the study finished, which might become a barrier to use |
The toolkit idea originated precisely because NHS managers have so little time The dial was changed (see above) Test sensitivity and specificity are reported in the toolkit The ‘market model’ needs to be worked out, but the study team intends to make it accessible |
Do you think your team will think it’s worthwhile – do they think that free-text comments should be better used? | Modal responses include ‘absolutely’, ‘definitely’, ‘no doubt about it’. However, some participants mentioned feeling ‘overwhelmed’ by so many systems to collect patient experience currently. Most want one system to process patient experience from different sources | The study team originally included the potential for users to upload other data (but see the DCE in Chapter 7) |
Would you trust the toolkit and other’s work using it – can you see people misusing it? | The majority of participants point out that this would be no different from any other system in terms of possible data misuse. Users could extract only data that would serve their purposes. The main trust issue concerns the need to be sure of the validity of the categories assigned to groups of comments |
Selective use is one reason that the toolkit does not enable a ‘report’ or customised printout/export facility Test sensitivity and specificity are reported in the toolkit |
Who would actually use this? Should there be different levels of access? | Participants all agreed that there should not be different levels of access and that transparency is key. ‘CPES is in the public domain anyway – why restrict access.’ Different levels of management may have different needs, but even so, everyone should have access. If too heavily password protected, one participant made the point that this may put health-care professionals off from using it |
During development, the study team moved away from a registration process, but passwords would be necessary if hospital or more fine-grained data are available Currently, the public cannot have access, as CPES respondents never envisaged this when they signed for consent |
Do you think that the use of the toolkit might be supported by your team – and by the organisation? | Modal responses were ‘absolutely’, definitely and ‘this data is invaluable’. Provisos included cost; data need to be provided in a timely manner; the dial needs to be improved first | See above |
Do you think that it will need a champion to allow the toolkit to be used in practice? | Participants were almost equally divided on this; some believe that users will decide for themselves after trying the system and some believe that a manager or the CCG needs to promote use. One person felt strongly that a champion is needed to use it and embed the use of it properly, citing the case of the Liverpool pathway as a really good tool that wasn’t used properly | Further exploration of implementation into practice is outside the scope of the study |
Would the toolkit encourage you to make better use of the free-text comments you receive from patients? Would you be able to implement any changes suggested by the free-text comments? Is the toolkit likely to support this (e.g. by giving data you can use)? | There was a mix of responses to this group of questions. Some believe that the themes would enable targeted improvement initiatives underpinned by good summaries of patients’ views. Others believe that the toolkit would be useful only if the data were timely, if you could drill down to local issues and not use it for ‘easy wins’ (one participant suggested that easy fixes could give better scores the following year). One participant suggested that evidence from the toolkit could be used to engender longer-term cultural changes | N/A |
Will you be able to access information about the effects (impact) of the toolkit? Will you be able to measure the differences it makes? | Most participants identified yearly audits, national benchmarking, annual toolkit results or other ‘run-chart’ over-time methods as useful impact measures of new initiatives. Some transformational changes require longer-term complex monitoring. Participants who currently use rapid, locally collected patient experience data felt that the effects of new initiatives could be gauged easily by follow-up local surveys | Run charts can be implemented into the toolkit once several years of data are accumulated (see Chapter 5) |
Usefulness of the Normalisation Process Theory
The NPT was helpful in determining how the toolkit might be used in practice and what adaptations it might need to enable this, but most participants felt that the NPT tool or e-HIT were not helpful in evaluating the potential workability and integration of interventions developed from the toolkit. A few participants felt that they would ‘add unnecessary extra work’. However, some participants thought that either might be useful for big projects and teams; they had seen NPT before, and were aware of organisations using it, although they would prefer bar chart output for ease of interpretation. One participant thought that the NPT radar output was similar to the 360-degree appraisal tool (see Appendix 7 for more quotations).
Diffusion of innovations
In the consideration of the diffusion of innovations, the study team focused on typing participants, as the use of NPT was intended to cover many of the other constructs in this theory. The intention was to follow-up the current study with an evaluation of implementation into practice once an implementation model had been developed. Most participants assessed themselves as innovator/early-adaptor types of manager (Figure 15). One participant assessed their organisation as having individuals who can innovate-adopt: the majority of participants worked in organisations where there was overarching authority or collective decision to innovate-adopt (Table 16).
FIGURE 15.
Diffusion of innovations types. Tech enthusiasts – the extreme tail of the curve (n = 2); bordering tech enthusiast and visionary categories (n = 4); range all the way from tech enthusiast to pragmatist – depending on what the work is – one of these said that they were also conservative at times (n = 3); bordering visionary–pragmatist (n = 2); visionary (n = 1); and pragmatist (n = 1).
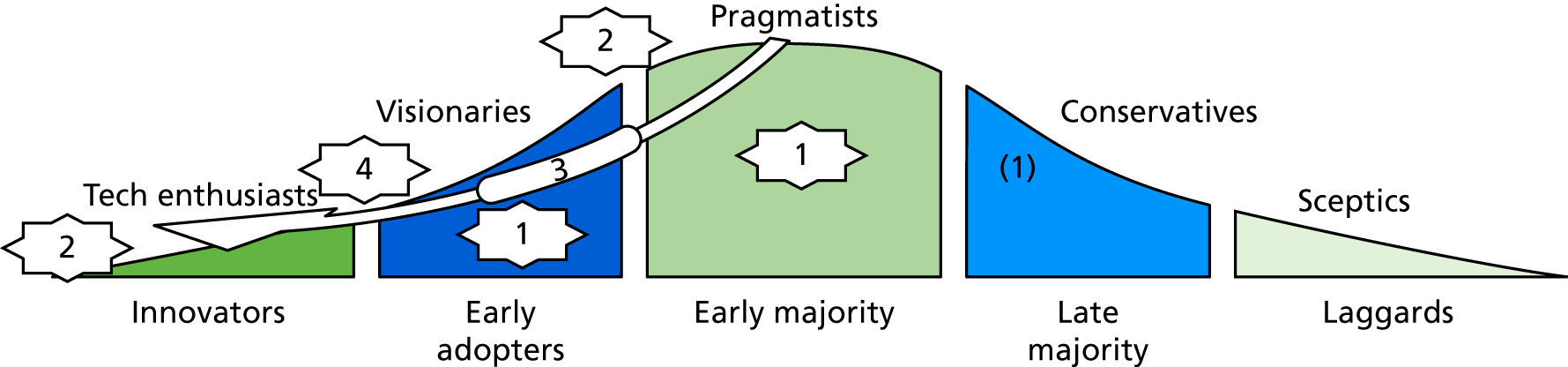
Organisation type | Definition | Number of participants | |
---|---|---|---|
Optional innovation decision | Made by an individual who is in some way distinguished from others | 1 | |
Collective innovation decision | Made collectively by all participants | 5 | }2 |
Authority innovation decision | Made for the entire social system by individuals in positions of influence or power | 5 |
Discussion
Overall, the toolkit evaluation approach that the study team used was successful in highlighting candidate areas for toolkit improvement. Its main strengths were that it enabled potential users to comment freely, to identify usability issues and to suggest solutions. This process will help to ensure that the toolkit is both usable by individuals and that it should meet their particular needs. Moreover, the methods that were used to surface any usability issues are structured methods, and are widely used in the industry. The fact that the study team was able to use them successfully in a health-care context is a contribution to the practice of system design in health care. Many changes were implemented as a result of this evaluation; some cannot be implemented until the system becomes live, as a result of the need for permissions and other current constraints.
The process used also highlighted weaknesses with current practices, and the need for and constraints to using systems such as this. There was wide recognition that patient-generated experiences (i.e. the free text delineating those experiences) should be used and that these can be more informative than quantitative data, to both patients and those providing care, in identifying areas where specific improvements need to be made. It was also agreed that current systems are slow, labour intensive, inaccurate and unstructured, and that with some small improvements – most of which the study team subsequently made – this approach could revolutionise patient experience free-text use.
Participants were nonetheless aware of the limitations of using data such as these, including the potential for misuse of the data (e.g. by selective reporting), and the need to demonstrate the accuracy of the analysis. The toolkit was seen by participants as not only providing information on patients’ experiences, but also highlighting where there were gaps in the knowledge as a result of non-responder bias, for example, when considering the ‘Sample characteristics: demographic overview’ page. A concern was raised that trusts could use the toolkit to achieve ‘quick wins’, but the unpredictable nature of patient-generated free-text comments could mitigate this. In any case, small nudge-type changes as a consequence of using the toolkit could have an important effect. 165
The NPT proved useful in structuring implementation questions, as expected. However, there were limitations in what the study team was able to explore with participants, because they were not presented with an implementation model, but simply the toolkit to trial. The original plan had been to link implementation into the NHS Cancer Dashboard, with the roll-out supported by Insight NHS England, but governance issues meant that the study team had to postpone this and, therefore, has been unable to explore it, which had a knock-on effect. At a meeting held in October 2017, Insight NHS England promised to consider the study in 2018, along with other studies in the same NIHR call (which explored other aspects of the use of patient feedback data).
The lack of enthusiasm for NPT or e-HIT links within the toolkit, as a way of enabling consideration of the workability of hypothetical future interventions, was not surprising, as this was not a use NPT or e-HIT tools had been developed for. The original plan, to link NPT radar plots to specific related themes was also not successful in earlier versions of the prototype. NPT has, however, been shown to be very useful when interventions are developed,52,53 and it is likely, therefore, that the use of NPT was attempted too early in the potential intervention development process. The diffusion of innovations theory provided a more general overview of the cultural issues of introducing technologies into the NHS workplace. Being self-assessment, this is, however, potentially open to bias, as people may have wanted to appear to the researcher as being more open to innovation than they actually are. Conversely, there may have been selection bias – the team may have recruited mainly innovators.
The main limitation of the approach was that the study team considered only the health-care professional version of the toolkit; however, the UAG commented that public users would use the toolkit only in a non-systematic, ad hoc manner that made this evaluation process unsuitable for them. In the initial study design, the team suggested methods for the evaluation of public use that could be incorporated into an evaluation of the system once it was ‘live’ (whether nationally or at selected sites for further evaluation and refinement). As a further possible limitation, only 13 participants were recruited. However, this is still a large number for this type of study,154 and it was not felt that new information was emerging from the last two completed interviews, so the team did not believe that this affected the usefulness of the data. It would also have been useful to have more commissioners, especially for the goal-directed questions, although sufficient participants were recruited in order for the study team to be confident about the validity and reliability of the evaluation.
How this contributes to knowledge
Heuristic evaluation and goal-directed design are validated structured methods that are widely used to uncover problems in usability (user interface design), and whether or not systems achieve user goals. They have not been used systematically in a health-care context before to the study team’s knowledge, and the study has produced evidence that the method is relevant and useful in this context. This is important because one of the major faults in system design, and especially in implementation in health-care settings generally and in the NHS in particular, is that although standard requirements analysis informs design, the experience of the user and the relationship of the system to the goals that the user wants to achieve are often, if not always, missing. This design and evaluation process is fast and inexpensive, and it uncovers potentially fatal flaws before they ever reach the workplace. Overall:
-
The process of evaluation with users when the designers were not present increased the users’ capacity to highlight errors and, in effect, co-design the system. This was a low-cost way of doing this, and it achieved a lot in just a few hours.
-
The discussions, added to the data from the group concept-mapping workshops and associated interviews, provided novel information about big data text processing and NLP, and how that information might be applied in future projects.
Chapter 10 Patient and public involvement
Patient and public involvement in health-care research is increasingly required by funding bodies. It was also an important part of the study, and so it is discussed in depth in this penultimate chapter. The chapter describes the effectiveness of PPI in the development of the technology-based complex intervention, a topic that might seem to be especially daunting to lay involvement. The challenges and issues, as well as the successes and the adoption of values and principles core to PPI work, are described and reflected on.
Introduction
Patient and public involvement is recognised as being important to health and social care research, in enabling researchers to take into account and incorporate the needs, wishes and experiences of those people whose health and well-being their research is intended to benefit. Brett et al. ,166 for example, in a systematic review, cite the advantages as ranging from more appropriate research objectives to more effective study recruitment and dissemination of findings. Staniszewska et al. 167 specify the benefits of improved quality, relevance and appropriateness, and the broader democratisation of research. As a consequence, PPI increases the likelihood of the effective translation of research findings and outputs into practice and their sustained use and benefit to patients and the public, as well as enhanced transparency, accountability and public ownership of the research. These are important factors in NIHR-funded research, which is supported by public money, and so NIHR, along with other UK funding bodies and research ethics committees, actively encourages PPI. 168 Significantly, in 1996, NIHR established INVOLVE as a national AG for PPI in health-care research.
Patient and public involvement was particularly important to the study, as this aimed to transform patient experience survey free text into a form that could drive health-care improvements through automated computer analyses. Including PPI throughout ensured that the study remained true to the purposes of the data, did not distort the patient voice and produced results that would improve the service experience per se.
Two projects funded by NIHR underpinned the consideration and the use of PPI in the work:
-
the ReseArch with Patient and Public invOlvement: a RealisT evaluation (RAPPORT) study,169 which resulted in an inductively and deductively derived list of the features of good PPI, using NPT to structure the analysis, just as NPT runs through this study
-
the framework of values and principles developed by INVOLVE. 168
The study team also followed the recommendations from Staniszewska et al. ,170 and the GRIPP (Guidance for Reporting Involvement of Patients and Public) checklist171 in this report.
Type of involvement
Tripp172 developed a typology of possible roles for PPI representatives, lying on a continuum from researcher in control, through shared control, to users in control. This makes plain the potential for PPI representatives to be involved in a range of activities from consenting, consulting and co-operation through to collaboration and collective action. The PPI in this study fits the consulting and collaboration roles and occurred at all stages of the research. It centred around group discussions of items supplied by the research team, preceded by updates on the progress and details of the study. On members’ request, a mailing group was established to enable communication outside the meetings.
Summary of involvement opportunities
Carers and patients were key partners in the research. Patients had cancer or had undergone major surgery for a condition different from cancer. This mix reflected both the study’s focus on a cancer survey, and the study’s commitment to making the approach transferable to other condition-specific and non-condition-specific surveys. The study team specified surgical non-cancer patients because the CPES includes comments across primary and secondary care, and this was a simple way of ensuring the same spectrum of experience across all members.
A PPI member was included as a co-investigator on the grant proposal, and two other members named in the proposal as lay PPI representation with roles on the SSC and AG. These three PPI representatives, who were all patients with cancer, helped to shape the research proposal and protocol through informal meetings and correspondence and consultation with other patients.
The study team also established a separate PPI reference group, referred to as the PPI Research Group (PPIRG), which emphasised its active role. Team members were in e-mail contact with members of the PPIRG throughout the early stages of the study. The PPIRG subsequently met approximately every 3 months over the last 10 months of the study; thus, there were four meetings in all. SSC and AG meetings always included a PPI agenda item.
The study team had intended to make use of the NIHR Research Design Service ‘Building Research Partnerships’ scheme to train PPIRG members, but the Research Design Service did not run a training event during the relevant time period. Macmillan Cancer Support had also stopped running their similar training programme, but all PPIRG members were invited to use its course content, which is available on its LearnZone web page [http://learnzone.org.uk/ (accessed 29 January 2019)] after free registration, and Macmillan Cancer Support also offered the use of experienced facilitators to optimise the use of this training. In the event, no PPIRG member wished to take up training; most already had some experience of PPI or similar tasks from work on other research projects or charity involvement.
At the final meeting, stakeholders were asked whether or not they wanted to continue being informed about the study. They stated that the study team should hold a feedback event 1 year later, so that they could determine the impact of their involvement.
Who was involved?
The study chief investigator attended all but one of the PPIRG meetings, which the lead programmer attended instead. The lead researcher attended all meetings and took notes, which she subsequently distributed to all PPIRG members for checking, amendment and finalisation. Only two research team members were included at meetings to ensure that PPIRG members were in the majority.
In addition to the research team members, the first meeting was attended by five patients, the second was attended by six patients, the third was attended by three patients and the fourth was attended by two patients. Meetings 1 and 3 took place at the University of Southampton and meetings 2 and 4 took place in central London.
Setting up the group and first meeting
The PPI co-applicant volunteered to recruit group members once the study was funded. However, because of time constraints, geographical distance and ongoing obligations to another study, this patient soon decided to withdraw his involvement. As a consequence, the recruitment strategy had to be revised. This also delayed the start of the PPIRG’s work. As he was the key PPIRG co-ordinator, for practical reasons, he had to be replaced in this role by the lead researcher. The lead researcher turned to the co-applicants, clinical networks and members of the advisory and steering groups that had already been set up, and asked for their support in reaching patients. A call was published on Macmillan’s Volunteer Village and Task Force Groups, as well as in the South East Coast Strategic Clinical Network Bulletin. Twelve patients responded, expressing their interest in joining the PPIRG. At this time, one of the steering group PPI representatives pulled out for health reasons and could not be replaced.
A Doodle poll (Doodle, Tamedia, Switzerland) was used to fix a suitable date for the first PPIRG meeting, which took place later than intended, 7 months into the project. Nonetheless, during this time, the three PPI representatives named on the proposal provided bridging input. This delay was only partly attributable to the recruitment issue and included the taking account of members’ summer holidays; some members were committed well before the first official PPIRG meeting and were invited to the study launch 3 months beforehand.
The PPIRG’s terms of involvement were agreed on at the first meeting, typed up by the research team and circulated among all members of the PPIRG for review. Further changes were incorporated.
To maximise attendance, dates were always agreed on using a Doodle poll. PPIRG member feedback on arrangements led us to alternate meetings between the University of Southampton and central London. This was agreed by members as being likely to give everyone the opportunity to attend at least half of the meetings. Skype™ (Microsoft Corporation, Redmond, WA, USA)/telephone contributions were also enabled throughout. Each meeting began with a summary of the study aims and a full update of progress from inception to ensure that all members were fully informed, regardless of previous participation. In all meetings, presentations included examples of the developing text-analytics and toolkit work, including interactive demonstrations and encouraging the input of ideas and comments.
All expenses incurred by the PPIRG members in attending the meetings were reimbursed; these were usually travel expenses and refreshments en route, but in one case it was agreed to pay for hotel accommodation. Lunch and refreshments were always provided.
Throughout the study, the team adhered to INVOLVE 2015 values.
Launch, infographic, newsletters, blogs and social media use
A talk by a cancer service user was a highlight of the study launch event and the final event, and the study team included informal workshops designed to help lay people to understand the more complex aspects of the study. This included an art workshop, a shared decision-making session in which teams were asked to design a PPI meal event and a hands-on workshop on what made for good infographics.
Prior to the first PPIRG meeting, the research team collaborated with a graphic designer to develop the study’s ‘storyline’ – an infographic resource to be used by PPIRG members, displayed during public dissemination events and placed on the website to explain the different stages of the study in an accessible way. This was circulated among the PPIRG members and discussed in the first meeting. The group emphasised the need for a clearer orientation. It was also argued that the images were trying to convey too much information at once and did not clearly indicate what was at the heart of the study or how it worked as a whole. The members also suggested a number of changes to the wording and visual improvements to particular elements of the images in order to increase clarity. It was also suggested that the word ‘toolkit’ may not be familiar to patients and should therefore be replaced by ‘website’. The feedback was shared with the designer, who worked on incorporating it into the storyline. This discussion helped to develop shared understandings between the researchers and the PPIRG members and was considered to be very rewarding.
To update all of the people involved or interested in the study about its progress, a PRESENT study Twitter account was created. It was used to share news about the research, publicise events, such as the study launch day, report on relevant conferences and seminars that the study team attended and link the study to other research in the area of health technology innovation or patient health-care experience. A Facebook (Facebook, Inc., Menlo Park, CA, USA; www.facebook.com) profile was created but abandoned, as it did not get any followers. The study team considered writing monthly newsletters, but opted instead for blogs about significant events, such as the participation in a group concept-mapping workshop in Sweden, the progress of the study’s workshops or talks and presentations given. It was felt that the combination of a blog and tweets would be a more rapid, engaging and manageable strategy than monthly newsletters, and blogs could still be printed out.
Impact of patient and public involvement
Two PPI members helped us to develop the study design and proposal. They:
-
Suggested improvements to the lay abstract, including SMOG (Simple Measure of Gobbledygook) testing.
-
Improved the study focus, which was initially too technology heavy; this had the potential to negatively affect the involvement of patients in the study itself and reduce the quality of outputs.
-
Simplified the study design; originally, group workshops were planned to process the outputs from the rule-based IR analysis using mindmapping scenarios and then more workshops were planned to undertake a separate concept-mapping task. The study team realised from PPI input that the tasks could be simplified and combined in one large workshop. This proved to be successful.
-
Strongly supported the plans to involve patients in the design of GATE rules because of their concerns that computer replacement of manual analysis might be detrimental, removing the patient voice. The validation stage was thus developed in accordance with a manual gold standard, partly to address their concerns.
-
Suggested that rule ideas (see Chapter 3) should be developed remotely through surveys (the study team had originally planned to hold a workshop). This had the further advantage, subsequently realised, of making the process easier to adopt by other groups wishing to use the study’s model process for other topics.
Within the PPIRG meetings, the group made several important contributions, including making the research accessible to the public, recruiting study participants, keeping the study’s focus on the patient experience and collaborating on the initial development of the toolkit prototype for discussion in phase 2 of the study. They also helped us to make sense of the research findings.
During the first meeting, the overall purpose of the toolkit was discussed, along with early ideas for its features. The group emphasised the importance of using simple language and diagrams, employing illustrations and large fonts on highly contrasting backgrounds to ensure that the toolkit was visually appealing and accessible, including for people with English as their second language. Suggestions were made with regard to themes (such as emotional support) that should be included in the toolkit and useful filtering options, such as sorting the comments by gender, age and the ethnicity of the author. The group also stressed the need to include an up-to-date list of websites and charities offering information and support. A few of the members expressed that they would like to be able to input their own feedback to contribute to the comments retrieved from the surveys, in an interactive forum, and to be able to track their own CPES comments to ensure that the rule-based IR system classified them correctly. This was ultimately decided to be outside the scope of the study, as the group agreed that it would require active facilitation for which the study team did not have funding.
During the second meeting, suggestions were made about introducing a word-search box to extract comments and changing the colours on the RAG-style dials. An initial design for the toolkit theme icons was presented and the team was advised that alternatives should be presented for stage 2 research participants to consider. This early PPIRG feedback was extremely useful for preparing the materials and topic guides for stage 2, in which user preferences would be explored via group concept-mapping workshops and associated interviews and surveys.
The group played an important role during the recruitment for stage 2. This included revising the flyer used for concept-mapping workshop recruitment to make it more engaging for patients and circulating the flyer among their networks. The study team also received useful comments on how to run the workshops. As a consequence, the number of comments the participants were to sort and rate in these workshops was reduced from 80 to 60, as the former was perceived to be too cognitively demanding.
Issues and challenges, with Patient and Public Involvement Research Group feedback and research team reflection
The withdrawal of the co-applicant patient and the subsequent need for a new recruitment strategy led to considerable delay in the setting up of the group. One of the participants withdrew before the first meeting as a result of ill health. There was a core group of seven patients who were very active and contributed to most of the meetings. Of the remaining five, one could not get involved until the last 5 months for personal reasons. The rest made no contact or contributions beyond their initial agreement to sit on the PPIRG, despite receiving meetings invitations, agendas and minutes. One issue was around compensation for time – all members were offered the INVOLVE recommended rate of £125 per day. For some potential PPI contributors, this was too big a stumbling block, as it would affect their disability allowance or other benefits. On the other hand, had the study team developed a suitable alternative and emphasised this to potential contributors, engagement might have been better.
The SSC and AGs were instrumental in facilitating recruitment for the PPIRG. Members of both groups circulated the call among their networks. Macmillan Cancer Support published the call on the Volunteer Village and forwarded the information to the leaders of its task forces and strategic lead. The AG offered useful advice on how to run the PPIRG meetings effectively. The group recommended that the materials shared with the PPIRG were rich in engaging visuals and clear, jargon-free language. It also asked the study team to carefully consider how the PPIRG might contribute to the study and to ensure that the tasks and questions for the group were set out clearly.
The majority of the members were already sitting on various other PPI or charity groups, and were thus a select group. The two members who were not affiliated with other groups were incidentally the ones who withdrew for health-related reasons and a change of circumstances. One of these patients doubted that she would be able to make a valuable contribution, given her lack of experience in PPI. She was reassured by the research team, but lack of confidence could have been a potential barrier in the recruitment of patients not already involved in PPI. Nevertheless, the feedback from the group members was that the group included people with a wealth of different experiences, knowledge and backgrounds.
It was found that some of the members had priorities based on their involvement with other organisations, and on their personal, sometimes upsetting, experiences as patients. They would sometimes pose expectations that lay outside the scope of the project. The group felt let down by the fact that patients will not have access to the original free-text comments on the toolkit. The members felt strongly that the public must have access to the feedback patients offer in PESs and made recommendations that appropriate consent procedures should be put into place to enable this.
Practical challenges were experienced in ensuring inclusion (explaining jargon, talk time in meetings and help with technical aspects). One of the members pointed out that some operational details of the toolkit became clear to him only at a late stage. Although the team members always tried to ensure that they explained the study in lay language, using visual materials to support the discussions, this feedback highlighted that the team members could have improved on this by undertaking a more adequate review of PPI members’ understanding of the study earlier on. It was sometimes easy to forget that PPI representatives were not colleagues but volunteers.
The overall feedback that the study team received verbally, on feedback forms and as solicited e-mail feedback, was very positive. The members felt that the meetings were well organised, with the materials circulated well in advance. Most importantly, they also pointed out that they felt that their contribution was always valued and acted on, as the group saw changes that they suggested being incorporated into new materials from meeting to meeting.
Discussion
Given the importance of co-design and the development of the toolkit with its users at the centre, it was felt that it was key that the research was understandable to the wider public. The PPIRG was imperative in helping us to achieve this aim by supporting us in the preparation of the public-facing materials and in the preparation for the group concept-mapping workshops. The group also played a key role in the recruitment of both patients and professionals for the study’s workshops and interviews, and designing the toolkit in such a way that the patient voice remained. Overall, it was found that PPI was useful in the development of this technology-based complex intervention. The PPI members also evinced appreciation of the efforts to determine their needs and satisfy these, the information provision and the transparency with which their contributions and inputs were used.
Engagement and contributions were good, and the study team followed the core values and principles of good PPI work. More could have been done, however, as some patients who expressed an interest failed to engage. The original facilitator, who had to drop out, might have helped us to resolve some of the challenges (Table 17).
What worked well | What the study team would do differently |
---|---|
Detailed planning | Vary the meeting formats |
Respectful support for stakeholders | Independent patient facilitator |
Negotiated terms of involvement | Determine suitable compensation with patients before start of the study |
Provision of engaging visual materials and examples illustrating the progress of the study during the meetings | Recruit more patients with no involvement with other PPI groups |
Keeping members up to date via Twitter and the study blog | Check if the members have a clear understanding of the study objectives, for instance, by asking them to highlight these in their own words |
PPI members involved at different stages of the study including the development of a grant proposal, designing dissemination materials, participant recruitment and initial development of the toolkit prototype |
Chapter 11 Overall discussion of findings and outputs and their strengths and limitations
The study team set out to achieve the following, and has been successful in completing all stages within the original projected timelines.

This section begins by considering how well the study team met the original brief and discussing the more innovative aspects of the study’s work. This is followed by subsections that consider the processes and outputs from the different parts of the study, and their benefits and challenges, and strengths and limitations. The chapter concludes with a consideration of the work that remains to be done and the possibilities that the study’s work has foregrounded.
Responding to the brief
Improving the use of patient feedback data
The NIHR call was intended to improve the use of patient feedback data within the NHS. The study team looked at PES free-text comments specifically, with the National CPES as the study’s first case. There is no systematic analysis and use of CPES free-text comments (or indeed, any free-text patient feedback) either within the trusts from which they arise or at a national or regional level. This makes it impossible to respond systematically to emergent themes from the comments or to understand how they relate to variation in care quality across NHS trusts. This study has made significant strides towards the development and validation of an approach that could automate the analysis of summary free-text data and present summaries in a visually engaging and useful way for the 150 NHS trusts that participate in the CPES. It has been shown how various challenges have been met to develop a model of the features a NHS toolkit should have to optimise the use of patient experience free text. This is the first evidence-based attempt that the study team knows of to represent PES free-text comments in ways that are useful to health-care professionals and that the public can understand, and the team members are eager for this to be further developed. As one outcome of the study, proof of concept is thus provided.
Assurances of acceptability and value
With its embedded co-design (using surveys and group concept-mapping workshops) and validation with stakeholders using established preference-based usability and acceptability techniques, the study also provides substantial reassurance that the approach has value for NHS staff. Through these approaches, the study has also shown keen patient interest once governance issues are addressed; until then, the value to patients is indirect, from improvements in the patient experience. The toolkit generated considerable excitement when it was shown to health-care professionals, who know of nothing like it and welcome its implementation into practice. They have declared that they would use it regularly if it has tailored options to adapt the settings to their workflow and themes relevant to their specific work environment and teams. This has been enabled by the study team.
Commissioners have also declared their needs independently, in commissioner events run by Macmillan Cancer Support,13 which are a good fit with what the study team has provided, confirming that the approach meets current demands. These include:
-
Awareness of the lack of data for some marginalised groups – the data summary tab (Figure 16) helps to show where data are missing. Summary demographic details of people with relevant comments can be viewed on the main toolkit page in a different format (Figure 17).
-
Annual and local comparisons as leverage at the board level.
-
Granularity, including access to raw CPES data sets.
-
A more systematic, easier and quicker way to analyse CPES free text, having had experience of its value when sorted into themes.
-
Online access rather than a report via e-mail.
-
Emphasis on priority areas [which the ranking of themes can achieve (Figure 18)].
FIGURE 16.
Part of a screenshot showing demographic details of respondents.
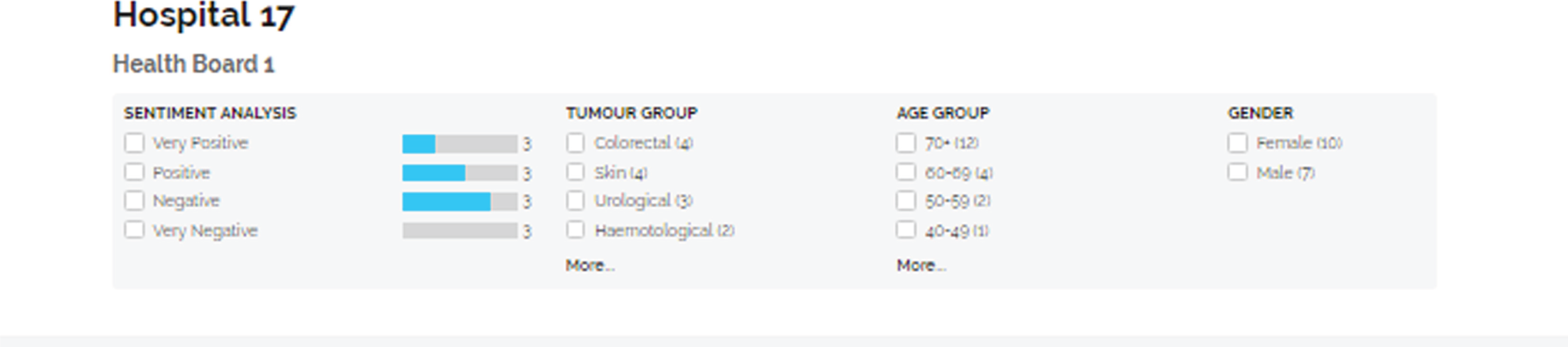
FIGURE 17.
Part of a screenshot of the toolkit highlighting how demographic details of patients making comments on a particular theme may be determined through filters.

FIGURE 18.
Overview of themes. As well as developing six high-priority default themes, a quick overview of all themes is provided. Note that the wording has changed slightly since this draft.

Commissioners in the Macmillan Cancer Support study13 also suggested an online forum for CCGs to compare and share examples of interventions for improvement, as well as peer support networks. The study’s own evidence confirms the value of such additions to the site [and a patient forum for the public-facing version (see Chapter 9)]. Neither of these has currently been included, as this would require some form of moderation or management, or a suitable filtering system to be set up, which needs further funding.
Patients were more likely to use the toolkit on a one-off basis to learn about treatment side effects and experiences of others suffering from the same condition, and to gain information on a site where they were to receive treatment.
Meaningful presentation of the data
The study has also addressed the point in the NIHR HSDR programme brief that there was still uncertainty as to how to present patient experience data in a meaningful and granular way that stimulates local action. An important result and advantage of the study’s approach is that it draws together very large and complex data sets into a thematically driven, simple visual display without loss of the nuances that other manually based methods can have, and it can still allow for exploration of the original text.
The study team is of the belief that:
Information is only useful if it is translated into knowledge and knowledge is only useful if it is used to improve the health of individual patients. One of the main reasons why big data have not fulfilled its[sic] full potential in health care is that small data are not adequately systematized to generate useful knowledge for future patients (research) and that big data are not used to improve health outcomes for individual patients (care).
The study team has achieved the potential systematisation of patient experience free-text comments in a way that has the potential to drive health-care improvements.
Good toolkit design has the following three requirements:
-
identification of what is being measured
-
connection to current strategic objectives
-
consideration of how the first can achieve the second, and how actionable outcomes can be achieved.
This third requirement was also the ethos of the study. The study team aimed to achieve this by establishing which dashboard or toolkit features were important from empirical evidence, and which were meaningful to individual users within the patient experience setting. This also indirectly satisfied the first and second requirements.
Transferability
The study’s approach is transferable with further work; it has been designed to enable this and has supporting documentation and code. The study’s testing on three very different health-care free-text data sets has elucidated some of the challenges with this and possible solutions.
Innovation
Using interdisciplinary approaches in innovative ways, the study successfully involved a range of salient stakeholders, whose feedback continued throughout the development process. This meant that the study team was accountable to them throughout for all of the team members’ design decisions. The study team considered the differences in perspective between them and facilitated their negotiation of these differences with each other. The evidence, in terms of the way the approach evolved in response to stakeholder input, makes it clear that novel interdisciplinary work such as ours is needed and has considerable benefits. Shared understandings, coherence and the facility to get meaning from data are required173 if feedback about care provision is to result in health-care improvements. Nonetheless, the differences between multidisciplinary data sources, such as the ones the study team has used, need consideration. In the study, these may have resulted from sampling bias/the participant mixtures (which users of the checklist should always consider), small numbers of study participants, biases caused by the methods chosen and real variation between the types of user. To determine which factors operate, more research is needed.
The dashboard-scoping survey and term and theme mindmapping survey enabled the different stakeholders in health care to contribute to the development of the work in a cheap, simple and efficient way. This approach could be readily replicated by teams with minimal effort during the design and development phase of complex interventions research as a way to engage the different stakeholders in iterative intervention development work more generally. However, it is prone to all of the standard limitations of surveys.
Concept mapping is usually used for the evaluation of interventions in health and social care and research and the use of this method in both co-design and intervention development work is innovative. The study showed how the process could be modified not only to help mixed groups of stakeholders to reach meaningful consensus face to face in a single session, but also to explore a topic (here patient experience) and representative themes in ways that considered and preserved the different stakeholder voices. Further consideration needs to be given to approaches to validation of the consensus themes. 174 The study has also shown the feasibility of adapting the software-based approach to concept mapping to enable full participation that can include people who are not computer literate. This technique could be used by others, not least to obtain information to modify the PRESENT rule-based IR-toolkit system for different conditions.
The walk-through methods that were used to surface any usability issues are structured methods, and widely used in the industry. The successful use of these methods in a health-care context is a contribution to the practice of system design in health care. Incorporation of NPT, although achieved, needs explicit implementation models to have been developed to maximise its potential (see Use of Normalisation Process Theory themes).
Overall, the innovative use of surveys, concept mapping work, structured walk-throughs and a DCE has led to particularly rich data on the needs, trade-offs, prioritisations, implementation moderators and toolkit and patient experience data usage, which can be translated into more general recommendations and checklists, as well as further in-depth analyses to be undertaken after completion of this report.
Small studies
Several authors47,49 emphasise the importance of small studies in the use of big data, and findings from these were an important element of the work.
Toolkit
The dashboard-scoping survey and scoping review showed that the focus of any clinical toolkit designer should be on ensuring minimum user effort and making role-specific tasks simple and quick to achieve. The survey suffered from sampling bias (see Chapter 3, New surveys). The review also had limitations. Although it was rigorous and systematic, given that it was a scoping review, it had a broad research question. Most studies considered the health-care professional user and few evaluated toolkits designed for patients. Many had top-down designs, so it is perhaps no surprise that many of the features elucidated are no different to more generalised good toolkit design features.
Nonetheless, the study team was able to use the findings from the dashboard-scoping survey and scoping review as the foundation for the prototype toolkit development and exploration in the group concept-mapping workshops, DCE and walk-throughs. Overall, these confirmed the scoping review and dashboard-scoping survey data and added further features. For example, the toolkit had to make patient care needs and service failures clear, for leverage in funding bids, care planning and – if a patient were to use it – treatment considerations. In addition, the toolkit had to enable comparisons between sites and years for these purposes. Searches and filters were important, but the suggested form that these should take differed depending on the approach that was used to explore them, as did the colours to use in the charts (RAG or a more neutral scheme) and the use of maps to indicate patterns in the data. Contradicting the dashboard-scoping review, web-page scrolling was deemed to be acceptable by participants across the study; possibly, this was because users did not envisage checking the toolkit on anything except for a desktop PC.
Differences need further exploration (see Transferability to other applications). However, there were more similarities than differences across methods and groups, enabling us to develop a list of recommendations for the evidence-based clinical toolkit design. This can be checked by researchers and users within health care when developing new toolkits, for optimisation.
These recommendations should not replace gestalt principles of design, which are constructed from preattentive processing (i.e. subconscious cognitive processes) and apply regardless of the use to which a toolkit is put. In the preliminary and developmental parts of the study (stages 1 and 2), the study team effectively asked stakeholders for their considered impressions of what they believe they are doing in preattentive processing, and this was explored in the scoping review of clinical digital toolkit design (see Chapter 2). It could be argued that the DCE work attempted to get at preattentive processing more objectively, although the caveats were also discussed (see Chapter 7). The checklist does not aim to replace gestalt principles, but the criteria that were developed should make it easier for designers who use these principles to appreciate health-care stakeholder needs for a digital dashboard or toolkit.
Themes
The group concept mapping was undertaken to enable mixed stakeholder co-design of the theme names and scope (drawing on the term and theme mindmapping survey and rapid review), as well as the toolkit. These goals were achieved, resulting in a draft evidence-based taxonomy of themes suited to driving improvements in the patient experience.
The study team was also able to record the conceptualisations of the different stakeholders through the way in which they sorted statements into themes. However, these data have limitations. The team considered only a small selection of statements, smaller than the number usually used (≥ 100) when the process is undertaken online. A total of 60 statements were purposively selected from the several thousands of comments in the data set, which inevitably included a wider selection of themes. The choices were influenced by findings from the term and theme mindmapping survey and rapid review of themes, and so carried across their limitations. For example, the rapid review of themes was undertaken by one researcher and was limited in scope, the sample sizes for the surveys were small and there was considerable sampling bias across the study.
Nonetheless, it is also a strength of this output that it drew on several data sources across the study, involving multiple stakeholder groups, as it is likely to represent the most significant themes in the health-care experience. The 66 comments considered in the workshops represented 26 out of the 36 themes (72%) that had been determined deductively before the workshops or that became evident inductively through further discussion within the workshops. Participants did not consider there to be major gaps in the themes used. The data had good construct validity. Larger numbers of workshop participants might have enhanced external validity, but the minimum number recommended for robust results was exceeded. 121
Overall, the study team is confident that the final taxonomy of themes had satisfactory external validity, as the stakeholders themselves determined theme names and rankings. Therefore, the taxonomy has meaning across the groups (being defined by them); moreover, themes were ranked by both importance and feasibility for health-care change. The taxonomy can be widely used within health-care studies and practice. The taxonomy was further refined in the last stage of the study and it will probably develop organically as it is used. It would be interesting to revisit it over time to see if priorities and needs change, in other words, to see if the patient experience changes and improves.
Information retrieval
Using a noun/verb-phrase approach to rule-based IR with gazetteer lookups informed by stakeholder mindmapped terms and phrases, the study team was able to group free-text data into themes with reasonable accuracy for the CPES. The free-text comments for the CPES almost exclusively refer to health-care services; data sets that included significant talk about home life or contained more expansive patient experience stories were less well analysed by the process. It is important to recognise that the study was exploring the ‘worst-case scenario’, in which no adaptations were made before analysing these further data sets. The study team was also conservative in scoring for the LAPCD data. In fact, the system can be easily modified to increase accuracy on the different sources of data; this is a work in progress. The ease with which rules can be tweaked was what led us to choose the approach we did in the first place. Nonetheless, it is important to acknowledge the inherent limitations with current IR and NLP technology (see Chapter 8, Results and discussion, and Realistic expectations).
The study team will need to explore whether or not modifications for transferability reduce the accuracy of the system for CPES data; the more complex rule-based approaches become, the more unstable they may be. Thus, it may be important to keep different uses packaged separately.
Opening up debates
Governance and big data
The study has served to open up or contribute to some significant debates, which was not anticipated. One of the most important is the current debate around the open use of big data and tensions with governance. This is explored further in Some remaining challenges.
Data misuse
A second debate is around data misuse. Each decision that is made from the moment a survey is designed, through the data collection phase, to its final display in digital form, influences the next. This means that users need to be aware of the different decisions that are made and the way in which they affect usage of the data. The study team has attempted to address this with a tab on the toolkit that describes the issues. For graphics from this tab, see Figures 19 and 20. This is good practice, as highlighted by the Government Statistical Service. 175 In this regard, it is to be noted that many commercial data-mining companies do not follow this practice, but offer NHS commissioners dashboards that have not been co-designed, to show data sets, such as the Friends and Family Test, in ways that they claim can be used as quality assurance measures in their own right. In fact, these data should always be used to contribute to the wider picture – albeit in a particularly useful, fine-grained and practical way – rather than as hard data. There are sufficient studies to show that each survey has limitations. 176–191 Potential users are often aware of these limitations already. A study by Macmillan Cancer Support13 showed that commissioners valued CPES free-text data, especially as a means of capturing broad information from patients and opening up conversations about patient experience between commissioners and providers. However, they would consider the qualitative data only alongside quantitative data and raised a number of issues with the CPES survey sampling and case mix, as well as the problem that transitory local difficulties, such as staff sickness, cannot be taken into account in national summaries. 13 The study’s participants were of the same mind.
FIGURE 19.
An example of how users are being alerted to consider the limitations as well as the strengths of all data sets, including the study’s own data set. Users can mine down to find more detail on each point. This graphic relates to PESs in general and not the CPES specifically.

FIGURE 20.
A draft of how the limitations of qualitative data may be highlighted. Such highlights may need to be customised to different uses of the system.

Realistic expectations
A third area in which we wish to open up debate is around managing expectations in a thematic analysis of large data sets and the reification of manual analysis. It has been shown how the system missed some theme annotations that seem obvious to a human, but often picked up themes that humans missed. When qualitative researchers undertake inter-rater reliability checks for manual thematic analyses, they often come up with a concordance (similar to the accuracy metric) of around 76%, which is considered to be acceptable. The accuracy figures, which are comparable (indeed slightly higher), are therefore a good achievement, although the study team will continue to work on improving them. Whether human or computer, there will always be some themes that are not accurately categorised, and thus the expectations of computational methods should be realistic.
Economic outcomes, strengths and limitations
The DCE shows purchasing behaviour to be very much dependent on the toolkit features, going from a 10% to a 90% probability to purchase (at £1500 a year) when the study team moved from using a baseline toolkit to using a fully featured one. However, extrapolating actual market demand from the experimental findings should be treated with caution. The study had a moderate retention rate of around 20% and the team members have no knowledge about the reasons for non-completion. It is known, however, that completers found the task difficult or wearying, which can lead them to answer at random or without full engagement, reducing internal validity. Even with full engagement in the task, completers may behave differently in real life; although the DCE is a relatively objective forced-choice approach, it depends on rational decision-making, when in fact humans often make irrational decisions. 192
In terms of the net benefit from the use of the toolkit, the DCE data provide only a lower-bound estimate, as non-monetary benefits are not included (such as improved health-care services, improved health outcomes, enhanced data availability and research, etc.). Such evaluation is outside the scope of this project and would need to be explored in future research.
Theory
The NPT was used throughout the study to structure data collection and design work and to drive the approach (i.e. to ensure that potential professional stakeholders in the patient experience could make sense of the free-text comments analysis in coherent ways that consider the contexts in which they work). However, there were limitations in what the study was able to explore with participants in the walk-through in terms of implementation, because they were not presented with an implementation model, but simply the toolkit to trial (see Chapter 9, Discussion).
The diffusion of innovations theory provided a more general overview of the cultural issues of introducing technologies into the NHS workplace. As self-assessments were used, this is, however, potentially open to bias, as people may have over-represented their innovativeness. Moreover, the study team considered only the aspects of this theory that did not overlap with NPT, as specified in the funding application.
Some remaining challenges
Notwithstanding the success of the project, some fine-grained aspects of what had been originally planned were not feasible.
A public-facing site
Although the study team developed HTML pages for a public-facing site, these are not currently useable as a result of governance limitations. These pages could be developed by the University of Southampton at a later date, with some more funding, once patient consent rules change. When this report was written, data regulations were in flux. Long-awaited results of the government consultation on the new health data security standards and consent/opt-out model for patient data had not been published. These were disseminated a month later in July 2017,193 and confirmed the recommendations of the independent review of data security, consent and opt-outs by the National Data Guardian, Dame Fiona Caldicott. 194 This review recommended:
-
new data security standards
-
a method of testing compliance with these standards
-
a new consent model for data sharing in health and social care.
The NHS still needs to implement these recommendations, which will take time.
In parallel, in late December 2015 the European Council, Commission and Parliament reached agreement on new data protection rules. A final draft of the new European Union General Data Protection Regulation came into force in mid-2018, and the UK’s withdrawal from the EU (Brexit) is unlikely to change this. The British government has announced that it will opt out of Article 43a of the General Data Protection Regulation, which requires there to be a mutual legal assistance treaty in place with the relevant country before a transfer of personal data can be made to that country to comply with a court order.
Given these changes, and the tightening of governance rules following NHS data breaches (unconnected to the project), NHS England restricted use of the CPES 2015 data. This is appropriate, as patients who completed the survey did not consent to new use of their data on public display. Although most study participants found this frustrating and believed that patients would be happy for this new use, a few agreed with us that it should not be done. It is important to record here that patients often felt that this was tantamount to concealment and said that they would not trust the NHS until data were fully shared and they could follow the journey of their own comments in the survey. This is something that has been acknowledged as being important in a Cabinet Office White Paper of 2013. 195
Use of Cancer Patient Experience Survey 2015 data
The same governance issues that precluded the launching of the public-facing site for CPES data also delayed the acquisition of these data, which before the study – and indeed until May 2016 – had been promised to us immediately on release. The study team did not get these data in time for the draft report. Although this did not compromise the study, it reduced the immediate benefits within the NHS. However, this should not be a problem in future years or with different data (it is also noted that these data can be obtained separately from each individual trust by local agreements, at the time of release).
Use of Normalisation Process Theory themes
In the first prototype, radar plots generated from completion of the NPT toolkit were included on the main dashboard page. It was felt that if professional users completed the NPT toolkit, this would enable them to reflect on the feasibility of implementing small-scale interventions as a response to the free-text data summaries. For example, if there were a great many negative comments about teamworking, and in the toolkit the score for interactional workability was poor, this might suggest both that the unit had an inherent problem with teamworking and that it was not practical to address this. Alternatively, it could be decided that the large number of negative comments relating to teamworking could be used as a lever to address deeper issues. However, the NPT toolkit was not designed or developed to drive change, but rather to understand the implementation, embedding and integration of new technology or complex interventions. NPT has, however, been shown to be very useful when interventions are developed,52,53 and it is likely, therefore, that the use of NPT was attempted too early in the potential intervention development process. This was, therefore, a high-risk application that did not work. Nonetheless, NPT has been retained as a link for users of the site to explore, given that the dashboard has now been developed into a broader toolkit. The toolkit explains that once health-care professionals have designed an intervention to improve the patient experience, they might explore its implementation using the NPT toolkit.
Final toolkit features
Some toolkit features were prepared by us, but could not be implemented either because of current governance restrictions or because the study team did not have > 1 year of data.
As an example, the current toolkit shows dials, even though there was consensus that these were not helpful, and currently additional data from other sources cannot be added, nor data reports exported or printed (although screens can be) – see Chapter 5, Back-end decisions and Software for a discussion. However, the study team has developed HTML pages to enable the chart style to be changed, and these other features to be included, once they can be used (Figure 21).
FIGURE 21.
Some alternative features that were developed for the dashboard. Note that as these were developed using WCPES data, health boards have been specified rather than trusts.
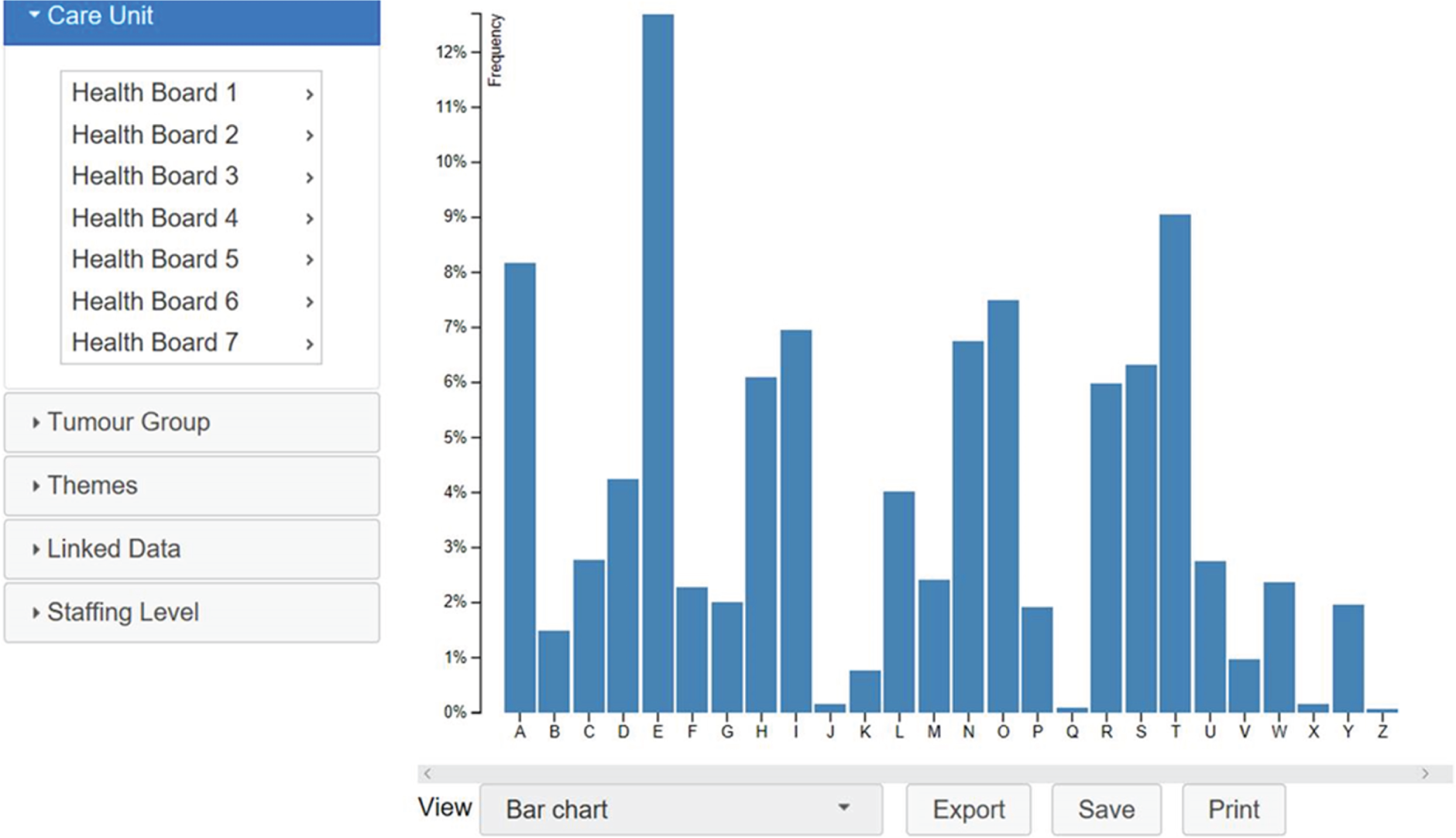
Recommendations for implementation into practice and the need for further research
Use for the Cancer Patient Experience Survey
Further steps are needed between this proof-of-concept study and real-world implementation into routine management.
At the start of the study, the study team discussed with Insight NHS England the possibility of embedding the approach within the management of the National CPES survey. However, discussions were frozen while governance issues are being attended to by Insight NHS England, and the full use of the system is not possible until CPES consent is changed. Implementation also requires more qualitative research and exploration of logistics, structured by implementation science theory, once an implementation model has been worked up. This should be the first research priority. If national roll-out is not embedded through Insight NHS England, and perhaps in any case as a preliminary to this, the first step is an exploration of local (small-scale) implementation into practice and potential sustainability of the rule-based IR toolkit approach for CPES data. One route forward could be to involve local strategic clinical networks13 in piloting the approach, once the necessary refinements to the work have been undertaken. It would be possible to use the rule-based IR process and toolkit for CPES in this way with only modest further financial input to undertake rule and toolkit refinement to suit the implementation model. The potential effect of the approach on practice itself could be examined, for example; a focused ethnography might be undertaken at a pilot site using actor–network theory. A multimodal evaluation of human–computer interaction would also be informative.
Any further study will need funding. Moreover, as indicated above, small refinements of the dashboard and IR for these studies require a small amount of funding for approximately 6 months of programmer and researcher time. This funding is being sought in 2018, with potential sources already identified. Further maintenance and updates, including the incorporation of an interactive forum as desired by stakeholders, are dependent on more funding or activation of a business model. A handover strategy has been developed, as the chief investigator has left the University of Southampton, and this is in the process of being worked through. See Transferability to other surveys.
Transferability to other surveys
The results have been less promising with non-CPES data than with CPES data, although the proof-of-concept work shows that transferability is possible with minor tweaking of the rules and the toolkit, which has been designed to be possible to do for someone with only basic technical know-how. Thus, a secondary priority is to further explore the use of the system with other health-care data sets to drive health-care improvements. More thorough testing of the technology on different data sets is critical if the approach is to be used extensively – as has already been shown, what works with some types of free text may work less well with other types, for example, with a more diffuse style of writing or with the conflation of clinical and personal experiences, as with the LAPCD data. If this further testing is not undertaken, the approach would still be useful for CPES data and similar free-text data, but it would be disappointing to limit it in this way.
On this basis, and with suitable documentation, the toolkit and the rule-based IR package will be placed on a new online repository targeted at health-care professionals, which the University of Southampton Faculty of Health Sciences is in the process of setting up. This will enable potential users to adopt and amend the toolkit freely, whereas the use of the rule-based IR package will probably need to incorporate a small maintenance fee depending on how it is intended for use; this is partly to ensure the integrity of the system, as rule-based approaches may cease to work well if they become overly complex. The processes in manuals and documentation have been operationalised to be accessed via this repository, whereby the system and the toolkit that have been developed could be transferred to other surveys or modified by other users. The study team also intends to use the system for further work of this nature with other data, subject to funding.
Transferability to other applications
The rule-based IR process as a stand-alone feature has considerable application in the processing of data for research and education use. The approach results in literal themes, the first stage of a thematic analysis, freeing up analyst time to focus on more conceptual theme development. Transferability has been built into the system, but further research needs to be done to explore how this works in the different settings of health care, research and teaching.
Engagement activities
More work needs to be done to determine how to engage members of the public with such approaches and how to enable single trusts or commissioners to use them. This might be facilitated through the involvement of charities and advocacy groups or the setting up of a dedicated group. Such a group could, for example, hold regular national training and discussion events developed locally within Wessex and then spread to other areas.
Methodological research
The co-design processes that were used could be further developed and refined to improve their usefulness, with a further exploration of the degree of concordance between the different approaches.
Information retrieval is a rapidly developing area, but for uses such as ours, more sophisticated processes are not needed. However, a scoping review of what is being undertaken and its underlying theoretical frameworks might be a useful way forward, so that guidelines on good practice can be developed. This might also inform the refinement of the analytical approach for better transferability.
Cost–benefit analysis
A detailed cost–benefit analysis is dependent on further research of the type detailed above.
Final conclusion
This study provides proof of concept for an approach that could automate the aggregation of patient feedback free-text data into themes and present summaries in a visually engaging and useful way. Novel application was made of existing multidisciplinary research approaches in ‘small studies’ (literature reviews, ‘mindmapping’ and scoping the design of outputs through surveys, group concept-mapping workshops, a DCE and structured walk-throughs) that complemented the more computational ‘big data’-style IR work. The use of these methods has provided us with rich data on the various perspectives of the different stakeholders and points of concordance and discordance. It has also provided the study team with a comparison of the types of evidence that these different methods provide, which the team hopes to explore further. These small studies ensured that the approach and outputs had meaning for diverse stakeholders in health care, including service users, so that they should be able to drive improvements in the patient experience.
The study team believes that the proof of concept is the first attempt of its kind in health care. Importantly, a modular approach has been used, which can be easily adapted for other surveys and disciplines, with supporting documentation. Nonetheless, further work is required to develop this work beyond the current prototype or pilot design and to embed the approach routinely within health-care processes. This is also true of the empirically derived taxonomy of themes and checklist of recommendations for the health-care dashboard and toolkit design, which need uptake, organic refinement and validation in use. Some further research is therefore needed. The approach has shown that routine adoption within health care of carefully automated analyses of free text and a move from annual to real-time feedback models are possible, once the process is refined and barriers to its use (such as governance issues) become resolved.
Acknowledgements
Contributions of authors
Carol Rivas (Associate Professor, Department of Social Science, formerly Senior Researcher, Health Sciences) was responsible for the conceptualisation of the study and led on all stages. She contributed significantly to all chapters and was the sole author of Chapters 1, 3 and 11.
Daria Tkacz (Postdoctoral Research Fellow, Health Sciences) contributed significantly to Chapters 2, 6 and 10, project managed the study, led on the main systematic review and was involved in all fieldwork.
Laurence Antao (Research Assistant, Health Sciences) undertook the major part of the rule-based IR work and led on Chapter 4.
Emmanouil Mentzakis (Associate Professor, Health Economics) was responsible for the conceptualisation and design of the DCE, its analysis and reporting, as well as the cost analysis, and wrote Chapter 7.
Margaret Gordon (Chief Executive Officer, PICOMEG Ltd, London, UK) led on the IR work and contributed to Chapter 4.
Sydney Anstee (Postdoctoral Research Fellow, Health Sciences) undertook the structured walk-through fieldwork and analyses, and wrote the first draft of Chapter 9.
Richard Giordano (Associate Professor, Health Sciences) supervised phase 3 and co-authored Chapter 9.
Contributions of others
Chun Borodzicz (Research Assistant, Health Sciences) contributed to the systematic review work and sensitivity checks of the text analytics.
Dr Mike Bracher (Postdoctoral Research Fellow, Health Sciences) contributed to the fieldwork and analysis for the group concept-mapping workshops and associated interviews.
Dr Luis Carrasqueiro (Chief Executive Officer, Healthtalk.org) helped with recruitment for stage 1 via the (then) Healthtalkonline website.
Dr Don Cruickshank (Senior Research Fellow, Electronics and Computer Science) helped to develop the dashboard and contributed to a part of Chapter 5.
Dr Adam Glaser (Medical Doctor and Professor of Medicine) facilitated the use and analysis of LAPCD survey data.
Ms Esther Irving (Research Assistant, Health Sciences) contributed to the systematic review work and early IR.
Dr Johana Nayoan (Postdoctoral Research Fellow, Health Sciences) contributed to the systematic review work and group concept-mapping workshops fieldwork.
David Simpson (Consultant, Nominet UK) provided support, advice and training on the use of GATE.
Dr Richard Wagland (Senior Research Fellow, Health Sciences) facilitated the use and analysis of LAPCD survey data and ran a PPI group related to this work.
Dr Mark Weal (Associate Professor in the Web and Internet Science Group in Electronics and Computer Science) edited Chapter 4 in the final report.
Peter West (Postgraduate Student, Health Sciences and Electronics and Computer Science) helped to interpret the phase 1 data and use this to develop the dashboard prototypes, and contributed to a large part of Chapter 9.
Dr Jenny Whitford, Hannah Hine and Selina Mehra (Macmillan Cancer Support team) supported the study with participant recruitment, location of venues and refreshments for meetings and other support and advice.
Dr Theresa Wiseman (Clinical Professor of Applied Health Research in Cancer Care at the University of Southampton and The Royal Marsden NHS Foundation Trust) provided recruitment support and edited drafts of the final report.
Professor Dame Jessica Corner (Health Sciences) and Professor Carl May (Health Sciences) commented on early drafts of the report.
Publications
Rivas C. Audio podcast of a talk at the Ninth International Conference on Social Science Methodology: https://soundcloud.com/mark-carrigan/automated-real-time-thematic-analysis-of-large-volume-surveyfree-text-responses (accessed October 2016).
Rivas C. Associated London School of Economics impact blog item: http://blogs.lse.ac.uk/impactofsocialsciences/2017/03/20/patient-experience-feedback-we-need-to-engagewith-the-issues-of-using-big-data-methods-to-capture-the-human-voice/ (accessed October 2016).
Rivas C. PRESENT: Patient Reported Experience Survey Engineering of Natural Text: Developing Practical Automated Analysis and Dashboard Representations of Cancer Survey Free-text Answers. HS&DR Welcome Meeting, Southampton, 2 February 2016.
Rivas C. No Time Like the PRESENT: Pragmatic Engineering of Themes from Large-volume Text to Improve Healthcare. Part of a session of linked talks conceived and run by Carol Rivas. The others were by Dr Richard Wagland on information retrieval and Professor Clive Seale on Wordsmith. Complex Healthcare Processes Research Group Seminar Series, Southampton, 1 March 2016.
Tkacz D, Rivas CA. Concept Mapping and PRESENT. Workshop presentation for the European Concept Mapping Group, Kristianstad, March 2016.
Rivas C. ‘I’m Seeing the Quack to Have Some Jungle Juice for my Morbid Growth’: How Different Stakeholders Talk About Cancer Care. British Sociological Association Annual Conference, Birmingham, 6–8 April 2016.
Rivas C. Analysis and Representations of Cancer Survey Free-text Answers. Invited Plenary, Day 1. Cancer Data and Outcomes Conference, Manchester, 13–14 June 2016.
Rivas C. Lifeworld Talk and its Role in the Pre-Judgment by Pharmacy Advisers of Smoker Success in Quitting. 14th International Communication, Medicine and Ethics conference (COMET), Aalborg, 4–7 July 2016.
Rivas C, Tkacz D, Cruickshank C. Quacks and Jungle Juice: Cancer Patients’ Views on Illness and Healthcare Through Metaphor and Slang. Poster presented at the 14th International Communication, Medicine and Ethics conference (COMET), Aalborg, 4–7 July 2016.
Rivas C. Automated ‘Real Time’ Thematic Analysis of Large Volume Survey Free-text Responses: Methodological Issues in Using an Intelligent System to Capture the Human Voice. The Ninth International Conference on Social Science Methodology, Leicester, 11–16 September 2016.
Rivas C. Challenges with Using Big Data Approaches for Patient Experience Data. Invited speaker and panel discussant, HealthTechXEurope, London, 20 June 2017.
Anstee S, Tkacz D, Cruickshank D, Rivas C. An Automated Approach to Analysing and Visualising Patient Experience Survey Free-Text Comments to Drive Service Improvements – Present Study: Development and Proof of Concept with Patients and Healthcare Professionals. Evidence Live, Oxford, 22 June 2017.
Data-sharing statement
All data requests (including dashboard HTML and programming code) should be submitted to the corresponding author for consideration. Please note that data originating from sources external to the project cannot be shared. Access to remaining anonymised data may be granted following review and subject to appropriate agreements being in place.
Patient data
This work uses data provided by patients and collected by the NHS as part of their care and support. Using patient data is vital to improve health and care for everyone. There is huge potential to make better use of information from people’s patient records, to understand more about disease, develop new treatments, monitor safety, and plan NHS services. Patient data should be kept safe and secure, to protect everyone’s privacy, and it’s important that there are safeguards to make sure that it is stored and used responsibly. Everyone should be able to find out about how patient data are used. #datasaveslives You can find out more about the background to this citation here: https://understandingpatientdata.org.uk/data-citation.
Disclaimers
This report presents independent research funded by the National Institute for Health Research (NIHR). The views and opinions expressed by authors in this publication are those of the authors and do not necessarily reflect those of the NHS, the NIHR, NETSCC, the HS&DR programme or the Department of Health and Social Care. If there are verbatim quotations included in this publication the views and opinions expressed by the interviewees are those of the interviewees and do not necessarily reflect those of the authors, those of the NHS, the NIHR, NETSCC, the HS&DR programme or the Department of Health and Social Care.
References
- Operating Framework for the NHS in England 2012/2013. London: Department of Health and Social Care and NHS Finance, Performance and Operations; 2011.
- Coulter A, Locock L, Ziebland S, Calabrese J. Collecting data on patient experience is not enough: they must be used to improve care. BMJ 2014;348. https://doi.org/10.1136/bmj.g2225.
- Using Patient Feedback. Oxford: The Picker Institute; 2009.
- Rust RT, Zahorik AJ, Kenningham TL. Return On Quality (ROQ): making service quality financially accountable. J Marketing 1995;59:58-70. https://doi.org/10.2307/1252073.
- Patient Experience Survey Programme: Outline Programme 2014/15 and 2015/16. Newcastle upon Tyne: Care Quality Commission; 2017.
- DeCourcy A, West E, Barron D. The National Adult Inpatient Survey conducted in the English National Health Service from 2002 to 2009: how have the data been used and what do we know as a result?. BMC Health Serv Res 2012;12. https://doi.org/10.1186/1472-6963-12-71.
- O’Cathain A, Thomas KJ. ‘Any other comments?’ Open questions on questionnaires – a bane or a bonus to research?. BMC Med Res Methodol 2004;4. https://doi.org/10.1186/1471-2288-4-25.
- Proceedings of the SAS® Global Forum 2010 Conference. Cary, NC: SAS Institute Inc.; 2010.
- Cancer Patient Experience Survey 2013: National Report. Leeds: Quality Health; 2013.
- de Silva D. Evidence Scan No.18 Measuring Patient Experience. London: The Health Foundation; 2013.
- Fowler FJ. Survey Research Methods. Thousand Oaks, CA: Sage Publications; 2013.
- Baranski A. Briefing: The Importance of the National Cancer Patient Experience Survey. London: Macmillan Cancer Support; 2013.
- Commissioner Events Summary Report June 2015. London: Macmillan Cancer Support; 2015.
- Wiseman T, Lucas G, Sangha A, Randolph A, Stapleton S, Pattison N, et al. Insights into the experiences of patients with cancer in London: framework analysis of free-text data from the National Cancer Patient Experience Survey 2012/2013 from the two London Integrated Cancer Systems. BMJ Open 2015;5. https://doi.org/10.1136/bmjopen-2015-007792.
- London Cancer Alliance Annual Report 2013/14. London: London Cancer Alliance; 2014.
- Darzi A. High Quality Care for All: NHS Next Stage Review Final Report. London: Department of Health and Social Care; 2008.
- Crossing the Quality Chasm: A New Health System for the 21st Century. Washington, DC: National Academy Press; 2001.
- Corner J, Wagland R, Glaser A, Richards SM. Qualitative analysis of patients’ feedback from a PROMs survey of cancer patients in England. BMJ Open 2013;3. https://doi.org/10.1136/bmjopen-2012-002316.
- Wagland R, Recio-Saucedo A, Simon M, Bracher M, Foster C, Hunt K, et al. Text-mining assisted qualitative analysis of free-text comments relating to quality of patient experiences of care within the National Cancer Experience Survey. BMJ Qual Saf 2014;25:1-2.
- Attanasio L, Kozhimannil KB, Jou J, McPherson ME, Camann W. Women’s experiences with neuraxial labor analgesia in the Listening to Mothers II Survey: a content analysis of open-ended responses. Anesth Analg 2015;121:974-80. https://doi.org/10.1213/ANE.0000000000000546.
- Bracher M, Wagland R, Corner J. Exploration and Analysis of Free-text Comments from the 2013 Wales Cancer Patient Experience Survey (WCPES). Southampton: University of Southampton; 2014.
- Cunningham M, Wells M. Scottish Cancer Patient Experience Survey 2015/16: Analysis of Free-text Comments. Edinburgh: Scottish Government; 2016.
- Cunningham M, Wells M. Qualitative analysis of 6961 free-text comments from the first National Cancer Patient Experience Survey in Scotland. BMJ Open 2017;7. https://doi.org/10.1136/bmjopen-2016-015726.
- Fradgley EA, Paul CL, Bryant J, Oldmeadow C. Getting right to the point: identifying Australian outpatients’ priorities and preferences for patient-centred quality improvement in chronic disease care. Crit Care Med 2011;39:1000-5.
- Hazzard E, Barone L, Mason M, Lambert K, McMahon A. Patient-centred dietetic care from the perspectives of older malnourished patients. J Hum Nutr Diet 2017;30:574-87. https://doi.org/10.1111/jhn.12478.
- Henrich NJ, Dodek P, Heyland D, Cook D, Rocker G, Kutsogiannis D, et al. Qualitative analysis of an intensive care unit family satisfaction survey. Int J Qual Health Care 2016;28:470-7. https://doi.org/10.1093/intqhc/mzw049.
- Iversen HH, Bjertnæs OA, Skudal KE. Patient evaluation of hospital outcomes: an analysis of open-ended comments from extreme clusters in a national survey. BMJ Open 2014;4. https://doi.org/10.1136/bmjopen-2014-004848.
- Lian OS, Hansen AH. Factors facilitating patient satisfaction among women with medically unexplained long-term fatigue: a relational perspective. Health 2016;20:308-26. https://doi.org/10.1177/1363459315583158.
- McLemore MR, Desai S, Freedman L, James EA, Taylor D. Women know best – findings from a thematic analysis of 5,214 surveys of abortion care experience. Womens Health Issues 2014;24:594-9. https://doi.org/10.1016/j.whi.2014.07.001.
- Poole R, Gamper A, Porter A, Egbunike J, Edwards A. Exploring patients’ self-reported experiences of out-of-hours primary care and their suggestions for improvement: a qualitative study. Fam Pract 2011;28:210-19. https://doi.org/10.1093/fampra/cmq090.
- Tippens KM, Chao MT, Connelly E, Locke A. Patient perspectives on care received at community acupuncture clinics: a qualitative thematic analysis. BMC Complement Altern Med 2013;13. https://doi.org/10.1186/1472-6882-13-293.
- Wagland R, Recio-Saucedo A, Simon M, Bracher M, Hunt K, Foster C, et al. Development and testing of a text-mining approach to analyse patients’ comments on their experiences of colorectal cancer care. BMJ Qual Saf 2016;25:604-14. https://doi.org/10.1136/bmjqs-2015-004063.
- York GS, Churchman R, Woodard B, Wainright C, Rau-Foster M. Free-text comments: understanding the value in family member descriptions of hospice caregiver relationships. Am J Hosp Palliat Care 2012;29:98-105. https://doi.org/10.1177/1049909111409564.
- McKinnon LC, Prosser SJ, Miller YD. What women want: qualitative analysis of consumer evaluations of maternity care in Queensland, Australia. BMC Pregnancy Childbirth 2014;14. https://doi.org/10.1186/s12884-014-0366-2.
- Cidell J. Content clouds as exploratory qualitative data analysis. Area 2010;42:514-23. https://doi.org/10.1111/j.1475-4762.2010.00952.x.
- Chowdhury SR, Saha H. Development of a FPGA based fuzzy neural network system for early diagnosis of critical health condition of a patient. Comput Biol Med 2010;40:190-20. https://doi.org/10.1016/j.compbiomed.2009.11.015.
- Kroeze JH, Matthee MC, Bothma TJD. Differentiating Data- and Text-Mining Terminology. Proceedings of the 2003 Annual Research Conference of the South African Institute of Computer Scientists and Information Technologists on Enablement Through Technology n.d.
- Winston P. Artificial Intelligence. Reading, MA: Addison Wesley; 1992.
- Chartier J-F, Meunier J-G. Text mining methods for social representation analysis in large corpora. Pap Soc Represent 2011;30:37.1-47.
- Herland M, Khoshgoftaar TM, Wald R. A review of data mining using big data in health informatics. J Big Data 2014;1:1-35.
- Ordenes FV, Burton J, Theodoulidis B, Gruber T, Zaki M. Analyzing customer experience feedback using text mining: a linguistics-based approach. J Serv Res 2014;17:278-95. https://doi.org/10.1177/1094670514524625.
- Gibbons C, Richards S, Valderas JM, Campbell J. Supervised machine learning algorithms can classify open-text feedback of doctor performance with human-level accuracy. J Med Internet Res 2017;19. https://doi.org/10.2196/jmir.6533.
- Maynard D, Roberts I, Greenwood MA, Rout D, Bontcheva K. A Framework for Real-time Semantic Social Media Analysis. Web Semantics: Science, Services and Agents on the World Wide Web. Sheffield: Department of Computer Science, University of Sheffield; 2017.
- Cunningham H, Tablan V, Roberts A, Bontcheva K. Getting more out of biomedical documents with GATE’s full lifecycle open source text analytics. PLOS Comput Biol 2013;9. https://doi.org/10.1371/journal.pcbi.1002854.
- Bhuiyan T, Yue X, Audun J. State-of-the-Art Review on Opinion Mining from Online Customers Feedback 2009:385-90.
- Ghazvinian A. Star Quality: Sentiment Categorization of Restaurant Reviews. Stanford, CA: Stanford University; 2011.
- Sacristán JA, Dilla T. No big data without small data: learning health care systems begin and end with the individual patient. J Eval Clin Pract 2015;21:1014-17. https://doi.org/10.1111/jep.12350.
- Wyly E. Automated (post) positivism. Urban Geog 2014;35:669-90. https://doi.org/10.1080/02723638.2014.923143.
- Halford S, Savage M. Reconceptualizing digital social inequality. Info Comm Soc 2010;13:937-55. https://doi.org/10.1080/1369118X.2010.499956.
- Giordano R, Bell D, Thomas J. Proceedings on the 2000 Conference on Universal Usability. New York, NY: Association for Computing Machinery; 2000.
- Thomson A, Rivas C, Giovannoni G. Multiple sclerosis outpatient future groups: improving the quality of participant interaction and ideation tools within service improvement activities. BMC Health Serv Res 2015;15. https://doi.org/10.1186/s12913-015-0773-8.
- May C. Towards a general theory of implementation. Implement Sci 2013;8. https://doi.org/10.1186/1748-5908-8-18.
- May CR, Eton DT, Boehmer K, Gallacher K, Hunt K, MacDonald S, et al. Rethinking the patient: using Burden of Treatment Theory to understand the changing dynamics of illness. BMC Health Serv Res 2014;14. https://doi.org/10.1186/1472-6963-14-281.
- Rumrill PD, Fitzgerald SM, Merchant WR. Using scoping literature reviews as a means of understanding and interpreting existing literature. Work 2010;35:399-404. https://doi.org/10.3233/WOR-2010-0998.
- Tufte ER. The Visual Display of Quantitative Information. Cheshire, CT: Graphics Press; 2001.
- Schwabe D, Rossi G. An object oriented approach to web-based applications design. Theor Pract Object Syst 1998;4:207-25. https://doi.org/10.1002/(SICI)1096-9942(1998)4:4<207::AID-TAPO2>3.0.CO;2-2.
- Shneiderman B. The Eyes Have It: A Task by Data Type Taxonomy for Information Visualizations n.d. https://doi.org/10.1109/VL.1996.545307.
- Shneiderman B, Plaisant C. Designing the User Interface: Strategies for Effective Human–Computer Interaction. Boston, MA: Addison-Wesley; 2004.
- Vessey I. Cognitive fit: a theory-based analysis of the graph versus tables literature. Decis Sci 1991;22:219-40. https://doi.org/10.1111/j.1540-5915.1991.tb00344.x.
- Nielsen J. F-Shaped Pattern for Reading Web Content 2006. www.nngroup.com/articles/f-shaped-pattern-reading-web-content-discovered/ (accessed 5 May 2018).
- La Grouw G. Effective Dashboard Design: Design Secrets to Getting More Value from Performance Dashboards. Auckland: Electrosmart; 2012.
- Broderick J, Devine T, Langhans E, Lemerise AJ, Lier S, Harris L. Designing Health Literate Mobile Apps. Washington, DC: Institute of Medicine; 2013.
- Edwards EA, Lumsden J, Rivas C, Steed L, Edwards LA, Thiyagarajan A, et al. Gamification for health promotion: systematic review of behaviour change techniques in smartphone apps. BMJ Open 2016;6. https://doi.org/10.1136/bmjopen-2016-012447.
- Clinical Dashboard Toolkit: Guide to Introducing Clinical Dashboards Within your Organisation. London: NHS; 2009.
- Wigley C. Quality Dashboard n.d.
- Gray J. Developing an Urgent Care Dashboard – Findings from a Rapid Literature Review. Yorkshire: North East Quality Observatory Service, Yorkshire & Humber Academic Health Science Network; 2016.
- Arksey H, O’Malley L. Scoping studies: towards a methodological framework. Int J Soc Res Methodol 2005;8:19-32. https://doi.org/10.1080/1364557032000119616.
- Levac D, Colquhoun H, O’Brien KK. Scoping studies: advancing the methodology. Implement Sci 2010;5. https://doi.org/10.1186/1748-5908-5-69.
- Daudt HM, van Mossel C, Scott SJ. Enhancing the scoping study methodology: a large, inter-professional team’s experience with Arksey and O’Malley’s framework. BMC Med Res Methodol 2013;13. https://doi.org/10.1186/1471-2288-13-48.
- Heneghan C, Badenoch D. Evidence-based Medicine Toolkit. London: BMJ Books; 2002.
- The Health Informatics Review: Report. London: Department of Health and Social Care; 2008.
- Durham J, McLauchlan L, Yuster R. Enabling a Common and Consistent Enterprise-Wide Terminology: An Initial Assessment of Available Tools n.d. https://doi.org/10.1109/WIIAT.2008.380.
- Croon RD, Klerkx J, Duval E. Design and Evaluation of an Interactive Proof-of-Concept Dashboard for General Practitioners n.d. https://doi.org/10.1109/ICHI.2015.25.
- Hartzler AL, Chaudhuri S, Fey BC, Flum DR, Lavallee D. Integrating patient-reported outcomes into spine surgical care through visual dashboards: lessons learned from human-centered design. EGEMS 2015;3. https://doi.org/10.13063/2327-9214.1133.
- Wolpin SE, Halpenny B, Whitman G, McReynolds J, Stewart M, Lober WB, et al. Development and usability testing of a web-based cancer symptom and quality-of-life support intervention. Health Informatics J 2015;21:10-23. https://doi.org/10.1177/1460458213495744.
- Brown B, Balatsoukas P, Williams R, Sperrin M, Buchan I. Interface design recommendations for computerised clinical audit and feedback: hybrid usability evidence from a research-led system. Int J Med Informatics 2016;94:191-206. https://doi.org/10.1016/j.ijmedinf.2016.07.010.
- Mishuris PG, Yoder J, Wilson D, Mann D. Integrating data from an online diabetes prevention program into an electronic health record and clinical workflow, a design phase usability study. BMC Med Inform Decis Mak 2016;16. https://doi.org/10.1186/s12911-016-0328-x.
- Mazor I, Heart T, Even A. Simulating the impact of an online digital dashboard in emergency departments on patients length of stay. J Decis Syst 2016;24:343-53. https://doi.org/10.1080/12460125.2016.1187422.
- Batley NJ, Osman HO, Kazzi AA, Musallam KM. Implementation of an emergency department computer system: design features that users value. J Emerg Med 2011;41:693-700. https://doi.org/10.1016/j.jemermed.2010.05.014.
- Colley A, Hulttu K, Harjumaa M, Oinas-Kukkonen H. Insights from the Design and Evaluation of a Personal Health Dashboard n.d. www.computer.org/csdl/proceedings/hicss/2016/5670/00/5670d483.pdf (accessed 30 May 2017).
- Crofts J, Moyo J, Ndebel W, Mhlanga S, Draycott T, Sibanda T. Adaptation and implementation of local maternity dashboard in a Zimbabwean hospital to drive clinical improvement. Bull World Health Organ 2014;91:146-52. https://doi.org/10.2471/BLT.13.124347.
- Forsman J, Anani N, Eghdam A, Falkenhav M, Koch S. Integrated information visualization to support decision making for use of antibiotics in intensive care: design and usability evaluation. Inform Health Soc Care 2013;38:330-53. https://doi.org/10.3109/17538157.2013.812649.
- McLaughlin N, Afsar-Manesh N, Ragland V, Buxey F, Martin NA. Tracking and sustaining improvement initiatives: leveraging quality dashboards to lead change in a neurosurgical department. Neurosurg 2013;74:235-44. https://doi.org/10.1227/NEU.0000000000000265.
- Reese K, Bessette R, Hancock P. KnowYourColors: Visual Dashboards for Blood Metrics and Healthcare Analytics n.d. https://doi.org/10.1109/ISSPIT.2013.6781845.
- Simpao AF, Ahumada LM, Desai BR, Bonafide CP, Gálvez JA, Rehman MA, et al. Optimization of drug-drug interaction alert rules in a pediatric hospital’s electronic health record system using a visual analytics dashboard. J Am Med Inform Assoc 2015;22:361-9.
- Horvath KJ, Alemu D, Danh T, Baker JV, Carrico AW. Creating effective mobile phone apps to optimize antiretroviral therapy adherence: perspectives from stimulant-using HIV-positive men who have sex with men. JMIR Mhealth Uhealth 2016;4. https://doi.org/10.2196/mhealth.5287.
- Kuijpers W, Groen WG, Oldenburg HS, Wouters MW, Aaronson NK, van Harten WH. Development of MijnAVL, an interactive portal to empower breast and lung cancer survivors: an iterative, multi-stakeholder approach. JMIR Res Protoc 2015;4. https://doi.org/10.2196/resprot.3796.
- Owens OL, Friedman DB, Brandt HM, Bernhardt JM, Hébert JR. An iterative process for developing and evaluating a computer-based prostate cancer decision aid for African American men. Health Promot Pract 2015;16:642-55. https://doi.org/10.1177/1524839915585737.
- Stellefson M, Chaney B, Chaney D, Paige S, Payne-Purvis C, Tennant B, et al. Engaging community stakeholders to evaluate the design, usability, and acceptability of a chronic obstructive pulmonary disease social media resource center. JMIR Res Protoc 2015;4. https://doi.org/10.2196/resprot.3959.
- Barbara AM, Dobbins M, Haynes RB, Iorio A, Lavis JN, Raina P, et al. The McMaster Optimal Aging Portal: usability evaluation of a unique evidence-based health information website. JMIR Hum Factors 2016;3. https://doi.org/10.2196/humanfactors.4800.
- Timmerman JG, Tönis TM, Dekker-van Weering MG, Stuiver MM, Wouters MW, van Harten WH, et al. Co-creation of an ICT-supported cancer rehabilitation application for resected lung cancer survivors: design and evaluation. BMC Health Serv Res 2016;16. https://doi.org/10.1186/s12913-016-1385-7.
- Kawamoto K, Houlihan CA, Balas EA, Lobach DF. Improving clinical practice using clinical decision support systems: a systematic review of trials to identify features critical to success. BMJ 2005;330. https://doi.org/10.1136/bmj.38398.500764.8F.
- Gray CS, Khan AI, Kuluski K, McKillop I, Sharpe S, Bierman AS, et al. Improving patient experience and primary care quality for patients with complex chronic disease using the electronic patient-reported outcomes tool: adopting qualitative methods into a user-centered design approach. JMIR Res Protoc 2016;18. https://doi.org/10.2196/resprot.5204.
- Sudram F, Hawken SJ, Stasiak K, Lucassen MFG, Fleming T, Shepherd M, et al. Tips and traps: lessons from codesigning a clinician e-monitoring tool for computerized cognitive behavioural therapy. JMIR Ment Health 2017;4. https://doi.org/10.2196/mental.5878.
- Dowding D, Randell R, Gardner P, Fitzpatrick G, Dykes P, Favela J, et al. Dashboards for improving patient care: review of the literature. Int J Med Inform 2015;84:87-100. https://doi.org/10.1016/j.ijmedinf.2014.10.001.
- McClellan MA, Karumur RP, Vogel RI, Petzel SV, Cragg J, Chan D, et al. Designing an educational website to improve quality of supportive oncology care for women with ovarian cancer: an expert usability review and analysis. Int J Hum Comput Interact 2016;32:297-30. https://doi.org/10.1080/10447318.2016.1140528.
- Wang J, Lam RW, Ho K, Attridge M, Lashewicz BM, Patten SB, et al. Preferred features of e-mental health programs for prevention of major depression in male workers: results from a Canadian national survey. J Med Internet Res 2016;18. https://doi.org/10.2196/jmir.5685.
- Alnosayan N, Chatterjee S, Alluhaidan A, Lee E, Houston Feenstra L. Design and usability of a heart failure mHealth system: a pilot study. JMIR Hum Factors 2017;4. https://doi.org/10.2196/humanfactors.6481.
- Hartzler AL, BlueSpruce J, Catz SL, McClure JB. Prioritizing the mHealth Design space: a mixed-methods analysis of smokers’ perspectives. JMIR Mhealth Uhealth 2016;4. https://doi.org/10.2196/mhealth.5742.
- Bruns EJ, Hyde KL, Sather A, Hook AN, Lyon AR. Applying user input to the design and testing of an electronic behavioral health information system for wraparound care coordination. Adm Policy Ment Health 2016;43:350-68. https://doi.org/10.1007/s10488-015-0658-5.
- Milward J, Khadjesari Z, Fincham-Campbell S, Deluca P, Watson R, Drummond C. User preferences for content, features, and style for an app to reduce harmful drinking in young adults: analysis of user feedback in app stores and focus group interviews. JMIR Mhealth Uhealth 2016;4. https://doi.org/10.2196/mhealth.5242.
- Ghazisaeidi M, Safdari R, Torabi M, Mirzaee M, Farzi J, Goodini A. Development of performance dashboards in healthcare sector: key practical issues. Acta Inform Med 2015;23:317-21. https://doi.org/10.5455/aim.2015.23.317-321.
- Hartzler AL, Izard JP, Dalkin BL, Mikles SP, Gore JL. Design and feasibility of integrating personalized PRO dashboards into prostate cancer care. J Am Med Inform Assoc 2016;23:38-47. https://doi.org/10.1093/jamia/ocv101.
- Coyne I, Prizeman G, Sheehan A, Malone H, While AE. An e-health intervention to support the transition of young people with long-term illnesses to adult healthcare services: design and early use. Patient Educ Couns 2016;99:1496-504. https://doi.org/10.1016/j.pec.2016.06.005.
- Durand MA, Alam S, Grande SW, Elwyn G. ‘Much clearer with pictures’: using community-based participatory research to design and test a Picture Option Grid for underserved patients with breast cancer. BMJ Open 2016;6. https://doi.org/10.1136/bmjopen-2015-010008.
- Lyles CR, Altschuler A, Chawla N, Kowalski C, McQuillan D, Bayliss E, et al. User-centered design of a tablet waiting room tool for complex patients to prioritize discussion topics for primary care visits. JMIR Mhealth Uhealth 2016;4. https://doi.org/10.2196/mhealth.6187.
- Ammerlaan JJ, Scholtus LW, Drossaert CH, van Os-Medendorp H, Prakken B, Kruize AA, et al. Feasibility of a website and a hospital-based online portal for young adults with juvenile idiopathic arthritis: views and experiences of patients. JMIR Res Protoc 2015;4. https://doi.org/10.2196/resprot.3952.
- Mirkovic J, Kaufman DR, Ruland CM. Supporting cancer patients in illness management: usability evaluation of a mobile app. JMIR Mhealth Uhealth 2014;2. https://doi.org/10.2196/mhealth.3359.
- Przezdziecki A, Alcorso J, Sherman KA. My changed body: background, development and acceptability of a self-compassion based writing activity for female survivors of breast cancer. Patient Educ Couns 2016;99:870-4. https://doi.org/10.1016/j.pec.2015.12.011.
- Bottorff JL, Oliffe JL, Sarbit G, Sharp P, Caperchione CM, Currie LM, et al. Evaluation of QuitNow Men: an online, men-centered smoking cessation intervention. J Med Internet Res 2016;18. https://doi.org/10.2196/jmir.5076.
- Winterling J, Wiklander M, Obol CM, Lampic C, Eriksson LE, Pelters B, et al. Development of a self-help web-based intervention targeting young cancer patients with sexual problems and fertility distress in collaboration with patient research partners. JMIR Res Protoc 2016;5. https://doi.org/10.2196/resprot.5499.
- Schall MC, Chen H, Pennathur PR, Cullen L. Development and evaluation of a health information technology dashboard of quality indicators. Proc Hum Factors Ergon Soc Ann Meeting 2015;59. https://doi.org/10.1177/1541931215591099.
- Rivas C, Seale C. Researching Society and Culture. London: Sage; 2017.
- Bontcheva K, Derczynski L, Roberts I, Ide N, Pustejovsky J. The Handbook of Linguistic Annotation. Dordrecht: Springer Scient+Business Media; 2013.
- Hochschild A. The Managed Heart: Commercialization of Human Feeling. Berkeley, CA: University of California Press; 1983.
- Cunningham H, Maynard D, Bontcheva K, Tablan V, Aswani N, Roberts I, et al. Text Processing with GATE (Version 6). Sheffield: University of Sheffield Department of Computer Science; 2011.
- Cunningham H, Hanbury A, Rüger S. Advances in Multidisciplinary Retrieval. Berlin: Springer; 2010.
- Bontcheva K, Derczynski L, Funk A, Greenwood MA, Maynard D, Aswani N. Proceedings of the International Conference on Recent Advances in Natural Language Processing. Cambridge, MA: Massachusetts Institute of Technology (MIT) Press; 2013.
- Tang J, Meng Z, Nguyen X, Mei Q, Zhang M. Understanding the Limiting Factors of Topic Modeling via Posterior Contraction Analysis n.d.
- Kane M, Trochim WMK. Concept Mapping for Planning and Evaluation. Thousand Oaks, CA: Sage Publications; 2007.
- Rosas SR. Group concept mapping methodology: toward an epistemology of group conceptualization, complexity, and emergence. Qual Quant 2017;51:1403-16. https://doi.org/10.1007/s11135-016-0340-3.
- Dalkey NC, Rourke DL, Dalkey NC, Rourke DL, Lewis R, Snyder D. Studies in the Quality of Life: Delphi and Decision-making. Lexington, MA: Lexington Books; 1972.
- Stoyanov S, Spoelstra H, Bennett D, Sweeney C, Van Huffel S, Shorten G, et al. Use of a group concept mapping approach to define learning outcomes for an interdisciplinary module in medicine. Perspect Med Educ 2014;3:245-53. https://doi.org/10.1007/s40037-013-0095-7.
- Rosas SR. The utility of concept mapping for actualizing participatory research. Cuadern Hispanoamericanos Psicol 2013;12:7-24.
- Kitzinger J. The methodology of focus groups: the importance of interaction between research participants. Soc Health Illness 1994;16:103-21. https://doi.org/10.1111/14679566.ep11347023.
- Kvale S. InterViews: An Introduction to Qualitative Research Interviewing. Thousand Oaks, CA: Sage Publications; 1996.
- Delacre M, Lakens D, Leys C. Why psychologists should by default use Welch’s t-test instead of Student’s t-test. Int Rev Soc Psychol 2015;30:92-101. https://doi.org/10.5334/irsp.82.
- Kruskal JB. Multidimensional scaling by optimizing goodness of fit to a nonmetric hypothesis. Psychometrika 1964;29:1-27. https://doi.org/10.1007/BF02289565.
- Sturrock K, Rocha J. A multidimensional scaling stress evaluation table. Field Methods 2000;12:49-60. https://doi.org/10.1177/1525822X0001200104.
- Rosas SR, Kane M. Quality and rigor of the concept mapping methodology: a pooled study analysis. Eval Program Plann 2012;35:236-45. https://doi.org/10.1016/j.evalprogplan.2011.10.003.
- Jackson KM, Trochim WMK. Concept mapping as an alternative approach for the analysis of open-ended survey responses. Org Res Methods 2002;5:307-36. https://doi.org/10.1177/109442802237114.
- Ryan GW, Bernard HR, Denzin NK, Lincoln YS. Handbook of Qualitative Research. Thousand Oaks, CA: Sage; 2000.
- Lancaster KJ. A new approach to consumer theory. J Political Econ 1966;74:132-57. https://doi.org/10.1086/259131.
- Rosen S. Hedonic prices and implicit markets: product differentiation in pure competition. J Political Econ 1974;82:34-55. https://doi.org/10.1086/260169.
- Hensher DA, Rose JM, Greene WH. Applied Choice Analysis: A Primer. Cambridge: Cambridge University Press; 2005.
- Dawes RM, Corrigan B. Linear models in decision making. Psychol Bull 1974;81:95-106. https://doi.org/10.1037/h0037613.
- Huber J, Zwerina K. The importance of utility balance in efficient choice design. J Marketing Res 1996;33:307-17. https://doi.org/10.2307/3152127.
- Zwerina K, Huber J, Kuhfeld WF. A General Method for Constructing Efficient Choice Designs. Durham, NC: Duke University; 1996.
- Kallas Z, Gil JM. A dual response choice experiments (DRCE) design to assess rabbit meat preference in Catalonia: a heteroscedastic extreme-value model. Brit Food J 2012;114:1394-413. https://doi.org/10.1108/00070701211262984.
- Manski CF. The structure of random utility models. Theory Decis 1977;8:229-54. https://doi.org/10.1007/BF00133443.
- Green W, Mills TC, Patterson K. Palgrave Handbook of Econometrics. Volume 2: Applied Econometrics. London: Palgrave; 2008.
- McFadden D, Zarembka P. Frontiers in Econometrics. New York, NY: Academic Press; 1974.
- Ryan M, Skåtun D. Modelling non-demanders in choice experiments. Health Econ 2004;13:397-402. https://doi.org/10.1002/hec.821.
- Lambooij M, Harmsen IA, Veldwijk J. Consistency between stated and revealed preferences: a discrete choice experiment and a behavioural experiment on vaccination behaviour compared. BMC Med Res Methodol 2015;15. https://doi.org/10.1186/s12874-015-0010-5.
- Swait J. A non-compensatory choice model incorporating attribute cutoffs. Transportation Res Part B 2001;35:903-28. https://doi.org/10.1016/S0191-2615(00)00030-8.
- de Palma A, Myers GM, Papageorgiou YY. Rational choice under an imperfect ability to choose. Am Econ Rev 1994;84:419-40.
- Swait J, Adamowicz JW. Choice complexity and decision strategy selection. J Consumer Res 2001;28:135-48. https://doi.org/10.1086/321952.
- DeShazo JR, Fermo G. Designing choice sets for stated preference methods: the effects of complexity on choice consistency. J Environ Econ Manag 2002;44:123-43. https://doi.org/10.1006/jeem.2001.1199.
- Swait J, Adamowicz W. Choice environment, market complexity and consumer behavior: a theoretical and empirical approach for incorporating decision complexity into 19 models of consumer choice. Organ Behav Hum Decis Process 2001;86:141-67. https://doi.org/10.1006/obhd.2000.2941.
- Elrod T, Johnson RD, White J. A new integrated model of noncompensatory and compensatory decision strategies. Organ Behav Hum Decis Process 2004;95:1-19. https://doi.org/10.1016/j.obhdp.2004.06.002.
- Downing A, Wright P, Wagland R, Watson E, Kearney T, Mottram R, et al. Protocol for a UK-wide patient-reported outcomes study. BMJ Open 2016;6. https://doi.org/10.1136/bmjopen-2016-013555.
- Nielsen J. Usability Engineering. Boston, MA: Academic Press; 1993.
- Pilke EM. Flow experiences in information technology use. Int J Hum Comput Stud 2004;61:347-57. https://doi.org/10.1016/j.ijhcs.2004.01.004.
- Nielsen J, Nielsen J, Mack RL. Usability Inspection Methods. New York, NY: Wiley; 1994.
- Nielsen J. Designing Web Usability. Indianapolis, IN: New Riders; 2000.
- Gerhardt-Powals J. Cognitive engineering principles for enhancing human–computer performance. Int J Hum Comput Interact 1996;8:189-211. https://doi.org/10.1080/10447319609526147.
- Nielsen J. Severity ratings for usability problems. Pap Essays 1995;54:1-2. www.nngroup.com/articles/how-to-rate-the-severity-of-usability-problems/ (accessed 2 June 2017).
- Van Lamsweerde A. Goal-Oriented Requirements Engineering: A Guided Tour n.d.:249-62.
- May CR, Johnson M, Finch T. Implementation, context and complexity. Implement Sci 2016;11. https://doi.org/10.1186/s13012-016-0506-3.
- Rogers EM. Diffusion of Innovations. New York, NY: Simon & Schuster; 2003.
- Greenhalgh T, Robert G, Macfarlane F, Bate P, Kyriakidou O. Diffusion of innovations in service organizations: systematic review and recommendations. Milbank Q 2004;82:581-629. https://doi.org/10.1111/j.0887-378X.2004.00325.x.
- O’Cathain A, Goode J, Drabble SJ, Thomas KJ, Rudolph A, Hewison J. Getting added value from using qualitative research with randomized controlled trials: a qualitative interview study. Trials 2014;15. https://doi.org/10.1186/1745-6215-15-215.
- O’Cathain A, Hoddinott P, Lewin S, Thomas KJ, Young B, Adamson J, et al. Maximising the impact of qualitative research in feasibility studies for randomised controlled trials: guidance for researchers. Pilot Feasibility Stud 2015;1. https://doi.org/10.1186/s40814-015-0026-y.
- Eakin JM. Educating critical qualitative health researchers in the land of the randomized controlled trial. Qual Inq 2016;22:107-18. https://doi.org/10.1177/1077800415617207.
- Perry C, Chhatralia K, Damesick D, Hobden S, Volpe L. Behavioural Insights in Health Care. London: The Health Foundation; 2015.
- Brett J, Staniszewska S, Mockford C, Herron-Marx S, Hughes J, Tysall C, et al. A systematic review of the impact of patient and public involvement on service users, researchers and communities. Patient 2014;7:387-95. https://doi.org/10.1007/s40271-014-0065-0.
- Staniszewska S, Thomas V, Seers K. Patient and public involvement in the implementation of evidence into practice. Evid Based Nurs 2013;16. https://doi.org/10.1136/eb-2013-101510.
- National Institute for Health Research (NIHR)-wide Learning and Development for Public Involvement: Working Group Report and Recommendations (2015). Southampton: INVOLVE; 2015.
- Wilson P, Mathie E, Keenan J, McNeilly E, Goodman C, Howe A, et al. ReseArch with Patient and Public invOlvement: a RealisT evaluation – the RAPPORT study. Health Serv Deliv Res 2015;3.
- Staniszewska S, Boardman F, Gunn L, Roberts J, Clay D, Seers K, et al. The Warwick Patient Experiences Framework: patient-based evidence in clinical guidelines. Int J Qual Health Care 2014;26:151-7. https://doi.org/10.1093/intqhc/mzu003.
- Staniszewska S, Brett J, Mockford C, Barber R. The GRIPP checklist: strengthening the quality of patient and public involvement reporting in research. Int J Technol Assess Health Care 2011;27:391-9. https://doi.org/10.1017/S0266462311000481.
- Tripp D, Shaklock G, Smyth J. Being Reflexive in Critical Educational and Social Research. London: Falmer Press; 1998.
- Johnson MJ, May CR. Promoting professional behaviour change in healthcare: what interventions work, and why? A theory-led overview of systematic reviews. BMJ Open 2015;5. https://doi.org/10.1136/bmjopen-2015-008592.
- Beckett K, Farr M, Kothari A, Wye L, Le May A. Embracing complexity and uncertainty to create impact: exploring the processes and transformative potential of co-produced research through development of a social impact model. Health Res Pol Syst 2018;16.
- Communicating Uncertainty and Change: Guidance for Official Statistics Producers. London: Government Statistical Service; 2014.
- Boyce MB, Browne JP. Does providing feedback on patient-reported outcomes to healthcare professionals result in better outcomes for patients? A systematic review. Qual Life Res 2013;22:2265-78. https://doi.org/10.1007/s11136-013-0390-0.
- Capuzzo M, Alvisi R. Is it possible to measure and improve patient satisfaction with anesthesia?. Anesthesiol Clin 2008;26:613-26. https://doi.org/10.1016/j.anclin.2008.07.008.
- Needham BR. The truth about patient experience: what we can learn from other industries, and how three Ps can improve health outcomes, strengthen brands, and delight customers. J Healthc Manag 2012;57:255-63. https://doi.org/10.1097/00115514-201207000-00006.
- Sen S, Fawson P, Cherrington G, Douglas K, Friedman N, Maljanian R, et al. Patient satisfaction measurement in the disease management industry. Dis Manag 2005;8:288-300. https://doi.org/10.1089/dis.2005.8.288.
- Abel GA, Saunders CL, Lyratzopoulos G. Cancer patient experience, hospital performance and case mix: evidence from England. Future Oncol 2014;10:1589-98. https://doi.org/10.2217/fon.13.266.
- Archer JC, McAvoy P. Factors that might undermine the validity of patient and multi-source feedback. Med Educ 2011;45:886-93. https://doi.org/10.1111/j.1365-2923.2011.04023.x.
- Ashley L, Jones H, Velikova G, Wright P. Cancer patients’ and clinicians’ opinions on the best time in secondary care to approach patients for recruitment to longitudinal questionnaire-based research. Support Care Cancer 2012;20:3365-72. https://doi.org/10.1007/s00520-012-1518-4.
- Byrne K, Sims-Gould J, Frazee K, Martin-Matthews A. ‘I’m satisfied … but’: clients’ and families’ contingent responses about home care. Home Health Care Serv 2011;30:161-77. https://doi.org/10.1080/01621424.2011.622242.
- Hargraves JL, Wilson IB, Zaslavsky A, James C, Walker JD, Rogers G, et al. Adjusting for patient characteristics when analyzing reports from patients about hospital care. Med Care 2001;39:635-41. https://doi.org/10.1097/00005650-200106000-00011.
- Lyratzopoulos G, Elliott M, Barbiere JM, Henderson A, Staetsky L, Paddison C, et al. Understanding ethnic and other socio-demographic differences in patient experience of primary care: evidence from the English General Practice Patient Survey. BMJ Qual Saf 2012;21:21-9. https://doi.org/10.1136/bmjqs-2011-000088.
- Marcinowicz L, Borzuchowska A, Grebowski R. Methodologic difficulties in measuring patient satisfaction – discrepancy coming from formulating questions. Wiad Lek 2002;55:335-40.
- Riiskjær E, Ammentorp J, Kofoed PE. The value of open-ended questions in surveys on patient experience: number of comments and perceived usefulness from a hospital perspective. Int J Qual Health Care 2012;24:509-16. https://doi.org/10.1093/intqhc/mzs039.
- Riiskjær E, Ammentorp J, Nielsen JF, Kofoed PE. Semi-customizing patient surveys: linking results and organizational conditions. Int J Qual Health Care 2011;23:284-91. https://doi.org/10.1093/intqhc/mzr001.
- Winter-Pfändler U, Morgenthaler C. Are surveys on quality improvement of healthcare chaplaincy emotionally distressing for patients? A pilot study. J Health Care Chaplain 2010;16:140-8. https://doi.org/10.1080/08854726.2010.480829.
- Weisman CS, Rich DE, Rogers J, Crawford KG, Grayson CE, Henderson JT. Gender and patient satisfaction with primary care: tuning in to women in quality measurement. J Womens Health Gend Based Med 2000;9:657-65. https://doi.org/10.1089/15246090050118189.
- Xiao J, Jiang C, Zhang M. Appropriate time for assessing patient satisfaction with cataract surgery care. J Cataract Refract Surg 2011;37. https://doi.org/10.1016/j.jcrs.2010.10.034.
- Lancsar E, Louviere J. Deleting ‘irrational’ responses from discrete choice experiments: a case of investigating or imposing preferences?. Health Econ 2006;15:797-811. https://doi.org/10.1002/hec.1104.
- Your Data: Better Security, Better Choice, Better Care. Government Response to the National Data Guardian for Health and Care’s Review of Data Security, Consent and Opt-Outs and the Care Quality Commission’s Review ‘Safe Data, Safe Care’. London: Department of Health and Social Care; 2017.
- Caldicott F. National Data Guardian for Health and Care: Review of Data Security, Consent and Opt-Outs. London: National Data Guardian for Health and Care; 2016.
- Open Data White Paper: Unleashing the Potential. London: HMSO; 2012.
Appendix 1 Search terms for the review reported in Chapter 2 and the rapid review reported in Chapter 3
Chapter 2: review of dashboard design principles search
Date range searched: from inception to 30 March 2017.
Date searched: 30 March 2017.
PubMed
Search terms
((dashboard*[Title/Abstract]) OR (website[Title/Abstract]) OR (internet[Title/Abstract]) OR (e?health[Title/Abstract]) OR (m?health[Title/Abstract]) OR (smartphone[Title/Abstract]) OR (decision?support[Title/Abstract]) OR (infographic*[Title/Abstract]) OR (score?card[Title/Abstract]) OR (Medical Informatics[MeSH]) OR (“medical Informatics”[Title/Abstract]) OR (“health?care Informatics”[Title/Abstract]) OR (“health Informatics”[Title/Abstract]))
AND
((digital[title/abstract]) OR (design[Title/Abstract]) OR (usability[Title/Abstract]) OR (accessibility[Title/Abstract]) OR (“real?time information”[Title/Abstract]) OR (interactiv*[Title/Abstract]) OR (output*[Title/Abstract]) OR (outcome*[Title/Abstract]) OR (user?friendly[Title/Abstract]) OR (visual*[Title/Abstract]) OR (metric*[Title/Abstract]) OR (dials[Title/Abstract]) OR (appearance[Title/Abstract]) OR (display[Title/Abstract]) OR (font[Title/Abstract]) OR (colour[Title/Abstract]) OR (icons[Title/Abstract]) OR (images[Title/Abstract]) OR (charts[Title/Abstract]) OR (maps[Title/Abstract])) AND ((“service improvement”[Title/Abstract]) OR (benchmarking[Title/Abstract]) OR (feedback[Title/Abstract]))
AND
((health[Title/Abstract]) OR (health?care[Title/Abstract]) OR (clinical[Title/Abstract])
Chapter 3: rapid review of possible themes searches
Date range searched: from inception to 5 June 2017.
Date searched: 5 June 2017.
PubMed
Search terms
“patient experience”[Title/Abstract] AND survey[Title/Abstract] AND (free*text[Title/Abstract] OR comments[Title/Abstract] OR open[Title/Abstract]) AND (“thematic analysis”[Title/Abstract] OR themes[Title/Abstract]) AND (“2007/06/07”[PDat] : “2017/06/03”[PDat])
Web of Science and Cumulative Index to Nursing and Allied Health Literature
Date range searched: 2007–17.
Search terms
((‘patient experience survey’ AND (free*text OR comment)) AND (‘thematic analysis’ OR theme)).
Appendix 2 Preferred Reporting Items for Systematic Reviews and Meta-Analyses 2009 flow diagram, scoping review for PRESENT (clinical digital toolkit design)
FIGURE 22.
Preferred Reporting Items for Systematic Reviews and Meta-Analyses 2009 flow diagram, scoping review for PRESENT (clinical digital toolkit design).

Appendix 3 Summary of the key features that health-care dashboards and toolkits should incorporate according to the literature only (see Chapter 2)
Feature category | Examples |
---|---|
Access |
Minimising workload burden Integrated into an already existing system/constantly in sight Registration absent or clear and short Engaging homepage with a clear statement of value Real-time access (e.g. during clinics) |
Flexibility and individualisation |
Personalisation features Alerts and reminders Information pins Dynamic movement of elements of the dashboard to fit around the user’s focus and workflow |
Usability and navigation |
URL simple, memorable and clearly associated with the focus of the dashboard Layout complexity matched to usage Applying colour to emphasise differentiations RAG traffic-light colour system Using bright, distinct and highly contrasting colours Large and clear black fonts on contrasting, light-coloured background Simple pages, key points at the top Giving users an option to view more detail Simple language free of abbreviations and jargon Use of first person in text, empowering, not patronising Definitions for dashboard elements that are not obvious Adopting ‘most common’ lists Browsing options clearly visible and well labelled No scrolling Information accessible in a few mouse clicks Left-to-right menu rather than top down Ensure reactive to different devices Layout logic and route that anticipates the user’s workflow |
Use of images and videos |
Informative, rather than decorative, images that reflect the user’s demographic profile Videos to instruct on how to use the dashboard |
Chart types |
Line and bar charts – well labelled – for analysing relationships, tables for extracting specific values and complex tasks; function to choose graphic type or table Including reference data points, such as national averages, for easier interpretation Several graphs on one screen |
Data interrogation |
Ability to filter the data in real time and to sort it by any level and quality indicator; filter parameters need to be practical, clearly defined and aligned with the user’s work Feedback that the page has changed after filtering Search box for interrogating the data/drop-down list or dictionary of suggested search terms |
Print and export | The option to print information, download data outputs |
Community features |
Patient stories and other narrative videos Forum, chat room or similar community feature Signposting to other sources of information and support |
Security and privacy | Security and privacy prioritised |
Offering recommendations and solutions | Highlighting problems, but also offering recommendations or solutions |
Appendix 4 Preferred Reporting Items for Systematic Reviews and Meta-Analyses 2009 flow diagram, rapid review for PRESENT (themes)
FIGURE 23.
Preferred Reporting Items for Systematic Reviews and Meta-Analyses 2009 flow diagram, rapid review for PRESENT (themes).

Appendix 5 Feedback from stakeholders on an early iteration of the dashboard and subsequent action taken
Desired features: first focus group’s data | Developer interpretation and comments for early iterations | Further co-design work and decision | The future |
---|---|---|---|
Highly customisable filtering was perceived to be crucial (i.e. participants wanted to be able to specify bespoke textual queries like ‘prostate cancer AND transport’ – although this form of Boolean search was unknown to some participants)
|
Filtering may be the practical solution, but there was a strong desire for textual entry of queries, as opposed to facets Implementation of both text-based and binary searches for comments Summaries as requested are possible, but only with site maintenance with a facilitator drawing out interesting facts or with some further NLP work that was considered to be outside the remit of the study |
Textual entry was discussed in subsequent groups and eventually determined to be problematic because of the limited number of comments that might be returned from some textual queries A compromise solution was reached whereby text entries for themes that drew from a limited range were possible |
As more data are included, textual entry could be possible Text searches for themes could do a simple word search of the data and this or more advanced NLP rules could be incorporated in the future, or the possibilities for more controlled returns expanded |
Bar charts should be rotated (i.e. so the date goes from left to right; in the first prototype they were presented with dates changing top to bottom) Mixed feelings on visualisation where there are a small number of comments – both perceived to be useful and misleading Show increase/decrease per year on the date chart |
As there was not more than 1 year of data, the comments were noted but were not included in the final version showing 1 year only | Discussions on small numbers continued through the focus groups as various solutions were tried. Ultimately, it was decided that, owing to considerable variation in opinion, visualisations would be shown where there were 10 or more comments, but all comments would be shown as text in the underlying layer | Respond to comments with amendments once more years of data are added |
Gauge visualisation had a few comments (e.g. it was not obvious that the size of the segment meant the number of comments; needle was considered to be more user-friendly than a line) It was considered to be essential to be able to choose between or customise graphs depending on the kinds of information the user wants, or to suit their expertise |
Variations on the gauge were tried and each one received a mixed response. Ultimately, it seemed that everyone preferred either pie charts (which were considered to be inaccurate) or bar charts. It was agreed that the best option, given the variation in opinion, was to give the user the option of personalising the chart type | This decision was ratified | Chart type may need to be revised once 5 years of data have been captured |
Generally, the Amazon-style chart – developed as a response to the first focus group – was perceived well, but the use of ‘stars’ was confusing (see next point) ‘Positive’/‘negative’ were preferred over ‘highly rated’ and ‘needs improvements’ (it was believed the last option to be more diplomatic and less polar, but all participants disagreed) Star ratings (to indicate very positive, positive, negative and very negative) were perceived to be ambiguous |
It was decided not to continue with the Amazon-style chart for practical reasons The star ratings were also dropped. The words positive and negative were used |
This choice was ratified in later focus groups in which health-care professionals suggested that it was appropriate for commercial purchases, but not for health-care decisions, which were driven by very different factors | No action |
Percentages were considered to be more meaningful than absolute numbers | This has been carried through all stages as was agreed | N/A | |
Red/green were perceived to be fine, although colour blindness needs to be considered | The developers experimented with different colours, as future groups considered the data to be too weak for there to be red alerts, but ultimately stuck with versions of red and green that were not too strident, as that was the dominant preference of all stakeholders | The issue of red alerts will be less problematic as more data accumulate | N/A |
Pictures/diagrams were considered to be important | Because of time constraints, preferences could not be explored further during the study, although an amendment was submitted that received ethics approval, to explore this in a survey with illustration choices | Needs more exploration | A possibility for the future, although preferences will depend on people’s backgrounds, so this may be something that should be reconsidered for each different application of the dashboard |
Appendix 6 Concept-mapping workshop participants
Role | Number of participants (%) |
---|---|
Patient | 14 (41.18) |
Carer | 1 (2.94) |
Consultant/specialist | 1 (2.94) |
GP | 1 (2.94) |
Nurse | 3 (8.82) |
Quality team member | 0 (0.00) |
Variety of commissioning roles | 2 (5.88) |
Budget holder | 0 (0.00) |
NED PPI lead | 1 (2.94) |
Policy-maker | 0 (0.00) |
Academic | 2 (5.88) |
Othera | 7 (20.59) |
Did not say | 2 (5.88) |
Total | 34 (100.00) |
Condition | Number of respondents (%) |
---|---|
Lung cancer | 0 (0.00) |
A cancer of the bladder, stomach, bowel, colon, pancreas, liver or kidneys | 3 (8.82) |
Cancer specific to men or to women (e.g. prostate, breast) | 2 (5.88) |
Leukaemia or lymphoma | 2 (5.88) |
Skin cancer | 0 (0.00) |
Brain cancer | 0 (0.00) |
Non-cancer muscular or skeletal condition | 0 (0.00) |
Diabetes mellitus | 1 (2.94) |
Lung problems that are not cancer | 0 (0.00) |
Eye problems | 1 (2.94) |
Gynaecological problems | 0 (0.00) |
Chronic pain not covered by any of the above | 0 (0.00) |
Othera | 21 (61.76) |
Did not say | 4 (11.76) |
Total | 34 (100.00) |
Gender | Number of participants (%) |
---|---|
Male | 8 (23.53) |
Female | 23 (67.65) |
I would rather not say | 0 (0.00) |
Did not say | 3 (8.82) |
Total | 34 (100.00) |
Statistic | Year of birth |
---|---|
Minimum | 1938 |
Maximum | 1988 |
Mean | 1965 |
Mode | 1984 |
SD (years) | 16.59 |
Did not say (n) | 6 |
Ethnicity | Number of participants (%) |
---|---|
White: English/Welsh/Scottish/Northern Irish/British | 18 (52.94) |
White: Irish | 1 (2.94) |
White: Gypsy or Irish Traveller | 0 (0.00) |
Any other white background | 3 (8.82) |
Mixed/multiple ethnic groups: white and black Caribbean | 1 (2.94) |
Mixed/multiple ethnic groups: white and black African | 1 (2.94) |
Mixed/multiple ethnic groups: white and Asian | 0 (0.00) |
Any other mixed/multiple ethnic background | 1 (2.94) |
Asian/Asian British: Indian | 1 (2.94) |
Asian/Asian British: Bangladeshi | 0 (0.00) |
Asian/Asian British: Pakistani | 0 (0.00) |
Asian/Asian British: Chinese | 1 (2.94) |
Any other Asian background | 2 (5.88) |
Black: African | 1 (2.94) |
Black: Caribbean | 1 (2.94) |
Any other black/African/Caribbean background | 0 (0.00) |
Arab | 0 (0.00) |
Any other ethnic group | 0 (0.00) |
Did not say | 3 (8.82) |
Total | 34 (100.00) |
Appendix 7 Summary of key findings concerning candidate design changes (opportunities and challenges) and the resultant refinements from stage 3
In this appendix, verbatim examples are provided of what participants said for the main findings from the structured walk-throughs. Note that many of these comments resulted in changes to the prototype, so they are no longer relevant to the study-end prototype.
Area of enquiry | Representative quotations from qualitative data relevant to ‘candidate design changes’ |
---|---|
Dashboard: usability | It’s so responsive currently, but with 75,000 comments you might need an indicator to show it’s working if it takes timeIt corresponds to the 2-week wait referrals and pathways, so that’s fineIt’s really easy to navigate; I guess the only thing I wasn’t sure about was the clicking on the dial thingHelp page is too wordyI can click and unclick the filters; that’s easyThere are no prompts at all. That’s one thing that is neededIt’s quite intuitiveYou don’t feel frightened of clicking on things and having a play with that and that’s what’s importantHalf pie chart things are confusing |
Dashboard: goals | To improve servicesIf running well, use positive messages to show this, if under-resourced could get patient quotes and other hospital comparisons to argue for resourcesExport and report, or printer-friendly version of pageNeed to think about transferability to other health areasNeed data denominators and better visualsClinicians need to meet certain targets and if they do that’s good, but meeting those targets may not be good for patientsWhat are the themes that come out as regular offendersIt would be good to input patient comments from other sources |
Dashboard: implementation | Most have managers sift and summarise by hand – most do no analysis – just summariesThe dashboard fits well with ‘Listening into Action’ movement in health care currentlySo potentially this is very excitingWhy ask probing questions if you’re not going to use the answers? (That is, important to use the data)I can see the purpose, and the remit, and the role, and I think it’s got a really important place and it’s a good bit of work – It just needs a little bit of fine-tuning . . .This would revolutionise thingsWho will host this then, once it’s up and running?People feel overwhelmed at the moment by so many systems |
Welcome page | Doctor and patient picture divides us and them – suggests distance dynamic‘Start’ should explain what’s coming on the dashboardImages too clichédWelcome page and next page don’t tell you about the dashboard, it tells you about the project – we need to work up to the dashboard |
About Us | I just want bullet points; importance of patient experience, blah, blah, blah. See all of that is just not necessaryNot About Us – take out ‘Us’Too wordy, maybe have links if people want more info |
Data | Just need volume, geography, when obtained and who fromNeed denominator – then absolute numbers of comment givers and percentages.Some of the key messages from analyses of the data and how they fit with national agenda and where the areas of need are |
Help | Help and prompts should be earlierHover over ‘help’ (on the dashboard)It says incorrectly that the dials give at a glance how well the hospital did – this is not true |
Other | It’s important for patients to know their free text is being usedInclude links to Macmillan, full (quantitative) CPES data, CRUK, NHS cancer dashboard, add NICE, CQC and other key target guidance. Could be applied to other health areas (dementia, safeguarding)It’s good to use positive comments to keep up morale |
Glossary
- F-score
- A statistic that shows the balance between precision (specificity) and sensitivity in text mining and information retrieval.
- General Architecture for Text Engineering
- A software framework and collection of resources that can be used for various natural language processing tasks. In this report, GATE (version 5.2.1; University of Sheffield, Sheffield, UK) is used to refer to two integrated programs: GATE Developer and GATE Embedded.
- Information retrieval
- A computational term for the extraction of structured data from unstructured or semistructured data.
- Knowledge extraction
- See the definition for information retrieval.
- Multidimensional scaling
- A statistical approach.
- Natural language processing
- A range of computational techniques theoretically developed from human language processing studies. One use of natural language processing is to analyse texts and get information from them.
- Normalisation Process Theory
- A theory used to understand the implementation, embedding and integration of new technology or complex interventions.
- Parsing
- Sequentially ‘reading’ or analysing natural language.
- Parts of speech
- Grammatical categories.
- Pipeline
- Computer code or modules in series.
- Tokenising
- Breaking text into its component parts, for example words, punctuation marks, numbers and other discrete features.
List of abbreviations
- AG
- advisory group
- API
- application programming interface
- CCG
- Clinical Commissioning Group
- CINAHL
- Cumulative Index to Nursing and Allied Health Literature
- CL
- conditional logit
- CPES
- Cancer Patient Experience Survey
- CQC
- Care Quality Commission
- CRUK
- Cancer Research UK
- CSS
- Cascading Style Sheets
- CSV
- comma-separated values
- DCE
- discrete choice experiment
- e-HIT
- e-Health Implementation Toolkit
- FN
- false negative
- FP
- false positive
- GATE
- General Architecture for Text Engineering
- GP
- general practitioner
- GUI
- graphical user interface
- HSDR
- Health Services and Delivery Research
- HTML
- Hypertext Markup Language
- IE
- information extraction
- IR
- information retrieval
- JAPE
- Java Annotation Patterns Engine
- JSON
- JavaScript Object Notation
- KNN
- k-nearest neighbour
- LAPCD
- Life After Prostate Cancer Diagnosis
- LDA
- latent Dirichlet allocation
- MDS
- multidimensional scaling
- MS
- multiple sclerosis
- NICE
- National Institute for Health and Care Excellence
- NIHR
- National Institute for Health Research
- NL
- nested logit
- NLP
- natural language processing
- NPT
- Normalisation Process Theory
- PES
- patient experience survey
- PICOT
- participants, intervention, comparison, outcomes, types of study
- POS
- part of speech
- PPI
- patient and public involvement
- PPIRG
- Patient and Public Involvement Research Group
- PR
- processing resource
- PRISMA
- Preferred Reporting Items for Systematic Reviews and Meta-Analyses
- QDA
- qualitative data analysis
- RAG
- red/amber/green
- RAM
- random-access memory
- SD
- standard deviation
- SPC
- statistical process control
- SSC
- study steering committee
- TDM
- treatment decision-making
- TN
- true negative
- TP
- true positive
- UAG
- user advisory group
- WCPES
- Wales Cancer Patient Experience Survey
- WTP
- willingness to pay
- XML
- Extensible Markup Language