Notes
Article history
The research reported in this issue of the journal was funded by the HS&DR programme or one of its preceding programmes as project number 14/194/21. The contractual start date was in May 2016. The final report began editorial review in May 2019 and was accepted for publication in October 2019. The authors have been wholly responsible for all data collection, analysis and interpretation, and for writing up their work. The HS&DR editors and production house have tried to ensure the accuracy of the authors’ report and would like to thank the reviewers for their constructive comments on the final report document. However, they do not accept liability for damages or losses arising from material published in this report.
Declared competing interests of authors
Peter Griffiths reports grants from the National Institute for Health Research (NIHR) during the conduct of the study [Health Services and Delivery Research (HSDR) 13/157/44, PR-ST-1115-10017 and HSDR 17/05/03)] and support from NIHR through a senior investigator award and as part of the NIHR Collaboration for Leadership in Applied Health Research (CLAHRC) Wessex. He is a member of the NHS Improvement safe staffing faculty steering group. The safe staffing faculty programme is intended to ensure that knowledge of the Safer Nursing Care Tool (SNCT), its development and its operational application is consistently applied across the NHS, using the evidence base underpinning the SNCT. This role attracts no remuneration. Jane E Ball reports grants from NIHR during the conduct of the study (PR-ST-1115-10017) and support from the NIHR CLAHRC Wessex. Rosemary Chable reports grants from NIHR during the conduct of the study (HSDR 13/157/44). Andrew Dimech reports that he was working on his clinical doctorate during the conduct of the study. Jeremy Jones reports grants from NIHR during the conduct of the study (II-LB-0814-20006, RP-PG-0610-10078 and HSDR 17/05/03). Thomas Monks reports grants from NIHR as part of NIHR CLAHRC Wessex during the conduct of the study.
Permissions
Copyright statement
© Queen’s Printer and Controller of HMSO 2020. This work was produced by Griffiths et al. under the terms of a commissioning contract issued by the Secretary of State for Health and Social Care. This issue may be freely reproduced for the purposes of private research and study and extracts (or indeed, the full report) may be included in professional journals provided that suitable acknowledgement is made and the reproduction is not associated with any form of advertising. Applications for commercial reproduction should be addressed to: NIHR Journals Library, National Institute for Health Research, Evaluation, Trials and Studies Coordinating Centre, Alpha House, University of Southampton Science Park, Southampton SO16 7NS, UK.
2020 Queen’s Printer and Controller of HMSO
Chapter 1 Introduction
Overview
Approximately 310,000 whole-time equivalent (WTE) registered nurses were employed in NHS hospital trusts in England during July–September 2018, with an estimated 11.6% vacancy rate; that is, there were > 40,000 WTE registered nurse vacancies. 1 Multiple reviews of research have established that higher registered nurse staffing levels in hospitals are associated with better patient outcomes and care quality. Reported associations include lower risks of in-hospital mortality, shorter lengths of stay and fewer omissions of necessary nursing care. 2–5 Newer studies6–9 exploring patient-level longitudinal relationships and causal mechanisms provide increasing confidence that the observed relationships are, at least in part, causal. However, beyond providing an injunction to invest in ‘more’ staff, such studies rarely offer a direct indication of how many staff are required in a given setting. Given the size of the nursing workforce and the shortages in supply, the ability to properly determine an ‘optimal’ staffing level is an imperative from the perspective of both quality and efficiency of care.
The Francis Inquiry10 into the failings of the Mid Staffordshire General Hospital (Mid Staffordshire NHS Foundation Trust) identified low staffing as a significant contributor to ‘conditions of appalling care’. A key recommendation of this inquiry was the development of guidance for nurse staffing, including:
. . . evidence-based tools for establishing what each service is likely to require as a minimum in terms of staff numbers and skill mix.
Francis. 10
The use of tools can vary to support a range of operational decisions, for example how many staff to employ on a ward, which staff are needed on particular shifts and whether or not staffing is adequate, and for billing purposes. Despite the large number of nurse staffing tools available, the determination of the hours required to maintain ‘adequate’ or ‘quality’ staffing remains problematic. Commissioned to develop national safe staffing guidelines for the NHS in the UK following the Francis Inquiry, the NHS advisory body the National Institute for Health and Care Excellence (NICE) concluded that:
There is a lack of research that assesses the effectiveness of using defined approaches or toolkits to determine nursing staff requirements and skill mix.
The evidence review undertaken by NICE was based on existing reviews and focused on the impact of staffing tools and methodologies on outcomes and quality,12 but other reviews of broader scope over many years have reached similar conclusions. 13–18
Despite the lack of evidence, soon after publishing its guidance in 2014, NICE endorsed the Safer Nursing Care Tool (SNCT) as a decision support tool for setting nurse staffing levels on adult inpatient wards to meet patient need, based on an acuity/dependency measure. 19–21 Already the most popular tool in use in the NHS, it is now used in the vast majority of acute NHS hospitals. 22 The present study explores the use of the SNCT and seeks to address a number of key questions about the tool, including (1) the extent to which the staffing requirements estimated using the tool provide staffing levels that accord with professional judgements about sufficient staffing to provide quality care, (2) the best approach to using the tool’s results to set the required staffing level and (3) the costs and likely consequences of staffing wards according to the levels prescribed by the tool, questions that, thus far, have been largely neglected in the literature about this or any other tool.
Nurse staffing levels and outcomes
Reviews of observational studies2–5,12,23,24 have consistently concluded that there is an association between higher registered nurse staffing levels and better outcomes for patients in acute hospitals. In 2007, a systematic review identified 96 studies and concluded from a meta-analysis of 28 that increased registered nurse staffing levels are associated with lower risks of in-hospital mortality (for intensive care, surgical and medical patients), shorter lengths of stay (for intensive care and surgical patients) and reductions in occurrences of various other adverse patient events. 4 The majority of the papers included in this 2007 meta-analysis were from the USA, but the association between nurse-to-patient ratios and in-hospital mortality has subsequently been confirmed in several other countries and regions, including in a large-scale cross-sectional study25 of more than 400 hospitals in many European countries.
Subsequent reviews have confirmed associations between nurse staffing and care quality across a range of outcomes and process measures, including omissions of essential nursing care, which has been identified as a key mechanism leading to adverse patient outcomes. 5 Because of the varying scope of material in the reviews, it is now hard to judge the full extent of the evidence, but an assumption that the size of the evidence base has more than doubled since Kane et al. ’s4 2007 review would probably be a highly conservative one. However, the evidence is, without exception, observational and, with few exceptions, cross-sectional, albeit based on large studies often involving hundreds of hospitals and millions of patients. 2
Causality
Although an overall association seems clear, it has been more difficult to establish that increased nurse staffing levels cause improved patient outcomes. In the abundant cross-sectional studies, it is conceivable that poor outcomes lead to a decision to increase staff levels, which may explain why associations with some of the most plausibly nurse sensitive outcomes, such as pressure ulcers, are least clearly supported. 2 It is also difficult to disentangle nurse staffing effects from wider hospital resources, including medical staffing levels, although there are now a number of studies that demonstrate a nurse staffing effect when controlling for levels of medical or other staff26–29 or that are otherwise largely immune from such confounding. 6,8,9
Kane et al. ’s4 meta-analysis provides evidence for causality assessed against the so-called ‘Bradford Hill’ criteria30 by, first, showing the consistency of results from multiple studies, second, showing that results are not sensitive to the study designs used, and, third, providing evidence of a dose–response relationship. More recent studies5–7,31,32 provide evidence for plausible causal mechanisms related to omissions of care by showing that omissions partially mediate the association between staffing levels and outcomes for both mortality and patient satisfaction. Recent studies6,8,9 have used a longitudinal rather than a cross-sectional design, showing that the association operates at the level of individual patient exposures to low nurse staffing on wards, as well as establishing the temporal order of events.
Cost-effectiveness and efficiency
Despite the plentiful evidence of the link between increased nurse staffing levels and improved patient outcomes, NICE found it insufficient to translate into specific staffing recommendations. 11 As NICE’s framework for evaluating service changes is to measure the potential cost benefit, for example the cost per life saved, reliable estimates of these measures are needed before evidence-based recommendations can be made. Existing international economic modelling studies have produced widely disparate estimates of cost-effectiveness. 2,33 Some studies suggest that increases in registered nurse staffing might fall within accepted cost-effectiveness thresholds and increases in skill mix could prove cost neutral, but there is substantial uncertainty associated with the finding. 6
Although the potential effects of changes in staffing levels have been addressed in many studies, the question of how many nurses are actually required, or how to make that determination, remains unanswered by such studies. Policies of mandatory staffing minimums have been much discussed and implemented in a number of jurisdictions, most notably California, USA. 34–36 These policies remain controversial and evidence of the benefits associated with them remains elusive, as well as being difficult to disentangle from the simple effects of staffing increases, making it unclear whether or not the specific staffing levels mandated are correct. 2,34 Furthermore, these policies have been criticised as providing an inflexible system that is potentially inefficient. 37 Even when mandatory minimum policies are implemented, a requirement remains that patient care needs over and above the minimum be assessed and staffing adjusted accordingly.
Overview of approaches to setting nurse staffing levels
Many approaches to setting nurse staffing levels have been described. Typologies to classify these approaches have been proposed by, for example, Hurst16 and Edwardson and Giovannetti. 15 We present the main types (using these proposed typologies with minor adjustments), along with examples, in Table 1. The number of nursing staff funded to work on a ward is called the nursing establishment. 11
Type of approach | Examplesa |
---|---|
Expert judgement | |
Patient volume |
|
Prototype/patient classification |
|
Task/timed task |
|
Indicator |
|
Regression |
|
Telford’s professional judgement method,38 which was first formally described in the UK in the 1970s and later documented by Hurst,16 shows how a shift-level staffing plan can be converted into a recommendation for the number of staff to employ. The method provides a framework for deliberation and consideration, but at its core the judgement of required staffing is based on the judgement of senior nurses, with no recourse to any direct calculation or formal use of an objective measure to determine need. 14 The calculations offered relate solely to the conversion of shift-level staffing plans to establishments or vice versa. In recent years this deliberative approach without formal measures appears to have been reflected in the US Veterans Administration staffing methodology. 39 Other approaches that could be characterised under this method include benchmarking approaches by which expert judgements are made about suitable comparators, and the staffing levels and achieved quality are compared between similar units.
Benchmarking exercises are often used to arrive at a nurse-to-patient ratio or equivalent (e.g. nursing hours per patient) to assign a minimum or fixed number of nursing staff per occupied bed. 16 Although characterised by Hurst as a distinct method, there is little initial formal assessment of patient requirements for nursing care, although consensus methods and expert judgement are often used when determining the staffing requirement for particular types of wards and selecting appropriate benchmarks.
Effectively, these approaches assume that all patients in similar wards have similar care needs, or that the average need is stable across patient groups for a given shift period, so that most of the time needs can be anticipated and met with a fixed roster. When demand is above this, it is expected that additional staffing requirements be determined. Although the benchmarking comparison with similar wards gives the appearance of objectivity, much depends on how the initial staffing levels were arrived at, and there is ample evidence that perceptions of staffing requirements are largely anchored to historical staffing levels. 40 Because some more recent approaches to monitoring workload extend this approach to take a wider view of activity, adding in admissions and discharge over and above the patient census, we will term these benchmarking and ratio approaches ‘patient volume’ approaches.
Other approaches attempt to account for variation in needs between patients, and these can be used instead of, or together with, minimum staffing levels. For example, patient classification systems (also referred to by Hurst as acuity/dependency systems) categorise patients according to their nursing care needs and recommend a staffing level for each category. 17 These categories may either be designed specially (e.g. classifications based on levels of acuity and/or dependency) or already be in use (e.g. diagnosis-related groups). 17 The SNCT is one such system, allocating patients to one of five acuity/dependency categories with a weighting (described as a ‘multiplier’) to indicate the required staff to employ associated with patients in each category. 19 On the other hand, the timed-task approach, rather than categorising patients, considers each new patient individually. The required staffing for a patient is calculated from time estimates for a list of the tasks and activities planned for that patient. 16 The commercial GRASP system, widely used in the USA, is one example. 15 In all of these approaches, the total staffing required is calculated by summing up the individual patient requirements, possibly with some overheads.
The approach to determining the required times for patient groups, specific tasks or broad areas of activity varies. The literature describes the use of both empirical observations and expert opinion to determine the average time associated with activities or patient groups. 41–43 In some cases, there is an explicit attempt to make workload/time allocations based on reaching some threshold of quality or optimum care. 44,45 Approaches to identifying the time required for work aside from directly caring for patients vary, but all approaches take this into account, often assigning a fixed percentage of time over and above that measured for patient contact.
Hurst16 also described regression-based approaches. These are based on models of the relationship between patient-, ward- and hospital-related variables, and the staffing level observed in ‘quality-assured’ wards. The regression coefficients, together with the values for each variable, are used to estimate the required staffing for other wards. Perhaps owing to the obvious complexity, there are relatively few examples, although Hoi et al. 46 provide one recent example, the workload intensity measurement system. In some respects, the regression-based models simply represent another approach to allocating time across a number of factors rather than directly observing time linked to specific activities. The RAFAELA system, widely used in the Nordic countries, although based on a relatively simple patient classification, also uses a regression-based approach to determine the staffing required to deliver an acceptable intensity of nursing work for a given set of patients in a given setting. 44,45,47
Edwardson and Giovannetti15 offer a slightly different typology, differentiating ‘prototype approaches’ from ‘indicator approaches’ and ‘task approaches’. Prototype and task approaches broadly resemble the patient classification and task approaches (respectively) previously described. Indicator approaches ultimately assign patients to categories but this is done based on ratings across a number of factors that are related to the time required to deliver patient care. These can include broad assessments of condition (e.g. ‘unstable’), states (e.g. ‘non-ambulatory’), specific activities (e.g. complex dressings) or needs (e.g. for emotional support or education). 15 The Oulu Patient Classification, part of the RAFAELA system, is one such example. Here patients are assigned to one of four classifications, representing different levels of care required, based on a weighted rating of care needs across six dimensions. 44 However, the inclusion of some specific activities in Edwardson and Giovannetti’s15 definition of indicator approaches makes it clear that the distinction from task-/activity-based systems is not an absolute one. Typically, however, task-based systems take many more elements into account: over 200 in some cases. 15
Overlap of approaches
Although these typologies are useful for setting out some common characteristics of nurse staffing approaches, it is not true that each approach belongs in only one category.
As an example, expert judgement-based approaches might involve benchmarking to set a fixed establishment for a ward, but based on an underlying staffing model that aims for a given nurse-to-patient ratio on each shift (and so might be classified as a patient volume-based approach). However, the original determination of that requirement might have involved a detailed appraisal of patient need on a given ward involving many factors, but no formal measurement.
On the other hand, although a patient classification system may set an establishment based on a measurement of individual patient needs, without the application of further expert judgement for deploying staff on shifts, the establishment, once set, implies that care needs are then met by a fixed nurse-to-bed ratio regardless of daily variation. Indeed, a simple prototype classification system, such as the SNCT, resembles a volume-based mandatory minimum staffing policy supplemented by assessment of variation above the base requirement, such as that implemented in California, because there is an implied absolute minimum staffing level per patient, associated with the lowest weighting.
Evaluation of staffing methods/tools
One early review of nurse staffing methods, published in 1973,18 included a bibliography of over 1000 studies but found no evidence concerning the relative costs or effectiveness of different staffing methods. It concluded that there was no substantive basis for choosing any one system over another, little evidence for validity beyond ‘face’ validity and scant evidence even of reliability, summing up that ‘Although the intent of the methodologies is admirable, all are weak’ (p. 57). 18
Subsequent reviews have had to embrace an ever-growing body of research, and yet they reach remarkably similar conclusions. A review13 undertaken for the then Department of Health and Social Services in the UK in 1982 identified over 400 different systems for determining staffing requirements. Writing in 1994, Edwardson and Giovannetti15 noted the absence of published scientific evidence for a number of commercial systems, such as GRASP or Medicus, which were in widespread use in North America. They also noted that, although different systems tended to produce results that were highly correlated, they could, nonetheless, produce substantially different estimates of the required level of nursing staff for a given patient or unit. 15 Edwardson and Giovannetti’s15 analysis concluded by asking a number of questions, which remained unanswered by the evidence they reviewed:
-
Do the results of workload measurement systems differ substantially from the staffing requirements judged by practising professional nurses?
-
Does the implementation of a staffing methodology or tool lead to altered staffing levels or, conversely do historical staffing levels influence the assessment of need?
-
Do workload measurement systems improve the quality of care?
-
Do workload measurement systems result in more efficient use of nursing personnel?
More recently, in 2010, Fasoli and Haddock17 reviewed 63 sources (primary research, theoretical articles and reviews) and also concluded that there was insufficient evidence of the validity of many current systems for measuring nursing workload and staffing requirements. They described the field as dominated by descriptive reports of locally developed approaches, with limited or weak evidence of validity for even widely used and commercially supported systems. 15,17 They concluded that systems in use may not be sufficiently accurate for resource allocation or decision-making. 17,48
There is little basis for preferring any one tool or approach over another based on the available evidence. Professional judgement-based approaches, despite being open to accusations of subjectivity, cannot be readily dismissed, as there is no substantial evidence that moving from a judgement-based staffing model to one informed by a tool has improved any outcomes or made more efficient staffing allocations. 2,13,14,16–18,40 One of the most comprehensively researched systems determines the staffing requirement by titration against a subjective report of work intensity. 44,47 Uncertainty over the best method might matter little if different approaches gave similar results. Although direct comparisons are few and far between, it is, however, clear that different systems can give vastly different estimates of required staffing. 49–52 In one study,52 the five systems tested provided estimates that correlated highly. However, they offered a wide range of average staffing requirements for the same sample of 256 patients, with the lowest average being < 60% of the highest [6.65 vs. 11.18 hours per patient-day (HPPD)].
The reviews discussed here, as well as others,2,13–18,40,48,53 give a clear indication of the vast literature on nurse staffing methodologies, but the field appears to have made little progress over many years. Most research reports on the underlying development of tools or assesses their implementation, but little research validates the resulting tools, and the reviews indicate that none has evaluated the impact of implementation on outcomes for staff or patients.
The Safer Nursing Care Tool
Following the Francis Inquiry report and the development of NICE guidelines, the SNCT was the first tool endorsed by NICE for setting nurse staffing levels. Following this, NICE endorsed three other tools. 54 Of these, one is a rostering system incorporating an acuity/dependency tool based on the SNCT (Health Roster/SafeCare®, Allocate Software, London, UK), one is a web-based tool that explicitly claims to use the SNCT criteria [iPAMS, formerly Albatross Financial Solutions; documentation found at https://docplayer.net/17204848-Ipams-integrated-patient-acuity-monitoring.html (accessed 2 November 2018); now IQVA UK/NHS Solutions] and one is a generic framework for reviewing establishments using benchmarking principles (Establishment Genie, Creative Lighthouse Ltd, Beaconsfield, UK).
The SNCT is a prototype tool. The documentation of the tool19 provides a framework for incorporating professional judgement and assessment of nursing-sensitive outcomes as part of a ‘triangulated approach’. However, the core of the tool is a method for determining the average patient need for a given ward based on assessing patients and allocating them to one of five acuity/dependency categories, each of which has a ‘multiplier’ corresponding to the typical workload involved in caring for a patient in that category. From these data, a ward establishment sufficient to provide staff to meet patient need is calculated, making provision for necessary ‘time out’, including study leave and expected sickness/absence rates.
The ubiquity of the SNCT is confirmed by surveys of English NHS acute hospital trusts. In 2010, as part of the RN4CAST study of the nursing workforce, we undertook a survey of a stratified random sample of 31 English acute hospital trusts. We found that a majority (59%) of these trusts used a formal acuity/dependency system to guide nurse staffing decisions. Most identified the tool used as the SNCT or its precursor (RN4CAST survey, 2010, unpublished data). Although the SNCT is designed to set nursing establishments based on periodic review, 36% of hospital trusts reported that they monitor acuity/dependency daily, making a responsive, flexible staffing policy potentially feasible. In 2017, we undertook a national survey of acute hospital trusts in England (91/148; 61% response rate) and found that > 80% were using the SNCT as part of their establishment-setting process. 22
Despite its endorsement and widespread use, there is no existing review of evidence about the SNCT.
Commissioning brief
This research was commissioned to address a number of key knowledge gaps identified by NICE and amplified in the commissioning brief 14/194 – ‘measuring nursing input, workload, activity and care’ from the National Institute for Health Research (NIHR) Health Services and Delivery Research programme (see the project web page at www.journalslibrary.nihr.ac.uk/programmes/hsdr/1419421/#/ for the full commissioning brief), specifically themes ii, ‘Reviewing and validating tools to measure nursing activity and workload’, and iv, ‘Making use of nurse staffing data in NHS organisations’.
Chapter summary
-
Evidence that establishes associations between nurse staffing levels and important patient outcomes, including mortality, indicates the likely importance of getting nurse staffing levels right, but does little to indicate what the correct level should be.
-
We identified a vast literature going back over many years, which described and purported to evaluate tools and systems for determining nurse staffing levels on hospital wards.
-
Nurse staffing tools and systems are used to inform the employment of staff (setting ward establishments) and the deployment of staff (both in terms of staffing plans and deployment on the day) and as evaluating the successful delivery of the staffing plan, and for billing purposes.
-
Although there are many distinct tools and systems, they tend to fall under one or more types of approach.
-
The main types of approach are professional judgement approaches, patient volume approaches, prototype/patient classification approaches, approaches that are based on assigning times to tasks on a care plan, and indicator and regression approaches, where several factors are considered.
-
Prototype/patient classification approaches, where an average time (or equivalent measure) is assigned to a patient ‘type’, are the most common and the most widely used.
-
In the UK, the SNCT is an example of a prototype approach, assigning patients to one of five categories based on an assessment of their acuity and dependency on nursing care.
-
The distinction between types of approach is not an absolute one and, for example, the SNCT relies heavily on professional judgement.
-
Despite the vast literature, a series of reviews have drawn very limited conclusions about the tools and are unable to provide unequivocal support for the validity, costs or effects of any tool, or type of approach.
Chapter 2 Literature reviews
We carried out two narrative reviews. In the first review, we looked at recent literature on what is known about nurse staffing methodologies in order to update the evidence base following the review of reviews undertaken in 2014 to support the development of the NICE safe staffing guidelines. For this, we considered papers on the use, development, reliability and usability of tools, papers comparing tools and papers evaluating the impact of using tools.
In the second review, we aimed to collate evidence about the SNCT in particular. This consisted of any material, including grey literature, describing the development or use of the SNCT and its forerunners.
Recent literature on staffing methodologies
Methods, scope and selection
We developed a search strategy that was specific and reasonably sensitive using the results of our comprehensive review for NICE as a test set. 12 We used the terms “Workload”[key word, MESH] or “Patient Classification”[key word] AND “Personnel Staffing and Scheduling” AND “Nurs*”[key word] or “Nursing”[Mesh] and limited using the Ovid MEDLINE sensitive limits for reviews, therapy, clinical prediction guides, costs or economics. We also searched across MEDLINE, Cumulative Index to Nursing and Allied Health Literature (CINAHL) (key word only) and The Cochrane Library. We performed additional searches for citations to existing reviews and the works of their authors on the assumption that new studies were likely to cite one or more of these. We also undertook focused searches on databases for works by key authors and on databases/the World Wide Web for specific terms associated with widely used tools. Searches were completed in mid-October 2018. We looked specifically for primary studies published from 2008 onwards (as the reviews we identified previously covered earlier material) and new reviews published from 2014 onwards (the date of our review for NICE). After removing duplicates, we had 392 sources to consider for this review.
We took a liberal approach to inclusion. First, we included primary studies that described the use, development, reliability or validity testing of systems or tools for measuring nursing workload or for predicting staffing requirements. Second, we included studies that compared the workload as assessed by different measures, or that used a tool as part of a wider study in such a way that it might usefully give some insight into the validity of tools or some other aspect of the determination of nurse staffing requirements. Third, we included studies that reported the costs and/or consequences of using a tool, including the impact on patient outcomes. We included only studies that were of direct relevance to calculating the staffing requirement on general acute adult inpatient units, and this excluded studies focusing on (for example) intensive or maternity care. However, had we identified material that illustrated a significant methodological advance or another insight, we would have been open to including it for illustrative purposes.
Review results
We found no new reviews and 36 primary sources published from 2008 onwards to consider (see Report Supplementary Material 1, Table 85, for fuller descriptions). We classified the sources according to the main purposes of the articles: description, comparison, development, operational research or evaluation. Some articles did not clearly sit in one or another category and were given a dual classification.
Description
Six sources19,20,39,55–57 simply described the use of a staffing system or tool (classified as ‘description’), although they also reported some data, generally exemplar graphs or charts of varying workload. In addition, three studies classified in this group sought to measure nursing workload or demand associated with different ward designs58 or diagnostic groups59 or to determine variability in patient need prior to developing a new workload management system. 60
These studies provide ample reminder that, however measured, there are many sources of variation in the requirement for nursing care on a ward. These arise from the number of patients and the numbers of admissions and discharges, individual patient characteristics and their specific needs, nursing skills and contextual factors such as the physical arrangement of the ward. These needs vary considerably between units and from day to day, although none of these studies provided a measure that allowed the variation to be directly quantified in terms of staff required from day to day.
Comparison
Four sources compared workload as assessed using different approaches, although two of these compared only patient volume-based approaches (different approaches to calculating HPPD, inclusive of turnover):61,62 one compared an acuity/dependency measure with a purely volume-based approach63 and the other compared a newly developed indicator system incorporating additional factors with the results of a pre-existing system. 46
Again, these studies emphasise the lessons determined from previous research: different methods arrive at different results, and methods that take into account more factors appear to arrive at higher workloads. By comparing nurse staffing tools, it has been shown that the inclusion/exclusion of some factors can make a great difference to the estimated workload. An unquantified statistically significant increase in workload from including patient turnover in a volume-based measure was noted in one study. 61 On the other hand, differences between alternative approaches to counting patients in methodologies using hours per patient-day seem to be of marginal overall practical significance. 61,62 However, an acuity/dependency system identified an additional 6 hours of care per day compared with a standard HPPD method. 63 Furthermore, a multifactorial indicator system led to an estimated nurse-to-patient ratio that was double that determined by an existing, less complex system. 46
Development
Perhaps most striking is that the second largest group of studies (13 in total) were those described as ‘development’, reporting on the full or partial development of a new measure or the adaptation of an existing measure. 41–43,46,60,64–71 These measures were often for local use only and most appeared to be subject to a single report only, although three papers42,43,66 related to a single programme of work. Rather than going into detail on the specifics of each of these tools, it is perhaps more instructive to note that all of the system types noted earlier, from professional judgement and volume-based approaches through to timed task, have appeared in these reports on developments from 2008 onwards.
Although these reports typically identify an average time or weighting associated with aspects of care/particular groups of patients, the variability in the underlying estimates is rarely reported or considered. A rare exception to this is the work of Myny et al. 42,43 in Belgium, where a few examples of variation in activities are presented as interquartile ranges (interquartile ranges for ‘settling a bedridden patient’ and ‘settling a non-bedridden patient’ are 5–25.75 minutes and 8–25.25 minutes, respectively). Some 95% confidence intervals for mean activity times are also reported; however, the underlying variation is unclear as sample sizes are not reported for these. The width of the confidence interval makes it clear that variation is, nonetheless, substantial. For example, the estimated standard time for ‘partial help with hygienic care in bed’ had a 95% confidence interval from 7.6 to 21.2 minutes. This was the only study that addressed the sample size required to gain accurate estimates of time. Over 40 days of observation, the authors were unable to reliably estimate the average care time (within ±10%) for the majority of the care tasks they considered. Large standard deviations and skewed distributions meant that the required sample sizes were unfeasible.
It may be that prototype approaches, whereby measures are based on the typical care needs of patients fitting a particular profile, are less subject to variation between individuals with the same classification, but we found no equivalent estimates of variation for such systems. One reason that such measures of variability rarely appear may be that, despite the external appearance of ‘objectivity’, the times or weights assigned within the system are often wholly or partly arrived at through an expert consensus exercise. 42,68,71 It is clear that professional judgement remains an important source of information and validation for any system.
Operational research
We identified four studies72–75 that emanated from the tradition of operational research methods. Although these papers are only examples of a much larger body of literature that focuses on nurse rostering as opposed to developing new workload measurement tools,76 they do serve to highlight one important issue: determining the average staffing requirement does not necessarily provide a solution to the base staffing required to meet varying patient need. Two studies,72,75 one using an acuity/dependency measure described as ‘informed’ by the work of the developers of the SNCT, determined that optimal staffing in the face of varying patient demand was higher than a level determined simply by staffing to meet the mean average demand. In one case the apparent ‘overstaffing’ was associated with net cost savings in modelling/simulations, in part because of the potential value of ‘excess’ staff who were available for re-deployment to understaffed units. 72 Other studies modelled the effects of the use of varying configurations of ‘float’ pools to meet fluctuations in demand. 73,74 The purpose of these studies seems to be primarily to generate ‘local’ solutions, rather than to provide directly generalisable answers. However, they do indicate that, even when a tool correctly determines average staffing requirements, the average does not necessarily provide the optimal answer to the question of how many staff should be employed or routinely deployed on a ward.
Evaluation
Many of the sources classified as ‘development’ were also classified as ‘evaluation’. In total, 16 sources were classified as evaluation based on reporting attempts to assess the reliability or usability/user experience of the system or data that might reflect on the validity or effectiveness of a system.
Reliability and usability
Reliability studies show that nurses can make reliable assessments using a range of classification systems,67,68,77 although achieving inter-rater agreement is not always straightforward and the reliability of ratings in a new setting should not be assumed. 78 Van Oostveen et al. ’s78 pre-implementation study for the RAFAELA system demonstrates that successful implementation of any system requires significant investment to engage and train staff. Even in the face of broad support, there was insufficient engagement with the measures of staffing adequacy required by the system, and satisfactory reliability proved hard to achieve, despite having been demonstrated in other studies. 78 Brennan and Daly79 also identified challenges in achieving reliable assessment and consensus on the validity of some important items of their indicator measure.
Taylor et al. 39 described the substantial challenges faced when implementing a professional judgement-based system for the US Veterans Administration. In concluding that their system could be implemented successfully, they highlighted nursing leadership and front-line staff buy-in as essential. They also emphasised the importance of staff training and the risk of cynicism if staff invest effort in a new system but see few tangible outcomes.
Validity and outcomes
A number of studies20,68–71 seek to demonstrate the validity of their measurement by showing that staffing or workload as measured by a tool corresponds with some other measure of workload or measure of acuity/dependency. In all but one of these examples, the criterion is, in effect, a professional judgement of some measure of demand for nursing care. In addition, the determination of the required staffing level in the RAFAELA system is based on identifying a level of Oulu Patient Classification weighting that is associated with nurses’ reports that staffing is ‘optimal’, further emphasising the continued role of professional judgement in determining validity. 55
We found no controlled prospective studies showing the effects of changing staffing models using tools. Three studies demonstrated that staffing below the level recommended by staffing tools was associated with adverse patient outcomes. A US study8 using an unspecified commercial patient classification system found that the hazard of death was increased by 2% on every occasion a patient was exposed to a shift with ≥ 8 hours below the target defined by the system. Mortality was also increased by exposure to shifts with unusually high patient turnover, suggesting that this might have been generating additional workload that was not measured by the system. Two studies80,81 used the ‘optimal’ staffing level defined by the Oulu Patient Classification, part of the RAFAELA system, as a reference. Both studies found that nursing workload above the defined ‘optimal’ level was associated with adverse patient outcomes, including increased mortality. However, nursing workload below the optimal level (higher staffing) was associated with further improvements in outcomes,80,81 which raises questions about whether or not the ‘optimal’ staffing level is correctly defined. Furthermore, the Oulu Patient Classification workload measure was not clearly superior to a simple patient-per-nurse measure, based on analysis of decision curves. 80
More recently, a UK study6 has shown that when patients were exposed to registered nurse staffing below the planned level, based on establishments determined using the SNCT, the hazard of death in one English hospital trust increased by 9%. This study also explored staffing level as a continuous variable and found that the relationship between mortality and registered nurse staffing levels appeared to be linear, whereas the relationship with support worker staffing was curvilinear. Deviation from the planned staffing level by nursing support workers was associated with worse outcomes, suggesting that the planned level of assistant staffing might indeed be ‘optimal’. 6,9
We found no evidence of the impact of implementing a staffing tool or system on costs, patient outcomes, quality of care or staff, other than the implementation of a ‘nursing hours per patient-day’ methodology in Australia. This methodology assigns a minimum staffing level (measured in HPPD) for six ward types, based on patient case mix and complexity. Improvements were seen over time in several patient outcomes, including mortality, although it is unclear if this was specifically associated with the system used or the resulting increases in staffing levels. 82
Consequently, although these studies give some indication of the validity of the tools used as workload measures, they do not provide clear evidence of the effectiveness of staffing using the tool as opposed to a simpler volume-based system, nor do they clearly show that the tools give an ‘optimal’ staffing level for registered nurses. In effect, the results show that increases in staffing result in improved outcomes. The tools may motivate increases in staffing, although results from using the RAFAELA system suggest that they could also restrict staffing at a level that is not clearly ‘optimal’, with further improvements achieved by higher staffing levels.
Discussion of recent research
Although recent years have seen a continued interest and a significant number of publications relating to systems and tools to determine nurse staffing requirements, the unanswered questions identified by Edwardson and Giovannetti15 in 1994 remain largely unanswered. The finding that outcomes improve as staffing levels rise above the ‘optimal’ level determined by the RAFAELA system80 is consistent with perceptions of adequate staffing being anchored by expectations set by current staffing levels, a phenomenon that has been widely noted and in turn is cited as a rationale for the use of formal systems. 38,39 It may well be that predetermined or historical staffing levels do alter the outcome of the workload measurement systems, despite the apparent objectivity.
Although there are perceptions of benefits, the effect of workload measurement systems on the quality of care remains unclear. In general, the results of a workload measurement system seem to correspond closely to professional judgement, although the magnitude and the significance of differences between the tool and professional judgement are hard to quantify in terms of implied staffing levels as they are generally not reported. However, different systems can give dramatically different results and so it is clear that there can be no single answer to the questions of whether or not workload measurement systems result in an improvement in the utilisation of nursing personnel.
Staffing decisions and the use of tools
A number of different decisions can be made using staffing systems, as summarised in Table 2. They all necessitate a way of quantifying demand for nursing staff (i.e. workload). The distinction between the uses of staffing systems is often unclear in much of the published literature and the relationship between these uses is implicit rather than explicit.
Prospective | Concurrent | Retrospective |
---|---|---|
|
|
|
Prospective decisions include how many nursing staff to employ on a ward (the nursing establishment) and the corresponding planned number of nursing staff to deploy each shift (by time of day and day of week). Although many of the descriptions of establishment-setting focus on doing this over a long-term horizon (with staffing plans reviewed only intermittently), tools can also be used on a shorter-term basis using data from current patients to predict staffing required in the immediate future (e.g. the next shift). Tools can also be used concurrently to determine current staffing adequacy and to alter staffing patterns in some way through re-deployment or balancing the workload between staff members. 68,79 Finally, tools can be used retrospectively to review the success of staffing plans – how well the plan met needs – or as a measure of resource use for pricing, budgeting or billing purposes. 57
Sources of variation
The methods described generally match staffing levels to the average (mean) demand associated with a particular patient group, factor or aspect of care when attempting to estimate current or future staffing requirements. However, the operational research studies make it clear that, in the face of variable demand, simplistic responses based on the average may not be the best way to use the results of measurement systems. Indeed, it is ironic that although much of the literature is concerned with measuring and identifying sources of variation, it is poor at quantifying such variation in a way that allows the assessment of decisions based on the mean. Reports rarely provide estimates of the variability, for example standard deviations around the mean, or even an indication of how certain the estimate of the mean is, in the form of a confidence interval. Furthermore, averaging occurs at multiple levels, for example average number of patients, average acuity/dependency per patient and average nursing time per patient in an acuity/dependency category, so considerable variation can be hidden. In Table 3 we summarise some major sources of variation in nurse staffing requirements.
Individual patient | Ward | Staff |
---|---|---|
Differing care needs
|
Patient census/occupancy
|
Sickness/absence
|
Varying time to deliver care
|
Turnover (admission/discharge)
|
Staff leave (holiday and study)
|
Ward layout
|
Vacancies
|
|
Varying time to deliver care
|
It may be appropriate to set the staffing level at the mean requirement if the workload distribution is approximately normal with low variability because normal distributions have 95% of values within 2 standard deviations of the mean. Provided there is some flexibility in a staff group’s work capacity, most patients will receive the care they need most of the time if standard deviations are small. However, it is unusual for staffing systems to state what constitutes an ‘acceptable’ level of variability. In a rare exception, the RAFAELA system does specify a 15% buffer around the mean,55 although the safety implications are unclear.
Despite the scarcity of data showing the extent of workload variation, it seems plausible that workload distributions could be highly variable and skewed. For example, there is evidence of left-skewed ward occupancy distributions,72 which would tend to lead to mean staffing requirements that are lower than the median. In this case, if staffing levels were set at the mean based on observations over a period of time, relative understaffing would occur > 50% of the time, although the significance of that depends on the degree of flexibility in staff capacity.
Furthermore, when planning workload in advance, it is clear that some sources of variation do not occur at random. As an example, to ensure that there are sufficient staff available to provide cover on wards, the literature describes the need to add an ‘uplift’ to establishments to allow for staff sickness. 16,38 However, staff sickness does not occur uniformly and tends to occur in clusters, with clear seasonal patterns and variation by day of the week. 83 The average uplift would be unlikely to be sufficient to accommodate for the absence of a member of staff on a given day.
Although the number of care tasks or aspects of care accounted for in different systems varies, it appears unlikely that any multifactorial system can fully encompass all aspects of the work associated with patients or the variation in time taken to complete tasks. Prototype systems may bypass this limitation because they are not reliant on estimates of specific care activities, but this remains untested. However, prototype systems cannot easily account for variation associated with activities that are not directly linked to the patient prototype and must instead rely on allowing some form of ‘overhead’ to account for such activities. In systems based on observing care delivered directly to patients, a large proportion of nursing time is unaccounted for (typically ≥ 50%), associated with indirect patient care or associated work, as opposed to direct care. This time is simply added as an overhead. 60
Other factors such as patient turnover are not easy to account for in a patient prototype because they occur at a fixed point in time for a patient and so must also be incorporated into an ‘overhead’ or else simply averaged across patient groups. Turnover clearly has significant potential to generate nursing work, as demonstrated by studies showing that volume measures of nursing workload incorporating this factor are strongly associated with patient outcomes. 6,8,9 Moreover, it is highly variable between and within wards, with some predictable sources of variation (e.g. day of the week) and the potential for substantially skewed distributions. 6 More complex indicator-based systems are better able to take this into account. For example, the RAFAELA system assesses the ‘planning and coordination of nursing care’ required for each patient,55 but again a mean average may not properly reflect varying requirements.
Systems based on local assessments of time required can take into account additional variation that arises from factors such as ward layout; however, systems that provide standard timings or similar to apply to patient types or tasks are, in general, unable to consider this. Although evidence that ward layout may alter staff requirements is limited,58 variation in travel distances and opportunity for patient surveillance are recognised as having the potential to generate considerable variation in workload associated with different ward configurations. 84,85
Although some systems are able to take into account some (but not all) sources of variation when estimating means, this does raise a final issue associated with the lack of quantification of variation in the literature. Even when a mean adequately allows staff to meet variable demand, it is unclear how much care needs to be observed to establish a reliable mean. As Myny et al. 42 point out, when variability is high, the samples needed to obtain reliable means are large.
Skill mix
The skill mix refers to the proportion of those in the total nursing workforce who are registered nurses. It is rarely addressed directly by nurse staffing methods,86 despite the significant evidence base that emphasises the specific association between registered nurse staffing levels or skill mix and patient outcomes. 87 One possible explanation is that many staffing approaches originated in settings where support staff make only a minor contribution to direct patient care (e.g. in the USA87). A further difficulty with recommending a suitable skill mix is that support workers’ roles can vary widely. 88 Thus, some staffing tools limit their scope to registered/licensed nurses, whereas others, such as the SNCT,19 plan the total nursing team size and recommend using professional judgement to decide on the skill mix.
‘Optimal’ staffing
Given that the human nature of nursing work means that it cannot be quantified simply, it is not easy to agree on the ‘right’ amount of time to spend with each patient/on each task. Underlying each staffing approach is an assumption about what constitutes ‘safe’, ‘adequate’ or ‘quality’ staffing; these assumptions are usually implicit. The parameters used in the approaches, for example the recommended length of tasks in the timed-task approach or the recommended time to care for each patient in patient volume/classification approaches, are obtained either from expert judgements or from staffing levels/observations in quality-assured wards. 16 Again, the quality criteria and thresholds are not always fully explained. This means that it is perhaps unsurprising when staffing tools arrive at different recommendations for staffing levels; they have been developed to meet different (implied) definitions of optimality.
The Safer Nursing Care Tool
In this section, we report specifically on research relating to the SNCT and its precursors. We considered all material that described or discussed the tool, although we have been selective in our use of purely descriptive accounts. We identified material by searching for the names of the tool and its precursors in databases (CINAHL, The Cochrane Library, MEDLINE, Scopus) and on the World Wide Web, and following paper references to find earlier documentation. We also enquired of the tool developers and members of our steering group. The searches were completed in November 2018. Our account of the tool’s development and history has also been informed by discussions with Keith Hurst and our advisory group member Ann Casey, two of the leading figures involved in developing and supporting the tool.
Review results
We found 23 sources in total that we used to inform our account of the history of and evidence for the SNCT. This includes accounts of some of the tool’s precursors and some simple accounts of planned development or implementations of the tool. We have also included reports of the tool’s use from national surveys.
It is difficult to fully characterise the underlying research because detail is often reported in multiple overlapping accounts, sometimes with a considerable lack of clarity. Although the disparate nature of the material makes a standardised approach to critical appraisal challenging, a striking feature is that some essential details for reports of work sampling studies, such as the numbers of observations and observers, are often missing from reports. 89
History and development
Prototype tools based on assessments of acuity/dependency are said to be the most widely used worldwide75 and this was confirmed in England by our recent surveys. 22 The SNCT falls into this category and a version of this tool was in use in > 80% of hospital trusts we surveyed in 2017. 22 Using the core acuity/dependency assessment of this tool, patients are allocated to one of five categories. These categories indicate requirements for nursing care based on an estimate of the time taken to care for patients in each category. 20 The acuity/dependency categories are associated with multipliers, used to estimate staffing requirements. These, in turn, have been derived from observations of care on approximately 1000 NHS wards. At the time of writing, the most recently published multipliers for general adult inpatient wards had been updated from 40,000 patient care episodes. 20,21 However, the underlying descriptive data used to derive the multipliers have not been systematically reported and so the number of patients, the mean times associated with each category and the variation around the mean cannot be determined from published reports.
The origins of the SNCT lie in the Southampton University Hospital NHS Trust (SUHT; now University Hospital Southampton NHS Trust) acuity and dependency measurement tool. 90 For the SUHT tool, Harrison90 adapted critical care acuity levels 0, 1, 2 and 391,92 and added the dependency on nurses element for application in general inpatient wards. Level 1 was subdivided into levels 1a (higher acuity than level 0) and 1b (higher dependency than level 0). The SUHT tool consists of the definitions of the levels, the detailed inclusion criteria for each level (e.g. medical conditions and admission types) and the dependencies associated with each level (e.g. specific care requirements and frequencies).
The SUHT tool suggested nurse staffing requirements, in WTEs per bed, for some of the acuity/dependency levels. Level 0 patients are those who need ‘normal’ levels of staffing; benchmark figures of 0.9 and 0.98 WTEs per bed were quoted based on the median in an Audit Commission database of ward staffing in general medical and specialist surgical wards, respectively. 90 Level 2 and level 3 requirements were 3.5–4.2 and 6.7 WTEs per bed, respectively, as then recommended by the Intensive Care Society. 93 It was left as future work to settle on appropriate WTEs per bed for the other levels. 90
These WTE per bed measures are known as multipliers because, when multiplied by the expected number of patients at each level, they enable the calculation of the total staffing establishment for a ward. Although the absence of suggested multipliers for level 1a and 1b patients means that no specific recommendation was offered for setting a total ward establishment, the use of WTE per bed (rather than patient) figures is notable in so far as bed occupancy may be < 100% and the variable patient load introduces an additional source of variation when determining how best to match staffing to variable need.
The SUHT tool became the basis for the University Trust Nurse Executive Forum Patient Care Portfolio Project, later renamed and launched as the Association of UK University Hospitals (AUKUH) Patient Care Portfolio in 2007. 94 Both descriptions and multipliers associated with each level of care were revised from the original SUHT tool; the multipliers for each level of patients were professionally agreed before being replaced by multipliers derived from observations of care contained in the Leeds University Acuity-Quality (LUA-Q) database of quality-assured wards (Table 4). 71,94
Level of care | SUHT tool | AUKUH original | AUKUH | SNCT (general) | SNCT (acute admissions units) |
---|---|---|---|---|---|
Level 0 | 0.9 for general medical and 0.98 for specialist surgical* | 0.9 | 0.79 | 0.99 | 1.27 |
Level 1a | Unspecified | 1.2 | 1.7 | 1.39 | 1.66 |
Level 1b | Unspecified | 1.5 | 1.86 | 1.72 | 2.08 |
Level 2 | 3.5–4.2** | 3.7 | 2.44 | 1.97 | 2.26 |
Level 3 | 6.7** | 6.5 | 6.51 | 5.96 | 5.96 |
Source of multipliers |
*Benchmarks based on median for non-London teaching hospital trusts in an Audit Commission database of ward staffing90 **Intensive Care Society recommendation93 |
Professionally agreed | Dual scoring used to convert LUA-Q multipliers into AUKUH multipliers. LUA-Q multipliers calculated from data for quality-assured wards | In October 2012, dual scoring used to convert LUA-Q multipliers into SNCT multipliers. LUA-Q multipliers calculated from data for quality-assured wards | In 2013, dual scoring used to convert LUA-Q multipliers into SNCT multipliers. LUA-Q multipliers calculated from data for quality-assured acute admissions units |
Reference | Harrison90 | Hurst et al.71 | Hurst,58 AUKUH94 | The Shelford Group19 | The Shelford Group19 |
The tool was adopted by the NHS Institute for Improvement and Innovation and became known as the SNCT before control passed back to AUKUH, now renamed the Shelford Group, when the Institute was disbanded. In 2012 and 2013, the multipliers were updated, again using estimates derived from the LUA-Q database. 19 At this stage, multipliers for acute assessment units were also published and a children and young people’s version published (Ann Casey, NHS Improvement, 2019, personal communication). Although the basic descriptors of the levels of care were largely unaltered (compare Report Supplementary Material 2, Table 86, with pages 5 and 6 of the SNCT guidance19), the elaboration and illustrative examples that accompanied them were extensively revised, informed by the revised Comprehensive Critical Care definitions, published in 2009 (Ann Casey, personal communication). 19
In 2014, following the publication of its guidance for safe staffing in adult inpatient wards,11 NICE endorsed the SNCT as a tool for setting nurse staffing levels on adult inpatient wards in the NHS, when used together with the NICE guideline. 21
The most recent multipliers, and any future versions, are subject to licensing restrictions for research and development users, but NHS users can access them free and a new training scheme for nurses is being piloted. 95
Deriving multipliers from the Leeds University Acuity-Quality database
The multipliers used to determine the required staffing in recent versions of the SNCT are based on empirical observations of patient care derived from the LUA-Q database containing information from about 2800 wards to date [Keith Hurst, 2018, personal communication]. Only wards that achieve at least a 70% quality score, deemed as representing ‘best practice’, were used in the SNCT multiplier calculations. The quality score is multidimensional, using 135 quality standards based on an updated version the Monitor Tool96 (Keith Hurst, 2018, personal communication), and is assessed by non-participant observers. 97 Independent observers carry out a work-sampling survey on each ward, whereby they record what each member of ward staff is doing at 10-minute intervals for two 24-hour periods. These data are used to make estimates of the time required to care for patients in each of four dependency categories. 97
To derive timings to be associated with the SNCT acuity dependency system (with its five acuity/dependency categories), a dual scoring exercise was undertaken. 20,71,97,98 The sample size for the dual scoring in 2012 was 40,000 patient episodes and 1600 acute admission unit patient episodes in 2013. 19 Rather than directly assessing time for each SNCT category, the LUA-Q multipliers are converted into SNCT multipliers. For each SNCT level, the proportion of patients with each LUA-Q level (across dual-scored patients) is calculated. 71,98 The average direct care time per patient hour for each SNCT level is obtained by weighting the average direct care times for each LUA-Q level. Although the size of the LUA-Q database and the dual scoring exercise suggests that the underlying mean care estimates are likely to be precise, the extent of variation at a patient level is not documented.
Using the Safer Nursing Care Tool to calculate the required staffing establishment
The ‘time per patient’ category is converted to ‘multipliers’ to obtain WTEs by, first, adding in average time spent on tasks other than direct care, including paid breaks; second, scaling by hours of the working week; and, third, adding a 22% ‘uplift’ to allow for sickness absence, study leave, annual leave and other leave. 19,20,98 The multiplier represents the number of WTE staff to employ to have enough staff available to provide the nursing care time observed in the quality-assured wards for one patient in a given category 24 hours per day over a whole year. Although not clearly documented, there is a deduction of 9.7% ‘ready-for-action time’ in the spreadsheet supplied to users of the tool,19,99 which may be problematic as this removes the slack/buffer for busy times from the system.
Thus, the multiplier is arrived at from an estimated direct care time, with additional allowance for unobserved indirect care and other activities but a reduction of (presumably) unproductive ‘ready-for-action time’, before adding on an additional uplift because employees are not available to provide care on every day for which they are paid.
The published guidance recommends that the data used to calculate the establishment for each ward be obtained from counts of patients per SNCT category once per day at 3 p.m. for at least 20 days Monday–Friday in January and June. 19,56,71 The average counts are multiplied by their multipliers and summed to obtain the recommended establishment. Although there is evidence to show some variation over time in the calculated staffing requirement,20,100 providing a rationale for periodic reassessment, the precision of estimates obtained by measuring over 20 days is not clear. No distributions are reported.
The documentation of the tool is largely silent on issues of skill mix and daily deployment; it describes these as a matter of professional judgement, although some guidance is provided in the spreadsheet supplied to users along with the resource pack. Skill mix can be specified by the user, with the default value in the spreadsheet being a 68 : 32 registered nurse-to-support worker ratio. The spreadsheet model also provides a default for the distribution of staff over 8- or 12-hour shifts. 99
Reliability and validity
Overall, there has been remarkably little evaluation of the tool’s use and we found few studies that had been conducted entirely independently of the team that developed the tool.
One account claims to report inter-rater reliability, giving a high Cronbach’s alpha (0.99). However, as Cronbach’s alpha is a measure of internal consistency, it is not entirely clear what was rated. It appears from the report that this statistic may refer to the correspondence between SNCT ratings and LUA-Q ratings for just one patient observed by 30 students. 20,71 In any case, it is limited and ambiguous evidence of reliability.
Data collection for the LUA-Q database is ongoing, and the multipliers are updated over time to reflect changes (e.g. owing to shorter patient stays and new staff roles19). In the original reports of the tool’s development, the procedure for using the LUA-Q database and associated timings as the source was justified by demonstrating statistically significant correlations between SNCT-rated acuity/dependency categories and activity of daily living ratings and subcategories in the Leeds database. 20,71,98 The reported Spearman’s correlations range from 0.55 to 0.73, with a correlation of 0.77 between the total scores. 98 However, it appears that these correlations may be between the ranked categories as opposed to the associated timed care, so all that this shows is that as the SNCT level increases so does the LUA-Q level and, in any case, even such high correlations suggest a substantial lack of fit. The coefficient of determination (R2) for the correlation between total scores is 0.59 (0.77). The coefficient of determination indicates the proportion of variation that is explained by the correlation – in this case, more than 40% of the variation is unexplained. So, despite the strong correlation, the correspondence between the two scores is quite loose.
We found no evidence establishing the costs or consequences of using the tool. One small-scale study provided a plot of adverse outcomes (measured by nurse-sensitive indicators) against staffing shortfall relative to the establishment (calculated using one of the tool’s precursors), but no measure of association is provided and visual inspection gives no obvious pattern. 100 Our own study of 138,000 patients in 32 wards in one NHS hospital over 3 years found that low registered nurse staffing relative to an establishment determined using the SNCT was associated with increased mortality. Over the first 5 days of hospital stay, each day a patient experienced lower than the planned registered nurse staffing level was associated with a 9% increase in the hazard of death. Furthermore, when nursing support worker staffing varied from the mean (which approximated to the planned establishment) in either direction, mortality was also increased. 6,9 By contrast, registered nurse staffing levels had a linear relationship with mortality. 6,9
Summary of evidence on the Safer Nursing Care Tool
We found very limited evidence about the SNCT, with most accounts focusing on its development and validation of the multipliers against another measure. Although staffing at the levels recommended by the SNCT is correlated with the staffing on wards that deliver quality care, we found no direct evidence that employing the SNCT-recommended establishment is associated with staffing that matches needs. Although staffing lower than levels planned using the SNCT was associated with increased risk of death, this does not establish the SNCT staffing level as ‘correct’. The non-linear relationship between support worker staffing and mortality does indicate that the level arrived at by the single site may well be ‘optimal’, but the SNCT leaves the determination of skill mix to professional judgement. Similar to the conclusions above about the RAFAELA system, there is no evidence that the registered nurse staffing level is optimal.
The removal of ‘ready-for-action time’ in arriving at the establishments associated with SNCT multipliers (outlined above) suggests that best-practice wards that provide data are staffed at higher levels than those recommended, as the ‘ready-for-action time’ is removed during the calculation of establishment. Because nursing work requests do not occur at a constant rate, having enough staff to deal with any urgent tasks in a timely manner means that there may be other times when some staff are legitimately unoccupied or could engage in what might be described as ‘discretionary’ work or work that is less time-critical. Examples of such work might include educating other members of staff and some aspects of patient support, counselling and relational care, which have been shown to be most often reported as ‘missed’ in the face of higher workloads, suggesting that this work is afforded a lower priority when workload in general is higher. 5
Furthermore, although the tool provides a method for estimating the number of employees required to provide the hours identified by observations, managers cannot distribute these hours as a continuous variable and instead have to employ and deploy whole people for whole shifts or reasonable fractions thereof. For example, a small ward with a mean of eight patients at level 0, four at level 1a and two at level 1b has an estimated establishment of 17.2 WTEs and a daily staffing requirement of 4.3 registered nurses for a 12-hour day shift. 99 It is unlikely that sensible working patterns and employment could arrive at a perfect fit under all circumstances every day. One strategy, namely rounding down to the nearest whole number of registered nurses, would leave the ward nearly 10% short of the predicted need.
Although authors emphasise the importance of ensuring reliable determination of the SNCT categories, the published data that suggest that reliability can be achieved are scant.
Literature review conclusions
The literature on staffing methodologies is vast and growing, suggesting continued interest. However, the conclusion of previous reviews that there is no substantial evidence on which to base staffing decisions or the selection of a decision support tool remains true. Despite the lack of evidence, it seems clear that an appetite for formal systems and tools exists. Although professional judgement remains the nearest to a gold standard, the desire to use a tool or another formal system to support, and indeed justify, such a judgement has remained a constant theme that can be traced back to Telford’s work in the 1970s in the UK,38 and no doubt further. The lack of evidence for existing tools seems to have propelled each generation (and, indeed, in some cases, each service) to develop a new tool. On the other hand, the widespread adoption of the SNCT in the UK might be indicative of a perceived validity, although NICE’s endorsement of it as an ‘evidence-based tool’ and the marketing efforts of e-roster companies that have embedded it may also explain its widespread uptake.
Although the time estimates for the SNCT appear to come from a large database, this is not well documented. However, in the face of rapidly changing care needs, it is not clear how long even such relatively extensive observation can be relied on, although the multipliers continue to be updated. Even if average nurse staffing requirements are accurately estimated, the costs and consequences of using the tool to base daily staffing on an average are unknown. Alternative approaches to setting required staffing levels using the tool’s measurement have not been explored.
Rather than continually invent new tools, it seems to be time to take a closer look at those already in use. Controlled trials may be problematic, but there are sufficient unanswered questions that much progress can be made before determining whether or not such a trial is strictly necessary. There is little evidence about many of the assumptions underlying the use of tools in general and, specifically for the UK, the SNCT. Questions about the extent to which the tool truly identifies a level of staffing sufficient to meet the needs of a ward of patients are unanswered, as are questions about the number of observations required to obtain an accurate baseline to estimate average need, or the costs and benefits of staffing according to the tool’s recommendations. The apparently simple assumption that staffing to meet average need is the optimal response to varying demand is also untested.
Aims and objectives of the present study
In the present study, undertaken on acute medical/surgical wards in four English NHS hospitals, we aim to assess a range of options for using the SNCT and to model the costs and consequences of various ward staffing policies based on acuity/dependency derived from the tool. Our study sets out to tackle several key knowledge gaps about the SNCT, and the use of staffing tools in general, to help local managers and decision-makers to more consistently achieve safe nurse staffing.
We will:
-
determine the required nursing establishment (daily staff equivalent) as measured by the tool using the recommended approach (based on the average acuity/dependency of every patient on the ward over a minimum of 20 days)
-
explore the variation in acuity/dependency of the patients as measured by the tool from day to day and within days
-
assess the number of observations required to determine a reliable baseline
-
determine how current staffing on the wards matches the staffing requirements measured by the tool
-
assess whether or not a mismatch between the staffing requirements measured by the tool and the staff available is associated with nurse perceptions of staffing adequacy as a ‘gold standard’ criterion
-
explore the influence of non-patient factors such as the admission/discharge rate
-
develop computer-based mathematical simulation models to explore scenarios with different approaches to using the tool and flexible staffing policies
-
model the costs and consequences of alternative staffing policies using different baseline staffing levels, considering opportunities to deploy staff from ‘overstaffed’ wards to ‘understaffed’ wards, the costs of bank/agency staff required to fill critical staffing deficits, the relative efficiencies of different staffing groups to meet patient need and the potential adverse outcomes associated with understaffing.
We focus on the SNCT because it is endorsed by NICE and appears to be the most widely used tool in England. However, the lessons learned from this study would have implications for the use of any similar tool and flexible staffing policies based on assessments made using them.
Chapter summary
-
Reviews published up to 2014 concluded that there was insufficient evidence to establish the reliability, validity or fitness for purpose of any of the many tools that are described.
-
Most studies were conducted on a small scale and reported local implementations.
-
There is no evidence of the costs or effectiveness of using any tool.
-
Our focused review of more recent literature confirmed this picture.
-
Recent studies report the development of new tools but do little to establish ‘reliability’.
-
There is some evidence that high workload as measured by some tools is associated with increased mortality.
-
The published evidence does not, however, give any clear evidence that tools are identifying ‘safe’ or ‘optimal’ staffing levels.
-
Professional judgement remains a key criterion against which staffing levels determined by tools are judged and, in some cases, forms the basis for setting the required staffing against the tool’s measure.
-
We found evidence that there may be considerable variation underlying the measures determined using tools.
-
We found evidence that setting staffing levels based on average demand could lead to understaffing for > 50% of the time.
-
The clinical significance of such understaffing is unclear, but a ‘tolerance’ level of 15% is suggested in the literature.
-
Our focused search for literature relating to the SNCT, a measure endorsed by NICE, confirmed that there is limited evidence about the tool.
-
We found no published evidence on daily variation and the reliability of staffing estimates derived from the tool.
-
We found some evidence that suggested that low staffing, relative to the tool’s recommended establishment, was associated with increased mortality.
-
We found no evidence that nurses working on wards judged the staffing levels determined using the tool to be sufficient.
Chapter 3 Methods
This is an observational study using linked routinely collected data from acute medical/surgical wards in four NHS hospital trusts. First, we used these data to assess the reliability and validity of the SNCT and its recommended use. To do this, we determined the association between staffing ‘shortfalls’ identified by the SNCT and nurse-reported assessments of staffing adequacy, and calculated the sample size needed for a stable estimate of the mean staffing requirement, and the variation in estimated staffing requirements within a day and between days of the week.
Second, we developed an in-depth simulation model that accounts for variability in patient acuity/dependency, patient numbers, and registered nurse and nursing support worker availability. We populated the simulation with patient, roster and cost data from each hospital trust, allowing us to compare alternative staffing scenarios using the SNCT to set total nursing establishments (registered nurses and nursing support workers).
Third, we estimated the cost per life saved for the alternative staffing scenarios using simulation experiment results, patient length of stay estimates and mortality coefficients from regression analyses derived from a recent longitudinal observational study9 measuring the effects of patient exposure to low staffing on general wards in one NHS hospital.
Setting
The study was undertaken using data from somatic acute adult care wards (excluding intensive care units) in one university hospital, two district general hospitals and one specialist cancer hospital trust based in London, south-east England and south-west England, with five hospital sites in total. The hospitals serve diverse populations, including those living in rural areas, deprived inner-city populations and specialist national referrals.
All four hospital trusts undertook regular periodic reviews of nurse staffing levels broadly in line with the approach recommended by NICE,11 with reviews undertaken two or three times per year. Two trusts had been using the SNCT as part of the process for some time and one had adopted it shortly before the study commenced, having not used a formal tool as part of the process previously. The other hospital trust had previously used the ‘patient requirements for nursing care’ tool, a multidimensional indicator-based system developed in Canada,101 but likewise had adopted the SNCT shortly before the study commenced. Three of the four trusts adopted a commercial system for daily reporting patient acuity dependency using the SNCT categories shortly before or during the study, with the fourth implementing a locally developed solution, initially with the primary intention of collecting data for the current study.
Over the course of a full year (2017), for each ward and for each day we sought to identify the staffing level actually deployed, the staffing level required as estimated using the SNCT, and nurses’ professional judgement about the adequacy of staffing.
Ward inclusion and exclusion criteria
The inclusion criteria for the study were:
-
ward provides 24-hour inpatient care for 7 days per week
-
adult somatic health population [i.e. medical or surgical (including oncology, cardiovascular, urology, neurosciences, emergency care, renal and transplant and medical assessment units)]
-
appropriate for SNCT according to the SNCT resource pack. 19
We excluded wards that provide highly specialised services and those that are clearly outside the scope of the SNCT (e.g. paediatrics, intensive care, maternity, neonatal and palliative), and any others with highly atypical staffing requirements (as determined by the local chief investigator, with documented reason). Across the four hospital trusts, our study included 81 adult medical/surgical wards with 2178 beds. This represents 74% of all beds across the four trusts. 102
Data sources
To address our aims, we need data on daily variation in staffing requirements as estimated by the SNCT, patient numbers, other ward factors that could affect workload, actual staffing, nurses’ judgements of the adequacy of that staffing and staff costs. The connections between the data requirements and the broad study aims (‘why needed’), as well as the data sources, are shown in Table 5.
Data | Measures | Why needed | Data sources |
---|---|---|---|
Actual staffing | Total/registered nurse/nursing support worker care hours per (patient) day, skill mix and distribution of staff over day | Validating the SNCT, simulation | Rosters, patient administration systems |
Estimated staffing requirements | Total/registered nurse/nursing support worker care hours per (patient) day, total/registered nurse/nursing support worker WTEs (per patient) | Assessing reliability and validity of the SNCT, simulation | Bedview® (CareView Communications Inc., Lewisville, TX, USA)/SafeCare® (Allocate Software, London, UK) software (for SNCT patient ratings and specialed patients’ reports), patient administration systems |
Deviation between estimated requirement and actual staffing | Apparent shortfall in HPPD, comparing required (estimated from SNCT) and worked hours | Validating the SNCT | Rosters, patient administration systems, Bedview/SafeCare software (for SNCT patient ratings and specialed patients’ reports) |
Nurse-reported staffing adequacy | Shift leader reports that they had enough staff for quality care, nursing care left undone, staff breaks missed and reported total staffing requirement | Validating the SNCT | Bedview/SafeCare software (for staffing adequacy questions) |
Other ward factors that could affect workload | Proportion single rooms, patient turnover, ward type | Validating the SNCT | Patient administration systems, ward profiles |
Staffing costs | Unit costs for substantive/agency/bank registered nurses and nursing support workers | Cost-effectiveness analyses | Unit Costs of Health and Social Care 103 |
For data field specifications for the hospital systems (patient administration systems, rosters and Bedview/SafeCare), see Appendix 1, Tables 28–30.
Patient administration systems
Patient administration systems contain records of patient ward stays, including times of admissions, transfers and discharges. These data are recorded primarily for administrative purposes. We used these data to estimate the numbers of patients on each ward, and the numbers of admissions and discharges.
These data are widely used and are likely to be valid at least at the day level. Although the times recorded may not be exactly accurate (as this is not needed for the original purpose), there is very little difference in the quantities derived from the variety of approaches to estimating daily patient load on wards, so any error and bias are likely to be small. 62
Rosters
All hospital trusts used electronic rostering systems. Hospitals collect these data mainly to facilitate rostering (assigning staff to shifts) and for financial purposes (including payroll). We used these data to identify the shifts worked by nursing staff, including their grades and whether the staff were substantive, bank or agency.
Because these data are used for payroll, they are subject to validation internally and thus are assumed to be accurate, although they may not reflect last-minute changes to work locations where a staff member has been moved to a different ward. Although we are led to believe that recording of such re-deployment was undertaken routinely in at least three of the four hospital trusts, we have no mechanism for validating this. At a ward level this can introduce error in the estimation of current staffing because the number of actual staff present might be greater or smaller than that recorded. Systematic bias can also be introduced at a ward level if particular wards are more likely to be staff donors than recipients (or vice versa). However, at a hospital level such biases would cancel out, leaving only a residual degree of error, which would be accounted for in the statistical models. For details of how we processed these data, extracting worked shifts and identifying worked hours, see Appendix 1.
Bedview and SafeCare
Developments in three of the hospital trusts independent of the study led them to implement daily collection of the SNCT for all patients using SafeCare, a commercial staffing system, shortly before the study commenced. We worked with the fourth trust to implement daily assessments of SNCT ratings for all patients on the local bed and discharge management system (Bedview). These systems are the sources of the SNCT ratings, specialed patients’ reports and nurse-reported staffing adequacy.
The SNCT ratings data consist of the numbers of patients in each acuity/dependency level (category). The SNCT ratings are collected routinely by ward shift leaders. Ward staff were given training in the use of the SNCT by senior staff in each hospital trust. As part of our pilot work in one trust, we determined that the SNCT could be completed with a high degree of inter-rater reliability between an expert and 15 trained shift leaders working across 14 wards. In total, 847 patients were rated independently by two raters across 81 shifts. At the patient level, there was 89% agreement on ratings (kappa 0.71: moderate agreement). At the shift level, based on the calculation of total staffing scores using the multipliers (which gives an estimated establishment WTE), there was an exact agreement on 65% of occasions and agreement within ±5% of the joint mean on 95% of occasions and a mean difference of ±1.9% in the estimated establishment.
Although the extensive nature of the study made it difficult to ensure that this level of reliability was achieved for every shift leader on every ward in each hospital trust, the circumstances of our study resemble routine use of the system in the ‘real world’ when, as is now becoming common, assessments are completed daily by shift leaders.
Because SafeCare requires only numbers of patients in each category (as opposed to tracking scores for individual patients), there is the possibility of inadvertent under- or over-reporting of the number of patients. The 7 a.m. patient count derived from patient administration systems was greater than the SNCT morning count by just over one patient per ward (unweighted hospital trust average), suggesting an under-reporting of patients on average. To correct for this, we used the total numbers of patients present on the ward derived from patient administration systems, and used the SNCT ratings solely for the proportions of patients in each level.
As well as core/standard nursing care, some patients, for example those at risk of falls and those with mental health problems, receive constant one-to-one care, generally called ‘specialing’ or ‘enhanced observations’. 104 Patients’ need for specialing is not directly accounted for in the standard SNCT and so this is an additional staffing requirement. In all cases, the systems used by the four hospital trusts recorded the number of patients requiring one-to-one specialing, either through creating a separate category to be reported alongside the SNCT ratings or as an additional report. Appendix 1 provides further details of how specials were recorded.
The SNCT guidance recommends collecting data at 3 p.m. 19 However, to maximise compliance and minimise the burden of additional work, we did not ask hospital trusts to change the times at which they routinely collect SNCT data. The SNCT ratings and answers to staffing adequacy questions were collected up to four times per day. All wards collected them in the morning and evening. We used the morning report as the primary data collection point. We explored the implications of the time of day the assessments are undertaken by comparing estimated establishments using data from these multiple assessments within each day.
Ward profiles
We obtained profiles of all wards, including the directorate, total beds, number of single rooms, planned registered nurses and nursing support worker staffing levels by shift, and a brief description of the patient population and/or specialty. Any changes to the wards taking place during the study period (e.g. changes in planned staffing levels, numbers of beds or types of patients) were recorded, together with the effective date. The data were factual and objective and so likely to be valid, although the dates of ward changes were, on occasion, difficult to establish with precision. Therefore, we cross-referenced the dates provided with patterns in the roster and patient administration system data.
National reference costs
The Unit Costs of Health and Social Care 2017103 report provides the hourly costs for staff at different bands. These data were originally produced for general research and policy questions, and include details of the assumptions made so that costs can be tailored appropriately. This document provides the best available estimate for costs of NHS registered nurses and nursing support workers across the UK. We used the national average (reference) cost for a non-elective bed-day105 to estimate the costs associated with any changes in length of stay, which is likely to be conservative assumption as it assumes that there are no specific treatment costs associated with extended stay. Costs for agency staff at each Agenda for Change band were derived from the NHS Improvement agency cap, specified in the Agency Rules106 guidance applicable to the study period.
Variables
Staffing levels
Our analyses consider ‘days’ from 7 a.m. to 7 a.m. on the next day with staffing distributed across 6-hour ‘shifts’ from 7 a.m. to 1 p.m. (‘morning’), from 1 p.m. to 7 p.m. (‘afternoon’), from 7 p.m. to 1 a.m. (‘evening’) and from 1 a.m. to 7 a.m. (‘night’). These 6-hour shifts successfully approximate major staffing changes and account for varying shift patterns and the mix of two- and three- shift systems in use across the hospitals. 6 We converted the individual staff shifts into aggregated hours per ward and 6-hour shift. Because we do not know when rest breaks were taken, we pro-rated breaks over the relevant 6-hour shifts. This allowed us to calculate the worked hours for each ward over each 24-hour period and 6-hour shift. We identified the hours worked by registered nurses (band 5 and above) and nursing support workers (bands 1 to 4) and also the hours worked by temporary bank and agency staff for each shift and each day.
We calculated patient-days, equivalent to one patient occupying one bed for 24 hours from 7 a.m. to 7 a.m. or two patients each occupying a bed for 12 hours during this time, and so on. We calculated individual patient hours using admission/transfer in and discharge/transfer out times from patient administration systems and summed them for each time period, dividing by 24 to give patient-days.
For each day, we used these data to derive care HPPD from the sum of hours worked divided by the sum of patient-days. In this way, we calculated registered nurse, nursing support worker and total care HPPD, as well as the equivalent measures for each shift.
Estimated staffing requirements
The SNCT categorises patients by their acuity/dependency level, and its multipliers are used to identify the WTE staff who would need to be employed to provide care to a given mix of patients. In our study, we also converted these WTE staff into the hours of staff time required on particular shifts and days. Throughout, we used the latest published multipliers;19 these are different for general wards and acute assessment units.
For each ward and day, we first calculated the establishment (including both registered nurses and nursing support workers) in WTEs. We determined the proportions of patients in each acuity/dependency level at the first recorded census, and the total patient count at 7 a.m. from the patient administration system. We then used the SNCT ratings and multipliers to calculate the weighted-average acuity/dependency multiplier per ward and day and multiplied this by the patient count (see Appendix 1, Equation 3). The weighted-average acuity/dependency multiplier can be interpreted as the daily average need of patients on a ward, measured in units of WTE staff member per patient. We considered a set of SNCT ratings ‘missing’ if no patients were recorded in any levels. If some patients were recorded, we assumed that any empty levels meant no patients in that category. When estimating the staffing requirements per 6-hour shift, we used the proportions of patients in each acuity/dependency level at the beginning of the shift (or the end of the shift if this was missing) and the number of patients present on the ward at the start of the 6-hour shift.
We converted WTE derived from the multipliers to daily hours, assuming a 37.5-hour week for 1 WTE. Because the SNCT multipliers account for the need to employ additional staff to cover annual leave and other absences from the ward (including sickness and staff training), the 22% ‘uplift’ must be removed when determining the hours that are needed to deliver patient care on the day (see Appendix 1, Equation 5).
Because the SNCT recommends using professional judgement to set the skill mix,19 we used the average observed daily skill mix per ward as a proxy for the planned skill mix. We estimated the registered nurse and the nursing support worker requirements (in hours per day) by applying this average observed skill mix for each ward to the total daily staffing requirements. As it is recognised that staffing requirements differ by time of day (leading to, for example, different staffing levels to care for the same patients during the day and at night), the SNCT guidance recommends using professional judgement to adjust staffing levels accordingly. 19 Therefore, analogous to our approach for skill mix, we used the average observed distribution of staff during a 24-hour period in each ward as a proxy for the planned distribution of staff. The total staffing requirement per shift was divided between registered nurses and nursing support workers using the average observed skill mix per ward for that shift (morning, afternoon, evening or night).
As the staffing deployed includes provision of specialing in addition to each patient’s care requirements according to the SNCT, we added this on as an extra level with the multiplier 5.47, equivalent to 24 HPPD for each patient who required ‘specialing’, that is one-to-one care (see Appendix 1, Equation 7). Where wards specialed patients in a cohort, we used four patients (the maximum permitted by the hospital trust’s policy) as an upper limit to calculate the number of specialed cohorts per ward and applied the same multiplier as for one-to-one specials to each cohort. We calculated the daily staffing requirements including these additional provisions for specialing.
In the same way as for actual staffing levels, we converted the estimated total care requirement in hours per day into HPPD using the actual time patients spent on the ward during that day or 6-hour shift (see Appendix 1, Equation 6). For reference, Table 31 (see Appendix 1) shows each multiplier with its corresponding staffing requirement in HPPD when patients are present on the ward for the full 24 hours.
The SNCT documentation recommends twice-yearly assessment with a sample of 20 weekdays in both June and January as the basis of the establishment review. We averaged 20 weekdays in June (or the next month if unavailable) to provide a base estimate of the establishment for use in the simulation models (see Appendix 1, Equation 4). Where wards had no data in the second half of the year, we used data from January and applied the average percentage increase/decrease from January for other wards at that hospital to obtain the base estimate.
Deviation between estimated requirement and actual staffing
Based on the above, for each day we were able to determine an estimate of the staffing requirement based on the SNCT and the nursing hours per patient actually deployed on the ward, both in HPPD. We then calculated the difference between the two (estimated requirement minus actual staffing). We also calculated the percentage difference, which recognises that the same apparent shortfall may be less problematic on a ward with higher overall workload. We consider shifts/days to be understaffed if their worked hours are more than 15% under the estimated staffing requirement in order to allow a buffer around the requirement, as is also done in the RAFAELA tool. 47
Nurse-reported staffing adequacy
Professional judgement remains a leading alternative approach to determining nurse staffing requirements20,75 and is seen as an essential adjunct to measurement systems, as recognised by NICE. 11 To determine professional judgements, we measured nurses’ perceptions of staffing adequacy using a microsurvey. The nurse in charge answered this survey at the same time as providing the SNCT ratings, directly inputting responses into the same system.
Two items were based on the RN4CAST survey (‘enough staff for quality’107,108 and ‘nursing care left undone’13). Both of these measures have been validated by relationships with patient care outcomes. 109–111 For example, nurse reports of missed care using the RN4CAST approach are associated with increased mortality. 31 Because nurses may prioritise completing care activities over taking personal breaks, creating additional staff time that may mitigate other effects from staffing shortfalls in the short term,112 we also asked about staff missing breaks. Finally, we asked staff to estimate the total numbers of registered nurses and nursing support workers needed.
Prior to implementation, we met with a group of staff consisting of eight matrons/ward sisters/charge nurses and an information technology (IT) systems expert at hospital trust A during July 2016 to discuss the proposed staffing adequacy questions and their implementation. We discussed the questions to ensure that interpretation was consistent across the group. In particular, we found a shared understanding of the concepts of ‘quality’ and ‘safety’, and tasks considered under ‘care left undone’ aligned with the goals of the study. Staff agreed that questions were the right ones to ask to check professional judgement about whether or not staffing is right/enough.
The questions were embedded in the electronic systems used to report SNCT ratings. Owing to system constraints in three of the four hospital trusts, nurses were presented with abbreviated versions of the questions with a laminated card to indicate the full question, as shown in Table 6. We undertook cognitive testing of both the full and the abbreviated questions with a group of ward staff and managers at one hospital trust before implementing the questions for a pilot period. Staff suggested minor changes to our originally proposed wording but seemed to understand the questions as intended.
Questions | Abbreviation |
---|---|
Were there enough nursing staff to provide quality care on the last shift? | Enough staff for quality |
Was necessary nursing care left undone (missed) on the last shift because there were too few nursing staff? | Nursing care left undone |
Were staff breaks missed on the last shift because there were too few nursing staff? | Staff breaks missed |
How many RNs were needed on the last shift? | Registered nurses needed |
How many HCAs were needed on the last shift? | Nursing support workers needed |
Other variables
Ward type
We classified wards as medical, surgical or mixed medical/surgical based on the ward descriptions and directorates provided in the ward profiles by our hospital trust partners and supplemented by research on hospital trust websites.
Proportion of single rooms
Ward layout is not accounted for in the SNCT but is thought to affect workload. 58 The proportion of single rooms is derived from the number of single rooms divided by the total beds, both of which were provided in ward profiles by our hospital trust partners. Because of the potential variety and lack of standardised descriptions, we did not attempt to consider other aspects of ward layout.
Patient turnover
The SNCT recommends taking patient turnover (throughput) into account when setting the ward establishment, although the mechanism for this is not built into the tool. 19 High patient turnover per nurse has been shown to be associated with negative patient outcomes, suggesting that it may significantly affect workload. 9 We calculated the turnover for each period (day or 6-hour shift) in terms of the patients arriving and leaving per worked hour. Specifically, we summed the numbers of patients entering and leaving wards (admissions, transfers in, discharges and transfers out) during that period and divided this by the total care hours provided during that period.
Staff costs
Hourly employment costs for substantive staff at each Agenda for Change band were estimated using the mean costs for each band reported in the Unit Costs of Health and Social Care 2017103 (see Appendix 1, Table 32). Salary and additional employer costs (superannuation and National Insurance) were included to derive a total employer cost for substantive and bank staff. Costs for agency staff at each Agenda for Change band were estimated using the NHS Improvement Agency Rules106 guidance applicable to the study period. Agency employment costs include the maximum 55% uplift on basic salaries (to include agency fee, framework and other fees, in addition to employers’ National Insurance contributions, allowances for employer pension contributions and holiday pay). The daily ward staffing costs include allowances for working unsociable hours, in accordance with the Agenda for Change framework, using multipliers applicable to the study period113 (see Appendix 1, Table 33).
Piloting and data collection period
We met with a group of staff consisting of eight matrons/ward sisters/charge nurses and an IT systems expert at hospital trust A during July 2016 to discuss the proposed staffing adequacy questions and their implementation. We discussed the questions to ensure that interpretation was consistent among the group. In particular, we found a shared understanding of the concepts of ‘quality’ and ‘safety’, and tasks considered under ‘care left undone’ aligned with the goals of the study. Staff agreed that the questions were the right ones to ask to check professional judgement about whether or not staffing is right/enough. At this meeting, ‘specialing’ was identified as a large consumer of nursing resource, so we decided to include this aspect in our study. We also identified an issue with IT that affected how questions were displayed, and started to address how to deal with them at this meeting. We shared findings from this meeting with the other hospital trusts, including ideas about how to increase levels of completion.
A pilot study of the staffing adequacy questions was run in November 2016 on 22 wards at hospital trust A and 17 wards at hospital trust C. Following our analysis of response rates and correspondence between questions, some less informative/relevant questions were dropped, although these were still asked internally at some of the trusts. We also analysed the data from the pilot at trust C to identify patterns in levels of completeness. The pilot study showed that the data could be collected on busy wards.
Following the pilot study, the data sets were collected for the period 1 January 2017 until 31 December 2017, with the exception of wards at hospital trust B, which began routinely recording SNCT ratings in February 2017.
Data transfers, storage, cleaning and processing
Data extracts from hospital systems were provided by each hospital trust via a secure file transfer service. All patient data were de-identified by pseudonymisation at source. Data were stored on secure encrypted servers at the University of Southampton and access was restricted to the project team.
Our study involves linking multiple data sets. As wards had different names in the different data sets, we gave them unique identifiers, allowing us to link the data sets by ward and period (deterministic linkage). When linking data sets, we checked the number of rows before and after in case any rows failed to link correctly.
Where there were major changes, such as ward moves, changes to the patient population or bed numbers, data for that ward were split into parts corresponding to before and after the change. In the reliability and validity testing, we considered each part. In the simulation modelling, we took the first part and assumed that it was there throughout. For some wards, data were recorded separately for their sub-wards; for a description of how we dealt with this, see Appendix 1. We identified missing data; the procedure for dealing with missing data is explained in the relevant sections about variables (see Variables) and methods (see Overview of analytical methods).
Data cleaning, processing and statistical analyses were carried out in R statistical software version 3.5.0 (The R Foundation for Statistical Computing, Vienna, Austria) because of its flexibility in easily repeating analyses with different parameters. Data cleaning included checking the range of values for each relevant data field, cross-checking data fields and standardising the data fields across the hospital trusts. We checked for duplicate records and removed these where appropriate.
We removed days with no patients, but otherwise kept all ward occupancy data. We identified extreme values of actual staffing and estimated staffing requirements by looking at actual staffing per patient-day and weighted-average multipliers respectively. We used a widespread definition of outliers, namely points outside the mean plus or minus 3 standard deviations. Actual staffing per patient may be atypical if the ward is not functioning as normal (e.g. over the Christmas period). Estimated staffing requirements may be unusual if there is an error in the recording of SNCT ratings. Anomalies can also arise during periods when wards move or merge temporarily, as most staff apparently are attached to the original ward name and patients and some staff are attached to the current physical location. Outliers were removed from the descriptive analyses and further modelling. As days during which there were daylight saving time changes have 1 more or 1 fewer hour than the usual 24, we removed these days from all analyses.
For staffing adequacy questions with yes/no answers (coded 1/0) we removed invalid answers (not 1 or 0). We considered answers to the question ‘registered nurses needed’ potentially valid if at least 1 was entered. We considered answers to the question ‘nursing support workers needed’ as potentially valid if at least one staff member in total (‘registered nurses needed’ plus ‘nursing support workers needed’) was entered. On days when it was reported that there were not enough staff for quality and more staff had been requested than worked on the ward during the preceding 24 hours (7 a.m.–7 a.m.), we calculated the increases in registered nurses, nursing support workers and total staff requested over the number working.
Compared with the other hospital trusts, trust D had a very low average percentage of shifts with ‘enough staff for quality’ per ward. On closer examination of the responses, we found evidence of consistent reverse coding (using 0 to mean yes and 1 to mean no) at all trust D wards except two for this question. We identified this issue by cross-referencing against other questions and using logical rules. As there were two wards where reverse coding did not happen, we ruled out a software issue; rather, this appears to be a training issue. Having highlighted this issue part way through the study, we decided against retraining as this could have ended in a mix of correctly and reverse-coded responses. Instead, we switched the responses (1 to 0 and 0 to 1) in all of the wards where we identified reverse coding.
Overview of analytical methods
Descriptive analyses
When describing the staffing levels, skill mix, temporary staff use, patient counts and estimated staffing requirements, we calculated daily measures for each ward. From these we calculated the mean daily measure and standard deviation for each ward. We summarised these at the hospital trust level using ‘mean ward’ measures, which are the means of the ward means.
Assessing the reliability and validity of the Safer Nursing Care Tool
Variation between different samples for setting ward establishments
We considered the appropriateness of the minimum recommended sample size of the SNCT data collection (20 days twice per year)19 by investigating the sample size needed to get a stable estimate of the mean staffing requirement (in WTE). For this we used two alternative approaches: bootstrapping114,115 and power calculations.
For the bootstrapping approach, starting from the recommended sample size of 20, for each ward, we took random samples of increasing size in steps of 5 up to 360 (or the size of the data set if smaller). From each, we took 1000 bootstrap samples (samples with replacement, of same size as the original sample). The mean of each sample represents the recommended establishment (see Appendix 1, Equations 3 and 4) based on the different samples. Where wards had undergone substantial changes in size or specialty, we treated them as two (or more) separate wards. This avoided sampling across periods where the establishment required was clearly different for reasons other than periodic fluctuations in acuity/dependency and patient numbers. For each sample size and ward, we calculated bias-corrected and accelerated 95% bootstrap intervals for the mean, which take into account any bias and skewness in the distribution of the bootstrapped means. 115
As an alternative approach, for each ward we calculated what sample size, N, would be required to obtain a 95% confidence interval that is, at most, 0.5, 1 or 2 WTEs wide. We used the standard deviation, s, of the whole data set for each ward (up to 363 data points), and applied the following standard formula for a two-tailed hypothesis for a single group mean,116 where d is the half-width of the confidence interval, α is 1 minus the confidence level, and Zα/2 is the inverse of the standard normal distribution at α/2:
For example, for a 95% confidence interval that is, at most, 0.5 WTEs wide (so the half-width is 0.25), the number of observations required is given using the following formula, where s2 is the variance:
Variation in calculated staffing requirements by time of day and day of week
We investigated the variation in estimated staffing requirements (in hours per day and, equivalently, WTE) within a day and between days of the week. We calculated intracluster correlation coefficients between morning and evening estimated staffing requirements to assess how well they agreed. Then we quantified how much staffing requirements changed from the morning to the evening by calculating the difference between the morning and the evening estimates on each day. Specifically, we were interested in the consequences of the time of the day of SNCT assessments on both recommended establishments and deployment decisions. We also calculated the average staffing requirements on different days of the week to show whether or not there were patterns in the numbers of patients and their acuity/dependency by day of the week.
Regression analyses: how does the Safer Nursing Care Tool compare with professional judgement of staffing adequacy?
Using multilevel modelling approaches, we assessed the relationship between deviation of actual staffing from the estimated staffing requirement (in HPPD) and nurse-reported measures of perceived staffing adequacy: ‘enough staff for quality’, ‘nursing care left undone’ and ‘missed breaks’. We nested the models at the ward and hospital trust levels using random intercepts.
We accounted for skill mix in our models by entering the deviation of actual registered nurse staffing and the deviation of actual nursing support worker staffing from their respective estimated requirements. We also fitted models using the total deviation across both staff groups, with skill mix as a separate variable. Furthermore, we assessed whether or not including other variables aside from deviations of actual staffing from estimated staffing requirements (Table 7) improved model fit. To assess non-linear staffing outcome relationships, we used quadratic terms for the registered nurse and nursing support worker staffing deviations. We investigated whether or not there were interactions between the registered nurse/nursing support worker staffing deviations and the other variables. Any periods missing the answer to the relevant staffing adequacy question(s), or any of the other variables, were excluded.
Predictor | Details |
---|---|
Registered nurse staffing deviation | Deviation of actual registered nurse staffing from the estimated registered nurse staffing requirement (in HPPD) (i.e. apparent registered nurse shortfall) |
Nursing support worker staffing deviation | Deviation of actual nursing support worker staffing from the estimated nursing support worker staffing requirement (in HPPD) (i.e. apparent nursing support worker shortfall) |
Staffing deviation | Deviation of total actual staffing from the total estimated staffing requirement (in HPPD) (i.e. apparent total shortfall) |
Skill mix | Actual registered nurse hours per day/total worked hours per day |
Squared registered nurse staffing deviation | To test for non-linear staffing effects |
Squared nursing support worker staffing deviation | As above |
Squared staffing deviation | As above |
Ward type | Dummy variable: surgical. Reference is other (medical or mixed medical/surgical) |
Proportion single rooms | Proxy for ward layout |
Day of the week | Dummy variables: Tuesday–Sunday. Reference is Monday |
Patient turnover | (admissions + transfers in + discharges + transfers out)/actual total care hours |
Shift | Used in the shift-level models. Dummy variables: morning, afternoon, evening. Reference is night |
We fitted multilevel logistic regression models for binary outcomes using the glmer (generalised linear mixed-effects regression) procedure from the lme4 package117 in R version 3.5.0. Its fitting method optimises a function of the log-likelihood using penalised iteratively reweighted least squares and evaluates the log-likelihood by the Laplace approximation. We checked the significance of variables using p-values from asymptotic Wald tests. We compared the fit of models using the Akaike information criterion and the Bayesian information criterion, the latter of which favours more parsimonious models.
Our core analyses use data aggregated to days. The day models used the first available SNCT rating per day (morning, or, if missing, a later time) for the proportions of patients in each level, the 7 a.m. patient count, the actual staffing and patient hours from 7 a.m. to 7 .a.m., and the staffing adequacy recorded in the morning of the next day. We also fitted models aggregated to 6-hour shifts. The 6-hour shift models used the SNCT rating corresponding to the start of the 6-hour shift for the proportions of patients in each level (or, if this was missing, the end of the 6-hour shift), the patient count at the start of the 6-hour shift, the actual staffing and patient hours for the duration of the 6-hour shift, and the staffing adequacy recorded during the next 6-hour shift. We used the average observed distribution of staff per ward and 6-hour period as a proxy for the required distribution of staff.
We performed the following sensitivity analyses. First, we refitted the models matching staffing adequacy reports to staffing and ward data on the same day/shift rather than the previous day/shift. Second, we refitted models keeping only the first section of data for any wards that were split into pseudo-wards. Third, we refitted the models predicting ‘enough staff for quality’ without hospital trust D’s data, the majority of which we suspected had been reverse-coded (i.e. 0 for yes and 1 for no).
Simulation modelling
The ward data collected were used as the empirical basis for an analysis of staffing policies by simulation. Our research question is ‘How do the costs and percentage understaffed shifts compare between scenarios that set flexible (low), standard and high ward establishments, and how do other factors affect the results?’. We assess this using a scenario-based analysis.
We used a Monte Carlo agent-based simulation118,119 framework to model each ward in a hospital. The simulation was developed in the multimethod simulation software AnyLogic researcher edition version 8.3.2. (The AnyLogic Company, Quorn, UK; www.anylogic.com; accessed 15 November 2017). We designed this simulation model for comparing alternative ways of using the SNCT for setting the nursing establishment (total nursing staff employed in WTE) on inpatient wards in a hospital. A video demonstration is available at https://eprints.soton.ac.uk/430632/.
Our simulation model consists of a hospital with a user-specified number of wards. These wards are the ‘agents’, which move between being understaffed, adequately staffed or overstaffed (their ‘states’) each shift, as shown in Figure 1. To understand how generalisable our conclusions might be, we developed models based on data from each of the four hospitals, with the number of wards and other parameters derived from each hospital.
FIGURE 1.
Ward state chart.

In the simulation model for each ward and on each shift, we assume that staffing is planned according to a recommended level, based on the SNCT. We modelled a number of scenarios using the SNCT in different ways to determine this baseline level. In addition to the conventional approach to using the SNCT, whereby the staffing establishment is based on the mean staffing requirement, we considered setting baseline staffing at higher or lower levels. On any given shift, a mismatch between the planned staffing and the staffing required could occur because of variation in patient need or because the planned staff were not available (e.g. because of sickness/absence).
We describe the simulation model following the Strengthening the Reporting of Empirical Simulation Studies – Agent Based Simulation (STRESS-ABS) guidelines. 120 A summary is below, and for full technical details including the model logic and assumptions see Appendix 1. In particular, for simulation input parameters see Appendix 1, Tables 34 and 35, and for logic diagrams see Appendix 1, Figures 8–10.
Scope, assumptions and input parameters
We determined the feasibility of filling any staffing shortfalls first from re-deployment within the hospital (wards with excess staff), second from using bank staff and third from using agency staff. We defined triggers for the point at which more resource is needed and priority rules for which ward receives extra resources if there are insufficient resources in total. Here, we assumed that wards with the highest shortfall as a proportion of their total staffing requirement for that shift would be prioritised.
We used estimates from hospital trust B of the proportion of bank and agency shifts filled to model the likelihood of filling a shift from bank/agency (see Appendix 1, Tables 36 and 37); these were not available for the other hospital trusts. We also tested the impact of different assumptions about the availability of temporary staff. We assessed the implications of lower efficiency for temporary staff on wards by using varying assumptions about the relative efficiency of temporary staff (e.g. 1 agency staff hour = 0.75 permanent staff hours). We included time of the day and day of the week patterns for ward occupancy and for the bank and agency request fulfilment rates.
We included a factor to allow for unanticipated absences on planned shifts. For this, we assumed that each rostered registered nurse (or support worker) had a 3% (or 4%) chance of being absent with insufficient lead time to rearrange the roster. We also recognised that when assigning staff to shifts there will be some rounding to deal with the mismatch between whole bodies and the staffing required.
For each shift, we generated the number of patients in each acuity/dependency level. Because we had no data on individual patient trajectories, time periods (6-hour shifts) in the model were treated as independent of one another; for example, the number of patients and their acuity/dependency levels in one period do not affect the number and levels in the next. We assume that work done or not done in one period does not affect the staffing requirements in the next period. We also assume that requirements in different wards are independent of one another, that is that neither the numbers of patients nor the acuity/dependency levels of patients in different wards interact. We assume that the actual (rather than the planned-for) needs of patients in the same acuity/dependency level vary (see Appendix 1, Figures 11 and 12).
Running the model
The model was run to simulate data for a period of 1 year for each hospital and for each comparative analysis, because hospital finances are typically planned over 1 year. We ran the model 10 times (i.e. for 10 independent years) for each scenario and calculated 95% confidence intervals around the mean to assess the error around the estimate. We chose this number of replications because it gave 95% confidence intervals that were narrow enough for our purposes (for the base scenario, widths were < £0.25 cost per patient-day at each hospital trust and < 0.7% understaffed patient shifts at all trusts). We also calculated the ranges to assess the variability of results in different years. 121
Outputs
The key performance measures that we used to compare scenarios were the percentage of patient shifts that are understaffed and the cost per patient-day. We considered shifts to be ‘understaffed’ if the total effective staffing (i.e. accounting for the lower efficiencies of temporary and re-deployed staff) was < 85% of the estimated requirement according to the SNCT for the shift. This allowed a buffer of 15% either side of the estimated requirement within which staffing is considered adequate, as is also used in the RAFAELA tool. 47 We looked at other outputs for some sets of scenarios, where relevant (see Appendix 1, Table 38, for descriptions of all of these performance measures). The simulation model records the necessary outputs for each ward (agent) and over the hospital (system).
Verification and validation
Throughout the simulation model development process, we performed verification (checking that the model is implemented correctly in the simulation software) and validation (checking that we built an appropriate and accurate enough model). As recommended by Sargent,122 we documented the conceptual validation, computer model verification, operational validation and data validation checks that we carried out (see Appendix 1, Tables 39–43). Conceptual validation refers to assessing whether or not the conceptual model represents the real world accurately enough for the purpose of the study. Computer model verification involves checking whether or not the computer model is programmed correctly to match the conceptual model. Operational validation means whether or not the simulation model results are similar enough between runs to allow conclusions to be drawn. Data validation consists of checking whether or not the data used in the simulation model are accurate enough for the purpose. In conclusion, we are highly confident about the operational validity and computer model correctness and are relatively confident about the conceptual validity and data validity.
Sensitivity analyses
We performed multiway sensitivity analyses to test the impact of varying parameters, particularly those for which we do not have data, on the key performance measures (percentage of understaffed shifts and cost per patient-day). We used a 2k factorial design and computed the average effect of each factor and the 95% confidence intervals to assess whether or not each effect is statistically significant. 123 We also tested for two-way interaction effects.
Scenarios
We investigated scenarios that differ in how they use the SNCT to set staffing establishments. The ‘standard’ approach calculates a staffing establishment based on the mean requirement over a data collection period. We compared the consequences and costs of using this approach to setting establishments with those of using an approach that relies more heavily on flexible staffing. This ‘low’ establishment is set to meet 80% of the mean requirement, with the intention that variable demand above this is met by internally re-deploying staff and/or by using temporary/flexible bank and agency staff. We contrasted this with a ‘high’ staffing approach, which sets staffing at the 90th percentile and hence staffs wards at a level that would be expected to match or exceed demand 90% of the time if that level of staff were deployed.
We explored the system complexity including running scenarios with different assumptions for temporary staff availability, and using data from different times of year to set the ward establishments (see Appendix 1, Table 44, for full descriptions of all scenarios. We ran all scenarios for all four hospital trusts separately, and ran some scenarios interacted with each other; for example, for each method for setting ward establishments, we ran all scenarios with different assumptions for the availability of temporary staff.
Cost-effectiveness analyses
We used the outputs of the simulation modelling to compare the cost-effectiveness of the three approaches to using the SNCT to set establishments: the ‘standard’ mean approach and our alternative ‘high’ and ‘flexible (low)’ establishment approaches, designed to meet demand on most days and to provide a low baseline staffing level, respectively. We estimated the hospital-level costs and the number of extra deaths due to short staffing using estimates of staffing/outcomes associations derived from published studies. We performed sensitivity analyses to see the effect of different assumptions and, in particular, examined the effect of differing assumptions about the availability of temporary staff because, as demonstrated in Chapter 6, it seems likely that substantive conclusions about the costs, the consequences (in terms of short staffing) and the effects of each strategy may be heavily dependent on this.
Sources of coefficients to estimate effects
We sought studies that could provide robust estimates of the effect of low staffing at the patient level on hospital wards in a context that was sufficiently close to that of the current study. In addition, we needed to be able to obtain valid estimates of baseline risk to link to our study hospitals. Despite the extensive body of research into this field, this limited the potential pool of studies.
We had originally identified Needleman et al. ’s8 study as a potential candidate for effectiveness coefficients because their measurement of individual patient exposure to low staffing in a longitudinal design provides a stronger basis for causal inference and a lower risk of bias than in cross-sectional studies. Unfortunately, the Needleman et al. study used an undocumented staffing system to determine the required registered nurse staffing levels only, and was undertaken in a very different context (a US tertiary academic medical centre), where baseline nurse staffing levels are typically very different from those within the NHS. The problem with not knowing the system used to determine nurse staffing requirements was that we could not assume that the SNCT would give comparable results. Indeed, given the very different staffing levels observed in apparently similar UK and US hospitals,124 it seems unlikely that the same staffing requirements would be estimated using systems designed in the different countries. Therefore, we had no way to measure a comparable absolute or proportional deviation from a baseline staffing requirement to determine what might constitute ‘low’ staffing. Other candidate studies we considered suffered similar limitations associated with different contexts and proved even more problematic to use because they reported coefficients related to absolute rather than relative staffing levels, using outcomes for which we could not obtain comparable baseline event data from the NHS (e.g. per-shift risk of one or more patient falls). 125
Consequently, we have used estimates for the association between low staffing and outcomes derived from our own recent NIHR-funded study. 6,9 Like Needleman et al. ’s8 study, this longitudinal study measured exposures to low staffing at the individual patient level. The study was based on data from 138,133 patients admitted to general medical and surgical wards of a large NHS general hospital between 2012 and 2015. In this study, staffing establishments for the wards in the hospital had been set using the SNCT and measures of exposure to ‘low’ staffing were defined relative to the ward mean staffing levels, an exposure variable that we were able to replicate in our simulation models. The study provided coefficients for the effect of exposure to low staffing over the first 5 days of the hospital stay, which covers the majority of the acute stay for the majority of patients. Low staffing was measured as the number of days below the mean (for risk of death) or the average nursing hours relative to the mean (for length of stay). We were also able to consider the effect of exposure to days of high (defined as > 1.5 hours per patient) temporary staffing from the coefficients derived in this study.
Sources to estimate baseline values
We identified baseline mortality rates for three of the four hospital trusts from the data used in the 2017 Standardised Hospital Mortality Indicator model. 126 This was not available for hospital trust C as this is a specialist hospital where the Standardised Hospital Mortality Indicator model would not apply. Furthermore, as the coefficients linking staffing to mortality and length of stay had been obtained from general hospitals, applying it to this population would, in any case, be unwarranted.
We obtained length of stay data for the 2017/18 financial year (April–March) from the Seven-day Services Length of Stay Indicator,127 which gives the distribution of patients staying on wards for (0) 1, 2, 3 or at least 4 days, as well as median length of stay for emergency admissions, who form the majority of admissions. We used this both to provide a hospital average length of stay for each hospital trust and to estimate an average number of days’ stay within 5 days (excluding zero-day stays), the period to which the effect estimates for exposure to low staffing applied. We extrapolated the available data for the first 4 days of the stay to estimate the average length of stay within 5 days.
We assumed that the trend in the number of patients experiencing stays of 0–4 days was continued in order to estimate how many of the patients who stayed for more than 3 days were discharged before day 5, and therefore how many also stayed for 5 (or more) days. More patients experienced a stay of 1 day than experienced a stay of 2 days, more stayed for 2 days than for 3 days and more stayed for 3 days than for 4 days. This allowed us to estimate the proportion of an admitted cohort who were discharged on each of days 0–3. We assumed that the observed trend continued with half the number of patients discharged after 4 days compared with after 3 days. Different assumptions about the trend made very little difference to the estimates (for the average number of days’ stay within 5 days). For example, assuming that the same number of patients were discharged on day 4 as day 3 reduced the estimate by ≤ 0.03 days for all hospital trusts. Assuming that no patients were discharged on day 4 increased the estimated average stay by ≤ 0.09 days. For coefficients and key parameters derived from external sources used in the cost-effectiveness models, see Table 45 in Appendix 1.
Temporary staffing
For each of the three approaches to using the SNCT to set establishments we tested [the ‘standard’ mean approach and our alternative ‘high’ and ‘flexible (low)’ establishment approaches, designed to meet demand on most days and to provide a low baseline staffing level, respectively], we used different assumptions about the availability of temporary staff and the use of internal re-deployment to cover any staffing shortfalls:
-
Assume that staffing deficits are not met.
-
Assume that staffing deficits are partially met, assuming limited availability of bank and agency staff. (We considered two alternatives here: first, 50% bank and 50% agency availability and, second, empirical availability estimated using data from one hospital trust for both – see Appendix 1, Tables 36 and 37.)
-
Assume that staffing deficits will be met by employing bank/agency staff, both registered and unregistered nurses, by assuming high availability of bank (50%) and agency (90%) staff with no internal re-deployment.
-
Assume that staffing deficits will be met initially by re-deployment from overstaffed wards, with bank/agency staff used only when no further re-deployment is possible, assuming high availability of bank (50%) and agency (90%) staff with internal re-deployment.
To generate data to estimate the consequences of each approach to setting establishments, we ran the simulation model for 1 year (for each set of assumptions and each hospital trust). We used data from the ‘standard’ average staffing scenario to determine a ward mean staffing level against which low staffing was judged when using the alternative approaches to setting establishments. We summed the days of low staffing and the days with > 1.5 hours per patient of temporary staffing across all wards. We calculated the mean change in staffing levels associated with each approach to using the SNCT. From data for each ward on each day, we calculated the overall risk of exposure to low staffing (or high temporary staffing).
The effects of each establishment relative to the ‘standard’ establishments were estimated using coefficients from relevant regression models. We divided the number of patient-days by the mean length of stay to estimate the patient population. We estimated the expected number of deaths using each hospital trust’s actual death rate (patient population times risk of death) and we assumed that this was the baseline mortality rate that would occur under the standard approach to staffing using the SNCT.
Because the regression estimates were derived from survival models that estimated the cumulative effect of exposure to variation in staffing, the effect of any change in staffing accumulates across the stay. That is to say, if the risk of exposure to low staffing is 50% then a patient who stays for 5 days will experience an average of 2.5 days of low staffing. The coefficients we used were based on the effect of exposures during the first 5 days of stay only. Therefore, we used our estimates of the mean stay within 5 days to estimate the mean exposure per patient.
To estimate effects, we multiplied differences in mean patient exposures relative to the exposures experienced under the standard establishment (baseline) by the relevant coefficients. The effect of staffing change on length of stay was estimated by multiplying the number of patients (β) by the average change in worked HPPD associated with the scenario (ΔHPPD × β) to estimate the bed-days saved (or added). For estimations involving mortality, we assumed that the hazard ratio approximated to a risk ratio, an assumption that is generally valid in the short term and when probabilities of events are small, which is the case with mortality in this study. 128,129 We estimated the risk of death for each establishment-setting approach by multiplying the observed risk by the hazard ratio associated with the change in exposure (to low staffing or high temporary staffing). We calculated the change in the number of deaths by subtracting the number of deaths associated with the ‘standard’ establishment scenario from the number of deaths estimated in the new scenario [(Δexposure × hazard ratio × baseline risk × population) – baseline death rate]. We estimated the ‘numbers needed to treat’ (NNT) or ‘numbers needed to harm’ (NNH) associated with each establishment (relative to the ‘standard’ average-based establishment) using the formulae NNT = 1/ARR or NNH = 1/ARI (where ARR and ARI are the absolute risk reduction and the absolute risk increase, respectively).
In our core analysis, we focused on the effect of low staffing on mortality alone, calculating a cost-effectiveness ratio (Δcost/Δmortality) comparing approaches to setting establishments that were associated with improved outcomes compared with the ‘standard’ approach to setting establishments. We also estimated net costs after taking into account the value of bed-days saved. As we had no other basis on which to attribute value or costs associated with these saved/additional bed-days, we used the 2017/18 national average (reference) cost for a non-elective bed-day,105 likely to be a conservative assumption as it assumes that there are no specific treatment costs associated with extended stay. We multiplied the change in bed-days associated with each establishment by the reference cost. This change was added to the staffing cost estimated as part of each model and we call the resulting figure the ‘net cost’. We also considered the consequence of adding the effect of exposures to high bank and agency staffing. We undertook sensitivity analyses exploring the effect of varying a number of key parameters, including the estimates of the effect of low staffing on mortality using the upper and lower bounds of the 95% confidence interval, the costs of agency staff and the effect of increases in the costs of assistant staff, as would be the case if the grade mix of such staff increased.
Because we were unable to estimate effects for hospital trust C, a specialist trust, this trust is omitted from the results. Because of the number of scenarios and parameters that are varied across the hospital trusts, we focus our reporting of results on the (unweighted) mean results across the three remaining hospital trusts. Because of different baseline absolute values in each trust, we give the percentage change (from ‘standard’ establishment) where relevant.
Expert and public involvement
Our multidisciplinary study team consists of experts in nursing research, operational research applied to health care, health economics and statistics, as well as senior nursing leads with responsibility for implementing staffing policies, from each of the four hospital trusts. Throughout the study, we held regular (fortnightly) internal team meetings and supplementary ad hoc meetings at which the team members providing the core analysis sought guidance from the relevant experts as appropriate. We also held regular meetings with the principal investigators at the hospital trusts, both face to face and via conference call, particularly in the set-up and data collection phases. A steering group of further experts who were independent of the study team oversaw the study. This group provided advice on the delivery of the project, including technical aspects and pertinent issues.
When developing our research proposal, we discussed appropriate patient/public involvement with Claire Ballinger [patient and public involvement lead for NIHR CLAHRC (Collaboration for Leadership in Applied Health Research and Care) Wessex] and Anya de Iongh (patient and public involvement champion for the ‘fundamental care in hospital’ theme of the CLAHRC). Following their guidance we sought no further direct patient/public involvement in prioritising or shaping the questions as these arose from the brief and need for technical assessment of the tool; instead, we focused on considering how the public could be involved in the proposed research and its governance and dissemination.
Based on this advice, we sought a lay member of our steering group with specific interest and expertise. To this end, we worked with Stephen Habgood, a lay member of the NICE safe staffing advisory committee for the development of guidelines in mental health, who agreed to participate in the project steering group. Stephen also has experience of staffing methodologies used in other sectors from his past work as a prison governor and is currently chairperson of a mental health charity. In addition, and guided by the advice from our patient and public involvement experts, we considered ward-based staff nurses as the potential end-users of our research in a way that is analogous to a patient receiving a treatment that might be recommended by an expert. Based on this, we have used multiple channels to connect with ward-based staff, including extensive use of social media and discussions with individual staff nurses at consultation events. These conversations have sensitised us to a number of issues in relation to our study, including several factors that we tested in the simulation modelling, such as requirements for nurses to have specific skills.
Ethics issues
Although results of the SNCT staffing tool may indicate staffing shortages and staff reports may also indicate concerns, hospital trusts already have established mechanisms for dealing with these issues. The University of Southampton Ethics Committee approved our study (reference 18809). The Health Research Authority approval number for our study is 190548. This study did not require NHS Research Ethics Committee approval because no data were collected directly from patients, only routine data were used and all patient data were de-identified at source.
Chapter summary
-
In our study, we used daily SNCT ratings of patients from acute general wards in four hospitals to investigate the tool’s reliability and validity and to model the costs and consequences of using the tool in different ways.
-
We explored how the estimated nursing establishments varied with different samples, exploring issues such as the sample size used (number of days), and the day of the week and the time of day at which ratings were collected.
-
We explored whether deviations from the staffing level indicated by the SNCT were associated with nurses’ reports of sufficient staff for quality, omissions of care or missed breaks.
-
We used the data to model different approaches to setting the establishment using the SNCT, comparing the ‘standard’ (mean-based) approach with a ‘high’ establishment (designed to meet assessed need 90% of the time) and a ‘flexible (low)’ establishment (designed to provide a minimum of core staff relying on flexible deployment to meet variable need).
-
We explored the consequences of these different approaches for the frequency of wards and patients experiencing substantial understaffing.
-
We explored the costs incurred by the hospitals in providing staff under these different approaches.
-
We modelled the cost-effectiveness (in terms of cost per life ‘saved’) of alternative approaches to setting establishments using estimates of the effects of low staffing and exposure to high levels of temporary staff from a recent study undertaken in the NHS.
Chapter 4 Descriptive analyses
This chapter provides a description of each of the key variables and the extent to which they varied between wards and hospitals.
Across all four hospital trusts, 81 out of 94 potentially suitable wards participated. Participation was at least 90% of wards in three of the four trusts. Trust D had a greater number of specialist wards for which the trust had judged the SNCT unsuitable and so it was not implemented. In this trust, 66% of all adult wards participated, but a number of specialist units, including cancer and cardiac wards, and including some high-dependency unit beds, did not (see Appendix 2, Table 46, for hospital-level participation).
For some wards, because of ward closures and changes we restricted the timespan of data or split wards into pseudo-wards (see Appendix 2, Table 47). (For ward profiles, see Appendix 2, Table 48; statistics at the ward level are provided in Report Supplementary Material 3, Tables 87–91 and Tables 93 and 94.)
The completeness of SNCT acuity/dependency ratings was high in participating wards; all hospital trusts completed over 85% of morning returns and, once ratings from later in the day were used to fill missing data, completeness ranged from 92% of possible ward-days (at hospital trust D) to 99% (at hospital trust A). In all, we obtained data linking SNCT ratings and staffing levels for 26,362 ward-days. Response rates for all staffing adequacy questions were also high. In two trusts (A and B), morning response rates for all items were > 90%. In trusts C and D, morning response rates were somewhat lower but still high (≥ 83% and 69%, respectively). (For full details, see Appendix 2, Table 49, for completeness of daily responses and Appendix 2, Table 52, for completeness of census/shift responses.)
Data validity for the staffing adequacy questions with yes or no answers (i.e. 1 or 0 answers) was above 99%. For the questions about numbers of staff needed, at least 80% were potentially valid (recording at least one registered nurse for ‘registered nurses needed’, or at least one staff member in total for ‘nursing support workers needed’). (See Appendix 2, Table 50, for data validity of morning/day responses and Appendix 2, Table 53 for all census/shift responses.)
When linking the deviation of actual staffing from the estimated requirement to the nurses’ responses to ‘enough staff for quality’, ‘nursing care left undone’ and ‘staff breaks missed’ questions, in each case about 15% of the larger data set was lost in linkage (as a result of invalid/missing data).
When linking the data set with the numbers of ‘registered nurses needed’ to that with the numbers of ‘nursing support workers needed’, < 1% (of the larger data set) was lost in the linkage (as a result of missing/invalid data in either data set). However, when further restricting data to days when it was reported that there were not ‘enough staff for quality’ and more total staff were needed (‘registered nurses needed’ plus ‘nursing support workers needed’) than worked on the ward during the preceding 24 hours (7 a.m.–7 a.m.), a relatively small data set remained (2522 observations, 13% of the previous data set). (See Appendix 2, Tables 51 and 54, for the numbers of records after linkage for daily responses and for census/shift responses, respectively.)
Ward size varied within and between hospitals. Typically, wards in hospital trust C were much smaller than those in the other trusts, with a mean of 12 patients per ward based on 7 a.m. census counts at trust C, and means of between 23 and 30 patients at the other trusts (see Appendix 2, Table 55). The mean within-ward standard deviation of patients at 7 a.m. for each trust is between 1.7 (trusts B and C) and 2.1 (trust A).
Staffing levels
Staff per day
Staffing levels varied considerably both between and within wards. Table 8 summarises the total daily staffing levels, skill mix and band mix for staff deployed on wards (substantive and temporary staff). Across all participating wards in all four hospital trusts, the mean ward staffing levels (i.e. the mean of ward means) were 4 registered nurse HPPD and 3 nursing support worker HPPD. Registered nurse HPPD varied substantially between trusts, from 3 (hospital trust D) to 8 (hospital trust C). On the other hand, mean ward nursing support worker levels were relatively stable across hospital trusts (about 3 HPPD in all hospital trusts). Within trusts, the range between the highest and lowest staffed wards was 60% of the mean.
Hospital trust | Total HPPD | Total hours | Skill mix, percentage registered nurses | Nursing support workers in bands 2, 3 and 4, respectively | Registered nurses in bands 5, 6, 7 and 8, respectively |
---|---|---|---|---|---|
A | 7.0 (5.4, 10.4) | 143 (73, 270) | 51% (42%, 70%) | 83%, 13%, 4% | 80%, 14%, 5%, < 1% |
B | 6.8 (5.0, 8.9) | 190 (70, 536) | 56% (39%, 79%) | 91%, 7%, 1% | 84%, 12%, 3%, < 1% |
C | 10.5 (7.5, 14.2) | 117 (79, 193) | 75% (70%, 78%) | 33%, 67%, < 1% | 31%, 64%, 5%, 0% |
D | 6.5 (5.2, 8.4) | 160 (101, 230) | 49% (40%, 63%) | 83%, 5%, 13% | 75%, 18%, 6%, < 1% |
The mean ward skill mix across all wards was 56% registered nurses; this varied between hospital trusts from 49% at hospital trust D to 75% at hospital trust C. Hospital trusts A, B and D had a similar spread of staff across bands, with most nursing support workers at band 2 and most registered nurses at band 5, while hospital trust C (the specialist cancer hospital trust) had a very different spread, with most nursing support workers at band 3 and most registered nurses at band 6.
Temporary staff
All wards made heavy use of temporary staff, with the ward average ranging from 21% of daily hours at hospital trust D to 24% at hospital trust B (Table 9). Overall, bank staff (overall ward average of 16% of all hours) were used much more often than agency staff (7%). Use of agency staff was more variable between trusts (4–9%) than overall use of temporary staff. The majority of agency staff are at band 2 or 5 at all hospital trusts. The skill mix of bank staff is lower than that of substantive staff. This is also true of agency staff in most hospital trusts, although the overall skill mix of agency staff more closely resembles that of substantive staff (see Appendix 2, Table 56).
Hospital trust | Bank staff | Agency staff | All temporary staff | Skill mix of temporary staff, percentage registered nurses |
---|---|---|---|---|
A | 17% | 5% | 22% | 44% |
B | 15% | 9% | 24% | 42% |
C | 19% | 4% | 23% | 62% |
D | 14% | 7% | 21% | 36% |
All wards | 16% | 7% | 23% | 44% |
Staffing by time of day
We described variation in staffing levels by time of day, using 6-hour shifts (7 a.m.–1 p.m., 1 p.m.–7 p.m., 7 p.m.–1 a.m. and 1 a.m.–7 a.m.) to approximately match major staffing changes. Overall, mean staffing levels were highest in the morning and lowest at night (Table 10), although in some wards the highest staffing levels were in the afternoon (see Report Supplementary Material 3, Table 88). In general, skill mix was lower at night, although the overall differences were not large.
Hospital trust | Total care hours per patient shift | Skill mix, percentage registered nurses | ||||||
---|---|---|---|---|---|---|---|---|
Morning | Afternoon | Evening | Night | Morning | Afternoon | Evening | Night | |
A | 2.2 | 2.0 | 1.5 | 1.3 | 51% | 50% | 51% | 50% |
B | 2.0 | 1.8 | 1.6 | 1.4 | 57% | 57% | 55% | 54% |
C | 3.1 | 3.1 | 2.4 | 2.0 | 74% | 71% | 74% | 63% |
D | 1.9 | 1.9 | 1.5 | 1.3 | 49% | 49% | 49% | 49% |
All wards | 2.2 | 2.1 | 1.7 | 1.4 | 56% | 56% | 55% | 53% |
Calculated staffing requirements
We used the reported numbers of patients in each SNCT category to estimate staffing requirements. For each hospital trust, the weighted-average multiplier without specialing requirements corresponds to an acuity/dependency level somewhere between SNCT levels 1a and 1b (Table 11). Across all wards, including specialing requirements added about 10% to the average estimated care HPPD, although this increase varied between trusts.
Hospital trust | Weighted-average multiplier without specialing requirements | Total HPPD without specialing requirements | Total HPPD with specialing requirements |
---|---|---|---|
A | 1.56 | 7.4 | 7.6 |
B | 1.60 | 7.3 | 8.2 |
C | 1.52 | 7.0 | 7.5 |
D | 1.55 | 7.0 | 8.2 |
All wards | 1.57 | 7.2 | 8.0 |
Because specials in hospital trust D were not allocated to one of the original SNCT categories, we tested the impact of our assumption that specials in trust D were in acuity/dependency level 1b, by instead using the average acuity/dependency level of patients on the ward when specials were requested. This made little difference, reducing the estimated staffing requirement by 2%.
The mean ward standard deviations of the calculated staffing requirement ranged from 0.41 HPPD (6% of the hospital trust mean) at hospital trust D to 0.93 HPPD (13% of the hospital trust mean) at hospital trust C. When including specialing requirements, the calculated staffing requirement per patient-day is more variable (with mean ward standard deviations ranging from 0.92 HPPD at hospital trust A to 1.49 HPPD at hospital trust C).
Deviation between calculated requirement and actual staffing
We compared the calculated staffing requirement with the actual staffing each day and on each ward by subtracting the actual staffing from the calculated requirement (Table 12). Hospital trust B had the greatest difference between calculated staffing requirements and actual staffing, with an apparent average staff shortage of 0.5 HPPD/18 hours per day. At the other end of the spectrum, hospital trust C had an apparent staff surplus of 3.6 HPPD/37 hours per day. Including specialing in the calculated staffing requirements more than doubles the percentage difference deviation in hospital trusts B and D.
Hospital trust | Total HPPD | Total hours per day | Percentage difference |
---|---|---|---|
Without specialing | |||
A | 0.4 (1.1) | 11.6 (19.9) | 4.7% (14.2%) |
B | 0.5 (0.9) | 18.1 (24) | 7.2% (11.9%) |
C | –3.6 (1.7) | –36.9 (16.3) | –53.2% (32.3%) |
D | 0.5 (0.8) | 15.5 (18.5) | 7.3% (11%) |
With specialing | |||
A | 0.6 (1.2) | 16.2 (22.7) | 7% (14.9%) |
B | 1.4 (1.2) | 42.5 (33.8) | 15.9% (13.2%) |
C | –3.1 (1.8) | –31.5 (16.4) | –44.4% (31.5%) |
D | 1.7 (1.3) | 44.9 (32.9) | 18.9% (12.7%) |
We used the 15% criterion derived from the RAFAELA system to determine potentially important deviations from the required staffing level. On average across all wards, 26% of days were classified as understaffed. Wards at hospital trusts A, B and D were ‘understaffed’ compared with the calculated requirement on about 30% of days (see Appendix 2, Table 57). By contrast, on average wards at hospital trust C were ‘overstaffed’ compared with the calculated requirement (over by more than 15% of the requirement) on more than 80% of days.
In hospital trust A, the majority of wards (13/19) were staffed within 15% of requirement on the majority of days (see Report Supplementary Material 3, Table 91). Five wards were understaffed compared with the calculated staffing requirement more than 50% of the time, while one was overstaffed compared with the calculated staffing requirement more than 50% of the time. In hospital trust B, 6 out of 31 wards were understaffed compared with the calculated staffing requirement more than half of the time, while the majority of wards (25/31) were staffed within 15% of requirement for the majority of days. In hospital trust C, most wards (10/12) tended to be overstaffed compared with the calculated staffing requirement (more than 50% of the time). In hospital trust D, 6 out of 19 wards were understaffed on more than 50% of days, and the majority of wards (13/19) were staffed within 15% of requirement on the majority of days.
Nurse-reported staffing adequacy
Enough staff for quality, care left undone and missed breaks
Table 13 summarises the valid morning responses for the staffing adequacy questions with binary 1/0 (yes/no) answers. Across all wards, a mean of 77.8% of reports suggested that there were enough staff for quality, on 5.4% of occasions it was reported that nursing care was left undone and on 5.3% of occasions it was reported that breaks were missed. For results for hospital trust D before correcting for reverse-coding, see Report Supplementary Material 3, Table 92.
Hospital trust | Shifts with ‘enough staff for quality’ (%) | Shifts with ‘nursing care left undone’ (%) | Shifts with ‘staff breaks missed’ (%) |
---|---|---|---|
A | 64.8 (24, 96.4) | 10.4 (0.3, 22.5) | 10.5 (0.6, 29.4) |
B | 81.2 (59.1, 97.5) | 5.5 (0, 24.5) | 5.3 (0, 16.7) |
C | 72.4 (44.2, 92.1) | 3.4 (0.6, 9) | 2.5 (0.6, 6.5) |
D | 88.5 (69.4, 100) | 1.6 (0, 16.1) | 1.9 (0, 16.2) |
All wards | 77.8 (24, 100) | 5.4 (0, 24.5) | 5.3 (0, 29.4) |
Reported staffing requirements
On days when it was reported that there were not enough staff for quality and more staff were needed than worked on the ward during the preceding 24 hours (7 a.m.–7 a.m.), we calculated the increases in registered nurses, nursing support workers and total staff needed (according to nurse reports) over the number working (Table 14). These are three relatively small data sets of between 2437 and 2522 observations and each about 13% of the potentially valid responses.
Hospital trusta | Registered nurses | Nursing support workersb | Total nursing staff |
---|---|---|---|
A | 1.1 (58.5%) | 1.2 (133.7%) | 1.6 (30.8%) |
B | 0.7 (25.5%) | 0.9 (71.8%) | 0.8 (12%) |
C | 1.2 (40.7%) | 0.7 (142.4%) | 1.5 (32.5%) |
D | 1.3 (50.8%) | 0.7 (27.8%) | 1.6 (26.2%) |
All wards | 1.0 (41%) | 1.0 (98.5%) | 1.3 (22.5%) |
The required increases in registered nurse staffing when nurses reported there were not enough staff for quality was between 0.7 and 1.3 (hospital-trust-level means) and the required increases in nursing support workers were between 0.7 and 1.2. Across all wards, an average of one extra registered nurse and one extra nursing support worker was requested.
Other factors potentially affecting workload
The proportion of single rooms per ward varied both between and within hospital trusts. Hospital trust D had relatively few single rooms, on average 12% per ward (see Appendix 2, Table 58). In trust C the proportion of single rooms was high, with a mean of 58%, reflecting a number of wards that were exclusively single rooms. Average daily turnover varied between trusts, from 0.04 admissions and discharges per care hour at trust C to 0.08 admissions and discharges per care hour at trust A, but it was much more variable between wards within trusts. Between the lowest turnover ward in trust B and the highest in trust A, there was a 29-fold difference (0.01–0.29 admissions and discharges per care hour).
Staffing costs
We estimated the costs of registered nurse and nursing support worker staffing levels observed on each ward in the study hospital trusts. To do this, we used the observed hours provided per day by substantive, bank and agency staff at each Agenda for Change band and we report these as staff costs per patient-day. Summary estimates for registered nurses and nursing support workers combined, and for each group, are reported in Table 15 (for more details, see Report Supplementary Material 3, Table 94).
Hospital trust | Total | Staff group | |
---|---|---|---|
Registered nurses | Nursing support workers | ||
A | 150 | 95 | 54 |
B | 148 | 98 | 49 |
C | 274 | 230 | 44 |
D | 141 | 85 | 56 |
All wards | 165 | 114 | 51 |
The mean ward cost in three of the hospital trusts is between about £140 and £150 per patient-day, while the fourth has a substantially higher mean cost (approximately double, at about £275 per patient-day). The relatively high cost for hospital trust C reflects the higher registered nurse staffing levels and skill mix.
There is substantial variation in staff cost per patient-day within wards and between wards in each of the hospital trusts. The mean cost per patient-day varies from £116 to £238, £112 to £203, £199 to £363 and £114 to £183 in hospital trusts A to D, respectively. The variation in cost arises from a combination of the underlying variability in staffing levels, skill mixes and band mixes shown in Table 8 (see Report Supplementary Material 3, Tables 87 and 88) and additional staffing costs arising from unsociable hours payments as well as from employing bank and agency staff.
Chapter summary
-
Across the four hospital trusts, 81 wards participated, with > 85% of potentially eligible general wards participating over 1 year.
-
We received SNCT ratings for 96% of possible ward-days, and on over 85% of ward-days we received answers to questions about staffing adequacy.
-
In all, we obtained data linking SNCT ratings and staffing levels for 26,362 ward-days.
-
Staffing levels and skill mix varied considerably within wards, between wards and between hospital trusts.
-
There was a large contrast between total (both registered nurse and support worker) staffing in the three general hospital trusts (a mean of 6.5–7 care HPPD) and that in the specialist trust (a mean of 10.5 HPPD).
-
Within hospital trusts, the range between the highest and lowest staffed wards was 60% of the mean.
-
Skill mix (the percentage of nursing staff who are registered nurses) also varied between and within trusts, with mean skill mix in the general hospital trusts (49–56%) lower than that in the specialist trust (75%).
-
All hospital trusts made significant use of temporary staff, with > 20% of all shifts worked by bank and agency staff in all trusts (range 21–24%).
-
The use of agency staff was more variable between trusts (4–9% of all shifts worked).
-
Required staffing estimated by the SNCT across all wards was, on average, 7.2 care HPPD, with calculated staffing slightly higher than the staffing we observed to be deployed in three general hospital trusts and considerably lower than staffing deployed in the specialist trust.
-
Using the criterion of 15% below the requirement for that day to define ‘understaffing’, we estimated that 26% of all ward-days were understaffed.
-
Nurses reported that they had enough staff to deliver quality care on 78% of shifts (according to morning responses).
-
When nurses reported that they did not have enough staff for quality, the additional staffing required varied by hospital trust, averaging between 0.7 and 1.3 registered nurses, or between 0.8 and 1.6 total additional staff required.
-
Mean hospital trust nurse staffing costs varied from £274 per patient-day in the specialist trust to between £141 and £150 per patient-day in the general hospital trusts.
Chapter 5 Precision, reliability and validity
Having described the underlying data, we now concentrate on exploring the use of the SNCT to generate ward establishments (for which it was designed) and to set staffing levels each day and shift (for which it is starting to be used).
We examine the variation in the recommended ward establishments generated by the SNCT when using different samples and sample sizes, and collecting data at different times of day and on different days of the week. In particular, we aim to answer the questions ‘what is the sample size required to get a stable estimate of the mean staffing requirement?’ and ‘does the time of day or day of the week on which SNCT ratings are collected matter?’.
In Chapter 6, we go on to investigate whether using the mean average is appropriate for setting staffing levels, or whether other measures such as percentiles may better match staffing to demand.
We measured the difference between the staffing requirement calculated using the SNCT and the actual staffing (both measured in HPPD), and we refer to this as the apparent ‘shortfall’. We compare nurses’ reports of staffing adequacy with the shortfall. This allows us to ascertain whether or not the required staffing level estimated using the SNCT accords with the judgement of staff on wards.
Variation between different samples for setting ward establishments
The SNCT guidance recommends calculating the average staffing required by collecting data on patients’ acuities and dependencies across at least 20 weekdays, at least twice per year (20 days in January and 20 days in June).
Our data set includes assessments of SNCT ratings for patients on each ward in scope of each hospital trust on different days of the year. After removing missing data and outliers, the sample size per ward averaged 325 days. From this, we calculated the estimated staffing requirements (in WTEs) for each day (see Report Supplementary Material 3, Table 89). We then compared the recommended establishments calculated by averaging different data samples. We used confidence intervals to assess how well these averages approximated the true average requirement. We calculated the precision of the estimate by dividing the half-width of the resulting confidence interval by the mean value. 130
Approach 1: bootstrapping
We use bootstrapping here so that we can derive confidence intervals for the average staffing requirement for different sample sizes. The bootstrap is a well-known resampling procedure that is used to generate the distribution of a statistic of interest. Rather than continually resampling from the system where the data were originally collected, the original sample – our best estimate of the population – is resampled, with replacement, to generate a large number (e.g. 1000) of new bootstrap data sets. For each data set, the statistic of interest (e.g. the mean) is recalculated. The resulting distribution is the sampling distribution of the statistic and can be used for inference. 114,131
Figure 2 illustrates the effect of sample size on the recommended staffing for ward 1 at hospital trust D (an arbitrary choice). The points show the bias-corrected and accelerated 95% confidence intervals for the mean for each sample size. The gridlines are spaced 0.25 WTEs apart. On this ward, for sample sizes below 100 data points, the widths of the 95% confidence intervals for the mean are more than 1 WTE, and for the largest possible sample size from our data, the width of the 95% confidence interval for the mean is about 0.5 WTEs.
FIGURE 2.
Bias-corrected and accelerated 95% confidence intervals for the mean calculated staffing requirement in ward 1 at hospital trust D for different sample sizes.
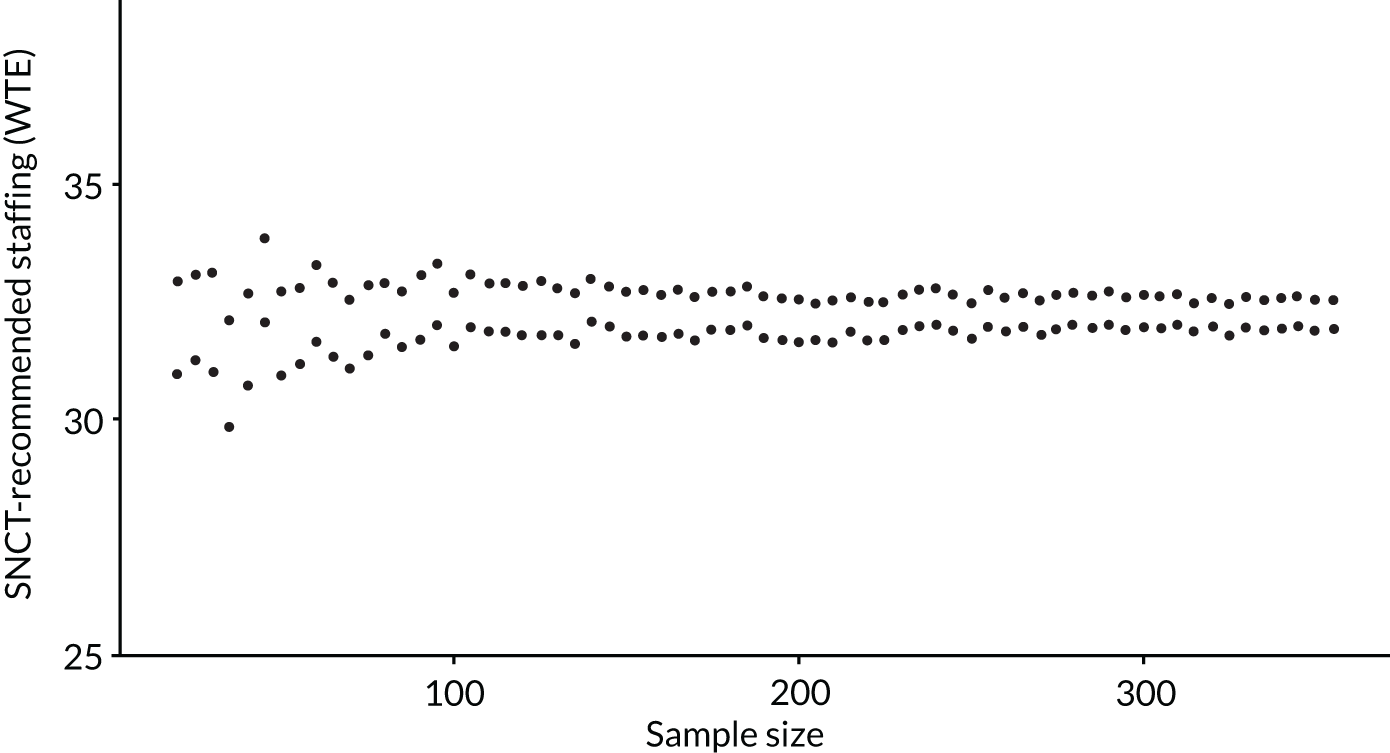
Across all wards, the average width of the 95% confidence intervals for the means using the recommended minimum of 20 data points is almost 3 WTEs, with an average precision (half the width of the 95% confidence interval expressed as a % of the mean) of 4.1%, and, even with 160 data points, the average width is more than 1 WTE (Table 16). In other words, the recommended establishment using 20 data points is highly variable.
Sample size | Average confidence interval width (WTE) | Average precision (%)a | Number of wards |
---|---|---|---|
20 | 2.9 | 4.1 | 86 |
40 | 2.1 | 3.0 | 86 |
60 | 1.7 | 2.5 | 86 |
80 | 1.5 | 2.1 | 86 |
100 | 1.3 | 1.9 | 82 |
120 | 1.2 | 1.8 | 81 |
140 | 1.1 | 1.6 | 81 |
160 | 1.1 | 1.5 | 80 |
180 | 1.0 | 1.4 | 77 |
200 | 0.9 | 1.3 | 77 |
220 | 0.9 | 1.3 | 77 |
240 | 0.8 | 1.2 | 73 |
260 | 0.8 | 1.2 | 72 |
280 | 0.7 | 1.1 | 69 |
300 | 0.7 | 1.1 | 63 |
320 | 0.7 | 1.2 | 43 |
340 | 0.7 | 1.1 | 32 |
360 | 0.6 | 0.8 | 3 |
Although 69 out of 86 wards (80%) achieved a confidence interval of width 1 WTE or less with a sufficiently large sample size, for 20% of wards the available data were insufficient to estimate the establishment with that degree of precision. However, in most cases the required sample size was much greater than the recommended minimum of 20, and only two wards provided a confidence interval width of 1 WTE with this sample size.
Approach 2: power calculation
As an alternative approach, we used a standard power calculation-based approach to derive the sample size requirement, given ward standard deviations of daily-calculated staffing requirements and the desired confidence interval width.
For the 20-day minimum sample size recommended in the SNCT guidelines to be sufficient, the standard deviation would need to be, at most, 1.14 WTEs for 95% confidence intervals of width 1. Most wards in this study had more variable staffing requirements than this (see Report Supplementary Material 3, Table 89) and so would need a sample size larger than 20 days to be able to estimate the mean value this precisely. For confidence interval widths of 0.5/2 WTEs, the standard deviations would need to be 0.57/2.28 WTEs, respectively.
The average sample size required for a 95% confidence interval width of 0.5 WTEs is almost 1000, although there is a wide range between wards. The average sample size requirement for a confidence interval 1 WTE wide is 240 points, and for a 2 WTE width the average sample is 60 (Table 17).
Desired width of 95% confidence interval of the mean | Average sample size | Minimum sample size | Maximum sample size |
---|---|---|---|
0.5 WTEs | 959 | 16 | 7215 |
1 WTE | 240 | 4 | 1804 |
2 WTEs | 60 | 1 | 451 |
Variation in calculated staffing requirements by time of day and day of week
Variation by time of day
Guidance on using the SNCT suggests that data for determining the establishment be collected at 3 p.m. We were unable to arrange data collection at this precise time. Ward staff reported that, instead, collecting data during periods (rather than precise times) was feasible, typically in the morning, in the evening and, in some settings, in the afternoon coinciding with handover. The variation in collection times allows us to investigate how collecting data at different times of day affects the staffing requirement that is calculated.
We identified the patient count for each of the four shifts (on average and at the start) and the weighted-average SNCT multiplier (see Appendix 3, Table 59). On average, the difference in patient counts between time points was small. In all hospital trusts the highest patient count was at 1 p.m., while the highest average patients present on ward per 6-hour shift was from 7 a.m. to 1 p.m. However, the range in average patient counts over the four shifts was less than one in all cases. Perhaps unsurprisingly, the weighted-average multiplier (i.e. the average patient acuity/dependency) was, on average, the same for all census points. While these results suggest that, on average, there may be little reason to adhere to the 3 p.m. census when determining the establishment, this does not mean that the staffing requirements determined at different time points remain constant.
We used intracluster correlation coefficients to assess the agreement between requirements calculated from morning and evening census points (Table 18). The mean intracluster correlation coefficients between morning and evening censuses per ward show moderate (≤ 0.5) or good (≤ 0.75) agreement for the weighted-average multiplier and moderate or good agreement for the total care hours per day. 132
Hospital trust | Weighted-average multiplier without specialing | Total care HPD without specialing | Total care HPD with specialing |
---|---|---|---|
A | 0.5 | 0.57 | 0.62 |
B | 0.77 | 0.53 | 0.76 |
C | 0.61 | 0.62 | 0.65 |
D | 0.61 | 0.64 | 0.71 |
All wards | 0.65 | 0.58 | 0.70 |
However, the intracluster correlation coefficients differ across wards. Whereas some wards have excellent agreement between results for each day calculated from morning and evening censuses (indicating minimal change in patient numbers and average acuity/dependency of these patients from morning to evening), for others agreement was poor (see Report Supplementary Material 3, Table 95).
We investigated the size of the deviations between morning and evening measurements, as the intracluster correlation coefficients do not tell us whether or not the disagreement between morning and evening censuses is large enough to be practically significant. For example, ward 1 at hospital trust A has only moderate agreement between morning and evening assessments (0.5 intracluster correlation coefficient) but the average size of the deviation is small (< 0.3%) and so would be unlikely to lead to different staffing establishment decisions. Table 19 shows the mean ward deviation between staffing requirements calculated from the morning and evening censuses.
Specialing requirements/hospital trust | Weighted-average multiplier absolute deviation | Weighted-average multiplier percentage deviation (%) | Total care HPD absolute deviation | Total care HPD percentage deviation (%) | Total required WTE absolute deviation |
---|---|---|---|---|---|
With specialing | |||||
A | 0.00 (0.13) | –0.17 (7.8) | 0.07 (19.11) | 0.46 (12.74) | 0.02 (4.35) |
B | 0.00 (0.12) | 0.02 (5.94) | –1.96 (20.89) | –0.06 (8.65) | –0.45 (4.76) |
C | –0.01 (0.25) | –0.34 (12.38) | 0.22 (15.4) | 0.17 (17.83) | 0.05 (3.51) |
D | –0.01 (0.21) | –0.21 (9.19) | –2.07 (26.96) | –0.83 (11.07) | –0.47 (6.14) |
Without specialing | |||||
A | 0 (0.09) | –0.16 (5.77) | 0.14 (16.24) | 0.48 (11.45) | 0.03 (3.7) |
B | 0 (0.05) | –0.03 (2.99) | –2.08 (14.11) | –0.11 (6.96) | –0.47 (3.21) |
C | 0 (0.1) | –0.29 (6.99) | 0.38 (11.11) | 0.22 (14.9) | 0.09 (2.53) |
D | 0 (0.05) | –0.07 (3.44) | –1.29 (11.76) | –0.69 (7.07) | –0.29 (2.68) |
Whereas on average for wards at hospital trusts A and C the staffing requirements (in total care hours per day or WTE) calculated from morning censuses are higher than those calculated from evening censuses, the opposite is true at hospital trusts B and D. On average, the absolute magnitude of differences is very small (< 1%). However, some wards have particularly large average differences between morning and evening requirements. For example, ward 9 at hospital trust B has an average difference of 26 hours between the total care hours per day calculated from the morning and evening censuses (including specialing requirements) largely due to variation in the numbers of patients.
Variation by day of the week
The day of the week makes little difference to the weighted-average multiplier (i.e. the average patient acuity/dependency) on average across wards (see Appendix 3, Table 60). In three hospital trusts (A, B and C), there is evidence that the highest average need per patient is at the weekend. However, owing to larger numbers of patients during the week, the overall ward (rather than individual patient) estimated nursing requirements tend to be lowest at the weekends. Using Monday as a reference, the weighted-average multiplier (without considering specials) varies by < 1% on other days, except in trust C, where the average multiplier is 1.3% lower on Thursday than on Monday. Similar variation is seen when specialing requirements are included, although the variation is slightly greater.
The required hours vary more because of variation in patient numbers, although, in general, the differences are small, except in hospital trust C, where staffing requirements on Wednesdays are 10.1% higher than on Mondays, with staffing requirement gradually falling across the remaining days of the week. In the other three hospital trusts, there is some variation between weekends and weekdays, with weekends generally having lower staffing requirements. However, the absolute differences are relatively small, with the largest observed difference being in trust A, with Saturday requiring 3.7% fewer staff hours than Monday.
How does the Safer Nursing Care Tool compare with professional judgement of staffing adequacy?
We assessed the extent to which apparent staffing shortfalls, measured by the difference between the deployed staffing and the daily requirement estimated using the SNCT (including specialing), were associated with the shift leader’s perception that staffing was adequate, using a number of different questions (asked at each census point). All analyses included random factors for ward and hospital trust. Multivariable analyses included control for other factors that may influence workload, including patient turnover, single rooms, ward type and day of the week.
Associations between daily staffing deviations from Safer Nursing Care Tool requirement and reported staffing adequacy
Differences between the staffing level deployed and the daily staffing requirement estimated using the SNCT for patients on the ward on that day are significantly associated with nurses’ perceptions of staffing adequacy (Table 20). In the multivariable models, when the registered nurse staffing level was 1 hour per patient-day below the required level estimated using the SNCT, the odds of the shift leader reporting that there were enough staff for quality were reduced by 11%, the odds of reporting nursing care left undone were increased by 14% and the odds of reporting staff missing breaks were increased by 12% (all p < 0.001). Findings are similar for shortfalls of nursing support workers. Models using overall care HPPD gave similar coefficients, with each care hour per patient-day of shortfall associated with a 12% reduction in the odds of reporting enough staff for quality (see Appendix 3, Table 62).
Variable | Enough staff for quality | Nursing care left undone | Staff breaks missed | |||
---|---|---|---|---|---|---|
Odds ratio (95% confidence interval) | p-value | Odds ratio (95% confidence interval) | p-value | Odds ratio (95% confidence interval) | p-value | |
Registered nurse shortfall (HPPD) | 0.89 (0.87 to 0.92) | < 0.001 | 1.14 (1.08 to 1.20) | < 0.001 | 1.12 (1.06 to 1.18) | < 0.001 |
Nursing support worker shortfall (HPPD) | 0.86 (0.83 to 0.89) | < 0.001 | 1.14 (1.07 to 1.20) | < 0.001 | 1.11 (1.05 to 1.17) | < 0.001 |
Turnover (per nursing hour) | 0.91 (0.30 to 2.75) | 0.863 | 3.36 (0.60 to 18.72) | 0.167 | 4.94 (0.95 to 25.78) | 0.058 |
Ward type: other | ||||||
Surgical | 0.54 (0.31 to 0.92) | 0.023 | 2.13 (1.19 to 3.82) | 0.011 | 2.15 (1.23 to 3.78) | 0.008 |
Proportion single rooms | 0.54 (0.18 to 1.66) | 0.283 | 3.01 (0.93 to 9.71) | 0.065 | 2.06 (0.65 to 6.56) | 0.221 |
Day of week: Monday | ||||||
Tuesday | 1.12 (0.99 to 1.26) | 0.079 | 0.86 (0.69 to 1.06) | 0.160 | 0.71 (0.58 to 0.88) | 0.001 |
Wednesday | 1.28 (1.13 to 1.45) | < 0.001 | 0.96 (0.78 to 1.18) | 0.684 | 0.61 (0.49 to 0.76) | < 0.001 |
Thursday | 1.08 (0.96 to 1.23) | 0.200 | 0.91 (0.73 to 1.13) | 0.383 | 0.81 (0.66 to 1.00) | 0.045 |
Friday | 1.03 (0.91 to 1.17) | 0.610 | 0.93 (0.74 to 1.15) | 0.488 | 0.79 (0.64 to 0.97) | 0.028 |
Saturday | 1.29 (1.14 to 1.47) | < 0.001 | 0.75 (0.60 to 0.95) | 0.016 | 0.50 (0.40 to 0.64) | < 0.001 |
Sunday | 1.02 (0.90 to 1.15) | 0.811 | 1.11 (0.90 to 1.37) | 0.310 | 0.82 (0.66 to 1.01) | 0.058 |
Standard deviation between wards | 1.06 | 1.08 | 1.05 | |||
Standard deviation between hospitals | 0.79 | 0.94 | 0.72 | |||
Akaike information criterion | 20,697 | 8377 | 8094 | |||
Bayesian information criterion | 20,809 | 8490 | 8206 |
Although there was some evidence that higher patient turnover was associated with perceptions of lower staffing adequacy in the univariable models (see Appendix 3, Table 61), turnover was not significantly associated with perceptions of lower staffing adequacy in the multivariable models. Nurses on surgical wards were less likely to perceive adequate staffing than nurses on other (medical or mixed) wards. For example, the odds of nurses reporting that there were enough staff for quality were 56% lower on surgical wards.
In wards with a higher proportion of single rooms, ward leaders were more likely to report care left undone and missed breaks, and less likely to report enough staff for quality, although the relationships were not statistically significant. However, the effect sizes were large.
Day of the week was significantly associated with perceptions of adequate staffing. In particular, after controlling for other factors, nurses were less likely to report that there were enough staff for quality and more likely to report missed care and missed breaks on Monday than on Saturday.
Non-linear effects and interactions
We tested for non-linear relationships with apparent registered nurse and nursing support worker shortfalls, as would be expected if the SNCT provides a threshold for adequate staffing. We estimated models using effects that were significant in the main effects models, adding a variable for staffing shortfall squared for each staff group. Overall, we found that only one non-linear term was statistically significant and all non-linear effects were too small to be of practical importance.
For nursing care left undone, the non-linear term for registered nurse staffing was statistically significant (p = 0.021; see Appendix 3, Table 64). Although there is some tendency for the odds of reporting care left undone to increase more as staffing shortfalls increase above 0 (the threshold for adequate staffing defined by our SNCT base estimates), when shortfall is negative (i.e. when staffing exceeds the estimated requirements), increases in staffing are still associated with substantial reductions in reports of care left undone (Figure 3). Including the non-linear term did not improve model fits over the models with significant variables only (compare Tables 63 and 64 in Appendix 3), apart from for ‘care left undone’, where the Akaike information criterion was reduced by a very small amount (from 8380 to 8378).
FIGURE 3.
Change in odds of reporting care left undone with change in staffing shortfalls.
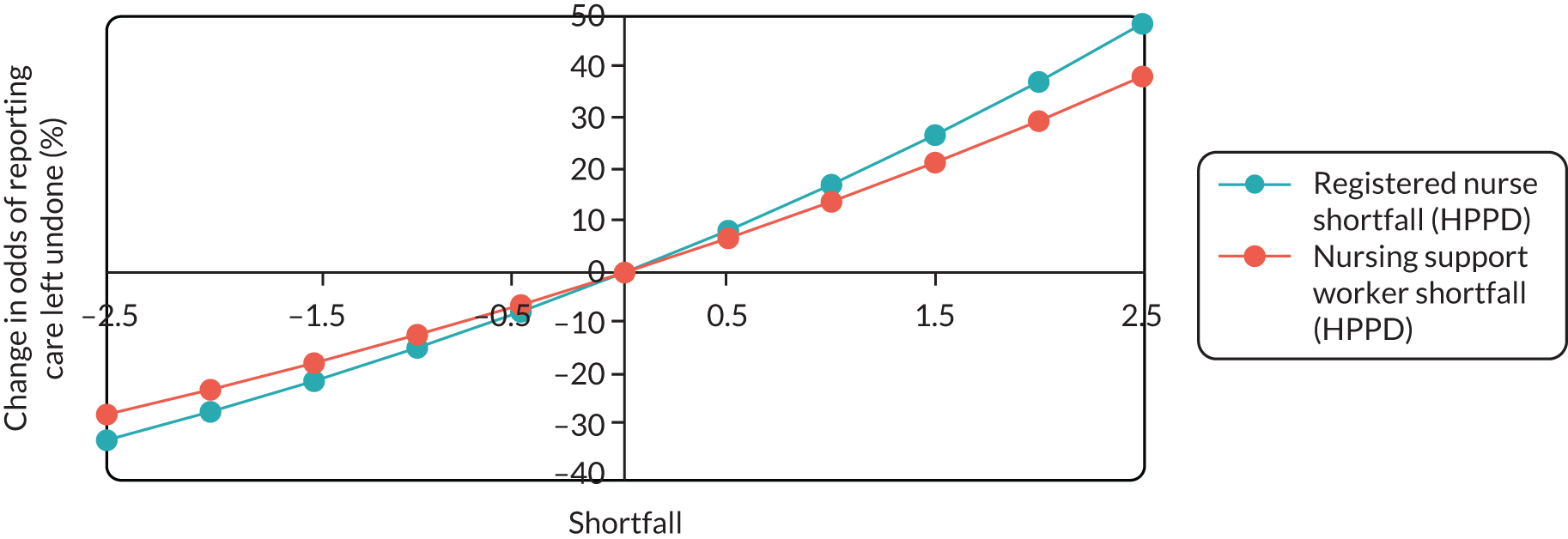
Next, we estimated models that included all statistically significant variables (including the non-linear term for registered nurse staffing shortfall for the nursing care left undone model) and the interactions between these variables. There were no significant interaction effects between apparent registered nurse shortfall and support worker shortfall, and both Akaike information criterion and Bayesian information criterion increased, indicating overall worse model fits (see Appendix 3, Table 65).
Shift-level analysis
We also investigated whether or not there is a relationship between the apparent shortfall and the ward leader’s perception of staffing adequacy in the previous 6-hour shift. As the SNCT recommends using professional judgement to apportion daily requirements into shifts, we used the average observed ward distributions of staff over the day as a proxy. Both registered nurse shortfall and nursing support worker shortfall during a shift are significantly associated with enough staff for quality, nursing care left undone and missed breaks as reported in the following shift.
Nurses were most likely to report that there were enough staff for quality on night shifts, followed by morning and then afternoon shifts (we did not have enough data to investigate evening shifts). Compared with night shifts, the odds of reporting enough staff for quality were 10% lower on morning shifts and 13% lower on afternoon shifts (see Appendix 3, Table 66). Similarly, nurses were least likely to report leaving care undone and missing breaks on night shifts, followed by afternoon and then morning shifts.
Although our day-level analysis found no evidence of important non-linear effects of staffing shortfall and no interaction between staff groups, in analysis at this level, a more complex picture emerges.
Including non-linear terms improves the fit of models (over the models with significant main effects only) as measured by the Akaike information criterion in all cases, and it improves the Bayesian information criterion for models predicting enough staff for quality and staff breaks missed (see Appendix 3, Tables 67 and 68). Both the registered nurse and nursing support worker shortfall squared terms are significant in most models (2/3 and 3/3 respectively) but their effects are in opposite directions.
Including interactions between shortfall and other predictors improves the model fits further for all three outcomes according to the Akaike information criterion (see Appendix 3, Table 69). The Bayesian information criterion is also improved for the model predicting staff breaks missed. There are several significant interaction effects in the shift models. In the model predicting enough staff for quality, the interaction between registered nurse and nursing support worker shortfall is statistically significant. To explore this interaction and the non-linear effects, we calculated the combined effects of the linear, non-linear and interaction terms for a range of plausible values of staffing shortfalls when all other variables in the model are held constant at mean or reference categories (Figure 4 and Appendix 3, Table 70).
FIGURE 4.
Combined effects of registered nurse and nursing support worker shortfall (change in odds of reporting ‘enough staff for quality’): registered nurse shortfall effect conditional on nursing support worker shortfall (shift-level analysis).
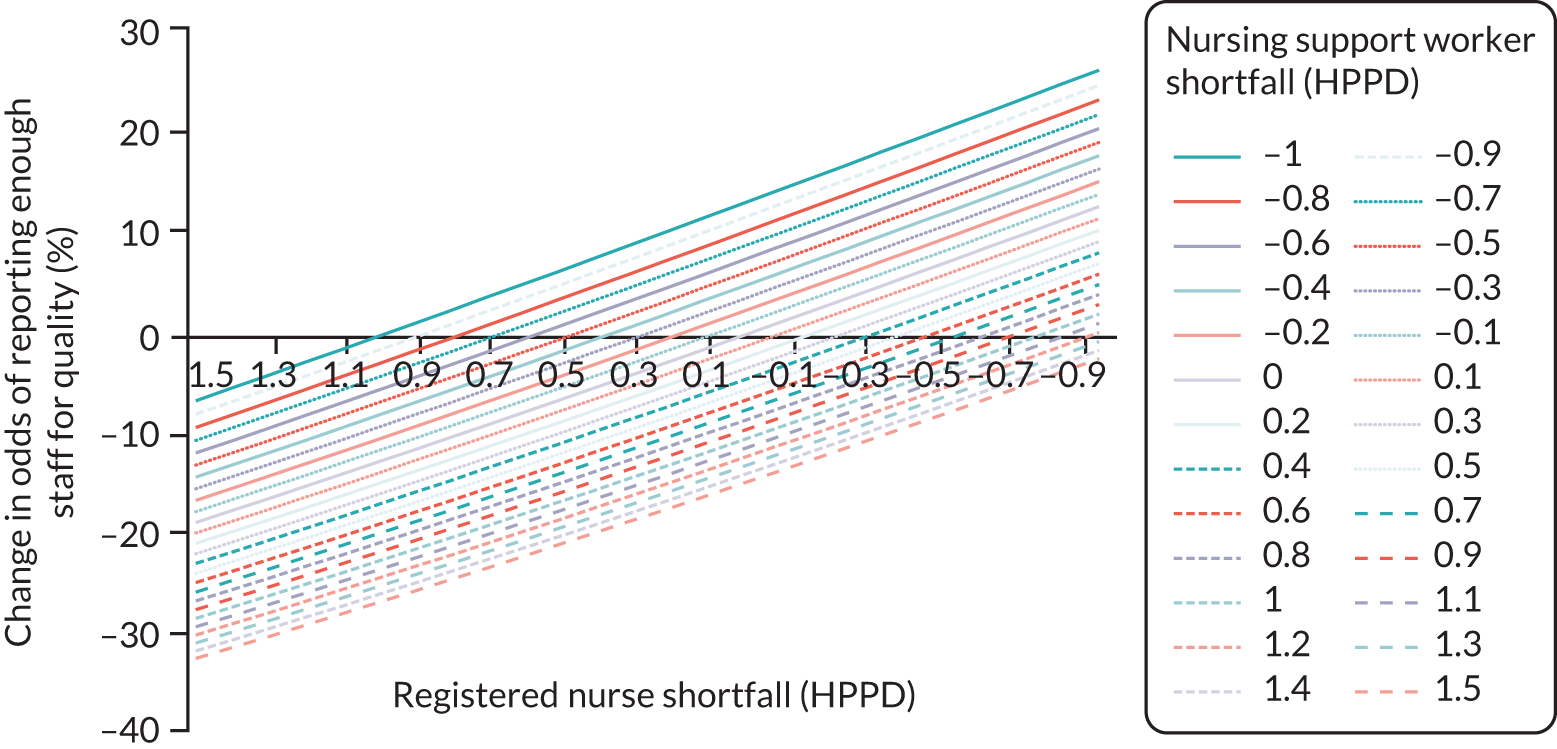
As deficits in registered nurse staffing reduce, the odds of reporting enough staff for quality are increased. The non-linear effect of registered nurse shortfall is very small and so over the plotted range the registered nurse shortfall effect is very close to being linear. The significant interaction effect appears to have marginal impact, with the plotted lines more or less parallel. For missed care and missed breaks, the relationship with registered nurse staffing appears to be linear, with no significant interaction effect. However, there are significant (but very small) non-linear effects for support worker staffing, which are difficult to interpret, suggesting benefit from both high and low staffing relative to assessed need but, in particular, benefits from staffing above the levels assessed by the SNCT.
The effect of shortfall on perceptions of staffing adequacy appears to vary to some extent by time of day. The interaction between nursing support worker shortfall and morning shifts has a statistically significant effect on reporting enough staff for quality. The interactions between registered nurse shortfall/nursing support worker shortfall and afternoon shifts have significant effects on reporting staff breaks missed and care left undone. Both of these interactions may play a part in explaining the complex non-linear relationships with support worker staffing shortfalls and it is possible that higher-order interactions exist. Given the relatively small observed effect sizes and the absence of clear theoretical propositions, we elected not to pursue this through modelling the current data as it seemed unlikely to yield insights related to our main aims and objectives.
Both registered nurse shortfall and nursing support worker shortfall have a larger effect on reporting staff breaks missed on surgical wards than on other wards. Registered nurse shortfall and nursing support worker shortfall lessen the impact of the proportion of single rooms on care left undone, and, in the case of nursing support worker shortfall, also on staff breaks missed; that is, if the shortfall is higher, the proportion of single rooms has less effect on perceived staffing adequacy.
Sensitivity analyses
Matching perceptions of staffing adequacy to the same day/6-hour shift gives a worse fit (higher Akaike information criterion) than matching to the previous day/6-hour shift, although the effects of staffing shortfalls are largely unaltered (see Report Supplementary Material 4, Tables 96–98).
As we split wards that had major changes into pseudo-wards, these wards count more than once towards the analyses. Therefore, we investigated the impact on results if these wards were entered only once. We arbitrarily chose to include the first part for each ward. This made little difference to the substantive statistical conclusions or estimated coefficients, which were almost identical for staffing shortfalls (see Report Supplementary Material 4, Table 99).
Given the potential problem with reverse coding at hospital trust D, we also fitted models predicting reports of enough staff for quality excluding this hospital trust. The variations between wards and between hospitals in the model fitted to data without hospital trust D were lower, but substantive conclusions and coefficients related to staffing shortfall were unaltered (see Report Supplementary Material 4, Table 100).
Chapter summary
-
The SNCT guidelines recommend a minimum sample size of 20 days’ worth of observations to estimate a ward’s required staffing establishment. On average across wards, the bootstrapped 95% confidence interval for this sample is almost 3 WTE staff members wide, with a precision of 4%.
-
On average across wards, a sample of 80 days gives a confidence interval that is 1.5 WTEs wide, with a precision of 2%.
-
We estimated that the sample required for a confidence interval width of 0.5 WTEs is over 900 days on average, although the required sample size varied considerably between wards.
-
On average, the time of day when SNCT assessments were made caused very little difference to the calculated establishment (< 1%), although, for some wards, admission/discharge patterns meant that there were large differences.
-
In general hospital trusts, there was little variation in the average staffing requirements between days of the week, although weekends had slightly lower staffing requirements. However, the magnitudes of differences were small (≤ 3.7%).
-
On days when nurse staffing fell below the daily staffing requirement estimated using the SNCT, the odds of reporting that there were enough staff for quality were reduced by 12% for each care hour per patient-day shortfall. Correspondingly, the odds of reporting that staff breaks and nursing care were missed were increased.
-
We found no evidence to indicate that the calculated staffing requirement represented a threshold, as staffing above this level was associated with increased chances of reporting that staffing was adequate (according to all three measures).
-
The effects of registered nurse and support worker shortfall appeared to be of a similar magnitude and largely independent of one another.
-
There is some evidence that non-linear effects and statistically significant interaction effects appear to result in little or no substantive changes to the overall results or conclusions.
-
After controlling for any shortfall between required and deployed staffing, nurses on wards with higher proportions of single rooms were less likely to respond positively to the staffing adequacy questions, although the relationships were not statistically significant.
Interpretation
In this chapter we assessed the appropriateness of the recommended sample size (20 days) and timing (3 p.m.) of collecting data for determining ward establishments. We found that, on most wards, larger samples than the currently recommended minimum of 20 days would be required to obtain accurate estimates of the required staff to employ within 1 WTE. This level of precision may be unachievable, but, on average, a precision of ±1 staff member can be achieved with a sample of 40 days. Wards with more variable staffing requirements need larger samples to estimate establishments accurately. In most wards, the time of day (morning or evening) made little difference to the calculated establishment required. This suggests that there is little basis for the current recommendation of using observations at 3 p.m.
We investigated whether or not ward leaders were more likely to report that they had enough staff when they had the number estimated by the SNCT. After taking into account other factors that could affect this relationship, we found that when there are fewer staff than the tool suggests are needed, the chance of ward leaders reporting ‘enough staff for quality’ is less, while the chance of them reporting ‘staff breaks missed’ and ‘nursing care left undone’ is more. However, other factors not directly measured in the tool (ward type, single rooms, day of the week, time of day) may also independently affect reported staffing adequacy. When there were more staff than recommended by the tool, there were further improvements in all three reported measures and the relationships we saw appeared to be effectively linear. So, although the tool does provide a measure that is associated with professional judgement of staffing adequacy, giving some indication of ‘validity’, it does not include all factors that seem to influence workload. Furthermore, it does not identify a staffing level that is always perceived as sufficient, nor does it identify a threshold staffing level above which staffing is much more likely to be seen as sufficient.
Chapter 6 Simulation model results
In this section, we present the results of the simulation models. We model the consequences of different approaches to setting ward establishments using the SNCT. These are:
-
The ‘standard’ staffing scenario. This scenario sets the establishment based on the mean value from a period of observation of SNCT ratings. This is the conventional approach to setting establishments using the SNCT. 19
-
The ‘flexible (low)’ staffing scenario. Under this scenario, we model a situation in which the establishment is set to provide a core staff group that would be sufficient on days of low demand, with a view that additional staff are re-deployed from other wards or employed from the hospital’s bank and/or an external agency to meet fluctuations in demand. NICE has identified staffing of more than 20% below plan as a possible red flag47 and therefore we used 80% of the average staffing requirement to set this level, based on the presumption that in the face of average demand this staffing level represents the bare minimum that is acceptable.
-
The ‘high’ staffing scenario. This scenario sets the establishment to match the 90th percentile (rather than the mean) of demand. This scenario models a situation in which staffing is set at a level such that it will be sufficient to meet predictable fluctuations in demand most of the time (i.e. 90% of the time).
-
In addition, we model a ‘vacancies’ scenario. This scenario assumes that the establishment is set at the mean required level but accepts that there are vacancies, set at 10% (reflecting the current national vacancy rate for nursing staff1).
For our core model, we used 20 weekdays in June (with adjustments if unavailable) to provide the data used to determine establishments.
Our core measure is understaffing, defined as staffing more than 15% below the staffing requirement measured each shift. The 15% criterion was selected as it remains some distance from NICE’s 20% trigger for a red flag, intended to highlight a safety risk. 11 Bounds of 15% are used in the RAFAELA system to define the tolerance limits for optimal staffing, recognising the variable and flexible capacity of staff to respond to need. 47
We go on to explore the potential influence of a number of factors including the variation in the availability of temporary staff to cover shifts at short notice, the influence of the sample used to set the ward establishments and the different criteria used to measure understaffing.
A single model run simulates 1 year at one hospital trust and we ran each model 10 times. The variation in results between runs is low for each scenario. Under the standard staffing scenario, the ranges between runs are 0.8%, 0.8%, 1.4% and 0.7% for the estimates of percentage understaffed shifts and £0.31, £0.21, £0.63 and £0.27 for the cost per patient-day at hospital trusts A, B, C and D, respectively. The widths of the corresponding 95% confidence intervals are 0.4%, 0.3%, 0.6% and 0.2% for the mean percentage understaffed shifts per year and £0.13, £0.09, £0.22 and £0.12 for the mean cost per patient-day across years. Therefore, for brevity we report the average result of the 10 runs rather than the ranges or confidence intervals. We report the percentage of understaffed patient shifts, as this reflects the chance that patients are affected by understaffing, although the percentage of understaffed ward shifts is almost identical (see Appendix 4, Table 71, for an example).
Ward establishments
Unsurprisingly, ward establishments are highest when set using the ‘high’ staffing approach, followed by the ‘standard’ and finally the ‘flexible (low)’ method (Table 21). Establishments varied both between and within hospitals largely because of the size of wards. Hospital trust B has the highest ward establishments on average, and trust C has the lowest. The minimum ward nursing support worker levels do not always follow the general pattern (‘high’ then ‘standard’ then ‘flexible’) because of rounding whole people/shifts and the requirement for at least one registered nurse to be present on each shift.
Staff group/establishment scenario | Hospital trust | |||
---|---|---|---|---|
A | B | C | D | |
All staff | ||||
High | 170 (72, 318) | 220 (84, 624) | 92 (48, 150) | 183 (120, 240) |
Standard | 155 (66, 288) | 207 (84, 576) | 78 (42, 126) | 171 (108, 228) |
Flexible (low) | 125 (48, 228) | 166 (66, 456) | 66 (30, 108) | 136 (84, 186) |
Registered nurses | ||||
High | 87 (48, 156) | 125 (42, 420) | 68 (36, 108) | 88 (60, 132) |
Standard | 77 (36, 132) | 117 (36, 396) | 57 (24, 96) | 82 (60, 126) |
Flexible (low) | 63 (36, 114) | 93 (36, 318) | 46 (24, 84) | 65 (42, 102) |
Nursing support workers | ||||
High | 83 (24, 168) | 95 (18, 204) | 24 (6, 42) | 95 (48, 126) |
Standard | 78 (30, 156) | 91 (24, 180) | 21 (6, 36) | 88 (48, 126) |
Flexible (low) | 62 (12, 114) | 72 (18, 138) | 20 (6, 30) | 71 (42, 102) |
Compared with the ‘standard’ (mean average) approach to setting the establishment, the ‘high’ approach to setting staffing (matching 90th percentile demand) results in an increased establishment of 10% on average across the four hospital trusts, although there is a greater increase at trust C. The ‘flexible (low)’ staffing approach, by definition, results in establishments that are approximately 20% lower, with differences arising as a result of rounding whole people/shifts.
Consequences of different approaches to setting establishments
Staff re-deployment and temporary staff
We examined the consequences of the different approaches to setting establishments for the use of re-deployed staff (i.e. staff moved from a ward with an excess to one with a shortfall), bank staff and agency staff (see Appendix 4, Table 72). Across all hospital trusts and all approaches to setting establishments, the proportion of hours worked by re-deployed staff was low. Staff re-deployment was highest with the ‘high’ establishment and lowest with the ‘flexible (low)’ establishment. By contrast, the use of bank and agency staff increased as the size of the baseline establishments decreased, with the ‘flexible (low)’ establishments necessitating high use of bank and agency staff, assuming that additional staff are sought to address staffing shortfalls.
With the ‘high’ staffing establishment, on average across the four hospital trusts < 1% of hours were provided by staff who had been re-deployed. Trust A had the highest level, at 1.4%. This could in part be explained by this trust having the greatest average number of wards per directorate across which staff are shared (3.8 wards per directorate at hospital trust A, compared with 2.8, 3 and 2.7 at hospital trusts B, C and D, respectively).
With the ‘high’ staffing establishment, the use of bank (2.3%) and agency (1.9%) staff was also low, at < 5% overall. This contrasts with the establishments set using the ‘standard’ SNCT approach, whereby the proportion of temporary staff used was, on average, 6.4% across the four hospital trusts, and the ‘flexible (low)’ establishments, whereby 14.4% of hours were worked by bank and agency staff. Bank staff are used slightly more than agency staff in all four scenarios and at all hospital trusts. As the availability of temporary staff to fill shifts at short notice in these scenarios is based on empirical data, which we were able to obtain only from trust B, we look at the impact of alternative assumptions in our scenario analyses (see Short-notice availability of temporary staff).
Understaffing and overstaffing
Applying the criterion of 15% below the estimated staffing requirement to define understaffing shows that understaffing is relatively common, irrespective of the approach taken to setting establishments (Table 22). This is because of variation in patient need and in availability of established staff (due to sickness absence), staff to re-deploy and temporary staff to hire. In all hospital trusts, the ‘high’ staffing establishment is associated with substantially fewer understaffed shifts than the ‘standard’ approach. Considering staffing adequacy by time of day, afternoons have the highest levels of understaffing, because the probability that requests for bank and agency staff will be fulfilled is lowest at this time of day in our empirical data (see Appendix 4, Table 73).
Staffing adequacy/establishment scenario | Hospital trust, % (relative risk) | Average across hospital trusts, % (relative risk) | |||
---|---|---|---|---|---|
A | B | C | D | ||
Understaffing | |||||
High | 7.5 (0.42) | 26.4 (0.73) | 16.3 (0.44) | 25.1 (0.73) | 18.8 (0.60) |
Standard | 17.9 | 35.9 | 37.3 | 34.2 | 31.3 |
Flexible (low) | 55.7 (3.11) | 69.8 (1.94) | 60.5 (1.62) | 67.0 (1.96) | 63.2 (2.02) |
Overstaffing | |||||
High | 18.8 (2.14) | 5.3 (2.39) | 30.8 (2.36) | 4.8 (2.04) | 14.9 (2.26) |
Standard | 8.8 | 2.2 | 13.1 | 2.3 | 6.6 |
Flexible (low) | 1.0 (0.11) | 0.3 (0.13) | 4.6 (0.35) | 0.2 (0.08) | 1.5 (0.23) |
Across all hospital trusts, ‘high’ establishments are associated with understaffing on 19% of patient shifts compared with 31% for the ‘standard’ mean-based establishment. The ‘flexible (low)’ staffing establishment is associated with 63% of patient shifts remaining understaffed, despite re-deployments and the use of bank and agency staff to cover staffing shortfalls.
The pattern is broadly the same at all hospital trusts. In all cases, both the absolute and the relative risk differences between the different establishments were substantial, although the magnitude differs between trusts. The relative risk reduction associated with a ‘high’ staffing establishment compared with ‘standard’ staffing was greatest in hospital trust A (relative risk 0.42) and smallest in trusts B and D (relative risk 0.73). Trust A also had the greatest relative risk increase associated with the ‘flexible (low)’ staffing strategy (relative risk 3.11), whereas the smallest increase was in trust C (relative risk 1.62).
Whereas understaffing (> 15% short) is common under most scenarios, overstaffing (> 15% surplus) is relatively rare under most scenarios in most hospital trusts. When establishments are set using the ‘standard’ staffing approach, hospital trusts B and D have 2% of patient shifts with overstaffing. On the other hand, in hospital trusts A and C, overstaffing is more common (9% and 13%, respectively), but still far less common than understaffing (on average 18% and 37%, respectively). Under the ‘high’ staffing scenario, overstaffing becomes more common, although the level relative to understaffing varies across trusts, remaining at low levels in trusts B and D but at higher levels than understaffing at trusts A and C. Under the ‘flexible (low)’ scenario, overstaffing is rare at all trusts (< 5%).
At ward level there was considerable variation in understaffing (the percentage of understaffed patient shifts ranged from 7% to 80% under the ‘standard’ staffing scenario) but less variation in overstaffing (the corresponding range was from 0% to 35%). There is a moderate negative correlation between the percentage of shifts that are overstaffed and the percentage of shifts that are understaffed (ρ = –0.460; p < 0.01). As overstaffing increases, understaffing decreases, but the relationship is not strong. Across all 82 wards in the simulation model, the standard approach to setting the establishment was associated with understaffing more than 50% of the time on 14 wards. These wards came from all four hospital trusts. Eleven of these wards were medical wards, of which nine were medicine for older people. In almost all of the 82 wards, understaffing was more common than overstaffing, and only two wards were overstaffed more than one-third of the time (34% and 35%). In three wards, understaffing and overstaffing were equally common (±1%), and in four wards overstaffing was more common than understaffing; no obvious pattern with regard to ward specialty emerged, although only two of these seven were medical wards. The rate of understaffing was not significantly correlated with ward size (measured as number of beds, ρ = –0.13; p = 0.239), whereas the rate of overstaffing showed a moderate negative correlation with the number of beds on the ward (ρ = –0.55; p < 0.001).
Costs
At all hospital trusts, total costs increase as the ward establishments increase (Table 23). This is because of the higher costs of employing permanent staff. Although the temporary staff costs change in the opposite direction (increased costs of hiring temporary staff with lower establishments), the changes in permanent staff costs have a greater effect, in part because of limited availability of temporary staff, an issue that we explore further in Short-notice availability of temporary staff. The cost per patient-day is variable between wards within trusts. Staffing costs associated with ‘high’ staffing establishments were, on average, £11 per patient-day (8%) higher than with ‘standard’ establishments, while staffing costs associated with ‘flexible (low)’ establishments were, on average, £15 per patient-day (11%) lower than with ‘standard’ establishments. The relationship between understaffed shifts, establishment and costs is similar at all trusts: the higher the establishment, the higher the cost, but the fewer shifts classified as understaffed (Figure 5).
Establishment scenario | Hospital trust | |||
---|---|---|---|---|
A | B | C | D | |
High | £136 (£114, £183) | £144 (£110, £173) | £169 (£142, £188) | £137 (£125, £152) |
Standard | £125 (£105, £162) | £138 (£96, £170) | £148 (£119, £177) | £132 (£120, £146) |
Flexible (low) | £110 (£91, £135) | £125 (£83, £155) | £130 (£111, £150) | £119 (£107, £133) |
FIGURE 5.
Costs vs. understaffing for alternative methods for setting ward establishments at different hospital trusts.
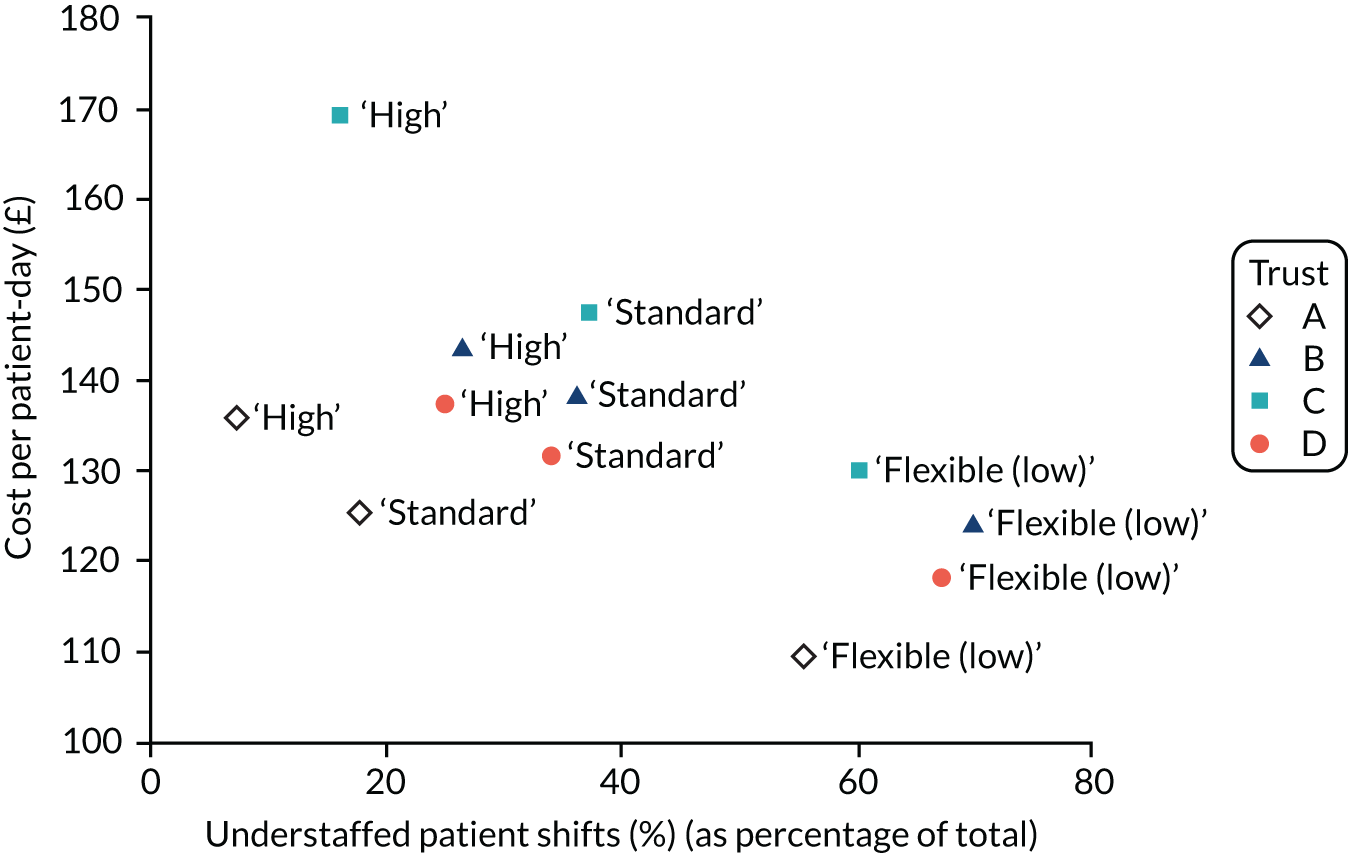
Scenario analyses
Short-notice availability of temporary staff
Given that we have data on the probabilities of bank and agency staff being available at short notice for only one hospital trust, we investigated the impact of alternative assumptions about the availability of temporary staff. We assessed the impacts of various combinations of assumptions under which bank and/or agency staff were more available with limited but still constrained availability (50%), largely unconstrained availability (90%) and unlimited availability (100%). This contrasts with a maximum empirical availability of 37% for bank shifts (for support workers on weekday evenings/nights) and 45% for agency staff (for registered nurses on weekday evening/nights) (see Appendix 1, Tables 36 and 37).
Regardless of the assumptions about temporary staff availability, the proportion of understaffed patient shifts increases as the permanent staffing levels decrease owing to the lower efficiencies (assumed) of re-deployed and temporary staff, as well as the buffer before additional staff are requested (Table 24). When a higher availability of bank and agency staff is assumed, the difference between scenarios reduces in terms of both the cost per patient-day and the percentage of understaffed patient shifts, although the general pattern remains the same. Increasing the availability of temporary staff also increases the costs of each scenario because of the cost of hiring additional temporary staff, and reduces the percentage of understaffed patient shifts.
Availability of temporary staff/establishment scenario | Hospital trust A | Hospital trust B | Hospital trust C | Hospital trust D | Average across hospital trusts | |||||
---|---|---|---|---|---|---|---|---|---|---|
Understaffed shifts (%) | Cost per patient-day (£) | Understaffed shifts (%) | Cost per patient-day (£) | Understaffed shifts (%) | Cost per patient-day (£) | Understaffed shifts (%) | Cost per patient-day (£) | Understaffed shifts (%) | Cost per patient-day (£) | |
Empirical | ||||||||||
High | 7.5 | 136 | 26.4 | 144 | 16.3 | 169 | 25.1 | 137 | 18.8 | 147 |
Standard | 17.9 | 125 | 35.9 | 138 | 37.3 | 148 | 34.2 | 132 | 31.3 | 136 |
Flexible (low) | 55.7 | 110 | 69.8 | 125 | 60.5 | 130 | 67.0 | 119 | 63.3 | 121 |
Bank and agency 50% | ||||||||||
High | 5.4 | 137 | 14.0 | 151 | 12.2 | 173 | 12.0 | 144 | 10.9 | 151 |
Standard | 12.3 | 128 | 19.9 | 147 | 28.3 | 155 | 17.5 | 141 | 19.5 | 143 |
Flexible (low) | 36.8 | 118 | 43.3 | 141 | 47.1 | 144 | 39.5 | 135 | 41.7 | 134 |
Empirical bank, agency 90% | ||||||||||
High | 4.9 | 137 | 8.5 | 155 | 11.1 | 174 | 5.9 | 149 | 7.6 | 154 |
Standard | 10.6 | 129 | 12.5 | 153 | 26.4 | 158 | 9.3 | 146 | 10.9 | 150 |
Flexible (low) | 29.7 | 123 | 28.5 | 152 | 44.4 | 149 | 24.6 | 145 | 31.8 | 142 |
Bank 50%, agency 90% | ||||||||||
High | 4.6 | 137 | 6.6 | 155 | 10.3 | 174 | 3.6 | 148 | 6.3 | 154 |
Standard | 9.9 | 129 | 9.9 | 153 | 24.0 | 159 | 5.8 | 146 | 12.4 | 147 |
Flexible (low) | 26.3 | 122 | 21.0 | 151 | 39.7 | 150 | 16.0 | 145 | 25.8 | 142 |
Bank 90%, agency 90% | ||||||||||
High | 4.2 | 138 | 4.7 | 156 | 8.9 | 175 | 1.3 | 149 | 4.8 | 154 |
Standard | 9.0 | 129 | 7.4 | 153 | 20.7 | 160 | 2.2 | 147 | 4.8 | 150 |
Flexible (low) | 21.9 | 123 | 13.6 | 152 | 32.6 | 152 | 6.2 | 146 | 18.6 | 143 |
Bank 50%, agency 100% | ||||||||||
High | 4.3 | 138 | 5.0 | 156 | 9.6 | 175 | 1.6 | 150 | 5.1 | 155 |
Standard | 9.3 | 129 | 7.8 | 154 | 22.6 | 159 | 2.8 | 147 | 10.6 | 147 |
Flexible (low) | 23.8 | 124 | 15.1 | 154 | 37.3 | 151 | 9.2 | 147 | 21.4 | 144 |
Overall, the smallest impact of varying temporary staff availability assumptions is for the ‘high’ staffing establishments. On average across the four hospital trusts, the scenario with highest cost for standard staffing levels assumed high availability of both bank and agency staff (set at 90% for each). For the ‘high’ staffing establishments, this reduced the percentage of understaffed patient shifts by 14% (from 18.8% to 4.8%) while increasing costs by £7 per patient–day (from £147 to £154). By contrast, this scenario reduced understaffed shifts associated with the ‘flexible (low)’ staffing establishment by 44.6% (from 63.2% to 18.6%) but increased costs by more than £22 per patient–day. However, this reduction in understaffed shifts relies on a somewhat unrealistic assumption about the availability of bank and agency staff. This ‘flexible (low)’ staffing approach combined with high temporary staff availability achieves much the same results at a similar cost to the ‘high’ staffing strategy under the assumptions about bank and agency staff availability based on empirical data.
No internal re-deployment
We explored the contribution of internal re-deployment to understaffing under the different staffing establishment scenarios and assumptions about temporary staff availability from the bank or agency. The contribution is small. Between the experiments with internal re-deployment (our baseline assumption) and those without, the percentage of understaffed patient shifts changed by fewer than 2 percentage points for all hospital trusts and under all scenarios (see Appendix 4, Table 76). There is an increase in cost (of less than £2 per patient-day for all hospital trusts and under all scenarios) when there is no internal re-deployment, as more money is spent on temporary staff instead.
Data sample to determine the establishment
We compared four alternative data samples used to set the ward establishments. Our core models used an establishment set using the first 20 available weekdays in June (or alternatives as specified in Chapter 3, Data sources, if June data were not available). To examine the effect of using this specific sample, we compared the results using a similar sample from data in January, an establishment based on the mean of all available data (‘all’) and a sample from the first two weekdays of each month (‘months’). Although not entirely consistent across all hospital trusts, establishments set using a sample of days in January resulted in establishments that were, on average, higher than using a sample of days in June (Table 25; see also Appendix 4, Table 74). The exception to this pattern, a specialist hospital trust (trust C), may be less subject to seasonal fluctuation. Establishments based on all available data corresponded closely with June establishments. On the other hand, establishments based on a 2-weekday sample per month, while always higher than establishments based on a June sample, were more variable, although in three of the four trusts they were, on average, within 0.6 WTE staff per ward of the estimate based on all data.
Data sample | Hospital trust | |||||||
---|---|---|---|---|---|---|---|---|
A | B | C | D | |||||
June | 35.4 | – | 47.2 | – | 17.8 | – | 38.8 | – |
January | 36.9 | 4.2% | 47.6 | 0.8% | 17.8 | 0.0% | 42.1 | 8.5% |
Months | 36 | 1.7% | 47.5 | 0.6% | 19.4 | 9.0% | 40.5 | 4.4% |
All | 35.4 | 0.0% | 47 | –0.4% | 17.9 | 0.6% | 40.2 | 3.6% |
Range between data samples | 1.5 | 0.6 | 1.6 | 3.3 |
The simulation results show that using different data samples for setting the establishment makes much less difference to the percentage of patient shifts that are understaffed than does using different methods to determine the establishment. Furthermore, in all cases, the pattern of results, and hence the substantive conclusions about the approach to using the SNCT sample to set an establishment, was largely unchanged (see Appendix 4, Table 75). Daily staffing cost differences associated with different samples used to set the establishments follow a similar pattern to the establishment size, although the larger differences are attenuated because larger establishments require fewer temporary staff. For example, in hospital trust D the standard approach to setting the establishment results in an 8.5% higher establishment when using a sample from January than when using a sample from June. However, costs are increased by only 4.7% and there are fewer understaffed shifts. A greater availability of temporary staff would further attenuate these differences.
What if a different criterion for ‘understaffing’ is used?
We compared our baseline understaffing measure (> 15% short) with two alternative criteria for understaffing: absolute understaffing and at least one person (6 hours) short. We also looked at the staff groups involved in understaffing (registered nurse shortfall, nursing support worker shortfall, both and either). The pattern of results, in terms of the relative effect of different approaches to determining the establishment, is unchanged (see Appendix 4, Table 77).
With the ‘standard’ (mean) approach to setting establishments using the SNCT, there is some degree of understaffing on 75% of patient shifts across all hospital trusts on average. This reflects the shapes of distributions of demand at ward level, with left-skewed distributions (where the median tends to be higher than the mean) in one-third of wards, as well as a range of real-world issues, including needing to roster whole people to shifts, variable patient need within SNCT acuity/dependency categories, unpredictable staff sickness and additional specialing requirements. Using the ‘flexible (low)’ (80% of the mean) approach to setting establishments results in 95% of patient shifts with some degree of understaffing on average across the four hospital trusts. Even with the ‘high’ establishment designed to meet need 90% of the time in the baseline period, 58% of shifts have some degree of understaffing because of the issues mentioned above.
Under some hospital trust scenarios, the percentage of understaffed patient shifts using the 15% criterion for understaffing is higher than the percentage of patient shifts with at least one person short, whereas under other hospital trust scenarios the opposite is true. This is because, in relatively small wards, the understaffing criterion will be reached before the ward is one person short, whereas the opposite is true in relatively large wards.
Because the overall criterion for understaffing makes no distinction between staff groups, we also applied the same criterion, of more than 15% below the requirement, to each staff group (registered nurses and nursing support workers) separately. The number of patient shifts that were understaffed by either staff group was substantially higher across all hospital trusts and all approaches to setting the establishment when using a criterion of 15% shortfall by one or other group as the criterion as opposed to an overall 15% shortfall. Crucially, registered nurse staffing of more than 15% below the requirement was also usually more common than low overall staffing. On the other hand, low staffing by both registered nurses and nursing support workers (i.e. both registered nurses and nursing support workers more than 15% below the requirement) was relatively rare under the ‘high’ establishment (< 10% on average) but remained frequent under other approaches (> 15% on average).
What if we use a fixed multiplier rather than distinguishing between acuity/dependency levels when setting establishments?
The average multiplier across all patients in all hospital trusts is 1.57 (1.57 at trust A, 1.58 at trust B, 1.53 at trust C and 1.56 at trust D). If ward establishments are determined by applying this multiplier to the number of patients, rather than using the level-specific multipliers, overall, there is little difference to the level of understaffing on average across wards except in trust C. In trusts A and B on an average ward understaffing is increased by 2 percentage points, whereas it is reduced by 2 percentage points in trust D and by 10 percentage points in trust C (see Appendix 4, Table 78). However, average ward overstaffing increases in all trusts, especially trusts A (by 6 percentage points) and C (by 7 percentage points). There is more variation at the ward level, with ranges between wards typically increased when using the fixed multiplier, reflecting a worse match to staffing requirements in individual wards.
Across all 82 wards, using the fixed multiplier increased the number of wards with understaffing more than 50% of the time to 17 (compared with 14). This group of wards with high levels of understaffing now includes four mixed medical/surgical wards, two surgical wards and 11 medical wards (mostly care of older people). Four wards (compared with two) were understaffed more than one-third of the time and overstaffing was as common as (two wards) or more common than (13 wards) understaffing in a total of 15 wards (compared with four).
Vacancies
Given the problems with high vacancy rates for nursing staff, we also investigated a scenario in which the ward establishments are set at the mean requirement across a baseline period (as for ‘standard’ staffing), but there are vacancies, set at 10%. This scenario leads to achieved ward establishments that are between ‘standard’ staffing and ‘flexible (low)’ staffing (see Appendix 4, Table 79).
Correspondingly, the average percentage of understaffed patient shifts and cost per patient-day also lie between the results for these two scenarios (Figure 6). However, when assuming high availability of temporary staff (90% rather than the empirical availability), for two of the hospital trusts (B and D), the ‘vacancies’ scenario costs the same as the ‘flexible (low)’ staffing scenario but with almost 10 percentage points fewer understaffed patient shifts (Figure 7). See Appendix 4, Table 80 for results for different availabilities of temporary staff.
FIGURE 6.
Costs vs. understaffing for ‘vacancies’ scenario vs. alternative methods for setting ward establishments at different hospital trusts.

FIGURE 7.
Costs vs. understaffing for alternative methods for setting ward establishments at different hospital trusts assuming high temporary staff availability (empirical bank and 90% agency availability).

Specific skills: intravenous-trained registered nurses
Our modelling of scenarios and counts of staff shortages has focused on a simple division of staff. However, wards often need to consider the presence of staff in the team who have specific skills. To illustrate these challenges, we repeated our core model, using the standard approach to setting the establishment using the SNCT, with a requirement that each shift had at least one intravenous (IV)-trained registered nurse (see Appendix 4, Table 81).
As the percentage of registered nurses that are IV-trained increases, the percentage of patient shifts with no IV-trained registered nurse decreases. The relationship is non-linear; there are diminishing returns as the percentage of IV-trained nurses increases. The same percentage of IV-trained registered nurses leads to a range of results across different hospital trusts. For example, having 10% of registered nurses trained in delivering IV drugs leads to between 53% and 75% of patient shifts with no IV-trained nurses. If 60% of registered nurses are IV-trained, the simulation results show that at all trusts, < 10% of patient shifts would not have an IV-trained registered nurse; for the general hospital trusts this was achieved with 40% of registered nurses trained.
Multiway sensitivity analysis
To understand the sensitivity of our models to the assumptions that we made, we tested the impact of seven factors on the percentage of understaffed patient shifts and the cost per patient-day using a 27 factorial design and calculating 95% confidence intervals for their effects. We ran the model across the four hospital trusts and found little variation between them. Consequently, we present average results from across all four trusts (see Appendix 4, Table 82).
Reducing the chances of short-notice absence for nursing support workers (from 4% to 3%) or increasing it for registered nurses (from 3% to 4%) made less than a 1% absolute difference to the rate of understaffed shifts. Changing the re-deployment pool from a limited pool (within the directorate) to the whole hospital reduced the rate of understaffing by < 0.5%. If bank staff are assumed 100% efficient (vs. 90% efficient) understaffing is reduced by 0.8%, and if we assume 100% efficiency of agency staff (vs. 75% efficiency) understaffing is reduced by 1.3%. We had no data about variation of patients’ actual staffing needs within acuity/dependency levels, such as the extent to which needs of patients categorised as level 1a differ from the level 1a multiplier. We made an assumption of modest variation (10% of the mean), but a difference between 0% and 25% had the effect of reducing understaffing by 1% on average.
Only the assumption about rounding, whereby requests for additional staff (permanent, re-deployed or temporary) were made in whole units (6 or 4 hours) and so requests were only triggered if at least 0.5 units of staff were needed, made a substantial difference to understaffing rates. For example, this meant that if there was a measured staffing deficit (≥ 15%) a request for more staff would not be made if less than half a person was needed to meet the shortfall. A request for 1.4 staff would be rounded down to 1 and a request for 1.6 staff would be rounded up to 2. Had we assumed that the required staff would always be rounded up (so 1.1 staff required would be rounded up to 2), there would have been a large reduction in understaffing (14.3%). This factor was also the only one that had any substantial effect on costs (increasing them by about £3.50 per patient-day on average). All two-way interaction effects between pairs of factors were small: at most 0.5% absolute change in percentage patient shifts and at most £0.11 change in cost per patient-day in any hospital trust (see Appendix 4, Table 83).
Chapter summary
-
Our simulation model used data from our observational study to model the consequences and costs of setting the establishment using the SNCT – ‘high’ establishments (set to match the level of demand observed on 90% of occasions) and ‘flexible (low)’ establishments (set to meet 80% of the average observed need) compared with the ‘standard’ approach whereby staffing is set to meet mean demand.
-
In all cases, we assumed that hospital trusts would attempt to make up staffing shortfalls using internal re-deployment of staff between wards, or hire from a bank or agency.
-
Compared with the ‘standard’ (mean average) approach to setting the establishment, the ‘high’ establishments were 10% higher, on average, across the four trusts.
-
The ‘flexible (low)’ staffing approach results in establishments that are approximately 20% lower than standard (mean) based establishments.
-
For our core models we used empirical data obtained from one hospital trust to estimate the availability of temporary staff.
-
Understaffing is common in all simulations.
-
Despite using temporary staff, the ‘flexible (low)’ establishments resulted in 63% of patient shifts falling ≤ 15% below the required staffing level on average across hospital trusts.
-
The ‘standard’ establishments resulted in 31% of patient shifts with understaffing.
-
The ‘high’ staffing establishments resulted in 19% of patient shifts with understaffing.
-
Staffing that was > 15% above the requirement was less common, occurring on 15% of patient shifts when using ‘high’ establishments, 7% of patient shifts when using ‘standard’ establishments and < 2% of patient shifts when using ‘flexible (low)’ establishments.
-
Increased staffing costs associated with the ‘high’ staffing establishments varied by hospital trust, but were, on average, £11 per patient-day higher (8%).
-
Low staffing establishments were associated with daily savings of £15 per patient-day (11%).
-
The differences in both understaffed shifts and costs between establishment scenarios reduce if greater availability of temporary staff is assumed.
-
If it is assumed that bank staff can fulfil 50% of late requests and there is unlimited availability of agency staff, ‘high’ staffing establishments are associated with understaffing on 5.1% of patient shifts and an increased staff cost of £8 per patient–day (5%).
-
Even with high availability of temporary staffing, ‘flexible (low)’ establishments are associated with high rates of understaffing because a threshold based on ‘whole people’ has to be reached to trigger a request for additional staff, actual patient need varies and we assume temporary staffing to be less efficient.
-
Assuming unlimited agency availability, ‘flexible (low)’ establishments are associated with understaffing on 21.4% of patient shifts and a cost saving of only £3 per patient-day (2%).
-
The use of internal re-deployment had a small (but mainly beneficial) effect on rates of understaffed patient shifts (< 2%) and daily costs (< £2 per patient-day).
-
We found that adding a requirement for a nurse with a specific skill (in this case training to administer IV drugs) meant that if 60% of nurses were trained at each hospital trust, > 90% of patient shifts were properly staffed.
-
Multiway sensitivity analysis indicated that results were only marginally affected by most of the assumptions made in the model.
-
Assumptions for short-notice absence rates, whole-hospital versus restricted re-deployment pool, relative efficiency of temporary staff, and variation in need around each acuity/dependency multiplier made small differences to the rates of understaffed patient shifts (all < 1.5%) and negligible differences to costs (at most £0.20 per patient-day) on average across the four hospital trusts.
-
Assumptions about the number of staff to request made quite large differences to the rate of understaffed patient shifts; if all requests were rounded up rather than rounding up/down to the nearest whole number, the rates of understaffing dropped by 14% in the ‘standard’ staffing scenario and the daily costs increased by £3.53, on average, across the four hospital trusts.
Interpretation
The tool’s guidelines suggest employing enough staff to meet demand on an average day. As hospitals have the potential to meet demand for nursing care with temporary staff when demand is higher than the establishment provides, and can re-deploy staff from potentially overstaffed wards to understaffed wards, we set out to compare how often understaffing might occur with different approaches to using the tool to set the establishment. We compared the standard approaches with a higher establishment (enough to meet demand on 90% of days) and with a lower establishment (to meet 80% of mean demand). We developed a computer program that simulates the staff required, staff available and staff reassigned to other wards, in addition to the hiring of extra temporary staff.
Employing enough staff to meet demand on an average day (the standard approach) led to about one-third of patient shifts being substantially understaffed in the simulation because there is limited availability of re-deployable and temporary staff, and they are less efficient than permanent staff. Mismatches also arise when demand is not a round number, as staff work specific shift lengths. Furthermore, the true demand is unknown at the start of the shift owing to different patient needs even within the same acuity/dependency level.
Employing fewer nursing staff than in the standard approach is cheaper, but substantial understaffing occurs on two-thirds of patient shifts because reassigned and temporary staff cannot make up shortfalls. The rate of understaffing remains high even when we assume that temporary staff are nearly always available to make up shortfalls, and in this situation cost savings become minimal.
Employing more nursing staff than in the standard approach is more expensive, but it substantially reduced the rate of understaffed shifts in the simulation. We look at the trade-off between the impacts of understaffing and costs in the next chapter.
Chapter 7 Cost-effectiveness
In this chapter, we seek to model the relative cost-effectiveness associated with different approaches to setting establishments using the SNCT under varying conditions. We investigate the impact of patients’ exposures to lower than average staffing and high (> 1.5 hours per day) temporary staffing on costs and mortality. We consider both staff costs and costs accounting for changes in lengths of stay due to staffing levels.
Effects of low staffing
Average results of the costs across the three hospital trusts for the consequences and cost-effectiveness of different approaches to using the SNCT to set establishments under varying assumptions about temporary staffing are summarised in Table 26. For more detailed reports with ranges between the three hospital trusts, see Report Supplementary Material 5, Table 101.
Parameter | Staff cost (%) | Bed-days (%) | Deaths (%) | NNT (NNHa) | Staff cost/life (£) | Net cost/life (£) | ||||||
---|---|---|---|---|---|---|---|---|---|---|---|---|
Establishment approach | High | Flexible (low) | High | Flexible (low) | High | Flexible (low) | High | Flexible (low) | High | Flexible (low) | High | Flexible (low) |
Availability of temporary staff and re-deployment | ||||||||||||
No temporary staff (NR) | 7.8 | –19.9 | –1.4 | 2.4 | –4.2 | 13.5 | 762 | (219) | 32,129 | –23,874 | 16,323 | –15,981 |
No temporary staff (WR) | 7.8 | –19.9 | –1.4 | 2.4 | –4.5 | 13.4 | 663 | (222) | 27,663 | –23,970 | 14,608 | –16,067 |
Empirical availability of temporary staff (NR) | 5.7 | –11.0 | –1.2 | 1.7 | –4.5 | 8.7 | 660 | (357) | 21,324 | –23,121 | 9586 | –14,214 |
Empirical availability of temporary staff (WR) | 5.5 | –10.8 | –1.2 | 1.7 | –4.5 | 8.3 | 665 | (361) | 20,423 | –22,910 | 9506 | –13,967 |
50% bank, 50% agency staff availability (NR) | 4.2 | –5.7 | –1.0 | 0.8 | –3.8 | 4.7 | 828 | (690) | 18,687 | –22,759 | 8390 | –14,621 |
50% bank, 50% agency staff availability (WR) | 4.0 | –5.5 | –1.0 | 0.8 | –3.8 | 4.4 | 873 | (719) | 18,278 | –22,956 | 8113 | –14,381 |
50% bank, 90% agency staff availability (NR) | 3.5 | –2.8 | –1.0 | 0.4 | –3.4 | 2.6 | 1058 | (1357) | 17,845 | –17,976 | 7436 | –10,409 |
50% bank, 90% agency staff availability (WR) | 3.2 | –2.4 | –0.9 | 0.4 | –3.1 | 2.4 | 1140 | (1666) | 17,454 | –16,803 | 6146 | –8301 |
50% bank, 100% agency staff availability (NR) | 3.2 | –2.2 | –0.9 | 0.3 | –3.2 | 2.3 | 1106 | (2372) | 17,100 | –12,989 | 6136 | –5646 |
50% bank, 100% agency staff availability (WR) | 2.9 | –1.6 | –0.9 | 0.3 | –3.0 | 1.9 | 1272 | (2828) | 16,464 | –10,347 | 5524 | –946 |
Using our baseline assumptions about temporary staffing availability – that is, based on the empirically observed availability – staff costs are increased by 5.5% when using ‘high’ staffing establishments and reduced by 10.8% when using the ‘flexible (low)’ establishments.
‘High’ establishments are associated with a 1.2% reduction in bed-days used, and a relative reduction of 4.5% in the risk of death, equating to one life ‘saved’ for every 665 patients admitted to a hospital (number needed to treat) using this establishment. The additional staff cost per life saved is £20,423, with a net cost (after taking account of the value of bed-days saved) of £9506 per life saved.
By contrast, ‘flexible (low)’ establishments are associated with an 8.3% increase in the risk of death, equating to one additional death for every 361 patients admitted (number needed to harm) with a negative incremental cost-effectiveness ratio. This negative ratio can be interpreted as the cost per life saved associated with the ‘standard’ (SNCT mean-based) establishment when compared with the ‘flexible (low)’ establishment. Staff cost per life saved is £22,910, with a net cost per life saved of £13,967. In very simple terms, the cost-effectiveness of ‘high’ establishments relative to ‘standard’ is similar, indeed marginally better, than that of the ‘standard’ compared with ‘flexible (low)’ establishments.
If no temporary staff were used and there were no re-deployments, the differences between the scenarios based on the three approaches to setting establishments are maximised because differences simply reflect the establishments themselves. Compared with the ‘standard’ establishment, staff costs would be increased by 7.8% for ‘high’ establishments and reduced by 19.9% for the ‘flexible (low)’ establishments.
Under these assumptions, ‘high’ establishments were associated with a 1.4% reduction in bed-days used and a 4.2% reduction in deaths, which equates to one life ‘saved’ for every 762 patients admitted (NNT 762). Relative to the ‘standard’ establishment scenario, the staff cost per life saved is £32,129 with a net cost per life saved of £16,323. By contrast, ‘flexible (low)’ staffing establishments were associated with a 19.9% reduction in staff costs, a 2.4% increase in bed-days used and a 13.5% increase in deaths – one additional death for every 219 admissions (NNH). The negative cost-effectiveness values reflecting the relative value of moving from the ‘flexible (low)’ establishment to ‘standard’ are £23,874 per life saved (staff costs only) or £15,981 net.
The assumption of no flexible staffing whatsoever is, of course, completely unrealistic. However, it does serve as a useful base against which to compare the effect of varying the assumptions about temporary staff availability and internal hospital re-deployments because, as we saw earlier, it is clear that conclusions may be highly sensitive to these assumptions, particularly in relation to the availability of temporary staff. As assumptions about the availability of temporary staff become more ‘generous’, there is a general trend for differences in costs and consequences between the different establishments to reduce.
Under the ‘flexible (low)’ establishment scenario, both associated harms and cost savings are substantially decreased for any assumptions about temporary staffing when compared with the zero availability assumptions. Whereas staff costs are reduced by 19.9% relative to the ‘standard’ establishment when there are no temporary staff – reflecting the simple reduction in the establishments – when we assume empirical availability of temporary staff, cost savings relative to the ‘standard’ establishment are decreased to 10.8% (allowing for internal re-deployment). There is also a lesser impact on the death rate (a rise of 8.3% relative to the ‘standard’ establishment, vs. 13.5%) with the number needed to harm increased to 361 versus 219.
The relative cost-effectiveness of the ‘standard’ establishment compared with the ‘flexible (low)’ establishment is largely unchanged in the face of limited availability of temporary staff (empirical or 50% availability for both bank and agency). However, with high availability of temporary staff, the cost per life saved associated with the ‘standard’ [vs. ‘flexible (low)’] establishment is considerably lower, reducing to £10,347 per life saved if 100% availability of agency staff (with internal re-deployment) is assumed (£946 net of length of stay savings).
For the ‘high’ establishments, there is a clear trend for both lower relative costs and lower relative benefits as higher availability of temporary staff is assumed, mirroring changes for the ‘flexible (low)’ establishment scenario. However, the effect of increasing availability of temporary staffing is less marked because the original high establishment meets needs more often. Under the scenario with the most opportunity for hiring additional staff above the employed establishment (50% availability of bank, 100% availability of agency with internal re-deployment), staff costs of the ‘high’ staffing establishment relative to the ‘standard’ establishment are increased by 2.9% and the staff cost per life saved is estimated to be £16,464 (£5524 net of length of stay savings).
Allowing re-deployment of permanent staff between wards (before requesting temporary staff) consistently leads to lower estimates of cost per life saved associated with ‘high’ establishments. However, the differences are relatively small (< £1000) for all assumptions except no availability of temporary staff.
Temporary staffing effects
In our core models reported above, we assumed that deployed temporary staff were as effective as deployed permanent staff. However, there is some evidence that such staff may be less effective, and some studies have reported that higher levels of temporary staff are associated with increased mortality risk. In our recent study from which the coefficients for the effect of low staffing in the core economic models were derived, we observed that there was evidence of increased mortality when patients experienced very high levels of temporary staffing from either registered nurses or health-care support workers (1.5 HPPD or more). In determining the likely effect of different staffing policies, especially the ‘flexible (low)’ staffing policy, this is important to consider, as temporary staff will be deployed more often and in greater numbers. Therefore, we added the effect of high levels of temporary staffing to revise the mortality estimate used in the models reported above.
The pattern of results is largely unchanged but the relative advantages of higher establishments is much greater when greater availability of temporary staff is assumed (Table 27). For example, with 50% availability of temporary staff the ‘high’ staffing establishment is associated with a 4.4% reduction in deaths, compared with 4.0% when modelling low staffing effects only. With higher availability of temporary staff (50% bank and 90% agency), the ‘high’ staffing establishment is associated with a 4.1% reduction in deaths, compared with 3.2% when modelling low staffing effects only.
Parameter | Deaths (%) | NNT (NNHa) | Staff cost/life (£) | Net cost/life (£) | ||||
---|---|---|---|---|---|---|---|---|
Establishment approach | High | Flexible (low) | High | Flexible (low) | High | Flexible (low) | High | Flexible (low) |
Availability of temporary staff (all with re-deployment) | ||||||||
No temporary staff | –4.7 | 14.6 | 632 | (204) | 26,423 | –22,053 | 13,803 | –14,782 |
Empirical availability of temporary staff | –4.7 | 9.9 | 627 | (304) | 19,390 | –19,173 | 8876 | –11,689 |
50% bank, 50% agency staff availability | –4.4 | 8.1 | 703 | (381) | 15,269 | –12,549 | 6369 | –8013 |
50% bank, 90% agency staff availability | –4.1 | 8.0 | 744 | (401) | 12,572 | –6366 | 3978 | –3932 |
50% bank, 100% agency staff availability | –4.1 | 8.1 | 766 | (405) | 11,305 | –4783 | 3292 | –3072 |
The relative harm of ‘flexible (low)’ establishment scenarios is increased to a much greater extent when considering adverse effects associated with high use of temporary staff. For 50% availability of temporary staff the increase in mortality associated with the ‘flexible (low)’ establishment is estimated as 8.1%, compared with 4.4% when modelling low staffing effects only.
Consequently, the estimates of cost-effectiveness become more favourable for higher establishments, especially when availability of temporary staff is high. For example, if 50% bank, 90% agency availability (with internal re-deployment) is assumed, the staff cost per life saved is £12,572 (net cost £3978) for the ‘high’ establishment scenario, compared with £17,454 (net cost £6146) when considering low staffing effects alone.
Costs and consequences of changing establishments at hospital trust level
To help understand what these results mean at a hospital trust level, we explored them in the context of each trust’s annual turnover. Trust A, which has an annual turnover of about £250M, would spend £1.7M (< 1% of turnover) more on nursing with ‘high’ establishments than with ‘standard’ establishments. We also predict that ‘high’ establishments would be associated with 67 fewer deaths per year than ‘standard’ establishments.
Hospital trust B, which has an annual turnover of > £500M, would spend £1.9M (< 0.5% of turnover) more on nursing with ‘high’ establishments than with ‘standard’ establishments. Here we predict that ‘high’ establishments would be associated with 126 fewer deaths per year than ‘standard’ establishments. In trust B, we found that in the 50% bank and 100% agency availability scenario, ‘flexible (low)’ establishments cost more (+£73,000) than ‘standard’ establishments, with 261 extra deaths predicted.
Finally, hospital trust D has an annual turnover of > £800M, and would spend < £1M (0.1% of turnover) more on ‘high’ than ‘standard’ establishments, with a corresponding 54 fewer deaths.
Sensitivity analyses
We focus reporting sensitivity analysis on the cost-effectiveness estimates derived from the low staffing (only) models, although estimates based on low staffing and high temporary staffing effects would in all cases show similar sensitivities.
Mortality estimates
Unsurprisingly, estimates of cost-effectiveness are sensitive to the precision of the effect estimates for mortality (see Appendix 5, Table 84). If the high estimate effect is used (based on the upper bound of the 95% confidence interval for effectiveness), the cost-effectiveness estimates for higher establishments are substantially more favourable. With empirical availability of temporary staff (with internal re-deployment), the staff cost per life saved for the ‘high’ establishment is £12,328 compared with the ‘standard’ establishment. Assuming 50% bank and 90% agency availability (with re-deployment), the estimated staff cost per life saved is reduced to £10,567.
If the lower confidence interval for the effect of low staffing on mortality is used, the cost per life saved for the ‘high’ establishment scenarios compared with ‘standard’ establishments exceeds £50,000 per life saved under all assumptions about temporary staffing availability. Under the empirical availability of temporary staffing, the staff cost is £62,257 per life saved. However, the net cost per life saved (accounting for the value of reduced hospital stays), if any availability of temporary staff is assumed, is below £30,000. Assuming 50% bank and 90% agency availability (with re-deployment), the net cost per life saved is £18,266.
Cost of additional bed-days
Increases in the estimated cost of additional days of stay lead to reduced estimates of the (net) cost per life saved. A 25% increase reduces the estimated net cost per life saved associated with the high establishment and empirical availability of bank and agency staff from £9175 to £6381. The relative decrease is greater with more availability of temporary staff, with the estimate reducing from £6146 to £3320 under the assumption of 50% availability of bank and 90% availability of agency (with internal re-deployment).
Cost of agency staff
If the costs of agency staff are increased by 25%, the relative increase in staff costs associated with higher establishments are reduced, albeit not substantially. For empirical availability of temporary staff, staff costs for the ‘high’ establishment are 5.3% higher than the ‘standard’ establishment (vs. 5.5% with base assumptions about agency staff costs). The net cost per life saved is reduced to £8636 (vs. £9506).
The effects of changing this cost assumption are greater with higher temporary staff availability. If 50% availability of bank and 100% availability of agency staff are assumed, the ‘high’ establishment costs 2.4% more than the ‘standard’ (vs. 2.9% using our base assumptions on costs of agency staff). The net cost per life saved in this model is £1212 (vs. £5524). Under this assumption, the ‘flexible (low)’ establishment is associated with a staff cost increase of 0.2% compared with the ‘standard’ staffing establishment (vs. a decrease of 1.6%).
Cost of bank staff
We assumed that bank staff would be cheaper than permanent staff as 50% might choose not to make pension contributions. This assumption does not substantively change conclusions, although it slightly reduces the cost-effectiveness estimates associated with higher establishments. For example, removing this assumption (i.e. assuming that bank staff cost the same as permanent staff) changes the net cost per life saved with the empirical estimate of temporary staff from £9506 to £9273. If 50% availability of bank staff is assumed (with 50% agency), the cost per life saved is estimated to be £7389 (vs. £8313 under base cost assumptions).
Use of bank or agency staff
To determine the effect of using agency staff rather than bank staff when modelling flexible staffing policies, we estimated the costs of ‘all bank staff’ scenarios. We did this by reducing the estimated cost of agency staff by 10% to approximate the cost of bank staff. This made little difference to substantive conclusions, although the effects were greater when assuming higher availability of agency staff. Under empirical availability of temporary staff, the staff cost increase of the ‘high’ establishment over the ‘standard’ establishment barely changed (cost increase changed from 5.5% to 5.6%) between using both bank and agency staff and using bank staff only, with little consequent impact on cost per life saved. Net cost per life saved is increased marginally to £9854 when using bank staff only (vs. £9506 under base assumption). The differences are greater with higher availability of temporary staff. In the 100% availability scenario, the net cost per life saved in the ‘high’ staffing establishment is increased to £7248 when using bank staff only (from £5524).
Cost of nursing support workers
Finally, as there is a move toward employing more and more highly trained assistant staff who might command higher salaries (e.g. nursing associates), we modelled the effect of a 10% increase in the pay of nursing support worker staff only. Again, the effect of this variation was small and had little impact on substantive conclusions, although the cost-effectiveness was slightly less favourable for higher establishments because they are associated with higher overall achieved staffing levels. For example, the estimated net cost per life saved for ‘high’ establishments increased from £9854 to £10,046 assuming empirical availability of temporary staff and from £5524 to £5769 assuming 50% availability of bank and 100% availability of agency staff.
Chapter summary
-
We modelled the costs, effects and cost-effectiveness (in terms of cost per life saved) for the three general hospital trusts, considering both gross (staff only) and net (including any savings associated with reduced length of stay) costs.
-
We used coefficients from a recent longitudinal observational study and considered the effects of low (below the mean) staffing, but also, as a secondary analysis, the potential adverse effects associated with high temporary staffing.
-
Our core models assumed a limited availability of temporary staff (based on empirical data from one hospital trust), and internal re-deployment of staff within directorates.
-
‘High’ establishments are associated with a reduction of 1.2% in bed-days used, and a relative reduction of 4.5% in the risk of death compared with ‘standard’ establishments, which equates to one life ‘saved’ for every 665 patients admitted to a hospital (number needed to treat).
-
The additional staff cost per life saved is £20,423, with a net cost (after taking account of the value of bed-days saved) of £9506 per life saved.
-
If the potential adverse effects of temporary staffing are included, the number needed to treat is lower (n = 627), as is the cost per life saved (£19,390 staff costs and £8876 net costs).
-
‘Flexible (low)’ establishments are associated with an 8.3% increase in the risk of death, equating to one additional death for every 361 patients admitted (number needed to harm).
-
The ‘standard’ (SNCT mean-based) establishment when compared with the ‘flexible (low)’ establishment gives a cost per life saved of £22,910 (net cost £13,967).
-
As availability of temporary staffing increases, the cost per life saved of higher relative to lower staffing establishments reduces.
-
As availability of temporary staffing increases, the impact of including an estimate of negative effects associated with temporary staffing also increases.
-
With highest availability of temporary staff and including negative effects from temporary staffing, the staff cost per life saved for ‘high’ establishments compared with ‘standard’ establishments is low, at £11,305 (£3292 net).
-
Sensitivity analysis shows that substantive conclusions are most sensitive to the estimates of the effect of low staffing on mortality.
-
If higher effects on mortality are assumed, the cost-effectiveness estimates for higher staff establishments become much more favourable, especially when there is low availability of temporary staff.
-
If lower effects of mortality are assumed, the cost-effectiveness for higher establishments becomes less favourable, although under all scenarios where temporary staff are available, net cost-effectiveness of ‘high’ establishments compared with ‘standard’ establishments is < £30,000 per life saved.
Interpretation
In this chapter, we assessed the cost-effectiveness of different approaches to setting ward nurse staffing establishments. We compared the standard approach (employing enough staff to meet demand on an average day) with lower and higher staffing levels. Previous studies have shown that understaffing and greater use of temporary staff increase the risk of in-hospital death and that lower staffing is associated with longer hospital stays. We used estimates for the sizes of these effects from a recent study undertaken in one of the participating hospital trusts. We considered both staffing costs and the costs of lengths of stay associated with changes in staffing levels. We calculated the trade-off between these costs and the lives saved.
Although more expensive, we found that employing more staff at the level needed to meet the demand observed on 90% of days could provide value for money compared with the standard approach. Under almost all assumptions, the additional cost of this higher establishment was < £30,000 per life saved, and under most assumptions the cost was much lower than this. Including the value of reductions in length of stay (bed-days saved) further improved the estimated cost-effectiveness, as did including the potential adverse effects of temporary staff on mortality rates and, importantly, increasing the availability of temporary staff. Under the circumstances where flexible staffing policies might seem most viable – where there is unlimited availability of temporary staff to meet any shortfall – we found that the higher establishment was still associated with fewer deaths, at an estimated cost per life saved (after taking into account savings from reduced length of stay) of £3292, than the standard establishment. At all hospital trusts, the investment in employing more staff would cost < 1% of their annual turnover.
On the other hand, using a smaller establishment and using more temporary staff to meet shortfalls would be associated with a substantial increase in patient deaths. Even assuming unlimited availability of temporary staff, and thus minimising staffing shortfalls under this scenario, we estimated that there would be up to one additional patient death for every 405 admissions, after considering the adverse effect of temporary staffing on patient outcomes. Where more realistic assumptions about temporary staffing were made, the increase in death rate was much higher. In all cases, the standard establishment appeared to represent a preferable, cost-effective alternative to this low ‘flexible’ establishment.
Chapter 8 Discussion and conclusion
Summary of main results
In this observational study, we successfully gathered data about daily nurse staffing requirements using the SNCT and the staffing levels deployed from 81 wards across four NHS hospital trusts on a total of 25,362 ward-days. We collected data on staff perceptions of staffing adequacy on more than 23,000 ward-days.
Actual and required staffing levels varied considerably between hospital trusts, between wards within trusts and within wards. We found that on many wards the variation in required staffing level was skewed, such that mean demand, used as the basis for setting establishments using the SNCT, was below the median. This means that when a ward is staffed to meet mean demand, staffing is below the measured requirement more than 50% of the time. We estimated that, on average, 26% of ward-days were substantially understaffed (more than 15% under the requirement). In morning reports, shift leaders reported that they had enough staff to deliver quality care 78% of the time. In all of the hospital trusts we studied, there was a high level of temporary staffing (over 20%), although the majority of temporary staffing was by trust-employed staff through the local bank.
When the SNCT is used to estimate the required ward establishment, the currently recommended minimum sample of 20 weekdays gives a reasonable precision in percentage terms overall but, on average, the 95% confidence interval of the estimate is more than one WTE staff member either side of the mean. Only two wards achieved precision of less than a whole staff member (±0.5 staff member) with the recommended minimum sample, and for many this could not be achieved with the available sample (up to 1 year of data). On average, a precision of ±1 staff member was achieved with a sample of 40 days. Overall, day of week and time of day used to sample staffing requirements made little difference to the estimated establishments, although on some wards the effect was substantial.
Using daily measurement of the SNCT allowed us to estimate the staffing required for each day on each ward and to assess whether or not staffing shortfalls were associated with professional judgements of staffing adequacy. On days when staffing fell below the estimated requirement, odds of staff reporting that there were not enough staff to deliver quality care were reduced and there were increased reports of missed staff breaks and care. The relationships we observed were, essentially, linear, with no obvious threshold as staffing rose above the estimated requirement and no important interaction between registered nurse and support worker shortfall. There was evidence that having a high proportion of single rooms and being a surgical ward were associated with decreased perceptions of staffing adequacy. High patient turnover was also associated with decreased perceptions of staffing adequacy, although the relationships were not statistically significant.
When we simulated different approaches to using the SNCT to set establishments, we found that ‘high’ establishments – a level set to meet estimated demand on 90% of occasions – led to a much lower rate of shifts on which patients would be exposed to substantial understaffing. Conversely, a lower ‘flexible’ establishment – whereby temporary staff are relied on to respond to most variation in demand – results in a very high rate of understaffing. In large part this is due to the limited availability of temporary staff but it is due also to the unmeasured variation in patient need, the lower efficiency of temporary staff and the need to request additional staff in ‘quanta’ representing a reasonable working shift. Although the number of understaffed shifts associated with a ‘flexible (low)’ staffing establishment is greatly reduced if higher availability of temporary staff is assumed, it is always at a level substantially higher than that achieved using the standard approach to setting the establishment, which is, in turn, associated with more understaffing than the ‘high’ establishments, irrespective of the availability of temporary staff.
We modelled cost-effectiveness under a range of different assumptions about temporary staff availability and considering a number of different factors. ‘Flexible (low)’ establishments are associated with lower costs but a substantial increase in the risk of death. As greater availability of temporary staff is assumed, the increased risks are reduced but the cost savings are greatly diminished and the ‘standard’ establishment delivers improved outcomes at low cost per life saved. ‘High’ establishments, set to meet need most of the time, are associated with substantial decreases in bed utilisation (1.2%) and mortality risk (4.5%; number needed to treat, n = 665), with a staff cost per life saved of £20,423 and a net cost of £9506 (accounting for the cost of bed-days saved). Higher temporary staff availability and adding estimates for the effect of temporary staff on mortality make the cost-effectiveness estimates for ‘high’ establishments considerably more favourable.
Staffing requirements estimated by the Safer Nursing Care Tool
In common with other studies, we observed that there was greater variation between wards within hospital trusts than between hospital trusts. 133 Much of the variation between hospital trusts arose from the difference between the general hospital trusts and the specialist hospital trust. When including this specialist trust, the magnitude of variation within trusts was even greater. Most studies on nurse staffing have focused on hospital-level averages, which may not reflect important sources of variation. This has been observed previously in Belgian hospitals133 and is likely to be the case elsewhere, and it may explain why some cross-sectional studies of nurse staffing at the hospital level have not found the expected associations with patient outcomes. Much of the difference between wards is, however, entirely warranted by variation in patient need. Across all wards in the study, the estimated nursing establishment required ranged from 5.9 to 10.2 (in HPPD equivalents).
Both deployed staffing and estimated staffing requirements varied considerably between days on the same ward. Even in the hospital trust with the lowest variability, the daily staffing requirement had a mean within-ward standard deviation that was 6% of the trust mean.
In part because of this variability, when using the SNCT to estimate mean demand, larger samples than currently recommended would be required to obtain estimates of the required staff to employ within 1 WTE. This level of precision may be unachievable, but, on average, a precision of ±1 staff member can be achieved with a sample of 40 days.
-
There is a limit to the degree of precision that can be achieved in practice.
-
Wards with more variable staffing requirements need larger samples to estimate establishments.
-
Although decisions still need to be made about base staffing levels, and the SNCT and NICE guidance recommend regular re-reassessment of the base establishment,11,19 this variability highlights the potential advantage of daily/shift-level measurement of nurse staffing requirements to ensure that deployed staffing matches variable need. For most wards, the time of day selected for the ratings used to estimate the establishment made little difference.
-
For establishment-setting, the time of day selected to determine staffing requirements may make little difference on most wards.
-
The specific current recommendation to undertake SNCT assessments at 3 p.m. is unwarranted.
-
However, on some wards the timing of the assessment makes a large difference, and so the time chosen needs to be considered carefully.
-
Similarly, variation in demand by day of the week makes little overall difference to the estimated establishments, but the samples chosen need to be mindful of patterns that might occur on particular wards.
However, intracluster correlation coefficients showed only moderate/good agreement between morning and evening measurements, and high variation in differences was observed on different wards. There were sometimes considerable differences in staffing required by time of day, irrespective of professionally determined within-day variation in staffing patterns. Events that cause a ward’s estimated staffing requirement to change are transfers, admissions, discharges and the worsening or improvement of a patient’s acuity/dependency rating. Near real-time data about the acuity/dependency of current patients could be beneficial, although the resources involved in additional data collection and input need to be measured against the potential benefits. Another finding of this study is that increased establishments to meet anticipated fluctuation in patient need may be cost-effective. Such strategies require fewer data than flexible strategies, which we found to be associated with increased harm and, often, little cost saving.
The standard SNCT takes no direct account of ‘specialing’, except in so far as this requirement is reflected in the staffing levels on the wards used to derive the multipliers. The inclusion of ‘specialing’ substantially increased the variability of requirements between days. The SNCT multipliers were developed from observations that may have included some specialing, although we have no way of knowing what proportion of observed patients were specials. Thus, the multipliers plan for the average level of observed specials at the time of the tool’s development, which is likely to be lower than it is today, as some patient groups requiring specialing, such as patients at risk of falls or those with severe mental health difficulties, are growing. 104
Understanding specialing requirements on wards is critical when planning nurse staffing levels
An approach to setting the establishment that assumed a symmetrical distribution of demand could be said to balance the risks of overstaffing with those of understaffing. Others have noted that variation in demand for nurse staffing on wards is not symmetrical around the mean. 72 We observed that the staffing requirement on many wards had a left-skewed distribution, meaning that mean staffing demand is likely to be exceeded more than 50% of the time. Thus, an establishment based on mean demand risks understaffing even before real-world challenges of matching staffing supply to demand, such as short-notice sickness absence and limited availability of temporary staff, are encountered. Furthermore, the risks associated with understaffing are potentially considerable, including the known risks of increased mortality2 and the increases in costs associated with adverse events and extended hospital stay. 134 On the other hand, ‘overstaffing’ may be associated with considerable ‘salvage value’ (to borrow a term associated with some operational research models). 72 Salvage value reflects the fact that staff who might appear to be excess to requirements still have value. This is in part because they could be deployed elsewhere, but, as we discuss below, ‘excess’ staff may also directly add value on their home ward, even when they are apparently surplus to requirements. This is because the measure used to determine the requirement does not identify an ‘optimum’ staffing level at which adding more staff does not improve care outcomes or there are substantial diminishing returns from adding more staff.
-
Planning establishments to meet average demand makes understaffing more likely than overstaffing.
-
The risks associated with understaffing are higher than those associated with overstaffing.
-
The ‘salvage value’ of apparently excess staff is considerable.
How does actual staffing compare with the estimated requirement?
We estimated that, on average, 26% of ward-days were substantially understaffed, that is, more than 15% below the calculated requirement. We found that, on average, the three general hospital trusts had relatively small apparent shortages of nursing staff (≤ 0.5 HPPD). It is possible that we have not reliably identified all non-clinical shifts (e.g. management or education shifts) to remove them from the deployed staffing, meaning that the staff shortages may be larger than they appear. Furthermore, including specialing requirements more than doubles the percentage difference between the estimated requirement and the actual staffing at hospital trusts A and D. This again highlights that specialing is a large extra demand that hospital trusts appear to be struggling to resource, although some trusts rely on the support of volunteers and family members to help with this, which is not shown in our data. 104 Overall, this picture is consistent with trusts attempting to staff to the SNCT requirements and experiencing staffing shortfalls due to sickness, vacancies and limited availability of temporary staff.
By contrast, hospital trust C had a large apparent surplus of staff, which may result from a perception that SNCT staffing levels were not suitable in the specialist wards, the large proportion of single rooms and the problem of staffing smaller wards where the required registered nurse/support worker staffing may be less than a whole person. Modelling studies have suggested that smaller units require larger (relative) workforces. 135 We found that wards with a higher proportion of single rooms were more likely to report missed care, consistent with these wards having a higher staffing requirement that is not reflected in patient prototypes, for example because of increased walking distances and the challenges of undertaking routine visual checks on patients’ conditions. 84,85 Certain ward types, including those with more single rooms, have also been shown to have higher levels of indirect care, including handover times, which is consistent with single rooms reducing the opportunity for staff-to-staff communication. 85
-
The SNCT multipliers may not properly reflect the staffing requirements of some units that are influenced by factors other than patient acuity/dependency.
-
Smaller units and those with more single rooms may require more care HPPD and higher ‘multipliers’ to provide adequate staffing to reliably meet patient need.
Staffing ‘shortfall’ and nurses’ perceptions of staffing adequacy
The differences between actual staffing and estimated requirements, the ‘shortfalls’, are significantly associated with nurses’ perceptions of staffing adequacy. For example, according to our multivariable models, if the registered nurse staffing level was 1 hour per patient-day below the required level estimated using the SNCT, the odds of the shift leader reporting that there were enough staff for quality were reduced by 11%, the odds of reporting nursing care left undone were increased by 14% and the odds of reporting staff missing breaks were increased by 12%. The results were similar for nursing support workers.
-
These results provide the first evidence, to our knowledge, that the SNCT assessments are related to nurses’ professional judgements of staffing requirements.
However, our models suggested that there are additional workloads not included in the SNCT multipliers, although they are, to some extent, recognised by the tool documentation as matters requiring professional judgement. 19 The effect of single rooms and, potentially, of other aspects of ward layout was noted above. In addition, nurses in charge of surgical wards were significantly more likely than those on medical/mixed wards to report nursing care left undone and missed breaks, and less likely to report enough staff for quality. This may reflect that nursing staff on surgical wards carry out different tasks from those on medical wards, such as changing dressings and taking out sutures from healed wounds, but it could also reflect different assumptions about what constitutes quality. Turnover per day was not significant in the models, although it is thought to have an effect on workload,6,8,9 and the non-significant effects we observed were consistent with higher turnover leading to increased workload.
After controlling for other variables, there was evidence that the day of the week affected perceptions of staffing adequacy. After controlling for the staffing shortfall, nurses were more likely to report that there were enough staff for quality and less likely to report missed care and missed breaks on Saturday than on Monday. Our determination of the required staffing assumed that a patient in any given acuity/dependency category required the same amount of care irrespective of the day of the week. However, rosters are often planned on the assumption that demand is lower at weekends, in part because some work that is required on weekdays is not required at weekends,6 including interactions with other departments that mainly function during the week, such as surgery and radiology. Many wards have lower staffing at weekends because of this. 6 However, although this evidence seems to lend support to the existing practice of tailoring staffing to such presumed variations in demand, we did not find a consistent pattern across days of the week, and Sundays were no more likely than Mondays to be seen as adequately staffed at any given level.
-
Simple assumptions that wards require less staffing at weekends may be increasingly outdated in the face of shorter hospital stays, increased acuity and an increasing push toward 7-day working in the NHS.
We also modelled associations between deviations of actual staffing from estimated requirements and reported staffing adequacy at the shift level. Nurses were less likely to report that there were enough staff for quality on morning and afternoon shifts than at night. Similarly, they were more likely to report leaving care undone and missing breaks, especially on the morning shift. When modelling shifts, we did adjust the required staffing to reflect each ward’s typical (mean average) distribution of staff over the day. This finding is consistent with differences in workload between morning, afternoon and night shifts that are greater than planned for or, alternatively, with less variable demand at night. Overall lower staffing levels at night may also necessitate relative overstaffing as the ability to match demand exactly is limited by shift lengths and the quantum of staff that can be requested.
-
The allocation of sufficient staff hours to night shifts may result in a relative shortage of staff during the day.
We explored these data for non-linear effects and interactions between the staff groups. If, for example, greater numbers of support workers enable registered nurses to work more effectively, we might see that the effect of a registered nursing shortfall was reduced when there were greater numbers of support workers. This complementarity is a key part of the rationale for adding support workers to the workforce, but, in common with a number of other studies,6,108,136,137 we see no substantial evidence of it occurring. In our models focusing on shift-level patterns, there was a small significant interaction between support worker shortfall and registered nurse shortfall, but when we plotted this it did not appear to have any substantial impact on the relationship with ‘enough staff for quality’. Similarly, although we saw some evidence of non-linear effects, these appeared to make little material difference. In particular, we did not observe that the chances of reporting that staffing was sufficient reduced only as shortfalls increased. Indeed, if anything, adding more staff above the required level estimated by the SNCT appeared to increase the chances of reporting enough staff for quality further. Results were similar for other measures of staffing adequacy and we saw no evidence of a threshold effect indicating reduced benefits from further staff increases.
For registered nurse staffing levels, this absence of a threshold has been observed previously. 9 The finding is consistent with a study showing that increases in staffing above the ‘optimal’ level using the RAFAELA tool were associated with further reductions in mortality. 80,81 Whereas SNCT multipliers are derived from wards that are assessed as meeting a quality threshold, many judgements of ‘quality’ are subjective and may be anchored to current expectations. 138 Equally, perceptions of ‘enough staff’ and ‘quality’ may be influenced by expectations, including historical staffing patterns, and so may not represent absolute judgements. We found that nurses reported enough staff for quality less often than they reported no missed care, which is consistent with a high quality threshold operating. However, the rates of missed care reported using our single-item measure were much lower than typically reported in studies that base overall missed care rates on responses across multiple items. 5 Therefore, this low rate of reporting may be an artefact based on the lesser ‘prompt’ for items to consider or it could arise from an expectation that certain items of care are no longer possible.
Although professional judgement may, in some respects, represent the ‘gold standard’ in judging staffing requirements, with no evidence that any tool provides a more accurate measure, both judgement and the tool are potentially subject to bias. 17 The SNCT acuity and dependency categories may provide a valid measure of varying demand without necessarily indicating the optimal level of staffing. The documentation of the tool as a whole recommends a ‘triangulated’ approach including professional judgements and outcomes. All are potentially subject to measurement error and subjectivity, but these findings do serve to emphasise the importance of not relying simply on a single measure.
-
Although staffing requirements measured by the SNCT may correspond with professional judgement, there is no basis on which to judge that they are ‘optimal’.
-
Benefits may continue to accrue at staffing levels above those recommended.
-
Although professional judgement may be subject to anchoring bias, it remains an important part of any system for judging required staffing.
Setting establishments using the Safer Nursing Care Tool
We simulated three alternative staffing strategies for planning establishments using the SNCT: ‘high’ staffing corresponding to the 90th percentile of demand, ‘standard’ staffing corresponding to average demand and ‘flexible (low)’ staffing corresponding to 80% of average demand. Under our baseline assumption, with limited availability of temporary staff, understaffing (more than 15% below the measured requirement) was relatively common regardless of the approach. Understaffing occurred much less often with ‘high’ establishments (19%) than with ‘standard’ establishments (31%) and ‘flexible (low)’ establishments (63%).
Given the variable nature of demand within wards, there are times when wards are overstaffed. This happens much less than understaffing. Overstaffing by more than 15% was relatively rare in most scenarios. Even with ‘high’ establishments, it occurred less often than understaffing in half of the hospital trusts, with 15% overstaffing compared with 19% understaffing on average across the four trusts. With ‘flexible (low)’ establishments, overstaffing was virtually eliminated.
As we noted above, the potential ‘salvage’ value associated with excess staff is potentially high, so any apparent ‘overstaffing’ should not be assumed to be inefficient and, consequently, the elimination of ‘overstaffing’ should not be assumed to be beneficial. Given the evidence suggesting that benefits may accrue when staffing is above the measured requirements, the presence of these extra staff on the ward may add direct value to patient care. Another potential benefit from ‘overstaffing’ is the opportunity to cover staffing shortfalls on other wards without recourse to bank or agency staff, often referred to as ‘floating’ staff between wards. 139
Internal re-deployment of staff (‘floating’)
Staff ‘floating’ between wards has been shown to be unpopular and associated with staff anxiety, especially when staff move outside their specialty area. 139–141 Despite the potential contribution, overall in our models we found that, across all scenarios and hospital trusts, fewer than 1.5% of the total hours were worked by staff re-deployed from one ward to another. Opportunities to float staff between wards are constrained by staff quanta (because staff cannot effectively be moved partially or for very short periods of time) and are less frequent with lower staffing establishments when the benefits might be greater, because understaffing is common whereas overstaffing is rare. In our modelling we assumed that re-deployments were restricted to within directorates, although wider sharing may be possible. Extending the potential re-deployments to the whole hospital made very little difference (0.3%) to the rate of understaffed shifts and, in our economic models for the general hospitals, the inclusion of any internal re-deployment made only a modest contribution to reducing understaffing and costs. Re-deployments outside staff members’ specialties are also more likely to be associated with reduced efficiency and effectiveness of staff. 141
-
Internal re-deployment (floating) of staff within directorates makes a modest contribution to reducing understaffing.
-
Wider re-deployments across the hospital have very little impact on understaffing, and such assignments are known to be unpopular with staff.
Availability of temporary staff and costs
The use of temporary staff varied between scenarios, with lower establishments associated with higher use of temporary staff, as one would expect: ‘high’, ‘standard’ and ‘flexible (low)’ staffing scenarios had, on average, < 5%, 6% and 14% temporary staff, respectively.
In our core model, at all hospital trusts, the higher the establishment the higher the cost, but the fewer shifts are understaffed. This is because of the lower efficiencies of re-deployed/temporary staff and the limited availability of temporary (bank and agency) staff who are able to cover shortfalls according to our empirical data. If we model high availability of temporary staff, the costs of ‘flexible (low)’ staffing establishments become close to those of the ‘standard’ establishments while the risk of leaving shifts understaffed remains higher. In some trusts, when assuming largely unlimited availability of temporary staff, the costs of the ‘low’ establishments were higher than those of the standard establishments owing to the higher cost of temporary staff.
-
The apparent cost savings of employing fewer staff are largely achieved by below-adequate staffing.
-
When temporary staff availability is higher, making ‘flexible (low)’ staffing more feasible, cost savings are eroded while understaffing remains relatively high.
Beyond care hours and registered nurse skill mix
The SNCT provides a measure of overall demand for care but provides staffing estimates that are (in essence) equivalent to an overall measure of care HPPD. While this overall measure is now reported as a benchmarking measure in the NHS,142 there is little research that supports the use of a combined measure of registered nurse and support worker hours. This is because the contributions to patient safety and quality appear to be different, to be independent of one another9 or to have a complex interaction, suggesting that benefits from adding support workers arise only if there are sufficient registered nurses to supervise them. 6,143
Using the SNCT, decisions about skill mix are deferred to ‘professional judgement’ and are not subject to measurement, which might be seen as a significant limitation, although the evidence we reviewed suggests that this professional judgement might still be regarded as the ‘gold standard’ for judging staffing requirements. We used the empirically observed skill mix for each ward as the basis of our models, assuming that this reflected professional judgement of the relative skill mix required. In our previous study,6 we determined that this assumption is broadly correct, although difficulty recruiting registered nurses may lead to a slightly lower skill mix than planned. The associations between perceptions of staffing adequacy and registered nurse/support worker shortfalls were similar, which lends some support to the assumption that observed skill mix serves as a useful proxy for the ‘correct’ skill mix.
However, skill mix is, in reality, more complex than a simple issue of registered versus unregistered staff. Existing literature has rarely addressed this issue at all, although some literature from the USA has considered three broad groups (aides, licensed practical nurses and registered nurses) rather than two. 137 To begin an exploration of this complexity, in addition to modelling registered nurse skill mix in our core simulations, we performed further experiments in which we investigated the impact of wards requiring staff with particular skills in more detail. Based on discussion with our advisory group, we used the example of registered nurses who are trained in administering IV drugs.
We considered the presence of at least one IV-trained nurse as a requirement on all wards, as our advisory group noted the disruption involved when staff had to be obtained from other wards simply to administer IV drugs. We found that as the percentage of registered nurses with IV training increases, the number of shifts with no IV-trained nurse decreases non-linearly. At a hospital level, we determined that once 60% of registered nurses were trained, the rate of shifts that were understaffed according to this criterion was low (< 10% in all hospital trusts and < 2% in the general hospital trusts). Although IV training may rapidly become a ubiquitous skill for registered nurses, currently in the UK, newly qualified nurses will not have had opportunity to demonstrate competence in administering IV medication. This approach to understanding the likely demand for staff training to ensure that staff are available to meet patient needs when additional or specific training is required illustrates that simple approaches based on mean demand are unlikely to succeed.
-
When taking a more ‘refined’ approach to skill mix, it is challenging to deliver specific skills to wards reliably unless a high proportion of staff are trained, even when the requirement is for a single member of staff with that skill.
-
Increasing the complexity of ‘skill mix’ may prove difficult to manage efficiently.
Differences between hospital trusts and assumptions made
In general, understaffing in hospital trust A was the most sensitive to staffing policy (i.e. it had the greatest relative risk increase/decrease associated with alternative establishments) and this trust had lower levels of understaffing than the other hospital trusts when there was limited availability of temporary staff. This may be explained partly by differences in specialing requirements, which varied between trusts. Another reason for trust differences is the size of wards: wards at hospital trusts B and D tended to be larger than at those at trust A, which are larger than those at hospital trust C. Hospital trusts B and D had similar understaffing results across staffing scenarios. In general, across most scenarios, the results from hospital trust C, a specialist hospital with small wards and a high proportion of single rooms, differed substantially from those from the other three. The differences between the deployed staff and our estimated staffing requirements are also much greater in this trust.
Although the absolute and relative differences varied by hospital trust, the pattern of results was common across all and so the qualitative conclusions apply to all. In general, we report our results at the hospital level. However, managers may be more interested in staffing adequacy and costs evaluated at the site or directorate level. Individual wards can be affected more dramatically than others by policy changes, although, again, the patterns observed generally apply at the ward level.
-
These results give general patterns that are likely to apply in other hospitals, but the extent to which any establishment delivers a high level of properly staffed shifts is variable between hospitals and between wards within hospitals.
-
Several strands of evidence from our study suggest that the current multipliers, however used, may often fail to deliver enough staff in a specialist hospital trust with many small wards and single rooms.
Given the limited or lack of data available for some parameters, and the need to make some simplifying assumptions, we investigated the effects of parameter uncertainty on the results. We found that changing the values of most uncertain parameters did not have a practically significant effect on the hospital costs or understaffing results. The exception to this was the ‘rounding’ parameter. We assumed that requests for staff were rounded up or down to the nearest whole person, a practice endorsed by our advisory group and recommended by guidance in Australia (although this guidance has recently been amended in the state of Victoria). 144 If instead we had assumed that requests were always rounded up, there would have been an absolute reduction in understaffing of 14.3% patient shifts and an increase in costs of £3.53 per patient-day on average.
The current national sickness rates for nursing staff are 4.5%,145 so we assumed rates of unanticipated absence from short-notice sickness of 4% for nursing support workers and 3% for registered nurses. This approximates known differences in sickness absence between registered nurses and support workers. 146 Although short-notice sickness absence is an important reason that shifts end up being understaffed, even when establishments are high, a large relative (1% absolute) change in the assumed rate led to a small (< 1% absolute) change in the rate of understaffed patient shifts.
Nursing vacancies are recognised as a current problem in the NHS, so we ran some illustrative simulation scenarios considering a 10% vacancy rate. 1 This scenario had achieved ward establishments between ‘standard’ staffing and ‘flexible (low)’ staffing. Correspondingly, the average percentage of understaffed patient shifts and cost per patient-day also lie between the results for these two scenarios. However, differences between scenarios based on different baseline establishments followed similar patterns.
Economic modelling of using the Safer Nursing Care Tool in different ways to set staffing levels
In the economic modelling, we used the results of the simulation from the three general hospital trusts, together with estimates of the effects of the resulting staffing levels on mortality and length of stay,6,9 to estimate the staff and net cost per life saved for ‘high’ and ‘flexible (low)’ establishments relative to the ‘standard’ establishment.
We found that higher staffing establishments – ‘high’ versus ‘standard’ and ‘standard’ versus ‘flexible (low)’ – are associated with improved outcomes (lives saved, cost per life saved and number needed to treat) under all assumptions about temporary staff availability. When we assume higher availability of temporary staff, the difference in outcomes (costs, bed-days and deaths) between alternative staffing establishments is reduced, but the cost-effectiveness for higher establishments is more favourable.
Using our core assumption – with limited availability of temporary staff based on empirical observations – the ‘high’ establishment is associated with a reduction of 1.2% in bed utilisation and a reduction in mortality of 4.5% compared with the ‘standard’ establishment. This equates to one life saved for every 665 admissions. Staff costs per life saved are £20,423 and costs net of the value of bed-days saved are £9506 per life saved. If unlimited availability of temporary staff is assumed, these estimates are reduced to £16,464/£5524 (staff/net cost).
Although there are no studies with which we could make a direct comparison, these figures are broadly comparable with the estimated cost-effectiveness of implementing the nursing HPPD staffing method in Western Australia, an approach that sets ward establishments and nurse staffing deployments at a level that is higher than typically observed in the UK. Extrapolating life-years saved based on mean life expectancy, Twigg et al. 33 estimated a cost of AU$8907 per life-year gained. Assuming that the paper was reporting 2010 costs, converting to Great British pounds (GBP) using a 2010 exchange rate [mean AUD to USD exchange 0.92 and USD to GBP exchange 1.55 (source: www.macrotrends.net; accessed 10 February 2020)] and applying Consumer Price Index inflation gives £6462 GBP in 2017 prices (source: www.in2013dollars.com/UK-inflation; accessed 10 February 2020). Applying the average life expectancy and approximate discount used (18%) gives approximately £33,500 per life saved.
Unless very pessimistic assumptions about life expectancy or utility of life gained were made for our estimates, it seems likely that the cost-effectiveness estimates for the cost per life saved of ‘high’ establishments would lead to costs per quality-adjusted life-year that sit well below generally accepted thresholds for defining cost-effectiveness. 147 Indeed, ‘high’ establishments are potentially very cost-effective. 147
On the other hand, because the ‘flexible (low)’ establishment is associated with worse outcomes than the ‘standard’ establishment under all assumptions about temporary staffing, it is easy to reject it simply because it is not an effective strategy. However, it is worth noting that under circumstances where ‘flexible (low)’ staffing establishments come closest to providing an effective alternative – when temporary staff availability is high – cost-effectiveness estimates associated with the ‘standard’ establishments approach the level at which the ‘standard’ establishment would dominate economic decision-making, because the cost per life saved associated with it is so low.
The results are sensitive to effect estimates for mortality, but if we take £30,000 per life saved as a threshold and account for length of stay savings, ‘high’ establishments are potentially cost-effective for both lower and upper (95% confidence interval) limits for estimates under all assumptions tested about temporary staff availability (except the unrealistic zero temporary staff scenario). Using the mean estimate or upper limit, the cost-effectiveness estimates are much more favourable than this, even when focusing just on staff costs.
Under low staffing establishments, high availability of temporary staff is needed to cover shifts left unfilled by permanent staff to avoid understaffing. However, the higher the availability of temporary staff, the stronger the case for higher establishments in terms of cost-effectiveness.
The conclusions are not very sensitive to different cost assumptions, although assuming an increased cost per bed-day would lead to ‘high’ establishments being even more cost-effective. If agency staff cost more, the cost savings associated with lower establishments are reduced and potentially even eliminated (under assumptions of high bank and agency staff availability).
-
‘High’ staffing establishments are associated with costs per life saved that are likely to be assessed as cost-effective under most assumptions about temporary staffing, and the conclusion is not sensitive to most assumptions made.
-
‘Flexible (low)’ staffing establishments are associated with worse outcomes and diminished cost savings when sufficient temporary staff are available to make them a viable option.
-
Investing in ‘high’ staffing establishments over ‘standard’ staffing establishments would cost < 1% of annual turnover at all hospital trusts studied.
Restrictions on decision-making are likely to arise because of budget constraints and, more significantly in the short term, limited supply of staff, particularly registered nurses. These findings, therefore, have implications as much for policy as for local practice and use of the SNCT. However, given the evidence that higher staffing levels and improved establishments associated with mandatory minimum staffing policies are associated with improved job satisfaction and retention,148–150 increases in establishment could still be associated with a cost-effective approach to managing the local pool of nursing staff if recruitment and retention of staff were improved as a result.
Other considerations
All hospital trusts involved in our study have moved towards routinely collecting SNCT ratings of patients at multiple time points each day. Although we have explored cost-effectiveness under realistic scenarios of limited availability of temporary staff, we have assumed that there would always be an attempt to make up staffing shortfalls. However, comparisons of our models with no availability of temporary staff with models assuming some availability provide support for a conclusion that this ‘tailored’ approach, rather than using a fixed daily staffing level, is cost-effective. However, this does not lend support to using these systems to set low base establishments that are flexed upwards as required. The results of this study emphasise the potential benefits of higher nursing establishments and show that using the SNCT to set establishments to meet predicted need 90% of the time, rather than to meet mean average need, could be cost-effective, substantially reducing the risk to patients that is associated with low staffing.
-
Our findings can be used to support a flexible staffing policy, but one that is based on high, not low, establishments.
In our models, we have assumed that there will be an attempt to fill measured staffing shortfalls if such staffing is available. Because we have generally assumed that there are limits to the availability of temporary staff, it may be that our model provides a reasonable facsimile for a system in which professional judgement might also determine that, on occasion, existing staff are best placed to manage, and so some requests for additional staff are averted. The findings of this study do not establish the superiority of measurement over professional judgement.
The SNCT is generally ‘mute’ on the matter of skill mix. However, although these findings support setting higher establishments, other research makes it clear that benefits are unlikely to accrue if the higher establishments erode skill mix. Indeed, in our recent study6 we did not model the effects of increases in nursing support worker staffing because this strategy delivered worse outcomes at higher costs.
-
Increasing establishments while decreasing skill mix is unlikely to be cost-effective.
In this study, we looked only at the SNCT. However, although the specific parameters that we estimated may not apply to other tools, our simulation models show the likely consequences of different approaches to using any measurement system, because needs vary around the measured means. These findings might be of particular relevance to US hospitals, where measurement systems are frequently used for real-time staffing deployment decisions. It is striking that, although much of the UK literature has been preoccupied with setting establishments (nurses to employ), and thus emphasises forward planning, the US literature seems to be much more focused on flexible staffing assignments using real-time or near real-time measurement. Our findings demonstrate that the success of any such approach requires that it operate with an appropriate baseline establishment; establishments are unlikely to be safe or cost-effective if they are set to meet only minimal demands.
Results in context
This study was undertaken at a time of shortages in the supply of registered nurses and increasing nurse vacancy rates in many NHS organisations. Although this may seem to render these results somewhat academic, the shortage of supply does not remove the need to quantify the demand properly. Over the lifetime of this project, the ‘crisis’ in nurse staffing across the UK emerged as a major policy focus, resulting in a number of initiatives to increase the supply of nurses and to improve staff retention. If these initiatives are, ultimately, to be successful, it is imperative that the conditions that nurses find themselves working in present them with a manageable workload that enables them to consistently deliver care of a high standard. Otherwise, efforts to improve supply may be thwarted by staff outflows in a competitive labour market. To use (and extend) an oft-quoted aphorism, it is hard to fill a leaky bucket, and, when planning to fix it, it is wise not to underestimate the size of the hole. A key challenge in achieving a stable workforce and improving retention is to ensure that we properly understand how many staff are actually needed to deliver quality care. Tools such as the SNCT have a role in this, although our study results point towards a baseline staffing requirement that may be higher than that identified by using the tool as currently recommended.
In common with other studies, our results indicate that registered nurse staffing levels that are higher than present levels and higher than those recommended by the SNCT might deliver additional benefits for patient outcomes, with a cost-effectiveness ratio that supports this approach. 6,33 In the face of ongoing budget constraints in the NHS, it may seem problematic to suggest additional investment in nursing. However, although it may be that policy-makers and the public choose to make other investments, it is important to understand the costs and consequences of options. Furthermore, although nurse vacancies are at a high level nationally, not all hospital trusts are equally affected, and the baseline staffing deployed varies considerably between trusts. In the absence of an understanding of how many nurses are required, it is hard to justify such variation. A simplistic model of economic efficiency focuses attention on hospitals with apparently high levels of nurse staffing, identifying them as potentially unproductive based on simple measures of HPPD. Our economic modelling results suggest that hospital trusts that have chosen to invest more heavily in nursing staff may be using their resources cost-effectively. Conversely, these results show that what might appear to be an efficient and effective response to nurse shortages – using low establishments and flexible deployment of staff – is unlikely to be a successful strategy. This strategy is likely to cause harm to patients, and, in the circumstances when it is most likely to be successful (which requires unfeasible assumptions about the availability of temporary staff), saves little, if any, money. In some cases, such a strategy costs more and increases harm.
In the face of staff shortages, it is tempting to consider alternative approaches for improving the effectiveness or efficiency of the current workforce. Although there is scant evidence for improved productivity arising from quality improvement initiatives, such as the Productive Ward programme,151,152 or structural changes, such as altering shift patterns,146 the importance of quality leadership and management in ensuring an effective and productive ward nursing team is largely beyond dispute. However, without dismissing the importance of such factors, they serve merely to remind that having enough staff is necessary but not sufficient. This is amply illustrated by one US study that showed little benefit from increasing staffing levels in hospitals that provided poor work environments. 153
Finally, this study has focused on the nursing workforce almost exclusively. The provision of health care is a multiprofessional endeavour. That we have focused on nursing does not mean that we underestimate the potential contribution of other professions to patient safety and quality of care. The current recognition of the importance of nurse staffing for patient safety in hospitals stands as a major correction to a historical tendency to overlook this, which was illustrated in recent times in the many pages of the Francis Report10 but certainly did not begin there. The evidence provided here relates to how best to plan the staffing of hospital wards by nurses. There may be potential to reconfigure ward establishments and to shift work between professional groups. However, simply including other staff on the nursing roster does not in itself lead to a redistribution of the work that is undertaken by nurses. Conversely, shifting resources from other groups towards nursing because of studies such as this one does not guarantee cost-effectiveness unless the costs and consequences of disinvestment in other services are also understood.
Limitations and future work
In this section, we identify some further research recommendations arising from the study limitations. These recommendations are summarised and prioritised in Box 1.
-
Undertake more extensive observational studies, considering the potential influence of the availability of other professions and using other patient/staff outcomes to identify tipping points at which there are ‘enough’ staff and more staff would not improve outcomes further.
-
Undertake prospective studies of the use of different approaches to determining staffing requirements, including assessment of patient outcomes.
-
Investigate the fit of the SNCT at ward level.
-
Study variability of workload within acuity/dependency levels.
-
Carry out simulation modelling of different priorities of nursing tasks and how much time is required as a buffer to have enough nurses when there are simultaneous urgent tasks.
-
Carry out more detailed investigation into specialing requirements.
-
Develop simulation models of nurse staffing that model interdependence of staffing requirements in different wards.
-
Study how much tolerance needs to be built in between the employment, deployment and rostering planning stages.
In our regression modelling, we were unable to identify clear ‘tipping points’ at which there was diminishing return from higher staffing levels and, thus, we could not identify ‘optimal’ levels. Therefore, our simulation is designed to achieve staffing levels that may still be below what is optimal. Our data from reports of numbers of nurses/support workers needed when staffing was low were too unreliable to fit similar models as a result of ambiguities around whether total staff needed or additional staff needed had been reported. However, repeating such surveys might help with identifying the ‘magic numbers’ of how many staff are required each shift, and modelling the relationship between this requirement and underlying factors (regression approach). It is possible that there is a range of acceptable staffing levels in a given situation (as we simulated using a buffer around acceptable staffing) and so ideas from fuzzy modelling and optimisation,154,155 which deal with ambiguity and vagueness, may be applicable.
In the simulation modelling, we assumed that requirements in different wards are independent of one another, that is that neither the numbers of patients nor the acuity/dependency levels of patients in different wards interact. This is unlikely to be upheld in practice. Although future work could relax this assumption, it introduces significant complexity to both the data collection and the modelling. As it seems most likely that periods of high demand in one ward would be associated with periods of high demand in others, the significance of internal re-deployment is likely to be overestimated by our current assumption. The impact on our results and conclusions is unlikely to be great because re-deploying staff between wards did not make substantial contributions to reducing low staffing and did not substantially alter cost-effectiveness estimates.
We did not take into account that there may be feedback loops whereby adverse effects of low staffing (increased risk of adverse events and longer hospital stays) could result in increased workloads for staff. It is difficult to determine whether or not such effects would make a measurable impact on overall results, but if results were influenced by such effects it would be in a manner consistent with our conclusions rather than serving to undermine them. Establishments already associated with high levels of understaffing would appear to be even more disadvantageous because of this positive feedback loop in which understaffing generates additional demand for staff.
The cost per patient-day is variable between wards because of different pay band mixes of the staff employed, which is accounted for in the modelling. These costs do not include temporary staff covering long-term/planned absences, and hence we observed higher costs in reality than in the simulation experiments. We did not have a breakdown of different amounts of ‘notice’ for sickness absence or enough data to estimate seasonality (clustering) of staff sickness absence and have not considered a reduction in availability of temporary staff due to their sickness.
We did not have data on the variability of workload within acuity/dependency levels. In our modelling, we assumed symmetric distributions and that each standard deviation was 10% of the respective multiplier, although it did not appear that our hospital-level conclusions were highly sensitive to this assumption. Future work could look at the impact of non-symmetric distributions, study the impacts of these distributions on ward results and collect data about the shape and variability of these distributions. This may also help confirm whether or not the categories 0, 1a, 1b, 2 and 3 are the best way of segmenting patients, and, if not, cluster analysis could be applied to generate categories that better group patients according to their nursing requirements.
In the process of deriving the SNCT multipliers from data on quality-assured wards, ready-for-action time was removed, but this means that recommended establishments are lower than those observed on the quality-assured wards. Nurses not only work on routine, plannable tasks, such as ward rounds, but also must react to emergent, urgent tasks, such as adverse reactions to infusions or emergency tasks involving responding to life-threatening incidents. 156 Simulation modelling or queuing theory could be used to investigate these dynamics, and how many staff are needed so that different priorities of tasks can be completed within appropriate time frames. However, as our work tends to point toward the overall benefits of higher establishments, this anomaly in the SNCT does not alter our conclusions, and the way in which the SNCT treats this ready-for-action time needs to be reviewed.
In this study, we limited our scope to setting ward establishments according to the SNCT and reacting to shortfalls each shift, that is, dealing with fluctuations in demand and unforeseen absences on the day. We did not investigate how to roster staff fairly157,158 or how to plan staffing levels to cover foreseen absences (e.g. leave, training and long-term sickness). There is interplay between different planning stages; the nearer to the start of the shift, the more information is known about the availability of nursing staff and the patients’ nursing requirements. Thus, further studies could focus on understanding the links between employment, deployment and rostering better, in particular how much tolerance needs to be built in between each planning stage (i.e. the ‘uplift’ for absences and flexibility to adjust rosters).
We found that specialing requirements are a major source of variability in demand. Future work could investigate specialing requirements in more detail, including the contribution of volunteers and relatives to specialing, whether or not this demand could be managed by a pool of staff at the hospital level (as fluctuations in demand are wide at the ward level) and perhaps a more detailed investigation into how much time specialing duties take (accounting for handovers and cohorts).
In our study, the judgement of staffing adequacy and ratings of the SNCT were undertaken by the same people or teams. Although it is difficult to picture a sensible way of routinely separating these two judgements to make them fully independent, the possibility that some degree of bias is induced cannot be discounted. However, the nature of the SNCT reports – numbers of patients in each category – is sufficiently distinct from the staffing adequacy questions that our findings are unlikely to be a simple product of common method bias.
We saw a degree of consistency in the results between the four participating hospital trusts but we cannot be sure that these results would generalise across all trusts. Certainly the quantitative estimates (rather than the qualitative relationships) do appear to differ by trust. Costs differ as a result of the bands of staff employed, and the ability of wards to match staffing to demand depends on factors such as ward size and specialing requirements. Therefore, the results may be different for hospital trusts with substantially different mixes of staff and ward types. Future research could look in more detail at the fit of the SNCT for particular types of wards. We have concentrated on hospital-trust-level results but our results do suggest that requirements may differ between medical and surgical wards, even when the measured staffing requirement appears to be the same based on the acuity/dependency rating. Our results also suggest that staffing requirements are much more variable on some wards than on others, which makes determining the correct establishment even more challenging.
We did not estimate directly the effect of low staffing on the sample of patients in the study. Although our effect estimates derived from a methodologically strong study that was undertaken in one of our participating hospital trusts, they were nonetheless derived from a single-site study. It is possible that the observational evidence used to estimate the outcomes association could be biased, although this coefficient is consistent with other estimates of effect, and most biasing mechanisms would tend to suggest an underestimation of the strength of association. 6 Cause and effect, although plausible and consistent with an increasingly large body of evidence, should not be assumed. Furthermore, we considered the impact of staffing levels on mortality only, but other aspects of care are likely to benefit from increased staffing, including patient experience,143 and staff outcomes such as sickness absence and turnover may also improve. Thus, decision-making based on mortality alone underestimates the likely benefit of increasing nurse staffing levels. Further observational studies are needed to extend this research to other trusts, and consider the influence of the availability of other staff groups (including other staff rostered on wards) and other outcomes. Different patient types may be affected to greater or lesser extents by understaffing, so this is another possible area to explore.
Finally, this was an observational study with modelling of scenarios. We cannot be sure that the simulated costs and consequences of different staffing establishments would hold in reality. To overcome the limitations of observational and simulation studies, experiments could be conducted whereby real ward establishments are systematically and purposefully changed. Although the challenges of undertaking such prospective intervention studies on sufficient scale are considerable, they are not insurmountable. Currently, the An Roinn Sláinte (Department of Health) in Ireland is backing an intervention study by supporting the increase of nurse staffing establishments to levels identified by a staffing system (www.trendcare.com.au/). The study will evaluate the effects on patient and staff outcomes. Likewise, the aforementioned study by Twigg et al. 33 was a prospective intervention study. However, neither of these studies is randomised, and undertaking such studies at a scale such as that achieved by the study we report here still seems unlikely. 159 While prospective and even randomised studies of different staffing strategies and methods for determining establishments are feasible, observational studies will retain a role, including in the search for a threshold that, in UK data at least, remains elusive.
Conclusions
The hospital trusts in this study have implemented the SNCT and have used it to guide establishment-setting. The staffing levels measured by the tool are associated with professional judgement of staffing adequacy and so the tool has some degree of validity, although we cannot show that the tool provides a measure that is superior to professional judgement. In modelling different approaches to using the data to set ward establishments, we have demonstrated that patient need can be better met by setting the ward establishment at a level that is above the mean. Other approaches risk patients being exposed to very frequent low staffing, requiring the use of bank and agency staff, who are not always available. Because of the consequences of low staffing, the higher establishments appear to be cost-effective when compared with lower ones. Our findings can be used to support flexible staffing policies guided by the SNCT, but such policies are cost-effective when based on establishments that are planned to meet patient need 90% of the time. A staffing policy that sets establishments based on mean demand is also cost-effective when compared with a staffing policy based on a ‘low’ core establishment (to which additional staff are added when required). This is in part because flexibility is constrained by the limited availability of bank and agency staff, but also because such a policy is likely to increase the unit costs of staff. Flexible staffing and low base establishments should not be equated, as low establishments are associated with increased patient harm even when temporary staff availability is high. Furthermore, when temporary staffing availability is high, cost savings are largely eliminated. Although the routine deployment of systems that allow real-time calculation of staffing requirements can help with ongoing monitoring of a ward’s staffing, for sufficient staffing to be delivered consistently, the priority remains correct determination of the necessary baseline. The best outcomes for patients are achieved at the highest establishments, provided that skill mix is maintained.
Acknowledgements
We would like to thank Clare Aspden (University Hospital Southampton NHS Foundation Trust), Tracey Cassar (Portsmouth Hospitals NHS Trust) and Shirley Hunter (Poole Hospital NHS Foundation Trust) who, despite not meeting all of the criteria for authorship, contributed to data collection and revisions of drafts.
We would also like to thank Francesca Lambert (Patient and Public Co-ordinator, Project Co-ordinator) for practical assistance throughout, including formatting the final report.
Furthermore, we would like to thank David Culliford and Antonello Maruotti for early statistical advice on the regression modelling.
Finally, we wish to acknowledge all the nurses who answered staffing adequacy questions and completed SNCT ratings, as well as those who contributed to pilot work.
Steering group membership
We would like to thank all the members of our steering group:
-
Professor Karen Spilsbury (chairperson), Chair of Nursing Research, University of York
-
Professor Chris Bojke, Professor of Health Economics, University of Leeds
-
Professor Sally Brailsford, Professor of Management Science, University of Southampton
-
Ann Casey MBE, Clinical Workforce Lead NHS Improvement/Assistant Chief Nurse, University College Hospital
-
Professor Jonathan Drennan, Professor of Professor of Nursing and Health Service Research, University College Cork
-
Stephen Habgood, patient and public involvement representative
-
Professor Alison Leary, Chair of Healthcare and Workforce Modelling, London South Bank University
-
Mr Trevor Murrells, Statistician, King’s College London
-
Ms Lorna Wilkinson, Director of Nursing, Salisbury NHS Foundation Trust.
Contributions of authors
Peter Griffiths (https://orcid.org/0000-0003-2439-2857) (Professor, Health Services Research) was principal investigator. He was responsible for the original conceptualisation of the study and study design, secured funding, oversaw acquisition of the data, data analysis (in particular economics), and interpreted the results and contributed to drafting the report.
Christina Saville (https://orcid.org/0000-0001-7718-5689) (Research Fellow, Operational Research) undertook descriptive and regression analyses, developed the simulation model and undertook simulation analyses, and drafted and revised the final report.
Jane E Ball (https://orcid.org/0000-0002-8655-2994) (Professor, Health Services Research) contributed to study design, acquisition of funding, interpretation of results and critical revision of the report.
Rosemary Chable (https://orcid.org/0000-0002-2598-0100) (Deputy Director of Nursing and Deputy Director of Education and Workforce, Nursing and Health Services Management) contributed to study design, acquisition of funding, acquisition of the data, interpretation of results and critical revision of the report.
Andrew Dimech (https://orcid.org/0000-0001-5212-1929) (Divisional Clinical Nurse Director, Nursing and Health Services Management) contributed to the original conceptualisation of the study and study design, secured funding, oversaw acquisition of the data, interpretation of results and critical revision of the report.
Jeremy Jones (https://orcid.org/0000-0002-2725-0937) (Principal Research Fellow, Health Economics) contributed to study design, acquisition of funding, oversaw acquisition of the data, data analysis (in particular processing), interpretation of results and critical revision of the report.
Yvonne Jeffrey (https://orcid.org/0000-0002-6001-7723) (Assistant Director of Nursing, Nursing and Health Services Management) contributed to study design, acquisition of funding, acquisition of the data, interpretation of results and critical revision of the report.
Natalie Pattison (https://orcid.org/0000-0002-6771-8733) (Clinical Professor, Nursing) contributed to study design, acquisition of funding, acquisition of the data, interpretation of results and critical revision of the report.
Alejandra Recio Saucedo (https://orcid.org/0000-0003-2823-4573) (Research Fellow, Health Services Evaluation) contributed to study design, acquisition of funding, interpretation of results and critical revision of the report.
Nicola Sinden (https://orcid.org/0000-0002-9497-6973) (Lead Nurse for Workforce, Nursing and Health Services Management) contributed to study design, acquisition of funding, acquisition of the data, interpretation of results and critical revision of the report.
Thomas Monks (https://orcid.org/0000-0003-2631-4481) (Principal Research Fellow, Operational Research and Data Science) contributed to study design, acquisition of funding, oversaw acquisition of the data, data analysis, interpretation of results and critical revision of the report.
Publications
Saville CE, Griffiths P, Ball JE, Monks, T. How many nurses do we need? A review and discussion of operational research techniques applied to nurse staffing. Int J Nurs Stud 2019;97:7–13.
Griffiths P, Saville C, Ball J, Jones J, Pattison N, Monks T. Nursing workload, nurse staffing methodologies and tools: a systematic scoping review and discussion. Int J Nurs Stud 2020;103:103487.
Data-sharing statement
The data set is available from the University of Southampton Institutional Research Repository (https://eprints.soton.ac.uk/435408/; accessed 25 February 2020) and does not contain individual-level or personal data. Further information, if required, can be obtained from the corresponding author.
Patient data
This work uses data provided by patients and collected by the NHS as part of their care and support. Using patient data is vital to improve health and care for everyone. There is huge potential to make better use of information from people’s patient records, to understand more about disease, develop new treatments, monitor safety, and plan NHS services. Patient data should be kept safe and secure, to protect everyone’s privacy, and it’s important that there are safeguards to make sure that it is stored and used responsibly. Everyone should be able to find out about how patient data are used. #datasaveslives You can find out more about the background to this citation here: https://understandingpatientdata.org.uk/data-citation.
Disclaimers
This report presents independent research funded by the National Institute for Health Research (NIHR). The views and opinions expressed by authors in this publication are those of the authors and do not necessarily reflect those of the NHS, the NIHR, NETSCC, the HS&DR programme or the Department of Health and Social Care. If there are verbatim quotations included in this publication the views and opinions expressed by the interviewees are those of the interviewees and do not necessarily reflect those of the authors, those of the NHS, the NIHR, NETSCC, the HS&DR programme or the Department of Health and Social Care.
References
- NHS Improvement . Performance of the NHS Provider Sector for the Quarter Ended 30 September 2018 2018. https://improvement.nhs.uk/documents/3520/Performance_of_the_NHS_provider_sector_for_the_month_ended_30_Sept_18_FINAL.pdf (accessed 25 February 2020).
- Griffiths P, Ball J, Drennan J, Dall’Ora C, Jones J, Maruotti A, et al. Nurse staffing and patient outcomes: strengths and limitations of the evidence to inform policy and practice. A review and discussion paper based on evidence reviewed for the National Institute for Health and Care Excellence Safe Staffing guideline development. Int J Nurs Stud 2016;63:213-25. https://doi.org/10.1016/j.ijnurstu.2016.03.012.
- Shekelle PG. Nurse–patient ratios as a patient safety strategy: a systematic review. Ann Intern Med 2013;158:404-9. https://doi.org/10.7326/0003-4819-158-5-201303051-00007.
- Kane RL, Shamliyan TA, Mueller C, Duval S, Wilt TJ. The association of registered nurse staffing levels and patient outcomes: systematic review and meta-analysis. Med Care 2007;45:1195-204. https://doi.org/10.1097/MLR.0b013e3181468ca3.
- Griffiths P, Recio-Saucedo A, Dall’Ora C, Briggs J, Maruotti A, Meredith P, et al. The association between nurse staffing and omissions in nursing care: a systematic review. J Adv Nurs 2018;74:1474-87. https://doi.org/10.1111/jan.13564.
- Griffiths P, Ball J, Bloor K, Böhning D, Briggs J, Dall’Ora C, et al. Nurse staffing levels, missed vital signs and mortality in hospitals: retrospective longitudinal observational study. Health Serv Deliv Res 2018;6. https://doi.org/10.3310/hsdr06380.
- Ball JE, Bruyneel L, Aiken LH, Sermeus W, Sloane DM, Rafferty AM, et al. Post-operative mortality, missed care and nurse staffing in nine countries: a cross-sectional study. Int J Nurs Stud 2018;78:10-5. https://doi.org/10.1016/j.ijnurstu.2017.08.004.
- Needleman J, Buerhaus P, Pankratz VS, Leibson CL, Stevens SR, Harris M. Nurse staffing and inpatient hospital mortality. N Engl J Med 2011;364:1037-45. https://doi.org/10.1056/NEJMsa1001025.
- Griffiths P, Maruotti A, Recio Saucedo A, Redfern OC, Ball JE, Briggs J, et al. Nurse staffing, nursing assistants and hospital mortality: retrospective longitudinal cohort study. BMJ Qual Saf 2019;28:609-17. https://doi.org/10.1136/bmjqs-2018-008043.
- Francis R. Report of the Mid Staffordshire NHS Foundation Trust Public Inquiry 2013.
- National Institute for Health and Care Excellence . Safe Staffing for Nursing in Adult Inpatient Wards in Acute Hospitals (Guidance) 2014. www.nice.org.uk/guidance/sg1 (accessed 25 February 2020).
- Griffiths P, Ball J, Drennan J, James L, Jones J, Recio-Saucedo A, et al. The Association Between Patient Safety Outcomes and Nurse/Healthcare Assistant Skill Mix and Staffing Levels and Factors that may Influence Staffing Requirements (NICE Evidence Review). University of Southampton Centre for innovation and Leadership in Health Sciences; 2014.
- Department of Health and Social Services Operational Research Service . Nurse Manpower Planning: Approaches and Techniques 1982.
- Arthur T, James N. Determining nurse staffing levels: a critical review of the literature. J Adv Nurs 1994;19:558-65. https://doi.org/10.1111/j.1365-2648.1994.tb01121.x.
- Edwardson SR, Giovannetti PB. Nursing workload measurement systems. Annu Rev Nurs Res 1994;12:95-123. https://doi.org/10.1891/0739-6686.12.1.95.
- Hurst K. Selecting and Applying Methods for Estimating the Size and Mix of Nursing Teams: A Systematic Review of the Literature Commissioned by the Department of Health. Nuffield Institute for Health; 2003.
- Fasoli DR, Haddock KS. Results of an integrative review of patient classification systems. Annu Rev Nurs Res 2010;28:295-316. https://doi.org/10.1891/0739-6686.28.295.
- Aydelotte MK. Nurse Staffing Methodology, a Review and Critique of Selected Literature. Washington, DC: US Department of Health, Education, and Welfare; 1973.
- The Shelford Group . Safer Nursing Care Tool Implementation Resource Pack 2014. http://shelfordgroup.org/library/documents/Shelford_Safer_Nursing_23May14a.pdf (accessed 29 September 2019).
- Smith S, Casey A, Hurst K, Fenton K, Scholefield H. Developing, testing and applying instruments for measuring rising dependency-acuity’s impact on ward staffing and quality. Int J Health Care Qual Assur 2009;22:30-9. https://doi.org/10.1108/09526860910927934.
- National Institute for Health and Care Excellence . Safer Nursing Care Tool (SNCT): Statement of Endorsement 2014. www.nice.org.uk/guidance/sg1/resources/endorsed-resource-the-safer-nursing-care-tool-snct-11943902 (accessed 25 February 2020).
- Ball J, Barker H, Griffiths P, Jones J, Lawless J, Burton CR, et al. Implementation, Impact and Costs of Policies for Safe Staffing In Acute Trusts. Report to Funders. Southampton: NIHR Policy Research programme; 2018.
- Lang TA, Hodge M, Olson V, Romano PS, Kravitz RL. Nurse-patient ratios: a systematic review on the effects of nurse staffing on patient, nurse employee, and hospital outcomes. J Nurs Adm 2004;34:326-37. https://doi.org/10.1097/00005110-200407000-00005.
- Lankshear AJ, Sheldon TA, Maynard A. Nurse staffing and healthcare outcomes: a systematic review of the international research evidence. ANS Adv Nurs Sci 2005;28:163-74. https://doi.org/10.1097/00012272-200504000-00008.
- Aiken LH, Sloane DM, Bruyneel L, Van den Heede K, Griffiths P, Busse R, et al. Nurse staffing and education and hospital mortality in nine European countries: a retrospective observational study. Lancet 2014;383:1824-30. https://doi.org/10.1016/S0140-6736(13)62631-8.
- Bond CA, Raehl CL, Pitterle ME, Franke T. Health care professional staffing, hospital characteristics, and hospital mortality rates. Pharmacotherapy 1999;19:130-8. https://doi.org/10.1592/phco.19.3.130.30915.
- Griffiths P, Ball J, Murrells T, Jones S, Rafferty AM. Registered nurse, healthcare support worker, medical staffing levels and mortality in English hospital trusts: a cross-sectional study. BMJ Open 2016;6. https://doi.org/10.1136/bmjopen-2015-008751.
- Ozdemir BA, Karthikesalingam A, Sinha S, Poloniecki JD, Vidal-Diez A, Hinchliffe RJ, et al. Association of hospital structures with mortality from ruptured abdominal aortic aneurysm. Br J Surg 2015;102:516-24. https://doi.org/10.1002/bjs.9759.
- Ozdemir BA, Sinha S, Karthikesalingam A, Poloniecki JD, Pearse RM, Grocott MP, et al. Mortality of emergency general surgical patients and associations with hospital structures and processes. Br J Anaesth 2016;116:54-62. https://doi.org/10.1093/bja/aev372.
- Hill AB. The environment and disease: association or causation?. Proc R Soc Med 1965;58:295-300. https://doi.org/10.1177/003591576505800503.
- Recio-Saucedo A, Dall’Ora C, Maruotti A, Ball J, Briggs J, Meredith P, et al. What impact does nursing care left undone have on patient outcomes? Review of the literature. J Clin Nurs 2018;27:2248-59. https://doi.org/10.1111/jocn.14058.
- Bruyneel L, Li B, Ausserhofer D, Lesaffre E, Dumitrescu I, Smith HL, et al. Organization of hospital nursing, provision of nursing care, and patient experiences with care in Europe. Med Care Res Rev 2015;72:643-64. https://doi.org/10.1177/1077558715589188.
- Twigg DE, Geelhoed EA, Bremner AP, Duffield CM. The economic benefits of increased levels of nursing care in the hospital setting. J Adv Nurs 2013;69:2253-61. https://doi.org/10.1111/jan.12109.
- Donaldson N, Shapiro S. Impact of California mandated acute care hospital nurse staffing ratios: a literature synthesis. Policy Polit Nurs Pract 2010;11:184-201. https://doi.org/10.1177/1527154410392240.
- Mark BA, Harless DW, Spetz J, Reiter KL, Pink GH. California’s minimum nurse staffing legislation: results from a natural experiment. Health Serv Res 2013;48:435-54. https://doi.org/10.1111/j.1475-6773.2012.01465.x.
- Royal College of Nursing . Policy Briefing: Mandatory Nurse Staffing Levels 2012.
- Buchan J. A certain ratio? The policy implications of minimum staffing ratios in nursing. J Health Serv Res Policy 2005;10:239-44. https://doi.org/10.1258/135581905774414204.
- Telford WA. Determining nursing establishments. Health Serv Manpow Rev 1979;5:11-7.
- Taylor B, Yankey N, Robinson C, Annis A, Haddock KS, Alt-White A, et al. Evaluating the Veterans Health Administration’s staffing methodology model: a reliable approach. Nurs Econ 2015;33.
- Twigg D, Duffield C. A review of workload measures: a context for a new staffing methodology in Western Australia. Int J Nurs Stud 2009;46:132-40. https://doi.org/10.1016/j.ijnurstu.2008.08.005.
- de Cordova PB, Lucero RJ, Hyun S, Quinlan P, Price K, Stone PW. Using the nursing interventions classification as a potential measure of nurse workload. J Nurs Care Qual 2010;25:39-45. https://doi.org/10.1097/NCQ.0b013e3181b3e69d.
- Myny D, Van Goubergen D, Limère V, Gobert M, Verhaeghe S, Defloor T. Determination of standard times of nursing activities based on a nursing minimum dataset. J Adv Nurs 2010;66:92-102. https://doi.org/10.1111/j.1365-2648.2009.05152.x.
- Myny D, De Bacquer D, Van Hecke A, Beeckman D, Verhaeghe S, Van Goubergen D. Validation of standard times and influencing factors during the development of the Workload Indicator for Nursing. J Adv Nurs 2014;70:674-86. https://doi.org/10.1111/jan.12232.
- Fagerström L, Rainio AK. Professional assessment of optimal nursing care intensity level: a new method of assessing personnel resources for nursing care. J Clin Nurs 1999;8:369-79. https://doi.org/10.1046/j.1365-2702.1999.00266.x.
- Fagerström L, Rauhala A. Benchmarking in nursing care by the RAFAELA patient classification system – a possibility for nurse managers. J Nurs Manag 2007;15:683-92. https://doi.org/10.1111/j.1365-2934.2006.00728.x.
- Hoi SY, Ismail N, Ong LC, Kang J. Determining nurse staffing needs: the workload intensity measurement system. J Nurs Manag 2010;18:44-53. https://doi.org/10.1111/j.1365-2834.2009.01045.x.
- Rauhala A, Fagerström L. Determining optimal nursing intensity: the RAFAELA method. J Adv Nurs 2004;45:351-9. https://doi.org/10.1046/j.1365-2648.2003.02918.x.
- Fasoli DR, Fincke BG, Haddock KS. Going beyond patient classification systems to create an evidence-based staffing methodology. J Nurs Adm 2011;41:434-9. https://doi.org/10.1097/NNA.0b013e31822edd8e.
- Jenkins-Clarke S. Measuring Nursing Workload: A Cautionary Tale. York: Centre for Health Economics, University of York; 1992.
- O’Brien-Pallas L, Cockerill R, Leatt P. A Comparison of Workload Estimates of Five Patient Classification Systems in Nursing: Final Report 1991.
- O’Brien-Pallas L, Leatt P, Deber R, Till J. A comparison of workload estimates using three methods of patient classification. Can J Nurs Adm 1989;2:16-23. https://doi.org/10.1016/S0840-4704(10)61225-2.
- O’Brien-Pallas L, Cockerill R, Leatt P. Different systems, different costs? An examination of the comparability of workload measurement systems. J Nurs Adm 1992;22:17-22. https://doi.org/10.1097/00005110-199212000-00007.
- Butler M, Collins R, Drennan J, Halligan P, O’Mathúna DP, Schultz TJ, et al. Hospital nurse staffing models and patient and staff-related outcomes. Cochrane Database Syst Rev 2011;7. https://doi.org/10.1002/14651858.CD007019.pub2.
- National Institute for Health and Care Excellence . Safe Staffing for Adult Inpatient Wards in Acute Hospitals: Tools and Resources 2014. www.nice.org.uk/guidance/sg1/resources (accessed 25 February 2020).
- Fagerström L, Lønning K, Andersen MH. The RAFAELA system: a workforce planning tool for nurse staffing and human resource management. Nurs Manag 2014;21:30-6. https://doi.org/10.7748/nm2014.04.21.2.30.e1199.
- Fenton K, Casey A. A tool to calculate safe nurse staffing levels. Nurs Times 2015;111:12-4.
- Kolakowski D. Constructing a nursing budget using a patient classification system. Nurs Manage 2016;47:14-6. https://doi.org/10.1097/01.NUMA.0000479449.43157.b5.
- Hurst K. UK ward design: patient dependency, nursing workload, staffing and quality-an observational study. Int J Nurs Stud 2008;45:370-81. https://doi.org/10.1016/j.ijnurstu.2006.09.007.
- Hurst K. Nursing and Payment By Results: Understanding the Cost of Care (RCN Policy Briefing: 11/2009). London: Royal College of Nursing; 2009.
- Gabbay U, Bukchin M. Does daily nurse staffing match ward workload variability? Three hospitals’ experiences. Int J Health Care Qual Assur 2009;22:625-41. https://doi.org/10.1108/09526860910986885.
- Beswick S, Hill PD, Anderson MA. Comparison of nurse workload approaches. J Nurs Manag 2010;18:592-8. https://doi.org/10.1111/j.1365-2834.2010.01124.x.
- Simon M, Yankovskyy E, Klaus S, Gajewski B, Dunton N. Midnight census revisited: reliability of patient day measurements in US hospital units. Int J Nurs Stud 2011;48:56-61. https://doi.org/10.1016/j.ijnurstu.2010.07.002.
- Rivera K. A Comparison of a Standardized Method of Identifying Nursing Staff Needs with Measures of Nursing Staff Needs by Patient Acuity [D.N.P.]. Ann Arbor, MI: The William Paterson University of New Jersey; 2017.
- Baernholdt M, Cox K, Scully K. Using clinical data to capture nurse workload: implications for staffing and safety. Comput Inform Nurs 2010;28:229-34. https://doi.org/10.1097/NCN.0b013e3181e1e57d.
- Ferguson-Paré M, Bandurchin A. The Ontario nursing workload demonstration projects: rethinking how we measure, cost and plan the work of nurses. Nurs Leadersh 2010;23:20-32. https://doi.org/10.12927/cjnl.2010.21745.
- Myny D, Van Hecke A, De Bacquer D, Verhaeghe S, Gobert M, Defloor T, et al. Determining a set of measurable and relevant factors affecting nursing workload in the acute care hospital setting: a cross-sectional study. Int J Nurs Stud 2012;49:427-36. https://doi.org/10.1016/j.ijnurstu.2011.10.005.
- Perroca MG. The new version of a patient classification instrument: assessment of psychometric properties. J Adv Nurs 2013;69:1862-8. https://doi.org/10.1111/jan.12038.
- Brennan CW, Daly BJ, Dawson NV, Higgins PA, Jones KR, Madigan E, et al. The oncology acuity tool: a reliable, valid method for measuring patient acuity for nurse assignment decisions. J Nurs Meas 2012;20:155-85. https://doi.org/10.1891/1061-3749.20.3.155.
- Larson EL, Cohen B, Liu J, Zachariah P, Yao D, Shang J. Assessing intensity of nursing care needs using electronically available data. Comput Inform Nurs 2017;35:617-23.
- Morales-Asencio JM, Porcel-Gálvez AM, Oliveros-Valenzuela R, Rodríguez-Gómez S, Sánchez-Extremera L, Serrano-López FA, et al. Design and validation of the INICIARE instrument, for the assessment of dependency level in acutely ill hospitalised patients. J Clin Nurs 2015;24:761-77. https://doi.org/10.1111/jocn.12690.
- Hurst K, Smith A, Casey A, Fenton K, Scholefield H, Smith S. Calculating staffing requirements. Nurs Manag 2008;15:26-34. https://doi.org/10.7748/nm2008.07.15.4.26.c6616.
- Davis A, Mehrotra S, Holl J, Daskin MS. Nurse staffing under demand uncertainty to reduce costs and enhance patient safety. Asia Pac J Oper Res 2014;31:1-19. https://doi.org/10.1142/S0217595914500055.
- Kortbeek N, Braaksma A, Burger C, Bakker P, Boucherie R. Flexible nurse staffing based on hourly bed census predictions. Int J Prod Econ 2015;161:167-80. https://doi.org/10.1016/j.ijpe.2014.12.007.
- Maenhout B, Vanhoucke M. An integrated nurse staffing and scheduling analysis for longer-term nursing staff allocation problems. Omega-Int J Manage S 2013;41:485-99. https://doi.org/10.1016/j.omega.2012.01.002.
- Harper PR, Powell NH, Williams JE. Modelling the size and skill-mix of hospital nursing teams. J Oper Res Soc 2010;61:768-79. https://doi.org/10.1057/jors.2009.43.
- Saville CE, Griffiths P, Ball JE, Monks T. How many nurses do we need? A review and discussion of operational research techniques applied to nurse staffing. Int J Nurs Stud 2019;97:7-13. https://doi.org/10.1016/j.ijnurstu.2019.04.015.
- Liljamo P, Kinnunen UM, Ohtonen P, Saranto K. Quality of nursing intensity data: inter-rater reliability of the patient classification after two decades in clinical use. J Adv Nurs 2017;73:2248-59. https://doi.org/10.1111/jan.13288.
- van Oostveen CJ, Ubbink DT, Mens MA, Pompe EA, Vermeulen H. Pre-implementation studies of a workforce planning tool for nurse staffing and human resource management in university hospitals. J Nurs Manag 2016;24:194-91. https://doi.org/10.1111/jonm.12297.
- Brennan CW, Daly BJ. Methodological challenges of validating a clinical decision-making tool in the practice environment. West J Nurs Res 2015;37:536-45. https://doi.org/10.1177/0193945914539738.
- Fagerström L, Kinnunen M, Saarela J. Nursing workload, patient safety incidents and mortality: an observational study from Finland. BMJ Open 2018;8. https://doi.org/10.1136/bmjopen-2017-016367.
- Junttila JK, Koivu A, Fagerström L, Haatainen K, Nykänen P. Hospital mortality and optimality of nursing workload: a study on the predictive validity of the RAFAELA Nursing Intensity and Staffing system. Int J Nurs Stud 2016;60:46-53. https://doi.org/10.1016/j.ijnurstu.2016.03.008.
- Twigg D, Duffield C, Bremner A, Rapley P, Finn J. The impact of the nursing hours per patient day (NHPPD) staffing method on patient outcomes: a retrospective analysis of patient and staffing data. Int J Nurs Stud 2011;48:540-8. https://doi.org/10.1016/j.ijnurstu.2010.07.013.
- Barham C, Begum N. Sickness absence from work in the UK. Labour Market Trends 2005;113:149-58.
- Maben J, Griffiths P, Penfold C, Simon M, Anderson JE, Robert G, et al. One size fits all? Mixed methods evaluation of the impact of 100% single-room accommodation on staff and patient experience, safety and costs. BMJ Qual Saf 2016;25:241-56. https://doi.org/10.1136/bmjqs-2015-004265.
- Maben J, Griffiths P, Penfold C, Simon M, Pizzo E, Anderson J, et al. Evaluating a major innovation in hospital design: workforce implications and impact on patient and staff experiences of all single room hospital accommodation. Health Serv Deliv Res 2015;3. https://doi.org/10.3310/hsdr03030.
- Buchan J, Ball J, O’May F. If changing skill mix is the answer, what is the question?. J Health Serv Res Policy 2001;6:233-8. https://doi.org/10.1258/1355819011927549.
- Aiken LH, Sloane D, Griffiths P, Rafferty AM, Bruyneel L, McHugh M, et al. Nursing skill mix in European hospitals: cross-sectional study of the association with mortality, patient ratings, and quality of care. BMJ Qual Saf 2017;26:559-68. https://doi.org/10.1136/bmjqs-2016-005567.
- Kessler I, Heron P, Dopson S, Magee H, Swain D, Askham J. The Nature and Consequences of Support Workers in a Hospital Setting. Southampton: NIHR Service Delivery and Organisation programme; 2010.
- Blay N, Duffield CM, Gallagher R, Roche M. Methodological integrative review of the work sampling technique used in nursing workload research. J Adv Nurs 2014;70:2434-49. https://doi.org/10.1111/jan.12466.
- Harrison J. Addressing increasing patient acuity and nursing workload. Nurs Manag 2004;11:20-5. https://doi.org/10.7748/nm2004.07.11.4.20.c1984.
- Department of Health and Social Care . Comprehensive Critical Care: A Review of Adult Critical Care Services 2000.
- Intensive Care Society . Levels of Critical Care for Adult Patients: Standards and Guidelines 2002.
- Intensive Care Society . Standards for Intensive Care Units 1997.
- AUKUH . AUKUH Acuity Dependency Tool Implementation Resource Pack 2007.
- Merrifield N. Exclusive: nurse training to be launched ‘to combat incorrect use of safe staffing tool’. Nursing Times 2018.
- Goldstone LA, Ball JA, Collier MM. Monitor: An Index of the Quality of Nursing Care for Acute Medical and Surgical Wards. Newcastle upon Tyne: Newcastle upon Tyne Polytechnic Products Ltd; 1983.
- Hurst K. Relationships between patient dependency, nursing workload and quality. Int J Nurs Stud 2005;42:75-84. https://doi.org/10.1016/j.ijnurstu.2004.05.011.
- Hurst K. Developing and Validating AUKUH’s WP–D System. Leeds: Leeds University; 2005.
- Hurst K. 2015. http://shelfordgroup.org/library/documents/SNCT_calculator_adult_May_2015.xls (accessed 19 November 2018).
- Smith J, Forde V, Goodman M, Cannaby AM, Radford M. How to keep score of acuity and dependency. Nurs Manag 2009;16. https://doi.org/10.7748/nm2009.12.16.8.14.c7392.
- Blastorah M, Alvarado K, Duhn L, Flint F, McGrath P, Vandevelde-Coke S. Development and evaluation of an RN/RPN utilization toolkit. Nurs Leadersh 2010;23:33-50. https://doi.org/10.12927/cjnl.2010.21733.
- NHS England . Bed Availability and Occupancy Data – Overnight n.d. www.england.nhs.uk/statistics/statistical-work-areas/bed-availability-and-occupancy/bed-data-overnight/ (accessed 25 February 2020).
- Curtis L, Burns A. Unit Costs of Health and Social Care 2017. Canterbury: Personal Social Services Research Unit, University of Kent; 2017.
- Wood VJ, Vindrola-Padros C, Swart N, McIntosh M, Crowe S, Morris S, et al. One to one specialling and sitters in acute care hospitals: a scoping review. Int J Nurs Stud 2018;84:61-77. https://doi.org/10.1016/j.ijnurstu.2018.04.018.
- NHS Improvement . Reference Costs (2017 18) 2018. https://improvement.nhs.uk/resources/reference-costs/#rc1718 (accessed 25 February 2020).
- NHS Improvement . Agency Rules 2018. https://improvement.nhs.uk/documents/2827/Agency_rules_-_2018_final_draft.pdf (accessed 25 February 2020).
- Sermeus W, Aiken LH, Van den Heede K, Rafferty AM, Griffiths P, Moreno-Casbas MT, et al. Nurse forecasting in Europe (RN4CAST): rationale, design and methodology. BMC Nurs 2011;10. https://doi.org/10.1186/1472-6955-10-6.
- Ball JE, Murrells T, Rafferty AM, Morrow E, Griffiths P. ‘Care left undone’ during nursing shifts: associations with workload and perceived quality of care. BMJ Qual Saf 2014;23:116-25. https://doi.org/10.1136/bmjqs-2012-001767.
- Smeds Alenius L, Tishelman C, Runesdotter S, Lindqvist R. Staffing and resource adequacy strongly related to RNs’ assessment of patient safety: a national study of RNs working in acute-care hospitals in Sweden. BMJ Qual Saf 2014;23:242-9. https://doi.org/10.1136/bmjqs-2012-001734.
- Gunnarsdóttir S, Clarke SP, Rafferty AM, Nutbeam D. Front-line management, staffing and nurse-doctor relationships as predictors of nurse and patient outcomes. A survey of Icelandic hospital nurses. Int J Nurs Stud 2009;46:920-7. https://doi.org/10.1016/j.ijnurstu.2006.11.007.
- Schubert M, Clarke SP, Aiken LH, de Geest S. Associations between rationing of nursing care and inpatient mortality in Swiss hospitals. Int J Qual Health Care 2012;24:230-8. https://doi.org/10.1093/intqhc/mzs009.
- Patterson J. The effects of nurse to patient ratios. Nurs Times 2011;107:22-5.
- Nursing Times . Unsocial Hours Pay Deal for Nurses Finally Tabled 2008. www.nursingtimes.net/ (accessed April 2019).
- Efron B, Tibshirani RJ. An Introduction to the Bootstrap. Chapman and Hall/CRC; 1994.
- Davison AC, Hinkley DV. Bootstrap Methods and Their Application. Cambridge: Cambridge University Press; 1999.
- Taylor S. Business Statistics for Non-mathematicians. Basingstoke: Palgrave Macmillan; 2007.
- Bates D, Maechler M, Bolker B, Walker S. Fitting linear mixed-effects models using lme4. J Stat Software 2015;67:1-48. https://doi.org/10.18637/jss.v067.i01.
- Macal CM. Everything you need to know about agent-based modelling and simulation. J Simul 2017;10:144-56. https://doi.org/10.1057/jos.2016.7.
- Macal CM, North MJ. Tutorial on agent-based modelling and simulation. J Simul 2017;4:151-62. https://doi.org/10.1057/jos.2010.3.
- Monks T, Currie CSM, Onggo BS, Robinson S, Kunc M, Taylor SJE. Strengthening the reporting of empirical simulation studies: introducing the STRESS guidelines. J Simul 2019;13:55-67. https://doi.org/10.1080/17477778.2018.1442155.
- Nelson BM. The MORE Plot: Displaying Measures of Risk and Error from Simulation Output n.d. https://doi.org/10.1109/WSC.2008.4736095.
- Sargent RG. Verification and validation of simulation models. J Simul 2013;7:12-24. https://doi.org/10.1057/jos.2012.20.
- Law AM. A Tutorial on Design of Experiments for Simulation Modeling n.d.
- Aiken LH, Sermeus W, Van den Heede K, Sloane DM, Busse R, McKee M, et al. Patient safety, satisfaction, and quality of hospital care: cross sectional surveys of nurses and patients in 12 countries in Europe and the United States. BMJ 2012;344. https://doi.org/10.1136/bmj.e1717.
- Patrician PA, Loan L, McCarthy M, Fridman M, Donaldson N, Bingham M, et al. The association of shift-level nurse staffing with adverse patient events. J Nurs Adm 2011;41:64-70. https://doi.org/10.1097/NNA.0b013e31820594bf.
- NHS Digital . Summary Hospital-Level Mortality Indicator (SHMI) – Deaths Associated With Hospitalisation, England, January 2017 – December 2017 2018. https://digital.nhs.uk/data-and-information/publications/clinical-indicators/shmi/archive/shmi-april-2017---march-2018 (accessed 25 February 2020).
- NHS Digital . Seven-Day Services Length of Stay Indicator (Data Set) 01 Apr 2017 to 31 Mar 2018 2018. https://digital.nhs.uk/data-and-information/publications/clinical-indicators/seven-day-services/archive/mi-seven-day-services---england-april-2017---march-2018 (accessed 25 February 2020).
- Nurminen M. To use or not to use the odds ratio in epidemiologic analyses?. Eur J Epidemiol 1995;11:365-71. https://doi.org/10.1007/BF01721219.
- Stare J, Maucort-Boulch D. Odds ratio, hazard ratio and relative risk. Metodoloski Zvezki 2016;13.
- Hoad K, Robinson S, Davies R. Automating DES Output Analysis: How Many Replications to Run n.d. https://doi.org/10.1109/WSC.2007.4419641.
- Cheng R. Non-standard Parametric Statistical Inference. New York, NY: Oxford University Press; 2017.
- Koo TK, Li MY. A Guideline of selecting and reporting intraclass correlation coefficients for reliability research. J Chiropr Med 2016;15:155-63. https://doi.org/10.1016/j.jcm.2016.02.012.
- Van den Heede K, Sermeus W, Diya L, Clarke SP, Lesaffre E, Vleugels A, et al. Nurse staffing and patient outcomes in Belgian acute hospitals: cross-sectional analysis of administrative data. Int J Nurs Stud 2009;46:928-39. https://doi.org/10.1016/j.ijnurstu.2008.05.007.
- Dall TM, Chen YJ, Seifert RF, Maddox PJ, Hogan PF. The economic value of professional nursing. Med Care 2009;47:97-104. https://doi.org/10.1097/MLR.0b013e3181844da8.
- Yankovic N, Green LV. Identifying good nursing levels: a queuing approach. Oper Res 2011;59:942-55. https://doi.org/10.1287/opre.1110.0943.
- Ball JE, Griffiths P, Rafferty AM, Lindqvist R, Murrells T, Tishelman C. A cross-sectional study of ‘care left undone’ on nursing shifts in hospitals. J Adv Nurs 2016;72:2086-97. https://doi.org/10.1111/jan.12976.
- Harless DW, Mark BA. Nurse staffing and quality of care with direct measurement of inpatient staffing. Med Care 2010;48:659-63. https://doi.org/10.1097/MLR.0b013e3181dbe200.
- Saposnik G, Redelmeier D, Ruff CC, Tobler PN. Cognitive biases associated with medical decisions: a systematic review. BMC Med Inform Decis Mak 2016;16. https://doi.org/10.1186/s12911-016-0377-1.
- Dziuba-Ellis J. Float pools and resource teams: a review of the literature. J Nurs Care Qual 2006;21:352-9. https://doi.org/10.1097/00001786-200610000-00013.
- Roberts D. Competence increases comfort for float nurses. Medsurg Nurs 2004;13.
- Dall’ora C, Griffiths P. Flexible nurse staffing in hospital wards: the effects on costs and patient outcomes. Health Work Evid Briefs 2017;3.
- NHS Improvement . Care Hours Per Patient Day (CHPPD) Data 2018. https://improvement.nhs.uk/resources/care-hours-patient-day-chppd-data/ (accessed 23 January 2019).
- Bridges J, Griffiths P, Oliver E, Pickering RM. Hospital nurse staffing and staff-patient interactions: an observational study. BMJ Qual Saf 2019;28:706-13. https://doi.org/10.1136/bmjqs-2018-008948.
- Department of Health and Human Services . Guide to Implementation of the Safe Patient Care (Nurse to Patient and Midwife to Patient Ratios) Amendment Act 2019 2019. www.anmfvic.asn.au/∼/media/files/anmf/vic%20branch%20policies%20and%20position%20statements/safe%20patient%20care%20act.pdf?la=en (accessed 25 February 2020).
- Moberly T. Sickness absence rates across the NHS. BMJ 2018;361. https://doi.org/10.1136/bmj.k2210.
- Dall’Ora C, Ball J, Redfern O, Recio-Saucedo A, Maruotti A, Meredith P, et al. Are long nursing shifts on hospital wards associated with sickness absence? A longitudinal retrospective observational study. J Nurs Manag 2019;27:19-26. https://doi.org/10.1111/jonm.12643.
- Marseille E, Larson B, Kazi DS, Kahn JG, Rosen S. Thresholds for the cost-effectiveness of interventions: alternative approaches. Bull World Health Organ 2015;93:118-24. https://doi.org/10.2471/BLT.14.138206.
- Spetz J, Chapman S, Herrera C, Kaiser J, Seago J, Dower C. Assessing the impact of California’s nurse staffing ratios on hospitals and patient care. Calif Health Care Found Issue Brief 2009:1-10.
- Chan ZC, Tam WS, Lung MK, Wong WY, Chau CW. A systematic literature review of nurse shortage and the intention to leave. J Nurs Manag 2013;21:605-13. https://doi.org/10.1111/j.1365-2834.2012.01437.x.
- Hayes LJ, O’Brien-Pallas L, Duffield C, Shamian J, Buchan J, Hughes F, et al. Nurse turnover: a literature review - an update. Int J Nurs Stud 2012;49:887-905. https://doi.org/10.1016/j.ijnurstu.2011.10.001.
- Sarre S, Maben J, Griffiths P, Chable R, Robert G. The 10-year impact of a ward-level quality improvement intervention in acute hospitals: a multiple methods study. Health Serv Deliv Res 2019;7. https://doi.org/10.3310/hsdr07280.
- Robert G, Sarre S, Maben J, Griffiths P, Chable R. Exploring the sustainability of quality improvement interventions in healthcare organisations: a multiple methods study of the 10-year impact of the ‘Productive Ward: Releasing Time to Care’ programme in English acute hospitals. BMJ Qual Saf 2020;29:31-40. https://doi.org/10.1136/bmjqs-2019-009457.
- Aiken LH, Cimiotti JP, Sloane DM, Smith HL, Flynn L, Neff DF. Effects of nurse staffing and nurse education on patient deaths in hospitals with different nurse work environments. Med Care 2011;49:1047-53. https://doi.org/10.1097/MLR.0b013e3182330b6e.
- Bezdek JC. Editorial. Fuzzy models –what are they, and why?. IEEE Trans Fuzzy Syst 1993;1:1-6. https://doi.org/10.1109/TFUZZ.1993.6027269.
- Tang J, Wang DW, Fung RY, Yung KL. Understanding of fuzzy optimization: theories and methods. J Syst Sci Comp 2004;17:117-36.
- Hendry C, Walker A. Priority setting in clinical nursing practice: literature review. J Adv Nurs 2004;47:427-36. https://doi.org/10.1111/j.1365-2648.2004.03120.x.
- Burke EK, De Causmaecker P, Berghe GV, Van Landeghem H. The state of the art of nurse rostering. J Scheduling 2004;7:441-99. https://doi.org/10.1023/B:JOSH.0000046076.75950.0b.
- de Causmaecker P, Vanden Berghe G. A categorisation of nurse rostering problems. J Scheduling 2011;14:3-16. https://doi.org/10.1007/s10951-010-0211-z.
- Needleman J, Shekelle PG. More ward nursing staff improves inpatient outcomes, but how much is enough?. BMJ Qual Saf 2019;28:703-5. https://doi.org/10.1136/bmjqs-2018-009266.
- Knuth DE. The Art of Computer Programming, Vol. 3: Sorting and Searching. Addison-Wesley; 1981.
- NHS Improvement . FOI: Agency Price Cap Overrides 2017. https://improvement.nhs.uk/about-us/corporate-publications/publications/FOI-agency-price-cap-overrides/ (accessed 1 March 2018).
- Nursing and Midwifery Council . What Is a Nursing Associate? 2019. www.nmc.org.uk/standards/nursing-associates/what-is-a-nursing-associate/ (accessed 10 May 2018).
Appendix 1 Methodological details
Data specifications
Variable | Content/format |
---|---|
Ward | Ward name or code (CHARACTER STRING) |
Date | Date for which census applies (DD/MM/YYYY) |
Time | Time for which census applies (HH:MM 24 hour) |
Total patient count reported | If collected (rather than calculated as sum category 0 to category 3) (INTEGER) |
Category 0 | Count of patients in category (INTEGER) |
Category 1a | Count of patients in category (INTEGER) |
Category 1b | Count of patients in category (INTEGER) |
Category 2 | Count of patients in category (INTEGER) |
Category 3 | Count of patients in category (INTEGER) |
Completed by | Indicator of who completed assessment |
Variable | Content/format |
---|---|
Ward | Ward name or code (CHARACTER STRING) |
Date | Date shift starts (DD/MM/YYYY) |
Start time | Time shift starts (HH:MM 24 hour) |
End time | Time shift ends (HH:MM 24 hour) |
Break | Length of scheduled break (HH:MM) |
Shift type | Designation (e.g. early/late/night). Must indicate whether shift worked on ward |
Grade | Agenda for Change bands or system codes (CHARACTER STRING) |
Staff type | Substantive/bank/agency (CHARACTER STRING) |
Staff ID | Indicator of person working this shift. Pseudonymised/ staff code (INTEGER) |
Data item | Content/format |
---|---|
Patient ID number | Pseudonymised |
Spell number | |
Episode number | Within spell |
Admission date | Date (DD/MM/YYYY) |
Admission time | Time (24hr HH:MM) |
Discharge date | Date (DD/MM/YYYY) |
Discharge time | Time (24hr HH:MM) |
Ward start date/time | Date/Time (DD/MM/YYYY HH:MM) |
Inward activity | Admission/transfer |
Outward activity | Discharge/transfer |
Ward code | Text |
Ward name | Text |
Extraction of worked shifts and identification of worked hours
We derived the staffing variables (total/registered nurse/nursing support worker hours per day, skill mix and distribution of staff over the day) from roster systems. Data provided from roster systems were in the form of one row per shift and staff member. The data fields included duty date, actual shift start and end times, planned shift start and end times, staff type (substantive, bank or agency), ward and break duration, as well as the post title and band either recorded together across multiple fields or contained within longer shift type descriptions. For hospital trust B, the roster data were provided from separate systems for substantive and non-substantive (bank and agency) staff, with different data fields.
The following shift inclusion and exclusion criteria were applied. Posts that we classed as nursing roles and so included in the analysis were different types of registered nurses and nursing support workers, called by a variety of names (staff nurses, senior staff nurses, course nurses, junior ward sisters, ward sisters, charge nurses, advanced nurse practitioners, nurse specialists, community nurses, specialist nurse practitioners, nursing support workers, community health-care assistants, assistant practitioners, bank support workers, patient support workers, acute coronary syndrome nurses, advanced clinical specialist nurses and phlebotomists). Although maternity wards were excluded from the analysis, we included registered midwives and maternity care assistants who worked as bank/agency staff on other wards at hospital trust A. Staff in non-nursing roles, such as ward clerks, housekeepers, administrative/clerical staff and therapists, were excluded. Trainees, students and honorary staff, when recorded in the data, were also excluded because they do not count towards the ward establishment. Our analyses focus on worked shifts, so any cancelled shifts, rest shifts and unfilled bank/agency shifts were excluded.
Agenda for Change bands were used throughout the analysis for costings and to distinguish between registered nurses (at least band 5) and nursing support workers (below band 5). These bands were extracted from text in the relevant data fields for each hospital trust. Bands were not recorded for some advanced nurse practitioners, patient support workers and bank support workers at hospital trust A, where they were assumed to be band 7, 3 and 3, respectively.
Actual shift start and end times were usually provided; however, where the actual end times were missing, we assumed that these were the same as the planned end times. Hospital trust B did not have breaks recorded for bank and agency staff. Therefore, we assumed that small positive differences (0–1 hour inclusive) between planned and actual work time were due to breaks, but that otherwise differences were due to a shift being worked of a different length from that planned.
Recording of requirements for ‘specialing’
Specialing staffing requirements are in addition to the requirement generated from the SNCT, and hospital trusts have made adjustments to the tool or use parallel processes to deal with specials. The four hospital trusts recorded specials in different ways. Hospital trust A provided reports of requests for staff to special patients, as specialing was covered by bank/agency staff. From September 2017 onward, these contained shift names rather than times. To deal with this, we assumed that the most common shift times from the earlier data (night shifts from 7 p.m. to 7.30 a.m., late shifts from 1.30 p.m. to 7.30 p.m., early shifts from 7 a.m. to 1 p.m. and long day shifts from 7 a.m. to 7.30 p.m.). We converted these shift times into numbers of specials present in each ward at the start of each 6-hour shift. Hospital trusts B and C provided the numbers of specials recorded by the nurse in charge at census times. Hospital trust B also recorded cohort specials. Hospital trust D has defined extra categories, levels 1c and 1d, for patients requiring specialing by nursing support workers or registered nurses, respectively. This means that these patients are not included in the standard levels, as they were for the other hospital trusts. We assume that they are in level 1b.
Staffing variables (calculations)
We calculated the specials multiplier by substituting 24 hours per day in Equation 5 and rearranging to get the total required daily establishment in WTE for one specialed patient:
Correspondence between Safer Nursing Care Tool multipliers and daily care hours
Level | General wards | Acute admissions units | ||
---|---|---|---|---|
Multipliera (WTE per patient) | Estimated total care requirementb (HPPD) | Multipliera (WTE per patient) | Estimated total care requirementb (HPPD) | |
0 | 0.99 | 4.35 | 1.27 | 5.58 |
1a | 1.39 | 6.10 | 1.66 | 7.29 |
1b | 1.72 | 7.55 | 2.08 | 9.13 |
2 | 1.97 | 8.65 | 2.26 | 9.92 |
3 | 5.96 | 26.2 | 5.96 | 26.2 |
Special/specialed cohort | 5.47 | 24 | 5.47 | 24 |
Dealing with sub-wards
Some wards consist of several sub-wards that each separately recorded the staffing adequacy and SNCT level counts, but are rostered and/or recorded in patient administration systems as one ward.
We summed up the numbers of patients in each level across sub-wards to obtain the ward level counts, and discarded instances where data for at least one sub-ward were missing. As sub-wards may care for different types of patients, estimating level counts for the whole ward from partial data may not be reliable.
When calculating the completeness of the variables ‘enough staff for quality’, ‘nursing care left undone’ and ‘staff breaks missed’, we considered data for sub-wards missing if they were missing for all sub-wards. For these staffing adequacy questions with yes/no responses, we considered data valid if they were valid for at least one sub-ward. We combined the answers to the questions at sub-ward level into an answer at the ward level by using majority vote of valid answers. If there were equal numbers of sub-wards answered ‘yes’ and ‘no’, we took the negative answer (‘no’ for ‘enough staff for quality’, ‘yes’ for ‘nursing care left undone’ and ‘yes’ for ‘staff breaks missed’).
For the reported total staffing requirement variable (the sum of the answers to the questions ‘nursing support workers needed’ and ‘registered nurses needed’), we considered data for sub-wards missing if they were missing for any sub-ward. We combined the answers to the questions at sub-ward level into an answer at the ward level by summing up the requested registered nurses/nursing support workers across sub-wards. We used the normal validity criteria on the whole ward answer.
Staff costs
Agenda for Change band | Substantive staff | Bank staff | Agency staff | ||||||
---|---|---|---|---|---|---|---|---|---|
Salarya | Employer oncost | Total cost per hourb | Salary | Employer oncostc | Total cost per hourb | Salaryd (agency cap maximum) | Employer oncost | Total cost per houre | |
1 | 15,516 | 8534 | 12.29 | ||||||
2 | 16,536 | 3548 | 12.63 | 16,536 | 2359 | 11.88 | 17,978 | 9888 | 14.24 |
3 | 18,333 | 4054 | 14.08 | 18,333 | 2736 | 13.25 | 19,655 | 10,810 | 15.56 |
4 | 20,279 | 4602 | 15.65 | 20,279 | 3144 | 14.73 | 22,458 | 12,352 | 17.79 |
5 | 26,038 | 6225 | 20.48 | 26,038 | 4353 | 19.3 | 28,462 | 15,654 | 22.55 |
6 | 32,342 | 8002 | 25.62 | 32,342 | 5676 | 24.14 | 35,225 | 19,374 | 27.9 |
7 | 38,801 | 9822 | 30.87 | 38,801 | 7032 | 29.1 | 41,373 | 22,755 | 32.77 |
8a | 45,544 | 11,722 | 36.36 | 45,544 | 8447 | 34.28 | 48,034 | 26,419 | 38.05 |
8b | 54,307 | 14,191 | 43.49 | 54,307 | 10,287 | 41.01 | 57,640 | 31,702 | 45.66 |
8c | 63,703 | 16,839 | 51.14 | 63,703 | 12,259 | 48.23 | 68,484 | 37,666 | 54.25 |
8d | 75,171 | 20,071 | 60.47 | 75,171 | 14,666 | 57.04 | 82,434 | 45,339 | 65.3 |
9 | 88,526 | 23,834 | 71.34 | 88,526 | 17,469 | 67.3 | 99,437 | 54,690 | 78.77 |
Agenda for Change band | Weekdays (8 p.m. to 6 a.m.) and any time Saturday | Any time Sunday and public holidays |
---|---|---|
1 | 1.5 | 2.00 |
2 | 1.44 | 1.88 |
3 | 1.37 | 1.74 |
4–9 | 1.30 | 1.60 |
Simulation model technical details
Implementation and experimentation
The simulation was developed on a Microsoft Windows® 10 (Microsoft Corporation, Redmond, WA, USA) Enterprise operating system, version 1709, build number 16299.726, in AnyLogic 8 researcher edition software version 8.3.2, build number 8.3.2.201807061745 x64. This used Java™ 2 Standard Edition 8.0 (Oracle Corporation, Redwood Shores, CA, USA) and Fluid library version 2.0.0 (The AnyLogic Company, Quorn, UK; www.anylogic.com).
We used the default random number generator in AnyLogic, an instance of the Java class Random, which is a linear congruential generator.
Time is modelled as fixed time steps. There are no concurrent events that are interdependent.
There is no warm-up period or model initialisation as each day/shift is independent of the next. The model is stochastic. The run length is 1 year, and the time units are 6-hour shifts (the model also works for days). The number of replications is 10.
Running one experiment (1 year, 10 runs) takes between 10 and 20 minutes for the different hospital trusts on a Dell Latitude (Dell Inc., Round Rock, TX, USA) laptop with an Intel® Core™ (Intel Corporation, Santa Clara, CA, USA) i5-7300U processor with 2.60-GHz CPU speed, 8-GB RAM and 32 GB ROM.
Computer model sharing statement
For a video demonstration see https://eprints.soton.ac.uk/430632/ (accessed 25 February 2020). The simulation model is available from the corresponding author on reasonable request. The AnyLogic simulation software can be downloaded from www.anylogic.com/downloads/ (accessed 25 February 2020).
Simulation model logic
An overview of the simulation logic is shown in Figure 8. Each stage is described in more detail in the following sections. The simulation was designed to work for any number of wards. The wards are ‘agents’ that interact with each other (within directorates) by lending/borrowing staff in an attempt to cover shortfalls of staffing in one ward with surplus in another. Outputs are updated at the end of each time step (day/shift) and aggregated at the end of each year.
FIGURE 8.
Simulation model overview diagram.

Before running the simulation
Before running the simulation for a particular hospital trust, information about its wards is imported from Microsoft Excel® (Microsoft Corporation, Redmond, WA, USA) files to fill database template tables within the simulation. The three files contain occupancy data, acuity/dependency data and other ward information. The occupancy distributions consist of the number of patients, labelled with the ward identification numbers (a contiguous number for internal purposes and the number used for external reporting), day of the week and 6-hour-shift. The acuity/dependency distributions consist of the proportions of patients in each SNCT acuity/dependency level and the proportion of patients who require specialing, labelled with the ward identification numbers and observation number (used when sampling a row). The other ward information consists of the baseline staffing levels for each scenario (in WTE), the skill mix (in the morning, afternoon, evening and night, and over a 24-hour period), the distribution of staff over 24 hours (proportion of staff deployed in the morning/afternoon/evening/night), the directorate number, whether or not the ward is an acute admissions unit and some purely descriptive information (directorate, medical or surgical).
The probabilities of requests for temporary staff being fulfilled is imported from a Microsoft Excel file into another database template table. The template allows probabilities to differ between bank and agency, up to three staff types, weekend and weekday, and time period (morning, afternoon, evening and night, and over a 24-hour period).
Next, further parameter values that apply globally to the whole hospital trust (the system) are set. These parameters relate to:
-
nursing staff requirements – acuity/dependency multipliers, specials multiplier, standard deviation of multipliers, minimum number of registered nurses needed (constraint), demand for registered nurses with a particular skill
-
permanent staff – the baseline staffing level, the data sample used to calculate the staffing level, the staff types, absence chance for each staff type, absence length, proportion of registered nurses with a particular skill
-
re-deployed staff – re-deployment rules, priority sequences for providing and receiving re-deployed staff, efficiency of re-deployed staff, re-deployed staff shift length
-
temporary staff – rules for requesting temporary staff, efficiency of bank and agency staff, temporary staff shift length
-
display settings – the understaffing criterion to plot in charts, e.g. 15% or more under requirement
-
general settings – step length, round-down bound used when converting required hours to requested hours.
At start of a run
At the start of a run, variables tracking time (the step number and the shift number) are reset to zero. The wards, staff types and sharing groups are counted. Occupancy distributions are created from the occupancy data table. Data are placed in arrays, which are convenient structures for working with multidimensional data.
Then, the establishment (number of staff employed in WTE) is converted to the number of planned deployed hours per time step (6-hour shift or day), including applying the skill mix, rounding to whole people and dealing with minimum constraints, as follows. Note that the establishment does not need to be a whole number as staff may work part time, but the planned number of staff to deploy on each 6-hour shift should be a whole number. As in our other analyses, we use Equation 5 for converting the planned staffing in WTE to the planned total care hours per day (see Appendix 1).
The planned skill mix (proportion of staff who are registered nurses) and distribution of staff over the day in each ward is set as the average observed for that ward. There is a constraint that there must be at least one registered nurse present on each ward, so if the registered nurse hours is under 6, this is rounded up to 6. Otherwise, the registered nurse hours are rounded up or down to the nearest 6 hours. The remaining planned hours are assigned to nursing support workers, and again are rounded up or down to the nearest 6 hours.
For example, suppose that the planned nursing hours for a morning shift on a particular ward are 18, and the skill mix is 50%. This is equivalent to 9 hours of registered nurse time, which is rounded up to 12 hours. There are 6 hours left to cover, which are assigned to nursing support workers.
The planned deployed hours per day (sum over the four shifts) are converted back into WTE to enable calculation of the cost of employing this number of permanent staff.
Before time step
Before each time step, that is before the simulation switches to the next period (6-hour shift or day), the variables for this period are updated. These variables are the time step, the shift (one to four), the day type (weekday, Saturday or Sunday/bank holiday) and the planned staffing level for this shift. The deployment array (numbers of staff from each source and of each staff type deployed on each ward in what capacity) is reset at zero ready to be filled in at the next stages.
Before time step, in each ward
Next, the required staffing for this period is calculated for each ward in turn. For this, first the number of patients on the ward is sampled from the occupancy distribution for that ward, day of week and shift (morning, afternoon, evening or night).
Second, the acuity/dependency profile is sampled from the acuity/dependency data. This is done by selecting a random observation for that ward (we assumed that there were no day of week or time of day patterns). For each patient, the probability of being in each acuity/dependency category and the probability of requiring specialing are the corresponding observed proportions. The required staffing per patient (in WTE) is sampled based on the patient’s acuity/dependency category and specialing requirements. This is converted into the required staffing level for this period using the skill mix, distribution of staff over the day and minimum constraints (as for the planned staffing levels), but is not rounded.
On time step, in each ward
On the time step, that is, immediately when the period begins, the number of hours of staffing provided by permanent staff in this period is calculated for each ward. For this, the number of planned staff who are not unexpectedly absent (i.e. at short notice) is calculated. The chance of being unexpectedly absent can differ between staff types in the model. All of these staff are allocated to their home ward to start with. The (absolute) shortfall for each staff type is calculated as required minus allocated hours. Where applicable, the simulation checks which of the registered nurses working are IV trained (sampled probabilistically).
Similarly, the spare hours (hours that could be re-deployed to another ward) for each staff type is calculated. This is the allocated minus the required hours, rounded down to the nearest multiple of ‘re-deployed hours chunk’, as staff can be re-deployed only for fixed time periods.
On time step
Next, staff are re-deployed within sharing groups (directorates) to attempt to cover shortfalls for each staff type, as shown in Figure 9. The shortfall is rounded up or down (depending on the round-down bound) to the nearest multiple of ‘re-deployment chunks’. Requests for extra staff are triggered if the rounded shortfall for that staff type is more than zero, and if either the total shortfall or the staff type shortfall is more than the trigger (6 hours). To decide the priority of re-deploying extra staff to wards, lists of wards are sorted using the bubble sort algorithm,160 which works by comparing the shortfall as a proportion of the requirement (or spare hours as a proportion of requirement) for adjacent wards in the list and swapping them if they are in the wrong order.
FIGURE 9.
Summary process flow of staff re-deployment.

Following this, for the wards that are still requesting extra staff, for each staff type, first bank and then agency staff are requested, as shown in Figure 10. The hours requested are the shortfall rounded up or down (depending on the round-down bound) to the nearest multiple of ‘external work time’. The probability of a request for temporary staff being fulfilled depends on the staff source, the staff type, whether it is a weekday or a weekend and the time period.
FIGURE 10.
Process flow of hiring temporary staff.

Then, the effective staffing on each ward, given that re-deployed and temporary staff are less efficient than permanent staff, is calculated. The effective shortfall on each ward is calculated.
The staffing adequacy (understaffed, adequately staffed or overstaffed) on each ward is assessed according to a range of criteria (e.g. understaffing can be measured as ‘more than 15% under the requirement’, ‘more than one person short’). Depending which staffing adequacy criterion was chosen to be displayed, the state chart for each ward is updated (see Figure 1). The running totals of understaffed, adequately staffed and overstaffed time periods per ward are updated, as are the charts tracking their relative numbers. The array of the total number of staff deployed on wards from different sources and of different types is updated.
At the end of run (1 year)
At the end of each run, the total permanent staff employed (in WTE) and the number of agency and bank hours worked across the whole hospital are summed by staff type.
The total yearly costs are calculated. For this, first, the costs of employing staff and hiring temporary staff at the standard rates are calculated. Then, bonuses for unsocial hours for substantive, bank and agency staff are added.
The results of the run at both the ward and the hospital level are exported to Microsoft Excel.
Simulation input parameters
Parameter | Description | Value/distribution | Source/rationale |
---|---|---|---|
Step length | Length of time steps. Demand, supply and reallocation calculations happen at the beginning of each time step | 6 hours | Six-hour shifts – captures main staffing changes over day |
Round-down bound |
Used for dealing with fractional people when requesting permanent staff, internal re-deployments or temporary staff The number of hours is rounded either up or down to the nearest assignment length (4 or 6 hours; see rows ‘Temporary staff shift length’ and ‘Re-deployed staff shift length’), depending on the round-down bound. 0 means always round down, 1 means always round up, 0.5 means round to nearest assignment length and if halfway between then round up For example, the hours are divided by 6. If the remainder divided by 6 is below the round-down bound, the hours are rounded down to the nearest 6; otherwise they are rounded up to the nearest 6 |
0.5 | Common way of rounding |
Multipliers | The SNCT multipliers |
For general wards: 0.99, 1.39, 1.72, 1.97 and 5.96 For acute admissions units: 1.27, 1.66, 2.08, 2.26 and 5.96 |
From the SNCT guidance19 |
Specials multiplier | The extra hours of care for a specialed patient | 5.4656 | Assumes additional 24-hour one-to-one care. Specials multiplier = (24 × 1.22 × 7)/37.5 |
Standard deviation of multipliers | Standard deviation of HPPD required within an acuity/dependency level | 10% of multiplier (0 for specials). Sample from normal distribution truncated at mean ± 2 standard deviations | Assumption. Given the lack of data on variability of patient nursing requirements within acuity/dependency levels, we investigated different values in sensitivity analyses |
Staffing level | Rule for setting the establishment from SNCT data | Varied across establishment scenarios – ‘flexible (low)’, ‘standard’, ‘high’ or ‘vacancy’ – and across data samples – ‘January’, ‘June’, ‘all’ or ‘months’ | |
Unanticipated absence chance | The chance that a registered nurse/nursing support worker will be absent at short notice | 4% for nursing support workers, 3% for registered nurses | Assumption. Sensitivity analysis. The total nurse absence rate was 4.5% in 2016/17,145 but it is not clear how much of this was anticipated and so could be dealt with by adapting the roster. According to our hospital trust partners, nursing support worker absence rate is higher than registered nurse absence rate |
Absence length | The length of an absence (time steps are independent of one another) | 6 hours | Half of a 12-hour shift. Experts (hospital trust PIs) agreed reasonable |
Temporary staff shift length | The length of time temporary staff (bank/agency) are deployed for | 6 hours | Half of a 12-hour shift. Experts (hospital trust PIs) agreed reasonable |
Re-deployed staff shift length | The length of time substantive staff are re-deployed to another ward | 4 hours for nursing support workers and 6 hours for registered nurses | Half of an 8- or 12-hour shift. Experts (hospital trust PIs) agreed reasonable |
Staff type allocation rules | When there are staffing shortfalls, the order in which different types of additional staff are allocated | Like-for-like. Bank before agency | Experts (hospital trust PIs) advised that these are reasonable general rules (although they will not always be true) |
Efficiency | The productivity of re-deployed/temporary staff |
Efficiency of re-deployed/bank staff – 90% Efficiency of agency staff – 75% |
Assumption. Recognises that agency staff, who work across different hospitals, will be less familiar with ward processes than re-deployed/bank staff who are familiar with hospital but not necessarily with ward |
Constraint | Minimum number of registered nurses present on each ward shift | One per ward and time step | Assumption. One registered nurse must always be present as there are some tasks only registered nurses can do and nursing support workers require supervision |
Prioritisation sequence | Prioritise wards with biggest proportion shortfall/spare (rule number 1) | Assumption. Recognises that will prioritise wards that are most in need for receiving extra staff/have most spare for providing extra staff. Experts (hospital trust PIs) agreed reasonable | |
Vacancy rate | 0% | Assumption. We also investigated the effect of a 10% vacancy rate in each ward | |
Wards that can lend/borrow staff | Share across directorate | Assumption. Recognises that staff are trained to work in particular areas | |
Probability of a request for temporary staff being fulfilled | Depends on the staff source, staff type, whether it is a weekday or a weekend and the time period | Empirical observed proportions | Based on data on previous-day and same-day staffing requests from hospital trust B |
Parameter | Description | Value/distribution |
---|---|---|
Number of patients | Each time step and, for each ward, the number of patients | Sampled (with replacement) from observed (empirical) distributions of patient counts at 7 a.m., 1 p.m., 7 p.m., 1 a.m. for that ward, separately for each day of the week |
Probabilities of patients being in each acuity/dependency level and that patient requires specialing | Each time step and, for each ward, the probabilities of patients being in each acuity/dependency level and of patient requiring specialing; for example, for one shift on one ward, probabilities are Prob(level 0) = 0.4, Prob(level 1a) = 0.4, Prob(level 1b) = 0.2, Prob(level 2) = 0, Prob(level 3) = 0 and Prob(special) = 0.05 | Sampled with replacement from observed acuity/dependency proportions for that ward and observed specials proportions for that ward |
Planned skill mix | The planned proportion of registered nurses for the morning, afternoon, evening and night 6-hour shifts | The average observed skill mix in each ward for each time period. Note that planned registered nurse/nursing support worker hours are subject to a minimum constraint of one registered nurse and rounded to the nearest 6 hours |
Required skill mix | Of the required staffing each 6-hour shift on each ward, how much is required to be done by registered nurses | The average observed skill mix in each ward for each time period but the required registered nurse/nursing support worker hours are also subject to a minimum constraint of one registered nurse (no rounding) |
Planned distribution of staff over the day | The proportion of planned staffing that occurs in each 6-hour shift (morning, afternoon, evening and night) | The average observed proportion of worked hours in each time period for each ward |
Required distribution of staff over the day | The proportion of daily staffing required in each 6-hour shift (morning, afternoon, evening and night) | As for planned distribution of staff over day |
Variability within acuity/dependency levels
We have no information about the shape and variability of nursing requirement distributions within acuity/dependency levels. Even with relatively small variability within acuity/dependency levels (standard deviation set at 10% of the mean), and assuming that they follow symmetric distributions, the required staffing for patients in levels 1a, 1b and 2 would overlap substantially, as shown in Figure 11, and even more so for a standard deviation at 25% of the mean, as shown in Figure 12.
FIGURE 11.
Histogram of required HPPD if standard deviations within acuity/dependency levels were 10% of the means (multipliers).

FIGURE 12.
Histogram of required HPPD if standard deviations within acuity/dependency levels were 25% of the means (multipliers).

Staff availability
Table 36 shows the empirical percentages of all requested temporary shifts (both bank and agency) that were filled by bank staff at hospital trust B (i.e. assuming that bank staff were asked first). Morning shifts are those that start between 7 a.m. and 1 p.m., afternoon shifts start between 1 p.m. and 7 p.m. and evening/night shifts start between 7 p.m. and 7 a.m. Table 37 shows the percentage of empirical percentages of agency requests that were filled at hospital trust B. We did not have equivalent data for the other hospital trusts.
Requests fulfilled by bank staff | Registered nurses | Nursing support workers |
---|---|---|
Weekday | ||
Morning | 28% | 28% |
Afternoon | 10% | 9% |
Evening/night | 19% | 37% |
Weekend | ||
Morning | 32% | 31% |
Afternoon | 13% | 17% |
Evening/night | 19% | 32% |
Requests fulfilled by agency staff | Registered nurses | Nursing support workers |
---|---|---|
Weekday | ||
Morning | 15% | 36% |
Afternoon | 9% | 5% |
Evening/night | 45% | 44% |
Weekend | ||
Morning | 19% | 43% |
Afternoon | 14% | 14% |
Evening/night | 37% | 39% |
Simulation outputs
Key performance measures | Description |
---|---|
Percentage understaffed patient shifts | We say a shift is ‘understaffed’ if the total effective staffing (i.e. accounting for the lower efficiencies of temporary and re-deployed staff) is < 85% of the estimated requirement according to the SNCT for the shift. This allows a buffer of 15% either side of the estimated requirement within which staffing is considered adequate, as is also done in the RAFAELA tool.47 We consider patient shifts rather than shifts to estimate how many understaffed shifts individual patients are exposed to. We express the understaffed patient shifts (the sum of the occupancies at the start of each understaffed shift) as a percentage of the total patient shifts (the sum of the occupancies at the start of each shift) |
Cost per patient-day | The annual staffing cost divided by the number of patient shifts, multiplied by four (in our model there are four 6-hour shifts in a day) |
Other performance measures | |
Ward establishments | The number of staff employed on each ward under a particular scenario. Measured in WTEs |
Percentage of hours worked by re-deployed/bank/agency staff | Calculated from hours of staff time provided by re-deployed/bank/ agency staff divided by total hours from all staff × 100 |
Percentage overstaffed patient shifts | We say a shift is ‘overstaffed’ if the total effective staffing (i.e. accounting for the lower efficiencies of temporary and re-deployed staff) is more than 15% over the estimated requirement according to the SNCT for the shift |
Percentage of patient shifts with no IV-trained registered nurse | Used only for the scenario looking at IV-trained nurses |
Alternative understaffing criteria | |
Percentage patient shifts with absolute understaffing | ‘Absolute understaffing’ means the total effective staffing is less than the estimated requirement for the shift |
Percentage patient shifts with at least one person short | ‘At least one person short’ means the total effective staffing is at least 6 hours below the estimated requirement for the shift |
Percentage patient shifts with registered nurse shortfall | ‘Registered nurse shortfall’ means the effective registered nurse staffing is < 85% of the estimated registered nurse requirement for the shift |
Percentage patient shifts with nursing support worker shortfall | ‘Nursing support worker shortfall’ means the effective nursing support worker staffing is < 85% of the estimated nursing support worker requirement for the shift |
Percentage patient shifts with both | ‘Both’ means there is both ‘registered nurse shortfall’ and ‘nursing support worker shortfall’ on this shift |
Percentage patient shifts with either | ‘Either’ means there is either ‘registered nurse shortfall’ or ‘nursing support worker shortfall’ on this shift |
Category | Item | Technique(s) used | Justification for technique used | Result/conclusion | Confidence in result |
---|---|---|---|---|---|
Collecting and maintaining data | SNCT data | Pilot study | For finding any issues | It was feasible to collect data on busy wards. Cognitive testing showed staffing adequacy questions were understood as intended | Medium |
Inter-rater reliability | For checking how well SNCT ratings agree between responders | At the shift level, based on the calculation of total staffing scores using the multipliers there was agreement within ±5% of the joint mean on 95% of occasions (in total 847 patients were rated independently by two raters across 81 shifts) | |||
Testing relationships within data | Checking aggregations correct | Checking numbers of records before and after merging data tables | Standard way | Numbers were consistent | High |
Screening for outliers and determining whether correct (and deciding whether to include in model) | Weighted-average multipliers |
Screening: points outside the mean plus or minus 3 standard deviations for weighted-average multipliers Whether or not correct: compared against ward change dates and known unusual times of year (e.g. Christmas and New Year) Deciding whether or not to include in model: removed because likely to be data error |
Standard technique combined with practical insights | Outliers dealt with appropriately | High |
Patient numbers |
Screening: looked at periods with small numbers of patients Whether or not correct: compared against ward change dates and known unusual times of year (e.g. Christmas and New Year) Deciding whether or not to include in model: removed small; numbers of patients during ward change periods but kept if due to unusual time of year (e.g. Christmas) |
Category | Item | Technique(s) used | Details | Justification for technique used | Result/conclusion | Confidence in result |
---|---|---|---|---|---|---|
Assumptions | Independence of requirements between time periods | Face validity | Team meeting, steering group | No data available on this | No data on how time periods may depend on one another, so best we can do | Low |
Independence of requirements in different wards | Face validity | Team meeting, steering group | No data available on this | No reason to suppose wards do interact | Medium | |
Order of re-deploying staff to wards | Face validity | Team meeting, steering group. Expert opinion on how would prioritise re-deploying staff | Because we want to generalise results, we are interested in a sensible likely method rather than capturing the details of how each hospital/directorate currently does this | After a few iterations, agreed internal re-deployment, then bank then agency, and like-for-like substitutions | Medium–high | |
Temporary staff shift length | Face validity | Team meeting, steering group | Because we want generalisable results, we want a sensible approximation | Agreed that using multiples of 6 hours would capture most shift patterns | Medium–high | |
Re-deployed staff shift length | Face validity | Team meeting, steering group | Because we want generalisable results, we want a sensible approximation | Agreed that using multiples of 6 hours for registered nurses and 4 hours for nursing support workers would capture most shift patterns | Medium–high | |
Absence length | Face validity | Team meeting, steering group | Because we want generalisable results, we want a sensible approximation | Agreed that using multiples of 6 hours would capture most shift patterns | Medium–high | |
Model representation | Rounding logic | Structured walkthrough | With PG and JJ, showed Microsoft Excel sheet with step-by-step calculations for translating baseline staffing in WTE into registered nurse and nursing support worker staffing per shift | To agree sensible logic for rounding staffing levels with other quantitative researchers | Agreed after a few iterations | High |
Structure, detail and aggregate relationships (wards and hospitals), logic, mathematical (e.g. calculating requirements) and causal relationships (e.g. understaffing leads to re-deploying staff) | Face validation | Showed logical steps of (various iterations of) model in presentations to steering group and to hospital trust PIs | To build credibility and gain expert opinion from steering group and trust PIs | Trust PIs and steering group agreed in general with the conceptual model, and suggested some changes (such as how staffing requirements are calculated for specials) that were incorporated in subsequent iterations of model development | High |
Category/item | Technique(s) used | Details | Justification for technique used | Result/conclusion | Confidence in result |
---|---|---|---|---|---|
Assumptions | Sensitivity analyses with design of experiments | 2k factorial design | Used to test how much outputs are influenced by parameters we do not have data for | Apart from the round-down bound, the other factors made less than a 5-percentage point difference to the percentage understaffed patient shifts and less than a £0.50 difference to cost per patient-day. We agreed with our hospital trust partners that rounding to the nearest whole person rather than always rounding up was more realistic | High |
Input–output relationships | Face validity | Circulated around team | Building credibility and expert opinion | No scenario simulation results were identified as unlikely/surprising | High |
Internal validity | Replications | Determining stochastic variability using 10 replications of 1 year | Standard technique | The between-year variation at hospital level is low enough that alternative scenarios have significantly different results | High |
Category/item | Technique(s) used | Justification for technique used | Result/conclusion | Confidence in result |
---|---|---|---|---|
Error-free simulation language, simulation language properly implemented | Trace | Used a simulation language so simulation debugger picked up most errors in the language. CS checked for problems (e.g. with array sizes) by tracing through calculations | Yes | High |
Model programmed correctly | Animation, operational graphics, trace |
Animation of numbers of staff from each source used to show whether re-deployments working correctly Operational graphics of understaffed/overstaffed wards over time used to quickly spot errors, e.g. all wards understaffed Put in actual staffing levels, occupancy and acuities. Compared understaffed shifts output of simulation with actual understaffed shifts |
Yes | High |
Pseudo random number generator properly implemented | Trace | Used standard AnyLogic in-built random number generator, so this has already been tested for correctness, so just needed to check that sampling was working correctly in our model | Yes | High |
Evaluation area | Overall conclusion | Justification for overall conclusion | Confidence in conclusion |
---|---|---|---|
Conceptual model validity | The model is a sufficiently accurate representation of the real world | Model developed in partnership with nursing experts from four hospital trusts (not high because some of the data needed to check assumptions are not readily available) | Medium to high |
Computer model verification | Computer model programmed correctly | Range of techniques used throughout model development process | High |
Operational validity | The simulation model results are similar enough between runs to be able to draw conclusions | Face validity was tested and variability between run results was low | High |
Data validity | Data sufficiently valid – improving data validity further unlikely to impact conclusions | Outliers were removed, although it is hard to distinguish between valid and invalid outliers. Inter-rater reliability between SNCT ratings was high | Medium to High |
Simulation scenarios
Establishment scenario | Scenario name | Description |
---|---|---|
Method for setting the baseline staffing | Standard staffing | This scenario corresponds to the current guidelines for using the SNCT.19 Here the ward establishments are the mean average of the staffing requirements according to the SNCT assessed over an observation period |
Flexible (low) staffing | In this scenario we model a situation in which establishments are set to provide a core staff group that would be sufficient on shifts with low demand, with the intention that, on shifts with higher demand, additional staff are re-deployed from other wards. NICE11 identified staffing of more than 20% below plan as a possible red flag. We set the staffing levels at 80% of the mean average requirements, presuming that, in the face of average demand, this staffing level represents a bare minimum that is acceptable | |
High staffing | This scenario is considered to see whether or not a higher than standard level of staffing could improve cost-effectiveness because fewer temporary staff are needed. This scenario sets the establishments at the 90th percentile of required staffing according to the SNCT over an observation period. This is calculated using the quantile procedure in R using type 7 (the default), which is equivalent to the PERCENTILE function in Microsoft Excel | |
Vacancies | This scenario assumes that the establishments are set as for standard staffing, but accepts that there are unfilled posts. It assumes that the establishments are set at the mean required levels according to the SNCT over an observation period, but that 10% of posts are unfilled, roughly the current national nursing staff vacancy rate1 | |
Scenarios exploring system complexity | ||
Short-notice availability of temporary staffa | Empirical | The chance of there being a temporary staff member available to cover shifts at short notice is based on empirical data from hospital trust B (see Tables 36 and 37). The probabilities differ by registered nurses/nursing support workers, weekday/weekend and shift |
Bank and agency 50% | Regardless of the staff type, day of week or shift, there is a 50% chance of bank staff being available to cover a shift at short notice. For each shift left unfilled by bank staff, there is a 50% chance of agency staff being available to cover | |
Bank 50%, agency 90% | Analogous to above | |
Empirical bank, agency 90% | Analogous to above | |
Bank 90%, agency 90% | Analogous to above | |
Data samples used to determine the establishmentb | June | First 20 available weekdays of June (or following month if unavailable) |
January | First 20 available weekdays of January (or following month if unavailable) | |
Months | First 2 available weekdays of each month | |
All | All available (up to 363 days) | |
Measuring patient nursing needs when setting establishment | Standard multiplier | Patients are assigned to SNCT acuity/dependency categories when setting baseline staffing levels |
Fixed (average) multiplier | Assumes all patients are assigned the average multiplier (across all hospital trusts) when setting baseline staffing levels | |
Use of re-deployed staff | Internal re-deployment | When extra staff are requested, staff are first re-deployed between wards in the same directorate before bank and then agency staff are requested |
No internal re-deployment | Staff are never re-deployed between wards | |
Percentage of registered nurses who are IV trained | 0%, 10%, 20%, 30%, 40%, 60%, 80% and 100% | Each shift, each registered nurse has this chance of being IV trained |
Costs | Core assumption | This scenario assumes that agency costs are capped and that the band mix of nursing support workers is the same as in empirical data |
Increased agency cost | Here agency staff cost 10% more than in core assumption. We chose to look at this because caps are regularly exceeded where necessary to ensure patient safety106,161 | |
Increased nursing support worker cost | Here nursing support workers cost 10% more than in core assumption. We investigated this scenario because of the new roles including nursing associates that are being introduced162 |
Coefficients and baseline parameters used in cost-effectiveness models
Parameter | Coefficient | Valuea | |
---|---|---|---|
Mortalityb effect (95% confidence interval) | |||
Day of low registered nurse staffing | HR | 1.03 | 1.01 to 1.06 |
Day of low nursing support worker staffing | HR | 1.04 | 1.02 to 1.07 |
Temporary staffing modelsc | |||
Day of low registered nurse staffing | HR | 1.03 | |
Day of low nursing support worker staffing | HR | 1.05 | |
Day of high temporary registered nurse staffing | HR | 1.12 | |
Day of high temporary nursing support worker staffing | HR | 1.05 | |
Baseline mortality rated | |||
Hospital trust A | % | 3.21 | |
Hospital trust B | % | 3.87 | |
Hospital trust C | % | – | |
Hospital trust D | % | 3.15 | |
Length of stay effectb | |||
Average registered nurse staffing relative to mean | Beta | –0.23 | |
Average nursing support worker staffing relative to mean | Beta | 0.076 | |
Mean length of stay (in 5 days)e | |||
Hospital trust A | Days | 3.65 | 2.89 |
Hospital trust B | Days | 6.33 | 3.74 |
Hospital trust C | Days | 4.80 | 3.14 |
Hospital trust D | Days | 5.05 | 3.15 |
Cost of excess bed-dayf | £ | 337 |
Appendix 2 Descriptive analysis details to supplement Chapter 4
Hospital trust | Participating wards (n) | Potentially eligible (n) | Percentage | Notes |
---|---|---|---|---|
A | 19 | 20 | 95% | One ward supplied no staffing adequacy data (so was deemed to have elected not to participate) |
B | 31 | 33 | 94% | Private patients ward did not use the SNCT plus one ward supplied staffing adequacy and SNCT data for only part of the rostered ward |
C | 12 | 12 | 100% | |
D | 19 | 29 | 64% | A number of specialist wards (e.g. oncology) where SNCT and multipliers were deemed unsuitable/not implemented by the hospital trust and mixed wards including high-dependency units did not provide data |
All | 81 | 94 | 86% |
Hospital trust | Ward | Date range | Reason |
---|---|---|---|
A | 10a | To 31 March 2017 | More beds in winter |
A | 10b | 1 April 2017–30 September 2017 | |
A | 10c | From 1 October 2017 | |
A | 11 | To 26 June 2017 | Ward closure |
A | 19 | To 31 May 2017 | Ward changed specialty (patients and staff). Did not take part after change |
A | 21a | To 23 June 2017 | Ward changed specialty |
A | 21b | From 10 September 2017 | |
B | All | From various dates in February 2017 | Data collection began late |
B | 5a | To 5 October 2017 | Ward changed specialty |
B | 5b | From 8 October 2017 | |
B | 9a | To 7 October 2017 | Ward changed specialty |
B | 9b | From 8 October 2017 | |
C | 4 | To 31 August 17 | Ward split into three and no longer took part in study |
C | 5 | To 31 July 17 and from 1 October 2017 | Wards 5 and 6 (neighbouring wards) appear to have been rostered together in August and September, but separately for the rest of the year, so we decided to remove these 2 months |
C | 6 | To 31 July 2017 and from 1 October 2017 | |
All | All | Removed 25 March 2017 and 28 October 2017 | Days (7 a.m.–7 a.m.) with daylight saving time changes |
Hospital trust | From date | To date | Ward IDa | Medical or surgical | Assessment unit | Total beds (n) | Single rooms (n) | Early (n) | Late (n) | Night (n) | Mainly 12-hour shifts? | |||
---|---|---|---|---|---|---|---|---|---|---|---|---|---|---|
Registered nurse | Nursing support worker | Registered nurse | Nursing support worker | Registered nurse | Nursing support worker | |||||||||
A | 1 January 2017 | 31 March 2017 | 1 | Medical | Yes | 30 | 6 | 7 | 5|6 | 7 | 4.5|5.5 | 5|6 | 1 | Yes |
A | 1 January 2017 | 30 September 2017 | 2 | Medical | 28 | 14 | 4|7 | 4|3 | 3|5 | 3 | 2|3 | 2|3 | Yes | |
A | 1 January 2017 | 31 December 2017 | 3 | Medical | 23 | 15 | 4 | 3 | 3 | 2 | 2 | 2 | Yes | |
A | 1 January 2017 | 31 December 2017 | 6 | Medical | 8 | 6 | 3 | 1 | 3 | 1 | 2 | 0 | Yes | |
A | 1 January 2017 | 31 December 2017 | 8 | Medical | 17 | 5 | 3 | 2 | 3 | 2 | 2 | 1 | Yes | |
A | 1 January 2017 | 31 March 2017 | 10a | Medical | 34 | 4 | 4 | 6 | 4 | 5 | 3 | 3 | Yes | |
A | 1 April 2017 | 30 September 2017 | 10b | Medical | 20 | 4 | 3 | 3 | 3 | 3 | 2 | 2 | Yes | |
A | 1 October 2017 | 31 December 2017 | 10c | Medical | 34 | 4 | 4 | 6 | 4 | 5 | 3 | 3 | Yes | |
A | 1 January 2017 | 26 June 2017 | 11 | Medical | 20 | 4 | 3 | 3 | 2 | 2 | 2 | 2 | Yes | |
A | 1 January 2017 | 31 December 2017 | 12 | Medical | 20 | 4 | 3 | 3 | 2 | 2 | 2 | 2 | Yes | |
A | 1 January 2017 | 31 December 2017 | 13 | Medical | 20 | 4 | 3 | 3 | 2 | 2 | 2 | 2 | Yes | |
A | 1 January 2017 | 31 December 2017 | 14 | Medical | 12 | 9 | 3 | 2 | 2 | 2 | 2 | 2 | Yes | |
A | 1 January 2017 | 31 December 2017 | 15 | Medical | Yes | 24 | 16 | 7 | 3 | 5 | 3 | 3 | 3 | Yes |
A | 1 January 2017 | 31 December 2017 | 16 | Medical/surgical | 20 | 8 | 3 | 3 | 3 | 3.5 | 2 | 1.5 | Yes | |
A | 1 January 2017 | 31 December 2017 | 17 | Medical | 42 | 11 | 6 | 8 | 5 | 7 | 5 | 4 | Yes | |
A | 1 January 2017 | 31 December 2017 | 18 | Surgical | Yes | 12 | 1 | 2 | 2 | 2 | 2 | 2 | 1 | Yes |
A | 1 January 2017 | 31 May 2017 | 19 | Surgical | 19 | 12 | 3 | 4 | 3 | 3 | 2 | 2 | Yes | |
A | 1 January 2017 | 31 December 2017 | 20 | Surgical | 24 | 4 | 4 | 3 | 4 | 2 | 2 | 2 | Yes | |
A | 1 January 2017 | 23 June 2017 | 21a | Surgical | 27 | 7 | 6 | 4 | 5 | 3 | 3 | 2 | Yes | |
A | 10 September 2017 | 31 December 2017 | 21b | Surgical | 28 | 13 | 4 | 5 | 4 | 3 | 3 | 3 | Yes | |
A | 1 January 2017 | 31 March 2017 | 22 | Surgical | 30 | 8 | 5|4 | 4|5 | 4 | 3 | 2|3 | 3 | Yes | |
A | 1 January 2017 | 17 June 2017 | 23 | Surgical | 30 | 8 | 5 | 4 | 4 | 3 | 3 | 2|3 | Yes | |
B | 6 February 2017 | 31 December 2017 | 1 | Medical/surgical | 23 | 7 | 5 | 3 | 5 | 3 | 3 | 2 | Combination | |
B | 6 February 2017 | 31 December 2017 | 2 | Medical | 36 | 12 | 7 | 3 | 7 | 3 | 4 | 3 | Combination | |
B | 6 February 2017 | 31 December 2017 | 3 | Surgical/Medical | 36 | 12 | 6 | 4 | 6 | 4 | 4 | 2 | Combination | |
B | 6 February 2017 | 5 October 2017 | 5a | Surgical | 28 | 4 | 5 | 3 | 5 | 3 | 4 | 3 | Combination | |
B | 8 October 2017 | 31 December 2017 | 5b | Medical | 28 | 4 | 4 | 5 | 4 | 5 | 3 | 4 | Combination | |
B | 6 February 2017 | 31 December 2017 | 6 | Medical | 30 | 4 | 5 | 3 | 5 | 3 | 4 | 2 | Combination | |
B | 7 February 2017 | 31 December 2017 | 7 | Medical | 34 | 4 | 6 | 4 | 6 | 4 | 4 | 2 | Combination | |
B | 6 February 2017 | 31 December 2017 | 8 | Surgical | 27 | 2 | 5 | 2 | 4 | 2 | 4 | 2 | Combination | |
B | 6 February 2017 | 7 October 2017 | 9a | Surgical | 36 | 4 | 6 | 4 | 6 | 4 | 5 | 3 | Combination | |
B | 8 October 2017 | 31 December 2017 | 9b | Surgical | 28 | 4 | 6 | 4 | 6 | 4 | 5 | 3 | Combination | |
B | 6 February 2017 | 31 December 2017 | 10 | Surgical | 36 | 4 | 7 | 5 | 7 | 5 | 4 | 3 | Combination | |
B | 6 February 2017 | 31 December 2017 | 11 | Medical/Surgical | 36 | 12 | 7 | 4 | 7 | 3 | 4 | 2 | Combination | |
B | 6 February 2017 | 31 December 2017 | 12 | Surgical | 27 | 12 | 6 | 2 | 6 | 2 | 3 | 2 | Combination | |
B | 6 February 2017 | 31 December 2017 | 13 | Surgical | 30 | 4 | 5 | 3 | 5 | 3 | 4 | 2 | Combination | |
B | 6 February 2017 | 31 December 2017 | 14 | Surgical | 34 | 4 | 6 | 3 | 6 | 3 | 4 | 2 | Combination | |
B | 6 February 2017 | 31 December 2017 | 15 | Medical | 18 | 12 | 3 | 3 | 3 | 3 | 2 | 3 | Combination | |
B | 9 February 2017 | 31 December 2017 | 16 | Medical | 40 | 0 | 8 | 4 | 8 | 4 | 7 | 3 | Combination | |
B | 6 February 2017 | 31 December 2017 | 17 | Medical | 36 | 12 | 6 | 4 | 6 | 4 | 4 | 2 | Combination | |
B | 6 February 2017 | 31 December 2017 | 18 | Medical | 13 | 4 | 2 | 3 | 2 | 3 | 2 | 1 | Combination | |
B | 6 February 2017 | 31 December 2017 | 19 | Medical | 30 | 4 | 6 | 4 | 5 | 4 | 4 | 2 | Combination | |
B | 6 February 2017 | 31 December 2017 | 20 | Medical | 29 | 2 | 6 | 4 | 5 | 4 | 4 | 2 | Combination | |
B | 6 February 2017 | 31 December 2017 | 21 | Medical | 34 | 4 | 8 | 5 | 8 | 5 | 7 | 5 | Combination | |
B | 6 February 2017 | 31 December 2017 | 22 | Medical/surgical | 44 | 21 | 9 | 3 | 34 | 9 | 7 | 3 | Combination | |
B | 7 February 2017 | 31 December 2017 | 23 | Medical | 23 | 11 | 4 | 3 | 4 | 3 | 3 | 2 | Combination | |
B | 6 February 2017 | 31 December 2017 | 24 | Medical | 29 | 2 | 6 | 4 | 5 | 4 | 4 | 2 | Combination | |
B | 7 February 2017 | 31 December 2017 | 25 | Medical | 30 | 10 | 6 | 4 | 5 | 4 | 4 | 2 | Combination | |
B | 6 February 2017 | 31 December 2017 | 26 | Medical | 21 | 7 | 3 | 3 | 3 | 3 | 3 | 2 | Combination | |
B | 6 February 2017 | 31 December 2017 | 28 | Medical/surgical | 14 | 3 | 4 | 1 | 4 | 1 | 3 | 1 | Combination | |
B | 6 February 2017 | 31 December 2017 | 29 | Medical/surgical | 22 | 5 | 6 | 3 | 6 | 3 | 5 | 2 | Combination | |
B | 6 February 2017 | 31 December 2017 | 30 | Medical/surgical | 14 | 2 | 4 | 2 | 4 | 2 | 3 | 1 | Combination | |
B | 6 February 2017 | 31 December 2017 | 31 | Surgical | Yes | 28 | 4 | 6 | 2 | 6 | 2 | 4 | 2 | Combination |
B | 6 February 2017 | 31 December 2017 | 32 | Surgical | 10 | 0 | 3 | 1 | 4 | 1 | 3 | 0 | Combination | |
B | 7 February 2017 | 31 December 2017 | 33 | Medical | Yes | 63 | 0 | 18 | 8 | 18 | 8 | 15 | 7 | Combination |
C | 1 January 2017 | 31 December 2017 | 1 | Medical/surgical | 11 | 3 | 3 | 1 | 3 | 1 | 2 | 1 | Yes | |
C | 1 January 2017 | 31 December 2017 | 2 | Medical/surgical | 16 | 6 | 5 | 2 | 4 | 2 | 2 | 1 | Yes | |
C | 1 January 2017 | 31 December 2017 | 3 | Medical/surgical | 13 | 13 | 5 | 1 | 4 | 1 | 2 | 1 | Yes | |
C | 1 January 2017 | 31 August 2017 | 4 | Medical/surgical | 21 | 21 | 8 | 2 | 7 | 2 | 6 | 1 | Yes | |
C | 1 January 2017 until 31 July 2017 | 1 October 2017 until 31 December 2017 | 5 | Medical | 16 | 16 | 7 | 2 | 6 | 2 | 4 | 1 | Yes | |
C | 1 January 2017 until 31 July 2017 | 1 October 2017 until 31 December 2017 | 6 | Medical | 12 | 4 | 5 | 2 | 4 | 2 | 2 | 1 | Yes | |
C | 1 January 2017 | 31 December 2017 | 8 | Medical/surgical | 17 | 17 | 7 | 2 | 6 | 2 | 5 | 1 | Yes | |
C | 1 January 2017 | 31 December 2017 | 9 | Medical | 14 | 2 | 4 | 1 | 4 | 1 | 3 | 0 | Yes | |
C | 1 January 2017 | 31 December 2017 | 10 | Medical/surgical | 15 | 1 | 4 | 1 | 4 | 1 | 3 | 0 | Yes | |
C | 1 January 2017 | 31 December 2017 | 11 | Medical/surgical | 16 | 1 | 5 | 1 | 4 | 0 | 3 | 0 | Yes | |
C | 1 January 2017 | 31 December 2017 | 12 | Medical/surgical | 8 | 6 | 3 | 1 | 3 | 1 | 3 | 1 | Yes | |
C | 1 January 2017 | 31 December 2017 | 13 | Medical | 8 | 8 | 3 | 1 | 3 | 1 | 2 | 1 | Yes | |
D | 1 January 2017 | 31 December 2017 | 1 | Medical/surgical | 23 | 8 | 5 | 3 | 4 | 3 | 2 | 2 | Combination | |
D | 1 January 2017 | 31 December 2017 | 3 | Medical | 20 | 6 | 5 | 2 | 5 | 2 | 4 | 1 | Combination | |
D | 1 January 2017 | 31 December 2017 | 5 | Medical/surgical | 36 | 0 | 7 | 3 | 7 | 2 | 4 | 2 | Combination | |
D | 1 January 2017 | 31 December 2017 | 6 | Medical/surgical | 28 | 4 | 5 | 3 | 4 | 3 | 3 | 2 | Combination | |
D | 1 January 2017 | 31 December 2017 | 7 | Medical/surgical | 34 | 4 | 6 | 4 | 5 | 4 | 4 | 2 | Combination | |
D | 1 January 2017 | 31 December 2017 | 8 | Medical/surgical | 30 | 4 | 5 | 3 | 5 | 3 | 4 | 2 | Combination | |
D | 1 January 2017 | 31 December 2017 | 9 | Medical | 28 | 4 | 5 | 3 | 4 | 3 | 3 | 2 | Combination | |
D | 1 January 2017 | 31 December 2017 | 11 | Medical | 26 | 4 | 4 | 4 | 4 | 3 | 3 | 3 | Combination | |
D | 1 January 2017 | 31 December 2017 | 12 | Medical | 28 | 0 | 4 | 4 | 4 | 3 | 2 | 2 | Combination | |
D | 1 January 2017 | 31 December 2017 | 13 | Medical | 26 | 4 | 4 | 4 | 4 | 2 | 3 | 2 | Combination | |
D | 1 January 2017 | 31 December 2017 | 14 | Medical/surgical | 28 | 4 | 4 | 5 | 4 | 5 | 3 | 3 | Combination | |
D | 1 January 2017 | 31 December 2017 | 15 | Medical/surgical | 24 | 4 | 5 | 2 | 5 | 2 | 4 | 2 | Combination | |
D | 1 January 2017 | 31 December 2017 | 16 | Surgical | 18 | 0 | 3 | 2 | 3 | 2 | 2 | 1 | Combination | |
D | 1 January 2017 | 31 December 2017 | 17 | Surgical | 18 | 2 | 3 | 2 | 3 | 2 | 2 | 1 | Combination | |
D | 1 January 2017 | 31 December 2017 | 18 | Surgical | 36 | 0 | 7 | 4 | 7 | 4 | 4 | 2 | Combination | |
D | 1 January 2017 | 31 December 2017 | 19 | Surgical | 29 | 4 | 6 | 2 | 6 | 1 | 3 | 1 | Combination | |
D | 1 January 2017 | 31 December 2017 | 20 | Surgical | 32 | 0 | 6 | 4 | 6 | 4 | 5 | 3 | Combination | |
D | 1 January 2017 | 31 December 2017 | 21 | Surgical | 34 | 4 | 5 | 5 | 5 | 4 | 4 | 4 | Combination | |
D | 1 January 2017 | 31 December 2017 | 22 | Surgical | 24 | 4 | 4 | 3 | 4 | 3 | 3 | 3 | Combination |
Hospital trust | Theoretical maximum | SNCT ratings | ‘Enough staff for quality’ | ‘Nursing care left undone’ | ‘Staff breaks missed’ | ‘Registered nurses needed’ | ‘Nursing support workers needed’ |
---|---|---|---|---|---|---|---|
A | 6419 | 6363 (99.1%) | 6017 (93.7%) | 5930 (92.4%) | 5922 (92.3%) | 6086 (94.8%) | 6072 (94.6%) |
B | 10,130 | 9802 (96.8%) | 9329 (92.1%) | 9329 (92.1%) | 9329 (92.1%) | 9182 (90.6%) | 9182 (90.6%) |
C | 4113 | 3858 (93.8%) | 3492 (84.9%) | 3492 (84.9%) | 3492 (84.9%) | 3433 (83.5%) | 3423 (83.2%) |
D | 6897 | 6339 (91.9%) | 4754 (68.9%) | 4735 (68.7%) | 4751 (68.9%) | 4753 (68.9%) | 4756 (69.0%) |
Total | 27,559 | 26,362 (95.7%) | 23,592 (85.6%) | 23,486 (85.2%) | 23,494 (85.2%) | 23,454 (85.1%) | 23,433 (85.0%) |
Hospital trust | Patient-days | SNCT ratings | ‘Enough staff for quality’ | ‘Nursing care left undone’ | ‘Staff breaks missed’ | ‘Registered nurses needed’ | ‘Nursing support workers needed’ | Deviation between calculated requirement and actual staffing |
---|---|---|---|---|---|---|---|---|
A | 6419 (100.0%) | 6307 (99.1%) | 5987 (99.5%) | 5924 (99.9%) | 5892 (99.5%) | 5407 (88.8%) | 5415 (89.2%) | 6245 (98.1%) |
B | 10,130 (100.0%) | 9715 (99.1%) | 9329 (100.0%) | 9329 (100.0%) | 9329 (100.0%) | 9119 (99.3%) | 9119 (99.3%) | 9669 (98.6%) |
C | 4080 (99.2%) | 3837 (99.5%) | 3483 (99.7%) | 3489 (99.9%) | 3482 (99.7%) | 2932 (85.4%) | 2934 (85.7%) | 3796 (98.4%) |
D | 6897 (100.0%) | 6295 (99.3%) | 4747 (99.9%) | 4730 (99.9%) | 4743 (99.8%) | 1358 (28.6%) | 1408 (29.6%) | 6251 (98.6%) |
Total | 27,526 (99.9%) | 26,154 (99.2%) | 23,546 (99.8%) | 23,472 (99.9%) | 23,446 (99.8%) | 18,816 (80.2%) | 18,876 (80.6%) | 25,961 (98.5%) |
Hospital trust | Total nursing staff needed | Increase in total nursing staff needed | Increase in ‘registered nurses needed’ | Increase in ‘nursing support workers needed’ | ‘Enough staff for quality’ and deviation between calculated requirement and actual staffing | ‘Nursing care left undone’ and deviation between calculated requirement and actual staffing | ‘Staff breaks missed’ and deviation between calculated requirement and actual staffing |
---|---|---|---|---|---|---|---|
A | 5392 (99.6%) | 1074 (19.9%) | 995 (18.5%) | 1020 (18.9%) | 5793 (92.8%) | 5733 (91.8%) | 5704 (91.3%) |
B | 9119 (100.0%) | 873 (9.6%) | 896 (9.8%) | 872 (9.6%) | 8852 (91.6%) | 8852 (91.6%) | 8852 (91.6%) |
C | 2921 (99.6%) | 335 (11.5%) | 316 (10.8%) | 343 (11.7%) | 3283 (86.5%) | 3290 (86.7%) | 3283 (86.5%) |
D | 1357 (96.4%) | 240 (17.7%) | 296 (21.8%) | 202 (14.9%) | 4436 (71.0%) | 4419 (70.7%) | 4432 (70.9%) |
Total | 18,789 (99.5%) | 2522 (13.4%) | 2503 (13.3%) | 2437 (13.0%) | 22,364 (86.1%) | 22,294 (85.9%) | 22,271 (85.8%) |
Sample sizes for shift-level data
Hospital trust | Theoretical maximum | SNCT ratings | Calculated staffing requirementa | ‘Enough staff for quality’ | ‘Nursing care left undone’ | ‘Staff breaks missed’ | ‘Registered nurses needed’ | ‘Nursing support workers needed’ |
---|---|---|---|---|---|---|---|---|
A | 25,676 | 13,047 (50.8%) | 24,490 (95.4%) | 12,478 (48.6%) | 12,115 (47.2%) | 12,078 (47.0%) | 12,666 (49.3%) | 12,651 (49.3%) |
B | 40,520 | 17,008 (42.0%) | 34,016 (83.9%) | 17,469 (43.1%) | 17,469 (43.1%) | 17,469 (43.1%) | 17,008 (42.0%) | 17,008 (42.0%) |
C | 16,452 | 10,114 (61.5%) | 14,152 (86.0%) | 9305 (56.6%) | 9310 (56.6%) | 9311 (56.6%) | 8933 (54.3%) | 8920 (54.2%) |
D | 27,588 | 16,231 (58.8%) | 22,860 (82.9%) | 11,987 (43.5%) | 11,936 (43.3%) | 11,996 (43.5%) | 11,991 (43.5%) | 11,994 (43.5%) |
Total | 110,236 | 56,400 (51.2%) | 95,518 (86.6%) | 51,239 (46.5%) | 50,830 (46.1%) | 50,854 (46.1%) | 50,598 (45.9%) | 50,573 (45.9%) |
Hospital trust | Patient-days | SNCT ratings | ‘Enough staff for quality’ | ‘Nursing care left undone’ | ‘Staff breaks missed’ | ‘Registered nurses needed’ | ‘Nursing support workers needed’ | Deviation between calculated requirement and actual staffing |
---|---|---|---|---|---|---|---|---|
A | 25,676 (100.0%) | 12,940 (99.2%) | 12,419 (99.5%) | 12,101 (99.9%) | 11,994 (99.3%) | 11,485 (90.7%) | 11,491 (90.8%) | 24,140 (98.6%) |
B | 40,520 (100.0%) | 16,853 (99.1%) | 17,469 (100.0%) | 17,469 (100.0%) | 17,469 (100.0%) | 16,908 (99.4%) | 16,908 (99.4%) | 33,585 (98.7%) |
C | 16,312 (99.1%) | 10,061 (99.5%) | 9283 (99.8%) | 9305 (99.9%) | 9268 (99.5%) | 7724 (86.5%) | 7747 (86.8%) | 14,000 (98.9%) |
D | 27,588 (100.0%) | 16,120 (99.3%) | 11,964 (99.8%) | 11,921 (99.9%) | 11,920 (99.4%) | 3768 (31.4%) | 3888 (32.4%) | 22,623 (99.0%) |
Total | 110,096 (99.9%) | 55,974 (99.2%) | 51,135 (99.8%) | 50,796 (99.9%) | 50,651 (99.6%) | 39,885 (78.8%) | 40,034 (79.2%) | 94,348 (98.8%) |
Hospital trust | Total nursing staff needed | ‘Enough staff for quality’ and deviation between calculated requirement and actual staffing | ‘Nursing care left undone’ and deviation between calculated requirement and actual staffing | ‘Staff breaks missed’ and deviation between calculated requirement and actual staffing |
---|---|---|---|---|
A | 11,461 (99.7%) | 12,026 (49.8%) | 11,716 (48.5%) | 11,613 (48.1%) |
B | 16,908 (100.0%) | 16,091 (47.9%) | 16,091 (47.9%) | 16,091 (47.9%) |
C | 7706 (99.5%) | 8761 (62.6%) | 8784 (62.7%) | 8747 (62.5%) |
D | 3763 (96.8%) | 11,377 (50.3%) | 11,335 (50.1%) | 11,334 (50.1%) |
Total | 39,838 (99.5%) | 48,255 (51.1%) | 47,926 (50.8%) | 47,785 (50.6%) |
Ward sizes and occupancy
Hospital trust | Ward beds range (minimum–maximum) | Mean ward patients at 7 a.m. | Mean within-ward standard deviation of patients at 7 a.m. |
---|---|---|---|
A | 8–42 | 22.6 | 2.1 |
B | 10–63 | 29.9 | 1.7 |
C | 8–21 | 12.0 | 1.7 |
D | 18–36 | 25.7 | 2.0 |
Skill mix and band mix for substantive, bank and agency staff
Hospital trust | Staff type | Skill mix (%) | Nursing support workers (%) | Registered nurses (%) | |||||
---|---|---|---|---|---|---|---|---|---|
Band 2 | Band 3 | Band 4 | Band 5 | Band 6 | Band 7 | Band 8 | |||
A | Substantive | 54 | 78 | 17 | 5 | 77 | 16 | 7 | < 1 |
Bank | 40 | 98 | 2 | < 1 | 88 | 11 | 1 | ||
Agency | 59 | 100 | 100 | ||||||
B | Substantive | 60 | 89 | 10 | 1 | 80 | 15 | 4 | |
Bank | 34 | 96 | 2 | 2 | 98 | 2 | < 1 | < 1 | |
Agency | 56 | 100 | 100 | ||||||
C | Substantive | 80 | 18 | 82 | 32 | 62 | 6 | ||
Bank | 62 | 54 | 46 | < 1 | 13 | 85 | 2 | ||
Agency | 54 | 96 | 4 | < 1 | 96 | 4 | |||
D | Substantive | 52 | 77 | 7 | 16 | 71 | 22 | 8 | < 1 |
Bank | 32 | 98 | 2 | 97 | 3 | ||||
Agency | 40 | 100 | 100 |
Days over and under the calculated care requirement
Hospital trust | ‘Understaffed’ (more than 15% under calculated requirement) | Under calculated requirement | Over calculated requirement | ‘Overstaffed’ (more than 15% over calculated requirement) |
---|---|---|---|---|
Without specialing | ||||
A | 32.1% | 67.9% | 32.1% | 16.1% |
B | 28.3% | 70.8% | 29.2% | 9.7% |
C | 1.6% | 8.5% | 94.3% | 82.9% |
D | 32.5% | 71% | 29% | 11.4% |
With specialing | ||||
A | 38.7% | 71.7% | 29.9% | 14.8% |
B | 55.7% | 84.4% | 15.6% | 6.9% |
C | 2.2% | 10.8% | 91% | 77.1% |
D | 63.2% | 86.9% | 13.8% | 6.5% |
Ward information summary by hospital trust
Hospital trust | Number of medical, surgical and mixed wards, respectively | Mean percentage single rooms (minimum, maximum) | Mean ward average daily turnover, as admissions and discharges per care-hour (minimum, maximum) | Mean ward standard deviation of daily turnover, as percentage of mean | ||
---|---|---|---|---|---|---|
A | 12 | 6 | 1 | 36% (8%, 75%) | 0.08 (0.02, 0.29) | 60% |
B | 15 | 9 | 7 | 22% (0%, 67%) | 0.06 (0.01, 0.24) | 67% |
C | 4 | 0 | 8 | 58% (6%, 100%) | 0.04 (0.02, 0.07) | 67% |
D | 5 | 7 | 7 | 12% (0%, 35%) | 0.05 (0.02, 0.13) | 57% |
All wards | 36 | 22 | 23 | 28% (0%, 100%) | 0.06 (0.01, 0.29) | 63% |
Appendix 3 Reliability and validity of the Safer Nursing Care Tool: details to supplement Chapter 5
Hospital trust | Average patient count over time period | Patient count at time point | ||||||
---|---|---|---|---|---|---|---|---|
7 a.m.–1 p.m. | 1 p.m.–7 p.m. | 7 p.m.–1 a.m. | 1 a.m.–7 a.m. | 7 a.m. | 1 p.m. | 7 p.m. | 1 a.m. | |
A | 21.8 | 21.2 | 21 | 21.5 | 22.6 | 23.7 | 22.6 | 21.9 |
B | 28.9 | 28.4 | 28 | 28.6 | 29.9 | 31 | 30.1 | 29.3 |
C | 11.5 | 11.4 | 11.5 | 11.5 | 11.9 | 12.7 | 11.8 | 11.4 |
D | 25.2 | 24.7 | 24.6 | 25.1 | 25.7 | 26.6 | 25.9 | 25.5 |
Day of week | With specialing | Without specialing | ||||
---|---|---|---|---|---|---|
Weighted-average multiplier ward mean (SD) | Total care HPD ward mean (SD) | Total required WTE ward mean (SD) | Weighted-average multiplier ward mean (SD) | Total care HPD ward mean (SD) | Total required WTE ward mean (SD) | |
Hospital trust A | ||||||
Monday | 1.61 (0.16) | 160.36 (21.36) | 36.52 (4.86) | 1.56 (0.09) | 155.54 (16.83) | 35.42 (3.83) |
Tuesday | 1.61 (0.16) | 164.14 (21.65) | 37.38 (4.93) | 1.56 (0.09) | 159.41 (16.63) | 36.3 (3.79) |
Wednesday | 1.61 (0.15) | 162.32 (20.64) | 36.97 (4.7) | 1.56 (0.08) | 157.5 (16) | 35.87 (3.64) |
Thursday | 1.6 (0.15) | 159.32 (21.05) | 36.28 (4.79) | 1.56 (0.09) | 154.97 (16.61) | 35.29 (3.78) |
Friday | 1.61 (0.15) | 158.9 (21.8) | 36.19 (4.97) | 1.56 (0.09) | 154.34 (17.78) | 35.15 (4.05) |
Saturday | 1.62 (0.16) | 154.41 (22.89) | 35.16 (5.21) | 1.57 (0.09) | 149.77 (18.15) | 34.11 (4.13) |
Sunday | 1.62 (0.16) | 155.21 (23.03) | 35.35 (5.24) | 1.56 (0.09) | 150.32 (18.27) | 34.23 (4.16) |
Hospital trust B | ||||||
Monday | 1.8 (0.23) | 232.37 (30.18) | 52.92 (6.87) | 1.6 (0.07) | 207.14 (13.71) | 47.17 (3.12) |
Tuesday | 1.79 (0.22) | 235.84 (30.13) | 53.71 (6.86) | 1.6 (0.07) | 211.15 (13.5) | 48.09 (3.07) |
Wednesday | 1.79 (0.22) | 234.72 (31.09) | 53.45 (7.08) | 1.6 (0.07) | 210.45 (13.87) | 47.93 (3.16) |
Thursday | 1.79 (0.21) | 234.16 (29.31) | 53.33 (6.68) | 1.6 (0.07) | 210.01 (13.53) | 47.83 (3.08) |
Friday | 1.78 (0.21) | 232.37 (29.12) | 52.92 (6.63) | 1.59 (0.07) | 208.51 (13.73) | 47.48 (3.13) |
Saturday | 1.79 (0.23) | 228.59 (30.82) | 52.06 (7.02) | 1.6 (0.07) | 204.48 (14.66) | 46.57 (3.34) |
Sunday | 1.8 (0.22) | 227.39 (30.31) | 51.78 (6.9) | 1.6 (0.07) | 202.69 (14.3) | 46.16 (3.26) |
Hospital trust C | ||||||
Monday | 1.65 (0.33) | 81.88 (18.82) | 18.65 (4.29) | 1.53 (0.14) | 76.27 (13.56) | 17.37 (3.09) |
Tuesday | 1.62 (0.27) | 86.38 (18.17) | 19.67 (4.14) | 1.52 (0.14) | 81.07 (13.74) | 18.46 (3.13) |
Wednesday | 1.64 (0.3) | 90.24 (18.24) | 20.55 (4.15) | 1.52 (0.13) | 83.96 (12.66) | 19.12 (2.88) |
Thursday | 1.63 (0.3) | 89.17 (17.85) | 20.31 (4.06) | 1.51 (0.13) | 83.08 (12.18) | 18.92 (2.77) |
Friday | 1.62 (0.26) | 87.44 (16.44) | 19.91 (3.74) | 1.52 (0.13) | 82.56 (12.56) | 18.8 (2.86) |
Saturday | 1.64 (0.27) | 84.66 (17.2) | 19.28 (3.92) | 1.53 (0.13) | 79.77 (12.69) | 18.17 (2.89) |
Sunday | 1.65 (0.3) | 79.94 (18.15) | 18.21 (4.13) | 1.54 (0.13) | 74.72 (13.13) | 17.02 (2.99) |
Hospital trust D | ||||||
Monday | 1.82 (0.26) | 206.68 (35.33) | 47.07 (8.05) | 1.55 (0.06) | 176.03 (16.35) | 40.09 (3.72) |
Tuesday | 1.8 (0.24) | 205.3 (33.1) | 46.75 (7.54) | 1.55 (0.06) | 176.57 (15.28) | 40.21 (3.48) |
Wednesday | 1.81 (0.28) | 206.05 (36.35) | 46.92 (8.28) | 1.55 (0.06) | 176.18 (14.31) | 40.12 (3.26) |
Thursday | 1.82 (0.28) | 205.57 (35.5) | 46.81 (8.08) | 1.55 (0.06) | 175.55 (14.54) | 39.98 (3.31) |
Friday | 1.81 (0.24) | 205.26 (32.76) | 46.75 (7.46) | 1.55 (0.06) | 175.92 (15.11) | 40.06 (3.44) |
Saturday | 1.81 (0.24) | 199.14 (32.19) | 45.35 (7.33) | 1.55 (0.06) | 171.14 (15.94) | 38.98 (3.63) |
Sunday | 1.82 (0.27) | 202.3 (34.89) | 46.07 (7.94) | 1.55 (0.06) | 172.76 (16.48) | 39.34 (3.75) |
Additional regression models
Variable | Enough staff for quality | Nursing care left undone | Staff breaks missed | |||
---|---|---|---|---|---|---|
Odds ratio (95% confidence interval) | p-value | Odds ratio (95% confidence interval) | p-value | Odds ratio (95% confidence interval) | p-value | |
Overall shortfall (HPPD) | 0.88 (0.86 to 0.90) | < 0.001 | 1.14 (1.09 to 1.19) | < 0.001 | 1.12 (1.07 to 1.17) | < 0.001 |
Registered nurse shortfall (HPPD) | 0.94 (0.91 to 0.96) | < 0.001 | 1.09 (1.04 to 1.15) | < 0.001 | 1.08 (1.03 to 1.13) | 0.001 |
Nursing support worker shortfall (HPPD) | 0.90 (0.87 to 0.92) | < 0.001 | 1.08 (1.03 to 1.14) | 0.003 | 1.06 (1.01 to 1.12) | 0.024 |
Skill mix (registered nurse HPPD/total HPPD) | 0.80 (0.61 to 1.05) | 0.107 | 0.74 (0.47 to 1.16) | 0.190 | 0.89 (0.57 to 1.39) | 0.610 |
Patient turnover (per care hour) | 0.35 (0.12 to 1.01) | 0.053 | 7.16 (1.35 to 37.9) | 0.021 | 3.85 (2.36 to 6.26) | < 0.001 |
Ward type: other (reference) | ||||||
Surgical | 0.57 (0.34 to 0.96) | 0.033 | 2.00 (1.12 to 3.58) | 0.020 | 2.13 (1.22 to 3.69) | 0.007 |
Proportion single rooms | 0.89 (0.30 to 2.68) | 0.840 | 1.58 (0.48 to 5.21) | 0.455 | 1.05 (0.32 to 3.44) | 0.932 |
Day of week: Monday (reference) | ||||||
Tuesday | 1.11 (0.99 to 1.26) | 0.084 | 0.86 (0.69 to 1.07) | 0.170 | 0.94 (0.82 to 1.09) | 0.432 |
Wednesday | 1.28 (1.13 to 1.45) | < 0.001 | 0.95 (0.77 to 1.17) | 0.634 | 1.01 (0.88 to 1.16) | 0.884 |
Thursday | 1.09 (0.96 to 1.23) | 0.182 | 0.90 (0.73 to 1.12) | 0.349 | 0.96 (0.83 to 1.11) | 0.583 |
Friday | 1.03 (0.91 to 1.17) | 0.637 | 0.93 (0.75 to 1.16) | 0.535 | 1.03 (0.89 to 1.19) | 0.689 |
Saturday | 1.28 (1.13 to 1.46) | < 0.001 | 0.74 (0.59 to 0.93) | 0.011 | 0.86 (0.75 to 1.00) | 0.054 |
Sunday | 1.02 (0.91 to 1.16) | 0.697 | 1.08 (0.88 to 1.33) | 0.468 | 0.84 (0.73 to 0.97) | 0.019 |
Variable | Enough staff for quality | Nursing care left undone | Staff breaks missed | |||
---|---|---|---|---|---|---|
Odds ratio (95% confidence interval) | p-value | Odds ratio (95% confidence interval) | p-value | Odds ratio (95% confidence interval) | p-value | |
Overall shortfall (HPPD) | 0.88 (0.86 to 0.90) | < 0.001 | 1.14 (1.09 to 1.19) | < 0.001 | 1.11 (1.07 to 1.16) | < 0.001 |
Skill mix (registered nurse HPPD/total HPPD) | 0.81 (0.62 to 1.06) | 0.117 | 0.74 (0.47 to 1.17) | 0.195 | 0.88 (0.57 to 1.38) | 0.586 |
Patient turnover (per care hour) | 0.91 (0.30 to 2.77) | 0.869 | 3.51 (0.63 to 19.65) | 0.153 | 4.99 (0.96 to 26.02) | 0.057 |
Ward type: other (reference) | ||||||
Surgical | 0.54 (0.31 to 0.93) | 0.025 | 2.14 (1.20 to 3.83) | 0.010 | 2.16 (1.23 to 3.80) | 0.007 |
Proportion single rooms | 0.53 (0.17 to 1.64) | 0.271 | 3.01 (0.93 to 9.69) | 0.065 | 2.06 (0.65 to 6.57) | 0.222 |
Day of week: Monday (reference) | ||||||
Tuesday | 1.12 (0.99 to 1.26) | 0.078 | 0.86 (0.69 to 1.06) | 0.159 | 0.71 (0.58 to 0.88) | 0.001 |
Wednesday | 1.28 (1.13 to 1.45) | < 0.001 | 0.96 (0.77 to 1.18) | 0.676 | 0.61 (0.49 to 0.76) | < 0.001 |
Thursday | 1.09 (0.96 to 1.23) | 0.195 | 0.91 (0.73 to 1.13) | 0.379 | 0.81 (0.66 to 1.00) | 0.045 |
Friday | 1.03 (0.91 to 1.17) | 0.604 | 0.92 (0.74 to 1.15) | 0.481 | 0.79 (0.64 to 0.97) | 0.028 |
Saturday | 1.30 (1.14 to 1.48) | < 0.001 | 0.75 (0.60 to 0.95) | 0.016 | 0.50 (0.40 to 0.64) | < 0.001 |
Sunday | 1.02 (0.90 to 1.15) | 0.795 | 1.11 (0.90 to 1.37) | 0.316 | 0.82 (0.66 to 1.01) | 0.058 |
Standard deviation between wards | 1.06 | 1.08 | 1.05 | |||
Standard deviation between hospitals | 0.77 | 0.95 | 0.72 | |||
Akaike information criterion | 20,700 | 8376 | 8094 | |||
Bayesian information criterion | 20,812 | 8488 | 8206 |
Variable | Enough staff for quality | Nursing care left undone | Staff breaks missed | |||
---|---|---|---|---|---|---|
Odds ratio (95% confidence interval) | p-value | Odds ratio (95% confidence interval) | p-value | Odds ratio (95% confidence interval) | p-value | |
Registered nurse shortfall (HPPD) | 0.90 (0.87 to 0.92) | < 0.001 | 1.14 (1.08 to 1.20) | < 0.001 | 1.12 (1.07 to 1.18) | < 0.001 |
Nursing support worker shortfall (HPPD) | 0.86 (0.83 to 0.89) | < 0.001 | 1.14 (1.07 to 1.20) | < 0.001 | 1.11 (1.05 to 1.18) | < 0.001 |
Day of week: Monday (reference) | ||||||
Tuesday | 0.71 (0.58 to 0.88) | 0.002 | ||||
Wednesday | 0.61 (0.49 to 0.76) | < 0.001 | ||||
Thursday | 0.81 (0.66 to 1.00) | 0.050 | ||||
Friday | 0.80 (0.65 to 0.99) | 0.039 | ||||
Saturday | 0.49 (0.39 to 0.62) | < 0.001 | ||||
Sunday | 0.80 (0.65 to 0.98) | 0.031 | ||||
Ward type: other (reference) | ||||||
Surgical | 0.56 (0.33 to 0.96) | 0.034 | 1.99 (1.11 to 3.56) | 0.021 | 2.10 (1.20 to 3.67) | 0.009 |
Proportion single rooms | ||||||
Standard deviation between wards | 1.06 | 1.10 | 1.07 | |||
Standard deviation between hospitals | 0.88 | 1.07 | 0.81 | |||
Akaike information criterion | 20,714 | 8380 | 8095 | |||
Bayesian information criterion | 20,762 | 8428 | 8191 |
Variable | Enough staff for quality | Nursing care left undone | Staff breaks missed | |||
---|---|---|---|---|---|---|
Odds ratio (95% confidence interval) | p-value | Odds ratio (95% confidence interval) | p-value | Odds ratio (95% confidence interval) | p-value | |
Registered nurse shortfall (HPPD) | 0.89 (0.86 to 0.92) | < 0.001 | 1.15 (1.09 to 1.21) | < 0.001 | 1.13 (1.08 to 1.19) | < 0.001 |
Nursing support worker shortfall (HPPD) | 0.85 (0.83 to 0.88) | < 0.001 | 1.15 (1.08 to 1.22) | < 0.001 | 1.12 (1.06 to 1.19) | < 0.001 |
Registered nurse shortfall squared | 1.00 (0.99 to 1.00) | 0.469 | 1.01 (1.00 to 1.02) | 0.021 | 1.00 (0.99 to 1.02) | 0.533 |
Nursing support worker shortfall squared | 1.01 (1.00 to 1.02) | 0.102 | 0.99 (0.97 to 1.01) | 0.194 | 0.99 (0.97 to 1.01) | 0.165 |
Day of week: Monday (reference) | ||||||
Tuesday | 0.71 (0.58 to 0.88) | 0.002 | ||||
Wednesday | 0.61 (0.49 to 0.75) | < 0.001 | ||||
Thursday | 0.82 (0.66 to 1.00) | 0.053 | ||||
Friday | 0.80 (0.65 to 0.99) | 0.042 | ||||
Saturday | 0.49 (0.39 to 0.62) | < 0.001 | ||||
Sunday | 0.80 (0.65 to 0.98) | 0.031 | ||||
Ward type: other (reference) | ||||||
Surgical | 0.57 (0.33 to 0.96) | 0.036 | 1.98 (1.10 to 3.54) | 0.022 | 2.07 (1.18 to 3.63) | 0.011 |
Proportion single rooms | ||||||
Standard deviation between wards | 1.06 | 1.10 | 1.07 | |||
Standard deviation between hospitals | 0.88 | 1.07 | 0.80 | |||
Akaike information criterion | 20,715 | 8378 | 8097 | |||
Bayesian information criterion | 20,779 | 8442 | 8209 |
Variable | Enough staff for quality | Nursing care left undone | Staff breaks missed | |||
---|---|---|---|---|---|---|
Odds ratio (95% confidence interval) | p-value | Odds ratio (95% confidence interval) | p-value | Odds ratio (95% confidence interval) | p-value | |
Registered nurse shortfall (HPPD) | 0.92 (0.89 to 0.95) | < 0.001 | 1.14 (1.07 to 1.21) | < 0.001 | 1.13 (1.02 to 1.25) | 0.019 |
Nursing support worker shortfall (HPPD) | 0.87 (0.84 to 0.90) | < 0.001 | 1.14 (1.06 to 1.22) | < 0.001 | 1.13 (1.00 to 1.28) | 0.049 |
Registered nurse shortfall squared (HPPD) | 1.01 (1.00 to 1.02) | 0.044 | ||||
Day of week: Monday (reference) | ||||||
Tuesday | 0.71 (0.57 to 0.90) | 0.005 | ||||
Wednesday | 0.60 (0.47 to 0.76) | < 0.001 | ||||
Thursday | 0.84 (0.67 to 1.05) | 0.135 | ||||
Friday | 0.87 (0.69 to 1.09) | 0.225 | ||||
Saturday | 0.50 (0.38 to 0.65) | < 0.001 | ||||
Sunday | 0.81 (0.64 to 1.01) | 0.064 | ||||
Ward type: other (reference) | ||||||
Surgical | 0.63 (0.37 to 1.08) | 0.096 | 2.03 (1.11 to 3.69) | 0.021 | 2.04 (1.15 to 3.63) | 0.015 |
Proportion single rooms | ||||||
Turnover (per nursing hour) | ||||||
Registered nurse shortfall: nursing support worker shortfall | 0.99 (0.98 to 1.01) | 0.315 | 1.00 (0.98 to 1.02) | 0.972 | 1.01 (0.99 to 1.03) | 0.202 |
Registered nurse shortfall: Tuesday | 0.98 (0.86 to 1.11) | 0.708 | ||||
Registered nurse shortfall: Wednesday | 0.99 (0.86 to 1.13) | 0.840 | ||||
Registered nurse shortfall: Thursday | 0.96 (0.84 to 1.09) | 0.540 | ||||
Registered nurse shortfall: Friday | 0.94 (0.82 to 1.07) | 0.332 | ||||
Registered nurse shortfall: Saturday | 1.05 (0.89 to 1.24) | 0.558 | ||||
Registered nurse shortfall: Sunday | 1.04 (0.91 to 1.20) | 0.566 | ||||
Registered nurse shortfall: surgical ward | 0.88 (0.82 to 0.95) | 0.001 | 1.00 (0.89 to 1.13) | 0.952 | 1.00 (0.89 to 1.12) | 0.961 |
Registered nurse shortfall: proportion single rooms | ||||||
Nursing support worker shortfall: Tuesday | 1.01 (0.85 to 1.20) | 0.905 | ||||
Nursing support worker shortfall: Wednesday | 1.04 (0.87 to 1.24) | 0.694 | ||||
Nursing support worker shortfall: Thursday | 0.95 (0.81 to 1.11) | 0.536 | ||||
Nursing support worker shortfall: Friday | 0.87 (0.74 to 1.02) | 0.097 | ||||
Nursing support worker shortfall: Saturday | 0.92 (0.76 to 1.11) | 0.390 | ||||
Nursing support worker shortfall: Sunday | 0.95 (0.80 to 1.12) | 0.522 | ||||
Nursing support worker shortfall: surgical ward | 0.95 (0.88 to 1.04) | 0.263 | 0.98 (0.86 to 1.11) | 0.766 | 1.07 (0.94 to 1.21) | 0.325 |
Nursing support worker shortfall: proportion single rooms | ||||||
Standard deviation between wards | 1.06 | 1.10 | 1.07 | |||
Standard deviation between hospitals | 0.86 | 1.07 | 0.81 | |||
Akaike information criterion | 20,708 | 8384 | 8116 | |||
Bayesian information criterion | 20,780 | 8464 | 8341 |
Variable | Enough staff for quality | Nursing care left undone | Staff breaks missed | |||
---|---|---|---|---|---|---|
Odds ratio (95% confidence interval) | p-value | Odds ratio (95% confidence interval) | p-value | Odds ratio (95% confidence interval) | p-value | |
Registered nurse shortfall (HPPD) | 0.90 (0.89 to 0.91) | < 0.001 | 1.21 (1.18 to 1.24) | < 0.001 | 1.19 (1.16 to 1.22) | < 0.001 |
Nursing support worker shortfall (HPPD) | 0.89 (0.88 to 0.91) | < 0.001 | 1.20 (1.17 to 1.24) | < 0.001 | 1.19 (1.16 to 1.22) | < 0.001 |
Time of day: night | ||||||
Morning | 0.90 (0.83 to 0.97) | 0.004 | 1.49 (1.28 to 1.75) | < 0.001 | 2.33 (2.00 to 2.71) | < 0.001 |
Afternoon | 0.87 (0.82 to 0.92) | < 0.001 | 1.12 (1.02 to 1.24) | 0.020 | 1.72 (1.58 to 1.88) | < 0.001 |
Day of week: Monday | ||||||
Tuesday | 1.03 (0.95 to 1.11) | 0.511 | 0.95 (0.83 to 1.10) | 0.501 | 0.88 (0.77 to 1.00) | 0.048 |
Wednesday | 1.15 (1.06 to 1.25) | 0.001 | 1.03 (0.89 to 1.18) | 0.720 | 0.95 (0.84 to 1.08) | 0.455 |
Thursday | 1.01 (0.93 to 1.10) | 0.802 | 0.98 (0.85 to 1.13) | 0.762 | 1.07 (0.95 to 1.22) | 0.276 |
Friday | 0.98 (0.90 to 1.07) | 0.664 | 1.05 (0.91 to 1.21) | 0.547 | 1.10 (0.97 to 1.25) | 0.141 |
Saturday | 1.12 (1.03 to 1.22) | 0.008 | 0.86 (0.74 to 1.00) | 0.044 | 0.80 (0.70 to 0.91) | 0.001 |
Sunday | 1.19 (1.09 to 1.29) | < 0.001 | 0.84 (0.72 to 0.97) | 0.018 | 0.68 (0.59 to 0.78) | < 0.001 |
Ward type: mixed | ||||||
Surgical | 0.62 (0.36 to 1.06) | 0.078 | 2.08 (1.21 to 3.57) | 0.008 | 2.18 (1.33 to 3.58) | 0.002 |
Proportion single rooms | 0.46 (0.15 to 1.40) | 0.171 | 3.32 (1.11 to 9.94) | 0.032 | 3.09 (1.13 to 8.46) | 0.028 |
Turnover (per nursing hour) | 1.44 (0.98 to 2.11) | 0.063 | 0.60 (0.32 to 1.10) | 0.097 | 1.30 (0.77 to 2.17) | 0.326 |
Standard deviation between wards | 1.07 | 1.04 | 0.97 | |||
Standard deviation between hospitals | 0.70 | 0.93 | 0.77 | |||
Akaike information criterion | 47,229 | 18,605 | 21,718 | |||
Bayesian information criterion | 47,370 | 18,746 | 21,858 |
Variable | Enough staff for quality | Nursing care left undone | Staff breaks missed | |||
---|---|---|---|---|---|---|
Odds ratio (95% confidence interval) | p-value | Odds ratio (95% confidence interval) | p-value | Odds ratio (95% confidence interval) | p-value | |
Registered nurse shortfall (HPPD) | 0.90 (0.89 to 0.92) | < 0.001 | 1.20 (1.17 to 1.23) | < 0.001 | 1.19 (1.16 to 1.22) | < 0.001 |
Nursing support worker shortfall (HPPD) | 0.90 (0.88 to 0.91) | < 0.001 | 1.20 (1.17 to 1.23) | < 0.001 | 1.19 (1.16 to 1.22) | < 0.001 |
Time of day: night | ||||||
Morning | 0.90 (0.83 to 0.97) | 0.006 | 1.50 (1.28 to 1.76) | < 0.001 | 2.32 (1.99 to 2.70) | < 0.001 |
Afternoon | 0.89 (0.85 to 0.93) | < 0.001 | 1.08 (0.99 to 1.18) | 0.079 | 1.75 (1.61 to 1.89) | < 0.001 |
Ward type: mixed | ||||||
Surgical | 2.04 (1.19 to 3.52) | 0.010 | 2.20 (1.34 to 3.61) | 0.002 | ||
Proportion single rooms | 3.35 (1.11 to 10.05) | 0.031 | 3.07 (1.13 to 8.40) | 0.028 | ||
Standard deviation between wards | 1.09 | 1.04 | 0.97 | |||
Standard deviation between hospitals | 0.77 | 0.93 | 0.77 | |||
Akaike information criterion | 47,255 | 18,608 | 21,780 | |||
Bayesian information criterion | 47,317 | 18,687 | 21,859 |
Variable | Enough staff for quality | Nursing care left undone | Staff breaks missed | |||
---|---|---|---|---|---|---|
Odds ratio (95% confidence interval) | p-value | Odds ratio (95% confidence interval) | p-value | Odds ratio (95% confidence interval) | p-value | |
Registered nurse shortfall (HPPD) | 0.89 (0.88 to 0.90) | < 0.001 | 1.21 (1.17 to 1.24) | < 0.001 | 1.21 (1.18 to 1.24) | < 0.001 |
Nursing support worker shortfall (HPPD) | 0.89 (0.88 to 0.91) | < 0.001 | 1.21 (1.18 to 1.26) | < 0.001 | 1.23 (1.19 to 1.27) | < 0.001 |
Scaled registered nurse shortfall squareda | 0.91 (0.87 to 0.95) | < 0.001 | 1.08 (0.97 to 1.20) | 0.144 | 1.13 (1.04 to 1.23) | 0.005 |
Scaled nursing support worker shortfall squareda | 1.12 (1.04 to 1.20) | 0.003 | 0.87 (0.77 to 0.99) | 0.041 | 0.72 (0.63 to 0.84) | < 0.001 |
Time of day: night | ||||||
Morning | 0.91 (0.84 to 0.98) | 0.010 | 1.50 (1.28 to 1.75) | < 0.001 | 2.31 (1.99 to 2.69) | < 0.001 |
Afternoon | 0.90 (0.85 to 0.94) | < 0.001 | 1.08 (0.98 to 1.17) | 0.105 | 1.73 (1.60 to 1.88) | < 0.001 |
Ward type: mixed | ||||||
Surgical | 2.03 (1.18 to 3.48) | 0.010 | 2.17 (1.32 to 3.57) | 0.002 | ||
Proportion single rooms | 3.35 (1.12 to 10.00) | 0.031 | 3.15 (1.15 to 8.64) | 0.026 | ||
Standard deviation between wards | 1.10 | 1.04 | 0.97 | |||
Standard deviation between hospitals | 0.77 | 0.92 | 0.77 | |||
Akaike information criterion | 47,234 | 18,606 | 21,755 | |||
Bayesian information criterion | 47,313 | 18,703 | 21,851 |
Variable | Enough staff for quality | Nursing care left undone | Staff breaks missed | |||
---|---|---|---|---|---|---|
Odds ratio (95% confidence interval) | p-value | Odds ratio (95% confidence interval) | p-value | Odds ratio (95% confidence interval) | p-value | |
Registered nurse shortfall (HPPD) | 0.88 (0.86 to 0.90) | < 0.001 | 1.23 (1.15 to 1.32) | < 0.001 | 1.09 (1.03 to 1.16) | 0.006 |
Nursing support worker shortfall (HPPD) | 0.89 (0.86 to 0.91) | < 0.001 | 1.18 (1.09 to 1.28) | < 0.001 | 1.11 (1.03 to 1.19) | 0.007 |
Scaled registered nurse shortfall squared | 0.90 (0.86 to 0.94) | < 0.001 | 0.99 (0.89 to 1.11) | 0.872 | ||
Scaled nursing support worker shortfall squared | 1.15 (1.06 to 1.24) | 0.001 | 0.81 (0.70 to 0.95) | 0.008 | 0.64 (0.54 to 0.77) | < 0.001 |
Time of day: night | ||||||
Morning | 0.93 (0.86 to 1.01) | 0.075 | 1.43 (1.22 to 1.68) | < 0.001 | 2.06 (1.76 to 2.41) | < 0.001 |
Afternoon | 0.90 (0.86 to 0.95) | < 0.001 | 0.90 (0.80 to 1.00) | 0.047 | 1.30 (1.18 to 1.44) | < 0.001 |
Ward type: mixed | ||||||
Surgical | 2.02 (1.15 to 3.52) | 0.014 | 1.79 (1.08 to 2.97) | 0.024 | ||
Proportion single rooms | 3.00 (0.99 to 9.09) | 0.053 | 2.73 (1.00 to 7.49) | 0.051 | ||
Registered nurse shortfall: Nursing support worker shortfall | 0.99 (0.99 to 1.00) | 0.011 | 1.00 (0.99 to 1.01) | 0.483 | 0.99 (0.98 to 1.00) | 0.237 |
Registered nurse shortfall: morning | 1.03 (1.00 to 1.06) | 0.067 | 1.04 (0.98 to 1.10) | 0.219 | 0.98 (0.93 to 1.04) | 0.582 |
Registered nurse shortfall: afternoon | 1.02 (0.99 to 1.04) | 0.129 | 1.11 (1.06 to 1.17) | < 0.001 | 1.20 (1.14 to 1.26) | < 0.001 |
Registered nurse shortfall: surgical | 0.95 (0.89 to 1.01) | 0.117 | 1.06 (1.00 to 1.13) | 0.040 | ||
Registered nurse shortfall: proportion single rooms | 0.83 (0.75 to 0.92) | < 0.001 | 0.93 (0.85 to 1.03) | 0.175 | ||
Nursing support worker shortfall: morning | 1.04 (1.00 to 1.08) | 0.040 | 1.03 (0.95 to 1.12) | 0.472 | 1.08 (1.00 to 1.17) | 0.064 |
Nursing support worker shortfall: afternoon | 1.00 (0.97 to 1.03) | 0.860 | 1.14 (1.07 to 1.21) | < 0.001 | 1.23 (1.16 to 1.30) | < 0.001 |
Nursing support worker shortfall: surgical | 1.02 (0.94 to 1.09) | 0.660 | 1.09 (1.02 to 1.17) | 0.007 | ||
Nursing support worker shortfall: proportion single rooms | 0.87 (0.77 to 0.99) | 0.030 | 0.87 (0.78 to 0.98) | 0.017 | ||
Standard deviation between wards | 1.10 | 1.06 | 0.97 | |||
Standard deviation between hospitals | 0.77 | 0.94 | 0.77 | |||
Akaike information criterion | 47,226 | 18,571 | 21,635 | |||
Bayesian information criterion | 47,349 | 18,738 | 21,810 |
Shortfall (HPPD) | Nursing support worker | ||||||||||||||||||||||||||
---|---|---|---|---|---|---|---|---|---|---|---|---|---|---|---|---|---|---|---|---|---|---|---|---|---|---|---|
1.5 | 1.4 | 1.3 | 1.2 | 1.1 | 1 | 0.9 | 0.8 | 0.7 | 0.6 | 0.5 | 0.4 | 0.3 | 0.2 | 0.1 | 0 | –0.1 | –0.2 | –0.3 | –0.4 | –0.5 | –0.6 | –0.7 | –0.8 | –0.9 | –1 | ||
Registered nurse | 1.5 | –27% | –29% | –30% | –32% | –33% | –34% | –35% | –36% | –36% | –37% | –37% | –37% | –37% | –36% | –36% | –35% | –34% | –33% | –31% | –30% | –28% | –26% | –24% | –21% | –18% | –15% |
1.4 | –23% | –26% | –27% | –29% | –30% | –31% | –32% | –33% | –34% | –34% | –34% | –34% | –34% | –33% | –33% | –32% | –31% | –30% | –28% | –27% | –25% | –23% | –20% | –17% | –14% | –11% | |
1.3 | –20% | –22% | –24% | –26% | –27% | –28% | –29% | –30% | –31% | –31% | –31% | –31% | –31% | –31% | –30% | –29% | –28% | –27% | –25% | –24% | –22% | –19% | –17% | –14% | –11% | –7% | |
1.2 | –17% | –19% | –21% | –23% | –24% | –25% | –26% | –27% | –28% | –28% | –28% | –28% | –28% | –28% | –27% | –26% | –25% | –24% | –22% | –21% | –19% | –16% | –14% | –11% | –7% | –4% | |
1.1 | –13% | –16% | –18% | –20% | –21% | –23% | –24% | –24% | –25% | –25% | –26% | –26% | –25% | –25% | –24% | –24% | –22% | –21% | –20% | –18% | –16% | –13% | –10% | –7% | –4% | 0% | |
1 | –10% | –13% | –15% | –17% | –18% | –20% | –21% | –22% | –22% | –23% | –23% | –23% | –23% | –22% | –22% | –21% | –20% | –18% | –17% | –15% | –13% | –10% | –7% | –4% | –1% | 3% | |
0.9 | –7% | –10% | –12% | –14% | –16% | –17% | –18% | –19% | –20% | –20% | –20% | –20% | –20% | –20% | –19% | –18% | –17% | –16% | –14% | –12% | –10% | –7% | –4% | –1% | 3% | 7% | |
0.8 | –4% | –7% | –9% | –11% | –13% | –14% | –16% | –16% | –17% | –18% | –18% | –18% | –18% | –17% | –17% | –16% | –14% | –13% | –11% | –9% | –7% | –4% | –1% | 2% | 6% | 10% | |
0.7 | –1% | –4% | –6% | –8% | –10% | –12% | –13% | –14% | –15% | –15% | –15% | –15% | –15% | –15% | –14% | –13% | –12% | –10% | –9% | –7% | –4% | –2% | 1% | 5% | 9% | 13% | |
0.6 | 2% | –1% | –4% | –6% | –8% | –9% | –11% | –12% | –12% | –13% | –13% | –13% | –13% | –13% | –12% | –11% | –10% | –8% | –6% | –4% | –2% | 1% | 4% | 8% | 12% | 16% | |
0.5 | 4% | 1% | –1% | –3% | –5% | –7% | –8% | –9% | –10% | –11% | –11% | –11% | –11% | –10% | –10% | –9% | –7% | –6% | –4% | –2% | 1% | 3% | 7% | 10% | 14% | 19% | |
0.4 | 7% | 4% | 1% | –1% | –3% | –5% | –6% | –7% | –8% | –9% | –9% | –9% | –9% | –8% | –8% | –7% | –5% | –4% | –2% | 0% | 3% | 6% | 9% | 13% | 17% | 21% | |
0.3 | 9% | 6% | 3% | 1% | –1% | –3% | –4% | –5% | –6% | –7% | –7% | –7% | –7% | –6% | –6% | –5% | –3% | –2% | 0% | 2% | 5% | 8% | 11% | 15% | 19% | 24% | |
0.2 | 11% | 8% | 5% | 3% | 1% | –1% | –2% | –3% | –4% | –5% | –5% | –5% | –5% | –5% | –4% | –3% | –2% | 0% | 2% | 4% | 7% | 10% | 13% | 17% | 21% | 26% | |
0.1 | 13% | 10% | 7% | 5% | 3% | 1% | –1% | –2% | –3% | –3% | –4% | –4% | –4% | –3% | –2% | –1% | 0% | 2% | 3% | 6% | 8% | 11% | 15% | 18% | 23% | 28% | |
0 | 15% | 12% | 9% | 6% | 4% | 2% | 1% | 0% | –1% | –2% | –2% | –2% | –2% | –2% | –1% | 0% | 1% | 3% | 5% | 7% | 10% | 13% | 16% | 20% | 24% | 29% | |
–0.1 | 17% | 13% | 10% | 8% | 6% | 4% | 2% | 1% | 0% | –1% | –1% | –1% | –1% | –1% | 0% | 1% | 2% | 4% | 6% | 8% | 11% | 14% | 17% | 21% | 26% | 31% | |
–0.2 | 18% | 14% | 11% | 9% | 7% | 5% | 3% | 2% | 1% | 0% | 0% | 0% | 0% | 0% | 1% | 2% | 3% | 5% | 7% | 9% | 12% | 15% | 19% | 22% | 27% | 32% | |
–0.3 | 19% | 15% | 12% | 10% | 8% | 6% | 4% | 3% | 2% | 1% | 1% | 1% | 1% | 1% | 2% | 3% | 4% | 6% | 8% | 10% | 13% | 16% | 19% | 23% | 28% | 33% | |
–0.4 | 20% | 16% | 13% | 11% | 8% | 6% | 5% | 3% | 2% | 2% | 1% | 1% | 1% | 2% | 2% | 3% | 5% | 6% | 8% | 11% | 13% | 16% | 20% | 24% | 28% | 33% | |
–0.5 | 20% | 17% | 14% | 11% | 9% | 7% | 5% | 4% | 3% | 2% | 2% | 2% | 2% | 2% | 3% | 4% | 5% | 7% | 9% | 11% | 14% | 17% | 20% | 24% | 29% | 33% | |
–0.6 | 21% | 17% | 14% | 11% | 9% | 7% | 5% | 4% | 3% | 2% | 2% | 2% | 2% | 2% | 3% | 4% | 5% | 7% | 9% | 11% | 14% | 17% | 20% | 24% | 29% | 34% | |
–0.7 | 21% | 17% | 14% | 11% | 9% | 7% | 5% | 4% | 3% | 2% | 2% | 2% | 2% | 2% | 3% | 4% | 5% | 7% | 9% | 11% | 14% | 17% | 20% | 24% | 28% | 33% | |
–0.8 | 21% | 17% | 14% | 11% | 9% | 7% | 5% | 4% | 3% | 2% | 2% | 1% | 1% | 2% | 3% | 4% | 5% | 6% | 8% | 11% | 13% | 16% | 20% | 24% | 28% | 33% | |
–0.9 | 20% | 17% | 13% | 11% | 8% | 6% | 5% | 3% | 2% | 2% | 1% | 1% | 1% | 1% | 2% | 3% | 4% | 6% | 8% | 10% | 13% | 16% | 19% | 23% | 27% | 32% | |
–1 | 19% | 16% | 13% | 10% | 8% | 6% | 4% | 3% | 2% | 1% | 0% | 0% | 0% | 1% | 1% | 2% | 4% | 5% | 7% | 9% | 12% | 15% | 18% | 22% | 26% | 31% |
Appendix 4 Detailed simulation results to supplement Chapter 6
Establishment scenario | Hospital trust | Average across hospital trusts | ||||||||
---|---|---|---|---|---|---|---|---|---|---|
A | B | C | D | |||||||
High | 7.5% | 7.6% | 26.4% | 26.9% | 16.3% | 16.5% | 25.1% | 24.3% | 18.8% | 18.8% |
Standard | 17.9% | 17.5% | 35.9% | 34.9% | 37.3% | 36.0% | 34.2% | 33.3% | 31.3% | 30.4% |
Flexible (low) | 55.7% | 54.8% | 69.8% | 68.3% | 60.5% | 57.2% | 67.0% | 66.1% | 63.3% | 61.6% |
Staff group/establishment scenario | Hospital trust | Average across hospital trusts | |||
---|---|---|---|---|---|
A | B | C | D | ||
Re-deployed staff | |||||
High | 1.4% | 0.7% | 0.9% | 0.6% | 0.9% |
Standard | 1.2% | 0.4% | 0.4% | 0.4% | 0.6% |
Flexible (low) | 0.5% | 0.1% | 0.4% | 0.1% | 0.3% |
Bank staff | |||||
High | 0.5% | 3.7% | 1.2% | 3.7% | 2.3% |
Standard | 1.2% | 4.8% | 2.7% | 5.1% | 3.5% |
Flexible (low) | 5.5% | 9.8% | 5.7% | 10.3% | 7.8% |
Agency staff | |||||
High | 0.4% | 3.1% | 1.0% | 3.1% | 1.9% |
Standard | 1.0% | 4.0% | 2.3% | 4.2% | 2.9% |
Flexible (low) | 4.4% | 8.6% | 4.6% | 8.8% | 6.6% |
Time of day/establishment scenario | Hospital trust | Average across hospital trusts | |||
---|---|---|---|---|---|
A | B | C | D | ||
Morning (7 a.m.–1 p.m.) | |||||
High | 4.6% | 22.9% | 13.4% | 21.6% | 15.6% |
Standard | 11.7% | 32.3% | 33.7% | 29.9% | 26.9% |
Flexible (low) | 49.1% | 69.7% | 59.3% | 65.4% | 60.9% |
Afternoon (1 p.m.–7 p.m.) | |||||
High | 11.4% | 40.9% | 22.0% | 37.4% | 27.9% |
Standard | 30.3% | 52.3% | 53.5% | 50.3% | 46.6% |
Flexible (low) | 73.6% | 87.7% | 74.5% | 86.3% | 80.5% |
Twilight (7 p.m.–1 a.m.) | |||||
High | 6.7% | 22.6% | 16.7% | 19.9% | 16.5% |
Standard | 16.1% | 32.0% | 27.5% | 27.1% | 25.7% |
Flexible (low) | 50.7% | 61.5% | 58.6% | 58.7% | 57.4% |
Night (1 a.m.–7 a.m.) | |||||
High | 7.1% | 18.1% | 13.1% | 20.8% | 14.8% |
Standard | 12.0% | 26.6% | 33.5% | 29.3% | 25.4% |
Flexible (low) | 47.8% | 60.0% | 48.5% | 56.5% | 53.2% |
Data sample/establishment scenario | Hospital trust | Average across hospital trusts | |||
---|---|---|---|---|---|
A | B | C | D | ||
June (reference) | |||||
High | 7.5% (0.42) | 26.4% (0.74) | 16.3% (0.44) | 25.1% (0.73) | 18.8% (0.60) |
Standard | 17.9% (–) | 35.9% (–) | 37.3% (–) | 34.2% (–) | 31.3% (–) |
Flexible (low) | 55.7% (3.11) | 69.8% (1.94) | 60.5% (1.62) | 67.0% (1.96) | 63.3% (2.02) |
January | |||||
High | 4.0% (0.34) | 24.3% (0.71) | 17.0% (0.47) | 17.7% (0.74) | 15.8% (0.59) |
Standard | 11.9% (–) | 34.4% (–) | 36.4% (–) | 23.9% (–) | 26.7% (–) |
Flexible (low) | 45.8% (3.85) | 69.0% (2.01) | 63.2% (1.74) | 57.7% (2.41) | 58.9% (2.21) |
Months | |||||
High | 6.7% (0.44) | 24.8% (0.71) | 13.1% (0.48) | 20.5% (0.71) | 16.3% (0.61) |
Standard | 15.4% (–) | 34.8% (–) | 27.2% (–) | 29.0% (–) | 26.6% (–) |
Flexible (low) | 53.8% (3.49) | 69.5% (2.00) | 54.0% (1.99) | 62.9% (2.17) | 60.1% (2.26) |
All | |||||
High | 5.5% (0.33) | 23.5% (0.64) | 12.7% (0.36) | 19.9% (0.67) | 15.4% (0.52) |
Standard | 16.9% (–) | 36.5% (–) | 35.0% (–) | 29.7% (–) | 29.5% (–) |
Flexible (low) | 55.5% (3.28) | 70.3% (1.93) | 60.4% (1.73) | 65.0% (2.19) | 62.8% (2.13) |
Data sample | Hospital trust | Average across hospital trusts | |||
---|---|---|---|---|---|
A | B | C | D | ||
June (reference) | |||||
January | £4.66 (3.7%) | £0.48 (0.3%) | £0.48 (0.3%) | £6.24 (4.7%) | £2.97 (–2.3%) |
Months | £1.56 (1.2%) | £0.31 (0.2%) | £9.72 (6.6%) | £2.90 (2.2%) | £3.62 (–2.6%) |
All | £0.04 (0.0%) | –£0.34 (–0.2%) | £0.85 (0.6%) | £2.49 (1.9%) | £0.76 (–0.8%) |
Availability of temporary staff/establishment scenario | Hospital trust | Average change across hospital trusts | ||||||||
---|---|---|---|---|---|---|---|---|---|---|
A | B | C | D | |||||||
No temporary staff | ||||||||||
High | +3.4% | £0.00 | +1.5% | £0.02 | +1.3% | –£0.11 | +1.4% | –£0.02 | +1.9% | –£0.03 |
Standard | +2.7% | £0.02 | +0.6% | £0.00 | +0.8% | –£0.06 | +0.7% | £0.04 | +1.2% | £0.00 |
Flexible (low) | +0.6% | –£0.02 | –0.0% | £0.01 | –0.1% | –£0.05 | –0.1% | –£0.01 | +0.1% | –£0.02 |
Empirical | ||||||||||
High | +1.7% | £0.97 | +1.0% | £0.31 | +0.8% | £0.80 | +0.9% | £0.84 | +1.1% | £0.73 |
Standard | +1.4% | £1.17 | +0.4% | £0.63 | +0.4% | £0.01 | +0.6% | £0.25 | +0.7% | £0.52 |
Flexible (low) | +0.3% | £0.16 | +0.1% | –£0.32 | –0.3% | £0.55 | 0.0% | –£0.49 | +0.0% | –£0.03 |
Bank 50%, agency 90% | ||||||||||
High | –0.2% | £1.94 | 0.0% | £1.13 | –0.3% | £1.66 | +0.1% | £1.03 | –0.1% | £1.44 |
Standard | –0.5% | £1.64 | –0.1% | £0.54 | 0.0% | £0.53 | +0.1% | £0.42 | –0.1% | £0.78 |
Flexible (low) | –0.3% | £0.69 | –0.1% | £0.16 | –0.4% | £0.50 | 0.0% | £0.02 | –0.2% | £0.34 |
Bank 50%, agency 100% | ||||||||||
High | –0.2% | £2.03 | –0.0% | £1.19 | –0.1% | £1.58 | +0.0% | £0.87 | –0.1% | £1.42 |
Standard | –0.6% | £1.68 | –0.1% | £0.61 | +0.0% | £0.96 | –0.0% | £0.66 | –0.2% | £0.98 |
Flexible (low) | –0.3% | £0.64 | –0.2% | £0.09 | –0.3% | £0.45 | –0.2% | £0.15 | –0.2% | £0.33 |
Low staffing criterion/establishment scenario | Hospital trust (%) | Average across hospital trusts (%) | |||||||||||||||||||||||
---|---|---|---|---|---|---|---|---|---|---|---|---|---|---|---|---|---|---|---|---|---|---|---|---|---|
A | B | C | D | ||||||||||||||||||||||
Overall | RN | NSW | Either | Both | Overall | RN | NSW | Either | Both | Overall | RN | NSW | Either | Both | Overall | RN | NSW | Either | Both | Overall | RN | NSW | Either | Both | |
Understaffing (more than 15% below requirement) | |||||||||||||||||||||||||
High | 7.5 | 10.5 | 17.4 | 25.5 | 2.5 | 26.3 | 29.7 | 31.6 | 46.1 | 15.1 | 16.4 | 19.7 | 30.1 | 44.9 | 5.0 | 25.0 | 30.7 | 30.2 | 47.2 | 13.7 | 18.8 | 22.7 | 27.3 | 40.9 | 9.1 |
Standard | 17.7 | 24.6 | 25.3 | 42.9 | 7.0 | 36.0 | 39.3 | 39.5 | 57.0 | 21.7 | 37.4 | 40.5 | 40.3 | 67.2 | 13.6 | 34.3 | 39.1 | 37.9 | 57.6 | 19.3 | 34.3 | 35.9 | 35.8 | 56.2 | 15.4 |
Flexible (low) | 55.6 | 55.3 | 53.2 | 79.7 | 28.8 | 69.9 | 70.2 | 62.6 | 85.8 | 46.9 | 60.6 | 66.5 | 42.1 | 83.6 | 25.0 | 66.9 | 68.1 | 60.1 | 86.3 | 41.9 | 66.9 | 65.0 | 54.5 | 83.9 | 35.7 |
Absolute understaffing (below requirement) | |||||||||||||||||||||||||
High | 44.4 | 39.9 | 48.3 | 65.1 | 23.1 | 73.0 | 67.5 | 70.9 | 84.8 | 53.6 | 43.3 | 41.9 | 44.5 | 68.1 | 18.3 | 71.2 | 65.4 | 67.4 | 84.5 | 48.4 | 58.0 | 53.7 | 57.8 | 75.6 | 35.9 |
Standard | 66.4 | 65.3 | 58.0 | 84.0 | 39.3 | 84.2 | 78.8 | 77.8 | 91.9 | 64.7 | 69.8 | 67.5 | 54.6 | 86.2 | 35.9 | 82.2 | 76.2 | 74.3 | 91.3 | 59.2 | 75.7 | 72.0 | 66.2 | 88.4 | 49.8 |
Flexible (low) | 95.0 | 86.9 | 85.1 | 98.0 | 73.9 | 98.3 | 95.5 | 90.6 | 99.3 | 86.8 | 87.7 | 87.8 | 58.0 | 95.7 | 50.1 | 98.2 | 93.0 | 89.3 | 99.4 | 83.0 | 94.8 | 90.8 | 80.8 | 98.1 | 73.5 |
At least one person short | |||||||||||||||||||||||||
High | 10.0 | 4.3 | 5.7 | 8.3 | 1.7 | 41.5 | 27.7 | 23.0 | 35.6 | 15.1 | 8.4 | 6.5 | 1.6 | 7.4 | 0.7 | 34.2 | 20.2 | 18.9 | 28.3 | 10.7 | 23.5 | 14.7 | 12.3 | 19.9 | 7.1 |
Standard | 21.8 | 10.4 | 10.3 | 16.3 | 4.3 | 51.6 | 35.4 | 29.6 | 44.4 | 20.6 | 19.4 | 12.6 | 4.3 | 15.4 | 1.5 | 43.1 | 24.4 | 25.5 | 35.4 | 14.5 | 34.0 | 20.7 | 17.4 | 27.9 | 10.2 |
Flexible (low) | 53.1 | 28.4 | 25.5 | 39.9 | 14.0 | 78.8 | 60.5 | 47.4 | 70.4 | 37.4 | 33.3 | 25.9 | 3.7 | 27.5 | 2.2 | 70.4 | 45.5 | 42.5 | 59.7 | 28.3 | 58.9 | 40.1 | 29.8 | 49.4 | 20.5 |
Multipliers used to set the establishment | Hospital trust | |||
---|---|---|---|---|
A | B | C | D | |
Fixed (average) multiplier | ||||
Understaffing | 21% (1%, 90%) | 38% (0%, 96%) | 29% (15%, 51%) | 33% (14%, 77%) |
Overstaffing | 17% (0%, 62%) | 4% (0%, 34%) | 20% (4%, 32%) | 3% (0%, 15%) |
Standard multipliers | ||||
Understaffing | 19% (7%, 52%) | 36% (7%, 80%) | 39% (17%, 53%) | 35% (14%, 76%) |
Overstaffing | 11% (1%, 35%) | 3% (0%, 16%) | 13% (4%, 27%) | 3% (0%, 11%) |
Absolute difference | ||||
Understaffing | +2% | +2% | –10% | –2% |
Overstaffing | +6% | +1% | +7% | +1% |
Staff group | Hospital trust | |||
---|---|---|---|---|
A | B | C | D | |
All | 138 (54, 258) | 187 (72, 516) | 74 (36, 120) | 154 (102, 204) |
Registered nurses | 71 (36, 126) | 106 (36, 348) | 52 (24, 84) | 74 (48, 114) |
Nursing support workers | 68 (18, 132) | 81 (24, 168) | 22 (12, 36) | 80 (42, 108) |
Availability of temporary staff | Hospital trust | Average across hospital trusts | ||||||||
---|---|---|---|---|---|---|---|---|---|---|
A | B | C | D | |||||||
Empirical | 36.9% | £116 | 52.5% | £131 | 45.7% | £140 | 49.9% | £125 | 46.3% | £128 |
Bank and agency 50% | 24.6% | £122 | 30.7% | £144 | 35.3% | £149 | 27.3% | £137 | 29.5% | £138 |
Bank 50%, agency 90% | 18.5% | £124 | 15.2% | £151 | 29.9% | £153 | 10.2% | £145 | 18.5% | £143 |
Empirical bank, agency 90% | 20.4% | £125 | 19.7% | £152 | 33.0% | £153 | 15.7% | £145 | 22.2% | £143 |
Bank 90%, agency 90% | 16.2% | £125 | 10.9% | £152 | 25.9% | £155 | 4.2% | £146 | 14.3% | £144 |
Registered nurses who are IV trained (%) | Hospital trust | |||
---|---|---|---|---|
A | B | C | D | |
0 | 100% | 100% | 100% | 100% |
10 | 66.4% | 52.8% | 75.1% | 65.9% |
20 | 38.5% | 23.3% | 52.8% | 38.4% |
30 | 19.5% | 8.7% | 34.7% | 19.6% |
40 | 9.2% | 2.9% | 21.9% | 9.0% |
50 | – | – | – | – |
60 | 1.6% | 0.4% | 7.8% | 1.2% |
70 | – | – | – | – |
80 | 0.2% | 0.0% | 2.3% | 0.0% |
90 | – | – | – | – |
100 | 0% | 0% | 0% | 0% |
Factor | Level | Understaffed patient shifts (%) | Cost per patient-day (£) | ||
---|---|---|---|---|---|
– | Baseline | + | |||
(a) Short-notice absence for nursing support workers | 3% | 4% | 0.5 (0.5 to 0.5) | 0.02 (0.02 to 0.03) | |
(b) Short-notice absence for registered nurses | 3% | 4% | 0.8 (0.8 to 0.8) | 0.03 (0.03 to 0.03) | |
(c) Rounding fractional parts of shifts | Round down for fractional parts < 0.5 | Always round up | –14.3 (–14.4 to –14.3) | 3.53 (3.53 to 3.54) | |
(d) Re-deploying staff | Across whole hospital | Within directorate | 0.3 (0.2 to 0.3) | 0.20 (0.20 to 0.20) | |
(e) Variation in multipliers (standard deviation as percentage of mean) | 0% | (10%) | 25% | 1.0 (1.0 to 1.1) | 0.00 (0.00 to 0.01) |
(f) Efficiency of re-deployed/bank staff | 90% | 100% | –0.8 (–0.9 to –0.8) | 0.00 (0.00 to 0.00) | |
(g) Efficiency of agency staff | 75% | 100% | –1.3 (–1.3 to –1.2) | 0.00 (–0.01 to 0.01) |
Interaction between factorsa | Understaffed patient shifts (%) | Cost per patient-day (£) |
---|---|---|
a:b | 0.0 (0.0 to 0.0) | 0.00 (0.00 to 0.00) |
a:c | 0.1 (0.1 to 0.1) | –0.01 (–0.01 to 0.00) |
a:d | 0.0 (0.0 to 0.0) | 0.00 (–0.01 to 0.00) |
a:e | 0.0 (0.0 to 0.0) | 0.00 (0.00 to 0.00) |
a:f | 0.0 (0.0 to 0.0) | 0.00 (0.00 to 0.00) |
a:g | 0.0 (–0.1 to 0.0) | 0.00 (0.00 to 0.00) |
b:c | 0.1 (0.0 to 0.1) | 0.01 (0.00 to 0.01) |
b:d | 0.0 (–0.1 to 0.0) | 0.00 (0.00 to 0.00) |
b:e | 0.0 (0.0 to 0.0) | 0.00 (0.00 to 0.00) |
b:f | 0.0 (–0.1 to 0.0) | 0.00 (0.00 to 0.00) |
b:g | 0.0 (–0.1 to 0.0) | 0.00 (0.00 to 0.00) |
c:d | –0.2 (–0.2 to –0.2) | –0.06 (–0.07 to –0.06) |
c:e | 0.1 (0.0 to 0.1) | 0.00 (0.00 to 0.00) |
c:f | –0.1 (–0.2 to –0.1) | 0.00 (0.00 to 0.00) |
c:g | –0.3 (–0.4 to –0.3) | 0.00 (0.00 to 0.00) |
d:e | –0.1 (–0.1 to 0.0) | 0.00 (0.00 to 0.00) |
d:f | 0.1 (0.0 to 0.1) | 0.00 (–0.01 to 0.00) |
d:g | –0.1 (–0.1 to 0.0) | 0.00 (–0.01 to 0.00) |
e:f | 0.0 (0.0 to 0.0) | 0.00 (0.00 to 0.00) |
e:g | 0.0 (0.0 to 0.0) | 0.00 (0.00 to 0.00) |
f:g | 0.0 (0.0 to 0.1) | 0.00 (0.00 to 0.00) |
Appendix 5 Economic modelling sensitivity analysis to supplement Chapter 7
Availability of temporary staff (re-deployment) | Mortality parameter/effect/establishment | |||||||
---|---|---|---|---|---|---|---|---|
Lower confidence limit | Upper confidence limit | |||||||
Staff cost/life (£) | Net cost/life (£) | Staff cost/life (£) | Net cost/life (£) | |||||
High | Flexible (low) | High | Flexible (low) | High | Flexible (low) | High | Flexible (low) | |
Zero temporary staff (NR) | 97,699 | –68,990 | 47,914 | –46,186 | 19,463 | –13,968 | 9958 | –9350 |
Empirical availability of temporary staff (WR) | 62,257 | –67,469 | 27,275 | –41,010 | 12,328 | –14,197 | 5588 | –8630 |
50% availability of bank and agency staff (WR) | 53,961 | –62,658 | 23,574 | –39,199 | 11,119 | –13,915 | 4944 | –8720 |
50% bank and 90% agency availability (NR) | 52,948 | –46,669 | 21,864 | –26,869 | 10,832 | –11,068 | 4515 | –6414 |
50% bank and 90% agency availability (WR) | 52,294 | –44,216 | 18,266 | –21,713 | 10,567 | –10,319 | 3722 | –5101 |
List of abbreviations
- AUKUH
- Association of UK University Hospitals
- CINAHL
- Cumulative Index to Nursing and Allied Health Literature
- CLAHRC
- Collaboration for Leadership in Applied Health Research and Care
- HPPD
- hours per patient-day
- IT
- information technology
- IV
- intravenous
- LUA-Q
- Leeds University Acuity-Quality
- NICE
- National Institute for Health and Care Excellence
- NIHR
- National Institute for Health Research
- NNH
- numbers needed to harm
- NNT
- numbers needed to treat
- SNCT
- Safer Nursing Care Tool
- SUHT
- Southampton University Hospital NHS Trust
- WTE
- whole-time equivalent
Notes
-
Associations between staffing shortfall and staffing adequacy: additional analyses
-
Costs, consequences and cost-effectiveness of ‘high’ and ‘flexible (low)’ staffing establishment scenarios versus ‘standard’ establishments
Supplementary material can be found on the NIHR Journals Library report page (https://doi.org/10.3310/hsdr08160).
Supplementary material has been provided by the authors to support the report and any files provided at submission will have been seen by peer reviewers, but not extensively reviewed. Any supplementary material provided at a later stage in the process may not have been peer reviewed.