Notes
Article history
The research reported in this issue of the journal was funded by the HS&DR programme or one of its preceding programmes as project number 15/71/06. The contractual start date was in January 2017. The final report began editorial review in July 2019 and was accepted for publication in November 2019. The authors have been wholly responsible for all data collection, analysis and interpretation, and for writing up their work. The HS&DR editors and production house have tried to ensure the accuracy of the authors’ report and would like to thank the reviewers for their constructive comments on the final report document. However, they do not accept liability for damages or losses arising from material published in this report.
Declared competing interests of authors
Iestyn Williams was a member of the Health Services and Delivery Research Prioritisation Committee (Commissioned) (2015–19). Magdalena Skrybant and Richard J Lilford are also supported by the National Institute for Health Research Applied Research Collaboration West Midlands.
Permissions
Copyright statement
© Queen’s Printer and Controller of HMSO 2020. This work was produced by Ayorinde et al. under the terms of a commissioning contract issued by the Secretary of State for Health and Social Care. This issue may be freely reproduced for the purposes of private research and study and extracts (or indeed, the full report) may be included in professional journals provided that suitable acknowledgement is made and the reproduction is not associated with any form of advertising. Applications for commercial reproduction should be addressed to: NIHR Journals Library, National Institute for Health Research, Evaluation, Trials and Studies Coordinating Centre, Alpha House, University of Southampton Science Park, Southampton SO16 7NS, UK.
2020 Queen’s Printer and Controller of HMSO
Chapter 1 Background
Publication and related bias
Publication bias refers to the phenomenon by which research findings that are statistically significant and/or are perceived to be interesting or favourable are more likely to be published than those that are not statistically significant or perceived to be interesting. Publication bias occurs at study level (i.e. when a study is not published at all owing to the nature of its findings). Similar bias may manifest at outcome level (i.e. a study may have evaluated several outcomes, but only the findings related to a subset of the outcomes that are statistically significant or judged to be worth noting are reported in its publication). This is termed ‘outcome reporting bias’. 1 Bias can also occur when researchers carry out analyses of data collected from research. For example, multiple analyses may be performed using different techniques or different subsets of the data until statistically significant results are obtained, which are then published. This is referred to as ‘data dredging’ or ‘p-hacking’. 2,3 These biases are a major threat to evidence-based decision-making, as they distort the full picture of the ‘true’ evidence gathered from research and may lead to misinformed decisions. Here we use the term ‘publication and related bias’ to refer to these biases collectively (Figure 1). Further bias may occur following the publication of research findings, such as citation bias and media attention bias, which alongside publication and related bias are collectively known as dissemination bias. 4 For the present study, we focus on publication and related bias that occurs up to the stage of publication (see Figure 1), as biases that occur following publication can largely be overcome through systematic literature search, which is becoming a standard practice when evidence is synthesised to support decision-making.
FIGURE 1.
Various forms of bias in the process of research publication. See Glossary for the definition of ‘winner’s curse’.

A large body of literature has demonstrated the existence of publication and related bias in clinical research. 1,4,5 In particular, several high-profile cases highlighting the non-publication and/or under-reporting of unfavourable results from clinical trials of new pharmaceuticals have raised ethical concerns over publication bias and have highlighted the potential harm it may cause to patients. 6–8 These concerns have led to mandatory clinical trial registration9,10 and, more recently, mandatory reporting of findings of registered trials. 11 By contrast, health services and delivery research (HSDR) has not been subject to similar levels of regulation and scrutiny, and the issue of publication bias appears to be largely ignored. Our preliminary scoping of the health services research literature found only a small number studies addressing this topic, which suggests the possible existence of publication bias. 12–15 The paucity of documented evidence was affirmed in our discussions with some leading experts in health services research.
The lack of published literature on publication and related bias in HSDR compared with clinical research is surprising, given that publication bias has also been documented in many other scientific disciplines, such as social science,16 management research17 and ecology. 18 As there is no obvious reason to believe that HSDR is immune to publication and related bias, and such bias could have substantial implications for health service decisions relying on HSDR, we set out to explore the existence and extent of publication and related bias in this field in order to inform best practice in the reporting and synthesis of evidence from HSDR.
Aims and objectives
The primary aims of this study were to gather prima facie evidence of the existence and potential impact of publication and related bias in HSDR, to examine current practice in systematic reviews of HSDR literature, and to explore common methods in relation to the detection and mitigation of the bias.
The above aims were to be achieved through five distinct but inter-related work packages (WPs), each with a specific objective:
-
WP 1 – a systematic review of empirical and methodological studies concerning the occurrence, potential impact and/or methodology related to publication bias in HSDR, to provide a summary of what is known from current literature.
-
WP 2 – an overview of systematic reviews of intervention and association studies in HSDR, to describe current practice and potential challenges in assessing publication bias during evidence synthesis.
-
WP 3 – in-depth case studies to evaluate the applicability of different methods for detecting and mitigating publication bias in HSDR and to provide guidance for future research and practice.
-
WP 4 – a retrospective study to follow up the publication status of cohorts of HSDR studies to directly observe publication bias in HSDR.
-
WP 5 – semistructured interviews with health services researchers and commissioners, journal editors and service managers, and a focus group discussion with patient representatives to explore their perception and experience related to publication bias.
These WPs complemented each other to provide a full picture of both empirical evidence and current practices related to publication bias in HSDR (Figure 2). Detailed methods for the WPs are described in the next chapter.
FIGURE 2.
Overview of the five complementary WPs included in this study. HSRProj, Health Services Research Projects in Progress; NIHR, National Institute for Health Research.

Structure of the report
The rest of this report is organised as follows: Chapter 2 details the scope and methods of each of the five WPs described above. Chapters 3–7 present the findings from each of the WPs. Chapter 8 includes discussions of specific issues related to methods and findings of individual WPs, and provides some discourse of findings across the WPs and wider issues related to use of evidence to inform decision-making in health services. The chapter concludes with issues worth considering by different stakeholders of HSDR in relation to publication and related bias, and recommendations for future research.
Chapter 2 Overview of methods
Scope
The subject area of HSDR is very broad. In order to draw a boundary that allowed a focused investigation, we used the definition adopted by the National Institute for Health Research (NIHR) and defined HSDR as ‘research that produces evidence on the quality, accessibility and organisation of health services’. In addition, we targeted two types of quantitative studies: (1) intervention studies, which were carried out to evaluate interventions to improve and optimise the effectiveness and/or efficiency of the delivery of health services; and (2) association studies, which were carried out to evaluate associations between different variables along the service delivery causal chain. 19 A large number of variables can be covered in association studies in HSDR. These include structure variables (e.g. characteristics of a hospital, nurse–patient ratio); generic processes (e.g. continuous professional development, institutional human resource policy); intervening variables (e.g. safety culture, staff knowledge and morale, etc., that could be influenced by structure and generic processes and then impact on many downstream processes);19 targeted processes (e.g. door-to-balloon time for treating myocardial infarction, adherence to guidelines for management of patients with diabetes); and health service utilisation, patient, carer or health care provider outcomes and context (e.g. weekdays vs. weekends, low- and middle-income countries vs. high-income countries). We recognised that intervention studies cannot be completely separated from association studies, as the former is a special case of the latter. Nevertheless, such classification reflected how research questions are often asked in HSDR (e.g. whether or not an intervention works vs. whether or not certain factors affect one another or influence outcomes in the health system). We hypothesised that association studies may be more vulnerable to selective publication and reporting than intervention studies, as a causal relationship is assumed between an intervention and outcomes, whereas relationships between different factors examined in association studies are exploratory and not necessarily causal. In addition, evaluation of interventions may be more likely to be specifically funded with a mandate from the funder to disseminate results, whereas association studies may be carried out without specific funding and related incentive for publication.
The criteria for selecting intervention and association studies were applied in conjunction with the definition of HSDR described in the previous paragraph. Eligible studies may focus on any aspects of health systems and health policy, health-care organisations, people who organise and deliver the health services, and users and carers of the services, as well as related processes, outcomes and contextual factors. Studies concerning clinical research and health technology assessment (i.e. those focusing on interventions applied directly to individual patients), disease epidemiology and genetic associations have previously been examined in detail4 and, therefore, were not included in this study. We were aware of potential grey areas in which the boundary between HSDR and non-HSDR studies may be vague. These were dealt with by consulting members of the Project Management Group and Study Steering Committee.
A wide variety of research designs, including quantitative, qualitative and mixed-methods research, have been used in HSDR. 20,21 This study focused on quantitative research and mixed-methods research that incorporated an element of quantitative estimation of intervention effects or association, although we acknowledged that qualitative research can also be subject to publication bias. 22 As the mechanisms and manifestation of publication bias for qualitative research are likely to be different, and methods for evaluating its occurrence and impact are not well developed, we felt that issues related to qualitative research were beyond the scope of the study and warranted a separate investigation. 23
Methods for assessing publication and related bias
Many methods have been developed in order to detect publication and related bias and estimate and/or mitigate its potential impact. 4,24,25 Methods used to detect publication bias can be broadly classified as either making indirect inference or employing direct observation.
Several statistical methods, such as funnel plots and related regression tests, can suggest that publication and related bias may be present during the synthesis of evidence across many studies. 24,25 However, these widely used methods allow only indirect inference, as they rely on the identification of specific patterns in the findings across studies that are suggestive of publication bias, but cannot rule out alternative causes. 26 As a result, evidence on publication and related bias obtained using these methods is generally weak and could be misleading if assumptions underlying these methods do not hold.
Direct evidence on publication and related bias can be obtained in two ways:
-
Identifying a cohort of studies (usually through a registry) and then following these up over time to determine whether or not they are published; this is sometimes described as an inception cohort study and is often undertaken retrospectively given the length of time between the inception of a research and publication of its findings (if published).
-
Consulting stakeholders who are involved in generating and/or disseminating research evidence through surveys or interviews to find out their experience.
These methods of direct observation are labour intensive, but may provide the strongest evidence on the presence (or absence) of publication and related bias. A major challenge in HSDR lies with the difficulty in identifying study cohorts owing to the lack of registries.
Among the five WPs included in this study, WP 1 systematically reviewed previous studies that set out to investigate publication and related bias in any substantive areas of HSDR, using either direct or indirect methods. WP 2 examined a random sample of systematic reviews of HSDR topics with regard to their practice in assessing publication and related bias. WP 3 explored issues surrounding commonly used methods for detecting publication and related bias in more depth using case studies. WP 4 identified four cohorts of HSDR studies from various sources and followed them up to check their publication status and to explore if this was associated with the direction or strength of study findings. WP 5 gathered perceptions and experiences of HSDR stakeholders on this issue through interviews and a focus group discussion. The methods for each WP are described in detail below.
Work package 1: a systematic review of empirical evidence on publication bias in HSDR
Protocol registration
The protocol of this systematic review was registered with PROSPERO, registration number CRD42016052333.
Search strategy
The diverse research disciplines, subject areas and terminologies related to HSDR pose a challenge for searching relevant literature. 27 We used a combination of different information sources and search methods to ensure that our coverage of literature was as comprehensive as possible and was inclusive of disciplines closely related to HSDR. These included searches of general and HSDR-specific electronic databases, citation search of key papers (snowballing), search of the internet and contact with experts.
Electronic database searches
We searched general databases, including MEDLINE, EMBASE, Health Management Information Consortium, Cumulative Index to Nursing and Allied Health Literature (CINAHL) and Web of Science™ (Clarivate Analytics, Philadelphia, PA, USA) (which includes Social Science Citation Index), using indexed terms and text words related to HSDR (defined broadly) and publication and related bias. We also searched HSDR-specific databases, including Health Systems Evidence and systematic reviews published by the Cochrane Effective Practice and Organisation of Care review group. Searches were undertaken in March 2017 and were updated in July/August 2018. The search terms for MEDLINE and EMBASE can be found in Appendix 1.
Forward and backward citation search (commonly known as ‘snowballing’)
Reference lists of all studies that met the inclusion criteria for this review (see Inclusion criteria) were examined. Google Scholar was used to locate other potentially relevant studies by examining articles subsequently published that have cited the included studies.
Internet searches
The importance of grey literature in health services research has been highlighted in a report funded by the US National Library of Medicine. 28 In order to locate grey literature, we searched websites of key organisations linked to HSDR, such as The Health Foundation, The King’s Fund, the Institute for Health Improvement, the Agency for Healthcare Research and Quality and the RAND Corporation. In addition, we searched the NIHR HSDR programme’s website and the US Health Services Research Projects in Progress (HSRProj)’s database for details of previously commissioned and ongoing studies.
Consultation with experts
We presented a draft study plan in a meeting associated with the Collaboration for Leadership in Applied Health Research and Care West Midlands, which was attended by international experts in HSDR. Members of the Study Steering Committees were consulted to identify any additional studies that may not have been captured by other means.
Study screening and selection
Records retrieved from electronic databases and subsequently obtained from other sources were imported into EndNote X9 (Clarivate Analytics) to facilitate identification and removal of duplicates. Titles and abstracts of de-duplicated records were screened to exclude clearly irrelevant records. Full-text publications were retrieved for the remaining records, and inclusion and exclusion decisions were made independently by two reviewers for each study based on the selection criteria described in the next paragraph. Screening of all records was conducted independently by two reviewers and any disagreements were resolved by discussion or through consultation with the wider research team.
Inclusion criteria
Studies of all designs that set out to examine any forms of publication and related bias in any fields of HSDR within the scope of this project were included. Specifically, a study needed to meet the following criteria:
-
have investigated data dredging/p-hacking, selective outcome reporting or publication bias, or evaluated methods for detecting these forms of bias
-
have provided empirical, quantitative or qualitative evidence (i.e. not just commentaries or opinions)
-
be concerned with HSDR-related topics.
Data extraction and risk-of-bias assessment
The following information were extracted from each included studies:
-
citation details
-
methods of selecting study sample and characteristics of the sample
-
methods for investigating publication bias
-
key findings, limitations and conclusions reported by the authors.
We were not aware of the availability of any suitable tools for assessing the risk of bias of the included studies, which adopted diverse methods. We therefore critically appraised individual studies based on epidemiological principles, such as representativeness of study sample, potential bias in the sampling and data collection processes, and issues related to confounding. Included studies were read by at least two authors. Key methodological weaknesses of each study were recorded and summarised. We expected that there will not be sufficiently large number of studies with comparable measures for conducting tests for small study effects and publication bias. However, we searched extensively for grey and unpublished literature.
Data synthesis and reporting
Study characteristics, methods and findings were tabulated and narrative summaries compiled. As anticipated at the beginning of the study, data were insufficient for quantitative synthesis.
Work package 2: overview of current practice and findings associated with publication and related bias in systematic reviews of intervention and association studies in HSDR
Systematic reviews have emerged in various fields, including HSDR, as a key tool for summarising the rapidly expanding evidence base in a way that maximises the completeness, while minimising potential bias in their coverage of relevant evidence. Steps to identify and reduce various types of bias are built into the process of a systematic review. The following steps are particularly relevant for publication and related bias:
-
comprehensive search of literature, including attempts to locate unpublished studies
-
assessment of outcome reporting bias of included studies
-
assessment of potential publication bias using funnel plots, related regression methods or other techniques.
This WP examined a random sample of 200 HSDR systematic reviews and inspected each review with regard to whether or not the above measures for minimising and detecting publication and related bias were considered and/or performed. Data were also collected on the methods used and findings from the assessment of publication and related bias. We focused on systematic reviews covering two main types of quantitative study: intervention studies and association studies (see Scope). We explored whether or not the practice of assessing publication and related bias differed between these two types of studies, and whether or not this was associated with various characteristics of the review and the journal in which it was published.
Protocol registration
The protocol for this methodological overview of systematic reviews (meta-epidemiological study) was registered with PROSPERO (registration number CRD42016052366).
Search and sampling strategies
Health services and delivery research systematic reviews examined in this WP were obtained from Health Systems Evidence,29 a database that focuses on evidence related to health systems and services research, and has a comprehensive coverage of systematic reviews, economic evaluations and policy documents related to HSDR. The database is continuously updated and covers several bibliographic databases, including MEDLINE and the Cochrane Database of Systematic Reviews, as its sources. 30 We downloaded (with permission from the database owner) records for all systematic reviews that were classified as ‘systematic reviews of effects’ (n = 4416) and ‘systematic reviews addressing other questions’ (n = 1505) in May 2017, and then assigned a random number to each record. We screened each record against our inclusion criteria, described in the next paragraph, in ascending order based on the assigned random number until the targeted number of reviews (100 each for intervention and association reviews) was reached. On the basis of a baseline rate of 32% for formally assessing or providing partial information with regard to publication bias reported in a previous meta-epidemiological study31 of systematic reviews published by the Cochrane Effective Practice and Organisation of Care review group, the sample size has 80% power to detect a 20% difference in the characteristics and findings between the two types of reviews.
Inclusion/exclusion criteria
For this project, a systematic review was defined as a literature review with explicit statements with regard to research question(s), a strategy for literature search and criteria for study selection. Review articles that did not meet this definition were excluded.
A systematic review needed to fulfil the following requirements to be included:
-
It focused on a topic related to HSDR, as defined for this project.
-
It examined quantitative data concerning intervention effects or associations between different factors.
-
It reported at least one quantitative effect estimate or one result (p-value) of a statistical test. These could be obtained from studies included in the review and it was not necessary for a review to have carried out meta-analyses to be included.
Systematic reviews that investigated the clinical effectiveness and cost-effectiveness of clinical interventions (i.e. those traditionally falling under the provenance of health technology assessment) and those exploring the association between risk factors and disease conditions (i.e. those falling under the provenance of clinical and genetic epidemiology) were excluded.
The eligibility check was carried out by one reviewer and checked by a second reviewer. Disagreements were resolved through discussions between the reviewers. The Project Management Group was also consulted when required.
Assessment of included systematic reviews
Included systematic reviews were examined with regard to the characteristics of the review, the methods used to assess potential publication and related bias, and findings and/or any issues raised concerning the assessment. The following data were extracted:
-
Key study question(s) for which quantitative estimates were sought (e.g. associations or intervention effects).
-
Databases searched and whether or not an attempt was made to search grey literature and unpublished reports, or reasons for not doing this.
-
Types of studies included, in terms of study design (whether or not the review included only controlled trials); whether or not a meta-analysis was carried out; and number of studies included in the review (≥ 10 vs. < 10, the minimum number recommended for the use of funnel plots and related methods for assessing publication bias). 26
-
Methods (if used at all) for detecting and/or mitigating potential publication bias (apart from comprehensive search), for example funnel plots and related regression methods, trim and fill.
-
Methods for assessing outcome reporting bias.
-
Findings of assessment of publication and outcome reporting biases, or reasons for not assessing these.
-
Whether or not the review authors reported adherence to systematic review guidelines, such as Preferred Reporting Items for Systematic Reviews and Meta-Analyses (PRISMA)32 and Meta analysis of Observational Studies in Epidemiology. 33 All Cochrane reviews were considered to have adhered to the Methodological Expectations of Cochrane Intervention Reviews standards,34 even if this was not explicitly stated.
-
Whether or not the review authors reported using Grading of Recommendations Assessment, Development and Evaluation (GRADE) for assessing overall quality of evidence. 35
In addition, we obtained the impact factor for the journal in which each review was published from the ISI Web of Knowledge based on the records for year 2016, or from the journal’s website if the former was not available. Each journal’s website was also searched to ascertain whether or not it explicitly endorsed systematic review guidelines.
Data extraction was carried out by one reviewer and checked by another, with any discrepancies resolved by discussion or by contacting authors for further information and clarification.
Data analysis
Descriptive statistics were compiled to summarise the characteristics of HSDR systematic reviews, the practice of assessing publication and related bias among the reviews, and their findings. Exploratory comparisons of review characteristics, practice and findings of assessing publication bias were made between intervention and association reviews, using both univariable and multivariable logistic regression.
Work package 3: case studies to explore the applicability of methods for detecting and dealing with publication and related bias
Several methods have been developed to facilitate the detection and potential adjustment of publication and related bias. Among these, funnel plots and related regression methods are the most widely used and have been adopted in many systematic reviews. The key assumption for these methods is that the precision of a study (mainly determined by its sample size) is not correlated with the actual size of the intervention effect or association being estimated and, hence, the results of smaller studies are scattered more widely due to random variation, forming an inverse funnel shape when plotted against precision. Asymmetry in a funnel plot would suggest possible publication bias. Figure 3 shows an example of an asymmetrical funnel plot compiled (by authors of this report) using data from a published systematic review of mortality risk associated with out-of-hours admissions in patients with myocardial infarction. 36
FIGURE 3.
An example of an asymmetrical funnel plot.
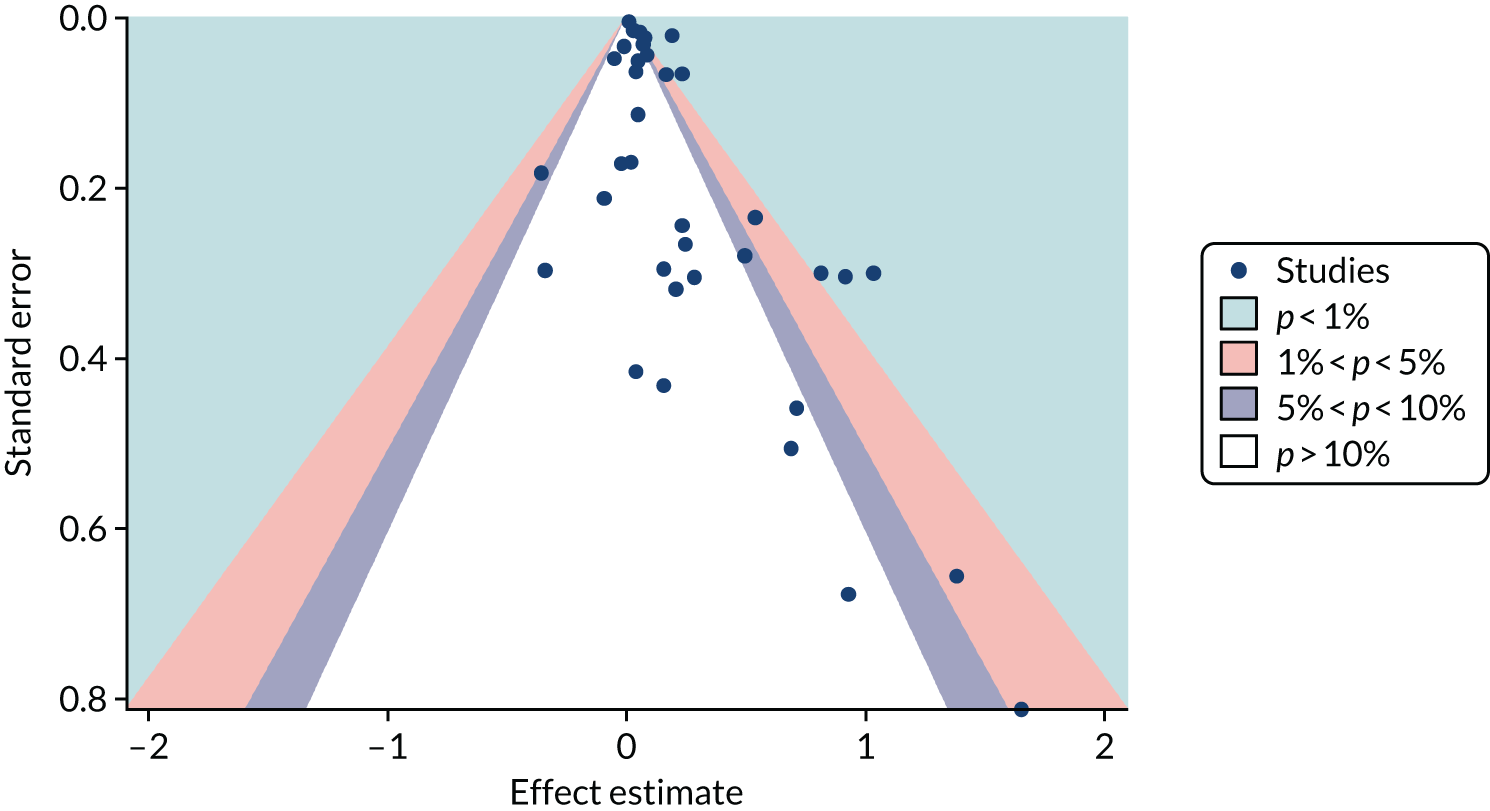
Although the assumption behind funnel plots and related regression methods holds for many clinical interventions, this is not necessarily true in many HSDR studies. For example, early evaluation of a quality improvement intervention in a small number of sites may observe a large intervention effect due to the expertise and dedication of the personnel and thoroughness of implementation, which may be difficult to maintain when the intervention is scaled up in a larger study. Alternatively, an intervention that appears to be highly effective in early small-scale studies may have an apparently diminished intervention effect by the time it is subject to a large-scale evaluation due to a system-wide improvement triggered by the same social pressure that prompted the intervention. 37 On the other hand, the availability of data from large databases covering nearly the whole population may render the influence of small studies negligible. These different types of heterogeneity arising from the complexity of HSDR interventions and associations, and the context in which they are deployed and observed, pose a potential threat for the validity of applying these conventional methods. In addition, funnel plots and related regression methods require a sufficiently large number of studies (e.g. ≥ 10), which may not be available for many topics in HSDR.
The WP 2 described above allowed us to obtain an overview of current practice of examining publication and related bias in systematic reviews of HSDR, including a description of if and what methods have been used. Nevertheless, when formal methods, such as funnel plots and related regression methods, have been used, there remain potential issues concerning the validity and applicability of these methods. 26,38 WP 3 aimed to address these issues through more detailed case studies. In addition, WP 3 offered an opportunity to explore novel methods, such as the p-curve for identifying p-hacking (see Investigation of p-hacking using p-curves), which could be very relevant for HSDR.
Selection of cases to be studied
Given that the purpose was to shed light on the applicability of existing methods to HSDR, we purposively sampled five systematic reviews to ensure reasonable coverage of this diverse field. The selection of cases was guided by the following considerations:
-
The review included a sufficiently large number of studies (≥ 10) to meet the minimal requirement for using funnel plots and regression methods.
-
Covering reviews of various sizes in terms of number of studies included.
-
Inclusion of both reviews that evaluate intervention effectiveness and those investigating associations.
-
Coverage of major issues and scenarios likely to be encountered during evidence synthesis of HSDR.
-
The topics were of general interest for health services researchers, practitioners and the general public.
We had to drop one of the sampled case studies due to practical considerations (see Deviations from the original protocol). The following four topics were subsequently chosen in consultation with the Study Steering Committee, taking into account the above criteria, possible saturation of issues and scenarios covered, and practicality within the project timeline:
-
case study 1– the association between weekend and weekday admissions and hospital mortality
-
case study 2 – the association between organisational culture and climate and nurses’ job satisfaction
-
case study 3 – the effectiveness of computerised physician order entry systems on medication errors and adverse events
-
case study 4 – the effectiveness of standardised hand-off protocols on information relay, patient, provider and organisational outcomes.
Three of the cases (case studies 2–4) were identified through WPs 1 and 2, and one case (case study 1) was built on a systematic review associated with another NIHR HSDR programme-funded project that we have been involved in.
Data collection and presentation
For each systematic review selected as a case study, we extracted information on the methods and findings related to publication bias from the original articles. The information was presented in a structured format, with commentary on the methods and findings related to publication bias provided, to highlight any issues particularly relevant to HSDR. In addition, we utilised detailed numerical data from a systematic review of association studies on the weekend effect available to the principal investigator of this project to carry out further analyses for case study 1, in which we explored commonly used methods for detecting publication bias, as described in the next paragraph.
A large number of tools have been developed to facilitate the assessment of risk and potential impact of publication and related biases. 25,39 We chose five of the techniques that are widely used and relatively easy to implement, and tested their applicability in case study 1: (1) funnel plots, (2) Egger’s regression test, (3) Begg and Mazumdar’s rank correlation test, (4) trim and fill and (5) meta-regression. 24 In addition, given the theoretical risk of p-hacking in analyses without predefined protocols, which may not be rare in HSDR, we also tried a relatively new technique of p-curves to explore its potential utility for detecting this practice. 2 Findings from the application of these statistical techniques were presented with detailed critiques, highlighting potential issues that could impact on the validity of these methods and the interpretation of their findings.
Funnel plots and related methods
We constructed a funnel plot for the primary meta-analysis of case study 1. Funnel plot asymmetry was assessed by Egger’s regression40 and the Begg and Mazumdar’s rank correlation test,41 and by visual inspection given the relatively low level of statistical power of the tests. In the Egger’s regression test, the effect size is regressed against its precision (inverse variance). In the absence of asymmetry, the regression line would pass through the origin (with an intercept equalling zero). A significant deviation of the intercept from zero signifies funnel plot asymmetry (p < 0.05 for the Egger’s regression test). In the Begg and Mazumdar’s test, a rank correlation test is conducted to explore the correlation between (standardised) effect sizes and their standard errors. A significant correlation (p < 0.05) for the Begg and Mazumdar’s rank correlation test suggests that effect sizes vary with standard errors (smaller studies tend to have larger standard errors), which indicates a small study effect and thus potential publication bias.
The ‘trim and fill’ method was used to estimate the potential impact of small study effects. 42 In this method, the asymmetry of a funnel plot is assumed to be caused by publication bias, and alternative estimates correcting for the bias are calculated first by trimming out smaller studies with more extreme effect size estimates causing the asymmetry, and then by reintroducing these studies along with their ‘missing’ counterparts. The method provides a way to estimate how sensitive the results of meta-analyses are to the small study effects. It is important to recognise that publication bias is just one potential cause of small study effects and to interpret findings with caution accordingly.
Evaluating the association between estimated effect sizes and other potential effect modifiers through meta-regression
The regression methods used alongside funnel plots essentially test the existence of an association between observed effect sizes and precision (or sample sizes) of studies. As described above, heterogeneity in the intervention components, study design, settings and context commonly seen in HSDR may confound this association. One approach to investigating this issue is to use multivariate meta-regression analyses, including both sample size and potential confounding factors (e.g. quality of study, or year of publication, as a proxy for changes in context) as covariates. If the association between observed effect sizes and sample sizes persists after adjusting for potential confounders, the likelihood of observed funnel plot asymmetry being caused by publication bias increases. In case study 1, we carried out meta-regression to explore whether any association found between effect sizes and sample sizes (or precision) could be attributed to other confounding factors.
Investigation of p-hacking using p-curves
Repeating analyses using different analytical approaches and data sets until a statistically significant result is obtained – so called ‘p-hacking’ – introduces a bias closely related to publication bias. 3 Recently, a novel methodology, termed ‘p-curve’, that allows the detection of p-hacking from published literature has been developed. 2 The method is based on the fact that, when the null hypothesis is true, the distribution of p-values is uniform and, therefore, should take the shape of a straight line when a collection of p-values from studies that declare statistical significance are plotted. When p-hacking exists, however, the distribution of p-values will be distorted and a spike in the region just below p = 0.05 would be observed. 3 The method has been tested within psychology and biology literature and demonstrated apparent p-hacking in these fields. 2,3 Although we were not aware of the application of p-curve in health services research, p-hacking is a possible threat in HSDR, particularly in the increasing number of analyses of data sets from routine databases. We therefore proposed to use p-curves to explore the potential occurrence of p-hacking in HSDR in case study 1, for which we had more detailed data. We first calculated z-scores for each individual effect estimate included in the meta-analysis of this case study. These were then entered into the tool developed by Simonsohn et al. 43 to generate p-curves.
Work package 4: follow-up of publication status of cohorts of health services research studies
The previous three WPs drew on crucial evidence on issues concerning the extent of publication bias and methods of detecting it in HSDR from the literature. Nevertheless, most of the evidence gathered was indirect in nature, as observations made (such as asymmetry in funnel plots and significant tests) were indicative of the existence of such bias, rather than confirmatory. WP 4 consisted of a retrospective investigation of cohorts of HSDR studies, which were followed over time to ascertain whether their publication status was associated with the statistical significance, or perceived ‘positivity’ or interest of their findings. The main objective was to provide a direct observation of the presence or absence of publication bias in HSDR, as measured by the presence or absence of an association between the publication status of HSDR projects and the statistical significance and perceived ‘positivity’ (see Extraction of study information and classification of study findings) of their findings. In addition, if publication bias was observed, whether it was associated with study design, study type (intervention vs. association) and/or sample size would be explored.
Selection of study cohorts
We initially planned to identify and follow two cohorts of 100 HSDR studies, which would provide confidence limits of under ± 10% for each cohort (assuming a publication rate of 60%) and an 88% power to detect a 20% difference between the two cohorts. To increase the diversity of HSDR covered in our sample, we subsequently added two cohorts of 50 studies, each from HSDR-related conferences. Further details are described in National Institute for Health Research cohort, HSRProj cohort, Health Services Research UK conference cohort and ISQua conference cohort.
Studies initially sampled for each of the cohorts were checked against the following criteria:
-
The research question fell within the scope of HSDR defined for this project.
-
The study included a quantitative component that was not one of the following: descriptive studies not making any comparisons or evaluating any associations; simulation and other studies that were mainly based on modelling or development of models; and methodological studies associated with development and validation of tools.
Studies that did not meet these inclusion criteria were discarded and replaced until the targeted sample size was achieved for each cohort.
National Institute for Health Research cohort
The only comprehensive database of UK HSDR studies that we were aware of is the project portfolio of the NIHR HSDR programme-funded projects, including those previously commissioned under the NIHR Service Delivery and Organisation (SDO) programme and the NIHR Health Services Research programme. These studies have gone through a highly competitive bidding and peer review selection process and are likely to be the most well-funded projects among HSDR studies. In addition, the NIHR has had a strong policy to mandate the publication of research findings and, indeed, the HSDR programme has been routinely publishing its funded studies submitted from July 2012 onwards in its Health Services and Delivery Research journal, which is published as part of the NIHR Journals Library. Studies included in the HSDR database are therefore ‘atypical’ and are least likely to be subject to publication bias. Nevertheless, given the prominence of this portfolio of studies, evaluating the presence or absence of publication bias and documenting the impact of the establishment of the HSDR journal series on the publication of these studies were both very important.
In July 2017 we requested records from the NIHR on projects that had been funded by the NIHR SDO, Health Services Research and HSDR programmes that had been completed by the end of 2014. A total of 338 projects were included in the records supplied. We initially screened all studies completed between 2009 and 2012 (n = 131), but the targeted sample size of 100 studies could not be achieved after excluding projects that were not a primary study including a quantitative component (e.g. evidence synthesis projects and those that adopted exclusively qualitative methods). Consequently, we extended the year range for project completion to between 2007 and 2014 for the final sample of 100 projects.
HSRProj cohort
In order to complement the cohort of studies funded by the NIHR, we identified another cohort of studies from the US-based HSRProj database [URL: wwwcf.nlm.nih.gov/hsr_project/home_proj.cfm (accessed 17 December 2019)]. The HSRProj is hosted within the US National Library of Medicine and is the largest (and the only one that we were aware of) publicly accessible prospective registry of health services and public health research that covers multiple institutions and funding bodies. As of 2017, the database held information on > 32,000 projects (including both ongoing and completed projects) undertaken by nearly 5000 organisations from > 50 countries (mainly from the USA). Although it is unlikely that projects registered with this database were representative of all HSDR, the coverage in terms of number of projects and types of studies made it one of the best alternative sources to assemble a cohort of HSDR studies.
The HSRProj database classifies its project records into three categories: ongoing, completed or archived. Records are archived 5 years after the project’s end date. We took a random sample of 100 studies from the 1531 studies recorded as being completed in 2012 (to allow sufficient time for publication). As the HSRProj had a broad scope (e.g. including public health projects and comparative effectiveness research), studies that were initially sampled but judged to be falling outside the scope of this project were excluded and replaced during the assembly of the study cohort.
Health Services Research UK conference cohort
We obtained all abstracts presented in the Health Services Research UK (HSRUK) conferences during 2012–14 from Universities UK. We aimed to sample a total of 50 studies, with equal numbers from oral and poster presentations. However, only 19 of available abstracts for poster presentations met the inclusion criteria and, therefore, we sampled 31 abstracts from oral presentations.
ISQua conference cohort
We randomly selected a total of 50 abstracts from the International Society for Quality in Health Care (ISQua) 2012 conference using the abstract books published by the conference, with equal number from poster and oral presentations (25 each).
Extraction of study information and classification of study findings
Information on title, authors, abstract and funding source and contact information for the lead investigator for the sampled studies were supplied by the NIHR, downloaded and imported from the website of HSRProj or obtained from conference abstracts. Each study was classified according to study type (intervention vs. association) and study design features [method of data collection (bespoke vs. routine data vs. mixed) and, for intervention studies, whether or not there was a concurrent control group].
We also classified each study according to statistical significance (with a p-value of ≤ 0.05 considered statistically significant). For studies focusing on one outcome or with one prespecified primary outcome, we coded statistical significance based on this outcome. When results were reported for more than one outcome or association and a main outcome could not be easily discerned, we classified the findings from each study as ‘all or mostly significant’, ‘mixed (one or more significant result, but for less than two-thirds of the outcomes/associations)’ or ‘all or mostly non-significant’.
Statistical significance may not be the main mechanism through which publication bias occurs. For example, the findings of a study may be regarded as positive or favourable if a cheaper way to deliver a service is as effective as a more costly option (i.e. no significant difference in outcomes between the options). We therefore adopted the method used by Song et al. 4 and classified the findings of each study as ‘positive’ or ‘non-positive’. Studies coded as positive included those that were considered (by the original study authors) as being ‘positive’, ‘favourable’, ‘significant’, ‘important’, ‘striking’, ‘showed effect’ and ‘confirmatory’. Non-positive result refers to other results labelled as being ‘negative’, ‘non-significant’, ‘less or not important’, ‘invalidating’, ‘inconclusive’, ‘questionable’, ‘null’ and ‘neutral’. The ‘positivity’ classification was used as a sensitivity analysis in place of ‘statistical significance’ given that the two measures are likely to be highly correlated.
Some of the larger HSDR projects had multiple components (e.g. quantitative and qualitative) and/or involved multiple stages (e.g. pilot study, process evaluation and effectiveness trial), and may have produced multiple publications. In such cases, we chose the quantitative component/publication that was considered to be closest to the stated primary aim(s) of the project, or chose the earliest publication for data collection and coding if the most relevant component or publication could not be determined. For a project encompassing multiple methods and stages, we focused on the publication or findings associated with the later stage (usually an effectiveness trial) of the project.
All data extraction and coding decisions were made by one reviewer and checked by a second reviewer. Any discrepancies were resolved through discussions.
Verification of publication status
The publication status of each study was verified firstly by searching PubMed and Google (Google Inc., Mountain View, CA, USA), using information on title and lead investigator/author. When no publication was identified, or when it was not clear if the identified publications were direct outputs from the selected project, we attempted to contact the investigators by e-mail to verify the status of publication and to request information on published articles or unpublished study results, and reasons for non-publication if this is the case. We sent up to two reminders when no response was received and other means (e.g. search of funding agency’s website) were pursued to enhance the completeness of follow-up. We classified publication status for each study as published (in academic journals), grey literature (available on the internet in a form other than an article published in academic journals, such as a technical report or working paper) or unpublished.
Data analysis
Descriptive statistics concerning study type, study design, findings and publication status were computed. Univariable and multivariable logistic regression were carried out to explore the association between publication status and statistical significance and positivity of study findings, controlling for potential confounders. Our prespecified variables were type of study (intervention vs. association), method of data collection (routine data, bespoke data collection or mixed), funding source [no specific funding, local funding, national funding (HSDR programme), national funding (others)], size of study (number of institutions or number of individuals) and, for intervention studies, studies with concurrent controls compared with before-and-after studies (including time series without a control group). We dropped two variables, funding source and study size, during data collection, as (1) the NIHR cohort has a single funding source by default and this information was not available for a large proportion of studies in the conference cohort; and (2) attributing a study size to a given study based on number of participating institutions, number of individual patients, number of observations or number of events could be arbitrary, and inclusion of study sizes based on different units could lead to difficulties in interpretation. In addtion, we collected information with regard to study design [randomised controlled trial (RCT) vs. non-RCT] among studies with concurrent controls, but did not include this in multivariable analyses, as all RCTs adopted bespoke data collection and had a concurrent control group by definition.
Work package 5: semistructured interviews and a focus group discussion with health services researchers, journal editors and other stakeholders
Work package 5 sought to complement direct evidence collected from the retrospective cohort study in WP 4 by exploring the perceptions and first-hand experiences of health services researchers and commissioners, journal editors, service managers and users, with regard to the occurrence and impact of publication and related bias. It contributes to the overall aim of obtaining (qualitative) evidence on the extent and existence of publication bias. It was also intended to contribute to the development of methods for the detection and mitigation of publication bias in HSDR. As well as generating important data on the perspectives of key actors in the HSDR process, this WP was designed to support analysis of results deriving from prior WPs.
Objectives
Interviews and the focus group were designed to:
-
enable qualitative exploration of quantitative findings derived from WPs 1–4, for example in relation to current rates and types of publication bias in HSDR
-
gauge the views of a sample of those currently commissioning, publishing or conducting HSDR as to the prevalence (or existence) and perceived impact of publication bias
-
identify and explore current and future strategies for prevention, detection and mitigation of any bias detected
-
explore the experiences and views of service managers and patient and user experts involved in HSDR.
Methods
Selection and recruitment of key informants
We undertook in-depth interviews with 24 key informants in the field of health services research to explore their perceptions, experience and preferred solutions to overcoming problems associated with publication bias in HSDR. We conducted a focus group with eight patient and service user representatives to explore these issues from a patient and service user perspective.
Potential interviewees were invited by the lead researcher for WP 5 (IW) via e-mail in the first instance. Invitation e-mails were tailored to the individual respondent (see Appendix 2) and included a short summary of the project, with further detail attached (i.e. participant information leaflet, see Appendix 3). Those agreeing to take part were requested to return a signed consent form (see Appendix 4), either by e-mail (scanned signed copy or provision of an electronic signature) or by post. Those not providing a consent form consented verbally to the audio-recording of the interview. Non-responders were sent a reminder e-mail within 2 weeks of the initial e-mail, and this was trailed in the initial invitation. Those declining to take part were asked to give reasons for declining. Of the 27 targeted respondents who did not take part, eight cited lack of available time, four indicated a lack of interest or expertise in the topic and 15 did not respond.
We sought to ensure that the sample included researchers from different epistemological traditions and at various stages in their careers. Researchers included in the sample were selected to include individuals:
-
with a track record of HSDR publication
-
at different stages of their careers (indicated by level of seniority)
-
specialising in aspects of HSDR, such as systematic review, improvement science, management, health sociology, health economics and operations research.
We did not require that those included had a specific research interest in publication bias, but instead designed our interview schedule to enable them to reflect on publication bias from their standpoints and experiences (see Appendix 5).
Editors and assistant editors of key UK health services journals were also included, along with journal editors from outside the UK. Three respondents were included primarily because of their roles at major funders of UK HSDR, and two interviewees were included as national and local decision-makers within the English NHS. Details of the final sample are presented in Table 1.
Research participant | Primary identifier | Secondary identifier | Total |
---|---|---|---|
Senior researcher | 7 | 7 | 14 |
Junior/mid-career researcher | 4 | 1 | 5 |
Journal editor | 7 | 3 | 10 |
Researcher funder/commissioner | 3 | 1 | 4 |
Patient/service user representative | 8 | 0 | 8 |
Manager | 2 | 0 | 2 |
Consultant evaluator | 1 | 0 | 1 |
Clinician | 0 | 5 | 5 |
Total | 32 | 17 | 49 |
Our final sample therefore included 14 senior researchers (with seven of these interviewed primarily in this capacity and seven in a secondary capacity), five junior mid-career researchers (four in this capacity and one in a secondary capacity), 10 editors (seven in this capacity and three in a secondary capacity), four funders (three in this capacity and one in a secondary capacity), two managers, one private consultant and eight patient and service user representatives. Five of these respondents were also practising clinicians.
Topic guides for the interviews and focus group were informed by previous phases of the study and focused on the informants’ perceptions and past experience of publication bias in HSDR, and their opinions on possible approaches to its mitigation.
We obtained ethics approval from the University of Warwick Biomedical and Scientific Research Ethics Committee (REGO-2017-1918 AM01). Although assurances were given that, when possible, all steps would be taken to ensure anonymity, it was made clear that in a relatively small sample of high-profile interviewees full anonymity may be compromised. In acknowledgement of the sensitivity of the subject material we put safeguards and assurances in place so that respondents felt able to speak freely and candidly. For example, we assured interviewees that, as well as anonymising transcripts, steps would be taken to ensure that any identifying details are redacted in subsequent reports. Participants were offered the opportunity to comment on a draft report of this WP so that they could be assured that all identifying features had been removed.
Data collection and analysis
Interview and focus group data were collected during the period September 2017–August 2018, with interviews conducted by a single member of the research team and the focus group facilitated by two members. All interviewees opted for a telephone interview format. Interviews ranged from 20 to 45 minutes in length and the focus group lasted 1.5 hours. Example interview schedules can be found in Appendix 5. Permission to voice-record the interviews was obtained in all cases (including the focus group), and recorded interviews were fully transcribed for subsequent analysis.
Data were analysed inductively to gauge participants’ perspectives and experiences within the framework provided by the research aims, as well as issues identified in prior WPs. Findings from earlier interviews were used to inform subsequent interviews to facilitate the exploration of different perceptions, experiences and opinions among the interviewees. For internal validity, all interviews were fully transcribed and we used qualitative coding software (NVivo version 11; QSR International, Warrington, UK) to facilitate data storage and retrieval during analysis. 44 Two members of the research team contributed to the building of thematic coding frames from qualitative data and shared independent coding of a data subset in order to ensure consistency. Identified themes were regularly discussed at meetings of the core project team. External validity and transferability of analysis were addressed through detailed description and data triangulation between WPs. 45
Saturation checks conducted during the final three interviews suggested that, although additional themes of interest were still forthcoming, these did not relate to the core research aims. 46 These are put forward as areas for possible future investigation in Chapter 8 of this report. Results are presented in Chapter 7, using verbatim quotes to illustrate main themes. 47
Patient and public involvement
This project involved two public contributors from its inception. One of the public representatives (MS) was a member of the Project Management Group and co-author of the report, and helped with planning and facilitating the focus group discussion for WP 5. Another public representative sat on the Study Steering Committee. Both members of the public regularly participated in project meetings and discussions, received meeting minutes, provided advice on all issues related to patient and public involvement (PPI) and dissemination of findings. The project also benefited from input from NIHR Collaboration for Leadership in Applied Health Research and Care West Midlands PPI Supervisory Committee Advisors.
Deviations from the original protocol
This 2-year project tackled a complex area of HSDR, which is very broad in scope and diverse in methods. Several challenges needed to be overcome in order to deliver the proposed research within the time and resources available. As a consequence, some amendments of the methodological approaches were made during the conduct of the project, which deviated from the original protocol. These are described below with rationale behind the changes explicated.
Work package 1
The original protocol noted that the systematic review will cover HSDR and ‘cognate fields’. Given the multidisciplinary nature of HSDR, many different fields, such as management, economics and psychology, could be considered as cognate. Although our literature searches retrieved some studies investigating publication and related bias in these fields, it became clear that it was not feasible in the scope of our study to systematically review all of this diverse literature, taking into account other WPs. We do, however, provide a brief description and discussion of these studies in Chapter 8.
Work package 2
The initial plan was to identify the required sample of HSDR systematic reviews through searches of general databases, such as MEDLINE, by combining HSDR-related terms with systematic review filters. Retrieved records would then be screened to verify whether or not they were systematic reviews of a HSDR topic. However, owing to the lack of specificity of HSDR-related terms, this approach resulted in the retrieval of a very large number of systematic reviews, many of which would fall outside the scope of HSDR defined in this project. The process for undertaking this step of screening would be very time-consuming and could substantially delay the progress of the project. We therefore obtained our sample of HSDR systematic reviews directly from the Health Systems Evidence database, after consulting the Study Steering Committee (see Search and sampling strategies).
We planned to collect information concerning the number and type of variables (e.g. structure, process, outcome or context) investigated by each of the included systematic reviews. Nevertheless, it became clear during data extraction that many HSDR systematic reviews covered diverse interventions in different settings, and reported findings for a large number of outcomes and/or associations. The variables and associations explored were often not clearly stated in the methods section, but were scattered in tables and text throughout the articles. We therefore had to drop this item from our data collection.
We were to classify the type of journal in which the review was published into four categories: medical, health services research and health policy, management and social science, and other. However, initial examination of sampled systematic reviews showed that very few reviews were published in management and social science journals and other outlets, and the distinction between ‘medical’ and ‘health services research and health policy’ categories could be vague. Therefore, on the advice of the Study Steering Committee, we classified journals according to whether or not they endorsed systematic review guidelines instead, as this would be a feature that might be associated with assessment of publication and related bias in published systematic reviews.
Work package 3
The original plan was to select 5–10 systematic reviews for in-depth examination, and to utilise data reported in these reviews to carry out further analyses and test different methods for detecting publication and related bias. However, it became apparent that detailed quantitative data on outcomes and coding on study-level variables that are likely to be effect modifiers were often not adequately reported in published reviews, and it would not be practical for the project team to locate and extract data from individual studies included in the reviews. Consequently, on the advice of the Study Steering Committee, we selected four systematic reviews to be included as case studies (case studies 1–4) and focused on one of them (case study 1), for which we were able to access original data to conduct detailed further analyses. We provided critiques of the methods and findings for the remaining three case studies (case studies 2–4). In addition, we obtained data and worked on another systematic review as a potential further case study. It was based on a data set that included 272 RCTs, supplied by colleagues from the Cochrane Collaboration in relation to an ongoing, updated systematic review on the effectiveness of quality improvement strategies for the management of diabetes that has previously been published. 12,48,49 Unfortunately, due to the substantial time required for obtaining and organising the data and for carrying out imputation of unavailable data (e.g. standard errors of effect estimates), and considering that the owners of the data set are yet to analyse and to publish main findings from the data at the conclusion of this project, we are unable to include this further case study in this report. Nevertheless, we have completed preliminary analyses and it is our intention to continue this effort and to publish the completed case study in collaboration with colleagues from the Cochrane Collaboration.
Work package 4
We planned to obtain cohorts of HSDR studies to be followed up from the NIHR’s registry of funded projects and the HSRProj database. These were carried out as planned. During one of the Study Steering Committee meetings, members of the committee pointed out that these samples are likely to be the most well-funded projects and may cover only the upper end of the spectrum of existing HSDR. They therefore recommended that the project team identify further cohorts from other sources, such as HSDR-related conferences. As a result, two additional cohorts drawing from the HSRUK and the ISQua were included.
Work package 5
We intended to carry out semistructured interviews for various groups of HSDR stakeholders, including health services researchers, journal editors, HSDR funders, service managers and patient and public representatives. Interviews were undertaken as planned for most of the stakeholders. However, considering the experience from initial interviews and following discussions with the PPI advisors of the project, we decided to hold a focus group discussion instead of individual interviews for the patients and public stakeholder group. This was because they were unlikely to have much previous exposure to the concept and terminology associated with publication and related bias, and thus might have difficulties in forming a clear and considered opinion. It was felt that the dynamics of a focus group discussion would assist participants to clarify their thoughts on salient topics. This change was approved by the University of Warwick’s Biomedical and Scientific Research Ethics Committee, which issued initial approval for the project, and was agreed by the NIHR HSDR programme.
Chapter 3 Findings of systematic review of empirical evidence on publication and related bias in HSDR
This chapter presents findings from WP 1 of the project, in which we systematically searched and reviewed empirical studies that set out to investigate the occurrence of publication and related bias in HSDR.
Literature search and study selection
Initial searches of electronic databases in March 2017 retrieved 7732 records. After removing duplicates, the titles and abstracts of 6155 records were screened. Of these, 422 records were considered potentially relevant and their full-text articles were retrieved. Six additional full-text articles were identified and obtained from other sources.
Of the 428 full-text articles examined, 188 were retained. Of these, four were methodological studies that set out specifically to investigate publication and related bias in HSDR13–15,50 and three were systematic reviews of substantive HSDR topics, in which evidence from published literature was compared with grey literature and unpublished studies (and thus provided direct evidence on publication bias). 51–53 These seven studies were examined and are described in detail in this chapter. The remaining 181 studies were systematic reviews of substantive HSDR topics, in which publication and outcome reporting bias was assessed as part of the review process. As these reviews provided only indirect evidence on publication bias, they are briefly described in this chapter and are summarised in Appendix 6.
Two hundred and forty retrieved full-text articles did not meet the inclusion criteria and were excluded. The primary reasons for exclusion were not mentioning publication and related bias at all (we examined systematic reviews that might have examined publication and related bias, even if this was not explicitly stated in the titles and abstracts); mentioning these biases but without assessing them; and topics being outside the definition of HSDR adopted for this project. A flow diagram for the literature retrieval and study selection process is shown in Figure 4. We carried out an updated search in July/August 2018. This retrieved 1328 new records, but no relevant methodological studies were identified.
FIGURE 4.
Flow diagram for literature search and study selection process for the systematic review. This figure has been reproduced from Ayorinde et al. 54 This is an Open Access article distributed in accordance with the terms of the Creative Commons Attribution (CC BY 4.0) license, which permits others to distribute, remix, adapt and build upon this work, for commercial use, provided the original work is properly cited. See: http://creativecommons.org/licenses/by/4.0/. The figure includes minor additions and formatting changes to the original text.

Methodological studies investigating publication and related bias in HSDR
Four studies specifically set out to investigate publication and related bias in a substantive topic area of HSDR. The objectives, methods, key findings and limitations of these studies are summarised in Table 2. Three studies investigated publication bias in health informatics research,14,15,50 and one study explored potential reporting bias or p-hacking arising from researchers competing for limited space of publication in high-impact journals in health economics and policy literature. 13
Study (HSDR topic) | Objective(s) | Method(s) | Key finding(s) |
---|---|---|---|
Machan et al. 200650 (health informatics) | To determine (1) the percentage of evaluation studies describing positive, mixed or negative results; (2) the possibility of statistical assessment of publication bias in health informatics; and (3) the quality of reviews and meta-analysis in health informatics with regard to publication bias |
Descriptive analysis of random sample of 86 evaluation studies and planned to construct funnel plot Examined characteristics and quality of reviews and meta-analyses (n = 54) in medical informatics |
For the primary studies, 69.8% positive results, 14% negative and 16.3% unclassified For the reviews 36.6% had a positive conclusion, 61.5% were inconclusive and only one review came to a negative conclusion |
Ammenwerth and de Keizer 200715 (health informatics) | To determine (1) the percentage of IT evaluation studies that are not published in international journals or proceedings; and (2) typical reasons for not publishing the results of an IT evaluation study | E-mail-based survey conducted in spring 2006. Participants were drawn from various working groups of the American Medical Informatics Association, the European Federation for Medical Informatics and the International Medical Informatics Association; and first authors of MEDLINE-indexed IT evaluation papers published between 2001 and 2006 (total n = 722) | Response rate 19% (136/722). 118 of the respondents reported completion of 217 evaluation studies. Of these studies, 47% (103/217) were published in peer-reviewed international journals, proceedings or books; 49% (107/217) were unpublished or published only locally. Common reasons for non-publication included ‘not of interest for others’, ‘no time for writing’, ‘limited scientific quality’, ‘political and legal reasons’ and ‘only meant for internal use’ |
Vawdrey and Hripcsak 201314 (health informatics) | To measure the rate of non-publication and assess possible publication bias in clinical trials of electronic health records | Follow-up of health informatics trials registered in ClinicalTrials.gov (2000–8) | Trials with positive results were more likely to be published (35/38, 92%) than trials with null results (10/14, 71%; p = 0.052); the study authors reported p < 0.001 |
Costa-Font et al. 201313 (health policy) | To examine the winner’s curse phenomenon (studies needing to have more extreme results to be published in high-impact journals) and publication selection bias, using quantitative findings on income and price elasticities, as reported in health economics research | Funnel plot and multivariate analysis to examine the association between estimated effect sizes (and their statistical significance) and the impact factors of the journals in which they were published | Meta-regression analysis demonstrated that both publication bias (reflected by positive correlation between effect size and standard error) and the winner’s curse (reflected by an independent association between effect size and journal impact factor) influence the estimated income/price elasticity |
Of the four studies,13–15,50 only one was an inception cohort study that tracked individual research projects from the start and thus provided direct evidence of publication bias. 14 Studies included in this cohort were clinical trials of electronic health records registered with ClinicalTrials.gov during 2000–8. Findings from 76% (47/62) of completed studies were published. Of these, 74% (35/47) reported predominantly positive findings, 21% (10/47) reported neutral results (no significant effects) and 4% (2/47) reported negative or harmful results. Of the 15 unpublished trials, three had positive findings and four had neutral results based on information supplied by the investigators. Findings for the remaining eight studies were unknown. The authors found that trials with positive findings were more likely to be published than those with neutral findings (see Table 2), but cautioned that the sample included in the cohort may be atypical of general studies in the field.
Another study15 in health informatics was an e-mail-based survey of people who were likely to be involved in the evaluation of health information systems. Participants were asked about (1) what information systems they had evaluated in the past 3 years; (2) where they published the results of the evaluation; and (3) the reasons for non-publication of the results, if this was the case. A response rate of 19% (136/722) was achieved, with 118 respondents reporting the completion of a total of 217 evaluation studies. Most of these respondents were from an academic background (92/118), with a small number from information technology management, industry and government institutions. Approximately half of the identified evaluation studies were published in peer-reviewed journals, proceedings or books, whereas the remaining half either were published only locally (e.g. internal reports) or were unpublished (see Table 2). Reasons cited for not publishing included: not of global interest (35%), publication in preparation (31%), no time for publication (22%), limited scientific quality (17%), political and legal reasons (14%) and for internal use only (13%).
A low response rate was the major limitation of this study. Nevertheless, the survey provided some insights concerning reasons behind non-publication. Like most surveys, the study findings could be influenced by sampling, response and recall bias. It is also worth noting that publication was still being considered or under way for about one-third of the unpublished studies at the time of the survey, and, therefore, the actual publication rate might be higher.
The third methodological study50 in health informatics utilised evaluation studies identified from a specialist health informatics database that covered literature published between 1982 to 2002, and adopted three different approaches to assessing publication bias: (1) statistical analyses of the small study effect; (2) examination of the percentage of evaluation studies with positive findings compared with percentage of studies with mixed or negative findings; and (3) examination of the percentage of systematic reviews reporting positive, neutral or negative results. The authors did not identify sufficient number of studies with the same outcome measures to carry out statistical analyses of the small study effect. Although the percentages of primary studies with negative findings and systematic reviews reporting negative results were low (see Table 2), these were not good indicators for the existence of publication bias, as there is no estimate of what the ‘unbiased’ proportion of negative findings should be for evaluation studies and reviews of health informatics interventions.
The fourth methodological study13 included in this review examined quantitative estimates of income elasticity of health care and price elasticity of prescription drugs reported in the published health economics literature. 13 The authors identified a positive correlation between effect sizes and the standard errors of income and price elasticity estimates using funnel plots and meta-regression, which suggested potential existence of publication bias. Having adjusted for this, they also found an independent association between effect size and journal impact factor. This suggested that studies reporting larger effect sizes (i.e. more striking findings) were more likely to be published in ‘high-impact’ journals. However, the finding still needs to be interpreted with caution, as other confounding factors could not be ruled out for these observed associations. In addition, the authors acknowledged that studies in the field concerned were often reported in grey literature, which was not examined in this study.
Systematic reviews of substantive HSDR topics providing evidence on publication and related bias
In addition to the four methodological studies13–15,50 that set out to investigate publication and related bias, we identified 184 systematic reviews of substantive HSDR topics, in which findings from assessment of publication bias and outcome reporting bias were reported. Therefore, these systematic reviews, although not undertaken to specifically investigate publication and related bias, also provided empirical evidence on this topic. In particular, three of these reviews51–53 provided direct evidence on publication bias by comparing evidence from studies published in academic journals with evidence from grey literature or unpublished studies. These reviews are described in detail below. The remaining 181 reviews provided only indirect evidence and are summarised briefly in the following section (and further details can be found in Appendix 6).
Health services research systematic reviews comparing published and grey and unpublished evidence
Table 3 provides a summary of the three HSDR systematic reviews51–53 that compared evidence from published and grey and unpublished literature. The first review53 set out to evaluate the effectiveness of mass mailings for increasing the utilisation of influenza vaccine. The review included evidence only from controlled trials. In addition to computerised bibliographic databases, the authors had access and were able to search a Health Care Quality Improvement Project database, which included records of projects carried out to improve Medicare-funded services. Of the six studies identified, one published study reported statistically significant intervention effects. However, five of the studies remained unpublished and they all reported clinically trivial intervention effects (no effect or an increase in uptake of less than two percentage points). The authors highlighted that even at the time when they were disseminating findings from this review, further mass mailing interventions were being considered by service planners on the basis of results from the first published study. The generalisability of the findings may be limited by the inclusion of a small number of trials identified from a single study registry and targeting a specific US population.
Study (HSDR topic) | Topic | Methods of identifying grey literature/unpublished studies | Key findings of comparison between published literature and grey literature/unpublished studies |
---|---|---|---|
Maglione et al. 200253 (immunisation programme) | Effectiveness of mass mailings to increase utilisation of influenza vaccine among Medicare beneficiaries | Search of the Medicare Peer Review Organisation Health Care Quality Improvement Project database | Six controlled trials were identified. Only one (earliest) trial reporting modest but statistically significant improvement in vaccination rate (2–8%, depending on the format of the letter and location of the study) was published. Five subsequent trials that found smaller, clinically trivial improvement in vaccination rate of no more than 2% remained unpublished |
Batt et al. 200451 (immunisation programme) | Costs, effects and cost-effectiveness of strategies to increase coverage of routine immunisations in low- and middle-income countries | Hand-searches in institutional documentation centres, including WHO and USAID; interviews with 28 international experts; search of grey literature databases; searches of the internet, conference proceedings and web pages of pertinent organisations | Quality of data on effect and cost-effectiveness was similar between published and grey literature, but the quality of costing data were poorer in grey literature. Inclusion of grey literature doubled the quantity of available evidence. Interventions examined in the grey literature were more up to date, associated with more complex interventions aimed at health systems, and better represented West Africa and the Middle East. Conclusions drawn from the two sets of literature therefore differed |
Fang 200752 (organisational studies) | Relationships between organisational culture, organisational climate, and nurses’ job satisfaction and turnover | Extensive search of 35 databases, ‘footnote chasing’ and searching by author | Of the 10 associations for which findings were compared between published articles and unpublished doctoral dissertations, significant differences were found for two of them: global climate and job satisfaction; and reward orientation climate and job satisfaction. Both differences were related to magnitude rather than direction of the estimated association |
The second review51 examined evidence from grey literature and compared it with an earlier review on the same topic that included only published literature. 55 The review evaluated the effectiveness and cost-effectiveness of strategies to improve immunisation coverage in developing countries. The authors observed that the quality and nature of evidence differed in some aspects between these two sources of evidence, and that the recommendations about the most cost-effective interventions would differ based on evidence summarised in these two reviews (see Table 3). The authors of the review acknowledged that the grey literature was mainly identified from international organisations and, therefore, would not have covered evidence from national governments. In addition, their literature searches were limited to English keywords and, thus, literature written in other languages was not examined.
The third review52 assessed 23 associations between various measures of organisational culture, organisational climate and nurses’ job satisfaction. The author searched for and included doctoral dissertations in the review, and made comparison between evidence from the dissertations and that from published journal articles for 10 of the associations. Statistically significant differences in the pooled estimates between these two types of literature were found in two of the 10 associations. This review was chosen as the basis of case study 2 of WP 3 and, therefore, further information concerning the methods and findings of the review can be found in Chapter 5.
Findings from other systematic reviews of substantive HSDR topics
Of the 181 remaining systematic reviews of specific HSDR topics, 100 examined potential publication bias across included studies, using funnel plots and related techniques, and 108 assessed outcome reporting bias within individual included studies, generally as part of the risk-of-bias assessment. The methods used in these reviews and key findings in relation to publication bias and outcome reporting bias are summarised in Appendix 6. Approximately half (51/100) of the 100 reviews that attempted to assess publication bias found some evidence of small-study effects.
Among the 108 reviews that assessed outcome reporting bias, reviewers frequently reported difficulties in judging outcome reporting bias due to the absence of a published protocol for the included studies. For example, a Cochrane review on the effectiveness of interventions to enhance medication adherence included 182 RCTs and judged eight and 32 of the RCTs to be at high and low risk for outcome reporting bias, respectively, but the authors could not make a clear judgement for the remaining 142 RCTs, primarily because of unavailability of protocols. 56 Comparison of outcomes specified in the methods section with those reported in the results section was a commonly adopted approach in the absence of a protocol for the included studies; and authors sometimes made subjective judgements on the extent to which all important outcomes were reported. All but one57 of the reviews that assessed outcome reporting bias used either the Cochrane risk-of-bias tool or bespoke tools derived from this. In the only exception,57 the authors undertook a sensitivity analysis by imputing zero effects (with average standard deviation) for studies that would have otherwise met the inclusion criteria except for not providing sufficient data on relevant outcomes and including them in the analysis. The authors found that the pooled effect was considerably reduced after including the imputed data, although it was still statistically significant. 57
Chapter 4 Overview of systematic reviews of intervention and association studies in HSDR
This chapter presents findings from WP 2 of the project, which was an overview of 200 randomly selected systematic reviews of intervention and association studies, as described in Chapter 2.
Characteristics of included intervention and association reviews
As planned, 200 systematic reviews (100 intervention reviews and 100 association reviews) were included in this meta-epidemiological study. The characteristics of the included systematic reviews are shown for both intervention and association reviews in Table 4. The majority of the 200 systematic reviews (79%) included at least 10 studies, with association reviews more likely than intervention reviews to include ≥ 10 studies (86% vs. 71%; p = 0.01). Only 22% of all the systematic reviews performed a meta-analysis, which was more common in the intervention reviews than in the association reviews (33% vs. 10%; p < 0.0001). Of the 157 reviews that did not include meta-analysis, 90 (57%) provided reasons for this (mainly heterogeneity between studies and a small number of comparable studies).
Characteristic | All (N = 200) | Association (N = 100), % | Intervention (N = 100), % | p-valuea |
---|---|---|---|---|
Number of included studies, n (%) | ||||
< 10 | 43 (21.5) | 14 | 29 | |
≥ 10 | 157 (78.5) | 86 | 71 | 0.01 |
Meta-analysis included, n (%) | ||||
No | 157 (78.5) | 90 | 67 | |
Yes | 43 (21.5) | 10 | 33 | < 0.0001 |
Design of included studies, n (%) | ||||
Mixed | 164 (82.0) | 99 | 65 | |
RCT and controlled trials | 36 (18.0) | 1 | 35 | < 0.0001 |
Searched grey/unpublished literature, n (%) | ||||
No | 97 (48.5) | 48 | 49 | |
Yes | 103 (51.5) | 52 | 51 | 0.887 |
Quality assessment performed, n (%) | ||||
No | 43 (21.5) | 30 | 13 | |
Yes | 157 (78.5) | 70 | 87 | 0.003 |
Authors mentioned using GRADE | ||||
No, n (%) | 177 (88.5) | 94 | 83 | |
Yes, n (%) | 23 (11.5) | 6 | 17 | 0.015 |
Journal impact factor, median (IQR) | 3.00 (2.26–5.10) | 2.66 (2.07–3.39) | 3.55 (2.30–7.08) | 0.002 |
Journal endorses systematic review guideline, n (%) | ||||
No | 60 (30.0) | 31 | 29 | |
Yes | 140 (70.0) | 69 | 71 | 0.758 |
Reviewers reported using systematic review reporting guideline | ||||
No, n (%) | 127 (63.5) | 72 | 55 | |
Yes, n (%) | 73 (36.5) | 28 | 45 | 0.013 |
AMSTAR rating (%), median (IQR) | 60 (44–73) | 50 (40–65) | 65 (50–82) | < 0.00001 |
Publication bias mentioned or assessed, n (%) | ||||
No | 115 (57.5) | 69 | 46 | |
Yes | 85 (42.5) | 31 | 54 | 0.001 |
Publication bias assessed, n (%) | ||||
No | 181 (90.5) | 95 | 86 | |
Yes | 19 (9.5) | 5 | 14 | 0.030 |
Outcome reporting bias mentioned and assessed, n (%) | ||||
No | 166 (83.0) | 96 | 70 | |
Yes | 34 (17.0) | 4 | 30 | < 0.0001 |
Mentioned or assessed publication bias and/or outcome reporting bias, n (%) | ||||
No | 105 (52.5) | 68 | 37 | |
Yes | 95 (47.5) | 32 | 63 | < 0.0001 |
Assessed publication bias and/or outcome reporting bias, n (%) | ||||
No | 151 (75.5) | 91 | 60 | |
Yes | 49 (24.5) | 9 | 40 | < 0.0001 |
Intervention and association reviews also differ significantly in several other characteristics, such as inclusion of study design beyond controlled trials, undertaking quality assessment of included studies, mentioning the use of systematic review reporting guidelines and GRADE, their A MeaSurement Tool to Assess systematic Reviews (AMSTAR) rating and the impact factors for the journals in which they were published (see Table 4). Just over half (52%) of the systematic reviews searched for grey and unpublished literature, and the proportions were similar in both association reviews (52%) and intervention reviews (51%). Seventy per cent of the journals in which the reviews were published endorsed a reporting guideline for systematic reviews, with similar proportions for both intervention reviews and association reviews (71% vs. 69%; p = 0.758) (see Table 4).
Publication bias
We found that 85 (43%) of the systematic reviews considered publication bias, and this was more common in intervention reviews than in association reviews (54% vs. 31%; p = 0.001). However, only about 10% (19/200) formally assessed publication bias through statistical analysis, mostly using funnel plots and related methods (Figure 5). Again, intervention reviews assessed publication bias more frequently than association reviews (14% vs. 5%; p = 0.03) (see Table 4). Five59–63 of the 19 reviews (26%) that assessed publication bias reported some evidence of publication bias. The remaining reviews mostly reported no risk or low risk of publication bias, and one reported that the funnel plot was not very informative owing to small numbers (the review included only four studies). Authors of reviews in which publication bias was mentioned but not assessed often reported conditions of using funnel plots not being met, especially insufficient number of studies and/or heterogeneity between included studies.
FIGURE 5.
Methods used to assess publication bias in sampled systematic reviews. a, Nineteen reviews assessed publication bias but some reviews used more than one method of assessment.

Factors associated with mentioning (including assessing) publication bias
Publication bias was more likely to be mentioned in intervention reviews than association reviews in the univariable analysis [odds ratio (OR) 2.61, 95% confidence interval (CI) 1.47 to 4.66]. The strongest association was observed in reviews that included meta-analysis, compared with those with no meta-analysis (OR 5.71, 95% CI 2.67 to 12.21). Mentioning publication bias was also associated with quality assessment of individual studies, authors reporting the use of GRADE, journal impact factor and reviewer reporting the use of systematic review guidelines (Table 5). Journal endorsement of systematic review reporting guidelines, search of grey and unpublished literature, design of included studies and number of included studies were not significantly associated with mentioning publication bias. In the multivariable analysis, only the inclusion of meta-analysis was statistically associated with mentioning or assessing publication bias (see Table 5). Intervention reviews were still more likely than association reviews to mention publication bias, although the relationship was no longer statistically significant (OR 1.63, 95% CI 0.85 to 3.15).
Factor | Mentioned publication bias, n (row %) | Univariable | Multivariable | |||
---|---|---|---|---|---|---|
Yes (N = 85) | No (N = 115) | OR (95% CI) | p-value | OR (95% CI) | p-value | |
Number of included studies | ||||||
< 10 (n = 43, 22%) | 19 (44) | 24 (56) | ||||
≥ 10 (n = 157, 78%) | 66 (42) | 91 (58) | 0.92 (0.46 to 1.81) | 0.801 | 1.16 (0.53 to 2.53) | 0.706 |
Meta-analysis included | ||||||
No (n = 157, 78%) | 53 (34) | 104 (66) | ||||
Yes (n = 43, 22%) | 32 (74) | 11 (26) | 5.71 (2.67 to 12.21) | < 0.0001 | 4.02 (1.76 to 9.15) | 0.001 |
Design of included studies | ||||||
Mixed (n = 164, 82%) | 65 (40) | 99 (60) | ||||
RCT and controlled trials (n = 36, 18%) | 20 (56) | 16 (44) | 1.90 (0.92 to 3.94) | 0.083 | Not included | |
Searched grey/unpublished literature | ||||||
No (n = 97, 49%) | 39 (40) | 58 (60) | ||||
Yes (n = 103, 51%) | 46 (45) | 57 (55) | 1.20 (0.68 to 2.10) | 0.524 | 1.16 (0.60 to 2.23) | 0.657 |
Quality assessment performed | ||||||
No (n = 43, 22%) | 10 (23) | 33 (77) | ||||
Yes (n = 157, 78%) | 75 (48) | 82 (52) | 3.02 (1.39 to 6.54) | 0.005 | 2.08 (0.88 to 4.90) | 0.094 |
Authors mentioned using GRADE | ||||||
No (n = 177, 88%) | 70 (40) | 107 (60) | ||||
Yes (n = 23, 12%) | 15 (65) | 8 (35) | 2.87 (1.15 to 7.12) | 0.023 | 1.58 (0.57 to 4.44) | 0.381 |
Journal impact factor, median (IQR) | 3.26 (2.27–6.01) | 2.74 (2.18–4.29) | 1.11 (1.02 to 1.22) | 0.017 | 1.04 (0.96 to 1.15) | 0.312 |
Journal endorses systematic review guideline | ||||||
No (n = 60, 30%) | 24 (40) | 36 (60) | ||||
Yes (n = 140, 70%) | 61 (44) | 79 (56) | 1.16 (0.67 to 2.14) | 0.64 | 0.94 (0.46 to 1.93) | 0.872 |
Reviewers reported using systematic review guideline | ||||||
No (n = 127, 63%) | 45 (35) | 82 (65) | ||||
Yes (n = 73, 37%) | 40 (55) | 33 (45) | 2.21 (1.23 to 3.97) | 0.008 | 1.35 (0.68 to 2.70) | 0.393 |
Type of review | ||||||
Association (n = 100, 50%) | 31 (31) | 69 (69) | ||||
Intervention (n = 100, 50%) | 54 (54) | 46 (46) | 2.61 (1.47 to 4.66) | 0.001 | 1.63 (0.85 to 3.15) | 0.143 |
Factors associated with assessing publication bias
As with mentioning of publication bias, assessment of publication bias was most strongly associated with the inclusion of meta-analysis (OR 112.32, 95% CI 14.35 to 879.03) in the univariable analysis. Intervention reviews were more likely than association reviews to include such an assessment (OR 3.09, 95% CI 1.07 to 8.95). Inclusion of only RCTs and controlled trials, journal impact factor and reviewers reporting the use of systematic review guidelines were also associated with the assessment of publication bias (Table 6). There was no significant association between the assessment of publication bias and the number of included studies, search of grey and unpublished literature, quality assessment of individual studies or journal endorsement of systematic review guidelines. In the multivariable analysis, only the inclusion of meta-analysis and reviewers reporting the use of systematic review guidelines were significantly associated with the assessment of publication bias (see Table 6). The relationship between types of review and assessment of publication bias diminished after they were adjusted for other factors (OR 0.94, 95% CI 0.20 to 4.55). As current methods for assessing publication bias primarily rely on constructing funnel plots and/or performing related statistical tests as part of meta-analyses in which data are available from ≥ 10 studies, we further explored and illustrate the influence of these factors in Figure 6.
Factor | Assessed publication bias, n (row %) | Univariable | Multivariable | |||
---|---|---|---|---|---|---|
Yes (N = 19) | No (N = 181) | OR (95% CI) | p-value | OR (95% CI) | p-value | |
Number of included studies, n (%) | ||||||
< 10 (n = 43, 22%) | 2 (5) | 41 (95) | ||||
≥ 10 (n = 157, 78%) | 17 (11) | 140 (89) | 2.49 (0.55 to 11.22) | 0.235 | 2.21 (0.32 to 15.27) | 0.422 |
Meta-analysis included, n (%) | ||||||
No (n = 157, 78%) | 1 (1) | 156 (99) | ||||
Yes (n = 43, 22%) | 18 (42) | 25 (58) | 112.32 (14.35 to 879.03) | < 0.0001 | 84.65 (9.56 to 749.49) | < 0.0001 |
Design of included studies, n (%) | ||||||
Mixed (n = 164, 82%) | 12 (7) | 152 (93) | ||||
RCT and controlled trials (n = 36, 18%) | 7 (19) | 29 (81) | 3.06 (1.11 to 8.42) | 0.031 | Not included | |
Searched grey/unpublished literature, n (%) | ||||||
No (n = 97, 49%) | 13 (13) | 84 (87) | ||||
Yes (n = 103, 51%) | 6 (6) | 97 (94) | 0.40 (0.15 to 1.10) | 0.075 | 0.34 (0.08 to 1.46) | 0.148 |
Quality assessment performed, n (%) | ||||||
No (n = 43, 22%) | 1 (2) | 42 (98) | ||||
Yes (n = 157, 78%) | 18 (11) | 139 (89) | 5.44 (0.71 to 41.96) | 0.104 | 5.29 (0.38 to 82.82) | 0.236 |
Authors mentioned using GRADE | ||||||
No (n = 177, 88%), n (%) | 17 (10) | 160 (90) | ||||
Yes (n = 23, 12%), n (%) | 2 (9) | 21 (91) | 0.90 (0.19 to 4.16) | 0.889 | 0.47 (0.07 to 3.38) | 0.453 |
Journal impact factor, median (IQR) | 3.85 (2.73–5.76) | 2.94 (2.14–4.98) | 1.09 (1.004 to 1.18) | 0.040 | 1.01 (0.90 to 1.13) | 0.848 |
Journal endorses systematic review guideline, n (%) | ||||||
No (n = 60, 30%) | 9 (15) | 51 (85) | ||||
Yes (n = 140, 70%) | 10 (7) | 130 (93) | 0.44 (0.17 to 1.34) | 0.089 | 0.22 (0.04 to 1.09) | 0.064 |
Reviewers reported using systematic review guideline, n (%) | ||||||
No (n = 127, 63%) | 5 (4) | 122 (96) | ||||
Yes (n = 73, 37%) | 14 (19) | 59 (81) | 5.79 (1.99 to 16.84) | 0.001 | 5.38 (1.19 to 24.23) | 0.029 |
Type of review, n (%) | ||||||
Association (n = 100, 50%) | 5 (5) | 95 (95) | ||||
Intervention (n = 100, 50%) | 14 (14) | 86 (86) | 3.09 (1.07 to 8.95) | 0.037 | 0.94 (0.20 to 4.55) | 0.941 |
FIGURE 6.
Assessment of publication bias in sampled reviews stratified by type of review, number of included studies and whether or not a meta-analysis was performed. (a) Intervention reviews; and (b) association reviews. PB, publication bias.


Outcome reporting bias
Outcome reporting bias was mentioned in 34 (17%) systematic reviews and, again, this was more frequent in intervention reviews than in association reviews (30% vs 4%; p < 0.0001). All of the 34 reviews stated that outcome reporting bias was assessed as part of quality assessment of included studies. The risk-of-bias tool used most often was the Cochrane’s risk-of-bias tool (28/34). 64 Two reviews used the Agency for Healthcare Research and Quality’s Methods Guide for Effectiveness and Comparative Effectiveness Reviews,65 one used the Amsterdam–Maastricht Consensus List for Quality Assessment, and the remaining three reviews used unspecified or bespoke tools. Three of the 34 reviews did not report the findings of outcome reporting bias assessment, despite having stated such an assessment in their methods section. Of the remaining 31 reviews that reported the findings, 35% (11/31) reported having at least one study with high risk of selective outcome reporting, 32% (10/31) judged all included studies as low risk, and the remaining 10 reviews (32%) had at least one study in which the authors were unable to judge the risk of bias and were classed as ‘unclear’.
Factors associated with assessing outcome reporting bias
Intervention reviews were more likely than association reviews to include an assessment of outcome reporting bias (OR 10.29, 95% CI 3.47 to 30.53). Authors mentioning the use of GRADE (OR 9.66, 95% CI 3.77 to 24.77) and inclusion of RCTs or controlled trials only (OR 7.74, 95% CI 3.39 to 17.75) were also strongly associated with assessment of outcome reporting bias. Other features found to be associated with assessing outcome reporting bias in the univariable analysis included the number of included studies, inclusion of meta-analysis, journal impact factor, journal endorsement of systematic review reporting guidelines and reviewers mentioning the use of systematic review guidelines (Table 7). Search of grey and unpublished literature was not associated with assessing outcome reporting bias. All the studies which assessed outcome reporting bias performed quality assessment of individual studies; therefore, the variable was not included in the regression analysis. In the multivariable analysis, two variables were found to be significantly associated with assessing outcome reporting bias: (1) author mentioning the use of GRADE and (2) being an intervention review (see Table 7). Lack of pre-registered protocols was frequently reported as a major barrier to adequately assessing outcome reporting bias.
Factor | Assessed outcome reporting bias, n (row %) | Univariable | Multivariable | |||
---|---|---|---|---|---|---|
Yes (N = 34) | No (N = 166) | OR (95% CI) | p-value | OR (95% CI) | p-value | |
Number of included studies, n (%) | ||||||
< 10 (n = 43, 22%) | 14 (33) | 29 (67) | ||||
≥ 10 (n = 157, 78%) | 20 (13) | 137 (87) | 0.30 (0.14 to 0.67) | 0.003 | 0.53 (0.20 to 1.43) | 0.209 |
Meta-analysis included, n (%) | ||||||
No (n = 157, 78%) | 21 (13) | 136 (87) | ||||
Yes (n = 43, 22%) | 13 (30) | 30 (70) | 2.81 (1.27 to 6.23) | 0.011 | 1.73 (0.65 to 4.59) | 0.271 |
Design of included studies, n (%) | ||||||
Mixed (n = 164, 82%) | 17 (10) | 147 (90) | ||||
RCT and controlled trials (n = 36, 18%) | 17 (47) | 19 (53) | 7.74 (3.39 to 17.75) | < 0.0001 | Not included | |
Searched grey/unpublished literature, n (%) | ||||||
No (n = 97, 49%) | 12 (12) | 85 (88) | ||||
Yes (n = 103, 51%) | 22 (21) | 81 (79) | 1.92 (0.89 to 4.14) | 0.094 | 1.33 (0.51 to 3.46) | 0.554 |
Quality assessment performed, n (%) | ||||||
No (n = 43, 22%) | 0 (0) | 43 (100) | Not included in regression analysis (all reviews which assessed outcome reporting bias performed quality assessment) | |||
Yes (n = 157, 78%) | 34 (22) | 123 (78) | ||||
Authors mentioned using GRADE | ||||||
No (n = 177, 88%), n (%) | 21 (12) | 156 (88) | ||||
Yes (n = 23, 12%), n (%) | 13 (57) | 10 (43) | 9.66 (3.77 to 24.77) | < 0.0001 | 5.18 (1.61 to 16.67) | 0.006 |
Journal impact factor, median (IQR) | 6.58 (2.63–7.08) | 2.77 (2.11–4.28) | 1.10 (1.01 to 1.19) | 0.022 | 1.04 (0.95 to 1.13) | 0.444 |
Journal endorses systematic review guideline, n (%) | ||||||
No (n = 60, 30%) | 5 (8) | 55 (92) | ||||
Yes (n = 140, 70%) | 29 (21) | 111 (79) | 2.87 (1.05 to 7.83) | 0.039 | 1.99 (0.65 to 6.12) | 0.231 |
Reviewers reported using systematic review guideline, n (%) | ||||||
No (n = 127, 63%) | 12 (9) | 115 (91) | ||||
Yes (n = 73, 37%) | 22 (30) | 51 (70) | 4.13 (1.90 to 8.99) | < 0.0001 | 1.97 (0.78 to 4.99) | 0.152 |
Type of review, n (%) | ||||||
Association (n = 100, 50%) | 4 (4) | 96 (96) | ||||
Intervention (n = 100, 50%) | 30 (30) | 70 (70) | 10.29 (3.47 to 30.53) | < 0.0001 | 6.44 (2.01 to 20.60) | 0.002 |
Chapter 5 In-depth case studies on the applicability of methods for detecting and mitigating publication and related biases in HSDR
In the absence of comprehensive registration of HSDR and accessible study protocols for direct identification and verification of publication and related biases, statistical methods remain key tools for facilitating the detection and mitigation of publication and related bias when quantitative evidence from HSDR is synthesised. Methods, such as funnel plots, are widely used in systematic reviews, including those of HSDR topics, as shown in Chapters 3 and 4. As with any statistical techniques, these methods are based on certain assumptions, the violation of which could impact on the validity of their results. Although there is a large volume of empirical evidence and methodological research in the use of these methods for detecting and mitigating publication and related biases in clinical research, our systematic review in Chapter 3 reveals that there is paucity of literature concerning the practical application of these methods in HSDR. This chapter aims to fill in this gap in evidence by exploring issues concerning the applicability of these methods in HSDR through the use of in-depth case studies.
Four case studies are described in this chapter:
-
case study 1 – association between weekend and weekday admissions and hospital mortality66
-
case study 2 – relationships between organisational culture, organisational climate and nurse work outcomes52
-
case study 3 – the effect of electronic prescribing on medication errors and adverse drug events67
-
case study 4 – effects of standardised hand-off protocols on information relay and patient, provider and organisational outcomes. 68
The cases were chosen from topics of general interest to HSDR stakeholders, with the aims of illustrating problems, issues and potential solutions pertinent to different aspects of health services research when applying statistical methods for assessing publication and related biases.
Case study 1: association between weekend/weekday admissions and hospital mortality (Chen et al.66)
Description of the case
This systematic review evaluated international literature on the ‘weekend effect’, defined as ‘differences in patient outcomes between weekend and weekday hospital admissions’. 66 The authors meta-analysed quantitative estimates of the weekend effect on mortality following hospital admissions, and explored factors that influence the magnitude of estimated weekend effect using meta-regression and subgroup analyses. The review focused on unselected (hospital-wide) admissions; studies that focused on admissions associated with specific disease condition(s) were excluded. This case study represents an association review drawing evidence primarily from observational studies carried out using routine administrative databases of health service activities.
The stated review question was:
What is the magnitude of the weekend effect associated with hospital admission, and what are the likely mechanisms through which differences in structures and processes of care between weekdays and weekends contribute to this effect?
Chen et al. 66
The review was carried out as part of a mixed-methods synthesis, which also incorporated a framework synthesis (a qualitative method that allows the development and refinement of a conceptual model using emerging findings from evidence being reviewed). 69
The review included 68 studies examining the weekend effect quantitatively on mortality, adverse events, length of stay and patient satisfaction. However, quantitative meta-analyses carried out in the review focused only on comparing the mortality following weekend admissions with that following weekday admissions.
Methods adopted in the original publication
Sources searched
Seven databases, MEDLINE, EMBASE, Cumulative Index to Nursing and Allied Health Literature (CINAHL), Health Management Information Consortium, Electronic Theses Online Service (EThOS), Conference Proceedings Citation Index (CPCI) and The Cochrane Library, were searched from year 2000 onwards in April 2015. The MEDLINE search was updated in November 2017.
Inclusion of grey and unpublished literature
Conference abstracts and grey literature were excluded because of the difficulty in assessing risk of bias.
Methods for assessing publication and reporting bias
A funnel plot was constructed to assess potential publication and reporting bias, and other small study effects. A data augmentation approach,70 in which studies are assumed to be missing with probabilities that are a function of their lack of statistical significance, was adopted in order to derive a pooled estimate ‘adjusted for’ publication bias caused by the assumed missing studies.
Main review findings and findings and statements related to publication and related bias
The pooled OR obtained from a Bayesian meta-analysis for weekend mortality effect was 1.16 [95% credible interval (CrI) 1.10 to 1.23; I2 16%, 95% CrI 0% to 62%]. The funnel plot for this analysis appeared asymmetrical and some statistical heterogeneity between studies of large sample sizes was noted. Given this apparent funnel plot asymmetry, a sensitivity analysis using a data augmentation approach (which ‘adjusted for’ the asymmetry assuming that it was caused by publication bias) was performed, and this lowered the pooled OR by a small amount, from 1.16 to 1.11 (95% CrI 1.08 to 1.13). The finding suggested that, if the observed funnel plot asymmetry was caused by publication bias and if those ‘missing’ unpublished studies were to be included in the analysis, the estimated increase in the odds of death associated with weekend admissions compared with weekday admissions would reduce from 16% to 11%.
The authors also carried out meta-regression and subgroup analyses to explore potential factors influencing the estimated weekend effect. Multivariate meta-regression indicated that the estimated weekend mortality effect was larger for studies including elective admissions and smaller for studies including maternity admissions, and that studies incorporating measures of acute physiology (which are likely to better reflect the urgency and severity of a patient’s condition at admission, but which are usually not available in health service databases for administrative purpose) tend to report smaller weekend mortality effects that are closer to null. Subgroup analyses using data from both between- and within-study comparisons corroborate these findings, but also highlight that substantial statistical heterogeneity exists within individual types of admissions. The authors of the review concluded that the estimates of the weekend effect were influenced by ‘many clinical (case mix), service (e.g. route of admission) and methodological (e.g. statistical adjustment and data completeness) factors’. 66 Outcome reporting bias was not assessed in this review.
Comments and further exploration of different methods
As the Bayesian meta-analysis and data augmentation approach used by the review authors are technically demanding, we reapply other commonly used (frequentist) statistical methods to explore their applicability in HSDR scenarios.
Egger’s test, Begg and Mazumdar’s rank correlation test, and trim and fill method
Egger’s regression test40 (in which the standardised effect estimate is regressed against its standard error) suggested no funnel plot asymmetry (p = 0.686), whereas Begg and Mazumdar’s rank correlation test41 (which is a test of correlation between the ranks of effect sizes and the ranks of their variances) indicated a strong small study effect (p = 0.004). These results are surprising, as the statistical power is usually greater for Egger’s regression test than for Begg and Mazumdar’s rank correlation test. The above results are likely due to the fact that most of the studies have very small standard errors (very large sample sizes). The lack of studies with large standard errors (small sample sizes) and, hence, a spread of studies with varied sample sizes makes the regression test unreliable but Begg and Mazumdar’s rank correlation test is less affected owing to its non-parametric nature. Application of the trim and fill procedure resulted in little change in the overall estimate (meta-analysis with missing studies filled, OR 1.15, 95% CI 1.11 to 1.20); this is probably because any effects of ‘filling’ missing studies would be minor considering the weight given to studies with very large sample sizes based on data from population databases.
The above example highlights an important technical requirement for the appropriate use of funnel plot and related methods, that is, the studies cannot be mostly of similar sample sizes. 26 Failure to meet this requirement may occur in HSDR, for which studies often rely on analyses of data from large administrative databases.
A further issue arises when studies draw data from population databases that essentially cover the entire population or health system, such as the English Hospital Episode Statistics. The concept of sampling error that underpins a funnel plot becomes irrelevant if a study has access to and utilises whole-population data. Publication bias associated with smaller studies (e.g. based on data from a region or a few hospitals within the population) may seem irrelevant, as these studies represent repeated analyses of a fraction of the same data (admissions) and could be regarded as redundant. However, an important issue to consider here is that, unlike RCTs of interventions, in which large sample sizes tend to confer a high level of methodological rigour and hence a low level of bias, observational studies based on whole-population data do not necessarily confer the same advantage over smaller studies that are based on bespoke data collected locally. Indeed, the contrary may be true. For example, the weekend mortality effect is hypothesised to reflect the effect of differential care quality between weekend and weekday, but this assertion is valid only if potential confounders, such as differential case mix in patients admitted between weekends and weekdays, have been accounted for. It has been speculated that the weekend mortality effect demonstrated in studies based on large administrative databases may be, at least in part, attributed to unmeasured (and thus unadjusted) differences in the frailty and urgency of patients admitted at weekends and on weekdays, and emerging evidence conducted by independent research groups has shown that the estimated weekend effect attenuates when data (not usually available in administrative databases) reflecting patients’ acute physiology or admission context are taken into account. 71–73
In such a scenario, in which an association may exist between estimated effect sizes and sample sizes, but for a different reason and in a different form from what is expected of funnel plot asymmetry caused by publication bias, use of funnel plot and related methods and interpretation of their findings require further caution.
A further issue, which is commonplace in HSDR, and which is another important consideration in the application of funnel plot and related methods, is the existence of heterogeneity between studies. 26 The authors of this weekend effect review focused on hospital-wide studies and excluded studies that examined only condition-specific hospital admissions. This might have helped to reduce statistical heterogeneity between studies. Nevertheless, heterogeneity associated with differences in clinical (e.g. emergency vs. elective vs. maternity admissions; different geographic locations) and methodological factors (e.g. variables included in statistical adjustment; variation in outcome measures, such as inhospital, 7- or 30-day mortality, definition of weekends, etc.) between the included studies is still evident, as shown in Figure 7a, in which many studies lie outside the 95% confidence limits, indicating that they were not measuring a common effect.
FIGURE 7.
Funnel plots for the meta-analysis of weekend mortality effect. (a) Funnel plot showing 95% confidence limits (dashed lines), centred at the fixed-effect pooled estimate and a fitted regression line for Egger’s test; and (b) contour-enhanced funnel plot showing contours of different levels of statistical significance, centred at null effect (OR = 1, or log-OR = 0 as shown here).


Heterogeneity poses an issue for the use of funnel plots and related methods, as it confounds the association between effect size and sample size. For example, studies that include only elective admissions are likely to have smaller sample sizes than studies that cover all types of admissions (both emergency and elective). If the weekend effect is more pronounced among elective admissions than other types of admissions, an association between sample size and effect size may be observed, even if there is no publication bias. Attributing the funnel plot asymmetry to publication bias and attempts to ‘correct’ for this purported bias could therefore be misleading.
There are a couple of ways to further investigate whether apparent funnel plot asymmetry may be attributed to publication and reporting bias, or is likely to be caused by other factors, such as heterogeneity. One is to use a contour-enhanced funnel plot (as shown in Figure 7b). For asymmetry caused by publication bias, the ‘missing’ (presumably unpublished) studies that could have made the funnel plot symmetric would have lain within the area corresponding to non-significant results (p > 0.05, the dark grey and white areas in Figure 7b), which seems likely here. In addition, p-hacking and reporting bias could also result in a higher density of (published) studies appearing in the area corresponding to borderline statistical significance (the area within and surrounding 1% < p < 5%), which is not apparent here. Another way is to produce funnel plots by study-level features that might create distinct subgroups contributing to the heterogeneity. Figures 8 and 9 show funnel plots in which studies are sorted by whether or not they included elective admissions and maternity admissions, respectively. Overall, the asymmetry appears to be more profound in studies including elective admissions and in studies excluding maternity admissions. These suggest that the degree of funnel plot asymmetry may differ within different types of admissions, but the asymmetry observed in the pooled analysis is unlikely to be purely caused by inclusion of different types of admissions.
FIGURE 8.
Funnel plot with pseudo-95% confidence limits of the weekend effect, sorted by whether individual studies include or exclude elective admissions.
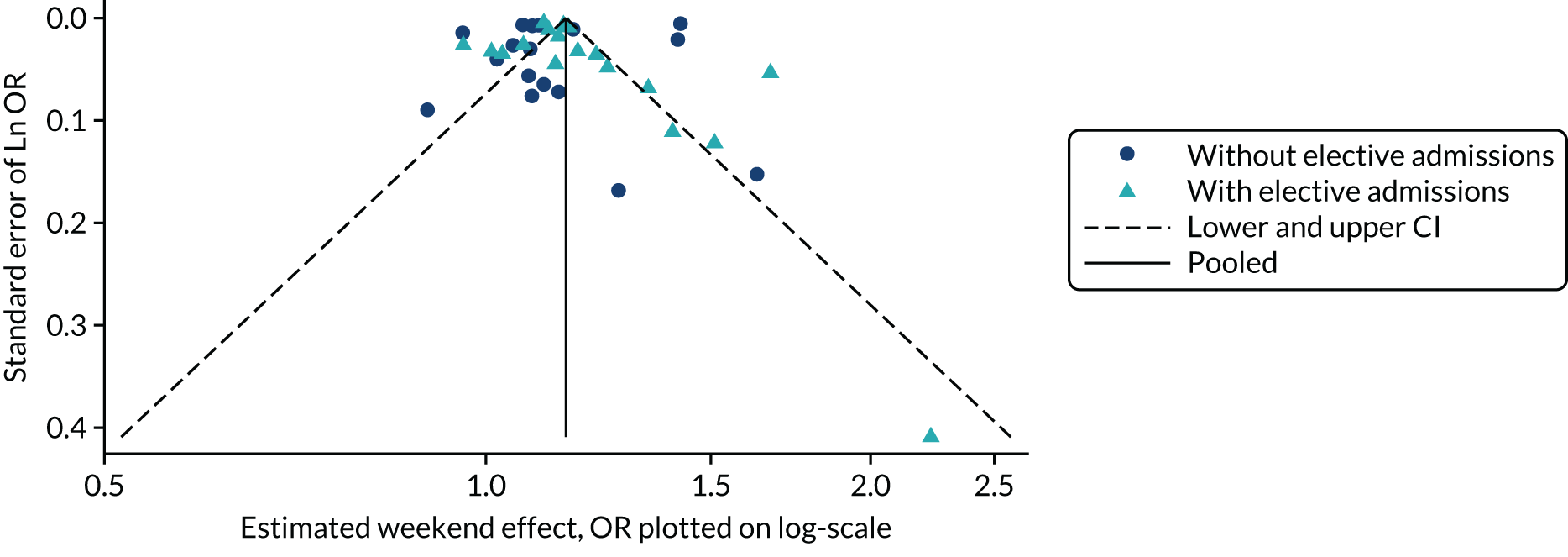
FIGURE 9.
Funnel plot with pseudo-95% confidence limits of the weekend effect, sorted by whether individual studies include or exclude maternity admissions.
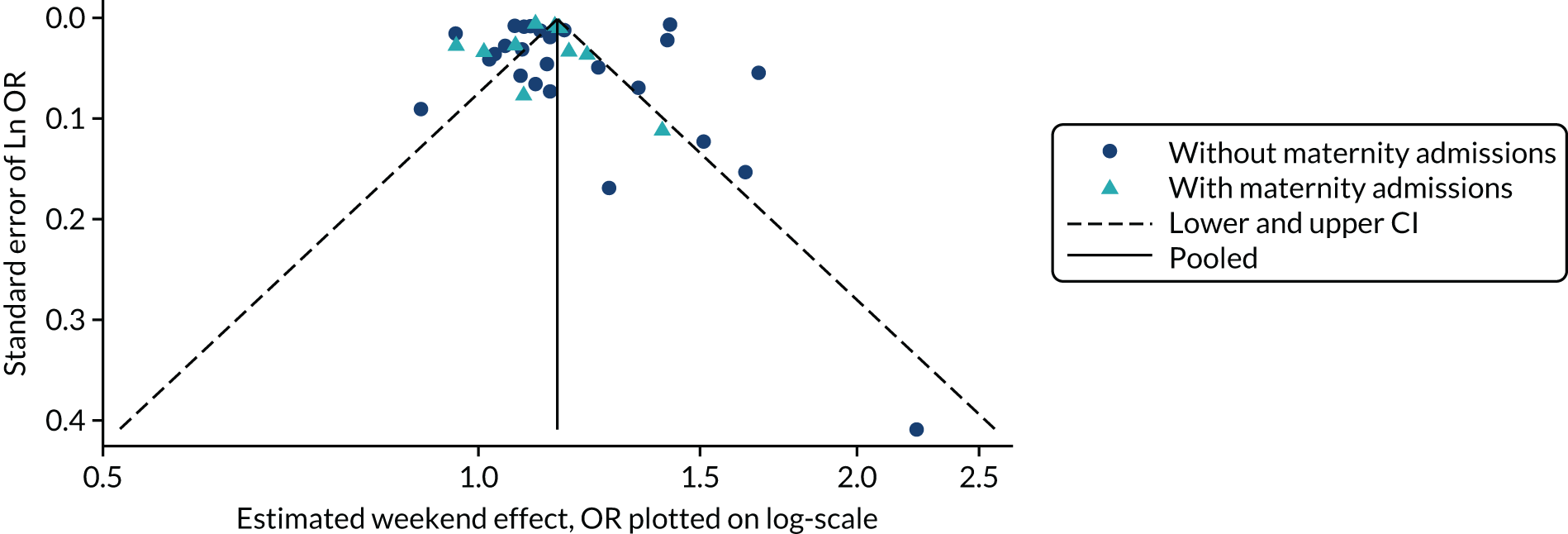
Meta-regression
A further approach to investigating publication and related bias is to use meta-regression to examine the presence or absence of an association of between effect sizes and the sample sizes of individual studies (this is similar to Egger’s regression test), while simultaneously controlling for other study-level variables. The authors of the systematic review carried out a meta-regression, although sample size was not included as one of the covariates in the analysis. We replicated the meta-regression, including variables initially included by the review authors, and then added the total study sample size as a binary variable (categorised into two groups of greater or less than 3 million hospital admissions). The findings are shown in Table 8.
Variable | Exponential (β) | Standard error | p-value | 95% CI |
---|---|---|---|---|
Sample size > 3 million admissionsa | 1.078 | 0.375 | 0.033 | 1.006 to 1.155 |
Statistical adjustment categoryb | ||||
1 and 2a | 1 | Reference | ||
2b | 1.047 | 0.091 | 0.601 | 0.881 to 1.243 |
3 | 1.125 | 0.964 | 0.171 | 0.950 to 1.334 |
4 | 1.123 | 0.094 | 0.168 | 0.952 to 1.325 |
Year | 1.001 | 0.003 | 0.769 | 0.994 to 1.008 |
Inclusion of elective admissions | 1.301 | 0.561 | < 0.001 | 1.194 to 1.417 |
Inclusion of surgical admissions | 0.984 | 0.072 | 0.822 | 0.850 to 1.138 |
Inclusion of maternity admissions | 0.824 | 0.032 | < 0.001 | 0.764 to 0.889 |
Intercept | 1.015 | 0.114 | 0.896 | 0.812 to 1.268 |
The analysis suggested that estimates obtained from larger studies with a total sample size of > 3 million hospital admissions showed a weekend effect approximately 8% larger than those obtained from studies of smaller sample sizes. This is in contrast with evidence from randomised trials, which tend to show that larger studies tend to report smaller effects. 12 A possible explanation is that smaller studies were able to make better statistical adjustment, with additional data not available from administrative databases. However, the completeness of statistical adjustment (based on review authors’ judgement) was taken into account in the meta-regression reported in the review. Although the level of statistical adjustment did not appear to significantly influence effect size in the analysis, it remains a possibility that the review authors’ judgement did not properly capture adequacy of statistical adjustment and, hence, the existence of residual confounding between bias related to adjustment, sample size and effect size. Finally, the finding from this meta-regression is exploratory. It could simply be a chance finding and, therefore, needs to be treated with great caution.
Application and applicability of p-curve
The risk of p-hacking may be particularly high in studies involving analysis of large data sets with many different variables, such as the ones included in the weekend effect systematic review. We therefore used the p-curve technique to explore the potential occurrence of p-hacking. 2 First, we calculated corresponding p-values for the 119 estimates (adjusted ORs and their CIs) of the weekend effect included in the above meta-regression, using the approximation method described by Altman and Bland,74 and then created a p-curve using the online application provided by Simonsohn et al. 43 The p-curve (Figure 10) suggests that, based on the estimates from included studies, the weekend effect does exist (the curve peaks on the left), but there is no evidence of p-hacking (there is no peak on the right close to p = 0.05).
FIGURE 10.
p-curve for estimates of the weekend effect included in meta-regression. The observed p-curve includes 82 statistically significant (p < 0.05) results, of which 78 are p < 0.025. There were 37 additional results entered but excluded from p-curve because they were p > 0.05.
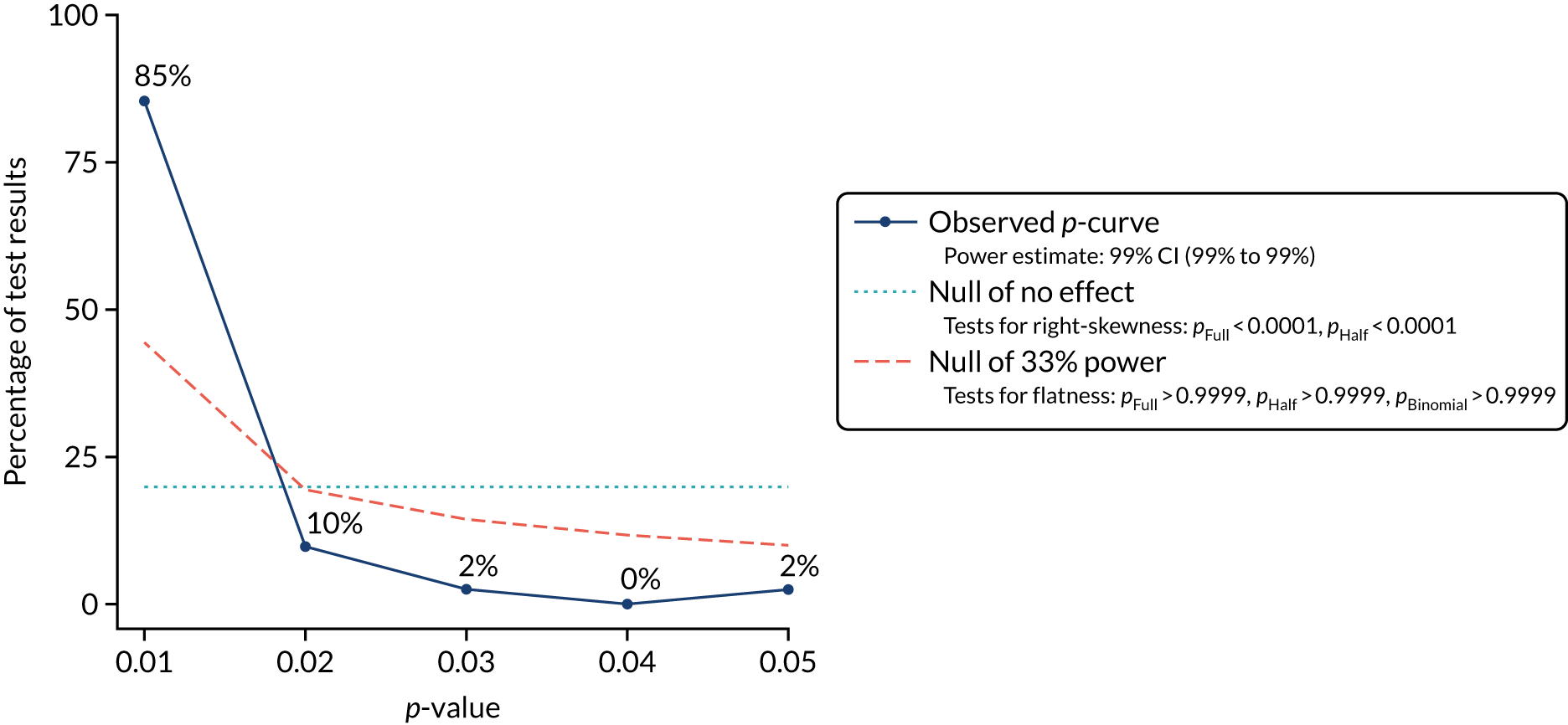
The p-curve is a relatively new tool and, thus, its validity and utility are still subject to debate and require further empirical testing. 75,76 Our exploratory analysis provides some assurance that p-hacking is not evident among the literature included in the weekend effect systematic review. It is plausible that with very large sample sizes available from administrative databases and myriad variables/groups that can be analysed, it would not be necessary to resort to p-hacking to obtain a statistically significant finding to report. On the other hand, the strong right-skewness does not necessarily confirm the existence of a true ‘weekend effect’; as Bruns and Ioannidis76 demonstrated in their simulation study, the p-curve cannot distinguish true effects from null effects in the presence of even a minimal level of unaccounted confounding, which is a likely scenario in the case of studies of the weekend effect.
Key summary points
-
This case study explores issues that might need to be considered when using statistical techniques to assess publication and reporting biases in systematic reviews in which most of the included studies are based on analyses of administrative databases of health service activities.
-
Funnel plots highlighted the lack of studies of smaller sample sizes and showed a high level of statistical heterogeneity between studies of very large sample sizes. Both features may compromise the validity of funnel plots as a tool for detecting publication bias.
-
Two commonly used tests (Egger’s regression test and Begg and Mazumdar’s rank correlation test) produced discrepant results. The use of Egger’s regression test might be particularly problematic given the lack of small studies.
-
In contrast to evidence from RCTs, in which larger studies tend to report smaller effects, studies of larger sample sizes tended to report larger effects in this case study. The possibility that large database studies might suffer from a higher level of bias (due to lack of required information to adequately adjust for confounders) than smaller studies (which may be able to access detailed clinical data) could not be ruled out.
-
Adjustment of pooled estimates based on imputation of ‘missing’ small studies had little impact, given the negligible weight assigned to them compared with large database studies. Such adjustment might also be inappropriate if the underlying assumption that funnel plot asymmetry was caused by publication bias was invalid.
-
Investigation of publication bias might be secondary to identification of the sources of statistical heterogeneity, which was of primary importance in the scenario described in the case study.
Case study 2: relationships between organisational culture, organisational climate and nurse work outcomes (Fang52)
Description of the case
This case study is based on a Doctor of Philosophy (PhD) dissertation, in which a systematic review was undertaken to assess the relationships between organisational culture, organisational climate and nurses’ job satisfaction and nurses’ turnover in hospitals the USA, and to examine moderators that influence such relationships.
Organisational culture was defined in the systematic review as ‘a pattern of shared assumptions among organizational members that guides the formation of managerial practices and members’ behaviours to achieve external adaptation and internal integration of an organization in order to maintain the organization’s survival and growth’. 52 To facilitate analyses, the author mapped different measures of organisational culture to the three cultural patterns identified by the Organisational Culture Inventory:77 (1) constructive culture, (2) passive and defensive culture, and (3) aggressive and defensive culture.
Organisational climate was defined as ‘members’ perceptions of managerial practices in the work unit that direct members’ work-related behaviours’ in an organisation. 52 Measures of organisational climate were mapped to the five elements described in the ‘model of climate, culture, and productivity’ proposed by Kopelman et al. :78 (1) goal emphasis, (2) means emphasis, (3) reward orientation, (4) task support and (5) socioemotional support. An additional measure of ‘global climate’ was also adopted.
Overall, the review covered 23 relationships between different measures of organisational culture and climate and job-related outcomes for nurses:
-
association between each of the three patterns of organisational culture described above and job satisfaction
-
association between each of the six elements/measures of organisational climate described above and nurse job satisfaction
-
association between each of the three patterns of organisational culture and nurse turnover, measured at two different levels (rate of turnover at hospital level and turnover intent at individual level), thus creating six pairs of association)
-
association between five of the six elements/measures of organisational climate (excluding goal emphasis climate as no study was found) and nurse turnover (all measured at individual level)
-
association between the three patterns of organisational culture and global climate (as no study was found for individual elements of organisational climate).
When data were available, the contribution of the various study characteristics and contextual factors towards variation in the magnitudes of these associations was explored using subgroup analyses. Factors that were examined included measurement instrument (Organisational Culture Inventory or other), publication year, whether or not hospital was under redesign, number of hospitals (one or more than one), professional level (registered nurse, manager or mixed), type of nursing unit (medical, surgical, intensive care unit or obstetrics, gynaecology or multiple), whether or not psychiatry units were included, US region, whether or not the survey was given by manager, whether or not the survey was collected at work, adoption of random sampling and quality rating of the study. Estimates of association from individual studies were transformed into a standardised effect size and pooled using both fixed- and random-effects modelling for each of the associations examined. Effect sizes were interpreted as < 0.30, small effect; between 0.30 and 0.49, medium effect; and ≥ 0.50, large effect.
Methods adopted in the original publication
Sources searched
Thirty-five computer databases were searched. Reference lists of primary studies included in the review were also checked. For publications in which the desired effect sizes were not provided, authors were searched in computer databases to identify other relevant publications and authors were also contacted by letter to request relevant data, when necessary.
Inclusion of grey and unpublished literature
The review included both published and unpublished studies, although unpublished studies were limited to doctoral dissertations. The dissertations were identified from computer databases. Overall, the review included 32 primary studies, covering 15 unpublished doctoral dissertations and 17 published journal articles.
Methods for assessing publication and reporting bias; subgroup and sensitivity analyses
When data were available, a funnel plot was constructed and a ‘fail-safe N’ was calculated for each of the associations examined. Fail-safe N is the number of studies with a null result (or a specified effect size) that would be required in a meta-analysis to render a statistically significant finding insignificant. 79 A large fail-safe N suggests that a large number of (unpublished) studies with null result (or a specified effect size) would be required to overturn the observed effect and association and, therefore the finding is robust and is unlikely to be influenced by publication bias (and vice versa). In addition, the author undertook subgroup meta-analyses based on the sources of included studies (journal articles vs. doctoral dissertations), and thus provided an opportunity for comparing published and unpublished studies.
Main review findings and findings and statements related to publication bias
Of the 23 associations examined, 14 were found to be statistically significant. Of these, the effect size was considered large for only one association (global climate and job satisfaction, effect size 0.51, 95% CI 0.41 to 0.60, I2 = 88%), medium for seven and small for six associations (see Appendix 7 for further details).
The total number of studies available for each association ranged from one to 12, and the original author was unable to formally assess publication bias for 13 of the associations owing to the small number of studies. For the remaining 10 associations, the author constructed a funnel plot for each of them and calculated a fail-safe N for which the pooled estimate of the association was found to be statistically significant. In addition, the author compared the pooled effect size from journal articles with that from (unpublished) doctoral dissertations in subgroup analyses and calculated a fail-safe N for each of these two subgroups. The findings are summarised in Table 9.
Association assessed | Number of studies (number of effect sizes)a included | Pooled effect size (95% CI) | Test of between-group homogeneity | Fail-safe N (all studies) | Fail-safe N | Funnel plotb | Original author’s comments |
---|---|---|---|---|---|---|---|
Constructive culture and job satisfaction | Journal: 5 (6) | 0.34 (0.23 to 0.43) | p = 0.49 | 894 | 289 | Symmetrical | No publication bias is suggested |
Dissertation: 5 (6) | 0.40 (0.24 to 0.55) | 161 | |||||
Passive/defensive culture and job satisfaction | Journal: 4 (5) | –0.29 (–0.45 to –0.12) | p = 0.08 | 152 | 134 | Asymmetrical | More studies are required |
Dissertation: 5 (6) | –0.02 (–0.27 to 0.22) | NAc | |||||
Aggressive/defensive culture and job satisfaction | Journal: 5 (6) | –0.22 (–0.28 to –0.16) | p = 0.53 | 204 | 105 | Symmetrical | No publication bias is suggested |
Dissertation: 3 (3) | –0.26 (–0.36 to –0.15) | 14 | |||||
Global climate and job satisfaction | Journal: 1 (1) | 0.34 (0.22 to 0.45) | p = 0.007 | 897 | NRd | Symmetrical | No publication bias is suggested |
Dissertation: 5 (5) | 0.54 (0.44 to 0.63) | 743 | |||||
Mean emphasis climate and job satisfaction | Journal: 6 (7) | 0.42 (0.23 to 0.58) | p = 0.63 | 1155 | 174 | Symmetrical | No publication bias is suggested |
Dissertation: 6 (7) | 0.37 (0.29 to 0.45) | 424 | |||||
Reward orientation climate and job satisfaction | Journal: 1 (2) | 0.34 (0.18 to 0.48) | p = 0.02 | 211 | NRd | Not an exact funnel shape | No publication bias is suggested |
Dissertation: 3 (3) | 0.52 (0.46 to 0.58) | 142 | |||||
Task support climate and job satisfaction | Journal: 1 (2) | 0.20 (0.03 to 0.36) | p = 0.46 | 133 | NRd | Not a funnel shape | No publication bias is suggested |
Dissertation: 3 (4) | 0.31 (0.08 to 0.51) | 96 | |||||
Socioemotional support climate and job satisfaction | Journal: 2 (3) | 0.25 (0.01 to 0.46) | p = 0.17 | 713 | 577 | Not a funnel shape | Except the subgroup of journal articles, no publication bias is suggested |
Dissertation: 6 (7) | 0.44 (0.28 to 0.58) | ||||||
Mean emphasis climate and turnover | Journal: 3 (3) | –0.22 (–0.56 to 0.20) | p = 0.63 | 12 | NAc | Missing smaller studies of lower effect sizes | Suggested presence for publication bias |
Dissertation: 1 (1) | –0.11 (–0.24 to 0.02) | NAc | |||||
Socioemotional support climate and turnover | Journal: 2 (2) | –0.04 (–0.15 to 0.07) | p = 0.37 | 0e | NAc | Missing smaller studies of lower effect sizes | Suggested presence for publication bias |
Dissertation: 1 (1) | 0.12 (–0.24 to 0.01) | NAc |
Of the 10 associations for which publication bias was assessed, the original author suggested that there was no publication bias for six of these, according to funnel plots, comparison of pooled estimates between journal articles and doctoral dissertations, and the values of fail-safe N calculated (see Table 9). Publication bias was suspected for three of the associations, and for one of the associations the author indicated that more studies were required. The pool estimates between published journal article(s) and doctoral dissertation(s) differ significantly (based on test of homogeneity between subgroups) for two of the 10 associations. However, in both cases there was only one study in the journal article subgroup and, therefore, the findings need to be interpreted with caution. In addition, in both cases the estimated effects were in the same direction (showing a positive association), and the estimates based on unpublished doctoral dissertations showed larger effects than those based on the published journal article. Consequently, publication bias was not suspected in either case. The author also cautioned that the meta-analyses might be susceptible to reporting bias.
Comments and further exploration of different methods
This case study illustrates a common scenario in HSDR, in which associations between abstract concepts, such as organisational culture, organisation climate and job satisfaction, are explored. As exemplified in the case, quantitative measurement of these concepts and synthesis of evidence from these association studies face many challenges, including diverse measurement tools that may cover different domains or components of a concept, measurements made at different levels (e.g. individual or hospital level), lack of standardised terminology, diverse contexts in which the associations are measured and the large number of factors that could potentially modify these associations. Consequently, evaluation of associations between an apparently small number of concepts could rapidly expand into examination of a large number of associations between variables that represent different domains and measurement approaches for these concepts. There are some key implications in relation to publication and related bias:
-
The large number of associations that may be examined in individual studies creates a theoretical risk of selective outcome reporting of only statistically significant findings. However, a possible counterargument would be that the examination of a large number of association increases the chance that at least one statistically significant finding would be observed and, thus, publication bias associated with completely null findings would be reduced. In addition, exploration of such associations is often guided by theories, and observations both conforming to and disagreeing with existing theories may be considered of interest, irrespective of statistical significance.
-
The large number of decisions that researchers have to make during the data analysis process, such as the choice of measurement instruments and unit, inclusion, exclusion and grouping of individuals in the collected sample, selection of variables for statistical adjustment and so forth, creates a ‘garden of forking paths’, as described by Gelman and Loken,80 in which researchers have a large, and potentially unknown, ‘degree of freedom’ in making decisions contingent on data in the process of data analyses, resulting in a problem similar to multiple comparison even without p-hacking. p-values (statistical significance) of analyses from such exploratory analyses should therefore be interpreted more cautiously.
-
Attempts to divide evidence and studies into more refined and homogeneous groups based on conceptual domains or contextual factors during evidence synthesis tend to result in a smaller number of studies being included in each meta-analysis. This reduces precision and increases uncertainty of individual effect estimate, and hampers the applicability and usefulness of statistical methods such as funnel plots, for detecting publication and reporting bias.
Given the above, caution is required in the interpretation of findings in the assessment of publication bias presented in this case study. First, for the associations for which a funnel plot was constructed by the original author, the number of studies included for each association was no more than 12, with several associations including less than the minimum number of 10 studies recommended for using funnel plot. 26 The determination of funnel plot symmetry was likely to be very subjective and potentially unreliable.
Second, the method of fail-safe N focuses primarily on statistical significance, and the calculation is heavily dependent on the assumed effect size of unpublished studies (zero in the current case study). Its use is therefore not recommended by the Cochrane Collaboration, and judgement of the presence and absence of publication bias based on fail-safe N was better avoided.
Despite these caveats, the original author undertook a very comprehensive search of the literature, and the comparison of data from published journal articles with unpublished doctoral dissertations provided a unique opportunity and stronger evidence for assessing potential publication bias. Although there appeared to be no indication of a high level of publication bias mediated by observed strength of association, the volume of evidence was inadequate for many of the associations examined and, therefore, the lack of evidence of publication bias should not be equated to evidence of no publication bias until further evidence has been accrued.
Case study 3: the effect of electronic prescribing on medication errors and adverse drug events (Ammenwerth et al.67)
Description of the case
This case study was based on a systematic review that aimed to determine the effect of electronic prescribing on the risk of medication errors and adverse drug events, and to analyse the factors contributing to those effects. The review covered all settings (e.g. inpatient and outpatient) and all patient groups, but focused on systems of which physicians were the primary users (i.e. excluding studies with pharmacists or nurses as the primary users). Studies which provided quantitative empirical evidence on the effectiveness of electronic prescribing systems on the outcomes of interest were included. RCTs, non-RCTs, before-and-after and time series were all eligible, whereas studies based on laboratory experiments and simulation, as well as those that did not focus primarily on medication errors or adverse drug events, were excluded. This case study provides an example from the prominent area of using technologies to improve service delivery. Components of such interventions may vary substantially between different locations and may evolve over time. The interventions may have a generic effect across different services and patients, but their effects may be difficult to measure owing to the infrequent occurrence of the events (which could have serious consequences) that they aim to prevent or reduce, such as medication errors and adverse events.
Methods adopted in the original publication
Sources searched
Electronic databases MEDLINE and EMBASE were searched, and the authors examined reference lists of relevant reviews identified from the Cochrane Database of Systematic Reviews. Three journals were also hand-searched: Journal of the American Medical Informatics Association, International Journal of Medical Informatics and Methods of Information in Medicine (1990–2006). In addition, reference lists of all retrieved articles were examined. Searches were conducted in 2006 without any language restriction.
Inclusion of grey and unpublished literature
The review included only published studies.
Methods for assessing publication and reporting bias; subgroup and sensitivity analyses
Methods of assessing publication bias were not described in the published article of the systematic review. However, the authors mentioned publication bias in their discussion and highlighted this as a potential issue in more detail in a separate publication. 81 Outcome reporting bias was not assessed.
Several subgroup analyses were performed for the outcome of medication errors using subgroups defined a priori, including the clinical setting (inpatient, outpatient or intensive care), patient group, type of drugs, type of system (home grown or commercial), functionality (no, limited or advanced decision support), study design and method to detect errors in order to explore factors that may influence intervention effectiveness.
Main review findings and findings and statements related to publication bias
The literature search identified 172 evaluation studies, but only 27 met the review’s inclusion criteria. Fifteen of these evaluated medication errors only, two assessed adverse drug events only and 10 reported on both medication errors and adverse drug events. The majority of the studies were before-and-after and time series studies. Only two included studies were RCTs.
Meta-analyses were not carried out due to substantial heterogeneity between included studies. Twenty-three studies (out of 25 studies) on medication errors showed statistically significant reduction with the intervention, but the risk ratios compared with control groups ranged from 0.01 (99% relative risk reduction) to 0.87 (13% relative risk reduction). Six out of nine studies on potential adverse drug events (defined as ‘medication errors with significant potential to harm a patient that may or may not actually reach a patient’) reported a statistically significant risk reduction, with risk ratios ranging from 0.02 to 0.65. Four of the six studies with data on adverse drug events reported a statistically significant risk reduction, with risk ratios ranging from 0.16 to 0.70. Although no meta-analysis was conducted, the authors plotted effect estimates for different subgroups separately in forest plots. These suggested that intervention effects may differ between home-grown and commercial systems, between electronic prescribing and hand-written ordering, between systems with different levels of decision support functions and manual chart review and automated database analysis as the methods for detecting medication errors. Nevertheless, substantial heterogeneity persisted within most of the subgroups.
No findings in relation to publication bias were reported in the review article, but the authors stated that ‘in our CPOE [computerised physician order entry] meta-analysis, the funnel plot showed a slight asymmetry, which may indicate a potential publication bias’ in the related publication. 81
Comments and further exploration of different methods
Electronic prescribing systems are one of the archetypal generic service interventions that have been evaluated in HSDR. Quantitative assessments of the effectiveness of such interventions and synthesis of this body of evidence are fraught with challenges, including lack of standardised terminology and outcome measures, inadequate quality of study design and reporting, and a high level of heterogeneity between studies, as the authors highlighted in their reflection of undertaking the systematic review. 81 In addition, they pointed out several pertinent issues which, in combination with or as a contributor towards publication bias, may lead literature included in such a review to largely represent the maximum effectiveness of the intervention in favourable conditions, rather than a representative range of possible (both positive and negative) effects. These include preferential publication and reporting of positive rather than negative results by developers or sponsors of the technologies due to conflict of interest; researchers reporting and publishing findings only after a (potentially long) optimisation process has been completed; and the tendency of published findings to be generated from atypical, so-called ‘alpha sites’,82 where staff are highly motivated and strong technical and financial support is available. Findings from meta-analyses of the evidence, if undertaken, are therefore likely to overestimate intervention effectiveness in a representative setting.
The high level of heterogeneity among studies included in this systematic review means that application of funnel plots and other statistical methods is unlikely to be informative. The number of available studies is too small for the application of techniques such as meta-regression. Consequently, being aware of the caveats highlighted above when interpreting evidence may be the best approach for evidence reviewers and users before a comprehensive study registry is established, which the authors of the systematic review advocated. 81
Case study 4: effects of standardised hand-off protocols on information relay, patient, provider and organisational outcomes (Keebler et al.68)
Description of the case
This systematic review was conducted to determine whether or not implementing a standardised hand-off (handover) protocol improves the process of hand-off (with regard to information being passed on between care providers), and to evaluate its effects on patient, care provider and organisational outcomes.
The authors did not place any restrictions on clinical areas, type of hand-off protocols (e.g. this could be mnemonics, checklists, protocols or computerised sign-out programs), study dates or study design, other than a requirement that a study needed to have described an empirical investigation of a standardised protocol and have reported sufficient data for calculating effect sizes.
Measures reported in studies included in the review were classified into four broad categories as defined below.
Hand-off outcomes
Measure of the amount of information passed between care providers (e.g. adequacy of patient information provided to on-call residents).
Patient outcomes
Outcomes related to patients’ health or their opinions about their care (e.g. cardiopulmonary bypass time, monitor alarms checked and adapted to patients’ status, mortality, length of stay and satisfaction).
Provider outcomes
Measures of providers’ performance or opinions, including their response to hand-off implementation (e.g. satisfaction with the sign-out process, time spent on tasks and effects on interruptions).
Organisational outcomes
Outcomes related to higher levels of the organisation, including measures of culture and organisation-wide improvements (e.g. leadership and culture to learn from errors).
Hedges’ g,83 which is one form of standardised mean difference calculated with a correction for bias associated with small sample sizes, was computed for all reported outcomes, including both continuous and binary measures. Meta-analyses were carried out using a random-effects model.
The review included 36 studies, all of which were of uncontrolled before-and-after design. The authors reported the total sample size in terms of ‘measurement data points’, with 106,724 pre-intervention measures and 97,642 post-intervention measures.
Methods adopted in the original publication
Sources searched
Four databases, MEDLINE, Sage, EMBASE and PsycINFO, were searched from inception up to and including March 2015.
Inclusion of grey and unpublished literature
The authors initially did not search for grey literature, but after finding only a small number of studies with null findings from the initial literature search they conducted another search on greylit.org, but found no relevant articles. The authors also reported searching the World Health Organization database of registered clinical trials, but found no study with relevant data.
Methods for assessing publication and reporting bias
Funnel plots were constructed to assess publication bias. The funnel plots were assessed visually and by Egger’s test.
Main review findings and findings and statements related to publication bias
Among the 36 included studies, 27 studies assessed hand-off information (with 273 different measures and variables reported among them), 16 studies assessed patient outcomes (with 66 different measures reported), 13 studies assessed provider outcomes (with 85 different measures reported) and three assessed organisational outcomes (with 26 different measures reported). Pooled estimates suggested that standardised protocols are effective in improving hand-off outcomes (Hedges’ g 0.71, 95% CI 0.63 to 0.79, I2 = 83%), patient outcomes (Hedges’ g 0.53, 95% CI 0.41 to 0.65, I2 = 97%), provider outcomes (Hedges’ g 0.51, 95% CI 0.41 to 0.60, I2 = 66%) and organisational outcomes (Hedges’ g 0.29, 95% CI 0.23 to 0.35, I2 = 14%).
The authors reported that funnel plots indicated publication bias for all four outcome categories. Results of Egger’s test were statistically significant for all four corresponding funnel plots (p < 0.001).
To further assess the potential impact of publication bias, the authors examined the articles included in the meta-analyses to assess negative outcomes reported in the studies. They found that, although many articles reported mainly positive effects, there are some instances in which the intervention resulted in negative outcomes. Negative outcomes are reported in < 20% of the total variables analysed for each outcome measure, 12% for hand-off information, 19% for provider outcomes, 18% for patient outcomes and 3% for organisational outcomes. It was not clear if low occurrence of negative outcomes were due to under-reporting of negative outcomes.
Comments and further exploration of different methods
This review represents a laudable effort to synthesise available evidence concerning the effectiveness of hand-off protocols on a comprehensive range of outcomes. The findings of predominantly positive effects on the handover of information from uncontrolled before-and-after studies agree with the findings of other systematic reviews on similar topics,84,85 but the overall effectiveness of these interventions, in particular their effects on patient outcomes, has been questioned, particularly when the quality of evidence is taken into account. It is beyond the scope of this case study to critically appraise these systematic reviews and underlying evidence. However, two issues related to the methodological approach adopted in the example selected for the case study merit further discussion. The first issue concerns the approach to meta-analyses and corresponding funnel plots and the second issue relates to the use of proportion of negative outcomes as an indicator for publication bias. We explicate these issues below.
It appears that, within each outcome category, the review authors had included multiple effect sizes for multiple outcome variables in the same meta-analysis and funnel plot. For example, although only three studies reported data for outcome variables within the organisational outcome category, the meta-analysis and funnel plot for this outcome category included 26 effect sizes associated with 26 different organisational outcome measures reported in these studies. Most (23/26) of the effect sizes and outcome measures were contributed by one of the three studies; the other two studies contributed only one and two effect sizes. This approach departs from the usual practice for meta-analysis, where each study contributes one effect size from one outcome measure towards the pooled estimate to ensure independence of individual observations and to avoid double counting a study due to reporting of multiple outcome measures. The pooled effect sizes reported in this review are likely to have been driven, at least in part, by studies that measured and reported a large number of items and outcome variables within a given outcome category and, therefore, were potentially inaccurate. Similarly, as data points included in the funnel plots were not independent, the shapes of their distributions and the results of Egger’s test based on the data could be unreliable.
The authors noted that only a very low proportion of results across all outcome categories were ‘negative’, and suspected publication bias as a potential reason behind this. As we highlighted in Chapter 3, the proportion of studies or outcomes with negative findings is not a reliable measure of publication and related bias, as this proportion depends on the nature of the intervention and outcome measure; one would expect an approximately equal split of positive and negative findings from a random sample of studies only if an intervention truly had no effect on the specific outcome. Nevertheless, the authors’ further examination of negative findings included in their meta-analyses unearthed the important issue of including heterogeneous outcome measures (within an outcome category) in the same meta-analysis, as we pointed out above. In this case, it appears that the negative findings were mainly associated with specific items or measures, such as delay and increased duration of handover activities, and omission of relevant information items not explicitly listed on the hand-off checklist. Rather than publication bias or selective outcome reporting being the reason behind the low proportion of negative findings, the low proportion of negative findings might have arisen from the standardised hand-off protocols having positive impacts on most aspects of measured processes or outcomes, or alternatively because few studies had measured the outcomes on which standardised hand-off protocols had negative impacts. Nevertheless, the findings showed that potentially unintended effects could have gone unnoticed had people focused on the pooled summary estimates derived from combining different outcome measures together.
Chapter 6 Follow-up of HSDR study cohorts for investigating publication bias
This chapter presents findings from WP 4, in which we followed up cohorts of HSDR studies to ascertain their publication status and its association with their findings. Four study cohorts were assembled from the studies funded by the NIHR HSDR programme and its predecessors (n = 100), studies registered with the HSRProj database (n = 100), abstracts presented in the HSRUK (n = 50) and ISQua (n = 50) conferences, as described in Chapter 2. Based on the nature of these cohorts, we also refer to the NIHR and HSRProj cohorts collectively as the ‘inception cohort’, and the HSRUK and ISQua cohorts collectively as the ‘conference cohort’.
Verification of publication status
After the initial online search for publications, we contacted investigators for 145 of the 300 studies in order to verify their publication status. Investigators for 34 of these studies were unreachable, as we were not able to find their contact details (n = 26) or the e-mails were undeliverable (n = 8). Thus, 111 investigators were contacted. The overall response rate was 60% (67/111) and this varied by cohorts: NIHR, 79% (33/42); HSRUK, 60% (6/10); HSRProj, 50% (23/46); and ISQua, 38% (5/13). Of the 26 investigators whose contact details we could not obtain, 23 were from the ISQua cohort. Organisers of the ISQua conference could not provide the contact details to us owing to data protection regulation, but agreed to forward our information request to the abstract presenters so that they could contact us directly. However, no response was received.
Characteristics of included cohorts of HSDR studies
The characteristics of included studies stratified by different cohorts are presented in Table 10. In summary, 140 (47%) of the selected studies were intervention studies (inception cohort, 44%; conference cohort, 53%). Of the intervention studies, just over one-third (36%) were RCTs (inception cohort, 44%; conference cohort, 23%), but nearly two-thirds (62%) had a concurrent control group (inception cohort, 77%; conference cohort, 38%). Two-thirds of studies (67%) used bespoke data sources rather than using data from databases. The majority of the studies (79%) reported statistically significant findings. Findings were judged to be positive in the same proportion (79%) of studies.
Characteristic | All included studies (N = 300), n (%) | All inception cohort (N = 200), n (%) | Cohort, n (%) | ||||
---|---|---|---|---|---|---|---|
HSRProj (N = 100) | NIHR (N = 100) | Conference (N = 100) | HSRUK (N = 50) | ISQua (N = 50) | |||
Type of study | |||||||
Association | 160 (53) | 113 (56.5) | 43 (43) | 70 (70) | 47 (47) | 23 (46) | 24 (48) |
Intervention | 140 (47) | 87 (43.5) | 57 (57) | 30 (30) | 53 (53) | 27 (54) | 26 (52) |
Of intervention studies | |||||||
Before and after | 51 (36)a,b | 19 (22)a | 11 (19)a | 8 (27) | 32 (60)b | 11 (41)b | 21 (81) |
With concurrent control | 87 (62)a,b | 67 (77)a | 45 (79)a | 22 (73) | 20 (38)b | 15 (56)b | 5 (19) |
Non-RCT | 90 (64) | 49 (56) | 25 (44) | 24 (80) | 41 (77) | 18 (63) | 23 (88) |
RCT | 50 (36) | 38 (44) | 32 (56) | 6 (20) | 12 (23) | 9 (33) | 3 (12) |
Data source | |||||||
Database and routine surveys | 96 (32)a | 69 (35)a | 37 (37)a | 32 (32) | 27 (27) | 16 (32) | 11 (22) |
Bespokec | 203 (67)a | 130 (65)a | 62 (62)a | 68 (68) | 73 (73) | 34 (68) | 39 (78) |
Statistical significance | |||||||
Non-significant | 41 (14) | 30 (15) | 20 (20) | 10 (10) | 11 (11) | 9 (18) | 2 (4) |
Significant | 237 (79) | 155 (77.5) | 66 (66) | 89 (89) | 82 (82) | 37 (74) | 45 (90) |
Unknown | 22 (7) | 15 (7.5) | 14 (14) | 1 (1) | 7 (7) | 4 (8) | 3 (6) |
Positivity of findings | |||||||
Non-positive | 52 (17) | 42 (21) | 18 (18) | 24 (24) | 10 (10) | 9 (18) | 1 (2) |
Positive | 237 (79) | 150 (75) | 74 (74) | 76 (76) | 87 (87) | 38 (76) | 49 (98) |
Unknown | 11 (4) | 8 (4) | 8 (8) | 0 (0) | 3 (3) | 3 (6) | 0 (0) |
Publication status of included HSDR studies
Of all 300 studies, 62% were published in academic journals (inception cohort, 70%; conference cohort, 48%). Findings for 20% of the 300 studies were available as grey literature (inception cohort, 26%; conference cohort, 8%). Fifty-three studies (18%) were unpublished (inception cohort, 4.5%; conference cohort, 44%). The pattern of publication varied between individual cohorts (Figure 11). The highest academic journal publication rate was observed in the HSRProj cohort (75%) and the lowest was in the ISQua cohort (26%). All studies from the NIHR cohort were published either in academic journals or as grey literature (technical reports available through the NIHR’s website), whereas results for 68% of the ISQua were not published.
FIGURE 11.
Publication status of selected studies at follow-up.

Factors associated with publication in academic journals
We explored factors associated with publication in academic journals for all studies and separately for intervention studies, using logistic regression. The results are shown in Table 11. For the analysis including all studies, being a study from the ISQua cohort was the only statistically significant factor associated with a reduced odds of publishing in an academic journal in univariable analysis. Neither statistical significance nor positivity of study finding was associated with publication in academic journals.
Factor | Univariable analysis | Multivariable analysis | ||
---|---|---|---|---|
OR (95% CI) | p-value | OR (95% CI)a | p-value | |
All studies | ||||
Study cohort | ||||
HSRProj | 1 (reference) | 1 (reference) | ||
NIHR | 0.593 (0.322 to 1.090) | 0.093 | 0.293 (0.138 to 0.619) | 0.001 |
HSRUK | 0.778 (0.365 to 1.656) | 0.514 | 0.569 (0.230 to 1.411) | 0.224 |
ISQua | 0.117 (0.054 to 0.255) | < 0.001 | 0.052 (0.021 to 0.131) | < 0.001 |
Intervention study (vs. association study) | 1.169 (0.731 to 1.869) | 0.514 | 1.248 (0.688 to 2.266) | 0.466 |
With bespoke data collection (vs. database and routine surveys) | 1.300 (0.790 to 2.137) | 0.302 | 2.137 (1.169 to 3.907) | 0.014 |
Statistically significant (vs. non-significant) | 0.999 (0.496 to 2.009) | 0.997 | 2.206 (0.979 to 4.971) | 0.056 |
Positive finding (vs. non-positive) | 1.586 (0.862 to 2.920) | 0.138 | 3.151 (1.542 to 6.435) | 0.002 |
Intervention studies | ||||
Study cohort | ||||
HSRProj | 1 (reference) | 1 (reference) | ||
NIHR | 0.413 (0.153 to 1.114) | 0.081 | 0.211 (0.060 to 0.750) | 0.016 |
HSRUK | 0.568 (0.198 to 1.633) | 0.294 | 0.437 (0.106 to 1.807) | 0.253 |
ISQua | 0.072 (0.023 to 0.221) | < 0.001 | 0.036 (0.008 to 0.158) | < 0.001 |
With bespoke data collection (vs. database and routine surveys) | 1.718 (0.761 to 3.877) | 0.193 | 3.041 (1.016 to 9.100) | 0.047 |
Statistically significant (vs. non-significant) | 0.926 (0.381 to 2.254) | 0.866 | 2.977 (0.927 to 9.558) | 0.067 |
Positive finding (vs. non-positive) | 1.242 (0.566 to 2.723) | 0.589 | 3.413 (1.198 to 9.730) | 0.022 |
With concurrent control (vs. before and after) | 4.718 (2.224 to 10.009) | < 0.001 | 2.453 (0.855 to 7.037) | 0.095 |
RCT (vs. non-RCT) | 3.200 (1.426 to 7.180) | 0.005 | Not includedb |
In multivariable analysis for all studies, studies from both the NIHR cohort and the ISQua cohort were significantly less likely to be published in academic journals (compared with studies from HSRProj cohort). In addition, studies with bespoke data collection were more likely to be published in an academic journal than those utilising data from routine databases or surveys. Statistical significance and positivity of finding also appeared to be associated with increased odds of publication in academic journals, having adjusted for the other factors.
Findings from analyses focusing on intervention studies identified similar factors associated with publication in academic journals, with having a concurrent control group being an additional strong predictor in univariable analysis and approaching statistical significance in multivariable analysis.
Factors associated with non-publication
In this subsection we examine factors associated with non-publication (i.e. when study findings were neither published in academic journals nor available as grey literature). Given that non-publication did not occur in the NIHR cohort, these studies were excluded from the analyses. Findings from univariable and multivariable logistic regression for all studies and for intervention studies are presented in Table 12.
Factor | Univariable analysis | Multivariable analysis | ||
---|---|---|---|---|
OR (95% CI) | p-value | OR (95% CI)a | p-value | |
All studies (N = 200) | ||||
Study cohort | ||||
HSRProj | 1 (reference) | 1 (reference) | ||
HSRUK | 2.528 (0.954 to 6.697) | 0.062 | 17.348 (1.890 to 159.216) | 0.012 |
ISQua | 21.486 (8.677 to 53.203) | < 0.001 | 425.985 (39.500 to 4593.998) | < 0.001 |
Intervention study (vs. association study) | 0.888 (0.473 to 1.667) | 0.711 | 1.356 (0.481 to 3.823) | 0.565 |
With bespoke data collection (vs. database and routine surveys) | 1.233 (0.618 to 2.461) | 0.552 | 0.322 (0.091 to 1.136) | 0.078 |
Statistically significant (vs. non-significant) | 1.492 (0.531 to 4.190) | 0.447 | 0.177 (0.034 to 0.924)a | 0.040 |
Positive finding (vs. non-positive) | 1.421 (0.506 to 3.994) | 0.505 | 0.213 (0.049 to 0.931)a | 0.040 |
Intervention studies | ||||
Study cohort | ||||
HSRProj | 1 (reference) | 1 (reference) | ||
HSRUK | 3.786 (0.969 to 14.785) | 0.055 | 6.725 (0.543 to 83.336) | 0.138 |
ISQua | 29.813 (8.012 to 110.927) | < 0.001 | 93.278 (6.918 to 1257.715) | 0.001 |
With bespoke data collection (vs. database and routine surveys) | 0.829 (0.266 to 2.586) | 0.747 | 0.314 (0.043 to 2.292) | 0.254 |
Statistically significant (vs. non-significant) | 2.069 (0.551 to 7.774) | 0.282 | 0.242 (0.030 to 1.968) | 0.185 |
Positive finding (vs. non-positive) | 2.145 (0.576 to 7.985) | 0.255 | 0.272 (0.036 to 2.046) | 0.206 |
With concurrent control (vs. before and after) | 0.087 (0.029 to 0.260) | < 0.001 | 0.147 (0.027 to 0.802) | 0.027 |
RCT (vs. non-RCT) | 0.175 (0.056 to 0.549) | 0.003 | Not includedb |
For the analyses across all (intervention and association) studies, the ISQua cohort was the only statistically significant predictor for non-publication in the univariable analysis. However, in the multivariable analysis, studies from both HSRUK and ISQua cohorts were significantly more likely than studies from the HSRProj cohort to remain unpublished, and statistical significance (or positivity of findings if the variable was entered in the model in place of statistical significance) also became a significant predictor of reduced risk of non-publication.
The influence of being from a conference cohort and bespoke data collection remains similar in the univariable and multivariable analyses focusing on intervention studies (although the analyses had less statistical power). However, having a concurrent control group or being a RCT appeared to be an additional independent factor associated with reduced odds of non-publication.
Chapter 7 Key informant interviews and focus group discussion to explore publication bias in HSDR
This chapter presents findings from WP 5. As outlined in Chapter 2, the results are intended to develop the themes from earlier WPs and to explore views of those involved in all aspects of HSDR. Findings are presented under broad themes, including the commissioning and funding of HSDR; journal and publisher decision-making; the influence of research design and researcher conduct in HSDR; distinctive features of HSDR; and the impact of publication bias. The final section explores these themes from the perspective of patients and members of the public.
Is publication bias a problem in HSDR?
The first issue we explored with interviewees was to what extent they believe that publication bias was a feature of HSDR. A striking finding of the study is that many felt unable to respond with any certainty. This included researchers, for whom typical answers included ‘I don’t know the answer to the question’ and ‘I can’t tell really, to be honest’. Of the 16 interviewees who expressed a view, 11 believed that publication bias was prevalent and five that it was not. However, within each of these groups there was varying levels of confidence in this assessment. For example, although some argued it to be ‘rampant’, others were circumspect (‘I don’t think that it should be, but I think that it creeps in’, ‘I think it is important, but I don’t think it’s the central issue’). Those arguing against the prevalence of publication bias were also hesitant in their judgement (‘I don’t think publication bias is an issue, to be honest with you’). These findings appear to mirror the complexity emerging from previous work and further exploration helped to unpick the reasons behind this. Subsequent questioning focused on aspects or stages of the publication process of most relevance to the interviewee and focus group respondents.
Commissioning and funding of HSDR
Interviewees generally agreed that the circumstances in which HSDR is commissioned and funded influence the likelihood of publication bias. For example, many believed that highly formalised programmes of research funding were less susceptible. Some cited the NIHR HSDR programme as an example of commissioners adopting criteria-based decision-making by autonomous panels, based on external peer review:
With the NIHR or research councils, you know, you put in your research proposal and they let you get on with the research.
Interviewee 12, senior researcher/clinician, UK
A key reason why such funders were seen as associated with low publication bias was the presence of formal publication requirements (including, for example, mandatory full reporting and making data publicly available), as well as conflict policies and adherence to study protocols. This in turn was associated with substantial research projects led by teams with a strong academic track record and motivation to produce published academic outputs. Commissioners in these circumstances were described as being largely ‘blind’ to considerations other than the rigour of research outputs.
This approach to the commissioning and funding of research was contrasted with end user sponsored research, characterised by high levels of interest and involvement of the commissioners in the conduct and reporting of results. One respondent, for example, felt that government bodies directly commissioning evaluations of their own programmes were often ‘desperate for those to be shown to be positive’ and another spoke of charitable foundations requiring striking findings in order to ‘feed their communications machine’:
A lot of the research will be funded by apparently objective, non-commercial funding agencies, but there will still be the management consultancies and charities, there will still be government involvement. So I don’t think we can be too complacent.
Interviewee 2, editor, UK
Many of the interviewees who worked as researchers in such circumstances nevertheless asserted the relative autonomy afforded to them, indicating that any subsequent bias was unlikely to be direct or overly coercive:
What I worry about is this subtle, indirect pulling of punches; so softening the edges of the messaging. Because you get comments coming back from policy officials saying things like ‘you’ve given us a kind of glass half empty story. Can’t you turn it round?’. And of course, often it is a matter of language and emphasis.
Interviewee 11, editor/senior researcher, UK
Interviewees indicated that pressure from funders was of a lesser magnitude than that generated in much clinical research, from their perceptions or experience, and that there were less likely to be major conflicts of interests:
There’s a danger of framing it in the same way as in medical research because the stakes are very high in medical research and there’s big commercial involvement through the pharmaceutical companies, who’ve been repeatedly caught ‘off side’, as it were. Whereas I think the same issues don’t apply, at least universally, in health service research.
Interviewee 16, funder/senior researcher, UK
I think because so much of the funding we have is competitive and most of it is won through committees that actually make it very difficult, I would imagine, for the result of the previous study to affect the funding. Whereas traditionally, you do a trial and the company wants to give you more money to do more research in the area. I’ve never been in that situation, [laughing] sadly.
Interviewee 11, editor/senior researcher UK
Despite this, the frequent absence of a formal requirement for peer-reviewed publications from such government bodies was identified as a potential source of bias:
It effectively leaves the onus on getting peer-reviewed publications up to us which is exactly when publication bias is more likely.
Interviewee 14, senior researcher/funder, UK
Interviewees noted that locally commissioned evaluations and audits are rarely intended to be reported beyond the immediate organisational context, and that therefore it is inappropriate for them to be subjected to the rigours of formal peer review before publication. Publication bias was therefore seen as a result of publishing of such data beyond the intended scope and setting of the data collection exercise.
Many interviewees made a distinction between research and other forms of knowledge:
I think the boundary between research and service improvement is really blurred and if you’re just using someone’s routinely collect data, not purposely collected for a research study, but just kind of scraped off their systems, and you’re using it for a model which will help them improve their systems, then I don’t call that research personally.
Interviewee 4, editor/senior researcher, UK
I suppose then it becomes a question of what counts as publication bias and where the borderline is with something that actually really isn’t research, or shouldn’t be judged as such, shouldn’t be kind of assessed as such. I think it entirely depends on how the project’s been set up . . . many small-scale quality improvement projects are not generalisable analytically or statistically and therefore I’m not terribly sure why you’d try to publish them other than using them in a local setting.
Interviewee 9, junior mid-career researcher, UK
One interviewee considered that ‘editorial control’ was the primary means of differentiating research and consultancy:
At [name of organisation] we did both consultancy and research, and the distinction was research meant that there was an explicit agreement up front, that we the researchers would be able to have editorial control so that we could seek publication in a journal. In other words, that the funder could not stop us publishing.
Interviewee 15, consultant/senior researcher, UK
Publication bias in journal decision-making
Within the sample, researchers were most likely to argue that publication bias was pervasive in academic publishing and especially in editorial decisions over whether to accept or reject HSDR journal submissions:
I think [positive effects] are of interest to all the journals and some are more explicit about it than others . . . All of the journals, despite their veneer of academic impartiality, are highly news oriented and they are kind of pushing for space in that crowded market place.
Interviewee 16, funder/senior researcher, UK
You would never ever send something non-significant to a top journal, that’s my feeling.
Interviewee 3, junior mid-career researcher, Germany
You do quite often find editors will say, ‘This is too similar to another paper that’s already published’.
Interviewee 4, editor/senior researcher, UK
The responses of journal editors within the sample were more mixed. For example, some argued that authors were primarily responsible for any publication bias at the analysis and write-up phase of the research process (see Research design and researcher conduct in HSDR). However, most conceded that factors other than scientific quality influenced publication decisions, and many of these factors coalesced around the requirement for novelty of some form. One editor of a medical journal used the term ‘saturation bias’ to describe the diminishing likelihood of publication of papers once ‘the message is already out’, irrespective of scientific merit. Another identified study scale and certainty of results as being important:
If you have a very clear-cut answer on either side, positive or negative, obviously any journal will be very interested. We’re not shying away from a negative study by any means, if it’s definitively negative. I think it’s when it’s non-definitive because the study is too small, or inadequately powered. I think we’re a little bit more hesitant with those.
Interviewee 13, editor/mid-senior researcher, USA
Editors of medical journals acknowledged that HSDR submissions, in general, were less likely to be accepted, especially when they did not follow a trial-based design. Editors of journals in subdisciplines of HSDR described a range of characteristics predisposing journal editors to accept HSDR manuscripts for publication. Along with methodological rigour and study scale, these included extent of methodological and theoretical innovation, and the political salience of the research topic or intervention:
I think [strength of effects] wouldn’t affect publication in an OR [operational research] journal, but then usually they would be looking for you to show that your method was better than somebody else’s method. And if in a particular case you had shown that: you know, you’d applied your method to some problem and it hadn’t really made any difference, I think that would be harder to publish.
Interviewee 4, editor/senior researcher, UK
I do think that there is a very strong relationship between, if you like, the size of the funded project and its chances of being published, irrespective of its findings.
Interviewee 5, mid-senior researcher/editor, UK
In our kind of publishing, if you don’t see any change, for example, that’s not a very interesting study theoretically. Nobody is really very keen to publish articles that explain why things stay the same and so, to that extent, there is some bias in that we think we know why things stay the same.
Interviewee 2, editor/senior researcher, Canada
These views and experiences were echoed in interviews with researchers:
There are some systematic biases there and I think people, especially editors and peer reviewers, are sort of denying them in a way. They’re looking for the best quality research and they’ve got this idea of what best quality research looks like. And they do privilege the very novel, you know, highly rigorous, high impact studies over those that are sort of more mundane but still important.
Interviewee 19, senior researcher/editor, UK
I think it’s absolutely right that poor or null effects are less interesting, but they’re only less interesting if it’s a topic where people really want it to work; if it’s a topic where people don’t want it to work, then our hypothesis is great and, you know, the [name of journal] will publish it. So I don’t think it’s a simple as just positive results.
Interviewee 9, junior mid-career researcher, UK
These factors were seen as diluting the influence of the magnitude and statistical significance of quantitative findings. By comparison, the latter were generally attributed higher importance in medical journal decision-making. This suggests that although HSDR journals may be less likely to exacerbate publication bias through their editorial practices, they may be substituting this with other forms of bias associated with preference for novelty.
Responses were less consistent with regard to the influence of the editor and peer review roles in publication bias. Although some editors referred to peer reviewers as ‘human funnel plots’, other interviewees believed that publication bias was rarely a major consideration in the peer review process:
Certainly as a reviewer and as an editor I don’t see many questions or prompts asking about the presence of publication bias. I don’t see many reviewers’ comments about publication bias unless they’re aware of particular studies that have been missed.
Interviewee 5, mid-senior researcher/editor, UK
However, the proliferation of peer-reviewed journals was seen as mitigating the threat of publication bias, for example in HSDR systematic reviews. This meant that the status of the journal was a secondary consideration:
I don’t think it matters a toss where it’s published as long as it’s published.
Interviewee 16, funder/senior researcher, UK
Obviously, there’s a certain level of prestige that comes through getting it in a high impact factor journal but, nowadays, everything is findable in PubMed so as long as it’s there, I’m much less bothered about where it goes.
Interviewee 7, senior researcher, UK
Research design and researcher conduct in HSDR
Interviewee perspectives were again mixed in relation to the role played by researchers in publication bias. Although some argued that in trial-based research, behaviours, such as selective reporting and ‘data hacking’, were no longer possible, others believed this to be commonplace, especially in association studies:
The more subtle and more common type [of publication bias] is the issue around hypothesis testing when you’re the statistician doing the analysis and when you do a lot of what you would, in your mind, neatly label ‘preliminary analysis’; a lot of unofficial hypothesis testing – ‘see if this works’ – and subgroup analysis . . . That is a massive problem.
Interviewee 8, mid-senior researcher, UK
Others believed there to be a temptation to search for positive results when researchers were in some way connected to the intervention being evaluated:
It’s very hard to find adverse effects of service delivery and part of that is either that the people who are introducing those models are sort of strongly advocating them, you know, it’s sort of like airing your dirty washing. And I suspect the other thing is also it’s very hard to identify unintended consequences because they have a lens that focuses on the evaluation framework and don’t look more widely at knock-on effects. So that would be a variation of selective outcome reporting bias but one particularly unique to Health Service and Delivery Research.
Interviewee 5, mid-career researcher/editor, UK
It depends if you think bias is the absence of the results or it’s the change in what people report. So people may have been trying, for example, reduce [hospital infection] rates and if in fact, they stay the same, but they manage to cut readmissions for whatever reason, they may then report, ‘Oh, this is an effective intervention to cut readmissions rates’, although it wasn’t actually designed that way in the first place.
Interviewee 7, senior researcher, UK
For example, when I evaluated [name of government initiative]. I didn’t care whether it worked or not but if I have been part of developing a particular service, so for example I’m developing a medical adherence intervention at the moment, there is going to be part of me invested in wanting that to be positive.
Interviewee 6, senior researcher/editor, UK
There was general support for the view that research teams might take longer to submit negative or null results for publication. This was seen to be related to general levels of ‘excitement’ associated with positive findings:
I’ve been involved in a couple of large implementation research trials and neither of them came out with positive or significant outcome findings . . . To motivate yourself to get that negative finding trial out there, it’s a real challenge. I think that there is something about not wanting to share something that you feel failed in some way, which is ridiculous.
Interviewee 18, funder/senior researcher, UK
The psychology is, you know, ‘we looked for something, we didn’t find it, that’s a bit disappointing. Can we be bothered to write it up? It may not get published anyway. Let’s go ahead and look at something else’, kind of thing. I think that’s understandable and human nature.
Interviewee 2, editor, UK
Some respondents identified a risk of publication bias when evaluators are responsible for developing the intervention, and many felt that publication bias was a danger within much service improvement research:
There’s strong movement within quality improvement, which is a very enthusiastic kind of movement, one that celebrates success in lots of ways. And some relatively large claims are made for some work that’s often done with relatively simple ways of looking at data . . . Now that is all maturing rapidly but my instincts are that because of that strong enthusiasm and the desire for things to be seen to work, there’s a risk.
Interviewee 17, funder/junior mid-researcher, UK
This problem was seen by the two managers in our sample to be compounded by a health-care environment that rewarded ‘good news’ stories about health-care innovations and improvements:
It’s a fear of failure and therefore you cannot be seen to try something that doesn’t work because that’s not celebrated.
Interviewee 20, manager, UK
Table 13 summarises the factors seen to increase or decrease the risk of publication bias in HSDR.
Stage | Reduced risk | Raised risk |
---|---|---|
Commissioning of HSDR |
Criteria-based decisions Autonomous panels External peer review Publication requirements Conflicts policies Study protocols |
Non-competitive allocation of funding Iterative research design Ongoing interaction with research teams Direct interest in outcomes No publication requirements |
Conduct of HSDR |
Trial-based design High number of incentives to publish High level of research expertise No involvement/interest in intervention development |
Association studies Low number of incentives to publish Low level of research expertise Involvement/interest in intervention development |
Publishing HSDR |
HSDR submissions to HSDR journals Low levels of institutional pressure Other novel features of publications |
HSDR submissions to medical journals High levels of institutional pressure No other novel features of publications |
It was clear from the interviews with researchers that, to the extent that they considered researchers guilty of publication bias, this was partly driven by the perceived expectations of journals and universities. Some argued that ‘job prospects’ are linked to high-impact journal publications. For example, junior researchers within our sample were more likely to believe publication bias to be widespread:
Even though we are all academics and we know about publication bias when it comes to our own work we’re thinking, ‘Well, which journal will be interested in these findings?’. If they’re positive ones it’s easier to get kind of excited about it, as it were, when thinking about likelihood of acceptance by a bigger journal. You know, if you’re evaluating some new policy and you find it doesn’t work then clearly that is vital but I think the threshold is higher for a negative finding.
Interviewee 8, mid-senior researcher, UK
A small number of respondents identified incentives to researcher-instigated publication that went beyond the individual’s interests:
Sometimes important parts of HSDR studies don’t get published because the organisations, universities, don’t see the value of that.
Interviewee 6, senior researcher/editor, UK
In the USA the amount of funding that’s available for health systems improvement work is quite limited so there’s definitely a competition aspect that plays in, which leads to issues with individual investigators; obviously they want to show that the work that they’re doing is worthwhile which increases the pressure to publish positive results.
Interviewee 23, editor/senior researcher, USA
For reasons outlined in the following section (see Distinctive features of HSDR), senior researchers were more likely to underplay the pressure to publish positive results:
Almost all of my studies show that whatever I’m looking at doesn’t work . . . The answer to your question is that, in my own personal research, it would not cross my mind not to publish something.
Interviewee 12, senior researcher/clinician, UK
Distinctive features of HSDR
As noted, a significant minority of researchers in the sample felt that, in broad terms, HSDR was less susceptible to publication bias than clinical research. They attributed this to characteristics they considered distinctive and typical of HSDR. These included the complex nature of interventions, which generated uncertainty in evaluation, and the context-specific determinants of outcomes, which meant that definitive conclusions were often difficult to draw:
I wonder whether epistemologically they are doing different things; essentially within the [Health Technology Assessment] community it’s much more of an aggregative process coming up with, you know, pooled overall effects, etc. But if you assume that there will be a great deal of heterogeneity in health service delivery research then perhaps epistemologically you’re trying to do much more about making sense of options and mapping different models. And linked to that is the interplay of context so that within an HTA [Health Technology Assessment] context there’s almost the assumption that a drug will work in a relatively similar way across multiple contexts, whereas a service delivery intervention is very context-specific. So maybe people are bringing different quality markers or a different sort of epistemological view.
Interviewee 5, mid-career researcher/editor, UK
They further argued that this complexity and contingency often prevented study randomisation and replication:
The sort of research I do, it is rarely possible to randomise the intervention, so if you’re evaluating the [name of national government initiative] for example, you can’t persuade NHS England to randomise it to practices. So much of the research designs have been observational.
Interviewee 12, senior researcher/clinician, UK
Allied to this was the ‘multifactorial’ and ‘multicomponent’ nature of much HSDR study design, and the simultaneous addressing of multiple research questions, so that strength and direction of findings was assigned less overall importance:
I think in a lot of clinical research the effectiveness question is the only question that people are really interested in. They still pretend to be interested in mechanisms of action, you know, why something didn’t work. There’s still a pretence that we’re interested in the cost-effectiveness but at the end of the day that effectiveness question is the absolute king. Whereas in health services research that’s less of an issue, I think. Usually, we do have an effectiveness question that we can rarely answer with a randomised control trial; we have to address with something lower down the hierarchy of evidence. And because those designs have less status than the RCT, there’s more of a balance in the status of the questions and the methods that are being used within health services research . . . You know, in meetings everybody’s talking about all the different components and how they’re going. You don’t have to fight to get any component onto the agenda.
Interviewee 6, senior researcher/editor, UK
However, some interviewees expressed frustration at the relative inattention paid to outcomes and the primacy given to implementation in HSDR publications, highlighting the challenges this posed for subsequent evidence synthesis:
We’ve done work on user engagement in major health service reforms and it’s kind of, ‘Well, what’s the outcome? Is it whether you actually got the change implemented or whether you did the right consultation?’. The fact that the change was or wasn’t implemented may not be the key outcome but that’s often the one that’s reported, with the description about how you got there. So you may not have information about what might be considered the key outcome.
Interviewee 10, senior researcher, UK
The equivocal nature of many HSDR results was cited as an additional reason for reduced levels of external scrutiny and verification from, for example, media, government and industry. This was seen as a further check on the pressure to find positive associations and effects.
Tackling publication bias
The range of views on the prevalence of the publication bias ‘problem’ was reflected in interviewee comments on potential ‘solutions’. All of those who considered publication bias to be widespread (and many of those who did not) expressed support for pre-registration of studies and the strict application of study protocols containing statements of pre-set outcome measures:
It’s very easy to do and I think it’s also a mark of sound intellectual practice to actually say what you’re going to study and why you’re going to study it, so we don’t end up seeing fishing expeditions.
Interviewee 1, editor/clinician, UK
A subgroup of interviewees believed this would result in unwarranted and inappropriate constraints on researcher conduct (‘feet of clay’). As well as the practical challenges in large, multistrand studies, interviewees argued that sensitivity to changes in context during the lifetime of a research programme would be compromised:
You’re not dealing with nature, you’re dealing with people. So you want to be able to take advantage of what you learn, not just think, ‘Alright, we’ll stick with the state of knowledge we had when we wrote the proposal’. But you need to be quite transparent about why you’ve changed what you’ve done.
Interviewee 15, consultant/senior researcher, UK
If you take a recent study which we’ve done in which we probably had about eight substudies. All using different methodologies. Are you going to register each of them? And difficult to register the programme as the whole, not least because the nature of the grant is that things change as your 5 years goes on. So I remain to be persuaded of the benefits of pre registration for HSR [health service research] trials principally because pre registration for drug trials is to get at the very clear problem which you’ve articulated, which I don’t think exists in quite the same way for us.
Interviewee 12, senior researcher/clinician, UK
Other strategies identified for tackling publication bias included mandatory publishing and development of repositories of null findings; training and awareness raising in publication bias; a general improvement in the rigour of HSDR studies (especially in the field of service improvement); strengthening of research teams (e.g. through partnering with senior academics); and greater incorporation of publication bias checks in journal peer review.
Impact of publication bias in HSDR
As noted, some interviewees believed publication bias to be common in HSDR and cited the potential distorting effects of this on syntheses of the evidence, for example through systematic review. However, others identified the proliferation of journals publishing HSDR as partly counteraction to this trend.
Interviews (including with service managers at senior and local levels) revealed some apparent contradictions in relation to the prevalence and impact of publication bias. On the one hand, there was a widespread view that decision-making was often carried out without due regard to the published evidence, such that publication bias was less of a concern. Interviewees were at pains to emphasise the weak relationship between evidence and the decision-making they observed:
You have to consider just how poor the access of NHS managers to research evidence is. That is my experience and when I came to realise there’s this whole world out there of people doing health services research it was a revelation. But it was something I had to discover for myself. There is a very low awareness of research work generally, I mean, in the management class. That’s a very broad statement, but that’s from my experience of having worked in it. The way you made decisions is very much based on what is in front of us and our conversations with colleagues, rather than through reference to literature.
Interviewee 24, manager/policy, UK
There might be a sad reason why publication bias would not be such a problem in health services research, because the decision-makers don’t pay attention to the evidence anyway.
Interviewee 22, senior researcher/clinician, Canada
On the other hand, respondents identified similar sources of bias generated from within the decision-making environment itself and perpetuated, for example, through professional periodicals and information sources:
It’s the same in my organisation. I can’t think of anyone who’s really stood up and said ‘We tried this and I’m gonna write it up and get it published because I don’t want anyone else going through this pain or spending energy on it’. We just don’t seem to have a culture of doing that . . . I think when you’re getting a decision from your governing body or your board to try something there is an expectation from them that it will work. My director says, ‘Oh, we’ve been asked for some good news stories’ and in my head I want to say ‘well the good news could be that we tried this and it didn’t work so we can’t go through it again’.
Interviewee 20, manager, UK
If you take something like [name of professional journal], most of the papers are subtitled ‘How my last two year project has transformed health care’ you know? They’re less frequently constructed as ‘How my last two year project proved that this didn’t work.
Interviewee 24, manager/policy, UK
If we run something like the [national health services initiative] and we’ve committed many billions of pounds to what is notionally a pilot project for other people to copy, it’s quite hard for the taxpayer to understand that at the end of spending many millions of pounds on testing something, we came to the conclusion we shouldn’t do it anymore because it’s a waste of money. Now, there is an acceptance in the drug industry that you have to kiss a lot of frogs before you find a prince and that’s not really the culture in management. The culture in management is everything needs to work every time.
Interviewee 24, manager/policy, UK
One researcher in the interview sample identified a further knock-on effect of this on the research community when deciding which interventions to evaluate:
There’s great reason to believe that the picture of what’s going on, for example, in the NHS is heavily influenced by reporting bias, in other words, people do a successful initiative and they inter-disseminate it. That doesn’t translate into quantitative bias but upstream if you think that that means that these interventions are then more likely to get funding to be explored, then that will lead to a sort of distortional bias in terms of interventions that are available.
Interviewee 5, mid-senior researcher/editor, UK
Patient and public views: findings from the focus group
The focus group was structured so as to enable discussion in three phases, starting with general discussion of evidence, followed by discussion of publication bias in clinical research and, finally, discussion of publication bias in HSDR. Examples were used to illustrate publication bias in the second and third phases. The over-riding aim was to gather perspectives on the importance of this topic from a patient and public perspective.
Participants described receiving an ‘avalanche’ of information, but said that they would search for more detailed information on the stories that piqued their interest (i.e. if it affected them, a family member or a friend). There was acknowledgement that news stories as presented might not be an accurate reflection of the research evidence and the need for critical reflection. Participants described the need to be ‘critical’ of sources and to consult and listen to varied perspectives. Participants also discussed different sources of information that they would consult, including social media (e.g. Twitter; Twitter, Inc., San Francisco, CA, USA), books (written by authors they trusted), to lectures and articles in peer-reviewed journals.
Key points of consensus included:
-
There is a need to identify ‘trustworthy’ sources that accurately summarise evidence.
-
Some people accept what is reported in the mainstream media unquestioningly, with associated risks and harms.
-
When reporting research findings the mainstream media typically provide only limited information on how the study is funded and conducted, making it impossible to judge extent of potential bias.
-
Some level of bias is unavoidable (e.g. ‘bias starts when you decide what you’re going to study’), so transparency of reporting is of the utmost importance.
The recent clinical example of reboxetine6 (Edronax®, Pfizer Inc., New York, NY, USA) (for anxiety and depression) was discussed. Participants used words, such as ‘worrying’, in relation to the example and raised ethical objections. For example, participants noted that patients participating in studies might not be aware that drug companies were not publishing all of the evidence. Participants also discussed the dangers of drug companies funding trials and the importance of patient involvement in setting research aims and outcome measures.
It’s worrying, yeah.
And it is worrying that there’s . . . because there’s always this issue of ethics, isn’t there, in medical studies and although people sign up and say, ‘This is an ethical study,’ where there is a benefit to, like, a drug company then I’m not sure you could ever think, ‘Well, they’d be unbiased,’ because I’d always think that, ‘Have they actually published all their findings from this study?’. So although you would hope such people, you would hope researchers would be ethical. If they’re being funded and their research is being funded then how difficult is it for them to be completely neutral?
A second example from HSDR, related to the use of mass mail-outs, was discussed (see Maglione et al. ,53 also described in Chapter 3, see Table 3). This prompted discussion of the need for public resources to be used as efficiently as possible, reducing waste and the threat posed to this by publication bias of this kind.
Does it matter if, for example, the NHS invests in things where the evidence is slightly biased . . . is that a problem?
It’s a waste of money.
Yeah.
It is if your resources are short, yes.
Across the two examples, participants made the following observations:
-
Much extra attention is given to new interventions (‘the latest thing’) that promise to solve major problems and the tendency, therefore, is to be biased towards positive findings for new interventions.
-
There are dangers in measuring and reporting only short-term outcomes and neglecting longer-term effects, whether positive or otherwise.
-
Participants were aware that researchers might be under pressure to publish interesting results and might be deemed a ‘failure’ if they fail to do so.
This led to a series of recommendations put forward by participants:
-
Critical appraisal – participants emphasised the importance of educating people at a young age to be critical of information they receive, especially in popular and social media.
-
Clear reporting of information about who funds should be compulsory, with any conflicts declared.
-
Addressing the pressures within universities to ‘get results and publish’ was seen as a priority. People should be allowed to ‘make mistakes’ (i.e. embark on studies resulting in null results) because these present ‘opportunities for learning’.
-
Participants argued that other aspects of studies (e.g. methods) might be of interest and warrant publication where results are unremarkable.
Participants also agreed that research should be able to be replicated and, therefore, all sources of data should be made fully available for future researchers:
Is the issue not because of the company that we don’t have access to the data?
Yes, yeah.
And all this would be solved if it was a legal requirement that every time you did an experiment, the data was held in some sort of archive accessible by anybody.
Overall, participants argued that having strong PPI throughout a study is critical. This needs to be carefully planned and inclusive of all groups, including those typically under-represented. They argued that PPI advisors should have roles in designing studies and ‘challenging’ the experts so that bad practices associated with publication were less likely to happen.
This chapter summarises diverse views on publication and related bias obtained from our interviews with a wide range of stakeholders of HSDR, including researchers of varied seniorities and specialties, journal editors, research funders and service managers. In addition, we present findings from a focus group discussion, which was planned with PPI involvement and highlighted service users’s and the public’s concerns about the potential impact of publication and related bias in terms of research waste and the influence of evidence on health service decision-making. We discuss the implications of these findings in relevant sections in Chapter 8.
Chapter 8 Discussion and conclusion
In this chapter we discuss findings from individual WPs presented in Chapters 3–7. These are followed by an overview of learnings across WPs and limitations of the project, and a brief discourse of wider issues surrounding publication and related bias, and use of evidence to inform health-care service delivery. We then provide some points for consideration for stakeholders who fund, generate, review, publish and use HSDR in relation to publication and related bias. The chapter ends with some recommendations for future research and an overall conclusion.
Work package 1: systematic review of empirical evidence on publication bias in HSDR
Our systematic review set out to examine available evidence on publication and related bias in HSDR. Despite an extensive literature search, we found only four methodological studies13–15,50 that specifically investigated this issue, with three14,15,50 of them focusing on health informatics. Although the evidence is very limited and there are methodological weaknesses in the identified studies, the available evidence does indicate the existence of publication bias: study findings were not always published; differences may exist between published and unpublished evidence; and examples, such as null or unfavourable findings being associated with non-publication,53 and magnitude of effect sizes being associated with publication in high-impact journals, have been reported. 13
Reasons for non-publication of HSDR findings described in the only survey we found concerning the publication of evaluation studies in health informatics appear to be similar to those for clinical research. 87 Lack of time and interest on the part of the researcher appears to be a major factor, which could be exacerbated when the study findings are perceived as uninteresting. Some of the reported reasons, such as ‘not of interest for others’ and ‘only meant for internal use’, highlighted the context-sensitive nature of evidence that is common for HSDR. These reasons for non-publication also highlight issues arising from the vague boundary between research and non-research (such as quality improvement projects and service evaluations) for many interventions and data collection activities undertaken in health-care organisations. Another reason for non-publication of particular relevance to HSDR is ‘political and legal reasons’. Publication bias arising from conflict of interest is well documented in clinical research,4 and similar situations may arise when funding for the evaluation is provided by commercial, charitable or health-care organisations which developed and/or implemented the service intervention. We did not identify methodological research specifically related to the impact of conflict of interest on publication of findings in HSDR, although anecdotal evidence of financial arrangements influencing editorial process exists88 and there are debates concerning the public’s access to information related to health services and policy. 89
Among systematic reviews of substantive HSDR topics that have reported findings of assessment of publication bias, most have used funnel plots and regression tests and approximately half of them found some evidence of bias. The required conditions for utilising these methods and caveats for interpretation of their findings,26,38 as illustrated and discussed in detail in case studies presented in Chapter 5, hold true here and warrant particular attention in view of heterogeneity commonly found among HSDR, resulting from the complexity and variability of service delivery interventions and the influence of contextual factors. 90,91
Searching grey literature and contacting researchers and funders who may be involved in research on the topic of interest remain important measures to reduce the risk of publication and related bias when undertaking evidence synthesis for HSDR, although these are often time and resource intensive, and to what extent the invested effort outweighs the negative impact of the bias requires careful evaluation. The findings reported by Batt et al. ,51 that published and grey literature from low- and middle-income countries may differ in volume, quality and geographical coverage, have important implications for stakeholders who are involved in the synthesis and use of HSDR related to global health.
This systematic review has several strengths, but also has some weaknesses. We used a wide range of search terms (see Appendix 1) and searched several databases and information sources. Nevertheless, we could not rule out the possibility that some evidence was missed in the process. In addition, we may not have covered all pertinent areas of HSDR owing to the definition that we adopted. We focused only on publication and related bias in quantitative studies and did not consider qualitative research, which plays an important role in HSDR. Given the limited evidence we found, which was mainly collected in the field of health informatics, the generalisability of the findings for other fields of HSDR is uncertain. Finally, despite our efforts in extensive literature search, the possibility that our findings are subject to publication and related bias cannot be ruled out.
Work package 2: overview of systematic reviews of intervention and association studies in HSDR
Work package 2 examined a random sample of 200 systematic reviews in HSDR in relation to reviewers’ practice in the assessment of publication bias and outcome reporting bias. Although 43% of the systematic reviews mentioned publication bias, only 10% formally assessed it. Outcome reporting bias was mentioned and assessed in 17% of the reviews. The proportions of reviews in which these biases were mentioned and assessed were significantly higher among intervention reviews than association reviews.
There are similarities and differences between our findings and those of previous meta-epidemiological studies on assessment of publication and outcome reporting biases in systematic reviews, mostly concerning clinical research (Table 14). Based on existing literature, approximately one-third to a half of systematic reviews mentioned publication bias and thus demonstrated awareness of this issue, with the notable exception of systematic reviews of genetic association studies, of which 70% mentioned publication bias. Mention of outcome reporting bias was lower than 30% across the board, with very low rates observed in reviews of HSDR association studies and reviews of epidemiological risk factors.
Study and nature of systematic reviews examined | Searched grey literature/unpublished studiesa | Included meta-analysis | Mentioned publication bias | Formally assessed publication bias | Mentioned outcome reporting bias | Outcome reporting bias assessed |
---|---|---|---|---|---|---|
Current review | ||||||
HSDR intervention (n = 100) | 51% | 33% | 54% | 14% | 30% | 30% |
HSDR association (n = 100) | 52% | 10% | 31% | 5% | 4% | 4% |
Li et al. 2015:31 health policy research (n = 99) | 67% judged to be comprehensive | 39% | 32%b | 9% | NR | NR |
Ziai et al. 2017:92 high-impact clinical journals (n = 203) | 64% | NR | 61% | 33% | NR | NR |
Herrmann et al. 2017:93 clinical oncology (n = 182) | 27% conference abstract; 8% trial registries | NR | 40% | 28% | NR | NR |
Chapman et al. 2017:94 high-impact surgical journals (n = 81 pre PRISMA, n = 201 post PRISMA) | 71% pre PRISMA, 90% post PRISMA judged to be comprehensive | 65% pre PRISMA, 78% post PRISMA | NR | 39% pre PRISMA, 53% post PRISMA | NR | NR |
Page et al. 2016:95 biomedical literature (n = 300) | 16% conference abstract, 19% trial registry | 63% | 47% | 31% | NR | 24% (n = 296) |
Song et al. 20104 | ||||||
Treatment effectiveness (n = 100) | 58% | 60% | 32% | 21% | 18% | NR |
Diagnostic accuracy (n = 50) | 36% | 82% | 48% | 24% | 14% | NR |
Epidemiological risk factors (n = 100) | 35% | 68% | 42% | 31% | 3% | NR |
Genetic association (n = 50) | 10% | 96% | 70% | 54% | 16% | NR |
Kirkham et al. 2010:96 Cochrane reviews of RCTs with well-defined primary outcome (n = 283) | NR | NR | NR | NR | 7% | NR |
Although the findings suggest that there is still substantial scope for improvement in raising awareness of these potential biases and in explicitly acknowledging them in the conduct of HSDR systematic reviews, a few inter-related issues warrant further consideration when interpreting these findings and the making recommendations for further actions. These relate to research traditions and the nature of evidence, as well as the requirement for research registration, which we explicate below.
The research traditions and nature of evidence in different subject disciplines may influence the perceived importance and relevance for considering publication and outcome reporting biases in the review process. These might have contributed to the apparently low prevalence of assessing and documenting these biases in HSDR reviews. For example, both our study and the other HSDR-related study31 concerning health policy research mentioned earlier found that a meta-analysis was conducted in < 40% of all reviews, and in our study the prevalence was found to be as low as 10% for reviews of association. In contrast, at least 60% of reviews of clinical research included meta-analysis (see Table 14). There is a general recognition that HSDR requires consideration of multiple factors in a complex health system,19 and that evidence generated from HSDR tends to be context specific. It is therefore possible that HSDR systematic reviews, and particularly those examining associations between the myriads of structure, process, outcome measures and contextual factors, may tend to adopt a more configurative, descriptive approach (as opposed to the more aggregative, meta-analytical approach in reviews of various types of clinical research). 97 As generating an overall estimate of a ‘true effect’ is not the main focus, the issue of publication and outcome reporting biases may be perceived as unimportant or irrelevant in reviews adopting configurative approaches. This may partly explain the strong association between inclusion of meta-analysis and assessment of publication bias in our multivariable analysis.
The diverse, context-specific nature of evidence in HSDR may have further impeded formal assessment of publication bias. Funnel plots and related techniques, the methods most commonly used in HSDR, as in other fields, require that at least 10 studies that are sufficiently similar can be included in an analysis. 26 The requirement has probably prevented many HSDR reviews from carrying out formal statistical assessment of publication bias, as the level of heterogeneity among studies included in HSDR systematic reviews is often high. Irrespective of the feasibility of adopting statistical methods, these techniques provide only indirect evidence suggestive of the presence or absence of publication bias, and their limitations have been well documented. 26
Irrespective of research approaches and nature of evidence, a major barrier for evaluating both publication and outcome reporting biases in HSDR is the lack of prospective registration of study protocols. Comprehensive study registration and accessible study protocols are essential for identifying unpublished studies and unreported outcomes, which in turn is a prerequisite for directly assessing publication and outcome reporting biases. As mandatory registration of research protocols has been enforced among clinical studies on human subjects only, lack of study registry and protocols is likely to have contributed to the low prevalence of assessing these biases, particularly among reviews of observational studies (e.g. 4% among HSDR association reviews in our study and 7% among epidemiological risk factor reviews examined by Song et al. 4). However, irrespective of evidence synthesis approaches and challenges, the potential threats from these biases do not dissipate as long as the intention is to quantify an intervention effect or an association. Pre-registration of study protocols, which is the ultimate safeguard against publication and outcome reporting biases, may therefore be worth serious consideration for both HSDR intervention studies and at least some types of association studies. Accessibility to pre-registered study protocols will also alleviate current difficulties in assessing outcome reporting bias.
Calls for comprehensive registration of research studies and their protocols are not new, but making further progress beyond clinical trials is likely to require careful debates and assessments with regard to feasibility and practical value for registering different types of studies, weighing potential benefits against costs and potential harms. Meanwhile, it is important to continue raising awareness of these biases and improving the levels of documenting the awareness when evidence from quantitative HSDR is synthesised. Our findings show that systematic reviews mentioning the use of a systematic review guideline are five times more likely to include an assessment of publication bias than those without a mention. A previous study also found that the proportion of systematic reviews which assessed publication bias was significantly higher after the publication of PRISMA (53%) than before PRISMA (39%). 94 Methodological standards, such as Cochrane Collaboration’s Methodological Expectations of Cochrane Intervention Reviews, and systematic reviews reporting guidelines, such as PRISMA and Meta analysis of Observational Studies in Epidemiology,33 are therefore likely to play an important role. Nevertheless, the suboptimal level of documenting awareness found in our study and others highlights that additional mechanisms may be required to enforce them.
For the assessment of outcome reporting bias, we noted that all the reviews which assessed this did so as part of quality assessment of individual studies. Currently, outcome reporting bias is a standard item in the Cochrane’s risk-of-bias tool,64 which is most widely used in intervention reviews. However, this item is not included in tools commonly used for assessing observational studies, such as the Newcastle–Ottawa Scale. 98 Given that the risk of outcome reporting bias is substantially higher for observational studies, this is an important deficit which developers of quality assessment tools for observational studies need to address in the future.
Finally, the search and inclusion of grey and unpublished literature remains a potentially important strategy in minimising the potential effect of publication bias. However, the practice varies widely between different types of reviews. Interestingly, our study showed that reviewers who searched for grey literature do not always assess or discuss the potential effect of publication bias. This suggests that some review authors might have followed the good practice of searching the grey and unpublished literature to ensure comprehensiveness, without considering minimising publication bias as a rationale behind this. Alternatively, these authors may consider it unnecessary to assess and/or discuss the potential impact of publication bias in addition to the efforts in locating unpublished studies. Limited evidence suggests that data included in published HSDR studies differ in quality and nature from those included in grey literature. 51 More empirical evidence is needed to guide future practice regarding search of grey and unpublished literature, taking into account the trade-off between biases averted and additional resources required.
One of the strengths of the current study is that the systematic reviews were randomly selected from the HSE database, which covers multiple sources of literature, and our selection was neither limited by a single source of literature nor restricted to highly ranked journals. We therefore believe that the sample is representative of quantitative HSDR systematic reviews. In addition, study selection and data extraction were carried out by one person and checked by another in order to ensure accuracy and completeness. We also evaluated factors which may influence the assessment of publication and outcome reporting bias. The main limitation of this study is that the results of the multivariable logistic regression analyses produced ORs with fairly wide CIs. We are aware that variables which we examined may interact in various ways, but believe that collinearity is not a major issue, judging from the broad consistency between the results of univariable and multivariable analyses.
In conclusion, this study has shown that publication and outcome reporting biases are not consistently considered or assessed in HSDR systematic reviews. Until a comprehensive registration of HSDR studies and their protocols becomes available, formal assessment of publication bias and outcome reporting biases may not always be possible. In such cases, review authors could still consider and acknowledge the potential implications. Developers of quality assessment tools for observational studies should consider including items for outcome reporting bias. Adherence to existing systematic review guidelines may also improve the consistency in assessment of these biases. The findings of this study would enhance awareness of publication and outcome reporting biases in HSDR systematic reviews and inform future systematic review methodologies and reporting.
Work package 3: in-depth case studies on the applicability of methods for detecting and mitigating publication and related biases in HSDR
In case study 1, described in Chapter 5, we utilised data from a systematic review of studies on the weekend mortality effect to explore in detail the applicability of common methods that have been used in clinical research to detect and/or adjust for publication bias. We highlighted several issues pertinent to the use of these methods in evidence synthesis in HSDR, and the findings may be useful in similar scenarios in which HSDR mainly relies on evidence from analyses of observational data obtained from routine administrative databases.
The case study shows that, although funnel plot and related methods can be used in HSDR as a tool to explore the presence of small-study effects (which are a common manifestation of publication/reporting bias), several features of HSDR based on observational databases could contradict requirements for their appropriate use and, in particular, could invalidate the crucial assumption that any observed small-study effects are caused by publication or reporting bias. Special attention needs to be paid to the underlying heterogeneity of studies included in a review. Factors that could define distinctive subgroups and settings (e.g. types of admissions, availability of clinical data) may be potential confounding factors that are independently associated with effect sizes and sample sizes and may therefore confound the assumed publication bias. Any findings from these statistical techniques will thus remain highly speculative, unless these potential confounding factors can be ruled out, which is likely to be difficult.
Our detailed exploration in this case study suggests that some level of publication or reporting bias is possible in the literature included in the chosen systematic review, but its effect on the pooled estimate of the weekend effect is limited. A few points are worth mentioning. First, the authors of the review excluded grey literature, such as conference abstracts and institutional reports, because it was difficult to assess their quality. This precluded direct assessment of potential publication bias by comparing published and grey literature. Second, the impact of publication bias may be relatively small when data from population databases are available. Nevertheless, caution is required in such circumstances, as precise estimates generated by large study samples are not necessarily accurate. Evaluating other sources of bias, such as the potential impact of unaccounted confounding, may be of higher priority and importance than publication bias. Finally, a deeper epistemological consideration is the utility of a ‘pooled effect’ in HSDR in general. In contrast to the priority of pursuing an accurate, universal estimate of the effect of a treatment or exposure in clinical research (however, this might change in the era of ‘precision medicine’), a pooled effect estimate, such as the weekend effect across various patient populations, health systems and geographical locations, may be of interest to no more than a small number of persons. Examining the mediators and contextual moderators of an effect is likely to play a more prominent role in HSDR. Funnel plot and related methods that are designed to assess the impact of publication bias surrounding a pooled estimate may therefore be of limited value, except in examples such as multivariate meta-regression, in which investigation of the impact of multiple factors can be carried out. Issues identified in case study 1, such as heterogeneity and potential confounding, were mirrored in other case studies included in the WP.
Work package 4: follow-up of HSDR study cohorts for investigating publication bias in HSDR
This WP collected direct, and thus the strongest, evidence on publication bias. Rates of publication among the selected HSDR cohorts are relatively high, except the ISQua cohort. This may reflect the nature of these cohorts, most comprising studies being well-funded research carried out by researchers. We noted that many of the studies from the ISQua cohort were reported by practitioners and were conducted in a single institution, as exemplified by the larger proportion of before-and-after studies without a control group. Many of these were likely to be studies of in-house improvement project and service evaluation that were not intended to be published.
The significantly lower rate of publication in this cohort than in other cohorts therefore suggests that the motivation for conducting these studies is likely to be one of the key factors influencing subsequent publication of the findings. Nonetheless, our multivariable analysis provided some indication that statistical significance and perceived positivity of the findings did appear to influence the likelihood of publication having adjusted for other factors associated with motivation, funding and methodological rigour. This finding should be considered tentative, given our limited sample size and potential subjectivity in classifying research findings. In addition, we were unable to obtain further information from investigators for 34 studies, with 23 of these from the ISQua cohort. This might have introduced some bias, although it could be argued that from the perspective of accessibility of evidence, any publications that might have existed but were missed by our own searches may be difficult to reach by decision-makers and other users of HSDR. Given the differential motivation, publication bias may be more profound in cohorts, such as the ISQua, than in cohorts of independently funded research. However, our sample size was too small to investigate this.
The 100% accessibility of study findings from the NIHR cohort demonstrates the important influence that research funders could have. Archives of historical projects accessible online and the now established NIHR Journals Library facilitate the dissemination of research findings irrespective of whether or not they are published in ‘traditional’ academic journals. An unexpected finding from this work was that the rate of publication in academic journals is lower for the NIHR cohort than for the HSRProj cohort. We could offer two plausible explanations among others. First, for consistency, we have classified all full reports from NIHR-funded projects as ‘grey literature’. These included project reports from the previous SDO and Health Service Research programme available online, as well as more recent monographs published in the Health Services and Delivery Research series of the NIHR Journals Library. If the latter had been classified as an academic journal, the publication rate would have appeared higher. Second, given that the journals included in the NIHR Journals Library are indexed in MEDLINE and are openly accessible, researchers might not perceive it necessary to publish the findings in other academic journals. In addition, during our communications with HSDR researchers, we were made aware that some academic journals in the field appear to be more reluctant to publish findings that would also be described and made available in NIHR Journals Library. Furthermore, as the production of the project final report as a monograph in the NIHR Journals Library requires substantial effort, this might have incurred some opportunity costs in relation to preparation of publications in other academic journals.
Work package 5: key informant interviews and focus group discussion to explore publication bias in HSDR
A notable finding from WP 5 was that many respondents were uncertain as to how significant a problem publication bias presented in HSDR. Although this is perhaps unsurprising in the case of those not directly involved in publishing, for example patients and health-care managers, it was also a feature of interviews with researchers, journal editors and research funders. Although the majority of these believed publication bias in some form to be present, few were able to refer to instances when this had been directly observed. This lack of clarity may reflect the apparently equivocal findings of previous WPs and our interviews therefore provide some potentially valuable qualitative insights.
One area that influenced views as to the presence of publication bias was the subdiscipline of HSDR to which respondents belonged. The researchers in our sample spanned diverse scholarly subfields, and this was reflected in the journals they targeted to publish their work. Although respondents sought to publish HSDR results in medical journals, many did so as a response to institutional pressures to publish in journals with high impact scores. In these circumstances, there was some consensus that significant (if not necessarily positive) results would be more likely to be (1) submitted and (2) accepted for publication. In contrast, many believed that other criteria were more important when seeking to publish in their disciplinary ‘home’ journals. Here, strength of results was believed to be secondary to other potential sources of ‘novelty’ in shaping publication outcomes.
As well as journal variation, interviewees drew a distinction between externally funded and peer-reviewed research on the one hand, and end user-funded quality improvement projects on the other, with the latter category attributing higher susceptibility to publication bias instigated by the research teams. Respondents also identified risks in ex post facto decisions to submit for publication, data which have been gathered primarily for other purposes. In the former category, there was general agreement that HSDR studies often contain more than summative assessments or measurements of associations. The typical presence of multiple study objectives, complex interventions, higher levels of ‘mess and noise’ in the data and their interpretation, was seen as reducing the importance ascribed to effect sizes and significance of associations. For this reason, a substantial minority of respondents were resistant to the proposal that all HSDR research be pre-registered.
There was some support in interview findings for the claim that forms of bias are linked to study type, with, for example, association studies considered more susceptible to p-hacking and selective outcome reporting, and evaluation studies at greater risk of funder pressure. However, respondents contrasted the level of external scrutiny (e.g. from industry and health systems) in clinical research with the tendency towards ‘lower stakes’ in HSDR, which was again seen as mitigating the incentive towards publication and related bias. Overall, interviewees considered incentives towards publication and related bias to be present but to a lesser degree.
The research cultures described by interviewees confirm the previously reported pressure to publish in high-impact journals. 99 However, in comparison with clinical research, HSDR is fragmented, and ‘subcultural’ factors may attenuate the drivers of publication and related bias. Other variables that warrant further investigation include researcher seniority (e.g. senior researchers in our sample were more likely to believe that negative or null results did not present an impediment to them publishing their work).
The interviews and focus group discussions with those not directly involved in publishing research (health-care decision-makers, patients and citizens) raise issues that go beyond the specific question of publication and related bias. These include the relatively weak relationship between HSDR and decision-making in health care, and the wider ethical implications of research waste, especially for participating patients and the allocation of scarce public resources.
Overall learning from the project and study limitations
Having described lessons learned from individual WPs above, we offer below some learning that emerges from across the WPs and highlight key limitations of this project.
Gleaning from the evidence collected across all WPs, it is reasonably clear that publication and related bias can and does occur in HSDR. However, it is currently difficult to gauge the true scale and impact of the bias given the sparse high-quality evidence, which in turn may be associated with difficulties in identifying study cohorts due to lack of comprehensive study registration. Solid evidence on publication bias and selective outcome reporting in clinical research has primarily been obtained from RCTs, as study protocols are made available in the trial registration process. 1 Stakeholders in our interviews generally supported registration of HSDR, albeit with some reservations, and quality improvement projects and implementation studies were identified as being potentially at high risk of these bias. As repositories of quality improvement projects emerge,100 HSDR and quality improvement communities will need to consider and evaluate the feasibility and values of adopting these practices for studies of varied types and purposes.
The lack of prospective study registration poses further challenges in assessing outcome reporting bias, which could be a greater concern for HSDR than clinical research, given the more exploratory approaches to examining a larger number of variables and associations in HSDR. Interestingly, our hypothesis that association HSDR studies based on observational evidence are more susceptible to publication and reporting bias was supported neither by the quantitative evidence that we collected so far, nor by recent research from the field of organisational studies (described in more detail in Publication and related bias in other cognate fields). 101–104 Nevertheless, the potential risk could still not be dismissed, as most of available evidence relies on methods that have some weakness. It is possible that the focus on formative evaluations rather than summative measures may have mitigated the bias to some extent.
Like many issues in HSDR, publication and related bias is the product of complex social processes and is likely be context dependent. Qualitative and quantitative data obtained from different WPs identified several potential factors (see Table 13) that may affect on the risk of its occurrence. Further empirical evidence needs to be collected to explore the relative influence of these factors and to ascertain whether interventions or policies targeting some of these factors can effectively reduce or prevent publication and related bias.
The strengths and limitations of individual pieces of work from this project have been discussed in detail in each of the WPs. Overall, although our findings have shed new light on our understanding of publication and related bias, the project and its findings have some important limitations:
-
We focus only on quantitative HSDR investigating interventions and associations.
-
Few studies have systematically examined publication and related bias in HSDR, limiting the conclusion that we can draw from review of the literature.
-
The numbers of HSDR systematic reviews that we evaluated in WP 2 and HSDR cohorts that we followed in WP 4 are still relatively small.
-
Although issues with the application of statistical methods to detect publication and related bias in HSDR are highlighted in WPs 2 and 3, novel methods need to be developed to overcome the issues identified.
-
Owing to lack of comprehensive registration of study protocols, we have not been able to collect direct evidence on publication and related bias concerning quality improvement projects, and to examine outcome reporting bias in study cohorts that we followed.
-
The sample size for our qualitative work in WP 5 is relatively small, and within the scope of the current project we have not explored some of the wider issues related to use of evidence from HSDR in making service decisions and transparency and public accountability of this process.
These limitations need to be addressed in future research.
Publication and related bias in other cognate fields
This project investigated publication and related bias in HSDR. A major challenge for the project was to draw a boundary between HSDR and other fields of research, given its multidisciplinary nature. In our systematic review presented in Chapter 3, we have focused on research in areas that fall under the definition of HSDR adopted in this project. However, we are aware that literature on publication and related bias has emerged in other scientific disciplines. Although it was not possible for us to systematically review this diffuse literature within the scope of this project, we provide below a brief description of key papers that may have some relevance to HSDR.
In our literature search for the systematic review described in Chapter 3, and through citation check, we identified several studies on publication and related bias in cognate fields. We describe these studies briefly in this section.
Organisational behaviour, organisational psychology and human resource management
Four studies examined publication and outcome reporting bias in the broad area of organisational behaviour, organisational psychology and human resource management. All four studies focused on measures of correlations and all found that publication and outcome reporting bias did not seem to be a major issue. The studies are described below.
Kepes et al. 101 evaluated publication bias in organisational sciences. Using data on employment interview validities (total sample size of 25,244 interviews and 160 correlation coefficients), the authors conducted multiple analyses, including trim and fill, contour-enhanced funnel plots, Egger’s regression test, Begg and Mazumdar’s rank correlation, meta-regression, cumulative meta-analysis and selection models. When all the samples were analysed together, many of the publication bias detection methods suggested potential publication bias, although the effect seems minimal and it would not have affected the conclusions. The authors anticipated that between-sample heterogeneity may have influenced the results and, therefore, created subgroups and assessed publication bias within the subgroups. This resulted in analyses of 40 distributions, in three of which the authors concluded that publication bias is likely to be present. Although most of the results from different methods were consistent, there were many occasions when at least one method indicated conflicting results to the other methods. Most of the disagreements may be related to problem of statistical power. Notwithstanding, the interpretation was inconclusive in only 6 out of 40 analyses performed. Using cumulative meta-analyses by year of publication, indication of potential time-lag bias was also observed: the two samples published in 1947 have a cumulative mean estimate of 0.67 and all eight samples published before 1970 have a cumulative estimate of 0.33, whereas the final meta-analytic estimate is 0.24. However, the data covered a 50-year time period and time-lag bias would not have changed the practical conclusions of the analyses. The authors concluded that publication bias has minimal effect in the data set assessed. They recommended that meta-analytic reviews include multiple publication bias methods that are based on different assumptions. To mitigate heterogeneity, potential moderating variables could be identified using meta-regression, in order to form more homogeneous subgroups before evaluating publication bias.
Dalton et al. 102 evaluated the extent of the file-drawer problem (an alternative term for publication and related bias frequently used in social sciences) in non-experimental research in organisational behaviour and human resource management, industrial and organisational psychology, and related fields. 102 They estimated the frequency and percentage of statistically non-significant correlations reported in correlation matrices of primary studies from four different sources: (1) randomly selected issues of three organisational management journals published between 1985 and 2009 (403 correlation matrices, including 37,970 correlations); (2) 51 published meta-analyses (6935 correlations); (3) unpublished manuscripts obtained from randomly selected faculty members of 30 schools of business and 30 industrial and organisational psychology programmes (167 correlation matrices, including 13,943 correlations); and (4) 50 randomly selected dissertations identified from the ProQuest Dissertations & Theses A&I Database (217 correlation matrices, including 20,860 correlations). They found that the percentages of statistically non-significant correlations were all in the range between 44% and 51%, with overlapping CIs. In addition, they compared the average magnitude of randomly selected 1002 correlations from published primary studies [i.e. source (1) above] with that of randomly selected 1224 correlations from unpublished dissertations [i.e. source (4) above] and found similar average magnitude in both groups (0.227 vs. 0.228, respectively). They therefore concluded that the file-drawer problem does not severely threaten the validity of conclusions derived from meta-analyses and does not lead to overestimation of effect sizes in meta-analyses, as commonly believed. However, they also acknowledged that they did not compare published and unpublished findings among studies of specific focal areas in the broad field.
Stemig and Sackett103 assessed the file-drawer issue in industrial and organisational psychology. The authors examined meta-analyses that compared the effect sizes from published with unpublished studies, for which publication status was examined as a potential moderator variable. The meta-analyses were selected from two journals that the authors deemed to be journals in which meta-analyses are most prevalent in industrial and organisation research: Journal of Applied Psychology (JAP) and Personnel Psychology. Of 181 meta-analyses from JAP, 145 (80.1%) reported inclusion of unpublished studies and only 16 (8.8%) analysed publication status as a moderator variable. Twenty out of 43 meta-analytic studies from Personnel Psychology included unpublished studies, but only four (9.3%) examined publication status as a moderator variable. Thus, 20 studies (16 from JAP and four from Personnel Psychology) presenting 84 comparisons of published and unpublished studies were included. The overall mean observed correlations in the published and unpublished studies were similar (0.221 vs. 0.224, respectively). Differences between mean correlations of published and unpublished studies were small in majority of the comparisons (60% were < 0.1; 24% were between 0.1 and 0.2; 12% were between 0.2 and 0.3; and 4% were > 0.3). Most of the supposedly large differences were not statistically significant. Significant differences between published and unpublished studies were observed in seven of the correlations, but only two of the significant differences exceeded 0.2. Interestingly, both of these showed larger mean effects in unpublished studies, which is contrary to what is expected for a file-drawer problem. The authors concluded that, although average effect sizes from published and unpublished studies are similar, there are instances when they produce different results. However, the authors acknowledge that the study had a relatively small sample size and that unpublished studies identified in the included meta-analyses may not be representative of all unpublished studies.
Paterson et al. 104 assessed the file-drawer problem in meta-analyses of organisational behaviour and human resources studies. The authors searched 30 management journals that were considered to have the most impact which had published at least one meta-analysis by 1 June 2012. The search identified 350 articles, from 11 journals, that included words relating to meta-analyses in their titles. The 350 articles were examined to identify those that (1) reported a meta-analytic relationship between two variables; (2) were based on three or more independent samples from at least two sets of authors; and (3) involved ‘micro’ domains of organisational behaviour or human resources, or industrial and organisational psychology (i.e. ‘macro’ domains, such as business policy and strategic management, were excluded). Ultimately, 258 meta-analyses which generated 776 meta-analytic conclusions were included. The study assessed file-drawer issues in two ways. First, the correlation between the effect size and proportion of unpublished studies was calculated for the 456 analyses in which the proportion of unpublished studies was reported. The result showed no significant relationship between effect size and proportion of unpublished studies (r = –0.065; p > 0.05). Second, the relationship between sample size and effect size was assessed and the result also showed no statistically significant relationship (r = −0.026; p > 0.05). Hence, the authors concluded that publication bias does not pose a major threat in the micro-oriented management literature. This conclusion is similar to the previous studies described above. However, the study focused on meta-analyses published in top management journals and so the sample may not be representative of all studies.
Social sciences
Utilising a Time-sharing Experiments for the Social Sciences programme sponsored by the US National Science Foundation, Franco et al. 16 undertook an inception cohort study to investigate publication bias in social sciences. Researchers submitted research proposals of survey-based experiments to be run on representative samples of American adults (e.g. to test the effect of questionnaire wording) to the Time-sharing Experiments for the Social Sciences programme. These were peer reviewed and grants were allocated on a competitive basis and, therefore, studies in the cohort assembled from the programme passed a certain quality threshold. The authors followed up 221 of 249 selected studies to verify the statistical significance of their findings and their publication status. Study findings were classified as strong (all or most of the hypotheses were supported by the statistical tests), null (all or most hypotheses were not supported) or mixed (the remainder of studies). They found a strong association (chi-squared test p < 0.001) between strength of findings and publication status, with > 60% of studies with strong findings published compared with around 20% of studies with null results. In addition, they found that the authors did not write up the findings in only 4% of studies with strong results compared with 65% of studies with null results, indicating that authors’ motivation is a key factor contributing to publication bias.
Points for consideration by stakeholders in relation to publication and related bias in HSDR
In this project we collected prima facie evidence of publication and related bias in quantitative HSDR using a variety of approaches that generated both direct and indirect evidence, as described in the previous chapters. Overall, our findings suggest that publication and related bias can and does occur in quantitative HSDR. However, there is substantial uncertainty with regard to the nature and extent of its occurrence and its impact on decision-making concerning health service delivery.
We presented our findings in a meeting convened at the end of the project, in which we invited a group of key stakeholders of HSDR (see Acknowledgements) to help us make sense of the evidence gathered in this project and formulate potential recommendations for future research practice. The attendees included senior researchers in HSDR and evidence synthesis, journal editors, funders and a PPI representative. Diverse views and perspectives were presented in the meeting, echoing the findings from our interviews. Taken in the round, the emerging consensus seemed to be that a nuanced interpretation, with due attention paid to the epistemological and methodological diversity in HSDR and the changing landscape of research publication, is needed rather than rushing to replicate measures (such as compulsory study registration in an attempt to reduce occurrence of this bias) that have been adopted in clinical research or dismissing the importance of this issue due to current lack of concrete evidence. Here, we highlight pertinent issues that different stakeholders might wish to consider when commissioning, undertaking, reviewing, publishing or using HSDR.
Epistemological and methodological diversity in HSDR
As stated in Chapter 2, we adopted a pragmatic definition of HSDR in line with that used by the NIHR HSDR programme for this project, and focused our attention on two specific types of quantitative studies: those evaluating the effectiveness of an intervention and those assessing the association between various structures, processes, outcomes and contexts along the service delivery causal chain. These allowed us to draw a boundary and maintain the practicality of our investigation. The findings of our project are naturally bounded by this chosen scope. They cannot be considered representative of the full spectrum of HSDR, which is broad and diverse in terms of subject areas, epistemological stances and methodological approaches. For example, the project did not consider publication bias in qualitative studies, case studies and simulation studies in operational research, all of which play an important role in HSDR. The mechanisms, occurrence (or lack of) and magnitude of publication and related bias may also differ between subspecialties of HSDR. Nevertheless, we contend that the two types of quantitative studies that we have focused on are important sources of information that can potentially influence health service delivery and policy and, therefore it is important for us to understand if and how publication and related bias might affect the dissemination and use of this evidence.
The methodological and epistemological diversity of HSDR has implications for the generation and interpretation of quantitative evidence, and corresponding bearing on publication and related bias. For example, mixed-methods studies are widely used in HSDR, in which evidence gathered from quantitative and qualitative methods is intended to provide a deeper understanding of the phenomenon than either methodological approach could offer alone. The appropriateness of separating quantitative data from such studies in order to combine them with data from other quantitative studies in a meta-analysis, and assessing the evidence for the presence of publication and related bias using the assumptions underpinning a quantitative research paradigm, may be questionable.
Changing landscape of research publication
A large volume of scientific literature on publication bias was accumulated in the middle to late twentieth century, when academic journals were the main, and sometimes the only, outlet for disseminating research findings. Publication bias in the form of selective publication of positive and/or statistically significant findings by academic journals was a major concern, as it would be very difficult for people other than the investigators themselves to locate and access ‘unpublished’ research findings. The situation has dramatically changed since the advent of the internet, through which research findings can be easily shared with other people all around the world in a variety of forms, such as technical reports, pre-publication manuscripts, discussion papers and so forth, even if they are not submitted or accepted for publication by academic journals. This has implications for the study and measurement of publication bias, which may increasingly focus on the extent and ease of online access to research findings, rather than availability in traditional peer-reviewed outlets.
Within academic research, mandatory publication of findings in open access platforms, such as the principle advocated by the Plan S initiative,105 is gaining strong momentum that could potentially minimise publication bias for funded research. The creation of journals which aim to provide a platform for publishing research findings according to methodological rigour, irrespective of the perceived interest of the findings, is a further response to well-documented concerns regarding publication bias in clinical research. The increasing availability of such journals (e.g. PLOS ONE and BMJ Open) may reduce potential publication bias across scientific disciplines, including HSDR. However, this creates other risks, including bias associated with the availability of funding to pay article processing charges, and conflicts of interest as, for example, motivation for publishing in such journals may be stronger when there is a potential for financial or reputational gains associated with the publication. 106 This development also diversifies the roles played by academic journals, which traditionally serve both as a competitive space for showcasing research excellence and as an archive of tested knowledge. Measures to reduce publication bias might therefore lead to the unintended introduction or exacerbation of other forms of bias and so should be adopted with caution.
The changing landscape of research publication and information dissemination also means that the definitions of terms, such as ‘publication’ and ‘grey literature’ are evolving, with the boundary between the two becoming increasingly vague. Careful selection, definition and use of appropriate terms will be required (see Terminology related to bias in reporting research findings).
Terminology related to bias in reporting research findings
Both publication bias that occurs at the study level and selective outcome reporting that occurs at individual outcome levels result in a distorted picture of the totality of evidence available to decision-makers. Given the rapid evolution of research publication described above and associated changes to the nature of biases, there is a move to adopt ‘non-reporting bias’ as the collective term to cover these biases by the Cochrane Collaboration (Professor James Thomas, University College London, 7 June 2019, personal communication). Consistent use of this term should help facilitate exploration and debate in future.
Prevention of publication and related bias in HSDR
A clear message from the findings of WP 4 (follow-up of HSDR cohorts) is that research funders can play a crucial role in minimising publication and related bias and the associated research waste through a clear policy of mandating the publication of findings of commissioned research, and by providing suitable platforms for archiving and disseminating funded research, such as those adopted by various research programmes of the UK NIHR.
However, implementation of such policy and arrangements requires substantial resources which may not be available to smaller-scale funders. In addition, many HSDR studies are motivated by the desire to understand service issues or to gauge the impact of efforts to improve services and are carried out with limited or no funding. Without the supporting infrastructure and incentives or sanctions from the funders, these studies may be more susceptible to publication and related bias. Although findings from WP 5 (key informant interviews) suggest that HSDR stakeholders generally support the practice of registering research protocols (or making them available in openly accessible repositories) when feasible, there are also concerns that inconsiderate and prescriptive adoption of these practices may stifle the creativity and diversity that are crucial for resolving challenges in health service delivery.
An area clearly requiring further attention is quality improvement projects and service evaluations. These activities often occupy a grey area between research and non-research, are often conducted locally and/or retrospectively, and are not (at least initially) carried out with an intention to produce generalisable knowledge. An isolated study included in our systematic review (WP 1) and findings from our interviews with HSDR stakeholders (WP 5) suggested that any such studies that subsequently appear in the academic literature may be those with the most favourable results. Therefore, caution may be required when studies of this nature are included in systematic reviews and evidence syntheses: policy-makers and service-planners should be aware that isolated quality improvement studies reported in the literature may represent a subset of locally evaluated interventions, rather than proven best practice that can be easily replicated or scaled up in other settings.
Comprehensive registration of quality improvement projects may reduce potential publication and reporting bias associated with these studies. It may further enhance the potential utility of evidence generated from them, providing an audit trail and a denominator for the totality of evidence, as well as improving the ease of locating and accessing these studies. However, these benefits need to be balanced against the resources required for the creation and maintenance of such registries; the opportunity costs of the efforts required by service planners and practitioners to complete the registration and the practicality against other service and policy imperatives; the quality and value of evidence that can be obtained from the registries; and the potential burden of examining this type of evidence in future systematic reviews of HSDR.
Assessment and mitigation of publication and related bias in systematic reviews of HSDR
Findings from our meta-epidemiological study of published HSDR systematic reviews (WP 2) showed a low level of documented awareness of the bias. It is difficult to ascertain whether the lack of documentation was due to reviewers genuinely lacking awareness of the bias or to their perception of the (lack of) importance and relevance of the bias, either in relation to the suitability of available methods for detecting and mitigating the bias or in relation to the aims and epistemological stance (e.g. configurative vs. aggregative) of the review. Our findings from WP 2 pointed to the latter, but further investigation is required.
In terms of assessment of publication bias and outcome reporting bias, funnel plots and related techniques remain the dominant methods used in the reviews in the absence of comprehensive study registries. As our case studies (WP 3) illustrated, application and interpretation of funnel plots and related techniques are often problematic owing to the inherent heterogeneity of evidence from HSDR and potentially incompatible assumptions arising from epistemological differences. These techniques are best used as part of broader efforts to investigate the source of statistical heterogeneity between studies, with extra caution needed in ascertaining the assumptions and requirements for appropriate application of these methods. In particular, injudicious interpretation of small-study effects illustrated by funnel plot asymmetry as direct evidence of publication bias should be avoided. 26 Further training of evidence reviewers on appropriate use of these techniques and development of novel methods may be required.
Limited evidence included in our systematic review (WP 1) suggested that important differences in the quantity, quality and nature of evidence may exist between published academic literature and other sources of (grey) literature. 51 Further documentation and accumulation of evidence on the extent to which search for and inclusion of non-academic literature affects the findings and conclusions of HSDR systematic reviews are needed.
It is also worth highlighting that publication and related bias is just one of many different biases that could affect the trustworthiness of research evidence. For example, case study 1 described in Chapter 5 (WP 3) illustrated that unaccounted confounding may be a greater concern in meta-analyses of HSDR studies based on analyses of administrative databases. Guidance on interpretation of overall quality of evidence, such as GRADE, may support reviewers to make appropriate judgements in interpreting the importance and relevance of publication and related bias relative to other factors. 35
Potential impact of publication and related bias in HSDR
Given the paucity of evidence and the challenges in establishing the occurrence and magnitude of publication and related bias in HSDR, it is currently not possible to estimate the impact of this bias on decision-making related to health services, or assess its further effects downstream (e.g. resulting service organisation and patient outcomes). One pertinent issue is that, although evidence-based decision-making has become an integrated part of health care for individual patients, the same may not be true when decisions on the organisation and delivery of health services are made. Indeed, emerging evidence has shown a very different decision-making process in the planning and organisation of health services. Qualitative research has shown that health-care commissioners’ decision-making process is highly pragmatic,107,108 with many sources of information used in a dynamic environment, including best practice guidance, the opinions of clinicians and service users, local data, conversations and story sharing within informal networks and innovations from elsewhere. Research evidence may therefore play only a minor part in informing service planning and policy. As a consequence, publication and related bias in research evidence may appear likely to have only a small, indirect, impact on SDO. However, although explicit use of research evidence may seem rare in decision-making concerning health-care services and policies, research evidence could also have influenced the decision-making process indirectly through tacit knowledge, precedent decisions based on research evidence and conceptual use of research. 109 Consequently, the importance of the issue of publication and related bias in HSDR should not be overlooked. In our focus group discussion, patients and members of the public attributed high importance to decision-making being based on unbiased evidence (WP 5).
Recommendations for future research
To the best of our knowledge, this project is the first to systematically investigate publication and related bias across different subdisciplines of HSDR. Overall, our findings highlight a paucity of evidence, with many gaps and weaknesses in the evidence base informing this subject. We present below our recommendations for future research that may help in bridging these gaps.
Prevention
-
To explore the feasibility, acceptability and sustainability of establishing new registries and/or making the best use of existing registries for various types of HSDR, including quality improvement projects and service evaluations. As measures related to prevention could reduce the need for subsequent efforts in the detection and mitigation of publication and related bias, exploration of these measures may deserve a high priority.
Occurrence, magnitude and relevance
-
To accumulate further empirical evidence on publication and related bias in different subject areas and for studies of different designs within HSDR, in particular through direct methods that allow verification of the actual occurrence of non-publication and/or selective outcome reporting, as well as reasons behind these in cohorts of HSDR studies for which a denominator (i.e. the total number of studies undertaken) is known.
-
To explore the existence, nature, relevance and impact of publication bias in qualitative and mixed-methods studies in HSDR and compare these with quantitative studies.
-
To characterise HSDR in relation to other disciplines of research and features of HSDR which might increase or mitigate the occurrence of publication and related bias.
-
To investigate what aspects of multicomponent HSDR studies are subsequently published in academic journals.
-
To explore the influence of the composition of the research team on the occurrence of publication and related bias.
Methods of detection and mitigation
-
To evaluate the utility and most efficient methods of including non-academic literature in systematic reviews of HSDR, taking into account the burden on reviewers and impact on the findings and conclusion of the reviews.
-
To explore reasons behind the apparent low levels of awareness of publication and related bias in HSDR systematic reviews.
-
To develop new tools for assessing the risk bias associated with potential non-reporting of data in HSDR.
Wider methodological development of HSDR
-
To explore the value of including findings from quality improvement projects and service evaluation in systematic reviews of HSDR.
Conclusion
This project collected prima facie evidence on publication and related bias in quantitative HSDR. Overall, our findings suggested that this bias can and does occur in this field, although literature on this topic is scant. The epistemological and methodological diversity of HSDR, the changing landscape of research publication and the boundary delineated by project scope all need to be taken into account when interpreting our findings.
The occurrence of publication and related bias and its impact are likely to vary between studies with different methodological designs for different purposes, with evaluations motivated by service improvement locally most susceptible to such bias. Variation in the practice of research publication may exist between different subspecialties in HSDR but this requires further exploration. The bias is likely to be most profound in relation to publication of HSDR findings in academic journals with a general medical orientation.
The precise magnitude of publication and related bias is difficult to quantify owing to the lack of comprehensive study registration and the vague boundary between research and activities undertaken for service improvement. Epistemology and methodological approaches that do not focus solely on summative assessments of outcomes or associations and that do not heavily rely on inferences based on statistical significance may to some extent mitigate the occurrence and influence of publication and related bias in HSDR.
Documentation of awareness of publication and related bias and formal assessment of the bias were low in HSDR systematic reviews, particularly in reviews of association studies. Methodological guidelines might have some positive impact on practice. Application of statistical tools, such as funnel plots, and related tests for detecting publication and related bias in HSDR is often problematic owing to the heterogeneity of studies and potential confounding in any associations observed between sample size (or precision of estimate) and effect size. These tools are best used, with great caution, as part of the broader effort in investigating potential modifiers of intervention effects or associations. Use of funnel plot asymmetry as a sole indicator of the presence or absence of publication and related bias should be avoided.
Further research to collect empirical evidence based on methods that allow for direct observation and inference of the occurrence of publication and related bias in various subdisciplines and study designs of HSDR is required. The utility and most efficient ways of including quality improvement projects and other evidence beyond academic literature in systematic reviews of HSDR need to be explored.
Acknowledgements
We are indebted to the following members of the Study Steering Committee for their advice and guidance throughout the project: Professor Stephen Sutton (chairperson), Dr Christopher Chiswell, Reverend Dr Barry Clark, Professor Jeremy Grimshaw, Professor Timothy Hofer, Mr Tim Sacks, Professor Kaveh Shojania and Professor James Thomas.
We thank the McMaster University and McMaster Health Forum for their permission for us to use records from the Health Systems Evidence database for our WP 2, and Dr Kaelan Moat for his assistance in this process. We thank the HSDR programme-funded High Intensity Specialist Led Acute Care (HiSLAC) project for permission to use the data set from the systematic review of the weekend effect on which case study 1, described in Chapter 5, was based. We thank Dr Ruth Pullen for providing data on projects funded by the NIHR SDO, Health Service Research and HSDR programmes, and Daniel Camero for supplying the abstracts of past HSRUK conferences for our WP 4. We also wish to thank Alice Davis for her help in data checking for WP 2.
We are grateful to all HSDR investigators who responded to our requests for further information concerning their research, and all participants in the interviews and the focus group discussion of this project.
We thank the following delegates who attended our project dissemination meeting in June 2019 and provided invaluable advice on the interpretation of the project findings and formulation of recommendations for research practice and future research: Dr Andrew Booth, Professor Dawn Craig, Professor Graham Martin, Helen Crisp, Professor James Raftery, Professor James Thomas, Professor Nick Mays, Tara Lamont and Professor Timothy Hofer.
Magdalena Skrybant and Richard J Lilford are also supported by the NIHR Applied Research Collaboration West Midlands.
Contributions of authors
Abimbola A Ayorinde (https://orcid.org/0000-0002-4915-5092) (Research Fellow, Evidence Synthesis) was the main researcher on the project and maintained day-to-day running of the project, involved in all stages of the work and drafted the report.
Iestyn Williams (https://orcid.org/0000-0002-9462-9488) (Reader, Health Policy Management) was the lead for WP 5 of the project, planned and carried out the interviews and led the focus group discussion, analysed the data and drafted WP 5-related sections.
Russell Mannion (https://orcid.org/0000-0002-0680-8049) (Professor, Health Systems) was a member of the Project Management Group and provided senior advice on health services research.
Fujian Song (https://orcid.org/0000-0002-4039-1531) (Professor, Research Synthesis) was a member of the Project Management Group and provided advice on statistics and publication bias-related issues.
Magdalena Skrybant (https://orcid.org/0000-0001-7119-2482) (PPI and Engagement Lead) was a member of the Project Management Group, provided advice and was involved in all aspects of PPI-related activities, and facilitated the focus group discussion.
Richard J Lilford (https://orcid.org/0000-0002-0634-984X) (Professor, Public Health) was a member of the Study Steering Committee and provided senior advice on methodology.
Yen-Fu Chen (https://orcid.org/0000-0002-9446-2761) (Associate Professor, Evidence Synthesis) was the principal investigator of the project, was involved in all stages of the work and drafted the report.
All authors commented on multiple drafts of report sections, helped to revise the report and approved its submission.
Publications
Williams I, Ayorinde AA, Mannion R, Song F, Skrybant M, Lilford RJ, Chen YF. Stakeholder views on publication bias in health services research. J Health Serv Res Pol 2020;25:162–71. https://doi.org/10.1177/1355819620902185
Ayorinde AA, Williams I, Mannion R, Song F, Skrybant M, Lilford RJ, Chen YF. Assessment of publication bias and outcome reporting bias in systematic reviews of health services and delivery research: a meta-epidemiological study. PLOS ONE 2020;15:e0227580. https://doi.org/10.1371/journal.pone.0227580
Ayorinde AA, Williams I, Mannion R, Song F, Skrybant M, Lilford RJ, Chen YF. Publication and related biases in health services research: a systematic review of empirical evidence. BMC Med Res Methodol 2020;20:137. https://doi.org/10.1186/s12874-020-01010-1
Data-sharing statement
Most data collected in this project have been presented in this report. All data requests should be submitted to the corresponding author for consideration. Access to available anonymised data may be granted following review.
Disclaimers
This report presents independent research funded by the National Institute for Health Research (NIHR). The views and opinions expressed by authors in this publication are those of the authors and do not necessarily reflect those of the NHS, the NIHR, NETSCC, the HS&DR programme or the Department of Health and Social Care. If there are verbatim quotations included in this publication the views and opinions expressed by the interviewees are those of the interviewees and do not necessarily reflect those of the authors, those of the NHS, the NIHR, NETSCC, the HS&DR programme or the Department of Health and Social Care.
References
- Dwan K, Gamble C, Williamson PR, Kirkham JJ. Reporting Bias Group . Systematic review of the empirical evidence of study publication bias and outcome reporting bias – an updated review. PLOS ONE 2013;8. https://doi.org/10.1371/journal.pone.0066844.
- Simonsohn U, Nelson LD, Simmons JP. P-curve: a key to the file-drawer. J Exp Psychol Gen 2014;143:534-47. https://doi.org/10.1037/a0033242.
- Head ML, Holman L, Lanfear R, Kahn AT, Jennions MD. The extent and consequences of p-hacking in science. PLOS Biol 2015;13. https://doi.org/10.1371/journal.pbio.1002106.
- Song F, Parekh S, Hooper L, Loke YK, Ryder J, Sutton AJ, et al. Dissemination and publication of research findings: an updated review of related biases. Health Technol Assess 2010;14. https://doi.org/10.3310/hta14080.
- Kicinski M, Springate DA, Kontopantelis E. Publication bias in meta-analyses from the Cochrane Database of Systematic Reviews. Stat Med 2015;34:2781-93. https://doi.org/10.1002/sim.6525.
- Eyding D, Lelgemann M, Grouven U, Härter M, Kromp M, Kaiser T, et al. Reboxetine for acute treatment of major depression: systematic review and meta-analysis of published and unpublished placebo and selective serotonin reuptake inhibitor controlled trials. BMJ 2010;341. https://doi.org/10.1136/bmj.c4737.
- Turner EH, Matthews AM, Linardatos E, Tell RA, Rosenthal R. Selective publication of antidepressant trials and its influence on apparent efficacy. N Engl J Med 2008;358:252-60. https://doi.org/10.1056/NEJMsa065779.
- Whittington CJ, Kendall T, Fonagy P, Cottrell D, Cotgrove A, Boddington E. Selective serotonin reuptake inhibitors in childhood depression: systematic review of published versus unpublished data. Lancet 2004;363:1341-5. https://doi.org/10.1016/S0140-6736(04)16043-1.
- Gülmezoglu AM, Pang T, Horton R, Dickersin K. WHO facilitates international collaboration in setting standards for clinical trial registration. Lancet 2005;365:1829-31. https://doi.org/10.1016/S0140-6736(05)66589-0.
- Phillips AT, Desai NR, Krumholz HM, Zou CX, Miller JE, Ross JS. Association of the FDA Amendment Act with trial registration, publication, and outcome reporting. Trials 2017;18. https://doi.org/10.1186/s13063-017-2068-3.
- Goldacre B, DeVito NJ, Heneghan C, Irving F, Bacon S, Fleminger J, et al. Compliance with requirement to report results on the EU Clinical Trials Register: cohort study and web resource. BMJ 2018;362. https://doi.org/10.1136/bmj.k3218.
- Shojania KG, Ranji SR, McDonald KM, Grimshaw JM, Sundaram V, Rushakoff RJ, et al. Effects of quality improvement strategies for type 2 diabetes on glycemic control: a meta-regression analysis. JAMA 2006;296:427-40. https://doi.org/10.1001/jama.296.4.427.
- Costa-Font J, McGuire A, Stanley T. Publication selection in health policy research: the winner’s curse hypothesis. Health Policy 2013;109:78-87. https://doi.org/https://doi.org/10.1016/j.healthpol.2012.10.015.
- Vawdrey DK, Hripcsak G. Publication bias in clinical trials of electronic health records. J Biomed Inform 2013;46:139-41. https://doi.org/10.1016/j.jbi.2012.08.007.
- Ammenwerth E, de Keizer N. A viewpoint on evidence-based health informatics, based on a pilot survey on evaluation studies in health care informatics. J Am Med Inform Assoc 2007;14:368-71. https://doi.org/10.1197/jamia.M2276.
- Franco A, Malhotra N, Simonovits G. Social science. Publication bias in the social sciences: unlocking the file drawer. Science 2014;345:1502-5. https://doi.org/10.1126/science.1255484.
- Harrison JS, Banks GC, Pollack JM, O’Boyle EH, Short J. Publication bias in strategic management research. J Manage 2017;43:400-25. https://doi.org/10.1177/0149206314535438.
- Jennions MD, Møller AP. Publication bias in ecology and evolution: an empirical assessment using the ‘trim and fill’ method. Biol Rev Camb Philos Soc 2002;77:211-22. https://doi.org/10.1017/s1464793101005875.
- Lilford RJ, Chilton PJ, Hemming K, Girling AJ, Taylor CA, Barach P. Evaluating policy and service interventions: framework to guide selection and interpretation of study end points. BMJ 2010;341. https://doi.org/10.1136/bmj.c4413.
- Brown C, Hofer T, Johal A, Thomson R, Nicholl J, Franklin BD, et al. An epistemology of patient safety research: a framework for study design and interpretation. Part 2. Study design. Qual Saf Health Care 2008;17:163-9. https://doi.org/10.1136/qshc.2007.023648.
- Brown C, Hofer T, Johal A, Thomson R, Nicholl J, Franklin BD, et al. An epistemology of patient safety research: a framework for study design and interpretation. Part 4. One size does not fit all. Qual Saf Health Care 2008;17:178-81. https://doi.org/10.1136/qshc.2007.023663.
- Petticrew M, Egan M, Thomson H, Hamilton V, Kunkler R, Roberts H. Publication bias in qualitative research: what becomes of qualitative research presented at conferences?. J Epidemiol Community Health 2008;62:552-4. https://doi.org/10.1136/jech.2006.059394.
- Lewin S, Glenton C, Munthe-Kaas H, Carlsen B, Colvin CJ, Gülmezoglu M, et al. Using qualitative evidence in decision making for health and social interventions: an approach to assess confidence in findings from qualitative evidence syntheses (GRADE-CERQual). PLOS Med 2015;12. https://doi.org/10.1371/journal.pmed.1001895.
- Rothstein HR, Sutton AJ, Borenstein M. Publication Bias in Meta-Analysis: Prevention, Assessment and Adjustments. Chichester: Wiley; 2005.
- Mueller KF, Meerpohl JJ, Briel M, Antes G, von Elm E, Lang B, et al. Methods for detecting, quantifying, and adjusting for dissemination bias in meta-analysis are described. J Clin Epidemiol 2016;80:25-33. https://doi.org/10.1016/j.jclinepi.2016.04.015.
- Sterne JA, Sutton AJ, Ioannidis JP, Terrin N, Jones DR, Lau J, et al. Recommendations for examining and interpreting funnel plot asymmetry in meta-analyses of randomised controlled trials. BMJ 2011;343. https://doi.org/10.1136/bmj.d4002.
- Wilczynski NL, Haynes RB, Lavis JN, Ramkissoonsingh R, Arnold-Oatley AE. HSR Hedges team . Optimal search strategies for detecting health services research studies in MEDLINE. CMAJ 2004;171:1179-85. https://doi.org/10.1503/cmaj.1040512.
- National Library of Medicine . Health Services Research and Health Policy Grey Literature Project: Summary Report 2006. www.nlm.nih.gov/nichsr/greylitreport_06.html (accessed 29 November 2015).
- McMaster Health Forum . Health Systems Evidence 2017. www.healthsystemsevidence.org/?lang=en (accessed 5 May 2017).
- Lavis JN, Wilson MG, Moat KA, Hammill AC, Boyko JA, Grimshaw JM, et al. Developing and refining the methods for a ‘one-stop shop’ for research evidence about health systems. Health Res Policy Syst 2015;13. https://doi.org/10.1186/1478-4505-13-10.
- Li X, Zheng Y, Chen TL, Yang KH, Zhang ZJ. The reporting characteristics and methodological quality of Cochrane reviews about health policy research. Health Policy 2015;119:503-10. https://doi.org/10.1016/j.healthpol.2014.09.002.
- Liberati A, Altman DG, Tetzlaff J, Mulrow C, Gøtzsche PC, Ioannidis JP, et al. The PRISMA statement for reporting systematic reviews and meta-analyses of studies that evaluate healthcare interventions: explanation and elaboration. BMJ 2009;339. https://doi.org/10.1136/bmj.b2700.
- Stroup DF, Berlin JA, Morton SC, Olkin I, Williamson GD, Rennie D, et al. Meta-analysis of observational studies in epidemiology: a proposal for reporting. Meta-analysis Of Observational Studies in Epidemiology (MOOSE) group. JAMA 2000;283:2008-12. https://doi.org/10.1001/jama.283.15.2008.
- Cochrane Methods . The Methodological Expectations of Cochrane Intervention Reviews (MECIR) 2018. https://methods.cochrane.org/mecir (accessed 9 May 2019).
- Guyatt GH, Oxman AD, Vist GE, Kunz R, Falck-Ytter Y, Alonso-Coello P, et al. GRADE: an emerging consensus on rating quality of evidence and strength of recommendations. BMJ 2008;336:924-6. https://doi.org/10.1136/bmj.39489.470347.AD.
- Sorita A, Ahmed A, Starr SR, Thompson KM, Reed DA, Prokop L, et al. Off-hour presentation and outcomes in patients with acute myocardial infarction: systematic review and meta-analysis. BMJ 2014;348. https://doi.org/10.1136/bmj.f7393.
- Chen YF, Hemming K, Stevens AJ, Lilford RJ. Secular trends and evaluation of complex interventions: the rising tide phenomenon. BMJ Qual Saf 2016;25:303-10. https://doi.org/10.1136/bmjqs-2015-004372.
- Lau J, Ioannidis JP, Terrin N, Schmid CH, Olkin I. The case of the misleading funnel plot. BMJ 2006;333:597-600. https://doi.org/10.1136/bmj.333.7568.597.
- Page MJ, McKenzie JE, Higgins JPT. Tools for assessing risk of reporting biases in studies and syntheses of studies: a systematic review. BMJ Open 2018;8. https://doi.org/10.1136/bmjopen-2017-019703.
- Egger M, Davey Smith G, Schneider M, Minder C. Bias in meta-analysis detected by a simple, graphical test. BMJ 1997;315:629-34. https://doi.org/10.1136/bmj.315.7109.629.
- Begg CB, Mazumdar M. Operating characteristics of a rank correlation test for publication bias. Biometrics 1994;50:1088-101. https://doi.org/10.2307/2533446.
- Duval S, Tweedie R. Trim and fill: A simple funnel-plot-based method of testing and adjusting for publication bias in meta-analysis. Biometrics 2000;56:455-63. https://doi.org/10.1111/j.0006-341x.2000.00455.x.
- Simonsohn U, Nelson L, Simmons J. P-Curve App 4.06 2017. www.p-curve.com/app4/ (accessed 28 June 2018).
- Gale NK, Heath G, Cameron E, Rashid S, Redwood S. Using the framework method for the analysis of qualitative data in multi-disciplinary health research. BMC Med Res Methodol 2013;13. https://doi.org/10.1186/1471-2288-13-117.
- Lincoln Y, Guba E, Lincoln Y, Guba E. Naturalistic Inquiry. Newbury Park, CA: Sage Publications; 1985.
- Francis JJ, Johnston M, Robertson C, Glidewell L, Entwistle V, Eccles MP, et al. What is an adequate sample size? Operationalising data saturation for theory-based interview studies. Psychol Health 2010;25:1229-45. https://doi.org/10.1080/08870440903194015.
- Pratt MG. From the Editors: for the lack of a boilerplate: tips on writing pp (and reviewing) qualitative research. Acad Manage J 2009;52:856-62. https://doi.org/10.5465/amj.2009.44632557.
- Tricco AC, Ivers NM, Grimshaw JM, Moher D, Turner L, Galipeau J, et al. Effectiveness of quality improvement strategies on the management of diabetes: a systematic review and meta-analysis. Lancet 2012;379:2252-61. https://doi.org/10.1016/S0140-6736(12)60480-2.
- Ivers N, Tricco AC, Trikalinos TA, Dahabreh IJ, Danko KJ, Moher D, et al. Seeing the forests and the trees – innovative approaches to exploring heterogeneity in systematic reviews of complex interventions to enhance health system decision-making: a protocol. Syst Rev 2014;3. https://doi.org/10.1186/2046-4053-3-88.
- Machan C, Ammenwerth E, Bodner T. Publication bias in medical informatics evaluation research: is it an issue or not?. Stud Health Technol Inform 2006;124:957-62.
- Batt K, Fox-Rushby JA, Castillo-Riquelme M. The costs, effects and cost-effectiveness of strategies to increase coverage of routine immunizations in low- and middle-income countries: systematic review of the grey literature. Bull World Health Organ 2004;82:689-96.
- Fang Y. A Meta-Analysis of Relationships Between Organizational Culture, Organizational Climate, and Nurse Work Outcomes. Baltimore, MD: University of Maryland, Baltimore; 2007.
- Maglione MA, Stone EG, Shekelle PG. Mass mailings have little effect on utilization of influenza vaccine among Medicare beneficiaries. Am J Prev Med 2002;23:43-6. https://doi.org/10.1016/S0749-3797(02)00443-9.
- Ayorinde AA, Williams I, Mannion R, Song F, Skrybant M, Lilford RJ, et al. Publication and related biases in health services research: a systematic review of empirical evidence. BMC Med Res Methodol 2020;20. https://doi.org/10.1186/s12874-020-01010-1.
- Pegurri E, Fox-Rushby JA, Damian W. The effects and costs of expanding the coverage of immunisation services in developing countries: a systematic literature review. Vaccine 2005;23:1624-35. https://doi.org/10.1016/j.vaccine.2004.02.029.
- Nieuwlaat R, Wilczynski N, Navarro T, Hobson N, Jeffery R, Keepanasseril A, et al. Interventions for enhancing medication adherence. Cochrane Database Syst Rev 2014;11. https://doi.org/10.1002/14651858.CD000011.pub4.
- Lu Z, Cao S, Chai Y, Liang Y, Bachmann M, Suhrcke M, et al. Effectiveness of interventions for hypertension care in the community – a meta-analysis of controlled studies in China. BMC Health Serv Res 2012;12. https://doi.org/10.1186/1472-6963-12-216.
- Ayorinde AA, Williams I, Mannion R, Song F, Skrybant M, Lilford RJ, et al. Assessment of publication bias and outcome reporting bias in systematic reviews of health services and delivery research: a meta-epidemiological study. PLOS ONE 2020;15. https://doi.org/10.1371/journal.pone.0227580.
- Donnelly NA, Hickey A, Burns A, Murphy P, Doyle F. Systematic review and meta-analysis of the impact of carer stress on subsequent institutionalisation of community-dwelling older people. PLOS ONE 2015;10. https://doi.org/10.1371/journal.pone.0128213.
- Eskander A, Merdad M, Irish JC, Hall SF, Groome PA, Freeman JL, et al. Volume-outcome associations in head & neck cancer treatment: a systematic review and meta-analysis. Head Neck 2014;36:1820-34. https://doi.org/10.1002/hed.23498.
- Nyamtema A, Urassa D, Roosmalen J. Maternal health interventions in resource limited countries: a systematic review of packages, impacts and factors for change. BMC Pregnancy Childbirth 2011;11:30-42. https://doi.org/10.1186/1471-2393-11-30.
- Santschi V, Chiolero A, Colosimo AL, Platt RW, Taffe P, Burnier M, et al. Improving blood pressure control through pharmacist interventions: a meta-analysis of randomized controlled trials. J Am Heart Assoc 2014;3. https://doi.org/10.1161/JAHA.113.000718.
- Su D, Zhou J, Kelley MS, Michaud TL, Siahpush M, Kim J, et al. Does telemedicine improve treatment outcomes for diabetes? A meta-analysis of results from 55 randomized controlled trials. Diabetes Res Clin Pract 2016;116:136-48. https://doi.org/10.1016/j.diabres.2016.04.019.
- Higgins JP, Altman DG, Gøtzsche PC, Jüni P, Moher D, Oxman AD, et al. The Cochrane Collaboration’s tool for assessing risk of bias in randomised trials. BMJ 2011;343. https://doi.org/10.1136/bmj.d5928.
- Agency for Healthcare Research and Quality . Methods Guide for Effectiveness and Comparative Effectiveness Reviews. 2008.
- Chen YF, Armoiry X, Higenbottam C, Cowley N, Basra R, Watson SI, et al. Magnitude and modifiers of the weekend effect in hospital admissions: a systematic review and meta-analysis. BMJ Open 2019;9. https://doi.org/10.1136/bmjopen-2018-025764.
- Ammenwerth E, Schnell-Inderst P, Machan C, Siebert U. The effect of electronic prescribing on medication errors and adverse drug events: a systematic review. J Am Med Inform Assoc 2008;15:585-600. https://doi.org/10.1197/jamia.M2667.
- Keebler JR, Lazzara EH, Patzer BS, Palmer EM, Plummer JP, Smith DC, et al. Meta-Analyses of the effects of standardized handoff protocols on patient, provider, and organizational outcomes. Hum Factors 2016;58:1187-205. https://doi.org/10.1177/0018720816672309.
- Dixon-Woods M. Using framework-based synthesis for conducting reviews of qualitative studies. BMC Med 2011;9. https://doi.org/10.1186/1741-7015-9-39.
- Givens GH, Smith DD, Tweedie RL. Publication bias in meta-analysis: a Bayesian data-augmentation approach to account for issues exemplified in the passive smoking debate. Stat Sci 1997;12:221-40. https://doi.org/10.1214/ss/1030037958.
- Meacock R, Anselmi L, Kristensen SR, Doran T, Sutton M. Higher mortality rates amongst emergency patients admitted to hospital at weekends reflect a lower probability of admission. J Health Serv Res Policy 2017;22:12-9. https://doi.org/10.1177/1355819616649630.
- Mohammed M, Faisal M, Richardson D, Howes R, Beatson K, Speed K, et al. Impact of the level of sickness on higher mortality in emergency medical admissions to hospital at weekends. J Health Serv Res Policy 2017;22:236-42. https://doi.org/10.1177/1355819617720955.
- Walker AS, Mason A, Quan TP, Fawcett NJ, Watkinson P, Llewelyn M, et al. Mortality risks associated with emergency admissions during weekends and public holidays: an analysis of electronic health records. Lancet 2017;390:62-7. https://doi.org/10.1016/S0140-6736(17)30782-1.
- Altman DG, Bland JM. How to obtain the P value from a confidence interval. BMJ 2011;343. https://doi.org/10.1136/bmj.d2304.
- Bishop DV, Thompson PA. Problems in using p-curve analysis and text-mining to detect rate of p-hacking and evidential value. PeerJ 2016;4. https://doi.org/10.7717/peerj.1715.
- Bruns SB, Ioannidis JP. p-Curve and p-Hacking in observational research. PLOS ONE 2016;11. https://doi.org/10.1371/journal.pone.0149144.
- Cooke RA, Szumal JL, Ashkanasy NM, Wilderom CPM, Peterson MF. Handbook of Organizational Culture & Climate. Los Angeles, CA: Sage; 2011.
- Kopelman RE, Brief AP, Guzzo RA, Scheiner B. Organizational Climate and Culture. San Francisco, CA: Jossey-Bass; 1990.
- Rosenthal R. The file drawer problem and tolerance for null results. Psychol Bull 1979;86:638-41. https://doi.org/10.1037/0033-2909.86.3.638.
- Gelman A, Loken E. The Garden of Forking Paths: Why Multiple Comparisons Can Be a Problem, Even When There Is No ‘Fishing Expedition’ or ‘P-Hacking’ and the Research Hypothesis Was Posited Ahead of Time 2013. www.stat.columbia.edu./~gelman/research/unpublished/p_hacking.pdf (accessed 16 May 2020).
- Ammenwerth E, Schnell-Inderst P, Siebert U. Vision and challenges of evidence-based health informatics: a case study of a CPOE meta-analysis. Int J Med Inform 2010;79:e83-8. https://doi.org/10.1016/j.ijmedinf.2008.11.003.
- Rigby M. Essential prerequisites to the safe and effective widespread roll-out of e-working in healthcare. Int J Med Inform 2006;75:138-47. https://doi.org/10.1016/j.ijmedinf.2005.06.006.
- Cooper H, Hedges LV, Valentine JC. Handbook of Research Synthesis and Meta-Analysis. New York, NY: Russell Sage Foundation; 2009.
- Pucher PH, Johnston MJ, Aggarwal R, Arora S, Darzi A. Effectiveness of interventions to improve patient handover in surgery: a systematic review. Surgery 2015;158:85-9. https://doi.org/10.1016/j.surg.2015.02.017.
- Robertson ER, Morgan L, Bird S, Catchpole K, McCulloch P. Interventions employed to improve intrahospital handover: a systematic review. BMJ Qual Saf 2014;23:600-7. https://doi.org/10.1136/bmjqs-2013-002309.
- Williams I, Ayorinde AA, Mannion R, Song F, Skrybant M, Lilford RJ, et al. Stakeholder views on publication bias in health services research. J Health Serv Res Pol 2020;25:162-71. https://doi.org/10.1177/1355819620902185.
- Song F, Loke Y, Hooper L. Why are medical and health-related studies not being published? A systematic review of reasons given by investigators. PLOS ONE 2014;9. https://doi.org/10.1371/journal.pone.0110418.
- Homedes N, Ugalde A. Are private interests clouding the peer-review process of the WHO bulletin? A case study. Account Res 2016;23:309-17. https://doi.org/10.1080/08989621.2016.1171150.
- Dyer C. Information commissioner condemns health secretary for failing to publish risk register. BMJ 2012;344. https://doi.org/10.1136/bmj.e3480.
- Long KM, McDermott F, Meadows GN. Being pragmatic about healthcare complexity: our experiences applying complexity theory and pragmatism to health services research. BMC Med 2018;16. https://doi.org/10.1186/s12916-018-1087-6.
- Greenhalgh T, Papoutsi C. Studying complexity in health services research: desperately seeking an overdue paradigm shift. BMC Med 2018;16. https://doi.org/10.1186/s12916-018-1089-4.
- Ziai H, Zhang R, Chan AW, Persaud N. Search for unpublished data by systematic reviewers: an audit. BMJ Open 2017;7. https://doi.org/10.1136/bmjopen-2017-017737.
- Herrmann D, Sinnett P, Holmes J, Khan S, Koller C, Vassar M. Statistical controversies in clinical research: publication bias evaluations are not routinely conducted in clinical oncology systematic reviews. Ann Oncol 2017;28:931-7. https://doi.org/10.1093/annonc/mdw691.
- Chapman SJ, Drake TM, Bolton WS, Barnard J, Bhangu A. Longitudinal analysis of reporting and quality of systematic reviews in high-impact surgical journals. Br J Surg 2017;104:198-204. https://doi.org/10.1002/bjs.10423.
- Page MJ, Shamseer L, Altman DG, Tetzlaff J, Sampson M, Tricco AC, et al. Epidemiology and reporting characteristics of systematic reviews of biomedical research: a cross-sectional study. PLOS Med 2016;13. https://doi.org/10.1371/journal.pmed.1002028.
- Kirkham JJ, Dwan KM, Altman DG, Gamble C, Dodd S, Smyth R, et al. The impact of outcome reporting bias in randomised controlled trials on a cohort of systematic reviews. BMJ 2010;340. https://doi.org/10.1136/bmj.c365.
- Gough D, Thomas J, Oliver S. Clarifying differences between review designs and methods. Syst Rev 2012;1. https://doi.org/10.1186/2046-4053-1-28.
- Wells GA, Shea B, O’Connell D, Peterson J, Welch V, Losos M, et al. The Newcastle-Ottawa Scale (NOS) for Assessing the Quality of Nonrandomised Studies in Meta-Analyses n.d. www.ohri.ca/programs/clinical_epidemiology/oxford.asp (accessed 13 February 2014).
- Tijdink JK, Schipper K, Bouter LM, Maclaine Pont P, de Jonge J, Smulders YM. How do scientists perceive the current publication culture? A qualitative focus group interview study among Dutch biomedical researchers. BMJ Open 2016;6. https://doi.org/10.1136/bmjopen-2015-008681.
- Bytautas JP, Gheihman G, Dobrow MJ. A scoping review of online repositories of quality improvement projects, interventions and initiatives in healthcare. BMJ Qual Saf 2017;26:296-303. https://doi.org/10.1136/bmjqs-2015-005092.
- Kepes S, Banks GC, McDaniel M, Whetzel DL. Publication bias in the organizational sciences. Organ Res Methods 2012;15:624-62. https://doi.org/10.1177/1094428112452760.
- Dalton DR, Aguinis H, Dalton CM, Bosco FA, Pierce CA. Revisiting the file drawer problem in meta-analysis: an assessment of published and nonpublished correlation matrices. Pers Psychol 2012;65:221-49. https://doi.org/10.1111/j.1744-6570.2012.01243.x.
- Stemig MS, Sackett PR. Another Look Into the File Drawer Problem in Meta-Analysis n.d.
- Paterson TA, Harms PD, Steel P, Crede M. An assessment of the magnitude of effect sizes: evidence from 30 years of meta-analysis in management. J Leadersh Organ Stud 2016;23:66-81. https://doi.org/10.1177/1548051815614321.
- Science Europe . Plan S: Making Full and Immediate Open Access a Reality 2019. www.coalition-s.org/ (accessed 18 October 2019).
- Kurien M, Sanders DS, Ashton JJ, Beattie RM. Should I publish in an open access journal?. BMJ 2019;365. https://doi.org/10.1136/bmj.l1544.
- Wye L, Brangan E, Cameron A, Gabbay J, Klein JH, Pope C. Evidence based policy making and the ‘art’ of commissioning – how English healthcare commissioners access and use information and academic research in ‘real life’ decision-making: an empirical qualitative study. BMC Health Serv Res 2015;15. https://doi.org/10.1186/s12913-015-1091-x.
- Cairney P, Kwiatkowski R. How to communicate effectively with policymakers: combine insights from psychology and policy studies. Palgrave Commun 2017;3. https://doi.org/10.1057/s41599-017-0046-8.
- Lavis JN, Ross SE, Hurley JE, Hohenadel JM, Stoddart GL, Woodward CA, et al. Examining the role of health services research in public policymaking. Milbank Q 2002;80:125-54. https://doi.org/10.1111/1468-0009.00005.
- Abdalla G, Moran-Atkin E, Chen G, Schweitzer MA, Magnuson TH, Steele KE. The effect of warm-up on surgical performance: a systematic review. Surg Endosc 2015;29:1259-69. https://doi.org/10.1007/s00464-014-3811-4.
- Akl EA, Kairouz VF, Sackett KM, Erdley WS, Mustafa RA, Fiander M, et al. Educational games for health professionals. Cochrane Database of Syst Rev 2013;3. https://doi.org/10.1002/14651858.CD006411.pub3.
- Algie CM, Mahar RK, Wasiak J, Batty L, Gruen RL, Mahar PD. Interventions for reducing wrong-site surgery and invasive clinical procedures. Cochrane Database Syst Rev 2015;3. https://doi.org/10.1002/14651858.CD009404.pub3.
- Al-Muhandis N, Hunter PR. The value of educational messages embedded in a community-based approach to combat dengue Fever: a systematic review and meta regression analysis. PLOS Negl Trop Dis 2011;5. https://doi.org/10.1371/journal.pntd.0001278.
- Aoyagi Y, Beck CR, Dingwall R, Nguyen-Van-Tam JS. Healthcare workers’ willingness to work during an influenza pandemic: a systematic review and meta-analysis. Influenza Other Respir Viruses 2015;9:120-30. https://doi.org/10.1111/irv.12310.
- Arditi C, Rège-Walther M, Wyatt JC, Durieux P, Burnand B. Computer-generated reminders delivered on paper to healthcare professionals; effects on professional practice and health care outcomes. Cochrane Database Syst Rev 2012;12. https://doi.org/10.1002/14651858.CD001175.pub3.
- Aubin M, Giguère A, Martin M, Verreault R, Fitch MI, Kazanjian A, et al. Interventions to improve continuity of care in the follow-up of patients with cancer. Cochrane Database Syst Rev 2012;7. https://doi.org/10.1002/14651858.CD007672.pub2.
- Badamgarav E, Weingarten SR, Henning JM, Knight K, Hasselblad V, Gano A, et al. Effectiveness of disease management programs in depression: a systematic review. Am J Psychiatry 2003;160:2080-90. https://doi.org/10.1176/appi.ajp.160.12.2080.
- Baker R, Camosso-Stefinovic J, Gillies C, Shaw EJ, Cheater F, Flottorp S, et al. Tailored interventions to address determinants of practice. Cochrane Database Syst Rev 2015;4. https://doi.org/10.1002/14651858.CD005470.pub3.
- Ballini L, Negro A, Maltoni S, Vignatelli L, Flodgren G, Simera I, et al. Interventions to reduce waiting times for elective procedures. Cochrane Database Syst Rev 2015;2. https://doi.org/10.1002/14651858.CD005610.pub2.
- Balogh R, McMorris CA, Lunsky Y, Ouellette-Kuntz H, Bourne L, Colantonio A, et al. Organising healthcare services for persons with an intellectual disability. Cochrane Database Syst Rev 2016;4. https://doi.org/10.1002/14651858.CD007492.pub2.
- Barnard S, Kim C, Park MH, Ngo TD. Doctors or mid-level providers for abortion. Cochrane Database Syst Rev 2015;7. https://doi.org/10.1002/14651858.CD011242.pub2.
- Baskerville NB, Liddy C, Hogg W. Systematic review and meta-analysis of practice facilitation within primary care settings. Ann Fam Med 2012;10:63-74. https://doi.org/10.1370/afm.1312.
- Berdot S, Gillaizeau F, Caruba T, Prognon P, Durieux P, Sabatier B. Drug administration errors in hospital inpatients: a systematic review. PLOS ONE 2013;8. https://doi.org/10.1371/journal.pone.0068856.
- Bos JM, van den Bemt PM, de Smet PA, Kramers C. The effect of prescriber education on medication-related patient harm in the hospital: a systematic review. Br J Clin Pharmacol 2017;83:953-61. https://doi.org/10.1111/bcp.13200.
- Bosch-Capblanch X, Liaqat S, Garner P. Managerial supervision to improve primary health care in low- and middle-income countries. Cochrane Database Syst Rev 2011;9. https://doi.org/10.1002/14651858.CD006413.pub2.
- Bradford DW, Cunningham NT, Slubicki MN, McDuffie JR, Kilbourne AM, Nagi A, et al. An evidence synthesis of care models to improve general medical outcomes for individuals with serious mental illness: a systematic review. J Clin Psychiatry 2013;74:e754-64. https://doi.org/10.4088/JCP.12r07666.
- Brasil PE, Braga JU. Meta-analysis of factors related to health services that predict treatment default by tuberculosis patients. Cad Saude Publica 2008;24:s485-502. https://doi.org/10.1590/S0102-311X2008001600003.
- Brocklehurst P, Price J, Glenny AM, Tickle M, Birch S, Mertz E, et al. The effect of different methods of remuneration on the behaviour of primary care dentists. Cochrane Database Syst Rev 2013;11. https://doi.org/10.1002/14651858.CD009853.pub2.
- Brown L, Forster A, Young J, Crocker T, Benham A, Langhorne P. Day Hospital Group . Medical day hospital care for older people versus alternative forms of care. Cochrane Database Syst Rev 2015;6. https://doi.org/10.1002/14651858.CD001730.pub3.
- Butler M, Collins R, Drennan J, Halligan P, O’Mathúna DP, Schultz TJ, et al. Hospital nurse staffing models and patient and staff-related outcomes. Cochrane Database Syst Rev 2011;7. https://doi.org/10.1002/14651858.CD007019.pub2.
- Campanella P, Lovato E, Marone C, Fallacara L, Mancuso A, Ricciardi W, et al. The impact of electronic health records on healthcare quality: a systematic review and meta-analysis. Eur J Public Health 2016;26:60-4. https://doi.org/10.1093/eurpub/ckv122.
- Campbell F, Biggs K, Aldiss SK, O’Neill PM, Clowes M, McDonagh J, et al. Transition of care for adolescents from paediatric services to adult health services. Cochrane Database Syst Rev 2016;4. https://doi.org/10.1002/14651858.CD009794.pub2.
- Cappuccio FP, Kerry SM, Forbes L, Donald A. Blood pressure control by home monitoring: meta-analysis of randomised trials. BMJ 2004;329. https://doi.org/10.1136/bmj.38121.684410.AE.
- Chaillet N, Dumont A. Evidence-based strategies for reducing cesarean section rates: a meta-analysis. Birth 2007;34:53-64. https://doi.org/10.1111/j.1523-536X.2006.00146.x.
- Chang CW, Shih SC, Wang HY, Chu CH, Wang TE, Hung CY, et al. Meta-analysis: the effect of patient education on bowel preparation for colonoscopy. Endosc Int Open 2015;3:E646-52. https://doi.org/10.1055/s-0034-1392365.
- Chen CC, Chen Y, Liu X, Wen Y, Ma DY, Huang YY, et al. The efficacy of a nurse-led disease management program in improving the quality of life for patients with chronic kidney disease: a meta-analysis. PLOS ONE 2016;11. https://doi.org/10.1371/journal.pone.0155890.
- Chen Y, Jiang J, Wu Y, Yan J, Chen H, Zhu X. Does hospital-based transitional care reduce the postoperative complication in patients with enterostomy? A meta-analysis. J Cancer Res Ther 2016;12:76-8. https://doi.org/10.4103/0973-1482.191637.
- Chen Z, King W, Pearcey R, Kerba M, Mackillop WJ. The relationship between waiting time for radiotherapy and clinical outcomes: a systematic review of the literature. Radiother Oncol 2008;87:3-16. https://doi.org/10.1016/j.radonc.2007.11.016.
- Chodosh J, Morton SC, Mojica W, Maglione M, Suttorp MJ, Hilton L, et al. Meta-analysis: chronic disease self-management programs for older adults. Ann Intern Med 2005;143:427-38. https://doi.org/10.7326/0003-4819-143-6-200509200-00007.
- Christensen M, Lundh A. Medication review in hospitalised patients to reduce morbidity and mortality. Cochrane Database Syst Rev 2016;2. https://doi.org/10.1002/14651858.CD008986.pub3.
- Connock M, Stevens C, Fry-Smith A, Jowett S, Fitzmaurice D, Moore D, et al. Clinical effectiveness and cost-effectiveness of different models of managing long-term oral anticoagulation therapy: a systematic review and economic modelling. Health Technol Assess 2007;11. https://doi.org/10.3310/hta11380.
- Cook O, McIntyre M, Recoche K. Exploration of the role of specialist nurses in the care of women with gynaecological cancer: a systematic review. J Clin Nurs 2015;24:683-95. https://doi.org/10.1111/jocn.12675.
- Corbett M, Heirs M, Rose M, Smith A, Stirk L, Richardson G, et al. The delivery of chemotherapy at home: an evidence synthesis. Health Services and Delivery Research 2015;3. https://doi.org/10.3310/hsdr03140.
- Costa-Font J, Gemmill M, Rubert G. Biases in the healthcare luxury good hypothesis?: a meta-regression analysis. J R Stat Soc A 2011;174:95-107. https://doi.org/10.1111/j.1467-985X.2010.00653.x.
- Cui M, Wu X, Mao J, Wang X, Nie M. T2DM Self-management via smartphone applications: a systematic review and meta-analysis. PLOS ONE 2016;11. https://doi.org/10.1371/journal.pone.0166718.
- Cuijpers P, Donker T, Johansson R, Mohr DC, van Straten A, Andersson G. Self-guided psychological treatment for depressive symptoms: a meta-analysis. PLOS ONE 2011;6. https://doi.org/10.1371/journal.pone.0021274.
- De Luca G, Biondi-Zoccai G, Marino P. Transferring patients with ST-segment elevation myocardial infarction for mechanical reperfusion: a meta-regression analysis of randomized trials. Ann Emerg Med 2008;52:665-76. https://doi.org/10.1016/j.annemergmed.2008.08.033.
- de Lusignan S, Mold F, Sheikh A, Majeed A, Wyatt JC, Quinn T, et al. Patients’ online access to their electronic health records and linked online services: a systematic interpretative review. BMJ Open 2014;4. https://doi.org/10.1136/bmjopen-2014-006021.
- de Roten Y, Zimmermann G, Ortega D, Despland JN. Meta-analysis of the effects of MI training on clinicians’ behavior. J Subst Abuse Treat 2013;45:155-62. https://doi.org/10.1016/j.jsat.2013.02.006.
- Demonceau J, Ruppar T, Kristanto P, Hughes DA, Fargher E, Kardas P, et al. Identification and assessment of adherence-enhancing interventions in studies assessing medication adherence through electronically compiled drug dosing histories: a systematic literature review and meta-analysis. Drugs 2013;73:545-62. https://doi.org/10.1007/s40265-013-0041-3.
- Donald F, Kilpatrick K, Reid K, Carter N, Martin-Misener R, Bryant-Lukosius D, et al. A systematic review of the cost-effectiveness of nurse practitioners and clinical nurse specialists: what is the quality of the evidence?. Nurs Res Pract 2014;2014. https://doi.org/10.1155/2014/896587.
- Dorresteijn JA, Kriegsman DM, Assendelft WJ, Valk GD. Patient education for preventing diabetic foot ulceration. Cochrane Database Syst Rev 2010;5. https://doi.org/10.1002/14651858.CD001488.pub3.
- Dudley L, Garner P. Strategies for integrating primary health services in low- and middle-income countries at the point of delivery. Cochrane Database Syst Rev 2011;7. https://doi.org/10.1002/14651858.CD003318.pub3.
- Dyer TA, Brocklehurst P, Glenny AM, Davies L, Tickle M, Issac A, et al. Dental auxiliaries for dental care traditionally provided by dentists. Cochrane Database Syst Rev 2014;8. https://doi.org/10.1002/14651858.CD010076.pub2.
- Easthall C, Watson S, Wright D, Wood J, Bhattacharya D. The impact of motivational interviewing (MI) as an intervention to improve medication adherence: a meta-analysis. International Journal of Pharmacy Practice 2012;20.
- Fiander M, McGowan J, Grad R, Pluye P, Hannes K, Labrecque M, et al. Interventions to increase the use of electronic health information by healthcare practitioners to improve clinical practice and patient outcomes. Cochrane Database Syst Rev 2015;3. https://doi.org/10.1002/14651858.CD004749.pub3.
- Fisher E, Law E, Palermo TM, Eccleston C. Psychological therapies (remotely delivered) for the management of chronic and recurrent pain in children and adolescents. Cochrane Database Syst Rev 2015;3. https://doi.org/10.1002/14651858.CD011118.pub2.
- Fletcher KE, Reed DA, Arora VM. Patient safety, resident education and resident well-being following implementation of the 2003 ACGME duty hour rules. J Gen Intern Med 2011;26:907-19. https://doi.org/10.1007/s11606-011-1657-1.
- Flodgren G, Deane K, Dickinson HO, Kirk S, Alberti H, Beyer FR, et al. Interventions to change the behaviour of health professionals and the organisation of care to promote weight reduction in overweight and obese people. Cochrane Database Syst Rev 2010;3. https://doi.org/10.1002/14651858.CD000984.pub2.
- Flodgren G, Parmelli E, Doumit G, Gattellari M, O’Brien MA, Grimshaw J, et al. Local opinion leaders: effects on professional practice and health care outcomes. Cochrane Database Syst Rev 2011;8. https://doi.org/10.1002/14651858.CD000125.pub4.
- Flodgren G, Pomey MP, Taber SA, Eccles MP. Effectiveness of external inspection of compliance with standards in improving healthcare organisation behaviour, healthcare professional behaviour or patient outcomes. Cochrane Database Syst Rev 2011;11. https://doi.org/10.1002/14651858.CD008992.pub2.
- Flodgren G, Conterno LO, Mayhew A, Omar O, Pereira CR, Shepperd S. Interventions to improve professional adherence to guidelines for prevention of device-related infections. Cochrane Database Syst Rev 2013;3. https://doi.org/10.1002/14651858.CD006559.pub2.
- Forsetlund L, Bjørndal A, Rashidian A, Jamtvedt G, O’Brien MA, Wolf F, et al. Continuing education meetings and workshops: effects on professional practice and health care outcomes. Cochrane Database Syst Rev 2009;2. https://doi.org/10.1002/14651858.CD003030.pub2.
- Foy R, Hempel S, Rubenstein L, Suttorp M, Seelig M, Shanman R, et al. Meta-analysis: effect of interactive communication between collaborating primary care physicians and specialists. Ann Intern Med 2010;152:247-58. https://doi.org/10.7326/0003-4819-152-4-201002160-00010.
- Free C, Phillips G, Galli L, Watson L, Felix L, Edwards P, et al. The effectiveness of mobile-health technology-based health behaviour change or disease management interventions for health care consumers: a systematic review. PLOS Med 2013;10. https://doi.org/10.1371/journal.pmed.1001362.
- French SD, Green S, Buchbinder R, Barnes H. Interventions for improving the appropriate use of imaging in people with musculoskeletal conditions. Cochrane Database Syst Rev 2010;1. https://doi.org/10.1002/14651858.CD006094.pub2.
- Gallagher RW. A Meta-Analysis of Cultural Competence Education in Professional Nurses and Nursing Students. Tampa, FL, USA: University of South Florida; 2011.
- Gardner MP, Adams A, Jeffreys M. Interventions to increase the uptake of mammography amongst low income women: a systematic review and meta-analysis. PLOS ONE 2013;8. https://doi.org/10.1371/journal.pone.0055574.
- Gemmill MC, Costa-Font J, McGuire A. In search of a corrected prescription drug elasticity estimate: a meta-regression approach. Health Econ 2007;16:627-43. https://doi.org/10.1002/hec.1190.
- Gensichen J, Beyer M, Muth C, Gerlach FM, Von Korff M, Ormel J. Case management to improve major depression in primary health care: a systematic review. Psychol Med 2006;36:7-14. https://doi.org/10.1017/S0033291705005568.
- Giguère A, Légaré F, Grimshaw J, Turcotte S, Fiander M, Grudniewicz A, et al. Printed educational materials: effects on professional practice and healthcare outcomes. Cochrane Database Syst Rev 2012;10. https://doi.org/10.1002/14651858.CD004398.pub3.
- Gillaizeau F, Chan E, Trinquart L, Colombet I, Walton RT, Rège-Walther M, et al. Computerized advice on drug dosage to improve prescribing practice. Cochrane Database Syst Rev 2013;11. https://doi.org/10.1002/14651858.CD002894.pub3.
- Gillies D, Buykx P, Parker AG, Hetrick SE. Consultation liaison in primary care for people with mental disorders. Cochrane Database Syst Rev 2015;9. https://doi.org/10.1002/14651858.CD007193.pub2.
- Göhler A, Januzzi JL, Worrell SS, Osterziel KJ, Gazelle GS, Dietz R, et al. A systematic meta-analysis of the efficacy and heterogeneity of disease management programs in congestive heart failure. J Card Fail 2006;12:554-67. https://doi.org/10.1016/j.cardfail.2006.03.003.
- Goldzweig CL, Orshansky G, Paige NM, Miake-Lye IM, Beroes JM, Ewing BA, et al. Electronic health record-based interventions for improving appropriate diagnostic imaging: a systematic review and meta-analysis. Ann Intern Med 2015;162:557-65. https://doi.org/10.7326/M14-2600.
- Gooiker GA, van Gijn W, Post PN, van de Velde CJ, Tollenaar RA, Wouters MW. A systematic review and meta-analysis of the volume-outcome relationship in the surgical treatment of breast cancer. Are breast cancer patients better of with a high volume provider?. Eur J Surg Oncol 2010;36:27-35. https://doi.org/10.1016/j.ejso.2010.06.024.
- Goossens-Laan CA, Gooiker GA, van Gijn W, Post PN, Bosch JL, Kil PJ, et al. A systematic review and meta-analysis of the relationship between hospital/surgeon volume and outcome for radical cystectomy: an update for the ongoing debate. Eur Urol 2011;59:775-83. https://doi.org/10.1016/j.eururo.2011.01.037.
- Green CJ, Maclure M, Fortin PM, Ramsay CR, Aaserud M, Bardal S. Pharmaceutical policies: effects of restrictions on reimbursement. Cochrane Database Syst Rev 2010;8. https://doi.org/10.1002/14651858.CD008654.
- Grobler L, Marais BJ, Mabunda S. Interventions for increasing the proportion of health professionals practising in rural and other underserved areas. Cochrane Database Syst Rev 2015;6. https://doi.org/10.1002/14651858.CD005314.pub3.
- Grochtdreis T, Brettschneider C, Wegener A, Watzke B, Riedel-Heller S, Härter M, et al. Cost-effectiveness of collaborative care for the treatment of depressive disorders in primary care: a systematic review. PLOS ONE 2015;10. https://doi.org/10.1371/journal.pone.0123078.
- Gurusamy KS, Vaughan J, Davidson BR. Formal education of patients about to undergo laparoscopic cholecystectomy. Cochrane Database Syst Rev 2014;2. https://doi.org/10.1002/14651858.CD009933.pub2.
- Han HR, Lee JE, Kim J, Hedlin HK, Song H, Kim MT. A meta-analysis of interventions to promote mammography among ethnic minority women. Nurs Res 2009;58:246-54. https://doi.org/10.1097/NNR.0b013e3181ac0f7f.
- Hata T, Motoi F, Ishida M, Naitoh T, Katayose Y, Egawa S, et al. Effect of hospital volume on surgical outcomes after pancreaticoduodenectomy: a systematic review and meta-analysis. Annals of Surgery 2016;263:664-72. https://doi.org/10.1097/SLA.0000000000001437.
- Hayhurst KP, Leitner M, Davies L, Flentje R, Millar T, Jones A, et al. The effectiveness and cost-effectiveness of diversion and aftercare programmes for offenders using class A drugs: a systematic review and economic evaluation. Health Technol Assess 2015;19. https://doi.org/10.3310/hta19060.
- Higginson IJ, Finlay IG, Goodwin DM, Hood K, Edwards AG, Cook A, et al. Is there evidence that palliative care teams alter end-of-life experiences of patients and their caregivers?. J Pain Symptom Manage 2003;25:150-68. https://doi.org/10.1016/S0885-3924(02)00599-7.
- Hodgkinson B, Haesler EJ, Nay R, O’Donnell MH, McAuliffe LP. Effectiveness of staffing models in residential, subacute, extended aged care settings on patient and staff outcomes. Cochrane Database Syst Rev 2011;6. https://doi.org/10.1002/14651858.CD006563.pub2.
- Horsley T, Hyde C, Santesso N, Parkes J, Milne R, Stewart R. Teaching critical appraisal skills in healthcare settings. Cochrane Database Syst Rev 2011;11. https://doi.org/10.1002/14651858.CD001270.pub2.
- Ivers N, Jamtvedt G, Flottorp S, Young JM, Odgaard-Jensen J, French SD, et al. Audit and feedback: effects on professional practice and healthcare outcomes. Cochrane Database Syst Rev 2012;6. https://doi.org/10.1002/14651858.CD000259.pub3.
- Jacobson Vann JC, Szilagyi P. Patient reminder and patient recall systems to improve immunization rates. Cochrane Database Syst Rev 2005;3. https://doi.org/10.1002/14651858.CD003941.pub2.
- Jamal MH, Doi SA, Rousseau M, Edwards M, Rao C, Barendregt JJ, et al. Systematic review and meta-analysis of the effect of North American working hours restrictions on mortality and morbidity in surgical patients. Br J Surg 2012;99:336-44. https://doi.org/10.1002/bjs.8657.
- Jia L, Yuan B, Huang F, Lu Y, Garner P, Meng Q. Strategies for expanding health insurance coverage in vulnerable populations. Cochrane Database Syst Rev 2014;11. https://doi.org/10.1002/14651858.CD008194.pub3.
- Kahn SR, Morrison DR, Cohen JM, Emed J, Tagalakis V, Roussin A, et al. Interventions for implementation of thromboprophylaxis in hospitalized medical and surgical patients at risk for venous thromboembolism. Cochrane Database Syst Rev 2013;7. https://doi.org/10.1002/14651858.CD008201.pub2.
- Karmali KN, Davies P, Taylor F, Beswick A, Martin N, Ebrahim S. Promoting patient uptake and adherence in cardiac rehabilitation. Cochrane Database Syst Rev 2014;6. https://doi.org/10.1002/14651858.CD007131.pub3.
- Kelley JM, Kraft-Todd G, Schapira L, Kossowsky J, Riess H. The influence of the patient-clinician relationship on healthcare outcomes: a systematic review and meta-analysis of randomized controlled trials. PLOS ONE 2014;9. https://doi.org/10.1371/journal.pone.0094207.
- Kerlin MP, Adhikari NK, Rose L, Wilcox ME, Bellamy CJ, Costa DK, et al. An official American Thoracic Society systematic review: the effect of nighttime intensivist staffing on mortality and length of stay among intensive care unit patients. Am J Respir Crit Care Med 2017;195:383-93. https://doi.org/10.1164/rccm.201611-2250ST.
- Ketelaar NABM, Faber MJ, Flottorp S, Rygh LH, Deane KHO, Eccles MP. Public release of performance data in changing the behaviour of healthcare consumers, professionals or organisations. Cochrane Database of Syst Rev 2011;11. https://doi.org/10.1002/14651858.CD004538.pub2.
- Khangura JK, Flodgren G, Perera R, Rowe BH, Shepperd S. Primary care professionals providing non-urgent care in hospital emergency departments. Cochrane Database Syst Rev 2012;11. https://doi.org/10.1002/14651858.CD002097.pub3.
- Khunpradit S, Tavender E, Lumbiganon P, Laopaiboon M, Wasiak J, Gruen RL. Non-clinical interventions for reducing unnecessary caesarean section. Cochrane Database Syst Rev 2011;6. https://doi.org/10.1002/14651858.CD005528.pub2.
- Kilpatrick K, Reid K, Carter N, Donald F, Bryant-Lukosius D, Martin-Misener R, et al. A systematic review of the cost-effectiveness of clinical nurse specialists and nurse practitioners in inpatient roles. Nurs Leadersh 2015;28:56-7.
- Kim YJ, Soeken KL. A meta-analysis of the effect of hospital-based case management on hospital length-of-stay and readmission. Nurs Res 2005;54:255-64. https://doi.org/10.1097/00006199-200507000-00007.
- Kroon FP, van der Burg LR, Buchbinder R, Osborne RH, Johnston RV, Pitt V. Self-management education programmes for osteoarthritis. Cochrane Database Syst Rev 2014;1. https://doi.org/10.1002/14651858.CD008963.pub2.
- Lambrinou E, Kalogirou F, Lamnisos D, Sourtzi P. Effectiveness of heart failure management programmes with nurse-led discharge planning in reducing re-admissions: a systematic review and meta-analysis. Int J Nurs Stud 2012;49:610-24. https://doi.org/10.1016/j.ijnurstu.2011.11.002.
- Lau D, Hu J, Majumdar SR, Storie DA, Rees SE, Johnson JA. Interventions to improve influenza and pneumococcal vaccination rates among community-dwelling adults: a systematic review and meta-analysis. Ann Fam Med 2012;10:538-46. https://doi.org/10.1370/afm.1405.
- Laver KE, Schoene D, Crotty M, George S, Lannin NA, Sherrington C. Telerehabilitation services for stroke. Cochrane Database of Syst Rev 2013;12. https://doi.org/10.1002/14651858.CD010255.pub2.
- Lin H, Wu X. Intervention strategies for improving patient adherence to follow-up in the era of mobile information technology: a systematic review and meta-analysis. PLOS ONE 2014;9. https://doi.org/10.1371/journal.pone.0104266.
- Luiza VL, Chaves LA, Silva RM, Emmerick IC, Chaves GC, Fonseca de Araújo SC, et al. Pharmaceutical policies: effects of cap and co-payment on rational use of medicines. Cochrane Database Syst Rev 2015;5. https://doi.org/10.1002/14651858.CD007017.pub2.
- Lutge EE, Wiysonge CS, Knight SE, Sinclair D, Volmink J. Incentives and enablers to improve adherence in tuberculosis. Cochrane Database Syst Rev 2015;9. https://doi.org/10.1002/14651858.CD007952.pub3.
- Lv L, Shao YF, Zhou YB. The enhanced recovery after surgery (ERAS) pathway for patients undergoing colorectal surgery: an update of meta-analysis of randomized controlled trials. Int J Colorectal Dis 2012;27:1549-54. https://doi.org/10.1007/s00384-012-1577-5.
- Ma N, Cameron A, Tivey D, Grae N, Roberts S, Morris A. Systematic review of a patient care bundle in reducing staphylococcal infections in cardiac and orthopaedic surgery. ANZ J Surg 2017;87:239-46. https://doi.org/10.1111/ans.13879.
- Maaskant JM, Vermeulen H, Apampa B, Fernando B, Ghaleb MA, Neubert A, et al. Interventions for reducing medication errors in children in hospital. Cochrane Database Syst Rev 2015;3. https://doi.org/10.1002/14651858.CD006208.pub3.
- McBain H, Mulligan K, Haddad M, Flood C, Jones J, Simpson A. Self management interventions for type 2 diabetes in adult people with severe mental illness. Cochrane Database Syst Rev 2016;4. https://doi.org/10.1002/14651858.CD011361.pub2.
- McGowan JL, Grad R, Pluye P, Hannes K, Deane K, Labrecque M, et al. Electronic retrieval of health information by healthcare providers to improve practice and patient care. Cochrane Database Syst Rev 2009;3. https://doi.org/10.1002/14651858.CD004749.pub2.
- Ming WK, Mackillop LH, Farmer AJ, Loerup L, Bartlett K, Levy JC, et al. Telemedicine Technologies for Diabetes in Pregnancy: A Systematic Review and Meta-Analysis. J Med Internet Res 2016;18. https://doi.org/10.2196/jmir.6556.
- Mitchell GK, Burridge L, Zhang J, Donald M, Scott IA, Dart J, et al. Systematic review of integrated models of health care delivered at the primary-secondary interface: how effective is it and what determines effectiveness?. Aust J Prim Health 2015;21:391-408. https://doi.org/10.1071/PY14172.
- Moja L, Kwag KH, Lytras T, Bertizzolo L, Brandt L, Pecoraro V, et al. Effectiveness of computerized decision support systems linked to electronic health records: a systematic review and meta-analysis. Am J Public Health 2014;104:e12-22. https://doi.org/10.2105/AJPH.2014.302164.
- Murphy SM, Irving CB, Adams CE, Waqar M. Crisis intervention for people with severe mental illnesses. Cochrane Database Syst Rev 2015;12. https://doi.org/10.1002/14651858.CD001087.pub5.
- Murthy L, Shepperd S, Clarke MJ, Garner SE, Lavis JN, Perrier L, et al. Interventions to improve the use of systematic reviews in decision-making by health system managers, policy makers and clinicians. Cochrane Database Syst Rev 2012;9. https://doi.org/10.1002/14651858.CD009401.pub2.
- Neumeyer-Gromen A, Lampert T, Stark K, Kallischnigg G. Disease management programs for depression: a systematic review and meta-analysis of randomized controlled trials. Med Care 2004;42:1211-21. https://doi.org/10.1097/00005650-200412000-00008.
- Nglazi MD, Bekker LG, Wood R, Hussey GD, Wiysonge CS. Mobile phone text messaging for promoting adherence to anti-tuberculosis treatment: a systematic review. BMC Infect Dis 2013;13. https://doi.org/10.1186/1471-2334-13-566.
- Oliver D, Hopper A, Seed P. Do hospital fall prevention programs work? A systematic review. J Am Geriatr Soc 2000;48:1679-89. https://doi.org/10.1111/j.1532-5415.2000.tb03883.x.
- Opiyo N, English M. In-service training for health professionals to improve care of seriously ill newborns and children in low-income countries. Cochrane Database Syst Rev 2015;5. https://doi.org/10.1002/14651858.CD007071.pub3.
- Opiyo N, Yamey G, Garner P. Subsidising artemisinin-based combination therapy in the private retail sector. Cochrane Database Syst Rev 2016;3. https://doi.org/10.1002/14651858.CD009926.pub2.
- O’Sullivan JW, Harvey RT, Glasziou PP, McCullough A. Written information for patients (or parents of child patients) to reduce the use of antibiotics for acute upper respiratory tract infections in primary care. Cochrane Database Syst Rev 2016;11. https://doi.org/10.1002/14651858.CD011360.pub2.
- Panagioti M, Richardson G, Murray E, Rodgers A, Kennedy A, Newman S, et al. Reducing care utilisation through self-management interventions (RECURSIVE): a systematic review and meta-analysis. Health Services and Delivery Research 2014;2. https://doi.org/10.3310/hsdr02540.
- Pande S, Hiller JE, Nkansah N, Bero L. The effect of pharmacist-provided non-dispensing services on patient outcomes, health service utilisation and costs in low- and middle-income countries. Cochrane Database Syst Rev 2013;2. https://doi.org/10.1002/14651858.CD010398.
- Parmelli E, Flodgren G, Fraser SG, Williams N, Rubin G, Eccles MP. Interventions to increase clinical incident reporting in health care. Cochrane Database Syst Rev 2012;8. https://doi.org/10.1002/14651858.CD005609.pub2.
- Peñaloza B, Pantoja T, Bastías G, Herrera C, Rada G. Interventions to reduce emigration of health care professionals from low- and middle-income countries. Cochrane Database Syst Rev 2011;9. https://doi.org/10.1002/14651858.CD007673.pub2.
- Perrier L, Mrklas K, Shepperd S, Dobbins M, McKibbon KA, Straus SE. Interventions encouraging the use of systematic reviews in clinical decision-making: a systematic review. J Gen Intern Med 2011;26:419-26. https://doi.org/10.1007/s11606-010-1506-7.
- Peterson AM, Takiya L, Finley R. Meta-analysis of trials of interventions to improve medication adherence. Am J Health Syst Pharm 2003;60:657-65. https://doi.org/10.1093/ajhp/60.7.657.
- Peytremann-Bridevaux I, Arditi C, Gex G, Bridevaux PO, Burnand B. Chronic disease management programmes for adults with asthma. Cochrane Database Syst Rev 2015;5. https://doi.org/10.1002/14651858.CD007988.pub2.
- Rachoin JS, Skaf J, Cerceo E, Fitzpatrick E, Milcarek B, Kupersmith E, et al. The impact of hospitalists on length of stay and costs: systematic review and meta-analysis. Am J Manag Care 2012;18:e23-30.
- Rashidian A, Omidvari AH, Vali Y, Sturm H, Oxman AD. Pharmaceutical policies: effects of financial incentives for prescribers. Cochrane Database Syst Rev 2015;8. https://doi.org/10.1002/14651858.CD006731.pub2.
- Reeves S, Perrier L, Goldman J, Freeth D, Zwarenstein M. Interprofessional education: effects on professional practice and healthcare outcomes (update). Cochrane Database Syst Rev 2013;3. https://doi.org/10.1002/14651858.CD002213.pub3.
- Robotham D, Satkunanathan S, Reynolds J, Stahl D, Wykes T. Using digital notifications to improve attendance in clinic: systematic review and meta-analysis. BMJ Open 2016;6. https://doi.org/10.1136/bmjopen-2016-012116.
- Rockers PC, Bärnighausen T. Interventions for hiring, retaining and training district health systems managers in low- and middle-income countries. Cochrane Database Syst Rev 2013;4. https://doi.org/10.1002/14651858.CD009035.pub2.
- Ross Middleton KM, Patidar SM, Perri MG. The impact of extended care on the long-term maintenance of weight loss: a systematic review and meta-analysis. Obes Rev 2012;13:509-17. https://doi.org/10.1111/j.1467-789X.2011.00972.x.
- Rotter T, Kinsman L, James E, Machotta A, Gothe H, Willis J, et al. Clinical pathways: effects on professional practice, patient outcomes, length of stay and hospital costs. Cochrane Database Syst Rev 2010;3. https://doi.org/10.1002/14651858.CD006632.pub2.
- Rubio-Valera M, Serrano-Blanco A, Magdalena-Belío J, Fernández A, García-Campayo J, Pujol MM, et al. Effectiveness of pharmacist care in the improvement of adherence to antidepressants: a systematic review and meta-analysis. Ann Pharmacother 2011;45:39-48. https://doi.org/10.1345/aph.1P429.
- Ruotsalainen JH, Verbeek JH, Mariné A, Serra C. Preventing occupational stress in healthcare workers. Cochrane Database Syst Rev 2015;4. https://doi.org/10.1002/14651858.CD002892.pub5.
- Ruppar TM, Cooper PS, Mehr DR, Delgado JM, Dunbar-Jacob JM. Medication adherence interventions improve heart failure mortality and readmission rates: systematic review and meta-analysis of controlled trials. J Am Heart Assoc 2016;5. https://doi.org/10.1161/JAHA.115.002606.
- Saffari M, Ghanizadeh G, Koenig HG. Health education via mobile text messaging for glycemic control in adults with type 2 diabetes: a systematic review and meta-analysis. Prim Care Diabetes 2014;8:275-85. https://doi.org/10.1016/j.pcd.2014.03.004.
- Salyers MP, Bonfils KA, Luther L, Firmin RL, White DA, Adams EL, et al. The relationship between professional burnout and quality and safety in healthcare: a meta-analysis. J Gen Int Med 2017;32:475-82. https://doi.org/10.1007/s11606-016-3886-9.
- Santo K, Kirkendall S, Laba TL, Thakkar J, Webster R, Chalmers J, et al. Interventions to improve medication adherence in coronary disease patients: A systematic review and meta-analysis of randomised controlled trials. Eur J Prev Cardiol 2016;23:1065-76. https://doi.org/10.1177/2047487316638501.
- Scott A, Sivey P, Ait Ouakrim D, Willenberg L, Naccarella L, Furler J, et al. The effect of financial incentives on the quality of health care provided by primary care physicians. Cochrane Database Syst Rev 2011;9. https://doi.org/10.1002/14651858.CD008451.pub2.
- Shamliyan TA, Duval S, Du J, Kane RL. Just what the doctor ordered. Review of the evidence of the impact of computerized physician order entry system on medication errors. Health Serv Res 2008;43:32-53. https://doi.org/10.1111/j.1475-6773.2007.00751.x.
- Shen YC, Eggleston K, Lau J, Schmid CH. Hospital ownership and financial performance: what explains the different findings in the empirical literature?. Inquiry 2007;44:41-68. https://doi.org/10.5034/inquiryjrnl_44.1.41.
- Shepperd S, Iliffe S, Doll HA, Clarke MJ, Kalra L, Wilson AD, et al. Admission avoidance hospital at home. Cochrane Database of Syst Rev 2016;9. https://doi.org/10.1002/14651858.CD007491.pub2.
- Shepperd S, Gonçalves-Bradley DC, Straus SE, Wee B. Hospital at home: home-based end-of-life care. Cochrane Database of Syst Rev 2016;2. https://doi.org/10.1002/14651858.CD009231.pub2.
- Shojania KG, Ranji SR, McDonald KM, Grimshaw JM, Sundaram V, Rushakoff RJ, et al. Effects of quality improvement strategies for type 2 diabetes on glycemic control: a meta-regression analysis. JAMA 2006;296:427-40.
- Singh S, Sedlack RE, Cook DA. Effects of simulation-based training in gastrointestinal endoscopy: a systematic review and meta-analysis. Clin Gastroenterol Hepatol 2014;12:1611-23.e4. https://doi.org/10.1016/j.cgh.2014.01.037.
- Smith SM, Wallace E, O’Dowd T, Fortin M. Interventions for improving outcomes in patients with multimorbidity in primary care and community settings. Cochrane Database Syst Rev 2016;3. https://doi.org/10.1002/14651858.CD006560.pub3.
- Stacey D, Légaré F, Col NF, Bennett CL, Barry MJ, Eden KB, et al. Decision aids for people facing health treatment or screening decisions. Cochrane Database Syst Rev 2014;1. https://doi.org/10.1002/14651858.CD001431.pub4.
- Stephens M, Hourigan LF, Appleyard M, Ostapowicz G, Schoeman M, Desmond PV, et al. Non-physician endoscopists: A systematic review. World J Gastroenterol 2015;21:5056-71. https://doi.org/10.3748/wjg.v21.i16.5056.
- Stroke Unit Trialists’ Collaboration . Collaborative systematic review of the randomised trials of organised inpatient (stroke unit) care after stroke. BMJ 1997;314:1151-9. https://doi.org/10.1136/bmj.314.7088.1151.
- Suphanchaimat R, Cetthakrikul N, Dalliston A, Putthasri W. The impact of rural-exposure strategies on the intention of dental students and dental graduates to practice in rural areas: a systematic review and meta-analysis. Adv Med Educ Pract 2016;7:623-33. https://doi.org/10.2147/AMEP.S116699.
- Teding van Berkhout E, van Berkhout ET, Malouff JM. The efficacy of empathy training: a meta-analysis of randomized controlled trials. J Couns Psychol 2016;63:32-41. https://doi.org/10.1037/cou0000093.
- Thakkar J, Kurup R, Laba TL, Santo K, Thiagalingam A, Rodgers A, et al. Mobile telephone text messaging for medication adherence in chronic disease: a meta-analysis. JAMA Intern Med 2016;176:340-9. https://doi.org/10.1001/jamainternmed.2015.7667.
- Thompson Coon J, Martin A, Abdul-Rahman AK, Boddy K, Whear R, Collinson A, et al. Interventions to reduce acute paediatric hospital admissions: a systematic review. Arch Dis Child 2012;97:304-11. https://doi.org/10.1136/archdischild-2011-301214.
- Thompson RL, Summerbell CD, Hooper L, Higgins JP, Little PS, Talbot D, et al. Dietary advice given by a dietitian versus other health professional or self-help resources to reduce blood cholesterol. Cochrane Database Syst Rev 2003;3. https://doi.org/10.1002/14651858.CD001366.
- Toma T, Athanasiou T, Harling L, Darzi A, Ashrafian H. Online social networking services in the management of patients with diabetes mellitus: systematic review and meta-analysis of randomised controlled trials. Diabetes Res Clin Pract 2014;106:200-11. https://doi.org/10.1016/j.diabres.2014.06.008.
- Tongsai S, Thamlikitkul V. The safety of early versus late ambulation in the management of patients after percutaneous coronary interventions: a meta-analysis. Int J Nurs Stud 2012;49:1084-90. https://doi.org/10.1016/j.ijnurstu.2012.03.012.
- Tsai AC, Morton SC, Mangione CM, Keeler EB. A meta-analysis of interventions to improve care for chronic illnesses. Am J Manag Care 2005;11:478-88.
- Tura G, Fantahun M, Worku A. The effect of health facility delivery on neonatal mortality: systematic review and meta-analysis. BMC Pregnancy Childbirth 2013;13. https://doi.org/10.1186/1471-2393-13-18.
- Tyson MD, Chang SS. Enhanced recovery pathways versus standard care after cystectomy: a meta-analysis of the effect on perioperative outcomes. Eur Urol 2016;70:995-1003. https://doi.org/10.1016/j.eururo.2016.05.031.
- Tzortziou Brown V, Underwood M, Mohamed N, Westwood O, Morrissey D. Professional interventions for general practitioners on the management of musculoskeletal conditions. Cochrane Database Syst Rev 2016;5. https://doi.org/10.1002/14651858.CD007495.pub2.
- Urquhart C, Currell R, Grant MJ, Hardiker NR. Nursing record systems: effects on nursing practice and healthcare outcomes. Cochrane Database Syst Rev 2009;1. https://doi.org/10.1002/14651858.CD002099.pub2.
- van Driel ML, Morledge MD, Ulep R, Shaffer JP, Davies P, Deichmann R. Interventions to improve adherence to lipid-lowering medication. Cochrane Database Syst Rev 2016;12. https://doi.org/10.1002/14651858.CD004371.pub4.
- van Ginneken N, Tharyan P, Lewin S, Rao GN, Meera SM, Pian J, et al. Non-specialist health worker interventions for the care of mental, neurological and substance-abuse disorders in low- and middle-income countries. Cochrane Database Syst Rev 2013;11. https://doi.org/10.1002/14651858.CD009149.pub2.
- van Straten A, Cuijpers P. Self-help therapy for insomnia: a meta-analysis. Sleep Med Rev 2009;13:61-7. https://doi.org/10.1016/j.smrv.2008.04.006.
- Vasilevska M, Ku J, Fisman DN. Factors associated with healthcare worker acceptance of vaccination: a systematic review and meta-analysis. Infect Control Hosp Epidemiol 2014;35:699-708. https://doi.org/10.1086/676427.
- Vaughan J, Gurusamy KS, Davidson BR. Day-surgery versus overnight stay surgery for laparoscopic cholecystectomy. Cochrane Database Syst Rev 2013;7. https://doi.org/10.1002/14651858.CD006798.pub4.
- Vernon SW, McQueen A, Tiro JA, del Junco DJ. Interventions to promote repeat breast cancer screening with mammography: a systematic review and meta-analysis. J Natl Cancer Inst 2010;102:1023-39. https://doi.org/10.1093/jnci/djq223.
- Virk SA, Bowman SRA, Chan L, Bannon PG, Aty W, French BG, et al. Equivalent outcomes after coronary artery bypass graft surgery performed by consultant versus trainee surgeons: a systematic review and meta-analysis. J Thorac Cardiovasc Surg 2016;151:647-54.e1. https://doi.org/10.1016/j.jtcvs.2015.11.006.
- von Meyenfeldt EM, Gooiker GA, van Gijn W, Post PN, van de Velde CJ, Tollenaar RA, et al. The relationship between volume or surgeon specialty and outcome in the surgical treatment of lung cancer: a systematic review and meta-analysis. J Thorac Oncol 2012;7:1170-8. https://doi.org/10.1097/JTO.0b013e318257cc45.
- Walsh CM, Sherlock ME, Ling SC, Carnahan H. Virtual reality simulation training for health professions trainees in gastrointestinal endoscopy. Cochrane Database Syst Rev 2012;6. https://doi.org/10.1002/14651858.CD008237.pub2.
- Warsi A, Wang PS, LaValley MP, Avorn J, Solomon DH. Self-management education programs in chronic disease: a systematic review and methodological critique of the literature. Arch Intern Med 2004;164:1641-9. https://doi.org/10.1001/archinte.164.15.1641.
- Watson SJ, Aldus CF, Bond C, Bhattacharya D. Systematic review of the health and societal effects of medication organisation devices. BMC Health Serv Res 2016;16. https://doi.org/10.1186/s12913-016-1446-y.
- Weeks G, George J, Maclure K, Stewart D. Non-medical prescribing versus medical prescribing for acute and chronic disease management in primary and secondary care. Cochrane Database Syst Rev 2016;11. https://doi.org/10.1002/14651858.CD011227.pub2.
- Wei I, Pappas Y, Car J, Sheikh A, Majeed A. Computer-assisted versus oral-and-written dietary history taking for diabetes mellitus. Cochrane Database Syst Rev 2011;12. https://doi.org/10.1002/14651858.CD008488.pub2.
- Weller CD, Buchbinder R, Johnston RV. Interventions for helping people adhere to compression treatments for venous leg ulceration. Cochrane Database Syst Rev 2016;3. https://doi.org/10.1002/14651858.CD008378.pub3.
- Witter S, Fretheim A, Kessy FL, Lindahl AK. Paying for performance to improve the delivery of health interventions in low- and middle-income countries. Cochrane Database Syst Rev 2012;2. https://doi.org/10.1002/14651858.CD007899.pub2.
- Wootton R. Twenty years of telemedicine in chronic disease management – an evidence synthesis. J Telemed Telecare 2012;18:211-20. https://doi.org/10.1258/jtt.2012.120219.
- Zhai YK, Zhu WJ, Cai YL, Sun DX, Zhao J. Clinical- and cost-effectiveness of telemedicine in type 2 diabetes mellitus: a systematic review and meta-analysis. Medicine 2014;93. https://doi.org/10.1097/MD.0000000000000312.
- Zhao FF, Suhonen R, Koskinen S, Leino-Kilpi H. Theory-based self-management educational interventions on patients with type 2 diabetes: a systematic review and meta-analysis of randomized controlled trials. J Adv Nurs 2017;73:812-33. https://doi.org/10.1111/jan.13163.
- Zhou S, Sheng XY, Xiang Q, Wang ZN, Zhou Y, Cui YM. Comparing the effectiveness of pharmacist-managed warfarin anticoagulation with other models: a systematic review and meta-analysis. J Clin Pharm Ther 2016;41:602-11. https://doi.org/10.1111/jcpt.12438.
Appendix 1 Search strategies for work package 1
MEDLINE
Date range searched: 1946 to 30 July 2018.
Date searched: 16 March 2017, updated 31 July 2018.
Search strategy
-
*Health Services Research/or health service$ research.mp.
-
service delivery.mp.
-
health system$.mp.
-
healthcare system$.mp.
-
Health Policy.mp. or *Health Policy/
-
((health care or healthcare or hospital$ or service$) adj1 (administration or organi$ation or management or structure or govern* or financ* or account* or accredit* or policy or efficiency)).mp.
-
exp Primary Health Care/and (administration or organi$ation or management or structure or govern* or financ* or account* or accredit* or policy or efficiency).mp.
-
exp Governing Board/
-
Patient Safety.mp. or exp Patient Safety/
-
exp Medical Errors/
-
((adverse event$ or infection$ or complication$) adj1 prevent*).mp.
-
Quality Improvement.mp. or exp Quality Improvement/
-
exp Total Quality Management/or *Risk Management/or change management.mp.
-
exp Organizational Culture/
-
leadership.mp. or exp Leadership/
-
exp Medical Records Systems, Computerized/
-
exp Operations Research/or operational research.mp.
-
exp “Appointments and Schedules”/or waiting time.mp.
-
exp Triage/or triage.mp.
-
((integrat* or pathway or continuity or access* or model*or transition) adj2 (care or service$)).mp.
-
(exp Health Personnel/or (staff or professional$ or healthcare worker$ or health care worker$ or health worker$ or workforce$ or nurse$ or doctor$ or physician$ or trainee$ or consultant$ or general practitioner$ or surgeon$ or dentist$ or therapist$ or pharmacist$ or radiologist$ or pathologist$ or technician$ or assistant$).mp.) and (skill$ or training or education or competence or morale or burnout or absenteeism or retention or deploy* or workforce).mp.
-
exp Patient Education as Topic/
-
exp Patient Satisfaction/
-
*Disease Management/
-
(variation$ adj2 (care or service$ or outcome$ or mortality or process*)).mp.
-
((organization* or organisation*) adj1 performance).mp.
-
implementation science$.mp.
-
*publication$/
-
bias$.mp. or “Bias (Epidemiology)”/
-
28 and 29
-
publication bias.mp. or exp Publication Bias/
-
(bias$ adj3 (publication$ or disseminat$ or language$ or reporting or grey or gray or citation$ or time delay or time lag or national or country or location or conference or abstract or duplicat$ or multiple publication$)).tw.
-
((reference$ or database$ or index$) adj2 bias$).tw.
-
(file adj1 drawer$).tw.
-
(time adj2 (completion or publication)).tw.
-
unpublished research.tw.
-
(fail$ adj2 publish$).tw.
-
(p-curve$ or p-hack*).tw.
-
(data adj2 dredg*).tw.
-
phishing.tw.
-
non-publication.tw.
-
selective report$.tw.
-
selective non report$.tw.
-
selective non-report$.tw.
-
outcome report$ bias.tw.
-
1 or 2 or 3 or 4 or 5 or 6 or 7 or 8 or 9 or 10 or 11 or 12 or 13 or 14 or 15 or 16 or 17 or 18 or 19 or 20 or 21 or 22 or 23 or 24 or 25 or 26 or 27
-
30 or 31 or 32 or 33 or 34 or 35 or 36 or 37 or 38 or 39 or 40 or 41 or 42 or 43 or 44 or 45
-
46 and 47
EMBASE
Date range searched: 1947 to 30 July 2018.
Date searched: 16 March 2017, updated 31 July 2018.
Search strategy
-
health services research/
-
health service$ research.mp.
-
service delivery.mp.
-
health system$.mp.
-
health care system/
-
healthcare system$.mp.
-
health care policy/
-
Health Policy.mp.
-
((health care or healthcare or hospital$ or service$) adj1 (administration or organi$ation or management or structure or govern* or financ* or account* or accredit* or policy or efficiency)).mp. [mp=title, abstract, heading word, drug trade name, original title, device manufacturer, drug manufacturer, device trade name, keyword, floating subheading word, candidate term word]
-
exp Primary Health Care/and (administration or organi$ation or management or structure or govern* or financ* or account* or accredit* or policy or efficiency).mp. [mp=title, abstract, heading word, drug trade name, original title, device manufacturer, drug manufacturer, device trade name, keyword, floating subheading word, candidate term word]
-
Governing Board.mp. or “board of trustees”/
-
exp patient safety/
-
patient safety.mp.
-
Medical Errors.mp. [mp=title, abstract, heading word, drug trade name, original title, device manufacturer, drug manufacturer, device trade name, keyword, floating subheading word, candidate term word]
-
((adverse event$ or infection$ or complication$) adj1 prevent*).mp. [mp=title, abstract, heading word, drug trade name, original title, device manufacturer, drug manufacturer, device trade name, keyword, floating subheading word, candidate term word]
-
Quality Improvement.mp. or total quality management/
-
Total Quality Management/or *Risk Management/or change management.mp. [mp=title, abstract, heading word, drug trade name, original title, device manufacturer, drug manufacturer, device trade name, keyword, floating subheading word, candidate term word]
-
Organizational Culture.mp.
-
Leadership.mp. or leadership/
-
electronic medical record/or medical record/or Medical Records Systems.mp.
-
Operations Research/or operational research.mp. [mp=title, abstract, heading word, drug trade name, original title, device manufacturer, drug manufacturer, device trade name, keyword, floating subheading word, candidate term word]
-
“Appointments and Schedules”/or waiting time.mp. [mp=title, abstract, heading word, drug trade name, original title, device manufacturer, drug manufacturer, device trade name, keyword, floating subheading word, candidate term word]
-
Triage.mp.
-
((integrat* or pathway or continuity or access* or model*or transition) adj2 (care or service$)).mp. [mp=title, abstract, heading word, drug trade name, original title, device manufacturer, drug manufacturer, device trade name, keyword, floating subheading word, candidate term word]
-
(exp Health Personnel/or (staff or professional$ or healthcare worker$ or health care worker$ or health worker$ or workforce$ or nurse$ or doctor$ or physician$ or trainee$ or consultant$ or general practitioner$ or surgeon$ or dentist$ or therapist$ or pharmacist$ or radiologist$ or pathologist$ or technician$ or assistant$).mp.) and (skill$ or training or education or competence or morale or burnout or absenteeism or retention or deploy* or workforce).mp. [mp=title, abstract, heading word, drug trade name, original title, device manufacturer, drug manufacturer, device trade name, keyword, floating subheading word, candidate term word]
-
exp patient education/
-
exp patient satisfaction/
-
disease management/
-
(variation$ adj2 (care or service$ or outcome$ or mortality or process*)).mp. [mp=title, abstract, heading word, drug trade name, original title, device manufacturer, drug manufacturer, device trade name, keyword, floating subheading word, candidate term word]
-
((organization* or organisation*) adj1 performance).mp. [mp=title, abstract, heading word, drug trade name, original title, device manufacturer, drug manufacturer, device trade name, keyword, floating subheading word, candidate term word]
-
implementation science$.mp.
-
publication bias.mp.
-
(bias$ adj3 (publication$ or disseminat$ or language$ or reporting or grey or gray or citation$ or time delay or time lag or national or country or location or conference or abstract or duplicat$ or multiple publication$)).tw,ot.
-
((reference$ or database$ or index$) adj2 bias$).tw.
-
(file adj1 drawer$).tw.
-
(time adj2 (completion or publication)).tw.
-
unpublished research.tw.
-
(fail$ adj2 publish$).tw.
-
(p-curve$ or p-hack*).tw.
-
(data adj2 dredg*).tw.
-
phishing.tw.
-
non-publication.tw.
-
*publication$/
-
bias$.mp.
-
43 and 44
-
selective report$.tw.
-
selective non report$.tw.
-
selective non-report$.tw.
-
outcome report$ bias.tw.
-
1 or 2 or 3 or 4 or 5 or 6 or 7 or 8 or 9 or 10 or 11 or 12 or 13 or 14 or 15 or 16 or 17 or 18 or 19 or 20 or 21 or 22 or 23 or 24 or 25 or 26 or 27 or 28 or 29 or 30 or 31
-
32 or 33 or 34 or 35 or 36 or 37 or 38 or 39 or 40 or 41 or 42 or 45 or 46 or 47 or 48 or 49
-
50 and 51
Parts of this appendix have been reproduced from Ayorinde et al. 54 This is an Open Access article distributed in accordance with the terms of the Creative Commons Attribution (CC BY 4.0) license, which permits others to distribute, remix, adapt and build upon this work, for commercial use, provided the original work is properly cited. See: http://creativecommons.org/licenses/by/4.0/. The text above includes minor additions and formatting changes to the original text.
Appendix 2 Invitation letter for potential interview participants for work package 5
Appendix 3 Participant information leaflet for interviews in work package 5
Appendix 4 Consent form for interviewees for work package 5
Appendix 5 Interview schedule for work package 5
Appendix 6 Methods used to investigate publication bias and outcome reporting bias in HSDR systematic reviews identified in work package 1
This appendix is reproduced from Ayorinde et al. 54 This is an Open Access article distributed in accordance with the terms of the Creative Commons Attribution (CC BY 4.0) license, which permits others to distribute, remix, adapt and build upon this work, for commercial use, provided the original work is properly cited. See: http://creativecommons.org/licenses/by/4.0/. The text below includes minor additions and formatting changes to the original text.
Study | Type of bias assessed | Funnel plot | Begg and Mazumdar’s rank correlation test | Egger’s test | Trim and fill | Fail-safe N | Reported evidence of publication bias | Cochrane’s (and related) risk-of bias-tool | Other approach |
---|---|---|---|---|---|---|---|---|---|
Abdalla 2015110 | ORB | ✓ | |||||||
Akl 2013111 | ORB | ✓ | |||||||
Algie 2015112 | ORB | ✓ | |||||||
Al-Muhandis 2011113 | PB | ✓ | |||||||
Aoyagi 2015114 | PB | ✓ | ✓ | ✓ | |||||
Arditi 2012115 | PB and ORB | ✓ | ✓ | ✓ | |||||
Aubin 2012116 | ORB | ✓ | |||||||
Badamgarav 2003117 | PB | ✓ | ✓ | ||||||
Baker 2015118 | ORB | ✓ | |||||||
Ballini 2015119 | ORB | ✓ | |||||||
Balogh 2016120 | ORB | ✓ | |||||||
Barnard 2015121 | ORB | ✓ | |||||||
Baskerville 2012122 | PB | ✓ | ✓ | ✓ | ✓ | ||||
Berdot 2013123 | PB | ✓ | ✓ | ||||||
Bos 2017124 | ORB | ✓ | |||||||
Bosch-Capblanch 2011125 | ORB | ✓ | |||||||
Bradford 2013126 | PB | Searched ClinicalTrials.gov completed unpublished studies and ongoing studies | |||||||
Brasil 2008127 | PB | ✓ | ✓ | ✓ | ✓ | ||||
Brocklehurst 2013128 | ORB | ✓ | |||||||
Brown 2015129 | PB and ORB | ✓ | ✓ | ||||||
Butler 2011130 | ORB | ✓ | |||||||
Campanella 2016131 | PB | ✓ | ✓ | ||||||
Campbell 2016132 | ORB | ✓ | |||||||
Cappuccio 2004133 | PB | ✓ | ✓ | ✓ | ✓ | ||||
Chaillet 2007134 | PB | ✓ | ✓ | ✓ | |||||
Chang 2015135 | PB | ✓ | ✓ | ✓ | |||||
Chen 2016136 | PB | ✓ | |||||||
Chen 2016137 | PB | ✓ | ✓ | ✓ | ✓ | ||||
Chen 2008138 | PB | ✓ | ✓ | ✓ | ✓ | ✓ | |||
Chodosh 2005139 | PB | ✓ | ✓ | ✓ | ✓ | ||||
Christensen 2016140 | PB and ORB | ✓ | ✓ | ||||||
Connock 2007141 | PB | ✓ | ✓ | ✓ | |||||
Cook 2015142 | ORB | ✓ | |||||||
Corbett 2015143 | ORB | ✓ | |||||||
Costa-Font 2011144 | PB | ✓ | ✓ | ✓ | |||||
Cui 2016145 | ORB | ✓ | |||||||
Cuijpers 2011146 | PB | ✓ | ✓ | ||||||
De Luca 2008147 | PB | ✓ | ✓ | ||||||
de Lusignan 2014148 | ORB | ✓ | |||||||
de Roten 2013149 | PB | ✓ | ✓ | ✓ | |||||
Demonceau 2013150 | PB and ORB | ✓ | ✓ | ✓ | ✓ | ||||
Donald 2014151 | ORB | ✓ | |||||||
Dorresteijn 2010152 | ORB | ✓ | |||||||
Dudley 2011153 | ORB | ✓ | |||||||
Dyer 2014154 | ORB | ✓ | |||||||
Easthall 2012155 | PB | ✓ | |||||||
Fiander 2015156 | ORB | ✓ | |||||||
Fisher 2015157 | ORB | ✓ | |||||||
Fletcher 2011158 | PB | ✓ | |||||||
Flodgren 2010159 | ORB | ✓ | |||||||
Flodgren 2011160 | ORB | ✓ | |||||||
Flodgren 2011161 | ORB | ✓ | |||||||
Flodgren 2013162 | ORB | ✓ | |||||||
Forsetlund 2009163 | PB | ✓ | ✓ | ||||||
Foy 2010164 | PB | ✓ | ✓ | ✓ | |||||
Free 2013165 | PB and ORB | ✓ | ✓ | ||||||
French 2010166 | ORB | ✓ | |||||||
Gallagher 2011167 | PB and ORB | ✓ | ✓ | ✓ | ✓ | ||||
Gardner 2013168 | PB and time lag bias | ✓ | ✓ | ✓ | Time lag bias assessed by comparing the time lag between the end of the intervention period and the date of publication (classified into ≤ 4 years and > 4 years) | ||||
Gemmill 2007169 | PB | ✓ | ✓ | ||||||
Gensichen 2006170 | PB | ✓ | ✓ | ✓ | |||||
Giguère 2012171 | ORB | ✓ | |||||||
Gillaizeau 2013172 | ORB | ✓ | |||||||
Gillies 2015173 | ORB | ✓ | |||||||
Göhler 2006174 | PB | ✓ | ✓ | ✓ | ✓ | ✓ | |||
Goldzweig 2015175 | PB | ✓ | ✓ | ||||||
Gooiker 2010176 | PB | ✓ | ✓ | ||||||
Goossens-Laan 2011177 | PB | ✓ | ✓ | ||||||
Green 2010178 | ORB | ✓ | |||||||
Grobler 2015179 | ORB | ✓ | |||||||
Grochtdreis 2015180 | ORB | ✓ | |||||||
Gurusamy 2014181 | ORB | ✓ | |||||||
Han 2009182 | PB | ✓ | ✓ | ✓ | |||||
Hata 2016183 | PB | ✓ | ✓ | ||||||
Hayhurst 2015184 | PB | ✓ | ✓ | ||||||
Higginson 2003185 | PB | ✓ | ✓ | ||||||
Hodgkinson 2011186 | ORB | ✓ | |||||||
Horsley 2011187 | ORB | ✓ | |||||||
Ivers 2012188 | ORB | ✓ | |||||||
Jacobson 2005189 | PB | ✓ | |||||||
Jamal 2012190 | PB | ✓ | ✓ | ✓ | |||||
Jia 2014191 | ORB | ✓ | |||||||
Kahn 2013192 | PB and ORB | ✓ | ✓ | ✓ | |||||
Karmali 2014193 | ORB | ✓ | |||||||
Keebler 201668 | PB | ✓ | ✓ | ✓ | |||||
Kelley 2014194 | PB and ORB | ✓ | ✓ | ✓ | ✓ | ✓ | |||
Kerlin 2017195 | PB and ORB | ✓ | ✓ | ✓ | Macaskill test | ||||
Ketelaar 2011196 | ORB | ✓ | |||||||
Khangura 2012197 | ORB | ✓ | |||||||
Khunpradit 2011198 | ORB | ✓ | |||||||
Kilpatrick 2015199 | ORB | ✓ | |||||||
Kim 2005200 | PB | ✓ | ✓ | ||||||
Kroon 2014201 | PB and ORB | ✓ | ✓ | ||||||
Lambrinou 2012202 | PB | ✓ | ✓ | ✓ | |||||
Lau 2012203 | PB | ✓ | Harbord’s test | ||||||
Laver 2013204 | ORB | ✓ | |||||||
Lin 2014205 | PB and ORB | ✓ | ✓ | ✓ | |||||
Lu 201257 | PB and ORB | ✓ | ✓ | ✓ | Peter’s method to assess the risk of possible outcome reporting bias, reviewers included studies that met the inclusion criteria but did not provide sufficient data on relevant outcomes | ||||
Luiza 2015206 | ORB | ✓ | |||||||
Lutge 2015207 | ORB | ✓ | |||||||
Lv 2012208 | ORB | ✓ | |||||||
Ma 2017209 | PB | ✓ | |||||||
Maaskant 2015210 | ORB | ✓ | |||||||
McBain 2016211 | ORB | ✓ | |||||||
McGowan 2009212 | ORB | ✓ | |||||||
Ming 2016213 | ORB | ✓ | |||||||
Mitchell 2015214 | ORB | ✓ | |||||||
Moja 2014215 | PB and ORB | ✓ | ✓ | ✓ | ✓ | ||||
Murphy 2015216 | ORB | ✓ | |||||||
Murthy 2012217 | ORB | ✓ | |||||||
Neumeyer-Gromen 2004218 | PB | ✓ | ✓ | ✓ | |||||
Nglazi 2013219 | ORB | ✓ | |||||||
Nieuwlaat 201456 | ORB | ✓ | |||||||
Oliver 2000220 | PB | ✓ | |||||||
Opiyo 2015221 | ORB | ✓ | |||||||
Opiyo 2016222 | ORB | ✓ | |||||||
O’Sullivan 2016223 | ORB | ✓ | |||||||
Panagioti 2014224 | PB | ✓ | ✓ | ✓ | |||||
Pande 2013225 | ORB | ✓ | |||||||
Parmelli 2012226 | ORB | ✓ | |||||||
Peñaloza 2011227 | ORB | ✓ | |||||||
Perrier 2011228 | ORB | ✓ | |||||||
Peterson 2003229 | PB | ✓ | ✓ | ||||||
Peytremann-Bridevaux 2015230 | PB and ORB | ✓ | ✓ | ||||||
Rachoin 2012231 | PB | ✓ | ✓ | ||||||
Rashidian 2015232 | ORB | ✓ | |||||||
Reeves 2013233 | ORB | ✓ | |||||||
Robotham 2016234 | PB | ✓ | ✓ | ✓ | |||||
Rockers 2013235 | ORB | ✓ | |||||||
Ross Middleton 2012236 | PB | ✓ | ✓ | ✓ | |||||
Rotter 2010237 | PB and ORB | ✓ | ✓ | ||||||
Rubio-Valera 2011238 | PB | ✓ | ✓ | ||||||
Ruotsalainen 2015239 | PB and ORB | ✓ | ✓ | ✓ | |||||
Ruppar 2016240 | PB | ✓ | Conducted moderator analyses to evaluate whether or not effect estimates were different based on factors, such as year of publication, publication method (e.g. journal article, dissertation) among other factors | ||||||
Saffari 2014241 | PB and ORB | ✓ | ✓ | ✓ | ✓ | ✓ | |||
Salyers 2017242 | PB | ✓ | ✓ | ||||||
Santo 2016243 | PB and ORB | ✓ | ✓ | ✓ | ✓ | ✓ | |||
Scott 2011244 | ORB | ✓ | |||||||
Shamliyan 2008245 | PB | ✓ | ✓ | Peter’s method | |||||
Shen 2007246 | PB | ✓ | Assessed publication bias by examining the relationship between the square root of degrees of freedom and the absolute value of t-statistics. They also ran a difference-in-difference regression using the absolute t-statistics as the dependent variables and square root of degrees of freedom, ownership-focus indicator and their interaction term as the independent variables | ||||||
Shepperd 2016247 | PB and ORB | ✓ | ✓ | ||||||
Shepperd 2016248 | ORB | ✓ | |||||||
Shojania 2006249 | PB | ✓ | ✓ | Univariate analysis of sample size and effect size | |||||
Singh 2014250 | PB | ✓ | ✓ | ✓ | ✓ | ||||
Smith 2016251 | ORB | ✓ | |||||||
Stacey 2014252 | PB and ORB | ✓ | ✓ | ||||||
Stephens 2015253 | ORB | ✓ | |||||||
Stroke Unit Triallists’ Collaboration 1997254 | PB | ✓ | Assessed publication bias by calculating how many randomised patients (with a similar baseline event rate as in the review) would have to be recruited from neutral trials (OR 1.0) to render the overall result non-significant to estimate the degree to which conclusions of the review would be overturned by missing neutral trials | ||||||
Suphanchaimat 2016255 | PB | ✓ | ✓ | ✓ | |||||
Teding van Berkhout 2016256 | PB | ✓ | ✓ | ✓ | ✓ | ||||
Thakkar 2016257 | PB | ✓ | ✓ | ✓ | ✓ | ||||
Thompson Coon 2012258 | ORB | ✓ | |||||||
Thompson 2003259 | PB | ✓ | ✓ | ||||||
Toma 2014260 | PB and ORB | ✓ | ✓ | ✓ | ✓ | ||||
Tongsai 2012261 | PB | ✓ | ✓ | ✓ | ✓ | ||||
Tricco 201248 | ORB | ✓ | |||||||
Tsai 2005262 | PB | ✓ | ✓ | ✓ | |||||
Tura 2013263 | PB | ✓ | ✓ | ✓ | ✓ | ✓ | |||
Tyson 2016264 | PB | ✓ | ✓ | ✓ | |||||
Tzortziou Brown 2016265 | ORB | ✓ | |||||||
Urquhart 2009266 | ORB | ✓ | |||||||
van Driel 2016267 | ORB | ✓ | |||||||
van Ginneken 2013268 | ORB | ✓ | |||||||
van Straten 2009269 | PB | ✓ | ✓ | ✓ | |||||
Vasilevska 2014270 | PB | ✓ | ✓ | ✓ | |||||
Vaughan 2013271 | ORB | ✓ | |||||||
Vernon 2010272 | PB | ✓ | ✓ | ✓ | |||||
Virk 2016273 | PB | ✓ | ✓ | ✓ | ✓ | ||||
Von Meyenfeldt 2012274 | PB | ✓ | |||||||
Walsh 2012275 | ORB | ✓ | |||||||
Warsi 2004276 | PB | ✓ | ✓ | ||||||
Watson 2016277 | ORB | ✓ | |||||||
Weeks 2016278 | PB and ORB | ✓ | ✓ | ✓ | |||||
Wei 2011279 | ORB | ✓ | |||||||
Weller 2016280 | ORB | ✓ | |||||||
Witter 2012281 | ORB | ✓ | |||||||
Wootton 2012282 | PB | ✓ | ✓ | ||||||
Zhai 2014283 | PB and ORB | ✓ | ✓ | ✓ | ✓ | ✓ | |||
Zhao 2017284 | PB and ORB | ✓ | ✓ | ✓ | ✓ | ||||
Zhou 2016285 | ORB | ✓ |
Appendix 7 Key findings for the systematic review described in Chapter 5, case study 3
Table 15 summarises key findings from the meta-analyses presented in the doctoral dissertation52 on which case study 3 was based.
Association examined | n | Pooled effect size (95% CI)a | p-value | I2 (%) |
---|---|---|---|---|
Organisational culture and job satisfaction | ||||
Constructive culture | 12 | 0.37 (0.28 to 0.45) | < 0.001 | 78 |
11b | 0.39 (0.31 to 0.46)b | < 0.001b | 76b | |
Passive/defensive culture | 11 | –0.16 (–0.32 to 0.01) | 0.06 | 91 |
10b | –0.23 (–0.36 to –0.09)b | 0.001b | 87b | |
Aggressive/defensive culture | 9 | –0.22 (–0.27 to –0.17) | < 0.001 | 15 |
Organisational climate and job satisfaction | ||||
Global climate | 6 | 0.51 (0.41 to 0.60) | < 0.001 | 88 |
Goal emphasis climate | 2 | 0.43 (0.09 to 0.67) | < 0.05 | 92 |
Mean emphasis climate | 14 | 0.39 (0.31 to 0.47) | < 0.001 | 77 |
Reward orientation climate | 5 | 0.47 (0.38 to 0.55) | < 0.001 | 46 |
Task support climate | 6 | 0.29 (0.11 to 0.45) | 0.002 | 89 |
Socioemotional support climate | 10 | 0.40 (0.26 to 0.52) | < 0.001 | 90 |
Organisational culture and turnover (individual-level measurement) | ||||
Constructive culture | 2 | –0.23 (–0.33 to –0.13) | < 0.001 | 0 |
Passive/defensive culture | 2 | 0.33 (0.23 to 0.42) | < 0.001 | 0 |
Aggressive/defensive culture | 2 | 0.27 (0.16 to 0.36) | < 0.001 | 0 |
Organisational culture and turnover (unit-level measurement) | ||||
Constructive culture | 2 | –0.21 (–0.39 to –0.01) | 0.04 | 0 |
Passive/defensive culture | 2 | 0.04 (–0.30 to 0.38) | 0.81 | 63 |
Aggressive/defensive culture | 1 | 0.12 (–0.13 to 0.35) | 0.36 | NA |
Organisational climate and job satisfaction | ||||
Global climate | 2 | –0.05 (–0.46 to 0.37) | 0.82 | 95 |
Mean emphasis climate | 4 | –0.19 (–0.41 to 0.06) | 0.14 | 88 |
3b | –0.06 (–0.15 to 0.02)b | 0.15b | 0b | |
Reward orientation climate | 2 | –0.13 (–0.31 to 0.06) | 0.17 | 39 |
Task support climate | 2 | –0.08 (–0.16 to 0.01) | 0.08 | 0 |
Socioemotional support climate | 3 | –0.07 (–0.16 to 0.01) | 0.09 | 0 |
Organisational culture and organisational climate (global) | ||||
Constructive culture | 2 | 0.32 (–0.12 to 0.65) | 0.15 | 93 |
Passive/defensive culture | 1 | –0.24 (–0.40 to –0.06) | 0.01 | NA |
Aggressive/defensive culture | 1 | 0.11 (–0.07 to 0.28) | 0.24 | NA |
Glossary
- Reproduced with permission from Cochrane Community. Glossary. URL: https://community.cochrane.org/glossary (accessed 2 January 2020).2020The Cochrane CollaborationFunnel plot
- A graphical display of some measure of study precision plotted against effect size that can be used to investigate whether or not there is a link between study size and treatment effect. One possible cause of an observed association is reporting bias (including publication bias). Reproduced with permission from Cochrane Community. (URL: https://community.cochrane.org/glossary; accessed 2 January 2020.)
- Grey literature
- Literature that is not formally published as academic journal articles and/or indexed in major bibliographic databases. Reports produced by governmental or non-governmental organisations (in print or online), discussion papers, theses and dissertations, and conference abstracts are all considered as grey literature in this report.
- Reproduced with permission from Cochrane Community. Glossary. URL: https://community.cochrane.org/glossary (accessed 2 January 2020).2020The Cochrane CollaborationHeterogeneity
- (1) Used in a general sense to describe the variation in, or diversity of, participants, interventions and measurement of outcomes across a set of studies, or the variation in internal validity of those studies. (2) Used specifically as statistical heterogeneity, to describe the degree of variation in the effect estimates from a set of studies; also used to indicate the presence of variability among studies beyond the amount expected due solely to the play of chance. Reproduced with permission from Cochrane Community. (URL: https://community.cochrane.org/glossary; accessed 2 January 2020.)
- Reproduced with permission from Cochrane Community. Glossary. URL: https://community.cochrane.org/glossary (accessed 2 January 2020).2020The Cochrane CollaborationI2
- A measure used to quantify heterogeneity. It describes the percentage of the variability in effect estimates that is due to heterogeneity rather than sampling error (chance). A value > 50% may be considered to represent substantial heterogeneity. Reproduced with permission from Cochrane Community. (URL: https://community.cochrane.org/glossary; accessed 2 January 2020.)
- Reproduced with permission from Cochrane Community. Glossary. URL: https://community.cochrane.org/glossary (accessed 2 January 2020).2020The Cochrane CollaborationMeta-regression
- A technique used to explore the relationship between study characteristics (e.g. concealment of allocation, baseline risk, timing of the intervention) and study results (the magnitude of effect observed in each study) in a systematic review. Reproduced with permission from Cochrane Community. (URL: https://community.cochrane.org/glossary; accessed 2 January 2020.)
- Winner’s curse
- A phenomenon by which authors need to report more extreme results in order to have their studies published in high-impact academic journals, as a result of the alleged preference for selecting and publishing studies with more spectacular findings by these journals.
List of abbreviations
- CI
- confidence interval
- CrI
- credible interval
- GRADE
- Grading of Recommendations Assessment, Development and Evaluation
- HSDR
- Health Services and Delivery Research
- HSRProj
- Health Services Research Projects in Progress
- HSRUK
- Health Services Research UK
- ISQua
- International Society for Quality in Health Care
- JAP
- Journal of Applied Psychology
- NIHR
- National Institute for Health Research
- OR
- odds ratio
- PPI
- patient and public involvement
- PRISMA
- Preferred Reporting Items for Systematic Reviews and Meta-Analyses
- RCT
- randomised controlled trial
- SDO
- Service Delivery and Organisation
- WP
- work package