Notes
Article history
The research reported in this issue of the journal was funded by the HS&DR programme or one of its preceding programmes as project number 12/128/17. The contractual start date was in February 2014. The final report began editorial review in February 2020 and was accepted for publication in October 2020. The authors have been wholly responsible for all data collection, analysis and interpretation, and for writing up their work. The HS&DR editors and production house have tried to ensure the accuracy of the authors’ report and would like to thank the reviewers for their constructive comments on the final report document. However, they do not accept liability for damages or losses arising from material published in this report.
Permissions
Copyright statement
© Queen’s Printer and Controller of HMSO 2021. This work was produced by Bion et al. under the terms of a commissioning contract issued by the Secretary of State for Health and Social Care. This issue may be freely reproduced for the purposes of private research and study and extracts (or indeed, the full report) may be included in professional journals provided that suitable acknowledgement is made and the reproduction is not associated with any form of advertising. Applications for commercial reproduction should be addressed to: NIHR Journals Library, National Institute for Health Research, Evaluation, Trials and Studies Coordinating Centre, Alpha House, University of Southampton Science Park, Southampton SO16 7NS, UK.
2021 Queen’s Printer and Controller of HMSO
Chapter 1 Introduction
Parts of this chapter have been reproduced from the study protocol with permission (June 2021). 1
In September 2012, the National Institute for Health Research (NIHR) Health Services and Delivery Research (HSDR) programme issued a commissioned call for research into the ‘organisation and delivery of 24/7 [24 hours a day, 7 days a week] health care’; the topic had been prioritised by service managers, clinical leaders, patients and researchers, with the overall aim of improving access to health care regardless of the time of day or the day of the week.
Background
The stimulus for the NIHR HSDR programme’s call was the increasing difficulty of providing satisfactory emergency health care in the NHS. The background to this problem was multifaceted. The European Working Time Directive in 2003 limited hours of work;2 the NHS responded by moving junior doctors to shift-working, introducing non-physician clinicians, and increasing the numbers of consultants on new contracts that explicitly identify and reimburse out-of-hours work. This increased staffing and costs, but did not improve team working, training or continuity of care. 3 At the same time, a new contract for family doctors [general practitioners (GPs)] allowed them to withdraw from providing out-of-hours services. 4 Emergency admissions (EAs) continued to rise every year at a rate similar to that of the increase in consultant staff and faster than that of the background population increase, particularly those involving frail elderly people,5 while policies to deliver more health care in the community reduced hospital beds6 without a sufficient compensatory expansion in social care funding. 7 This increased the number of delayed hospital discharges, which adversely affected care quality8 and blocked beds, making it even more difficult to accommodate the growing numbers of EAs. 9 Temporal changes in medical staffing, emergency department (ED) attendances, EAs and hospital beds are shown in Figure 1. We discuss the apparent improvement in reducing delayed discharges of care in Chapter 4.
FIGURE 1.
Trends in NHS England emergency care workload, staffing and beds: 2011/12–2018/19. NHS England overall monthly average number of delayed days; number of overnight beds; annual average number of consultants and specialists, and other doctors; ED total attendance rate (% population); EA rate (% population); and percentage of ED attendances admitted. DTOC, delayed transfer of care; FTE, full time equivalent.
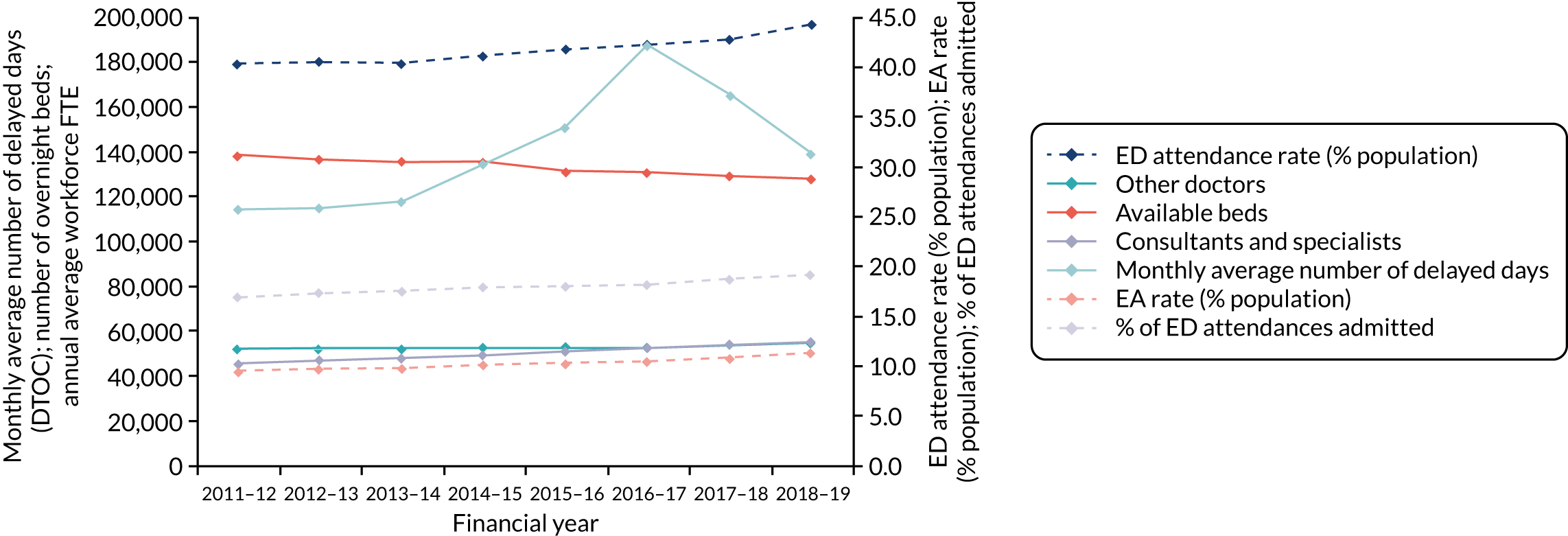
Public concern about the deteriorating quality of hospital care was exacerbated by failings in professional standards identified by the Mid-Staffordshire NHS Foundation Trust Public Inquiry. 10,11 There was also a longstanding perception that hospital care out of hours (at nights and weekends) was unreliable and potentially unsafe because of evidence showing the ‘weekend effect’. The Royal College of Physicians and the Society of Acute Medicine’s survey12 found that only 20% of hospital specialists were available at weekends for periods exceeding 8 hours, 18% reported never attending hospitals at weekends and 73% of acute physicians were not contractually obliged to provide medical care at weekends; only 19% of responding hospitals reported having a formalised rapid response team for acutely ill patients. Only 39% of specialists working in acute medical units (AMUs) reported having protected time for this work free of other duties, and providing care for blocks of time greater than a single day. Suboptimal specialist input had also been identified in the National Confidential Enquiry into Patient Outcome and Death (NCEPOD) audit of deaths following emergency hospital admission:13 at 12 hours following admission, 40% of patients had not been seen by a consultant, and in 12.4% of cases there was no documented evidence of consultant review. Of the 95 cases in which the assessors considered the delay in consultant review to have been unacceptable, the delay was considered to have adversely affected the accuracy or timeliness of diagnosis in 32.6% of cases, and may have contributed to adverse outcomes [intensive care unit (ICU) admission, worsening prognosis or death] in 49.5% of cases.
The profession responded to these findings with proposals for the reconfiguration of hospital services,14,15 and the development of enhanced standards of consultant practice. The Academy of Medical Royal Colleges convened a working group to develop standards for daily consultant review. 15 In the absence of strong direct evidence, the working group arrived at a consensus recommendation, namely that all hospitalised patients should be reviewed by a consultant every day unless prior review had determined that this was not necessary. The Academy of Medical Royal Colleges’ report concluded that systematic evaluation of the standards was required, within a research framework.
Seven-day services
Initiatives to improve access to emergency care were brought together under the UK government’s policy of ‘7-day services’,16 with the development of 10 clinical standards for emergency care,17 six of which directly or indirectly required front-line consultant involvement for delivery. Initially, NHS England required these standards to be introduced at scale and pace within 3–4 years, backed by incentives, rewards and sanctions. This was subsequently diluted to achieving four ‘priority standards’ across the NHS by 2020 (quotations in this paragraph contain public sector information licensed under the Open Government Licence v3.0, URL: http://nationalarchives.gov.uk/doc/non-commercial-government-licence/version/2/). 16 These are:
-
standard 2 – EAs assessed by a consultant within 14 hours
-
standard 5 – timely access to diagnostic services according to urgency
-
standard 6 – access to consultant-directed interventions 24/7
-
standard 8 – consultant review of patients in acute medical, surgical and intensive care units twice daily by consultants working blocks of days; daily consultant review of ward patients.
Whether or not these standards were the correct solution to problems of access, and how they were to be measured, monitored and funded, became a source of tension between the professions on one side18 and the government and Department of Health and Social Care19 on the other, with NHS England in the middle. A publication by Freemantle et al. 20 in 2015 presented a surplus weekend admission mortality of 16% as justification for 7-day services as the ‘solution’. During negotiations to change NHS doctors’ contracts to facilitate the introduction of 7-day services,21 the then Secretary of State for Health and Social Care also linked weekend deaths to doctor numbers,22 stating that ‘. . . someone is 15% more likely to die if admitted on a Sunday than on a Wednesday because we do not have as many doctors in our hospitals at the weekends as we have mid-week’ (contains Parliamentary information licensed under the Open Parliament Licence v3.0, URL: https://parliament.uk/site-information/copyright-parliament/open-parliament-licence/) and referring to the weekend effect as a ‘global scandal’. This precipitated a vigorous antipathetic response to 7-day services on social media (Figure 2) and contributed to a threat of strike action by junior doctors. 23 The ‘weekend effect’ seemed to have evolved from a problem requiring scientific exploration into a political tool for implementing health policy. It was against this background that the High-intensity Specialist-Led Acute Care (HiSLAC) project was established.
FIGURE 2.
Frequency of citations of the ‘weekend effect’, 7-day services and integrated care. a, Twitter, Inc., San Francisco, CA, USA (www.twitter.com); b, up to 31 October 2019.
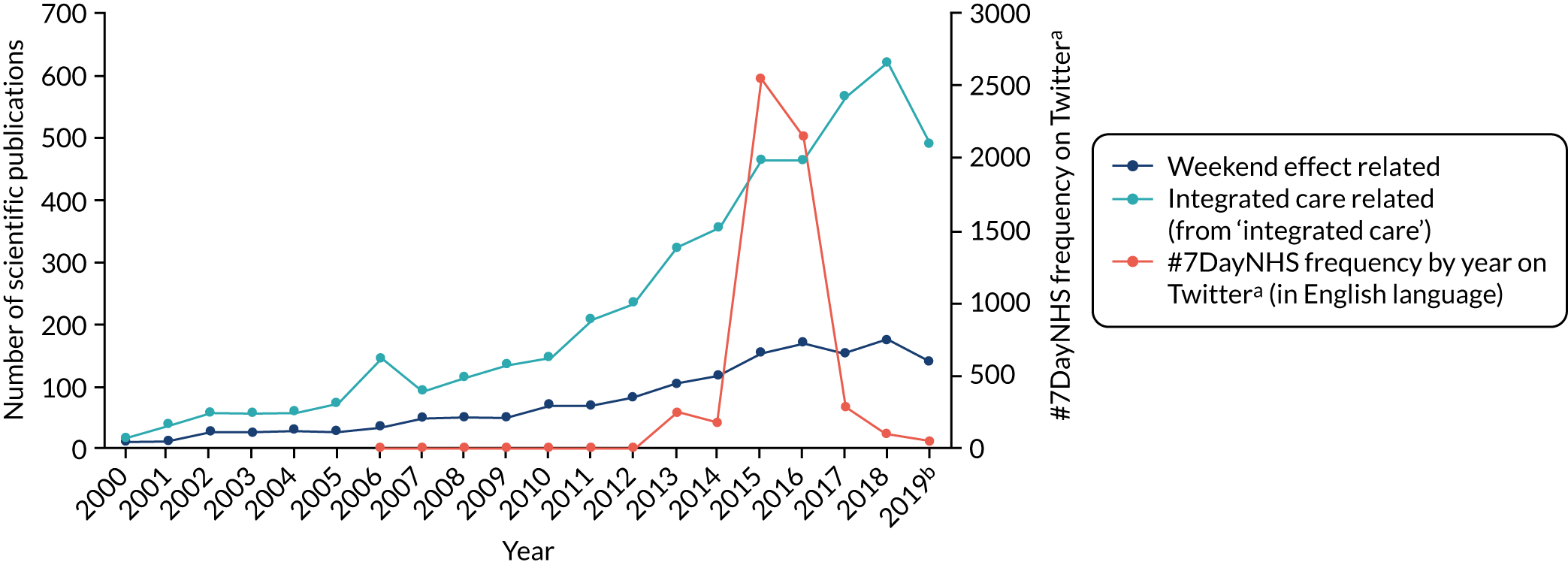
The ‘weekend effect’
The weekend effect was first reported by Bell and Redelmeier in 2001,24 who reported a significantly higher mortality rate associated with EA to hospital at weekends for 23 of the 100 leading causes of death in Canada. The authors were unable to exclude the possibility that patients admitted at the weekend were sicker, but hypothesised that the cause for the surplus mortality was the reduction in medical staffing, particularly senior doctors, in hospital at weekends. In a perceptive accompanying editorial to that paper, Halm and Chassin25 stated that:
Disentangling the potential causal pathways would require painstaking detective work . . . of first accounting for the biologic and social determinants of risk and then identifying the precise differences in processes of clinical care that explain the differences in risk-adjusted outcomes.
Halm and Chassin25
However, few investigators attempted this detective work in the intervening years. Although the literature quantifying the magnitude of the weekend effect continued to accumulate, only one paper had attempted to investigate the cause: a single-centre 8-year study from Dublin that found that patients admitted at weekends were sicker,26 based on adjustment using abnormal biochemistry results. The HiSLAC project therefore chose to focus on Halm and Chassin’s challenge, as the weekend effect was of importance to patients, health professionals, policy-makers and politicians.
How is the weekend effect calculated?
The weekend effect is a complex metric, a ratio of two ratios: the weekend mortality rate divided by the weekday mortality rate. This means that there are four primary mechanisms by which an excess mortality might be generated, as shown in Figure 3.
FIGURE 3.
Potential primary mechanisms of the weekend effect.
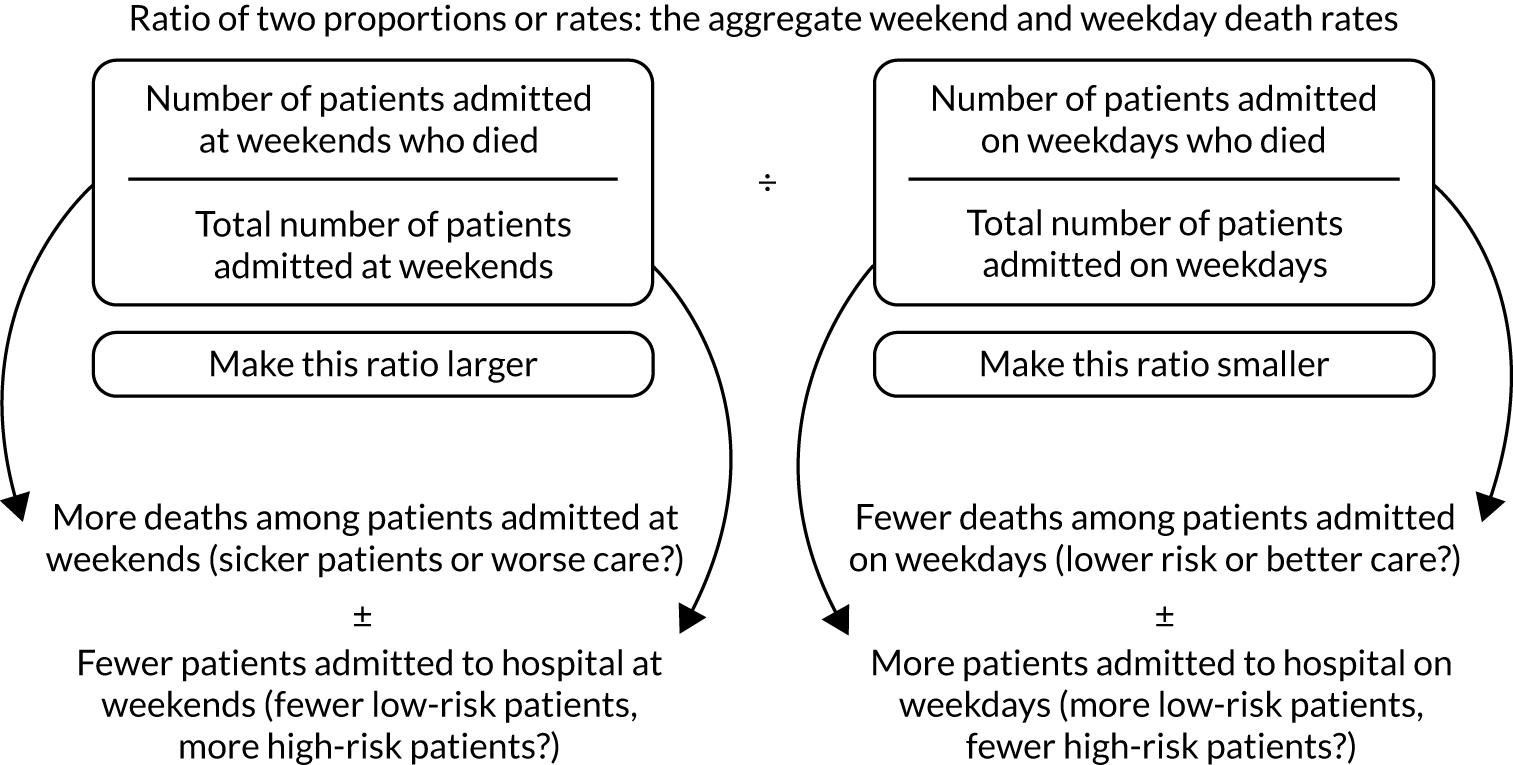
The definition of ‘weekend’ requires some elaboration. In the Christian tradition, Sunday was a day for worship and rest from labour; the weekend now usually incorporates Saturday and Sunday in the western world. The Jewish Sabbath is from sunset on Friday to sunset on Saturday; the Muslim days of rest in some countries was from Thursday to Friday, but increasingly now spans Friday to Saturday. The most frequent definition of ‘weekend’ in the scientific literature is that period of the week from midnight on Friday until midnight on Sunday. This is the definition we use in the HiSLAC project. In our literature reviews we have used the data as published in the source documents.
The potential causal pathway for the weekend effect
The causal pathway could include a number of independent or interlinked underlying causes that could be community or hospital based, and could be related to case mix, clinical care quality or measurement artefact (Figure 4).
FIGURE 4.
The acutely ill patient pathway and factors potentially influencing weekend–weekday admission mortality differences. Reproduced with permission from Chen et al. 27 © Author(s) (or their employer(s)) 2019. Re-use permitted under CC BY. Published by BMJ. This is an open access article distributed in accordance with the Creative Commons Attribution 4.0 Unported (CC BY 4.0) license, which permits others to copy, redistribute, remix, transform and build upon this work for any purpose, provided the original work is properly cited, a link to the licence is given, and indication of whether changes were made. See: https://creativecommons.org/licenses/by/4.0/. This figure includes minor additions and formatting changes to the original figure.
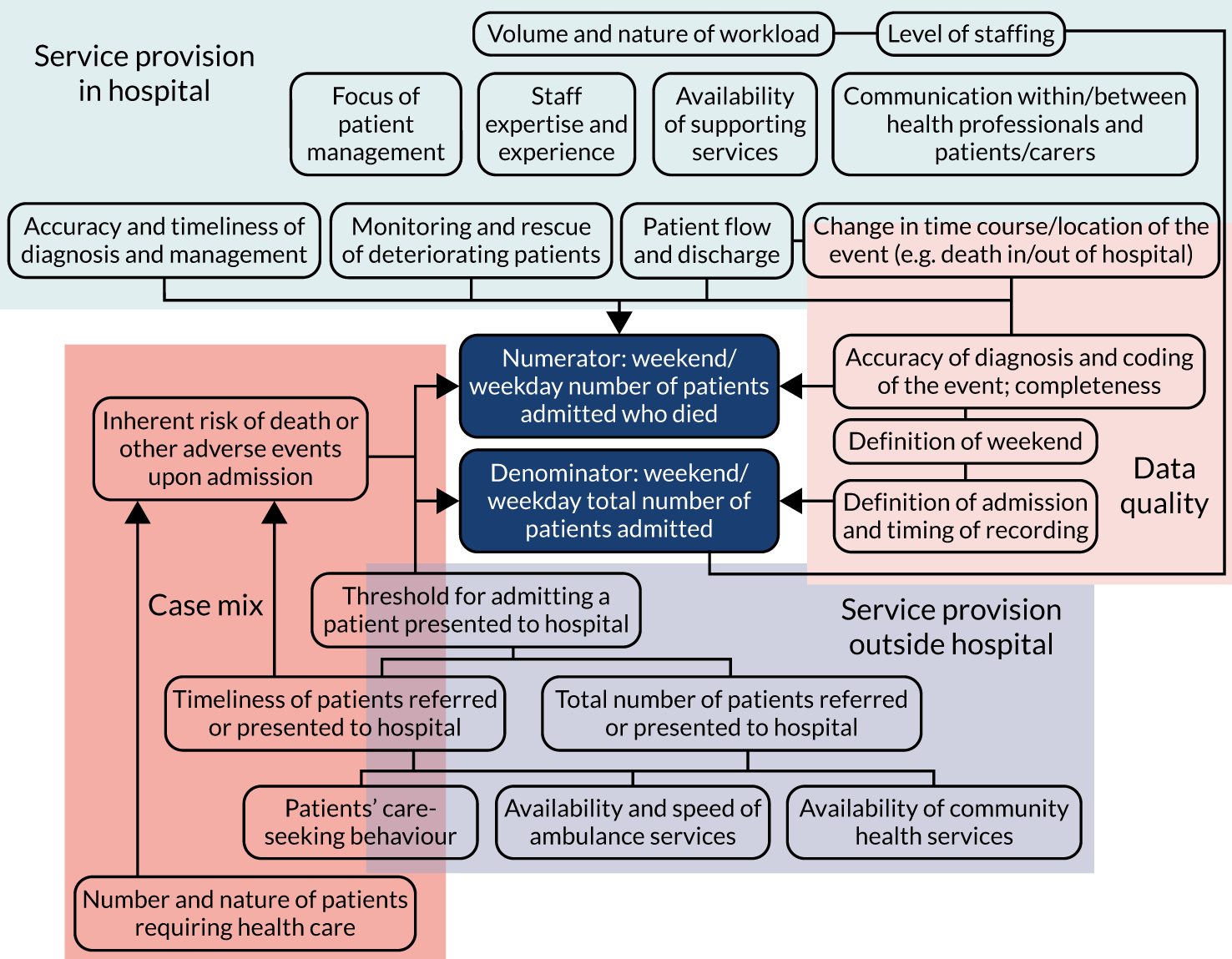
Rationale for and design of the HiSLAC project
When the Academy of Medical Royal Colleges’ working party produced its recommendations for daily consultant review,28 it did so recognising that this was a consensus opinion across all royal colleges, and that the supporting scientific evidence was largely indirect and observational in nature. Given the estimated cost of implementing 7-day services (including increased consultant presence at weekends) of between £1.07B and £1.43B,29 research using empirical data incorporating health economics modelling was needed. It was self-evidently impossible to conduct a conventional prospective randomised controlled trial of high- compared with low-intensity consultant staffing, but the planned roll-out of 7-day services provided an unique opportunity for a ‘natural experiment’ conducted under real-world conditions to test the hypothesis that increasing the numbers of (senior) doctors would result in better quality of care for patients following EA to hospital, with weekend admission mortality rates as one of the key indicators.
Doing this required the development of measures of specialist (consultant grade) doctors’ input across the health service, and metrics for care quality that would allow us to map 7-day service penetration and impact over time. At the time of implementation of 7-day services, it was not clear how the uptake of the standards would be measured and monitored. In the event, NHS Improvement required trusts to perform board-assured audits based on local case record reviews. However, the methodology changed in subsequent years, making analysis of secular trends difficult, and the data did not allow for the calculation of the ‘dose’ of specialist input. The 7-day service standard of greatest relevance to potential consultant impact on the weekend effect is standard 2, ‘emergency admissions assessed by a consultant within 14 hours’. For 2017 and 2018, the methodology for standard 2 was based on local case record review of 20 weekday and 10 weekend admissions; the results were presented as a single figure of percentage compliance across the 7 days of the week. 30 In 2019, the methodology was changed to a binary ‘yes’ or ‘no’ response based on each trust’s self-assessment of whether or not 90% of their audited patients had been reviewed by a consultant on weekends and weekdays combined. 31 Neither method permitted the separation of weekend from weekday performance without access to trust-specific data, which we were unable to obtain from NHS Improvement. Moreover, the definition of consultant review is not specified; it could mean direct bedside review by the consultant, remote review (e.g. a ‘board round’), or that a patient had been reviewed by a junior doctor working under the supervision of a consultant present in the hospital but not at the patient’s bedside.
Consultant numbers were available by trust, but trusts provided no information on how those consultants were deployed during the week, in hours or out of hours, or in caring for EAs.
The first phase of the HiSLAC project, therefore, required us to develop measures of the ‘dose’ of specialist input. We also had to acquire sufficient preliminary data (phase 1) to demonstrate that a project extending over several years was achievable (phase 2). Although HiSLAC was initially conceived as a 3-year project, the HSDR programme board proposed that we extend this to 5 years to allow sufficient duration to determine secular change. Appropriate stewardship of public funds was assured by establishing an independent Governance and Oversight Board to control ‘decision gates’ based on meeting specific progress goals.
An overview of the study is presented in Figure 5 and the initial research plan design is shown in Figure 6. We chose two key methods to strengthen the observational nature of the proposed research: triangulation and difference-in-difference analyses. Triangulation employs concurrent use of different (and preferably independent) measures of a particular phenomenon; if similar observations are made using different methods, this increases the confidence in the credibility of those observations. We did this by using quantitative and qualitative (mixed-methods) approaches, and by combining whole-system measurements across NHS England with in-depth examinations of a subgroup of 20 hospitals. Difference-in-difference analyses strengthen causal inferences by reducing the impact of confounding variables (which indirectly influence associations between dependent and independent variables) in studies where random assignment to intervention or control is infeasible. 32 We used this approach in comparing trust-level differences between weekends and weekdays (specialist intensity, admission mortality, error and adverse event rates, and judgements of care quality) and then comparing those differences with change over time (a ‘triple’ difference).
FIGURE 5.
Overview of the HiSLAC project.
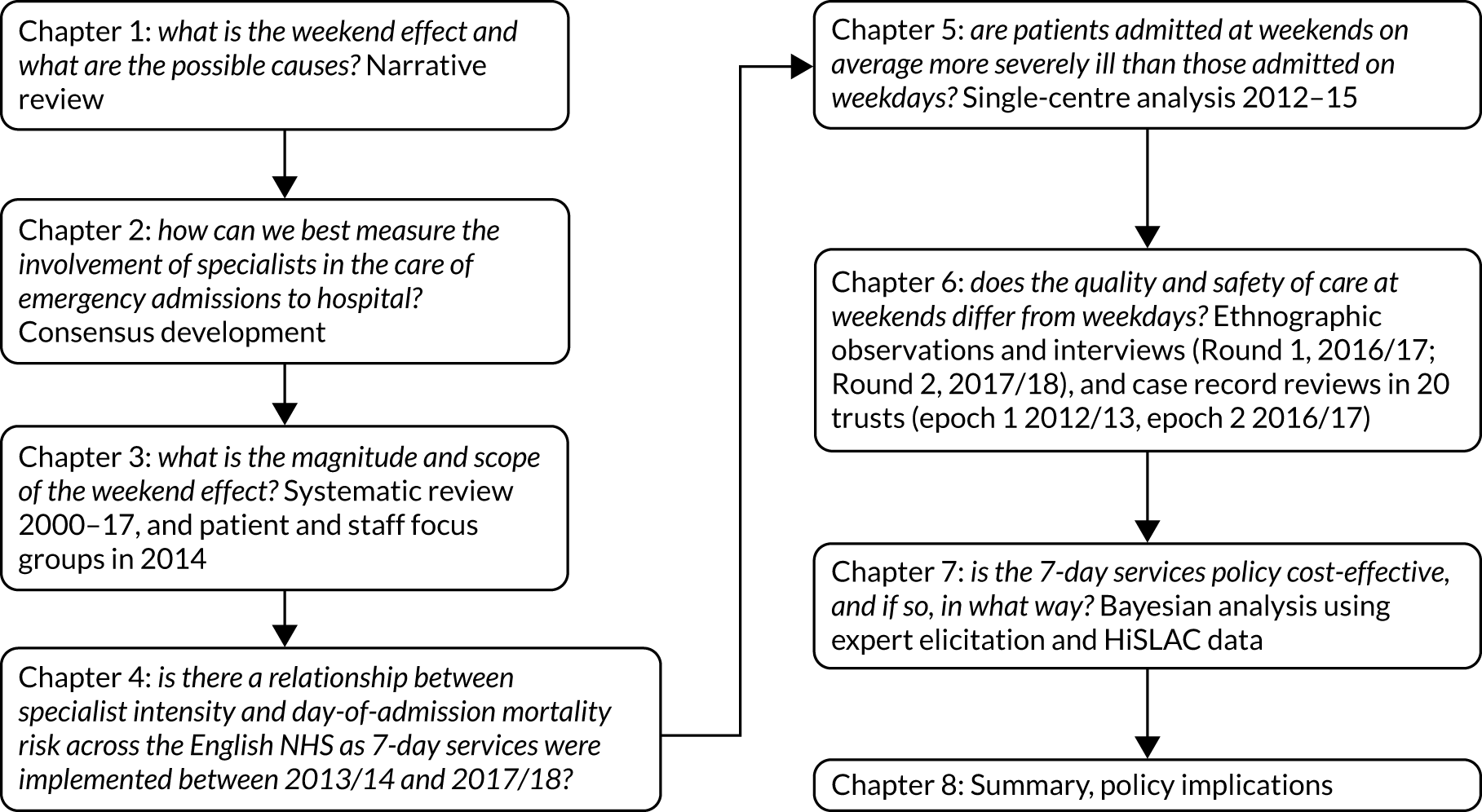
FIGURE 6.
The initial research plan flowsheet for the HiSLAC project. CPR, cardiopulmonary resuscitation; LoSLAC, Low-intensity Specialist-Led Acute Care; ICNARC-CMP, Intensive care national audit & research centre – case mix programme; NICE, National Institute for Health and Care Excellence; NHS(E), NHS England; NHS-IQ, NHS Improving Quality; non-op, non-operative; ONS, Office for National Statistics; PAS, Patient Administration System; QA, quality assurance.
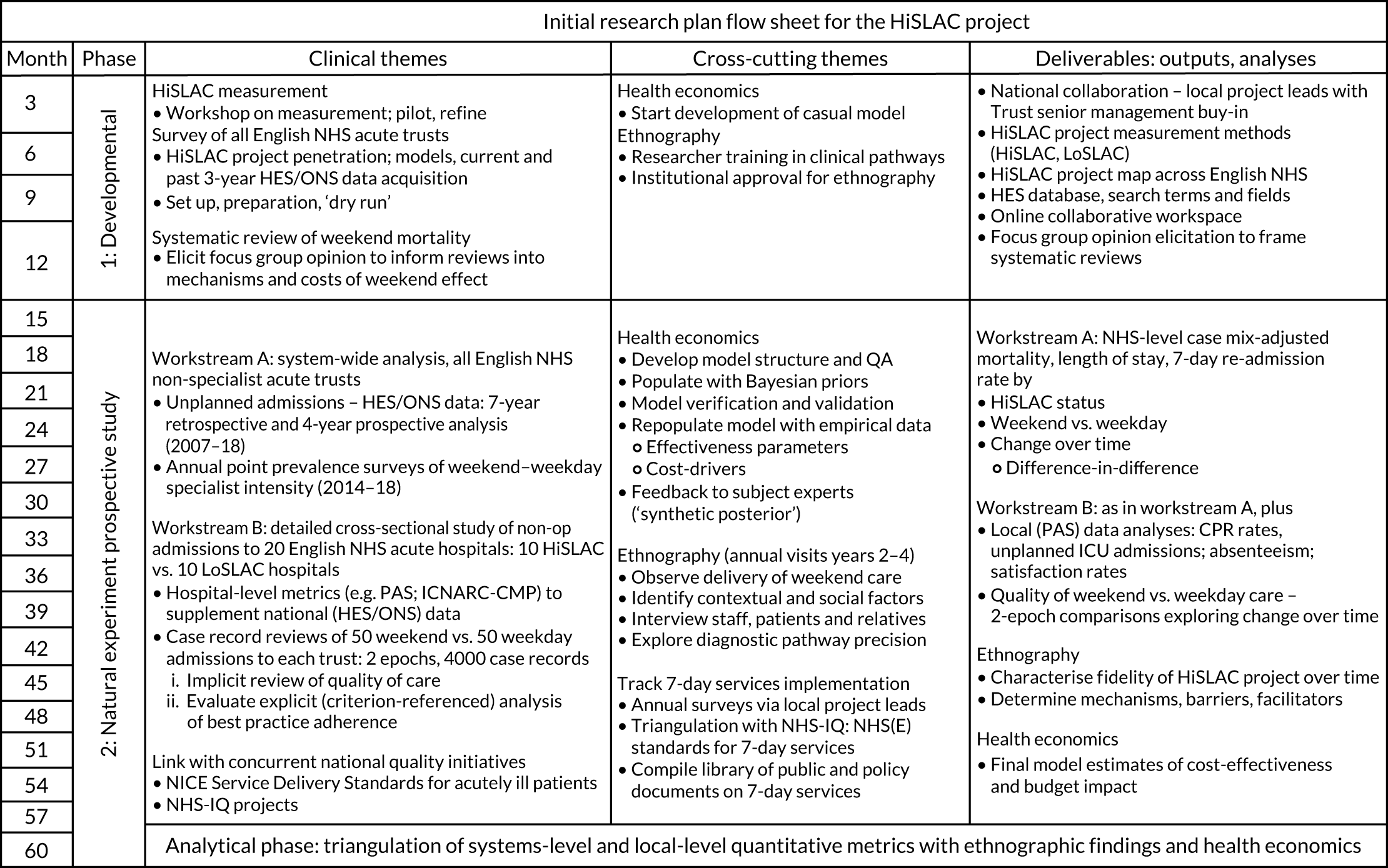
The research plan was broadly retained over the 5 years of the project, with two modifications. The first modification was that, despite repeated efforts, we were unable to realise the proposed collaboration with NHS Improving Quality [renamed NHS Improvement] when it assumed the regulatory roles previously held by the Trust Development Authority (for NHS trusts) and Monitor (for NHS foundation trusts) in 2016. Now merged with NHS England, NHS Improvement holds the annual self-reported audit data returns from each trust for implementing 7-day services. These data are reported by individual trusts and are aggregated by NHS Digital for each standard in binary form as having been attained or not, but the original raw data were not made available to the HiSLAC project; therefore, we were unable to compare HiSLAC data on specialist intensity with each trust’s self-reported 7-day service standards. In addition, NHS Improvement’s methodology for collecting 7-day service data and the definitions employed have changed over time, making secular changes difficult to assess.
The second modification was the decision to perform an additional study to test the hypothesis that patients admitted to hospital at weekends were sicker. As this required detailed information, including physiology (vital signs measurements), that is not collected nationally in a standardised format, we performed a single-centre study using the clinical information system available at a large urban university teaching hospital.
Aims and objectives
The aim of the HiSLAC project was to determine whether or not increasing the intensity of specialist-led care at weekends improves outcomes for patients admitted to hospital as emergencies at weekends.
The objectives of the HiSLAC project were to:
-
quantify specialist input into the care of EAs and map changes in provision over time
-
compare the quality of care in hospitals with high levels of weekend specialist cover with that of hospitals with lower levels, using mixed methods
-
determine whether or not the case mix of patients admitted at weekends differs from that of patients admitted on weekdays
-
develop a health economics model to estimate the costs and health outcomes [quality-adjusted life-years (QALYs)] associated with increased intensity of specialist provision.
Chapter 2 Methodology and metric development
In this chapter, we describe the general methodology of the HiSLAC project.
Phase 1, the first year of the HiSLAC project (2014), was dedicated to three goals:
-
establishment of the HiSLAC national collaboration of acute trusts across England
-
acquiring data on the case mix of and outcomes for adult EAs
-
developing and piloting a metric for measuring and tracking specialist intensity over 5 years.
Phase 2 continued for a further 4 years from 2015 to 2019. The specific methods for each study are presented in Chapters 3–7.
Establishment of the HiSLAC national collaboration
We wished to engage the participation of as many adult acute non-specialist hospital trusts in England as possible. We therefore sought and obtained endorsement of the HiSLAC project from NHS England, the NHS Confederation and the Academy of Medical Royal Colleges in the form of a joint letter to trust chief executives and medical directors asking them to support the project. In the absence of a centralised and accessible list of names and contact details, the project team telephoned the personal assistant to the chief executive of every acute trust in England to obtain this information, which was provided with varying degrees of confidence and alacrity. The supporting letters and a description of the project were then sent by e-mail in February 2014, with follow-up e-mails and telephone calls to non-responders. We asked each chief executive to appoint a local HiSLAC project lead for the trust. Of 141 acute hospital trusts in England, 127 agreed to participate and 115 trusts contributed data to subsequent surveys.
Acquiring data on adult emergency admissions across acute non-specialist trusts in England
We applied to NHS Digital for all acute admission data for English hospitals from 1 April 2007 until 31 March 2018. The data set, Hospital Episode Statistics (HES), is a mandated commissioning minimum data set in which all admitted patient care activity is captured in all English hospitals. The data set is applied consistently across the service, backed by appropriate training in the various coding systems it uses. With the exception of minor modifications, the data set is constant over time. The units of data are ‘episodes’ of care spent by one patient under the care of one consultant. Typically, one spell in hospital consists of just one episode of care, although complex cases can involve several sequential episodes. HES is highly comprehensive, capturing administrative, demographic and clinical information, most of which is coded. There are comprehensive coding and classification systems for diagnoses, procedures, units of administrative geography, specialties, NHS organisations and case-mix categories, among others. Although largely designed for administrative purposes, given the large number of clinical data it contains, HES is commonly used in observational studies of clinical outcomes such as mortality and emergency re-admission.
The HES data sets were stored on a dedicated secure server in the University of Birmingham and processed using Microsoft Structured Query Language (SQL) Server 2008 (Microsoft Corporation, Redmond, WA, USA), which is database software capable of handling large numbers of data. From an initial data set of 220 million patient records, we extracted those records relating to EAs over the decade.
Once in our data warehouse, we added a number of derived variables to the data set. We linked the primary diagnosis on discharge (captured using the International Statistical Classification of Diseases and Related Health Problems, Tenth Revision33) to the Clinical Classifications Software category to obtain a case-mix variable. We mapped the secondary diagnoses to the Charlson Comorbidity Index as a further measure of case severity. We also attached income deprivation scores to the data. This is a domain of The English Indices of Deprivation 2010,34 which is the standard measure of area deprivation used by the UK government. Data on variables such as income, unemployment education and crime are collected at a small level of census geography called a lower-level super output area. Income deprivation, the domain used in this study to adjust for socioeconomic status, is a score based on the proportion of residents of a lower-level super output area whose income is ≤ 60% of the national median income. As this domain uses more up-to-date data than other domains of the score, we chose this as our socioeconomic status indicator.
Hospital Episode Statistics were used in several phases of the study and several iterations of analysis were completed, each differing slightly in objectives, methods and inclusion criteria, depending on the requirement of the study. First, we performed a detailed analysis of in-hospital mortality in financial year 2013/14. In this part of the study (subsequently published),35 we examined the discharge episodes of all patients admitted as emergencies to a list of hospitals that we had previously identified as belonging to non-specialist acute trusts. It was important to understand mortality trends over time in this study, as the introduction of the 7-day services policy was implemented over time and in the context of changes in the underlying epidemiology of needs for emergency care. For this reason, we needed to examine secular trends in hospital mortality. To do this, we analysed mortality data for EAs between financial years 2007/8 and 2017/18. For the longitudinal analyses, EA data were analysed for those trusts that participated in the point prevalence survey (PPS).
For some components of the study, we would have preferred to use HES, but the data were not available. HES are typically made available to the research community (in a cleaned format) approximately 6 months in arrears. To select cases for our case note review, we needed data more quickly than this, so we arranged for participating hospitals to send us pseudonymised extracts from their patient administration system. These data are effectively the raw material of HES, as they are uploaded to NHS Digital, where they are cleaned and a number of derived variables are added. Using data in this ‘raw’ format was problematic as extra work was required to clean the data, including eliminating duplicate records and, in some cases, censoring data that were insufficiently complete. This had to be done for 20 participating hospitals, all of which had slight differences in formatting and data quality. The data were managed similarly to HES in a standalone secure server, where they were uploaded to the Microsoft SQL Server platform. These data were then used to randomly select cases of adult non-surgical EAs to be included in the case note review phase of the study.
Developing and piloting a specialist intensity metric
Nominal Group
We convened a moderated 19-member Nominal Group comprising those with expertise in hospital medicine, human resources, health services research, and patient and public representatives. We defined specialists as consultants or associate specialists with a certificate of completion of specialist training or equivalent. We employed a two-step approach. A briefing paper was prepared and circulated before the plenary meeting (step 1), which used moderated group dialogue to explore the main issues and define more clearly the areas of certainty and uncertainty. The content of these discussions was captured by two rapporteurs. In step 2, the proposals from step 1 were presented to the group for further refinement by iterative e-mail discussion until consensus was reached.
Participants were asked to focus on three main questions:
-
The numerator – what measure(s) would best characterise high-intensity specialist-led acute care (HiSLAC)?
-
The denominator – what measure(s) would permit standardised comparisons to be made between different hospitals or patient populations?
-
Information source – how should hospital information be accessed?
Nominal Group members considered the possible options and limitations of currently available data, such as contractual time, actual time spent on direct care, numbers of specialists available, or perceived or actual adherence to national standards (Box 1). The relationship between contracted time and delivered service would be variable and likely to be an underestimate of the actual time spent. Contracts vary between specialists, even in the same discipline, and details of individual contracts may not be held by each hospital in an easily accessible centralised database. Multitasking is common. Some specialists work across different locations within or between institutions. Contracted hours allocated to emergency or out-of-hours care may represent a composite of weekday nights, weekend nights, and weekend days, complicating the link between contacted time and weekend working.
Information is likely to be available for location-specific urgent care disciplines (the ED, the AMU, the ICU), but not for general ward care or support services. For example, specialists may be rostered as the duty consultant for acute admissions while still providing routine outpatient clinics, or radiologists may undertake reporting of elective as well as emergency CT scans.
The average or minimum number of hours a specialist is present on the wardThis is a location-specific metric that is employed in certain specialty standards, for example in acute medicine,39 but for general ward care does not differentiate emergency from elective admissions, or the care of patients in the convalescent phase of their illness.
The frequency of specialist ward roundsA daily ward round in an ICU or an AMU would indicate that all or nearly all patients are likely to be reviewed, but this is not the case for ordinary wards, where specialists may see only those patients for whom they are responsible twice per week, with care between these intervals delivered by doctors in training. The Academy of Medical Royal Colleges’ standards do not use ward rounds as a metric.
The total number of specialists contracted to deliver direct clinical care to patients undergoing emergency admission to hospital at weekendsThis requires counting the number of specialists contributing to rotas at weekends. Data could be collected at Directorate level from published clinical rotas, which would allow differentiation of weekend duties from night duties, as both are classed as ‘out-of-hours’ work. However, it does not provide an indication of ‘dose’ of specialist involvement (one specialist or several concurrently), provide the extent to which contractual commitments are realised in practice or continuing care responsibilities for emergency patients now in a convalescent phase, or distinguish specialists delivering care in a single location from those covering several disciplines and clinical areas concurrently.
The total number of contractual professional activities allocated to direct clinical care of emergency admissionsThis metric focuses on contractual time allocation rather than the number of specialists. It requires inspection of individual contracts unless all specialists in a given area/specialty work the same hours. This metric has the merit of identifying clinical work delivered at nights or at weekends by counting ‘premium rate’ professional activities, which are 3 hours in duration, compared with 4 hours for weekday daytime work. However, it will not distinguish premium rate professional activities delivered at weekends from those allocated to weekday night duties. Moreover, the contractual time allocation may bear little resemblance to the time actually delivered.
Compliance or non-compliance with national standards for specialist staffing: gap analysesPublished standards for 7-day consultant staffing are available for EDs (16 hours per day), AMUs (12 hours per day) and ICUs (twice-daily ward rounds; all new admissions reviewed in person by consultant in intensive care medicine within 12 hours) and general medicine (Academy of Medical Royal Colleges). Drawbacks of a compliance metric include its binary and un-nuanced nature (compliant/non-compliant), and the requirement for local audit.
Individual specialist self-reported hours spent providing care for acute admissionsAn annual PPS of every specialist in the hospital, asking them to identify whether or not they had delivered direct patient care (in hospital, not remotely) to EAs on a Sunday and Wednesday, and the estimated number of hours they had provided that care. This would source data from as close to the bedside as possible, but would also be a new metric, requiring a web-based survey of all hospital specialists.
Denominator Number of acute hospital bedsThe total number of acute hospital beds is generally accessible information. However, although bed occupancy averages 90% across the NHS, the number of beds may be no guide to workload or the volume of admissions.
The number of emergency admissions (weekend, weekday, total)This can be obtained from HES data, avoiding the need to trouble hospital administrative staff. If necessary, non-operative EAs can be distinguished from surgical admissions by their coding.
The number of patients reviewed each day by a specialistThis would require local knowledge (most likely an estimate, rarely original data). It would need to differentiate new EAs from those later in their hospital stay, and to distinguish emergency from elective admissions.
AMU, acute medical unit; CT, computerised tomography.
Source references for Nominal Group
The group agreed that the ideal metric or set of metrics would be unambiguous, accurately reflect the specialist care of patients admitted as emergencies, and be available from existing data sets within trusts or relatively easy to collect without substantial additional work being required. Because outcomes would more likely be influenced by events occurring early in the admission pathway, metrics for specialist intensity should permit a comparison between weekends and weekdays to allow a difference-in-difference analysis to mitigate baseline variations in hospital type or case mix. The group noted that the absence of a difference could indicate either an equivalently good or an equivalently poor standard of staffing across the 7 days.
Options for measuring specialist intensity
The project team assembled a range of options for the Nominal Group to consider. These are listed in Box 1.
Final formulation of the specialist intensity metric
Following the plenary meeting, there were two discussion rounds by e-mail. The Nominal Group unanimously selected the self-reported estimated number of specialist hours per 10 EAs on Sunday and on Wednesday as the primary measure of specialist intensity; this was to be obtained using a web-based survey (Table 1) distributed via local project leads in each acute trust. Sunday and Wednesday were chosen because they represented the most extreme values for the weekend effect, and for survey simplicity. Response rates would be calculated from the number of specialists contacted by the local project leads. For the denominator, the Nominal Group prioritised the mean number of EAs for each trust on Sundays and Wednesdays throughout the financial year, with the data being obtained from HES. A parsimonious question set was considered essential to maximise response rates. To take into account variable response rates between specialists and trusts, estimates of total specialist hours from the survey would be scaled up using the reciprocals of the response rates in each trust. It should be noted that this adjustment has no effect on Sunday-to-Wednesday ratios.
PPS questions: all specialist staff in the trust [specialists = all CCT holders (consultants and associate specialists)] | Were you providing direct clinical care to EAs? | If yes . . . ↓ | Approximately how long did you spend providing direct care? (Maximum of 12 hours) | Were you the specialist responsible for continuing care of these patients? (i.e. ‘named consultant’) | Which of the following locations best describe where you spent most time delivering direct patient care? (Maximum of two choices for each period) | |
---|---|---|---|---|---|---|
1. Last Sunday [date] were you physically present in the hospital at any point between 08:00 and 20:00 hours providing direct clinical care to patients who had been admitted for their current admission episode as an emergency to an in-patient bed including CDUs/observation wards? | Yes/no | If yes . . . ↓ |
Yes Some No |
Acute medical unit Acute surgical unit Combined medical/surgical acute unit Adult medical wards Adult surgical wards Specialist wards or units High-dependency unit Obstetrics or gynaecology |
Intensive care unit Operating theatre Radiology Endoscopy Laboratories Paediatrics wards/unit ED clinical decision unit Other |
|
2. Last Wednesday [date] were you physically present in the hospital at any point between 08:00 and 20:00 hours providing direct clinical care to patients who had been admitted for their current admission episode as an emergency to an inpatient bed including CDUs/observation wards? | Yes/no | If yes . . . ↓ |
Yes Some No |
Acute medical unit Acute surgical unit Combined medical/surgery acute unit Adult medical wards Adult surgical wards Specialist wards or units High-dependency unit Obstetrics or gynaecology |
Intensive care unit Operating theatre Radiology Endoscopy Laboratories Paediatrics wards/unit ED clinical decision unit Other |
|
3. Please identify your main specialty (only one choice) Please answer this question even if you had no clinical duties on the 2 days identified above |
Acute internal medicine Allergy Anaesthetics Cardiology Cardiothoracic surgery Chemical pathology Clinical genetics Clinical neurophysiology Clinical pharmacology and therapeutics Dermatology Emergency medicine |
Endocrinology and diabetes Gastroenterology General surgery Genitourinary medicine Geriatric medicine General (internal) medicine Haematology Histopathology Immunology Infectious diseases Intensive care medicine Medical microbiology |
Oncology Ophthalmology Otolaryngology Oral and maxillofacial surgery Obstetrics and gynaecology Neurology Neurosurgery Nuclear medicine Paediatrics Palliative medicine Pharmaceutical medicine Plastic surgery |
Radiology Rehabilitation medicine Renal medicine Respiratory medicine Rheumatology Sport and exercise medicine Trauma and orthopaedic surgery Tropical medicine Urology Other |
The weekend-to-weekday intensity ratio is derived as follows:
An alternative formulation of this ratio is:
The Nominal Group also proposed a directorate-level managerial questionnaire as a second option for evaluation (see Appendix 1). Directed to the divisional director or clinical service lead for each specialty participating in EAs, this questionnaire requested information for Sundays and Wednesdays on the numbers of specialists providing direct patient care, the average hours of direct care, the way clinical duties were delivered (single days, blocks of days) and the frequency of consultant review.
Piloting the surveys across acute trusts in England
We asked local project leads to circulate the web-enabled PPS to all specialists in the participating hospitals on Sunday 15 June and Wednesday 18 June 2014; these dates avoided major holidays and winter pressures. Respondents were asked to confirm specialist status, and state whether or not they were in the hospital and providing care to patients admitted as emergencies from 08:00 to 20:00 on either of these days, and, if so, for how long. Local project leads were given response rates and asked to issue weekly reminders over the following 4 weeks before the survey closed.
The directorate-level questionnaire was sent via trust local project leads to the medical and managerial service leads of the four disciplines most focused on acute and emergency medical care: emergency medicine, acute medicine, general internal medicine and intensive care medicine. The questionnaire asked respondents to estimate the number of specialists and the number of specialist hours, allowing comparison with the PPS. Respondents were also asked if there were gaps in the consultant rota filled by locums, and whether or not there were routine consultant-led ward rounds on a Sunday, as a marker of progress towards one of the standards for 7-day services.
The overall response rate to the PPS was 45%. Complete responses to the directorate-level questionnaire were obtained from 31% of trusts. We found a moderate correlation between these two estimates of specialist intensity (Wednesday: r = 0.406, p = 0.0002; Sunday: r = 0.480, p < 0.0001) (see Appendix 2). As the PPS was directed to all specialists, while the directorate level survey focused on four acute specialties only, we considered this level of agreement to be acceptable and chose the PPS as the most authentic method with the highest response rate.
Ethics
HiSLAC was approved by the Health Research Authority [Integrated Research Application System (IRAS) project ID 139089] and by the Welsh Research Ethics Committee (reference 13/WA/0372) as service evaluation of an existing form of health-care delivery without collecting patient-identifiable data. The committee noted that informed consent was not required for accessing anonymised patient records and only the qualitative research observations and interviews needed consent. Consent for this component (involving focus groups, interviews, and observations employing elements of an ethnographic approach) was obtained from participants after they received the participant information sheet (see Report Supplementary Material 1).
There were no variations in the ethics status of this research during the study.
Chapter 3 The magnitude and mechanisms of the weekend effect in hospital admissions: a mixed-methods systematic review
Introduction
The aim of this mixed-methods review was to quantify the magnitude of the weekend effect on hospital mortality and, concurrently, to conduct a qualitative narrative synthesis of the literature on the mechanisms of the weekend effect. In practice, however, we were not able to identify literature that provided sound evidence on the mechanisms that underpinned the weekend effect. We therefore conducted an exploratory qualitative study consisting of focus groups to explore staff and patient perceptions of how differences in structures and processes of care between weekdays and weekends might contribute to the weekend effect. The methodology was planned and published as a unified parallel process42 and the results were published as two separate papers. 27,43 We provide a summary here.
Methods
This review was registered with PROSPERO (registration number CRD42016036487). MEDLINE, CINAHL (Cumulative Index to Nursing and Allied Health Literature), HMIC (Health Management Information Consortium), EMBASE™ (Elsevier, Amsterdam, the Netherlands), EThOS (Electronic Theses Online Service), CPCI (Conference Proceedings Citation Index; Clarivate Analytics, Philadelphia, PA, USA) and the Cochrane Library were searched from January 2000 to April 2015, with the MEDLINE search updated to November 2017, using terms related to ‘weekends or out-of-hours’ and ‘hospital admissions’. 42 Records were screened by the review team for two component reviews: a systematic review of the magnitude of the weekend effect and the proposed qualitative analysis of the mechanisms of the weekend effect.
Studies reporting predominantly unselected hospital admissions were included in the reviews, covering emergency and elective adult, paediatric, medical, surgical and obstetric admissions. For studies that reported both aggregated and condition-specific weekend effects, only the aggregated data were used in the quantitative analyses of the systematic review. We chose to focus on unselected, rather than condition-specific, admissions to avoid duplicating meta-analyses focusing on condition-specific admissions. 42,44–46 We did not include studies that compared night-time with daytime admissions only. Independent duplicate coding of potentially relevant studies was performed for the first 450 (40%) potentially relevant records to maximise consistency of approach; the remaining studies were then assessed by single reviewers. Final study selection was determined by two reviewers. Any discrepancies in study coding and selection were resolved by discussion or seeking further opinion from other review team members. Risk-of-bias assessment performed independently by two reviewers focused on the level of statistical adjustment categorised as ‘comprehensive’, ‘adequate’ [(1) adjustment for acute physiology; and (2) adjustment for context using other measures of urgency or severity], ‘partial’ and ‘inadequate’.
For the quantitative review, the primary outcome was the weekend effect on mortality. Secondary outcomes included adverse events, length of hospital stay and patient experience. The data were meta-analysed using a Bayesian random-effects model allowing within-study variation and between-study heterogeneity. Analyses were undertaken using (log)-adjusted odds ratios (ORs) (or hazard ratios or rate ratios if ORs were not reported) and the reported standard errors or equivalent. Studies were therefore implicitly weighted by the estimated variance of individual effect estimates. Where multiple estimates based on different reference day(s) were reported, we used the estimate based on or including Wednesday as the reference group. The primary meta-analysis included all types of admissions. Exploratory subgroup analyses were performed for mixed, emergency, elective and maternity admissions. We calculated the I2 statistic to quantify statistical heterogeneity between studies (with I2 > 50% indicating a substantial degree of heterogeneity). 47 The overall quality of evidence for the primary and secondary outcomes was assessed using the GRADE (Grading of Recommendations, Assessment, Development and Evaluations) framework. 48
Screening of papers for the review of mechanisms of the weekend effect did not identify any high-quality studies with robust evidence of the mechanisms of the weekend effect. As a result, we undertook a qualitative study to explore the views of health-care staff and patients on how the quality of hospital care differed between weekends and weekdays, and how this could contribute to the weekend effect. Quality of care was conceptualised using Darzi’s three elements of effectiveness, safety and patient experience. 49,50 Patients and staff were recruited from three hospital trusts in the Midlands, the north-east and the south-west of England. Patients were recruited through two existing acute-care patient and public involvement groups and through face-to-face recruitment of patients and their relatives during the patient’s stay in the acute medical wards. Focus group moderators and scribes, and interviewers, were trained qualitative researchers. Focus groups were audio-recorded and transcribed verbatim. Data analysis employed thematic analysis,51 supported by NVivo (QSR International, Warrington, UK) qualitative data analysis software, to explore perceptions of quality and safety of care at weekends and the impact on patient outcomes.
Results
Between January 2000 and November 2017, we identified 6441 records, 613 of which passed initial screening. Of these, 319 were subsequently excluded, 224 were considered for qualitative analysis and 68 met the criteria for the quantitative review. None of the papers met inclusion/quality criteria for the qualitative review, but the most relevant were used to inform the topic guides for the qualitative study.
Quantitative review and Bayesian meta-analysis results
Parts of this section have been reproduced with permission from Chen et al. 27 © Author(s) (or their employer(s)) 2019. Re-use permitted under CC BY. Published by BMJ. This is an open access article distributed in accordance with the Creative Commons Attribution 4.0 Unported (CC BY 4.0) license, which permits others to copy, redistribute, remix, transform and build upon this work for any purpose, provided the original work is properly cited, a link to the licence is given, and indication of whether changes were made. See: https://creativecommons.org/licenses/by/4.0/ The text below includes minor additions and formatting changes to the original text.
The 68 selected studies (see Appendix 3) encompassed more than 640 million admissions (range 824 admissions from a single hospital to 351,170,803 admissions from a national database). 52,53 Patient populations included unselected general admissions (n = 11), medical admissions (n = 1), surgical admissions (n = 3), EAs (n = 34), elective surgical admission (n = 5) and maternity (n = 13).
Definitions of ‘weekend’ varied. The majority (n = 28) of studies defined the weekend as including Saturday and Sunday, whereas 14 provided no definition. Nineteen variably included Friday evening, Monday morning or both, and seven included Friday daytime. Mortality timing included death in hospital, and at 7 and 30 days post admission. These differences may contribute to statistical heterogeneity, although bias is limited by the metric that employs the difference in weekend admission with the difference in weekday admission (as described in Chapter 1).
For the primary outcome of mortality, we found only one study54 that had adjusted comprehensively for potential sources of bias, including acute laboratory tests and source of admission. Three other studies had adjusted for acute physiology in regression models (to which we can now add the HiSLAC study, published subsequently and described in this report; see Chapter 5). 26,55–57 Adjustment using other non-physiological measures related to patient acuity or dependence (e.g. route of admission) had been used in 17 studies. Of the remaining studies, adjustment was partially achieved in 20 and inadequate in 27.
Bayesian meta-analysis of all types of admissions provided a pooled estimate for the odds of death for weekend admissions of 1.16% [95% credible interval (CrI) 1.10% to 1.23%] (Figure 7). There was wide variation in the estimated weekend effect between studies and subpopulations; although the value for I2 (measuring between-study variance relative to total variance) is low, there is substantial uncertainty (16%, 95% CrI 0% to 62%). The posterior predictive interval suggests that a new study would have an estimated OR of between 1 (no weekend effect) and 1.34 (odds of death 34% higher).
FIGURE 7.
Bayesian meta-analysis of the weekend effect on mortality following hospital admissions. Mohammed et al. 75 and Ruiz et al. 59 contributed to two estimates for each country, as the weekend effect was estimated separately for different subpopulations (e.g. emergency and elective admissions). ‘Posterior predictive’ indicates the predictive interval (see Quantitative review and Bayesian meta-analysis results) obtained from the Bayesian meta-analysis. I2 = 16% (95% CrI for I2 0% to 62%). The I2 represents the ratio of between-study variance to total variance in this three-level model. The apparently low I2 could be attributed to the between-study variance being relatively small compared with the between-estimate variance within individual studies. As the wide CrI indicates, the I2 statistic was estimated with substantial uncertainty. Several studies included in the review were not included in this meta-analysis because of substantial overlap of data between studies, in which case studies judged to have adopted the most comprehensive statistical adjustment were selected.
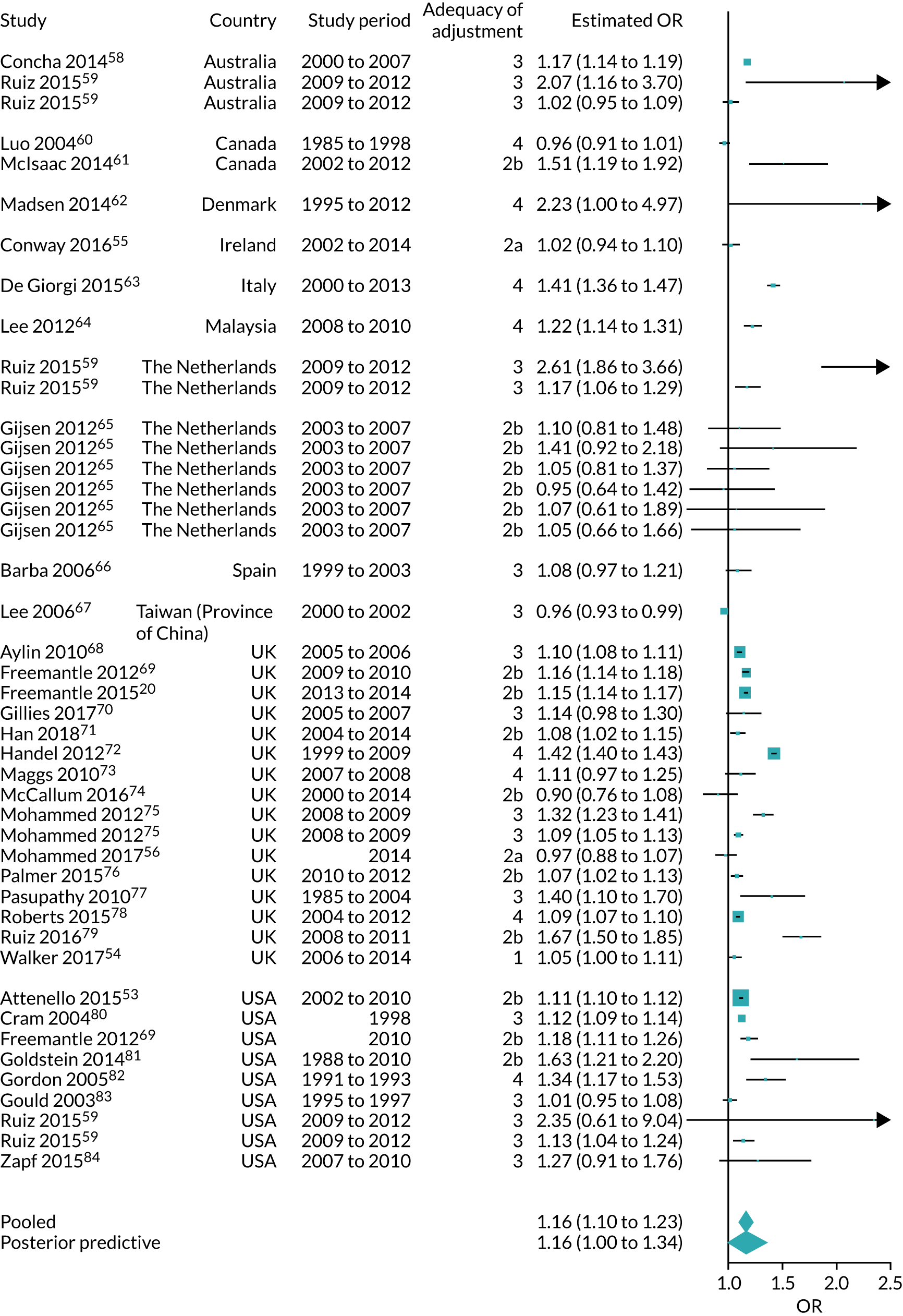
The multivariate metaregression shows that including measures of acute physiology in statistical adjustment produced estimates of the weekend effect close to null, with ORs for mortality approximately 15% lower than those of other studies (see Appendix 4). The weekend effect is greater for studies including elective admissions (ratio of ORs 1.31, 95% CrI 1.23 to 1.38) and is lower for studies including maternity admissions (ratio of ORs 0.84, 95% CrI 0.77 to 0.90). Finally, across all studies, there did not appear to be a secular trend: the magnitude of the weekend effect was similar over the 17 years. However, a study from the USA based on the National Inpatient Sample reported a reduction in the weekend effect on mortality between 2003 and 2013. 85
Nineteen studies examined the impact of weekend admission on the risk of adverse events. 52,53,65,76,81,84,86–97 There was heterogeneity in populations and types of adverse events, and no consistent direction of effect or association with the magnitude of the weekend effect. No study adjusted for physiological severity of illness, even though sicker patients and non-survivors are more susceptible to adverse events. 98 Only one study examined patient perceptions in relation to EA timing, and found greater satisfaction with information provided in the ED for weekend admissions. 99
Focus group study results
Four focus groups, two with clinicians (n = 15) and two with patients (n = 10), were supplemented with individual interviews with four clinicians and two patients. We invited participants to consider their experiences of safety and quality of hospital care at weekends. Four themes emerged: rescue and stabilisation of sick patients, monitoring and responding to deterioration, timely and effective management of the therapeutic pathway, and resilience and risk of error.
Rescue and stabilisation of sick patients
Staff and patients said that the sickest patients were prioritised at weekends, but that others might experience delays in investigation, a lack of special expertise and less continuity of care:
I think my admission during the week was actually less efficient and worse than . . . the one at the weekend [when critically ill].
Patient focus group
It’s the less sick [that] probably have a worse experience [at weekends] because they’re being pushed down the list and waiting longer.
Junior doctor interview
Monitoring and responding to deterioration
Deteriorating patients were perceived as being at greater risk at weekends as a result of delays in detection and response. These delays were associated with reduced staffing levels, staff being unfamiliar with patients who were nominally under the care of teams not on duty in the hospital at that time, the prioritisation of sicker new admissions and the selective identification of certain patients for regular review:
You’ve got less experienced people, you’ve got more bank staff, you’ve got fewer doctors – it’s the whole thing.
Patient focus group
The advantage of the weekdays is the team in that ward knows those patients if anything goes wrong, whereas on a weekend, you are going to call someone who doesn’t know these patients.
Clinician focus group
At a weekend it was really quick to get a consultant review in the morning, the plan was in place, everything was fine, [but] that patient really deteriorated throughout the day and I really struggled to get a senior review on this patient. I was calling people and they were too busy in resus[citation] or whatever.
Clinician group
Timely and effective management of the therapeutic pathway
Patient ‘flow’ includes both the patients’ therapeutic progress from initial acute diagnosis and treatment towards rehabilitation and recovery, and their physical transfer between locations: from an acute medical unit (AMU) to a general ward or specialty areas and elderly care, and thence to discharge back to the community. These transitions between teams and locations were seen as associated with discontinuities in care, presenting a risk to patients. At weekends, the pace of care was perceived to slow markedly, in part because of the decrement in senior medical and specialist input, or because the on-call staff deferred treatment decisions until the named consultant team was back on the Monday:
You don’t have necessarily all the specialisms you need represented, so you need an extra specialism, that person may or may not be on call. There is a delay in getting that input.
Patient group
It’s more like a babysitting service over the weekend.
Clinician focus group
Access to laboratory and radiology investigations could also be problematic at weekends, despite these departments nominally providing a 7-day service:
There may be one or two slots [for scans] for the whole weekend, for the most urgent of patients, and you have to beg, barter, plead, to get your patient seen.
Junior doctor interview
Discharge to the community was similarly impeded, particularly for the frail elderly:
When you have an elderly frail lady or man, 80-plus, who requires [a] package of care [in the community] . . . you have to wait until Monday to get it started.
Consultant interview
Delays in discharging patients would then add to the difficulties of admitting acutely ill patients from the ED.
Resilience and risk of error
Patients and staff regarded weekend health care as less resilient outside high-care areas, such as AMU and ICUs. Error trapping was less likely, handovers and communication were more hurried and less thorough, fewer support staff were available, and patients and families experienced greater difficulty arranging meetings with senior decision-makers:
A patient had the wrong medicines prescribed on a Saturday, got moved to a ward that didn’t have pharmacy cover and [only had] medication reconciliation done 3 days later.
Clinician focus group
You can’t get information as a relative on a ward on a weekend in the same way as you can [in the week] . . . it’s really infuriating.
Patient focus group
Discussion
This mixed-methods examination of the weekend effect is novel in that it combines what is, to our knowledge, the largest quantitative analysis to date with a detailed exploration of care processes at weekends. The use of Bayesian methods for the quantitative review provided a point estimate for the weekend effect (16%), which is in line with other studies, but a wider CrI, which reflects appropriately the variations reported both within and between studies. This higher mortality associated with weekend admission to hospital is a consistent finding across health systems and over time. The qualitative study identified four key deficiencies in processes of care at weekends that are relevant to the health service in England. However, convincing evidence that hospitals are responsible for a causal relationship between processes of care and outcome is elusive for unselected hospital admissions.
Moreover, there is evidence that patients presenting at weekends are sicker than those on weekdays, and both staff and patients observe that the initial care of more severely ill patients following weekend admission is at least as good as, and possibly better than, the care provided on weekdays. Sicker patients might be explained by fewer patients presenting to the ED or being admitted (i.e. a change in the denominator), but could also be a consequence of delays in care of patients in the community pre admission. Reduced community services at weekends were identified by clinical staff as impeding patient discharge, with secondary consequences for timely admission.
We do not imply that the absence of a proven relationship between hospital care processes and weekend admission outcomes means that there is no relationship. The process deficiencies identified by the qualitative study have at least the potential for patient harm, and certainly add to workplace stresses for staff. However, it seems likely that the relationship is complex. For example, standardisation of stroke care has improved very considerably over the last decade, with the development of a centralised hyperacute stroke unit model in London and an integrated model in Greater Manchester;100 however, despite evidence of substantial improvement in reliability of best practice in both centres,101 a secular trend for a reduction in stroke-associated weekend effects between 2008 and 2014 occurred nationally and did not particularly favour London’s centralised model. 102 We conclude, therefore, that insufficient progress has been made since Halm and Chassin called for ‘painstaking detective work’25 to unravel the causal relationships, and that this research needs to examine the whole of the causal pathway, from community to hospital discharge.
Chapter 4 Cross-sectional and longitudinal 5-year study of weekend–weekday specialist intensity and emergency admission mortality
Introduction
As described in Chapter 1, during 2012 and 2013, several initiatives were broadly focused on improving equity of access to quality health care in the NHS across all days of the week, particularly (but not exclusively) for emergency care. There was a degree of consensus between the professions and health policy-makers that weekend services were suboptimal, and that front-line medical leadership (GPs as well as hospital consultants) was central to achieving improvements. There were lower levels of agreement about how this could be funded and delivered, and, in 2015, relationships between politicians and health-care professionals deteriorated when, in the midst of contract negotiations, the Department of Health and Social Care19 and the Secretary of State for Health and Social Care22 made public statements linking the weekend effect to a reduction in specialist availability in hospitals at weekends. There were two problems with implying a causative relationship. The first was that there was no evidence to support the assertion. The second was the absence of any objective measure of specialist availability. The HiSLAC project’s 5-year longitudinal study was under way by this stage, and, given the need for objective evidence, we decided to publish the first year’s data in a cross-sectional study35 while continuing to collect data through to 2019. We describe both the published study and the longitudinal study here.
Methods
We described earlier (see Chapter 2) the establishment of the HiSLAC collaboration, the acquisition of HES and the development of the specialist intensity metric based on specialist hours per 10 EAs obtained from a web-based annual PPS on a Sunday and the following Wednesday in June each year. The parallel directorate-level survey used to provide assurance for the PPS is described in Chapter 2; it also offered the opportunity to obtain information on consultant rotas and vacant posts, which we report below.
Over 5 years, 13 hospital sites were subject to trust mergers. In eight cases, the data collected after a merger refer to a combination of hospitals that had previously provided separate data. In the case of three hospitals subject to trust mergers, it was possible to preserve the integrity of the data by treating these as coming from separate sites, whereas, for the remaining two hospitals, the post-merger data were treated as being derived from a separate independent organisation.
Statistical analysis
Trust size was represented each year by bed numbers, which were acquired by calculating the annual mean of NHS England’s (KH03) quarterly submissions. 103 PPS data were summarised using the proportions of specialists present and the average time spent with emergency cases. The specialist intensity measure (specialist hours per 10 EAs) was computed for each trust for both Sunday and Wednesday. Raw estimates were scaled up by the reciprocal of the response rate to correct for the incompleteness of the survey data. The Sunday-to-Wednesday intensity ratio (a quantity unaffected by the scaling correction) was used to quantify the weekend deficit at trust level.
The analysis of trust survey responses over time is based on a weighted analysis of variance of the trust-specific Sunday-to-Wednesday ratio of hours per 10 EAs. Fixed effects were estimated for trust and year. The weights were designed to capture variation generated by the incompleteness of the survey data, together with trust-level variation within the sampling epochs (see Appendix 5). Differences associated with hospital size were analysed using the average number of beds per trust (‘bed-size’) computed across those years during which the trust participated. Quintiles of bed-size were derived from these data.
The analysis of in-hospital mortality of adult EAs was carried out in a logistic regression model with adjustments made for diagnostic category [as represented by the English NHS Summary Hospital-level Mortality Indicator (SHMI) diagnostic grouping],104 patient age (using a restricted cubic spline with five knots), comorbidity and the income deprivation component of The English Indices of Deprivation 2010. 34 In the 2013/14 cross-sectional study,35 each diagnostic category was represented by the full Clinical Classifications Software code. This approach is similar to that of Freemantle et al. 69 The model was refitted with additional terms for hospital trusts and for trust-specific weekend effects (i.e. the average of Saturday and Sunday effects, minus the average over all weekdays within each trust). Meta-regression was used to analyse the model estimates of the trust-specific weekend effects over time, using standard errors from the logistic regression. Association with the intensity deficit ratios was investigated using correlation methods. The focus on weekend-to-weekday ratios at trust level – rather than absolute levels of weekend mortality and specialist intensity – minimises the impact of unmeasured differences in case mix and other potential trust-level confounders.
Comparisons of specialist intensity and admission mortality are based on data from the trusts participating in the annual PPS. The analysis of mortality trends since 2007 is carried out using HES data from the slightly larger cohort of all acute non-specialist trusts in England.
Results
The 2013/14 cross-sectional study35
Specialist intensity
Of 141 acute hospital trusts in England receiving unselected emergency medical admissions, 127 agreed to participate and 115 (81.6% of total eligible trusts) contributed data to the survey, providing 15,537 responses. Of these responses, 1003 (6.5%) were from doctors who did not hold a specialist accreditation, and, therefore, these were excluded, leaving 14,534 eligible responses for analysis. The mean response rate was 45% (range 16–79%) and exceeded 40% in two-thirds of trusts.
There were substantially fewer specialists present and providing care to EAs on Sunday (n = 1667, 11.5%) than on Wednesday (n = 6105, 42.0%). This difference was partly offset by the greater average time spent caring for acutely admitted patients on Sunday (5.74 hours) than on Wednesday (3.97 hours). These patterns were consistent across the quintiles of trust size.
For both Sunday and Wednesday, there was a clear relationship between the sum of specialist hours delivered by each trust and the numbers of EAs on that day averaged across the year: as expected, larger hospitals had more specialists and more admissions (Figures 8a and c).
FIGURE 8.
(a) Total number of Wednesday specialist hours and average number of Wednesday EAs; (b) Wednesday specialist hours per 10 EAs and average Wednesday EAs; (c) total number of Sunday specialist hours and average Sunday EAs, 2013/14; and (d) Sunday specialist hours per 10 EAs and average Sunday EAs, 2013/14.
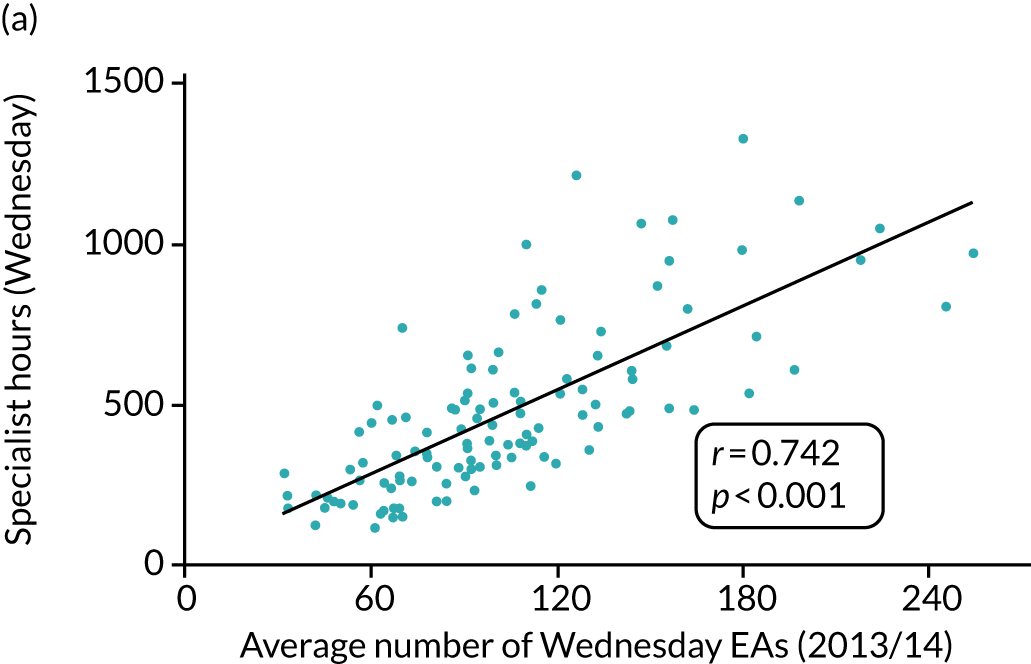
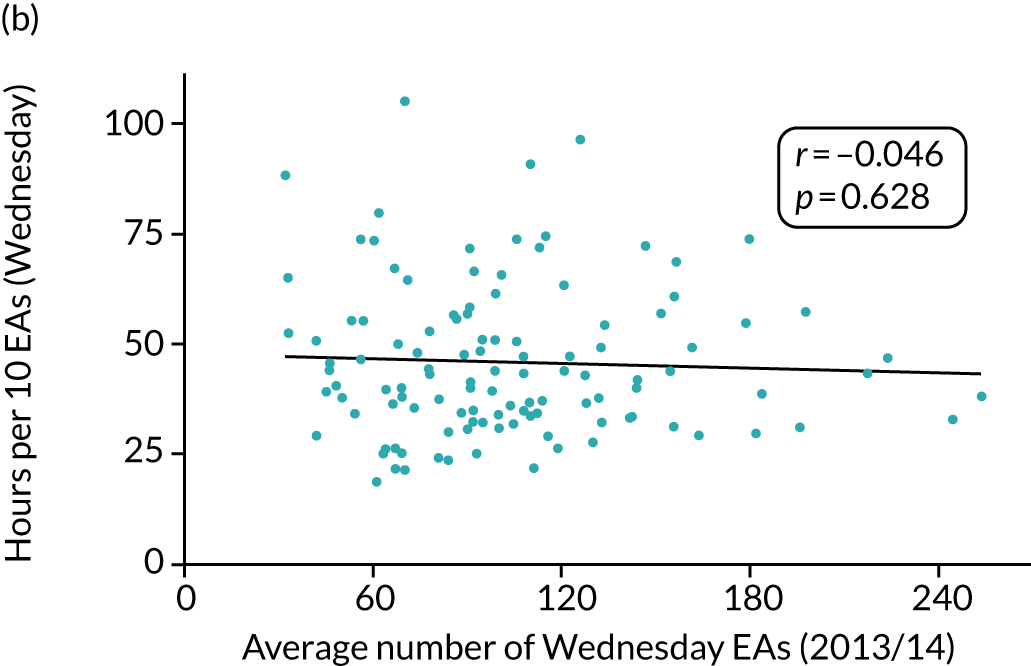


There was substantial variation between trusts when specialist hours were expressed per 10 EAs (see Figures 8b and d), suggesting that factors other than emergency workload influence the amount of specialist time delivered to EAs in any given trust. The median intensity ratio (Sunday divided by Wednesday) was 48% across all trusts, with similar results across quintiles of trust size, as measured using bed numbers for 2013/14. There was no trust for which the Sunday-to-Wednesday ratio was > 1, and in 90% of trusts the ratio was < 0.7 (Figure 9).
FIGURE 9.
Sunday-to-Wednesday specialist hours and ratio per 10 EAs by trust.

Mortality
Using 2013/14 HES data, the logistic model for all 141 acute hospital trusts provided a surplus weekend admission mortality risk of 9%. Trust-specific weekend odds ratios (WEORs) for the 115 trusts contributing to the survey ranged from 0.82 to 1.35, with 96 (83%) trusts recording an OR > 1 (Figure 10). There was no systematic relationship between weekend mortality effect and trust size.
FIGURE 10.
Trust-specific weekend effect ORs with confidence intervals from the logistic regression model, 2013/14.

Specialist intensity and admission day mortality
The correlation between the weekend mortality ORs and the Sunday-to-Wednesday specialist intensity ratio was very low (r = –0.015) and is not suggestive of a clear relationship (Figure 11).
FIGURE 11.
Weekend-to-weekday EA mortality OR and Sunday-to-Wednesday specialist intensity ratio by trust, 2013/14.

Directorate-level questionnaire
Clinical directors reported that the daily review of all patients was the norm on Sundays (Wednesdays) for 50% (86%) of AMUs, 100% (100%) of ICUs and 27% (58%) of acute wards. Consultant vacancies with gaps in cover were reported by 52% of responding EDs, 71% of AMUs, 65% of acute wards and 30% of ICUs.
Conclusion
This cross-sectional study showed a substantial weekend–weekday difference in specialist involvement in the care of patients admitted as emergencies to acute hospitals in England. There was no sign that the variation in the weekend-to-weekday specialist hours ratio was associated with the variation in the weekend-to-weekday admission mortality ratio. This suggests the need for caution in attributing the weekend effect primarily to a lack of consultants at weekends.
Longitudinal study results
Point prevalence survey responses
A total of 116 participating sites contributed 548 sets of survey results during the 5-year period, of which 96 sites contributed data in every year (thus five times in all). The primary analysis was conducted using all available data from the 116 trusts. A total of 66,425 analysable responses were received. The response rate by trust varied from 7% to 93% and declined by 9.4% during the study period, from 45.2% in 2014 to 35.8% in 2018. The average number of specialist responses from which relevant data could be drawn was 121.2 per trust (Table 2).
Year | Total | |||||
---|---|---|---|---|---|---|
2014 | 2015 | 2016 | 2017 | 2018 | ||
Participating trusts | 112 | 108 | 110 | 106 | 112 | 116a |
Total number surveyed | 34,374 | 32,978 | 36,462 | 35,573 | 39,357 | 178,744 |
Average number per trust | 306.9 | 305.4 | 331.5 | 335.6 | 351.4 | 326.2 |
Total number of clean responses | 15,535 | 13,369 | 13,492 | 13,841 | 14,097 | 70,334 |
Average number per trust | 138.7 | 123.8 | 122.7 | 130.6 | 125.9 | 128.3 |
Response rate (f)b | 0.452 | 0.405 | 0.370 | 0.389 | 0.358 | 0.393 |
Total number of specialist responders | 14,532 | 12,657 | 12,846 | 12,974 | 13,416 | 66,425 |
Average number per trust | 129.8 | 117.2 | 116.8 | 122.4 | 119.8 | 121.2 |
Emergency admissions
Between 2013/14 and 2017/18, EA rates increased by 14.7% (all acute trusts in England), continuing the background trend (see Appendix 6). The increase in EAs per day was greater on weekdays (15.7%) than at weekends (11.3%) (mean weekend-to-weekday ratio 0.78).
Specialist intensity
Between 2013/14 and 2017/18, there was an increase in whole-time equivalent consultant staff of 14.6% across the NHS in England and of 4% among non-consultant doctors (see Figure 1).
The PPS data from 116 trusts are summarised in Table 3, alongside bed numbers and EAs. The results for specialist hours are shown both with and without correction for response rate. Importantly, no adjustment is necessary for the Sunday-to-Wednesday intensity ratio, as the numerator and denominator are affected equally by any such correction. Therefore, any change in the intensity ratio over time is likely to represent a true effect.
Year | All | |||||
---|---|---|---|---|---|---|
2014 | 2015 | 2016 | 2017 | 2018 | ||
Number of specialists present on Sunday per trust | 14.9 | 12.8 | 13.3 | 12.8 | 12.3 | 13.2 |
Number of specialists present on Wednesday per trust | 54.5 | 44.7 | 45.0 | 42.8 | 39.2 | 45.3 |
Total number of hours on Sunday per trust | 85.4 | 79.7 | 85.6 | 83.2 | 80.0 | 82.8 |
Corrected for overall response ratea | 188.9 | 196.8 | 231.4 | 213.9 | 223.5 | 210.7 |
Total number of hours on Wednesday per trust | 216.6 | 201.8 | 220.3 | 205.8 | 189.9 | 206.9 |
Corrected for overall response ratea | 479.2 | 498.3 | 595.4 | 529.0 | 530.4 | 526.5 |
Average number of hospital bed numbers per trust | 774.9 | 758.9 | 792.2 | 788.5 | 777.5 | 778.4 |
Mean number of EAs on Sunday per trust | 87.1 | 89.5 | 95.7 | 94.8 | 97.7 | 93.0 |
Mean number of EAs on Wednesday per trust | 106.9 | 110.8 | 119.1 | 122.4 | 128.0 | 117.4 |
Average number of hours per 10 EAs: Sundayb | 9.81 | 8.90 | 8.95 | 8.78 | 8.19 | 8.91 |
Corrected for overall response ratea | 21.70 | 21.98 | 24.19 | 22.57 | 22.88 | 22.67 |
Average number of hours per 10 EAs: Wednesdayb | 20.26 | 18.22 | 18.50 | 16.82 | 14.84 | 17.62 |
Corrected for overall response ratea | 44.82 | 44.99 | 50.00 | 43.24 | 41.45 | 44.83 |
Sunday-to-Wednesday intensity ratio (hours per 10 EAs) | 0.484 | 0.489 | 0.484 | 0.522 | 0.552 | 0.506 |
There was a significant change in the intensity ratio over time (p = 0.0081). The response curve is flat to begin with (2014–16) and then rises over the next 2 years (2017 and 2018), as shown in Figure 12 (data are provided in Appendix 7). The variation over time was equivalent to an average annual increase in the intensity ratio of approximately 1.5% (standard error 0.46; p = 0.0011) per year, or about six percentage points during the period of the study. There is a significant difference (p < 0.0001) between trusts in the (time-averaged) intensity ratio (Figure 13). Furthermore, there is some evidence (p = 0.0035) of differences in rates of improvement over time. However, it is not clear how, if at all, these differences are related to the size of the trusts and we were unable to identify any other potential causative mechanisms.
FIGURE 12.
Sunday-to-Wednesday intensity ratios and adjusted weekend-to-weekday mortality ORs, 2013/14 to 2017/18.
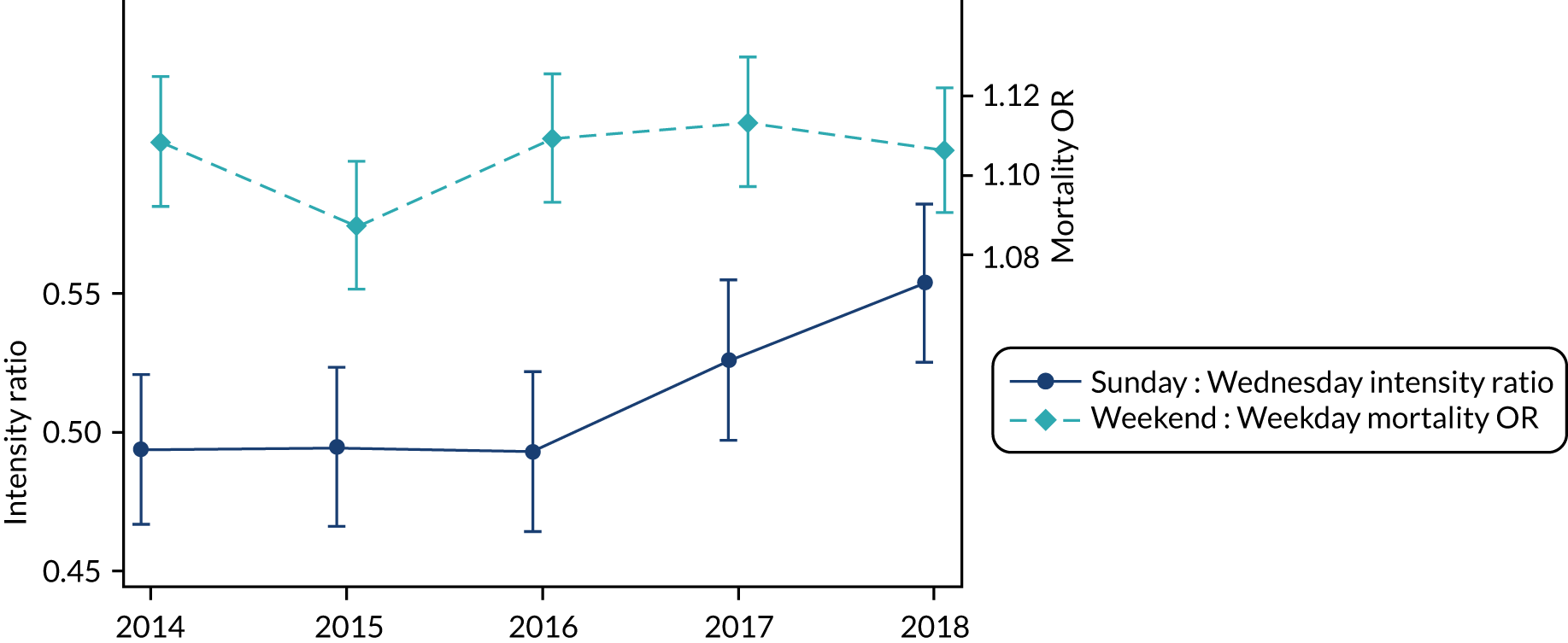
FIGURE 13.
Sunday-to-Wednesday intensity ratios by trust (mean and 95% confidence interval).

How was the ‘improvement’ in the Sunday-to-Wednesday specialist intensity ratio achieved? If the 7-day services policy initiative were to have had its desired effect, it would have stimulated trusts to increase specialist numbers and, hence, specialist hours on Sundays, either through employing more specialists committed to service delivery at weekends or by redirecting specialist activities from weekdays to weekends. Specialist hours per 10 EAs would increase if more specialists were delivering care to the same number of patients, remain stable if the increase in specialist hours were accompanied by a parallel increase in EAs, or diminish if the increase in EAs outstripped the increase in specialist input or if specialist input actually fell.
Figure 14 shows that, over the 5 years, there was only a very modest overall increase in specialist hours per 10 EAs on Sundays, and a marked reduction on Wednesdays. As shown above, between 2013/14 and 2017/18, EAs increased by 14.7% overall, by 11.3% at weekends and by 15.7% on weekdays (see Appendix 6), and the increase in consultant staff during the same period was 14.6% (see Figure 1). If additional consultant input had been distributed uniformly across all days of the week, it would, therefore, seem likely that, although the increase in consultant staffing would have exceeded the increase in EAs at weekends, it would have been outstripped by the larger increase in EAs on weekdays.
FIGURE 14.
Specialist hours per 10 EAs adjusted for response rates and Sunday-to-Wednesday specialist intensity ratio. Specialist hours are corrected for response rate using the formula n × (1/response rate).
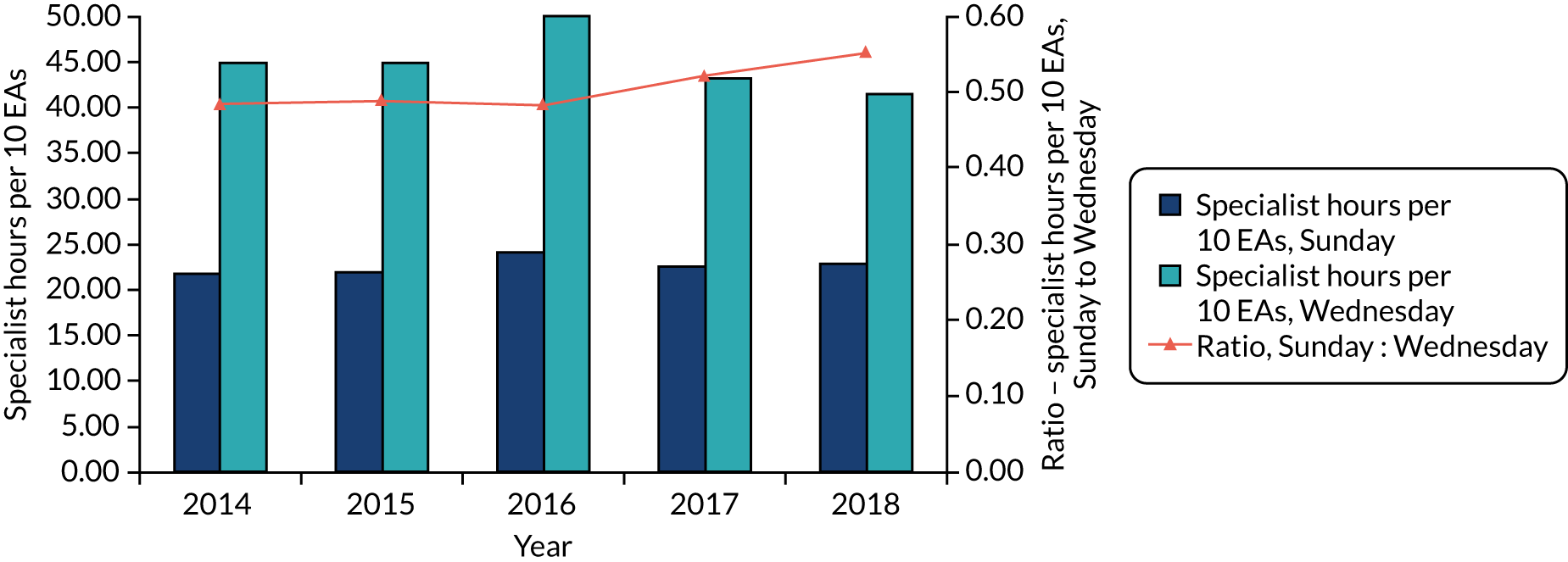
To explore this relationship between specialist hours and EAs in more detail, we present each trust’s mean annual percentage change in specialist intensity for Sundays against Wednesdays in Figure 15. The majority of trusts (n = 71, 62%) achieved an increase in the Sunday-to-Wednesday intensity ratio (i.e. a positive correlation between intensity ratio and year). In this group, 23 trusts increased specialist intensity for both Sundays and Wednesdays, 22 increased Sunday intensity while reducing intensity on Wednesdays, and a further 26 showed a reduction in both, but which was more marked for Wednesdays than for Sundays. Forty-four trusts showed a reduction in specialist intensity ratios over time; of these, 10 had marginally increased Sunday and Wednesday intensity, 15 had increased Wednesday intensity only, and 19 showed a reduction in both Sunday and Wednesday intensity. However, it would be misleading to conclude that actual specialist hours declined during the period. Indeed, there is evidence of a general increase in hours in the majority of trusts for both Wednesdays (63%) and Sundays (61%) (see Figure 15).
FIGURE 15.
Trends in specialist involvement in EAs, 2014–18. (a) Sunday and Wednesday specialist intensity (specialist hours per 10 EAs); and (b) Sunday and Wednesday specialist hours. The average proportional changes per year are computed from trust-level regression analyses of (log-transformed) intensity values. In (a), the 71 (62%) trusts above the diagonal line showed an improvement in the Sunday-to-Wednesday intensity ratio (i.e. positive correlation between intensity ratio and year). Data are from 115 trusts, one of which was excluded because it contributed PPS data for 1 year only.

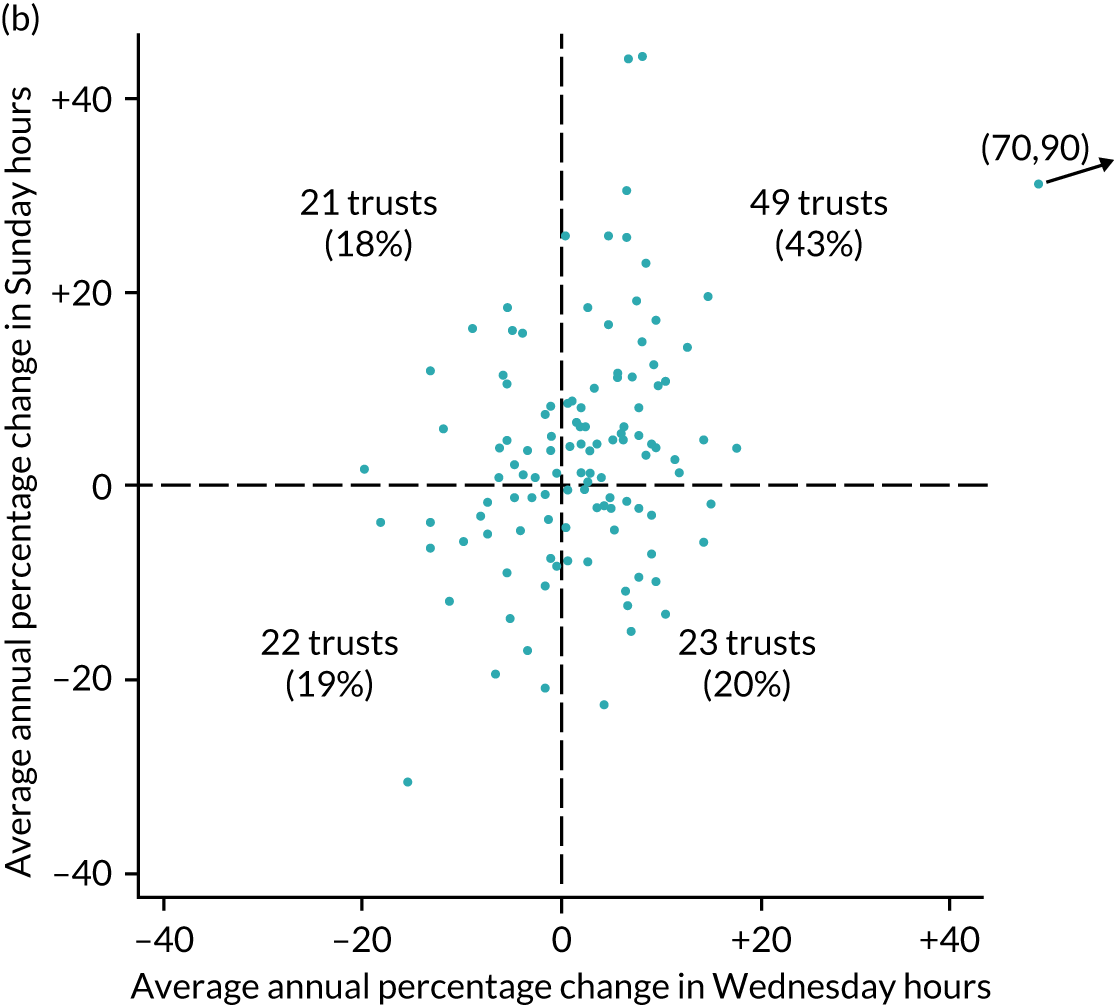
The final step in elucidating the causes for the change in specialist intensity ratios is to examine the ratio of supply (specialist hours) to demand (EAs) across the 115 trusts. Here, we use the alternative formulation of the weekend-to-weekday specialist intensity ratio:
During the study, each component of this expression increased by about 7%. Using data from Table 3, the ratio of Sunday to Wednesday specialist hours increased from 0.39 (= 85.4 ÷ 216.6) in 2013/14 to 0.42 (= 80.0 ÷ 189.9) in 2017/18, and the ratio of Wednesday to Sunday EAs increased from 1.23 (= 106.9 ÷ 87.1) to 1.31 (= 128.0 ÷ 97.7) during the same period. Together, these changes produced an overall increase of 14% in the intensity ratio, from 0.484 (2013/14) to 0.552 (2017/18). The general increase in EAs was more pronounced for Wednesdays (19.7%) than for Sundays (12.1%), and this change in the distribution of EAs across the week accounts for half of the improvement in the intensity ratio. It reflects a change in the pattern of demand on the service rather than a redirection of specialist resources.
In summary, the increase in the specialist intensity ratio appears to have been achieved by a general increase in specialist hours, which has been more pronounced at weekends, and a general rise in EAs, which has been more pronounced during the week.
Mortality and associations with specialist intensity
Hospital and 30-day post-admission EA mortality rates for all acute trusts in England reduced each year (30-day post-admission mortality reduced from 6.43% in 2007/8 to 5.47% in 2013/14), with weekend and weekday admission mortality rates falling in parallel. From 2014, overall mortality rates stabilised, but, in 2017/18, the weekend 30-day post-admission mortality rate increased from 5.8 to 6.06 and the unadjusted mortality ratio increased from 1.08 to 1.15. Adjustment for baseline characteristics abolishes this apparent increase (see Appendix 8), indicating that the rise in mortality rates is attributable to case-mix differences. The difference between hospital and 30-day post-admission mortality rates increased over these 11 years (Figure 16) for both weekend and weekday admissions, so that the ratio of hospital to 30-day mortality rates diminished. Figure 1 shows that, in the last 2 years, the progressive rise in delayed transfers of care has been reversed, indicating that patients are being discharged more rapidly back to the community. Taken together, these data suggest that an increasing proportion of the mortality risk is being transferred to the community post discharge (see Figure 16 and Appendix 8).
FIGURE 16.
Ratio of in-hospital to 30-day post-admission mortality and overall 30-day post-admission mortality.

The weekend mortality effects (i.e. the adjusted weekend-to-weekday mortality ORs) are shown in Appendix 9 and have been plotted alongside the specialist intensity ratios for each year of the study in Figure 12.
There is no evidence for any change in the weekend admission mortality ratios during this period (p = 0.1971). The weekend effect may differ between trusts (p = 0.0034), but these differences do not appear to relate to hospital size. Details are given in Appendix 10. We found no apparent correlation (r = 0.077) between the weekend mortality effect and the Sunday-to-Wednesday intensity ratio.
Discussion
We have shown that patients admitted as emergencies to acute hospital trusts in England on a Sunday collectively receive, on average, half as much specialist time (hours per 10 EAs) as patients admitted on a Wednesday. There is considerable variation between trusts in specialist intensity, which is independent of hospital size. Over the 5 years of the study, the Sunday-to-Wednesday specialist intensity ratio increased, and this appears to have occurred through several mechanisms: a modest increase in specialist input on Sundays; some redistribution of specialist hours in favour of Sundays; and an increase in the number of EAs, which was more marked for Wednesdays than for Sundays. It seems likely that the 7-day service policy had some effect by transferring specialist time from weekdays to weekends, and increasing consultant numbers, but the impact has been obscured by the increase in EAs during this period.
We have been unable to identify a relationship between weekend-to-weekday specialist intensity ratios and trust-level weekend admission mortality effects in either the cross-sectional analysis or the longitudinal study. Trusts with a narrower Sunday-to-Wednesday specialist intensity ratio do not seem to have a correspondingly smaller weekend effect.
Hospital admissions have continued to increase during the past 11 years. However, there was a progressive reduction in mortality rates until 2013/14, when the improvement in survival appeared to have plateaued and the 30-day mortality rate for weekend admissions increased (see Figure 16). Adjustment for baseline characteristics shows that this increase in mortality is attributable to case-mix differences (see Appendix 8). However, the increasing gap between hospital and 30-day mortality rates suggests that there has been a progressive transition in the place of death from hospital to the community. The reduction in length of hospital stay during the same period would be consistent with this interpretation. Whether this represents a desirable effort to allow those destined to die to do so in the peace of their own homes, or a failure to prevent avoidable deaths through, for example, effective rehabilitation of frail elderly patients who are admitted to hospital at weekends, requires further research at the intersection of community and hospital care at discharge, not just at admission.
The conventional narrative about the perceived – and now measured – gap in specialist input between weekends and weekdays is that patients admitted at weekends are being ‘short-changed’ in some way: that half as much specialist input means half as good care. An alternative interpretation might be that patients newly admitted at weekends and on weekdays are receiving the urgent care they need for this acute phase of their illness, while on weekdays specialists are additionally providing the less time-critical components of acute care targeted at refining treatments and promoting recovery. The decrement in specialist input at weekends might result in delays in progressing patients along the therapeutic pathway, but without contributing additional mortality risk.
Limitations of our study relate to the inherent weaknesses of surveys, namely variable response rates and subjective data recall. We mitigated the response rate issue by comparing weekends with weekdays in each trust. Other factors unrelated to specialist intensity might include unmeasured case-mix differences;26 variation in support services in hospital, including ‘failure to rescue’ (the inability of the system to respond promptly to patient deterioration), which has been shown to explain the difference in surgical outcomes between high- and low-volume centres;89 or a difference in outcomes from patient safety incidents at weekends. 105 We explore these factors in the following chapters, starting with an examination of patient case mix (see Chapter 5) and following with an evaluation of hospital care quality (see Chapter 6).
Chapter 5 Case-mix differences between weekend and weekday emergency admissions to a large hospital trust
Parts of this chapter have been reproduced with permission from Sun et al. 57 © Author(s) (or their employer(s) 2019. Re-use permitted under CC BY. Published by BMJ. This is an open access article distributed in accordance with the Creative Commons Attribution 4.0 Unported (CC BY 4.0) license, which permits others to copy, redistribute, remix, transform and build upon this work for any purpose, provided the original work is properly cited, a link to the licence is given, and indication of whether changes were made. See: http://creativecommons.org/licenses/by/4.0/. The text below includes minor additions and formatting changes to the original text.
Introduction
The HiSLAC systematic review (see Chapter 3) identified three studies showing that patients admitted as emergencies to hospitals at weekends tended to be more severely ill, on average, than those admitted on weekdays. 26,55,56 However, it was not clear whether this was actually attributable to greater severity of illness, or the effect was caused by a reduction in the proportion of less severely ill patients presenting to hospitals. 106 Making this judgement required access to certain specific indicators: the ED attendance rate; the ‘conversion rate’ of attendances to admissions; a dynamic measure of physiological severity of illness, not just ‘static’ measures such as age and comorbidities; and the ability to track the initial stages of patients’ journeys in hospital as an indicator of acuity and dependence. The last two indicators can be obtained at trust level from those trusts that have a sufficiently sophisticated electronic patient record only. As there is no standardised national system for electronic patient records, this work can be performed at the local trust level only. We therefore obtained permission to access the database of one of the largest urban tertiary referral centres in the UK, which cares for > 800,000 patients every year and serves a large and diverse local patient population. The results of this study have been published. 57
Methods
This was a retrospective analysis of data that had been prospectively collected between January 2012 and December 2015 from the trust’s Microsoft SQL electronic clinical databases. We identified all adult EAs during this 4-year period, extracting demographic information [age, sex, ethnicity, deprivation, principal diagnosis (International Classification of Diseases and Related Health Problems, Tenth Revision33), comorbidities] and outcome (survival at 30 days post admission and hospital discharge). Re-admissions were classified as new hospital spells. Charlson Comorbidity Index score was calculated and categorised according to the SHMI. 104 Principal diagnosis was grouped using SHMI groupings. Income deprivation index was based on the 2015 Index of Multiple Deprivation,107 which was based on the patient’s residential postcode.
We supplemented these standard measures of case mix with National Early Warning Score (NEWS) data calculated from physiological vital signs (respiration rate, oxygen saturation, temperature, systolic blood pressure, pulse rate, level of consciousness and supplemental oxygen). 108 NEWS was calculated using primary variables for the first full set of vital signs available within the 24 hours following admission; we have termed this ‘NEWS24’. Post-admission severity was also derived from whether or not patients had been transferred to the ICU. At the time of this study (2012–15), vital signs of admitted patients were recorded electronically, except in the ICU, where the complex electronic ICU chart was still in development. We were therefore unable to calculate NEWS for the majority of patients transferred directly to the ICU from the ED. Therefore, the patients were classified into six risk categories using ICU status and NEWS24 values. The (putative) highest-risk category consisted of patients admitted to an intensive care unit within 24 hours (ICU24). The remaining (non-ICU24) patients were assigned, where possible, to one of four NEWS severity bands given by NEWS24 (≥ 7, 5–6, 1–4 and 0). The sixth category consisted of those non-ICU24 patients for whom data for calculating NEWS24 were missing.
Weekend admissions were defined as those admitted on or after midnight on Friday until midnight on Sunday. Admission time was the point of admission into the hospital, not the time when the patient first presented to the ED. Length of stay was calculated by subtracting the day of admission from the day of discharge. Admitted patients who either were discharged or died before midnight on the day of admission are classified as having zero length of stay (Z-LOS).
Statistical analysis
Descriptive statistics for demographic data, routes of admission, ICU transfer, length of stay and NEWS status included means/standard deviations (SDs), medians/interquartile ranges (IQRs) and percentages. Logistic regression methods were used to analyse mortality 30 days post admission. Outcome was linked to each specific admission for patients with multiple admissions. We used multivariable logistic regression to estimate the weekend-to-weekday OR of death following admission [weekend OR (WEOR)]. The ‘standard model’ employed conventional risk adjustment variables (age – restricted cubic spline with five knots, sex, ethnicity, day of the year, admission source; diagnostic category – SHMI grouping, income deprivation, number of previous admissions within 30 days; and comorbidity – Charlson Comorbidity Index score category). As the data set includes 4 years’ data, calendar year is also included as an adjustment variable. This approach is similar to that of Freemantle et al. 20,69 Then, a second set of models was developed incorporating patient risk strata (ICU admission and NEWS band) into the standard adjustment model, and the WEOR of each group was calculated. Finally, we examined the effect of severity of illness on weekend-to-weekday admission mortality by adjusting crude mortality for NEWS alone. The data extraction and cleaning process was carried out in Microsoft SQL. The statistical analyses were performed using Stata®/SE 14 (StataCorp LP, College Station, TX, USA). The p-values are two-sided.
Results
Population and pathway characteristics are shown in Table 4.
Characteristic | Weekend | Weekday | Overall | p-valuea |
---|---|---|---|---|
ED attendances, N (mean/day) | 113,913 (273) | 282,422 (271) | 396,335 (271) | |
Admissions, n (mean/day; % of attendances) | 37,979 (91; 27.2) | 125,149 (120; 28.6) | 163,128 (112; 28.2) | |
Admission route, n (mean/day) | ||||
ED | 31,023 (74) | 80,759 (77) | 111,782 (77) | < 0.001 |
GP plus bed bureau | 3197 (8) | 26,596 (25) | 29,793 (20) | < 0.001 |
Outpatient clinic | 859 (2) | 7690 (7) | 8549 (6) | < 0.001 |
Other providers | 2900 (7) | 10,104 (10) | 13,004 (9) | 0.006 |
Sex, n (%) | ||||
Male | 19,360 (51.0) | 62,375 (49.8) | 81,735 (50.1) | < 0.001 |
Female | 18,619 (49.0) | 62,774 (50.2) | 81,393 (49.9) | < 0.001 |
Age (years) | ||||
Median (IQR) | 60 (40–78) | 60 (42–77) | 60 (41–77) | 0.295 |
Mean (SD) | 58.2 (22.239) | 58.5 (21.393) | 58.4 (21.593) | 0.056 |
Ethnicity, n (%) | ||||
White | 28,947 (76.2) | 96,339 (77.0) | 125,286 (76.8) | 0.002 |
Asian | 4681 (12.3) | 15,515 (12.4) | 20,196 (12.4) | 0.709 |
Black | 1622 (4.3) | 5322 (4.3) | 6944 (4.3) | 0.877 |
Mixed | 507 (1.3) | 1511 (1.2) | 2018 (1.2) | 0.049 |
Other | 1253 (3.3) | 3294 (2.6) | 4547 (2.8) | < 0.001 |
Not stated | 969 (2.6) | 3168 (2.5) | 4137 (2.5) | 0.828 |
Charlson Comorbidity Index score category, n (%) | ||||
0 | 18,626 (49.0) | 61,393 (49.1) | 80,019 (49.1) | 0.733 |
1–5 | 6716 (17.7) | 21,646 (17.3) | 28,362 (17.4) | 0.072 |
> 5 | 12,637 (33.3) | 42,110 (33.6) | 54,747 (33.6) | 0.278 |
Previous admissions,b n (%) | ||||
0 | 33,330 (87.8) | 110,028 (87.9) | 143,358 (87.9) | 0.601 |
1 | 3943 (10.4) | 13,071 (10.4) | 17,014 (10.4) | 1.000 |
2 | 575 (1.5) | 1696 (1.4) | 2271 (1.4) | 0.150 |
≥ 3 | 131 (0.3) | 354 (0.3) | 485 (0.3) | 1.000 |
NEWS24 value | ||||
Median (IQR) | 1 (0–3) | 1 (0–2) | 1 (0–2) | < 0.001 |
Mean (SD) | 1.8 (2.049) | 1.7 (1.969) | 1.7 (1.989) | 0.008 |
ICU transfers | ||||
N (% total); n/day | 1579 (4.2); 3.8/day | 3781 (3); 3.6/day | 5360 (3.3); 3.7/day | < 0.001; < 0.001 |
Direct, n (% of weekend/weekday total admissions); % of weekend/weekday ICU24 cases) | 1202 (3.2; 76.1) | 2469 (2; 65.3) | 3761 (2.3; 68.5) | < 0.001; < 0.001 |
Indirect, n (% of weekend/weekday total admissions); % of weekend/weekday ICU24 cases) | 377 (1; 23.9) | 1312 (1; 34.7) | 1689 (1; 31.5) | < 0.001; < 0.001 |
Length of stay (days) | ||||
Median (IQR) | 3 (1–8) | 2 (1–7) | 2 (1–7) | < 0.001 |
Mean (SD) | 7.1 (13.129) | 6.9 (13.248) | 7.0 (13.221) | 0.008 |
Z-LOS, n (%) | 6529 (17.2) | 27,390 (21.9) | 33,919 (20.8) | < 0.001 |
Income deprivation decile | ||||
Median (IQR) | 4 (1–6) | 4 (1–6) | 4 (1–6) | 0.858 |
Mean (SD) | 4.2 (2.788) | 4.2 (2.770) | 4.2 (2.774) | 0.621 |
Demographics
Complete data on 163,128 EAs were obtained between 1 January 2012 and 31 December 2015. The median age of those admitted was 60 years and did not vary by day of the week. Overall, there were no differences between weekend and weekday admitted populations in burden of comorbid disease, income deprivation or previous admissions within 30 days.
Admission pathways
Of 163,128 EAs, 37,979 (23.3%) occurred at weekends; 111,782 presented though the ED as self-referrals or ambulance transfer, and 38,342 were admitted directly to a hospital ward as referrals from GPs, the bed bureau or outpatient clinics. The ED attendance rate did not vary by day of the week (mean attendances of 273 per day at weekends and 271 per day on weekdays). However, the ‘conversion rate’ (the mean daily hospital admission rate) was lower at weekends than on weekdays (91 vs. 120), mainly because there were fewer referrals from GPs and the bed bureau (eight per day at weekends and 25 per day on weekdays), and from outpatient clinics (two per day at weekends and seven per day on weekdays) than self-presentations and emergency ambulance transfers (74 per day at weekends and 77 per day on weekdays). There was no evidence of a secular trend between 2012 and 2015.
Intensive care transfers
During the 4-year study, 5360 (3.3%) patients were transferred to intensive care following emergency hospital admission. More patients (both the absolute number and the proportion of hospital admissions) were transferred to the ICU within 24 hours at weekends (4.2%; 3.8 per day) than on weekdays (3.0%; 3.6 per day) (see Appendix 11). Among these patients transferred to ICU, more were transferred directly from the ED at weekends than on weekdays (2.9 per weekend day, 76.1%, vs. 2.4 per weekday, 65.3%).
Length of stay
The median and mean lengths of hospital stay were greater for EAs at weekends than on weekdays [3.0 and 7.1 days, respectively, at weekends, compared with 2 and 6.9 days, respectively, on weekdays (p = 0.008 for median and p < 0.001 for mean)]. Z-LOS patients (those who were discharged or died before midnight on the day of admission) constituted 20.8% of admissions (see Table 4); the proportion of such patients was smaller at weekends (17.2%) than on weekdays (21.9%).
National Early Warning Scores
It was possible to calculate NEWS within the first 24 hours for 90% of those admitted. The documentation of vital signs improved from 86% of admissions in 2012 to 92% of admissions in 2015, and was more complete for weekend admissions (91.7%) than for weekday admissions (89.5%) (Figure 17). NEWS could be calculated within 4 hours of admission for 72.5% of patients and within 8 hours of admission for 83% of patients; completion rates were higher at weekends. As expected, the vital signs required for calculation of NEWS were more likely to be missing for patients transferred to ICU within 24 hours of hospital admission (63.4%) than for patients not transferred to ICU within 24 hours (8.2%), particularly for those transferred directly to ICU from the ED (83.5% missing NEWS) rather than indirectly from another hospital location (19.8% missing NEWS).
FIGURE 17.
The NEWS distribution in first 24 hours of admission and the ratio of the distribution of each NEWS band weekend to weekday, 2012–15.

Weekend admissions had a higher NEWS than those admitted on weekdays (see Table 4) (mean NEWS 1.8 weekend, 1.7 weekday; p = 0.008), and a greater proportion of weekend admissions than weekday admissions were in the higher NEWS bands (see Figure 17). Among patients for whom it was recorded, ICU admission within 24 hours was associated with a higher mean NEWS (2.98) than non-ICU admission (1.70). In the non-ICU population, increasing NEWS values were associated positively with increasing age and length of hospital stay (see Appendix 12).
Mortality rates and mortality adjustment
Crude 30-day post-admission mortality rates are summarised in Table 5. The overall mortality rate was 5.1%, and it was higher for weekend admissions (5.6%) than weekday admissions (5.0%) [crude, unadjusted WEOR 1.13, 95% confidence interval (CI) 1.08 to 1.19]. As expected, mortality was much higher among ICU24 admissions (17.7%) than among non-ICU24 admissions (4.7%; p < 0.001), and there was a significant upward trend in mortality with increasing NEWS band (p < 0.001) among non-ICU24 admissions for whom NEWS was available, ranging from 1.5% (NEWS 0) to 29.1% (NEWS ≥ 7). The mortality rate for non-ICU24 admissions who had missing NEWS was 2.7%, which falls within the range of the two lowest NEWS bands.
Admission group | Crude mortality, n/N (%) | WEOR (95% CI) | ||
---|---|---|---|---|
Weekend | Weekday | Total | ||
All admissions | 2140/37,979 (5.6) | 6257/125,149 (5.0) | 8397/163,128 (5.1) | 1.13 (1.08 to 1.19) |
ICU24 | 275/1579 (17.4) | 673/3781 (17.8) | 948/5360 (17.7) | 0.97 (0.83 to 1.14) |
No ICU24 | ||||
NEWS ≥ 7 | 390/1257 (31.0) | 1033/3627 (28.5) | 1423/4884 (29.1) | 1.13 (0.98 to 1.30) |
NEWS 5–6 | 325/2091 (15.5) | 862/6008 (14.3) | 1187/8099 (14.7) | 1.10 (0.96 to 1.26) |
NEWS 1–4 | 895/20,535 (4.4) | 2895/66,297 (4.4) | 3790/86,832 (4.4) | 1.00 (0.92 to 1.08) |
NEWS 0 | 164/10,467 (1.6) | 532/34,578 (1.5) | 696/45,045 (1.5) | 1.02 (0.85 to 1.22) |
NEWS missing | 91/2050 (4.4) | 262/10,858 (2.4) | 353/12,908 (2.7) | 1.88 (1.47 to 2.40) |
Crude WEORs within strata are shown in the final column of Table 5 and in Figure 18. For five of the six risk strata (i.e. ICU24 and the four non-ICU bands with NEWS available), the WEOR CI includes the value 1, which is consistent with the hypothesis that risk stratification has eliminated the weekend effect. The size of the WEOR (1.88; p < 0.001) in the non-ICU24 plus missing NEWS group presents an anomaly. Despite the low average mortality rate (2.7%), it appears that the risk is significantly higher for weekend admissions than for weekday admissions in this group.
FIGURE 18.
Rates of 30-day crude mortality (%) and adjusted WEOR (95% CI): effect of non-availability of NEWS, increasing NEWS band, and ICU transfer within 24 hours.

Among the 146,822 (90%) admissions for whom NEWS could be calculated, the 30-day mortality rate was 1825/34,833 = 5.2% at weekends and 5506/111,989 = 4.9% on weekdays (unadjusted WEOR 1.07, 95% CI 1.01 to 1.13). Adjustment using the standard model had little impact (Table 6 and Figure 19). Adjustment using the NEWS alone annulled the weekend effect (WEOR 1.02, 95% CI 0.96 to 1.08), with no additional contribution when standard adjustment variables were included (see Table 6).
Unadjusted WEOR (95% CI) | With standard adjustment (95% CI) | Adjustment by NEWSa only (95% CI) | Standard adjustment plus NEWSa (95% CI) | |
---|---|---|---|---|
Admissions with NEWS available (n = 146,822) | 1.07 (1.01 to 1.13) | 1.07 (1.01 to 1.14) | 1.02 (0.96 to 1.08) | 1.04 (0.98 to 1.11) |
All admissions (n = 163,128) | 1.13 (1.08 to 1.19)b | 1.11 (1.05 to 1.17) | (Standard adjustment plus ICU24/NEWS strata) | 1.08 (1.02 to 1.14) |
ICU24 | 0.97 (0.83 to 1.14)b | 1.09 (0.92 to 1.30) | ||
No ICU24 | ||||
NEWS ≥ 7 | 1.13 (0.98 to 1.30)b | 1.14 (0.97 to 1.33) | ||
NEWS 5–6 | 1.10 (0.96 to 1.26)b | 1.07 (0.92 to 1.25) | ||
NEWS 1–4 | 1.00 (0.92 to 1.08)b | 1.03 (0.95 to 1.11) | ||
NEWS 0 | 1.02 (0.85 to 1.22)b | 1.03 (0.86 to 1.24) | ||
NEWS missing | 1.88 (1.47 to 2.40)b | 1.73 (1.33 to 2.25) |
FIGURE 19.
The 30-day WEOR (95% CI) for all patients and those with NEWS available: impact of adjustment variables. a, Risk stratas: (1) ICU24; (2) no ICU24 and NEWS ≥ 7; (3) no ICU and NEWS 5–6; (4) no ICU24 and NEWS 1–4; (5) no ICU24 and NEWS 0; and (6) No ICU24 and NEWS missing.
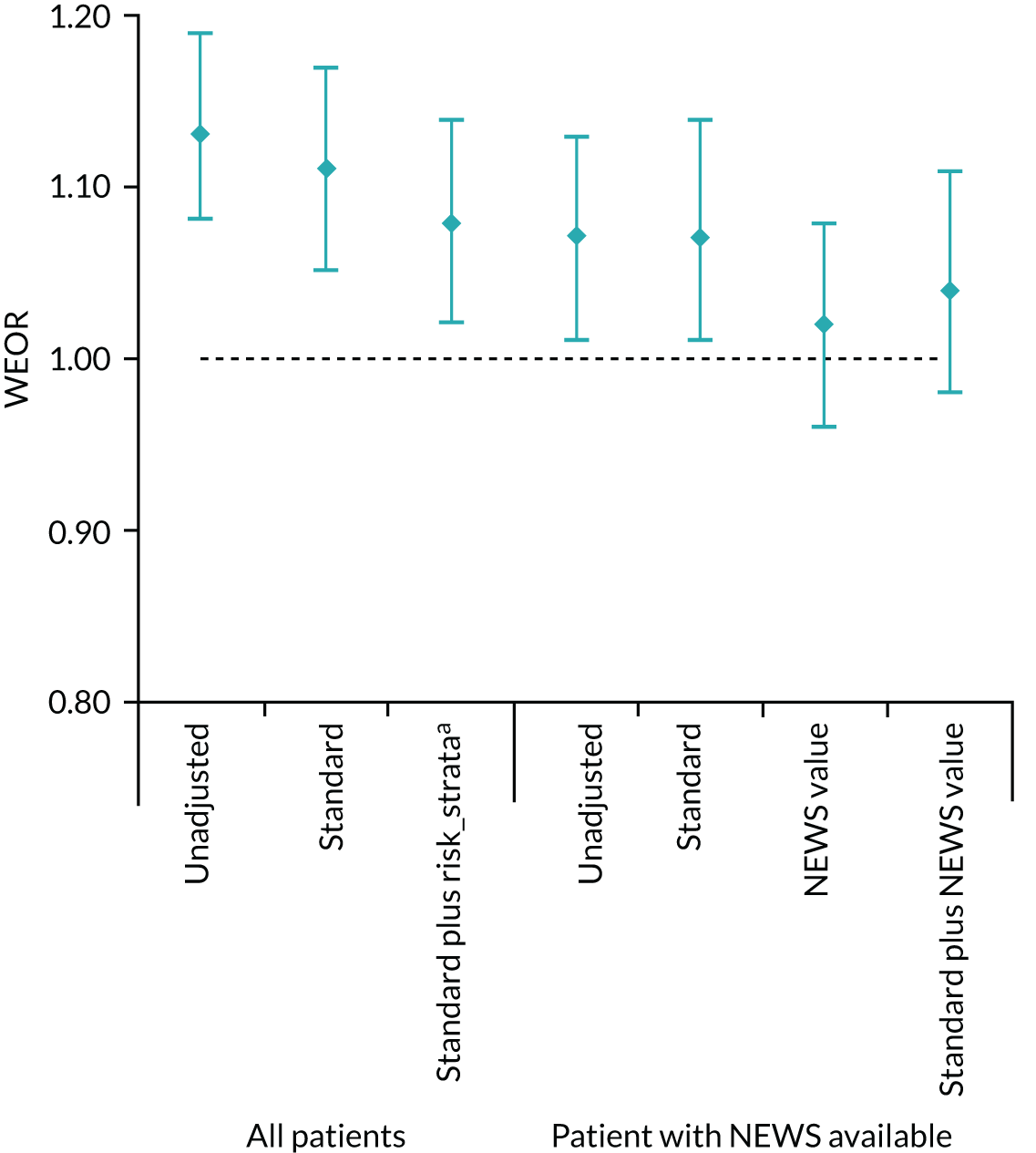
For the full sample of admissions (including those with missing NEWS), stratified estimates of the WEOR can be calculated using the ICU24/NEWS categories, with allowance for standard adjustment variables as well. The results are presented in Table 6. This approach leads to a modest attenuation of the WEOR from 1.13 to 1.08 (95% CI 1.02 to 1.14), but it does not appear to eliminate the weekend effect altogether, a consequence of the very high WEOR value (1.88) in the non-ICU, missing-NEWS stratum.
Discussion
We have shown that weekend admissions have a higher 30-day mortality rate that attenuates only modestly with standard case-mix adjustment, but effectively disappears when adjusted for physiological severity (NEWS) in the 90% of patients for whom complete NEWS variables could be calculated within 24 hours of admission. This shows that patients admitted to hospital at weekends are sicker – more acutely ill – than those admitted on weekdays. This is not just a matter of proportions, that is that the weekend cohort appears sicker because fewer patients who are less ill are admitted; a substantially larger number and larger proportion of weekend admissions required transfer to intensive care in the first 24 hours following admission, and more of these were transferred directly from the ED at weekends, again indicating that weekend admissions are more severely ill at hospital presentation. Patients admitted at weekends remain in hospital longer than those admitted on weekdays, which is another potential indicator of severity. Importantly, we find no clinically important differences in age, gender or comorbid disease between weekends and weekday admissions; patients admitted at the weekend are more acutely ill, not more chronically ill.
At weekends, more patients are transferred to the ICU directly from the ED, whereas on weekdays those referred to the ICU are more likely to follow an indirect route. This suggests that weekend care is more efficient for sicker patients. Delays in patient flow on weekdays may be a consequence of competition with elective admissions. Indirect (and potentially delayed) admission to intensive care is associated with worse outcomes. 109 Rates of NEWS completion rates for admissions at weekends are slightly better than those for admissions on weekdays. Taken together, these findings suggest that weekend emergency care is more efficient and more reliable than weekday emergency care.
We have also shown that, despite a constant daily ED attendance rate, fewer patients are admitted to hospital at weekends, mainly because of the reduction in community referrals from GPs (family doctors). This is consistent with the finding from England-wide data by Meacock et al. 106 that ‘direct’ hospital admission rates are lower at weekends. The smaller numbers of Z-LOS patients, combined with the higher mortality rate of these patients, suggest that this group contains frail or elderly patients who are more difficult to discharge back to the community at weekends. Taken together, these findings suggest that the search for the causes of the weekend effect needs to include the whole patient pathway, particularly referral patterns, and the organisation of care in the community, not just care in hospital.
Limitations of this study include the constraint that data with this amount of detail could be obtained from a single trust only. Concerns about generalisability are mitigated by the trust’s case mix (large and diverse), the fact that measurement was over several years, and the fact that the effects identified are in the same direction as those in other studies. The ‘missing NEWS’ values could have been addressed using imputation, but this requires that data be missing at random, and, as we have shown, there was a systematic reason for the non-availability of NEWS. Had we used imputation, we would not have identified the link with ICU admission.
Although the sample is large and covers 4 years, the finding that in-hospital care processes at weekends may be more reliable, not less reliable, requires confirmation across a larger sample of hospitals before the weekend effect can be attributed to pre-hospital factors. We therefore undertook a series of mixed-methods studies across 20 acute hospital trusts in England to investigate the quality of care of patients admitted as emergencies at weekends and on weekdays.
Chapter 6 Safety and quality of weekend care in hospital: a mixed-methods evaluation
Introduction
The preliminary theory underpinning the 7-day services policy initiative was that the higher hospital mortality risk associated with weekend admission could be mitigated by increasing specialist input into the care of patients admitted at weekends. In the preceding chapters, we have confirmed the magnitude of the weekend effect in the worldwide literature, characterised patients’ and staff’s views about deficiencies in the quality of hospital care at weekends, and shown that consultant input into the care of EAs at weekends across acute trusts in England is half of that on weekdays. However, we have been unable to show that this decrement in specialist input is linked to weekend admission mortality risk, or that a modest shift in consultant input over 5 years from weekdays to weekends has reduced that risk. In a single-centre study, we have shown that patients admitted at weekends are sicker than those admitted on weekdays, and that those care processes available for study were performed at least as reliably and efficiently at weekends as on weekdays. Moreover, we found some preliminary evidence of deficiencies in pre-hospital community care at weekends that could contribute to the weekend effect. Our logic model is, therefore, becoming more nuanced and more complex.
To explore these findings in more detail, we undertook a mixed-methods evaluation of the safety and quality of care of patients admitted at weekends to 20 acute hospital trusts. Two rounds of qualitative research were conducted, the first involving all 20 trusts (round 1) and the second involving a subset of eight trusts (round 2). The qualitative research included observations of acute-care delivery up to, including, and following a weekend in each participating hospital, and interviews with front-line staff and senior executives in each site. In parallel, we evaluated error and adverse event rates, and global care quality from the case records of 4000 EAs at weekends and on weekdays to these 20 trusts in two epochs, 2012–13 and 2016–17, representing a period before and a period during the implementation of 7-day services.
Methods
The protocols of these studies have been published. 110,111
Selection of 20 hospital trusts
We invited 20 of the 115 acute hospital trusts in England participating in the HiSLAC project to contribute to a more detailed analysis, involving qualitative research and case record review. First, trusts were classified into quintiles of size (acute beds) and then four were selected from each quintile, two with the highest (HiSLAC) and two with the lowest (LoSLAC; low-intensity specialist-led acute care) Sunday specialist intensity (2014 data). Data on specialist intensity (hours of consultant time per 10 EAs) were derived from the 2014 HiSLAC national PPS.
Qualitative research
The aims of the qualitative research (involving interviews, and observations employing elements of an ethnographic approach) were to:
-
describe the role of specialist input in the quality of care delivery
-
identify the features of service delivery that have an impact on the quality of care organisation and delivery at weekends for acute medical admissions, and how these differ across trusts
-
describe how hospitals organise specialist staffing and reviews of medical admissions at weekends, compared with on weekdays
-
describe how hospitals have responded to policies for increasing specialist intensity on weekends, and what challenges they have faced.
Qualitative research round 1: 20 trusts
We used 7-day service standards17 and guidance on acute care from the Royal College of Physicians112 to develop semistructured topic guides to explore features of the quality of service delivery at weekends. The structured observation guide provided an a priori framework that established the aims and limits of the domains of enquiry in each of the very different sites (see Appendix 13). Fieldwork was conducted by a team of six experienced qualitative researchers who had been trained in research practice and were equipped with research passports. Researchers attended a half-day briefing session to prepare them for fieldwork. All researchers were blinded to which sites were classed as high or low specialist intensity at weekends. Four-day visits spanning a weekend were co-ordinated by a fieldwork manager working with each local project lead (a senior consultant in each trust). Visits and interviews took place between April 2016 and April 2017. Qualitative researchers were supplied with a standardised pack containing all materials required for site visits, including an information leaflet to distribute to patients and staff (see Report Supplementary Material 1). Working practices relating to the care of EAs were observed in EDs, AMUs, general wards and ICUs. Semistructured, audio-recorded interviews (either in person or over the telephone, and with written consent) were held with local project leads, patients and ward staff.
Team debriefings were held as soon as possible following visits. Audio-recordings of interviews and observations were transcribed and anonymised, and imported into NVivo for coding and analysis. We conducted a thematic analysis to characterise the role of specialists in delivering high-quality care. For the analysis of quality of care delivery at weekends, we coded data to a framework of characteristics derived from the documents used to develop the topic guide. We also generated narrative case study reports for each of the 20 trusts, describing how acute medical care was organised and delivered at the weekend, and the contextual influences on this. Case studies were sent to the local project leads for comment, clarification or factual correction where required. These reports, along with the data coded to the framework, formed the basis of subsequent comparative analysis across sites. Two anonymised case study examples are provided in Appendix 14. The overall picture of weekend service delivery for each trust was represented visually, based on reference examples from the qualitative data, using a ‘red/amber/green’ (RAG) rating: red – severe problems evident, amber – some limitations and problems, or green – satisfactory. Colours were then weighted for subsequent quantitative analysis (red, 2; amber, 1; green, 0) to produce a ‘RAG score’.
Qualitative research round 2: subset of eight hospital trusts
We purposively selected 8 of the 20 trusts for an in-depth examination of their organisational response to 7-day service policy standards. We accessed NHS Improvement data on progress of implementing the four priority 7-day service standards. 113 We conducted focused observational visits and semistructured interviews with five or six members of staff in each of the eight trusts, including board-level staff (medical directors, financial directors and chief medical officers), acute medical consultants, acute consultant rota co-ordinators and senior nurses. Visits and interviews took place between November 2017 and February 2018.
The interview topic guide (see Appendix 15) included questions about the organisation’s response to the new standards and how it had fared in implementing the priority standards. The competing values framework,114,115 a model of different organisational cultures for change, was used to stimulate discussion and reflection on organisational and team culture, and how this shaped responses to the 7-day service standards. Interviews were conducted in person or over the telephone. Interviews lasted between 30 minutes and 1 hour and were audio-recorded with the consent of all participants. Interviews and observation field notes were audio-recorded, transcribed and anonymised. Transcripts were entered into NVivo for analysis, and a thematic analysis was conducted to explore influences on, challenges to and facilitators of implementation of the 7-day service standards. We used case summaries and cross-case narratives to interpret the findings. Throughout the findings, quotations are labelled as follows: 01OBS refers to hospital 1, observation; 01IV03 refers to hospital 1, interview 3; and so on.
Case record review
In parallel with the round 1 qualitative research, we examined errors, error-related adverse events and global care quality across the participating 20 trusts through a case record review of EAs. We based our approach to obtaining case records on the method used for the evaluation of the Safer Patient Initiative. 116 Following an on-site initiation visit, each trust was asked to provide an anonymised and hash-encrypted Patient Administration System data set for all admissions during two epochs, financial years 1 April 2012–31 March 2013 and 1 April 2016–31 March 2017, from which 200 non-operative admissions were randomly identified (without replacement) for each trust, 100 from each epoch, each with 50 weekend and 50 weekday admissions, giving a total of 4000 unique admissions. We chose not to confine the study to mortality reviews to avoid endogenous selection bias (from the outcome influencing the sample) and to ensure that the study population was representative. Oversampling was used to allow trusts to compensate for missing records. Trusts were reimbursed £600 for staff to copy and scan the case records, masking patient identifiers (name, address, age, postcode); records were censored for lengths of stay exceeding 7 days. Record completeness was assured using a checklist. Files were transferred using a file-sharing programme to a central repository at the University of Birmingham and checked for anonymisation.
Complete records were uploaded to Research Electronic Data Capture (REDCap; Vanderbilt University, Nashville, TN, USA) and allocated randomly to 79 case record reviewers (consultants and senior registrars in acute medical specialties). Reviewers attended one of three centralised half-day training sessions in case record reviewing, focusing on the typology of errors and adverse events, judgements of care quality and forms of bias, and practising case record evaluation, followed by plenary discussion. Following training, each reviewer independently accessed the password-protected case records online in their own time. Progress was monitored every 2 weeks, with group reminders and personal contacts if required. An honorarium of £10 per completed review was paid at the end of the project. Reviewers used structured judgement review to identify and characterise errors and associated adverse events. 117–119 Reviewers were not blinded to dates as this would have prevented them from determining the timeliness of specialist reviews. Error typologies were based on those used by Hogan et al. 117 Reviewers then gave a free-text description of the error; more than one typology could be chosen per error. Error-related adverse events were graded for preventability using a six-point scale. 120 Error-related adverse events (corresponding to ‘preventable adverse events’) distinguish adverse events preceded by an error from those attributable to the underlying disease(s). Reviewers gave a global assessment of care quality based on their view of the extent to which the patient received best practice care, on a five-point scale from ‘completely’ to ‘not at all’. The data collection fields are presented in Appendix 16.
Statistical analysis
Of the 4000 case records, 800 (40 from each trust, of which 20 were from each epoch) were randomly selected for repeat review by two randomly allocated reviewers. Reviewer reliability coefficients were computed from these repeat reviews. Intraclass correlation coefficients (with class = case record) were used for errors and adverse events, and a (quadratically) weighted kappa coefficient for the five-point quality-of-care Likert scale. The reliability of aggregated assessments (within trusts and epochs) was estimated using the Spearman–Brown formula. 121
Errors and adverse events per case record were analysed using mixed-effects generalised linear models: negative binomial models (for the numbers of errors) and logistic regression models (for the presence or absence of an error or adverse event). Mixed-effects ordinal logistic models were used for the quality-of-care Likert scale. In all models, fixed effects were fitted for hospital trust, day of week (weekend/weekday) and time epoch; random effects were fitted for reviewers. Changes in the weekend effects over time were captured by adding day-by-epoch interaction terms to the mixed-effects models. These interaction terms constitute a ‘difference-in-difference’ analysis of rates on a logarithmic scale. Trust-level effects were extracted from these analyses for correlation analysis with qualitative research RAG ratings and estimates of specialist involvement (specialist hours per 10 EAs) from the PPS. Nested models to allow for repeat reviews of the same case note proved computationally infeasible for the full data set, but were successfully fitted to the 20% of case notes with repeat reviews. The estimated variance components were used to compute approximate adjustment factors for the confidence limits of the ORs and rate ratios in the full analysis. The indicated adjustments were small (< 1%) in every case.
Results
Qualitative research: views of front-line staff, patients, relatives and executives on barriers to, facilitators of, and potential impact of 7-day service standards
In this section, we present three key sets of findings: (1) the role of consultants in the organisation and delivery of care at weekends (qualitative research data round 1); (2) the quality and outcomes of care at weekends (case note review and qualitative research data round 1); and (3) the challenges and facilitators of implementation of 7-day standards (qualitative research data round 2).
The role of consultants in the organisation and delivery of care at weekends
In round 1, six qualitative researchers undertook 826 hours of observations of care delivery, spanning weekends, at all 20 sites. A total of 93 interviews were conducted with staff and patients; the professionals interviewed at each site are listed in Table 7.
Site | Consultants | Registrars | Junior doctors | Nursing | Other | Patients | Total |
---|---|---|---|---|---|---|---|
1 | 1 | 1 | 1 | 3 | |||
2 | 1 | 2 | 3 | ||||
3 | 3 | 2 | 1 | 6 | |||
4 | 2 | 1 | 1 | 4 | |||
5 | 2 | 1 | 2 | 2 | 7 | ||
6 | 1 | 1 | 1 | 3 | |||
7 | 1 | 1 | 2 | ||||
8 | 1 | 1 | 1 | 3 | |||
9 | 1 | 1 | 1 | 1 | 4 | ||
10 | 1 | 2 | 3 | ||||
11 | 4 | 1 | 4 | 9 | |||
12 | 1 | 2 | 1 | 3 | 7 | ||
13 | 1 | 1 | 1 | 3 | |||
14 | 1 | 1 | 1 | 3 | |||
15 | 1 | 1 | 1 | 3 | |||
16 | 2 | 1 | 1 | 1 | 5 | ||
17 | 2 | 2 | 3 | 7 | |||
18 | 2 | 1 | 3 | 1 | 3 | 10 | |
19 | 2 | 3 | 5 | ||||
20 | 1 | 1 | 1 | 3 | |||
Total | 31 | 6 | 22 | 20 | 1 | 13 | 93 |
Decision-making: authority and expertise
Consultants are the key decision-makers, both at the individual level and organisationally, from admission to discharge. They categorise and allocate patients to the most appropriate specialty, and ensure that diagnosis and treatment can begin, be amended where necessary and be completed satisfactorily. Consultants use their seniority and expertise to prioritise and advance diagnosis and treatment decisions.
All interviewed staff believed that consultants were crucial in getting decisions made, and that this was one important means through which having more consultant availability could improve weekend care delivery. Observations revealed the extent and breadth of the decisions in which consultants were involved. They included decisions made at the individual patient level and those at an organisational level, particularly important in ensuring patient flow throughout the hospital. At the patient level, consultants were characterised as responsible for determining a diagnosis, deciding which tests are required and determining how urgent these were (and hence making prioritisation decisions). Their presence at weekends and their authority helped expedite decision-making and action on the next steps in a patient’s journey:
And the consultant said that definitely try and get him endoscoped today because they really need to find out what’s happening . . . So he explained to the registrar actually to try and organise this straight away with the gastro[enterology] team. He said there’s a gastro[enterology] list on a Saturday morning, let’s see if we can add this particular patient onto the end of this list.
07OBS
Consultants acted as overseers and co-ordinators of patient pathways, and held responsibility over the weekend for decisions that would alter the pathway of treatment or progress through the hospital system for a patient. Although there were many cases where the specialty of the consultant was not critical to their decision-making, in some cases, access to specialist expertise from someone with the authority of a consultant who could make high-stakes decisions was seen as critical to ensuring optimal patient care:
[Consultant] says to [researcher] ‘So you’ve witnessed a lovely example of why it is good to have consultants in the weekend. My colleague from the ICU needed a specialist input, and my input did make a change for this patient, it did change the patient plan. They were not sure of what to do from a respiratory point of view and I offered them my advice . . . The consultant-to-consultant discussion was very useful in this case’.
08IV03
We observed nurse-led discharge in one of the sites at weekends, but, in the rest, consultants had overall responsibility for making discharge decisions. Consultants were seen as responsible for determining discharge planning and this was facilitated by the availability of consultants at weekends.
The involvement of a consultant could expedite decision-making and patient progress because of the expertise and authority they could bring to bear. Consultant input was seen as particularly important in ‘high-stakes’ decisions, for example decisions to escalate or withdraw treatment, and when patients were acutely unwell.
Consultants used their higher-level overview of patient management plans, along with their authority, to ensure that the steps required in patient care took place and to push for actions to be taken. This included using their authority to access tests that they felt were essential, or to negotiate for patient transfers or access to equipment. There were examples of consultants making assertive orders, making it clear that certain things had to happen, and suggesting that staff could call on them to use their authority to overcome any obstacles:
The consultant had asked one of the junior doctors, a FY1 [Foundation Year 1], to get a patient . . . a MRI [magnetic resonance imaging] so that they could get her discharged. But the junior doctor came back and said we might not get a MRI today, because neurology wants to come and see this patient. But the consultant on the AMU said ring them back and tell them this MRI is the only thing that’s holding up discharge, so we need to get it today. And he said get the name of the neurology consultant who wants to see the patient, with an implication that if there was any hold-up, the consultant would step in and talk to the other consultant and make sure that they didn’t hold up this patient’s discharge.
06OBS
Support for junior staff
Along with patient-level and organisational decision-making, consultants played a key role in monitoring, supporting and teaching junior colleagues, and performing ‘scaffolding’ roles, such as providing supervision, guidance and support to junior doctors. Managing the flow of work included managing juniors, making sure that they completed their tasks in a timely fashion and ensuring that all patient reviews were conducted in a timely manner. Without consultant input, momentum could be lost over the weekend:
If there’s no reason for them to be reviewed [by a consultant at the weekend], the jobs that have been written down aren’t necessarily chased appropriately by the relevant doctors. So things can sometimes get missed . . . I don’t think you get that kind of consistency that you get during the week.
19IV04
Role of consultant limited by infrastructure and resources at weekends
Our analysis identified that the extent to which increased specialist input can improve the quality of service delivery is constrained by broader factors related to the resources available to support them both within the hospital and in the community. The value of having consultants available was limited if junior doctors were not available to action decisions; when other specialist and allied services within the hospital, such as physiotherapy or speech and language therapy services, were in short supply; and where organisational infrastructure was not supportive (e.g. when hospital pharmacies had limited opening hours at weekends). Perhaps most importantly, the ability of consultants to progress patient care through to discharge was limited by the availability of support services in the community:
Thinking about what the problems are at the weekend in hospitals, [consultant] said it’s not the lack of consultants or junior doctors that’s the problem at the weekend. It’s a whole list of things which he reeled off. Inappropriate admissions [. . .]. Lack of social services at the weekend to provide the services that patients need to be discharged.
02OBS
You can’t have that weekend service, that weekend review, unless you put everything else in place, you know we can’t get patient flow without pharmacists. We can’t get people out of hospital without physiotherapists, occupational therapists, social workers. Improving doctors on their own won’t improve flow.
04IV04
Quality and outcomes of care at weekends
We next outline our findings on quality of care and outcomes at the weekend, bringing together case note review and analysis of qualitative research data. We start by describing variation between hospitals in quality of care at the weekend. We then report the findings from the case note review, which triangulates the quantitative findings on quality of care with the findings from the qualitative data.
Observations and views of front-line staff, patients and relatives
In round 1, along with the 826 hours of observations of care delivery spanning weekends in 20 sites, a total of 93 interviews were conducted with staff and patients. The spread of interviewees by site is shown in Table 7. An additional set of telephone interviews was conducted with local project leads in 19 sites between the two rounds of fieldwork. In one site, the project lead failed to respond, despite repeated requests.
An overview of how each site organised and delivered acute medical care at the weekend was produced in the form of a case study (see Appendix 14 for examples). Analysis was conducted by exploring case by case which quality domains were frequently identified as important within each site or reported/observed to be lacking, with reference to the 7-day service standards17 and guidance on acute care from the Royal College of Physicians. 112 A framework analysis was then produced that enabled a cross-case comparison of each domain. The analysis was drawn from both observation field notes and interviews with front-line staff and patients. The key domains identified were:
-
staffing levels for consultants, junior doctors and nurses
-
the extent of senior oversight on medical wards
-
the access to senior expertise
-
continuity of care on AMU over the weekend and into the week
-
handover and communication
-
patient flow (at admission, general bed capacity, and at discharge).
There was substantial variation between trusts in staff perceptions and qualitative researcher observations of the quality of weekend care. Examples are provided in Table 8.
Staffing levels: consultants | |
---|---|
Good:We do OK for consultant cover over the weekends, I think we’re well staffed. They have several people providing the acute medicine cover over the weekend, endoscopy cover for GI bleeds, a cardiologist who comes in to do a ward round on Sunday. So a lot of issues can be dealt with then02OBS | Limited:We need 16 or 18 consultants in A&E. But we’re about 50% short of what we need03INT01 |
Junior doctor staffing | |
Good:I don’t know if you’ve been in to other hospitals, but [our] Trust is completely out of the ordinary, it’s unlike any other hospital at the weekend: there are a ridiculous number of junior doctors here in at the weekend06OBS | Limited:You have got access to the doctors who are on the on-call rota but they are very, very stretched. The registrar and the SHO are mainly clerking in new patients in the AMU, [but there are only] two FY1 doctors running around the whole hospital trying to deal with the sick patients and you know, they’re just so stretched11OBS |
Nurse staffing | |
Good:I’ve got four staff nurses on today [weekend day] so they’ve each got a bay and a side room [. . .] So this morning, we’ve got a staff nurse in each bay and then a HCA taking a bay and a half each16IV02 | Limited:It’s not very good, from a nursing perspective, on the ward during the weekend: mainly agency staff and that wasn’t safe and even with the agency staff [the ward sister] still has to see if she can get somebody else to come and help on a weekend07OBS |
Senior oversight on wards | |
Good:At the weekend each medical speciality [sic] has one consultant to go round in the morning seeing the patients that were flagged up for review over the weekend, just in their speciality [sic]. They don’t look after all the medical wards, just their own speciality [sic]16OBS | Limited:There are no consultants in the ward at a weekend. The only doctor is the on-call junior covering the [whole of the] third floor08OBS |
Access to specialist expertise | |
Good:Across the wards, so for every speciality [sic], so elderly care or renal or respiratory, so on there is a consultant that comes in and [. . .] see[s] anybody who is unwell, anybody who is new to their ward, anybody who they are concerned about, anybody who is going to be discharged and do a sort of selective ward round with some of the patients16IV04 | Limited:The neurologists . . . don’t work over the weekend. And we don’t necessarily have neurology back-up, in terms of AED[s], antiepileptic drugs05IV01 |
Continuity of care on AMU | |
Good:The AMU keeps [junior] doctors on the same area as much as possible, during the week and at the weekend, so they have some continuity. And the consultants for the week try to keep that continuity, so they are following the same cohort of patients06OBS | Limited:So you start on the Saturday not knowing them, and you may see them on Sunday and overnight, but then come Monday they’re no longer your patient, they’re back to another team. So there’s a break in continuity from Friday to Saturday and Saturday to Sunday . . . which can be risky for the patient . . . because things are missed01INT03 |
Handover and communication | |
Good:The AMU has the same pattern of handover 7 days a week. The 8 a.m. morning handover from night to day is quite long; they review the AMU patients, sick patients on base wards, patients on ITU that they need to be aware of, and this all gets handed over electronically. 3.30 p.m. on Friday, they go through all the patients quickly and put patients and tasks on the review list and a junior doctor would be on a computer updating it. So, their pattern is 8 a.m. big handover, 3.30 p.m. catch-up, 8.30 p.m. big handover again to the night. And that’s the pattern 7 days a week02OBS | Limited:Sometimes it’s very hard to interpret the jobs that are handed over by the week team that are basically a job list that they can access through their computer system. ‘Patient X review and manage if needed.’ But manage what? So they lose a lot of time because the handovers from the week team are not clear08OBS |
Flow: front door | |
Good:They have systems and structures to avoid admission. The care of the elderly clinic [allows] older patients who come into A&E to go for short-term management to avoid admission. In some cases, the acute medical consultant on call will see patients who are still in the emergency department, so if a patient’s been allocated to the AMU, but they haven’t actually been transferred yet, the acute medical consultant will come back and see that patient as part of their ward round06OBS | Limited:Actually the difference at the weekend is that they also don’t have that short-stay unit open, where the patients who have been referred to the hospital via the GP route were going on during the weekday. That isn’t open on a weekend17OBS |
Flow: beds | |
Good:They have non-clinical administrators who’ve been employed in the Trust for quite a long time with really good tacit knowledge about what can be done and how to push for things to happen. They know the workarounds and who to ask about things; they provide a lot of continuity, because they’re on 7 days a week. They would sit in on handovers, and come on ward rounds, and chase things, particularly patients’ care packages and facilitating discharges to happen06OBS | Limited:I just watched a game of musical beds. There were no spare beds, so patients were stacking up in the corridors, which isn’t good for dignity when you do examinations. Some patients were in chairs. Patients were being swapped between the majors section, which is where I was, and resus[citation]. There were no beds on AMU04OBS |
Flow: discharge | |
Good:The consultant in charge was the discharge doctor of the day [on Saturday], to go round and to review all the patients that had been flagged for discharge on a whiteboard that was in the doctors’ office on the ward. There was a star against each of the patients who had been considered potentially suitable for discharge today, and the consultant’s job was to review them and make decisions for discharge06OBS | Limited:The emphasis on [Sunday] was not to discharge people, whereas on the Friday, [it] was looking through the list, can this patient go home or does this patient have to stay17OBS |
Services to support flow: in-house | |
Good:Therapists, we have on-call therapists rather than the sort of the full complement, which does limit the ability, have to then be a bit more focused on those things, but it’s still there01IV01 | Limited:The consultant said, from his point of view, that the biggest issue at the weekend is the fact that patient flow is obstructed because of the lack of allied diagnostic and therapeutic services at the weekend. So he said, again, that patient who needs a [speech and language assessment], Friday it’s difficult, Saturday no, Sunday no. So nothing is going to happen02OBS |
Services to support flow: social and community services | |
Good/limited:One of the good things about the trust is that they don’t have major problems with flow over the weekend because some of the community services, for example the district nurses, the COPD nurses, are employed by the trust, not by the council, and this means that they keep working, they keep functioning over the weekend as well05OBS | Poor:Also outside of hospital, that’s where a lot of the blockage comes from. So a lot of patients actually stuck in hospital and cannot go out because the services aren’t there to assist them outside, there is no social services for instance, there is nobody to receive them out in the community or to organise stuff014IV02 |
Qualitative researcher ratings of weekend care quality
A RAG rating was produced that identified the key features of the quality of service delivery at weekends. This was conducted by mapping which domains (outlined earlier) appeared to be operating well or were limited in each site. Sites were rated as green (good) on the domain if an element of the service or care was identified as happening as intended, or as not presenting a threat to quality at the weekend. Sites were rated as amber when there was reduced capacity within the domains. Sites were rated as red when there was clear evidence of a problem of quality of service delivery within a domain or where it was clear that the service or care was not happening as intended. To enable triangulation of the findings from the qualitative research with the case record review, colours were weighted for subsequent quantitative analysis (red, 2; amber, 1; and green, 0) to produce a RAG score (Table 9).
Trust | Staffing | Senior oversight on wards | Access to expertise: specialist availability | Continuity of care in the AMU (nights and weekends) | Handover and communication | Flow: evidence of action taken | Services to support flow | Total weighted score for each trust | |||||
---|---|---|---|---|---|---|---|---|---|---|---|---|---|
Consultants | Junior doctors | Nurses | To reduce admissions | To manage beds | To facilitate hospital discharge | In house facilities, diagnostics, pharmacy | In the community | ||||||
1 | 0 | 1 | 0 | 1 | 0 | 1 | 1 | 0 | 0 | 0 | 0 | 2 | 6 |
2 | 0 | 1 | 1 | 0 | 1 | 0 | 0 | 1 | 2 | 2 | 2 | 1 | 11 |
3 | 1 | 1 | 1 | 1 | 0 | 0 | 1 | 0 | 2 | 1 | 0 | 2 | 10 |
4 | 0 | 2 | 0 | 0 | 1 | 2 | 1 | 1 | 2 | 2 | 2 | 2 | 15 |
5 | 0 | 1 | 0 | 2 | 1 | 0 | 0 | 0 | 0 | 0 | 0 | 1 | 5 |
6 | 0 | 0 | 0 | 1 | 1 | 0 | 0 | 0 | 0 | 0 | 0 | 1 | 3 |
7 | 0 | 1 | 2 | 2 | 2 | 1 | 1 | 0 | 1 | 2 | 1 | 1 | 14 |
8 | 1 | 2 | 2 | 2 | 1 | 0 | 2 | 2 | 2 | 2 | 2 | 2 | 20 |
9 | 0 | 2 | 1 | 0 | 0 | 1 | 1 | 1 | 1 | 1 | 2 | 1 | 11 |
10 | 1 | 2 | 1 | 2 | 2 | 1 | 2 | 2 | 2 | 1 | 2 | 1 | 19 |
11 | 1 | 2 | 0 | 1 | 1 | 1 | 1 | 1 | 2 | 2 | 1 | 2 | 15 |
12 | 2 | 1 | 1 | 1 | 1 | 1 | 1 | 1 | 2 | 1 | 1 | 2 | 15 |
13 | 2 | 2 | 1 | 2 | 1 | 1 | 1 | 1 | 1 | 1 | 2 | 2 | 17 |
14 | 1 | 2 | 0 | 1 | 1 | 1 | 0 | 1 | 1 | 1 | 1 | 2 | 12 |
15 | 0 | 2 | 1 | 1 | 1 | 0 | 1 | 1 | 1 | 1 | 1 | 2 | 12 |
16 | 0 | 0 | 0 | 0 | 0 | 0 | 1 | 0 | 0 | 0 | 1 | 2 | 4 |
17 | 2 | 2 | 2 | 2 | 1 | 1 | 1 | 2 | 2 | 1 | 2 | 2 | 20 |
18 | 0 | 1 | 0 | 0 | 0 | 1 | 1 | 0 | 1 | 0 | 1 | 2 | 7 |
19 | 0 | 1 | 0 | 0 | 0 | 1 | 0 | 2 | 2 | 1 | 2 | 2 | 11 |
20 | 1 | 2 | 0 | 2 | 1 | 1 | 1 | 0 | 0 | 2 | 2 | 1 | 13 |
Sum of domain scores | 11 | 28 | 13 | 20 | 15 | 14 | 17 | 16 | 23 | 20 | 24 | 33 |
Case record review: results
Four thousand case records were retrieved. The characteristics of the randomly selected study population were representative of the total hospital admitted population in England (see Appendix 17). Seventy-nine reviewers participated; the mean number of reviews per reviewer was 61 (20–69 reviews), with 800 records reviewed in duplicate. Of 4800 case reviews, in 37 the reviewer found that the case record contained insufficient documentation. This left 4763 case reviews available for analysis, of which 1584 could be used for inter-reviewer reliability assessment (Figure 20). There were also 37 reviews where no global care assessment was offered. Unless otherwise stated, analyses are based on available reviews, not on available case records.
FIGURE 20.
Case record review data acquisition and processing. PAS, Patient Administration System; UoB, University of Birmingham.
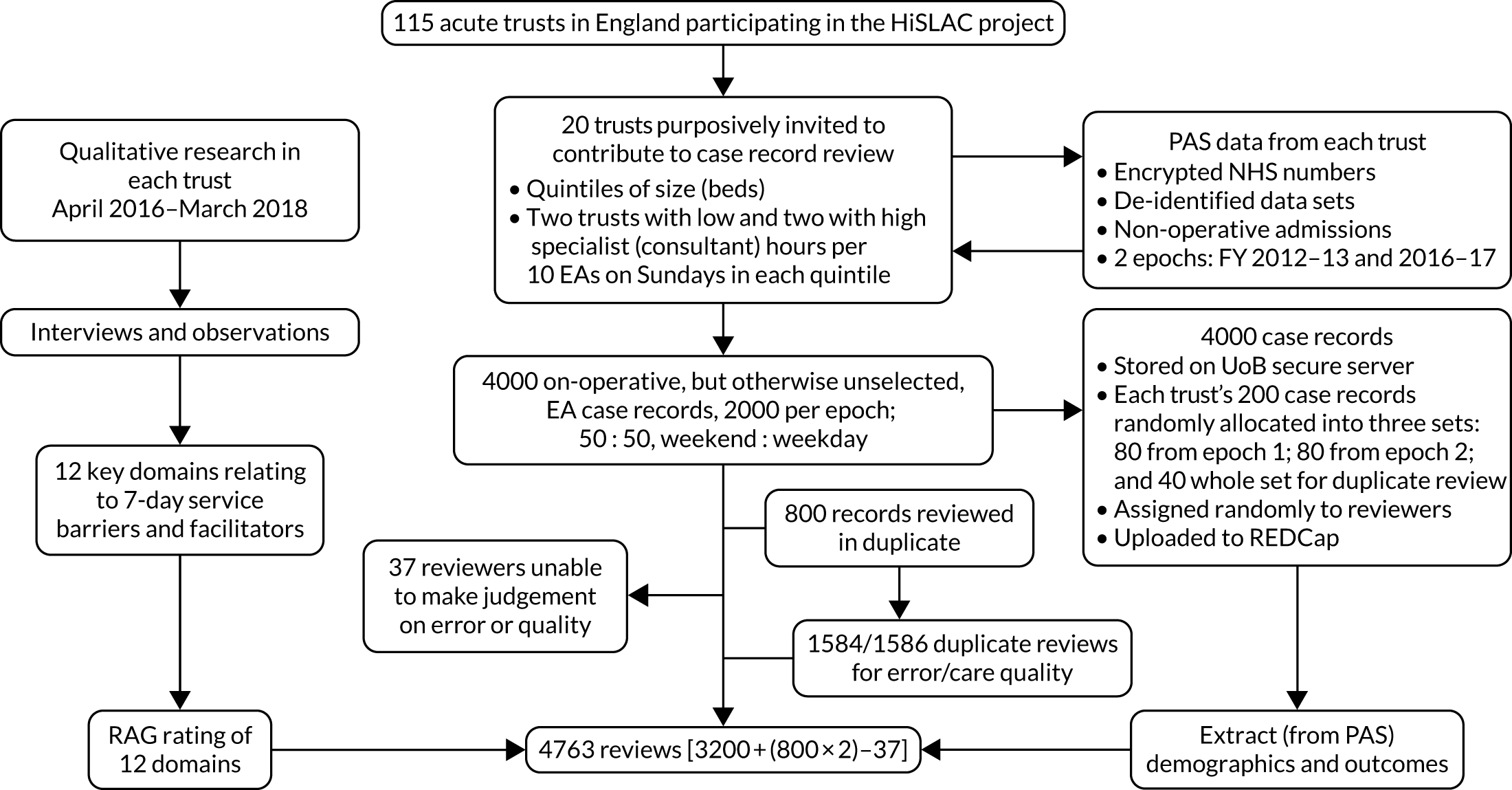
Errors, error-related adverse events and global quality of care
Of the 4763 case reviews (50% weekend admissions, 50% weekday admissions), 1178 (24.7%) showed one or more errors in care: 1909 errors were identified in total. Single errors were identified for 15.8% of reviews, and two or more errors were identified for 8.7% (Table 10). The most frequently identified category of error was ‘clinical assessment, investigation or diagnosis’ (31.7%), followed by ‘treatment and management’ (28.9%), ‘communication’ (15.5%) and ‘medication’ (13.3%). In total, 149 error-related adverse events were identified in 120 case reviews (2.52% of reviews, 7.8% of errors), of which 110 (73.8%) were judged to have a > 50% chance of being preventable. Reviewers judged that best practice care had been provided completely in 1891 (39.7%) reviews, substantially in 2002 (42.0%) reviews, partially in 740 (15.4%) reviews, very little in 99 (2.1%) reviews and not at all in 31 (0.6%) reviews.
Both epochs, n (%) | Epoch 1, n (%) | Epoch 2, n (%) | |||||||
---|---|---|---|---|---|---|---|---|---|
Total | Weekend | Weekday | Total | Weekend | Weekday | Total | Weekend | Weekday | |
Total (N) | 4800 | 2395 | 2405 | 2400 | 1199 | 1201 | 2400 | 1196 | 1204 |
Number of errors | |||||||||
No error | 3585 (74.7) | 1807 (75.4) | 1778 (73.9) | 1752 (73) | 880 (73.4) | 872 (72.6) | 1833 (76.4) | 927 (77.5) | 906 (75.2) |
1 | 760 (15.8) | 375 (15.7) | 385 (16) | 400 (16.7) | 207 (17.3) | 193 (16.1) | 360 (15) | 168 (14) | 192 (15.9) |
2 | 256 (5.3) | 120 (5) | 136 (5.7) | 144 (6) | 62 (5.2) | 82 (6.8) | 112 (4.7) | 58 (4.8) | 54 (4.5) |
3 | 91 (1.9) | 40 (1.7) | 51 (2.1) | 46 (1.9) | 20 (1.7) | 26 (2.2) | 45 (1.9) | 20 (1.7) | 25 (2.1) |
4 | 39 (0.8) | 20 (0.8) | 19 (0.8) | 26 (1.1) | 14 (1.2) | 12 (1) | 13 (0.5) | 6 (0.5) | 7 (0.6) |
5 | 14 (0.3) | 9 (0.4) | 5 (0.2) | 7 (0.3) | 5 (0.4) | 2 (0.2) | 7 (0.3) | 4 (0.3) | 3 (0.2) |
6 | 10 (0.2) | 4 (0.2) | 6 (0.2) | 5 (0.2) | 1 (0.1) | 4 (0.3) | 5 (0.2) | 3 (0.3) | 2 (0.2) |
7 | 3 (0.1) | 1 (0) | 2 (0.1) | 2 (0.1) | 0 (0) | 2 (0.2) | 1 (0) | 1 (0.1) | 0 (0) |
8 | 2 (0.0) | 1 (0) | 1 (0) | 2 (0.1) | 1 (0.1) | 1 (0.1) | 0 (0) | 0 (0) | 0 (0) |
13 | 2 (0.0) | 0 (0) | 2 (0.1) | 2 (0.1) | 0 (0) | 2 (0.2) | 0 (0) | 0 (0) | 0 (0) |
15 | 1 (0.0) | 1 (0) | 0 (0) | 1 (0) | 1 (0.1) | 0 (0) | 0 (0) | 0 (0) | 0 (0) |
Missing | 37 (0.8) | 17 (0.7) | 20 (0.8) | 13 (0.5) | 8 (0.7) | 5 (0.4) | 24 (1) | 9 (0.8) | 15 (1.2) |
Error in care | |||||||||
Yes | 1178 (24.5) | 571 (23.8) | 607 (25.2) | 635 (26.5) | 311 (25.9) | 324 (27.0) | 543 (22.6) | 260 (21.7) | 283 (23.5) |
No | 3585 (74.7) | 1807 (75.4) | 1778 (73.9) | 1752 (73.0) | 880 (73.4) | 872 (72.6) | 1833 (76.4) | 927 (77.5) | 906 (75.2) |
Missing | 37 (0.8) | 17 (0.7) | 20 (0.8) | 13 (0.5) | 8 (0.7) | 5 (0.4) | 24 (1.0) | 9 (0.8) | 15 (1.2) |
Total number of errors | |||||||||
1909 | 914 | 995 | 1066 | 501 | 565 | 843 | 413 | 430 | |
Mean number of errors per patient admissiona | |||||||||
0.4 | 0.38 | 0.42 | 0.45 | 0.42 | 0.47 | 0.35 | 0.35 | 0.36 |
Weekend–weekday differences
Of the 1909 identified errors, 914 were in weekend admissions and 995 were in weekday admissions (see Table 10). The mean rate of errors per case review was numerically smaller for weekend admissions (0.384) than for weekday admissions (0.417), but this did not achieve statistical significance (rate ratio 0.92, 95% CI 0.84 to 1.02; p = 0.1029) (Table 11). Similarly, there was no difference in adverse event rates, or in global care quality assessments, between weekend and weekday admissions. Nor was there any evidence for temporal change in weekend–weekday differences over time (see Table 11).
Errors, adverse events and global quality | Epochs (epoch 2 : epoch 1), RR/OR (95% CI) | Day of admission (weekend : weekday), RR/OR (95% CI) | Weekend : weekday (between epochs), RR/OR (95% CI) |
---|---|---|---|
Errors | |||
Assessment, investigation or diagnosis | 0.75 (0.66 to 0.86) | 0.88 (0.78 to 1.00) | 1.03 (0.72 to 1.48) |
Treatment and management | 0.75 (0.66 to 0.85) | 0.93 (0.80 to 1.09) | 1.07 (0.79 to 1.44) |
Communication | 0.82 (0.67 to 0.99) | 1.02 (0.85 to 1.24) | 1.42 (0.99 to 2.04) |
Medication | 0.76 (0.63 to 0.94) | 0.93 (0.74 to 1.17) | 0.73 (0.44 to 1.24) |
Monitoring | 0.81 (0.58 to 1.14) | 0.88 (0.68 to 1.14) | 0.75 (0.35 to 1.63) |
Resuscitation | 0.72 (0.42 to 1.23) | 1.74 (0.81 to 3.73) | 1.28 (0.36 to 4.64) |
Infection | 2.16 (0.93 to 5.02) | 0.70 (0.27 to 1.79) | 1.26 (0.19 to 8.25) |
Invasive procedures | 0.46 (0.20 to 1.07) | 1.62 (0.70 to 3.74) | 0.99 (0.19 to 5.2) |
Other | 0.54 (0.32 to 0.89) | 1.11 (0.72 to 1.71) | 0.96 (0.40 to 2.29) |
Any errora | 0.80 (0.73 to 0.87); p < 0.0001 | 0.92 (0.84 to 1.02); p = 0.1029 | 1.03 (0.83 to 1.29); p = 0.7762 |
Adverse eventa | 0.55 (0.39 to 0.78); p = 0.0008 | 0.99 (0.69 to 1.41); p = 0.9417 | 1.61 (0.77 to 3.39); p = 0.2063 |
Global quality of carea | 0.82 (0.74 to 0.91); p = 0.0001 | 0.96 (0.86 to 1.08); p = 0.4786 | 0.96 (0.77 to 1.2); p = 0.7307 |
Temporal trends between epochs (2012–13 and 2016–17)
Error rates between epochs diminished significantly overall (rate ratio 0.80, 95% CI 0.73 to 0.87; p < 0.0001), and also for the four most frequently identified error categories (see Table 11); there was also a significant reduction in error-related adverse events (100 in epoch 1, 49 in epoch 2, OR 0.55, 95% CI 0.39 to 0.78; p = 0.0008) and in care quality (p = 0.0001) (see Table 11): the proportion of reviews attracting the two highest care quality assessments (‘completely’ and ‘substantially’) rose from 79.8% in epoch 1 to 82.4% in epoch 2.
Inter-reviewer differences
Reviewers varied in their rates of error identification (see Appendix 18) and in their judgements of errors and global care quality (Table 12). Reviewer reliability coefficients were low for the assessment of any error (0.026), but higher for treatment (0.131), communication (0.058) and medication errors (0.072), and for global quality (0.142) (see Table 12). However, the study does not aim to establish the quality of care for any particular patient. Rather, it is concerned with aggregate data in individual trusts and the ratio of weekend to weekday error rates. The lowest meaningful level of aggregation is based on the (approximately) 50 case notes representing the condition of a particular trust in a particular epoch for weekend or weekday admissions. The reliability of such aggregates estimated using the Spearman–Brown formula is much higher, as shown in Table 12.
Error category and global quality | All reviews (N = 4763) | Errors per review | Repeat reviews (N = 1584 = 2 × 792 reviews in total) | ||||
---|---|---|---|---|---|---|---|
Errors (n) | Mean (n) | SD | Maximum (n) | Errors (n) | Individual-level reliability | Trust-level reliabilitya | |
Assessment | 903 | 0.19 | 0.58 | 12 | 272 | 0.003 | 0.138 |
Treatment | 824 | 0.17 | 0.54 | 10 | 265 | 0.131 | 0.883 |
Communication | 442 | 0.09 | 0.44 | 14 | 140 | 0.058 | 0.753 |
Medication | 380 | 0.08 | 0.34 | 8 | 129 | 0.072 | 0.794 |
Monitoring | 138 | 0.03 | 0.19 | 4 | 47 | b | b |
Resuscitation | 38 | 0.01 | 0.10 | 2 | 12 | b | b |
Infection | 26 | 0.01 | 0.08 | 2 | 11 | b | b |
Invasive | 25 | 0.01 | 0.08 | 2 | 5 | b | b |
Other | 75 | 0.02 | 0.13 | 2 | 25 | b | b |
Any error | 1909 | 0.40 | 0.92 | 15 | 606 | 0.026 | 0.568 |
Global quality of care | NA | NA | NA | NA | NA | 0.142 | 0.892 |
Trust-level aggregate quality measure
The relationship between error rates and global quality of care identified from the case record review at trust level is represented in Figure 21, with trust-specific ORs (for ‘any error’ and ‘global quality’ of care) obtained from the models reported in Table 11. The overall correlation is close to zero (r = 0.06), but this masks an evident – and plausible – relationship between higher error rates and lower global quality of care assessments for 18 of the 20 trusts. Two trusts (labelled 10 and 15 in Figure 21a) did not follow this pattern, recording the lowest error rates alongside relatively poor global care assessments.
FIGURE 21.
Trust-level aggregate analysis. (a) Error and global care quality; and (b) global care quality and qualitative research quality score. The ORs for the presence of (any) error and the ORs for (worse) quality of care are plotted on a logarithmic scale, with a value of 1 corresponding to the average trust.

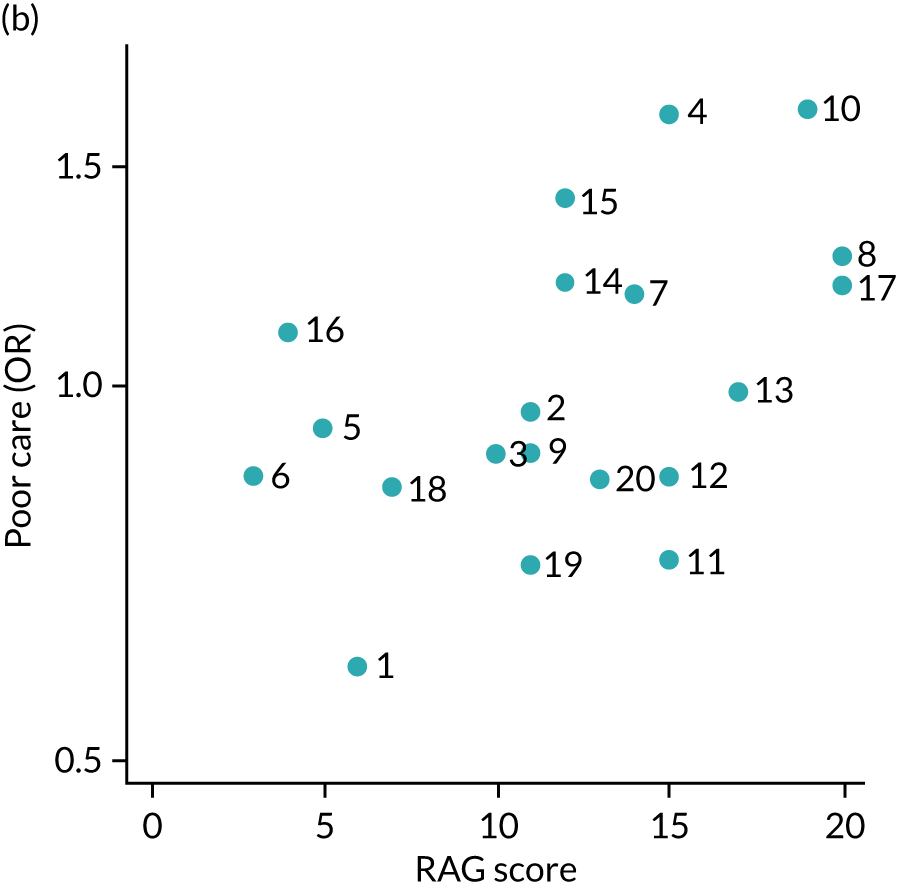
We explored this further using the qualitative research RAG-rated evaluations of quality of weekend care. The RAG score ranged from 3 (good) to 20 (poor) over the 20 trusts, with a mean value of 12.0. Trust 10 had a high (19, adverse) weighted RAG score, and trusts 14 and 15 had an intermediate score (12) (see Table 9). The RAG score is moderately correlated (r = 0.49; p = 0.0273) with the reviewers’ trust-specific global assessment scores (see Figure 21b). This suggests that the case record reviewers and the qualitative researchers in the field are detecting quality signals that may have something in common, but that less clearly correlate with clinical errors (see Figure 21a).
Of the 12 qualitative research weekend care quality criteria, the one that attracted adverse scores across all 20 trusts was support services in the community for patient flow (impacting on admission and discharge) (see Table 9). Junior doctor staffing and workload were also identified as particular sources of concern at weekends.
Between epochs and specialist intensity
When weekend–weekday differences are aggregated across the entire study period, there is little evident correlation between trust-level weekend effects for quality of care (i.e. weekend-to-weekday ratios pooled over both epochs) and the corresponding ratios for the measure of specialist intensity, as computed over the 5 years of the PPS from 2013/14 to 2017/18 (r = –0.23, p = 0.3341 in Figure 22a). Nevertheless, this does not rule out the possibility that changes in staffing policy over time in an individual trust might have an impact on the weekend-to-weekday ratios of care quality in that trust. To examine this further, the PPS data from 2013/14 were matched with the case-note reviews from epoch 1, and the PPS data from 2016/17 were matched with the reviews from epoch 2. In this way, a ratio of weekend effects between epochs could be calculated for each trust both for global quality of care and for specialist intensity. These ratios (corresponding to differences in differences on the log scale) are shown in Figure 22b. The negative correlation here (r = –0.53) indicates that improved compliance with 7-day consultant care in individual trusts was accompanied by a corresponding equalisation of standards of care across the week, although it does not establish a causal connection. A similar analysis using trust-level weekend effects for the presence of error in place of poor care quality (figure not shown) produced a somewhat smaller negative correlation (r = –0.34).
FIGURE 22.
Trust-level weekend effects for quality of care and specialist intensity. (a) Pooled over both epochs and all PPS time points; and (b) relative changes between the two epochs. In (a) values above (below) 1 on the vertical axis represent care that is worse (better) than average. In (b), ratios greater (less) than 1 indicate that care had worsened (improved) from epoch 1 to epoch 2.


Patient admission pathways
Data on the pre- and post-admission phases are summarised in Table 13.
Both epochs, n (%) | Epoch 1, n (%) | Epoch 2, n (%) | |||||||
---|---|---|---|---|---|---|---|---|---|
Total | Weekend | Weekday | Total | Weekend | Weekday | Total | Weekend | Weekday | |
Total (N) | 4800 | 2395 | 2405 | 2400 | 1199 | 1201 | 2400 | 1196 | 1204 |
Source of admission | |||||||||
Own home | 4059 (84.6) | 2052 (85.7) | 2007 (83.5) | 2052 (85.5) | 1038 (86.6) | 1014 (84.4) | 2007 (83.6) | 1014 (84.8) | 993 (82.5) |
Nursing or residential care home | 314 (6.5) | 149 (6.2) | 165 (6.9) | 160 (6.7) | 73 (6.1) | 87 (7.2) | 154 (6.4) | 76 (6.4) | 78 (6.5) |
No information available | 291 (6.1) | 127 (5.3) | 164 (6.8) | 119 (5) | 52 (4.3) | 67 (5.6) | 172 (7.2) | 75 (6.3) | 97 (8.1) |
Another hospital | 40 (0.8) | 20 (0.8) | 20 (0.8) | 15 (0.6) | 8 (0.7) | 7 (0.6) | 25 (1.0) | 12 (1.0) | 13 (1.1) |
No fixed abode | 88 (1.8) | 43 (1.8) | 45 (1.9) | 51 (2.1) | 26 (2.2) | 25 (2.1) | 37 (1.5) | 17 (1.4) | 20 (1.7) |
Missing | 8 (0.2) | 4 (0.2) | 4 (0.2) | 3 (0.1) | 2 (0.2) | 1 (0.1) | 5 (0.2) | 2 (0.2) | 3 (0.2) |
Patient condition before admission | |||||||||
Independent | 3142 (65.5) | 1552 (64.8) | 1590 (66.1) | 1605 (66.9) | 804 (67.1) | 801 (66.7) | 1537 (64) | 748 (62.5) | 789 (65.5) |
Needing help with some ADL | 789 (16.4) | 394 (16.5) | 395 (16.4) | 404 (16.8) | 194 (16.2) | 210 (17.5) | 385 (16) | 200 (16.7) | 185 (15.4) |
Dependent on others for most/all ADL, including personal hygiene | 476 (9.9) | 270 (11.3) | 206 (8.6) | 226 (9.4) | 128 (10.7) | 98 (8.2) | 250 (10.4) | 142 (11.9) | 108 (9.0) |
Unable to determine; no relevant information in notes | 384 (8.0) | 174 (7.3) | 210 (8.7) | 162 (6.8) | 71 (5.9) | 91 (7.6) | 222 (9.3) | 103 (8.6) | 119 (9.9) |
Missing | 9 (0.2) | 5 (0.2) | 4 (0.2) | 3 (0.1) | 2 (0.2) | 1 (0.1) | 6 (0.3) | 3 (0.3) | 3 (0.2) |
Referral to hospital mechanism | |||||||||
999/ambulance transfer to ED | 2261 (47.1) | 1253 (52.3) | 1008 (41.9) | 1197 (49.9) | 662 (55.2) | 535 (44.5) | 1064 (44.3) | 591 (49.4) | 473 (39.3) |
Self-presentation to ED (walk in/own transport) | 1022 (21.3) | 543 (22.7) | 479 (19.9) | 489 (20.4) | 263 (21.9) | 226 (18.8) | 533 (22.2) | 280 (23.4) | 253 (21.0) |
GP or deputising service referral (documented letter or telephone call) | 667 (13.9) | 195 (8.1) | 472 (19.6) | 344 (14.3) | 102 (8.5) | 242 (20.1) | 323 (13.5) | 93 (7.8) | 230 (19.1) |
Unable to determine | 644 (13.4) | 322 (13.4) | 322 (13.4) | 271 (11.3) | 141 (11.8) | 130 (10.8) | 373 (15.5) | 181 (15.1) | 192 (15.9) |
Patient instructed by GP to attend ED, no formal evidence of referral | 116 (2.4) | 34 (1.4) | 82 (3.4) | 64 (2.7) | 18 (1.5) | 46 (3.8) | 52 (2.2) | 16 (1.3) | 36 (3) |
Urgent care centre or walk-in centre referral | 55 (1.1) | 33 (1.4) | 22 (0.9) | 20 (0.8) | 7 (0.6) | 13 (1.1) | 35 (1.5) | 26 (2.2) | 9 (0.7) |
Self-presentation, patient instructed to attend by 111 service | 23 (0.5) | 9 (0.4) | 14 (0.6) | 9 (0.4) | 3 (0.3) | 6 (0.5) | 14 (0.6) | 6 (0.5) | 8 (0.7) |
Missing | 12 (0.3) | 6 (0.3) | 6 (0.2) | 6 (0.3) | 3 (0.3) | 3 (0.2) | 6 (0.3) | 3 (0.3) | 3 (0.2) |
Admission pathway | |||||||||
ED/pre-admission area | 4016 (83.7) | 2110 (88.1) | 1906 (79.3) | 1990 (82.9) | 1048 (87.4) | 942 (78.4) | 2026 (84.4) | 1062 (88.8) | 964 (80.1) |
Direct admission to acute ward | 555 (11.6) | 189 (7.9) | 366 (15.2) | 304 (12.7) | 104 (8.7) | 200 (16.7) | 251 (10.5) | 85 (7.1) | 166 (13.8) |
Unable to determine | 220 (4.6) | 91 (3.8) | 129 (5.4) | 103 (4.3) | 45 (3.8) | 58 (4.8) | 117 (4.9) | 46 (3.8) | 71 (5.9) |
Missing | 9 (0.2) | 5 (0.2) | 4 (0.2) | 3 (0.1) | 2 (0.2) | 1 (0.1) | 6 (0.3) | 3 (0.3) | 3 (0.2) |
Pre-admission vital signs documentation | |||||||||
Yes, full set, and a NEWS | 2113 (44.0) | 1098 (45.8) | 1015 (42.2) | 918 (38.3) | 474 (39.5) | 444 (37.0) | 1195 (49.8) | 624 (52.2) | 571 (47.4) |
Full set but no NEWS calculated | 1546 (32.2) | 796 (33.2) | 750 (31.2) | 935 (39) | 475 (39.6) | 460 (38.3) | 611 (25.5) | 321 (26.8) | 290 (24.1) |
Incomplete vital signs or time missing | 377 (7.9) | 180 (7.5) | 197 (8.2) | 223 (9.3) | 107 (8.9) | 116 (9.7) | 154 (6.4) | 73 (6.1) | 81 (6.7) |
No vital signs documented | 436 (9.1) | 212 (8.9) | 224 (9.3) | 159 (6.6) | 84 (7.0) | 75 (6.2) | 277 (11.5) | 128 (10.7) | 149 (12.4) |
Not applicable: patient not admitted via ED: direct admission to ward | 313 (6.5) | 99 (4.1) | 214 (8.9) | 160 (6.7) | 55 (4.6) | 105 (8.7) | 153 (6.4) | 44 (3.7) | 109 (9.1) |
Missing | 15 (0.3) | 10 (0.4) | 5 (0.2) | 5 (0.2) | 4 (0.3) | 1 (0.1) | 10 (0.4) | 6 (0.5) | 4 (0.3) |
First location post admission | |||||||||
Acute medical unit (AMU/MAU) | 1676 (34.9) | 822 (34.3) | 854 (35.5) | 842 (35.1) | 392 (32.7) | 450 (37.5) | 834 (34.8) | 430 (36.0) | 404 (33.6) |
Unable to determine ward type | 804 (16.8) | 404 (16.9) | 400 (16.6) | 395 (16.5) | 212 (17.7) | 183 (15.2) | 409 (17.0) | 192 (16.1) | 217 (18.0) |
Clinical decision unit or short-stay ward | 782 (16.3) | 420 (17.5) | 362 (15.1) | 419 (17.5) | 220 (18.3) | 199 (16.6) | 363 (15.1) | 200 (16.7) | 163 (13.5) |
Other (please specify) | 513 (10.7) | 234 (9.8) | 279 (11.6) | 244 (10.2) | 120 (10) | 124 (10.3) | 269 (11.2) | 114 (9.5) | 155 (12.9) |
General surgerya | 452 (9.4) | 221 (9.2) | 231 (9.6) | 214 (8.9) | 117 (9.8) | 97 (8.1) | 238 (9.9) | 104 (8.7) | 134 (11.1) |
Medical subspecialties including high careb | 257 (5.4) | 128 (5.3) | 129 (5.4) | 136 (5.7) | 54 (4.5) | 82 (6.8) | 121 (5.0) | 74 (6.2) | 47 (3.9) |
General medical ward | 199 (4.1) | 97 (4.1) | 102 (4.2) | 103 (4.3) | 55 (4.6) | 48 (4.0) | 96 (4) | 42 (3.5) | 54 (4.5) |
Older people’s medicine/elderly care unit | 56 (1.2) | 34 (1.4) | 22 (0.9) | 22 (0.9) | 14 (1.2) | 8 (0.7) | 34 (1.4) | 20 (1.7) | 14 (1.2) |
Critical care unit/ICUc | 36 (0.8) | 23 (1.0) | 13 (0.5) | 18 (0.8) | 11 (0.9) | 7 (0.6) | 18 (0.8) | 12 (1) | 6 (0.5) |
Missing | 20 (0.4) | 10 (0.4) | 10 (0.4) | 5 (0.2) | 3 (0.3) | 2 (0.2) | 15 (0.6) | 7 (0.6) | 8 (0.7) |
Rehabilitation | 5 (0.1) | 2 (0.1) | 3 (0.1) | 2 (0.1) | 1 (0.1) | 1 (0.1) | 3 (0.1) | 1 (0.1) | 2 (0.2) |
Location appropriateness | |||||||||
Yes, definitely appropriate | 2952 (61.5) | 1452 (60.6) | 1500 (62.4) | 1483 (61.8) | 723 (60.3) | 760 (63.3) | 1469 (61.2) | 729 (61.0) | 740 (61.5) |
Probably appropriate | 1041 (21.7) | 532 (22.2) | 509 (21.2) | 539 (22.5) | 274 (22.9) | 265 (22.1) | 502 (20.9) | 258 (21.6) | 244 (20.3) |
Unable to determine | 643 (13.4) | 332 (13.9) | 311 (12.9) | 295 (12.3) | 163 (13.6) | 132 (11) | 348 (14.5) | 169 (14.1) | 179 (14.9) |
No | 137 (2.9) | 66 (2.8) | 71 (3) | 73 (3) | 33 (2.8) | 40 (3.3) | 64 (2.7) | 33 (2.8) | 31 (2.6) |
Unknown | 27 (0.6) | 13 (0.5) | 14 (0.6) | 10 (0.4) | 6 (0.5) | 4 (0.3) | 17 (0.7) | 7 (0.6) | 10 (0.8) |
Post-admission vital signs documentation | |||||||||
Yes, full set, and a NEWS | 2324 (48.4) | 1203 (50.2) | 1121 (46.6) | 1108 (46.2) | 573 (47.8) | 535 (44.5) | 1216 (50.7) | 630 (52.7) | 586 (48.7) |
No vital signs documented | 1151 (24.0) | 538 (22.5) | 613 (25.5) | 501 (20.9) | 242 (20.2) | 259 (21.6) | 650 (27.1) | 296 (24.7) | 354 (29.4) |
Full set but no NEWS calculated | 932 (19.4) | 459 (19.2) | 473 (19.7) | 586 (24.4) | 281 (23.4) | 305 (25.4) | 346 (14.4) | 178 (14.9) | 168 (14.0) |
Incomplete vital signs | 353 (7.4) | 175 (7.3) | 178 (7.4) | 190 (7.9) | 93 (7.8) | 97 (8.1) | 163 (6.8) | 82 (6.9) | 81 (6.7) |
Missing | 40 (0.8) | 20 (0.8) | 20 (0.8) | 15 (0.6) | 10 (0.8) | 5 (0.4) | 25 (1) | 10 (0.8) | 15 (1.2) |
Initial consultant review | |||||||||
Consultant review documented | 2259 (47.1) | 1107 (46.2) | 1152 (47.9) | 1088 (45.3) | 512 (42.7) | 576 (48) | 1171 (48.8) | 595 (49.7) | 576 (47.8) |
No evidence for consultant review in first 24 hours | 1512 (31.5) | 776 (32.4) | 736 (30.6) | 708 (29.5) | 395 (32.9) | 313 (26.1) | 804 (33.5) | 381 (31.9) | 423 (35.1) |
Consultant review, time not documented, but case record suggests < 14 hours after admission | 373 (7.8) | 170 (7.1) | 203 (8.4) | 232 (9.7) | 103 (8.6) | 129 (10.7) | 141 (5.9) | 67 (5.6) | 74 (6.1) |
Probable consultant review but status of doctor uncertain | 342 (7.1) | 187 (7.8) | 155 (6.4) | 201 (8.4) | 106 (8.8) | 95 (7.9) | 141 (5.9) | 81 (6.8) | 60 (5.0) |
Unlikely that consultant review occurred during first 24 hours | 196 (4.1) | 107 (4.5) | 89 (3.7) | 103 (4.3) | 55 (4.6) | 48 (4.0) | 93 (3.9) | 52 (4.3) | 41 (3.4) |
Consultant review, time not documented, but case record suggests > 14 hours after admission | 75 (1.6) | 27 (1.1) | 48 (2) | 49 (2) | 16 (1.3) | 33 (2.7) | 26 (1.1) | 11 (0.9) | 15 (1.2) |
Missing | 43 (0.9) | 21 (0.9) | 22 (0.9) | 19 (0.8) | 12 (1) | 7 (0.6) | 24 (1.0) | 9 (0.8) | 15 (1.2) |
Palliative care discussed | |||||||||
No, not required, patient appropriate for full treatment | 3476 (72.4) | 1702 (71.1) | 1774 (73.8) | 1765 (73.5) | 871 (72.6) | 894 (74.4) | 1711 (71.3) | 831 (69.5) | 880 (73.1) |
No, but would probably have been appropriate to consider some form of treatment limitation | 526 (11.0) | 255 (10.6) | 271 (11.3) | 291 (12.1) | 144 (12.0) | 147 (12.2) | 235 (9.8) | 111 (9.3) | 124 (10.3) |
Yes | 321 (6.7) | 190 (7.9) | 131 (5.4) | 136 (5.7) | 81 (6.8) | 55 (4.6) | 185 (7.7) | 109 (9.1) | 76 (6.3) |
No, but would definitely have been appropriate to limit treatment | 300 (6.3) | 158 (6.6) | 142 (5.9) | 164 (6.8) | 79 (6.6) | 85 (7.1) | 136 (5.7) | 79 (6.6) | 57 (4.7) |
DNACPR already in place prior to admission | 142 (3.0) | 73 (3.0) | 69 (2.9) | 29 (1.2) | 15 (1.3) | 14 (1.2) | 113 (4.7) | 58 (4.8) | 55 (4.6) |
(Sub)total of above three categoriesd | 763 (16.0) | 421 (17.5) | 342 (14.2) | 329 (13.7) | 175 (14.6) | 154 (12.8) | 434 (18.1) | 246 (20.6) | 188 (15.6) |
Missing | 35 (0.7) | 17 (0.7) | 18 (0.7) | 15 (0.6) | 9 (0.8) | 6 (0.5) | 20 (0.8) | 8 (0.7) | 12 (1.0) |
Palliative care decision appropriateness (of those discussed) | |||||||||
Yes, appropriate decision | 318 (99.1) | 189 (99.5) | 129 (98.5) | 135 (99.3) | 81 (100) | 54 (98.2) | 183 (98.9) | 108 (99.1) | 75 (98.7) |
Yes, but patient might have benefited from escalation | 2 (0.6) | 0 (0) | 2 (1.5) | 1 (0.7) | 0 (0) | 1 (1.8) | 1 (0.5) | 0 (0) | 1 (1.3) |
Missing | 1 (0.3) | 1 (0.5) | 0 (0) | 0 (0) | 0 (0) | 0 (0) | 1 (0.5) | 1 (0.9) | 0 (0) |
Palliative care referral | |||||||||
No | 4613 (96.1) | 2289 (95.6) | 2324 (96.6) | 2317 (96.5) | 1149 (95.8) | 1168 (97.3) | 2296 (95.7) | 1140 (95.3) | 1156 (96) |
Yes | 104 (2.2) | 62 (2.6) | 42 (1.7) | 46 (1.9) | 29 (2.4) | 17 (1.4) | 58 (2.4) | 33 (2.8) | 25 (2.1) |
Missing | 83 (1.7) | 44 (1.8) | 39 (1.6) | 37 (1.5) | 21 (1.8) | 16 (1.3) | 46 (1.9) | 23 (1.9) | 23 (1.9) |
Admission avoidable | |||||||||
No | 3493 (72.8) | 1787 (74.6) | 1706 (70.9) | 1769 (73.7) | 896 (74.7) | 873 (72.7) | 1724 (71.8) | 891 (74.5) | 833 (69.2) |
Possibly | 939 (19.6) | 434 (18.1) | 505 (21.0) | 463 (19.3) | 218 (18.2) | 245 (20.4) | 476 (19.8) | 216 (18.1) | 260 (21.6) |
Yes | 335 (7.0) | 156 (6.5) | 179 (7.4) | 156 (6.5) | 76 (6.3) | 80 (6.7) | 179 (7.5) | 80 (6.7) | 99 (8.2) |
Missing | 33 (0.7) | 18 (0.8) | 15 (0.6) | 12 (0.5) | 9 (0.8) | 3 (0.2) | 21 (0.9) | 9 (0.8) | 12 (1) |
Pre admission
The majority of patients were admitted from home. Weekend admissions were substantially more likely to be classed as dependent on others for activities of daily living (weekend 11.3% vs. weekday 8.6%) and to have been brought to hospital by ambulance following an emergency (999) call (52.3% vs. 41.9%), and were less likely to have been referred by a GP (8.1% vs. 19.6%) or to have been admitted directly to an acute ward, bypassing the ED (7.9% vs. 15.2%). These weekend–weekday differences were more marked for epoch 2 than for epoch 1. Weekend admissions were more likely to include patients for whom a palliative care decision was already in place, had been applied at the time of admission, or, in the opinion of the reviewer, should definitely have been made (17.6% vs. 14.2%), with a marked increase between epochs (13.7% vs. 18.1%). The reviewers considered a smaller proportion of weekend than weekday admissions to be definitely avoidable (6.5% vs. 7.4%).
Post admission
The initial location of admission (usually the AMU) was considered by the reviewers to have been appropriate for the majority of admissions, regardless of the day of admission. The initial and subsequent documentation of vital signs and NEWS was more complete at weekends, and better in epoch 2 than in epoch 1. Documentation of an initial review of the patient by a consultant was marginally less likely for weekend (46.2%) than for weekday (47.9%) admissions, but increased during epoch 2 for weekend admissions (49.7%) to a level above that of weekday admissions, which remained constant. Consultant review within 14 hours of admission was identified in 22.9% (weekend) and 24.9% (weekday) of case reviews for epoch 1, increasing in epoch 2 more markedly for weekend than for weekday admissions (29.5% vs. 27.2%, respectively). Combining ‘documented’ with ‘probable’ consultant reviews within 24 hours of admission indicated that this had occurred in 54.2% of cases overall.
Implementing 7-day standards: challenges and facilitators
Round 2 of the qualitative research involved exploring board-level and front-line staff’s views on implementing 7-day standards and services, and conducting focused observations. Here we report the findings from interviews with 43 front-line and executive-level staff in eight sites (Table 14).
Site | Board level (n) | Consultants (n) | Senior nursing (n) | Rota co-ordinators (n) | Total (n) |
---|---|---|---|---|---|
3 | 2 | 3 | 1 | 6 | |
7 | 2 | 2 | 1 | 1 | 6 |
10 | 1 | 3 | 4 | ||
11 | 2 | 2 | 1 | 1 | 6 |
12 | 1 | 2 | 1 | 4 | |
16 | 1 | 3 | 4 | ||
17 | 1 | 2 | 1 | 2 | 6 |
18 | 2 | 2 | 1 | 2 | 7 |
Total | 12 | 19 | 5 | 7 | 43 |
Progress in implementing 7-day standards
Interviewees in each site concentrated on discussing the extent to which they believed they were meeting the four priority standards. These were:
-
standard 2 – EAs assessed by a consultant within 14 hours
-
standard 5 – timely access to diagnostic services according to urgency
-
standard 6 – access to consultant-directed interventions 24/7
-
standard 8 – consultant review of patients in acute medical, surgical and intensive care units twice daily by consultants working blocks of days; daily consultant review of ward patients.
Trusts’ performances on meeting the four quality standards (based on national audit data)16 are shown in Table 15.
Site | Weekend results (March 2017) (%) | Weekend results (March 2018) (%) | ||||||
---|---|---|---|---|---|---|---|---|
Standard 2 | Standard 5 | Standard 6 | Standard 8 | Standard 2 | Standard 5 | Standard 6 | Standard 8 | |
3 | 79 | 100 | 89 | 62 | 86 | 87 | 89 | N/A |
7 | 64 | 82 | 89 | 60 | 94 | 87 | 89 | 79 |
10 | 49 | 100 | 89 | 53 | 78 | 95 | 100 | 77 |
11 | 87 | 87 | 100 | 92 | 93 | 100 | 100 | 94 |
12 | 55 | 100 | 100 | 63 | 83 | 100 | 100 | 79 |
16 | 81 | 100 | 100 | 97 | 89 | 100 | 100 | 85 |
17 | 74 | 68 | 78 | 69 | 83 | 73 | 100 | 77 |
18 | 68 | 100 | 100 | 83 | 66 | 100 | 100 | 83 |
Although most participants believed that they had made some progress in delivering 7-day standards, many recognised that they still had much more that they wanted to achieve and acknowledged the difficulty of delivering the standards with their existing resources. Implementing the standards was challenged by questions about the legitimacy of the standards. Contextual and resource issues affected the extent to which sites could deliver the standards. These included organisational instability such as mergers or changes of leadership. Where sites were struggling with financial and quality pressures, the need to address weekend working remained secondary to improving their overall quality indicators.
Challenges in implementation
Implementing standards was challenged by four concerns: the legitimacy of focusing mainly on increasing consultant intensity at weekends; the evidence that this would in fact reduce mortality; the risk of reducing safety during the week by changing working patterns; and the need to improve services across the board as opposed to focusing primarily on the weekend:
We need to rethink what it is that 7-day process is going to bring us, and what is it that we’re trying to achieve . . . If [condition-specific mortality] goes down from 1.2% to 1.1%, is that worth the investment and the issues that would arise? I don’t know the answer, I definitely ask the question.
03INT01
The implementation of 7-day services most often involved changing the working patterns of existing staff groups. Engagement across the staff body within organisations was sometimes seen as variable. Certain staff groups or ‘clans’ had their own ways of working and could be seen as harder to engage, particularly those who were used to working in an individual and autonomous way:
Actually . . . talking particularly about doctors I think there are some clans if you like or big groups of doctors that are much less likely to embrace change and [. . .] are much more likely to want to carry on doing things their own way as they see fit, which is the way that they, they’ve done it.
11IV03
Different staff groups with differing experiences had different values when it came to working weekends. Some consultants, for example, were seen as reluctant to take on weekend working, having already ‘paid their dues’ as a junior.
The most debilitating constraint on implementing 7-day services was a lack of staff and the ability to pay for them. This was particularly the case for two sites in ‘special measures’, one with a large financial deficit and the other with both financial and quality issues. Both sites had real problems in recruiting and retaining staff and, thus, in delivering a 7-day service. Justifying the costs to commissioners of employing extra consultants to facilitate patient flow was also difficult. This left little room for proactive efforts to implement 7-day services:
I get the impression that it is not so much working towards a real 7-day service as an effort to keep patients safe with the bare minimum staffing levels because, as I am sure you are aware, staffing recruitment, retention is a huge issue with the trust and . . . it is unrealistic to I think that at the current situation, it is unrealistic to expect a genuine 7-day service.
17IV04
One organisation (site 10) had lost middle-grade trainees after they had been reallocated to one of the other hospitals in the trust. This meant that the site had to use its consultants differently to cover the out-of-hours rotas. The lack of middle-grade doctors was seen as particularly problematic for some sites aiming to make optimal use of their consultant hours:
I think the other thing that’s hitting everyone and us included is of course the situation in relation to juniors gaps because so, gaps in middle-grade rota, particularly in middle-grade rotas during out-of-hours periods as a result of some posts being unfilled, again this is a national problem. And of course that has a knock-on effect on consultants . . . If the consultant ends up doing a resident shift then clearly that will compromise their work the next day.
12IV01
All of the sites had difficulty in ensuring patient flow, and, for some, this was seen as the main area of weakness in their ability to provide high-quality care at weekends. Community and social work services were typically unavailable over the weekend and, as a result, consultant-led ward rounds were not felt to be needed at the weekend. Indeed, even others who were performing relatively well on meeting the standards commented that much of the inability to deliver 7-day standards and services was owing to the lack of support from social and community services. The problem of providing good-quality 7-day services often lay beyond their control:
Theoretically we could [conduct geriatrician ward rounds at the weekend]. But the reality is that social services don’t work on the weekends. And nursing homes don’t do assessments on the weekends, so actually there’s no point. There are services that we don’t run 24/7 because there’s no point, because you’d just be paying people to come in and say nothing’s changed since Friday.
18IV04
Sites that considered themselves to have embraced the 7-day standards described strong leadership as playing a key role in overcoming resistance to changing ways of working. The way that staff were communicated with was considered crucial to encouraging their investment in delivering 7-day services. Indeed, an organisation appearing to listen to its front-line staff was fundamental in encouraging a perception of unity and willingness to work together to achieve weekend working. Taking time to encourage and explain helped to gain staff participation in weekend working, particularly among specialisms:
There was quite a big change to get to 7-day working, and the kind of the 12-hour shifts, and actually, it was a strong leader who did bring people together and . . . Because they were tight, quite a tight group of clinicians, brought them on board so that they did it.
07IV01
Management support and investment, whether in time or in additional staff, was critical to creating the conditions for implementing external standards:
They’ve invested in the acute services quite heavily in the past 4 [or] 5 years, because we’ve gone from four to nine consultants, we’ve gone from four- to six-bedded area, to additional space for ambulatory growth and for this assessment area if you like.
03IV01
Ultimately, however, sites that were more receptive to change were those that had the greatest ‘organisational slack’; they were more likely to have a history of higher performance and to be facing fewer resource and infrastructure challenges. 122
Discussion
We have adopted the relatively novel approach in mixed-methods evaluation of presenting qualitative themed assessments (the RAG rating of progress towards 7-day standards; see Table 9) in a form that has allowed comparison with a completely independent set of assessments from the case record review. The RAG rating was developed through facilitated discussion between the qualitative researchers, who were blinded to the outputs of the case record review. This binocular approach strengthens our conclusions.
Our analysis of the role of consultants at weekends highlighted the mechanisms through which senior specialist input to patient care could contribute to optimising the quality of service delivery at weekends. Consultants have overall responsibility for the diagnosis and treatment of individual patients, conducting or organising patient reviews, and organising and allocating tasks to junior doctors and other members of their service’s teams. They have organisational oversight, contributing to maintaining momentum, and facilitating discharge, helping to maintain patient flow. They also play a crucial scaffolding role, supervising and supporting junior staff. Our analysis also identified, however, that the impact of consultant input to care at weekends was limited when there was a lack of a supportive infrastructure to ensure decisions could be actioned, where key in-hospital services were not available, and when lack of services in the community slowed discharge-planning.
Weekends in hospital differ from weekdays in terms of available resources, care processes and access to expertise, but there is also wide variation between hospitals, with some having developed innovative ways of closing the weekend–weekday gap. The gap seems to affect already-hospitalised patients more than those presenting acutely. At weekends, EAs may even receive more timely care than on weekdays. It is of interest that the qualitative researchers’ assessments in the workplace of weekend care quality should be associated with the global quality judgements of the case record reviewers: a shared perspective emerging from two distinct and independent sources of information.
In terms of care quality, we find no evidence that the quality of in-hospital medical care of patients undergoing EA is lower at weekends than on weekdays. However, we do find strong evidence of a temporal trend towards improved hospital care between epochs: there was a statistically significant improvement in error rates, error-related adverse events and global quality of care assessments. The error-related adverse event rate of 2.5% compares favourably with a recent systematic review finding of 6% across 70 studies between 2000 and 2018. 123 Our findings triangulate well with improvements in in-hospital processes of care between epochs, such as documentation of initial consultant review and recording of NEWS. They are also aligned with the ‘rising tide’ phenomenon of secular improvements in care processes, such as those identified by the evaluation of the Safer Patients Initiative in the previous decade. 124,125
Seven-day services is clearly a complex intervention. Trusts varied in their approaches to introducing this change in service provision. Commitment-orientated approaches and a collaborative culture would seem to be advantageous. The case record review shows a reduction in error rates between epochs, and particularly in those forms of error that are most influenced by doctors: assessment, diagnosis, treatment, prescribing and communication. It seems possible that this may be a consequence of more timely consultant reviews across all days of the week, not just at weekends.
The improvement of in-hospital care processes are in marked contrast to the pre-hospital data, which indicate deficiencies in the provision of community services at weekends, services which have deteriorated with time. The qualitative researchers also identified the interface between hospital and community services, and the decrement in provision of community services at weekends, as distinctly problematic. Compared with weekday admissions, patients admitted at weekends were more likely to be dependent, have a palliative care decision in place and arrive by ambulance to the ED; they were much less likely to have been reviewed and referred by a GP in the community, as would usually be the case. All of these indicators had deteriorated further by epoch 2 (2016–17). These findings are consistent with those of other studies showing that weekend admissions from the community are sicker than those admitted on weekdays56,57 and there are fewer GP referrals at weekends;57,106 and with the documented reduction in social care funding despite increasing demand. 126
Case record review lacks precision when using a single review of a single record by an expert reviewer. 127 Consensus-based joint reviews create an illusory improvement in reliability. 128 Averaging across multiple reviews improves reliability;129 the approach we have adopted, with 4000 case records in total and 4763 usable reviews covering 20 trusts in two epochs, has led to one of the largest reviews undertaken, and distinguishes reliably between trusts (Spearman–Brown coefficients 0.8–0.9). Our conclusions are enhanced by triangulation with qualitative research (involving interviews, and observations employing elements of an ethnographic approach) and employing a difference-in-difference analysis that minimises confounding from variation in case mix. Our analysis of the implementation of 7-day standards identifies the challenges to and facilitators of increasing specialist intensity.
In summary, we describe the role of consultants in providing quality of service delivery in hospitals at weekends, and highlight how the impact of consultants on quality of care is moderated by the availability of supportive infrastructure and resources. Essential pre-conditions for improvement include the perceived legitimacy of the standards, leadership and organisational ‘slack’. However, we find no evidence to support the contention that the weekend effect is attributable to worse in-hospital care at weekends. In-hospital care improved between 2012/13 and 2016/17, and, at trust level, qualitative researchers’ findings on weekend care quality concurred with the case record reviewers’ judgements of global quality. By contrast, we find evidence that community care performs less well at weekends, has deteriorated between epochs and could be the source of the weekend effect. Standards to improve weekend care had a mixed reception in practice and there were significant challenges to implementation, including resource constraints and a lack of focus on improving community services provision. Policy-makers should focus their efforts to improve acute and emergency care on a ‘whole-system’ 7-day approach that integrates social, community and secondary health-care organisation and delivery.
The final stage in the HiSLAC programme was to determine whether or not increasing consultant intensity at weekends would be cost-effective. We address this issue in the next chapter.
Chapter 7 Health economics evaluation of increasing the weekend-to-weekday specialist intensity ratio in hospitals in England
Introduction
In 2013, NHS England published The NHS Belongs to the People: A Call to Action,130 in which it called for a public debate on how to develop new ways of working in the health service to respond to the financial crisis, given a funding gap of around £30B between projected spending requirements and resources available between 2013/14 and 2020/21. In the event, the government’s 2015 spending review indicated that additional funding for the NHS, part of which was intended to facilitate the introduction of 7-day services, would have to be accompanied by savings of £22B, suggesting that trusts were likely to be expected to find at least some of the funding for 7-day services from existing resources. 131 However, the true cost (including opportunity costs) of implementing 7-day services was unknown, and the potential benefits are uncertain and unquantified. The cost of training a doctor to the level of consultant was estimated to be £510,411 in 2016, with an annual basic salary of £89,046 and cost per hour of £105. 132 Given the emphasis in the 7-day service standards on expanding consultant input at weekends, it was clear that a major cost component for individual trusts to consider would be doctors’ salaries. The HiSLAC study provided an opportunity to examine the cost-effectiveness of this element of 7-day services on the quality of emergency care.
Economic evaluation of medical products and technologies is standard practice in developed health-care systems,133,134 generally extrapolating effectiveness estimates from randomised controlled trials to generic health end points, such as QALYs. Historically, less attention has been paid to service delivery interventions affecting staffing and system organisation, although this is now starting to change. 135 Service delivery interventions are complex, act further upstream of the patient than drugs or devices, and may have diffuse ‘downstream’ effects. They therefore require large sample sizes for accurate estimation and pose potential difficulties for causal interpretation. 136
The level of specialist intensity is a good example of a complex service delivery intervention acting through multiple causal pathways. It has both direct and indirect effects, that is acting through a mediating variable or not, respectively. 137 Specialist presence affects, for example, both the acute phase of stabilisation, diagnosis and treatment at admission, and the subsequent risk of experiencing preventable harm. Indeed, it was this mechanism that motivated the policy. As discussed in Chapter 1, the observed increased risk of mortality among weekend admissions was most frequently assumed to be a consequence of a reduction in care quality. However, the level of specialist presence is also likely to be correlated with the health of the patient population. 106 A single regression model of mortality on day of admission, as much of the weekend effect literature is based on, cannot separate these two effects, a problem discussed widely at the time. 138 However, a structural equation modelling approach can be used to specify models based on causal hypotheses of the relationships between observed and latent variables. 139–141
In this chapter, we present results from a cost–benefit analysis of a policy of changing the specialist-to-patient ratio, based on a structural equation modelling method. Our objective in using this approach was to develop, compare and estimate models that accounted for the different possible pathways through which specialists might affect the relevant outcomes. By jointly estimating models from across the causal pathway we could separate out the effects of unobserved patient health from those needed for cost–benefit analysis, subject to some key assumptions. We took a Bayesian approach to model specification, estimation and interpretation, which permits the incorporation of prior information and knowledge, typically affords the estimation of more complex joint and hierarchical models, and naturally fits into a decision-making framework.
Aim
The aim was to determine whether or not implementing a policy of increasing specialist intensity at weekends to the level observed in ‘high-intensity’ hospitals is cost-effective.
Objectives
-
Develop candidate qualitative causal models.
-
Estimate and compare structural equation models derived from the causal models.
-
Estimate the expected net benefits of increasing specialist intensity.
-
Determine the probability that net benefits are positive at different cost-effectiveness thresholds.
Methods
Data
Quality of care was assessed from the case record review (see Chapter 6, Results). For each case record, a binary indicator was derived for whether or not each patient experienced each of the nine different types of error, and whether or not they were judged to have experienced an adverse event. Age, sex and mode of arrival at the hospital were also extracted. Mode of arrival has been previously shown to be an important indicator of patient severity, particularly in studies of the weekend effect. 142 Where case records were reviewed twice, we randomly selected one review for inclusion. Overall, 4000 patients were included in the data set (as opposed to all 4800 reviews in Chapter 6). Mortality and length of stay for each patient were extracted from the HES database for each patient. Information on specialist intensity and change over time was taken from the HiSLAC annual PPSs (2013/14 to 2017/18) (see Chapter 4). The level of specialist intensity for the appropriate epoch, day of admission and hospital were matched to each patient.
Cost–benefit analysis
The ultimate outcome for this study was the expected net benefits of shifting from a low level of specialist intensity to a high level (‘high’ and ‘low’ were defined as the weekday and weekend averages, respectively). The expected net benefits are the change in benefits to patients resulting from this shift, measured in QALYs and valued at the societal willingness to pay per QALY, minus the change in costs. If the expected net benefits are positive, then this indicates that the policy generates more benefit in monetary terms than it costs and would be considered cost-effective.
Patient QALYs were not captured by our data, so we specify a distribution of possible QALY losses associated with the outcomes we do observe, namely adverse events and mortality, following the approach of Yao et al. 143 Adverse events were divided into different severity categories based on a common classification. 144 We used published data identified by systematic review to specify a distribution for the proportion of adverse events falling within each category, as well as for their duration and severity. 145–147 The results of this exercise are shown in Table 16.
Category | Non-fatal, mean (95% CrI) | Fatal, mean (95% CrI) | ||
---|---|---|---|---|
Minimal harm | Temporary disability | Permanent disability | Death | |
Probability | 0.41 (0.37 to 0.44) | 0.46 (0.43 to 0.49) | 0.10 (0.09 to 0.12) | 0.03 (0.03 to 0.04) |
Incremental QALY loss | 0.00 (0.00 to 0.00) | 0.04 (0.00 to 0.22) | 0.51 (0.06 to 1.65) | 3.61 (0.42 to 11.93) |
Counterfactual life expectancies were required to estimate the duration of life lost associated with a fatal adverse event. One study was identified that provided estimates of these counterfactuals. 148 The same distribution was used for permanent disability as the effects would be expected to last for the remainder of an individual’s life. For temporary disability, in the absence of further information, we specified a distribution with a median of 3 months and with 95% of patients recovered by 1 year. For minimal harm, we specified that the duration is uniformly distributed between 1 day and 1 month. The quality-of-life value associated with each year lost associated with mortality was equivalent to reported quality-of-life values at the age the patient would have otherwise attained, which we estimated from Ham. 149 A sample weighted by these age groups was taken from the Health Survey for England150 for the years in which the survey reported EQ-5D-derived health-related quality-of-life weights (1996, 2003–6, and 2008). The mean (SD) quality-of-life weight was 0.64 (0.25). Quality-of-life weights for non-fatal adverse events were based on evidence from studies of representative conditions.
For the cost side of the equation, there were both direct and indirect costs to consider. The direct cost is the additional expenditure required to employ specialists at a ‘high’ level. To specify a distribution for this, we considered the pay scales (approximately £80,000–110,000), clinical excellence award levels (approximately £3000–80,000) and superannuation costs (21%) for specialists in NHS England. 151 There is no information in the public domain about the distribution of specialists across pay scales or clinical excellence awards. We therefore take the top end of the pay scale (with superannuation costs) as a mean point, and allow variation to cover the range of possible pay and awards. Based on a whole-time equivalent of 40 hours per week for 46 weeks per year, we specified that an additional hour of specialist presence has a mean cost of £77 and SD of £10. The indirect cost is the change in the cost of treatment due to an adverse event. We based this on any estimated change in length of stay due to an adverse event multiplied by the mean cost of a non-elective excess bed-day reported in the most recent publication of the NHS 2017/18 Reference Costs,152 which was £373.
Model development
To support the development of the model, an expert workshop and focus group was convened initially to discuss the mechanisms by which increasing the intensity of specialist input at weekends might affect patient outcomes. Based on this discussion and on previous literature, a candidate set of three models of increasing complexity was developed (Figure 23). All of the models included the causal effect of specialists on the risk of experiencing each of the nine types of error and a direct effect on length of stay due to early or delayed discharge. Model 1 has these two pathways only, so that the only mechanism by which specialists could influence adverse events and mortality is by affecting the risk of an error. Unobserved patient health was also included, which had an effect on the risk of mortality and overall length of stay only. However, model 1 may be unrealistic, sicker patients may be taken to hospitals with greater specialist presence or, conversely, less severely ill patients may not be admitted when specialist levels are lower. Model 2, therefore, extends model 1 by allowing for a relationship between the specialist-to-patient ratio and patient health. So, for example, an increase in specialist presence could affect length of stay through three mechanisms: changing the risk of errors, changing the risk of delayed discharge or correlating with the health of the patient population. Model 3 adds an additional extension by allowing for a relationship between patient health and the risk of each type of error and the risk of experiencing an adverse event. More severely ill patients may be at higher risk of experiencing an error as they are exposed to a greater number of interventions and have more complex conditions.
FIGURE 23.
Three candidate models.
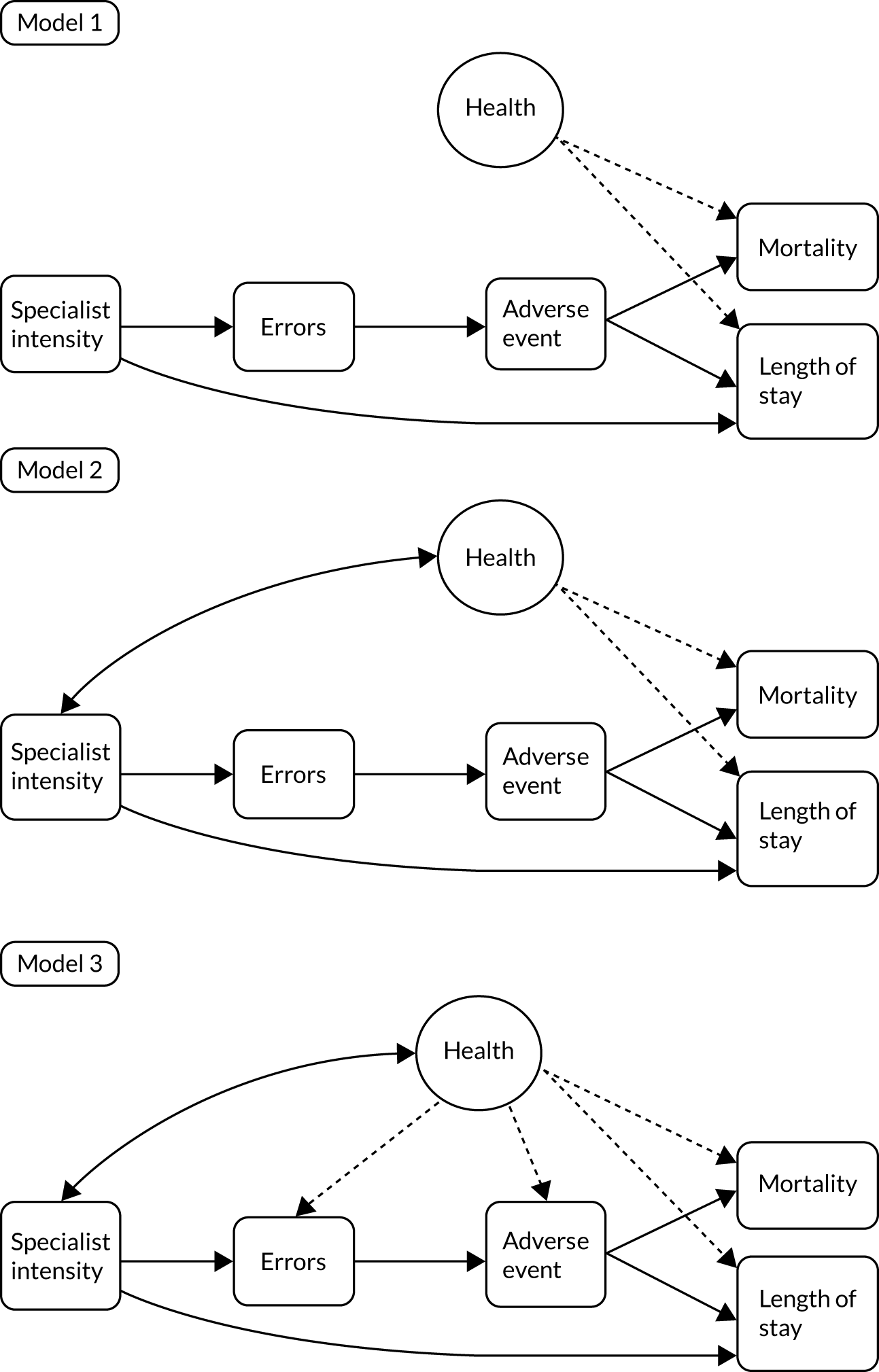
Structural equation model
We used a Bayesian approach to model design, specification and estimation. A Bayesian statistical model has two components: a model for the data – the likelihood – and prior distributions of the model parameters. The posterior distribution of the model parameters, which are used for inference, is directly proportional to the product of the likelihood and prior distribution. The likelihood here is a structural equation model and the qualitative causal models can be used to specify the structural equation model. A structural equation model can be considered a joint regression model for a number of different outcomes with some shared parameters or latent variables. For example, in the models above, the same latent health term, α, would appear in models for outcomes including mortality and length of stay. For each outcome, we can specify what goes into its constituent part of the joint model by examining all of the other variables whose arrows point into it. In model 1, for example, the model for mortality has an indicator for adverse events and patient health.
For dichotomous outcomes (mortality, adverse event, error of each type), we specified binomial-logistic models; we used a Poisson regression model for length of stay and a linear model for specialist intensity. Where health appears in a model, we included patient sex, age, age squared and an indicator of whether or not the mode of arrival was by ambulance. We also included the hospital- and individual-level random effects (i.e. latent health effects). To allow for differing effects of the latent health variables in each model, these were multiplied by a model-specific parameter, typically known as ‘factor loadings’. In addition, as the hospital-level effects may change over time, we allowed for the random effect to be different but correlated in each epoch; this was specified as an auto-regressive relationship (of order 1).
The relationship between specialist intensity and either the risk of the various errors or length of stay was unlikely to be linear. For example, it is possible that there is a diminishing marginal benefit to additional specialists. However, there was little prior evidence on the ‘shape’ of this relationship. A flexible, non-parametric approach was therefore used for specialist intensity, which let the data dictate the shape of the function relating specialist intensity to each outcome. In particular, we used natural cubic splines. To ensure that this function was smooth (i.e. that it did not vary dramatically for small changes in intensity), we limited the degrees of freedom to 3. Internal knots were placed at the upper and lower thirds of the range of intensity.
Prior distributions
Prior distributions are used to capture knowledge about model parameters and quantify what values are plausible. For example, we know that it is completely implausible for the risk of death to be 10-fold higher among women than among men or vice versa. It is highly likely that any difference in risk between men and women will be less than 20 percentage points. The more precise the prior distributions, the more influence they have over the posterior distributions and, hence, our inferences about parameter values. We wanted to specify prior distributions that ruled out extreme, implausible values but had little influence on the posterior distributions within the plausible range to let the data ‘do the talking’. These are sometimes referred to as ‘weakly informative’ prior distributions. 153 They also facilitate computation and model estimation, compared with so-called non-informative prior distributions that allow for extreme and implausible values.
The splines used to flexibly model the effect of specialist intensity have two parameters that dictate their shape. However, little prior information exists on the effect of specialist presence on patient safety outcomes, although recent evidence suggests that it may be negligible. 154 Our priors for the effect of specialist intensity are partially informed by an expert elicitation exercise that we conducted on 20 September 2018 with 19 specialists in acute medicine to ascertain expert beliefs about the magnitude of the effect of shifting specialist intensity. The elicited beliefs do not map directly onto model parameters for the effect of specialist intensity as the parameters of the spline function do not have any ‘natural’ interpretation. However, the elicited information did inform us that any changes in specialist intensity are unlikely to be associated with large changes in the risk of errors or changes to length of stay. For example, the expert panel expected the effect of doubling specialist intensity from weekend to weekday averages to lead to a 25% (95% CrI 10% to 40%) reduction in the risk of a diagnostic error and a 5% (95% CrI –5% to 15%) reduction in length of stay. On this basis, we specify standard normal priors for the spline parameters, which rule out large shifts in risk for small changes in specialist intensity but allow for a range of functional shapes, including increases, decreases and no change in risk. For the remaining model parameters, we adopted weakly informative N(0, 5) prior distributions and, for the SDs of random-effect terms, we specified an inverse-gamma distribution with shape and rate parameters of 1.
Model comparisons
Three candidate structural equation models were specified from the qualitative models. Although the more complex model might be more realistic, it might be too complex and have too many parameters for the data to tell us anything meaningful about their values. A very complex model with a large number of parameters may be able to successfully replicate the data used to estimate it, given the flexibility afforded by the sheer number of parameters, but be very poor at predicting new data. This problem is sometimes referred to as ‘overfitting’. We therefore compare the models using two statistics. First, the widely applicable information criterion assesses how closely predictions from a fitted model align with the data, but adds a penalty term for the number of parameters to avoid overfitting. Second, the leave-one-out cross-validation score examines the out-of-sample predictive ability of the model by determining how well the model would predict each data point if it were estimated with all of the remaining data (hence ‘leave-one-out’). In the protocol we stated that we would use posterior predictive model checks; however, these checks are poor at detecting overfitting and so were considered to be a bad choice for the required model comparison.
Expected net benefits
From the best-fitting model, we determined the estimated change in adverse events, mortality and length of stay associated with a change in specialist intensity, while adjusting for unobserved patient health. On this basis, we calculated the expected net benefits. The expected net benefits should be interpreted as being for a fixed patient population, that is what might happen if the level of specialist intensity were changed but there was no change in the patients admitted to a hospital. We considered the expected net benefits of, first, increasing mean weekend specialist intensity levels to the weekday level and, second, increasing specialist intensity incrementally to identify potential minimum and optimum levels.
Results
Summary statistics
Using data from the PPS, Figure 24 shows the levels of specialist intensity at the participating sites on Sundays and Wednesdays in the two epochs. Although the majority of sites were estimated to have increased their level of specialist presence at the weekend, most also reduced their specialist intensity on a weekday. Across the 20 trusts, the mean level of specialist intensity at the weekend increased from 25.1 hours per 10 EAs to 27.7 hours, but on weekdays intensity declined (52.7 and 49.8 hours per 10 EAs, respectively). As we have shown (see Chapter 4), the reduction in weekday intensity is explained partly by a disproportionately greater increase in Wednesday EAs than in Sunday admissions between the two epochs, but it is likely that specialists have transferred a proportion of their weekday work to weekends.
FIGURE 24.
Mean specialist intensity by trust and epoch, hours per 10 EAs.
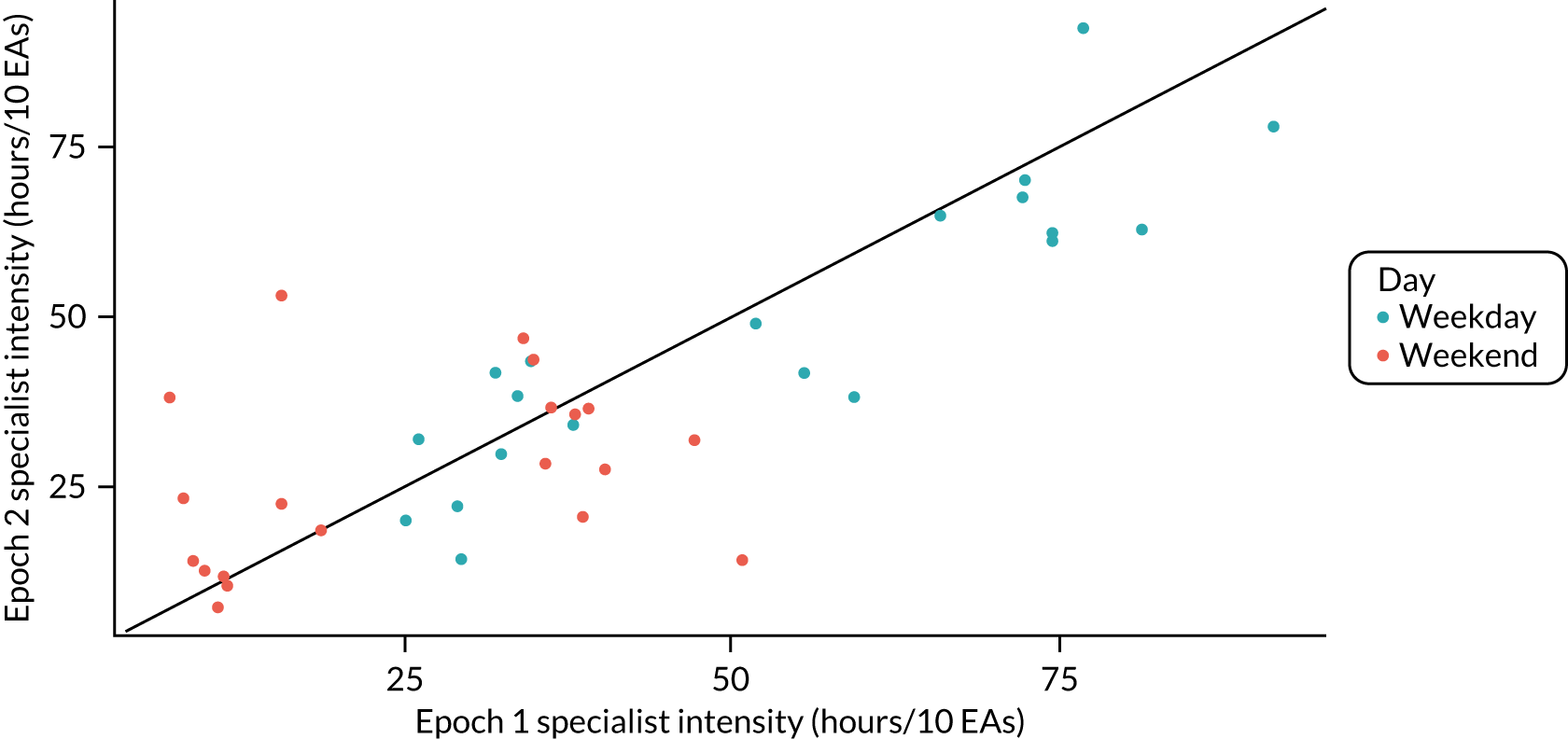
Summary statistics of the sample of 4000 admissions by epoch and day of admission are shown in Table 17. There were some changes in the patient population over the two epochs. The proportion of patients arriving by ambulance declined at weekends and on weekdays over time, although it remained relatively larger at the weekend. The proportion of patients experiencing almost all types of error also declined over time; there was no clear pattern showing that the number of errors or adverse events were consistently larger for weekend or weekday admissions. Mortality among weekend admissions was higher than that among weekday admissions in both epochs.
Epoch 1 | Epoch 2 | |||
---|---|---|---|---|
Weekend | Weekday | Weekend | Weekday | |
Hospital level | ||||
n | 20 | 20 | 20 | 20 |
Specialists (hours/10 EAs) | 25.5 (15.0) | 52.7 (22.0) | 26.9 (13.2) | 48.4 (20.7) |
Individual level | ||||
n | 993 | 996 | 992 | 988 |
Age (years), mean (SD) | 60.5 (22.5) | 60.7 (22.2) | 62.8 (22.2) | 60.4 (22.3) |
Female (%) | 53.8 | 51.3 | 52.4 | 55.6 |
Arrival by ambulance (%) | 55.7 | 44.9 | 49.0 | 39.8 |
Communication error (%) | 6.8 | 8.3 | 6.9 | 5.7 |
CPR error (%) | 1 | 0.5 | 0.9 | 0.4 |
Diagnostic error (%) | 15.7 | 16.3 | 12.2 | 11.9 |
Infection error (%) | 0.3 | 0.4 | 0.8 | 0.8 |
Invasive procedure error (%) | 0.9 | 0.6 | 0.5 | 0.3 |
Medication error (%) | 8 | 7.4 | 5.4 | 5.9 |
Monitoring error (%) | 2.7 | 2.9 | 2.1 | 2.3 |
Other error (%) | 2 | 1.7 | 1.1 | 1 |
Treatment or management error (%) | 14.1 | 14.9 | 11.3 | 10.6 |
Adverse event (%) | 3.2 | 3.5 | 2.2 | 1.5 |
In-hospital mortality (%) | 4.9 | 4.2 | 4.1 | 3.6 |
Length of stay, median (IQR) | 2 (1–5) | 2 (0–5) | 2 (0–5) | 1 (0–4) |
We can examine the relationship between specialist intensity and markers of patient health to support or refute different models. Figure 25 shows the association between specialist intensity and patient age and the proportion of patients arriving by ambulance. There is evidently a relationship, which would initially refute model 1, as it provides evidence that patient health is associated with level of specialist intensity.
FIGURE 25.
(a) Relationship between specialist intensity and patient age (b) and the proportion of patients arriving by ambulance.


Model comparisons
Model 3 was the preferred model under both comparison statistics. Model 2 was a large improvement over model 1, and model 3 was a (smaller) improvement over model 2. Figure 25 shows the estimated adjusted relationships between specialist intensity and the risk of each of the nine types of error from the three models. For models 1 and 2, which do not allow for patient health to affect the risk of error, there is little evidence of a relationship between specialist intensity and the risk of each type of error (see Appendix 19). For model 3, higher levels of specialist intensity are associated with a reduced risk of diagnostic, communication, medication, monitoring and management errors. For example, an increase in specialist intensity from 25 to 50 hours per 10 EAs is associated with a reduction in the odds of a diagnostic error of 26% (95% CrI 2% to 45%). These results suggest that there is a degree of confounding in models 1 and 2 as we expected; higher levels of specialist intensity are associated with both a lower risk of error and poorer health among patients, and poorer health is associated with a higher risk of error.
Little evidence of a relationship was seen between specialist intensity and the risk of experiencing an adverse event, and there was a large degree of uncertainty about any effects. The risk of an adverse event was very low (1.35%; see Table 17). Diagnostic errors were the most likely to lead to harm; the risk of an adverse event was approximately 10% with a diagnostic error, compared with the next most common error, treatment/management errors, which had a risk of < 1%. As a result, the doubling of specialist intensity from 25 to 50 hours per EAs was associated with a reduction in the number of patients experiencing adverse events only by approximately 2 in 1000. For the same reason, there was little evidence of a relationship between specialist intensity and mortality. Although experiencing an adverse event was associated with an increased risk of mortality in all models, given the relatively low risk of both mortality and adverse events, the effects of specialist intensity are very small. For the same doubling of specialists described above, we would expect five fewer deaths per 10,000 EAs on average.
Figure 26 shows the posterior predictive distribution of length of stay compared with specialist intensity. Both models 2 and 3, which allowed for latent health to be correlated with specialist intensity, mortality and length of stay, showed a shorter expected length of stay with higher levels of specialists. Indeed, the effects were, qualitatively, highly similar, although they were associated with wide CrIs. Most of the observed effect was from the direct effect of specialists rather than from a reduced risk of adverse events.
FIGURE 26.
Posterior predictive distribution of length of stay and specialist intensity: all models.
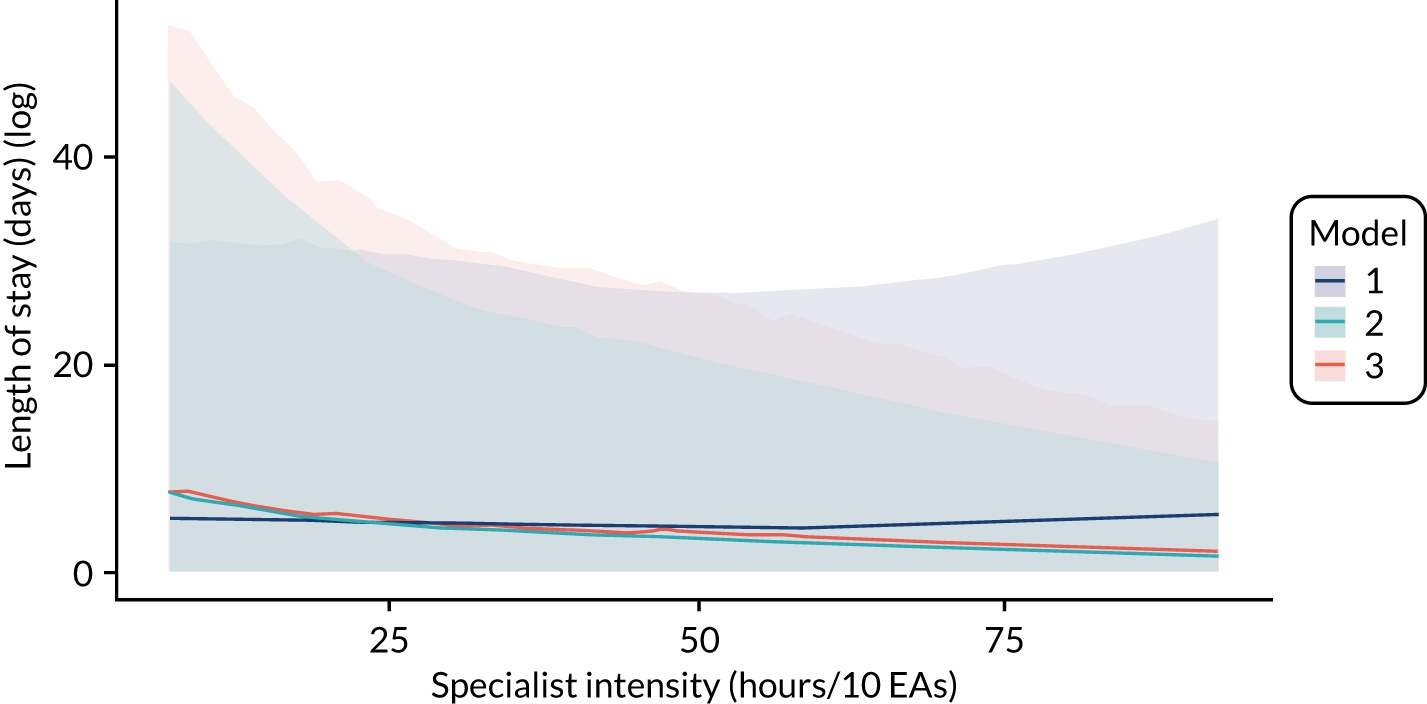
Cost–benefit analysis
Model 3 was selected as the best-fitting model and was therefore used for the cost–benefit analysis. As Figure 24 and Table 17 show, the 7-day services policy had little discernible effect on the level of specialist intensity in these 20 trusts. However, we can examine the expected net benefits of the policy, had it been implemented with greater fidelity, by predicting the outcomes from model 3 at a level of specialist intensity of 26.9 hours per 10 EAs (the weekend average) and 48.4 hours per 10 EAs (the weekday average). The posterior mean expected net benefit was £177, indicating that the policy is cost-effective at a willingness to pay per QALY of £20,000.
On average, the policy would cost £167 per EA, generate £5 per EA in QALYs from the reduced risk of an adverse event, and save £349 as a result of a reduced length of stay. Model 3 predicted that the length of stay would be reduced by an average of 0.9 days under this policy. Given that QALY gains made a relatively small contribution to the overall expected net benefits, the model was not affected by the choice of cost-effectiveness threshold.
To consider more generally the cost–benefit of increasing specialist intensity from this model, we next examined the effects of increasing specialist intensity incrementally from its lowest to highest observed values. Figure 27 shows the expected net benefits of increasing the level of specialists by 10 hours per 10 EAs. The expected net benefits were greater than zero across the range of specialist intensity. However, they were flat and close to zero above approximately 35 hours per 10 EAs, and the 95% CrI was fairly wide, with a substantial area below zero. Below 35 hours per 10 EAs, there is much stronger evidence that increasing specialist intensity is cost-effective.
FIGURE 27.
Posterior predictive distribution of expected net benefits of raising specialist intensity by 10 hours per 10 EAs with 95% CrI.

Discussion
As described above, one of the main aims of the 7-day services policy was to improve care quality for patients admitted to hospital at the weekend. However, the confounding of the relationship between specialist intensity and care quality by patient health status complicates the task of identifying the effect of the policy from observational data. Indeed, this is a general problem in the evaluation of service delivery interventions, which, by definition, have effects that are mediated by other services and have clinical processes that are diffuse but affect a large proportion of the patient population. 136 The policy was not implemented as designed, and so the expected within-hospital variation in specialist intensity that we planned to use to identify the effects of the policy was not present. However, significant variation in specialist intensity levels between hospitals and by day of admission was present, and this enabled us to examine the relationship of this variation and patient outcomes. Implicit, however, in these analyses is the assumption that any other changes in care quality by day of admission were constant across hospitals in each epoch, which may be a strong assumption.
For this study, we developed structural equation models for this type of evaluation, which enabled us to disentangle the different causal pathways. In doing so, our evidence supports the hypothesis that an increase in the level of specialist intensity is associated with reductions in the risk of errors and adverse events, which is confounded by patient health. However, the effect is small and certainly not large enough to justify the potential outlay required to implement the policy. This agrees with a number of previous studies and discussions that have been published based on cruder analyses. 29 A more significant benefit is estimated in the reduced length of stay associated with increases in specialist intensity, which is potentially large enough to make the policy cost-effective, particularly when specialist intensity is low.
We estimated that increasing specialist intensity at the weekend to weekday levels would reduce the average length of stay by almost a day, thereby significantly reducing the cost of patient care. The qualitative analysis (see Chapter 6) also identified delayed discharge as a key issue at the weekend that results from a lack of specialists. However, the system-level implications of reducing length of stay have not been explored in detail. Overall patient volume and bed occupancy would be unlikely to reduce, but throughput would increase. This would imply an improved efficiency of the health service and potentially improved patient experience as a result of less unnecessary time spent in the hospital, although we did not account for patient experience in our analysis here. Any improvement in care quality would also affect the patient experience, although we reiterate that significant adverse events were exceedingly rare. The types of error that the analysis suggested would be impacted by specialist presence also aligned with those identified in our focus group exercise, further validating the evidence.
There has been growing interest in the evaluation of service delivery interventions. 155,156 Watson and Lilford,156 Lilford et al. 136 and Watson et al. 157 have discussed the necessity of capturing and integrating data from across the causal chain to estimate policy relevant effects and conduct economic evaluations. The analyses in this chapter exemplify how this might be done in practice using a structural equation modelling approach. However, we caution that, in the absence of an experimental methodology, the results cannot necessarily be interpreted as causal effects. Even the more complex models are simplifications of the health system and may miss issues of confounding or reverse causality. However, as we discussed, the data become less and less informative about model parameters as complexity increases. A method of principled Bayesian analysis using qualitative model development and comparison aims to deal with this problem. 158 We believe that our analysis provides the most reliable evidence to date of the cost–benefit of increasing specialist intensity.
We reiterate the issues with the data discussed in previous chapters. Response rates to the PPS were variable and often low. The consequences of this could be significant in terms of bias if the response rate were systematically dependent on other key variables, such as patient health or specialist intensity. However, we do not have reason to believe that this is the case and the results have strong face validity. Although the inter-reviewer reliability of subjective case note review was low at the individual level (see Chapter 6, Case record review: results), it was high when aggregated at trust level and by epoch. By the same reasoning, we expect the ‘noise’ to cancel out in the aggregate. Our results here focus on average effects, and the effect of the high variation in case note review is to increase our uncertainty around these effects rather than to introduce any systematic bias.
Had the 7-day services policy been implemented as designed, the evidence presented in this chapter suggests a high probability that it would have been cost-effective. This may seem to contradict previous findings and discussions (e.g. Godlee,138 Meacock et al. 29), which determined that improvements in quality and reductions in mortality would be unlikely to justify the policy on cost-effectiveness grounds. We would agree with this assessment, but disagree with the overall conclusions, as through reductions in length of stay the policy would likely be justifiable. Thus, we would tentatively conclude that the 7-day services policy was right, but for the wrong reasons.
Chapter 8 Discussion
Overview
In this 5-year programme of research, we used quantitative and qualitative methodologies to investigate why the risk of death seems to be higher for patients admitted to hospitals at weekends than for those admitted during the week. The answer turns out to be complex. Contrary to our initial hypothesis, we find no evidence that this situation is related to deficiencies in hospital medical staffing. We do find circumstantial evidence that the causes may lie in the community, upstream of hospital admission. Importantly, we find that, although the overall quality of hospital care in general is not worse at weekends, and improved between 2013/14 and 2017/18, the limited measures available to us suggest that community care is worse at weekends and seems to have deteriorated with time.
The 7-day services policy was – and is – a commendable package of measures intended to improve the overall quality of care for acutely ill patients and EAs to hospital, but the use of the weekend effect as an argument to effect change was unhelpful. As the weekend effect has been reported for 20 years from most health systems worldwide, the cause or causes needed to be identified to inform policy, rather than merely attributing it to deficiencies in hospital medical staffing.
Determining causation in health services usually relies on interpreting associations, strengthened by the use of instrumental variables, where these can be identified. We took the opportunity to use the introduction of 7-day services as an (uncontrolled) intervention to test the hypothesis that increasing the availability of hospital specialists (consultants, attendings) at weekends would mitigate the weekend effect. As this was, in effect, a ‘natural experiment’, we wished to strengthen the interpretation of the findings using triangulation: looking for similar signals from different and independent sources. We also wanted to ensure that we had adequate representation from those on the front line, namely patients and staff. We therefore used qualitative methods in addition to quantitative measures. We also examined changes over time. By comparing secular changes in weekend and weekday differences within individual trusts, we were able to reduce confounding from between-trust comparisons, for example in case mix, or service delivery and organisation.
Summary of main findings
Here we summarise our main findings and then consider their implications.
Emergency admissions and post-hospital mortality rates are increasing (2007–18)
-
Emergency department attendances and hospital admissions have continued to increase each year, while the number of hospital beds has decreased.
-
Hospital mortality rates fell progressively between 2007/8 and 2013/14, but the rate of reduction slowed thereafter. The weekend admission mortality increased in 2017/18, but the adjusted weekend-to-weekday admission mortality ratio did not increase. The increase in crude mortality is, therefore, attributable to case-mix differences, probably more patients with multimorbidity (five or more health conditions) and palliative care admissions. This finding is consistent with work by The Health Foundation showing that elderly people (aged > 85 years) and those with multimorbidity account for a large proportion of the increase in acute admissions. 5
-
There has been a progressive widening of the gap between hospital mortality and 30-day mortality. It is possible that efforts to reverse the increase in delayed discharges from hospital could have resulted in the mortality risk being transferred from hospitals to the community. For example, other studies have shown that frail elderly people are particularly susceptible to adverse outcomes following hospital discharge. 159–163 Whether this change in location at death represents avoidable mortality from the premature discharge of frail patients or a desirable substitution of home care for those destined to die requires further research at the intersection of hospital and community care at the time of discharge.
Hospital specialist availability is not the cause of the weekend effect
-
Consultants are key clinical decision-makers from admission to discharge. Effective consultants contribute diagnostic accuracy and therapeutic specificity, provide visible on-site leadership for and support to junior doctors and other members of the multidisciplinary team, and promote timely hospital discharge.
-
The intensity of consultant input into the care of EAs at weekends across acute trusts in England is, on average, half that of weekdays.
-
The weekend-to-weekday specialist intensity difference has diminished (i.e. improved) in the last 2 years. This appears to be attributable to a modest increase in specialist hours throughout the 7 days, which has been masked by the proportionately greater increase in EAs, particularly on weekdays.
-
The difference in weekend-to-weekday specialist input is not associated with the difference in weekend-to-weekday admission mortality risk; the weekend effect is not linked to the availability of consultants at weekends.
-
There has not been a parallel increase in the numbers of non-consultant grade (junior) doctors to support the growing emergency workload.
Contextual factors influence local adoption of the 7-day services policy
-
Organisational slack: those trusts that had more resources and fewer infrastructure challenges exhibited greater flexibility and responsiveness to policy imperatives.
-
Trust ‘culture’ matters: visible and clear leadership, combined with a collaborative approach to listening to staff, responding to concerns and involving them in decision-making, promoted engagement with the policy.
-
‘Clan’ cultures inhibited clinician engagement with the policy in the absence of shared interpretation of the data used to identify the problem to which 7-day services was the proposed solution.
-
Community services that were poorly integrated with secondary care acted as a barrier to clinical and managerial staff trying to introduce the policy.
Increasing specialist intensity at weekends may be cost-effective by promoting timely patient discharge from hospital
-
Health economics modelling suggests that 7-day services would be cost-effective if weekend specialist intensity were to achieve parity with that currently provided on weekdays; however, the mechanism of benefit is reducing the length of hospital stay by promoting earlier discharge, not by influencing the care quality provided to EAs at weekends. Community services would need to be engaged for this effect to be fully realised.
Care quality of emergency admissions in hospital has improved over time, but may be deteriorating in the community
-
Patients and staff could identify deficiencies in hospital weekend care processes and quality for already-admitted patients and convalescent patients awaiting discharge back into the community, but considered that newly admitted emergency patients could receive more timely care at the weekend than those admitted on weekdays.
-
These perceptions are supported by evidence that hospital care processes for emergency admissions are more reliable at weekends than on weekdays. It is likely that, on weekdays, elective admissions (e.g. from clinics or operating theatres) compete with EAs for scarce resources.
-
Case record reviewer judgements of global care quality aggregated at trust level concurred with the independent observations of trust weekend care quality that qualitative researchers made on site. This form of triangulation strengthens the validity of our observations.
-
Error rates, error-related adverse event rates and global care quality are similar for weekend and weekday admissions; all have improved further with time. By contrast, indicators of community care quality (sicker patients, more patients have chronic disease, more patients require palliative care, fewer GP referrals preceding admission) are worse at weekends and have deteriorated further with time.
The causal pathway for the weekend effect includes community health care preceding hospital admission
-
Being admitted to hospital at a weekend is consistently associated with a 16% higher mortality rate relative to weekday admission in both UK and international literature.
-
The case mix of patients admitted to hospital at weekends differs from that of patients admitted on weekdays: patients admitted at the weekend are more severely ill, which accounts for most of the surplus mortality of weekend admission. They also have more comorbid conditions, are more likely to be candidates for palliative care, and are less likely to be discharged into the community before midnight on the day of their admission. These adverse features of weekend case mix had become more frequent by 2016/17.
-
Although the same numbers of patients are presenting to EDs at weekends and on weekdays, fewer patients are admitted at weekends. This contributes to the weekend effect by reducing the denominator of the weekend mortality rate.
-
The reduction in admissions is attributable partly to a reduction of two-thirds in the proportion of patients referred directly to hospital at weekends by their family doctor (GP). This reduction in GP referrals at weekends has become more marked over time.
Implications for policy and practice
The main implication of our research is that efforts to improve the care of patients who require EAs to hospital must be system-wide and occur across all days of the week. The focus on mortality associated with hospital admission has distracted attention from deficits in community health services at weekends; moreover, mortality is not a good indicator of care quality. 129,164 Our findings provide evidence to support many of the recommendations in National Institute for Health and Care Excellence guideline 94 entitled Emergency and Acute Medical Care in Over 16s: Service Delivery and Organisation on emergency and acute medical care135 and are consistent with a recent systematic review of models of integrated care. 165 They also provide further justification for the promotion of the development of integrated care systems in the NHS149 through Sustainability and Transformation Partnerships166 and acute and emergency care collaborations,167 such as the one in London that incorporates the development of seamless partnerships across social and primary and secondary health care. 168 New research being commissioned by the NIHR Applied Research Collaboration West Midlands169 has a focus on integrated care and a subtheme of acute care. The emergency care pathways for frail elderly people and those with multimorbidity are an important target for further research.
Pre hospital
The adequacy and availability of community care at weekends need to be examined. The earlier application of more sophisticated interventions for acute illness in the community might prevent a proportion of hospital admissions. As proposed in National Institute for Health and Care Excellence guideline 94 entitled Emergency and Acute Medical Care in Over 16s: Service Delivery and Organisation,135 these interventions include paramedics who have enhanced competencies, acute hospital-at-home services, and enhanced advanced care-planning and palliative care provision to ensure that patients’ wishes are known and respected. This will involve access to data, as well as sharing information and patient care responsibilities 24/7 among the various agencies involved in community health care: general practice, community nursing, community palliative care, the emergency services and hospital-based community outreach services.
In hospital
Improvements in hospital care processes should be focused on all 7 days, not just the weekends; key decision-makers (usually consultants) need to be visible, and supported by managers and an effective multidisciplinary team using standardised and structured systems of care to optimise safety and efficiency. An expansion in consultant numbers alone is unlikely to solve a system-wide problem. The ease with which new ways of working are adopted is enhanced by a collaborative local culture, which is set by the attitudes and behaviours of senior leaders (trust executives, managers, senior clinical staff).
Post hospital
The timely discharge of a patient from hospital involves a (multidisciplinary) judgement about the impact that the transition from a supported to a less supported or unsupported environment will have on the patient’s current health status. Rehabilitation starting in hospital may need to be continued after the patient’s transfer back into the community to ensure that efforts to promote timely discharge are invested effectively. Hospital-at-home services and physiotherapy have the potential to bridge the gap between secondary and community care, but the effectiveness of these interventions will only be realised with the integration of information and care processes throughout the patient pathway.
Recommendations for future research
The global burden of emergency medical diseases has been estimated to cause 28.3 million deaths and to contribute to 50.7% of all deaths worldwide. 170 The need for a system-wide integrated approach to improving outcomes through research is well recognised in the UK. 169,171 The HiSLAC study demonstrates that signals from performance indicators conventionally attributed to hospital practice may originate in the community, both before and after discharge from hospital. Our research recommendations, therefore, take this wider view of exploring quality of care throughout the patient pathway.
How does decision-making by senior doctors differ from less experienced or non-physician staff to promote effective and timely patient-centred care?
The HiSLAC study has shown that hospital specialists are regarded as key decision-makers from admission to discharge, influencing not just the quality of care but also patient ‘flow’, and that they are regarded as more effective than junior doctors or other professionals. The health economics model indicates that increasing specialist input at weekends may be cost-effective, primarily by promoting the timely discharge of patients from hospital. It might, therefore, have been expected that specialists would have a shared mental model when evaluating the quality of care of individual patients from case records; this is true in aggregate, but not at the level of individual patients, as we have shown in the case record review. Therefore, we would propose studies that examine medical decision-making in relation to experience, professional status, values and beliefs, combining qualitative research observations of practice with ‘think-aloud’ experiments. 172 This should involve not just hospital specialists, but, crucially, also GPs. The outputs of such research would help to inform the development of both physician and allied health professional training programmes, and promote the efficient transit of patients through the health system.
Is there a causative association between the lower rates of general practitioner emergency referrals to hospital at weekends and the weekend effect?
The reduction in the proportion of GP referrals at weekends has become more marked over time. Possible causes include the introduction of the 111 urgent call service, although this should have had the same influence on weekdays as at weekends; the progressive withdrawal of GPs from providing emergency care services; GPs instructing patients who contact them urgently to call the emergency number (999) for an ambulance if they themselves are overloaded with emergency calls; or GPs failing to act as ‘filters’ of EAs to hospital specifically at weekends, thereby permitting an increase in the proportion of patients admitted who have palliative care decisions or multimorbidity. Exploring these possibilities requires the evaluation and linkage of primary care and HES data sets, direct observation of emergency practice at weekends and on weekdays, and qualitative research involving practitioners and patients.
What is the cost-effectiveness of integrated care initiatives focused on preventing acute deterioration in the community?
The HiSLAC study has shown that, compared with those admitted on weekdays, patients admitted at weekends are more severely ill, have more comorbid diseases, have a higher likelihood of receiving palliative care, are less likely to be discharged home on the day of admission and are more likely to be transferred directly to intensive care following hospital admission. This raises the question of whether or not opportunities to intervene earlier in the community are being missed. A number of initiatives are already being implemented in England that partially address this issue; some of the 50 vanguard sites173 are focused on improving acute and emergency care, with the aim of reducing avoidable ED attendances and admissions, but evaluation of these has so far been incomplete174 and at least one intervention, a new hospital specifically for emergency care, has resulted in an increase in ED attendances. 175 NHS England has funded seven ‘accelerator’ sites since April 2020 to establish rapid response teams intended to respond within 2 hours to acute deterioration in the health of older people and those with complex care needs to enable them to remain at home instead of being transferred to hospital. 176 There are no details at present on how these patients might be identified and assessed; acute physiology (vital signs, NEWS) monitoring by health-care professionals (whether directly through more frequent visits or using telemedicine) is feasible but the impact is uncertain. 177 This important initiative provides an opportunity for a collaborative mixed-methods evaluation. The work would be complemented by international comparisons of other health systems to determine how social and primary care structures and processes are engaged in responding to the deteriorating patient in the community.
Is the increase in post-discharge mortality rates since 2007 linked to the increase in emergency admission rates of frail elderly people and those with multimorbidity?
Are these avoidable deaths, part of planned palliative care, or appropriate but unexpected deaths? Could the care of these patients be improved by more intensive rehabilitation before discharge or by more intensive support in the community following discharge? Are the admissions preventable in the first place, through new ways of delivering care in the community or by better sharing of information about end-of-life care preferences, or does active medical care need to be expedited and more timely during ‘off hours’ such as weekends? During the patient journey, before admission or after discharge, are there gaps or deficiencies in data access, discussions about risks and benefits of treatment, detection of acute deterioration when this occurs, decision-making by informed and competent individuals, discharge decisions or destination following discharge, and, if so, how might these gaps or deficiencies be addressed?
Conclusions and lessons learned
The HiSLAC study has shown that the weekend effect is most likely to be a system-wide pathway problem that has its origins in community services. Contributory factors in the community include budgetary constraints during the period of austerity that followed the financial crisis of 2008, and the progressive increase in health service utilisation, accompanied by persistent demands for greater efficiencies and reductions in hospital length of stay. The improvements in hospital safety and care quality between 2012/13 and 2016/17 contrast with the deterioration in community care metrics. Although direct comparisons of secondary and primary care were not possible in this study, the evidence we have presented here is consistent with the hypothesis that the closer integration of social, primary and secondary care will provide benefits to patients. 178,179
The marked reduction in hospital errors and adverse events, and the improvement in global quality identified between epochs by the HiSLAC case record reviewers has parallels with the MERIT study,180 the Safer Patients’ Initiative116,125 and the Matching Michigan study;181 it has been described as the ‘rising tide’ phenomenon. 124 The reduction in errors and adverse events demonstrated in HiSLAC could be a manifestation of a non-specific long-term secular trend, but it is also consistent with being driven by the salience of the 7-day services policy, in particular the six standards that require increased consultant involvement in the acute-care pathway. If this were the case, then the 7-day services policy should be regarded as having benefited patients across all days of the week.
Lessons learned
We focused our attention primarily on in-hospital care because this was the main focus of the 7-day services policy. However, an inspection of Figure 3, which shows the possible mechanisms for generating the weekend effect, draws attention to the potential contribution of variation in community health service provision. It would have been helpful to have included community services in the HiSLAC project from the start.
Negotiations to obtain standard access to HES through NHS Digital were extremely prolonged and could have compromised the timely conclusion of the entire project. We hope that new processes at NHS Digital will accelerate access for research and permit better data linkage between secondary and primary health-care data sets.
The 20-hospital case record review (see Chapter 6) required each trust to obtain, copy, redact and digitally convert 200 case records. The time it took for different trusts to complete this task varied considerably, although funding was available for this purpose and site visits were undertaken with all those involved in facilitating access to case records for research purposes. The difference between trusts seemed to be related to local leadership, staff engagement and competing priorities. Encouraging local teams to provide the case records consumed a considerable amount of the project team’s time and resources, and delayed completion of this phase. The gradual transition in the NHS from paper to electronic case records should facilitate research, but, at present, the variation in local clinical information systems does not necessarily make the task of collation easier.
To conduct a research programme across the NHS in England for 5 years, we had to gain the support of senior leadership centrally, locally in each trust, and at the level of individual clinicians. We were fortunate to have the support of the Academy of Medical Royal Colleges and individual Royal Colleges and faculties, and of NHS England and NHS Confederation. The majority of trust chief executives and medical directors were supportive, and we were grateful to them and to each local project lead for the time they gave to the project, as well as to each consultant who completed the annual survey. We maintained contact with this diverse group through a newsletter and social media, but we were well aware of the competing demands on their time, not least in implementing the 7-day services policy.
Acknowledgements
Oversight and Governance Committee
Michael Rawlins (chairperson), Jennifer Dixon, Peter Lees, Paddy Storrie, Alastair Henderson, Matt Sutton and Naomi Fulop.
Local project leads from participating NHS trusts
Dr Tim Felton and Dr Tim Cooksley, Manchester University NHS Foundation Trust; Dr Victor Lawrence, Isle of Wight NHS Trust; Dr Michelle Gallagher, Royal Surrey NHS Foundation Trust; Dr Rebecca Thorpe, University Hospitals Bristol NHS Foundation Trust; Dr Andrew Griffiths, Torbay and South Devon NHS Foundation Trust; Professor John Kinnear, Southend University Hospital NHS Foundation Trust; Dr Rupert Negus, Royal Free London NHS Foundation Trust; Dr Achim Schwenk and Dr Cathy Cale, North Middlesex University Hospital NHS Trust; Dr Fiona Wisniacki, The Hillingdon Hospitals NHS Foundation Trust; Miss Jane Wilson, Kingston Hospital NHS Foundation Trust; Dr Matthew Hayman, Taunton and Somerset NHS Foundation Trust; Dr Richard Jee, Dorset County Hospital NHS Foundation Trust; Dr Mark Lipton, Wirral University Teaching Hospital NHS Foundation Trust; Dr Shirley Hammersley, Mid Cheshire Hospitals NHS Foundation Trust; Dr Roope Manhas, Northern Devon Healthcare NHS Trust; Dr Shwe Zin Chit Pan, Bedford Hospitals NHS Foundation Trust; Dr Peter Albert, Bedfordshire Hospitals NHS Foundation Trust; Dr Andrew Alldred, Dr Peter Hammond and Dr David Scullion, Harrogate and District NHS Foundation Trust; Dr Franco Guarasci, Airedale NHS Foundation Trust; Dr Mark Blunt, The Queen Elizabeth Hospital King’s Lynn NHS Foundation Trust; Dr Tina Tamm, Heatherwood and Wexham Park Hospitals NHS Foundation Trust; Dr Ian Reckless, Milton Keynes University Hospital NHS Foundation Trust; Dr Marcus Pittman, Basildon and Thurrock University Hospitals NHS Foundation Trust; Dr Allan Harkness, Colchester Hospital University NHS Foundation Trust; Dr Earl Williams, The Royal Bournemouth and Christchurch Hospitals NHS Foundation Trust; Dr Mark Daly and Dr David Friedericksen, Royal Cornwall Hospitals NHS Trust; Dr John Hollingsworth, Aintree University Hospital NHS Foundation Trust; Dr Magda Smith, Barking, Havering and Redbridge University Hospitals NHS Trust; Professor Anil Hormis and Dr David Harling, The Rotherham NHS Foundation Trust; Dr Anitha Mathews, Dr Suzanne Hamilton and Dr Callum Gardner, North West Anglia NHS Foundation Trust; Dr Paul Molyneux, West Suffolk NHS Foundation Trust; Dr Chris Adcock, Cambridge University Hospitals NHS Foundation Trust; Mr James Hobbs and Mr Martin Cooper, Royal Devon and Exeter NHS Foundation Trust; Dr Mike Clancy, University Hospital Southampton NHS Foundation Trust; Dr Andrew Gibson, Sheffield Teaching Hospitals NHS Foundation Trust; Dr Phil Young, Portsmouth Hospitals University NHS Trust; Dr Emma Vaux, Royal Berkshire NHS Foundation Trust; Dr David Wood, Guy’s and St Thomas’ NHS Foundation Trust; Dr Mehool Patel and Dr David Sulch, Lewisham and Greenwich NHS Trust; Dr Nnenna Osuji, Croydon Health Services NHS Trust; Dr Jane Evans, St George’s University Hospitals NHS Foundation Trust; Dr Viviana Elliott and Dr Jyothi Nippani, South Warwickshire NHS Foundation Trust; Dr Nick Coleman, University Hospitals of North Midlands NHS Trust; Mrs Deborah Price and Dr Andrew Willis, Burton Hospitals NHS Foundation Trust; Dr Sanjeev Srivastava, East Cheshire NHS Trust; Dr Frank Joseph, Countess of Chester Hospital NHS Foundation Trust; Dr William Bernal, King’s College Hospital NHS Foundation Trust; Dr Andrew Haynes, Sherwood Forest Hospitals NHS Foundation Trust; Dr Sam Waddy, University Hospitals Plymouth NHS Trust; Dr Richard de Boer and Dr Mike Iredale, University Hospitals Coventry and Warwickshire NHS Trust; Dr Clarissa Murdoch, The Whittington Hospital NHS Trust; Dr Lee Dowson, The Royal Wolverhampton NHS Trust; Dr Martyn Farrer, City Hospitals Sunderland NHS Foundation Trust; Ms Jo Sampson and Dr Rupert Ransford, Wye Valley NHS Trust; Dr Catherine Free and Dr Gordon Wood, George Eliot Hospital NHS Trust; Professor Erika Denton, Dr Neil Burgess and Mr Peter Chapman, Norfolk and Norwich University Hospitals NHS Foundation Trust; Dr Richard Warner, Salford Royal NHS Foundation Trust; Dr Natalie Walker and Dr Leonidas Zaccharides, Bolton NHS Foundation Trust; Dr Martin Pattrick, Tameside and Glossop Integrated Care NHS Foundation Trust; Dr Debesh Mukherjee, Great Western Hospitals NHS Foundation Trust; Mr Ben Cresswell, Hampshire Hospitals NHS Foundation Trust; Dr Aref Rastegar and Dr Lanitha Srikugan, Dartford and Gravesham NHS Trust; Dr Hassan Paraiso, The Dudley Group NHS Foundation Trust; Dr Rod Harpin, North Cumbria University Hospitals NHS Trust; Dr Raja Reddy, Kettering General Hospital NHS Foundation Trust; Dr Raghu Govindan and Dr Amanda Bisset, Northampton General Hospital NHS Trust; Dr Stuart Henderson, Salisbury NHS Foundation Trust; Dr Timothy Noble, Doncaster and Bassetlaw Teaching Hospitals NHS Foundation Trust; Dr Graeme Sanders, Medway NHS Foundation Trust; Dr Peter Burnham and Dr Kathryn Clark, Royal Liverpool and Broadgreen University Hospitals NHS Trust; Dr Jayachandran Radhakrishnan, Mid and South Essex Hospital Services NHS Trust; Dr Emma Rowlandson, Chelsea and Westminster Hospital NHS Foundation Trust; Dr Andy Morris, The Princess Alexandra Hospital NHS Trust; Dr Emma Rowland, Homerton University Hospital NHS Foundation Trust; Dr Rifat Rashid and Professor Matthew Cooke, Heart of England NHS Foundation Trust; Dr Kilimangalam Narayanan and Dr Christopher Scott, Gateshead Health NHS Foundation Trust; Dr Graham Johnson, Leeds Teaching Hospitals NHS Trust; Dr Catherine Snelson and Dr Mike Berry, University Hospitals Birmingham NHS Foundation Trust; Dr Samer Elkhodair, Dr Nicoletta Gaston, Dr Breid O’Brien and Ms Simone Akuffo-Akoto, University College London Hospitals NHS Foundation Trust; Dr Sean Elyan, Gloucestershire Hospitals NHS Foundation Trust; Dr Eliot Sykes, Northumbria Healthcare NHS Foundation Trust; Professor Richard Donnelly, Derby Teaching Hospitals NHS Foundation Trust; Dr James Price, Oxford University Hospitals NHS Foundation Trust; Mr Pasha Nisar, Ashford and St Peter’s Hospitals NHS Foundation Trust; Dr Sath Nag, South Tees Hospitals NHS Foundation Trust; Dr Andrew Higham, University Hospitals of Morecambe Bay NHS Foundation Trust; Dr Kiaran Flanagan, North Bristol NHS Trust; Dr Pauline Swift, Dr Aik Guam Lim and Dr Steve Hyer, Epsom and St Helier University Hospitals NHS Trust; Dr Paul Stevens, East Kent Hospitals University NHS Foundation Trust; Dr Nick Roper, North Tees and Hartlepool Hospitals NHS Foundation Trust; Dr Patrick McDonald, Southport and Ormskirk Hospital NHS Trust; Dr Nick Tierney, Pennine Acute Hospitals NHS Trust; Dr Sanjiv Jain, United Lincolnshire Hospitals NHS Trust; Dr Mark Ardron, University Hospitals of Leicester NHS Trust; Dr Vinay Bhatia and Dr Clive Lawson, Maidstone and Tunbridge Wells NHS Trust; Dr Michael Van Der Watt, West Hertfordshire Hospitals NHS Trust; Professor Phillip Smith, Miss Jane McCue and Mr Neil Harris, East and North Hertfordshire NHS Trust; Dr Colin Wasson and Dr James Catania, Stockport NHS Foundation Trust; Ms Anna Walker, Worcestershire Acute Hospitals NHS Trust; Dr Rehan Naseer, Calderdale and Huddersfield NHS Foundation Trust; Dr Keith Girling, Nottingham University Hospitals NHS Trust; Dr James Wilkinson, East Sussex Healthcare NHS Trust; Mr Ian Carr, The Mid Yorkshire Hospitals NHS Trust; Dr Heather Brown and Dr Wendy Munro, Brighton and Sussex University Hospitals NHS Trust; Dr David Carruthers, Sandwell and West Birmingham Hospitals NHS Trust; Professor Mark O’Donnell, Blackpool Teaching Hospitals NHS Foundation Trust; Dr Paul Peter and Dr Mike Jones, County Durham and Darlington NHS Foundation Trust; Dr Andrew Huang; Buckinghamshire Healthcare NHS Trust; Mr Charles Thomson, East Lancashire Hospitals NHS Trust; Dr Richard Heinink, Shrewsbury and Telford Hospital NHS Trust; Professor Julian Redhead and Dr William Oldfield, Imperial College Healthcare NHS Trust; Dr Tim Taylor and Dr Rob Haigh, Western Sussex Hospitals NHS Foundation Trust; and Dr Faris Al-Sultan, Weston Area Health NHS Trust.
Case note reviewers
Randeep Mullhi, Jonathon Coates, Neil Patel, Emma Carver, Zoe Kimbley, Nicholas Price, Akshay Nair, Sheena Sikka, Navinee Gilliat, Greg Packer, Anna Blackwell, Emma Christmas, Adebola Adebowale, Sebastien Ellis, Rebecca Mallinson, Farhad Peerally, Daniel Forster, Simmi Krishnan, Vishal Patil, Richard Hopkins, Ruth Todd, Sofia Cavill, James Murray, Imran Ghafoor, Katey Flowers, Paul Dean, Christopher Wright, Shreena Shah, Jonarthan Thevanayagam, Richard Hunt, Karwai Tsang, Mohammed Yusuf, Mark Whitsey, Brian Hogan, Amina Jaffer, Emma Bryden, Rebecca Powell, William Wareing, Yasir Elhassan, Parijat De, Manoj Viegas, Christopher Wharton, Michael Duffy, Richard Heinink, Chris Beet, Elin Roddy, Anna Szczerbinska, Annabel Makan, Manohasandra Majuran Manohasandra, Neel Patel, Behrad Baharlo, James Brown, Jun Jack Wong, Salmaan Mughal, Musarat Hussain, Nick Murch, Georgina Barrows, Ryan Boyle, Syed Habib Zaidi, Ivan Collin, Caroline Kibbler, Monica Bowa-Nkhoma, Clare Hughes, Muniswamy Hemavathi, Thomas Cozens, Khin Nyo, Debbie Beck, Claire Peplow, Devasena Subramayam, Tom McLellan, Gautam Bagchi, David Hartin, Stewart McMorran, Chung Thong Lim, Hiba Mohamed, John Ong, Hoi-Ping Mok, Ayesha Khaliq, Abigail Ford and Alison McInerney.
Additional acknowledgements
Felicity Evison for statistical support with Chapter 5.
Professor Nick Black and Dr Helen Hogan for access to their case record review methodology.
Emma Lancashire, Kam Gareja, Claire Price and Olivia Brookes for project support.
Elisa Liberati, David Kocman, Sarah Chew, Jen Jones and Emma Angell for conducting qualitative research visits to hospital sites (particular thanks go to Emma Angell for her role supporting project management).
Liz Shaw for her contribution to the analysis of qualitative data.
Xavier Armoiry, Caroline Higenbottam, Nicholas Cowley, Ranjna Basra, Chia-Wei Wu and Amy Gosling for their contribution to the systematic review.
Sue Pargeter, Manager, NIHR HSDR programme.
In memoriam
Professor Tim Evans: an outstanding researcher, collaborator, leader, clinician and good friend.
Contributions of authors
Julian Bion (https://orcid.org/0000-0003-0344-5403) (Professor of Intensive Care Medicine) was the HiSLAC project chief investigator. He developed the original design and contributed to methodology development, analysis of data and writing of the manuscript.
Cassie Aldridge (https://orcid.org/0000-0002-9162-3478) (HiSLAC Project Manager) contributed to data collection, methodology development, analysis of data and writing of the manuscript.
Chris Beet (https://orcid.org/0000-0002-9259-9852) (Specialty Registrar in Intensive Care Medicine) contributed to methodology development, development of the case record review and reviewer training materials, and the writing of the manuscript.
Amunpreet Boyal (https://orcid.org/0000-0002-5156-2869) (Research Associate) contributed to data collection, methodology development and writing of the manuscript.
Yen-Fu Chen (https://orcid.org/0000-0002-9446-2761) (Associate Professor, Population Evidence and Technologies) contributed to the systematic review, methodology development and the writing of the manuscript.
Michael Clancy (https://orcid.org/0000-0001-8669-5226) (Consultant Emergency Physician) contributed to methodology development and the writing of the manuscript.
Alan Girling (https://orcid.org/0000-0003-2022-045X) (Honorary Reader in Medical Statistics) contributed to data collection, methodology development, analysis of HES data, statistical analysis and the writing of the manuscript.
Timothy Hofer (https://orcid.org/0000-0003-0434-8787) (Professor of General Medicine) contributed to methodology development and the writing of the manuscript.
Joanne Lord (https://orcid.org/0000-0003-1086-1624) (Director and Professorial Fellow in Health Economics) conducted the health economics analysis and contributed to the writing of the manuscript.
Russell Mannion (https://orcid.org/0000-0002-0680-8049) (Professor of Health Systems) contributed to methodology development and the writing of the manuscript.
Peter Rees (https://orcid.org/0000-0001-5654-6254) (Patient and Public Involvement Lead) contributed to methodology development and the writing of the manuscript.
Chris Roseveare (https://orcid.org/0000-0002-9767-7725) [Consultant in Acute and General (Internal) Medicine] contributed to methodology development and the writing of the manuscript.
Louise Rowan (https://orcid.org/0000-0001-6211-3869) (Communications Fellow) contributed to collaborator engagement, dissemination of findings and the writing of the manuscript.
Gavin Rudge (https://orcid.org/0000-0002-9857-6547) (Research Fellow, Applied Health Research) contributed to data collection, methodology development, analysis of HES data, statistical analysis and the writing of the manuscript.
Jianxia Sun (https://orcid.org/0000-0003-4029-6515) (Statistical Intelligence Analyst) contributed to data collection, methodology development, analysis of HES data, statistical analysis and the writing of the manuscript.
Elizabeth Sutton (https://orcid.org/0000-0002-6691-6287) (Research Associate, Health Sciences) was involved in the qualitative research design and contributed to organising and facilitating the focus groups and interviews, analysis of data and the writing of the manuscript.
Carolyn Tarrant (https://orcid.org/0000-0001-7356-5342) [Professor and Research Group Lead of the Social Science Applied to Healthcare Improvement Research (SAPPHIRE) Group] devised and led on the qualitative research design, and contributed to analysis of data and the writing of the manuscript.
Mark Temple (https://orcid.org/0000-0003-1600-851X) (Consultant Nephrologist) contributed to methodology development and the writing of the manuscript.
Sam Watson (https://orcid.org/0000-0002-8972-769X) (Senior Research Fellow, Population Evidence and Technologies) contributed to the statistical methodology development for the cost–benefit analysis, conducted the health economics analysis and contributed to writing of the manuscript.
Janet Willars (https://orcid.org/0000-0002-7886-3223) (Honorary Visiting Fellow, Health Sciences) contributed to organising and facilitating the focus groups and interviews, analysis and writing of the manuscript.
Richard Lilford (https://orcid.org/0000-0002-0634-984X) (Director of NIHR Applied Research Centre West Midlands, and Research Methodology Lead for HiSLAC) contributed to the writing of the application, design of the project, health economics analysis and the writing of the manuscript.
Publications
Aldridge C, Bion J, Boyal A, Chen YF, Clancy M, Evans T, et al. Weekend specialist intensity and admission mortality in acute hospital trusts in England: a cross-sectional study. Lancet 2016;388:178–86.
Chen YF, Boyal A, Sutton E, Armoiry X, Watson S, Bion J, et al. The magnitude and mechanisms of the weekend effect in hospital admissions: a protocol for a mixed methods review incorporating a systematic review and framework synthesis. Syst Rev 2016;5:84.
Bion J, Aldridge CP, Girling A, Rudge G, Beet C, Evans T, et al. Two-epoch cross-sectional case record review protocol comparing quality of care of hospital emergency admissions at weekends versus weekdays. BMJ Open 2017;7:e018747.
Tarrant C, Sutton E, Angell E, Aldridge CP, Boyal A, Bion J, et al. The ‘weekend effect’ in acute medicine: a protocol for a team-based ethnography of weekend care for medical patients in acute hospital settings. BMJ Open 2017;7:e016755.
Sutton E, Bion J, Aldridge C, Boyal A, Willars J, Tarrant C. Quality and safety of in-hospital care for acute medical patients at weekends: a qualitative study. BMC Health Serv Res 2018;18:1015.
Watson SI, Chen YF, Bion JF, Aldridge CP, Girling A, Lilford RJ, et al. Protocol for the health economic evaluation of increasing the weekend specialist to patient ratio in hospitals in England. BMJ Open 2018;8:e015561.
Chen YF, Armoiry X, Higenbottam C, Cowley N, Basra R, Watson SI, et al. Magnitude and modifiers of the weekend effect in hospital admissions: a systematic review and meta-analysis. BMJ Open 2019;9:e025764.
Sun J, Girling AJ, Aldridge C, Evison F, Beet C, Boyal A, et al. Sicker patients account for the weekend mortality effect among adult emergency admissions to a large hospital trust. BMJ Qual Saf 2019;28:223–30.
Bion J, Aldridge C, Girling AJ, Rudge G, Sun J, Tarrant C, et al. Changes in weekend and weekday care quality of emergency medical admissions to 20 hospitals in England during implementation of the 7-day services national health policy [published online ahead of print October 28 2020]. BMJ Qual Saf 2020.
Data-sharing statement
All data requests should be submitted to the corresponding author for consideration. Access to available anonymised data may be granted following review.
Patient data
This work uses data provided by patients and collected by the NHS as part of their care and support. Using patient data is vital to improve health and care for everyone. There is huge potential to make better use of information from people’s patient records, to understand more about disease, develop new treatments, monitor safety, and plan NHS services. Patient data should be kept safe and secure, to protect everyone’s privacy, and it’s important that there are safeguards to make sure that it is stored and used responsibly. Everyone should be able to find out about how patient data are used. #datasaveslives You can find out more about the background to this citation here: https://understandingpatientdata.org.uk/data-citation.
Disclaimers
This report presents independent research funded by the National Institute for Health Research (NIHR). The views and opinions expressed by authors in this publication are those of the authors and do not necessarily reflect those of the NHS, the NIHR, NETSCC, the HS&DR programme or the Department of Health and Social Care. If there are verbatim quotations included in this publication the views and opinions expressed by the interviewees are those of the interviewees and do not necessarily reflect those of the authors, those of the NHS, the NIHR, NETSCC, the HS&DR programme or the Department of Health and Social Care.
References
- Bion J, Roseveare C, Dixon J, Clancy M, Temple R, Girling A, et al. Prospective Evaluation of the Impact of High-Intensity Specialist-Led Acute Care (HiSLAC) on Emergency Medical Admissions to NHS Hospitals at Weekends. Protocol: V4 18 05 2015 2015. www.journalslibrary.nihr.ac.uk/programmes/hsdr/1212817/#/ (accessed 31 January 2020).
- European Union . Directive 2003 88 EC of the European Parliament and of the Council of 4 November 2003 Concerning Certain Aspects of the Organisation of Working Time 2003.
- Lambert TW, Smith F, Goldacre MJ. The impact of the European Working Time Directive 10 years on: views of the UK medical graduates of 2002 surveyed in 2013–2014. JRSM Open 2016;7. https://doi.org/10.1177/2054270416632703.
- House of Commons Health Committee . GP Out-of-Hours Services. Fifth Report of Session 2003–04 2004.
- Steventon A, Deeny S, Friebel R, Gardner T, Thorlby R. Emergency Hospital Admissions in England: Which May be Avoidable and How?. London: The Health Foundation; 2018.
- Ewbank L, Thompson J, McKenna H. NHS Hospital Bed Numbers: Past, Present, Future. London: The King’s Fund; 2017.
- Gershlick B, Kraindler J, Idriss O, Charlesworth A. Health and Social Care Funding General Election 2019: Priorities for the Next Government. London: The Health Foundation; 2019.
- Rojas-García A, Turner S, Pizzo E, Hudson E, Thomas J, Raine R. Impact and experiences of delayed discharge: a mixed-studies systematic review. Health Expect 2018;21:41-56. https://doi.org/10.1111/hex.12619.
- The King’s Fund . Delayed Transfers of Care: A Quick Guide 2018.
- Commission for Healthcare Audit and Inspection . Investigation into Mid Staffordshire NHS Foundation Trust 2009.
- Francis R. Report of the Mid Staffordshire NHS Foundation Trust Public Inquiry 2013.
- Royal College of Physicians and Society for Acute Medicine . An Evaluation of Consultant Input into Acute Medical Admissions Management in England, Wales and Northern Ireland 2011.
- Martin IC, Mason DG, Stewart J, Mason M, Smith NCE, Gill K. Emergency Admissions: A Journey in the Right Direction. London: National Confidential Enquiry into Patient Outcome and Death; 2007.
- Royal College of Physicians . Hospitals on the Edge? The Time for Action 2012.
- Royal College of Physicians . Future Hospitals Commission 2013.
- NHS England . Seven Day Hospital Services. Our Ambition n.d. www.england.nhs.uk/seven-day-hospital-services/our-ambition/ (accessed January 2020).
- NHS . NHS England: Seven Day Services 2017. https://improvement.nhs.uk/resources/seven-day-services/ (accessed January 2020).
- Porter M. Seven Day Services: Our Letter to Government. London: British Medical Association; 2016.
- Department of Health and Social Care . 7-Day NHS Services: A Factsheet 2015. www.gov.uk/government/publications/7-day-nhs-services-a-factsheet/7-day-nhs-services-a-factsheet (accessed 5 February 2020).
- Freemantle N, Ray D, McNulty D, Rosser D, Bennett S, Keogh BE, et al. Increased mortality associated with weekend hospital admission: a case for expanded seven day services?. BMJ 2015;351. https://doi.org/10.1136/bmj.h4596.
- Neville S. Jeremy Hunt ready to push through 7 day NHS consultants’ terms. Financial Times 2015.
- UK Parliament . Oral Answers to Questions, Health, Seven-Day NHS Services. Volume 600: Debated on Tuesday 13 October 2015 2015.
- National Health Executive . Junior Doctor Contracts Dispute Timeline: Key Events 2015. www.nationalhealthexecutive.com/Health-Service-Focus/junior-doctor-contracts-dispute-timeline-key-events (accessed 5 February 2020).
- Bell CM, Redelmeier DA. Mortality among patients admitted to hospitals on weekends as compared with weekdays. N Engl J Med 2001;345:663-8. https://doi.org/10.1056/NEJMsa003376.
- Halm EA, Chassin MR. Why do hospital death rates vary?. N Engl J Med 2001;345:692-4. https://doi.org/10.1056/NEJM200108303450911.
- Mikulich O, Callaly E, Bennett K, O’Riordan D, Silke B. The increased mortality associated with a weekend emergency admission is due to increased illness severity and altered case-mix. Acute Med 2011;10:182-7.
- Chen YF, Armoiry X, Higenbottam C, Cowley N, Basra R, Watson SI, et al. Magnitude and modifiers of the weekend effect in hospital admissions: a systematic review and meta-analysis. BMJ Open 2019;9. https://doi.org/10.1136/bmjopen-2018-025764.
- Academy of Medical Royal Colleges . Seven Day Consultant Present Care 2012.
- Meacock R, Doran T, Sutton M. What are the costs and benefits of providing comprehensive seven-day services for emergency hospital admissions?. Health Econ 2015;24:907-12. https://doi.org/10.1002/hec.3207.
- NHS England . Survey Results for Individual Trust Performance for 7 Day Hospital Service 2017.
- NHS England . 7-Day Hospital Services Self Assessment Results 2019. www.england.nhs.uk/publication/7-day-hospital-services-self-assessment-results/ (accessed 5 February 2020).
- Wing C, Simon K, Bello-Gomez RA. Designing difference in difference studies: best practices for public health policy research. Annu Rev Public Health 2018;39:453-69. https://doi.org/10.1146/annurev-publhealth-040617-013507.
- World Health Organization . International Statistical Classification of Diseases and Related Health Problems. 2004.
- Ministry of Housing, Communities and Local Government . The English Indices of Deprivation 2010 2010. www.gov.uk/government/statistics/english-indices-of-deprivation-2010 (accessed 26 February 2021).
- Aldridge C, Bion J, Boyal A, Chen YF, Clancy M, Evans T, et al. Weekend specialist intensity and admission mortality in acute hospital trusts in England: a cross-sectional study. Lancet 2016;388:178-86. https://doi.org/10.1016/S0140-6736(16)30442-1.
- Royal College of Emergency Medicine . Emergency Medicine Consultants Workforce Recommendations 2010. www.rcem.ac.uk/docs/Workforce/CEM5324-Emergency-Medicine-Consultants---CEM-Workforce-Recommendations-Apr-2010.pdf (accessed 31 January 2020).
- NHS London Health Programmes . Quality and Safety Programme Emergency Departments: Case for Change 2013. www.england.nhs.uk/wp-content/uploads/2013/08/lon-qual-stands.pdf (accessed 31 January 2020).
- West Midlands Quality Review Service and the Society for Acute Medicine . Quality Standards for Acute Medical Units 2012. www.acutemedicine.org.uk/wp-content/uploads/2010/04/wmqrs-sam%20am%20qss%20v2%2020120610%201.pdf (accessed 31 January 2020).
- Royal College of Physicians . Acute Care Toolkit 4: Delivering a 12-Hour, 7-Day Consultant Presence on the Acute Medical Unit 2012. www.rcplondon.ac.uk/guidelines-policy/acute-care-toolkit-4-delivering-12-hour-7-day-consultant-presence-acute-medical-unit (accessed 31 January 2020).
- Bell D, Lambourne A, Percival F, Laverty AA, Ward DK. Consultant input in acute medical admissions and patient outcomes in hospitals in England: a multivariate analysis. PLOS ONE 2013;8. https://doi.org/10.1371/journal.pone.0061476.
- Faculty of Intensive Care Medicine . Core Standards for Intensive Care Units 2013. www.ficm.ac.uk/sites/default/files/Core%20Standards%20for%20ICUs%20Ed.1%20(2013).pdf (accessed 31 January 2020).
- Chen YF, Boyal A, Sutton E, Armoiry X, Watson S, Bion J, et al. HiSLAC Collaborative . The magnitude and mechanisms of the weekend effect in hospital admissions: a protocol for a mixed methods review incorporating a systematic review and framework synthesis. Syst Rev 2016;5. https://doi.org/10.1186/s13643-016-0260-2.
- Sutton E, Bion J, Aldridge C, Boyal A, Willars J, Tarrant C. Quality and safety of in-hospital care for acute medical patients at weekends: a qualitative study. BMC Health Serv Res 2018;18. https://doi.org/10.1186/s12913-018-3833-z.
- Sorita A, Ahmed A, Starr SR, Thompson KM, Reed DA, Dabrh AM, et al. Off-hour presentation and outcomes in patients with acute ischemic stroke: a systematic review and meta-analysis. Eur J Intern Med 2014;25:394-400. https://doi.org/10.1016/j.ejim.2014.03.012.
- Sorita A, Ahmed A, Starr SR, Thompson KM, Reed DA, Prokop L, et al. Off-hour presentation and outcomes in patients with acute myocardial infarction: systematic review and meta-analysis. BMJ 2014;348. https://doi.org/10.1136/bmj.f7393.
- Zhou Y, Li W, Herath C, Xia J, Hu B, Song F, et al. Off-hour admission and mortality risk for 28 specific diseases: a systematic review and meta-analysis of 251 cohorts. J Am Heart Assoc 2016;5. https://doi.org/10.1161/JAHA.115.003102.
- Higgins JP, Thompson SG, Deeks JJ, Altman DG. Measuring inconsistency in meta-analyses. BMJ 2003;327:557-60. https://doi.org/10.1136/bmj.327.7414.557.
- Guyatt GH, Oxman AD, Vist GE, Kunz R, Falck-Ytter Y, Alonso-Coello P, et al. GRADE: an emerging consensus on rating quality of evidence and strength of recommendations. BMJ 2008;336:924-6. https://doi.org/10.1136/bmj.39489.470347.AD.
- Darzi A. High Quality Care for All: NHS Next Stage Review Final Report 2008.
- Department of Health and Social Care . Quality Governance in the NHS: A Guide for Provider Boards 2011.
- Braun V, Clarke V. Using thematic analysis in psychology. Qual Res Psychol 2006;3:77-101. https://doi.org/10.1191/1478088706qp063oa.
- Khanna R, Wachsberg K, Marouni A, Feinglass J, Williams MV, Wayne DB. The association between night or weekend admission and hospitalization-relevant patient outcomes. J Hosp Med 2011;6:10-4. https://doi.org/10.1002/jhm.833.
- Attenello FJ, Wen T, Cen SY, Ng A, Kim-Tenser M, Sanossian N, et al. Incidence of ‘never events’ among weekend admissions versus weekday admissions to US hospitals: national analysis. BMJ 2015;350. https://doi.org/10.1136/bmj.h1460.
- Walker AS, Mason A, Quan TP, Fawcett NJ, Watkinson P, Llewelyn M, et al. Mortality risks associated with emergency admissions during weekends and public holidays: an analysis of electronic health records. Lancet 2017;390:62-7. https://doi.org/10.1016/S0140-6736(17)30782-1.
- Conway R, Cournane S, Byrne D, O’Riordan D, Silke B. Time patterns in mortality after an emergency medical admission; relationship to weekday or weekend admission. Eur J Intern Med 2016;36:44-9. https://doi.org/10.1016/j.ejim.2016.08.010.
- Mohammed M, Faisal M, Richardson D, Howes R, Beatson K, Speed K, et al. Impact of the level of sickness on higher mortality in emergency medical admissions to hospital at weekends. J Health Serv Res Policy 2017;22:236-42. https://doi.org/10.1177/1355819617720955.
- Sun J, Girling AJ, Aldridge C, Evison F, Beet C, Boyal A, et al. Sicker patients account for the weekend mortality effect among adult emergency admissions to a large hospital trust. BMJ Qual Saf 2019;28:223-30. https://doi.org/10.1136/bmjqs-2018-008219.
- Concha OP, Gallego B, Hillman K, Delaney GP, Coiera E. Do variations in hospital mortality patterns after weekend admission reflect reduced quality of care or different patient cohorts? A population-based study. BMJ Qual Saf 2014;23:215-22. https://doi.org/10.1136/bmjqs-2013-002218.
- Ruiz M, Bottle A, Aylin PP. The Global Comparators project: international comparison of 30-day in-hospital mortality by day of the week. BMJ Qual Saf 2015;24:492-504. https://doi.org/10.1136/bmjqs-2014-003467.
- Luo ZC, Liu S, Wilkins R, Kramer MS. Fetal and Infant Health Study Group of the Canadian Perinatal Surveillance System . Risks of stillbirth and early neonatal death by day of week. CMAJ 2004;170:337-41. https://doi.org/10.1097/00006254-200407000-00010.
- McIsaac DI, Bryson GL, van Walraven C. Elective, major noncardiac surgery on the weekend: a population-based cohort study of 30-day mortality. Med Care 2014;52:557-64. https://doi.org/10.1097/MLR.0000000000000137.
- Madsen F, Ladelund S, Linneberg A. High levels of bed occupancy associated with increased inpatient and thirty-day hospital mortality in Denmark. Health Aff 2014;33:1236-44. https://doi.org/10.1377/hlthaff.2013.1303.
- De Giorgi A, Fabbian F, Tiseo R, Misurati E, Boari B, Zucchi B, et al. Weekend hospitalization and inhospital mortality: a gender effect?. Am J Emerg Med 2015;33:1701-3. https://doi.org/10.1016/j.ajem.2015.07.068.
- Lee KG, Indralingam V. A study of weekend and off-hour effect on mortality in a public hospital in Malaysia. Med J Malaysia 2012;67:478-82.
- Gijsen R, Hukkelhoven CW, Schipper CM, Ogbu UC, de Bruin-Kooistra M, Westert GP. Effects of hospital delivery during off-hours on perinatal outcome in several subgroups: a retrospective cohort study. BMC Pregnancy Childbirth 2012;12. https://doi.org/10.1186/1471-2393-12-92.
- Barba R, Losa JE, Velasco M, Guijarro C, García de Casasola G, Zapatero A. Mortality among adult patients admitted to the hospital on weekends. Eur J Intern Med 2006;17:322-4. https://doi.org/10.1016/j.ejim.2006.01.003.
- Lee LH, Hsueh YS, Yang CH. The relationship between admission on weekends or holidays and treatment outcome. Taiwan J Public Health 2006;25:107-14.
- Aylin P, Yunus A, Bottle A, Majeed A, Bell D. Weekend mortality for emergency admissions. A large, multicentre study. Qual Saf Health Care 2010;19:213-7. https://doi.org/10.1136/qshc.2008.028639.
- Freemantle N, Richardson M, Wood J, Ray D, Khosla S, Shahian D, et al. Weekend hospitalization and additional risk of death: an analysis of inpatient data. J R Soc Med 2012;105:74-8. https://doi.org/10.1258/jrsm.2012.120009.
- Gillies MA, Lone NI, Pearse RM, Haddow C, Smyth L, Parks RW, et al. Effect of day of the week on short- and long-term mortality after emergency general surgery. Br J Surg 2017;104:936-45. https://doi.org/10.1002/bjs.10507.
- Han L, Sutton M, Clough S, Warner R, Doran T. Impact of out-of-hours admission on patient mortality: longitudinal analysis in a tertiary acute hospital. BMJ Qual Saf 2018;27:445-54. https://doi.org/10.1136/bmjqs-2017-006784.
- Handel AE, Patel SV, Skingsley A, Bramley K, Sobieski R, Ramagopalan SV. Weekend admissions as an independent predictor of mortality: an analysis of Scottish hospital admissions. BMJ Open 2012;2. https://doi.org/10.1136/bmjopen-2012-001789.
- Maggs F, Mallet M. Mortality in out-of-hours emergency medical admissions – more than just a weekend effect. J R Coll Physicians Edinb 2010;40:115-18. https://doi.org/10.4997/JRCPE.2010.205.
- McCallum IJ, McLean RC, Dixon S, O’Loughlin P. Retrospective analysis of 30-day mortality for emergency general surgery admissions evaluating the weekend effect. Br J Surg 2016;103:1557-65. https://doi.org/10.1002/bjs.10261.
- Mohammed MA, Sidhu KS, Rudge G, Stevens AJ. Weekend admission to hospital has a higher risk of death in the elective setting than in the emergency setting: a retrospective database study of national health service hospitals in England. BMC Health Serv Res 2012;12. https://doi.org/10.1186/1472-6963-12-87.
- Palmer WL, Bottle A, Aylin P. Association between day of delivery and obstetric outcomes: observational study. BMJ 2015;351. https://doi.org/10.1136/bmj.h5774.
- Pasupathy D, Wood AM, Pell JP, Fleming M, Smith GC. Time of birth and risk of neonatal death at term: retrospective cohort study. BMJ 2010;341. https://doi.org/10.1136/bmj.c3498.
- Roberts SE, Thorne K, Akbari A, Samuel DG, Williams JG. Weekend emergency admissions and mortality in England and Wales. Lancet 2015;385. https://doi.org/10.1016/S0140-6736(15)60580-3.
- Ruiz M, Bottle A, Aylin PP. Exploring the impact of consultants’ experience on hospital mortality by day of the week: a retrospective analysis of Hospital Episode Statistics. BMJ Qual Saf 2016;25:337-44. https://doi.org/10.1136/bmjqs-2015-004105.
- Cram P, Hillis SL, Barnett M, Rosenthal GE. Effects of weekend admission and hospital teaching status on in-hospital mortality. Am J Med 2004;117:151-7. https://doi.org/10.1016/j.amjmed.2004.02.035.
- Goldstein SD, Papandria DJ, Aboagye J, Salazar JH, Van Arendonk K, Al-Omar K, et al. The ‘weekend effect’ in pediatric surgery – increased mortality for children undergoing urgent surgery during the weekend. J Pediatr Surg 2014;49:1087-91. https://doi.org/10.1016/j.jpedsurg.2014.01.001.
- Gordon HS, Johnson ML, Wray NP, Petersen NJ, Henderson WG, Khuri SF, et al. Mortality after noncardiac surgery: prediction from administrative versus clinical data. Med Care 2005;43:159-67. https://doi.org/10.1097/00005650-200502000-00009.
- Gould JB, Qin C, Marks AR, Chavez G. Neonatal mortality in weekend vs. weekday births. JAMA 2003;289:2958-62. https://doi.org/10.1001/jama.289.22.2958.
- Zapf MA, Kothari AN, Markossian T, Gupta GN, Blackwell RH, Wai PY, et al. The ‘weekend effect’ in urgent general operative procedures. Surgery 2015;158:508-14. https://doi.org/10.1016/j.surg.2015.02.024.
- An R. Impact of weekend admission on in-hospital mortality among U.S. adults, 2003–2013. Ann Epidemiol 2017;27:790-5. https://doi.org/10.1016/j.annepidem.2017.10.001.
- Auger KA, Davis MM. Pediatric weekend admission and increased unplanned readmission rates. J Hosp Med 2015;10:743-5. https://doi.org/10.1002/jhm.2426.
- Bendavid E, Kaganova Y, Needleman J, Gruenberg L, Weissman JS. Complication rates on weekends and weekdays in US hospitals. Am J Med 2007;120:422-8. https://doi.org/10.1016/j.amjmed.2006.05.067.
- Ozrazgat-Baslanti T, Blanc P, Thottakkara P, Ruppert M, Rashidi P, Momcilovic P, et al. Preoperative assessment of the risk for multiple complications after surgery. Surgery 2016;160:463-72. https://doi.org/10.1016/j.surg.2016.04.013.
- Ricciardi R, Nelson J, Francone TD, Roberts PL, Read TE, Hall JF, et al. Do patient safety indicators explain increased weekend mortality?. J Surg Res 2016;200:164-70. https://doi.org/10.1016/j.jss.2015.07.030.
- Dubois L, Vogt K, Vinden C, Winick-Ng J, McClure JA, Roshanov PS, et al. Association between day of the week of elective surgery and postoperative mortality. CMAJ 2017;189:E303-E309. https://doi.org/10.1503/cmaj.160511.
- Zare MM, Itani KM, Schifftner TL, Henderson WG, Khuri SF. Mortality after nonemergent major surgery performed on Friday versus Monday through Wednesday. Ann Surg 2007;246:866-74. https://doi.org/10.1097/SLA.0b013e3180cc2e60.
- de Graaf JP, Ravelli AC, Visser GH, Hukkelhoven C, Tong WH, Bonsel GJ, et al. Increased adverse perinatal outcome of hospital delivery at night. BJOG 2010;117:1098-107. https://doi.org/10.1111/j.1471-0528.2010.02611.x.
- Frank-Wolf M, Tovbin J, Wiener Y, Neeman O, Kurzweil Y, Maymon R. Is there a correlation between time of delivery and newborn cord pH?. J Matern Fetal Neonatal Med 2017;30:1637-40. https://doi.org/10.1080/14767058.2016.1220526.
- Lyndon A, Lee HC, Gay C, Gilbert WM, Gould JB, Lee KA. Effect of time of birth on maternal morbidity during childbirth hospitalization in California. Am J Obstet Gynecol 2015;213:705.e1-11. https://doi.org/10.1016/j.ajog.2015.07.018.
- Snowden JM, Kozhimannil KB, Muoto I, Caughey AB, McConnell KJ. A ‘busy day’ effect on perinatal complications of delivery on weekends: a retrospective cohort study. BMJ Qual Saf 2017;26. https://doi.org/10.1136/bmjqs-2016-005257.
- Wu YW, Pham TN, Danielsen B, Towner D, Smith L, Johnston SC. Nighttime delivery and risk of neonatal encephalopathy. Am J Obstet Gynecol 2011;204:37.e1-6. https://doi.org/10.1016/j.ajog.2010.09.022.
- Buckley D, Bulger D. Trends and weekly and seasonal cycles in the rate of errors in the clinical management of hospitalized patients. Chronobiol Int 2012;29:947-54. https://doi.org/10.3109/07420528.2012.672265.
- Baines RJ, Langelaan M, de Bruijne MC, Wagner C. Is researching adverse events in hospital deaths a good way to describe patient safety in hospitals: a retrospective patient record review study. BMJ Open 2015;5. https://doi.org/10.1136/bmjopen-2014-007380.
- Graham C. People’s experiences of hospital care on the weekend: secondary analysis of data from two national patient surveys. BMJ Qual Saf 2018;27:455-63. https://doi.org/10.1136/bmjqs-2016-006349.
- Hunter RM, Fulop NJ, Boaden RJ, McKevitt C, Perry C, Ramsay AIG, et al. The potential role of cost-utility analysis in the decision to implement major system change in acute stroke services in metropolitan areas in England. Health Res Policy Syst 2018;16. https://doi.org/10.1186/s12961-018-0301-5.
- Melnychuk M, Morris S, Black G, Ramsay AIG, Eng J, Rudd A, et al. Variation in quality of acute stroke care by day and time of admission: prospective cohort study of weekday and weekend centralised hyperacute stroke unit care and non-centralised services. BMJ Open 2019;9. https://doi.org/10.1136/bmjopen-2018-025366.
- Balinskaite V, Bottle A, Shaw LJ, Majeed A, Aylin P. Reorganisation of stroke care and impact on mortality in patients admitted during weekends: a national descriptive study based on administrative data. BMJ Qual Saf 2018;27:611-18. https://doi.org/10.1136/bmjqs-2017-006681.
- NHS England . Bed Availability and Occupancy Data – Overnight n.d. www.england.nhs.uk/statistics/statistical-work-areas/bed-availability-and-occupancy/bed-data-overnight/ (accessed 5 February 2020).
- NHS Digital . Summary Hospital-Level Mortality Indicator (SHMI) Indicator Specification 2019. https://files.digital.nhs.uk/93/E9232B/SHMI%20specification%20v1.31.pdf (accessed 5 February 2020).
- Wu TC, Chuah SK, Chang KC, Wu CK, Kuo CH, Wu KL, et al. Outcome of holiday and nonholiday admission patients with acute peptic ulcer bleeding: a real-world report from southern Taiwan. Biomed Res Int 2014;2014. https://doi.org/10.1155/2014/906531.
- Meacock R, Anselmi L, Kristensen SR, Doran T, Sutton M. Higher mortality rates amongst emergency patients admitted to hospital at weekends reflect a lower probability of admission. J Health Serv Res Policy 2017;22:12-9. https://doi.org/10.1177/1355819616649630.
- Ministry of Housing, Communities and Local Government . The English Indices of Deprivation 2015 2015. www.gov.uk/government/statistics/english-indices-of-deprivation-2015 (accessed 26 February 2021).
- Royal College of Physicians . National Early Warning Score (NEWS) – Standardising the Assessment of Acute-Illness Severity in the NHS 2012.
- Levy MM, Artigas A, Phillips GS, Rhodes A, Beale R, Osborn T, et al. Outcomes of the Surviving Sepsis Campaign in intensive care units in the USA and Europe: a prospective cohort study. Lancet Infect Dis 2012;12:919-24. https://doi.org/10.1016/S1473-3099(12)70239-6.
- Tarrant C, Sutton E, Angell E, Aldridge CP, Boyal A, Bion J. HiSLAC study . The ‘weekend effect’ in acute medicine: a protocol for a team-based ethnography of weekend care for medical patients in acute hospital settings. BMJ Open 2017;7. https://doi.org/10.1136/bmjopen-2017-016755.
- Bion J, Aldridge CP, Girling A, Rudge G, Beet C, Evans T, et al. Two-epoch cross-sectional case record review protocol comparing quality of care of hospital emergency admissions at weekends versus weekdays. BMJ Open 2017;7. https://doi.org/10.1136/bmjopen-2017-018747.
- Royal College of Physicians . Acute Care Toolkit 2011. www.rcplondon.ac.uk/projects/acute-care-toolkits (accessed January 2020).
- NHS Digital . Seven-Day Services n.d. https://digital.nhs.uk/data-and-information/publications/clinical-indicators/seven-day-services (accessed 12 February 2020).
- Cameron KS. Cultural congruence, strength, and type: relationships to effectiveness. Rev Higher Educ 1985;9. https://doi.org/10.1353/rhe.1985.0034.
- Mannion R, Harrison S, Jacobs R, Konteh F, Walshe K, Davies HT. From cultural cohesion to rules and competition: the trajectory of senior management culture in English NHS hospitals, 2001–2008. J R Soc Med 2009;102:332-6. https://doi.org/10.1258/jrsm.2009.090066.
- Benning A, Ghaleb M, Suokas A, Dixon-Woods M, Dawson J, Barber N, et al. Large scale organisational intervention to improve patient safety in four UK hospitals: mixed method evaluation. BMJ 2011;342. https://doi.org/10.1136/bmj.d195.
- Hogan H, Zipfel R, Neuburger J, Hutchings A, Darzi A, Black N. Avoidability of hospital deaths and association with hospital-wide mortality ratios: retrospective case record review and regression analysis. BMJ 2015;351. https://doi.org/10.1136/bmj.h3239.
- Hutchinson A, Coster JE, Cooper KL, Pearson M, McIntosh A, Bath PA. A structured judgement method to enhance mortality case note review: development and evaluation. BMJ Qual Saf 2013;22:1032-40. https://doi.org/10.1136/bmjqs-2013-001839.
- Gibson A. Using the Structured Judgement Review Method – A Clinical Governance Guide to Mortality Case Record Reviews. London: Royal College of Physicians; 2016.
- Mayor S, Baines E, Vincent C, Lankshear A, Edwards A, Aylward M, et al. Measuring harm and informing quality improvement in the Welsh NHS: the longitudinal Welsh national adverse events study. Health Serv Deliv Res 2017;5. https://doi.org/10.3310/hsdr05090.
- de Vet HCW, Mokkink LB, Mosmuller DG, Terwee CB. Spearman-Brown prophecy formula and Cronbach’s alpha: different faces of reliability and opportunities for new applications. J Clin Epidemiol 2017;85:45-9. https://doi.org/10.1016/j.jclinepi.2017.01.013.
- Jones L, Pomeroy L, Robert G, Burnett S, Anderson JE, Morris S, et al. Explaining organisational responses to a board-level quality improvement intervention: findings from an evaluation in six providers in the English National Health Service. BMJ Qual Saf 2019;28:198-204. https://doi.org/10.1136/bmjqs-2018-008291.
- Panagioti M, Khan K, Keers RN, Abuzour A, Phipps D, Kontopantelis E, et al. Prevalence, severity, and nature of preventable patient harm across medical care settings: systematic review and meta-analysis. BMJ 2019;366. https://doi.org/10.1136/bmj.l4185.
- Chen YF, Hemming K, Stevens AJ, Lilford RJ. Secular trends and evaluation of complex interventions: the rising tide phenomenon. BMJ Qual Saf 2016;25:303-10. https://doi.org/10.1136/bmjqs-2015-004372.
- Benning A, Dixon-Woods M, Nwulu U, Ghaleb M, Dawson J, Barber N, et al. Multiple component patient safety intervention in English hospitals: controlled evaluation of second phase. BMJ 2011;342. https://doi.org/10.1136/bmj.d199.
- Bottery S, Ward D, Fenney D. Social Care 360. London: The King’s Fund; 2019.
- Hofer TP, Asch SM, Hayward RA, Rubenstein LV, Hogan MM, Adams J, et al. Profiling quality of care: is there a role for peer review?. BMC Health Serv Res 2004;4. https://doi.org/10.1186/1472-6963-4-9.
- Hofer TP, Bernstein SJ, DeMonner S, Hayward RA. Discussion between reviewers does not improve reliability of peer review of hospital quality. Med Care 2000;38:152-61. https://doi.org/10.1097/00005650-200002000-00005.
- Manaseki-Holland S, Lilford RJ, Te AP, Chen YF, Gupta KK, Chilton PJ, et al. Ranking hospitals based on preventable hospital death rates: a systematic review with implications for both direct measurement and indirect measurement through standardized mortality rates. Milbank Q 2019;97:228-84. https://doi.org/10.1111/1468-0009.12375.
- NHS England . The NHS Belongs to the People: A Call to Action 2013. www.england.nhs.uk/wp-content/uploads/2013/07/nhs-belongs.pdf.
- HM Treasury . Spending Review and Autumn Statement 2015 2015.
- Curtis L, Burns A. Unit Costs of Health and Social Care 2016 2016. www.pssru.ac.uk/project-pages/unit-costs/unit-costs-2016/ (accessed 31 January 2020).
- Drummond M, Sculpher M, Claxton K, Stoddart G, Torrance G. Methods for the Economic Evaluation of Health Care Programmes. Oxford: Oxford University Press; 2015.
- National Institute for Health and Care Excellence . Developing NICE Guidelines: The Manual 2018.
- National Institute for Health and Care Excellence . Emergency and Acute Medical Care in Over 16s: Service Delivery and Organisation 2018.
- Lilford RJ, Chilton PJ, Hemming K, Girling AJ, Taylor CA, Barach P. Evaluating policy and service interventions: framework to guide selection and interpretation of study end points. BMJ 2010;341. https://doi.org/10.1136/bmj.c4413.
- Pearl J, Hoyle RH. Handbook of Structural Equation Modeling. New York, NY: Guilford Press; 2012.
- Godlee F. The ‘weekend effect’. BMJ 2016;353. https://doi.org/10.1136/bmj.i2801.
- Greenland S. Causal analysis in the health sciences. J Am Stat Assoc 2000;95:286-9. https://doi.org/10.1080/01621459.2000.10473924.
- Greenland S, Brumback B. An overview of relations among causal modelling methods. Int J Epidemiol 2002;31:1030-7. https://doi.org/10.1093/ije/31.5.1030.
- Sobel ME. Causal inference in the social sciences. J Am Stat Assoc 2000;95:647-51. https://doi.org/10.1080/01621459.2000.10474243.
- Anselmi L, Meacock R, Kristensen SR, Doran T, Sutton M. Arrival by ambulance explains variation in mortality by time of admission: retrospective study of admissions to hospital following emergency department attendance in England. BMJ Qual Saf 2017;26:613-21. https://doi.org/10.1136/bmjqs-2016-005680.
- Yao GL, Novielli N, Manaseki-Holland S, Chen YF, van der Klink M, Barach P, et al. Evaluation of a predevelopment service delivery intervention: an application to improve clinical handovers. BMJ Qual Saf 2012;21:i29-38. https://doi.org/10.1136/bmjqs-2012-001210.
- Brennan TA, Leape LL, Laird NM, Hebert L, Localio AR, Lawthers AG, et al. Incidence of adverse events and negligence in hospitalized patients. N Engl J Med 1991;324:370-6. https://doi.org/10.1056/NEJM199102073240604.
- Kennerly DA, Kudyakov R, da Graca B, Saldaña M, Compton J, Nicewander D, et al. Characterization of adverse events detected in a large health care delivery system using an enhanced global trigger tool over a five-year interval. Health Serv Res 2014;49:1407-25. https://doi.org/10.1111/1475-6773.12163.
- Landrigan CP, Parry GJ, Bones CB, Hackbarth AD, Goldmann DA, Sharek PJ. Temporal trends in rates of patient harm resulting from medical care. N Engl J Med 2010;363:2124-34. https://doi.org/10.1056/NEJMsa1004404.
- Hoonhout LH, de Bruijne MC, Wagner C, Zegers M, Waaijman R, Spreeuwenberg P, et al. Direct medical costs of adverse events in Dutch hospitals. BMC Health Serv Res 2009;9. https://doi.org/10.1186/1472-6963-9-27.
- Zegers M, de Bruijne MC, Wagner C, Hoonhout LH, Waaijman R, Smits M, et al. Adverse events and potentially preventable deaths in Dutch hospitals: results of a retrospective patient record review study. Qual Saf Health Care 2009;18:297-302. https://doi.org/10.1136/qshc.2007.025924.
- Ham C. Making Sense of Integrated Care Systems. London: The King’s Fund; 2018.
- NHS Digital . Health Survey for England n.d. https://digital.nhs.uk/data-and-information/publications/statistical/health-survey-for-england (accessed 5 May 2021).
- British Medical Association . Pay Scales for Consultants in England 2019. www.bma.org.uk/pay-and-contracts/pay/consultants-pay-scales/pay-scales-for-consultants-in-england (accessed 8 February 2020).
- NHS Improvement . 2017/18/Reference/Costs n.d. https://webarchive.nationalarchives.gov.uk/20200501111106/https://improvement.nhs.uk/resources/reference-costs/ (accessed 5 May 2021).
- Gelman A, Jakulin A, Pittau MG, Su YS. A weakly informative default prior distribution for logistic and other regression models. Ann Appl Stat 2008;2:1360-83. https://doi.org/10.1214/08-AOAS191.
- Meacock R, Sutton M. Elevated mortality among weekend hospital admissions is not associated with adoption of seven day clinical standards. Emerg Med J 2018;35:108-13. https://doi.org/10.1136/emermed-2017-206740.
- Sutton M, Garfield-Birkbeck S, Martin G, Meacock M, Sculpher M, Street A, et al. Economic analysis of service and delivery interventions in health care. Health Serv Deliv Res 2018;6. https://doi.org/10.3310/hsdr06050.
- Watson S, Lilford R, Raine R, Fitzpatrick R, Barratt H, Bevan G, et al. Health Serv Deliv Res. 2016.
- Watson SI, Chen YF, Bion JF, Aldridge CP, Girling A, Lilford RJ. HiSLAC Collaboration . Protocol for the health economic evaluation of increasing the weekend specialist to patient ratio in hospitals in England. BMJ Open 2018;8. https://doi.org/10.1136/bmjopen-2016-015561.
- Gabry J, Simpson D, Vehtari A, Betancourt M, Gelman A. Visualization in Bayesian workflow. J R Stat Soc A 2019;182:389-402. https://doi.org/10.1111/rssa.12378.
- Hubbard RE, Peel NM, Samanta M, Gray LC, Mitnitski A, Rockwood K. Frailty status at admission to hospital predicts multiple adverse outcomes. Age Ageing 2017;46:801-6. https://doi.org/10.1093/ageing/afx081.
- Kahlon S, Pederson J, Majumdar SR, Belga S, Lau D, Fradette M, et al. Association between frailty and 30-day outcomes after discharge from hospital. CMAJ 2015;187:799-804. https://doi.org/10.1503/cmaj.150100.
- Andreasen J, Gobbens RJJ, Eriksen HH, Overvad K. Health-related quality of life at hospital discharge as a predictor for 6-month unplanned readmission and all-cause mortality of acutely admitted older medical patients. Qual Life Res 2019;28:3015-24. https://doi.org/10.1007/s11136-019-02259-w.
- Keeble E, Roberts HC, Williams CD, Van Oppen J, Conroy SP. Outcomes of hospital admissions among frail older people: a 2-year cohort study. Br J Gen Pract 2019;69:e555-e560. https://doi.org/10.3399/bjgp19X704621.
- van Rijn M, Buurman BM, MacNeil-Vroomen JL, Suijker JJ, ter Riet G, Moll van Charante EP, et al. Changes in the in-hospital mortality and 30-day post-discharge mortality in acutely admitted older patients: retrospective observational study. Age Ageing 2016;45:41-7. https://doi.org/10.1093/ageing/afv165.
- Girling AJ, Hofer TP, Wu J, Chilton PJ, Nicholl JP, Mohammed MA, et al. Case-mix adjusted hospital mortality is a poor proxy for preventable mortality: a modelling study. BMJ Qual Saf 2012;21:1052-6. https://doi.org/10.1136/bmjqs-2012-001202.
- Baxter S, Johnson M, Chambers D, Sutton A, Goyder E, Booth A. Understanding new models of integrated care in developed countries: a systematic review. Health Serv Deliv Res 2018;6. https://doi.org/10.3310/hsdr06290.
- NHS England . Sustainability and Transformation Partnerships 2020.
- NHS England . No Hospital Is an Island – Learning from the Acute Care Collaborations 2018.
- Healthy London Partnership . Case Study: Collaboratively Transforming Urgent and Emergency Care in London 2019.
- University of Warwick . NIHR Applied Research Collaboration (ARC) West Midlands 2020. https://warwick.ac.uk/fac/sci/med/about/centres/arc-wm/ (accessed 5 August 2020).
- Razzak J, Usmani MF, Bhutta ZA. Global, regional and national burden of emergency medical diseases using specific emergency disease indicators: analysis of the 2015 Global Burden of Disease Study. BMJ Glob Health 2019;4. https://doi.org/10.1136/bmjgh-2018-000733.
- National Institute for Health Research . Funding Opportunities; Themed Call 2020. www.nihr.ac.uk/researchers/funding-opportunities/?custom_in_Workstream=9443 (accessed 5 August 2020).
- Güss CD. What is going through your mind? Thinking aloud as a method in cross-cultural psychology. Front Psychol 2018;9. https://doi.org/10.3389/fpsyg.2018.01292.
- NHS England . Models of Care 2020. www.england.nhs.uk/new-care-models/about/ (accessed 6 February 2020).
- Morse SA. Developing New Care Models Through NHS Vanguards. London: National Audit Office; 2018.
- O’Neill S, Wolters A, Steventon A. The Impact of Redesigning Urgent and Emergency Care in Northumberland: Health Foundation Consideration of Findings from the Improvement Analytics Unit. London: The Health Foundation; 2017.
- NHS England . Rapid NHS Response Teams to Help People Stay Well at Home 2020. www.england.nhs.uk/2020/01/rapid-nhs-response-teams-to-help-people-stay-well-at-home/.
- Finnikin S, Hayward G, Wilson F, Lasserson D. Are referrals to hospital from out-of-hours primary care associated with National Early Warning Scores?. Emerg Med J 2020;37:279-85. https://doi.org/10.1136/emermed-2019-209069.
- Baxter S, Johnson M, Chambers D, Sutton A, Goyder E, Booth A. The effects of integrated care: a systematic review of UK and international evidence. BMC Health Serv Res 2018;18. https://doi.org/10.1186/s12913-018-3161-3.
- Liljas AEM, Brattström F, Burström B, Schön P, Agerholm J. Impact of integrated care on patient-related outcomes among older people – a systematic review. Int J Integr Care 2019;19. https://doi.org/10.5334/ijic.4632.
- Hillman K, Chen J, Cretikos M, Bellomo R, Brown D, Doig G, et al. Introduction of the medical emergency team (MET) system: a cluster-randomised controlled trial. Lancet 2005;365:2091-7. https://doi.org/10.1016/S0140-6736(05)66733-5.
- Bion J, Richardson A, Hibbert P, Beer J, Abrusci T, McCutcheon M, et al. ‘Matching Michigan’: a 2-year stepped interventional programme to minimise central venous catheter-blood stream infections in intensive care units in England. BMJ Qual Saf 2013;22:110-23. https://doi.org/10.1136/bmjqs-2012-001325.
- Coiera E, Wang Y, Magrabi F, Concha OP, Gallego B, Runciman W. Predicting the cumulative risk of death during hospitalization by modeling weekend, weekday and diurnal mortality risks. BMC Health Serv Res 2014;14. https://doi.org/10.1186/1472-6963-14-226.
- Earnest A, Chen MI, Seow E. Exploring if day and time of admission is associated with average length of stay among inpatients from a tertiary hospital in Singapore: an analytic study based on routine admission data. BMC Health Serv Res 2006;6. https://doi.org/10.1186/1472-6963-6-6.
- Ricciardi R, Roberts PL, Read TE, Baxter NN, Marcello PW, Schoetz DJ. Mortality rate after nonelective hospital admission. Arch Surg 2011;146:545-51. https://doi.org/10.1001/archsurg.2011.106.
- Ricciardi R, Nelson J, Roberts PL, Marcello PW, Read TE, Schoetz DJ. Is the presence of medical trainees associated with increased mortality with weekend admission?. BMC Med Educ 2014;14. https://doi.org/10.1186/1472-6920-14-4.
- Sharp AL, Choi H, Hayward RA. Don’t get sick on the weekend: an evaluation of the weekend effect on mortality for patients visiting US EDs. Am J Emerg Med 2013;31:835-7. https://doi.org/10.1016/j.ajem.2013.01.006.
- Shiue I, McMeekin P, Price C. Retrospective observational study of emergency admission, readmission and the ‘weekend effect’. BMJ Open 2017;7. https://doi.org/10.1136/bmjopen-2016-012493.
- Sullivan C, Staib A, Eley R, Griffin B, Cattell R, Flores J, et al. Who is less likely to die in association with improved National Emergency Access Target (NEAT) compliance for emergency admissions in a tertiary referral hospital?. Australian Health Rev 2016;40:149-54. https://doi.org/10.1071/AH14242.
- Conway R, Cournane S, Byrne D, O’Riordan D, Silke B. Improved mortality outcomes over time for weekend emergency medical admissions. Ir J Med Sci 2018;187:5-11. https://doi.org/10.1007/s11845-017-1627-7.
- Conway R, Cournane S, Byrne D, O’Riordan D, Silke B. Survival analysis of weekend emergency medical admissions. QJM 2017;110:291-7. https://doi.org/10.1093/qjmed/hcw219.
- Vest-Hansen B, Riis AH, Sørensen HT, Christiansen CF. Out-of-hours and weekend admissions to Danish medical departments: admission rates and 30-day mortality for 20 common medical conditions. BMJ Open 2015;5. https://doi.org/10.1136/bmjopen-2014-006731.
- Beecher S, O’Leary DP, McLaughlin R. Increased risk environment for emergency general surgery in the context of regionalization and specialization. Int J Surg 2015;21:112-14. https://doi.org/10.1016/j.ijsu.2015.06.070.
- Ozdemir BA, Sinha S, Karthikesalingam A, Poloniecki JD, Pearse RM, Grocott MP, et al. Mortality of emergency general surgical patients and associations with hospital structures and processes. Br J Anaesth 2016;116:54-62. https://doi.org/10.1093/bja/aev372.
- Aylin P, Alexandrescu R, Jen MH, Mayer EK, Bottle A. Day of week of procedure and 30 day mortality for elective surgery: retrospective analysis of hospital episode statistics. BMJ 2013;346. https://doi.org/10.1136/bmj.f2424.
- Hamilton P, Restrepo E. Sociodemographic factors associated with weekend birth and increased risk of neonatal mortality. J Obstet Gynecol Neonatal Nurs 2006;35:208-14. https://doi.org/10.1111/j.1552-6909.2006.00034.x.
- Salihu HM, Ibrahimou B, August EM, Dagne G. Risk of infant mortality with weekend versus weekday births: a population-based study. J Obstet Gynaecol Res 2012;38:973-9. https://doi.org/10.1111/j.1447-0756.2011.01818.x.
- Snowden JM, Darney BG, Cheng YW, McConnell KJ, Caughey AB. Systems factors in obstetric care: the role of daily obstetric volume. Obstet Gynecol 2013;122:851-7. https://doi.org/10.1097/AOG.0b013e3182a2dd93.
Appendix 1 Directorate-level questionnaire
Appendix 2 Comparison of directorate-level questionnaire and point prevalence survey data on specialist hours per 10 emergency admissions for (a) Wednesday and (b) Sunday


Appendix 3 Characteristics of studies included in the quantitative systematic review reported
Study [rating of statistical adjustment]a | Country [number of hospitals] | Study period | Sample size (admissions, unless otherwise stated) | Type of admission (emergency, elective) | Type of procedure (medical, surgical, maternity) | Population | Mortality outcomes | Other outcomes: adverse events, length of stay, patient satisfaction |
---|---|---|---|---|---|---|---|---|
All admissions (including both medical and surgical, emergency and elective admissions) | ||||||||
Attenello 201553 [3] | USA (nationwide) [NR] | 2002–10 | 351,170,803 | Emergency and elective | Medical and surgical | All patients | In hospital | Hospital-acquired conditions |
Auger 201586 [4] | USA (Michigan) [1] | 2006–12 | 55,383 | Emergency and elective | Medical and surgical | Children only | – | Unplanned re-admission |
Coiera 2014182 [3] | Australia (New South Wales) [501] | 2000–7 | 11,732,260 | Emergency and elective | Medical, surgical and maternity | All patients | In/out of hospital up to 7 days post dischargeb | – |
Cram 200480 [2b or 3]c | USA (California) [NR] | 1998 | 1,100,984 | Emergency and elective | Medical and surgical | Adults only | In hospital | – |
Earnest 2006183 [4] | Singapore [1] | 2003–4 | 45,395 | Emergency and elective | Medical and surgical | Adults only | – | Length of stay |
Freemantle 201269 [2b] | UK (England) [NR] and USA (United Health Care System) [254] | 2009–10 | 14,217,640 | Emergency and elective | Medical, surgical and maternity | All patients | In/out of hospital (30 days); also in hospital (30 days) and 3 days | – |
Freemantle 201520 [2b] | UK (England) [NR] | 2013–14 | 14,818,374 | Emergency and elective | Medical, surgical and maternity | All patients | In/out of hospital (30-day) | Length of stay |
Graham 201899 [4] | UK (England) (NR) | 2014 | 59,083 | Emergency and elective | Medical and surgical | Adults only | – | Patient satisfaction |
Lee 201264 [4] | Malaysia (Perak) [1] | 2008–10 | 126,627 | Emergency and elective | Medical and surgical | All patients | In hospital | – |
Mohammed 201275 [3] | UK (England) [NR] | 2008–9 | 4,640,516 | Emergency and elective | Medical and surgical | Adults only | In hospital (at discharge) | |
Ruiz 201559 [3] | International: UK (England) [11], Australia [6], the Netherlands [6], USA [5] | 2009–12 | 2,982,570 | Emergency (all) and elective (surgical only) | Medical and surgical | All patients | In hospital (30 days) | – |
Medical admissions | ||||||||
Madsen 201462 [4] | Denmark (nationwide) [72] | 1995–2012 | 2,651,021 | Emergency and elective | Medical | Adults only | In hospital and 30 daysb | – |
Surgical admissions | ||||||||
Bendavid 200787 [3] | USA (New York, Massachusetts, North Carolina) [NR] | 1999–2001 | 4,967,114 | Emergency and elective | Surgical and obstetric | All patients | – | AHRQ Patient Safety Indicator (surgical and birth complications) |
Gordon 200582 [4] | USA (VA hospital) [44] | 1991–3 | 78,546 | Emergency and elective | Surgical (non-cardiac) | Adults | In hospital (30 days) | – |
Ozrazgat-Baslanti 201688 [3] | USA (Florida) [1] | 2000–10 | 50,314 | Emergency and elective | Surgical | Adults only | – | Post-operative complications |
Emergency admissions: medical and surgical | ||||||||
Aldridge 201635 [3] | UK (England) [141] | 2013–14 | 4,422,387 | Emergency | Medical and surgical | Adults only | In hospital | – |
An 201785 [3] | USA (nationwide) [NR] | 2003–13 | 51,762,178 | Non-elective | Medical, surgical and maternity | Adults only | In hospital | Length of stay |
Anselmi 2017142 [2b] | UK (England) [140] | 2013–14 | 3,027,946 | Emergency | Medical and surgical | Not stated | In hospital (30 days) | – |
Aylin 201068 [3] | UK (England) [163] | 2005–6 | 4,317,866 | Emergency | Medical and surgical | Adults and children | In hospital | – |
Barba 200666 [3] | Spain (Madrid) [1] | 1999–2003 | 35,993 | Emergency | Medical and surgical | Adults only | In hospital (2 days and any) | – |
Bell 200124 [4]d | Canada (Ontario) [NR] | 1988–97 | 3,789,917 | Emergency | Medical and surgical | Adults and children | In hospitalb | – |
De Giorgi 201563 [4] | Italy (Ferrara) [1] | 2000–13 | 411,588 | Emergency | Medical and surgical | All patients | In hospital (30 days) | – |
Han 201871 [2b] | UK (Salford) [1] | 2004–14 | 246,350 | Emergency | Medical and surgical | Adults only | In hospital, 7 days and 30 days | – |
Handel 201272 [4] | UK (Scotland) [NR] | 1999–2009 | 5,271,327 | Emergency | Medical and surgical | All patients | In hospital | – |
Lee 200667 [3] | Taiwan (province of China) (nationwide) [NR] | 2000–2 | 712,787e | Emergency | Medical and surgical | Adults and children | In/out of hospital (24 hours, 48 hours, 30 days) | – |
Meacock 201529 [4] | UK (England) [NR] | 2010–11 | 5,212,973 | Emergency | Medical and surgical | Not stated | In/out of hospital (30 days)b | – |
Meacock 2017106 [2b] | UK (England) [140] | 2013–14 | 4,656,586 | Emergency | Medical and surgical | All patients | In hospital (30 days) | – |
Meacock 2018154 [3] | UK (England) [123] | 2013–16 | Not stated | Emergency (also included supplementary data on all admissions) | Medical and surgical | Not stated | In/out of hospital (30 days) | – |
Perez Concha 201458 [3] | Australia (New South Wales) [501] | 2000–7 | 3,381,962 | Emergency | Medical and surgical | All patients | In/out of hospital (7 days); also reported in hospital and post discharge separately | – |
Ricciardi 2011184 [3] | USA (nationwide) [1000] | 2003–7 | 29,991,621 | Emergency | Medical and surgical | All patients | In hospital (vital status at discharge) | – |
Ricciardi 2014185 [3] | USA (nationwide) [1000] | 2003–8 | 26,051,775 | Emergency | Medical and surgical | All patients | In hospital (vital status at discharge)b | – |
Ricciardi 201689 [4] | USA (nationwide) [1000] | 2003–8 | 28,236,749 | Emergency | Medical and surgical | All patients | In hospital (vital status at discharge) | Patient safety indicators |
Roberts 201578 [4] | UK (England and Wales) [NR] | 2004–12 | 32,628,333 | Emergency | Medical and surgical | Not stated | 30 days (location not specified) | – |
Sharp 2013186 [4] | USA (nationwide) [NR] | 2008 | 4,225,973 | Emergency | Medical and surgical | Adults only | Mortality (not specified) | – |
Shiue 2017187 [4] | UK (Newcastle Upon Tyne) [1] | 2010–15 | 148,996 | Emergency | Medical and surgical | Not stated | In/out of hospital (30 days) | – |
Sullivan 2016188 [4] | Australia (Queensland) [1] | 2011 and 2013 | 34,184 | Emergency | Medical and surgical | All patients | In hospital (timing not specified) | – |
Walker 201754 [1] | UK (Oxford) [4] | 2006–14 | 503,938 | Emergency | Medical and surgical | All patients | In/out of hospital (30 days) | Admission to ICU |
Emergency medical admissions | ||||||||
Conway 2016,55 2017,189 2017190 [2a or 4]f | Ireland (Dublin) [1] | 2002–14 | 82,368 | Emergency | Medical | All patients | In hospital (30 days) | – |
Khanna 201152 [2b] | USA (Chicago) [1] | 2008 | 824 | Emergency | Medical | All patients | – | Need for ICU, 30-day ED revisit, 30-day re-admission, poor outcomes in the first 24 hours |
Maggs 201073 [4] | UK (Bath) [1] | 2007–8 | 15,594 | Emergency | Medical | Adults only | In hospital and ‘late’ mortality (in-hospital death beyond the first 7 days) | – |
Mikulich 201126 [2a] | Ireland (Dublin) [1] | 2002–9 | 25,833 | Emergency | Medical | Adults only | In hospital (30 days) | Length of stay |
Mohammed 201756 [2a] | UK (Yorkshire and Humberside) [4] | 2014 | 47,117 | Emergency | Medical | Adults only | In hospital (vital status at discharge) | Length of stay |
Vest-Hansen 2015191 [4] | Denmark (nationwide) [NR] | 2010 | 174,192 | Emergency | Medical | Adults only | In/out of hospital (30 days) | Length of stay |
Emergency surgical admissions | ||||||||
Beecher 2015192 [4] | Ireland (Galway) [1] | 2012-13 | 7041 | Emergency | Surgical | Not stated | – | Length of stay |
Gillies 201770 [3] | UK (Scotland) [NR] | 2005–7 | 50,844 | Emergency | Surgical | Adults only | In hospital or within 30 days; overall survival (4 years) | – |
Goldstein 201481 [2b] | USA (nationwide) [NR] | 1988–2010 | 439,457 | Emergency | Surgical | Children only | In hospital | Various surgical complications; length of stay |
McCallum 201674 [2b] | UK (Northern England) [NR] | 2000–14 | 370,671 | Emergency | Surgical | Adults only | In hospital (30 days) | Length of stay |
Ozdemir 2016193 [3] | UK (England) [156] | 2005–10 | 294,602 | Emergency | General surgical | All patients | In/out of hospital (30 days and 90 days) | – |
Zapf 201584 [3] | USA (Florida) [NR] | 2007–10 | 80,861 | Emergency | Surgical | All patients | In hospital (timing not specified) | Post-operative complications, length of stay |
Elective surgical admissions | ||||||||
Aylin 2013194 [2b] | UK (England) [163] | 2008–11 | 4,133,346 | Elective | Surgical | Adults and children | In/out hospital, (30 days and 2 days) | – |
Dubois 201790 [2b] | Canada (Ontario) [NR] | 2002–12 | 402,899 | Elective | Surgical (day of surgery) | Adults only | In/out of hospital (30 days), also 2 days and 90 days and in hospital | Admission to ICU; re-admission (30 days); reoperation (30 days); length of stay |
McIsaac 201461 [2b] | Canada (Ontario) [NR] | 2002–12 | 333,344 | Elective | Surgical (non-cardiac) | Adults only (aged ≥ 40 years) | In/out of hospital (30 days and 2 days) | – |
Ruiz 201679 [2b] | UK (England) [163] | 2008–11 | 3,922,091 | Elective | Surgical | Adults and children | In/out of hospital (30 days) | – |
Zare 200791 [2b] | USA (VA hospitals) [124] | 2000–4 | 188,212 | Elective | Surgical | Adults only | 30 days (location not specified) | Post-operative morbidity (complications) |
Maternity admissions | ||||||||
de Graaf 201092 [2b] | The Netherlands (nationwide) [99] | 2000–6 | 764,406 | Spontaneous and elective | Medical and surgical | Maternity | Intrapartum and early neonatal (within 7 days of birth) | 5-minute Apgar score of < 7; transfer to neonatal ICU |
Frank-Wolf 201793 [4] | Israel [1] | 2005–14 | 56,428 | Spontaneous and elective | Medical and surgical | Maternity | – | Cord blood pH of < 7; 5-minute Apgar score of < 7 |
Gijsen 201265 [2b] | The Netherlands (nationwide) [NR] | 2003–7 | 449,714 | Spontaneous | Medical and surgical | Maternity | Intrapartum and early neonatal (within 7 days of birth) | 5-minute Apgar score of < 7; a composite measure of adverse outcomes |
Gould 200383 [3] | USA (California) [NR] | 1995–7 | 1,615,041 | Spontaneous and elective | Medical and surgical | Maternity | Neonatal mortality (within 28 days of birth) | – |
Hamilton 2006195 [4] | USA (Texas) [NR] | 1999–2001 | 923,905 | Not stated | Not stated | Maternity | Neonatal mortality (within 27 days of birth)b | – |
Luo 200460 [4] | Canada (nationwide, excluding Ontario) [NR] | 1985–98 | 3,239,972 | Spontaneous and elective | Medical and surgical | Maternity | Neonatal mortality (within 6 days of birth) | – |
Lyndon 201594 [4] | USA (California) [NR] | 2005–7 | 1,475,593 | Spontaneous and elective | Medical and surgical | Maternity | – | Pelvic morbidity, severe maternal morbidity |
Palmer 201576 [2b] | UK (England) [NR] | 2010–12 | 1,332,835 maternity admissions and 1,349,599 births | Spontaneous and elective | Medical and surgical | Maternity and neonates | In-hospital perinatal mortality | Maternal and neonatal infections, emergency re-admissions and injuries |
Pasupathy 201077 [3] | UK (Scotland) [NR] | 1985–2004 | 1,039,560 | Spontaneous and elective | Medical and surgical | Maternity and neonates | Neonatal mortality (within first week of birth) | – |
Salihu 2012196 [4] | USA (Missouri) | 1989–97 | Not stated | Spontaneous and elective | Medical and surgical | Maternity and neonates | Neonatal, post-neonatal and infant death | –g |
Snowden 2013197 [4] | USA (California) [257] | 2006 | 462,322 | Spontaneous and elective | Medical and surgical | Maternity | – | Birth asphyxia |
Snowden 201795 [4] | USA (California) [214] | 2009–10 | 724,967 | Spontaneous and elective | Medical and surgical | Maternity | Neonatal death (timing not specified) | Adverse maternal and neonatal outcomes (including prolonged length of stay) |
Wu 201196 [4] | USA (California) [NR] | 1999–2002 | 1,864,766 | Spontaneous and elective | Medical and surgical | Maternity | – | Neonatal encephalopathy |
Other | ||||||||
Buckley 201297 [4] | Australia (New South Wales) [63] | 2006–10 | 4370 clinical management incidents | Unclear | Unclear | Unclear | – | Adverse events |
Appendix 4 Results of meta-regressions of the weekend effect on mortality for the quantitative systematic review
Reproduced from Chen et al. 27 with permission. © Author(s) (or their employer(s)) 2019. Re-use permitted under CC BY. Published by BMJ. This is an open access article distributed in accordance with the Creative Commons Attribution 4.0 Unported (CC BY 4.0) license, which permits others to copy, redistribute, remix, transform and build upon this work for any purpose, provided the original work is properly cited, a link to the licence is given, and indication of whether changes were made. See: https://creativecommons.org/licenses/by/4.0/. The text below includes minor additions and formatting changes to the original text.
Parameter | Number of estimates in category | Estimate (95% CrI) | Percentage difference in OR (compared with baseline/reference category) (95% CrI) |
---|---|---|---|
Intercept | – | 0.05 (–0.10 to 0.20) | (Baseline/reference category OR) 1.05 (0.90 to 1.22) |
Adequacy of statistical adjustment | |||
1 or 2a: adjustment including measures of acute physiology | 5 | Reference | Reference |
2b: adequate adjustment of main and contextual factors | 40 | 0.13 (–0.03 to 0.30) | 14 (–3 to 35) |
3: partial adjustment | 40 | 0.13 (–0.03 to 0.29) | 14 (–3 to 34) |
4: inadequate adjustment | 34 | 0.15 (–0.01 to 0.31) | 16 (–1 to 37) |
Surgical admissions, yes | 81 | –0.04 (–0.14 to 0.06) | –4 (–13 to 6) |
Elective admissions, yes | 27 | 0.27 (0.21 to 0.32) | 31 (24 to 38) |
Maternity admissions, yes | 23 | –0.18 (–0.26 to –0.10) | –17 (–23 to –10) |
Time (linear trend) | 119 | 0.00 (0.00 to 0.00) | 0 (0 to 0) |
Total number of observations/estimates | 119 |
Time (year) was selected as the mid-point of the data collection period. Categories 1 (comprehensive adjustment) and 2a (adequate adjustment including measures of acute physiology) were combined because of the small number of studies in these categories. Estimates can be interpreted as approximate percentage increase in the estimate of WEOR. Meta-regressions also have country random effect (varying intercept for countries). Individual studies can contribute to multiple estimates of the weekend effect, for example by individual years, different patient subgroups and individual weekdays/weekend days (e.g. Saturday vs. Wednesday and Sunday vs. Wednesday).
Appendix 5 Within-samples variance function
For each of the 548 survey samples (i.e. available combinations of site and year), the SD of the average number of hours worked on a particular day per responder is estimated using a finite population correction based on the response rate. For a sample with N responders:
where f is the response rate in that particular sample (f = clean responses ÷ total surveyed) and SD is the standard deviation of the number of hours worked.
For a sample of size N, the coefficient of variation of the ratio of Sunday hours to Wednesday hours is estimated using the approximate formula:
where the values for the means (and SDs) of the reported hours per site are as follows: Wednesday, 1.707 hours (3.057 hours), and Sunday, 0.683 hours (2.257 hours). The SDs (which are pooled across the 548 samples) are much larger than the corresponding means. This reflects the substantial proportion of zeros in the samples, when a particular specialist was not present in the hospital on the specified date. The pooled correlation (RSW) between Sunday and Wednesday hours worked by an individual specialist was estimated as 0.556.
The formula in Equation 5 ignores errors arising from imprecise recollection of numbers of hours worked, which were not recorded by the respondents at the time. In practice, the associated component of variance is likely to be dominated by the within-sample variance of the numbers of hours actually worked. A more exact approximation would replace f with f ÷ (1 + K) in Equation 5, where K is the ratio of these components. The value of K cannot be estimated directly from our data, although it is likely to be small and, thus, to have minimal impact on the calculations.
From the above formula, the standard error of the Sunday-to-Wednesday intensity ratio of hours per 10 EAs (= RSW) is approximated by:
where µR is the expected value of the ratio.
This expression is designed to capture variation in the intensity ratio associated with non-response to the survey. In practice, there is an additional term (denoted by σST2) reflecting day-to-day variation in staffing levels in each site at the time of the survey, and other local factors. Thus, a more complete version of the variance expression takes the form:
This form of the variance was used to generate a site-specific weighting function, W ∝ V–1, for use in a site by year analysis of variance of the ratio RSW. The quantity µR was calculated for each site as a pooled estimate of RSW across all available years. The additional variance term σST2 was obtained from a metaregression analysis of the intensity ratios, with standard errors from Equation 6. The resulting value of 0.01168 represents 43.7% of the average variance in RSW attributable to site-level variation, leaving 56.3% attributable to survey-related sampling error.
Appendix 6 Emergency admissions weekend and weekday, all non-specialists acute trusts in England, 2007/8 to 2017/18

Appendix 7 (Sequential) analysis of variance of Sunday-to-Wednesday intensity ratio by trust and year (n = 548)
Source | df | Sum of squares | Mean square | F | p-value |
---|---|---|---|---|---|
Model | 119 | 5.0808 | 0.0427 | 1.91 | < 0.0001 |
Size quintile (number of beds) | 4 | 0.4190 | 0.1047 | 2.67a | 0.0357 |
Trusts within quintiles | 111 | 4.3493 | 0.0392 | ||
Trusts | 115 | 4.7683 | 0.0415 | 1.85 | < 0.0001 |
Year (linear) | 1 | 0.2430 | 0.2430 | 10.85 | 0.0011 |
Year (non-linear) | 3 | 0.0695 | 0.0232 | ||
Between years | 4 | 0.3126 | 0.0781 | 3.49 | 0.0081 |
Residual | 428 | 9.5908 | 0.0224 |
Appendix 8 Thirty-day post-admission crude and adjusted mortality
Financial year | Crude mortality, n/N (%) | Crude WEOR (95% CI) | Adjusted WEOR (95% CI) | ||
---|---|---|---|---|---|
Weekend | Weekday | Overall | |||
2007/8 | 61,195/902,820 (6.78) | 186,238/2,946,122 (6.32) | 247,433/3,848,942 (6.43) | 1.08 (1.07 to 1.09) | 1.12 (1.11 to 1.13) |
2008/9 | 63,608/952,667 (6.68) | 193,622/3,137,365 (6.17) | 257,230/4,090,032 (6.29) | 1.09 (1.08 to 1.10) | 1.11 (1.10 to 1.12) |
2009/10 | 63,064/1,019,418 (6.19) | 191,179/3,338,566 (5.73) | 254,243/4,357,984 (5.83) | 1.09 (1.08 to 1.10) | 1.12 (1.10 to 1.13) |
2010/11 | 64,100/1,053,126 (6.09) | 189,770/3,405,395 (5.57) | 253,870/4,458,521 (5.69) | 1.10 (1.09 to 1.11) | 1.13 (1.11 to 1.14) |
2011/12 | 63,843/1,066,867 (5.98) | 187,349/3,395,259 (5.52) | 251,192/4,462,126 (5.63) | 1.09 (1.08 to 1.10) | 1.13 (1.11 to 1.14) |
2012/13 | 67,532/1,097,338 (6.15) | 195,920/3,440,563 (5.69) | 263,452/4,537,901 (5.81) | 1.09 (1.08 to 1.10) | 1.11 (1.10 to 1.12) |
2013/14 | 65,171/1,124,401 (5.80) | 188,843/3,515,969 (5.37) | 254,014/4,640,370 (5.47) | 1.08 (1.07 to 1.09) | 1.09 (1.08 to 1.10) |
2014/15 | 70,777/1,176,978 (6.01) | 201,422/3,664,658 (5.50) | 272,199/4,841,636 (5.62) | 1.10 (1.09 to 1.11) | 1.09 (1.08 to 1.10) |
2015/16 | 70,809/1,198,545 (5.91) | 201,095/3,787,652 (5.31) | 271,904/4,986,197 (5.45) | 1.12 (1.11 to 1.13) | 1.11 (1.10 to 1.12) |
2016/17 | 73,632/1,206,804 (6.10 | 208,892/3,896,015 (5.36) | 282,524/5,102,819 (5.54) | 1.15 (1.14 to 1.16) | 1.12 (1.11 to 1.13) |
2017/18 | 75,948/1,251,016 (6.07) | 216,249/4,069,184 (5.31) | 292,197/5,320,200 (5.49) | 1.15 (1.14 to 1.16) | 1.10 (1.09 to 1.12) |
Appendix 9 Risk-adjusted weekend-to-weekday mortality odds ratios
Year | OR | 95% CI |
---|---|---|
2014 | 1.107 | 1.093 to 1.122 |
2015 | 1.087 | 1.073 to 1.101 |
2016 | 1.107 | 1.094 to 1.121 |
2017 | 1.110 | 1.097 to 1.124 |
2018 | 1.104 | 1.090 to 1.117 |
Overall | 1.103 | 1.097 to 1.109 |
Appendix 10 (Sequential) analysis of variance of risk-adjusted weekend-to-weekday mortality odds ratio by trust and year (n = 548)
Source | df | Sum of squares | Mean square | F | p-value |
---|---|---|---|---|---|
Model | 119 | 1.0352 | 0.0087 | 1.47 | 0.0030 |
Quintile of bed size | 4 | 0.0312 | 0.0078 | 0.90 | 0.4685 |
Sites within quintiles | 111 | 0.9681 | 0.0087 | ||
Sites | 115 | 0.9994 | 0.0087 | 1.47 | 0.0034 |
Time (linear) | 1 | 0.0041 | 0.0041 | 0.70 | 0.4040 |
Time (non-linear) | 3 | 0.0317 | 0.0106 | 1.79 | 0.1491 |
Time | 4 | 0.0358 | 0.0090 | 1.51 | 0.1971 |
Residual | 428 | 2.5317 | 0.0059 |
Appendix 11 Case characteristics: NEWS24 status, ICU24-NEWS24 status, zero length of stay and associated mortality
Characteristics | Weekend | Weekday | Overall | p-valuea |
---|---|---|---|---|
Total, N (average per day) | 37,979 (91) | 125,149 (120) | 163,128 (112) | |
NEWS24 status, n (%) | ||||
Missing | 3146 (8.3) | 13,160 (10.5) | 16,306 (10.0) | < 0.001 |
Available | 34,833 (91.7) | 111,989 (89.5) | 146,822 (0.9) | < 0.001 |
ICU24 transfer cases, n (% of weekend/weekday total; % of the category; average per day) | ||||
NEWS24 missing | 1096 (69.4; 34.8; 2.6) | 2302 (60.9; 17.5; 2.2) | 3398 (63.4; 20.8; 2.3) | < 0.001; < 0.001 |
NEWS24 available | 483 (30.6; 1.4; 1.2) | 1479 (39.1; 1.3; 1.4) | 1962 (36.6; 1.3; 1.3) | < 0.001; 0.154 |
Total | 1579 (100.0; 4.2; 3.8) | 3781 (100.0; 3.0; 3.6) | 5360 (100.0; 3.3; 3.7) | –; < 0.001 |
Direct/indirect ICU,b n (% of weekend/weekday total; % of weekend/weekday ICU24 cases) | ||||
Direct ITU | 1202 (3.2; 76.1) | 2469 (2.0; 65.3) | 3671 (2.3; 68.5) | < 0.001; < 0.001 |
Indirect ITU | 377 (1.0; 23.9) | 1312 (1.0; 34.7) | 1689 (1.0; 31.5) | < 0.001; < 0.001 |
Direct ICU by NEWS status,b n (%) | ||||
NEWS missing | 1022 (85.0) | 2042 (82.7) | 3064 (83.5) | 0.078 |
NEWS available | 180 (15.0) | 427 (17.3) | 607 (16.5) | 0.078 |
Indirect ICU by NEWS status,b n (%) | ||||
NEWS missing | 74 (19.6) | 260 (19.8) | 334 (19.8) | 0.932 |
NEWS available | 303 (80.4) | 1052 (80.2) | 1355 (80.2) | 0.932 |
No ICU24 transfer cases, n (% of weekend/weekday total; % of the category; average per day) | ||||
NEWS24 missing | 2050 (5.6; 65.2; 4.9) | 10,858 (8.9; 82.5; 10.4) | 12,908 (8.2; 79.2; 8.8) | < 0.001; < 0.001 |
NEWS24 available | 34,350 (94.4; 98.6; 82.4) | 110,510 (91.1; 98.7; 105.9) | 144,860 (91.8; 98.7; 99.2) | < 0.001; 0.154 |
Total | 36,400 (100.0; 95.8; 87.3) | 121,368 (100.0; 97.0; 116.3) | 157,768 (100.0; 96.7; 108.0) | –; < 0.001 |
By NEWS band or ICU, n (%)b | ||||
ICU24 | 1579 (4.2) | 3781 (3.0) | 5360 (3.3) | < 0.001 |
No ICU24, NEWS ≥ 7 | 1257 (3.3) | 3627 (2.9) | 4884 (3.0) | < 0.001 |
No ICU24, NEWS 5–6 | 2091 (5.5) | 6008 (4.8) | 8099 (5.0) | < 0.001 |
No ICU24, NEWS 1–4 | 20,535 (54.1) | 66,297 (53.0) | 86,832 (53.2) | < 0.001 |
No ICU24, NEWS 0 | 10,467 (27.6) | 34,578 (27.6) | 45,045 (27.6) | 1.000 |
No ICU24, NEWS missing | 2050 (5.4) | 10,858 (8.7) | 12,908 (7.9) | < 0.001 |
Z-LOS, n (%) | ||||
ICU24 | 45 (2.8) | 92 (2.4) | 137 (2.6) | 0.394 |
No ICU24, NEWS ≥ 7 | 64 (5.1) | 191 (5.3) | 255 (5.2) | 0.784 |
No ICU24, NEWS 5–6 | 116 (5.5) | 351 (5.8) | 467 (5.8) | 0.611 |
No ICU24, NEWS 1–4 | 3073 (15.0) | 11,222 (16.9) | 14,295 (16.5) | < 0.001 |
No ICU24, NEWS 0 | 1952 (18.6) | 7652 (22.1) | 9604 (21.3) | < 0.001 |
No ICU24, NEWS missing | 1279 (62.4) | 7882 (72.6) | 9161 (71.0) | < 0.001 |
Total | 6529 (17.2) | 27,390 (21.9) | 33,919 (20.8) | < 0.001 |
Died at discharge of Z-LOS cases, n (%) | ||||
ICU24 | 31 (68.9) | 68 (73.9) | 99 (72.3) | 0.539 |
No ICU24, NEWS ≥ 7 | 41 (64.1) | 77 (40.3) | 118 (46.3) | 0.001 |
No ICU24, NEWS 5–6 | 8 (6.9) | 15 (4.3) | 23 (4.9) | 0.263 |
No ICU24, NEWS 1–4 | 2 (0.1) | 22 (0.2) | 24 (0.2) | 0.247 |
No ICU24, NEWS 0 | 3 (0.2) | 4 (0.1) | 7 (0.1) | 0.255 |
No ICU24, NEWS missing | 28 (2.2) | 73 (0.9) | 101 (1.1) | < 0.001 |
Total | 113 (1.7) | 259 (0.9) | 372 (1.1) | < 0.001 |
Appendix 12 Characteristics in subgroups: stratified by intensive care unit and NEWS status within 24 hours post admission
Characteristics | Weekend | Weekday | Overall | p-valuea |
---|---|---|---|---|
Total, N (average per day) | 37,979 (91) | 125,149 (120) | 163,128 (112) | |
Age (years), median (IQR) | ||||
No ICU24, NEWS missing | 51 (34–67) | 53 (37–69) | 53 (36–69) | < 0.001 |
No ICU24, NEWS 0 | 56 (37–76) | 57 (39–74) | 57 (39–74) | 0.541 |
No ICU24, NEWS 1–4 | 61 (41–78) | 62 (43–78) | 61 (42–78) | 0.083 |
No ICU24, NEWS 5–6 | 73 (56–83) | 71 (56–83) | 71 (56–83) | 0.125 |
No ICU24, NEWS ≥ 7 | 75 (62–84) | 74 (62–84) | 74 (62–84) | 0.316 |
ICU24 | 50 (33–66) | 54 (39–67) | 54 (37–67) | < 0.001 |
Age (years), mean (SD) | ||||
No ICU24, NEWS missing | 51.2 (20.296) | 53.4 (20.116) | 53.1 (20.160) | 0.000 |
No ICU24, NEWS 0 | 56.0 (22.309) | 56.2 (21.261) | 56.2 (21.509) | 0.462 |
No ICU24, NEWS 1–4 | 58.9 (22.350) | 59.2 (21.632) | 59.2 (21.805) | 0.018 |
No ICU24, NEWS 5–6 | 68.0 (19.592) | 67.5 (19.065) | 67.7 (19.202) | 0.361 |
No ICU24, NEWS ≥ 7 | 71.3 (17.285) | 70.8 (17.308) | 71.0 (17.301) | 0.376 |
ICU24 | 50.3 (19.815) | 53.3 (18.616) | 52.4 (19.024) | < 0.001 |
Sex (male), n (%) | ||||
No ICU24, NEWS missing | 1063 (51.8) | 5108 (47.0) | 6171 (47.8) | < 0.001 |
No ICU24, NEWS 0 | 5464 (52.2) | 17,670 (51.1) | 23,134 (51.4) | 0.008 |
No ICU24, NEWS 1–4 | 10,190 (49.6) | 32,664 (49.3) | 42,854 (49.4) | 0.395 |
No ICU24, NEWS 5–6 | 1005 (48.0) | 2877 (47.9) | 3882 (47.9) | 0.679 |
No ICU24, NEWS ≥ 7 | 634 (50.4) | 1681 (46.4) | 2315 (47.4) | 0.000 |
ICU24 | 1004 (63.6) | 2375 (62.8) | 3379 (63.0) | 0.053 |
Length of stay (days), median (IQR) | ||||
No ICU24, NEWS missing | 0 (0–1) | 0 (0–1) | 0 (0–1) | < 0.001 |
No ICU24, NEWS 0 | 2 (1–5) | 2 (1–5) | 2 (1–5) | 0.001 |
No ICU24, NEWS 1–4 | 3 (1–8) | 3 (1–8) | 3 (1–8) | 0.437 |
No ICU24, NEWS 5–6 | 6 (2–13) | 6 (2–14) | 6 (2–14) | 0.167 |
No ICU24, NEWS ≥ 7 | 6 (3–13) | 7 (3–14) | 7 (3–14) | 0.012 |
ICU24 | 11 (5–23) | 11 (6–24) | 11 (6–24) | 0.875 |
Length of stay (days), mean (SD) | ||||
No ICU24, NEWS missing | 1.8 (6.515) | 1.2 (5.373) | 1.3 (5.574) | < 0.001 |
No ICU24, NEWS 0 | 5.2 (10.341) | 5.3 (10.767) | 5.3 (10.670) | 0.573 |
No ICU24, NEWS 1–4 | 7.1 (12.983) | 7.3 (12.982) | 7.2 (12.982) | 0.200 |
No ICU24, NEWS 5–6 | 10.7 (14.385) | 11.6 (16.657) | 11.3 (16.105) | 0.035 |
No ICU24, NEWS ≥ 7 | 10.5 (13.257) | 12.0 (15.925) | 11.6 (15.295) | 0.004 |
ICU24 | 19.0 (23.445) | 19.8 (26.172) | 19.5 (25.399) | 0.334 |
Charlson Comorbidity Index, n (%) | ||||
No ICU24, NEWS missing | ||||
0 | 1273 (62.1) | 7029 (64.7) | 8302 (64.3) | 0.022 |
1–5 | 322 (15.7) | 1725 (15.9) | 2047 (15.9) | 0.838 |
> 5 | 455 (22.2) | 2104 (19.4) | 2559 (19.8) | 0.003 |
No ICU24, NEWS 0 | ||||
0 | 5926 (56.6) | 19,550 (56.5) | 25,476 (56.6) | 0.885 |
1–5 | 1747 (16.7) | 5701 (16.5) | 7448 (16.5) | 0.629 |
> 5 | 2794 (26.7) | 9327 (27.0) | 12,121 (26.9) | 0.571 |
No ICU24, NEWS 1–4 | ||||
0 | 9630 (46.9) | 30,480 (46.0) | 40,110 (46.2) | 0.020 |
1–5 | 3714 (18.1) | 11,699 (17.7) | 15,413 (17.8) | 0.149 |
> 5 | 7191 (35.0) | 24,118 (36.4) | 31,309 (36.1) | < 0.001 |
No ICU24, NEWS 5–6 | ||||
0 | 629 (30.1) | 1722 (28.7) | 2351 (29.0) | 0.218 |
1–5 | 409 (19.6) | 1222 (20.3) | 1631 (20.1) | 0.444 |
> 5 | 1053 (50.4) | 3064 (51.0) | 4117 (50.8) | 0.614 |
No ICU24, NEWS ≥ 7 | ||||
0 | 315 (25.1) | 826 (22.8) | 1141 (23.4) | 0.098 |
1–5 | 285 (22.7) | 729 (20.1) | 1014 (20.8) | 0.053 |
> 5 | 657 (52.3) | 2072 (57.1) | 2729 (55.9) | 0.003 |
ICU24 | ||||
0 | 853 (54.0) | 1786 (47.2) | 2639 (49.2) | < 0.001 |
1–5 | 239 (15.1) | 570 (15.1) | 809 (15.1) | 0.955 |
> 5 | 487 (30.8) | 1425 (37.7) | 1912 (35.7) | < 0.001 |
Income deprivation, median (IQR) | ||||
No ICU24, NEWS missing | 4 (1–7) | 4 (1–6) | 4 (1–6) | 0.150 |
No ICU24, NEWS 0 | 4 (1–6) | 4 (1–6) | 4 (1–6) | 0.178 |
No ICU24, NEWS 1–4 | 4 (1–6) | 4 (1–6) | 4 (1–6) | 0.231 |
No ICU24, NEWS 5–6 | 4 (1–6) | 4 (1–6) | 4 (1–6) | 0.252 |
No ICU24, NEWS ≥ 7 | 4 (1–6) | 4 (1–6) | 4 (1–6) | 0.485 |
ICU24 | 4 (1–7) | 4 (1–7) | 4 (1–7) | 0.826 |
Income deprivation, mean (SD) | ||||
No ICU24, NEWS missing | 4.3 (2.885) | 4.2 (2.749) | 4.1 (2.772) | 0.973 |
No ICU24, NEWS 0 | 4.2 (2.798) | 4.3 (2.812) | 4.3 (2.809) | 0.085 |
No ICU24, NEWS 1–4 | 4.2 (2.768) | 4.2 (2.753) | 4.2 (2.756) | 0.915 |
No ICU24, NEWS 5–6 | 4.0 (2.688) | 4.1 (2.621) | 4.0 (2.638) | 0.194 |
No ICU24, NEWS ≥ 7 | 4.0 (2.623) | 4.1 (2.616) | 4.0 (2.618) | 0.234 |
ICU24 | 4.5 (3.049) | 4.6 (3.065) | 4.6 (3.060) | 0.391 |
Previous admissions, n (%) | ||||
No ICU24, NEWS missing | ||||
0 | 1861 (90.8) | 9810 (90.4) | 11,671 (90.4) | 0.541 |
1 | 160 (7.8) | 916 (8.4) | 1076 (8.3) | 0.336 |
2 | 21 (1.0) | 109 (1.0) | 130 (1.0) | 0.934 |
≥ 3 | 8 (0.4) | 23 (0.2) | 31 (0.2) | 0.126 |
No ICU24, NEWS 0 | ||||
0 | 9329 (89.1) | 30,723 (88.9) | 40,052 (88.9) | 0.424 |
1 | 969 (9.3) | 3326 (9.6) | 4295 (9.5) | 0.272 |
2 | 145 (1.4) | 423 (1.2) | 568 (1.3) | 0.172 |
≥ 3 | 24 (0.2) | 106 (0.3) | 130 (0.3) | 0.183 |
No ICU24, NEWS 1–4 | ||||
0 | 17,822 (86.8) | 57,807 (87.2) | 75,629 (87.1) | 0.135 |
1 | 2279 (11.1) | 7329 (11.1) | 9608 (11.1) | 0.842 |
2 | 349 (1.7) | 967 (1.5) | 1316 (1.5) | 0.014 |
≥ 3 | 85 (0.4) | 194 (0.3) | 279 (0.3) | 0.008 |
No ICU24, NEWS 5–6 | ||||
0 | 1756 (84.0) | 5116 (85.2) | 6872 (84.9) | 0.199 |
1 | 294 (14.1) | 776 (12.9) | 1070 (13.2) | 0.185 |
2 | 33 (1.6) | 103 (1.7) | 136 (1.7) | 0.690 |
≥ 3 | 8 (0.4) | 13 (0.2) | 21 (0.3) | 0.217 |
No ICU24, NEWS ≥ 7 | ||||
0 | 1061 (84.4) | 3070 (84.6) | 4131 (84.6) | 0.846 |
1 | 171 (13.6) | 480 (13.2) | 651 (13.3) | 0.739 |
2 | 20 (1.6) | 68 (1.9) | 88 (1.8) | 0.520 |
≥ 3 | 5 (0.4) | 9 (0.3) | 14 (0.3) | 0.393 |
ICU24 | ||||
0 | 1501 (95.1) | 3502 (92.6) | 5003 (93.3) | 0.001 |
1 | 70 (4.4) | 244 (6.5) | 314 (5.9) | 0.004 |
2 | 7 (0.4) | 26 (0.7) | 33 (0.6) | 0.286 |
≥ 3 | 1 (0.1) | 9 (0.2) | 10 (0.2) | 0.165 |
Z-LOS, n (%) | ||||
No ICU24, NEWS missing | 1279 (62.4) | 7882 (72.6) | 9161 (71.0) | < 0.001 |
No ICU24, NEWS 0 | 1952 (18.6) | 7652 (22.1) | 9604 (21.3) | < 0.001 |
No ICU24, NEWS 1–4 | 3073 (15.0) | 11,222 (16.9) | 14,295 (16.5) | < 0.001 |
No ICU24, NEWS 5–6 | 116 (5.5) | 351 (5.8) | 467 (5.8) | 0.611 |
No ICU24, NEWS ≥ 7 | 64 (5.1) | 191 (5.3) | 255 (5.2) | 0.784 |
ICU24 | 45 (2.8) | 92 (2.4) | 137 (2.6) | 0.394 |
Appendix 13 Observation guide, HiSLAC qualitative research (involving interviews, and observations employing elements of an ethnographic approach): round 1
Aims
To describe how each theme covered below affects the operation of the hospital at weekends and how it differs from weekdays, and describe the impact that it may have for patients, with a focus on urgent and emergency medical patients.
Themes
Staffing levels
-
In each area visited, explore how senior doctor staffing is co-ordinated over the weekend and how this compares to weekdays. Look for: numbers and specialties of senior doctors who are available; whether doctors are working in blocks of multiple days.
-
In each area visited, explore levels of staffing across all roles and grades – senior, mid-grade, junior doctors, nurses, HCAs (health-care assistants) and admin (e.g. ward clerks); numbers of agency and locum staff; whether there are skills shortages at weekends.
Emergency department/clinical decision unit/acute medical unit: case mix and demand
-
Are the demographics of medical patients being admitted at weekends different to the week? In what way, and why? What impact does this have?
-
What particular pressures does the hospital face at the weekend (e.g. demand on ED)?
Clinical decision unit/acute medical unit: patient review
-
Describe how consultant review is co-ordinated in clinical decision unit/AMU.
-
Who reviews medical patients – which specialty are they from? How soon do patients get reviewed by a consultant once admitted to clinical decision unit? How often? Is this different at weekends to weekdays?
-
-
What happens when patients are moved from clinical decision unit/AMU on to a ward? Are arrangements made to make sure they are reviewed within 24 hours?
Medical wards: medical cover
-
What form does consultant input take at weekends? Is this different to weekdays?
-
Are consultants on or off site?
-
Are consultants covering the area they usually cover during the week or is this different?
-
Is the consultant ‘passing through’ or do they remain on the ward for a set time period?
-
-
How is junior doctor input organised over the weekend? Are there ‘on-call doctors’ – what areas do they cover? What are on-call/junior doctors doing at the weekend?
Medical wards: (specialist) patient review
-
Describe arrangements for specialist review of patients on medical wards over the weekends, what form review takes (e.g. consultant led ward rounds), and how frequently it happens.
-
Who reviews these patients (consultant, senior house officer . . .)?
-
Are patients reviewed by doctors from the matched specialty (e.g. respiratory patients by respiratory consultants)?
-
-
How is the system for review managed?
-
Is it proactive (i.e. is there an active list of patients each day for review), or are patients just reviewed reactively (e.g. if they deteriorate)?
-
Is it based on inclusion (see only those identified as requiring review) or exclusion (i.e. see all patients unless identified as not requiring review)?
-
-
How are decisions made about who is reviewed and who is not reviewed? Who makes these decisions? What are the prompts for patient review at the weekend, e.g:
-
newly admitted
-
unstable/uncertain management plan
-
outstanding tasks
-
concerned relatives
-
potential discharge.
-
-
-
Is the system for review different across specialties?
-
How does patient review at the weekend compare to weekday?
Specialist input
-
What exactly are specialists doing, and what difference does specialist input (or lack of specialist input) make? Collect examples of this (e.g. spotting missed diagnosis, expediting tests).
-
How are consultant decisions actioned at the weekend? Do any problems arise?
-
How easy is it for junior doctors/nurses to get input and advice from a more senior doctor, or from a specific specialty at the weekend? Do junior doctors seem comfortable seeking senior support/input?
Handovers and communication
-
Describe how handovers happen at weekend, and from the weekend to the weekday. Is this different from weekday handovers?
Who’s involved – are consultants involved in weekend handovers? Where do they take place, how are they structured and what is handed over?
-
Describe quality of communication and multidisciplinary working.
Are patient records on paper or electronic, and what electronic systems do they have (e.g. e-prescribing)?
What is communication between doctors and nurses like? Is there evidence of multidisciplinary working at weekends?
Deteriorating patients and urgency
-
How are deteriorating patients detected and responded to over weekends?
Look for/ask: What happens if staff become worried about a patient? How does the local Early Warning Score system (EWS) work? Is it a paper or electronic system (are there automatic flags); who is responsible for responding (e.g. outreach team)? How well does the system work?
Problems with transfers to ICU at the weekend?
-
Probe for staff understanding of ‘emergency/urgent/non-urgent’ in relation to requests for consultant review and for diagnostic tests/therapy. Do delays at weekends for less urgent things really matter? Why?
Access to diagnostics, therapy, and other services
Describe issues that arise relating to availability of services at weekends as compared to weekdays:
-
diagnostic services, e.g. X-ray, cardio testing, CT (computerised tomography), blood tests and results (does this differ for urgent cases and for less urgent cases?)
-
therapy services and allied health professionals (e.g. physiotherapy, occupational therapy, nutrition, speech and language therapy, microbiologist, drug and alcohol services, mental health services)
-
pharmacy for ordering medication/pharmacist input (e.g. medication reconciliations)
-
resources or systems to support discharge at the weekend [e.g. do they have a discharge team, access to community services (at weekend)]
-
access to palliative care at weekends both within and outside the hospital?
Safety and quality of care
-
Is there evidence of risks to the quality or safety of patient management or care due to problems at the weekend?
-
Evidence of differences in tempo and flow at weekends; evidence of delays in diagnosis, or treatment; problems with patient flow.
-
Problems that arise around transitions [e.g. between AMU and wards, into ITU (intensive therapy unit), discharge].
-
Problems due to patient deterioration; or other problems with patient care arising because of the nature of weekend working.
-
-
Is there evidence of a weekend work ethic, or problems being taken for granted because it’s the weekend? Is there evidence of staff informally compensating for or working round problems that arise over the weekend?
-
Any evidence of systematic safety-focused activities (e.g. advance planning for the weekend/safety briefs/safety huddles)?
-
How do staff approach management on Mondays (e.g. do they prioritise the sickest patients first on ward rounds)?
Patient experience
-
Is there evidence of staff taking time to talk to patients, keeping them informed, involving in decisions? Is this different at the weekend to weekdays?
-
Researcher to chat informally to patients and relatives about:
-
How has it been over the weekend in hospital?
-
Has a doctor been to see you? Do you know which specialty they are from and whether they are a consultant, junior, etc.?
-
How much have staff talked to you about what’s going on?
-
Have you had enough chance to ask questions?
-
How quickly have things been sorted out for you, and have there been any delays or problems?
-
Is there anything you have felt worried or unhappy about?
-
How secure and confident have you felt during your stay at the weekend?
-
Appendix 14 Two anonymised case study examples
This appendix comprises field notes and thus the case studies are in note form.
HiSLAC case study 1
Background and context
This is a small district general hospital: an old 1960s building. There are a number of specialty wards. It feels friendly; staff know each other and have a good rapport. It does not feel as clinical and high-tech as some other hospitals that we have visited. There is not much negative hierarchy. First names are used. Juniors are comfortable with their consultants (OBS003).
There is a sister hospital with which it shares some specialties. There are two tertiary centres nearby that can deal with cardiac emergencies, stroke patients, neurology, renal and oncology (OBS001).
The hospital has just introduced a new IT (information technology) system, a computerised online record system that can pull up patient details and generate a list of patients who need a junior or consultant review over the weekend (OBS001). The same weekend, patients are being diverted from location 2 so the hospital is very busy and there is a rush to discharge patients before the weekend (OBS001).
There is accident and emergency (A&E) on the ground floor with an urgent care centre (a GP-run clinic as an alternative to A&E), AMU with an ambulatory care unit next to it. The ambulatory care unit is called RAMAC (rapid access medical assessment clinic) and takes a lot of the GP referrals but according to one consultant it is not very helpful in taking the pressure off A&E. The consultant says that the unit is very variable in what it will take and it is difficult to get the consultants down to A&E to see patients and so it is easier to admit patients to AMU, where they will be seen (OBS001).
During this weekend of observations, location 2 is on divert to this hospital, so it is taking patients from other areas and the GP cannot access patient notes from location 2, making it harder to get patients discharged. The hospital is very busy because of the extra patients (OBS003).
Case mix and demand
In A&E there is a mix of patients, majors and minors and a few major traumas, but they are mostly sick, elderly, medical patients. There is no real difference in case mix at weekends, although they do get more alcohol-related issues (OBS001). There is an increased number of chest patients (more than usual) because of the time of year (OBS002). There are a lot of GP referrals on a Friday afternoon and there is a perception that these are people who are unable to manage at home (IV02).
There are 14–20 patients in ambulatory care each day and it used to be that most of these (90%) would be treated and go home without needing to be admitted. Now the figure is more like 70% because the hospital receives more GP referrals who need to be admitted (OBS001).
Consultant cover
The AMU weekend rota is covered by 4.8 FTE (full-time equivalent) acute-care physicians (OBS001). There are three consultants in AMU at the weekend, each called ‘physician of the day’. Physicians of the day 1 and 3 are based in AMU and also look after ambulatory care and medical admissions. Physician of the day 2 covers the base wards, accompanied by two junior doctors, and reviews the patients on the wards (OBS001).
The physician of the day is also described as short-day consultant, long-day consultant and all-weekend consultant. On Saturday and Sunday the short-day consultant works from 8 a.m. to 2 p.m. and does the take for half of the AMU beds. He or she also goes on all of the wards and gets a list of all the patients for the long-day consultant to review; these patients will be outliers from AMU and will need to be seen by a consultant because they are ready for discharge, or because they are unwell, or because they have been transferred to the ward without first having been seen by a consultant (this can happen on busy nights), and they are described as patients who have not had a ‘ward round’ (INT03). The short-day consultant will then go home.
The long-day consultant does the other half of the AMU beds and then covers ambulatory care. The all-weekend consultant is responsible for all acute medicine from 8 a.m. on Saturday to 12 noon on Monday and reviews all patients flagged up for review (this takes until lunchtime) and then takes charge of the take on AMU (OBS001).
At the weekend, once the AMU ward round is done in the morning, one consultant goes home and one goes to the ambulatory care unit, leaving the weekend consultant to cover AMU. This consultant tends to leave in the afternoon and leaves the registrar and junior doctors to clerk in the patients and the consultant will come back later and review these patients as a batch. The consultant is available on call for advice (OBS002).
There is reported to be little difference in consultant staffing levels between weekends and weekdays. During the week in the AMU three consultants are on all the time, whereas at the weekend only two consultants are on at a time (INT03).
Cardiologists come in on a Sunday from 8 a.m. to 2 p.m. to this hospital and on Saturday they cover the sister hospital to review their own patients and give advice on other patients. They are available at the end of a telephone. Gastroenterology provides a ‘bleeder rota’, so if there is an upper gastrointestinal bleed the gastrointestinal team can be telephoned and will decide whether or not the patient needs an endoscopy. No other specialties provide an on-call service at the weekend. During the week respiratory, cardiology, endocrine and diabetes and gastroenterology services are available. Haematology, dermatology and surgical specialties are available at the weekend over the telephone (INT03).
Some staff are concerned about workloads on the AMU at weekends and the potential for mistakes to arise:
[Person x] had 60 patients to review on AMU & the wards which they said was unmanageable and that is when mistakes happen.
OBS001
There is also concern about the lack of continuity of care at the weekend, which is felt to be inefficient and increase workload, with some believing that it would be better if the specialists always looked after their own patients rather than acute medicine physicians providing cover out of hours and at weekends (INT03).
Review of medical patients
New admissions to AMU at the weekend are clerked in by the junior doctor, accompanied by a nurse, who takes notes and also carries out a nursing assessment. These admissions are seen later by the consultant (OBS002).
Patients on the wards will get a consultant review if they are taken straight to the ward on admission and have not been seen by a consultant (this sometimes happens if there is pressure on beds in the AMU), or if they have been flagged for consultant review because they are unwell, or if they are to be discharged. On a Friday each ward will identify which patients will need a review over the weekend and which level of review will be required; for example, the junior doctor (F1) will do basic tasks such as take bloods, the senior house officer will review slightly unwell patients, and the physician of the day will do any post-takes and will see more poorly patients and potential discharges (OBS003).
Patients needing a cardiology review are seen by the physician of the day on a Saturday and by the cardiology consultant on a Sunday. This causes some confusion about who is responsible for reviewing a patient on a Saturday, with our observer noting the potential for some patients to ‘fall through the net’ (OBS003).
At weekends a GP is stationed in the AMU who is able to access records, notes and letters from other hospitals. This GP’s role is to reduce the need for repeat and unnecessary scans and interventions. In one example, a patient had suspected epilepsy and because the GP could see the discharge letter from another hospital they could see that the patient experienced pseudo-seizures and so did not need a scan or epilepsy medication (OBS002).
The new nerve centre provides an electronic system into which patients can be entered for a review. It provides options to select the level of review required and doctors can print off a list to take with them on their rounds. The doctors can also access the information from iPads (Apple Inc., Cupertino, CA, USA), where there are details of the patient’s care plan and next steps (OBS001).
The handover in AMU is the same 7 days a week. At 8 a.m. each day the night team has quite a long handover to the day team – they will review all patients on AMU and sick patients on the wards and in ITU. There is a 3.30 p.m. quick catch-up and another long handover at 8.30 p.m. (OBS001).
Deteriorating patients and escalation
Patients with an early warning score above 5 will automatically be reviewed by the nurse practitioner (the nurses can also alert for patients who are unwell but not scoring) and the nurse practitioner can escalate to the registrar. There is also a senior house officer on the wards and an on-call consultant (INT03). The MET (medical emergency team) is a brand new service that supports ‘back of house’ (i.e. wards) and sees any patients on the wards, including paediatric patient and neonates. They support the nurses and junior doctors 24/7. Jobs are allocated to them via the nerve centre, and a nurse practitioner, a band 3 health-care assistant and a junior doctor decide who is most appropriate to do certain tasks. These might include inserting cannulas, prescribing medication and reviewing unwell patients. This service was created after a few instances of failure to rescue, the aim being to provide general support for everyone so that patients receive interventions earlier (INT03).
Dealing with emergency calls is flagged as an issue at the weekend when there are fewer junior doctors. On AMU there is one registrar and two junior doctors, and this was described by more than one person as a problem when there is a MET (emergency call). The registrar and junior doctors will attend the emergency, which could be anywhere in the hospital, and might be gone for some time, leaving the consultant on their own in the AMU. There are plans to obtain funding for a junior doctor to stay on the AMU to clerk patients in from 8 a.m. to 4.30 p.m. (OBS001).
Allied services
The hospital is paired with another in the same trust and some services are split across both sites. For example, the 24/7 on-call endoscopy service covers both sites, and cardiac emergencies on a Saturday will be transferred to the nearby tertiary centre with 24/7 access. There is also 24/7 access to renal and neurology at the same tertiary location. All stroke patients go to a tertiary centre at a third location, where stroke consultants are available 24/7. Oncology services are available 24/7 at the tertiary centre and the oncology nurse comes to provide advice and support to cancer patients (OBS001).
One difficulty appears to be that IT support for the new software and patient record system in AMU is lacking at weekends. Diagnostic services such as computed tomography, magnetic resonance imaging and biochemistry can be harder to access at weekends, and radiotherapy is limited. Fewer allied staff, such as porters and speech and language therapy teams, are available. Physiotherapy is also limited at weekends, although it supposed to be available 7 days a week (OBS001). Pharmacy is open only on Saturday morning and all prescriptions are required by 11 a.m. This results in delayed discharge (OBS001, INT03). There is an on-call pharmacist, but interview data suggest that if the medication is not essential the patient has to wait, or what is needed will be borrowed from another ward (INT02). The tissue viability service might not be available over the weekend, and there is no intravenous cannulation service at the weekend (OBS001). Blood tests are available for acute patients but not for the ongoing management of longer-term conditions. (OBS003). A mental health crisis team is available at weekends until about 9 p.m. and advice can be obtained but the team will not always come in to see a patient and it is hard to get a crisis intervention at night (OBS001). Microbiology provides a consultant on call for advice and there is a microbiology technician on all weekend. There are no specialist nurses available at the weekend, for example palliative care, percutaneous endoscopic gastronomy, i.v. (intravenous), respiratory nurses, and discharge assessments for elderly people are harder to obtain, especially if the patient needs to be followed up in the community (INT03).
Flow
There appeared to be a lack of patient flow throughout the system and there were reported failures to meet the 4-hour target in A&E due to high demand (OBS001).
This hospital admits patients from two locations and patients from one of the locations have better access to social & community care and are easier to discharge. Patients from the other location might have to stay in AMU for longer until a care package is organised for them (OBS001). However, there is a district nurse in the hospital at the weekend who knows about the district nurse community service and nursing home step down beds that patients can be discharged to (OBS002).
There used to be a winter pressures ward that could take extra patients when there were pressures on the system, but this is now a cardiology ward and there is no flex in the system. In addition, they have checklists and guidelines that slow things up (OBS001).
Junior doctors can produce discharge letters, but in one instance a new junior doctor was reluctant to do this because they were unfamiliar with the system. The letters take quite a long time to do and the junior doctors are often stretched (OBS003). The consultant does discharge patients at the weekend but will err on the side of caution because they are not a patient’s own specialist.
HiSLAC case study 2
Background and context
The hospital is a district general hospital and serves a geographically large, rural area.
Because of its location, the hospital has a particular problem recruiting staff; for example, in A&E it needs eight junior doctors (it has six) and 12 registrars (it has eight). It relies a lot on locum staff out of hours and at weekends. The staffing situation is worse on the wards because staff in A&E are able call on staff from the rest of the hospital, leaving the wards short-staffed.
There is a shortage of acute physicians working in AMU. The job is seen as less prestigious than other specialties and is hard work. They depend on specialty consultants to help on the AMU rota.
Junior doctors are given a lot of responsibility. One junior doctor is on call for the whole hospital at weekends, along with a senior house officer, a nurse practitioner and a registrar, who covers the take.
Case mix and demand
A junior doctor says numbers of patients at weekends can be unpredictable, but the case mix is the same. This is borne out by observations of a very hectic shift and a very quiet one. Mondays and Fridays are described as the busiest days due to a combination of increased GP referrals on a Friday and people delaying coming into hospital over the weekend until Monday.
According to one member of staff there are fewer patients at the weekend and AMU can be quieter because there is no ambulatory care clinic, although another member of staff thought that the case mix at weekends was very different. A GP service is open at the weekend for emergency treatments that require GP input only, but it is not used properly (although it is not clear why).
Consultant cover
There are six full-time equivalent respiratory physicians; two of those will be on the ward at any one time, another will cover outliers and referrals (without any junior doctor support), one will be on leave and the remaining two will be doing administration and SPA (supporting professional activities) work, clinics and multidisciplinary team meetings, etc. They each conduct one in 13 of the acute medical take rotas – at weekends this means starting at 2 p.m. to 8/10 p.m. on a Saturday post-taking patients and then coming in on the Sunday at 8 a.m. to 12/1 p.m. to post-take all patients seen the day before and new patients who came in overnight. There is a break until the evening, when there is a further post-take ward round lasting 3–5 hours. There is another post-take ward round on Monday between 8 a.m. and noon. Following our visit, this system changed. The on-call consultant starts the post-take ward round at 8 a.m. and sees all patients who have been clerked still in A&E or on an unlinked medical ward. This rarely goes beyond 9.30 a.m. The AMU consultant will see those patients on the AMU. Occasionally (usually owing to sickness, although occasionally owing to uncovered leave), the on-call consultant has to also see the post-take ward round patients on the AMU/clinical decision unit. At weekends they do not cover respiratory; the role is that of acute general physician. When they are on call out of hours at the weekend, it is for the whole hospital. There are two general acute consultants and the rest are specialists, and they all take part in the on-take rota. For the consultants there is very little difference in the roles between being on-take or on-call.
The on-take (AMU) and the cover team (the wards) share a consultant and registrar. The weekend rota starts on Friday, when there are two specialist consultants: one covers patients who are admitted from 8 a.m. to 6 p.m., and the other covers 6 p.m. on Friday to 8 a.m. on Saturday. The consultant covering the take during the day on Friday will also be there on Saturday to review all the patients they admitted, no matter where they are in the hospital. Although there is a formal weekend rota, the consultants often agree their working patterns between themselves. Gastroenterology and cardiology consultants do not take part in the on-take rota as they provide a 24-hour on call service for any patient who has been admitted under any specialty. The other specialties (respiratory, care of the elderly, renal, endocrinology) take part in the on-take rota and so do not run an on-call service as gastroenterology and cardiology do, but there is a referral system if specialist input is required.
Review of medical patients
There are three main routes into the hospital. (1) A&E – the patient is seen by an A&E doctor who bleeps the AMU medical registrar, and the patient is then sent to AMU, where they are triaged. Their details are put on the whiteboard and they are marked as urgent or non-urgent. They are then clerked by a junior doctor and subsequently reviewed by the consultant. Their stay in AMU should be no longer than 24 hours. From AMU the patient will go to either a specialty ward or the short-stay ward (for up to 48 hours). (2) GP – the GP refers the patient to the central co-ordination centre and from there to AMU or the ambulatory care centre depending on their condition. (3) Various other routes (e.g. referral from the oncology centre).
Occasionally, AMU can be bypassed; for example, a patient with chronic obstructive pulmonary disease needing non-invasive ventilation was taken straight to the respiratory ward. Patients admitted to AMU can expect a senior review and a similar number of doctors to during the week but patients on the wards will not be seen every day (patients on AMU are seen at a weekend only if they form part of the post-take ward round; if not, then they are not reviewed). In this respect, their care is no different from the care of patients on the wards over a weekend (except that there are doctors on the ward, and so nurses find it easier to get patients reviewed if needed). There are only three doctors covering the rest of the hospital. The clinical decisions unit is part of A&E and is (or should be) used for patients who do not need to be kept in A&E but just need some more treatment, such as occupational therapy or physiotherapy. However, the clinical decision unit is also used for outliers when there are not enough beds in the AMU or the wards. Patients coming in by ambulance are always escorted in by the ambulance crew, who hand over to the nurse co-ordinator in A&E. The triage nurse can also send people to the urgent care centre: a walk-in area for people needing GP-type treatment. The A&E registrar cannot refer patients directly to the ITU. Surgical patients are reviewed by the surgeons. All patients are seen by a consultant either on the day of their admission or the day after. Patients admitted on a Friday or Saturday and not seen by a consultant will always be seen by a consultant the next morning, irrespective of which ward they are on. Patients admitted on a Friday or Saturday but seen by a consultant on the day of their admission are usually seen by a consultant also the following day irrespective of which ward they are on – this is what is expected (some consultants will not re-review their patients, however, unless the clinical situation demands it). Patients admitted Sunday to Thursday will be seen by a consultant the day after admission (whether or not they are seen on the day of their admission): if in A&E, then by the on-call consultant; if in AMU/clinical decision unit, then by the AMU consultant; if on a ward, then by the specialty consultant covering that ward. At the weekend the on-take consultant is responsible for the patients they have admitted the previous day and will conduct a review no matter where the patient is, which can be complicated. The administration lags behind and the consultant has to remember who the patients are and then find them, and sometimes the patient may have been seen by another consultant in the meantime, but the on-take consultant sees it as their responsibility to make sure that the ward team has accepted responsibility for the patient. So for this post-take review the patient may be reviewed not by the specialty they will ultimately come under, but by whichever consultant covered the on-take. However, if the patient is already on the specialty ward, then the post-take review will be by the specialty consultant on the ward. There is a chance that a patient could miss their post-take review – one patient nearly missed their review because they had gone for an X-ray – and then it would be the specialty consultant’s responsibility to ensure that the patient is reviewed.
The on-call/on-take team is responsible for all the acute patients who are admitted; the on-cover team looks after the wards. However, the on-call/on-take team and the on-cover team share a consultant and registrar – and the job of the registrar is particularly stressful. At the weekend there are frequent backlogs of patients waiting for their post-take review and patients who were admitted the day before might not be seen until the afternoon. Patients on the wards might be reviewed by the senior house officer or junior doctor depending on how they have split the ward cover between them. At the weekends the wards are covered by a senior house officer, a junior doctor and an advanced nurse practitioner (the on-cover team) and they may also take one of the doctors for a discharge round as well as doing all the routine jobs on the wards. There is also a cardiac arrest team.
The on-cover team does not work at night on the wards; the wards are covered by the advanced nurse practitioner out-of-hours team (hospital-at-night team), which is supported by the on-call team. Neurological advice (out of hours) is provided by a regional on-call centre.
Deteriorating patients and escalation
There is a bleep system for emergencies and a cardiac arrest team.
Transfers within the hospital can happen at the weekend (as in the case of a patient transferred to the critical care unit), but the observers have been told more than once that this is quite rare. In one example, having a cardiologist on site would have expedited the transfer of a patient to a specialist unit.
Treatment is described as safe but it is silver not gold standard (e.g. consultants can treat different conditions and patients with no problem, but it might not be their area of expertise). Gastroenterology and cardiology procedures often require special expertise and that is why these departments provide a 24-hour on-call service. Lack of specialist input is a problem (i.e. consultants on call can be reached but it causes delays). Patients in AMU admitted on Friday or before are not reviewed proactively on a Sunday and they would benefit from a review because the condition of these acute patients can change. One ward nurse is of the opinion that there are sufficient safety nets at the weekend (consultants and specialists on call and the on-call team)
Culturally there do not seem to be barriers to contacting consultants and specialists, but there can be delays in getting advice, and there is evidence that staff try not to overburden specialisms at the weekend with non-urgent cases, which can delay treatment. Junior doctors and nurses are happy to escalate, but at weekends it can take longer to escalate care for deteriorating patients. On the wards, the nurses need to be experienced and confident that they can escalate because the junior doctors are given a lot of responsibility there.
Allied services
The hospital is a small district general hospital and does not house all of the specialist services. The problem is if an acute cardiac or stroke patient needs to be transferred to the specialist location within the trust, which can be challenging and time-consuming, although junior doctors do not normally have a problem getting a transfer accepted.
The radiology department is good over the weekend – it prioritises urgent scans, then semi-urgent ones and will even mop up the routine scans that could wait until Monday, although one junior doctor says input is needed from a consultant if CT scans and imaging are to take place out of hours. Since the initial visit, radiology on-call after 8 p.m. has been outsourced to an external company, and it is in the company’s best interests to accept almost all requests, meaning that consultant-to-consultant referral for scans is rarely asked for.
There is a limited phlebotomy service and an on-call physiotherapy service at weekends. There is no social worker department at the weekend. Pharmacy is limited to Saturday until noon and on call after that. There is no diabetic team.
There is an intermediate care service, which normally includes an occupational therapist, a physiotherapist and various social services. Over the weekend, only a physiotherapist is available and so does not really provide the same service as the team that they have over the weekdays. The function of this team is to ensure that the patient can be discharged safely.
Flow
Discharges are worse at the weekend; there are not enough doctors and they are not allowed to do nurse-led discharges (OBS002). There used to be a dedicated on-call discharge team, but this was available only intermittently and not ‘rota’d’. This led one member of staff to believe that it had been stopped by the trust.
Doctors are less keen to discharge someone else’s patient. It is also harder to discharge at weekends because there is no social work department. However, there is a process in place so that if a patient needs a diagnostic (e.g. magnetic resonance imaging) but does not have to be in hospital they can be discharged and come back on Monday to ambulatory care. Reduced physiotherapy, occupational therapy, pharmacy services, etc., make discharge at the weekend more challenging. Diabetic patients cannot always be discharged until they are seen by the diabetic team on Monday, otherwise there is a risk of inappropriate discharge (OBS002).
Case studies were compiled based on observations and interviews completed between April 2016 and March 2018. Local project leads in each participating hospital were sent a draft version in June 2018, and were asked to check and correct or update to ensure the case studies reflected the current situation in their hospital.
Appendix 15 The focus group interview topic guide
Preamble
-
The facilitator will introduce themselves, including their position within the HiSLAC project.
-
Explain the objectives of HiSLAC and the focus group.
-
Ensure that participants have read and understand the information sheet and have been given the opportunity to ask any questions.
-
State we will digitally record the focus groups so that we capture the thoughts, opinions and ideas from the group. No names will be attached to the focus groups and the recordings will be destroyed as per University of Birmingham guidelines.
-
Remind participants that all information will be treated in confidence and taking part in the focus group is voluntary.
-
Remind participants to please keep any information shared in the group confidential, and not discuss outside the focus group.
-
Time frame – inform participants that the focus group is likely to take around two hours and lunch will be provided after the meeting.
-
Iterate that the HiSLAC team will either email or post a one page summary with the key themes/topics and search terms generated from the focus group. Participants will then be asked to comment if any important areas have been excluded from the summary.
-
Inform participants that the role of the facilitator is to guide the discussion and that there are no right or wrong answers, only differing points of view.
Warm up questions
Clinicians
-
Can you tell me how long have you been working in the NHS and your job title?
-
What department do you work in?
Main questions
Reiterate the area of interest – there is evidence that mortality rates are higher for patients admitted to hospital at weekends than weekdays – the so-called ‘weekend effect’. We are interested in the ‘in hospital’ factors that might result in this weekend–weekday mortality gap.
-
Do you think there are differences between the way care is organised and delivered at the weekend as opposed to a weekday? What differences are there?
-
What impact does this have on patients who are admitted over the weekend?
-
Can you tell me any stories from your own experience, about the care of patients admitted to hospital at weekends, and what the problems can be?
-
What factors do you think have an effect on patient mortality, for patients admitted at the weekends? (Discuss, then agree a list using a flipchart.)
-
Prompt: staffing (lack of specialists; nurse numbers and expertise; overall staffing levels); access to services and other expertise (such as X-rays/surgery/pharmacist); care processes (e.g. patient review/ward rounds/handovers/documentation); delays in care; quality and speed of decision-making; errors; poor organisation of care/transfers.
-
-
Can you explain how you think each of these factors can contribute to increasing mortality? (what are the underlying mechanisms).
-
Are any of the factors linked with each other (e.g. lack of specialists and quality of decision-making) (use flip chart to illustrate links)
-
Which of the factors do you think have the most impact?
-
Are there any factors that you think mitigate or reduce the weekend effect (e.g. care planning, escalation plans)? What are these and why?
-
What changes could improve care for patients admitted at weekends?
Concluding remarks
-
Ask if participants have any questions.
-
Remind participants that we will circulate (either by email or post) a one page summary with the key themes/topics and search terms that were generated from the focus group. Participants will then be asked to comment if any important areas have been excluded from the summary.
-
Ensure participants are happy with the way the focus group has been conducted.
-
Thank participants for their participation in the focus group and adjourn for lunch.
Appendix 16 HiSLAC case record review data collection form
Appendix 17 Characteristics of study population compared with background population
Characteristics | Case notes review data, n (%) | All trusts/hospitals data,a n (%) | ||||
---|---|---|---|---|---|---|
Total | Weekend | Weekday | Total | Weekend | Weekday | |
Total, N | 4000 | 2000 | 2000 | 5,818,430 | 1,411,394 | 4,407,036 |
Age | ||||||
Mean (SD) | 61 (22.310) | 62 (22.379) | 61 (22.271) | 61 (22.350) | 61 (22.780) | 61 (22.190) |
Median (IQR) | 65 (43–81) | 66 (44–81) | 64 (42–80) | 65 (43–80) | 66 (43–81) | 65 (43–80) |
Gender, n (%) | ||||||
Male | 1869 (46.7) | 938 (46.9) | 931 (46.6) | 2,639,489 (45.4) | 645,166 (45.7) | 1,994,323 (45.3) |
Female | 2131 (53.3) | 1062 (53.1) | 1069 (53.5) | 3,178,941 (54.6) | 766,228 (54.3) | 2,412,713 (54.7) |
Ethnicity, n (%) | ||||||
White | 3140 (78.5) | 1584 (79.2) | 1556 (77.8) | 4,918,775 (84.5) | 1,191,464 (84.4) | 3,727,311 (84.6) |
Non-white | 818 (20.5) | 396 (19.8) | 422 (21.1) | 526,384 (9.0) | 128,736 (9.1) | 397,648 (9.0) |
Unknown | 42 (1.1) | 20 (1.0) | 22 (1.1) | 373,271 (6.4) | 91,194 (6.5) | 282,077 (6.4) |
Top five primary discharge diagnoses (SHMI grouping), n (%) | ||||||
Non-specific chest pain | 227 (5.7) | 116 (5.8) | 111 (5.6) | 326,876 (5.6) | 72,314 (5.1) | 254,562 (5.8) |
Pneumonia (excluding TB/STD) | 186 (4.7) | 105 (5.3) | 81 (4.1) | 322,014 (5.5) | 85,643 (6.1) | 236,371 (5.4) |
Urinary tract infections | 176 (4.4) | 100 (5.0) | 76 (3.8) | 235,612 (4.0) | 64,076 (4.5) | 171,536 (3.9) |
Abdominal pain | 156 (3.9) | 70 (3.5) | 86 (4.3) | 243,013 (4.2) | 54,697 (3.9) | 188,316 (4.3) |
COPD and bronchiectasis | 128 (3.2) | 67 (3.4) | 61 (3.1) | 193,168 (3.3) | 48,288 (3.4) | 144,880 (3.3) |
Other | 3127 (78.2) | 1542 (77.1) | 1585 (79.3) | 4,497,747 (77.3) | 1,086,376 (77.0) | 3,411,371 (77.4) |
Length of stay (days) | ||||||
Median (IQR) | 2 (0–5) | 2 (0–5) | 1 (0–4) | 1 (0–5) | 2 (0–5) | 1 (0–5) |
Z-LOS, n (%) | ||||||
1107 (27.7) | 512 (25.6) | 595 (29.8) | 1,739,129 (29.9) | 388,274 (27.5) | 1,350,757 (30.7) | |
In-hospital mortality, n (%) | ||||||
Died | 168 (4.2) | 90 (4.5) | 78 (3.9) | 239,719 (4.1) | 63,372 (4.5) | 176,281 (4.0) |
Appendix 18 Mean number of errors identified per case note by reviewer
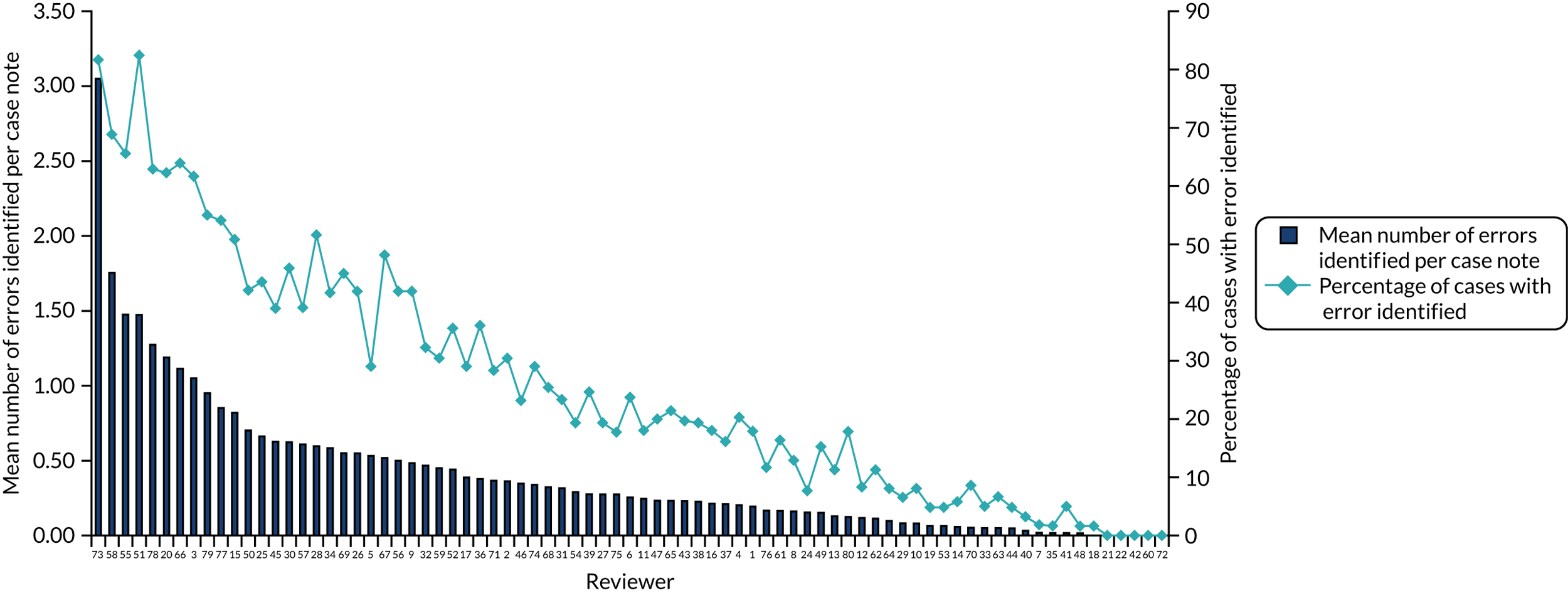
Appendix 19 Posterior estimate and 95% credible interval of specialist intensity function for each error type by model

Glossary
- Analysis of variance
- A statistical method in which the variation in a set of observations is divided into distinct components.
- English Indices of Deprivation
- The standard measure of area deprivation used by the UK government. Data on variables, such as income, unemployment education and crime, are collected at a small level of census geography called a lower-level super output area.
- Health Services and Delivery Research
- A programme of research funded by the National Institute for Health Research.
- ICU24
- Patients admitted to ICU within 24 hours following hospital admission.
- NEWS24
- The National Early Warning Score calculated using the primary variables for the first full set of vital signs available within 24 hours of admission.
- Odds ratio
- A value of > 1 indicates a higher than expected value, in this case of the risk of death.
- Point prevalence survey
- In this report, an annual survey of specialists carried out by High-intensity Specialist-Led Acute Care (HiSLAC) project researchers.
List of abbreviations
- 24/7
- 24 hours a day, 7 days a week
- A&E
- accident and emergency
- AMU
- acute medical unit
- CI
- confidence interval
- CrI
- credible interval
- CT
- computerised tomography
- EA
- emergency admission
- ED
- emergency department
- GP
- general practitioner
- HES
- Hospital Episode Statistics
- HiSLAC
- High-intensity Specialist-Led Acute Care
- HSDR
- Health Services and Delivery Research
- ICU
- intensive care unit
- IQR
- interquartile range
- IT
- information technology
- ITU
- intensive therapy unit
- MET
- medical emergency team
- NEWS
- National Early Warning Score
- NIHR
- National Institute for Health Research
- OR
- odds ratio
- PPS
- point prevalence survey
- QALY
- quality-adjusted life-year
- RAG
- red/amber/green
- REDCap
- Research Electronic Data Capture
- SD
- standard deviation
- SHMI
- Summary Hospital-level Mortality Indicator
- SQL
- Structured Query Language
- WEOR
- weekend odds ratio
- Z-LOS
- zero length of stay
Notes
-
Qualitative study of weekend care for emergency medical admission patients and staff information leaflet
Supplementary material can be found on the NIHR Journals Library report page (https://doi.org/10.3310/hsdr09130).
Supplementary material has been provided by the authors to support the report and any files provided at submission will have been seen by peer reviewers, but not extensively reviewed. Any supplementary material provided at a later stage in the process may not have been peer reviewed.