Notes
Article history
The research reported in this issue of the journal was funded by the HSDR programme or one of its preceding programmes as project number 17/05/03. The contractual start date was in September 2016. The final report began editorial review in September 2021 and was accepted for publication in May 2023. The authors have been wholly responsible for all data collection, analysis and interpretation, and for writing up their work. The HSDR editors and production house have tried to ensure the accuracy of the authors’ manuscript and would like to thank the reviewers for their constructive comments on the final manuscript document. However, they do not accept liability for damages or losses arising from material published in this manuscript.
Permissions
Copyright statement
Copyright © 2024 Briggs et al. This work was produced by Briggs et al. under the terms of a commissioning contract issued by the Secretary of State for Health and Social Care. This is an Open Access publication distributed under the terms of the Creative Commons Attribution CC BY 4.0 licence, which permits unrestricted use, distribution, reproduction and adaptation in any medium and for any purpose provided that it is properly attributed. See: https://creativecommons.org/licenses/by/4.0/. For attribution the title, original author(s), the publication source – NIHR Journals Library, and the DOI of the publication must be cited.
2024 Briggs et al.
Chapter 1 Introduction and context
Background
‘Vital signs’ is the term for a group of important physiological characteristics that indicate the status of the body’s vital (i.e. life-sustaining) functions. They include such measures as blood pressure (BP), heart (pulse) rate, oxygen saturation and breathing (respiration) rate.
The frequency at which patients should have their vital signs measured on general medical and surgical wards is currently unknown. Monitoring protocols in use at present are based on expert opinion,1–3 but supported by little empirical evidence. 4 The National Institute for Health and Care Excellence (NICE) recommends that all patients in acute hospitals are observed at least every 12 hours and more frequently if abnormal physiology is detected. 2 Early warning score (EWS) systems, such as the National Early Warning Score (NEWS),1 provide the means to quantify that abnormality by combining observations into a single score. However, while there is some evidence to support the use of EWSs to identify patients most at risk of adverse outcomes [e.g. death, cardiac arrest, unanticipated intensive care unit (ICU) admission],5–7 the associated monitoring protocols are currently based solely on expert consensus. 1,4 The absence of data to inform clinical practice is a major patient-safety issue, as treatment will be delayed if deterioration is missed due to under-observation. 8–10 Likewise, over-observation redirects nursing time away from other essential aspects of patient care. Indeed, several studies have shown that adherence to current monitoring protocols is often poor,11–14 with many observations missed, particularly at night. This is in part due to available nursing resources. 15–17 One solution would be to continuously monitor all patients, as is the case on high-dependency wards. However, at present this is costly4 and a 2016 systematic review of clinical trials concluded that current evidence is ‘insufficient to recommend continuous vital signs monitoring in general wards as routine practice’. 18
Rationale
What is the problem being addressed?
In 2007, NICE recommended that all patients in acute hospitals should have their vital signs measured and recorded at least every 12 hours and more frequently if abnormal vital signs are observed. 2 In addition, they advised the use of a ‘system’ to identify patients at risk of deterioration. In response to this, UK hospitals have tended to use EWS as the ‘system’.
In 2012, the Royal College of Physicians London (RCP) introduced the NEWS1 to encourage a consistent approach across the NHS. EWS systems, NEWS included, permit a set of vital signs observations to be converted into a single integer score, quantifying a patient’s overall level of physiological disturbance. As part of the NEWS system, the NEWS score is also used to guide how frequently the patient should be monitored. 1 While there is an evidence base to support the use of NEWS to predict which patients are more likely to experience adverse outcomes (in-hospital mortality, cardiac arrest, unanticipated ICU admission),5–7,19 the measurement frequency of vital signs in general hospital wards has been described as ‘an evidence-free zone’. 4 A later revision of the protocol (NEWS220) made no significant changes to these monitoring schedules. With no data to support clinical practice, monitoring protocols still vary widely across NHS trusts and may well waste limited staff resources.
This study aims to recommend observation frequency based on the risk of missing significant deterioration in the intervening period. Prospective observations of nursing staff will allow us to quantify the cost of different monitoring protocols, based on varying thresholds of risk.
Why is it important?
Implications for patient safety
There is now substantial evidence that inadequate monitoring is a major patient-safety issue, contributing to avoidable deaths and other significant adverse outcomes. 3,8,13 The Keogh review into 14 hospital trusts with high mortality rates noted that ‘a consistent theme throughout almost all of the organisations reviewed was the management of complex deteriorating patients and the monitoring of Early Warning Scores’. 9 The Keogh report is not unique in identifying mismanagement of physiological deterioration of patients in hospital. Numerous reports on patient safety and avoidable deaths have identified the failure to observe or respond to patient deterioration as a significant contributory factor. 1,8,9,21 This is often referred to as ‘failure to rescue’ and is thought to be sensitive to the quality of care provided, including levels of nurse staffing. 15–17,22,23 While progress in statistical modelling techniques and development of electronic medical records will likely lead to more sophisticated and accurate risk-prediction algorithms to detect deterioration from vital signs and other clinical data,24–26 they will not directly provide evidence on how frequently these measurements should be obtained.
Implications for staff resources
Whereas under-observation can delay the opportunity to detect patient deterioration and initiate remedial treatment,8,9 over-observation uses valuable nursing resources that could be better deployed to other essential aspects of patient care. Indeed, compliance with current monitoring protocols is often poor, with many observations missed or delayed. 11–14 This is particularly evident at night,12,27,28 when staffing is at its lowest. Results from our HS&DR ‘Missed Care’ study (ISRCTN: 17930973) show that compliance with vital signs observations is significantly (p < 0.05) associated with the level of available nursing staff. 29,30 Estimates of the time required to take observations are scarce, but a survey of 2917 registered nurses (RNs) across 46 acute hospitals in England showed that one-third had felt unable to undertake all necessary patient surveillance due to lack of time on their last shift. 15 However, even when staffing is sufficient, there are valid clinical reasons why nursing staff will deviate from protocols. Therefore, it is essential to have a monitoring protocol that is achievable on the ward and does not compromise other aspects of care.
With this in mind, and by adapting techniques from our previous work,15,31,32 part of this study is a prospective observation of recording vital signs across a range of wards. This will allow us to understand better the impact on staff workload and factors that might affect compliance with patient surveillance.
Evidence explaining why this research is needed now
Current UK professional guidance1,2,13,33,34 suggests that the frequency of monitoring should be determined by some measure of physiological disturbance. One such measure is the NEWS,1 which provides a simple integer score based on the degree to which a patient’s vital signs are outside the normal range. There is now some evidence to support its ability to predict a patient’s risk of adverse outcomes,5,7,35–37 albeit with high false-positive rates. However, we were unable to identify any large studies to support the vital sign monitoring intervals suggested by the original guidelines from the Royal College of Physicians. 1 For example, the NEWS Development and Integration Group (NEWSDIG) recommended at least hourly observations for any patient with acuity of NEWS 5 or more. To put this recommendation into context, the surveillance protocol for patients with NEWS 5 at Portsmouth Hospitals was 4 hourly in the period to 2018, and there was only 50–70% compliance with protocol at this frequency, for a number of potentially legitimate reasons. Without an evidence base, compliance is seen mainly as a superficial mechanistic proxy for quality of care.
As discussed above, under-observing patients run the risk of missing early signs of deterioration, but taking repeated measurements in patients is also a drain on valuable staff resources. Obtaining vital sign measurements is one part of the ‘chain of prevention’,38 necessary to effectively recognise and manage the deteriorating patient. For example, a national enquiry into patients who underwent cardiopulmonary resuscitation in hospital8 showed 20–40% did not have a clear monitoring plan in the 48 hours prior to the event, despite over 70% having significant physiological abnormalities. It is therefore crucial for safety that patients’ observations are correctly targeted and escalated appropriately. However, this must be balanced with other negative consequences. For example, obtaining observations can be disturbing for patients39 and might be associated with negative health outcomes if they contribute to sleep disruption. 28,40 Monitoring protocols that demand observations when staff deem it futile in certain situations, in particular if these may have adverse effects, risk delegitimising the EWS protocol.
One solution would be to implement continuous monitoring for all patients. However, systems for continuous monitoring are at present costly4 and there is little evidence that they improve patient outcomes. 18,41,42 There are also legitimate concerns that continuous monitoring might introduce other risks, such as loss of nurse interaction with patients to pick up soft signs, alarm fatigue and technology failures (e.g. detached monitoring devices). A recent systematic review and meta-analysis18 of randomised trials of continuous and intermittent observation concluded there was ‘insufficient evidence to recommend continuous vital signs monitoring in general wards as routine practice’. Despite a number of trials of wearable devices to measure vital signs outside intensive care,43,44 no device is capable of measuring all vital signs required to generate the NEWS (e.g. BP is rarely possible). In the UK, we found one recent randomised controlled trial (ISRCTN: 60999823) of a continuous monitoring device. 45 A prospective cohort study (SNAP40-ED) trialled the use of a wearable device to detect deterioration in patients in the emergency department. 46
Aim and objectives
This study aimed to investigate observation frequencies based on the risk of missing significant deterioration in the intervening period.
The objectives included to:
-
Develop a data warehouse of linked admission records with information on patient demographics, vital signs observations and adverse events (cardiac arrests, unanticipated ICU admissions, in-hospital mortality).
-
Estimate the rate of clinically significant changes in vital signs over the course of patients’ admission.
-
Explore the relationship between vital signs and adverse outcomes over time.
-
Determine the extent to which relationships between the time to deterioration and risk of adverse outcomes vary across different patient groups.
-
Organise stakeholder (nurse, doctor, patient) events to identify additional factors relevant for selecting an optimal monitoring protocol.
-
Undertake prospective observations of nursing care to estimate the time taken to obtain and respond to vital signs observations, using techniques adapted from previous work.
-
Derive a set of simple monitoring protocols by identifying any threshold effects between the risks over time predicted by our models.
-
Use estimates from our observational work to model the marginal costs and consequences for all protocols with better or equal performance compared to the current protocols for detecting deterioration.
Structure of the team, the project and this report
This project was a collaborative effort involving a large team of people from four organisations:
-
University of Portsmouth (lead organisation)
-
University of Oxford
-
University of Southampton
-
Portsmouth Hospitals University NHS Trust (PHU).
The project was organised into six work packages (denoted WP0–5) reflecting the major aspects of the work. While work packages 0 (administration) and 5 (dissemination) were purely functional, it is useful to understand how the others contributed to the totality of the work. The major tasks were:
-
the retrospective study of historic data on patient admissions to explore the relationships between vital signs and outcome (WP1 to create the warehouse of data extracted from the participating hospitals; WP2 to carry out the analysis of that data);
-
the observational study of nursing staff to ascertain the time taken to perform vital sign observations (WP3).
These were both supplemented by WP4, which addressed stakeholder engagement and experiences with patient observation and monitoring in hospital. It also allowed us to validate our results with stakeholders. WP5 coordinated the production of this report and a number of papers (specified later).
The relationship between the main work packages is shown in Figure 1. In particular, WP4 was designed to inform the later stages of the retrospective study in identifying factors to be considered in monitoring protocols.
FIGURE 1.
Study flow chart.

In this report, we first of all describe the results of our literature reviews. We completed two reviews, one related to each major task. These were: a scoping study (described in Evidence for best vital-sign monitoring practice: a scoping review) to determine what evidence already existed on the optimum frequency for monitoring vital signs; and a systematic review (described in What is the nursing time and workload involved in taking and recording patients’ vital signs? A systematic review) of evidence regarding the time nurses take to monitor and record vital signs observations.
We then describe our observational study. This followed nursing staff as they conducted vital signs observations to measure how long they took and what factors influenced the time taken. This was a prerequisite to informing estimates of the costs and effects of changes to practice. Chapter 3 describes the methods adopted and Chapter 4 describes the results.
Following that, we describe our retrospective study. This analysed data from two hospital trusts to assess the relationships that the time between vital sign observations has with the patient’s outcome. Chapter 5 describes the methods adopted and Chapter 6 describes the results.
Chapter 7 describes how our stakeholder engagement work was integrated into protocol recommendations.
The project conclusions are discussed in Chapter 8.
Chapter 2 Literature review
Evidence for best vital sign monitoring practice: a scoping review
The objective of this scoping review is to map existing evidence and identify gaps in our current knowledge on the optimum frequency for monitoring vital signs of patients admitted to hospital to inform future research into vital sign monitoring and the development of new monitoring protocols.
Methods
Scoping reviews are ideally suited to map the scope and size of existing research in a field of interest and to identify knowledge gaps in the literature. 47 Compared to systematic reviews, scoping reviews have a less structured approach to data extraction. They permit inclusion of different study designs, do not necessarily assess the quality of included studies and typically use a qualitative synthesis of the evidence. 48–50
We followed the scoping review methodology by Arksey and O’Malley,51 including the following stages: (1) identifying the research question; (2) identifying relevant studies; (3) study selection; (4) charting the data; and (5) collecting, summarising and reporting the results. The methodology summarises existing literature on a topic in terms of volume, nature and characteristics of the primary research and identifies gaps in existing literature.
Research question
The review aims to address the question: ‘How often should vital signs be measured as part of routine monitoring of patients in hospital?’ Our search strategy for identifying and selection of studies is outlined below. The studies were divided into the following categories based on similarities in their design, main objectives and themes: primary research, review articles.
Identifying relevant studies
The initial literature search was conducted in November 2019 only including articles in English in the following databases: MEDLINE and EMBASE. The database search was expanded to remove language restrictions in April 2020. We checked for newly published relevant studies in April and October 2021 by screening papers citing studies already included in this review.
The search strategy was developed together with an academic librarian at Bodleian Health Care Libraries at the University of Oxford through a series of preliminary searches. Our search strategy included two key elements: (1) terms describing timing, frequency and intervals; and (2) terms related to routine monitoring of patients using EWSs or medical emergency team (MET) systems, as well additional search terms to exclude studies on non-adult patient populations as well as conference abstracts. We included primary research and relevant review articles in this scoping review because the latter further highlighted the existing evidence gap. The full list of resulting search terms is provided in Appendix 1.
Study selection
The initial screening for inclusion was based on the title and abstract of studies to eliminate studies that did not meet the minimum inclusion criteria. Two of the project team members (CK and IK) reviewed titles and abstracts generated by the original search against agreed inclusion and exclusion criteria. Any disagreements regarding inclusions were resolved through discussion, or, in the case of continued disagreement, by a third team member (OR). A full-text copy of each study was assessed against eligibility criteria by CK and IK to decide on final inclusion. Reference sections of selected studies were checked manually to identify additional relevant studies that were potentially missed in the database search. Relevant references as well as studies identified through other sources then underwent the same two-stage screening process to be included in this review.
Inclusion criteria: This review focuses on the monitoring of adult patients admitted to hospital wards. This review included publications in any language that reported on the speed of patient deterioration or the impact of patient monitoring on outcomes. Review articles addressing either of these questions were also included.
Exclusion criteria: Case studies, studies limited to outpatient care or care provision in community or rehab centres as well as studies on paediatric, pregnant or post-partum patients and patients directly admitted to ICU (without any monitoring on general wards) were excluded. Primary research studies were excluded if the article did not specify which frequency or protocol was used to monitor patients. Studies focusing on the escalation of care once deterioration was detected were also excluded, as were studies assessing compliance with an existing monitoring protocol. For primary studies that did not compare different monitoring regimes, studies were excluded if they did not present longitudinal vital sign data and hence did not provide information on the speed of deterioration which could potentially be used to guide the development of future monitoring protocols.
Charting data
Data from each article meeting the eligibility criteria were extracted separately by the two reviewers using a standardised data-extraction sheet. Collection included the following items: authors, year of publication, journal, type of study, study design and setting, patient population, vital signs and EWS monitored, monitoring protocols in intervention and control group (if applicable), methods and interventions, outcome measures, summary of results, key conclusions, recommendations, source. Identified studies were grouped into key themes based on similarities in their study design.
Results
Search results
Initial searches yielded 515 studies (after removal of duplicates), of which 77 were selected for full-text review. Eleven papers met eligibility criteria and an additional eight were added through citation tracking and other sources before undergoing data extraction [see Preferred Reporting Items for Systematic Reviews and Meta-Analyses (PRISMA) flow diagram, Figure 2]. The selected papers ranged from 2006 to 2020. The methodological quality of the studies was not formally assessed in line with the framework of scoping reviews. Meta-analysis was not feasible due to heterogeneity of study design, study populations and outcome measures.
FIGURE 2.
PRISMA flow diagram for the scoping review.
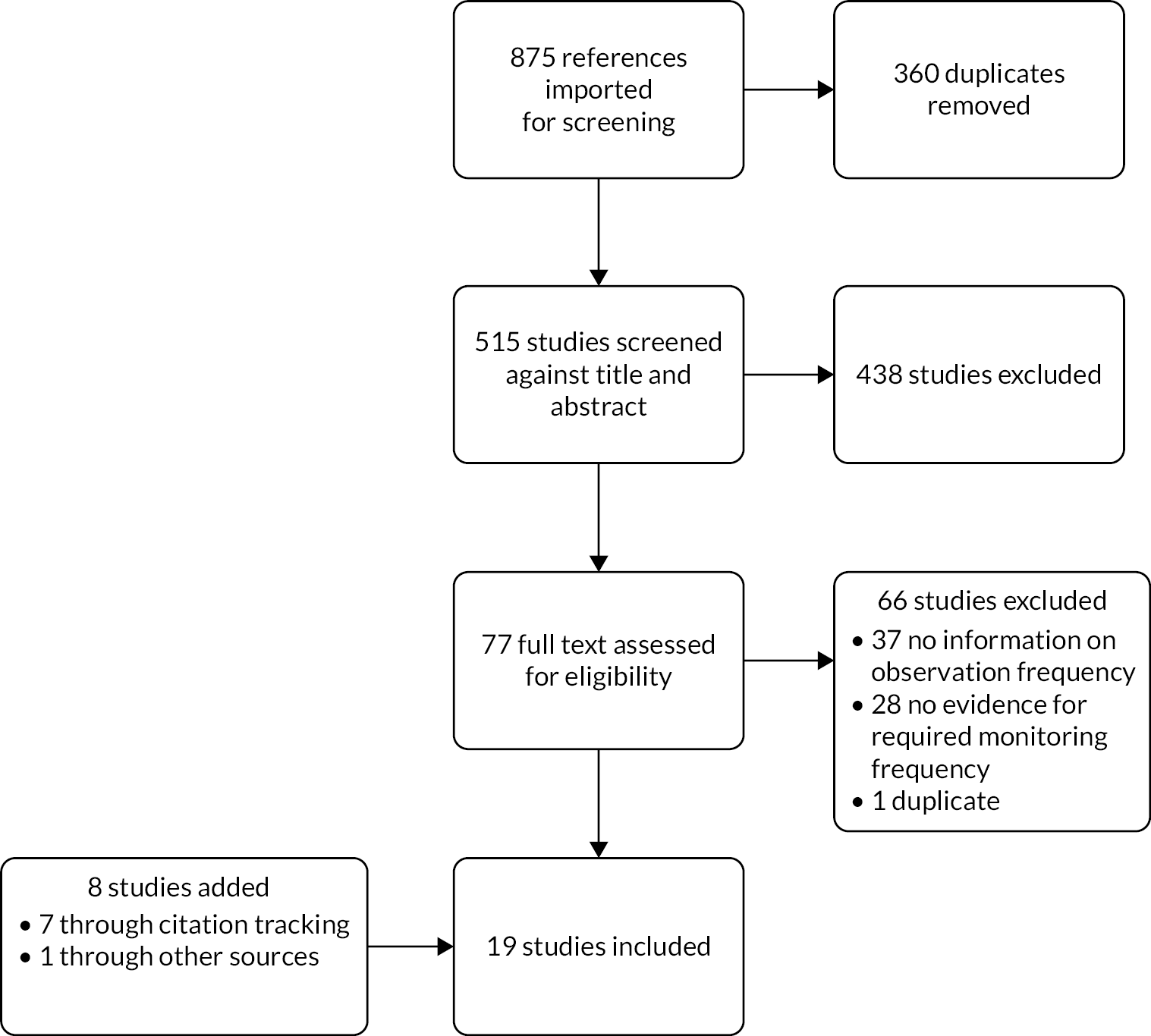
Of the 66 articles excluded during full-text assessment, 28 did not provide sufficient detail on the monitoring frequency used to collect data and a further 37 neither compared different monitoring protocols nor presented longitudinal vital sign or EWS data with high temporal resolution. We also excluded one study by Petersen et al. 52 that merely summarised, and hence duplicated, the results from an already included study53 as part of a published PhD thesis.
General characteristics of included studies
The majority of identified articles (68.4%) were published in 2016 or later. We identified two groups of articles:
-
twelve primary research studies (see Appendix 2),41,45,53–62 of which one was a survey of hospital policies on the management of the deteriorating patient;55
-
seven review articles (see Appendix 3)3,4,18,63–66 including one editorial4 and one opinion piece. 64
Key characteristics of primary research studies and review articles are detailed separately below.
Primary research
Of the 12 primary research studies identified (see Appendix 2):
-
three were randomised controlled trials (RCTs),45,53,67 of which two were cluster/ward randomised;45,53
-
seven were observational before-and-after studies or parallel group studies comparing different monitoring practice;56–62
-
one was a retrospective observational study, assessing trends in EWS observations;54
-
one was a qualitative review of hospital policies and guidelines on managing patient deterioration which is summarised separately below. 55
Articles reporting primary research originated from a variety of countries: three each from the USA, UK and Denmark, and one each from the Netherlands, Greece and Australia.
The majority of studies were carried out at a single institution: only one study involved two hospitals (one public and one private56). Study duration ranged from 8 weeks to 24 months, with six studies lasting between 3 and 7 months and four studies lasting 16 months or more.
The 11 quantitative studies involved a wide range of different study populations. One study focused on patients with acute exacerbated chronic obstructive pulmonary disease. 57 Five studies involved both medical and surgical patients41,53,57,61,62 and five involved different groups of surgical patients at different stages of their treatment journey. 45,56,58–60 The latter included one study of postoperative patients,56 one study of patients in a surgical trauma step-down unit,58 one with neurosurgical patients,59 one study of general elective patients45 and one study of elderly patients (≥ 65 years) with elective major abdominal cancer surgery. 60
Ten out of the 12 primary research studies examined interventions created by implementing or trialling new monitoring practice involving a change in monitoring frequency. Most commonly, studies compared intermittent and continuous monitoring practice (seven studies),41,45,57–61 followed by comparisons of different intermittent monitoring protocols (three studies). 53,56,62
Most studies involving continuous monitoring showed that continuous monitoring was able to identify more periods of deranged physiology;57–60 however, only one study investigated whether this had an impact on clinical outcomes. 60
Five studies investigated the effect of changes to monitoring regimes on adverse events or clinical outcomes such as length of stay (LOS), mortality, time to intervention of rapid response team (RRT) activation and ICU admission. 41,45,53,59,61 Evidence for the benefit of changing to continuous monitoring was varied. While some studies observed reduction in LOS either in hospital45 or in ICU61 and reduction of event rates including cardiac arrest, sepsis, mortality, ICU admission45,61 or RRT activation,59 others did not find significant differences in outcome rates such as mortality, cardiac arrest or transfer to high care. 41,59 One study (focused on the impact of different intermittent monitoring protocols in low-risk patients) found no benefit in increasing monitoring frequency to more than twice daily in this patient group. 53
Some studies highlighted technical problems and patient compliance with wearing continuous-monitoring devices as real issues. 41,45
One study showed that protocolised monitoring (three times daily) improved compliance over a clinical judgement model. 62
Two studies presented deterioration rates or patterns, providing indirect evidence for optimal monitoring frequency. 54,58 One study showed that 4-hourly monitoring of an EWS can provide an indication of a patient’s ICU LOS and mortality. 54
The paper by Freathy et al. 55 was a qualitative review of policies on the response to deterioration from 55 hospitals; 65% of hospitals used NEWS and all hospitals collected vital sign observations at a minimum frequency of two times a day, although observation protocols varied widely even when the same EWS was used. The study also revealed that policies often lacked specifics such as out-of-hour guidelines (missing in 40% of policies), and rarely specified the maximum permitted response time once deterioration was identified. It suggested that clear dissemination of service information to clinical staff is required and that hospitals need to review their policies and operating procedures, including guidance for whole 24-hour periods.
Included reviews
Seven of the articles we included were reviews, of which three were systematic18,65,66 and four were narrative reviews,3,4,63,64 reports from consensus conferences or opinion pieces (see Appendix 3). All but two3,65 were published in 2016 or later. Systematic reviews covered from 14 to 22 studies, while narrative reviews covered between 7 and 149 references.
One article pointed out that current monitoring practice is often based on tradition or clinical judgement. 64
Five articles discussed both continuous and intermittent monitoring,18 while one review focused on intermittent monitoring only63 and another considered vital signs measurements on ‘a routine basis’. 65
Four review articles concluded that there is some evidence that monitoring frequency has clinical relevance. 4,18,65,66 However, reviews presented varying evidence regarding the impact of continuous monitoring on clinical outcomes when compared to existing intermittent-monitoring practice. Cardona-Morell et al. 18 found no significant evidence for reduction in mortality with continuous versus intermittent monitoring but significant impact between different intermittent-monitoring practices. Sun et al. 66 concluded that the clinical evidence showed that continuous monitoring can reduce mortality, LOS, ICU admission and RRT activation, and Smith et al. 4 suggested that changes in monitoring practice can affect outcomes. Four review articles concluded that there is some evidence that monitoring frequency has clinical relevance. 4,18,65,66 However, reviews presented varying evidence regarding the impact of continuous monitoring on clinical outcomes when compared to existing intermittent-monitoring practice. Cardona-Morell et al. 18 found no significant evidence for reduction in mortality with continuous versus intermittent monitoring but significant impact between different intermittent-monitoring practices. Sun et al. 66 concluded that the clinical evidence showed that continuous monitoring can reduce mortality, LOS, ICU admission and RRT activation, and Smith et al. 4 found that changes in monitoring practice generally affect outcomes.
All selected review articles highlighted the lack of clinical evidence regarding optimum monitoring practice. They called for more research, particularly large clinical trials, into the clinical benefit of different monitoring frequencies, including the benefit of continuous monitoring.
One review pointed out that compliance with protocolised, intermittent-monitoring frequency is poor. There was no consensus on whether the introduction of protocolised monitoring (intermittent or continuous) improves adherence to monitoring schedules, with one article supporting this hypothesis18 and one suggesting evidence was inconclusive. 4
Recommendations regarding monitoring frequency
Overall, the studies we identified presented little robust evidence and few recommendations to support the development of evidence-based monitoring protocols. Almost all studies called for further research into the clinical effectiveness of different monitoring frequencies, with studies highlighting a lack of evidence supporting either currently implemented intermittent-monitoring regimes or the benefit of continuous monitoring.
The most specific recommendations identified through this scoping review are listed below:
-
Monitoring should be protocolised rather than based on tradition or clinical judgement, as this results in better compliance and more reliable RRT activation. 62 At the moment, however, intermittent monitoring of patients is predominantly tradition-based56,64 with large variations across NHS trusts. 55
-
Some evidence suggests that there is no benefit in monitoring low-risk patients more frequently than twice daily, that is 12 hourly. 53
-
Clinical consensus is that the minimum observation frequency is 12 hourly. However, this recommendation is not evidence-based.
-
Clinical consensus also suggests that patients should be monitored with individual intermittent-monitoring schedules based on their risk. 3,64
-
There is large variation in vital sign monitoring and scheduling across different hospitals, and guidelines often lack specifics. 55
-
There are very few studies comparing two different intermittent-monitoring protocols and most focus on compliance rather than outcomes or clinical effectiveness.
-
Most comparisons of different monitoring frequencies compare intermittent with continuous monitoring, often focusing on staff workload and alarm fatigue.
-
Where studies investigate the clinical benefit or effectiveness of continuous monitoring compared to intermittent monitoring, the evidence is inconclusive or controversial. 41,45,58,59
Discussion
The review revealed a general shift from studies describing the development, implementation and validation of EWSs68–71 to studies assessing the effectiveness of their associated escalation and monitoring protocols. 12,72–75
Lack of evidence on monitoring frequencies identified
The first paper to address the question of monitoring frequency was published by DeVita et al. 3 in 2010. The report from a consensus conference set out recommendations for identification and treatment of deteriorating patients. They highlighted that there was no evidence for the optimum monitoring frequency of patients in hospital but agreed that the assessment of vital signs should be made every 12 hours as a minimum. This review largely confirmed the lack of evidence regarding vital signs monitoring practices and timing of observations. A 2017 editorial by Smith et al. 4 stressed the need for research into best vital sign monitoring practice and required compliance with protocol. Storm-Versloot et al. 65 assessed the clinical relevance of routine vital signs observations and also indicated a lack of evidence. Other studies have also described the lack of consensus regarding necessary observation frequency but highlighted the importance of considering the nurse workload generated by monitoring demands. 74 This is also reflected in large variations in hospital policies guiding the observation of vital signs. 55
Protocolisation improves monitoring frequency
In an observational study of 804 surgical patients, Ludikhuize et al. 76 showed better detection of deteriorating patients with a regime for three sets of observations daily rather than nurse discretion. Their findings agree with several other studies showing that protocolised monitoring increased the number of observations taken and resulted in reduced adverse outcomes compared to monitoring based on clinical expertise or tradition. McGregor et al. 77 found standardised monitoring improved its reliability and was a step towards a patient-centred approach, while van Galen et al. 72 found the introduction of a protocolised EWS assessment was a good screen for major adverse events in the hospital population. In 2013, Hands et al. 12 found there was only partial adherence to the observation protocol with definite morning/evening observation peaks indicating observation rounds, although time to next observation (TTNO) decreased with increasing VitalPAC Early Warning Score (ViEWS), but not necessarily in line with the protocol. While these studies support the shift from monitoring guided by clinical expertise to protocolised monitoring, they were largely outside the scope of this review because they either did not provide enough information on the monitoring protocol used or did not investigate the impact of different intermittent monitoring schedules.
The evidence regarding the clinical benefit of an intermittent protocol is extremely scarce. This review identified only one observational study and one ward-level randomised non-blinded control trial53,62 that compared different monitoring regimes and investigated outcomes. Petersen et al. 53 showed that for low-risk patients observations made twice daily were as effective as three times per day in identifying clinical deterioration. However, they did not address the question of varying observation frequency based on patient risk. We did not find any patient-level RCTs investigating vital sign monitoring schedules.
Continuous versus intermittent monitoring
A number of recent studies have investigated devices to automate the observation of patient vital signs in an effort to gather evidence that supports widespread continuous monitoring of patients. Studies largely focus on the accuracy of these devices compared to manual observations by nursing staff,78 but some also investigated the comfort of wearable devices. 59,79–81 However, not all patients found the device acceptable and nearly a quarter of those in the study did not wear the monitoring device for the required time. 45 One study by Mestrom et al. 82 showed that automated systems can result in better compliance with protocols but results did not show an impact on outcomes in high-risk surgical patients.
The majority of studies identified in this review compared intermittent and continuous monitoring,41,57–61 exploring the potential benefits and drawbacks associated with continuous monitoring outside the ICU, and concluded that overall more research was deemed necessary. Watkinson et al. 41 found mandated electronic vital signs monitoring had no effect on adverse events or mortality in high-risk medical and surgical patients. A recent study by Areia et al. 79 identified benefits of continuous monitoring but suggested its use should be carefully considered given limitations such as excess noise, comfort, potential impact on patient mobility and direct nurse–patient contact should not be replaced.
Another potential issue associated with automated and/or continuous monitoring is the fact that, while evidence suggests that it improves vital sign documentation,82 it might reduce recognition and escalation as technology cannot replace clinical knowledge and acumen. 83 Introduction of continuous monitoring bears the risk of a reduction/limitation of nurse–patient interaction. Recognising the importance of nurse–patient interaction, which is often driven by vital sign observation, Klepstad et al. 179 recommended a maximum interval of 12 hours between observations, in line with DeVita et al. 3 and a suggestion by our stakeholders (see Chapter 7).
Our review showed that while continuous monitoring has the potential to play a major role at some point in the future, current evidence supporting the effectiveness of continuous monitoring is scarce41,45,59,66 and existing studies have identified issues with patient compliance45,59,61 and high alarm rates. 45,58,59,84,85
Compliance with existing protocols
While outside the scope of this review, we would like to highlight that any future evidence-based monitoring protocol will have to take into account that compliance with observation schedules will be limited. Hands et al. 12 found the vital signs monitoring protocol was only partially followed, although sicker patients were more likely to have overnight observations. Pimental et al. 86 reported that most deviations from protocol were attributable to various factors such as clinical judgement, the wish to avoid disturbing the patient at night and escalation might be more sensitive to nurse staffing levels than monitoring, which all need to be considered when developing new monitoring protocols. Similarly, Toth et al. 87 suggested avoiding waking low-risk patients at night might improve outcome (and patient satisfaction) as well as boost the efficiency of healthcare provision.
In summary, no evidence was found describing the best regime for intermittent monitoring, although for low-risk patients there was no support for assessing vital signs more than twice per day. There are trends towards the use of continuous monitoring and automation but benefits to support this outside of ICU are limited, while drawbacks include staff alarm fatigue and loss of nurse–patient contact. Therefore, further research into how different regimes for evidence-based intermittent vital signs monitoring is required.
What is the nursing time and workload involved in taking and recording patients’ vital signs? A systematic review
Before conducting our observational study to establish how long it takes nursing staff to perform and record vital signs, we carried out a systematic review of the literature on that topic.
The results of that review were published as:
-
Dall’ora C, Griffiths P, Hope J, Barker H, Smith G. What is the nursing time and workload involved in taking and recording patients’ vital signs? A systematic review. J Clin Nurs 2020;29(13–14);2053–68. https://doi.org/10.1111/jocn.1520288
The following summarises the contents of that paper (reproduced with permission).
Introduction
Patients’ vital signs and associated trends are accurate predictors of clinical deterioration,89–91 and a failure to monitor them is associated with adverse patient outcomes, including death. 13,92 Often the measured vital signs values are used within aggregate early warning scoring systems to provide a single numerical assessment of the patient’s risk of deterioration – an EWS (e.g. the NEWS). 20 Measuring and recording vital signs and calculating an EWS are fundamental aspects of nursing work in acute care hospitals. 93,94 However, these activities are often incomplete10,74,95 or sometimes omitted completely,96–99 with inadequate nurse staffing29,95,100 or long nursing shifts101 cited as possible underlying reasons.
This raises the question of what the workload associated with taking vital signs observations is, and it highlights the importance of understanding the costs and benefits of changes in vital signs observation frequency. A recent systematic review found that implementing continuous monitoring in acute wards outside of ICU is feasible and may improve patient safety; however, the cost-effectiveness of such an approach is still unknown. 102 Current guidance on the recommended frequency of vital signs collection is supported by minimal empirical evidence,4 and has largely been based on expert opinion. 2,3,103 While the evidence broadly points towards benefits from more frequent observations, the absence of precise guidance combined with uncertainty about the resources required makes comparison between alternative strategies difficult.
The precise contribution that measuring and recording vital signs makes to overall nurse and nursing assistant workloads is unknown. However, it will depend upon (1) the time taken to collect and document the vital signs; (2) the number of patients in a given clinical area needing to have vital signs measured at any one time; and (3) the chosen frequency of measurements for individual patients, which is dictated by clinical opinion and/or national policy. 2,4 This is summarised in Figure 3.
FIGURE 3.
Process of taking vital signs observations. aRepeated measurements sometimes required in order to check accuracy of the measured value. bThis calculation might be automatic following the entry of vital sign data.

Methods
Search strategy
We undertook a literature search up to 17 December 2019 to identify quantitative studies reporting the time spent by members of the nursing workforce (i.e. registered and licensed nurses, nursing assistants and equivalent roles – henceforth referred to as ‘nursing staff’) in undertaking vital signs observations, the length of time to take a set of observations or factors that influenced the time taken. The study methods were compliant with the PRISMA checklist. We searched CINAHL, MEDLINE, EMBASE and the Cochrane Library using the following search terms: vital signs; monitoring; surveillance; observation; recording; EWS; workload; time; and nursing (see Appendix 4). The search strategy was agreed by all authors and one author conducted the search.
Inclusion and exclusion criteria
We included studies that provided an evaluation of the time spent by members of the nursing workforce in gathering and recording any of the following vital signs (which are those included in NEWS). 20 These are heart (or pulse) rate; respiration rate (RR); body temperature; BP; level of consciousness; peripheral oxygen saturation (SpO2), and the inspired gas (air or oxygen) at the time of SpO2 measurement. 20 Because we anticipated that we would find limited evidence, we decided not to exclude studies that were not explicit in reporting which vital signs were being measured, provided that the focus appeared to be on these ‘standard’ observations. We focused on adult secondary and tertiary care ward settings, excluding studies exclusively in paediatric or maternity settings, as the necessary vital signs measurements are often different for these populations. We excluded qualitative-only studies, as our review question was quantitative in nature (i.e. time involved in vital signs activities). We retained studies that included other observations (e.g. patient weight, urine output) within the total times offered, as long as any or all of the components of NEWS were measured.
Data selection
One reviewer conducted the first screening of titles and abstracts for relevance. Two reviewers independently assessed the list of potentially relevant studies and identified studies for inclusion; any disagreements were resolved by discussion.
For quality appraisal, we focused on describing key aspects of the study likely to affect the validity of the results, including design, the methods of observation and recording, the vital signs observed and the setting and sample sizes, using a framework based on the Joanna Briggs Institute Critical Appraisal checklist for descriptive/case series. 104 Items that were not applicable to the main study question (such as confounding) were omitted from the checklist. The checklist comprises some items relating to risk of bias, and some concerning adequate reporting and statistical analysis, and poses questions to which possible answers are ‘no’, ‘yes’ and ‘unclear’. A response of ‘no’ or ‘unclear’ to any of the questions implies lower quality or else insufficient detail to judge the quality of a study. The checklist was completed by two reviewers, and one further reviewer resolved any disagreements. We did not exclude any studies based on their quality.
Data extraction
We extracted the following data from included studies: country; study design; sample size and setting; methods of vital signs measuring and recording; data collection; results; vital signs definition; mean (minutes); standard deviation (minutes).
Data analysis
Where authors reported only mean and 95% confidence intervals (CIs), we calculated the standard deviation as: (√n*(upper limit – lower limit)/t-value*2), where n is the sample size, upper limit and lower limit are those from confidence intervals. If the sample size is > 100, the 95% confidence interval is 3.92 standard errors wide. We initially considered conducting a meta-analysis, but the high heterogeneity between studies, in terms of sample sizes, settings and vital signs timing measurements, rendered this unfeasible.
Results
The database search retrieved 1277 papers, of which 11 studies met the inclusion criteria. An additional five studies were identified from the reference lists of papers accessed in full text (n = 59). The article screening and selection process is reported in Figure 4. The results of all 16 included studies are summarised in Appendix 5.
FIGURE 4.
Article screening and selection process.
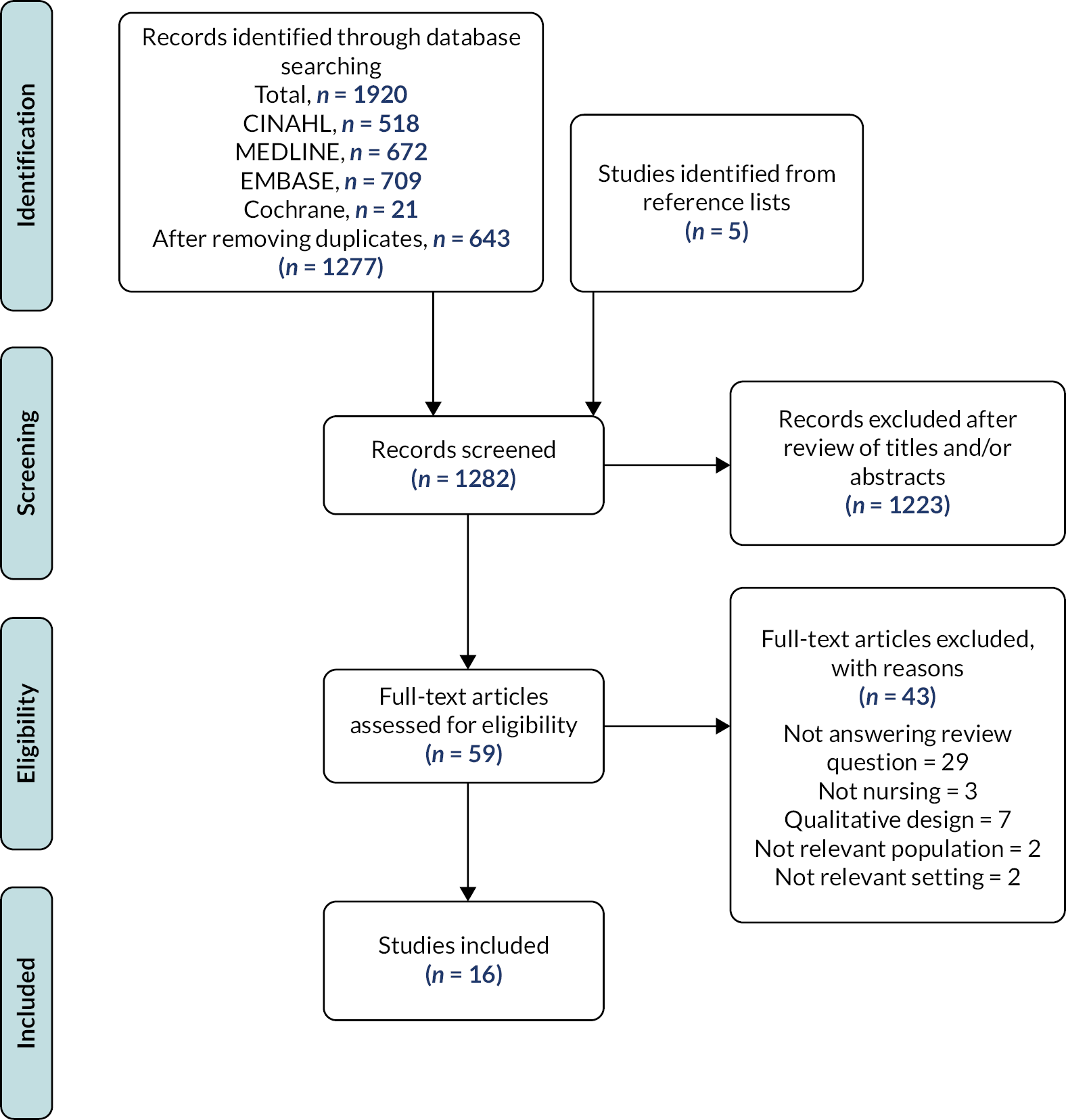
Overall, the quality of the reports was low, with unclear reporting and significant limitations across many items in most studies (Table 1). No study reported any reliability assessment of their measure of time, and no study scored a positive response to all remaining items on the checklist.
Study | Random or representative sample from defined population? | Clear inclusion/exclusion criteria? | Clear description of methods of assessment (time)? | Clear description of what was included/excluded in vital signs? | Was the assessment reliable? | Was information given to determine the precision of the estimate? |
---|---|---|---|---|---|---|
Bellomo et al. 2012 | U | N | N | Y | U | Y |
Clarke 2006 | N | ?N | Y | Y | U | Y |
Ito et al. 1997 | N | N | Y | N | U | N |
Kimura et al. 2016 | N | N | N | Y | U | Y |
McGrath et al. 2019 | N | N | Y | Y | U | N |
Travers 1999 | Y | Y | Y | Y | U | N |
Wager et al. 2010 | U | N | N | Y | U | Y |
Wong et al. 2017 | U | N | Y | Y | U | Y |
Zeitz 2005 | U | N | N | Y | U | Y |
Zeitz et al. 2006 | U | N | N | Y | U | Y |
Adomat and Hicks 2003 | N | N | Y | N | U | N |
Erb and Coble 1989 | N | N | N | Y | U | N |
Fuller et al. 2018 | N | N | N | Y | U | N |
Hendrich et al. 2008 | Y | Y | Y | N | U | N |
Hoi et al. 2010 | Y | Y | Y | N | U | N |
Yeung et al. 2012 | U | Y | Y | Y | U | Y |
Design of studies
Five publications were described as before-and-after studies,32,105–108 mostly evaluating the impact of introducing automatic electronic vital signs systems or continuous vital signs monitoring. Ten studies were classified as descriptive observational,56,109–116 and one study was a pilot study of a bedside clinical-information system. 117
Most (n = 11) used time-and-motion methodologies. 32,56,106,108,109,111,112,114–116,118 In eight studies, researchers collected data by directly observing nursing staff. 32,56,108,112,114–116,118 One study used data from a video recording of 48 continuous shifts. 109 Another plotted the number of steps that nurses took from the bedside to the computer when documenting vital signs in addition to measuring the time taken to complete and record vital signs observations using time-and-motion methodology. 106 In three studies, nurses were asked to estimate the time they had taken to complete vital signs observations. 107,110,111 In one of these studies, nurses noted the time taken to complete vital signs for each patient and calculated the total time spent on this activity. 107 In one time-sampling study, nurses were asked to report the activity they were engaged in when a personal digital assistant they were carrying vibrated at random times during the shift. 111 As indicated in Table 1, seven studies did not provide a clear description of how time to complete a set of vital signs was assessed; among these, three studies did not give any meaningful detail about how the time to complete a set of vital sign observations had been collected. 105,113,117 Among these, one study was available as abstract only. 113
Setting of studies
Coverage of settings ranged from 1 to 36 hospital wards of various types, namely: acute surgical/medical general wards, ICU, an emergency department, a cardiovascular unit, a trauma ward and a radiology unit. One study did not specify the type of hospital or which wards were included. 113
Methods of vital signs measurement
The 16 studies generally described taking vital signs observations as the time to measure/collect vital signs and the time to record/document vital signs. However, the specific set of vital signs chosen for measurement differed by study, which inevitably affected the overall time taken. Some included seven different physiological signs in a complete vital-sign set,32 while others included only four. 113 All studies reporting physiological signs measured temperature, heart rate (HR), RR and BP. 32,56,105,106,108,114–118 Some studies offered no specific description of the vital signs collected. 107,109,111,112 Also, studies did not always specify whether only complete sets of vital signs had been included for analysis. A number of studies included additional observational and assessment activities in the time taken to complete vital signs, such as completing fluid balance charts, checking an infusion pump and weighing the patient. 105,117 The measurement tools did not vary substantially across the years.
Methods of time recording
In general, the time involved in vital signs recording and documenting was reported in two different ways. A number of studies reported a mean time for taking a vital-sign set, mean time to record vital signs on charts or both. 32,56,105,107,108,110,113,114,116,118 Other studies reported the amount of time that nursing staff spent taking vital signs and/or recording them over a shift, per hour, per patient or over an amount of time (e.g. over 44.5 hours). 106,109,111,112,115,117 None attempted to disentangle the time taken to collect or document each vital sign (i.e. BP or oxygen saturations or RR) or EWS value separately.
Studies reporting mean times
Ten studies provided a total of 12 samples to estimate mean times for taking and/or recording vital signs (Table 2).
Study | Mean (minutes) | Standard deviation | Vital signs included | Vital signs activities assessed |
---|---|---|---|---|
Studies involving taking and documenting vital signs | ||||
Bellomo et al. 2012 | 4.10 | 1.3 | Temperature, HR, RR, BP, oxygen saturations, consciousness, urine output | Measure vital signs and document them with paper |
Bellomo et al. 2012 | 2.50 | 0.5 | Temperature, HR, RR, BP, oxygen saturations, consciousness, urine output | Measure vital signs and document them electronically at bedside |
Clarke 2006 | 5.80 | 3.72 | Cardiovascular, respiratory, body temperature data | Measure vital signs and document them with paper |
McGrath et al. 2019 | 2.15 | Not reported | Temperature, HR, RR, BP, oxygen saturations | Continuous monitoring (multiple monitors) and document vital signs electronically at bedside |
McGrath et al. 2019 | 2.98 | Not reported | Temperature, HR, RR, BP, oxygen saturations | Continuous monitoring (single monitor) and document vital signs electronically outside bed space |
Travers 1999 | 4 | Not reported | BP, pulse, RR, tympanic temperature | Measure vital signs observations |
Wong et al. 2017 | 3.58 | 8.9a | Temperature, HR, RR, BP, oxygen saturations, oxygen therapy, consciousness | Measure vital signs and document them with paper |
Wong et al. 2017 | 2.50 | 0.74a | Temperature, HR, RR, BP, oxygen saturations, oxygen therapy, consciousness | Measure vital signs and document them electronically at bedside |
Zeitz 2005 | 5.80 | 2.56 | Temperature, HR, RR, BP | Measure vital signs and document them with paper |
Zeitz et al. 2006 | 5.80 | 2.56 | Temperature, HR, RR, BP | Measure vital signs and document them with paper |
Studies reporting documentation only | ||||
Ito et al. 1997 | 0.90 | Not reported | Not specified | Document vital signs electronically at bedside using RFID from continuous monitor |
Ito et al. 1997 | 2.02 | Not reported | Not specified | Document vital signs electronically outside bed space |
Kimura et al. 2016 | 1.27 | 0.55 | Body temperature, oxygen saturations, HR, BP | Document vital signs electronically at bedside with hand-held device |
Kimura et al. 2016 | 1.47 | 0.62 | Body temperature, oxygen saturations, HR, BP | Document vital signs electronically outside bed space |
Wager et al. 2010 | 1.24 | 2.17 | BP, temperature, HR, SpO2, RR | Mean time difference between the time vital signs were taken and when the data were recorded on paper in the patient’s record (paper to paper) |
Wager et al. 2010 | 9.15 | 7.25 | BP, temperature, HR, SpO2, RR | Mean time difference between the time vital signs were taken on paper and when the data were recorded on a computer on wheels |
Wager et al. 2010 | 0.59 | 1.42 | BP, temperature, HR, SpO2, RR | Mean time difference between the time vital signs were taken on a vital-sign monitor and when the data were recorded on PC tablet |
When studies investigated the time involved in measuring and documenting vital signs using pen-and-paper methods, mean times ranged from 3.58 minutes32 to 5.80 minutes. 56,116 When documentation was performed using electronic systems, mean times for measurement and documentation were lower, at 2.50 minutes in both studies. 32,105 We did not find any differences in mean times involved in vital signs measuring and documenting that could be attributed to different clinical settings, nor to different nursing personnel (i.e. RNs vs. nurse assistants). Differences in mean times appeared to be related to the combination of vital signs included within the recorded data set; the method(s) of vital signs measurement; the timing of entry into the patient record; the method of recording; the calculation of EWS values. In addition to mean times being variable between studies, it was clear that there was considerable variation in the time taken within some studies, as standard deviations were high.
All studies where reported times focused only on vital signs documentation involved continuous patient monitoring and focused on electronic systems of data transfer to the patient record. Electronic systems where vital signs were entered at the bedside seemed to be associated with reduced time. The mean times to document vital signs observations electronically at the bedside ranged from 0.90107 to 1.27 minutes,113 and the mean time for documenting vital signs outside the bed space was between 1.47 minutes113 and 2.02 minutes. 107 One study focused on the mean time difference between the time vital signs were taken and when the data were recorded in the patient’s record, and found that when staff were recording data on a vital signs monitor at the bedside and transferring them to a PC tablet the mean difference was 0.59 minutes; when vital signs observations were transcribed from handwritten notes to patient notes the mean difference was 1.24 minutes; and for handwritten observations to be transferred to a computer on wheels outside the bed space, the latency time was 9.15 minutes. 114
Studies which do not provide mean time estimates
Four studies reported the time involved in collecting and recording vital signs by hour or by nursing shift. 109,111,112,115 Hoi et al. found it took 144 minutes of total nursing time per day for a patient in the most highly acute and dependent category, where assistance with all care needs and multiple treatments were often required. 112 Adomat and Hicks showed that charting observations and record-keeping in two units ranged from a mean average of 5.44 to 10.78 minutes per hour in an HDU and from 10.66 to 17.43 min/hour in ICU. 109 Hendrich et al. showed that vital signs took up 7.2% of nursing time or 30.9 minutes in a 10-hour shift. 111 According to Yeung et al., the total time spent by each nurse performing vital signs observations was on average 12 minutes, albeit the unit of observation was not reported (i.e. per hour, per shift or per patient). 115
Erb and Coble reported vital signs documentation on a new automated system, where nurses record all vital signs at the bedside using a monitor that measures BP, pulse rate and temperature. 117 Vital signs data are stored on a computer at the nurse station unit, and the bedside unit and nurse station unit are connected directly. The authors compared this system to an older manual system, and found that it offered an overall mean time saving per nurse per shift of 11.86 minutes. 117 Fuller et al. reported that the time taken to document vital signs in a computer was 7 minutes per 10 patients,106 suggesting a mean time below that of any study reporting a per patient time.
Discussion
This is the first systematic review of evidence to identify the amount of nursing time required to take vital signs observations. We found 16 studies that evaluated the time taken by nursing staff to perform and/or record vital signs observations. Studies varied considerably in their time estimates, although most estimates demonstrate the potential for this activity to occupy a considerable amount of nursing time, especially if undertaken with high frequency. However, this variation and uncertainty in the evidence means that we were unable to give a reliable estimate of time taken. A variety of factors influence the times taken to complete vital signs observations and, while the studies illustrate these factors, they are inconsistently and incompletely recorded in the literature, making direct comparisons of their influence on total times difficult or impossible.
We identified a number of key variables related to overall times recorded in the studies:
-
the combination of vital signs included within the recorded data set;
-
the method(s) of vital signs measurement;
-
the timing of entry into the patient record;
-
the method of recording;
-
the calculation of EWS values.
Across studies, there was variation in the data set of vital signs measured each time, as these are often determined by local guidance. There was also variation in the methods of measurement of vital signs observations. Some vital signs (e.g. HR and respiratory rate) can be measured manually (i.e. without the use of equipment) or automatically using devices/monitors. Some parameters (e.g. consciousness level) can only be measured on general wards using manual techniques, while others (e.g. SpO2) can only be measured using an electronic monitor. Studies considered in this review either involved a mixture of manual and automatic methods of measuring vital signs or did not clearly report them. 107,109,111,112
A further source of variation seen in the papers described in this review was the timing of entry of measured vital signs data into charting systems. Nursing staff entered data either in real time at the patient’s bedside105–108,117 or after leaving the bedside (i.e. delayed). 32,114,115 In some studies, vital signs data were entered in real time at the bedside on paper charts, and in others they were manually entered on electronic or paper medical records after collection. However, in the papers reviewed here, when data were recorded at the bedside, it was mostly done using hand-held electronic devices, where data were either uploaded automatically or required the nurse to physically transfer data to a central database using a wired system. Results from studies where real-time electronic systems had been introduced showed a reduction in the time involved in vital signs monitoring and recording compared to traditional paper-based methods, especially if the latter required further transcribing at the end of the observation sessions.
In the papers we studied, there was little indication of the approach to the calculation of EWS values even though determination of risk based on vital signs is now seen as an important function of taking the observations. 4 It would be possible for these to be calculated manually (i.e. without using a device), using a device such as a calculator, within a free-standing, mobile app, or automatically using a hand-held device or as part of the data measurement/entry system. In this review, two studies reported that the electronic systems being piloted were designed to calculate EWS values automatically after the entry of vital signs data. 32,105 In both studies, the EWS values were displayed on the electronic systems alongside clinical advice (e.g. escalation to a doctor or RRT) based on the automatically calculated EWS value. Previous studies reported that calculation of EWS with hand-held devices improves accuracy of EWS values119 and saves nursing time, with one study reporting that using a programmed digital assistant (i.e. VitalPAC™) was on average 1.6 times faster than using the traditional pen and paper method. 120
The workload involved in vital signs activities for nursing staff is potentially significant56,110,116 with important clinical consequences. However, we have shown that there is a very limited body of research that might inform workload planning. The studies surveyed here also highlight that there is currently no standardised way of measuring vital signs workload or interpreting it. Several publications affirm that failure to engage with vital signs activity leads to adverse patient outcomes, including mortality. 90,121,122 Nursing staff have previously reported that workload is an important factor in the timeliness and ability to observe patients regularly,74,123 so that the absence of reliable evidence to determine the workload involved with vital signs observations is surprising. This is of particular importance, especially as vital signs monitoring and recording is regarded as a fundamental component of nursing care. Clinical guidelines recommending the frequency of vital signs observations do not take into account the time required to complete them. 2,20
If nursing staff perceive the vital signs workload as excessive, they may choose to prioritise other activities and follow their clinical judgement rather than an observation schedule dictated by a protocol124 At present, there is no evidence to determine whether the nursing workforce is sufficient to accommodate existing demand – or potentially an increase in demand – arising from increasing compliance with current observation protocols, or from changing such protocols because the demand is not clearly quantified. Based on the current literature we cannot yet tell whether observations for all patients in a 30-bed unit might require an hour of work (2 minutes per patient) or two and a half hours (5 minutes per patient) or indeed considerably more or less if these estimates are inaccurate, or suboptimal systems are in place.
The investigation of time and workload involved in taking vital signs observations activities has focused mainly on reporting average times. However, mean times varied substantially due to different physiological parameters being measured across studies and, where reported, different methods of measurement and vital signs documenting. Future research that can determine the workload associated with nurses’ activities around vital signs observations is warranted. Future studies should be more explicit in describing contexts and systems in use. In particular, for new electronic systems, it would be worthwhile establishing how accessible it is for nursing staff to observe vital signs observation trends of their patients and how accessible these systems are for temporary staff.
Limitations
In appraising studies, we applied a checklist based on the Joanna Briggs Institute Critical Appraisal checklist for descriptive/case series,104 and all studies were of low quality. We highlighted key omissions of important details, for example which vital signs were included and how they were measured and recorded, or how nurses and/or patients were sampled. The results of the review illustrate the variety of factors that may influence the time estimates derived from the studies and demonstrate where information is missing. While we used a reproducible search strategy searching MEDLINE, CINAHL, EMBASE and the Cochrane Library, it is possible that we did not identify studies indexed elsewhere and not cited by the included studies. It seems unlikely that these exist in sufficient quantity to substantively change our conclusions.
Conclusions
There is currently insufficient robust evidence around the time nurses require to perform vital signs activities. To increase consistency and impact, we propose a framework for future studies to adopt measuring the time and workload involved in vital signs observations that includes (1) the methods of measurements, (2) the timing of entry of measured data into the charting system and (3) the approach to calculation of EWS values. This categorisation would be suitable for vital signs measured on an individual patient basis, or on the basis of vital signs ‘rounds’, where the mean time for each patient on the round could be calculated.
Recommendations for vital signs observations need to consider the workload involved and include consideration of the potential opportunity costs if observations are given higher priority at the expense of other aspects of nursing work. Vital signs observations are considered to be a fundamental aspect of nursing work and key to ensure early detection of patient deterioration. The lack of robust evidence means that those making clinical and managerial decisions about resource allocations, including workload planning around vital signs observations, must make these in the face of considerable uncertainty. Uncertainty means that the workload associated with changes to the frequency of observations associated with EWSs is unknown. At a system level, the costs from changes in policy such as the shift from NEWS to NEWS2, which increased the frequency of observations for some patient groups, are unquantifiable. On a ward level, the feasibility of implementing such changes and integrating them into existing work is uncertain. On another hand, workload reductions associated with the introduction of technology that facilitates continuous monitoring, which might reduce requirement for nurses to take vital signs observations, are equally uncertain. Measuring patients’ vital signs at times that are appropriate is key to avoiding patient deterioration and adverse outcomes. In the interest of patient safety, further research that uses vital signs and patient objective data aiming to define the optimal frequency of vital signs observations should be conducted.
Chapter 3 Methods: prospective observational study
Adapted with permission from Dall’ora C, Griffiths P, Hope J, Briggs J, Jones J, Gerry S, Redfern OC. How long do nursing staff take to measure and record patients’ vital signs observations in hospital? A time-and-motion study. Int J Nurs Stud 2021;118:103921. https://doi.org/10.1016/j.ijnurstu.2021.103921125
Study setting
The observational ‘time and motion’ study aimed to provide reliable estimates of the nursing work involved in measuring vital signs and to identify factors influencing the time involved with measuring and recording vital signs. It was undertaken in 16 adult general wards at 4 acute general NHS hospitals in four trusts. The sample of hospitals was largely one of convenience informed by geographical location in the Southeast/South Central Region, but we sought variation in the approach to recording vital signs observations when we approached potential participants beyond the two sites participating in the rest of the study.
Sample and recruitment
All hospitals used NEWS2 to guide the frequency at which patients are observed. Three hospitals used electronic systems to record vital signs. These electronic systems differed in each hospital, but they shared the embedded algorithm to calculate NEWS2 scores. In one hospital, vital signs were recorded on paper charts at the patient’s bedside and the NEWS2 was calculated manually.
Eligible wards were those classified as adult general (medical/surgical) wards. As there is no generally accepted precise definition of this, we asked participating trusts to identify wards against the following criteria:
-
Adult (18+) patients. Wards that occasionally admit adolescents of younger age would remain eligible provided this was not a common occurrence.
-
Open at weekends with most patients experiencing overnight stays of 1 day or more.
-
Ward provides Levels of Care 0, or 0 and 1.
[Note: Level 0 care: patients whose needs can be met through normal ward care in an acute hospital and patients at risk of their condition deteriorating. Level 1 care: patients recently relocated from higher levels of care, whose needs can be met on an acute ward with additional advice and support from the critical care team. Levels of care above 1 (levels 2 and 3) are delivered for the most part in specified critical care areas. The majority of patients receiving care above level 1 will require support for one or more major organ systems. 126]
Exclusions:
-
wards which routinely cater for large numbers of younger people (< 18);
-
wards in which a significant proportion of patients require protective isolation;
-
wards that are intended to exclusively provide Level 1+ care;
-
wards where the risk of acute deterioration is low or where the primary reason for continued hospital stay is not medical/surgical treatment/recovery (e.g. rehabilitation units, other post-acute wards);
-
wards where full vital signs measurements are not routinely taken;
-
small wards.
We then randomly selected four wards in each hospital. If a ward manager did not agree to participate, we randomly selected another ward. In each ward, the ward manager provided consent for the research on behalf of the ward staff, and we sought to recruit all nursing staff [i.e. RNs, healthcare assistants (HCAs), nursing associates, student nurses] working on the ward at the time of each observation session. Staff were given the chance to opt out of the research when the observation session began and when approached during the session. Their consent to participate was implicit – we chose not to record it in writing because that would have imposed additional workload on the staff concerned. Patients and relatives were informed about the study through posters displayed on the ward and through explanation given by the researchers and/or nursing staff when patients were having their vital signs monitored. We explained to patients that they were not the focus of our studies and that we were observing nursing staff only, and gave them the option to opt out in case they did not want to have researchers near their bed space, which only occurred twice. These procedures were reviewed and approved by the relevant ethics committees (NHS REC and University of Southampton).
There were few existing data to help calculate the required sample size and estimation was further complicated by clustering of observations in nurses and units. As a guide, we used the confidence intervals reported by Wong and colleagues,32 a study with a similar clustering structure to our planned study, to provide an estimate. Based on their 95% confidence intervals, we inferred a standard error of 10.96 [standard error = 95% CI/(2*1.96)]. Based on their standard error, in a sample of 280 observations from wards with electronic recording of vital signs, we calculated that a sample of 640 sets of observations would give an estimated mean with a precision of ± < 10% if each set of observations took 3 minutes. For each ward, we planned to observe for a total of 8 hours, spread across four sessions, aiming to observe a minimum of 10 sets of vital signs measurements per session.
Data collection
Data were collected by trained non-participating observers using the Quality of Interactions (QI) tool, bespoke software on an Android tablet that enables users to enter data in real time. The QI tool also enabled us to collect contextual data, including the total number of patients on the ward, and numbers of RNs, nursing assistants and student nurses on shift during the observation session as well as qualitative observations on factors influencing the measurement and recording of vital signs, including reasons for, and the nature of, interruptions. In order to achieve the required sample size sessions were scheduled at times ward staff reported that we could expect to see a lot of vital signs activity. At the end of each 2-hour session, researchers also asked staff whether they had modified their practice as a result of being observed. The general approach to observation and the tool have been used previously. 127 We adapted the approach to focus specifically on activities related to taking vital signs and associated interruptions.
The vital signs-related activities recorded during observations are reported in Appendix 6. Observers recorded start and finish times for vital signs rounds, individual vital signs measurements and interruptions. We were interested in the preparation time occurring at the beginning of the observations round, as this is work associated with vital signs, and we wanted to take interruptions into account if they contained any vital signs-related activity. A vital signs round was deemed to start every time nursing staff sourced vital signs equipment or vital signs documentation, and to finish when one or more sets of vital signs observations were taken and the vital signs equipment and/or documentation were replaced. A vital signs observation set was deemed to start when a nurse entered the bed space and measured one or more of the six physiological parameters of the NEWS2 scoring system, and to end when the nurse left the bed space and the measuring of vital signs as defined by NEWS2 had finished.
Observers were trained using an observation guide. The guide was found to be easy to use and led to a high level of inter-rater agreement, with a mean difference between raters of only 3 seconds per set of vital signs observation (mean observation estimate 3 minutes 47 seconds) and limits of agreement from + 19 to −13 seconds. The cumulative difference over 4 hours of observation during the training sessions was less than 2% of the total time observed. See Dall’ora et al. 128 for more detail on the development of the standardised approach, observer training and reliability testing.
Data management
After each observation session, data were uploaded onto the QI tool server. This server and the associated databases were located at, and managed by the IT team of, the raters’ institution. We did not store any personal data. Before data analysis, one member of the team checked all data extracts for any errors: for example, if an observer had indicated that the timing of an interaction was incorrect (e.g. where there had been an inadvertent delay in recording the end of the observation). These instances were rare (n = 37) and were corrected, and all interruptions were coded as vital-sign-related or not vital signs related.
Statistical analysis
Our data comprised sets of vital sign observations within observation rounds. As well as the time taken to measure and record vital signs, each round included preparation time and interruptions. For this reason, we estimated the time taken to perform a set of vital sign observations in three ways:
-
Our first estimate (E1) was calculated by dividing the length of the round by the number of vital signs observation sets.
-
Our second estimate (E2) was the time taken at the patient’s bedside, between when the nurse entered and left the bed space.
-
Variants of the E1 and E2 estimates were calculated by removing time associated with some or all interruptions (e.g. non-vital signs related such as discussions with relatives).
To account for heterogeneity within hospitals and wards, we used mixed-effects models with nested random-effects terms for wards within each study hospital. To test whether the time taken to obtain observations was influenced by nursing staff grade (i.e. RN, nursing assistant and student nurse) or study hospital, we fitted a second model where nursing staff grade and study hospital were set as fixed effects. Coefficients from these models were used to estimate adjusted (conditional) means for each hospital and staff grade. Model-based estimates were compared to raw means, with confidence intervals calculated using bootstrapping (2000 samples).
To determine whether any efficiency was gained by measuring individual observations within a round, we fitted a mixed model to estimate the total time of the round, with the number of observations as a fixed effect and nested random-effects terms for wards within each study hospital. Conditional means from this model allowed us to estimate the marginal effects from changing the length of rounds. All analyses were performed with R. 129
Qualitative data analysis
A wide range of different kinds of activities that delayed or paused vital signs measurement were recorded during our observations. Through thematic coding (following the approach described by Lofland et al. 130) each interruption was given a first-order (index) code and these codes were organised under six second-order codes. Third-order theoretical coding was used to explore how different kinds of interruptions influenced the measurement of vital signs observations and, in particular, the scheduling of care. Coding, themes and third-order theoretical coding were developed iteratively with a lead researcher (JH) making initial proposals that were then discussed with a wider group including researchers who were qualified health professions (CDO, PG, OR) until consensus was reached.
Chapter 4 Results of the observational study
The results presented here are adapted from Dall’ora C, Griffiths P, Hope J, Briggs J, Jones J, Gerry S, Redfern OC. How long do nursing staff take to measure and record patients’ vital signs observations in hospital? A time-and-motion study. Int J Nurs Stud 2021;118:103921. https://doi.org/10.1016/j.ijnurstu.2021.103921125
Length of time
We undertook 64 sessions in four hospitals. In hospitals 1, 2 and 3 vital signs observations were recorded on electronic devices. Hospital 4 recorded vital signs observations on paper charts after which nurses manually calculated the NEWS2. In total, we observed 715 sets of vital signs measurements (181 in Hospital 1, 219 in Hospital 2, 140 in Hospital 3 and 175 in Hospital 4). Of these, 680 (95%) were complete sets where all six vital signs were measured and recorded. These observations were clustered in 260 rounds, with a median of two observations per round (interquartile range: 1–4; range: 1–11).
Registered nurses performed 355 observations (50%) nested in 122 rounds (47%). Healthcare assistants performed 217 observations (30%) nested in 81 rounds (31%). Student nurses performed 143 observations (20%) nested in 57 rounds (22%). There was little evidence that observation changed behaviours, although in five sessions a staff member reported they felt their behaviour had changed as a result of being observed. The number and proportions of vital signs sets performed by staff group per hospital are shown in Table 3.
Number of vital sign sets | Hospital 1 N (%) |
Hospital 2 N (%) |
Hospital 3 N (%) |
Hospital 4 N (%) |
Total N (%) |
---|---|---|---|---|---|
RNs | 54 (30) | 163 (74) | 116 (83) | 22 (13) | 355 (50) |
HCA | 63 (35) | 32 (15) | 1 (1) | 121 (69) | 217 (30) |
Student nurse | 64 (35) | 24 (11) | 23 (16) | 32 (18) | 143 (20) |
Total | 181 (100) | 219 (100) | 140 (100) | 175 (100) | 715 (100) |
The proportion of vital signs taken by registered nurses ranged from 13% in Hospital 4 to 83% in Hospital 3. In Hospitals 2 and 3, RNs took the majority of the vital signs observations, whereas HCAs and student nurses did so in Hospitals 1 and 4.
When considering estimates from rounds, where we calculated the time as total round length divided by the number of observations within that round, the mean time per observation set excluding non-vital-sign-related interruptions was 5 minutes and 1 second (5:01) (95% CI = 4:39 to 5:24).
When considering the time at the patient bedside only (i.e. vital signs observation sets without the preparatory time that is shared out across a round), the mean time after excluding non-vital-sign-related interruptions was 3:45 (95% CI 3:32 to 3:58). Table 4 summarises all marginal means from the mixed-effects models. The raw estimates did not differ substantially from the mixed-effects models’ estimates. There was some positive (right) skew in the distribution of time taken, with means slightly higher than the median although the distributions were not grossly asymmetrical.
Including interruptions (minutes:seconds), mean (95% CI) | Excluding non-vital-sign interruptions (minutes:seconds), mean (95% CI) |
Excluding all interruptions (minutes:seconds), mean (95% CI) |
---|---|---|
From rounds | ||
6:26 (6:01 to 6:50) | 5:01 (4:39 to 5:24) | 4:15 (4:04 to 4:27) |
At the patient bedside only | ||
4:24 (4:11 to 4:38) | 3:45 (3:32 to 3:58) | 3:31 (3:22 to 3:40) |
Differences between hospitals and staff groups were small and there was no substantial variation associated with wards. Table 5 shows the marginal mean estimates (excluding non-vital signs observations) for staff groups and hospital. Round-based estimates ranged from 4:53 for RNs to 5:16 for student nurses. For hospitals, estimates ranged from 4:38 per set of observations to 5:41. In both cases, there was substantial overlap between the confidence intervals. Differences based on bedside-only observations were much smaller.
Estimates from rounds (minutes:seconds), mean (95% CI) | Estimates from time at the patient bedside only (minutes:seconds), mean (95% CI) | |
---|---|---|
Staff type | ||
RN (n = 355) | 4:53 (4:26 to 5:20) | 3:42 (3:27 to 3:58) |
HCA (n = 217) | 5:08 (4:35 to 5:41) | 3:48 (3:29 to 4:07) |
Student nurse (n = 143) | 5:16 (4:41 to 5:51) | 3:51 (3:31 to 4:11) |
Hospital | ||
Hospital 1 | 4:55 (4:17 to 5:33) | 3:43 (3:18 to 4:08) |
Hospital 2 | 5:08 (4:27 to 5:50) | 3:41 (3:16 to 4:06) |
Hospital 3 | 5:41 (4:57 to 6:26) | 4:02 (3:34 to 4:30) |
Hospital 4 | 4:38 (3:58 to 5:17) | 3:42 (3:16 to 4:08) |
Are there efficiencies to be gained from batching observations of multiple patients in the same round? We found substantial efficiency gains from undertaking observations as part of a round as opposed to observing a single patient (effectively a round comprising one set of vital signs observations). However, marginal gains from longer (as opposed to shorter) rounds were low. Using estimates excluding non-vital signs observations, we estimated that a round where two patients are observed takes 7% less time per person observed than observing a single patient. This occurs because preparatory time such as sourcing equipment and travel to the patient care area is divided across more than one patient. Marginal gains reduce as round length increases. A round where five patients are observed takes 12% less time per patient compared to observing a single patient, while rounds of 10 patients give a time saving of 13% per patient (Figure 5).
FIGURE 5.
Time per set of observations vs. number of patients observed in a round.

Since the rounds-based estimate, excluding interruptions, best represents the direct work associated with measuring and recording vital signs, we use this estimate (5:01 minutes) as our primary estimate in the economic analysis (see Economic analysis).
Limitations
Our data were collected during periods of time when staff had told us we could observe vital signs observations being taken in hospital wards. We observed only on weekdays between 9 a.m. and 5 p.m. However, there is no a priori reason to assume that time taken differs at night, although this is possible due to increased time taken to wake patients up and/or the challenges associated with measuring and recording vital signs observations in low light conditions. Our sample is also based on UK hospitals only so our results may not generalise to other countries; however, our sample is bigger than most previous studies, which have been mainly conducted in a single hospital. 108–110 In common with other studies the presence of observers and awareness of being observed might have influenced the behaviours of staff, although we explained carefully to participants that the aim of the study was to achieve a realistic picture of vital signs observations and not to measure their performance. In most cases, staff reported that they did not feel observation influenced behaviour although we cannot discount unconscious changes. The direction of any resulting bias is unpredictable as it could lead to either slower (more careful/diligent) observation or faster (more focused/efficient) performance. We have presented arithmetic means because our main purpose is to give an estimate of the work involved which can be summed across activities and used to estimate the effect of changes. Other measures of central tendency might better reflect the typical time taken because the distributions are asymmetrical, but such measures cannot be used arithmetically to quantify the effect of changes on the required staff time. Because of the large sample, according to the central limit theorem, estimates and inferences (including 95% CIs) are likely to be robust because sample means are likely to be normally distributed.
While these data can show us what kinds of other activities interrupted vital signs, we cannot know from these data how often vital signs observations were taken in preference to other kinds of care that were left undone. However, we observed some instances of nurses taking vital signs who declined to support their colleagues’ requests for collaborative care, as described below.
Interruptions to vital signs observations
Despite all wards being in hospitals where NEWS protocols for individualised monitoring frequencies were in place, 15 out of 16 ward leaders planned to take their vital signs in rounds which were expected to happen at certain times of day. A wide range of different kinds of activities were recorded during our observations that delayed or paused vital signs observations being taken. Through thematic coding (following the approach described by Lofland et al. 130) these were sorted into six themed areas. Each had a different kind of impact on vital signs observations and care, which are explored here.
Fixed care
Fixed care must be accomplished at a set time. These tasks happened at set times every day, for example washing, meals and associated tasks, doctors’ rounds, medication rounds. Fixed care tasks tended to delay the start of vital signs rounds or reduce available pockets of time to complete them. If the window between two fixed care tasks narrowed too much (e.g. between doctors’ rounds and lunchtime), rounds were often delayed until a larger window of time became available. Our observation findings showed that despite ward leaders planning for vital signs rounds to take place at a certain time of day, they would be delayed if they clashed with fixed care tasks.
Bundled care
Bundled care comprised tasks that needed to be completed during the course of a day or by (but not at) a particular time, which were then ‘bundled’ with vital signs observation rounds. This included completing food orders, discharge planning and other kinds of assessments (e.g. pain and nausea). Some of these tasks were part of other protocols, such as postoperative checks, neurological observations (post falls) and cognitive assessments on some wards.
While these extended the time at the bedside with patients, they were likely to represent a time saving through reducing overheads of time, for example in approaching patients, gathering equipment and taking notes. Indeed, some of these assessments were built into EWS software and undertaken alongside NEWS observations.
Collaborative care
Collaborative care consisted of key communications and activities with other health professionals (other members of the nursing team, doctors, physios, specialist nurses, etc.). They involve care planning, asking for medication to be prescribed, passing on important information about where staff or patients are, outcomes of assessments, planned investigations, checking if colleagues need help, colleagues asking for help, responding to requests to help move another patient and so on. This has been described as the ‘organising work’ of nurses in managing patient trajectories through collaboration with a range of health professionals. 131 These tend to be shorter verbal interruptions and staff decided whether requests were urgent or not in responding to them, so were less disruptive and time-consuming than care that took staff away from the bed space.
Reactive care
Care classified as reactive was emergencies that required a quick response – responding to patient crisis, for example unresponsive patient, supporting a patient to move to the toilet, reassuring an agitated patient, changing soiled bedding. We know from our data that staff did not always stop taking vital signs observations to respond to a ‘collaborative care’ request or direct patient request to deal with some forms of reactive care all the time (e.g. toileting or changing soiled bedding). These kinds of interruptions take more time but are less common than other kinds of interruptions found in our data.
Proximity-related care
Care classified as proximity-related was care that would not necessarily occur if a member of staff was not already with the patient doing vital signs observations (or some other bedside task). This includes responding to patient and visitor questions (e.g. about care, treatment, symptoms, when the doctor is coming), doing fundamental care tasks (e.g. noticing a patient needs a blanket, getting water for them, supporting/encouraging them to drink water), helping patients find dropped or lost items, getting pain medication or chatting with the patient. While not sharing the same urgency as reactive care, proximity-related care could also involve responding to important emergent needs, for example changing soiled bedding if a member of staff was already with a patient to carry out vital signs observations. Proximity-related care tended to increase the quality of care for the individual, and increased patient and relatives’ involvement and the timely meeting of fundamental care needs.
Patient absent
Sometimes observations could not be taken as a patient was absent from their bed or unavailable due a range of reasons (eating, speaking to a doctor, on the phone, having a scan or physio).
Prioritising vital signs versus other kinds of care
We observed that nurses attempted to protect vital signs observations by setting regular observation rounds during the day (in 15 of the 16 hospital wards we observed). However, during normal working hours (9 a.m.–5 p.m.), vital signs observations became more flexible in their timing as they had to compete with care tasks led by others, both fixed and flexible, which tended to be prioritised above vital signs observations and other forms of flexible, nurse-led care. There were two kinds of prioritisation happening on wards during these times. Reactive care that related to medical emergencies or managing risk (such as preventing someone at risk of falls from standing up) did interrupt vital signs observations but was relatively uncommon in this data set. For all other kinds of care, the timing of care and who was leading the care interacted to create four different categories of care that were prioritised differently.
Timing of care related to whether care was done at a fixed time every day or whether it was done at a variable time. Tasks done at a fixed time were routinely done close to the same time every day. Tasks done at a variable time were those that tended to be fitted around the fixed-time tasks. Where care was led by others (not nurses) it tended to be prioritised ahead of nursing tasks, particularly if the care was being carried out by someone with seniority or who had limited time to spend on the ward. In Figure 6, we show how the different kinds of care described in the previous section tended to relate to these four categories. It also shows how vital signs observations fitted into two different categories of care, depending on the time of day.
FIGURE 6.
Scheduling and care leadership interactions with vital signs interruptions.

Fixed-time care led by others tends to be done around the same time each day according to others’ fixed schedules and limits other kinds of care. This included input into patient care by doctors, who visited the ward on a fixed schedule each day for ward rounds, and meal deliveries. At these times these activities took precedence unless there was a medical emergency. For instance, we observed that vital signs observation rounds did not take place until after ward rounds had been completed and were not undertaken during meal delivery times. Nursing staff would try to organise rounds around these times, so would tell us, for example, that they were not taking a round too close to mealtimes starting.
Fixed-time, nursing-led care tends to be done at the same time every day by the nursing team but can be interrupted by other kinds of care. These are often done as rounds, and either are more likely to be adhered to due to medical consequences of failing to do them approximately on time (medication rounds), being part of the routine of the ward (e.g. washing rounds – although even these could be delayed), or are done in reliably quieter times when fewer interruptions are happening (morning/evening vital signs observations).
Variable-time care led by others tended to be prioritised ahead of other variable care according to others’/externally set schedules. Such care includes responding to colleagues’ input where the colleagues’ availability was limited and/or they had seniority.
In contrast, variable-time, nursing-led care tends to be missed more often – done around other care or when possible. This was fitted in ‘around’ the other forms of care, which either took place at a fixed time and/or were led by others or were prioritised as forms of reactive care. Variable-time, nursing-led care included fundamental care, scheduled daytime observations, proximity-related care and reactive care, when staff deemed care urgent enough to stop taking vital signs observations (e.g. medical emergencies but not always assistance to visit the toilet).
What are the implications for scheduling vital signs observations?
-
During 9 a.m.–5 p.m. hours, it is difficult for nursing teams to schedule observations whose timing is dictated by the NEWS protocol. These vital signs observations are treated as variable and nurse-led, making it hard for them to be prioritised above activities led by others, especially if these are fixed activities like meal deliveries and ward rounds.
-
It is easier for nurses to complete vital signs observations as rounds outside the 9–5 window, particularly first thing in the morning and in the evening as there are fewer competing care tasks led by others during this time – in this case, they are treated as fixed-time, nurse-led activities.
-
The more frequently vital signs need to be taken between 9 and 5, the more likely it is they will be delayed or missed by the need to meet other demands.
-
If frequency of observations is reduced, it is possible that vital signs observations may be completed in a more timely way between 9 and 5, although they are still likely to be prioritised below activities led by others, particularly fixed-time care activities.
-
Collaborative care interruptions tend to be short and verbal, and staff decide whether requests are urgent or not in responding to them. Thus, they are less disruptive overall than other forms of care. These are therefore unlikely to have much impact on how quickly vital signs observations are completed.
-
Bundled care is flexible and nurse-led but is prone to the same deprioritisation as other forms of variable-time, nurse-led care. It is possible that any reduction in vital signs observations might lead to more missed care in this area (e.g. missing other assessments like pain and nausea), particularly when this is included in software used to prompt and record vital signs readings. However, there are some forms of care that are required to be done by a certain time of day (like menu planning) and it is possible vital signs observations might be bundled with these between 9 and 5. Any increase in vital signs observations might lead to an increase in other bundled forms of assessment (e.g. pain assessment), especially if these are included in software used to prompt and record vital signs readings.
Proximity-related care was another form of variable-time, nurse-led care, which often improved the quality of care received by patients, making it more person-centred and responsive to patient needs. Patients in our discussion group also noted that taking vital signs provided an opportunity to raise questions. They reported they would not call a nurse over specifically to ask this kind of question. All forms of proximity-related care are likely to reduce with fewer observations or increase with greater observations, which would have an impact on the quality of care, patient involvement and the meeting of fundamental care needs. Emergent care needs that come to the nurse’s attention because of proximity are likely to be missed or met in a less timely fashion.
Chapter 5 Methods for the retrospective study
Study design
This is a retrospective longitudinal observational study using routinely collected data from two acute care NHS hospitals to study the association between frequency of vital sign observation and risk of adverse events. Additionally, the study models the optimum observation intervals for any given outcome as well as associated costs.
Setting
The study took place at two of the collaborating NHS Trusts: PHU and OUH.
PHU provides acute services from a single large district general hospital with approximately 1250 beds and 7200 staff, providing a full range of elective and emergency medical and surgical services to a local population of around 675,000. OUH provides inpatient services from four hospitals with a combined total of approximately 1465 beds and 12,000 staff, serving a local population of around 655,000. Three of the hospitals are in Oxford: the John Radcliffe Hospital (a teaching hospital which is a tertiary referral centre for trauma, cardiology and neurosurgery as well as providing general acute medical and surgical services), the Churchill Hospital (providing a specialist renal transplant and cancer referral centre) and the Nuffield Orthopaedic Centre (specialising in orthopaedics, rheumatology and rehabilitation). The fourth hospital is an acute district general hospital, the Horton General Hospital in Banbury, north Oxfordshire.
Data collection
The data in the retrospective part of this study were vital signs measurements from routine nurse observations and their associated EWS along with subsequent outcomes for all adult patients.
In both study hospitals, patient vital signs data were taken from adult general medical and surgical wards where they were recorded electronically at the bedside. For OUH, the source system was SEND,132 whereas for PHU it was System C ‘CareFlow Vitals’133 for inpatient observations and Servelec Oceano134 for observations taken in the emergency department.
The monitoring systems demand that each observation set contains all parameters required to calculate the NEWS or its successor NEWS2. The structure of NEWS2 differs from NEWS by the inclusion of a specific oxygen-saturation scale for use in patients at risk of type II respiratory failure and the addition of ‘new confusion’ (C) to the AVPU scale. NEWS or NEWS2 values used in our analyses were those calculated and displayed by the monitoring software in accordance with the original guidance from the RCP. 1,20 For over 95% of patients, NEWS and NEWS2 are effectively identical, and we therefore did not distinguish between the two and used whichever was exported from the monitoring systems.
The EWS value also determines the time to the next vital signs observation (TTNO), that is when the patient should next be monitored. Once a patient’s vital sign observations have been entered by the hospital staff, the monitoring systems automatically display the EWS value and when the next set of observation should be taken, based on the hospital’s clinical escalation protocol.
With regard to the frequency of monitoring, slightly different escalation protocols were in operation at PHU during the study period (Table 6). The original version of NEWS was deployed at PHU in 2011 and had the schedule shown in the first column. It was updated in November 2016 to increase the frequency of observation for patients with a NEWS of 6. Finally, it was updated in February 2019 with the implementation of NEWS2.
NEWS/NEWS2 value | Minimum interval between observations (minutes) | ||
---|---|---|---|
Pre December 2016 | December 2016–January 2019, inclusive | From February 2019 | |
0–1 | 360 or 720a | 360 or 720a | 360 |
2 | 360 | 360 | 360 |
3–4 | 240 | 240 | 240 |
5 | 240 | 240 | 60 |
6 | 240 | 120 | 60 |
7–8 | 60 | 60 | 30 |
≥ 9 | 30 | 30 | 30 |
In OUH, the SEND EWS135 was in use throughout the study period. Minimum observation frequencies were 720 minutes for patients with a SEND score of 0, 240 minutes for a score of 1 or 2 and 60 minutes for any higher score.
Data sources for the retrospective study
Data linkage
The requirement was to link these vital signs at the patient level to the patient administration, ICU and cardiac arrest data for outcome events. At Oxford, much of the data were available from a Trust data warehouse updated from their Cerner Millennium Electronic Patient Record (EPR), whereas in Portsmouth there was no integrated EPR at the time and the data were extracted from separate information systems.
The study protocol identified EWS values, unanticipated ICU admissions, cardiac arrests and deaths as indicators of deterioration. It is known from previous work that blood results as well as vital signs can be predictors of deterioration. Other relevant factors are demographics, comorbidities and surgical interventions. Considering this information generated a first-cut conceptual data model (shown in Appendix 7).
The model is complicated by the separation of emergency department (ED) attendance data and inpatient admission data. These two types of healthcare episodes are reported separately in activity reports although clinically many inpatient admissions directly follow an ED attendance. In Portsmouth, these ED attendance and admissions data are recorded in different information systems. This study is not concerned with ED attendances which are not followed by an admission, although any ED attendance data preceding an admission are relevant.
In PHU, as well as the vital signs data, the nurses record the ‘Observation Status’, which identifies whether the patient is on the standard schedule of vital signs monitoring determined by their previous NEWS or whether they are not for standard observations, usually because they are on an end-of-life pathway. In OUH systems, an electronic clinical note is created to identify patients on an end-of-life pathway.
An admission episode can have many consultant episodes – periods of time when the patient is under the care of a particular consultant team for a particular specialty. Any diagnoses and any patient procedures recorded in the medical case notes during a consultant episode are coded by clinical coders as the International Classification of Diseases (ICD-10) and NHS Office of Population Censuses and Surveys 4th revision (OPCS-4) codes respectively after the patient has been discharged.
Theatre visits identify interventions which alter risk and also commonly alter vital signs monitoring schedules for an interval after the patient returns to a ward. The theatre’s record has the operative procedures carried out in coded form (OPCS-4136).
An earlier study, HAVEN,137 identified blood tests that were of interest in the physiological monitoring of acute patients (see Appendix 9).
It was decided to use common names and data types for the extracted data taken from the two contributing trusts to facilitate data analysis and model-building.
Records from the different data sources were linked by patient identifiers and by dates and times according to the Patient Administration System (PAS) admission records.
Data extraction
Data extraction was subject to local information governance approvals at both trusts involving a data protection impact assessment and due diligence. Patient data were extracted with Health Research Authority Confidentiality Advisory Group (HRA CAG) approval (ref: 19/CAG/0132). For both trusts, patient records were filtered to remove people who had registered with the NHS national opt-out. Patient admissions that did not meet the study inclusion criteria were also removed before the data sets were made available to the research team.
Portsmouth data extraction
In PHU, the extraction was performed by the Research Data Management Team. Extraction proceeded by identifying adult admissions from PAS for the period of interest for patients who had not registered with the NHS opt-out. Extraction of records from other sources was then constrained to link to this cohort of retained admissions. Real-world and source-system patient identifiers were removed and replaced with a patient study identifier.
There were two extractions. The first covered admissions from January 2014 to December 2018. A second extraction covered admissions from January 2019 to August 2020 (357,852 admissions and 3,511,486 observations).
Oxford data extraction
In OUH, data extraction was performed by the local Clinical Data Warehouse team in collaboration with two members of the study team (who hold honorary contracts with the Trust). Data for patients admitted and discharged between 2017 and 2020 were extracted. Patients registered with the NHS opt-out were first excluded, followed by admissions that met the study exclusion criteria. We extracted at total of 614,930 admissions from 259,047 unique patients from OUH.
Data cleaning and validation
The counts of the numbers of records extracted from each source were sense-checked and broken down by year to check there were no gaps. Primary keys were defined to identify and remove any duplicate records. Referential integrity checks were made to ensure there were no orphan records, for example diagnoses without an owning consultant episode. Checks on the proportions of missing values for key data items were made and investigated if more than expected.
Participants
The following data were excluded from our analysis:
-
vital sign observations outside of the recorded admission and discharge periods (due to recording errors in the latter);
-
admissions of patients with missing information on their gender (only two patients);
-
admissions of patients discharged alive from hospital before midnight on the day of admission;
-
admissions to maternity or paediatric specialties;
-
partial and ED vital sign observations;
-
vital sign measurements recorded after midnight on 30 June 2019, to avoid any data potentially contaminated by undetected COVID-19 infections.
Where duplicate sets of vital signs existed at a given date/time, we assumed that the last recorded set was ‘correct’ and removed all others. Analyses of PHU data were undertaken using either NEWS or NEWS2 values, whichever was source data. For OUH data where the SEND score was in use throughout the study period, NEWS values were calculated retrospectively.
The study database also included patient demographics (e.g. patient gender and age) and admission specialty (i.e. medical, surgical, other) data, and a range of patient outcomes (e.g. LOS, in-hospital mortality).
We created the following three study cohorts:
-
development data set: all hospital admissions to PHU between 1 January 2014 and 30 June 2017
-
internal validation data set: all hospital admissions to PHU between 1 July 2017 and 30 June 2019
-
external validation data set: all hospital admissions to OUH between 1 July 2017 and 30 June 2019.
Patient subgroups
Data sets also contained observations taken according to protocols associated with specific therapies or procedures. We therefore repeated the analysis for different patient subgroups to assess whether these were monitored at different frequencies:
-
admissions to medical specialties
-
admissions to surgical specialties
-
admissions to ‘elderly medicine’
-
admissions with and without visit to operating theatre
-
elective admissions versus emergency admissions
-
admissions with and without an observation in the last 24 hours
-
patients aged 80 or older and patients aged 30 or younger.
Medical, surgical and other groups were identified based on their admission specialty codes (see Appendix 7). This classification approach was chosen instead of an analysis by ward type because ward allocations changed over time and were difficult to track based on the available data. Specialty codes therefore provided a more accurate description of a patient’s condition.
Vital sign observations for admission with operating-theatre visit were further categorised into pre- and postoperative observations.
Equality, diversity and inclusion
The study data sets consisted of consecutive admissions so there was no selection bias. Patients without electronically recorded vital signs were excluded, these being maternity, paediatric, day case and regular day attenders. This exclusion was valid in a study concerned with inpatient deterioration. All the outcome end points apart from cardiac arrest are reliably recorded. It is well known that records of cardiac arrest, rather than arrest team calls, are incomplete. The cardiac arrest outcome was not separately modelled but formed part of a combined outcome.
Our methods ensured that our participant population was very representative of the patient populations of the two study hospitals. We know that the population was representative of gender in the wider population. We do not know whether it was representative of ethnicity or any other protected characteristic since data on that were not available to us.
Analysis
All data were analysed using R statistical computing and graphics software.
Quantitative variables
Continuous variables were not dichotomised or grouped for descriptive analysis or modelling. The only exception was that NEWS observations were grouped into three risk groups (low, medium, high) for the assessment of observation timing (see details below).
Statistical methods
Descriptive analysis and outcomes
Patient demographics, admission characteristics, observation frequency, average NEWS values and patient outcomes were compared across the three patient cohorts (development, internal validation and external validation). Descriptive statistics were calculated including counts, means (±SD), medians (IQR, Q1–Q3) and proportions. We further estimated outcome rates for each of the patient subgroups described above in both the PHU and OUH data sets.
We considered the following adverse events as outcomes in our analysis and modelling:
-
DEATH: in-hospital death
-
COMB: combined outcome of either in-hospital death or cardiac arrest or unexpected admission to a high-care ward, whichever comes first
-
NEWS7: any of the events included in the combined outcome or a NEWS value greater than 6, and lastly
-
NEWSINC: combined outcome or NEWS > 6 or a NEWS increase of 2 or more.
The numbers of admissions to each of six admission categories were calculated for every month within the study period. The six admission categories were emergency and elective admissions to medical, surgical and ‘other’ admission specialties, respectively.
Observation patterns
We calculated median NEWS, LOS and observation frequencies for each of the patient subgroups described above in both the PHU and OUH data sets.
For the analysis of observation patterns and protocol compliance, observations taken within 1 minute were considered duplicated and only the second was used.
Each vital signs set was allocated to an hour of the day, with the time for each set being labelled as the current hour at the moment of the timestamp, for example timestamps between 7:00 and 7:59 were labelled as 7:00. We calculated the number of vital signs sets collected each hour, as a proportion of the total number of vital signs sets collected in the 24-hour period, and stratified them by NEWS value grouped from ‘0’ to ‘9 or more’. We also calculated the number of vital signs sets collected each hour, expressed as a proportion of the total collected in the day, stratified by day of the week. We plotted the mean TTNO for each hour of the day, categorised by NEWS for each year in the study period.
We defined vital signs recordings as ‘late’ if they were in the latest 20% of TTNO for a given NEWS value and as ‘early’ if they were in the bottom 20% (Figure 7). The middle 60% were regarded as ‘on-time’. Stratifying compliance by NEWS allows assessment of the adherence with monitoring protocols independent of the increased frequency demanded for increases in severity of illness, that is, NEWS. The range of TTNO that was classified as ‘late’ and ‘early’ for each NEWS value is given in Table 7. We calculated the proportion of early, late and on-time observations for each month between February 2014 and June 2019 (January 2014 removed to avoid artefacts from data extraction). We further categorised the proportion of early, late and on-time observations by low (NEWS 0–2), medium (NEWS 3–5) and high (NEWS ≥ 6) severity of illness for each year within the study period.
FIGURE 7.
Distribution of observed time intervals between NEWS observations for three severity groups [low (NEWS 0–2), medium (3–5) and high risk (≥ 6)]. For each NEWS the earliest 20% of observation intervals were classified as ‘early’ and the latest 20% were classified as ‘late’.

NEWS | Expected observation interval (2017–9) | Early: min interval (h) |
Early: max interval (h) |
Late: min interval (h) |
Late: max interval (h) |
---|---|---|---|---|---|
0 | 6 | 0.0 | 4.2 | 11.7 | 24.0 |
1 | 6 | 0.0 | 3.9 | 9.5 | 24.0 |
2 | 6 | 0.0 | 3.2 | 8.3 | 24.0 |
3 | 4 | 0.0 | 2.4 | 6.6 | 24.0 |
4 | 4 | 0.0 | 2.2 | 6.3 | 24.0 |
5 | 4 | 0.0 | 2.0 | 5.9 | 23.9 |
6 | 2 | 0.0 | 1.6 | 5.4 | 23.9 |
7 | 1 | 0.0 | 1.0 | 3.7 | 24.0 |
8 | 1 | 0.0 | 0.9 | 3.4 | 23.7 |
9+ | 0.5 | 0.0 | 0.6 | 1.5 | 24.0 |
Deterioration over time
To better understand changes in NEWS over the course of a patient’s hospital admission, we calculated the mean NEWS and average risk of adverse events (for outcome definition see Descriptive analysis and outcomes) for the first 10 days after hospital admission (day 0). We excluded patients without an observation in the last 24 hours of their stay who are presumed not to be monitored because they are on an end-of-life pathway. We then calculated a mean NEWS and mean NEWS component for individual vital sign for each 24-hour interval leading up to one of five outcomes: in-hospital death, combined outcome, NEWS7, NEWSINC and discharge alive.
Using the area under the receiver operating characteristics curve (AUROC), we evaluated the ability of individual vital signs to discriminate any of the adverse outcomes. We calculated AUROCs for the NEWS components (not vital sign measurements) of temperature, systolic BP, HR, breathing rate and oxygen saturation to account for non-linear relationships with outcome.
Model development and validation
Using the PHU development data, we estimated the risk of deterioration (defined by any of the four outcomes described in Descriptive analysis and outcomes) for each of the patient subgroups using Cox models with random observation sampling. We used a sampling approach to avoid bias towards admissions that had more vital sign observations. Firstly, we sampled a random point in time during each admission and selected the closest vital sign observation. NEWS values greater than 14 were truncated. We then fitted strata for each NEWS value. The fitted survival curves were used to estimate the risk of each outcome at 15-minute intervals over a 24-hour period. This process was repeated 2000 times to estimate the average probability of patient deterioration, that is, risk of adverse outcome.
The model was validated internally, using a separate data set from PHU, and externally, using data from OUH. In addition, model performance was assessed in the patient subgroups described above using the PHU internal validation data. We randomly sampled a single observation from each admission in any of our validation cohorts and assessed model calibration and discrimination at three time points: 6 hours, 12 hours and 24 hours after NEWS observation.
For model calibration, the median probability of an outcome for NEWS ≤ 14 at the three time points was calculated from the randomly sampled data and graphically compared against the predicted risk of outcome estimated by the Cox model. 138 Linear regressions were fitted to the data to estimate the linear intercept and slope. 139 The intercept is a measure of calibration-in-the-large and has a target of 0, with positive values suggesting underestimation and negative values suggesting overestimation. The slope assesses the spread of risk estimates (target value: 1). Slopes < 1 suggest that the model produces risk estimates that are too extreme, that is, too high for high-risk patients and too low for low-risk patients, and, vice versa, that risk estimates are too conservative if the slope is > 1.
We determined AUROCs for each of the 2000 random samples to evaluate model discrimination.
Designing new monitoring regimes
Monitoring protocols were created by setting a consistent risk threshold before which the next observation should be taken, given a NEWS value. We developed a user interface (Shiny App140) to explore the various constraints of the optimisation routine. Possible constraints were:
-
acceptable risk thresholds for a selected outcome;
-
generated workload relative to workload in the development cohort; and
-
the proportion of observations that will be missed.
An observation interval of 15 minutes was recommended whenever the accepted threshold risk of deterioration was exceeded within 1 hour. The 15-minute interval was used as a proxy for continuous monitoring of the patient but counted (and costed) as four observations per hour. Based on consultations with stakeholders, we added further optional constraints to the optimisation routine to ensure the resulting monitoring protocol is practical. These additional constraints force observation intervals to either any integer value between 1 hour and 24 hours or one of the following intervals: 1 hour, 2 hours, 4 hours, 6 hours, 8 hours, 12 hours. Once appropriate observation intervals for each NEWS value were selected, we calculated the expected risk of outcome for individual NEWS values as well as the average across the entire patient populations, for each of the four outcomes. As a first test, we estimated the optimal observation interval, using the average risk of combined outcome, including death, unplanned ICU admission and cardiac arrest (COMB) in the development data set as maximum risk threshold, without any constraints on the choice of observation interval (see Alternative monitoring regimes). While the resulting monitoring protocol is not feasible in clinical practice, it helped us to identify major weaknesses in the existing monitoring protocol.
For the purpose of this report, we first tested how risk and workload change if the current maximum risk of COMB of 1.2% observed for NEWS = 10 was applied to all NEWS values. We used this example scenario to show the differences in using optimised non-integer observation intervals and compared them to a protocol where we only allowed integer hours (1 hour, 2 hours, 4 hours, 6 hours, 8 hours, 12 hours).
We then explored the effect of three alternative monitoring regimes on the risk of outcome, nursing workload and associated cost (details below). Possible observation intervals were again limited to integer hours (1 hour, 2 hours, 4 hours, 6 hours, 8 hours, 12 hours) and risk thresholds were varied as follows:
-
maximum risk threshold for combined outcome was set to 0.5%, below the maximum risk of COMB (1.2%) observed in the development data set;
-
reduced COMB risk threshold of 0.1%;
-
a maximum risk threshold for NEWSINC (combined outcome including NEWS ≥ 7 and NEWS increase of 2 or more) of 4%.
Economic analysis
Workload associated with alternative monitoring regimes
Data from the retrospective and prospective observational studies were used to estimate the workload and staffing costs associated with alternative observation schedules.
Patient numbers, and their distribution across EWS categories, were used to model the expected number of vital signs observations to be undertaken under two candidate observation protocols. Combining the expected number of observations and the average time taken to complete an observation, we calculated the nursing time required for vital signs observations and the nursing cost per patient day under each alternative protocol. Expected numbers of vital signs observations were estimated by sampling patients on wards each day and calculating the daily number of observations associated with the vital signs observed in the protocol. To estimate the expected number of observations per day, we selected a random time (hour) each day and identified which patients were present, according to the patient administration system. For each time point, we extracted each patient’s most recent EWS and mapped this to the observation frequency (in minutes) in the protocol. We calculated the expected number of observations for each patient by dividing 1440 (the number of minutes in a day) by the expected observation frequency. Although an individual’s vital signs might change throughout the day (leading to a change in frequency), daily sampling for a ward meant that individual changes averaged out. We assessed the impact of different strategies for sampling within the day (e.g. at fixed hours of the day) and found that there was minimal impact of the daily estimates of numbers of observations.
For the base-case analysis, we considered the efficiency and patient risk of each protocol under an assumption that no scheduled vital signs observations were missed. Empirical evidence suggests this is not the case. 12,101,141
Cost associated with new monitoring regimes
The nurse time for undertaking vital signs observations was derived from our observational study. This included estimates of the time taken (5 minutes) per patient to complete an observation with an additional overhead to allow for preparation of equipment, hand-washing and documentation (see Chapter 4). Staffing costs were estimated by applying representative unit costs for substantive RN and healthcare support workers. 142 The cost estimates were weighted to reflect the proportion of vital signs observations undertaken by the respective grades of nurse staff, based on data from our observational study in which approximately 50% (355/715) of observations were conducted by RNs.
Incremental costs associated with different protocols were compared and used to determine the benefit [in terms of lives saved and quality-adjusted life-years (QALYs)] that would need to accrue from a particular regime for it to be judged as cost-effective, using a range of thresholds including average gross domestic product (GDP) per QALY143 (approximate £40,000) and a lower threshold for the NHS of £10,000 per QALY,144 reflecting opportunity costs associated with adopting new treatments in a resource-constrained system.
Cost-effectiveness analysis
We further evaluated the cost-effectiveness of each monitoring protocol with higher resource demand than the PHU reference protocol by calculating the cost of each life saved, based on estimates of the number and cost of QALYs associated with change in mortality.
To estimate the expected QALYs associated with changes in mortality we used the discounted and quality-adjusted life expectancy (DANQALE) tariffs reported by Meacock et al. 145 We took the weighted average tariff based on the age and sex profile of the patients who died in the development data set (patients admitted to PHU for whom the reference NEWS protocols apply) and assumed that each death averted would expect to gain associated QALYs. The estimated mean DANQALE for patients who died in the PHU development data set was 6.28 (95% empirical CI from 2000 bootstrap samples 6.04 to 6.54). As a sensitivity analysis we considered QALYs per life saved assuming 50% of the point estimate (3.1 QALYs per life saved) and at the point estimate + 50% (9.3 QALY per life saved).
Using these QALY per life gained estimates we calculated the proportion of deaths that would have to be prevented in order for any new regime to be cost-effective using three thresholds: £10,000 per QALY, £20,000 per QALY and £30,000 per QALY. This range spans recognised cost-effectiveness thresholds from the national per capita GDP143 (£29,147 in 2020) to the level considered by NICE as representing ‘exceptional value for money’ for new drugs (£10,000),144 with the mid estimate approximating to the threshold recommended for UK health policy purposes146 (£12,936 in 2008 approximates to £17,705 in 2021).
We determined a threshold for a ‘plausible’ impact on death which would therefore allow us to identify a regime as potentially cost-effective and thus worthy of further investigation. Large-scale studies estimate that between 3% and 6% of inpatient deaths are preventable. 147 In the UK, it has been estimated that approximately one-third of the 5.2% preventable deaths are attributable to failures in clinical monitoring. 92 This gives an absolute upper bound of plausible effect from improvements in monitoring of 2% of deaths averted. However, as alternative regimes are likely to give only a marginal improvement on the current NEWS-based regimes, this 2% threshold is unrealistic and so we set 1% as a threshold for considering any regime as potentially cost-effective (recognising that this is still a very large effect for such an intervention). Scenarios involving either equal or reduced costs are assumed potentially cost-effective and worth further consideration as they potentially improve outcomes at lower cost.
Chapter 6 Results of the retrospective study
In this chapter, we present the results of our analysis of the retrospective data.
The results are in four parts:
-
a descriptive analysis of our three data sets, covering patient demographics and vital sign observation patterns;
-
an analysis of how patients deteriorate over time;
-
the development of a model of patient deterioration over time;
-
an analysis of the impact of alternative monitoring regimes.
In the interests of brevity, we omit some tables of data where their contents are broadly similar to others.
Descriptive analysis
Patients
What are the characteristics of the patients in our study data sets?
Summary of different data sets
After exclusions, the development data set comprised 174,290 hospital admissions to PHU from January 2014 to June 2017 aged > 16 years with at least one complete NEWS observation. The internal validation set included 98,109 admissions to PHU from July 2017 to June 2019, while the external validation set included 139,891 admissions to OUH over the same period between July 2017 and June 2019.
For both PHU data sets, approximately 53% of patients were female and the median age was 67 and 68 (development and internal validation data). Fifty-one per cent of patients admitted to OUH were female with a median age of 65. Just over 40% of admissions and observations were from patients over 80 years old.
The majority (71.2–75.3%) of admissions in all three data sets were emergency admissions and more than 61% were admitted to medical specialties. The proportion of admissions to surgical specialties varied from 29.7% at OUH to 35% in the PHU development data set. Around 1% of admissions were to ‘elderly medicine’. The proportion of patients who had a theatre visit was comparable for both validation sets (18.1% and 19.4%) but slightly higher in the development data (23%). While the exact reason for this drop remains unknown, it could be explained by the fact that an increasing proportion of surgeries were performed in a nearby private hospital (Spire Portsmouth Hospital). Only a small fraction of patients received a blood transfusion (< 0.5%) or chemotherapy (< 0.8%) during their stay, after exclusion of admissions who only had observations on the day they received treatment.
Median LOS and mortality rate were lower at Oxford than in either PHU data set. OUH LOS was 1.6 days with a mortality rate of 2.6%, whereas LOS of PHU was 2.4 and 2.7 days (development and internal validation data respectively) with a mortality rate of almost 4%. The data set from OUH included data from four different hospitals, one of which is an orthopaedic hospital, potentially explaining the lower LOS and mortality. Outcome rates for NEWS7 (combined outcome of first occurrence of death, unplanned intensive care admission, or cardiac arrest in hospital, or recorded NEWS of 7 or more) and NEWSINC (combined outcome of first occurrence of death, unplanned intensive care admission, or cardiac arrest in hospital, or recorded NEWS of 7 or more, or an increase in NEWS of 2 or more) are more consistent across data sets. Even though NEWS7 and NEWSINC are additive outcomes also including death, unplanned ICU admission and cardiac arrest, occurrences of high NEWS or NEWS increase are much more frequent, dominating these outcomes. NEWS7 and NEWSINC are therefore a better representation of deterioration, also capturing events when other outcomes (death, ICU admission, cardiac arrest) were avoided and were more consistent across patient populations.
Table 8 provides details on patient demographics and outcomes for development and validation data sets.
FIGURE 8.
Age distribution of admission to PHU in the development data set, split by sex.

Data set | Model development | Internal validation | External validation |
---|---|---|---|
Hospital | PHU | PHU | OUH |
Study period | Jan 2014 Jun 2017 |
Jul 2017 Jun 2019 |
Jul 2017 Jun 2019 |
Patients, N | 91,577 | 57,061 | 84,401 |
Admissions, N | 174,290 | 98,109 | 139,891 |
Of which emergency, N (%) | 124,172 (71.2) | 73,911 (75.3) | 102,214 (73.1) |
Admissions to medical specialties (%) | 112,903 (64.8) | 67,470 (68.8) | 85,979 (61.5) |
Admissions to ‘elderly medicine’ specialty (%) | 1054 (0.6) | 557 (0.6) | 247 (0.2) |
Admissions to surgical specialties (%) | 60,932 (35) | 30,345 (30.9) | 41,482 (29.7) |
Female, N (%) | 92,957 (53.3) | 52,536 (53.5) | 71,028 (50.8) |
Age (years), median (IQR) | 67 (50–80) | 68 (51–81) | 65 (47–79) |
Admissions with theatre visits, N (%) | 40,079 (23) | 17,776 (18.1) | 27,175 (19.4) |
Admissions with blood transfusion, N (%) | 205 (0.1) | 114 (0.1) | 604 (0.4) |
Admissions with chemotherapy, N (%) | 1103 (0.6) | 622 (0.6) | 1021 (0.7) |
Admissions without observations in last 24 hours, N (%) | 7659 (4.4) | 4118 (4.2) | 5538 (4) |
Vital sign/NEWS observations | |||
Observations, N | 4,134,572 | 2,489,507 | 2,525,851 |
Observations/day, median (IQR) | 4.1 (3.2–5.4) | 4.1 (3.3–5.4) | 4.5 (3.4–6.2) |
Admission NEWS, median (IQR) | 1 (0–3) | 1 (0–2) | 1 (0–2) |
Average NEWS, median (IQR) | 1 (0–3) | 1 (0–2) | 1 (1–3) |
Outcomes | |||
LOS (days), median (IQR) | 2.4 (1–6.9) | 2.7 (1–7.2) | 1.6 (0.4–4.8) |
DEATH,a N (%) | 6722 (3.9) | 3684 (3.8) | 3705 (2.6) |
COMB,a N (%) | 7032 (4.0) | 3818 (3.9) | 4031 (2.9) |
NEWS7,a N (%) | 21,777 (12.5) | 11,159 (11.4) | 16,943 (12.1) |
NEWSINC,a N (%) | 103,696 (59.5) | 58,048 (59.2) | 73,444 (52.5) |
Total admission numbers to PHU were fairly constant throughout the study period, with a slow increase in emergency admissions to medical specialties (Figure 9). In comparison, surgical admissions decreased slightly over time. Combined monthly admissions to the four data sets included in the OUH data set were about 50% higher than admissions to PHU (see Figure 9). The increase in admissions over the study period was also driven by an increase in emergency admissions to medical specialties – the blue dashed line in the figures.
FIGURE 9.
Time series of admissions per month to different specialties. Top: admissions to PHU between January 2014 and June 2019; bottom: admissions to OUH between January 2017 and June 2019.

There was a noticeable difference in the distribution of NEWS values in data sets collected at PHU and OUH (Figure 10). While in both data sets from PHU, used for model development and internal validation, NEWS 0 was the most common occurrence, NEWS 1 was most prominent in the OUH data set, indicating a clear difference in the patient population. These differences were consistent across all patient subgroups.
FIGURE 10.
Distribution of NEWS observations in the development, internal validation and external validation data sets, expressed as a percentage of the total number of observations in each data set.

For the majority of admissions in all data sets, LOS was shorter than 72 hours. At OUH (external validation data), more patients were discharged in the first 24 hours compared to PHU (Figure 11).
FIGURE 11.
Distribution of admission LOS in the development, internal validation and external validation data sets, expressed as a percentage of the total number in each data set.

Patient subgroups
In addition to the overall patient population, we also considered specific subgroups of patients in order to ascertain whether their characteristics might make them candidates for different monitoring regimes.
Outcome rates varied significantly between different patient subgroups. Table 9 shows outcome rates for the PHU development data set and key differences in outcome rates in either validation data set are discussed below. As expected, elective admissions had lower adverse outcome rates compared to emergency admissions across all outcome measures. For example, mortality was 0.3% in elective admissions while it was 5.3% for emergency admissions. Admissions without an observation in the last 24 hours had the highest outcome rates compared to any other patient groups (mortality: 34.9%). There are two possible reasons for missing observations at the end of an admission: (1) system failure, such as hardware or software malfunction, or (2) patients were put on an end-of-life pathway, when vital signs are not monitored. As system failure is a relatively rare event, admissions without observation in the last 24 hours were therefore assumed to be on an end-of-life pathway. This was supported by a 43% 30-day mortality rate in this patient group, indicating that these patients die either in hospital or in the community. Admissions to surgical specialties had lower outcome rates compared to admissions to medical specialties, with highest outcomes in the cohort admitted under specialty code ‘elderly medicine’ (mortality 13.1%). Patients receiving blood transfusion and chemotherapy had generally lower than average outcome rates, with mortality rates < 3.3%.
Patient subgroup | Admissions, N |
DEATH, N (%) |
COMB, N (%) |
NEWS7, N (%) |
NEWSINC, N (%) |
---|---|---|---|---|---|
PHU development data set | 174,290 | 6722 (3.9) | 7027 (4) | 21,775 (12.5) | 103,696 (59.5) |
Emergency admissions | 124,172 | 6585 (5.3) | 6846 (5.5) | 20,303 (16.4) | 82,486 (66.4) |
Elective admissions | 50,118 | 137 (0.3) | 181 (0.4) | 1472 (2.9) | 21,210 (42.3) |
Admissions with observation in last 24 hours | 166,631 | 4047 (2.4) | 4330 (2.6) | 18,185 (10.9) | 97,460 (58.5) |
Admissions without observation in last 24 hours | 7659 | 2675 (34.9) | 2697 (35.2) | 3590 (46.9) | 6236 (81.4) |
Admissions to medical specialties | 112,903 | 5,876 (5.2) | 6,020 (5.3) | 17,517 (15.5) | 63,695 (56.4) |
Admissions to elderly medicine specialty | 1054 | 138 (13.1) | 138 (13.1) | 271 (25.7) | 922 (87.5) |
Admissions to surgical specialties | 60,932 | 844 (1.4) | 1005 (1.6) | 4242 (7) | 39,843 (65.4) |
Admissions without operating-theatre visit | 134,211 | 6242 (4.7) | 6368 (4.7) | 18,567 (13.8) | 75,261 (56.1) |
Admissions with operating-theatre visit | 40,079 | 480 (1.2) | 659 (1.6) | 3208 (8) | 28,435 (70.9) |
Admissions receiving blood transfusion | 205 | 5 (2.4) | 6 (2.9) | 14 (6.8) | 88 (42.9) |
Admissions receiving chemotherapy | 1103 | 35 (3.2) | 37 (3.4) | 136 (12.3) | 739 (67) |
Admissions older than 80 years | 44,354 | 4060 (9.2) | 4139 (9.3) | 9753 (22) | 33,417 (75.3) |
Admissions younger than 30 years | 15,258 | 8 (0.1) | 15 (0.1) | 402 (2.6) | 6,499 (42.6) |
Outcome rates in the internal validation set (detailed data provided in Appendix 10) were comparable to the development data set. The most notable change was a reduction in mortality in admissions to elderly medicine, that is, admission with associated admission specialty code ‘430’ (from 13.1% to 9.2%) as well as in admissions of patients aged 80 or older, admitted to any specialty (9.7–8.3%).
In comparison, the external validation data set (detailed data provided in Appendix 10) had overall lower rates of mortality and combined outcome, but when including NEWS values of 7 or more, or considering an increase in NEWS by 2 or more points, rates were similar (DEATH, COMB, NEWS7 and NEWSINC respectively) (see Table 8). In particular, mortality was lower in emergency admissions (3.5%), admissions to medical specialties (3.6%) and ‘elderly medicine’ (4.0%), those receiving blood transfusions (0.8%) and admissions that did not include a visit to the operating theatre (3.0%). In contrast, mortality rates were higher in admissions receiving chemotherapy (3.8%).
Several patient subgroups (elective admissions, those younger than 30 years old, admissions to ‘elderly medicine’ and admissions receiving either blood transfusions or chemotherapy) had very low admission and/or outcome rates (see Table 9), limiting subsequent robust analysis. While these patients were included in model development (see Model development) as they contribute to the overall monitoring and hence nursing workload, findings from the descriptive analysis for these patient subgroups are omitted from detailed discussion. Full results for these patient subgroups have been provided in Appendix 10. Note that admissions to elderly medicine are included in the medical cohort.
Vital sign observation patterns
How often were patients’ vital signs observed?
Observation frequency in patient subgroups
In the PHU development data set, observation frequency, defined as median number of observations per day, varied across subgroups of patients even though median NEWS was comparable (Table 10). Patients without an observation in the last 24 hours of their stay had a higher median NEWS compared to average in the entire cohort in all three data sets, when considering their entire hospital admission period. That means, on average, these patients had a higher severity of illness, supporting the assumption that a proportion of these patients might have been on an end-of-life pathway. In the external validation data set, admissions to all medical specialties and elderly medicine also showed an elevated median NEWS (data not shown).
Patient subgroup | Observations, N | Average NEWS, median (IQR) | Average observation period (hours), median (IQR) | Recorded observations per day, median (IQR) |
---|---|---|---|---|
PHU development cohort | 4,134,572 | 1 (0–3) | 2 (1–7) | 4.05 (3.18–5.35) |
Emergency admissions | 3,484,573 | 1 (0–3) | 3 (1–9) | 3.77 (3.05–4.69) |
Admissions with observation in last 24 hours | 3,782,272 | 1 (0–3) | 2 (1–6) | 4.11 (3.25–5.43) |
Admissions without observation in last 24 hours | 352,300 | 2 (0–4) | 13 (4–28) | 2.41 (1.38–3.43) |
Admissions to medical specialties | 2,736,247 | 1 (0–3) | 2 (1–8) | 3.97 (3.12–5.50) |
Admissions to surgical specialties | 1,393,534 | 1 (0–2) | 2 (1–6) | 4.17 (3.31–5.22) |
Admissions without operating-theatre visits | 2,995,031 | 1 (0–3) | 2 (1–7) | 3.95 (3.11–5.28) |
Admissions with operating-theatre visits | 1,139,541 | 1 (0–2) | 3 (1–7) | 4.37 (3.47–5.51) |
Preoperative period | 164,763 | 1 (0–2) | 1 (0–2) | 4.36 (3.43–5.67) |
Postoperative period | 972,344 | 1 (0–2) | 2 (1–6) | 4.66 (3.60–6.09) |
Admissions older than 80 years | 1,666,951 | 1 (0–3) | 6 (2–15) | 3.59 (2.92–4.49) |
For each admission, we calculated the average number of observations per day (obs/day) and then calculated the median observation rate for each patient subgroup. In both PHU data sets, the average observation frequency was 4.1 obs/day; it was slightly higher (4.5 obs/day) in the OUH data set (data not shown). Lowest observation frequencies were found in patients without observation in the last 24 hours, with an average of 2.4 obs/day over the entire admission, reflecting the fact that patients on an end-of-life pathway are not monitored routinely. Other patient groups with reduced observation rates were those over 80, who make up almost 40% of admissions and observations (see Figure 8). Reduced observation rates are potentially driven by significantly longer average observation periods as well as a high proportion of patients on an end-of-life pathway. In contrast, the proportion of elderly patients in the OUH data set was smaller (around 30% of observations for patients over 80), with an observation rate of 4.3 obs/day and median LOS of 2 days, significantly closer to the average for the entire external validation cohort (data not shown).
In both PHU data sets, where observations of admissions with an operating-theatre visit could be split into pre- and postoperative periods, the observation frequency increased after surgery, probably due to increased monitoring in the immediate postoperative recovery period. After excluding observations taken in the first 48 hours after surgery, postoperative observation rates fell to preoperative levels (data not shown).
Variation in number of observations within 24-hour period
When vital signs sets were stratified by NEWS value and collection hour and expressed as a proportion of the total number of observations collected, the pattern of vital sign recording at PHU exhibited peaks of activity at 06:00, 11:00 and 16:00, and between 20:00 and 23:00 (Figure 12). However, peaks became less distinct from 2016 onwards, showing a slightly more even distribution of observations across a 24-hour period. This trend was sustained throughout the data collection period included in the validation data set and observed in all subgroups included in the detailed analysis (see Patient subgroups). There was no significant difference between weekdays and weekends.
FIGURE 12.
Recorded vital signs observation sets, stratified by NEWS value and collection hour and expressed as a proportion of the total number collected during the 24-hour period for each year included in the two PHU data sets. The development data set covers January 2014 to June 2017, the internal validation data set covers July 2017 to June 2019.

Observation patterns between the two hospitals were remarkably similar given the difference in observation schedules (see Data collection), with peak activity at OUH at 06:00, 10:00, 16:00 and 20:00 (Figure 13). Overall, a smaller proportion of observations were taken at night (23:00–04:00) at OUH, compared to PHU (up 1%).
FIGURE 13.
Recorded vital signs observation sets, stratified by NEWS value and collection hour and expressed as a proportion of the total number collected during the 24-hour period for each year in the OUH external validation data sets.

Similarly, we analysed daily patterns of the mean TTNO for each NEWS value. For data collected at PHU, this showed less variation across a 24-hour period from 2016 onwards, compared to data from 2014 to 2015 (Figure 14). From 2017 onwards, TTNO patterns show groupings that reflect the escalation protocols deployed in Vitalpac.
FIGURE 14.
Mean TTNO, stratified by NEWS value and collection hour for each year included in the two PHU data sets. The development data set covers January 2014 to June 2017, the internal validation data set covers July 2017 to June 2019.

Figure 15 shows slightly increased variability in TTNO over the course of a day at OUH compared to PHU, with a rapid increase in mean TTNO between 20:00 and 24:00. This is presumably because observations were not taken overnight for low- to medium-risk patients, regardless of NEWS value.
FIGURE 15.
Mean TTNO, stratified by NEWS value and collection hour for each year included in the OUH external validation data set.

Monitoring compliance
We classified the timing of observations as follows: the shortest 20% of observation intervals for each NEWS value were classified as ‘early’, the longest 20% of observation intervals were classified as ‘late’ with the remainder being labelled ‘on time’. For PHU data, there was a clear and sustained improvement in compliance from May 2015, with an increase in the proportion of ‘on time’ NEWS observations and simultaneous decrease of ‘late’ observations (Figure 16).
FIGURE 16.
Monthly time series of the proportion of observations that were taken on time, early or late for both PHU data sets, for model development and internal validation.

When observations are categorised by NEWS value, the proportion of ‘late’ observations was smaller for severely ill patients (NEWS of 6 or greater) than for low-risk patients (with a NEWS between 0 and 2) from 2016 onwards (Figure 17). In 2019, there was a noticeable decrease in the proportion of observations that were classified as ‘late’, from 17.9% in 2018 to 12.2% in 2019, for patients with a NEWS ≤ 6. A new monitoring protocol was introduced in February 2019 to accompany the roll-out of NEWS2. While the new monitoring protocol lowered the expected observation interval from 2 hours to 1 hour for NEWS 6, from 4 hours to 1 hour for NEWS 5, and from 1 hour to 30 minutes for NEWS ≥ 7, it also endorsed the use of customised observation schedules for certain groups of patients where authorised by an appropriate clinician and recorded in the patient notes. The two main uses of the customised scheduling were for elderly patients who were medically fit for discharge but awaiting a package of care, and some patients for whom it was normal to receive oxygen therapy. For both these groups the usual observation schedule was lengthened where approved.
FIGURE 17.
Proportion of ‘on time’, ‘early’ and ‘late’ observations categorised by risk groups – low (NEWS 0–2), medium (NEWS 3–5) and high risk (NEWS 6 or above) – for each year within the PHU data sets. The development data set covers the period from January 2014 to June 2017, the internal validation data set includes data from July 2017 to June 2019.

The proportion of ‘on time’ observations in the external validation data set collected at OUH was around 60% throughout the study period. From August 2018, there was a small decrease in observations classified as ‘late’ (data not shown). Note that OUH did not use NEWS during the study period and that the monitoring protocol included only three observation frequencies (12 hourly, 4 hourly and 1 hourly observations). In contrast to PHU, there was no difference in the distribution of ‘early’, ‘on time’ and ‘late’ observations with patients’ risk (Figure 18), presumably because of less variable observation frequencies dictated by the OUH monitoring protocol.
FIGURE 18.
Proportion of ‘on time’, ‘early’ and ‘late’ observations categorised by risk groups – low (NEWS 0–2), medium (NEWS 3–5) and high risk (NEWS 6 or above) – for each year within the OUH external validation data set.

Deterioration over time
How quickly (or slowly) do patients deteriorate, as measured by their NEWS score?
Trends post admission
Firstly, we analysed how a patient’s NEWS score changes from the time of their admission to hospital. Later we looked at the trends from the viewpoint of the period leading up to their outcome. Outcomes of interest (as specified in Descriptive analysis and outcomes) were DEATH, COMB (combined outcome of in-hospital death, unplanned admission to intensive care, or in-hospital cardiac arrest), NEWS7 (combined outcome of in-hospital death, unplanned admission to intensive care, or in-hospital cardiac arrest, or a NEWS value of 7 or above) or NEWSINC (combined outcome of in-hospital death, unplanned admission to intensive care, or in-hospital cardiac arrest, or a NEWS value of 7 or above, or an increase in NEWS of 2 or more).
Figure 19 shows that, on average, NEWS values were highest in the first days after admission. Both mean NEWS and the proportion of outcomes decreased over the course of hospital admission (Figure 20).
FIGURE 19.
Mean NEWS value for any given 24-hour period in the first 10 days after admission to PHU (development data set).

FIGURE 20.
Distribution of four outcomes over the first 10 days after admission, expressed as proportion of total number of a given outcome in the data set (PHU development data set).

In the first 4 days (96 hours) after admission, the risk of NEWS7 and NEWSINC (both defined above) was higher compared to the risk of death or combined outcome, suggesting that a significant proportion of patients continue to deteriorate after admission to hospital. This is supported further by the fact that the risk of combined outcome (COMB) exceeded the risk of death in the first 48 hours, indicating that a large proportion of unplanned admissions to ICU take place during this period.
Trends prior to adverse outcome
Just as importantly, it is necessary to consider the trends in the patient’s condition in the days prior to their outcome.
We further assessed the change in NEWS in the lead-up to one of five outcomes: death, COMB, NEWS7, NEWSINC or discharge without occurrence of an adverse outcome at any point during the admission. Only the first outcome for any admission was included and admissions without a NEWS observation in the last 24 hours were removed from the analysis.
For in-hospital death and the combined outcome (COMB), average NEWS increased noticeably from NEWS of 3 (9 days prior to the adverse event) to NEWS greater than 6 (within 24 hours of the event) (Figure 21). Average NEWS also increased for the outcomes NEWS7 and NEWSINC; however, values were overall lower, with maximum values < 2 (NEWSINC) and < 4 (NEWS7), reflecting the lower acuity prior to these outcomes. In contrast, during admissions without an adverse event, the mean NEWS decreased within the last 5 days of a patient’s hospital stay, from NEWS 2 to NEWS 1. Interestingly, patients who were discharged without any adverse outcome had a higher average NEWS compared to patients whose NEWS value was about to increase by 2 or more, up until 24 hours prior to the event.
FIGURE 21.
Average NEWS for a given 24-hour period leading up to one of five outcomes: death, COMB, NEWS7, NEWSINC or discharge. Only the first outcome for each admission was included in this analysis. The graph shows data from the PHU development data set (2014–7). Admissions without an observation in last 24 hours of their stay were excluded.

Repeating the same analysis for each NEWS component, five vital sign measurements showed similar trends (Figure 22). Breathing rate and oxygen saturation contributed most to the high NEWS values prior to outcomes DEATH and COMB, and breathing rate also showed the highest increase over the 10-day period leading up to either outcome.
FIGURE 22.
Average NEWS component for five vital sign measurements for a given 24-hour period leading up to one of five outcomes: DEATH, COMB, NEWS7, NEWSINC or discharge with no outcome. Only the first outcome for each admission in the PHU development data set was included in this analysis. Admissions without an observation in last 24 hours of their stay were excluded.

Area under the receiver operating characteristic curve values (AUROCs) for individual vital signs support these findings. Table 11 shows that breathing rate was most predictive of outcomes DEATH and COMB (AUROC > 0.87), followed by oxygen saturation (AUROC > 0.76). The ability of vital sign NEWS components to discriminate outcome NEWSINC was expectedly poor, with a maximum AUROC of 0.629 for oxygen saturation.
NEWS component | AUROC (CI) | |||
---|---|---|---|---|
DEATH | COMB | NEWS7 | NEWSINC | |
HR | 0.779 (0.777–0.780) | 0.766 (0.764–0.768) | 0.699 (0.698–0.700) | 0.595 (0.595–0.596) |
BP | 0.678 (0.676–0.680) | 0.671 (0.669–0.673) | 0.613 (0.612–0.614) | 0.626 (0.625–0.627) |
Temperature | 0.725 (0.723–0.727) | 0.713 (0.711–0.715) | 0.600 (0.599–0.601) | 0.570 (0.569–0.571) |
Respiratory rate | 0.891 (0.890–0.892) | 0.870 (0.868–0.871) | 0.754 (0.753–0.754) | 0.619 (0.619–0.620) |
Oxygen saturation | 0.780 (0.779–0.782) | 0.765 (0.763–0.766) | 0.768 (0.767–0.769) | 0.629 (0.628–0.630) |
Modelling risk of outcome over time
We set out to devise a statistical model that would allow us to analyse a patient’s risk over time.
Model development
The survival models describing the risk of outcome in relationship to NEWS and time since observations were fitted to the entire PHU development data set. Fitted survival models showed an increase in risk of outcome over the 24-hour period following NEWS observation as well as with increasing NEWS value for each of the four adverse outcomes. Figure 23 shows how risk varies over time for different levels of NEWS score. There was not much difference in risk between 4-hourly and 24-hourly observations for patients with NEWS ≤ 2, meaning that the increase in risk due to longer periods between observations is small.
FIGURE 23.
Percentage risk of adverse outcome over a 24-hour period post NEWS observations, for four different NEWS values (NEWS 0, NEWS 2, NEWS 4, NEWS 6). Note the different scales on y-axes.

Figure 24 shows the same thing but represented as how risk varies according to NEWS score for different periods since the patient’s last observation. After a rapid increase in risk for NEWS ≤ 7, the risk of NEWS7 or NEWSINC is relatively stable for NEWS values > 8.
FIGURE 24.
Percentage risk of adverse outcome for a given NEWS values, at four different times after NEWS observation (4, 6, 12, 24 hours).

Note the risk of NEWSINC was higher for NEWS 0 than for NEWS 2. This is because an observation of NEWS 0 can only be followed by the same or a higher NEWS, while for NEWS greater than 0 it can go down (see Figures 23 and 24).
Model validation
Model calibration
Model calibration is a measure of the accuracy of risk estimates, that is, how they compare to observed risk. Details on the different metrics for judging model performance are provided in Model development and validation. Figure 25 shows model calibration in the internal validation data set, with relatively consistent performance for NEWS values up to and including 14. This cut-off was chosen to avoid bias in the results for very high NEWS values where observation numbers are small. In addition, patients with a NEWS of 15 or higher are likely to be so severely ill that they are admitted to ICU and/or continuously monitored, that is, intermittent monitoring regimes that are the focus of this investigation do not apply. For in-hospital death, calibration suggested a combination of slight overestimation, with intercepts ranging from −0.09 to −0.27, and slightly extreme risk prediction, with slopes ranging from 0.92 to 0.94 (Table 12). Model performance improved with increasing distance from NEWS observation, with similar results for the combined outcome. For outcome NEWS7 model performance was very consistent across the three time points at which calibration was assessed. The model had a slight tendency to underestimate risk for outcome NEWSINC.
FIGURE 25.
Model calibration for outcomes DEATH, COMB, NEWS7 and NEWSINC at 6, 12 and 24 hours after a NEWS observation for all NEWS ≤ 14 in the internal validation data sets, collected at PHU.

Internal validation | DEATH | COMB | NEWS7 | NEWSINC | ||||
---|---|---|---|---|---|---|---|---|
Time since observation | Slope | Intercept | Slope | Intercept | Slope | Intercept | Slope | Intercept |
6 hours | 0.92 | −0.27 | 0.91 | −0.33 | 0.98 | 0.02 | 1.04 | 0.04 |
12 hours | 0.93 | −0.15 | 0.94 | −0.14 | 0.99 | 0.02 | 1.02 | 0.01 |
24 hours | 0.94 | −0.09 | 0.94 | −0.10 | 0.98 | 0.02 | 1.00 | −0.03 |
Model calibration for emergency admissions, admissions to medical specialties and admissions without theatre visit and patients aged 80 and older was broadly comparable to the entire internal validation cohort (Table 13). Considering all admissions with an observation in the last 24 hours, the intercept was ≥ 0.30 for all outcomes, demonstrating an expected substantial underestimation of average risk (i.e. calibration-in-the-large). Calibration slopes greater than 1 indicated that the model tended to produce risk estimates for DEATH and COMB that were too moderate. This was particularly noticeable in the surgical cohort.
24 hours since observation | DEATH | COMB | NEWS7 | NEWSINC | ||||
---|---|---|---|---|---|---|---|---|
Patient cohort | Slope | Intercept | Slope | Intercept | Slope | Intercept | Slope | Intercept |
Entire internal validation | 0.94 | −0.09 | 0.94 | −0.10 | 0.98 | 0.02 | 1.00 | −0.03 |
Emergency admissions | 0.89 | −0.13 | 0.90 | −0.13 | 0.94 | 0.06 | 0.95 | 0.00 |
Admissions with observation in last 24 hours | 1.04 | 0.46 | 1.05 | 0.45 | 1.08 | 0.30 | 1.25 | 0.37 |
Admissions without observation in last 24 hours | 0.32 | −4.78 | 0.30 | −3.66 | 0.49 | −1.08 | 0.18 | −1.27 |
Admissions to medical specialties | 0.89 | −0.1 | 0.90 | −0.15 | 0.96 | 0.08 | 1.02 | −0.04 |
Admissions to surgical specialties | 1.14 | 0.13 | 1.11 | 0.09 | 1.02 | −0.40 | 1.02 | 0.07 |
Admissions without operating-theatre visit | 0.91 | −0.08 | 0.92 | 0.08 | 0.97 | 0.08 | 1.04 | −0.02 |
Admissions with operating-theatre visit | – | – | – | – | – | – | – | – |
Admissions older than 80 years | 0.84 | 0.19 | 0.86 | 0.19 | 0.87 | 0.10 | 0.88 | −0.02 |
Model performance decreased in the external validation set, consistently overestimating risk due to the lower outcome rates observed in this cohort (Figure 26, Table 14). Intercepts indicate that calibration-in-the-large is poorer compared to the internal validation, particularly for outcomes DEATH and COMB, which could be explained by the difference in outcome rates between the two sites. For the outcomes NEWS7 and NEWSINC, calibration coefficients < 1 suggest that risk estimates are also too extreme.
FIGURE 26.
Model calibration for outcomes DEATH, COMB, NEWS7 and NEWSINC at 6, 12 and 24 hours after a NEWS observation for all NEWS ≤ 14 in the external validation data sets, collected at OUH.

External validation | DEATH | COMB | NEWS7 | NEWSINC | ||||
---|---|---|---|---|---|---|---|---|
Time since observation | Slope | Intercept | Slope | Intercept | Slope | Intercept | Slope | Intercept |
6 hours | 1.18 | −0.96 | 1.25 | −0.96 | 0.90 | −0.49 | 0.79 | −0.57 |
12 hours | 1.11 | −0.68 | 1.15 | −0.70 | 0.93 | −0.43 | 0.86 | −0.38 |
24 hours | 1.07 | −0.71 | 1.09 | −0.73 | 0.94 | −0.46 | 0.88 | −0.33 |
Model discrimination
Discrimination evaluates the ability of a model to distinguish between patients who have an outcome and those who do not. The ability of our survival models to discriminate outcome was very good for outcomes DEATH, COMB and NEWS7, with AUROCs > 0.890 (Table 15). For NEWSINC, AUROCs were significantly lower, with AUROCs ≤ 0.671 in the internal validation data and ≤ 0.599 in the external validation data. Unexpectedly, discrimination was slightly higher in OUH data than PHU data for outcomes DEATH and COMB, suggesting better model discrimination in the external data set. This was consistent with findings from previous studies. 137,148 Discrimination generally decreased the more time passed between NEWS observation and outcome.
Time since observation | DEATH | COMB | NEWS7 | NEWSINC |
---|---|---|---|---|
Internal validation | ||||
6 hours | 0.922 | 0.897 | 0.946 | 0.617 |
12 hours | 0.913 | 0.900 | 0.927 | 0.575 |
24 hours | 0.902 | 0.893 | 0.908 | 0.566 |
External validation | ||||
6 hours | 0.964 | 0.964 | 0.926 | 0.599 |
12 hours | 0.944 | 0.943 | 0.909 | 0.582 |
24 hours | 0.934 | 0.933 | 0.897 | 0.585 |
Alternative monitoring regimes
In this section, we consider the impact that different monitoring regimes might have on patient outcome. We start off by analysing the state of affairs due to the existing monitoring protocol in our development hospital and then consider what would happen if the protocol was adjusted to achieve different goals.
The monitoring regimes discussed below differ slightly from monitoring protocols presented to stakeholders (see Results) due to changes to the model parameterisation in the final stages of the data analysis. Using survival models to predict the risk outcome based on the value of NEWS and the time that passed since NEWS observations, we selected an observation interval for each NEWS value during which the risk of outcome did not exceed a chosen threshold.
Existing protocol
First, we assessed the risk of outcome before the next NEWS observation for any given NEWS value in the development data set. We chose the monitoring protocol that was used to collect NEWS observations at PHU between 2016 and 2019 (see Table 6) as reference protocol. The maximum risk of outcome before the next NEWS observation was 0.68% for death, 1.19% for the combined outcome of in-hospital death, unplanned admission to intensive care, or in-hospital cardiac arrest, and 6.9% for both NEWS7 (combined outcome including NEWS 7 or higher) and NEWSINC (combined outcome including NEWS 7 or higher or NEWS increase by 2 or more). However, the risk was unevenly distributed across different NEWS values, with lower risk for NEWS 0 or 1 and increased risk for high NEWS values (Table 16).
PHU protocol (2016–2019) | Risk of adverse outcome (%)a | ||||
---|---|---|---|---|---|
NEWS | Observation interval (hours) |
COMB | DEATH | NEWS7 | NEWSINC |
0 | 6 | 0.02 | 0.01 | 0.04 | 4.23 |
1 | 6 | 0.04 | 0.02 | 0.10 | 3.45 |
2 | 6 | 0.09 | 0.06 | 0.32 | 3.88 |
3 | 4 | 0.12 | 0.08 | 0.59 | 2.92 |
4 | 4 | 0.32 | 0.20 | 1.46 | 3.04 |
5 | 4 | 0.67 | 0.49 | 3.72 | 3.72 |
6 | 2 | 0.43 | 0.27 | 3.06 | 3.06 |
7 | 1 | 0.44 | 0.25 | 4.39 | 4.39 |
8 | 1 | 0.89 | 0.24 | 6.90 | 6.90 |
9 | 0.5 | 0.67 | 0.68 | 4.18 | 4.18 |
10 | 0.5 | 1.19 | 0.34 | 5.13 | 5.13 |
The monitoring protocol that was used at PHU from February 2019 reduced the observation interval for patients with a NEWS of 5 or 6 to 1 hour, from 4 hours (NEWS = 5) and 2 hours (NEWS = 6). This required an additional 14% of resources, requiring an additional 15,000 hours of nursing time at an annual cost of over £500,000 (see Table 22). Alongside the introduction of the new protocol, the hospital endorsed personalised observation scheduling. This allowed the target observation interval to be altered (typically extended) on a patient-by-patient basis where authorised by the medical team.
Optimisation for maximum risk of combined outcome
For comparison, we distributed the risk more evenly across all NEWS values by selecting a maximum observation interval for which the risk of combined outcome did not exceed a specified threshold. In the examples presented here, we chose to optimise monitoring regimes based on combined outcome because it is a more comprehensive measure of deterioration and includes more ‘salvageable’ patients than hospital death alone. Even though NEWS7 and NEWSINC are even more comprehensive measures of deterioration, they are not completely independent of NEWS observations. We provide an example of a monitoring regime based on maximum risk of NEWSINC in Alternative regime 3. Evaluating model performance using combined outcome COMB is also in line with previous work in this area. 5,7
As a starting point, applying the same maximum risk threshold of 1.2% for COMB to all NEWS values, increases the time between observations for NEWS values < 8 (Table 17), significantly reducing monitoring workload for nursing staff. In turn, however, the risk of all outcomes for low NEWS increased significantly. Approximately three-quarters of observations have a NEWS value of 2 or lower, meaning that the observation regime in Table 17 will result in a significantly increased average risk of outcome across the entire hospital population.
COMB risk threshold: 1.2% | Risk of adverse outcome (%) | ||||
---|---|---|---|---|---|
NEWS | Observation interval (hours) |
COMB | DEATH | NEWS7 | NEWSINC |
0 | 24.0 | 0.07 | 0.05 | 0.23 | 16.99 |
1 | 24.0 | 0.15 | 0.12 | 0.50 | 13.07 |
2 | 24.0 | 0.37 | 0.33 | 1.58 | 14.26 |
3 | 24.0 | 0.90 | 0.83 | 4.39 | 16.42 |
4 | 14.1 | 1.20 | 1.05 | 7.06 | 13.29 |
5 | 6.4 | 1.20 | 0.97 | 7.75 | 7.75 |
6 | 3.7 | 1.18 | 0.90 | 7.77 | 7.77 |
7 | 2.1 | 1.18 | 0.77 | 14.64 | 14.64 |
8 | 1.2 | 1.14 | 0.48 | 10.17 | 10.17 |
9 | 1.1 | 1.16 | 1.17 | 15.02 | 15.02 |
10 | 0.5 | 1.19 | 0.34 | 5.13 | 5.13 |
When devising alternative monitoring regimes, we restricted possible observation intervals, rounding down to the closest of these commonly used values: 12 hours, 8 hours, 6 hours, 4 hours, 2 hours and 1 hour, plus 0.25 hour as a proxy for continuous monitoring. These intervals were chosen because they allow for vital sign observations to fit in with other clinical work. The maximum observation interval was set to 12 hours, based on feedback from our clinical stakeholders who felt that all patients should be monitored at least twice daily. This gives the adaptation shown in Table 18.
COMB risk threshold: 1.2% | Risk of adverse outcome (%) | ||||
---|---|---|---|---|---|
NEWS | Observation interval (hours) |
COMB | DEATH | NEWS7 | NEWSINC |
0 | 12.0 | 0.03 | 0.02 | 0.10 | 10.09 |
1 | 12.0 | 0.08 | 0.06 | 0.26 | 8.12 |
2 | 12.0 | 0.19 | 0.16 | 0.83 | 9.14 |
3 | 12.0 | 0.49 | 0.41 | 2.54 | 10.92 |
4 | 12.0 | 1.01 | 0.87 | 6.14 | 11.81 |
5 | 6.0 | 1.14 | 0.91 | 7.23 | 7.23 |
6 | 2.0 | 0.43 | 0.27 | 3.06 | 3.06 |
7 | 2.0 | 1.10 | 0.71 | 13.99 | 13.99 |
8 | 1.0 | 0.89 | 0.24 | 6.90 | 6.90 |
9 | 1.0 | 1.08 | 1.08 | 13.34 | 13.34 |
10 | 0.25 | 0.38 | 0.06 | 1.70 | 1.70 |
Alternative regime 1
In an attempt to reduce the average risk of outcome to levels observed with the existing monitoring protocol, three alternative regimes with reduced risk thresholds were considered.
The first alternative regime we considered was derived using a maximum risk threshold for COMB (first occurrence of in-hospital death, unplanned admission to intensive care, or in-hospital cardiac arrest) of 0.5%, which would be a 58% reduction from the maximum observed risk (1.2%). Overall, regime 1 reduced annual workload by 31,321 hours alongside a reduction in maximum risk of death (0. 62%) and NEWS7 (4.39%). Observation frequencies increased compared to the PHU protocol for NEWS ≥ 5 (Table 19). However, for NEWS 0–3, the observation interval increased by 4 hours or 6 hours to 12 hours, which resulted in an increase in risk for all outcomes for these low-risk patients. As a result, alternative regime 1 is likely to lead to a net increase in risk across the entire hospital population that could be significant, even though the exact magnitude and its impact on patient outcomes remain unknown.
COMB risk threshold: 0.5% | Risk of adverse outcome (%) | ||||
---|---|---|---|---|---|
NEWS | Observation interval (hours) | COMB | DEATH | NEWS7 | NEWSINC |
0 | 12 | 0.03 | 0.02 | 0.10 | 10.09 |
1 | 12 | 0.08 | 0.06 | 0.26 | 8.12 |
2 | 12 | 0.19 | 0.16 | 0.83 | 9.14 |
3 | 12 | 0.49 | 0.41 | 2.54 | 10.92 |
4 | 4 | 0.32 | 0.20 | 1.46 | 3.04 |
5 | 2 | 0.27 | 0.13 | 1.20 | 1.20 |
6 | 2 | 0.43 | 0.27 | 3.06 | 3.06 |
7 | 1 | 0.44 | 0.25 | 4.39 | 4.39 |
8 | 0.25 | 0.20 | 0.07 | 0.90 | 0.90 |
9 | 0.25 | 0.35 | 0.62 | 1.41 | 1.41 |
10 | 0.25 | 0.38 | 0.06 | 1.70 | 1.70 |
Alternative regime 2
We further reduced the risk threshold for COMB to 0.1% for alternative regime 2, which resulted in a significantly increased observation frequency for NEWS ≥ 3 (Table 20). The maximum risk of death and NEWS7 also reduced dramatically, while the maximum risk of NEWSINC between two NEWS observations increased to 10.1%. Regime 2 increases the annual workload at PHU by 54,000 working hours, with significant implications for cost-effectiveness (see Economic analysis).
COMB risk threshold: 0.5% | Risk of adverse outcome (%) | ||||
---|---|---|---|---|---|
NEWS | Observation interval (hours) | COMB | DEATH | NEWS7 | NEWSINC |
0 | 12 | 0.03 | 0.02 | 0.10 | 10.09 |
1 | 12 | 0.08 | 0.06 | 0.26 | 8.12 |
2 | 6 | 0.09 | 0.06 | 0.32 | 3.88 |
3 | 2 | 0.05 | 0.04 | 0.17 | 0.93 |
4 | 1 | 0.07 | 0.01 | 0.19 | 0.39 |
5 | 0.25 | 0.00 | 0.00 | 0.03 | 0.03 |
6 | 0.25 | 0.00 | 0.02 | 0.11 | 0.11 |
7 | 0.25 | 0.19 | 0.14 | 0.70 | 0.70 |
8 | 0.25 | 0.20 | 0.07 | 0.90 | 0.90 |
9 | 0.25 | 0.35 | 0.62 | 1.41 | 1.41 |
10 | 0.25 | 0.38 | 0.06 | 1.70 | 1.70 |
Alternative regime 3
Lastly, we considered a monitoring schedule designed to reduce the risk of NEWSINC to less than 4%. Using alternative regime 3, the maximum risk of COMB was 0.67%, risk of death was 0.49%, while the risk of NEWS7 was 3.72%, which is a reduction of risk across all outcomes compared to the existing PHU protocol (Table 21). Observation frequencies were more consistent over different NEWS values, with 4-hour and 6-hour intervals for NEWS ≤ 5 and continuous monitoring for NEWS ≥ 7. There was more variation in risk of DEATH, COMB and NEWS7 across NEWS values, compared to the monitoring schedules discussed above, while the annual workload increased by 27,000 hours.
NEWSINC risk threshold: 4% | Risk of adverse outcome (%) | ||||
---|---|---|---|---|---|
NEWS | Observation interval (hours) | COMB | DEATH | NEWS7 | NEWSINC |
0 | 4 | 0.01 | 0.00 | 0.02 | 2.23 |
1 | 6 | 0.04 | 0.02 | 0.10 | 3.45 |
2 | 6 | 0.09 | 0.06 | 0.32 | 3.88 |
3 | 4 | 0.12 | 0.08 | 0.59 | 2.92 |
4 | 4 | 0.32 | 0.20 | 1.46 | 3.04 |
5 | 4 | 0.67 | 0.49 | 3.72 | 3.72 |
6 | 2 | 0.43 | 0.27 | 3.06 | 3.06 |
7 | 0.25 | 0.19 | 0.14 | 0.70 | 0.70 |
8 | 0.25 | 0.20 | 0.07 | 0.90 | 0.90 |
9 | 0.25 | 0.35 | 0.62 | 1.41 | 1.41 |
10 | 0.25 | 0.38 | 0.06 | 1.70 | 1.70 |
Economic analysis
What are the economic implications of these different regimes?
For this analysis, we assumed the following:
-
Observations are performed taking the NEWS protocol (used at PHU between 2016 and 2019) as a baseline.
-
Fifty per cent of observations are undertaken by RNs and 5 minutes is taken per observation set, as identified by the prospective work in this study (see Chapter 4).
-
There is 100% compliance with monitoring protocols (though we know this is not strictly true but it is still useful for making comparisons between protocols).
On this basis, there are a total of 3572 sets of vital signs observations per day (equating to 0.4-hour care time per patient) at a total cost of £10,717 per day.
We first considered the alternative regime 1 with a risk threshold for COMB (combined outcome of death, unplanned ICU admission or cardiac arrest before discharge) of 0.5%. The reduction in resource use associated with this regime equates to savings of 36,700 hours and £1.5 million per year at PHU (Table 22). Alternative regime 2, based on a lower risk threshold of 0.1% risk of combined outcome before the next observation is due, incurs a 65% increase in resource use (£2.5M at PHU).
Monitoring regime | Obs per patient per day | Hours per patient per day | Cost per patient per day | Annual hours increase (PHU) | Annual cost increase (PHU) |
Change in resource use (%) |
---|---|---|---|---|---|---|
PHU protocol (pre Feb 2019) | 4.62 | 0.38 | £13.85 | – | – | 0 |
PHU protocol (from Feb 2019) | 5.26 | 0.44 | £15.79 | 15,183 | £546,570 | 14 |
Example 1 (1.2% risk of COMB) |
1.79 | 0.15 | £5.37 | −66,509 | −£2,394,320 | −61 |
Example 2 (1.2% risk of COMB sequence) |
2.82 | 0.24 | £8.47 | −42,208 | −£1,519,473 | −39 |
Alternative 1 (0.5% risk of COMB) |
3.06 | 0.25 | £9.17 | −36,702 | −£1,321,284 | −34 |
Alternative 2 (0.1% risk of COMB) |
7.60 | 0.63 | £22.79 | 70,077 | £2,522,784 | 65 |
Alternative 3 (4% risk of NEWSINC) |
6.16 | 0.51 | £18.49 | 36,344 | £1,308,396 | 33 |
Potential cost-effectiveness
We estimated the percentage of deaths to be avoided by a new monitoring regime to be judged cost-effective at differing thresholds with varying assumptions about the QALYs associated with each death averted (50% more than estimated or 50% of the estimate, see Cost-effectiveness analysis for details). A monitoring protocol was deemed to be cost-effective when the maximum proportion of deaths that might be averted through changing the observations regime was less than 1% (see Cost-effectiveness analysis for details).
Despite lowering the threshold for risk of COMB compared to the observed risk in the development cohort, alternative regime 1 is associated with a reduction in workload and, hence, cost. Table 23 shows that alternative regime 2 is potentially cost-effective at a threshold of £20,000 or £30,000 per QALY although this is sensitive to assumptions about QALYs per life gained and the required effect is large (0.85% of deaths averted). Alternative regime 3 is more likely to be cost-effective within the range of cost-effectiveness thresholds considered, requiring less than 1% of deaths to be averted to achieve cost-effectiveness at the medium threshold (£20,000) although not for the conservative assumption (−50%) about QALYs per life saved.
Cost-effectiveness threshold | ||||
---|---|---|---|---|
£10,000 (%) | £20,000 (%) | £30,000 (%) | ||
Alternative regime 2 | ||||
QALY per life | 3.1 | 5.12 | 2.56 | 1.71 |
6.2 | 2.56 | 1.28 | 0.85a | |
9.3 | 1.71 | 0.85a | 0.57a | |
Alternative regime 3 | ||||
QALY per life | 3.1 | 2.65 | 1.33 | 0.88a |
6.2 | 1.33 | 0.66a | 0.44a | |
9.3 | 0.88a | 0.44a | 0.29a |
As suggested by stakeholder discussion, we further investigated whether a change in skill-mix can help to make alternative regime 2 cost-effective. However, even if all vital sign monitoring were done by a HCA instead of a RN this would still not be sufficient to make regime 2 cost-effective with 1.88% to be avoided, using the assumption of the most conservative threshold (£10,000) and an estimated QALY per life saved of 6.2. Using a cost-effectiveness threshold of £20,000 in combination with 6.2 QALYs per life saved, regime 2 would be cost-effective only if at least 92% of observations were taken by HCAs.
Discussion
This retrospective study developed methods to design evidence-based monitoring protocols that use NEWS (or any other risk predictor) to distribute risk more equally across scores. We developed survival models to predict the risk of different outcomes (as markers for deterioration) based on NEWS value and depending on the time from observation. This allowed us to select observation intervals at which the risk of outcome did not exceed a specified risk threshold.
We developed our model on 3.5 years of data from a large NHS trust (PHU) and successfully validated it in a separate data set from the same trust as well as an independent data set (OUH). Model performance was overall acceptable and any shortcoming of the model could be explained by differences in the patient case-mix and differences in existing monitoring practice.
In addition, we developed tools to evaluate the cost-effectiveness of any new monitoring regime by calculating expected workload and associated costs. Results showed that redistribution of risk by limiting the risk of the combined outcome between two observations to 0.5% resulted in a decrease in workload and cost by 34%, potentially saving over £1.3M in staff costs in one of our study sites.
Our study addresses many of the shortcomings of previous studies. It is a large multicentre study that directly links different intermittent monitoring regimes and a range of clinical outcomes, including death and unplanned ICU admission, as well as other markers of deterioration such as high NEWS and a rapid increase in NEWS. It is also the first study to develop the methodology to generate a monitoring protocol from clinical data and link these to nursing workload and economic impact. This allows us to rule out any protocols that would require unfeasible changes in outcomes or costs and helps to identify plausible candidate protocols whose actual performance could then be assessed in clinical trials.
A major strength of this study was the difference in case-mix across the two study sites. We are able to show acceptable model performance across both hospitals, suggesting that any monitoring regime developed based on these models is suitable for most adult patients. This is important because a single hospital-wide monitoring schedule is a huge operational advantage (e.g. simplifying patients and staff transferring from one ward to another without the need to adjust to different monitoring schedules). Additionally, using a consistent risk threshold for all NEWS values distributes the risk more evenly across all NEWS values, making care more equitable.
There is currently no consensus on an acceptable risk threshold – this is both a clinical and an operational decision. However, the tools presented in this study can be used to investigate the impact of any risk threshold on healthcare cost and, hence, support the design of future evidence-based monitoring regimes.
While there is room to further improve model parameterisation and performance, any shortcomings of the models were expected and can be explained by our methodology. In the majority of patient subgroups, the model tended to slightly overestimate risk, which will result in overly conservative observation intervals, that is, reducing the risk of adverse outcome for the individual patient.
While our study was able to overcome many limitations of previous research, it remains an observational study with limitations inherent to this type of study. As a retrospective study, it did not predict the actual impact of any new monitoring regime on clinical outcomes in practice. However, our findings highlight the real opportunity to improve efficiencies while maintaining or even improving patient safety. We need prospective studies to assess and gather additional evidence on the impact of different intermittent monitoring regimes on clinical outcomes.
There were certain limitations to the creation of different patient subgroups based on the data available to us. For example, elderly patients will often be admitted under the specialty code ‘general medicine’ and are later transferred to ‘elderly medicine’, which is reflected in significantly (up to 12 times) higher numbers discharged from that specialty code than admitted. We chose to distinguish patient subgroups by admission specialty to reflect the information available to clinical staff on the wards.
There are a number of different approaches that can be used to optimise observation protocols based on the risk of outcome. In this study we explored the use of maximum risk thresholds that would not be exceeded before the next vital sign observations. While this strategy allows risk to be spread more evenly across all NEWS values, it does not evaluate the impact on net risk across the entire hospital population. The approach has the potential to shift the risk from a small group of high-risk patients to a large group of low-risk patients, as low-risk patients (NEWS ≤ 2) make up the population for around 75% of all observations. For example, alternative strategies for determining observation frequencies could be to optimise based on lowest risk thresholds or average risk across the hospital population. Future work is required to explore these options.
The approach chosen to estimate QALYs gained is based on a series of assumptions, each potentially introducing uncertainty and assumptions. Estimates are liable to underestimation because future years are discounted and because it assumes the average QALY expectancy for a life saved whereas younger and fitter patients might experience potentially reversible deteriorations. At the same time, QALYs gained are likely to be overestimated because of the assumption that expectancy and quality is the same as in the general population. The net bias remains unknown.
Numerous studies have highlighted the lack of clinical evidence for monitoring protocols as well as the lack of consensus on optimum monitoring frequency. 3,4,64 We are aware of only one study, by Petersen et al.,53 into the benefits of different intermittent monitoring regimes. The ward-level randomised, non-blinded controlled trial included 1346 low-risk medical and surgical patients who were admitted to a Danish urban university hospital. The research assessed the impact of increasing observations from twice daily to three times a day. Results showed no significant difference in clinical outcome rates between the different regimes. That study does not consider varying monitoring frequency based on risk of outcome.
With the development of advanced monitoring technology and tools, there has been growing interest in continuous monitoring of patients in recent years. 57–60,149 However, there is limited evidence for clinical benefits of continuous monitoring over intermittent monitoring. 41,56,61 Currently, there is a lack of cost-effective continuous monitoring systems and concerns that automated monitoring will reduce patient interaction, which is important for picking up signs of deterioration not captured by vital sign measurements. In addition, some studies have reported a risk of alarm fatigue as a result of significantly increased alarm rates, which will impact on patient experience and clinical workload, potentially reducing quality of care. 59,149 Intermittent monitoring will therefore be an important part of routine hospital care for the foreseeable future and findings presented here are therefore highly relevant and timely.
Conclusions
This retrospective study developed the methodology to design evidence-based monitoring schedules by:
-
generating monitoring protocols based on predicted risk (using NEWS, but methodology could be adapted to include other score/risk model);
-
spreading risk consistently across a range of scores, potentially making monitoring more equitable;
-
linking new protocols to economic models to evaluate potential cost-effectiveness.
We were able to show that spreading the risk of adverse outcome consistently across NEWS values reduces overall resource use. This means that revising a monitoring schedule has the potential to reduce the risk of outcome while maintaining (or even lowering) the current workload and staffing costs.
We presented a system for deriving and evaluating new monitoring regimes based on a survival model. The model was found to be representative of patient cohorts from two different hospitals with different case-mix, as well as different patient subgroups, suggesting that a single monitoring schedule could be appropriate for the monitoring of the general adult hospital patient population. This has significant operational advantages, for example, simplifying patients and staff transferring from one ward to another without the need to adjust to different monitoring schedules.
Additionally, our models were designed ‘fail-safe’ with a tendency to overestimate risk in most patient groups and, hence, suggest overly conservative observation intervals. We therefore conclude that new monitoring schedules developed using this model are appropriate for the majority of patients admitted to hospital.
This study has provided the tools and methodology to design and evaluate future monitoring tools. However, ultimately it is a clinical and operational decision as to what is deemed an acceptable risk – given that there is always some residual risk.
Chapter 7 Stakeholder evaluations
Background
Patient and public involvement (PPI), now more commonly also including engagement (PPIE), is a key part of research projects funded by the National Institute for Health and Care Research (NIHR). 150 Other funding bodies also require good-quality PPIE prior to submission of applications, and recommend this should be an ongoing feature of funded projects although uncertainties about how this should be achieved remain. 151 The definition of PPI varies but most accept the NIHR definition, which is that involvement means ‘research being carried out ‘with’ or ‘by’ members of the public rather than ‘to’, ‘about’ or ‘for’ them’. 152 Rationale for public involvement is also varied but there is widespread agreement that including different perspectives and experiences can improve research quality. More directly, there are clear advantages to including the views of those who could be affected by any potential change as a result of the research findings. Acceptance and implementation of change are much more likely if relevant stakeholders have been involved. This is especially true of institutional systems change, where there are several examples of healthcare informatics systems that have failed. 153,154
Despite having been an acknowledged feature of clinical research since the late 1990s, the impact of PPI is still poorly understood,155 and there are conflicting opinions on how impact that relates to PPI should be recognised and measured. 156 It appears the medical science community has been slow to adopt participatory models157 and undertaking good-quality, effective PPI that is truly embedded within the research structure is acknowledged to be difficult. 158 Nevertheless, there is a cohort of support for involving patients more widely than simply reviewing patient-facing materials159 and the evidence base for PPI is growing with a recognition that integration with the research team may yield the best results. 160
Aims
The aim of work package four (WP4) was to organise stakeholder events to identify factors relevant to the selection of an optimal monitoring protocol. We defined stakeholders as anyone with experience of physiological monitoring in hospital. Stakeholders for this project included patients and their carers, and healthcare staff who were responsible for either taking vital signs, escalating patients for more senior clinical review or introducing monitoring systems into one or more institutions.
Methods
When considering potential changes to the way patients’ care is delivered when in hospital, it is important to recognise the lived experiences of patients, their families, carers and visitors, and those of the professional healthcare staff who care for them. During the preparation of the funding application, we involved two experienced expert patients. They were asked to provide insight into a patient’s perspective on the issues. Issues raised included:
-
A patient might deteriorate quite significantly in the gap between observations, but to keep patients under too frequent observation would create a high workload for nursing staff as well as inconvenience to patients (especially if they have to be woken up during the night). What are the trade-offs between frequency and sleep?
-
There are issues surrounding a patient’s understanding of why they are getting more observations (or not as many as the person in the next bed). How is this best communicated?
-
Future work should look at ways in which observations can be made without disturbing the patient at all but, for the moment, we will focus on minimising this.
-
Seeking patients’ and clinicians’ views should be an important part of the study.
These two patient experts continued to be involved in the project for its duration: one as an active member of the project management group, and one who attended meetings of the project oversight group. A third person was recruited as a member of the project oversight group.
The role of this oversight group was to support the management group and to provide overall supervision for the project on behalf of the project sponsor and project funder. The oversight group also included representatives from other relevant stakeholder groups who could be affected by changes in patient monitoring scheduling (nurses, trainee doctors and medical consultants). This subgroup of subject-matter experts from the oversight group provided central stakeholder involvement. We also drew on external groups for additional insight.
We drew from the principles of experience-based co-design161 and participatory research practices,162,163 as well as qualitative research skills from within the project team. Stakeholder interactions most closely match definitions of natural group interviews (patient consultation), consensus panels and focus groups (healthcare professional consultations). 164 As per other co-production projects,165 stakeholder contributors were not considered research participants. Written informed consent was therefore not required. All contributors supporting the project agreed that their anonymised quotes could be used in project reports and outputs.
Patient stakeholder consultation
We organised two discussion groups for patients to identify the factors that they believe should be taken into account in designing monitoring protocols. One group (March 2019) included patients, their relatives, and healthcare professionals with experience of intensive care and hospital admissions. The other group (May 2019) included patients, relatives and carers with experience of general hospital admission and also interested lay members of the public.
We asked both groups to consider the following questions:
-
What factors influence their thinking on how frequently a patient should be monitored?
-
How can clinical staff best communicate to a patient the factors affecting their monitoring frequency and differences they might notice from that of other patients?
-
How should monitoring protocols strike a balance between the patient’s safety and their inconvenience (e.g. waking the patient from sleep to measure their vital signs)?
-
What can be done in the future to improve how observations are made?
-
Is there a difference to be made between how frequently vital signs should be taken and how frequently escalations in care should happen?
Group discussion was led by the work package lead (JLD). Live notes were taken by several members of the research team during the discussion sessions. These notes were subsequently reviewed and combined to create a single summary resource that captured key factors in response to the questions above. The summary draft was created by JLD, and other researchers within the team who were present at the meetings (JE, JH, OR, VW) were given the opportunity to comment and reflect on the summary. All members of the team agree the final version is an accurate summary of the discussions.
The results of these early discussions were used to inform the design and refinement of initial test monitoring protocols.
Healthcare professional stakeholder consultation
Restrictions enforced by the SARS-CoV-2 pandemic meant we were unable to hold face-to-face meetings after mid-March 2020. We therefore held an online discussion in November 2020. This was organised via the @WeNurses Twitter account. This online interaction included representation from key clinical stakeholder groups (nurses, doctors, HCAs). During the discussion, they identified additional factors they felt should be considered when designing and implementing physiological deterioration monitoring for patients in hospital. We asked them to consider the following questions:
-
When do you think patients should be monitored more or less than NEWS/NEWS2 suggests?
-
How do you prioritise patient monitoring when it is not possible to take observations as suggested?
-
What do you tell patients (if anything) about how often they will be monitored and why?
-
How can we strike a balance between patients’ well-being and other factors?
-
What can be done to improve how observations are scheduled?
Following the online discussion, the transcript of the chat was made available for analysis. This was reviewed by the work package lead (JLD), who summarised a draft of the discussion. This draft was reviewed for accuracy and sense by another researcher in the team (JH) with qualitative experience and who was also participating in the online discussion. All members of the team agree the final version is an accurate summary of the discussions.
Results from this discussion were used to support algorithm development alongside workload and costings information from work package three (WP3).
Towards the end of the project (May 2021), an online group discussion was hosted to discuss proposed observation protocols with our core stakeholders. We shared some specific scheduling options that had been generated by the WP2 algorithm. The online discussion format allowed the group to consider the ‘facilitators and barriers’ to implementation at scale. We addressed the following questions:
-
What are the potential technical barriers to implementing these protocols, given the range of systems for recording vital signs observations (both electronic and paper-based)?
-
What are the potential barriers to integrating these protocols into current nursing workflows and workloads?
-
What modifications to the protocols could facilitate implementation?
Live notes were taken by several members of the research team. After the session, these were aggregated and a final summary report of the meeting was circulated by the work package lead (JLD). This draft was reviewed for accuracy by two other researchers who attended the online discussion (IK, CK). All members of the team agree the final version is an accurate summary of the discussions.
Results from this discussion were used to finalise the algorithms to generate optimal schedules for monitoring patients when in hospital.
End of project stakeholder evaluation
At the end of the project, we interviewed a consultant physician (specialty: acute medicine) and a lay member of the project oversight group. These interviews were conducted individually and remotely (online and via the telephone) by the lead researcher for work package 4 (JLD). During the conversation, the interviewees were asked to consider the practicalities of delivering a protocolised vital signs observation schedule and also the implications that might be associated with specific revised schedules proposed by the algorithm generated through work package 2.
Live notes were taken by JLD during the interviews. These notes formed the basis of a written summary of the conversation. Information from these conversations was used to clarify the calculations that underlie the algorithm and subsequent interval setting, and to simplify points of confusion for dissemination purposes.
Data synthesis
The mixed-methods approach enables triangulation of the qualitative data collection, developing a richer understanding of the process and experiences of patient monitoring. Qualitative and quantitative data were collected sequentially, allowing each to influence the other (see Figure 1).
The interaction between qualitative and quantitative data across the study as a whole can be described as a multistage evaluation design. 166 We collected and analysed both qualitative and quantitative data at the beginning of the study in a convergent design. 166 Work package 2 (WP2) focused on quantitative data from EPRs, and work package 3 (WP3) conducted an integral convergent design study to assess the practical realities of conducting vital signs observations in the hospital setting. Work package 4 (WP4) completed initial group discussions with patients. We identified key themes from these early discussions with patients. We expanded on themes with later discussions, integrating new information as it became available. We returned to these core stakeholder views throughout the study to support algorithm development.
The initial qualitative data were shared with the algorithm development team early in the project. The early retrospective data analysis completed by the WP2 team enabled the WP4 team to understand the complexity of patient vital signs monitoring better. This understanding helped to iterate the topic guide used for the Twitter chat.
Later-stage stakeholder discussions offered opportunities for the algorithm development team (WP2) to explore specific features and functionalities in more detail. The expert guidance at this stage steered focus for final protocol proposals that were designed and presented to the final stakeholder panel at the end of the project.
Results
Oxford adult intensive care unit patient forum: 18 March 2019
Contributors: patients, relatives/carers, lay members of the public, healthcare staff.
Summary of discussion
-
Patients continue to be flabbergasted that the interval timing of vital signs observations has no evidence base: ‘I’m surprised there isn’t the equivalent of a minimum service level’.
-
There was an overwhelming response from patients that if it is necessary to disturb them at 2 a.m., they are OK with that: ‘Taking blood pressure is just as important as a good night’s sleep’, but they were also clear that observations should be done because they are needed.
-
Patients reported they were largely unaware of time passing when in hospital.
-
For patients, the knowledge that someone will check on them (even to just say ‘hi’) was just as important as actually having their vital signs taken/recorded.
-
Staff also valued simple conversation and thought that this could be a trigger to taking new measures outside of a protocolised schedule ‘if a patient tells you they feel rough’.
-
Both patients and staff mentioned that individuality was likely to be important to a patient’s need for monitoring (baseline pre-hospital values of low HR or high BP, etc.), as well as reason for admission, with different monitoring needs for post-surgery versus mental health patients for instance.
-
‘Individuality’ should include the physical location of patients – those patients in single rooms may need closer observation/monitoring than those in multiple-bay spaces where it became obvious that patients are (informally) part of the escalation practice: ‘each patient looks after the others’.
-
It was acknowledged that although patients should each have their own schedule for monitoring, the reality tended to be that the timing of monitoring rounds is somewhat standardised and linked to system convenience rather than individual patient need (10 p.m., 2–3 a.m., 4–6 a.m., etc.).
-
Patients confirmed that they associated more frequent care with higher levels of sickness and would ‘consider themselves lucky’ if they were being monitored every 12 hours compared to a patient in their bay on a 4-hourly monitoring schedule, although most patients couldn’t remember anyone having their vital signs taken anyway.
-
Staff (more than patients) were concerned about missing deterioration (although this was linked to ‘missing the required observations’ and being investigated for not following guidelines), although some patients felt it was ‘better to be safe than sorry’.
-
Patients could not remember ever being told why they were having their vital signs taken and would have liked more information at the time.
Many of these points were also raised by the second group (see below) so, for brevity, we will consider them together.
Southampton CLAHRC patient group meeting: 30 May 2019
Contributors: patients, relatives/carers, lay members of the public, healthcare staff.
Summary of discussion
Many points raised were similar to those discussed with the Oxford (post-ICU) patient group.
From both groups it was clear that communication and common sense should prevail when considering when to take vital signs observations. Both groups also welcomed the contact time with the clinical staff and expressed the view that the observation-taking was more than simply checking physiological health status. The concept of ‘individualising’ the monitoring schedule to each patient was addressed in both conversations and in both groups’ patients were happy to be disturbed when resting if this was clinically necessary. The patients who had not been admitted to intensive care were more strongly vocal in being allowed to sleep rather than be woken for vital signs.
The key difference between the groups was that the post-ICU group (Oxford) was largely unaware of time passing, whereas the non-ICU group (Southampton) was much more aware of boredom and the need to punctuate the day with periodic moments.
All patients in both groups expressed surprise that the evidence for 2-hour/4-hour/12-hour observation frequency, etc. is limited.
Night-time observations: waking patients to carry out observations was felt to be inappropriate in most situations – although the group universally agreed that when this was likely to be absolutely necessary they would be happy to be woken up if they had been told in advance that they would be (‘If you tell me, I’m not going to mind’). It was also suggested that patients should be grouped by their observation needs as, although disturbances from personal observations were accepted, other people having their observations done was highly disturbing, and much less well tolerated, especially overnight.
Timing: there was unanimous agreement that observations should not be taken during ‘care’ times – especially mealtimes. It was acknowledged that a rigid adherence to a schedule was unlikely to be appropriate in the majority of cases. A 12-hourly schedule should not include routine observations set between 10 p.m. and 6 a.m. Most patients liked the predictability of observation times (‘It breaks the day up’; ‘Being in hospital is so boring’). All patients felt that staff should have good awareness of previous observations (‘you get woken up at the end of the night shift, then the day staff take them again’; ‘you can get a bit fed up about constantly having vitals done – can start to feel “oh no, not again!” …’). There was general agreement that better synchronisation between ward activities and observation rounds would benefit both staff and patients.
Individualised care: it was considered appropriate to monitor fewer individual vital signs for patients where deterioration is unlikely (orthopaedic patients with no additional comorbidities were proposed as a group where simple HR or BP might suffice) and there was broad support for patients admitted to hospital for different reasons to have different schedules of observations frequency and/or to utilise HCA resources more for patients who are largely ‘well’.
Self-directed care: there was widespread support for patients being able to take their own observations if they felt ill, although it was also acknowledged that some patients might lie about the results, or take observations too frequently. Some patients expressed that they would like to be consulted about the frequency of their observation schedule (‘I would like to say if I want to be disturbed or not’). Some patients wanted to know how they could take steps to improve their vital signs to speed up their recovery to be able to go home.
Circadian rhythm: a few patients suggested that EWS limits should be different depending on the time of day due to natural fluctuations in (for example) HR and BP.
Practicalities: a couple of patients mentioned the inconvenience of being connected to monitoring systems for periods of time and nursing staff being apparently unaware of the difficulties moving around when attached to monitoring equipment.
Technology: the group was aware of some of the capabilities of modern technology and volunteered information about camera monitoring (‘I would like to see this technology fast-tracked for night-time observations’) and simple continuous monitoring devices such as Fitbits. 167 It was queried if other factors (e.g. blood results) could be (or should be) part of the EWS.
Opportunistic conversation: quite a lot of the patients agreed that the observation time was a good opportunity to talk to clinical staff and suggested that patients would wait for the observations before asking questions about their care/treatment/plans to go home. Several patients were concerned that observation frequency could drop to very low levels and this would deprive patients of human contact and company during the day. They were keen to stress that, from their point of view, the observation rounds were more than simply a check on their physical health.
Escalation: almost all patients in the room were keen to be given feedback from the nurse/HCA about their vital signs (‘it’s nice to be told ‘you’re doing well’’) Most patients wanted some information about what would happen next and when the next set of observations would be taken, especially if the vital signs were ‘abnormal’ (‘I’d like to be told the course of action’). Some patients wanted more detail about their observations and asked for contextualisation (e.g. ‘is my BP high compared to a national trend, or high from a few hours ago?’).
Summary of early consultations with patients and lay contributors
-
Patients understand that they will be monitored more frequently if they are more unwell. This was more obvious with the ICU group, which may be related to information they are given as they are monitored less in the ICU in preparation for ward transfer.
-
Patients should be treated as individuals with personalised vital signs schedules (and thresholds).
-
Patients should be involved in agreeing their frequency schedule if at all possible and it should be clear when the next set of scheduled observations will be.
-
Contextualised feedback to patients following vital signs being taken is perhaps more important than staff realise.
-
Patients like the security of regular monitoring and welcome the opportunity to have a conversation.
-
Overnight monitoring is accepted but patients need to be alerted to the need for it at the start of the night.
-
No patient on long frequency schedules (e.g. 8 hours or more between observation sets) should have routine observations taken overnight (e.g. between 10 p.m. and 6 a.m.).
-
Patients support the use/development of monitoring systems that release nursing staff from ‘observation rounds’ (this includes new technology and HCAs taking observations as long as they are subsequently reviewed by qualified nurses).
-
Post-ICU patients do not remember much from their time on the general wards, whereas the non-ICU patient group felt that the observation rounds broke the day up into manageable segments.
-
Observations may be missed (without penalty) to accommodate mealtimes, although better synchronisation between ward activities and observation schedules should largely resolve inappropriate clashes.
-
‘Cohorting’ patients (putting beds in the same physical space) by their frequency of observation needs may prevent non-frequently monitored patients from unnecessary disturbance.
-
Staff (from ICU-group meeting) expressed concern about missing required observations and being investigated for not following guidelines.
-
Blood pressure monitoring is consistently reported as intrusive and uncomfortable.
Feedback for algorithm development and project outputs
-
Advised physically grouping patients together according to observation frequency requirements.
-
Consider the need for BP monitoring at every vital signs observation opportunity.
-
Consider developing personalised observation frequencies.
-
No observations between 10 p.m. and 6 a.m. for patients not requiring frequent observation.
-
If overnight observations are required, tell patients when these will be taken.
-
Always report back to the patient after vitals taken with information about results and what they mean (‘next steps’).
TwitterChat hosted by @WeNurses: 20 October 2020
Contributors: 47 individuals; total tweets between 20:00 and 21:00, 361; total reach, 7,853,244.
Summary of discussion
We used Twitter as a forum to collect views about vital signs observation practices from clinical professionals.
Over the course of an hour, five questions were posted:
-
When do you think patients should be monitored more or less than NEWS/NEWS2 suggests?
-
How do you prioritise patient monitoring when it is not possible to take observations as suggested?
-
What do you tell patients (if anything) about how often they will be monitored and why?
-
How can we strike a balance between patients’ well-being and other factors?
-
What can be done to improve how observations are scheduled?
These questions were designed to complement insights from patients and carers, notes made by the work package three (WP3) team during their time on wards observing ward staff take patients’ vital signs (see Chapters 3 and 4: observational study methods and results), the original questions proposed in the funding application, and quantitative data available from work package two (WP2).
The full chat, including transcript and engagement stats, was exported from the WeCommunities website and subsequently analysed to discern common themes.
Several themes were apparent in responses, and to some extent these map to the patient and carer experiences outlined above. The ‘representativeness’ of patients and careers who are motivated to be part of wider hospital improvement groups was also discussed. This is a valid point that we need to be mindful of. At the same time, the project can only ever collect views from this type of patient and so this remains the best source for patient experiences that we can explore directly.
Two clear points from the patient and carer discussions were that overnight observations should be minimised and that patients should be included in the decision-making processes to agree how frequently a patient should be monitored. To some degree the TwitterChat group agreed with both these points but also expressed reservations about the extent to which this was possible.
Overnight observations were largely felt to be inappropriate [‘who decided that 2 a.m. and 6 a.m. were good times to wake a patient up?’; ‘it’s like ‘here’s some melatonin to help you sleep and get time oriented’ but also ‘we will be hitting you with that blood pressure cuff at 4 a.m.’’; ‘if medically stable (patient scoring 0) then reducing the frequency of observations, especially overnight, may be more beneficial for the patient holistically’] and this was balanced with a strong overarching belief that clinical expertise should guide decisions to observe patients outside of NEWS/NEWS2 defined protocols (‘I was always taught NEWS was to guide you but your own intuition overrules with monitoring’; ‘if your gut feels wrong, increase the monitoring’; ‘NEWS/NEWS2 does not replace the eyes and ears of the clinicians physically looking at the patient’).
Clinical expertise was referred to in responses to all questions and was a key factor when considering which patients were differently normal and who should therefore be observed ‘off-protocol’. These included patients being treated palliatively (‘replace NEWS with a symptoms chart to monitor for comfort’), those being investigated for possible stroke [‘escalate if blood pressure is high, even if not scoring (on the chart) so doctors can prescribe (anti)hypertensives’], or those with a sudden behavioural change (‘consider a patient who is usually a night owl, who is suddenly sleeping’) where holistic awareness of the patient including medication changes should guide interpretation of clinical signs. There was confusion about NEWS/NEWS2 protocol frequency due to limited evidence about observations generally (‘how do we provide information to our service users that variance from NEWS/NEWS2 was done with sound clinical judgement?’).
There was some discussion that electronic monitoring was reducing clinical skills (‘machines have taken away a lot of the touch and visual assessment; too focused on screens rather than the patient’) but at the same time the group acknowledged that with an electronic kit ‘anyone can take vital signs’ and that HCAs are asked to ‘continue routine observations on stable patients’ which allows more senior nurses ‘to focus attention on unstable patients’. This again picked up the topic of clinical expertise and ability/understanding to interpret observations, not just recording the measures, and the additional benefits that manual observations have over electronic that go beyond the (lack of) availability of kit and patients declining (‘we need to look, listen, and feel … to support decisions on frequency of observations’; ‘you can assess a patient just by looking at them’). Limited availability and accuracy of kit was a clear frustration with the participating group (‘you spend so long to find things’; ‘many a time the dynamap [electronic monitoring equipment] has given a wildly inaccurate reading’).
There was a small but consistent theme running through the questions that electronic monitoring is common outside of healthcare (‘every other industry and customer service has been doing it for 20 years, offering better leaner engagement and output at lower cost’) and that automated systems should run alongside manual systems to support efficiencies of care (‘I love a bit of technology but there is a process of learning and thinking when we put pen to paper which does not occur with electronic and automatic systems’).
Many participants in the group agreed that patients could be the best guide to observation frequency (‘we need to talk more to patients about observation so they can make informed choices’; ‘for many of them this isn’t their first rodeo and they know when they’re ‘not right’’). This view was somewhat juxtaposed against the rather limited ways in which patients seem to be included in decisions about their routine care where patients are rarely included in discussions about observation frequency, and really only told about frequency at all if on a high-frequency plan [‘I’m ashamed to say I only tend to do this if it’s very frequent’; ‘I put it in their care plan and explain that it will be BD (twice daily) in the first 72 hours of admission’; ‘I tend to tell patients how often I will be monitoring them and why when I’m doing frequent observations like hourly’]. A short side discussion on consent and engagement between patient and health professional suggests that patients are not often active participants in their care (‘I don’t think we do this enough and presume a patient consents’; ‘can’t say I’ve ever had this conversation unless the patient is on more frequent monitoring’; ‘sometimes nurses will explain why while they’re giving the obs but I think we get a bit complacent and assume everyone knows about observations or has heard it before’). There were mixed views on the observations round with some considering this ‘essential’, others advocating a patient-based approach, ‘chaotic at times but … not based on what suits us as nurses’.
Alongside clinical expertise, the importance of team communication was highlighted during the discussion, particularly with reference to when observation frequency is different from NEWS/NEWS2 protocol suggestion [‘rationale needs to be documented and communicated. There’s no point one person doing one thing with someone else doing another. Obs will become meaningless, misleading, and potentially put the patient at risk’; ‘ensure (off-protocol obs frequency) is documented clearly in notes and handed over’].
A small number of additional ‘vital signs’ were suggested to identify deterioration:
-
glucose (especially for patients with diabetes)
-
oxygen flow rate
-
urine output (especially for cardiac patients)
-
strength of heartbeat
-
rhythm of heartbeat.
Feedback for algorithm development
-
(Outside of scope for project) but it is clear that electronic monitoring is only as effective if wards have adequate kit.
-
Consider recognition of clinical expertise of individual taking observations and adjust guidance on frequency/escalation accordingly.
-
Highlight the importance of including patient in decision-making.
-
Ascertain ‘normal for patient’ early in admission to allow adjustments to scoring and frequency.
-
Require confirmation of ‘off-protocol’ frequency.
-
Make evidence behind the algorithm/s clear to users to facilitate better discussion with patients and clinical team.
-
Consider the need for BP at same frequency as other less intrusive observations, especially overnight.
-
Consider alternative/additional vital signs for some groups of patients.
Stakeholder group discussion: 19 May 2021
Contributors: three senior nurses, two lay patient experts.
Summary of discussion
Work completed to date has recognised that vital signs observations (also referred to as ‘obs’) are conducted through two potentially conflicting patterns of work. Most of the clinical staff we have spoken to acknowledge that the NEWS escalation protocol creates an individualised schedule for each patient, dependent on their clinical response to the NEWS trigger thresholds at each time point. However, they also acknowledged that this can be difficult to deliver on a wide scale across a whole ward and the most common approach is to run ward rounds at specific times. This was observed by the WP3 team when they conducted their ward-based work.
We have also discovered that most clinical staff feel the NEWS system is not a ‘one-size-fits-all’ approach to monitoring and recognising physiological deterioration. Groups of patients who may not ‘fit’ the standardised practice include:
-
Patients with diabetes as there is a general feeling that their glucose measures should be included in their observation sets.
-
Renal and cardiac patients require fluid monitoring in addition to vital signs.
-
Patients with COPD have differing oxygen requirements due to prolonged acclimatisation to living with low levels (this is partly addressed with NEWS2).
-
Younger patients, and also the very old.
-
Most people we have spoken to also want to see a difference drawn between surgical and medical patients.
Based on a prototype algorithm developed by the WP2 team, we presented first estimates of a risk-adjusted schedule of observation frequencies. Following technical adjustments to the algorithm, the specifics of both the current protocol in use at Portsmouth and alternative monitoring regimes changed. The underlying principles of identifying ‘acceptable risk thresholds’ remain valid and the stakeholder discussion highlighted clinical priorities that persist, with the final results presented in Chapter 6. Data presented to the group illustrated differences between workforce and health economic calculations between two schedules, or protocols for collecting patients’ vital signs.
The first protocol presented was based on the current EWS system in use at PHU. Data presented showed that patients admitted to hospital had an overall risk of death before their next observation of 0.41%; a risk of a combination of death, unplanned ICU admission, or cardiac arrest of 0.96%; a risk of combination of death, unplanned ICU admission, cardiac arrest, or an EWS above 7 of 4.69% and a risk of 6.1% of a combination of death, unplanned ICU admission, cardiac arrest, an EWS above 7, or their EWS increasing by two or more points.
Frequency of observations range from every 15 minutes (continuous) for patients with EWS 9 and above to every 6 hours for patients with scores 2 and below.
The assumed cost of this is £8756.70 per day when calculated with an equal division of workload between RNs and HCAs. This baseline cost also assumes no missed/late observations. We acknowledge that a significant proportion of observations are either completed late or missed altogether. 168 A comparison baseline cost of £11,140.90 was suggested for an observation schedule completed in full every 4 hours for all patients, regardless of their EWS.
We then presented an alternative scheduling system, determined by the WP2 algorithm, which was programmed to ‘optimise’ risk across all patients. This allows individual patients’ risk of the combined outcome of death, unplanned ICU admission, cardiac arrest, an EWS above 7, or their EWS increasing by 2 or more points to rise from 6.1% to 25.4% while maintaining the risk of death at 0.41% without increasing the existing workload.
Under the optimised approach the risk of a combination of death, unplanned ICU admission, or cardiac arrest reduces from 0.96% to 0.50%. The combination of death, unplanned ICU admission, cardiac arrest, or an EWS greater than 7 reduces from 4.69% to 2.58%.
As with the original data presented, this optimised schedule assumes no missed or late observations and that the workload is divided equally between RNs and HCAs. The optimised schedule indicates a reduced workload of 99.6 hours/day, compared with 243.2 hours/day for the baseline schedule. This suggests a cost reduction of £837,698 per annum.
The two protocols were discussed by the group in terms of how they differ, and how practical it would be to deliver the revised ‘optimised’ version in comparison to current practice. Some general points raised are summarised here:
-
The group thought that 12-hourly (twice daily) observations should be a minimum for all patients.
-
The group also thought that the threshold for increasing observation frequency should be an EWS > 3.
-
The group concluded that patients with EWS > 6 could have their vital signs taken every 2 hours (although they recognised that the Royal College of Physicians recommendation is to repeat observation sets every hour for patients with NEWS/NEWS2 values of 5 and above).
-
There was a strong feeling in the group that practical delivery of individualised patient scheduling might only be feasible with electronic systems.
-
The general consensus in the group was that HCAs complete the majority of observations and therefore should contribute > 50% of the workload for the purposes of assessing costs. The group also thought they would be faster than RNs (although we discovered this isn’t the case from WP3 data which indicates ~4 minutes/set regardless of if completed by RN or HCA, or if on paper vs. electronic system).
Further discussion explored the proposed scheduling of the optimised protocol: specifically, how precise this should be and how ward staff could best remain aware of individual patient schedules.
-
There was unanimous agreement that ‘no one thinks in 10-hour blocks’, proposing that (especially outside of electronic systems) timings should be rounded to the closest frequency matching common perceptions of divisions of time (e.g. 1, 2, 3, 4, 6 or 12 hours).
-
However, the group agreed that showing 1.8 or 1.2 would allow ward teams to decide if ‘every hour’ or ‘every 2 hours’ was most appropriate on an individual basis. They felt this would be easily managed with automated systems that can alert staff when next observations are due. Traffic-light indicators at bed spaces were suggested as an extension to the electronic record system to alert ward teams of the urgency for observations.
-
Visualisation of trends was suggested as a useful additional aid when considering individualised schedules.
-
Clinical members of the group proposed that the ‘science should be separated from the social interaction’, suggesting a check-in approach with lower-scoring patients. This would give patients the contact they need as part of the observation schedule without the additional burden of having the observations taken. This approach was welcomed by the lay members (‘it’s nice to know someone has half an eye on you’).
-
The group had a more focused discussion on the increased risk for patients whose EWS rises by > 2 between observation sets. Although at first glance this increased risk (6.1% to 25.4%) looked alarming, the group agreed that the absolute risk of any significant untoward event was likely to be low as the majority of these patients would be moving from scores of 0 or 1 to scores of 2 or 3. The group thought that the corresponding reduction in risk (4.69% to 2.58%) of patients’ EWS increasing to more than 7 was more important.
-
The patients acknowledged they might be concerned if they noticed they were having fewer observations than other patients (‘this would worry me, but that’s not a rational response’). This could be mitigated through discussion with the ward team.
Feedback for algorithm development
-
Clinical variation between some specific groups of patients is believed likely: recommendation for WP2 to assess differences/similarities where possible (acknowledging that not all data fields are available in sufficient numbers to derive fully working deterioration prediction).
-
‘Baseline’ workload and associated costs to be offered for 100% HCA and 100% RN and other divisions of workload in addition to the equal 50/50 split currently proposed.
-
There was widespread support for the project providing an evidence base for intervals. To maximise impact (implementation in the real world), these intervals need to map roughly to generalised concepts of time on the hospital ward (continuous, every hour, every 2 hours, every 4 hours, every 6 hours, every 12 hours) but being clear about exact suggested intervals (e.g. 1.8/1.2 rather than round to 1 or 2 hours) will support individual decision-making.
-
All patients should be reviewed at least twice each day.
-
Patients scoring 1 or less on the NEWS/NEWS2 scale could have an additional check-in (without observation set being taken) each shift.
-
Propose additional ‘vital signs’ for specific patient groups for NEWS3 development.
Overall recommendations
Our stakeholders (patients, carers and professional staff) came up with several recommendations for optimised evidence-based scheduling of vital signs observations for patients in hospital.
Stakeholder recommendations specific to WP2 output
-
Minimum interval between two observation sets to be 12 hours.
-
Adjust schedule for patients scoring 0 or 1 on the NEWS/NEWS2 scale to avoid overnight monitoring requirements between 10 p.m. and 6 a.m. (this is likely to mean bringing ‘scheduled’ observations forward to between 8 and 10 p.m.).
-
Initial suggested intervals to be accurate to within 0.25 of an hour (15 minutes) but allow automated rounding to commonly understood intervals (hour, 2 hours, 4 hours, 6 hours, 12 hours) for simplicity when electronic systems are not in use.
-
Add an interim check-in for patients scoring 1 or less on the NEWS/NEWS2 scale where an observation set isn’t required but patient has contact with care team.
-
For health economics analysis, run costs associated with different division of workload between RNs and HCAs.
-
Evaluate baseline and optimised protocols for the following patient groups if possible:
-
surgical patients
-
medical patients
-
patients with diabetes
-
renal patients
-
cardiac patients
-
patients with COPD
-
young patients (e.g. < 50 and 16–35 as potential subgroups)
-
very old patients (e.g. > 85).
-
Stakeholder recommendations for general project output
-
propose additional ‘vital signs’ for specific patient groups for NEWS3 development (e.g. glucose, urine output, oxygen flow rate, strength and rhythm of heart beats)
-
recommendation to include in all data recording systems a reminder to discuss observation frequency with patients especially if overnight monitoring is suggested
-
recommendation for all electronic vital signs systems to include visual representation of trends
-
recommendation to allow local clinical staff to ‘adjust for normal’ on an individual patient basis where this information is available.
Final stakeholder feedback: 7 September 2021
Lay expert (retired nurse, currently volunteer at local hospital): approx. 1 hour
Conversation focused on the practicalities of delivering care to multiple patients with differing requirements and how individual clinical needs change over time: ‘you’ve got your fairly well and robust patients – not far from being discharged […] once/twice a day is fine. Then you go incrementally and end up with those who’ve come back from theatre […] every 15–30 minutes […] rigorous checks during their first day then increase duration between observations’. Views on physiological individuality were similar to the patients and stakeholders consulted earlier in the project in that ‘the 60–70-year-old who’s really ill will need more monitoring than the 25–35-year-old after their RTA [road traffic accident]’.
During the conversation the interviewee introduced the idea that practice change over time now means that nursing skills are more specialised, leading to less generalisation. Specifically ‘we used to have one nurse who did nothing else except observations on surgery day’ and that overnight care was overseen by a ‘fire and brimstone senior sister’. Concerns were raised that junior healthcare staff were no longer able to ‘spot ill’ patients without electronic monitoring.
The thought that patients should be grouped together according to their monitoring requirements and allowed to rest overnight (both points raised earlier in the project) was given provenance in that the interviewee described this historical practice from her own experiences ‘[we were] attuned to the fact that there were certain sections of the ward that needed rigorous observation’ and ‘we used to sneak up on people at 2 a.m. and check their respiration and pulse and only wake them up if they were ‘not right’’.
Clinical opinion (NHS consultant physician): 13 September 2021, approx. 1 hour and 45 minutes
This interview highlighted the importance of presenting supporting information behind electronic calculations. Much of the conversation centred on how to present the differences between current practice (NEWS/NEWS2) and the revised interval scheduling suggested by the algorithm work. Specifically, the interviewee wanted to know how many patients would come to harm in the various scenarios proposed and if there was evidence of a ‘gap in patients getting sicker and being captured later’, also summarised in terms of unknown uncertainty between observation sets (‘how do you know what happens to a patient if you don’t look at the patient?’). The current focus on economics as the main illustration of outcome was questioned as ‘money only follows after you have understood the amplitude of the difference in deaths < or other outcome measure as agreed >’.
The specific proposal offered by the ‘optimised algorithm’ that all patients with scores of 7 or higher should be monitored continuously was welcomed as a sensible approach. This would in their opinion ‘release observation time for nursing care’ and counterbalance the risks of missing or late observations at the more frequent rates. This assumption would appear to be consistent with the workload analysis presented alongside the risks of adverse outcome. The clinical view was that most people die with EWSs of 4–6, suggesting that more time for these patients might improve their outcomes.
The interviewee felt that evidence was needed for the decision to include ‘risk of increase in EWS by 2’ as part of the composite outcome (‘Why plus 2? Why not plus 3 or plus 4?’). They were also looking for evidence that other methods of analysis had been considered, such as trend recognition (‘Is there a difference between patients who run 5/6/7/6/8/7/8/6/ etc. and those who run 3/2/1/2/1/2/3/6?’) and simple < 4 hours or > 4 hours (‘can we run comparative analyses of outcomes for those seen under and over 4 hours after triggering?’). They also wanted clarification on how ‘every 15 minute’ monitoring had been costed for the economics analysis as it was not obvious from the information presented for review.
When thinking about presenting differences between schedules the following were suggested ‘to make it real’:
-
number of deaths per 1000 people;
-
number of people who currently go to ICU versus the number of people who might potentially go to ICU under alternative schedules.
These final thoughts were given to the algorithm development team to help clarify the reporting of the algorithm.
Discussion
Stakeholder feedback guided the research team throughout the project. At all stages of algorithm development, stakeholder experiences of physiological monitoring in hospital (as patient, carer or healthcare provider) were considered alongside the objective data from EPR systems. The insights from our stakeholders were also integral to understanding what could be considered the hidden complexities of patient monitoring in hospital.
We recognise that we did not conduct one-to-one interviews which might be expected as per other experience-based co-design projects. We felt that the advantages offered through group discussion were beneficial to this project. Specifically, group interviews are an efficient approach to generate an understanding of a broad range of ideas. We were also aware of the benefits of working with groups of people who are already comfortable in each other’s company.
Although stakeholder interactions were embedded formally within the research design, and the project team was in agreement that group interviews would be appropriate, in common with other research teams,158 we still found it time-consuming and more complicated than expected to involve people outside the core research team. Specifically, we struggled to arrange meetings that were convenient for everyone. We overcame this initially by choosing to attend meetings with established patient and carer groups. The advantages of this approach were two-fold. Firstly, the key contributors (the members of the patient and carer groups) were already familiar to each other. This familiarity meant the group was comfortable sharing what could be very personal medical information. Secondly, the group was meeting anyway, and the research team was able to slot into their timeline without incurring additional time or cost burden to the contributors.
It was harder to arrange group meetings with healthcare professionals due to busy and conflicting work schedules. This was further affected by the SARS-CoV-2 pandemic. The decision to host the Twitter chat through the @WeNurses account, rather than run this independently, was influenced by the @WeNurses established practice of weekly online chats. Having been able to see similar sessions over time, we were confident we would attract enough participants to generate useful discussion.
Later-stage stakeholder discussion was held with members of the project oversight group.
The primary aim of the stakeholder discussions was to understand the potential ‘facilitators and barriers’ to delivering current NEWS/NEWS2 schedules and how these might be influenced by alternative interval timing protocols. The stakeholder interactions also revealed some of the wider issues associated with vital signs monitoring, separate from the direct clinical interpretation of vital signs information. These related concerns included practical considerations such as availability and maintenance of equipment, cultural expectations with regard to the acceptability of interrupting monitoring on the wards,125 and the concept of shared decision-making between clinicians and patients.
While the remit of the project is restricted to the background algorithm calculations, it was clear that for most people the mental model169 of patient monitoring also included data capture, data visualisation and escalation processes. These are all aspects of patient monitoring that need to be recognised and taken into account when considering change to the current guidelines. Implementing change at scale requires an understanding of socio-cultural norms that facilitate engagement and support. 170,171
Of relevance when evaluating the evidence base for optimal intervals between sets of vital signs is the foundation that NEWS/NEWS2 has created. Stakeholders within the team were fully aware that the NEWS/NEWS2 guidelines were agreed by consensus, hence the need for our study to develop evidence-based scheduling. Despite this understanding, there remains a clear dependence on what has become accepted practice. From this we learn that significant deviation from current guidelines (especially where this would result in a lengthening of periods between observations) could be ignored and not implemented if it was in conflict with accepted practice. There is then a potential conflict between objective interpretation of the data and qualitative understanding of the real world. Our pragmatic solution is a proposed schedule that does not perform worse than current usual care. This recognises that a different schedule might be better when considered objectively, but at the risk of widespread non-adherence, thus nullifying any improvement that might otherwise be possible. To facilitate adoption of any variation from accepted practice we recognise the clinical advice from the end of the project that risks and benefits need to be presented clearly and in ways that are easily interpreted.
We also found that most healthcare providers struggle at different times and in different ways to adhere to the ‘one size fits all’ model that is assumed by NEWS/NEWS2. The inherent individualisation that is central to patient care can be difficult to reconcile with the generic thresholds within current EWS systems. We identified conflicts of intent where stakeholders both upheld the guidelines of NEWS/NEWS2 while simultaneously reporting that they were happy to circumvent the recommendation when they felt it appropriate clinically. In line with findings from other research,172 the freedom to deviate from protocol was felt to be particularly important for those with more experience. There was a widespread belief that NEWS/NEWS2 was not appropriate for some patient groups. Despite reports of general positive attitudes towards a standardised EWS,173,174 this divergence of opinion has been recognised elsewhere, with lower adherence to recommended frequency reported in specialist treatment units and specific patient populations, as well as reduced adherence to vital signs observation frequency overnight. 124,172
The study data set did not include sufficient data to confirm equitable risk estimates for different observation frequencies for all of the subgroups suggested by the stakeholders. Mostly the data set indicated very low numbers of patients discharged having experienced an outcome of interest. This was particularly the case with patients under the age of 30. Other groups that were not represented sufficiently in the data set include those admitted with diabetes, patients admitted with renal or cardiac conditions, and those with severe respiratory conditions such as COPD. It was also not within this study remit to design a new early warning scoring system by including ‘alternative’ vital signs such as glucose, oxygen flow rate, urine output, strength or rhythm of heartbeat. However, prediction model validation methods allowed the WP2 team to evaluate some of the proposed patient subgroups (specifically, surgical patients, medical patients and older patients over the age of 80 years). Other subgroups assessed include emergency admissions, patients who did not have a surgical procedure during their hospital stay, and those patients with no vital signs observations recorded in the last 24 hours of their hospital stay. This validation work (see Table 13) indicated that alternative frequency scheduling and resulting risks for the various outcomes were similar for most patients admitted to hospital.
Throughout all the stakeholder interactions we identified this same widespread conceptualisation of hospitalised patients that not all patients are alike. For patients this meant they could be disturbed by monitoring of other patients, and they found this particularly troubling overnight. For professional healthcare workers this meant they were forced to recognise the illogicality of conducting ‘observation rounds’ at specific times of the day rather than tailor the individual patient intervals according to NEWS/NEWS2 protocol. That hospital beds used to be arranged within the ward space according to patient acuity suggests a rather more direct link than is often supposed between historic nursing practices and modern day EWSs used to detect deterioration.
Patient and carer participants were particularly keen to be included in decisions relating to their care. We acknowledge that patients and carers in our stakeholder groups may not be representative of the wider patient population. Simply by being members of existing stakeholder groups they have demonstrated a high level of interest in being involved with healthcare improvement and change. As such, it is likely they may represent a cohort of the patient population with a higher-than-average desire to be consulted about their care. We acknowledge that not all patients feel this way. That said, there is a growing interest across health care generally to improve communication between healthcare providers and patients by promoting patient-centred care approaches175 (see, e.g. the Choosing Wisely initiative that supports patients and clinicians to have conversations that are more informed176). Most healthcare professionals we spoke to advocated discussion with patients but admitted that in reality conversations surrounding physiological monitoring were not extensive. It is likely that few healthcare providers consider all seven principles of decision-making and consent177 each time they measure patients’ vital signs.
Conclusions
The mixed-methods approach to stakeholder involvement enabled us to collect and analyse different types of data over the course of the project. This variance of view allowed the research team to develop a rich understanding of the process and experiences of patient monitoring. This in turn enhanced the interpretation of the quantitative results from other phases of the project. Specifically we identified some key priorities and perceptions of patient monitoring that allowed us to interpret better the practical realities of delivering health care in a busy hospital ward. We also recognise that some apparent barriers to adherence can be viewed as facilitators to personalised care.
It is clear that stakeholder input influenced project team decisions. Future research would enable evaluation of the practicability of introducing alternative physiological monitoring protocols into routine clinical care alongside assessing their long-term impact on patient outcomes. We would recommend that any future evaluation of EWSs incorporates significant stakeholder input through participatory research methods and co-design practices that include both patients and professional healthcare staff.
Chapter 8 Conclusions
Recap of context
When we embarked on the project it was anticipated that we might conclude by suggesting protocol modifications for frequency of vital sign observations for specific groups of patients, rather than suggesting wholesale changes. By applying analytical techniques to large data sets of observations and related patient data, we anticipated that we might identify some patient groups whose care would not be compromised by less-frequent observation, and, perhaps, other groups who would benefit from more-frequent checks.
We noted in our funding application that while there is an evidence base to support the use of the NEWS to predict which patients are more likely to experience adverse outcomes (in-hospital mortality, cardiac arrest, unanticipated ICU admission),5–7,19 the measurement frequency of vital signs in general hospital wards has been described as ‘an evidence-free zone’. 4
It is an important issue. There is substantial evidence that inadequate monitoring is a major patient safety issue, contributing to avoidable deaths and other significant adverse outcomes. 3,8,13 The time was thus right for this piece of work.
Summary of observational study
One major part of our work was an observational study on the measurement and recording of a patient’s vital signs.
Across the four hospitals we studied, we found a variety of practices around who takes the observations, with two hospitals having RNs take the majority of them, while the other two hospitals most often left it to HCAs or student nurses. However, we found that whoever took the observations spent roughly the same length of time, an average of 5 minutes and 1 second per observation over a ‘round’. While RNs tended to be the quickest, there was a significant variation within each staff type: 95% of observations took between 4:39 and 5:24.
The 5:01 time included the preparation time associated with locating and preparing the equipment and travelling to the patient area. An average of 3:45 was spent at each patient bedside (excluding interruptions not related to the vital sign taking). If all interruptions were included, the average rose to 4:24 at the bedside and 6:26 per patient in a round.
We found substantial efficiencies in grouping observations into a round (i.e. doing more than one patient sequentially). Figure 5 shows, however, that the marginal gains from longer rounds are low. A round where five patients are observed takes 12% less time per patient compared to observing a single patient, while rounds of 10 patients give a time saving of 13% per patient. This is to be expected because set-up and tear-down of the equipment is a fixed overhead largely independent of round size.
Part of our work involved analysing the reasons for interruptions and we characterised six different types of interruption:
-
fixed care – things that have to be done at set times of the day;
-
bundled care – things convenient to do at the same time as vital signs observations;
-
collaborative care – mainly communication with other health professionals;
-
reactive care – emergencies that require a quick response;
-
proximity-related care – things that might not necessarily occur unless the member of staff was already with the patient;
-
patient absent or unavailable.
These factor in to an analysis of priorities: do vital sign observations take precedence over other activities? Our conclusion is that generally no they don’t during core time (9 a.m.–5 p.m.) but staff make up for this by scheduling the main rounds of observations around the main shift-change times (early morning and early evening). During daytime hours, vital signs tend to have to fit in around meals, washing and doctors’ ward rounds. 12 The more frequently vital signs need to be taken between 9 and 5, the more likely it is they will be delayed or missed by the need to meet other demands. Vital signs rounds tend to be put off until after those sorts of activities have been completed. Other nursing care activities that can be done at variable times tend also to be put off and perhaps missed. A recent study into the impact of the COVID pandemic on adherence to vital sign monitoring schedules suggested that the reduced workload due to a significant reduction in hospital admissions might have improved compliance with the existing protocol. 178 This supports our findings that nursing staff appreciate the importance of vital sign monitoring and will take observations according to schedule when their workload allows it.
However, there are periods at the start and end of the day (i.e. first thing in the morning and in the evening) when there are fewer competing tasks and consequently nurses have more opportunity to do important nurse-led tasks, such as vital sign observation. This is in line with previous observational studies that showed peak observation activity coinciding with nurse observation rounds. 12,178
If a protocol is proposed that reduces the frequency of observations, it may make it easier to fit in those fewer observations that need to be made between 9 and 5, though they would probably still be prioritised below fixed-time activities.
Where aspects of care are bundled together, there might be advantages if one aspect is judged to be higher priority – then other elements of the bundle would effectively have that higher priority too.
Any change to existing protocols might have knock-on effects on proximity-related care. For example, a reduced frequency of observations would reduce the opportunities for the patient to ask a question or benefit from other interaction with the nurse. However, an increased frequency of observation would increase these opportunities, and thus tend to improve the quality of care provided. Our stakeholders proposed that ‘social interaction’ should be separated from the science of vital sign observations, with a short ‘check-in’ approach adopted for lower-risk patients.
It is important to recognise that while the individual components of observation work are themselves quite small, when aggregated over many patients and multiple observations per patient per day it all adds up to a substantial amount of daily nursing workload that has to be integrated, prioritised and, of course, allocated resource.
Summary of retrospective study
The other major part of our work was the retrospective analysis of patient vital signs data from two hospital trusts. We had available to us data on a total of over 400,000 patient admissions and over 9 million vital sign observations.
Patient subgroups
We noticed that there were certain patient subgroups where patients’ outcomes and monitoring frequency were different from others:
-
Elective patients were (as expected) much less likely to have an adverse outcome than emergency patients.
-
Patients who were not monitored in the last 24 hours of their admission had the highest adverse outcome rates, but this is explained by the protocol of not monitoring patients who were on an end-of-life pathway. Consequently, this group of patients had the lowest average observation frequency. This group also had the highest average NEWS score.
-
Outcome rates were lowest in admissions to surgical specialties, followed by admissions to medical specialties (which include geriatric specialties) and highest in elderly patients (> 80 years old).
-
Inpatients (i.e. those who stayed in hospital for at least 1 night) receiving blood transfusions or chemotherapy had lower observation frequencies but were expected to be on different monitoring schedules.
Diurnal patterns
We analysed what time of day vital sign observations were taken:
-
There were definite peaks of activity at around 06:00, 11:00 and 16:00, and between 20:00 and 23:00. This indicates that a significant proportion of vital sign observations were taken at common times. This ties in with the data from our observational study (above) that showed that nursing staff tend to fit vital sign observations to avoid mealtimes and other regular activities.
-
In one of the hospitals, the peaks in activity became less distinct after 2015. There was also a reduction in the number of vital sign observations taken ‘late’ and a consequent increase in the number taken ‘on time’. This can be explained. At around that time, our source hospital undertook an initiative to reduce the number of patients whose vital signs were taken late. The data indicate the success of that. Data from the other hospital over a 2-year period from 2017 to 2019 did not show a reduction in peak activity, but showed a small reduction in ‘late’ observations in the first 6 months of 2019.
-
The data also showed that, after the initiative, there were fewer ‘late’ observations among higher-risk patients (those with NEWS ≥ 6) than lower-risk ones. This shows that staff were usually appropriately prioritising high-risk patients, though a change in monitoring protocol may have contributed to that too.
Trends over a patient’s admission
An important step in being able to consider the frequency of observation is to understand how patients’ vital signs (and NEWS score) vary over time.
By analysing trends in the data, we were able to see that:
-
There were differences between the two hospital trusts in terms of LOS. While at both hospitals the majority of patients were discharged within 72 hours, at our external validation data set hospital more patients were discharged in the first 24 hours than at our development-data set hospital. We do not have a full explanation for this except to speculate that it is possibly down to the development-data set hospital having an ambulatory clinic which deals with patients who do not need a bed and hence only the ‘sicker’ patients get admitted and stay overnight (and are included in our data).
-
When classified by NEWS score, the highest number of observations in our development-data set hospital was associated with NEWS 0, then monotonically decreasing for higher NEWS values. At our external-validation hospital, more patient observations had a score of 1 than any other value, but again there was a monotonically decreasing trend of numbers with higher scores than that. The difference between hospitals is probably down to local factors outside the scope of this study.
-
Our data showed that NEWS was on average highest in the first 96 hours after admission. During this period, patients were at the highest risk of a deteriorating score that reached NEWS 7 or higher. This indicates that a significant proportion of patients continue to deteriorate after admission to hospital. An alternative way to look at this is that it can take about 4 days for a patient to stabilise and improve.
-
Looking at trends from the perspective of the adverse outcome, Figure 21 shows that, as expected, NEWS increases in the days prior to that. The trend, particularly for the patients with the worst outcomes, seems to start up to 9 days prior to the event, with accelerated increase in the last 48 hours or so.
-
It is also noticeable that the patients with the worst outcomes (death, unanticipated ICU admission) average the highest NEWS scores and have the earliest increases. Of course this analysis is limited in that not all patients will have been in hospital for several days before their adverse outcome.
-
The charts in Figure 22 show similar trends for some of the components of NEWS. It is significant to note that breathing rate and oxygen saturation show the steepest and longest climbs. This suggests that staff should take particular note of deterioration in these two vital signs.
There are only limited conclusions that can be drawn about observation frequency from analysis of trends over days. Therefore, we also consider changes over periods of hours since the patient was last observed.
We looked at how risk varies over time for different levels of NEWS score and how risk varies according to NEWS score for different periods since the patient’s last observation (see Figures 23 and 24).
Our insights from trends over time are:
-
The risk that grows fastest since last observation is the risk that their NEWS score will go up by 2 or more.
-
It is only high-risk patients (NEWS ≥ 6) whose risk rises rapidly in the first few hours after an observation. For patients with scores of 0 or 2, the risk does not build for many hours, except the risk of their NEWS rising by 2.
Our insights from trends based on NEWS score are:
-
The growth rate of risk is highest for high-risk patients.
-
For low-risk patients, the increased risk due to longer periods between observations is small. There is not much of difference in risk between 4-hourly and 24-hourly observations for patients with NEWS ≤ 2.
Comparing protocols
So what are the consequences of changing an observation protocol?
We considered a number of different scenarios based on how ‘risk’ could be managed in different ways.
In our development data set, the maximum risk of the ‘combined outcome’ (death, unanticipated ICU admission, cardiac arrest) before the next observation was 0.89%. However, risk was unevenly distributed across different NEWS values, with lower risk for NEWS 0 or 1 and increased risk for high NEWS values (see Table 16). We examined this from five perspectives (each shown in a column in Table 24):
-
In column 1, we first of all considered a scenario where observations were redistributed by selecting a maximum observation interval for which the average risk of combined outcome did not exceed the maximum observed risk of 0.89%.
-
Noting feedback from our stakeholders that irregular frequencies would not be likely to gain adoption, in column 2 we ‘rounded down’ the above to one of 12 hours, 8 hours, 6 hours, 2 hours and 1 hour plus 0.25 as a proxy for continuous monitoring.
-
What could we do to reduce the risk of adverse outcomes occurring? Column 3 shows the frequencies of observations needed to reduce the overall maximum average risk from 0.89% to 0.5% (proportionate risk reduction = 0.44).
-
Column 4 shows what could happen if the aim was to reduce the risk to only 0.1% (proportionate risk reduction = 0.89).
-
Finally, column 5 shows what would happen if instead of trying to reduce the risk of the combined outcome, we aimed to reduce the risk of NEWSINC (NEWS score increasing by 2 or more) to < 4%.
NEWS | 0 | 1 | 2 | 3 | 4 | 5 |
---|---|---|---|---|---|---|
Dev site protocol | COMB = 0.89% | COMB = 0.89% (rounded down) | COMB = 0.5% | COMB = 0.1% | NEWSINC = 4% | |
0 | 6 | 24.0 | 12 | 12 | 12 | 4 |
1 | 6 | 24.0 | 12 | 12 | 12 | 6 |
2 | 6 | 24.0 | 12 | 12 | 6 | 6 |
3 | 4 | 23.7 | 12 | 12 | 2 | 4 |
4 | 4 | 10.0 | 8 | 4 | 1 | 4 |
5 | 4 | 4.8 | 4 | 2 | 0.25 | 4 |
6 | 2 | 2.9 | 2 | 2 | 0.25 | 2 |
7 | 1 | 1.4 | 1 | 1 | 0.25 | 0.25 |
8 | 1 | 0.25 | 0.25 | 0.25 | 0.25 | 0.25 |
9 | 0.25 | 0.25 | 0.25 | 0.25 | 0.25 | 0.25 |
10 | 0.25 | 0.25 | 0.25 | 0.25 | 0.25 | 0.25 |
Relative resource |
0 | −62% | −40% | −35% | +61% | +31% |
For comparison, column 0 shows the protocol used in the hospital during the period over which data was analysed.
A colour coding is then used to show how each value compares to the existing protocol:
-
higher frequency than the protocol in use;
-
same frequency as the protocol in use;
-
lower frequency than the protocol in use.
The final row in the table shows the cost of each scenario relative to the existing protocol. This shows that:
-
The existing risk to patients may be managed differently and reduce costs (Scenarios 1 and 2).
-
Reducing the risk of certain patients does not need to cost more (Scenario 3), but further reductions could cost more (Scenarios 4 and 5).
However, our analysis in Economic analysis showed that even Scenarios 4 and 5 could be judged to be cost-effective when judged against the QALYs per life gained, though this is sensitive to assumptions.
General discussion
By synthesising results from our different strands of work, we have been able to draw conclusions about the factors that contribute to safe and effective vital signs monitoring. Our PPI and stakeholder engagement has informed our studies, and the results obtained from our observational study provided input into our economic analysis. All our results were then discussed with stakeholders to obtain their feedback.
This is the first study to develop evidence-based monitoring regimes for intermittent vital sign observations using the NEWS. To date, existing monitoring protocols have been based on clinical expertise and tradition. 3,56,64,65 While the association between observation rates and clinical outcomes has been studied, the focus has been on the impact of introducing EWS and associated monitoring protocols where they had not been used previously. 62
Studies comparing different monitoring regimes have predominantly addressed the question of whether continuous monitoring is better than intermittent monitoring. 57–60,149 Furthermore, most assessments of continuous monitoring have focused on detection of physiological abnormalities and did not investigate the effect on clinical outcomes. We are aware of only one study, by Petersen et al.,53 into the benefits of different intermittent monitoring regimes. The ward-level randomised, non-blinded controlled trial included 1346 low-risk medical and surgical patients who were admitted to a Danish urban university hospital. The research assessed the impact of increasing observations from twice daily to three times a day. Results showed no significant difference in clinical outcome rates between the different regimes.
We have to be careful with our conclusions since there are limitations on our analysis.
Since reducing the risk to some patients (by observing them more frequently) is offset by a theoretical increase in risk to others (by observing them less frequently), we cannot be certain that the net effect is positive (though this is likely). Our analysis focuses on the risk of a patient deteriorating significantly and unnoticed before their next vital sign observations are taken. This is not the same as reducing the overall risk to the patient of the outcome of their admission being adverse.
Our results aggregate patients with similar NEWS scores. Not all patients with the same score are on the same trajectory (some are going up and – with the exception of NEWS 0 – some are going down). By no means will all patients within a group respond similarly. Each group contains patients with a very wide variety of conditions. We do not know for certain whether there may be patients with particular conditions who have a significantly different risk profile from the ‘average patient’, but our subgroup analysis has shown that differences in outcome rates are as might be expected.
We have developed an evidence-based framework, but we were unable to test the impact of any monitoring protocol on outcomes in practice in this observational work. It is up to professional bodies to evaluate potential candidate protocols (beyond the ones presented in this report), test one or more prospectively and then recommend one for use nationally.
Reflection
It is worth reflecting on our results from the patient’s perspective.
Patients are in hospital for a variety of reasons, but typically they all reduce to the fact that hospital is judged to be the safest place for them to be at that particular point in time. While there, they expect to be helped to get better (unless they or their representatives agree that they are on an end-of-life pathway). It is part of the informal contract between patient and clinician that the clinicians will monitor the patient and take appropriate actions to resolve any problems.
Thus, patients expect to be monitored. While vital signs observations are an important part of that, it is worth noting that other forms of observation take place, such as looking at and speaking to the patient, and sometimes noting that they have not eaten their food, been to the toilet or performed some other activity that is deemed ‘normal’. However, vital signs monitoring is designed to give objective evidence of the patient’s physiological state and is an important part of in-hospital care.
We have discussed the relative priorities of taking vital signs versus other activities that go on, from the nursing staff’s perspective. The patient’s point of view might be the same (prioritising eating, washing, talking to a doctor) or different, depending on their attitude and state of mind. We referred to the pros and cons of waking a patient up during the night to take their vitals (see Results). Some patients accept that more than others.
As an aside, we can report that the lead author of this study undertook an impromptu field test of vital sign observations during the later stages of the project. He was admitted to hospital for two nights after suffering a concussion. He had a NEWS of 0 from the initial assessment by the ambulance crew and on every occasion when his vital signs were taken during his stay in hospital. Despite this, he was woken up hourly during the first night after admission so that staff could check whether his pupils were dilated. The vital signs that make up NEWS do not cover all aspects of patient observation. Discussions with other patients and healthcare professionals during the project highlighted some signs not included in NEWS that they considered important for monitoring patients’ ongoing health.
Patients often relish the attention that nursing staff give them. It can become boring lying in a bed or sitting in the same seat all day, especially if your fellow patients are not sociable. Also, with time on your hands, it is easy to become unnecessarily anxious about your condition. The moment when the nurse or HCA comes to measure your vital signs is often a welcome opportunity to have a conversation, either seeking reassurance about your condition and care or whatever else is on your mind. That interaction is important too from the clinician’s perspective because of the insight it gives into the patient’s state of mind and concerns.
If there is a solid conclusion to be drawn from our retrospective data analysis, it is that often vital sign observations are done more frequently than are necessary from a bald assessment of the risk to the patient. Reducing their frequency would, however, detract from the other aspects of nursing that take place during those interactions. It is of course hard to measure the benefits from ‘incidental interactions’, but it does seem clear that they are important to patient well-being and hence outcomes. It might be necessary to take proactive steps to compensate for a reduction in vital signs observations.
Summary of contributions to knowledge and implications for decision-makers
Our main contribution from this work is not that there needs to be a radical shake-up of the frequency with which vital sign observations are taken. We can say that our work supports the approach proposed by the RCP20 and used by many hospitals, whereby a patient’s NEWS2 score guides observation frequency. We have not found evidence that patients really should be observed more frequently. The one exception might be for patients with a NEWS of 5, where 2-hourly observations would cap their risk of deterioration before the next observation to a level commensurate with patients with other scores. Our study hospital has already moved to 1-hourly observations for patients with a NEWS of 5 or 6.
Existing practice is to observe higher-risk patients more frequently. We have shown that this is objectively justified and we have attempted to quantify the risk associated with patients at each level of deterioration (i.e. NEWS value).
We have provided a framework by which anyone proposing to review observation schedules can assess the impact of changes. By showing how risk rises with time since the previous observation, we have enabled a discourse to happen about the consequences of changes to practice.
Finally, we have shown that to achieve improved patient outcomes does not necessarily demand an increase in nursing costs. The existing resources may be redeployed within a changed protocol to achieve better outcomes for some patients without compromising the safety of the rest.
We drew on opinions and experiences from patients and healthcare professionals to help us understand the wider implications of vital signs observation in hospital. This interaction ensured that the alternative protocols we have proposed are in line with their expectations and perceived benefits. We hope that our conclusions contribute to future discussions about how vital sign observations should be scheduled. Ultimately, it is for the relevant professionals to collectively decide how our work should be used. At a national level, this could be done by the RCP in reviewing NEWS; at an individual hospital level, it could be done by clinicians and managers combining to make decisions about local protocols and practice.
It may well be that, in the foreseeable future, frequency of observations will become an irrelevant issue because all patients will have all their vital signs continuously monitored. However, at the moment this is not effective or practicable for the general hospital population. The benefits of continuous monitoring need to be offset against any additional inconvenience or restrictions that it may place on the comfort or mobility of the patient. Of course, if there is no additional inconvenience or restriction, then continuous monitoring would logically seem to be a good idea.
Recommendations for future research
We have developed a framework that proposes some observation protocols and a way of evaluating them both clinically (in terms of patient outcome) and economically (possible cost-effectiveness). The obvious next step is to conduct some form of trial to see whether our framework is valid in practice. This could perhaps be implemented initially at the level of a single ward, perhaps expanding its scope to an entire hospital. A comparison between hospitals might be possible, but we are aware that inter-hospital differences in practice or culture might obscure any evaluation.
There are several additional aspects of this work that would be useful to investigate further.
-
We should look at trends in a patient’s NEWS scores or specific vital signs. For example, what difference is there between a patient whose sequence of NEWS values runs 5,6,7,6,8,7,8,6 and one whose runs 3,2,1,2,1,2,3,6? They both have a most recent NEWS of 6 but reached that point on different trajectories and at different rates of change. Can we discern information from the sequence to better understand how the patient’s condition might develop in the future?
-
What aspect of risk are we aiming to optimise? In this study, we employed maximum risk thresholds that would not be exceeded before the next vital sign observations. It would also be possible to optimise based on lowest risk thresholds or average risk across the hospital population. These nuances ought to be investigated in case they produce radically different results.
-
Before going much further, there is a need for a tool to make it easier to weigh the benefits and risks of changing a protocol. We said that we had provided a framework by which anyone proposing to review observation schedules can assess the impact of changes. While that is true, we have not necessarily made it easy to do so. It would be nice to have a tool whereby nursing managers or other senior staff could try out scenarios of their own devising, and assess the impact of them both in terms of patient outcome and cost. We have developed a prototype of such a tool to help us answer the questions posed by our scenarios, but it is not suitable for more widespread use. It would need some software development effort to convert it into a production tool that could import data about existing monitoring schedules, staff skill mix, protocol compliance and risk of outcomes, and permit the user to define constraints for any future monitoring regime.
-
Our final stakeholder feedback raised the question of whether an increase in NEWS of 2 was an appropriate outcome to consider: why not 1 or 3 or 4? Also, is going from NEWS 1 to 3 the same as going from 4 to 6 or even 12 to 14? It would be useful to have evidence to answer those questions to formulate the most appropriate analyses in future.
-
Our stakeholders proposed that additional ‘vital signs’ might be collected for specific patient groups (e.g. glucose, urine output, oxygen flow rate, strength and rhythm of heartbeats) and incorporated in future EWSs. The feasibility of collecting the data needs to be evaluated as well as the effectiveness of integrating those data into more sophisticated models that predict outcome.
-
Our analysis showed that breathing rate and oxygen saturation are often the earliest indicators of deterioration. Work to establish more precisely the relationship between measure and outcome, and to incorporate that into revised systems, would be valuable.
-
Our stakeholders recommended that all data-recording systems incorporate a reminder to discuss observation frequency with patients especially if overnight monitoring is suggested. It would be interesting to see how well that was accepted by both patients and hospital staff.
-
Our stakeholders also recommended that all electronic vital signs systems include a visual representation of trends. Our study did not look at how clinicians consider trends, though anecdotal evidence suggests that it is commonly done. Incorporating a visual representation of a trend in a user interface display would be expected to ‘train’ users to think about it in their practice. It might be interesting to evaluate this.
-
Our stakeholders recommended that protocols allow local clinical staff to ‘adjust for normal’ on an individual patient basis. This would require knowledge of what ‘normal’ is for a particular patient, which might not always be available. It would be necessary to establish procedures for how and when those decisions could be made, and some means of recording the adjustment would be essential to communicate it to other staff. Such procedures may already exist on a small scale but may not yet have been evaluated. It would be challenging to do that in such a way that the procedures could be rolled out on a larger scale.
Additional information
Acknowledgements
We would like to thank all the members of our Project Oversight Group for their invaluable contributions:
Christian Subbe (chair), Ysbyty Gwynedd
Rachel Binks, Airedale NHS Foundation Trust
Candice Downey, Leeds Teaching Hospitals NHS Trust
Lesley Durham, North of England Critical Care Network
Ruth Endacott, NIHR (formerly University of Plymouth)
Mohammed Mohammed, University of Bradford
Faith Ponsonby, PPI representative
Peter Thomas, formerly Bournemouth University
We would also like to express our thanks to Rachel Henning, Pauline Jeffs and Verity Westgate, who made major contributions to the administration of the project.
We would also like to acknowledge the contributions of Jody Ede, Antonio Maruotti, Paul Schmidt and Gary Smith to the project. In addition, Yvonne Alton contributed her PPI perspective. Alice Mortlock and Antonia Baker assisted with aspects of the project at Portsmouth Hospitals University NHS Trust.
Contributions of authors
Jim Briggs (https://orcid.org/0000-0003-1559-4879) (Professor, Health Informatics) led the study, interpreted data and drafted and revised the final report.
Ina Kostakis (https://orcid.org/0000-0003-1167-2227) (Research Fellow, Health Informatics) conducted much of the retrospective study analysis work, interpreting all aspects of analysis, and contributed significantly to drafting and revising the final report.
Paul Meredith (https://orcid.org/0000-0002-5464-371X) (Data Manager, Healthcare Research) contributed to the design of the study and led on the extraction of data from trust systems, contributed to the interpretation of data and commented on drafts leading to revisions of the final report.
Chiara Dall’ora (https://orcid.org/0000-0002-6858-3535) (Lecturer, Health Sciences) contributed to the acquisition of observational data, contributed to the analysis and interpretation of data and contributed to drafting the final report.
Julie Darbyshire (https://orcid.org/0000-0002-7655-1963) (Research Programme Manager, Critical Care) contributed to the design of the study and the project management of it, led the stakeholder engagement work and contributed significantly to drafting and revising the final report.
Stephen Gerry (https://orcid.org/0000-0003-4654-7311) (Senior Medical Statistician, Statistics in Medicine) contributed to the retrospective study analysis work.
Peter Griffiths (https://orcid.org/0000-0003-2439-2857) (Professor, Health Services Research) contributed to the design of the study, led the observational study, interpreted data and contributed to drafting and revising the final report.
Jo Hope (https://orcid.org/0000-0002-8939-7045) (Lecturer, Health Sciences) contributed to the acquisition of observational data, contributed to the analysis and interpretation of data, contributed to stakeholder engagement and contributed to drafting the final report.
Jeremy Jones (https://orcid.org/0000-0002-2725-0937) (Principal Research Fellow, Health Economics) contributed to the design of the economic aspects of the study and interpretation of the economic analysis, and commented on drafts leading to revisions of the final report.
Caroline Kovacs (https://orcid.org/0000-0002-3548-8603) (Senior Research Associate, Health Informatics) contributed to the scoping review and commented on drafts leading to revisions of the final report.
Rob Lawrence (https://orcid.org/0000-0001-8641-2820) (Public Patient Involvement Representative) contributed to interpretation of the data, contributed to the stakeholder engagement and contributed to revisions of the report, including the plain language summary.
David Prytherch (https://orcid.org/0000-0003-2621-6451) (Professor, Health Informatics) contributed to the design of the study and analysis/interpretation of the data, and commented on drafts leading to revisions of the final report.
Peter Watkinson (https://orcid.org/0000-0003-1023-3927) (Associate Professor, Intensive Care Medicine) contributed to the design of the study and provided clinical interpretation of the analysis.
Oliver Redfern (https://orcid.org/0000-0002-3596-3806) (Clinical Researcher, Health Informatics) contributed to the design of the study, led the retrospective study analysis work and contributed to drafting the report and interpreting all aspects of the analysis.
Use of patient data
This work uses data provided by patients and collected by the NHS as part of their care and support. Using patient data is vital to improve health and care for everyone. There is huge potential to make better use of information from people’s patient records, to understand more about disease, develop new treatments, monitor safety and plan NHS services. Patient data should be kept safe and secure, to protect everyone’s privacy, and it is important that there are safeguards to make sure that they are stored and used responsibly. Everyone should be able to find out about how patient data are used. #datasaveslives. You can find out more about the background to this citation here: https://understandingpatientdata.org.uk/data-citation.
This research has been conducted using the Oxford University Hospitals NHS Foundation Trust Clinical Data Warehouse, which is supported by the NIHR Oxford Biomedical Research Centre and Oxford University Hospitals NHS Foundation Trust.
Data-sharing statement
Owing to the nature of this study and the conditions attached to original data agreements, there are no data available for wider use. All queries should be submitted to the corresponding author in the first instance.
Department of Health and Social Care disclaimer
This publication presents independent research commissioned by the National Institute for Health and Care Research (NIHR). The views and opinions expressed by authors in this publication are those of the authors and do not necessarily reflect those of the NHS, the NIHR, MRC, NIHR Coordinating Centre, the HSDR programme or the Department of Health and Social Care.
Publications
Dall’ora C, Griffiths P, Hope J, Barker H, Smith G. What is the nursing time and workload involved in taking and recording patients’ vital signs? A systematic review. J Clin Nurs 2020;29(13–14):2053–68. https://doi.org/10.1111/jocn.15202
Dall’ora C, Hope J, Bridges J, Griffiths P. Development and validation of a methodology to measure the time taken by hospital nurses to make vital signs observations. Nurse Res 2020. https://doi.org/10.7748/nr.2020.e1716
Dall’ora C, Griffiths P, Hope J, Briggs J, Jones J, Gerry S, Redfern OC. How long do nursing staff take to measure and record patients’ vital signs observations in hospital? A time-and-motion study. Int J Nurs Stud 2021 Jun 1;118:103921. https://doi.org/10.1016/j.ijnurstu.2021.103921
Dall’ora C, Saville C. (editor) How long do nurses take to measure patients’ vital signs, and does it matter? Evidence Brief, University of Southampton. August 2021.
Disclaimers
This manuscript presents independent research funded by the National Institute for Health and Care Research (NIHR). The views and opinions expressed by authors in this publication are those of the authors and do not necessarily reflect those of the NHS, the NIHR, the HSDR programme or the Department of Health and Social Care. If there are verbatim quotations included in this publication the views and opinions expressed by the interviewees are those of the interviewees and do not necessarily reflect those of the authors, those of the NHS, the NIHR, the HSDR programme or the Department of Health and Social Care.
References
- Royal College of Physicians . National Early Warning Score (NEWS): Standardising the Assessment of Acute Illness Severity in the NHS. Report of a Working Party 2012.
- National Institute for Health Care Excellence . Acutely Ill Patients in Hospital: Recognition of and Response to Acute Illness in Adults in Hospital 2007.
- DeVita MA, Smith GB, Adam SK, Adams-Pizarro I, Buist M, Bellomo R, et al. ‘Identifying the hospitalised patient in crisis’: a consensus conference on the afferent limb of rapid response systems. Resuscitation 2010;81:375-82.
- Smith GB, Recio-Saucedo A, Griffiths P. The measurement frequency and completeness of vital signs in general hospital wards: an evidence free zone?. Int J Nurs Stud 2017;74:A1-4.
- Smith GB, Prytherch DR, Meredith P, Schmidt PE, Featherstone PI. The ability of the National Early Warning Score (NEWS) to discriminate patients at risk of early cardiac arrest, unanticipated intensive care unit admission, and death. Resuscitation 2013;84:465-70.
- Abbott TEF, Torrance HDT, Cron N, Vaid N, Emmanuel J. A single-centre cohort study of National Early Warning Score (NEWS) and near patient testing in acute medical admissions. Eur J Intern Med 2016;35:78-82.
- Kovacs C, Jarvis SW, Prytherch DR, Meredith P, Schmidt PE, Briggs JS, et al. Comparison of the National Early Warning Score in non-elective medical and surgical patients. Br J Surg 2016;103:1385-93.
- National Confidential Enquiry into Patient Outcomes and Death . Time to Intervene? A Review of Patients Who Underwent Cardiopulmonary Resuscitation As a Result of an In-Hospital Cardiorespiratory Arrest 2012.
- Keogh B. Review into the Quality of Care and Treatment Provided by 14 Hospital Trusts in England: Overview Report 2013.
- National Patient Safety Agency . Safer Care for the Acutely Ill Patient: Learning from Serious Incidents. The Fifth Report from the Patient Safety Observatory 2007.
- Cardona-Morrell M, Prgomet M, Lake R, Nicholson M, Harrison R, Long J, et al. Vital signs monitoring and nurse–patient interaction: a qualitative observational study of hospital practice. Int J Nurs Stud 2015;56:9-16.
- Hands C, Reid E, Meredith P, Smith GB, Prytherch DR, Schmidt PE, et al. Patterns in the recording of vital signs and early warning scores: compliance with a clinical escalation protocol. BMJ Qual Saf 2013;22:719-26.
- National Patient Safety Agency . Recognising and Responding Appropriately to Early Signs of Deterioration in Hospitalised Patients 2007. www.patientsafetyoxford.org/wp-content/uploads/2018/03/NPSA-DeteriorPatients.pdf (accessed 15 October 2021).
- National Confidential Enquiry into Perioperative Deaths . The NCEPOD Classification of Intervention 2004.
- Ball JE, Murrells T, Rafferty AM, Morrow E, Griffiths P. ‘Care left undone’ during nursing shifts: associations with workload and perceived quality of care. BMJ Qual Saf 2014;23:116-25.
- Silber JH, Williams SV, Krakauer H, Schwartz JS. Hospital and patient characteristics associated with death after surgery. A study of adverse occurrence and failure to rescue. Med Care 1992;30:615-29.
- Aiken LH, Sloane DM, Bruyneel L, Heede K, Griffiths P, Busse R, et al. Nurse staffing and education and hospital mortality in nine European countries: a retrospective observational study. Lancet 2014;383:1824-30.
- Cardona-Morrell M, Prgomet M, Turner RM, Nicholson M, Hillman K. Effectiveness of continuous or intermittent vital signs monitoring in preventing adverse events on general wards: a systematic review and meta-analysis. Int J Clin Pract 2016;70:806-24.
- Hodgson LE, Dimitrov BD, Congleton J, Venn R, Forni LG, Roderick PJ. A validation of the National Early Warning Score to predict outcome in patients with COPD exacerbation. Thorax 2016;72:23-30.
- Royal College of Physicians . National Early Warning Score (NEWS) 2: Standardising the Assessment of Acute-Illness Severity in the NHS 2017;17.
- Francis R. Report of the Mid Staffordshire NHS Foundation Trust Public Inquiry: Executive Summary 2013;3. www.gov.uk/government/publications/report-of-the-mid-staffordshire-nhs-foundation-trust-public-inquiry (accessed 15 October 2021).
- Silber JH, Romano PS, Rosen AK, Wang Y, Even-Shoshan O, Volpp KG. Failure-to-rescue: comparing definitions to measure quality of care. Med Care 2007;45:918-25. https://pubmed.ncbi.nlm.nih.gov/17890988/ (accessed 15 October 2021).
- Griffiths P, Jones S, Bottle A. Is ‘failure to rescue’ derived from administrative data in England a nurse sensitive patient safety indicator for surgical care? Observational study. Int J Nurs Stud. 2013;50:292-300. https://pubmed.ncbi.nlm.nih.gov/23195407/ (accessed 15 October 2021).
- Herasevich V, Pieper MS, Pulido J, Gajic O. Enrollment into a time sensitive clinical study in the critical care setting: results from computerized septic shock sniffer implementation. J Am Med Inform Assoc 2011;18:639-44.
- Henry KE, Hager DN, Pronovost PJ, Saria S. A targeted real-time early warning score (TREWScore) for septic shock. Sci Transl Med 2015;7.
- Churpek MM, Yuen TC, Winslow C, Robicsek AA, Meltzer DO, Gibbons RD, et al. Multicenter development and validation of a risk stratification tool for ward patients. Am J Respir Crit Care Med. 2014;190:649-55.
- Yoder JC, Yuen TC, Churpek MM, Arora VM, Edelson DP. A prospective study of nighttime vital sign monitoring frequency and risk of clinical deterioration. JAMA Intern Med 2013;173.
- Griffiths P, Recio-Saucedo A, Schmidt P, Smith G. Vital signs monitoring in hospitals at night. Nurs Times 2015;111:16-7.
- Griffiths P, Recio-Saucedo A, Dall’ora C, Briggs J, Maruotti A, Meredith P, et al. Missed Care Study Group . The association between nurse staffing and omissions in nursing care: a systematic review. J Adv Nurs 2018;74:1474-87. www.ncbi.nlm.nih.gov/pubmed/29517813 (accessed 15 October 2021).
- Recio-Saucedo A, Dall’ora C, Maruotti A, Ball J, Briggs J, Meredith P, et al. What impact does nursing care left undone have on patient outcomes? Review of the literature. J Clin Nurs 2018;27:2248-59.
- Barker HR, Griffiths P, Mesa-Eguiagaray I, Pickering R, Gould L, Bridges J. Quantity and quality of interaction between staff and older patients in UK hospital wards: a descriptive study. Int J Nurs Stud 2016;62:100-7.
- Wong D, Bonnici T, Knight J, Gerry S, Turton J, Watkinson P. A ward-based time study of paper and electronic documentation for recording vital sign observations. J Am Med Inform Assoc 2017;24:717-21.
- The Royal College of Surgeons of England, Department of Health . The Higher Risk General Surgical Patient: Towards Improved Care for a Forgotten Group 2011. www.rcseng.ac.uk/library-and-publications/rcs-publications/docs/the-higher-risk-general-surgical-patient/ (accessed 15 October 2021).
- McGaughey J, Blackwood B, Halloran P, Trinder TJ, Porter S. Realistic Evaluation of Early Warning Systems and the Acute Life-threatening Events – Recognition and Treatment Training course for early recognition and management of deteriorating ward-based patients: research protocol. J Adv Nurs 2010;66:923-32.
- Alam N, Vegting IL, Houben E, van Berkel B, Vaughan L, Kramer MHH, et al. Exploring the performance of the National Early Warning Score (NEWS) in a European emergency department. Resuscitation 2015;90:111-5.
- Sbiti-Rohr D, Kutz A, Christ-Crain M, Thomann R, Zimmerli W, Hoess C, et al. ProHOSP Study Group . The National Early Warning Score (NEWS) for outcome prediction in emergency department patients with community-acquired pneumonia: results from a 6-year prospective cohort study. BMJ Open 2016;6.
- Abbott TEF, Vaid N, Ip D, Cron N, Wells M, Torrance HDT, et al. A single-centre observational cohort study of admission National Early Warning Score (NEWS). Resuscitation 2015;92:89-93.
- Smith GB. In-hospital cardiac arrest: is it time for an in-hospital ‘chain of prevention’?. Resuscitation 2010;81:1209-11.
- Freedman NS, Kotzer N, Schwab RJ. Patient perception of sleep quality and etiology of sleep disruption in the intensive care unit. Am J Respir Crit Care Med 1999;159:1155-62.
- Figueroa-Ramos MI, Arroyo-Novoa CM, Lee KA, Padilla G, Puntillo KA. Sleep and delirium in ICU patients: a review of mechanisms and manifestations. Intensive Care Med 2009;35:781-95.
- Watkinson PJ, Barber VS, Price JD, Hann A, Tarassenko L, Young JD. A randomised controlled trial of the effect of continuous electronic physiological monitoring on the adverse event rate in high risk medical and surgical patients. Anaesthesia 2006;61:1031-9.
- Loon K, Zaane B, Bosch EJ, Kalkman CJ, Peelen LM. Non-invasive continuous respiratory monitoring on general hospital wards: a systematic review. PLOS ONE 2015;10.
- Kroll RR, Boyd JG, Maslove DM. Accuracy of a wrist-worn wearable device for monitoring heart rates in hospital inpatients: a prospective observational study. J Med Internet Res 2016;18.
- Jeffs E, Vollam S, Young JD, Horsington L, Lynch B, Watkinson PJ. Wearable monitors for patients following discharge from an intensive care unit: practical lessons learnt from an observational study. J Adv Nurs 2016;72:1851-62.
- Downey C, Randell R, Brown J, Jayne DG. Continuous versus intermittent vital signs monitoring using a wearable, wireless patch in patients admitted to surgical wards: pilot cluster randomized controlled trial. J Med Internet Res 2018;20. www.jmir.org/2018/12/e10802 (accessed 15 October 2021).
- Reed MJ, McGrath M, Black PL, Lewis S, McCann C, Whiting S, et al. Detection of physiological deterioration by the SNAP40 wearable device compared to standard monitoring devices in the emergency department: the SNAP40-ED study. Diagn Progn Res 2018;2. https://pubmed.ncbi.nlm.nih.gov/31093566/ (accessed 12 October 2021).
- Grant MJ, Booth A. A typology of reviews: an analysis of 14 review types and associated methodologies. Health Info Libr J 2009;26:91-108.
- Peters M, Godfrey C, McInerney P, Soares C, Khalil H, Parker D. The Joanna Briggs Institute Reviewers’ Manual 2015: Methodology for JBI Scoping Reviews. Adelaide, South Australia: The Joanna Briggs Institute; 2015.
- Levac D, Colquhoun H, O’Brien KK. Scoping studies: advancing the methodology. Implement Sci 2010;5:1-9.
- Munn Z, Peters MDJ, Stern C, Tufanaru C, McArthur A, Aromataris E. Systematic review or scoping review? Guidance for authors when choosing between a systematic or scoping review approach. BMC Med Res Methodol 2018;18:1-7.
- Arksey H, O’Malley L. Scoping studies: towards a methodological framework. Int J Soc Res Methodol 2005;8:19-32.
- Petersen JA. Early warning score challenges and opportunities in the care of deteriorating patients. Dan Med J 2018;65.
- Petersen JA, Antonsen K, Rasmussen LS. Frequency of early warning score assessment and clinical deterioration in hospitalized patients: a randomized trial. Resuscitation 2016;101:91-6.
- Zografakis-Sfakianakis M, De Bree E, Linardakis M, Messaritaki A, Askitopoulou H, Papaioannou A, et al. The value of the modified early warning score for unplanned intensive care unit admissions of patients treated in hospital general wards. Int J Nurs Pract 2018;24:e12632-8.
- Freathy S, Smith GB, Schoonhoven L, Westwood G. The response to patient deterioration in the UK National Health Service – a survey of acute hospital policies. Resuscitation 2019;139:152-8.
- Zeitz K, McCutcheon H. Observations and vital signs: ritual or vital for the monitoring of postoperative patients?. Appl Nurs Res 2006;19:204-11. www.ncbi.nlm.nih.gov/pubmed/17098158 (accessed 15 October 2021).
- Elvekjaer M, Aasvang EK, Olsen RM, Sørensen HBD, Porsbjerg CM, Jensen JU, et al. WARD-Project Group . Physiological abnormalities in patients admitted with acute exacerbation of COPD: an observational study with continuous monitoring. J Clin Monit Comput 2019;34:1051-60.
- Chen L, Olufunmilayo O, Clermont G, Hravnak M, Pinsky MR, Dubrawski AW. Dynamic and personalized risk forecast in step-down units: implications for monitoring paradigms. Ann Am Thorac Soc 2017;14:384-91.
- Weller RS, Foard KL, Harwood TN. Evaluation of a wireless, portable, wearable multi-parameter vital signs monitor in hospitalized neurological and neurosurgical patients. J Clin Monit Comput 2018;32:945-51.
- Duus CL, Aasvang EK, Olsen RM, Sørensen HBD, Jørgensen LN, Achiam MP, et al. Continuous vital sign monitoring after major abdominal surgery-quantification of micro events. Acta Anaesthesiol Scand 2018;62:1200-8.
- Brown H, Terrence J, Vasquez P, Bates DW, Zimlichman E. Continuous monitoring in an inpatient medical-surgical unit: a controlled clinical trial. Am J Med 2014;127:226-32.
- Ludikhuize J, Borgert M, Binnekade J, Subbe C, Dongelmans D, Goossens A. Standardized measurement of the Modified Early Warning Score results in enhanced implementation of a Rapid Response System: a quasi-experimental study. Resuscitation 2014;85:676-82.
- Credland N, Dyson J, Johnson MJ. What are the patterns of compliance with Early Warning Track and Trigger Tools: a narrative review. Appl Nurs Res 2018;44:39-47.
- Kellett J, Sebat F. Make vital signs great again – a call for action. Eur J Intern Med 2017;45:13-9.
- Storm-Versloot MN, Verweij L, Lucas C, Ludikhuize J, Goslings JC, Legemate DA, et al. Clinical relevance of routinely measured vital signs in hospitalized patients: a systematic review. J Nurs Scholarsh 2014;46:39-4.
- Sun L, Joshi M, Khan SN, Ashrafian H, Darzi A. Clinical impact of multi-parameter continuous non-invasive monitoring in hospital wards: a systematic review and meta-analysis. J R Soc Med 2020;113:217-24.
- Redfern OC, Pimentel MAF, Prytherch D, Meredith P, Clifton DA, Tarassenko L, et al. Predicting in-hospital mortality and unanticipated admissions to the intensive care unit using routinely collected blood tests and vital signs: development and validation of a multivariable model. Resuscitation 2018;133:75-81.
- Goldhill DR, McNarry AF, Mandersloot G, McGinley A. A physiologically-based early warning score for ward patients: the association between score and outcome. Anaesthesia 2005;60:547-53.
- Tarassenko L, Clifton DA, Pinsky MR, Hravnak MT, Woods JR, Watkinson PJ. Centile-based early warning scores derived from statistical distributions of vital signs. Resuscitation 2011;82:1013-8.
- Subbe CP, Slater A, Menon D, Gemmell L. Validation of physiological scoring systems in the accident and emergency department. Emerg Med J 2006;23:841-5.
- Prytherch DR, Smith GB, Schmidt PE, Featherstone PI. ViEWS – towards a national early warning score for detecting adult inpatient deterioration. Resuscitation 2010;81:932-7.
- van Galen LS, Dijkstra CC, Ludikhuize J, Kramer MHH, Nanayakkara PWB. A protocolised once a day Modified Early Warning Score (MEWS) measurement is an appropriate screening tool for major adverse events in a general hospital population. PLOS ONE 2016;11.
- Ede J, Jeffs E, Vollam S, Watkinson P. A qualitative exploration of escalation of care in the acute ward setting. Nurs Crit Care 2019;25:171-8.
- Mok W, Wang W, Cooper S, Ang ENK, Liaw SY. Attitudes towards vital signs monitoring in the detection of clinical deterioration: scale development and survey of ward nurses. Int J Qual Health Care 2015;27:207-13. www.ncbi.nlm.nih.gov/pubmed/25888564 (accessed 15 October 2021).
- Petersen JA, Mackel R, Antonsen K, Rasmussen LS. Serious adverse events in a hospital using early warning score – what went wrong?. Resuscitation 2014;85:1699-703.
- Ludikhuize J, Smorenburg SM, de Rooij SE, de Jonge E. Identification of deteriorating patients on general wards; measurement of vital parameters and potential effectiveness of the Modified Early Warning Score. J Crit Care 2012;27:424.e7-13.
- McGregor C, Chohan S, O’Reilly J. Collaborative approach to reducing cardiac arrests in an acute medical unit. BMJ Open Qual 2017;6.
- Taenzer AH, Pyke J, Herrick MD, Dodds TM, McGrath SP. A comparison of oxygen saturation data in inpatients with low oxygen saturation using automated continuous monitoring and intermittent manual data charting. Anesth Analg 2014;118:326-31.
- Areia C, King E, Ede J, Young L, Tarassenko L, Watkinson P, et al. Experiences of current vital signs monitoring practices and views of wearable monitoring: a qualitative study in patients and nurses. J Adv Nurs 2021;78:810-22.
- Elvekjaer M, Carlsson CJ, Rasmussen SM, Porsbjerg CM, Gronbæk KK, Haahr-Raunkjær C, et al. Agreement between wireless and standard measurements of vital signs in acute exacerbation of chronic obstructive pulmonary disease: a clinical validation study. Physiol Meas 2021;42.
- Järvelä K, Takala P, Michard F, Vikatmaa L. Clinical evaluation of a wearable sensor for mobile monitoring of respiratory rate on hospital wards. J Clin Monit Comput 2021;36:81-6.
- Mestrom E, De Bie A, van de Steeg M, Driessen M, Atallah L, Bezemer R, et al. Implementation of an automated early warning scoring system in a surgical ward: practical use and effects on patient outcomes. PLOS ONE 2019;14.
- Tirkkonen J, Ylä-Mattila J, Olkkola KT, Huhtala H, Tenhunen J, Hoppu S. Factors associated with delayed activation of medical emergency team and excess mortality: an Utstein-style analysis. Resuscitation 2013;84:173-8.
- Khanna AK, Ahuja S, Weller R, Harwood TN. Post-Operative Ward Monitoring – Why; What Now? Best Practice & Research Clinical Anaesthesiology. Amsterdam: Elsevier; 2019.
- Blankush JM, Freeman R, McIlvaine J, Tran T, Nassani S, Leitman IM. Implementation of a novel postoperative monitoring system using automated Modified Early Warning Scores (MEWS) incorporating end-tidal capnography. J Clin Monit Comput 2017;31:1081-92.
- Pimentel MAF, Redfern OC, Hatch R, Young JD, Tarassenko L, Watkinson PJ. Trajectories of vital signs in patients with COVID-19. Resuscitation 2020;156:99-106.
- Tóth V, Meytlis M, Barnaby DP, Bock KR, Oppenheim MI, Abed Y, et al. Let Sleeping Patients Lie, avoiding unnecessary overnight vitals monitoring using a clinically based deep-learning model. NPJ Digit Med 2020;3.
- Dall’ora C, Griffiths P, Hope J, Barker H, Smith GB. What is the nursing time and workload involved in taking and recording patients’ vital signs? A systematic review. J Clin Nurs 2020;29:2053-68. https://onlinelibrary.wiley.com/doi/full/10.1111/jocn.15202 (accessed 12 November 2021).
- Brekke IJ, Puntervoll LH, Pedersen PB, Kellett J, Brabrand M. The value of vital sign trends in predicting and monitoring clinical deterioration: a systematic review. PLOS ONE 2019;14. https://doi.org/10.1371/journal.pone.0210875.
- Churpek MM, Adhikari R, Edelson DP. The value of vital sign trends for detecting clinical deterioration on the wards. Resuscitation 2016;102:1-5. www.ncbi.nlm.nih.gov/pubmed/26898412 (accessed 15 October 2021).
- Kause J, Smith G, Prytherch D, Parr M, Flabouris A, Hillman K. Intensive Care Society (UK) . A comparison of antecedents to cardiac arrests, deaths and emergency intensive care admissions in Australia and New Zealand, and the United Kingdom – the ACADEMIA study. Resuscitation 2004;62:275-82. www.ncbi.nlm.nih.gov/pubmed/15325446 (accessed 27 February 2020).
- Hogan H, Healey F, Neale G, Thomson R, Vincent C, Black N. Preventable deaths due to problems in care in English acute hospitals: a retrospective case record review study. BMJ Qual Saf 2012;21:737-45.
- Odell M, Victor C, Oliver D. Nurses’ role in detecting deterioration in ward patients: systematic literature review. J Adv Nurs 2009;65:1992-2006. www.ncbi.nlm.nih.gov/pubmed/20568317 (accessed 15 October 2021).
- Rose L, Clarke SP. Vital signs. Am J Nurs 2010;110. www.ncbi.nlm.nih.gov/pubmed/20520095 (accessed 12 November 2021).
- Odell M. Detection and management of the deteriorating ward patient: an evaluation of nursing practice. J Clin Nurs 2015;24:173-82. www.ncbi.nlm.nih.gov/pubmed/25039312 (accessed 15 October 2021).
- Palese A, Ambrosi E, Prosperi L, Guarnier A, Barelli P, Zambiasi P, et al. Missed nursing care and predicting factors in the Italian medical care setting. Intern Emerg Med 2015;10:693-702. www.ncbi.nlm.nih.gov/pubmed/25840678 (accessed 15 October 2021).
- Schubert M, Ausserhofer D, Desmedt M, Schwendimann R, Lesaffre E, Li B, et al. Levels and correlates of implicit rationing of nursing care in Swiss acute care hospitals – a cross sectional study. Int J Nurs Stud 2013;50:230-9. www.ncbi.nlm.nih.gov/pubmed/23084600 (accessed 15 October 2021).
- Wood C, Chaboyer W, Carr P. How do nurses use early warning scoring systems to detect and act on patient deterioration to ensure patient safety? A scoping review. Int J Nurs Stud 2019;94:166-78. www.ncbi.nlm.nih.gov/pubmed/31002971 (accessed 15 October 2021).
- Zander B, Dobler L, Baumler M, Busse R. [Nursing tasks left undone in German acute care hospitals: results from the international study RN4Cast]. Gesundheitswesen 2014;76:727-34. www.ncbi.nlm.nih.gov/pubmed/24771101 (accessed 15 October 2021).
- Griffiths P, Ball J, Bloor K, Böhning D, Briggs J, Dall’ora C, et al. Nurse staffing levels, missed vital signs and mortality in hospitals: retrospective longitudinal observational study. Health Serv Deliv Res 2018;6. https://doi.org/10.3310/hsdr06380.
- Dall’ora C, Griffiths P, Redfern O, Recio-Saucedo A, Meredith P, Ball J. Missed Care Study Group . Nurses’ 12-hour shifts and missed or delayed vital signs observations on hospital wards: retrospective observational study. BMJ Open 2019;9. www.ncbi.nlm.nih.gov/pubmed/30782743 (accessed 15 October 2021).
- Downey CL, Chapman S, Randell R, Brown JM, Jayne DG. The impact of continuous versus intermittent vital signs monitoring in hospitals: a systematic review and narrative synthesis. Int J Nurs Stud 2018;84:19-27. www.ncbi.nlm.nih.gov/pubmed/29729558 (accessed 15 October 2021).
- Miltner RS, Johnson KD, Deierhoi R. Exploring the frequency of blood pressure documentation in emergency departments. J Nurs Scholarsh 2014;46:98-105. www.ncbi.nlm.nih.gov/pubmed/24355058 (accessed 15 October 2021).
- Munn Z, Moola S, Riitano D, Lisy K. The development of a critical appraisal tool for use in systematic reviews addressing questions of prevalence. Int J Health Policy Manag 2014;3:123-8. www.ncbi.nlm.nih.gov/pubmed/25197676 (accessed 15 October 2021).
- Bellomo R, Ackerman M, Bailey M, Beale R, Clancy G, Danesh V, et al. Vital Signs to Identify, Target, and Assess Level of Care Study (VITAL Care Study) Investigators . A controlled trial of electronic automated advisory vital signs monitoring in general hospital wards. Crit Care Med 2012;40:2349-61. www.ncbi.nlm.nih.gov/pubmed/22809908 (accessed 15 October 2021).
- Fuller T, Fox B, Lake D, Crawford K. Improving real-time vital signs documentation. Nurs Manage 2018;49:28-33. www.ncbi.nlm.nih.gov/pubmed/29287047 (accessed 15 October 2021).
- Ito C, Satoh I, Michiya H, Kitayama Y, Miyazaki K, Ota S, et al. Reducing nurses’. Workload using a computerized nursing support system linked to the hospital information system. Stud Health Technol Inform 1997;46:527-32.
- McGrath SP, Perreard IM, Garland MD, Converse KA, Mackenzie TA. Improving patient safety and clinician workflow in the general care setting with enhanced surveillance monitoring. IEEE J Biomed Health Inform 2019;23:857-66. www.ncbi.nlm.nih.gov/pubmed/29993903 (accessed 15 October 2021).
- Adomat R, Hicks C. Measuring nursing workload in intensive care: an observational study using closed circuit video cameras. J Adv Nurs 2003;42:402-12.
- Clarke MF. Exploration of Nursing Intensity in a Sample of Acute Care Cardiovascular Patients Using the Nursing Interventions Classification (NIC). Iowa: University of Iowa; 2006.
- Hendrich A, Chow MP, Skierczynski BA, Lu Z. A 36-hospital time and motion study: how do medical-surgical nurses spend their time?. Perm J 2008;12:25-34. www.ncbi.nlm.nih.gov/pmc/articles/PMC3037121/pdf/i1552-5775-12-3-25.pdf (accessed 15 October 2021).
- Hoi SY, Ismail N, Ong LC, Kang J. Determining nurse staffing needs: the workload intensity measurement system. J Nurs Manag 2010;18:44-53. www.ncbi.nlm.nih.gov/pubmed/20465728 (accessed 15 October 2021).
- Kimura E, Nakai M, Ishihara K. Evaluation of efficiency improvement in vital documentation using RFID devices. Stud Health Technol Inform 2016;225.
- Wager KA, Schaffner MJ, Foulois B, Kazley AS, Parker C, Walo H. Comparison of the quality and timeliness of vital signs data using three different data-entry devices. Comput Inform Nurs 2010;28:205-12. www.ncbi.nlm.nih.gov/pubmed/20571371 (accessed 15 October 2021).
- Yeung MS, Lapinsky SE, Granton JT, Doran DM, Cafazzo JA. Examining nursing vital signs documentation workflow: barriers and opportunities in general internal medicine units. J Clin Nurs 2012;21:975-82. www.ncbi.nlm.nih.gov/pubmed/22243491 (accessed 15 October 2021).
- Zeitz K. Nursing observations during the first 24 hours after a surgical procedure: what do we do?. J Clin Nurs 2005;14:334-43. www.ncbi.nlm.nih.gov/pubmed/15707444 (accessed 15 October 2021).
- Erb PS, Coble D. Vital signs measured with nursing system. Comput Healthc 1989;10:32-4. www.ncbi.nlm.nih.gov/pubmed/10292004 (accessed 15 October 2021).
- Travers D. Triage: how long does it take? How long should it take?. J Emerg Nurs 1999;25:238-40. http://search.ebscohost.com/login.aspx?direct=true&db=rzh&AN=107198396&site=ehost-live (accessed 15 October 2021).
- Mohammed M, Hayton R, Clements G, Smith G, Prytherch D. Improving accuracy and efficiency of early warning scores in acute care. Br J Nurs 2009;18:18-24. www.ncbi.nlm.nih.gov/pubmed/19127227 (accessed 15 October 2021).
- Prytherch DR, Smith GB, Schmidt P, Featherstone PI, Stewart K, Knight D, et al. Calculating early warning scores – a classroom comparison of pen and paper and hand-held computer methods. Resuscitation 2006;70:173-8.
- Devita MA, Bellomo R, Hillman K, Kellum J, Rotondi A, Teres D, et al. Findings of the first consensus conference on medical emergency teams. Crit Care Med 2006;34:2463-78. www.ncbi.nlm.nih.gov/pubmed/16878033 (accessed 15 October 2021).
- Osborne S, Douglas C, Reid C, Jones L, Gardner G. RBWH Patient Assessment Research Council . The primacy of vital signs – acute care nurses’ and midwives’ use of physical assessment skills: a cross sectional study. Int J Nurs Stud 2015;52:951-62. www.ncbi.nlm.nih.gov/pubmed/25704371 (accessed 15 October 2021).
- Smith DJ, Aitken LM. Use of a single parameter track and trigger chart and the perceived barriers and facilitators to escalation of a deteriorating ward patient: a mixed methods study. J Clin Nurs 2016;25:175-85. www.ncbi.nlm.nih.gov/pubmed/26769205 (accessed 15 October 2021).
- Hope J, Recio-Saucedo A, Fogg C, Griffiths P, Smith GB, Westwood G, et al. A fundamental conflict of care: nurses’ accounts of balancing patients’ sleep with taking vital sign observations at night. J Clin Nurs [Internet] 2018;27:1860-71. www.ncbi.nlm.nih.gov/pubmed/29266489 (accessed 15 October 2021).
- Dall’ora C, Griffiths P, Hope J, Briggs J, Jeremy J, Gerry S, et al. How long do nursing staff take to measure and record patients’ vital signs observations in hospital? A time-and-motion study. Int J Nurs Stud 2021;118.
- Intensive Care Society . Levels of Adult Critical Care: Consensus Statement 2021. www.ics.ac.uk/Society/Patients_and_Relatives/Levels_of_Care (accessed 15 October 2021).
- Bridges J, Pickering RM, Barker H, Chable R, Fuller A, Gould L, et al. Implementing the Creating Learning Environments for Compassionate Care (CLECC) programme in acute hospital settings: a pilot RCT and feasibility study. Health Serv Deliv Res 2018. https://eprints.soton.ac.uk/416200/ (accessed 15 October 2021).
- Dall’ora C, Hope J, Bridges J, Griffiths P. Development and validation of a methodology to measure the time taken by hospital nurses to make vital signs observations. Nurse Res 2020;28:52-8. https://doi.org/10.7748/nr.2020.e1716.
- R Development Core Team . R: A Language and Environment for Statistical Computing, Version 3.6.3 2020. www.r-project.org/ (accessed 15 October 2021).
- Lofland J, Snow D, Anderson L, Lofland LH. Analyzing Social Settings: A Guide to Qualitative Observation and Analysis. London: Wadsworth; 2006.
- Allen D. Making visible the unseen elements of nursing. Nurs Times 2015;111:17-20. https://europepmc.org/article/med/26689055 (accessed 2 November 2021).
- Wong D, Bonnici T, Knight J, Morgan L, Coombes P, Watkinson P. SEND: a system for electronic notification and documentation of vital sign observations. BMC Med Inform Decis Mak 2015;15.
- System C . System C: E-Observations n.d. www.systemc.com/solutions/epr/e-observations/ (accessed 23 July 2021).
- Oceano . Patient Administration System (PAS). NHS Patient Management Portal n.d. www.servelec.co.uk/product-range/oceano/ (accessed 23 July 2021).
- Watkinson PJ, Pimentel MAF, Clifton DA, Tarassenko L. Manual centile-based early warning scores derived from statistical distributions of observational vital-sign data. Resuscitation 2018;129:55-60.
- NHS Data Model and Dictionary . OPCS Classification of Interventions and Procedures 2021. https://datadictionary.nhs.uk/supporting_information/opcs_classification_of_interventions_and_procedures.html (accessed 23 July 2021).
- Pimentel MA, Redfern OC, Malycha J, Meredith P, Prytherch D, Briggs J, et al. Detecting deteriorating patients in the hospital: development and validation of a novel scoring system. Am J Respir Crit Care Med 2021;204:44-52. www.atsjournals.org (accessed 23 July 2021).
- Austin PC, Harrell FE, van Klaveren D. Graphical calibration curves and the integrated calibration index (ICI) for survival models. Stat Med 2020;39:2714-42.
- Van Calster B, McLernon DJ, van Smeden M, Wynants L, Steyerberg EW, Bossuyt P. Topic Group ‘Evaluating diagnostic tests and prediction models’ of the STRATOS initiative. Calibration: the Achilles heel of predictive analytics. BMC Med 2019;17:1-7.
- RStudio . Shiny n.d. https://shiny.rstudio.com/ (accessed 1 September 2021).
- Jones S, Mullally M, Ingleby S, Buist M, Bailey M, Eddleston JM. Bedside electronic capture of clinical observations and automated clinical alerts to improve compliance with an early warning score protocol. Crit Care Resusc 2011;13:83-8.
- Curtis L, Burns A. Unit Costs of Health and Social Care 2019. Kent: Personal Social Services Research Unit, University of Kent, Canterbury; 2019.
- Marseille E, Larson B, Kazi DS, Kahn JG, Rosen S. Thresholds for the cost-effectiveness of interventions: alternative approaches. Bull World Health Organ 2015;93:118-24.
- National Institute for Health and Care Excellence (NICE) . Changes to NICE Drug Appraisals: What You Need to Know 2017.
- Meacock R, Kristensen SR, Sutton M. The cost-effectiveness of using financial incentives to improve provider quality: a framework and application. Health Econ 2014;23:1-13.
- Claxton K, Martin S, Soares M, Rice N, Spackman E, Hinde S, et al. Methods for the estimation of the National Institute for Health and Care Excellence cost-effectiveness threshold. Health Technol Assess 2015;19:1-503.
- Manaseki-Holland S, Lilford RJ, Te AP, Chen Y-F, Gupta KK, Chilton PJ, et al. Ranking hospitals based on preventable hospital death rates: a systematic review with implications for both direct measurement and indirect measurement through standardized mortality rates. Milbank Q 2019;97:228-84.
- Smith GB, Redfern OC, Pimentel MA, Gerry S, Collins GS, Malycha J, et al. The National Early Warning Score 2 (NEWS2). Clin Med (Lond) 2019;19. https://pubmed.ncbi.nlm.nih.gov/31092526/ (accessed 12 November 2021).
- Downey CL, Croft J, Buckley H, Randell R, Brown JM, Jayne DG. Trial of Remote Continuous versus Intermittent NEWS monitoring after major surgery (TRaCINg): protocol for a feasibility randomised controlled trial. Pilot Feasibility Stud 2018;4.
- National Institute for Health and Care Research (NIHR) . Involve Patients n.d. www.nihr.ac.uk/health-and-care-professionals/engagement-and-participation-in-research/involve-patients.htm (accessed 27 October 2021).
- Locock L, Boaz A. Drawing straight lines along blurred boundaries: qualitative research, patient and public involvement in medical research, co-production and co-design. Evid Policy 2019;15:409-21.
- UK Public Involvement Standards Development Partnership . UK Standards for Public Involvement 2019. www.invo.org.uk/wp-content/uploads/2019/11/UK-standards-for-public-involvement-v6.pdf (accessed 15 October 2021).
- Liew MS, Zhang J, See J, Ong YL. Usability challenges for health and wellness mobile apps: mixed-methods study among mhealth experts and consumers. JMIR Mhealth Uhealth 2019;7. https://mhealth.jmir.org/2019/1/e12160/ (accessed 2 August 2021).
- Hirschtritt ME, Hirschtritt DB. Improving usability of health information technology. JAMA 2019;322:364-5. https://jamanetwork.com/journals/jama/fullarticle/2738538 (accessed 2 August 2021).
- Crocker JC, Boylan AM, Bostock J, Locock L. Is it worth it? Patient and public views on the impact of their involvement in health research and its assessment: a UK-based qualitative interview study. Health Expect 2017;20:519-28.
- Staniszewska S, Adebajo A, Barber R, Beresford P, Brady LM, Brett J, et al. Developing the evidence base of patient and public involvement in health and social care research: the case for measuring impact. Int J Consum Stud 2011;35:628-32. https://onlinelibrary.wiley.com/doi/full/10.1111/j.1470-6431.2011.01020.x (accessed 3 August 2021).
- Boaz A, Biri D, McKevitt C. Rethinking the relationship between science and society: has there been a shift in attitudes to patient and public involvement and public engagement in science in the United Kingdom?. Health Expect 2016;19:592-601. https://onlinelibrary.wiley.com/doi/full/10.1111/hex.12295 (accessed 15 October 2021).
- Boylan AM, Locock L, Thomson R, Staniszewska S. ‘About sixty per cent I want to do it’: health researchers’ attitudes to, and experiences of, patient and public involvement (PPI) – a qualitative interview study. Health Expect 2019;22:721-30. https://onlinelibrary.wiley.com/doi/full/10.1111/hex.12883 (accessed 3 August 2021).
- Holmes L, Cresswell K, Williams S, Parsons S, Keane A, Wilson C, et al. Innovating public engagement and patient involvement through strategic collaboration and practice. Res Involv Engagem 2019;5:1-12. https://researchinvolvement.biomedcentral.com/articles/10.1186/s40900-019-0160-4 (accessed 27 October 2021).
- McCarron TL, Clement F, Rasiah J, Moran C, Moffat K, Gonzalez A, et al. Patients as partners in health research: a scoping review. Health Expect 2021;24:1378-90. https://onlinelibrary.wiley.com/doi/full/10.1111/hex.13272 (accessed 27 October 2021).
- Bate P, Robert G. Experience-based design: from redesigning the system around the patient to co-designing services with the patient. Qual Saf Health Care 2006;15:307-10. https://pubmed.ncbi.nlm.nih.gov/17074863/ (accessed 3 August 2021).
- MacDonald C. Understanding participatory action research: a qualitative research methodology option. Can J Action Res 2012;13:34-50. https://journals.nipissingu.ca/index.php/cjar/article/view/37 (accessed 3 August 2021).
- Baum F, MacDougall C, Smith D. Participatory action research. J Epidemiol Community Health 2006;60:854-7. https://jech.bmj.com/content/60/10/854 (accessed 3 August 2021).
- Green J, Thorogood N. Qualitative Methods for Health Research 2018. https://us.sagepub.com/en-us/nam/qualitative-methods-for-health-research/book254905 (accessed 15 October 2021).
- Locock L, Kirkpatrick S, Brading L, Sturmey G, Cornwell J, Churchill N, et al. Involving service users in the qualitative analysis of patient narratives to support healthcare quality improvement. Res Involv Engagem 2019;5:1-11. https://researchinvolvement.biomedcentral.com/articles/10.1186/s40900-018-0133-z (accessed 15 October 2021).
- Creswell J. A Concise Introduction to Mixed Methods Research. Thousand Oaks, CA: SAGE Publications Ltd; 2014.
- Fitbit Official Site for Activity Trackers and More n.d. www.fitbit.com/ (accessed 2 August 2021).
- Redfern OC, Griffiths P, Maruotti A, Saucedo AR, Smith GB. Missed Care Study Group. The association between nurse staffing levels and the timeliness of vital signs monitoring: a retrospective observational study in the UK. BMJ Open 2019;9. https://bmjopen.bmj.com/content/9/9/e032157 (accessed 15 October 2021).
- Jones NA, Ross H, Lynam T, Perez P, Leitch A. Mental models: an interdisciplinary synthesis of theory and methods. Ecol Soc 2011;16.
- Greenhalgh T, Wherton J, Papoutsi C, Lynch J, Hughes G, Court C, et al. Beyond adoption: a new framework for theorizing and evaluating nonadoption, abandonment, and challenges to the scale-up, spread, and sustainability of health and care technologies. J Med Internet Res 2017;19. https://pubmed.ncbi.nlm.nih.gov/29092808/ (accessed 15 October 2021).
- Rogers EM. Diffusion of Innovations. New York, NY: Free Press; 2003.
- Spångfors M, Molt M, Samuelson K. National Early Warning Score: a survey of registered nurses’ perceptions, experiences and barriers. J Clin Nurs 2020;29:1187-94. https://onlinelibrary.wiley.com/doi/full/10.1111/jocn.15167 (accessed 15 October 2021).
- Petersen JA, Rasmussen LS, Rydahl-Hansen S. Barriers and facilitating factors related to use of early warning score among acute care nurses: a qualitative study. BMC Emerg Med 2017;17:1-9. https://bmcemergmed.biomedcentral.com/articles/10.1186/s12873-017-0147-0 (accessed 15 October 2021).
- Fox A, Elliott N. Early warning scores: a sign of deterioration in patients and systems. Nurs Manag (Harrow) 2015;22:26-31. https://pubmed.ncbi.nlm.nih.gov/25806463/ (accessed 15 October 2021).
- Dwamena F, Rovner M, Gaulden CM, Jorgenson S, Sadigh G, Sikorskii A, et al. Interventions for providers to promote a patient‐centred approach in clinical consultations. Cochrane Database Syst Rev 2012;12. www.cochranelibrary.com/cdsr/doi/10.1002/14651858.CD003267.pub2/full (accessed 15 October 2021).
- ChoosingWisely UK 2017. www.choosingwisely.co.uk/ (accessed 3 August 2021).
- General Medical Council . Decision Making and Consent: Guidance on Professional Standards and Ethics for Doctors 2020. www.gmc-uk.org/guidance (accessed 3 August 2021).
- Kostakis I, Smith GB, Prytherch D, Meredith P, Price C, Chauhan A. Portsmouth Academic ConsortIum For Investigating COVID-19 (PACIFIC-19) . Impact of the coronavirus pandemic on the patterns of vital signs recording and staff compliance with expected monitoring schedules on general wards. Resuscitation 2021;158:30-8.
- Klepstad PK, Nordseth T, Sikora N, Klepstad P. Use of National Early Warning Score for observation for increased risk for clinical deterioration during post-ICU care at a surgical ward. Ther Clin Risk Manag 2019;15:315-22. https://dx.doi.org/10.2147/TCRM.S192630.
Appendix 1 Scoping review database search strategy
MEDLINE search
Search terms | Results (n) | |
---|---|---|
1 | *HOSPITAL RAPID RESPONSE TEAM | 604 |
2 | “rapid response team* “.ti. | 343 |
3 | “medical emergency team* “.ti. | 267 |
4 | “MET call* “.ti. | 3 |
5 | ‘MET criteria’.ti. 4 | 4 |
6 | 1 or 2 or 3 or 4 or 5 | 964 |
7 | (trigger* or activat* or speed or delay* or ‘call* criteri*’).ab,ti. | 2,300,624 |
8 | 6 and 7 | 347 |
9 | (pediatric* or paediatric* or PICU or child* or infant* or newborn* or neonat*).ti. | 1,205,806 |
10 | 8 and 9 | 35 |
11 | limit 8 to (‘all infant (birth to 23 months)’ or ‘all child (0 to 18 years)’) | 49 |
12 | 10 or 11 | 57 |
13 | 8 not 12 | 290 |
EMBASE search
Search terms | Results (n) | |
---|---|---|
1 | Warning scor* “.af. | 1721 |
2 | ‘modified early warning’.af. | 501 |
3 | ‘national early warning’.af. | 475 |
4 | 1 or 2 or 3 | 1821 |
5 | TIME FACTORS/ | 18,238 |
6 | “time factor* “.af. | 34,692 |
7 | (timing or timely or timeliness or timeline*).af. | 269,683 |
8 | (hour* or minute* or hr or hrs).af. | 1,668,671 |
9 | (day or days or daily or night or nights or nightly or week*).af. | 4,487,754 |
10 | (continuous* or intermittent* or ongoing).af. | 1,009,333 |
11 | (frequent* or infrequent* or frequency).af. | 2,258,574 |
12 | ‘time of day’.af. | 12,887 |
13 | “time interval* “.af. | 60,617 |
14 | “time span* “.af. | 6200 |
15 | “time period* “.af. | 84,299 |
16 | ‘how often’.af. | 9166 |
17 | ‘how many times’.af. | 580 |
18 | 5 or 6 or 7 or 8 or 9 or 10 or 11 or 12 or 13 or 14 or 15 or 16 or 17 | 8,062,165 |
19 | 4 and 18 | 1095 |
20 | (pediatric* or paediatric* or PICU or child* or infant* or newborn* or neonat*).ti. | 1,374,844 |
21 | 19 and 20 | 139 |
22 | limit 19 to (infant < to one year > or child < unspecified age > or preschool child < 1 to 6 years > or school child < 7 to 12 years > or adolescent < 13 to 17 years>) | 165 |
23 | 21 or 22 | 200 |
24 | 19 not 23 | 895 |
25 | limit 25 to conference abstracts | 430 |
26 | 25 not 26 | 446 |
Appendix 2 Summary of identified primary research studies
First author | Year | Country | Type of study | Population | Monitoring | Outcome | Key findings | Recommendations |
---|---|---|---|---|---|---|---|---|
Brown61 | 2014 | USA | Observational | General medical, trauma and surgical patients (n = 7643) |
Continuous and intermittent: HR RR |
Primary: unanticipated ICU admission and LOS ICU Secondary: APACHE II score, number cardiac arrest and unexpected death |
– Intervention showed decreased ICU LOS, reduced CA rate – Faster transfer to ICU not significant |
Further research is needed: confirm findings, assess effect on mortality, and provide a better understanding regarding mechanisms for effect |
Chen58 | 2017 | USA | Observational, proof of concept | Surgical trauma step-down patients (n = 1971 adm) |
Continuous: HR, RR, SpO2 Intermittent: systolic blood pressure, diastolic blood pressure |
Risk of cardiorespiratory insufficiency (CRI) | – Analysis technique can identify which patients likely to become unstable and when – Potential to identify those most likely to deteriorate quickly – Risk escalation patterns, risk trends and initial stable period can help manage allocation of monitoring resources |
Continuous monitoring for high granularity required to study trends in vital sign data |
Downey45 | 2018 | UK | Cluster RCT | Elective surgical patients (n = 226) | Continuous: HR, RR, and Temp Intermittent: BP, PR, Temp, RR, SpO2 (per NEWS) |
Primary: time to antibiotics post first evidence of sepsis Secondary: in-hospital mortality, LOS, ICU adm, hospital readm within 30 days |
Continuous monitoring resulted in: – Shorter LOS – Reduced risk of readmission – Reduced event rates: sepsis, mortality or ICU adm (potentially due to high proportion of low-risk patients) – Nearly 24% continuous monitoring patients did not wear patch for entire adm – Initial high alert problems solved by range adjustment |
Further research required: demonstrate clinical benefit of continuous monitoring, including high-risk patients, individually randomised and stratified Outcomes relevant to all patients such as LOS, better than rarer outcomes such as mortality |
Duus60 | 2018 | DK | Observational | Elderly patients (≥ 65) with elective major abdominal cancer surgery (n = 50) |
Continuous: SpO2, HR, RR Intermittent: EWS |
Detection of abnormal vital signs (micro-events) | – Continuous monitoring identified more micro-events in all parameters than EWS | Future research required: evaluate clinical effect of continuous vs. intermittent monitoring Contact between nurse and patient important and should not be eliminated |
Elvekjaer57 | 2019 | DK | Observational pilot | Acute exacerbations of chronic obstructive pulmonary disease (AECOPD) patients (n = 50) |
Continuous: SpO2, HR, RR, BP Intermittent: EWS |
Micro-events: vital signs or EWS deranged for ≥ 60 s | – Continuous monitoring detects more events than EWS for all vital signs – Some desaturation events captured by EWS and not continuous monitoring due to missing data – Issues with data completeness due to technical problems and patient non-compliance |
Need for prospective trials into effectiveness of EWS and sophisticated algorithms for vital sign trend analysis |
Ludikhuize62 | 2014 | NL | Quasi-experimental observational | Medical and surgical patients (n = 804) | Intermittent MEWS: 3× daily (protocolised) vs. when clinically indicated (control) |
Implementation and compliance with set monitoring standards Outcomes: delayed doctor notification and RRT activation for MEWS ≥ 3 |
– Better compliance with protocol MEWS 3× daily and RRT activation more reliable, although both regimes increased compliance with MEWS and RRT activation (potential Hawthorne effect) – Observation: decrease in adverse events (study not designed to analyse clinical outcome) |
Electronic record-keeping, partial automation of response and standard operating procedures to reduce unnoticed deteriorating patient rate |
Petersen53 | 2016 | DK | Ward-level randomised, non-blinded, controlled trial | Low-risk adult medical and surgical patients (adm EWS 0 or 1) (n = 1346) |
Intermittent NEWS-based (12 vs. 8 hourly) | Primary: EWS ≥ 2 24 hours post adm Secondary: EWS ≥ 5 or CA, ICU adm, MET review, LOS, mortality (72 hours, 30 days) |
– No significant difference in clinical deteriorating between 3× vs. 2× daily monitoring in low-risk patients (EWS ≤ 2) within 24 hours post adm | No support for increased intermittent monitoring more than twice daily for patients with low EWS (NEWS < 2) |
Watkinson41 | 2006 | UK | Non-blinded RCT | High-risk adult medical and surgical patients (n = 402) |
Continuous vs. intermittent: HR, RR, BP, SpO2, PR, Temp |
Primary: major adverse rate: CA, higher care level, death, in 96 hours of randomisation Secondary: proportion of patients requiring treatment changes, 30-day and hospital LOS |
– Mandated electronic vital signs monitoring of high-risk patients had no effect on outcomes including intervention and LOS – Only 16% monitored for full 72-hour period |
More research required: combination of physiological monitoring, smart alarms and mandated responses may have clinical benefit |
Weller59 | 2018 | USA | Prospective, observational pilot | Adult neurological/neurosurgical patients (n = 736) |
Continuous vs. intermittent (4 hourly): BP, HR, RR, SpO2 |
Alarm rates, RRT calls, ICU adms, unexpected death | – RRT call rate significantly reduced – No significant evidence for reducing ICU transfers and mortality – Continuous monitoring can only be of benefit if no increase in work |
Need to minimise false alarms to avoid desensitisation Further research required: effect of continuous monitoring on LOS and outcomes |
Zeitz56 | 2006 | AUS | Observational | Postoperative surgical patients (n = 144) | Intermittent: HR, RR, BP, temp traditional vs. clinical judgement model |
Detection of post-op complications in 24-hour period after return to ward | – Routine vital sign collection tradition-based – Lack of evidence to support recommendations improving outcomes – Incomplete compliance with either monitoring regime |
Future research required: effect of different monitoring approaches on patient outcomes, should include the relationship between staffing levels and skills mix |
Zografakis- Sfakianakis54 |
2018 | GR | Observational, retrospective/prospective | Patients admitted to ICU from general wards > 24 hours post hospital adm (n = 153) |
Intermittent MEWS: RR, temp, HR, BP, cons level, urine output (five observations in 20-hour period up to outcome) |
Risk category prior to event, ICU LOS, ICU mortality (in-hospital, 90 day) | – Surgical patients: longer LOS prior to uICU – Higher MEWS correlated with increased ICU LOS – MEWS value immediately prior to ICU adm with ICU mortality – Trends have prognostic value for LOS and mortality – Deterioration mostly seen in 8 hours pre uICU |
Deterioration of patients in general wards is a progressive process 4-hourly monitoring for trajectory pre ICU adm indicative of ICU LOS and mortality |
Appendix 3 Summary of identified review articles
First authors | Year | Review type | No. of studies included | Publication years of included studies (of lit search) |
Review question | Monitoring regime | Key findings | Recommendations | Source |
---|---|---|---|---|---|---|---|---|---|
Cardona-Morrell18 | 2016 | Systematic | 22 (9 continuous, 13 intermittent) |
2003–14 [Jan 1980 – Jun 2014] | What strategies, intermittent or continuous, are implemented to improve vital signs monitoring in general wards? | Continuous and intermittent | – Interventions improved monitoring adherence and recording – Continuous interventions: no significant reduction in mortality – Intermittent interventions: sign reduction in mortality |
More research and evidence of the effectiveness of continuous monitoring on general wards and the impact patient outcomes required | Citation (Downey et al. 2018) |
Credland63 | 2018 | Narrative | 7 | 2001–15 [Jan 2000 – Jul 2018] | What are the patterns of compliance with Early Warning Track and Trigger systems? | Intermittent: EWS | Poor compliance with EWS calculation accuracy, monitoring frequency and clinical response | Need for more evidence on social, cultural and inter-professional factors limiting compliance – to improve compliance | Lit search |
DeVita3 | 2010 | Narrative/consensus conference | 65 | 1987–2009 | What is the consensus on best ways to detect patient deterioration and patient monitoring? | Continuous and intermittent | – Patient should have individual monitoring plans with variables to be measured, frequency of assessment, and values that require action. – A minimum standard for vital sign assessment is every 12 hours |
Research is required to establish the optimum frequency of monitoring and to demonstrate the presumed promise of continuous monitoring if practical and affordable, all patients should be monitored continuously | Citation (Kellett and Sebat 2017) |
Kellett64 | 2017 | Narrative/opinion piece | 149 | 1846–2017 | What is the value of routine monitoring of patient vital signs? | Continuous and intermittent | – Current monitoring protocols based on clinical expertise rather than evidence and might increase workload unnecessarily – Monitoring frequency should be determined based on a patient-centred approach to care – Optimal monitoring frequency for low-risk patients unknown |
Need for research into how to respond to routine monitoring and develop rational management protocols (incl. for continuous monitoring) Calls for professional societies and regulatory bodies to address this problem |
Lit search |
Smith4 | 2017 | Narrative/editorial | 2003–17 | Is current monitoring practice evidence based? | Continuous and intermittent | Existing evidence supports impact on clinical outcomes but not monitoring frequency and compliance | Research into best vital sign monitoring practice needed | Citation (Freathy et al. 2019) |
|
Storm-Versloot65 | 2014 | Systematic | 15 | 1986–2012 [< Jan 2013] |
Assess effectiveness of routinely measured vital signs on the early detection of increased probability of adverse events | Routine monitoring of individual vital signs | Lack of well-designed diagnostic and large observational studies specifically intended to investigate the clinical relevance of routine vital sign monitoring, incl. trigger thresholds and generalisability | More evidence needed for effectiveness of routine daily vital sign measurements and contribution of individual vital signs to prediction of adverse events | Citation (Kellett and Sebat 2017) |
Sun66 | 2020 | Systematic | 14 (5 association with clinical parameters, 9 impact on clinical outcomes) |
2003–18 [< Jun 2019] |
What is the clinical impact of multiparameter continuous non-invasive monitoring? | Continuous vs. intermittent | Evidence of clinical benefit of continuous monitoring in general adult patients supporting use of multiparameter monitoring outside of ICU: reduced mortality, LOS, ICU transfers and RRT activations | Further research required: larger-scale RCTs | Other |
Appendix 4 Systematic review search strategy
Search terms | Results (n) | ||
---|---|---|---|
CINAHL with full text via EBSCO | 1 | (MH “Vital Signs+”) | 16,161 |
2 | vital N5 sign* | 8034 | |
3 | S1 OR S2 | 21,597 | |
4 | monitor* | 159,043 | |
5 | Surveill* | 56,790 | |
6 | observ* | 384,258 | |
7 | record* | 283,819 | |
8 | (MH “Monitoring, Physiologic+”) | 92,650 | |
9 | early warning s* | 2348 | |
10 | EWS | 232 | |
11 | S4 OR S5 OR S6 OR S7 OR S8 OR S9 OR S10 | 826,739 | |
12 | workload | 21,779 | |
13 | time | 674,090 | |
14 | length | 104,896 | |
15 | S12 OR S13 OR S14 | 760,537 | |
16 | nurs* | 841,214 | |
17 | S3 AND S11 AND S15 AND S16 | 518 | |
MEDLINE via Ovid | 1 | (vital adj2 sign*).mp. | 12,330 |
2 | Exp Vital Signs/ | 403,473 | |
3 | 1 or 2 | 413,180 | |
4 | monitor*.mp. | 817,977 | |
5 | surveillance.mp. | 193,784 | |
6 | observ*.mp. | 2,965,582 | |
7 | record*.mp. | 985,514 | |
8 | early warning scor*.mp. | 617 | |
9 | EWS.mp. | 1992 | |
10 | 4 or 5 or 6 or 7 or 8 or 9 | 4,507,596 | |
11 | workload.mp. or exp Workload/ | 35,442 | |
12 | time.mp. or “Time and Motion Studies”/ | 3,475,006 | |
13 | length.mp. | 564,952 | |
14 | 11 or 12 or 13 | 3,919,463 | |
15 | nurs*.mp. | 67,451 | |
16 | 3 and 10 and 14 and 15 | 672 | |
EMBASE | 1 | (vital adj2 sign*).mp. | 31,425 |
2 | Exp Vital Signs/ | 21,792 | |
3 | 1 or 2 | 31,425 | |
4 | monitor*.mp. | 1,120,388 | |
5 | surveillance.mp. | 257,516 | |
6 | observ*.mp. | 3,553,351 | |
7 | record*.mp. | 1,338,773 | |
8 | early warning scor*.mp. | 1656 | |
9 | EWS.mp. | 3352 | |
10 | 4 or 5 or 6 or 7 or 8 or 9 | 5,598,085 | |
11 | workload.mp. or exp Workload/ | 47,161 | |
12 | time.mp. or “Time and Motion Studies”/ | 4,087,053 | |
13 | length.mp. | 743,879 | |
14 | 11 or 12 or 13 | 4,663,345 | |
15 | nurs*.mp. | 531,189 | |
16 | 3 and 10 and 14 and 15 | 709 | |
Cochrane Library | 1 | Vital signs | 21 |
2 | Early Warning Score | 1 | |
3 | 1 OR 2 | 21 | |
4 | Time | 3395 | |
5 | Nursing | 562 | |
6 | 3 AND 4 AND 5 | 21 |
Appendix 5 Summary of selected studies
Sixteen papers were selected
Author(s), year and country | Study design | Sample size and setting | Vital signs measuring and recording | Data collection | Results | Vital signs definition |
---|---|---|---|---|---|---|
Studies reporting mean times of vital signs measurements and/or recording | ||||||
Bellomo et al., 2012 USA, Sweden, UK, The Netherlands and Australia |
Before-and-after controlled trial | 10 hospitals in 5 countries, 74 nurses. The number of observation hours is unspecified | Measurement: Enhanced surveillance system: manual Mode of recording and documentation timing: Enhanced surveillance system: nurse obtains oxygen saturations, HR, BP, temperature and these are automatically transferred and displayed by direct physical link with the monitoring devices. RR and conscious state are input by the nurse. Calculation of EWS value: The electronic automated advisory vital signs system automatically calculates EWS. When EWS is calculated, it displays a colour-coded message to the nurse (red range prompted the need for increased frequency of monitoring or escalation; safe range in white; observe range in yellow; warning range in orange) |
Information on how vital signs data were collected before the introduction of the automated advisory vital signs monitors was not reported | After the introduction of continuous monitoring, time required to complete and record a set of vital signs decreased from on average 4.1 minutes (SD 1.3) to 2.5 minutes (SD 0.5) (difference 1.6; 95% CI 1.4 to 1.8; p < 0.0001) | Temperature, HR, RR, BP, oxygen saturations, consciousness, urine output |
Clarke, 2006 USA |
Descriptive observational study | 200 patients, 1 community hospital, 1 cardiovascular unit. A total of 10,645 nursing interventions [classified with Nursing Intervention Classifications (NIC)] were reported during a 1-month data collection period |
Measurement: Not specified Mode of recording and documentation timing: Not specified Calculation of EWS value: Not specified |
Nurses were asked to self-report the number of times the NIC intervention was used during the shift, estimate the average time each intervention took to complete, and identify the education level that was required to accomplish each intervention | Vital signs monitoring occurred on 814 occasions during the 1-month data-collection period. Mean time for vital signs monitoring: 5.8 minutes (SD: 3.72; 95% CI 5.54 to 6.06) | According to NIC definition: ‘Collection and analysis of cardiovascular, respiratory, and body temperature data to determine and prevent complications’ |
Ito et al., 1997 Japan |
Before-and-after study with time-motion methodology | 1 hospital, 1 radiology ward, 23 nurses working day shifts | Measurement: Mixture of automatic and manual Mode of recording and documentation timing: Nurses enter vital signs into the hand-held computer at the bedside. Vital signs data are then transferred from the hand-held computers to the desktop computers with a cable. The information is then sent automatically to the data server Calculation of EWS value: Not specified |
Nurses had to note the time taken to complete vital signs with each patient and calculate total time spent on each task. This was done before and after introduction of hand-held computers for use by bedside | Mean time required to measure vital signs and fill in vital signs documentation was reduced from 2.02 to 0.90 minutes (p < 0.01), because information transfer directly from hand-held computers to the desktop computer eliminated the need to duplicate data entry | Not specified |
Kimura et al., 2016 Japan (abstract) |
Descriptive observational study | 1 hospital | Measurement: Automatic: the radio-frequency identification (RFID) reader on the patient automatically transfers data to the electronic medical records. Mode of recording and documentation timing: Automatic: the RFID reader on the patient automatically transfers data to the electronic medical records. Calculation of EWS value: Not specified |
There were no details on how the time required to obtain a set of vital signs was captured | Time for information to be transferred from the patient tags to the device: Cart: 1.47 minutes (SD: 0.55) per person Bed: 1.27 minutes (SD: 0.62) per person |
Body temperature, oxygen saturations, HR, BP |
McGrath et al., 2019 USA |
Before-and-after study | 1 hospital. Enhanced surveillance system trialled in 2 surgical units, 71 beds. Control were 3 medical-surgical units, 61 beds | Measurement: Enhanced surveillance system: continuous monitoring for oxygen saturations, HR, BP, temperature. RR is calculated by observation Control: a mixture of automatic and manual instruments. Mode of recording and documentation timing: Enhanced surveillance system: all vital signs automatically transferred to electronic medical records by pressing a button on patient’s monitor Control: each vital sign entered manually into medical record after collection Calculation of EWS value: Not specified |
One hundred samples of vital signs assessment were collected in the before and after periods of the study units by direct observation of licensed nurse assistants over 12 months. Time to collect vital signs and enter data into the medical record manually or electronically was measured using an electronic stopwatch. Observations were conducted at various times on multiple days |
Mean vital signs assessment times were 2.98 minutes before implementation and 2.15 minutes after implementation | Temperature, HR, RR, BP, oxygen saturations |
Travers, 1999 USA |
Observational time-and-motion study | 16 nurses, 1 emergency department | Measurement: Not specified Mode of recording and documentation timing: Not specified Calculation of EWS value: Not specified |
Nurses observed over 10 days. Research assistants performed prospective, direct observations of triage, using stopwatches to measure triage start and stop times | Mean time of vital signs taken at triage: 4 minutes (range 1.9–11.1) | BP, pulse, respiratory rate, and tympanic temperature |
Wager et al., 2010 USA |
Observational descriptive | 1 hospital, 4 inpatient medical/surgical units, 270 vital signs sets | Measurement: Not specified Mode of recording and documentation timing: (1) a paper medical record system where vital signs were handwritten on a piece of paper and then transcribed to the patient’s record (paper to paper) (2) a clinical documentation system with a ‘computer on wheels’ workstation outside the patient’s room where vital signs were handwritten on a piece of paper and then transcribed into a computer on wheels (paper to computer) (3) a clinical documentation system with a tablet PC affixed to the vital-sign monitor, a machine where vital signs were immediately transcribed from the vital signs monitor to the tablet PC (machine to computer) Calculation of EWS value: Not specified |
Observers record the date and time the vital signs were taken and the time the vital signs were entered into the patient’s record | Mean time difference between the time vital signs were taken and when the data were recorded in the patient’s record: (1) with paper records: mean time: 1.24 minutes (SD: 2.17 minutes) (2) computer on wheels: 9.15 minutes (SD 7:25 minutes) (3) tablet PC: mean time: 35 seconds (SD 1:42 minutes) |
BP, temperature, HR, SpO2, and RR |
Wong et al., 2017 UK |
Before-and-after study | 606 sets of vital signs observed. Two university teaching hospitals, three medical wards | Measurement: Mixture of manual and automatic Mode of recording and documentation timing: Before e-Obs (i.e. a system that allows vital signs to be recorded on a hand-held device) system was introduced: notes After e-Obs system was introduced: vital signs are manually entered using the tablet Calculation of EWS value: Before e-Obs: not specified After e-Obs: Automatic on electronic chart |
Nursing staff were observed. Observers recorded start and end times of: (1) view chart (locating and opening chart) (2) take vital signs (measuring and documenting vital signs). Interruptions were timed and subtracted from the measured process duration |
Mean time to view chart: 0.3 minutes on paper: 0.21 minutes on e-Obs Mean time to take a complete set of vital signs: 3.58 minutes (SD 8.9) on paper; 2.50 minutes (SD: 0.74) on e-Obs |
Temperature, HR, RR, BP, oxygen saturations, oxygen therapy, consciousness |
Zeitz 2005 Australia |
Descriptive observational study | 282 hours of observation, 81 patients, 2 hospitals, 2 surgical units | Measurement: Not specified Mode of recording and documentation timing: Not specified Calculation of EWS value: Not specified |
Non-participant observation of nursing practice: observation of postoperative patients in the first 24 hours after returning to the ward over an 8-week period | Mean time to take vital signs and any other activities alongside was 5.8 minutes (SD: 2.56; range: 1–15) | Temperature, HR, RR, BP |
Zeitz et al., 2006 Australia |
Descriptive observational study | 282 hours of observation, 81 patients, 2 hospitals, 2 surgical units | Measurement: Not specified Mode of recording and documentation timing: Not specified Calculation of EWS value: Not specified |
Non-participant observation of nursing practice: observation of postoperative patients in the first 24 hours after returning to the ward over an 8-week period | Mean time to take vital signs and any other activities alongside was 5.8 minutes (SD: 2.56; range: 1–15) | Temperature, HR, RR, BP |
Studies which do not provide mean time measurement estimates | ||||||
Adomat and Hicks, 2003 UK |
Descriptive observational study |
360 hours of observation. 1 hospital, 2 ICUs | Measurement: Not specified Mode of recording and documentation timing: Not specified Calculation of EWS value: Not specified |
Video recorder documented nurse activity for 48 continuous shifts | Charting observations/record-keeping. ICU A high-dependency unit (HDU) patients: 10.78 minutes/hour ICU patients: 17.43 minutes/hour ICU B HDU patients: 5.44 minutes/hour; ICU patients: 10.66 minutes/hour |
Not specified |
Erb and Coble, 1989 USA |
Pilot study | 1 general hospital, 1 trauma unit with 31 beds; 1 cardiac catheter unit with 26 beds | Measurement: Mixture of automatic and manual Mode of recording and documentation timing: The nurse records all vital signs at the bedside unit, which is a system that records BP, pulse rate and thermometer. Vital-sign data are stored on the nurse station unit, which is a computer at the nurses’ station. The bedside unit and nurse station unit are connected by existing telephone wires. Calculation of EWS value: Not specified |
There were no details on how the time required to obtain a set of vital signs was captured other than to say the results came from a management study of nursing activities | On average, each nurse saved 11.86 minutes per shift by using the automated system to record vital signs (length of shift was unspecified). This equated to a 63% decrease in overall nursing time on vital-sign collection | BP, arterial pulse rate, respiration, temperature, intake/output and patient’s weight |
Fuller et al., 2018 USA |
Before-and-after study | A 32-bed medical telemetry unit with 54 nurses and unlicensed assistive personnel | Measurement: Mixture of automatic and manual Mode of recording and documentation timing: Nurses enter vital signs into the mobile machines directly at the bedside. Vital signs are automatically transferred to electronic health record (real time). Calculation of EWS value: Not specified |
A time-and-motion study plotted the number of steps and time taken to document vital signs data | Time to document vital signs before the introduction of mobile vital signs machines interfaced with the electronic health record: 7 minutes/10 patients. After the introduction it was 0 seconds | BP, HR, respirations, temperature, and oxygen saturation |
Hendrich et al., 2008 USA |
Descriptive observational study | 382 nurses over 1083 shifts, within 17 healthcare systems in 15 states, 36 medical-surgical units | Measurement: Not specified Mode of recording and documentation timing: Not specified Calculation of EWS value: Not specified |
Data were collected for seven consecutive 24-hour days using time-motion methodology. Nurses self-reported what they were doing by recording the activity in which they were engaged when a pager vibrated during the shift. ‘Vital signs’ was a categorised activity | Assessments and vital signs observations took on average 30.9 minutes (7.2%) in a 10-hour shift | Not specified |
Hoi et al., 2010 Singapore |
Descriptive observational study | 1596 hours of observation. 1 acute care hospital, 19 general wards | Measurement: Mixture of manual and automatic Mode of recording and documentation timing: Not specified Calculation of EWS value: Not specified |
All nursing staff were observed adopting a work sampling technique. Each staff member was coded using a coloured lanyard, activities performed were observed and documented at 5-minute intervals | Observation and monitoring (i.e. vital signs and other assessments including urinary catheter care): 16.5 minutes for very low acuity and dependency patients 40.5 minutes for very high acuity and dependency patients per day |
Not specified |
Yeung et al., 2012 Canada |
Descriptive observational study | 44.5 hours of observations, 24 nurses, 88 patients, 3 tertiary care hospitals, 5 general medical wards | Measurement: Not specified Mode of recording and documentation timing: Two hospitals: pen and paper, some directly on charts before leaving the bedside One hospital: pen and paper, transcribed on electronic documentation after leaving bedside Calculation of EWS value: Not specified |
Nurses were observed. Time-motion methodology to measure the duration of and the time between clinical activities i.e. vital signs assessment and documentation | Measuring vital signs: 12 minutes per nurse over 44.5 hours for measuring vital signs. Total mean documentation time at the electronic-documentation-based hospital: 53.2 minutes (SD 27.1) Total mean documentation time at the paper-only-based hospitals: 17.2 minutes (SD 13.2 minutes) over 44.5 hours |
Temperature, HR, RR, BP, oxygen saturations |
Appendix 6 Vital signs-related activities recorded in the QI tool
These are the data collected as part of the observational study.
Vital sign | One or more of the six physiological parameters that form the basis of the NEWS2 scoring system (Royal College of Physicians, 2017): RR, oxygen saturation, systolic BP, pulse rate, temperature and level of consciousness or new confusion. |
Vital signs equipment | Sphygmomanometer, manual BP cuff, stethoscope, oxygen pulse oximeter, thermometer, electronic recording system for vital signs, tablets. |
Vital signs documentation | Observing previous vital signs trends electronically or on paper, patient notes, track and trigger charts, other vital signs observation charts. |
Vital signs observation set | The collected acts of taking and recording the measurements of vital signs and sourcing vital signs equipment per patient. These can be complete (i.e. there is no reason for the observer to suppose that the six physiological parameters of vital signs as outlined in NEWS2 have not all been completed) or incomplete (i.e. it is obvious to the observer that not all six physiological vital signs have been completed). |
Vital signs round | One or more sets of individual vital signs observations taken sequentially by a single nurse are clustered in a vital signs round. Captures all parts of taking vital signs observations including using ‘Vital signs documentation’ and ‘Vital signs equipment’ as well as carrying out ‘Vital signs observation sets’. |
Interruption | An act outside the process of ‘Vital signs’, and ‘Vital signs documentation’ – see ‘Vital signs-related interruption’ and ‘Non-vital-signs-related interruption’ for more information. |
Vital signs-related interruption | Interruptions that would not have occurred unless vital signs were being taken (e.g. cleaning vital signs monitoring equipment, sourcing alternative equipment when equipment was faulty, escalation to other staff members if vital signs were abnormal). |
Non-vital-signs related interruption | Interruptions that are outside of the process of ‘Vital signs round’, for example the provision of personal care; the nurse leaving the patient to speak to someone else or to attend to other patients. |
Appendix 7 Categorisation of admissions into medical, surgical and ‘other’ groups
These lists show the specialty codes associated with each category.
Medicine | Surgery | Other |
---|---|---|
Emergency Medicine (180) | General Surgery (100) | Anaesthetics (190) |
Pain Management (191) | Urology (101) | Intensive Care Medicine (192) |
General Internal Medicine (300) | Transplant Surgery (102) | Audio Vestibular Medicine (310) |
Gastroenterology (301) | Breast Surgery (103) | Respiratory Physiology (341) |
Endocrinology (302) | Colorectal Surgery (104) | Paediatric (420) |
Clinical Haematology (303) | Hepatobiliary and Pancreatic Surgery (105) | Obstetrics (501) |
Hepatology (306) | Upper Gastrointestinal Surgery (106) | Midwifery (560) |
Rehabilitation Medicine (314) | Vascular Surgery (107) | Adult Mental Health (710) |
Cardiology (320) | Bariatric Surgery (109) | Interventional Radiology (811) |
Acute Internal Medicine (326) | Trauma and Orthopaedic (110) | Not a treatment function (820) |
Stroke Medicine (328) | ENT (120) | |
Transient Ischaemic Attack (329) | Ophthalmology (130) | |
Dermatology (330) | Oral Surgery (140) | |
Respiratory Medicine (340) | Restorative Dentistry (141) | |
Specialist Rehabilitation (345) | Orthodontic (143) | |
Renal Medicine (361) | Maxillofacial Surgery (144) | |
Medical Oncology (370) | Plastic Surgery (160) | |
Neurology (400) | Thoracic Surgery (177) | |
Rheumatology (410) | Gynaecology (502) | |
Elderly Medicine (430) | ||
Clinical Oncology (800) |
Appendix 8 Conceptual data model

Appendix 9 Blood tests of interest
Acute kidney injury | Eosinophils | Sodium |
Albumin | Fasting flag | Neutrophils |
Alkaline phosphatase | Serum glucose | Natriuretic peptide (NT-proBNP) |
Alanine transaminase | Glucose (fluid) | Phosphate |
Automated nucleated red count | Globulin fraction calculated | Platelet count (PLT) |
Activated partial thromboplastin time ratio (APTR) | Haematocrit | Red cell count |
Activated partial thromboplastin time (ACL TOP clot time) | Haemoglobin | Red cell distribution width (RDW) |
Basophils | International normalised ratio (INR) | Total bilirubin |
Calcium | Potassium | Total protein |
Adjusted calcium | Lymphocytes | Troponin I |
Creatinine | Mean cell haemoglobin | Urea |
C-reactive protein | Mean cell volume | White cells |
Plasma D-dimer | Monocytes | |
Estimated glomerular filtration rate (eGFR) (MDRD calculation) | Mean platelet volume |
Appendix 10 Results of retrospective analysis
Descriptive analysis
Patient subgroups
Summary of outcomes for different patient subgroups in the PHU internal validation data set
Patient subgroup | Admissions, N | DEATH, N (%) | COMB, N (%) | NEWS7, N (%) | NEWSINC, N (%) |
---|---|---|---|---|---|
PHU internal validation data set | 98,109 | 3684 (3.8) | 3818 (3.9) | 11,159 (11.4) | 58,048 (59.2) |
Emergency admissions | 73,911 | 3637 (4.9) | 3754 (5.1) | 10,680 (14.4) | 48,762 (66) |
Elective admissions | 24,198 | 47 (0.2) | 64 (0.3) | 479 (2) | 9286 (38.4) |
Admissions with observation in last 24 hours | 93,991 | 2086 (2.2) | 2215 (2.4) | 9147 (9.7) | 54,771 (58.3) |
Admissions without observation in last 24 hours | 4118 | 1598 (38.8) | 1603 (38.9) | 2012 (48.9) | 3277 (79.6) |
Admissions to medical specialties | 67,470 | 3279 (4.9) | 3351 (5) | 9307 (13.8) | 38,325 (56.8) |
Admissions to elderly medicine specialty | 557 | 54 (9.7) | 54 (9.7) | 106 (19) | 430 (77.2) |
Admissions to surgical specialties | 30,345 | 404 (1.3) | 466 (1.5) | 1839 (6.1) | 19,612 (64.6) |
Admissions without operating-theatre visit | 80,333 | 3514 (4.4) | 3583 (4.5) | 10,031 (12.5) | 45,472 (56.6) |
Admissions with operating-theatre visit | 17,776 | 170 (1) | 235 (1.3) | 1128 (6.3) | 12,576 (70.7) |
Admissions receiving blood transfusion | 114 | 2 (1.8) | 2 (1.8) | 7 (6.1) | 52 (45.6) |
Admissions receiving chemotherapy | 622 | 24 (3.9) | 24 (3.9) | 81 (13) | 464 (74.6) |
Admissions older than 80 years | 26,682 | 2207 (8.3) | 2239 (8.4) | 4967 (18.6) | 19,747 (74) |
Admissions younger than 30 years | 7965 | 4 (0.1) | 9 (0.1) | 199 (2.5) | 3211 (40.3) |
Summary of outcomes for different patient subgroups in the OUH external validation data set
Patient subgroup | Admissions, N | DEATH, N (%) | COMB, N (%) | NEWS7, N (%) | NEWSINC, N (%) |
---|---|---|---|---|---|
OUH external validation data set | 139,891 | 3705 (2.6) | 4031 (2.9) | 16,943 (12.1) | 73,444 (52.5) |
Emergency admissions | 102,214 | 3594 (3.5) | 3855 (3.8) | 13,946 (13.6) | 53,583 (52.4) |
Elective admissions | 37,677 | 111 (0.3) | 176 (0.5) | 2997 (8) | 19,861 (52.7) |
Admissions with observation in last 24 hours | 134,353 | 1735 (1.3) | 2056 (1.5) | 14,571 (10.8) | 70,054 (52.1) |
Admissions without observation in last 24 hours | 5538 | 1970 (35.6) | 1975 (35.7) | 2372 (42.8) | 3390 (61.2) |
Admissions to medical specialties | 85,979 | 3083 (3.6) | 3353 (3.9) | 11,207 (13) | 37,204 (43.3) |
Admissions to elderly medicine specialty | 247 | 10 (4) | 10 (4) | 30 (12.1) | 92 (37.2) |
Admissions to surgical specialties | 41,482 | 451 (1.1) | 492 (1.2) | 4305 (10.4) | 27,791 (67) |
Admissions without operating-theatre visit | 112,716 | 3353 (3) | 3651 (3.2) | 13,887 (12.3) | 52,522 (46.6) |
Admissions with operating-theatre visit | 27,175 | 352 (1.3) | 380 (1.4) | 3056 (11.2) | 20,922 (77) |
Admissions receiving blood transfusion | 604 | 5 (0.8) | 7 (1.2) | 99 (16.4) | 507 (83.9) |
Admissions receiving chemotherapy | 1021 | 39 (3.8) | 42 (4.1) | 154 (15.1) | 723 (70.8) |
Admissions older than 80 years | 32,726 | 2098 (6.4) | 2172 (6.6) | 6528 (19.9) | 20,061 (61.3) |
Admissions younger than 30 years | 14,832 | 18 (0.1) | 19 (0.1) | 654 (4.4) | 5760 (38.8) |
Vital sign observations patterns
Observation frequency in patient subgroups
Patient groups with lower-than-average observation rates were patients receiving blood transfusions or chemotherapy as well as patients admitted to geriatric specialties.
Highest median observation rates were found in the elective cohort, which could to some extent be explained by the shorter than average LOS of elective admissions and that for many patients who do not suffer an adverse outcome their observations are ‘front loaded’, that is, earlier in their stay.
Patient subgroup | Observations, N | Average NEWS, median (IQR) | Average observation period, median (IQR) | Recorded observations per day, median (IQR) |
---|---|---|---|---|
PHU development cohort | 4,134,572 | 1 (0–3) | 2 (1–7) | 4.05 (3.18–5.35) |
Elective admissions | 649,999 | 1 (0–2) | 1 (0–3) | 5.50 (3.87–14.26) |
Admissions to geriatric specialty | 50,028 | 1 (0–2) | 12 (6–22) | 2.94 (2.55–3.44) |
Admissions receiving blood transfusion | 3554 | 1 (0–2) | 2 (1–4) | 3.53 (2.37–4.67) |
Admissions receiving chemotherapy | 56,878 | 1 (0–2) | 5 (4–15) | 3.52 (2.93–4.22) |
Admissions younger than 30 years | 150,508 | 1 (0–2) | 1 (1–2) | 4.2 (3.19–5.69) |
Variation in number of observations within 24-hour period
Peaks of observation activity were slightly less pronounced in the elective cohort as well as in patients younger than 30 years old. Both groups have shorter than average LOS and by result observations were more likely taken independently from ward observation rounds. We observed more noise in the daily patterns of observations of geriatric patients due to the small size of the data set. Patients receiving blood transfusions and chemotherapy were not analysed separately because observations taken on the same day as treatment were excluded from the analysis, hence, skewing the results.
We observed slightly more variation across a 24-hour period in elective patients, with an overall lower mean TTNO (≤ 5 hours) but more distinct peaks compared to the entire PHU cohort at 06:00–07:00 and 20:00–23:00, where TTNO increased to 7 hours and 9 hours respectively. For small subgroups, that is, geriatric patients and patients ≤ 30 years old, patterns were too noisy to analyse, particularly in the PHU internal validation cohort (data not shown).
Modelling risk of outcome over time
Model calibration
We were unable to quantify model calibration in patients admitted to geriatric specialties, elective admissions, admissions with a theatre visit and patients ≤ 30 years because admission numbers and/or outcome rates were too small for robust regression.
List of abbreviations
- AUROC
- area under the receiver operating characteristics curve
- BP
- blood pressure
- COMB
- combined outcome of first occurrence of death, unplanned intensive care admission, or cardiac arrest in hospital
- DANQALE
- discounted and quality-adjusted life expectancy
- DEATH
- in-hospital death
- ED
- emergency department
- EPR
- electronic patient record
- EWS
- early warning score
- GDP
- gross domestic product
- HCA
- healthcare assistant
- HR
- heart rate
- ICU
- intensive care unit
- LOS
- length of stay
- MET
- medical emergency team
- NEWS
- National Early Warning Score
- NEWS7
- combined outcome of first occurrence of death, unplanned intensive care admission, or cardiac arrest in hospital, or recorded NEWS of 7 or more
- NEWSINC
- combined outcome of first occurrence of death, unplanned intensive care admission, or cardiac arrest in hospital, or recorded NEWS of 7 or more, or an increase in NEWS of 2 or more
- NHS
- National Health Service
- NICE
- National Institute for Health and Care Excellence
- OUH
- Oxford University Hospitals NHS Trust
- PHU
- Portsmouth Hospitals University NHS Trust
- PRISMA
- Preferred Reporting Items for Systematic Reviews and Meta-Analyses
- QALY
- quality-adjusted life-year
- RCP
- Royal College of Physicians London
- RCT
- randomised controlled trial
- RN
- registered nurse
- RR
- respiration (breathing) rate
- RRT
- rapid response team
- TTNO
- time to next observation