Notes
Article history
The research reported in this issue of the journal was funded by the HSDR programme or one of its preceding programmes as project number 15/145/04. The contractual start date was in March 2017. The final report began editorial review in September 2022 and was accepted for publication in July 2023. The authors have been wholly responsible for all data collection, analysis and interpretation, and for writing up their work. The HSDR editors and production house have tried to ensure the accuracy of the authors’ manuscript and would like to thank the reviewers for their constructive comments on the final manuscript document. However, they do not accept liability for damages or losses arising from material published in this manuscript.
Permissions
Copyright statement
Copyright © 2024 Davies et al. This work was produced by Davies et al. under the terms of a commissioning contract issued by the Secretary of State for Health and Social Care. This is an Open Access publication distributed under the terms of the Creative Commons Attribution CC BY 4.0 licence, which permits unrestricted use, distribution, reproduction and adaptation in any medium and for any purpose provided that it is properly attributed. See: https://creativecommons.org/licenses/by/4.0/. For attribution the title, original author(s), the publication source – NIHR Journals Library, and the DOI of the publication must be cited.
2024 Davies et al.
Chapter 1 Introduction
Why develop general practitioner services in the emergency department?
The urgent and emergency care (UEC) system includes emergency departments (EDs), ambulance services, NHS 111 and urgent GP appointments. 1 Currently, there is a significant mismatch between capacity and demand, with attendances at Type 1 EDs increasing year on year and reaching record levels. 1,2 This increasing demand, together with high hospital bed occupancy, leads to ‘exit block’ in the ED, creating crowded EDs and delays to treatment. 3 These challenging conditions make it difficult to provide safe care and contribute to staff burnout and failure to retain highly skilled professionals. 2,3
To meet demand, we need to ensure that patients can see the right person at the right time to meet their needs. A proportion of patients attends the ED with problems that could be effectively managed in community primary care. 2 Some EDs deal with this group by redirecting patients from the ED back to their own general practitioner (GP). Other hospitals have employed GPs to work in or alongside the ED to see these patients.
There has been growing international interest in using members of the primary care team in or alongside EDs. 4 Urgent and emergency care services are highly variable and have often been described using inconsistent terminology. 5 The 2010 Primary Care Foundation report identified three main GP-ED models used in the UK: GPs working geographically ‘adjacent’ to EDs; GPs serving in a triage and screening capacity; and GPs fully ‘integrated’ into ED service provision. 6 Ablard et al. 7 later highlighted additional models including ‘co-located’ urgent care centres and GP out-of-hours (OOH) services.
The 2014 NHS Five Year Forward view8 suggested UEC services should be redesigned to integrate EDs with primary care and in 2017 a significant NHS England funding initiative allowed EDs to apply for one-off payments to develop new or existing services to support streaming patients to GPs. 9 However, the evidence base for these interventions is underdeveloped. A 2018 Cochrane review identified only four studies for inclusion, concluding there was insufficient evidence to conclude whether primary care services in the ED (referred to as GP-ED models in this report) were effective or safe, with the impact on patient flow and waiting times remaining uncertain. 10 Due to the paucity of evidence available, NICE chose not to make any recommendations for practice regarding GP-ED models. 11
Important concerns about potentially negative impacts of GP-ED models include causing an unintended increase in ED attendances due to ‘provider-induced demand’,12 or because the primary care workforce is finite and already struggling to manage increasing workload and GP-ED models may lead to decreased community primary care capacity. 13 The training needs of staff working in GP-ED models to ensure safe care and the cost effectiveness of these services are also unknown.
The commissioning brief
This study was designed and conducted in response to a commissioning call from the National Institute of Health and Care Research (NIHR) to evaluate the effectiveness, safety, patient experience and system implications of the different models of GPs working in or alongside EDs.
While the commissioning brief focused on the role of GPs in EDs, during the course of the study it became apparent that primary care services in EDs are being delivered by a range of staff including GPs and both nursing and paramedic advanced clinical practitioners (ACPs). While the specific role for GPs in EDs remained a focus of the project, where our findings were also relevant to the wider primary care teamworking within/alongside the ED, we indicate this within the report. 4
Aims of the study
Four key research questions were identified:
-
What models are in place? How are they staffed/funded/operationally managed/performance managed? On what scale are they delivered?
-
How do the models work (in comparison with standard practice)? Is the way they work in line with the intentions of those who set them up?
-
What are the outcomes of each model in terms of: percentages of ED attendances seen; admissions; reattendance rates; waiting times; costs; patient safety; patient experience; team impact and sustainability?
-
What are the relationships between these contexts, mechanisms and outcomes, and is the evidence base transferable to commissioning, service improvement and cost-effective delivery in other settings?
The research approach
The study used a realist evaluation approach collecting mixed-methods data across three phases (see Figure 1 for summary). Realist evaluation (described in detail in Chapter 2) is an iterative approach, in which theories about how, why, where and for whom interventions work are developed, tested and refined.
FIGURE 1.
Three phases of data collection and analysis.
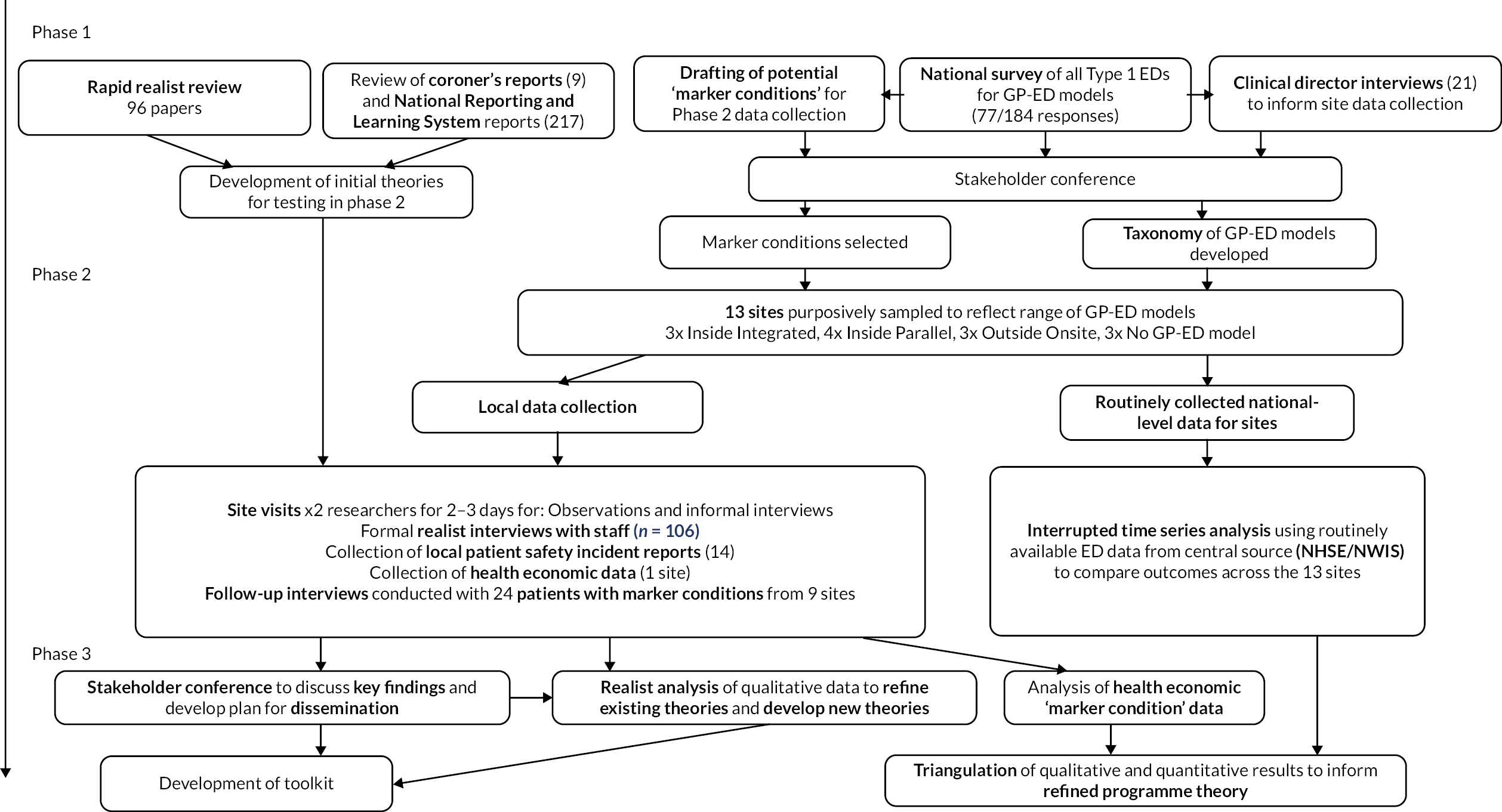
Preliminary preparatory activities in the pre-funding period (Phase 0) included: identifying ‘marker conditions’ which could be used to focus the later data collection and analysis (see Chapter 6); analysing existing patient safety incident reports from the National Reporting and Learning System (NRLS) which related to GP-ED models;14 analysing Coroners avoiding future deaths reports relating to GP-ED models. 14
Phase 1 activities focused on developing theories about how and why different GP-ED models worked for testing in the later phases. Theory development activities included:
-
a rapid realist review (RRR) of the existing literature (including 96 studies, Chapter 3)15
-
a service evaluation of current GP-ED models [including a national survey and follow-up interviews with selected clinical directors (CDs); Chapter 3].
In Phase 2, we collected and analysed mixed-methods data at 13 case study sites (10 with a GP ED model and 3 without GPs) to test our theories, including:
-
qualitative data from case study sites (observations, interviews with patients and staff, local patient safety incident reports; see Chapter 4)
-
centrally held routinely collected ED data for each site accessed via NHS Digital/Digital Health and Care Wales (DHCW) (for interrupted time series and cost–consequence analyses, see Chapter 5)
-
additional data for health economic analysis at a selected exemplar site (see Chapter 6).
Phase 3 involved the triangulation of each data set to refine our theories and develop our programme theory along with a toolkit for stakeholders based on our findings (see Chapter 7).
Deviations from the original protocol
Limitations in the availability of data necessitated some changes to the original protocol:
-
Emergency care data set (ECDS) data were not available for the case sites. Routine data were therefore collected from hospital episode statistics [accident and emergency (HES-AE) and admitted patient care (HES-APC), via NHS digital] for case study sites in England and emergency department data set (EDDS) and patient episode database for Wales (PEDW) data sets [via secure anonymised information linkage (SAIL)] for one study site in Wales.
-
The planned medical record reviews for marker condition analysis were found to be too time consuming with hard-to-decipher handwritten medical notes. The co-applicants opted for local case site data collection as an alternative. Pandemic and other constraints meant only one site was able to share these data for analysis.
-
The health economic analysis was changed from activity-based costing methods to cost–consequences analysis (CCA) following consultation with the steering committee with an awareness of data quality.
-
To improve patient recruitment for interviews, we added another ‘marker condition’ and recruited patients onsite as well as via mail.
-
Our second stakeholder conference was well attended in December 2019 by a wide range of stakeholders. This was used for some validation of findings since the planned second survey was not conducted due to the COVID pandemic.
Public involvement
Two experienced public contributors (JH and BH) were recruited from the Health and Care Research Wales Public Involvement Network to join the research development group to plan the study. They took part in all discussions about the research questions and proposed methods and drafted the plain English summary for the proposal. They shared responsibility for the bid sections about proposed public involvement, including reviewing the proposed budget (total and for public involvement). They were named co-applicants in the outline and full applications. After funding confirmation, two further public contributors were appointed to the Steering Committee, responsible for independent oversight of the study.
We were committed to continuing our relationship with the two public contributor co-applicants throughout the study as equal members among all co-applicants within the research management team. We named an academic co-applicant in the funded post of Public Involvement Lead and tasked her with supporting and facilitating public involvement.
This team developed a detailed role description at study outset, approved by the research management team. This set out how public involvement, summarised in the research application, would be undertaken in practice. Throughout the study, the team communicated regularly and met annually to review the activities carried out and update documentation on public involvement roles and responsibilities.
We describe below public involvement roles and activities in each phase of the study and compare these to our original public involvement plan developed in our project proposal (Table 1). A paper describing our involvement processes, impacts and learning has been published (see Project web page). 16
Involvement plan | Change | Variation or addition |
---|---|---|
Study management and delivery | ||
Two public contributors in research management group – strategic and operational responsibility over study | No change | Undertaken in line with plan |
Two public contributors in four subgroups: data collection; review findings; plan stakeholder events; dissemination | Change | Six subgroups convened and included public contributors. Additional groups: manage RRR; review interview data |
Support public contributors at two stakeholder events | Change | Additional roles undertaken by public contributors: recruiting public contributors; co-planning the agenda and room layout to address public needs; facilitating discussion groups; co-presenting |
Dissemination – lead public strand | Change | Dissemination activities extended across all aspects of the study and included:
|
Study oversight and advice | ||
Two public contributors of Study Steering Committee | No change | Undertaken in line with plan |
Additional public input | ||
Seven public contributors (excluding study public contributors) at two stakeholder events | Change | Fifteen public contributors attended the two stakeholder events; 6 attended the first; 10 attended the second; 1 of these people attended both |
Public involvement processes and effects | ||
Named academic lead for public involvement to support public contributors | No change | Undertaken in line with plan |
New | Public Involvement Team meetings to plan, review and operationalise public involvement throughout the study | |
New | Public contributors conducted audit of public involvement in the study, by reference to UK National Standards, and amended processes in light of results | |
New | Public contributors collected data on processes and effects of public involvement in the study and reported these |
Phase 1
Public co-applicants were involved in all aspects of work in this phase including:
-
reviewing all participant information and data collection tools and processes which were submitted for Research Ethics Committee approval.
-
membership of working groups, helping to plan and undertake:
-
planning and delivering the first stakeholder event including recruiting public participants (n = 6 attended), designing elements of delivery and materials, facilitating workshop discussions and presenting results alongside researchers (see Chapter 3)
-
proposing additional marker conditions based on feedback from stakeholder event.
During Phase 1, public co-applicants also undertook a Public Involvement audit to assess activity in line with the recently published UK Public Involvement Standards. 17 Changes to improve practice related to:
-
recruiting more diverse public participants at stakeholder events
-
improving communication
-
undertaking training reviews
-
strengthening involvement in dissemination.
To improve communication and current awareness of study activity, public co-applicants requested monthly updates from the Project Team. These regular, succinct reports kept them informed of actions and discussions between management meetings.
Following the audit, the public co-applicants proposed recording impact of public contributors as the study progressed. Co-applicants agreed that the regular Public Involvement agenda item (at Study Management Group meetings) should also include a ‘Public Involvement Impact’ box where all co-applicants could regularly record evidence of impact.
Phase 2
During this phase, the public co-applicants were particularly involved in discussions regarding patient interviews and identified ways to address poor response rates, including rewording patient information sheets and recruitment letters, using hospital letterheads and offering gift tokens to respondents. 18 These were implemented and resulted in an improved acceptance rate.
Public co-applicants reviewed transcripts of interviews with patients and CDs. 19,20 They identified themes, which they discussed with qualitative researchers and contributed to data synthesis. They contributed ideas about why patients might have responded the way they did and possible reasons for not participating in interviews. The themes they identified were in line with those put forward by the researchers. The public co-applicants reported that this exercise gave them a real feel for the research on the ground and greater insight into data quality, patient experience and complexity of the models which helped inform their involvement. In turn, the researchers found it useful to have their initial theories confirmed by the public co-applicants.
Public co-applicants were actively involved in planning the second Stakeholder Event. Changes implemented after contributions by public co-applicants at planning meetings included:
-
written clarification of the scope of the event and the meaningful role of the patient attendees
-
amendment to the recruitment method, letter, instructions for attendees and timetable
-
membership of discussion groups to include a mix of researchers and public contributors.
Barbara Harrington and Julie Hepburn co-presented and co-facilitated a workshop based on findings relating to patients’ acceptability of primary care streaming on the day. Eleven public contributors also attended, alongside other stakeholders.
Phase 3
This phase included the submission and publication of papers relating to study findings and public involvement. The public co-applicants co-conceived and co-authored papers about public involvement and drafted this section of the final report. They also contributed as co-authors on other publications. The public co-applicants were particularly keen to ensure that the findings of this study are accessible to a lay audience. To support this, they wrote lay summaries for each of the published papers. These are listed with the full papers on the website and in the study ‘newsletter’ [see GPs in EDs (PRIME Centre Wales)].
To ensure that study results will be disseminated to the public, public co-applicants recommended widening the Dissemination and Publication Strategy to include Engagement. To identify ways of improving public dissemination, one of the public co-applicants (JH) collected views from a public and patient group linked to Primary and Emergency Care Research (PRIME) Centre Wales21 and used their feedback to produce an outline plan. This co-applicant also approached the Communications experts within Health and Care Research Wales Public Involvement and Engagement them, who agreed to facilitate media engagement.
The public co-applicants contributed to the design and development of a toolkit (see Chapter 8) offering practical suggestions on improvements EDs can make based on findings from this research.
Finally, the public co-applicants initiated, planned and conducted a group interview of study co-applicants to reflect on experiences of public involvement in the project. This was transcribed and analysed using thematic analysis. These findings, plus records of public involvement activity and impact, have been published. 16
Conclusion
Public contributors were actively involved in data collection, analysis and dissemination. They attended management, scrutiny, planning and task meetings. In addition to their role in study management, delivery and dissemination, the public co-applicants developed and facilitated public involvement in the study identifying further opportunities for them to contribute and critically reviewing the effectiveness of their involvement as the study progressed.
The public co-applicants contributed directly to the management and delivery of the study and helped maintain awareness of patients’ perspectives among the whole co-applicant team. In addition, we have reflected on public involvement in this project to identify factors enabling effective involvement so that other studies can learn from our experience. 16
Collaboration with University of West England general practitioner–emergency department study
The NIHR funded two separate projects following the commissioning call. The GP-ED study was led by Professor Jonathan Benger, University of West England (UWE). 22
The two study teams identified the value of collaboration across the two projects to maximise learning by sharing information across two projects, minimise unnecessary duplication and ensure consistent terminology across GP-ED research was used by both projects.
Joint activities conducted included:
-
initial survey (Cardiff Team led) supplemented by additional national data collection (UWE led) to form a single data set describing current GP-ED models in place at UWE 22 March 2018
-
taxonomy development (Cardiff-led)
-
stakeholder events – Cardiff-led events attended by UWE team (27 February 2018 and 3 December 2019) and UWE-led events attended by Cardiff team (16 November 2018 and 20 October 2020).
Changes in health care following the COVID-19 pandemic
Most data collection for this study was completed in April 2019, before the global COVID-19 pandemic. The pandemic hugely impacted all areas of health and social care. In response, new models of working were rapidly introduced, including a move to telephone consultation before or instead of face-to-face assessment, in community primary care and at some EDs. Despite the changes in healthcare delivery since the study was conducted, our findings remain highly relevant and can usefully inform new ways of working including:
-
how to safely and effectively redirect the right patients away from ED care and towards primary care
-
how patients experience alternative models of delivering urgent care
-
ensuring new service models do not drive additional demand
-
understanding what primary care staff working in ED settings need to practice safely and effectively.
Structure of the report
Chapter 2 describes the realist methodology and its application in this study. It also describes the preparatory activities undertaken as part of Phase 0.
Chapter 3 describes the Phase 1 activities including the RRR and service evaluation. It also includes information about the Phase 1 stakeholder event and the production of an agreed taxonomy of GP-ED models as a key Phase 1 output.
Chapter 4 describes the methods used for qualitative data collection in Phase 2 at the case study sites. It includes the realist analysis of these data, which develops the Phase 1 theories further.
Chapters 5 and 6 cover the methods and findings from the quantitative data analysis of the routinely collected data obtained for Phase 2 sites. They also include the ‘marker condition’ analysis completed for a single site.
Chapter 7 describes Phase 3 of the project in which the qualitative and quantitative results were triangulated to further refine our theories.
In Chapter 8, we discuss our findings and describe the toolkit produced.
Chapter 2 Realist methodology
What is realist evaluation?
The realist evaluation approach was developed by Pawson and Tilley23 in response to the recognised complexity of evaluating new policies and has increasingly been used in health services research. It applies a realist philosophical stance to policy research. Realism is often described as sitting between positivist and constructivist approaches. While realists accept that a ‘real’ world exists, they also recognise that our understanding of reality will only ever be partial, and this understanding can be refined as new information is obtained. Realists recognise the interplay between structure (e.g. the way ED work is organised) and agency (the decision-making of individuals involved), in understanding causality.
In applying realist philosophy to evaluation, Pawson and Tilley23 advocate a theory-driven approach, recognising that interventions work differently when applied in different settings, generating a range of expected and unexpected results. Therefore, realist research questions explore what works, for whom and in what circumstances. Researchers then develop, test and refine theories which explain how and why interventions work.
A core premise in realist evaluation is that interventions work or do not work because of the way people respond to them that is, outcomes are generated by what realists call ‘mechanisms’. Furthermore, there is recognition that these responses to the resources an intervention offers may differ depending on the context into which interventions are delivered. Many factors such as individual views and preferences, the existence of competing interventions and the history of the organisation are recognised to have the potential to influence the success or failure of a new programme. 24
Realist research therefore focuses not only on what outcomes occur in which contexts, but also on exploring how and why those outcomes occur in those settings by identifying the generative mechanisms. These mechanisms are often described in two parts, recognising that they include both the resources offered by the intervention and also the reasoning processes of the individual’s involved. 25
The product of realist research is a programme theory which describes the way in which interventions delivered into a given context produce outcomes via a mechanism. Results of realist research are therefore usually expressed as context–mechanism–outcome configurations (CMOCs).
The overall aim of realist research is to generate transferable findings. While CMOCs configurations may initially be generated at the level of an individual or site, during analysis, researchers work to increase the level of abstraction away from individual data extracts and towards developing theories that are more ‘middle range’ in nature – that is, they may be relevant across a number of different settings.
‘Methodological pluralism’23 is typical of realist research. No single method is favoured; rather, methods are chosen for their ability to help develop, test and refine theories. For example, observations may provide useful contextual information about communication patterns between different professional groups, interview data can help to explore the reasoning processes used by staff selecting patients for review by a GP and waiting times data might be used to understand the desired outcome of moving patients efficiently through the department. No single type of data collection method is seen as inherently superior to another; data are instead judged based on their relevance to the theories under development and the rigour of the approach used. 26 This approach allows for ‘nuggets of information’27 gained from a variety of sources to be brought together to offer a more complete explanation of causality.
Why use realist methods here?
Introducing GPs to work in EDs is a complex intervention, comprising multiple strategies (e.g. streaming and triage processes, individual clinical decision-making) and actors (including staff and patients). While several potential outcomes for GP-ED models have been suggested (see Chapter 1), we did not identify any existing logic model or theory of change which set out how the intervention was expected to create its effects. It is accepted that the evaluation of complex interventions benefits from a theory-driven approach, mapping out the expected pathways through which interventions are expected to generate outcomes. 28 Exploring local contextual factors, at clinician–patient level (microsystem), team and organisational level (mesosystem) or wider level (macrosystem), is becoming an increasing focus of healthcare quality improvement to explain the outcomes. 29
We therefore selected realist evaluation as an approach which would allow us to explore how a range of stakeholders expected that GP-ED models might work, and then to test these ‘folk conjectures’30 in a detailed and systematic fashion. Realist evaluation is well suited to using data from multiple sources and perspectives and synthesises these to produce explanatory theories.
We identified the importance of highlighting and exploring the complexity of implementing GP-ED models, as part of our evaluation, to allow commissioners and policy-makers to understand the range of potential impacts that such an intervention might have in their own setting.
By focusing on understanding the role of context, readers can assess the transferability of our findings to their own settings, potentially pre-empting barriers identified, and targeting delivery to settings where they may be most likely to succeed (see Project web page).
While the realist approach encouraged us to explore context in detail to understand causal pathways, the process of theory generation, testing and refinement also aimed to produce explanations that were adequately abstracted to be broadly useful and informative. See Table 2 for a list of realist terminology used within this report.
Context (C) | Pre-existing conditions which may be at the individual, team or organisational level and influence the success or failure of different interventions |
Mechanism (M) | The way in which the intervention works, generally comprises the resources that the intervention offers and the reasoning responses it generates |
Outcome (O) | The intended or unintended results of the intervention caused by mechanism/s operating within a particular context |
If–then–because statement | Statements developed during realist analysis as an accessible way to start thinking about causality when working with data. For example, if staff are experienced, then they are more likely to stream patients to a GP, because they have developed a good understanding of cases appropriate for GP care |
CMOC | Realist theories are described as context–mechanism–outcome configurations (CMOCs) to demonstrate how mechanisms ‘fire’ in certain settings to generate the results described |
Initial rough theory | An early theory, informed by available evidence, about how, why, for whom and in what circumstances the intervention is thought to work, described using a CMOC. Requires further testing |
Refined theory | An initial theory that has been refined using primary or secondary evidence |
Programme theory | An overall high-level theory summarising how the intervention works, using the theories developed from the data |
Formal theory | Existing social theories used as a lens through which to examine the data. Also known as middle range theory or substantive theory (e.g. Crosskerry’s dual process model of clinical reasoning31 used in Chapter 4) |
Realist interviews | Use a ‘teacher–learner’ approach in which the researchers’ theories are openly discussed. Teaching the interviewee about the theories being tested allows the researcher to learn from the interviewee’s response |
How we have taken a realist approach to the evaluation
We have applied a realist lens throughout the evaluation process, which influenced data collection and analysis in a number of ways highlighted below. The specific details of the methods used at each stage of the study are described in detail in the chapters that follow.
We designed a mixed-methods study with several phases, including in-depth case studies at multiple sites to adequately explore context. The wide range of data sources used (highlighted in Figure 1) recognises the value of different types of data for both theory building and theory testing.
We engaged early and often with key stakeholders. At the outset of the project, we harnessed their understanding of how GP-ED models work ‘in real life’ to aid theory development, while two national stakeholder events during the data collection and later data analysis stages meant their input continued to shape theory development and refinement. This should help to maximise the relevance of our findings in practice, ensuring we are addressing the questions that are important to staff involved in implementing these models.
The realist approach encouraged us to focus on causality, trying to understand how and why outcomes were produced throughout the study. This meant that we started our theorising and analysis early in the project and continued several iterations of the realist evaluation cycle throughout the study (theorising, data collection and testing, refining and further theorising). 23
For example, undertaking our realist review (see Chapter 3) enabled us build theories which were taken forward for testing at the case study sites. These sites were purposively selected, not only to represent a range of different settings (urban and rural, different GP-ED models etc.) but also on the basis of their ability to provide rich explanatory data. This was accomplished by interviewing CDs before case study site selection, targeting sites which in the survey had described33 particular successes and failures they had observed using GP-ED models (see also Chapter 3).
Our initial theories provided the researchers with a way to structure their interactions with staff, using a teacher–learner approach in which our developing ideas were shared for staff to expand upon or challenge. 34 As the project progressed, and the researchers collecting the data were also involved in ongoing data analysis and theory development, it was possible to focus later observations and interviews towards trying to expand upon and contextualise findings from earlier sites. Our ongoing focus on understanding causality encouraged the researchers to tailor data collection to explore relevant areas of theory emerging, for example, exploring how teamwork and communication was encouraged across different GP-ED models.
Our theory-driven qualitative data analysis (described in detail in Chapter 4) used both deductive reasoning (by comparing the data gathered to our initial rough theories) and inductive reasoning (developing new theories from the data), as well as the realist process of ‘retroductive reasoning’. Retroduction is an interpretive process used by realist researchers to understand causality. It involves identifying or suggesting the causal mechanisms which could explain events or patterns seen in the data. 33,35 As causal factors may often be unobservable (e.g. status, power) researchers must interpret the data to make sense of the observations made. This process was done both by individual researchers as they developed if–then–because statements from the data, and also collaboratively as a team when refined context–mechanism–outcome (CMO) statements were developed.
We also chose to use formal theory as a further analytical lens during the data analysis stage. 36 Pawson and Tilley advocate for the application of existing formal theory in realist evaluation. 23 Formal theory can be useful to confirm the coherence of the theories developed during the evaluation. 37 When a match can be identified between the empirical data collected and the relationships already described in a formal theory, the causal explanations generated can be strengthened. 38 Generally, realist evaluations utilise theories developed to explain a specific phenomenon39 (e.g. Croskerry’s dual process model of clinical reasoning;31 see Chapter 4) and we used several different formal theories to aid our interpretation of the data (also principally Chapter 4).
Chapter 7 describes how we used the quantitative data described in Chapter 5 to further test our developing theories. This triangulation process helped to increase our confidence in some of the theories developed, while challenging others and identifying areas where we could not be as confident in our findings.
Finally, as with all realist studies, a key output is the refined programme theory of GP-ED models, described in detail in Chapter 7. The RAMESES guidance on the reporting of realist evaluation studies has been followed in the production of this report.
Marker conditions
At the outset, we identified that many of the routinely collected outcome data we planned to analyse were at a departmental level, for example overall attendances and admissions. However, one of the key premises of GP-ED models is that changes at a departmental level may occur because GPs might manage certain groups of patients presenting to the ED with particular ‘marker conditions’ differently to ED clinicians (e.g. differences in use of investigations, time spent observing patients in the department, admission rates). The aim of selecting marker conditions was to provide further data to test our theories that some conditions may be managed differently by GPs and ED clinicians and to explore whether the management was influenced by the GP-ED model in place. We also chose conditions that contributed information about resource use (investigations/consultation times/admissions) and patient experience to supplement the routinely collected outcome data. We wanted to consider both conditions that GPs managed well in the ED setting and others that they may not manage so well.
We planned to use these marker conditions at our case study sites to identify patients (or parents/carers) to interview (see Chapter 4). The marker conditions were occasionally used when discussing theories during the staff interviews. We also collected local data about investigation use and hospital admissions for these conditions at one site for health economic analysis (see Chapter 6).
To identify potential marker conditions, we initially undertook a scoping review of the literature on ED relevant ambulatory care sensitive conditions (ACSCs) (described below). The shortlist generated was then subsequently refined using additional data from our national survey, a ranking exercise within the co-applicant team and a further consensus exercise at our first stakeholder event (all described in Chapter 3). We looked to identify marker conditions that could potentially be seen by a GP or ED clinician in any GP-ED model.
Scoping the literature on emergency department relevant ambulatory care sensitive conditions
Ambulatory care sensitive conditions are conditions where effective community care and case management can help prevent the need for hospital admission. This work was conducted in July and August 2017 by a medical student (FH) with support from the team (AC and FD) and an information specialist.
A MEDLINE search generated 477 hits, of which 347 were relevant after abstract screening. Of these 347 papers, 224 used a defined list of ACSCs, with most referencing 9 core papers. 40–48 From the 224 papers, 206 ACSC terms were identified. These 206 conditions were reviewed by 2 academic GPs (AC and FD) to identify potential marker conditions. Acute confusion was not included in the list but was included following discussion. Similar conditions were grouped together, for example, acute exacerbation of asthma and chronic obstructive pulmonary disease (COPD). Nine groups of diagnoses were generated. These were matched with an appropriate presenting complaint described in the ECDS and are shown in Table 3.
Diagnosis | ECDS chief complaint |
---|---|
1. Acute confusion | Drowsy (altered level of consciousness) Confusion Hallucinations/delusions |
2. Acute exacerbation of asthma Acute exacerbation of COPD Influenza and pneumonia |
Short of breath Difficulty breathing Noisy breathing |
3. Musculoskeletal back pain | Pain in back/trunk (no injury) |
4. Cellulitis | Localised swelling/redness/lumps/bumps |
5. Anxiety/depression | Depressive disorder Anxiety disorder |
6. Otitis media Tonsillitis Upper respiratory tract infection |
Fever Ear pain Sore throat |
7. Gastroenteritis | Abdominal pain Diarrhoea Vomiting +/− nausea |
8. Migraine | Headache |
9. Pyelonephritis Urinary tract infection |
Pain on passing urine Frequent urination |
This list of conditions was used as a starting point for discussion during Phase 1 and was subsequently refined at our first stakeholder event (described in Chapter 3) seeking to identify up to five conditions. We intended that this number of conditions would be adequately diverse to represent differing experiences for patients, while also providing adequate data about each specific condition to allow for helpful comparisons to be made within and between GP-ED models.
Conclusion
This chapter has outlined the rationale for using realist methods and described our application of a realist approach. The chapters that follow now describe the specific methods used at each stage, alongside the results.
Chapter 3 Phase 1
Overview of Phase 1 work
This chapter describes the work undertaken in Phase 1 of the project, which were later used to inform data collection in Phase 2. We summarise five key pieces of work: a rapid realist literature review, a service evaluation of current GP-ED models (undertaken via online survey and key informant interviews), development of a taxonomy of GP-ED models, selection of a set of potential marker conditions and an analysis of existing safety reports relating to GP-ED models.
Rapid realist review
Rationale for rapid realist review
The focus of our review was to develop initial theories about the contexts within and mechanisms by which the different GP-ED models produce the intended and unintended outcomes. Although there had been several systematic reviews undertaken exploring GP-ED models, their narrow inclusion criteria limited the evidence included and their conclusions. We sought to glean explanatory information from a wider range of data sources including policy documents, case study reports and opinion pieces to contribute to our theory building process. We used the previous recent reviews12,49,50 as a starting point for a RRR that focused on theory development. We did not seek to use the realist review for theory testing because we planned to collect primary data in Phase 2.
Methods
A detailed description of the methods used for the review is published elsewhere. 15 In brief, we followed the steps for RRR outlined by Saul et al. 51 in which we used our co-applicants as an expert reference group. Our review was registered on the PROSPERO database. 15 The review was carried out between April and September 2017. An initial scoping exercise of four UK papers52–55 identified in a previous systematic review12 and two policy documents6,56 was used to generate a set of 27 initial theories (grouped into themes) which were developed and refined following discussions with our expert group. To further develop these initial theories, we then sourced the papers included in the earlier reviews and combined the search terms used in these reviews to identify more recent studies (see search strategy in Report Supplementary Material 1). 15 We performed forward and backward citation tracking of included studies and asked our expert group to contribute papers.
There were no restrictions on the type of study included. Data were judged at the level of the individual extracts based on their relevance to the developing theories and the rigour of the methods used to generate the data. 26 Papers were excluded if they lacked explanatory power or were unavailable in English. Two authors extracted data into NVivo 11 (QSR International, Warrington, UK) and met weekly to discuss their interpretation. A co-applicant working group (AP, PA, BAE, BH, JD and ACS) met 6-weekly to discuss review progress and guide priority areas of focus for further searches and data extraction. As the review progressed, limitations of the available evidence were recognised, with notable gaps in some areas (e.g. impact of GP-ED models on the wider healthcare system). The expert group considered this was more likely to represent a lack of available evidence rather than a deficiency in the search strategy used. As such, at the end of the review process, many of the candidate theories remained untested or only weakly supported by the available evidence. We published our findings in a paper entitled ‘The impact of general practitioners working in or alongside emergency departments: a rapid realist review’15 (published via CC BY 4.0.), extracts from which are reproduced in part in the section below.
Results
The sources of papers contributing to the review are outlined in Figure 2.
Most of the included papers were British (n = 44), and a significant proportion were Dutch (n = 17). Other studies included came from Ireland, Belgium, Switzerland, Sweden, Italy, Finland, Australia, USA, Canada, Singapore and New Zealand.
Included articles were largely observational studies (n = 74) but also included organisational reports (n = 7), controlled studies (n = 5), qualitative studies (n = 5), opinion pieces (n = 3) and modelling studies (n = 2).
Results of the review
The initial scoping work and subsequent expert group discussion identified 27 candidate theories, categorised into five themes: streaming and flow, the GP role, patient satisfaction, patient safety implications and wider system implications.
Those which we were able to refine, or were supported by the review data, are discussed below, together with the CMOC developed. The remaining candidate theories stayed on file for the research team to refer to during the Phase 2 data collection.
Streaming and flow
Candidate theories about streaming and flow explored ideas about how staff made streaming decisions, who was the best person to perform streaming and how streaming might lead to duplicate assessments.
Using the literature, the CMOC developed was:
General practitioners and ED staff use their own personal experience and expectation (C) when interpreting streaming guidance (M) to influence which patients are streamed to GPs (O). 6,53,54,57,58
The process of streaming patients to the GP service was observed to influence service effectiveness. Variable streaming rates were described due to differences in guidelines and also how the guidance was interpreted by ED clinical and non-clinical staff of varying experience. 7,58–61 Staff were sometimes described as being unclear which patients GPs could deal with,7,55,54,59,62–64 or being more familiar with ED work so favouring ED referral,7,58,59,63,65,66 even over-ruling the guidelines if they felt that the patient would require specific investigations63 or admission. 58 When there was a good relationship between the GPs and ED nurses,62 and when the GPs were directly involved in the streaming process, increased streaming rates were reported. 67,68 GPs were also noted to over-ride nurse decisions to select patients that suited their own interests or perceived skills. 69
The role of the general practitioner
Our candidate theories about the roles GPs undertook in EDs explored how the characteristics of individual clinicians and the way in which they were expected to work could influence their approach.
Using the literature, the following CMOC was developed:
When GPs working in the ED maintain a ‘traditional role’ using the same approach taken in the primary care setting (M) to treat patients with primary care problems (C),7,66,70–73 investigations, admissions and process times will reduce (O). 54–56,61,72–78 However, if GPs adopt an ‘emergency clinician role’ working as another pair of hands (‘going native’) because of their personal interest or experience or because they feel this is the correct way to work in this setting (M), there will be no difference in the rate of investigations and admissions (O). 7,62,66,79
Material in this section has been reproduced from Cooper et al. 15 This is an open access article distributed under the terms of the Creative Commons CC-BY licence, which permits unrestricted use, distribution and reproduction in any medium, provided the original work is properly cited.
The traditional GP approach was described by many authors as a different approach to risk management and diagnostic uncertainty, with less reliance on acute investigations. 7,66,71–73 This approach was maintained in a variety of different settings including when GPs worked in parallel to the ED in their own room54,55 and also when GPs worked in a more integrated model, alongside ED clinicians. 61,72,73 There were multiple examples of GPs managing non-urgent patients using this approach to divert attendances from ED staff. 59,64,67,68,80–93 Several services used GPs specifically to see ‘non-urgent’ paediatric patients. 67,74,81,94 In some settings where the GPs were less experienced, this was associated with increased antibiotic prescribing. 61,79
Data indicating that GPs took on an ‘emergency clinician’ role were more limited. 7 There was evidence that GPs working in or alongside EDs saw more acutely unwell patients7,95 and minor injuries7,54,59,63,64,96 than when working in community primary care. The implications of this on their required skillset and learning needs were not explored. One Irish study suggested inexperienced GPs working in a setting without a robust triage process appeared to adopt a more ‘diagnosis-driven’ emergency clinician approach. 79 Other articles described GPs at the front door of the ED redirecting patients to other services. 97,98 The wider literature provided examples of both good practice (e.g. a comprehensive assessment with vital signs recorded)99–103 and potential concerns (e.g. low sensitivity of triage criteria in infants)104 relating to redirection. The influence of GPs’ special interests, experience in emergency medicine or the effect of staff shortages on the roles GPs adopted were not described.
Patient satisfaction
Our candidate theories described how satisfaction could be linked to the expectations that patients attend with (e.g. relating to waiting times and investigations required). Following the review, we developed the following CMOC:
Patients with primary care problems that present to EDs (C) and are seen by GPs, are more satisfied with the care they receive (O) if the experience exceeds expectation (M), but if they do not perceive any difference in the care they received compared with what they expected (M), there is no difference in satisfaction (O). 56,61,66,72,105–109
Data to support this theory were limited, with an increase in satisfaction for patients seen by GPs generally associated with shorter waiting times,72,105 rather than expectation of investigation and treatment. 66 The GPs were sometimes supernumerary which may have contributed towards this. 56,72 Other studies demonstrated that GPs focused more on patient education and counselling than ED clinicians with some improvement in satisfaction rates. 110,111 In more fully integrated models, the patient was often unaware that they had seen a GP rather than an ED clinician and there was no difference in patient satisfaction. 66,70,56,106
Patient safety implications
Our candidate theories about patient safety discussed the potential differences between patients presenting to EDs and community primary care, how communication within and between services could impact on safety and how a GP service might improve safety in the ED for the sickest patients.
Following our review, we developed the following CMOC:
In EDs, where there are delayed patient transfers to wards or inadequate staffing (C), GPs seeing patients with primary care type problems (M) may not free up ED staff to care for the sickest patients (O). 67,112–118
There was a lack of evidence that GPs working in or alongside EDs directly or indirectly improved care and safety for the sickest patients. An Australian study reported a reduced mean time taken to see more seriously ill patients,113 and a Canadian study of over 4 million attendances reported that low-complexity ED patients did not increase time to first physician contact for high-complexity patients. 114 Other studies also described how diverting non-urgent patients did not improve the high-level care required by others, and that influences such as delayed transfer of patients to the ward were more likely to contribute to overcrowding. 71,115–117
There were minimal data on the safety implications of GPs working in ED settings. Several studies used ED reattendance as a marker of safety, with no increase among patients seen by GPs compared with the usual ED staff. 53,56,61,119,120 For GPs working inside the ED, good communication and integration were described in some studies,7,54,56,95 with anecdotal reports of poor communication negatively affecting care quality in others. 80
Wider system implications
We developed several candidate theories relating to a range of implications of GP-ED models on the wider system. These considered the cost effectiveness of the approach and whether it would act to create additional demand. We also considered how the models might impact on ED staff as well as on the primary care workforce. Although we drafted candidate theories around other potential broader impacts (e.g. widening access to primary care for homeless people), some of these were subsequently shelved as they were too far beyond the scope of our project.
Cost effectiveness
If there is a demand for patients with primary care problems presenting to EDs (C), and they are streamed to onsite GPs and managed using a traditional GP approach (M), the service is cost-effective due to fewer referrals, admissions, investigations and better outcomes compared with usual services (O). 56,60,77
Data to support this theory were limited, but supported by three economic evaluations (UK, Ireland and the Netherlands) where non-urgent patients were streamed to GPs during normal daytime hours. 56,60,77 The comparator was ‘business as usual’ with no GP service. The UK and Irish studies were published in 1996 and may not represent current ED staffing models.
Three other studies of ‘out-of-hours’ patients did not find the addition of a primary care service to be cost-saving as patients and staff were not comfortable with redirection,58 preferred to attend another convenient location62 or because minimum staffing levels had to be maintained at the ED. 93
Risk of provider-induced demand
If patients with primary care-type problems present to EDs (C) and are streamed to indistinct primary care services, without patient awareness or choice (M), there is no provider-induced demand (O). 53,54,82,83 However, distinct urgent primary care services may offer convenient access to primary care (M), resulting in provider-induced demand (O). 84,103,121–127
Four articles described fully integrated models, where non-urgent patients were streamed directly to GPs inside the ED without provider-induced demand. 53,54,82,83 Here, there was no patient choice offered and often a lack of patient awareness. Another 10 articles described distinct urgent primary care services, often in separate buildings outside the EDs, as duplicating services and creating their own demand, increasing patient presentation rates directly or at nearby services, rather than relieving pressure on the ED. 103,121–131
Impact on staff
Limited evidence from the included studies prevented us from refining theories on the implications on ED staff or the wider primary care system.
Two articles did highlight the potential reduction in learning opportunities for junior doctors in EDs. 78,95 There were no reports of ED clinicians changing their approach as a result of working alongside GPs, but some reports of GPs in management positions influencing system changes. 132,133
There was limited evidence that working in an ED setting led to increased job satisfaction for some UK GPs with a special interest in emergency care. 7,133 However, reduced satisfaction was also described because the job was outside the scope of the usual general practice,7,76 possibly contributing towards recruitment problems. 7,134
Summary of rapid realist review findings
The review demonstrated that GPs are working in a variety of ways in and alongside EDs. Important influences on the way in which these models operate at the level of individual clinicians (level of experience, personal interests) and wider service design (e.g. visibility of the service) were identified.
We found evidence describing ED staff uncertainty about the role of GPs impacting on streaming to primary care services, GPs being able to maintain a traditional GP role while working in EDs, and how highly visible services generate their own demand. Less evidence was available around safety implications and whether these services represent value for money.
The review has highlighted the importance of understanding local context, including how the GP-ED model sits within the wider provision of urgent care locally when trying to understand outcome patterns, which we now explore further in key informant interviews, and later in Phase 2. It also emphasises the need to represent complexity in any categorisation of GP models while also allowing for meaningful comparison across sites, which informed the development of our taxonomy described later in this chapter.
Service evaluation
To understand what GP-ED models were currently being used, and how they were working, we undertook a service evaluation in two parts, firstly a national survey, followed by telephone interviews with key informants identified from the survey.
National online survey
Methods
We carried out a national online survey of all Type 1 EDs in England and Wales in 2017 (see Project web page) to gather information including:
-
the aims of their GP-ED service; the extent to which the EDs consider these aims are being achieved in practice; perceived successes or failures of GP-ED services; date(s) of changes to their GP models; patient volume, case mix, funding, governance and training processes
-
where there was no GP service, to identify the reasons for this.
The survey was developed in collaboration with our co-applicants and the GP-ED study team from University of West England, informed also by our initial scoping of the literature (influenced particularly by a previous survey conducted by the Primary Care Foundation). 6 We used a combination of fixed-response multiple-choice questions and open-ended questions with free-text responses. The survey was delivered to ED CDs using Online Surveys. Research and Development departments for all EDs were provided with details of the study. We obtained ethical approval for the survey and follow-up interviews as a service evaluation from Cardiff University School of Medicine Ethics Committee (17/45). We obtained contact details for CDs through networks of contacts (co-applicants, UWE collaborators) and internet searches. We sent invitations to all CDs of Type 1 EDs in England (n = 171) and Wales (n = 13) by post and e-mail. Initial invitations were sent in September 2017, with follow-up invitations 2 weeks later. We recontacted non-responders to encourage participation until the survey closed in February 2018.
Results
We received 77 responses out of 184 invitees (41.3%). Fifty-one (66%) respondents used a GP model in their ED, and 26 (34%) did not. At 21 sites (27%), GPs were integrated within the ED, at 23 sites they operated inside the ED but in parallel to the ED service (e.g. seeing patients in a separate room), at 7 (9%) sites the GP service was located outside the ED elsewhere on the hospital site.
Aims of services
We asked respondents to indicate the aims of introducing GPs in or alongside their ED. Among those who had introduced GPs, the most common aims selected were reduce ED waiting times (n = 40; 78%), reduce ED patient volume (n = 36; 71%) and better use of available ED resources (n = 35; 69%), improve the quality of care for specific cases (n = 31; 61%) and improve patient experience (n = 29, 57%). Less frequently cited aims included to ‘educate’ patients by sending them to the right place, to meet a national or other directive, to reduce admissions or to save costs. Free-text responses related to a change in OOHs GP service, tariff applied to patients seen in urgent care centre and difficulties in recruiting ED doctors.
Respondents were also asked to what extent various pre-selected aims were achieved. Most frequently reported aims achieved were improved quality of care for certain groups (all, most or some of the time n = 40/46, 86%), improved patient experience (n = 38/46, 82%), reduced ED patient volume (n = 38/47, 81%), better use of available ED resources (n = 39/47, 79%) and reduced ED waiting times (n = 39/50, 78%). Those aims least commonly achieved were reducing hospital admissions (rarely or never n = 29/47, 62%) and saving costs (n = 23/42, 55%).
Workforce
The number of GPs working in the surveyed EDs each week ranged from 1 to over 15, with most EDs using 1–3 GPs per week. EDs reported using a variety of primary care clinicians including using sessional locum GPs working ad hoc shifts (n = 41; 80%); salaried GPs working fixed shifts (n = 22; 43%); advanced nurse practitioners (ANPs) working ad hoc shifts (n = 15; 29%); ANPs working fixed shifts (n = 15; 29%); other nurses working ad hoc shifts (n = 4; 8%) and other nurses working fixed shifts (n = 8; 16%).
General practitioner workload
At some EDs, up to 40% of patients (average 13% across all sites) were reported as being seen by a GP or primary care clinician in ED. The types of patients seen by GPs varied both across and within sites and included patients with primary care problems (n = 47; 92%); low-acuity patients including minor trauma (n = 26; 50%) and undifferentiated patients, that is, same case mix as ED clinicians (n = 6; 12%).
A range of staff members selected which patients should be seen by a GP, most commonly ED nurses (n = 34; 67%), GPs themselves (n = 24; 47%) and ED doctors (n = 14, 27%). Others involved included primary care nurses, telephone triage services, paramedics and patients themselves. Decisions about selecting patients to be seen by a GP were informed by clinical judgement (n = 36; 71%), locally developed criteria (n = 35; 69%) and national triage/streaming tools (n = 11; 22%) including Manchester triage tool, NEWS, Luton Streaming Tool or adaptations of these.
Training
Induction and training for GPs working in or alongside EDs were provided by a range of organisations including the hospital (n = 21; 41%), the primary care provider (n = 16; 31%) and as a shared responsibility between the hospital and primary care provider (n = 10; 20%).
Marker conditions
Conditions the survey respondents felt that the GPs managed well included chronic pain/illness (n = 18; 35%), non-urgent paediatric patients (n = 15; 29%), dermatology problems (n = 12; 24%), ear, nose and throat problems (n = 7; 14%) and gynaecological problems (n = 6; 12%).
Conditions the respondents felt that GPs did not manage so well included trauma (n = 23; 45%), chest pain (n = 16; 31%), injuries (n = 12; 24%) and sepsis (n = 6; 12%).
Using findings from free-text survey responses
The survey included a free-text response question about barriers and enablers identified in relation to setting up the service and achieving the intended aims. We coded the responses to these questions into key themes and used our analysis to purposively select ED CDs to interview for maximum variation.
Key themes that emerged included:
-
motivations for setting up service (staffing shortages, policy directive, increasing demand)
-
providing a consistent service (issues obtaining funding for GPs, finding staff to provide a consistent service)
-
variation in GPs skillset (scope of practice, number of patients they see, risk taking)
-
agreeing governance structures
-
space for GPs to work from (physical structure of the department, capacity to create work areas for GPs)
-
variation in demand for primary care and concerns about creating additional demand
-
variation in streaming methods.
Key informant interviews
We identified a purposive sample of 30 EDs as potential case study sites. We looked for sites with a GP-ED model implemented since 2010 (required for case site time series analysis) spread across England and Wales representing a variety of settings (urban and rural, smaller and larger EDs), GP-ED models (location of GPs, access to investigations, range of patients seen) and experiences of the GP-ED model. We invited the CDs of all 30 EDs for a follow-up interview; 9 declined to take part or did not respond.
We included participants from 21 EDs (19 England, 2 Wales). Eleven had GPs working in separate primary care services (seven within the ED and four outside), five EDs had GPs integrated in the ED and three EDs did not employ GPs (two in Wales).
Interview guide
We developed individualised interview guides informed by our survey responses and the initial theories derived from our RRR (see Project web page). 15 We aimed to:
-
obtain more detail about participants’ survey responses relating to the aims of the primary care service; enablers and barriers to implementing and delivering a service; GPs’ roles; streaming; patient demand and flow
-
gain a better understanding of the local context and explore ideas about what factors impacted on way their service worked and their perceptions of the outcomes of the GP-ED model to contribute to later theory refinement.
Data collection and analysis
We interviewed 18 CDs by telephone and three in person (March 2018 to March 2019). All signed a consent form before interview. Interviews were audio-recorded and transcribed verbatim. In our first stage of analysis, we created an initial thematic coding framework in NVivo 11 based on the research aims, findings from the RRR and the survey responses. We coded themes and subthemes within the thematic framework (ME/DP), also allowing for new themes to be identified. When it was established, there were no additional concepts observed, then sampling was terminated. This initial analysis was used to inform case site selection and orientated the researchers to important local contextual factors for further exploration during the Phase 2 case site visits. It also fed into the development of a taxonomy of GP-ED models (see below). 5 The interview data were then incorporated into the data set for each of the case sites for further realist analysis alongside the other qualitative data collected at each site in Phase 2.
Results of initial analysis
The full set of findings from the interviews can be found in a published paper. 15 Three main themes were identified, described in turn below.
Achieving the aims of implementing and delivering a general practitioner–emergency department service
Sites with separate primary care services (either within or alongside the ED) aimed to reduce waiting times and improve flow, by streaming patients identified to have primary care needs away from the main area of the ED. Some CDs perceived that they had reduced waiting times and improved flow in the ED, enabling primary care patients to be seen quickly without investigations and enabling ED doctors to focus on more acutely unwell patients.
Sites with integrated primary care services aimed to: (1) improve waiting times and flow by focusing on primary care patients and sometimes also patients with minor injury or more acutely unwell patients; (2) reduce admissions by focusing on frail/elderly patients or (3) work in the ED as a middle-grade ED doctor seeing undifferentiated patients (if experienced in emergency care) to fill gaps in ED staff recruitment. GPs caseloads sometimes included a wide range of acuity and primary care streaming was not always used. However, having GPs with skills, experience and special interests and a multidisciplinary team of staff with a range of skills helped manage demand; provided opportunities for sharing advice and learning between GPs and ED clinicians; and was perceived as potentially better value.
Challenges in implementing and delivering a general practitioner–emergency department service
Some factors preventing implementation were a lack of space for GPs and a potential reduction in ED space, which might negatively impact ED flow; competing funding priorities for extended community primary care services; and previously unsuccessful pilot services. Some participants were concerned that if GPs worked inside the ED, they might be tempted to work beyond their expected role and see emergency care patients, potentially leading to quality and safety concerns. In Wales, there were no policy or funding initiatives for primary care services in EDs; two departments had ED staff with previous experience of working as GPs but who were employed in a middle grade ED doctor role. These hospitals had previously piloted models with GPs to focus on primary care type patients but there was no longer funding available.
Some participants commented that the ‘Luton and Dunstable model’135 promoted by NHS England (where suitable patients are streamed by a nurse to a separate GP service within the ED) was not appropriate for their service. Low demand for primary care was attributed to local demographics or easily accessible and good-quality community-based primary care and ambulatory care services. Some ED primary care services were reported not to be cost-effective due to the high costs of GPs, low demand and little or no impact on waiting times, flow or use of investigations. Consequently, some primary care services were scaled down or discontinued.
There were concerns that implementing a new primary care service could cause ‘provider-induced demand’ by attracting additional ED attendances (see Chapter 4 for further analysis of findings relating to demand).
Material in this section has been reproduced from Edwards et al. 19 This is an open access article distributed under the terms of the Creative Commons CC-BY license, which permits unrestricted use, distribution and reproduction in any medium, provided the original work is properly cited.
Facilitators and barriers to primary care streaming
Facilitators to primary care streaming were described as consistent demand from primary care patients; experienced and confident nurses; clear guidelines and protocols (CD interview sites 4, 6, 10, 11, 13, 16 and 20); NHS or shared clinical governance (CD interview site 3); training, evaluation and improvement workshops (CD interview sites 6 and 20) mentoring and support from senior ED staff was perceived to improve streaming (CD interview sites 4, 10 and 16). The monitoring and supervision of streaming by senior ED clinicians also facilitated more patients to be streamed to a GP (CD interview sites 3, 4, 15 and 20).
Establishing governance pathways and policies and reaching an agreement on which types of patients a GP should see was reported as a key challenge (CD interview sites 4, 6, 18). Sometimes, the working relationships and teamworking between NHS commissioned ED service and a primary care service operated by an independent contractor were not well established and presented a barrier to setting assessment time targets and developing streaming criteria (CD interview sites 6 and 18), meaning that primary care patients were seen by ED clinicians or moved across streams. Where primary care demand was low, streaming was difficult to implement and sustain (CD interview sites 4 and 14).
Stakeholder event
Our Phase 1 stakeholder event was held in Bristol in February 2018. We presented findings from our RRR and the national survey which highlighted the complexity of service models. This event then focused on gathering stakeholder feedback on two main topics; the development of a taxonomy of GP-ED models and identifying appropriate ‘marker conditions’ to guide data collection at Phase 2 case sites.
Attendees were commissioners and policy-makers (n = 6); clinical leads and ED clinicians (n = 8); GPs and nurse practitioners (n = 6); public contributors (n = 8) and research team members (n = 14) from England and Wales.
Taxonomy of general practitioner–emergency department models
The need for taxonomy
An array of different terminology describing the ways in which GPs work in EDs was identified during our realist review and service evaluation. Sometimes, different terms were used to describe the same model, while on other occasions the same term was used to mean different things.
As researchers, we needed clear definitions to be able to make useful comparisons and ensure that we grouped services together appropriately. As described earlier, the NIHR funded two projects simultaneously to examine the role of GPs in EDs (NIHR HS&DR 15/145/04 and 15/145/06). It was important that these did not cause further confusion by using different terminology. The two teams therefore agreed to develop a shared taxonomy for characterising the ways in which primary care services worked in or alongside EDs.
Development process
The development of our shared taxonomy of GP-ED models has been described in a publication (see Project web page). 5
We contributed data from our Phase 1 activities described above (RRR, national survey and telephone interviews), as well as early data from the first five case sites selected for Phase 2. The role of a taxonomy and what it should include was also discussed at our stakeholder event.
Additional data from the UWE GP-ED team informing the taxonomy development included: 38 telephone interviews conducted with senior staff at sites which had applied for capital funding for GP streaming in 2017, findings from 5 early case study sites and results of searches of routinely collected data and publicly available documents. This collaboration enabled us to obtain data for 41 English EDs from additional sources [NHS England, Care Quality Commission (CQC) reports] totalling information on 62% of (n = 118/189) all Type 1 EDs in England and Wales. 5
The data demonstrated the complexity of models in use and inconsistency in the language being used to describe the service models. Stakeholders recognised the importance of any taxonomy being able to effectively represent the complexity of GP-ED models. A key concept identified was the difficulty of defining GP-ED models using distinct categories when there was a wide spectrum of how well integrated GPs were into EDs and the level of integration of different processes could vary within individual sites.
The two research teams used the data to collaboratively develop a taxonomy with two parts, the first describing the ‘form’ of the GP-ED model in terms of where patients were seen, and the second describing a spectrum of integration across a variety of constructs which could influence how the model ‘functioned’. 5
Taxonomy produced
Part 1 describes the form of GP-ED models:
First, the GP-ED model was classified as being either inside or outside the ED. These categories were then subdivided into the following groups:
-
Inside–integrated: GPs are fully integrated with the emergency medicine service.
-
Inside–parallel: GPs provide a separate service within the ED, for patients with primary care type problems.
-
Outside–onsite: The GP service is elsewhere on the hospital site.
-
Outside–offsite: The primary care service is off-site and may include telephone advice via 111, or pharmacies, dentists, opticians, urgent care centres or registered in-hours or OOHs primary care services. As these services are distinct from ED provision, they were not represented further in the study. 5
Part 2 describes several constructs which influence how GP-ED models function, represented on a spectrum of integration with a typical ED service at one end and a typical primary care service at the other, outlined in Figure 3.
FIGURE 3.
Conceptual model identifying constructs that influence the function of primary care services in or alongside EDs from Cooper et al. 5 published via CC BY 4.0.

We used this taxonomy to categorise our case study sites (though position on some elements of the spectrum may vary day to day), inform case site sampling and to develop a toolkit for EDs (see Chapter 8).
Marker conditions
As outlined in Chapter 2, our early scoping of the literature identified nine groups of conditions that could potentially be used as ‘marker conditions’ during Phase 2. Before our stakeholder meeting, we discussed the findings of the scoping review and survey within our co-applicant team exploring the pros and cons of including individual conditions. Three additional conditions identified by the survey respondents were added (menorrhagia, chest pain and ankle injury). Following the discussions, the co-applicants independently selected their top five conditions. The ACSC work focused on diagnosis rather than presenting complaint. The team considered it important to view the clinician’s management of undifferentiated patients; therefore, the presenting complaint was equally important. The top 10 presenting complaints with associated exemplar diagnoses following the co-applicant ranking were presented at the stakeholder conference for discussion in a modified nominal group technique exercise. 136 The list had been circulated before the event with explanatory information following input from the co-applicant public contributors. Attendees were invited to complete their top five rankings at registration. The results were presented and discussed, with some conditions then added as a result of the discussions (e.g. abdominal pain). Attendees independently ranked the conditions again at the end (32 ranked forms collected). Conditions were given a score out of 5 based on the perceived likelihood of providing useful data to test our theories about potential management differences between GPs and ED doctors with the highest scoring conditions being: child with a fever; adult with cough/shortness of breath, abdominal pain, back pain or chest pain. These were selected for use in Phase 2 data collection. Due to difficulties in patient recruitment, a sixth condition was added at a co-applicant meeting in November 2018 (while all case sites were still open to patient recruitment). Headache (one of the conditions ranked next highest by the stakeholders) was chosen as, if not investigated appropriately, it could lead to a missed serious diagnosis. 137
Learning from previous patient safety incidents
The final activity undertaken in Phase 1 focused on the safety of GP-ED models. Our rapid review had identified limited data specific to patient safety. Several members of our research team have significant expertise in patient safety research and identified two sources of additional data which could be used to develop further theories for testing in Phase 2. This process has been fully described in our published paper (see Project web page)14 and is summarised briefly below.
Data sources
Coroners’ reports
The Chief Coroner publishes Reports to Prevent Future Deaths made by coroners as part of their statutory duty when they believe future action should be taken. 138 We searched those published between 2013 and August 2018 and identified 9 of the 1347 reports identified related to a GP-ED service.
National Reporting and Learning System patient safety incident reports
This database holds over 18 million incident reports, usually completed by healthcare staff in England and Wales. These anonymised reports include structured sections (e.g. demographics) and unstructured sections (descriptions of what happened, potential contributors, ideas about future prevention). We searched reports from 3 January 2005 to 30 November 2015 to identify reports relating to diagnostic errors made in GP-ED services. 14 We focused our search on diagnostic errors as these were a common theme among the Coroner’s reports. We identified 217 relevant reports from the 1878 reports screened.
Data analysis
We used the PISA framework for classifying patient safety incident reports as a first step to code the data139 and summarised the findings using descriptive statistics. We then thematically analysed reports, grouped by features identified by this classification process, to identify key themes and potential learning. We used the realist process of retroduction140 to infer why incidents may have occurred when this was not explicit in the reports and developed CMOCs from these data. These CMOCs were validated through discussions within our co-applicant group.
Results
Three CMOs were developed using these reports, described in turn below.
Difficulty with triage and streaming processes
If patients presenting to the ED (C) are assessed for streaming but the streaming nurse is unclear which patients are appropriate (due to unclear guidance or inexperience) (M) or the initial assessment is inadequate (limited history or lack of physiological observations) (M), then higher-risk patients may be streamed to the GP service (O).
Data from one Coroner’s Report and 29 NRLS reports were used to develop this theory which expands our RRR theory about how clinicians use their own personal experience and expectations when interpreting streaming guidance. They highlighted a lack of understanding of which patients were appropriate for the GP service (n = 7), delays in the initial assessment of patients streamed to the GP service (n = 14) and inadequate assessment including basic observations (n = 13), as contributing to safety events. Common presentations included patients presenting with chest pain (n = 10) and unwell children (n = 7).
Material in this chapter/section has been reproduced from Edwards et al. 19 This is an open access article distributed under the terms of the Creative Commons CC-BY license, which permits unrestricted use, distribution and reproduction in any medium, provided the original work is properly cited.
Errors in clinical decision-making
If patients present to the ED with a condition not usually dealt with in primary care (C) and are seen by a GP who may have inadequate knowledge or skillset for the condition (M), the patient may be at risk of a mismanagement (O).
This new theory was developed directly from these data. Six Coroners’ reports and 59 NRLS reports described under-investigation of patients with serious conditions who were seen in GP-ED models. Fractures were the most reported condition in this group (n = 27), followed by headache (n = 10), deep-vein thrombosis (n = 5), acute coronary syndrome (n = 4) and unwell infants (n = 4). Eighty-seven NRLS reports related to GPs interpreting X-rays incorrectly (a task outside their usual role in the community) when the fractures were subsequently identified through radiology reporting systems at a later date. Five NRLS reports highlighted specific concerns about a lack of knowledge of ED child safeguarding procedures.
Inadequate referral pathways and communication between services
If there is poor communication between the GP service and the ED service (C) because of a lack of awareness about capacity (M), a lack of awareness that investigations have already been requested (M) or inadequate referral pathways (M), then patient assessment and treatment may be delayed (O).
One study identified in the RRR described a lack of communication between the GP and ED services delaying patient assessment and treatment. 7 One Coroner’s report and 21 NRLS reports described inadequate referral pathways from community or ED GP services as contributing towards diagnostic errors. Five other NRLS reports described miscommunication between services about capacity (sometimes caused by incompatible IT systems) causing patients to get ‘lost’ in the system, sometimes leading to treatment delays. One Coroner’s and one NRLS report identified understaffing as contributing towards delayed assessment and diagnosis. Another Coroner’s report described how a lack of communication with the patient’s usual GP about attendances at a walk-in centre may have contributed towards the death of a baby. Inferences were made that some patients may choose these settings as a first point of call rather than their registered GP, affecting communication between services and continuity of care.
Summary of Phase 1 findings
Our RRR of 96 papers developed 6 key theories for further testing relating to the effectiveness of streaming, role of the GP, patient satisfaction, safety, cost effectiveness and provider-induced demand. Our service evaluation described how GP-ED models were being used (or not) at 77 EDs across England and Wales, with our follow-up interviews providing additional detail about 21 sites. These data informed the development of a taxonomy of GP-ED models and a list of ‘marker conditions’ which might be managed differently by GPs and ED doctors. Analysis of Coroners’ and NRLS reports enabled us to develop three additional theories about the safety of GP ED models. Chapter 4 describes how case study sites were selected and qualitative data collected to further test the theories from Phase 1.
Chapter 4 Phase 2 qualitative results
Overview of Phase 2 qualitative work
This chapter describes the collection and analysis of qualitative data from 13 ED case sites and how these data were used to further develop and refine our theories.
Data collection methods
Case site selection
Phase 1 data (survey and interviews) were used to identify potential case sites from the three different GP-ED models described in our taxonomy and potential control sites with no GP-ED model. We selected 13 of the 21 potential sites for maximum diversity looking to represent a variety of geographical locations, size of ED and experiences of the GP-ED service resulting in our sample of 3 inside–integrated sites, 4 inside–parallel sites (1 was reclassified following the visit), 3 outside–onsite sites and 3 control sites (with no GP-ED model). 5
Data collection at case sites
Two researchers (AC, ME) visited each site (2–4 days) with a GP-ED model to complete fieldwork (see Appendix 1 for researcher characteristics). One researcher attended the control sites for a 1-day visit (ME). Ahead of visits, we provided sites with information leaflets and posters for staff to inform them about the study and planned research activities. We requested to review relevant patient safety incident reports regarding the associated GP service during our visit. Before the visit, the two researchers familiarised themselves with the survey and CD interview data already held on that ED, also the most recent CQC report if available, to consider which of our theories might be useful to test and refine at each site.
Observations
We met the CD on arrival for an introductory tour to orientate us to the department and meet key staff members. We then independently observed various areas of the ED (e.g. triage and streaming areas) and spoke informally to a wide variety of staff, making field-notes and documenting key findings. Discussion topics were guided by the theories to which the staff member could most helpfully contribute. We met regularly during the day to discuss findings and prioritise further topics to explore. Eleven visits were conducted mid-week (usually Monday–Wednesday) with six including observations into the evening. Two visits were conducted over a weekend. Most GP-ED services were not 24 hours; therefore, night observations were not conducted. Two researchers spent approximately 8 hours per day in the ED. We completed 23 sets of field notes (2 for each GP-ED site and 1 for each control site).
Interviews with staff
We interviewed key staff members at each case site to explore, test and refine theories developed from Phase 1 and those emerging throughout the site visits. We carried out formal realist interviews,34 structured around the theories most relevant to that individual and their role during our case study visits using a tailored interview guides for each staff group (see Project web page). Most staff approached agreed to an interview; however, at some EDs, we experienced difficulties in recruitment because the department was very busy (e.g. Parallel-3).
We used a realist teacher–learner interview technique, sharing our theories with the interviewee for discussion. 34 In total, we interviewed 106 members of ED staff (including 26 GPs) across all 13 sites34 (see Tables 21 and 22 in Appendix 2 for a summary of staff interviewed at each site). Interviews lasted 10–60 minutes and were audio-recorded and transcribed. Interview data from CDs collected during Phase 1 were also taken forward into the Phase 2 analysis.
Patient safety incident reports
In total, 14 anonymised reports were provided from 6 sites (either during the visit or in response to 3 follow-up reminders). The reports were uploaded to a remotely accessible secure computer platform (PISA platform) at Cardiff University for analysis. Reports were included if there was evidence of a patient safety incident involving the GP-ED model.
Patient interviews
We aimed to interview up to 12 patients per case site (6 seen by an ED doctor and 6 seen by a GP). The sampling frame included patients who had one of the six marker conditions identified in Phase 1 (see Chapter 3). We used two methods of recruitment:
-
By mail – NHS research nurses screened electronic records to identify patients who had attended the ED in the last 3 months, with a marker condition. Eligible patients were mailed study information and consent forms were returned to the research team to arrange a telephone appointment.
-
At the hospital – During site visits, a member of hospital staff would identify eligible patients while they were in the ED and provide them with participant information and consent form (see Project web page). Patients were asked to return the consent form to the research team and an interview was then arranged. Researchers also took consent from some patients at the ED and arranged a telephone interview.
Overall, we invited 748 patients to take part in a telephone interview. Forty-three patients (6%) provided initial consent, but 19 were subsequently uncontactable. Twenty-four patients (3%) were subsequently interviewed (see Table 23 in Appendix 3 for summary of participant characteristics). We faced several challenges with recruiting patients, which we have reported in a published paper to maximise learning for future studies. 18 Eligibility criteria limited the number of patients who could be invited for an interview, and complicated IT systems made identifying eligible patients difficult and time-consuming. At some sites, there was no or little research support available to recruit patients, and a lack of personal interaction between researchers and patients may have limited recruitment.
The interview guide (see Project web page) explored patients’ motivations for attending the ED and their expectations and experiences in terms of seeing an ED clinician or a primary care clinician to develop our theories from Phase 1. We (ME) interviewed 24 patients by telephone from 1 week to 3 months after their ED visit. Nine of the 13 case study sites were represented (including all models). We included data from two patients at an additional site with no GP model which were collected when the site was being considered for inclusion as a case study site (CD interview site 5). Interviews lasted between 9 and 39 minutes (average 16 minutes).
Ethical approval
Ethical approval for Phase 2 data collection was provided by South Wales Research Ethics Committee REC reference 17/WA/0328.
Data analysis
Local patient safety incident ‘Datix’ reports
We (AC) coded reports that described an incident involving the GP service in or alongside the ED using the multiaxial PISA coding framework. 139 This identifies the primary incident type closest to the patient, and any contributing incidents using the principles of recursive analysis. The reports received described instances of:
-
inadequate patient management (n = 3)
-
triage or streaming-related errors (n = 3)
-
errors with handwritten prescriptions (n = 3)
-
problems with investigation follow-up (n = 2)
-
diagnostic error (n = 1)
-
referral delay (n = 1).
Findings from the incident report analysis fed into the realist interviews to explore mechanisms that led to the unintended outcomes.
Observation and interview data
Our audio-recorded interviews were transcribed verbatim. We created NVivo 11 project files for each GP-ED model (Integrated, Parallel, Outside and Control) which included all qualitative data available (Phase 1 interviews with CD, Phase 2 field notes and staff and patient interviews) for each site. We then created codes for each theory area (e.g. GP role, patient safety).
Developing and refining theories
We used the coded data to generate ‘if, then, because statements’. These statements were used to begin the theorising process at the level of individual data extracts. They were exported to Microsoft Excel spreadsheets, one page for each model type, and consolidated these using the three questions, based on the questions described by Pearson et al.:141
-
Is this statement novel (and can therefore be imported directly into the CMO)? and/or
-
If the statement was not novel, does this challenge the explanations made in related statements? and/or
-
Does this statement add important refinements to the understanding of contexts, mechanisms or outcomes?
Once we had developed CMOCs for each service model, we created a spreadsheet for each of the main themes to map and analyse CMOCs between service models. For the patient safety theme, the local patient safety incident ‘Datix’ report data were used to support existing CMOCs or generate new ones.
The CMOCs were then synthesised using Pawson’s theory-building processes. 23 For example, adjudication principles were used to evaluate the relative strength of the different evidence sources. Based on meta-ethnography principles, we rated data obtained during observations as most trustworthy, followed by participants’ descriptions of their own behaviour, then participants’ accounts of the behaviour of others and finally our own interpretations of the data. 23 Reconciliation principles were used to explain contextual differences that may have resulted in contradicting outcomes. Juxtaposition principles were used when reported outcomes could be explained by observation or interview data. Theories that resulted in the same outcome could be consolidated across service models into a multifaceted explanation, rival explanations could be situated depending on which mechanisms were activated in which contexts. Independent researchers took the lead for different themes with findings presented and discussed weekly within the study team. (Please see Appendix 4 for an outline of the steps in the realist analysis.)
Engaging with our co-applicant group
We presented our CMOCs to study co-applicants in regular meetings (five 2-hour meetings between May 2017 and November 2018) and discussed our interpretations. During these meetings, members of the group contributed their expert knowledge to help in refining our theories.
Second stakeholder event
This full-day event was held in Bristol on 3 December 2019 after all case site visits had been completed (see Project web page). There were 70 attendees (13 public contributors, 11 NHS managers, 8 commissioners/policy makers, 8 ED doctors, 6 GPs, 2 nurses, 19 academic staff from this study and the GP-ED study and 3 admin/support staff). Theories refined from qualitative data about GP role and streaming mechanisms were presented. Small group work with 8–10 participants, facilitated by a co-applicant, focused on validation of the patient experience and safety findings with stakeholder feedback on quality improvement ideas and potential measurements of safe care. The facilitators’ notes were collected, and findings fed back in a plenary session. Stakeholder input for these areas was sought, particularly, due to the poor patient recruitment for interviews and the low numbers of patient safety incident reports collected.
Using middle-range theories to interpret findings and create programme theories
Realist studies use knowledge from middle-range theories of social phenomena to develop CMOCs and to help explain causation in a programme theory. 23,142 We searched for theories and conceptual frameworks from a wide range of literature relating to medical decision-making, emergency care nursing, patient experience of emergency care, health economics and health service delivery. This was an informal process in which the researchers used their existing knowledge and targeted electronic searches to explain recurring phenomena identified during the data analysis. When we identified potentially suitable theories or frameworks, we discussed them as a research team and with co-applicants with specialist knowledge to evaluate their relevance. Once selected, we used them to help interpret our findings and develop our theories as outlined below.
Results
Overview of case sites
Table 4 provides an overview of the characteristics of the sites visited. Sites were spread across England [North (4), Central (4), South (4) and Wales (1)].
Primary care model | Site reference | Hospital size and serving population | Setting | ED attendances per year | GP service commissioning organisation | GP streaming | GP access to acute investigations | Date GP model introduced | Hours of coverage per week in GP service | Staff mix in GP service |
---|---|---|---|---|---|---|---|---|---|---|
No GP service | Control-1 | 350 beds; trust serves 500,000 people in 4 hospitals | Town | 65,000 | ||||||
Control-2 | 500 beds for the 2 hospitals in trust; population 250,000 people | Town | 55,000 | |||||||
Control-3 | 800 beds; population 600,000 people | City | 84,000 | |||||||
Inside–integrated | Integrated-1 | 550 beds; population 350,000 people | Small town in rural area | 65,000 | NHS Trust | Yes, and GPs self-select | Yes | 2017 | 8 a.m.–11 p.m., 7 days a week; > 80 hours | GPs |
Integrated-2 | 150 beds; population 120,000 people | Rural area | 20,000 | NHS Trust | No | Yes | 2017 | 8 a.m.–6 p.m., 3–4 days per week; 33–40 hours | GPs | |
Integrated-3 | 450 beds; population 450,000 people | Small town | 78,000 | NHS Trust | No | Yes | 2009–10 | 10 a.m.–10 p.m., 7 days a week; 65–72 hours | GPs | |
Inside–parallel | Parallel-1 | 400 beds; population 200,000 people | Rural area | 56,000 | Primary care provider |
Yes | Yes, but encouraged not to use | 2015 |
6 a.m.–11 p.m. weekdays, 10 a.m.–10 p.m. weekends; 49–56 hours |
GPs and ANPs |
Parallel-2 | 600 beds; population 200,000 people | Large town | 115,000 |
Primary care provider. GPs hold honorary contracts with NHS Trust. | Yes, plus 111 and walk-in patients | Yes, but encouraged not to use | 2015 | 10 a.m.–10 p.m. 7 days a week; 80 hours | GPs and ANPs | |
Parallel-3 | 1000 beds; population 1.3 million people | Large city | 165,000 | Primary care provider | Model 1: yes Model 2: no |
Model 1: no Model 2: yes | 2012 | 8 a.m.–9 p.m., 5 days a week; 57–64 hours | GPs | |
Parallel-4 | 700 beds; population 500,000 people | City | 84,000 | Primary care provider until May 2018 then NHS Trust | Yes, plus 111 and walk-in patients | No | 2014 | 10 a.m.–10 p.m., 7 days a week; 80 hours | GPs, ANPs, paramedics | |
Outside–onsite | Outside-1 | 600 beds; population 400,000 people | Town | 89,000 | Local GP federation | Yes, plus 111 and walk-in patients | No | 2017 | 24 hours a day (includes OOH contract), 7 days a week; > 80 hours | GPs and ANPs |
Outside-2 | 800 beds; population 500,000 people | Large city hospital adults only | 140,000 | NHS Trust and a Locum agency | Yes, plus 111 and walk-in patients | No | 2005 | 8 a.m.–10 p.m., 7 days per week; 73–80 hours | Locum GPs, mainly ANPs | |
Outside-3 | 550 beds; population 250,000 people | City | 70,000 | NHS Trust |
Yes, plus 111 patients (no walk-ins) | Yes | 2017 | 10 a.m.–10 p.m., 5 days per week; 41–48 hours | GPs and ED ANPs |
In addition to the variation shown in Table 4, our site visits revealed additional differences in how primary care services operated within each model, as briefly described below.
Inside–integrated sites
One small rural site (Integrated-2) employed GPs who were interested and experienced in emergency medicine to work in the role of middle-grade emergency medicine clinicians due to difficulties in recruiting ED clinicians. Another site (Integrated-1) described an ‘integrated front door’ with nurses streaming to GPs who worked as part of a wider team of clinicians (including specialist nurses, an emergency care practitioner, physiotherapists) providing a 24-hour service. There was an ethos of ‘sorting patients out’ once they attended the ED, due in part to the wide geographical area it served, rather than referring patients back to community primary care. There, GPs were supported to pursue their own interests, using either a traditional GP approach or managing ‘emergency medicine’ type patients. At Integrated-3, the GPs were led by a GP consultant, and saw elderly 999 admissions and some referrals for additional investigations from community primary care in the day and primary care type patients in the evenings.
Inside–parallel sites
Two sites (Parallel-1, Parallel-3) were observed to favour redirecting patients to community primary care when possible. Parallel-3 had a non-clinical staff member in a specific role (‘navigator’) to enable this and Parallel-1 favoured sending patients to the co-located GP OOH service rather than them being seen in the ED by a GP. The GP-ED service at Parallel-1 operated only during the OOH period at the time of the visit and has since ceased. In contrast, at Parallel-2, where an ED consultant worked alongside GPs in a former walk-in centre, there was a preference for addressing patients’ problems in the ED. At Parallel-1, Parallel-3 and Parallel-4, GPs were noted to be seeing low numbers of patients. This related to a limited number of sessions (Parallel-3), and strict streaming criteria limiting the number of eligible patients (Parallel-1 and Parallel-4). GPs at Parallel-4 were also expected to supervise and support prescribing among the ACPs in the primary care service. At Parallel-2, there were concerns that when the GP-ED model, which seemed to generate its own demand, closed in the evening, patients were then seen in the ED which increased their workload.
Outside–onsite sites
Triage and streaming processes varied across these sites. It was possible to ‘walk into’ the GP-ED service at sites Outside-1 and Outside-2, and at Outside-2 patients were then assessed by a primary care nurse. At Outside-1, most patients were not triaged (with the exception of under-16s and people with mental health problems) but could be advised to attend the main ED next door by the receptionist if they were presenting with urgent complaints, for example, chest pain. Patients at Outside-3 went through two streaming processes, first being streamed to attend the GP-ED service from the ED or 111, and then being assessed again by a primary care nurse using a computer algorithm. At Outside-3, there was little distinct primary care identity, with ED doctors and ED ANPs also working in the service. The service was nurse led at Outside-2, supported by a GP locum. At Outside-1, a stronger GP identity was identified, facilitated by clear leadership, induction and appraisal processes and an IT system shared with community primary care.
Control sites
All three control sites had previously used GPs in the department in some capacity (closely linked to the GP OOH service in Control-1, in an ED clinician role in Control-3). Control-2 reported a lack of demand for a specific GP-ED service. Concerns were also raised about the suitability of ED patients for streaming to a GP service from nursing staff (Control-1) and senior ED clinicians (Control-3). At two sites (Control-1 and Control-2), patients were often referred having already seen their own GP in the community. At Control-3, it was reported that ‘exit block’ (transferring patients from ED to hospital wards) was the main challenge in managing flow through the department rather than patients attending with primary care type problems.
Ethos and purpose of the service
As identified in our taxonomy (see Chapter 3), a GP-ED model may aim to provide more of an EM service or more of a primary care service. 5 While this often followed the form (e.g. more integrated – more EM; more separate – more primary care), there were also examples of crossover (e.g. an outside–onsite model which had access to ED investigations) where the form of the service did not always align with the proposed functions.
Our taxonomy recognised the importance of local leadership and culture in influencing whether clinicians took on an emergency medicine or primary care type role (see Chapter 3). 5 During case site visits, we developed an understanding of local culture and ‘ethos’ of the service, often seemingly driven by the way in which the CD viewed the role of the GP-ED model and varied significantly.
At some sites, there was a clear vision that everything possible should be done when patients attended the ED (Integrated-1, Parallel-2).
We wouldn’t just be a fob off service, so we weren’t just going to see people and say ‘you need to go back and see your GP about that’, no, we were going to sort you out.
Clinical Director, Parallel-2
At two EDs located in a rural location, redirecting patients back to community service was not feasible because patients would have to travel too far (Integrated-1 and Integrated-2). However, at some sites, a strong gatekeeping ethos was necessary and primary care patients were redirected to community primary care. This occurred where there was less consistent GP cover (Parallel-1), or the hospital was in a densely populated area with a high volume of demand (Parallel-3), or a high level of walk-in urgent care attendances (Outside-1 and Outside-2) or a high rate of 111 referrals (Parallel-2).
We will navigate patients and either suggest they see the GP that’s standing here with us, but that’s only a couple of mornings a week, or we will navigate them back to their own GP.
Clinical Director, Parallel-3
Additionally, there were examples of sites where the scope of the work of the GP was strictly limited by either the ED (Parallel-1) or the private provider of the GP service (Parallel-4) due to concerns about the perceived risks of the GP-ED model.
Funding the service
Interview data identified complicated funding arrangements for GP-ED models. Concerns were raised that the distance between clinical commissioning groups (CCGs) and the services they funded meant that some CCGs were funding multiple services to perform largely similar functions, while multiple CCGs might fund different services serving the same community. 143 Separate funding schemes were seen to disincentivise co-operation across services, particularly at Integrated models where this would increase outgoings. Views were mixed on more integrated funding models. Staff from the control sites cited a lack of dedicated funds as a barrier to implementing GP-ED models, integrated funding models were seen as favourable at the Parallel sites, while the Outside models reported successfully developing single health economies in which Hospital Trusts jointly funded and governed community and urgent care.
Managers at the control sites suggested that employing Advanced Practitioners and Physician Associates might be more cost-effective than recruiting GPs. However, managers at the sites with GP-ED models recognised that the increased workload for ED doctors associated with supervising these staff groups was a potential concern.
Staffing the service
There were GPs with a range of experience across all models of service. Those working in parallel and outside models mostly also had community GP roles in addition to working in the ED, while integrated models had mostly experienced GPs with a special interest in emergency medicine who only worked in the ED (see Appendix 3).
Recruiting local GPs was seen as desirable because they understood the local community and had a ‘vested interest’ in providing high-quality care at the ED. However, some services struggled to recruit because multiple services were trying to access the same pool of local staff.
Many sites had experiences of using private providers of GP services. However, there were problems with recruitment and staffing, which meant that ED staff had to cover their work. Another challenge with using private providers was a lack of accountability due to convoluted commissioning procedures.
We have kind of a strange arrangement whereby the CCG pay an external company … [to] run the in hours service. But then they sublet the out-of-hours service to yet another external provider … they probably ran the whole year at about two thirds of our slots being filled … there seems very little that the CCG could kind of hold [company name] to account.
Finance Manager, Parallel-3
Drivers of demand
Rising demand at EDs was seen as inevitable, relating to multiple factors including the aging population with associated increased complexity of patients presenting at ED. The proportion of demand related to patients with primary care type problems was seen to vary in relation to several factors including the characteristics of the local population (e.g. transient populations unable to access community primary care) and the capacity and performance of local community primary care.
At some sites, there was a perception that people were generally good judges of when they required ED level care, with staff noting that the probability of serious illness among people attending the ED is greater than that among patients attending primary care. One CD attributed this to the local largely well-educated middle-class population selecting services appropriately (Integrated-1). However, at other sites, staff reported patients choosing to attend the ED for convenience, reporting an increased societal expectation of being able to address any health problem immediately.
It sort of reflects current society, that we need everything done now, so you get somebody coming in at 10 am because they couldn’t get a GP appointment until 4 pm for their chronic problem.
Clinical Director, CD interview site 16 – no GPs
In some areas, overstretched local primary care services referred patients to the ED, while staff at other sites believed that the capacity available in community primary care was responsible for attendances by people with primary care type problems. The 111 service also referred people directly to EDs, with some staff reporting concerns that ED attendance was not always necessary. A lack of access to community primary care or a culture of attending hospitals rather than GP services among some groups was also noted to drive demand.
There’s a huge student population who don’t tend to use their GPs, 15,000 patients apparently don’t have an allocated GP, there’s a big problem with the homeless, and a big problem with different ethnic groups … if your culture is that when your child is ill you bring them to a hospital, not take them to a GP, then it’s hard to overcome that cultural difference.
Clinical Director, Outside-2
I observed a patient come into ED triage with a headache and concerns because she had previously was investigated for stroke-like symptoms. She told the nurse that she had come to the ED because it was hard to get an appointment with her GP. After checking her obs (which were normal), she was streamed to be seen for minor illness in the walk-in-centre.
Observation of triage, Parallel-2
Service developments driving demand
There was concern at several case sites that publicising a new GP-ED model would drive demand. At one site (Parallel-2), staff believed increased attendances were related to the significant publicity around a new building opening.
Our attendance rate went up by 30%. The trust wanted to go big bang into the press … We started to see whole populations coming to us which never came before.
ED consultant, Parallel-2
Two other sites intentionally limited the visibility of their GP-ED model to avoid generating additional demand (Integrated-1 and Parallel-4).
Improvements in the 111 service in one area (Parallel-2) enabled operators to review waiting times in urgent care services across the region and refer patients to services with shorter waiting times. This created additional demand from patients who might have otherwise attended a service elsewhere and this was a source of frustration for the ED staff.
Theory development and refinement across four themes
We now present data under four key themes:
-
streaming and flow
-
the role of the GP and how it influences clinical decision-making
-
communication between GP-ED models and the ED
-
patient experience.
Patient safety was recognised to be a key outcome in the first three themes and so data relating to safety are reported in these sections, rather than in a separate theme. For each theme, we explain the findings from Phase 1 to be explored, and present the data gathered to help develop and refine these theories during Phase 2. For each theme, we present CMOCs outlining our refined theories based on the qualitative data analysis.
Theme 1: streaming and flow
Our initial theory developed in Phase 1 described how staff involved in streaming interpret available guidance based on their own experience to influence which patients are streamed to GP-ED models.
We observed three main pathways where patients are streamed to ED clinicians or primary care clinicians: front door streaming (patients streamed by a nurse at the front door of the ED – before being booked in at reception); streaming inside the ED during a more complex assessment (including triage and streaming – after being booked in at reception); no primary care streaming (usual triage, with GPs self-selecting patients). 144 These pathways were influenced by whether the primary care service was ‘inside’ or ‘outside’ the ED and were often adapted based on local circumstances such as the department layout, patient demand levels, skill mix and interests of primary care staff and the accessibility of community primary care services. Varied approaches to streaming were also implemented for specific patient groups (e.g. older people, children). Pathways were also in place to redirect patients with non-urgent primary care problems to community-based services. Adaptations to protocols were employed, based on staffing, patient demand and links to community primary care (see Project web page).
In this section, we discuss our theories relating to the quality of streaming decisions, the efficiency of moving patients through the ED and the ways in which oversight of the streaming process can improve the service.
Data sources
The new and refined theories presented in this chapter were developed from interviews with the ED staff and GPs and observations of primary care streaming.
Applying formal theory
We used a middle-range theory that has previously been applied to triage decision-making to aid interpretation of our findings (the Revised Cognitive Continuum Theory). 145 This helped explain how experienced and senior nurses stream patients from the ED front door using their intuitive and reflective judgement to make quick decisions. In EDs where nurses carry out more complex assessments inside the ED, they mostly make use of decision tools and computerised triage systems using system-aided judgement (requiring less intuition).
Findings
Experience of the staff member involved in streaming
During the RRR, we developed two candidate theories outlining possible advantages and disadvantages of GPs performing streaming at the ED front door. During our site visits, there was no evidence of GPs routinely acting in a streaming role. However, at Integrated-3, the lead GP sometimes saw patients during a triage assessment when the department was very busy. He reported that he was able to improve patient flow by ‘seeing and treating’ patients during their initial assessment. However, it was not felt to be sustainable nor a desirable role for other GPs due to the intensity of the decision-making and a higher patient safety risk.
it really helps just clear the triage stream for a bit, but what we’ve found with that is it’s very labour intensive, it’s hard work, difficult … it is high pressure, it’s high turnover, and it’s quite high risk, because you are making very quick decisions.
GP, Integrated-3
All outside models and one parallel model (Parallel-3) in a large hospital with high attendances used senior nurses and nurse practitioners in front door streaming. Clinicians at these sites recognised the importance experienced streaming nurses at the front door.
We have to have experience up front because it’s an extremely important job getting them in the right place.
ED Dr, Outside-1
Where streaming was inside the ED (most parallel models and Integrated-1), there was often a mix of senior nurses, nurse practitioners and less experienced nurses making streaming decisions during a complex triage assessment.
Highly experienced nurses were reported to make quicker and better-quality streaming decisions, allowing patients to move through the ED more quickly. They appeared to use both intuitive and reflective judgement in their decision-making and to have more confidence in their decision-making, leading to more effective streaming (patients streamed to the service/clinician most appropriate for their needs). Other ED clinicians reported that senior nursing staff were also more confident about redirecting patients with non-urgent problems elsewhere and communicating their reasoning with patients, helping improvements in patient flow.
they’ll be used to making those decisions about discharging patients, so they’ll often feel comfortable to say, ‘that’s such a minor injury, you don’t need to wait and be seen’, or ‘that’s very much (a community) primary care condition, we’re not going to be able to help you with that …’
Clinical Director, Parallel-1
Less experienced nurses took longer to carry out assessments and were more cautious in making streaming decisions. They were sometimes reported to order unnecessary investigations, so some patients waited longer to be seen. They also experienced delays in obtaining support with their decisions because they were less confident in communicating with senior ED staff and GPs to influence their decisions about which patients might be appropriate for them to see. Thus, there were fewer opportunities for peer-aided judgment leading to poorer patient flow.
… they will go in too deep to why people have come, which then takes time … they have less influence … some of them don’t know how to approach sort of people … and talk to them in a medical way that speeds this transition along.
Advanced Nurse Practitioner, Outside-3
They appeared to rely more on system-aided judgement (e.g. guidelines and algorithms) to inform their decisions. Where streaming guidance was not well developed, less experienced nurses were thought to be more at risk of making errors in their streaming decisions. Some GPs reported that less experienced nurses lacked knowledge of the types of patients that GPs manage and their scope of practice in terms of access to investigations and making referrals.
Sometimes, the right decisions aren’t necessarily being made … there just isn’t that experience and that intuitive knowledge … .
Emergency Nurse Practitioner, Integrated-1
At some sites, non-clinical staff became involved in the triage and streaming process. At Parallel-4, understaffing meant that the streaming nurse also had to administer treatments. Consequently, receptionists with no clinical training had a list of patients’ presenting complaints about which to call the triage nurse to attempt to avoid delays.
Streaming guidelines and training
Emergency department doctors, nurses and GPs reported that some streaming nurses had difficulty identifying patients with appropriate conditions for the GP service (Parallel-1, Parallel-2, Parallel-3 and Outside-1). GPs also reported that sometimes the nurse did not appear to be aware which presenting conditions were appropriate for the service.
It’s a bit hit and miss, it depends on what the help of the triage nurse is, there’s no set system … sometimes patients you’re seeing are inappropriate … but there’s lots of things that I could be seeing which I don’t end up seeing, because they’re deemed to be an A&E case.
GP, Parallel-1
Locally adapted guidance, protocols and training supported nurses making streaming decisions (promoting system-aided judgement), aiming to ensure patients were streamed to the most appropriate clinician or service.
We have our streaming assessment workbook … they undergo numerous assessments and then they have a final assessment and then when we’re happy that they are competent they are signed off and they can do it themselves.
Senior nurse, Parallel-4
Guidance relevant to the local primary care service was felt important but an experienced streaming nurse using clinical judgement was felt to be essential (Parallel-3, Parallel-4, Outside-1). Relying too heavily on guidance could be problematic: for example, junior nurses sometimes were not experienced enough to ask intuitive questions, leading to patients being inappropriately streamed.
It’s criteria led, but I think experience comes into it a lot … when a junior member of staff sees a patient in the streaming cubicle, they normally only hear the duration and the problem, the actual history of why they’ve come today gets missed.
Advanced Nurse Practitioner, Outside-3
With no clear guidance available to support their judgement and decision-making, less experienced nurses were reported to have sometimes missed ‘red flags’, and inappropriately streamed patients needing emergency care to a primary care service, causing risk of healthcare-associated harm. Thus, when junior nurses made streaming decisions based solely on their limited clinical experience (intuitive and reflective judgements), there was considered more scope for error with potential for delays in assessment and appropriate treatment when patients needed to be redirected back to the ED.
A patient presented at a triage assessment with a rash on his leg and was streamed to the out-of-hours GP. The GP sent him back to wait to be seen in the emergency department for further investigations with suspected deep-vein thrombosis.
Observation of triage, Parallel-1
Where there was no clear streaming guidance established, some of the ED staff perceived GPs to be selective and seeing fewer patients than expected. Conversely, where guidance was too strict and GPs did not have flexibility about which patients they could see, the GP-ED model was also perceived to have less impact on overall patient flow within the department.
Material in this section has been reproduced from Edwards et al. 146 This is an open access article distributed under the terms of the Creative Commons CC-BY license, which permits unrestricted use, distribution and reproduction in any medium, provided the original work is properly cited.
Teamworking and communication
When the ED and primary care staff had positive teamworking relationships, there was good communication between the ED and primary care team. This enabled ED nurses to develop better knowledge of the skillset in the primary care team and which patients they could stream to them, so patients were more appropriately streamed.
it’s developing an understanding between ourselves and the urgent care team about what can and can’t be seen
Nurse, Parallel-2
Our RRR identified that GPs were just one part of the wider ED and hospital team and recognised that where ED staffing was inadequate or transferring patients to wards was delayed, a GP-ED model was unlikely to influence flow through the department. This theory was supported by data from a control site where a GP-ED model had previously been ineffective.
So we’re throwing extra resource at the front door when actually it’s the back end of the hospital where the biggest problem is, and ironically if community care was better which is part of the GP remit, then we might not have such a big problem at the front door.
Clinical Director, Control-3
We developed two new theories based on these data. The first describes the interaction between the experience of the streamer, their understanding of the GP-ED service and the availability of relevant streaming guidelines. The second describes further inefficiencies in streaming systems that we identified.
Refined theory – quality of streaming decisions
Staff performing streaming to primary care integrate their clinical experience, knowledge of the local GP-ED service and local guidelines to make streaming decisions. When staff are highly experienced (C), they are competent and confident in evaluating how to use this information (M) resulting in high-quality streaming decisions that ensure patients are appropriately directed to optimise patient flow through the department (O). If staff have the skills (C) to identify appropriate investigations for patients during the streaming process (M) and can arrange these, this may improve efficiency (O).
When staff have less clinical experience, less understanding of the GP-ED model or less clear guidance with which to work (C), they may take longer to assess patients, or miss important information (M), resulting in poorer-quality streaming decisions, and delays in patient care (O). If inexperienced staff (C) request unnecessary investigations during streaming (M), this can decrease efficiency (O).
Refined theory – efficiency in moving patients through the department
If there is no clear guidance about which patients primary care staff see or the guidance is too strict (C), then primary care staff may not see all patients that could potentially be seen by a primary care clinician (M), and streaming may not be effective in improving waiting times and patient flow in the ED (O). Additionally, when EDs are short staffed or experience ‘exit block’ (C), then GP-ED models (M) may not improve overall flow (O). If patients are inappropriately assessed and streamed to one service and then need to be (re-) streamed back to another service (C), they spend more time waiting to be seen (O) and may experience delays in receiving appropriate care and treatment (O).
Oversight of the streaming process
Good communication between services allowed streaming nurses to understand the capacity of the different streams which influenced streaming decisions (Outside-1).
In EDs where streaming was inside the ED, a senior staff member (e.g. ED consultant) screened the notes of patients already streamed to the ED to identify patients that could be changed to the primary care stream to improve flow (Integrated-1, Parallel-1, Parallel-4). At Outside-3, where streaming was from the front door, a (non-clinical) operational manager made decisions about suspending streaming to urgent care and redeploying ANPs from the front door to the Urgent Care Centre to see patients when the demand for primary care was high and capacity in the service was limited. This was to prevent primary care patients from waiting for extended periods of time and to enhance overall flow.
I’ll oversee exactly how many patients are in there, how many patients are going down there per hour and whether the GP is struggling or not.
Operations Manager, Outside-3
On Wednesday at 1.40 streaming to the UCC has been put on pause because the GP has 10 patients waiting. The operations manager has authorised this pause. All patients are now to be seen in the ED.
Observation of streaming Outside-3
Service improvements
Many case sites were happy to share learning about how and why the streaming process worked well in their experience and how it had been developed and modified to ensure appropriate patients were streamed to the GPs.
Strategies such as training and mentoring for nurses, local-level evaluation, developing local guidance and streaming pathways and creating physical spaces from which nurses can stream and to which patients can be streamed aimed to help improve flow.
We run rapid improvement workshops … we spot bits of the processing that aren’t right and think about how we could make it better …
Medical Director, Parallel-2
One site described how they felt the streaming guidance for young children was inadequate and after a ‘near miss’, the process was modified to include paediatric-specific early warning scores and healthcare assistant support, to ensure basic measurements could be taken (Integrated-1).
New theory: service improvements
On a day-to-day basis, if staff performing streaming or those with oversight of the streaming system have a good understanding of the current capacity and skillset available in the GP-ED model (C) and can adapt to these by changing which patients are streamed or by redeploying staff (M), then delays in patient care may be reduced (O).
In the longer term, when departments have systems in place (C) to learn from problems identified in the streaming process and to make changes to the process (M), appropriate patients can be safely streamed to the GP-ED service, and flow through the department may improve (O).
Theme summary
We have described the contextual factors and mechanisms that are perceived to affect whether patients are efficiently and safely streamed to primary care or emergency care clinicians when attending an ED. Figure 4 shows the connections between contexts, mechanisms and outcomes and illustrates how certain outcomes (e.g. streaming to the most appropriate service and improvement in patient flow) also influence patient safety. Strategic and operational management also influenced other contextual factors such as the experiences of nurses, the implementation of guidance, teamwork and communication. 146 We discuss how these findings can inform service design in our toolkit (see Project web page).
Theme 2: general practitioner role and influence on clinical decision-making
Our RRR identified that GPs could take on a range of different roles in GP-ED models, including acting more like ‘traditional’ GPs or more like emergency medicine clinicians. 15 Similarly, our taxonomy identified multiple different influences on whether the GP-ED model operated more like an emergency medicine or a primary care service. 5
This section now describes how our case site data helped to refine our initial theory about the role of the GP in GP-ED models.
Data sources
The theories in this section were largely developed from the first-hand accounts of GPs working in GP-ED models, supported by accounts from other primary care clinicians and our own observations at sites. One formal theory, Croskerry’s dual process model of clinical reasoning,31 was used to aid the interpretation of the results around diagnostic decision-making.
Findings
Contextual factors influencing general practitioner role
Our initial theory suggested that it was individual clinician factors that influenced the role GPs took on. However, our qualitative case study data also described the influence of wider system and department-level factors.
Hospitals where the GPs were employed by the NHS Trust, rather than a primary care provider, often functioned more like an emergency medicine service (Integrated-1, Integrated-2, Integrated-3). At one site, this related to a shortage of emergency medicine clinicians (Integrated-2). At one site where GPs had the autonomy to select their own patients, the CD described how GPs were perceived to improve flow within the department because they required no supervision.
[GPs] are senior decision makers, they’re autonomous … they’re not coming back to ask how to manage patients … they can just crack on and knock through the patients, so the amount of time the A&E consultant is spending down doing queue busting has massively dropped, and we can focus on the resus majors patients.
Clinical Director, Integrated-1
General practitioners were also observed to adopt an ED clinician role at one model (Outside-3) where geographical distance was overcome by GPs sending patients back to the ED for acute investigations including blood tests, X-rays and ultrasound scans. This service was also staffed by ED ANPs who were able to deal with minor injuries and therefore the unit was more integrated with the ED than other ‘outside’ services.
There were some sites where GPs were employed by the NHS Trust but delivered more traditional GP services (Parallel-4, Outside-2). At these sites, department-level organisational factors appeared to be the main drivers of the GP role. For example, services that were physically distanced from the ED, with no access to acute investigations, functioned more as traditional GP services (Parallel-4, Outside-1, Outside-2). Strict streaming protocols and different computer systems meant the GPs at some sites were only able to see patients who had been assessed as appropriate for GP management, supporting a traditional GP role (Parallel-4, Outside-1, Outside-2); this was facilitated by strong GP clinical leadership (Parallel-2, Outside-1). At another site, the ED CD’s leadership allowed flexibility of the role of the GPs, depending on the skillset and interest of the individual GPs (Integrated-1). Where governance responsibilities lay and whether GPs should follow ED protocols or treat the patient as they would in a community primary care setting was unclear at one inside–parallel service (Parallel-1).
I’m an autonomous practitioner, but then I’m working in A&E under another consultant effectively, so there are those problems there as well … It’s just who’s in charge isn’t it, and how you work, whether you follow A&E protocol or you follow your GP hat.
GP, Parallel-1
As highlighted in our original theory, individual GPs’ interests and expectations were also described to influence their roles and the function of services. At some integrated sites, GPs described how they had chosen to work there because of their interest in emergency medicine. At another integrated site (Integrated-3), GPs were encouraged to maintain a traditional GP approach in the evening when the department experienced more walk-in patients. Individual GPs’ experience was perceived to facilitate a traditional GP role in this context, with this site (Integrated-3) reporting that they actively recruited experienced GPs.
How clinicians decide their role
We used our case site interviews with GPs to explore how they felt that working in ED settings influenced (or not) their clinical decision-making.
Using a general practitioner approach
Some GPs who worked in a service where they had no access to acute investigations and often a strict streaming process, reported that the patient cohort they managed was very similar to usual primary care.
If they’ve self-selected to come to hospital because they perceive that they’re really ill and in need of something urgent, I suppose they are at high risk but quite a lot are redirected here by NHS 111, or the practice receptionist is most common now.
GP, Outside-2
They reported that sick patients may also present to community primary care and the GPs could therefore manage the patients with their usual GP approach (Parallel-1, Parallel-2, Parallel-3, Outside-1, Outside-2). Skills that they reported to rely on included having confidence in clinical skills, being comfortable with uncertainty, having a threshold to admit patients for further investigation if necessary and using recognised ‘safety netting’ techniques, so that patients were aware when they should seek medical help again. 147
Clear guidance and expectation of the role of the GP were reported to facilitate this approach, supported by strong GP and emergency clinician leadership.
The general theme we say to our GPs is we shouldn’t work any differently here than we would do if we sat in our practices, just because we’re in a hospital, we don’t do anything differently … we’re not trying to be a mini A&E here.
GP, Outside-1
More cautious general practitioner approach
Other GPs, some at the same hospitals as above, perceived that they saw a different cohort of higher-risk patients than in usual primary care and incorporated this into their clinical decision-making (Integrated-1, Integrated-3, Parallel-1, Parallel-2, Parallel-3)
Working in an urgent care A&E environment, you’re going to see potentially a lot sicker patients, you’re going to see the sub-arachnoid bleeds, potentially the subdurals after head injuries … so again, in my experience the sort of characters that tend to do GP work in an A&E department are quite au fait with these conditions.
GP, Parallel-3
They described using additional clinical skills to manage these patients including longer consultations to take a more thorough clinical history to establish chronology of symptoms and events; being aware of their role and limitations; and having a different threshold for admitting patients for further investigation.
An expectation to adopt an emergency medicine approach
Some GPs, however, described internal conflict about when it was appropriate or not to use acute investigations. Sometimes the decision had been taken away because the patient had already had investigations requested at triage which needed to be interpreted. Some clinicians described not being aware that tests had already been requested (Integrated-1, Parallel-1).
It’s very frustrating because then you’ve only found out because the patient’s told you, literally as they’re going out the door ‘oh, did my blood tests come back?’
Primary care ANP, Parallel-1
Expectation of the department, loss of confidence in clinical skills and medicolegal concerns were all described to influence the GPs’ use of investigations when they felt they would not have done so in usual primary care.
Thinking about defence, if you don’t do tests when they’re right next to you, and something were to happen … you would have to be able to stand up to that and defend yourself and say why you didn’t do those tests, so it’s tricky.
GP, Integrated-3
The choice to use a GP or emergency medicine approach
Some GPs working in integrated services described how even if they did have access to acute investigations, then many patients could still be managed by a traditional GP approach using the clinical skills described above. Ability to select the right patients and experience of the GP were felt to facilitate this approach.
If you select the right patients to see, as a GP in the department, you should be able to deal with them in a similar way to you do in primary care, but always just having that slight radar on to think okay, is there something else going on, do we need to do that little bit more?
GP, Integrated-3
Categorising patients into those that needed investigations and those that did not was also described, with investigations being used as a decision-making tool when patients could not be confidently categorised as either ‘safe to see and treat’ or ‘definitely needing admission’.
There’s the group in the middle where you’re uncertain whether this patient needs urgent admission or not, and you use the investigations as a tool to help in that decision-making.
GP, Integrated-1
General practitioners described being able to use acute investigations for patients when clinically indicated, because they were confident in interpreting results; were aware of protocols for high-risk conditions; they wanted to prevent unnecessary hospital admissions; and had the support of ED staff to provide this level of service. X-ray reporting systems were described as a useful backup to advise patients that a radiologist would also be reviewing the films while dealing with minor fractures.
These data were used to develop the following two theories about the roles taken on by GPs.
Refined theory: maintaining a general practitioner approach
If GPs who are experienced (C) and confident in their clinical skills (C) work in GP-ED models where a primary care role is clearly defined by departmental systems and structures, they are able (M) to use their usual ‘GP approach’ to care in the ED setting (O). However, if GPs work in EDs with access to investigations (C) where investigations are requested during triage, there is an expectation to follow ED protocols (C) or governance responsibility is unclear (C), GPs may feel that they are required to use these investigations (M), become less confident in their clinical skills (M) or have medicolegal concerns (M), resulting in more investigations being requested for patients in GP-ED models than in usual primary care (O). 15
Refined theory: flexibility in approach
If GP-ED models involve GPs seeing a cohort of patients they perceive to be higher risk (C), GPs may manage this risk by adapting their usual approach, for example, longer consultations (M) or a different threshold for admission or using investigations (M), to safely manage patient care (O). If GPs with additional emergency medicine skills and experience (C) work in EDs seeing a wider range of patients (C) with access to acute investigations (C), they can assess (M) which patients can be safely managed by a usual GP approach and which patients require an emergency medicine approach including acute investigations (O).
Potential risks associated with a ‘general practitioner’ approach in the emergency department
One theory developed in Phase 1 (see Chapter 3) described the potential risks associated with GPs seeing patients outside their usual remit which they had inadequate skills to manage.
Clinical directors from 9 of the 10 hospitals with a GP service had no patient safety concerns related to the GP service. The CD of an inside–parallel service had concerns about the skillset of primary care practitioners working in the ED and which organisation held governance responsibilities, following two local patient safety incidents (missed cervical spine fracture and a wound managed inappropriately) (Parallel-1).
The CD from one control site that no longer had GPs working in the department (Control-3) discussed a historical case of diagnostic error involving a child seen by a GP and expressed concerns that GPs treat patients differently to ED clinicians.
Well, we got it wrong, we streamed the wrong patients to them, and they got treated as GP patients and they weren’t. We had one … a litigation case … an under 1, unwell, horrible obs, went to the GP. The GP didn’t look at the obs, looked at the child and went “they look okay,” went away, came back in and was really septic and almost died.
Clinical Director, Control-3
Three ‘Datix’ reports referred to inadequate management, two of which related to a lack of knowledge about specific ED protocols.
ED protocol not followed, child with suspected non-accidental injury not admitted to paediatrics and sent home for outpatient follow-up.
Datix report, Integrated-1
Mitigating the risks of being a general practitioner in emergency department
There were examples of how strong clinical leadership could promote patient safety, with good practices including clear induction and appraisal processes, and periodically auditing a sample of GPs’ medical records for evidence of appropriate clinical decision-making and safety netting (Outside-1).
We used Croskerry’s framework highlighting the risks of cognitive errors at different stages of the diagnostic process (hypothesis generation; hypothesis evaluation; and hypothesis verification) to structure and explain our findings about how GPs described strategies to mitigate such errors. 148,149 In line with Croskerry’s model,148 the GPs interviewed described how they began generating hypotheses before the clinical encounter began, based on the written triage and streaming notes. Even at this early stage, some GPs reported referring patients back to the ED if they felt the patient was not suitable for a GP consultation.
Croskerry’s model describes factors that are thought to be important in hypothesis generation as: acuity (severity) of the patient’s condition; disease prevalence and heuristics. 148 Croskerry suggests that the acuity of the patient’s condition is most relevant when ED doctors generate hypotheses. 148 GPs working in ED settings reported categorising patients into those that required immediate medical attention or investigation and those that did not, rather than focusing on a specific diagnosis. As described above, GPs’ perception of the prevalence of serious disease and whether the cohort of patients was similar to usual primary care or a higher risk was described to impact their clinical decision-making. GPs who perceived the cohort of patients to be at higher risk, described a different level of concern and managing risk in the ED than in usual primary care.
I think that the group of patients I see in A&E is very different to the patients that I see in general practice … I’m quicker to be concerned with an A&E patient … statistically the chances of it being something more significant are much higher in A&E.
GP, Integrated-1, inside–integrated
General practitioner experience and their associated confidence in clinical skills was a common theme across the case sites for why services were successful and patient care was safe.
Initial information gathering from the patient, to understand why they had presented to the ED that day and the background of the presenting complaint, was described by some experienced GPs as key to diagnostic decision-making.
Hypothesis evaluation
As described above, while some GPs felt confident in using their clinical skills to eliminate the potential for serious disease, others felt that the ED setting necessitated a more cautious approach. The level of caution GPs displayed did not clearly relate to the type of service model within which they worked, with some more cautious clinicians identified across all models.
Some GPs who did have access to acute investigations described how they used their clinical skills to manage risk and choose which patients to manage with a GP approach and which to investigate. Croskerry discusses that the threshold for managing risk and when to request further investigation is influenced not only by experience but also by personal traits of the individual. Contextual factors were also noted to influence this behaviour, including investigation availability and departmental expectation. Some GPs working in more integrated models expressed that time could be used as a risk management tool in the ED, which was not possible in the usual primary care consultation.
Sometimes we just give them a bit of time, I call it the ‘cup of tea test’. Often, they look better after a cup of tea which helps the decision.
GP comment, Field-notes, Integrated-3
Hypothesis verification
General practitioners reported that often their priority was to exclude serious disease rather than making an actual diagnosis. There was also an acceptance of the limitations of the service and that the diagnostic process may be incomplete.
I don’t have access to all of your notes … I can’t organise blood tests, I can’t organise scans … I’ll have to send you back to your GP.
GP, Parallel-4
General practitioners described the strategy of ‘safety netting’ as good practice to help manage diagnostic uncertainty. 147 These findings contributed to the following new theory.
New theory: actions to maintain safe patient care
General practitioners who work in EDs with clear governance processes, who are aware of their intended role and expectation depending on their experience, skillset and patient demand (C), use communication skills to effectively gather patient information (M), actively consider prevalence of more serious diseases that may present to the ED setting (M), use clinical skills to rule out serious diagnoses (M), refer to guidance when acute investigation/referral may be necessary to exclude serious disease (M) and use safety netting to help manage diagnostic uncertainty (M) to deliver safer patient care (O).
Material in this section has been reproduced from Cooper et al. 32 This is an open access article distributed under the terms of the Creative Commons CC-BY license, which permits unrestricted use, distribution and reproduction in any medium, provided the original work is properly cited.
Theme summary
Multiple local contextual factors at the wider system, department and individual clinician level can influence GPs to maintain a traditional GP role or adopt an emergency medicine role when working in GP-ED models. GPs described how this influenced their clinical decision-making: no change to their usual GP approach; a more cautious GP approach; the choice to treat patients with a GP approach or to adopt an emergency medicine approach; or an expectation to adopt an emergency medicine approach. Structuring findings with formal cognitive theory highlighted areas with risk of cognitive biases and how GPs perceived these were mitigated to deliver safer patient care.
Theme 3: communication within and between services
As identified in Phase 1, inadequate referral pathways and poor communication between services were implicated in a number of patient safety incidents (see Chapter 3). This section explores communication both within the ED and between the ED (according to type of GP-ED model) and community primary care.
Data sources
To develop this theory further, we used data from local ‘Datix’ reports, observations at case study sites and interviews with ED staff and patients.
Findings
Communication between the general practitioner–emergency department model and the emergency department
Some case study sites were observed or reported to have limited communication between the GP-ED service and the ED. Incompatible computer systems between services were linked directly to patient safety incidents in three local incident reports where patient assessment and treatment had been delayed, and one patient had become ‘lost’ in the system (Integrated-1, Parallel-2, Outside-2). Receptionists at one site described how they had three different computer systems to operate (for the ED, the GP-ED service and the GP OOHs service) which led to duplicate patient entries on different systems and again increased the likelihood of patients becoming lost in or between the different systems (Parallel-4).
Physical distance between services limited face-to-face communication and was felt to contribute to a lack of communication between services at one site.
We’re not very integrated with the ED and we don’t, we don’t feel very integrated, it still feels a bit us and them.
GP, Outside-2
Another site, however, with a separate GP service, reported good communication through the senior nursing team reviewing on-the-day capacity and skillset and moving staff between services to meet patient demand (Outside-3). At sites where local GPs worked regularly within the ED, there was a perception that this also facilitated better communication (e.g. Parallel-4).
An ‘us and them’ culture was observed at another parallel site where there was good opportunity for face-to-face communication. At this site, juniors were not encouraged to ask the GPs for advice (Parallel-1). The personality of an individual GP as preferring lone working was felt to be significant at another site (Parallel-2). Poor communication between the private providers running GP services and the ED at Parallel and Outside models was perceived to create an inhospitable working environment for the GPs, which acted as a disincentive to working for the service.
The integrated sites reported good communication, which was perceived to promote interprofessional learning. Two CDs reported that since GPs had been working in the department, overall patient safety had improved because more experienced, permanent GPs could also give advice to other staff members (Integrated-1 and Integrated-2).
a positive I suppose is the fact that we’ve got someone with experience that is staffing and supporting our rota, which makes it a more safe department to work in.
Clinical Director, Integrated-2
At the integrated sites, the GPs were permanent staff members and there was good opportunity for face-to-face communication. GPs were described not only to give clinical advice but also to provide advice on primary care referral pathways which several ED staff reported as helpful. We observed a sense of multidisciplinary respect and teamwork with clear ED clinical leadership (Integrated-1, Integrated-2, Integrated-3)
One of the biggest things we didn’t expect is the effect of education, that there’s a GP sitting in the department, so they’re seeing a frail elderly patient, the F2 is sitting next to them seeing a similar patient, and the F2 is going ‘why are you sending your patient home and I’m admitting mine?’, and the amount of cross-fertilisation knowledge and support … we’ve really benefited from.
Clinical Director, Integrated-3
Strong GP leadership was also seen at several case sites which was reported to improve communication between the services and perceived to improve patient safety (Integrated-1, Integrated-3, Outside-1).
These findings contributed to the following new theory:
New theory: Having service models with strong clinical leadership, employing experienced, regular GPs with opportunity for face-to-face communication between services and compatible computer systems (C) with a culture that encourages formal and informal inter-professional communication and learning (M) and clinical leadership that promotes mutual respect (M), encourages communication between services and teamwork to facilitate safer patient care (O).
Communication with community primary care
There was limited evidence to explore patient safety outcomes from inadequate referral and communication pathways to and from local primary care (which had been highlighted as a potential issue in Phase 1). Collecting data from community primary care was outside the scope of the current project. The lead GP at one site (Outside-1) described good virtual communication with local primary care where both services used the same computer system and were able to access consultation notes. Additionally, she felt this assisted both services to unite antibiotic stewardship messages – if patients chose to present to a different service seeking antibiotics for a viral illness that the local GP had advised were not appropriate, this was clearly documented on computer system and could be taken into consideration. Inadequate discharge summary communication with community primary care was described in one local incident report (Outside-1, Table 25 in Appendix 5).
Theme summary
Communication between the ED and the GP-ED model was important for safer patient care. This appeared to be achieved most consistently in the most integrated models. Barriers to communication at a system level (e.g. IT and department geography) were identified. Senior staff managing the process could overcome some of these problems. Even in services with an apparently favourable setting, the preferences of individuals (e.g. for lone working or about involving GPs in ED care) could hamper communication.
Theme 4: patient experience
In our RRR, we described how patients’ expectations of the care they receive at the ED shaped their satisfaction with the experience of a GP-ED model.
Data sources
Semi-structured interviews with 24 patients/carers of patients who visited the ED with one of six marker conditions (see Chapter 3). We also reflected on feedback from 56 attendees at our second stakeholder event (see Chapter 3) to support our analysis.
Applying formal theory: Sonis et al. developed a conceptual model of patient experience in EDs which is intended as a framework for improving patient experience in EDs, by linking existing problems, potential interventions, resulting outcomes and mechanisms for incorporating feedback. 150
We used Sonis’ conceptual model as a framework to help us understand how patient experiences could be improved. This is explored in detail in our published paper (see Project web page). 20
Findings
Patients who were seen by a GP, regardless of the GP-ED model or their condition, generally reported being pleased with the quality of care received from staff members. Patients reported that staff were professional, helpful and caring, and emphasis was placed on the importance of this in an ED setting:
… they were particularly brilliant and professional and very caring with my mental state … so I would say that’s paramount really, to a really good service.
Patient with back pain seen by GP, Integrated-1
While many patients were unaware that they had seen a GP, when asked how they felt about GPs working in EDs, patients generally responded positively. Patients appreciated the experience and knowledge that GPs have, and how this can contribute to better patient care in ED.
I think it’s useful, if they have the time and resources, funding or whatever, I think it’s a great idea, because I think they can work together with their experiences.
Patient with back pain seen by a GP, Integrated-1
Clinicians reported that when patients are not aware that there is a primary care service at the ED, but an explanation is given about why they are being streamed to a GP, as an appropriate clinician with skills and expertise to see them, they seem to find being streamed to a GP acceptable.
Meeting expectations about timeliness and level of care
Timely care
Some patients (8/24), regardless of the GP-ED model or their condition, reported that they perceived their condition as an emergency, which required immediate ED care. Some followed advice to attend the ED from the 111 service or their GP (11/24), others attended after difficulty in accessing, or receiving timely and satisfactory care from community primary care services and expected to attend the ED to receive assessment, diagnosis and treatment on the same day (7/24).
I just needed somebody to give me sort of help … where it come from it didn’t really matter … Getting to the hospital, you’ve obviously come to the right place … and I wasn’t getting any advice at all off my local GP.
Patient with cough and breathlessness seen by a GP, Outside-3
Some patients suggested that if there was better access to community primary care services, there would be a reduced need for GPs in EDs, as patients would feel able to access timely appointments in their local community primary care service.
Some of the patients interviewed expected a long wait in the ED and appreciated that by seeing a GP in the ED rather than an ED clinician, they were seen more quickly, and therefore felt positive about GP-ED models. Our stakeholders suggested that timely access, and seeing a clinician on the same day, may be more important to patients than the level of investigations received. Our public contributors placed particular emphasis on the importance of the amount of time spent in ED, and how this is more important than the type of clinician they are seen by. They agreed that if seeing a GP in ED reduces waiting time and allows patients to see a clinician on the same day, then streaming to a primary care clinician in the ED is likely to be acceptable to patients. Patients who attended the ED because they believed they perceived their complaint as an emergency, and were streamed to a primary care clinician, generally found this acceptable if their complaint was dealt with in a timely manner and led to appropriate treatment.
I think it is good because otherwise we would’ve had to wait however long to see a general doctor but … the GP seemed a little bit more knowledgeable.
Parent of febrile child seen by a GP, Outside-3
However, if patients who were streamed to a primary care clinician in the ED did not receive treatment which resolved their issue, and had to seek further treatment elsewhere, this could make the process of primary care streaming less acceptable to patients.
The right level of care
Our initial theory about investigations related to patients being dissatisfied if they did not receive the level of investigations they expected in the ED. We did not find any evidence of patients reporting dissatisfaction relating to investigations. Some patients expressed satisfaction when they felt they had received appropriate investigations and had results explained to them during their visit, by a GP.
… we were happy … to be seen by a GP, and I think for me, as well, knowing that he’d been seen … he’d had his levels checked, and then sort of recovered himself and she was happy for him to go with … the instructions for further medication if things do deteriorate.
Parent of a child with cough and breathlessness seen by a GP, Integrated-1
Patients reported that previous experiences of attending the ED had given them an expectation of the kinds of tests and investigations that they would receive, and reported therefore feeling satisfied when they had received these same tests and investigations by a GP in the ED.
I had learned from my previous experience with my son that … they would do the standard observations, tests, like checking temperature, monitoring the heart rate and so on, and that was all handled.
Parent of a child with fever seen by a GP, Parallel-3
Using these data, we refined our initial theory describing how a GP-ED model that meets expectations results in patient satisfaction.
New theory: meeting expectations
Patients attend the ED with expectations around timeliness of care and level of care (e.g. investigations) they require but without specific expectations about who would provide this care, sometimes in the context of having failed to access care they feel they need elsewhere (C). When they are seen in a GP-ED model which meets or exceeds their expectations of being assessed and treated in a timely fashion (M), they are satisfied with experience (O).
Redirection
At some outside–onsite models, patients were streamed to a primary care service to be triaged, before then being redirected to a community primary care service. This meant they had to wait and be assessed twice. One streaming nurse suggested that the streaming system would be more efficient and less frustrating for patients if patients could be discharged and redirected to the community primary care service directly from the ED, as this would reduce waiting time for patients (Outside-3).
At another outside–onsite model, a GP described frustration with the system:
If it’s not for them they [ED] send it here, as their default even if it’s something completely that we’re not going to help them with, … they wait three hours and then they get told we can’t help you which is not great.
GP, Outside-2
Our stakeholders suggested that being sent away may lead to patients feeling dismissed or that they should not have attended ED in the first place but acknowledged that good communication and reassurance are important in these scenarios. In EDs where primary care patients were redirected to booked appointments in community services, patients avoided a potentially long wait in ED to be seen by a GP and can go home and be seen that same day by their own GP. Staff at these sites perceived that patients were likely to feel like they are being helped, rather than sent away to seek care elsewhere themselves, particularly as community services may be closer to their home than the ED. Stakeholder feedback emphasised the importance to patients of seeing a clinician quickly, even if they are sent away from the ED for an appointment in the community.
We developed a second theory specific to the acceptability of redirection.
New theory: redirection
If a patient attends the ED with a problem that could be dealt with in primary care (C), and the ED facilitates access to an appointment in the community which avoids a long wait in ED (M), this is likely to be seen as acceptable (O). However, if the patient is referred to a GP-ED model and this process involves duplicated assessments and long waiting times but does not result in definitive care for their problem (M), then the patient may be frustrated (O).
Theme summary
We found that patients generally find it acceptable to be streamed to a primary care clinician in the ED if they have no expectations of which clinician they should be seen by, trust in the initial assessment process and believe that they will receive good advice at the hospital. Patients reported a positive experience when they felt their complaint had been dealt with in a timely manner, and when they received the level investigations and treatments which they expected.
Summary of Phase 2 qualitative results
The qualitative data collected in Phase 2 have allowed us to generate and refine our theories on: how streaming processes were influenced by nurse experience, guidance and overall operational and strategic management; how GPs found working in EDs influenced their clinical decision-making to maintain a usual GP approach or adopt an ED clinician approach, mitigating safety risks; factors that facilitate teamwork and communication between GP-ED services and the ED; and patients’ expectations and experiences of using services.
Chapter 5 Routine data analysis to examine clinical effectiveness and cost consequences of different general practitioner–emergency department models
Introduction
This chapter summarises the analysis of routinely collected data available for the different GP-ED models.
Study aim; chapter aims and objectives
The overall aim of the study is to determine clinical effectiveness and the resources, costs and consequences of different GP-ED models compared to others (including controls) with respect to key outcomes, and to further understand the ways in which service design and setting (context) generate variations in outcomes. The study is based on 13 purposively selected sites representing a range of GP-EDs models (3 inside–integrated, 4 inside–parallel, 3 outside–onsite) including Control (3 with no GP-ED model). A combined statistical and health economic analysis plan was produced prior to analysis and available from the study authors (see Report Supplementary Material 2).
Clinical effectiveness analysis
Methods
Patient-level routinely collected data relating to ED attendances and subsequent hospital admissions were obtained from HES AE and HES APC data sets (via NHS Digital) for study sites located in England; and from EDDS and PEDW data sets (via SAIL) for study sites located in Wales. The attendance-level data are summarised as time series (per site, aggregating data for each study fortnight) for the following variables:
-
counts of ED attendances
-
reattendance at same ED
-
ED attendance leading to a hospital admission (defined by patient record appearing in the APC data set)
-
investigations undertaken during an ED attendance
-
treatments delivered during an ED attendance
-
average time (minutes) of an ED attendance
-
length of stay (LOS) (days) of hospital admission.
Table 5 shows study fortnights for which routine data were provisioned at study sites with the first study fortnight with a GP model (intervention) in place; these define the maximum number of aggregated values for variables, before and after introduction of GP model.
Site | Intervention date | Study fortnight | ED data | Inpatient data |
---|---|---|---|---|
Integrated-1 | 1 January 2017 | 164 | t = 1 to t = 209 (n = 209) | t = 66 to t = 209 (n = 144) |
Parallel-1 | 1 October 2015 | 131 | t = 1 to t = 209 (n = 209) | t = 66 to t = 209 (n = 144) |
Outside-1 | 1 October 2017 | 183 | t = 1 to t = 209 (n = 209) | t = 66 to t = 209 (n = 144) |
Control-1 | N/A | N/A | t = 1 to t = 209 (n = 209) | t = 1 to t = 209 (n = 209) |
Integrated-2 | 28 March 2017 | 170 | t = 1 to t = 209 (n = 209) | t = 66 to t = 209 (n = 144) |
Parallel-2 | 4 February 2015 | 114 | t = 46 to t = 209 (n = 154) | t = 66 to t = 209 (n = 144) |
Outside-2 | 1 October 2005 | 1 | t = 170 to t = 209 (n = 40) | t = 170 to t = 209 (n = 40) |
Control-2 | N/A | N/A | t = 1 to t = 209 (n = 209) | t = 66 to t = 209 (n = 144) |
Integrated-3 | 1 October 2010 | 1 | t = 1 to t = 209 (n = 209) | t = 66 to t = 209 (n = 144) |
Parallel-3 | 1 September 2012 | 51 | t = 1 to t = 209 (n = 209) | t = 66 to t = 209 (n = 144) |
Outside-3 | 1 October 2017 | 183 | t = 1 to t = 209 (n = 209) | t = 66 to t = 209 (n = 144) |
Control-3 | N/A | N/A | t = 1 to t = 209 (n = 209) | t = 1 to t = 209 (n = 209) |
Parallel-4 | 1 April 2018 | 196 | t = 1 to t = 209 (n = 209) | t = 66 to t = 209 (n = 144) |
Data from 1 October 2010 to 30 September 2018 were eligible for inclusion. This study window was intended to allow acquisition of data covering at least 1 year before and after the GP-ED model was implemented at a study site. Including both start and end days, this study window comprises 2922 days, including two ‘extra days’ – 29 February – in 2012 and 2016. For the purposes of data aggregation, the study window divides into 208 complete fortnightly periods (starting with 1 October 2010–14 October 2010, 15 October 2010–28 October 2010 and ending with 7 September 2018–20 September 2018) and a single 10-day period (21 September 2018–30 September 2018).
The date of introduction of GP models varied between 1 October 2005 (Outside-2) and 1 April 2018 (Parallel-4); the study window did not include 1 year’s data both before and after introduction of the GP model at these two study sites or at Integrated-3. Emergency department data were available for the full study window for 11 sites (Parallel-2 and Outside-2 were the exceptions); in contrast, Inpatient data (i.e. HES APC) were only available for the full study window at one site (Control-3).
Data processing
We first cleaned the routine data provided, linked ED and inpatient data, and assigned eligible attendance-level data to study sites; summarising the quantity of data per site, and documenting issues noted. Each attendance was further categorised as (1) a reattendance; (2) an attendance leading to hospital admission; (3) an attendance at which a medical investigation was undertaken and (4) an attendance at which a medical treatment was delivered. We also recorded the time in ED (in minutes), and, where an admission was recorded in that hospital’s inpatient records, the LOS (in days). We then aggregated data using study fortnights, and documented adjustments made in producing aggregated data – for example, to accommodate missing data or incomplete study fortnights.
We are aware that various factors may affect the quality of data assigned to study sites and produce variations in data assigned to a study site over time. These include, inter alia, site-specific factors such as staffing levels and local practices, which then affect one or more outcome measures. We first assessed data quality, checking for completeness and the presence of unexpected features (e.g. ‘spikes’) or changes in trends. These explorations were presented and interpreted in the context of each site’s known history over the study window, which may provide reasons for such data ‘spikes’, omissions or unexpected variations over time attributable to local circumstances (e.g. NHS reconfigurations) or complexities.
We initially summarised the quality of the base variable (count of ED attendances per study fortnight), categorised as A1 (good); A2 (intermediate) or A3 (poor). Data regarded as A1 are included in formal analyses. Where data are categorised as A2, we either (1) noted subsets of data deemed to be anomalous and exclude these from analysis, or (2) noted limitations within the data (typically associated with coding in underlying routine data), included data in analyses and interpreted results in the light of these limitations. Data categorised as A3 are regarded as too poor in quality or quantity to be included in analyses.
We then assessed the quality of other variables in turn, documenting cases where data issues were deemed to require further data exclusions.
Variable definition
Key variables (counts of ED attendances; reattendances at the same ED) are generated directly from an ED attendance recorded in routine data; this high-level information was generally recorded to reasonable quality. Reattendances (further attendance at the same ED within 28 days) were expressed as proportions of all ED attendances per study fortnight.
Other variables depend on further information recorded at sites (e.g. investigations; treatments; disposition from ED) and the recording of this information was expected to be of variable quality, both across time; and across sites. We recorded the proportion of ED attendances leading to hospital admission; we derived one measure using ED Disposal codes (with better completeness over time), and a second measure based on hospital inpatient records (requiring linkage between ED and inpatient data).
We recorded the proportion of ED attendances for which any medical investigation was undertaken during the ED attendance, and the proportion of ED attendances during which any medical treatments were delivered. For these variables, variation in recording practices across time and sites precluded using records on specific subgroups of investigations or treatments.
We recorded an average time (in minutes) of ED attendances per study fortnight in ED, and an average LOS (in days) of hospital admission, with the convention that admission and discharge on the same day is recorded as a stay of 0.5-day duration.
Analysis
For all variables, we used standard time series analysis methods [time plots, correlograms of autocorrelation functions (ACFs), trend lines] to assess the nature and extent of linear trends and seasonality in data; (3). We fit ordinary least squares (OLS) linear trends to capture trends, and hence the change in trend before and after an intervention point at intervention sites, rather than time series methods (such as differencing) which accounts for a wider range of non-stationarity in data but lacks a useful parametric basis for capturing change in trend.
Since a seasonal component may be present in some variables, we defined a seasonality value for each study fortnight as follows. We first used a sine function with a period of 365.25 days to calculate a daily seasonality value, with the maximum value +1 in mid-July, the minimum value −1 in mid-January and zeroes around the spring and autumn equinoxes. For each fortnightly period, we then defined the seasonality factor to be the average of the 14 values for the days in that fortnight (with appropriate adjustment at the end of the study window).
Where the quality and quantity of available data allowed, we summarised the structure in a time series at an intervention study site by: (1) a pre-intervention trend coefficient, denoted by b0, (2) a post-intervention trend coefficient, denoted by b1, (3) the change (post–pre) in trend, denoted by D and (4) a seasonality coefficient, denoted by bs. For control study sites, these summaries comprised: (1) a single trend coefficient, denoted by b0 and (2) a seasonality coefficient (bs). We noted where change in trends align with dates of known NHS reconfigurations (and hence a change in data recording scope and/or protocols), which may also have been confounded with the introduction of a GP-ED model. To facilitate interpretation of results, we analysed proportions as percentages.
We describe trends as upwards (associated with a positive coefficient) or downwards (negative coefficient), and where possible, focus on change in trend, which can itself be negative or positive. Some care, therefore, is needed with interpretation, since a positive coefficient, and hence an upward trend, are not necessarily associated with good or desirable healthcare outcomes. We emphasise changes in trend sufficiently large enough to be deemed statistically significant but note that the assessment of statistical significance here depends inter alia on numbers of study fortnights in pre- and post-intervention phases. Although assessment of seasonal variation is secondary here, similar remarks apply to interpretation of the seasonal coefficient; a positive value for this coefficient implies generally higher values of variable of interest in the summer (over winter), and a negative coefficient the reverse.
In all sites, we determined the extent of the underlying temporal dependency within a time series (after allowing for trend and seasonality) by assessing and summarising (via the first three values, denoted r1, r2, r3) its ACF, which indicates the strength of any remaining temporal dependence or structure within de-trended and de-seasonalised data. Details on this short-term correlation structure are reported in Report Supplementary Material 3; this structure allows an initial assessment of the appropriateness or otherwise of the assumption of a simple linear trend and may also be used to inform further formal analyses.
Results
We assessed the quality of the base variables (ED attendance) to be A1 in seven study sites (spread across all four subgroups); A2 in five study sites and A3 in one study site (Outside-2), where the general unavailability in much of the study window was compounded by a lack of clarity linking ED attendances to that ED. There were generally good-quality data at study control sites and across Integrated sites, and a small reduction in data quality in Parallel sites; there is, however, a further reduction in data quality across the Outside sites, which hampers discussion of this subgroup. Due to the poor quality of data at Outside-2, this site has been largely excluded from descriptive analyses presented below. Further issues and limitations associated with specific variables are noted in the relevant Extended Tables (see Report Supplementary Material 3).
The quality of data on other variables was generally consistent with the categorisation of the main variable (ED attendance), although further issues were noted for selected combinations of variables, sites and time points. Such issues were particularly noticeable in the two variables which rely on records of activities undertaken during an ED attendance (medical investigations undertaken; medical treatments received), where some time plots indicate relatively large and sudden changes in variables’ values. These changes, consistent with a change in recording protocols or staff, are sometimes prolonged, but sometimes of only a few fortnights’ duration, while some appear to be temporary (so followed by reversion to previous levels), while others are seemingly more permanent.
The remainder of this section examines the variables in turn; in each case, we provide examples of the data at one intervention study site and one control study site. Using the data quality assessments above, we use either Control-1 or Control-2 and focus on Integrated or Parallel intervention sites. We also provide a table summarising results for all study sites, with a brief commentary on these results. With data at study control sites providing some overall context, we outline the extent to which patterns or themes emerge from an assessment of the quantitative data for study sites with any GP-ED model; and then look at the consistency, similarities and differences within subgroups of study intervention sites.
Emergency department attendances
Figures 5 and 6 illustrate ED attendance data at two example study sites: Integrated-1 and Control-3.
FIGURE 5.
Emergency department attendances per study fortnight and OLS trend lines for Integrated-1. Note: Vertical orange line indicates approximate fortnight of GP model introduction at this site.

FIGURE 6.
Emergency department attendances per study fortnight and OLS trend line for Control-3.

For Integrated-1 (see Figure 5), we observe a strong upward trend and regular seasonality, with evidence of an increased trend post intervention. This interpretation is consistent with the coefficient values b0 = 2.988, b1 = 10.759, D = 7.771 and bs = 118.4; see Table 5. We note that the de-trended/de-seasonalised data show only moderate short-term correlation; this supports the use of linear trendlines to capture the structural temporal variation in this variable.
For Control-3 (see Figure 6), we observed a consistent downward trend over the study window, and clear evidence of seasonality; this is consistent with the coefficient values b0 = –1.236 and bs = 145.9; see Table 6. We again note that the de-trended/de-seasonalised data show moderate short-term correlation; this supports the use of a linear trendline to capture the structural temporal variation in this variable.
Site | Integrated-1 | Parallel-1 | Outside-1 | Control-1 |
---|---|---|---|---|
Data quality | A1 | A1 | A2 | A1 |
Trend | ||||
b 0 | 2.988 | 0.008 | 1.092 | 2.812 |
b 1 | 10.760 | 3.034 | –5.430 | |
D | 7.771* | 3.026* | N/A | |
Seasonality bs | 118.4 | 88.9 | N/A | 81.7 |
Site | Integrated-2 | Parallel-2 | Outside-2 | Control-2 |
Data quality | A1 | A2 | A3 | A2 |
Trend | ||||
b 0 | –1.037 | –3.681 | N/A | 0.354 |
b 1 | –1.116 | 2.716 | N/A | |
D | –0.079 | 6.398 | N/A | |
Seasonality bs | 71.6 | 19.6 | N/A | 66.2 |
Site | Integrated-3 | Parallel-3 | Outside-3 | Control-3 |
Data quality | A2 | A2 | A1 | A1 |
Trend | ||||
b 0 | N/A | 10.853 | 1.716 | –1.236 |
b 1 | 2.604 | 5.719 | 15.518 | |
D | N/A | –5.134 | 13.442* | |
Seasonality bs | 316.8 | 54.8 | 93.2 | 145.9 |
Site | Parallel-4 | |||
Data quality | A1 | |||
Trend | ||||
b 0 | 0.950 | |||
b 1 | 6.732 | |||
D | 5.782 | |||
Seasonality bs | 73.4 |
Table 6 summarises this outcome with a mixed picture across study control sites, with two upward trends and one downwards. This mixed picture also extends to study intervention sites and subgroups of intervention sites, but the general picture is of attendances showing increasingly upwards trends over time, as exemplified by three statistically significant positive changes in trend, at Integrated-1, Parallel-1 and Outside-3. Four other intervention sites show changes in trend that are not statistically significant; and there are insufficient data to make an assessment at the other remaining three sites.
Extended Table 6a (see Report Supplementary Material 2) provides further details on data quality categorisations A2 and A3.
Emergency department reattendances
Figures 7 and 8 illustrate reattendance data at two example study sites: Parallel-3 and Control-1.
FIGURE 7.
Reattendance per study fortnight and OLS trend lines for Parallel-3. Note: Vertical orange line indicates approximate fortnight of GP model introduction at this site.
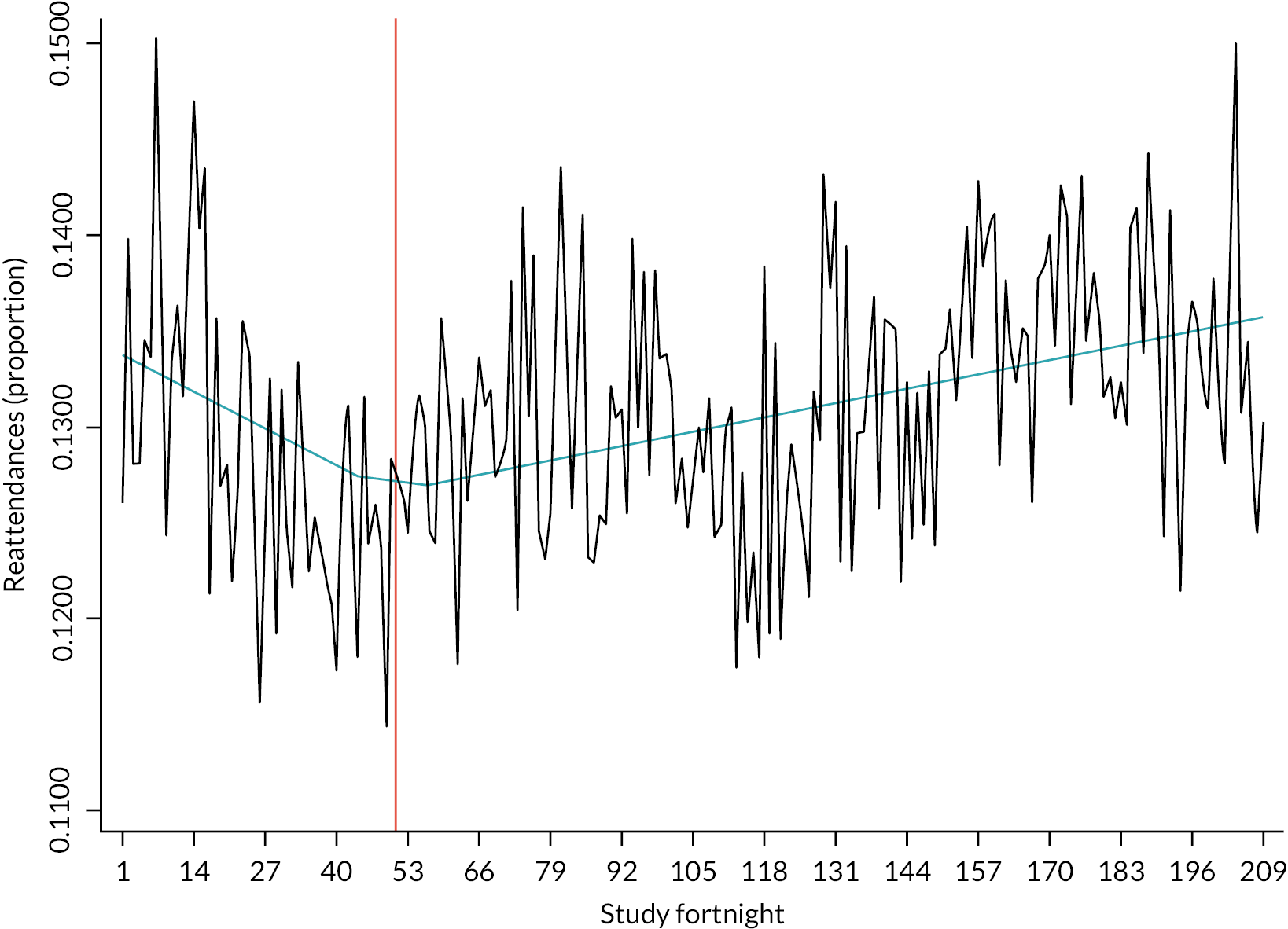
FIGURE 8.
Reattendances per study fortnight and OLS trends for Control-1.

For Parallel-3 (see Figure 7), we observe a downward trend in the pre-intervention phase, followed an upward trend in the post-intervention phase; no distinct seasonality is present. This interpretation is consistent with the coefficient values b0 = −0.015, b1 = 0.006, D = 0.021 and bs = 0.035; see Table 7. We note that the de-trended/de-seasonalised data show only moderate short-term correlation; this supports the use of linear trendlines to capture the structural temporal variation in this variable.
Site | Integrated-1 | Parallel-1 | Outside-1 | Control-1 |
---|---|---|---|---|
Trend | ||||
b 0 | 0.009 | –0.006 | 0.005 | 0.009 |
b 1 | 0.027 | 0.009 | 0.080 | |
D | 0.018* | 0.015** | N/A | |
Seasonality bs | 0.087 | 0.016 | N/A | 0.043 |
Site | Integrated-2 | Parallel-2 | Outside-2 | Control-2 |
Trend | ||||
b 0 | 0.002 | –0.040 | N/A | 0.027 |
b 1 | –0.059 | 0.008 | N/A | |
D | –0.061** | 0.048 | N/A | |
Seasonality bs | –0.191 | 0.247 | N/A | –0.196 |
Site | Integrated-3 | Parallel-3 | Outside-3 | Control-3 |
Trend | ||||
b 0 | N/A | –0.015 | 0.021 | –0.001 |
b 1 | 0.004 | 0.006 | 0.185 | |
D | N/A | 0.021** | 0.164** | |
Seasonality bs | 0.056 | 0.035 | –0.185 | 0.732 |
Site | Parallel-4 | |||
Trend | ||||
b 0 | –0.004 | |||
b 1 | 0.131 | |||
D | 0.135** | |||
Seasonality bs | 0.137 |
For Control-1 (see Figure 8), we observed a consistent upward trend over the study window, but no clear evidence of seasonality; this is consistent with the coefficient values b0 = 0.009 and bs = 0.043; see Table 7. We again note that the de-trended/de-seasonalised data show only moderate short-term correlation; this supports the use of a linear trendline to capture the structural temporal variation.
Report Supplementary Material 3 (Extended Table7a) provides details on further data issues.
Table 7 shows a mixed picture across study control sites and pre-intervention phases in study intervention sites, with roughly equal numbers of upward and downward trends. A clearer picture emerges in the post-intervention phases in study intervention sites; where eight of nine sites show an upward trend, and, in six of these cases, this trend has increased over that in the pre-intervention phase. Data quality issues make interpretation difficult for the Outside sites, while the Integrated sites include the one site with a change from upward to downward trend. However, a more uniform pattern is observed across Parallel sites, where a downward trend pre intervention is followed by an upward trend post intervention, with changes found statistically significant in three of the four sites.
We note that the de-trended/de-seasonalised data show reasonably moderate short-term correlation; this provides some support for the use of linear trendlines to capture the structural temporal variation.
Hospital admissions
Given the extended coverage ED data for this variable, we present here data based on emergency department disposition (EDD) codes. Figures 9 and 10 illustrate hospital admissions (EDD) data at two example study sites: Parallel-1 and Control-1.
FIGURE 9.
Hospital admissions per study fortnight and OLS trend lines for Parallel-1. Note: Vertical orange line indicates approximate fortnight of GP model introduction at this site.
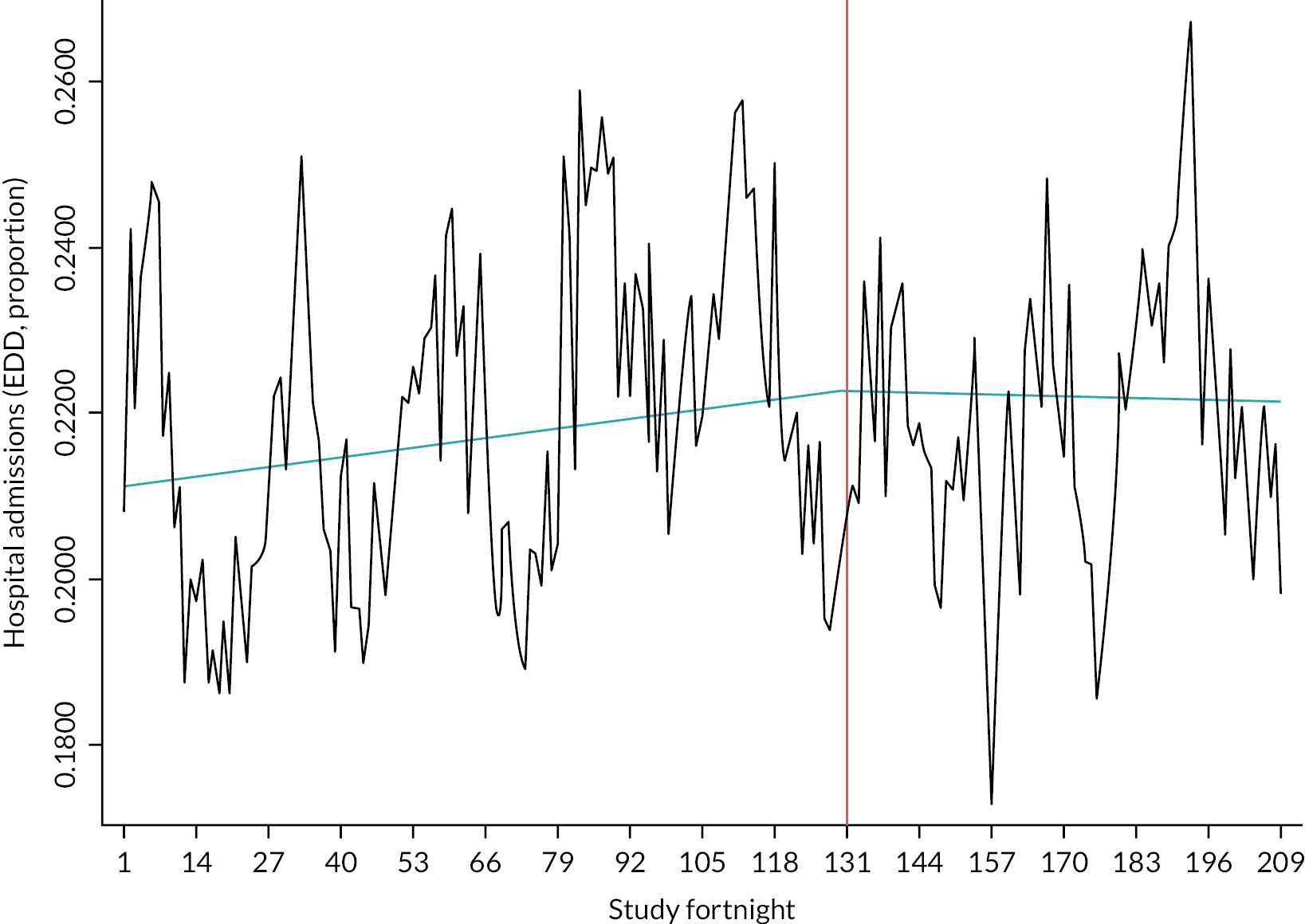
FIGURE 10.
Hospital admissions per study fortnight and OLS trend lines for Control-1.
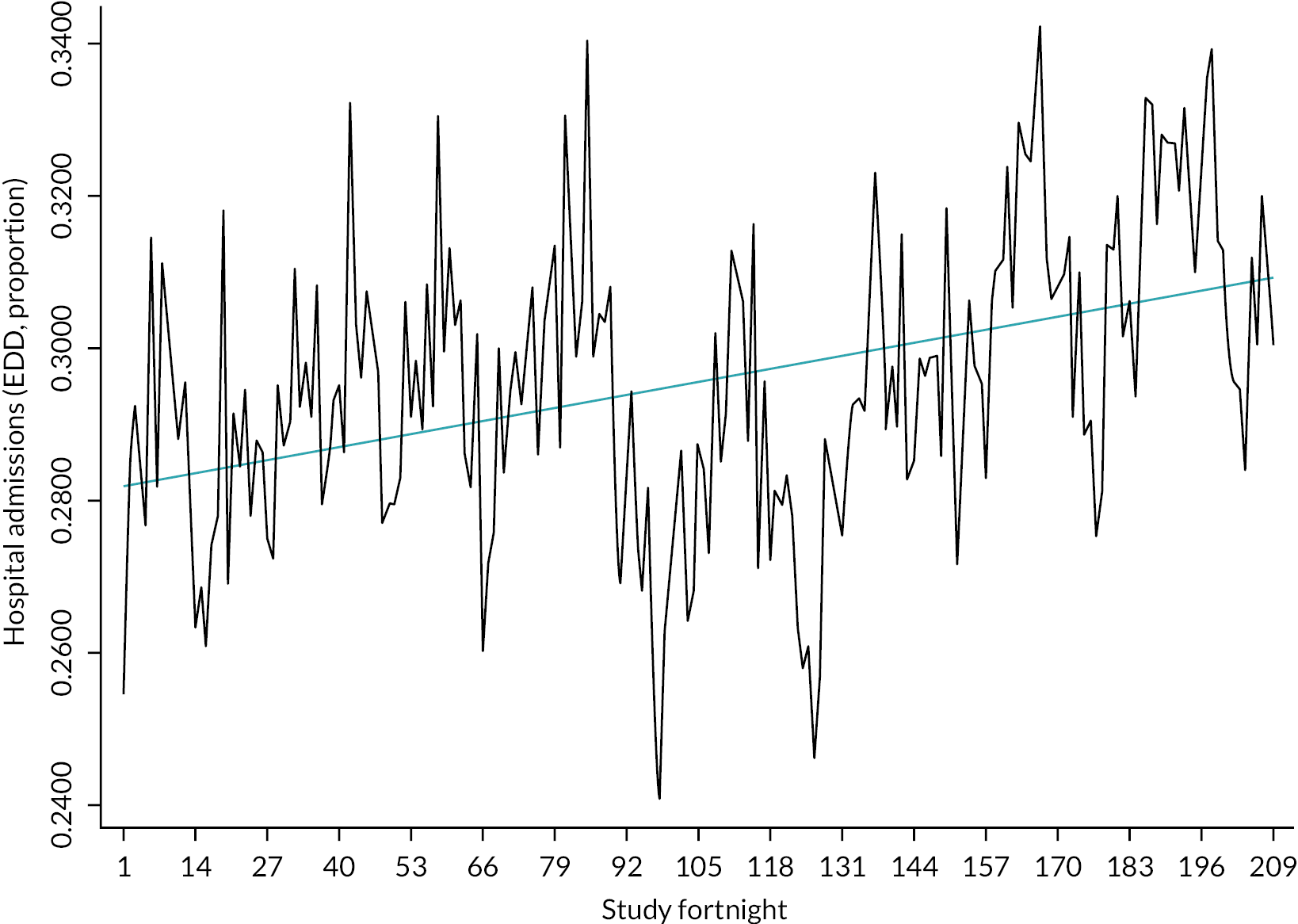
For Parallel-1 (see Figure 9), we observe an upward trend in the pre-intervention phase, followed an essentially flat trend line in the post-intervention phase; there is some evidence of seasonality. This interpretation is consistent with the coefficient values b0 = 0.009, b1 = –0.001, D = 0.010 and bs = –1.613; see Table 8. We note that the de-trended/de-seasonalised data show reasonably moderate short-term correlation; this provides some support for the use of linear trendlines to capture the structural temporal variation.
Site | Integrated-1 | Parallel-1 | Outside-1 | Control-1 |
---|---|---|---|---|
Trend | ||||
b 0 | 0.010 | 0.009 | 0.030 | 0.013 |
b 1 | 0.142 | –0.001 | 0.107 | |
D | 0.132* | –0.010 | N/A | |
Seasonality bs | –2.235 | –1.613 | N/A | –1.156 |
Site | Integrated-2 | Parallel-2 | Outside-2 | Control-2 |
Trend | ||||
b 0 | –0.023 | 0.045 | N/A | 0.053 |
b 1 | –0.069 | 0.013 | N/A | |
D | –0.046 | –0.032 | N/A | |
Seasonality bs | –2.250 | –1.047 | N/A | –1.648 |
Site | Integrated-3 | Parallel-3 | Outside-3 | Control-3 |
Trend | ||||
b 0 | N/A | –0.121 | –0.013 | 0.019 |
b 1 | –0.019 | 0.039 | –0.062 | |
D | N/A | 0.159** | –0.049 | |
Seasonality bs | –1.836 | –0.459 | –1.241 | –1.501 |
Site | Parallel-4 | |||
Trend | ||||
b 0 | 0.019 | |||
b 1 | –0.319 | |||
D | –0.339* | |||
Seasonality bs | –0.754 |
For Control-1 (see Figure 10), we observed a consistent upward trend over the study window, but no clear evidence of seasonality; this is consistent with the coefficient values b0 = 0.013 and bs = –0.156; see Table 8. Here, the de-trended/de-seasonalised data show evidence of more persistent short-term correlation; a linear trend is best regarded only as a first step in capturing the structural temporal variation.
Report Supplementary Material 3 (Extended Table 8a) provides details on further data issues.
Table 8 indicates upward trends in all three control study sites; with a more mixed picture across the intervention study sites, where there are examples of upward and downward trends in both pre-intervention and post-intervention phases. Changes in trend are largely downward (as observed in five of seven sites), and with reversal of trend direction from upward to downward observed at in two sites (Parallel-1 and Parallel-4), and from downward to upward (Parallel-3). Changes in trend are deemed to be statistically significant at two sites; Parallel-4 (as discussed above) and Integrated-1, with an increase in the upward trend. The mixed picture seen within the Parallel subgroup of sites also extends to the Integrated subgroup; as above, data issues affect the interpretation of results in the Outside subgroup.
Medical investigations undertaken
Figures 11 and 12 illustrate data on medical investigations undertaken at two example study sites: Parallel-1 and Control-3.
FIGURE 11.
The proportion of ED attendances where a medical investigation was undertaken per study fortnight for Parallel-1. Note: Vertical orange line indicates approximate fortnight of GP model introduction at this site.

FIGURE 12.
The proportion of ED attendances where a medical investigation was undertaken per study fortnight for Control-3.

For Parallel-1 (see Figure 11), we observe a downward trend in the pre-intervention phase, which broadly continues, with a slight further decrease, in the post-intervention phase; there is little obvious evidence of seasonality. This interpretation is consistent with the coefficient values b0 = −0.007, b1 = –0.012, D = –0.005 and bs = –0.620; see Table 9. We note that the de-trended/de-seasonalised data show reasonably moderate short-term correlation; this provides some support for the use of linear trendlines to capture the structural temporal variation.
Site | Integrated-1 | Parallel-1 | Outside-1 | Control-1 |
---|---|---|---|---|
Trend | ||||
b 0 | –0.001 | –0.007 | 0.048 | 0.089 |
b 1 | 0.121 | –0.012 | 0.238 | |
D | 0.122* | –0.005 | 0.191 | |
Seasonality bs | –0.305 | –0.620 | –0.486 | –0.477 |
Site | Integrated-2 | Parallel-2 | Outside-2 | Control-2 |
Trend | ||||
b 0 | 0.028 | 0.003 | N/A | 0.020 |
b 1 | –0.145 | 0.148 | N/A | |
D | –0.173 | 0.145 | N/A | |
Seasonality bs | –1.862 | 0.419 | N/A | –0.300 |
Site | Integrated-3 | Parallel-3 | Outside-3 | Control-3 |
Trend | ||||
b 0 | N/A | –0.116 | –0.026 | 0.000 |
b 1 | 0.045 | –0.020 | –0.025 | |
D | N/A | 0.096*** | 0.001 | |
Seasonality bs | –1.737 | 0.554 | 0.230 | –0.336 |
Site | Parallel-4 | |||
Trend | ||||
b 0 | 0.010 | |||
b 1 | –0.835 | |||
D | –0.845*** | |||
Seasonality bs | 0.226 |
For Control-3 (see Figure 12), we observe no simple trend pattern over the study window, or clear evidence of seasonality; interpretation of the corresponding coefficient values b0 = 0.089 and bs = –0.477 (see Table 9) therefore requires some care. Here, the de-trended/de-seasonalised data show evidence of persistent short-term correlation; this is consistent with a more complex form of non-stationarity in the time plot, emphasising that a linear trend is best regarded only as a first step in capturing this structural temporal variation.
Table 9 shows flat or upward overall trends in the three control sites, but with some strong evidence that linear trends do not fully capture the more complex patterns of structural temporal variation. In the intervention sites overall and within subgroups of these sites, the picture is generally more mixed in terms of upwards and downwards trends pre and post intervention; there is some indication of reversal in the initial trends at three sites. Statistically significant changes in trend were indicated at three sites: Integrated-1, with reversal of a slight downward trend to an upward trend; Parallel-4, with another reversal, but this time from upward to downward; Parallel-3, with an initial downward trend followed by an even stronger downward trend. However, interpretation of these findings also needs to reflect the strong evidence that linear trendlines do not fully capture the more complex patterns of structural temporal variation observed.
Medical treatment delivered
Figures 13 and 14 illustrate data on medical treatments delivered at two example study sites: Outside-3 and Control-1.
FIGURE 13.
The proportion of ED attendances where a medical treatment was delivered per study fortnight for Outside-3. Note: Vertical orange line indicates approximate fortnight of GP model introduction at this site.

FIGURE 14.
The proportion of ED attendances where a medical treatment was delivered per study fortnight for Control-1.

For Outside-3 (see Figure 13), we observe an upward trend in the pre-intervention phase, which is then reversed in the post-intervention phase; there is little obvious evidence of seasonality. This interpretation is consistent with the coefficient values b0 = 0.015, b1 = –0.615, D = −0.630 and bs = 0.737; see Table 10. We note that the de-trended/de-seasonalised data show strong short-term correlation; this shows that the use of linear trendlines may not fully capture the structural temporal variation.
Site | Integrated-1 | Parallel-1 | Outside-1 | Control-1 |
---|---|---|---|---|
Trend | ||||
b 0 | 0.075 | 0.087 | 0.361 | 0.170 |
b 1 | –0.997 | –0.312 | –0.104 | |
D | –1.072*** | –0.399*** | –0.465 | |
Seasonality bs | 2.159 | 1.202 | –0.375 | –0.709 |
Site | Integrated-2 | Parallel-2 | Outside-2 | Control-2 |
Trend | ||||
b 0 | 0.058 | 0.419 | N/A | 0.074 |
b 1 | 0.040 | –0.277 | N/A | |
D | –0.019 | –0.696 | N/A | |
Seasonality bs | –0.386 | 4.457 | N/A | –0.971 |
Site | Integrated-3 | Parallel-3 | Outside-3 | Control-3 |
Trend | ||||
b 0 | N/A | 0.151 | 0.015 | –0.001 |
b 1 | 0.373 | –0.115 | –0.615 | |
D | N/A | –0.266*** | –0.630 | |
Seasonality bs | 0.559 | 0.151 | 0.737 | 0.163 |
Site | Parallel-4 | |||
Trend | ||||
b 0 | 0.022 | |||
b 1 | –0.240 | |||
D | –0.262 | |||
Seasonality bs | 0.794 |
For Control-1 (see Figure 14), we observe a complex pattern over the study window, with no clear evidence of seasonality; interpretation of the corresponding coefficient values b0 = 0.170 and bs = –0.709 (see Table 10) therefore requires some care. Here, the de-trended/de-seasonalised data show evidence of persistent short-term correlation; this is consistent with a more complex form of non-stationarity in the time plot, emphasising that a linear trend is best regarded only as a first step in capturing this structural temporal variation.
Table 10 shows flat or upward overall trends in the three control sites, but with some strong evidence that linear trends do not fully explain the more complex patterns of structural temporal variation observed in time plots. In the intervention sites overall, there is a consistent pattern of upward trends in pre-intervention phases followed by downward trends in post-intervention phases, with changes at three sites (Integrated-1; Parallel-1; Parallel-3) sufficiently large to be flagged as statistically significant. However, interpretation of these results also needs to reflect strong and widespread evidence that linear trendlines do not fully capture the more complex patterns of structural temporal variation observed in this variable at study sites.
Average time in emergency department
Figures 15 and 16 illustrate data on average time in ED at two example study sites: Parallel-1 and Control-3.
FIGURE 15.
The average time in ED per study fortnight for Parallel-1. Note: Vertical orange line indicates approximate fortnight of GP model introduction at this site.
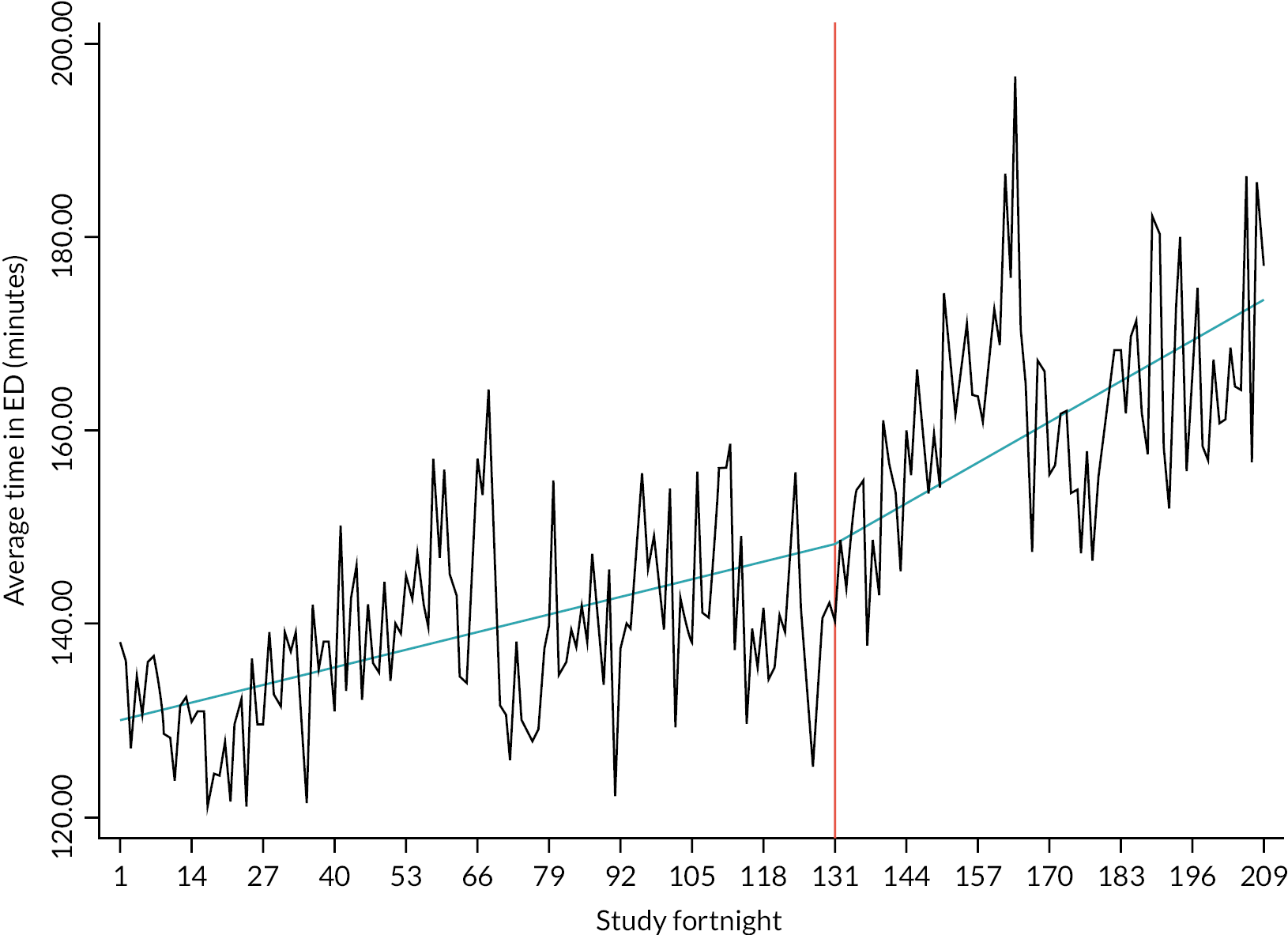
FIGURE 16.
The average time in ED per study fortnight for Control-3.
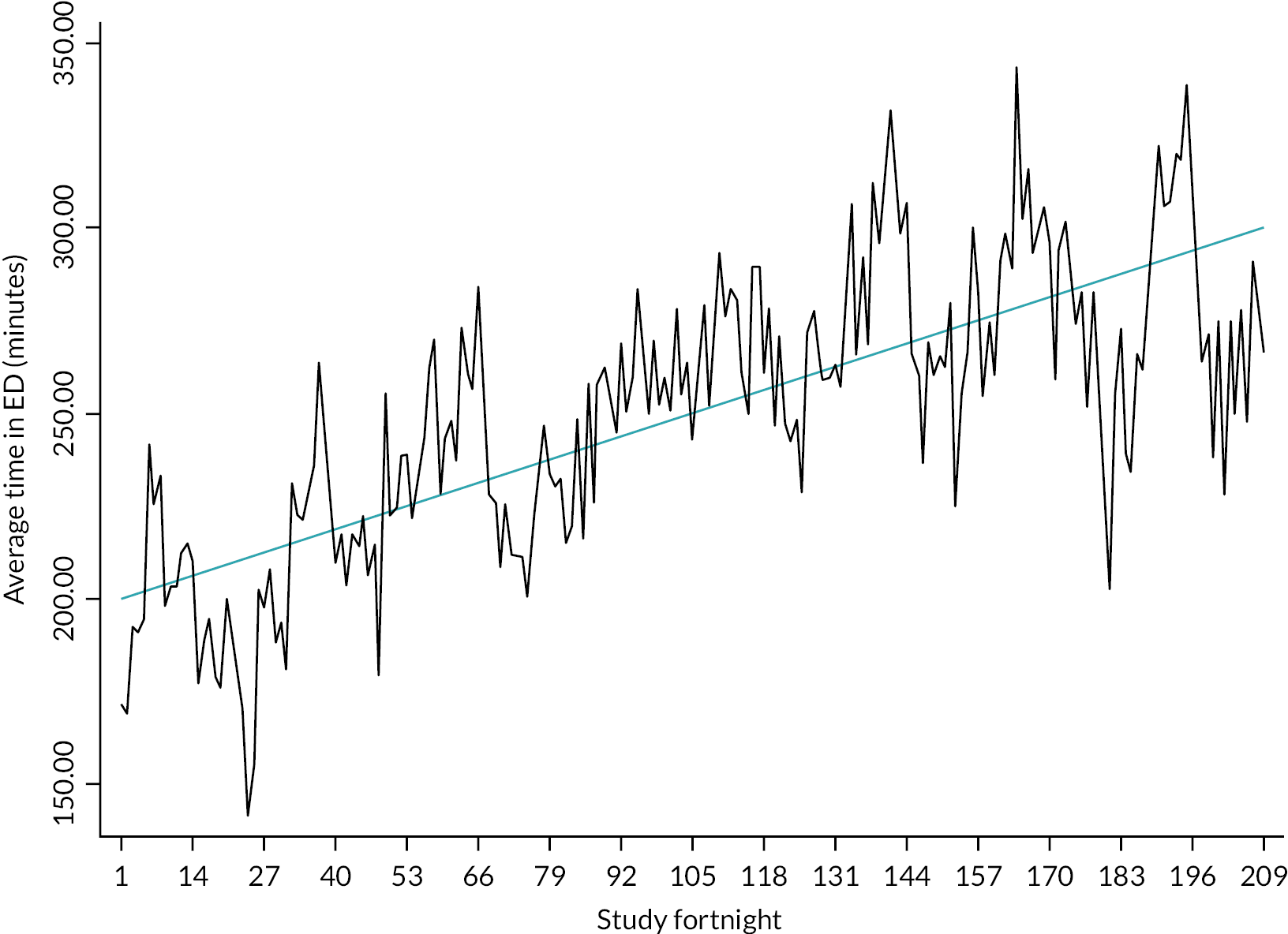
For Parallel-1 (see Figure 15), we observe an upward trend in the pre-intervention phase, which continues, with a further increase, in the post-intervention phase; there is little obvious evidence of seasonality. This interpretation is consistent with the coefficient values b0 = 0.139, b1 = 0.324, D = 0.185 and bs = −3.02; see Table 11. We note that the de-trended/de-seasonalised data show only moderate short-term correlation; this provides support for the use of linear trendlines to capture the structural temporal variation.
Site | Integrated-1 | Parallel-1 | Outside-1 | Control-1 |
---|---|---|---|---|
Trend | ||||
b 0 | 0.197 | 0.139 | 0.294 | 0.126 |
b 1 | 0.246 | 0.324 | 0.578 | |
D | 0.049 | 0.185 | 0.384 | |
Seasonality bs | –2.36 | –3.02 | –3.04 | –12.90 |
Site | Integrated-2 | Parallel-2 | Outside-2 | Control-2 |
Trend | ||||
b 0 | –0.020 | 0.922 | N/A | 0.543 |
b 1 | 0.029 | 0.119 | N/A | |
D | 0.050 | –0.803* | N/A | |
Seasonality bs | –4.48 | –7.43 | N/A | –14.14 |
Site | Integrated-3 | Parallel-3 | Outside-3 | Control-3 |
Trend | ||||
b 0 | N/A | 0.131 | 0.231 | 0.486 |
b 1 | 0.230 | 0.338 | –0.424 | |
D | N/A | 0.208 | –0.605*** | |
Seasonality bs | –3.99 | –9.58 | –7.10 | –18.86 |
Site | Parallel-4 | |||
Trend | ||||
b 0 | 0.368 | |||
b 1 | 0.583 | |||
D | 0.216 | |||
Seasonality bs | –11.94 |
For Control-1 (see Figure 16), we observed a consistent upward trend over the study window, and some evidence of seasonality; this is consistent with the coefficient values b0 = 0.126 and bs = −12.90; see Table 11. Here, the de-trended/de-seasonalised data show evidence of moderate short-term correlation; this supports a linear trend as a first step in capturing the structural temporal variation.
Table 11 shows upward overall trends in the three control sites, and with evidence that linear trends capture the structural temporal variation observed in time plots. In the intervention sites overall, there is generally a consistent pattern of upward trends in both pre- and post-intervention phases, balanced by evidence of reversals in upwards trends at two sites (Parallel-2; Outside-3). However, interpretation of some of these results also needs to reflect evidence that linear trendlines sometimes do not fully capture the more complex patterns of structural temporal variation observed at some study sites (Integrated-1; Control-2), although doing so to a reasonable extent at the others.
Average hospital length of stay
Figures 17 and 18 illustrate data on average hospital LOS (in days) for admissions following an ED attendance at two example study sites: Outside-1 and Control-3.
FIGURE 17.
The average hospital LOS per study fortnight for Outside-1. Note: Vertical orange line indicates approximate fortnight of GP model introduction at this site.
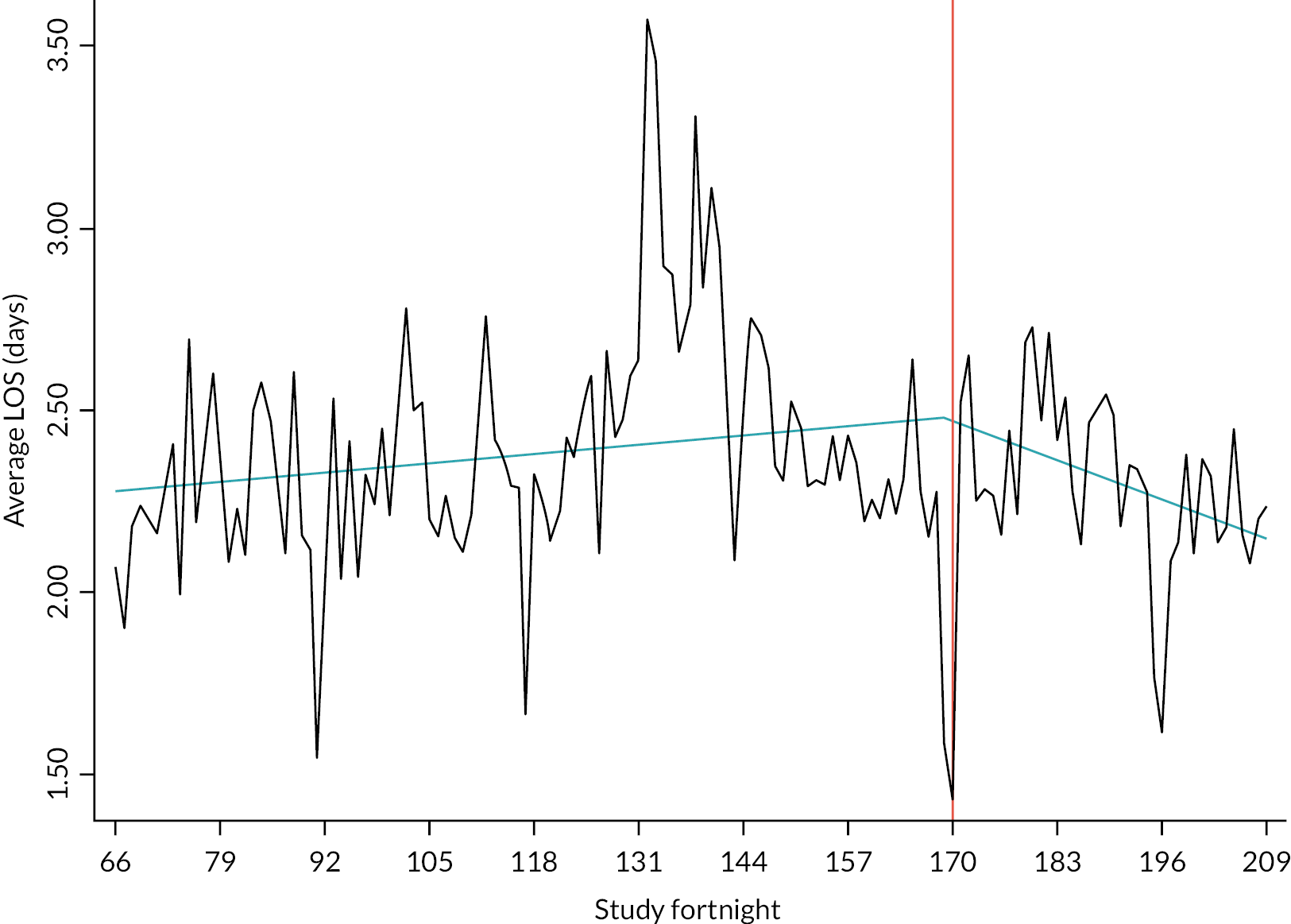
FIGURE 18.
The average hospital LOS per study fortnight for Control-3.
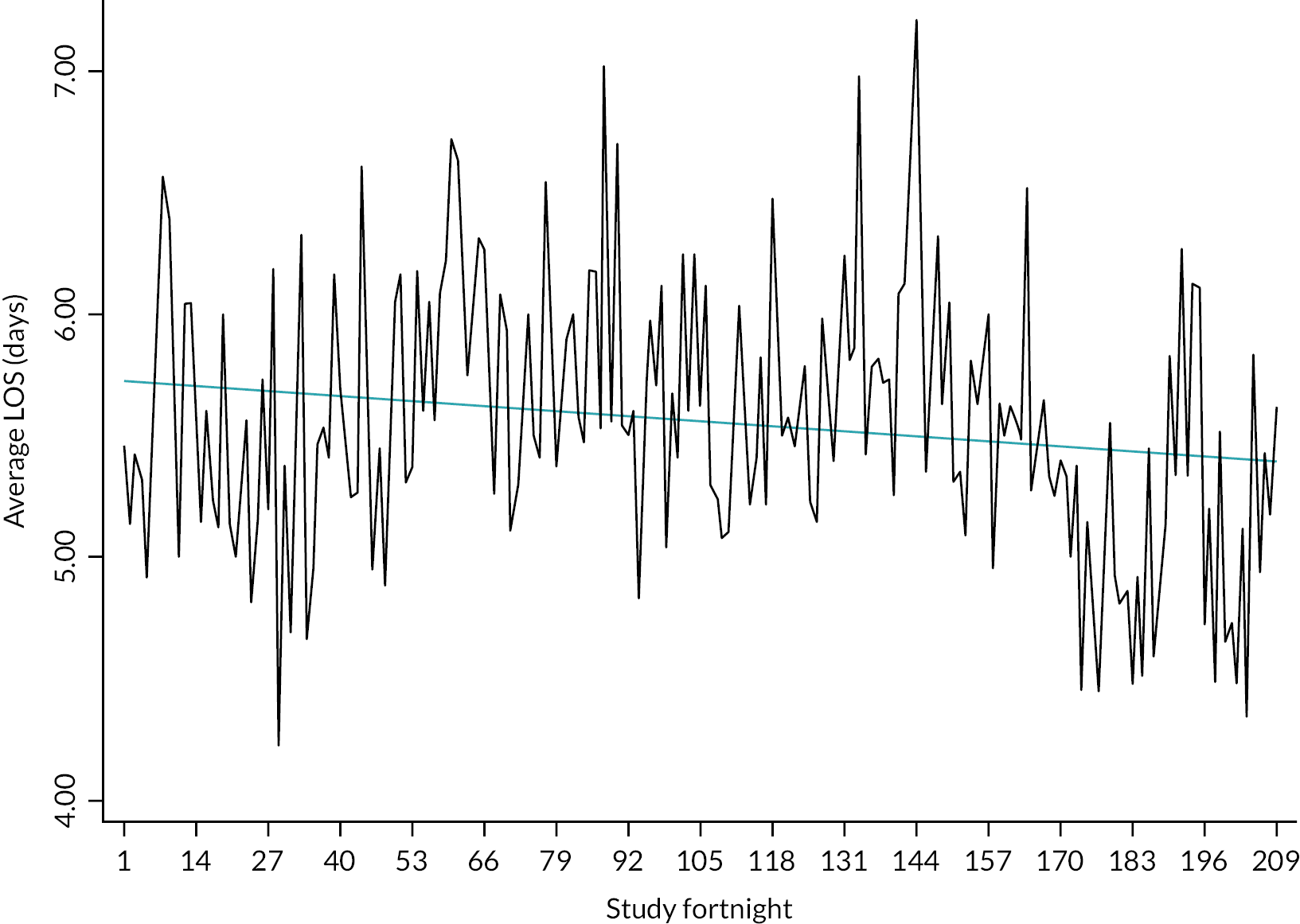
For Outside-1 (see Figure 17), we observe an upward trend in the pre-intervention phase, followed by a downward trend in the post-intervention phase; there is little obvious evidence of seasonality. This interpretation is consistent with the coefficient values b0 = 0.002, b1 = –0.008, D = –0.010 and bs = –0.004; see Table 12. We note that the de-trended/de-seasonalised data show some moderate short-term correlation; this supports for the use of linear trendlines to capture the structural temporal variation.
Site | Integrated-1 | Parallel-1 | Outside-1 | Control-1 |
---|---|---|---|---|
Trend | ||||
b 0 | –0.002 | –0.009 | 0.002 | –0.005 |
b 1 | 0.000 | 0.007 | –0.008 | |
D | 0.002 | 0.015*** | –0.010** | |
Seasonality bs | –0.005 | –0.063 | –0.004 | –0.001 |
Site | Integrated-2 | Parallel-2 | Outside-2 | Control-2 |
Trend | ||||
b 0 | 0.000 | –0.008 | N/A | 0.004 |
b 1 | 0.007 | –0.003 | N/A | |
D | 0.007 | 0.005 | N/A | |
Seasonality bs | –0.084 | 0.013 | N/A | –0.167 |
Site | Integrated-3 | Parallel-3 | Outside-3 | Control-3 |
Trend | ||||
b 0 | N/A | N/A | 0.002 | –0.002 |
b 1 | –0.003 | –0.014 | 0.000 | |
D | N/A | N/A | –0.001 | |
Seasonality bs | 0.021 | 0.002 | 0.000 | –0.171 |
Site | Parallel-4 | |||
Trend | ||||
b 0 | –0.002 | |||
b 1 | –0.054 | |||
D | –0.052*** | |||
Seasonality | ||||
b s | 0.028 |
For Control-3 (see Figure 18), we observed a consistent downward trend over the study window, and limited evidence of seasonality; this is consistent with the coefficient values b0 = –0.002 and bs = –0.171; see Table 12. Here, the de-trended/de-seasonalised data show evidence of weak short-term correlation; this supports a linear trend as a first step in capturing the structural temporal variation.
Table 12 shows a mixed picture in the control sites, with two downward trends and one upwards; there is some evidence that linear trends capture the structural temporal variation observed in time plots. There is also a mixed picture in intervention sites overall and in some subgroups, with some trendlines essentially flat (e.g. Integrated-1; Integrated-2; Outside-3). Change in trend is deemed statistically significant at three sites: Parallel-1, where an initial downward trend is reversed; Outside-1, where an initial upward trend is reversed (see Figure 17); and Parallel-4, where an initial downward trend is followed by a greater downward trend. As Figures 17 and 18 illustrate, this variable also displays a greater degree of random noise than is observed in other variables.
Summary of routine data analysis
Data quality
Data quality across sites was variable, tending to decrease as the level of integration between GP-ED model and the ED decreased. It also varied across the variables studied, with more inconsistency in those variables defined via details coded in routine data. Limitations in the routine data provided effectively precluded the inclusion of one study site (Outside-2) in analyses. We are more confident in interpretations on analyses for some outcomes (attendances, reattendances, admissions, LOS) than others (investigations, treatments, average time in ED) due to data quality issues.
We note that some variables initially under consideration (e.g. time to treatment in the ED; receipt of particular treatments) were excluded from analyses due to further significant issues within routine data. We also note the possibility of extending the list of variables to be investigated (e.g. by refining the reattendance period, or by considering further summary measures for some outcomes).
Summary of findings
The routine data indicated that, in general, at the GP-ED model sites, attendances increased over time, with accelerated increases at some sites in the post-intervention phase; see Table 6. Reattendances within 28 days in the post-intervention period increased over that in the pre-intervention period in 8 (of 9) sites assessed. Overall, intervention sites also demonstrated a consistent trend of increasing average time in the ED across both the pre- and post-intervention phases, with (non-significant) accelerations at 6 (of 8) sites. Data on investigation use at intervention sites demonstrated a mixed picture, difficult to interpret due to the complex patterns of structural temporal variation. This complexity was also observed in data relating to treatments delivered: overall, there was a pattern of increasing treatments delivered pre intervention, followed by decreasing treatments delivered post intervention – the significance of the identified trends is uncertain due to the non-linear nature of trends in data. A mixed picture was seen in relation to hospital admissions in the Integrated and Parallel sites, with data quality issues at the Outside sites, with examples of both increases and decreases both pre and post intervention. Similarly, average length of hospital stay showed a mixed picture both over all intervention sites and within models with some trendlines remaining essentially flat.
Assessment of trend methodology
The adoption of linear trendlines to assess temporal trends and changes in those trends was effective for some variables (including key variables related to ED attendances and hence reattendances) but less so for others, including those which depended on details coded in routine data. Possible reasons for this include changes in recording policies and protocols over time at sites; time plots include examples of non-linear non-stationarity for the two variables summarising medical investigations undertaken and medical treatments received. Findings and limitations for specific variables are summarised in the relevant Tables, and then briefly discussed.
Implications for advanced times series modelling
Prospects for more advanced time series models for data from subgroups of study sites (and, therefore, a single model for data from all study sites) appear to be somewhat limited. The main objection to fitting models which include a single subgroup intervention parameter is the mixed picture in trends and changes in trend observed across sites for most variables. Thus, we would intuitively expect a mixed picture across sites to yield an overall estimate in which (for example) a positive trend at one site largely cancelled out a negative trend at another site, leading to an overall estimate which failed to capture behaviour observed at either site, with a poorer fit than that obtained from two separate analyses.
Cost–consequences analysis
A CCA is an economic evaluation where the costs and outcomes (consequences) are reported without aggregation or weighting, presenting them separately, allowing the reader to form their own opinion and apply their own preferences and respective importance to their decision-making processes. The CCA approach is often recommended for complex interventions with a broad range of effects, which are difficult to measure in a single common unit. A CCA uses a balance sheet to help give a comprehensive but uncomplicated set of information on costs and outcomes for decision-makers.
Method
Costing the HES AE, HES APC, EDDS and PEDW data sets
The study variables were derived from the linked data sets with Healthcare Resource Group (HRG) codes already coded for each individual activity record. Healthcare Resource Group codes group patient events that use similar resource to each other for the purposes of hospital payments; for most events, these HRG codes include staff costs, investigations and treatments bundled into one, with certain procedures (e.g. rehabilitation) and high-cost drugs excluded.
These data were then matched on the HRG codes to the relevant annual NHS Reference costs (e.g. 2017–8). Emergency department data (HES AE and EDDS) were costed using the accident and emergency (A&E) lookup data based on the HRG code and whether the patient was admitted to hospital or discharged; inpatients (HES APC and PEDW) were costed using the EL (Elective Inpatient), NEL (Non-Elective Inpatient), NES (Non-Elective Short Stay) and DC (Day Case) lookup data based on the patient’s admission route (emergency or elective) and their LOS.
Where costs could not be identified, we imputed costs were using average costs for the year of that attendance/admission. Costs were valued in Great British pounds at 2019 prices, inflating previous year’s costs at a rate of 3.5% per annum to reflect the study time horizon of over 12 months. This methodological approach enables the presentation of resource use and the related costs, based on the recorded routine data for individual patients.
Intervention costs
Intervention costs (the costs of the GP-ED models) were obtained from published resources and directly from one of the participating hospitals (also used in Chapter 6).
Perspective
The costs of GP-ED models were considered from a healthcare provider (UK NHS) perspective over a 9-year period between 1 April 2010 and 31 March 2019.
Cost–consequences analysis
Descriptive statistics were reported for ED attendances and inpatient admissions. Using the dates of when each participating site implemented the intervention, patient attendances were classified into pre and post intervention; costs were reported for both periods for each of the model types. As control sites had no intervention, costs were reported over the whole study period. By subtracting mean resource use, per patient, for each of the model types from the control site, incremental differences were calculated and reported as mean cost (£)/time (minutes) saving per episode (ED attendance and inpatient admission).
Results
The results of the CCA are presented below. The healthcare costs (Table 13) are described for each model type within the study, with incremental differences reported in the cost–consequence balance sheets (Tables 14 and 15).
ED attendances | ||||
---|---|---|---|---|
Model | Statistic | Pre intervention | Post intervention | Control |
All GP models | n | 2,520,174 | 2,426,062 | |
Mean (95% CI) | £162.93 (£162.85 to £163.01) | £163.59 (£163.50 to £163.68) | ||
Median | £166.01 | £166.01 | ||
Standard deviation | £63.15 | £73.51 | ||
IQR (Q1–Q3) | £87.26 9 (£110.08–197.34) | £89.90 (£107.54–197.44) | ||
Inside–integrated | n | 514,523 | 1,224,912 | |
Mean (95% CI) | £163.71 (£163.54 to £163.88) | £154.16 (£154.05 to £154.28) | ||
Median | £166.01 | £160.22 | ||
Standard deviation | £61.38 | £66.09 | ||
IQR (Q1–Q3) | £87.26 (£110.08–197.34) | £82.98 (£93.11–176.09) | ||
Inside–parallel | n | 1,045,156 | 1,059,502 | |
Mean (95% CI) | £170.64 (£170.51 to £170.76) | £172.40 (£172.25 to £172.55) | ||
Median | £166.29 | £166.29 | ||
Standard deviation | £66.51 | £79.52 | ||
IQR (Q1–Q3) | £85.63 (£113.27–198.90) | £106.87 (£109.83–216.70) | ||
Outside–onsite | n | 960,495 | 141,648 | |
Mean (95% CI) | £154.13 (£154.02 to £154.25) | £179.19 (£178.79 to £179.59) | ||
Median | £160.22 | £169.91 | ||
Standard deviation | £59.07 | £76.77 | ||
IQR (Q1–Q3) | £65.72 (£109.83–175.55) | £111.98 (£113.29–225.27) | ||
Control | n | 1,558,841 | ||
Mean (95% CI) | £162.89 (£162.81 to £162.98) | |||
Median | £142.37 | |||
Standard deviation | £55.02 | |||
IQR (Q1–Q3) | £60.05 (£129.41–189.46) | |||
Inpatient admissions | ||||
Model | Statistic | Pre intervention | Post intervention | Control |
All GP models | n | 399,903 | 549,647 | |
Mean (95% CI) | £2285 (£2281 to £2289) | £2130 (£2126 to £2134) | ||
Median | £1927 | £1927 | ||
Standard deviation | £1196 | £1542 | ||
IQR (Q1–Q3) | £488 (£1859–2348) | £836 (£1512–2348) | ||
Inside–integrated | n | 85,719 | 254,303 | |
Mean (95% CI) | £2383 (£2376 to £2390) | £2107 (£2102 to £2113) | ||
Median | £1927 | £1927 | ||
Standard deviation | £1037 | £21,432 | ||
IQR (Q1–Q3) | £488 (£1859–2348) | £836 (£1512–2348) | ||
Inside–parallel | n | 162,403 | 251,367 | |
Mean (95% CI) | £2244 (£2237 to £2250) | £2177 (£2171 to £2183) | ||
Median | £1927 | £1927 | ||
Standard deviation | £1329 | £1552 | ||
IQR (Q1–Q3) | £488 (£1859–2348) | £488 (£1859–2348) | ||
Outside–onsite | n | 151,580 | 43,934 | |
Mean (95% CI) | £2272 (£2266 to £2278) | £1987 (£1969 to £2006) | ||
Median | £1927 | £1616 | ||
Standard deviation | £1122 | £2007 | ||
IQR (Q1–Q3) | £488 (£1859–2348) | £2124 (£565–2689) | ||
Control | n | 579,593 | ||
Mean (95% CI) | £2202 (£2197 to £2207) | |||
Median | £1927 | |||
Standard deviation | £1815 | |||
IQR (Q1–Q3) | £1575 (£1859–3434) |
Intervention costs | |||
---|---|---|---|
GP in ED cost scenarios | Unit cost per hour | Unit cost per minute | |
GPs employed directly by the NHS Trust in ED paid at the same rate as salaried GPs in primary care practices with same benefits | Per hour of GMS activity in primary care (excluding direct care staff costs, with qualification costs). Cost source: PSSRU 2019 | £136 | £2.26 |
GPs employed directly by the NHS Trust not as employees but as Locums | Locum rate paid direct to a GP in ED in 2019. Cost source: Clinical of a participating ED (Integrated-2) | £65 | £1.08 |
GPs employed directly by the NHS Trust not as employees but as Locums [with employers’ National insurance contribution (NIC) and employers pension contribution at 2019 rates] | Locum rate paid direct to a GP in ED in 2019. Cost source: Participating ED Integrated-2. Employers NIC at 13.8% and pension at 20.6% | £65 + £8.97 (NIC) + £13.39 (Pension) = £87.36 | £1.46 |
ED clinician cost scenarios | |||
Registrar (working alone) | Cost per working hour Cost source: PSSRU 2019 |
£47 | 0.78 |
Registrar (with 10 minutes consultant advice for 10% patients – assumption based on ED expert co-investigator) | Cost per working hour of registrar + 10 minutes per hour of senior consultant grade advice (£18). Cost source: PSSRU 2019 | £65 |
Post-intervention costs | ||||||||||
---|---|---|---|---|---|---|---|---|---|---|
Mean resource use per patient | Incremental mean resource use per model (vs. Control) | |||||||||
Control | All GP models | Inside–integrated | Inside–parallel | Outside–onsite | Control | All GP models | Inside–integrated | Inside–parallel | Outside–onsite | |
ED – all | ||||||||||
Cost (£) | 162.89 | 163.59 | 154.16 | 172.40 | 179.19 | – | 0.70 | –8.73 | 9.51 | 16.3 |
Inpatient admissions | ||||||||||
Cost (£) | 2202 | 2130 | 2107 | 2177 | 1987 | – | –72 | –95 | –25 | –215 |
Post intervention – consequences | ||||||||||
ED – all | ||||||||||
Time (in minutes) | 178.2 | 160.4 | 174.7 | 154.7 | – | –17.8 | –3.5 | –23.5 | ||
Inpatient admissions | ||||||||||
LOS (in days) | 3.0 | 1.8 | 1.9 | 1.6 | – | –1.2 | –1.1 | –1.4 |
Analysis of healthcare resource use and costs
The mean ED and other secondary healthcare resource use for each model type are shown in Table 13. There were 7,447,626 ED attendances in total across the different sites over the study period. Mean post-intervention ED visit estimated costs for the intervention sites were £163.59 [standard deviation (SD) £73.51] with mean costs associated with inpatient admissions were £2130 (SD £1542) while for the control site mean costs were £162.89 (SD £55.02) and £2202 (SD £1815) for ED visits and inpatient admission, respectively.
Mean post-intervention ED costs ranged from £154.16 (SD £66.09) in the inside–integrated model to £179.19 (SD £76.77) in outside–onsite, while mean inpatient costs ranged from £1987 (SD £2007) in the outside–onsite model to £2177 (SD £1552) in the inside–parallel model.
Negligible incremental ED attendance cost differences were observed between the model types and the control (see Table 14) with an extremely small increased cost (£0.70) observed when looking at all models combined compared with control sites, though a saving of £72 when looking at inpatient admissions. When looking at ED visits by individual models, the inside–integrated model saw a small cost saving (£8.73), but small cost increases were seen in the inside–parallel (£9.51) and outside–onsite model (£16.30). With respect to inpatient admissions, all models saw small cost savings (£25–215) with the outside–onsite model seeing the largest saving (£215). In addition to the negligible cost differences in the ED, there were also very small time savings (range 3.5–23.5 minutes) compared to the control.
Resource use data were costed individually; it is therefore possible for costs to vary depending on what the ED visit or hospital admission entailed, that is, more complicated visits incur a greater cost than a less complicated visit of the same duration; in this situation, it is possible for one model type to have less, or equal, resource use than the other model type, but still have higher costs.
Most of the costs incurred came from hospital admissions. Not only were individual costs higher than ED visit costs, the total costs are greater for inpatient admissions also. The proportion of admissions across the model types varied. Admission costs increase as a patient’s stay in hospital increases, especially when the admission is an emergency admission via ED, and therefore reducing emergency admissions and LOS, where possible, results in cost savings for the hospital. Each of the different GP-ED models saw small savings in LOS (1.1–1.2 days), which is possibly where the cost savings occurred. While each of the GP-ED model types had reduced admission LOS and costs compared to the control, it is an assumption that the case mix for each of the model types was the same (but possible that the type of patients seen and admitted are different, thus resulting in different lengths of stay and costs).
In Chapter 7, we explore specific aspects of the quantitative data with further context provided by known events and initiatives at case sites to refine our theories. The five themes explored there are:
-
effectiveness of streaming
-
GP role
-
effective teamwork and communication
-
patient experience
-
provider-induced demand.
Chapter 6 Investigating the economic impact of general practitioners in emergency departments: case study in an Inside–Parallel Emergency Department with selected (‘marker’) conditions that may be managed by emergency medicine or primary care colleagues
Introduction
The experience and training that a qualified GP brings to an ED are seen as strengths in terms of patient management. When appropriately used, a GP within the ED could potentially enable prudent use of investigations and lower inappropriate admissions. As described in Chapter 3, two studies from the UK56 and Ireland,70 published in 1996 and a later Dutch study77 suggest that GPs working within the ED managing patients with primary care type problems leads to cost savings from the hospital perspective. In these studies, patients were allocated to the GP by an ED triage nurse, compared with ‘business as usual’ – that is, no GP care. The costs savings were due to fewer referrals and admissions and lower investigation rates by the GPs, assumed due to a different approach to patient management. Cost savings were noted despite the GPs giving longer consultation times, and having higher salaries compared with ED clinicians. The key driver of the cost reduction was identified as reduction in admission to hospital by the GPs, compared with an ED clinician. 56 Other studies without economic evaluations reported that GPs had reduced ‘process times’ in the ED for patients with primary care type problems. 56,73,74,76–78 None of the studies identified in our RRR were full economic evaluations and may not be representative of current ways of working, financing models or current service pressures.
In Chapter 3, we described an initial theory explaining how engaging GPs to work in EDs to see patients with primary care problems might be a cost-effective option. However, as the research progressed it became clearer that there was no single outcome for a cost-effectiveness analysis that would be of greatest relevance for the stakeholders of this research. The health economic analysis was therefore reframed to be a CCA, with the following refinement to our initial theory.
If there is demand for patients with primary care type problems (C), GPs can see these patients in the ED (M), which is economically attractive (O) compared with patients being seen by ED clinicians, due to fewer referrals and admissions and lower investigation rates by the GPs despite the GPs having higher salary costs (M).
During the analysis, we were also mindful of an additional candidate theory which had suggested that GP-ED models might help to free up ED staff for more seriously ill patients. Our RRR suggested this might not occur in settings where there are delays in transferring patients to wards or inadequate staffing.
Aim
We aimed to investigate the economic impact of GPs on ED resource use and the related costs of clinical management when a GP within the ED treats a patient with primary care problems compared with an ED clinician.
Methods
To support the testing of the theories developed by the realist review, the methodological approach used for the economic analyses was CCA. 151 CCA considers the costs and resource consequences resulting from, or associated with, the use of an intervention (in this case GPs managing patients) and its alternative (ED clinicians managing the patient). The range of costs and resource consequences to be included in the analysis depends on the characteristics of interventions and their comparators. Generally, the estimates of resource use and of benefits are separate domains of the evaluation. Estimates of resource use may also include the comparative value of healthcare service use outcomes (such as length of hospital stay, or number of hospitalisations, outpatient or primary care consultations) associated with the intervention or its comparators. The analysis plan was incorporated into the combined statistical and health economics plan (see Report Supplementary Material 2). The perspective taken for the CCA is an NHS perspective and the time horizon was 1 year.
Marker condition selection
Testing our theories required that we have a clear concept of which ‘primary care problems’ could potentially be managed by either a GP or an ED clinician. As described in Chapter 3, six representative (‘marker’) conditions were identified to inform sampling for both the qualitative and quantitative data collection.
For the analyses described in this chapter, marker conditions were defined using the coded presenting complaint (Table 16).
Marker condition | Presenting complaint as coded |
---|---|
1. Abdominal pain | 1. Abdomen pain non-trauma |
2. Back pain | 2. Back pain non-trauma |
3. Chest pain | 3. Chest pain non-trauma |
4. Headache | 4. Headache/head pain non-trauma |
5. Cough and shortness of breath | 5. Cough; short of breath; noisy breathing; difficulty breathing |
6. Child under 10 years with fever | 6. Child < 10 years of age with a fever |
Site availability
All 13 research sites were invited to contribute to the quantitative investigation of the management of marker conditions in the ED. Most agreed to support this aspect of the research and investigate how to undertake the extraction of the data from their ED databases, using a ‘trial extraction’ based on the local activity variables available for the back pain marker condition. Unfortunately, some EDs found it difficult to devote staff time to support provision of data or did not have access to data of sufficient quality at this stage in the research (October 2019–January 2020). Subsequently, the COVID-19 pandemic profoundly affected the ability of most EDs to support the research. This left only one centre – Parallel-2 – able to provide a full data set for analysis.
Data collection and processing
Anonymised patient-level data extracts from the Parallel-2 ED database were obtained. The data extracts included information on presenting complaint and final diagnosis and whether a GP or ED clinician was responsible for management of the patients. As the data are based on HRG codes, specific information on diagnostic tests and treatments was not available. Data were cleaned to ensure standardisation of each marker condition data set.
The time in ED was categorised into three distinct periods and extracted in line with these periods:
-
arrival (T1) to treatment (T2)
-
treatment (T2) to discharge (T3)
-
overall time in ED (T1–T3).
The HRG codes were used to determine investigation and treatment categories as proxies for severity and complexity. Table 17 provides examples of the investigations and treatments included in each category. Table 18 shows the HRG codes and their associated treatment and investigation combinations. 152
Investigation category level | Example investigations | Treatment category level | Example treatments |
---|---|---|---|
1 | Urinalysis, electrocardiogram, pregnancy test, some blood tests (e.g. biochemistry) | 1 | Observations, IV cannula |
2 | Plain film X-ray, some blood tests (e.g. haematology, blood cultures) | 2 | Local anaesthesia, physiotherapy |
3 | CT scan, MRI scan, ultrasound scan | 3 | Urinary catheter, incision and drainage. |
4 | Chest drain, intubation |
HRG code | Definition | Severity/complexity |
---|---|---|
VB02Z | Investigation category 3 and treatment category 4 | Most |
VB03Z | Investigation category 3 and treatment category 1–3 | Most |
VB04Z | Investigation category 2 and treatment category 4 | Most |
VB05Z | Investigation category 2 and treatment category 3 | Most |
VB06Z | Investigation category 1 and treatment category 3–4 | Most |
VB07Z | Investigation category 2 and treatment category 2 | Medium |
VB08Z | Investigation category 2 and treatment category 1 | Medium |
VB09Z | Investigation category 1 and treatment category 1–2 | Least |
VB11Z | No investigations of treatment | Least |
Analysis
For each marker condition, descriptive analyses were undertaken, reporting frequencies and percentages for categorical data, and measures of central tendency for numerical data. Pearson’s chi-squared test was used to compare proportions of patients admitted to hospital and discharged home, while the non-parametric Mann–Whitney U-test was used to compare time. Data were stratified by whether the patient had been seen by ED clinician or by a GP. Generalised linear regression models (GLM) were then used to investigate the effect of clinician seen on patient time within the ED (see Report Supplementary Material 4). Time was included as the dependent variable; categorical variables, such as marker condition, gender or being seen by a GP or ED clinician, were included as fixed variables; and continuous variables, such as age, were included as covariates. A backward enter stepwise approach was taken with non-statistically significant variables (p > 0.05) removed.
We undertook both base-case analysis and sensitivity analysis, in order to investigate the influence of how GPs impact the time spent by patients in the ED.
-
Base case. The base-case analysis was obtained from running the economic analysis with assumptions and inputs that represents the most usual circumstances in the ED setting and is analogous to an ‘intention-to-treat’ approach. In practice, this included all patients who attended with one of the relevant identified presenting complaints, representing ‘real life’ ED as the diagnosis is not known at the time of arrival.
-
Sensitivity analysis: The purpose of undertaking this analysis was to understand whether and how effective streaming might influence the CCA. Of all patients with one of the selected presenting complaints, the subsequent diagnostic code recorded was reviewed by an ED clinician/co-investigator supporting the research. For this analysis, patients with a diagnosis that would not be expected to be managed by a GP in ED, and should not have been streamed to a GP, were excluded. For example, this excludes patients presenting with chest pain and subsequently diagnosed with conditions including myocardial infarction, supraventricular tachycardia and tension pneumothorax (see Report Supplementary Material 3).
Consequences
The ‘consequences’ of interest for this analysis of management of patients with the marker conditions, using their ED data, are the relative differences observed in:
For each marker condition:
-
Numbers (%) treated by GP versus ED clinician.
-
Severity/complexity (derived from HRG investigation and treatment categories).
-
Admission (to inpatient care) rate.
-
Overall mean time in ED (arrival to discharge): T-overall.
-
Mean waiting time from arrival (booking in at reception) to being seen by a treating clinician (treatment): T-1.
-
Mean time from treatment (seen by treating clinician) to discharge: T-2.
For all marker conditions:
-
Discharge destination.
-
Reattendance at ED with same complaint.
Costs
At the Parallel-2 site, the costs of the GP sessions or service were not available as the CCG paid the local provider for the service delivery. The cost of a GP delivering ED sessions was provided by Integrated-2 (a different site) with information about funding sources and strategies from other sites also providing context. NHS Reference costs 2019153 were used to provide costs for the investigations and treatment categories.
The CCA has a 12-month time horizon and so no discounting was applied to either costs or consequences.
Results
Parallel-2 site, setting and context
At the time of the case site visits (between June 2018 and July 2019), Parallel-2 had an Emergency Care Centre, comprising the ED, Emergency Admissions Unit and an Urgent Care Centre (see Chapter 4, Table 4 for further site characteristics). The urgent care centre and the ED are adjacent to each other but are separate work areas (corridors known as ‘pods’). Streaming to each service is undertaken by the triage nursing team. The GPs do not provide a service overnight – all patients will be seen by ED staff during this period and in daytime hours the service was not always fully staffed. The GPs were encouraged to act as GPs and deal with primary care problems streamed to them.
Marker conditions analysis
Below we present brief summaries of the base-case and sensitivity analyses undertaken for all six marker conditions. Tables presenting the full results are available in Report Supplementary Material 4. Table 19 summarises the number of patients with each marker condition through the 12-month period (1 July 2018 through 30 June 2019).
Marker condition | Total patients, N | Male (%) | Female (%) | Mean age (SD) | Median age (IQR) | Age range (minimum–maximum) |
---|---|---|---|---|---|---|
Back pain | 1929 | 847 (43.9) | 1082 (56.1) | 45.7 (18.6) | 45 (31–59) | 2–98 |
Chest pain | 4747 | 2339 (49.3) | 2407 (50.7) | 47.0 (19.4) | 47 (31–61) | 0–96 |
Cough and breathlessness | 7485 | 3505 (46.8) | 3979 (53.2) | 35.5 (28.8) | 33 (4–61) | 0–100 |
Fever (< 10s) | 2528 | 1305 (51.6) | 1223 (48.4) | 2.1 (2.1) | 1 (1–3) | 0–9 |
Abdominal pain | 7824 | 2891 (37.0) | 4931 (63.0) | 39.1 (21.0) | 35 (24–54) | 0–98 |
Headache | 1907 | 784 (41.1) | 1122 (58.8) | 37.0 (21.5) | 33 (21–53) | 0–96 |
Back pain
Of the 1929 patients presenting with back pain with a median age 45 years of age, 56% were female, 44% male and most (78%) were seen by ED clinicians, the rest (22%) by GPs.
Using the HRG investigations and treatment category groupings (see Table 17) as a proxy for severity, the more severe patients were more often seen by ED clinicians with the proportion of patients being seen by GPs increasing as severity decreased. While inpatient admission rates were higher when the patients were seen by ED clinicians, this aligned with severity. For patients who were least severe (and seen by ED clinicians (69%), inpatient admissions were higher (p = 0.047) when the patient was seen by ED clinicians, compared with GPs.
Once registered, for patients who were put into the GP stream on arrival to the ED, the average overall time (T1–T3) spent in the ED was shorter than if they were streamed to the ED clinician (a difference of 47 minutes, p < 0.001). The driver of that difference was the time between initiation of treatment and departure (T2–T3) from the ED (a difference of 45 minutes, p < 0.001). The less severe patients spent less time in the period between treatment to departure but a difference in duration between ED clinicians and GPs was maintained (13 minutes, p < 0.001 shorter time if seen by GPs).
Sensitivity analysis
The population used in the sensitivity analysis was 1339 (69.4%) of the base-case population. For those patients with a presenting complaint of back pain (non-trauma), categorised as likely to have been suitable for streaming to a GP based on their subsequent diagnosis, the average age was similar (45 years of age, 54% female). Most (77%) were seen by ED clinicians, the rest (23%) treated by GPs.
Using the HRG category groupings (see Table 17), the more severe patients were more often seen by an ED clinician with the proportion of patients being seen by GPs increasing as severity decreased. While inpatient admission rates were higher when the patients were seen by ED clinicians, this aligned with severity. For patients who were least severe and seen by ED clinicians (57.9%), inpatient admissions were higher for ED clinicians compared with GPs, although the difference was not statistically significant (p = 0.091).
For the patients allocated to the GP stream on arrival at the ED, the time (T1–T3) spent in the ED was shorter than if they were streamed to the ED clinician (a difference of 46 minutes, p < 0.001). The driver of that difference was the time (T2–T3) between initiating treatment and departure from the ED (a difference of 46 minutes, p < 0.001). The less severe patients spent less time in the period between treatment to departure but a difference in duration between ED clinicians and GPs was maintained (16 minutes shorter time if seen by the GP vs. ED clinician, p < 0.001).
Chest pain
Of the 4747 patients attending the ED with a presenting complaint of chest pain, 50.7% were female and 49.3% male. Most (93.5%) patients were treated by an ED clinician and 6.5% treated by a GP. Patients seen by the ED clinicians were significantly older than those seen by the GP (median age 48 vs. 31, p < 0.001) which may reflect some age-related selection bias.
Using HRG investigation and treatment categories as proxies for severity, most severe patients (see Table 17) were more often seen and treated by the ED clinicians (99.4% vs. 0.6%), with the proportion of patients seen by GPs increasing as severity decreased.
Overall, the ED clinicians admitted a significantly higher proportion of patients to hospital than GPs (27.1% vs. 3.6%, χ² = 83.9, p < 0.001), consistent with seeing more severe and complicated patients than the GPs. Of the less severe patients, inpatient admissions were higher when the patient was seen by the ED clinician compared with the GP (3.0% vs. 0.7%, χ² = 4.4, p = 0.043). Emergency department clinicians treated the majority of severe patients, where most hospital admissions originated, and there were not enough patients in the GP group to test the difference in the proportions of inpatient admissions.
Once booked in, patients who were treated by GPs spent less time (T-overall 61 minutes less) before discharge than those treated by ED clinicians (mean 2 hours 4 minutes vs. 3 hours 5 minutes, p < 0.001). This time includes the waiting time to be seen before treatment (T1–T3). There was no significant difference in the waiting time (mean 1 hour 26 minutes vs. 1 hour 29 minutes, p = 0.317) before being treated; the significant time difference was between treatment and discharge (T2–T3) (1 hour 38 minutes vs. 35 minutes, p < 0.001) indicating that, once seen by a doctor, the GPs’ patients took less time before discharge.
The main time difference between initial treatment and discharge (T2–T3) was in the least severe patients (43 minutes for ED clinicians and 29 minutes for GPs, p < 0.001) and the medium severity patients (1 hour 45 minutes for ED clinicians and 1 hour 18 minutes for GPs, p = 0.016).
Sensitivity analysis
The matched population for the sensitivity analysis was 3915 (82.5% of base-case population). For patients with a presenting complaint of chest pain and subsequent diagnosis indicating likely suitability for streaming to a GP, the median age was 47 years of age though, again, the ED clinicians saw significantly older patients than GPs (median age 48 vs. 30 years, p < 0.001). Most patients (92.9%) were seen by ED clinicians, and 7.1% were treated by GPs.
Using the HRG category groupings (see Table 17), the more severe patients were more often seen by an ED clinician with the proportion of patients being seen by GPs increasing as severity decreased. Inpatient admissions were only significantly different between ED clinicians and GPs for the least severe patients (3.5% vs. 0.8%, χ² = 4.9, p = 0.027).
For patients streamed to GPs on arrival to the ED, the overall time (T1–T3) spent in the ED was shorter if they were streamed to the GP (a difference of 64 minutes, p < 0.001). The driver of that difference was the time between initiating treatment and departure (T2–T3) from the ED (a difference of 65 minutes, p < 0.001). The less severe patients spent less time from treatment to departure (T2–T3) but a difference in duration between ED clinicians and GPs was maintained (21 minutes shorter time if seen by GPs, p < 0.001).
Cough and breathlessness
Of the 7485 patients with a presenting complaint of cough or breathlessness, 53.2% were female and 46.8% were male. Most (81.2%) patients were treated by an ED clinician and 18.8% treated by a GP. The median age of those treated by ED clinicians compared with those treated by GPs was 34 years of age compared with 32 years of age.
Using HRG investigation and treatment categories as proxies for severity (see Table 17), most severe patients were more often seen and treated by the ED clinicians (96.5% vs. 3.5%), with the proportion of patients seen by GPs increasing as severity decreased.
Overall, the ED clinicians admitted a significantly higher proportion of patients to hospital than GPs (29.0% vs. 2.8%, χ² = 429.0, p < 0.001), consistent with seeing more severe and complicated patients than GPs. A significant difference in the proportions of patient admissions existed in the least severe patients (admissions 5.9% vs. 1.8%, χ² = 32.4 p < 0.001) and the most severe patients (admissions 64.6% vs. 8.3%, χ² = 94.2 p < 0.001), while in the middle group no difference was observed (p = 0.229).
Once booked in, patients seeing a GP spent less time (T1–T3) (47 minutes) than those treated by ED clinicians (mean 2 hours 5 minutes vs. 2 hours 52 minutes, p < 0.001). Comparing the time between initial treatment and discharge, there was a small but significant difference in the waiting time (mean 1 hour 16 minutes vs. 1 hour 26 minutes, p < 0.001) in favour of seeing ED clinicians (T1–T2), but a larger, significant, time difference between treatment and discharge (T2–T3) (mean 1 hour 36 minutes vs. 38 minutes, p < 0.001) in favour (shorter time) of seeing GPs.
When stratified by severity, again there was no difference in waiting times between ED clinicians and GPs (T1–T2) for the most severe and the medium severity cough and breathlessness patients (p = 0.053 and p = 0.178, respectively) though for the least severe patients there was a small, but significant, difference in wait time (T1–T2) (1 hour 13 minutes vs. 1 hour 26 minutes, p < 0.001) favouring those seen by an ED clinician (shorter time). For each severity category, the time between initial treatment and discharge (T2–T3) was significantly shorter for patients seeing a GP compared with an ED clinician, with the time difference ranging from 16 minutes (least severe, p < 0.001) to 1 hour and 35 minutes (most severe, p < 0.001).
Sensitivity analysis
The matched population for the sensitivity analysis was 7001 (93.5% of the base-case population). When the patients had both a presenting complaint of breathlessness and a subsequent diagnosis suggesting they were suitable for streaming to a GP, the median age was 33 years (median age 34 years ED clinicians vs. 32 years GPs, p = 0.033). Most patients (80.9%) were seen by ED clinicians and 19.1% were treated by GPs.
Using the HRG category groupings (see Table 17), the more severe patients were more often seen by an ED clinician with the proportion of patients being seen by GPs increasing as severity decreased. Inpatient admissions were higher among ED clinicians than GPs for the most severe (65.0% vs. 9.1%, χ² = 85.9, p < 0.001) and the least severe patients (6.3% vs. 1.6%, χ² = 38.7, p < 0.001), though no difference was observed in the medium severity patients (p = 0.067).
For patients streamed to GPs on arrival to the ED, the time (T1–T3) spent in the ED was shorter than if they were streamed to the ED clinician (a difference of 52 minutes, p < 0.001). The driver of that difference was the time between initiating treatment and departure (T2–T3) from the ED (a difference of 61 minutes, p < 0.001). The least severe patients spent less time between arrival and treatment (T1–T2) (10 minutes shorter, p < 0.001) when seen by the ED staff, but less time between treatment to departure (T2–T3) (21 minutes shorter, p < 0.001) when seen by a GP. Significantly shorter times were observed between treatment and discharge (T2–T3) for both the most severe (1 hour 35 minutes shorter (p < 0.001) and the medium severe patients (35 minutes shorter, p < 0.001) when seen by GPs compared with ED clinicians.
Fever in children under 10 years of age
Of the 2528 children with a presenting complaint of fever, 48.4% were female and 51.6% were male. Most (71.7%) patients were treated by an ED clinician and 28.3% treated by a GP. No statistically significant difference in age was observed between those seen by ED clinicians (median age 1 year) and those seen by GPs (2 years of age) (p = 0.057), respectively.
Using HRG investigation and treatment categories as proxies for severity, most severe patients (see Table 17) were more often seen and treated by the ED clinicians (98.6% vs. 1.4%), with the proportion of patients seen by GPs increasing as severity decreased.
Overall, the ED clinicians admitted a significantly higher proportion of patients to hospital than GPs (13.8% vs. 2.4%, χ² = 71.1, p < 0.001), though the significant difference in the proportion of admissions was most evident in the least severe patients (admissions 9.0% vs. 2.4%, χ² = 31.6, p < 0.001) and the medium severity patients (admissions 30.1% vs. 2.7%, χ² = 12.5, p = 0.001).
Once booked in, patients treated by GPs spent less time (19 minutes) in ED (T1–T3) than those treated by ED clinicians (mean 2 hours 16 minutes vs. 2 hours 35 minutes, p < 0.001). However, this time includes the waiting time to be seen before treatment (T1–T2). The significant differences in time were those between the initial arrival and treatment (T1–T2) (mean 1 hour 37 minutes vs. 1 hour 19 minutes, p < 0.001) – shorter for those seen by ED clinician than GPs, and the time between treatment and departure (T2–T3) (mean 38 minutes vs. 1 hour 16 minutes, p < 0.001), which was shorter for those seen by GPs.
When stratified by severity, the time between initial treatment and discharge (T2–T3) was significantly shorter for those treated by GPs, in both the least severe patients (1 hour 9 minutes vs. 38 minutes, p < 0.001) and the medium severity patients (1 hour 43 minutes vs. 49 minutes, p < 0.001).
Sensitivity analysis
The matched population for the sensitivity analysis was 2405 (95.1% of the base-case population). When the patients, aged under 10 years, had both a presenting complaint of fever and a subsequent diagnosis indicating that they were likely to have been suitable for streaming to a GP, the median age was 1 year for those seen by the ED clinicians compared with median age 2 years for GPs (p = 0.074). Most patients (71.7%) were seen by ED clinicians and 28.3% treated by GPs.
Using the HRG category groupings (see Table 17), the more severe patients were more often seen by an ED clinician with the proportions of patients being seen by GPs increasing as severity decreased, though the vast majority of patients were of the least severe category. Inpatient admissions were higher between ED clinicians and GPs only for the least severe patients (9.1% vs. 2.2%, χ² = 33.3, p < 0.001).
For the patients who were streamed to GPs on arrival to the ED, the overall time (T1–T3) spent in the ED was shorter than if they were streamed to ED clinicians (a difference of 23 minutes, p < 0.001). The driver of that difference was the time between initiating treatment and discharge (T2–T3) from the ED (a difference of 40 minutes, p < 0.001). The least severe patients spent less time between arrival and treatment (T1–T2, 15 minutes shorter, p < 0.001) when seen by the ED staff, but less time between treatment to departure (T2–T3, 34 minutes shorter, p < 0.001) when seen by a GP. Significantly shorter times were observed between arrival and treatment (T1–T2, 31 minutes shorter, p < 0.001) if seen by ED clinicians, though shorter times between treatment and discharge (T2–T3), for the medium severe patients, (61 minutes shorter, p < 0.001) when seen by GPs compared with ED clinicians.
Abdominal pain
Of the 7824 patients attending the ED with a presenting complaint of abdominal pain, 63.0% were female and 37.0% were male. Most patients (85.8%) were treated by an ED clinician and 14.2% by a GP. There was a statistically significant difference in age of 7 years between those seen by the ED clinician and those seen by the GP (median age 36 vs. 29 years, p < 0.001) with the ED clinician seeing older patients which suggests some selection bias.
Using HRG investigation and treatment categories as proxies for severity, the most severe patients (see Table 17) were more often seen and treated by the ED clinicians (99.4% vs. 0.6%), with the proportion of patients seen by GPs increasing as severity decreased.
Overall, ED clinicians admitted a significantly higher proportion of patients to hospital than GPs (30.2% vs. 4.8%, χ² = 315.0, p < 0.001). This was driven by significant differences in the proportions of patient admissions among medium severity patients (admissions 17.3% vs. 9.0%, χ² = 6.2, p = 0.013), with the difference in admissions between ED clinicians and GPs for the least severe patients showing no significant difference (5.4% vs. 3.9%, χ² = 2.5, p = 0.116).
Most severe patients, where most hospital admissions originated, were seen and treated by the ED clinicians and there were not enough patients in the GP group to test the difference in proportions of patients admitted.
Once booked in, patients treated by GPs with a presenting complaint of abdominal pain experienced a shorter overall time (T1–T3) before discharge than those treated by ED clinicians (mean 2 hour 6 minutes vs. 3 hours 14 minutes, p < 0.001). This was most evident between treatment and discharge (T2–T3) (mean 37 minutes for GPs vs. 1 hour 45 minutes for ED clinicians, p < 0.001).
When stratified by severity, there was a small, but significant, difference in overall waiting times (T1–T3) between ED clinicians and GPs for the least severe patients (1 hour 18 minutes vs. 1 hour 28 minutes, p < 0.001), while there was no difference between ED clinicians and GPs seeing the medium severity and most severe abdominal pain patients (p = 0.588 and 0.315, respectively). Time between initial treatment and discharge (T2–T3) was significantly shorter for all patients of all severities who saw GPs with differences ranging from 15 minutes (p < 0.001) for the least severe patients to 49 minutes (p = 0.030) for the most severe patients.
Sensitivity analysis
The matched population for the sensitivity analysis was 6967 (89.0% of the base-case population). When the patients had both a presenting complaint of abdominal pain and a subsequent diagnosis likely to have been suitable for streaming to GPs, the median age was 35 years with a significant difference between those seen by ED clinicians compared to GPs (median age 36 vs. 29 years, p < 0.001). Most patients (85.3%) were seen by ED clinicians and 14.7% by GPs.
Using the HRG category groupings (see Table 17), the more severe patients were more often seen by an ED clinician with the proportions of patients being seen by GPs increasing as severity decreased. Inpatient admissions were significantly higher between ED clinicians and GPs for all patient severities (most severe: 62.4% vs. 25.0%, χ² = 7.1, p = 0.008; medium severe: 16.3% vs. 7.9%, χ² = 6.3, p = 0.012; and least severe: 5.8% vs. 3.4%, χ² = 6.3, p = 0.012).
The overall time (T1–T3) spent in the ED was shorter for patients streamed to the GPs (a difference of 1 hour 10 minutes, p < 0.001). The driver of that difference was the time between initiating treatment and discharge (T2–T3) from the ED (a difference of 1 hour 9 minutes, p < 0.001). The least severe patients spent less time between arrival and treatment (T1, 8 minutes shorter, p = 0.001) when seen by the ED staff, but less time between treatment to discharge (T2–T3, 23 minutes shorter, p < 0.001) when seen by a GP. Significantly shorter times were only otherwise observed between treatment and discharge (T2–T3) for the medium severity patients (32 minutes shorter, p < 0.001) when seen by GPs compared with ED clinicians.
Headache
Of the 1907 patients attending the ED with a presenting complaint of headache, 59% were female and 41% were male. Most patients (82%) were treated by an ED clinician and 18% by a GP. Those seen by the ED clinician were older than those seen by the GP (median age 34 vs. 31 years, p = 0.002), respectively, which suggests some selection bias.
Using HRG investigation and treatment categories as proxies for severity, most severe patients (see Table 17) were more often seen and treated by the ED clinicians (99.3% vs. 0.7%), with the proportion of patients seen by GPs increasing as severity decreased.
Overall, the ED clinicians admitted a significantly higher proportion of patients to hospital than GPs (18.1% vs. 2.7%, χ² = 50.5, p < 0.001). When patients were stratified by severity, there were no significant differences in the proportion of patient admissions between GPs and ED clinicians, but sample sizes limited power of analysis.
Once booked in, patients treated by GPs with a primary complaint of headache spent a shorter (T1–T3) time (48 minutes) before discharge than those treated by ED clinicians (2 hours 9 minutes vs. mean 2 hours 57 minutes, p < 0.001). The time between treatment and discharge (T2–T3) (mean 33 minutes vs. 1 hour 26 minutes, p < 0.001) was also shorter when the patient was treated by GPs.
When stratified by severity, there was a small, significant difference in waiting times (T1–T2) between ED clinicians and GPs for the least severe patients (1 hour 24 minutes vs. 1 hour 35 minutes, p = 0.010), but no difference for the medium or severe patients (p = 0.435 and p = 0.894, respectively). Time between initial treatment and discharge (T2–T3) was significantly shorter in both the least severe (43 vs. 31 minutes, p < 0.001) and medium severity patients (1 hour 13 minutes vs. 34 minutes, p < 0.001) if seen by GPs compared with ED clinicians.
Sensitivity analysis
The matched population for the sensitivity analysis was 1361 (71.4% of the base-case population). When the patients had both a presenting complaint of headache and a subsequent diagnosis categorised as likely to have been suitable for GP streaming, the median age was 34 years with a significant difference between those seen by the ED clinicians compared to GPs (median age 35 vs. 30 years, p < 0.001). Most patients (81.1%) were seen by an ED clinician and 18.9% by a GP.
Using the HRG category groupings (see Table 17), the more severe patients were more often seen by an ED clinician with the proportion of patients being seen by GPs increasing as severity decreased. No differences in inpatient admissions were observed between ED clinicians and GPs for any patient severity (most severe, p = 0.852; medium severe, p = 0.157 and least severe, p = 0.117).
The overall time (T1–T3) spent in the ED was shorter for patients streamed to GPs (difference 1 hour 1 minute, p < 0.001). The driver of that difference was the time between initiating treatment and departure (T2–T3) from the ED (1 hour 5 minutes less, p < 0.001). Time between treatment and discharge (T2–T3) was shorter for both the least severe (20 minutes shorter, p < 0.001) and medium severe patients (45 minutes shorter, p < 0.001) when seen by GPs compared with ED clinicians.
Discharge destination
Across all marker conditions, for those treated by ED staff and GPs, most patients were discharged home after their ED visit, though some patients were referred to other healthcare providers (ED 3.5–9.3%; GPs 3.3–8.1%). There was a small proportion of patients who self-discharged having been seen by either ED clinician [during the treatment to discharge (T2–T3) interval; 0.7–1.8%] or GPs (0.0–0.9%), while some did not wait for treatment (ED 1.6–2.8%; GPs 0.7–3.3%).
In the case of the sensitivity analyses, across all marker conditions, for those treated by ED clinicians and GPs, most patients were discharged home after their ED visit, though some patients were referred to other healthcare providers (ED 2.0–7.0%; GPs 2.3–6.6%). There was a small proportion of patients who self-discharged having been seen by either ED clinicians (0.3–0.9%) or GPs (0.0–0.8%), and some did not wait for treatment (ED 0.3–0.9%; GPs 0.0–0.8%).
Reattendance to emergency department with the same complaint
One of the concerns when comparing GP and ED clinician management of patients overall, and specifically the marker conditions, is that suboptimal management may result in reattendance to the ED (with the same condition). A reattendance with the same presenting complaint within 7 days was considered a reasonable time period in which to attribute a relationship between the original and the reattendance. In Chapter 7, we discuss reattendance as a potential marker of patient safety. Here, we investigate it because of its cost consequences. We have investigated the reattendance rate (with the same condition) across all the patients in our marker condition cohorts in the first 7 days after discharge.
Overall, there were 24,834 initial attendances across all marker conditions; 83% (n = 20,658) of these were seen by ED staff, and 17% (n = 4176) were seen by GPs. Of these, 1586 (6%) reattended within the first 7 days after discharge. Of those seen by ED staff, 1319 (6%) reattended, while of those seen by GPs 267 (6%) reattended [χ² (1) = 0.0004, p = 0.983]. There were no significant differences in the proportion of reattendances between physician types for any individual marker condition.
Multiple regression analysis
These analyses were undertaken with several variables included to investigate their effect on patient time within the ED. Time was included as the dependent variable; categorical variables, such as condition, gender or being seen by GPs or ED clinicians, were included as fixed variables; and continuous variables, such as age, were included as covariates. Time in ED was categorised into time from arrival to treatment (T1–T2), time from treatment to discharge (T2–T3) and overall time (T1–T3).
Time from arrival to treatment (T1–T2)
The patients seen by ED clinicians spent less time waiting to be seen and treated than those by GPs (9.9 minutes, p < 0.001). This model gave a baseline intercept value of 98.8 minutes after adjusting for treating doctor (ED or GP), condition type, age, discharge status and investigations and treatment. However, this model accounted for 1.9% of the variability meaning that unknown variables accounted for a further 98.1%.
Time from initial treatment to discharge (T2–T3)
Patients seen by the GP spent 17.5 fewer minutes being treated than those seen by ED clinicians (p < 0.001) after adjusting for treating doctor (ED or GP), condition type, age, discharge status and investigations and treatment. This model accounted for 42.9% of the variability meaning that unknown variables accounted for a further 57.1%.
Overall time in emergency department (T1–T3)
Overall, after adjusting for treating doctor (ED or GP), condition type, age, discharge status and investigations and treatment, patients spent a baseline value of 247 minutes in ED between arrival and departure. Those patients seen by GPs spent 7.7 fewer minutes in ED than those seen by ED clinicians (p < 0.001). This model accounted for 33.3% of the variability meaning that unknown variables accounted for a further 66.7%.
Costs
The costs of GPs in EDs vary depending on the type of funding model adopted by individual trusts. In some cases where the service is provided through a contract placed with a private provider, there are no data available to estimate the cost per GP session or an hourly rate. This has meant that the actual cost of a GP has been difficult to ascertain. Table 13 in Chapter 5 outlines different scenarios for the cost of the GP in ED. In some scenarios, GPs cost less than ED staff, while in others they cost more.
We used HRG codes to categorise the severity of the attendance, and analyses were adjusted for severity where possible. HRG codes are derived from EDs and ignore data about the treating physician; this makes the use of HRG codes to cost the hourly rate of the respective clinicians impossible.
Discussion
Our CCA to investigate the economic impact of GPs working at study site Parallel-2 suggests that GPs can provide a time and investigation sparing service to patients with the marker conditions selected for this research, without increasing admission or reattendance rates. Our results from the analysis of the outcomes of management of all marker conditions and the related sensitivity analyses suggest that these GPs may manage the patients presenting with the marker conditions more quickly, the main driver of this difference being the time taken from treatment to discharge, rather than the time from admission to the ED to being seen for treatment. However, in this single-site observational study, caution must be exercised in overinterpreting patient conditions where the disparity in age of patients streamed to GP compared to ED clinician, for some marker conditions (chest pain, abdominal pain), suggests potential selection bias, with ED clinicians seeing older higher-risk groups.
The GPs completed patient management (i.e. T2–T3) more quickly, compared with ED clinicians, in both the base-case and sensitivity analyses. However, there may be other factors affecting these time differences. To adjust for these other factors, a regression analysis was undertaken on the overall population (all marker conditions). Adjusting for the potential influencing factors (treating doctor; GP vs. ED clinician, the marker condition, patient age, whether admitted or discharged, investigation and treatment category and triage category, patients spent 7.7 fewer minutes overall in ED but from the time when treatment is initiated, to discharge (T2–T3) patients with marker conditions had 17.5 fewer minutes being treated by GPs than ED clinicians. This is a potentially meaningful amount of time, at a stage of treatment where clinician time is a scarce resource and the time saved may be useful for deployment elsewhere if aggregated across a shift or day. However, given high unexplained variance for this analysis, caution is required in interpretation of its significance. Furthermore, it is important to consider the impact of the way of working in this site; the GPs work from a separate area and can be relatively undisturbed when they are seeing patients and the ED clinician may be managing more than one patient and/or being interrupted.
This economic analysis did not set out to examine the effectiveness of the streaming service. Our results suggest streaming at Parallel-2 effectively directs lower severity and less complex patients to the GP service, potentially enabling shorter treatment times (and improved patient experience) than if the patient with the same marker condition severity were seen by ED clinicians.
Across all marker conditions, the trend was for GPs to admit fewer patients than ED clinicians. This trend was more evident (and for some reaching statistical significance) among those requiring the lowest levels of intervention. Reassuringly, the reattendance rate for patients with the same marker condition at 7 days was the same for both GPs and ED clinicians and patients’ destination at discharge did not vary between the treating clinicians. The Parallel-2 study site which provided the data for analysis could provide costs of providing the GP service. This was because the funding for the service was provided by the local CCG as part of a wider package that supported urgent care in the region across several sites and the salary data were not available to the CD. However, across all study sites, we gained a reasonable sense of the different ways in which the GPs providing the service were paid and the pros and cons of these approaches (see Chapter 5, Table 14). 150 One site (Integrated-2) provided hourly rates of £65 for GPs employed in the ED, and we used this as our base case and undertook a sensitivity analysis using other information. From the cost scenarios we put together in Table 14 it seems that the GP service, however funded, is likely to cost more per hour of provision than middle-grade ED clinicians seeing patients that GPs might see. There are trade-offs – again potentially unique to each ED – in that while there may be resource release for ED clinicians, these must be offset against potentially increased admissions, investigations and treatments for patients managed by ED clinicians. Caution is required in interpreting this as there may be selection bias for types of patients treated in the ED (even at given severity levels).
As each GP in ED service is likely to have individual characteristics and sources of funding and our research here is based on only one site – an inside–parallel model – the findings of the study, both costs and consequences should be interpreted and applied with caution as different service models may not see the same outcomes. This research should be contextualised by individual trusts or CCGs for potential application, to decide whether the time savings observed when using GPs in EDs are also likely to result in cost savings or resource release or a better way of managing flow and patient care, as results at this case site suggest. Any service model must also be viewed in the light of its local funding model and where the costs are borne.
We have therefore refined our initial theory as follows:
New theory: If a patient attends an ED with a primary care type problem and is triaged and streamed appropriately to a GP service (C), the GP can use their skills and experience to manage the patient appropriately (M). In this setting, the GP can take less time to manage the patient than an ED clinician (O). The GP is also likely to use fewer investigations and treatments and admit fewer patients (M) without a negative impact on reattendances for the same problem (O). This service may incur relatively higher salary costs (O) which may (or may not) be offset by costs saved through reductions in activity outcomes, but GPs efficiently managing primary care type problems (M) may enable ED clinicians to see more seriously ill patients (O).
Strengths and limitations
Our analysis of the management of marker conditions has strengths and weaknesses which affect interpretation.
The strength of this study is that the data were comprehensive, reasonably complete (at this site) and supported the CCA (see Chapter 5). The hospital collects high-quality ED data and was an early adopter of the ECDS and invests in data management and analysis. The service had a modern well-resourced Urgent Care service staffed at least 10 hours per day. However, this site will not function as other GP services do, so our findings may not be generalisable or replicable.
As a service that has matured, and the 12-month period selected for our data extract was viewed as the service running at ‘steady state’, we believe our findings to be valuable. However, insights from the qualitative research (see Chapter 4) suggest that the performance of the service is influenced by ‘intangible’ factors; for example, it was suggested that the type of GP is important – ‘our sort of people’ – who fit well with aims of the service and ways of working and it took time to reach this situation. The service is also committed to ‘sorting the patient out’ once they have attended rather than redirecting them to a potentially more appropriate place of care.
The findings revealed a clear pattern of GPs seeing patients in the less severe/less complex HRG categories and the ED clinicians seeing patients who have more severe and complex HRG investigation and treatment categories. However, this should be interpreted with caution: If the ED clinicians overtreat and investigate patients who are in fact less complex and severe, then we are miscategorising these patients as severe and may have underestimated differences in admission rates between ED and GP clinicians.
Although some significant differences were observed in admission rates between GPs and ED clinicians, most differences were not significant. It is possible that there are true differences between the clinician groups, but because of small sample size for admission rates within conditions and severity levels, this difference could not be observed.
Further research
The original aim of the study was to undertake the analysis presented here in all the contributing centres. We conceived that either availability or quality of the data may preclude some centres contributing data. The COVID-19 pandemic made further enquiries for data to examine the impact of GP models on ED resources impossible. As this is an analysis based on a single centre, although potentially representative of some EDs in metropolitan areas, further research is necessary in a range of EDs and typologies, as originally conceived for this research to assess generalisability. This would deliver a better assessment of whether and how a GP service supports the care of patients attending EDs with primary care problems.
The process of defining the analysis was iterative, supported by the site CD and data analyst and the ED clinician co-investigators. Feedback about the data analysis for the ED and its GP service was deemed very useful, providing new insights. This feedback suggests that undertaking similar analyses on a regular basis may enable EDs with GPs supporting the service to understand the contribution that GPs make and how patient management is impacting the service as ECDS data collection matures.
Chapter 7 Mixed-methods analysis and overall programme theory
Introduction
In line with realist methodology, there is an understanding that using different data sources is likely to be most valuable to explore different elements of context, mechanism and outcome. For example, data about context may be obtained from observations, about mechanisms from interviews and about outcomes from quantitative data collection. Bringing together of all these data sources can support a comprehensive understanding of the way complex phenomena work and explain why things might work differently in different settings and contexts.
Multiple forms of triangulation were built into the Phase 2 qualitative data collection (see Chapter 4) and analysis including triangulation of data collection methods and formal theories. In the final stage of our analysis, theories derived from the Phase 2 qualitative results were examined using the data generated from the quantitative analyses.
Methods
We initially planned a detailed exploration of which elements of context, mechanism and outcome of each theory could be tested using each data source and considered using matrices for identifying areas of agreement and disagreement for each piece of evidence collected across the different data sets as described elsewhere. 154 However, we were limited by the fact that our qualitative data and theories are largely based around individual reasoning (clinicians and patients), whereas only whole department-level data were available for quantitative analysis, meaning there were no ‘matching’ pieces of evidence in many areas for tabulation. We therefore elected to adopt a ‘following the thread’ approach. 155 Each data set was analysed separately using appropriate methods (as outlined in Chapters 3–6). Key themes and questions arising following these analyses were then identified, and following the identification of questions in one data set, others were examined to provide a multifaceted explanation. We first identified questions raised through the qualitative data analysis explored through quantitative data analyses (see Approach 1), then identified noteworthy findings from the statistical analysis and cross-checked with both the qualitative data set and the marker conditions analysis (see Approach 2).
Approach 1 – identifying questions raised by the qualitative data
The team involved in the Phase 2 qualitative analysis met to review the theories developed, discussing each element of identified context, mechanism and outcome. We considered what we needed to know from the quantitative data to support, refute or refine the theories developed and identified key questions to address using the quantitative data and analyses.
Theory area 1 – streaming and patient flow
Our three theories about streaming and patient flow identified characteristics of individuals (experience, confidence, understanding of the GP-ED model) and systems (streaming guidance, staffing levels, ‘exit block’, oversight of the streaming process) which resulted in effective or less effective streaming. These were in turn understood to impact on patient flow in terms of patients being appropriately streamed to a GP, being seen more quickly, spending less time in the ED and ED doctors being able to more efficiently focus their time on ED patients. We characterised sites as having more or less robust streaming systems based on these factors using our qualitative data, grouping them into three categories (those with most, some or few features of an effective streaming system) and used the routine data and the marker condition analyses to explore whether those with systems which appeared most effective resulted in shorter waiting times when compared to other sites with less of the identified features. The four sites categorised as having most features of effective streaming were Integrated-1, Parallel-2, Outside-1 and Outside-3.
Routinely collected data
As discussed in Chapter 5, time series analysis was conducted using routine data from all the case sites including three control sites over 8 years.
Data on waiting time to treatment were not available and were generally poorly and inconsistently recorded. However, we did have data on the length of time patients who visited the ED stayed in the department. These data include all patients who visited the department (including those who were seen by ED clinicians or primary care clinicians). The results of the analysis showed that there was an upward trend in length of time in the department at most study sites (including the three control sites) over the study period both pre and post intervention.
Two sites showed a statistically significant decrease post intervention, both of which (Parallel-2, Outside-3) we had identified as having more features of effective streaming (e.g. senior trained nurses streaming at the front door, good oversight of the streaming system). Outside-3 also had a separate unit for geriatric emergency care which may have influenced LOS in the ED. The other sites where we categorised streaming as appearing most effective (Integrated-1, Outside-1) showed a trend towards increase in the length of time all patients spent in the department. Another site (Parallel-3) which had also established a system for redirecting patients to community primary care services also showed a non-significant increase in time in the ED after the GP model was introduced.
Marker conditions
Time spent in the department for the less severely unwell patients (those requiring lowest levels of investigation and treatment – see Chapter 6) streamed to GPs was less than those patients seen by ED clinicians across all marker conditions: back pain (a difference of 46 minutes, p < 0.001), chest pain (a difference of 64 minutes, p < 0.001), cough/breathlessness (a difference of 52 minutes, p < 0.001); child with a fever (a difference of 23 minutes, p < 0.001); abdominal pain (a difference of 1 hour 10 minutes, p < 0.001); headache (a difference of 1 hour 1 minute, p < 0.001).
Summary
The marker condition analysis did indicate the potential for GPs to improve the flow of the least unwell patients through the ED. However, at the whole ED level examined by the routine data, despite some sites demonstrating established and robust streaming systems and ED staff perceptions that streaming patients to a primary care clinician helped improve flow and decrease waiting times in the ED, the analysis demonstrated increased time in the ED for patients at most sites. Therefore, our theories related to streaming and patient flow have limited support from quantitative data and remain mostly based on qualitative evidence reported by ED staff and patients.
Theory area 2 – the general practitioner role
We were interested in the extent to which the roles GPs took on related to the models they were working within. Our qualitative data suggested individual reasoning such as decision-making approaches and attitudes to risk played a large part, possibly more so than model type. However, we also recognised that model type could influence and constrain individuals’ behaviours (e.g. when investigations were not available). We had identified that a ‘GP approach’ might result in use of fewer investigations, less ‘observation time’ in the ED and possibly fewer acute hospital admissions while a more ‘EM approach’ might be associated with GPs using more hospital investigations and acute admissions. We identified two sites (Parallel-4 and Outside-1) to test this theory with no access to acute investigations, clear guidance on the role of the GP, strong leadership supporting a GP role and governance processes which supported a GP approach.
Routine data
Time in the department
As described above, there was a positive trend for increasing time in the department over time for all three control sites, and most GP-ED model sites. Time in the department showed a trend towards increasing both pre and post intervention at the two sites of interest where the GP role appeared well supported (Parallel-4 and Outside-1) (no statistically significant change post intervention).
Investigations
The use of overall investigations within the ED was analysed. Data were non-stationary suggesting poor quality and that rates were influenced by coding/recording practices. Findings were a mixed picture of the use of investigations across control sites and all GP-ED models. There were three sites where the GPs had no access to acute investigations (Parallel-4, Outside-1, Outside-2) where we expected the ‘usual GP approach’ to be enabled, one site (Parallel-4) showed a statistically significant change in trend from increasing investigations (pre intervention) to decreasing investigations (post intervention). Data quality at Outside-2 precluded analysis.
Admissions
For acute hospital admissions, there were increasing admissions over time for all the control sites and a mixed picture across the intervention sites. Changes in trend were largely towards fewer admissions. Two changes reached statistical significance. At Parallel-4 (identified as an example of a site facilitating ‘the GP role’), there was a reversal in trend from increasing to decreasing admissions post intervention. At Integrated-1, where a more EM role would be expected, there was an increase on the background upward trend in admissions post intervention.
Reattendances
Reattendances from routine data were measured at 28 days in contrast to the marker conditions data where they were measured at 7 days. There was a trend demonstrating increased reattendances for most control sites and intervention sites. All four parallel sites demonstrated a downward trend pre intervention, with a subsequent increase in reattendances post intervention (three of these reached statistical significance including Parallel-4, a site of particular interest for facilitating the GP role). Data quality at Outside-1 prevented analysis.
Marker conditions
As described in Chapter 6, the marker conditions data were analysed from a single case study site (Parallel-2), where GPs and ANPs saw patients streamed to the primary care service and were encouraged to use a ‘usual GP approach’.
Across all conditions, the GPs saw fewer patients in total than the ED clinicians and the patients seen required lower levels of investigation and treatment. As described above, time in the department for the less severely unwell patients (defined as those requiring lowest levels of investigation and treatment) streamed to GPs was less than for those patients seen by ED clinicians across all marker conditions, due to a shorter period between seeing a clinician and discharge. Due to data quality, it was not possible to compare use of investigations between GPs and ED clinicians.
For patients who were less severely unwell, for three of the marker conditions (those with breathlessness, children with a fever and abdominal pain), GPs made statistically significantly fewer acute hospital admissions than ED clinicians.
For all marker conditions, there were no significant differences in the proportion of reattendances to the ED within 7 days. Overall, there were 24,834 initial attendances across all marker conditions; 83% (n = 20,658) of these were seen by ED staff, and 17% (n = 4176) were seen by GPs. Of these, 1586 (6%) reattended within the first 7 days after discharge. Of those who were seen by ED staff, 1319 (6%) reattended, while of those that were seen by a GP, 267 (6%) reattended [χ² (1) = 0.0004, p > 0.05]. Using reattendance within 7 days as a marker of safety, these data support the theory that GPs managing less severely unwell patients in an ED setting does not lead to safety issues, compared with ED clinicians.
Summary
Marker conditions data from a single parallel case site (where GPs were encouraged to maintain a GP approach seeing less severely unwell patients streamed to a GP service), showed that patients were likely to spend less time in the department and less likely to be admitted with no difference in reattendance rates at 7 days. Data on investigation use were of poor quality and not included in the analysis.
Routine data for all intervention sites and three control sites, also showed poor quality of investigation data creating unreliable findings. General trends for time in the department and reattendance rates at 28 days were increasing across most intervention and control sites (though not always reaching statistical significance). Any influence on admission rates and time in the department receiving care described in the marker condition analysis was not seen consistently in the wider routine data set.
While the marker conditions analysis supports the theory that a GP approach in an ED setting can be different to an ED clinician approach, it remains unclear whether this is due to individual clinicians’ management behaviours or service-level differences in ways of working. The quantitative data did not help us to identify particular models which were associated with favourable outcomes that could be attributed to facilitating the ‘GP role’.
Theory area 3 – teamwork and communication
Our theory about teamwork described that where there is effective teamwork, safer care will be delivered. We therefore classified sites according to the presence of features identified in our earlier theory building in relation to strong teamworking (ED leadership/culture with a positive view of GP contribution, compatible computer systems, physical proximity/informal face-to-face communication opportunities with ED team, clear formal system for allocating patients, evidence of interprofessional learning/supporting junior staff, strong leadership of the GP team, employing regular GPs and strong communication within the primary care team). Three sites with particularly strong teamwork and communication were identified (Integrated-1, Integrated-3, Parallel-2).
Routine data
The routine data showed a mixed picture in the pre-intervention phases for the reattendance outcome at 28 days, with a clearer picture post intervention where eight of the nine sites demonstrated a trend towards an increase. At the ‘strong’ teamwork and communication sites (one showed a statistically significant trend for increased reattendances post intervention (Integrated-1), one showed a non-statistically significant increase (Parallel-2) and the third had inadequate data for calculation (Integrated-3).
Marker conditions
The site for marker conditions analysis was one of the sites we identified as demonstrating strong teamwork and communication (Parallel-2). The marker conditions analysis did not identify any difference in reattendance rates between patients consulting with GPs or ED clinicians at 7 days at this site.
Summary
The routine data indicated increasing rates of reattendance across most intervention sites and the control sites. This may be due to changes in levels of morbidity among the population, public behaviours or service configurations (including availability of services elsewhere, notably in-hours primary care) rather than necessarily representing a change in the quality of the care provided at the ED. The marker conditions data suggested that GP care in the ED appeared to be as safe as ED clinician care using reattendances as a proxy. We have not identified data that support or further develop our theory related to the strength of communication and teamworking within the ED.
Theory area 4 – patient experience
Two theories were developed from the qualitative data around patient experience. These related to the relationship between patients’ expectations of ED care and subsequent satisfaction with the service provided, and how patients experienced being redirected away from the ED.
None of the quantitative data collected related to redirection, so this theory could not be further refined.
Our qualitative findings indicated that some patients access the ED because they expect to be seen by a doctor on that day (even though they expect a long wait) and found it acceptable to be streamed to a GP. If it also meant that their time in the ED was shorter than they expected, then they seem to be satisfied with their care. However, these findings were based on a small number of patients and there were insufficient qualitative data available on satisfaction to draw meaningful conclusions about differences in satisfaction across the different GP-ED models.
Routine data
Our routine data did not identify any model which was consistently associated with shorter stays in the ED; in fact, overall time in the department increased at most sites over the study period. We did not have any data quantifying patient satisfaction across the different sites to test our theory of a relationship between waiting times and satisfaction.
Marker conditions
Our marker conditions analysis did suggest that for patients requiring the lowest level of investigation and treatment, being seen by a GP was quicker than being seen by an ED doctor.
Summary
While the marker conditions analysis indicated that patients attending ED who required the fewest investigations and treatments might be more satisfied by being seen by a GP due to a shorter ED stay, we could not identify any particular GP-ED model that appeared most likely to consistently decrease LOS.
Theory area 5 – economic implications: provider-induced demand
Theories developed on the economic implications of the GP-ED models centred around three themes – that GPs would arrange fewer investigations and admissions (as discussed in Theory area 2), that GPs would free up ED staff to care for sicker patients, so improving patient flow through the department (as discussed in Theory area 1) and that GP-ED models might create ‘provider-induced demand’. 150
Our qualitative data (see Chapter 4) indicated that in some EDs, Parallel-2 being the strongest example, the staff perceived that when the primary care service started, more people started attending the ED for primary care because the service was visible and easily accessible. There was also qualitative evidence from patients that they may choose to attend the primary care service in the ED because it may offer them more convenient and quicker access to a GP than in their usual GP service.
To inform review of the routine data, we classified our intervention sites based on features that increased their visibility to the public (signage over a separate entrance, patients able to self-present, 111 redirecting to the GP-ED service, patients being informed they are being streamed to GP, media/press coverage of unit/local awareness). We identified five sites which we classified as ‘highly visible’ (Parallel 2, 4 and Outside 1, 2 and 3).
Routine data
Attendance rates showed a mixed picture across control sites, across all intervention sites and within GP-ED models, but with a general picture of attendances increasing over time. At one of the ‘highly visible’ sites of interest (Outside-3), this reached statistical significance, with statistically significant increases post intervention also seen at two other sites (Integrated-1, Parallel-1). However, the quantitative data measured all ED attendances (with no focus on primary care attendances) and the qualitative data described staff perceptions based on their experiences of individual patients attending with primary care needs.
Marker conditions
The marker conditions analysis did not set out to address questions around provider-induced demand.
Summary
Our theory about provider-induced demand in distinct and visible services is not supported by the data we have on ED attendances over the study period, but our qualitative findings suggest that there is a perceived additional demand for primary care at an ED in services where the primary care service is visible, easily accessible and more known about within the local area.
Approach 2 – identifying questions raised by the quantitative data
Exploring key insights from the statistical analysis
Noteworthy findings from the statistical analysis were then identified and cross-checked with both the qualitative data set and the marker conditions analysis (Table 20) in order to attempt to explain any signals in the data that may not have been explored in depth as a result of the theory-driven analysis above.
Outcome measured | Key insights from the statistical analysis | Possible explanation from the qualitative data | Fit with marker condition data |
---|---|---|---|
ED attendances over time (2010–8) | No evidence of reduction in attendances, with increased attendances in all intervention sites where change could be assessed with confidence (some statistically significant). | Perception at some sites that increases in primary care demand have been triggered by the visibility, accessibility and local awareness of the GP-ED model. | Not assessed. |
ED reattendances within 28 days | Controls all show increases over time. At intervention sites, all that could be calculated show increased reattendances post intervention except for Integrated-2 (statistically significant decrease). | Integrated-2 was a very small ED and saw a limited range of patients with more unwell patients being taken to an alternative hospital. (After the study period, this ED was downgraded to an urgent care centre.) | Consistent – no difference in reattendance rates between patients seen by GP or ED clinician was identified. |
Average time in the ED | Increased at all three control sites during study period and most intervention sites. Two intervention sites (Outside-3, Parallel-2) identified with evidence of reversal in upward trends in average time in the ED. | There was a new frailty unit at Outside-3 introduced at the same time as the GP-ED model. Note this site also showed a negative trend for admissions. | Consistent. Parallel-2 showed a reversal of the pre-intervention upward trend for time spent in the ED in the post-intervention period. The number of patients seen by the GP-ED model will determine the impact on department level averages. |
Investigation use | Mixed picture of investigation use from control sites (flat or upward trends). Also, a mixed picture within each model. Two sites of interest showing statistically significant decreases in investigations post intervention (Parallel-3, Parallel-4). | At Parallel-4, GPs had no access to investigations. At Parallel-3, there was a structured pathway for redirecting patients to community primary care. GP-ED model saw only small proportion of overall ED attendances limiting potential impact. |
Our marker condition analysis used investigation use to define the severity of the condition. This makes it challenging to also use the analysis to interpret the appropriateness of investigation use across different patient and clinician groups. |
Admissions | All three control sites have upward trends, mixed picture across intervention sites. Statistically significant post-intervention changes identified at two sites. Parallel-4 showed a reversal of direction of trend from increasing to decreasing admissions. Integrated-1 showed increased admissions post intervention. |
At Parallel-4, ‘the GP role’ was supported and staff gave examples of GPs managing paediatric patients without the need for admission. At Integrated-1, staff perceived that demand was increasing due to new housing developments in the area. |
In the marker condition analysis for five of the six marker conditions, in the lowest severity group, GPs made fewer admissions than ED staff. There was a trend for decreasing admissions in the routine data for this site, but this was not statistically significant. |
Moving towards a refined programme theory
During our qualitative analysis, we described the theories through the core mechanisms identified along the patient journey through a GP-ED model, focusing on understanding how the models worked. However, when considering the presentation of our refined theory, we felt that it would be helpful to put more emphasis on the differences observed in ‘what was working where’ and identifying the contexts which facilitated or inhibited the mechanisms we had identified. We adopted this approach because it was felt most likely to help address the key question of most interest to commissioners and practitioners, that is, if you were going to implement a GP-ED model, which one should you choose (where and why)?
The refined programme theory diagram below is shown in three parts. Each diagram shows the same central pathway of the patient journey through the ED: being selected to see a GP; being provided with care; and leaving the ED having received a certain level of investigation and treatment; resulting in a level of satisfaction with the experience for the individual, and patient flow through the department.
We provide three different versions of the diagram to highlight similarities and differences between the three GP-ED models we studied. We recognise that each site visited had some unique characteristics, so these diagrams represent a high-level summary of key features for a given model, rather than an exhaustive description of every possible variation.
The patient journey through the ED is depicted with grey arrows. Context is labelled in blue, mechanisms within the green arrows and outcomes in red circles. We are most confident in the data supporting the links towards the left side of the diagram(s) which explore individual level decision-making amongst streaming staff and GPs. The links between these processes and patient- or departmental-level outcomes shown on the right of the diagrams remain unproven by the available quantitative data.
Compared to the other models, the streaming process is less influential at inside–integrated sites (Figure 19). The ‘invisibility’ of the GP service means its impact on patient expectations is also more limited. Unlike in other models, inside–integrated models allow for GPs to take on supervision of junior ED doctors which was felt to be a particular asset at some sites.
FIGURE 19.
Programme theory to describe contexts, mechanisms and outcomes in inside–integrated GP-ED models.

At outside–onsite models (Figure 20), the process of ensuring that the right patient got to see the GP was more complex, and so more open to potential problems. Their high visibility and accessibility were likely to have a greater impact on patients’ expectations and experiences. At these sites, GPs sometimes took on a supervisory role for a wider primary care team including other health professionals.
FIGURE 20.
Programme theory to describe contexts, mechanisms and outcomes in outside–onsite GP-ED models.
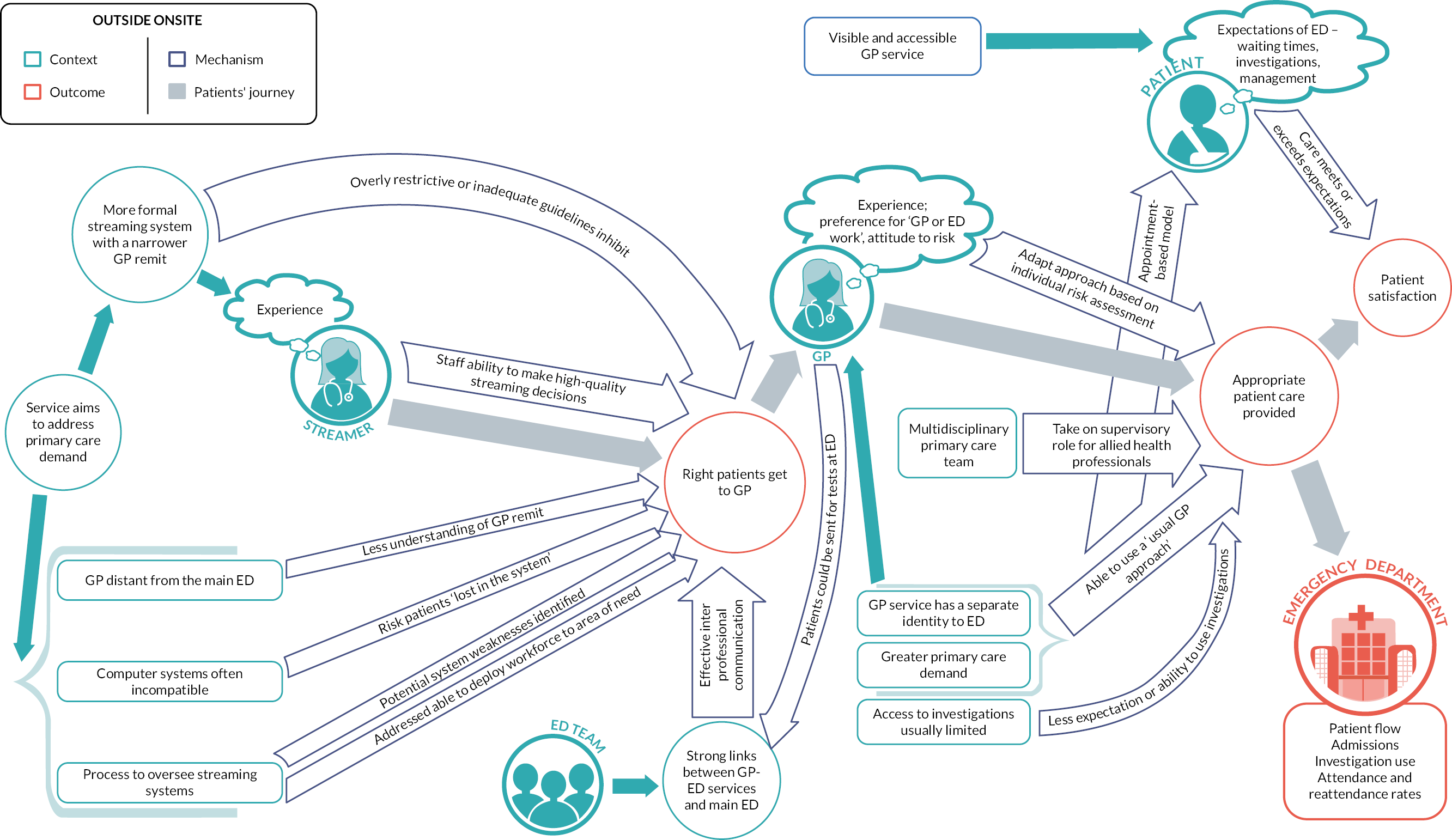
Inside–parallel models (Figure 21) showed the most variation in the way the services were set up, some were more similar to inside–integrated models, others more distinct as inside–parallel models. The lack of clarity around the breadth of the GP role could be a particular concern in these models though this might be overcome in settings with strong clinical leadership.
FIGURE 21.
Programme theory to describe contexts, mechanisms and outcomes in inside–parallel GP-ED models.
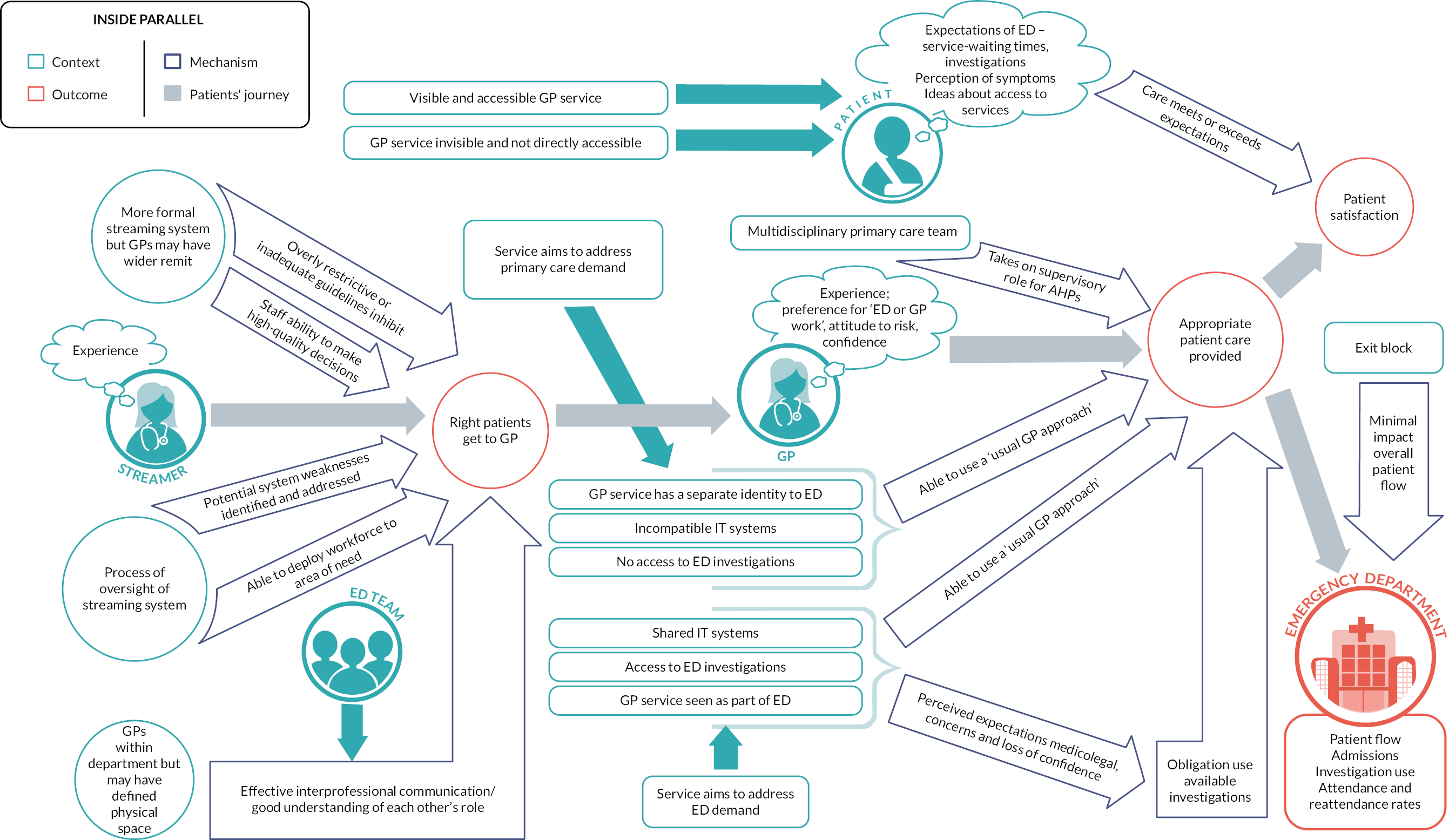
Conclusions
We took a structured approach to triangulating our different data sets, using both inductive and deductive approaches to maximise the insights generated.
We identified uncertainty in the links between context, mechanism and outcome identified in the theories our qualitative work generated as well as some conflicting findings between the routine data analysis and the local marker conditions analysis (both for the marker condition site and in general). Our qualitative data did offer some explanation for some patterns observed in the routine data analysis. We focused our refined programme theory on highlighting the differences in behaviours noted (largely at an individual level) between the three GP-ED models studied. This may be helpful when needing to consider how individuals may respond to a certain situation/model. We have focused our theories on explaining the areas where we are most confident in the data (links between context and mechanism) and identify where uncertainty remains (particularly around confirming links between context and outcomes and mechanisms and outcomes). We can be less confident in predicting outcome patterns at a department level for factors such as patient flow through department and investigation use, with some of this uncertainty being driven by the quality of data available for analysis.
Chapter 8 Discussion
Principal findings
The research questions addressed are discussed below.
What models are in place? How and on what scale are they delivered? (CONTEXT)
This study showed that while GPs are commonly working in EDs (66% of respondents to our initial survey used some form of GP-ED model), delivery models vary widely in scope of the GP role and scale of the GP service. Service objectives most frequently included reducing waiting times, reducing ED patient volume and making better use of available ED resources.
After developing a taxonomy to classify the ways in which GPs work in and alongside EDs, we identified three main models of working which were explored in depth (see Chapter 4). We found sites where GPs were working within the ED in a fully integrated fashion, sites where GPs worked in the ED providing a parallel service alongside the ED team and sites where the GP service was provided outside the ED but still within the same hospital location. 5 Significant variation within models was also identified, in relation to (inter alia): streaming systems; level of oversight of the GP model; remit of the GP; access to investigations; level of integration and communication; and IT system compatibility (see Chapter 4). 19 Models of GP employment varied but could broadly be divided into: ‘in-house’ by the NHS Trust or by a separate primary care provider (which could result in a stable workforce) or by external providers sometimes with unstable locum staffed services. 150 While our focus was on GPs in EDs, several of our case study sites also used wider primary care teams involving a range of professionals to meet the needs of patients attending the ED.
While variation within models was observed, there were however key features identified and commonly associated with each of the three service designs. Integrated models were typically used at sites with lower levels of ‘primary care demand’ at the ED, often funded from within the same NHS Trust as the ED. GPs were fully integrated members of the ED team with access to the same IT system and investigations as other ED staff and viewed and valued as members of the ED team but acting in extended GP roles.
At the other end of the spectrum, outside–onsite models typically aimed to deal specifically with primary care demand and had a clearly defined identity in a separate physical location. As a result, there were usually agreed criteria and pathways for accessing the service and GPs’ remit generally remained similar to that of community primary care (without access to ED investigations).
The inside–parallel models were the most varied and sometimes had features from each of the other two models. For example, at these sites, the level of primary care demand (and aim of addressing this) varied. While streaming guidelines often existed, there was sometimes flexibility for GPs to take on a wider role, with variable access to ED level investigations and differing perceptions about how integrated the GP service and the ED were intended to be at different sites.
How do the models work (in comparison with standard practice)? Does the way they work align with the intentions of those who set them up? (MECHANISM)
In our refined programme theory (see Chapter 7), we identified pathways through which GP models were observed to operate. The first step in all models related to the decisions taken about which patients would be directed to the GPs within the ED (often through a formal streaming system, but sometimes based on individual clinicians’ preferences). 156 We identified settings where high-quality streaming decisions were facilitated by experienced staff (usually nurses), adequately detailed guidelines, the streamer’s understanding of GP skills, communication between the streamer and the GP service and oversight and active quality improvement of the streaming set-up. 146 When such contextual factors were lacking, there were risks of patients being streamed either inappropriately to GPs (risking redirection back to ED after review) or inappropriately to the ED (meaning the GP model made less impact). The system for streaming was more influential at inside–parallel and outside–onsite models where GPs acted differently to ED doctors, than in the inside–integrated models where more overlap in approach was expected.
The second step in our theory discussed how GPs worked within the deployed model to deliver patient care. We observed that GPs used a variety of different approaches, sometimes working in very similar manner to community primary care, sometimes taking on an ED clinician role and sometimes working flexibly using different approaches for different patients. 32 Role boundaries were generally clearer in the outside–onsite models. The GP role could be restricted or expanded due to the level of resources available within the model (e.g. access to ED level investigations) and for personal characteristics of individual clinicians, including preferred ways of working, confidence levels and medicolegal concerns. Techniques described by GPs (see Chapter 4) as ways to mitigate risks of ED working included longer appointments, different thresholds for investigation or admission and developing supplementary ED skills. 32
The third stage of our theory refers to the end outcomes for both patients and the wider ED. While our patient data were limited, we identified how patients’ expectations of an ED visit, and whether these were met or exceeded appeared linked to subsequent satisfaction. 20 In addition to patient-level factors influencing these expectations, the visibility and accessibility of the GP-ED model also shaped expectations. 157 Reports from staff indicated that models where patients were redirected to the GP-ED model but could not then receive definitive care (observed in outside–onsite models) were frustrating for patients and staff alike. 146
Our theory also highlighted the importance of culture in underpinning successful GP-ED models, with strong clinical leadership aiding the development of a culture which encourages interprofessional communication and mutual respect to enabling safe care provision.
What are the outcomes of each model in terms of: percentages of emergency department attendances seen; admissions; reattendance rates; waiting times; costs; patient safety; patient experience; team impact and sustainability? (OUTCOMES)
Effectiveness
Routine data quality across sites was variable. Our marker conditions analysis at a single inside–parallel site with strict primary care streaming criteria showed that when treating the least unwell patients (those requiring no investigations or treatment at the ED) those seeing GPs generally spent less overall time in the department than those seeing an ED clinician. The routine data identified only two sites with statistically significant evidence of reversal in the existing upwards trends in time spent in the ED.
The routine data indicated that in general, at the GP-ED model sites, attendances increased over time. Reattendances within 28 days in the post-intervention period increased over that in the pre-intervention period in 8/9 sites assessed. Data on investigation use at intervention sites demonstrated a mixed picture which was difficult to interpret. This complexity was also identified in the data relating to treatments delivered although overall there was a pattern of increasing treatments delivered pre intervention, followed by decreasing treatments delivered post intervention, but the significance of this is uncertain. A mixed picture was seen in relation to hospital admissions in the Integrated and Parallel sites, with data quality issues at the Outside sites, with examples of both increases and decreases both pre and post intervention. Similarly, average length of hospital stay showed a mixed picture both over all intervention sites and within models with some trendlines remaining essentially flat.
Patient experience
In many instances, we found descriptions of how GP-ED models could meet or exceed patients’ expectations about the level and timeliness of care provided in the ED. Our qualitative data identified the potential for patient dissatisfaction with GP-ED models that redirected patients elsewhere after they had waited to be seen rather than providing definitive treatment. Given the small numbers of patients participating in the study, we were not able to identify a single GP model most likely to improve patient experience. 20
Safety
Clinical directors, from 11 of the 13 case study sites visited, had no patient safety concerns and did not describe any patient safety experiences related to GP-ED service models. The CD of a parallel service (Parallel-1) had concerns about which organisation held governance responsibilities and the skillset of GPs working in the ED following two local patient safety incidents. Two CDs perceived that since GPs had been working in the department, overall patient safety had improved because the experienced, permanent GPs could also give advice to other staff members (Integrated-1, Integrated-2). Small numbers of local patient safety incident reports regarding the GP-ED services were identified (n = 14 from all case study sites). The qualitative data provided examples of departmental and organisational factors that were identified to improve the safety of care delivered notably: appropriate streaming processes, led by an experienced nurse; communication and teamwork between the services; and clear governance processes for the GP-ED service. We described how individual GPs attempted to mitigate the risks of ED work in their clinical decision-making. 32
Marker conditions data analysis suggested that there was no increase in reattendances within 7 days seen among patients treated at one site (Parallel-2), which we used as a marker of the safety of the care provided. Routine data analysis indicated upward trends in reattendances within 28 days at most intervention sites.
Resource use
When looking at ED visits by individual models, the inside–integrated model saw a small cost saving (£8.73), but small cost increases were seen in the inside–parallel model (£9.51) and the outside–onsite model (£16.30). With respect to inpatient admissions, all models saw small cost savings (£25–215) with the outside–onsite model seeing the largest saving (£215). While each model type had reduced admission LOS and costs compared to the controls, this assumes that case mix for each model type was the same, though it is possible that the type of patients seen and admitted are different thus resulting in different lengths of stay and costs. There is also considerable variation in approaches to financing GP services. 146 In addition to negligible cost differences in the ED, there were also small time savings (range 3.5–23.5 minutes) compared to the controls.
Our CCA to investigate the economic impact of GPs working in a ‘parallel’ service model at Parallel-2 study site (see Chapter 6) suggested that GPs could provide a time and investigation sparing service to the patients with selected ‘marker conditions’ and who required the lowest levels of intervention, without increasing admission or reattendance rates.
What are the relationships between context, mechanism and outcomes, and is the evidence base transferable to commissioning, service improvement and cost-effective delivery in other settings?
Our refined programme theory aims to summarise our findings, highlighting the mechanisms acting in the three different models and how these may produce differing results. When reviewing each of the models they each have strengths and weaknesses, a single ‘best’ model for every site cannot be identified, and will depend on the local context and priorities of the service, which should be examined and clarified,5 as discussed in our implementation toolkit (see Project web page). We have noted that a clearer ED identity (inside–integrated) or GP identity (outside–onsite) generally meant service design aligned with aims, whereas there was more scope for variation and possible confusion at the Integrated-Parallel models.
We recognise that we have a large body of qualitative evidence describing mechanisms at the level of individual clinicians and teams, and the contexts in which these mechanisms are likely to occur (see Chapter 4). We have not demonstrated that these mechanisms lead to outcomes visible at the department level in routinely collected data (see Chapter 5).
Complex data and temporal variation, local variation in service provision and general lack of clarity regarding funding of GP-ED models meant that the relative balance between costs and effects is unclear. The analysis of the management of marker conditions using data provided by a single site cannot be generalised to all parallel sites but indicates potentially how GPs can make a difference in some EDs.
Toolkit development
Our research teamworked with study co-applicants and a stakeholder group of CDs to translate the research findings into actionable findings to be included in a ‘toolkit’ (see Project web page). This seeks to support ED CDs to appraise and reflect on how their GP-ED service functions and make operational plans for practice improvements in four key areas:
-
Managing patient demand – including understanding patient-level, department-level and wider system-level influences on demand, information and education strategies for addressing demand and recommendations for evaluating demand.
-
Improving patient flow – including strategic plans for improving nurses’ knowledge of primary care-type conditions (for streaming), training GPs to work in an ED, implementing streaming guidance; and operationally managing streaming and redirection to primary care.
-
Improving patients’ experiences (and expectation) of accessing urgent care –including education and information to improve understanding and acceptability of streaming, considerations in redirecting patients to community primary care and suggestions for patient surveys to help inform service improvements.
-
Improving patient safety – including supporting streaming to an appropriate clinician, clinical decision-making and communication between GP and ED teams.
The toolkit enables directors to identify what strategies they can implement in their service and provides a quality improvement tool and action plan to support service improvements in the four key areas.
Comparison with existing literature
Our RRR of 96 articles (see Chapter 3) developed candidate theories under five key themes: streaming and flow, the GP role, patient satisfaction, patient safety implications and wider system implications (including cost effectiveness). 15
Streaming and flow
Our review findings (see Chapter 3) emphasised the importance of effective streaming, identifying influences including levels of available guidance, understanding GPs’ remit and effective interprofessional communication. These aligned both with our own findings146 from case study sites (see Chapter 4) and with the findings from another recent qualitative exploration of GP-ED streaming. 158 However, our quantitative data did not demonstrate relationships between the quality of the streaming process and the rate of flow of patients through the department at our case study sites. Similarly, another NIHR-funded GP-ED study recently published findings from a 1-year analysis of HES data from 40 sites with GP-ED models and detected no statistically significant effect on the 4-hour wait target. 159
The general practitioner role
Our initial review identified the concept of ‘the GP role’, which was particularly associated with the use of fewer investigations and admissions. Although GPs working in an ‘ED clinician’ role has also been identified, this was explored in a more limited way. Our qualitative data, particularly from inside–integrated sites, provide a fuller description of the range of tasks additional to the ‘usual’ GP role that GPs may undertake in EDs. We also identified descriptions of how GPs adapt their approach to meet the needs of the patient they are seeing. Potential barriers to maintaining the ‘GP role’ in ED were also described. In keeping with our findings, the recent large qualitative study described by Scantelbury et al. 160 described how GPs are more ‘risk tolerant’ as a key premise of GP ED models, but that this was contested by some staff and service leaders. They also identified differing views on what level of access to investigations would make a GP-ED model most effective, with some services feeling access was essential, while others remained concerned about the risk of ‘GPs going native’ and consequently limiting access. Our marker conditions analysis (see Chapter 6) suggested that those GPs may use a different approach to ED clinicians when both groups treated patients who required the lowest level of investigation or treatment. However, our routine data analysis failed to demonstrate this impact at the department level. This is consistent with Gaughan et al. 159 who found no statistically significant effects of GP-ED services on 4-hour waits, patients leaving without being seen or hospital admissions.
Patient satisfaction
Our initial review findings suggested that any improved satisfaction from seeing a GP in the ED would likely be derived from care exceeding initial expectations, particularly in relation to waiting time. It also highlighted that patients in more integrated models may be unaware they have seen a GP, without any resultant impact on satisfaction. Our qualitative data supported this, describing that people were often unaware they had seen a GP, were broadly supportive of the idea of GP-ED models and satisfied with timely care. 20 Access to timely care is one key reason that patients choose to attend the ED for more minor problems. 161,162 Our marker conditions analysis suggested that people most likely to have minor problems (requiring no investigations or treatment) would be seen in a more timely fashion by GPs than by ED clinicians. However, this impact did not extend beyond this small proportion of overall ED attendances to influence LOS in the ED for the whole department.
Patient safety implications
There was no evidence in our initial RRR15 (see Chapter 3) that GP-ED service models improved care of the sickest patients by freeing up ED staff. This is in keeping with our quantitative findings that there were no reductions in average patient time in the department, as found also by Gaughan et al. with no impact on 4-hour waits. 159
Using reattendance as a marker of safety, there was no evidence in the review15 of more reattendances for GPs than for ED staff. This was reflected in our marker condition analysis at a single site and again in keeping with Gaughan et al. 159 who found GPs working in/alongside the ED was associated with a small reduction in unplanned reattendances within 7 days. Our routine data indicated upward trends across intervention sites for reattendance within 28 days, largely irrespective of GP-ED model intervention, although this is outside the usual timescale for assessing safety. 163
There were some anecdotal reports in the review about poor communication impacting quality of care – also a theme in the small number of Coroners’ reports, NRLS and local patient safety incident reports identified. 14,164 GPs are recognised as low patient safety incident reporters, which may have contributed to the low number of reports identified. 146 Communication failures, exacerbated by hierarchical differences and conflicting roles and role ambiguity, are known to be associated with patient safety incidents,165,166 while interventions to improve communication between healthcare professionals such as briefings, or ‘huddles’, are associated with improved patient safety outcomes. 167,168 Our qualitative data identified factors that inhibited and facilitated communication between the GP-ED and ED services. Strong clinical leadership was observed at the sites with a stronger sense of professional respect, teamworking and collaboration. Clinician involvement in leadership positions in hospitals is associated with improved quality of patient care. 169
The patient safety incident reports described how high-risk patients could be incorrectly streamed to the GP service and that GPs may mismanage conditions outside of their usual skillset. 14 There is little national guidance on which ED patients should be streamed to GP services. 170 This study’s work supports an experienced senior nurse over algorithmic methods. 14,171 There is little national guidance on which ED patients should be streamed to GP services. 170 This study’s work supports an experienced senior nurse over algorithmic methods. 171 Our qualitative data improved our understanding of the strategies GPs in EDs used to try to ensure quality and safety of the care they provided, with findings structured around Croskerry’s dual-process model of reasoning (see Chapter 4). 31,32
Wider system implications – cost effectiveness and provider-induced demand
Our RRR did not identify any research reporting an economic assessment, whether CCA, a full economic evaluation or a detailed analysis of GP activity and outcomes where the presenting complaint is a typical primary care problem, but seen in an ED.
In broad terms, our findings from the single-site marker conditions analysis support the existing UK-based research56,61 and other research that has not included an economic assessment,72,73,76–78,96,172 suggesting that GPs do have a role in managing some patients attending ED and they may admit fewer patients, take less time and use fewer investigations and treatments compared with ED clinicians, particularly for lower acuity presentations. This saving, however, may be offset against the GP higher employment costs compared with ED clinicians seeing equivalent patients. Nonetheless, releasing ED clinicians to see patients that they are more skilled at managing has potential value for the EDs. Our qualitative data provide additional detail to the current literature about the influence of context, and how this strongly influences how GP may practice compared with ED clinicians.
Our CCA using routine data suggests all models saw small cost savings, largely driven by reductions in hospital admissions (largest savings in the outside–onsite group). This conflicts with the data from Gaughan et al.,159 which did not identify reductions in admissions at sites using GP-ED models. However, the savings identified should be interpreted with caution as the cost of employing GPs may vary considerably compared with the cost of employing GPs that we used. 143
Our RRR identified studies suggesting that visible services may create their own demand, rather than relieving ED pressures, while this was not reported at sites where an integrated model was ‘invisible’. 15 This fitted with our qualitative findings, with staff describing examples of what they perceived to be provider-induced demand at more ‘visible’ sites. 157 However, our routine data analysis found that demand increased over time at all study sites including controls regardless of the visibility of services. The wider literature highlights other drivers of demand at the ED, in particular identifying the attractiveness of promptly available tests. 162 While patient-reported reasons for attending EDs are often related to limited or poor access to primary care, an analysis of routine data found that there was no statistically significant relationship between the number of GPs in a primary care trust area and the number of emergency admissions. 162,173 While patient-reported reasons for attending EDs are often related to limited or poor access to primary care, an analysis of routine data found that there was no statistically significant relationship between the number of GPs in a primary care trust area and the number of emergency admissions. 173 However, in deprived areas, a higher number of GPs was associated with reduced emergency admission.
Strengths and limitations identified
From the outset, our realist approach focused the study on understanding not just the outcomes of GP-ED models, but also the reasons why different models may lead to different outcome patterns. The use of mixed methods in both data collection and analysis has strengthened our ability to investigate and demonstrate the true complexity of current GP-ED models in operation (see Figure 1 for study summary). The taxonomy developed in Phase 1 provided an easily applied method for classifying GP-ED models while also recognising the variability seen within models and how they function. 5 We gathered data from a wide variety of services across the two study phases, adding breadth to our descriptions of how these models operate. We have engaged regularly and meaningfully with both public contributors and wider stakeholders and ED staff to ensure the relevance of our findings to policy and practice. We identified key actionable findings (see below) along with a toolkit (see Project web page) to help those working in EDs to develop or improve their GP-ED models based on the evidence gathered.
Several key limitations were identified. Firstly, study recruitment was challenging at various levels. Our narrow recruitment criteria both for case study sites (due to the requirements for our Interrupted Time Series Analysis) and individual patients (due to attempting to identify patients with the chosen ‘marker conditions’) limited the potential pool of participants. While we reached our target number of case study sites, we did not recruit as many patients as intended. 18 Governance issues at two sites prevented us recruiting patients. We have published our reflections on the challenges of patient recruitment in ED research for wider learning (see Project web page). 18 Additionally, recruitment of staff participants was particularly challenging at some of the busiest sites. We recognise that those services under the most pressure may not have been well represented in our sample.
Our second major challenge related to the quality of the routinely collected data available. This is a recognised issue in emergency care research. 174 Accessing the available data from NHS Digital involved lengthy delays (including pre pandemic), meaning the qualitative and quantitative data analysis did not run concurrently as intended. Thus, we did not have the opportunity to collect further explanatory (and contemporaneous) qualitative data to explain patterns identified from the quantitative analysis. The quality of the data was also often concerning, with some data, such as those on time-to-treatment and investigation use, being particularly unreliable. Some of our remaining uncertainty about the potential role of GPs in EDs exists due to these data quality issues.
We were also limited in the data we could collect from local sites for our marker conditions analysis, successfully gathering data from just 1 of our 10 intervention sites. The pragmatic approach used in this analysis was also shaped by the limitations of the data, particularly around assessing acuity of presenting condition. We relied on investigations and treatments received as a proxy for severity of condition but recognise that this makes it problematic to assess appropriateness of treatment and investigation for patients at different severity levels.
Our third concern related to how well the data allowed us to refine our initial theories. Our qualitative data relied heavily on self-report from clinicians and patients, and no GP consultations were observed. Further triangulation of these data with observed encounters would have been useful. Our quantitative data were largely limited to whole department-level statistics, which made it difficult to be confident of potential links to reasoning at the individual level, that we had identified through our fieldwork. Our refined programme theory diagrams highlight these areas of uncertainty.
Finally, it is important to acknowledge the shifting ED landscape over the period of the study. There has been a rapid evolution of models of UEC with further disruptive innovation during and after the COVID-19 pandemic. We identified huge complexities in service models, with every case site having set up their service somewhat differently, and in several settings the services were continually evolving. Furthermore, the COVID-19 pandemic impacted both how individuals access health care and how services design their systems. New approaches such as ‘telephone first’ and ‘total triage’ approaches in both EDs and community primary care were much less widespread at the time of data collection and their relevance to our findings remains unexplored.
Equality, diversity and inclusion
Chapter 1 describes the role of our public contributors throughout the study in ensuring inclusivity and accessibility at all stages. While our use of routinely collected data from sites across England and Wales should represent all ED attenders, we faced challenges with patient recruitment for our qualitative work and our small sample is unlikely to be fully representative. We reflected on these recruitment difficulties and suggested possible mitigations (e.g. amended recruitment materials and the provision of incentives) in a published paper. 18 Our research team brought a range of expertise and levels of experience. More junior team members were provided with training opportunities and encouraged to take the lead in specific areas of work, resulting in a large number of peer-reviewed publications and several new first authors.
Implications for policy and practice/key actionable findings
We have not identified a single GP-ED model that appears to represent ‘best practice’. Rather, we emphasise the need to understand individual patient-level, department-level and wider system-level influences on demand for service design features (including signage, layout, resources, staffing streaming/redirection policies), developing patient education materials, managing public awareness and working with community-based primary care to ensure patients seek care from the most appropriate service for their need.
To manage their expectations, patients need to be kept informed of which type of clinician they are being streamed to and why they are being streamed to primary care clinicians or redirected to another service, along with reassurance of primary care clinicians’ expertise and role in the ED. Decisions to stream patients to a clinician should also be collaborative, with patients feeling involved in this decision. Patient surveys would be helpful to gather service-user feedback and provide insights into what makes primary care streaming or redirection to community primary care more or less acceptable to patients.
To ensure that patients are efficiently and safely streamed to an appropriate clinician for their needs, nurses should be trained and experienced, and streaming pathways/protocols need to be co-produced by primary care and ED teams and clearly defined. To support this, there also need to be governance processes in place that reflect the aim of the service and how primary care clinicians are expected to work. A strong teamworking and communication culture can support clinicians develop an understanding of how individual clinicians and teams work. Operationally managing streaming can help monitor attendances and capacity and deploy staff to manage peaks in demand.
General practitioners should be recruited in line with the intended role of the service (and skillset required) with ongoing reflective training with a senior GP or ED clinician. GPs working in a role where they are seeing mostly primary care patients need to consider that they are seeing higher-risk patients and patients may require additional clinical skills (e.g. history taking, examination, safety netting) to ensure serious illness is excluded. 32 GPs seeing emergency care patients require tailored professional developmental opportunities relevant to their role.
Adding GPs to the skill mix in EDs increases processing capacity in the department. However, if the ED’s main challenge is exit block, as frequently reported currently, then the effectiveness at resolving the overall problem of patient flow will be limited. Potential locality-based opportunity costs of using GPs in EDs must also be considered.
While our marker conditions analysis did show some promise that the single GP-ED model explored (inside–parallel) could result in the least sick patients being seen more efficiently, we recognise that for this to have an impact at a department level, there need to be enough patients needing this level of care. This was not the case at all case study sites (e.g. where GP remit was narrow) and we saw GPs extending their role seeing sicker patients, but benefits of this at the ED level were not demonstrated from the routinely collected data.
Further work needed
Routine data collection and quality
The quality and extent of routine ED data collection during the study period limited the conclusions we could draw. The ECDS has since been introduced across England to standardise data collection and this should facilitate further research in this area. Patient experience data were notably very limited in this study (mainly in relation to experience of streaming) and we would advocate for the systematic capture of patient experience measures (PREM) as part of routine care. However, we also recognise that there is currently no agreed patient-reported outcome measure (PROM)175 for use in the ED and further work on the development of relevant PROMs and PREMs would be valuable. The relationships between patients’ expectations and those experience and outcome measures are also important to examine further. We recognise the tension that exists between maximising patient satisfaction and managing demand appropriately in a system under pressure.
To improve our understanding of the safety of GP ED models, there would be benefits for linking data between primary and secondary care to understand the whole patient journey. Currently it remains challenging to understand how attendances at ED relate to use of primary care and other elements of the urgent care system. It would be helpful to understand how frequently patients attending one service reattend elsewhere, and whether patients who are redirected from a service do go on to access appropriate care in another service.
With better quality data from EDs and other secondary care or primary care domains, it is important to conduct well-designed comparative effectiveness and cost-effectiveness studies based upon more rigorous quantitative epidemiological and statistical methods than has been possible with the data available to date. Such research is essential to guide future policy regarding GPs in EDs.
Workforce
It was beyond the scope of this study to explore the impact of GP-ED models on local community primary care. Potential effects might include EDs competing for primary care staff from an already limited pool,176 with a potential increase in workload for the remaining community workforce. They might also see their workload increased by redirection policies. Exploring these issues from the perspective of community primary care and the whole system would be helpful.
This study focused specifically on the role of GPs in EDs; however, we recognise that often GPs are working as part of wider primary care teams staffed by professionals from a variety of backgrounds. While some of the insights we have gained will be transferrable to other professional groups, there is a need to further explore how these multidisciplinary teams may operate differently. We note the ongoing NIHR-funded study177 (NIHR131356) which addresses this topic for non-medical practitioners. Teamworking across the ED is an important dimension to that evaluation.
Specific to the role of GPs in EDs, more could be done to support (and evaluate) the development of the GP with a special interest in ED role, including the development of a core set of competencies for ED work, and as adapted for different GP-ED models. Standardised minimum requirements could support training programme design, continuing professional development and governance of these services.
Similarly, we suggested that basic minimum requirements for the assessments made during the process of streaming to a GP (e.g. recording of observations) could be considered, recognising again that more robust streaming systems will be required for models operating outside the ED. Finally, we recognise that our qualitative data about what GPs do in consultations are based on self-report. Other methods such as consultation observations and activity tracking could provide helpful additional evidence about differences in practice.
Conclusion
As supported by national policy, GPs commonly work in emergency care, within or alongside emergency medicine doctors. A range of GP-ED models exist, with varying levels of integration, communication and training. We developed a taxonomy to describe GP service models (integrated with the ED service, parallel within the ED, outside the ED on the hospital site) and present a programme theory that describes how these service models were observed to operate. Routine data were of variable quality, limiting our analysis. We did not find clear or consistent effects on processes or outcomes of care although findings were mixed – this precludes definitive evidence about clinical or operational benefits. Time series analysis demonstrated trends across intervention sites for increased time spent in the ED, increased ED attendances and reattendances and mixed findings for hospital admissions. Findings suggest that GP-ED models do not meet the aim of reducing ED waiting times and improving patient flow with limited evidence of cost savings. Evidence on patient experience was limited but broadly supportive; we identified department-level processes to optimise the safety of GP-ED models. Qualitative data indicated GPs were often valued members of the wider ED team, with significant appetite at some sites to use their specific skillset to provide care in the ED. We developed a toolkit, based on our findings, to provide guidance for implementing and delivering GP-ED services. Better-quality routine data from EDs are needed to conduct well-designed comparative effectiveness and cost-effectiveness studies and to guide future policy regarding GPs in EDs.
Additional information
Acknowledgements
The co-authors would like to acknowledge the significant contributions made to the project by Nigel Pearson – Project Officer (2017–20) and Charlotte Bonner-Evans (Project Officer 2019). We thank Professor Deborah Fitzsimmons, Swansea University for supporting the health economics elements of the project. We also wish to thank particularly staff and patients at the 13 selected case study sites who participated in interviews and other elements of the study, and participants from other sites who took part in the baseline survey and selected interviews.
We thank staff at NHS Digital and SAIL Databank for assistance with provisioning data (Case number DARS-NIC-267223-D4Q3F, with data sharing agreement; all patient data were anonymised). We thank South Wales Research Ethics Committee for advice, support and approval for the study (IRAS 217765). We thank colleagues at the University of West of England and elsewhere in the parallel NIHR study of GPs in EDs, led by Professor Jonathan Benger, with whom we collaborated for the survey, Taxonomy and in selecting different study sites (and with data-sharing agreement). We also thank all members of the Study Steering Committee and project managers at HSDR for their support and advice throughout the study duration.
Contributions of authors
Freya Davies (https://orcid.org/0000-0002-6956-1100) (Senior Clinical Research Fellow, Primary Care) was the realist methods lead and led final report drafting.
Michelle Edwards (https://orcid.org/0000-0001-7432-2828) (Research Fellow, Qualitative Methods) conducted ethical approvals, qualitative data collection and analysis.
Delyth Price (https://orcid.org/0000-0002-4659-2908) (Research Assistant) conducted qualitative data analysis.
Pippa Anderson (https://orcid.org/0000-0003-2959-2671) (Associate Professor, Health Economics) was the methodological lead for health economics.
Damon Berridge (Late Professor of Statistics) led for time-series analysis design; RIP.
Andrew Carson-Stevens (https://orcid.org/0000-0002-7580-7699) (Clinical Professor of Patient Safety and Quality Improvement) was an academic GP and methodological lead for patient safety incident report analysis.
Mazhar Choudhry (https://orcid.org/0000-0001-8052-3490) (Former Cardiff University Medical Student) conducted qualitative data analysis of finance manager interviews.
Matthew Cooke (https://orcid.org/0000-0002-1038-3821) (Professor of Clinical Systems Design) conducted study design and provided emergency medicine policy and delivery expertise.
Jeremy Dale (https://orcid.org/0000-0001-9256-3553) (Professor of Primary Care) was an academic GP and conducted study design and provided unscheduled care research expertise.
Liam Donaldson (https://orcid.org/0000-0001-9170-6057) (Professor, WHO Patient Safety Envoy) conducted study design and provided National and International Patient Safety Policy context.
Bridie Angela Evans (https://orcid.org/0000-0003-0293-0888) (Research Officer, Public Involvement) was the public involvement lead.
Barbara Harrington (Welsh Government Involving people in research network) was a public contributor.
Shaun Harris (https://orcid.org/0000-0001-7724-6621) (Research Officer, Health Economics) conducted health economics data collection and analysis.
Julie Hepburn (Welsh Government Involving people in research network) was a public contributor.
Peter Hibbert (https://orcid.org/0000-0001-7865-343X) (Honorary Associate Professor, Patient Safety) conducted study design and provided patient safety expertise.
Thomas Hughes (https://orcid.org/0000-0001-5490-1267) (Consultant in Emergency Medicine) provided clinical and Emergency Care Data Set expertise.
Faris Hussain (https://orcid.org/0000-0002-7840-6815) (Former Cardiff University Medical Student) conducted the ambulatory care sensitive condition literature review.
Saiful Islam (https://orcid.org/0000-0003-3182-8487) (Statistician) conducted quantitative data analysis.
Rhys Pockett (https://orcid.org/0000-0003-4135-7383) (Senior Lecturer, Health Economics) conducted health economics data analysis.
Alison Porter (https://orcid.org/0000-0002-3408-7007) (Associate Professor, Health Services Research) provided unscheduled care and qualitative methods expertise.
Tim Rainer (Consultant in Emergency Medicine) provided unscheduled care research expertise.
Aloysius Niroshan Siriwardena (https://orcid.org/0000-0003-2484-8201) (Professor of Primary and Pre-Hospital Health Care) was an academic GP and provided unscheduled and pre-hospital care research expertise.
Helen Snooks (https://orcid.org/0000-0003-0173-8843) (Professor of Health Services Research) conducted study design and provided unscheduled care research expertise.
Alan Watkins (https://orcid.org/0000-0003-3804-1943) (Professor of Statistics) led routine data collection and analysis.
Adrian Edwards (https://orcid.org/0000-0002-6228-4446) (Professor of Primary Care) was the Principal Investigator, an academic GP and provided mixed methods expertise applied to unscheduled care
Alison Cooper (https://orcid.org/0000-0001-8660-6721) (Clinical Research Fellow, Primary Care) conducted study design, was the study management group lead researcher, and conducted qualitative and patient safety data collection and analysis.
Disclosure of interests
Full disclosure of interests: Completed ICMJE forms for all authors, including all related interests, are available in the toolkit on the NIHR journals Library report publication page at https://doi.org/10.3310/JWQZ5348.
Primary conflicts of interest: Liam Donaldson declares his role on the Integrated Care Board for North East and North Cumbria. Bridie Angela Evans is a co-applicant on the following NIHR-funded studies: Randomised trial of clinical and cost effectiveness of Administration of Prehospital fascia Iliaca compartment block for emergency Pre-Hospital hip fracture care Delivery (RAPID 2); Predictive RIsk Stratification Models: Assessment of Implementation Consequences (PRISMATIC 2). Julie Hepburn declares the receipt of honoraria and travel allowances for attending meetings as a public contributor. Thomas Hughes declares his role as NHS Clinical Lead for the implementation of the Emergency Care Data Set; Aloysius Niroshan Siriwardena declares involvement in grants from NIHR for unrelated studies; Alan Watkins declares his role as a member, NIHR HS&DR Funding Committee 2018–22 and as a member, NIHR College of Experts 2020–21; Helen Snooks is a current member of the NIHR HTA and EME Editorial Board, and is an NIHR Journals Library Editor. Alison Cooper reports sustenance costs covered by Cardiff University for qualitative data collection at case site visits. All other authors declare no conflicts of interest.
Patient data statement
This work uses data provided by patients and collected by the NHS as part of their care and support. Using patient data is vital to improve health and care for everyone. There is huge potential to make better use of information from people’s patient records, to understand more about disease, develop new treatments, monitor safety and plan NHS services. Patient data should be kept safe and secure, to protect everyone’s privacy, and it is important that there are safeguards to make sure that they are stored and used responsibly. Everyone should be able to find out about how patient data are used. #datasaveslives You can find out more about the background to this citation here: https://understandingpatientdata.org.uk/data-citation
Data-sharing statement
All data requests should be submitted to the corresponding author for consideration. Access to anonymised data may be granted following review.
Ethics statement
Ethical approval for Phase One activities was provided by Cardiff University School of Medicine Research Ethics Committee (REF 17/45). Ethical approval for Phase 2 activities was provided by the South Wales Research Ethics Committee REC reference 17/WA/0328.
Information governance statement
Cardiff University is committed to handling all personal information in line with the UK Data Protection Act (2018) and the General Data Protection Regulation (EU GDPR) 2016/679. Under the Data Protection legislation, Cardiff University is the Data Controller, and you can find out more about how we handle personal data, including how to exercise your individual rights and the contact details for our Data Protection Officer here (www.cardiff.ac.uk/public-information/policies-and-procedures/data-protection).
Department of Health and Social Care disclaimer
This publication presents independent research commissioned by the National Institute for Health and Care Research (NIHR). The views and opinions expressed by the interviewees in this publication are those of the interviewees and do not necessarily reflect those of the authors, those of the NHS, the NIHR, MRC, NIHR Coordinating Centre, the HSDR programme or the Department of Health and Social Care.
This monograph was published based on current knowledge at the time and date of publication. NIHR is committed to being inclusive and will continually monitor best practice and guidance in relation to terminology and language to ensure that we remain relevant to our stakeholders.
Disclaimers
This manuscript presents independent research funded by the National Institute for Health and Care Research (NIHR). The views and opinions expressed by authors in this publication are those of the authors and do not necessarily reflect those of the NHS, the NIHR, the HSDR programme or the Department of Health and Social Care. If there are verbatim quotations included in this publication the views and opinions expressed by the interviewees are those of the interviewees and do not necessarily reflect those of the authors, those of the NHS, the NIHR, the HSDR programme or the Department of Health and Social Care.
References
- Davies J. Urgent and emergency care: quality watch indicator n.d. https://www.england.nhs.uk/urgent-emergency-care/about-uec/ (accessed 30 March 2023).
- Royal College of Emergency Medicine . RCEM CARES: The Next Phase 2021 n.d. https://rcem.ac.uk/wp-content/uploads/2021/11/CARES_3_Final.pdf (accessed 13 December 2021).
- Boyle A, Higginson I, Sarsfield K, Kumari P. RCEM Acute Insight Series: Crowding and Its Consequences. London: Royal College of Emergency Medicine; 2021.
- Jeyaraman MM, Copstein L, Al-Yousif N, Alder RN, Kirkland SW, Al-Yousif Y, et al. Interventions and strategies involving primary healthcare professionals to manage emergency department overcrowding: a scoping review. BMJ Open 2021;11.
- Cooper A, Edwards M, Brandling J, Cooke M, Davies F, Hughes T, et al. Taxonomy of the form and function of primary care services in or alongside emergency departments: concepts paper. Emerg Med J 2019;36:625-30.
- Carson D, Clay H, Stern R. Primary Care and Emergency Departments 2010.
- Ablard S, O’Keeffe C, Ramlakhan S, Mason SM. Primary care services co-located with Emergency Departments across a UK region: early views on their development. Emerg Med J 2017;34:672-6.
- NHS England . Five Year Forward View 2014 n.d. www.england.nhs.uk/wp-content/uploads/2014/10/5yfv-web.pdf (accessed 28 February 2017).
- Department of Health and Social Care . A&Amp;E Departments to Get More Funding 2017 n.d. www.gov.uk/government/news/ae-departments-to-get-more-funding (accessed 13 December 2021).
- Goncalves-Bradley D, Khangura JK, Flodgren G, Perera R, Rowe BH, Shepperd S. Primary care professionals providing non-urgent care in hospital emergency departments. Cochrane Database Syst Rev 2018;2.
- National Institute for Health and Care Excellence . Chapter 17 GPs Within or on the Same Site As Emergency Departments: NICE Guideline 94 2018. www.nice.org.uk/guidance/ng94/evidence/17gps-within-or-on-the-same-site-as-emergency-departments-pdf-172397464604 (accessed 13 December 2021).
- Ramlakhan S, Mason S, O’Keeffe C, Ramtahal A, Ablard S, Burke D, et al. Primary care services located with EDs: a review of effectiveness. Emerg Med J 2016;33:495-503.
- NHS England . General Practice Forward View 2016. www.england.nhs.uk/wp-content/uploads/2016/04/gpfv.pdf (accessed 30 March 2023).
- Cooper A, Carson-Stevens A, Cooke M, Hibbert P, Hughes T, Hussain F, et al. Learning from diagnostic errors to improve patient safety when GPs work in or alongside emergency departments: incorporating realist methodology into patient safety incident report analysis. BMC Emerg Med 2021;21.
- Cooper A, Davies F, Edwards M, Anderson P, Carson-Stevens A, Cooke MW, et al. The impact of general practitioners working in or alongside emergency departments: a rapid realist review. BMJ Open 2019;9.
- Evans BA, Carson-Stevens A, Cooper A, Davies F, Edwards M, Harrington B, et al. Implementing public involvement throughout the research process – experience and learning from the GPs in EDs study. Health Expect 2022;25:2471-84.
- The UK Public Involvement Standards Development Partnership . UK Standards for Public Involvement 2019. https://sites.google.com/nihr.ac.uk/pi-standards/standards (accessed 20 December 2019).
- Price D, Edwards M, Carson-Stevens A, Cooper A, Davies F, Evans B, et al. Challenges of recruiting emergency department patients to a qualitative study: a thematic analysis of researchers’ experiences. BMC Med Res Methodol 2020;20.
- Edwards M, Cooper A, Davies F, Sherlock R, Carson-Stevens A, Price D, et al. Emergency department clinical leads’ experiences of implementing primary care services where GPs work in or alongside emergency departments in the UK: a qualitative study. BMC Emerg Med 2020;20.
- Price D, Edwards M, Davies F, Cooper A, McFadzean J, Carson-Stevens A, et al. Patients’ experiences of attending emergency departments where primary care services are located: qualitative findings from patient and clinician interviews from a realist evaluation. BMC Emerg Med 2022;22.
- Evans BA, Gallanders J, Griffiths L, Harris-Mayes R, James M, Jones S, et al. SUPER Group and PRIME Centre Wales . Public involvement and engagement in primary and emergency care research: the story from PRIME Centre Wales. Int J Popul Data Sci 2020;5.
- Morton K, Voss S, Adamson J, Baxter H, Bloor K, Brandling J, et al. General practitioners and emergency departments (GPED) – efficient models of care: a mixed-methods study protocol. BMJ Open 2018;8.
- Pawson R, Tilley N. Realistic Evaluation. London: SAGE Publications Ltd; 1997.
- Pawson R. The Science of Evaluation: A Realist Manifesto. London: SAGE Publications Ltd; 2013.
- Dalkin SM, Greenhalgh J, Jones D, Cunningham B, Lhussier M. What’s in a mechanism? Development of a key concept in realist evaluation. Implement Sci 2015;10.
- Pawson R. Evidence-Based Policy: A Realist Perspective. London: SAGE Publications Ltd; 2006.
- Pawson R. Digging for nuggets: how ‘bad’ research can yield ‘good’ evidence. Int J Soc Res Methodol 2006;9:127-42.
- Fletcher A, Jamal F, Moore G, Evans RE, Murphy S, Bonell C. Realist complex intervention science: applying realist principles across all phases of the Medical Research Council framework for developing and evaluating complex interventions. Evaluation 2016;22:286-303.
- Kaplan HC, Provost LP, Froehle CM, Margolis PA. The Model for Understanding Success in Quality (MUSIQ): building a theory of context in healthcare quality improvement. BMJ Qual Saf 2012;21:13-20.
- Pawson R, Manzano-Santaella A. A realist diagnostic workshop. Evaluation 2012;18:176-91.
- Croskerry P. A universal model of diagnostic reasoning. Acad Med 2009;84:1022-8.
- Cooper A, Carson-Stevens A, Edwards M, Davies F, Donaldson L, Anderson P, et al. Identifying safe care processes when GPs work in or alongside emergency departments: realist evaluation. Br J Gen Pract 2021;71:e931-40.
- Olsen W. Triangulation in social research: qualitative and quantitative methods can really be mixed. Develop Sociol 2004;20:103-18.
- Manzano A. The craft of interviewing in realist evaluation. Evaluation 2016;22:342-60.
- Sayer A. Realism and Social Science. London: SAGE Publications Ltd; 2000.
- Astbury B. Doing Realist Research. London: SAGE Publications Ltd; 2018.
- Haig BD, Evers C. Realist Inquiry in Social Science. London: SAGE Publications Ltd; 2016.
- Yin RK. Case Study Research: Design and Methods. Thousand Oaks, CA: SAGE Publications Ltd; 2014.
- Greenhalgh T, Pawson R, Wong G, Westhorp G, Greenhalgh J, Manzano A, et al. ‘Theory’ in Realist Evaluation: The RAMESES II Project 2017. www.ramesesproject.org/pgm-download_media.php?name=RAMESES_II_Theory_in_realist_evaluation.pdf (accessed 23 April 2019).
- Department of Health . The NHS Outcomes Framework 2011 12 2010.
- Agency for Healthcare Research and Quality . Organizing Quality Measures by Domains of Health Care Quality 2016. www.ahrq.gov/talkingquality/translate/organize/quality-domain.html#_ftn2 (accessed 18 May 2020).
- Weissman JS, Gatsonis C, Epstein AM. Rates of avoidable hospitalization by insurance status in Massachusetts and Maryland. JAMA 1992;268:2388-94.
- Sanderson C, Dixon J. Conditions for which onset or hospital admission is potentially preventable by timely end effective ambulatory care. J Health Serv Res Policy 2000;5:222-30.
- Bardsley M, Blunt I, Davies S, Dixon J. Is secondary preventive care improving? Observational study of 10-year trends in emergency admissions for conditions amenable to ambulatory care. BMJ Open 2013;3.
- Caminal J, Starfield B, Sánchez E, Casanova C, Morales M. The role of primary care in preventing ambulatory care sensitive conditions. Eur J Public Health 2004;14:246-51.
- Brown AD, Goldacre MJ, Hicks N, Rourke JT, McMurtry RY, Brown JD, et al. Hospitalization for ambulatory care-sensitive conditions: a method for comparative access and quality studies using routinely collected statistics. Can J Public Health 2001;92:155-9.
- Sundmacher L, Fischbach D, Schuettig W, Naumann C, Augustin U, Faisst C. Which hospitalisations are ambulatory care-sensitive, to what degree, and how could the rates be reduced? Results of a group consensus study in Germany. Health Policy 2015;119:1415-23.
- Purdy S, Griffin T, Salisbury C, Sharp D. Ambulatory care sensitive conditions: terminology and disease coding need to be more specific to aid policy makers and clinicians. Public Health 2009;123:169-73.
- Turner J, Coster J, Chambers D, Phung VH, Knowles E, Bradbury D, et al. What evidence is there on the effectiveness of different models of delivering urgent care? A rapid review. Health Soc Care Deliv Res 2015;3:1-134.
- Khangura JK, Flodgren G, Perera R, Rowe BH, Shepperd S. Primary care professionals providing non‐urgent care in hospital emergency departments. Cochrane Database Syst Rev 2012;11.
- Saul JE, Willis CD, Bitz J, Best A. A time-responsive tool for informing policy making: rapid realist review. Implement Sci 2013;8.
- Salisbury C, Hollinghurst S, Montgomery A, Cooke M, Munro J, Sharp D, et al. The impact of co-located NHS walk-in centres on emergency departments. Emerg Med J 2007;24:265-9.
- Ward P, Huddy J, Hargreaves S, Touquet R, Hurley J, Fothergill J. Primary care in London: an evaluation of general practitioners working in an inner city accident and emergency department. Emerg Med J 1996;13:11-5.
- Dale J, Green J, Reid F, Glucksman E, Higgs R. Primary care in the accident and emergency department: II – comparison of general practitioners and hospital doctors. BMJ 1995;311:427-30.
- Dale J, Lang H, Roberts JA, Green J, Glucksman E. Cost effectiveness of treating primary care patients in accident and emergency: a comparison between general practitioners, senior house officers, and registrars. BMJ 1996;312:1340-4.
- Primary Care in Emergency Departments . A Guide to Good Practice: NHS Interim Mangement and Support 2015. https://www.nhsimas.nhs.uk/fileadmin/Files/IST/Emergency_care_conference_2014/Primary_Care_in_A_E_Guidance_Feb_2015.pdf (accessed 5 January 2024).
- Thijssen W, Wijnen-van Houts M, Koetsenruijter J, Giesen P, Wensing M. The impact on emergency department utilization and patient flows after integrating with a general practitioner cooperative: an observational study. Emerg Med Int 2013;2013.
- van Veen M, ten Wolde F, Poley MJ, Ruige M, van Meurs AH, Hablé C, et al. Referral of nonurgent children from the emergency department to general practice: compliance and cost savings. Eur J Emerg Med 2012;19:14-9.
- van Gils-van Rooij ESJ, Meijboom BR, Broekman SM, Yzermans CJ, de Bakker DH. Is patient flow more efficient in Urgent Care Collaborations?. Eur J Emerg Med 2018;25:58-64.
- Bolton P, Thompson L. The reasons for, and lessons learned from, the closure of the Canterbury GP after-hours service. Aust Health Rev 2001;24:66-73.
- Murphy A, Bury G, Plunkett PK, Gibney D, Smith M, Mullan E, et al. Randomised controlled trial of general practitioner versus usual medical care in an urban accident and emergency department: process, outcome, and comparative cost. BMJ 1996;312:1135-42.
- Bickerton J, Dewan V, Allan T. Streaming A&E patients to walk-in centre services. Emerg Nurse 2005;13:20-3.
- van der Straten LM, van Stel HF, Spee FJ, Vreeburg ME, Schrijvers AJ, Sturms LM. Safety and efficiency of triaging low urgent self-referred patients to a general practitioner at an acute care post: an observational study. Emerg Med J 2012;29:877-81.
- Chmiel C, Wang M, Sidler P, Eichler K, Rosemann T, Senn O. Implementation of a hospital-integrated general practice: a successful way to reduce the burden of inappropriate emergency-department use. Swiss Med Wkly 2016;146:w142-84.
- Carson D, Clay H, Stern R. Urgent Care Centres: what works best?. Prim Care Found 2012:1-23.
- Dale J. Primary Care in Accident and Emergency Departments: The Cost Effectiveness and Applicability of a New Model of Care. London: London School of Hygiene & Tropical Medicine; 1998.
- Gnani S, Morton S, Ramzan F, Davison M, Ladbrooke T, Majeed A, et al. Healthcare use among preschool children attending GP-led urgent care centres: a descriptive, observational study. BMJ Open 2016;6.
- Cowling T, Ramzan F, Ladbrooke T, Millington H, Majeed A, Gnani S. Referral outcomes of attendances at general practitioner-led urgent care centres in London, England: retrospective analysis of hospital administrative data. Emerg Med J 2016;33:200-7.
- Freeman GK, Meakin RP, Lawrenson RA, Leydon GM, Craig G. Primary care units in A and E departments in North Thames in the 1990s: initial experience and future implications. Br J Gen Pract 1999;49:107-10.
- Murphy AW, Bury G, Plunkett PK, Gibney D, Smith M, Mullan E, et al. Randomised controlled trial of general practitioner versus usual medical care in an urban accident and emergency department: process, outcome, and comparative cost. BMJ 1996;312:1135-42.
- Wilson H. Co-locating primary care facilities within emergency departments: brilliant innovation or unwelcome intervention into clinical care?. N Z Med J 2005;118.
- Boeke AJ, van Randwijck-Jacobze ME, de Lange-Klerk EM, Grol SM, Kramer MH, van der Horst HE. Effectiveness of GPs in accident and emergency departments. Br J Gen Pract 2010;60:378-84.
- Wang M, Wild S, Hilfiker G, Chmiel C, Sidler P, Eichler K, et al. Hospital-integrated general practice: a promising way to manage walk-in patients in emergency departments. J Eval Clin Pract 2014;20:20-6.
- Smith L, Narang Y, Ibarz Pavon AB, Edwardson K, Bowers S, Jones K, et al. To GP or not to GP: a natural experiment in children triaged to see a GP in a tertiary paediatric emergency department (ED). BMJ Qual Saf 2018;27:521-8.
- Huibers L, Thijssen W, Koetsenruijter J, Giesen P, Grol R, Wensing M. GP cooperative and emergency department: an exploration of patient flows. J Eval Clin Pract 2013;19:243-9.
- Kool RB, Homberg DJ, Kamphuis HCM. Towards integration of general practitioner posts and accident and emergency departments: a case study of two integrated emergency posts in the Netherlands. BMC Health Serv Res 2008;8.
- Bosmans JE, Boeke AJ, van Randwijck-Jacobze ME, Grol SM, Kramer MH, van der Horst HE, et al. Addition of a general practitioner to the accident and emergency department: a cost-effective innovation in emergency care. Emerg Med J 2012;29:192-6.
- Hess S, Sidler P, Chmiel C, Bögli K, Senn O, Eichler K. Satisfaction of health professionals after implementation of a primary care hospital emergency centre in Switzerland: a prospective before-after study. Int Emerg Nurs 2015;23:286-93.
- Gibney D, Murphy AW, Barton D, Byrne C, Smith M, Bury G, et al. Randomized controlled trial of general practitioner versus usual medical care in a suburban accident and emergency department using an informal triage system. Br J Gen Pract 1999;49:43-4.
- Thijssen W. Emergency Departments in the Netherlands the Influence of General Practitioner Cooperatives 2017. https://repository.ubn.ru.nl/bitstream/handle/2066/134183/134183.pdf (accessed 9 September 2022).
- Clancy E, Mayo A. Launching a social enterprise see-and-treat service. Emerg Nurse 2009;17:22-4.
- van Uden CJT, Crebolder HFJM. Does setting up out of hours primary care cooperatives outside a hospital reduce demand for emergency care?. Emerg Med J 2004;21:722-3.
- van Uden C, Winkens R, Wesseling G, Fiolet H, van Schayck O, Crebolder H. The impact of a primary care physician cooperative on the caseload of an emergency department: the Maastricht integrated out-of-hours service. J Gen Intern Med 2005;20:612-7.
- O’Kelly F, Teljeur C, Carter I, Plunkett P. Impact of a GP cooperative on lower acuity emergency department attendances. Emerg Med J 2010;27:770-3.
- van Gils-van Rooij ESJ, Yzermans CJ, Broekman SM, Meijboom BR, Welling GP, de Bakker DH. Out-of-hours care collaboration between general practitioners and hospital emergency departments in the Netherlands. J Am Board Fam Med 2015;28:807-15.
- Brand CL, Linden MC, Veelen MJ, Reijnen R. Effects of a general practitioner cooperative co-located with an emergency department on patient throughput. World J Emerg Med 2016;7:270-3.
- Arain M, Campbell MJ, Nicholl JP. Impact of a GP-led walk-in centre on NHS emergency departments. Emerg Med J 2015;32:295-300.
- van Uden CJ, Winkens RA, Wesseling GJ, Crebolder HF, van Schayck CP. Use of out of hours services: a comparison between two organisations. Emerg Med J 2003;20:184-7.
- Johnson SJ. Evidence for primary care services at A&E. BMJ 2015;350.
- Grant C, Nicholas R, Moore L, Salisbury C. An observational study comparing quality of care in walk-in centres with general practice and NHS Direct using standardised patients. BMJ 2002;324.
- Schols A, Stevens F, Zeijen C, Dinant G, van Vugt C, Cals J. Access to diagnostic tests during GP out-of-hours care: a cross-sectional study of all GP out-of-hours services in the Netherlands. Eur J Gen Pract 2016;22:176-81.
- Posocco A, Scapinello MP, De Ronch I, Castrogiovanni F, Lollo G, Sergi G, et al. Role of out of hours primary care service in limiting inappropriate access to emergency department. Intern Emerg Med 2017;13:549-55.
- van Uden CJ, Ament AJ, Voss GB, Wesseling G, Winkens RA, van Schayck OC, et al. Out-of-hours primary care: implications of organisation on costs. BMC Fam Pract 2006;7.
- Gritz A, Sen A, Hiles S, Mackenzie G, Blair M. More under-fives now seen in urgent care centre than A&E: should we shift our focus?. Arch Dis Child 2016;101:A132.1-A132.
- Morton S, Igantowicz A, Gnani S, Majeed A, Greenfield G. Describing team development within a novel GP-led urgent care centre model: a qualitative study. BMJ Open 2016;6.
- Dale J, Reid F, Green J, Glucksman E. Primary care in the accident and emergency department: I – prospective identification of patients. BMJ 1995;311:423-6.
- Harris T, McDonald K. How do clinicians with different training backgrounds manage walk-in patients in the ED setting?. Emerg Med J 2014;31:975-9.
- Begum F, Khan H, Moss P. Solving the A&E Crisis Using GP Lead Triage and Redirection Evaluation of GP-Lead Service to Identify and Re-Direct Patients from A&Amp;E to Primary Care Services 2017. https://www.transformationpartners.nhs.uk/wp-content/uploads/2017/10/30.-Solving-the-AE-crisis-using-GP-lead-triage-and-redirection.pdf (accessed 9 September 2022).
- Rivara F, Wall H, Worley P, James K. Pediatric nurse triage: it’s efficacy, safety and implications for care. J Pediatr Health Care 1991;5:291-8.
- Derlet RW, Kinser D, Ray L, Hamilton B, McKenzie J. Prospective identification and triage of nonemergency patients out of an emergency department: a 5-year study. Ann Emerg Med 1995;25:215-23.
- Ellbrant J, Akeson J, Akeson PK. Pediatric emergency department management benefits from appropriate early redirection of nonurgent visits. Pediatr Emerg Care 2015;31:95-100.
- Bentley JA, Thakore S, Morrison W, Wang W. Emergency Department redirection to primary care: a prospective evaluation of practice. Scott Med J 2017;62:2-10.
- Anantharaman V. Impact of health care system interventions on emergency department utilization and overcrowding in Singapore. Int J Emerg Med 2008;1:11-20.
- van Veen M, Steyerberg EW, Lettinga L, Ruige M, van Meurs AHJ, van der Lei J, et al. Safety of the Manchester Triage System to identify less urgent patients in paediatric emergence care: a prospective observational study. Arch Dis Child 2011;96:513-8.
- Arain M, Nicholl J, Campbell M. Patients’ experience and satisfaction with GP led walk-in centres in the UK; a cross sectional study. BMC Health Serv Res 2013;13.
- Chalder M, Montgomery A, Hollinghurst S, Cooke M, Munro J, Lattimer V, et al. Comparing care at walk-in centres and at accident and emergency departments: an exploration of patient choice, preference and satisfaction. Emerg Med J 2007;24:260-4.
- Hunter C, Chew-Graham C, Langer S, Stenhoff A, Drinkwater J, Guthrie E, et al. A qualitative study of patient choices in using emergency health care for long-term conditions: the importance of candidacy and recursivity. Patient Educ Couns 2013;93:335-41.
- Rajpar SF, Smith MA, Cooke MW. Study of choice between accident and emergency departments and general practice centres for out of hours primary care problems. J Accid Emerg Med 2000;17:18-21.
- Hutchinson A, Galvin K. Presentation to the emergency department by breathless patients: a qualitative study. Eur Respir J 2016;48.
- Dale J, Sandhu H, Lall R, Glucksman E. The patient, the doctor and the emergency department: a cross-sectional study of patient-centredness in 1990 and 2005. Patient Educ Couns 2008;72:320-9.
- Sandhu H, Dale J, Stallard N, Crouch R, Glucksman E. Emergency nurse practitioners and doctors consulting with patients in an emergency department: a comparison of communication skills and satisfaction. Emerg Med J 2009;26:400-4.
- Lengu D, Kobbacy K, Sapountzis S, Kagioglou M. Application of Simulation and Modelling in Managing Unplanned Healthcare Demand. Salford: University of Salford; 2012.
- Sharma A, Inder B. Impact of co-located general practitioner (GP) clinics and patient choice on duration of wait in the emergency department. Emerg Med J 2011;28:658-61.
- Schull MJ, Kiss A, Szalai JP. The effect of low-complexity patients on emergency department waiting times. Ann Emerg Med 2007;49:257-64, 264.e1.
- Vertesi L. Does the Canadian Emergency Department Triage and Acuity Scale identify non-urgent patients who can be triaged away from the emergency department?. Can J Emerg Med 2004;6:337-42.
- Richardson DB, Mountain D. Myths versus facts in emergency department overcrowding and hospital access block. Med J Aust 2009;190:369-74.
- Allen P, Cheek C, Foster S, Ruigrok M, Wilson D, Shires L. Low acuity and general practice-type presentations to emergency departments: a rural perspective. Emerg Med Australas 2015;27:113-8.
- O’Cathain A, Knowles E, Turner J, Hirst E, Goodacre S, Nicholl J. Variation in avoidable emergency admissions: multiple case studies of emergency and urgent care systems. J Health Serv Res Policy 2016;21:5-14.
- Murphy AW, Plunkett PK, Bury G, Leonard C. Effect of patients seeing a general practitioner in accident and emergency on their subsequent reattendance: cohort study. Br Med J 2000;320:903-4.
- Doran KM, Colucci AC, Hessler RA, Ngai CK, Williams ND, Wallach AB, et al. An intervention connecting low-acuity emergency department patients with primary care: effect on future primary care linkage. Ann Emerg Med 2013;61:312-321.e7.
- Arain M, Baxter S, Nicholl J. Perceptions of healthcare professionals and managers regarding the effectiveness of GP-led walk-in centres in the UK. BMJ Open 2015;5.
- Kork AA, Vakkuri J. Improving access and managing healthcare demand with walk-in clinic. Int J Public Sect Manag 2016;29:148-63.
- Greenfield G, Ignatowicz A, Gnani S, Bucktowonsing M, Ladbrooke T, Millington H, et al. Staff perceptions on patient motives for attending GP-led urgent care centres in London: a qualitative study. BMJ Open 2016;6:e0076-83.
- Tammes P, Morris RW, Brangan E, Checkland K, England H, Huntley A, et al. Exploring the relationship between general practice characteristics, and attendance at walk-in centres, minor injuries units and EDs in England 2012/2013: a cross-sectional study. Emerg Med J 2016;33:702-8.
- Colliers A, Remmen R, Streffer ML, Michiels B, Bartholomeeusen S, Monsieurs KG, et al. Implementation of a general practitioner cooperative adjacent to the emergency department of a hospital increases the caseload for the GPC but not for the emergency department. Acta Clin Belg 2017;72:49-54.
- Krakau I, Hassler E. Provision for clinic patients in the ED produces more nonemergency visits. Am J Emerg Med 1999;17:18-20.
- Maheswaran R, Pearson T, Jiwa M. Repeat attenders at National Health Service walk-in centres: a descriptive study using routine data. Public Health 2009;123:506-10.
- Hsu RT, Lambert PC, Dixon-Woods M, Kurinczuk JJ. Effect of NHS walk-in centre on local primary healthcare services: before and after observational study. BMJ 2003;326.
- Salisbury C, Chalder M, Scott TM, Pope C, Moore L. What is the role of walk-in centres in the NHS?. BMJ 2002;324:399-402.
- Desborough J, Parker R, Forrest L. Development and implementation of a nurse-led walk-in centre: evidence lost in translation?. J Health Serv Res Policy 2013;18:174-8.
- Pope C, Chalder M, Moore L, Salisbury C. What do other local providers think of NHS walk-in centres? Results of a postal survey. Public Health 2005;119:39-44.
- Chew-Graham C, Rogers A, May C, Sheaf R, Ball E. A new role for the general practitioner? Reframing ‘inappropriate attenders’ to inappropriate services. Prim Health Care Res Dev 2004;5:60-7.
- Dale J, Russell R, Harkness F, Wilkie V, Aiello M. Extended training to prepare GPs for future workforce needs: a qualitative investigation of a 1-year fellowship in urgent care. Br J Gen Pract 2017;67:e659-67.
- Van den Heede K, Quentin W, Dubois C, Devriese S, Van de Voorde C. The 2016 proposal for the reorganisation of urgent care provision in Belgium: a political struggle to co-locate primary care providers and emergency departments. Health Policy 2017;121:339-45.
- NHS England . Primary Care Streaming: Roll Out to September 2017 2017.
- Fink A, Kosecoff J, Chassin M, Brook RH. Consensus methods: characteristics and guidelines for use. Am J Public Health 1984;74:979-83.
- Edlow JA. Diagnosis of subarachnoid hemorrhage. Neurocritical Care 2005;2:99-109.
- Courts and Tribunals Judiciary . Reports to Prevent Future Deaths 2022. www.judiciary.uk/related-offices-and-bodies/office-chief-coroner/https-www-judiciary-uk-subject-community-health-care-and-emergency-services-related-deaths/ (accessed 7 March 2022).
- Carson-Stevens A, Hibbert P, Williams H, Evans HP, Cooper A, Rees P, et al. Characterising the Nature of Primary Care Patient Safety Incident Reports in the England and Wales National Reporting and Learning System: A Mixed-methods Agenda-setting Study for General Practice. Southampton, UK: NIHR Journals Library; 2016.
- The RAMESES II Project . Retroduction in Realist Evaluation 2017. www.ramesesproject.org/pgm-download_media.php?name=RAMESES_II_Retroduction.pdf (accessed 17 January 2018).
- Pearson M, Brand S, Quinn C, Shaw J, Maguire M, Michie S, et al. Using realist review to inform intervention development: methodological illustration and conceptual platform for collaborative care in offender mental health. Implement Sci 2015;10:1-12.
- Merton RK. Social Theory and Social Structure. New York: Simon & Schuster, The Free Pressl; 1949.
- Choudhry M, Edwards M, Cooper A, Anderson P, Carson-Stevens A, Cooke M, et al. Senior clinical and business managers’ perspectives on the influence of different funding mechanisms, and barriers and enablers to implementing models of employing general practitioners in or alongside emergency departments: qualitative study. Health Policy 2021;125:482-8.
- Edwards M, Cooper A, Hughes T, Davies F, Sherlock R, Anderson P, et al. A classification of primary care streaming pathways in UK emergency departments: findings from a multi-methods study comprising cross-sectional survey; site visits with observations, semi-structured and informal interviews. Int Emerg Nurs 2021;56.
- Standing M. Clinical judgement and decision‐making in nursing–nine modes of practice in a revised cognitive continuum. J Adv Nurs 2008;62:124-34.
- Edwards M, Cooper A, Hughes T, Davies F, Price D, Anderson P, et al. The effectiveness of primary care streaming in emergency departments on decision-making and patient flow and safety: a realist evaluation. Int Emerg Nurs 2022;62.
- Neighbour R. Safety netting: now doctors need it too. Br J Gen Pract 2018;68:214-5.
- Kovacs G, Croskerry P. Clinical decision making: an emergency medicine perspective. Acad Emerg Med 1999;6:947-52.
- Kassirer JP, Kopelman RI. Cognitive errors in diagnosis: instantiation, classification, and consequences. Am J Med 1989;86:433-41.
- Sonis JD, Aaronson EL, Castagna A, White B. A conceptual model for emergency department patient experience. J Patient Exp 2019;6:173-8.
- National Institute for Health and Care Excellence . Medical Technologies Evaluation Programme Methods Guide 2017. www.nice.org.uk/process/pmg33/chapter/evidence-synthesis-and-cost-consequence-analysis (accessed 9 September 2022).
- NHS Digital . HRG4 + 2017/18/Local/Payment/Grouper 2017. https://digital.nhs.uk/services/national-casemix-office/downloads-groupers-and-tools/payment-hrg4-2017-18-local-payment-grouper (accessed 9 September 2022).
- NHS England . National Schedule of NHS Costs. https://www.england.nhs.uk/publication/2020-21-national-cost-collection-data-publication/ (accessed 9 September 2022).
- Tonkin-Crine S, Anthierens S, Hood K, Yardley L, Cals JWL, Francis NA, et al. Discrepancies between qualitative and quantitative evaluation of randomised controlled trial results: achieving clarity through mixed methods triangulation. Implement Sci 2016;11.
- Moran-Ellis J, Alexander VD, Cronin A, Dickinson M, Fielding J, Sleney J, et al. Triangulation and integration: processes, claims and implications. Qual Res 2006;6:45-59.
- Edwards M, Cooper A, Freya D, Carson-Stevens A, Hughes T, Siriwardena N, et al. A classification of primary care pathways in emergency departments: a multi-methods study comprising cross-sectional survey; site visits with observations; semi-structured and informal interviews. Emerg Med J 2020;37:841.2-842.
- McFadzean I, Edwards M, Davies F, Cooper A, Price D, Carson-Stevens A, et al. Realist analysis of whether emergency departments with primary care services generate ‘provider-induced demand’. BMC Emerg Med 2022;22.
- Anderson H, Scantlebury A, Leggett H, Brant H, Salisbury C, Benger J, et al. Factors influencing streaming to General Practitioners in emergency departments: a qualitative study. Int J Nurs Stud 2021;120.
- Gaughan J, Liu D, Gutacker N, Bloor K, Doran T, Benger JR. Does the presence of general practitioners in emergency departments affect quality and safety in English NHS hospitals? A retrospective observational study. BMJ Open 2022;12.
- Scantlebury A, Brant H, Anderson H, Leggett H, Salisbury C, Cowlishaw S, et al. Potential impacts of general practitioners working in or alongside emergency departments in England: initial qualitative findings from a national mixed-methods evaluation. BMJ Open 2021;11.
- Butun A, Linden M, Lynn F, McGaughey J. Exploring parents’ reasons for attending the emergency department for children with minor illnesses: a mixed methods systematic review. Emerg Med J 2019;36:39-46.
- O’Cathain A, Simpson R, Phillips M, Knowles E. Tendency to call an ambulance or attend an emergency department for minor or non-urgent problems: a vignette-based population survey in Britain. Emerg Med J 2022;39:436-42.
- Rising KL, Victor TW, Hollander JE, Carr BG. Patient returns to the emergency department: the time-to-return curve. Acad Emerg Med 2014;21:864-71.
- Hussain F, Cooper A, Carson-Stevens A, Donaldson L, Hibbert P, Hughes T, et al. Diagnostic error in the emergency department: learning from national patient safety incident report analysis. BMC Emerg Med 2019;19.
- Leonard M, Graham S, Bonacum D. The human factor: the critical importance of effective teamwork and communication in providing safe care. Qual Saf Health Care 2004;13:i85-90.
- Sutcliffe KM, Lewton E, Rosenthal MM. Communication failures: an insidious contributor to medical mishaps. Acad Med 2004;79:186-94.
- Edelson DP, Litzinger B, Arora V, Walsh D, Kim S, Lauderdale DS, et al. Improving in-hospital cardiac arrest process and outcomes with performance debriefing. Arch Intern Med 2008;168:1063-9.
- Makary MA, Mukherjee A, Sexton JB, Syin D, Goodrich E, Hartmann E, et al. Operating room briefings and wrong-site surgery. J Am Coll Surg 2007;204:236-43.
- Sarto F, Veronesi G. Clinical leadership and hospital performance: assessing the evidence base. BMC Health Serv Res 2016;16.
- Cooper A, Carson-Stevens A, Hughes T, Edwards A. Is streaming patients in emergency departments to primary care services effective and safe?. The BMJ 2020;368:m462-11.
- Iversen AKS, Kristensen M, Østervig RM, Køber L, Sölétormos G, Lundager Forberg J, et al. A simple clinical assessment is superior to systematic triage in prediction of mortality in the emergency department. Emerg Med J 2019;36:66-71.
- Smith L, Narang Y, Pavon A, Edwardson K, Bowers S, Jones K, et al. To GP or not to GP: evaluation of children triaged to see a GP in a tertiary paediatric emergency department. BMJ Qual Saf 2017:1-8.
- Nicodemo C, McCormick B, Wittenberg R, Hobbs FR. Are more GPs associated with a reduction in emergency hospital admissions? A quantitative study on GP referral in England. Br J Gen Pract 2021;71:e287-95.
- BBC News . A&Amp;E Crisis Plans ‘Not Good Enough’, MPs Say n.d. www.bbc.co.uk/news/health-23423796 (accessed 9 September 2022).
- Poulsen NR, Schougaard LMV, Søvsø MB, Leutscher PDC, Pedersen MK. Patient-reported outcome measures in the emergency department: a scoping review protocol. JBI Evid Synth 2021;19:3102-12.
- Anderson H, Scantlebury A, Leggett H, Salisbury C, Benger J, Adamson J. Perspectives of GPs working in or alongside emergency departments in England: qualitative findings from the GPs and Emergency Departments Study. Br J Gen Pract 2022;72:e764-72.
- Halter M. Implementation of the non-medical practitioner workforce into the urgent and emergency care system skill-mix in England: a mixed methods study of configurations and impact. NIHR 2021. https://fundingawards.nihr.ac.uk/award/NIHR131356 (accessed 9 September 2022).
Appendix 1 Qualitative research team characteristics
All qualitative data were collected and analysed by Dr Alison Cooper (she/her) and Dr Michelle Edwards (she/her)
Dr Alison Cooper (Clinical Research Fellow) is a co-applicant for the study and works in an academic research role and as a GP. Dr Cooper has advanced level training in realist methods and patient safety research expertise. At the time of the study, Dr Cooper was completing a PhD using realist methodology, ‘Exploring opportunities to improve patient safety when GPs work in or alongside emergency departments’. Dr Cooper interviewed all GPs and some other clinical staff during the fieldwork, drawing on her knowledge and experience from community general practice. She declared her interest in GP roles in EDs and patient safety and her role as a GP to clinical staff when carrying out observations and interviews.
Dr Michelle Edwards (Research Fellow) is social scientist with a degree in psychology, MSc in social science research methods (sociology) and PhD in medicine using observation and interview methods and has over 10 years of qualitative research expertise and advanced level training in realist methods. Dr Edwards was responsible for recruiting research sites and had established connections with Emergency Department Clinical Leads before interviews and site visits. Dr Edwards interviewed some clinical staff and all patients and declared her interest in medical sociology and health service delivery to clinical staff and her interest in patient’s reported experiences of health care to patients.
Some analysis of clinical director interviews and patient interviews was undertaken by Miss Delyth Price (she/her)
Miss Delyth Price is currently a Research Assistant with a degree in sociology and a MSc in health psychology background with experience of analysing qualitative data. At the time of the study, Miss Price had a project management role and helped set up research permission and access to research sites, produced and prepared study documentation and facilitated co-applicant and stakeholder meetings. She had the opportunity to receive training and supervision to analyse data and contribute to sections of the report relating to Phase 1 clinical lead interviews, methodology (patient recruitment) and patient interviews.
Data analysis and triangulation of qualitative and quantitative data were carried out by Dr Freya Davies (she/her)
Dr Freya Davies (Senior Clinical Research Fellow) works in an academic research role and a clinical role as a GP and has a PhD in medicine, with training, experience, and expertise in realist methods.
Appendix 2 Details of staff interviewed
Site | Participant number | Model | Staff group | Duration of interview | Individual or group interview |
---|---|---|---|---|---|
Integrated-1 | GPED03 ACP01 | Inside–integrated | Advanced Care Practitioner | 25 minutes | Individual interview |
Integrated-1 | GPED03 EDDR01 | Inside–integrated | Emergency Department Doctor | 15 minutes | Individual interview |
Integrated-1 | GPED03 EDF101 | Inside–integrated | Foundation 1 Doctor | 7 minutes | Individual interview |
Integrated-1 | GPED03 ENP01 | Inside–integrated | Emergency Nurse Practitioner (ENP) | 16 minutes | Individual interview |
Integrated-1 | GPED03 ENP02 | Inside–integrated | Emergency Nurse Practitioner | 19 minutes | Individual interview |
Integrated-1 | GPED03 GP01 | Inside-integrated | General Practitioner | 15 minutes | Individual interview |
Integrated-1 | GPED03 GP02 | Inside–integrated | General Practitioner | 17 minutes | Individual interview |
Integrated-1 | GPED03 GP03 | Inside–integrated | General Practitioner | 31 minutes | Individual interview |
Integrated-1 | GPED03 GP04 | Inside–integrated | General Practitioner | 37 minutes | Individual interview |
Integrated-1 | GPED03 SRN01 | Inside–integrated | Senior Nurse | 6 minutes | Individual interview |
Integrated-1 | GPED03 SN01 | Inside–integrated | Staff Nurse | 11 minutes | Individual interview |
Integrated-1 | GPED03 CD01 | Inside–integrated | Clinical Director | 78 and 24 minutes | |
Integrated-1 | GPED03 SFM01 | Inside–integrated | Service/Financial Manager | Unknown | Group interview |
Integrated-1 | GPED03 SFM02 | Inside–integrated | Service/Financial Manager | ||
Integrated-1 | GPED03 SFM03 | Inside–integrated | Service/Financial Manager | ||
Integrated-1 | GPED03 SFM04 | Inside–integrated | Service/Financial Manager | ||
Integrated-2 | GPED08 GP01 | Inside–integrated | General Practitioner | 25 minutes | Individual interview |
Integrated-2 | GPED08 GP02 | Inside–integrated | General Practitioner | 16 minutes | Individual interview |
Integrated-2 | GPED08 CD01 | Inside–integrated | Clinical Director | 37 and 26 minutes | Individual interview |
Integrated-3 | GPED14 GP01 | Inside–integrated | General Practitioner | 19 minutes | Individual interview |
Integrated-3 | GPED14 GP02 | Inside–integrated | General Practitioner | 12 minutes | Individual interview |
Integrated-3 | GPED14 EDDR01 | Inside–integrated | Emergency Department Doctor | 16 minutes | Individual interview |
Integrated-3 | GPED14 CD01 | Inside–integrated | Clinical Director | 25 minutes | Individual interview |
Integrated-3 | GPED14 GPCons01 | Inside–integrated | GP Consultant | 58 minutes | Individual interview |
Integrated-3 | GPED14 FM01 | Inside–integrated | Finance Manager | 60 minutes | Group interview |
Integrated-3 | GPED14 FM02 | Inside–integrated | Finance Manager | ||
Integrated-3 | GPED14 FM03 | Inside–integrated | Finance Manager | ||
Parallel-1 | GPED04 ANP01 | Inside–parallel | Advanced Nurse Practitioner | 27 minutes | Individual interview |
Parallel-1 | GPED04 GP01 | Inside–parallel | General Practitioner | 8 minutes | Individual interview |
Parallel-1 | GPED04 GP02 | Inside–parallel | General Practitioner | 15 minutes | Individual interview |
Parallel-1 | GPED04 EDDR01 | Inside–parallel | Emergency Department Doctor | 22 minutes | Individual interview |
Parallel-1 | GPED04 EDDR02 | Inside–parallel | Emergency Department Doctor | 4 minutes | Individual interview |
Parallel-1 | GPED04 EDDR03 | Inside–parallel | Emergency Department Doctor | 32 minutes | Individual interview |
Parallel-1 | GPED04 EDDR04 | Inside–parallel | Emergency Department Doctor | 35 minutes | Individual interview |
Parallel-1 | GPED04 ENP01 | Inside–parallel | Emergency Nurse Practitioner | 17 minutes | Individual interview |
Parallel-1 | GPED04 ENP02 | Inside–parallel | Emergency Nurse Practitioner | 17 minutes | Individual interview |
Parallel-1 | GPED04 CT101 | Inside–parallel | Core Training 1 Doctor | 12 minutes | Individual interview |
Parallel-1 | GPED04 HCSW01 | Inside–parallel | Healthcare Support Worker | 9 minutes | Individual interview |
Parallel-1 | GPED04 CD01 | Inside–parallel | Clinical Director | 72 minutes | Group interview |
Parallel-1 | GPED04 SFM01 | Inside–parallel | Service/Financial Manager | ||
Parallel-2 | GPED06 CD01 | Inside–parallel | Clinical Director | Unknown and 56 minutes | Individual interview |
Parallel-2 | GPED06 EDDR01 | Inside–parallel | Emergency Department Doctor | 20 minutes | Individual interview |
Parallel-2 | GPED06 EDDR02 | Inside–parallel | Emergency Department Doctor | 27 minutes | Individual interview |
Parallel-2 | GPED06 GP01 | Inside–parallel | General Practitioner | 45 minutes | Individual interview |
Parallel-2 | GPED06 GP02 | Inside–parallel | General Practitioner | 28 minutes | Individual interview |
Parallel-2 | GPED06 GP03 | Inside–parallel | General Practitioner | 12 minutes | Individual interview |
Parallel-2 | GPED06 GP04 | Inside–parallel | General Practitioner | 16 minutes | Individual interview |
Parallel-2 | GPED06 ENP01 | Inside–parallel | Emergency Nurse Practitioner | 9 minutes | Individual interview |
Parallel-2 | GPED06 SFM01 | Inside–parallel | Service/Financial Manager | 72 minutes | Group interview |
Parallel-2 | GPED06 SFM02 | Inside–parallel | Service/Financial Manager | ||
Parallel-3 | GPED09 EDDR01 | Inside–parallel | Emergency Department Doctor | 18 minutes | Individual interview |
Parallel-3 | GPED09 GP01 | Inside–parallel | General Practitioner | 22 minutes | Individual interview |
Parallel-3 | GPED09 GP03 | Inside–parallel | General Practitioner | 22 minutes | Individual interview |
Parallel-3 | GPED09 CD1 | Inside–parallel | Clinical Director | 22 and 11 minutes | Individual interview |
Parallel-3 | GPED09 FM01 | Inside–parallel | Finance Manager | 24 minutes | Individual interview |
Parallel-3 | GPED09 FM02 | Inside–parallel | Finance Manager | Unknown | Individual interview |
Parallel-4 | GPED07 SRN01 | Inside–parallel | Senior Nurse | 32 minutes | Individual interview |
Parallel-4 | GPED07 MN01 | Inside–parallel | Matron | 46 minutes | Individual interview |
Parallel-4 | GPED07 GP01 | Inside–parallel | General Practitioner | 24 minutes | Individual interview |
Parallel-4 | GPED07 EDDR01 | Inside–parallel | Emergency Department Doctor | 24 minutes | Individual interview |
Parallel-4 | GPED07 CD01 | Inside–parallel | Clinical Director | 32 minutes | Individual interview |
Parallel-4 | GPED07 FM01 | Inside–parallel | Finance Manager | 41 minutes | Group interview |
Parallel-4 | GPED07 FM02 | Inside–parallel | Finance Manager | ||
Parallel-4 | GPED07 FM03 | Inside–parallel | Finance Manager | ||
Parallel-4 | GPED07 FM04 | Inside–parallel | Finance Manager | ||
Parallel-4 | GPED07 FM05 | Inside–parallel | Finance Manager | ||
Parallel-4 | GPED07 FM06 | Inside–parallel | Finance Manager | 23 minutes | Individual interview |
Outside-1 | GPED10 EDDR01 | Outside–onsite | Emergency Department Doctor | 31 minutes | Individual interview |
Outside-1 | GPED10 GP01 | Outside–onsite | General Practitioner | 16 minutes | Individual interview |
Outside-1 | GPED10 GP02 | Outside–onsite | General Practitioner | 19 minutes | Individual interview |
Outside-1 | GPED10 GP03 | Outside–onsite | General Practitioner | 29 minutes | Individual interview |
Outside-1 | GPED10 GP04 | Outside–onsite | General Practitioner | 13 minutes | Individual interview |
Outside-1 | GPED10 CD01 | Outside–onsite | Clinical Director | 26 minutes | Individual interview |
Outside-1 | GPED10 FM01 | Outside–onsite | Finance Manager | 39 minutes | Group interview |
Outside-1 | GPED10 FM02 | Outside–onsite | Finance Manager | ||
Outside-1 | GPED10 FM03 | Outside–onsite | Finance Manager | ||
Outside-1 | GPED10 FM04 | Outside–onsite | Finance Manager | ||
Outside-2 | GPED11 GP01 | Outside–onsite | General Practitioner | 16 minutes | Individual interview |
Outside-2 | GPED11 GP03 | Outside–onsite | General Practitioner | 8 minutes | Individual interview |
Outside-2 | GPED11 GP04 | Outside–onsite | General Practitioner | 14 minutes | Individual interview |
Outside-2 | GPED11 NP01 | Outside–onsite | Nurse Practitioner | 15 minutes | Individual interview |
Outside-2 | GPED11 FM01 | Outside–onsite | Finance Manager | 42 minutes | Group interview |
Outside-2 | GPED11 FM02 | Outside–onsite | Finance Manager | ||
Outside-2 | GPED11 FM03 | Outside–onsite | Finance Manager | ||
Outside-3 | GPED13 GP02 | Outside–onsite | General Practitioner | 17 minutes | Individual interview |
Outside-3 | GPED13 ANP01 | Outside–onsite | Advanced Nurse Practitioner | 19 minutes | Individual interview |
Outside-3 | GPED13 OM01 | Outside–onsite | Operations Manager | 13 minutes | Individual interview |
Outside-3 | GPED13 FM01 | Outside–onsite | Finance Manager | 41 minutes | Group interview |
Outside-3 | GPED13 FM02 | Outside–onsite | Finance Manager | ||
Outside-3 | GPED13 FM03 | Outside–onsite | Finance Manager | ||
Control-1 | GPED12 FM01 | Control | Finance Manager | 37 minutes | Group interview |
Control-1 | GPED12 FM02 | Control | Finance Manager | ||
Control-2 | GPED15 CD01 | Control | Clinical Director | Unknown | Individual interview |
Control-2 | GPED02 CD01 | Control | Clinical Director | 51 and 34 minutes | Individual interview |
Control-2 | GPED02 GP01 | Control | General Practitioner | 21 minutes | Individual interview |
Control-2 | GPED02 PCCD | Control | Primary Care Clinical Director | 14 minutes | Individual interview |
CD interview site 1 | GPED01 CD01 | Non-case study site | Clinical Director | 30 minutes | Individual interview |
CD interview site 5 | GPED05 CD01 | Non-case study site | Clinical Director | 28 minutes | Individual interview |
CD interview site 16 | GPED16 CD01 | Non-case study site | Clinical Director | 41 minutes | Individual interview |
CD interview site 17 | GPED17 CD01 | Non-case study site | Clinical Director | 34 minutes | Individual interview |
CD interview site 18 | GPED18 CD01 | Non-case study site | Clinical Director | 28 minutes | Individual interview |
CD interview site 19 | GPED19 CD01 | Non-case study site | Clinical Director | 24 minutes | Individual interview |
CD interview site 20 | GPED20 CD01 | Non-case study site | Clinical Director | 38 minutes | Individual interview |
CD interview site 21 | GPED21 CD01 | Non-case study site | Clinical Director | 31 minutes | Individual interview |
Model | Number of GP interviews | GP gender | GP experiencea | GPs with a current community role |
---|---|---|---|---|
Inside–integrated (II) |
9 | 4 male 5 female |
Experienced including 2 × GP leads (n = 8) Newly qualified (n = 1) |
1 |
Inside–parallel (IP) |
9 | 6 male 3 female |
Experienced including 1 × GP lead (n = 7) Newly qualified (n = 2) |
7 |
Outside–onsite (OO) |
8 | 6 male 2 female |
Experienced including 1 × GP lead (n = 8) | 6 |
Appendix 3 Details of patients interviewed
Site | Condition | Seen by | Gender | Duration of interview |
---|---|---|---|---|
Integrated-1 | Back pain | GP | Female | 20 minutes |
Integrated-1 | Abdominal pain | GP | Male | 30 minutes |
Integrated-1 | Cough and breathlessness | ED Dr | Female | 16 minutes |
Integrated-1 | Cough and breathlessness | GP | Female | 20 minutes |
Integrated-3 | Abdominal pain | ED Dr | Female | 13 minutes |
Integrated-3 | Abdominal pain | ED Dr | Female | 15 minutes |
Parallel-1 | Back pain | GP | Female | 14 minutes |
Parallel-1 | Cough and breathlessness | ED Dr | Female | 11 minutes |
Parallel-1 | Child with fever | ED Dr | Female child | 17 minutes |
Parallel-1 | Child with fever | ED Dr | Male child | 14 minutes |
Parallel-2 | Back pain | ED Dr | Female | 21 minutes |
Parallel-2 | Abdominal pain | GP | Female | 19 minutes |
Parallel-2 | Cough and breathlessness | ED Dr | Male | 10 minutes |
Parallel-2 | Cough and breathlessness | GP | Male | 18 minutes |
Parallel-3 | Cough and breathlessness | GP | Female child | 20 minutes |
Outside-1 | Cough and breathlessness | GP | Male | 19 minutes |
Outside-1 | Chest pain | ED Dr | Male | 10 minutes |
Outside-2 | Back pain | GP | Female | 18 minutes |
Outside-2 | Chest pain | ED Dr | Male | 11 minutes |
Outside-3 | Cough and breathlessness | GP | Male | 22 minutes |
Outside-3 | Child with fever | GP | Female child | 14 minutes |
Control-3 | Chest pain | ED Dr | Male | 40 minutes |
CD interview site 5 (parallel) | Back pain | ED Dr | Female | 11 minutes |
CD interview site 5 (parallel) | Cough and breathlessness | GP | Male | 16 minutes |
Appendix 4 An outline of steps in realist analysis
-
A NVivo 11 (QSR International) folder was created for each GP service model (inside–integrated, inside–parallel, outside–onsite and control) which included all observation and interview data for each site.
-
The CD interviews were coded using a framework of the themes from initial rough theories and planned to be used for each service model folder. Folders were created for each service model type and analysed separately, one case site at a time, starting with the inside–integrated models then the outside–onsite models and then inside–parallel sites.
-
Data were coded using ‘if, then, because statements’. These are statements to explain the minutiae and nuances of different mechanisms in different contexts that produce outcomes, evident from the data. The statements were grouped into positive and negative outcomes under the main theory theme headings in the coding framework – for example, ‘if, then, because’ statements describing why streaming statements were perceived to be appropriate or not were grouped under the theme ‘streaming’. Data that supported an ‘if, then, because’ statement already created were coded under the same statement but due to the detail of each statement, multiple ‘if then because’ statements were generated.
-
‘If, then, because’ statements were coded into high-level themes and positive and negative outcomes, grouped with mechanisms at the individual level, the department level and the wider system level.
-
The level of evidence was classified using a hierarchy based on meta-ethnography principles.
-
Level 1: Observations
-
Level 2: How participants say they behave
-
Level 3: How participants say others behave
-
Level 4: My interpretation
-
All ‘if, then, because’ statements generated were exported into a Microsoft Excel document, one page for each model type, to develop into CMO configurations – the ‘if’ became the context, the ‘then’ became the outcome and the ‘because’ became the mechanism(s).
-
CMO configurations were consolidated using the three questions, based on the conceptual platform by Person et al.:2,155
-
Is this account novel (and can therefore be imported directly into the CMO)?
-
If the account was not novel, does this challenge the explanations made in related accounts?; or
-
Does this account add important refinements to the understanding of contexts, mechanisms or outcomes?
-
The CMOs were then synthesised using Pawson’s theory-building processes.
A master file was created to populate the evidence for theories (where available) for refined CMO development (see Table 24 for an example)
Theme | Initial CMO from pilot lit review and expert group | Refined CMO following RRR | Refined CMO following qualitative case site analysis | If–then–because statement | Quote |
---|---|---|---|---|---|
GPs at risk of under investigation | Primary care practitioners working in or alongside EDs that usually work in a community setting where there is a lower probability of serious illness (C), may incorporate this lower probability into their diagnostic reasoning (M), and may therefore be at risk of under-referral for further investigation and missing serious illness (O) | No data to refine | Primary care practitioners working in or alongside EDs that usually work in a community setting where there is a lower probability of serious illness (C), may incorporate this lower probability into their diagnostic reasoning (M), and may therefore be at risk of under-referral for further investigation and missing serious illness (O) | If sick patients are streamed to the GP in ED, then the GP may under investigate the patient and there may be a diagnostic error | ‘Well, we got it wrong, we streamed the wrong patients to them and they got treated as GP patients and they weren’t. We had one that’s actually come back across my desk this year, a litigation case of a child who had already be seen by OOH twice, and presented to us as an under 1, unwell, horrible obs, went to the GP, the GP didn’t look at the obs, looked at the child and went “they look okay”, went away, came back in and was really septic and almost died, and they’ve got brain injuries and things like that. So, we’ve got a litigation ongoing. And that was one of the main ones that we said “actually, this isn’t working for either of us,” and I think that’s probably what stopped it, that was in 2005’. CD GPED02 control |
Appendix 5 Datix reports
GP service model | Site reference | Incident reports available | Number of reports relevant to the GP service | Primary incident type | Incident free text (key information extracted with minor edits for spelling and abbreviations only) | Patient harm (WHO definitions) |
---|---|---|---|---|---|---|
Inside–integrated | Integrated-1 | 134 (3-month period December 2017 – March 2018, excluding pressure ulcers) | 3 | Triage/streaming error | ‘Patient triaged to (GP stream) and seen out of order due to lower position on computer (waited an hour longer than other patients with the same priority). Patient deteriorated and was transferred to majors’. | Moderate |
Inadequate management | ‘ED protocol not followed, child with suspected NAI (non-accidental injury) not admitted to paeds (paediatrics) and sent home for OPD (outpatient) follow up’. | Unknown | ||||
Inadequate management | ‘Patient sent to ED on advice of CAMHS (child and adolescent mental health service) as OOH (out of hours) and thought to be at risk of self-harm … GP unaware of policy should have been admitted for MH (mental health) assessment’. | Unknown | ||||
Integrated-2 | Not available on site. CD reported none involving GPs working in ED | N/A | ||||
Integrated-3 | Not available on site and no response to 3 × follow-up e-mails | N/A | ||||
Inside–parallel | Parallel-1 | 1162 reports, 430 reports excluding pressure ulcers (from 1 April 2017 to 31 March 2018) | 2 | Diagnostic error | ‘Patient seen by agency (primary care) NP (nurse practitioner). Had fallen downstairs C/O (complaining of) neck pain Diagnosis muscular injury Returned today Multiple unstable fractures of C1 and C2 (neck fractures)’. | Unknown |
Inadequate management | ‘GP was gluing the wound on the patient’s forehead the glue inadvertently dripped down into the patients right eye gluing his eyelids shut’. | Low | ||||
Parallel-2 | 365 reports in 2017 (majors 254, minors 111) | 1 | Triage/Streaming error | ‘Patient triaged to UTC. As shift lead I allocated patient to see an OOH GP. This patient was later discharged from (OOH) (adastra system) and subsequently discharged from medway (ED computer system). However, the patient in question was still in the department’. | Unknown | |
Parallel-3 | Not available on site. CD reported none involving GPs working in ED | N/A | ||||
Parallel-4 | 7 reports only since change of provider 1 month ago | 1 | Inaccurate documentation | ‘Patient admitted to PAU (paediatric assessment unit) from UTC (urgent treatment centre). Nurse handed over that patient’s DOB (date of birth) was wrong on their system which would make her 3 when she is 2’. | Unknown | |
Outside–onsite | Outside-1 | 68 complaints, 150 incidents (April 2017 to first 2 quarters 2018) | 1 | Investigation follow-up | ‘Positive MSU (mid-stream urine) reports filed without action being taken - if action needed this is now highlighted to community GP’. | Unknown |
Outside-2 | 11 WIC reports (24 February 2018–16 October 2018) | 6 | Investigation follow-up | ‘After waiting in accident and emergency department for over 2 hours, 2 patients were inappropriately referred to the WIC (walk-in centre) from A&E when the WIC opened at 8am. Both patients had blood tests performed by A&E. The WIC nurse practitioners and locum GP are unable to, and not here to review A&E investigations … Having requested these investigations to not have them reviewed poses potential risk to patient safety’. | Unknown | |
Triage/streaming error | ‘One hour after triage the patient was transferred to WIC on symphony, but the patient claims she was not directed to go to the WIC by any one from A&E. The WIC nurses discharged patient as called no reply as patient was not in walk in centre. After waiting 5 hours the patient asked A&E reception and she was directed to the WIC’. | Unknown | ||||
Referral delay | ‘Patient seen at WIC? torsion of testicle requiring urgent Urology review. Unable to contact Urology core-trainee, middle or consultant through Vocare, just keep getting put through to switch who say nothing they can do to contact anyone from Urology’. | Unknown | ||||
Prescribing error | ‘Locum doctor prescribed Mirtazapine for a patient with depression. On the prescription he did not specify the quantity’. | Low | ||||
Prescribing error | ‘Patient returned today with handwritten prescription. Patient said pharmacist said prescription was not legible so advised patient to return to the walk-in centre to have prescription re written. Clinical notes checked and patient re-examined, and further prescription was issued’. | Low | ||||
Prescribing error | ‘A patient was given a handwritten FP10 prescription for a community pharmacy with the wrong patient details’. | Unknown | ||||
Outside-3 | Not available on site and no response to 3 × follow-up e-mails | N/A |
List of abbreviations
- ACF
- autocorrelation function
- ACP
- advanced clinical practitioner
- ACSC
- ambulatory care sensitive condition
- ANP
- advanced nurse practitioner
- CCA
- cost–consequences analysis
- CCG
- clinical commissioning group
- CD
- clinical director
- CMOC
- context–mechanism–outcome configuration
- COPD
- obstructive pulmonary disease
- CQC
- Care Quality Commission
- ECDS
- emergency care data set
- ED
- emergency department
- EDD
- emergency department disposition
- EDDS
- emergency department data set
- GP
- general practitioner
- HES
- hospital episode statistics
- HES AE
- hospital episode statistics accident and emergency
- HES APC
- hospital episode statistics admitted patient care
- HRG
- Healthcare Resource Group
- LOS
- length of stay
- NIHR
- National Institute of Health and Care Research
- NRLS
- National Reporting and Learning System
- OLS
- ordinary least squares
- OOH
- out-of-hours (GP service)
- PEDW
- patient episode database for Wales
- RRR
- rapid realist review
- SAIL
- secure anonymised information linkage
- UEC
- urgent and emergency care
Notes
Supplementary material can be found on the NIHR Journals Library report page (https://doi.org/10.3310/JWQZ5348).
Supplementary material has been provided by the authors to support the report and any files provided at submission will have been seen by peer reviewers, but not extensively reviewed. Any supplementary material provided at a later stage in the process may not have been peer reviewed.