Notes
Article history
The research reported in this issue of the journal was funded by the HSDR programme or one of its preceding programmes as project number 16/04/06. The contractual start date was in October 2017. The final report began editorial review in January 2020 and was accepted for publication in November 2021. The authors have been wholly responsible for all data collection, analysis and interpretation, and for writing up their work. The HSDR editors and production house have tried to ensure the accuracy of the authors’ report and would like to thank the reviewers for their constructive comments on the final report document. However, they do not accept liability for damages or losses arising from material published in this report.
Disclaimer
This report contains transcripts of interviews conducted in the course of the research and contains language that may offend some readers.
Permissions
Copyright statement
Copyright © 2022 Randell et al. This work was produced by Randell et al. under the terms of a commissioning contract issued by the Secretary of State for Health and Social Care. This is an Open Access publication distributed under the terms of the Creative Commons Attribution CC BY 4.0 licence, which permits unrestricted use, distribution, reproduction and adaption in any medium and for any purpose provided that it is properly attributed. See: https://creativecommons.org/licenses/by/4.0/. For attribution the title, original author(s), the publication source – NIHR Journals Library, and the DOI of the publication must be cited.
2022 Randell et al.
Chapter 1 Introduction
Overview
This study involved the design and evaluation of a quality dashboard (QualDash) for exploring national clinical audit (NCA) data. This chapter provides the background to the study, describing what NCAs are and explaining what is meant by a quality dashboard. We then define the research aim and objectives. We conclude the chapter by outlining the structure of the remainder of the report.
Background
National clinical audits
In the UK, the Healthcare Quality Improvement Partnership (HQIP) centrally develops and manages a programme of NCAs each year through the National Clinical Audit and Patient Outcomes Programme (NCAPOP). The number of NCAs included within the NCAPOP varies year to year. At the time of writing (November 2020), there were 61 NCAs included in the NCAPOP. The NHS standard contract requires all health-care organisations that provide NHS services to participate in the NCAPOP NCAs and gives commissioners the power to impose penalties on NHS trusts that fail to participate. 1 Since 2012, trusts have been responsible for funding a proportion of NCAPOP costs through subscription funding, whereby trusts pay an annual amount to HQIP (and this amount has remained at £10,000 since 2016). In addition, there are 82 independent NCAs, not part of the NCAPOP, funded either through subscription, by a charity or professional body or by NHS England (London, UK). Each year, the NHS England Quality Accounts list advises trusts which NCAs they should prioritise for participation.
Each NCA focuses on a particular clinical area or condition and aims to produce a publicly available report annually. The objective of NCAs is to systematically measure the quality of care delivered by clinical teams and health-care organisations and to stimulate quality improvement (QI). 2 Although there is evidence of positive impacts of NCAs,3–5 and previous research suggests that clinicians consider NCA annual reports important for identifying QI opportunities,6 there is variation within and between trusts in the extent to which they engage with NCA data. 6,7 A number of NCAs provide trusts with online access to more recent data and allow users to download data for local analysis. For example, the National Hip Fracture Database provides live data on how hospitals are meeting key performance indicators, plus interactive online charts, dashboards and benchmarking summaries. However, with a recognised shortage of data analysis skills within the NHS,8,9 the use of NCA data poses challenges for some trusts. 7 Consequently, NCA data are substantially underutilised and the potential for NCA data to inform QI is not being realised fully.
Dashboards
Dashboards use data visualisation techniques to provide information to individuals, services or organisations in either a paper or a computer-based format. 10 Use of such techniques is thought to improve data comprehension11 and reduce cognitive load,12 resulting in more efficient and effective decision-making. 13 A distinction can be made between clinical dashboards and quality dashboards. Clinical dashboards may provide data at the level of the patient, the clinician (showing all patients they are caring for and comparing them with their peers and national benchmarks) or allow the user to move between viewing information at both of these levels,14 with the aim of informing decisions about, and thereby improving, patient care. 15 By contrast, quality dashboards show performance at the ward or organisational level (and ideally will provide feedback to be used at both levels16,17) to inform operational decision-making and QI efforts. 18 Visualisations provided by quality dashboards can inform QI by supporting the identification of previously unnoticed patterns in the data. 19
Health-care providers, in the UK and internationally, are increasingly using dashboards to measure care quality and as the basis for QI initiatives. Lord Darzi’s next-stage review20 and the health informatics review,21 which were both published in 2008, recommended greater use of dashboards by NHS organisations. Two distinct strands of thinking permeated these reviews and subsequent policies and guidance on dashboards. One strand of thinking emphasised the need for summary real-time information for use by clinicians in their clinical work. The former NHS Connecting for Health agency encouraged these developments through its clinical dashboard project. 22–24 This was followed by an emphasis on the need for integrated real-time information on care quality, which could be interrogated by clinical teams for the purpose of learning. 25 The other strand of thinking focused on the need for trust boards and regulators to have summary performance information. NHS foundation trusts already published performance dashboards, required by Monitor, but otherwise trusts did not use dashboards for their own internal reporting, or to report to the Care Quality Commission (CQC) or (as they were then) primary care trusts. Developments in this area were prompted by two major reports in 2013, that is, the second Francis report on substandard care provided at Mid Staffordshire NHS Foundation Trust26 and the post-Francis Keogh review of 14 NHS trusts with persistently high mortality rates. 8
An empirical study of quality dashboard use in acute trusts in England was published in 2018. 16 A survey of 15 trusts found that most trusts had some form of quality dashboard in place for reporting measures, such as those included in the NHS Safety Thermometer. 27 However, there was significant variation in dashboard sophistication, the term being used to refer both to information technology (IT) systems and to the outputs from these IT systems, typically in the form of printed reports. The majority of dashboards still depended on resource-intensive manual collation of information from a number of systems by central performance management teams, with retrospective reports then being circulated to wards, directorates and trust boards. However, the study also revealed a clear ‘direction of travel’,16 with a desire for real-time quality dashboards.
Despite the enthusiasm for quality dashboards, most empirical literature has focused on clinical dashboards, with the consequence that little is known about how and in what contexts computer-based quality dashboards drive improvement. A review of studies of the use of computer-based quality and clinical dashboards published between 1996 and 2012 included 11 studies that evaluated their impact on care quality. 10 Only one dashboard met the definition of a quality dashboard,28 with the other 10 dashboards being clinical dashboards. Chapter 3 provides an update to this review, focusing on quality dashboards.
Aim and objectives
The study aim was to develop and evaluate QualDash, an interactive web-based quality dashboard that supports clinical teams, quality subcommittees and NHS trust boards to better understand and make use of NCA data, thereby leading to improved care quality and clinical outcomes. The study had the following research objectives:
-
to develop a programme theory that explains how and in what contexts use of QualDash will lead to improvements in care quality
-
to use the programme theory to co-design QualDash
-
to use the programme theory to co-design an adoption strategy for QualDash
-
to understand how and in what contexts QualDash leads to improvements in care quality
-
to assess the feasibility of conducting a cluster randomised controlled trial (RCT) of QualDash.
Structure of the remainder of the report
Chapter 2 provides the details of the study design and research methods. (Note that details of the study management, including public and patient involvement, are provided in Appendix 1.) Chapter 3 provides an update to the review of computerised dashboards described above, focusing on quality dashboards, while also presenting a summary of substantive theories relevant to understanding how and in what contexts quality dashboards lead to improvements in care quality. Chapter 4 presents our biography of NCAs and the resulting programme theory of how and in what contexts NCA feedback influences QI, drawing primarily on interviews across five NHS trusts, in which we sought to understand what supports and constrains NCA data use. Chapter 5 addresses the first and second objectives. Chapter 5 begins our biography of QualDash, describing functional requirements elicited from the interviews, how these were translated into the design of QualDash through a co-design process with staff from one NHS trust, and the associated programme theory of how and in what contexts QualDash could lead to improvements in care quality. Chapter 6 addresses the third objective. Chapter 6 continues the QualDash biography, describing strategies for supporting adoption, developed with staff from five NHS trusts, additions to the QualDash programme theory resulting from this and the process of undertaking some of these activities prior to installation of QualDash. Chapters 7 and 8 address the fourth objective, reporting the introduction and use of QualDash within the five trusts, the subsequent impacts and the resulting revisions to the QualDash programme theory. Chapter 9 describes new requirements for QualDash that emerged in response to the COVID-19 pandemic. Chapter 10 concludes the report by reflecting on the implications of the study findings and outlining future research priorities.
Chapter 2 Study design and methods
Overview
This chapter begins by describing the two approaches used (i.e. realist evaluation and the biography of artefacts). We then summarise deviations to the protocol and reasons for these deviations. The remainder of the chapter is organised according to the study phases (Figure 1).
FIGURE 1.
Overview of study phases.
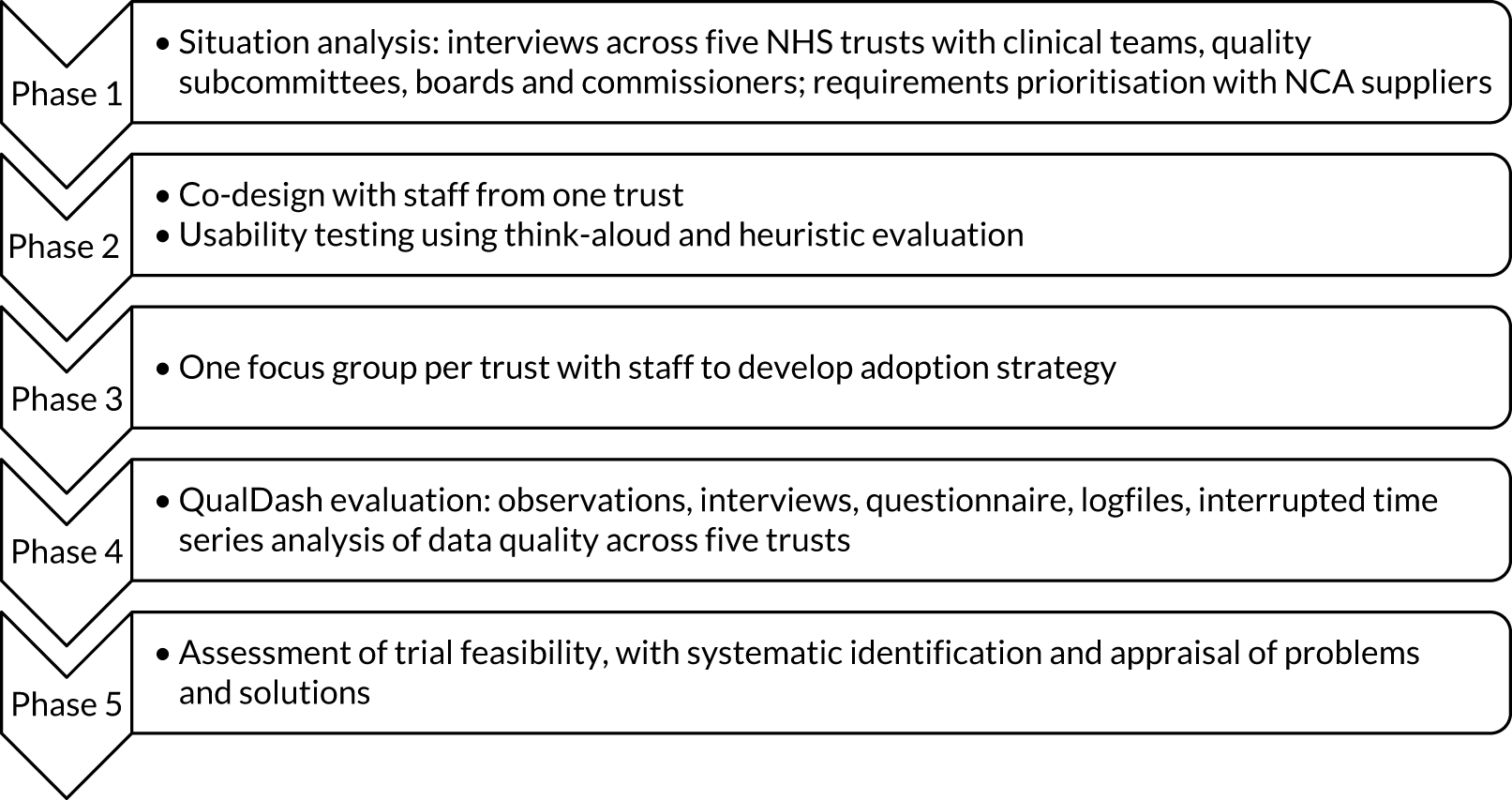
Realist evaluation
The Medical Research Council guidance for the design and evaluation of complex interventions emphasises that new interventions should have a coherent theoretical basis, with theory being used systematically in their development. 29 In addition, in the field of audit and feedback,25,30,31 and QI more generally,32–34 the need for theory-informed research is well recognised. Therefore, we used realist evaluation35 as a framework for data collection and analysis, an approach that has been recommended for studying QI. 36
Realist evaluation involves building, testing and refining programme theories composed of one or more context–mechanism–outcome (CMO) configurations. These CMO configurations explain how and why an intervention is supposed to work, for whom and in what circumstances. This is because interventions in and of themselves are not seen as determining outcomes. Rather, interventions are considered to offer resources to recipients and outcomes depend on how those recipients make use (or not) of those resources, which will vary according to context. Although theories may sometimes be considered to be abstract, irrelevant and separate from the everyday experience of practitioners, the term can also be used to refer to practitioners’ ideas about how an intervention works,33 and this is how the term is used in realist evaluation. 33,35
Although realist evaluation has been used for studying the introduction and impact of a number of complex interventions in health care,37,38 including large-scale QI programmes,39 there is growing acknowledgement of the value of realist approaches for design. 40–42 In this study, we have used co-design to develop QualDash, as the principles of realist evaluation and co-design have been demonstrated to be complementary. 40
Biography of artefacts
The biography of artefacts approach43 is located within the tradition of science and technology studies. The biography of artefacts approach is concerned with capturing how particular contexts and appropriations of a technology lead to different processes and generate different outcomes, analogous to realist evaluation’s concern with contexts, mechanisms and outcomes. 44 It is an approach that has been used successfully in a number of studies of health information technology (HIT)45,46 and one that we found to be useful in a previous study of dashboards. 16 The biography of artefacts approach involves longitudinal ‘strategic ethnography’43 where data collection is guided by a provisional understanding of the moments and locales in which a technology and associated practices evolve. 44 Although, initially, we intended to use the biography of artefacts approach only for the evaluation of QualDash, it influenced our thinking throughout the study because it enabled us to capture the challenges experienced by both the research team and the intended QualDash users. This helped us to understand and explain why the study unfolded and evolved as it did, with users’ early experiences with QualDash being important contextual factors that shaped subsequent responses. We begin the presentation of our findings through a biography of NCAs before moving on to present the biography of the design, installation, adoption and use of QualDash.
Protocol deviations
This study was completed during the period of the COVID-19 pandemic, which meant that we were unable to undertake all of the activities as outlined in the study protocol. These changes are described below; however, for transparency, we summarise these changes here.
Phase 4: multisite case study
In March 2020, installation of QualDash v2.1 was in progress, with the intention that observations would resume once it had been installed in all sites. However, at this time, all on-site research in hospitals was suspended in response to the COVID-19 pandemic and, therefore, installation of QualDash v2.1 and observations were discontinued. This meant that 148.5 hours of observations were undertaken, rather than the planned 384 hours (see Sampling for further details). It also meant that we were unable to undertake teacher–learner cycle interviews with staff (see Data collection). We notified the National Institute for Health and Care Research (NIHR) of these challenges in our progress report submitted in March 2020.
Phase 4: interrupted time series study
We had intended to consider a range of measures (see Analysis). However, by January 2020, limited QualDash use within our study sites meant that QualDash use could not be hypothesised to have had an impact on these measures. Therefore, we agreed with our Study Steering Committee (SSC) in January 2020 that, if use did not increase, it was not appropriate to use those measures, instead focusing on impact on data quality, for which there was some qualitative evidence. We had planned to undertake further adoption activities following installation of QualDash v2.1, but we were unable to do so because on-site research in hospitals was suspended. Consequently, use did not increase and analysis considered measures of data quality. We notified NIHR of this change in our progress report submitted in March 2020. An additional change was that we had intended to undertake a controlled interrupted time series (ITS) study. By the time that data were to be received for analysis, only three sites had issued research and development (R&D) approval, with delays as a result of prioritising COVID-19 studies and restarting studies that had stopped because of the COVID-19 pandemic. Of the three control sites that had issued R&D approval, only one submitted data, with the failure of the other sites to do so presumably because of service pressures at that time. Given that data for only one control site were received and the matching was not relevant to the measures used in the final analysis, control data were not used, with time providing the control instead (see Sampling). Service pressures meant that site D did not submit data and, therefore, analysis was limited to sites A, B, C and E. We notified NIHR of potential challenges of obtaining data during the COVID-19 pandemic in our progress report submitted in March 2020.
Phase 5: assessment of wider applicability
Part of our plan for phase 5 of the study had been to assess the extent to which QualDash is suitable for different NCAs and the extent to which QualDash meets the needs of commissioners, through three focus groups. However, given the international focus on COVID-19 at the time, and a request from a region-wide Gold Command, we sought, instead, to understand the requirements of such a body (which included commissioners) and how QualDash could be revised to support their work, using interviews for this purpose (see Assessing the wider applicability of QualDash). At this time, we also received a request from one of our sites for us to adapt QualDash to monitor cardiology activity during COVID-19, which we did (see Chapter 9, Adapting QualDash to support daily monitoring). We notified NIHR of this additional work in our progress report submitted in March 2020, which was positively received.
Phase 1: situation analysis
The aim of phase 1 was to undertake a situation analysis (i.e. to identify the nature and extent of the opportunities and problems to be addressed by an intervention and the context within which it will operate). 47 Interviews were used to identify critical elements of context that support and constrain mechanisms underpinning NCA data use for QI, enabling us to establish requirements for the design and introduction of QualDash, while also developing an understanding of the contextual factors that may support or hinder its use. 40 A workshop with suppliers of both NCAPOP and independent NCAs and representatives of HQIP was carried out to prioritise and assess the generalisability of the identified requirements.
Interview study
Settings and participants
Throughout the study, we focused on two NCAs: the Myocardial Ischaemia National Audit Project (MINAP) and the Paediatric Intensive Care Audit Network (PICANet). The decision to work with two audits was motivated by the desire to ensure that the study would generate findings generalisable beyond a single NCA. Both MINAP and PICANet are part of the NCAPOP; however, they are delivered by different suppliers and involve different clinical specialties working with different patient groups and multiple professional groups (e.g. medical and nursing), and include multiple types of metrics (e.g. structure, process and outcome). MINAP and PICANet also differ in data quality and completeness. Further information about these audits is provided in Chapter 4. To increase the generalisability of our findings, in phase 1, we also gathered data on independent audits that have different funding arrangements [i.e. the National Audit of Cardiac Rehabilitation, which is funded by the British Heart Foundation (London, UK), and the Elective Surgery National Patient Reported Outcome Measures Programme, which is funded by NHS England]. There are a number of NCAs for which participation is at individual clinician, rather than trust, level. To understand the impact of this difference, in phase 1, we also gathered data about British Cardiovascular Intervention Society (BCIS) (Lutterworth, UK) and British Association of Urological Surgeons (London, UK) audits.
Throughout the study, we worked with three NHS acute trusts (i.e. sites A–C), which participate in both MINAP and PICANet. These trusts were identified at the time of preparing the study proposal and were selected to ensure variation in key outcome measures [MINAP: 30-day mortality for ST-elevation myocardial infarction (STEMI) patients; PICANet: risk-adjusted standardised mortality ratio (SMR)], using data reported in the most recently published MINAP and PICANet reports (i.e. the MINAP 2014 report, using data from April 2011 to March 2014,48 and the PICANet 2015 annual report, using data from 201449). Given that trusts that participate in PICANet tend to be larger and to be teaching hospitals, they are not representative of the range of trusts that participate in MINAP. Therefore, MINAP use was also studied in two district general hospitals (DGHs) (i.e. sites D and E) without a paediatric intensive care unit (PICU). These were selected to ensure variation in the same key MINAP measure. To further ensure generalisability of our findings, we selected trusts with and without foundation status. Those trusts with PICUs also varied in the number of PICU patients treated per year. We selected one trust that was included in the Keogh8 review of 14 trusts with persistently high mortality.
To identify interviewees, a combination of purposive and snowball sampling was used. In each site, the clinical contact for the study (typically a MINAP or PICANet lead) was interviewed first. These contacts were then asked to identify others who were involved with NCAs, enabling us to map the networks through which NCA data were captured, accessed and analysed. We interviewed participants from a range of professional groups and with a variety of roles. Fifty-four interviews were conducted between November 2017 and June 2018. The number of interviews per site is provided in Table 1.
Role | Teaching hospital site | DGH site | Total | |||
---|---|---|---|---|---|---|
A | B | C | D | E | ||
Doctor | 5 | 2 | 2 | 4 | 1 | 14 |
Nurse | 1 | 2 | 7 | 2 | 2 | 14 |
Audit support staff | 1 | 1 | 2 | 0 | 0 | 4 |
Trust board and committee member | 2 | 1 | 0 | 1 | 0 | 4 |
Quality and safety staff | 2 | 1 | 2 | 1 | 1 | 7 |
Information staff | 3 | 1 | 1 | 0 | 1 | 6 |
Other | 3 | 1 | 0 | 0 | 1 | 5 |
Total | 17 | 9 | 14 | 8 | 6 | 54 |
Data collection
Realist ‘theory gleaning’ interviews were undertaken, whereby interviewees are asked to articulate how their contextual circumstances influence their behaviour. 50 The research team developed a semistructured interview topic guide. Questions sought to understand NCA data use in the broader context of processes for monitoring and improving care quality, how and in what contexts NCA data were used for QI, and how and in what contexts interviewees considered a quality dashboard could provide benefit. The interview topic guide was reviewed by the study Lay Advisory Group (LAG) and revised based on their feedback to ensure that the interviews explored topics that matter to patients (see Report Supplementary Material 1 for the interview topic guide). Interviews were audio-recorded and transcribed verbatim. Interviews ranged from 33 minutes to 1 hour 29 minutes, with an average (mean) duration of 54 minutes.
Analysis
Interview transcripts were checked for accuracy against the audio-recordings and anonymised. Framework analysis was used to analyse the transcripts. 51 Informed by the phase 1 objectives (to identify requirements for QualDash and construct programme theories) and the interview topic guide, and through reading preliminary interview transcripts, codes for data indexing were identified and agreed by four members of the research team (NA, LM, RR and ME). Themes and subthemes were incorporated into the framework that reflected realist concepts of context (operationalised as characteristics of staff, culture or infrastructure that appeared to support or constrain NCA data use), mechanism (operationalised as the ways in which people responded to NCA data and why) and outcome (operationalised as the impact of these responses, with a focus on service changes intended to improve performance). Five transcripts were then indexed in NVivo 11 (QSR International, Warrington, UK) to test the applicability of the codes and assess agreement. Where there was variation in indexing, codes were refined and definitions clarified. Refined codes were applied to all transcripts.
To construct CMOs, we explored the coded data to identify instances where NCA data were reported to have been used. Of primary interest was where use of NCA data had stimulated QI in the form of practice changes. However, we also sought to capture other end points, for example where it was used for assurance (i.e. confirming performance complies with certain standards). The data were then interrogated to understand how and why NCA data were used in these ways and what supported or constrained the use of NCA data across sites.
To elicit QualDash requirements, study team members (ME, RAR, NA, LM and RR) discussed and grouped data indexed under ‘quality dashboard’, which captured what participants reported they would want from a quality dashboard and what they thought would support its introduction and use. In addition, Mai Elshehaly analysed the interview transcripts to generate an initial list of tasks that users of QualDash might want to undertake based on questions they described asking of NCA data. The resulting requirements were brought together into a software requirements specification (SRS).
Workshop with national clinical audit suppliers
Participants
A purposive sample of participants was recruited, comprising suppliers representing NCAPOP and independent NCAs, including audits where participation is at the individual clinician level, and representatives of HQIP. At the time of organising the workshop, NCAPOP and independent NCAs were delivered by 37 suppliers, with some responsible for up to five audits. With HQIP’s permission, we used information about NCA suppliers listed on the HQIP website to contact potential participants, sending them an invitation by e-mail to register for the workshop. Where a supplier delivered more than one NCA, we approached the national clinical lead for each NCA. We also advertised the workshop in the HQIP newsletter. Twenty-nine participants registered and 21 attended on the day, with representation from 19 NCAs and HQIP (see Appendix 2 for a list of participating audits).
Data collection
Ahead of the workshop, participants were sent the requirements. During the workshop, participants worked in groups comprising three to five participants, facilitated by members of the QualDash team (NA, LM, ME, RAR and RR). All activities were audio-recorded.
To identify which requirements were generalisable across NCAs, a variation of the nominal group technique (NGT) was used based on methods used by Rebecca Randell for a similar purpose,52 and involving card-sorting activities recommended for co-design. 53 NGT is a highly structured group process that can be used for identifying requirements and establishing priorities. NGT enables a substantial amount of work to be achieved in a relatively short space of time, providing immediate results with no requirement for further work. 54 The technique has previously been used successfully in the development of other complex interventions. 55 The first activity focused on prioritising functional requirements, which were grouped into categories of visualisation, interaction, data quality, reporting and notification. Participants had 2 hours to complete this activity. To make efficient use of time, interaction requirements were considered jointly with visualisation requirements, and reporting requirements with notification requirements (data quality was considered individually because phase 1 interviews had highlighted its significance and complexity). Groups varied the order in which they considered the categories of requirements to ensure that information was gathered about all of them (in case any group was unable to complete the task for all categories).
The first step was ‘silent generation of ideas’ (a concept used in NGT). This step involved each participant reviewing a set of cards describing the functional requirements and selecting those that they considered to be essential. Participants were also asked to write down on blank cards any additional essential functional requirements that they could think of. Facilitators introduced the task with the question ‘If QualDash were available to the users of your audit, what does it need to be able to do in relation to [interaction and visualisation; reporting and notifications; data quality] to enable/encourage users to make better use of audit data for QI?’. Then, the group merged their requirements, creating an ‘agreed’ and ‘not agreed’ list on a flip chart. The ‘agreed’ list comprised functional requirements that were considered to be essential by all group members and the ‘not agreed’ list comprised requirements that were considered to be essential by some (or none) of the members. A weighting was given to each ‘not agreed’ item based on how many people (if any) considered this requirement to be essential. The lists also included any additional requirements identified by participants. Finally, participants were asked to select individually, and rank in order of priority, the three functional requirements that they considered to be the most important. To allow participants to select the most important requirements for their audits, they could select from both the ‘agreed’ and the ‘not agreed’ lists. Participants were then invited to feed back to the group their highest priority requirements and explain why this was so, in the context of their specific audits, for discussion in the group as a whole. This was repeated for each set of requirements.
The second activity sought to build context around the tasks identified from the interviews and map them to a taxonomic classification to ensure coverage of the space of possible tasks and, subsequently, the space of possible visualisation design alternatives. We designed a scenario-generation activity in which each group of participants was presented chronologically with three task taxonomic dimensions (i.e. task granularity, data type cardinality and task target) in the form of an example scenario. 56 To account for different QualDash contexts of use, two of the groups were assigned an exploratory analysis scenario, two groups were assigned a confirmatory analysis scenario and one group was given an information presentation scenario. These three types of scenario were inspired from the levels of the task ‘goal’ dimension of the task space defined by Schultz et al. 57 In each group, participants were given an activity sheet that described the example scenario in a stepwise fashion and asked them to write down similar details relevant to their audit(s). After developing their own individual scenarios, the group discussed them. Whatever the focus of the worksheet (e.g. explorer, confirmer or presenter), the worksheets asked participants to record:
-
important metrics for their audits
-
the granularity [i.e. level of detail for patient data (whether at individual patient, organisation, regional or global – for the entire audit – level) and time data (e.g. daily, weekly, monthly or annual)] needed to explore, confirm or present the data effectively
-
the type cardinality, which defines the most useful types of information (e.g. specific values, trends over time or frequency) needed to explore, confirm or present the data effectively
-
the number and combination of variables required (e.g. single, two, three or more).
Finally, the target information required in each scenario was elicited by presenting alternative possibilities (e.g. trends over time, distribution and association) and leading a discussion with participants to select the targets that they thought were the most critical.
In the third activity, for which 1.5 hours was allocated, adoption requirements were prioritised. Requirements were grouped into categories of awareness, training, monitoring and support, and access. Activity 3 followed largely the same format as activity 1, moving from silent generation of ideas (based this time on the question ‘If QualDash were available to the users of your audit, what would need to happen to enable/encourage users to use it for QI?’) to merging and ranking requirements. As the task involved reviewing fewer categories than in activity 1, more time was available at each step, giving participants the opportunity to rank the 10 – rather than three – requirements they considered most important.
Analysis
The lists created by the groups for activities 1 and 3 were analysed quantitatively to identify the functional and adoption requirements that were considered to be essential by all groups. Next, the final ranking of priorities was combined to produce a list of functional requirements and a list of adoption requirements ordered by priority.
Participants’ responses to the visualisation worksheets for activity 2 were used to identify tasks QualDash users may want to undertake using NCA data and these were added to the tasks identified through analysis of the interview data. The tasks were analysed in terms of the structural characteristics of granularity, type cardinality and target. This allowed a characterisation of a subset of the task space that covered the most relevant combinations of granularity, type cardinality and target information levels included. This characterisation was used to inform a task sampling process to select a representative subset of tasks, which was taken forward to the co-design activities in phase 2.
To capture the richness of participants’ observations and to provide context to the ranked priorities, we had intended to transcribe the audio-recordings of the sessions and analyse them using framework analysis. However, we decided not to, given the poor quality of the recordings, owing to overall noise levels in the room and because we obtained clear results from the quantitative analysis.
Phase 2a: design of QualDash
A co-design approach was used for design of QualDash, following Bate and Robert’s53 four-stage model for experience-based co-design: (1) reflection, analysis, diagnosis and description; (2) imagination and visualisation; (3) modelling, planning and prototyping; and (4) action and implementation. Phase 1 represented the reflection, analysis, diagnosis and description co-design stage. Phase 2 represented the imagination and visualisation and the modelling, planning and prototyping co-design stages. QualDash was co-designed with NHS staff through two co-design workshops. In addition, observation of meetings at which audit data were discussed was undertaken to provide further information to inform design. The QualDash prototype was then evaluated using heuristic evaluation and the think-aloud protocol (described in Phase 2b: usability evaluation of QualDash). Alongside the design process, team members regularly discussed the QualDash requirements and their understanding of how, for whom and in what contexts the associated features would support use of NCA data to develop the QualDash programme theory. In doing so, we drew on understandings gained from phase 1 and the phase 2 co-design workshops and observations, while Mai Elshehaly and Roy A Ruddle used their expertise in information visualisation to present their own literature-informed theories. 58,59
Co-design workshops
Settings and participants
We intentionally involved staff from one trust only (i.e. site A) in phase 2 to help us to assess, in phase 4, the extent to which the success of QualDash was dependent on this level of staff involvement in the design process. We held two co-design workshops and in both we included users of MINAP and PICANet data and representatives from other trust groups (e.g. information managers) on the basis that bringing together a diverse group would support identification of similarities and differences in the needs and preferences of different stakeholders. There were seven participants in each co-design workshop, including both clinical and non-clinical staff (Table 2). The first co-design workshop was held in September 2018 and the second in February 2019.
Role | Workshop 1 | Workshop 2 |
---|---|---|
Doctor | 2 (paediatricians) | 1 (paediatrician) |
Nurse | 1 (cardiology nurse specialist) | 1 (cardiology nurse specialist) |
Audit co-ordinator | 1 (PICANet) | 1 (PICANet) |
Information manager | 2 | 1 |
Clinical outcomes and information manager | 1 | 1 |
Clinical effectiveness and compliance manager | 0 | 2 |
Total | 7 | 7 |
Data collection
Each co-design workshop lasted 3 hours. In the first workshop, we divided participants into two groups, depending on which audit they were the most familiar with, and assigned each group a task set corresponding to one of the audits. Two study team members facilitated the discussion with each group over the course of two co-design activities.
The first activity was a ‘story generation’ activity that was inspired by approaches used in information visualisation design. 59 We asked participants to answer questions about why tasks are important, how tasks are currently performed, what information needs to be available to perform a task (i.e. input) and what information might arise after completing it (i.e. output). To understand the impact of context, we also asked who can benefit most from a task. To facilitate discussion around these four questions, co-design participants were presented with a set of ‘task cards’. Each card focused on a single task and was subdivided into four main sections. Three sections were designed to elicit information about how this task is performed in current practice: (1) the data elements used, (2) the time it takes and (3) any textual information that might be involved. The majority of space on the card was dedicated to the fourth section, labelled ‘sketches’. This section provided space for participants to sketch out the processes involved in performing the task and visualisations (if any) used in the same context. The header of a card contained an empty box for participants to assign a relevance score. Participants were presented with a set of task cards corresponding to the subset of tasks developed in phase 1. We also gave each participant a set of blank cards. Participants were given the freedom to select task cards that they deemed relevant to their current practice or to write their own on a blank card. For each task, participants were asked to solve the what and how questions individually on paper while thinking about the why and who questions for a later group discussion. During the discussion, we asked participants to justify the relevance scores that they assigned to each task and elaborate on the details that they had written on the cards. Finally, we asked them to sort the cards depending on who they believed this task was most relevant to.
The second activity was designed to identify ‘entry-point’ tasks and sequences of follow-up tasks. We returned the prioritised and grouped task cards from activity 1 to participants and asked them to (1) select the most pressing questions to be answered at a glance at a dashboard, (2) sketch the layout of a static dashboard that could provide the minimally sufficient information for answering these tasks and (3) select/add follow-up tasks that arise from these entry-point tasks.
Between the first and the second co-design workshops, the results of the first workshop were used to develop a QualDash prototype. To ensure that the prototype did not unintentionally move away from the needs of our intended users, we regularly discussed our ideas with participants from site A in a sequence of nine one-to-one meetings. Participants in these meetings were two cardiologists, a paediatrician and two members of audit support staff.
The second co-design workshop involved two activities. The first activity made use of a paper prototype to free participants from learning the software. 60 Participants were divided into three groups. Each group was handed paper prototypes for a set of metric cards. For each metric card, the paper prototype included a task believed to be of high relevance, screen printouts of metric card(s) that address the task and a list of audit measures used to generate the cards. A group facilitator led the discussion of each metric and encouraged participants to change or add more tasks, comment on the data granularity used and sketch ideas to improve the visualisations. The second activity involved interaction with the QualDash software and is described in Phase 2b: usability evaluation of QualDash.
Observation of meetings
The phase 1 interviews identified a range of meetings, at different levels of trusts, at which quality and safety are discussed and QualDash could potentially be used. The research team considered, and the SSC agreed, that it would be useful to observe these meetings to provide further insight into how data were used to inform the design of QualDash. Therefore, meeting observations were added to phase 2.
Sampling
The intention was to observe at least one meeting at the ward level, quality subcommittee level and trust board level per site. However, the phase 1 analysis and adoption focus groups suggested that QualDash was most likely to be used within clinical team and directorate meetings, rather than at quality subcommittee or trust board meetings, and, therefore, we focused observations on those. In total, seven meetings were observed between October and December 2018 (Table 3), representing 13 hours of data collection. Having observed these meetings, the research team concluded that they had sufficient information about such contexts and that there was no need for further observations.
Meeting type | Site | Total | ||||
---|---|---|---|---|---|---|
A | B | C | D | E | ||
Directorate meeting | 0 | 1 | 0 | 0 | 0 | 1 |
Clinical governance/morbidity and mortality meetings | 2 | 1 | 0 | 1 | 0 | 4 |
Business meeting | 0 | 0 | 1 | 1 | 0 | 2 |
Total | 2 | 2 | 1 | 2 | 0 | 7 |
Data collection and analysis
The researchers observed the meetings and recorded observations in fieldnotes. These notes were written up in detail as soon as possible post observation using a framework developed in collaboration with Mai Elshehaly and Roy A Ruddle (i.e. the team members responsible for software development). The framework was used to capture details that would allow us to better understand user requirements relating to, for example, which data were used in the meeting (variables and metrics), how they were visualised and for what purpose.
An iterative approach to data collection and analysis was taken. Observation notes were read closely by Natasha Alvarado and Lynn McVey (i.e. the qualitative researchers) and Mai Elshehaly, who then met to discuss the findings and identify areas in which further information was required. Summary sections in the observation notes were used to reflect on what the data added to the situation analysis, which, in turn, informed programme theory development, providing insight into motivations for data use within meeting contexts and potential supports and constraints on QualDash use.
Phase 2b: usability evaluation of QualDash
Originally, the study design included a controlled user experiment with a mixed factorial experimental design, designed to compare the visualisations offered through QualDash with how NCA data are currently visualised, on the assumption that QualDash would incorporate novel visualisation techniques. However, nearly all tasks identified in phase 1 contained only two variables, for which standard visualisations, such as bar graphs, are more appropriate, particularly as users would already be familiar with such visualisations. Therefore, it was decided that the design of QualDash would focus on providing benefit through its interactive capabilities and the ability to look at multiple visualisations, rather than through novel visualisations. Consequently, a different approach to user evaluation, which evaluated QualDash’s interactive capabilities, was needed. We decided to use two usability inspection methods: (1) the think-aloud technique and (2) heuristic evaluation. The think-aloud technique is an appropriate fit for the realist approach given that it provides access to participants’ reasoning. We anticipated identifying most usability issues through use of the think-aloud technique, to be conducted with the intended QualDash users, whereas heuristic evaluation was used as a check for any significant usability issues that may have been missed. The System Usability Scale (SUS), a quick and easy questionnaire designed to assess any technology, consisting of 10 statements scored on a five-point scale of strength of agreement, had been proposed for the controlled user experiment. 61 We retained this as a quantitative assessment of usability.
Think-aloud technique
Sample
Two rounds of the think-aloud technique were undertaken. The first round was undertaken during the second co-design workshop, whereas the second round was undertaken during one of the adoption focus groups (see Phase 3: QualDash adoption). To allow time for revisions to be made to the QualDash prototype on the basis of the first round, the second round was undertaken approximately 1 month later. This was undertaken with participants from one of the four trusts not involved in the co-design workshops. These participants were selected to ensure a different level of familiarity with, and confidence in, engaging with NCA data. Seven participants (see Table 2 for roles) took part in round 1 and five participants took part in round 2. Participants in round 2 included a PICANet audit clerk, a PICU matron, a PICU nurse, a senior cardiology information analyst and a data manager.
Data collection
Participants were asked to think aloud as they used the QualDash prototype to complete a series of tasks. Tasks were designed to enable them to explore key QualDash functionality. To have consistency in the tasks that users completed, all participants used the PICANet version of QualDash, which was populated with simulated data. For the first round, conducted at the University of Leeds, participants used QualDash concurrently and worked either alone or in pairs, with one researcher with each individual/pair. The researchers gave a short demonstration of the prototype. As an initial training exercise, the researchers asked participants to reproduce a screen that was printed on paper. This exercise was intended to familiarise participants with the dashboard and the think-aloud technique. Following this step, the researchers presented a sequence of tasks to participants and observed their interactions with the dashboard to perform the tasks. Either video- or audio-recording was used with each individual/pair to capture participants’ comments as they conducted the tasks, with the video providing additional data regarding participants’ interaction with QualDash.
For the second round, conducted at site B, QualDash was run on a laptop with Morae software (TechSmith, Okemos, MI, USA). Participants took it in turns to use QualDash, with Morae software used for recording participants’ interaction with QualDash and capturing audio, video and on-screen activity, and keyboard and mouse input. The researcher provided a short demonstration to the group as part of the adoption workshop, before asking individual participants to complete a sequence of tasks as part of the think-aloud exercise.
In both rounds, following completion of tasks on the prototype, participants were asked to complete the SUS questionnaire.
Analysis
Descriptive statistics were produced for the SUS score. We analysed recordings from all sessions and divided feedback into five categories:
-
a task-related category [capturing (mis)matches between participants’ intended task sequences and view compositions supported in the dashboard]
-
a data-related category (capturing comments relating to the variables and aggregation rules used to generate visualisations)
-
a visualisation-related category (capturing feedback on the choice of visualisation in each view)
-
a graphical user interface-related category (capturing comments on the interface usability)
-
an ‘other’ category (capturing any further comments).
From this, a prioritised list of revisions to be made to QualDash prior to installation in the five sites was generated.
Heuristic evaluation
Sample
Heuristic evaluation is a form of expert review. Following recommendations for the conduct of heuristic evaluation,62 we aimed to involve three to five experts. The selection of experts sought to ensure that their collective expertise spanned the areas of human–computer interaction, visualisation and clinical audit. Five experts agreed to participate, four of whom undertook the task and returned the documentation. Two participants had expertise in human–computer interaction and health informatics (with one participant also having medical training), one participant had expertise in human–computer interaction and visualisation, and one participant was a clinician with expertise in clinical audit.
Data collection
The experts were provided with (1) a list of tasks designed to enable them to explore the functionality of QualDash, (2) a heuristic evaluation checklist and (3) a set of heuristics for determining the potential utility of visualisations (see Report Supplementary Material 2).
The heuristic evaluation checklist was developed and validated by a member of the study team (DD) as part of previous research to develop and evaluate a dashboard. 63 The checklist consists of seven general usability principles derived from Nielsen’s usability principles,64 consisting of 40 usability heuristics, and three visualisation-specific usability principles (i.e. spatial organisation, information coding and orientation), consisting of nine usability heuristics. Although some of the heuristics within the heuristic evaluation checklist were not appropriate for QualDash, we retained them because the questionnaire had already been validated and each item allowed an answer of ‘not applicable’. The experts were instructed to give a score of 1 (yes) if the heuristic was met and a score of 0 (no) if the heuristic was not met.
The set of heuristics to assess the potential utility of the dashboard come from the visualisation literature. 65 The set is based on the visualisation value framework, which contains four components that relate to (1) time savings a visualisation provides, (2) insights and insightful questions it spurs, (3) the overall essence of the data it conveys and (4) the confidence it inspires in the data and the domain. There are 10 heuristics (two related to time, three related to insight, two related to essence and three related to confidence). Each heuristic is rated on a seven-point scale, ranging from 1 (strongly disagree) to 7 (strongly agree). The participant can also answer ‘not applicable’.
Analysis
Output from the heuristic evaluation checklist was a score for each usability principle, along with a list of usability problems identified by the experts. Scores were calculated by dividing the total number of heuristics met (points) awarded by the total number available. Where all participants selected ‘not applicable’, this heuristic was removed from the analysis. Where three of the four participants selected ‘not applicable’, we came to a decision as a team about whether or not that heuristic was applicable. If the heuristic was considered as not applicable, we reduced the total number of heuristics available for that principle by one. For all other cases where one or more participants selected ‘not applicable’, we gave a score of 1 on the basis that the participant had not identified any problems in relation to that heuristic. The higher the score (i.e. the percentage of points awarded compared with total number available), then the greater the system usability.
For the visualisation value heuristics, we calculated the average score for each of the four components.
Phase 3: QualDash adoption
The aim of phase 3 was to agree a QualDash adoption strategy with each site. Within health services research, the term implementation is used to refer to putting an intervention into practice, using strategies to support and encourage the use of that intervention in ways that will lead to the desired impact,66 and it is a key element in the Medical Research Council guidance on development and evaluation of complex interventions. 29 However, the term has variable meanings within software engineering and is sometimes used to refer to the programming involved in translating a requirements specification into a piece of software. Therefore, being a multidisciplinary team, we have used the term adoption, which is familiar to software engineers, to avoid confusion. Although an understanding of adoption requirements was developed in phase 1 interviews and prioritised with NCA suppliers in the phase 1 workshop, it is important that adoption strategies are tailored to the local context. 36 Adoption strategies are also most likely to be successful if stakeholders are involved in their design. 67
Sample
One focus group was held at each site and sought to involve intended QualDash users. Potential participants were provided with an information sheet about the focus group following their participation in either the phase 1 interviews or, for those at site A, following their participation in the co-design workshops. Potential participants were contacted by e-mail to see if they were willing to participate and, if so, to identify a suitable date. In addition, where contacts were identified, IT staff were invited to attend. Details of participants in each focus group are provided in Table 4. Focus groups were conducted between February and April 2019.
Role | Site | Total | ||||
---|---|---|---|---|---|---|
A | B | C | D | E | ||
Cardiologist | 0 | 0 | 0 | 1 | 0 | 1 |
Paediatrician | 1 | 0 | 1 | N/A | N/A | 2 |
Nurse | 1 (MINAP) | 2 (clinical/quality, matron) | 1 (cardiology) | 2 (cardiology, n = 1; radiology, n = 1) | 1 (cardiology) | 7 |
Audit clerk | 1 (PICANet) | 1 (PICANet) | 1 (MINAP) | 3 | ||
Managers | 2 (clinical effectiveness) | 1 (data) | 2 (information, n = 1; clinical effectiveness, n = 1) | 1 (patient service) | 1 (clinical audit) | 7 |
IT | 0 | 1 (information analyst) | 1 | 0 | 1 | 3 |
Total | 5 | 5 | 6 | 4 | 3 | 23 |
Data collection
Each focus group was facilitated by two researchers, who presented a study summary and provided a demonstration of QualDash. Post demonstration, the adoption strategies and associated activities that were identified and prioritised in phase 1 were presented to the group. Participants were encouraged to discuss why they thought a particular activity would work (or not), for which groups of staff within their trust and how the activity should be delivered. Each focus group was audio-recorded and researchers also made notes during the discussion. Each session was scheduled to last for 90 minutes and the discussion lasted between 41 minutes and 1 hour 9 minutes, with an average (mean) duration of 50 minutes.
Analysis
Audio-recordings were transcribed verbatim, checked for accuracy, anonymised and uploaded into NVivo for indexing. Codes used for indexing transcripts were devised by three researchers (NA, LM and RR), with the intention of categorising data related to assumptions about adoption, dashboard functionality and current/future NCA data use. Close reading of transcripts, indexing and a review of researcher notes were used to identify consistencies and variations across sites regarding participants’ theories about activities that would support adoption. For each site, the researchers summarised discussion of each strategy, including how it should be delivered and why participants felt that it might work to support QualDash uptake and use. These documents became a blueprint for adoption within each site.
Focus group analysis was also used to further inform the QualDash programme theory. The programme theory developed in phase 2 hypothesised potential QualDash impacts. In their original form, these hypotheses assumed that QualDash would be adopted successfully. The adoption focus groups analysis enabled theorisation around how and why use and uptake would be successful, which was subsequently added to the QualDash programme theory.
Phase 4: QualDash evaluation
Phase 4 was designed to test and refine the QualDash programme theory following introduction of QualDash into the five sites. Realist evaluation does not employ particular methods of data collection, but is explicitly a mixed-methods approach. 68 Outcome data were collected and analysed in an ITS study, whereas a multisite case study69 provided insight into the contexts and mechanisms that led to those outcomes, as well as providing data on intermediate outcomes, such as increased use of NCA data. Data for the multisite case study were captured through a combination of ethnographic observations, informal interviews, log files and a questionnaire. Audit and feedback interventions, and QI interventions more generally, require longitudinal evaluation to allow sufficient time for staff to implement changes and incorporate them into practice. 70–72 Similarly, evaluation of HIT should allow time for staff to integrate the technology into their practices and evolve those practices to take advantage of the functionality offered by the technology. 45 Therefore, the intention was to collect data over a 12-month period.
Multisite case study
Sampling
The intention was that in sites A–C we would undertake a minimum of 24 4-hour periods of observation per trust and in sites D and E we would undertake a minimum of 12 4-hour periods of observation per trust (the difference reflecting that data were to be collected in two clinical areas in sites A–C, but in only one clinical area in sites D and E), totalling 384 hours. The researchers were to return to each trust monthly to understand how QualDash use changed over time, but spending more time in the first few months following the introduction of QualDash, as this is when users are most likely to engage with and explore affordances of QualDash and establish new practices around it, generating information with implications for system enhancement and future adoption strategies. 44 Although observations began in June 2019, they were paused in February 2020 while revisions to QualDash were made. The intention was to have a focused period of data analysis and resume observations once a revised version of QualDash, which addressed user feedback, had been installed. QualDash v2.0 was installed in three sites, but issues were raised when demonstrating QualDash v2.0 and, therefore, further revisions were required. Unfortunately, as installation of QualDash v2.1 was in progress, all on-site research in hospitals was suspended in response to the COVID-19 pandemic and, therefore, installation of QualDash v2.1 and observations were discontinued. In total, 148.5 hours of observations was undertaken (Table 5). Although attempts were made to organise ward observations in site C’s cardiology department, this did not prove possible, but other data collection provided the opportunity to learn about the department’s approaches to MINAP and wider data processes.
Type of observation | Site | Total | ||||
---|---|---|---|---|---|---|
A | B | C | D | E | ||
QualDash installation and customisation meetings | 9 | 8 | 16 | 7 | 6.5 | 46.5 |
Ward and ‘back office’ observations, including data collection and validation | 25 | 24.5 | 17 | 2 | 13.5 | 82 |
Meeting observations and informal interviews | 7.5 | 3 | 4 | 3 | 2.5 | 20 |
Total | 41.5 | 35.5 | 37 | 12 | 22.5 | 148.5 |
The questionnaire was e-mailed to 35 participants who were known either to have used QualDash themselves or to have seen it demonstrated or used in meetings. Twenty-three participants completed the questionnaire.
Data collection
The primary method of data collection for the multisite case study was ethnographic observation. Ethnography is well suited to realist evaluation because it involves observing phenomena in context, supporting understanding of how context influences the response to an intervention. 73 An initial phase of general observation provided an opportunity for researchers to become familiar with the setting and for those in the setting to become familiar with the presence of the researchers. Following a previous study of dashboards,16 observations were undertaken in clinical areas to understand clinical teams’ working practices and to capture ‘corridor committees’ where issues of quality and safety are discussed more informally,74 as well as to record general details of the setting that may influence QualDash use, such as staffing levels and availability of computers. These initial observations were undertaken in cardiology wards, catheterisation laboratories, PICUs and paediatric high-dependency units (HDUs).
After this initial phase, observation was guided by the QualDash programme theory. In addition to observing clinical and directorate meetings at which NCA data tend to be discussed and used, observation involved shadowing staff members as they undertook particular activities, including collection and entry of NCA data to see if and how this changed over time; accessing and interrogating NCA data, whether using QualDash or another means; preparation of reports and/or presentations using NCA data, again whether using QualDash or another means. Researchers also observed meetings between Mai Elshehaly and site staff to install and test QualDash and discuss how it could be customised to meet user needs more closely. These meetings provided opportunities to explore participants’ working practices and reasoning about QualDash. An observation schedule was developed to support the recording of fieldnotes (see Report Supplementary Material 3). Researchers kept this with them as an aide-memoire, with fieldnotes written in a notebook. Fieldnotes were written up in detail as soon after data collection as possible, using the observation schedule as a template.
Informal interviews were conducted with clinicians and audit staff while undertaking observations. Our intention had been to undertake longer semistructured interviews, using the teacher–learner cycle, with staff later in the evaluation to discuss revisions to our CMO configurations. In teacher–learner cycle interviews, the theories under investigation are made explicit to the interviewee so that the interviewee can use their experiences to refine the researcher’s understanding. 75 Being concerned with the reasoning of intervention recipients, mechanisms are often not observable76 and, therefore, these longer interviews would also have provided the opportunity to explore staff reasoning about QualDash. However, owing to the COVID-19 pandemic, data collection stopped before we were able to undertake such interviews.
For the duration of the evaluation period, log files automatically recorded information about QualDash use at each site, including information about the user (e.g. job title), data used (e.g. audit and year), time spent interacting with different QualCards and functionality used.
At the beginning of August 2020, a link to the questionnaire was e-mailed to participants. The questionnaire (see Report Supplementary Material 4) is based on the technology acceptance model (TAM), using well-validated items used in numerous previous studies of HIT77 and previous studies of dashboards. 78 The TAM consists of 12 statements, with six statements concerned with usefulness and six statements concerned with ease of use, which respondents rate on a scale of 1 to 5, where 1 indicates strong disagreement and 5 indicates strong agreement. In addition to the TAM items, the questionnaire included questions regarding how frequently respondents used NCA data, how frequently respondents used QualDash during the evaluation period and how likely respondents would be to continue to use QualDash after the evaluation period. Following the initial e-mail, two reminder e-mails were sent. The survey closed at the beginning of September 2020.
Analysis
An iterative approach to data collection and analysis was taken to enable ongoing testing and refinement of the CMO configurations, gathering of further data in the light of such revisions and refinement of QualDash in response to participants’ feedback. Fieldnotes were entered into NVivo and were analysed thematically. Having developed the thematic framework, based on initial reading of the data, Natasha Alvarado and Lynn McVey analysed four sets of ethnographic fieldnotes to test the applicability of the codes and assess agreement. Codes were refined and definitions clarified where there was variation, and refined codes were applied to all fieldnotes, using NVivo. To begin to develop a ‘biography’ of QualDash, we developed narratives that linked cognate themes, enabling us to examine practices within and across cases, and to explore convergence and divergence in participants’ responses. In line with the biography of artefacts approach, we ensured that these narratives traced changes over time. The narratives were compared with the QualDash programme theory to determine whether the findings supported, refuted or suggested a revision or addition to the theory.
Analysis of fieldnotes was supplemented with analysis of the log files and questionnaire data, with the findings, again, being used to assess to what extent they supported or refuted the QualDash programme theory. Log file data were post processed in Microsoft Excel® (Microsoft Corporation, Redmond, WA, USA), removing entries generated by members of the research team undertaking on-site testing of the software. Using timestamps for each login, the number of sessions per audit per month from installation to the end of July 2020 were determined for each site. Where a login occurred less than 20 minutes after the last timestamp and appeared to be the same user (based on the audit and year selected and the job title entered), this was treated as a continuation of the previous session. We also used the login information to determine the breakdown of users by role and audit for each site.
Questionnaire data were analysed in Excel to produce summary statistics for each TAM item, calculating mean and range, for both all respondents and just those who reported having used QualDash, broken down by audit and role. Following previous studies,78 ratings of ≥ 3 were taken to indicate a positive response.
Interrupted time series study
Sampling
The intention was to collect data across the five sites, receiving MINAP data for all sites and PICANet data for the three sites that participated in PICANet, with two control sites per intervention site. This required 10 control sites for MINAP and six control sites for PICANet. Matching of sites was based on the initial measures to be used (described in Analysis below). For MINAP, potential control sites were identified using the most recently published MINAP data (covering 2013–16) and matched according to size (i.e. number of nom-STEMI patients per year), percentage of admissions where door to balloon was < 90 minutes and percentage of patients who received gold-standard drugs at discharge. For PICANet, potential control sites were identified using the most recently published PICANet data (covering 2013–17) and matched according to size (i.e. number of admissions per year and number of beds), number of emergency re-admissions within 48 hours per year and number of accidental extubations per year. A total of 20 potential control sites were approached. Nine control sites for MINAP agreed to participate and five control sites agreed to participate for PICANet. One site, however, subsequently withdrew. By the time that data were to be received for analysis, only three sites had issued R&D approval, with delays as a result of prioritising COVID-19 studies and restarting studies that had stopped because of the COVID-19 pandemic. Of the three control sites that had issued R&D approval, only one submitted data (the failure of the other sites to do so presumably owing to service pressures at that time). Similarly, service pressures meant that site D did not submit data, although data were received for sites A, B, C and E.
Given that data for only one control site were received and the matching was not relevant to the measures used in the final analysis (described in Analysis below), control data were not used, with time, instead, providing the control.
Data collection
An ITS study requires data for a minimum of three time points pre intervention and three time points post intervention for robust estimation of effects and, ideally, will also allow for estimation of seasonal effect on the outcomes. 79 Sites were requested to provide MINAP and, where appropriate, PICANet data for 24 months pre intervention and 12 months post intervention. Consequently, for each site, there were 24 data points prior to introduction and 12 data points post intervention.
Analysis
Given the study intention to determine the feasibility of, and inform the design of, a trial, we intended to consider a range of measures. Our thoughts regarding which measures were appropriate evolved as the study progressed. Initially, we selected two process measures (i.e. one for MINAP and one for PICANet). For MINAP, we selected the composite process measure of cumulative missed opportunities for care. This measure has nine components [pre-hospital electrocardiography (ECG), acute use of aspirin, timely perfusion, referral for cardiac rehabilitation and prescription at hospital discharge of what are considered to be the gold-standard drugs (i.e. aspirin, thienopyridine inhibitor, angiotensin-converting enzyme inhibitor, β-hydroxy β-methylglutaryl coenzyme A reductase inhibitor and beta-blockers)] and is inversely associated with mortality. 80 Given that some of these components, such as pre-hospital ECG, are outside direct control of the trust, we also intended to explore the impact of QualDash on the individual measures that make up cumulative missed opportunities for care. On the basis of the measures that cardiology clinicians described in the interviews as being important for measuring care quality, we intended to also look at the percentage of patients who undergo angiography within 72 hours from first admission to hospital, which is part of the Best Practice Tariff (BPT) financial incentive scheme, and, for those hospitals that provide percutaneous coronary intervention (PCI), the proportion of patients who have a door-to-balloon time (i.e. the time from arrival at the hospital to PCI) of < 60 minutes.
For PICANet, we selected use of non-invasive ventilation first for patients requiring ventilation, which has been shown to be associated with reduced mortality. 81 However, this was not raised as an area of concern in interviews with PICU clinicians. On the basis of this, and two additional considerations, that is (1) it would require loading additional data into QualDash, which would reduce the performance of QualDash in terms of speed, and (2) it would require computation of the data, but the focus of QualDash is on visualising the data, a QualCard was not created for this metric. Although we still intended to include this measure in the ITS, we hypothesised that it would not change, unless other sources of information, such as the PICANet annual report, drew a PICU team’s attention to it. However, accidental extubation and unplanned readmission within 48 hours were identified in our interviews with PICU clinicians as being important indicators of care quality and, therefore, we intended to include these two measures in the ITS.
Limited QualDash use within our study sites meant that QualDash use could not be hypothesised to have had an impact on these measures and, therefore, we agreed with our SSC that it was inappropriate to use these measures. There was some qualitative evidence that QualDash positively impacted data quality and, therefore, it was agreed that analysis would focus on this. For MINAP, we looked at the precision of arrival time (as a measure of accuracy) and whether or not ethnicity was recorded (as a measure of completeness). For PICANet, we looked at the precision of admission time and discharge time and whether or not ethnicity was recorded. The decision to focus on the precision of arrival/admission and discharge times was based on discussions with PICANet about their own checks for data quality and we followed the methods that they used, where times are considered precise if they are not on the hour or at half past the hour. In addition, at the time of undertaking the analysis, we were in discussion with the Leeds Institute for Clinical Trials Research (Leeds, UK) regarding the design of a trial of QualDash in collaboration with PICANet (see Appendix 13), with emergency readmission within 48 hours as the primary outcome, for which the precise admission and discharge times would be needed, further motivating our focus on these measures. Ethnicity was chosen for assessing completeness, as this was known to be a field where there was variation in completeness.
For both NCAs, the outcome was regressed on time and intervention (QualDash). Sites were analysed separately to allow for different effects of QualDash in different sites and variation of all other effects by site. Binominal regression was used, meaning that pooling would provide little advantage. Plotting the proportion of precise variables and known ethnicity showed no obvious seasonal effects or trends and, therefore, seasonal and trend effects were not added to the models. A simple fixed model with QualDash as the sole predictive variable was used across all sites and, therefore, variation is between sites and not between models. The effect of QualDash was anticipated to build up over the first few months of implementation and so a 25% impact is modelled in August 2019, 50% in September 2019, 75% in October 2019 and 100% thereafter. R software version 4.0.0 (The R Foundation for Statistical Computing, Vienna, Austria) was used to analyse the data (the R code is provided in Report Supplementary Material 5 and the data are provided in Report Supplementary Material 6). We report the effect of QualDash on a log-odds scale, with the standard error and p-value from Wald test. Plots show the effect on a probability scale.
Phase 5: assessment of trial feasibility and wider applicability of QualDash
Trial feasibility and design
Phase 5 assessed the feasibility of a cluster RCT of QualDash, using the following three progression criteria: (1) QualDash used by ≥ 50% of intended users, (2) NCA data completeness improves or remains the same and (3) participants perceive QualDash to be useful and intend to continue using it after the study. Criteria 1 and 3 are concerned with the acceptability and uptake of the intervention and, therefore, have implications for recruitment to a trial. The second criterion is concerned with ensuring that the intervention does not have unintended negative consequences, which would affect both the success of the intervention (as QualDash will be less useful if data completeness is reduced) and the feasibility of outcome assessment. The third criterion is also concerned with participants’ perceptions of impact of QualDash on care.
As these progression criteria were met (although there were limitations in the data used for assessing whether or not the criteria were met), we drew on our findings from the multisite case study to determine which NCAs should be included in a trial. Having identified PICANet as a suitable NCA, we worked with the Leeds Institute of Clinical Trials Research to design an appropriate trial.
Assessing the wider applicability of QualDash
Part of our plan for phase 5 of the study had been to assess the extent to which QualDash is suitable for different NCAs and the extent to which QualDash meets the needs of commissioners, through three focus groups. However, given the international focus on COVID-19 at the time and a request from a region-wide Gold Command, we sought, instead, to understand the requirements for such a body (which included commissioners) and how QualDash could be revised to support their work, using interviews for this purpose.
Sample
Although it was not possible to interview those individuals who sit on Gold Command because of pressures on their time, access was provided to individuals who support the work of Gold Command members and would have an understanding of their requirements for a quality dashboard. Seven interviews were conducted. Four participants worked part time as general practitioners (GPs) in large community practices and also had more strategic roles, working within the Clinical Commissioning Group (CCG) or a primary care network, bringing together different general practices within the region. From the remaining participants, one participant worked as a chief operating officer for the primary care network, one participant was head of business intelligence for the CCG and one participant was an associate clinical director for the CCG.
Data collection
A semistructured interview topic guide was developed by the research team. After clarifying the participant’s role, questions were asked about the sorts of decisions to be made, the time pressure involved in making these decisions, the information used in making these decisions and how that information would be obtained. A demonstration of QualDash was then given and participants were asked for their feedback. Interviews were conducted using videoconference software, typically by Mai Elshehaly and either Natasha Alvarado or Lynn McVey, and were recorded. Audio-recordings of the interviews were transcribed verbatim.
Analysis
Interview transcripts were checked for accuracy against the audio-recordings and anonymised. Analysis was based on framework analysis. Natasha Alvarado, Lynn McVey and Rebecca Randell read three of the interview transcripts and, based on this, agreed codes for indexing. Transcripts were then indexed in NVivo. From this, narratives were written that linked cognate themes, drawing attention to convergence and divergence in participants’ responses.
A note on presentation of the findings
Qualitative data collection, through interviews and observations, was an important part of the study methods. Limits on report length mean that, in the following chapters, we have not been able to present the number of interview quotations that we would have liked, particularly when reporting phase 1 findings. However, such quotations are included in other publications reporting these findings. 82–84
Chapter 3 Literature review
Overview
This chapter presents an update of our previous review of studies of dashboard use. 10 For reasons of space, we focus on studies that provided quantitative data on the impact of quality dashboards. The full review update will be published separately. Methods for this review update are provided in Appendix 3. We then consider substantive theories that may help to understand how and in what contexts quality dashboards lead to care QI.
Review results
Literature on dashboard use has grown substantially in 2012–20. Sixty-five studies were identified that evaluated the impact of dashboards on either quality or clinical outcomes, providing a total of 76 studies when combined with studies from the original review. However, emphasis has remained on clinical dashboards, and 49 of the 76 studies are of clinical dashboards, 24 studies are of quality dashboards and three studies are of dashboards that incorporated features of both clinical and quality dashboards.
Study characteristics
The 27 studies of quality dashboards were from Australia (n = 185), Canada (n = 386–88), Italy (n = 189), Malta (n = 190), the Netherlands (n = 391–93), Nigeria (n = 194), the UK (n = 427,28,95,96) and the USA (n = 1397–109). The dashboards were used in the hospital setting, including maternity and outpatient settings (n = 2227,85–91,93,95,97–108), mental health care (n = 128), primary care (n = 394,96,109) and care homes (n = 192). The dashboards incorporated a wide range of metrics, covering topics such as medication (n = 695,97–101), nutritional care (n = 192), care quality for specific conditions (n = 589,91,104,107,108) or at specific points in the patient pathway (n = 1105), organisation-wide care quality (n = 327,85,109), organisational performance (e.g. admission and discharge rates) (n = 285,90) and documentation and data quality (n = 685,96,102,103,105,106), with some dashboards covering more than one of these areas. A variety of study designs were used, including a cluster RCT (n = 1105), a controlled before-and-after study (n = 1101), before-and-after studies without controls (n = 885,89,94,99,100,102–104), ITS analyses (n = 286,97), qualitative studies with data collected through interviews and/or focus groups (n = 627,87,88,90,91,106) and surveys (n = 592,96,107–109).
Effect on outcomes and care processes
Thirteen studies85,86,94,95,97–105 provided quantitative data on the impact of quality dashboards, nine85,94,95,97–99,102–104 of which showed positive impacts. The other four studies86,100,101,105 showed a mixed impact, achieving improvement in some measures but not in others. Six95,97–101 of the 13 studies were concerned with medication, four95,97–99 of which showed positive impacts. Coleman et al. 95 evaluated a dashboard that showed ward performance levels of overdue medication doses that were available to view by clinical and managerial staff. Alongside introduction of the dashboard, weekly e-mails based on directorate-level information were sent to divisional directors and managers, with escalation to executive level if unacceptable thresholds were reached. Missed antibiotic doses decreased significantly [0.60 percentage points, 95% confidence interval (CI) 0.26 to 0.95 percentage points; p = 0.001], as did missed non-antibiotic doses (0.41 percentage points, 95% CI 0.11 to 0.70 percentage points; p = 0.007). 95
Lin et al. 97 evaluated an opioid safety initiative dashboard across 141 veterans’ health administration facilities. Although there was already a decreasing trend in high-dosage opioid prescribing, the dashboard was associated with an additional decrease of 331 (95% CI –378 to –284) patients per month receiving opioids of > 100 morphine equivalents, a decrease of 164 (95% CI –186 to –142) patients per month receiving opioids of > 200 morphine equivalents and a decrease of 781 (95% CI –969 to –593) patients per month receiving concurrent benzodiazepines. A champion at each facility was responsible for reviewing the dashboard, identifying prescribing variation and taking action to promote patient safety.
Michtalik et al. 98 evaluated a combined clinical and quality dashboard that displayed venous thromboembolism prophylaxis rates at individual and group levels. Monthly venous thromboembolism prophylaxis compliance rates were 86% (95% CI 85% to 88%) at baseline and 90% (95% CI 88% to 93%) during the dashboard period, representing a significant improvement in compliance (p = 0.01). At monthly meetings, the dashboard, group results and trends were discussed.
Simpao et al. 99 report on the development and evaluation of a dashboard displaying drug–drug interaction alerts and over-ride rates within their hospital electronic health records (EHRs). Dashboard use enabled the hospital’s Clinical Decision Support Committee, made up of clinicians and pharmacy representatives, to identify a series of alerts for deactivation. For pharmacists, the median alert rate prior to first deactivation was 58.74 [interquartile range (IQR) 54.98–60.48] alerts per 100 orders and 25.11 (IQR 23.45–26.57) alerts per 100 orders following the deactivations (p < 0.001). For clinicians, the baseline median alert rate was 19.73 (IQR 18.66–20.24) alerts per 100 orders and 15.11 (IQR 14.44–15.49) alerts per 100 orders following the deactivations (p < 0.001). Pharmacists’ over-ride rates for drug–drug interaction alerts not modified in the system decreased from a median of 93.06 (IQR 91.96–94.33) over-rides per 100 alerts to 85.68 (IQR 84.29–87.15) over-rides per 100 alerts (p < 0.001). The medication serious safety rate decreased during the study period, and no serious safety events were reported in association with deactivated alert rules.
Two studies100,101 concerned with medication showed mixed effects. Trinh et al. 100 evaluated a dashboard used by pharmacy managers and administrators that displayed adherence rates to antimicrobial and enoxaparin guidelines. Individualised performance feedback was provided to the pharmacists based on trends identified by the dashboard. The overall adherence rate increased significantly post intervention (90.1% vs. 91.9%; p = 0.040). The rate of documentation for non-adherent orders increased significantly post intervention (57.1% vs. 68.5%; p = 0.019). The percentage of pharmacists with at least 90% overall adherence increased post intervention, but was not statistically significant (52.9% vs. 70.6%; p = 0.103) and time to verification was similar before and after the study intervention.
Graber et al. 101 evaluated an interactive web-based antimicrobial dashboard across US Veterans Affairs medical centres. Intervention sites demonstrated a 2.1% decrease (95% CI –5.7% to 1.6%) in total antimicrobial use compared with a 2.5% increase (95% CI 0.8% to 4.1%) at non-intervention sites (absolute difference 4.6%; p = 0.025). Anti-pseudomonal antimicrobial use decreased by 3.4% (95% CI –8.2% to 1.7%) at intervention sites compared with a 3.6% increase (95% CI 0.8% to 6.5%) at non-intervention sites (absolute difference 7.0%; p = 0.018). Antimethicillin-resistant Staphylococcus aureus antimicrobial use decreased by 11.3% (95% CI –16.0% to 6.3%) at intervention sites compared with a 6.6% decrease (95% CI –9.1% to –3.9%) at non-intervention sites, but this was not statistically significant (absolute difference 4.7%; p = 0.092). Dashboard use was supported by a range of strategies, including a kick-off lecture to medical staff to promote antimicrobial stewardship, monthly learning collaborative calls with antimicrobial stewards and sharing of ‘lessons learned’ among stewards regarding effective use of information gleaned from the dashboard.
Five studies85,94,102,103,105 looked at the impact of quality dashboards on documentation and all found a positive impact. Clark et al. 85 evaluated a dashboard that provided real-time display of clinical, performance and patient-flow parameters at organisation and ward level. Although statistical significance was not reported in this before-and-after study, there was an increase in percentage of patients with estimated date of discharge recorded within 12 hours of admission (82% vs. 90%), a management plan documented and communicated to the patient within 24 hours of admission (78% vs. 100%), criteria for discharge parameters documented and developed by multidisciplinary team within 24 hours of admission (76% vs. 92%), a discharge plan communicated to patient 24 hours before discharge (48% vs. 86%) and a pharmacy script written 24 hours before discharge (62% vs. 84%).
Etamesor et al. 94 evaluated a dashboard displaying key routine immunisation indicators by local government area or health facility used by primary health workers in Nigeria. In this before-and-after study, report completeness increased (53% vs. 81%; statistical significance was not reported). The authors94 also state that report timeliness increased, although details are not provided.
Hagaman et al. 102 evaluated a dashboard showing compliance with a preoperative documentation standard. In this before-and-after study, there were two intervention periods. In phase 1, preoperative evaluation clinic (PEC) staff were given training on standard and monthly feedback via e-mail. In phase 2, the dashboard was introduced. PEC managers were given dashboard access. In addition, the metric was included in quarterly performance evaluations for PEC clinicians. Baseline adherence by PEC clinicians was 0%, increasing to 44.7% in phase 1 and to 91.5% in phase 2 (p < 0.0001). Partial adherence by PEC clinicians increased from 0.1% at baseline to 64.8% in phase 1 and to 98.2% in phase 2 (p < 0.001). Adherence by non-PEC clinicians who did not have access to the dashboard increased from 0.20% at baseline to 2.4% in phase 1 and to 12.8% in phase 2 (p < 0.0001). Partial adherence by non-PEC clinicians increased from 5.8% at baseline to 17.6% in phase 1 and to 36.0% in phase 2 (p < 0.001).
Patel et al. 105 evaluated the impact of a team-based dashboard and weekly in-person review of team data on medication reconciliation, a high-quality after-visit summary, a timely discharge summary and a composite discharge metric (i.e. a Discharge Mix Index). Completion of the Discharge Mix Index was achieved for 79.3% of patients in intervention group compared with 63.2% of patients in the control group (p < 0.0001). However, in the washout period, when in-person reviews were removed, there was no significant difference in performance between the two groups.
Dolan et al. 103 evaluated the impact of an anaesthesia opioid documentation discrepancy dashboard. In this before-and-after study, the dashboard was the last intervention in a series of QI interventions introduced. Prior to dashboard introduction, the rate of controlled substance documentation discrepancies was 1.14%. Following dashboard introduction, the rate reduced to 0.87% (i.e. a reduction of 24%; statistical significance was not reported).
One study104 provided quantitative data on the impact of condition-specific quality dashboards. Banerjee et al. 104 evaluated a heart failure dashboard that displayed aggregate data for all patients with a primary diagnosis of heart failure on discharge to support an ongoing QI initiative. Implementation of the QI initiative led to a reduction in 30-day index hospital all-cause heart failure admission rates from 18.2% at baseline to 14%. This was further reduced to 10.1% after dashboard introduction (p-value for trend = 0.0001).
Finally, one study86 provided quantitative data on the impact of a quality dashboard in maternity care. Weiss et al. 86 evaluated the Ontario maternal newborn dashboard in an ITS study. The results were mixed. There were statistically significant absolute decreases in rates of episiotomy [a decrease of 1.5 (95% CI 0.64 to 2.39) per 100 women], induction for postdates in women who were < 41 weeks at delivery [a decrease of 11.7 (95% CI 7.4 to 16.0) per 100 women] and repeat caesarean delivery in low-risk women performed before 39 weeks [a decrease of 10.4 (95% CI 9.3 to 11.5) per 100 women]. There was also an absolute increase in rate of appropriately timed group B streptococcus screening [an increase of 2.8 (95% CI 2.2 to 3.5) per 100 women]. However, rates of unsatisfactory newborn screening blood samples or formula supplementation at discharge were not significantly affected. There was also varying success between sites in achieving practice change.
Relevant substantive theories
The studies above reveal variation in the impact of quality dashboards, even when looking at a particular topic, such as medication. Relevant substantive theories can potentially help to explain this variation.
Theories of audit and feedback
Quality dashboards are a form of audit and feedback. With origins in psychology, audit and feedback theories tend to focus on how audit and feedback interventions are intended to change the reasoning and behaviour of individuals and, therefore, operate at the individual or ‘micro’ level. Audit and feedback theories are premised on the idea that clinicians have an intrinsic motivation to improve and that, if presented with evidence that there is a discrepancy between their own performance and that of a target or standard, they will make efforts to improve their performance. Therefore, these theories focus on how characteristics of the audit and feedback intervention itself might trigger an individual’s intrinsic desire to improve. For example, contextual feedback intervention theory (CFIT) suggests that the standard must be perceived as desirable and achievable, and feedback about the discrepancy between their performance and the standard must be perceived as accurate. 110 CFIT contends that such feedback is more likely to change behaviour if it is timely, frequent, cognitively simple (e.g. presented graphically), unambiguous and provides concrete suggestions of how to improve performance. Hysong et al. ’s111 model of actionable feedback suggests that clinicians are more likely to respond to audit data if the data are perceived to be timely and non-punitive and if clinicians receive feedback about their own individual performance rather than aggregated data about overall performance of the organisation. If clinicians are able to customise how they view the data, then this leads to active engagement in sense-making, further increasing the likelihood that the data will be acted on. Clinical performance feedback intervention theory (CP-FIT) is a more recent addition to theories of audit and feedback, and goes beyond previous theories by drawing on both psychological and sociological theories to consider both individual characteristics and organisation or team characteristics that influence response to feedback. 112 Organisation or team characteristics considered to influence response to feedback are resource, competing priorities, leadership support, champions, teamwork, intraorganisational networks and extraorganisational networks. The three propositions of CP-FIT are (1) health-care professionals and organisations have a finite capacity to engage with and respond to feedback and, therefore, interventions that require less work, supply additive resource or are considered worthwhile enough to justify investment are more effective; (2) health-care professionals and organisations have strong beliefs regarding how patient care should be provided, which influence their interactions with feedback, such that those that align with and enhance those aspects are most effective; and (3) feedback interventions that successfully and directly support clinical behaviours for individual behaviours are most effective.
Although the audit and feedback literature is largely concerned with feedback to individual clinicians,113 the majority of NCAs provide feedback at the clinical team or provider level. Studies of national audits suggest that audit and feedback will have an impact only if (1) clinical teams are given adequate time to engage with and use the data7 and (2) clinical teams perceive that they have the power to make changes,114 emphasising the importance of contextual factors at the organisational or ‘meso’ level. At this level, theories of benchmarking can provide useful insight. Benchmarking refers to the identification, sharing and implementation of practices at team or organisational level that lead to excellent performance. 115 Van Helden and Tilemma’s116 model of benchmarking hypothesises that an organisation’s response to benchmarking is determined by different types of institutional pressures exerted on them by government, professional groups, interest groups and the general public. An organisation’s response pattern, which can vary from passive compliance to proactive manipulation of pressures, depends on its willingness and ability to conform to institutional pressures, which, in turn, is shaped by which stakeholders exert pressure and how, stakeholders’ reasons for exerting pressure, what the pressures are and the context in which the pressure is exerted.
Van Helden and Tilemma’s116 model of benchmarking draws on institutional theory and resource dependency theory, both of which emphasise the importance of contextual factors at the ‘macro’ level. Institutional theory focuses on problems associated with traditional top-down regulation and emergence of regulation through networks of actors. An underlying thread in institutional theory is the concept of legitimacy, with the institutional environment creating not only legal structures but also social and mental structures that actors must work within if they are to be accepted as legitimate actors. 117 At this level, we can also consider theories of ‘new professionalism’ that draw on Foucauldian concepts of disciplinary power and governmentality. 118,119 Governmentality, which refers to forms of self-surveillance that ensure that performance meets expectations, negating the need for managerial surveillance,74 has been found to be relevant to understanding QI in the NHS. For example, Martin et al. 119 revealed the importance of clinical leaders in championing quality and safety, and regular meetings that bring clinicians together to discuss quality and safety constructively. This theoretical lens would suggest that professional community is important to the success of NCAs, with social obligations among members of the community offering opportunities for critical reflection and improvement. 34,119
Theories of technology adoption
Successful introduction of technology involves interactions between individual clinicians and their work environment until the technology becomes embedded (i.e. routinely incorporated into everyday work) and integrated (i.e. sustained over time) into routine practice, which is a process known as ‘normalisation’. 120 A number of theories seek to explicate the contextual factors that influence this process. At the micro level, it is necessary to consider the relationship between the technology and the work practice. 121 For example, Fit between Individuals, Task and Technology is based on the idea that adoption of HIT depends on fit between user attributes (e.g. computer anxiety, motivation), technology attributes (e.g. usability, functionality, performance) and work practice attributes (e.g. organisation, task complexity). The TAM, which is widely used in HIT research, draws particular attention to perceived usefulness and perceived ease of use as contextual factors that influence a user’s attitude towards a technology and, consequently, their intention to use it. 77 In considering user attributes, attention should be given to the user’s ability to interpret different forms of data visualisation, with studies suggesting an individual’s ability to understand information presented in dashboards is often determined by their level of graph literacy. 122,123 For example, Gaissmaier et al. 122 found that individuals with low graph literacy understood numerical information better if it was presented as numbers, whereas individuals with high graph literacy had better comprehension when the same information was presented in a graphical format. 122
At the organisation (meso) level, it is necessary to consider the process through which the technology was developed and introduced. For example, normalisation process theory (NPT) suggests that, for successful integration to occur, the following four key constructs need to be considered: (1) coherence (i.e. sense-making, where individuals make sense of the new technology and how it differs from existing practice); (2) cognitive participation (i.e. the process of engaging individuals with the introduction of the technology); (3) collective action (i.e. how the work processes are adapted and altered to make the intervention happen); and (4) reflexive monitoring (i.e. the formal and informal appraisal of the benefits and costs of the intervention). 120,124,125 This suggests that it is more likely to become embedded into practice if clinical teams have been able to ‘make sense’ of a quality dashboard, have been engaged in the adoption process, have been able to adapt their work processes and are able to identify potential benefits to its introduction.
At the policy (macro) level, we can, again, turn to the class of theories discussed above that focus on governance arrangements. A quality dashboard could potentially increase access to NCA data, enabling greater scrutiny of clinicians’ practices by managers and of trust performance by commissioners. 118,119 If perceived this way, clinicians’ views regarding the appropriateness and legitimacy of this could serve to justify non-participation. 74 Therefore, power relations between clinical teams, quality subcommittees, trust boards and CCGs form an important macro context, shaping how quality dashboards are perceived and used and subsequent impacts. A second class of theories relevant at this level are historical institutional approaches. 43,45 Historical institutional approaches emphasise the extent to which current HITs are rarely self-contained, but are linked to one another in ‘e-infrastructures’. 44 Quality dashboards, and NCAs more generally, depend on NHS e-infrastructures that have evolved over time, such as the IT infrastructure that allows trusts to upload large data sets to regulators and other bodies, and the working practices that have evolved with them.
Discussion
The studies in our updated review reveal variation in the impact of quality dashboards, even when concerned with the same area of practice. The exception to this was with dashboards that sought to improve documentation quality, although the quality of these studies was variable. More generally, the review results reveal a lack of rigorous studies of the impact of quality dashboards, with only one cluster RCT. 105
The studies report a range of strategies used to support the adoption of quality dashboards, including champions, e-mails to managerial staff and monthly meetings to review data. In several studies, the dashboard seems to work by providing information to one person – a champion, a manager – who then encourages others to take action to improve the metrics. Other studies did not include such strategies, but, rather, the dashboard was introduced to support ongoing QI initiatives, suggesting that there was existing motivation to improve the metrics the dashboard presented.
We chose to update our previous review10 of studies of computer-based dashboards, rather than using a different review approach. The search revealed many ‘lessons-learned’ reports, recounting organisations’ experiences of implementing a dashboard, which did not meet our inclusion criteria. The growth in literature on dashboards, heterogeneity in their impact and availability of reports providing stakeholders’ theories suggests that a realist review may be a more appropriate method of synthesis, drawing together the available literature and supplementing it with the substantive theories described above to strengthen our understanding of how and in what contexts dashboards lead to improvements in care quality and safety. 126
Chapter 4 A biography of national clinical audits
Overview
This chapter presents our biography of NCAs. We begin by presenting a brief history of NCAs and of the two NCAs that we focus on in this study, before presenting our findings and the resulting programme theory of how and in what contexts NCA data influences QI.
A history of national clinical audits
Chapter 1 provided an introduction to NCAs. NCAs are well established in the NHS, having first been introduced in the 1990s. Although early NCAs were developed by clinicians working in specific clinical areas, typically in response to recognition of inadequate or variable care quality, the idea of a programme of audits was the Government’s response to a perceived need to re-establish public trust in the NHS127 following a number of scandals and subsequent inquiries, including those related to excess mortality in paediatric cardiac surgery at Bristol Royal Infirmary; Rodney Ledward, the gynaecologist removed from the medical register by the General Medical Council for poor practice; and GP and convicted murderer Harold Shipman. 128 The Department of Health (now the Department of Health and Social Care) commissioned a series of ‘sentinel’ audits,129 with the first of these being launched by the Royal College of Physicians (London, UK) in 1998. 130
Over this period, wider developments in regulation also had an impact the role of audit within the NHS. The 1997 Department of Health white paper The New NHS: Modern, Dependable131 emphasised the importance of clinical governance and announced the creation of the National Institute of Clinical Excellence (now the National Institute for Health and Care Excellence), which would be responsible for producing audits, and the Commission for Health Improvement (CHI), which would support and oversee the quality of clinical governance. Although CHI undertook inspections of health-care providers, the Commission for Healthcare Audit and Inspection, also known as the Healthcare Commission, which replaced CHI in 2004, initiated the NCAPOP and NCAs being seen as a means of targeted and proportionate review. 128 The 2005/6 NCAPOP included 27 projects at varying stages of development. 132
In 2006, the Department of Health’s white paper Our Health, Our Care, Our Say: A New Direction for Community Services133 confirmed the intention to merge the Healthcare Commission and Commission for Social Care Inspection as part of a wider review of regulation, subsequently leading to the creation of the CQC. HQIP was set up in 2008 by a consortium of the Academy of Medical Royal Colleges (London, UK), Royal College of Nursing (London, UK) and National Voices (London, UK). When CQC took over the responsibilities of the Healthcare Commission in 2009, the Department of Health contracted HQIP to manage the NCAPOP. The Health Act (2009)134 and the NHS (Quality Accounts) Regulations (2010)135 increased the significance of NCAs by requiring reporting of NCA participation in trusts’ quality accounts. 1
The MINAP was developed in 1998 by the Royal College of Physicians to provide participating hospitals with a common mechanism for auditing performance against standards defined in the Department of Health’s National Service Framework (NSF) for coronary heart disease. 136 The NSF standards related to areas such as delays between a call for help, and access to a defibrillator and reperfusion treatment, and also required an annual audit of prescription of beta-blockers, aspirin and lipid-lowering treatment for secondary prevention of infarction. 137 MINAP has been running continuously since 2000 and since 2006 has been delivered by the National Institute for Cardiovascular Outcomes Research (NICOR) (London, UK). MINAP is one of six audits run by NICOR as part of the National Cardiac Audit Programme. Data are contributed by all hospitals in England, Wales and Northern Ireland that admit patients with acute coronary syndromes. At its inception, the data set comprised 53 items, capturing data on those aspects of care incorporated in the NSF, primarily drugs prescribed to patients following a myocardial infarction, but then expanded to include interventions, as well as expanding to cover all other acute coronary syndromes. 138 The data set is revised every 2 years136 and currently comprises 130 data fields. 139
The PICANet was set up in 2001 by the universities of Leeds, Leicester and Sheffield in collaboration with the Paediatric Intensive Care Society (London, UK), following a competitive tender put out by the DHSC to develop and run a national paediatric intensive care database. 140 Creation of the database was prompted by the death of 10-year-old Nicholas Geldard in 1995 and the subsequent inquiry commissioned by the North West Regional Health Authority. Since November 2002, PICANet has collected data from all NHS PICUs in England and Wales outside the Pan Thames region, as well as from services that transport critically ill children from DGHs to PICUs. The Pan Thames region units began data collection in March 2003 and, subsequently, PICUs in Scotland, Northern Ireland and Ireland, as well as private PICUs in England, have joined PICANet. Since 2006, PICANet has been run by the universities of Leeds and Leicester. The data set has been revised regularly since it was established. Currently, between 100 and 150 items will be completed on the PICANet admission form for each patient.
Use of national clinical audit data in the NHS
Collection of national clinical audit data
Interviews revealed variation between sites in the resources available for recording NCA data, particularly in terms of audit support staff and technology (Table 6). This variation had a number of impacts. In some sites, where NCA data were stored in local databases and staff had the necessary skills and time, reports and visualisations of the data were generated, increasing accessibility of the data for other staff members. This generation of reports and visualisations was reported in the cardiology departments at sites A, B and C and in the PICUs at sites A and C. However, this was not an option in sites that did not have these resources. Variation in resources also had an impact on timeliness of data collection. In some sites, data would be entered with only a day’s lag, whereas in one site it was reported that, although the site submits MINAP data regularly, these data relate to past patients and it can take them 6 months to obtain this information. Consequently the timeliness of data collection affected staff confidence of the data being accurate reflection of service performance. For example, at the PICU in site A, trust in the audit co-ordinator’s skills and experience meant that clinical colleagues and service managers were confident that their PICANet data were an accurate measure of service performance (more so than data stored in other trust systems).
Site | MINAP | PICANet |
---|---|---|
A |
Staff: a full-time nurse specialist is dedicated to MINAP (and BCIS) data collection, entry, submission and validation Technology: data recorded on Excel spreadsheets and an in-house database, from which the trust IT department upload MINAP data to NICOR monthly |
Staff: a full-time audit co-ordinator is dedicated to PICANet data collection, entry, submission and validation. A PICANet lead/consultant oversees data quality Technology: an in-house Microsoft Access® database (Microsoft Corporation, Redmond, WA, USA) (10 years old). Some more recent PICANet fields are not included in the database and so when an upload is carried out these data have to be entered manually |
B |
Staff: a non-clinical cardiology information analyst leads MINAP data collection, entry, submission and validation, assisted by another team member (the team is also responsible for five other NICOR audits, including BCIS) Technology: an in-house Access database |
Staff: an audit clerk is responsible for PICANet data collection, entry, submission and validation Technology: there is no dedicated PICU database and so the audit clerk has to copy and paste clinical data from the trust’s patient data management system for critical care services directly into the PICANet online portal |
C |
Staff: a non-clinical primary PCI project assistant collects and enters MINAP data for STEMI patients. Non-STEMI data are collected and entered by two acute chest pain specialist nurses, in addition to their clinical roles Technology: an in-house database from which the trust IT team upload MINAP data to NICOR monthly |
Staff: a full-time database manager is responsible for PICANet data collection, entry, submission and validation (with support from a part-time clinical research nurse) Technology: data are entered onto an Excel spreadsheet |
D |
Staff: clinicians, including chest pain nurses, are responsible for MINAP data collection, entry, submission and validation as part of their overall workload Technology: a third-party database |
N/A |
E |
Staff: a cardiac assessment nurse, working with two other nurses, is responsible for MINAP data collection, entry, submission and validation, alongside clinical roles Technology: there is no local database and so data are entered direct to NICOR’s web portal after discharge |
N/A |
Interviewees reported little change over time in the IT systems used for recording data, despite that some changes would have been desirable, such as the addition of fields as new fields were added to the NCA. Whether or not such changes were made depended on a mixture of whether or not the database was in house and, if in house, the extent to which the trust’s IT department supported that database and the extent to which the trust’s unit or service has the range of skills necessary to make the required changes themselves. For example, the database used by the PICU in site A was developed over 10 years ago by a junior doctor and was not maintained by the trust, nor did the PICU staff have the skills to make these changes. Consequently, new fields added to PICANet could not be recorded in this database. In contrast, in the cardiology department at site B, the information analyst had the skills to make revisions to the structure of the database and would do so when NICOR introduced a new field.
Staff changes were, in some cases, reported to affect the data collection process. For example, in site B, one clinician suggested that the quality of their PICANet data was poor, and attributed this to high turnover of audit support staff in the unit. Some sites also described a reduction in resources over time to support data collection. In site B, MINAP data collection and entry used to be carried out at assessment by nurses, but the nurses had been reassigned. Subsequently, data entry was carried out by a non-clinical information analyst and another team member, gathering data from patient notes. The non-clinical information analyst and the other team member were unable to submit data for all eligible patients (reporting having around 66% case ascertainment) and entered data for mandatory fields only, owing to lack of time. In site D, MINAP data collection used to be carried out contemporaneously by chest pain nurses at the bedside, using laptops connected wirelessly to the database; however, the nurses no longer carried out this role and, instead, data tended to be entered retrospectively from the notes, often after patients had been discharged.
The work involved in NCA data collection became more fully apparent during the phase 4 observations. Double data entry and use of paper data collection forms were common practices. For example, in the cardiology department at site A, each day the nurse specialist would print a ‘day sheet’ to identify which patients needed to be entered and then completed a MINAP data collection form for each patient, based on the latest MINAP data set. The nurse specialist gathered information from patients’ paper medical notes that they collected from the wards and the trust’s EHR to populate the form and then entered the data in the cardiology database. When the nurse specialist had completed data input for the month, they informed the trust’s IT department and it uploaded the data to NICOR, normally at the end of each month. Once uploaded, NICOR sent an error report to the trust IT department, which was passed to the nurse specialist for resolution. The nurse specialist checked and cross-referenced anomalies using patient notes, the local database and the trust’s EHR. It could take some time to resolve problems and some errors could not be resolved. For example, if ambulance staff had omitted to record the time at which patients arrived in the hospital, door-to-angiography times could not be calculated. Similarly, PICANet data collection in site A began in the PICU when patients were admitted to the ward. Junior doctors and nurses entered the data available to them on a paper form. The audit co-ordinator compared information in the forms with information in the admissions book and handover sheet, before entering the data on the database. On the ward, there was a large whiteboard on which staff recorded outstanding ‘jobs’ to be carried out for each patient. The audit co-ordinator wrote any queries about the forms on the board so that staff were aware of them and resolved them. The audit co-ordinator also obtained data and cross-referenced information against other trust systems, such as the hospital’s in-house EHR and patient administration system. This need to cross-check data across multiple systems was a feature of NCA data collection across sites. Further details of the work involved in NCA data collection are reported elsewhere. 141
Access and use of national clinical audit data by clinical teams
Interviewees at the clinical team level discussed three main channels through which they accessed NCA data: (1) the national report produced by the supplier, usually on an annual basis but in some cases less frequently; (2) data requests or online through the supplier’s website (a service offered by both MINAP and PICANet); and (3) local databases, if available (as described above).
Interviewees across all sites reported a designated NCA lead, typically a consultant, and received a copy of the national report. When discussing the value of national reports, data quality was raised frequently, with key issues being timeliness and validity. A key constraint in use of national reports for QI was that interviewees often perceived the data to be out of date and so could not be used to support an argument for change. For example, data in the September 2019 MINAP report were at least 18 months old on publication. Timely data, reflecting current performance, were considered essential if they were to inform QI.
Interviewees reported that, for clinical teams to engage with NCA data for QI, it was important that they trusted the quality of the data. The confidence that sites had in their own data depended on the resources available to them to support data collection, which varied, as described above. However, even when sites did trust their own data, they did not necessarily have confidence in the data submitted by other trusts nationally. Inconsistent or inaccurate coding was reported to have a negative impact on interviewees’ trust in MINAP data and on their ability to make meaningful comparisons with other trusts. Owing to inconsistent coding, one interviewee likened such assessments to ‘comparing apples and oranges’ (cardiologist, site E). A cardiologist at site D considered this a consequence of the expansion of data collected for MINAP, leading to it becoming ‘a tick box exercise of collecting data for the sake of collecting data with no effective feedback’ (cardiologist, site D).
Although more timely data could be accessed via requests to the supplier or the supplier website, in practice, interviewees reported challenges using these channels of access, particularly the time taken to receive data from the supplier. Equally, clinicians reported constraints on their time to log onto supplier websites to review metrics. However, some sites did make use of the supplier website. For example, the PICU at site A was accustomed to accessing PICANet data through the website because although they had their own local database, the website provided additional information. Specifically, the clinical lead reported monitoring the PICU’s SMR using the website, which provides a funnel plot visualisation.
Despite perceived limitations to accessing data from suppliers, the value of national comparator data was clearly recognised, something that clinical teams did not have when relying on data within their local databases. National comparisons were seen to offer opportunities to identify services performing well, which could act as examples of good practice from which clinical teams could learn. Making such comparisons was important to all sites, but perhaps especially within the PICUs, which, in contrast to cardiology services, did not have evidence-based standards against which to assess their performance and, therefore, felt the need to benchmark themselves against other units to assess their performance. (Although there are quality standards for PICUs, the standards relate to the organisation of care, covering areas such as staffing levels, facilities and equipment, the presence of guidelines and protocols, and the provision of information to families,142 in contrast to the specific patient-level and often time-sensitive targets set within cardiology.) National comparator data were also seen as important for those trusts wanting to attract patient referrals, potentially stimulating practice change if the service was not performing well compared with peers.
Sites that had local databases and audit support staff with the skills and time to generate visualisations of NCA data more frequently engaged with those data. Regular use of NCA data was reported in both the cardiology departments and the PICUs at sites A and C. For example, in the cardiology department at site A, the nurse specialist described receiving requests for reports on MINAP data from multiple sources, including both clinicians and managers. The nurse specialist reported that they ran monthly PCI clinical governance meetings, lasting around 3–4 hours, which were attended by cardiologists from the site and referring trusts. MINAP data were reviewed at these meetings, as well as deaths and complications for individual patients. These and other regularly requested ad hoc reports were produced by the IT department at the nurse specialist’s request, using MINAP data in the local database and generated via Structured Query Language (SQL) queries. There was little use of generic reports from the NICOR website, which the nurse specialist attributed to staff’s confidence in their own data. Examples of in-house ad hoc reports included activity levels within the catheterisation laboratories, numbers of procedures and door-to-angiography and call-to-balloon times. Where reports were wanted that could not be generated from the local database, the nurse specialist produced them themselves using Excel. For example, the nurse specialist generated a regular report on delays in treatment times and how many related to issues outside the service’s control.
In site C, the PCI project assistant used MINAP data to produce reports. Historically, these data were reviewed quarterly in clinical governance meetings, which were attended by other sites, and during which the different site data were compared. Although at the time of interviewing these meetings had been cancelled for some time because of organisational constraints, the project assistant continued to produce the reports for clinical staff to review separately (e.g. when requested as evidence to inform performance reviews). The acute chest pain nurses described how MINAP data had been used to support an improvement initiative, where they had noticed delays occurring in patients accessing the catheterisation laboratory within the target time, owing to the length of time that it took to receive an ECG beforehand. To address this, a new process was put in place to ensure that all patients presenting with chest pain were given an immediate ECG.
In the cardiology department at site B, a cardiologist reported that they tended to use BCIS data in their monthly morbidity and mortality meetings, which were attended by consultants and junior staff, because BCIS proactively sent, on a monthly basis, Microsoft PowerPoint® (Microsoft Corporation, Redmond, WA, USA) slides displaying data in an attractive and concise way. This tension between MINAP and BCIS came up across sites. Although MINAP considers the pathway of care and captures data at the clinical team and organisational level, BCIS captures individual operator data. Interviewees noted that because these data reflected specifically on the standards of care provided by individuals, those individuals took ownership of these data and were more motivated to monitor these data routinely to ensure that they themselves were meeting required standards and that these data accurately reflected their performance. Similar comments were made by interviewees who participated in the British Association of Urological Surgeons audit, which also collects data at individual operator level. In considering differences between MINAP and PICANet, interviews suggested that PICANet appeared to generate greater levels of engagement among medical staff than MINAP, except among those doctors who used MINAP for research, rather than QI.
In the cardiology department at site D, clinical staff did not describe using their third-party database to produce reports. Instead, staff’s main interaction with MINAP data was via the national report. When that report was published, the MINAP lead reported that they presented the results and action plans at a monthly clinical audit meeting, which was attended by the clinical audit facilitator, consultants and nurses. In this site, constraints on resources, including staff being able to enter data in a timely way and limited clinician time to analyse and interact those data, presented challenges to more frequent data use. In addition, the MINAP lead reported a lack of support from the trust for QI, reducing motivation for engaging with MINAP data.
At site E, the chest pain nurse responsible for uploading MINAP data reported that they did not typically download their data from the NICOR website, unless they received a query from one of the doctors. Therefore, MINAP data were used in this service but were used to address specific queries as part of internal audit or reviews, rather than for routine monitoring.
In the PICU at site A, the PICANet clinical lead described the service as ‘obsessed’ with data. In addition to accessing data from the supplier website, the audit co-ordinator generated reports from their local database for review at monthly clinical governance meetings. The reports contained bar charts showing month-by-month data for performance measures, including those data reported as part of PICANet (e.g. readmissions within 48 hours of discharge, mortality and unplanned extubations), enabling PICU staff to monitor variation in the number of incidents over time.
At the PICU at site C, the database manager used an Excel spreadsheet to produce graphs for monthly multidisciplinary business meetings that were attended by the directorate manager, doctors, nurses, allied health professionals (e.g. pharmacists) and support staff. The metrics that were monitored overlapped with those at site A, although the database manager highlighted that extracting data to evidence unit activity according to Healthcare Resource Group (HRG) codes was also a priority, in preparation for Payment by Results (i.e. a system under which commissioners would pay health-care providers for each patient seen or treated, taking into account complexity of needs).
In terms of motivation for engaging with NCA data for QI, clinical teams with the necessary resources saw it as part of their role in caring for patients, ensuring that they provided safe and effective care. However, as indicated above, financial incentives also played a role.
In those sites that used their local NCA data, we identified two key ways in which clinical teams assessed their performance: (1) assessment of performance against evidence-based standards known to improve patient outcomes, as described above by the PCI project assistant at site C, and (2) monitoring trends over time. This type of monitoring was particularly important for clinical areas for which there were no evidence-based standards against which to compare service performance, as is the case for PICUs. Consequently, we found that clinical teams would use PICANet data to monitor trends over time, as described above. A paediatrician at site A described how monitoring PICANet data in this way led to a QI initiative. Through monitoring data, the site noticed an increase in the number of unplanned extubations and introduced training for nursing staff in taping and checking the tube position on a chest X-ray.
Although the example of QI above points specifically to an issue related to nursing practice, there was generally less engagement with PICANet data among nursing staff. For example, when asked about quality markers, a site A PICU nurse responded that they would tend to consider ‘many things, yeah, for example, central line infections, looking at those, ventilator-associated infections, problems with sedation and weaning’. In the PICU in site C, the nurses who were interviewed described robust processes for monitoring service quality, including ‘quality care rounds’ that audited aspects of care, such as infection control and the patient environment. A ‘harm-free care’ initiative was also used to assess patient safety, which included reviewing the number of inpatient falls, and ‘patient experience’ was captured using a patient care tracker. Consequently, nursing staff had access to other sources of data that provided information on nursing-sensitive care quality indicators. These data were said to overlap with only one PICANet metric considered a potential harm (i.e. unplanned extubations). By contrast, nursing staff in cardiology units were more likely to perceive MINAP metrics as relevant to the care that they delivered.
The above analysis points to resources in terms of local databases, audit support staff and the presence or absence of evidence-based guidelines as contextual factors that influenced the extent of NCA data use and how NCA data were used. Interviewees also described ways in which NCA data use varied over time and highlighted contextual factors that shaped those variations. One source of variation was national priorities. For example, historically, the collection and use of MINAP data had been supported by the political context, with the introduction of the NSF for coronary heart disease being motivated, in part, by what was considered an unacceptable variation in outcomes,143 leading to introduction of regional initiatives, such as regular regional meetings at which practice was shared. Clinicians described how MINAP had an impact on practice during this time. For example, when primary angioplasty was introduced, clinicians were able to see the number of patients treated and improvements in patient outcomes. Other clinicians reported how MINAP supported improvements in secondary prevention and treatment. However, the priority accorded to MINAP diminished once the original changes that it was designed to promote had been adopted widely across trusts. The changing political context appeared to have an impact on resources and infrastructure for collection and engagement with NCA data (e.g. reduction in resources to support data collection for MINAP at site D and the absence of clinical governance meetings in the cardiology department at site C), alongside an end to the regional networks more generally.
Engagement was also influenced by national targets that changed over time. MINAP includes a metric that is part of the BPT, which aims to improve quality by reducing unexplained variation using financial incentives, whereas PICANet includes measures that are part of Commissioning for Quality and Innovation (CQUIN). Introduced in 2009, the CQUIN system makes a proportion of a provider’s income conditional on demonstrating improvements in specified areas of patient care. When discussing with clinicians which metrics they prioritised, some included BPT and CQUIN measures.
Engagement was also found to vary over time depending on a trust’s prior performance in relation to a particular audit. In the PICU in site B, PICANet data access was via the supplier website. A consultant at this site explained that they did not routinely monitor their service performance using the PICANet website because this PICU’s mortality rates tended to be well within, if not below, the expected rates. This PICU was relatively small and clinicians felt that they would know immediately if the mortality rate changed. Therefore, the consultant no longer monitored the SMR as closely as they once did, and their main interaction with PICANet data was through the national report. This was in contrast to the PICU in site A, which, having previously been identified as an outlier, routinely used the PICANet website to monitor their SMR, as described above.
Access and use of national clinical audit data by divisional managers
Clinical teams in the five sites sat within divisions or clinical service units (CSUs), which, in several trusts, were headed by a ‘triumvirate’ that comprised a senior doctor, a nurse and a manager. At this level, there was responsibility for overseeing compliance with NCAs. Processes for this varied between trusts.
A clinical audit manager in site E described how site E confirmed which NCAs its CSU would be participating in. In response to the numerous mandatory and voluntary audits that a CSU might participate in, systems were put in place to (1) ensure that NCA data were submitted to the audit supplier and (2) monitor compliance and audit outputs. This clinical audit manager made sure that appropriate individuals within the CSU were aware of the publication of the NCA report and monitored their response to the report. A cardiologist in site E explained their process of review and dissemination of the NCA report:
As the [MINAP] lead I will summarise what I think are the main points and what I think the main changes that we might recommend from it. So, they don’t have to trawl through 100 pages or whatever. They’ll get the full version and then I will usually just send an e-mail saying: these are the main points, I think, from this and then the comment is usually: can you please just give us your clinical review of it and . . . what are the take-home messages and what changes, potentially might be needed as a consequence? . . . So, I send it back to [the trust’s clinical audit manager], to [a cardiac assessment nurse, whose role involves co-ordinating and validating MINAP data collection] and then it goes to . . . they have a clinical effectiveness board, a group of people who monitor all of these audits to make sure that the hospital is performing as it should be, in these big important areas because it is obviously publicly available documents and they want to make sure the trust is being portrayed well.
Site E, cardiologist
Summaries, like the one described above, aimed to disseminate learning from the NCA and assure those in governance roles that the service was responding appropriately to the audit and that the trust would not receive negative publicity as a result of any concerns raised in the NCA report and lose public (and commissioner) confidence. This trust had been identified in the Keogh8 review of trusts with persistently high mortality rates and, although this was not discussed in interviews at this site, this may have provided added motivation for ensuring that such processes were in place.
In site A, there were similar monitoring structures and the corporate quality governance team provided a standard template for clinical staff to summarise their response to NCA reports, recording areas where the service was performing well, any areas where the trust was an outlier and actions taken to resolve such issues.
Across the five sites, in addition to such monitoring processes, there were regular meetings at this level that considered NCA data (see Appendix 4, Table 16). These meetings tended to maintain general oversight of national NCA reports, checking that the reports had been responded to and considering any resultant issues, including business cases for expenditure for QI, which might be based on NCA data. It did not appear that NCA data were used in detail in these meetings, unless a particularly significant issue was identified that required higher-level input and, possibly, escalation to organisational levels.
Access and use of national clinical audit data by trust boards and subcommittees
Above the level of divisions or CSUs in trusts was the organisational level, where boards that governed trusts and their subcommittees were located. Trust board priorities were reported to be heavily influenced by CQC. A chief operating officer at site D provided insight into some service performance metrics that they reviewed:
Our dashboard has got our national performance metrics on it, so that’ll be A&E [accident and emergency], cancer waits, 18-week waits, they’re aligned to the CQC values of safe effective caring, responsive, so there are key metrics under all of the CQC requirements, so our performance dashboard is measured against those, so well led, so that would have the safe staffing, the attendance, the mandatory training, sickness profile of staff, which is why they all align.
Board members reported that they did not receive detailed reports on NCA data. Detailed analysis of this kind was generally delegated to clinical teams or (for high-risk areas) board subcommittees. The only routine way in which board members described encountering NCA data and reports was during their annual consideration of their trust’s quality accounts. However, there was provision, by exception, for boards to review performance in specific clinical areas in which they had been identified as outliers. Even then, boards did not review data in detail, but focused on approving action plans. Interviewees were clear that it was not possible to approve all recommendations arising from NCA reports, owing to financial constraints and, where recommendations related to employing new staff, to difficulties in recruiting people to some roles.
The remits of board subcommittees varied among sites, although there was always at least one remit with a specific role to assure care quality. Appendix 4, Table 17, summarises the meetings at this level across the five sites. Board subcommittees did not review NCA data in the same level of detail as clinical teams; however, board subcommittees might be involved in more in-depth reviews where particular risks were identified (e.g. when a unit appeared as an ‘outlier’ in a NCA report). Where such issues were raised, the relevant subcommittees may establish a QI project and ask for a report and action plan from the clinical area concerned. In site E, for example, a divisional clinical effectiveness committee reported action plans to address issues arising from NCA national reports to a trust-level effectiveness committee, which reported, in turn, to the trust board. If outliers were identified that represented a risk to the trust, then these were also reported to the trust’s medical director. In site A – a large trust – a quality management group with nine subcommittees reported to a quality assurance committee, which reported directly to the trust board. One of the quality assurance committee’s subcommittees (i.e. the safety and outcomes group) reviewed a quarterly audit report that showed trust-wide progress in submitting data and responding to NCA reports. NCA data were reviewed at different levels within this structure in site A, depending on the severity and sensitivity of the issue raised and the level of associated risk.
Interviewees explained that quality and safety subcommittees were supported in their work by NCA data that were as contemporaneous as possible, reflecting the current care quality context, and, ideally, that were no more than 1–3 months old. The work of quality and safety subcommittees was constrained by older data, especially when data were over 1 year old. The clinical audit manager in site E, for example, talked about the benefits for quality monitoring and improvement when NCAs, such as the National Hip Fracture Database, offered real-time access to data, which recalibrated each time users submitted new information.
By contrast, a quality subcommittee member in site D described problems associated with being unable to access recent NCA data when the subcommittee had needed to follow up action taken by a particular clinical area in which the mortality rate was out of line. In this case, NCA data were available from the supplier only every 6 months, which represented a significant constraint. The interviewee concluded that, given patient turnover, even 3-month-old data could constrain effectiveness of both clinical- and organisational-level QI processes.
Another point raised by several interviewees at this level illustrates the limits in confidence between different levels of trusts, relating to lack of timely data, which was seen to have an impact on their ability to engage with clinical teams about quality issues. Interviewees reported that clinicians seemed sometimes to use timeliness as a reason to dismiss data that put their service in a poor light, while disregarding the fact that data were old when they reflected well on their service.
There were concerns about NCAs that asked for what staff at this level considered too much data, without due regard to the resource implications for trusts or the utility of those data at either the clinical team or the organisational level. Particularly frustrating was when NCA suppliers significantly changed or added to their metrics, which generated a need for corresponding changes to local and third-party data storage systems. These changes could have unwelcome financial implications. Third-party suppliers, for example, might charge trusts to upgrade their software to reflect the changes and additional staffing might be needed for data input. Consequently, some staff called for more co-ordination of NCAs nationally and for more consultation with trusts about their resource implications. A trust board member in site A also described a lack of confidence at the organisational level in certain standards in some NCA reports, referring to them as ‘gold-plated’ (i.e. being regarded as unrealistic and unaffordable). This perceived lack of legitimacy meant that there was some reluctance among board members to approve expenditure arising from clinicians’ recommendations on NCA reports, especially when such expenditure was not connected directly to patient care.
Access and use of national clinical audit data by commissioners
As we were able to interview participants from only three CCGs (associated with sites A, B and E), the following account is necessarily limited and practice may vary in other CCGs. However, CCGs do include QI in their remit. For example, at the CCG associated with site A, one of their strategic objectives was ‘to transform care and drive continuous improvement in quality and safety,’ whereas the interviewee at the CCG associated with site E described how they worked with general practices to improve quality in primary care.
An interviewee in the CCG associated with site A described how issues of quality were considered from the contracting stage. When commissioning a service, the relevant quality manager would be involved in the process, developing, with the procurement team, questions relating to quality, which those bidding to provide the service would have to answer. Once a contract was established with a health-care provider for a particular service, the requirements relating to care quality would be written into that contract and the progress in meeting those requirements would be monitored, achieved, in part, through regular meetings with the provider. In the three CCGs interviewed, regular meetings with providers (including trusts) to monitor quality were described, using a range of metrics. In site A, for example, a clinical quality review group met bimonthly, with a remit to gain assurance that the care commissioned was of high quality, with a focus on clinical effectiveness. Meetings of the group were chaired by the CCG’s director of nursing and quality or by a senior trust representative, and the meetings were attended by senior clinicians and managers from the trust and CCG. The group received a quality report from the provider at each meeting. The report set out compliance with contractual performance requirements, which might relate to issues such as numbers of pressure ulcers, health-care-associated infections, falls, complaints, the Friends and Family Test and CQUINs, as well as compliance with national standards, such as National Institute for Health and Care Excellence guidance. In terms of NCAs, an interviewee at site A reported that CCGs were mainly interested in participation rates, rather than actual results. If there were concerns about care quality, then these concerns would be escalated to the contract management board and the CCG’s quality and safety committee (i.e. a statutory subcommittee of the CCG governing body). The quality and safety committee used an integrated quality and performance report, accompanied by an integrated quality and performance report dashboard. The printed report included a table of quality and safety performance measures, showing the number of incidences for each trust in the period and in the year to date, using red, amber and green colour-coding. In both interviews undertaken at the CCG for site A, interviewees emphasised the importance of the integrated quality and performance report data for their work. A similar (monthly) quality monitoring meeting took place in site B, to which providers submitted reports.
It was clear that commissioners used data extensively within quality monitoring and QI processes, but made relatively little use of NCA data. This was particularly the case for PICANet, as children’s paediatric intensive care is commissioned directly by NHS England specialist commissioning and not through CCGs. However, commissioners were always looking for new data sources to inform their work. Generally, CCGs welcomed data sources that could help them to assure and improve the quality of services that they commissioned, and used NCA data where this was the case. For example, an NCA mentioned by two of the CCGs was the National Diabetes Audit, which is used to monitor primary care performance. Within this, CCGs appeared to use data from only NCA national reports, which, as noted above, were often over 1 year out of date. Commissioners recognised that the need for more recent data was dependent on how data were going to be used. Commissioners acknowledged that there was little that they needed to measure in real time (other than issues such as accident and emergency waiting times) and, therefore, in some cases, year-old data were acceptable. However, when monitoring and improving current care, data that did not reflect the current care context represented a constraint. Data that were as close to ‘live’ as possible – for example, refreshed monthly – supported these processes. Finally, like interviewees from the other groups, commissioners talked about problems in trusting data perceived to be out of date.
A programme theory of national clinical audits
In summary, there were multiple mechanisms operating within the clinical team and at higher levels of trusts that explained why staff might use NCA data for QI. For clinical teams, a key mechanism underpinning monitoring of specific metrics can be termed professionalism, which relates to the use of data to monitor whether or not patients receive safe and effective care as part of their professional responsibility. Another mechanism is incentives, with the monitoring of some metrics being underpinned by financial incentives (e.g. as part of CQUINs and the BPT). Competition was also identified as a mechanism and related to how some services use NCA data to demonstrate good performance in comparison with peer organisations to attract patient referrals from feeder centres. A key mechanism that appeared to operate at the higher levels of trusts (as opposed to within clinical teams) was reputation, which was tied to the public nature of the national report. These mechanisms are captured in our NCA programme theory, which is constructed of CMO configurations that hypothesise the use and impact of NCA data (Table 7). Following Dalkin et al. ,76 we separate out resources and reasoning within the mechanism, drawing attention to the particular resources provided by NCAs.
Context | Mechanism | Outcome | |
---|---|---|---|
Resource | Response | ||
Trust boards and their subcommittees that have oversight of clinical services across their organisation, particularly where service previously identified as an outlier | The NCA national report, which shows if a service is an outlier | Reputation: to preserve trust reputation for providing safe and effective care, particularly in response to measures considered publicly sensitive in nature, processes are put in place to identify where service is an outlier | If a service is identified as an outlier, then data are interrogated to establish cause of the outlier status and may lead to more frequent monitoring of the clinical service for assurance |
Clinicians who trust that data are accurate, but do not have resources to monitor metrics routinely | The NCA national report offers national benchmarks against which to compare service performance | Professionalism: clinicians incorporate the NCA report into the service’s clinical governance processes to assess service performance and where improvements can be made | Clinical services introduce QI initiatives to improve their performance, where resources allow |
Tertiary centres that compete with other organisations for patient referrals from district hospitals | The NCA national report enables services to benchmark their performance against peer organisations in target-based measures | Competition: the clinical service uses the NCA report to evidence competitive performance to feeder services |
Feeder services may choose to refer more patients to the centre Clinical teams may act to improve performance to attract patient referrals |
Clinical services resourced to collect accurate and timely data and to maintain local databases where NCA data are stored prior to upload to the NCA supplier | Audit support staff create visualisations of NCA data that visualise service performance over time | Measures considered important for professionalism and/or to obtain incentives (e.g. financial or accreditation) are integrated into the service’s routine monitoring processes | Clinical staff can quickly identify rises in unwanted incidents or delays in treatment times, introduce changes to improve performance where resources allow and establish the impact of those changes |
The national context, including government priorities and public opinion, had an impact on how, and the extent to which, NCA data were used within trust groups, resonating with the ideas of governmentality and self-regulation, as discussed in Chapter 3. For example, organisations had established processes to participate in mandatory NCAs and ensure that learning from the national report was disseminated to appropriate groups. Furthermore, NHS England used initiatives, such as the BPTs and CQUINs, that financially incentivised performance, and these were found to underpin routine monitoring of some metrics. Historic performance in the national report was also found to shape current engagement with NCA data. Trust groups (clinical and quality and safety committees) worked to ensure that their organisation did not reappear as an outlier in the public report based on past experience. However, although the national context influenced ‘self-regulation’ in terms of data collection, submission and monitoring, action in response to feedback could be dependent on organisational support to resource QI efforts, which clinical staff reported was not always forthcoming. This suggests that, although a dashboard could be designed to support NHS staff to use NCA data in monitoring clinical service performance, acting on the data for QI might be dependent on whether or not the priorities of the trust board and its subcommittees align with those of specific clinical services.
In general terms, clinical teams expressed more motivation to use NCA data within routine practice than other groups, such as the trust board, that had a broader remit for care quality monitoring. Broadly, it can be said that those at the organisational level perceived an imbalance between the benefits to their organisations from NCAs and the substantial resources consumed by participating in them. However, several factors were reported to influence routine use of NCA data by clinical teams, such as data timeliness and accuracy, whether feedback was provided at the individual or service level, and the ability to customise reports for local relevance. This finding overlaps with some theories of audit and feedback discussed in Chapter 3 (e.g. CFIT110 and the model of actionable feedback111), which focus on how the nature and format of feedback affects recipients’ response and action. Our findings suggest that QualDash would need to display data that were considered timely and, importantly, were trusted to be accurate by clinical staff and metrics relevant to service needs. In addition, access to NCA data should be quick and easy, as clinical staff reported time constraints on accessing feedback via supplier websites. With these attributes in place, the mechanisms in Table 7 help explain why staff may interact with QualDash and then act on the data for QI. However, this must also be understood in the organisational context, in which the power and resource to support a QI initiative (depending on its nature) may lie outside the clinical team.
Figure 2 summarises these contextual factors and illustrates the complex interplay of contextual factors at different levels.
FIGURE 2.
Contextual factors that support and constrain NCA use.

Given the interacting nature of the contextual factors that influence engagement with NCA data, which are difficult to represent within the structure of CMO configurations, we also provide a summary our programme theory of NCAs in narrative form (see Appendix 5). We hope to have captured in this programme theory the idea that activation of a mechanism should not be thought of as an on/off switch. 76 Rather, there is a continuum of activation where the intensity of the response and the subsequent outcomes vary according to the evolving context (e.g. that a NCA includes metrics that relate to financial incentives is not a guarantee clinical team members will engage with NCA data, but inclusion may increase engagement).
Discussion
In this chapter, we have presented our analysis of data collected across five NHS trusts and our resulting programme theory of NCAs. The programme theory highlights mechanisms underpinning NCA data use. These mechanisms were linked to metrics that staff felt represented safe and effective care or were of significance in other ways and, for this reason, could be used to inform dashboard design. Furthermore, the programme theory highlights a number of contextual factors that support and constrain use of NCA data, some of which can potentially be influenced by a quality dashboard (e.g. variation in ability to generate visualisations of NCA data and timeliness of such visualisations). Other mechanisms are less amenable to change, such as organisational support for QI, or are outside the scope of this study, such as the choice of metrics included in a NCA.
Our sampling strategy captured variation in NCAs, trusts and health-care professionals, enabling us to explore how different trusts, services and professional groups interacted with NCA data and for what purpose. However, a limitation of our NCA programme theory is that it is based largely on interviews and, therefore, remains to be empirically tested.
Chapter 5 Designing QualDash
Overview
This chapter begins our QualDash biography. The chapter describes the functional requirements for QualDash elicited from the phase 1 interviews, the prioritisation of those requirements with NCA suppliers, the outcome of the co-design of QualDash and the results of the usability evaluation. We conclude the chapter by presenting the QualDash programme theory, which describes how and in what contexts we expected QualDash to lead to improvements in care quality.
Requirements for a quality dashboard
The analysis presented in Chapter 4 highlighted constraints on the use of NCA data that a quality dashboard would need to address. Key among these constraints, and a concern that was at nearly all levels, was timeliness of data. At certain sites, NCA data use was constrained by (1) the absence of a local database from which staff could generate visualisations of the data and/or (2) the lack of audit support staff with the time and skills to generate such visualisations. Therefore, requirements for QualDash included the presentation of timely data and automatic generation of visualisations that support assessment of performance. The way in which NCA data were used to assess performance in some sites suggested that these visualisations should allow assessment against evidence-based standards and easy identification of trends over time.
In addition to exploring how NCA data were used to understand the constraints that QualDash would need to address, we also explicitly asked interviewees about the functionality that they would want from a quality dashboard (i.e. functional requirements) and what they perceived was needed to support adoption of a quality dashboard (i.e. adoption requirements, presented in Chapter 6). Functional requirements could be broken down into five main categories: (1) visualisation requirements, concerning the data to be displayed and how; (2) interaction requirements, concerning how users would interact with QualDash; (3) data quality requirements, concerning data timeliness, completeness and correctness; (4) reporting requirements, concerning the ability to generate reports; and (5) notification requirements, concerning functionality to make users aware of certain information. Each of these categories of requirements is briefly described in more detail below. As described in Chapter 2, we also analysed the interview data to determine the tasks that users of QualDash might want to undertake (see Visualisation requirements).
Visualisation requirements
Interviewees described wanting a dashboard that, on first access, showed static visualisations of key performance metrics, displaying data for all patients. As to which metrics should be displayed, we asked interviewees about which metrics they considered to be important indicators of care quality, which linked to those identified in the mechanisms underpinning NCA data use in the NCA programme theory. For MINAP, prioritised metrics related to the delivery of evidence-based recommendations. For PICANet, emergency readmission within 48 hours and unplanned extubation were repeatedly described as important indicators of care quality; however, SMR was also considered to be a key metric (as part of the mechanism labelled ‘reputation’) and the one of greatest concern at organisational levels within trusts. Interviewees also prioritised metrics linked to financial incentives for both NCAs. Key metrics that were described for the two NCAs, and the reasons given, are summarised in Table 8. In line with current practice at the time, interviewees wanted QualDash to allow them to monitor their performance against particular targets/benchmarks and against national averages. However, interviewees also wanted to compare themselves with trusts that they considered to be similar to them in size and/or case mix.
MINAP | PICANet | ||
---|---|---|---|
Metric | Significance | Metric | Significance |
Call to balloon time | Target of 150 minutes | Emergency readmission within 48 hours | An emergency readmission within 48 hours could suggest that a patient has been discharged too early or has not received adequate or appropriate care in the unit. Related to CQUINs |
Door to balloon time |
|
Unplanned extubation | Unplanned extubation should be avoided for optimum patient care |
Time to angiography |
|
SMR | SMR is a headline metric of concern to trust management, especially if the PICU has previously been an outlier. Related to CQUINs |
Medication on discharge | Based on evidence-based guidelines | Length of stay | Ideally, the PICU is not somewhere patients should be for prolonged periods of time |
Patient seen by a cardiologist | Based on evidence-based guidelines | Admissions (planned or unplanned) | Admissions is concerned with bed occupancy (i.e. whether or not a service is acting at full capacity). Unplanned admission may reflect complications or quality of surgery/care received in other wards |
Number of follow-up appointments | Referral to/uptake of cardiac rehabilitation, which improves outcomes and medication adherence | Refusal of admission | A trust is allowed to refuse only a certain percentage of patients. Related to CQUINs |
Although key metrics should be shown when first logging in, interviewees also wanted access to metrics from other areas of the organisation to understand how their performance interacted with, and was affected by, those areas. For example, if a PICU received a large number of emergency readmissions from the HDU, then they might also want to look at metrics for the HDU. This was part of a broader desire for the visualisation of patient pathways to see metrics presented chronologically in terms of where they occurred within the pathway.
In looking at the questions that interviewees described wanting to ask of NCA data, we identified 124 tasks. The majority of these tasks involved only two variables, suggesting that simple visualisation techniques that users were already familiar with, such as bar graphs and pie charts, should be used, rather than developing novel visualisation techniques.
Interaction requirements
A key interaction requirement was that QualDash allowed users to quickly access, ‘at the touch of a button’, frequently reviewed data, which is in relation to the requirement described above that key metrics should be visible when users first access QualDash.
In discussing the choice of metrics to be displayed, interviewees talked about wanting a customisable dashboard, enabling them to select what metrics were displayed. Such an approach would enable users to select metrics that they perceived to be important signifiers of care quality.
Interviewees also described wanting to be able to customise the time period over which a particular metric was displayed. For example, for metrics for which users might expect to see fluctuations over a year, then it would be necessary to view data for several years. Interviewees also wanted to be able to select certain groups of patients (e.g. based on age or condition). For example, with MINAP, for some metrics, interviewees wanted to be able to look at data for non-STEMI patients only, whereas for other metrics, interviewees wanted to see data for STEMI patients only.
Selecting certain groups of patients to look at was part of the ability, also required by interviewees, to ‘drill down’ into the data to understand the reasons behind the numbers. Having assessed performance, a quality dashboard should support clinical teams to identify causes for metrics that raised concern. This might go as far as looking at details of individual patients.
Some interviewees within clinical teams talked about wanting a dashboard that could support simultaneous interaction by multiple users so as to be able to drill down into the data and discussed these within a meeting. These interviewees felt that, through this, QualDash could enhance the ability of teams to engage flexibly and in depth with NCA data.
Data quality
Data timeliness was considered to be a key requirement. For some interviewees, this meant that data should ideally be in ‘real time’. However, other interviewees considered that, for data to be used for QI, the most recent data should be no more than 3 months old. It was also felt to be important to notify users when incomplete data were displayed to ensure that users did not make erroneous assumptions based on incomplete data.
Reporting
Interviewees talked about wanting to generate reports that were customisable to their specific requirements that could also be edited at a later date (i.e. without having to start again if they needed to change something). Interviewees wanted to be able to cut and paste information from QualDash into Word and Excel to create their own reports. Given that the most common use of NCA data was to produce a summary of national reports to provide to other levels within the organisation, interviewees also wanted QualDash to produce reports that summarised the national report and compared the service’s performance with those results.
Notifications
There was a desire that QualDash made users aware of areas for improvement and of concern. This was both at the clinical team level, where clinical teams wanted to identify, seek to understand and resolve problems in advance of the national report, and at the higher levels of the organisation, where there was a focus on identifying outliers. A quality dashboard could support use of such data at organisational levels by enabling easy identification of a clinical area’s outlier status within an audit. One suggestion for achieving this was use of the red-amber-green rating system. This system was favoured by several interviewees because it was used frequently within health-care organisations and enabled users to identify potential problems at a glance. However, several interviewees also pointed out limitations with such an approach, most notably that it did not capture nuances within data, such as when a standard has not been achieved for sound clinical reasons. It was also felt that it would be useful if QualDash issued alerts (e.g. triggering an e-mail when performance drifted out of the normal range).
Prioritising requirements
The functional requirements were prioritised in the NCA suppliers’ workshop. Box 1 shows the functional requirements that were considered to be essential by all workshop participants.
Targets and benchmarks: QualDash should enable users to monitor their performance against particular targets/benchmarks.
InteractionChoose time period: QualDash should allow the user to select the time period over which the data are displayed.
Patient selection: QualDash should allow the user to select certain groups of patients to look at (e.g. based on age or condition).
ReportingCut and paste: QualDash should allow the user to cut and paste information from QualDash-produced reports into Word and Excel.
NotificationAlert via QualDash: QualDash should make the user aware of areas of improvement or concern (e.g. using ‘traffic light’ colours).
Data qualityNotification of incomplete data: QualDash should make the user aware when incomplete data are displayed.
The top three functional requirements for each category are shown in Box 2.
Targets and benchmarks: QualDash should enable users to monitor their performance against particular targets/benchmarks.
Data of all patients: QualDash should initially display data for all patients.
Key metrics: QualDash should display static visualisations that depict key metrics.
InteractionChoose the data displayed: QualDash should allow the user to choose what data are displayed.
Choose the time period: QualDash should allow the user to select the time period over which the data are displayed.
Patient selection: QualDash should allow the user to select certain groups of patients to look at (e.g. based on age or condition).
Reporting and notificationsCustomised reports: QualDash should allow the user to produce and save customised reports.
Annual report summary: QualDash should produce reports that summarise the annual report and compare the service’s/organisation’s performance with those results.
Cut and paste: QualDash should allow the user to cut and paste information from QualDash-produced reports into Word and Excel.
Data qualityNotification of incomplete data: QualDash should make the user aware when incomplete data are displayed.
Real-time data: QualDash should display ‘real-time’ data.
Validated data: QualDash should show only validated data.
From the visualisation activity that the participants undertook in the workshop, 49 tasks in addition to those captured in the interviews were identified, involving 78 different variables. As with the tasks identified in the interviews, the majority of tasks involved only two variables. Further details about the findings of this activity are reported elsewhere. 56
From requirements to design
Given constraints on time and resources for developing QualDash, the research team decided that the initial version of QualDash would focus on the visualisation and interaction requirements, which, in turn, informed the activities undertaken in the co-design workshops.
Co-design workshop 1
In the first workshop, we not only gave participants task cards based on the tasks identified through the phase 1 interviews and the workshop with NCA suppliers, but also allowed participants to write their own tasks. The next activity involved identifying the order in which users would want to use the data to answer certain questions, which can be referred to as ‘task sequences’. Figure 3 is an example of the output from this activity. Figure 3 shows the series of visualisations that one group of participants considered to be necessary for answering questions about call-to-balloon time, first looking at the overall achievement of the target, then seeing the achievement of the target over several months, and last looking at the breakdown of admissions by whether they were direct or indirect.
FIGURE 3.
Example output from co-design workshop 1, activity 2.
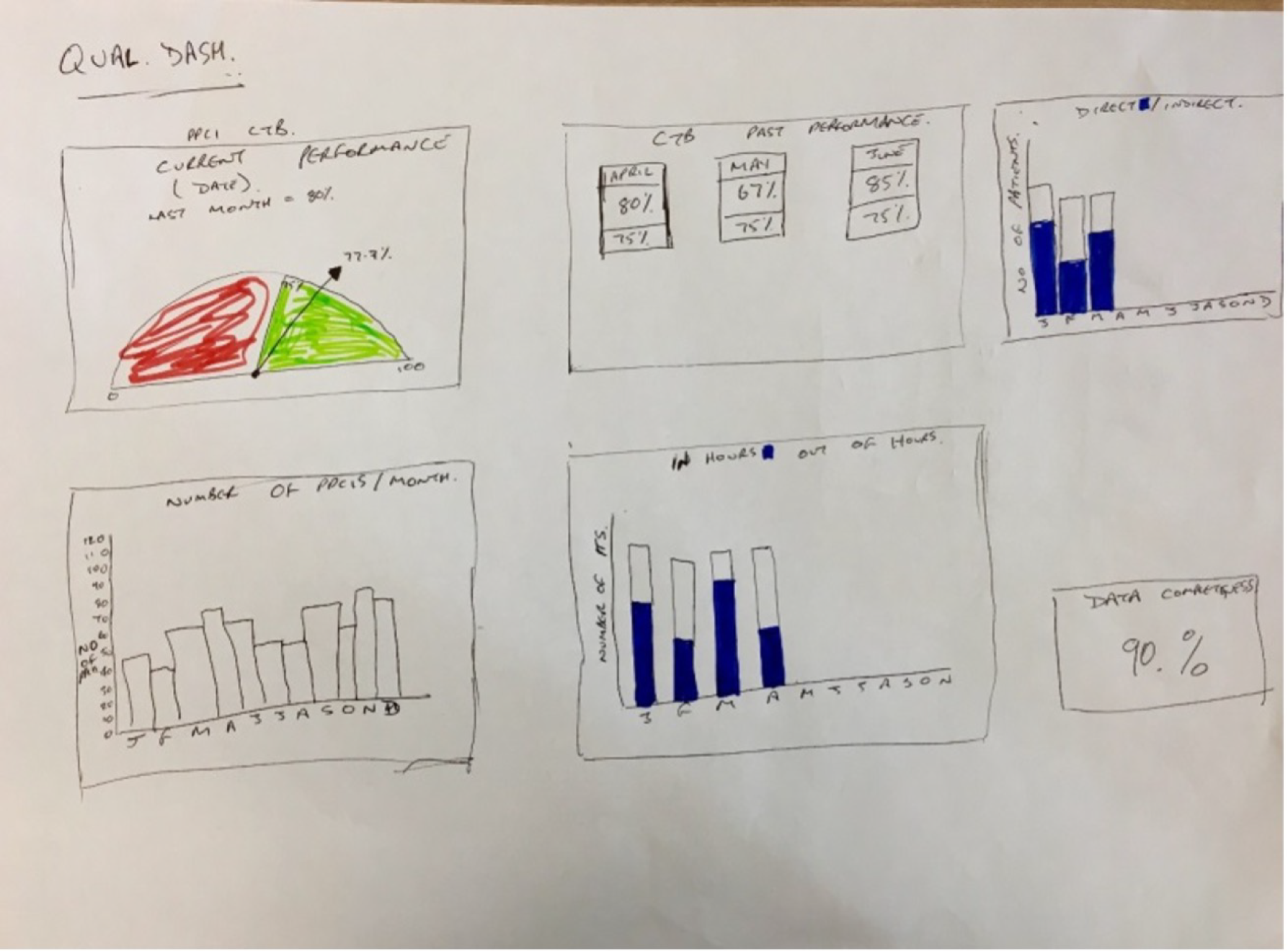
This activity revealed several key findings with implications for the design of QualDash:
-
For each metric, there are what we can refer to as ‘entry-point tasks’. These ‘entry-point tasks’ are primary tasks that a user will want to undertake in relation to the metric, which involve monitoring a small number of measures over time.
-
Investigation of further detail of a metric involves one or more of three subtasks: (1) breaking down the measure(s) for patient subcategories, (2) linking with other metric-related measures and (3) expanding in time to include different temporal granularities.
-
Individual metrics have independent task sequences (i.e. what a user will want to explore after the entry-point tasks will vary according to the metric).
Although we intentionally involved staff from only one site in the co-design activities, it was reassuring to note that these findings reflected broader points made by participants in the interviews.
Prototype development
Following on from this first co-design workshop, we began to develop a QualDash prototype, seeking feedback from key clinical collaborators and audit support staff through this process. Details of the discussions during these meetings and their implications for the design of QualDash are reported elsewhere. 144
QualDash was constructed as a web-enabled dashboard generation engine, dynamically generated and rendered from a configuration file. QualDash was designed to address the key constraints of the use of NCA data captured in our NCA programme theory, while also incorporating requirements elicited from the phase 1 interviews and the learning about task sequences gathered from the first co-design workshop:
-
QualDash provided immediate visualisations of key metrics to create a ‘level playing field’ between sites that did and did not have the resources of a local database and/or audit support staff able to produce visualisations. A QualCard was generated for each key metric, providing a quick view of all key metrics on accessing QualDash. It was not possible to produce visualisations of all metrics that participants identified as important. Specifically, data about unplanned extubation were not currently captured in the PICANet data, but, instead, unplanned extubation was inferred by detecting sequences of intubation followed by no intubation and then intubation again. QualDash included an R script that performed pre-processing steps, including calculating derived fields that were not readily available in the NCA data; however, it would have been computationally expensive and infeasible to run data validation procedures if we were to adapt the R script to detect the sequence used for inferring unplanned extubation.
-
The QualCards could be expanded, providing three customisable visualisations (i.e. one for categories, one for quantities and one showing 3 years’ worth of data) to support tasks associated with the key metric. The initial version of the prototype was developed for PICANet. An expanded PICANet QualCard included in the prototype is shown in Figure 4 and all other QualCards included in the prototype are shown in Appendix 6, Figures 20–23. Users could customise the measures shown in the categories and quantities visualisations via graphical user interface buttons. The times subview used a small multiples view, with small line charts in each multiple linking the same time unit across years. A button enabled users to toggle between this alternative and a traditional multiseries line chart. QualDash was also designed in such a way that it would be easy to make customisations to QualCards, including creating new QualCards and adapting QualCards for a different NCA.
-
To support users to drill down into the data easily, moving the cursor over a segment of the pie chart in the categories subview highlighted the distribution of the corresponding category over time in the main measures view. Similarly, moving the cursor over one or more month(s) in the bar chart caused the pie chart to respond and display the distribution of categories within the selected cohort.
-
The grey area that outlined a QualCard acted as a handle that can be used to drag and reposition the card on the grid or expand it (when double-clicked).
-
Given concerns with data timeliness, QualDash sought to improve access to timely data, providing users with a means to visualise data that they collected for the NCAs, without having to wait for data to be returned to them from the NCAs. Therefore, QualDash would sit on servers within each site, giving users control over how often data were uploaded. This was different from what was anticipated at the beginning of the study. Originally it was thought that QualDash would be hosted at the University of Leeds, with data received from NCA suppliers and users having web-based access to QualDash. A compromise of locating QualDash on local servers was that users would not have access to data from other trusts against which to compare their performance. This was considered to be a reasonable compromise, given that this was not included as one of the prioritised requirements at the end of phase 1 (see Box 2).
FIGURE 4.
Expanded PICANet mortality QualCard in QualDash prototype (using simulated data).
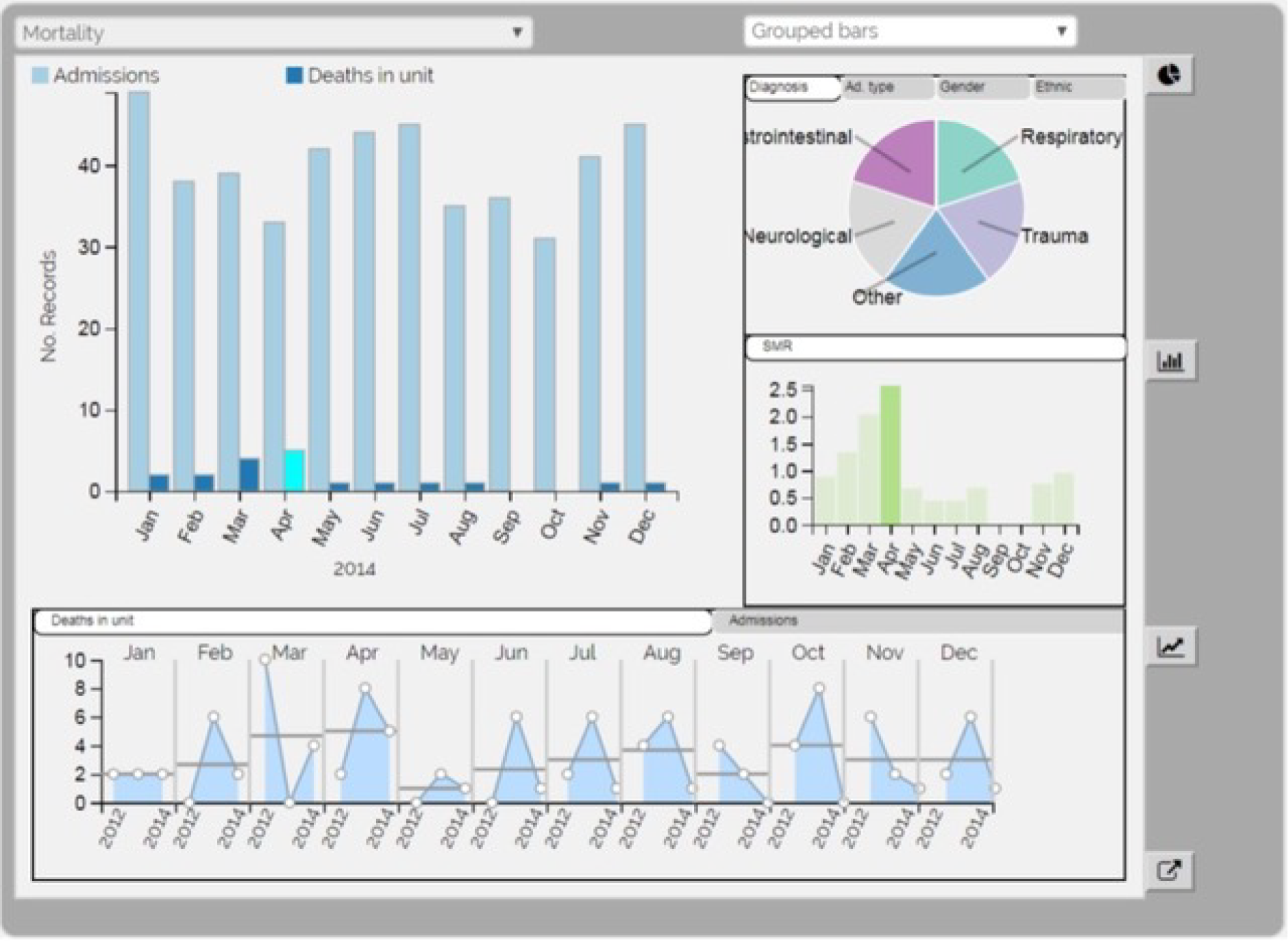
Usability evaluation and revision
In the second co-design workshop, we sought participants’ feedback on the QualDash prototype. From the activities undertaken in this workshop, combined with the second think-aloud session undertaken at site B, a total of 90 comments (both positive and negative) were captured. Forty comments were elicited from the paper-based activity and a further 50 comments from the think-aloud sessions, although there was overlap in the comments from these different activities. Key comments and changes are summarised in Appendix 7. The SUS scores from the second co-design workshop (n = 7) and the second think-aloud session (n = 5) were 74 and 89.5, respectively, indicating very good usability. Results of the heuristic evaluation are presented in Appendix 8.
QualDash v1.0
The QualDash prototype was revised based on feedback from the usability evaluation, resulting in QualDash v1.0 (Figures 5 and 6).
FIGURE 5.
QualDash v1.0 main dashboard view for PICANet (using simulated data).
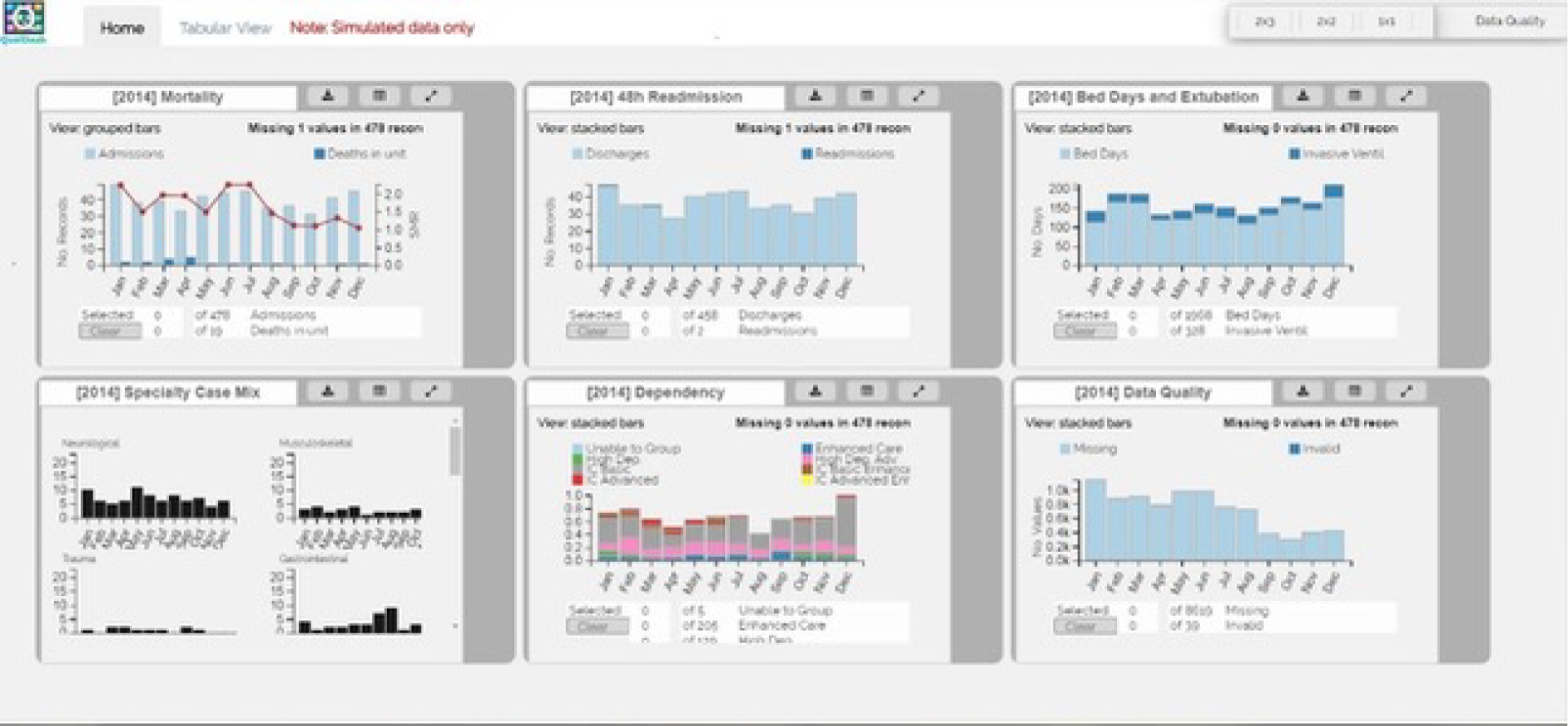
FIGURE 6.
QualDash v1.0 main dashboard view for PICANet with the mortality QualCard expanded (using simulated data).
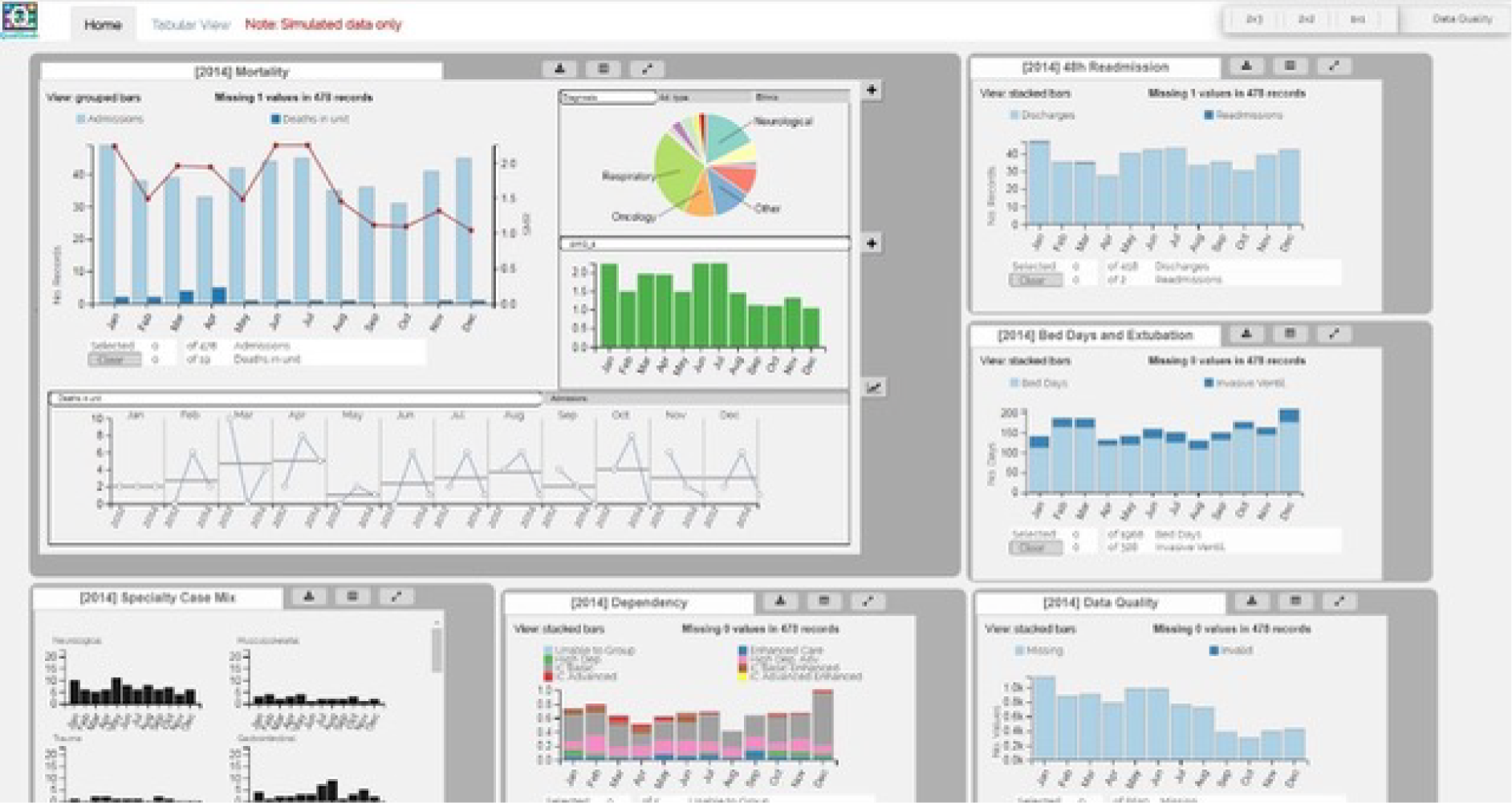
Feedback from the adoption focus groups
Feedback was gathered from the adoption focus groups, which, apart from at site A, were the first time that participants had seen QualDash. There were also a series of e-mail exchanges with clinicians following the adoption focus groups to clarify their comments. Although participants were positive about QualDash, a cardiologist in site D highlighted that the MINAP measures would need to be adapted for the cardiology service. In particular, the QualCard ‘call-to-balloon’ was not considered to be useful for site D, as the site typically did not provide the primary PCI treatment to which the metric relates.
Following on from this, effort was invested in clarifying what measures participating DGHs (i.e. sites D and E) wanted displayed on the MINAP dashboard through discussion with cardiologists at these sites. Discussions confirmed that most measures displayed were of interest to the service, but ‘call-to-balloon’ could be removed. The QualCards confirmed for installation of QualDash v1.0 are presented in Table 9.
NCA | Metric installed |
---|---|
MINAP sites A–C |
Mortality Call-to-balloon Door-to-angio Discharge on gold-standard drugs Referral for cardiac rehabilitation Acute use of aspirin |
MINAP sites D and E |
Mortality Door-to-angio Discharge on gold-standard drugs Referral for cardiac rehabilitation Acute use of aspirin |
PICANet |
Mortality (with SMR) Invasive ventilation Readmission within 48 hours Dependency 1 Dependency 2 Data quality |
The initial QualDash programme theory
The QualDash programme theory was formalised alongside the development of QualDash as it became clear what functions and resources it would offer to sites. Building on the NCA programme theory, the metrics that underpinned or motivated the use of NCA data were incorporated into QualDash in the form of QualCards. Design choices focused on the development of QualDash in ways that could help to overcome constraints on NCA data use and our ideas about potential benefits (e.g. facilitation of report production and improving data quality). Mai Elshehaly and Roy A Ruddle, drawing on their expertise in information visualisation, provided literature-informed theories regarding why certain features of QualDash would provide benefit to users. 58,59
Table 10 provides our initial QualDash programme theory presented as a series of CMO configurations. On the basis of phase 1 interviews and the NCA programme theory, we hypothesised that benefits perceived from using QualDash would vary between sites, with under-resourced sites that previously made little use of NCA data for QI perceiving greater impact than those sites that already had the means to use NCA data for QI, and this is reflected in our CMO configurations. As noted in Chapter 4, there were also constraints on use of NCA data for QI that QualDash was not able to address, but would probably impact on its use, and, again, we reflected these within our CMO configurations.
Number | Context | + | Mechanism | = | Outcome | |
---|---|---|---|---|---|---|
Resource | Response | |||||
1 |
Teams have processes in place (e.g. clinical governance meetings) for monitoring service performance using data available to them Teams are constrained in their ability to use NCA data routinely because data are not considered timely, accurate and/or complete |
+ | Easy access to visualisations of key metrics | Data quality: teams interact with QualDash to utilise data, underpinned by the mechanisms of professionalism, incentives and competition. If data displayed are not timely, accurate and/or complete, then efforts are made to adjust data collection processes to benefit from QualDash | = |
Improvement in data timeliness, accuracy and completeness As data quality improves, QualDash use increases |
2 |
Teams have processes in place (e.g. clinical governance meetings) for monitoring that patient care is safe and effective and/or for monitoring performance in relation to service funding Teams resourced to use NCA data routinely (e.g. audit support staff) are available to produce reports that are timely and trusted to be accurate by clinical staff |
+ | Web-based access, visualisation of key metrics and ability to interrogate data |
Facilitation: teams use QualDash in meetings because it facilitates direct access and monthly monitoring of metrics that are considered markers of safe and effective care (underpinned by the mechanism of professionalism) and/or are linked to service funding (underpinned by the mechanism of incentives) Automation: audit support staff use QualDash to produce reports when requested by clinical and managerial staff, as it automates the calculation and visualisation of metrics |
= | Reduced time spent by clinical and audit support staff in accessing, and generating visualisations of, NCA data |
3 |
Teams have processes in place (e.g. monthly clinical governance meetings) for monitoring service performance Teams that do not, or are not able to, monitor key NCA metrics routinely (e.g. owing to constraints in resources) |
+ | Web-based access to key metrics considered to be markers of safe and effective care | Integration: teams use QualDash because it facilitates access to NCA data that are considered to be key markers of care quality and provide visualisations that highlight discrepancies between performance and targets (e.g. in MINAP) and/or changes in performance month by month (e.g. in MINAP and PICANet), which they take action to address, underpinned by the mechanisms of professionalism and incentives | = |
NCA data integrated into routine service monitoring processes Introduction of QI initiatives in relation to key metrics, where resources allow Over time, improvement in those metrics |
4 | Teams have processes in place (e.g. monthly clinical governance meetings) for monitoring service performance, but existing systems produce static reports and do not provide functions to interact with, and drill down into, NCA data | + | Ability to interact with NCA data and explore relationships between variables | Data interrogation: teams use QualDash in meetings instead of traditional static reports because it enables them to interrogate anomalies in the data, underpinned by the mechanisms of professionalism and/or incentives, helping them to understand what has affected performance and to identify appropriate QI initiatives | = |
Introduction of appropriate QI initiatives where performance is not in line with expectations, where resources allow Improvement in metrics that QI initiatives target over time |
5 | Teams asked to produce reports and recommendations for managers and other groups about service performance (e.g. at time of publication of NCA national report) | + | Easy access to NCA data and visualisations that can be exported into reports | Reporting: teams choose to use QualDash to produce reports requested by other groups because they see that it removes the manual work involved | = |
Reduced time spent by team in report preparation Use of NCA data at divisional and organisational levels via QualDash outputs Over time, divisional and organisational QualDash use increases as teams become aware that they can access the data they require directly |
6 | Teams receive data requests from managers | + | Web-based access for multiple users | Access: managers will use QualDash to access the information they need quickly and easily | = |
Streamlines use of NCA data for managers Reduced time spent by audit support staff/team in producing data reports for managers |
7 | Teams need to evidence performance to managers and other groups to support a case for practice change (e.g. in business meetings with managers or in the NCA national report summary) | + | Visualises key metrics that can also be exported into reports and presentations | Evidence for change: teams use QualDash to monitor service performance, underpinned by the mechanisms of professionalism and/or incentives, and, when they identify a QI need that requires additional resources, then they use QualDash to report evidence that can convince trust groups with power to provide resource | = | Trust groups with power to allocate additional resource are convinced of need for change, particularly where key metrics relate to trust priorities (e.g. relating to trust reputation or avoiding penalties/receiving incentives) |
Discussion
This chapter has described the process of designing QualDash and the development of the QualDash programme theory. There are limitations to this work. First, although we were able to elicit requirements from the interviews, many interviewees found it difficult to define what they would want from a quality dashboard, reflecting the well-acknowledged challenge of eliciting requirements. 145 We also found it necessary to conduct observations during phase 2 to better understand data use because the phase 1 interviews, although a good method for capturing perceptions of what supports or constrains NCA data use, did not provide the level of detail necessary for design. We would recommend that future studies use a combination of observation and interviews. This would not only provide further data for informing design, but also provide greater insight into contexts and mechanisms for the purpose of developing programme theory.
A further limitation is that the requirements elicited were focused on two NCAs. Although we sought to ensure the generalisability of these requirements through the workshop with NCA suppliers, the perspectives of suppliers may differ from those who engage with those different NCAs.
In designing QualDash, we initially had limited engagement of cardiologists. No cardiologists attended the co-design workshops or participated in the second round of think-aloud sessions, although we did consult with two cardiologists between the two co-design workshops. This limited engagement delayed the identification of differences between sites in what were considered to be relevant metrics. More broadly, these differences between sites point to the limitations of undertaking co-design with staff from just one site.
The number of participants involved in the usability evaluation was small. In total, 12 participants took part in the think-aloud session and only four were clinicians. However, such sample sizes are in line with recommendations for usability testing. The purpose is to identify usability problems for correction in future iterations. Eighty per cent of usability problems are detected with four or five participants, and the number of additional problems identified per participant decreases as the number of participants increases. In addition, the most severe usability problems tend to be detected by the first few participants. 146
Chapter 6 Designing the QualDash adoption strategy
Overview
This chapter continues the QualDash biography, describing the identification, prioritisation and refinement of strategies for supporting the adoption of QualDash. We conclude the chapter by presenting the resulting additions to the QualDash programme theory.
Adoption requirements
In the phase 1 interviews, we asked staff what activities should be undertaken to support the uptake and adoption of QualDash. We were able to group these adoption requirements into four categories: (1) awareness, (2) training, (3) support and monitoring, and (4) access. For example, interviewees discussed that staff must first be made aware of what QualDash was, how it could be accessed and how it could benefit their practice and patients. Suggestions to raise awareness included sending an e-mail or e-bulletin to potential user groups, engaging a champion to promote and advertise QualDash to colleagues, and displaying posters. Interviewees also discussed a need for training so that staff could learn how to use QualDash and learn how to make use of the data for QI:
. . . helping us understand the benefits, how to use it, when to use it, what to do with some of the data that we’d find.
Site D, trust board member
There was a preference for ‘short snappy training’ (i.e. a format and mode of delivery that could be accommodated by the busy workloads of health-care professionals). Training suggestions included online delivery or on-site drop-in sessions ‘to have a face-to-face person to talk to’ and answer queries at their convenience. One interviewee suggested that online delivery or on-site drop-in sessions would also be beneficial for the research team to build relationships to encourage engagement beyond information dissemination via e-mails:
Coming in and doing like little workshops are good, because then people remember you. You see them face to face and you can catch them. They will pretend they don’t read their e-mails . . .
Site C, PICU nurse
An important point, echoed by several interviewees, was that QualDash use should be intuitive so that minimal training was required. One interviewee suggested that an intuitive design should negate the need for formal training altogether, which staff would perceive as a burden:
I think if you need training you won’t get people engaged, they won’t have the time to take the training even if it’s a lead, it would be a burden on them almost, just because of time, everything is about time.
Site D, cardiology patient service manager
Therefore, an intuitive design and training that is convenient for time-limited staff were understood to be important requirements to support QualDash use. Furthermore, interviewees perceived adoption as not a ‘one-off thing’. Interviewees advised that, after raising awareness and providing appropriate training, ongoing support and monitoring would be necessary to maintain QualDash use. This requirement included assessing how staff were using QualDash and providing support to overcome any difficulties experienced. Suggestions to fulfil this requirement included feedback (e.g. the research team could monitor how sites were using QualDash, for what purpose and with what impact). Furthermore, if used, a champion could support ongoing use, for example by identifying new staff in need of training, particularly junior doctors on rotation who were expected to undertake audits. A telephone helpline, user manual and online forum were also suggested to support users over the duration of the project.
A key requirement was that QualDash should be accessible from any computer on site. There was some desire for authorised individuals to be able to access QualDash off site. The intention was that QualDash would be web based and several interviewees commented that a web-based dashboard would be beneficial, allowing flexibility in where it could be accessed. It was noted that QualDash should be accessible without the need for multiple different logins and passwords. In addition, some participants wanted to have access via mobile devices, whereas others felt that, depending how it was being used, staff would be more likely to use QualDash on a personal computer (PC):
And I think when they want to look at their data and drill down to it, they wouldn’t necessarily do it on a handheld, they would be sitting in their office, they would be . . . They would have time and they would be wanting to look at something specifically, so they wouldn’t be doing it on the go.
Site A, clinical information and outcomes manager
In summary, four key categories of adoption requirements were identified in the phase 1 interviews (i.e. awareness, training, support and monitoring, and access). The suggestion to engage a champion crossed requirement categories and stood out as a key strategy to support adoption.
Prioritising adoption requirements
Adoption requirements elicited from the interviews were prioritised with NCA suppliers. All participants considered it to be essential that there was a champion who would promote QualDash and encourage and support its ongoing use, that QualDash would be intuitive to use and that online training was available. Box 3 lists the top three requirements for awareness, training, and monitoring and support. In relation to access, there was strong support for both on- and off-site access if data were appropriately secure.
Champion: a champion should be selected to promote and advertise QualDash.
E-bulletin: information about QualDash should be included in an e-bulletin.
E-mail: information about QualDash should be sent via e-mail to relevant parties.
TrainingQualDash should be intuitive to use so that staff can use it with minimal or no training.
Training should be provided online (e.g. via a webinar).
Training and guidance should provided on using audit data for QI.
Support and monitoringA champion should encourage and support ongoing use of QualDash.
Feedback regarding how QualDash is being used should be collected and provided.
Support should be made available in an online forum.
Developing adoption strategies
The prioritised requirements were discussed with staff in the phase 3 adoption focus groups. We anticipated adapting the adoption strategy for each trust, and discussion focused on if participants felt that the activities/interventions that were suggested would work within their trust or if a different approach was needed. Through discussion, we were able to elicit further detail regarding how these activities/interventions could be operationalised to support adoption.
Champion
In discussing the attributes of a ‘champion’, the research team initially expressed the role (based on phase 1 analysis) as someone who would encourage use of and disseminate information about QualDash. However, participants suggested that the role could encompass teaching other staff how to use it and how to overcome problems to support and maintain use, acting as ‘super users’. To undertake this role, champions would need to be trained to use QualDash and feel confident in doing so. In terms of delivery to impact adoption, participants discussed that a clinical champion was key, with some staff indicating that a ‘senior consultant’ would have the most impact. The rationale was that a senior clinical champion would have the authority, in comparison with IT or audit support staff, to lead the use of QualDash (e.g. put QualDash on the agenda of clinical governance meetings and then lead its use within meetings). Furthermore, a senior clinical champion was thought to have influence over the behaviour of their colleagues (i.e. their use of QualDash ‘lends weight’ to its benefits as a tool to support data use and would encourage others’ uptake).
It was agreed, based on focus group discussion, that at least one clinical champion from each participating cardiology department and PICU would be identified. To support a clinical champion, it was suggested that there could be two or more champions to support QualDash use within a clinical area (e.g. a clinician and an audit support staff member). Although staff did not see the role of champion as a large task, having more than one champion was viewed to be a way to share the load.
Awareness
Participants were also supportive of the use of an e-bulletin to raise awareness of QualDash, how QualDash could be accessed and how QualDash could benefit the service. It was suggested that responses to the e-bulletin (whether or not people read it) would depend on who sent it, suggesting that influence of the clinical champion was, again, key. However, it was noted that some staff would not read it regardless of who sent it. In terms of timing of e-mails, participants discussed sending them a couple of weeks in advance of installation of QualDash and again when QualDash was live as a prompt/reminder. Participants also advocated providing demonstrations of QualDash at appropriate meetings to disseminate information to stakeholders. For example, at site C, it was suggested that a presentation was given at the quarterly audit for the clinical effectiveness meeting. This meeting was protected time for clinicians and viewed as an efficient way to deliver information to a number of clinical staff at one time. Other suggestions were clinical governance meetings, multidisciplinary team meetings and sisters’ meetings.
Training
As in phase 1, participants suggested that QualDash should be intuitive to use and, therefore, require minimal training, a requirement operationalised in the design of the dashboard. In addition, participants supported the idea of online training on the basis that it would be difficult to find a time when clinicians could come together for face-to-face training. People could undertake online training at a time convenient for them and return to the session if they needed a refresher. Online training was also considered to be useful resource for new staff. Participants explained that any training materials offered should be in the form of quick reference tools, such as crib sheets, that could be easily accessed at the convenience of busy staff. However, a more fundamental point was noted during one focus group:
I think the most important thing when you’re introducing anything to clinicians is that it works. If you introduce it and it only works 80% of the time or 90% of the time, it’s a bit crap.
You lost interest.
The clinicians will tar it with a dodgy brush and they’re going to slag it off, and nobody will use it . . .
Based on lived experience of similar initiatives, participants highlighted that initial experiences of using QualDash needed to be positive (i.e. it had to work as intended when accessed by staff) to maintain their interest in and use of the technology.
Additional training needs
When asked about whether or not training in data interpretation was necessary, some participants referred to training materials already online, whereas others felt that interpretation was straightforward. However, the role that some audit support staff play in supporting data interpretation became apparent in terms of understanding data definitions. Training for uploading data into QualDash also emerged as a training need when the decision to locate QualDash on local servers was made. The strategy required for this varied according to the individual. For example, at site C, one of the IT staff commented that:
I suppose, from our point of view, because the person that would be doing that, is very technical, I don’t think it really matters. As long as there is a well thought out set of rules, then we’re happy to conform to them.
In contrast, at site A, where it was anticipated that the audit co-ordinator would carry out the upload, their preference was for someone to come and show them how to do it.
Support and monitoring
There was variation among participants in the level of enthusiasm for the idea of feedback. We had anticipated that it would be the research team feeding back use and impact to the service, but the idea of clinicians giving examples of questions that they had and were able to answer using QualDash was proposed, tying in with their capacity to influence others. It was suggested that feedback could work to maintain enthusiasm by letting people know that QualDash use was making a difference. Providing feedback via meetings routinely held within the services was suggested to fit in with existing structures and processes.
Similarly, participants varied in their views of the benefits of an online forum. It was suggested that, in addition to leaving a question for response in a forum, users could share how they have used QualDash, linking to the idea of feedback. The advantage would be that participants across sites could share their experiences. Participants described similar forums run by NCAs in which individuals could seek clarification (e.g. how to identify the patient sample for a particular audit). It was noted that MINAP used to have an online forum and staff had found that useful. At site C, a member of IT staff commented that such forums are used a lot in the IT community, with benefits including that it is an asynchronous forum of communication and, therefore, does not depend on staff being in a particular meeting and could be searched through at any point in the future. However, some participants questioned whether or not staff would realistically have time to contribute to and make use of such a forum. As a source of support, the asynchronous nature of an online forum was perceived to be a disadvantage by some, as users would want an answer to their question straight away rather than waiting for someone to respond. The limited number of sites involved in the study was seen to affect this, in contrast to the MINAP forum, which could be accessed by every trust.
Implementing the adoption strategies
Adoption strategies for each site were developed. These adoption strategies addressed the requirements highlighted as key for underpinning uptake and adoption and were also feasible given the resources available to the research team. We considered key strategies to be (1) the use of a clinical champion (a consultant if possible) who would work to raise awareness, engage users and provide support for ongoing use and (2) to design QualDash to be as intuitive as possible so that minimal formal training was required and to ensure that it worked as intended to avoid being tarred with the ‘dodgy brush’. Secondary requirements were the use of an e-bulletin and site demonstrations to advertise QualDash to a wide range of staff and the development of a training video and crib sheets as quick reference tools to learn how to (1) use QualDash functions and (2) upload data into QualDash.
Recruiting champions and data uploaders
Informal agreement to become a champion from appropriate staff, for example consultants (usually our local collaborators), was often obtained at the adoption focus groups or, alternatively, focus group participants suggested appropriate colleagues. Prior to the installation of QualDash, potential champions were contacted by e-mail. Potential champions were provided with a description of the types of activities they would be asked to support as champion. The following list of activities was included in the e-mail:
-
lead use of QualDash in appropriate settings (e.g. in meetings and report preparation)
-
advocate the use of QualDash to other potential users
-
support others to use QualDash if/when necessary
-
act as point of contact for the QualDash team.
Appendix 9, Table 20, provides a list of the champions confirmed by site. In all sites, apart from the cardiology department at site C, clinical champions were identified. In most cases, champions were the local collaborator. Therefore, champions were already knowledgeable and supportive of the study aims and familiar with the research team.
Staff who would take responsibility for uploading data into QualDash were identified and confirmed in the same way as the champions and, in some cases, this role was performed by those acting as non-clinical champions (e.g. audit support staff) (details of who took on the role in each site are available in Appendix 9, Table 21). In the PICUs, staff who collected and uploaded data to the PICANet web agreed to upload data into QualDash. Staff who agreed to support MINAP data upload were based within and outside the cardiology service and performed different roles. Variation in background and experience of staff who agreed to upload data meant that they required different levels of support from the research team to learn how to complete the upload process.
E-bulletin and site demonstrations
To deliver information to potential users, in support of the champions’ efforts to raise awareness, two modes of dissemination were used. First, QualDash demonstrations were given in appropriate meetings that brought together clinicians (e.g. doctors and nurses) and other staff (e.g. service managers. Demonstrations were sometimes given by members of the research team, whereas in other cases the QualDash champions chose to provide a demonstration (see Appendix 9, Table 22, for overview). Second, an e-bulletin was drafted with the intention of providing it to the champions for them to disseminate via e-mail. However, this activity was suspended because of issues that emerged on installation of the dashboard (see Chapter 7).
Training materials
Crib sheets were produced that, with screenshots, provided step-by-step instructions to use the QualDash functions (e.g. how to add and remove variables from the subview and how to export visualisations). The crib sheets also included tips on interpreting data, including not making general claims based on small or unrepresentative samples, not mistaking correlation with causation and trying not to let preconceptions influence interpretation. The crib sheets (version 1) were sent out to one site when the installation of QualDash v1.0 was completed. However, based on user feedback, dissemination of the crib sheets was suspended to develop further iterations (e.g. to provide more information about each QualCard).
The research team also prepared a script for a training video and began recording the video to show the use of QualDash functions and to provide further guidance on data interpretation. However, as QualDash was introduced into each site, user feedback led to customisation of the QualCards (described in Chapter 7), meaning that a generic video would be potentially confusing if what it showed was different from what users saw when using QualDash. (QualDash v2.0, described in Chapter 7, included a documentation page with a demonstration video.)
A ‘QualDash set-up’ document was created with instructions for uploading data into QualDash, which was sent to relevant staff in advance of the installation visits.
Extending the QualDash programme theory
Chapter 5 presented our initial QualDash programme theory from which we hypothesised how staff would respond to QualDash and how this response would be shaped by the local context, without consideration of how it might also be shaped by the way in which QualDash was introduced. Adoption focus groups allowed us to build on the QualDash programme theory to include adoption requirements. In realist teams, we understood that the research team would need to engage champions to the extent that they would lead the use of QualDash in quality monitoring and improvement activities, and engage colleagues in its uptake and use via their influence as senior clinicians. The research team were also expected to become expert users who could train others and troubleshoot problems to maintain QualDash use. We thought of the e-bulletin and site demonstrations as resources that would support the work of the site champion. These resources would provide information about what QualDash was, how to access QualDash and the potential benefits of QualDash across a broad range of users – a mechanism that we termed ‘awareness’. It was expected that ‘awareness’ would prompt early interaction with and exploration of QualDash from which potential users could assess its utility. The training materials provided were expected to offer users the knowledge needed to develop skills to utilise QualDash to its full potential, in a user-friendly and appealing format, so they could become confident users themselves.
Important to note was that a starting assumption in developing the adoption strategy was that QualDash would be developed and maintained to a standard that would not discourage further use or ‘tar it with a dodgy brush’. As dashboard installation unfolded (described further in Chapter 7), it quickly became apparent that a significant amount of work was needed to get to this level of development, something that the adoption strategy had not fully considered.
Table 11 provides CMO configurations that, together, explain how and in what contexts adoption activities would support the uptake and adoption of QualDash.
Number | Context | + | Mechanism | = | Outcome | |
---|---|---|---|---|---|---|
Resource | Response | |||||
1 |
Clinical services in which NCA data use is desirable but variable because of constrained resources QualDash successfully installed, accessible and works as intended |
+ | Clinical champion and e-bulletin and/or meetings | Awareness: teams are informed about QualDash (i.e. how it can be accessed, its functions and potential benefits) and log on to explore its utility | = | Clinical staff with interest in the NCA (e.g. service managers) make assessment of if and how they can use QualDash to facilitate work processes |
2 |
Clinical services in which NCA data use is desirable but variable because of constrained resources QualDash successfully installed, accessible and works as intended |
+ | Clinical (senior) staff engaged as champions for QualDash |
Leadership: clinical champions add QualDash to meeting agendas and lead its use in the meeting Influence: the clinical champion’s colleagues are encouraged/inspired to use QualDash themselves based on the champion’s example |
= |
QualDash trialled in settings where there is potential for integration into routine practices The champion’s colleagues use QualDash to assess if and how it can be useful to them |
3 |
QualDash successfully installed, accessible and works as intended Early interactions have established role for QualDash in use of NCA data |
+ |
QualDash is intuitive to use Quick reference training materials functions Champions available to support use |
Confident users: teams learn quickly how to use the dashboard functions to support and benefit their work processes | = | Sustained and optimised QualDash use (QualDash not ‘tarred with the dodgy brush’) |
4 | Upload of data to QualDash undertaken by IT staff who have the expertise necessary to complete such tasks with minimum training | + | Quick reference training materials | IT staff quickly learn how, and feel confident, to upload data to QualDash without further support | = | Data are uploaded to QualDash routinely, providing access to timely data to maintain QualDash use |
5 | Upload of data to QualDash by non-technical staff | + |
In-person guidance for first upload Quick reference training materials |
Audit support staff learn and are supported to upload data to QualDash | = | Data are uploaded to QualDash routinely, providing access to timely data to maintain QualDash use |
Appendix 10, Table 23, brings together the CMO configurations that made up the initial QualDash programme theory, which was presented in Chapter 5, with the additional CMO configurations relating to adoption, providing an extended QualDash programme theory.
Discussion
This chapter has described how we combined several data collection methods to iteratively develop strategies intended to support the adoption of QualDash. However, as with the design of QualDash, we had limited engagement from cardiologists, with only one cardiologist participating in the adoption focus groups. Reflection on the adoption strategies is provided in Chapter 7 in the light of how they worked (or not) to support adoption of QualDash and the reasons for this.
Chapter 7 Installation and initial use of QualDash
Overview
QualDash v1.0 was successfully installed at four sites between June and July 2019 and in the fifth site in December 2019. This chapter presents the next phase of the QualDash biography, describing the process of installation, feedback from users based on initial experiences of using QualDash and the customisations made to QualDash in response.
The process of installation
As described in Chapter 5, owing to the emphasis placed on the importance of timely data for QI, QualDash was hosted on site servers to give staff control over how frequently they uploaded data to QualDash. Placing QualDash on trusts’ servers was also perceived to have other benefits. A limitation of hosting QualDash at the University of Leeds was that site staff would need to have a university account to log in to the university systems, which would limit access to particular users and could have acted as a barrier to use. However, this meant that there needed to be a process of installing QualDash into each site (Appendix 11 describes this process and the challenges experienced). In this chapter, we focus on a challenge the intended QualDash users were witness to – the initial uploading of data – highlighting contextual factors that influenced the installation process. From a realist perspective, such early experiences themselves are important contextual factors, potentially shaping later responses to QualDash.
Part of the installation process was to upload 3 years of real PICANet and/or MINAP data for the first time. Even in sites in which installation was completed by the end of July 2019, challenges were experienced that delayed the process and affected routines for maintaining QualDash post installation, as Box 4 illustrates.
QualDash was configured in the site A IT office in June 2019. On 20 June 2019, the research team visited the PICU offices to upload PICANet data into QualDash and complete installation. Mai Elshehaly installed RStudio for the audit co-ordinator. However, for reasons not initially apparent, the download of RStudio did not support the required functionality to install software packages necessary to run the R script, which meant that data could not be uploaded at this visit. As a temporary measure, PICANet data were sent to the clinical informatics office, at which the correct version of RStudio was installed and MINAP data were uploaded. PICANet and MINAP data were uploaded in this office by Mai Elshehaly on 21 June 2019. Liaison between Mai Elshehaly and trust IT clarified that the packages could not be installed on the audit co-ordinator’s PC due to ‘firewall ‘issues. Although the IT department managed to install a different version of RStudio, the research team then encountered a new challenge. The audit co-ordinator’s computer was an old 32-bit Windows machine (Microsoft Corporation, Redmond, WA, USA), which meant searching historic archives to find the correct version of RStudio (current versions of RStudio do not support 32-bit machines). Although PICANet data could be uploaded by clinical informatics, the PICU team were keen to have control over how frequently data were uploaded. Therefore, it was suggested that RStudio be installed on a PC belonging to a paediatrician (also a champion) in the same office as the audit co-ordinator. Installation of RStudio was confirmed by the paediatrician on 18 September 2019. Subsequently, Mai Elshehaly visited the PICU office on 3 October 2019 to show the audit co-ordinator and paediatrician how to upload data. The data were run through the R script at this visit. However, the data could not be uploaded into QualDash because there was no access to the necessary server from this PC. Therefore, further liaison with the IT department was necessary to see if they would be happy to receive and upload the formatted data at this time. Subsequently, IT established a shared location accessible over the site intranet and the R script was enabled to deposit the data into this shared location from which QualDash reads directly.
The example in Box 4 highlights that, although the server in site A was set up in June, the installation of QualDash was not completed until July because of unforeseen challenges with RStudio and service PCs. Furthermore, although installation was completed within expected timelines, a routine for uploading PICANet data that would enable the PICU to view timely data was still not established in October 2019. Here we see that, although the PICU at site A had resources to support engagement with NCA data in terms of audit support staff and a local database providing access to their own data, a contextual factor that constrained the installation of QualDash was a lack of resources in terms of IT.
User experience and feedback
Post installation of QualDash v1.0, champions were sent an e-mail notifying them of how they could access QualDash. In all three PICUs and in the cardiology departments at sites A, B and D, access was via a URL that they could copy and paste into Google Chrome (Google Inc., Mountain View, CA, USA). In the cardiology department at sites C and E, shortcuts were placed by site IT staff on the intranet home pages of the trust and service, respectively. In this way, all staff on trust premises could potentially access and use QualDash, as long as Google Chrome was installed on their PCs. Champions were asked to trial the dashboard and feedback to the research team any issues.
Initial issues and their resolution
QualDash was developed and tested using simulated data. Therefore, the first time that QualDash was used with real MINAP and PICANet data was following installation and we anticipated that more validation and debugging would be required at that point. Two key sources of error were anticipated: (1) different data formats (e.g. time and date formats) supported on different server machines and (2) special cases that are encountered in only real-world data and are impossible to fabricate in simulated data. With new software, new requirements are also expected to arise as users start to use the software and, therefore, the research team anticipated that they would need to work to address new requirements to improve QualDash functionality for users.
The research team prepared to work with our champions to address major issues before wider dissemination of QualDash to avoid it being tarred with a ‘dodgy brush’ by users. To address issues efficiently, a process needed to be established for receiving and responding to user feedback. Hosting QualDash on local servers affected this process. Over 40 issues (a combination of bugs, where the software did not act as expected, and new requirements) were reported across sites between August and December 2019. Issues affecting correct data interpretation were considered to be top priorities. Figure 7 provides an overview of the process through which issues were identified and resolved by Mai Elshehaly, with the support of site staff and the research team.
FIGURE 7.
Process for identifying and resolving issues.
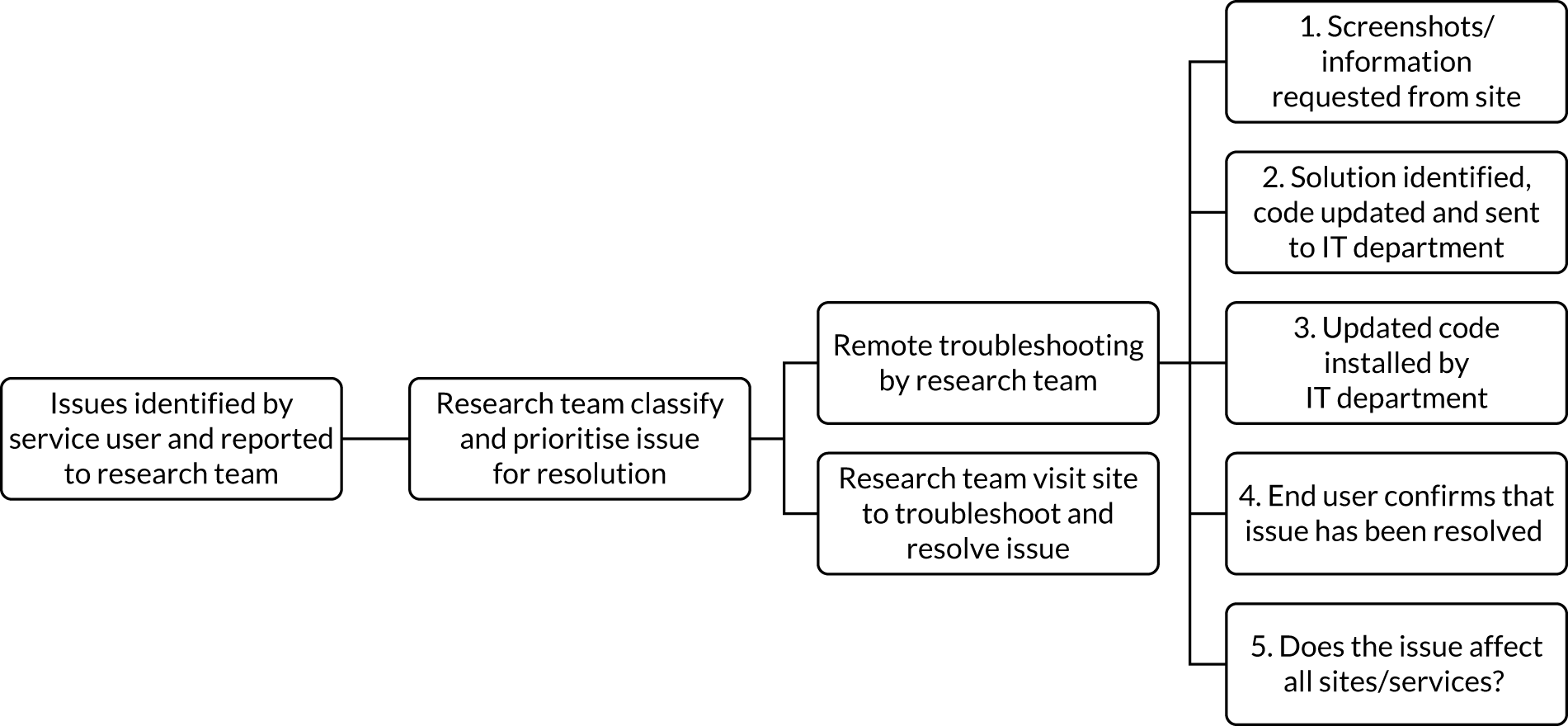
The research team could not access the QualDash software without being on site. Consequently, resolving reported issues had to be completed remotely, which could be a time-consuming and complex process, or Mai Elshehaly had to visit the site, which involved co-ordination with IT staff and/or clinical staff. Often, a site visit was required if the issue could not be satisfactorily resolved remotely. An example of this is provided in Box 5.
On 26 July 2019, a cardiologist in site E reported via e-mail that the numbers displayed in the door-to-angio QualCard appeared too small. To troubleshoot the problem, the cardiologist was asked to explain the problem in more detail and provide a screenshot. This information was received by the research team on 30 July 2019. A potential explanation for the problem, suggested by Mai Elshehaly, was that the date formats in the fields from which the measure was calculated might be different from the R script. Mai Elshehaly requested a single anonymised row of data to investigate the date formats. The line of data was received on 1 August 2018 and was found to be in line with the R script. The next investigation was the field ‘2.01 initial diagnosis’, which determines whether a patient is STEMI or NSTEMI. Mai Elshehaly noted that numbers in the QualCard may appear small if data are not being entered as expected in this field, and suggested that a ‘quick fix’ would be to remove the ‘NSTEMI only’ from the measure to display all patient records. The cardiologist confirmed that this fix could be applied as a temporary measure but the BPT is based on NSTEMIs only, so it was not the best option long term. Mai Elshehaly sent instructions to the IT department on 2 August 2019 to update the server. The update included adding ‘diagnosis’ as the first tab in the pie chart subview so it could be used to highlight NSTEMI records in the main bar chart. Mai Elshehaly then visited the site and discovered the problem was that MINAP does not only have non-unified headers but also non-unified values (categories) in each field. In this case, the STEMI/NSTEMI values were stored differently in this site than in some other sites, with the categories being using to filter the view spelled differently (extra capitalisation and extra spaces inserted).
NSTEMI, non-ST elevation myocardial infarction.
In addition, there was the challenge that when remote resolution required an update to the code that this was dependent on IT staff installing the updated code. For example, staff in the IT department at site B were sent an update for the PICANet dashboard in late October 2019, but at the end of November 2019 PICU staff reported that this update was not yet available to them.
Intuitive use compared with intuitive data interpretation
A feature of QualDash that was anticipated to support uptake and use, as formulated in the QualDash programme theory, was that it was intuitive to use. As described in Chapter 5, usability was rated as very good and observations in the think-aloud sessions suggested that participants found use to be intuitive. Therefore, there was confidence that QualDash enabled a certain level of intuitive use. Installation of QualDash v1.0 enabled site staff to view QualDash with real data for the first time. This prompted them to scrutinise QualDash in a way that they had not previously. For example, QualDash determined the labels for the pie chart segments based on what was stored within the data file, with the consequence that some pie chart segments were labelled with numbers as opposed to descriptors. QualDash’s interactive functions (e.g. using the pie chart segments to highlight the distribution of the variable across the months in the main bar chart) worked well. However, the labelling issue meant that users could not understand what the segment represented. This problem was a constraint on the use of the data interrogation functions, an attribute of QualDash linked in the programme theory to QI. As this had the potential to affect data interpretation across sites, the issue was prioritised for resolution. It was resolved by the creation of data definition files that translated these to actual labels wherever those labels were not made available by the audit file.
Alongside labelling, site staff familiar with service data identified what they perceived as ‘anomalies’ in the metrics displayed, which often transpired to be examples of where the configuration (i.e. metric calculation) did not meet their expectations. The programme theory hypothesised that, through displaying key metrics (and associated with the mechanisms) by site staff for monitoring and improving quality, QualDash would engage site staff in the use of NCA data and, therefore, the QualCard metrics were originally configured using variables and definitions provided by participants (from site A) at the phase 2 co-design workshops. These issues were not identified in the design workshops or adoption focus groups because simulated data were used to populate the dashboard. However, when champions scrutinised QualDash using real data, they identified discrepancies between the QualCard measure and their understanding of service performance. Examples of this are provided in Boxes 6 and 7.
On 24 July 2019 (post installation of QualDash), the audit clerk at site B sent an e-mail to the research team stating that the data on the mortality graph showed that a patient had passed away in March, when in fact they were admitted in March and passed away in May. It was explained to the audit clerk that the QualCard shows mortality by month of admission. Data from the co-design workshop had indicated that it would be helpful to view the measure in this way, but the QualCard could be customised to show mortality in the month in which it took place, if required by users. The measure was discussed further with site B staff at a customisation meeting held on 18 October 2019 at which it was confirmed that it was preferable to the PICU to view death by month of event. As a result, the two other participating PICUs, one of which was involved in the original co-design, were consulted about the mortality QualCard and both requested the same change.
At a visit to the PICU in site A on 4 September 2019, QualDash was discussed with the audit co-ordinator. They noted that counts in the bed-days and extubation QualCard were different from the graphs that they had created for the same months. They explained that this discrepancy might have occurred because QualDash shows bed-days and ventilation by date of admission rather than the month in which the events occurred. This discrepancy was reported to Mai Elshehaly, who met with the audit co-ordinator and a paediatrician (PICANet lead and champion) to discuss the measures further. Discussion revealed that it was impossible to calculate ventilation days by month of event without a manual calculation. Owing to the way the data were captured in the PICANet dataset, generating the metric requested required significant input from Mai Elshehaly to rebuild the data model and to adapt the R script to iterate through daily activity data, capturing the start and end dates of ventilation events. The measures were discussed with site staff at the two other participating PICUs, and across all PICUs the QualCards were adapted in this way.
Scrutinising QualDash with real data was an important part of the design and development process, surfacing the specific variables needed to calculate the key metrics that users wanted to use for quality monitoring. Adapting the QualCards became an important part of adoption, with learning from one site prompting discussion with, and revision of QualDash in, other sites.
Adoption activities around QualDash v1.0
Appendix 9, Table 22, lists the demonstrations of QualDash given, with some before and some after installation of QualDash v1.0. However, these demonstrations did not always go as planned. The site B information analyst described trying to publicise QualDash:
They presented QualDash at a consultants’ meeting on 18 July 2019 but was unable to bring the dashboard up on the screen, owing to a local connectivity issue that was not to do with QualDash. Nevertheless, they talked about the dashboard to the consultants and there was general interest, especially from their departmental manager.
Site B
This excerpt shows a site champion in action as envisaged, trying to enthuse potential users within a consultants’ meeting. Unfortunately, owing to a site technology issue, QualDash could not be demonstrated. Furthermore, by the time of observations in November, there had not been another opportunity to present QualDash in such a meeting.
Delivery of the e-bulletin and crib sheets was intended to take place post installation of QualDash v1.0. The materials were sent to the site E champion on 15 October 2019 because this champion confirmed that the issues that they had reported with QualDash v1.0 had been resolved. However, the e-bulletin was not sent to the remaining sites at this time, owing to a combination of events and learning post installation. Although the customisations to QualCards that were made can be thought of as a form of iterative development, typical in software design, the QualCard configration issues represented a signifcant concern. QualDash was accessible to all site staff, not all of whom would recognise discrepencies between a QualCard and the metric typically used in service monitoring. The research team was awaiting confirmation from local collaborators/champions in the remaining cardiology services that they had, at least, trialled the dashboard before disseminating the e-bulletin. The site A cardiology champion did not have Google Chrome installed on his PC, despite requests to the trust IT department, and, therefore, the champion was unable to open QualDash and provide this confirmation nearly 2 months post installation. A response to a request for feedback had not been received from site C and in site D QualDash was not installed until December 2019. Furthermore, in response to queries regarding the QualCard metric configurations and concerns about clarity of labelling, the research team decided to add QualCard descriptions to the crib sheets. These descriptions were considered to be additions that where necessary to support users to interpret the data correctly, an issue that had been raised in the adoption focus groups with regard to enabling a wide range of users to access data easily.
QualDash v2.0
The issues identified, including the metric queries that affected all sites, informed the development of QualDash v2.0, which sought to address issues and improve QualDash use. The potential impact on data interpretation emphasised the importance of clearly labelling QualCards so that users were made aware which measures they were interacting with and how they had been configured. Therefore, QualDash v2.0 provided ‘pop-up’ labels, incorporating a description of the card, that would appear when users hovered their mouse over the QualCard title. A ‘help tab’ and training video were also added. Other changes responded to requests from users. For example, in site C, the PCI project assistant enquired whether or not it was possible to view data by financial, rather than calendar, year, as this was how their service reported data and, therefore, QualDash v2.0 allowed users to see data by calendar or financial year. Images of QualDash v2.0 are provided in Figures 8 and 9.
FIGURE 8.
PICANet dashboard v2.0 (using simulated data).
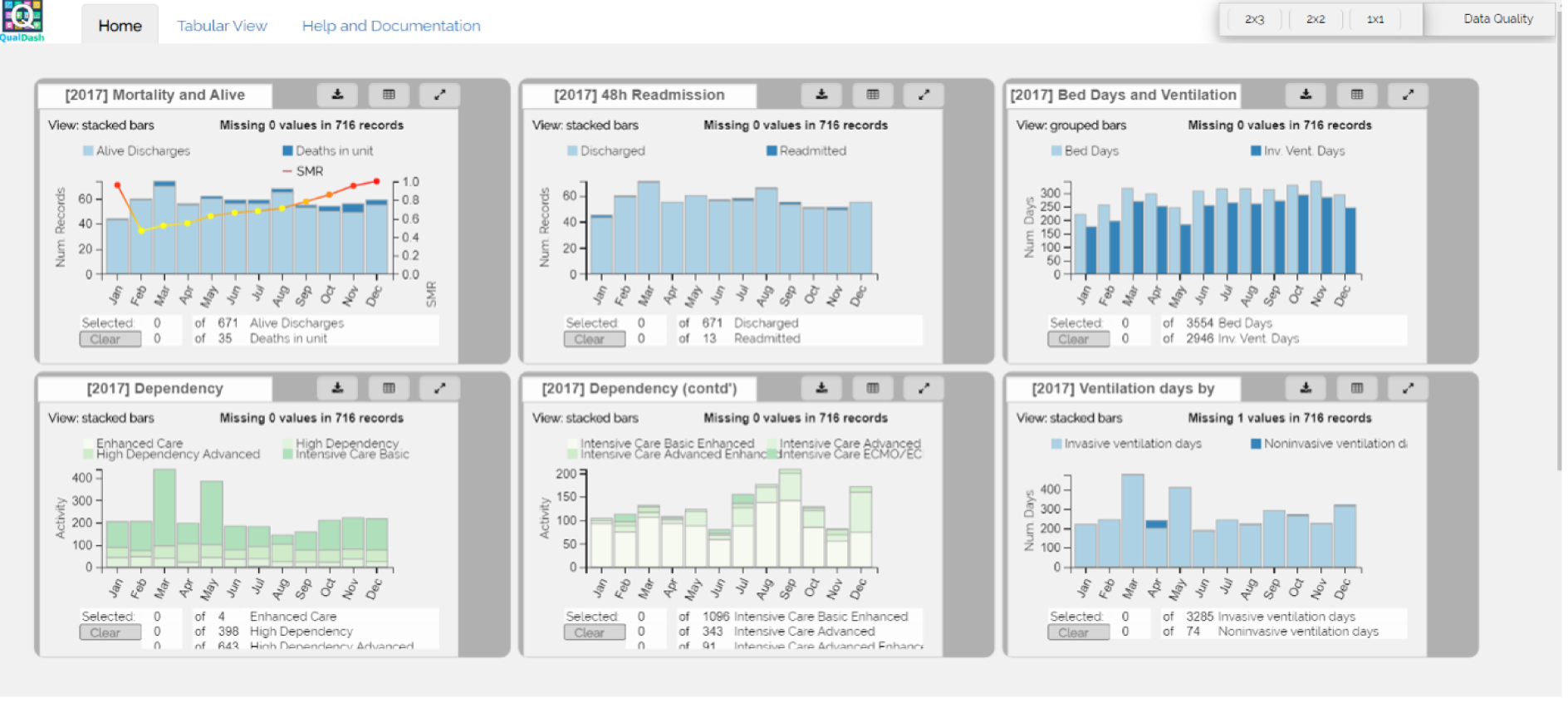
FIGURE 9.
MINAP dashboard v2.0 (using simulated data).

In January 2020, QualDash v2.0 was installed in sites A, C and D, and the updates were demonstrated to site staff. Installation of QualDash v2.0 represented an opportunity to disseminate the e-bulletin and updated crib sheets within sites, with confidence that the dashboard and updated crib sheets supported data interpretation. The e-bulletin and crib sheets were sent to a group of core users in site D. However, demonstrations of QualDash v2.0 revealed that further adaptations were needed before QualDash offered users the measures expected and most useful for quality monitoring.
In the cardiology department at site C, the PCI project assistant and service manager discussed that it would be useful to be able to view ‘call-to-balloon’ with a target time of 150 minutes (rather than 120 minutes) and door-to-angio with a target time of 90 minutes, as these were measures reviewed within the service. Again, customisation was found to be a key attribute of QualDash, as Mai Elshehaly was able to adapt QualDash in the way requested at the time of installation. However, there were limitations to what customisation could be offered. For example, the PCI project assistant reported that the service reviewed median ‘call-to-balloon’ times, but QualDash could not be adapted to calculate medians. Furthermore, a cardiologist (the local collaborator) asked to view data for a neighbouring service within the same trust to compare performance. This request was documented but could not be completed because of time constraints.
In the PICU in site C, the research team was not able to access 3 years of PICANet data and the R version installed on the server was outdated. These issues meant that the SMR, as requested by the site champion, was not displayed on the dashboard and that only four out of six QualCards were populated with data.
During demonstration of QualDash v2.0 at site D, a cardiologist familiar with the data set reported concern with the counts in the discharge on gold-standard drugs QualCard. This feedback led to a thorough investigation of the QualCard and how STEMI/non-STEMI was defined, as described in Box 8.
The research team met with the site D champions on 15 January 2020 to discuss the QualCards displayed in QualDash v2.0. A cardiologist (also the local collaborator) noted that the number of patients receiving gold-standard drugs on discharge appeared to be small. The cardiologist requested that the measure was calculated using patients discharged with a diagnosis of NSTEMI, STEMI, acute coronary syndrome, unstable angina, and angina only to rectify the counts. Mai Elshehaly updated the R script to show those patients as requested. However, the discrepancy prompted two further activities: (1) the cardiologist asked that all QualCards undergo a ‘sense check’ by site clinicians for the team to have confidence that the visualisations were meaningful and were configured as expected by the service before wider dissemination, and (2) the research team began to consult with other cardiology services to understand whether or not they also wanted the gold-standard drugs on discharge QualCard adapted to show patients with specific diagnoses. E-mails were sent to the cardiology local collaborator at site A detailing the adaptation requested at site D and asking whether or not they required a similar change. Concurrently, the research team worked to establish how the metric was calculated by NICOR in the national report, as this could provide clarity on the universally accepted form of the metric, from which customisations could be made. These enquiries highlighted a need to clarify how NICOR defined NSTEMI and STEMI (currently QualDash used definitions provided at the co-design workshop). Therefore, an e-mail was sent to NICOR asking which patients should be included in gold-standard drugs on discharge and how they defined STEMI and NSTEMI for the MINAP report. A response was received from NICOR on 28 February 2020 stating that ‘In reporting drugs on discharge we have combined all acute myocardial infarction patients – i.e. all those with a final discharge diagnosis of either STEMI or NSTEMI (4.02 option 1 and option 4)’ and explaining how gold-standard drugs on discharge was calculated. This information was also confirmed at a NICOR meeting attended by the research team on the 6 March 2020. It was decided to provide the NICOR version of the metric, that is what was acceptable nationally, and also to consult clinicians and add customisations, if requested.
NSTEMI, non-ST elevation myocardial infarction.
This vignette (see Box 8) highlights that, similar to PICANet metrics, clarifying how metrics should be calculated for MINAP required end users to scrutinise QualDash with real data. In most cases, the customisations were supported by QualDash. However, further details on the configuration needed to be collected, which could be challenging and time-consuming for the research team. Furthermore, ensuring that each metric reflected the relevant patient cohorts was necessary prior to further dissemination.
The queries received following the installation of QualDash v2.0 were accompanied by reservations from site champions about undertaking further dissemination work before the issues and uncertainties had been resolved, illustrated in the fieldnote extracts from the demonstrations below:
Paediatrician [and champion] requested addition of a label to clarify whether the SMR is the ‘raw’ or ‘adjusted’ calculation. The paediatrician says that it is important users know what they are looking at to have confidence in the integrity of the display. ME [Mai Elshehaly] later clarified it is the adjusted SMR.
Site A, PICU customisation meeting 16 January 2020
[Referring to the gold-standard drugs QualCard configuration] cardiologists comment they have come across this sort of thing before with new systems developed by people outside their service: the data going in are correct, but it doesn’t make sense, and time is needed to check it to make sure the data are interpreted correctly. They know what their own data should look like, though people outside the service, including managers from their trust, don’t always have that understanding and can misinterpret data that aren’t displayed correctly. They stress that it’s worth putting the time in to make sure the data are displayed and therefore interpreted in a meaningful way, otherwise the systems are not useful to them.
Site D, observation 15 January 2020
As two QualCards were not populated with data and the SMR was not displayed at all, the paediatrician [and champion] thought that it might be best to delay wider dissemination of QualDash, as in its current state it might be ‘a bit of an anticlimax’.
Site C, PICU demonstration of v2.0 9 January 2020
Owing to these reservations, it was decided that dissemination of the e-bulletin and crib sheets would resume after these issues had been investigated and any updates to QualDash (QualDash v2.1) had been successfully installed across sites.
Demonstrations of QualDash v2.0 also highlighted to the research team that some of the issues identified with QualDash v1.0 persisted, for example some labels in the default subviews continued to display numbers rather descriptors (in sites where a description field was not available in the data). In some cases, we hard-coded textual interpretations in configuration files, but it was not feasible to do this for every individual field in both audits. To help to cultivate user confidence, systematic checks of the dashboard functions were performed at site A, identifying issues that needed resolving prior to installing QualDash v2.1. These checks covered issues such as interaction between the main and subviews, exporting graphs and raw data, and the labelling in the main view and default subviews. Where possible, issues were resolved by Mai Elshehaly and a troubleshooting page was added to the crib sheets to help users to overcome minor problems that could not be addressed in the software, with the intention that adoption activities would resume once this work was completed. Site A was used to perform these checks and make updates to QualDash, as the research team had regular access to the server and real data, and was supported by the trust informatics team. The validation process, whereby counts in the visualisations were compared with the raw data, was repeated after the updates were completed. Installation of updates and validations were completed in site A on 13 March 2020. However, this coincided with lockdown measures in response to the COVID-19 pandemic across the UK. On 16 March 2020, the research team made the decision to pause installation of QualDash v2.1 because of COVID-19. The crib sheets were sent to the site A champions for dissemination. However, following government and university advice to work from home where possible, on 17 March 2020 all further on-site research activity was suspended.
Refining and extending the QualDash programme theory
In developing the QualDash programme theory, a starting assumption was that QualDash would be developed and maintained to a standard that would not discourage further use or ‘tar it with a dodgy brush’. As the installation process unfolded and initial use took place, it quickly became apparent that a significant amount of work was still needed to get to this point in development. A further assumption was that QualDash maintenance (e.g. data upload and software updates) would not be problematic or act as a constraint on its use. However, installation and initial use revealed that choice of server location and unforeseen complexity in metric calculations created challenges that undermined those assumptions, disrupting the planned adoption work. In practical terms, this meant that the research team’s efforts post installation became focused on continued dashboard development, overcoming challenges of updating trust servers from a remote location and working to support the establishment of data upload routines, rather than supporting champions in their role. Further development work was expected as a usual process in technology development, but the specific work required here was such that some champions were reluctant to engage colleagues in its use and/or unwilling to use QualDash themselves until they were satisfied that certain changes had been made. This response made the research team cautious about dissemination in sites that had not expressed the same concerns until they had confirmed that they had scrutinised the issue and were satisfied with all QualCards.
Normalisation process theory can help with the interpretation of our findings regarding adoption. NPT explains the work undertaken to implement new processes and technologies. As described in Chapter 3, NPT consists of four constructs: (1) coherence (i.e. sense-making), (2) cognitive participation (i.e. engagement/buy-in), (3) collective action (i.e. operational work to enact practices) and (4) reflexive monitoring (i.e. appraisal of the benefits and costs). Figure 10 illustrates how these constructs resonate with the planned adoption strategies and their intended impacts.
FIGURE 10.
Planned adoption strategies according to NPT constructs.

The QualDash adoption strategy utilised interventions that aimed to stimulate the work described in the four constructs. In this study, clinical champions were also study collaborators and, for this reason, they understood the aims of QualDash and were committed to supporting those aims, indicating good levels of coherence and cognitive participation within this user group. Post installation, champions began work to operationalise QualDash (e.g. using its interface and functions to explore the NCA data set), which is a subconcept of collective action known as interactional workability. It was in these early interactions that champions reported concerns about the configuration of some QualCards. These concerns were not easily or quickly resolved within or across sites because of QualDash being located on local servers. This meant that assistance from IT and support staff was needed to update and maintain QualDash to support its uptake and adoption. How to engage these staff in this work, a subconcept of cognitive participation known as enrolment, was not fully accounted for in the adoption strategy. Without resolution of the key concerns, clinicians’ confidence to champion QualDash, a subconcept of collective action known as relational integration, was diminished, resulting in a suspension of adoption activities. This finding spoke to a practitioner theory identified in the adoption focus groups, that is, novel technologies can be tarred with a ‘dodgy brush’ and go unused if they do not work as intended on first interactions. Although this idea does not explain the entirety or complexity of our findings, it captures a user experience that appeared to be occurring in some services.
Installing QualDash presented a number of challenges, particularly a lack of computing resources within sites constraining the process of data upload. Feedback from initial use of QualDash provided important lessons about the process of designing a quality dashboard and the requirements for such a dashboard. The usability evaluation of QualDash involved participants from sites A and B, using the QualDash prototype populated with simulated data. When staff used QualDash with real data from their own site, staff raised issues that they had not previously noted, concerning configuration of metrics. This suggests that it is important to use real data for usability evaluations such as these, carried out with staff with the expertise to interrogate the metric, particularly audit support staff. Appendix 12, Table 26, shows an extended and refined QualDash programme theory. The theory has been extended to include the issue of design (CMO configuration D1), although this is a CMO configuration that remains to be tested, and it has been refined to indicate that computing resources are a contextual factor needed for the process of data upload (CMO configuration A5).
Initial use of QualDash also highlighted that labelling of the metrics needed to state clearly which variables were displayed to support users in accurate data interpretation. Providing clarity about how the metrics were calculated via labelling was necessary for champions to have confidence to use and disseminate QualDash. This had implications for the CMO configurations related to adoption and those related to features of QualDash that supported engagement with NCA data. These refinements are shown in Appendix 12, Table 26.
Discussion
This chapter has described the process of installing QualDash and the initial responses. Some of the challenges described in this chapter can be considered as limitations of the design process, specifically the use of simulated data when undertaking the usability evaluation of QualDash. They can also be considered as limitations of the development of adoption strategies (e.g. inadequate engagement of IT staff in this process and perhaps naive assumptions about the ability of IT teams and the available resources to enable QualDash use). Spending time at the sites as part of the design process may have highlighted the reality of these constraints.
The findings also point to a tension between the iterative nature of development (i.e. the need for revisions to QualDash was expected by the computer scientists on the team) and the need to avoid new technologies being ‘tarred with the dodgy brush’. This tension has long been recognised within the computing literature and resolving it might require a fundamentally different approach to the design process. 147 Although we used a co-design approach, in the computing literature a co-realisation approach has been argued for, where there is a continuous cycle of design and revised work practice, taking place within the work setting, with the aim of enabling users to grow into a technology. 148,149 The emphasis is on ‘simple’ technology, in the sense that the system should provide only the necessary functionality and that, where possible, use should be made of technologies that are already available. The intention is not to be a provider of ‘bells and whistles’, but the provider of something that will afford work. 148 In this way, the dodgy brush can be avoided. As users became familiar with the technology and new requirements arise, the technology can be further developed. However, questions remain about how such an approach can be employed across multiple sites and issues of the resources required to facilitate such an approach.
Finally, it is necessary to reflect on the relationship between the research team and the champions. Over the course of the project, the researchers built up strong relationships with the champions. In some cases, the champions were local collaborators and, therefore, were committed to the project before it even began. Although champions would not promote QualDash because of their concerns, we may not have been able to maintain their ongoing commitment to QualDash without those strong relationships.
Chapter 8 Evaluating QualDash
Overview
This chapter addresses the fourth objective of the study, which sought to understand how and in what contexts QualDash might lead to improvements in care quality. As described in Chapter 7, throughout the period of observations between June 2019 and March 2020, customisation and refinement of QualDash was ongoing and delivery of the planned adoption activities was constrained. Nevertheless, QualDash was available across most sites by August 2019 and, therefore, observations to understand the impact of QualDash began. This was complemented by log file data from installation to end of July 2020, a survey of perceived usefulness conducted in August 2020 and analysis of MINAP and PICANet data.
Extent of use
Log files provided data on extent of QualDash use. Total number of uses per clinical area by site, as well as use of the functionality of saving the QualDash visualisations and exporting data to a table, are provided in Table 12, whereas Figure 11 shows how use in cardiology departments varied over time and Figure 12 shows how use in the PICUs varied over time. Although the numbers do not reveal any patterns in how the extent of use changed over time, there is clear variation in extent of use across sites and clinical areas. In the PICU in site A, QualDash was not used throughout the evaluation period, whereas use in cardiology departments started in March 2020. Greatest use was in the PICU at site B and in the cardiology department at site E, although in site E six of the 13 uses occurred in July 2019, suggesting an initial exploration of QualDash functionality. In the remainder of this chapter, we consider reasons for this variation, looking at the practices surrounding QualDash use, the actual and anticipated benefits perceived by QualDash users and the broader perceptions of QualDash. We conclude the chapter by reflecting on the extent to which the patterns of use provide support for, or reasons to reject or refine, our QualDash programme theory.
Site | Clinical area | Total number of uses (n) | Use of save functionality (n) | Use of table export functionality (n) |
---|---|---|---|---|
A | Cardiology | 3 | 3 | 1 |
PICU | 0 | 0 | 0 | |
B | Cardiology | 9 | 9 | 4 |
PICU | 41 | 0 | 3 | |
C | Cardiology | 10 | 3 | 3 |
PICU | 3 | 0 | 0 | |
D | Cardiology | 6 | 3 | 1 |
E | Cardiology | 13 | 3 | 2 |
FIGURE 11.
QualDash use in cardiology departments over the evaluation period.

FIGURE 12.
QualDash use in the PICUs over the evaluation period.
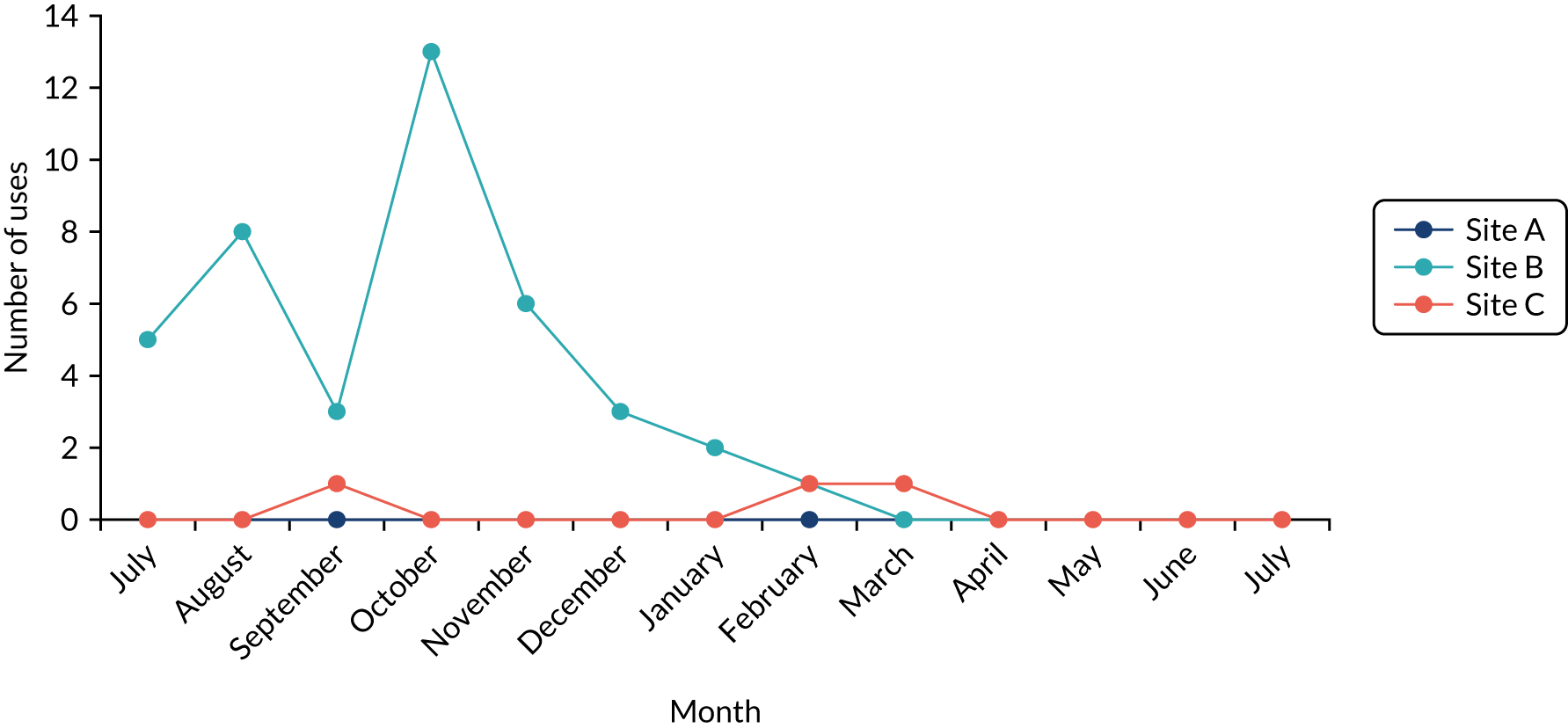
Practices surrounding QualDash use
Routines for data upload
Following initial upload of 3 years’ data into QualDash as part of the installation process, the intention was that those who had agreed to take on the role of data upload would learn to complete data upload autonomously and establish a routine for doing so, thereby providing users with access to data that was as timely as possible. The most routine and regular upload of data was in the PICU in site B, where the audit clerk reported uploading the data weekly. Despite not having an IT background, the audit clerk learned how to complete the data upload process with support from Mai Elshehaly. QualDash facilitated the reporting that the audit clerk had to do (described further in Time savings from using QualDash) and so they were motivated to learn how to complete data upload. In most sites, staff reported the intention to upload data monthly. However, staff changes complicated this. In the PICU at site C and in the cardiology departments at sites D and E, the person who originally agreed to complete the upload either left the organisation or moved to another role. In site E, data upload was further complicated by data extraction and upload not being completed by the same person (i.e. a clinical audit and effectiveness manager downloaded data from the supplier website and sent it to a member of staff in trust IT department to upload, neither of whom were users of QualDash).
Use of QualDash for monitoring care quality
QualDash use in cardiology departments
When adequately resourced with audit support staff, cardiology services tended to have well-established and semi-automated processes for generating performance reports. Therefore, QualDash ideally needed to facilitate production of the reports requested routinely. As described in Chapter 7, in the cardiology department at site C, several changes were made to QualDash to match the sites’ reporting requirements, including the ability to view data by financial year, incorporated into QualDash v2.0, and then local customisations to QualDash v2.0, changing the call-to-balloon target time to 150 minutes and the door-to-angio target time to 90 minutes. Even so, at a follow-up visit the PCI project assistant discussed:
I would really love to be able to use [QualDash], but it just doesn’t show me, you know, everything I need, [ . . . ] it just doesn’t do exactly what I need. But maybe if you spoke to someone like, the lead consultant, and you showed them what was available, and you said, does this answer, you know, some of your queries? But like the thing they’ve asked me to do, to look at the patients that have failed [within specific time points]. So can I . . . how do I look at those patients? Is it this, export?
The request to which the assistant refers was to see data for patients treated between specific time points (i.e. data that QualDash does not present in QualCards). The MINAP assistant used a number of data sets, one of which was MINAP, to produce reports when requested and further explained:
. . . it’s [QualDash] not got the exact criteria I need, I’d probably just do a quick pivot [table], and I’d get what I need straightaway.
The PCI project assistant perceived that it was easier for them to use existing systems and processes rather than QualDash. However, the assistant indicated potential users of QualDash, including the MINAP lead and the chest pain nurses:
I feel like [ . . . ] this is good for [chest pain nurse], because they are not good with Excel. So once they know how to use this, they can probably, you know, pick what they need.
These assumptions are supported by the log file data, with 7 of the 10 uses of QualDash in the cardiology department at site C being by nursing staff (there was also one use by audit support staff, one use by a doctor and one use where it was not possible to determine their role).
Compared with the cardiology department at site C, the cardiology nurse specialist in site A who supported audit participation and report production was unable to have Google Chrome installed on his PC to access QualDash. Therefore, the cardiology nurse specialist continued to use the service database and an Excel spreadsheet that they had created to produce reports when requested, not using QualDash at any point throughout the evaluation period. In cardiology department at site A, QualDash was used by a cardiologist who was motivated by a desire to understand how COVID-19 was affecting performance (discussed further in Chapter 9).
In the cardiology departments at sites B and E, QualDash was reported to be used in routine reporting and this is supported by the log file data. In site B, eight of the nine uses were by audit support staff (it was not possible to determine the role of the user for the other use).
In site E, phase 1 interviews revealed that MINAP data were not used routinely, but a cardiologist (a local collaborator and champion) was keen to introduce more frequent use of the data via QualDash. It was anticipated, therefore, that the cardiologist would lead use of QualDash. However, post installation of QualDash v1.0, QualDash use was discussed with the cardiologist:
I ask [cardiologist] if they have been using QualDash since its installation. The consultant says that they haven’t used QualDash since we first e-mailed the link to them in the ‘installation’ e-mail. When asked why, they explain that they haven’t integrated QualDash into their routines yet. They say that they are not used to having access to MINAP data in this way. Therefore, they sometimes forget that it is there to look at and use via QualDash. However, they say that there is no reason why they shouldn’t use it and that one way in which they could try and integrate it into their practice is in their ‘performance meetings’ where such data could be reviewed.
The cardiologist added QualDash to the agenda for the next directorate meeting. The meeting was used as an opportunity to demonstrate what QualDash could offer to the group (predominantly other cardiologists) rather than to monitor service performance. Although some improvement was noted by the cardiologist in data completeness, and meeting attendees responded positively to being able to make more use of the data, the meeting chairperson queried the accuracy of the data:
The meeting chair[person] queries the data displayed. They say that all the graphs steadily decline and ‘that can’t be accurate’. They ask [cardiologist who is QualDash champion] why the graphs are like that, who responds that the audit team must be behind with data collection. The chair[person] says it is ‘amazing’ to have access to real time data, and they should be able to make more use of MINAP data using QualDash. This comment ends the QualDash presentation and [cardiologist who is QualDash champion] closes QualDash and logs off the PC.
Log files from site E captured use by nursing staff (n = 5), a consultant (n = 2) and the clinical audit and effectiveness manager (n = 1). However, it was not possible to determine the role of the user for the other five uses.
QualDash use in paediatric intensive care units
All PICUs in the study were resourced with audit support staff who supported data collection and submission to PICANet and who reported quarterly to NHS England [using specialised services quality dashboards (SSQDs), which are used by NHS England specialised services commissioners to understand the quality and outcomes of services and reasons for excellent performance, and CQUINs]. In sites A and C, audit support staff produced reports for clinicians and managers and for review within clinical governance meetings. Reports included visualisations, such as bar charts that depicted performance, typically month by month, in specific measures of interest. In September 2019, the PICANet audit co-ordinator in site A was observed while updating reports routinely requested from them by clinical staff (within and outside the PICU) and managers. The reports were listed in a Word document with notes about what data were needed and how frequently these reports were required, categorised as ‘monthly’, ‘bimonthly’ and ‘intermittent’:
The audit co-ordinator explains that they update all reports monthly (a) so the data are less than a month old should anyone request it unexpectedly, and (b) because some of the measures reported have to be calculated manually, as the system hasn’t been programmed to calculate them automatically, e.g. for bed-days, the system aggregates the total number according to the patients’ admission date, rather than spreading the bed-days across the months that the patient was in the PICU. Therefore, the audit co-ordinator manually adds and subtracts bed-days to the appropriate months (by looking at admission and discharge dates of patients and counting the days in each using pen and paper) so that their report, which visualises bed-days month by month and over a 3-year period, are accurate.
The excerpt reveals the manual work involved in report production, using systems that were slow because of age:
The audit co-ordinator tells me they entered the query (to update the reports) into the Access database when first arriving at the office that day, as it can take over an hour to run.
When asked about QualDash at the end of the observation, the co-ordinator commented that it took a while to open on their PC. The co-ordinator described, as presented in Chapter 7, the discrepancy between the bed-days and extubation QualCard that calculated events by month of admission and the report requested from them that calculated the measure by month of event. Furthermore, QualDash had not been updated since July because of challenges establishing a data upload routine (see Chapter 7) and, therefore, the use of the Access database was necessary to produce the reports required.
Unplanned extubation was a key metric discussed in phase 1. Audit support staff enquired how QualDash could be used to access this metric post installation of QualDash v1.0. Unfortunately, as described in Chapter 5, owing to how PICANet captured these data, QualDash could not offer this metric as a QualCard bar chart. As an alternative, the measure was provided as the total number of unplanned extubations across the year in the subview pie chart (which did not interact with the main bar chart, as in other cards) of the bed-days and extubation QualCard. This meant that those who generated reports on unplanned extubation (a metric that is reported to NHS England quarterly by all PICUs) needed to use systems other than QualDash to generate the report. A similar constraint was discussed by the database manager in site C in October 2019:
The database manager says that the service database contains more detail than PICANet, e.g. when they produce reports for the business meeting, which includes CQUINs and HRG, they include information about what ward the patients have been referred from and where the patient was discharged to. [ . . . ] They also get ‘ad hoc’ requests for data on a ‘regular basis’ – these are often from [ . . . ] the CSU lead, the service manager, and the operations manager. The service database is updated daily, therefore it contains data that are more timely than PICANet and it has more detail about the patient pathway (e.g. referral ward and discharge destination). The database clerk says that clinicians often want more detail after an initial enquiry, e.g. if they ask for number of admissions, they then want to know next where those admissions came from.
QualDash, using only PICANet data, was unable to provide this information reported routinely by this database manager. Therefore, despite the manual work involved in generating reports at sites A and C, audit support staff did not use QualDash, as it was not perceived to facilitate their processes. This remained true throughout the observation period.
The PICU at site B had not used PICANet data routinely and formally in meetings before the introduction of QualDash. Staff at the site explained that this was because the unit was small and staff tended to discuss matters informally between themselves. However, like the other PICUs, the site did have to report data to NHS England, and, owing to not having a local database like sites A and C, the audit clerk used QualDash to prepare these reports, partly explaining the higher level of QualDash use in this site (25 of the 41 uses were by audit support staff, with the other 16 uses by consultants). The benefits that the audit clerk experienced from this are described further in Time savings from using QualDash. The matron also expressed an interest in using reports from QualDash in monthly sisters’ meetings, senior line managers’ meetings and ward meetings. The ward meetings, in particular, were seen as an important context in which to engage nurses with such data, as nurses tended to have little awareness of, or involvement with, PICANet in this unit.
Benefits of QualDash
Although Practices surrounding QualDash use described constraints on QualDash use, some users reported anticipated or perceived benefits of QualDash, relating to data quality and time savings. We discuss these further below.
Impact of QualDash on data quality
In line with the QualDash programme theory, a number of users anticipated that QualDash would have a positive impact on data quality. For example, the information analyst and champion in the cardiology department at site B wanted to enthuse consultants to use QualDash on the basis that they might complete MINAP data more fully if they use the data more, which would make the information analyst’s job easier. The information analyst also wanted to use QualDash to monitor and report on data quality, which could help them get more resources if they could provide evidence to managers of data being incomplete.
Similarly, the champion in site E, a cardiologist, highlighted positive impacts of QualDash on data quality:
The cardiologist says that when QualDash was first installed it enabled them to see where there was missing data and the audit team ‘cleaned up the data’ in response. Therefore, QualDash has already helped the service to get their data ‘a bit cleaner’.
The ITS analysis provided an opportunity to explore the impact of QualDash on data quality in terms of data accuracy and completeness. As described in Chapter 2, for MINAP, we looked at precision of arrival time (as a measure of accuracy) and whether or not ethnicity was recorded (as a measure of completeness). For PICANet, we looked at precision of admission time and discharge time and whether or not ethnicity was recorded. Times were considered precise if they were not on the hour or at half past the hour. The results are reported in Table 13. Analysis of MINAP ethnicity data for site E is not included, as there was only one record for which ethnicity was not known and, therefore, it was not appropriate for statical modelling of this kind. Similarly, analysis of PICANet ethnicity data for site A is not included, as ethnicity data were recorded for all patients, both before and after introduction of QualDash.
NCA | Dependent variable | Site | Parameter estimate (%) and SE | p-value |
---|---|---|---|---|
MINAP | Precision of arrival time | A | –0.281 (0.116) | 0.016 |
B | 0.437 (0.182) | 0.016 | ||
C | –0.492 (0.187) | 0.008 | ||
E | –0.192 (0.229) | 0.402 | ||
Recording of ethnicity | A | –0.435 (0.086) | < 0.001 | |
B | 2.471 (0.588) | < 0.001 | ||
C | 0.200 (0.357) | 0.574 | ||
PICANet | Precision of admission time | A | –0.073 (0.101) | 0.472 |
B | 0.423 (0.236) | 0.074 | ||
C | –0.399 (0.088) | < 0.001 | ||
Precision of discharge time | A | 0.390 (0.104) | < 0.001 | |
B | 0.259 (0.181) | 0.155 | ||
C | –1.020 (0.105) | < 0.001 | ||
Recording of ethnicity | B | 0.368 (0.246) | 0.135 | |
C | –0.614 (0.205) | 0.003 |
For MINAP, the proportion of records with a precise arrival time did not change following introduction of QualDash in site E, but rose in site B and declined in sites A and C (Figure 13). The proportion of records with ethnicity recorded did not change in site C, but rose in site B and declined in site A (Figure 14). These outcome patterns can to some extent – but not fully – be explained by drawing on the qualitative and log-file data. In site A, there was very limited QualDash use and, therefore, we would not expect to see an impact of QualDash, positive or negative, in this site, whereas in site B there was use of QualDash and an expressed intention to use QualDash to improve data quality. Although levels of QualDash use were similar in site C, our experience on the ground was that there was very little engagement with QualDash, in terms of both the dashboard and the study as a whole and, therefore, again, we would not expect to see an impact of QualDash, positive or negative, in this site. In site E, the champion perceived that QualDash did lead to an improvement in data quality, but this was not supported by analysis of the MINAP fields that we considered. However, we also know that MINAP data were not used routinely in this site and it may be that the absence of a routine that integrated QualDash into the work practices of the clinical team constrained the impact of QualDash.
FIGURE 13.
Proportion of precise MINAP arrival times for (a) site A; (b) site B; (c) site C; and (d) site E. Dotted orange line indicates installation of QualDash. Aqua line indicates the trend.
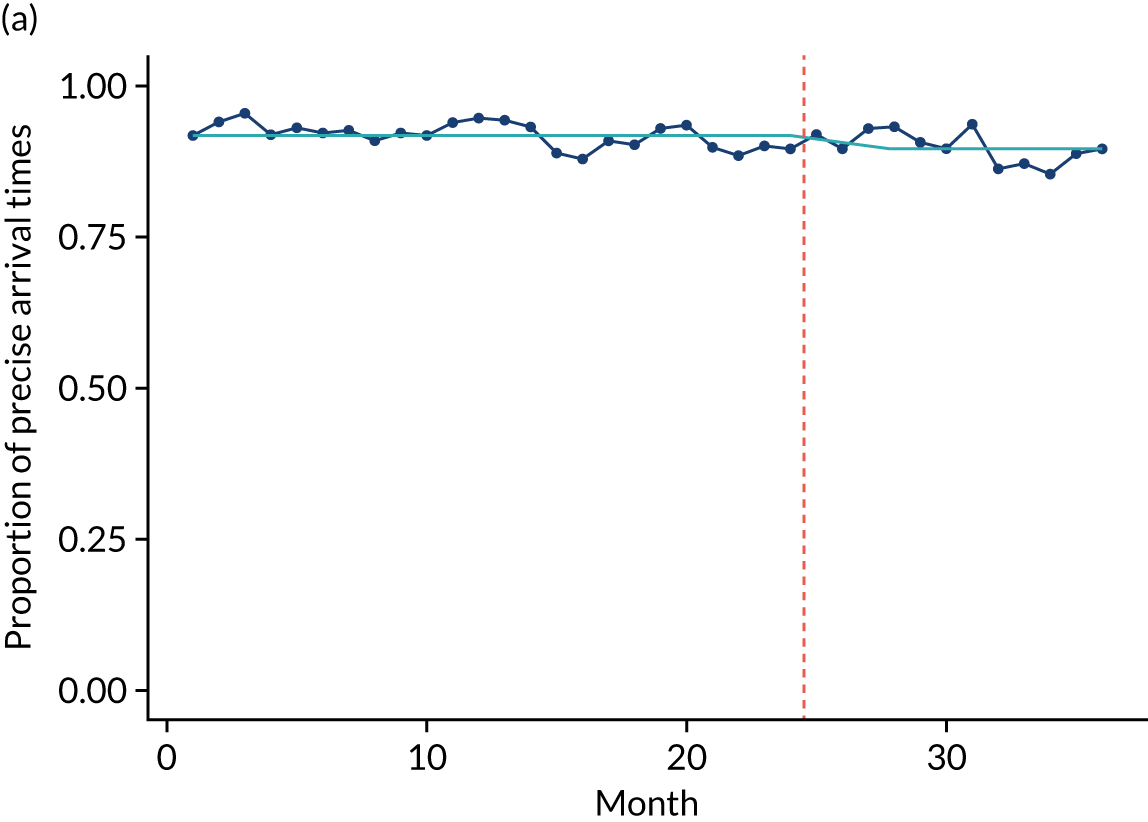
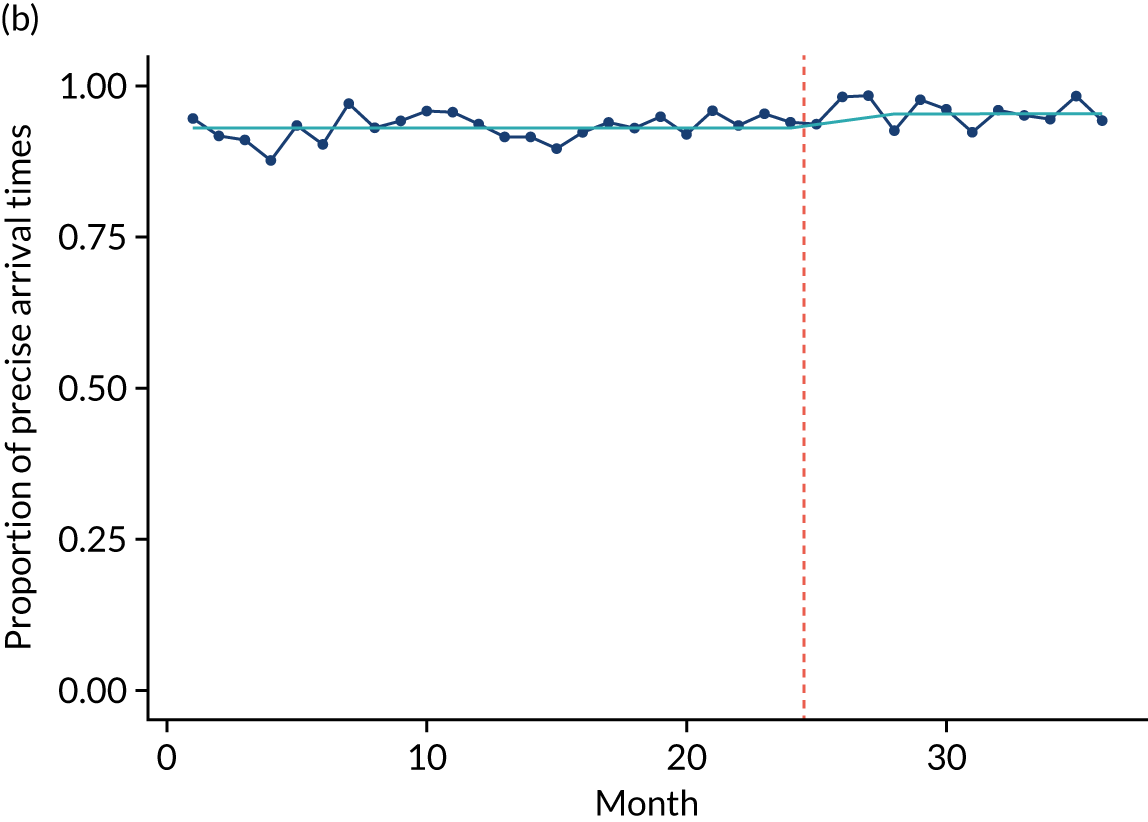


FIGURE 14.
Proportion of MINAP patients with known ethnicity for (a) site A; (b) site B; and (c) site C. Dotted orange line indicates installation of QualDash. Aqua line indicates the trend.

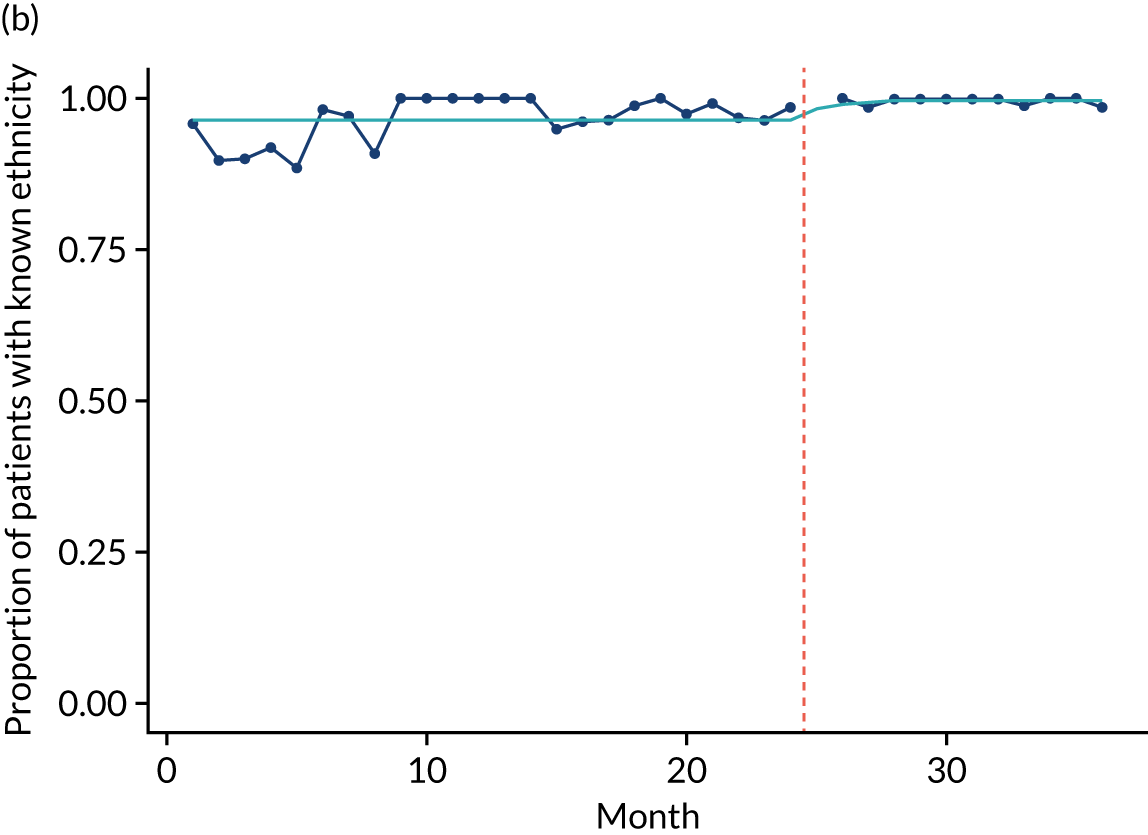
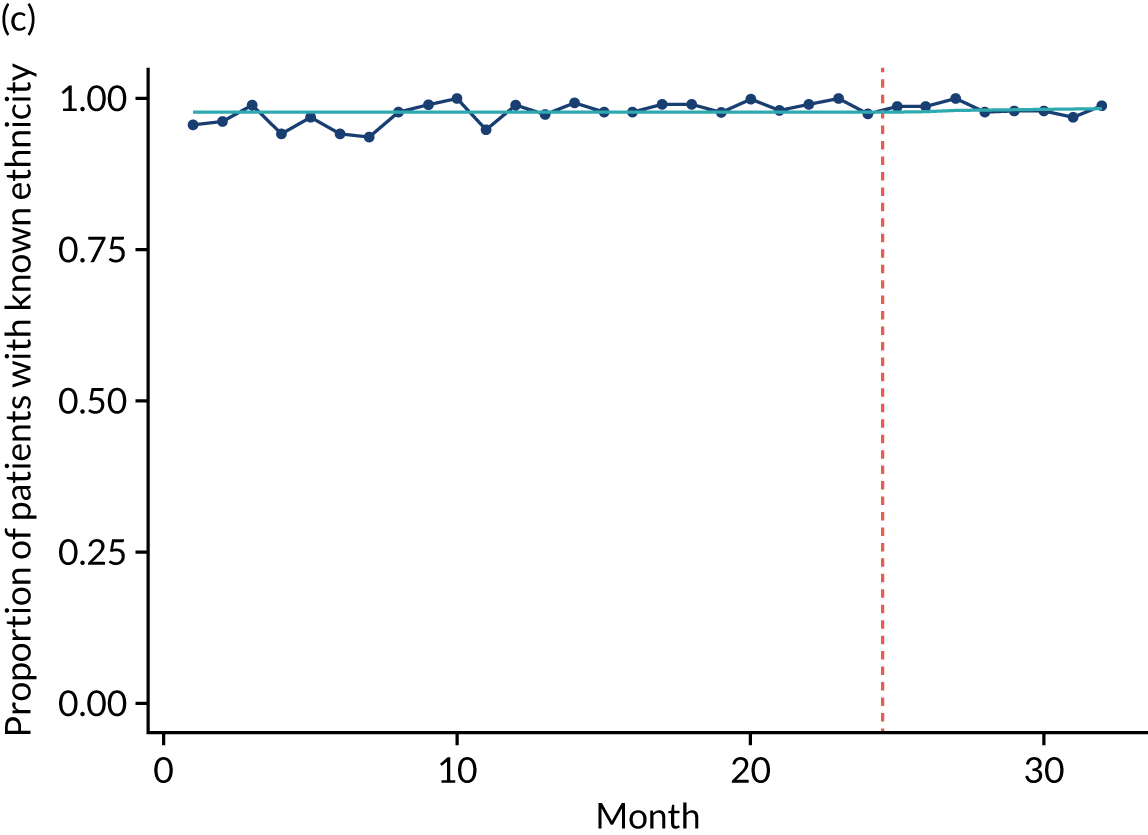
For PICANet, the proportion of records with a precise admission time did not change following introduction of QualDash in sites A and B, but declined in site C (Figure 15). The proportion of records with a precise discharge time did not change in site B, but rose in site A and declined in site C (Figure 16). The proportion of records with ethnicity recorded did not change in site B, but declined in site C (Figure 17). The PICU at site B is where we would expect to see an increase in data quality, as this is the site in which staff previously did not have access to data and in which there was significant QualDash use, yet the analysis does not support this hypothesis. The PICU at site A did not use QualDash and QualDash use in the PICU at site C was significantly limited and, therefore, we would not expect to see an impact of QualDash, positive or negative, in these sites. The decrease in data quality in site C is possibly explained by a change in who was responsible for entering the PICANet data that occurred during the study period.
FIGURE 15.
Proportion of precise PICANet admission times for (a) site A; (b) site B; and (c) site C. Dotted orange line indicates installation of QualDash. Aqua line indicates the trend.
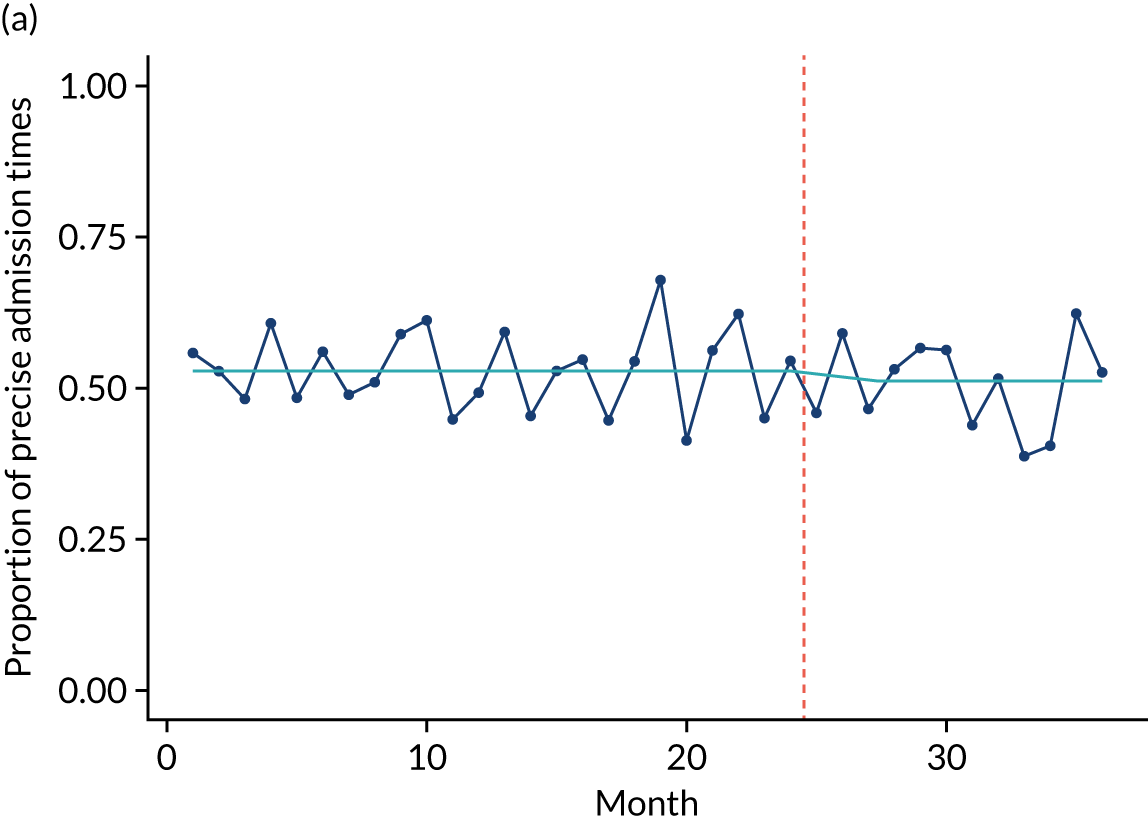


FIGURE 16.
Proportion of precise PICANet discharge times for (a) site A; (b) site B; and (c) site C. Dotted orange line indicates installation of QualDash. Aqua line indicates the trend.



FIGURE 17.
Proportion of PICANet patients with known ethnicity for (a) site B; and (b) site C. Dotted orange line indicates installation of QualDash. Aqua line indicates the trend.

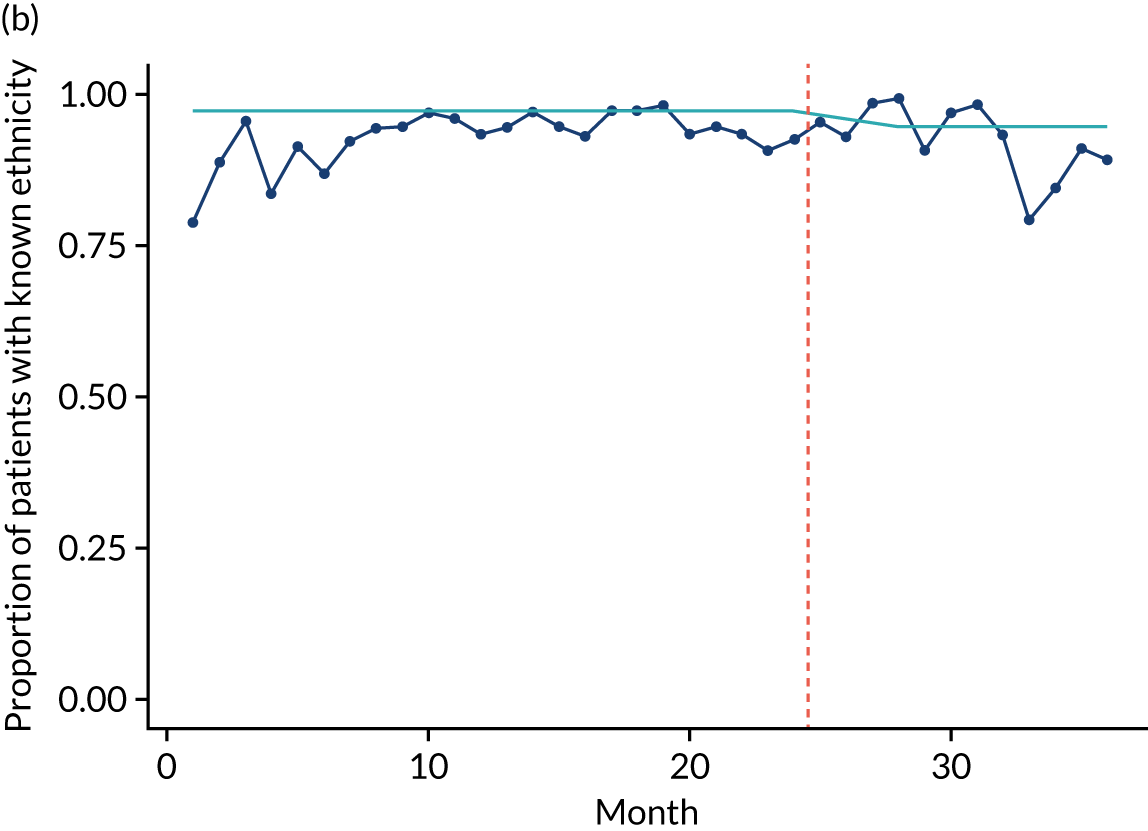
Time savings from using QualDash
As described above, the PICU at site B did not use PICANet data routinely for quality monitoring or improvement prior to introduction of QualDash. A major way in which the data were used, however, was to populate fields in the mandatory quarterly NHS England SSQDs. Before QualDash, collecting this information involved the audit clerk consulting a number of hard-copy and electronic data sources, including the ward diary, the ward admissions books and the patient administration system. The audit clerk also had to obtain bed occupancy rates from the matron and unplanned extubation rates from consultants. It used to take the audit clerk a half-day to collect these data (one-third of their part-time working week); however, following installation of QualDash, it took a few minutes. Not all SSQD information was included within PICANet (and, therefore, within QualDash) and some manual work was, therefore, still needed to finalise the submission, but QualDash’s introduction meant that, for example, the audit clerk no longer had to use a calculator to laboriously work out bed-days, 48-hour readmission rates and mortality rates. The audit clerk expressed their delight in an e-mail to the research team, following the 2019 SSQD data submission:
Just wanted to say QualDash has saved me hours of work with regards to data I submit to NHS England. It’s fab . . . Took me about 2 mins [minutes] to extract what I needed whereas before would have taken me an afternoon. So thank you.
Perceived usefulness and ease of use
The questionnaire, sent to 35 participants at the end of the evaluation period, received 23 responses (i.e. a 66% response rate). Eighteen of the 23 respondents (78%) had experience of using QualDash. Table 14 shows the average rating of each of the TAM statements. Although the average rating for all statements was positive, ratings were higher among those who had used QualDash than among those who had not used QualDash and ratings were higher for ease of use (i.e. statements 7–12) than for usefulness (i.e. statements 1–6).
Statement | Average rating (all respondents, n = 23) | Average rating (QualDash users, n = 18) |
---|---|---|
Using QualDash in my job enables me to accomplish tasks more quickly | 3.35 | 3.44 |
Using QualDash improves my job performance | 3.30 | 3.38 |
Using QualDash in my job increases my productivity | 3.22 | 3.31 |
Using QualDash enhances my effectiveness on the job | 3.26 | 3.31 |
Using QualDash makes it easier to do my job | 3.30 | 3.44 |
I find QualDash useful in my job | 3.65 | 3.88 |
Learning to use QualDash has been easy for me | 3.74 | 4.00 |
I find it easy to get QualDash to do what I want it to do | 3.48 | 3.75 |
My interaction with QualDash is clear and understandable | 3.74 | 3.88 |
I find QualDash to be flexible to interact with | 3.48 | 3.69 |
It has been easy for me to become skilful at using QualDash | 3.48 | 3.63 |
I find QualDash easy to use | 3.70 | 3.94 |
Although the anonymous nature of the questionnaire means that we are unable to link responses to particular sites, we are able to analyse them according to clinical area and role. Perceptions of QualDash were slightly more positive among PICU staff than among cardiology staff, with an average rating of 3.59 compared with 3.41 for all respondents (PICU staff, n = 9; cardiology staff, n = 12) and an average rating of 3.76 compared with 3.52 among QualDash users (PICU staff , n = 7; cardiology staff, n = 8). Figure 18 shows responses to the usefulness statements according to role, suggesting that audit support staff (n = 6) and doctors (n = 9) found QualDash more useful than nursing staff (n = 6), which is probably a reflection of nurses’ lower engagement with MINAP and PICANet.
FIGURE 18.
Response to usefulness statements according to role.
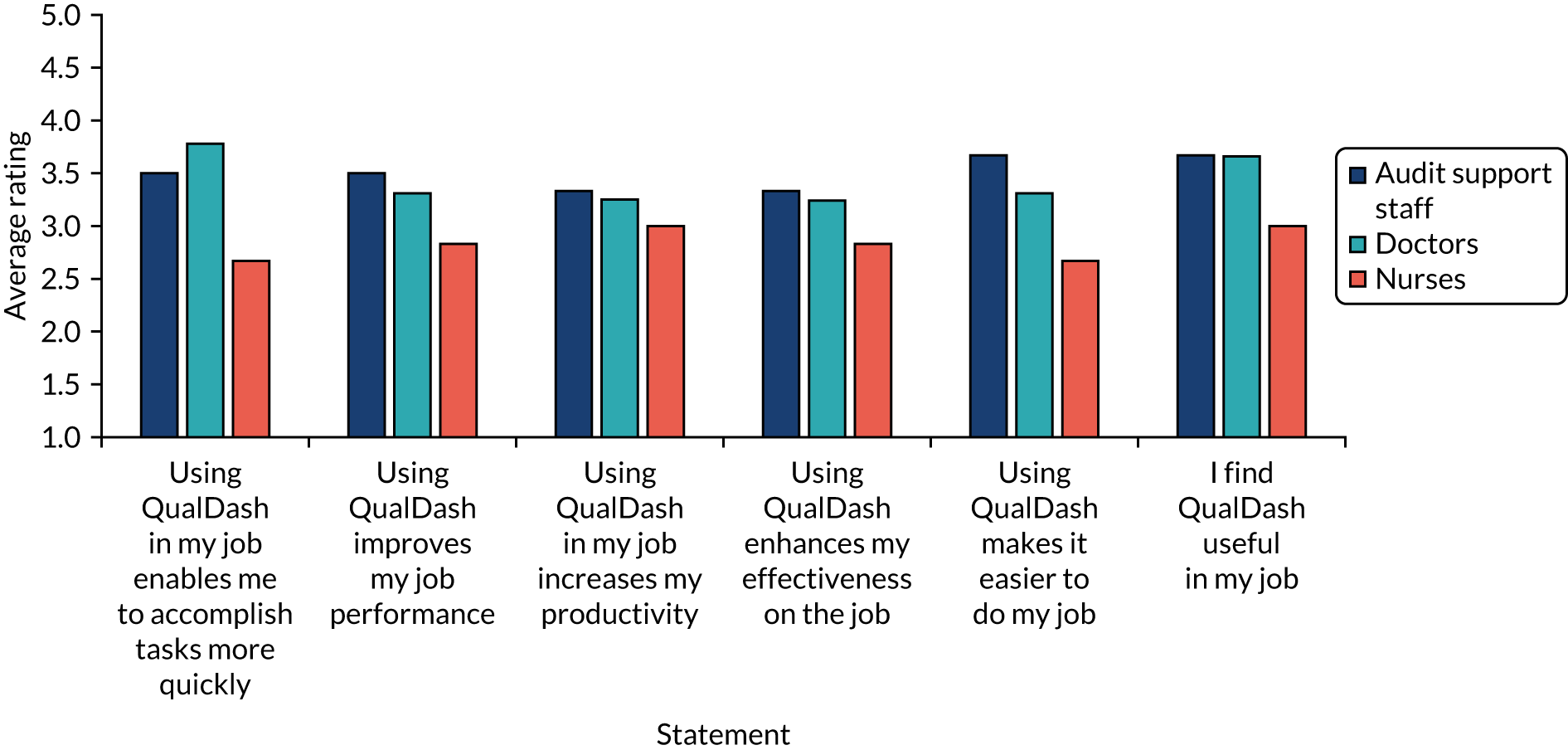
Fifteen respondents (65%) said that they would consider using QualDash beyond the evaluation period, including two participants who had not yet used it.
Refining the QualDash programme theory
The preceding sections have described how interactions with QualDash unfolded post installation of versions 1.0 and 2.0 across the five participating sites. Below we summarise these findings to refine the extended QualDash programme theory (as presented in Appendix 12, Table 26). To widen the applicability of this refined programme theory, we have revised the language to refer to quality dashboards more generally, with a final programme theory of quality dashboards provided in Table 15.
CMO configuration | Context | + | Mechanism | = | Outcome | |
---|---|---|---|---|---|---|
Resource | Response | |||||
D1 | Staff who already use audit data and are expert in its interpretation | + | Usability evaluation using real data | Expertise: owing to their knowledge of the audit, the performance of their service in that audit and how the metrics are normally presented within their service, those who are expert in the audit are easily able to identify discrepancies between how metrics are displayed and their expectations | = | Dashboard revised prior to introduction so that metrics fit with user expectations |
A1a |
Teams already use audit data, facilitated by audit support staff Dashboard is successfully installed, is accessible and works as intended Audit support staff have confidence that metrics are calculated appropriately Necessary computing resources |
+ |
Functionality to support current queries and tasks Promotion of dashboard directly to audit support staff, emphasising how it supports current queries and tasks |
Awareness: audit support staff are aware of the dashboard and can see that it will allow them to undertake their work more quickly or easily | = | Audit support staff are motivated to use the dashboard |
A1b |
Clinical services where audit data use is desirable but variable because of constrained resources Dashboard is successfully installed, is accessible and works as intended Champions have confidence that metrics are calculated appropriately |
+ | Clinical champion and e-bulletin and/or meetings |
Awareness: teams are informed about dashboard (i.e. how it can be accessed, its functions and potential benefits) and log on to explore its utility Leadership and influence: teams trust the champion’s opinion and can see potential benefits of QualDash and so are willing to use it |
= | Clinical staff with interest in the audit (e.g. service managers) make assessment of if and how they can use QualDash to facilitate work processes |
A2 |
Clinical services in which audit data use is desirable but variable because of constrained resources Dashboard is successfully installed, is accessible and works as intended |
+ | Clinical (senior) staff engaged as champions for dashboard |
Leadership: clinical champions add dashboard to meeting agendas and lead its use in the meeting Influence: clinical champion’s colleagues are encouraged/inspired to use dashboard themselves based on the champion’s example |
= |
QualDash trialled in settings in which there is potential for integration into routine practices Champion’s colleagues use dashboard to assess if and how it can be useful to them |
A3 |
Dashboard is successfully installed, is accessible and works as intended Early interactions have established a role for the dashboard in use of audit data Routines for using audit data |
+ |
Dashboard is intuitive to use Quick reference training materials Champions available to support use |
Confident users: teams learn quickly how to use dashboard functions to support and benefit their work processes | = | Sustained and optimised dashboard use (i.e. dashboard not ‘tarred with the dodgy brush’) |
A4 | Upload of data to dashboard undertaken by IT staff who have the expertise necessary to complete such tasks with minimum training | + | Quick reference training materials | IT staff quickly learn how, and feel confident, to upload data to dashboard without further support | = | Data uploaded to dashboard routinely, providing access to timely data to maintain dashboard use |
A5 |
Upload of data to dashboard by non-technical staff Necessary computing resources |
+ |
In-person guidance for first upload Quick reference training materials |
Audit support staff learn and are supported to upload data to dashboard | = | Data uploaded to dashboard routinely, providing access to timely data to maintain dashboard use |
Q1 |
Teams have processes in place (e.g. clinical governance meetings) for monitoring service performance using data available to them Teams constrained in ability to use audit data routinely because data are not considered timely, accurate and/or complete Teams motivated to use dashboard and feel confident in ability to do so Data are uploaded to dashboard, providing access to recent data |
+ |
Easy access to visualisations of key metrics Clear labelling of metrics |
Data quality: teams interact with dashboard to utilise data, underpinned by the mechanisms of professionalism, incentives and competition. As the teams understand the metrics, they can determine whether or not data displayed are timely, accurate and/or complete. If not, efforts are made to adjust data collection processes to benefit from dashboard Teams use the dashboard to embed audit data within their monitoring processes |
= |
Improvement in data timeliness, accuracy and completeness, although impact is dependent on resources allocated to audit participation As data quality improves, dashboard use increases |
Q2 |
Teams have processes in place (e.g. clinical governance meetings) for monitoring that patient care is safe and effective and/or for monitoring performance in relation to service funding Teams resourced to use audit data routinely (e.g. audit support staff are available to produce reports that are timely and trusted as accurate by clinical staff) Teams motivated to use the dashboard and feel confident in ability to do so Data are uploaded to dashboard, providing access to recent data Necessary computing resources |
+ |
Visualisation of key metrics are in line with existing visualisations and staff ability to interrogate data Clear labelling of metrics |
Facilitation: teams use the dashboard in meetings because it facilitates direct access and monthly monitoring of safe and effective care (underpinned by the mechanism of professionalism) and/or are linked to service funding (underpinned by the mechanism of incentives) because they are confident that the calculation of the metrics matches their expectations Automation: audit support staff are confident that calculation of metrics matches their expectations and use the dashboard to produce reports when requested by clinical and managerial staff, as the dashboard automates the calculation and visualisation of metrics |
= | Reduced time spent by clinical and audit support staff in accessing, and generating visualisations of, audit data |
Q3 |
Teams have processes in place (e.g. monthly clinical governance meetings) for monitoring service performance Teams that do not, or are not able to, monitor key metrics routinely (e.g. because of constraints in resources to produce reports using audit data) Teams motivated to use the dashboard and feel confident in ability to do so Data uploaded to dashboard, providing access to recent data |
+ |
Web-based access to key metrics considered markers of safe and effective care Clear labelling of metrics |
Interrogation: teams use the dashboard because it facilitates access to audit data considered key markers of care quality, they understand the metrics and visualisations highlight discrepancies between performance and targets and/or changes in performance month by month, which they take action to address (underpinned by the mechanisms of professionalism and incentives) | = |
NCA data integrated into routine service monitoring processes Introduction of QI initiatives in relation to key metrics where resources allow Over time, improvement in those metrics |
Q4 |
Teams have processes in place (e.g. monthly clinical governance meetings) for monitoring service performance, but existing systems produce static reports and do not provide functions to interact with and drill down into audit data Teams are resourced to make practice changes Teams are motivated to use QualDash and feel confident in their ability to do so Data uploaded to QualDash, providing access to recent data |
+ |
Ability to interact with audit data and explore relationships between variables Clear labelling of metrics |
Data interrogation: teams use the dashboard in meetings, instead of traditional static reports, because they understand the metrics and the dashboard enables them to interrogate anomalies in the data (underpinned by the mechanisms of professionalism and/or incentives), helping them to understand what has affected performance and enabling them to identify appropriate QI initiatives | = |
Introduction of appropriate QI initiatives where performance is not in line with expectations, where resources allow Over time, improvement in metrics that QI initiatives target |
Q5 |
Teams asked to produce reports and recommendations for managers and other groups about service performance Teams motivated to use dashboard and feel confident in ability to do so Data are uploaded to QualDash, providing access to recent data Necessary computing resources |
+ |
Easy access to audit data and visualisations that match existing visualisations and can be exported into reports Clear labelling of metrics |
Reporting: teams choose to use the dashboard to produce performance reports requested by other groups because they see that it removes the manual work involved. Teams are confident that the calculation of the metrics matches their expectations | = |
Reduced time spent by team in report preparation Use of audit data at divisional and organisational levels via dashboard outputs Over time, divisional and organisational dashboard use increases as teams become aware that they can access the data they require directly |
Q6 |
Teams receive data requests from managers Managers are motivated to use the dashboard and feel confident in their ability to do so Data are uploaded to dashboard, providing access to recent data |
+ |
Web-based access for multiple users Clear labelling of metrics |
Access: managers will use the dashboard to access the information they need quickly and easily. In addition, managers are confident that they understand the metrics | = |
The dashboard streamlines use of audit data for managers The dashboard reduces time spent by audit support staff/team in producing data reports for managers |
Q7 |
Teams need to evidence performance to managers and other groups to support a case for practice change (e.g. in business meetings with managers) Teams are motivated to use the dashboard and feel confident in ability to do so Data are uploaded to the dashboard, providing access to recent data Necessary computing resources |
+ |
The dashboard visualises key metrics, which match existing visualisations, and these visualisation can be exported into reports and presentations Clear labelling of metrics |
Evidence for change: teams use the dashboard to monitor service performance, underpinned by the mechanisms of professionalism and/or incentives. When teams identify a QI need that requires additional resources, they use the dashboard to report evidence, which can convince trust groups with power to provide resource for the change is needed. Teams are confident that the calculation of the metrics matches their expectations | = | Trust groups with power to allocate additional resource to teams are convinced of need for change, particularly where key metrics relate to trust priorities (e.g. relating to trust reputation or avoiding penalties/receiving incentives) |
Adoption activities for increasing uptake
The QualDash programme theory was expanded to include a number of CMO configurations that hypothesised how and why certain activities would support uptake and adoption of QualDash. We recruited champions and, in collaboration with them, QualDash was promoted at relevant meetings. The QualDash programme theory hypothesised that use of champions and promotional activities would support the adoption of QualDash, underpinned by leadership in its use, influencing others to use it by raising awareness about what QualDash offered and how it can benefit work processes. Owing to issues in dashboard design, we found that champions across most sites did not choose to lead use of QualDash because they wanted reassurance that metrics were configured as expected (in the case of consultants) before they used their influence to promote wider use or because they did not perceive benefits to their role (in the case of audit support staff). However, the idea that a clinical champion could lead QualDash use in appropriate settings was supported by qualitative data from site E where a clinical champion put QualDash on the agenda for their directorate meeting and presented QualDash within the meeting itself; however, based on the log files, this did not appear to prompt use by other staff. This may be because support for data collection was constrained, meaning that what was displayed was not an accurate measure of performance and, therefore, staff did not perceive it as optimally useful for QI.
Some support for CMO configuration A3 is provided by those cases where staff were motivated to use QualDash and did use QualDash, notably audit support staff in the cardiology department and the PICU at site B. These audit support staff appeared to find QualDash intuitive to use and this intuitive use is a feature of QualDash that is supported by the survey findings. However, these were staff who already had routines for using NCA data. By contrast, the clinical champion at site E was motivated to use QualDash but did not, reflecting that, not previously having access to MINAP data, they were not in the habit of doing so. This suggests that routines for using NCA data need to be established for QualDash to be used. In the revised programme theory, we have captured this additional contextual factor.
In CMO configuration A1, as a contextual factor, we did not initially include differences in resources in terms of (1) access to NCA data and (2) having audit support staff with the time and skills to produce visualisations of NCA data. However, our findings suggest that those sites that already have systems and processes in place for engaging with NCA data will be less likely to use a quality dashboard, such as QualDash. It is also questionable whether or not clinical staff would take over this work (vs. having audit support staff undertake this work). This suggests that the adoption strategy for such users should be directed at audit support staff, as QualDash should support the specific queries and tasks that these staff currently undertake, and the adoption strategy should not only involve promoting the features of QualDash but also show how it allows audit support staff to undertake their work more easily or quickly. Configuration A1 is also dependent on having the necessary computing resources, in terms of both hardware and software. Consequently, we break configuration A1 down into two CMO configurations to reflect such differences in context.
Configurations A4 and A5 are concerned with uploading data to QualDash. Again, our findings point to the need for necessary computing resources for audit support staff to be able to do this. As described in Chapter 7, the audit clerk in site A had agreed to upload data to QualDash, but was unable to.
Improving data quality and timeliness
In CMO configuration Q1, we hypothesised that, in certain contexts, QualDash may result in improved data quality and timeliness. Although some sites identified issues with data quality through using QualDash and sought to then improve data quality, our ITS analysis of audit data does not support the hypothesis that QualDash use results in an improvement in data quality. Although we identified the impact of reduced resources on the ability to engage with NCA data and saw QualDash as a way to address this, such reduced resources will also have an impact on the ability of teams to improve data quality.
Report generation
In CMO configuration Q2, we hypothesised that QualDash would be used because it facilitates report generation in sites that already produce reports using NCA and other data sources (e.g. in the cardiology departments and the PICUs in sites A and C). In practice, audit support staff in these sites chose to use their existing systems. This choice was influenced by the metrics that QualDash initially displayed, some of which were not configured as typically used by the service, and because QualDash was unable to display some metrics typically reported. In addition, difficulties in uploading data to QualDash meant that, for the PICU at site A, QualDash did not provide them with timely data. Again, computing resources also presented a constraint in terms of speed and having the necessary browser.
In CMO configurations Q5 and Q7, we hypothesised that teams would use QualDash to produce reports requested by groups outside the immediate clinical service team because of the ease of accessing NCA data and the ability to export visualisations, resulting in reduced time spent in report preparation and increased use of NCA data at divisional and organisational levels. Within our observations, we did not see the production of reports for divisional and organisational level staff, either using QualDash or by other means. The log files reveal that the functionality for exporting visualisations was used in all five cardiology departments, although we do not know if and how these visualisations were used. There was no evidence of this functionality being used in the PICUs and, based on our observations, it is likely that audit support staff in the PICUs in sites A and C would use their existing systems and processes for producing such reports, as they do for reports used within the PICU. For these audit support staff to use QualDash for this purpose, again our findings would suggest the need for visualisations that match the visualisations they currently prepare and the necessary computing resources. However, QualDash did lead to reduced time spent in preparation of reports to NHS England in the PICU at site B.
Reducing variation in extent of use of national clinical audit data
In CMO configurations Q3 and Q4, we hypothesised how and why QualDash may work to introduce NCA data into routine monitoring processes in sites where NCA data were used in a more limited capacity (i.e. sites B, D and E). In sites B and E, our findings present some evidence to support these hypotheses, suggesting that quality dashboards can reduce variation in the extent to which sites engage with NCA data. However, what we were not able to observe over the study period was whether or not this led to the introduction of any QI initiatives.
Optimising number and range of users
In CMO configuration Q6, we hypothesised that, via web-based access using a URL on any trust PC, QualDash would enable a greater range of staff to interact with NCA data. In sites where managers requested data reports from audit support staff, QualDash could be used by these staff to access the data directly. Although observations and informal conversations indicated that there may be a number of potential user groups beyond site champions and local collaborators, ongoing dashboard development was prioritised over delivery of dissemination activities planned to engage a wider range of users, which was subsequently prevented because of the COVID-19 pandemic. Consequently, our ability to test this hypothesis was limited.
Discussion
This chapter has described our realist evaluation of QualDash. Although the data captured for this allowed us to further refine our theories, the COVID-19 pandemic meant that we were unable to capture as much data as we wanted. Consequently, we were unable to introduce QualDash v2.1 and assess the impact of this version, or undertake further work to support its adoption. We were also unable to work with our participants to refine our theories through teacher–learner cycle interviews.
In addition to the shortening of the data collection period, the ITS was weakened by the absence of control sites. The sample size for the survey was small and probably suffered from response bias, with those who were more positive about QualDash being more likely to complete the questionnaire. However, we did obtain a reasonable response rate of 66%. Despite these limitations, the use of multiple methods of data collection did allow us to gain insight into the factors that constrained QualDash use, providing learning for the design of future quality dashboards.
It is important to reflect on the position of the research team as both designers and evaluators of QualDash. The two qualitative researchers, Natasha Alvarado and Lynn McVey, supported Mai Elshehaly in the design and development of QualDash, including facilitating co-design workshops and liaising with site staff to inform ongoing development work. Natasha Alvarado and Lynn McVey also led data collection and analysis for the realist evaluation. There was a risk that their involvement in both software development support and evaluation could have introduced bias into the evaluation process because of a vested interest in QualDash’s success. The researchers attempted to minimise such bias by discussing their feelings about their roles, and the ethics implications, with each other regularly during the project, and reflecting critically on how their position may have an impact on interpretations of findings and reporting. Natasha Alvarado and Lynn McVey recognised that working closely with Mai Elshehaly gave them an appreciation of the significant levels of work invested in dashboard development and the strenuous efforts made to address user needs. At the same time, however, Natasha Alvarado and Lynn McVey also developed strong working relationships with potential QualDash users in the study sites, and were keen to support QualDash development so that it would be a useful tool for these staff. In this sense, then, the researchers felt themselves to be part of a team, comprising both users and QualDash project staff. Natasha Alvarado and Lynn McVey were committed to evaluating QualDash fully, and this had the potential to generate tension between their desire to ‘do right’ by the team and the evaluation process. Honest and supportive relationships within the project team helped to address this tension (e.g. RR, NA, LM and ME met weekly to discuss challenges and progress within the project). As a result, this report, although acknowledging the tremendous skill and hard work that Mai Elshehaly devoted to QualDash, also reflects transparently on the finding that despite this work, which included ongoing customisation to better meet user needs, for the most part, participants did not respond in the ways hypothesised in the CMO configurations.
Despite participants not responding to QualDash in the way that we had hypothesised, it could be argued that all three of our progression criteria for a trial were met. Although uptake was limited, it was used by > 50% of the intended users, having been used by 78% of the survey respondents, and the log files reveal use within seven of the eight services that we worked with across the five trusts. Data completeness improved or remained the same in those sites where there was greater QualDash use and, as shown by the survey, participants perceived it to be useful, with 65% of participants expressing some intention to continue using it. However, we have already noted the potential for bias in the survey results. Another source of data about the intention of sites to continue using QualDash comes from their response to an offer of receiving QualDash v3.0 (described in Chapter 9), with four of the eight services we worked with expressing the desire to have this version installed.
Chapter 9 QualDash in COVID-19
Overview
Although the COVID-19 pandemic stopped data collection for the QualDash evaluation, development work continued in response to new requirements that emerged at this time. Following a request from a region-wide Gold Command, we also sought to understand the requirements of such a body and how QualDash could be revised to support their work. In this chapter, we describe the new requirements that emerged from our sites, the changes that were made to QualDash in response, the challenges that occurred in introducing this new version of QualDash into our sites and the additional further developments to QualDash that this required. We then describe findings from the phase 5 interviews and the implications for the design of a quality dashboard to support a body such as Gold Command.
Impact of the COVID-19 pandemic
Installation and validation of QualDash v2.1 was completed in site A on 13 March 2020. The intention was to install QualDash v2.1 in all sites in March and April so that staff would have an opportunity to interact with the updated version before the evaluation ended and, having discussed with our SSC the limited uptake of QualDash, we planned to undertake further activities to support adoption, particularly:
-
continuing to discuss with the champions what could be undertaken to increase uptake and, where feasible, undertaking and/or supporting them to undertake these activities
-
sitting with intended users to demonstrate QualDash and show them the NCA data set, discussing with them what might encourage their use of QualDash and/or the data
-
discussing with users if and how the constraints we identified could be addressed, supporting them in implementing solutions where feasible and sharing lessons from other sites
-
discussing the NCA national reports with site staff, particularly the most recent PICANet report, how they consider their unit ‘performs’ and whether or not any activities (e.g. internal reporting) and/or changes occurred as a result.
However, the outbreak of COVID-19 meant that on 16 March 2020 installation activities were paused and on 17 March 2020, following government and university advice, all face-to-face data collection was postponed.
Adapting QualDash to support daily monitoring
QualDash was designed to support QI, with study participants telling us that to use QualDash for this purpose they wanted to see data broken down by month. However, the outbreak of COVID-19 led to interest in how QualDash could be used for more frequently monitoring care quality. An e-mail was received on 26 March 2020 from site A that said that they wished to use QualDash to assess the impact of COVID-19 on cardiology care. This was in response to data from China that suggested that people were less likely to present to hospital with a suspected heart attack during the pandemic and that people experienced delays to treatment when they did present to hospital (with such delays being directly associated with increased mortality). Mai Elshehaly discussed with a local collaborator at site A the adaptations to QualDash that would be required so that it could support this. There was a need to adapt QualDash so that the cardiology service activity could be reviewed daily during the COVID-19 pandemic. Therefore, Mai Elshehaly revised QualDash so that, in the history subview, data could be presented broken down by days, weeks, months, quarters or years (Figure 19).
FIGURE 19.
QualDash revision to support more frequent monitoring (using simulated data).
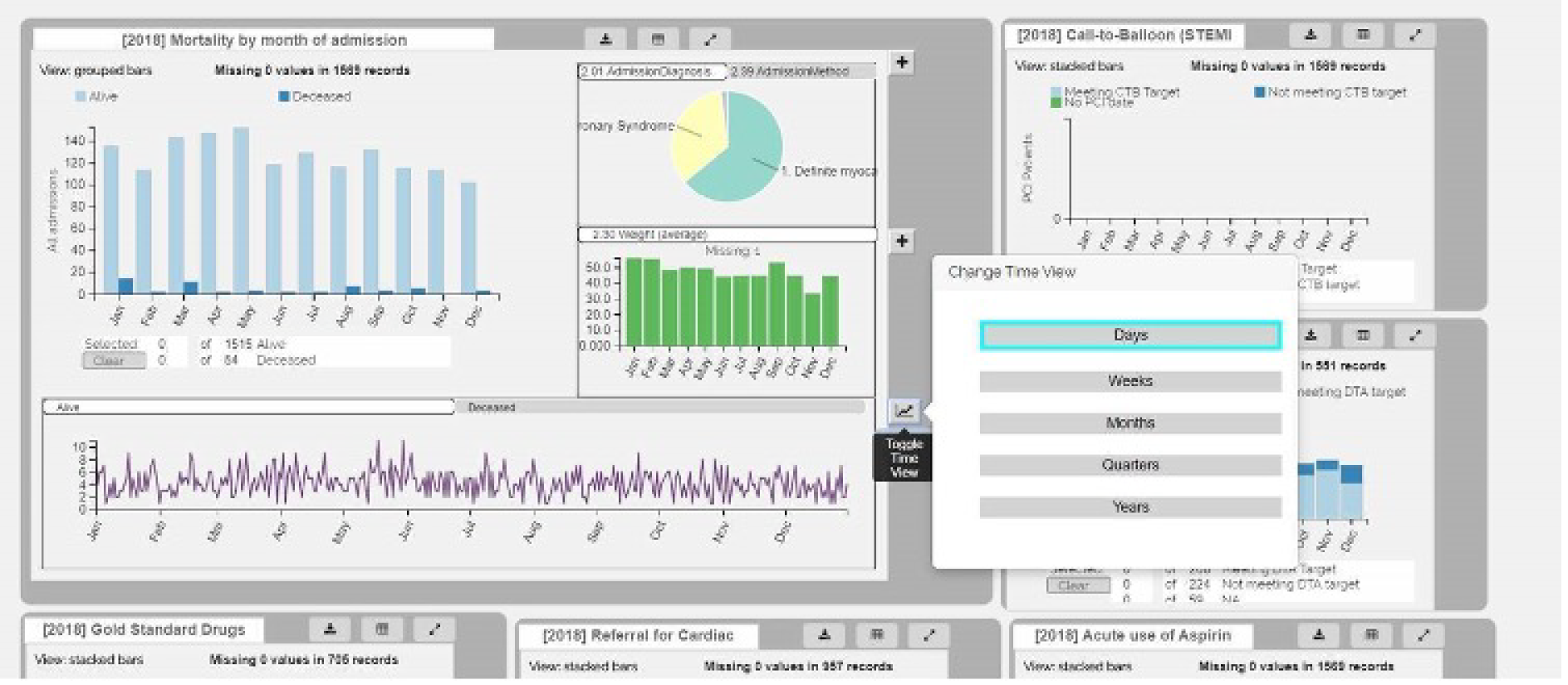
Owing to university concerns about, and restrictions on, accessing NHS sites, a request was made to site A for remote access to the server to install and validate the updated version of QualDash, but remote access was not possible. Although the IT team at site A would have been willing to install the update, our experience from earlier installations showed that thorough validation was required. Using QualDash to monitor care on a daily basis would also require data to be uploaded to QualDash on a daily basis and, therefore, there was a need to make this as simple as possible. Therefore, the decision was made to make additions to QualDash to automate validation and to enable automatic data upload so that data could be uploaded through a web browser without the need for RStudio. This additional development work was supported by the University of Bradford (Bradford, UK) through funding to enable staff to undertake research that would support the COVID-19 response, with this funding employing a software developer for 3 months.
All sites were approached to see if they would be interested in receiving this updated version (i.e. QualDash v3.0). The local collaborator at site E expressed a desire to receive this update, as did PICU staff at sites A and C. The local collaborator at site D felt that their site was not resourced to update MINAP data on such a regular basis or to make use of QualDash. At the time of writing, the functionality for web upload is complete and the functionality for automating validation is being developed.
Adapting QualDash to support population health decision-making
In April 2020, we were approached about adapting QualDash for a different context and data set, supporting the work of a region-wide Gold Command in responding to the COVID-19 pandemic through visualising a data set that links patient data across primary care, secondary care, community care and social care.
Interviewees described the membership and activities of Gold Command. Gold Command brings together the chief executive officers of health and social care services to inform the region’s COVID-19 response. One interviewee described Gold Command as ‘COVID focused’ and further discussed:
[Gold Command is] probably, in some ways, a little bit reactive to things, so almost this idea that . . . we’ve been focused on a lot of issues, so say PPE [personal protective equipment] and how we get that through, or the testing and those sort of things. I think going forward, the group that’s been our Gold Command, which is pretty much our exec[utive] boards anyway, our system exec[utive] boards, will continue to meet, but with a slightly different focus, which is more run on some of the big population issues, and setting out strategy and prioritisation of what we do together.
Interviewee 1
As we found in our phase 1 interviews with commissioners, our interviewees were accustomed to working with data and saw it as essential to their decision-making, suggesting a different context to that of the more limited and variable use found with MINAP and PICANet data. The interviewees described working with a wide range of data sources and were already using tools for this purpose. The interviewees had recently started using RAIDR (Rapid Actionable Insight Driving Reform), which is a dashboard and reporting platform developed by North of England Commissioning Support Unit (Durham, UK), and were also looking to use Microsoft’s Power BI (Microsoft Corporation, Redmond, WA, USA), which allows users to create their own dashboards and reports.
Dashboard requirements
Data requirements
There were several similarities with the requirements identified for QualDash. Data timeliness was seen as important if NCA data were to be used for QI and this was especially important in the context of population health monitoring:
We do get all the graphs and pie charts and visualisations and everything but it’s what is feeding into those graphs and charts [ . . . ] the graph will be as good as the information that’s feeding into it. So if we’re not getting live information from each and every sector then how do we know . . . When it comes to digging down, to, OK if something is not working, let’s say that suddenly there is a surge of elderly patients going into A&E [accident and emergency], and we want to know that we have commissioned a virtual ward, we have commissioned community matrons, we have commissioned district nurses, all these services – where is the problem? Where is there a sudden surge? Where do we need to fix . . . what do we need to fix? And we can’t fix until . . . we won’t have the information feeding into a system that evaluates that information.
Interviewee 2
There was also a desire to bring together different data sources, as the following quote illustrates. Some interviewees, but not all, wanted a single dashboard to address the workload involved in using several separate systems. One interviewee described longing for ‘complete information’:
So at the moment there are loads of dashboards, there’s a CCG dashboard, there’s a public health dashboard, there is the Institute of Health Research doing their thing, then every organisation has got their own business intelligence and analytics. So what we want to create, we want to create a system BI function, well, that we say is a single version of truth – so a single dashboard, a system-level dashboard. [ . . . ] The information is not there to facilitate the system to make those decisions at the system level. So what we want to do now is to create that information that gives the system leaders that can facilitate the system-level decision making. [ . . . ] Let’s say there is a system that is gathering all the information from the front end, not just to health but also about the care, about the deprivation, about the inequalities, all that information, something’s gathering that information; creating that, turning it into intelligence and presenting it to the CCG or to the system where we will know: OK, these are the needs of our population. And decisions will be made what to commission, what not to commission, where to put money, where not to put money.
Interviewee 2
In terms of what Gold Command would need to look at, there was an emphasis on vulnerable groups and reducing health inequalities, suggesting the need to be able to look at different cohorts. Similar to the cardiology department at site A, there was a recognised need to monitor changes in accessing health care during the COVID-19 pandemic. Another area considered important to monitor was accident & emergency admissions.
Interaction requirements
The desire for ‘complete’ information also meant that there was a need to help users know what to focus on. Similar to the way in which NCA users at organisational levels wanted a dashboard that enabled easy identification of clinical areas that are outliers, interviewees described wanting a dashboard that would help them identify priorities:
Recently, the CCG has produced masses of data. They’ve probably got about 20 bar charts and graphs and all sorts of stuff, and I look at it and I can’t make head nor tail of it. [ . . . ] Partly because there’s too much information. I’m sure it is all valid when you look at it and go well, that’s interesting and it’s telling us what’s happening, but they are presented all individually on their own. There’s a lot to be said for sharing that information but then actually extracting some of the key points from these, because ultimately lots of the graphs and tables interlink and there’s a point from that one and there’s a point from this one.
Interviewee 5
This is what you’d hope the dashboard might direct people to, is that this is your killer priority, if you could make a move in this direction, you could have a massive impact down the line, and not just patch people up.
Interviewee 1
When designing QualDash, our participants were able to define key metrics for MINAP and PICANet and questions that they wanted to ask in relation to those metrics. By contrast, when talking about population health management, interviewees described often not knowing what variables they needed to look at or what questions to ask when they began the analysis, suggesting the need for functionality to support exploratory analysis:
The key is that people cannot define what the question is 99% of the time that they want the data to be able to provide them with the answer for. That’s the really difficult starting point.
Interviewee 6
There was a need for functionality that allowed users to start wide, in an exploratory analysis mode, and then ‘drill down’:
And I guess we sort of start at that sort of population level, start to look at trends, and then break down further from that. So, then we would start quite wide and then narrow down in our questioning [ . . . ]. The ability to be able to take it up and down, depending on the size of the intervention, or the scale of the intervention, but to be able to really target to the specifics as well, would be something that would be quite amazing to be able to have a tool that allows you to bring all of that data, and have the breadth and that population perspective very high level, but then to be able to almost delve down and dig into the bits that you feel may need that greater depth of understanding.
Interviewee 3
Finally, as with QualDash, there was an emphasis on the need for a dashboard to be intuitive to use.
Discussion
This chapter has described how we adapted QualDash and our project plans in the light of the COVID-19 pandemic. Although at the outset of the project we had intended to use the final phase to consider how QualDash could be adapted to support use of other NCA data for QI, we, instead, adapted QualDash for monitoring on a more regular basis and considered what would be needed to adapt QualDash for exploring a different data set to support population health decision-making. The sample size for the interviews undertaken in this phase was small (i.e. all from one locality) and we interviewed those who engaged with Gold Command, rather than members of Gold Command themselves. Nonetheless, we identified requirements that QualDash could support, but also important differences, particularly the need to be able to explore the data in a way not required with NCA data where there are key metrics of interest.
Chapter 10 Discussion and conclusion
Revisiting the objectives
The study had five objectives:
-
to develop a programme theory that explains how and in what contexts the use of QualDash will lead to improvements in care quality
-
to use the programme theory to co-design QualDash
-
to use the programme theory to co-design an adoption strategy for QualDash
-
to understand how and in what contexts QualDash leads to improvements in care quality
-
to assess the feasibility of conducting a cluster RCT of QualDash.
We discuss each of these objectives and the extent to which they have been met below.
A programme theory of QualDash
We developed a programme theory of QualDash, as presented in Chapter 5. The QualDash programme theory built on the mechanisms, and contextual supports and constraints, elicited in phase 1 that explained the use and impact of NCA data. We described the resources that QualDash would offer to clinical teams when inserted into varying contexts, how we anticipated users would respond to these resources, how those responses would vary according to the user’s context and the subsequent impacts. Therefore, although the ordering of our objectives suggests that we would develop a programme theory focused on QualDash from the outset to inform dashboard design, the QualDash programme theory and CMO hypotheses could be formalised only after there was clarity regarding what functionality QualDash would provide.
Using programme theory to co-design QualDash
Developing a programme theory of NCAs enabled us to identify the metrics that engaged NHS staff in interactions with NCA data and what supported or constrained these interactions across sites. The NCA programme theory provided insight into what metrics should be prioritised for visualisation and how QualDash could incorporate functionality to address constraints on data use across sites, particularly variation in the ability to generate visualisations of NCA data and timeliness of such visualisations. Explicit direction regarding how these constraints should be overcome was not provided by the programme theory. Here, we drew on a combination of (1) our understanding from the phase 1 interviews of how the intended users already visualised NCA data, (2) the ideas and feedback interviewees provided and (3) the information visualisation expertise of team members. These details enabled us to move to development of the QualDash programme theory. This programme theory indicates the functionality that should be included in quality dashboards, separate from the underlying technology, providing guidance for future design of quality dashboards. Specifically, our findings suggest that those designing quality dashboards to support engagement with NCA data may find it beneficial to include the following:
-
‘at-a-glance’ visualisation of key metrics considered markers of safe and effective care on first logging into the dashboard
-
simple visualisations, such as bar graphs and pie charts, configured in line with existing visualisations used by teams, with clear labelling of metrics
-
functionality that supports current queries and tasks, including creation of reports and presentations
-
the ability to interrogate the data, including exploring relationships between variables and drilling down to look at specific subgroups of patients.
Although the NCA programme theory highlighted areas where QualDash could provide benefit, it also revealed contextual factors that constrain the use of NCA data, which QualDash would not be able to address. Our focus was specifically on presentation of NCA data, with what data are collected, and how, being outside the scope of the study. However, as Chapter 4 reveals, the nature of the data collected and processes for data collection are intimately linked to how those data are subsequently used. Stewart et al. ,130 reporting on the state of NCAs in 2016, note that early NCAs were perceived to be relevant to doctors only and not to policy-makers, managers or other clinicians. Although some NCAs are notably interdisciplinary, PICANet was perceived by nursing staff to be of little relevance to them, in part, because it did not capture what they considered to be important markers of care quality. This has implications for the design of a quality dashboard, suggesting that the decision of which metrics should be displayed should be an interdisciplinary decision to ensure that the metrics have relevance to all members of the multidisciplinary team. Achieving this may also mean drawing on multiple data sources. This finding also has implications for NCAs more broadly, in terms of considering who they involve in making decisions about which data are to be collected. Although we found some use of NCA data at the organisational level, staff at this level perceived an imbalance between the benefits to their trusts from NCAs and the substantial resources consumed by participating in them, leading them to question the legitimacy of NCAs. This also has implications for NCAs more broadly. Possible measures to enhance the perceived legitimacy of NCAs could include NCAs moving from an emphasis on cumulative retrospective reports to real-time reporting, clearly presenting the ‘headline’ outcomes important to organisational level staff; further negotiation between trusts and NCAs about the nature and number of data to be collected; wider use by hospitals of routine clinical data to populate NCA data fields; and further use of technologies, such as dashboards, that help staff to explore and report audit data in meaningful ways.
Other learning that has come from this study is the critical role that audit support staff can play in supporting engagement with NCA data, through labour-intensive work to ensure data quality and the visualisation of data, and the importance of engaging audit support staff and drawing on their expertise in the process of design. As we move to a paperless NHS and a likely move to automatic gathering of NCA data from EHRs, our work is a timely reminder of the skilful work that goes into ensuring the quality of NCA data, enabling clinicians to trust the data and be willing to use it for QI. This is not to argue against automatic gathering. Rather, we suggest two factors that can facilitate this while maintaining data quality and clinicians’ trust in the data. First, the data that feed such systems must be accurate to avoid the problem of ‘garbage in–garbage out’,150 which may still require some of the time-consuming cross-checking that we identified. Second, software and data exchange interfaces between linked systems must be appropriately defined, both technically and semantically, and the complexity of the links between them navigated effectively. Crucially, both factors need input not only from IT specialists, but also from the audit support staff who understand the data and their contexts, meanings, dependencies, provenances, quality and limitations.
This study contributes to understanding the role that realist methods can play in informing intervention design. We would argue that developing a programme theory of the current programme, as we did with the programme theory of NCAs, is an important step because it provides an understanding of the constraints that need to be overcome. It should not be considered a weakness that the programme theory will not necessarily provide guidance on how those constraints should be addressed, as this provides the space to co-design solutions with intended users and draw on relevant subject expertise.
A benefit of developing the QualDash programme theory was that it made our reasoning explicit, providing a programme theory that could be tested and refined through testing of the CMO configurations in the evaluation. In this way, realist evaluation provides a way for design knowledge, in terms of what works and what does not, to cumulate. Most HITs are based on designers’ implicit theories about why certain features will provide benefit to users. If we become explicit about the theories that underlie our designs, we can then test those theories, using the refined theories to inform subsequent designs.
Using programme theory to co-design an adoption strategy
As with the second objective, it was not that the QualDash programme theory enabled us to develop the adoption strategy, but rather through the ideas elicited from stakeholders in phase 1 and working with the stakeholders to refine these ideas in phase 3, we were able to identify activities and their associated mechanisms that we hypothesised would support uptake and adoption. These activities and associated mechanisms were then incorporated into the QualDash programme theory. Again, this provided us with CMO configurations that could be tested and refined to allow knowledge of what works and what does not work to cumulate.
Our findings related to adoption fit with findings of other studies of dashboard use. A recent dashboard design study reported the need to add detailed definitions of the metrics used and the need to clarify section headers to improve comprehension and trust. 151 Our champions’ belief that QualDash should not be shared more widely before initial issues were addressed is supported by a study in which early issues created negative impressions that discouraged further use. 152 In the study by Bersani et al. ,152 it was felt that future efforts should focus on strong adoption planning and increased stakeholder engagement.
Phases 1 and 3 captured stakeholders’ perspectives on what is needed to successfully introduce a quality dashboard. Although our ability to fully test these theories was constrained by the COVID-19 pandemic, our findings suggest that, for health-care organisations seeking to introduce a quality dashboard, the following strategies may be beneficial.
Clinical champion
If a clinical champion promotes use of the quality dashboard, highlighting its benefits (e.g. via an e-bulletin and/or demonstrating its use in meetings), then staff will be willing to use it because of their trust in the champion’s opinion.
Avoiding the ‘dodgy brush’
The dashboard should be tested with real data prior to roll-out. The dashboard should be tested by staff who already use the data presented by the dashboard and who are expert in its interpretation. The dashboard should be revised prior to roll-out so that the metrics that are displayed fit with user expectations. This will give champions confidence that metrics are calculated appropriately and, therefore, they will be willing to promote dashboard use.
Routines for using audit data
If data presented by the dashboard are not already used routinely, then routines for integrating dashboard use into work practices of clinical teams should be established.
Involvement of audit support staff
If clinical teams are already using the data the dashboard displays, supported by audit support staff, adoption activities should focus on audit support staff, promoting not just the features of the dashboard but showing how it allows audit support staff to undertake their work more easily or quickly.
Customisation as design
The process of customising the dashboard to meet local user expectations should be seen as part of the adoption strategy.
However, our findings also highlight the challenges of introducing technology into the NHS, with old computers and difficulties in seemingly simple tasks, such as installing Google Chrome or RStudio, having the potential to get in the way of adoption efforts and constraining uptake and use.
It is questionable whether or not all of these strategies would be feasible for a NCA when seeking to roll out a dashboard nationally and, therefore, how to support adoption in such a context remains to be explored. The role of a clinical champion could be taken on by the NCA clinical lead, as this is what typically (but not always) happened within our sites. How NCAs can encourage and support clinical teams to establish routines for using NCA data is less clear, although the literature does suggest some possibilities. For example, in Oxfordshire, a dashboard has been developed based on the National Diabetes Audit, with data shown at individual GP level, locality level and CCG level. 153 The dashboard was reviewed in twice-yearly general practice visits by a diabetologist and community diabetes specialist nurse and discussed in diabetes locality meetings, where the dashboard was used to identify practices that had significantly improved diabetes care. Such locality meetings appear similar to the cardiology regional network meetings that our participants described, which no longer happen.
A number of NCAs do have dashboards. Our experience suggests the need to understand to what extent such dashboards are used and, if they are being used, what strategies NCAs are using to encourage uptake.
Understanding how and in what contexts QualDash leads to improvements in care quality
The COVID-19 pandemic meant that we were unable to implement and evaluate a revised version of QualDash, limiting the opportunity to test and refine our QualDash programme theory. However, our study has provided the following insights into possible impacts of QualDash and the contexts in which they may occur:
-
In contexts where clinical teams have been constrained in their ability to use NCA data, but where they have adequate resource to improve data quality, QualDash can support efforts to improve data quality via visualisation of missing data.
-
In contexts where clinical teams have been constrained in their ability to use NCA data, QualDash can reduce the time spent in preparation of reports via automation of measure calculation and visualisation.
-
QualDash can lead to reduced variation between sites in the extent to which sites engage with NCA data, due to clinical teams that have been constrained in their ability to use NCA data now engaging with those data via automatically generated visualisations.
However, what we were not able to observe over the study period was whether or not increased engagement with NCA data led to the introduction of any QI initiatives.
Feasibility of a trial of QualDash
As described in Chapter 8, it could be argued that all three of our progression criteria for a trial were met. Our findings have provided insight into a number of issues that need to be addressed for a successful trial of QualDash:
-
QualDash would need to be hosted by a NCA supplier (or similar body), as hosting it within trusts presented challenges when working across five trusts and would not be feasible for wider roll-out. A particular challenge was inconsistency of data formats and labelling at individual NHS sites. Although this is not a challenge if QualDash is to be hosted by a NCA, it does, nevertheless, suggest a need for more work to standardise data formats and labelling if trusts want to procure software for exploring NCA data.
-
QualDash uptake was limited, suggesting that encouraging QualDash use more widely could be challenging. However, this study has provided understanding of what constrains use of a quality dashboard, which can be categorised as those constraints that (1) could potentially be overcome by strategies to support adoption, (2) could potentially be overcome through functionality and features of the dashboard (including constraints that revisions to QualDash have already sought to address) and (3) cannot be overcome by a quality dashboard and need to be addressed by other means (e.g. lack of timely data as a result of human and technological resource constraints). Given the need for further research on effective adoption strategies that can be used by NCAs, we propose a trial that will not only assess the impact of QualDash, but will also provide rigorous evaluation of the adoption strategy. The design of such a trial is presented in Appendix 13.
-
NCA data are complex, with the consequence that, even if a quality dashboard is intuitive to use, data interpretation is not necessarily intuitive. Although this may be addressed, in part, through clear labelling, we would seek to also evaluate the impact of training as part of a trial.
Our updated literature review suggests the need for more rigorous evaluation of the impact of computer-based quality dashboards on quality and safety of care. However, conscious of the resources that a trial of QualDash would require, the limited uptake and limitations of the data gathered to demonstrate that the progression criteria were met, we consider it is necessary to first refine QualDash and the adoption strategy to provide greatest potential for positive impact.
Implications for future research
We suggest that the following areas would be fruitful for future research:
-
investigation of the extent to which NCA dashboards are used and the strategies NCAs are using to encourage uptake
-
a realist review of the impact of computer-based dashboards on quality and safety of care
-
rigorous evaluation of the impact of computer-based quality dashboards on the processes and outcomes of care
-
rigorous evaluation of the effectiveness of different strategies for encouraging use of dashboards, particularly for NCAs.
Acknowledgements
We would like to thank all of the NHS staff who participated in this research for generously giving their time to be interviewed and for their willingness to have their work practice observed. We are also very grateful to the IT staff who supported the introduction of QualDash to enable this research to take place. We would also like to thank Professor Justin Keen and Dr Roger Parslow (co-applicants) who made important contributions to the study design and data analysis in the earlier phases of the study. In addition we thank the reviewers of this report for their helpful feedback.
We are very grateful to the members of our LAG: Pauline Bland, Peter Dransfield, Rosie Hassman, Susan Hodgson, Gus Ibeguna, Alan Keys, Manoj Mistry, Graham Prestwich, Martin Rathfelder, Lucy Wheeler and Laurence Wood.
We thank our SSC for the support and guidance given over the course of the project: Sam Butterfield, Professor Alex Bottle, Andrew Fleming, Dr Ana Manzano, Professor Graham Martin and Dr Kieran Mullen.
Contributions of authors
Rebecca Randell (https://orcid.org/0000-0002-5856-4912) (Professor in Digital Innovations in Healthcare, Design and Evaluation of HIT) was the principal investigator, led the design of the study, contributed to data collection and analysis, and is lead author of this report.
Natasha Alvarado (https://orcid.org/0000-0001-9422-4483) (Research Fellow, theory-driven evaluation) was responsible for day-to-day management of the project, undertook data collection and was involved in the analysis and preparation of this report.
Mai Elshehaly (https://orcid.org/0000-0002-5867-6121) (Research Fellow, information visualisation) led the co-design and development of the QualDash software, contributed to data analysis and was involved in the preparation of this report.
Lynn McVey (https://orcid.org/0000-0003-2009-7682) (Research Assistant, qualitative research) undertook data collection and was involved in the analysis and preparation of this report.
Robert M West (https://orcid.org/0000-0001-7305-3654) (Professor of Biostatistics, statistics) was co-applicant, designed the ITS study and undertook the ITS analysis, and was involved in the preparation of this report.
Patrick Doherty (https://orcid.org/0000-0002-1887-0237) (Professor of Cardiovascular Health, clinical audit) was involved in study design, analysis and preparation of this report.
Dawn Dowding (https://orcid.org/0000-0001-5672-8605) (Professor in Clinical Decision-Making, nursing informatics) was co-applicant and was involved in study design, analysis and preparation of this report.
Amanda J Farrin (https://orcid.org/0000-0002-2876-0584) (Professor of Clinical Trials and Evaluation of Complex Interventions, clinical trials) was co-applicant and was involved in study design (particularly trial feasibility assessment), analysis and preparation of this report.
Richard G Feltbower (https://orcid.org/0000-0002-1728-9408) (Senior Lecturer, PICANet) was involved in study design, analysis and preparation of this report.
Chris P Gale (https://orcid.org/0000-0003-4732-382X) (Professor of Cardiovascular Medicine, MINAP) was co-applicant and was involved in study design, analysis and preparation of this report. He contributed expertise in MINAP and a clinician perspective.
Joanne Greenhalgh (https://orcid.org/0000-0003-2189-8879) (Professor of Applied Social Research Methodology, realist methods) was co-applicant and was involved in study design (particularly in relation to realist methods), analysis and preparation of this report.
Julia Lake (https://orcid.org/0000-0002-5013-7086) (Clinical Information and Outcomes Manager, clinical audit) was co-applicant and was involved in study design, analysis and preparation of this report. She contributed an NHS perspective.
Mamas Mamas (https://orcid.org/0000-0001-9241-8890) (Professor of Cardiology, MINAP) was co-applicant and was involved in study design, analysis and preparation of this report. He contributed expertise in MINAP and a clinician perspective.
Rebecca Walwyn (https://orcid.org/0000-0001-9120-1438) (Associate Professor, clinical trials methodology) contributed to trial feasibility assessment, designed the QualDash trial and was involved in preparation of this report.
Roy A Ruddle (https://orcid.org/0000-0001-8662-8103) (Professor of Computing, information visualisation) was co-applicant and contributed to study design, oversaw the design and development of the QualDash software and was involved in analysis and preparation of this report.
Publications
Elshehaly M, Alvarado N, McVey L, Randell R, Mamas M, Ruddle RA. From Taxonomy to Requirements: A Task Space Partitioning Approach. Proceedings of the IEEE VIS Workshop on Evaluation and Beyond–Methodological Approaches for Visualization (BELIV), Berlin, Germany, 21 October 2018, abstract no. 2478.
Dowding D, Alvarado N, McVey L, Mamas M, Randell R. Variation in national clinical audit data capture: is using routine data the answer? Stud Health Technol Inform 2019;264:1658–9.
Randell R, Alvarado N, McVey L, Ruddle R, Doherty P, Gale C, et al. Requirements for a Quality Dashboard: Lessons from National Clinical Audits. Proceedings of the AMIA 2019 Annual Symposium, Washington, DC, USA, 16–20 November 2019.
Alvarado N, McVey L, Greenhalgh J, Dowding D, Mamas M, Gale C, et al. Exploring variation in the use of feedback from national clinical audits: a realist investigation. BMC Health Serv Res 2020;20:859.
Randell R, Alvarado N, McVey L, Greenhalgh J, West R, Farrin A, et al. How, in what contexts, and why do quality dashboards lead to improvements in care quality in acute hospitals? Protocol for a realist feasibility evaluation. BMJ Open 2020;10:e033208.
Alvarado N, McVey L, Elshehaly M, Greenhalgh J, Dowding D, Ruddle R, et al. Analysis of a web-based dashboard to support the use of national audit data in quality improvement: realist evaluation. J Med Internet Res 2021;23:e28854.
Elshehaly M, Randell R, Brehmer M, McVey L, Alvarado N, Gale C, Ruddle R. QualDash: adaptable generation of visualisation dashboards for healthcare quality improvement. IEEE Trans Vis Comput Graph 2021;27.
McVey L, Alvarado N, Greenhalgh J, Elshehaly M, Gale C, Lake J, et al. Hidden labour: the skilful work of clinical audit data collection and its implications for secondary use of data via integrated health IT. BMC Health Serv Res 2021;21.
McVey L, Alvarado N, Keen J, Greenhalgh J, Mamas M, Gale C, et al. Institutional use of National Clinical Audits by healthcare providers. J Eval Clin Pract 2021;27:143–50.
Data-sharing statement
The data used for the ITS is provided in Report Supplementary Material 6. All other data will be kept until June 2030 and can be accessed by other researchers during this time, subject to the necessary ethics approvals being obtained. Requests for access to these data should be addressed to the corresponding author.
Patient data
This work uses data provided by patients and collected by the NHS as part of their care and support. Using patient data is vital to improve health and care for everyone. There is huge potential to make better use of information from people’s patient records, to understand more about disease, develop new treatments, monitor safety, and plan NHS services. Patient data should be kept safe and secure, to protect everyone’s privacy, and it’s important that there are safeguards to make sure that it is stored and used responsibly. Everyone should be able to find out about how patient data are used. #datasaveslives You can find out more about the background to this citation here: https://understandingpatientdata.org.uk/data-citation.
Disclaimers
This report presents independent research funded by the National Institute for Health and Care Research (NIHR). The views and opinions expressed by authors in this publication are those of the authors and do not necessarily reflect those of the NHS, the NIHR, the HSDR programme or the Department of Health and Social Care. If there are verbatim quotations included in this publication the views and opinions expressed by the interviewees are those of the interviewees and do not necessarily reflect those of the authors, those of the NHS, the NIHR, the HSDR programme or the Department of Health and Social Care.
References
- Healthcare Quality Improvement Partnership . Guidance on Quality Accounts, the Quality Accounts List, and Healthcare Service Provider Requirements 2018.
- Phekoo KJ, Clements J, Bell D. National Clinical Audit Quality Assessment – Overview of the Self-Assessment Survey: ‘Audit of Audits’. London: Healthcare Quality Improvement Partnership; 2014.
- Birkhead JS, Walker L, Pearson M, Weston C, Cunningham AD, Rickards AF. National Audit of Myocardial Infarction Project Steering Group . Improving care for patients with acute coronary syndromes: initial results from the National Audit of Myocardial Infarction Project (MINAP). Heart 2004;90:1004-9. https://doi.org/10.1136/hrt.2004.034470.
- Bridgewater B, Grayson AD, Brooks N, Grotte G, Fabri BM, Au J, et al. Has the publication of cardiac surgery outcome data been associated with changes in practice in northwest England: an analysis of 25,730 patients undergoing CABG surgery under 30 surgeons over eight years. Heart 2007;93:744-8. https://doi.org/10.1136/hrt.2006.106393.
- Neuburger J, Currie C, Wakeman R, Tsang C, Plant F, De Stavola B, et al. The impact of a national clinician-led audit initiative on care and mortality after hip fracture in England: an external evaluation using time trends in non-audit data. Med Care 2015;53:686-91. https://doi.org/10.1097/MLR.0000000000000383.
- Taylor A, Neuburger J, Walker K, Cromwell D, Groene O. How is feedback from national clinical audits used? Views from English National Health Service trust audit leads. J Health Serv Res Policy 2016;21:91-100. https://doi.org/10.1177/1355819615612826.
- Allwood D. Engaging Clinicians in Quality Improvement through National Clinical Audit. London: Healthcare Quality Improvement Partnership; 2014.
- Keogh B. Review into the Quality of Care and Treatment Provided by 14 Hospital Trusts in England. London: NHS; 2013.
- National Advisory Group on the Safety of Patients in England . A Promise to Learn – A Commitment to Act: Improving the Safety of Patients in England 2013.
- Dowding D, Randell R, Gardner P, Fitzpatrick G, Dykes P, Favela J, et al. Dashboards for improving patient care: review of the literature. Int J Med Inform 2015;84:87-100. https://doi.org/10.1016/j.ijmedinf.2014.10.001.
- Van Der Meulen M, Logie RH, Freer Y, Sykes C, McIntosh N, Hunter J. When a graph is poorer than 100 words: a comparison of computerised natural language generation, human generated descriptions and graphical displays in neonatal intensive care. Appl Cogn Psychol 2010;24:77-89. https://doi.org/10.1002/acp.1545.
- Hutchinson J, Alba JW, Eisenstein EM. Heuristics and biases in data-based decision making: effects of experience, training, and graphical data displays. J Mark Res 2010;47:627-42. https://doi.org/10.1509/jmkr.47.4.627.
- Vessey I. Cognitive fit: a theory-based analysis of the graphs versus tables literature. Decis Sci 1991;22:219-40. https://doi.org/10.1111/j.1540-5915.1991.tb00344.x.
- Linder JA, Schnipper JL, Tsurikova R, Yu DT, Volk LA, Melnikas AJ, et al. Electronic health record feedback to improve antibiotic prescribing for acute respiratory infections. Am J Manag Care 2010;16:e311-9.
- Dowding D, Merrill J, Russell D. Using feedback intervention theory to guide clinical dashboard design. AMIA Annu Symp Proc 2018;2018:395-403.
- Keen J, Nicklin E, Long A, Randell R, Wickramasekera N, Gates C, et al. Quality and safety between ward and board: a biography of artefacts study. Health Serv Deliv Res 2018;6. https://doi.org/10.3310/hsdr06220.
- Kroch E, Vaughn T, Koepke M, Roman S, Foster D, Sinha S, et al. Hospital boards and quality dashboards. J Patient Saf 2006;2:10-9.
- Pauwels K, Ambler T, Clark BH, LaPointe P, Reibstein D, Skiera B, et al. Dashboards as a service why, what, how, and what research is needed?. J Serv Res 2009;12:175-89. https://doi.org/10.1177/1094670509344213.
- Tukey JW. Exploratory Data Analysis. Boston, MA: Addison-Wesley; 1977.
- Department of Health and Social Care (DHSC) . High Quality Care For All: NHS Next Stage Review Final Report 2008.
- Department of Health and Social Care (DHSC) . Health Informatics Review Report 2008.
- NHS Connecting for Health . Clinicians Driving Clinical Dashboards Forward: A Case Study With Dr Anne Talbot n.d.
- NHS Connecting for Health . Clinical Dashboards Case Study: NHS Bolton Staff Dash to Clinical Improvement n.d.
- NHS Connecting for Health . Implementing Clinical Dashboards: Older Person Mental Health Services n.d.
- Foy R, Eccles MP, Jamtvedt G, Young J, Grimshaw JM, Baker R. What do we know about how to do audit and feedback? Pitfalls in applying evidence from a systematic review. BMC Health Serv Res 2005;5. https://doi.org/10.1186/1472-6963-5-50.
- GOV.UK . Report of the Mid Staffordshire NHS Foundation Trust Public Inquiry 2013.
- Randell R, Keen J, Gates C, Ferguson E, Long A, Ginn C, et al. Managing quality and safety in real time? Evidence from an interview study. Stud Health Technol Inform 2016;228:23-7.
- Daley K, Richardson J, James I, Chambers A, Corbett D. Clinical dashboard: use in older adult mental health wards. Psychiatr 2013;37:85-8. https://doi.org/10.1192/pb.bp.111.035899.
- Medical Research Council . Developing and Evaluating Complex Interventions: New Guidance 2008.
- Ivers NM, Grimshaw JM, Jamtvedt G, Flottorp S, O’Brien MA, French SD, et al. Growing literature, stagnant science? Systematic review, meta-regression and cumulative analysis of audit and feedback interventions in health care. J Gen Intern Med 2014;29:1534-41. https://doi.org/10.1007/s11606-014-2913-y.
- Ivers NM, Sales A, Colquhoun H, Michie S, Foy R, Francis JJ, et al. No more ‘business as usual’ with audit and feedback interventions: towards an agenda for a reinvigorated intervention. Implement Sci 2014;9. https://doi.org/10.1186/1748-5908-9-14.
- Grol RP, Bosch MC, Hulscher ME, Eccles MP, Wensing M. Planning and studying improvement in patient care: the use of theoretical perspectives. Milbank Q 2007;85:93-138. https://doi.org/10.1111/j.1468-0009.2007.00478.x.
- Davidoff F, Dixon-Woods M, Leviton L, Michie S. Demystifying theory and its use in improvement. BMJ Qual Saf 2015;24:228-38. https://doi.org/10.1136/bmjqs-2014-003627.
- Dixon-Woods M, Bosk CL, Aveling EL, Goeschel CA, Pronovost PJ. Explaining Michigan: developing an ex post theory of a quality improvement program. Milbank Q 2011;89:167-205. https://doi.org/10.1111/j.1468-0009.2011.00625.x.
- Pawson R, Tilley N. Realistic Evaluation. London: SAGE Publications Ltd; 1997.
- Robert G, Fulop N. Perspectives on Context. A Selection of Essays Considering the Role of Context in Successful Quality Improvement. London: The Health Foundation; 2014.
- Byng R, Norman I, Redfern S, Jones R. Exposing the key functions of a complex intervention for shared care in mental health: case study of a process evaluation. BMC Health Serv Res 2008;8. https://doi.org/10.1186/1472-6963-8-274.
- Ranmuthugala G, Cunningham FC, Plumb JJ, Long J, Georgiou A, Westbrook JI, et al. A realist evaluation of the role of communities of practice in changing healthcare practice. Implement Sci 2011;6. https://doi.org/10.1186/1748-5908-6-49.
- Greenhalgh T, Humphrey C, Hughes J, Macfarlane F, Butler C, Pawson R. How do you modernize a health service? A realist evaluation of whole-scale transformation in London. Milbank Q 2009;87:391-416. https://doi.org/10.1111/j.1468-0009.2009.00562.x.
- Westhorp G, Stevens K, Rogers PJ. Using realist action research for service redesign. Evaluation 2016;22:361-79. https://doi.org/10.1177/1356389016656514.
- Fletcher A, Jamal F, Moore G, Evans RE, Murphy S, Bonell C. Realist complex intervention science: applying realist principles across all phases of the Medical Research Council framework for developing and evaluating complex interventions. Evaluation 2016;22:286-303. https://doi.org/10.1177/1356389016652743.
- Pearson M, Brand SL, Quinn C, Shaw J, Maguire M, Michie S, et al. Using realist review to inform intervention development: methodological illustration and conceptual platform for collaborative care in offender mental health. Implement Sci 2015;10. https://doi.org/10.1186/s13012-015-0321-2.
- Pollock N, Williams R. Software and Organisations: The Biography of the Enterprise-Wide System or How SAP Conquered the World. New York, NY: Routledge; 2008.
- Pollock N, Williams R. e-Infrastructures: how do we know and understand them? Strategic ethnography and the biography of artefacts. CSCW Conf Comput Support Coop Work 2010;19:521-56. https://doi.org/10.1007/s10606-010-9129-4.
- Hyysalo S. Health Technology Development and Use: From Practice-Bound Imagination to Evolving Impacts. New York, NY: Routledge; 2010.
- Mozaffar H, Cresswell KM, Lee L, Williams R, Sheikh A. NIHR ePrescribing Programme Team . Taxonomy of delays in the implementation of hospital computerized physician order entry and clinical decision support systems for prescribing: a longitudinal qualitative study. BMC Med Inform Decis Mak 2016;16. https://doi.org/10.1186/s12911-016-0263-x.
- Funnell SC, Rogers PJ. Purposeful Program Theory: Effective Use of Theories of Change and Logic Models. San Francisco, CA: John Wiley & Sons; 2011.
- Myocardial Ischemia National Audit Project . How the NHS Cares for Patients With Heart Attack: Annual Public Report April 2013 to March 2014 2014.
- Paediatric Intensive Care Audit Network . 2015 Annual Report 2015.
- Manzano A. The craft of interviewing in realist evaluation. Evaluation 2016;22:342-60. https://doi.org/10.1177/1356389016638615.
- Ritchie J, Spencer L, Bryman A, Burgess RG. Analyzing Qualitative Data. London: Routledge; 1994.
- Galliers J, Wilson S, Randell R, Woodward P. Final Report on Safer Handover 2009.
- Bate P, Robert G. Bringing User Experience to Healthcare Improvement: The Concepts, Methods and Practices of Experience-Based Design. Oxford: Radcliffe Publishing; 2007.
- Carney O, McIntosh J, Worth A. The use of the nominal group technique in research with community nurses. J Adv Nurs 1996;23:1024-9. https://doi.org/10.1046/j.1365-2648.1996.09623.x.
- Waugh A, Austin A, Manthorpe J, Fox C, Stephens B, Robinson L, et al. Designing a complex intervention for dementia case management in primary care. BMC Fam Pract 2013;14. https://doi.org/10.1186/1471-2296-14-101.
- Elshehaly M, Alvarado N, McVey L, Randell R, Mamas M, Ruddle RA. From Taxonomy to Requirements: A Task Space Partitioning Approach n.d. https://doi.org/10.1109/BELIV.2018.8634027.
- Schulz H, Nocke T, Heitzler M, Schumann H. A design space of visualization tasks. IEEE Trans Vis Comput Graph 2013;19:2366-75. https://doi.org/10.1109/TVCG.2013.120.
- Andrienko N, Andrienko G. Exploratory Analysis of Spatial and Temporal Data: A Systematic Approach. Berlin: Springer Science & Business Media; 2006.
- Brehmer M, Munzner T. A multi-level typology of abstract visualization tasks. IEEE Trans Vis Comput Graph 2013;19:2376-85. https://doi.org/10.1109/TVCG.2013.124.
- Lloyd D, Dykes J. Human-centered approaches in geovisualization design: investigating multiple methods through a long-term case study. IEEE Trans Vis Comput Graph 2011;17:2498-507. https://doi.org/10.1109/TVCG.2011.209.
- Burns Wendell J, Holtzblatt K, Wood S. Rapid Contextual Design: A How to Guide to Key Techniques for User-Centered Design. Burlington, MA: Morgan Kaufman; 2004.
- Rogers Y, Sharp H, Preece J. Interaction Design: Beyond Human–Computer Interaction. Chichester: John Wiley & Sons; 2011.
- Dowding D, Merrill JA. The development of heuristics for evaluation of dashboard visualizations. Appl Clin Inform 2018;9:511-18. https://doi.org/10.1055/s-0038-1666842.
- Nielsen J. 10 Usability Heuristics for Interface Design 1995. www.nngroup.com/articles/ten-usability-heuristics/ (accessed 25 January 2022).
- Wall E, Agnihotri M, Matzen L, Divis K, Haass M, Endert A, et al. A heuristic approach to value-driven evaluation of visualizations. IEEE Trans Vis Comput Graph 2019;25:491-500. https://doi.org/10.1109/TVCG.2018.2865146.
- Skolarus TA, Sales AE, Richards DA, Hallberg IR. Complex Interventions in Health: An Overview of Research Methods. Oxford: Routledge; 2015.
- McCormack B, Richards DA, Hallberg IR. Complex Interventions in Health: An Overview of Research Methods. Oxford: Routledge; 2015.
- Pawson R, Manzano-Santaella A. A realist diagnostic workshop. Evaluation 2012;18:176-91. https://doi.org/10.1177/1356389012440912.
- Yin RK. Case Study Research: Design and Methods. Thousand Oaks, CA: SAGE Publications Ltd; 2003.
- Benn J, Burnett S, Parand A, Pinto A, Iskander S, Vincent C. Studying large-scale programmes to improve patient safety in whole care systems: challenges for research. Soc Sci Med 2009;69:1767-76. https://doi.org/10.1016/j.socscimed.2009.09.051.
- Øvretveit J, Gustafson D. Evaluation of quality improvement programmes. Qual Saf Health Care 2002;11:270-5. https://doi.org/10.1136/qhc.11.3.270.
- Ramsay AI, Turner S, Cavell G, Oborne CA, Thomas RE, Cookson G, et al. Governing patient safety: lessons learned from a mixed methods evaluation of implementing a ward-level medication safety scorecard in two English NHS hospitals. BMJ Qual Saf 2014;23:136-46. https://doi.org/10.1136/bmjqs-2012-001730.
- Dainty KN, Golden BR, Hannam R, Webster F, Browne G, Mittmann N, et al. A realist evaluation of value-based care delivery in home care: the influence of actors, autonomy and accountability. Soc Sci Med 2018;206:100-9. https://doi.org/10.1016/j.socscimed.2018.04.006.
- Waring J. Adaptive regulation or governmentality: patient safety and the changing regulation of medicine. Sociol Health Illn 2007;29:163-79. https://doi.org/10.1111/j.1467-9566.2007.00527.x.
- Pawson R. Theorizing the interview. Br J Sociol 1996;47:295-314. https://doi.org/10.2307/591728.
- Dalkin S, Greenhalgh J, Jones D, Cunningham B, Lhussier M. What’s in a mechanism? Development of a key concept in realist evaluation. Implement Sci 2015;10. https://doi.org/10.1186/s13012-015-0237-x.
- Holden RJ, Karsh BT. The technology acceptance model: its past and its future in health care. J Biomed Inform 2010;43:159-72. https://doi.org/10.1016/j.jbi.2009.07.002.
- Lee K, Jung SY, Hwang H, Yoo S, Baek HY, Baek RM, et al. A novel concept for integrating and delivering health information using a comprehensive digital dashboard: an analysis of healthcare professionals’ intention to adopt a new system and the trend of its real usage. Int J Med Inform 2017;97:98-108. https://doi.org/10.1016/j.ijmedinf.2016.10.001.
- Cochrane Effective Practice and Organisation of Care Group . What Study Designs Should Be Inlcuded in an EPOC Review and What Should They Be Called? 2013.
- Simms AD, Baxter PD, Cattle BA, Batin PD, Wilson JI, West RM, et al. An assessment of composite measures of hospital performance and associated mortality for patients with acute myocardial infarction. Analysis of individual hospital performance and outcome for the National Institute for Cardiovascular Outcomes Research (NICOR). Eur Heart J Acute Cardiovasc Care 2013;2:9-18. https://doi.org/10.1177/2048872612469132.
- Morris JV, Ramnarayan P, Parslow RC, Fleming SJ. Outcomes for children receiving noninvasive ventilation as the first-line mode of mechanical ventilation at intensive care admission: a propensity score-matched cohort study. Crit Care Med 2017;45:1045-53. https://doi.org/10.1097/CCM.0000000000002369.
- Alvarado N, McVey L, Greenhalgh J, Dowding D, Mamas M, Gale C, et al. Exploring variation in the use of feedback from national clinical audits: a realist investigation. BMC Health Serv Res 2020;20. https://doi.org/10.1186/s12913-020-05661-0.
- McVey L, Alvarado N, Keen J, Greenhalgh J, Mamas M, Gale C, et al. Institutional use of National Clinical Audits by healthcare providers. J Eval Clin Pract 2021;27:143-50. https://doi.org/10.1111/jep.13403.
- Randell R, Alvarado N, McVey L, Ruddle RA, Doherty P, Gale C, et al. Requirements for a Quality Dashboard: Lessons from National Clinical Audits n.d.
- Clark KW, Whiting E, Rowland J, Thompson LE, Missenden I, Schellein G. Breaking the mould without breaking the system: the development and pilot of a clinical dashboard at The Prince Charles Hospital. Aust Health Rev 2013;37:304-8. https://doi.org/10.1071/AH12018.
- Weiss D, Dunn SI, Sprague AE, Fell DB, Grimshaw JM, Darling E, et al. Effect of a population-level performance dashboard intervention on maternal-newborn outcomes: an interrupted time series study. BMJ Qual Saf 2018;27:425-36. https://doi.org/10.1136/bmjqs-2017-007361.
- Jeff L, Beswick S, Lo J, Lai Y, Chhun A, Campbell H. Insights from staff nurses and managers on unit-specific nursing performance dashboards: a qualitative study. BMJ Qual Saf 2014;23:1001-6. https://doi.org/10.1136/bmjqs-2013-002595.
- Reszel J, Dunn SI, Sprague AE, Graham ID, Grimshaw JM, Peterson WE, et al. Use of a maternal newborn audit and feedback system in Ontario: a collective case study. BMJ Qual Saf 2019;28:635-44. https://doi.org/10.1136/bmjqs-2018-008354.
- Dagliati A, Sacchi L, Tibollo V, Cogni G, Teliti M, Martinez-Millana A, et al. A dashboard-based system for supporting diabetes care. J Am Med Inform Assoc 2018;25:538-47. https://doi.org/10.1093/jamia/ocx159.
- Pace A, Buttigieg SC. Can hospital dashboards provide visibility of information from bedside to board? A case study approach. J Health Organ Manag 2017;31:142-61. https://doi.org/10.1108/JHOM-11-2016-0229.
- Webers C, Beckers E, Boonen A, van Eijk-Hustings Y, Vonkeman H, van de Laar M, et al. Development, usability and acceptability of an integrated eHealth system for spondyloarthritis in the Netherlands (SpA-Net). RMD Open 2019;5.
- Meijers JM, Halfens RJ, Mijnarends DM, Mostert H, Schols JM. A feedback system to improve the quality of nutritional care. Nutrition 2013;29:1037-41. https://doi.org/10.1016/j.nut.2013.02.007.
- Gude WT, Roos-Blom MJ, van der Veer SN, Dongelmans DA, de Jonge E, Francis JJ, et al. Health professionals' perceptions about their clinical performance and the influence of audit and feedback on their intentions to improve practice: a theory-based study in Dutch intensive care units. Implement Sci 2018;13. https://doi.org/10.1186/s13012-018-0727-8.
- Etamesor S, Ottih C, Salihu IN, Okpani AI. Data for decision making: using a dashboard to strengthen routine immunisation in Nigeria. BMJ Glob Health 2018;3. https://doi.org/10.1136/bmjgh-2018-000807.
- Coleman JJ, Hodson J, Brooks HL, Rosser D. Missed medication doses in hospitalised patients: a descriptive account of quality improvement measures and time series analysis. Int J Qual Health Care 2013;25:564-72. https://doi.org/10.1093/intqhc/mzt044.
- Pathirannehelage S, Kumarapeli P, Byford R, Yonova I, Ferreira F, de Lusignan S. Uptake of a dashboard designed to give realtime feedback to a sentinel network about key data required for influenza vaccine effectiveness studies. Stud Health Technol Inform 2018;247:161-5.
- Lin LA, Bohnert ASB, Kerns RD, Clay MA, Ganoczy D, Ilgen MA. Impact of the opioid safety initiative on opioid-related prescribing in veterans. Pain 2017;158:833-9. https://doi.org/10.1097/j.pain.0000000000000837.
- Michtalik HJ, Carolan HT, Haut ER, Lau BD, Streiff MB, Finkelstein J, et al. Use of provider-level dashboards and pay-for-performance in venous thromboembolism prophylaxis. J Hosp Med 2015;10:172-8. https://doi.org/10.1002/jhm.2303.
- Simpao AF, Ahumada LM, Desai BR, Bonafide CP, Galvez JA, Rehman MA, et al. Optimization of drug–drug interaction alert rules in a pediatric hospital’s electronic health record system using a visual analytics dashboard. J Am Med Inform Assoc 2015;22:361-9. https://doi.org/10.1136/amiajnl-2013-002538.
- Trinh LD, Roach EM, Vogan ED, Lam SW, Eggers GG. Impact of a quality-assessment dashboard on the comprehensive review of pharmacist performance. Am J Health Syst Pharm 2017;74:S75-S83. https://doi.org/10.2146/ajhp160556.
- Graber CJ, Jones MM, Goetz MB, Madaras-Kelly K, Zhang Y, Butler JM, et al. Decreases in antimicrobial use associated with multihospital implementation of electronic antimicrobial stewardship tools. Clin Infect Dis 2019;1. https://doi.org/10.1093/cid/ciz941.
- Hagaman DH, Ehrenfeld JM, Terekhov M, Kla KM, Hamm J, Brumley M, et al. Compliance is contagious: using informatics methods to measure the spread of a documentation standard from a preoperative clinic. J Perianesth Nurs 2018;33:436-43. https://doi.org/10.1016/j.jopan.2016.08.016.
- Dolan JE, Lonsdale H, Ahumada LM, Patel A, Samuel J, Jalali A, et al. Quality initiative using theory of change and visual analytics to improve controlled substance documentation discrepancies in the operating room. Appl Clin Inform 2019;10:543-51. https://doi.org/10.1055/s-0039-1693688.
- Banerjee D, Thompson C, Kell C, Shetty R, Vetteth Y, Grossman H, et al. An informatics-based approach to reducing heart failure all-cause readmissions: the Stanford heart failure dashboard. J Am Med Inform Assoc 2017;24:550-5. https://doi.org/10.1093/jamia/ocw150.
- Patel S, Rajkomar A, Harrison JD, Prasad PA, Valencia V, Ranji SR, et al. Next-generation audit and feedback for inpatient quality improvement using electronic health record data: a cluster randomised controlled trial. BMJ Qual Saf 2018;27:691-9. https://doi.org/10.1136/bmjqs-2017-007393.
- Fenton SH, Smith DH. Evidence-based operations management in health information management: a case study. Perspect Health Inf Manag 2019:1-13.
- Fischer MJ, Kourany WM, Sovern K, Forrester K, Griffin C, Lightner N, et al. Development, implementation and user experience of the Veterans Health Administration (VHA) dialysis dashboard. BMC Nephrol 2020;21:1-12. https://doi.org/10.1186/s12882-020-01798-6.
- Wu DTY, Vennemeyer S, Brown K, Revalee J, Murdock P, Salomone S, et al. Usability testing of an interactive dashboard for surgical quality improvement in a large congenital heart center. Appl Clin Inform 2019;10:859-69. https://doi.org/10.1055/s-0039-1698466.
- Ward CE, Morella L, Ashburner JM, Atlas SJ. An interactive, all-payer, multidomain primary care performance dashboard. J Ambul Care Manag 2014;37:339-48. https://doi.org/10.1097/JAC.0000000000000044.
- Bickman L, Riemer M, Breda C, Kelley S. CFIT: a system to provide a continuous quality improvement infrastructure through organizational responsiveness, measurement, training, and feedback. Rep Emot Behav Disord Youth 2006;6:86-7.
- Hysong SJ, Best RG, Pugh JA. Audit and feedback and clinical practice guideline adherence: making feedback actionable. Implement Sci 2006;1. https://doi.org/10.1186/1748-5908-1-9.
- Brown B, Gude WT, Blakeman T, van der Veer SN, Ivers N, Francis JJ, et al. Clinical Performance Feedback Intervention Theory (CP-FIT): a new theory for designing, implementing, and evaluating feedback in health care based on a systematic review and meta-synthesis of qualitative research. Implement Sci 2019;14. https://doi.org/10.1186/s13012-019-0883-5.
- Hysong SJ, Knox MK, Haidet P. Examining clinical performance feedback in patient-aligned care teams. J Gen Intern Med 2014;29:667-74. https://doi.org/10.1007/s11606-013-2707-7.
- Eldh AC, Fredriksson M, Vengberg S, Halford C, Wallin L, Dahlström T, et al. Depicting the interplay between organisational tiers in the use of a national quality registry to develop quality of care in Sweden. BMC Health Serv Res 2015;15. https://doi.org/10.1186/s12913-015-1188-2.
- Meade P. A Guide to Benchmarking. Dunedin, New Zealand: University of Otago; 2007.
- Van Helden GJ, Tillema S. In search of a benchmarking theory for the public sector. Financ Account Manage 2005;21:337-61. https://doi.org/10.1111/j.0267-4424.2005.00224.x.
- Furusten S. Institutional Theory and Organizational Change. Cheltenham: Edward Elgar Publishing; 2013.
- Martin GP, Armstrong N, Aveling EL, Herbert G, Dixon-Woods M. Professionalism redundant, reshaped, or reinvigorated? Realizing the ‘third logic’ in contemporary health care. J Health Soc Behav 2015;56:378-97. https://doi.org/10.1177/0022146515596353.
- Martin GP, Leslie M, Minion J, Willars J, Dixon-Woods M. Between surveillance and subjectification: professionals and the governance of quality and patient safety in English hospitals. Soc Sci Med 2013;99:80-8. https://doi.org/10.1016/j.socscimed.2013.10.018.
- Finch TL, Mair FS, O’Donnell C, Murray E, May CR. From theory to ‘measurement’ in complex interventions: methodological lessons from the development of an e-health normalisation instrument. BMC Med Res Methodol 2012;12. https://doi.org/10.1186/1471-2288-12-69.
- Allen D. The importance, challenges and prospects of taking work practices into account for healthcare quality improvement: nursing work and patient status at a glance white boards. J Health Organ Manag 2016;30:672-89. https://doi.org/10.1108/JHOM-04-2014-0062.
- Gaissmaier W, Wegwarth O, Skopec D, Müller AS, Broschinski S, Politi MC. Numbers can be worth a thousand pictures: individual differences in understanding graphical and numerical representations of health-related information. Health Psychol 2012;31:286-96. https://doi.org/10.1037/a0024850.
- Okan Y, Garcia-Retamero R, Cokely ET, Maldonado A. Individual differences in graph literacy: overcoming denominator neglect in risk comprehension. J Behav Decis Mak 2012;25:390-401. https://doi.org/10.1002/bdm.751.
- May C, Finch T. Implementing, embedding, and integrating practices: an outline of normalization process theory. Sociology 2009;43:535-54. https://doi.org/10.1177/0038038509103208.
- Murray E, Treweek S, Pope C, MacFarlane A, Ballini L, Dowrick C, et al. Normalisation process theory: a framework for developing, evaluating and implementing complex interventions. BMC Med 2010;8. https://doi.org/10.1186/1741-7015-8-63.
- Pawson R. Evidence-Based Policy: A Realist Perspective. London: SAGE Publications Ltd; 2006.
- National Institute for Health and Care Excellence . Principles for Best Practice in Clinical Audit 2002.
- Bevan G. Changing paradigms of governance and regulation of quality of healthcare in England. Health Risk Soc 2008;10:85-101. https://doi.org/10.1080/13698570701782494.
- Cloud G, Hoffman A, Rudd A. Intercollegiate Stroke Working Party . National sentinel stroke audit 1998–2011. Clin Med 2013;13:444-8. https://doi.org/10.7861/clinmedicine.13-5-444.
- Stewart K, Bray B, Buckingham R. Improving quality of care through national clinical audit. Future Hosp J 2016;3:203-6. https://doi.org/10.7861/futurehosp.3-3-203.
- Department of Health and Social Care (DHSC) . The New NHS: Modern, Dependable 1997.
- Haslam D. What is the Healthcare Commission trying to achieve?. J R Soc Med 2007;100:15-8. https://doi.org/10.1177/014107680710000109.
- Department of Health and Social Care (DHSC) . Our Health, Our Care, Our Say: A New Direction for Community Services 2006.
- Great Britain . Health Act 2009 2009.
- Great Britain . National Health Service (Quality Accounts) Regulations 2010 2010.
- Herrett E, Smeeth L, Walker L, Weston C. MINAP Academic Group . The Myocardial Ischaemia National Audit Project (MINAP). Heart 2010;96:1264-7. https://doi.org/10.1136/hrt.2009.192328.
- Birkhead JS. Responding to the requirements of the national service framework for coronary disease: a core data set for myocardial infarction. Heart 2000;84:116-17. https://doi.org/10.1136/heart.84.2.116.
- Birkhead J, Pearson M, Norris RM, Rickards AF, Georgiou A. The national audit of myocardial infarction: a new development in the audit process. J Clinical Excell 2002;4:379-85.
- Wilkinson C, Weston C, Timmis A, Quinn T, Keys A, Gale CP. The Myocardial Ischaemia National Audit Project (MINAP). Eur Heart J 2019;6:19-22. https://doi.org/10.1093/ehjqcco/qcz052.
- Paediatric Intensive Care Audit Network . PICANet: A Decade of Data 2014.
- McVey L, Alvarado N, Greenhalgh J, Elshehaly M, Gale CP, Lake J, et al. Hidden labour: the skilful work of clinical audit data collection and its implications for secondary use of data via integrated health IT. BMC Health Serv Res 2021;21. https://doi.org/10.1186/s12913-021-06657-0.
- Paediatric Intensive Care Society . Quality Standards for the Care of Critically Ill Children 2015.
- Department of Health and Social Care (DHSC) . National Service Framework for Coronary Heart Disease 2000.
- Elshehaly M, Randell R, Brehmer M, McVey L, Alvarado N, Gale CP, et al. QualDash: adaptable generation of visualisation dashboards for healthcare quality improvement. IEEE Trans Vis Comput Graph 2021;27:689-99. https://doi.org/10.1109/TVCG.2020.3030424.
- Crabtree A. Designing Collaborative Systems: A Practical Guide to Ethnography. London: Springer; 2003.
- Virzi RA. Refining the Test phase of usability evaluation: how many subjects is enough?. Hum Factors 1992;34:457-68. https://doi.org/10.1177/001872089203400407.
- Shapiro D, Tauber M, Traunmüller R, Shapiro D, Tauber M, Traunmüller R. The Design of Computer Supported Cooperative Work and Groupware Systems. Amsterdam: Elsevier; 1996.
- Büscher M, Shapiro D, Hartswood M, Procter R. Promises, Premises and Risks: Sharing Responsibilities, Working Up Trust and Sustaining Commitment in Participatory Design Projects n.d.
- Hartswood M, Procter R, Slack R, Soutter J, Voß A, Rouncefield M. The Benefits of a Long Engagement: From Contextual Design to the Co-Realisation of Work Affording Artefacts n.d. https://doi.org/10.1145/572020.572066.
- Karsh BT, Weinger MB, Abbott PA, Wears RL. Health information technology: fallacies and sober realities. J Am Med Inform Assoc 2010;17:617-23. https://doi.org/10.1136/jamia.2010.005637.
- van Deen WK, Cho ES, Pustolski K, Wixon D, Lamb S, Valente TW, et al. Involving end-users in the design of an audit and feedback intervention in the emergency department setting – a mixed methods study. BMC Health Serv Res 2019;19. https://doi.org/10.1186/s12913-019-4084-3.
- Bersani K, Fuller TE, Garabedian P, Espares J, Mlaver E, Businger A, et al. Use, perceived usability, and barriers to implementation of a patient safety dashboard integrated within a vendor EHR. Appl Clin Inform 2020;11:34-45. https://doi.org/10.1055/s-0039-3402756.
- Rea RD, Lumb A, Tan GD, Owen K, Thanabalasingham G, Latif A, et al. Using data to improve the care of people with diabetes across Oxfordshire. Pract Diabetes 2020;37:27-31. https://doi.org/10.1002/pdi.2257.
- Rodgers M, Sowden A, Petticrew M, Arai L, Roberts H, Britten N, et al. Testing methodological guidance on the conduct of narrative synthesis in systematic reviews: effectiveness of interventions to promote smoke alarm ownership and function. Evaluation 2009;15:49-73. https://doi.org/10.1177/1356389008097871.
- Randell R, Greenhalgh J, Hindmarsh J, Dowding D, Jayne D, Pearman A, et al. Integration of robotic surgery into routine practice and impacts on communication, collaboration, and decision making: a realist process evaluation protocol. Implement Sci 2014;9. https://doi.org/10.1186/1748-5908-9-52.
- Paediatric Intensive Care Audit Network . Paediatric Intensive Care Audit Network Annual Report: Summary Report, Data Collection Period January 2016–December 2018 2019.
- Sundin PT, Crespi CM. Power Analysis for Stepped Wedge Trials With Two Treatments 2020. www.medrxiv.org/content/10.1101/2020.10.05.20206003v2 (accessed 25 January 2022).
Appendix 1 Study management
The study was undertaken by a multidisciplinary Project Management Group, comprising academics in the fields of health services research, health informatics, computer science, sociology, statistics and clinical trials, both clinical and non-clinical NHS staff and the researchers. Three researchers were employed on the project. The two researchers (NA and LM) who undertook the qualitative research were trained in realist methods. Natasha Alvarado has a PhD in realist evaluation, supervised by Ray Pawson, and Lynn McVey attended a 2-day ‘Introduction to Realist Methodology’ course at the Centre for Advancement in Realist Evaluation and Synthesis, University of Liverpool (Liverpool, UK), in addition to receiving guidance from Rebecca Randell, Natasha Alvarado and Joanne Greenhalgh.
A SSC was convened, which met with members of the Project Management Group at four points over the course of the project. The SSC provided advice on the design and conduct of the study.
Patient and public involvement
Eleven people were recruited to take part in the QualDash LAG and two members agreed to represent the LAG at alternate SSC meetings. The LAG met four times over the course of the project to (1) provide feedback about the phase 1 interview schedule to ensure that it addressed issues that matter to patients and carers; (2) discuss the NCAs under investigation; (3) provide feedback on the SRS in terms of its understandability; and (4) trial the QualDash prototype and provide feedback about usability and usefulness from the lay perspective. The interview schedule and SRS were modified because of the feedback received and we acted on LAG advice to write a post for a patient safety blog and publish findings regarding use of NCA data by trust boards and subcommittees. The final LAG meeting was cancelled because of the COVID-19 pandemic. We e-mailed the LAG in March 2020 to notify them of this cancellation and update them about study progress and plans and again in October 2020 to provide a summary of their contributions and ask for feedback about their involvement in QualDash.
Appendix 2 National clinical audits represented at the supplier workshop
NCA | HQIP/independent/individual |
---|---|
Assessment of Risk for Cardiothoracic Intensive Care | Independent (charity) |
Cleft Registry and Audit Network | Independent (funded through NHS specialist service commissioners) |
Intensive Care National Audit and Research Centre Case Mix Programme | Independent (charity) |
Irish National Intensive Care Unit Audit | Independent (charity) |
National Cardiac Arrest Audit | Independent (charity) |
National Audit Project on Perioperative Anaphylaxis | Independent [managed by the National Institute of Academic Anaesthesia Health Services Research Centre (London, UK)]. Individual |
National Audit of Dementia | HQIP |
National Bariatric Surgery Registry | Independent [managed by the British Obesity and Metabolic Surgery Society (Lichfield, UK)]. Individual |
National Cancer Diagnosis Audit | Independent [managed by Cancer Research UK (London, UK)/charity)] |
National Clinical Audit of Anxiety and Depression | HQIP |
National Comparative Audit of Blood Transfusion | Independent (funded by NHS Blood and Transplant through a blood pricing mechanism) |
National Confidential Enquiry into Patient Outcome and Death | HQIP |
National Emergency Laparotomy Audit | HQIP |
National Maternity and Perinatal Audit | HQIP |
National Mortality Case Record Review Programme | HQIP |
National Ophthalmology Database Audit | HQIP |
National Vascular Registry | HQIP |
Perioperative Quality Improvement Programme | Independent (managed by the National Institute of Academic Anaesthesia Health Services Research Centre, working on behalf of a number of royal colleges, faculties and professional specialist societies). Individual |
UK Cystic Fibrosis Registry | Independent (sponsored and managed by Cystic Fibrosis Trust/charity) |
UK Parkinson’s Audit | Independent [managed by Parkinson’s UK (London, UK)/charity] |
Appendix 3 Methods of literature review update
We updated a previously conducted rapid review of literature related to quality and clinical dashboards, replicating the methods used for the original review.
Study inclusion criteria
Intervention
Studies were included in the review if they described an evaluation of the use and impact of clinical or quality dashboards. We defined clinical dashboards as dashboards that use visualisation to inform the practice of health-care professionals and dashboards that may provide data at the level of the patient and health-care professional (showing all patients who they are caring for and comparing them with their peers and national benchmarks) or may allow the user to move between viewing information at both of these levels. 14 We defined quality dashboards as dashboards that provide a visual display of quality indicators at the ward or organisational level to inform operational decision-making and QI efforts. 18
The dashboard had to include some form of visualisation, such as graphical displays (e.g. bar graphs and pie charts) and/or colour coding of information. Dashboards that simply displayed information without the use of such techniques were excluded. A dashboard may be viewed on a computer screen or via another form of display, such as an interactive whiteboard. We did not include paper-based systems or systems that generated only a paper-based report.
Participants
All health-care professionals and managers at the organisational and/or ward/unit level within health-care organisations using the dashboard. Studies involving only health-care students were excluded.
Study design
All study designs were included in the review as long as they took place within a health-care organisation.
Performance and outcome measures
All reported performance and outcome measures were considered. This included qualitative and quantitative data on both measurable impacts and staff/patient perceptions. Studies were excluded where a dashboard was one part of an intervention and where it was not possible from the results to distinguish between the impact of the dashboard and the other intervention components.
Search strategy
We searched Cumulative Index to Nursing and Allied Health Literature, MEDLINE, MEDLINE In-Process & Other Non-Indexed Citations, EMBASE, The Cochrane Library (Wiley), PsycINFO, Science Direct and the Association for Computing Machinery Digital Library for the dates 2012 to May 2020. Search strategies were developed using ‘dashboard’ as a free-text term and, for non-health-care databases, domain terms such as ‘health’ and ‘nursing’. Owing to limited time and resources available for translation, the search was restricted to studies in English. All search results were collated in an EndNote (Clarivate Analytics, Philadelphia, PA, USA) library, where duplicate references were identified and removed.
Study selection
All retrieved records were screened based on title and abstract. Full-text copies of potentially eligible papers were retrieved and re-screened. A ‘liberal accelerated’ approach to both rounds of screening was taken, where one reviewer reviewed all records/full-text papers and a second reviewer reviewed records/full-text papers excluded by the first reviewer.
Data extraction, analysis and synthesis
Data extraction for included studies was undertaken by a single reviewer who used a matrix constructed on an Excel spreadsheet to collate information for each study on:
-
study design, sample type and size, and setting
-
nature of the intervention and (where present) control condition and any changes made to the intervention during the period of the study
-
any reported process and outcome measures
-
specific features of the dashboard used (e.g. nature and type of visualisation of data)
-
factors influencing implementation or outcomes.
Following this, a short summary of each study was written, promoting greater engagement with the data and enabling us to capture in detail any aspects of the studies that did not appear relevant at the start of the synthesis, while also providing an opportunity to check the previous stages for accuracy. 154
Appendix 4 Meetings where national clinical audit data are considered
Site | Divisional/CSU meetings | NCA data use |
---|---|---|
A | ‘Triumvirate’ of lead clinician, head nurse and general manager in CSU forward action plans to address NCA reports in their area to organisational level, using a standard pro forma | Summaries of NCA reports are considered |
B | Divisional governance meeting | Considers NCA data if a problem is identified |
C | Divisional/hospital clinical effectiveness committee to be replaced under new structure by a quality and safety committee | New quality and safety dashboard being introduced. Unclear to what extent NCA data will be included, if at all |
D | Divisional clinical governance meetings | Divisional managers assure themselves that relevant NCA reports are being reviewed and acted on |
E | Divisional effectiveness committee and integrated care meeting for medicine and community services to discuss effectiveness and audit agenda | Divisional managers assure themselves that NCA reports are being reviewed and acted on |
Site | Meeting | NCA data use |
---|---|---|
A |
The trust board has six statutory subcommittees: (1) risk management committee, (2) research, education and training committee, (3) finance and performance committee, (4) quality assurance committee, (5) audit committee and (6) remuneration/nomination committee The quality assurance committee has a quality management group with nine subcommittees, including a safety and outcomes group, which has oversight of the trust’s clinical audit programme, including NCAs |
A similar pattern across trusts: summary of response to NCAs included in quality accounts, considered by trust board once a year NCA data considered by exception, especially by subcommittees with specific remit to assure the organisation’s response to NCAs |
B | The trust board has a range of subcommittees, including a risk management panel and a quality and safety forum. The trust board meets monthly and receives governance reports from each division | |
C | Since restructure, several trusts have merged to form a new trust governed by a board of directors to which several subcommittees report, including a group risk management committee and a trust quality and safety committee. The trust comprises several hospitals, each headed by its own board with a range of subcommittees, such as a clinical effectiveness board (which has a clinical audit subcommittee) | |
D | The trust board has a series of statutory subcommittees, including a ‘tier one’ quality committee, which is the senior committee in the trust, with a remit relating to clinical audit. A number of (tier two) subcommittees report to the quality committee, including a mortality group and a patient experience group | |
E | The trust board has a trust effectiveness committee, which has oversight of the trust’s response to NCA reports. NCA reports or summaries of reports may be reviewed at the trust effectiveness committee |
Appendix 5 National clinical audit programme theory in narrative form
In services with a local database and, in addition, audit support staff who have the time and skills to enter NCA data accurately and in a timely manner to generate visualisations of the data when requested by clinical and managerial staff (context), audit support staff create visualisations of NCA data that visualise service performance over time (resource). Clinical teams trust the data and find the visualisations of the metrics of interest easy to interpret and, therefore, they embed NCA data within their monitoring processes, underpinned by the mechanisms of professionalism, incentives and competition (response). This leads to the introduction of QI initiatives in relation to metrics that the team considers to be important and where performance is not in line with expectations (outcome). Over time, this leads to improvement in metrics that the QI initiatives target (outcome). As performance improves (context), interest in these metrics may decline (response) and there may be a decrease in engagement with NCA data (outcome). Equally, previously poor performance (context) is likely to lead to NCA data (resource) to be more closely monitored at both the organisational and the clinical team level (response). If the NCA is mandatory, includes metrics that relate to financial incentives and/or includes metrics that are perceived to be particularly sensitive (context), interest at the organisational and clinical team level is also likely to be increased (response).
Appendix 6 The QualDash prototype
FIGURE 20.
Expanded PICANet 48-hour readmission QualCard in QualDash prototype (using simulated data).
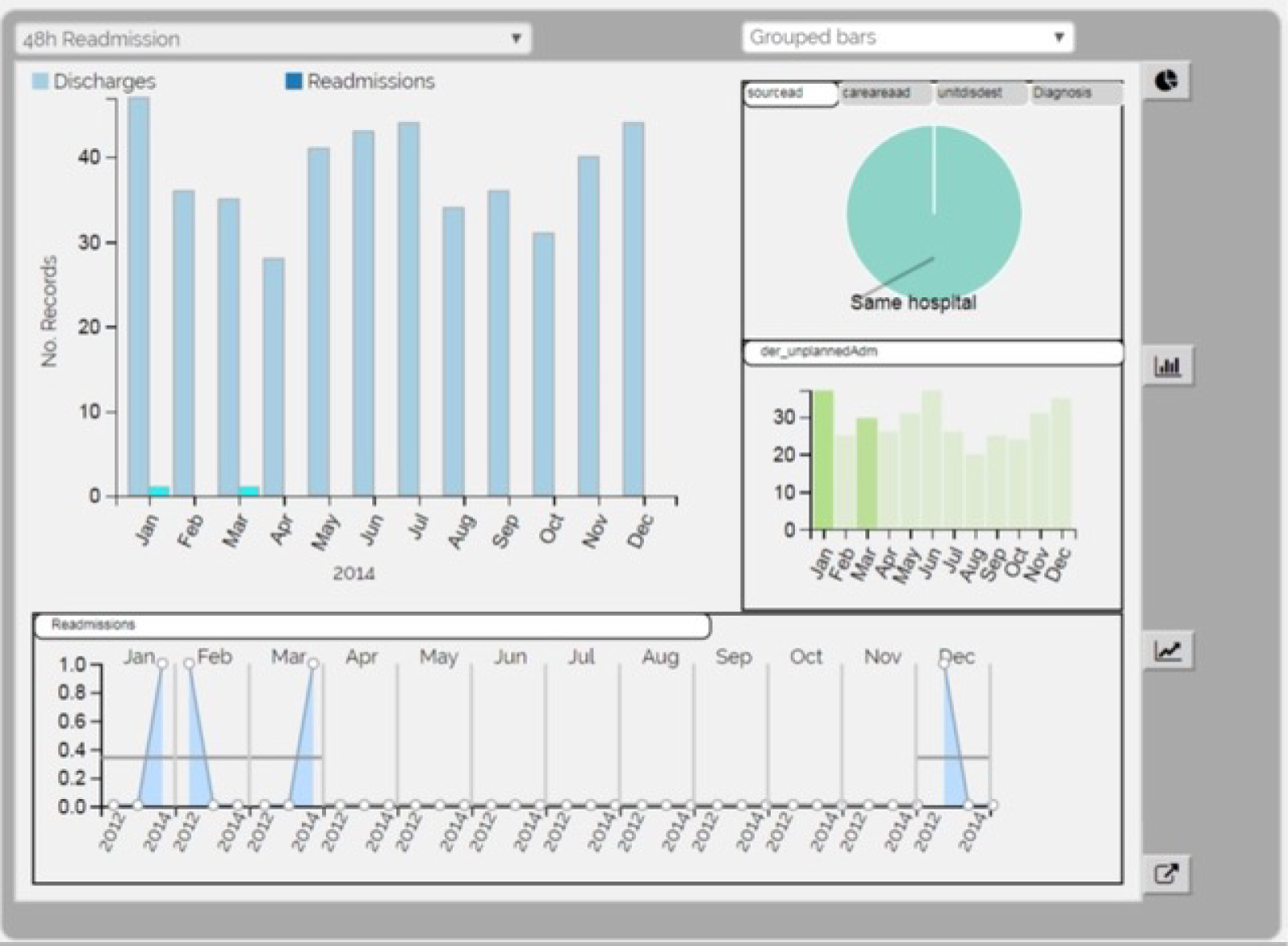
FIGURE 21.
Expanded PICANet bed-days and extubation QualCard in QualDash prototype (using simulated data).

FIGURE 22.
Expanded PICANet dependency QualCard in QualDash prototype (using simulated data).
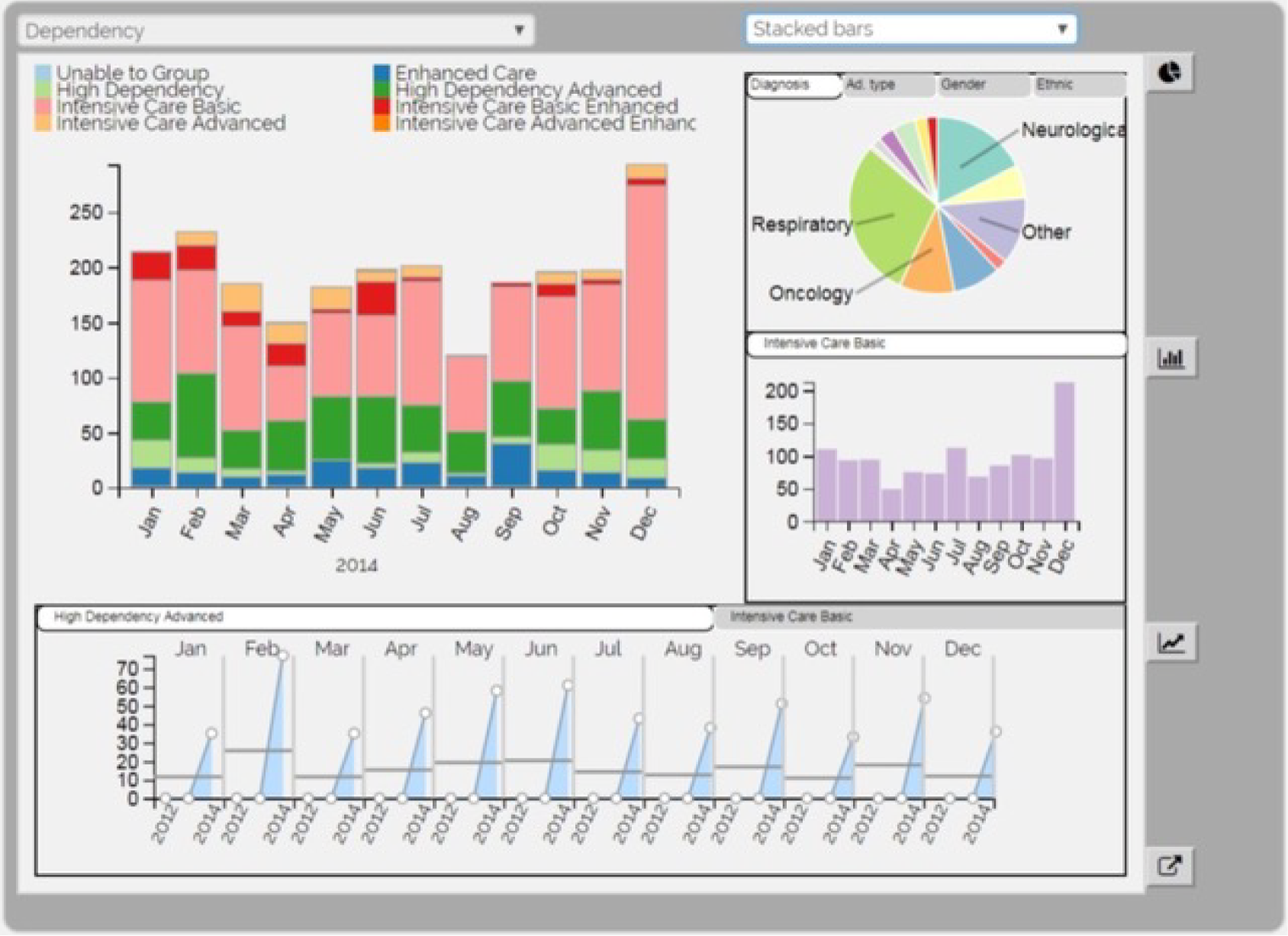
FIGURE 23.
Expanded PICANet data quality QualCard in QualDash prototype (using simulated data).

Appendix 7 User feedback
Visualisation feedback
An important change that resulted from the feedback was that the SMR was included in the main bar chart for the mortality QualCard, reflecting its importance as a metric (whereas on the prototype it was a subview). Other changes were ensuring that a printer-safe colour palette was used and, for the dependency bar chart, using a stacked bar chart for the default view.
Graphical user interface feedback
Feedback related to labelling included using black font for the metric names and having the metric card titles in bold. It was suggested that it would be good to have an expand button to complement the double-click behaviour for card expansion. One participant requested a ‘refresh’ button, as they were concerned that, having filtered down to look at a subset of data, they would move on to a different task without first clearing the filters on the data. To address this concern, at the bottom of each QualCard the number of selected records was shown and a ‘clear’ button was added to clear the selections.
Data-related feedback
Data-related feedback at this stage of evaluation largely focused on issues such as data validation and timeliness, rather than on choice of data elements used to generate the QualCards. However, a number of comments regarding aggregation rules were captured. For example, it was noted that the SMR should be displayed as a cumulative aggregate.
Task-related feedback
Task-related comments were nearly all positive, suggesting that there was limited mismatch between participants’ tasks and QualDash.
Appendix 8 Heuristic evaluation results
Table 18 shows the results from analysis of responses to the heuristic evaluation checklist. Two heuristics were assessed by all participants as not being applicable and, therefore, were not included in the analysis (i.e. ‘Do users have the option of either clicking on menu items with a mouse or using a touchscreen/stylus?’ and ‘On menus, do users have the option of either clicking directly on a menu item or using a touchscreen/stylus?’). One heuristic was assessed by three participants as not being applicable (i.e. ‘Can the users enter default or baseline ranges?’) and we agreed that this was not applicable and, therefore, this was also excluded from the analysis.
Usability principle | Maximum score | Mean score (n = 4) | Result (%) |
---|---|---|---|
Visibility of system status | 6 | 5.75 | 96 |
Match between system and the real world | 5 | 4.5 | 90 |
User control and freedom | 4 | 3.75 | 94 |
Consistency and standards | 6 | 5 | 83 |
Recognition rather than recall | 4 | 4 | 100 |
Flexibility and efficiency of use | 5 | 4.25 | 85 |
Aesthetic and minimalist design/remove the extraneous (ink) | 7 | 5 | 71 |
Spatial organisation | 3 | 1.75 | 58 |
Information coding | 2 | 2 | 100 |
Orientation | 4 | 3 | 75 |
Total | 46 | 38.75 | 85 |
Few usability issues were identified in the categories of visibility of system status, match between the system and the real world, user control and freedom, consistency and standards, recognition rather than recall, flexibility and efficiency of use, and information coding. The main issues related to spatial organisation. Three participants gave an overall rating of ‘minor usability problem’ for this category, and one participant gave an overall rating of ‘major usability problem’. Comments were given in relation to the factor of ‘Are all information elements clear and visible?’ (noting that text labels on some of the pie charts overlapped, making them difficult to read) and ‘Does the information provide detail on the context and detail associated with the data element?’ (noting that information on missing values did not change with the choice of category). QualDash was revised to address the issue of the overlapping text labels. For example, for pie charts where there are many segments, text labels are shown only for larger segments, with text labels for other segments appearing when users hover over them. Revising the information on missing values to change with choice of category was not considered a high priority change, as this information had not been requested by the clinicians and audit support staff we worked with in designing QualDash.
Average scores (on a scale of 1–7) for the four components of the visualisation value heuristics are presented in Table 19. The results suggest that participants thought that QualDash could provide insight in an efficient manner. However, participants were less certain about the extent to which QualDash communicated the essence of the data set with respect to overview and context (essence) and how QualDash helped the user feel confident in their understanding of the data set in terms of the quality of the data and quality of the visualisation (confidence). The range of scores shown in Table 19 indicates that there was significant variability among participants in the ratings they gave for the components of time, essence and confidence.
Component | Average score (range) (n = 4) |
---|---|
Insight | 5.9 (5.3–6.3) |
Time | 5.5 (3–7) |
Essence | 4.89 (3–6) |
Confidence | 4.92 (3–7) |
Appendix 9 Champions, staff responsible for data upload and demonstrations
Site | Cardiology staff | PICU staff | ||
---|---|---|---|---|
Clinical | Other | Clinical | Other | |
A | A cardiology nurse specialist (local collaborator and MINAP data collection, analysis and reporting) | The service’s clinical lead and manager agreed to support dissemination activities | Two paediatricians (one was the local collaborator and the other was the PICANet lead) | An audit coordinator |
B | The local collaborator (cardiologist) took on elements of the champion role, but was unable to take on the role fully because of the particular nature of his clinical involvement at site 5 | An information analyst | Two PICU nurses (with support from a paediatrician/local collaborator) | An audit clerk |
C | The local collaborator (cardiologist) was asked to perform this role, but no response was received | A service manager and a NICOR project assistant assisted in dissemination activities | Paediatrician (also the local collaborator) | N/A |
D | A cardiologist (also local collaborator) and two cardiac nurses | A patient service manager | N/A | N/A |
E | A cardiologist (also the local collaborator) | N/A | N/A | N/A |
Site | Service | Staff responsible for data upload |
---|---|---|
A | Cardiology | Trust data analyst |
PICU | Audit coordinator (champion) | |
B | Cardiology | Information analyst (champion) |
PICU | Audit clerk (champion) | |
C | Cardiology | Cardiology IT manager |
PICU | PICU database manager | |
D | Cardiology | Cardiology nurse (champion) |
E | Cardiology | Audit and clinical effectiveness team member |
Site | Service | Demonstration provided at | By whom | When |
---|---|---|---|---|
A | Cardiology | Clinical governance meeting | Research team | Pre installation (13 June 2019) |
PICU | Clinical governance meeting | Research team | Pre installation (14 June 2019) | |
B | Cardiology | Consultants meeting (information analyst) | Champion (information analyst) | Post installation (18 July 2019) |
PICU | Multidisciplinary team meeting | Champion (paediatrician) | Post installation (23 July 2019) | |
C | Cardiology | Audit and clinical effectiveness meeting | Research team | Pre installation (5 June 2019) |
PICU | None | |||
D | Cardiology | Clinical governance meeting | Research team | Pre installation (17 July 2019) |
E | Cardiology | Clinical governance meeting | Champion (cardiologist) | Post installation (11 December 2019) |
Appendix 10 An extended QualDash programme theory
To enable the reader to track how the theories develop over the course of the study, the numbering of the CMO configurations remains the same as in Chapter 5; however, CMO configurations concerned with adoption are labelled A and CMO configurations concerned with the resources provided by QualDash are labelled Q (i.e. CMO configuration 1 in Table 10 is CMO configuration Q1 in Table 23).
Configuration | Context | + | Mechanism | = | Outcome | |
---|---|---|---|---|---|---|
Resource | Response | |||||
A1 |
Clinical services where NCA data use is desirable but variable because of constrained resources QualDash is successfully installed, is accessible and works as intended |
+ | Clinical champion and e-bulletin and/or meetings | Awareness: teams are informed about QualDash (i.e. how it can be accessed its functions and potential benefits) and log on to explore its utility | = | Clinical staff with interest in the NCA (e.g. service managers) make an assessment about if and how they can use QualDash to facilitate work processes |
A2 |
Clinical services where NCA data use is desirable but variable because of constrained resources QualDash is successfully installed, is accessible and works as intended |
+ | Clinical (senior) staff engaged as champions for QualDash |
Leadership: clinical champions add QualDash to meeting agendas and lead its use in the meeting Influence: clinical champion’s colleagues are encouraged/inspired to use QualDash themselves based on the champion’s example |
= |
QualDash is trialled in settings where there is potential for integration into routine practices Champion’s colleagues use QualDash to assess if and how it can be useful to them |
A3 |
QualDash is successfully installed, is accessible and works as intended Early interactions have established a role for QualDash in use of NCA data |
+ |
QualDash intuitive to use Quick reference training materials provided to support use of dashboard functions Champions available to support use |
Confident users: teams learn quickly how to use the dashboard functions to support and benefit their work processes | = | Sustained and optimised QualDash use (i.e. QualDash not ‘tarred with the dodgy brush’) |
A4 | Upload of data to QualDash undertaken by IT staff who have the expertise necessary to complete such tasks with minimum training | + | Quick reference training materials | IT staff quickly learn how, and feel confident, to upload data to QualDash without further support | = | Data uploaded to QualDash routinely, providing access to timely data to maintain QualDash use |
A5 | Upload of data to QualDash by non-technical staff | + |
In-person guidance for first upload Quick reference training materials |
Audit support staff learn and are supported to upload data to QualDash | = | Data uploaded to QualDash routinely, providing access to timely data to maintain QualDash use |
Q1 |
Teams have processes in place (e.g. clinical governance meetings) for monitoring service performance using data available to them Teams constrained in ability to use NCA data routinely because data not considered timely, accurate and/or complete Teams motivated to use QualDash and feel confident in ability to do so Data uploaded to QualDash, providing access to recent data |
+ | Easy access to visualisations of key metrics | Data quality: teams interact with QualDash to utilise data, underpinned by the mechanisms of professionalism, incentives and competition. If data displayed are not timely, accurate and/or complete, then efforts are made to adjust data collection processes in order to benefit from QualDash | = |
Improvement in data timeliness, accuracy and completeness As data quality improves, QualDash use increases |
Q2 |
Teams have processes in place (e.g. clinical governance meetings) for monitoring that patient care is safe and effective and/or for monitoring performance in relation to service funding Teams are resourced to use NCA data routinely (e.g. audit support staff are available to produce reports that are timely and trusted as accurate by clinical staff) Teams motivated to use QualDash and feel confident in ability to do so Data are uploaded to QualDash, providing access to recent data |
+ | Visualisation of key metrics and ability to interrogate data |
Facilitation: teams use QualDash in meetings because it facilitates direct access and monthly monitoring of metrics that are considered markers of safe and effective care (underpinned by the mechanism of professionalism) and/or are linked to service funding (underpinned by the mechanism of incentives) Automation: audit support staff use QualDash to produce reports when requested by clinical and managerial staff, as it automates the calculation and visualisation of metrics |
= | Reduced time spent by clinical and audit support staff in accessing, and generating visualisations of, NCA data |
Q3 |
Teams have processes in place (e.g. monthly clinical governance meetings) for monitoring service performance Teams that do not, or are not able to, monitor key metrics routinely (e.g. due to constraints in resources). Teams motivated to use QualDash and feel confident in ability to do so Data are uploaded to QualDash, providing access to recent data |
+ | Web-based access to key metrics considered markers of safe and effective care | Integration: teams use QualDash because it facilitates access to NCA data that are considered to be key markers of care quality and provides visualisations that highlight discrepancies between performance and targets (e.g. in MINAP) and/or changes in performance month by month (e.g. in MINAP and PICANet), which they take action to address, underpinned by the mechanisms of professionalism and incentives | = |
NCA data integrated into routine service monitoring processes Introduction of QI initiatives in relation to key metrics, where resources allow Over time, improvement in those metrics |
Q4 |
Teams have processes in place (e.g. monthly clinical governance meetings) for monitoring service performance, but existing systems produce static reports and do not provide functions to interact with and drill down into NCA data Teams are motivated to use QualDash and feel confident in ability to do so Data are uploaded to QualDash, providing access to recent data |
+ | Ability to interact with NCA data and explore relationships between variables | Data interrogation: teams use QualDash in meetings instead of traditional static reports because QualDash enables teams to interrogate anomalies in the data, underpinned by the mechanisms of professionalism and/or incentives, helping teams to understand what has affected performance and identify appropriate QI initiatives | = |
Introduction of appropriate QI initiatives where performance is not in line with expectations, where resources allow Over time, improvement in metrics that QI initiatives target |
Q5 |
Teams are asked to produce reports and provide recommendations for managers and other groups about service performance (e.g. at time of publication of NCA national report) Teams are motivated to use QualDash and feel confident in ability to do so Data are uploaded to QualDash, providing access to recent data |
+ | Easy access to NCA data and visualisations that can be exported into reports | Reporting: teams choose to use QualDash to produce reports requested by other groups because they see that QualDash removes the manual work involved | = |
Reduced time spent by team in report preparation Use of NCA data at divisional and organisational levels via QualDash outputs Over time, divisional and organisational QualDash use increases as teams become aware that they can access the data they require directly |
Q6 |
Teams receive data requests from managers Managers are motivated to use QualDash and feel confident in ability to do so Data are uploaded to QualDash, providing access to recent data |
+ | Web-based access for multiple users | Access: managers will use QualDash to access the information they need quickly and easily | = |
Streamlines use of NCA data for managers Reduced time spent by audit support staff/team in producing data reports for managers |
Q7 |
Teams need to evidence performance to managers and other groups to support a case for practice change (e.g. in business meetings with managers or in NCA national report summary) Teams are motivated to use QualDash and feel confident in ability to do so Data are uploaded to QualDash, providing access to recent data |
+ | QualDash visualises key metrics which can also be exported into reports and presentations | Evidence for change: teams use QualDash to monitor service performance, underpinned by the mechanisms of professionalism and/or incentives, and, when they identify a QI need that requires additional resources, they use QualDash to report evidence that can convince trust groups with power to provide resource | = | Trust groups with power to allocate additional resource are convinced of the need for change, particularly where key metrics relate to trust priorities (e.g. relating to trust reputation or avoiding penalties/receiving incentives) |
Appendix 11 QualDash installation process
Table 24 summarises the process of installing QualDash into the sites. A consequence of the decision to host QualDash on trust servers was that site responsibilities, particularly for IT staff, were extended beyond the scope originally anticipated.
Step | Process | Purpose | Requirement |
---|---|---|---|
1 | Server-side installation of WAMP stack | Runs the software stack for the QualDash server |
Install Bitnami WAMP stack Download QualDash from GitHub (GitHub, Inc., San Francisco, CA, USA) |
2 | Client-side installation of RStudio | An open-source integrated development environment to run R scripts that automatically pre-process and upload data into the specified location on the QualDash server data in the correct folder for upload into QualDash | Install RStudio on the PC from which data are uploaded into QualDash |
3 | Download of 3 years of audit data, with column headers | Data uploaded and displayed on the dashboard |
Three years of data accessed via the same PC on which RStudio is installed Data need to be run through the R script Column headers needed (if data set is different from the official audit’s description) for the R script to read when formatting the data |
4 | Validate QualDash | To ensure that data displayed on the dashboard are accurate |
Research team use raw data to manually recreate the graphs displayed in QualDash Counts in manually created graphs compared with counts in QualDash |
5 | Installation of Google Chrome | QualDash was designed and tested using Google Chrome and is not necessarily compatible with other web browsers | Site staff wanting to use QualDash need Google Chrome installed on their PC |
Communication and engagement with information technology staff
The research team spoke to relevant IT staff to describe the implications in terms of the IT resources required to host QualDash and to obtain staff agreement. These conversations were with senior IT managers within the sites who then transferred responsibility for managing the process to another member of IT staff. However, with site D, initial attempts to contact IT staff were unsuccessful.
After the server-side installation of the software stack was undertaken by IT staff (see step 1 in Table 24), completing the installation required the configuration of QualDash and upload of NCA data, both of which were completed by Mai Elshehaly with support from site staff. Variation across sites in IT infrastructure influenced the process of installation and level of involvement required by different staff members to support the process. Sites A, B and E allowed access to the server via PCs in the IT department only. Consequently, visits to configure (and later to update) QualDash were arranged and managed via IT contacts, whereas data upload was completed in a different location with support from different staff. In contrast, IT staff in sites C and D created ‘virtual servers’ that allowed Mai Elshehaly access, with administrator rights, to QualDash via service PCs. Therefore, staff within the clinical area, typically those supporting the data upload process, were contacted for site visits to configure and upload data into QualDash. Table 25 provides an overview of staff who supported installation across sites.
Site | QualDash server | QualDash data upload | Installation completed | ||||
---|---|---|---|---|---|---|---|
Accessed via | Contact | Cardiology | PICU | ||||
Data accessed via | Contact | Data accessed via | Staff | ||||
A | IT office | Trust IT | Service database | Downloaded and uploaded by trust data analyst | Supplier database | Downloaded and uploaded by PICU audit clerk | 26 July 2019 |
B | IT office | Trust IT | Service database | Downloaded and uploaded by cardiology information analyst | Supplier database | Downloaded and uploaded by PICU audit clerk | 17 July 2019 |
C | Virtual server and so could be accessed within the cardiology department/PICU | Cardiology IT/PICU database manager | Service database | Downloaded and uploaded by cardiology IT | Supplier database | Downloaded and uploaded by PICU database manager |
8 July 2019 (PICANet) 15 July 2019 (MINAP) |
D | Virtual server and so could be accessed within the cardiology department | Cardiology nurse | Service database | Downloaded and uploaded by cardiology nurse | N/A | N/A | 5 December 2019 |
E | IT office | Trust IT | Supplier database | Downloaded by audit and effectiveness manager and uploaded by trust IT | N/A | N/A | 25 July 2019 |
To complete the installation process, site visits had to be carefully arranged at the convenience of IT and/or clinical service staff. Arranging an appropriate date and time to visit was often time-consuming, as IT and clinical service staff had their main duties and responsibilities to perform and, in some cases, coordination between two clinical services and IT departments were required to complete the installation. For example, in site C, two separate visits were organised to install QualDash (one visit to the cardiology department and one visit to the PICU). The longest delay in installation was at site D where trust approvals, additional to R&D approvals, were required. The vignette in Box 9 provides the details of this process.
The installation process began on 23 May 2019 when an IT contact was finally identified. However, unlike other sites, the IT contact had concerns over who would maintain QualDash, stating typically software updates were managed by the ‘supplier’ and explaining QualDash would need to be approved by the trust’s IM&T steering group.
The research team investigated whether the research approvals in place were sufficient (without IM&T approval) to progress the installation, as QualDash was developed for research. With support from our local collaborator at the site, the research team identified and supplied more technical information to a member of the IM&T steering group, hoping this would progress the installation. However, on 16 July 2019 the IT contact reaffirmed that, although they had created the software stack, QualDash would need to be approved by the IM&T group. In response, the research team further clarified what information was required, which included who would maintain and update QualDash. The information was collated in a document that was submitted for review at the next IM&T meeting held on 11 September 2019, where study approval was received.
Liaison between the research team and site IT confirmed that the next convenient date for an installation visit was 10 October 2019. However, at this visit Mai Elshehaly experienced issues using the virtual server to configure QualDash and RStudio was not available for download on the PC from which data would be uploaded. Mai Elshehaly was unable to contact the IT department for support during the site visit, with the consequence that installation could not be completed.
Subsequently, efforts were made to contact site IT to rearrange the visit, but no response was received. On 8 November 2019, the local collaborator advised the research team to contact a member of the IM&T steering group to help progress the installation and a message from the research team was sent on 14 November 2019. On 25 November 2019, the IT contact responded to the research team, indicating it had been a busy period for them, but they could now arrange a date to support the installation; this visit took place and installation was completed on 5 December 2019.
IM&T, information management and technology.
In most sites, IT staff undertook the server-side installation of the software stack (see step 1 in Table 24) and allowed the research team to access trust PCs to configure QualDash when convenient. However, the vignette in Box 9 highlights that successfully installing QualDash in a timely way, when located on local servers, was entirely dependent on support from site IT departments. Furthermore, it demonstrates that to obtain that support, IT departments differed in the information they required to formally approve the process, even in the context of research.
Variation in data sets
A further challenge identified during installation, with potential to have an impact on ongoing QualDash use, was lack of consistency across cardiology services in column headers in MINAP data sets, as described in the vignette in Box 10.
The research team visited the clinical informatics office in site A to upload MINAP data and complete installation of QualDash on 21 June 2019. However, the MINAP data set available did not include column headers, which meant it could not be fed into the R script and uploaded into QualDash at the visit. The research team later contacted the data analyst, who was supporting upload, to request the column headers. On receipt of the headers they were found to differ from those used in the R script; for example ‘2.10 Initial Diagnosis’ in the MINAP schema was ‘AdmissionDiagnosis’ in the file and ‘4.02 Discharge Diagnosis’ was ‘FinalDiagnosis’. The same problem was experienced in sites B and E and meant header titles in the R script needed to be re-coded to match those in the data set.
The R script was originally coded using the MINAP data set version 10.3.2 available on the NICOR website. However, sites A, B, C and D extracted data from local databases for upload into QualDash, and the versions extracted varied as NICOR allow different versions to be uploaded and data to be uploaded without headers. Site E, by contrast, downloaded the data directly from NICOR. Variation in column headers and its impact was an ongoing problem for the MINAP dashboard, with variation not just between sites but also within sites, as was the case in site B, with different column headers used for different uploads. In comparison with MINAP, the column headers in the PICANet data sets used were consistent, as they were downloaded at all sites from PICANet web.
Appendix 12 An extended and refined QualDash programme theory
Configuration | Context | + | Mechanism | = | Outcome | |
---|---|---|---|---|---|---|
Resource | Response | |||||
D1 | Staff who already use NCA data and who are expert in its interpretation | + | Usability evaluation using real data | Expertise: because of their knowledge of the NCA, the performance of their service in that NCA and how the metrics are normally presented within their service, those staff who are expert in the NCA are easily able to identify discrepancies between how metrics are displayed and their expectations | = | QualDash revised prior to introduction so that QualCards fit with user expectations |
A1 |
Clinical services where NCA data use is desirable but variable because of constrained resources QualDash is successfully installed, is accessible and works as intended Champions have confidence metrics are calculated appropriately |
+ | Clinical champion and e-bulletin and/or meetings |
Awareness: teams are informed about QualDash (i.e. how it can be accessed, its functions and potential benefits) and log on to explore its utility Leadership and Influence: teams trust champion’s opinion and can see the potential benefits of QualDash and so are willing to use it |
= | Clinical staff with an interest in the NCA (e.g. service managers) make an assessment of if and how they can use QualDash to facilitate work processes |
A2 |
Clinical services where NCA data use is desirable but variable because of constrained resources QualDash is successfully installed, is accessible and works as intended |
+ | Clinical (senior) staff engaged as champions for QualDash |
Leadership: clinical champions add QualDash to meeting agendas and lead its use in the meeting Influence: the clinical champion’s colleagues are encouraged/inspired to use QualDash themselves based on champion’s example |
= |
QualDash is trialled in settings where there is potential for integration into routine practices The champion’s colleagues use QualDash to assess if and how it can be useful to them |
A3 |
QualDash is successfully installed, is accessible and works as intended Early interactions have established a role for QualDash in use of NCA data |
+ |
QualDash is intuitive to use Quick reference training materials Champions available to support use |
Confident users: teams learn quickly how to use the dashboard functions to support and benefit their work processes | = | Sustained and optimised QualDash use (i.e. QualDash not ‘tarred with the dodgy brush’) |
A4 | Upload of data to QualDash undertaken by IT staff who have the expertise necessary to complete such tasks with minimum training | + | Quick reference training materials | IT staff quickly learn how, and feel confident, to upload data to QualDash without further support | = | Data are uploaded to QualDash routinely, providing access to timely data to maintain QualDash use |
A5 |
Upload of data to QualDash by non-technical staff Necessary computing resources |
+ |
In-person guidance for first upload Quick reference training materials provided to support ongoing data upload |
Audit support staff learn and are supported to upload data to QualDash | = | Data are uploaded to QualDash routinely, providing access to timely data to maintain QualDash use |
Q1 |
Teams have processes in place (e.g. clinical governance meetings) for monitoring service performance using data available to them Teams are constrained in ability to use NCA data because data are not considered timely, accurate and/or complete Teams are motivated to use QualDash and feel confident in ability to do so Data are uploaded to QualDash, providing access to recent data |
+ |
Easy access to visualisations of key metrics Clear labelling of metrics |
Data quality: teams interact with QualDash to utilise data, underpinned by the mechanisms of professionalism, incentives and competition. As staff understand the metrics, they can determine whether or not data displayed are timely, accurate and/or complete. If not, efforts are made to adjust data collection processes to benefit from QualDash Teams use QualDash to embed NCA data within their monitoring processes |
= |
Improvement in data timeliness, accuracy and completeness As data quality improves, QualDash use increases |
Q2 |
Teams have processes in place (e.g. clinical governance meetings) for monitoring that patient care is safe and effective and/or for monitoring performance in relation to service funding Teams resourced to use NCA data routinely (e.g. audit support staff) are available to produce reports that are timely and trusted as accurate by clinical staff Teams are motivated to use QualDash and feel confident in ability to do so Data are uploaded to QualDash, providing access to recent data |
+ |
Visualisation of key metrics and ability to interrogate data Clear labelling of metrics |
Facilitation: teams use QualDash in meetings because it facilitates direct access and monthly monitoring of metrics that are considered markers of safe and effective care (underpinned by the mechanism of professionalism) and/or are linked to service funding (underpinned by the mechanism of Incentives). Staff are confident that the calculation of the metrics matches their expectations Automation: audit support staff who are confident that the calculation of the metrics matches their expectations use QualDash to produce reports when requested by clinical and managerial staff, as QualDash automates the calculation and visualisation of metrics |
= | Reduced time spent by clinical and audit support staff in accessing, and generating visualisations of, NCA data |
Q3 |
Teams have processes in place (e.g. monthly clinical governance meetings) for monitoring service performance Teams that do not, or are not able to, monitor key metrics routinely (e.g. because of constraints in resources to produce reports using NCA data) Teams are motivated to use QualDash and feel confident in ability to do so Data are uploaded to QualDash, providing access to recent data |
+ |
Web-based access to key metrics that are considered markers of safe and effective care Clear labelling of metrics |
Integration: teams use QualDash because it facilitates access to NCA data that are considered to be key markers of care quality, they understand the metrics, and visualisations highlight discrepancies between performance and targets (e.g. in MINAP) and/or changes in performance month by month (e.g. in MINAP and PICANet), which staff then take action to address, underpinned by the mechanisms of professionalism and incentives | = |
NCA data are integrated into routine service monitoring processes Introduction of QI initiatives in relation to key metrics, where resources allow Over time, improvement in those metrics |
Q4 |
Teams have processes (e.g. monthly clinical governance meetings) in place for monitoring service performance, but existing systems produce static reports and do not provide functions to interact with and drill down into NCA data Teams are motivated to use QualDash and feel confident in ability to do so Data are uploaded to QualDash, providing access to recent data |
+ |
Ability to interact with NCA data and explore relationships between variables Clear labelling of metrics |
Data interrogation: teams use QualDash in meetings, instead of traditional static reports, because teams understand the metrics and QualDash enables them to interrogate anomalies in the data, underpinned by the mechanisms of professionalism and/or incentives, helping teams to understand what has affected performance and enabling them to identify appropriate QI initiatives | = |
Introduction of appropriate QI initiatives where performance is not in line with expectations, where resources allow Over time, improvement in metrics that QI initiatives target |
Q5 |
Teams are asked to produce reports and provide recommendations for managers and other groups about service performance (e.g. at time of publication of NCA national report) Teams are motivated to use QualDash and feel confident in ability to do so Data are uploaded to QualDash, providing access to recent data |
+ |
Easy access to NCA data and visualisations that can be exported into reports Clear labelling of metrics |
Reporting: teams choose to use QualDash to produce performance reports requested by other groups, as they are confident that the calculation of the metrics matches their expectations and because they see it removes the manual work involved | = |
Reduced time spent by team in report preparation Use of NCA data at divisional and organisational levels via QualDash outputs Over time, divisional and organisational QualDash use increases as teams become aware that they can access the data they require directly |
Q6 |
Teams receive data requests from managers Managers are motivated to use QualDash and feel confident in ability to do so Data are uploaded to QualDash, providing access to recent data |
+ |
Web-based access for multiple users Clear labelling of metrics |
Access: managers will use QualDash to access the information they need quickly and easily and are confident that they understand the metrics | = |
Streamlines use of NCA data for managers Reduced time spent by audit support staff/team in producing data reports for managers |
Q7 |
Teams need to evidence performance to managers and other groups to support a case for practice change (e.g. in business meetings with managers or in NCA national report summary) Teams are motivated to use QualDash and feel confident in ability to do so Data are uploaded to QualDash, providing access to recent data |
+ |
QualDash visualises key metrics that can also be exported into reports and presentations Clear labelling of metrics |
Evidence for change: teams use QualDash to monitor service performance, underpinned by the mechanisms of professionalism and/or incentives, and, when they identify a QI need that requires additional resources, they use QualDash to report evidence that can convince trust groups with power to provide resource. Staff are confident the calculation of the metrics matches their expectations | = | Trust groups with power to allocate additional resource to teams are convinced of the need for change, particularly where key metrics relate to trust priorities (e.g. relating to trust reputation or avoiding penalties/receiving incentives) |
Appendix 13 QualDash main trial design
We explored the feasibility of undertaking a trial of QualDash in collaboration with PICANet (MINAP seems a less appropriate option given cardiology consultants’ preference for BCIS as a source of audit and feedback). This trial would focus on use at the clinical team level, as our findings reveal that this is where most use of NCA data takes place. Working with the Leeds Institute of Clinical Trials Research, we have developed the following trial design for QualDash.
Trial design
A stepped wedge factorial design randomised at the PICU level, with a repeated cross-sectional design in which outcomes are assessed on different children in each cluster in each time period. Alongside this, we would undertake a realist process evaluation,155 allowing further refinement of the QualDash programme theory.
Interventions
-
Dashboard compared with no dashboard.
-
Adoption and training compared with no adoption and training.
Primary outcome
The primary outcome would be emergency readmission within 48 hours of discharge (yes/no). This metric is considered an important indicator of care by PICU clinicians and one where there is substantial unexplained variation between PICUs.
Randomisation
Paediatric intensive care units would be randomised by the Clinical Trials Research Unit to one of eight groups (Table 27), using block randomisation with equal allocation to determine what intervention they receive in years 1–5 of the trial. Following randomisation, the Clinical Trials Research Unit would inform the chief investigator and PICANet of what each PICU will receive on a year-by-year basis, 1 year at a time, who will, in turn, inform the PICUs. A log will be maintained of who is unblinded to what at specific points in the trial.
Group | Year | ||||
---|---|---|---|---|---|
1 | 2 | 3 | 4 | 5 | |
1 | 0 | 1 | 1 + 2 | 1 + 2 | 1 + 2 |
2 | 0 | 1 | 1 | 1 + 2 | 1 + 2 |
3 | 0 | 0 | 1 | 1 | 1 + 2 |
4 | 0 | 0 | 0 | 1 | 1 |
5 | 0 | 0 | 0 | 2 | 2 |
6 | 0 | 0 | 0 | 2 | 1 + 2 |
7 | 0 | 0 | 2 | 2 | 1 + 2 |
8 | 0 | 2 | 2 | 1 + 2 | 1 + 2 |
Sample size
There are two principal comparisons of interest (dashboard vs. no dashboard and adoption and training vs. no adoption and training) that relate to the two main effects of the 2 × 2 factorial design. Assuming, based on the PICANet annual report (2019),156 that an average of 1.66% of admissions per year result in an emergency readmission within 48 hours of discharge in the control group with an intracluster correlation coefficient of 0.003846, then 32 PICUs each with an average of 627 admissions per year would provide 90% power to detect a reduction to 1.524% in the presence of a small synergistic interaction, with a two-sided 5% significance level.
Statistical analysis
The primary analyses will be conducted on an intention-to-treat basis using all available follow-up data from all consecutive admissions, imputing unavailable data where possible. The child-level binary primary outcome of emergency readmission within 48 hours of discharge will be analysed using the mixed-effects model suggested by Sundin and Crespi,157 with a random intercept for PICU, a fixed effect for time, treatment effects for interventions 1 and 2 and their interaction, and a residual. Consideration will be given as to whether or not to decompose the PICU-level variance into time-varying and time-invariant components. Supportive analyses will be conducted to assess the sensitivity of the conclusions of the primary analysis to assumptions about the missing data.
Assumptions for sample size calculation
Total number of readmissions: 327 (2016) + 334 (2017) + 340 (2018) = 1001 (2016–18).
Total number of admissions: 20,269 (2016) + 19,849 (2017) + 20,145 (2018) = 60,263 (2016–18).
So proportions are: 327/20269 = 0.0161; 334/19849 = 0.0168 and 340/20145 = 0.0169.
Overall: 1001/60,263 = 0.0166, and so approximately 1.7%.
Intracluster correlation coefficient estimate: 0.003846.
Sample size calculations in R
Using code provided by Sundin and Crespi157 for standardised effect sizes and then adding assumptions for QualDash:
##QUALDASH
delta_1 <- delta_2 <- delta_3 <- 0.048
n.clusters <- 32
n.periods <- 5
n.individuals <- 627
typeIerror <- 0.05
RhoW <- RhoA <- 0.003846
ModelChoice <- “RCS”
IAC <- 0
Sequence_Tx1 <- c(2,2,3,4,NA,5,5,4)
Sequence_Tx2 <- c(3,4,5,NA,4,4,3,2)
PowerFunction_SWFD(RhoW, ModelChoice,n.individuals, delta_1, delta_2,delta_3, RhoA, IAC, typeIerror, Sequence_Tx1,Sequence_Tx2)

##QUALDASH
delta_1 <- delta_2 <- delta_3 <- 0.042
n.clusters <- 32
n.periods <- 5
n.individuals <- 627
typeIerror <- 0.05
RhoW <- RhoA <- 0.003846
ModelChoice <- “RCS”
IAC <- 0
Sequence_Tx1 <- c(2,2,3,4,NA,5,5,4)
Sequence_Tx2 <- c(3,4,5,NA,4,4,3,2)
PowerFunction_SWFD(RhoW, ModelChoice,n.individuals, delta_1, delta_2,delta_3, RhoA, IAC, typeIerror, Sequence_Tx1,Sequence_Tx2)
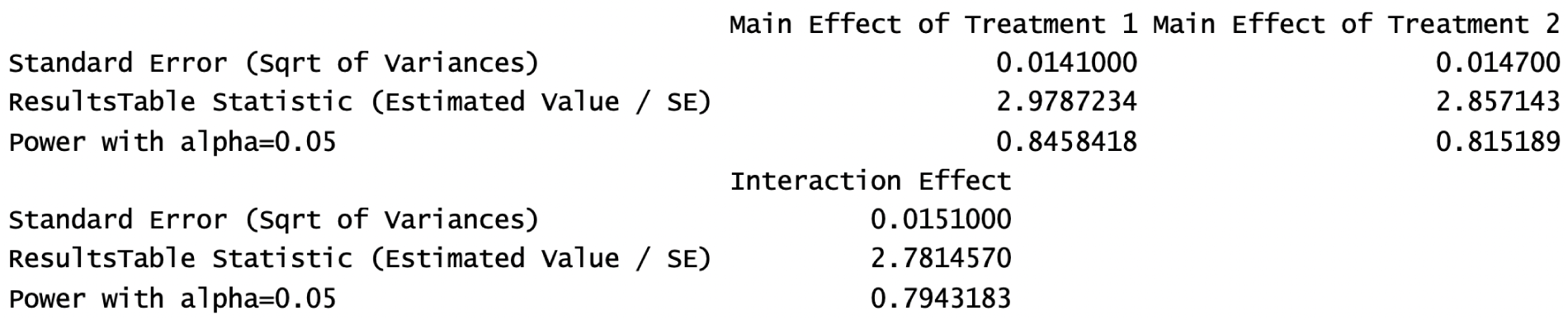
Transfer standardised effects sizes into odds ratios
URL: https://campbellcollaboration.org/escalc/html/EffectSizeCalculator-OR5.php (accessed 27 January 2022).
Delta = 0.042 -> OR = 1.0792
Delta = 0.048 -> OR = 1.0910
Use NQuery to see what the minimum effect we would be able to detect.
0.0166 versus 0.0156 leads to OR = 1.065
0.0166 versus 0.0155 leads to OR = 1.072
0.0166 versus 0.01524 leads to OR = 1.091
Therefore, it is possible to detect a minimum change to 1.55% with 80% power and to 1.524% with 90% power.
List of abbreviations
- BCIS
- British Cardiovascular Intervention Society
- BPT
- Best Practice Tariff
- CCG
- Clinical Commissioning Group
- CFIT
- contextual feedback intervention theory
- CHI
- Commission for Health Improvement
- CI
- confidence interval
- CMO
- context–mechanism–outcome
- CQC
- Care Quality Commission
- CQUIN
- Commissioning for Quality and Innovation
- CSU
- clinical service unit
- DGH
- district general hospital
- DHSC
- Department of Health and Social Care
- ECG
- electrocardiography
- EHR
- electronic health record
- GP
- general practitioner
- HDU
- high-dependency unit
- HIT
- health information technology
- HQIP
- Healthcare Quality Improvement Partnership
- HRG
- Healthcare Resource Group
- IQR
- interquartile range
- IT
- information technology
- ITS
- interrupted time series
- LAG
- Lay Advisory Group
- MINAP
- Myocardial Ischaemia National Audit Project
- NCA
- national clinical audit
- NCAPOP
- National Clinical Audit and Patient Outcomes Programme
- NGT
- nominal group technique
- NICOR
- National Institute for Cardiovascular Outcomes Research
- NIHR
- National Institute for Health and Care Research
- NPT
- normalisation process theory
- NSF
- National Service Framework
- PC
- personal computer
- PCI
- percutaneous coronary intervention
- PEC
- preoperative evaluation clinic
- PICANet
- Paediatric Intensive Care Audit Network
- PICU
- paediatric intensive care unit
- QI
- quality improvement
- R&D
- research and development
- RCT
- randomised controlled trial
- SMR
- standardised mortality ratio
- SRS
- software requirements specification
- SSC
- Study Steering Committee
- SSQD
- Specialised Services Quality Dashboard
- STEMI
- ST-elevation myocardial infarction
- SUS
- System Usability Scale
- TAM
- technology acceptance model
Notes
-
QualDash: designing and evaluating an interactive dashboard to improve quality of care – evaluation questionnaire
Supplementary material can be found on the NIHR Journals Library report page (https://doi.org/10.3310/WBKW4927).
Supplementary material has been provided by the authors to support the report and any files provided at submission will have been seen by peer reviewers, but not extensively reviewed. Any supplementary material provided at a later stage in the process may not have been peer reviewed.