Notes
Article history
The research reported in this issue of the journal was funded by the HTA programme as award number NIHR127454. The contractual start date was in July 2019. The draft report began editorial review in January 2022 and was accepted for publication in September 2022. The authors have been wholly responsible for all data collection, analysis and interpretation, and for writing up their work. The HTA editors and publisher have tried to ensure the accuracy of the authors’ manuscript and would like to thank the reviewers for their constructive comments on the draft document. However, they do not accept liability for damages or losses arising from material published in this manuscript.
Permissions
Copyright statement
Copyright © 2024 Horner et al. This work was produced by Horner et al. under the terms of a commissioning contract issued by the Secretary of State for Health and Social Care. This is an Open Access publication distributed under the terms of the Creative Commons Attribution CC BY 4.0 licence, which permits unrestricted use, distribution, reproduction and adaptation in any medium and for any purpose provided that it is properly attributed. See: https://creativecommons.org/licenses/by/4.0/. For attribution the title, original author(s), the publication source – NIHR Journals Library, and the DOI of the publication must be cited.
2024 Horner et al.
Chapter 1 Introduction
The problem – preventing hospital-acquired venous thromboembolism
Venous thromboembolism (VTE) remains a major worldwide health burden, with an annual incidence of between 0.75 and 2.69 per 1000 individuals in the population. 1 At least half of all VTE occurs during hospitalisation, or up to 90 days following discharge; such cases are described as hospital-associated VTE or hospital-acquired thrombosis (HAT). 2 Many HAT cases are potentially preventable, through the use of thromboprophylaxis during the initial hospital stay and patient education. 3–5 Despite the widespread availability of evidence-based prevention strategies, HAT has been described as the number one patient safety issue for individuals requiring hospital admission, worldwide. 6 Previous evidence suggests that HAT accounts for more deaths and disability than nosocomial pneumonia, catheter-related bloodstream infections or adverse drug events in low- and middle-income countries. 7
Both pharmacological and mechanical thromboprophylaxis can reduce the risk of VTE. However, pharmacological thromboprophylaxis potentially increases the risk of bleeding and exposes patients to iatrogenic adverse events. Therefore, the decision to provide thromboprophylaxis involves consideration of the risks of VTE and bleeding, along with the costs of providing thromboprophylaxis and treating the consequences of VTE and bleeding.
VTE risk assessment models (RAMs) are used to estimate the risk of VTE and hence the benefit of providing thromboprophylaxis. Using a VTE RAM to target those with higher risk should improve cost-effectiveness. However, this cannot be assumed. If thromboprophylaxis is very effective, then it may be cost-effective to treat everyone rather than treating only those at higher risk. If it is not very effective, then it may not be cost-effective to treat anyone.
The cost-effectiveness of VTE risk assessment tools: uncertainty and evidence deficit
Many VTE RAMs have been developed and some have been validated by estimating the prognostic accuracy for VTE. Systematic reviews have examined VTE RAMs for acutely ill medical patients (11 studies), hospitalised non-surgical patients (11 studies) and all hospitalised populations (22 studies). 8–10 The reviews identified many RAMs but with limited evaluation. The primary studies had important methodological limitations and were generally judged as low quality. There was substantial variation between the primary studies in terms of populations, methods and outcomes used, which precluded meta-analysis and limited comparison between RAMs. Overall, the studies suggested that the identified RAMs had modest prognostic value with most reporting C-statistics around 0.6–0.7. Sensitivity and specificity depended upon the threshold used but high sensitivity could only be achieved by substantial loss of specificity. An ideal RAM could not be recommended by any of the reviews. However, further primary research has been published since these systematic reviews were undertaken, so there may be some benefit from updating these reviews.
To date, limited research has explored the trade-off between the risks of VTE and thromboprophylaxis. This trade-off is essential in determining whether a RAM will be cost-effective and, if so, the threshold of risk or balance of sensitivity and specificity that should be used in decision-making. Le et al. used decision-analytic modelling to estimate a risk threshold for prophylaxis in hospitalised medical patients. 11 Undertaken from a US health system perspective with a willingness to pay threshold of $100,000 per quality-adjusted life-year (QALY) gained, the analysis showed that pharmacological thromboprophylaxis was cost-effective for an average medical patient with a VTE risk exceeding 1.0%. Decision-analytic modelling undertaken from a NHS perspective using thresholds advised by the National Institute for Health and Care Excellence (NICE) could inform NHS use of VTE prophylaxis.
There has been very little primary research into VTE RAMS in the NHS. Ideally, the preferred approach in this situation would be to derive and validate VTE RAMs in a typical setting. However, the established effectiveness of VTE prophylaxis in hospital inpatients presents a substantial challenge for studies estimating the accuracy of VTE RAMs. Given our current understanding, it would be unethical to withhold prophylaxis from patients who are at risk of VTE. The fundamental aim of risk assessment is to identify patients where VTE could be prevented by thromboprophylaxis. Over 70% of medical patients in the UK receive thromboprophylaxis when the Department of Health (now Department of Health and Social Care) risk assessment tool is used (NICE 2018). 8 In this situation, around half of all VTE will have been prevented and any prognostic model derived from observational data will largely be predicting VTE that would not be prevented with thromboprophylaxis. 4,12 Any prognostic model derived in this setting would, therefore, be based on factors that predict non-preventable VTE while underestimating (or missing) those that predict preventable VTE. A RAM that predicted non-preventable VTE while failing to predict preventable VTE would be of no clinical benefit and could potentially increase harm.
These uncertainties are highlighted in recent NICE guidance within a UK setting, which now advocates use of any RAM published by a national UK body, professional network or peer-reviewed journal. 8 Australasian guidance is similar. 13 However, North American guidelines offer variable messaging on risk assessment. The American Society of Haematology guidelines avoid any recommendations on RAM use, but advise that clinicians ‘integrate VTE and bleeding risk assessments into clinical decision-making processes’. 14 Alternatively, the American College of Chest Physician guidelines dichotomise non-surgical patients into low and high risk within their recommendations, basing assessment on a specific RAM (the Padua prediction score). 15,16 All guidelines highlight the limitations within the evidence base for RAMs. Such disparity in recommendations invites variation in practice and ongoing uncertainty for clinicians and patients.
Chapter 2 Rationale and objectives
Rationale
Recent systematic reviews have shown the limited evaluation of VTE RAMs to date and we have highlighted the challenge of obtaining robust primary data. 8–10 However, it would be worth updating recent systematic reviews to avoid research waste and ensure that further research is based on the best currently available data. Decision-analytic modelling of the published evidence can also be used to estimate the cost-effectiveness of VTE RAMs for hospital inpatients. It can determine whether risk assessment has the potential to be cost-effective compared with thromboprophylaxis for all and, if so, what threshold of risk should be used for providing thromboprophylaxis and what trade-off between sensitivity and specificity would be optimal. It can, therefore, increase the insights available from the existing evidence and identify areas of uncertainty that require further research. This is particularly valuable when ethical considerations limit the design of primary research.
Given that the widespread use of VTE prophylaxis limits the potential to undertake prognostic accuracy studies of VTE RAMs, future primary research may need to evaluate the comparative effectiveness and cost-effectiveness of implementing alternative approaches to risk assessment. This research could involve individual patient or cluster randomised interventional trial designs, quasi-randomised methods or observational methods. These methods could all benefit from using an efficient design, in which routine data sources are used to measure VTE and bleeding outcomes, and potentially also to record or implement RAMs as part of an evaluation. Efficient methods could increase the available sample size for future primary research and support a study comparing different risk-assessment methods. This could increase the potential range of feasible study designs and the information generated by future primary research. However, research is required to determine whether efficient methods are feasible for comparative evaluation of VTE RAMs. Specifically:
-
In order to be used in future research designs, routine data sources need to accurately record VTE and bleeding outcomes.
-
Efficient methods for recording or implementing RAMs should build upon existing methods of delivering risk assessment within a hospital setting.
-
Future research comparing RAMS would need to ensure that different patients (and different proportions of patients) received prophylaxis, otherwise any differences in outcome would be difficult to attribute to the RAM used.
Objectives
We aimed to evaluate the cost-effectiveness of VTE RAMs in hospital inpatients, determine the optimal approach to providing thromboprophylaxis and determine how changing the risk threshold for prophylaxis affects cost-effectiveness. We also sought to evaluate the potential performance of different RAMs in an NHS setting, assess the use of efficient methods to capture data on risk characteristics and to determine the accuracy of efficient methods to capture relevant outcomes in a future trial. Our specific objectives were as follows:
-
Update recent systematic reviews to identify VTE RAMs for hospital inpatients and estimate prognostic accuracy.
-
Undertake decision-analytic modelling to determine the cost-effectiveness of VTE risk assessment compared to thromboprophylaxis for all and thromboprophylaxis for none, specifically determining the risk threshold that optimises effectiveness (QALYs) and cost-effectiveness (i.e. maximises net benefit assuming willingness to pay according to NICE thresholds).
-
Use the decision-analytic model to identify key areas of uncertainty and determine the value of gathering additional information to reduce uncertainty.
-
Pilot the use of efficient methods alongside routine practice to determine the feasibility of a future implementation study of VTE risk assessment tools in hospital inpatients.
-
Estimate key parameters for planning a future implementation study.
Objectives 1, 2 and 3 are addressed by workstream 1 (systematic review and economic modelling). Objectives 4 and 5 are addressed by workstream 2 (cohort study).
Chapter 3 Workstream 1 – systematic review
A systematic review of the literature was undertaken to determine the comparative accuracy of individual RAMs for predicting the risk of developing VTE in hospital inpatients. Sections of this chapter have been reproduced from Pandor et al. under licence CC BY 4.0. 17
This review was undertaken in accordance with the general principles recommended in the Preferred Reporting Items for Systematic Reviews and Meta-Analyses (PRISMA) statement18 and was registered on the International Prospective Register of Systematic Reviews (PROSPERO) database (CRD42020165778).
Methods
Eligibility criteria
We sought studies evaluating RAMs which could be applied to a general inpatient population (medical, surgical or trauma), rather than disease-specific models. All primary validation studies (as derivation studies may give an overoptimistic assessment of model performance measures) that evaluated the accuracy (e.g. sensitivity, specificity, concordance statistic) of a multivariable RAM (or scoring system) for predicting the risk of developing VTE were eligible for inclusion. We selected studies that included validation of the model in a group of patients that were not involved in model derivation. This involved either splitting the study cohort (internal) or using a new cohort (external). The study could have reported derivation of the model, but we only used the validation data to estimate accuracy. The study population consisted of hospital inpatients including those who required medical care, undergoing any surgery (excluding day surgery) or received care following an injury. Studies that primarily focused on children (aged under 16 years), women admitted to hospital for pregnancy-related reasons and any patient admitted to a level 2 or above critical care environment (e.g. patients requiring more detailed observation or intervention including support for a single failing organ system or postoperative care and those ‘stepping down’ from higher levels of care) were excluded. These patient groups have VTE risk profiles that differ markedly from the general inpatient population, making the use of a generic model inappropriate.
Data sources and searches
Potentially relevant studies were identified by searching the following electronic databases and research registers:
-
Ovid MEDLINE® Epub Ahead of Print, In-process and Other Non-indexed Citations, Ovid MEDLINE® Daily, MEDLINE and Versions® (OvidSP) 1946 to February 2021
-
EMBASE (OvidSP) 1974 to February 2021
-
Cochrane Database of Systematic Reviews (www.cochranelibrary.com/) 1996 to February 2021
-
Cochrane Central Register of Controlled Trials (www.cochranelibrary.com/) 1898 to April 2017
-
ClinicalTrials.gov (US NIH) 2000 to February 2021
-
International Clinical Trials Registry Platform (WHO) 1990 to February 2021
The search strategy used free text and thesaurus terms and combined synonyms relating to the condition (e.g. VTE in medical inpatients) with risk prediction modelling terms. No language restrictions were used. However, as the current review updated three previous systematic reviews,8,9,10 searches were limited by date from 2017 (last search date from earlier reviews)8 to February 2021. Searches were supplemented by hand-searching the reference lists of all relevant studies (including existing systematic reviews); forward citation searching of included studies (using the Web of Science Citation Index Expanded and Conference Proceedings Citation Index – Science) to identify articles that cite the relevant articles; contacting key experts in the field; and undertaking targeted searches of the World Wide Web using the Google search engine. Further details on the search strategy can be found in Appendix 1.
All identified citations from the electronic searches and other resources were imported into and managed using the EndNote bibliographic software (version X8; Clarivate Analytics, Philadelphia, PA, USA).
Study selection process
The inclusion of potentially relevant articles was undertaken using a two-step process. First, all titles were examined for inclusion by one reviewer Katie Sworn (KS) and any citations that clearly did not meet the inclusion criteria (e.g. non-human, unrelated to VTE inpatients) were excluded. Second, all abstracts and full-text articles were then examined independently by two reviewers (KS and AP). Any disagreements in the selection process were resolved through discussion or if necessary, arbitration by a third reviewer (SG) and included by consensus.
Data abstraction and quality assessment strategy
Data relating to study design, methodological quality and outcomes were extracted by one reviewer (KS) into a standardised data extraction form and independently checked for accuracy by a second [AP or Michael Tonkins (MT)]. Any discrepancies were resolved through discussion or if necessary, arbitration by a third reviewer (SG) and included by consensus. Where multiple publications of the same study were identified, data were extracted and reported as a single study.
The methodological quality of each included study was assessed using PROBAST (Prediction model Risk Of Bias Assessment Tool). 19,20 This instrument evaluates four key domains: patient selection, predictors, outcome and analysis. Each domain is assessed in terms of risk of bias and the concern regarding applicability to the review (first three domains only). To guide the overall domain-level judgement about whether a study is at high, low or an unclear (in the event of insufficient data in the publication to answer the corresponding question) risk of bias, subdomains within each domain include a number of signalling questions to help judge with bias and applicability concerns. An overall risk of bias for each individual study was defined as low risk when all domains were judged as low; and high risk of bias when one or more domains were considered as high. Studies were assigned an unclear risk of bias if one or more domains were unclear and all other domains were low. Further details on the PROBAST tool can be found in Appendix 1, Table 20.
The methodological quality of each included study was independently evaluated by two reviewers (KS and AP). Any discrepancies were resolved through discussion or if necessary, with involvement of a third reviewer (MT). Blinding of the quality assessor to author, institution or journal was not considered necessary.
Data synthesis and analysis
We were unable to perform meta-analysis due to significant levels of heterogeneity between studies (participants, inclusion criteria, clinical condition) and variable reporting of items. As a result, a pre-specified narrative synthesis approach21,22 was undertaken, with data being summarised in tables with accompanying narrative summaries that included a description of the included variables, statistical methods and performance measures [e.g. sensitivity, specificity and C-statistic (a value between 0.7–0.8 and > 0.8 indicated good and excellent discrimination, respectively; and values < 0.7 were considered weak23)], where applicable. All analyses were conducted using Microsoft Excel 2010 (Microsoft Corporation, Redmond, WA, USA).
Patient and public involvement
Patients and the public were not involved in the design of this systematic review. However, all patient representatives reviewed the most widely validated risk models identified, to comment on the appropriateness of criteria, acceptability and potential for face-to-face and remote (patient-led) completion.
Results
Quantity and quality of research available
The literature searches identified 6355 citations. Of these, 51 studies15,24–73 investigating 24 unique RAMs met the inclusion criteria. A flow chart describing the process of identifying relevant literature can be found in Figure 1. A total of 60 full-text articles were excluded as they did not meet all the pre-specified inclusion criteria. The majority of the articles were excluded primarily for not using a RAM for predicting the risk of developing VTE, having no useable or relevant outcome data or an inappropriate study design (e.g. derivation study, reviews, commentaries or editorials). A full list of excluded studies with reasons for exclusion is presented in Report Supplementary Material 1.
FIGURE 1.
Study flow chart (adapted from free online resources hosted by the EQUATOR network https://www.equator-network.org).

Description of included studies (design and patient characteristics)
The design and participant characteristics of the 51 included studies15,24–73 that provided data on the comparative accuracy of RAMs for predicting VTE in patients admitted to hospital is summarised in Table 1. All studies were published between 2003 and 2020 and were undertaken in North America (n = 24),26,27,35–42,45,49,50,54–61,67,70,71 Asia (n = 13),31,32,44,46–48,62–65,69,72,73 Europe (n = 9),15,25,28–30,33,51,53,68 the Middle-East (n = 2),24,65 South America (n = 1),34 Australia (n = 1)43 and one study was intercontinental. 52 Sample sizes ranged from 7042 to 1,099,09345 patients in 37 observational cohort studies [11 prospective15,24,25,30,31,34,46,53,54 (5 of which were multicentre) and 26 retrospective26–29,35,36,38–43,45,48,51,52,55–57,60,61,64,65,67,70,71 (16 of which were multicentre) in design]. Sample sizes in 14 case–control studies32,33,37,44,47,49,50,59,62,63,68,69,72,73 (4 of which were multicentre) ranged from 14863 to 19,21759 patients.
Author, year | Country | Design | Single/multicentre | Sample size | Population | Mean age (years) | Female | VTE prophylaxis | Risk assessment model | Target condition (risk period) |
Incidence |
---|---|---|---|---|---|---|---|---|---|---|---|
Autar, 200325 | UK | P, CS | Single | 148 | Hospitalised patients from orthopaedic, medical and surgical specialties | NR | NR | 50% | Novel (Autar 2003) | DVT, not defined (90 days) | 18.9% |
Rogers, 200758 | USA | P, CS | Multi | 91,308 | Hospitalised surgical patients (undergoing vascular and general surgery) | NR | NR | NR | Novel (Rogers 2007) | VTE (30 days) | 0.6% |
Abdel-Razeq, 201024 | Jordan | P, CS | Single | 606 | Hospitalised (> 24 hours) cancer patients aged ≥ 18 years | 51 | 49% | 55% | Caprini (modified) | VTE, symptomatic (60 days) | 3.5% |
Bahl, 201026 | USA | R, CS | Multi | 8216 | Hospitalised surgical patients (undergoing general, vascular and urologic surgery) | NR | NR | NR | Caprini | VTE, (30 days) | 1.4% |
Barbar, 201015 | Italy | P, CS | Single | 1180 | Hospitalised medical patients | NR | 53% | 16% | Padua | VTE, symptomatic (90 days) | 3.1% |
Rothberg, 201160 | USA | R, CS | Multi | 48,540 | Hospitalised (≥ 3 days) medical patients aged ≥ 18 years | NR | NR | 30% | Novel (Rothberg 2011) | VTE, hospital-associated (NR) | 0.5% |
Woller, 201171 | USA | R, CS | Multi | 46,856 | Hospitalised medical patients aged ≥ 18 years | 61 | 54% | NR | Intermountain Kucher |
VTE, defined by ICD-9 codes (90 days) | 4.5% |
Pannucci, 201255 | USA and Canada | R, CS | Multi | 5761 | Hospitalised (> 2 days) patients with a burn injury aged ≥ 18 years | 46 | 31% | NR | Novel (Panunucci 2012) | VTE, not defined (NR) | 1.0% |
Rogers, 201257 | USA | R, CS | Multi | 234,032 | Hospitalised trauma patients | NR | NR | NR | TESS | VTE (NR) | NR |
Bilimoria, 201327 | USA | R, CS | Multi | 88,053 | Hospitalised surgical patients (undergoing colorectal surgery) | NR | NR | NR | ACS NSQIP – Colon-specific ACS NSQIP – Universal |
DVT, not defined (30 days) | 2.3% |
Hegsted, 201341 | USA | R, CS | Single | 2281 | Hospitalised (≥ 2 days) trauma patients aged ≥ 13 years | 45 | 30% | NR | RAP | DVT, not defined or PE (NR) | DVT: 10.5% PE: 1.5% |
Vardi, 201366 | Israel | P, CS | Single | 1080 | Hospitalised (≥ 2 days) sepsis patients aged > 18 years | 75 | 48% | 18% | Padua | VTE, hospital associated (NR) | 1.3% |
Ho, 201443 | Australia | R, CS | Single | 357 | Hospitalised major trauma patients | NR | 25% | NR | TESS | VTE, symptomatic (NR) | 20.7% |
Liu, 201446 | China | P, CS | Single | 287 | Hospitalised acute stroke patients aged > 18 years | NR | 37% | 22% | Post-stroke DVT Prediction System | DVT (14 ± 3 days) | 10.5% |
Mahan, 201449 | USA | CC | Multi | 417 | Hospitalised (≥ 3 days) medical patients aged ≥ 18 years | NR | 51% | NR | IMPROVE (7-factor) | VTE, hospital-associated (92 days) | NA |
Nendaz, 201453 | Switzerland | P, CS | Multi | 1478 | Hospitalised (> 24 hours) medical patients aged ≥ 18 years | 65 | 47% | 57% | Geneva Padua |
VTE, symptomatic including PE or DVT (90 days) | 2.0% |
Pannucci, 201454 | USA | P, CS | Multi | 3576 | Hospitalised surgical patients aged ≥ 18 years | NR | NR | 66% | Novel (Panunucci, 2014) | VTE (90 days) | 1.4% |
Rosenberg, 201459 | USA | CC | Multi | 19,217 | Hospitalised (≥ 3 days) medical patients aged ≥ 18 years | NR | 53% | 43% | IMPROVE (7-factor) | VTE, defined by ICD-9 codes (90 days) | NA |
Zhou 201473 | China | CC | Single | 998 | Hospitalised (≥ 2 days) medical patients aged > 18 years | NR | 42% | 15% | Caprini Padua |
VTE, defined by ICD-10 codes (NR) | NA |
Hewes, 201542 | USA | R, CS | Single | 70 | Hospitalised cancer patients (undergoing oesophagectomy) | NR | 17% | 96% | Caprini (modified) | VTE (60 days) | 14.3% |
de Bastos, 201634 | Brazil | P, CS | Single | 11,091 | Hospitalised medical patients aged > 18 years | 50 | 39% | 0% | Caprini | VTE, symptomatic (NR) | 0.3% |
Grant, 201638 | USA | R, CS | Multi | 63,548 | Hospitalised (≥ 2 days) medical patients aged ≥ 18 years | 66 | 55% | 61% | Caprini | VTE, hospital associated (90 days) | 1.1% |
Greene, 201639 | USA | R, CS | Multi | 63,548 | Acutely ill, hospitalised (≥ 2 days) medical patients aged ≥ 18 years | 66 | 55% | 61% | IMPROVE (4-factor) Intermountain Kucher Padua |
VTE, hospital associated (90 days) | 1.1% |
Hachey, 201640 | USA | R, CS | Single | 232 | Hospitalised surgical patients (undergoing segmentectomy, lobectomy or pneumonectomy for lung cancer) | NR | 57% | 92% | Caprini | VTE (60 days) | 5.2% |
Lui, 201647 | China | CC | Single | 640 | Hospitalised (> 2 days) medical patients aged ≥ 18 years | NR | 48% | NR | Caprini Padua |
VTE (NR) | N/A |
Lobastov, 201648 | Russia | R, CSa | Multi | 140 | Hospitalised high-risk emergency surgery patients (undergoing general and neurosurgery) | 69 | 51% | 100% | Caprini | DVT or PE, new (NR) | 27.9% |
Shaikh, 201661 | USA | R, CS | Multi | 1598 | Hospitalised surgical patients (undergoing plastic surgery) | 50 | 81% | 34% | Caprini | VTE, not defined (30 days) | 1.5% |
Elias, 201736 | USA | R, CS | Single | 30,726 | Hospitalised (> 2 days) medical and surgical patients | NR | 56% | 21% | Padua | VTE, defined by ICD-9 codes (NR) | 0.8% |
Frankel, 2017 (abstract)37 | USA | CC | NR | 149 | Hospitalised surgical patients aged ≥ 18 years (undergoing robotic-assisted laparoscopic prostatectomy) | NR | NR | NR | Caprini | VTE, not defined (90 days) | NA |
Krasnow, 2017 (abstract)45 | USA | R, CS | Multi | 1,099,093 | Hospitalised surgical patients (major urological cancer surgery) | NR | NR | NR | Caprini | VTE, symptomatic (90 days) | 1.2% |
Patell, 201756 | USA | R, CS | Single | 2780 | Hospitalised (> 24 hours) cancer patients aged > 18 years | 62 (median) | 44% | 65% | Khorana | VTE, defined by ICD-9 codes (NR) | 3.8% |
Winoker, 201770 | USA | R, CS | Multi | 300 | Hospitalised surgical patients (undergoing urological surgery using robot-assisted partial nephrectomy) | 61 (median) | 38% | NR | ACS NSQIP – Universal | VTE, not defined (NR) | 0.3% |
Blondon, 201830 | Switzerland | P, CS | Multi | 1478 | Hospitalised (> 24h) medical patients aged ≥ 18 years | 65 | 47% | 59% | IMPROVE (7-factor) Genevab Paduab |
VTE, symptomatic including PE or DVT (90 days) | 2.0% |
Chen, 201832 | China | CC | Single | 390 | Hospitalised (> 2 days) patients aged ≥ 18 years with and without DVT | NR | 49% | 41% | Caprini Padua |
DVT (NR) | NA |
Dornbus, 2018 (abstract)35 | USA | R, CS | NR | 2830 | Hospitalised surgical patients (undergoing neurosurgery) | NR | NR | NR | Caprini | VTE, not defined (NR) | NR |
Vaziri, 201867 | USA | R, CS | Single | 1006 | Hospitalised surgical patients (undergoing neurosurgery) | NR | 54% | NR | ACS NSQIP – Universal | VTE, not defined (NR) | 1.3% |
Vincentelli, 201868 | Italy | CC | Multi | 1215 | Acutely ill, hospitalised medical patients aged > 18 years | NR | 56% | NR | Chopard Kucher Padua |
VTE (NR) | NA |
Zhou, 201872 | China | CC | Single | 1804 | Hospitalised (≥ 2 days) medical patients aged > 18 years | NR | 41% | 5% | Caprini Padua |
VTE, defined by ICD-10 codes (NR) | NA |
Blondon, 2019a3 | Italy | R, CSa | Single | 1180 | Hospitalised medical patients | 72 | 53% | 20% | Geneva (simplified) | VTE, symptomatic (90 days) | 3.1% |
Blondon, 2019b (abstract)29 | Switzerland | R, CSa | Multi | 991 | Hospitalised elderly medical patients | 75 | 45% | NR | Geneva (simplified) IMPROVE (NR) Padua |
VTE, symptomatic (NR) | 15.0% |
Cobben, 201933 | Netherlands | CC | Multi | 556 | Hospitalised (> 24 hours) medical patients | NR | 48% | NR | Caprini Geneva IMPROVE (4-factor) IMPROVE (7-factor) Intermountain Kucher Lecumberri NAVAL NICE Guideline Padua PRETEMED Guideline Zakai et al. (model 2) |
VTE (NR) | NA |
Tachino, 201964 | Japan | R, CS | Multi | 859 | Hospitalised (> 24 hours) trauma patients aged ≥ 18 years | NR | 36% | NR | RAP Quick RAP |
VTE (NR) | 3.0% |
Tian, 201965 | China | R, CS | Single | 533 | Hospitalised surgical patients (undergoing thoracic surgery) | 53 | 47% | 0% | Caprini Khorana Padua Novel (Rogers, 2007) |
VTE (NR) | 8.4% |
Bo, 202031 | China | P, CS | Multi | 24,524 | Hospitalised (≥ 2 days) patients from medical and surgical specialties aged ≥ 18 years | 57 | 43% | NR | Caprini | DVT (NR) | 0.9% |
Hu, 202044 | China | CC | Single | 442 | Hospitalised (≥ 2 days) cancer patients aged ≥ 18 years | NR | 38% | 3.8 | Caprini Khorana |
VTE, defined by ICD-10 codes (NR) | NA |
Mlaver, 202050 | USA | CC | Single | 189 | Hospitalised surgical patients (undergoing hepatobiliary, colorectal, endocrine, plastic, transplant or general surgery) | NR | NR | NR | Caprini Padua |
VTE, not defined (NR) | NA |
Moumneh, 202051 | France | R, CSa | Multi | 14,660 | Acutely ill, hospitalised (≥ 2 days) medical patients aged ≥ 40 years | 73 | 50% | 46.1 | Caprini Padua IMPROVE (7 factor) |
VTE, symptomatic including PE or DVT (90 days) | 1.8% |
Nafee, 202052 | 35 countries | R, CSa | Multi | 6459 | Hospitalised medical patients | 76 | 55% | 100 | IMPROVE (NR) Novel (Nafee, 2020a) Novel (Nafee, 2020b) |
VTE (77 days) | 6.3% |
Shang, 202062 | China | CC | Single | 2878 | Hospitalised (≥ 2 days) cancer patients aged ≥ 18 years | 56 | 53% | NR | Caprini (2009) Caprini (2013) |
VTE, (NR) | NA |
Shen, 202063 | China | CC | Single | 148 | Hospitalised (≥ 2 days) medical patients aged ≥ 18 years | NR | NR | 0 | Novel (Shen 2020) | VTE, not defined (NR) | NA |
Wang, 202069 | China | CC | Single | 1579 | Hospitalised (≥ 3 days) medical patients aged ≥ 18 years | 53 | 43% | NR | Padua | VTE (NR) | NA |
The vast majority of studies evaluated VTE risk assessment in hospital inpatients who required medical care (n = 21),15,28–30,33,34,38,39,47,49,51,53,59,60,63,68,69,71–73 were undergoing surgery (n = 15)26,27,35,37,40,42,45,48,50,54,58,61,65,67,70 or were a mixed medical and surgical cohort (n = 4). 25,31,32,36 The remaining studies focused on patients receiving care for trauma (n = 4),41,43,57,64 cancer (n = 4),24,44,56,62 stroke (n = 1),46 burn injuries (n = 1)55 and sepsis (n = 1). 66 The mean age ranged from 45 years41 to 76 years52 (not reported in 29 studies)15,25–27,32,33,35,37,40,42–47,49,50,54,57–60,63,64,67,68,72,73 and the proportion of female subjects ranged from 17%42 to 81%61 (not reported in 12 studies). 25–27,35,37,45,50,54,57,58,60,63
The majority of studies (n = 37)15,24,26,28–34,38,40,42–49,51,54,57–60,62,64–66,68,69,72,73 defined the VTE end point [deep-vein thrombosis (DVT) and or pulmonary embolism (PE)] as being objectively confirmed. Of the remainder, three studies36,56,71 had no objective confirmation of VTE and 11 studies25,27,35,37,41,50,55,61,63,67,68 did not report the methods for diagnosis confirmation. In terms of VTE risk period, half of the studies (n = 23)15,24–27,28,30,37–40,42,45,46,49,51–54,58,59,61,71 utilised the RAMs to predict the occurrence of VTE within 3 months of the index hospitalisation. The remaining studies did not report the VTE risk period. The reported incidence of VTE ranged widely from 0.3%39,70 to 27.9%48 depending on definition, study design and study participants (e.g. medical, surgical or trauma).
The studies included in this review evaluated 24 validated unique RAMs. The most widely evaluated models were the Caprini RAM (22 studies),24,26,31–35,37,38,40,42,44,45,47,48,50,51,61,62,65,72,73 Padua Prediction Score (16 studies),15,29,30,32,33,36,39,47,50,51,65,66,68,69,72,74 International Medical Prevention Registry on Venous Thromboembolism (IMPROVE) models (8 studies),29,30,33,39,49,51,52,59 the Geneva risk score (4 studies)28,29,30,33 and the Kucher score (4 studies). 33,39,68,71 A summary of their associated characteristics and composite clinical variables is provided in Appendix 1, Table 21. Our patient and public involvement (PPI) group reviewed the most widely validated RAMs and identified all as appropriate and acceptable, in the context of face-to-face risk assessment. They did not feel patient-led completion of any RAM in isolation, to be feasible.
Statistical methods varied significantly between studies. Most studies reported the discrimination of the RAMs using a combination of the C-statistic and sensitivity or specificity.
Thromboprophylaxis was employed in about half (n = 25) of the studies, with the proportion receiving thromboprophylaxis ranging from 3.8% to 100%. It was not employed in three studies, and 23 studies did not report on thromboprophylaxis use. The use of thromboprophylaxis may lead to underestimation of predictive accuracy if a given RAM were to predict VTE events that were subsequently prevented by thromboprophylaxis.
Risk of bias and applicability assessments of included studies
The overall methodological quality of the 51 included studies15,24–73 is summarised in Table 2 and Figures 2 and 3. The methodological quality of the included studies was variable, with most studies having high or unclear risk of bias in at least one item of the PROBAST tool. The main sources of potential bias were related to the following domains: (1) patient selection factors, such as retrospective data collection, incomplete patient enrolment or unclear criteria for patients receiving VTE prophylaxis; (2) predictor and outcome bias arising from inappropriate inclusion of predictors within RAMs, unclear methods of outcome definition, low event rates and missing predictor or outcome data; (3) analysis factors, such as small sample sizes, inappropriate handling of missing data and failure in reporting relevant performance measures such as calibration.
Author, year | Risk of bias | Concern regarding applicability | Overall judgement | ||||||
---|---|---|---|---|---|---|---|---|---|
Participants | Predictors | Outcome | Analysis | Participants | Predictors | Outcome | Risk of bias | Applicability | |
Abdel-Razeq, 201024 | High | High | High | High | High | High | High | High | High |
Autar, 200325 | High | High | High | High | High | High | High | High | High |
Bahl, 201026 | High | High | High | High | Unclear | Unclear | Unclear | High | Unclear |
Barbar, 201015 | Low | Unclear | Unclear | High | Low | Unclear | Unclear | High | Unclear |
Bilimoria, 201327 | Low | Low | Low | High | Low | Low | Low | High | Low |
Blondon, 2019a28 | Low | Unclear | High | High | Low | Low | Low | High | Low |
Blondon, 2019b (abstract)29 | Unclear | Unclear | Unclear | Unclear | Unclear | Unclear | Unclear | Unclear | Unclear |
Blondon, 201830 | Low | Unclear | Unclear | High | Unclear | Low | Unclear | High | Unclear |
Bo, 202031 | Low | Unclear | Unclear | Unclear | High | Low | Low | Unclear | High |
Chen, 201832 | High | High | High | High | Unclear | High | High | High | High |
Cobben, 201933 | Unclear | Unclear | High | High | Unclear | Low | Unclear | High | Unclear |
de Bastos, 201634 | High | Low | High | High | High | Low | Low | High | High |
Dornbus, 2018 (abstract)35 | High | Unclear | High | Unclear | Unclear | Unclear | Unclear | High | Unclear |
Elias, 201736 | High | Unclear | High | High | Low | Low | High | High | High |
Frankel, 2017 (abstract)37 | High | Unclear | Unclear | High | High | Unclear | Unclear | High | High |
Grant, 201637 | High | Unclear | Unclear | Unclear | Low | Low | Low | High | Low |
Greene, 201639 | Unclear | Unclear | Unclear | Unclear | Low | Low | Low | Unclear | Low |
Hachey, 201638 | High | Unclear | Unclear | High | High | Low | High | High | High |
Hegsted, 201341 | High | Unclear | High | High | High | Low | Unclear | High | High |
Hewes, 201542 | High | Unclear | Unclear | High | High | Unclear | Low | High | High |
Ho, 201443 | Unclear | Unclear | Unclear | High | High | Unclear | Unclear | High | High |
Hu, 202044 | Unclear | Unclear | Unclear | Unclear | High | Unclear | Unclear | Unclear | High |
Krasnow, 2017 (abstract)45 | Unclear | Unclear | Unclear | Unclear | High | Unclear | Unclear | Unclear | High |
Liu, 201446 | Low | Low | Unclear | Unclear | High | High | High | Unclear | High |
Liu, 201647 | High | Unclear | High | High | High | Low | Low | High | High |
Lobastov, 201648 | Unclear | Unclear | Unclear | High | High | Low | High | High | High |
Mahan, 201449 | Low | Unclear | Unclear | Unclear | High | Low | Unclear | Unclear | High |
Mlaver, 202050 | Unclear | Unclear | Unclear | Unclear | High | Unclear | Unclear | Unclear | High |
Moumneh, 202051 | High | Unclear | Unclear | Low | High | Low | Low | High | High |
Nafee, 202052 | Unclear | Low | Low | Low | Unclear | Low | Low | Unclear | Unclear |
Nendaz, 201453 | Low | Unclear | Low | High | Low | Unclear | Low | High | Unclear |
Pannucci, 201255 | High | Unclear | Unclear | High | High | High | Unclear | High | High |
Pannucci, 201454 | Low | Unclear | High | High | High | Low | Low | High | High |
Patell, 201756 | High | Unclear | Unclear | High | High | Unclear | Unclear | High | High |
Rogers, 200758 | Unclear | Unclear | Unclear | High | Low | Unclear | Unclear | High | Unclear |
Rogers, 201257 | High | High | Unclear | High | High | High | Unclear | High | High |
Rosenberg, 201459 | Low | Unclear | Unclear | Unclear | Unclear | Unclear | Unclear | Unclear | Unclear |
Rothberg, 201160 | High | Unclear | Unclear | High | Low | Unclear | Unclear | High | Unclear |
Shaikh, 201661 | High | Unclear | High | High | High | Unclear | High | High | High |
Shang, 202062 | Low | Unclear | Unclear | Unclear | High | Unclear | Unclear | Unclear | High |
Shen, 202063 | Unclear | High | Unclear | Unclear | High | Unclear | Unclear | High | High |
Tachino, 201964 | High | Unclear | Unclear | High | High | Unclear | Unclear | High | High |
Tian, 201965 | High | Unclear | High | High | High | High | High | High | High |
Vardi, 201366 | Unclear | Low | Low | High | High | Low | Low | High | High |
Vaziri, 201867 | Unclear | Unclear | Unclear | High | High | Unclear | Unclear | High | High |
Vincentelli, 201868 | High | Low | Unclear | High | High | Low | Unclear | High | High |
Wang, 202069 | Low | Unclear | Unclear | Unclear | High | Unclear | Unclear | Unclear | High |
Winoker, 201770 | High | Unclear | Unclear | High | High | High | High | High | High |
Woller, 201171 | High | High | Unclear | High | Unclear | Unclear | Unclear | High | Unclear |
Zhou 201473 | Unclear | Unclear | Unclear | High | High | Unclear | Unclear | High | High |
Zhou, 201872 | Low | High | High | High | High | Unclear | Unclear | High | High |
FIGURE 2.
PROBAST assessment summary graph – review authors’ judgements on risk of bias.

FIGURE 3.
PROBAST assessment summary graph – review authors’ judgements on applicability.

Assessment of applicability to the review question led to the majority of studies being classed either as high (n = 35)24,25,31,32,34,36,37,40–51,54–57,61–70,72,73 or unclear (n = 12)15,26,29,30,33,35,52,53,58,60,71 risk of inapplicability. These assessments were generally related to patient selection (highly selected study populations, e.g. single pathologies, single site settings), predictors (inconsistency in definition, assessment or timing of predictors) and outcome determination.
Quantitative data synthesis (summary of results)
As there were a reasonable number of studies to compare, a summary of the C-statistics for studies involving medical, surgical and trauma patients, respectively, is presented in Figure 4, with the results grouped by RAM. Results of other hospital inpatients are presented in Appendix 1, Table 22. C-statistics varied markedly between these studies and between models, with no RAM performing obviously better than other models. In studies evaluating a single model, C-statistics23 were sometimes weak (< 0.7; 10 studies with 17 data points), often good (0.7–0.8; 17 studies with 20 data points) and a few were excellent (> 0.8; 5 studies with 5 data points). There was marked heterogeneity between multiple studies evaluating the same model. Studies evaluating multiple (more than 3) models33,39 tended to report weak accuracy across all the models (C-statistic < 0.7; 2 studies with 16 data points).
FIGURE 4.
C-statistics by model for studies involving (a) medical, (b) surgical and (c) trauma inpatients.
ACS NSQIP, American College of Surgeons National Surgical Quality Improvement Program; CI, confidence interval; NR, not reported; RAP, risk assessment profile.
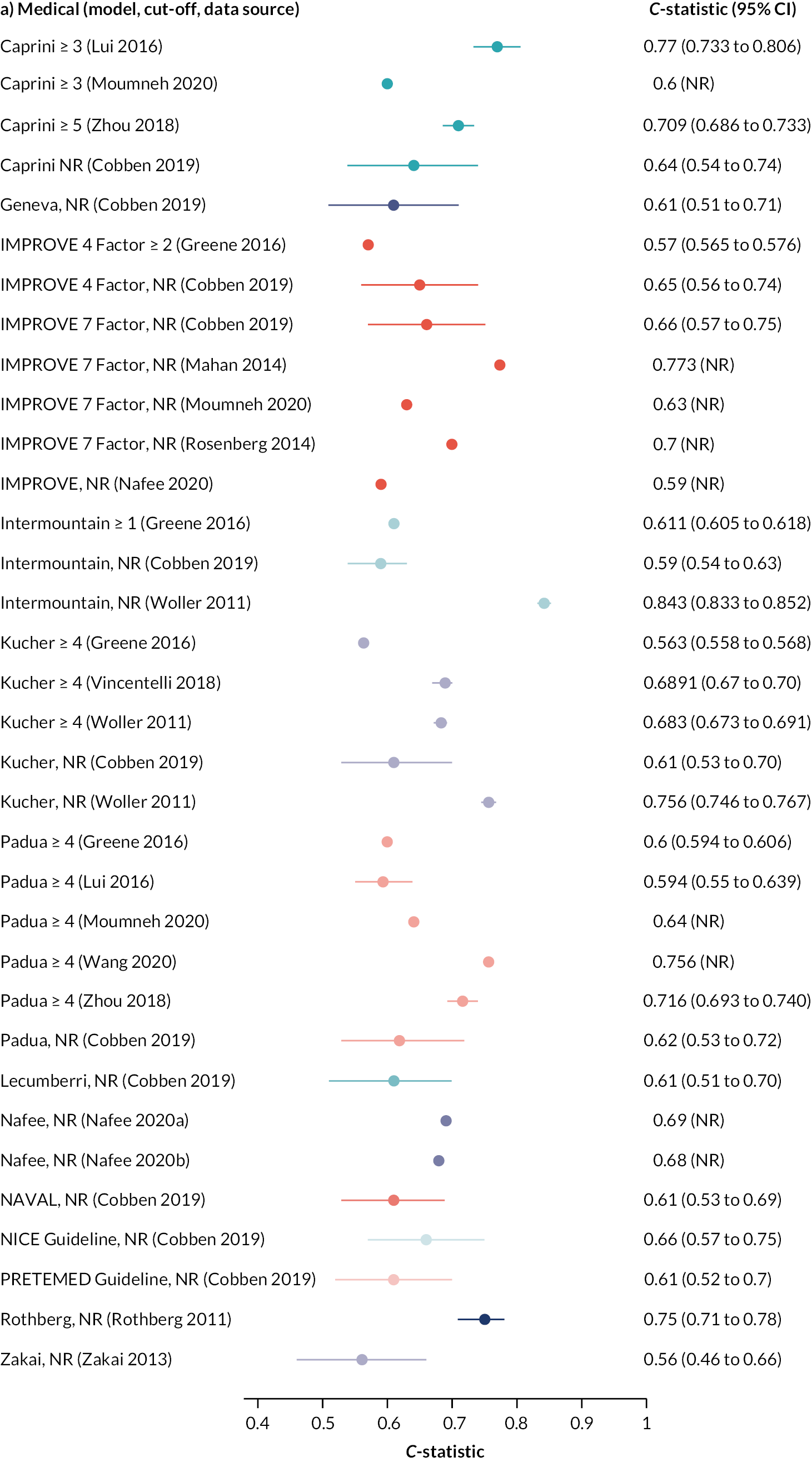

Table 3 shows the sensitivity and specificity at various thresholds for studies involving medical, surgical and trauma patients, respectively, with the results grouped by RAM. Interpretation was again limited by marked heterogeneity, which was exacerbated when different thresholds were reported by different studies evaluating the same model. Model accuracy was generally poor, with high sensitivity usually reflecting a threshold effect, as evidenced by corresponding low specificity (and vice versa).
Risk assessment models | Threshold or cut-off | End point | Data source | Sensitivity (95% CI) | Specificity (95% CI) |
---|---|---|---|---|---|
Medical inpatients | |||||
Caprini (7 studies) | Risk score ≥ 3 | VTE | Lui 201647 | 70.9% (NR) | 73.4% (NR) |
Risk score ≥ 3 | VTE | Moumneh 202051 | 98.1% (95.6 to 99.4) | 7.5% (7.1 to 8.0) | |
Risk score ≥ 3 | VTE | Zhou 201473 | 82.3% (NR) | 60.4% (NR) | |
Risk score ≥ 3 | VTE | Zhou 201872 | 84.3% (NR) | 33.5% (NR) | |
Risk score ≥ 5 | VTE | Zhou 201872 | 57.1% (NR) | 75.4% (NR) | |
Risk score ≥ 5 | VTE | Grant 201638 | 69.7% (NR) | 50.28% (NR) | |
Risk score ≥ 7 | VTE | Grant 201638 | 42.69% (NR) | 74.71% (NR) | |
Risk score ≥ 9 | VTE | Grant 201638 | 18.51% (NR) | 89.03% (NR) | |
NRa | VTE | de Bastos 201634 | 86.5% (NR) | 47.0% (NR) | |
NR | VTE | Cobben 201933 | 88.6% (NR) | 21.4% (NR) | |
Chopard (1 study) | Risk score ≥ 3 | VTE | Vincentelli 201868 | 64.2% (38.4 to 81.9) | 57.7% (63.9 to 79.4) |
Geneva models (4 studies) | Risk score ≥ 3 | VTE | Blondon 2018;30 Nendaz 201453 | All patients: 90.0% (73.5 to 97.9) |
All patients: 35.3% (32.8 to 37.8) |
No prophylaxis: 85% (NR) |
No prophylaxis: NR | ||||
NR | VTE | Cobben 201933 | 75.0% (NR) | 34.1% (NR) | |
Simplified model: Risk score ≥ 3 |
VTE | Blondon 2019a28 | 95.0% (NR) | 44.0% (NR) | |
Simplified model: NR |
VTE | Blondon 2019b (abstract)29 | 86.4% (NR) | NR | |
IMPROVE models (4 studies) | 4-factor model: NR |
VTE | Cobben 201933 | 27.9% (NR) | 85.4% (NR) |
7-factor model: Risk score ≥ 2 |
VTE | Moumneh 202051 | 73.8% (68.0 to 79.0) | 47.1% (46.3 to 47.9) | |
7-factor model: Risk score 2–3 |
VTE | Blondon 2018;30 Nendaz 201453 | All patients: 87% (NR) |
All patients: NR | |
No prophylaxis: 85% (NR) |
No prophylaxis: NR | ||||
7-factor model: Risk score ≥ 3 |
VTE | Blondon 2018;30 Nendaz 201453 | All patients: 73% (NR) |
All patients: NR | |
No prophylaxis: 54% (NR) |
No prophylaxis: NR | ||||
7-factor model: Risk score ≥ 4 |
VTE | Moumneh 202051 | 24.7% (19.6 to 30.4) | 85.5% (84.9, 86.1) | |
7-factor model: NR |
VTE | Cobben 201933 | 63.3% (NR) | 70.7% (NR) | |
NR | VTE | Blondon 2019b (abstract)29 | 57.6% (NR) | NR | |
Intermountain (1 study) | NR | VTE | Cobben 201933 | 26.4% (NR) | 90.2% (NR) |
Kucher (2 studies) | Risk score ≥ 4 | VTE | Vincentelli 201868 | 25.1% (17.0 to 55.1) | 92.9% (81.0, 95.4) |
NR | VTE | Cobben 201933 | 28.0% (NR) | 85.7% (NR) | |
Lecumberri (1 study) | NR | VTE | Cobben 201933 | 61.6% (NR) | 46.3% (NR) |
NAVAL (1 study) | NR | VTE | Cobben 201933 | 19.0% (NR) | 92.7% (NR) |
NICE Guidelines (1 study) | NR | VTE | Cobben 201933 | 77.6% (NR) | 39.0% (NR) |
Padua (10 studies) | Risk score ≥ 4 | VTE | Barbar 201015 | 94.6% (NR) | 62.0% (NR) |
Risk score ≥ 4 | VTE | Blondon 2018;30 Nendaz 201453 | All patients: 73.3% (54.1 to 87.7) |
All patients: 51.9% (49.3, 54.5) | |
No prophylaxis: 62% (NR) |
No prophylaxis: NR | ||||
Risk score ≥ 4 | VTE | Lui 201647 | 23.4% (NR) | 85.6% (NR) | |
Risk score ≥ 4 | VTE | Moumneh 202051 | 91.6% (87.6 to 94.7) | 25.6% (24.9, 26.3) | |
Risk score ≥ 4 | VTE | Zhou 201473 | 30.1% (NR) | 12.7% (NR) | |
Risk score ≥ 4 | VTE | Zhou 201872 | 49.1% (NR) | 83.8% (NR) | |
Risk score ≥ 4 | VTE | Vincentelli 201868 | 52.4% (38.4 to 81.9) | 72.3% (63.9, 79.4) | |
Risk score ≥ 4 | VTE | Wang 202069 | 76.2% (NR) | 61.6% (NR) | |
NR | VTE | Blondon 2019b (abstract)29 | 72.7% (NR) | NR | |
NR | VTE | Cobben 201933 | 61.8% (NR) | 48.8% (NR) | |
PRETEMED Guidelines (1 study) | NR | VTE | Cobben 201933 | 81.6% (NR) | 24.4% (NR) |
Shen 2020 (1 study) | NR | VTE | Shen 202063 | 77.8% (NR) | 84.7% (NR) |
Zakai 2013 (1 study) | Model 2: NR | VTE | Cobben 201933 | 63.8% (NR) | 31.7% (NR) |
Surgical inpatients | |||||
Caprini (8 studies) | Risk score > 5 | VTE | Hachey 201640 | 100% (100 to 100) | 7.2% (4.1, 11.0) |
Risk score ≥ 5 | VTE | Mlaver 202050 | 88.9% (NR) | 32.7% (NR) | |
Risk score > 5 | VTE | Shaikh 201661 | 70.8% (48.9 to 87.4) | 39.39% (37.0, 41.9) | |
Youden index > 5.5 | VTE | Tian 201965 | 76.0% (NR) | 64.0% (NR) | |
Risk score > 6 | VTE | Frankel 2017 (abstract)37 | 61.5% (NR) | 59.8% (NR) | |
Risk score > 6 | VTE | Shaikh 201661 | 58.3% (36.6 to 77.9) | 60.1% (57.6, 62.5) | |
Risk score > 7 | VTE | Hachey 201640 | 100% (100 to 100) | 31.4% (25, 37.3) | |
Risk score > 9 | VTE | Hachey 201640 | 83.3% (58.3 to 100) | 60.5% (54.4, 67.3) | |
Risk score > 9 | VTE | Shaikh 201661 | 16.7% (NR) | 93.3% (NR) | |
Risk score > 10 | VTE | Hachey 201640 | 75.0% (50 to 100) | 69.6% (64.6, 76.4) | |
Risk score > 10 | VTE | Dornbus 2018 (abstract)24 | 78.9% (NR) | 60.9% (NR) | |
Risk score > 10.5 | DVT or PE | Lobastov 201648 | 95.0% (NR) | 73.0% (NR) | |
Risk score > 15b | VTE | Hewes 201542 | 100% (100 to 100) | 66.7% (55.0, 78.3) | |
Khorana (1 study) | Youden index > 0.5 | VTE | Tian 201965 | 78.0% (NR) | 48.0% (NR) |
Padua (2 studies) | Risk score ≥ 4 | VTE | Mlaver 202050 | 61.1% (NR) | 47.4% (NR) |
Youden index > 3.5 | VTE | Tian 201965 | 36.0% (NR) | 93.0% (NR) | |
Rogers 2007 (1 study) | Youden index > 14.5 | VTE | Tian 201965 | 53.0% (NR) | 54.0% (NR) |
Trauma patients | |||||
RAP (2 studies) | Risk score ≥ 5 | VTE | Tachino 201964 | 100% (86.8 to 100) | 37.9% (34.6, 41.3) |
Risk score 5 to ≤ 14 | DVT or PE | Hegsted 201341 | DVT: 82.0% (77 to 87) PE: 71.0% (55 to 86) |
DVT: 57.0% (55, 59) PE: 53.0% (51, 56) |
|
Risk score > 14 | DVT or PE | Hegsted 201341 | DVT: 15.0% (11 to 20) PE: 12.0% (1 to 23) |
DVT: 97.0% (97, 98) PE: 96.0% (95, 97) |
|
TESS (2 studies) | Risk score ≥ 5 | VTE | Rogers 201257 | 77.4% (NR) | 75.6% (NR) |
Risk score < 9 | VTE | Ho 201443 | All VTE: 97.0% (91 to 99) |
All VTE: 27.0% (22, 32) |
|
Risk score < 9 | VTE | Ho 201443 | Fatal and non-fatal PE: 97.0% (87 to 99) | Fatal and non-fatal PE: 24.0% (20, 29) | |
Risk score < 9 | VTE | Ho 201443 | Fatal PE only: 100% (81 to 100) |
Fatal PE only: 20.0% (13, 28) |
Summary of key findings
-
A number of RAMs have been evaluated for potential VTE risk stratification of hospitalised adult patients.
-
In general, external validation studies have poor designs and limited generalisability.
-
Available data suggest that RAMs have generally weak predictive accuracy for VTE.
-
Use of VTE prophylaxis varied across the study populations and was not reported for many studies.
-
There is insufficient evidence and too much heterogeneity to recommend the use of any particular RAM for predicting the risk of developing VTE in hospital inpatients.
Chapter 4 Workstream 1 – economic evaluation
Decision problem
Aim
The aim of the independent economic evaluation is to assess the cost-effectiveness of different strategies for selecting hospitalised patients for pharmacological thromboprophylaxis including prophylaxis for all, prophylaxis for none and prophylaxis based on a VTE RAM. Sections of this chapter have been reproduced from Pandor et al. under licence CC BY 4.0. 74
Population
The target population is hospital inpatients, including medical, surgical and trauma patients but excluding critical care patients, children and women admitted to hospital for pregnancy-related reasons. In order to allow for differences in patient characteristics and risks within this very broad population, the economic analysis considered two broad groups as follows:
-
acutely ill medical inpatients
-
surgical inpatients including both elective and emergency surgery.
The analysis focused on those populations where NG89 (NICE Guideline 89: Venous thromboembolism in over 16s: reducing the risk of hospital-acquired DVT or PE)8 recommends the use of thromboprophylaxis based on the balance of VTE and bleeding risks. The analysis is not expected to be applicable to very specialist patient groups, such as neurosurgical patients, where the decision whether to use prophylaxis is based on a very individualised approach and the use of a RAM designed for general hospitalised patients is unlikely to be relevant.
Patients identified to be at increased risk of bleeding, such as those identified on the Department of Health risk assessment tool, are assumed not to receive pharmacological thromboprophylaxis under any strategy and are excluded from the model. Therefore, when we refer to a strategy of ‘prophylaxis for all’, this does not mean offering pharmacological thromboprophylaxis to those identified as being at high risk of bleeding.
We considered whether to model elective and emergency surgical patients separately. However, one of the more established risk-scoring tools for surgical patients, Caprini, has been validated in a mixed population of elective and emergency surgical patients and includes questions that would differentiate emergency surgical patients, such as those who have had fractures, into higher-risk groups. It therefore seemed reasonable to evaluate RAMs for use across the broad category of surgical inpatients and to treat the reason for surgery as a risk factor rather than modelling separate decision-making processes in elective and emergency surgical inpatients.
Trauma patients are not modelled as an explicit subgroup as trauma patients are highly complex, often have critical site bleeding injuries and are not managed within a specific trauma speciality in the UK. Managing speciality is often determined by their main complaint, which means they usually fall under a neurosurgical, spinal, general or orthopaedic speciality and, therefore, fall within the emergency surgical population. In addition, many of the RAMs which have been developed specifically for trauma patients, have been developed and validated in countries where trauma patients are treated within a critical care environment; this cohort is excluded from project scope.
Patients having elective hip or knee replacements are examined as a specific subset within the surgical inpatient cohort because of procedure-specific national recommendations (NG89); these patients are recommended to have a longer duration of prophylaxis than is typical in other surgical populations.
Strategies for prophylaxis
The prophylaxis interventions examined were chosen based on the recommendations in NG89 as these were selected based on cost-effectiveness modelling and, therefore, should represent the most cost-effective strategies. For most types of surgery, NG89 recommends that low-molecular-weight-heparin (LMWH) is considered if the person’s VTE risk outweighs their risk of bleeding. Although the use of mechanical prophylaxis in the form of antiembolism stockings (AES) is generally recommended for surgical patients within NG89, a recent study has shown that LMWH alone is non-inferior to LMWH with AES in elective surgical patients who were assessed as being at moderate or high risk of VTE. 75 Given that it is the use of LMWH that is being driven by risk assessment and not the use of AES, the comparison of interest for the modelling is LMWH versus no LMWH. The conclusions from the modelling should apply equally to patients having mechanical prophylaxis or no mechanical prophylaxis. This is because the model estimates whether it is cost-effective to use a RAM to decide whether to add LMWH to the treatment of patients who would not otherwise receive it and that is not dependent on any underlying care being given, provided that the underlying care is not altered by the addition of LMWH. Further discussion of mechanical thromboprophylaxis was, therefore, considered to be outside the scope of the modelling.
For acutely ill medical patients, LMWH is recommended in NG89 but mechanical prophylaxis is not recommended in acutely ill medical patients. Therefore, for acutely ill medical patients we compare LMWH to no prophylaxis.
When LMWH is used, we assume that it is given for the duration of admission. Although NG89 recommends that LMWH is given for a minimum of 7 days, information from the National VTE Exemplar Centres Network suggests that a pragmatic approach has been taken to the implementation of NG89 with LMWH generally not being prescribed for post-discharge use. 76 Therefore, we assume in the base-case analysis that LMWH is given for the duration of admission, but we also explore a scenario analysis in which it is given for 7 days even if this means post-discharge administration.
In higher-risk populations, such as elective hip and knee replacement, an extended form of pharmacological prophylaxis is recommended in NG89. Therefore, we have modelled these groups as specific subsets of the surgical population in separate analyses, described in Model inputs for the elective hip replacement and elective knee replacement scenarios, to determine if the threshold for cost-effective intervention is different in these groups. In addition, NG89 recommends that clinicians should ‘consider extending pharmacological VTE prophylaxis to 28 days postoperatively for people who have had major cancer surgery in the abdomen’. Therefore, a scenario analysis is conducted to determine if the threshold for cost-effective intervention is different in general surgical patients having 28 days of LMWH.
In summary, the main comparisons being modelled are:
-
acutely ill medical inpatients – LMWH for duration of admission versus no prophylaxis
-
surgical inpatients (excluding elective hip and knee replacement) – LMWH for duration of admission (without or without AES) versus no pharmacological prophylaxis (with or without AES).
Although the recommendations in NG89 were informed by modelling to determine the most cost-effective thromboprophylaxis strategy, the analysis did not explore the use of RAMs to determine which patients should be offered prophylaxis. The RAMs have been selected based on the review in the previous chapter. For medical patients, the primary analysis compares a group of several RAMs that have been externally validated within a single cohort as this minimises the risk of introducing bias from comparing tools that have been studied in populations with different characteristics. A secondary analysis then compares the remaining tools that have been externally validated in different medical cohorts. The number of RAMs for surgical patients was more limited. Therefore, for surgical patients we have included in the primary analysis all tools externally validated in either a surgical or mixed medical and surgical population. In all analyses, the comparator strategies of LMWH for all and LMWH for none are included. The tools evaluated are summarised in Table 4.
Population | Tools in primary analysis | Tools in secondary analysis |
---|---|---|
Medical | Caprini (Grant 2016)38 Kucher (Greene 2016)39 Padua (Greene 2016) IMPROVE (Greene 2016) Intermountain (Greene 2016) |
Padua (Blondon 2018)30 IMPROVE (Blondon 2018) Geneva (Blondon 2018) Simplified Geneva (Blondon 2019a)29 Kucher (Woller 2011)71 Intermountain (Woller 2011) Rothberg (Rothberg 2011)60 |
Surgical | Modified Caprini (Bahl 2010)26 Pannucci (Pannucci 2014)54 |
|
Mixed medical and surgical | Padua (Elias 2017)36 |
Modelling methods
Context
The model estimates lifetime costs and QALYs for the different thromboprophylaxis strategies and the comparator of no thromboprophylaxis under a NHS and Personal Social Services perspective. Future costs and benefits are both discounted to their net present value at a rate of 3.5% per annum in accordance with the NICE guide to the methods of technology appraisal. 77 Costs are reported in Great British pounds based on 2020 prices. To achieve this, historical prices used as model inputs were uplifted using the hospital and community health services pay and prices index up to 2016 and the NHS cost inflation index thereafter. 78
Conceptual model for medical inpatients
The model consists of a decision tree (see Figure 5) to capture short-term outcomes followed by a lifetime state transition (Markov) model (see Figure 6) to capture the impact of outcomes that result in death or ongoing morbidity. The model estimates outcomes for a cohort of identical patients with average characteristics. In reality, the application of RAMS may lead to treated and untreated patients having different characteristics. Based on clinical advice, age was the patient characteristic considered most likely to result in variation in outcomes within the cohort being risk assessed. We conducted a scenario analysis to explore whether incorporating patient heterogeneity into the model would affect the conclusions by varying the age of the cohort. From this we concluded that the cohort-level modelling approach was adequate and would not introduce significant bias as the optimal strategy was fairly robust to changes in the age.
FIGURE 5.
Short-term (6-month) decision tree model structure.

FIGURE 6.
Long-term Markov model.

The decision tree is used to estimate for each strategy: the number of patients receiving thromboprophylaxis, the impact of thromboprophylaxis on VTE outcomes (PEs and DVTs) and the incidence of major bleeds during either thromboprophylaxis or VTE treatment with anticoagulants. Major bleeds were considered to be those meeting the International Society for Thrombosis and Haemostasis (ISTH) definitions for major bleeding for surgical and non-surgical (medical) patients. 79,80 Major bleeds were divided into fatal bleeds, non-fatal intracranial haemorrhages (ICHs) and other major bleeds (referred to as non-fatal non-ICH major bleeds). Gastrointestinal (GI) bleeds were assumed to be a reasonable proxy for non-fatal non-ICH major bleeds and these were assumed to have no impact on costs or quality of life after 1 month. PEs were divided into fatal and non-fatal events. DVTs were divided first into symptomatic and asymptomatic DVTs and then into proximal and distal DVTs. Symptomatic DVTs and non-fatal PEs are assumed to result in 3 months of anticoagulant treatment. Patients having major bleeds during either prophylaxis or VTE treatment are assumed to stop their anticoagulant medication at the time of the bleed. In our previous analysis of thromboprophylaxis strategies in patients having lower limb immobilisation following injury, we found that the prevention of post-thrombotic syndrome (PTS) following asymptomatic DVT was an important driver of both cost-effectiveness and decision uncertainty due to asymptomatic DVTs being more common than symptomatic DVTs but their long-term consequences being more uncertain. 74 So while asymptomatic DVTs are assumed to remain undetected and untreated, it is important to capture these DVTs in the decision tree phase of the model in order to capture any ongoing morbidity due to PTS in the long-term state-transition model. In the decision tree, any VTE event that is related to the hospital admission is assumed to occur within 90 days of hospital admission. Therefore, a 6-month time frame was considered sufficient to capture the period of VTE risk associated with the hospital admission and the period of treatment following VTE (3 months). Diagnosis of PTS and chronic thromboembolic pulmonary hypertension (CTEPH) is assumed not to occur until the end of the decision-tree phase of the model, as it is difficult to distinguish these chronic complications from acute symptoms during the first 3 months after VTE. The decision tree allows for the possibility that patients not having anticoagulation may have a major bleed during their hospitalisation. The likelihood of VTE and the likelihood of bleeding during treatment for VTE is assumed to be independent of patient characteristics (e.g. age and sex) and independent of whether the patient had major bleeding during hospitalisation. Clinically relevant non-major bleeding (CRNMB) is excluded in the base-case analysis as it was considered that CRNMB occurring during hospitalisation would have a minimal impact on costs and quality of life in patients already hospitalised. Heparin-induced thrombocytopenia (HIT) was not included in the model because the most important consequence of HIT is the increased risk of VTE, but any increase in VTE related to HIT in the LMWH arms of the clinical trials would be included within the efficacy estimates for LMWH versus placebo and would, therefore, already be accounted for in the model.
The key model assumptions for the decision tree phase are as follows:
-
Bleeding events following hospitalisation are possible in both those having thromboprophylaxis and those having no thromboprophylaxis.
-
Bleeds associated with thromboprophylaxis are assumed to occur during prophylaxis and, therefore, before hospital discharge.
-
VTE associated with hospitalisation is assumed to occur within 90 days of admission.
-
Patients who have major bleeding will stop thromboprophylaxis immediately, but the treatment effect of thromboprophylaxis is assumed to be the same as for those who completed treatment, as patients who bleed are assumed to be adequately anticoagulated.
-
The risk of VTE is the same whether or not thromboprophylaxis caused bleeding.
-
All patients with symptomatic DVT receive accurate diagnosis and initiate treatment with anticoagulants [3 months of either direct oral anticoagulants (DOACs) or phased anticoagulation].
-
Asymptomatic DVTs are not detected and are not treated.
-
All PEs are symptomatic and lead to detection and treatment (3 months of either DOACs or phased anticoagulation) in all cases.
-
Patients treated for symptomatic DVT and PE have a bleed risk associated with treatment which is assumed to occur during the 3-month treatment period.
-
Chronic complications of VTE (CTEPH following PE and PTS following DVT) are assumed to be diagnosed at least 3 months after VTE and therefore occur after any bleeds associated with VTE treatment.
-
Deaths due to PE occur before any bleeding associated with the treatment of PE.
-
Risk of bleeding during treatment of VTE is independent of whether the patient bled during prophylaxis.
-
Risk of VTE, risk of bleeding and risk of PTS/CTEPH are based on average patient characteristics (e.g. age and sex) for the cohort being risk assessed.
A state-transition model (see Figure 6) was then used to extrapolate lifetime outcomes including overall survival and ongoing morbidity related to either bleeds or VTE. The health states included within the state-transition model capture the risk of PTS following VTE and the risk of CTEPH following PE. The risk of PTS is dependent on whether the DVT is symptomatic and treated or asymptomatic and untreated, and also whether the DVT is proximal or distal. All patients with PTS are combined in a single health state as costs, utilities and survival are not expected to be affected by whether PTS occurred following proximal or distal DVT. The PTS health state is not split into different severity levels as the utility estimates are based on the average utility across severity levels and the costs are not expected to differ by severity. The CTEPH health state is divided according to whether patients receive medical or surgical management to allow for differential costs and survival between these groups. There is also a post-ICH state to capture ongoing morbidity following ICHs. Further adverse outcomes (PTS, CTEPH) are not modelled following ICH, as lifetime costs and QALYs are assumed to be predominantly determined by morbidity related to ICH. Recurrent VTEs are not modelled as it is unclear whether any recurrent VTE would be related to the VTE provoked by hospital admission or whether it is related to underlying risk factors and would therefore have occurred whether or not prophylaxis was initiated during the hospital admission. The state-transition model has one 6-month cycle to extrapolate the outcomes of the decision tree up to 1 year, followed by annual cycles thereafter. All-cause mortality during the first year is applied at exactly 6 months. Thereafter, the health state occupancy is half-cycle corrected such that all transitions between states, including mortality, are assumed to occur mid-cycle. The key model assumptions during the state-transition phase are as follows:
-
All symptomatic DVTs are associated with a risk of PTS but the rate is allowed to differ depending on whether the DVT is distal or proximal and whether it is symptomatic and treated or asymptomatic and untreated.
-
There is no risk of PTS following PE and CTEPH is possible only after PE.
-
Further outcomes (i.e. VTE, CTEPH and PTS) are not modelled for those who experience ICH as lifetime cost and QALYs will be determined predominantly by disability related to the ICH.
-
All-cause mortality is applied to all transition states except CTEPH and post-ICH which have state-specific mortality rates.
-
Recurrent VTE is not modelled.
Conceptual model for surgical patients
We considered whether it was necessary to add additional wound-related outcomes to the model for surgical patients, such as surgical site bleeding or surgical site infections associated with wound haematoma. The clinical experts advised that the vast majority of major bleeds in surgical patients are distant from the surgical site. In addition, any complications related to surgical site bleeding that require patients to return to theatre or that result in prolonged hospitalisation would fall under the ISTH definition of major bleeding in surgical patients. 79 Therefore, these would already be captured within the decision-tree model as non-fatal non-ICH major bleeds. For these reasons, the conceptual model for surgical patients was kept identical to that for medical inpatients, with the caveat that the definition of major bleeding is different for surgical patients. Extended duration thromboprophylaxis in clinical subgroups (such as surgical patients with abdominal cancer) was evaluated using the same conceptual model with a separate scenario analysis (as per Strategies for prophylaxis).
Data sources
The input parameters used in a previous analysis of thromboprophylaxis during lower limb immobilisation were examined to identify any that were less relevant to patients receiving prophylaxis during hospitalisation. The data related to the population characteristics (age, sex and life expectancy), the incidence of VTE, the incidence of bleeding and the costs of prophylaxis were updated to use data specific to our target population. Other data such as the incidence of chronic sequelae (PTS and CTEPH) following VTE, and the costs and utility values for patients experiencing adverse outcomes were generally based on the same sources with costs updated to reflect changes in prices. One exception is the utility data for DVT and PE which have been updated because a more detailed analysis of the study used previously is now available.
Clinical input parameters are described below in Population characteristics to Mortality risks for adverse outcomes. Those that are specific to the medical and surgical inpatient populations are summarised in Table 5, with full details of all clinical input parameters provided in Appendix 2, Table 23. Cost inputs are described below in Cost of prophylaxis to Costs of managing PTS and CTEPH and summarised in Appendix 2, Table 24 with a detailed breakdown of drug costs for treating VTE and resource use for diagnosing and treating VTE provided in Appendix 2, Tables 25 and 26. Utility inputs are described below in Utility values and summarised in Appendix 2, Table 27 for the decision tree phase of the model and Appendix 2, Table 28 for the state-transition phase of the model. Probabilistic distributions for cost and utility inputs are summarised in Appendix 2, Table 29. The assumed timings of utility inputs are described in Timing and duration of utility decrements applied in the decision tree. Sensitivity and specificity data for the alternative RAMs are described in Sensitivity and specificity of RAMs.
Parameter | Medical | Surgical | Report section |
---|---|---|---|
Average age at admission | 65.8 | 54.2 | Population characteristics |
% male | 45% | 46% | Population characteristics |
Duration of prophylaxis (assumed to be given till discharge) | 5 days | 5 days | Cost of prophylaxis |
Absolute risk of PE without prophylaxis | 1.38% | 0.62% | Risk of VTE in medical inpatients to Risk of VTE in surgical inpatients |
Absolute risk of symptomatic DVT without prophylaxis | 2.02% | 0.78% | Risk of VTE in medical inpatients to Risk of VTE in surgical inpatients |
Absolute risk of asymptomatic DVT without prophylaxis | 30.46% | 12.61% | Risk of VTE in medical inpatients to Risk of VTE in surgical inpatients |
RR of VTE for prophylaxis (LMWH) vs. no prophylaxis | 0.49 | 0.29 | Risk of VTE in medical inpatients to Risk of VTE in surgical inpatients |
Absolute risk of PE with prophylaxis (LMWH) | 0.68% | 0.18% | Risk of VTE in medical inpatients to Risk of VTE in surgical inpatients |
Absolute risk of symptomatic DVT with prophylaxis (LMWH) | 0.99% | 0.23% | Risk of VTE in medical inpatients to Risk of VTE in surgical inpatients |
Absolute risk of asymptomatic DVT with prophylaxis (LMWH) | 14.93% | 3.65% | Risk of VTE in medical inpatients to Risk of VTE in surgical inpatients |
Absolute risk of major bleeding without prophylaxis (including fatal, ICH and other) | 0.67% | 1.24% | Risk of major bleeding during prophylaxis in medical inpatients to Risk of major bleeding during prophylaxis for surgical inpatients |
Major bleed risk by type for patients without prophylaxis | Risk of major bleeding during prophylaxis in medical inpatients to Risk of major bleeding during prophylaxis for surgical inpatients | ||
Fatal major bleeding | 0.10% | 0.01% | |
ICH | 0.06% | 0.02% | |
Other major bleeding | 0.51% | 1.21% (including 0.16% surgical site bleeding requiring return to theatre) |
|
RR of bleeding for prophylaxis (LMWH) vs. no prophylaxis | 1.53 | 2.98 | Risk of major bleeding during prophylaxis in medical inpatients to Risk of major bleeding during prophylaxis for surgical inpatients |
Absolute risk of any major bleeding when receiving prophylaxis with LMWH (including fatal, ICH and other) | 1.02% | 3.70% | Risk of major bleeding during prophylaxis in medical inpatients to Risk of major bleeding during prophylaxis for surgical inpatients |
Major bleed risk by type for patients having prophylaxis | Risk of major bleeding during prophylaxis in medical inpatients to Risk of major bleeding during prophylaxis for surgical inpatients | ||
Fatal major bleeding | 0.15% | 0.03% | |
ICH | 0.09% | 0.07% | |
Other major bleeding | 0.79% | 3.60% (including 0.48% surgical site bleeding requiring return to theatre) |
|
Case-fatality rate for PE | 26.8% | 6% | Mortality risks for adverse outcomes |
Standardised mortality ratio vs. general population in the year following admission | 9.4 | 5.0 | All-cause mortality |
Population characteristics
The cohort of patients reported in the papers by Greene et al. and Grant et al. was selected as being representative of the medical inpatient population. 38,39 This cohort was selected as it is the cohort used to estimate the sensitivity and specificity for five RAMs in the primary analysis for medical inpatients and the inclusion criteria were not considered to be overly restrictive compared with the target population. The starting age in the model was, therefore, set to be 65.8 years of age with 44.5% of the starting population being male.
The pooled mean age of surgical patients in the UK in 2015 was reported by Fowler et al. to be 54.2 years based on analysis of hospital episode statistics (HES) data. 81 This sample included both day-case procedures (45.6%) and regular inpatient admissions and did not distinguish between elective and emergency surgery. Of these 45.8% were surgical admissions in men. A cohort study examining emergency surgical admissions across six UK acute surgical units reported a mean age of 54 with 56% being female. 82 Based on these two sources we have assumed that the surgical cohort being modelled has an average age of 54 years of age and that 46% are male.
All-cause mortality
Life expectancy is known to vary between men and women of the same age, and therefore any QALY gains from deaths prevented would be dependent on sex. Rather than modelling male and female patients separately to account for their different mortality risks, we used age and sex-specific general population mortality data to calculate the average risk of mortality for the cohort as a whole based on their age at baseline and the proportion of males and females at baseline. The weighting of the mortality risk across males and females was allowed to vary over the course of the model to allow for the fact that lower mortality risks in females lead to a slight increase in the proportion alive who are female over time.
Having calculated the all-cause mortality for an age- and sex-matched cohort with general population mortality risks, it was then necessary to consider the increased risk of mortality for hospitalised patients relative to the general population. A study by Moore et al. reported that medical inpatients have a ninefold risk of mortality in the year following admission when compared to an age- and sex-matched general population cohort. 83 We have therefore applied relative risk (RR) of 9.4 to the estimate of general population all-cause mortality in the first year of the model. This gives a 10% absolute risk of death in the first year of the model, which is substantially lower than the absolute mortality rate observed by Moore et al. , which was 22.4% at 1 year. However, it is unclear whether the absolute risk of mortality reported by Moore et al. is representative of current practice across the UK. The 30-day risk reported by Moore et al. is 6.5%, which is higher than the 30-day mortality rate for non-elective inpatients reported in the Summary Hospital-level Mortality Indicator (Standard Hospital Mortality Indicator) tables for England, which was 3.4% in 2018/19. 84 We have conducted a sensitivity analysis in which an absolute mortality rate of 22.4% is applied in the first year of the model for all-cause mortality to see whether uncertainty around all-cause mortality is important in determining the optimal thromboprophylaxis strategy.
In the surgical population, the RR from Clark et al. for all-cause mortality in the year after hospitalisation for medical versus surgical patients (RR = 1.9, 95% CI 1.7 to 2.0) is applied to estimate the all-cause mortality in surgical patients. 85 This gives a RR for surgical patients versus the general population of 4.9 in the first year after hospitalisation. Clark et al. did not distinguish between emergency and elective surgery. A comparison of crude 30-day mortality rates for elective and emergency admissions, suggests that mortality is lower for elective admissions (1% vs. 3.4%)84 but as the emergency admissions are not reported separately for medical and surgical patients it is unclear how much of this is due to the inclusion of acutely ill medical patients in the emergency admission cohort. To explore whether a lower risk of mortality in elective surgical patients could lead to different recommendations for thromboprophylaxis, we have conducted a sensitivity analysis in which we apply general population mortality rates to surgical patients.
Moore et al. also present information on the mortality risk over the course of the first year and this shows that the rate of mortality is high initially and then decreases over time, although they do not report whether it reaches general population levels of mortality by the end of the first year. To allow for the fact that the risk of all-cause mortality decreases with time since discharge, we have not included any increased risk of mortality in year 2 and beyond for hospitalised medical and surgical patients relative to the general population.
Risk of VTE in medical inpatients
The risk of VTE in medical inpatients not receiving pharmacological prophylaxis has been taken from a prospective observational study by Barbar et al. , which was the derivation cohort for the Padua RAM. 15 The paper reports the rate of VTEs in high- and low-risk patients according to whether or not they received prophylaxis. Therefore, it is possible to estimate the risk of VTE in those not receiving prophylaxis across both low and high-risk patients. However, a weakness of this study design is that prophylaxis is more likely to be offered to higher-risk patients. Therefore, this study does not give an estimate of the rate of VTEs in an unselected population who do not receive prophylaxis. However, only 39.7% of the higher-risk group (defined as those with a Padua score ≥ 4) received prophylaxis. Higher-risk patients not receiving prophylaxis were younger, less likely to have reduced mobility, were less likely to have heart and/or respiratory failure and were less likely to have acute infection or rheumatological disorder. Despite this the risk of VTE in higher-risk patients not receiving prophylaxis was 11.8%, which is considerably higher than the risk of 2.2% in higher-risk patients receiving prophylaxis. We cannot know what the risk would have been in the latter group had prophylaxis not been administered. However, the majority of the patients in this cohort did not receive prophylaxis, even in the higher-risk group, and this paper allows us to estimate the risks of VTE in those not receiving prophylaxis. The risks of VTE in those not receiving prophylaxis within the cohort report by Barbar et al. was 1.38% for PE, and 2.02% for symptomatic DVT, giving a total risk of 3.40% for symptomatic VTE.
Using data from this prospective cohort was considered preferable to using data from the placebo arms of randomised controlled trials (RCTs) for two reasons. Firstly, many of the RCTs comparing prophylaxis against placebo are old and may not be representative of the risks of VTE within current clinical practice. Secondly, many RCTs have restrictive inclusion and exclusion criteria which results in a selective population that may over or under-estimate the risk of VTE in real-world clinical practice. The rates of VTE from the subset of patients reported by Barbar et al. who did not receive prophylaxis were applied in the base case but scenario analyses exploring higher rates of VTE are also presented as it is unclear whether the risk of VTE would be higher in an unselected population not receiving prophylaxis.
Barbar et al. did not conduct any form of screening to identify asymptomatic DVT. The economic model which informed the 2009 NICE guidelines on VTE prevention in hospitalised patients (CG92) used the ratio of asymptomatic to symptomatic DVTs from those RCTs reporting both distal and proximal asymptomatic DVTs across all patient groups excluding total hip and total knee replacement. 86 This gave a ratio of 40 symptomatic DVTs to 604 asymptomatic DVTs. From this we calculated that the incidence of asymptomatic DVTs in the cohort reported by Barber et al. would be 30.46%. Therefore, the overall rate of VTE including asymptomatic DVTs would be 33.85%. We also needed to determine the proportion of DVTs which are proximal as distal DVTs are assumed to have different consequences, such as a lower risk of PTS. In the model that informed CG92, the ratio of distal to proximal DVTs was based on all RCTs included in their systematic review which reported the incidence of both. Based on this, the proportion of all DVTs which are proximal was estimated to be 31% and this was assumed to be constant across both symptomatic and asymptomatic DVTs.
The RR of VTE was estimated from the RCTs included in the review for NG89, which reported relevant outcomes. 8 For acutely ill medical inpatients there are 12 studies described in NG89. Of these only four compared LMWH (standard dose/standard duration) to placebo,87–90 but one of these only reported mortality outcomes89 (all-cause mortality and fatal-PE), leaving three studies reporting VTE outcomes. All three RCTs reported the incidence of VTE including both PE and DVT. Two of these studies88,90 included both symptomatic DVT and asymptomatic DVT in their VTE incidence, although one included only asymptomatic proximal DVT. 88 The third study87 did not assess patients for asymptomatic DVT and, therefore, only reported symptomatic DVT or PE. We conducted a random-effects meta-analysis of VTE outcomes from these three studies to obtain an estimate of the RR (0.49, 95% CI 0.37 to 0.67) and applied this to all VTE outcomes within the model. Details of the numbers analysed are included in Appendix 2, Figure 27.
Risk of VTE in surgical inpatients
The risk of VTE in surgical inpatients was taken from the risk reported by Pannucci et al. in the derivation cohort for the Pannucci RAM. 54 This cohort was chosen as PE rates (0.62%) were reported separately in addition to the overall incidence of VTE at 90 days (1.40%). The overall incidence of VTE in the validation cohort, reported by Pannucci et al. , and in the validation cohort for the Caprini RAM, reported by Bahl et al. were similar to that reported in the derivation cohort by Pannucci et al. (1.40% at 90 days and 1.44% at 30 days, respectively), but details regarding the split between PE and DVTs were not provided for these validation cohorts. 26,54 The data from the Pannucci et al. cohort were in an exclusively non-emergent surgical population, whereas Bahl et al. included both elective and emergency surgical patients, but it seems reasonable to use the data from Pannucci et al. for all surgical patients, given that the overall rate of VTE is similar in Bahl et al. In both the derivation and validation cohorts reported by Pannucci et al. , a similar proportion of the cohort received no pharmacological prophylaxis (34% in both cases). However, the risk of VTE is not reported separately for those not receiving prophylaxis by Pannucci et al. , meaning that the risk of VTE in a cohort receiving no prophylaxis may have been underestimated. Bahl et al. did attempt to adjust for adherence to the local prophylaxis protocol, but they only reported what the VTE rate would have been for a cohort with full adherence, rather than the rate of VTE expected without any thromboprophylaxis. Therefore, the incidence from Pannucci et al. was applied in the model, albeit with the limitation that the use of pharmacological prophylaxis in around two-thirds of cases in this cohort may have underestimated the risk in a cohort without any pharmacological prophylaxis. Given this limitation, sensitivity analyses were conducted to explore whether the most cost-effectiveness strategy for pharmacological prophylaxis would be different if the VTE level risk was higher or lower than observed by Pannucci et al. The risk of asymptomatic DVT was estimated using the same assumption as applied in medical inpatients (i.e. that 6.2% of all DVTs are symptomatic), giving a risk of asymptomatic DVT of 12.6%. The same ratio of proximal to distal DVTs as applied in medical inpatients was also applied to elective surgical inpatients (i.e. 31% of all DVTs are proximal).
The RR of VTE in surgical inpatients for LMWH in combination with AES compared with AES alone was not readily available from the review reported in NG89. Separate network meta-analyses (NMA) are reported for elective hip replacement, elective knee replacement and abdominal surgery in NG89, with the latter being considered most generalisable to our target population (as discussed in Population). However, the NMA conducted for NG89 separated studies according to the timing and duration of LMWH, and separated studies according to the exact form of mechanical prophylaxis used meaning that the efficacy estimates for individual prophylaxis strategies are based on very few studies. As an alternative to using the data from NG89, we used data from a systematic review and economic analysis by Wade et al. which aimed to determine the relative effectiveness of knee versus thigh length AES when used in combination with pharmacological prophylaxis. 91 The base-case NMA conducted by Wade et al. made the simplifying assumption that the treatment effect for heparin with AES versus AES alone would be similar to the treatment effect of heparin versus no prophylaxis. This assumption is in part supported by the recent GAPS study, not included in the NMA but which showed no clinical benefit from adjunctive graduated compression stockings in addition to LMWH in moderate risk surgical patients. 75,92 Wade et al. also combined studies using LMWH, UFH and low-dose heparin within the network into a single heparin comparator. Both of these simplifying assumptions had the effect of allowing the estimate of treatment effect to draw on more studies than the approach used in NG89, which tended to split studies according to the exact prophylaxis regimen rather than lump them together. In addition, the analysis by Wade et al. did not distinguish between different types of surgical patients in their base case. Although scenario analyses were conducted excluding orthopaedic surgery, the economic analysis assumed that the efficacy estimates were common across all surgical patients. Wade et al. report that the median OR for heparin versus no heparin was 0.26 [95% credible interval (CrI) 0.09 to 0.87] in the whole surgical population for the outcome of DVT. In the economic model reported by Wade et al. , the efficacy estimates for DVT were applied to all VTE outcomes as there was no evidence of differential effects by VTE event type (i.e. DVT and PE). Similarly, we have applied this efficacy estimate for all VTE outcomes in surgical patients.
Risk of major bleeding during prophylaxis in medical inpatients
When estimating the risk of major bleeding in the model, the absolute risk in those having LMWH is likely to be estimated more precisely from empirical data sources than the absolute risk in those not having prophylaxis as it will be based on more events. We, therefore, decided to estimate the absolute risk of major bleeding in those having LMWH in the model and then use a RR to estimate the risk in those having no prophylaxis.
The current NICE guideline for preventing VTE in hospitalised patients (NG89) provides an estimate of the risk of major bleeding for patients randomised to either LMWH or placebo based on 4051 patients enrolled in three RCTs. 87,88,90 The RR of major bleeding for LMWH compared to placebo (1.53, 95% CI 0.8 to 2.92) was taken from the meta-analysis of three RCTs provided in N89 (Table 88 of NG89).
The three RCTs included in NG89 gave a risk of 1.02% for patients receiving LMWH. We considered whether it would be better to use data from a prospective cohort study such as the study by Barbar et al. which was used to estimate the absolute risk of VTE in those having prophylaxis. 15 However, the number of patients having prophylaxis in the Barbar et al. cohort was only 238, making the estimate of risk less precise than that provided by the RCTs included in NG89. In addition, the concern that patients enrolled in RCTs would be a selective group was considered less important for bleeding outcomes as inclusion/exclusion criteria for RCTs would be more likely to focus on VTE risk than bleeding risk. Therefore, we decided to use the data from NG89 based on the three RCTs to estimate bleeding risks in medical inpatients in the model.
The three RCTs used to estimate the risk of major bleeding did not provide sufficient information to determine the breakdown of major bleeding events into fatal bleeds, non-fatal ICHs and other major bleeds. For this information we used data from the IMPROVE registry, which was a multinational, observational study designed to examine VTE prophylaxis patterns and clinical outcomes in patients hospitalised with an acute medical illness. 93 Decousus et al. report bleeding outcomes from 10,866 patients of which 5231 (48.1%) received pharmacological prophylaxis including 4172 (38.4%) who received LMWH. The risk of major bleeding was 1.2% in the IMPROVE cohort overall. The type of bleeding is not reported according to whether or not prophylaxis was given or the type of prophylaxis used. However, the study does report data in sufficient detail to calculate the proportion of major bleeds that were fatal (14%) and the proportion of non-fatal major bleeds that were ICHs (10%). We have, therefore, assumed that these proportions are constant regardless of whether the bleeding occurred during LMWH or in patients not receiving LMWH.
Risk of major bleeding during prophylaxis for surgical inpatients
In NG89, the risk of bleeding for LMWH in surgical patients was estimated from five RCTs which compared LMWH either to placebo or to mechanical prophylaxis (which is not thought to increase the risk of bleeding) in patients having abdominal surgery. 94–98 The absolute risk of major bleeding across the 297 patients who received LMWH across the five RCTs was 3.7% (11/297). Neither of the studies reporting validation cohorts for RAMs in surgical populations reported the risk of bleeding in the population receiving prophylaxis. 26,54 Therefore, the data from NG89 for patients having abdominal surgery were used to inform the absolute risk in those receiving LMWH.
In NG89, the RR for major bleeding in patients having abdominal surgery obtained from the NMA was 2.98 (95% CI 0.88 to 14.80) for LMWH (postoperatively, standard duration, standard dose) versus placebo or mechanical prophylaxis. This RR was applied in the model for surgical patients.
The five RCTs used to estimate the absolute risk of major bleeding in surgical patients do not provide consistent information on the site of bleeding making it difficult to estimate the proportion of major bleeds that are ICHs. The data from the IMPROVE registry, which was conducted exclusively in medical inpatients, were not considered applicable to patients having surgery due to differences in the ISTH definitions of major bleeding for surgical and non-surgical patients. 79,80
We identified a number of registries/cohort studies that reported the rate of major bleeding in surgical patients receiving thromboprophylaxis through ad hoc searches using Google Scholar. All of the identified registry/cohort studies recruited either elective hip replacement patients,99 elective knee replacement patients100 or both. 101–103 As discussed in Population, we decided to model the use of thromboprophylaxis in patients having elective hip or knee replacement separately as the recommended duration of prophylaxis is greater in these patients than in most other surgical groups. However, we were unable to find any registry studies describing the site of major bleeding (i.e. ICH vs. non-ICH major bleeding) in non-orthopaedic populations having thromboprophylaxis. Our clinical experts advised that most major bleeds in surgical patients are distant from the surgical site and, therefore, information on the proportion of major bleeds that are fatal or ICHs is likely to be transferable from the orthopaedic population to the non-orthopaedic population.
From the registry/cohort studies identified, we chose to use the data from the XAMOS Xarelto® in the prophylaxis of post-surgical venous thromboembolism after elective major orthopedic surgery of hip or knee (XAMOS) cohort study103 because it provided a detailed breakdown of the number of patients meeting various criteria for major bleeding, which allowed us to identify which bleeds would fall within the ISTH definition of major bleeding. We did this by taking the number meeting the EMA definition (122 for the adjusted safety population) and removing patients whose only reason for being categorised as having major bleeding was bleeding leading to treatment cessation (13 for the adjusted safety population) as this is not part of the ISTH criteria. The XAMOS study compared rivaroxaban to ‘standard care’, which included a variety of different thromboprophylaxis interventions, but a high proportion (81.7%) received LMWHs. Therefore, the major bleeding events in the standard-of-care arm were considered to be broadly representative of what would be expected for patients receiving LMWH. From the data reported from the XAMOX cohort, we estimated that 0.9% (1 in 109) of major bleeds meeting the ISTH definition are fatal and that 1.9% of non-fatal major bleeds are ICH (2 in 108) in patients having orthopaedic surgery. The proportion of non-fatal non-ICH major bleeds requiring reoperation is 13%. These proportions were applied in the model to the number of major bleeds for surgical patients to estimate the number of fatal bleeds and non-fatal ICHs.
Risk of major bleeding during treatment for VTE
The risk of bleeding during treatment for VTE has been taken from a paper by Kooiman et al. which reports the incidence of bleeding at 6 months in a cohort of patients having treatment for VTE [LMWH followed by vitamin K antagonist (VKA)] stratified by Hypertension, Abnormal renal/liver function, Stroke, Bleeding history or predisposition, Labile international normalised ratio, Elderly, Drugs/alcohol concomitantly (HAS-BLED) score. 104 This was used to calculate the incidence in those with a HAS-BLED score of 0 to 1 which was 0.9% (3/335). The rationale for using data from a population with a low HAS-BLED score is that inpatients would be unlikely to be considered for prophylaxis if they had significant risk factors for bleeding and, therefore, the population with a low HAS-BLED score is more representative of the population being modelled than an unselected population. The risk in the whole cohort starting treatment from the same study was 2%. For comparison, the risks in other unselected cohorts were 1.5% at 6 months in the Prevention of Thromboembolic Events – European Registry in Venous Thromboembolism (PREFER-VTE) registry and 2.24% at 3 months in the Computerized Registry of Patients with Venous Thromboembolism (RIETE) registry. 105,106 The risk reported by Carrier et al. in a systematic review of RCTs and observational cohorts was 1.6% at 3 months. 107 Therefore, the risk in the cohort reported by Kooiman appears to be similar to other cohorts receiving VTE treatment. 104
The proportion of major bleeds that are fatal (25%) and the proportion of non-fatal major bleeds that are ICHs (9%) was based on data from the RIETE registry, which provides a detailed breakdown of major bleeds in patients receiving treatment for VTE.
Risk of chronic complications
The risk of CTEPH in patients having PE was taken from a systematic review by Ende-Verhaar et al. 108 A cumulative incidence of 3.2% (95% CI 2.0 to 4.4) over 2 years was reported in those who survived the initial 3-month period after PE and this was applied to the model giving a risk of 1.6% per annum. We assumed no risk of new CTEPH beyond 2 years based on a study with a median follow-up of 94 months which reported no new cases of CTEPH after 2 years. 109
The risk of PTS in patients with symptomatic DVT in the model was estimated from a prospective registry [Thrombosis and Pulmonary Embolism in Out-Patients (TULIPA) PLUS], reported by Hach-Wunderler et al. 110 This source was chosen as the study design excluded patients with previous DVT or signs of PTS prior to the index DVT. The incidence is reported separately for proximal and distal DVT and is stratified into mild, moderate and severe PTS using a validated scoring system (Villalta scale), which is the scale preferred by the ISTH. The prevalence of symptomatic PTS at 3 years was 24.4% for all DVTs, 32.4% for proximal DVT and 15.6% for distal DVT, with 70% being mild, 24% being moderate and 6% being severe (average across both proximal and distal). Although the TULIPA registry recruited outpatients, 63.7% of the cohort were considered to have had provoked DVT, with 23.7% having had surgery in the last 12 weeks and 14.8% having had acute illness in the past 4 weeks. Given that we are assuming that DVT occurs post discharge and the majority of DVTs are managed as outpatients, it seemed reasonable to use data from this outpatient population in the current analysis.
Given that patients with asymptomatic DVT will remain undiagnosed and untreated, the incidence for asymptomatic proximal DVT was inflated using data from van Dongen et al. which reported an odds ratio of 2.71 (95% CI 1.44 to 5.10) for the risk of PTS in patients with symptomatic proximal DVT with good versus poor anticoagulation [defined as spending more than 50% of their time outside of the target international normalised ratio (INR) range]. 111 The rate of PTS in asymptomatic distal DVT was not inflated because this would have increased the incidence to a level similar to that seen in symptomatic proximal DVT and the clinical experts believed that the rate in untreated distal DVT should be lower than for treated proximal DVT. The timing of PTS was based on information from van Dongen et al. , which reported the cumulative incidence over 4 years. This data was used to distribute the cumulative incidence reported by Hach-Wunderler et al. over 4 years. As van Dongen et al. reported no further incidence beyond 4 years we assumed no new incidence of PTS beyond 4 years in the model.
Mortality risks for adverse outcomes
Patients who survive the first 28 days after having an ICH have an increased risk of mortality in the long term. Folgelholm et al. report a standardised mortality ratio (SMR) of 4.5 in the first year and 2.2 in years two to six for patients who survived 28 days after ICH, compared to age- and sex-matched controls. 112 We have applied the latter in years 2–6 of the state-transition model to capture the increased risk of death in the long term for patients having non-fatal ICH. However, the SMR reported for the first year for patients having non-fatal ICH is lower than the SMR of 9.4 for medical inpatients and 4.9 for surgical inpatients. 83,85 Therefore, we have not applied any additional mortality risks for patients having non-fatal ICH in the first year as it did not seem logical that the mortality risks would be lower for those having ICH than for other medical or surgical inpatients not having ICH.
Two of the RCTs of LMWH versus placebo in medical inpatients reported the incidence of symptomatic PEs and the incidence of fatal PEs allowing the case-fatality rate to be calculated. 88,90 Overall, across both the LMWH and the placebo arms, 29% (5/17) of the PEs that occurred were fatal PEs. This is substantially higher than the case-fatality rate reported in registries of patients presenting with VTE, such as the RIETE registry where the case-fatality rate was 3.2% overall, with a rate of 4.1% in the inpatient population and 2.9% in the outpatient population. 113 However, given that the risk of death in the first 30 days reported by Moore et al. for all medical inpatients was 6.5%, it seems reasonable that the risk of death in those having PE would be higher than in all medical inpatients. It is also important to consider the fact that the RIETE registry only included patients diagnosed with PE and would miss cases where patients died suddenly and PE was only detected post-mortem. Studies that include PE diagnosed post-mortem have found a high rate of mortality even when excluding cases where autopsy discovered PE is classified as non-causal for death. Heit et al. report a 7-day survival rate of only 71.1% when using this approach, but a survival rate of 93% when excluding immediately fatal PEs or PEs first diagnosed at autopsy. 114 This suggests that registries that recruit patients only after confirming PE using objective tests are likely to miss a substantial proportion of fatal PEs. Heit et al. also found that patients having a PE while in hospital had a higher risk of death than those having PE in a community setting, and those having surgery in the 90 days prior to their PE had a lower risk of death than those not having previous surgery. This suggests that we should expect higher case-fatality rates for inpatients compared to outpatients and higher case-fatality rates for medical inpatients compared to surgical inpatients. However, Heit et al. do not report an absolute case-fatality rate specifically for medical or surgical inpatients. An estimate of the case-fatality rate for PE for all surgical (6%) and medical patients (44.7%) is provided in CG92 (Table 4-3 of CG92) based on the average across all RCTs. These figures represent the ratio of fatal PE to symptomatic PE across multiple RCTs included in the systematic reviews that informed CG92. These figures were used in the model, in preference to rates from registry studies, to reflect the higher expected case-fatality rate for PEs in hospital inpatients, particularly in medical inpatients. However, the case-fatality rate for medical patients was re-estimated after excluding a single study which included sudden unexplained deaths in its calculation of fatal PEs, and which had a high rate of case-fatality (67%). Including this study was not considered appropriate in the calculation of the case-fatality rate as in the model the case-fatality rate is applied to confirmed PEs. After excluding this study, the average case-fatality rate across the remaining five RCTs was 26.8% and this was applied to medical inpatients in the base case. 88,90,115–117 However, as there is considerable uncertainty regarding the appropriate case-fatality rate we have explored the range of estimates observed in the RCTs (13% to 67%) for medical inpatients in sensitivity analyses. 88,118 We have also explored the impact of applying the higher average rate observed in medical inpatients (26.8%) to the surgical cohort.
Mortality risks in patients with CTEPH having either medical or surgical management were based on survival curves reported by Goodacre et al. , which were estimated from an international prospective registry of patients with CTEPH. 119 Deaths related to PE occurring within 6 months of PE are already accounted for in the model. 120 For this reason, the hazard of death for patients with CTEPH is only applied from 1 year onwards, with PE-specific mortality applied up to 6 months and all-cause mortality applied from 6 months to 1 year. To ensure that the risk of death in the CTEPH group was not artificially low compared with the risk of death in the general population, general population mortality risks were applied whenever these were higher than the risk in the CTEPH population, based on the survival curves.
Cost of prophylaxis
We have assumed that pharmacological prophylaxis is LMWH. Although NG89 recommends that LMWH is given for a minimum of 7 days, a survey of 25 exemplar centres suggests that the majority of hospitals give LMWH for the duration of admission, which is typically less than 7 days. 76 We have, therefore, assumed that LMWH is given only for the duration of admission.
The mean length of stay in admitted patients based on HES data is 5 days when excluding day cases (HES: Hospital Admitted Patient Care Activity 2018–19). 84 We were unable to obtain an estimate of the mean length of stay specifically for medical inpatients, as the breakdown is given by main speciality and some specialities include both medical and surgical patients. However, we note that the mean length of stay is similar for general medical patients and general surgical patients, and these are the two most common categories, suggesting that it is reasonable to apply the same estimate for both medical and surgical patients. We also identified a study by Fowler et al. which reported that the pooled mean duration of stay for surgical patients (excluding day cases) was 4.8 days in 2015, suggesting that this estimate of 5 days is reasonable at least for surgical inpatients. 81 We, therefore, assume that both medical and surgical patients receive 5 days of LMWH.
It is assumed that the lowest cost preparation of LMWH is prescribed. Therefore, drug costs for LMWH were based on the cost of dalteparin (5000 units given every 24 hours by subcutaneous injection) as this had a cost of £14.12 over 5 days, which was lower than the cost for equivalent doses of enoxaparin (£15.14 for 40 mg every 24 hours) or tinzaparin (£17.82 for 4500 for units every 24 hours). In the base-case analysis, we assumed that LMWH is only continued until discharge and, therefore, the treatment can be administered by a hospital nurse. We have assumed that each administration requires 2.5 minutes of time for a band 6 hospital nurse, with a unit cost of £47 per working hour, giving an administration cost of £9.79 over 5 days. 78 Therefore, the total cost of prophylaxis is £23.91 for both medical and surgical inpatients.
We have also provided results for a scenario analysis in which LMWH is continued 2 days post-discharge to give a total of 7 days of LMWH, as this is recommended in NG89 as the minimum treatment duration. For this analysis, we have applied the costs for 2 days of outpatient administration of LMWH by adjusting the costs estimated by Menakaya et al. for outpatient LMWH. 121 The costs estimated by Menakaya et al. included clinical time for counselling and blood taking (including a 5-day platelet count), pharmacy dispensing and initial administration. These estimates include the costs of administration by a district nurse in 4% of patients to reflect the fact that not all patients will be able to self-administer. The costs were adjusted to reflect the shorter duration of outpatient administration in this case (2 days vs. 46 days). In this scenario analysis the costs of administration are increased to £81.26 and the drug costs are increased to £19.76, such that the total cost of prophylaxis is £101.03.
In the strategies examining the use of prophylaxis based on RAMs, we have assumed that the risk assessment will require 5 minutes of time spent by a hospital consultant, which is a cost of £9.08 when applying a unit cost per hour of £109. 78 This cost is applied to all strategies involving the use of a RAM, but not to the comparator strategies of prophylaxis for all and prophylaxis for none. A sensitivity analysis has also been conducted to explore whether the optimal strategy would be different if it could be assumed that the risk assessment would result in no additional cost, with the aim of exploring whether the cost of the risk assessment itself is a significant source of decision uncertainty.
Costs of treating VTE
The average time to VTE in medical inpatients reported by Barbar et al. was 42 days but this estimate is based on only 37 events. 15 The Million Women study reported by Sweetland et al. shows that postoperative VTE risk in women peaks at 3 weeks although it remains raised up to 12 weeks and the average time to VTE is not reported. 122 However, both these figures suggest that the majority of VTE occurs after discharge in both medical and surgical patients. Therefore, treatment costs for VTE are based on the same assumptions applied in a previous cost-effectiveness analysis of prophylaxis in patients having outpatient lower limb immobilisation. These include healthcare contacts for diagnosis, a short-stay inpatient admission where necessary, and 3 months of outpatient anticoagulant treatment. Resource use for diagnosis and admissions are summarised in Appendix 2, Table 26.
It is assumed that any VTE occurring prior to discharge, would extend hospital length of stay by up to 2 days and would, therefore, have a similar cost to that incurred by patients having VTE as an outpatient who require a short stay admission of up to 2 days. To determine whether the model is sensitive to uncertainties regarding the proportion of VTEs occurring pre-discharge and the likelihood that these will delay discharge, we have conducted a sensitivity analysis in which we assume that all VTEs occur pre-discharge and these VTEs result in no discharge delays.
Treatment for VTE is assumed to consist of 3 months of either a VKA (warfarin) or DOAC. Patients having treatment with a VKA are also assumed to have initial anticoagulation with LMWH until VKA treatment is established. It is assumed within the base-case model that 40% of patients will have a DOAC based on the usage of DOACs in the PREFER-VTE cohort from within those countries where DOACs had been launched. 105 An additional scenario analysis has been conducted to examine the potential impact of increased DOAC prescribing in the years since its launch. The costs for administering LMWH are based on the costs reported by Menakaya, but these were adjusted to reflect the shorter duration of treatment (7 days compared with 42 days in Menakaya). Administration costs for oral anticoagulants include nine attendances at an anticoagulation service over 3 months for those receiving VKA, and a nurse-led telephone consultation at 10 days for DOACs. In addition, all patients are assumed to require an appointment with a consultant after 3 months to assess whether ongoing treatment is needed.
Costs of treating major bleeding
The cost over 90 days of fatal haemorrhagic stroke provided by Luengo-Fernandez et al. was uplifted to current prices and applied as the cost of fatal bleeds in the decision tree phase of the model. 123,124 This paper also provided costs over 90 days for non-fatal haemorrhagic stroke stratified by the level of disability. A weighted average cost was calculated across non-disabling, moderately disabling and totally disabling haemorrhagic strokes. This was then uplifted to current prices and applied as the cost of non-fatal ICH in the decision tree phase of the model. Luengo-Fernandez et al. also report the average costs per annum from 90 days to 5 years post-stroke, but these are not reported separately for haemorrhagic stroke. The costs of GP care and emergency care are reported to be statistically significantly higher post-stroke compared to the year before stroke. In addition, they report the cost of residential care in patients not living in residential care prior to their stroke. The total post-acute (beyond 90 days) costs for primary care, emergency care and residential care were calculated and uplifted to current prices and applied in the state-transition phase of the model to those having non-fatal ICH. A pro rata cost is also applied to those having stroke more than 90 days before the end of the decision tree phase of the model.
The cost of non-fatal non-ICH major bleeds was assumed to be similar to the cost of GI bleeds. This was estimated based on a weighted average cost for non-elective inpatient and non-elective short-stay management of GI bleeds using NHS reference costs for bleeds requiring single, multiple or no interventions (HRG codes FD03A to FD03H). 125 This was based on clinical expert advice that in surgical patients, the majority of major bleeds are distant from the site of surgery and, therefore, GI bleeding is a reasonable proxy for the cost of major bleeding even in surgical patients having non-GI surgery. In addition, the costs of GI bleeding are considered to be a reasonable proxy for the management of major bleeding in surgical patients, as the NHS reference cost data show that the majority of GI bleeds (87%) are managed without intervention, which is consistent with the data from XAMOS which shows that the majority of major bleeds in surgical patients are managed without a return to theatre. 103
No costs are applied in the base-case analysis for CRNMB because it is assumed that these will be managed within the existing cost of inpatient care for those experiencing a CRNMB during inpatient prophylaxis. However, in the scenario analysis examining the use of LMWH for up to 7 days, a cost for ED attendance without admission is applied to a proportion of those having prophylaxis based on the ratio of CRNMBs to all major bleeds (2.05) reported in the PREFER-VTE registry. 105
Costs of managing PTS and CTEPH
The management of PTS is assumed to involve one first and one follow-up vascular surgery outpatient appointment in the first year after diagnosis and two follow-up GP appointments every year thereafter. This is consistent with the assumption applied in a previous analysis. 74 An alternative cost based on the burden estimated in a US cohort is considered in a sensitivity analysis. 126
Drug costs for medical management of CTEPH were based on the costs used in CG92, which were uplifted to give a cost of £18,507 per year. The costs for medically managed patients are applied each year to those surviving with CTEPH. The proportion of patients having surgical management for CTEPH (59%) is based on data from Delcroix et al. 120 The cost for surgical management of CTEPH is based on a weighted average of the reference costs for complex thoracic procedures (DZ02H/J/K) giving an average cost of £8175. A proportion of patients having surgical management (29%) are assumed to require medical treatment as a bridging therapy (average of 4.6 months). Including these costs brings the total cost in the first year to £10,237 for surgical management. No costs are applied beyond the first year for those managed surgically.
Utility values
In our previous modelling of VTE prevention in lower limb injury, we identified a systematic review of utility values in VTE by Lubberts et al. 127 Forward citation searching was used to identify more recent systematic reviews on this topic and this identified a more recent review by Ghanima et al. but this yielded no more recent studies with relevant data. 128 Further forwards and backwards citation searching from the review by Ghanima identified one paper by Utne et al. which reported EQ-5D outcomes by trial arm in patients being treated with either rivaroxaban or warfarin for DVT. 129 Additional ad hoc searches using Google Scholar, which were limited to 2017 onwards, identified two more recent publications of health-related quality of life (HRQoL) data from the PREFER-VTE registry study, which had been previously used to obtain utility estimates in the model for lower limb injury. 113,130 The new data from this paper were extracted and used to calculate updated utility multipliers for DVT and PE giving utility multipliers of 0.962 and 0.960 for the first 6 months after DVT and PE, respectively. The utility multipliers in the second 6 months were 1.00 and 0.99, respectively. For PE these are higher than the multipliers applied in the previous model because the utility values reported in the recent paper were much higher than those reported previously from the same study. This is because the final publication reported results separately for patients with and without active cancer and we selected the data for those without cancer for use in the model. However, it should be noted that the difference in utility between those with and without active cancer is fairly constant across time for PE, suggesting that the utility decrement attributable to PE would be similar, but that there is an additional decrement due to active cancer. In the previous model, the average utility for PE over 6 months was compared to the utility achieved 6 months after DVT to determine the multiplier on the assumption that patients with DVT will have returned to full utility by 6 months. Whereas in the analysis of the new data, the utility multiplier was compared against the norm value index score for an age-/gender-matched general population (0.838 for PE and 0.852 for DVT) provided in the more recent papers. This method is preferable as it is not affected by differences in the characteristics of those experiencing PE and DVT.
Using forward citation searching from the review by Lubberts et al. we identified a systematic review of studies reporting HRQoL after adverse events associated with antithrombotic therapy for primary or secondary prevention of VTE. However, only four studies were identified in this review and they related to VTE prevention in populations with atrial fibrillation or following acute myocardial infarction. Two of these studies reported EQ-5D in patients with and without a bleeding adverse event,131,132 but only one reported the EQ-5D for patients with major bleeding compared to no bleeding. 132 After adjusting for baseline differences in EQ-5D, Amin et al. (2016) report that the EQ-5D decrement for major bleeding (BRAC bleeding type 2–4) in the past 6 months compared to no bleeding in the past 6 months was (−0.0445, 95% CI 0.073 to 0.016, p = 0.0022). As the utility in those without bleeding was 0.86 at both baseline and 6 months, this decrement is equivalent to a 5% proportional decrement. In our previous analysis for lower limb immobilisations, we assumed that the utility in the month following a GI bleed would be equivalent to the utility in the month following PE. Using data from the more recent publication of the PREFER-VTE registry130 would give a 16% decrement (0.718 compared to 0.838) when making this same assumption. The difficulty here is that the utility decrement associated with a major bleed is likely to reduce over time which is why we previously stated that any decrement would only persist for 1 month after a major bleed. Therefore, the study by Amin et al. , which is focused on bleeding at any time in the past 6 months, may miss the maximum period of utility decrement. For this reason, we have chosen to make the same assumption as before and to apply the utility decrement in the first month post-PE to the first month post-major bleeding using data from the more recent PREFER-VTE publication. 130
Utility values following ICH were based on data from 5-year follow-up of the Oxford Vascular Study (OXVASC) study as these data were previously applied in our analysis of prophylaxis during lower limb injury. 123 A decrement of 0.22 was assumed in the decision tree part of the model where time since stroke was < 6 months and a decrement of 0.09 was assumed in the long-term part of the model. This study was chosen as the source of utility values previously as the duration of follow-up allowed time since stroke to be accounted for, and a comparison was made against general population norms.
In our previous analysis of prophylaxis during lower limb injury (Pandor et al. 201974), we used utility data from the CaVenT study to estimate the utility decrement in patients with PTS. 133 An estimate of 10% was applied from diagnosis onwards, which was obtained from the CaVenT study by comparing the EQ-5D scores in those with and without PTS at 2 years. The CaVenT study did not stratify the utility estimates by severity of PTS, so this estimate was applied to all patients with PTS in the model regardless of severity. This may overestimate the utility decrement if the proportion of patients having severe PTS is lower in the modelled population than in the CaVenT study which recruited patients with acute iliofemoral DVT. A study by Lenert and Soetikno reported utility estimates for mild and severe PTS (0.98 and 0.93, respectively) obtained by using health state descriptions and a standard gamble valuation technique in a sample of volunteers. 134 These were not used in the base case as utility measured using the EQ-5D in patients with the condition is preferable to utility measured using standard gamble in volunteers based on descriptions of the condition. However, a scenario analysis was conducted in which the data from Lenert and Soetikno were combined with data on the proportion of PTS that is severe (6%) from Hach-Wunderle et al. to estimate a utility decrement of 2% across all patients with PTS. In the current analysis we have taken the same approach and have applied a 10% decrement in the base case and a 2% decrement in a scenario analysis.
The utility decrement in patients with CTEPH was estimated from a study by Meads et al. by comparing the utility in patients having CTEPH (0.56) and the utility in patients with disease categorised as New York Heart Association (NYHA) class 1 (0.89) in which the quality-of-life impact of symptoms would be expected to be minimal. 135 This gave a utility multiplier of 0.63 or a 37% decrement. This decrement is applied lifelong in the model to those having medical management of CTEPH, but only for 1 year in those having surgical management who have the utility multiplier for PE applied thereafter.
In our previous analysis of thromboprophylaxis during lower limb injury we identified several sources which estimated the utility decrement associated with VTE prophylaxis or VTE treatment. 74 The study selected for use in the previous model was a study by Marchetti et al. which reported that patients would be willing to trade 2.7 of 365 days to avoid treatment with LMWH and 4 of 365 days to avoid treatment with warfarin. 136 These data were previously used to estimate utility decrements of 0.007 for thromboprophylaxis and 0.011 for VTE treatment. These same decrements have been applied in this model.
Utility values for patients not experiencing any utility decrement due to prophylaxis, treatment, symptomatic VTE events, bleeding events (ICH or other major bleeds), long-term sequelae following VTE (PTS or CTEPH) or death are based on general population norms for a cohort of the same age and this is allowed to vary as the cohort ages during the model. 137
Timing and duration of utility decrements applied in the decision tree
In order to calculate the QALYs gained by patients having different paths through the decision tree, it is necessary to make some assumptions regarding the timing of events as these are not explicitly modelled in a decision tree. We made the following assumptions when estimating QALYs in the decision tree:
-
Baseline utilities using general population utility values for the starting age are applied to those not having treatment and not having any clinical events (e.g. VTE, bleeds).
-
Bleeds during prophylaxis are assumed to occur halfway through the hospital admission (i.e. 2.5 days after starting prophylaxis).
-
VTE is assumed to occur within 90 days of hospital admission and utility decrements for PE and DVT are applied from 42 days until the end of the decision tree period.
-
Bleeds occurring during treatment for VTE are assumed to occur at 13, 32 and 12 days post diagnosis of VTE for fatal, ICH and other major bleeds, respectively (based on data from the RIETE registry reported by Nieto et al. ).
-
Disutilities for ICH are applied lifelong but separate disutilities are applied in decision tree and state-transition phases of the model (i.e. during the first 6 months after admission and beyond 6 months after admission).
-
Disutility of non-fatal non-ICH major bleeding is assumed to last a maximum of 28 days.
-
Disutility of prophylaxis applies for the duration of prophylaxis (5 days) and is therefore less in those stopping early due to major bleeding.
-
Disutility of treatment for VTE applies for the duration of treatment (3 months) and is therefore less in those stopping early due to major bleeding.
Although the utility decrements for VTE are assumed to apply from 42 days onwards based on the mean time to VTE reported by Barbar et al.,15 there is likely to be considerable variability in the timing of VTE, which could occur before discharge or as late as 90 days after admission. Therefore, a sensitivity analysis was conducted in which the timing of VTE was varied from 3 to 90 days to determine if the model was sensitive to the assumption regarding the timing of VTE. We made the following assumptions when estimating QALYs in the state-transition model:
-
Utility values for patents without any long-term sequelae (ICH, CTEPH, PTS) are taken from general population values and decrease as patients age in the model.
-
All other utility values are applied as multipliers such that the absolute utility value decreases due to ageing in all patients.
-
Utility decrements continue in the state-transition model for the remainder of the patient’s lifetime for PE but not for DVT where patients are assumed to return to general population utility values at 6 months.
-
Patients with CTEPH who are treated medically have a lifelong utility decrement, whereas those treated surgically return after 1 year to the same utility as those surviving PE without CTEPH.
-
Patients with PTS have the same utility decrement from diagnosis to death.
-
Patients with ICH have the same utility decrement from 6 months (i.e. the start of state-transition model) to death.
Sensitivity and specificity of RAMs
Sensitivity and specificity data have been extracted from the RAM studies listed in Chapter 3. 29,30,36,38,39,71 For many of the RAM studies we wished to examine in the model, the authors themselves have not reported sensitivity and specificity data. In this case, estimates of sensitivity and specificity have been extracted using reports of the incidence by risk score and graphical data, where possible. In some cases, estimates of sensitivity and specificity were extracted digitally from receiver operating characteristic (ROC) curves where these were reported.
Figure 7 shows the sensitivity and specificity data for RAMS in medical inpatients extracted from various studies. Overall, there is data reported for seven RAMs (Caprini, Kucher, Padua, IMPROVE, Intermountain, Geneva, Rothberg) and all except one (Rothberg) are reported in more than one cohort. It can be seen that there is not always consistency in the estimates of sensitivity and specificity for a particular RAM when reported across different cohorts. In particular, the estimates for IMPROVE and Intermountain obtained by Blondon et al. are more favourable than the estimates from Greene et al. However, several RAMs appear to have similar performance when estimates of sensitivity and specificity were taken from single cohort, as reported in papers by Greene et al. and Grant et al.
FIGURE 7.
ROC curve for multiple risk assessment models evaluated in cohorts of medical inpatients.

Figure 8 shows the sensitivity and specificity data for two RAMs in surgical inpatients. There were data on the Pannucci score reported by Pannucci et al. and data on the Caprini score reported by Bahl et al.
FIGURE 8.
ROC curve for multiple risk assessment models evaluated in cohorts of surgical inpatients.

Figure 9 shows the sensitivity and specificity data available for mixed populations of both surgical and medical inpatients. Only one paper reported this information for a mixed population (Elias et al. 201736). Elias et al. provide ROC curves for patients having prophylaxis and patients not having prophylaxis. They also provide a single estimate of sensitivity and specificity for the cohort as a whole.
FIGURE 9.
ROC curve for the Padua RAM in a mixed cohort of medical and surgical patients reported by Elias et al. 36 [with/without prophylaxis (PPX) and all].
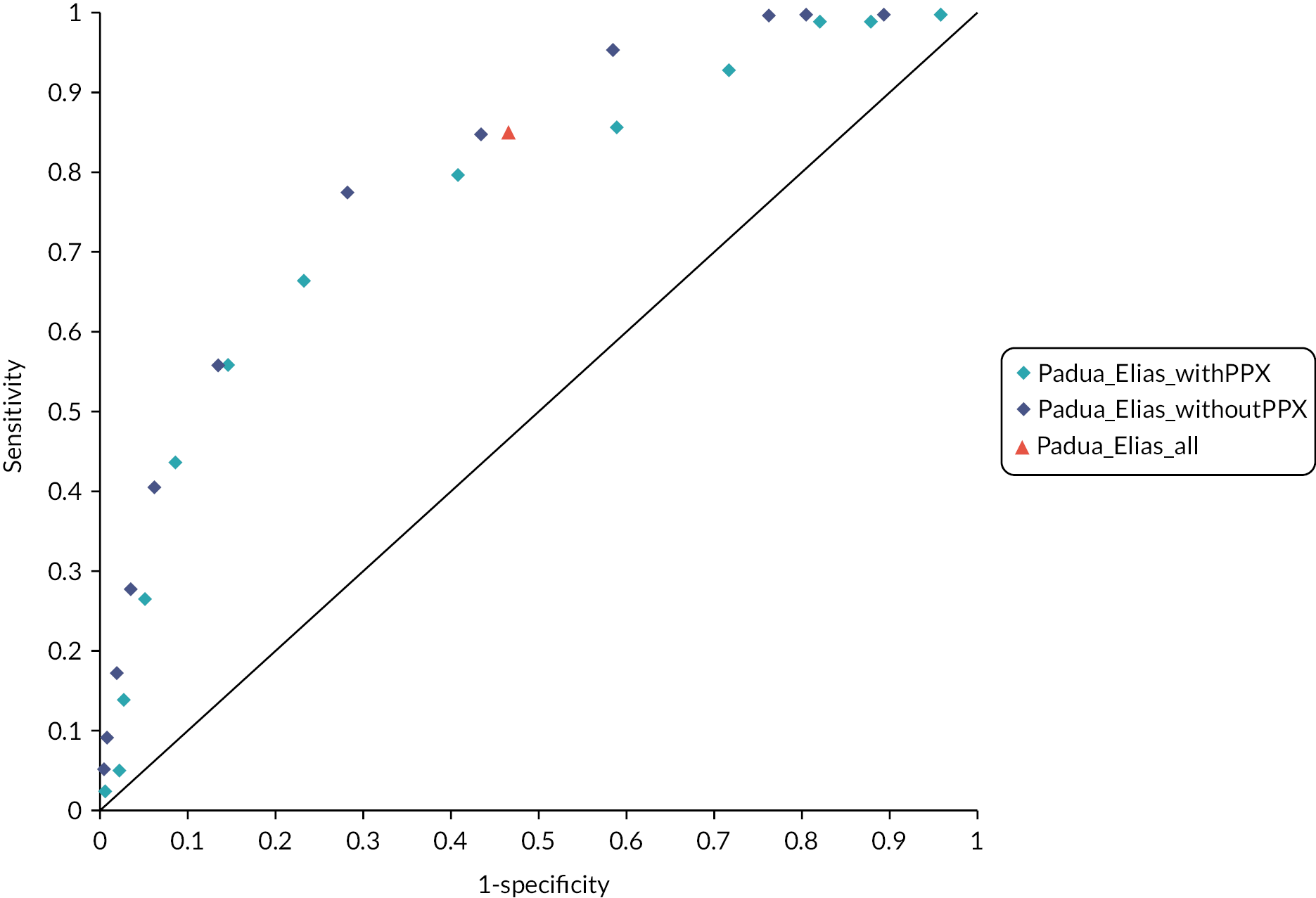
The base-case deterministic analysis estimates the expected costs and QALYs for all available RAMs where performance data are available in that specific population (i.e. surgical or medical patients). In addition, because the usage of prophylaxis within the cohorts will tend to result in RAM performance being underestimated, we have also conducted a secondary analysis using the data from Elias et al. which was estimated in the subset of the cohort not receiving prophylaxis. This cohort included both surgical and medical inpatients and is therefore not used in the base-case scenario for either group.
Available data suggest that RAMs have generally weak predictive accuracy for VTE. There is insufficient evidence and too much heterogeneity to recommend one RAM over another. Hence, we have focused on using data from studies of RAMs to explore the trade-off between sensitivity and specificity, rather than try to identify the most cost-effective RAM. To do this we have used regression to estimate the trade-off between sensitivity and specificity for a typical RAM and have used this to explore how this trade-off affects the cost-effectiveness of using RAMs to select patients for prophylaxis.
Figure 10 shows the sensitivity and specificity data available across all populations with trend lines fitted firstly to all of the estimates across the multiple RAMS reported by Greene et al. and Grant et al. which were estimated in a single cohort, and secondly to the estimates from Elias et al. , which were estimated in the subset of the cohort not having pharmacological prophylaxis. The trend lines have been fitted by assuming that there is a linear relationship between logit (sensitivity) and logit (1-specificity). It should be noted that for the line fitting against the data from Greene et al. and Grant et al. , the fitted line assumes that each point is equally valid and does not take into account (1) the uncertainty around each pair of sensitivity and specificity estimates, (2) the relationship between multiple points that represent the ROC curve for a single RAM in a single study or (3) any relationship between estimates for different RAMs from the same study. The estimates from the Elias cohort were extracted for use in a sensitivity analysis exploring the potential for a more accurate RAM to be cost-effective. This was undertaken specifically to address the concern that use of VTE prophylaxis could lead to underestimation of the accuracy of the RAMs. It should be noted, however, that the Elias study reported higher accuracy for the Padua RAM than other studies in cohorts with and without prophylaxis. It is, therefore, unclear why accuracy was better in the Elias study, but it probably reflects features of the study design.
FIGURE 10.
ROC curve for all studies (surgical, medical and mixed) with trend lines fitted.
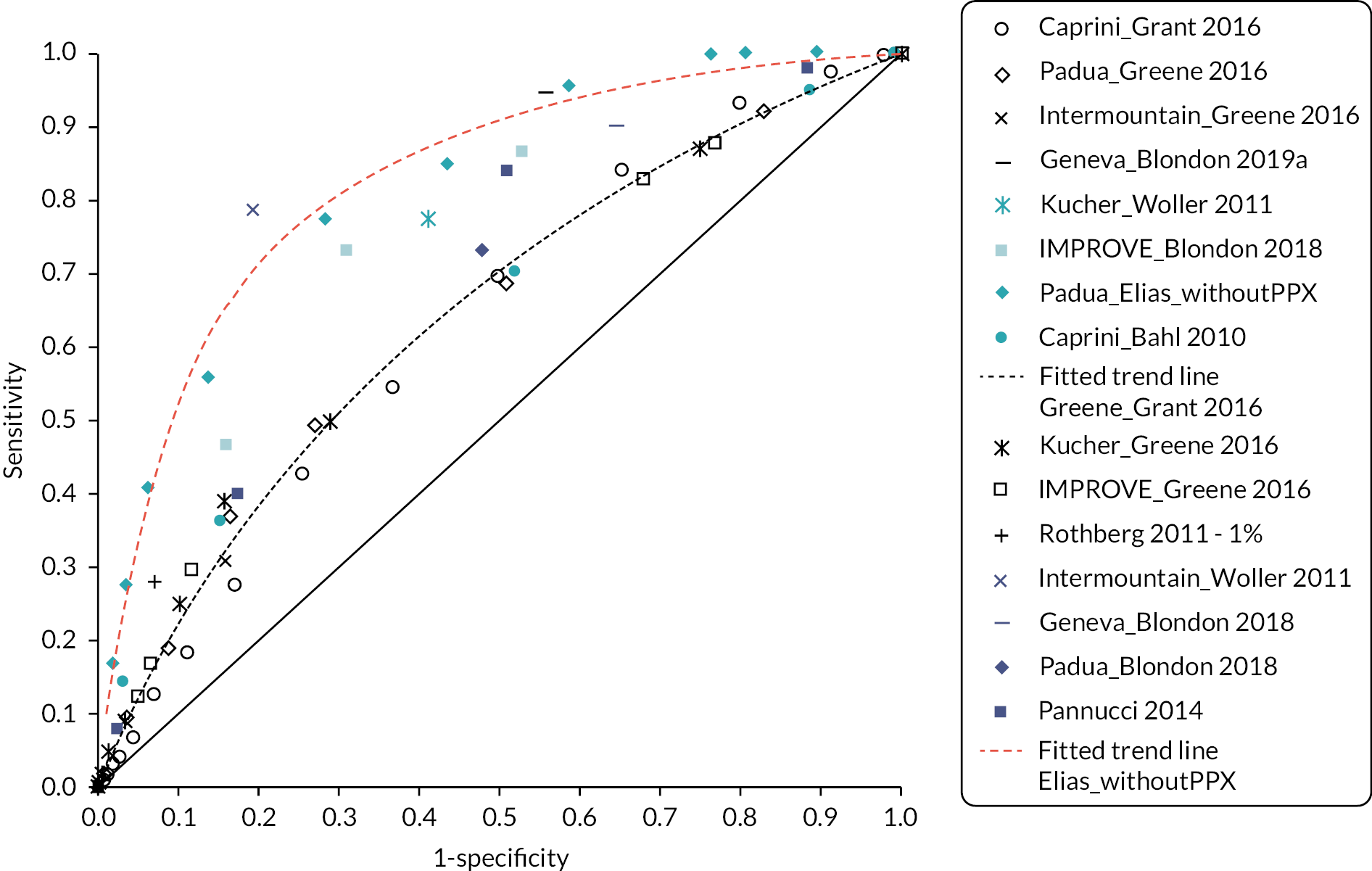
Model inputs for the elective hip replacement and elective knee replacement scenarios
In the main analysis for surgical inpatients, we excluded patients having elective hip and knee replacement surgery as the NICE guideline (NG89) recommends a more extended form of thromboprophylaxis in these groups. In knee replacement patients NG89 recommends LMWH for 14 days. In hip replacement patients NG89 recommends LMWH for 28 days. For comparison, in the majority of other categories of surgical patients, the duration of prophylaxis with LMWH recommended in NG89 is a minimum of 7 days.
If a single RAM is to be used across a broad range of surgical patients, encompassing populations that have a longer duration of prophylaxis, it is important to know whether the longer duration of prophylaxis in these groups would result in a different threshold for cost-effective use of prophylaxis. Therefore, we have conducted scenario analyses to determine what the risk threshold for thromboprophylaxis would be for elective hip and elective knee replacement patients and whether a prophylaxis for all strategies would be better in these specific populations.
In the scenario analyses for the two specific surgical populations of elective hip replacement patients and elective knee replacements, the overall modelling approach was the same as in the analysis for the general surgical cohort, but specific parameters were changed to make them more relevant to these groups. To identify relevant parameters, we used the cost-effectiveness models described for these populations in NG89. The key parameters for these populations are summarised in Table 6.
Parameter | Elective hip replacement | Elective knee replacement |
---|---|---|
Average age at admission | 68.7 | 69.2 |
% male | 40% | 44% |
Duration of prophylaxis with LMWH | 28 days | 14 days |
Absolute risk of PE without prophylaxis | 1.42% | 2.48% |
Absolute risk of symptomatic DVT without prophylaxis | 2.04% | 1.35% |
Absolute risk of asymptomatic DVT without prophylaxis | 10.01% | 28.62% |
RR of VTE for prophylaxis (LMWH) vs. no prophylaxis | 0.33 | 0.47 |
Absolute risk of PE with prophylaxis (LMWH) | 1.08% | 1.17% |
Absolute of symptomatic DVT with prophylaxis (LMWH) | 1.71% | 0.63% |
Absolute risk of symptomatic DVT with prophylaxis (LMWH) | 3.30% | 13.45% |
Absolute risk of major bleeding without prophylaxis (including fatal, ICH and other) | 1.26% | 1.13% |
Major bleed risk by type for patients without prophylaxis | ||
Fatal major bleeding | 0.01% | 0.01% |
ICH | 0.02% | 0.02% |
Other major bleeding | 1.23% (including 0.16% surgical site bleeding requiring return to theatre) |
1.10% (including 0.15% surgical site bleeding requiring return to theatre) |
RR of bleeding for prophylaxis (LMWH) vs. no prophylaxis | 2.55 | 1.09 |
Absolute risk of any major bleeding when receiving prophylaxis with LMWH (including fatal, ICH and other) | 3.21% | 1.23% |
Major bleed risk by type for patients having prophylaxis | ||
Fatal major bleeding | 0.03% | 0.01% |
ICH | 0.06% | 0.02% |
Other major bleeding | 3.12% (including 0.41% surgical site bleeding requiring return to theatre) |
1.20% (including 0.16% surgical site bleeding requiring return to theatre) |
Case-fatality rate for PE | 17% | 6% |
SMR vs. general population in the year following admission | 4.3 | 3.0 |
The absolute risks of VTE in elective hip and knee replacement patients were taken from the models in NG89. In NG89 these were estimated by combining information on the risk of symptomatic VTE in patients having prophylaxis taken from the National Joint Registry estimates of the ratio of asymptomatic to symptomatic DVTs provided by Quinlan et al. and information on the efficacy of prophylaxis taken from the NMAs conducted for NG89. 99,100,138
The bleeding risk for elective hip and knee replacement patients has been taken from the model inputs for NG89. In the elective knee replacement population, the risk of major bleeding with LMWH was 1.23% (0.64% major bleeding at surgical site, 0.39% GI and ICH, 0.2% other major) in the NG89 model. For elective hip replacement patients, the baseline risk of major bleeding in those having standard duration LMWH was 3.21% in the NG89 model (2.29% major surgical site bleeding, 0.72% ICH and GI, and 0.2% other major). This rate of major bleeding was applied to elective hip replacement patients having extended duration LMWH in the model, as although the NMA in NG89 predicted a lower risk of major bleeding for extended LMWH versus standard duration LMWH (0.78, 95% CI 0.11 to 3.85), the difference was not statistically significant and applying a lower risk of bleeding for a longer duration of prophylaxis was considered to lack clinical face validity.
The proportion of major bleeds that were fatal or non-fatal ICHs was taken from the same source as used in the main surgical cohort as this was a study of patients having major orthopaedic surgery. 103 Age at baseline and the proportion who are male were based on the estimates from the National Joint Registry used in NG89. The standardised mortality rates versus the general population were adjusted to give the same mortality rate at 12 months as applied in the NG89 model (1.49% for hip and 1.05% for knee), which again were based on data from the National Joint Registry. In hip replacement patients the case-fatality rate for PEs (17%) was taken from the model in NG89 and this was derived from the RCTs of elective hip replacement patients included in the reviews for NG89. However, in NG89 no equivalent data were available for knee replacement patients, so it was assumed that the case-fatality rate for knee replacement patients would be the same as for general surgical patients (6%).
The type of pharmacological prophylaxis used is assumed to be LMWH, but the duration of prophylaxis is assumed to be longer at 14 and 28 days, respectively, for knee and hip replacement patients compared with up to 7 days for the main surgical cohort. Also, because of the need for a longer duration of prophylaxis, it is assumed that prophylaxis will continue after discharge with outpatient prophylaxis used after discharge. For general surgical patients, in the scenario analysis in which we assumed that prophylaxis continues for 7 days, including 2 days of post-discharge prophylaxis, the total cost was £101.03. This total includes £67.55 for outpatient administration, which covers the cost of teaching patients to self-administer prophylaxis after discharge and the need for district nurse administration for a minority of patients (4%). In the elective knee replacement population, with a total of 14 days of LMWH, the total cost is increased to £141.71 due to the additional 7 days of LMWH. In the elective hip replacement population, with a total 28 days of LMWH, the total cost is £223.09.
NG89 also recommends prophylaxis with aspirin or DOACs as alternatives to LMWH in patients having elective hip or knee replacement. Therefore, we have conducted sensitivity analyses to see if adjusting the costs to reflect these alternative prophylaxis regimens, while assuming equivalent efficacy, results in different conclusions regarding the optimal use of RAMs in these cohorts. For the knee replacement population, the alternative regimens modelled consist or 14 days of rivaroxaban, at a cost of £25.20 or 14 days of aspirin, at a cost of £0.69. For the hip replacement population, the alternatives modelled consist of 35 days of rivaroxaban, at a cost of £63.00, or 10 days of LMWH followed by 28 days of aspirin, at a cost of £119.84.
Economic modelling results
Clinical outcomes predicted by the model with and without prophylaxis
Table 7 shows the clinical outcomes predicted by the model with and without prophylaxis in each of the modelled populations.
Outcomes at 6 months per 10,000 patients | Outcomes at 5 years per 10,000 patients | |||||||||||
---|---|---|---|---|---|---|---|---|---|---|---|---|
Fatal PE | Fatal bleed | Non-fatal ICH | Other major bleeda | Non-fatal PE | Symptomatic DVT | Asymptomatic DVT | PTS | PE survivor with CTEPH | PE survivor without CTEPH | ICH survivor | Dead (any cause) | |
Medical inpatients | ||||||||||||
No prophylaxis | 37 | 10 | 6 | 53 | 101 | 201 | 3041 | 787 | 2 | 83 | 5 | 1498 |
Prophylaxis | 18 | 15 | 9 | 79 | 49 | 98 | 1489 | 385 | 1 | 41 | 7 | 1486 |
Surgical inpatientsb | ||||||||||||
No prophylaxis | 4 | 1 | 2 | 122 | 58 | 78 | 1260 | 367 | 1 | 54 | 2 | 353 |
Prophylaxis | 1 | 3 | 7 | 360 | 17 | 23 | 365 | 107 | 0 | 16 | 6 | 352 |
Elective hip replacement inpatients | ||||||||||||
No prophylaxis | 24 | 2 | 2 | 124 | 118 | 204 | 1000 | 294 | 3 | 100 | 2 | 1225 |
Prophylaxis | 8 | 3 | 6 | 312 | 39 | 68 | 334 | 98 | 1 | 33 | 5 | 1212 |
Elective knee replacement inpatients | ||||||||||||
No prophylaxis | 16 | 2 | 2 | 112 | 247 | 134 | 2861 | 764 | 6 | 214 | 2 | 1079 |
Prophylaxis | 7 | 2 | 2 | 121 | 116 | 63 | 1337 | 357 | 3 | 100 | 2 | 1070 |
In medical inpatients, prophylaxis reduces serious adverse outcomes (fatal PEs, fatal bleeds and non-fatal ICHs) from 53 per 10,000 to 42 per 10,000. The reduction in symptomatic DVTs and non-fatal PEs is also higher than the increase in other bleed types. In the long-term outcomes (presented in Table 7) at 5 years, there is a large reduction in the number of patients experiencing PTS from prophylaxis from 787 per 10,000 to 385 per 10,000, but minimal difference in overall survival. Therefore, in medical inpatients, although prophylaxis increases the risk of major bleeding, this is more than outweighed by the number of VTE events avoided and there are additional benefits from preventing long-term VTE-related complications such as PTS.
In surgical inpatients, the risk of a serious adverse outcome (fatal PEs, fatal bleeds and ICH) is low at 7 per 10,000 but it is increased slightly by prophylaxis to 11 per 10,000 due to the additional risk of fatal bleeding or non-fatal ICH. Although the risk of any symptomatic VTE is reduced from 140 per 10,000 to 41 per 10,000, the risk of any major bleeding is increased from 125 per 10,000 to 370 per 10,000. Prophylaxis still reduces the risk of PTS in surgical inpatients, from 367 per 10,000 to 107 per 10,000, but PTS is less common in the surgical cohort than in the medical cohort due to the lower risk of DVT. Therefore, in the surgical population there is a more explicit trade-off between the short-term risk of major bleeding and the benefits of preventing VTEs and their long-term consequence.
In elective hip replacement patients, the risk of a serious adverse clinical outcome (fatal PEs, fatal bleeds and non-fatal ICH) is reduced by prophylaxis from 28 per 10,000 to 17 per 10,000. The risk of any symptomatic VTE is reduced from 346 per 10,000 to 116 per 10,000 and the risk of any major bleeding is increased from 128 per 10,000 to 321 per 10,000. The reduction in PTS is similar to that observed in the general surgical inpatient population (294 per 10,000 reduced to 98 per 10,000 by prophylaxis). Therefore, in the elective hip replacement population, the increase in rates of major bleeding is similar to the decrease in rates of symptomatic VTE, but overall there is a reduction in serious adverse clinical outcomes as only a very small minority of major bleeds are fatal or intracranial.
In elective knee replacement patients, the risk of a serious adverse clinical outcome (fatal PEs, fatal bleeds and non-fatal ICH) is reduced from 20 per 10,000 to 11 per 10,000 by prophylaxis. The risk of any symptomatic VTE is reduced from 397 per 10,000 to 186 per 10,000 but the increase in the risk of any major bleeding is smaller (116 per 10,000 to 125 per 10,000). The reduction in PTS is more similar to that observed in the medical inpatient population (764 per 10,000 reduced to 357 per 10,000), which is largely being driven by the high risk of asymptomatic DVT in the elective knee replacement population which is more similar to that observed in the medical inpatient population.
The 5-year survival is higher in surgical inpatients than in medical inpatients which is to be expected given the lower starting age of 54 years in surgical inpatients compared with 64 years in medical inpatients. In both the elective knee replacement population and the elective hip replacement population, all-cause mortality at 5 years is higher than in the general surgical population due to a higher starting age of 69 years in both groups. However, the impact of prophylaxis on all-cause mortality at 5 years is small regardless of the population selected.
Balance of benefits and harms of prophylaxis at different levels of risk
It can be seen from the clinical outcomes predicted by the model in Table 7, that there is always a trade-off between the benefits of using prophylaxis to reduce VTE risks and the potential harms that may occur due to the increased risk of bleeding. These differ between the medical and surgical populations largely because these groups have different average risks of VTE and major bleeding but also because some specific types of VTE outcomes and major bleeding events are more likely in some populations than others. It would, therefore, be useful to explore the overall impact of prophylaxis on health at differing levels of risk so that the balance of benefits and harms in individuals who have a higher or lower than average risk can be assessed. Figures 11 and 12 present information on whether the overall impact of prophylaxis on QALYs over the patient’s remaining lifetime is positive or negative for different levels of VTE risks and major bleeding risks. Where the overall impact of prophylaxis on lifetime QALYs is positive for a given level of VTE and major bleeding risk, the cell is coloured green and where it is negative the cell is coloured red. It should be noted that the steps in risks between rows and columns are not even across the table. In addition, although the risk levels presented for VTE without prophylaxis, and for major bleeding with prophylaxis are the same in Figures 11 and 12, the risk levels for VTE with prophylaxis and major bleeding without prophylaxis differ because the RRs for these outcomes are different in medical and surgical inpatients. The square outlined in bold is the average level of risk assumed for that population in the base-case analysis.
FIGURE 11.
Balance of benefits and harms for medical inpatients with different levels of risk (blue and red indicate overall QALY gain and loss, respectively).
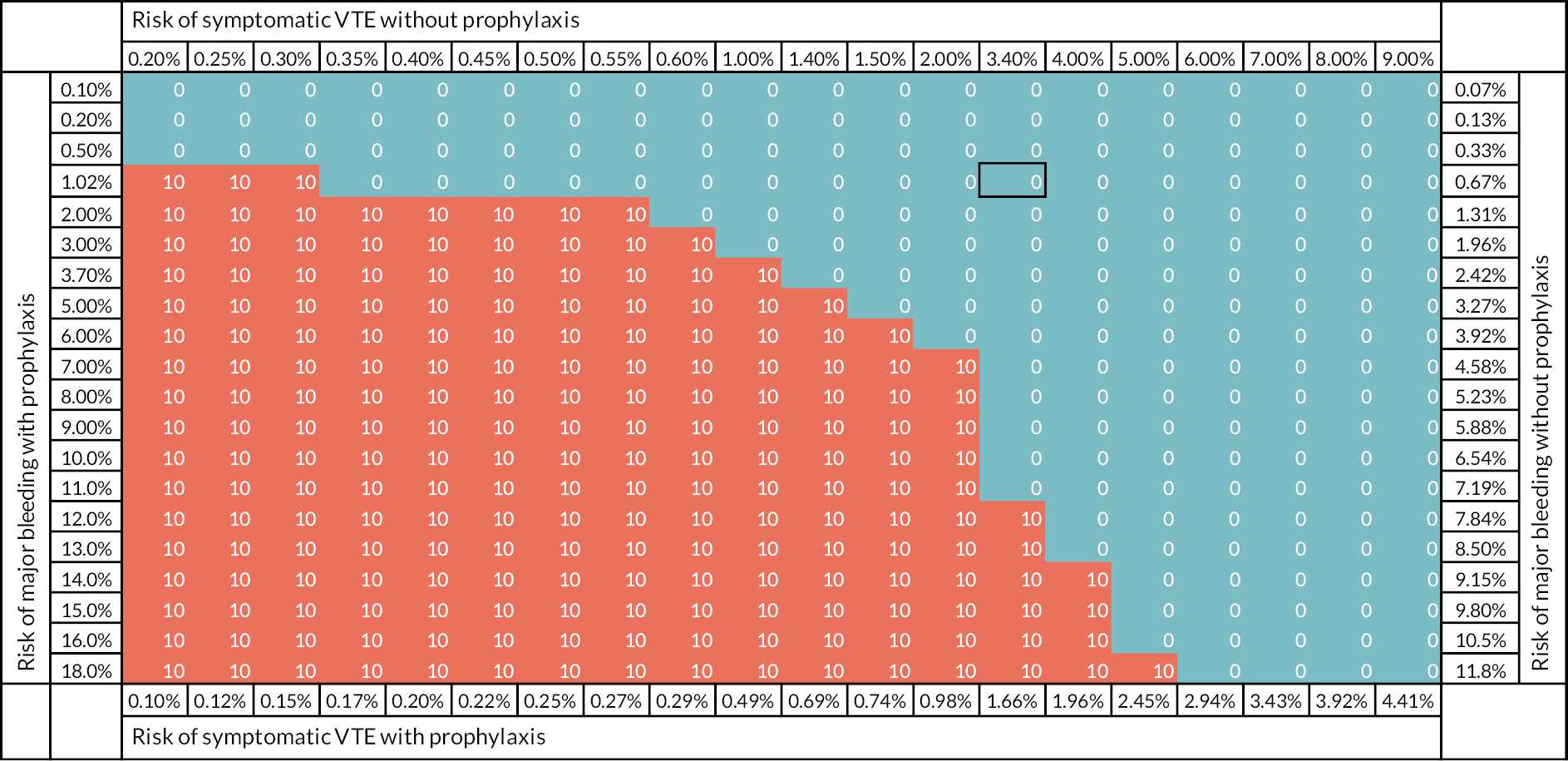
FIGURE 12.
Balance of benefits and harms for surgical inpatients with different levels of risk (blue and red indicate overall QALY gain and loss, respectively).

In Figure 11, it can be seen that prophylaxis for all results in QALY gains for medical inpatients with average levels of VTE and bleeding risks (3.4% and 1.02%, respectively). Prophylaxis for none would result in greater QALY gains if either the average VTE risk in the medical cohort was much lower (≤ 0.35%) or the average risk of bleeding in the medical cohort was much higher (≥ 11%). However, in a cohort of patients with both a higher risk of bleeding and a lower risk of VTE, for example, a 6% bleeding risk and a 1.5% VTE risk, prophylaxis would also do more harm than good.
In Figure 12, it can be seen that prophylaxis results in overall QALY gains over a much broader range of VTE and bleeding risks in surgical inpatients than in medical inpatients. In surgical inpatients a much lower proportion of major bleeding is fatal bleeding or a non-fatal ICH and, therefore, the overall impact of prophylaxis is positive over a broader range of bleeding risks. In addition, the average age assumed for patients in the surgical population is lower than the average age for patients assumed in the medical population. Therefore, any QALY losses due to long-term consequences of VTE, such as PTS, will be larger as they will have an impact over a longer time frame.
Figures 11 and 12 show exploratory analyses and should not be used to guide decision-making. The analysis assumes that the RR of bleeding with prophylaxis is constant and does not increase as the baseline bleeding risk increases, whereas it is conceivable that risk factors for bleeding also increase the RR of bleeding with prophylaxis. Furthermore, VTE and bleeding risk factors are often inter-related and there may be interactions between risk factors and between risks. These issues mean that the decision to prescribe prophylaxis for an individual patient with an increased bleeding risk is complex and unlikely to lend itself to determination by a simple trade-off.
Figures 11 and 12 show the balance of benefits and harms for prophylaxis in terms of the impact on overall lifetime QALYs, but they do not address the question of whether it is cost-effective to use prophylaxis at different levels of risk and whether it is cost-effective to use a RAM to select patients at higher risk for prophylaxis. These questions are addressed in Deterministic base-case cost-effectiveness results for medical inpatients to Deterministic scenario analyses for elective hip and knee replacement populations below.
Deterministic base-case cost-effectiveness results for medical inpatients
The deterministic base-case results obtained when applying the midpoint parameter estimates to the base-case scenario for medical inpatients using data for the five RAMS that were evaluated in a single cohort of patients (Green et al. 2016; Grant et al. 2016) are presented in Figure 13. For each RAM, several points are plotted which represent the costs and QALYs that can be achieved for different RAM cut-off points. It can be seen in Figure 13 that the strategy of prophylaxis for all dominates all other options because it has a larger expected QALY gain and it also has a lower expected cost. Figure 13 also shows the expected costs and QALYs for a RAM which has the average characteristics of all the RAMS evaluated in the cohort reported by Greene et al. and Grant et al. using the ROC curve regression described in Sensitivity and specificity of RAMs.
Figure 14 shows the secondary analysis introducing the data from RAMs evaluated in other cohorts. For some of these RAMs, only a single point is plotted as sensitive and specificity was only reported at a single threshold but for others multiple points are plotted showing different RAM thresholds. Several of these RAMs appear to perform better than the regression based on the average RAM evaluated in the cohort reported by Greene et al. and Grant et al. in that they provide a similar number of QALYs at a lower cost. The best-performing RAM reported in an alternative cohort, is the Geneva score with a cut-off of ≥ 3 (sensitivity of 94.6% and specificity of 44%) reported by Blondon et al. 29 However, prophylaxis for all would still be more cost-effective than using a Geneva score cut-off of ≥ 3, even when applying the performance data from Blondon et al.
Also shown in Figure 14 is a sensitivity analysis using the Padua RAM performance data from Elias et al. which was obtained in the subgroup of the cohort not having prophylaxis. 36 When including this data, the optimal strategy would be a Padua score of ≥ 3 (sensitivity 99.9% and specificity of 23.7%), as this has slightly higher QALYs and slightly lower costs than prophylaxis for all. However, the caveat here is that Elias et al. recruited a mixed population of medical and surgical patients and, therefore, it is unclear if this RAM would perform equally well in an exclusively medical cohort. When the Padua score was evaluated by Greene et al. in an exclusively medical cohort, the performance was not as good, with a Padua score of ≥ 3 having a sensitivity and specificity of 49.3% and 73.0%, respectively. However, this sensitivity analysis suggests that if a RAM with sufficiently good performance could be developed and validated in an exclusively medical cohort, then prophylaxis for all would not be the optimal strategy in medical patients. Also shown are the expected costs and QALYs when using a regression fitted to the data points from Elias et al. It can be seen from this that the performance based on the regression is not as good as when using the observed sensitivity and specificity for the actual Padua RAM scores obtained by Elias et al. This is because the regression is not a good fit to the ROC curve for low RAM scores.
Probabilistic base-case results for medical inpatients
Based on these deterministic analyses, the probabilistic model was run for the strategies of no prophylaxis, prophylaxis for all and for the various cut-offs of the Padua score (assuming the RAM performance observed in Greene et al. ). It should be noted that this probabilistic analysis does not explore the uncertainty regarding which RAM is optimal as this would require comparing all of the cut-offs from all of the various RAMs simultaneously. Instead, it uses the Padua RAM as an example RAM to answer the question of what the optimal strategy would be if the RAM chosen performed similarly to the Padua RAM.
The results based on the mean outcomes from 10,000 probabilistic model runs are summarised in Table 8. The broad conclusions are the same, in that prophylaxis for all is the optimal strategy when applying valuing a QALY at £20,000–30,000.
% PPX | Sensitivity | Specificity | Absolute costs, £ | Absolute QALYs | Cost vs. no PPX, £ | QALYs vs. no PPX | ICER vs. PPX for none, £ | INMB vs. PPX for none at £20K, £a | INMB vs. PPX for none at £30K, £a | |
---|---|---|---|---|---|---|---|---|---|---|
PPX for none | 0% | 0 | 1 | 244.93 | 9.0033 | - | NA | NA | NA | NA |
PPX for Paduab ≥ 7 | 3% | 5% | 98% | 251.40 | 9.0061 | 6.47 | 0.0028 | 2295.40 | 49.89 | 78.07 |
PPX for Paduab ≥ 6 | 6% | 10% | 96% | 249.09 | 9.0087 | 4.16 | 0.0055 | 762.55 | 104.91 | 159.44 |
PPX for Paduab ≥ 5 | 12% | 19% | 91% | 244.81 | 9.0141 | −0.12 | 0.0108 | Dominates | 215.61 | 323.36 |
PPX for Paduab ≥ 4 | 23% | 37% | 84% | 235.91 | 9.0243 | −9.02 | 0.0210 | Dominates | 428.46 | 638.19 |
PPX for Paduab ≥ 3 | 35% | 49% | 73% | 231.70 | 9.0311 | −13.23 | 0.0279 | Dominates | 570.57 | 849.23 |
PPX for Paduab ≥ 2 | 57% | 69% | 49% | 227.79 | 9.0417 | −17.14 | 0.0384 | Dominates | 786.04 | 1170.49 |
PPX for Paduab ≥ 1 | 86% | 92% | 17% | 224.43 | 9.0544 | −20.50 | 0.0511 | Dominates | 1042.68 | 1553.77 |
PPX for all | 100% | 1 | 0 | 216.49 | 9.0585 | −28.44 | 0.0552 | Dominates | 1132.39 | 1684.36 |
Figure 15 shows that while the average cost saving is £28.44 and the average QALY gain is 0.055 QALYs for prophylaxis for all versus prophylaxis for none, there are a broad range of estimates with 79% of samples resulting in a cost saving and the incremental cost having a CrI of £−113.66 to £46.28. The incremental QALY estimate has a CrI of 0.02 to 0.11, with 99.9% of samples resulting in a QALY gain compared to prophylaxis for none.
FIGURE 15.
Cost-effectiveness plane for prophylaxis for all versus prophylaxis for none in medical inpatients.

The cost-effectiveness acceptability curve (CEAC) is presented in Figure 16 for medical inpatients. There is < 1% chance that prophylaxis for all is not the optimal strategy when valuing a QALY at £20,000. In addition, because prophylaxis for all is cost-saving compared to even the next most effective strategy, there is a 76% chance that it would be the optimal strategy when valuing a QALY at £0.
FIGURE 16.
Cost-effectiveness acceptability curve (CEAC) for medical inpatients (applying performance of Padua RAM from Greene et al. 39). Strategies with < 1% probability across all values of a QALY are not plotted.

The overall expected value of perfect information (EVPI) associated with all parameters included in the probabilistic sensitivity analysis (PSA) when valuing a QALY at £20,000 was £0.06 per patient per year (£59.33 per 1000 patients per year) due to the high probability that prophylaxis for all is the optimal strategy. This suggests that there is minimal decision uncertainty regarding whether a RAM should be used in medical inpatients, when assuming the RAM will perform according to the performance of the Padua RAM in the Greene et al. cohort.
However, the results in Figure 14 suggest that the optimal strategy may not be prophylaxis for all if a better-performing RAM could be developed in medical patients. Therefore, we decided to re-run the EVPI analysis for the medical cohort when using the sensitivity and specificity data from the mixed cohort of medical and surgical patients reported by Elias et al. When using this sensitivity and specific data for medical inpatients, the EVPI is £2.42 per person per year (£2422.90 per 1000 patients per year). Offering prophylaxis at a RAM score of ≥ 3 has a 76.6% chance of being optimal and prophylaxis for all has a 17.6% chance of being optimal when valuing a QALY at £20,000. In addition, a large proportion of the global EVPI is attributable to the uncertainty around the RR of bleeding for prophylaxis versus no prophylaxis; expected value of partial perfect information (EVPPI) of £2.01 for this parameter with no other parameter having an EVPPI > £0.01. This sensitivity analysis suggests that if a better-performing RAM could be developed for medical inpatients, then the decision uncertainty would largely relate to the uncertainty around the increased risk of bleeding attributable to prophylaxis.
Deterministic scenario and sensitivity analyses for medical inpatients
Deterministic scenario and sensitivity analyses were conducted to explore which model assumptions and inputs were key drivers of decision uncertainty. To do this, deterministic results were generated using midpoint parameter inputs when varying individual model inputs or assumptions. These analyses assume that the RAM used is Padua and that it performs as observed in the cohort reported by Greene et al.
The results presented in Table 9 show that the broad conclusion that prophylaxis for all is the optimal strategy (defined as most cost-effective when valuing a QALY at £20,000) does not vary under most of the scenarios and sensitivity analyses considered. The exceptions were scenarios that explored large changes to the risk of VTE and bleeding during PPX, with a sixfold decrease in VTE risk or a sixfold increase in risk of major bleeding needed to alter the optimal strategy.
Scenario | Parameter varied | Parameter value in base-case scenario | Parameter value in current scenario | Optimal strategy in current scenario |
---|---|---|---|---|
Base case | N/A | N/A | N/A | PPX for all |
Younger patients | Age | 65.8 | 20 | PPX for all |
Older patients | Age | 65.8 | 80 | PPX for all |
Double utility decrement for PPX | Utility decrement | 0.007 | 0.015 | PPX for all |
Low discounting rate for costs and QALYs | Discount rate | 3.5% | 1.5% | PPX for all |
High discounting rate for costs and QALYs | Discount rate | 3.5% | 6.0% | PPX for all |
Utility decrement of PTS is halved | Utility decrement | 10% | 5% | PPX for all |
Utility decrement of PTS from Lenert et al. | Utility decrement | 10% | 2% | PPX for all |
Quadruple cost of administering RAM | Cost of RAM administration | £9.08 | £36.33 | PPX for all |
No PTS risk in asymptomatic DVT (proximal or distal) | Risk of PTS in: | PPX for all | ||
Proximal | 57.5% | 0% | ||
Distal | 15.6% | 0% | ||
Low risk of VTE (one-sixth of baseline risk) | VTE risk | Padua ≥ 2 | ||
Symptomatic | 3.4% | 0.6% | ||
Asymptomatic | 30.5% | 5.1% | ||
PTS cost from Caprini | Year 1 cost | £293 | £1022 | PPX for all |
Year 2 cost | £78 | £423 | ||
No asymptomatic DVTs | Asymptomatic DVT risk | 30.5% | 0% | PPX for all |
7 days of LMWH (including 2 days post discharge) | Cost of PPX | £23.91 | £101.03 | PPX for all |
Higher risk of death in year of admission | Risk of death in year 1 | 10% | 22% | PPX for all |
Bleed risk during VTE treatment reflects any HAS-BLED score | Risk of major bleeding during anticoagulation | 0.9% | 2.0% | PPX for all |
Higher bleed risk during PPX (six times higher) | Risk of bleeding during PPX | 1.02% | 6.12% | Padua ≥ 1 |
No additional cost for administering RAM | Cost of RAM administration | £9.08 | £0 | PPX for all |
Lower case-fatality rate of PE | Case-fatality rate of PE | 26.8% | 13% | PPX for all |
Higher case-fatality rate of PE | Case-fatality rate of PE | 26.8% | 67% | PPX for all |
No increase in inpatient care due to VTE | Distal DVT cost | £644.88 | £386.10 | PPX for all |
Proximal DVT cost | £765.05 | £386.10 | ||
PE cost | £1850.68 | £476.57 | ||
VTE occurs 3 days after admission | Time of VTE | 42 | 3 | PPX for all |
VTE occurs 90 days after admission | Time of VTE | 42 | 90 | PPX for all |
Increased use of DOACs to treat VTE | % of VTE treatment that is DOACs | 40% | 100% | PPX for all |
On the basis of this, further analyses were conducted to explore how the optimal strategy varies when changing the average risks of VTE and major bleeding in the medical cohort. Figure 17 shows that the optimum strategy is prophylaxis for all when the risk of VTE is high and the bleeding risk is low, but the optimum strategy is pharmacological prophylaxis for none when the risk of VTE is low and the bleeding risk is high. There is a band of combined VTE and bleeding risks where using a RAM to target prophylaxis at higher-risk patients would be optimal instead of a blanket strategy of either prophylaxis for all or prophylaxis for none. This suggests that it is important to gather good evidence on the average risks of VTE and major bleeding in the medical cohort as a whole in order to determine whether a RAM should be used to direct prophylaxis.
FIGURE 17.
Optimal risk assessment strategy for cohorts of medical inpatients with varying levels of baseline risks (box indicates base-case scenario). It should be noted that the rows and columns do not represent equal steps in risk; red (10) indicates prophylaxis for none is optimal and blue (0) indicates prophylaxis for all is optimal; the numbers in cells of other colours represent the optimal Padua RAM score at which prophylaxis should be offered at that level of risk to maximise incremental net monetary benefit when valuing a QALY at £20,000.
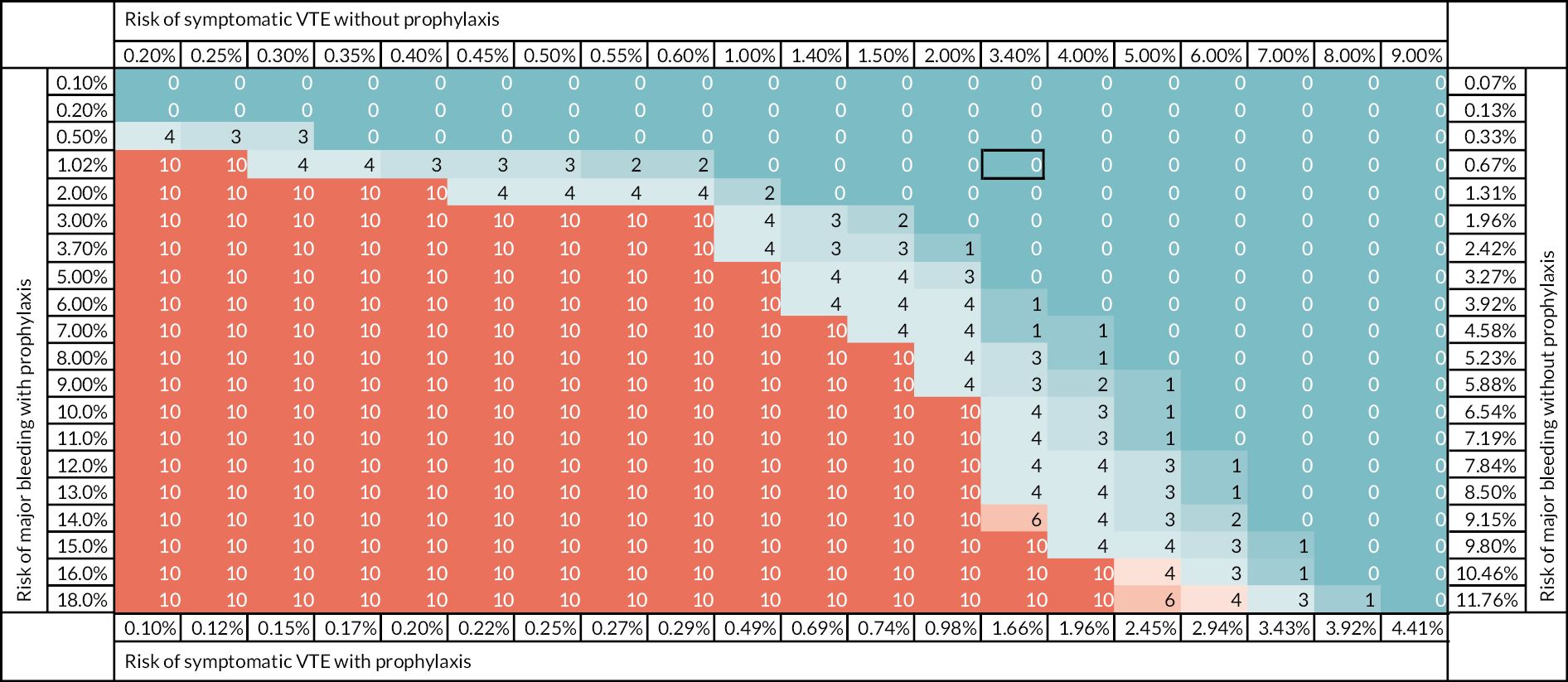
The sensitivity and scenario analyses also suggest that various individual factors about which there was some uncertainty have no impact on the optimal strategy. These include the incidence of PTS after asymptomatic DVT, the costs and utility losses attributable to PTS, the case-fatality rate for PE and the exact timing of VTE within the 90-day post-admission period. In addition, it was found that the optimal strategy did not change when assuming no additional cost for administering the RAM or when assuming that patients continue LMWH for 7 days including 2 days of post-discharge administration.
Deterministic base-case results for surgical inpatients
The deterministic base-case results obtained when applying the midpoint parameter estimates to the base-case scenario for surgical inpatients using data for the two RAMS that were evaluated in either a surgical population (Caprini by Bahl et al. ,26 Pannucci by Pannucci et al. 54) are presented in Figure 18. For each RAM, several points are plotted which represent the costs and QALYs that can be achieved for different RAM cut-off points. For the Pannucci RAM, the cut-offs shown are ≥ 6, ≥ 3 and ≥ 1. For the Caprini score the cut-offs shown are ≥ 7, ≥ 5, ≥ 3 and ≥ 2. From this it can be seen that the optimal strategy is pharmacological prophylaxis for all, if the analysis is restricted to RAMs evaluated exclusively in this cohort. Figure 18 also includes results for the Padua RAM from the mixed cohort of both surgical and medical inpatients reported by Elias et al. When including the performance data from the Padua score evaluated by Elias et al. , the optimal strategy is a Padua score of ≥ 3 (sensitivity of 99.9% and specificity of 23.7%), which achieves more QALYs at a reduced cost compared to prophylaxis for all.
Figure 18 also shows the expected cost-effectiveness of a RAM whose performance is based on a regression fitted to the data from the ROC curve for Padua using the data observed in the cohort not having thromboprophylaxis reported Elias et al. The data for the lower Padua score cut-offs (≥ 1 to ≥ 3) were excluded from this regression as they did not fit the assumption that there is a linear relationship between logit (sensitivity) and logit (1-specificity). Therefore, the regression does not closely follow the performance of the Padua score for the cut-offs below 4 and the Padua score is predicted by the regression to perform less well than observed at cut-offs below 4. This results in thromboprophylaxis for all being the optimal strategy when assuming that the RAM would follow the ROC curve predicted by the regression. Based on this, we can say that a RAM would need to have a very high sensitivity, similar to that observed by Elias et al. , to make using a RAM more cost-effective than offering prophylaxis to all.
Probabilistic base-case results for surgical inpatients
Based on these deterministic analyses, the probabilistic model was run for the strategies of no pharmacological thromboprophylaxis, prophylaxis for all and for the various cut-offs of the Pannucci score. It should be noted that this does not capture uncertainty related to which RAM is optimal as this would require a simultaneous comparison of all of the various cut-offs for all three RAMs.
The results based on the mean outcomes from 10,000 probabilistic model runs are summarised in Table 10. The broad conclusions are the same, in that offering prophylaxis to surgical patients with a Pannucci score ≥ 3 is the optimal strategy when applying valuing a QALY at £20,000 to £30,000.
% PPX | Sensitivity | Specificity | Absolute costs, £ | Absolute QALYs | Cost vs. no PPX £ | QALYs vs. no PPX | ICER vs. PPX for none, £ | INMB vs. PPX for none at £20K, £a | INMB vs. PPX for none at £30K, £a | |
---|---|---|---|---|---|---|---|---|---|---|
PPX for none | 0% | 0% | 100% | 159.13 | 13.9214 | NA | NA | NA | NA | NA |
PPX for Pannuccib ≥ 6 | 20% | 40% | 83% | 165.89 | 13.9362 | 6.76 | 0.0148 | 457.59 | 288.62 | 436.31 |
PPX for Pannuccib ≥ 3 | 55% | 84% | 49% | 176.99 | 13.9519 | 17.86 | 0.0306 | 584.51 | 593.17 | 898.68 |
PPX for Pannuccib ≥ 1 | 90% | 98% | 12% | 206.09 | 13.9561 | 46.96 | 0.0347 | 1353.16 | 647.13 | 994.17 |
PPX for all | 100% | 100% | 0% | 207.01 | 13.9565 | 47.88 | 0.0351 | 1363.99 | 654.11 | 1005.11 |
Figure 19 shows the incremental costs and QALYs for pharmacological thromboprophylaxis for all versus prophylaxis for none in surgical inpatients. It can be seen that while the average cost saving is £47.88 and the average QALY gain is 0.035 QALYs, there are a broad range of estimates with 24% of PSA samples resulting in a cost saving and the incremental cost having a CrI of −£96 to £254. The incremental QALY estimate has a CrI of 0.002 to 0.080 with 98% of samples resulting in a QALY gain compared to prophylaxis for none.
FIGURE 19.
Cost-effectiveness plane for prophylaxis for all versus prophylaxis for none in surgical inpatients.
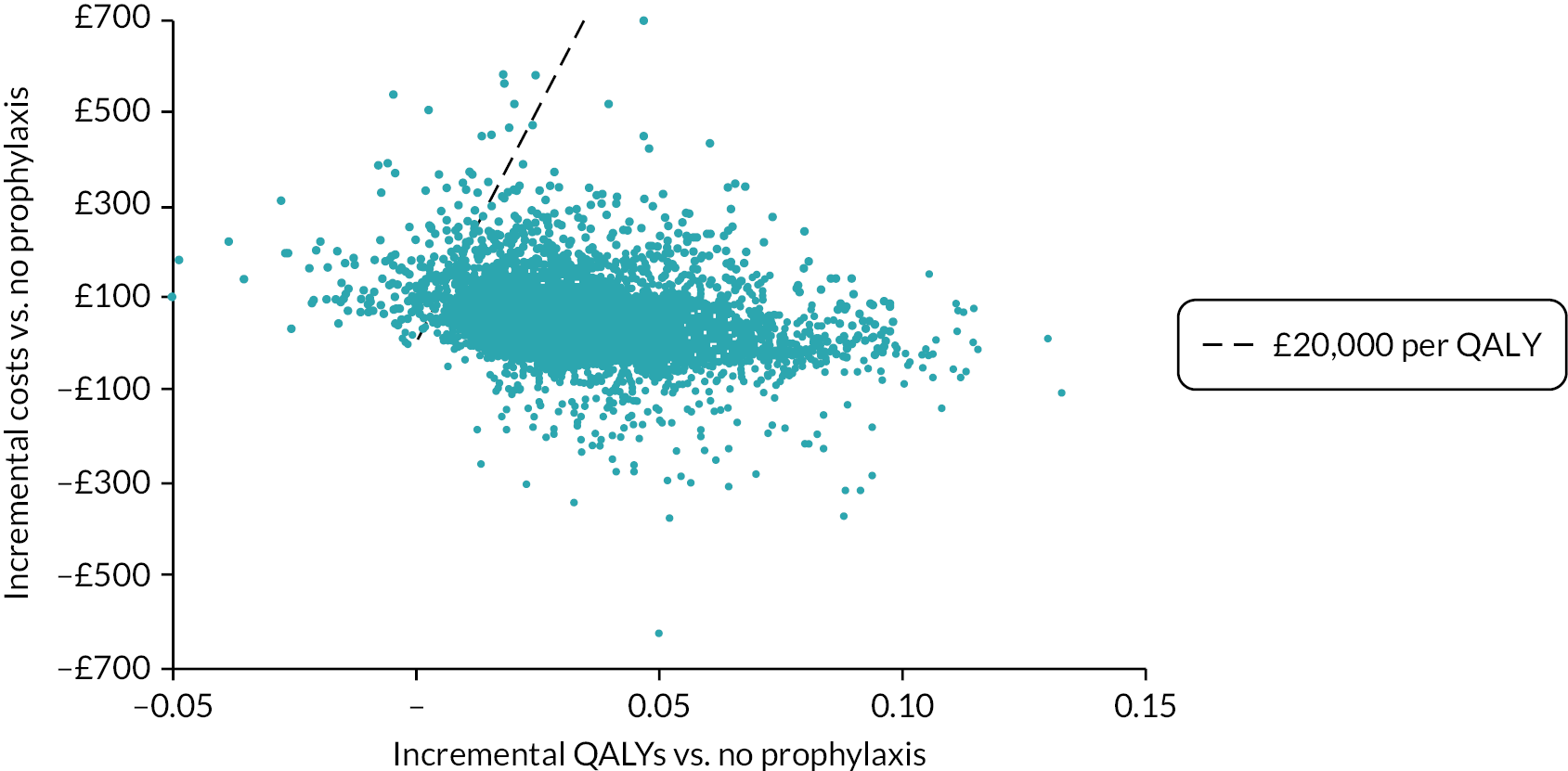
The CEAC is presented in Figure 20 for surgical inpatients. It can be seen from this that giving prophylaxis to all patients has the highest probability of being cost-effective at £20,000 per QALY, with a 70% likelihood of being optimal, but there is also a 17% likelihood that using a Pannucci score of ≥ 3 would be optimal and a 9% likelihood that using a Pannucci score of ≥ 1 would be optimal.
FIGURE 20.
CEAC for surgical inpatients (assuming the RAM used is Pannucci).

The overall EVPI associated with all parameters included in the PSA when valuing a QALY at £20,000 was £16.35 per person. This is calculated across all strategies including no prophylaxis, Pannucci scores of ≥ 6, ≥ 3 and ≥ 1 and thromboprophylaxis for all. It does not include the uncertainty of considering which RAM to use as the EVPPI has been run assuming that the Pannucci score is used. In addition, it should be noted that the EVPI analysis does not include any uncertainty not captured in the PSA such as uncertainty around the model assumptions or the choice of data sources, or uncertainty around the estimates of sensitivity and specificity.
In the analysis of parameter EVPI (EVPPI), only six individual parameters were found to have an EVPPI of more than £0.01 per person (see Table 11 EVPI results for surgical in patients when using Pannucci RAM based on sensitivity and specificity from Pannucci et al. ). The parameter with the largest EVPPI was the RR of VTE for patients having thromboprophylaxis compared to patients not having thromboprophylaxis. Other slightly less important parameters were the likelihood that major bleeding is intracranial (ICH) or fatal, the absolute and RRs of bleeding and the utility of patients experiencing PTS following DVT. These findings reinforce the idea that it is the trade-off between the short-term risks of bleeding in patients receiving prophylaxis and the long-term risks of chronic complications that are most important to the decision regarding which surgical inpatients should be offered prophylaxis.
Parameter | Per person EVPPI (£) | Standard error | Indexed to overall EVPI = 1.00 |
---|---|---|---|
RR of VTE for prophylaxis vs. no prophylaxis | 5.95 | 0.99 | 0.36 |
% fatal if major bleed during prophylaxis | 0.95 | 1.58 | 0.06 |
% of non-fatal major bleeds that are ICH during prophylaxis | 0.59 | 1.32 | 0.04 |
RR of major bleed for prophylaxis vs. no prophylaxis | 0.33 | 2.03 | 0.02 |
Absolute risk of major bleeding in patients having prophylaxis | 0.13 | 1.49 | 0.01 |
Utility of PTS | 0.01 | 1.05 | < 0.01 |
We also ran the PSA and EVPI analysis assuming that the Padua RAM is used and that it performs as seen in subset of the cohort reported by Elias et al. who did not receive prophylaxis. This was to explore whether further research would be more worthwhile if a RAM could be identified that performed better than the Pannucci RAM in surgical inpatients. The CEAC when assuming the RAM performance for Padua reported by Elias et al. is shown in Figure 21. When using the sensitivity and specificity data from Elias et al. the overall EVPI was £15.90 and the same set of parameters was identified as being the most important drivers of uncertainty.
Deterministic scenario and sensitivity analyses for surgical inpatients
Deterministic scenario and sensitivity analyses were conducted to determine whether the optimal strategy (when valuing a QALY at £20,000) varies under different assumptions or alternative sources of evidence. These analyses assume that the RAM used is Pannucci. The optimal strategy is defined as the most cost-effective strategy when valuing a QALY at £20,000.
These analyses (see Table 12) identified that prophylaxis for all is no longer the optimal strategy when the risk of VTE is halved, or when the risk of bleeding is doubled. It is also no longer optimal when the utility decrement of PTS is halved or estimated from alternative sources,134 or when assuming there is no risk of PTS in asymptomatic DVTs. It is also no longer optimal when assuming that there are no asymptomatic DVTs. These analyses suggest that the cost-effectiveness of offering prophylaxis to surgical patients is being partly driven by the prevention of PTS in patients having asymptomatic DVT. The scenario exploring an increase in age to 80 changed the optimal strategy to a RAM score of ≥ 3, which suggests that the optimal strategy may differ for patients who have lower life expectancy and lower quality of life prior to admission as these patients have less potential to benefit from reducing the risk of long-term complications. An increase in the duration of prophylaxis to 7 days to cover 5 days of admission and 2 days of post-discharge prophylaxis also altered the optimal strategy to offering prophylaxis at a RAM score of ≥ 1. However, this was largely attributable to the additional 2 days of prophylaxis provided after discharge, as the duration of in-hospital prophylaxis had to be increased to 16 days before the optimal strategy changed. The use of extended prophylaxis for 28 days also resulted in an optimal strategy of offering prophylaxis for those with a RAM score ≥ 3.
Scenario | Parameter varied | Parameter value in base-case scenario | Parameter value in current scenario | Optimal strategy in current scenario |
---|---|---|---|---|
Base case | N/A | N/A | N/A | PPX for all |
Younger patients | Age | 54.2 | 20 | PPX for all |
Older patients | Age | 54.2 | 80 | Pannucci ≥ 3 |
Double utility decrement for PPX | Utility decrement | 0.007 | 0.015 | PPX for all |
Low discounting rate (same for costs and QALYs) | Discount rate | 3.5% | 1.5% | PPX for all |
High discounting rate (same for costs and QALYs) | Discount rate | 3.5% | 6.0% | PPX for all |
Utility decrement of PTS is halved | Utility decrement | 10% | 5% | Pannucci ≥ 1 |
Utility decrement of PTS from Lenert | Utility decrement | 10% | 2% | Pannucci ≥ 3 |
Quadruple time for administering RAM (5–20 minutes) |
Cost of RAM administration | £9.08 | £36.33 | PPX for all |
No PTS risk in asymptomatic DVT (proximal or distal) | Risk of PTS in: | Pannucci ≥ 6 | ||
Proximal | 57.5% | 0% | ||
Distal | 15.6% | 0% | ||
No PTS risk in asymptomatic distal DVT | Risk of PTS in: | Pannucci ≥ 1 | ||
Proximal | 57.5% | 57.5% | ||
Distal | 15.6% | 0% | ||
No PTS risk in asymptomatic proximal DVT | Risk of PTS in: | Pannucci ≥ 3 | ||
Proximal | 57.5% | 0% | ||
Distal | 15.6% | 15.6% | ||
Lower risk of VTE (halving rate vs. base case) | VTE risk | Pannucci ≥ 3 | ||
Symptomatic | 1.4% | 0.7% | ||
Asymptomatic | 12.6% | 6.3% | ||
PTS cost from Caprini | Year 1 cost | £293 | £1022 | PPX for all |
Year 2 cost | £78 | £423 | ||
No asymptomatic DVTs | Asymptomatic DVT risk | 12.6% | 0% | Pannucci ≥ 6 |
7 days of LMWH (including 2 days post discharge) | Cost of PPX | £23.91 | £101.03 | Pannucci ≥ 1 |
Longer duration of admission (16 days) | Cost of PPX | £23.91 | £62.16 | Pannucci ≥ 1 |
Higher risk of death in year of admission | Risk of death in year of admission | 2% | 12% | PPX for all |
General population risk of mortality in year of admission | Risk of death in year of admission | 2% | 0.3% | PPX for all |
Bleed risk during VTE treatment reflects any HAS-BLED score | 0.9% | 2.0% | 0.9% | PPX for all |
Higher bleed risk during PPX | Risk of bleeding during PPX | 3.7% | 7.4% | Pannucci ≥ 3 |
No additional cost for administering RAM | Cost of RAM administration | £9.08 | £0 | Pannucci ≥ 1 |
Higher case-fatality rate for PE | Case-fatality rate | 6% | 26.8% | PPX for all |
Use of extended prophylaxis | Duration of prophylaxis | 5 | 28 | Pannucci ≥ 3 |
No increase in inpatient care due to VTE | Distal DVT cost | £644.88 | £386.10 | PPX for all |
Proximal DVT cost | £765.05 | £386.10 | ||
PE cost | £1850.68 | £476.57 | ||
VTE occurs 3 days after admission | Time of VTE | 42 | 3 | PPX for all |
VTE occurs 90 days after admission | Time of VTE | 42 | 90 | PPX for all |
Increased use of DOACs to treat VTE | % of VTE treatment that is DOACs | 40% | 100% | PPX for all |
The sensitivity and scenario analyses also suggest that various individual factors about which there was some uncertainty have no impact on the optimal strategy. These include the costs attributable to PTS, the case-fatality rate for PE and the exact timing of VTE within the 90-day post-admission period.
As these one-way scenario analyses identified that the conclusions were sensitive to the average risks of VTE and major bleeding in the cohort, we conducted a two-way scenario analysis to determine whether the optimal strategy would be different if the risk of VTE or the risk of major bleeding had been underestimated or overestimated in the base-case analysis. Figure 22 shows, although prophylaxis for all is the optimal strategy at the exact level of VTE and bleed risk assumed in the base-case scenario, the use of a RAM would be optimal across a large range of VTE and bleeding risks if that RAM performed similarly to the Pannucci RAM. This suggests that obtaining good evidence on the average baseline risks of VTE and major bleeding in the surgical cohort being offered risk assessment is important for determining which patients should be offered thromboprophylaxis.
FIGURE 22.
Optimal risk assessment strategy for cohorts of surgical inpatients with varying levels of baseline risks (box indicates base-case scenario). It should be noted that the rows and columns do not represent equal steps in risk; red (10) indicates prophylaxis for none is optimal and blue (0) indicates prophylaxis for all is optimal; the numbers in cells of other colours represent the optimal Pannucci RAM score at which prophylaxis should be offered at that level of risk to maximise incremental net monetary benefit when valuing a QALY at £20,000.

Deterministic scenario analyses for elective hip and knee replacement populations
Figure 23 shows the incremental costs and QALYs versus no prophylaxis for each RAM cut-off point. For each series of points, the point closest to the origin shows the costs and QALYs for a Pannucci ≥ 6, and then each successive point shows the next RAM cut-off (≥ 3, ≥ 1 etc.) until the last point shows prophylaxis for all. Separate lines show the results for the main surgical population and for the two specific populations of patients having either elective hip replacement or elective knee replacement. For the main surgical population, two alternative assumptions regarding whether thromboprophylaxis is stopped at discharge or continued post-discharge are shown for comparison.
FIGURE 23.
Incremental costs and QALYs versus no prophylaxis for various RAM cut-off points (Pannucci ≥ 6, Pannucci ≥ 3, Pannucci ≥ 1, PPX for all) for populations requiring PPX after discharge.

The optimal strategy in the base case for the main surgical population, where LMWH is assumed to stop on discharge at 5 days, is prophylaxis for all. This is also the optimal strategy in the knee replacement population where LMWH is assumed to continue post discharge up to 14 days. For the main surgical population, when allowing patients to continue prophylaxis post discharge up to a maximum of 7 days, the optimal strategy (when valuing a QALY at £20,000) is offering prophylaxis using a RAM score cut-off of ≥ 1. The optimal strategy in the hip replacement population where LMWH is assumed to continue post discharge up to 28 days is offering prophylaxis using a RAM score cut-off of ≥ 3.
When applying the costs for the alternative pharmacological prophylaxis strategies using either aspirin or DOACs, but assuming equivalent efficacy to LMWH, the incremental costs were lower than the base-case scenario as these thromboprophylaxis strategies had lower costs. In the case of elective knee replacement patients, the optimal strategy was thromboprophylaxis for all when assuming either 14 days of aspirin or 14 days of a DOAC were offered. In hip replacement patients, thromboprophylaxis for all was optimal when assuming that DOACs were offered instead of LMWH and offering prophylaxis at a Pannucci score of ≥ 1 was optimal when assuming that prophylaxis consisted of 10 days of LMWH was followed by 28 days of aspirin.
The additional costs of offering extended duration prophylaxis, particularly with LMWH instead of DOACs or aspirin, mean that using a RAM to reduce the population being offered prophylaxis is optimal in patients having an elective hip replacement. However, in patients having elective knee replacement, when the duration of prophylaxis is only 14 days, the optimal strategy is the same as in the main surgical cohort where prophylaxis is recommended up to 7 days.
Summary of key findings
-
For medical inpatients, pharmacological prophylaxis for all patients without contraindications is likely to be the more cost-effective strategy, when valuing a QALY at £20,000. This assumes that any RAM applied in medical inpatients would perform in a similar manner to the average RAM performance estimated in a single cohort of medical inpatients.
-
The optimal prophylaxis strategy was fairly robust under sensitivity analysis in the medical inpatient cohort. The key factors determining cost-effectiveness appear to be the baseline risks of VTE and major bleeding and the increased risk of major bleeding associated with prophylaxis.
-
For surgical inpatients, pharmacological prophylaxis for all patients without contraindications is likely to be the more cost-effective strategy, when valuing a QALY at £20,000 and assuming that any RAM applied in a surgical population performs similarly to either the Pannucci or Caprini RAM.
-
In the surgical inpatient cohort, the most cost-effective strategy was sensitive to many of the individual model inputs and assumptions tested, with the optimal strategy being a Pannucci score of ≥ 6 (sensitivity of 40% and specificity of 82%) when assuming no risk of PTS after asymptomatic proximal or distal DVT.
-
In specific surgical populations who require longer durations of prophylaxis, it was found that pharmacological prophylaxis for all eligible patients is optimal in those having 14 days of LMWH following elective knee replacement whereas offering prophylaxis at a Pannucci score of ≥ 3 (sensitivity of 84% and specificity of 49%) was optimal when offering 28 days of LMWH to patients having elective hip replacement.
-
Using a RAM to select patients for pharmacological prophylaxis in medical or surgical inpatients would only be optimal if that RAM had a high sensitivity, similar to that observed for the Padua RAM with a cut-off of ≥ 3 in a mixed cohort of medical and surgical patients (sensitivity 99.9%, specificity 23.7%).
Chapter 5 Workstream 2 – assessing the feasibility of efficient data methods for use in future implementation research
The accuracy of clinical outcome data on VTE events and bleeding is key to any future study of RAM performance and/or validation. However, any such studies are likely to require a large sample size, given the potential for low event rates and small absolute risk impact. The practical challenges and costs of individual patient contact and routine follow-up in such large studies may be prohibitive.
In this study, we sought to pilot the use of efficient methods alongside routine practice to determine the feasibility of a future implementation study of VTE risk assessment tools in hospital inpatients. Our main aim was to compare the accuracy and completeness of available coding data, local registry data and efficient data collection methods to determine clinically relevant VTE and bleeding outcomes against case note review by trained research assistants. We also collated further information on delivery methods for VTE risk assessment during routine care and the potential for granular data collection on individual risk variables, which could allow efficient RAM comparison in future work. Finally, we explored the ethical and regulatory acceptability of a data-enabled approach to any future potential implementation study.
We conducted this study in accordance with international in accordance with Enhancing the QUAlity and Transparency Of health Research (EQUATOR) guidelines. A completed STrengthening the Reporting of OBservational studies in Epidemiology (STROBE) reporting checklist was used throughout to inform design, conduct and analysis of this observational cohort study and has been submitted with the report.
Methods
A multicentre observational, cohort study with prespecified feasibility criteria. We approached four sites to participate in this study: Salford Royal NHS Foundation Trust, Manchester University Hospitals NHS Foundation Trust, the Northern General Hospital in Sheffield and St Thomas’ NHS Foundation Trust. All sites agreed to participate, approve and deliver this feasibility study over a 12-month period from August 2020 to 2021 inclusive.
Population
We identified a consecutive, unselected cohort of general medical and surgical patients requiring hospitalisation at each site during the calendar year from 1 January to 31 December 2019. We chose 2019 because of concern that patients admitted during the COVID-19 pandemic might have been assessed for use of thromboprophylaxis differently to prior guidelines. We collated data on all risk assessments performed prospectively at the point of hospital admission, then scrambled episodes into randomly assorted batches of 50 (‘A’ batches) to ensure diversity in speciality presentation and mitigate seasonal bias. We excluded paediatric patients (age < 16), anyone requiring critical care admission (defined as level 2 care or above) and pregnant/post-partum patients due to differential risk, as outlined in the wider study protocol.
Study design
For each patient episode in each batch, we extracted basic speciality data using business intelligence and VTE risk assessments completed prospectively by clinical teams during the provision of routine care. Risk assessments were captured differently at each site, including the use of paper proformas, dichotomous output on electronic prescribing record, or through a detailed structured note within the electronic healthcare record. Example images/screenshots for each site can be found in Appendix 3, Figure 28. All four sites recommended the Department of Health tool in local guidelines, to facilitate initial VTE risk assessment.
Research assistants at each site were subsequently employed to undertake retrospective case note review for each patient episode through shared primary and secondary care electronic health records (EHRs). We extracted descriptive data on relevant clinical outcomes such as the subsequent diagnosis of HAT, major bleeding and CRNMB events as per internationally agreed definitions. 79,80 Data extractors were trained in identification of these variables/outcomes and followed a detailed workflow diagram (see Appendix 3, Figure 29). Data abstractors were blinded to batch allocation, International Classification of Disease, Tenth Revision (ICD-10) coding/HAT database entries and the final analysis plan.
Following case note review, we collated multiple routine data sources for each patient episode to determine the accuracy of these methods in identifying relevant clinical outcomes, identified by research assistants. Data sources for interrogation were identified a priori and included the following: ICD-10 diagnostic codes judged relevant to thrombosis or bleeding by the project management group (PMG) (see Appendix 3, Table 30); Emergency Care Data Set/Systematised Nomenclature of Medicine (SNOMED) codes relevant to thrombosis or bleeding; and local HAT database entries. HAT database entries are co-ordinated by local thrombosis committees and include a contemporary register of all patients diagnosed with acute VTE (using radiology/pathology data), who are subsequently identified as HAT following local root cause analysis. All data sources were interrogated for the duration of hospital stay and up to 90 days post discharge, for each patient episode. Data sources were obtained through routine local business intelligence requests or direct approach to local coding teams. HAT database entries were obtained through local thrombosis committees, where feasible.
Given the potential for very low numbers of outcome events in the wider study population, we augmented the sample with likely positive thrombosis and bleeding cases. We obtained positive cases through ICD-10 coding identification for VTE events (V batches) and bleeding events (B batches) in accordance with the previously selected code list (see Appendix 3, Table 30) and local HAT database entries (H batches) within the relevant study period. Positive cases were scrambled, batched and reviewed in accordance with the general protocol.
The following feasibility criteria were proposed within our original protocol to determine success and accuracy of efficient data methods to identify relevant clinical outcomes:
-
ethical and regulatory approval for proposed methods
-
proportion of outcome events identified by routine data sources that are confirmed by record review (target 100%)
-
proportion of cases with no outcome event identified by routine data sources that have an event identified on record review (target 0%)
-
proportion of each RAM and individual risk variables collectible using efficient data methods (target 90%)
-
proportion of inpatients with data collected (target 90%).
In addition, we proposed using this sample of patients to estimate key parameters for any future implementation study, including the primary outcome event rates for HAT and major bleeding.
Statistical analysis
The accuracy of routinely recorded HAT and bleeding events was compared against direct case note review data for the cohort. Case note review determination of events was assumed to be the gold standard. Data are presented in contingency tables with sensitivity, specificity, positive and negative predictive values along with confidence intervals calculated using the Wilson score method. 139
The primary analysis included patients identified from all sources (A, V, B or H batches) with bleeding and HAT assumed to have not occurred unless coded as such in the relevant data, or detected following case note review. In addition, two pre-planned sensitivity analyses were undertaken:
-
Inclusion was limited to cases identified in routine case review (i.e. ‘A batch patients’ only), with exclusion of all augmented sample cases.
-
Inclusion limited to participants for whom bleeding or HAT was definitively recorded.
We used case note review identification of HAT and bleeding events as the reference standard and report contingency tables for diagnostic test characteristics of all preidentified efficient data sources. We took a conservative approach and interpreted missing or unknown end points as ‘no event’.
The proposed sample size was reliant on an initial iterative developmental phase, including database refinement, user acceptability testing and site trial. We originally planned to identify 3000 inpatients across 4 hospitals during a 12-month study period within the 2-year project plan, dependent on appointment of research assistants and time required for outcome ascertainment. This sample size was designed to allow key parameters to be estimated with a high degree of precision across the whole cohort (standard error < 1%).
Ethical aspects
The study received a favourable opinion from the Proportionate Review Sub-committee of the London – West London & Gene Therapy Advisory Committee (GTAC) Research Ethics Committee (REC) and approval from the HRA and Care Research Wales (HCRW) on 18 September 2019 (reference 19/LO/1303, IRAS project ID 262220).
Participating sites identified members of the clinical care team (research nurses or assistants predominately) to access patient records and extract clinical data using a predesigned and protected Microsoft Excel database with embedded macro function, hosted at site. All data subsequently underwent local de-identification following completion and were exported to an independent team of statisticians at the Clinical Trials Research Unit (CTRU) in Sheffield, for collation and analysis. The REC approved this process and, as researchers outside the clinical care team only used anonymised data, Clinical Advisory Group approval was not required. We were able to access HES through the sites, and we were, therefore, able to undertake the original proposed analysis without involving NHS Digital.
All aspects of the data collection process, export, analysis and oversight were regularly reviewed by the internal PMG including CTRU representation, and an external Trial Steering Committee (TSC), throughout the duration of the project.
Patient and public involvement
Representatives of two PPI groups, Thrombosis UK and Sheffield Emergency Care Forum (SECF) joined the research team and were involved in developing the initial proposal and undertaking the wider study.
The SECF is a patient and public representative group with an interest in emergency care research. The forum has provided PPI for many emergency care research projects over the last 10 years. 140 Thrombosis UK is a charity that aims to identify, inform and partner with the NHS, healthcare providers and individuals to work to improve prevention of VTE and the management and care of VTE events (see https://www.thrombosisuk.org/).
The PPI members were involved in determining the study design and ensuring that the proposal addressed the needs of patients and the NHS, while respecting the needs of potential participants. Their input regarding the importance of providing thromboprophylaxis for potential participants of any prospective cohort study and the need for such a study to yield reliable findings was instrumental in determining our approach to answering the research question. The PPI members also provided input at project management meetings and, where required, in day-to-day running of the project. The members used meetings and surveys of their wider PPI groups to enhance PPI in the project.
In relation to this particular substudy, the PPI members had specific roles in ensuring the acceptability of methods explored in the feasibility study. The efficient methods required to deliver an implementation study involve use of patient data without consent. We planned our methods so that they are compatible with ethical principles and data protection regulation, and would be acceptable to our PPI representatives. However, the feasibility study involved piloting these methods and the potential to adapt the methods to ensure efficiency. The PPI representatives were involved in project management, monitoring of data collection and specifically considered the acceptability of any proposed changes to the methodology throughout the project.
Results
Baseline sampling and augmentation
Over the 12-month feasibility study period we successfully set up and delivered the project across four sites. Patients (2115) were identified and selected for analysis with an original hospital admission occurring in the calendar year 2019. Of these, 107 patient episodes were ineligible due to being pregnant or post-partum women (n = 49); admitted to a critical care environment of level 2 or above (n = 38); children aged under 16 (n = 13) or for unrecorded reasons (n = 7) leaving 2008 episodes for analysis. All episodes were suitable for data extraction and comparison to routine data sources. Ethical and regulatory approvals were secured for all sites. All sites failed to meet their sample target of 750 for reasons mainly related to the SARS-CoV-2 pandemic, including redeployment of research staff to clinical care, delayed local approvals secondary to prioritised pandemic research and a longer than anticipated time for individual outcome ascertainment per case review. Data were missing or the outcome unknown for fewer than 2% of participants for each outcome/site.
Patient episodes showed an even balance of medical and surgical cases, but with a focus on emergency (73.7%) rather than elective (25.8%) admissions. A broad range of subspeciality interests were represented within the cohort. Median length of stay was 3 days [interquartile range (IQR) 3–8] and mean length of stay 7.75 days [standard deviation (SD) 16.5]. Speciality groups with frequencies and percentages are shown in Table 13. The vast majority of patient episodes (1809, 90.1%) were taken from ‘A’ batches, through patients identified as having a VTE risk assessment. The total sample was augmented by 45 (2.2%) patients with potential bleeding events (one B batch) and 154 (7.7%) patients with potential VTE events (one V and two H batches). Sites contributed evenly to the sample with the exception of Manchester; numbers at this site reflect a significant delay to institutional approval during the pandemic, with this research deprioritised. Site and batch numbers are shown in Table 14.
Frequency | Percentage | |
---|---|---|
Admission type | ||
Missing | 1 | 0.05 |
Elective | 518 | 25.80 |
Emergency | 1480 | 73.71 |
Unknown | 9 | 0.45 |
Total | 2008 | 100 |
Speciality group | ||
Missing | 9 | 0.45 |
Medical | 902 | 44.92 |
Surgical | 951 | 47.36 |
Tertiary speciality | 146 | 7.27 |
Total | 2008 | 100 |
Clinical category | ||
Missing | 9 | 0.45 |
Acute medicine | 340 | 16.93 |
Ageing and complex medicine | 133 | 6.62 |
Cardiology | 41 | 2.04 |
Cardiothoracic surgery | 87 | 4.33 |
Dermatology | 2 | 0.1 |
Emergency medicine | 87 | 4.33 |
Gastroenterology | 61 | 3.04 |
General surgery | 285 | 14.19 |
Medical: other | 169 | 8.42 |
Neurology | 10 | 0.5 |
Neurorehabilitation | 2 | 0.1 |
Neurosurgery | 39 | 1.94 |
Gynaecology | 57 | 2.84 |
Renal medicine | 26 | 1.29 |
Respiratory | 63 | 3.14 |
Rheumatology | 2 | 0.1 |
Trauma and orthopaedics | 158 | 7.87 |
Upper GI surgery | 13 | 0.65 |
Urology | 107 | 5.33 |
Surgery: other | 170 | 8.47 |
Tertiary speciality: other | 147 | 7.32 |
Total | 2008 | 100 |
Batch | |||||
---|---|---|---|---|---|
A | B | H | V | Total | |
GSTT | 504 | 0 | 21 | 0 | 525 |
Manchester | 241 | 0 | 0 | 0 | 241 |
Salford | 570 | 45 | 44 | 46 | 705 |
Sheffield | 494 | 0 | 43 | 0 | 537 |
Total | 1809 | 45 | 108 | 46 | 2008 |
Accuracy of efficient data methods compared to case note review for ascertainment of key clinical outcomes
The accuracy of routine data sources for HAT and major bleeding events compared to case note review are shown in Table 15. The proportion of outcome events identified by routine data sources that were confirmed by case note review was 71% (95% CI 63% to 79%). The proportion of cases with no HAT outcome event identified by routine data sources that had an event identified on record review (target 0%) was 3% (95% CI 2 to 4) for ICD-10/SNOMED coding and 2% (95% CI 1 to 2) for local HAT database entries.
HAT from case note review | ||||
---|---|---|---|---|
Yes | No | |||
HAT from ICD-10/SNOMED codes | Yes | 95 (A) | 39 (B) | PPV 71% (95% CI 63% to 79%) |
No | 59 (C) | 1815 (D) | NPV 96.9% (95% CI 96% to 97%) | |
Sensitivity 62% (95% CI 54% to 69%) |
Specificity 98% (95% CI 97% to 99%) |
(N = 2008) | ||
Yes | No | |||
HAT from HAT RCA database | Yes | 122 (A) | 0 (B) | PPV 100% (95% CI 97% to 100%) |
No | 29 (C) | 1616 (D) | NPV 98% (95% CI 98% to 99%) | |
Sensitivity 81% (95% CI 75% to 87%) |
Specificity 100% (95% CI 99.8% to 100%) |
(N = 1767) | ||
Major bleed from case note review | ||||
Yes | No | |||
Major bleed from ICD-10/SNOMED codes | Yes | 25 (A) | 98 (B) | PPV 20% (95% CI 13% to 27%) |
No | 40 (C) | 1845 (D) | NPV 98% (95% CI 97% to 98%) | |
Sensitivity 38% (95% CI 27% to 50%) |
Specificity 95% (95% CI 94% to 96%) |
(N = 2008) |
The sensitivity was 62% (95% CI 54% to 69%) for the use of ICD-10/SNOMED coding data to detect HAT events and 81% (95% CI 75% to 87%) for local HAT database entries. Sensitivity by individual site ranged from 45% (95% CI 28% to 63%) to 72% (95% CI 61% to 82%) using ICD-10/SNOMED coding and 68% (95% CI 51% to 84%) to 94% (95% CI 87% to 100%) using local HAT database entries. The sensitivity of ICD-10/SNOMED coding to detect major bleeding events identified by case note review was 38% (95% CI 27% to 50%). Sensitivity by individual site ranged from 22% (95% CI 0% to 49%) to 56% (95% CI 37% to 75%).
These sensitivity values imply moderate rates of missed HAT and major bleeding events through the use of efficient data methods in isolation. Specificity values were > 90% for both outcomes at all sites, implying reasonable accuracy when an event was identified by efficient data methods.
Sensitivity analyses and estimation of key parameters for future implementation work
A sensitivity analysis was conducted using only patient episodes obtained through ‘A’ batches, to remove augmentation of the sample and mitigate bias. The sensitivity of efficient data methods to detect key outcomes identified at case note review remained poor. These results are summarised in Table 16. This analysis also facilitated estimation of key parameters for any future implementation study. We found the HAT event rate on case note review to be 29/1809 (1.6%, 95% CI 1.0 to 2.2) and the major bleeding event rate to be 45/1809 (2.5%, 95% CI 1.8 to 3.2) within this large cohort of hospitalised patients receiving risk assessment and thromboprophylaxis in the context of routine care.
HAT from case note review | ||||
---|---|---|---|---|
Yes | No | |||
HAT from ICD-10/SNOMED codes | Yes | 18 (A) | 18 (B) | PPV 50% (95% CI 34% to 66%) |
No | 11 (C) | 1762 (D) | NPV 99% (95% CI 99% to 100%) | |
Sensitivity 62% (95% CI 44% to 80%) |
Specificity 99% (95% CI 99% to 99%) |
(N = 1809) | ||
Yes | No | |||
HAT from HAT RCA database | Yes | 7 (A) | 0 (B) | PPV 100% (95% CI 100% to 100%) |
No | 19 (C) | 1542 (D) | NPV 99% (95% CI 99% to 99%) | |
Sensitivity 27% (95% CI 12% to 48%) |
Specificity 100% (95% CI 100% to 100%) |
(N = 1568) | ||
Major bleed from case note review | ||||
Yes | No | |||
Bleed from ICD-10/SNOMED codes | Yes | 14 (A) | 68 (B) | PPV 17% (95% CI 11% to 25%) |
No | 31 (C) | 1696 (D) | NPV 98% (95% CI 98% to 99%) | |
Sensitivity 31% (95% CI 18% to 47%) |
Specificity 96% (95% CI 95% to 97%) |
(N = 1809) |
Key feasibility outcomes
Regarding key feasibility outcomes, we achieved ethical and regulatory approvals and the design of the study was acceptable to all sites. The proportion of HAT events identified by routine data sources that were confirmed by record review (target 100%) was 71% (95% CI 63% to 79%) for ICD-10/SNOMED coding and 100% (95% CI 97% to 100%) for local HAT database entries. The proportion of cases with no HAT outcome event identified by routine data sources that had an event identified on record review (target 0%) was 3% (95% CI 2% to 4%) for ICD-10/SNOMED coding and 2% (95% CI 1% to 2%) for local HAT database entries. The proportion of outcome major bleeding events identified by routine data sources that were confirmed by record review (target 100%) was 20% (95% CI 13% to 27%) for ICD-10/SNOMED coding. The proportion of cases with no major bleeding outcome event identified by routine data sources that have an event identified on record review (target 0%) was 2% (95% CI 1% to 3%) for ICD-10/SNOMED coding.
We were unable to use efficient data methods to collect individual risk variables in three out of four sites, due to use of either paper proformas or dichotomous risk assessment without granular data. As such we did not meet the feasibility target of using routine data to collect individual variables for 90% of patients. We were able to collect outcome data in 1745/2008 (87%) of inpatients (target 90%). This was mainly due to inability at the Manchester site to access the local HAT database. Excluding this issue, the other three sites all managed to collect relevant outcome data in > 98% of patient episodes.
Summary and key findings
-
In this multicentre cohort study, routine and efficient data methods used to identify HAT events and major bleeding events performed poorly when compared to direct case note review by trained research staff.
-
We were able to demonstrate ethical and regulatory approvals for our chosen design across four NHS sites.
-
We were unable to demonstrate overall feasibility of an efficient design and did not meet several prespecified criteria set out in the original research proposal:
-
Proportion of outcome events identified by routine data sources that are confirmed by record review (target 100%); VTE 71%, bleeding 20%: Criteria not met.
-
Proportion of cases with no outcome event identified by routine data sources that have an event identified on record review (target 0%); VTE 3%, bleeding 2%: Criteria not met.
-
Proportion of inpatients with data collected (target 90%); 87% across all sites, 98% across three sites: Partially met.
-
Proportion of each predictor variable recorded (target 90%); only routinely recorded at one site: Criteria not met.
-
Proportion of each risk assessment tool completed using available data (target 90%); only routinely recorded at one site: Criteria not met.
-
-
These findings suggest a future data-enabled study using efficient methods would significantly under-report events, leading to inaccurate RAM evaluation.
-
In this multicentre cohort of hospitalised inpatients, the HAT event rate was 1.6% (95% CI 1.0% to 2.2%) and the major bleeding event rate was 2.5% (95% CI 1.8% to 3.2%).
Chapter 6 Workstream 2 – estimating key parameters for future implementation research
In a further substudy, we sought to compare the proportion of hospital patients recommended for pharmacological thromboprophylaxis using multiple RAMs identified by our systematic review in workstream one, alongside current practice. We included RAMs with attempted external validation for this comparison. We specifically aimed to determine the following parameters for a potential future implementation study:
-
The current proportion of hospital inpatients receiving pharmacological prophylaxis in a UK NHS setting after local risk assessment, using the Department of Health tool.
-
The variable proportion of patients who would potentially receive prophylaxis if alternative RAMs were used.
We conducted this substudy in accordance with international EQUATOR guidelines. A completed STROBE reporting checklist was used throughout to inform design, conduct and analysis of this observational cohort study and has been submitted with the report.
Methods
We approached four sites engaging with the wider Venous ThromboEmbolism Assessment Methods (VTEAM) project to participate in this substudy. We originally intended to explore methods of efficient data collection for risk factors across two sites through an iterative process, then further pilot a final data collection tool across all sites. However, only one site maintained an EHR with prospective capture of individual VTE risk characteristics following clinical assessment. Other sites lacked the IT infrastructure to support efficient data collection, using either paper proformas to record risk assessment or dichotomous electronic methods [recording only the capture of a risk assessment (yes/no) and the prescribing intention, as shown in Appendix 3, Figure 28].
We considered adaptive design during the project and developing a paper case note review process, but encountered multiple barriers to delivery of workstream 2 during the project timeline; access to clinical settings was limited by infection control measures during the pandemic; redeployment of clinically trained research staff limited our available resources; pandemic research was rightly prioritised by local research teams; and NHS service pressures impacted on the project. In addition, working from home guidance further limited the ability to retrieve paper case notes or develop an efficient data collection tool. As such, we focused efforts on data capture from a single site which used a comprehensive EHR with contemporaneous data collection on risk factors at the point of assessment.
Population
We identified a consecutive cohort of general medical and surgical patients requiring hospitalisation during the calendar year from 1 January to 31 December 2019. We chose 2019 because of concern that patients admitted during the COVID-19 pandemic might have been assessed for VTE prophylaxis differently to prior guidelines. 141 We collated data on all risk assessments performed prospectively at the point of hospital admission, then scrambled episodes into randomly assorted batches of 50 to ensure diversity in speciality presentation and mitigate potential seasonal bias. We excluded all patients with an index presentation of suspected VTE, anticoagulated patients and patients with a hospital stay < 24 hours as they were unlikely to require risk assessment or thromboprophylaxis. We also excluded patients requiring critical care admission, children (< 16) and pregnant/post-partum patients due to differential risk, in keeping with the wider study protocol.
Study design
A single-centre observational cohort study, conducted at Salford Royal NHS Foundation Trust. We extracted initial clinical data on patient episodes using hospital admission records and VTE risk assessments completed prospectively by clinical teams during the provision of routine care. All risk assessments were captured through a structured note within the electronic healthcare record, with granular data capture accessible through business intelligence. The structured note mirrors the Department of Health VTE Risk assessment tool (see Appendix 3, Figure 28).
We identified additional necessary variables a priori to enable further completion and comparison of 6 prespecified RAMs for each patient episode, in addition to the Department of Health tool. These variables are listed in Table 17. We subsequently undertook case note review for each patient episode through shared primary and secondary care EHRs up to 90 days following hospital discharge. We extracted data points necessary to facilitate retrospective completion of the Padua, Caprini, Geneva, Kucher, IMPROVE predictive and IMPROVE associative RAMs. We also extracted descriptive data on relevant clinical outcomes, such as the real-world use of pharmacological thromboprophylaxis, subsequent diagnosis of HAT, length of stay and survival to hospital discharge. Data were also extracted on episodes of major and CRNMB as per internationally agreed definitions. 79,80 Data extractors were trained in identification of these variables/outcomes and followed a detailed workflow diagram (see Appendix 3, Figure 29). Data abstractors were blinded to the analysis plan.
DOH RAM | Padua RAM | IMPROVE Associative RAM |
Geneva RAM | Caprini RAM | Kucher RAM | IMPROVE Predictive RAM |
---|---|---|---|---|---|---|
Age | Cardiac failure | Paralysis of the lower extremities |
Myeloproliferative syndrome |
Sex | Nil additional | Nil additional |
Active cancer | Respiratory failure | Immobilisation > 7 days |
Nephrotic syndrome |
Acute pneumonia (< 1/12) |
||
Dehydration | Acute myocardial infarction (< 1/12) |
Recent travel > 6 hours | Immobilising plaster cast |
|||
Obesity | Acute ischaemic stroke (< 3/12) |
Chronic venous insufficiency |
Multiple trauma (< 1/12) |
|||
One or more significant medical comorbidities | Acute infectious disease (< 1/12) |
Current swollen legs |
||||
Personal history of first-degree relative with a VTE history | Acute rheumatological disorder (< 1/12) |
Current central venous access |
||||
Use of HRT | Inflammatory bowel disease |
|||||
Use of oestrogen-containing COCP | Other risk factor | |||||
Varicose veins with phlebitis | Major surgery (< 1/12) |
|||||
Pregnancy or < 6 weeks post-partum | Hip, pelvis or leg fracture (< 1/12) |
|||||
Mobility limited > 3/7 | ||||||
Acute surgical admission | ||||||
Critical care admission | ||||||
Specific surgical features |
Statistical analysis methods
The primary analysis was a comparison of the proportion of patients within the cohort in whom pharmacological thromboprophylaxis would be recommended during hospitalisation, by each RAM. We applied conventional prescribing thresholds for each RAM to the cohort in order to estimate the proportion of patients who would require medication, prior to any assessment of bleeding risk. Prescribing thresholds were taken from author recommendations, international guidelines and nationally endorsed summary tools for practical use. 8,15,16,28,34,49,53,59,142–149 We used ordinal scores to create distribution plots, clustered histograms and to facilitate descriptive analysis. We used dichotomous prescribing thresholds (as above) to calculate proportions with 95% confidence intervals and allow head-to-head RAM comparison. We used the Wilson method to estimate confidence intervals around a proportion, adapted by Newcombe. 139
Missing data were assumed to be negative (risk factor not present) for the primary analysis. A sensitivity analysis was planned with best-case-worse-case imputation for missing data when calculating the percentage of patients who would meet the criteria for prescribing thromboprophylaxis. We estimated that 80% of admitted patients would be classified as eligible for pharmacological thromboprophylaxis, according to the Department of Health VTE risk assessment tool. 8,145 A sample of 250 patients would, therefore, be sufficient to provide estimates of prescribing with a confidence interval range approaching 10%. We proposed a data review following completion of at least 500 case episodes (10 batches).
Ethical aspects
The study received a favourable opinion from the Proportionate Review Sub-committee of the London – West London & GTAC REC and approval from the HRA and Care Research Wales (HCRW) on 18 September 2019 (reference 19/LO/1303, IRAS project ID 262220).
Patient and public involvement
Representatives of two PPI groups, Thrombosis UK and SECF joined the research team and were involved in developing the proposal and undertaking the study.
The SECF is a patient and public representative group with an interest in emergency care research. The forum has provided PPI for many emergency care research projects over the last 10 years (see https://secf.org.uk/ and https://emj.bmj.com/content/33/9/665). Thrombosis UK is a charity that aims to identify, inform and partner with the NHS, healthcare providers and individuals to work to improve prevention of VTE and the management and care of VTE events (see www.thrombosisuk.org/).
The PPI members were involved in determining the study design and ensuring that the proposal addressed the needs of patients and the NHS, while respecting the needs of potential participants. Their input regarding the importance of providing VTE prophylaxis for potential participants of any prospective cohort study and the need for such a study to yield reliable findings was instrumental in determining our approach to answering the research question. The PPI members also provided PPI at project management meetings and, where required, in day-to-day running of the project. The members used meetings and surveys of their wider PPI groups to enhance PPI in the project.
Results
Comparative risk assessment and prescribing outcomes
We analysed 543 hospital episodes with VTE risk assessment performed during 2019. Two-hundred and eighty-nine episodes met exclusion criteria, leaving 254 episodes suitable for data extraction and comparative analysis.
Distribution plots of ordinal scoring for each RAM are shown in Figure 24. The Caprini and Department of Health RAMs appeared to be normally distributed, with the former showing the widest dispersion. All others were skewed to the left, indicating a higher attribution of low risk.
FIGURE 24.
Histograms for ordinal score (x-axis) by RAM using patient numbers (y-axis), with recommended prescribing thresholds (dashed blue lines). Source: National Institute for Health and Care Excellence8; Barbar et al. 15; Blondon et al. 28; Caprini et al. 142; Chopard et al. 143; Kucher et al. 146; Spyropoulos et al. 148


Overall recommendations for prescribing varied substantially between RAMs, ranging from 13% for the IMPROVE associative score to 91% for the Department of Health VTE risk assessment tool. Variation persisted throughout medical (15–92%) and surgical subgroups (10–88%). Key results and estimates of recommended prescribing by speciality (with 95% CIs) are shown in Table 18. Of note, all RAMs recommended higher rates of prescribing in medically unwell patients within this patient cohort. A bar and dot chart of prescribing recommendations with comparative 95% confidence intervals is shown in Figure 25. The Department of Health VTE risk assessment tool was an outlier within this cohort, recommending pharmacological thromboprophylaxis for over 90% of patients with a confidence interval that did not overlap those of any other RAM.
RAM and published prescribing threshold | All patients (n = 254) |
Medical admissions (n = 143) |
Surgical admissions (n = 111) |
---|---|---|---|
Department of Health VTE risk assessment tool | |||
Median value (IQR) | 2 (1–3) | 3 (2–4) | 2 (1–3) |
Prescribing threshold (score ≥ 1)a | 230 (91%, 95% CI 86.3 to 93.6) | 132 (92%, 95% CI 86.8 to 95.7) | 98 (88%, 95% CI 81.0 to 93.0) |
Padua score | |||
Median value (IQR) | 2 (1–4) | 3 (1–4) | 1 (0–3) |
Prescribing threshold (score ≥ 4) | 77 (30%, 95% CI 25.0 to 36.2) | 56 (39%, 95% CI 31.5 to 47.3) | 21 (19%, 95% CI 12.7 to 27.2) |
Caprini score | |||
Median value (IQR) | 5 (3–7) | 6 (4–8) | 4 (2–5) |
Prescribing threshold (score ≥ 5) | (56%, 95% CI 49.8 to 61.9) | 96 (67%, 95% CI 59.1 to 74.3) | 46 (41%, 95% CI 32.7 to 50.7) |
Geneva risk score | |||
Median value (IQR) | 2 (1–4) | 3 (2–5) | 2 (0–3) |
Prescribing threshold (score ≥ 3) | 111 (44%, 95% CI 37.7 to 49.9) | 81 (57%, 95% CI 48.5 to 64.5) | 30 (27%, 95% CI 19.6 to 36.0) |
Kucher score | |||
Median value (IQR) | 1 (1–2) | 1 (1–2) | 1 (0–2) |
Prescribing threshold (score ≥ 4) | 26 (10%, 95% CI 7.1 to 14.6) | 16 (11%, 95% CI 7.0 to 17.4) | 10 (9%, 95% CI 5.0 to 15.8) |
IMPROVE predictive score | |||
Median value (IQR) | 1 (0–1) | 1 (0.5–1) | 0 (0–1) |
Prescribing threshold (score ≥ 1) | 155 (61%, 95% CI 54.9 to 66.8) | 107 (75%, 95% CI 67.1 to 81.2) | 48 (43%, 95% CI 34.4 to 52.5) |
IMPROVE associative score | |||
Median value (IQR) | 1 (0–1) | 1 (1–1) | 0 (0–1) |
Prescribing threshold (score ≥ 3) | 32 (13%, 95% CI 9.1 to 17.2) | 21 (15%, 95% CI 9.8 to 21.4) | 11 (10%, 95% CI 5.6 to 16.9) |
On descriptive analysis, the variables used by the Department of Health tool with the highest prevalence within our cohort were as follows: one or more significant medical co-morbidities (76%); age > 60 (57%); dehydration (38%); significantly reduced mobility (29%); obesity (20%). These 5 variables were responsible for > 90% cumulative prescribing decisions within the cohort, with negligible addition from the other 14 variables.
All tools were subject by missing data with up to 12% of patients being partly scored, largely due to BMI being unavailable in 22 (9%) of patients. This affected all scores other than the two IMPROVE scores which were scored in all bar four (2%) patients. The impact of this was minimal in terms of the percentage of patients that would be recommended for thromboprophylaxis, since the missing data generally could not overturn a ‘do not recommend’ into ‘recommend’. For the Padua score, a further seven patients would meet the cut-off of 4 points if all missing data were assumed to be abnormal, taking the percentage from recommended from 30% to 33%. The equivalent assumption on other tools led to increases of less than 2%.
Temporary reference list for Figures 24 and 25 and Table 18:
-
Barbar S, Noventa F, Rossetto V, Ferrari A, Brandolin B, Perlati M, et al. A risk assessment model for the identification of hospitalized medical patients at risk for venous thromboembolism: the Padua Prediction Score. J Thromb Haemost 2010;8:2450–7. https://doi.org/10.1111/j.1538-7836.2010.04044.x (PADUA)
-
Blondon M, Righini M, Nendaz M, Glauser F, Robert-Ebadi H, Prandoni P, et al. External validation of the simplified Geneva risk assessment model for hospital-associated venous thromboembolism in the Padua cohort. J Thromb Haemost 2020;18:676–80. https://doi.org/10.1111/jth.14688 (GENEVA)
-
Caprini JA, Arcelus JI, Hasty JH, Tamhane AC, Fabrega F. Clinical assessment of venous thromboembolic risk in surgical patients. Semin Thromb Hemost 1991;17 Suppl 3:304–12. (CAPRINI)
-
Chopard P, Spirk D, Bounameaux H. Identifying acutely ill medical patients requiring thromboprophylaxis. J Thromb Haemost 2006;4:915–6. https://doi.org/10.1111/j.1538-7836.2006.01818.x (IMPROVE PREDICTIVE)
-
Kucher N, Koo S, Quiroz R, Cooper JM, Paterno MD, Soukonnikov B, et al. Electronic alerts to prevent venous thromboembolism among hospitalized patients. N Engl J Med 2005;352:969–77. https://doi.org/10.1056/NEJMoa041533 (KUCHER)
-
Spyropoulos AC, Anderson FA, Jr., FitzGerald G, Decousus H, Pini M, Chong BH, et al. Predictive and associative models to identify hospitalized medical patients at risk for VTE. Chest 2011;140:706–14. https://doi.org/10.1378/chest.10-1944 (IMPROVE ASSOCIATIVE)
-
National Institute for Health and Care Excellence. Venous thromboembolism in over 16s: reducing the risk of hospital-acquired deep vein thrombosis or pulmonary embolism (NG89). nice.org.uk: NICE; 2018. URL: www.nice.org.uk/guidance/ng89 (accessed 22 July 2022). (DOH SCORE)
We found no cases where an alternate RAM recommended thromboprophylaxis but the Department of Health tool did not, in keeping with the low threshold (≥ 1 risk factor) of the latter tool. Results for each alternate RAM compared against the Department of Health tool are shown in Appendix 3, Figure 30.
Estimation of other key parameters for future implementation research
Baseline demographics and relevant clinical outcomes for the final cohort of patients are shown in Table 19, by speciality. In this cohort, surgical patients appeared to be younger with lower rates of medical comorbidity and lower rates of significant mobility reduction than medical patients.
All patients (n = 254) |
Medical admissions (n = 143) |
Surgical admissions (n = 111) |
|
---|---|---|---|
Demographics | |||
Age | 62.6 (22.3)a | 70.4 (20.7)a | 52.7 (20.3)a |
Female sex | 139 (54.7) | 82 (57.3) | 57 (51.4) |
BMI | 25.7 (6.5)a | 24.4 (7.0)a | 27.3 (5.5)a |
Active cancer | 15 (5.9) | 9 (6.3) | 6 (5.4) |
Significant medical comorbidity | 192 (75.6) | 127 (88.8) | 65 (58.6) |
Personal history of VTE | 16 (6.3) | 8 (5.6) | 8 (7.2) |
Family history of VTE | 0 (0) | 0 (0) | 0 (0) |
Significantly reduced mobility for > 3 days | 74 (29.1) | 55 (38.5) | 19 (17.1) |
Active bleeding on admission | 12 (4.7) | 1 (0.7) | 11 (9.9) |
Emergency admission | 251 (98.8) | 143 (100) | 108 (97.3) |
Clinical outcomes | |||
Pharmacological prophylaxis | 219 (86.2) | 115 (80.4) | 104 (93.7) |
Hospital length of stay | 5 (2–11)b | 6 (1–16)b | 4 (2–6)b |
Hospital-acquired thrombosis | 6 | 4 | 2 |
(HAT) event rate | (2.4, 1.1–5.1) | (2.8, 1.1–7.0) | (1.8, 0.5–6.3) |
PE | 1 (0.4) | 0 (0) | 1 (0.9) |
Proximal DVT | 4 (1.6) | 3 (2.1) | 1 (0.9) |
Distal DVT | 1 (0.4) | 1 (0.7) | 0 (0) |
Major bleeding event rate | 3 | 1 | 2 |
(1.2, 0.4–3.4) | (0.7, 0.1–3.9) | (1.8, 0.5–6.3) | |
Fall in Hb > 2 g/dl | 1 (0.4) | 0 (0) | 1 (0.9) |
Critical site | 1 (0.4) | 1 (0.7) | 0 (0) |
Requiring surgical intervention | 1 (0.4) | 0 (0) | 1 (0.9) |
Clinically relevant non-major bleeding event rate | 36 | 18 | 18 |
(14.2, 10.4–19.0) | (12.6, 8.1–19.0) | (16.2, 10.5–24.2) | |
Requiring medical intervention by a professional | 3 (1.2) | 1 (0.7) | 2 (1.8) |
Leading to hospitalisation or increased level of care | 5 (2.0) | 2 (1.4) | 3 (2.7) |
Prompting face-to-face assessment | 28 (11.0) | 15 (10.5) | 13 (11.7) |
Alive at hospital discharge | 244 (96.5) | 135 (94.4) | 109 (98.2) |
We found six cases of HAT within this cohort, with a subsequent overall event rate of 2.4% (95% CI 1.1% to 5.1%). All six of the HAT cases in this cohort were diagnosed in patients who were prescribed thromboprophylaxis during hospital admission in line with an initial assessment using the Department of Health tool. It is, therefore, impossible to estimate whether application of other RAMs would have affected relevant clinical outcomes; all patients were receiving thromboprophylaxis.
Summary and key findings
-
The majority of sites approached within this substudy had no mechanism for accessing individual variables recorded at VTE risk assessment, other than case note review of written notes. Only one site recorded risk variables electronically. This raises significant challenges for future implementation research looking to compare RAMs using routine data or through retrospective methodology.
-
In this single-centre cohort of predominately emergency admissions, the proportion of patients receiving thromboprophylaxis in current practice was 86.2% (95% CI 81.2% to 90.1%). Over 90% of surgical inpatients received prophylaxis in the context of routine care.
-
Prior to evaluation of bleeding risk, existing RAMs produce highly variable rates of recommended pharmacological prophylaxis when applied to the same cohort of medical and surgical hospital inpatients, ranging from 13% (95% CI 9.1% to 17.2%) to 91% (95% CI 86.3% to 93.6%).
-
The national UK Department of Health VTE risk assessment tool conferred higher rates of potential prescribing in this cohort than any other RAM, by > 25%.
Chapter 7 Workstream 2 – national survey of VTE leads regarding current practice and willingness to participate in future implementation research
During this period of research, a number of external topic experts delivered a concurrent national survey of VTE leads, endorsed and supported by the ‘Getting it Right First Time (GIRFT)’ national project team. This survey has since been published as an online report and quantifies many aspects of current practice relevant to the VTEAM study. 150
Our PMG chose not to repeat this work, due to the scale and credibility of the GIRFT project and likelihood of survey fatigue in the relevant clinical population. We opted instead to supplement GIRFT data through a further abbreviated national survey of VTE leads at acute NHS hospitals across the UK, focused on practicalities of risk assessment and future research proposals. In particular, we sought to determine (1) local methods used to record VTE risk-assessment data and (2) willingness to participate in an implementation study of risk-assessment tools, in line with our original protocol.
Methods
A national cross-sectional online survey reported using the consensus-based Checklist for Reporting of Survey Studies (CROSS). 151
Population
We identified local VTE leads through the National Nursing and Midwifery Network, VTE exemplar centre network and the collated list utilised by the recently published GIRFT project. VTE leads represented a range of clinical disciplines, including anticoagulation pharmacists, specialist nurses, doctors and ward managers.
Survey design and dissemination
We designed the survey using Smartsurvey.co.uk. We utilised the VTEAM PMG to refine the questions through iterative development. The final survey included free text answers, radio button responses and multiselect options. Regarding VTE risk assessment, we included questions on positive characteristics (recording the definitive presence of a risk factor) and negative characteristics (recording the definitive absence of a risk factor). The survey was trialled on three members of the PMG prior to circulation, with incorporated feedback. A copy of the survey is available in Appendix 4.
We used single-stage sampling over a four-week period, from 20 October 2021 to 17 November 2021 inclusive. We disseminated the survey by personal e-mail, with facilitated access through a weblink, QR code and direct web address. We sent weekly reminders to all potential participants throughout the survey duration. We asked for trust identification to guard against multiple participant responses. Given the time frame, need for site identification and direct personal contact through an established list of VTE leads, we did not add security measures to the survey. We did not seek specific ethical approval for this survey work and assumed implied consent from clinicians for participation in the survey.
Statistical analysis
We did not perform a sample size calculation for this survey. All analyses were proportional or descriptive. No weighting was applied to any variables.
Results
We received 65 total responses to the survey, with 25 full completions. The survey was sent originally to 105 potential participants, representing a partial response rate of 61.9% and a total response rate of 23.8%. A summary of responding sites is included in Appendix 4.
Methods of VTE risk assessment and data capture
The majority of respondents (48%) reported using EHR to conduct VTE risk assessment within an NHS setting, although 20% respondents reported using a hybrid approach (paper prof orma and EHR) at site dependent on ward environment. A large proportion of sites (32%) reported use of a paper pro forma only.
The majority of respondents noted their site method of risk assessment to report positive VTE characteristics (57% for paper pro forma, 73% for EHR) and the final decision on thromboprophylaxis (57% for paper pro forma, 64% for EHR). Routine capture of negative risk characteristics was lower overall, with only 19% of sites using paper pro forma and 32% of sites reporting capture.
The majority of sites (52%) responding reported that they were uncertain as to whether they could access granular data from VTE risk assessments conducted within routine care, through local business intelligence requests.
Willingness to participate in future research
Enthusiasm appeared higher for participation in future research overall. More respondents were willing to participate in future observational research on VTE risk assessment (100%) compared to interventional research, including randomisation to different models of assessment (72%). When further questioned on potential methodologies for future interventional research, most respondents favoured either allocation of the whole trust site or certain wards to intervention/control within the context of a wider national cluster RCT, above a longitudinal step wedge design or individual patient randomisation. This preference continued through scenarios involving comparison of RAMs, higher dosing strategies for higher-risk patients and opt-out strategies for default prescribing. Pie chart summaries of proportional response are shown in Figure 26 below.
FIGURE 26.
Proportional summary preferences for research design methods on future prophylaxis studies.

Thematic analysis of free text responses
We received 21 free text responses, listed in Appendix 4. Themes of response included support for potential study designs evaluating opt-out strategies for prophylaxis/mandatory prescribing, higher dosing strategies for higher-risk patients and further refinement of bleeding risk scores. Several responses highlighted support for cluster or broad implementation study designs in this area, citing the existing challenges of standardisation and the potential for individual randomisation strategies to increase confusion. In particular, several respondents referred to the ‘tick box’ nature of VTE risk assessment and highlighted the increasing medical scrutiny on the value of complex risk assessment.
Several respondents highlighted areas of VTE risk assessment they felt required further specific research: exclusion of medical patients at ‘baseline’ mobility; better clarification of dehydration and other subjective risks; evidence that routine care follows national guidance on assessment and prescribing; validity of the Department of Health risk assessment tool; defining higher-risk categories.
Concerns were highlighted regarding the variation in care by speciality group, and the potential for more nuanced risk assessment to benefit certain elective surgical groups. In addition, several responders highlighted the potential delays in adapting EHRs as a concern for future research designs looking to change the manner/standard of risk assessment. Finally, several respondents noted concern about an opt-out strategy as moving away from current evidence themes on individualisation of approach.
Summary and key findings
-
Recently published GIRFT data suggest that the vast majority of UK sites (87%) continue to use the Department of Health VTE risk assessment tool to guide pharmacological prophylaxis decisions on admission to hospital. A high proportion (80%) of these sites also use weight-adjusted prescribing strategies.
-
Less than half of respondents to our survey report consistent use of an EHR to facilitate and record VTE risk assessment. Less than a third of sites record the absence of VTE risk characteristics. Half of our respondents were uncertain as to whether they could access granular data on risk characteristics through local business intelligence/information technology support services. This implies future studies using routinely collected data on individual VTE risk characteristics at admission are likely to be untenable.
-
VTE leads appear enthusiastic for further research in this area. Regarding interventional research, respondents appeared to prefer implementation designs (whole clinical areas randomised to a new intervention) rather than an individual patient randomised design.
Chapter 8 Discussion
Statement of principal findings
Systematic review of RAMs
Sections of this chapter have been reproduced from Pandor et al. under licence CC BY 4.0. 17 In a systematic review of 51 observational studies evaluating RAMs for predicting the risk of developing VTE in hospital inpatients, we found that VTE RAMs have generally weak predictive accuracy. The studies validating these models are heterogeneous and most have a high risk of bias. Lack of methodological clarity was common, leading to difficulty in assessing the applicability of the individual study results. VTE prophylaxis was used in around half the study populations, with markedly varying frequency, and was not reported in most of the other studies. This is an important potential source of bias and heterogeneity between studies.
Our systematic review updated previous reviews to include more recent studies, but did not fundamentally alter the conclusions that the available evidence shows weak predictive accuracy and has significant methodological limitations. 8–10 VTE RAMs may be useful, despite the limited evidence and apparently weak accuracy, if they can identify patients with a higher risk of VTE who are more likely to benefit from prophylaxis. Decision-analytic modelling allowed us to explore whether VTE RAMs can provide more cost-effective use of VTE prophylaxis.
Economic evaluation
Using decision-analytic modelling to address the cost-effectiveness of VTE risk assessment, we made several key findings. For medical inpatients, the balance of VTE risk and risk of major bleeding, combined with the performance observed for RAMs in medical cohorts, means that prophylaxis for all is the optimal strategy within the model. The key factors determining cost-effectiveness in the medical cohort appear to be the baseline risks of VTE and major bleeding and the increased risk of major bleeding associated with prophylaxis. In the analysis for surgical inpatients, the optimal strategy within the model is to offer prophylaxis to all. However, the scenario analyses found that the optimal strategy was sensitive to many of the individual model inputs and assumptions tested, with the optimal strategy varying from a Pannucci score of ≥ 6 (sensitivity of 40% and specificity of 82%) to offering prophylaxis for all.
In the scenario analyses exploring specific surgical populations who require longer durations of prophylaxis, it was found that prophylaxis for all is optimal in patients having elective knee replacement whereas offering prophylaxis at a Pannucci score of ≥ 3 (sensitivity of 84% and specificity of 49%) was optimal when offering extended duration prophylaxis with LMWH to patients having elective hip replacement. The difference in optimal strategy between the elective hip replacement population and the main surgical cohort appear to be driven in part by the high cost of providing extended-duration prophylaxis, as the scenario analysis assuming 28 days of LMWH in the general surgical population also resulted in a Pannucci score of ≥ 3 being optimal. In the elective knee replacement population, the increased risk of bleeding associated with LMWH was lower meaning that there was less of a trade-off between a reduced VTE risk and an increased bleed risk in this group.
Our findings differ slightly from the previous cost-effectiveness analysis from a US health system perspective by Le et al. , which found that LMWH is cost-effective for medical inpatients when the risk of VTE is over 1%. 11 Le et al. discussed the use of RAMs to select patients with a risk of < 1% but did not explicitly model using a RAM to identify patients with a risk of < 1%. The difference is explained by the fact that Le et al. only included proximal symptomatic DVT and PE within their analysis and their average VTE risk in medical inpatients was assumed to be 1.4%. Under these conditions, the optimal strategy using our model would be a Padua score of ≥ 3 (when valuing a QALY at £20,000 or £30,000). Green et al. report a risk of < 1% in those with a Padua score < 3, suggesting the analyses are consistent when the VTE risk is defined in a similar manner. 39
Assessing the feasibility of efficient data methods and estimating key parameters for future implementation research
The findings from our cohort study suggest that currently available, routine and efficient data methods which can potentially identify VTE or major bleeding events during hospital admission or within 90 days of discharge, are not sufficiently sensitive for use in a large data-enabled study. We failed to achieve feasibility for a number of predefined metrics and concluded that use of routine data to identify outcomes would be highly likely to miss important VTE and bleeding events, and may erroneously identify false-positive events. We also identify significant limitations in the ability of efficient methods to identify individual risk variables and facilitate RAM comparison at scale in any future work. The majority of our sites did not collect contemporaneous data on risk assessment in a digital, or easily accessible format.
In addition, we provide estimates of key parameters for any future implementation study, based on an observational cohort study of > 2000 patients across four NHS sites. We report a HAT event rate of 1.6% (95% CI 1.0% to 2.2%) and a major bleeding event rate of 2.5% (95% CI 1.8% to 3.2%) in the context of routine NHS care for hospital inpatients. We also identify high rates of pharmacological prophylaxis in the context of routine care and risk assessment using the Department of Health tool, noting prescribing rates of 86.2%, 80.4% and 93.7% for all, medical and surgical patients, respectively. When comparing RAMS with attempted validation, we demonstrate highly variable rates of potential pharmacological thromboprophylaxis within the same cohort of general medical and surgical patients, in a UK NHS setting. Prescribing recommendation rates at published thresholds varied from 13% to 91% between RAMs. These data provide key estimates of current and potential prescribing rates, and potential event rates for any future studies looking to compare VTE RAMs, or assess implementation of novel strategies.
Finally, recent survey work suggests that > 80% of UK NHS sites continue to use the Department of Health tool for VTE risk assessment and that a high proportion use weight-adjusted dosing of pharmacological thromboprophylaxis. Our survey data also found that many sites continue to conduct risk assessment via paper proforma only and that very few sites record the absence of risk characteristics. These findings suggest that further comparative research on individualised risk assessment reliant on routine data collection, is unlikely to be feasible or reliable. These are important points to consider in the design of any future study.
Strengths and limitations
Systematic review of RAMs
Our systematic review work has a number of strengths. The review was conducted with robust methodology in accordance with the PRISMA statement and the protocol was registered with the PROSPERO register. Clinical experts were involved throughout as checkers and to assess the validity and applicability of research during the review. We reported descriptive statistics to provide insight into the limited evidence base applicable to the subject matter, and the scientific concerns regarding validity of the data. However, there are a number of potential weaknesses. Decisions on study relevance, information gathering and validity were unblinded and could potentially have been influenced by pre-formed opinions. However, masking is resource intensive with uncertain benefits. The studies of risk prediction were a combination of prospective cohorts and retrospective health database registries. Both have significant limitations. Retrospective studies of health database registries may have large numbers but may be limited by poor data quality and failure to accurately ascertain outcomes. Prospective cohorts may have better quality data but with smaller numbers lack statistical power.
We were unable to undertake meta-analysis or statistical examination of the causes of the observed heterogeneity. Potential sources of heterogeneity include variation in study design, the study population, how RAMs are implemented, outcome definition and measurement and the use of thromboprophylaxis. The latter point is highlighted above as a key finding.
Economic evaluation
The main strength of the economic evaluation is that it is able to evaluate the trade-off between costs, benefits and harms in a systematic manner based on the existing evidence base. One of the key uncertainties in the modelling is the incidence of VTE in cohorts not having thromboprophylaxis and the incidence of major bleeds outside of clinical trials. To address this uncertainty, the two-way scenario analyses on VTE and bleeding risk provide information on how robust the conclusions would be if the average risk of bleeding and the average risk of VTE across the medical and surgical cohorts has been underestimated or overestimated. However, these analyses do not necessarily provide information on whether a different strategy would be optimal in a select cohort of patients known to have higher or lower risks of bleeding or VTE. This is because the performance of the RAMs has been measured in broad cohorts of patients and the RAMs may not perform as well in very select cohorts chosen to have a very high or very low risk of VTE or major bleeding.
The economic analysis assumed that the population did not have characteristics associated with an increased risk of bleeding, and therefore had a low risk of bleeding overall. The findings, and specifically the conclusion that prophylaxis for all is the optimal strategy, do not, therefore, apply to populations or individual patients with an increased bleeding risk. The analyses showing whether the overall health impact is positive or negative at varying levels of VTE and bleeding risk (see Figures 11 and 12) are intended to show how bleeding and VTE risks might interact but should not be used to guide individualised decisions for patients. Bleeding and VTE often share the same risk factors, and interactions may occur between risks and between risk factors. Furthermore, our analyses assume that the RR for bleeding associated with prophylaxis is constant and increases in proportion to baseline bleeding risk. It is conceivable, however, that the risk factors for bleeding could increase the RR associated with bleeding. Taken together, these issues mean that the decision to provide VTE prophylaxis in the presence of increased bleeding risk is extremely complex, and best undertaken on an individual patient basis by an experienced clinical decision-maker. In this scenario, there remains a potential option to use mechanical thromboprophylaxis through graduated compression stockings or intermittent pneumatic compression devices. However, the evidence to support use of either device in medically ill patients is limited. We opted not to evaluate alternative methods of mechanical prophylaxis for patients at high risk of bleeding within the model, for the reasons outlined in Chapter 4.
A key limitation of the economic analysis is the heterogeneity in the estimates of RAM performance across the various cohorts identified in the systematic review. The secondary analysis using performance data for the Padua RAM from the cohort reported by Elias et al. provides different conclusions about the optimal strategy than found in the base-case analysis, due to the better performance of the Padua RAM in this cohort compared to the performance of this and other RAMs in other cohorts. The Padua RAM did not perform consistently better than other RAMs in other studies, so the higher accuracy in the Elias study probably reflects study characteristics rather than superiority of the Padua RAM. Our use of these data was intended to explore the potential for an accurate RAM to be cost-effective, rather than draw conclusions regarding a specific RAM or population.
Estimating key parameters for future implementation research and assessing the feasibility of efficient data methods
We engaged a combination of digitally mature and paper-based NHS sites within our cohort study and supplemented the findings with survey work, to obtain an accurate picture of VTE risk assessment conduct in a contemporary UK setting. We based key parameter estimation on an updated systematic review (workstream 1) and strict consensus definitions for VTE and bleeding events. We used statistical support, topic experts and research staff to iteratively develop our data collection tool and designed a clear workflow diagram to ensure compliance with data collection methods and limit subjective interpretation of case note data.
However, there are a number of potential limitations with design which could impact on the outcomes from workstream 2. We recruited only teaching hospital sites with university affiliation, two of which were VTE exemplar centres and three of which were tertiary centres, which may limit the external validity of our key parameter estimates. Regarding the evaluation of risk variables and estimation of key parameters, we opted for a cohort design on the early assumption that VTE risk assessments would be completed prospectively and accurately at all sites, in line with NICE guidance and NHS contract standards,86 and that these data would be retained within the healthcare record. Limitations in electronic data capture at several chosen sites led to this aspect of the project becoming single centre. As such, we were unable to undertake detailed work to determine whether other sites could implement efficient methods of recording risk assessment data, but the issues identified with limited IT and service pressures suggested that substantial work would have been required and success was unlikely.
In addition, the complexity of identified RAMs for comparison necessitated retrospective case note review to identify additional variables. This may have introduced bias in assessment and downgrading of other RAMs due to absence of inquiry and information in the case notes. Other limitations include a relatively small sample size and differential ascertainment for the Department of Health VTE risk tool (collected in real time by clinicians) compared to other RAMs (collected through case note review by research teams). In addition, we do not report estimation of bleeding risk within this work. Although this is a vital aspect of VTE risk assessment, the six RAMs presented for comparison do not integrate any form of bleeding assessment. It is therefore unclear how these RAMs would perform comparatively within a real-world population of medical and surgical inpatients. It is likely that the proportions of patients receiving prophylaxis in practice would be lower than those recommended once bleeding risk is taken into account. The exclusion criteria applied to this cohort limit the generalisability of our results.
Regarding the feasibility of efficient data methods, research assistants across sites varied in seniority and clinical experience. Although all sites had a principal investigator with clinical expertise available for queries and strict working definitions for outcome events, this may have introduced variation in reporting. Finally, we did not achieve our intended target of 3000 patients. Reasons for this are detailed in the above chapters. However, it is important to note that the overall results within our cohort of 2008 patients are well outside of feasibility targets and that sensitivity values using routine data sources to identify key outcomes are universally poor. We do not envisage that further cases would have significantly affected these values to the point of reaching feasibility, even with a high level of accuracy.
Research in context
Systematic review of RAMs
The present review is the largest and most comprehensive systematic review in this field to date. It includes 18 recent studies published since the completion of the previous systematic review. These studies are consistent with the previous literature in that they report modest performance of the assessed RAMs, with limitations in methodology and reporting preventing further analysis. The conclusion of this review therefore concurs with previous systematic reviews: there is insufficient evidence to recommend one RAM over another.
Economic evaluation
Although the optimal strategy of prophylaxis for all is the same in the medical and surgical cohorts, there is a more evident trade-off between the benefits of reduced VTE and the risks of increased bleeding for surgical patients as shown by the table of clinical outcomes in Table 4. This is why the optimal strategy is more sensitive to changes in the model inputs and assumptions in the surgical population. Parameters related to the long-term benefits of preventing VTE, such as the risk of PTS and the utility decrement of PTS, were identified as being important in the EVPPI analysis and sensitivity analyses for the surgical cohort. Factors related to long-term risks of chronic complications such as PTS do not appear to be important in determining the optimal strategy in the medical cohort, which may be because the medical cohort is older and, therefore, has a shorter life expectancy over which to accrue benefits from avoiding long-term complications. It is also important to recognise that while the risk of any major bleeding is higher in the surgical population, the risk of fatal bleeding or a non-fatal ICH is higher in the medical population. Medical inpatients are also more likely to die following a PE, and more likely to die in the year following admission even without experiencing VTE or major bleeding. However, sensitivity analyses applying a lower case-fatality rate for PE in medical inpatients or a higher case-fatality rate for PE in surgical inpatients do not result in a change in the optimal strategy.
In the secondary analysis using RAM performance data from the study by Elias et al. , the optimal strategy was to use a RAM in both medical and surgical patients. This is because RAM performance from Elias et al. was better than assumed in the base case for either the medical or surgical patients. For example, Elias et al. reported a sensitivity of 99.9% and a specificity of 23.7% for a Padua score of ≥ 3, whereas the equivalent values reported by Greene et al. in an exclusively medical cohort, were 49.3% and 73.0%, respectively. Performance data for the Padua RAM were not evaluated in an exclusively surgical cohort so a direct comparison cannot be made. These results suggest that if a RAM were to be useful for determining which patients should receive prophylaxis, it would need to have very high sensitivity similar to that observed for the Padua RAM in the study by Elias et al. If a future RAM performed similar to the Padua score ≥ 3 and a cut-off of 3 was applied, the model predicts that we would expect 80% of surgical patients and 84% of medical patients to receive prophylaxis. NICE reported that according to national figures, over 70% of medical patients receive prophylaxis using the Department of Health Risk Assessment tool, with some trusts offering prophylaxis to over 90% of medical inpatients. 8
Estimating key parameters for future implementation research and assessing the feasibility of efficient data methods
Our findings are in line with previous comparative research on VTE risk assessment. Systematic review data have reported prescribing recommendation rates ranging from 30% to 90% for medical patients with the application of different RAMs. 10,145,152,153 We have not identified previous work describing the comparative prescribing rates of different RAMS in a cohort of surgical patients and therefore provide new comparisons in this area. The baseline data on hospital length of stay, HAT event rates, major bleeding rates and prescribing rates are all in keeping with published evidence reviews, suggesting internal validity of our cohort. 8
The findings in our study highlight the ongoing variation in clinical care, of both medical and surgical patients, when different RAMs are applied to similar populations. In addition, our findings support previous research regarding the risk thresholds employed using the Department of Health VTE risk tool; this RAM conferred the highest proportion of pharmacological prophylaxis to both medical and surgical patients than any of the other six RAMs, with a 36% increase in recommended prescribing for surgical patients, and a 21% increase in medical patients, compared to the next highest prescribing model. Such high prescribing recommendations invite two key questions. First, does this tool provide better clinical or cost-effectiveness compared to others? Second, if over 90% of patients are likely to receive pharmacological thromboprophylaxis following use of the RAM, is there any real value in asking clinical teams to perform subjective and complex risk assessment? Switching to an ‘opt-out’ approach based on contraindications and bleeding risk has the potential to confer advantage, through mitigation of time and opportunity costs while concurrently encouraging default and reliable prescribing options. Such changes could also mitigate the key recurring issues with preventable HAT, most commonly resulting from errors in assessment, linked prescribing or drug administration. 150
Our findings of limited sensitivity using routine data methods within a UK setting are consistent with previous international work in this area. An early comparison of HES data to general practice records in England reported in 2012, initially concluded reliable identification of vascular disease using HES data (derived from ICD-10 coding data). 154 However, this analysis was restricted to PE from a VTE perspective and sought only to correlate disease states, rather than identify new case episodes. Several authors have also used primary care research data sets correlated to evidence of anticoagulation or other secondary care data to identify VTE events, with reported reliable capture. This work does not seek to discriminate between index presentation of VTE and HAT. 155,156 A systematic review, with searches run in July 2010, published in 2012, summarised findings on this topic from 19 studies. The positive predictive value (PPV) for PE ICD codes ranged from 24% to 92%, with higher values from certain combinations of codes. PPV values for DVT codes ranged from 31% to 97%. More recently, a cross-sectional North American study compared ICD-10 codes for VTE in hospitalised medical patients to a ‘gold standard’ manual review of clinical data in 4000 patients. 157 The authors report a sensitivity of 63%/PPV of 43% for any DVT and a sensitivity of 83%/PPV of 70% for PE, implying further discrepancy between types of VTE.
Several authors have also experimented with composite data sets and diagnostic/procedural/disease coding combinations. One study combined ICD codes for VTE with a common procedural terminology code for a VTE Diagnostic Study plus at least one of the following within 30 days of diagnosis: pharmacy script for anticoagulation, placement of an inferior vena cava filter, or death. 158 This algorithm still lacked sensitivity, reporting a value of 0.67 (0.60, 0.73), a specificity of 0.99 (0.98, 0.99), a PPV of 0.92 (0.87, 0.96) and a NPV of 0.94 (0.93, 0.95). Alotaibi et al. subsequently combined routinely collected ICD-10 coding data with imaging procedure codes to identify VTE events over a 10-year period compared to case note review and reported highly specific results but limited sensitivity, in line with our findings [74.83% (95% CI 67.01 to 81.62) and 75.24% (95% CI 65.86 to 83.14) for PE and DVT, respectively]. 159 Verma et al. report findings using natural language processing (NLP) algorithms for digital interrogation of radiology reports in a large cohort of hospitalised medical patients. 157 The authors conclude a high level of accuracy for NLP algorithms, reporting sensitivities of 94%/91% and PPVs of 90%/89% for DVT and PE, respectively. Such algorithms would require external validation in a UK setting and would be unable to distinguish HAT from index presentation of VTE, in isolation. Reliance on such algorithms in the context of low event rates would also lead to potential bias from false-negative results.
In 2017, Baumgartner et al. highlighted further issues through interrogation of an administrative coding database, looking to determine the accuracy of ICD coding for new episodes of recurrent VTE in patients with a prior history. 160 Only 31.1% of coded encounters were verified by reviewers as true recurrent VTE. More recently, Pellathy et al. have conducted similar work to VTEAM within the USA, comparing accuracy of HAT diagnoses made through administrative coding to manual case note and radiology review. 161 The authors report only 40% of HAT cases identified through routine coding were confirmed by case note review and 45% of HAT confirmed through diagnostic test records lacked corresponding ICD codes. This body of evidence, now including our recent work, suggests caution with the use of routinely collected outcome data within any future study where HAT events are an outcome of significant importance.
There are multiple potential explanations for the limited performance of routine data to identify HAT. The condition is a temporal phenomenon and routine coding data can therefore mistake index presentation with VTE as HAT, or fail to code subsequent HAT following index admission with alternative pathology; there are clear international guidelines supporting outpatient diagnosis and management of VTE, so genuine cases of HAT may not necessarily require hospital admission or relevant administrative coding; and prior diagnosis of VTE can often be coded during repeat hospital attendance and mistaken as HAT. Many UK hospitals attempting root cause analysis of HAT cases in line with NHS contract standards have developed pathways to mitigate these issues and improve identification, through local reporting arrangements with radiology, ultrasound and pathology services. Such arrangements often work well transiently but are reliant on individuals and reporting systems subject to human error. These issues are reflected in our findings from workstream 2, which report a PPV of 100% for HAT RCA database findings, but limited sensitivity (implying missed cases still occur despite a systematic approach).
More generally, these findings raise questions about the current enthusiasm for data-enabled trials and epidemiological research using outcome measures reliant on routine data methods. 162 Such concepts are inherently attractive to researchers and patients, particularly in topic areas with low event rates. However, complex outcome measures which require temporal evaluation and qualification against prior disease states are unlikely to be reliably delivered through use of routinely collected data in isolation. Several explanations have been proposed for current demonstrations of inaccuracy. Relevant data may contain coding errors arising from ambiguous documentation by physicians and inconsistent handling of recurrent cases. 162,164 Recent case studies have reported significant amounts of missing data and poor interobserver agreement between routinely collected EHR data accessible through HES and case report form (CRF) evaluation. 165 Electronic records contain an abundance of free text, but often lack necessary intelligence to classify patient episodes appropriately, or allow processing and comparison of routinely collected data. 166 Recent evidence also suggests that more complex outcomes will be less easily identified through routine coding; a registry study of Medicare claims following mitral valve repair compared to formal adjudication, reported a PPV for mortality of 97%, heart failure requiring hospitalisation of 69%, bleeding of 40% and renal failure of 19%. 167 In addition, the time and effort needed to acquire necessary permissions for national routine coding data or to orchestrate data linkage can be substantial. A UK clinical trials unit recently reported a digital request to facilitate routine data comparison to CRFs in the context of a RCT, highlighting a negotiation process over consent that took several years and receipt of data 15 months following application. 168 Such time frames may only be realistic within the context of continually adaptive design trials and add further concern as to whether the juice is actually worth the squeeze.
In their call to action, Sydes et al. discuss supplementation of trial-specific follow-up as an option to realise the full potential of data-enabled research. 162 Such an approach has potential merit to attempt identification of potential HAT, given the high PPV and high specificity of routine data sources. These cases would require subsequent adjudication and this approach would still fail to address the low sensitivity reported using routine data methods. Our findings suggest the use of routine data sources in isolation would be insufficiently accurate to capture complex VTE and bleeding outcomes within the context of a pragmatic trial context, but supplementation may be a viable use of resources. In addition, routine data sources may have a role in other research contexts, such as identification of cases for qualitative work, case–control studies, targeted individual follow-up or downstream survey work.
Chapter 9 Conclusions
Implications for patients, clinicians and policy-makers
The updated systematic review within workstream 1 highlights the lack of supporting evidence for RAMs in this area and clarifies the limitations of previous work. In particular, our systematic review provides contemporary evidence that no method of risk assessment has been extensively validated, or methodically studied by implementation research. 17 This work supports recommendations within recent current UK NICE guidance, which highlight the lack of evidence for an ‘optimal RAM’ and support use of any RAM published by a national UK body, professional network or peer reviewed-journal to aid risk assessment in medical and surgical inpatients. 8 However, our cohort study within workstream 2 draws attention to the potential impact on practice of such a broad recommendation. Applying the RAMs identified by systematic review to a cohort of medical and surgical NHS patients within a single centre resulted in overall recommendations for pharmacological prophylaxis ranging from 91% (95% CI 88.6% to 95.2%) to as low as 13% (95% CI 9.4% to 17.7%). Such potential variation has clinical, educational and medico-legal implications for clinicians and policy-makers. This variation would also clearly have a significant impact on patients. Multiple thrombosis societies and patient-facing organisations have worked hard to raise awareness on VTE risks over the last decade and empower patients to self-advocate for risk assessment and appropriately challenge medical decision-making. 169 If the strategy of risk assessment is variable and the supporting evidence limited, participation in shared decision-making may be difficult.
Furthermore, decision-analytic modelling suggests that routine VTE prophylaxis is likely to be more cost-effective for medical and surgical patients requiring hospital admission than a strategy based on any currently available RAM. When taken in context with the lack of supporting evidence for available RAMs and ongoing errors/omissions leading to preventable cases of hospital-acquired VTE,150 these findings have significant implications for policy-makers. There is reasonable evidence for the clinical effectiveness of pharmacological prophylaxis (subject to contraindications) for prevention of VTE in all medical and surgical patients admitted to hospital. 4,13 If pharmacological prophylaxis for all is likely to also be the most cost-effective strategy, despite drug costs and potential harms, recommendation of unvalidated RAMs may be inappropriate. Based on our findings, it may be preferable for policy-makers to consider evaluating a new paradigm of ‘opt-out’ VTE prevention, in which all eligible patients are offered pharmacological prophylaxis on hospital admission without complex risk assessment. In this circumstance, patients who are ineligible for pharmacological prophylaxis due to contraindications, high bleeding risk or personal choice could still utilise mechanical thromboprophylaxis in accordance with current evidence.
In addition, during the course of this project we identified several pragmatic issues with RAM use and application which can lead to repeated system errors or disengagement. Several RAMs have subjective components with poor inter-rater reliability;170,171 RAMs may not be completed accurately (or at all) by busy clinical teams if they are deemed unhelpful to the encounter; clinicians may complete RAMs in a biased fashion due to experience (or inexperience) based on seniority; RAM completion and identification of high VTE risk may not translate to reliable prescribing; multifactorial RAMs applied to every patient accrue a significant work burden; and some multifactorial and complex RAMs will actually accrue decision-making points from only a small number of key variables. Although these issues do not feature widely in the current literature due to lack of implementation research, several studies have highlighted the numerous pragmatic challenges of RAM use. 51,145,153 These cumulative issues also potentially support a paradigm shift towards an ‘opt-out’ strategy.
There are limitations in translating these research findings to practice. Our systematic review focused on RAMs which evaluate thrombosis risk (rather than bleeding risk). As such, our economic modelling work is also based on estimations in a population without an increased risk of bleeding. In addition, workstream 1 focused on population-level estimates. In reality, each individual patient encounter is likely to have a different risk profile regarding VTE and bleeding; practicalities of implementation may be different from the model and it may still be necessary to perform a degree of risk assessment, or to evaluate the contraindications for pharmacological prophylaxis in detail.
Finally, we highlight the limitations of using routine data methods in the context of future research. Such systems run the risk of identifying both false-negative and false-positive VTE cases, through failure to identify ambulatory (uncoded) cases and overdiagnosis of previous disease. Many of these issues reflect the changing approach to modern VTE management and would be challenging to mitigate within any future research studies. Our findings were similar with regard to bleeding events, showing poor sensitivity of ICD-10 coding data and multiple false-positive events identified across four NHS sites. These findings have implications for funders looking to support further work in this area and suggest large studies reliant on routine data collection methods for outcomes are likely to be inaccurate and therefore unfeasible.
Our PPI group highlighted at several points during the project that risk assessment in the UK setting still seems an uncertain process, with many clinicians underestimating the consequences of VTE and the importance of prophylaxis. In addition, several respondents in our survey of national leads (see Chapter 7) highlighted the ‘tick box’ nature of VTE risk assessment; many clinicians feel they have already made the decision to prescribe prophylaxis prior to any risk assessment, and therefore consider the assessment itself to be a futile exercise. These findings also potentially support a more implicit use of prophylaxis at the point of hospital admission, with a clinical focus on reliable and standardised implementation, summarised by the ‘opt out’ approach described above, in contrast to current practice (complex risk assessment followed by opting in). However, the PPI group was also clear that lack of information and discussion about VTE risk during hospital admission or in the periprocedural period, was an ongoing concern. It was further highlighted by the steering committee that risk assessment, for all its faults, offers a clear opportunity to educate junior clinicians and discuss the risk of VTE with patients and service users, raising awareness of the condition and improving dialogue on the topic. These softer benefits may be potentially lost through any process of routine and unqualified thromboprophylaxis prescribing.
Suggested research priorities
Similar to our previous work, we identified that the current evidence base for RAMs looking to select appropriate hospitalised patients for pharmacological thromboprophylaxis is very limited and estimates of prognostic accuracy are subject to substantial uncertainty. 74 However, any future research on RAMs would be subject to the same challenges faced by this project, of VTE risk assessment as a contract standard within NHS practice and subsequent high rates of prophylaxis, supported by clear NICE guidance. 8 It would therefore be unethical to expose patients to implementation research on RAMs incorporating any control arm without risk assessment, or thromboprophylaxis.
Alternatively, given our findings on cost-effectiveness it may be reasonable to consider implementation research focusing on assessment of a novel opt-out strategy, regarding pharmacological prophylaxis. Such work could be conducted in any NHS setting and allocate patients to an intervention arm (default prophylaxis subject to contraindications or high bleeding risk only) compared to standard care (formal risk assessment and tailored prophylaxis as per national guidance). Patients ineligible for pharmacological prophylaxis in either group could also be offered alternative mechanical prophylaxis using evidence-based strategies, to further evaluate impact. Our survey work would suggest such an approach to be of interest to the national VTE community, with more support for a cluster randomised design (rather than individual patient randomisation) at hospital level. Such work would need to consider the challenges of reliable follow-up and outcome ascertainment given our findings from workstream 2. Proposals could include adjudicated co-primary outcome definitions for VTE and major bleeding, evaluated using a hybrid approach of digital patient follow-up, routine data sources and local VTE identification methods.
Within the context of such an implementation study (including high numbers of patients receiving pharmacological thromboprophylaxis), a large observational cohort could also be evaluated to determine which risk predictors are associated with potential thromboprophylaxis failure. Given the challenges identified by this project regarding the completion methods and accuracy of risk assessment, risk predictor variables would likely require reliable, real-time collection by research teams on admission, outside of clinical assessment. Serial evaluation over the first 48 hours would allow evaluation of temporal RAM trends and novel biomarkers (D-dimer, CRP) on risk profile, building on recent systematic review work. 172 Indeed, recently derived RAMs are heavily focused on objective biomarker variables at an early admission stage and echo concerns regarding inter-rater reliability with subjective RAM components. 173 Multivariate analysis could subsequently identify which of these features during the first 48 hours of admission best identify likely thromboprophylaxis failure, allowing further risk stratification during hospital admission. If validated, this approach could lead to future trials of enhanced thromboprophylaxis strategies for higher-risk populations, in keeping with the ‘individualised approach’ relevant to specific subgroups, already proposed by several topic experts. 174–176
The same logic could be applied to patients at the lower end of the risk spectrum. Within the context of an implementation study, patients who do not receive pharmacological thromboprophylaxis during routine care could be followed in a similar fashion to evaluate risk characteristics, temporal trends and outcomes. These data could facilitate multivariate analysis and subsequent identification of patients at a particularly low risk of VTE, who could be considered for early cessation of prophylaxis.
Taken together, the above projects could evaluate a paradigm shift in clinical VTE risk management; evaluation of a novel opt-out approach on hospital admission with the majority of medical and surgical patients immediately commenced on a weight-adjusted standard dose of pharmacological thromboprophylaxis without detailed risk assessment; a further evaluation at 48 hours using enhanced risk prediction, temporal trends in clinical progress and biomarker data; and a subsequent ‘rationalisation’ of prophylaxis at this time point – to increase, to continue or to cease. Such an approach would obviate complex risk assessment at the front door, when information, time and accuracy of diagnosis are limited and the chances of error are high, and facilitate early reliable use of prophylaxis followed by considered transition.
We found limited evidence on the accuracy or validity of bleeding risk assessment tools for hospitalised medical and surgical patients. Given we found bleeding risk to be a key factor in determining cost-effectiveness, further studies of bleeding risk and outcomes in patients receiving pharmacological thromboprophylaxis are urgently required. The high number of patients receiving prophylaxis in routine care could facilitate the rapid development of large observational cohort studies in this area, designed to accurately capture risk variables at presentation and evaluate their association with standardised major and CRNMB risk during hospital admission. Our findings in workstream 2 suggest that such a study could not rely on routine data collection methods to determine outcomes of relevance and may require significant research support to ensure diligent and accurate follow-up.
Last, our work is restricted primarily to medical, surgical and orthopaedic patients. We did not evaluate bespoke RAMs in specific patient subgroups, such as cancer or neurosurgery. Further studies of prophylaxis in specific patient populations are warranted, in particular where risk profile may differ significantly due to higher risk of critical site bleeding. A recent commissioned call for research into prophylaxis for patients with traumatic brain injury exemplifies this point. 177 Future studies in bespoke populations are likely best delivered through RCTs, informed by our work on the accuracy of routine data regarding relevant outcomes in thrombosis research.
Acknowledgements
The authors are grateful to Heather Dakin and Ben Thomas for clerical support, study management and manuscript formatting. We are also grateful to Mike Bradshaw (statistician) for his support with data analysis in workstream 2. The authors would also like to thank all members of the PPI group and the Study Steering Group for engagement with the project advice and comment throughout. The authors would also like to thank Emma Gee in particular, for assistance with dissemination of the relevant survey details to national VTE leads through the Nursing and Midwifery National Network and the GIRFT thrombosis contributors.
Contributions of authors
Daniel Edward Horner (https://orcid.org/0000-0002-0400-2017) (Consultant in Emergency and Critical Care Medicine) contributed to the design of the study, led workstream 2 as co-chief investigator and drafted the monograph.
Sarah Davis (https://orcid.org/0000-0002-6609-4287) (Health economist and reader in decision science) contributed to the design of the study, contributed to the Project Management Group throughout the study, conducted the analysis of economic models and critically revised the work for important intellectual content.
Abdullah Pandor (https://orcid.org/0000-0003-2552-5260) (Information Specialist) led the systematic review in workstream 1 and critically revised the work for important intellectual content.
Helen Shulver (https://orcid.org/0000-0001-7152-4125) (Study Manager) contributed to the design of the study, contributed to the Project Management Group throughout the study and provided project leadership for a substantial portion of the project.
Steve Goodacre (https://orcid.org/0000-0003-0803-8444) (Emergency Medicine) contributed to the design of the study, led workstream 1 as co-chief investigator and critically revised the work for important intellectual content.
Daniel Hind (https://orcid.org/0000-0002-6409-4793) (Clinical Trials) contributed to the design of the study, contributed to the Project Management Group throughout the study and critically revised the work for important intellectual content.
Saleema Rex (https://orcid.org/0000-0002-0318-6125) (statistics) provided statistical input to the project management group and was involved in the interpretation of data.
Michael Gillett (https://orcid.org/0000-0001-9243-3556) (statistics) provided statistical input to the project management group and was involved in the interpretation of data.
Matthew Bursnall (https://orcid.org/0000-0002-6519-3558) (statistics) provided statistical input to the project management group and was involved in the interpretation of data.
Xavier Griffin (https://orcid.org/0000-0003-2976-7523) (Trauma and Orthopaedics) contributed to the design of the study, contributed to the Project Management Group throughout the study and critically revised the work for important intellectual content.
Mark Holland (https://orcid.org/0000-0001-8336-5336) (Acute Medicine) contributed to the design of the study, contributed to the Project Management Group throughout the study and critically revised the work for important intellectual content.
Beverley Jane Hunt (https://orcid.org/0000-0002-4709-0774) (Thrombosis and Haemostasis) contributed to the design of the study, contributed to the Project Management Group throughout the study and critically revised the work for important intellectual content.
Kerstin de Wit (https://orcid.org/0000-0003-2763-6474) (Emergency Medicine and Thrombosis) contributed to the design of the study, contributed to the Project Management Group throughout the study and critically revised the work for important intellectual content.
Shan Bennett (patient representative) contributed to the design of the study, contributed to the project management group throughout the study and provided patient and public involvement throughout the study.
Robin Pierce-Williams (patient representative) contributed to the design of the study, contributed to the project management group throughout the study and provided patient and public involvement throughout the study.
All authors were involved in the final approval of the version to be published.
All authors agree to be accountable for all aspects of the work in ensuring that questions relating to the accuracy or integrity of any part of the work are appropriately investigated and resolved.
Publications
Pandor A, Tonkins M, Goodacre S, et al. Risk assessment models for venous thromboembolism in hospitalised adult patients: a systematic review. BMJ Open 2021;11:e045672. https://doi.org/10.1136/bmjopen-2020-045672
Horner D, Rex S, Reynard C, et al. Accuracy of efficient data methods to determine the incidence of hospital-acquired thrombosis and major bleeding in medical and surgical inpatients: a multicentre observational cohort study in four UK hospitals. BMJ Open 2023;13:e069244. https://doi.org/10.1136/bmjopen-2022-069244
Davis S, Goodacre S, Horner D et al. Decision-analysis modelling of effectiveness and cost-effectiveness of pharmacologic thromboprophylaxis for surgical inpatients using variable risk assessment models or other strategies. Journal of Thrombosis and Haemostasis 2023;21:1580-91.
Davis S, Goodacre S, Horner D et al. Effectiveness and cost effectiveness of pharmacological thromboprophylaxis for medical inpatients: decision analysis modelling study. BMJ Medicine 2024;3:e000408. https://doi.org/10.1136/bmjmed-2022-000408
Acknowledging the use of patient data
This work uses data provided by patients and collected by the NHS as part of their care and support. Using patient data is vital to improve health and care for everyone. There is huge potential to make better use of information from people’s patient records, to understand more about disease, develop new treatments, monitor safety and plan NHS services. Patient data should be kept safe and secure, to protect everyone’s privacy, and it is important that there are safeguards to make sure that they are stored and used responsibly. Everyone should be able to find out about how patient data are used. #datasaveslives You can find out more about the background to this citation here: https://understandingpatientdata.org.uk/data-citation.
Data-sharing statement
All data requests regarding primary research within this project should be submitted to ctru@sheffield.ac.uk for consideration. Access to anonymised data may be granted following review. Requests for access to data regarding secondary research within this project should be addressed to the corresponding author or to the data custodian (if known).
Ethics statement
Full HRA and ethical approval was granted for the Venous ThromboEmbolism Assessment Methods (VTEAM) study (Rec Ref: 19/LO/1303). The protocol for the full study is available online. Completed EQUATOR checklists have been submitted for each chapter as relevant.
Department of Health and Social Care disclaimer
This publication presents independent research commissioned by the National Institute for Health and Care Research (NIHR). The views and opinions expressed by authors in this publication are those of the authors and do not necessarily reflect those of the NHS, the NIHR, MRC, NIHR Coordinating Centre, the HTA programme or the Department of Health and Social Care.
This monograph was published based on current knowledge at the time and date of publication. NIHR is committed to being inclusive and will continually monitor best practice and guidance in relation to terminology and language to ensure that we remain relevant to our stakeholders.
Disclaimers
This manuscript presents independent research funded by the National Institute for Health and Care Research (NIHR). The views and opinions expressed by authors in this publication are those of the authors and do not necessarily reflect those of the NHS, the NIHR, the HTA programme or the Department of Health and Social Care. If there are verbatim quotations included in this publication the views and opinions expressed by the interviewees are those of the interviewees and do not necessarily reflect those of the authors, those of the NHS, the NIHR, the HTA programme or the Department of Health and Social Care.
References
- ISTH Steering Committee for World Thrombosis Day . Thrombosis: a major contributor to the global disease burden. J Thromb Haemost 2014;12:1580-90. https://doi.org/10.1111/jth.12698.
- Heit JA, O’Fallon WM, Petterson TM, Lohse CM, Silverstein MD, Mohr DN, et al. Relative impact of risk factors for deep vein thrombosis and pulmonary embolism: a population-based study. Arch Intern Med 2002;162:1245-8. https://doi.org/10.1001/archinte.162.11.1245.
- Roberts LN, Porter G, Barker RD, Yorke R, Bonner L, Patel RK, et al. Comprehensive VTE prevention program incorporating mandatory risk assessment reduces the incidence of hospital-associated thrombosis. Chest 2013;144:1276-81. https://doi.org/10.1378/chest.13-0267.
- Mismetti P, Laporte-Simitsidis S, Tardy B, Cucherat M, Buchmuller A, Juillard-Delsart D, et al. Prevention of venous thromboembolism in internal medicine with unfractionated or low-molecular-weight heparins: a meta-analysis of randomised clinical trials. Thromb Haemost 2000;83:14-9.
- Hunt BJ. Preventing hospital associated venous thromboembolism. BMJ 2019;365. https://doi.org/10.1136/bmj.l4239.
- Shojania KG, Duncan BW, McDonald KM, Wachter RM, Markowitz AJ. Making health care safer: a critical analysis of patient safety practices. Evid Rep Technol Assess (Summ) 2001:i-x. https://pubmed.ncbi.nlm.nih.gov/11510252/.
- Jha AK, Larizgoitia I, Audera-Lopez C, Prasopa-Plaizier N, Waters H, Bates DW. The global burden of unsafe medical care: analytic modelling of observational studies. BMJ Qual Saf 2013;22:809-15. https://doi.org/10.1136/bmjqs-2012-001748.
- National Institute for Health and Care Excellence . Venous Thromboembolism in Over 16s: Reducing the Risk of Hospital-Acquired Deep Vein Thrombosis or Pulmonary Embolism (NG89) 2018. www.nice.org.uk/guidance/ng89 (accessed 22 July 2022).
- Huang W, Anderson FA, Spencer FA, Gallus A, Goldberg RJ. Risk-assessment models for predicting venous thromboembolism among hospitalized non-surgical patients: a systematic review. J Thromb Thrombolysis 2013;35:67-80. https://doi.org/10.1007/s11239-012-0780-0.
- Stuck AK, Spirk D, Schaudt J, Kucher N. Risk assessment models for venous thromboembolism in acutely ill medical patients. A systematic review. Thromb Haemost 2017;117:801-8. https://doi.org/10.1160/TH16-08-0631.
- Le P, Martinez KA, Pappas MA, Rothberg MB. A decision model to estimate a risk threshold for venous thromboembolism prophylaxis in hospitalized medical patients. J Thromb Haemost 2017;15:1132-41. https://doi.org/10.1111/jth.13687.
- Mismetti P, Laporte S, Darmon JY, Buchmuller A, Decousus H. Meta-analysis of low molecular weight heparin in the prevention of venous thromboembolism in general surgery. Br J Surg 2001;88:913-30. https://doi.org/10.1046/j.0007-1323.2001.01800.x.
- Australian Commission on Safety and Quality in Healthcare . Venous Thromboembolism Prevention - Clinical Care Standard 2020. www.safetyandquality.gov.au/sites/default/files/2020-01/venous_thromboembolism_prevention_clinical_care_standard_-_jan_2020_2.pdf (accessed 20 August 2021).
- Schunemann HJ, Cushman M, Burnett AE, Kahn SR, Beyer-Westendorf J, Spencer FA, et al. American Society of Hematology 2018 guidelines for management of venous thromboembolism: prophylaxis for hospitalized and nonhospitalized medical patients. Blood Adv 2018;2:3198-225. https://doi.org/10.1182/bloodadvances.2018022954.
- Barbar S, Noventa F, Rossetto V, Ferrari A, Brandolin B, Perlati M, et al. A risk assessment model for the identification of hospitalized medical patients at risk for venous thromboembolism: the Padua prediction score. J Thromb Haemost 2010;8:2450-7. https://doi.org/10.1111/j.1538-7836.2010.04044.x.
- Kahn SR, Lim W, Dunn AS, Cushman M, Dentali F, Akl EA, et al. Prevention of VTE in nonsurgical patients: Antithrombotic Therapy and Prevention of Thrombosis, 9th ed: American College of Chest Physicians Evidence-Based Clinical Practice Guidelines. Chest 2012;141:e195S-226S. https://doi.org/10.1378/chest.11-2296.
- Pandor A, Tonkins M, Goodacre S, Sworn K, Clowes M, Griffin XL, et al. Risk assessment models for venous thromboembolism in hospitalised adult patients: a systematic review. BMJ Open 2021;11. https://doi.org/10.1136/bmjopen-2020-045672.
- Moher D, Liberati A, Tetzlaff J, Altman DG. PRISMA Group . Preferred reporting items for systematic reviews and meta-analyses: the PRISMA statement. Ann Intern Med 2009;151:264-9. https://doi.org/10.7326/0003-4819-151-4-200908180-00135.
- Moons KGM, Wolff RF, Riley RD, Whiting PF, Westwood M, Collins GS, et al. PROBAST: a tool to assess risk of bias and applicability of prediction model studies: explanation and elaboration. Ann Intern Med 2019;170:W1-33. https://doi.org/10.7326/m18-1377.
- Wolff RF, Moons KGM, Riley RD, Whiting PF, Westwood M, Collins GS, et al. PROBAST Group. PROBAST: a tool to assess the risk of bias and applicability of prediction model studies. Ann Intern Med 2019;170:51-8. https://doi.org/10.7326/m18-1376.
- Centre for Reviews and Dissemination . Systematic Reviews: CRD’s Guidance for Undertaking Reviews in Health Care 2009.
- McKenzie JE, Brennan SE, Ryan RE, Thomson HJ, Johnston RV. Chapter 9: Summarizing study characteristics and preparing for synthesis. Cochrane Handbook for Systematic Reviews of Interventions Version 6.0 2019.
- Hosmer DW, Lemeshow S. Applied Logistic Regression. New York, NY: John Wiley & Sons; 2000.
- Abdel-Razeq HN, Hijjawi SB, Jallad SG, Ababneh BA. Venous thromboembolism risk stratification in medically-ill hospitalized cancer patients. A comprehensive cancer center experience. J Thromb Thrombolysis 2010;30:286-93. https://doi.org/10.1007/s11239-010-0445-9.
- Autar R. The management of deep vein thrombosis: the Autar DVT risk assessment scale re-visited. J Orthop Nurs 2003;7:114-24.
- Bahl V, Hu HM, Henke PK, Wakefield TW, Campbell DA, Caprini JA. A validation study of a retrospective venous thromboembolism risk scoring method. Ann Surg 2010;251:344-50. https://doi.org/10.1097/SLA.0b013e3181b7fca6.
- Bilimoria KY, Liu Y, Paruch JL, Zhou L, Kmiecik TE, Ko CY, et al. Development and evaluation of the universal ACS NSQIP surgical risk calculator: a decision aid and informed consent tool for patients and surgeons. J Am Coll Surg 2013;217:833-42.e1. https://doi.org/https://doi.org/10.1016/j.jamcollsurg.2013.07.385.
- Blondon M, Righini M, Nendaz M, Glauser F, Robert-Ebadi H, Prandoni P, et al. External validation of the simplified Geneva risk assessment model for hospital-associated venous thromboembolism in the Padua cohort. J Thromb Haemost 2019;18:676-80. https://doi.org/doi: 10.1111/jth.14688.
- Blondon M, Limacher A, Righini M, Aujesky D, Mean M. Adequacy of hospital thromboprophylaxis and risk assessment models in the SWITCO65+ cohort. Res Pract Thromb Haemost 2019;3. http://dx.doi.org/10.1002/rth2.12229.
- Blondon M, Spirk D, Kucher N, Aujesky D, Hayoz D, Beer JH, et al. Comparative performance of clinical risk assessment models for hospital-acquired venous thromboembolism in medical patients. Thromb Haemost 2018;118:82-9. https://doi.org/https://dx.doi.org/10.1160/TH17-06-0403.
- Bo H, Li Y, Liu G, Ma Y, Li Z, Cao J, et al. Assessing the risk for development of deep vein thrombosis among Chinese patients using the 2010 Caprini risk assessment model: a prospective multicenter study. J Atheroscler Thromb 2020;27:801-8. https://doi.org/https://dx.doi.org/10.5551/jat.51359.
- Chen X, Pan L, Deng H, Zhang J, Tong X, Huang H, et al. Risk assessment in Chinese hospitalized patients comparing the Padua and Caprini Scoring algorithms. Clin Appl Thromb Hemost 2018;24:127S-135S. https://dx.doi.org/10.1177/1076029618797465.
- Cobben MRR, Nemeth B, Lijfering WM, Cannegieter SC. Validation of risk assessment models for venous thrombosis in hospitalized medical patients. Res Pract Thromb Haemost 2019;3:217-25. http://dx.doi.org/10.1002/rth2.12181.
- de Bastos M, Barreto SM, Caiafa JS, Boguchi T, Silva JLP, Rezende SM. Derivation of a risk assessment model for hospital-acquired venous thrombosis: the NAVAL score. J Thromb Thrombolysis 2016;41:628-35. http://dx.doi.org/10.1007/s11239-015-1277-4.
- Dornbos DL, Shah V, Priddy B, Schunemann V, Nimjee S, Powers C. Predicting venous thromboembolic complications following neurological surgery procedures. J Neurosurg 2018;128. http://dx.doi.org/10.3171/2018.4.JNS.AANS2018abstracts.
- Elias P, Khanna R, Dudley A, Davies J, Jacolbia R, McArthur K, et al. Automating venous thromboembolism risk calculation using electronic health record data upon hospital admission: The automated Padua prediction score. J Hosp Med 2017;12:231-7. https://dx.doi.org/10.12788/jhm.2714.
- Frankel JK, Belanger M, Tortora J, McLaughlin T, Staff I, Wagner J. Caprini score predicts venous thromboembolic events in patients undergoing robotic assisted prostatectomy. J Urol 2017;197.
- Grant PJ, Greene MT, Chopra V, Bernstein SJ, Hofer TP, Flanders SA. Assessing the Caprini score for risk assessment of venous thromboembolism in hospitalized medical patients results. Am J Med 2016;129:528-35. http://dx.doi.org/10.1016/j.amjmed.2015.10.027.
- Greene MT, Spyropoulos AC, Chopra V, Grant PJ, Kaatz S, Bernstein SJ, et al. Validation of risk assessment models of venous thromboembolism in hospitalized medical patients. Am J Med 2016;129:1001.e91001-1001.e18. http://dx.doi.org/10.1016/j.amjmed.2016.03.031.
- Hachey KJ, Hewes PD, Porter LP, Ridyard DG, Rosenkranz P, McAneny D, et al. Caprini venous thromboembolism risk assessment permits selection for postdischarge prophylactic anticoagulation in patients with resectable lung cancer. J Thorac Cardiovasc Surg 2016;151:37-44.e1. https://doi.org/10.1016/j.jtcvs.2015.08.039.
- Hegsted D, Gritsiouk Y, Schlesinger P, Gardiner S, Dean Gubler K. Utility of the risk assessment profile for risk stratification of venous thrombotic events for trauma patients. Am J Surg 2013;205:517-20. https://doi.org/10.1016/j.amjsurg.2013.01.022.
- Hewes PD, Hachey KJ, Zhang XW, Tripodis Y, Rosenkranz P, Ebright MI, et al. Evaluation of the Caprini Model for venothromboembolism in esophagectomy patients. Ann Thorac Surg 2015;100:2072-8. https://doi.org/10.1016/j.athoracsur.2015.05.098.
- Ho KM, Rao S, Rittenhouse KJ, Rogers FB. Use of the Trauma Embolic Scoring System (TESS) to predict symptomatic deep vein thrombosis and fatal and non-fatal pulmonary embolism in severely injured patients. Anaesth Intensive Care 2014;42:709-14. https://doi.org/10.1177/0310057x1404200605.
- Hu Y, Li X, Zhou H, Lin P, Zhang J, Huang D, et al. Comparison between the Khorana prediction score and Caprini risk assessment models for assessing the risk of venous thromboembolism in hospitalized patients with cancer: a retrospective case control study. Interact Cardiovasc Thorac Surg 2020;31:454-60. https://dx.doi.org/10.1093/icvts/ivaa137.
- Krasnow R, Preston M, Chung B, Kibel A, Chang S. Validation of venous thromboembolism risk assessment score in major urologic cancer surgery: a population based study. J Urol 2017;197.
- Liu L-P, Zheng H-G, Wang DZ, Wang Y-L, Hussain M, Sun H-X, et al. Risk assessment of deep-vein thrombosis after acute stroke: a prospective study using clinical factors. CNS Neurosci Ther 2014;20:403-10. https://doi.org/10.1111/cns.12227.
- Liu X, Liu C, Chen X, Wu W, Lu G. Comparison between Caprini and Padua risk assessment models for hospitalized medical patients at risk for venous thromboembolism: a retrospective study. Interact Cardiovasc Thorac Surg 2016;23:538-43. http://dx.doi.org/10.1093/icvts/ivw158.
- Lobastov K, Barinov V, Schastlivtsev I, Laberko L, Rodoman G, Boyarintsev V. Validation of the Caprini risk assessment model for venous thromboembolism in high-risk surgical patients in the background of standard prophylaxis. J Vasc Surg Venous Lymphat Disord 2016;4:153-60. https://doi.org/10.1016/j.jvsv.2015.09.004.
- Mahan CE, Liu Y, Turpie AG, Vu JT, Heddle N, Cook RJ, et al. External validation of a risk assessment model for venous thromboembolism in the hospitalised acutely-ill medical patient (VTE-VALOURR). Thromb Haemost 2014;112:692-9. https://doi.org/10.1160/TH14-03-0239.
- Mlaver E, Lynde GC, Gallion C, Sweeney JF, Sharma J. Development of a novel preoperative venous thromboembolism risk assessment model. Am Surg 2020;86:1098-105. https://dx.doi.org/10.1177/0003134820943556.
- Moumneh T, Riou J, Douillet D, Henni S, Mottier D, Tritschler T, et al. Validation of risk assessment models predicting venous thromboembolism in acutely ill medical inpatients: a cohort study. J Thromb Haemost 2020;18:1398-407. https://dx.doi.org/10.1111/jth.14796.
- Nafee T, Gibson CM, Travis R, Yee MK, Kerneis M, Chi G, et al. Machine learning to predict venous thrombosis in acutely ill medical patients. Res Pract Thromb Haemost 2020;4:230-7. https://dx.doi.org/10.1002/rth2.12292.
- Nendaz M, Spirk D, Kucher N, Aujesky D, Hayoz D, Beer JH, et al. Multicentre validation of the Geneva Risk Score for hospitalised medical patients at risk of venous thromboembolism. Explicit ASsessment of Thromboembolic RIsk and Prophylaxis for Medical PATients in SwitzErland (ESTIMATE). Thromb Haemost 2014;111:531-8. https://doi.org/10.1160/TH13-05-0427.
- Pannucci CJ, Laird S, Dimick JB, Campbell DA, Henke PK. A validated risk model to predict 90-day VTE events in postsurgical patients. Chest 2014;145:567-73. https://doi.org/10.1378/chest.13-1553.
- Pannucci CJ, Osborne NH, Wahl WL. Creation and validation of a simple venous thromboembolism risk scoring tool for thermally injured patients: analysis of the National Burn Repository. J Burn Care Res 2012;33:20-5. https://doi.org/10.1097/BCR.0b013e318234d8b5.
- Patell R, Rybicki L, McCrae KR, Khorana AA. Predicting risk of venous thromboembolism in hospitalized cancer patients: utility of a risk assessment tool. Am J Hematol 2017;92:501-7. https://doi.org/https://dx.doi.org/10.1002/ajh.24700.
- Rogers FB, Shackford SR, Horst MA, Miller JA, Wu D, Bradburn E, et al. Determining venous thromboembolic risk assessment for patients with trauma: the Trauma Embolic Scoring System. J Trauma Acute Care Surg 2012;73:511-5.
- Rogers SO, Kilaru RK, Hosokawa P, Henderson WG, Zinner MJ, Khuri SF. Multivariable predictors of postoperative venous thromboembolic events after general and vascular surgery: results from the patient safety in surgery study. J Am Coll Surg 2007;204:1211-21.
- Rosenberg D, Eichorn A, Alarcon M, McCullagh L, McGinn T, Spyropoulos AC. External validation of the risk assessment model of the International Medical Prevention Registry on Venous Thromboembolism (IMPROVE) for medical patients in a tertiary health system. J Am Heart Assoc 2014;3. https://doi.org/10.1161/JAHA.114.001152.
- Rothberg MB, Lindenauer PK, Lahti M, Pekow PS, Selker HP. Risk factor model to predict venous thromboembolism in hospitalized medical patients. J Hosp Med 2011;6:202-9. https://doi.org/10.1002/jhm.888.
- Shaikh M-A, Jeong HS, Mastro A, Davis K, Lysikowski J, Kenkel JM. Analysis of the American Society of Anesthesiologists Physical Status Classification System and Caprini Risk Assessment Model in predicting venous thromboembolic outcomes in plastic surgery patients. Aesthet Surg J 2016;36:497-505. https://doi.org/10.1093/asj/sjv198.
- Shang M-M, Yan R, Wang X-L, Gong W-P, Guo Z-Q. Comparison of 2013 and 2009 versions of Caprini risk assessment models for predicting VTE in Chinese cancer patients: a retrospective study. J Thromb Thrombolysis 2020;50:446-51. https://dx.doi.org/10.1007/s11239-020-02038-2.
- Shen C, Ge B, Liu X, Chen H, Qin Y, Shen H. Predicting the occurrence of venous thromboembolism: construction and verification of risk warning model. BMC Cardiovasc Disord 2020;20. https://dx.doi.org/10.1186/s12872-020-01519-9.
- Tachino J, Yamamoto K, Shimizu K, Shintani A, Kimura A, Ogura H, et al. Quick risk assessment profile (qRAP) is a prediction model for post-traumatic venous thromboembolism. Injury 2019;50:1540-4. https://dx.doi.org/10.1016/j.injury.2019.06.020.
- Tian B, Li H, Cui S, Song C, Li T, Hu B. A novel risk assessment model for venous thromboembolism after major thoracic surgery: a Chinese single-center study. J Thorac Dis 2019;11:1903-10. https://dx.doi.org/10.21037/jtd.2019.05.11.
- Vardi M, Ghanem-Zoubi NO, Zidan R, Yurin V, Bitterman H. Venous thromboembolism and the utility of the Padua prediction score in patients with sepsis admitted to internal medicine departments. J Thromb Haemost 2013;11:467-73. https://doi.org/10.1111/jth.12108.
- Vaziri S, Wilson J, Abbatematteo J, Kubilis P, Chakraborty S, Kshitij K, et al. Predictive performance of the American College of Surgeons universal risk calculator in neurosurgical patients. J Neurosurg 2018;128:942-7. https://dx.doi.org/10.3171/2016.11.JNS161377.
- Vincentelli GM, Timpone S, Murdolo G, Fusco Moffa I, L’Angiocola PD, Borgognoni F, et al. A new risk assessment model for the stratification of the thromboembolism risk in medical patients: the TEVere Score. Minerva Medica 2018;109:436-42. https://dx.doi.org/10.23736/S0026-4806.18.05689-6.
- Wang X, Yang Y-Q, Liu S-H, Hong X-Y, Sun X-F, Shi J-H. Comparing different venous thromboembolism risk assessment machine learning models in Chinese patients. J Eval Clin Pract 2020;26:26-34. https://dx.doi.org/10.1111/jep.13324.
- Winoker JS, Paulucci DJ, Anastos H, Waingankar N, Abaza R, Eun DD, et al. Predicting complications following robot-assisted partial nephrectomy with the ACS NSQIP universal surgical risk calculator. J Urol 2017;198:803-9. https://dx.doi.org/10.1016/j.juro.2017.04.021.
- Woller SC, Stevens SM, Jones JP, Lloyd JF, Evans RS, Aston VT, et al. Derivation and validation of a simple model to identify venous thromboembolism risk in medical patients. Am J Med 2011;124:947-954.e2. https://doi.org/10.1016/j.amjmed.2011.06.004.
- Zhou H, Hu Y, Li X, Wang L, Wang M, Xiao J, et al. Assessment of the risk of venous thromboembolism in medical inpatients using the Padua prediction score and Caprini risk assessment model. J Atheroscler Thromb 2018;25:1091-104. https://dx.doi.org/10.5551/jat.43653.
- Zhou H, Wang L, Wu X, Tang Y, Yang J, Wang B, et al. Validation of a venous thromboembolism risk assessment model in hospitalized Chinese patients: a case–control study. J Atheroscler Thromb 2014;21:261-72. https://doi.org/10.5551/jat.20891.
- Pandor A, Horner D, Davis S, Goodacre S, Stevens JW, Clowes M, et al. Different strategies for pharmacological thromboprophylaxis for lower-limb immobilisation after injury: systematic review and economic evaluation. Health Technol Assess 2019;23:1-190. https://doi.org/10.3310/hta23630.
- Shalhoub J, Lawton R, Hudson J, Baker C, Bradbury A, Dhillon K, et al. GAPS trial investigators. Graduated compression stockings as adjuvant to pharmaco-thromboprophylaxis in elective surgical patients (GAPS study): randomised controlled trial. BMJ 2020;369. https://doi.org/10.1136/bmj.m1309.
- Gee E. The National VTE Exemplar Centres Network response to implementation of updated NICE guidance: venous thromboembolism in over 16s: reducing the risk of hospital-acquired deep vein thrombosis or pulmonary embolism (NG89). Br J Haematol 2019;186:792-3. https://doi.org/10.1111/bjh.16010.
- National Institute for Health and Care Excellence . Guide to the Methods of Technology Appraisal 2013.
- Curtis X, Burns X. Unit Costs of Health and Social Care. UK: Canterbury; 2019.
- Schulman S, Angeras U, Bergqvist D, Eriksson B, Lassen MR, Fisher W, et al. Subcommittee on Control of Anticoagulation of the Scientific and Standardization Committee of the International Society on Thrombosis and Haemostasis. Definition of major bleeding in clinical investigations of antihemostatic medicinal products in surgical patients. J Thromb Haemost 2010;8:202-4. https://doi.org/10.1111/j.1538-7836.2009.03678.x.
- Schulman S, Kearon C. Subcommittee on Control of Anticoagulation of the Scientific and Standardization Committee of the International Society on Thrombosis and Haemostasis. Definition of major bleeding in clinical investigations of antihemostatic medicinal products in non-surgical patients. J Thromb Haemost 2005;3:692-4. https://doi.org/10.1111/j.1538-7836.2005.01204.x.
- Fowler AJ, Abbott TEF, Prowle J, Pearse RM. Age of patients undergoing surgery. Br J Surg 2019;106:1012-8. https://doi.org/10.1002/bjs.11148.
- Hewitt J, Carter B, McCarthy K, Pearce L, Law J, Wilson FV, et al. Frailty predicts mortality in all emergency surgical admissions regardless of age. An observational study. Age Ageing 2019;48:388-94. https://doi.org/10.1093/ageing/afy217.
- Moore E, Munoz-Arroyo R, Schofield L, Radley A, Clark D, Isles C. Death within 1 year among emergency medical admissions to Scottish hospitals: incident cohort study. BMJ Open 2018;8. https://doi.org/10.1136/bmjopen-2017-021432.
- Digital N. Summary Hospital-level Mortality Indicator (SHMI) – Deaths associated with hospitalisation, England, December 2018–November 2019. Journal 2020. https://digital.nhs.uk/data-and-information/publications/statistical/shmi/2020-04/shmi-december-2018---november-2019 (accessed 9 April 2020).
- Clark D, Schofield L, Graham F, Isles C, Gott M, Jarlbaek L. Likelihood of death within one year among a National Cohort of Hospital Inpatients in Scotland. J Pain Symptom Manage 2016;52:E2-4. https://doi.org/10.1016/j.jpainsymman.2016.05.007.
- National Institute for Health and Care Excellence . Venous Thromboembolism: Reducing the Risk of Venous Thromboembolism (Deep Vein Thrombosis and Pulmonary Embolism) in Patients Admitted to Hospital 2018.
- Lederle FA, Sacks JM, Fiore L, Landefeld CS, Steinberg N, Peters RW, et al. The prophylaxis of medical patients for thromboembolism pilot study. Am J Med 2006;119:54-9. https://doi.org/10.1016/j.amjmed.2005.03.049.
- Leizorovicz A, Cohen AT, Turpie AG, Olsson CG, Vaitkus PT, Goldhaber SZ, et al. PREVENT Medical Thromboprophylaxis Study Group . Randomized, placebo-controlled trial of dalteparin for the prevention of venous thromboembolism in acutely ill medical patients. Circulation 2004;110:874-9. https://doi.org/10.1161/01.CIR.0000138928.83266.24.
- Mahe I, Bergmann JF, d’Azemar P, Vaissie JJ, Caulin C. Lack of effect of a low-molecular-weight heparin (nadroparin) on mortality in bedridden medical in-patients: a prospective randomized double-blind study. Eur J Clin Pharmacol 2005;61:347-51. https://doi.org/10.1007/s00228-005-0944-3.
- Samama MM, Cohen AT, Darmon JY, Desjardins L, Eldor A, Janbon C, et al. A comparison of enoxaparin with placebo for the prevention of venous thromboembolism in acutely ill medical patients. Prophylaxis in Medical Patients with Enoxaparin Study Group. N Engl J Med 1999;341:793-800. https://doi.org/10.1056/NEJM199909093411103.
- Wade R, Sideris E, Paton F, Rice S, Palmer S, Fox D, et al. Graduated compression stockings for the prevention of deep-vein thrombosis in postoperative surgical patients: a systematic review and economic model with a value of information analysis. Health Technol Assess 2015;19:1-220. https://doi.org/10.3310/hta19980.
- Shalhoub J, Lawton R, Hudson J, Baker C, Bradbury A, Dhillon K, et al. Compression stockings in addition to low-molecular-weight heparin to prevent venous thromboembolism in surgical inpatients requiring pharmacoprophylaxis: the GAPS non-inferiority RCT. Health Technol Assess 2020;24:1-80. https://doi.org/10.3310/hta24690.
- Decousus H, Tapson VF, Bergmann JF, Chong BH, Froehlich JB, Kakkar AK, et al. IMPROVE Investigators. Factors at admission associated with bleeding risk in medical patients: findings from the IMPROVE investigators. Chest 2011;139:69-7. https://doi.org/10.1378/chest.09-3081.
- Bergqvist D, Flordal PA, Friberg B, Frisell J, Hedberg M, Ljungstrom KG, et al. Thromboprophylaxis with a low molecular weight heparin (tinzaparin) in emergency abdominal surgery. A double-blind multicenter trial. Vasa 1996;25:156-60.
- Nagata C, Tanabe H, Takakura S, Narui C, Saito M, Yanaihara N, et al. Randomized controlled trial of enoxaparin versus intermittent pneumatic compression for venous thromboembolism prevention in Japanese surgical patients with gynecologic malignancy. J Obstet Gynaecol Res 2015;41:1440-8. https://doi.org/10.1111/jog.12740.
- Osman Y, Kamal M, Soliman S, Sheashaa H, Shokeir A, Shehab el-Dein AB. Necessity of routine postoperative heparinization in non-risky live-donor renal transplantation: results of a prospective randomized trial. Urology 2007;69:647-51. https://doi.org/10.1016/j.urology.2006.12.017.
- Sakon M, Kobayashi T, Shimazui T. Efficacy and safety of enoxaparin in Japanese patients undergoing curative abdominal or pelvic cancer surgery: results from a multicenter, randomized, open-label study. Thromb Res 2010;125:e65-70. https://doi.org/10.1016/j.thromres.2009.09.009.
- Song KY, Yoo HM, Kim EY, Kim JI, Yim HW, Jeon HM, et al. Optimal prophylactic method of venous thromboembolism for gastrectomy in Korean patients: an interim analysis of prospective randomized trial. Ann Surg Oncol 2014;21:4232-8. https://doi.org/10.1245/s10434-014-3893-1.
- Jameson SS, Charman SC, Gregg PJ, Reed MR, van der Meulen JH. The effect of aspirin and low-molecular-weight heparin on venous thromboembolism after hip replacement: a non-randomised comparison from information in the National Joint Registry. J Bone Joint Surg Br 2011;93:1465-70. https://doi.org/10.1302/0301-620X.93B11.27622.
- Jameson SS, Baker PN, Charman SC, Deehan DJ, Reed MR, Gregg PJ, et al. The effect of aspirin and low-molecular-weight heparin on venous thromboembolism after knee replacement: a non-randomised comparison using National Joint Registry Data. J Bone Joint Surg Br 2012;94:914-8. https://doi.org/10.1302/0301-620X.94B7.29129.
- Cushner F, Agnelli G, FitzGerald G, Warwick D. Complications and functional outcomes after total hip arthroplasty and total knee arthroplasty: results from the Global Orthopaedic Registry (GLORY). Am J Orthop (Belle Mead NJ) 2010;39:22-8.
- Pedersen AB, Mehnert F, Sorensen HT, Emmeluth C, Overgaard S, Johnsen SP. The risk of venous thromboembolism, myocardial infarction, stroke, major bleeding and death in patients undergoing total hip and knee replacement: a 15-year retrospective cohort study of routine clinical practice. Bone Joint J 2014;96-B:479-85. https://doi.org/10.1302/0301-620X.96B4.33209.
- Turpie AG, Haas S, Kreutz R, Mantovani LG, Pattanayak CW, Holberg G, et al. A non-interventional comparison of rivaroxaban with standard of care for thromboprophylaxis after major orthopaedic surgery in 17,701 patients with propensity score adjustment. Thromb Haemost 2014;111:94-102. https://doi.org/10.1160/TH13-08-0666.
- Kooiman J, van Hagen N, Iglesias Del Sol A, Planken EV, Lip GY, van der Meer FJ, et al. The HAS-BLED score identifies patients with acute venous thromboembolism at high risk of major bleeding complications during the first six months of anticoagulant treatment. PLOS ONE 2015;10. https://doi.org/10.1371/journal.pone.0122520.
- Cohen AT, Gitt AK, Bauersachs R, Fronk EM, Laeis P, Mismetti P, et al. The management of acute venous thromboembolism in clinical practice. Results from the European PREFER in VTE Registry. Thromb Haemost 2017;117:1326-37. https://doi.org/10.1160/TH16-10-0793.
- Nieto JA, Solano R, Ruiz-Ribo MD, Ruiz-Gimenez N, Prandoni P, Kearon C, et al. RIETE Investigators . Fatal bleeding in patients receiving anticoagulant therapy for venous thromboembolism: findings from the RIETE registry. J Thromb Haemost 2010;8:1216-22. https://doi.org/10.1111/j.1538-7836.2010.03852.x.
- Carrier M, Le Gal G, Wells PS, Rodger MA. Systematic review: case-fatality rates of recurrent venous thromboembolism and major bleeding events among patients treated for venous thromboembolism. Ann Intern Med 2010;152:578-89. https://doi.org/10.7326/0003-4819-152-9-201005040-00008.
- Ende-Verhaar YM, Cannegieter SC, Vonk Noordegraaf A, Delcroix M, Pruszczyk P, Mairuhu AT, et al. Incidence of chronic thromboembolic pulmonary hypertension after acute pulmonary embolism: a contemporary view of the published literature. Eur Respir J 2017;49. https://doi.org/10.1183/13993003.01792-2016.
- Pengo V, Lensing AW, Prins MH, Marchiori A, Davidson BL, Tiozzo F, et al. Thromboembolic Pulmonary Hypertension Study Group. Incidence of chronic thromboembolic pulmonary hypertension after pulmonary embolism. N Engl J Med 2004;350:2257-64. https://doi.org/10.1056/NEJMoa032274.
- Hach-Wunderle V, Bauersachs R, Gerlach HE, Eberle S, Schellong S, Riess H, et al. Postthrombotic syndrome 3 years after deep venous thrombosis in the Thrombosis and Pulmonary Embolism in Out-patients (TULIPA) PLUS Registry. J Vasc Surg Venous Lymphat Disord 2013;1:5-12. https://doi.org/10.1016/j.jvsv.2012.07.003.
- van Dongen CJ, Prandoni P, Frulla M, Marchiori A, Prins MH, Hutten BA. Relation between quality of anticoagulant treatment and the development of the postthrombotic syndrome. J Thromb Haemost 2005;3:939-42. https://doi.org/10.1111/j.1538-7836.2005.01333.x.
- Fogelholm R, Murros K, Rissanen A, Avikainen S. Long term survival after primary intracerebral haemorrhage: a retrospective population based study. J Neurol Neurosurg Psychiatry 2005;76:1534-8. https://doi.org/10.1136/jnnp.2004.055145.
- Monreal M, Agnelli G, Chuang LH, Cohen AT, Gumbs PD, Bauersachs R, et al. Deep vein t in Europe-health-related quality of life and mortality. Clin Appl Thromb Hemost 2019;25. https://doi.org/10.1177/1076029619883946.
- Heit JA, Silverstein MD, Mohr DN, Petterson TM, O’Fallon WM, Melton LJ. Predictors of survival after deep vein thrombosis and pulmonary embolism: a population-based, cohort study. Arch Intern Med 1999;159:445-53. https://doi.org/10.1001/archinte.159.5.445.
- Harenberg J, Roebruck P, Heene DL. Subcutaneous low-molecular-weight heparin versus standard heparin and the prevention of thromboembolism in medical inpatients. The Heparin Study in Internal Medicine Group. Haemostasis 1996;26:127-39. https://doi.org/10.1159/000217198.
- Hull RD, Schellong SM, Tapson VF, Monreal M, Samama MM, Nicol P, et al. EXCLAIM (Extended Prophylaxis for Venous ThromboEmbolism in Acutely Ill Medical Patients With Prolonged Immobilization) study. Extended-duration venous thromboembolism prophylaxis in acutely ill medical patients with recently reduced mobility: a randomized trial. Ann Intern Med 2010;153:8-18. https://doi.org/10.7326/0003-4819-153-1-201007060-00004.
- Kleber FX, Witt C, Vogel G, Koppenhagen K, Schomaker U, Flosbach CW, et al. THE-PRINCE Study Group . Randomized comparison of enoxaparin with unfractionated heparin for the prevention of venous thromboembolism in medical patients with heart failure or severe respiratory disease. Am Heart J 2003;145:614-21. https://doi.org/10.1067/mhj.2003.189.
- Cohen AT, Davidson BL, Gallus AS, Lassen MR, Prins MH, Tomkowski W, et al. ARTEMIS Investigators . Efficacy and safety of fondaparinux for the prevention of venous thromboembolism in older acute medical patients: randomised placebo controlled trial. BMJ 2006;332:325-9. https://doi.org/10.1136/bmj.38733.466748.7C.
- Goodacre S, Horspool K, Shephard N, Pollard D, Hunt BJ, Fuller G, et al. Selecting pregnant or postpartum women with suspected pulmonary embolism for diagnostic imaging: the DiPEP diagnostic study with decision-analysis modelling. Health Technol Assess 2018;22:1-230. https://doi.org/10.3310/hta22470.
- Delcroix M, Lang I, Pepke-Zaba J, Jansa P, D’Armini AM, Snijder R, et al. Long-term outcome of patients with chronic thromboembolic pulmonary hypertension: results from an international prospective registry. Circulation 2016;133:859-71. https://doi.org/10.1161/CIRCULATIONAHA.115.016522.
- Menakaya CU, Pennington N, Muthukumar N, Joel J, Ramirez Jimenez AJ, Shaw CJ, et al. The cost of outpatient venous thromboembolism prophylaxis following lower limb injuries. Bone Joint J 2013;95-B:673-7. https://doi.org/10.1302/0301-620X.95B5.30555.
- Sweetland S, Green J, Liu B, Berrington de Gonzalez A, Canonico M, Reeves G, et al. Million Women Study collaborators. Duration and magnitude of the postoperative risk of venous thromboembolism in middle aged women: prospective cohort study. BMJ 2009;339. https://doi.org/10.1136/bmj.b4583.
- Luengo-Fernandez R, Gray AM, Bull L, Welch S, Cuthbertson F, Rothwell PM, et al. Oxford Vascular Study . Quality of life after TIA and stroke: ten-year results of the Oxford Vascular Study. Neurology 2013;81:1588-95. https://doi.org/10.1212/WNL.0b013e3182a9f45f.
- Luengo-Fernandez R, Yiin GS, Gray AM, Rothwell PM. Population-based study of acute- and long-term care costs after stroke in patients with AF. Int J Stroke 2013;8:308-14. https://doi.org/10.1111/j.1747-4949.2012.00812.x.
- NHS Improvement . National Cost Collection: National Schedule of NHS Costs – Year 2018-19 - NHS Trust and NHS Foundation 2021.
- Caprini JA, Botteman MF, Stephens JM, Nadipelli V, Ewing MM, Brandt S, et al. Economic burden of long-term complications of deep vein thrombosis after total hip replacement surgery in the United States. Value Health 2003;6:59-74. https://doi.org/10.1046/j.1524-4733.2003.00204.x.
- Lubberts B, Paulino Pereira NR, Kabrhel C, Kuter DJ, DiGiovanni CW. What is the effect of venous thromboembolism and related complications on patient reported health-related quality of life? A meta-analysis. Thromb Haemost 2016;116:417-31. https://doi.org/10.1160/TH16-02-0152.
- Ghanima W, Wik HS, Tavoly M, Enden T, Jelsness-Jorgensen LP. Late consequences of venous thromboembolism: measuring quality of life after deep vein thrombosis and pulmonary embolism. Thromb Res 2018;164:170-6. https://doi.org/10.1016/j.thromres.2017.07.025.
- Utne KK, Dahm A, Wik HS, Jelsness-Jorgensen LP, Sandset PM, Ghanima W. Rivaroxaban versus warfarin for the prevention of post-thrombotic syndrome. Thromb Res 2018;163:6-11. https://doi.org/10.1016/j.thromres.2018.01.013.
- Chuang LH, Gumbs P, van Hout B, Agnelli G, Kroep S, Monreal M, et al. Health-related quality of life and mortality in patients with pulmonary embolism: a prospective cohort study in seven European countries. Qual Life Res 2019;28:2111-24. https://doi.org/10.1007/s11136-019-02175-z.
- Amin AP, Bachuwar A, Reid KJ, Chhatriwalla AK, Salisbury AC, Yeh RW, et al. Nuisance bleeding with prolonged dual antiplatelet therapy after acute myocardial infarction and its impact on health status. J Am Coll Cardiol 2013;61:2130-8. https://doi.org/10.1016/j.jacc.2013.02.044.
- Amin AP, Wang TY, McCoy L, Bach RG, Effron MB, Peterson ED, et al. Impact of bleeding on quality of life in patients on DAPT: insights from TRANSLATE-ACS. J Am Coll Cardiol 2016;67:59-65. https://doi.org/10.1016/j.jacc.2015.10.034.
- Enden T, Wik HS, Kvam AK, Haig Y, Klow NE, Sandset PM. Health-related quality of life after catheter-directed thrombolysis for deep vein thrombosis: secondary outcomes of the randomised, non-blinded, parallel-group CaVenT study. BMJ Open 2013;3. https://doi.org/10.1136/bmjopen-2013-002984.
- Lenert LA, Soetikno RM. Automated computer interviews to elicit utilities: potential applications in the treatment of deep venous thrombosis. J Am Med Inform Assoc 1997;4:49-56. https://doi.org/10.1136/jamia.1997.0040049.
- Meads DM, McKenna SP, Doughty N, Das C, Gin-Sing W, Langley J, et al. The responsiveness and validity of the CAMPHOR Utility Index. Eur Respir J 2008;32:1513-9. https://doi.org/10.1183/09031936.00069708.
- Marchetti M, Pistorio A, Barone M, Serafini S, Barosi G. Low-molecular-weight heparin versus warfarin for secondary prophylaxis of venous thromboembolism: a cost-effectiveness analysis. Am J Med 2001;111:130-9. https://doi.org/10.1016/s0002-9343(01)00793-8.
- Ara R, Brazier JE. Using health state utility values from the general population to approximate baselines in decision analytic models when condition-specific data are not available. Value Health 2011;14:539-45. https://doi.org/10.1016/j.jval.2010.10.029.
- Quinlan DJ, Eikelboom JW, Dahl OE, Eriksson BI, Sidhu PS, Hirsh J. Association between asymptomatic deep vein thrombosis detected by venography and symptomatic venous thromboembolism in patients undergoing elective hip or knee surgery. J Thromb Haemost 2007;5:1438-43. https://doi.org/10.1111/j.1538-7836.2007.02571.x.
- Newcombe RG. Two-sided confidence intervals for the single proportion: comparison of seven methods. Stat Med 1998;17:857-72. https://doi.org/10.1002/(sici)1097-0258(19980430)17:8<857::aid-sim777>3.0.co;2-e.
- Hirst E, Irving A, Goodacre S. Patient and public involvement in emergency care research. Emerg Med J 2016;33:665-70. https://doi.org/10.1136/emermed-2016-205700.
- National Institute for Health and Care Excellence . COVID-19 Rapid Guideline [NG186]: Reducing the Risk of Venous Thromboembolism in Over 16s With COVID-19 2020. www.nice.org.uk/guidance/ng186 (accessed 22 July 2022).
- Caprini JA, Arcelus JI, Hasty JH, Tamhane AC, Fabrega F. Clinical assessment of venous thromboembolic risk in surgical patients. Semin Thromb Hemost 1991;17:304-12.
- Chopard P, Spirk D, Bounameaux H. Identifying acutely ill medical patients requiring thromboprophylaxis. J Thromb Haemost 2006;4:915-6. https://doi.org/10.1111/j.1538-7836.2006.01818.x.
- Geerts WH, Pineo GF, Heit JA, Bergqvist D, Lassen MR, Colwell CW, et al. Prevention of venous thromboembolism: the Seventh ACCP Conference on Antithrombotic and Thrombolytic Therapy. Chest 2004;126:338S-400S. https://doi.org/10.1378/chest.126.3_suppl.338S.
- Horner D, Goodacre S, Davis S, Burton N, Hunt BJ. Which is the best model to assess risk for venous thromboembolism in hospitalised patients?. BMJ 2021;373. https://doi.org/10.1136/bmj.n1106.
- Kucher N, Koo S, Quiroz R, Cooper JM, Paterno MD, Soukonnikov B, et al. Electronic alerts to prevent venous thromboembolism among hospitalized patients. N Engl J Med 2005;352:969-77. https://doi.org/10.1056/NEJMoa041533.
- Rosenberg DJ, Press A, Fishbein J, Lesser M, McCullagh L, McGinn T, et al. External validation of the IMPROVE Bleeding Risk Assessment Model in medical patients. Thromb Haemost 2016;116:530-6. https://doi.org/10.1160/TH16-01-0003.
- Spyropoulos AC, Anderson FA, FitzGerald G, Decousus H, Pini M, Chong BH, et al. IMPROVE Investigators . Predictive and associative models to identify hospitalized medical patients at risk for VTE. Chest 2011;140:706-14. https://doi.org/10.1378/chest.10-1944.
- Tapson VF, Decousus H, Pini M, Chong BH, Froehlich JB, Monreal M, et al. IMPROVE Investigators . Venous thromboembolism prophylaxis in acutely ill hospitalized medical patients: findings from the International Medical Prevention Registry on Venous Thromboembolism. Chest 2007;132:936-45. https://doi.org/10.1378/chest.06-2993.
- Roberts LN, Hunt B, Arya R, Briggs T. National Thrombosis Survey 2021. https://thrombosisuk.org/ (accessed 22 July 2022).
- Sharma A, Minh Duc NT, Luu Lam Thang T, Nam NH, Ng SJ, Abbas KS, et al. A Consensus-Based Checklist for Reporting of Survey Studies (CROSS). J Gen Intern Med 2021;36:3179-87. https://doi.org/10.1007/s11606-021-06737-1.
- Cobben M, Nemeth B, Lijfering W. Validation of risk assessment models for venous thrombosis in hospitalised medical inpatients. Res Pract Thromb Haemost 2019;3:217-25. https://doi.org/10.1002/rth2.12181.
- Darzi AJ, Karam SG, Spencer FA, Spyropoulos AC, Mbuagbaw L, Woller SC, et al. Risk models for VTE and bleeding in medical inpatients: systematic identification and expert assessment. Blood Adv 2020;4:2557-66. https://doi.org/10.1182/bloodadvances.2020001937.
- Wright FL, Green J, Canoy D, Cairns BJ, Balkwill A, Beral V, et al. Million Women Study Collaborators. Vascular disease in women: comparison of diagnoses in hospital episode statistics and general practice records in England. BMC Med Res Methodol 2012;12. https://doi.org/10.1186/1471-2288-12-161.
- Abdul Sultan A, Tata LJ, Grainge MJ, West J. The incidence of first venous thromboembolism in and around pregnancy using linked primary and secondary care data: a population based cohort study from England and comparative meta-analysis. PLOS ONE 2013;8. https://doi.org/10.1371/journal.pone.0070310.
- Lawrenson R, Todd JC, Leydon GM, Williams TJ, Farmer RD. Validation of the diagnosis of venous thromboembolism in general practice database studies. Br J Clin Pharmacol 2000;49:591-6. https://doi.org/10.1046/j.1365-2125.2000.00199.x.
- Verma AA, Masoom H, Pou-Prom C, Shin S, Guerzhoy M, Fralick M, et al. Developing and validating natural language processing algorithms for radiology reports compared to ICD-10 codes for identifying venous thromboembolism in hospitalized medical patients. Thromb Res 2021;209:51-8. https://doi.org/10.1016/j.thromres.2021.11.020.
- Sanfilippo KM, Wang TF, Gage BF, Liu W, Carson KR. Improving accuracy of International Classification of Diseases codes for venous thromboembolism in administrative data. Thromb Res 2015;135:616-20. https://doi.org/10.1016/j.thromres.2015.01.012.
- Alotaibi GS, Wu C, Senthilselvan A, McMurtry MS. The validity of ICD codes coupled with imaging procedure codes for identifying acute venous thromboembolism using administrative data. Vasc Med 2015;20:364-8. https://doi.org/10.1177/1358863X15573839.
- Baumgartner C, Go AS, Fan D, Sung SH, Witt DM, Schmelzer JR, et al. Administrative codes inaccurately identify recurrent venous thromboembolism: The CVRN VTE study. Thromb Res 2020;189:112-8. https://doi.org/10.1016/j.thromres.2020.02.023.
- Pellathy T, Saul M, Clermont G, Dubrawski AW, Pinsky MR, Hravnak M. Accuracy of identifying hospital acquired venous thromboembolism by administrative coding: implications for big data and machine learning research. J Clin Monit Comput 2021;36:397-405. https://doi.org/10.1007/s10877-021-00664-6.
- Sydes MR, Barbachano Y, Bowman L, Denwood T, Farmer A, Garfield-Birkbeck S, et al. Data Enabled Trials Group Workshop Group members. Realising the full potential of data-enabled trials in the UK: a call for action. BMJ Open 2021;11. https://doi.org/10.1136/bmjopen-2020-043906.
- Spencer FA, Emery C, Lessard D, Anderson F, Emani S, Aragam J, et al. The Worcester Venous Thromboembolism study: a population-based study of the clinical epidemiology of venous thromboembolism. J Gen Intern Med 2006;21:722-7. https://doi.org/10.1111/j.1525-1497.2006.00458.x.
- Burles K, Innes G, Senior K, Lang E, McRae A. Limitations of pulmonary embolism ICD-10 codes in emergency department administrative data: let the buyer beware. BMC Med Res Methodol 2017;17. https://doi.org/10.1186/s12874-017-0361-1.
- Powell GA, Bonnett LJ, Smith CT, Hughes DA, Williamson PR, Marson AG. Using routinely recorded data in a UK RCT: a comparison to standard prospective data collection methods. Trials 2021;22. https://doi.org/10.1186/s13063-021-05294-6.
- Liaw ST, Chen HY, Maneze D, Taggart J, Dennis S, Vagholkar S, et al. Health reform: is routinely collected electronic information fit for purpose?. Emerg Med Australas 2012;24:57-63. https://doi.org/10.1111/j.1742-6723.2011.01486.x.
- Lowenstern A, Lippmann SJ, Brennan JM, Wang TY, Curtis LH, Feldman T, et al. Use of Medicare claims to identify adverse clinical outcomes after mitral valve repair. Circ Cardiovasc Interv 2019;12. https://doi.org/10.1161/CIRCINTERVENTIONS.118.007451.
- Macnair A, Love SB, Murray ML, Gilbert DC, Parmar MKB, Denwood T, et al. Accessing routinely collected health data to improve clinical trials: recent experience of access. Trials 2021;22. https://doi.org/10.1186/s13063-021-05295-5.
- Jerome J. Thrombosis UK 2021. https://thrombosisuk.org (accessed 6 December 2021).
- Beck MJ, Haidet P, Todoric K, Lehman E, Sciamanna C. Reliability of a point-based VTE risk assessment tool in the hands of medical residents. J Hosp Med 2011;6:195-201. https://doi.org/10.1002/jhm.860.
- Vyas D. Variations in risk assessment models may contribute to the existing gap between venous thromboembolism prophylaxis guidelines and adherence. Springerplus 2012;1. https://doi.org/10.1186/2193-1801-1-60.
- Darzi AJ, Karam SG, Charide R, Etxeandia-Ikobaltzeta I, Cushman M, Gould MK, et al. Prognostic factors for VTE and bleeding in hospitalized medical patients: a systematic review and meta-analysis. Blood 2020;135:1788-810. https://doi.org/10.1182/blood.2019003603.
- Zakai NA, Koh I, Wilkinson K, Roetker N, Sparks A, Repp A, et al. Risk assessing medical inpatients for hospital-acquired venous thrombosis: The Medical Inpatients Thrombosis and Hemostasis (MITH) Study. Blood 2021;138.
- Nemeth B, Cannegieter SC. Venous thrombosis following lower-leg cast immobilization and knee arthroscopy: from a population-based approach to individualized therapy. Thromb Res 2019;174:62-75. https://doi.org/10.1016/j.thromres.2018.11.030.
- Nemeth B, Nelissen R, Arya R, Cannegieter S. Preventing VTE following total hip and knee arthroplasty: is prediction the future?. J Thromb Haemost 2021;19:41-5. https://doi.org/10.1111/jth.15132.
- Nemeth B, van Adrichem R, Nelissen R, le Cessie S, Cannegieter SC. Individualized thromboprophylaxis in patients with lower-leg cast immobilization – a validation and subgroup analysis in the POT-CAST Trial. Thromb Haemost 2019;119:1508-16. https://doi.org/10.1055/s-0039-1693410.
- NIHR . 21/588/Timing/of/Pharmacological/Thromboprophylaxis/in/Traumatic/Brain/Injury/Commissioning/Brief 2021. www.nihr.ac.uk/documents/21588-timing-of-pharmacological-thromboprophylaxis-in-traumatic-brain-injury-commissioning-brief/29197 (accessed 6 December 2021).
- Goodacre S, Horspool K, Shephard N, Pollard D, Hunt BJ, Fuller G, et al. Selecting pregnant or postpartum women with suspected pulmonary embolism for diagnostic imaging: the DiPEP diagnostic study with decision-analysis modelling. Health Technol Assess 2018;22:1-230. https://doi.org/10.3310/hta22470.
- Department of Health . National Schedule of Reference Costs – Year 2015–16 – NHS Trust and NHS Foundation Trusts 2016.
- Joint Formulary Committee . British National Formulary (online) [Drug Tariff] n.d. www.medicinescomplete.com (accessed 24 January 2018).
- Curtis L, Burns A. Unit Costs of Health and Social Care 2017. Canterbury, UK: Personal Social Services Research Unit; 2017.
- National Clinical Guideline Centre – Acute and Chronic Conditions (UK) . Venous Thromboembolism: Reducing the Risk of Venous Thromboembolism (Deep Vein Thrombosis and Pulmonary Embolism) in Patients Admitted to Hospital 2010. www.ncbi.nlm.nih.gov/books/NBK116518/ (accessed 4 March 2018).
Appendix 1 Literature search strategy and additional materials for the systematic review of RAMs for VTE in hospital inpatients
Database searched: | Ovid MEDLINE® Epub Ahead of Print, In-Process and Other Non-Indexed Citations, Ovid MEDLINE® Daily, Ovid MEDLINE and Versions® |
Platform or provider used: | Ovid SP |
Date of coverage: | 1946 to February 2021 |
Search undertaken: | February 2021 |
-
pulmonary embolism/ or thromboembolism/ or venous thromboembolism/ or venous thrombosis/ or upper extremity deep vein thrombosis/
-
(((venous or vein) adj (thrombosis or thromboses or thrombus or thromboembolism)) or (dvt or vte) or ((pulmonary or lung) adj3 (embolism or emboli or embolus or emboliz* or thromboembolism))).ti,ab.
-
1 or 2
-
letter/ or editorial/ or news/ or exp historical article/ or anecdotes as topic/ or comment/ or case report/ or (letter or comment).ti.
-
randomized controlled trial/ or random*.ti,ab.
-
4 not 5
-
animals/ not humans/
-
exp animals, laboratory/
-
exp animal experimentation/
-
exp models, animal/
-
exp rodentia/
-
(rat or rats or mouse or mice).ti.
-
6 or 7 or 8 or 9 or 10 or 11 or 12
-
3 not 13
-
(risk* adj2 assess*).ti,ab.
-
((score* or scoring) adj2 (tool* or system*)).ti,ab.
-
((risk* or predict* or prognos*) adj4 (tool* or rule* or index* or indices or score* or scoring or scale* or model* or system* or algorithm* or stratif* or criteria or calculat*)).ti,ab.
-
(vienna adj5 cats).ti,ab.
-
(vienna cancer and thrombosis study).ti,ab.
-
trauma embolic scoring.ti,ab.
-
tess.ti,ab.
-
(roger* or caprini* or kucher* or cohen* or padua* or khorana* or autar).ti,ab.
-
(well* adj2 (score* or scoring)).ti,ab.
-
department of health.ti,ab,au.
-
or/15-24
-
14 and 25
-
limit 26 to yr=‘2017 -Current’
Databases searched: | EMBASE |
Platform or provider used: | Ovid SP |
Date of coverage: | 1974 to February 2021 |
Search undertaken: | February 2021 |
-
thromboembolism/ or venous thromboembolism/ or vein thrombosis/ or deep veinthrombosis/ or leg thrombosis/ or lower extremity deep vein thrombosis/ or postoperativethrombosis/ or lung embolism/ or upper extremity deep vein thrombosis/
-
(((venous or vein) adj (thrombosis or thromboses or thrombus or thromboembolism)) or (dvt or vte) or ((pulmonary or lung) adj3 (embolism or emboli or embolus or emboliz* or thromboembolism))).ti,ab.
-
1 or 2
-
letter.pt. or letter/ or note.pt. or editorial.pt. or case report/ or case study/ or (letter or comment*).ti.
-
randomized controlled trial/ or random*.ti,ab.
-
4 not 5
-
animal/ not human/
-
nonhuman/
-
exp animal experiment/ or exp experimental animal/
-
animal model/
-
exp rodent/
-
(rat or rats or mouse or mice).ti.
-
6 or 7 or 8 or 9 or 10 or 11 or 12
-
3 not 13
-
(risk* adj2 assess*).ti,ab.
-
((score* or scoring) adj2 (tool* or system*)).ti,ab.
-
((risk* or predict* or prognos*) adj4 (tool* or rule* or index* or indices or score* or scoring or scale* or model* or system* or algorithm* or stratif* or criteria or calculat*)).ti,ab.
-
(vienna adj5 cats).ti,ab.
-
(vienna cancer and thrombosis study).ti,ab.
-
trauma embolic scoring.ti,ab.
-
tess.ti,ab.
-
(roger* or caprini* or kucher* or cohen* or padua* or khorana* or autar).ti,ab.
-
(well* adj2 (score* or scoring)).ti,ab.
-
department of health.ti,ab,au.
-
or/15-24
-
14 and 25
-
limit 26 to yr=‘2017 -Current’
Databases searched: | Cochrane CENTRAL Register of Randomised Controlled Trials & Cochrane Database of Systematic Reviews |
Platform or provider used: | www.thecochranelibrary.com |
Date of coverage: | Inception to February 2021 |
Search undertaken: | February 2021 |
-
MeSH descriptor: [venous thromboembolism] this term only
-
MeSH descriptor: [pulmonary embolism] this term only
-
MeSH descriptor: [venous thrombosis] this term only
-
MeSH descriptor: [thromboembolism] this term only
-
MeSH descriptor: [upper extremity deep vein thrombosis] this term only
-
((*venous or *vein) next (thrombosis or thromboses or thrombus or thromboembolism) or dvt or vte or (pulmonary or lung) near/3 (embolism or emboli or embolus or emboliz* or thromboembolism)):ti,ab
-
#1 or #2 or #3 or #4 or #6
-
(risk* near/2 assess*):ti,ab
-
((score* or scoring) near/2 (tool* or system*)):ti,ab
-
((risk* or predict* or prognos*) near/4 (tool* or rule* or index* or indices or score* or scoring or scale* or model* or system* or algorithm* or stratif* or criteria or calculat*)):ti,ab
-
(vienna near/5 cats):ti,ab
-
(vienna cancer and thrombosis study):ti,ab
-
trauma embolic scoring:ti,ab
-
tess:ti,ab
-
(roger* or caprini* or kucher* or cohen* or padua* or khorana* or autar):ti,ab
-
(well* near/2 (score* or scoring)):ti,ab
-
(department of health):ti,ab
-
(or #8-#17)
-
Domains | |||
---|---|---|---|
Patient selection | Predictors | Outcome | Analysis |
Signalling questions a | |||
1.1 Were appropriate data sources used, e.g. cohort, RCT or nested case–control study data? | 2.1 Were predictors defined and assessed in a similar way for all participants? | 3.1 Was the outcome determined appropriately? | 4.1 Were there a reasonable number of participants with the outcome? |
1.2 Were all inclusions and exclusions of participants appropriate? | 2.2 Were predictor assessments made without knowledge of outcome data? | 3.2 Was a pre-specified or standard outcome definition used? | 4.2 Were continuous and categorical predictors handled appropriately? |
– | 2.3 Are all predictors available at the time the model is intended to be used? | 3.3 Were predictors excluded from the outcome definition? | 4.3 Were all enrolled participants included in the analysis? |
– | – | 3.4 Was the outcome defined and determined in a similar way for all participants? | 4.4 Were participants with missing data handled appropriately? |
– | – | 3.5 Was the outcome determined without knowledge of predictor information? | Development studies only 4.5 Was selection of predictors based on univariable analysis avoided? |
– | – | 3.6 Was the time interval between predictor assessment and outcome determination appropriate? | 4.6 Were complexities in the data (e.g. censoring, competing risks, sampling of controls) accounted for appropriately? |
– | – | – | 4.7 Were relevant model performance measures evaluated appropriately? |
– | – | – | Development studies only 4.8 Was model overfitting, underfitting and optimism in model performance accounted for? |
– | – | – | Development studies only 4.9 Do predictors and their assigned weights in the final model correspond to the results from the reported multivariable analysis? |
RISK OF BIAS b | |||
Could the selection of participants have introduced bias? | Could the selection of predictors or their assessments have introduced bias? | Could the selection of outcomes or its determination have introduced bias? | Could the statistical analysis have introduced bias? |
APPLICABILITY c | |||
Is there concern that the included participants and setting do not match the review question? | Is there concern that the definition, assessment, or timing of predictors in the model does not match the review question? | Is there concern that the outcome, its definition, timing, or determination does not match the review question? | – |
Characteristics | Name of VTE risk assessment model | |||||
---|---|---|---|---|---|---|
Caprini score |
Padua prediction score |
IMPROVE predictive score |
IMPROVE associative score |
Geneva risk score |
Kucher score |
|
General | ||||||
Author, year | Caprini 2005142 | Barbar 201015 | Tapson 2007149 | Spyropoulos 2011148 | Chopard 2006143 | Kucher 2005146 |
Applicable cohort | Surgical and medical | Medical | Medical | Medical | Medical | Surgical and medical |
Design | Ordinal with cumulative score | Dichotomous variables with cumulative score | Dichotomous variables with VTE probability estimate | Dichotomous variables with VTE probability estimate | Dichotomous variables with cumulative score | Dichotomous variables with cumulative score |
Number of VTE risk variables | 31 | 11 | 4 | 7 | 19 | 8 |
C-statistic (range) across medical, surgical and trauma cohorts | 0.53–0.87 (12 studies) |
0.594–0.756 (7 studies) |
0.57–0.65 (2 studies) |
0.63–0.7731 (4 studies) |
0.61 (1 study) |
0.563–0.756 (4 studies) |
When is pharmacological thromboprophylaxis recommended? | Score ≥ 5 | Score ≥ 4 | No specific threshold Identified |
No specific threshold identified | Score ≥ 3 | Score ≥ 4 |
Clinical variables | ||||||
Patient related | ||||||
Active cancer | Yes | Yes | Yes | Yes | Yes | Yes (major risk) |
Age | Yes | Yes (≥ 70) | Yes (≥ 60) | Yes (≥ 60) | Yes (≥ 60) | Yes (≥ 70 minor risk) |
Dehydration | No | No | No | No | Yes | No |
Thrombophilia | Yes (generic and named conditions) | Yes (generic) | Yes (generic) | Yes (generic) | Yes (generic) | Yes (major risk) |
Obesity | Yes (≥ 25 kg/m2) | Yes (≥ 30 kg/m2) | No | No | Yes (≥ 30 kg/m2) | Yes (≥ 30 kg/m2 minor risk) |
Comorbidity | Yes (1–5 points for individual comorbidities) | Yes (1 point each for several individual comorbidities) | No | No | Yes (2 points each for several individual comorbidities) | No |
Prior VTE | Yes | Yes | Yes | Yes | Yes | Yes (major risk) |
Family history of VTE | Yes | No | No | No | No | No |
Use of HRT | Yes | Yes | No | No | Yes | Yes (minor risk) |
Use of oestrogen-containing contraceptive therapy | Yes | Yes | No | No | Yes | Yes (minor risk) |
Varicose veins | Yes | No | No | No | No | No |
Pregnancy or post-partum period | No | No | No | No | Yes | No |
Unexplained stillbirth or spontaneous abortions | Yes (≥ 3 spontaneous abortions) | No | No | No | No | No |
Current swollen legs | Yes | No | No | No | Yes | No |
Current central venous access | Yes | No | No | No | No | No |
Recent major surgery | Yes (< 1 month) | Yes (< 1 month) | No | No | No | No |
Recent use of plaster cast immobilisation | Yes (< 1 month) | No | No | No | No | No |
Lower limb paralysis | Yes | No | No | Yes | No | No |
Travel related | No | No | No | No | Yes (> 6 hours) | No |
Admission related | ||||||
Reduced mobility | Yes (variable points) | Yes | No | Yes (≥ 7 days) | Yes (≥ 3 days) | Yes (minor risk) |
Arthroplasty surgery | Yes | No | No | No | No | No |
Hip fracture | Yes | No | No | No | No | No |
Pelvic or lower limb surgery | Yes (arthroscopic) | No | No | No | No | No |
Total anaesthetic and surgical time | Yes (≥ 45 minutes) | No | No | No | No | Yes (≥ 60 minutes intermediate risk) |
Acute surgical admission | No | No | No | No | No | No |
Acute infection | No | Yes | No | No | Yes | No |
Acute rheumatological disorder | No | Yes | No | No | Yes | No |
Critical care admission | No | No | No | Yes | No | No |
Surgery leading to reduced mobility | Yes | No | No | No | No | No |
Other | ||||||
‘Other risk factors’ | Yes | No | No | No | No | No |
Data source (Author, year) | End point | Incidence | Risk assessment model | Threshold or cut-off | Predictive performance | ||||
---|---|---|---|---|---|---|---|---|---|
C-statistic (95% CI) | Sensitivity (95% CI) | Specificity (95% CI) | |||||||
Mixed inpatients | |||||||||
Autar 200325 | DVT | 18.9% | Novel (Autar 200326) | Risk score ≤ 6 | NR | 100% (NR) | 100% (NR) | ||
Novel (Autar 200326) | Risk score 7–10 | NR | 86.0% (NR) | 68.0% (NR) | |||||
Novel (Autar 200326) | Risk score 11–14 | NR | 68.0% (NR) | 31.0% (NR) | |||||
Novel (Autar 200326) | Risk score ≥ 15 | NR | 25.0% (NR) | 10.0% (NR) | |||||
Elias 201736 | VTE | 0.8% | Padua (automated) | Risk score ≥ 5 | 0.81 (0.79 to 0.83) | 85.4% (NR) | 53.3% (NR) | ||
Chen 201832 | DVT | NA | Caprini | Risk score ≥ 4 | NR | 73.8% (NR) | 64.7% (NR) | ||
Caprini | Risk score ≥ 5 | NR | 62.8% (NR) | 82.6% (NR) | |||||
Padua | Risk score ≥ 3 | NR | 53.5% (NR) | 82.1% (NR) | |||||
Padua | Risk score ≥ 4 | NR | 42.1% (NR) | 92.5% (NR) | |||||
Bo 202031 | DVT | 0.9% | Caprini | Risk score ≥ 3.5 | 0.74 (0.71 to 0.77) | 75.0% (NR) | 62.0% (NR) | ||
Cancer inpatients | |||||||||
Abdel-Razeq 201024 | VTE | 3.5% | Caprini (modified) | Risk score ≥ 3 | NR | 100% (NR) | 9.2% (NR) | ||
Caprini (modified) | Risk score ≥ 5 | NR | 57.1% (NR) | 53.2% (NR) | |||||
Patell 201756 | VTE | 3.8% | Khorana | Risk score ≥ 3 | NR | 18.9% (NR) | 87.2% (NR) | ||
Hu 202044 | VTE | NA | Caprini | Risk score ≥ 5 | 0.71 (0.66 to 0.75) | 82.4% (NR) | 46.2% (NR) | ||
Khorana | Risk score ≥ 2 | 0.58 (0.53 to 0.63) | 35.3% (NR) | 78.7% (NR) | |||||
Shang 202062 | VTE | NA | Caprini 2009 | Risk score ≥ 3 | 0.72 (0.70 to 0.74) | 83.5% (NR) | 52.7% (NR) | ||
Caprini 2013 | Risk score ≥ 5 | 0.80 (0.78 to 0.82) | 80.9% (NR) | 65.9% (NR) | |||||
Burns inpatients | |||||||||
Pannucci 201255 | VTE | 1.0% | Novel (Pannucci 201256) | NR | 0.75 | NR | NR | ||
Post-stroke inpatients | |||||||||
Liu 201446 | DVT | 10.5% | Post-stroke DVT Prediction System | NR | 0.65 (0.59 to 0.70) | NR | NR | ||
Sepsis inpatients | |||||||||
Vardi 201366 | VTE | 1.3% | Padua | NR | All patients 0.58 (0.43 to 0.73) |
All patients NR |
All patients NR |
||
Padua | NR | No prophylaxis 0.54 (0.37 to 0.71) |
No prophylaxis NR |
No prophylaxis NR |
Appendix 2 Supplementary material from the economic evaluation
Parameter description | Midpoint value | Uncertainty measure | Distribution | Source | ||
---|---|---|---|---|---|---|
Sensitivity of decision tools | See Figure 6 | Not reported – assumed fixed in PSA | Not applicable | Systematic review of RAMs | ||
Specificity of decision tools | See Figure 6 | Not reported – assumed fixed | Not applicable | Systematic review of RAMs | ||
Probability of PE in medical inpatients | 1.38% | 95% CI 0.7% to 2.2% | Beta (13, 929) | Barbar et al.15 | ||
Probability of symptomatic DVT in medical inpatients | 2.02% | 95% CI 1.2% to 3.0% | Beta (19, 923) | Barbar et al.15 | ||
Probability of PE in (elective) surgical inpatients | 0.62% | 95% CI 0.45% to 0.82% | Beta (42, 6726) | Pannucci et al.54 | ||
Probability of symptomatic DVT in (elective) surgical inpatients | 0.78% | 95% CI 0.59% to 1.01% | Beta (53, 6715) | Pannucci et al.54 | ||
Proportion of all DVTs that are symptomatic | 6.21% | 95% CI 4.4% to 8.2% | Beta (40, 604) | CG92 [This results in an incidence of asymptomatic DVT of 12.2% (95% CI 7.9% to 18.%) in medical inpatients and 31.4% (95% CI 16.9% to 51.9%) in surgical inpatients] |
||
Proportion of DVTs that are distal (same proportion applied for symptomatic and asymptomatic DVTs) | 69% | 95% CI 67% to 71% | =1-Beta (1991, 32,713)/ Beta (6467, 28,789) |
CG92 reports that 31% of all DVTs were proximal as estimated from the RCTs in their review that reported the incidence of both: (1991/34,704)/(6467/35,256) = (6%/18%) = 31% | ||
Effectiveness of prophylaxis in acutely ill medical inpatients – Risk ratio (RR) for VTE |
0.49 | 95% CI 0.37 to 0.67 | Lognormal (−0.40, 0.15) |
Meta-analysis of VTE events in the three RCTs included in NG89 for LMWH (standard dose/standard duration) vs. placebo in acutely ill medical patients | ||
Effectiveness of prophylaxis in surgical inpatients – Risk ratio (OR) for VTE |
0.26 | 95% CI 0.09 to 0.87 | Lognormal (−1.34, 0.58) |
Network meta-analysis by Wade et al.98 – estimate for heparin vs. no heparin | ||
Risk of major bleeding for PPX in medical inpatients | 1.02% | 95 CI 0.65% to 1.47% | Beta (23, 2236) | Incidence of bleeding across the LMWH arms of three RCTs which reported bleeding risk in the systematic review of LMWH vs. placebo for acutely ill medical inpatients reported in NG89 | ||
Risk of major bleeding for PPX in inpatients having elective surgery | 3.70% | 95 CI 1.87% to 6.13% | Beta (11, 286) | Incidence of bleeding across the LMWH arms of five RCTs which reported bleeding risk in the systematic review of LMWH vs. placebo/mechanical prophylaxis for abdominal surgery in NG89 | ||
Proportion of major bleeding during PPX that is fatal for medical inpatients | 14% | 95% CI 8% to 23% | Beta (12, 71) | Bleeds occurring within 14 days of hospitalisation for medical inpatient (minimum length of stay of 3 days) from IMPROVE registry – average across cohort regardless of use of PPX or not (Decousus et al.100) | ||
Proportion of major bleeding during PPX that is fatal for surgical inpatients | 0.9% | 95% CI 0.02% to 3.36% | Beta (1, 108) | Proportion of major bleeds that were fatal in cohort of patients having elective hip or knee replacements receiving standard care of which 81.7% received LMWH (Turpie et al.110) | ||
Proportion of non-fatal major bleeding during PPX that is ICH for medical inpatients | 10% | 95% CI 4% to 18% | Beta (7, 64) | Bleeds occurring within 14 days of hospitalisation for medical inpatient (minimum length of stay of 3 days) from IMPROVE registry – average across cohort regardless of use of PPX or not (Decousus et al.100) | ||
Proportion of non-fatal major bleeding during PPX that is ICH for surgical inpatients | 1.9% | 95% CI 0.23% to 5.10% | Beta (2, 106) | Proportion of non-fatal major bleeds that were ICH in cohort of patients having elective hip or knee replacements receiving standard care of which 81.7% received LMWH (Turpie et al.110) | ||
RR of bleeding for prophylaxis vs. none in medical inpatients – HR | 1.53 | 95% CI 0.90 to 2.53 | Lognormal (0.43, 0.33) | Meta-analysis of VTE events in the three RCTs included in NG89 for LMWH (standard dose/standard duration) vs. placebo in acutely ill medical patients | ||
RR of bleeding for prophylaxis vs. none in elective surgical inpatients – HR | 2.98 | 95% CI 0.88 to 14.80 | Lognormal (1.01, 0.72) | Network meta-analysis of major bleeding for LMWH (standard dose/standard duration) vs. placebo/mechanical prophylaxis in patients having abdominal surgery from NG89 | ||
Risk of bleeding during 3-month anticoagulant treatment for VTE | 0.8% | 95% CI 0.2% to 2.0% | Beta (3, 352) | 6-month incidence pooled across patients with HAS-BLED score of 0 or 1 from Kooiman et al.104 | ||
Proportion of major bleeds during VTE treatment that are fatal | 25% | 95% CI 21% to 28% | Beta (135, 411) | Based on case-fatality rates for major bleeds within the RIETE registry (Nieto et al.)106 | ||
Proportion of non-fatal major bleeds during VTE treatment that are ICH | 9% | 95% CI 6.5% to 11.9% | Beta (37, 374) | Based on proportion of major non-fatal bleeds within RIETE registry that were ICH (Nieto et al.)106 | ||
All-cause (non-VTE related) mortality for general population not in hospital | Varies by age | Assumed fixed | Not applicable | ONS lifetables Risk applied each year is based on current age and is not adjusted to account for contribution of VTE to population mortality. |
||
SMR for deaths in emergency medical inpatients in year after admission compared with deaths in age- and sex-matched general population | 9.43 | Ratio of two sampled death rates 11.7 (95% CI 11.6 to 11.8) in general population 108 (95% CI 104.4 to 116.5) in hospitalised medical patients |
Norm (11.7, 0.05) Norm (108, 3.09) |
Moore et al.90 | ||
Mortality in year after admission for surgical inpatients compared to medical inpatients − HR | 1.9 | 95% CI 1.7 to 2.0 | Lognormal (0.64, 0.04) |
Clark et al.85 | ||
SMR for patients surviving ICH compared with general population | SMR from Fogelholm et al.119 applied for years 2 to 6 and then assumed no increased mortality risk Increased risk in year after ICH is assumed to be the same as for all hospital inpatients as the SMR for ICH is lower than for the SMR for all medical and surgical inpatients Confidence intervals around SMR not reported so have assumed ± 20% on the log scale |
|||||
– Year 1 after ICH | NA | Same as for all hospitalised patients | ||||
– Years 2–6 after ICH | 2.2 | 95% CI 1.8 to 2.7 | Log (SMR) = Norm (0.8, 0.1) | |||
Probability of PE being fatal in general medical inpatients | 26.8% | 95% CI 11.3% to 33.1% | Beta (11, 30) | Average case-fatality rate across five RCTs reporting both PE and fatal PE incidence in NG89 (Samama et al.,97 Leizorovicz et al.,95 Hull et al.,123 Harenberg et al.,122 Kleber et al.124) | ||
Probability of PE being fatal in general surgical inpatients | 6.0% | 95% CI 5.3% to 13.4% | Beta (11, 173) | Average case-fatality rate across RCTs of surgical patients included in reviews in CG92 | ||
Cumulative risk of PTS for treated symptomatic DVT at 3 years | Cumulative incidence at 3 years based on the TULIPA PLUS registry reported by Hach-Wunderle et al.110 Distribution of risk across years 1–3 based on van Dongen et al.111 Zero risk assumed from year 4 onwards | |||||
Proximal | 32.4% | 95% CI 22.1% to 43.6% | Beta (23, 48) | |||
Distal | 15.6% | 95% CI 7.9% to 25.3% | Beta (10, 54) | |||
OR for PTS in asymptomatic untreated proximal DVT vs. treated proximal DVT | 2.71 | 95% CI 1.44 to 5.1 | Log (OR) = Norm (0.99, 0.32) | OR from van Dongen et al.111 OR applied to risk for treated asymptomatic DVT to get incidence at 3 years of 56.6% for proximal [This gives a PTS risk of 56.5% (95%CI 29.0% to 79.8%) in asymptomatic untreated proximal DVT] |
||
OR for PTS in asymptomatic distal DVT | 1 | Fixed | Not applicable | Assumed no increased risk for asymptomatic in distal DVT. | ||
Incidence of CTEPH at 2 years (converted to annual risk of 1.6%) | 3.2% | 95% CI 2.0 % to 4.4% | Beta (32, 967) | Ende-Verhaar et al.108 based on incidence in those surviving the initial treatment period of 3–6 months Assumed no risk beyond 2 years based on Pengo et al.109 |
||
Proportion of CTEPH treated surgically | 59.5% | 95% CI 55.8% to 63.2% | Beta (404, 275) | Delcroix et al.120 | ||
Proportion of CTEPH that are surgically treated who also received bridging medical care | 30.0% | 95% CI 24.6% to 33.5% | Beta (117, 287) | Delcroix et al.120 | ||
Mean hazard for exponential survival curve in medically treated patients with CTEPH | 0.1168 | SE = 0.0123 | Norm (0.1168, 0.0123) | Original data from Delcroix et al.120 but curves taken from Goodacre et al.178 (If the death hazard falls below general population values, then general population values apply) |
||
Mean and SD for lognormal survival curve in surgically treated patients with CTEPH | Mean = 5.08 SD = 3.34 |
SE of mean = 0.574 SE of SD = 0.399 |
Multivariate normal | Original data from Delcroix et al.120 but curves taken from Goodacre et al.178 (If the death hazard falls below general population values, then general population values apply) Variance–covariance matrix |
||
Mean log | SD log | |||||
Mean log | 0.017708 | −0.05572 | ||||
SD log | −0.05572 | 0.230935 | ||||
Age | −0.0001728 | SE = 0.0003737 | Multivariate normal | |||
Age × Age | −0.000034 | SE = 3.96 × 10−6 | ||||
Constant | 0.9584588 | SE = 0.0077431 |
Parameter description | Mean value | 95% CIa | Source | Notes |
---|---|---|---|---|
Application of RAM to patient | £9.08 | Fixed | Curtis et al.178 | Cost for 5 minutes of hospital consultant time |
Prophylaxis for medical/surgical inpatients 5 days of LMWH (Dalteparin) administered by hospital nurse |
£23.91 | NA | Admin costs from Curtis et al.178 Drug costs based on Drug Tariff |
Dalteparin is lowest-cost formulation of LMWH based on current Drug Tariff prices. |
Treatment of symptomatic proximal DVT | £763.12 | £748.04 to £795.10 | NHS reference costs Drug tariff |
Clinical expert discussion regarding likelihood resource use, combined with NHS reference cost data for healthcare contacts and drug tariff costs for treatments (see Table 30 for more detailed costing breakdown) |
Treatment of symptomatic distal DVT | £642.95 | £621.76 to £668.61 | NHS reference costs180 Drug tariff181 |
Clinical expert discussion regarding likelihood resource use, combined with NHS reference cost data for healthcare contacts and drug tariff costs for treatments (see Table 30 for more detailed costing breakdown) |
Treatment of non-fatal PE | £1848.75 | £1816.98 to £1884.53 | NHS reference costs180 Drug tariff181 |
Clinical expert discussion regarding likelihood resource use, combined with NHS reference cost data for healthcare contacts and drug tariff costs for treatments (see Table 30 for more detailed costing breakdown) |
Fatal PE | £1517.13 | £1491.37 to £1542.99 | NHS reference costs180 | As per non-fatal minus drug therapy for PE |
Fatal bleed | £1865.51 | £678.86 to £3698.12 | Luengo-Fernandez et al.124 | Costs of fatal haemorrhagic stroke from OXVASC subgroup with atrial fibrillation. Uplifted to current prices using inflation indices179 |
Non-fatal non-ICH bleed | £1209.75 | £1199.79 to £1220.07 | NHS reference costs 2015–2016180 | Weighted average of reference costs for gastrointestinal bleed (HRG codes FZ38G – FZ38P) |
Post non-fatal ICH – first 90 days | £21,987.80 | £17,413.48 to £27,302.45 | Luengo-Fernandez et al.124 | Weighted average of costs for non-fatal haemorrhagic strokes Uplifted to current prices using inflation indices179 |
Post non-fatal ICH – post acute (beyond 90 days) costs per annum | £8292.83 | £5,57.42 to £11,613.69 | Luengo-Fernandez et al.124 | Average costs across all stroke types (haemorrhagic not reported separately). Includes GP and ED costs and long-term care cost Uplifted to current prices using inflation indices179 |
PTS cost per annum – year 1 Mild/moderate Severe |
£293.16 in year 1 | £279.90 to £306.40 | NHS reference costs 2015–2016180 | One first and one follow-up vascular surgery outpatient appointments Weighted average of consultant-led and non-consultant-led outpatient appointments for non-admitted face-to-face first attendance (WF01B) and follow-up (WF01A) for vascular surgery (service code 107) |
PTS cost per annum – year 2 Mild/moderate Severe |
£78.00 in each subsequent year | Fixed | Curtis et al.179 | 2 × GP surgery consultations with qualification costs including direct care staff costs at £37 per appointment |
CTEPH cost per annum Medically managed |
£18,569.53 each year | Fixed | NICE CG92182 | Cost in CG92 was £1219 per 4 weeks in 2008–09 prices.182 This was uplifted to 2016–17 prices using inflation indices.181 Assume treatment lifelong |
CTEPH cost per annum Surgically managed |
£10,236.60 in year 1 and zero in Y2 onwards | £9932.52 to £10,557.20 | NHS reference costs 2015–2016180 | Average of DZ02H, DZ02J and DZ02K ‘Complex thoracic procedures’ relating to procedure code L041 ‘Pulmonary thromboendodartectomy’ for elective inpatients including excess bed-days In addition, 29% of surgically treated patients require medical bridging therapy for 4.6 months (average cost £1992) |
Drug | Dosing and delivery | Product and cost | Drug cost per course | Monitoring/administration cost | Proportion using treatment |
---|---|---|---|---|---|
Apixaban | Initially 10 mg twice daily for 7 days, orally. Followed by 5 mg twice daily, orally for the remainder of the 3-month (91 days) treatment period | Apixaban 5 mg = £53.20 for 56 tablets (cost per tablet is same for 28 tablet pack size) | £186.20 | £73a | 20% (half of the 40% using DOACs) |
Rivaroxaban | Initially 15 mg twice daily for 21 days, to be taken orally with food. Followed by 20 mg once daily, to be taken orally with food for the remainder of the 3-month (91 days) treatment period | Rivaroxaban 20 mg = £50.40 for 28 tablets (cost per tablet is same for 15 mg and larger and smaller pack sizes) | £201.60 | £73a | 20% (half of the 40% using DOACs) |
Enoxaparin | 1.5 mg/kg every 24 hours by subcutaneous injection until adequate oral anticoagulation established (7 days), i.e. 120 mg if assuming weight of 80 kg |
Clexane Forte 120 mg/0.8 ml solution (Sanofi) – £87.93 for 10 pre-filled syringes Prescription only medicine assumed for other drugs |
£61.55 | £72.71b | 30% (45% of heparin use) |
Dalteparin | 15,000 units (assuming body weight of 80 kg) once daily until adequate oral anticoagulation established (7 days) | Dalteparin sodium 15,000 units/0.6 ml solution (Pfizer Ltd/Ennogen Healthcare Ltd/JM McGill Ltd) – £42.34 for five pre-filled syringes | £59.28 | £72.71b | 18% (35% of heparin use) |
Tinzaparin | 175 units/kg once daily until adequate oral anticoagulation established (7 days), i.e. 14,000 units if assuming 80 kg | Innohep 14,000 units/0.7ml solution (LEO Pharma) – £83.30 for 10 pre-filled syringes | £58.31 | £72.71b | 6% (20% of heparin use) |
Warfarin | 5 mg once daily orally for 3 months (91 days) | Warfarin sodium 5mg (various suppliers) = £0.70 for 28 tablets | £3.22 | £238.84c | 60% |
Average across those using DOACs and those using LMWH/VKA | £115.55 | £216.07 | Total: £331.63 |
Proportion using resource | |||||
---|---|---|---|---|---|
Non-fatal PE | Symptomatic proximal DVT | Symptomatic distal DVT | Unit cost per patient using this resource | Description | |
Healthcare contacts/admission | |||||
GP visit | 20% | 50% | 50% | £39 | GP cost per surgery consultation with qualification costs including direct care staff costs |
Ambulance transfer to Emergency Department | 60% | 10% | 0% | £257 | NHS Schedule for Reference Costs 2018–19 ‘See and treat and convey’, code ASS02 |
Emergency Department visit leading to admission | 60% | 10% | 0% | £279 | NHS Schedule for Reference Costs 2018–19 VB05Z Type 01 Admitted (Category 2 investigation with Category 3 treatment) |
Emergency Department without admission | 40% | 90% | 100% | £239 | NHS Schedule for Reference Costs 2018–19 VB05Z Type 01 Non-admitted (Category 2 investigation with Category 3 treatment) |
Short-stay admission for PE | 60% | 0% | 0% | £1410 | NHS Schedule for Reference Costs 2018–19 Weighted average cost of non-elective inpatient (short and long stay with excess bed-days) for ‘Pulmonary Embolus with Interventions’, codes DZ09J to DZ09N & DZ09P and DZ09Q |
Short-stay admission for DVT | 0% | 10% | 0% | £904 | NHS Schedule for Reference Costs 2018–19 Weighted average cost of non-elective inpatient (short and long-stay with excess bed-days) for ‘Deep-Vein Thrombosis’ CC score 0–12+, codes YQ51A to YQ51E. |
Critical care unit stay | 10% | 0% | 0% | £1028 | NHS Schedule for Reference Costs 2018–19 Weighted average cost of adult Critical Care, 0–6 or more organs Supported, codes XC01Z to XC01Z |
Subtotal for healthcare contacts | £1374 | £379 | £259 | ||
Diagnostic costs | |||||
Risk assessment tool (Wells score) | Included in Emergency Department episode so not costed separately | ||||
D-Dimer | |||||
ECG | |||||
Chest X-ray | |||||
Proximal leg vein ultrasound | 0% | 100% | 100% | £53 | NHS Schedule for Reference Costs 2015–16.180 RD40Z outpatient ultrasound scan with duration of less than 20 minutes, without contrast £55 [RD47Z may be more relevant for diagnosis of distal DVTs but cost is similar (£58)] |
CTPA | 90% | 0% | 0% | £108 | NHS Schedule for Reference Costs 2015–16.180 RD21A outpatient computerised tomography scan of one area, with post contrast only, 19 years and over |
V/Q SPECT | 5% | 0% | 0% | £287 | NHS Schedule for Reference Costs 2015–16.180 RN08A outpatient single photon emission computed tomography (SPECT), 19 years and over |
V/Q planar | 5% | 0% | 0% | £321 | NHS Schedule for Reference Costs 2015–16.180 RN18A outpatient lung ventilation or perfusion scan, 19 years and over |
Echocardiogram | 20% | 0% | 0% | £76 | NHS Schedule for Reference Costs 2015–16.180 RD51A outpatient simple echocardiogram |
Subtotal for unbundled diagnostics | £143 | £53 | £53 | ||
Subtotal for drug treatment | £332 | £332 | £332 | ||
Total | £1,849a | £763 | £643 |
Absolute utility value | Absolute utility value | Range | Source | Notes |
---|---|---|---|---|
Well/asymptomatic DVT without prophylaxis | Ara and Brazier137 | Population mean utility values based on average age and sex mix at baseline | ||
Medical inpatients | 0.800 | 0.799–0.801 | ||
Surgical inpatients | 0.849 | 0.847–0.851 | ||
Symptomatic proximal or distal DVT | Monreal et al.113 | 3.8% reduction relative to well patients based on comparison of average utility over 6 months for DVT (0.820) vs. PE vs. utility of matched population norms (0.852) | ||
Medical | 0.769 | 0.756–0.779 | ||
Surgical | 0.817 | 0.802–0.828 | ||
Non-fatal PE | Chuang et al.130 | 4.0% reduction relative to well patients based on comparison of average utility over 6 months (0.804) for PE vs. utility of matched population norms (0.838) | ||
Medical | 0.769 | 0.756–0.779 | ||
Surgical | 0.815 | 0.803–0.827 | ||
Non-fatal ICH | Luengo-Fernandez et al.123 | Absolute decrement of 0.22 measured at 1 month | ||
Medical | 0.580 | 0.540–0.619 | ||
Surgical | 0.629 | 0.589–0.669 | ||
Non-fatal non-ICH bleed | Chuang et al.130 | Assumed same utility decrement for PE and GI bleeds at 1 month. 14% reduction based on utility for PE at 1 month (0.718) vs. utility of matched population norms (0.838) from Chuang et al.130 |
||
Medical | 0.685 | 0.684–0.686 | ||
Surgical | 0.727 | 0.725–0.729 | ||
Prophylaxis – absolute decrement applied to utility values of well/asymptomatic DVT | 0.007 | 0.000–0.050 | Marchetti et al.136 | Patients willing to trade average of 2.7 days per year to avoid treatment with LMWH |
Treatment – absolute decrement applied to utility values for non-fatal PE or symptomatic DVT | 0.011 | 0.000–0.083 | Marchetti et al.136 | Patients willing to trade average of 4 days per year to avoid treatment with warfarin |
Fatal PE | 0 | NA | Assumption |
Health state(s) | Utility multiplier relative to well | Range | Source | Notes |
---|---|---|---|---|
PE survivor without CTEPH and PE survivor more than 1 year after surgery for CTEPH | 1.000 | 0.998–1.000 | Chuang et al.130 | Average over 6–12 months following PE compared to matched general population norms |
Any DVT without PTS | 1 | NA | Assumption | Supported by Lubberts et al.127 systematic review finding no significant HRQoL decrement in nine long-term studies based on SF-36 outcomes |
Non-fatal ICH | Luengo-Fernandez et al.123 | Multiplier calculated based on absolute decrement of 0.09 at 5 years (utility values stable from 6 months to 5 years) relative to absolute utility for well state | ||
Medical | 0.888 | 0.837–0.937 | ||
Surgical | 0.894 | 0.847–0.941 | ||
PTS | 0.895 | 0.816–0.952 | Enden et al.133 | Multiplier calculated based on absolute decrement of 0.09 relative to absolute utility for well state of 0.86 |
CTEPH –first year for surgically managed and every year for medically managed | 0.629 | 0.579–0.690 | Meads et al.135 | Multiplier calculated based on comparison of utility for CTEPH (0.56) vs. utility for NYHA class I (0.89) |
Dead | 0 | Assumption |
Parameter description | Midpoint value | Uncertainty measure | Distribution | Source | |||
---|---|---|---|---|---|---|---|
Ambulance transfer to ED | £257 | SE = £11 | Gamma (551, 0.47) | NHS Schedule for Reference Costs 2018–19. HRG code, ASS02 See and treat and convey |
|||
ED visit leading to admission | £279 | SE = £6 | Gamma (2210, 0.15) | NHS Schedule for Reference Costs 2018–19. HRG code: Type 01, leading to admission, VB05Z Emergency Medicine, Category 2 Investigation with Category 3 Treatment |
|||
ED visit not leading to admission | £239 | SE = £4 | Gamma (3204, 0.07) | NHS Schedule for Reference Costs 2018–19. HRG code: Type 01, not leading to admission, VB05Z Emergency Medicine, Category 2 Investigation with Category 3 Treatment |
|||
DVT admission – weighted average of following HRG costs: | NHS Schedule for Reference Costs 2018–19. Non-elective inpatient (NEI) and non-elective short stay (NESS) costs for HRG codes covering deep-vein thrombosis with CC scores ranging from 0 to 12+ |
||||||
YQ51A – NEI (N = 1377) | £4017 | SE = £198 | Gamma (412, 9.7) | ||||
YQ51A – NESS (N = 492) | £564 | SE = £33 | Gamma (288, 2.0) | ||||
YQ51B – NEI (N = 1183) | £2873 | SE = £129 | Gamma (495, 5.8) | ||||
YQ51B – NESS (N = 895) | £470 | SE = £13 | Gamma (1237, 0.4) | ||||
YQ51C – NEI (N = 1665) | £2433 | SE = £78 | Gamma (973, 2.5) | ||||
YQ51C – NESS (N = 2391) | £418 | SE = £11 | Gamma (1433, 0.3) | ||||
YQ51D – NEI (N = 1686) | £2020 | SE = £46 | Gamma (1903, 1.1) | ||||
YQ51D – NESS (N = 6249) | £384 | SE = £9 | Gamma (1822, 0.2) | ||||
YQ51E – NEI (N = 908) | £1772 | SE = £42 | Gamma (1814, 1.0) | ||||
YQ51E- NESS (N = 11,731) | £320 | SE = 9 | Gamma (1330, 0.2) | ||||
PE admission – weighted average of following HRG costs: | NHS Schedule for Reference Costs 2018–19. Non-elective inpatient (NEI) costs and non-elective short stay (NESS) costs for HRG codes covering pulmonary embolus with and without interventions with CC score from 0 to 12+ |
||||||
DZ09J – NEI (N = 888) | £5450 | SE = £277 | Gamma (338, 14) | ||||
DZ09J – NESS (N = 62) | £1280 | SE = £168 | Gamma (58, 22) | ||||
DZ09K – NEI (N = 585) | £3384 | SE = £130 | Gamma (676, 5.0) | ||||
DZ09K – NESS (N = 65) | £790 | SE = £56 | Gamma (199, 4.0) | ||||
DZ09L – NEI (N = 3160) | £3522 | SE = £140 | Gamma (663, 5.5) | ||||
DZ09L – NESS (N = 1181) | £667 | SE = £21 | Gamma (1026, 0.7) | ||||
DZ09M – NEI (N = 3716) | £2671 | SE = £75 | Gamma (1255, 2.1) | ||||
DZ09M – NESS (N = 2197) | £577 | SE = 18 | Gamma (1054, 0.6) | ||||
DZ09N – NEI (N = 5105) | £2201 | SE = £45 | Gamma (2358, 0.9) | ||||
DZ09N – NESS (N = 4374) | £533 | SE = 12 | Gamma (2091, 0.3) | ||||
DZ09P – NEI (N = 6126) | £1845 | SE = £38 | Gamma (2417, 0.8) | ||||
DZ09P – NESS (N = 8768) | £488 | SE = £12 | Gamma (1595, 0.3) | ||||
DZ09Q – NEI (N = 3226) | £1584 | SE = £29 | Gamma (2989, 0.5) | ||||
DZ09Q – NESS (N = 9048) | £448 | SE = 9 | Gamma (2376, 0.2) | ||||
Critical care – weighted average of HRG costs for codes: | NHS Schedule for Reference Costs 2018–19. HRG codes for Adult Critical Care for 0–6 organs supported |
||||||
XC01Z | £1673 | N = 1 | Fixed | ||||
XC02Z | £1574 | SE = £152 | Gamma (107, 14.7) | ||||
XC03Z | £1655 | SE = £114 | Gamma (211, 7.9) | ||||
XC04Z | £1640 | SE = £67 | Gamma (605, 2.7) | ||||
XC05Z | £1450 | SE = £49 | Gamma (884, 1.7) | ||||
XC06Z | £792 | SE = £78 | Gamma (104, 7.6) | ||||
XC07Z | £516 | SE = £129 | Gamma (16.0, 32.2) | ||||
Proximal leg vein ultrasound | £53 | SE = £1 | Gamma (2135, 0.03) | NHS Schedule for Reference Costs 2018–19 | |||
CTPA | £108 | SE = £4 | Gamma (635, 0.17) | NHS Schedule for Reference Costs 2018–19 RD21A outpatient computerised tomography scan of one area, with post contrast only, 19 years and over |
|||
V/Q SPECT | £287 | SE = £20 | Gamma (202, 1.42) | NHS Schedule for Reference Costs 2018–19 RN08A, outpatient single photon Emission computed tomography (SPECT), 19 years and over |
|||
V/Q planar | £321 | SE = £10 | Gamma (1045, 0.31) | NHS Schedule for Reference Costs 2018–19 RN18A outpatient lung ventilation or perfusion scan, 19 years and over |
|||
Echocardiogram | £76 | SE = £6 | Gamma (146, 0.52) | NHS Schedule for Reference Costs 2018–19 RD51A outpatient simple echocardiogram, 19 years and over |
|||
Proportion receiving LMWH who need district nurse administration | 4% | 95% CI 1.3% to 7.8% | Beta (5123) | Menakaya et al.121 | |||
Fatal bleed | £1592 | SD = 1886, N = 8 | Gamma (5.70, 279) | Luengo-Fernandez et al.124 (cost before inflation) | |||
Acute costs for non-fatal ICH (first 90 days) – weighted average of: | Luengo-Fernandez et al.124 (cost before inflation) | ||||||
Non-disabling non-fatal stroke | £9903 | SD = 4510, N = 5 | Gamma (24, 411) | ||||
Moderately disabling non-fatal stroke | £25,442 | SD = 9635, N = 3 | Gamma (21, 1216) | ||||
Totally disabling non-fatal stroke | £43,036 | SD = NA, N = 1 | Fixed | ||||
Residential costs for non-fatal ICH (first 90 days) | £6880 | SD = £15,600, N = 136 | Gamma (26, 260) | Luengo-Fernandez et al.124 (cost before inflation) | |||
GP costs for non-fatal ICH (first 90 days) | £98 | 95% CI £27 to £169 | Norm (98, 36) | Luengo-Fernandez et al.124 (cost before inflation) | |||
Emergency care costs for non-fatal ICH (first 90 days) | £99 | 95% CI £56 to £141 | Norm (99, 22) | Luengo-Fernandez et al.124 (cost before inflation) | |||
Non-fatal non-ICH bleed (weighted average of HRG costs): | NHS Schedule for Reference Costs 2018–19 HRG codes for GI bleed without interventions, with single interventions and with multiple interventions. |
||||||
FD03A – NEI (N = 1110) | £5377 | SE = £201 | Gamma (714, 7.5) | ||||
FD03A – NESS (N = 30) | £2360 | SE = £310 | Gamma (58, 41) | ||||
FD03B– NEI (N = 885) | £3510 | SE = £131 | Gamma (722, 4.9) | ||||
FD03B– NSS (N = 16) | £2088 | SE = £1109 | Gamma (3.6, 590) | ||||
FD03C – NEI (N = 1642) | £3866 | SE = £171 | Gamma (514, 7.5) | ||||
FD03C– NSS (N = 41) | £1345 | SE = £105 | Gamma (166, 8.1) | ||||
FD03D – NEI (N = 2329) | £2796 | SE = £92 | Gamma (913, 3.0) | ||||
FD03D– NSS (N = 46) | £2360 | SE = £156 | Gamma (229, 10) | ||||
FD03E – NEI (N = 5481) | £2247 | SE = £47 | Gamma (2331, 1.0) | ||||
FD03E – NEI (N = 108) | £1089 | SE = £82 | Gamma (£178, 6.1) | ||||
FD03F – NEI (N = 2891) | £2818 | SE = £100 | Gamma (792, 3.6) | ||||
FD03F – NEI (N = 2213) | £591 | SE = £19 | Gamma (1000, 0.6) | ||||
FD03G – NEI (N = 7278) | £2198 | SE = £41 | Gamma (2931, 0.8) | ||||
FD03G – NEI (N = 8830) | £541 | SE = £15 | Gamma (1221, 0.4) | ||||
FD03H – NEI (N = 16,290) | £1575 | SE = £27 | Gamma (3523, 0.8) | ||||
FD03H – NEI (N = 40,167) | £438 | SE = 11 | Gamma (1640, 0.3) | ||||
Anticoagulant service face-to-face follow-up consultant led | £53 | SE = £5 | Norm (53, 5.3) with minimum of zero | NHS Schedule for Reference Costs 2018–19 Service code 324 – WF01A non-admitted |
|||
Anticoagulant service face-to-face follow-up non-consultant led | £20 | SE = £2 | Norm (20, 2.0) with minimum of zero | NHS Schedule for Reference Costs 2018–19 Service code 324 – WF01A non-admitted |
|||
Anticoagulant service first face-to-face attendance non-consultant led | £26 | SE = £3 | Norm (26, 2.6) with minimum of zero | NHS Schedule for Reference Costs 2018–19 Service code 324 – WF01B non-admitted |
|||
Anticoagulant service non-face-to-face follow-up non-consultant led | £20 | SE = £20 | Norm (20, 2.0) with minimum of zero | NHS Schedule for Reference Costs 2018–19 Service code 324 – WF01C non-admitted |
|||
Vascular surgery first appointment face to face consultant led | £165 | SE = £6 | Gamma (759, 0.22) | NHS Schedule for Reference Costs 2018–19 Service code 107 – WF01B non-admitted |
|||
Vascular surgery follow-up appointment face-to-face, consultant led | £134 | SE = £4 | Gamma (942, 0.14) | NHS Schedule for Reference Costs 2018–19 Service code 107 – WF01A non-admitted |
|||
Vascular surgery first appointment face-to-face non-consultant led | £132 | SE = £11 | Gamma (132, 1.0) | NHS Schedule for Reference Costs 2018–19 Service code 107 – WF01B non-admitted |
|||
Vascular surgery follow-up appointment face-to-face, non-consultant led | £121 | SE = £14 | Gamma (79, 1.53) | NHS Schedule for Reference Costs 2018–19 Service code 107 – WF01A non-admitted |
|||
Surgical management of CTEPH – average of following HRG costs; | NHS Schedule for Reference Costs 2018–19 HRG codes for complex thoracic procedures, 19 years and over, with CC Score ranging from 0 to 6+ |
||||||
DZ02H | £9782 | SE = £363 | Gamma (723, 13.5) | ||||
DZ02J | £7500 | SE = £300 | Gamma (627, 12.0) | ||||
DZ02K | £6506 | SE = £270 | Gamma (579, 11.2) | ||||
Disutility for stroke up to 6 months | −0.22 | 95% CI −0.26 to −0.18 | Norm (−0.22, 0.02) | Luengo-Fernandez et al.123 | |||
Disutility for stroke from 6 months | −0.09 | 95% CI −0.13 to −0.05 | Norm (−0.09, 0.02) | Luengo-Fernandez et al.123 | |||
Utility immediately after DVT | 0.72 | SE = 0.006 | Beta (3977, 1565) | Monreal et al.113 | |||
Utility immediately after PE | 0.72 | SE = 0.007 | Beta (2741, 1080) | Chuang et al.130 (assumed same SD as observed for patients having DVT in Monreal et al.113) |
|||
Utility for DVT without PTS | 0.86 | 95% CI 0.823 to 0.903 | Beta (248, 40.3) | Enden et al.133 | |||
Disutility for PTS vs. no PTS after DVT | 0.09 | 95% CI 0.03 to 0.15 | Beta (7.78, 78.6) | Enden et al.133 | |||
Utility for CTEPH | 0.56 | SD = 0.29, N = 308 | Beta (505, 397) | Meads et al.135 | |||
Utility for NYHA class 1 | 0.86 | SD = 0.17, N = 35 | Beta (105, 12.9) | Meads et al.135 | |||
Utility for LMWH | 0.993 | SD = 0.016 | Beta (27.5, 0.205) | Marchetti et al.136 | |||
Utility for warfarin | 0.989 | SD = 0.024 | Beta (17.6, 0.195) | Marchetti et al.136 | |||
Utility regression for age-related decrement – coefficients for | Ara and Brazier137 | ||||||
Age | −0.0001728 | SE = 0.0003737 | Multivariate normal | Variance–covariance matrix | |||
Age × Age | −0.000034 | SE = 3.96 × 10−6 | Age | Age × Age | Constant | ||
Constant | 0.9584588 | SE = 0.0077431 | Age | 1.4 × 10−7 | |||
Age × Age | −1.5 × 10−9 | 1.6 × 10−11 | |||||
Constant | −2.80 × 10−6 | 2.8 × 10−8 | 6 × 10−5 |
FIGURE 27.
Meta-analysis of VTE outcomes in medical inpatients.
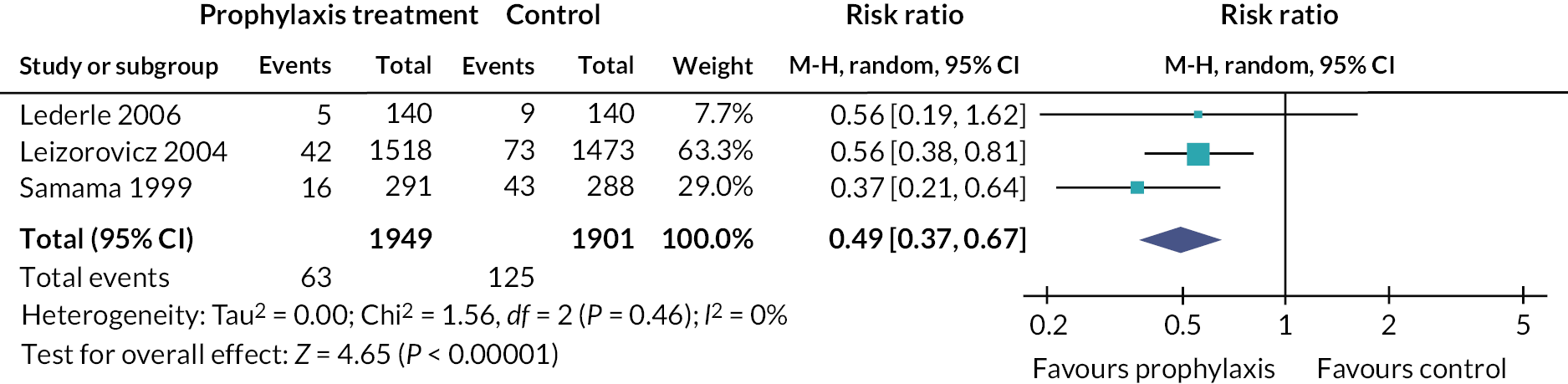
-
Lederle FA, Sacks JM, Fiore L, Landefeld CS, Steinberg N, Peters RW, et al. The prophylaxis of medical patients for thromboembolism pilot study. Am J Med 2006;119:54–9. https://doi.org/10.1016/j.amjmed.2005.03.049
-
Leizorovicz A, Cohen AT, Turpie AG, Olsson CG, Vaitkus PT, Goldhaber SZ, et al. Randomized, placebo-controlled trial of dalteparin for the prevention of venous thromboembolism in acutely ill medical patients. Circulation 2004;110:874–9. https://doi.org/10.1161/01.CIR.0000138928.83266.24
-
Samama MM, Cohen AT, Darmon JY, Desjardins L, Eldor A, Janbon C, et al. A comparison of enoxaparin with placebo for the prevention of venous thromboembolism in acutely ill medical patients. Prophylaxis in Medical Patients with Enoxaparin Study Group. N Engl J Med 1999;341:793–800. https://doi.org/10.1056/NEJM199909093411103
Appendix 3 Additional data from the multicentre observational cohort study
FIGURE 28.
Examples of electronic and paper documentation aids for RAM completion.
Site 1 – Structured note completed through Electronic Health Record and designed to aid VTE risk assessment at the point of hospital admission, using the Department of Health RAM (Salford Royal NHS Foundation Trust)
Site 2 – Paper risk assessment proforma with linked prescribing (Manchester NHS Foundation Trust)
Site 3 – Paper risk assessment proforma without linked prescribing (Sheffield Teaching Hospitals NHS Foundation Trust)
Site 4 – Electronic form within prescribing electronic health record designed to trigger consideration of VTE risk assessment at the point of hospital admission and prompt prescribing in accordance with local guidelines (St Thomas’ NHS Foundation Trust).)
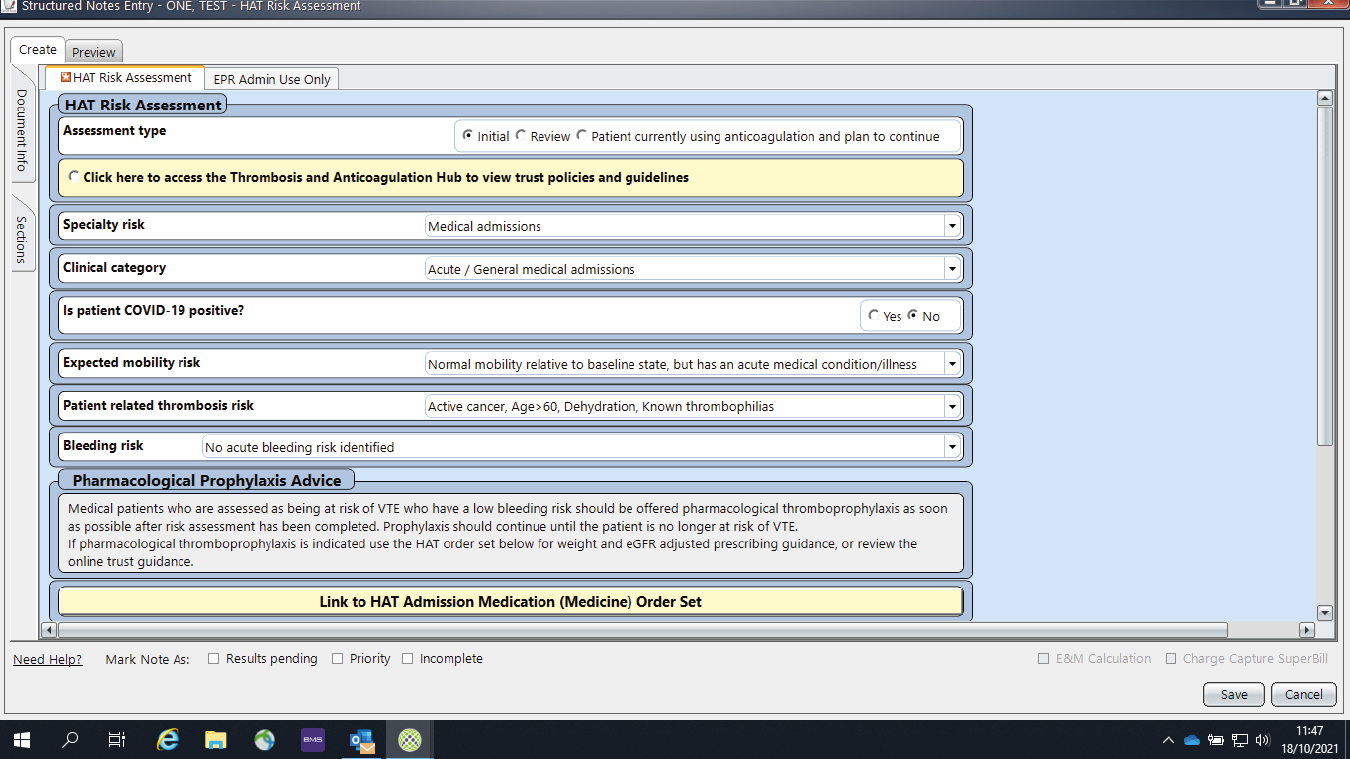

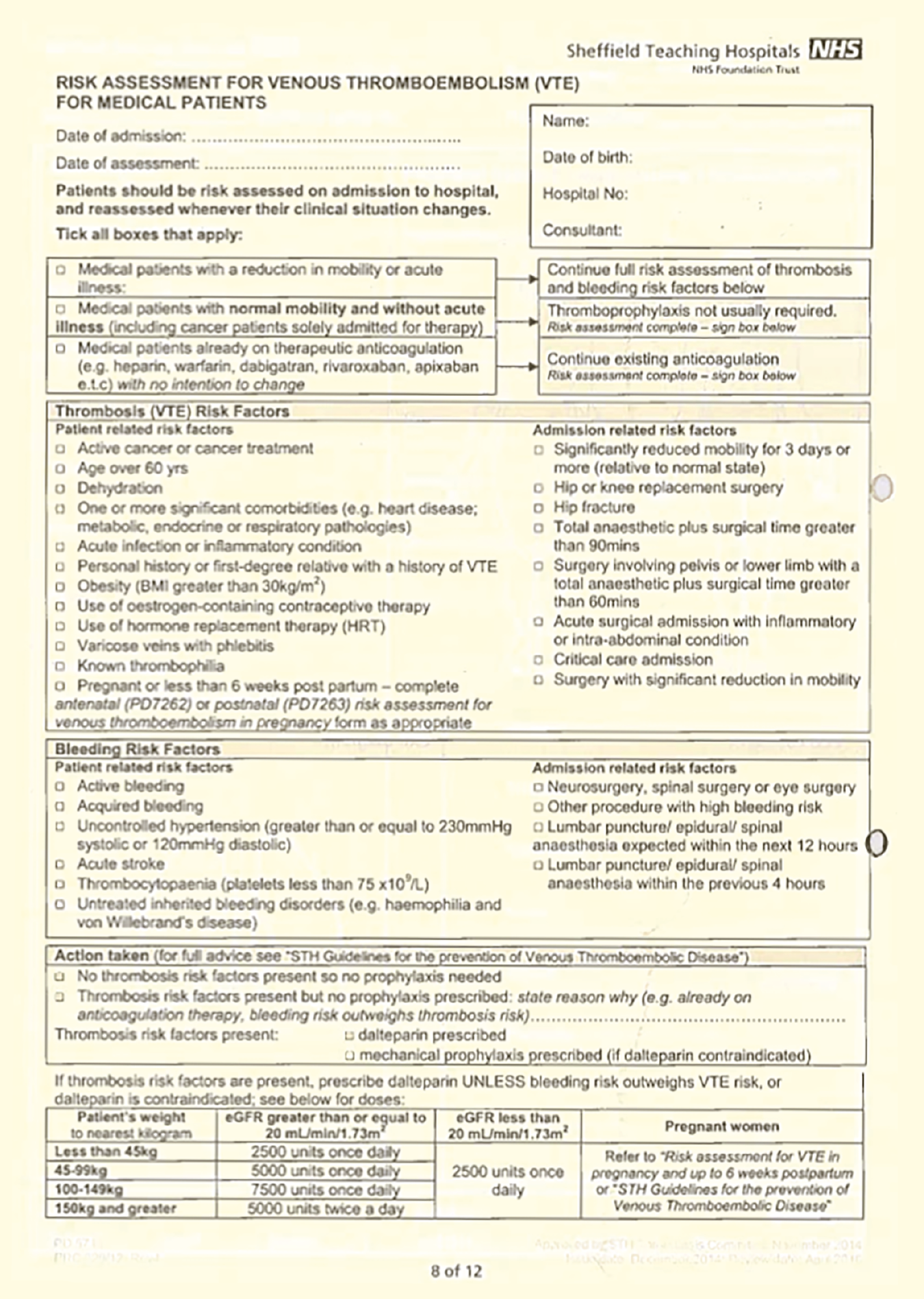


FIGURE 29.
Workflow diagram.
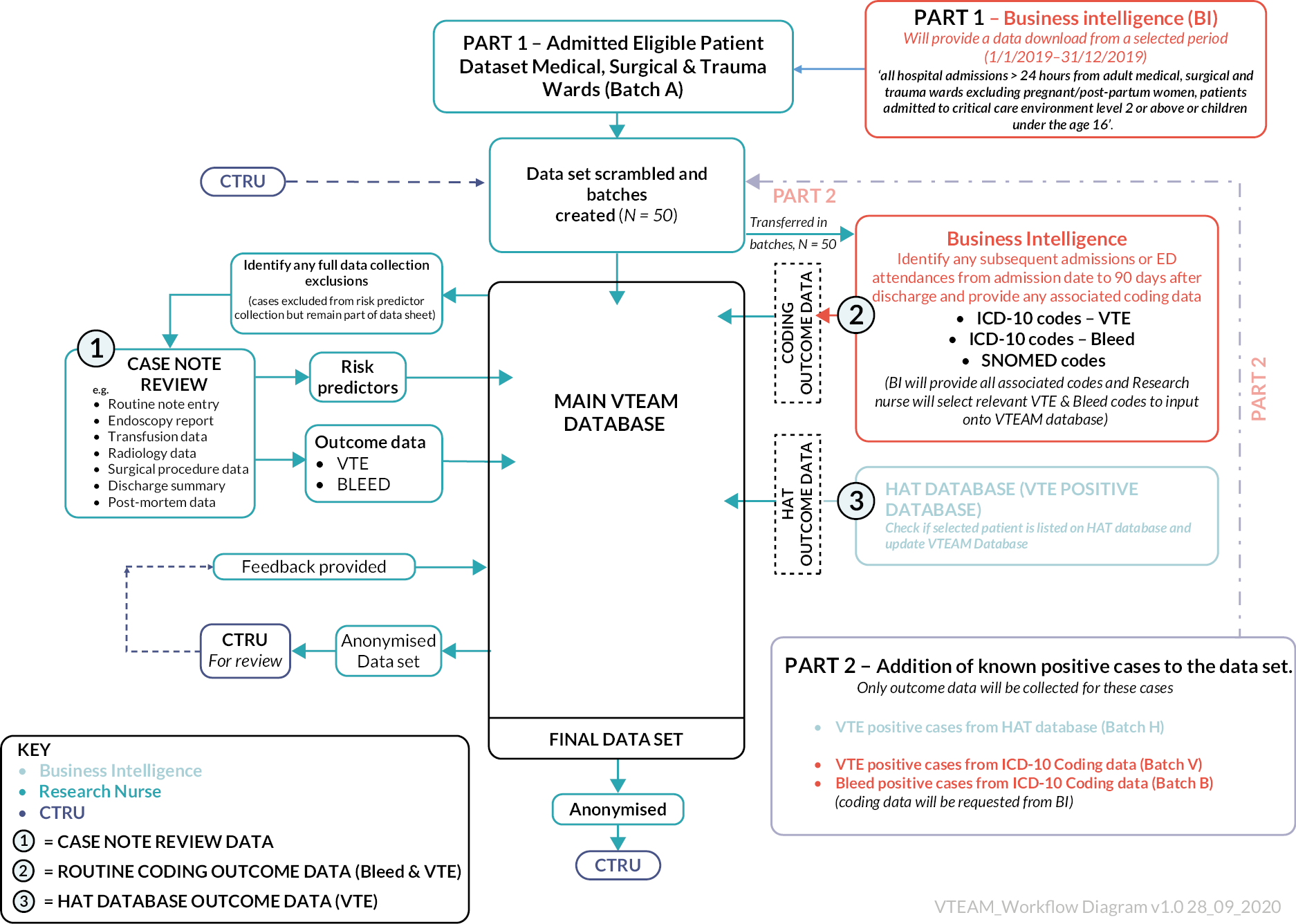
FIGURE 30.
Agreement between recommendations using the Department of Health VTE risk assessment tool and other RAMs.
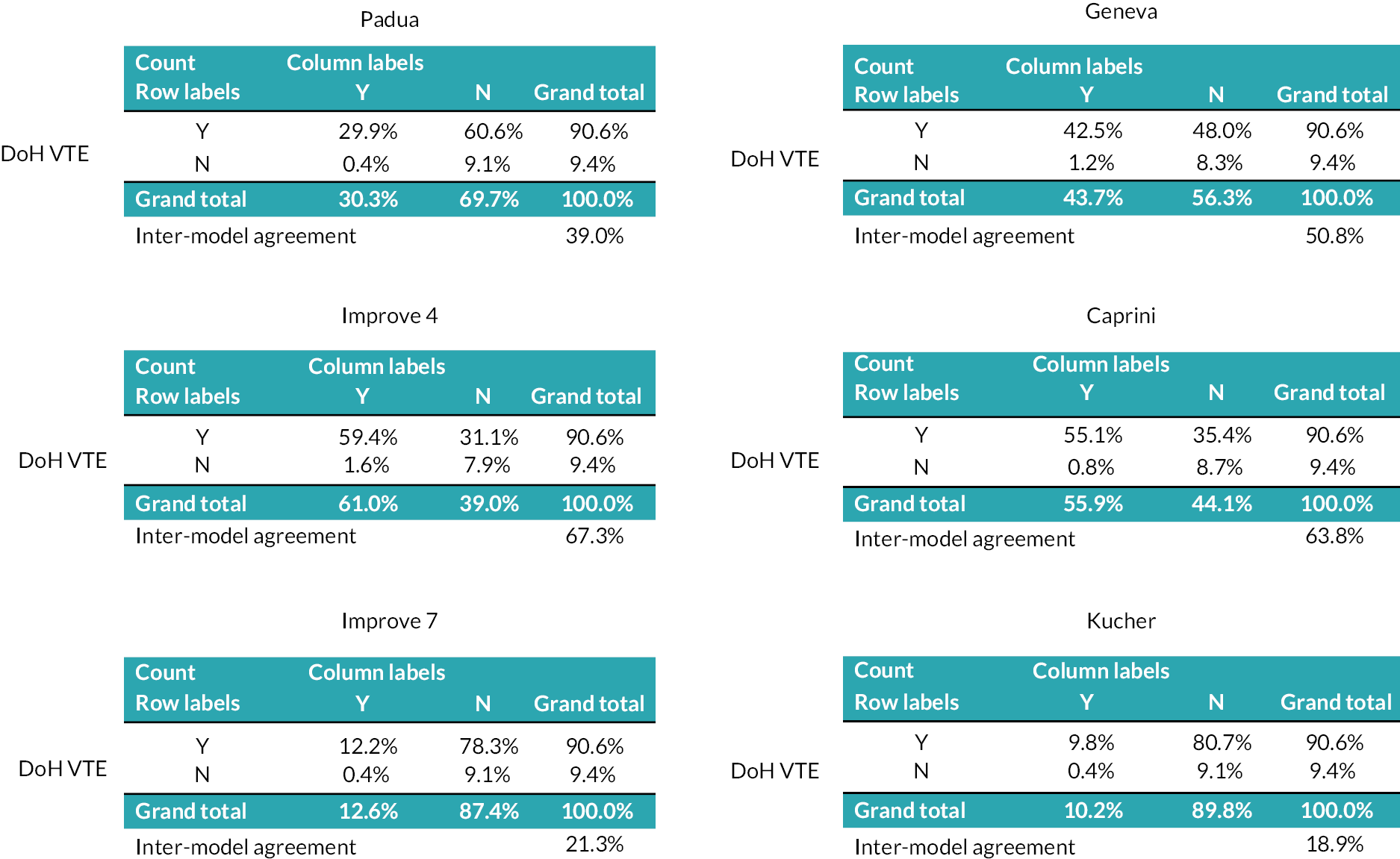
ICD-10 – 4 digit | Three character description | Four character description | All diagnoses | Main diagnosis | Category | Subcategory | Final selection |
---|---|---|---|---|---|---|---|
I26.0 | Pulmonary embolism | Pulmonary embolism with mention of acute cor pulmonale | 4031 | 2353 | VTE | Yes | |
I26.9 | Pulmonary embolism | Pulmonary embolism without mention of acute cor pulmonale | 108,637 | 53,273 | VTE | Yes | |
I80.1 | Phlebitis and thrombophlebitis | Phlebitis and thrombophlebitis of femoral vein | 10,156 | 4294 | VTE | Yes | |
I80.2 | Phlebitis and thrombophlebitis | Phlebitis and thrombophlebitis of other deep vessels of lower extremities | 61,647 | 24,297 | VTE | Yes | |
I80.3 | Phlebitis and thrombophlebitis | Phlebitis and thrombophlebitis of lower extremities, unspecified | 3971 | 1876 | VTE | Yes | |
I80.9 | Phlebitis and thrombophlebitis | Phlebitis and thrombophlebitis of unspecified site | 2906 | 524 | VTE | Yes | |
I82.2 | Other venous embolism and thrombosis | Embolism and thrombosis of vena cava | 3891 | 543 | VTE | Yes | |
I82.8 | Other venous embolism and thrombosis | Embolism and thrombosis of other specified veins | 10,001 | 1870 | VTE | Yes | |
I82.9 | Other venous embolism and thrombosis | Embolism and thrombosis of unspecified vein | 1124 | 215 | VTE | Yes | |
I60.0 | Subarachnoid haemorrhage | Subarachnoid haemorrhage from carotid siphon and bifurcation | 226 | 212 | Bleeding | Intracranial bleed | Yes |
I60.1 | Subarachnoid haemorrhage | Subarachnoid haemorrhage from middle cerebral artery | 1125 | 1014 | Bleeding | Intracranial bleed | Yes |
I60.2 | Subarachnoid haemorrhage | Subarachnoid haemorrhage from anterior communicating artery | 1731 | 1599 | Bleeding | Intracranial bleed | Yes |
I60.3 | Subarachnoid haemorrhage | Subarachnoid haemorrhage from posterior communicating artery | 899 | 838 | Bleeding | Intracranial bleed | Yes |
I60.4 | Subarachnoid haemorrhage | Subarachnoid haemorrhage from basilar artery | 384 | 324 | Bleeding | Intracranial bleed | Yes |
I60.5 | Subarachnoid haemorrhage | Subarachnoid haemorrhage from vertebral artery | 101 | 87 | Bleeding | Intracranial bleed | Yes |
I60.6 | Subarachnoid haemorrhage | Subarachnoid haemorrhage from other intracranial arteries | 564 | 513 | Bleeding | Intracranial bleed | Yes |
I60.7 | Subarachnoid haemorrhage | Subarachnoid haemorrhage from intracranial artery, unspecified | 426 | 319 | Bleeding | Intracranial bleed | Yes |
I60.8 | Subarachnoid haemorrhage | Other subarachnoid haemorrhage | 977 | 737 | Bleeding | Intracranial bleed | Yes |
I60.9 | Subarachnoid haemorrhage | Subarachnoid haemorrhage, unspecified | 7642 | 4585 | Bleeding | Intracranial bleed | Yes |
I61.0 | Intracerebral haemorrhage | Intracerebral haemorrhage in hemisphere, subcortical | 4996 | 4396 | Bleeding | Intracranial bleed | Yes |
I61.1 | Intracerebral haemorrhage | Intracerebral haemorrhage in hemisphere, cortical | 5439 | 4254 | Bleeding | Intracranial bleed | Yes |
I61.2 | Intracerebral haemorrhage | Intracerebral haemorrhage in hemisphere, unspecified | 1431 | 1157 | Bleeding | Intracranial bleed | Yes |
I61.3 | Intracerebral haemorrhage | Intracerebral haemorrhage in brain stem | 1029 | 866 | Bleeding | Intracranial bleed | Yes |
I61.4 | Intracerebral haemorrhage | Intracerebral haemorrhage in cerebellum | 1901 | 1508 | Bleeding | Intracranial bleed | Yes |
I61.5 | Intracerebral haemorrhage | Intracerebral haemorrhage, intraventricular | 3678 | 1886 | Bleeding | Intracranial bleed | Yes |
I61.6 | Intracerebral haemorrhage | Intracerebral haemorrhage, multiple localised | 764 | 561 | Bleeding | Intracranial bleed | Yes |
I61.8 | Intracerebral haemorrhage | Other intracerebral haemorrhage | 3854 | 3103 | Bleeding | Intracranial bleed | Yes |
I61.9 | Intracerebral haemorrhage | Intracerebral haemorrhage, unspecified | 11,863 | 9028 | Bleeding | Intracranial bleed | Yes |
I62.0 | Other nontraumatic intracranial haemorrhage | Subdural haemorrhage (acute)(nontraumatic) | 17,161 | 8197 | Bleeding | Intracranial bleed | Yes |
I62.1 | Other nontraumatic intracranial haemorrhage | Nontraumatic extradural haemorrhage | 318 | 100 | Bleeding | Intracranial bleed | Yes |
I62.9 | Other nontraumatic intracranial haemorrhage | Intracranial haemorrhage (nontraumatic), unspecified | 3230 | 2383 | Bleeding | Intracranial bleed | Yes |
I85.0 | Oesophageal varices | Oesophageal varices with bleeding | 4074 | 2876 | Bleeding | Gastrointestinal | Yes |
K22.6 | Other diseases of oesophagus | Gastro-oesophageal laceration-haemorrhage syndrome | 7232 | 3237 | Bleeding | Yes | |
K25.0 | Gastric ulcer | Gastric ulcer – acute with haemorrhage | 2077 | 1469 | Bleeding | Gastrointestinal | Yes |
K25.2 | Gastric ulcer | Gastric ulcer – acute with both haemorrhage and perforation | 49 | 20 | Bleeding | Gastrointestinal | Yes |
K25.4 | Gastric ulcer | Gastric ulcer – chronic or unspecified with haemorrhage | 4742 | 2951 | Bleeding | Gastrointestinal | Yes |
K25.6 | Gastric ulcer | Gastric ulcer – chronic or unspecified with both haemorrhage and perforation | 145 | 74 | Bleeding | Gastrointestinal | Yes |
K26.0 | Duodenal ulcer | Duodenal ulcer – acute with haemorrhage | 2955 | 2161 | Bleeding | Gastrointestinal | Yes |
K26.2 | Duodenal ulcer | Duodenal ulcer – acute with both haemorrhage and perforation | 126 | 96 | Bleeding | Gastrointestinal | Yes |
K26.4 | Duodenal ulcer | Duodenal ulcer – chronic or unspecified with haemorrhage | 7607 | 4972 | Bleeding | Gastrointestinal | Yes |
K26.6 | Duodenal ulcer | Duodenal ulcer – chronic or unspecified with both haemorrhage and perforation | 386 | 263 | Bleeding | Gastrointestinal | Yes |
K27.0 | Peptic ulcer, site unspecified | Peptic ulcer, site unspecified – acute with haemorrhage | 78 | 32 | Bleeding | Gastrointestinal | Yes |
K27.2 | Peptic ulcer, site unspecified | Peptic ulcer, site unspecified – acute with both haemorrhage and perforation | 4 | 1 | Bleeding | Gastrointestinal | Yes |
K27.4 | Peptic ulcer, site unspecified | Peptic ulcer, site unspecified – chronic or unspecified with haemorrhage | 231 | 116 | Bleeding | Gastrointestinal | Yes |
K27.6 | Peptic ulcer, site unspecified | Peptic ulcer, site unspecified – chronic or unspecified with both haemorrhage and perforation | 29 | 9 | Bleeding | Gastrointestinal | Yes |
K28.0 | Gastrojejunal ulcer | Gastrojejunal ulcer – acute with haemorrhage | 29 | 24 | Bleeding | Gastrointestinal | Yes |
K28.2 | Gastrojejunal ulcer | Gastrojejunal ulcer – acute with both haemorrhage and perforation | 3 | 3 | Bleeding | Gastrointestinal | Yes |
K28.4 | Gastrojejunal ulcer | Gastrojejunal ulcer – chronic or unspecified with haemorrhage | 149 | 96 | Bleeding | Gastrointestinal | Yes |
K28.6 | Gastrojejunal ulcer | Gastrojejunal ulcer – chronic or unspecified with both haemorrhage and perforation | 11 | 6 | Bleeding | Gastrointestinal | Yes |
K29.0 | Gastritis and duodenitis | Acute haemorrhagic gastritis | 3340 | 1365 | Bleeding | Gastrointestinal | Yes |
K62.5 | Other diseases of anus and rectum | Haemorrhage of anus and rectum | 37,545 | 21,106 | Bleeding | Gastrointestinal | Yes |
K66.1 | Other disorders of peritoneum | Haemoperitoneum | 3317 | 642 | Bleeding | Gastrointestinal | Yes |
K92.0 | Other diseases of digestive system | Haematemesis | 67,589 | 27,503 | Bleeding | Gastrointestinal | Yes |
K92.1 | Other diseases of digestive system | Melaena | 67,036 | 22,979 | Bleeding | Gastrointestinal | Yes |
K92.2 | Other diseases of digestive system | Gastrointestinal haemorrhage, unspecified | 192,053 | 97,428 | Bleeding | Gastrointestinal | Yes |
M25.0 | Other joint disorders, not elsewhere classified | Haemarthrosis | 3362 | 1730 | Bleeding | Yes | |
N02.0 | Recurrent and persistent haematuria | Recurrent and persistent haematuria – minor glomerular abnormality | 48 | 21 | Bleeding | Yes | |
N02.1 | Recurrent and persistent haematuria | Recurrent and persistent haematuria – focal and segmental glomerular lesions | 286 | 76 | Bleeding | Yes | |
N02.2 | Recurrent and persistent haematuria | Recurrent and persistent haematuria – diffuse membranous glomerulonephritis | 1858 | 517 | Bleeding | Yes | |
N02.3 | Recurrent and persistent haematuria | Recurrent and persistent haematuria – diffuse mesangial proliferative glomerulonephritis | 160 | 49 | Bleeding | Yes | |
N02.4 | Recurrent and persistent haematuria | Recurrent and persistent haematuria – diffuse endocapillary proliferative glomerulonephritis | 10 | 4 | Bleeding | Yes | |
N02.5 | Recurrent and persistent haematuria | Recurrent and persistent haematuria – diffuse mesangiocapillary glomerulonephritis | 47 | 12 | Bleeding | Yes | |
N02.6 | Recurrent and persistent haematuria | Recurrent and persistent haematuria – dense deposit disease | 9 | 1 | Bleeding | Yes | |
N02.7 | Recurrent and persistent haematuria | Recurrent and persistent haematuria – diffuse crescentic glomerulonephritis | 164 | 48 | Bleeding | Yes | |
N02.8 | Recurrent and persistent haematuria | Recurrent and persistent haematuria – other | 10,852 | 1289 | Bleeding | Yes | |
N02.9 | Recurrent and persistent haematuria | Recurrent and persistent haematuria – unspecified | 2902 | 1331 | Bleeding | Yes | |
N93.8 | Other abnormal uterine and vaginal bleeding | Other specified abnormal uterine and vaginal bleeding | 4801 | 2899 | Bleeding | Other bleed | Yes |
N93.9 | Other abnormal uterine and vaginal bleeding | Abnormal uterine and vaginal bleeding, unspecified | 24,423 | 11,036 | Bleeding | Other bleed | Yes |
R04.0 | Haemorrhage from respiratory passages | Epistaxis | 48,741 | 24,610 | Bleeding | Other bleed | Yes |
R04.1 | Haemorrhage from respiratory passages | Haemorrhage from throat | 191 | 69 | Bleeding | Other bleed | Yes |
R04.2 | Haemorrhage from respiratory passages | Haemoptysis | 32,143 | 12,743 | Bleeding | Other bleed | Yes |
R04.8 | Haemorrhage from respiratory passages | Haemorrhage from other sites in respiratory passages | 1332 | 222 | Bleeding | Other bleed | Yes |
R04.9 | Haemorrhage from respiratory passages | Haemorrhage from respiratory passages, unspecified | 83 | 23 | Bleeding | Other bleed | Yes |
R23.3 | Other skin changes | Spontaneous ecchymoses | 10,624 | 2774 | Bleeding | Yes | |
R58.X | Haemorrhage, not elsewhere classified | Haemorrhage, not elsewhere classified | 2747 | 408 | Bleeding | Yes | |
T81.0 | Complications of procedures, not elsewhere classified | Haemorrhage and haematoma complicating a procedure, not elsewhere classified | 67,338 | 28,601 | Bleeding | Yes | |
T81.7 | Complications of procedures, not elsewhere classified | Vascular complications following a procedure, not elsewhere classified | 1000 | 201 | Bleeding | Yes |
Appendix 4 Additional data from the national survey of VTE leads
Survey transcript
VTEAM methodology survey 2021
Introduction
Thank you for clicking the link and considering our survey.
We are writing to you as the project management group of the VTEAM study (NIHR 127454). This project is looking to assess the cost-effectiveness of different venous thromboembolism risk assessment tools for hospitalised inpatients within an NHS care setting.
Our project has several workstreams. Workstream 1 is an evidence synthesis and decision-analytic modelling exercise designed to collate risk assessment models and evaluate clinical effectiveness, then use these data to calculate the comparative cost-effectiveness of different models in practice.
Workstream 2 is a feasibility study, designed to evaluate the use of efficient data methods to collect routine outcome data on VTE and bleeding episodes. Within this workstream we are recording inter- and intra-hospital variation in risk assessment methodology, documentation and information access. Several outputs from the VTEAM study already summarise some of our early findings:
Systematic review of validated VTE risk assessment models – https://bmjopen.bmj.com/content/11/7/e045672
BMJ Uncertainties article; VTE risk assessment for hospitalised patients – www.bmj.com/content/373/bmj.n1106
In this survey, we would like you to provide information on the method of risk assessment used at your trust and how this information is stored locally. We would also like to gauge your opinion as VTE leads about participation in future research evaluating different methods of risk assessment and/or VTE prevention.
There are 10 questions within this survey and completion time is < 10 minutes. Please answer all questions as best you can and provide free text for us at the final question if you have suggestions you feel are within the remit of the project, but not identified within the questions.
Many thanks for your time on this survey, and your leadership in VTE prevention.
-
Daniel Horner
-
Steve Goodacre
-
Beverley Hunt
-
Kerstin de Wit
-
Xavier Griffen
-
Mark Holland
Local methods of VTE risk assessment and data storage
Which hospital trust, organisation or site are you representing?
How do clinical teams currently conduct a VTE risk assessment for hospitalised inpatients at your trust?
Paper pro forma | |
Within an electronic health record | |
A combination of the both within the hospital, dependent on ward environment |
If you use a paper pro forma, which of the following fields are recorded?
Positive VTE risk characteristics | |
Negative VTE risk characteristics (do you record the absence of a risk characteristic) | |
Final decision on pharmacological thromboprophylaxis | |
Not applicable (do not use paper pro forma) |
When completing an electronic VTE risk assessment, which of the following fields do you record?
Positive VTE risk characteristics | |
Negative VTE risk characteristics (do you actively record the absence of a VTE risk factor?) | |
Final decision regarding pharmacological thromboprophylaxis | |
Not applicable (do not use electronic methods of risk assessment) |
Would you be able to access granular data from your VTE risk assessments efficiently through a request to local business information/intelligence/data teams, in order to report the presence or absence of risk characteristics in a large cohort of patients?
Yes | |
No | |
Uncertain |
Potential design preferences and willingness to participate in future studies on VTE risk assessment.
Would you be potentially willing to participate in future observational research on VTE risk assessment methods, collecting data on individual risk characteristics and relevant outcomes up to 90 days post discharge?
Yes | |
No |
Would you be potentially willing to participate in future interventional research on VTE risk assessment methods, collecting data on individual risk characteristics and randomising patients to different risk assessment models at hospital admission?
Yes | |
No |
Our preliminary findings suggest there is significant uncertainty about the best approach to prophylaxis in patients identified at low risk for VTE events during hospitalisation.
Assuming your trust was properly supported to deliver a trial looking to compare other risk assessment models against current practice, which of the following designs would be acceptable to you as trust VTE lead?
Potential allocation of your site to a new risk assessment model within the context of a wider national cluster randomised trial for a set study duration | |
Potential allocation of certain local wards to a new risk assessment model, within the context of a wider national ‘cluster’ randomised trial for a set study duration | |
Potential allocation of your site to two discrete periods of risk assessment, with an interim washout period, where you were to introduce a new risk assessment model at a certain time period and continue standard practice during the other study period, with ongoing data collection on relevant outcomes | |
Individual patient randomisation with fully informed consent | |
I do not think any of these designs could be implemented at my trust |
Other authors have suggested an individualised approach is likely to be preferably regarding VTE risk assessment and thromboprophylaxis, including higher dosing regimens for patients at higher risk of VTE.
Assuming your trust was properly supported to deliver a trial looking to compare this strategy against current practice, which of the following designs would be acceptable to you as trust VTE lead?
Potential allocation of your site to a new dosing strategy in higher-risk patients, within the context of a wider national cluster randomised trial for a set study duration | |
Potential allocation of certain local wards within your site to a new dosing strategy in higher-risk patients, within the context of a wider national ‘cluster’ randomised trial for a set study duration | |
Potential allocation of your site to two discrete periods of dosing strategy for higher-risk patients, with an interim washout period, where you were to introduce a new dosing strategy for higher-risk patients at a certain time period and continue standard practice during the other study period, with ongoing data collection on relevant outcomes | |
Individual patient randomisation with fully informed consent | |
I do not think any of these designs could be implemented at my trust |
Taking the population as a whole, our preliminary findings suggest it may be most cost-effective to deliver pharmacological thromboprophylaxis as an ‘opt out’ strategy, unless contraindicated. In this situation, initial assessment would focus primarily on identification of bleeding risks only.
Assuming your trust was properly supported to deliver a trial looking to compare this strategy against current practice, which of the following designs would be acceptable to you as trust VTE lead?
Potential allocation of your site to a new ‘opt-out’ strategy, within the context of a wider national cluster randomised trial for a set study duration | |
Potential allocation of certain local wards within your site to a new ‘opt-out’ strategy, within the context of a wider national ‘cluster’ randomised trial for a set study duration | |
Potential allocation of your site to two discrete periods of VTE risk assessment strategy, with an interim washout period, where you introduce a new opt-out strategy for one period and continue standard practice during the other, with ongoing data collection on relevant outcomes | |
Individual patient randomisation with fully informed consent | |
I do not think any of these designs could be implemented at my trust |
Please also tell us what you think the priority research question should be regarding VTE risk assessment within the current NHS infrastructure. Please also use this opportunity to highlight any issues, queries or uncertainties with regard to the preceding questions and information.
Responding sites
-
St Peters Hospital
-
Sheffield Teaching Hospital NHS Foundation Trust
-
University of Leicester NHS Trust
-
North Bristol Trust
-
Norfolk and Norwich University Hospitals
-
University Hospitals Plymouth NHS Trust
-
Stockport NHS Foundation Trust
-
The Royal Orthopaedic Hospital Birmingham
-
University Hospital of North Midlands
-
Kings College Hospital NHS Foundation Trust
-
Salisbury NHS Healthcare Trust
-
Princess of Wales Hospital, Glamorgan
-
South Tyneside NHS Foundation Trust
-
Royal Cornwall NHS Foundation Trust
-
Princess Alexandra NHS Trust
-
Spire Hospital, Alexandra
-
Oxford University Hospitals NHS Foundation Trust
-
St Georges Hospital
-
Cambridge University Hospital NHS Foundation Trust
-
Ashford and St Peters Hospitals NHS Foundation Trust
-
Salford Royal NHS Foundation Trust
Free text responses
25 | As a site we would need adequate support to participate as our current establishment would not support a trial of this scale. | 05/11/2021 12:00 PM ID: 178398863 |
24 | n/a | 04/11/2021 15:46 PM ID: 178339995 |
23 | Prescribers think VTE risk assessment if a tick box exercise. | 04/11/2021 08:37 AM ID: 178291192 |
22 | No question. | 02/11/2021 17:39 PM ID: 178174756 |
21 | What would be the criteria for defining ‘higher-risk patients?’ I am slightly concerned that changing the criteria might cause some confusion. | 02/11/2021 17:25 PM ID: 178173203 |
20 | Happy to be involved with any research and discussions around this area. | 02/11/2021 11:06 AM ID: 178128769 |
19 | Identifying high-risk patients – benefit of higher dose thromboprophylaxis – validation of current RA tool. | 01/11/2021 10:45 AM ID: 178037304 |
18 | We are a low-risk private elective surgery hospital unsure of priority research question. | 28/10/2021 11:44 AM ID: 177822796 |
17 | Re Q10 Taking the population as a whole, our preliminary findings suggest it may be most cost-effective to deliver pharmacological thromboprophylaxis as an ‘opt out’ strategy, unless contraindicated. In this situation, initial assessment would focus primarily on identification of bleeding risks only. I agree the focus should be on the correct identification of bleeding risks. | 28/10/2021 09:29 AM ID: 177807213 |
16 | Whether VTE risk assessment actually has any positive impact on VTE prophylaxis prescribing rates and clinical outcomes in regard to HA-VTE. | 28/10/2021 09:12 AM ID: 177806366 |
15 | Identifying patients who need prophylaxis (depending upon risk stratification – mechanical or chemical) and duration of prophylaxis, reasons for delay in start of prophylaxis tool to flag daily risk assessment (when clinical condition changes). | 28/10/2021 09:02 AM ID: 177805185 |
14 | Is there evidence a VTE RA has been undertaken on admission and appropriate TP prescribed? I am happy to get involved but my biggest problem is I work alone managing a large service and therefore don’t always have enough time! | 27/10/2021 10:40 AM ID: 177742113 |
13 | Should patients be reviewed prior to stopping VTE prophylaxis, especially if mobility is still restricted? | 27/10/2021 10:12 AM ID: 177739844 |
12 | n/a | 27/10/2021 09:11 AM ID: 177734851 |
11 | producing a revised risk assessment tool that better identifies medical patients who need thromboprophylaxis. | 27/10/2021 08:38 AM ID: 177732485 |
10 | Other authors have suggested an individualised approach is likely to be preferably regarding VTE risk assessment and thromboprophylaxis, including higher dosing regimens for patients at higher risk of VTE. | 27/10/2021 07:47 AM ID: 177730926 |
9 | Questions we receive are significance of nil by mouth period and reduced mobility, i.e. is dehydration only risk if bloods demonstrate clinical dehydration. Re-assessment window – latest guidance is time of consultant review or if condition changes. If post-op what would optional time for review? | 26/10/2021 21:58 PM ID: 177720950 |
8 | In the current risk assessment, patients (medical) whose mobility is unlikely to be changed during admission are considered as not for further risk assessment. But in my opinion even if they are still mobile, there is a risk of dehydration, less mobile as in the limited space in hospital compared to their own residence, make them more prone to thrombosis. There are patients who are bed-bound and have no change to their mobility since admission, but deficiently are at a high risk for thrombosis as inpatients. Can this be reviewed again? | 18/10/2021 10:58 AM ID: 176826830 |
7 | How we define mobility assumption seems to be if they mobilise to bathroom that counts as mobile. | 14/10/2021 16:19 PM ID: 176648271 |
6 | For the three above questions our risk assessment is via an electronic system and it is very difficult to change it or add to it. If this can be overcome, then the options for research with different risk assessments are possible. | 14/10/2021 08:52 AM ID: 176598234 |
5 | I think that choosing certain wards for the potential change in assessing and prescribing TP would be best as change is complicated and not often greeted with enthusiasm. In order for a trial to work it must be robust. | 13/10/2021 14:54 PM ID: 176551827 |
4 | N/A | 13/10/2021 13:16 PM ID: 176539276 |
3 | Priority: Identifying those whose risk of harm associated with chemical thromboprophylaxis outweighs the potential benefits (i.e. refining bleeding scores- elderly, frail, falls risk etc.). Define cohorts at high risk of VTE who would benefit from extended chemical thromboprophylaxis. Study the delivery of thrombosis prevention strategies within organisations. Regarding proposed studies above, they will need to be carefully designed so they can be delivered. If including emergency admissions, with a variety of junior medical staff involved (ED, acute, generalists and specialities) care will need to be taken if to have ‘certain wards’ allocated to specific treatments. In our organisation, patients can move from ED, to MAU, to speciality wards. Therefore, for ‘acute’ care, allocation of the site to a specific strategy may work best. BUT for some specialities, (orthopaedic spinal, stroke, cancer surgery), specific guidance will be required. Keen to contribute | 13/10/2021 11:30 AM ID: 176520022 |
2 | VTE risk assessment and therefore the prophylaxis is often delayed and in many cases relevant risks are not ticked. I wonder if it would make a difference if it was done during the first doctor’s assessment and if highlighting the risks was mandatory. There is also another problem, I find it frustrating when the pharmacists find issues and in many cases, their notes to doctors are not acted on. I agree that research into higher doses of pharmacological prophylaxis for higher-risk patients is needed but also if a patient is in a higher risk category and his weight is at higher end of a specific range, then the dosing could be adjusted, for example: a person weighing 97 kg, could get dosing required for over 100 kg. | 13/10/2021 09:59 AM ID: 176510305 |
1 | I think the opt-out question is the most interesting one – happy to participate in a national trial on this, if funded and supported. | 12/10/2021 09:31 AM ID: 176411960 |
Glossary
- Cost-effectiveness acceptability curve
- A way of illustrating cost-effectiveness results by plotting the probability that the intervention is cost-effective (y-axis) against the maximum that society is willing to pay for an improvement in health (x-axis).
- Cost-effectiveness plane
- A way of illustrating cost-effectiveness results by plotting the mean incremental cost and effectiveness on a four-quadrant graph. Interventions that are more costly and more effective fall in the north-east quadrant.
- Deep-vein thrombosis
- A blood clot that develops within a deep vein in the body, most commonly in the leg.
- Hospital-acquired thrombosis
- Any new episode of venous thromboembolism (deep-vein thrombosis or pulmonary embolism) diagnosed during hospitalisation or within 90 days of discharge following an inpatient stay of at least 24 hours, or following a surgical procedure under general or regional anaesthesia.
- Incremental cost-effectiveness ratio
- The difference in the mean costs in the population of interest divided by the differences in the mean outcomes in the population of interest.
- Major bleeding
- Serious or fatal bleeding episodes, as defined by the International Society for Thrombosis and Haemostasis.
- Clinically relevant non-major bleeding
- Bleeding episodes which are not major, but require clinical assessment and potential intervention, as defined by the International Society for Thrombosis and Haemostasis.
- Post-thrombotic syndrome
- Pain, swelling, itching, skin discolouration and leg ulcers occurring after a deep-vein thrombosis, caused by damage to the valves in the leg veins that prevent backflow of blood.
- Prophylaxis
- A measure taken to prevent a disease.
- Pulmonary embolism
- A blood clot that breaks off from the deep veins and travels around the circulation to block the pulmonary arteries (arteries in the lung). Most deaths arising from deep-vein thrombosis are caused by pulmonary embolism.
- Quality-adjusted life-year
- A measure of the benefit of healthcare that combines the impact of both the expected length of life and quality of life.
- Risk assessment models
- A set of criteria that aims to estimate the risk of a particular condition/complication and often used by clinicians to inform individual patient decisions on medical interventions.
- Thromboprophylaxis
- A measure taken to reduce the risk of thrombosis; prophylaxis against thrombosis. This measure can be undertaken using drugs (pharmacological thromboprophylaxis) or using compression devices (mechanical thromboprophylaxis).
- Venous thromboembolism
- Thrombosis is the blocking of a blood vessel by a blood clot. This clot may be dislodged fully or partly from its site of origin and travel downstream to lodge in a vital organ, a process described as embolisation. Clots formed in the deep veins of the legs are known as deep-vein thromboses and when fragments break off they travel through the body to block pulmonary arteries. This process is termed pulmonary embolism. Venous thromboembolism is a composite term to describe all the above, including both deep-vein thrombosis and pulmonary embolism.
List of abbreviations
- AES
- antiembolism stockings
- CEAC
- cost-effectiveness acceptability curve
- CI
- confidence interval
- CRNMB
- clinically relevant non-major bleeding
- CRF
- case report form
- CrI
- credible interval
- CTEPH
- chronic thromboembolic pulmonary hypertension
- CTRU
- Clinical Trials Research Unit
- DOAC
- direct oral anticoagulant
- DVT
- deep-vein thrombosis
- EHR
- electronic health record
- EVPI
- expected value of perfect information
- EVPPI
- expected value of partial perfect information
- EQUATOR
- Enhancing the QUAlity and Transparency Of health Research
- GI
- gastrointestinal
- GTAC
- Gene Therapy Advisory Committee
- HAT
- hospital-acquired thrombosis
- HAS-BLED
- Hypertension, Abnormal renal/liver function, Stroke, Bleeding history or predisposition, Labile international normalised ratio, Elderly, Drugs/alcohol concomitantly
- HES
- hospital episodes statistics
- HIT
- heparin-induced thrombocytopenia
- HRA
- Health Research Authority
- HRQoL
- health-related quality of life
- HTA
- Health Technology Assessment
- ICD-10
- International Classification of Diseases, Tenth Revision
- ICH
- intracranial haemorrhage
- IQR
- interquartile range
- IMPROVE
- International Medical Prevention Registry on Venous Thromboembolism
- LMWH
- low-molecular-weight heparin
- NICE
- National Institute for Health and Care Excellence
- NLP
- natural language processing
- NMA
- network meta-analysis
- NSQIP
- National Surgical Quality Improvement Programme
- NYHA
- New York Heart Association
- OXVASC
- Oxford Vascular Study
- PE
- pulmonary embolism
- PMG
- project management group
- PPI
- patient and public involvement
- PSA
- probabilistic sensitivity analysis
- PREFER-VTE
- Prevention of Thromboembolic Events – European Registry in Venous Thromboembolisma
- PRISMA
- Preferred Reporting Items for Systematic Review and Meta-analyses
- PROBAST
- prediction model risk of bias assessment tool
- PROSPERO
- Prospective Register of Systematic Reviews
- PTS
- post-thrombotic syndrome
- QALYs
- quality-adjusted life-years
- RAM
- risk assessment model
- RCT
- randomised controlled trial
- REC
- Research Ethics Committee
- RIETE
- Computerized Registry of Patients with Venous Thromboembolism
- ROC
- receiver operating characteristic
- SD
- standard deviation
- SECF
- Sheffield Emergency Care Forum
- SMR
- standardised mortality ratio
- SNOMED
- Systematised Nomenclature of Medicine
- STROBE
- STrengthening the Reporting of OBservational studies in Epidemiology
- TESS
- Trauma Embolic Scoring System
- VKA
- vitamin K antagonist
- VTE
- venous thromboembolism
- VTEAM
- Venous ThromboEmbolism Assessment Methods
- XAMOS
- Xarelto® in the prophylaxis of post-surgical venous thromboembolism after elective major orthopedic surgery of hip or knee
Notes
Supplementary material can be found on the NIHR Journals Library report page (https://doi.org/10.3310/AWTW6200).
Supplementary material has been provided by the authors to support the report and any files provided at submission will have been seen by peer reviewers, but not extensively reviewed. Any supplementary material provided at a later stage in the process may not have been peer reviewed.