Notes
Article history
This issue of the Health Technology Assessment journal series contains a project commissioned by the MRC–NIHR Methodology Research Programme (MRP). MRP aims to improve efficiency, quality and impact across the entire spectrum of biomedical and health- related research. In addition to the MRC and NIHR funding partners, MRP takes into account the needs of other stakeholders including the devolved administrations, industry R&D, and regulatory/advisory agencies and other public bodies. MRP supports investigator-led methodology research from across the UK that maximises benefits for researchers, patients and the general population – improving the methods available to ensure health research, decisions and policy are built on the best possible evidence.
To improve availability and uptake of methodological innovation, MRC and NIHR jointly supported a series of workshops to develop guidance in specified areas of methodological controversy or uncertainty (Methodology State-of-the-Art Workshop Programme).
Workshops were commissioned by open calls for applications led by UK-based researchers. Workshop outputs are incorporated into this report, and MRC and NIHR endorse the methodological recommendations as state-of-the-art guidance at time of publication.
The authors have been wholly responsible for all data collection, analysis and interpretation, and for writing up their work. The HTA editors and publisher have tried to ensure the accuracy of the authors’ report and would like to thank the reviewers for their constructive comments on the draft document. However, they do not accept liability for damages or losses arising from material published in this report.
Permissions
Copyright statement
Copyright © 2023 Totton et al. This work was produced by Totton et al. under the terms of a commissioning contract issued by the Secretary of State for Health and Social Care. This is an Open Access publication distributed under the terms of the Creative Commons Attribution CC BY 4.0 licence, which permits unrestricted use, distribution, reproduction and adaptation in any medium and for any purpose provided that it is properly attributed. See: https://creativecommons.org/licenses/by/4.0/. For attribution the title, original author(s), the publication source – NIHR Journals Library, and the DOI of the publication must be cited.
2023 Totton et al.
Chapter 1 Introduction
Background
Randomised controlled trials (RCTs) are an essential methodology for an unbiased assessment of different health technologies in a real-world setting. RCT results are used to inform patient treatment decisions. In National Institute for Health and Care Research (NIHR) Health Technology Assessment (HTA) programme-funded RCTs, the comparison is often between a new health technology and an existing one that is already part of regular practice. In these cases, the benefit (i.e. superiority) of the new health technology may be not a health outcome, but instead an improvement in the safety profile, cost or convenience of delivery. However, it remains important that the RCT demonstrates that it is similar or at least non-inferior to the existing health technology for the desired health outcome to ensure that it would be acceptable for implementation in regular practice (assuming its superiority on another outcome).
Instead of the RCT being designed on a superiority basis, the trial may have an equivalence or non-inferiority design. Equivalence implies that the two health technologies are ‘equal’ to each other with respect to the primary outcome, whereas non-inferiority implies that the ‘new intervention is “not unacceptably worse” than the intervention used as the control’. 1 In this report, key factors that influence the trial design are outlined to aid the decision of which design is most appropriate.
Certain key elements of the trial, such as the sample size, depend on the trial objective chosen (i.e. superiority, equivalence or non-inferiority), which is usually based solely on the primary outcome. However, this singular focus does not reflect the complexity of policy decisions when information on multiple outcomes is of interest. For example, as well as the clinical effectiveness of a new health technology, there is a need to compare the effect of the technology on safety, cost(-effectiveness) and convenience with that of the existing technology when deciding if the former should be used in regular practice in the NHS.
There are existing statistical methodologies to account for the issue of more than one outcome of interest, such as the use of multiple primary outcomes,2 composite outcomes3 or multivariate analysis (i.e. where two or more outcomes are analysed simultaneously). These methods may be appropriate to use when multiple outcomes are expected to be improved by the treatment or when there is a clear ranking of health states in terms of their importance. However, they still require implicit judgement about how the outcomes relate and careful interpretation when the outcomes are in competing directions. For example, if a new health technology is clinically better than current practice, but results in an increase in the number or severity of adverse events, a method to identify the overall best health technology is required.
Benefit–risk (B–R) approaches are a group of methodologies that can be used in such situations to evaluate the net clinical benefit and allow a direct comparison of competing health technologies. B–R approaches are already used in the regulatory setting, where it is important that regulators can evaluate the benefits of a drug against its harms. In addition, the B–R trade-off is an important element of portfolio management decisions. 4 A European consortium B–R methodology project [named Pharmacoepidemiological Research on Outcomes of Therapeutics by a European Consortium (PROTECT)], which had a goal to improve the evaluation of benefit and risks related to medicines, is now complete. 5 However, despite their utility, the methods have yet to be fully applied to publicly funded RCTs, such as NIHR HTA trials.
Aims and objectives
The overall aim of this project is to provide consensus-driven recommendations for the inclusion of B–R approaches in the design of NIHR HTA programme-funded RCTs.
The objectives involved in achieving this aim are to:
-
describe the factors affecting selection of the appropriate design for a trial (i.e. superiority, non-inferiority or equivalence)
-
evaluate when it may be appropriate to apply B–R methods
-
explore the different B–R methods that could be included within NIHR HTA RCTs
-
define the information related to B–R that should be included in trial proposals, protocols and reports.
Scope of the report
This report discusses trial design in relation to superiority, non-inferiority or equivalence, but does not discuss the differences in other trial design features, such as crossover trials, network analyses or indirect comparisons. The focus of this report is predominantly on the parallel-group design in the first instance; in the future, work could be extended to assess the appropriateness of other designs.
In addition, this report considered clinical trials in which outcomes are assessed at the individual level. Again, this work could be extended in the future to contain population-level outcomes, for example including studies that aim to estimate prevalence effects.
Development of the recommendations
The Benefit–Risk Assessment to Inform Non-Inferiority and Superiority Study Design (BRAINS) project consisted of three key research stages, leading to the development of the recommendations. The stages were as follows.
Survey (work package 1)
Between March 2019 and July 2019, a survey of current practice relating to B–R was circulated to researchers within the field of B–R and/or publicly funded RCTs. The aim of this was to elicit how and why B–R has been used to date (if applicable) and respondents’ desire for information relating to B–R.
In total, 64 people took part in the survey, with most being from academic backgrounds in the UK. The majority (64%) did not have experience with B–R methods, but were interested in learning about the methodology. The most prevalent response was to include recommendations on how to select an appropriate trial design, which informed the focus of this topic in the workshop. Further details can be found in Appendix 1.
Rapid review (work package 2)
A rapid literature review was completed to assess available methods for B–R analysis in RCTs. This used a pearl-growing technique, starting with relevant papers known to the research team, to identify key words and medical subject headings (MeSH) to inform our formal search strategy. We performed two iterations of searches using MEDLINE and Web of Science™ (Clarivate Analytics, Philadelphia, PA, USA), plus a citation review. The foci of the review were on methods papers and building on previous, known reviews, with a different focus for data extraction.
Relevant information was extracted from 76 identified articles; 97 different methods were found, which have been categorised into six groups, ranging from an overarching framework to quantitative methods. These papers were then evaluated for context, reason, strengths and weaknesses associated with using the methods, as well as identifying those methods that can be completed with the data currently collected in publicly funded RCTs and those methods that would require additional information (see Appendix 2).
Consensus workshop (work package 3)
Information gathered from the survey and literature review was discussed at a 2-day consensus workshop that was held in September 2019 in Sheffield, UK. This included the use of presentations, the nominal group technique (NGT)6 and open discussions, followed by thematic analysis7 of the transcriptions (see Appendix 3 for further information).
The workshop was attended by 15 researchers, representing a mix of disciplines and sectors. A 19-item list of factors affecting the decision on the appropriate trial design to use was created at the workshop using the NGT. Themes from the discussions were extracted to provide reasons that would indicate the potential for use of a B–R method. A checklist was then produced to be used when reporting B–R information; the checklist contains five items at the trial design stage and seven items at the trial results stage.
Chapter 2 Selecting a trial design
Definitions
This chapter will further define the different types of trial objective (superiority, non-inferiority and equivalence) mentioned in Chapter 1 (Figure 1 presents a visual representation), before providing a list of factors that affect the decision of which design is the most appropriate for a trial. This was the item in the survey that most researchers said that they wanted guidance on (see Appendix 1).
FIGURE 1.
Demonstration of intervention effects for a health technology, with 95% confidence intervals, in relation to superiority, non-inferiority and equivalence. Adapted from Hahn et al. 8 This is an Open Access article distributed in accordance with the terms of the Creative Commons Attribution Non-Commercial (CC BY-NC 3.0) license, which permits others to distribute, remix, adapt and build upon this work, for non-commercial use, provided the original work is properly cited. See: https://creativecommons.org/licenses/by-nc/3.0/.

Superiority
Superiority trials are designed to ‘detect a difference between treatments’. 9 The null hypothesis is that there is no difference between the comparison groups, and the statistical tests aim to identify whether or not the observed difference could have occurred by chance if this assumption of no difference is true. If a test concludes with a p-value of < 0.05 (by convention) on a two-sided test, then this is considered evidence that the null hypothesis can be rejected and that there is evidence of a statistical difference between the comparison groups. Typically, this is based on a power level of either 80% or 90%. 10,11
The majority of clinical trials use a superiority design, as the aim is to show an improvement from current practice. The sample size for superiority trials is that required to ensure that a predetermined target difference of interest (δ)11 can be statistically detected within the data, if it is present.
Equivalence
Equivalence trials are designed to show that the health technologies differ only by a predefined value (δE) that has been deemed clinically not relevant. This provides a lower and upper bound around zero for the difference between the groups, which may be viewed as equivalent. The null hypothesis is that the treatment difference is of a size that would be considered clinically meaningful. To reject this, the point estimate and the two-sided 95% confidence interval must be within the limits of both the positive and the negative value (–δE, δE).
Equivalence trials have been used to show that a new health technology is not only no worse than the existing health technology, but also no better (within given limits). For example, researchers may wish to design an intervention to increase screening contacts in certain subgroups, but with the total number of invitations remaining the same across all patients (see Appendix 4 for more details). This may be important in a real-world setting.
Non-inferiority
Non-inferiority trials are similar to equivalence trials, but focus on a single direction and, therefore, in terms of the analysis, on one side of the confidence interval for the treatment effect only. The null hypothesis is that there is a treatment difference in favour of the comparator and the result relies on the one-tailed 97.5% confidence interval (lower limit) being inside the predefined non-inferiority margin (δNI). This margin can be based on the same principle as the equivalence margin, with the key difference being that the trial ignores whether or not the intervention is better than the comparator.
Non-inferiority trials are particularly used when new health technologies are tested against standard care and the benefit is on a secondary outcome, such as lower cost or reduced patient burden. In these cases, showing that the health technology is no worse than standard care suggests that it would be worth implementing this in practice.
Outcome measure selection
Selecting the outcome measures to be used in a trial is often based on the clinical decision of which aspects of health and well-being the health technology will affect. The primary outcome should be the most important outcome related to the health technology and the most important to patients. In addition, the Core Outcome Measures in Effectiveness Trials Initiative12 collates Core Outcome Sets to inform the decision of which key outcomes to include depending on the disease area and/or health technology. It is important to gather a range of opinions from various stakeholders, including patients, when selecting important outcomes for the trial to ensure that their considerations are included. 13
Factors for selecting a trial design
Through the workshop, a list of factors that inform the selection of the trial design was compiled that aims to assist decision-making when a study is being planned. These 19 items have been summarised in Table 1 and further elaboration is provided in the subsequent section. This list of factors will be illustrated further through worked examples.
Item | Trial designs | |
---|---|---|
Superiority | Equivalence/non-inferiority | |
1: Population | ||
1a: What is the trial population? | Low-disease-burden population | High-disease-burden population |
1b: What are the subpopulations of interest? | Superiority across all subpopulations | Different trial design on different subpopulations |
2: Intervention | ||
2a: What is the intervention? | Intervention is different to comparator in terms of mechanism, dose, method of administration, etc. | Intervention is similar to comparator in terms of mechanism, dose, method of administration, etc. |
3: Comparator | ||
3a: What is the comparator? | Placebo or no treatment (active comparator possible) | Active comparator |
3b: What is current practice or standard care? | Current practice is more effective than the chosen comparator | Current practice used as comparator |
3c: What evidence is there in relation to the comparator? | Poor-quality evidence that shows little benefit over placebo/no treatment | Good-quality evidence that shows a large benefit over placebo/no treatment |
4: Outcomes | ||
4a: What are the priorities of different outcomes? | Highest priority outcome is improved by intervention | Highest priority outcome is not necessarily improved by intervention |
4b: How many clinical outcomes are key to eventual decision-making? | One outcome | Multiple outcomes are key to eventual decision-making, such as quality of life, safety |
4c: What are the superiority outcomes? | Primary outcome is superiority outcome | At least one secondary outcome is viewed as superior (must be defined), but the primary outcome is not |
4d: Are the costs of the comparator and intervention being considered? | Higher expected costs for intervention than for comparator | Similar or lower expected costs for intervention than for comparator |
4e: Is non-inferiority or equivalence on the primary outcome plausible? | Not plausible that the intervention is within a reasonable limit compared with the comparator | Plausible that the intervention is within a reasonable limit compared with the comparator |
4f: What are the health economic outcomes? | Positive incremental net benefits expected | Cost-minimisation expected |
5: Feasibility | ||
5a: What is the proposed sample size? | Infeasible to achieve sample size for alternative trial design | Highest sample size is associated with equivalence, so if it is not possible to achieve the sample size, use non-inferiority |
5b: What is the VoI for the different trial designs? | Value of additional information from larger sample size does not provide good value for money | Value of additional information from larger sample size provides good value for money |
6: Perspectives | ||
6a: What ethics considerations are there? | Not ethical to observe any reduction on a particular outcome | Ethical to allow for some inferiority on the outcome, provided that there is an expected benefit on another outcome |
6b: What is the perspective of patients and service users? | Want to see superiority on chosen outcome to make treatment attractive | Secondary superiority outcome means patients are willing for equivalence or non-inferiority result on primary outcome |
6c: What is the perspective of decision-makers? | ||
6d: what is the perspective of clinicians? | ||
6e: What is the impact on different sectors (e.g. health, education, social care)? | Potential negative impact on another sector (e.g. increased workload) | Potential positive impact on another sector (e.g. reduced workload) |
There is a degree of overlap between some of the factors mentioned. However, based on the workshop, where the importance of items was voted on, the factors remain separated to reiterate or provide nuance to points when necessary. Those items in italics in the table represent factors that would necessitate choosing the given trial design. Other factors will influence the trial design, but do not dictate it.
This list mirrors both the population, intervention, comparison, outcome (PICO) format, which is used to appropriately frame research questions,14 and the estimand framework, which was an addendum to the International Council for Harmonisation (ICH) E9 guidance. 15 The aim of this framework was to clearly describe the trial objectives and, ultimately, ensure appropriate analysis, reporting and interpretation in relation to these.
Elaboration and explanation
Each of the items in Table 1 is explained further in the subsequent sections and examples are provided (one in Example: superiority and two further examples in Appendix 4).
Population
1a: What is the trial population?
The trial populations with the highest level of disease burden are likely to trade off gains in one element of burden for slight losses in another; here, the use of an equivalence or non-inferiority study design may be more appropriate. Those with the least burden are less likely to have such trade-offs and would be more focused on improvements; hence, a superiority trial is likely to be appropriate.
1b: What are the subpopulations of interest?
In any given trial, there may be subgroups of patients for whom superiority is hypothesised and others for whom equivalence/non-inferiority would be hypothesised. The importance of each of these subgroups and their impact on the overall results will inform the main research question to ensure that the design accurately reflects the most important or prevalent subgroup.
Intervention
2a: What is the intervention?
If the intervention is similar (e.g. in terms of mechanism of effect or method of administration) to the comparator, demonstrating superiority may not be feasible. In addition, the selection of the outcome measure used to assess clinical effectiveness (which, in turn, influences trial design; see Outcomes) is based on existing trial data or other data pertaining to the intervention.
Comparator
3a: What is the comparator?
If the comparator (i.e. control group) is a placebo or no treatment, it is conventional for the trial to adopt a superiority design. However, if the comparator is an active treatment, then equivalence or non-inferiority designs could be considered, depending on the expected treatment effect (see 2a: What is the intervention?). There may be a situation in which a trial compares an active treatment given in addition to the active control, in which case the comparator is the active control on its own (i.e. evaluating the active treatment as an ‘add-on’); equivalence or non-inferiority could also be considered in this situation.
3b: What is current practice or standard care?
If an active treatment is currently used in practice and a placebo or no treatment is being considered as the comparator, the trial should be on a superiority basis. The chosen value of delta should be at least the clinical effectiveness between the active treatment used in practice and control being used in this trial. However, use of the current standard practice as the comparator is most commonly recommended for HTA trials. If this is an active treatment, then the trial design is determined by target treatment effects. 11
3c: What evidence is there in relation to the comparator?
Evidence for the comparator treatment that suggests a large benefit of the active control against placebo/no treatment could warrant the use of a non-inferiority design, as the improvement has already been demonstrated. However, it is also important to consider the quality of this evidence, as, if this has a large margin of error, then the confidence in the improvement may be limited. Without this evidence, proving non-inferiority against a poorly judged active control would not be a worthwhile result and, therefore, a superiority design would be required.
Outcomes
4a: What are the priorities of different outcomes?
The prioritisation of outcomes will provide guidance on the selection of the primary outcome (and the key secondary outcomes), which will, in turn, determine which trial design is selected. This prioritisation should be justified and considered with input from patient representatives. The highest priority outcome can then be evaluated on either a superiority or an equivalence/non-inferiority basis.
4b: How many clinical outcomes are key to eventual decision-making?
Within a trial, there may be one key outcome of interest that is by far the most important, as it is related to the disease and its treatment (e.g. mortality). With only one important outcome, the trial would probably be considered on a superiority basis. If there are multiple outcomes of relevance, then an equivalence or non-inferiority design may be considered.
4c: What are the superiority outcomes?
Often the chosen primary outcome will be considered on a superiority basis and, therefore, no further consideration needs to be made. However, superiority may be a secondary outcome, such as cost or patient burden, meaning that the primary outcome could be designed on an equivalence or non-inferiority basis. When equivalence or non-inferiority designs have been selected, it is essential that the superiority outcomes and the method for evaluating these is clear.
4d: Are the costs of the comparator and intervention being considered?
If the consideration of the incremental costs of care is essential for decision-making about treatment success, this can affect which trial design is chosen. If the a priori expectation is that costs associated with the new health technology are higher than those associated with the comparator, then the trial will normally have to show superiority on health outcomes for this technology to be considered worthwhile in practice. By contrast, if one of the key benefits is the reduced cost of the new health technology, then the main outcome may be considered on an equivalence or non-inferiority basis; however, in any economic analysis, the joint uncertainty in costs and outcomes should always be considered alongside cost-effectiveness.
4e: Is non-inferiority or equivalence on the primary outcome plausible?
When considering some outcomes, it can be deemed implausible that a new health technology would be considered equivalent or non-inferior if the evidence to date suggests that, for this outcome, the comparator may be better than the intervention group. Depending on the importance of this outcome, this would require a superiority trial on another outcome to mitigate this potential negative effect.
Distinguishing between non-inferiority and equivalence designs relies on the acceptability of an improvement in the outcome due to the intervention. In some cases, logistical and/or resourcing constraints may prevent an improvement in the outcome and, therefore, equivalence must be shown rather than non-inferiority.
4f: What are the health economic outcomes?
Cost-effectiveness analyses are conducted as part of most NIHR HTA clinical trials. These are usually based on the cost of the intervention (or comparator) plus all other related resource use costs and the expected number of quality-adjusted life-years (QALYs) experienced. If the primary outcome is also a health economic outcome, the expected positive incremental net benefits would suggest a superiority design. An equivalence or non-inferiority basis might apply in exceptional instances of cost-minimisation.
Feasibility
5a: What is the sample size?
The sample size calculation for superiority trials often results in a smaller number than that for a non-inferiority or equivalence trial. This is driven by the decreased size of the margin (δ) that is usually used in non-inferiority/equivalence trials. 16 Furthermore, the equivalence design requires a larger sample size because it requires two tests to be carried out, which requires an adjustment to the type II error within the calculation. Although a particular trial design should not be chosen because it requires a larger sample size, it may be that an equivalence study would not be feasible in the population of interest if an extremely large sample size were required. This issue could lead to a non-inferiority design being chosen if the limit on the upper confidence interval (reflecting superiority) is not necessary.
5b: What is the value of information for the different trial designs?
Value of information (VoI) analysis is a ‘quantitative method to estimate the return on investment in proposed research projects’17 that could be used when selecting a trial design. Owing to the increased sample size required for non-inferiority and equivalence trials, there is an additional cost to running these trials. A VoI analysis could assess the information gained against the cost of each trial design and, therefore, identify the design that represents the best value for money. Methods to estimate the sample size based on VoI analysis may be useful. 18
Perspectives
6a: What ethics considerations are there?
In some trials, it may be unethical for patients to receive no treatment or a placebo when a standard active treatment is commonly available. In this case, a non-inferiority trial design may be the most appropriate design as it allows comparison against the current active treatment. However, in certain contexts, non-inferiority/equivalence trials could be unethical, as any reduction in a particular outcome would not be ethical to implement in practice. 19
6b: What is the perspective of patients and service users?
Patients and service users play an essential role when designing research, including clinical trials. NIHR actively promotes public involvement20 in studies to ensure that the public perspective is taken into account. In this context, patients can have a key role in defining the trial design that should be used. It will be clear to them, as users of the service, if they would be willing to see an equivalence or non-inferior result on an outcome or if it would be essential that the new health technology be superior for there to be uptake. Consulting with patient representatives early in the trial design will help to inform this and ensure that engagement with the trial is satisfactory.
6c: What is the perspective of decision-makers?
Convincing key decision-makers, such as those who run the service [NHS, the National Institute for Health and Care Excellence (NICE), the Medicines and Healthcare products Regulatory Agency (MHRA), etc.], of the quality of the evidence is essential, as this evidence will be used to inform recommended practice. If they require evidence of superiority of the health technology, a non-inferiority or equivalence trial may not be worthwhile. However, if they are convinced by a secondary outcome of superiority that can also be shown within the trial, then this design would be attractive to them if there is a key benefit to changing health technologies.
6d: What is the perspective of clinicians?
Clinicians need to be convinced by the results of a study to ensure that they would be willing to implement the health technology in practice. This means that they have an important input, alongside that of patients, to the outcomes of interest and their prioritisation. These decisions then dictate the trial design, depending on whether the clinicians feel they would require evidence of superiority on these outcomes or the existing evidence for benefit is sufficient but that non-inferiority or equivalence of a key health outcome must be shown.
6e: What is the impact on different sectors (e.g. health, education, social care)?
Some interventions may have an impact (unintended or otherwise) on different sectors, for example social care, that should be taken into account. If there is going to be a negative impact on a different sector (i.e. increased burden), it may be important to consider the trial using a superiority outcome, otherwise there would not be sufficient evidence to implement the intervention in practice. However, the fact that there may be a positive impact on a different sector is a reason to consider a non-inferiority or equivalence design.
Example 1: superiority
A RCT is being used to compare the clinical effectiveness and cost-effectiveness of two management strategies for non-acute anterior cruciate ligament (ACL) injury. 21 Box 1 presents the completed list of factors that have an impact on the trial design.
As there are two active health technologies to be assessed, an argument could be made for a non-inferiority trial design. However, there is no basis for suggesting that any of the secondary outcomes (in addition to knee health) would be superior for the intervention over the comparator; therefore, showing superiority on knee health is essential. Furthermore, as the comparison is between two management strategies, the desire is to identify which is more clinically effective. Owing to the lack of evidence regarding the health technologies, the main aim of the study is to define which is more clinically effective for treating knee health (i.e. a superiority design).
Demonstrating superiority on the primary outcome is particularly important when trying to convince clinicians that rehabilitation should be used instead of surgical management, which is currently the most commonly used treatment. Given all of this information, the trial was designed on a superiority basis.
The trial population is patients with non-acute ACL deficiency; the patients may be experiencing issues beyond the specific injury (i.e. effect on quality of life).
1b: What are the subpopulations of interest?There are no key subpopulations of interest to consider.
2: Intervention 2a: What is the intervention?The intervention is non-surgical management (i.e. ACL rehabilitation), which is sometimes used in practice, but the available evidence for its clinical effectiveness is weak and may lead to surgical management in the future.
3: Comparator 3a: What is the comparator?The comparator is surgery (i.e. ACL reconstruction), which has the same purpose as the intervention, but uses a different mode of action to achieve this.
3b: What is current practice or standard care?Surgery (i.e. ACL reconstruction) is currently used in 80% of non-acute patients and this is the comparator.
3c: What evidence is there in relation to the comparator?There is good evidence that surgery is beneficial for ACL injuries in some instances, but there is uncertainty between two potential management strategies (i.e. surgery vs. rehabilitation with the possibility of later surgery).
4: Outcomes 4a: What are the priorities of different outcomes?The most important outcome for these health technologies is knee health; the aim of the trial is to assess the most superior technology in relation to this outcome.
4b: How many clinical outcomes are key to eventual decision-making?There is one key clinical outcome (knee health), as well as cost-effectiveness and quality of life as important outcomes. Therefore, there is more than one outcome of interest.
4c: What are the superiority outcomes?The key clinical outcome (knee health) is to be assessed on a superiority basis; the impact on quality of life and cost-effectiveness is unknown.
4d: Are the costs of the comparator and intervention being considered?The cost of rehabilitation (i.e. the intervention) could be lower than the cost of surgery (i.e. usual care). However, some patients may still require surgery after rehabilitation, thereby increasing costs overall. This is important to assess in the trial, but does not form part of the a priori hypothesis.
4e: Is non-inferiority or equivalence on the primary outcome plausible?The two underlying health technologies are very different. Furthermore, the study is comparing management strategies, rather than individual health technologies. The study premise does not fit the classic non-inferiority/equivalence paradigms.
4f: What are the health economic outcomes?Cost-effectiveness is a key outcome of the trial, but it is in addition to the health outcome, not in place of it.
5: Feasibility 5a: What is the sample size?The calculated sample size of 320 participants for a superiority study has been deemed achievable within the patient population.
5b: What is the VoI for the different trial designs?Showing non-inferiority in this trial design would not change practice and, therefore, the value of undertaking such a trial does not represent good value for money.
6: Perspectives 6a: What ethics considerations are there?As surgery is currently the most common management practice, it would be unethical to have no treatment as the comparator.
6b: What is the perspective of patients and service users?Patients often prefer to avoid surgery, if possible, so the option for rehabilitation first is an attractive treatment as long as it works as well as surgery does.
6c: What is the perspective of decision-makers?The trial was funded by the NIHR HTA programme, which provides evidence for health technologies within the NHS. Therefore, improving the outcome of patients and the potential cost saving arising from a reduction in post-treatment requirements would be more cost-effective and is of interest to the decision-makers.
6d: What is the perspective of clinicians?Clinicians may be more convinced of the clinical efficacy of a surgical treatment than rehabilitation and, therefore, a superiority result may be required on the outcome of knee health to change clinical practice.
6e: What is the impact on different sectors (e.g. health, education, social care)?The impact should remain within the health sector for this trial.
Chapter 3 Benefit–risk inclusion in randomised controlled trials
Benefit–risk dependent on trial design
The considerations that take place when selecting the most appropriate trial design can also have an impact on whether or not a B–R method is appropriate. In the case of a superiority trial design, in which the only consideration is the primary outcome (and, potentially, other superiority secondary outcomes, as measured in the trial), trial conclusions could be considered self-evident and so a B–R method would be unnecessary. 22 One workshop participant said:
. . . for others [trials] it probably is quite benign treatments. You’ll just go with the most effective one or the most cost-effective one, other considerations might not be as important.
Workshop participant
However, there are numerous scenarios in which it could be useful to include a B–R method in a clinical trial, especially when an equivalence or a non-inferiority trial design has been selected.
Reason for inclusion
The key reasons for using a B–R method were identified from the survey, literature review and workshop and were collated into a few specific themes that are described in the following sections and summarised in Box 2.
-
The success of the trial depends on more than one outcome.
-
Important outcomes in the trial are in competing directions (i.e. a health technology is expected to be better on one outcome but worse on another).
-
To allow patient preferences to be included and directly influence trial results.
-
To provide transparency on subjective recommendations from a trial.
-
To provide consistency in the approach to presenting results from a trial.
-
To synthesise multiple outcomes into a single metric.
Trial success
The most common reason for including a more formal B–R method was that the overall success of the trial depended on not only the results of the designated primary outcome, but also the ‘totality of evidence’ (workshop participant). One participant phrased it as:
When you move away from the efficacy trials and you start doing effectiveness, you want to incorporate as many of these things [outcomes] that would be relevant.
Workshop participant
An indicator of this would be if the selected target difference or non-inferiority limit (δ) in the sample size has been adjusted based on other outcomes. This would suggest that the secondary outcomes have an important influence on the primary outcome and, therefore, have an impact on the success of the trial.
The importance of other outcomes in addition to the primary outcome is particularly pronounced in equivalence/non-inferiority studies in which showing equivalence or non-inferiority on the primary outcome is not sufficient. In these cases, equivalence or non-inferiority must be accompanied by an important benefit on another outcome. In these circumstances, by definition, the success of an intervention depends on more than one outcome.
Conflicting outcomes
In addition to multiple outcomes, there are cases in which the outcomes are conflicting (i.e. a health technology results in an improvement in one outcome, but has a detrimental effect on another outcome). B–R methods can consider multiple outcomes simultaneously in a formal trade-off to make an overall decision about a treatment. One participant stated:
If one of your outcomes gets better, if your key secondary outcome gets worse, then how do you say which one [health technology] is better overall? And so it’s being able to assess those things in one framework, whether that be qualitatively or quantitatively.
Workshop participant
At present, the decision as to which is the overall ‘best’ treatment in this context is typically subjective and is taken by the chief investigator when reporting the trial results. Using B–R methods could help to improve this by including:
. . . some explicit statements, supported by some analytical framework, of the benefits and risks, rather than making it implicit based on the text around the primary outcome and reporting of adverse events, which is what happens now.
Workshop participant
Patient preference
The inclusion of patient preferences is a key advantage of using B–R methods. One workshop participant stated:
[In] the HTA, we put a lot of emphasis on patient involvement in the design and deciding on the primary and secondary outcomes. People talk about using the patients to help with interpretation, but I don’t think we use them that much. So, potentially, that aspect is missing and it would maybe bring patient involvement right through the whole process.
Workshop participant
As patient and public involvement (PPI) and engagement are important elements of all NIHR projects from an early stage, any discussions with patients regarding their opinions of the relationship between outcomes would indicate that the trial could benefit from including a preference elicitation method.
An additional use of patient preference could be to reduce the number of treatment options in a multiarm study prior to starting a clinical trial. One workshop participant suggested that this could be a more ‘cost-effective way of doing the experiment’.
Transparency
Transparency is important within NIHR. 23 This transparency can be improved using B–R methods in a few different ways:
-
First, defining ‘upfront what your important outcomes really are’ (workshop participant) will improve the transparency of trial results, as instead of focusing on defining only a primary outcome, all important outcomes will be identified a priori, preventing undue focus on only the beneficial outcomes in the results.
-
Second, using a B–R framework, even if this is qualitative in nature, provides a transparent method of presenting all relevant data and information that will be used for decision-making. This ensures that everyone has the same objective information when making subjective decisions, thus providing ‘rational and transparent approaches to decision making’. 24
-
Last, if a quantitative B–R method is used, a transparent approach will have been used to create the overall trade-off metric. All of the methods, outcomes, weightings and related uncertainty can (and should) be described to provide clarity on the information used.
Consistency
A consistent approach to presenting information and results, which is the basis of many B–R frameworks, provides a structure to communicate the results of clinical trials and treatment recommendations. Ouellet25 suggests that ‘[a] more systematic approach of this trade-off would enhance our understanding of therapeutic index’.
Single metric
The reason for the use of some B–R methods is the ability to ‘score qualitative information’26 and, therefore, synthesise multiple benefits and risks into one metric. Many B–R methods use quantitative data as evidence, but summarise these in a qualitative way (e.g. by placing point estimates and confidence intervals from outcomes into a summary table). An extension of this is to quantify all of the relevant information (including weighting of the important outcomes) and combine this into a single quantitative metric that represents the overall trade-off between the benefits and risks of the health technology. A positive value would represent an overall beneficial health technology and values for each of the health technologies can be directly compared.
Although a quantitative method is not always desired or necessary, being able to quantify qualitative information can ensure that all important information is included in the analysis and results in a systematic way.
Chapter 4 Using benefit–risk methods
Definition
Benefit–risk assessment is a group of methods to compare or trade off favourable and unfavourable effects of a treatment. 27 This could be a subjective judgement or one achieved through more complex, quantitative, methods, but the overarching idea is that information related to multiple outcomes is taken into account simultaneously. The aim of this may be to assess if a single treatment has a positive B–R balance (i.e. the favourable effects outweigh the unfavourable effects) or to see which treatment has the best overall B–R balance, and thereby inform clinical practice.
Methods
Numerous methods are captured under the umbrella of B–R assessment; other reviews28–30 and the PROTECT group’s website5 provide a useful overview of these, as well as further detail.
The rapid review identified 92 appropriate methods, and these methods, along with information gained from the workshop, were split into seven groups. An overview of these methods is provided in this section and further details, including examples of specific methods, are reported in Appendix 2.
Overarching framework
Many of these methods are frameworks that are used from the planning of the evaluation to the point when an end judgement is made. As this report focused on NIHR HTA trials, the process of defining the problem is, naturally, included in the process of designing the study and submitting the proposal, although this step-by-step approach may still be helpful for providing an overview of the problem.
Narrative summary
Although it is not a formal method, workshop participants felt that it was important to include a narrative summary of the benefits and risks found in a trial as an option. A narrative synthesis of the (qualitative or quantitative) information on relevant outcomes would provide clarity around the decision-making and judgements that have been made based on the final trial conclusions.
Summary table
A table in which all important outcomes (defined a priori) are included (split into favourable or unfavourable events), along with the quantitative results and related uncertainty, would facilitate transparency in the reporting of trial results. One participant described this as follows:
. . . it’s putting all the outcomes together and saying ‘Which ones have shown benefit and which ones have shown harm?’. So it’s more formal . . . about trying to put all your evidence together.
Workshop participant
Quantitative trade-off
Formal quantitative methods take favourable and unfavourable outcomes and assign weights to them to evaluate an overall B–R balance (i.e. either positive or negative). Numerous methods could be used for this within a RCT; however, some of these would require the collection of additional data (see Appendix 2), especially if preferences for outcomes are taken into account. Many NIHR HTA trials with an economic evaluation will include an economic model. This, in effect, maps out the benefits and harms in a structured framework (e.g. decision analysis), maps out the weighted outcomes by utilities and considers the probabilities of occurrence. Although the outcome is typically the number of QALYs gained, this could be extended to express outcomes in alternative ways.
Preference elicitation
Preference elicitation for outcomes can be an essential part of a B–R analysis to provide a representative assessment of health technologies. In most NIHR HTA trials, the stakeholder of interest will be patients, but there can be a range of opinions among patients. The choice of which patients or other stakeholders to include can have a significant impact on the results and should be given sufficient consideration to ensure that the stakeholder group is appropriate to provide an answer to the intended research question.
Uncertainty estimation
In B–R quantitative trade-offs, as in many trial analyses, it is important to assess the robustness of the results. This can relate to model assumptions, uncertainties in the data and the included preferences, which could vary. Estimating the uncertainty in the data provides a better characterisation of the results and, therefore, provides greater validity; however, additional data collection may be required.
Visualisations
Generally, in RCTs, visualisations are used to support the understanding of the results for all readers. This can be even more worthwhile when a trade-off is present. The visualisations can provide the reader with information quickly and easily to show the trade-off that is being made and ‘facilitates understanding of information on multiple points’. 31 Numerous options of visualisations are available, many of which are consistent with those already used in NIHR HTA RCTs, and there are visualisations ‘available to suit specific methodologies or tasks’. 32
Applications of methods
During the workshop, there was universal agreement, supported by the literature,22,33 that there is not one method that best fits all situations. The scope of this project was not to assess the intricacies of which specific method to use. Rather, this section will suggest which of the group of methods outlined in Chapter 4, Methods, may be appropriate for each reason. Case studies that implement a range of methods are summarised in Appendix 4 for further information.
All National Institute for Health and Care Research Health Technology Assessment randomised controlled trials
Members of the workshop considered a narrative summary of the benefits and risks to be appropriate in every publicly funded RCT. Providing this narrative of the totality of evidence provides assurance that the importance and likelihood of harms have been considered against the potential benefits of the treatment.
In addition, visualisations are often used in the reporting of RCTs and can be very useful for expressing information. Therefore, visual methods could be considered in any situation and the type of visualisation could be chosen based on how much information it is important to convey.
Trial success depending on more than one outcome, outcomes in competing directions and transparency and consistency
Most of the reasons for applying B–R methods can be satisfied using a summary table that collates the information gathered in the trial. This would allow readers ‘to be able to at least look and [at] compare’ (workshop participant) the information objectively. The use of summary tables is supported by the grey literature as the minimum requirement when submitting for regulatory approval. As it ensures that everyone is making judgements based on the same information and all relevant information is clear and accessible (see Appendix 2 for further details). This approach is also consistent with cost–consequence analyses, which report the breadth of costs and outcomes in economic evaluations. This could then be followed by a narrative summary of the B–R conclusion that has been made, subjectively, by the team.
When there are multiple important outcomes in a trial, the delta value (i.e. superiority, equivalence or non-inferiority margin) in the sample size calculation may have been amended based on another outcome. This indicates that trade-offs are being considered (even subjectively) in a quantitative manner and it may be useful to use quantitative methods.
Patient preference
Preference elicitation methods are useful to quantify the extent to which patients are willing to trade-off different outcomes. For example, how much of a reduction in benefit might a patient be willing to accept for a reduction in an adverse effect associated with a treatment? This can be used as a form of sensitivity analysis ‘to ascertain whether weighting outcomes by patient preferences for those outcomes result in different rank ordering of treatments when compared with unweighted outcomes directly from the trial data’ (survey participant). This was the case in the SANAD (Standard And New Antiepileptic Drug) trial, in which the rank order of treatment based on patient preferences diffrered from the results based on clinical effectiveness from the trial. 34
For patient preferences to be included explicitly in a B–R analysis, quantitative methods, such as discrete choice experiments (DCEs), can be used to elicit stated preferences. This information can then be used to weight the outcomes of interest.
Create a single metric from multiple outcomes
If the reason for using B–R methods is related to the need for a single metric that represents multiple outcomes, then quantitative methods that provide a formal trade-off would be required. Examples of these can be found in Appendices 2 and 4. Sensitivity analyses are recommended35 and already commonly used in NIHR HTA RCTs to assess the robustness of the results; this extends to B–R methods, and uncertainty estimation could be considered if quantitative trade-offs have already been carried out.
Implementation of methods within randomised controlled trials
Within an individual trial, multiple B–R methods could be appropriate at different stages. In addition, there is an additive nature to the methods, meaning that multiple methods could also be appropriate at the same stage. For example, when using a quantitative trade-off, it could still be useful to present the results in a summary table and provide a narrative summary of the final decision and reason. As the complexity of the method increases, simpler methods could often be used to support the presentation of information.
There are also many situations when more than one method is required, for example the use of uncertainty estimation when quantitative trade-offs have been completed. A diagram showing how the methods interact is included in Appendix 2.
To assess the use of methods at different stages of the study, the roadmap,5,36 which contains five steps and was created by the PROTECT group, has been mapped onto the stages of a typical, individual NIHR HTA RCT (Figure 2). Potential method groups that could be appropriate at each of these stages, along with their purpose, are described in Table 2. In addition, as previously discussed, a descriptive framework may be useful, spanning all stages of the RCT. Examples of studies that have used B–R methods are provided in Appendix 4.
FIGURE 2.
Mapping of the PROTECT roadmap (reference 5 and 36) to an individual ranomdised controlled trial.

Stage | Method group | Purpose |
---|---|---|
1. Trial design | Summary table | Identify key variables that are important a priori as favourable and unfavourable effects using data available in the literature |
2. Trial conduct and data collection | Preference elicitation | Elicit stakeholder preferences based on the key outcome variables |
3. Analysis | Quantitative trade-off | In addition to usual RCT analysis, quantitative trade-off between key variables can be undertaken, including a weighting for each variable to indicate importance |
4. Sensitivity and post hoc analysis | Uncertainty estimation | Assess the robustness of the results to assumptions, uncertainties and weightings |
5. Conclusions and dissemination | Summary table | Summary tables transparently and consistently display the data used to make any final conclusions |
Visualisations | Visualisations provide transparency and clarity to the results gathered |
General considerations
Given the nature of B–R methods, there are a few additional considerations that should be taken into account.
Distinction between evidence and judgement
When discussing B–R ideas, it is essential to distinguish between evidence and judgement in decisions. The evidence relates directly to the data that have been collected and evaluated, including effect point estimates and variability. The judgement of the B–R balance is taken based on these results. Quantitative trade-off methods use subjective elements to quantify the weightings used. By concluding with a single metric, it can give the impression of being non-subjective. Therefore, it is important to be transparent about the use of subjective elements and consider the metrics created accordingly.
This distinction is also reflected in the assessment of the clinical importance of any observed numerical differences in RCTs, even if the differences are statistically demonstrated. Various methods have been used to evaluate which values of a specific outcome measure can be considered clinically important11 in the context of RCTs, but these values are ultimately based on clinical judgement.
Difficulty of assigning weights
The weights used in B–R methods can have a large effect on the outcome of the analysis. Research shows that patients’ risk perception can be skewed towards assigning more weight to negative effects in a basic trade-off,37 so it is important to include appropriate methods to account for this.
Perspectives
The choice of perspective can affect the trial results and whom the results most accurately represent. One participant stated:
It depends who’s on the committee. It could be the trial steering committee making the weights or it could be the PPI group, it depends who you choose.
Workshop participant
It is important to plan for this in the design stage of the B–R assessment, to ensure that the relevant stakeholders have been considered (i.e. NICE, patients, clinicians) and the information gathered from them is in line with the research question. Clarity and transparency regarding the perspectives that have been taken are essential.
Sufficient statistical power
As discussed in Chapter 1, Background, typically, clinical trials are powered on a single primary outcome. However, if a B–R method is used, the trial will use multiple outcomes. Therefore, consideration needs to be taken regarding whether or not the trial is sufficiently powered (or has sufficient precision) for the proposed B–R analysis.
Intervention type
The type of intervention being investigated must be considered during the B–R analysis, especially in relation to the selection of key outcomes. As a lot of the B–R research to date was completed in the regulatory setting,38 much of the research is related to drugs. However, some research has been completed on surgery39 and other medical health technologies. 40 When considering publicly funded studies, many health technologies are considered complex interventions that, unlike other interventions, have more than one component to them and the potential for these components to interact with each other. 41 There may be additional considerations because of the multidimensionality of this type of intervention.
External data requirements
According to one workshop participant, the choice of B–R method should depend on ‘whether it’s an individual trial, whether it’s an evidence synthesis, whether it’s a company making a case to NICE’.
This report is focused on the use of B–R methods in one individual trial; however, it may be possible to utilise other information within some of the models to achieve more robust results.
Considering costs in the trade-off
It may be intuitive to consider cost savings as a benefit and costs as a disbenefit (i.e. risk); however, in the context of resource allocation and maximising health, subject to budget constraints, the correct approach for assessing the B–R in the context of costs is to conduct a formal economic evaluation. The decision rules with reference to cost-effectiveness thresholds determine whether the incremental net benefits are positive (which indicates cost-effectiveness) or negative (which indicates that the health opportunity cost exceeds that gained by the beneficiaries of the intervention assessed in the trial).
Sometimes there is a conflict when benefits, harms and monetary costs are considered at the same time and a pragmatic approach may be required for a sequential analysis. For example, when assessing two drugs known to have differing efficacy and adverse effects, we may want to evaluate the B–R balance of the drugs first, before asessing their cost-effectiveness. The choice of what to assess first will depend on the study research question, that is whether the focus is principally on clinical effectiveness or cost-effectiveness.
Utilising quality-adjusted life-years
The use of QALYs within NIHR-funded trials is common and, in itself, a form of single-metric outcome that could be used in a B–R assessment; however, QALYs are not usually interpreted from a B–R perspective. Presenting this differently, such as disaggregating the quality of life that are gained and lost from the life years gained and lost, may provide more information; however, this can be difficult owing to how/when the data are collected and the extent of modelled extrapolation, etc.:
Within single trials, you collect utilities often at regular clinic visits. If they coincide with an adverse drug reaction or a particular benefit event, then all’s well and good but chances are it’s not going to be the case.
Workshop participant
Nevertheless, utility or disutility assigned to events can be presented separately as QALYs gained and QALYs lost (which sum to the overall QALYs per treatment), providing further information and nuance to the QALY result in a RCT from a B–R perspective. Utilities benefit from incorporating societal weights for dimensions of health outcome. The individual-level utility may be considered more appropriate for personalised B–R assessments42 because of the difference in utilities between patients;43 nevertheless, for group-level decisions (i.e. consistent with interpreting the average effect of an intervention), group-level value-sets remain more relevant.
Chapter 5 Reporting information
When a B–R method is intended to be/has been used, it important to include all relevant information when reporting the trial design or the results of the trial. We recommend that this information is included in a standalone paragraph collating all relevant information, as this will ensure consistency and transparency.
As with any RCT, the plan for the assessment should be made when designing the trial; therefore, a checklist for information to include at this stage is separate from the checklist for reporting the results of the trial. The specification of planned B–R analysis should follow other RCT conventions and be defined a priori in the protocol and also in an analysis plan.
The checklists, including explanations, are included in Chapter 6, Checklist for Reporting on Trial Design and Results, and a worked example is included in Chapter 6, Example. A printable version of the checklists is available in Appendix 5.
Checklist for reporting on trial design and results
The sections below provide checklists for the reporting of B–R assessments. These are split between the information to include when reporting the trial design, for instance in a funding application or protocol, and reporting on the results of a study (e.g. in the final trial report or a journal article containing the results). When reporting the trial design, this information is likely to be found in the methods section of the documents; however, when reporting the results of a trial, the information may need to be split across the methods and results sections of the document.
Reporting on trial design
1a: A heading labelled ‘benefit–risk’
A specific section should be provided in the report that is labelled ‘benefit–risk’ and includes all relevant information.
1b: Explicit use of the term ‘benefit–risk’
When any B–R methods are being considered, the term should be explicitly used. This will allow recognition of the fact that there are potential trade-offs in the study, whether this be narratively or quantitatively.
1c: Plan for benefit–risk assessment
As with any report, it is important to define a plan for the method of assessment a priori. This ensures that appropriate methods are being used to answer the research question.
1d: Anticipated benefits and risks
A list or table of the anticipated benefits and risks in the trial is essential for providing transparency. By defining these a priori, this provides clarity on the important outcomes in the trial that would be used to make any final judgements over and above the primary outcome.
1e: Discuss benefit–risk balance with patient representatives
Patient and public involvement and engagement is a key part of any trial design. Discussing the main benefits and risks with patients to understand their perspective on the balance would aid the trial design, as well as ensuring that the trial is worthwhile.
Reporting on trial results
2a: A heading labelled ‘benefit–risk’
A specific section should be provided in the report that is labelled ‘benefit–risk’ and includes all relevant information.
2b: Explicit use of the term ‘benefit–risk’
When any B–R methods have been used, the term should be explicitly used. This will allow recognition of the fact that trade-offs have been made in the study, whether this be narratively or quantitatively.
2c: Benefit–risk assessment used
A description of the B–R methods that have been used in the trial and/or analysis.
2d: Summary table of benefit–risk
A summary table, if applicable, containing all of the key outcomes defined at the trial design stage should be presented, including all relevant data and information gathered from the trial.
2e: Reporting quality-adjusted life-years in terms of benefit–risk
If an economic evaluation has taken place in the trial, report of the QALYs can be included within the B–R section. If it is possible to disaggregate QALYs gained and QALYs lost, this would be presented here.
2f: Realised risks (adverse events)
Information relating to the harms that were realised in the trial should be formally reported. This is, first, to compare the anticipated risks from the trial design stage with the realised risks. This will also ensure that any unfavourable effects that have occurred during the trial are properly considered and reported in the study. This should be supported by the Consolidated Standards of Reporting Trials (CONSORT) extension for harms. 44
2g: Consider benefit–risk judgement with patient representatives
All of the information gained related to the B–R assessment should be discussed with the patient representatives; this is especially important if patient preference has not been formally captured in the chosen methods. Patient representatives should have access to all summary data in a format appropriate for laypeople and provide their judgement on whether or not the benefits outweigh the risks.
Example
This example has been created by following the checklist to ensure that all appropriate information is included. Although the example is a real trial, the results presented are fictional and do not represent actual trial results.
The MAGIC (Melatonin for Anxiety prior to General anaesthesia In Children) trial is a multicentre, parallel RCT, aiming to compare the use of melatonin and midazolam as premedication for anxious children attending for elective surgery under general anaesthesia. This trial has a primary outcome to assess children’s anxiety on a non-inferiority basis using the modified Yale Preoperative Anxiety Scale (mYPAS) outcome measure.
Reporting the trial design
Benefit–risk assessment
The trial has multiple outcomes of interest and is designed on a non-inferiority basis for the primary outcome of children’s anxiety. Two active drugs are used in the trial, and side effects and recovery times are also important; therefore, a B–R assessment will take place to assess the overall best treatment. The Benefit–Risk Action Team (BRAT) key B–R summary table will be used to collate all information on important outcomes and inform a judgement on the comparative B–R balance of the two drugs.
The anticipated benefits are focused around cost-effectiveness and, particularly, quality of life. The anticipated harms are anxiety, pain, recovery, safety, anaesthetic turnaround time and anaesthetic failure. In addition, the costs of the drugs will be considered.
It is hypothesised that the intervention drug will be superior on recovery, pain, safety and cost-effectiveness. The intervention drug aims to be non-inferior on anxiety, anaesthetic turnaround time and anaesthetic failure within a pre-defined limit. The potential benefits of recovery and better safety have been discussed with patient representatives, who feel that this would outweigh the efficacy of the drug on anxiety; however, it is important that it does not increase anaesthetic failure rates.
Reporting the trial results
Benefit–risk assessment
The trial had multiple outcomes of interest and was designed on a non-inferiority basis of the primary outcome of children’s anxiety. Two active drugs were used in the trial, and side effects and recovery times were also important; therefore, a B–R assessment took place to assess the overall best treatment.
The BRAT key B–R summary table was used to collate all information on important outcomes (Table 3) and inform a judgement on the B–R balance of the two drugs. Furthermore, QALYs were separated into QALY component parts of QALYs gained and QALYs lost for each of the drugs (Table 4).
Outcome | Control: midazolam (N = 346) | Intervention: melatonin (N = 348) | Differencea (95% CI) |
---|---|---|---|
Favourable outcomes | |||
Cost-effectiveness, mean (SD) | |||
QALYs | 0.83 (0.19) | 0.87 (0.24) | 0.04 (0.01 to 0.07) |
Unfavourable outcomes | |||
Anxiety (primary outcome), mean (SD) | |||
mYPAS score | 45.8 (13.0) | 46.7 (19.1) | 0.9 (–1.5 to 3.3) |
Anaesthetic turnaround, mean (SD) | |||
Turnaround time (minutes) | 28.6 (11.7) | 32.4 (12.4) | 3.8 (2.0 to 5.6) |
Cost-effectiveness, mean (SD) | |||
Cost (£) | 18,432.71 (1274.34) | 17,347.60 (1180.48) | –1085.11 (–1268.22 to 902.00) |
Recovery, mean (SD) | |||
Paediatric Anaesthesia Emergence Delirium scale score | 15.4 (5.4) | 10.4 (3.6) | –5 (–5.7 to –4.3) |
Time to recovery (hours) | 4.7 (1.3) | 3.5 (1.2) | –1.2 (–1.3 to –1.0) |
Pain, mean (SD) | |||
Faces Pain Scale score | 4.3 (2.3) | 4.1 (2.9) | –0.2 (–0.5 to 0.2) |
Safety, n (%) | |||
Serious adverse events | 12 (3) | 11 (3) | 0.9 (0.4 to 2.09) |
Mild/moderate adverse events | 67 (19) | 54 (16) | 0.76 (0.52 to 1.13) |
Anaesthetic failure, n (%) | |||
Failure rate | 17 (4.9) | 19 (5.5) | 1.12 (0.57 to 2.19) |
QALYs | Control: midazolam (N = 346) | Intervention: melatonin (N = 348) | Difference (95% CI) |
---|---|---|---|
Gained | 0.89 (0.18) | 0.91 (0.21) | 0.02 (–0.01 to 0.05) |
Lost | 0.06 (0.01) | 0.04 (0.01) | 0.02 (0.01 to 0.02) |
Overall | 0.83 (0.19) | 0.87 (0.24) | 0.04 (0.01 to 0.07) |
The realised risks relate to the adverse events that are shown in the summary table (see Table 3). The rates of serious and mild/moderate adverse events were similar in both arms but were slightly lower in the intervention arm than in the control arm. The adverse event rate in both arms was as expected and in line with that in the general population; therefore, increased risks were not present in this trial. There were no adverse events in the trial that were not expected a priori.
In the trial, most outcomes favoured the intervention drug, apart from anxiety, turnaround time rate and failure rate. However, these were within the predefined non-inferiority limits set out and the confidence intervals spanned zero. Patient representatives felt that, because the loss of efficacy in reducing anxiety and the change in failure rate were small, the intervention drug offered a better B–R balance.
Chapter 6 Conclusions
Selecting the most appropriate trial design (i.e. superiority, equivalence or non-inferiority) can be difficult and requires consideration of many elements. A list of 19 factors, in six categories, was created to aid researchers in making this decision. This follows the PICO format that is already commonly used and, in addition, includes feasibility and consideration of a range of perspectives.
Six key reasons have been identified for when B–R methods could be used. These are focused around the idea of multiple important outcomes being present in a trial (commonly the case with equivalence and non-inferiority studies), the possibility of including patient preferences regarding health technologies, and the consistency and transparency that the methods provide. All of these factors promote robust evidence in trials.
Benefit–risk methods can be split into seven groups that could be used at different stages of a trial; however, some of these methods require the collection of additional information. The methods range from simple (e.g. narrative summary) to complex (e.g. quantitative trade-off and uncertainty estimation). There is justification, supported by the regulatory agency recommendations,45 that a summary table containing all relevant quantitative information may be sufficient in many cases and will improve the transparency and consistency of the required results. This will also be followed by a narrative summary of the information presented in the table and a judgement on the trade-off being made.
When using a B–R method in a trial, there are five pieces of information that should be included when reporting on the trial design:
-
heading of ‘benefit–risk’
-
explicit use of the term ‘benefit–risk’
-
plan for a B–R assessment
-
anticipated benefits and risks
-
discussion of the B–R balance with PPI.
A further two items should be included when reporting the results of the trial:
-
summary table of benefits and risks
-
reporting of QALYs in terms of B–R.
Use of this checklist of items will ensure the consistency and transparency of the trial results.
Implications for practice
Findings from this research suggest that there are circumstances in which B–R methods would be useful in publicly funded clinical trials to assess the overall effects of treatments that depend on multiple outcomes. If one of the six key reasons that were identified applies to the trial, the team should consider including a B–R method. Funding panels can also use the list to assess the appropriateness of the research plan when reviewing applications.
The available methods vary widely in complexity and processes; appropriate methods should be chosen based on which of the reason(s) are relevant to the trial. Again, trial teams and funding panels can be informed by the results of this work.
Limitations
The limited scope of this report means that there may be additional considerations required if other, more complex, design features are also present. This work would need to be evaluated to ensure that it remains applicable to these situations.
In addition, the sample size used in this work was small, which may have an impact on the representativeness of the results. However, the breadth of expertise was felt to be suitable for the recommendations produced.
Recommendations for future research
This project has focused on assessing when a B–R method, in any form, is applicable; however, detail on the individual methods has not been included, beyond using the methods as examples. Future research should create resources on specific methods and provide detail on how they can be integrated into a publicly funded clinical trial to support future research teams.
Acknowledgements
The team would like to thank the Medical Research Council (MRC), particularly the MRC–NIHR Methodology panel, which commissioned the call for this piece of work.
The authors would also like to thank those who took part in any stage of the research and would particularly like to acknowledge the following people for their valued input, whether that be through attendance at conference-based workshops, attendance at the consensus workshop and/or review and feedback on results: Dr Simon Bond, Mr Michael Bradburn, Professor Julia Brown, Professor Andrew Farmer, Dr Laura Flight, Ms Poushali Ganguli, Dr Necdet Gunsoy, Mr Rajendra Kadel, Dr Shahrul Mt-Isa, Veronique Robert, Mr Sam Rowley, Dr Praveen Thokala and Dr Ed Waddingham.
Patient and public involvement
Patient and public involvement was not included within this project as the focus of the work was to gain expert opinion to help design clinical trials. The participants included were selected based on their expertise and the methodology selected was trialled with similar experts before use to ensure its appropriateness.
Contributions of authors
Nikki Totton (https://orcid.org/0000-0002-1900-2773) (Research Fellow, Medical Statistics) designed the study, conducted the survey, rapid review and consensus workshop, analysed all collected data, and drafted and prepared the final manuscript.
Steven A Julious (https://orcid.org/0000-0002-9917-7636) (Professor of Medical Statistics) designed the study, provided oversight for the conduct of the study and read, contributed to and approved the final manuscript.
Elizabeth Coates (https://orcid.org/0000-0002-2388-6102) (Research Fellow, Qualitative Research) designed and ran the consensus workshop, prepared the workshop results for publication and read, commented on and approved the final manuscript.
Dyfrig A Hughes (https://orcid.org/0000-0001-8247-7459) (Professor of Pharmacoeconomics, Health Economics) designed the study, provided oversight for the conduct of the study and read, contributed to and approved the final manuscript.
Jonathan A Cook (https://orcid.org/0000-0002-4156-6989) (Professor, Medical Statistics) designed the study, provided oversight for the conduct of the study and read, contributed to and approved the final manuscript.
Katie Biggs (https://orcid.org/0000-0003-4468-7417) (Assistant Director, Literature Reviews) designed and conducted the rapid literature review, contributed to the workshop content, prepared the review results for publication and read, commented on and approved the final manuscript.
Catherine Hewitt (https://orcid.org/0000-0002-0415-3536) (Professor of Trials and Statistics) designed the study, contributed to the workshop content, contributed throughout the study and read, commented on and approved the final manuscript.
Simon Day (https://orcid.org/0000-0002-5672-6818) (Director, Clinical Trials and Statistics) designed the study, contributed throughout the study and read, commented on and approved the final manuscript.
Andrew Cook (https://orcid.org/0000-0002-6680-439X) (Consultant in Public Health Medicine and Fellow in HTA) designed the study, contributed throughout the study and read, commented on and approved the final manuscript.
Publication
Totton N, Julious S, Hughes D, Cook J, Biggs K, Coates L, et al. Utilising benefit-risk assessments within clinical trials – a protocol for the BRAINS project. Trials 2021;22.
Data-sharing statement
All data requests should be submitted to the corresponding author for consideration. Access to anonymised data may be granted following review if appropriate.
Disclaimers
This report presents independent research funded under a MRC–NIHR partnership. The views and opinions expressed by authors in this publication are those of the authors and do not necessarily reflect those of the NHS, the NIHR, the MRC, the HTA programme or the Department of Health and Social Care. If there are verbatim quotations included in this publication the views and opinions expressed by the interviewees are those of the interviewees and do not necessarily reflect those of the authors, those of the NHS, the NIHR, the MRC, the HTA programme or the Department of Health and Social Care.
References
- Schumi J, Wittes JT. Through the looking glass: understanding non-inferiority. Trials 2011;12. https://doi.org/10.1186/1745-6215-12-106.
- Qian HL. Evaluating co-primary endpoints collectively in clinical trials. Bio J 2009;51:137-45. https://doi.org/10.1002/bimj.200710497.
- Freemantle N, Calvert M, Wood J, Eastaugh J, Griffin C. Composite outcomes in randomized trials: greater precision but with greater uncertainty?. J Am Med Assoc 2003;289:2554-9. https://doi.org/10.1001/jama.289.19.2554.
- Saint-Hilary G, Robert V, Gasparini M. Decision-making in drug development using a composite definition of success. Pharm Stat 2018;17:555-69. https://doi.org/10.1002/pst.1870.
- PROTECT . Pharmacoepidemiological Research on Outcomes of Therapeutics by a European Consortium 2016. http://protectbenefitrisk.eu/aboutus.html (accessed 13 February 2020).
- Van de Ven AH, Delbecq AL. The nominal group as a research instrument for exploratory health studies. Am J Public Health 1972;62:337-42. https://doi.org/10.2105/AJPH.62.3.337.
- Braun V, Clarke V. Using thematic analysis in psychology. Qual Res Psychol 2006;3:77-101. https://doi.org/10.1191/1478088706qp063oa.
- Hahn S. Understanding noninferiority trials. Korean J Pediatr 2012;55:403-7. https://doi.org/10.3345/kjp.2012.55.11.403.
- Murray GD. Points to consider on switching between superiority and non-inferiority. Br J Clin Pharmacol 2001;52. https://doi.org/10.1046/j.0306-5251.2001.01397.x.
- Wittes J. Sample size calculations for randomized controlled trials. Epidemiol Rev 2002;24:39-53. https://doi.org/10.1093/epirev/24.1.39.
- Cook JA, Julious SA, Sones W, Hampson LV, Hewitt C, Berlin JA, et al. DELTA2 guidance on choosing the target difference and undertaking and reporting the sample size calculation for a randomised controlled trial. Trials 2018;19. https://doi.org/10.1186/s13063-018-2884-0.
- COMET Initiative . Core Outcome Measures in Effectiveness Trials n.d. www.comet-initiative.org/ (accessed 18 June 2020).
- Prinsen CA, Vohra S, Rose MR, Boers M, Tugwell P, Clarke M, et al. How to select outcome measurement instruments for outcomes included in a ‘Core Outcome Set’ – a practical guideline. Trials 2016;17. https://doi.org/10.1186/s13063-016-1555-2.
- Miller SA, Forrest JL. Enhancing your practice through evidence-based decision making: PICO, learning how to ask good questions. J Evid Based Dent Pract 2001;1:136-41. https://doi.org/10.1016/S1532-3382(01)70024-3.
- European Medicines Agency . ICH E9 (R1) Addendum on Estimands and Sensitivity Analysis in Clinical Trials to the Guideline on Statistical Principles for Clinical Trials 2020. www.ema.europa.eu/en/documents/scientific-guideline/ich-e9-r1-addendum-estimands-sensitivity-analysis-clinical-trials-guideline-statistical-principles_en.pdf (accessed 24 January 2022).
- Pocock SJ, Clayton TC, Stone GW. Challenging issues in clinical trial design: part 4 of a 4-part series on statistics for clinical trials. J Am Coll Cardiol 2015;66:2886-98. https://doi.org/10.1016/j.jacc.2015.10.051.
- Wilson EC. A practical guide to value of information analysis. PharmacoEconomics 2015;33:105-21. https://doi.org/10.1007/s40273-014-0219-x.
- Bader C, Cossin S, Maillard A, Bénard A. A new approach for sample size calculation in cost-effectiveness studies based on value of information. BMC Med Res Methodol 2018;18. https://doi.org/10.1186/s12874-018-0571-1.
- Hersh AM, Walter RJ, Abberegg SK. Use of mortality as an endpoint in noninferiority trials may lead to ethically problematic conclusions. J Gen Intern Med 2019;34:618-23. https://doi.org/10.1007/s11606-018-4813-z.
- INVOLVE . INVOLVE 2020. www.invo.org.uk/ (accessed 19 June 2020).
- Davies L, Cook J, Leal J, Areia CM, Shirkey B, Jackson W, et al. Comparison of the clinical and cost effectiveness of two management strategies (rehabilitation versus surgical reconstruction) for non-acute anterior cruciate ligament (ACL) injury: study protocol for the ACL SNNAP randomised controlled trial. Trials 2020;21. https://doi.org/10.1186/s13063-020-04298-y.
- Pignatti F, Ashby D, Brass EP, Eichler HG, Frey P, Hillege HL, et al. Structured frameworks to increase the transparency of the assessment of benefits and risks of medicines: current status and possible future directions. Clin Pharmacol Ther 2015;98:522-33. https://doi.org/10.1002/cpt.203.
- NIHR . NIHR Policy on Clinical Trial Registration and Disclosure of Results 2019. www.nihr.ac.uk/documents/nihr-policy-on-clinical-trial-registration-and-disclosure-of-results/12252 (accessed 13 August 2020).
- Goetghebeur MM, Wagner M, Khoury H, Levitt RJ, Erickson LJ, Rindress D. Evidence and Value: Impact on DEcisionMaking – the EVIDEM framework and potential applications. BMC Health Serv Res 2008;8. https://doi.org/10.1186/1472-6963-8-270.
- Ouellet D. Benefit-risk assessment: the use of clinical utility index. Expert Opin Drug Saf 2010;9:289-300. https://doi.org/10.1517/14740330903499265.
- Agapova M, Devine EB, Bresnahan BW, Higashi MK, Garrison LP. Applying quantitative benefit-risk analysis to aid regulatory decision making in diagnostic imaging: methods, challenges, and opportunities. Acad Radiol 2014;21:1138-43. https://doi.org/10.1016/j.acra.2014.05.006.
- PROTECT . What Is Benefit-Risk Assessment? n.d. http://protectbenefitrisk.eu/PPI2.html (accessed 17 September 2020).
- Guo JJ, Pandey S, Doyle J, Bian B, Lis Y, Raisch DW. A review of quantitative risk-benefit methodologies for assessing drug safety and efficacy – report of the ISPOR risk-benefit management working group. Value Health 2010;13:657-66. https://doi.org/10.1111/j.1524-4733.2010.00725.x.
- Mt-Isa S, Hallgreen CE, Wang N, Callréus T, Genov G, Hirsch I, et al. Balancing benefit and risk of medicines: a systematic review and classification of available methodologies. Pharmacoepidemiol Drug Saf 2014;23:667-78. https://doi.org/10.1002/pds.3636.
- Hallgreen CE, Mt-Isa S, Lieftucht A, Phillips LD, Hughes D, Talbot S, et al. Literature review of visual representation of the results of benefit-risk assessments of medicinal products. Pharmacoepidemiol Drug Saf 2016;25:238-50. https://doi.org/10.1002/pds.3880.
- Levitan B. A concise display of multiple end points for benefit-risk assessment. Clin Pharmacol Ther 2011;89:56-9. https://doi.org/10.1038/clpt.2010.251.
- Hughes D, Waddingham E, Mt-Isa S, Goginsky A, Chan E, Downey GF, et al. Recommendations for benefit-risk assessment methodologies and visual representations. Pharmacoepidemiol Drug Saf 2016;25:251-62. https://doi.org/10.1002/pds.3958.
- Mühlbacher AC, Juhnke C, Beyer AR, Garner S. Patient-focused benefit-risk analysis to inform regulatory decisions: the European Union perspective. Value Health 2016;19:734-40. https://doi.org/10.1016/j.jval.2016.04.006.
- Holmes EAF, Plumpton C, Baker GA, Jacoby A, Ring A, Williamson P, et al. Patient-focused drug development methods for benefit-risk assessments: a case study using a discrete choice experiment for antiepileptic drugs. Clin Pharmacol Ther 2019;105:672-83. https://doi.org/10.1002/cpt.1231.
- Thabane L, Mbuagbaw L, Zhang S, Samaan Z, Marcucci M, Ye C, et al. A tutorial on sensitivity analyses in clinical trials: the what, why, when and how. BMC Med Res Methodol 2013;13. https://doi.org/10.1186/1471-2288-13-92.
- Greenberg M, Simondon F, Saadatian-Elahi M. Perspectives on benefit-risk decision-making in vaccinology: conference report. Hum Vaccin Immunother 2016;12:176-81. https://doi.org/10.1080/21645515.2015.1075679.
- Bellanti F, van Wijk RC, Danhof M, Della Pasqua O. Integration of PKPD relationships into benefit-risk analysis. Br J Clin Pharmacol 2015;80:979-91. https://doi.org/10.1111/bcp.12674.
- Juhaeri J. Benefit–risk evaluation: the past, present and future. Ther Adv Drug Saf 2019;10:1-10. https://doi.org/10.1177/2042098619871180.
- Urban P, Gregson J, Owen R, Mehran R, Windecker S, Valgimigli M, et al. Assessing the risks of bleeding vs. thrombotic events in patients at high bleeding risk after coronary stent implantation: the ARC-high bleeding risk trade-off model. JAMA Cardiol 2021;6:410-19. https://doi.org/10.1001/jamacardio.2020.6814.
- Lerner H, Whang J, Nipper R. Benefit–risk paradigm for clinical trial design of obesity devices: FDA proposal. Surg Endosc 2013;27:702-7. https://doi.org/10.1007/s00464-012-2724-3.
- Medical Research Council . Developing and Evaluating Complex Interventions: New Guidance 2006. https://mrc.ukri.org/documents/pdf/complex-interventions-guidance/ (accessed 20 September 2020).
- Devlin NJ, Shah KK, Mulhern BJ, Pantiri K, van Hout B. A new method for valuing health: directly eliciting personal utility functions. Eur J Health Econ 2019;20:257-70. https://doi.org/10.1007/s10198-018-0993-z.
- Cowen ME, Miles BJ, Cahill DF, Giesler RB, Beck JR, Kattan MW. The danger of applying group-level utilities in decision analyses of the treatment of localized prostate cancer in individual patients. Med Decis Making 1998;18:376-80. https://doi.org/10.1177/0272989X9801800404.
- Ioannidis JP, Evans SJ, Gøtzsche PC, O’Neill RT, Altman DG, Schulz K, et al. Better reporting of harms in randomized trials: an extension of the CONSORT statement. Ann Intern Med 2004;141:781-8. https://doi.org/10.7326/0003-4819-141-10-200411160-00009.
- European Medicines Agency . Benefit-Risk Methodology 2011.
- Qualtrics . Qualtrics 2019. www.qualtrics.com/ (accessed 20 September 2020).
- Biggs K, Totton N, Hind D, Hughes D, Julious S. A Rapid Review of Benefit-Risk Assessment Methodologies Within Clinical Trials 2019. www.crd.york.ac.uk/PROSPERO/display_record.php?RecordID=144882 (accessed 15 September 2020).
- The Pharmaceutical Benefits Board (Tandvårds- och läkemedelsförmånsverket) . General Guidelines for Economic Evaluations from the Pharmaceutical Benefits Board 2003. www.tlv.se/download/18.2e53241415e842ce95514e9/1510316396792/Guidelines-for-economic-evaluations-LFNAR-2003-2.pdf (accessed 20 September 2020).
- European Medicines Agency . Guidance Document on the Content of the Rapporteur Day Critical Assessment Report 2016. www.ema.europa.eu/docs/en_GB/document_library/Regulatory_and_procedural_guideline/2016/05/WC500206989.pdf (accessed 20 September 2020).
- European Medicines Agency . ICH Guideline E2C (R2) on Periodic Benefit-Risk Evaluation Report (PBRER) 2013. www.ema.europa.eu/docs/en_GB/document_library/Regulatory_and_procedural_guideline/2012/12/WC500136402.pdf (accessed 20 September 2020).
- European Medicines Agency . Benefit-Risk Methodology Project – Project Description 2009. www.ema.europa.eu/en/documents/report/benefit-risk-methodology-project_en.pdf (accessed 24 January 2022).
- European Medicines Agency . Reflection Paper on Benefit-Risk Assessment Methods in the Context of the Evaluation of Marketing Authorisation Applications of Medicinal Products for Human Use 2008. www.ema.europa.eu/en/documents/regulatory-procedural-guideline/report-chmp-working-group-benefit-risk-assessment-models-methods_en.pdf (accessed 24 January 2022).
- Food and Drug Administration . Factors to Consider When Making Benefit-Risk Determinations in Medical Device Premarket Approval and De Novo Classifications 2012.
- Food and Drug Administration . Structured Approach to Benefit–Risk Assessment in Drug Regulatory Decision-Making: PDUFA V Draft Implementation Plan: February 2013 2013. www.fda.gov/files/about%20fda/published/PDUFA-V-Implementation-Plan--Structured-Approach-to-Benefit-Risk-Assessment-in-Drug-Regulatory-Decision-Making-%28Draft%29.pdf (accessed 24 January 2022).
- Food and Drug Administration . Benefit Risk Assessment in Drug Regulatory Decision Making 2018. www.fda.gov/files/about%20fda/published/Benefit-Risk-Assessment-in-Drug-Regulatory-Decision-Making.pdf (accessed 24 January 2022).
- Food and Drug Administration . Guidance for Industry Premarketing Risk Assessment 2005. www.fda.gov/media/71650/download (accessed 24 January 2022).
- Fischoff B, Brewer NT, Downs J. Communicating Risks and Benefits: An Evidence-Based User’s Guide n.d. www.fda.gov/media/81597/download (accessed 24 January 2022).
- Health Canada . Reader’s Guide to the Phase II Summary Basis of Decision (SBD) – Drugs 2012. www.canada.ca/en/health-canada/services/drugs-health-products/drug-products/summary-basis-decision/reader-guide-phase-2-summary-basis-decision-drugs.html (accessed 20 September 2020).
- Health Sciences Authority . Clinical Trials Guidance. Expedited Safety Reporting Requirements for Clinical Trials 2021. www.hsa.gov.sg/docs/default-source/hprg-io-ctb/hsa_gn-ioctb-10_safety_reporting_1mar2021.pdf (accessed 24 January 2022).
- Health Sciences Authority . Guidelines for Industry. Post-Marketing Vigilance Requirements For Therapeutic Products and Cell, Tissue and Gene Therapy Products 2021. www.hsa.gov.sg/docs/default-source/hprg-vcb/guidance-document/guidance-for-industry–post-marketing-vigilance-requirements-for-therapeutic-products-and-cell-tissue-and-gene-therapy-products_v3_01mar2021.pdf (accessed 24 January 2022).
- International Council for Harmonisation of Technical Requirements for Pharmaceuticals for Human Use . ICH Harmonised Tripartite Guideline: Statistical Principles for Clinical Trials 1998. www.ich.org/fileadmin/Public_Web_Site/ICH_Products/Guidelines/Efficacy/E9/Step4/E9_Guideline.pdf (accessed 20 September 2020).
- International Risk Governance Center . Introduction to the IRGC Risk Governance Framework, Revised Version 2017. https://infoscience.epfl.ch/record/233739/files/IRGC.%20%282017%29.%20An%20introduction%20to%20the%20IRGC%20Risk%20Governance%20Framework.%20Revised%20version.pdf (accessed 24 January 2022).
- Institut für Qualität und Wirtschaftlichkeit im Gesundheitswesen (IQWiG) . IQWiG – General Methods – Version 4.2 2015. www.iqwig.de/methoden/iqwig_general_methods_version_204-2.pdf (accessed 24 January 2022).
- Medsafe . How to Change the Legal Classification of a Medicine in New Zealand: Guidance Document 2019. www.medsafe.govt.nz/downloads/How_to_change_medicine_classification.pdf (accessed 24 January 2022).
- Pharmaceuticals and Medical Devices Agency . Risk Management Plan Guidance. Pharmaceuticals and Medical Devices Agency 2012. www.pmda.go.jp/files/000153333.pdf (accessed 24 January 2022).
- Therapeutic Goods Administration . Australian Public Assessment Report For Prescription Medicines 2013. www.tga.gov.au/australian-public-assessment-report-auspar-guidance (accessed 24 January 2022).
- Broadbent DM, Sampson CJ, Wang A, Howard L, Williams AE, Howlin SU, et al. Individualised screening for diabetic retinopathy: the ISDR study – design and methodology for a randomised controlled trial comparing annual and individualised risk-based variable-interval screening. BMJ Open 2019;9. https://doi.org/10.1136/bmjopen-2018-025788.
- Saxon D, Ashley K, Bishop-Edwards L, Connell J, Harrison P, Ohlsen S, et al. A pragmatic randomised controlled trial assessing the non-inferiority of counselling for depression versus cognitive-behaviour therapy for patients in primary care meeting a diagnosis of moderate or severe depression (PRaCTICED): study protocol for a randomised controlled trial. Trials 2017;18:1-14. https://doi.org/10.1186/s13063-017-1834-6.
- National Institute for Health and Care Excellence (NICE) . Depression in Adults: Recognition and Management 2009.
- Advani PP, Ballman KV, Dockter TJ, Colon-Otero G, Perez EA. Long-term cardiac safety analysis of NCCTG N9831 (alliance) adjuvant trastuzumab trial. J Clin Oncol 2016;34:581-7. https://doi.org/10.1200/JCO.2015.61.8413.
- Marson AG, Al-Kharusi AM, Alwaidh M, Appleton R, Baker GA, Chadwick DW, et al. The SANAD study of effectiveness of carbamazepine, gabapentin, lamptrigine, oxcarbazepine, or topiramate for treatment of partial epilepsy: an unblinded randomised controlled trial. Lancet 2007;369:1000-15. https://doi.org/10.1016/S0140-6736(07)60460-7.
- Levitan BS, Andrews EB, Gilsenan A, Ferguson J, Noel RA, Coplan PM, et al. Application of the BRAT framework to case studies: observations and insights. Clin Pharmacol Ther 2011;89:217-24. https://doi.org/10.1038/clpt.2010.280.
- Micaleff A, Callreus T, Phillips L, Hughes D, Hockley K, Wang N, et al. IMI Work Package 5: Report 1:b:Iii Benefit–Risk Wave 1 Case Study Report: Raptiva® (efalizumab) 2012. www.imi-protect.eu/documents/Micaleff_et_al_Benefit_Risk_Wave_Case_study_Report_Efalizumab_Feb_2013.pdf (accessed 20 September 2020).
- Feinn R, Curtis B, Kranzler HR. Balancing risk and benefit in heavy drinkers treated with topiramate: implications for personalized care. J Clin Psychiatry 2016;77:e278-82. https://doi.org/10.4088/JCP.15m10053.
- Garrison LP, Towse A, Bresnahan BW. Assessing a structured, quantitative health outcomes approach to drug risk-benefit analysis. Health Aff 2007;26:684-95. https://doi.org/10.1377/hlthaff.26.3.684.
- Zozaya N, Martínez-Galdeano L, Alcalá B, Armario-Hita JC, Carmona C, Carrascosa JM, et al. Determining the value of two biologic drugs for chronic inflammatory skin diseases: results of a multi-criteria decision analysis. BioDrugs 2018;32:281-91. https://doi.org/10.1007/s40259-018-0284-3.
- Tervonen T, van Valkenhoef G, Buskens E, Hillege HL, Postmus D. A stochastic multicriteria model for evidence-based decision making in drug benefit-risk analysis. Stat Med 2011;30:1419-28. https://doi.org/10.1002/sim.4194.
- Lynd LD, O’Brien BJ. Advances in risk-benefit evaluation using probabilistic simulation methods: an application to the prophylaxis of deep vein thrombosis. J Clin Epidemiol 2004;57:795-803. https://doi.org/10.1016/j.jclinepi.2003.12.012.
Appendix 1 Survey of current practice (work package 1)
Methods
The survey was hosted on the Qualtrics platform46 between May 2019 and August 2019. The link was sent through the UK Clinical Research Collaboration Clinical Trials Network Lead Statisticians, Health Econ on JISCMail and Medical Research Centre Hubs for Trial Methodology Research (Trials Methodology Research Partnership) distribution lists, as well as through the steering group to known networks or researchers in the area.
Results
The demographics of the survey participants are shown in Table 5.
N (%) Total = 64 | |
---|---|
Institution | |
Academia | 54 (84%) |
Industry | 5 (8%) |
NHS | 2 (3%) |
Other | 2 (3%) |
Missing | 1 (2%) |
Job role | |
Epidemiologist | 1 (2%) |
Funder | 1 (2%) |
Health economist | 11 (17%) |
Investigator | 2 (3%) |
Statistician | 40 (62%) |
Other | 7 (11%) |
Missing | 2 (3%) |
Location | |
Australasia | 1 (2%) |
Canada | 2 (3%) |
Other European country | 1 (2%) |
Switzerland | 1 (2%) |
United Kingdom | 55 (86%) |
United States | 2 (3%) |
Missing | 2 (3%) |
The data collected showed that most survey participants did not have experience with B–R methods. Those who did had mostly worked on publicly funded superiority trials testing a drug (Table 6); however, this finding reflects the demographics of the participants and is, therefore, not necessarily generalisable.
Experience | Number (%) of participants (N = 64) |
---|---|
Experience of B–R | |
No | 41 (64) |
Yes | 22 (34) |
Missing | 1 (2) |
Of those who answered yes, n (%) ( N = 22) a | |
Trial design experience | |
Superiority | 15 (68) |
Equivalence | 3 (14) |
Non-inferiority | 6 (27) |
Funding | |
Charity | 3 (14) |
Industry | 6 (27) |
Public | 8 (36) |
Missing | 7 (32) |
Intervention type | |
Complex intervention | 6 (27) |
Device | 3 (14) |
Drug | 9 (41) |
Therapy | 1 (5) |
Other | 1 (5) |
Missing | 8 (36) |
The reasons provided for carrying out a B–R assessment were that showing efficacy is not enough, to consider patient preferences in treatment decisions and because health economics were required in the trial, prompting the use of B–R methods. These were combined with the transcripts from the workshop to assess the key reasons for completing these designs. Out of eight respondents, only one had considered the B–R assessment in the design of the trial, although some respondents suggested that it was an important element to consider.
When asked, 44% of respondents stated that they would like recommendations on how to select the appropriate trial design, and 41% stated that they would like recommendations on how to select the end points, both of which have, therefore, been incorporated into the report (Selecting a trial design and Outcome measure selection, respectively). Other items were included where possible, but further work is needed in this area to provide further details and recommendations (Figure 3).
FIGURE 3.
Items requested within the survey for advice and recommendations.
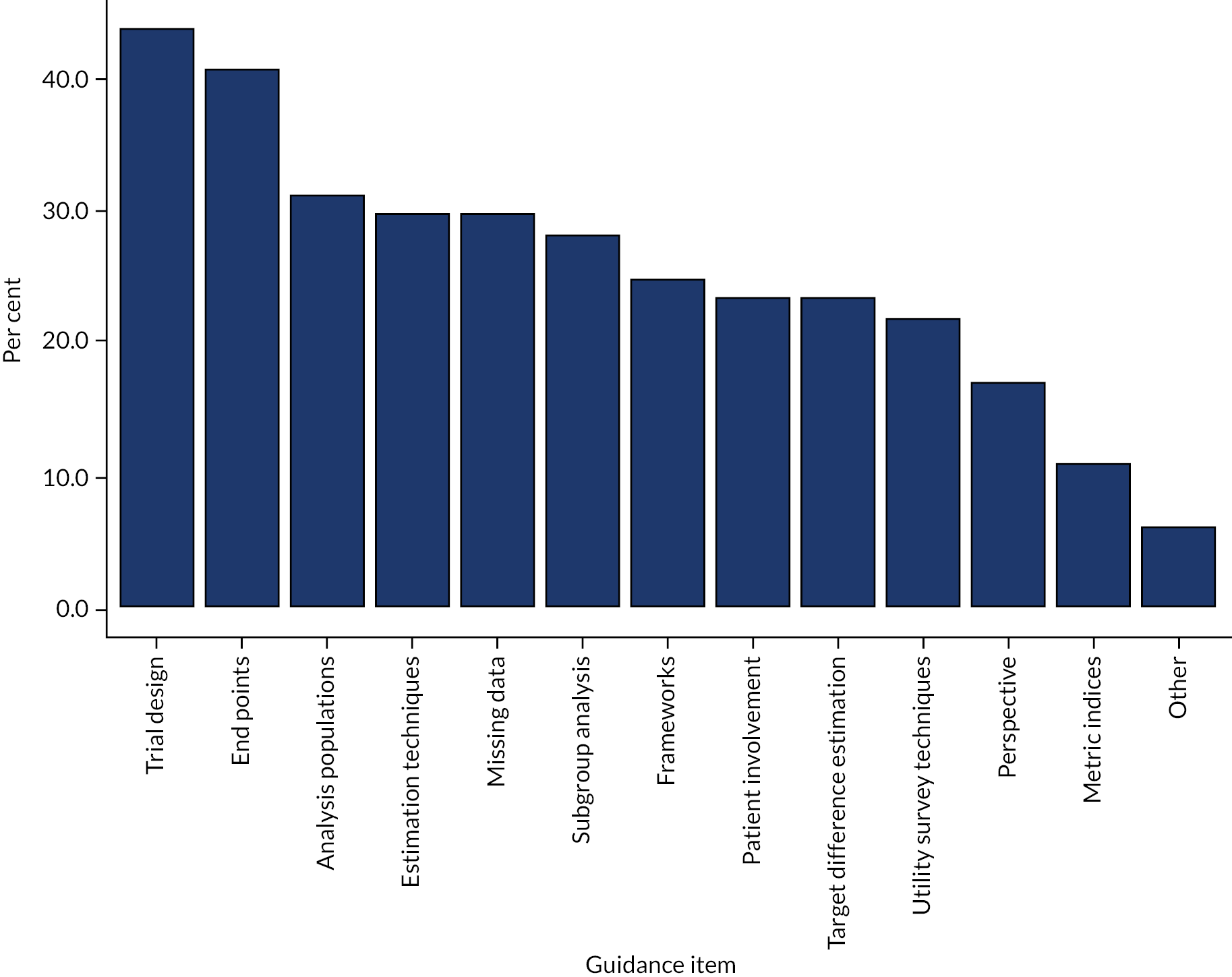
Limitations
A key limitation of the survey is that we were not able to estimate a response rate because of the use of mailing lists and personal networks to promote the survey. Therefore, we are unable to assess the potential bias in the selection of respondents; the focus on publicly funded trials suggests that the responses are not representative of all researchers using B–R methods.
Appendix 2 Literature review of available methods (work package 2)
Methods
Full details of the rapid review methods are given on the PROSPERO web page. 47 The rapid review has been registered with PROSPERO (PROSPERO reference CRD42019144882).
Search
A rapid review was conducted using systematic and pragmatic search strategies to gather information on the methods and guidance available relating to B–R methodology in clinical trials. To identify research articles, we performed two iterations of database searches (using PubMed) and conducted a citation search of eight key articles (four from iteration 1 and four from iteration 2, identified by the review team). A search of grey literature was undertaken using Google (Google Inc., Mountain View, CA, USA) for guidelines on B–R assessment in clinical trials.
For iteration 1 we searched for ‘benefit-risk’ in the title, or ‘benefit-risk assessment’ or ‘benefit-risk analysis’ in the abstract, combined with ‘methodology’ or ‘methodologies’ in the abstract. For iteration 2, we reviewed the key words of papers identified in iteration 1 and added the MeSH ‘Decision Support Techniques’ and searched for ‘method*’ in the title. Both iterations were limited to English-language papers and those published from 1999 onwards.
Inclusion and exclusion
Publication titles and abstracts were reviewed by two reviewers (NT and KB) in EndNote (Clarivate Analytics, Philadelphia, PA, USA), and 10% of the papers excluded at this stage were reviewed independently. Nikki Totton reviewed all full-text papers for inclusion and included papers discussing B–R methods in clinical trials; papers excluded at this stage were reviewed by Katie Biggs.
Data extraction
Nikki Totton extracted data from the included publications. Data were extracted on paper characteristics (e.g. location, type, year, stakeholder), the context of B–R (e.g. intervention, trial design), the reason for the use of the B–R method, brief explanations of methods, and the strengths and limitations of each approach (as detailed in the publication).
Results
In total, 1196 articles were identified, with 76 extracted (Figure 4). The 97 methods that were identified were categorised into seven groups; common examples are presented in Table 7, along with information about whether or not additional data would be required on top of usual data collection to include these in a NIHR HTA RCT. Figure 5 shows how these groups of methods interact and indicates the split when additional data would be required.
FIGURE 4.
The Preferred Reporting Items for Systematic Reviews and Meta-Analyses (PRISMA) flow chart from article identification to inclusion for the rapid review.
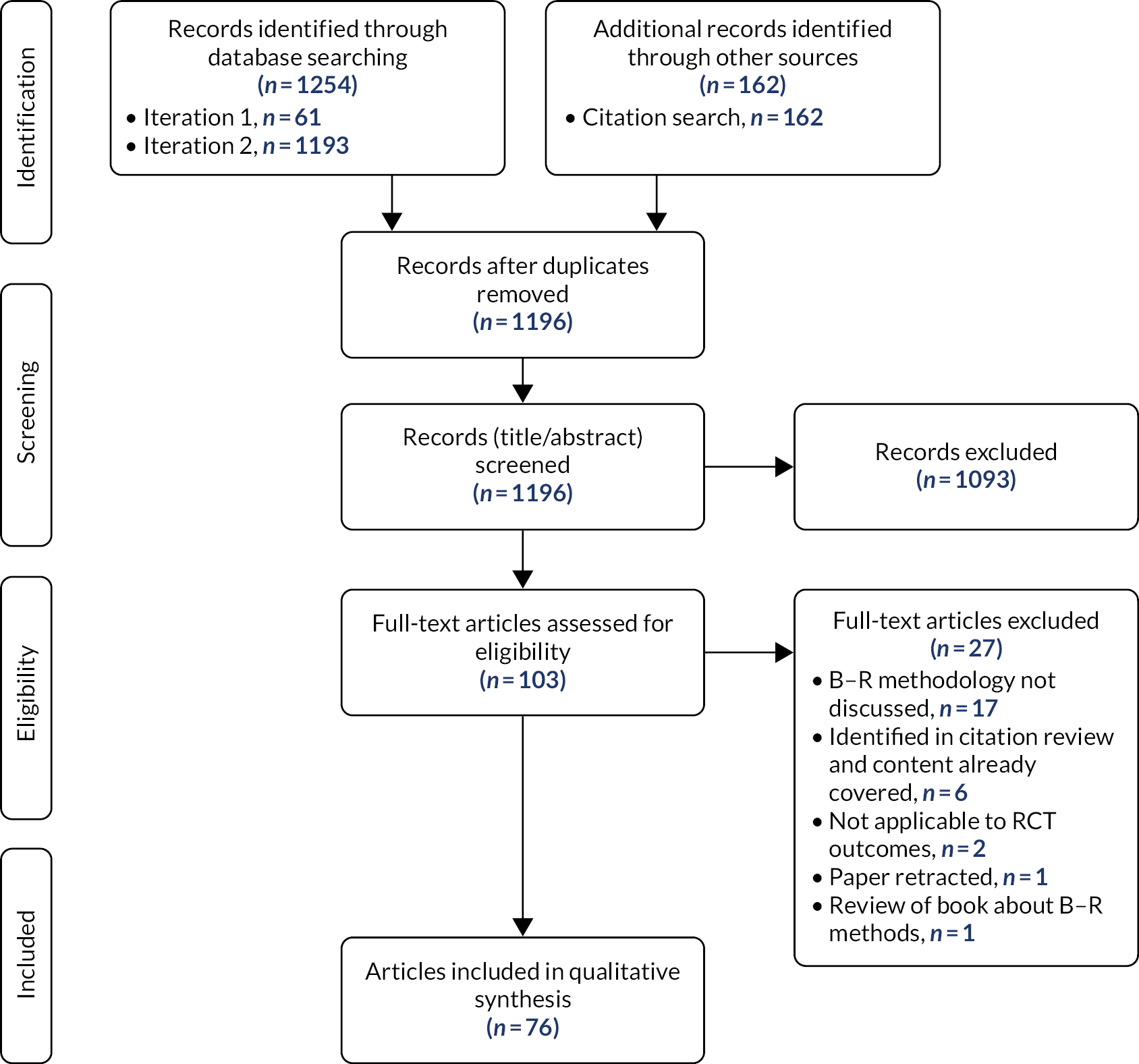
Method group | Example methods | Reason | Additional data required? |
---|---|---|---|
Overarching framework | BRAT framework PrOACT-URL framework |
Overview of the whole B–R process. Provides consistency and transparency in the process | No |
Summary table | BRAT key B–R summary table PrOACT-URL effects table |
When multiple outcomes of interest are present in a trial, the use of a quantitative summary table provides transparency and consistency with the reporting of the trial results and the subjective decision made on the trade-offs between outcomes | No |
Preference elicitation | DCE | To elicit the preferences of key stakeholders, who often include patients | Yes |
Quantitative trade-off | Number needed to treat QALYs Incremental net health benefit Multi-criteria decision analysis Stochastic multiattribute acceptability analysis |
To be used when multiple outcomes of interest are contained in the trial and the conversion to provide directly comparable metrics or synthesis into a single metric is desired By using an official process for this, it provides consistency and transparency to the final comparison between health technologies on multiple outcomes and removes the subjectivity of the trial team |
In some cases depending on the method |
Uncertainty estimation | Probabilistic simulation method Monte Carlo simulations |
To provide transparency on the uncertainty in treatment comparisons and results | In some cases depending on the method |
Visualisations | Decision tree Forest plot |
Provides consistency and transparency on the data gathered in the trial that contribute to the final treatment recommendations | No |
FIGURE 5.
Benefit–risk method groups from the rapid review and their interactions.
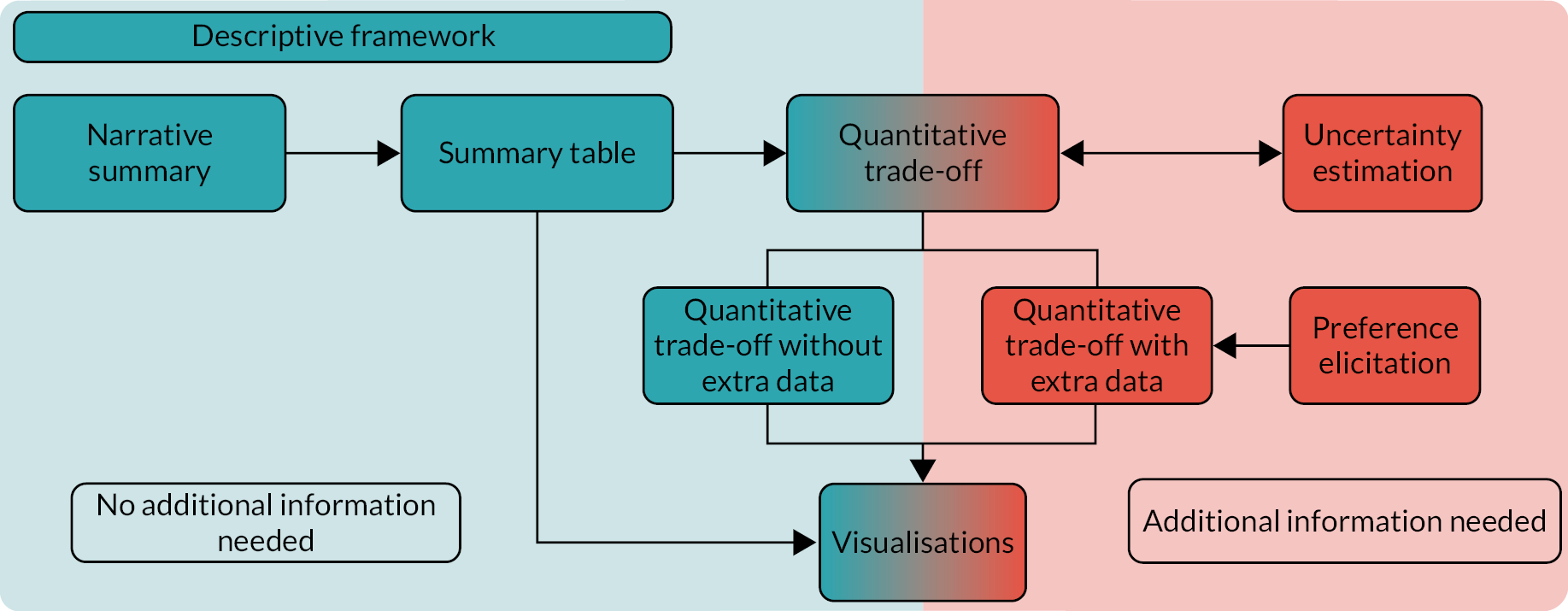
Key recommendations from regulatory agencies, which commonly use B–R methods to assess the balance of drugs, are summarised in Table 8 and have been used to suggest which methods may be appropriate in a range of circumstances.
Organisation | Location | Recommendations |
---|---|---|
Tandvårds- och läkemedelsförmånsverket48 | Sweden |
|
European Medicines Agency49–52 | Europe |
|
Food and Drug Administration53–57 | USA |
|
Health Canada58 | Canada |
|
Health Sciences Authority Singapore59,60 | Singapore |
|
International Council for Harmonisation of Technical Requirements for Pharmaceuticals for Human Use61 | Worldwide |
|
International Risk Governance Centre62 | Switzerland |
|
Institut für Qualität und Wirtschaftlichkeit im Gesundheitswesen63 | Germany |
|
Medsafe64 | New Zealand |
|
Pharmaceuticals and Medical Devices Agency65 | Japan |
|
Therapeutic Goods Administration66 | Australia |
|
Limitations
The use of a rapid literature review was selected because of the limit on time and resources in this project. This is not as thorough as a full systematic review,29 which could have resulted in less representative results. However, comparing the results of the rapid review with another published systematic review showed that more methods were identified in our review and none were omitted, providing some reassurance.
Appendix 3 Expert consensus workshop (work package 3)
Methods
Experts were invited to join the consensus workshop through several avenues:
-
Respondents to the survey who expressed interest in the workshop were provided with further information.
-
All co-applicants of the BRAINS project were invited.
-
Experts from NIHR funding panels were provided with information about the workshop.
-
Prominent experts in the area were invited directly.
The workshop contained presentations over the 2 days to support the discussions and, ultimately, the recommendations produced. The presentations were based on the findings from the two previous work packages (WPs) and included:
-
background to the project and its focus, highlighting the perspective of the funder who commissioned the work
-
results from the survey
-
background to the different trial designs (i.e. superiority, equivalence and non-inferiority), with examples
-
results from the rapid literature review
-
an overview of available B–R methods
-
background to the differences between the regulatory and publicly funded setting
-
an introduction to other checklists, also highlighting the purpose of improving reporting.
Two different techniques were used in the workshop to answer the research objectives. NGT was used as a consensus methodology to enable the generation of item lists, and open discussions were used to produce themes.
The NGT is an interactive multistage methodology through which group consensus can be sought during a face-to-face meeting. 6 This structured approach has several benefits, as it encourages equal and wide-ranging contributions from all participants and helps to reduce conflict and the potential for the discussion to be dominated by some participants. In addition, it can be completed in a relatively short time.
The potential use of B–R in clinical trials has additional complexities because of the number of methods, reasons for use and perspectives to consider. Therefore, an open discussion was held to ensure that no restrictions on points of discussion were in place. In addition, other relevant discussions ensued during the workshop following the presentations. Each of the workshop sessions was recorded and the recordings from the open discussion regarding the appropriateness of when to use B–R methods, as well as other relevant discussions, were transcribed verbatim. These transcripts were analysed thematically to identify key themes. 7
Results
The key participant characteristics are shown in Table 9. Some participants spanned multiple sectors, but their primary purpose for being at the workshop was recorded. The workshop attendees provided a good mix of those early in their career, developing in their career and in senior positions. This provided an overview of experiences within the group.
Characteristic | Number (%) (N = 15) |
---|---|
Sector | |
Academia | 10 (66) |
Funding body | 3 (20) |
Industry | 1 (7) |
NHS | 1 (7) |
Discipline | |
Statistics | 8 (53) |
Health economics | 4 (27) |
Trial/programme management | 2 (13) |
Clinical | 1 (7) |
Trial design factors
Twenty-eight discrete items were suggested in the brainstorming round and were subsequently categorised into six overarching categories. After two voting rounds, there was consensus to include 16 of the items. A further three items were included when the results were discussed with the oversight committee, resulting in the 19-item list in Factors for selecting a trial design.
Benefit–risk inclusion
Six themes were extracted from the transcripts, supported by the literature review findings that provide reasons for using B–R methods. A further six items have been included as general considerations for using B–R methods; a seventh item was added following review from the oversight team. In addition, information was gathered about whether or not the selected trial design affected the B–R method that was used to support data from the survey and literature review.
Benefit–risk reporting items
Twelve items were suggested during the brainstorming round. Five items were identified when reporting the design of the trial. For consistency, these five items, plus an additional two items, were identified for inclusion when reporting results of the trial. After both rounds of voting, there was consensus to include all items, resulting in the five- and seven-item checklists in Checklist for reporting on trial design and results.
Limitations
One limitation relates to the relatively small number of people who took part in this stage of the research. Owing to the limited use of B–R methods in the publicly funded setting, it was always going to be challenging to achieve a large sample size. Unfortunately, this means that the workshop is unlikely to have been widely representative; however, participants in the consensus workshop represented a range of specialties and perspectives, which helped to make up for the small number. As the aim was to achieve expert consensus, the representation achieved was suitable to reach the required conclusions. In addition, the draft results were shared with other researchers in the area, who were unable to attend the workshop, for input and confirmation.
Appendix 4 Trial design: further examples
Example 2: equivalence
The Individualised Screening for Diabetic Retinopathy (ISDR) study67 is funded by NIHR to evaluate standard annual screening against individualised, risk-based, variable-interval screening for people with diabetes. Box 3 presents the completed trial selection considerations.
The trial population is patients with diabetes attending screening clinics for diabetic retinopathy. As this is a screening visit, the population may be influenced by other factors, such as ease of access, which will have an impact on their attendance.
1b: What are the subpopulations of interest?There are three retinopathy groups for estimated risk of developing sight-threatening diabetic retinopathy. The results should be consistent in direction for all of these groups; however, subgroup analyses may suggest that the intervention is most effective for certain subgroups.
2: Intervention 2a: What is the intervention?The intervention is an individualised, risk-based, variable-interval screening (at 6, 12 or 14 months). As a screening plan, this is similar to the comparator, but the idea of the intervention being more individualised may make patients more willing to comply with the schedule.
3: Comparator 3a: What is the comparator?The comparator is annual screening visits for all patients.
3b: What is current practice or standard care?Annual screening visits is being used as the comparator.
3c: What evidence is there in relation to the comparator?‘Previous studies have shown that screening for diabetic retinopathy is a highly cost-effective intervention’;67 however, the use of annual visits was determined by clinical expert opinion and not direct evidence.
4: Outcomes 4a: What are the priorities of different outcomes?The most important outcome is the attendance rate for screening, to ensure that the intervention is not reducing the number of patients who are attending or, overall, increasing the number of appointments to an unfeasible level. The detection of sight-threatening diabetic retinopathy is also important, to ensure that the incidence rate of this is not made worse.
4b: How many clinical outcomes are key to eventual decision-making?The aims are to evaluate the safety, acceptability and cost-effectiveness, which relates to many outcomes, namely attendance rate, detection of sight-threatening diabetic retinopathy cases and cost per QALY. Therefore, multiple outcomes are important for eventual decisions.
4c: What are the superiority outcomes?It is hypothesised that the intervention will be more cost-effective than the control, as patients at high risk will be seen more often and, therefore, are likely to have any issues identified early. For those at lower risk, the benefit is they will have a longer interval between follow-ups.
4d: Are the costs of the comparator and intervention being considered?The costs of the two health technologies are, potentially, comparable, should the overall attendance rates be deemed equivalent in each arm, but this is to be assessed in the trial.
4e: Is non-inferiority or equivalence on the primary outcome plausible?Owing to the current evidence that screening is effective, but without any detail on the frequency of the screening, it is plausible that the two health technologies would be equivalent. An increase in attendance rates is not desired because of the funding and resourcing; therefore, an equivalence design is required, rather than a non-inferiority design.
4f: What are the health economic outcomes?Cost-effectiveness is the key superiority outcome; however, this must be supported by clinical information on the attendance rates and detection of sight-threatening diabetic retinopathy.
5: Feasibility 5a: What is the sample size?The sample size for an equivalence study is 4460 patients, which is felt to be feasible within a reasonable time frame (i.e. 18 months).
5b: What is the VoI for the different trial designs?The evidence does not support a superiority trial, so the value of the additional information related to an equivalence/non-inferiority design would be worthwhile for the additional costs that this would incur. As resourcing is a considerable issue, ensuring that attendance is constrained would be important within the trial as, otherwise, the intervention may not be implemented; therefore, the use of an equivalence design could be worthwhile.
6: Perspectives 6a: What ethics considerations are there?Ethically, it is important that this trial shows at least non-inferiority on the outcomes of attendance and detection of sight-threatening diabetic retinopathy, as a screening package is already in place in regular practice.
6b: What is the perspective of patients and service users?Patients played an integral part in the design and felt that it was important to consider the attendance rates and detection of sight-threatening diabetic retinopathy. They were convinced by the idea of an equivalence or non-inferiority trial design for the benefit of a risk-based screening programme, and a personalised screening schedule based on risk may be attractive to patients.
6c: What is the perspective of decision-makers?The trial was funded by the NIHR Programme Grants for Applied Research (PGfAR) programme. Cost-effectiveness is a key outcome for the NHS, and an improvement in this would be attractive; therefore, it would be important to show equivalence/non-inferiority on the health outcomes to ensure that these are not being affected.
6d: What is the perspective of clinicians?Clinicians may need strong evidence of equivalence/non-inferiority to implement a strategy whereby some patients are seen less often; however, the idea of seeing higher-risk patients more often may be attractive to them. However, this would need to be shown to be cost-effective in the long run to make this increase worthwhile.
6e: What is the impact on different sectors (e.g. health, education, social care)?There is no impact on other sectors that is considered to be important.
Given the lack of evidence around the timing of screening and the similarity between the groups, there is limited evidence that superiority would be seen on any of the health or screening attendance outcomes. These are the two key outcomes to assess in the trial, alongside the cost-effectiveness, meaning that consideration of equivalence or non-inferiority should be considered.
There is a resource constraint in the health-care system around the number of screening visits, which suggests that equivalence would be required on this outcome, whereas non-inferiority would be required for detection of sight-threatening diabetic retinopathy.
Although an equivalence design would require a larger sample size, it was felt that it was feasible to achieve such a sample in the trial population. In addition, the equivalence restriction is essential to answering the research question and, therefore, the increased sample size (and, thus, cost) was felt to be good value for money.
Example 3: non-inferiority
PRaCTICED (Pragmatic Randomised Controlled Trial assessing the non-Inferiority of Counselling and its Effectiveness for Depression)68 aimed to assess counselling for depression (CfD) against cognitive–behavioural therapy (CBT) in primary care patients who had suffered with depression. Box 4 considers the factors that influenced the selection of the trial design.
The population is patients with a diagnosis of moderate or severe depression accessing Improving Access to Psychological Therapies services. Depression is a difficult condition that can affect many aspects of a patient’s life and, therefore, there is good reason to believe that there would be multiple outcomes of interest.
1b: What are the subpopulations of interest?Subgroups have been identified as important based on the severity of diagnosis and the time to starting therapy. There are no hypotheses about some subgroups reacting in different directions to others, but this is being included for investigation.
2: Intervention 2a: What is the intervention?CfD is similar to the comparator in that they both aim to help those with depression through therapy, although they differ in their frameworks. Current evidence does not suggest that superiority over the comparator is plausible.
3: Comparator 3a: What is the comparator?The comparator is CBT, which is an active treatment.
3b: What is current practice or standard care?CBT was recommended as a front-line psychological intervention by a NICE review69 and so is commonly used in practice. This is the comparator in the trial; therefore, superiority regarding effectiveness may not be plausible.
3c: What evidence is there in relation to the comparator?There is a robust evidence base for the benefit of CBT for patients with depression and, therefore, a non-inferiority or equivalence design to compare against this as the comparator would be appropriate.
4: Outcomes 4a: What are the priorities of different outcomes?The key outcome is the patient’s depression and whether or not this is being treated effectively.
4b: How many clinical outcomes are key to eventual decision-making?There are three key outcomes that could influence the eventual treatment decision: (1) depression, (2) patient preference (and, therefore, uptake of treatment) and (3) cost-effectiveness.
4c: What are the superiority outcomes?CfD (the intervention) requires fewer formal qualifications so a larger number of therapists are available to deliver the intervention; therefore, costs and cost-effectiveness may be improved. However, there is little evidence to suggest that superiority would be found for the outcome of depression.
4d: Are the costs of the comparator and intervention being considered?The cost of delivering the intervention is hypothesised to be marginally cheaper and this is an important evaluation in the study.
4e: Is non-inferiority or equivalence on the primary outcome plausible?Non-inferiority of the two therapies for the outcome of depression is plausible, and previous evidence suggests that a non-inferiority design is appropriate. The need for equivalence is not required here, as improvement in the outcome of depression is desirable and there is no reason for it to be limited.
4f: What are the health economic outcomes?Cost-effectiveness is a key outcome of the trial, but is in addition to the health outcome.
5: Feasibility 5a: What is the sample size?A sample size of 550 patients has been calculated for the non-inferiority design, and the team believes that it is possible to recruit this number within 36 months.
5b: What is the VoI for the different trial designs?The previous literature suggested that a superiority outcome between the two health technologies is not realistic. An equivalence study would require a larger sample size and it is not required for CfD to be non-superior in this case; therefore, the additional information provided is not of value.
6: Perspectives 6a: What ethics considerations are there?It would not be ethical to test against no treatment, as CBT is routinely offered and recommended.
6b: What is the perspective of patients and service users?The preference of patients is a key element in this trial, as some may have a preference for one of the two therapies. Evidence is required to show the comparison between the two therapies as, if they are similar, then patient preference can be taken into consideration in treatment decisions.
6c: What is the perspective of decision-makers?If more cost-effective, the treatment would be attractive to funders. However, it is important for the treatment to be non-inferior on patient outcomes of depression; otherwise, it would not be considered.
6d: What is the perspective of clinicians?Clinicians may have a preference for one treatment method over the other and, therefore, robust evidence of a difference in efficacy between the two is required. As CBT is the currently recommended treatment, CfD would need to be non-inferior for clinicians to consider changing their practice, and patient preference information would need to indicate that this change would be desired by patients.
6e: What is the impact on different sectors (e.g. health, education, social care)?No other impact outside this sector is considered to be important.
The primary outcome for this trial must be the patient’s depression, to ensure that this is sufficiently treated by the interventions. Owing to the similarity of the two interventions, there is no evidence base to suggest that the intervention would be superior to the comparator; however, it may be cheaper and/or preferred by patients. As patient preference is often related to the efficacy of a treatment and not cost-effectiveness, this suggests that it would be important to demonstrate non-inferiority on the key outcome (i.e. depression). To change practice, the delta value chosen for the non-inferiority margin may need to be small in order for patients, clinicians and decision-makers to see this as a worthwhile treatment.
There is no restriction on an improvement in the outcome of depression for the intervention and, therefore, the added complexity of an equivalence design is not required. This trial was designed, using depression as the primary outcome, on a non-inferiority basis.
Appendix 5 Checklists for reporting
Checklist for reporting the trial design
Checklist item | Present? |
---|---|
1: Reporting on trial design | |
1a: A heading labelled ‘benefit–risk’ | □ |
A specific section within the report that is labelled ‘benefit–risk’ and has all relevant information collated in it | |
1b: Explicit use of the term ‘benefit–risk’ | □ |
1c: Plan for B–R assessment | □ |
A plan for the method of assessment defined a priori | |
1d: Anticipated benefits and risks | □ |
A list or table of the anticipated benefits and risks within the trial defined a priori | |
1e: Discuss B–R balance with PPI representatives | □ |
Confirmation that patients feel that the B–R trade-off is acceptable to them |
Checklist for reporting the trial results
Checklist item | Present? |
---|---|
2: Reporting on results | |
2a: A heading labelled ‘benefit–risk’ | □ |
A specific section within the report that is labelled ‘benefit–risk’ and has all relevant information collated within it | |
2b: Explicit use of the term ‘benefit–risk’ | □ |
2c: B–R methods used | □ |
A description of the B–R methods that have been used in the trial and/or analysis | |
2d: Summary table of B–R | □ |
A summary table, if applicable, containing all of the key outcomes defined at the trial design stage | |
2e: Reporting QALYs in terms of B–R | □ |
Reporting of the QALYs, disaggregated into QALYs gained and QALYs lost, if possible | |
2f: Realised risks (adverse events) | □ |
Information of the harms that were realised in the trial, supported by the CONSORT extension for harms | |
2g: Consider B–R judgement with patient representatives | □ |
Patient judgement on the B–R balance; this is especially important if patient preference has not been formally included in the analysis |
Appendix 6 Benefit–risk examples
Case studies of some of the mentioned B–R methods in Appendix 2 are shown in Table 10, including references for further detail, if required.
Description of study | Method used |
---|---|
Narrative summary | |
Herceptin (trastuzumab) use in HER2-positive breast cancer treatment. The drug improves survival outcomes, but also increases the risk of cardiac toxicity | Narrative text:The cumulative incidence of CE at 6 years was slightly higher with the addition of trastuzumab; however, the late development of CE is infrequent. Trastuzumab (in the context of anthracycline- and taxane-based therapy) continues to have a favourable benefit-risk ratio Advani et al.70 |
Evaluation of the effectiveness of carbamazepine, gabapentin, lamotrigine, oxcarbazepine or topiramate for treatment of partial epilepsy. Assessment of time to treatment failure, 12-month remission, QALYs and cost-effectiveness on each drug to evaluate which is the best overall | Narrative text:We have found lamotrigine to be significantly better for time to treatment failure than the current standard treatment, carbamazepine, and the newer drugs gabapentin and topiramate. For time to 12-month remission from seizures, lamotrigine was non-inferior to carbamazepine Marson et al.71 |
Summary table and overarching framework | |
Comparison of two different triptan drugs. Benefits of the drugs were reduced pain, sensitivity, function and nausea; risks were other adverse events. The comparison aimed to find which of the two drugs was best at dealing with the benefits, without an increase in risks | BRAT framework: the example followed the six steps of the framework, including creating a value tree and a key B–R table This table provided results for each of the important outcomes for the two drugs; odds ratios of the comparison, with confidence intervals; and a forest plot for the results72 |
Comparison of efalizumab with placebo for the treatment of moderate to severe plaque psoriasis. The expected benefit is an improvement on the Psoriasis Area and Severity Index; however, there is the possibility of an increase in progressive multifocal leucoencephalopathy or other adverse events | PrOACT-URL framework: the example used the 12-step framework, which uses a table format to evaluate all of the relevant information needed to make a subjective decision73 |
Quantitative trade-off | |
Evaluating the use of topiramate against placebo for the reduction of heavy alcohol intake, adjusting for the additional presence of adverse events. Alcohol intake was measured using the number of heavy-drinking days and abstinent days; adverse events were considered overall, as well as being split into moderate and severe | NNT: the NNT was calculated for topiramate to assess the number of patients needed to find a successful outcome in one patient. The NNT was adjusted for moderate and severe adverse events separately to assess their impact on this outcome74 |
Evaluate a new product against placebo aimed to assist with weight loss. Adverse events that have been identified are nausea, diarrhoea and, in a very few cases, cardiovascular events. All of this information, along with the population epidemiology and the QALY impact, was considered important | INHB: QALYs for the expected benefits and risks were calculated separately and the INHB (i.e. net QALY) per patient was calculated as the difference between the two. These values were multiplied by the population entering the treatment annually to obtain the annual INHB75 |
Treatment of moderate to severe atopic dermatitis and plaque psoriasis using dupilumab or secukinumab, based on 13 key criteria, including disease severity, clinical effectiveness, safety, cost consequences and expert consensus | MCDA: the 13 criteria were used to obtain an overall value of the interventions for direct comparison. An expert panel assigned weights to each criterion; using data on the two drugs, experts allocate a score to each criterion for each drug. These criteria were used to gain an overall value, which was transformed on the 0–1 scale. A forest plot for the differences on each criterion (including variation) was then created and included the overall value estimate to show the preferred drug76 |
Assessment of using venlafaxine and fluoxetine to treat depression. Benefits were a reduction in depression symptoms based on the Hamilton Depression Rating Scale; risks were three key adverse events (i.e. nausea, insomnia and anxiety) | SMAA: using the four criteria, risk differences were evaluated for each drug, along with scaling vectors which are used to gain parameter values and, ultimately, rank acceptability indices. This was completed with and without preference information77 |
Preference elicitation | |
Elicit the preference regarding five antiepileptic drugs (i.e. carbamazepine, gabapentin, topiramate, valproate and lamotrigine), based on six attributes to assess the acceptable trade-off of the adverse events that come with an improvement in seizures. The beneficial attributes were seizure prevention and seizure reduction. The harmful attributes were memory issues, depression, aggression and pregnancy issues | DCE: experts were used to elicit the attributes in the first stage; these were then included in a survey, with trade-offs based on the attributes at different levels (assessed using appropriate data). The trade-offs chosen by respondents allowed the calculation of the maximum acceptable level of risk for each risk based on an improvement in the benefits. The preference-weighted outcomes were then applied to the data from the four drugs and the total utility calculated as the sum of the weighted outcomes to assess the treatment preferred by patients overall34 |
Uncertainty estimation | |
Compare two heparin options (i.e. low molecular weight and low-dose unfractionated) as prophylaxis against deep-vein thrombosis for the treatment of high-risk patients. There is a need to assess the potential increased risk of a major bleed | PSM: information on the estimated probability of deep-vein thrombosis and a major bleed is taken, along with measures of uncertainty for both. A probabilistic model was created that uses this information and Monte Carlo simulations were run to provide a joint uncertainty of the risks and benefits. These results were plotted on a B–R plane and the chance of each quadrant of benefit against risk was evaluated78 |
Visualisation | |
Evaluate the efficacy and safety of two antipsychotic drugs (i.e. olanzapine and perphenazine) for schizophrenic patients. A total of 14 key outcomes were considered. Efficacy outcomes were related to discontinuation, whereas safety outcomes were based on adverse events, hospitalisation, the use of further medication and weight gain | Forest plot: the risk differences between the two drugs for each of the 14 outcomes were plotted on a forest plot. This includes the confidence interval to show the uncertainty within each data point. The line used at 0 shows which side will favour each drug and the bars are ordered in decreasing effect size and shaded with two different colours to show the benefits and the risks, separately31 |
List of abbreviations
- ACL
- anterior cruciate ligament
- B–R
- benefit–risk
- BRAINS
- Benefit–Risk Assessment to Inform Non-Inferiority and Superiority Study Design
- BRAT
- Benefit–Risk Action Team
- CBT
- cognitive–behavioural therapy
- CfD
- counselling for depression
- CONSORT
- Consolidated Standards of Reporting Trials
- DCE
- discrete choice experiment
- HTA
- Health Technology Assessment
- MeSH
- medical subject heading
- MRC
- Medical Research Council
- mYPAS
- modified Yale Preoperative Anxiety Scale
- NGT
- nominal group technique
- NICE
- National Institute for Health and Care Excellence
- NIHR
- National Institute for Health and Care Research
- PICO
- population, intervention, comparison, outcome
- PPI
- patient and public involvement
- PROTECT
- Pharmacoepidemiological Research on Outcomes of Therapeutics by a European Consortium
- QALY
- quality-adjusted life-year
- RCT
- randomised controlled trial
- VoI
- value of information
- WP
- work package