Notes
Article history
The research reported in this issue of the journal was commissioned by the National Coordinating Centre for Research Methodology (NCCRM), and was formally transferred to the HTA programme in April 2007 under the newly established NIHR Methodology Panel. The HTA programme project number is 06/97/04. The contractual start date was in October 2008. The draft report began editorial review in June 2011 and was accepted for publication in January 2012. The commissioning brief was devised by the NCCRM who specified the research question and study design.The authors have been wholly responsible for all data collection, analysis and interpretation, and for writing up their work. The HTA editors and publisher have tried to ensure the accuracy of the authors’ report and would like to thank the referees for their constructive comments on the draft document. However, they do not accept liability for damages or losses arising from material published in this report.
Declared competing interests of authors
none
Permissions
Copyright statement
© Queen’s Printer and Controller of HMSO 2012. This work was produced by Brazier et al. under the terms of a commissioning contract issued by the Secretary of State for Health. This journal is a member of and subscribes to the principles of the Committee on Publication Ethics (COPE) (http://www.publicationethics.org/). This journal may be freely reproduced for the purposes of private research and study and may be included in professional journals provided that suitable acknowledgement is made and the reproduction is not associated with any form of advertising. Applications for commercial reproduction should be addressed to: NETSCC, Health Technology Assessment, Alpha House, University of Southampton Science Park, Southampton SO16 7NS, UK.
2012 Queen’s Printer and Controller of HMSO
Chapter 1 Introduction and background
This report is concerned with a range of issues around the development, testing and use of condition-specific preference-based measures (CSPBMs) of health for estimating quality-adjusted life-years (QALYs) for use in economic evaluation of health-care interventions. Although the focus is on CSPBMs, many of the issues raised are relevant to preference-based measures in general, including other types of population-specific measures and generic measures. We begin this chapter by providing the rationale for using CSPBMs for this purpose, and then we outline the key stages of their development and set out the key methodological issues addressed in the report.
Rationale
The last decade has seen the increasing use of economics evaluation around the world to inform the allocation of resources between competing health-care interventions and particularly the use of cost-effectiveness, in which context interventions are assessed in terms of their cost per QALY gained. The QALY provides a way of measuring the benefits of health-care interventions in terms of improvements in health-related quality of life (HRQoL) and survival. QALYs are increasingly being calculated using health-state utility values provided by generic preference-based measures (preference-based measures) of health, such as the EQ-5D,1 SF-6D2,3 or HUI-3. 4 It has been claimed that ‘generic’ preference-based measures are applicable to all interventions and patient groups. This claim has support in many conditions for which they has been shown to be reliable, valid and responsive. 5 Many reimbursement agencies request that QALYs are estimated using a generic preference-based measure for economic evaluation submissions on pharmaceuticals, and the National Institute for Health and Clinical Excellence (NICE) in England and Wales specifies a preference for the EQ-5D. 6
Clinical studies of pharmaceuticals and other health-care interventions often use condition-specific measures (condition-specific measure) of health, which are typically not preference-based, but not generic preference-based, measures. This is partly attributable to concerns about the appropriateness of generic measures in some conditions. Generic preference-based measures have been show to perform poorly in terms of validity or responsiveness to change in some conditions, such as the EQ-5D in visual impairment in macular degeneration,7 hearing loss,8 leg ulcers9 and schizophrenia. 10,11 Whether or not there are genuine concerns with generic measures, researchers are keen to reduce patient burden and cost, meaning often that only a condition-specific measure is included in key studies. Furthermore, many pharmaceutical trials are designed for obtaining licensing approval from the US Food and Drug Administration (FDA), European Medical Agency (EMA) and similar licensing authorities around the world that do not require generic or preference-based measures. Indeed, guidelines published by the FDA on the use of patient-reported outcome measures (PROMs) (in support of labelling claims) have increased the pressure to use condition-specific measures that have specifically been developed in the patient groups in which they are going to be used and are typically not preference based. 12 Condition-specific measures are going to continue to be an important potential source of evidence on effectiveness. To limit the evidence used to populate economic models to generic measures in many cases would exclude valuable evidence on the effectiveness of an intervention. However, the use of condition-specific measures in economic evaluation is severely limited because these measures were not designed for this purpose and, unless they are preference based, cannot be used to calculate QALYs. 13 Another challenge is the focus of condition-specific measures: HRQoL and symptoms or HRQoL only related to the symptoms of the condition. QALYs are typically assumed to reflect HRQoL rather than symptoms, although many preference-based measures used to produce QALYs are a combination of these, for example the EQ-5D has one symptom dimension of pain but all remaining dimensions (mobility, self-care, usual activities, anxiety/depression) arguably measure HRQoL.
One solution to this problem has been to try to ‘map’ from the condition-specific measure on to one of the generic preference-based measures using a data set containing the non-preference-based condition-specific measure and generic preference-based measures using regression techniques. The mapping algorithm is then applied to the clinical study data containing the non-preference-based condition-specific measure to predict utility values for the generic preference-based measures. A review of 28 mapping studies found that the performance of these mapping functions in terms of model fit and predictions varied considerably, with the root-mean-square error (RMSE) ranging between 0.084 and 0.2. 14 These errors are all large as, for example, an error of 0.2 could mean that an observed value of 0.5 could have a predicted value as low as 0.3 or as high as 0.7 on the 1–0 full health–dead scale. More concerning has been the tendency for some models to overpredict in more severe cases and underpredict in very mild cases. 15,16 Mapping methods are fundamentally limited by the degree of overlap between the classification systems of the two measures. Where there are important dimensions of one instrument not covered by the other, then this may well undermine the model. It has been found, for example, that attempts to map SF-36 dimension scores on to the EQ-5D preference-based index yield small and often non-significant coefficients for the vitality or energy dimension. 15 This is not surprising, as the EQ-5D classification system does not contain this dimension. Mapping does not overcome inadequacies in the classification system of the generic measure and is appropriate only if the measure is appropriate for that condition and patient population.
An alternative approach is to construct bespoke vignettes or scenarios to describe different states of health rather than to use standardised measures of health. 17 This approach was widely used in the 1970s and 1980s before the advent of generic preference-based measures, and it continues to be used, such as in submissions to NICE. 18 The vignettes are typically constructed from interviews with clinical ‘experts’ or sometimes patients. They provide an opportunity to be more flexible about the content of the health states and so make them more relevant to a condition and its treatment than a generic measure. The downside is that they have little or no quantitative linkage to clinical trial or other sources of evidence of effectiveness and do not reflect the variability in outcomes commonly found in clinical studies. The construction of these types of vignettes is highly subjective and is prone to manipulation.
For these reasons there has been interest in the development of CSPBMs. 19 CSPBMs can either be developed de novo, i.e. as an entirely new measure,20,21 or developed from existing condition-specific measures. 22,23 Development from existing measures has the advantage that utility scores can be generated for respondents completing the existing measure in existing and future data sets. This means that data collected on the existing measure can be used for a variety of purposes including producing QALY estimates, and respondent burden and cost can be reduced if a generic preference-based measure is not also required. This report examines the methodological issues in developing preference-based measures, and their testing and application. The rest of this chapter provides an overview of the key stages in developing a preference-based condition-specific measure and the methodological issues addressed in this report.
The problem
Condition-specific measures are standardised multi-item questionnaires used to assess patient health across different areas of self-perceived health. The areas covered may include symptoms, physical functioning, work and social activities, and mental well-being. They are designed for patient populations with a specific medical condition. They can be unidimensional or multidimensional. Responses to items are combined into a score using a range of possible methods. The most commonly used scoring system remains a simple summation of responses to produce dimension scores. For example, the AQLQ is designed to assess HRQoL in patients with asthma. 24,25 The AQLQ consists of 32 items that measure HRQoL across four dimensions: symptoms (12 items), activity limitations (11 items), emotional function (five items) and environmental stimuli (four items). For each item in the AQLQ, respondents are asked to choose from a series of seven responses ranging from extreme problems to no problems to obtain a score out of seven for each item. Item scores are then summed to obtain a dimension score and an overall score across all 32 items. The original measure has no preference weightings of the items or dimensions and so no basis for producing a health-state value for calculating QALYs.
One problem that is faced when deriving preference-based indices from existing condition-specific measures, such AQLQ, is that they are large and complex. With multiple dimensions and numerous items they define many millions of potential health states, and each of these states involve too much information for valuation by respondents. Researchers at the University of Sheffield have been developing methods for dealing with this problem by developing health-state classifications from the measures. A health-state classification system consists of multiple dimensions, each with a number of severity levels: for example, the EQ-5D has five dimensions, each containing three severity levels, and is able to define 243 states. The precise number of dimensions and the levels per dimension may vary. However, even with a classification such as the EQ-5D there are too many states to value in a survey and so only a sample are valued and the remainder are then estimated by econometric modelling. More importantly, the health states themselves contain a limited number of statements (five in the case of the EQ-5D) to describe the state of health. Evidence suggests that the most respondents can value is between five and nine statements, and health states of this size have been shown to be amenable to valuation by respondents from the general population. Researchers at the University of Sheffield have applied the approach of developing a health-state classification from a larger instrument to a number of non-preference-based measures over the last 10 years, including SF-36 and SF-12,2,3,26 menopausal health questionnaire,23 atopic dermatitis,27 AQLQ,28,29 OAB-q,30,31 King’s Health Questionnaire,32 Sexual Quality of Life Questionnaire33 and European Organisation for Research and Treatment of Cancer Quality of Life Questionnaire 30 (EORTC QLQ-C30). 34
This ‘health-state classification approach’ aims to produce a new, reduced health-state classification that is amenable to valuation by respondents with a minimum loss of information and is subject to the constraint that responses to the original instrument can be unambiguously mapped on to it. This implies that the text of the items should be altered as little as possible. The task is therefore to determine the dimensions and select items and severity levels for the new classification and this is described next.
An overview of methods for deriving condition-specific preference-based measures from existing patient-reported outcome measures
Introduction
The methods described here cover the six stages (Figure 1) that can be used for deriving a CSPBM from an existing non-preference-based measure of HRQoL using traditional psychometric analysis and Rasch analysis. Item response theory can be used as an alternative to Rasch analysis. These stages are a guide to the key components in the development of a preference-based measure rather than a prescriptive methodology, as it is not always practical or possible to follow each stage separately or sequentially and the precise technique used may differ depending on the size and structure of the original instrument.
FIGURE 1.
The six stages for deriving a preference-based HRQoL measure.
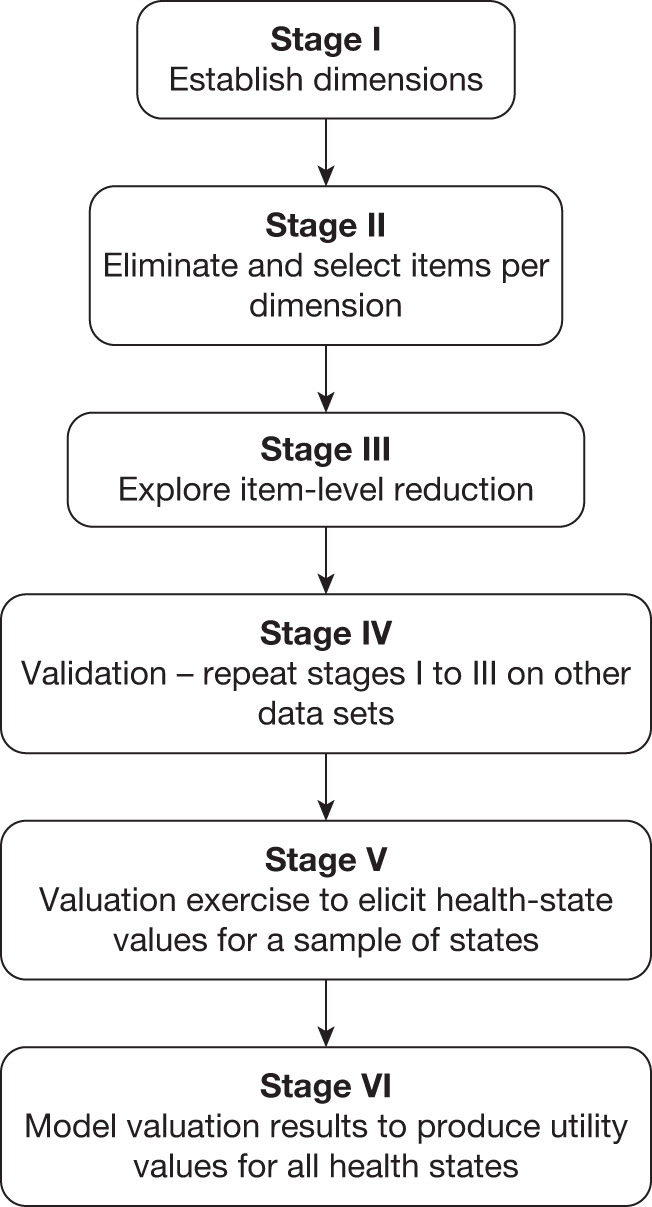
Stage I: establishing dimensions
Conventional preference-based measures of health use a health-state classification system in which the dimensions are structurally independent in order to avoid nonsensical health states generated by the statistical design or multi-attribute utility theory as described in stage VI. In other words, there must be a low correlation between the dimensions. One technique for identifying structurally independent dimensions is factor analysis (confirmatory or exploratory, and this can use orthogonal or oblique solutions), although other techniques can be used. Factor analysis can be helpful in confirming the original dimensional structure of a measure or show where dimensions are not sufficiently independent and suggest ways to reduce the number of dimensions. It can also be used to suggest a possible dimensional structure when none was proposed by the original instrument developer. 27,31 Alternatively, it can suggest modifications to the dimensional structure proposed by the instrument developer; for example, in a study deriving the AQL-5D from the AQLQ, factor analysis suggested that there were five dimensions, whereas the original instrument had four. 28 Factor analysis needs to be used with care, however, as the factors it suggests may not make conceptual sense. Another technique that has been used is cluster analysis. 35 The extent to which items belong to a single dimension can also be examined using Rasch analysis (see Stage II: selecting items, below).
Stage II: selecting items
A dimension of a health-state classification system of preference-based measures is usually represented by one item, or occasionally two items, from the original instrument. The selection of items must be undertaken with great care. This process has been assisted in past research by a combination of conventional psychometric analysis, Rasch analysis and preference data,29,31 although other techniques such as item response theory can be used. A technique that has proven helpful in the process of item selection is Rasch analysis. This is a mathematical technique that converts qualitative (categorical) responses to a continuous (unmeasured) latent scale using a logit model. 36,37 The intuition underlying this approach is that the probability of an affirmative response to each item (or each response to each item) depends on the degree of difficulty of the item (or severity in the case of health) and the ability of the respondent. In the development of a health-state classification system, Rasch analysis can be used to eliminate items that poorly represent the underlying latent scale (for a brief overview of the concept of Rasch analysis, see Appendix 1).
The process of selecting items in a number of studies has been broken down into two stages. The first has been the elimination of poorly performing items that do not meet key criteria. 31 However, for larger measures of HRQoL this will leave a number of items in some dimensions and so the second stage involves selecting the best item for each dimension.
Eliminating items per dimension
For multidimensional measures separate Rasch models should be fitted to each of the dimensions established in stage I. In deriving the AQL-5D from the AQLQ, five Rasch models were fitted and items were eliminated using three criteria:
-
Items that are not suitable for item-level ordering should be eliminated from consideration in the classification system, as it is not possible to distinguish between response levels for these items.
-
Differential item functioning (DIF) establishes whether respondents with different characteristics respond differently to items. Items that display DIF are of limited value in preference-based measures when using them across subgroups of patients defined by the characteristic (as often would be required in an economic evaluation) and are therefore usually excluded.
-
Items that do not fit the underlying Rasch model should be eliminated, as they do not represent the underlying dimension; these items can be identified using Rasch model goodness-of-fit statistics.
Selecting the best item per dimension
Once items have been eliminated from the selection process, Rasch/item response theory and traditional psychometric methods are applied to select the ‘best’ items for the health-state classification. The item selection criterion typically includes at least some of the following:
-
item-level coverage across the latent space using the Rasch model
-
item goodness of fit using the Rasch model
-
feasibility (level of missing data)
-
internal consistency (correlation between item and dimension scores)
-
distribution of responses to identify floor and ceiling effects
-
responsiveness between two time points (e.g. standardised response mean).
Stage III: exploring item-level reduction
In practice, patients may not be able to distinguish between item response choices. 5 Therefore, we recommend including this stage as an exploratory stage in examining the possibility of reducing the number of item levels in stage II. Item threshold probability curves from Rasch and response frequencies can be examined when selecting potential item levels for collapsing. Items for the AQL-5D, for example, were collapsed from 7 to 5 using evidence from the Rasch analysis. Cognitive debriefing of respondents by researchers may also help to inform this process.
Stage IV: validation
The application of stages I–III derives a health-state classification system that is small enough for valuation. However, before proceeding with the valuation process, we recommend validating the selected items by repeating the analysis on an alternative sample from the same data set that was used in stages I–III, an alternative time point from the data set used in stages I–III or an alternative data set.
Stage V: valuation survey
It is infeasible for members of the population to value all health states generated by most health-state classification systems, which typically define several thousands of health states. Therefore, a sample of health states is selected from the classification system for valuation. Two alternative approaches are used to sample health states and subsequently estimate utility values for all states (described below, see Stage VI: model health-state values): the decomposed approach and the composite approach. The decomposed approach uses multi-attribute utility theory to select states to determine the functional form (usually multiplicative or additive). This involves three stages: valuing each dimension separately to estimate single dimension utility functions; valuing ‘corner states’, where, for example, one dimension has extreme problems and all other dimensions have no problems; and valuing a selection of non-corner states. The composite approach involves the valuation of a sample of states chosen using a statistical design such as an orthogonal array and uses regression techniques to estimate values for all states defined by the classification. Both approaches generate states that contain combinations of levels across the dimensions, many of which will involve high levels on some dimensions and low levels for others. The problem this creates is discussed later and is one of the issues addressed in this research.
For use in economic evaluation health-state values must be valued on a common scale with an upper anchor of one at full (or perfect) health and a lower anchor at zero that is assumed equivalent to being ‘dead’. Once the health states have been selected they can then be valued by a sample of the population of interest, usually the general population, using valuation techniques such as time trade-off (TTO), standard gamble, visual analogue scales (VASs)5 or combinations of these. These valuation techniques are individual valuations of health states that require the individual to imagine themselves in the health state of interest. Social valuations of health states can also be used, such as person trade-off where individuals are asked to make a societal judgement of how many patients in one health state they would need to cure to be equivalent to curing a specified number of patients in another group. Individual valuation techniques have been typically used to elicit values for health state, although some researchers argue that societal valuation techniques may be more appropriate.
Stage VI: model health-state values
The decomposed approach produces utility values for all health states by solving a system of equations to generate preference weights for each dimension and any interactions specified in the model. 38 This approach was used to estimate utility values for the HUI-2 and HUI-34,39 and CSPBMs in rhinitis and asthma. 20,21
The composite approach uses regression techniques to estimate utility values for all health states valued. The standard model uses individual-level data, for which the value of a health state is defined as:
where i = 1, 2 … n represents individual health-state values and j = 1, 2 … m represents respondents. The dependent variable is the value for health state i valued by respondent j and X is a vector of dummy explanatory variables for each level λ of dimension ∂ of the health-state classification, where level λ = 1 acts as a baseline for each dimension. Beta represents the coefficients on X. ‘εij’ is the error term that is subdivided, εij = uj + eij, where uj is respondent-specific variation and eij is an error term for the ith health-state valuation of the jth individual, and this is assumed to be random across observations.
A number of alternative models are usually fitted to the data and the preferred model is selected based on goodness-of-fit statistics including mean absolute error (MAE), adjusted R2, and the number of health states with errors of > 0.05 and 0.1. The algorithm from the preferred model can then be used by others to obtain utility values for the preference-based measures.
Methodological problems in the development of condition-specific preference-based measures
Below are a set of methodological problems that this research project sought to address.
Lack of structural independence between dimensions
The approach of developing health-state classification systems has been successfully applied to a number of instruments, but one problem that has emerged is that some condition-specific measures do not have a clear multidimensional structure. This arises where items are found to be highly correlated. The items could be seen as unidimensional, although they may nonetheless tap important nuances in the impact of the condition. A condition may impact on a number of related areas of life, and a richer picture is provided by using more than one item. A lack of independence between items in a health-state classification system creates problems in the valuation and modelling stages. Many of the states that need to be valued for the modelling, using for example an orthogonal design, would involve combinations of dimension levels that would not be credible (e.g. feeling downhearted and low and happy most of the time). This problem is more likely to arise with condition-specific measures, as they tend to define a narrower range of domains.
One approach to this problem is to construct a sample of representative health states without using a health-state classification system. This involves defining health states that represent patients with particular severity levels of a health problem. This approach was pioneered by Sugar, Lenert and others using κ-means cluster analysis to break up the data into states. In one study they identified patterns of the physical and mental health summary scores of the SF-12 into models with varying numbers of discrete states. 40 They selected six states from the SF-12 data in a sample of depressed patients (i.e. near normal, mild mental and physical health impairment, severe physical health impairment, severe mental health impairment, severe mental and moderate physical impairment, and severe mental and physical impairment). These were defined in terms of scores, so a process of turning the score distributions of each state into words taken from the original 12 items to define the states had to be developed based on expert judgement. This is an interesting approach but it suffers from three limitations. First, the derivation of the states uses essentially arbitrary cut-offs in the cluster analysis. Second, it uses dimension scores that then need to be related to the item descriptions to generate the states and this uses expert judgement. Although some expert judgement is always needed in this type of work, it should be minimised where possible. Finally, this method has only been used to value a small sample of states and it is likely that it is not possible to allocate all patients to these states (in contrast to the inclusive approach of the health-state classification).
An alternative approach examined in this report avoiding these problems is to use the results of Rasch modelling to generate states to describe typical respondents at different points along the latent variable. The last decade has seen the increasing use of Rasch modelling in the development and testing of PROMs. As well as providing a method for assisting in the selection of items, we have pioneered its use in selecting health states for valuation that represent different levels of severity. This ‘Rasch vignette approach’ generates logical states based on the natural occurrence of states in the data set and avoids the infeasible combinations generated by statistical designs (e.g. an orthogonal array) from a health-state classification system.
A potential disadvantage with the Rasch vignette approach, as with clustering, is that it generates a small subset of potential states for valuation and so leaves a large number unvalued. A solution examined in this report is to estimate the relationship between the health-state utility values and the latent variable produced by the Rasch model using regression techniques. This permits the estimation of utility values for other points on the latent variable and hence other states generated by the items. This method is explored in Chapter 3.
Naming the condition
Many condition-specific measures state the cause of the health problems being assessed in the instrument (‘Your asthma interferes with getting a good night’s sleep all of the time’). There have been a number of studies looking at the effect of naming the condition on health-state values. 5 Evidence suggests that naming the condition has an impact in some, although not all, cases.
The reason why a condition-specific measure names the medical condition is probably to improve its precision, and avoid unrelated problems. On the other hand, by knowing the name of the medical condition non-patient respondents may bring their prejudices to the valuation exercise (e.g. ‘being limited in pursuing hobbies or other leisure time activities due to cancer’). Furthermore, this relies on the correct attribution by the patient. Patients may not be able to disentangle the impact of a given health condition from other possible conditions and non-health problems in their life. The usual practice in valuing generic measures (as being generic means, by definition, that disease label is not mentioned) is to avoid anything suggesting specific diseases. In the AQL-5D valuation, however, the disease labels were maintained because it was necessary to make sure what is valued in AQL-5D is the same as what patients report about their health using AQLQ, and thus it was important to minimise changes to wording. But, because of the danger of respondents bringing their own ideas to bear, maintaining the same wording does not really guarantee that what patients mean by a given statement and what non-patients understand by the same statement will be the same, and the impact this may have had on the valuation is not known. This report will examine the impact of naming the condition in valuation studies in Chapter 4.
Side effects
A well-known concern with condition-specific measures is that they do not cover potentially important side effects of treatment. When designing a study, one solution to this problem has been to include both generic measures and condition-specific measures in a trial. The problem for economic evaluation is that it is not possible to trade-off two measures to assess overall effectiveness. Economic evaluation requires a preference-based single index measure of health that is decision specific rather than condition specific, and captures the impact on the condition and any side effects. 41 This could be achieved either by taking a condition-specific measure and adding additional dimensions to cover any known side effects or by taking a generic measure that is known to cover side effects and adding dimensions to cover aspects of the condition. In Chapter 5 we examine the former strategy, by adding an additional generic dimension to two CSPBMs and valuing the classification systems with and without the add-on dimension. For one CSPBM we then apply the preference weights for the CSPBMs with and without the add-on dimension to a patient data set and examine the impact.
Comorbidities
Even assuming there are no side effects, the achievement of comparability between specific instruments requires an additional assumption, namely that the impact of different dimensions on preferences is additive, whether or not they are included in the classification system. The impact of breathlessness on asthma health-state values, for example, must be the same whether or not the patient has other health problems not covered by the condition-specific measure, such as pain in joints. Provided the intervention only alters the dimensions covered in the specific instrument then the estimated change in health-state value would be correct. However, it assumes preference independence between dimensions included in the classification system of the specific measure and those dimensions not included (i.e. for a health state with six dimensions, in which the level of each dimension is indicated by a digit, then the difference between state XXX and YYY should be the same as the difference between XXXZ and YYYZ).
Some degree of preference interaction has been shown to exist,2,4,42 with the impact of a problem on one dimension of health being reduced or exacerbated by the existence of a problem on another dimension. This is likely to create a larger problem in condition-specific measures that focus on a narrow range of health dimensions compared with generic measures that cover a broader range of health dimensions (although the problem will also exist for generic health measures, as they too exclude many potentially important dimensions). This problem of preference interaction will also be examined in Chapter 5.
One solution to the problem of preference interaction is to keep on adding extra dimensions to the condition-specific measure. However, this will reduce the usefulness of the new preference-based measure, as it cannot be applied to existing data sets containing the condition-specific measure because it requires additional information to be collected. Furthermore, respondents in valuation studies struggle if there are too many pieces of information at once and so there is a practical constraint on the size of classification systems designed for valuation. Any classification system that is to be amenable to valuation is likely to have a limited number of dimensions of health. For use in cross-programme comparison, it is ultimately a trade-off between having measures that are relevant and sensitive to those things that matter to patients with a particular condition (including side effects) and the potential size of the preference dependence between dimensions. The relative importance of the different arguments in this trade-off will vary between conditions. In Chapter 5, we examine the extent of this problem for two conditions (asthma and common mental health problems).
Information loss compared with original measure
The derivation of health states based on a small subset of items of the original measure inevitably involves a loss of information. The original measures had multiple items in order to improve reliability and so achieve better psychometric performance in terms of validity and responsiveness. Given that the original rationale for using a condition-specific measure is to use its greater relevance and sensitivity, it is important to ensure that it retains this informational advantage in the process of becoming an index.
The extent of information loss can be examined using conventional psychometric tests of validity and responsiveness. 43 In this report we compare the preference-based condition-specific measure with the original full measure in terms of construct validity by examining scores between severity groups and responsiveness to change over time. Any loss in information needs to be balanced against the ability of preference-based measures to generate quality adjustment weights for QALYs, but a substantial loss might suggest that the whole process of selecting a few items inevitably reduces the psychometric performance of the measure. Chapter 7 looks at this empirically for four CSPBMs.
Do preference-based measures derived from condition-specific measures really offer an improvement over generic measures?
An important question is whether preference-based measures derived from condition-specific measures really do offer an improvement over existing generic measures in terms of their psychometric properties, and whether they generate sufficiently different values for differences between states and for changes over time to be important for the results of economic evaluation. This is a further development of the previous section (see Information loss compared with original measure) and is addressed in Chapter 6 using similar methods on data sets that contain the condition-specific measure and one or more of the generic preference-based measures.
What are policy implications of using condition-specific measures?
One of the reasons for health economists being reluctant to use condition-specific measures has been a view that they cannot be used to make cross-programme comparisons. In Chapter 7 of this report we review these arguments in the light of the findings of the research presented in this report.
Aims and objectives of the report
The overall aim is to critically review and test methods for deriving preference-based measures of health from condition-specific measures of health (and other non-preference-based measure of health) in order to provide guidance on when and how to produce CSPBMs and to identify areas for further research.
The specific objectives are as follows:
-
to identify and review the existing literature on current methods for deriving a preference-based measure of health from non-preference-based measures of health in order to develop a framework
-
to examine and test a new method for generating health states – the Rasch-based vignette approach – from non-preference-based measures using Rasch modelling
-
to assess the impact of referring to the medical condition (or disease) in the descriptions on health-state values
-
to assess the impact on health-state utility values of attempting to capture side effects using CSPBMs
-
to assess the impact of comorbidities by testing the additivity assumption and the extent of any violation across two conditions (asthma and common mental health problems)
-
to examine the degree of information loss of moving from the original instrument to the preference-based index
-
to compare preference-based measures derived from the condition-specific measures with generic preference-based measures (EQ-5D and SF-6D) in order to examine the degree of agreement and the extent of any gain in psychometric performance
-
to propose a set of conditions that should be satisfied in order to justify the development and valuation of a CSPBMs for use in economic evaluation
-
to examine whether CSPBMs can be used to inform resource allocation decisions.
There are other issues to address in the development of preference-based measures, such as the methods of valuation and response shift, but these are not specific to CSPBMs and are therefore not addressed in this project.
Chapter 2 A review of studies developing condition-specific preference-based measures of quality of life to produce quality-adjusted life-years
Introduction
Over the last two decades there has been interest in the development of CSPBMs from existing condition-specific measures as this has the advantage that QALYs can be directly calculated using the data originally collected in the trial or study. Alternatively, CSPBMs can be developed ‘de novo’ to produce an entirely new measure. Yet unlike non-preference-based patient-reported outcome measures, there are no guidelines on the derivation of CSPBMs developed either from an existing measure or ‘de novo’. Surprisingly little research to date has been conducted on the methodological development of CSPBMs to inform best practice. The aims of this chapter are first to describe the methods used in previous studies that produce CSPBMs, and second from these findings to identify the main methodological issues in the development of these measures, in particular any issues that are additional to those identified in Chapter 1.
This chapter presents a review of the existing literature reporting on the derivation of a CSPBM. A CSPBM is defined as consisting of both (1) a classification system that can be used to categorise all patients with the condition of interest and (2) a means of obtaining a utility score for all states defined by the system. In Chapter 1 we outlined a six-stage approach for deriving a CSPBM from an existing measure and use this to provide the structure for this review. We use this structure both for measures developed ‘de novo’ and measures developed from existing measures as arguably the same process applies to both types of measures. We describe the studies undertaken to date and critically review these methods. We then use these results to outline methodological challenges and areas requiring further research. To retain focus and application the review is concerned only with papers deriving CSPBMs from an existing condition-specific measure or ‘de novo’. To illustrate, different concerns may arise in the development of generic and population-specific preference-based measures, for example deriving a measure for children or for the elderly poses population-specific considerations, and generic preference-based measures will face different concerns regarding focus and content.
Methods
Search strategy
Current methods for developing preference-based measures of quality of life from condition-specific measures or ‘de novo’ that were published in English were identified using a literature search conducted in December 2010. The literature search was undertaken on MEDLINE, EMBASE, Health Technology Assessment (HTA) database, Cochrane Central Register of Controlled Trials (CENTRAL), Web of Science (including Science Citation Index, Social Sciences Citation Index, Conference Proceedings), Cochrane Database of Systematic Reviews (CDSR), Cochrane Methodology Register, Database of Abstracts of Reviews of Effects (DARE). The following search strategy was used:
-
qol/hrqol/qaly/”Quality of Life”/quality of life/Quality Adjusted Life Year AND
-
utility/utilities/preference with based/index/measure within 4 words of 1 AND
-
transform*/translat*/transfer*/conver*/map*/deriv*.
The search identified 4093 papers; 104 papers remained after a title and abstract sift. The search strategy meant that the review included only journal papers published in or before December 2010.
Exclusion criteria
Of the remaining 104 papers, 78 papers were excluded at the full-paper stage for the following reasons: 30 reported the derivation and/or valuation of vignettes; 18 were either summaries or analyses of patient-valued utility data of own health, for example using standard gamble or TTO or existing utility measures in a patient group; eight reported the methodology used to develop a generic measure; five reported on measures that use patient-reported own health to produce utility scores for a selection of states, with no modelling of a tariff to produce values for all states; four were mapping studies (either mapping between measures, between valuation techniques or between a valuation technique and summary score); four were population-specific rather than condition-specific; three had no utility score (they either summarised values derived only from individuals, the scoring system was not preference based, or a tariff has not as yet been produced for the measure); two were treatment specific, not condition specific; one measured global quality of life rather than HRQoL and was not condition specific; one involved non-health aspects such as cost; and one measured relative improvement in HRQoL rather than HRQoL. This left 26 papers for inclusion in the final review.
Data extraction
The review examined the methodology used to produce the CSPBMs, focusing on the six-stage approach outlined in Chapter 1, where stages I–III produce the classification system, stage IV validates the classification system, stage V elicits health-state utility values and stage VI models the utility data to produce utilities for all health states defined by the classification system. The review was concerned with the motivation behind the study; the conditions examined; for stages I–III the method of construction and the number and composition of items and dimensions in the classification system; for stage IV, whether and how the classification system was validated; for stage V, whose values were used, how values were obtained, and whether utilities were valued on to a 1–0 full health–dead scale; and for stage VI, how utilities were estimated for every states and the accuracy of this process. Data extraction was undertaken by one member of the research team and summarised in Microsoft Excel (Microsoft Corporation, Redmond, WA, USA) using items summarised in Table 1 that were previously agreed by the team.
General | Author name |
Title of paper | |
Journal | |
Condition | |
Condition-specific measure | |
CSPBM | |
How/why chose original instrument | |
Reasons for deriving preference-based measures | |
Classification system | Dimensions and items in condition-specific measure |
Dimensions and items in CSPBMs classification | |
No. of states defined by system | |
Method for reducing condition-specific measure to CSPBMs classification/producing CSPBMs classification | |
Data used | |
Testing/validation of classification system | |
How dealt with multiple versions | |
Valuation | Population, method of recruitment and setting |
Sample size and selection of sample size | |
Preference elicitation technique | |
Anchors | |
No. of states valued | |
Sampling technique for states | |
Condition mentioned | |
Modelling preference data | Model type |
Dependent variable | |
Main effects variables | |
Interaction terms | |
Sociodemographics | |
Constant | |
Other variables | |
Transformations | |
Preferred model | |
Proportion of regression coefficients p < 0.05 | |
Proportion of regression coefficients with unexpected sign, and p < 0.05 | |
Proportion of inconsistent regression coefficients and p < 0.05 | |
R2-value and adjusted R2-value | |
Mean error | |
MAE and 95% CI | |
Proportion MAE > 0.05, > 0.10 | |
MAE as a percentage of observed range of the dependent variable | |
RMSE | |
Maximum predicted score compared with observed | |
Minimum predicted score compared with observed | |
Correlation | |
Plots | |
Other goodness-of-fit measures |
Results
Included papers
A brief summary of the 26 papers included in the review is presented in Table 2. Out of these 26 papers, 17 were published in non-clinical journals, including Quality of Life Research (five papers), Health and Quality of Life Outcomes (3), Pharmacoeconomics (3), Health Economics (1), International Journal for Quality in Health Care (1), Journal of ClinicalEpidemiology (1), Medical Decision Making (1), Quality in Health Care (1) and Value in Health (1). The remaining nine papers are each published in separate clinical journals: Amyotrophic Lateral SclerosisandOther Motor Neuron Disorders, British Journal of Cancer, British Journal of Dermatology, British Journal of Obstetrics and Gynaecology, Chest, Investigative Ophthalmology and Visual Science, Journal of Oral & Maxillofacial Surgery, Ophthalmic Epidemiology and Optometry and Vision Science.
First author | Year | Journal | Condition | Non-preference-based measure | Classification developed ‘de novo’ | Preference-based measure |
---|---|---|---|---|---|---|
Beusterien44 | 2005 | Amyotrophic Lateral Sclerosis and Other Motor Neuron Disorders | ALS | ALSFRS-R | No | ALS Utility Index |
Brazier23 | 2005 | Health and Quality of Life Outcomes | Menopause | Menopause-specific quality-of-life questionnaire | ||
Brazier32 | 2008 | Medical Decision Making | Urinary incontinence | The King’s Health Questionnaire (used for urinary incontinence and lower urinary tract symptoms) | No | |
Burr45 | 2007 | Optometry and Vision Science | Glaucoma | Glaucoma Profile Instrument | Yes | GUI |
Chiou46 | 2005 | International Journal for Quality in Health Care | Paediatric asthma | N/A | Yes | PAHOM |
Goodey47 | 2000 | Journal of Oral & Maxillofacial Surgery | Minor oral surgery | N/A | Yes | |
Harwood48 | 1994 | Quality in Health Care | Handicap | International Classification of Impairments, Disabilities, and Handicaps (ICIDH) | No | London Handicap Scale – designed for completion or self-completion in postal surveys |
Hodder49 | 1997 | British Journal of Cancer | Head and neck cancer | N/A | Yes | |
Kind50 | 2005 | Pharmacoeconomics | Lung cancer | FACT-L | No | |
Lamers51 | 2007 | Pharmacoeconomics | Lung cancer | FACT-L | No | |
McKenna52 | 2008 | Health and Quality of Life Outcomes | Pulmonary hypertension | Cambridge Pulmonary Hypertension Outcome Review (CAMPHOR) | No | |
Misajon53 | 2005 | Investigative Ophthalmology & Visual Science | Vision/visual impairment | N/A | Yes | VisQoL/AQoL-7D |
Peacock54 | 2008 | Ophthalmic Epidemiology | Vision/visual impairment | N/A | Yes | VisQoL/AQoL-7D |
Palmer55 | 2000 | Quality of Life Research | Parkinson’s disease | N/A | Yes | |
Poissant56 | 2003 | Health and Quality of Life Outcome | Stroke | N/A | Yes | |
Ratcliffe33 | 2009 | Health Economics | Sexual quality of life | SQoL | No | SQoL-3D |
Revicki21 | 1998 | Chest | Asthma | N/A | Yes | ASUI |
Revicki20 | 1998 | Quality of Life Research | Rhinitis | N/A | Yes | RSUI |
Shaw57 | 1998 | British Journal of Obstetrics and Gynaecology | Menorrhagia | N/A | Yes | |
Stevens27 | 2005 | British Journal of Dermatology | Paediatric atopic dermatitis | Un-named questionnaire on atopic dermatitis | No | |
Stolk22 | 2003 | Quality of Life Research | Erectile (dys)functioning | IIEF | No | |
Sundaram58 | 2009 | Journal of Clinical Epidemiology | Diabetes | ADDQoL plus additional items | No | Diabetes Utility Index |
Sundaram59 | 2010 | Pharmacoeconomics | Diabetes | ADDQoL plus additional items | No | Diabetes Utility Index |
Yang30 | 2009 | Value in Health | Overactive bladder | OAB-q | No | OAB-5D |
Young31 | 2009 | Quality of Life Research | Overactive bladder | OAB-q | No | OAB-5D |
Young60 | 2010 | Quality of Life Research | Flushing | FSQ | No |
Conditions
The range of conditions is broad, covering amyotrophic lateral sclerosis (ALS), asthma and paediatric asthma, erectile (dys)functioning, diabetes, flushing, glaucoma, handicap, head and neck cancer, lung cancer, menopause, menorrhagia, minor oral surgery, overactive bladder, paediatric atopic dermatitis, Parkinson’s disease, pulmonary hypertension, rhinitis, sexual quality of life, stroke, urinary incontinence, visual impairment. However, several measures cover similar conditions: asthma (two measures), cancer (two measures), vision (three measures), bladder (two measures), and sexual functioning (two measures). The papers discuss the derivation of 22 measures (four measures are each described using two papers, one of which has two sets of preference weights derived in two different countries).
Stages of developing preference-based measure
Stages I–III: deriving the classification system
Three papers reported only the valuation component30,54,59 of deriving a measure and hence are not discussed in this section, as the derivation of the classification systems was undertaken in separate papers. 31,53,58
Derivation of classification system from existing measure
Fourteen papers reported on measures that derived the classification system for the preference-based measure from an existing measure of HRQoL. All but one of these studies derived the classification system using a subset of items from the existing measure as the existing measure was considered too large to be amenable to valuation. One study supplemented the existing measure using other condition-specific items. The one study that used the classification system of the non-preference-based measure without modification actually derived the non-preference-based measure in the same study, and is therefore discussed in the section below on deriving measures ‘de novo’. 45
Six papers provide a clear and detailed description of their chosen methodology, and all analysed the performance of items from the non-preference-based measure using existing data and used a selection of psychometric criteria to select a subset of items. 31,50–52,58,60 Two papers also used qualitative analysis alongside the psychometric analysis. 50,51 The methods varied considerably between studies but all relied on the judgement of the researchers and/or experts, with varying degrees of usage of psychometric methods including factor analysis, Rasch analysis and classical psychometric methods of validity and responsiveness to determine both dimensionality and item selection.
Kind and Macran50 and Lamers et al. 51 reported on the same classification system. The method involved the use of factor analysis to determine the underlying structure and principal items in each dimension. The analysis was conducted on data from two clinical trials (n = 363) conducted for the condition of interest. Members of the research team independently qualitatively reviewed items and subscales from the existing measure for suitability and importance, and interviewed specialists, finding that these results were largely in agreement with the psychometric analysis.
McKenna et al. 52 selected items using the following criteria: percentage affirmation of item (not very small or very large); reasonable spread of item severity using the logit location in the Rasch model; significant coefficient in a model regressing items on to the general health question (‘very good/good/fair/poor health’); and content of items to ensure coverage of a range of issues. The analysis was conducted on responses of 201 patients.
Young et al. 31 selected items using the following process: first, factor analysis was used to establish instrument dimensionality; second, Rasch analysis was used to exclude items on the basis of item-level ordering, DIF and goodness of fit; third, Rasch and other psychometric analysis (e.g. low ceiling effects, low floor effects, low missing data, standardised response mean, correlation with domain score) was used to select items; fourth, Rasch analysis was used to collapse levels; and finally results were validated using other data. The analysis was conducted on data from a trial (n = 391) and validated on remaining patients in the trial (n = 746) and a separate trial (n = 793). Young et al. 60 also followed this approach using responses of patients suffering from the condition (n = 1270). Sundaram et al. 58 followed a similar approach using factor and Rasch analysis (but with different exclusion and selection criteria focusing on unidimensionality, interval-level measurement, additivity and sample-free measurement) on several data sets (n = 385, 52, 65, 111) and selected items for inclusion using both psychometric analysis and expert opinion.
The remaining seven papers use similar methods to the papers outlined above, but although they made reference to some criteria used to select items they do not fully outline the process used to derive the classification system. 22,23,27,32,33,44,48 For example, one paper23 stated that the most robust item per domain was selected, but the process used to determine robustness was not detailed. A further paper stated that items are selected that have the best coverage and responsiveness to change while ensuring the selected items represent different types of impact and that are related to disease severity, but the methods and results were not provided. 27 Brazier et al. 32 selected items using the following criteria: relevance to quality of life; percentage completed; avoidance of redundancy; face validity; distribution of scores (avoidance of floor/ceiling effects); construct validity; and responsiveness. Despite the detailed criteria the exact methods and data used to conduct the analysis was not reported. Another paper22 chose two items as they were considered primary end points, but this was not justified or explained.
Derivation of classification system ‘de novo’
Ten papers generated a classification system from scratch or ‘de novo’. Three papers used qualitative research,47,49,57 two papers used a literature review,46,55 three papers used a combination of literature review, patient interviews and expert opinion,20,21,45 one paper used a combination of qualitative research and a variety of psychometric analyses53 and one paper used psychometric analysis of a battery of existing items from the literature. 56
All three papers that used qualitative research to determine the classification system used a similar approach and conducted the process using recommendations by Babbie. 61 Goodey et al. 47 conducted semistructured interviews on 77 patients to identify domains patients believed were affected by surgery. Results were classified into ‘areas of concern’ by a panel of experts including patients and then categorised into domains taking into account frequency. The panel then constructed levels for these domains. Hodder et al. 49 conducted semistructured interviews on 25 patients and five experts. A Delphi panel including experts and a researcher was used to produce domains and the panel constructed levels for these domains. Shaw et al. 57 conducted unstructured interviews on 40 patients to identify the effects of the condition on different areas of their life. A panel consisting of experts and researchers derived domains and the panel constructed levels for the domains.
Chiou46 conducted a literature review of existing non-preference-based measures and a team including a psychologist and two specialists chose attributes based on patterns observed across the measures. Palmer et al. 55 identified dimensions using data from clinical trials, literature review and review of existing measures. Health states were reviewed by clinicians and researchers, piloted and subsequently revised.
The three papers that used a combination of literature review and qualitative research using patients provide little detail in their papers. Burr et al. 45 conducted focus groups with patients to explore their views of the effects of the condition and treatment on quality of life (guided by results from the literature and expert opinion) and the results were analysed using framework methodology to identify key domains for inclusion in the measure. Two papers used a combination of literature review, patient interviews and expert opinion,20,21 where the qualitative data were collected using 10 patient interviews to identify troublesome or distressing symptoms and problems and their relative importance.
Misajon et al. 53 conducted focus groups of patients to elicit attributes, guided by their previously validated questionnaire. Items and dimensions were generated using the focus groups and previous research and items were administered to 70 patients and 86 respondents without the condition. The number of items were reduced using psychometric criteria, factor analysis and reliability analysis, item response theory and structural equation modelling. The classification was confirmed by administering it to a second sample of 218 participants, 35% of whom were patients.
Poissant et al. 56 conducted telephone interviews on 493 patients, and 442 members of the general population matched on the basis of age and city district on a battery of existing measures and some additional items. Items were retained for consideration in the classification on the basis of prevalence and ability to capture effects of the condition. A subset of patients was subsequently asked to rate each item in terms of difficulty (i.e. severity) and importance and the results were used to select items. Three levels were selected per item and VAS on a convenience sample of 29 students was used to examine ordinality, and levels were reworded if necessary. The final classification was tested in a pilot study.
Content of health-state classifications
Table 3 outlines the number of dimensions and severity levels in each classification system and the number of health states defined by the classification. The number of dimensions varied: two measures had two dimensions; two measures had three dimensions, three measures had four dimensions, seven measures had five dimensions, five measures had six dimensions, three single measures had seven, eight and 10 dimensions each. The number of severity levels varied from 2 to 10 and often varied for different dimensions within a measure. The number of health states varied greatly from 10 to 390,625. Table 3 outlines the dimensions for each measure. It is worth noting that the focus of the dimensions differed by measure. Some measures had attributes that capture only symptoms or quality of life only related to the symptoms of the condition,22,31,60 whereas others incorporated dimensions that are likely to capture side effects and comorbidities covering both symptoms and HRQoL (e.g. Brazier et al. ,23 Kind and Macran50 and Lamers et al. 51). The measures suggest that there is not a single coherent underlying concept of HRQoL that is common to these measures, even for measures within a condition or International Classification of Diseases (ICD) classification.
First author | Condition | No. of dimensions | Severity levels | No. of states defined by system | Dimensions |
---|---|---|---|---|---|
Beusterien44 | ALS | 4 | 5–6 | 750 | Speech and swallowing; eating, dressing and bathing; leg function; respiratory function |
Brazier23 | Menopause | 7 | 3–5 | 6075 | Hot flushes; aching joints/muscles; anxious/frightened feelings; breast tenderness; bleeding; vaginal dryness; undesirable androgenic signs |
Brazier32 | Urinary incontinence | 5 | 4 | 1024 | Role limitations; physical limitations; social limitations/family life; emotions; sleep/energy |
Burr45 | Glaucoma | 6 | 4 | 4096 | Central and near vision; lighting and glare; mobility; activities of daily living; eye discomfort; other effects |
Chiou46 | Paediatric asthma | 3 | 2–3 | 12 – but only 10 are valid | Symptoms; emotion; activity |
Goodey47 | Minor oral surgery | 5 | 4 | 1024 | General health and well-being; health and comfort of mouth, teeth, and gums; impact on home/social life; impact on job/studies; appearance |
Harwood48 | Handicap | 6 | 6 | 46,656 | Handicap mobility; occupation; physical independence; social integration; orientation; economic self sufficiency |
Hodder49 | Head and neck cancer | 8 | 5 | 390,625 | Social function; pain; physical appearance; eating problems; speech problems; nausea; donor site problems; shoulder function |
Kind50 and Lamers51 | Lung cancer | 6 | 2 | 64 | Physical; social/family; emotional; functional; symptoms – general: symptoms – specific |
McKenna52 | Pulmonary hypertension | 4 | 2–3 | 36 | Social activities; travelling; dependence; communication |
Misajon53 | Vision/visual impairment | 6 | 5–7 | 45,360 | Physical well-being; independence; social well-being; emotional well-being; self-actualisation; planning and organisation |
Palmer55 | Parkinson’s disease | 2 | 2–5 | 10 | Disease severity; proportion of the day with ‘off-time’ (impact on quality of life due to condition covering domains: social function, ability to carry out daily activities, psychological function) |
Poissant56 | Stroke | 10 | 3 | 59,049 | Walking; climbing stairs; physical activities/sports; recreational activities; work; driving; speech; memory; coping; self-esteem |
Ratcliffe33 | Sexual quality of life | 3 | 4 | 64 | Sexual performance, sexual relationship, sexual anxiety |
Revicki20 | Asthma | 5 | 10 | 100,000 | Cough; wheeze; shortness of breath; awakening at night; side effects of asthma treatment |
Revicki19 | Rhinitis | 5 | 10 | 100,000 | Stuffy or blocked nose; runny nose; sneezing; itchy watery eyes; itchy nose or throat |
Shaw57 | Menorrhagia | 6 | 4 | 4096 | Practical difficulties; social life; psychological health; physical health; working life; family life |
Stevens27 | Paediatric atopic dermatitis | 4 | 2 | 16 | Activities; mood; settled; sleep |
Stolk22 | Erectile (dys)functioning | 2 | 5 | 25 | Ability to attain an erection sufficient for satisfactory sexual performance; ability to maintain an erection sufficient for satisfactory sexual performance |
Sundaram58 | Diabetes | 5 | 3–4 | 768 | Physical ability and energy level; relationships; mood and feelings; enjoyment of diet; satisfaction with managing diabetes |
Young31 | Overactive bladder | 5 | 5 | 3125 | Urge to urinate; urine loss; sleep; coping; concern |
Young60 | Flushing | 5 | 4–5 | 2500 | Redness of skin; warmth of skin; tingling of skin; itching of skin; difficulty sleeping |
Stage IV: validation of classification system
Few papers mention whether and how the classification system has been validated,20,21,31,45,56 and where validation of the classification system is mentioned the meaning of validation is interpreted differently depending on the study. One paper31 replicates the analysis used to construct the classification system using a different data set and different time-points of the data set used in the initial analysis and this can be interpreted as validation of the classification system. The remaining four papers20,21,45,56 do not validate the classification system but validate their measure using discriminative validity, by examining how the measure performs across subgroups of patients with different severity levels of the condition. These papers (with the exception of Burr et al. 45) also examine the agreement of their measure with a generic utility measure and a non-preference-based measure (Poissant et al. 56 used a generic measure, whereas Revicki et al. 20,21 used a condition-specific measure).
Stage V: valuation to elicit health-state values
The valuation component of each of the studies is outlined in Table 4. Three papers31,53,58 report only the classification component of deriving a measure and hence are not discussed in this section.
First author | Preference elicitation technique | Population | Administration | Sample size | No. of states | States valued per respondent | Sampling technique for states | Condition mentioned |
---|---|---|---|---|---|---|---|---|
Beusterien44 | VAS (for both each level of each dimension alone and health states) and standard gamble | Random US population sample | Self-administered via internet | 1108 for modelling, 1374 for descriptive analysis | 9 | 5 | Unclear | No |
Brazier23 | TTO | Women aged 45–60 years, randomly selected from GP lists in UK | Self-complete questionnaire undertaken in groups with two interviewers | 229 | 96 | 8 plus own health | Orthogonal array plus additional states | Yes |
Brazier32 | Standard gamble | UK patients with condition | Interview | 110 | 49 | 9 | Orthogonal array | Referred to as ‘bladder problem’ |
Burr45 | DCE | UK people with glaucoma | Postal survey | 286 used in analysis | 64 (32 pair-wise comparisons) | 32 | Fractional factorial design | Yes |
Chiou46 | VAS, standard gamble (asked to respond for children) | Random US population sample | Unclear | 94 for VAS, 101 for standard gamble | 10 in VAS, 5 in standard gamble | Unclear | VAS – all valid states, standard gamble – unclear | Unclear |
Goodey47 | Two tasks: distribute 100 counters across the dimensions in proportion to their importance; VAS of the levels per dimension | UK patients | Interview | 100 | N/A | N/A | N/A | Yes |
Harwood48 | VAS | UK patients aged 55–74 years | Interview | 79 | 30 | 30 | Conjoint analysis, but reduced number of levels for valuation | Unclear |
Hodder49 | VAS for dimensions relative to each other and VAS for the levels per dimension | UK surgeons | Unclear | 10 | N/A | N/A | N/A | Yes |
Kind50 | VAS | UK general population sample | Postal survey | 433 | Items for classification split into two sets, 10 states for each set | 10 plus own health | Orthogonal array | Yes |
Lamers51 | VAS | Dutch general population sample | Internet survey | 961 | Items for classification split into two sets, 10 states for each set | 10 plus own health | Orthogonal array | Yes |
McKenna52 | TTO | UK general population | Interview | 249 | 36 (all states) | 9 | All | Unclear |
Peacock54 | TTO and VAS for the levels per dimension | Unclear | Interview | Not included | 7 | 7 for TTO | Corner states (item worst responses with all other items at best level, e.g. 511111 and worst state) | Unclear |
Palmer55 | VAS and standard gamble | US patients | Interview | 59 for VAS, 58 for standard gamble | 10 (all) | 10 | All | Yes |
Poissant56 | VAS | Canadian patients and caregivers | Interview | 32 stroke patients, 28 caregivers | 11 | 11 | All best, all worst, corner states (item worst responses with all other items at best level) | Yes |
Ratcliffe33 | TTO, ranking, DCE | UK general population | TTO and ranking interview, DCE postal survey | TTO and ranking 207, DCE 102 | 64 (all) for TTO and ranking, 24 states across 12 pair-wise choices for DCE | TTO and ranking – 9, DCE – 12 (six choices) | TTO and rank used all states, DCE used optimal statistical design | Yes |
Revicki21 | Standard gambles and VAS both for states and for the levels per dimension | US patients | Interview | 161 | 10 | 10 | Corner and multisymptom states | Yes |
Revicki20 | Standard gamble and VAS both for states and for the levels per dimension | US patients | Interview | 100 | 10 | 10 | Corner and multisymptom states | Yes |
Shaw57 | Two tasks: distribute 21 counters across the dimensions in proportion to their importance; VAS of the levels per dimension | UK patients | Interview | 100 | N/A | N/A | N/A | Yes |
Stevens27 | Standard gamble (asked to respond for children) | UK general population | Interview | 137 | 16 (all) | 10 | All | No |
Stolk22 | TTO | Dutch general population and students | Group interviews for general population, interviews in groups and individually for students | 265 | 24 (all, excluding best state) | 24 | All (excluding best state) | Yes |
Sundaram59 | Standard gamble and VAS | US patients | Interview | 100 | 19 | VAS – 19, standard gamble – 4 | Corner, multisymptom and anchor states | Yes |
Yang30 | TTO | UK general population | Interview | 311 | 99 | 8 | Balanced design | Yes |
Young60 | TTO | UK general population | Interview | 147 | 16 | 8 | Rasch analysis to select plausible health states | Yes |
Elicitation technique
Out of the remaining 23 papers covering 23 valuation studies, five elicited values using VAS alone, five elicited values using only the TTO technique, six used both VAS and standard gamble, two used both VAS and the distribution of counters to indicate importance, two used standard gamble alone, one used discrete choice experiment (DCE) alone, one used TTO and VAS, and one used TTO, DCE and ranking. In total, VAS was used in 14 studies, TTO was used in seven studies, standard gamble was used in eight studies, DCE was used in two studies and ranking was used in one study. Although VAS was the most commonly used technique, its usage differs across studies. The VAS was used to value health states in 10 studies but six of these used VAS to predict standard gamble values using a mapping function. Furthermore, VAS was used to value severity levels within a dimension in seven studies and of these used to value the different dimensions in two studies.
Health-state selection
Nineteen studies elicited values for health states. The number of states included in the valuation studies varied from 0.01% of states to the inclusion of all states. The sampling technique used to select states varied by study, and this is affected by the valuation technique, sample size and the size of the classification system. Six papers valued all health states (one of these also used a statistical design to produce states for DCE), four used an orthogonal array, two used a fractional factorial design, five used corner states and multisymptom states (although the exact selection varied), one used a balanced design, one used Rasch analysis, and one paper selected a small number of states alongside levels of each dimension but the selection process is unclear. Three papers elicited values for levels and dimensions of the classification system directly and therefore did not value any health states. Only one study examined the issue of lack of independence between items, where some health states defined by the classification system may be infeasible,60 and this approach is reported in further detail in Chapter 3.
Population
The majority of valuation studies, 13 studies, were conducted in the UK. In addition, six studies were conducted in the USA, two in the Netherlands and one in Canada. Ten valuation studies elicited values from patients, nine studies elicited values for the general population, one study elicited values from both the general population and students, one study elicited values from patients and caregivers and one study elicited values from surgeons. For one study the population is unclear. 54 Sample size varies by study from 10 to 1374. The majority of valuation studies mentioned the condition. Two valuation studies did not mention the condition in their survey and whether a condition was mentioned was unclear for four studies.
Mode of administration
Interviews were the most popular mode of administration, with 17 studies using interviews. Of these 17 studies, 15 involved interviews undertaken individually, one study involved interviews undertaken in groups and one study involved both individual and group interviews. Other modes of administration were used, with three studies using postal surveys (one of which also used interviews) and two studies using internet surveys. For two studies the mode of administration is unclear. Mode of administration can affect response rates and the demographic composition of the sample. Historically most valuation surveys were untaken by interview, but in recent years the use of internet surveys is gaining popularity as it is cheaper and quicker, but may mean that the sample is not representative of the population of interest, for example with fewer elderly respondents. There is little published evidence examining the impact of all modes of administration on survey results.
Stage VI: modelling health-state utility data
The methods used to obtain health-state values for all states defined by the classification system are shown in Table 5. Three papers valued all health states defined by the classification and therefore did not undertake any form of modelling of these values. One study valued all states using VAS and converted these to standard gamble using a power function. Nine studies applied a composite approach using statistical analysis involving regression analyses used to estimate an additive function. The utility value of a health state is calculated as the sum of the coefficients of the appropriate levels of each dimension. Eight papers used a decomposed approach, which uses multi-attribute utility theory as the basis for the modelling, with five papers estimating a multiplicative function and three papers estimating an additive function. The exact process used in the papers reported here differs across different studies. Another study mapped Rasch logit scores generated for health states on to mean observed health-state values using regression analysis to produce a mapping function enabling utility values for all states to be estimated using the Rasch logit scores of each health state. 60 The methodology for one study was unclear. 56
First author | Preference elicitation technique | Method of extrapolation | Anchors | Anchored at dead = 0 |
---|---|---|---|---|
Beusterien44 | VAS (for both each level of each dimension alone and health states) and standard gamble | Decomposed – multiplicative | 0 = dead, 1 = full health | Yes |
Brazier23 | TTO | Composite – additive | 0 = dead, 1 = full health | Yes |
Brazier32 | Standard gamble | Composite – additive | 0 = dead, 1 = full health | Yes |
Burr45 | DCE | Composite – additive | 0 = worst state, 1 = best state | No |
Chiou46 | VAS and standard gamble (asked to respond for children) | Power function used to convert VAS to standard gamble, all states valued using VAS | 0 = death, 1 = full health | Yes |
Goodey47 | Two tasks: distribute 100 counters across the dimensions in proportion to their importance; VAS of the levels per dimension | Decomposed – additive | 0 = worst state, 100 = best state | No |
Harwood48 | VAS | Composite – additive | 0 = worst state, 1 = best state | No |
Hodder49 | VAS for dimensions relative to each other and VAS for the levels per dimension | Decomposed – additive | 0 = worst state, 100 = best state | No |
Kind50 | VAS | Composite, one model for each classification system, merged to obtain overall weights | 0 = dead,1 = full health | Yes |
Lamers51 | VAS | Composite, one model for each classification system, merged to obtain overall weights | 0 = dead,1 = full health | Yes |
McKenna52 | TTO | Composite – additive | 0 = dead,1 = full health | Yes |
Peacock54 | TTO and VAS for the levels per dimension | Decomposed – multiplicative | 0 = dead,1 = full health | Yes |
Palmer55 | VAS and standard gamble | All states valued | 0 = dead,1 = full health | Yes |
Poissant56 | VAS | Unclear | 0 = worst state, 1 = best state | No |
Ratcliffe33 | TTO, ranking and DCE | Composite – additive | 0 = dead,1 = full health | Yes |
Revicki21 | Standard gamble and VAS both for states and for the levels per dimension | Decomposed – multiplicative | 0 = worst state, 1 = best state | No |
Revicki20 | Standard gamble and VAS both for states and for the levels per dimension | Decomposed – multiplicative | 0 = worst state, 1 = best state | No |
Shaw57 | Two tasks: distribute 21 counters across the dimensions in proportion to their importance; VAS of the levels per dimension | Decomposed – additive | 0 = worst state, 100 = best state | No |
Stevens27 | Standard gamble (asked to respond for children) | All states valued | 0 = dead,1 = full health | Yes |
Stolk22 | TTO | All states valued | 0 = dead, 1 = absence of condition | Yes |
Sundaram59 | VAS and standard gamble | Decomposed – multiplicative | 0 = dead, 1 = full health | Yes |
Yang30 | TTO | Composite – additive | 0 = dead, 1 = full health | Yes |
Young60 | TTO | Maps Rasch logit scores onto mean utilities – additive | 0 = dead, 1 = full health | Yes |
Anchors
The majority of studies (14 papers) anchored the utility values on to the 0–1 dead–full health (referred to in some papers as ‘perfect health’ or ‘healthy’) scale required for QALY analysis. One further paper defines ‘1’ as absence of the condition, which may be interpreted as full health. 22 However, eight papers used alternative anchors: five papers anchored on to a 0–1 worst state–best state scale and three papers anchored on to a 0–100 worst state–best state scale, meaning that these measures cannot be used in their current form to estimate QALYs.
Discussion
The papers used a variety of methodologies at each stage of the development of the measure. There was no common method used to develop a CSPBM across all stages of the development process. However, several papers reported similar methodologies for some of the six stages of development of a condition-specific measure from an existing measure as outlined in Chapter 1. For example, six measures involved the derivation of the classification system from an existing measure using psychometric criteria, elicited health states from the general population and used statistical modelling with an additive function to produce utility values for all states anchored on to a 0–1 dead–full health scale. 23,30–33,50–52 However, even across these studies there are differences in the methodology used, including the psychometric criteria used to obtain the classification for stages I–III, and valuation technique and selection of health states for valuation for stage V.
The number of measures where the classification system was derived from an existing measure was similar to those developed de novo. Only half of the papers detailing the methodology used to derive the classification from an existing measure provided sufficient detail on the psychometric analysis, and some papers also used qualitative analysis. The majority of measures developed de novo involved the use of qualitative analysis, yet some studies again provided little detail of the methodology used. The lack of detail and clarity in many of the papers presents a barrier to enabling methodology in the derivation of the classification system from being better understood and evolving to become more scientifically rigorous. Future papers published in this area must be better explained and provide more detail to enable rigour and development in research in this field.
The content, composition and size of the classification systems varied widely across the measures. CSPBMs have been criticised for their narrow focus and inability to capture side effects and comorbidities, which raises the issue of accuracy when these measures are used to measure the effectiveness of treatments in economic evaluation. CSPBMs may also face criticism for their focus on symptoms (e.g. the measure for rhinitis20) rather than HRQoL. The narrow scope and focus of CSPBMs raises three important issues that are explored further in this report.
First, the use of preference-based measures to generate QALYs to inform resource allocation across patient groups and treatments may require that the utility values capture HRQoL rather than symptoms. The issue of whether CSPBMs should be used only in economic evaluation for agencies, such as NICE, if they measure HRQoL rather than symptoms alone has been considered elsewhere. 62 However, different agencies have different requirements and some may prefer measures with narrow focus. This is an important issue that will be explored further in Chapter 7. The performance of a selection of measures, some of which focus on symptoms and others which have a broader scope of HRQoL, in comparison with generic preference-based measures is examined in Chapter 6 and is used to provide further information on this issue.
The second issue is whether CSPBMs can and do capture side effects and comorbidities. The focus and scope of the measures included in the review varied so widely that it is likely that the classification systems of some measures are able to capture side effects and comorbidities (e.g. the measures for lung cancer50,51) and urinary incontinence. 23 However, this is something requiring further research regarding the performance of the measures when used in appropriate patient populations. The issue of exploring whether CSPBMs can be adapted to capture known side effects and comorbidities is examined in Chapter 5.
The third issue is whether the methodology used in the literature is appropriate for the development of a preference-base measured where the original measure is either unidimensional or has a unidimensional component. This has implications for all development stages covering both the classification system and the stages covering valuation and modelling of the utility values where it is assumed that all states are feasible. This is an issue particular to CSPBMs rather than generic preference-based measures but is only addressed in one paper in the literature review. 60 This issue is explored for the development of a condition-specific measure for common mental health problems in Chapter 3.
Stage IV was absent in most studies as the majority of papers did not validate the development of the classification system or examine the performance of the measure in their development paper. It is recommended that where possible the classification system is validated at the time of development, preferably repeating stages I–III using an independent data set (where appropriate for the methodology used in stages I–III) to test the reproducibility of the health-state classification system from the original measure, yet this was only mentioned in one paper. 30
For stage V, the valuation surveys used to elicit health-state values vary by technique, selection of whether to value health states or dimensions and levels, selection of health states, population used to elicit values and mode of administration. Ten of the 22 measures are valued by members of the general population in accordance with recommendations from agencies such as NICE6 and The Washington Panel of Cost Effectiveness63 for values used in economic evaluation. The remainder of the measures elicit values from patients, caregivers and surgeons. The majority of studies mention the condition in the valuation study, and this may affect the utility values elicited if respondents have prior experience or preconceptions of the condition, even if general population respondents are used. This issue is explored further in Chapter 4.
For stage VI, the techniques used to estimate values for all health states defined by the classification system are varied. The most commonly used technique is the use of statistical modelling in the composite approach that uses a regression function to estimate the relationship between the elicited values and the classification system. The majority of papers assume that the relationship between dimensions is additive, yet four papers assume that the relationship is multiplicative. This raises the question of whether the true relationship is additive, multiplicative or a more complex functional form across dimensions, or whether the relationship varies by factors such as classification system, condition or valuation technique. The issue of whether the relationship between dimensions is multiplicative or additive is not particular to CSPBMs and is equally relevant for generic and population-specific measures. This is an area where consensus has not been reached in the literature, but as the issue applies to all preference-based measures rather than CSPBMs per se this is beyond the scope of this project. However, the nature of the relationship also has implications for the selection of the size, composition and focus of the classification system. For example, the impact of missing or absent dimensions capturing side effects and comorbidities is affected by whether the relationship between dimensions is additive or multiplicative. This issue is explored further in Chapter 5.
The anchoring of the value set for all health states is an important issue as nine measures do not anchor on the 0–1 dead–full health scale required for QALY estimation. This means that these measures either cannot be used to generate QALYs, or must be adapted to be able to be used to generate QALYs. This begs the question of the usage of preference-based measures that are not anchored on to a 0–1 dead–full health scale, as these measures cannot be used for economic evaluation submissions for agencies such as NICE. However, these measures may have desirable properties, for example for informing clinical practice, social care or research examining patient experience. This issue is further explored in Chapter 7.
Once the measure is developed it should be validated and examined for responsiveness when applied to a patient population. Information loss may occur during the process of deriving the measure from the original condition-specific measure meaning the preference-based measure may not retain the desirable psychometric properties of the original measure. Only four papers compared the performance of the CSPBMs with a generic preference-based measure in the development paper, although the performance of the other measures may have been examined elsewhere. The performance of the CSPBMs in comparison with a generic preference-based measures and the original measure requires further research and is examined in Chapter 6.
Conclusion
This chapter presents a review of published studies producing a CSPBM. The review found 26 papers across 22 different measures published prior to January 2011. A variety of methodologies is used at each stage of the development of the measure covering the development of the classification system, the valuation survey to elicit health-state values and the extrapolation of these values to produce health-state values for all states defined by the classification system. The development of methodologies for producing a classification system is varied, yet it is clear that psychometric performance of items and dimensions is important when deriving classification systems from existing measures. The review found that many studies poorly described the methodology used to develop the CSPBMs, especially at the stage of developing the classification system. Clear reporting of methodology is important and this is something that should be taken into account for future publications in this area of research. Few papers validated the classification system either in terms of replicating the analysis used to derive the classification system or examining the performance of the preference-based measure in an independent data set. Testing of the classification system and final preference-based measure is important and will be explored further in Chapter 6. Further research examining best practice and providing recommendations for the development of CSPBMs is examined in the remaining chapters as outlined in Chapter 1.
Chapter 3 Developing a methodology for deriving measures with a unidimensional component: the Rasch vignette approach
Introduction
Chapter 1 provided an overview of the six stages to developing a CSPBM and referred to a number of examples where it had been successfully applied. Stages I–VI concerned the derivation of a health-state classification system that, in most cases, generates too many states for them all to be valued (e.g. AQL-5D generates 3125 states). Stage V involves the valuation of a sample of these states and then stage VI models the health-state valuations in order to value all states defined by the classification system. A crucial component of stage V is the generation of states for valuation.
The designs used to generate samples of states, such as an orthogonal design for statistical modelling and states required for applying multi-attribute utility theory, often include combinations of dimension levels that would not be credible (e.g. feeling downhearted and low and happy most of the time). The unusual combinations are required in order to model independent preference weights for each dimension, and although it is possible to ‘back off’ from more extreme clashes, as was done for HUI2 (Torrance et al. 39), this can undermine the model. This undermining may happen because the unusual combinations undermine the method that generated these states for valuation, or because the model still predicts values for unusual states, questioning the credibility of the whole value set. This problem arises to some extent with generic classification systems, such as SF-6D and EQ-5D, but is more likely to arise with CSPBMs as they tend to define a set of closely related domains. Indeed, in many cases, the domains may be found to be tapping the same underlying construct according to Rasch analysis. One solution would be to select just one item from the measure, but this would reduce reliability and lose important nuances in the impact of the condition. A condition may impact on a number of related areas of life, and a richer picture is provided by using more than one item.
The problem of highly correlated items was found in the development of a CSPBM from a measure of common mental heatlth problems: the CORE-OM. The CORE-OM is a valid and reliable measure of psychological health. All of the items in the CORE-OM provide a description of the impact of common mental health problems on the lives of patients and these are all psychological apart from one physical health item. Although items are grouped into domains reflecting different aspects of psychological health, Rasch analysis found that they were unidimensional. This provided the basis for exploring a new approach that uses the results of the Rasch to generate the sample of states for valuation. This ‘Rasch-based vignette approach’ generates credible and feasible states based on the natural occurrence of states in the data set and avoids infeasible combinations generated by statistical designs. For stage VI, the approach reported here uses the novel solution of estimating the relationship between the latent Rasch logit score of each state and corresponding utility value from the valuation survey. This permits the estimation of preference values for other points on the Rasch logit scale and hence other states generated by the items. This approach has been used for the development of two other preference-based measures, one for flushing symptoms60 and the other for vision. 67
This chapter presents an overview of the six stages to derive the CORE-6D preference-based measure from the CORE-OM, focusing on the methodological contribution of this approach through its application of Rasch analysis to select health states for valuation and further to produce utility values for all states (i.e. stages V and VI). First, the methods and results for stages I–IV obtaining the classification system are presented and, second, the methods and results of stages V and VI obtaining utility values for all health states are presented. Further details of the derivation of the classification system and preference weights are available elsewhere. 68,69
Methods for stages I–IV: classification system
Clinical Outcomes in Routine Evaluation – Outcome Measure
The CORE-OM has been developed to assess the outcomes of psychological interventions for people with common mental health problems. 70,71 It has 34 self-report items covering the domains of subjective well-being, symptoms, function and risk, outlined in Table 6. Each item has five levels (‘not at all’ through to ‘most or all of the time’). It has been shown to be reliable, valid and sensitive to change in clinical samples. 72 It has become one of the most widely used mental health outcome measures for psychological services in the NHS and is being used in a number of clinical trials as well as in Service Development and Organisation (SDO) programme evaluations of service delivery. However the CORE-OM cannot be currently used to produce QALYs as its scoring system is not preference based. The number of items in the CORE-OM also means that it is not amenable to health-state valuation in its current form.
Domain | Item no. | Item | |
---|---|---|---|
Subjective well-being | 4 | I have felt OK about myself | |
14 | I have felt like crying | ||
17 | I have felt overwhelmed by my problems | ||
31 | I have felt optimistic about my future | ||
Symptoms | Anxiety | 2 | I have felt tense, anxious or nervous |
11 | Tension/anxiety have prevented me doing important things | ||
15 | I have felt panic or terror | ||
20 | My problems have been impossible to put to one side | ||
Depression | 5 | I have felt totally lacking in energy and enthusiasm | |
23 | I have felt despairing or hopeless | ||
27 | I have felt unhappy | ||
30 | I have thought I am to blame for my problems and difficulties | ||
Physical | 8 | I have been troubled by aches, pains and physical problems | |
18 | I have had difficulty getting to sleep or staying asleep | ||
Trauma | 13 | I have been disturbed by unwanted thoughts and feelings | |
28 | Unwanted images or memories have been distressing me | ||
Functioning | General | 7 | I have felt able to cope when things go wrong |
12 | I have been happy with the things I have done | ||
21 | I have been able to do most things I needed to | ||
32 | I have achieved the things I wanted to | ||
Close relationships | 1 | I have felt terribly alone and isolated | |
3 | I have felt I have somebody to turn to for support when needed | ||
19 | I have felt warmth or affection for someone | ||
26 | I have thought I have no friends | ||
Social relationships | 10 | Talking to people has felt too much for me | |
25 | I have felt criticised by other people | ||
29 | I have been irritable when with other people | ||
33 | I have felt humiliated or shamed by other people | ||
Risk | Harm to self | 9 | I have thought of hurting myself |
16 | I made plans to end my life | ||
24 | I have thought it would be better if I were dead | ||
34 | I have hurt myself physically or taken risks with my health | ||
Harm to others | 6 | I have been physically violent to others | |
22 | I have threatened or intimidated another person |
Developing the health-state classification
Clinical Outcomes in Routine Evaluation - Outcome Measure: patient data set
The database used to derive the classification system contained data from 33 NHS primary care services in the UK (see Evans et al. 72 for further details). The data set contained 1500 primary care clients, and this was used for the conventional psychometric analysis. For the Rasch analysis, a random subsample of 400 respondents was used, as there is evidence that some Rasch fit statistics for polytomous scales such as the CORE-OM are dependent on sample size and larger samples can have a higher chance of type 1 errors. 73 The Rasch results were validated on an additional random subsample of 400 respondents.
The methodology for deriving the health-state classification from the CORE-OM uses a combination of classical psychometric and Rasch analysis. Rasch Unidimensional Measurement Models (RUMM2020; RUMM Laboratory Pty Ltd, Duncraig, Western Australia, 1997–2004) were used for the Rasch analysis and psychometric and statistical analysis was undertaken in SPSS 11.5 (SPSS Inc., Chicago, IL, USA).
Stage I: establishing dimensionality
The unidimensionality of the CORE-OM was examined using Rasch analysis using a partial-credit model.
Stages II and III: item exclusion and selection and exploration of item-level reduction per dimension
The following Rasch analysis criteria was used to exclude the following items: poor goodness of fit when items were ordered (measured using overall and item fit statistics, where items were excluded if fit residuals were > 2.5 or < –2.5 and/or chi-squared statistics were significant at the 1% level after Bonferroni adjustment); and DIF according to age, gender or ethnicity, as this indicates that items have different characteristics across populations. Items were excluded one at a time, on the basis that the poorest performing item (using criteria and expert opinion) was excluded first and the model re-estimated.
Following the exclusion of items meeting the criteria outlined above, the Rasch model was re-estimated. Additional criteria summarised below were applied to the remaining items to inform further item selection in order to construct a concise final measure based on the best-performing items. The aim was to construct a classification with the following properties: parsimonious and containing items representing the conceptual domains of CORE-OM with maximum of one item per domain; best possible model fit reported by the model statistics as this indicates unidimensionality; identical response levels for all items; response levels increasing in severity have higher Rasch logit scores; health state coverage across the full range of severity observed in the sample. An additional test proposed by Smith74 and recommended in the Rasch literature75,76 was used to confirm the unidimensionality of the scale, by examining the item fit residuals to determine whether or not there is more than one residual factor. Testing of a range of combinations of items was explored alongside a reduction in item levels.
Conventional psychometric criteria were also used to inform item exclusion and selection: percentage of missing data; correlation of item to dimension score using Spearman’s non-parametric ρ-values; and responsiveness to treatment measured using standardised response mean which is calculated using the mean change in item score before and after treatment divided by the standard deviation (SD) of the change score.
Although Rasch analysis was undertaken with the intention to develop a unidimensional scale capturing emotional aspects of HRQoL, CORE-OM also includes a domain with items covering physical aspects of health, which was considered to be essential for inclusion in the classification system capturing both physical and mental health problems. Thus, the classification system was expected to consist of a unidimensional emotional component, plus a physical health dimension (represented by one item) that is independent and not highly correlated to the unidimensional component.
Stage IV: validation of classification system
The classification system was validated using a random subsample of 400 respondents, examining overall and item fit statistics, DIF, unidimensionality and item–response combinations.
Results for stages I–IV: classification system
Health-state classification
Stages I–III: dimensions, items and item response levels
The results are summarised here and further details can be found elsewhere. 68 Out of the 34 CORE-OM items, 26 had disordered item thresholds, meaning that adjacent item levels were merged to obtain threshold ordering for these items. Two items (6. ‘I have been violent to others’ and 22. ‘I have threatened or intimidated another person’) were excluded from the analysis as they were judged irrelevant for a preference-based measure of HRQoL, and another item (34. ‘I have hurt myself physically or taken risks with my health’) was excluded as it was judged as being ambiguous. The application of the Rasch criteria and psychometric analysis led to the exclusion of a further 14 items (3, 5, 8, 9, 14, 18, 19, 23, 24, 27–31).
The remaining 17 items represent the items available for selection for the unidimensional emotional component of the classification system. Table 7 details the performance of these items using Rasch statistics and psychometric analysis. Following this model, items were excluded one at a time and the model was re-estimated for various combinations of five items, one from each of the remaining five conceptual domains (symptoms – anxiety; functioning – general; functioning – close relationships; functioning – social relationships; risk/harm to self) that were considered major domains for people with common mental health problems and thus requiring representation in the final classification system. The final set of items selected to represent the emotional component were items 1, 15, 16, 21 and 33, each with three-item response levels (not at all; only occasionally or sometimes; often, most or all the time). The results of the additional test proposed by Smith74 confirmed the unidimensionality of the emotional component. Figure 2 presents the item threshold map for the Rasch model estimated on the selected emotional component and is discussed further below.
Item | Rasch analysis | Psychometric analysis | ||||
---|---|---|---|---|---|---|
Residual | χ2 | p-value | Standardised response mean | Missing data | Spearman’s p-value | |
1. I have felt terribly alone and isolated | 1.415 | 10.118 | 0.072 | 0.99 | 0.4 | 0.714 |
2. I have felt tense, anxious or nervous | –0.373 | 2.658 | 0.752 | 1.18 | 0.3 | 0.603 |
4. I have felt OK about myself | –0.107 | 2.326 | 0.802 | 1.00 | 0.6 | 0.646 |
7. I have felt able to cope when things go wrong | 0.371 | 5.829 | 0.323 | 0.78 | 0.6 | 0.594 |
10. Talking to people has felt too much for me | 0.546 | 4.614 | 0.465 | 0.81 | 0.7 | 0.548 |
11. Tension/anxiety have prevented me doing important things | –0.191 | 6.021 | 0.304 | 0.89 | 0.8 | 0.642 |
12. I have been happy with the things I have done | 0.708 | 1.848 | 0.870 | 0.85 | 0.8 | 0.624 |
13. I have been disturbed by unwanted thoughts and feelings | 2.376 | 10.195 | 0.070 | 0.95 | 0.5 | 0.564 |
15. I have felt panic or terror | 0.133 | 5.590 | 0.348 | 0.84 | 0.4 | 0.576 |
16. I made plans to end my life | –0.485 | 4.897 | 0.428 | 0.29 | 1.0 | 0.436 |
17. I have felt overwhelmed by my problems | –2.084 | 11.369 | 0.045 | 1.09 | 1.0 | 0.744 |
20. My problems have been impossible to put to one side | 0.254 | 1.877 | 0.866 | 1.04 | 0.9 | 0.629 |
21. I have been able to do most things I needed to | 1.424 | 3.410 | 0.637 | 0.69 | 0.8 | 0.568 |
25. I have felt criticised by other people | 0.918 | 3.362 | 0.644 | 0.70 | 0.8 | 0.558 |
26. I have thought I have no friends | 0.742 | 8.993 | 0.110 | 0.65 | 0.9 | 0.595 |
32. I have achieved the things I wanted to | 0.799 | 1.426 | 0.921 | 0.86 | 1.5 | 0.590 |
33. I have felt humiliated or shamed by other people | –0.899 | 7.809 | 0.167 | 0.61 | 1.1 | 0.557 |
FIGURE 2.
Rasch item-threshold map of the emotional component of CORE-6D. Severity levels: 0, not at all; 1, only occasionally or sometimes; 2, often, most or all the time – with the exception that the response levels are reversed for the positively worded item ‘I have been able to do most things I needed to’. Source: Mavranezouli I, Brazier J, Young A, Barkham M. Using Rasch analysis to form plausible health states amenable to valuation: the development of the CORE-6D from a measure of common mental health problems (CORE-OM). Qual Life Res 2011;20:321–33.

Item 8 (‘I have been troubled by aches, pains, physical problems’) was excluded from the emotional component, as its fitting was poor, as expected, as it represents physical health rather than mental health. This item was considered important for inclusion in the classification system, as physical symptoms constitute an important dimension in their own right. Item 8 therefore was combined with the items selected for the emotional component to form an additional physical health dimension.
Health-state classification
Table 8 presents the classification system in which a health state is made up of six sentences and hence has a six-digit identifier, from best state 000000 to worst state 222222. This system generates a total of 729 health states, although it is likely that many of these health states are not plausible owing to the unidimensionality of the emotional component.
Item | Level | |
---|---|---|
Emotional component | ||
1 |
I never feel terribly alone and isolated I feel terribly alone and isolated only occasionally or sometimes I feel terribly alone and isolated often, most or all the time |
0 1 2 |
2 |
I never feel panic or terror I feel panic or terror only occasionally or sometimes I feel panic or terror often, most or all the time |
0 1 2 |
3 |
I never feel humiliated or shamed by other people I feel humiliated or shamed by other people only occasionally or sometimes I feel humiliated or shamed by other people often, most or all the time |
0 1 2 |
4 |
I am able to do most things I need to often, most or all the time I am able to do most things I need to only occasionally or sometimes I am not able to do the things I need to |
0 1 2 |
5 |
I never make plans to end my life I make plans to end my life only occasionally or sometimes I make plans to end my life often, most or all the time |
0 1 2 |
Physical health | ||
6 |
I am never troubled by aches, pains, physical problems I am troubled by aches, pains, physical problems only occasionally or sometimes I am troubled by aches, pains, physical problems often, most or all the time |
0 1 2 |
Stage IV: validation
The classification system was confirmed in validation analysis using another random sample of 400 patients. The Rasch model for the selected items had satisfactory model fit, item-fit and no DIF was observed.
Methods for stages V and VI: valuation
Stage V: health-state selection and valuation study
Health-state selection
Rasch analysis was used to select health states for the unidimensional emotional component of the classification system. Health states were selected using the item threshold map for the unidimensional emotional component of the classification system, termed the ‘Rasch vignette approach’. When all items are ordered the item threshold map shows the most likely item-response combinations, ‘health states’, across the Rasch logit scale that increases as symptom severity increases. This was used to select frequently observed, plausible health states amenable to valuation, as it identifies the most likely combinations of item responses expected for a range of locations across the latent Rasch logit scale capturing underlying health severity. This approach produced health states experienced by the study population across the full range of symptoms for the emotional component, rather than, say, simply health states that are most commonly appearing, as these would not represent the full severity range. Health states selected using the Rasch vignette approach, the ‘emotional states’, were combined with different response levels of the physical health dimension for use in the valuation survey to obtain the full health state. These health states were selected to ensure the emotional component could be mapped onto the Rasch logit score in stage VI and that the additional decrement of the physical health dimension could be estimated.
Valuation study
A valuation study was conducted using face-to-face interviews on a sample of the UK general population. Households were sampled using the AFD Names & Numbers version 3.1.25 database (AFD Software Ltd, Ramsey, UK) and balanced to the UK general population using geodemographic ACORN profiles. All respondents were interviewed in their own homes by trained interviewers with experience working on previous valuation surveys, including the HU-I210 and OAB-5D. 11 The project was approved by the ScHARR Research Ethics Committee at the University of Sheffield.
The study was designed to value 22 plausible health states to ensure an adequate mix of states with plausible combinations using the unidimensional emotional component and physical health dimension. Health states were divided into three card blocs of eight health states, where each bloc contained one state common to all blocks plus seven unique states. Each respondent valued health states from one card bloc. The valuation of these 22 states had two aims: first, to produce mean estimates for 18 health states containing the unidimensional component and physical health dimension to enable these to be mapped onto Rasch logit values in stage VI and produce decrements for the levels of the physical health dimension; and, second, to compare mean values between states with and without the physical health dimension using simple t-tests to determine the impact of removing the physical health dimension (results analysed in Chapter 5).
At the start of the interview respondents self-completed the EQ-5D and the classification system derived from the CORE-OM for their own health. This was to familiarise respondents with the idea of describing states, as well as with the items and response levels of the classification system. Respondents then ranked four health states alongside full health and dead and valued these states using the Measurement and Valuation of Health (MVH) group version of TTO using ‘full health’ as the upper anchor and including the visual prop designed by the MVH group (University of York). 41,77 Respondents then repeated the ranking and TTO tasks using another four health states.
The separation of the rank and TTO exercises into two halves was chosen as one of the card blocs consisted of four states describing health states containing only the unidimensional emotional component and four states describing the same health states plus the independent physical health dimension. For this card bloc the four states containing only the unidimensional component were valued in the first rank and TTO tasks, and the four states containing full CORE-6D states were valued in the second ranking and TTO tasks. Responses to this card bloc were used to inform the research outlined in Chapter 5 exploring the impact of the inclusion of an additional dimension on the elicited preferences of the other dimensions. Prior to the first TTO exercise, respondents valued an additional practice health state using TTO to familiarise themselves with the exercise. Finally respondents self-completed questions covering their health, sociodemographic characteristics and how difficult they found the valuation tasks. All respondents were strongly recommended to seek appropriate professional support if the interview raised personal issues for them, both in the participant information sheet provided before the interview and in a thank-you note handed out at the end of the interview.
Respondents were excluded from the following analysis on the TTO data if: all states were valued as identical and less than one; the worst possible health state was valued higher than every other state; or all states were valued as worse than dead. Responses to the four health states containing only the unidimensional emotional component were excluded from all analyses reported in this chapter, as these values do not represent the full classification system, and are analysed in Chapter 5.
Stage VI: modelling to produce preference weights for all states
This methodology builds on the approach undertaken for a unidimensional measure where health-state utility values for all health states defined by a classification system were produced using the relationship between Rasch model logit values and mean observed TTO values for a sample of health states. 78 This study develops the new approach further, as it contains both a unidimensional component and an additional independent dimension. The preference weights for the emotional component were estimated using the methodology outlined in Young et al. 62 and the preference weights for the additional physical health dimension were estimated using dummy variables following the standard approach outlined in Chapter 1 (e.g. Brazier et al. ,2 Yang et al. 30 and Dolan42).
Regression analysis was used to estimate the relationship between the elicited TTO values for each health state and the Rasch model logit value corresponding to the emotional component of the state (the Rasch health state) and the response level of the physical health dimension. The standard linear model specification was:
where y represents mean TTO value for state i, R represents the rescaled Rasch logit value (linearly rescaled to match the range of y), P is a dummy variable for response level j of the physical health dimension and ε is the error term. The inclusion of quadratic and cubic terms for the rescaled Rasch logit value was explored. Estimation was via ordinary least squares.
Model fit was assessed using R2 and RMSE. The model with the best fit was selected and used to predict health-state utility values for all health states described by the classification system using their respective Rasch model logit value and the response level of the physical health dimension.
Results for stages V and VI
Stage V: selected health states and valuation study
Health states
The item threshold map of the emotional component of CORE-6D is shown in Figure 2. The lowest Rasch logit scores are associated with the highest underlying trait (i.e. good) of HRQoL. The three response levels have been shaded in order of severity. Rasch health states for the emotional component are read from the map using the combinations of levels for each item observed across the Rasch logit scale. For example, at –3 on the Rasch logit scale the frequently observed plausible health state is 00000, and as we move towards –2.5 on the Rasch logit scale this state changes to 10000. Eleven health states are observed on this continuum, covering 37% of response combinations observed in the study sample (after excluding cases with one or more responses missing). In comparison, health states for the emotional component selected for valuation using an orthogonal array cover only 7% of responses in the same sample. The 11 emotional health states combine with the three response levels of the physical health item to produce a two-dimensional set of 11 × 3 = 33 plausible health states.
Table 9 shows that emotional health state 10 (22221) was not observed in the study sample and was therefore not selected for valuation. All remaining 10 emotional health states were combined with the physical health item at response level zero (never troubled by physical problems) for valuation. To assess the impact of physical health on utility values, four emotional health states (best state 00000, worst state 22222 and two intermediate states 11000 and 22110) were also combined with response levels 1 and 2 of the physical health item. These four emotional states were selected first to cover the full severity range captured by CORE-6D using their Rasch logit value from the item-threshold map in Figure 2 and second to represent frequently observed states in the study sample as shown in Table 9. In total, 18 plausible CORE-6D health states were selected for the valuation survey, plus four emotional health states with no reference to the physical health item (analysed in Chapter 5).
Item | Health state | ||||||||||
---|---|---|---|---|---|---|---|---|---|---|---|
1 | 2 | 3 | 4 | 5 | 6 | 7 | 8 | 9 | 10 | 11 | |
I have felt terribly alone and isolated | 0 | 1 | 1 | 1 | 1 | 2 | 2 | 2 | 2 | 2 | 2 |
I have felt panic or terror | 0 | 0 | 1 | 1 | 1 | 1 | 2 | 2 | 2 | 2 | 2 |
I have felt humiliated or shamed by other people | 0 | 0 | 0 | 1 | 1 | 1 | 1 | 2 | 2 | 2 | 2 |
I have been able to do most things I needed to | 0 | 0 | 0 | 0 | 1 | 1 | 1 | 1 | 1 | 2 | 2 |
I made plans to end my life | 0 | 0 | 0 | 0 | 0 | 0 | 0 | 0 | 1 | 1 | 2 |
Frequency of each health state in the study sample (%) | 5.3 | 5.9 | 6.2 | 5.0 | 5.6 | 2.7 | 2.7 | 1.5 | 1.5 | 0.0 | 0.6 |
Valuation study
The sample contained responses from 225 respondents from South Yorkshire. All responses were included in the analysis as no respondents met the exclusion criteria. The response rate was 45.7% of respondents answering their door at the time of the interview, with a TTO completion rate of 99.7% across all interviews. Table 10 compares the valuation sample with the general population in South Yorkshire and England. Overall the study sample had a higher average age and a higher proportion of females, home owners and retired individuals and a lower proportion of employed/self-employed individuals.
Characteristics | Respondents (n = 225) | South Yorkshirea | Englanda |
---|---|---|---|
Mean age (SD) (years) | 48.86 (17.16) | – | – |
Age distribution (years) | |||
18–40 | 32.7% | 41.2% | 41.6% |
41–65 | 48.0% | 39.1% | 39.1% |
> 65 | 19.3% | 19.7% | 19.3% |
Female | 58.7% | 51.2% | 51.3% |
Married/partner | 69.8% | N/A | – |
Employed or self-employed | 51.3% | 56.1% | 60.9% |
Unemployed | 3.1% | 4.1% | 3.4% |
Long-term sick | 5.4% | 7.7% | 5.3% |
Full-time student | 5.4% | 7.5% | 7.3% |
Retired | 22.3% | 14.4% | 13.5% |
Own home outright or with a mortgage | 81.0% | 64.0% | 68.7% |
Renting property | 20.0% | 36.0% | 31.3% |
Secondary school is highest level of education | 37.9% | N/A | – |
Average EQ-5D score (SD) | 0.83 (0.28) | N/A | 0.86 (0.23)b |
TTO completion rate | 99.7% | – | – |
Table 11 presents descriptive statistics for elicited utility values for each health state valued. Mean TTO values range from 0.96 for the best state (000000) to 0.10 for the worst state (222222). Increasing severity for the physical health dimension, although keeping the emotional component unchanged, leads to decreased mean utility values with higher SDs, for example moving from state 000000 to 000001. Similarly, increasing severity for the emotional component also leads to lower mean utility values with higher SDs, for example moving from 000000 to 100000. The only exception is moving from state 100000 to state 110000, where the mean TTO value increases from 0.87 to 0.88 despite the increasing severity of the emotional component. One explanation for this inconsistency is that these health states were included in different card blocs and hence were valued by different respondents.
State | n | Mean (SD) | Median | 25th percentile | 75th percentile |
---|---|---|---|---|---|
000000 | 75 | 0.96 (0.13) | 1.00 | 0.99 | 1.00 |
000001 | 75 | 0.93 (0.14) | 1.00 | 0.93 | 1.00 |
000002 | 76 | 0.82 (0.32) | 0.93 | 0.78 | 1.00 |
100000 | 74 | 0.87 (0.22) | 1.00 | 0.84 | 1.00 |
110000 | 75 | 0.88 (0.25) | 1.00 | 0.85 | 1.00 |
110001 | 76 | 0.86 (0.27) | 0.96 | 0.80 | 1.00 |
110002 | 75 | 0.74 (0.31) | 0.83 | 0.57 | 1.00 |
111000 | 74 | 0.79 (0.29) | 0.93 | 0.69 | 1.00 |
111100 | 74 | 0.76 (0.33) | 0.93 | 0.53 | 1.00 |
211100 | 75 | 0.66 (0.35) | 0.73 | 0.50 | 1.00 |
221100 | 76 | 0.57 (0.44) | 0.63 | 0.45 | 0.93 |
221101 | 74 | 0.49 (0.47) | 0.50 | 0.30 | 0.88 |
221102 | 74 | 0.40 (0.49) | 0.44 | 0.14 | 0.83 |
222100 | 74 | 0.47 (0.43) | 0.50 | 0.20 | 0.84 |
222110 | 75 | 0.38 (0.45) | 0.44 | 0.08 | 0.70 |
222220 | 225 | 0.23 (0.52) | 0.30 | 0.00 | 0.53 |
222221 | 74 | 0.21 (0.50) | 0.23 | –0.08 | 0.50 |
222222 | 75 | 0.10 (0.53) | 0.10 | –0.33 | 0.48 |
Stage VI: modelling health-state values
The results of the regression analysis are presented in Table 12. The dummy variable for the physical health dimension at level 1 was insignificant for all models; however, the dummy variable for level 2 of the physical health dimension is significant at the 5% level in all models. Across all models the coefficients for the rescaled Rasch logit score and squared and cubic terms for this score are significant at the 5% level, suggesting the appropriateness of using model 7 as the preferred model that contains all these terms. R2 and RMSE also confirm that this model performed better than the other models and was therefore used to produce estimated utility values for every state defined by the CORE-6D using the rescaled Rasch logit score for each state.
Model | α | β 1 | β 2 | β 3 | γ 1 | γ 2 | R 2 | Adjusted R2 | RMSE |
---|---|---|---|---|---|---|---|---|---|
Model 1: y = α + β1R + γ1P1 + γ2P2 | 0.008 (0.833) | 1.057 (0.000) | –0.044 (0.189) | –0.151 (0.000) | 0.968 | 0.961 | 0.0533 | ||
Model 2: y = α + β2R 2 + γ1P1 + γ2P2 | 0.302 (0.000) | 0.844 (0.000) | –0.070 (0.219) | –0.177 (0.006) | 0.906 | 0.886 | 0.0916 | ||
Model 3: y = α + β3R 3 + γ1P1 + γ2P2 | 0.416 (0.000) | 0.779 (0.000) | –0.085 (0.284) | –0.193 (0.025) | 0.813 | 0.773 | 0.1292 | ||
Model 4: y = α + β1R + γ2R 2 + γ1P1 + γ2P2 | –0.130 (0.100) | 1.585 (0.000) | –0.443 (0.056) | –0.029 (0.329) | –0.137 (0.000) | 0.976 | 0.969 | 0.0478 | |
Model 5: y = α + β1R + γ3R 3 + γ1P1 + γ2P2 | –0.108 (0.072) | 1.388 (0.000) | –0.282 (0.025) | –0.028 (0.329) | –0.135 (0.000) | 0.979 | 0.972 | 0.0452 | |
Model 6: y = α + β2R 2 + γ3R 3 + γ1P1 + γ2P2 | 0.099 (0.002) | 2.624 (0.000) | –1.758 (0.000) | –0.029 (0.170) | –0.137 (0.000) | 0.989 | 0.985 | 0.0331 | |
Model 7: y = α + β1R + γ2R2 + γ3R3 + γ1P1 + γ2P2 | 0.366 (0.004) | –1.695 (0.022) | 5.712 (0.000) | –3.446 (0.000) | –0.033 (0.069) | –0.141 (0.000) | 0.993 | 0.990 | 0.0275 |
Discussion
This chapter outlines the development of CORE-6D, a preference-based measure for common mental health problems derived from the CORE-OM. The development of this measure required a modification of the conventional approach used to derive preference-based measures from existing measures, as several of the emotional domains of the original measure were not independent. Existing techniques used to select health states for valuation require that all health states are plausible and select health states in order to enable the estimation of an additive regression model where the preference weight of each level of each dimension can be derived regardless of the level of severity of all other dimensions. Yet if dimensions are not independent some health states are implausible and the preference weighting of each level of each dimension cannot be assumed to be independent of the severity levels of the other dimensions. Several CORE-OM domains are highly correlated and are not independent, meaning that the standard approach is not appropriate.
The derivation of the CORE-6D modified stages I–IV of the six-stage approach is outlined in Chapter 1 to derive the health-state classification system, which consisted of a unidimensional emotional component and a physical health dimension. The new Rasch vignette approach was applied for stages V and VI, which were used to sample health states for valuation, value these health states and then use these values to produce utility values for all states defined by the classification system. The classification system contains 729 health states, which was too many to be amenable to and feasible for valuation. A sample of health states for the emotional component was selected using the item-threshold map produced by Rasch analysis, and these were combined with a range of responses to the physical health dimension. One potential criticism of the approach is the relatively small number of states selected for valuation, given the large number of states defined by the classification system. However an orthogonal array generated in SPSS also selects 18 states for valuation from the CORE-6D. The Rasch vignette approach offers the advantage that it has larger coverage in terms of the proportion of patients in the patient sample that were in the health states (37% compared with 7% for the orthogonal array).
Health states were valued using TTO by the general population and used to estimate mean values that were consistent with the classification system. Regression analysis was used to estimate utility values for all health states using the Rasch logit score for the emotional component and the physical health response. This stage builds on an approach previously undertaken for a unidimensional measure. 78 This study successfully adapted this approach by incorporating dummy variables for the independent physical health dimension in the regression model to account for the different severity levels of this dimension. This is a standard approach used to model utility values for multidimensional measures where all dimensions are independent. The mixed approach applied here can be used to estimate utility values for multidimensional measures that include one or more unidimensional components. The selected regression model had good predictive ability (RMSE 0.0275) and fits the data well, suggesting that the selected modelling approach is suitable for these data.
The Rasch vignette approach offers a useful approach when the original non-preference-based condition-specific measure has a unidimensional component. The advantage is that it enables utilities to be produced for a measure that has a large focus on one dimension, thus enabling precision in producing utility values for the unidimensional component. Yet the CSPBMs may face the criticism that, owing to its narrow dimensionality and focus, it may not be able to capture comorbidities and side effects of treatment. However, the CORE-6D measure may not suffer from this criticism owing to its physical health dimension that may capture both comorbidities and side effects affecting the physical health of the patient. The validity and responsiveness of the CORE-6D in comparison with CORE-OM and the generic EQ-5D and SF-6D is assessed in Chapter 6.
Conclusion
Existing techniques used to derive preference-based measures from existing measures are appropriate only if the domains captured by the existing measure and the dimensions for the new preference-based measure are independent. This chapter presented a modification of the six-stage approach outlined in Chapter 1, which can be used when some of the domains captured by the existing measure are not independent. The approach was used to derive the CORE-6D, a preference-based measure for common mental health problems derived from the CORE-OM. The classification system contains a unidimensional emotional component consisting of five emotional domains and a physical health dimension. This new Rasch vignette approach was successfully used with the Flushing Symptom Questionnaire, in which the items were tapping closely related symptoms. It has also been adopted to develop a preference-based index from the visual HRQoL measure ‘VFQ-25’,67 for which the domains are highly correlated, as they stem from a single cause. The Rasch vignette approach is a useful approach to develop CSPBMs when the content is largely unidimensional, and offers an advantage in that it utilises the qualitative depth of the original measure from using a number of items to represent the unidimensional component.
Chapter 4 The impact of labelling on health-state values
Introduction
Generic PBMs are valued by members of the general population who do not know which condition has caused the health state. This assumes that it is the health state that is important, and that the condition that caused the state or its prognosis is irrelevant. In contrast, non-preference-based condition-specific measures typically name the condition in their items. This can enable greater precision for assessing changes in quality of life due to that condition and the relevant intervention that has a significant advantage, for example for use in drug-labelling claims. As many CSPBMs are derived from non-preference-based measures, they also typically state the cause of the health problems being assessed in the classification system. Often the condition is embedded within the classification system derived from the non-preference-based measure, meaning the system cannot be valued without labelling the condition. For example, for the asthma-specific AQL-5D measure derived from the AQLQ,29,79 the mention of asthma cannot be removed without changing the meaning of the dimension ‘experience asthma symptoms as a result of air pollution’. However, the inclusion of a condition label may affect utility values elicited from the general population owing to, for example, prior preconceptions about the condition.
Consensus has not been reached in the literature regarding how condition labels in health-state descriptions impact on elicited utility values. The majority of studies examining the impact of labelling include more than one condition label and find that the results differ according to the specific condition. Five studies have found that the inclusion of condition labels has lowered health-state values,80–84 for at least one condition label. For example, one found lower health-state values associated with the explicit use of mental health labels including mental handicap, schizophrenia and dementia. 82 Another study found that labelling breast cancer states reduced health-state values,83 yet another two studies found that it did not affect values80,82 with the exception of scenarios written in the third person. 80 The finding that cancer labels have no impact in two out of three studies is surprising given that many people have mostly negative prior knowledge and preconceptions of cancer. For example, cancer treatments can have severe treatment side effects with low quality of life and cancer is widely known as one of the world’s largest killers, especially in developed countries. However, the impact of condition labels may depend on how the label appears and the framing of the question, for example whether the condition is mentioned in each dimension or just once for each health state, which can vary across studies.
This literature is limited in a number of ways. Typically, the studies have small sample size (e.g. the Robinson and Bryan83 study has 26 respondents, and Rabin et al. 82 has 42 respondents) meaning it is difficult to test statistical significance, and ask respondents to value states using a large number of different condition labels (e.g. in the Robinson and Bryan83 study one group value states across nine conditions and the other control group value states with no condition label). All studies ask respondents to value states with changing descriptions (owing to framing or labelling) and assess whether values change accordingly. This within-subject study design means that there may be a focusing effect, whereby changing the condition label means respondents give it more attention. This may cause respondents to purposefully consider their prior knowledge and preconceptions of the condition and change their values accordingly. Furthermore, the health states presented do not cover a wide range of severity meaning that the results are specific to the small number of states with small severity range that were valued. To address these limitations we undertook a between-subject study comparing health-state values from samples of respondents who valued a range of health states of differing severity with only one or no condition label. The study design and findings are summarised here (for further details see Rowen et al. 85).
This chapter assesses how the inclusion of medical condition labels in health-state descriptions impacts on utility values elicited from members of the general population. We undertook a valuation study where respondents valued health states featuring only one of three different labels: no label, irritable bowel syndrome (IBS) label and cancer label. Each respondent valued identical health-state descriptions with the exception of the condition label. The analysis explores whether health-state severity, respondent sociodemographic characteristics and prior experience of the relevant condition impact on elicited utility values.
Methods
Health-state description
Health-state descriptions were generated using the classification system from EORTC-8D, a preference-based measure for cancer derived from the EORTC QLQ-C30. 34 Box 1 presents the EORTC-8D classification. There are eight dimensions each with four or five severity levels: physical functioning, role functioning, pain, emotional functioning, social functioning, fatigue and sleep disturbance, nausea, and constipation and diarrhoea. The classification system defines a total of 81,920 health states. The original valuation study used to elicit preference weights for all states defined by the classification system did not include a condition label34 and the meaning of dimensions remains unchanged if labels are added or removed from the classification system. We chose condition labels that respondents could reasonably perceive accounted for EORTC-8D health states. We chose cancer and IBS as the condition labels, as the measure is designed for cancer, and consultation with several clinicians and doctors showed that the EORTC-8D classification system would accurately describe IBS. Advantages of having cancer and IBS as the condition labels are that experience and preconceptions of these conditions is likely to differ, and whereas cancer can be terminal IBS is non-fatal.
You had no trouble taking a long walk
You had a little trouble taking a long walk
You had quite a bit of trouble taking a long walk
You had very much trouble taking a long walk
You had very much trouble taking a short walk outside of the house
Role functioningYou were not limited in pursuing your hobbies or other leisure-time activities
You were limited a little in pursuing your hobbies or other leisure-time activities
You were limited quite a bit in pursuing your hobbies or other leisure-time activities
You were limited very much in pursuing your hobbies or other leisure-time activities
Social functioningYour physical condition or medical treatment did not interfere with your social activities
Your physical condition or medical treatment interfered a little with your social activities
Your physical condition or medical treatment interfered quite a bit with your social activities
Your physical condition or medical treatment interfered very much with your social activities
PainPain did not interfere with your daily activities
Pain interfered a little with your daily activities
Pain interfered quite a bit with your daily activities
Pain interfered very much with your daily activities
Emotional functioningYou did not feel depressed
You felt a little depressed
You felt quite a bit depressed
You felt depressed very much
Fatigue and sleep disturbanceYou were not tired
You were a little tired
You were quite a bit tired
You were tired very much
Constipation and diarrhoeaYou were not constipated and did not have diarrhoea
You were constipated and/or had diarrhoea a little
You were constipated and/or had diarrhoea quite a bit
You were constipated and/or had diarrhoea very much
NauseaYou did not feel nauseated
You felt a little nauseated
You felt nauseated quite a bit
You felt nauseated very much
Valuation survey
A valuation study was conducted where members of the UK general population each valued eight health states from EORTC-8D using TTO. The sample was divided into three groups: no label, IBS label and cancer label. All respondents valued the same health states, differing for each group only by the condition label used at the heading of the health state. The original valuation study asked each respondent to value eight health states, and hence eight health states were also selected in this study to be valued by each respondent and therefore by each group. Sample size was chosen to ensure sufficient power for comparison of mean health-state values across the three groups using simple t-tests. This required a total of 219 completed interviews containing 73 health-state values per state per group, assuming a power of 0.8, significance level of 0.05, SD of 0.3 and an expected difference of 0.1.
The sampling strategy used two steps to ensure that the sociodemographic characteristics of each group were the same and were representative of the UK general population. First, households were sampled using the AFD Names & Numbers database, and, using geodemographic ACORN profiles, the sample was balanced to the UK general population. Second, every unique postcode in the sample was divided across the three labelling groups to produce separate samples for each group. Respondents were interviewed in their own home by trained interviewers who had worked on previous valuation surveys including the HU1-286 and OAB-5D. 30 The project was approved by the ScHARR Research Ethics Committee at the University of Sheffield.
All interviews in the no-label group were conducted before respondents from the IBS-label group were contacted and, subsequently, all interviews in the IBS-label group were conducted before respondents from the cancer-label group were contacted. Using this design meant that respondents in the cancer and IBS-label groups could be informed of the relevant condition in the cover letter sent requesting their participation and in the participant information sheet. This design was selected owing to ethical concerns that respondents should be informed of the condition that would feature in their interview but should be unaware of the other conditions featured in this study in case this affects responses.
Selection of health states
Health states were selected to represent a range of health states for the EORTC-8D, using the results of the original valuation study to inform the selection. The original valuation study valued 85 health states across 12 ‘card blocs’, each containing the worst state plus seven other states. The best card bloc was selected for this study using: minimum prediction error per card bloc, largest range of mean TTO distribution per card bloc, smallest missing data per card bloc, and general ‘feasibility’ of states. Box 2 presents an example health state (24432411) for each label group. Level 1 represents no problems in that dimension, whereas level 4 (level 5 for physical functioning) is the most severe level for each dimension.
You have a little trouble taking a long walk
You are limited very much in pursuing your hobbies or other leisure time activities
Your physical condition or medical treatment interferes very much with your social activities
Pain interferes quite a bit with your daily activities
You feel depressed a little
You are tired very much
You are not constipated and do not have diarrhoea
You do not feel nauseated
Owing to IBSYou have a little trouble taking a long walk
You are limited very much in pursuing your hobbies or other leisure time activities
Your physical condition or medical treatment interferes very much with your social activities
Pain interferes quite a bit with your daily activities
You feel depressed a little
You are tired very much
You are not constipated and do not have diarrhoea
You do not feel nauseated
Owing to having cancerYou have a little trouble taking a long walk
You are limited very much in pursuing your hobbies or other leisure time activities
Your physical condition or medical treatment interferes very much with your social activities
Pain interferes quite a bit with your daily activities
You feel depressed a little
You are tired very much
You are not constipated and do not have diarrhoea
You do not feel nauseated
For health-state 11111111 the condition heading was altered to ‘despite having cancer/IBS’.
The interview began with respondents self-completing the EQ-5D. Respondents in the cancer and IBS-label groups were then shown an information sheet about the relevant condition (see Rowen et al. 85). Respondents completed the EORTC-8D classification system for themselves if they had the condition, or otherwise completed the classification for someone they knew/imagining someone with the condition to ensure that respondents were familiar with the system. Respondents in the no-label group self-completed the EORTC-8D for themselves. Respondents undertook a ranking exercise of the eight health states alongside ‘full health’ and ‘dead’, then valued these eight states using the MVH study version of TTO including a visual prop designed by the MVH Group (University of York)42,77 using ‘full health’ as the upper anchor. Respondents valued an additional practice state (22332322) before valuing the eight ranked health states to familiarise them with the TTO task. Finally, respondents self-completed questions covering sociodemographics, health service usage and experience of the labelled condition (where applicable).
Respondents were excluded from the TTO analysis if they valued all states as identical and less than one, valued the worst state higher than every other state or valued all states as worse than dead.
Analysis
Factorial analysis of variance (ANOVA) was estimated using a generalised linear model to determine significant differences in respondent characteristics across label groups. Simple t-tests were used to compare mean health-state values across the three label groups. The impact on elicited utility values from the inclusion of each condition label in the health-state description is analysed using regression analysis. The model specification is:
where i represents individual respondents and j represents the eight health states. The dependent variable, y, represents the TTO utility value, x represents the vector of dummies for the health states, q represents the vector of dummies to capture labelling effects, r represents the vector of interaction terms to capture labelling and severity effects, z represents the vector of sociodemographic characteristics including experience with the labelled condition, and εij represents the error term. Random effects generalised-least-squares (GLS) models were used, as they are appropriate for the structure of these data for which all respondents have multiple observations87 and have been used in similar valuation surveys (see, for example, Brazier et al. 2 and Brazier and Roberts3). Stata version 9 (StataCorp LP, College Station, TX, USA) was used for all regression analysis and SPSS version 15 was used for the descriptive statistical analysis.
The data
The sample contains responses from 241 respondents from northern England, with a response rate of 39% answering their door at time of interview, and completion rate of 99% across all TTO tasks. The response rate for the cancer-label group was 38%, whereas the response rate for the no-label and IBS-label groups was larger at 40%. No respondents met the exclusion criteria. The sample is largely comparable with the population of South Yorkshire and England (see Rowen et al. 85 for further details). Sample characteristics for each label group and comparison of the characteristics using ANOVA are shown in below (see Table 13). Respondent characteristics are significantly different at the 5% level for respondents aged 18–40 years and full-time students. These differences should be taken into account when modelling the data. IBS and cancer-label groups have different proportions of respondents with experience of the relevant condition both in their family and in caring for others.
Health state | Original study85 (n = 344), modelled utility value | No label (n = 81), mean (SD) | IBS label (n = 79–80)a, mean (SD) | Cancer label (n = 79–80)a, mean (SD) |
---|---|---|---|---|
11111111 | 1 | 0.96 (0.13) | 0.99 (0.06) | 0.96 (0.12) |
31212241 | 0.75 | 0.74 (0.32) | 0.81 (0.23) | 0.80 (0.22) |
13423411 | 0.72 | 0.67 (0.30) | 0.71 (0.37) | 0.64 (0.36) |
44321321 | 0.65 | 0.66 (0.35) | 0.68 (0.37) | 0.56 (0.50) |
23141224 | 0.64 | 0.63 (0.36) | 0.69 (0.36) | 0.57 (0.45) |
24432411 | 0.64 | 0.66 (0.33) | 0.65 (0.40) | 0.54 (0.44) |
51224434 | 0.51 | 0.49 (0.41) | 0.53 (0.42) | 0.41 (0.49) |
54444444 | 0.29 | 0.20 (0.49) | 0.17 (0.49) | –0.03 (0.50) |
Results
Descriptive statistics
Table 13 presents descriptive statistics of health-state values elicited for each label group and the modelled utility values estimated using regression analysis in the original valuation study. For six of the eight health states the mean value is highest for the IBS label; for six of eight states the mean value is lowest for the cancer label, and these are for the more severe health states. Health-state values for the cancer-label group were significantly different from health-state values for both the no-label group (t-test p-value = 0.01) and the IBS-label group (p-value < 0.001) but there were no significant differences between the no-label groups and IBS-label groups (p-value = 0.28).
Regression analysis
Regression analysis examining the relationship between elicited health-state utility values, health-state descriptions, condition labels and sociodemographic characteristics of respondents is presented in Table 14. Model (1) includes as predictor variables state level dummy variables, label dummy variables and sociodemographic variables; model (2), in addition, includes experience of the labelled condition; model (3) adds to model (1) interaction terms to reflect the interaction between the specific health-state and labelled condition; and model (4) adds to model (1) both interaction terms and experience of the labelled condition. Models using a range of sociodemographic and experience variables as predictors were estimated and the best models (using diagnostics, correlations and proportion of significant coefficients) are presented here. Only two sociodemographic variables are significant: where students have lower utility values and unemployed respondents have higher utility values than the employed/self-employed reference group. Goodness-of-fit measures report that models (3) and (4), which include interaction effects for cancer states, perform better than models (1) and (2), in which only experience variables and a simple additive labelling variable are included.
Variable | Model | |||
---|---|---|---|---|
(1) | (2) | (3) | (4) | |
State | ||||
31212241 | –0.187*** | –0.187*** | –0.197*** | –0.197*** |
13423411 | –0.297*** | –0.297*** | –0.284*** | –0.284*** |
44321321 | –0.340*** | –0.340*** | –0.304*** | –0.304*** |
23141224 | –0.343*** | –0.343*** | –0.313*** | –0.313*** |
24432411 | –0.354*** | –0.354*** | –0.317*** | –0.317*** |
51224434 | –0.489*** | –0.489*** | –0.456*** | –0.456*** |
54444444 | –0.856*** | –0.856*** | –0.785*** | –0.785*** |
Labelling | ||||
IBS | 0.009 | 0.009 | 0.009 | 0.008 |
Cancer | –0.088** | –0.118** | ||
Experience of labelled condition | ||||
Cancer in themselves | –0.156* | –0.157* | ||
Cancer in caring for others | 0.134** | 0.134** | ||
IBS in themselves | –0.036 | –0.036 | ||
IBS in caring for others | 0.064 | 0.064 | ||
Cancer interaction terms | ||||
11111111 × cancer | –0.011 | –0.041 | ||
31212241 × cancer | 0.022 | –0.007 | ||
13423411 × cancer | –0.050 | –0.079 | ||
44321321 × cancer | –0.118** | –0.147** | ||
23141224 × cancer | –0.099* | –0.128** | ||
24432411 × cancer | –0.119** | –0.148** | ||
51224434 × cancer | –0.107** | –0.136** | ||
54444444 × cancer | –0.224*** | –0.254*** | ||
Sociodemographics | ||||
Female | 0.012 | 0.008 | 0.012 | 0.008 |
Married | 0.046 | 0.046 | 0.046 | 0.046 |
Retired | –0.020 | –0.010 | –0.020 | –0.010 |
Unemployed | 0.131 | 0.160** | 0.131 | 0.160** |
Student | –0.270*** | –0.268*** | –0.271*** | –0.269*** |
Housework | 0.001 | 0.009 | 0.001 | 0.009 |
Long-term sick | 0.079 | 0.107 | 0.079 | 0.107 |
Secondary school is highest level of education | –0.055 | –0.051 | –0.055 | –0.051 |
Constant | 1.000*** | 0.993*** | 0.974*** | 0.967*** |
Observations | 1910 | 1910 | 1910 | 1910 |
No. of respondents | 241 | 241 | 241 | 241 |
Within R2 | 0.453 | 0.453 | 0.462 | 0.462 |
Between R2 | 0.122 | 0.152 | 0.122 | 0.152 |
Overall R2 | 0.122 | 0.152 | 0.122 | 0.152 |
RMSE | 0.272 | 0.272 | 0.270 | 0.270 |
Sigma u | 0.243 | 0.238 | 0.243 | 0.238 |
Sigma e | 0.271 | 0.271 | 0.269 | 0.269 |
Rho | 0.445 | 0.436 | 0.449 | 0.439 |
Wald chi-squared test | 1399.684 | 1406.617 | 1447.403 | 1453.937 |
Health-state dummy variables are significant at the 1% level in all models and the size of coefficients is consistent, as the decrement in the elicited utility value is larger for more severe health states (as reported using the modelled utility values from the original valuation study). The IBS label is never significant, whereas the cancer label is significant in the models when it is appropriate for inclusion (where interaction effects are not also included, as this variable is perfectly collinear with interaction variable 11111111 × cancer).
The inclusion of experience variables improves the model using within and between R2. Respondents in the IBS-label group with experience of IBS in themselves or in caring for others did not, on average, give significantly different responses to other respondents. In contrast, respondents in the cancer-label group with experience of cancer in themselves gave, on average, lower utilities for health states, whereas participants in this group with experience of cancer in caring for others gave higher utilities.
Interaction terms reflecting the interaction between the specific health state and the cancer label have negative coefficients, meaning that the inclusion of the interaction term reduces the utility value for that state. The inclusion of the interaction terms reduces the absolute size of coefficients for the state variables. The only exception is the positive coefficient for the interaction term for state 31212241 in model (3). Coefficients for the interaction terms were insignificant for the three mildest states and significant at the 5% level for the remaining five more severe states. Coefficients for these more severe states (44321321, 23141224, 24432411 and 51224434) have little variation, ranging from –0.128 to –0.148 in model (4) with the exception of the much larger coefficient for the most severe state (54444444) at –0.254 in model (4). Models were explored with the inclusion of interaction terms to reflect the interaction between the specific health state and the IBS label, but were never significant and did not improve the model.
Discussion
The literature has not reached consensus regarding how condition labels in health-state descriptions impact on elicited utility values. Some studies found that condition labels affected utility values, others found the reverse, although this may, in part, be due to framing effects. We found that the inclusion of a condition label in a health-state description can affect health-state values, but that this is dependent on the specific condition. This is in accordance with the literature. Yet contrary to previous studies our results demonstrated that the inclusion of a cancer label in the health-state description affected elicited utility values. Our results further indicated that this impact was dependent on the severity of the state. We found that the cancer label did not have a significant impact on utility values for milder states, but had a statistically significant impact for more severe states [with coefficients varying from 0.128 to 0.148 in model (4)], with a much larger reduction for the most severe state [coefficient of 0.254 in model (4)]. In contrast, the inclusion of an IBS condition label had no significant impact on health-state values in comparison with values for the same health states featuring no condition label.
There are many explanations for the differential impact on elicited utility values arising when different labels are used. One view is that the difference in condition that is causing the health state also impacts on the HRQoL associated with that state. For example, difficulty in taking a long walk owing to, say, needing to be near a toilet or owing to psychological problems, may be valued differently to difficulty taking a long walk because you do not have the strength and ability in your legs to do so. Differences in utility values may be a result of more precise estimates enabled through the use of condition labels.
Another possibility is that each condition is associated with differing prior knowledge and preconceptions, and that this may affect health-state values despite the identical description of the HRQoL in that state. For example, cancer covers a wide range of different conditions affecting different parts of the body, whereas IBS affects the digestive system. Cancer can be terminal, whereas IBS is a non-fatal long-term chronic disorder. IBS is generally regarded as mild and not widely publicised, whereas cancer is often associated with fear and dread. All of these differences in knowledge and preconceptions may impact on elicited utility values. We found that experience of the condition in respondents themselves and in caring for others significantly affected utility values in the regression analysis for respondents valuing cancer states, but not for IBS states. Experience of cancer in themselves led to lower utility values, contrary to the literature finding that patients provide higher utility values than the general population. 88 In contrast, experience of cancer in caring for others led to higher utility values, meaning greater unwillingness to sacrifice years of life in return for increased quality of life.
The TTO protocol was selected to ensure that the utility values were elicited using the same protocol as the UK EQ-5D valuation. However, the time frame of 10 years may have impacted on the utility values, as values may have been prone to maximum endurable time whereby states become worse with duration. However, this would be equivalent across condition labels for a given level of severity.
Qualitative research is recommended to enable better understanding of the reasoning behind the differences in utility values associated with different condition labels. Although there are persuasive arguments that differences in values should be taken into consideration as they represent real differences in the quality of life experienced in health states caused by different conditions, without qualitative research we cannot conclude that these differences are not distortions arising from prior knowledge, preconceptions or irrelevant information. Until further information is available, we recommend avoiding condition labels to avoid any potential distortion in values.
The finding that condition labels can impact on health-state utility values further raises the question of whether utility values used to inform resource allocation decisions should reflect this difference. We argue that they should not. Resource allocation distributes resources across different conditions and different groups, meaning there must be comparability in health-state descriptions irrespective of the underlying condition causing that health state. The same health state experienced by a patient with cancer, IBS, heart disease, depression or diabetes should be given the same preference weighting in terms of its impact on utility.
This recommendation poses difficulties for preference-based measures with health-state classification systems derived from existing condition-specific measures that mention the condition within the items. One option is to remove the condition label from the health-state classification system, but the preference-based measure would then not be aligned with responses to the original measure. Another option is to develop a classification system de novo rather than deriving the system from an existing measure. Neither option is ideal, as preference-based measures are often derived from existing measures owing to their wide usage and established reliability and validity. A remaining option is to retain the condition label and accept the potential distortion in utility values.
Conclusion
The inclusion of condition labels in health-state descriptions can impact on elicited utility values but this is dependent on the specific condition and severity of the health state. Further research is required to determine which condition labels may affect elicited utility values. Until this information is available, we recommend the exclusion of condition labels from health-state descriptions, where practical, to ensure that utility values elicited for use in economic evaluation are not distorted by prior knowledge, experience or preconceptions of the condition. This will enable comparability in economic evaluations undertaken across different patient groups and conditions.
Chapter 5 Adaptation of condition-specific measures to examine the impact of side effects and comorbidities on preference-based condition-specific measures
Introduction
In Chapter 1, it was argued that even where CSPBMs have been valued using the same set of methods other problems remain, which undermine their use in making cross-programme comparisons, including the potential impact of side effects and comorbidities. The failure to pick up important side effects of treatment is the rationale in clinical research for using a generic measure alongside a condition-specific measure in a trial. Another solution would be to add an extra dimension or dimensions to the condition-specific measure to take (known) side effects into account. The extra dimension could be treated in an additive fashion, i.e. assuming no interaction with the other dimensions. However, this assumption may not hold true. A similar problem may arise with comorbidities. In the case of asthma, for example, the impact of breathlessness on health-state values may not be the same where there are comorbidities such as pain (perhaps from rheumatic disease). The extra dimensions associated with side effects and/or comorbidities may interact in some way with the dimensions that are related to the main condition. This issue is explored in this chapter by examining the impact of adding on extra dimensions to two CSPBMs.
This chapter reports on two studies. The first study examines the impact of adding (or removing) the physical dimension to the five-dimensional emotional component of the preference-based CORE-6D developed in Chapter 3 from the CORE-OM outcome measure for common mental health problems. The second examines the impact of adding a pain and discomfort dimension to the AQL-5D that has been developed from the AQLQ measure for asthma. 28,29 This measure was selected owing to its lack of generic dimensions owing to its focus on HRQoL associated with asthma symptoms. A general population sample was asked to value a selection of health states defined by these instruments using TTO, with and without the extra dimension. The results are compared between the original and enhanced version of the health states and for the AQL-6D there were sufficient data to estimate an enhanced overall model of preference weights for the full classification system. The consequences for comparability between preference-based measures are discussed, as well as the implications for ‘add-on’ studies more generally.
Methods
CORE-6D study
The CORE-6D is a preference-based measure of health derived from the original CORE-OM outcome measure for common mental health problems. 70 Its derivation from the original CORE-OM has been summarised in Chapter 3 and described in detail elsewhere. 68 The health-state classification of the CORE-6D contains five emotional domains and a single physical-health dimension (see Table 13). The classification defines 729 states in all. Although the full CORE-6D does contain the physical health dimension, the study reported in Chapter 3 valued states with and without this dimension. The valuation of this instrument presented an opportunity to examine the impact of adding on a physical dimension to the five domains of the emotional component of the CORE-6D.
This add-on component ‘piggybacks’ on to the CORE-6D valuation survey. In the main study, there were 18 full CORE-6D states valued. In this add-on component, a further four states were valued and these were five-dimensional ‘emotional’ states that did not mention physical health at all: best state (00000), worst state (22222), 11000 and 22110. The valuation study design is detailed in Chapter 3 for all health states, although that chapter only reports the results for the 18 CORE-6D states. The 22 health states were divided into three ‘card blocs’. Card bloc 1 included the four emotional states and four full CORE-6D states. The other two card blocs only included eight full CORE-6D states each. All three blocs included the CORE-6D state 222220. This design results in the four emotional only states being matched to 12 CORE-6D states in terms of the emotional component. They differ only in having a physical dimension at either at level 0, 1 or 2. Emotional state 11000, for example, is matched to the CORE-6D states 110000, 110001 and 110002.
Bloc 1 allowed us to undertake paired t-tests between the mean values of the four emotional states and the four with the physical dimension. The sample size calculation found that a power of 0.8, significance of 0.05, a SD of 0.3 and an expected difference of 0.1 requires a sample of 73, and this was achieved for all states in the survey. However, given there are another eight independent comparisons between matched states, and a series of further comparisons between states with different levels on the physical health dimension, it was decided to undertake an ANOVA of mean health-state values in order to establish the significance of the impact of the physical health dimension.
AQL-5D study
The AQL-5D is a five-dimension five-level preference-based measure for asthma29 derived from the AQLQ24 using Rasch and conventional psychometric analysis as described in Chapter 1. 31 The five dimensions are concern about asthma, shortness of breath, weather and pollution stimuli, sleep impact, and activity limitations (Box 3). Each dimension has five levels of severity, with level 1 denoting no problems and level 5 indicating extreme problems. In the study reported in this paper, a reduced AQL-5D health-state classification system is valued where each dimension has three levels of severity: level 1 denoting no problems, level 2 denoting some problems and level 3 denoting extreme problems (see Table 13). These relate to levels 1, 3 and 5 in the original classification system. The reduced classification was chosen primarily to limit the size of the valuation survey required to address the study aim.
Feel concerned about having asthma none of the time
Feel concerned about having asthma some of the time
Feel concerned about having asthma all of the time
Shortness of breathFeel short of breath as a result of asthma none of the time
Feel short of breath as a result of asthma some of the time
Feel short of breath as a result of asthma all of the time
Weather and pollutionExperience asthma symptoms as a result of air pollution none of the time
Experience asthma symptoms as a result of air pollution some of the time
Experience asthma symptoms as a result of air pollution all of the time
SleepAsthma interferes with getting a good night’s sleep none of the time
Asthma interferes with getting a good night’s sleep some of the time
Asthma interferes with getting a good night’s sleep all of the time
ActivitiesOverall, not at all limited in any activity done owing to asthma
Overall, moderate or some limitation in every activity done owing to asthma
Overall, totally limited in every activity done owing to asthma
Sixth dimension included in AQL-6D only (EQ-5D pain/discomfort dimension): Pain and discomfortHave no pain or discomfort
Have moderate pain or discomfort
Have extreme pain or discomfort
The pain/discomfort dimension from the EQ-5D was added at the end to the AQL-5D, which also has three levels, in effect making it AQL-6D1 (see Box 3). This extra dimension was chosen to ensure little overlap and correlation with the existing dimensions while being able to capture potential comorbidities and/or side effects.
This was a more ambitious study than the CORE study, in that the aim was to estimate full models with and without the extra dimension. Therefore, health states for the AQL-5D and AQL-6D were selected using an orthogonal array in SPSS version 15. Sixteen health states were selected for AQL-5D, one of which was a repeated state (11111). Eighteen health states were selected for AQL-6D with no repeats. The worst state for each measure (33333 and 333333) was added, taking the number of unique health states to 16 for AQL-5D and 19 for AQL-6D. These included four health states that were matched across the two classification systems in terms of the level of the non-pain dimensions: states 11111 and 111111; 12132 and 121323; 23131 and 231311; and 33333 and 333333.
Health states were divided into three ‘card blocs’ of eight states for each measure, making six blocs or combinations of states in all. Different respondents valued the AQL-5D and the AQL-6D, although they were randomly selected from the same sampling frame. The worst state appeared in all card blocs and the remaining matched health states appeared in two card blocs to improve power. Other states repeated across more than one bloc for AQL-5D and AQL-6D were chosen to reflect a range of severity (using summed levels and dimensions) and levels for each dimension. Combinations of states within card blocs were chosen to reflect a range of severity (using summed levels and dimensions) and to ensure each card bloc included each level of each dimension.
The impact of adding pain/discomfort to AQL-5D was examined in two ways. First, the mean values for the matched states were compared using independent sample t-tests. Secondly, TTO values were modelled and the asthma-specific coefficients were compared across AQL-5D and AQL-6D. Third, the significance of the pain coefficients in the AQL-6D model was examined.
Modelling
Regression analysis was used to estimate the disutility associated with each level of each dimension, in order to enable utility scores to be estimated for all health states described by the classification system. Models have been estimated for the AQL-5D and the AQL-6D using a GLS regression with a random effects component to allow for repeated health-state values from the same respondent. Given the limitations in sample size, it was possible to only estimate additive models when each dimension level other than level 1 is entered as a dummy variable. The data set is not designed to formally examine interactions within the AQL-5D; however, we did examine a ‘N3’ dummy variable to pick up possible interactions between the worst levels across the dimensions. It assumes a value of ‘1’ when any dimension is at the worst level.
Model performance was assessed in terms of adjusted R2 (where available), the likelihood ratio and the size and significance of individual parameter estimates. Predictive ability was assessed by the individual level RMSE and the MAE at the state level (i.e. the difference between predicted and actual mean values at the state level). Plots were used to illustrate possible patterns of predicted errors. The coefficients on the non-pain dimensions of the models were compared using the z-score test for each dimension, where an absolute z-score of 1.96 or more would indicate a significant difference at the 5% level. Stata version 9 was used for all regression analysis and SPSS version 15 was used for the descriptive statistical analysis.
Valuation surveys
The two studies used the same valuation methods.
Respondents
Members of the general population valued eight health states from either the CORE-6D with or without physical health or the AQL-5D with or without pain/discomfort using TTO. The sampling for all households to be contacted in the study was undertaken using the AFD Names & Numbers database for South Yorkshire. The sample for each study was balanced to the UK population according to geodemographic profiles. Letters introducing the relevant survey and information sheets were sent by post to sampled addresses and later interviewers knocked on doors to request participation in the survey at multiple time points at different times and/or different days. Respondents were interviewed in their own homes by trained interviewers who had worked on previous valuation surveys including the HUI-286 and OAB-5D. 30 The project was approved by the ScHARR Research Ethics Committee at the University of Sheffield.
Interview
The interview began with respondents reading and self-completing both the EQ-5D and the CORE-6D or AQL-5D, to familiarise themselves with each classification system. Respondents then undertook a warm-up rank task ranking eight health states alongside two generic states – ‘full health’ and ‘dead’. Respondents then completed a practice TTO question for a separate state, followed by TTO questions valuing all of the eight health states seen in the rank task. For bloc 1 for the CORE-6D study, these tasks were undertaken on two sets of four states: one without the physical health dimension and the other with. The protocol used the York MVH study version of TTO,42,77 including the visual prop with generic full health for the alternative scenario (not instrument specific full health). TTO and the MVH protocol were selected to ensure that the elicited values were similar to UK EQ-5D values. At the end of the interview, respondents were asked to complete questions covering their demographic and socioeconomic characteristics.
Time trade-off values were estimated using the conventional transformations for states better and worse than dead to ensure a potential range of 1.0 to –1.0. 42 Three exclusion criteria were applied to the data to remove those respondents that do not appear to understand the task. Respondents were excluded from the analyses for valuing all states as identical and ‘< 1’. Valuing all states as ‘1’ may not reflect a lack of understanding, but rather an unwillingness to trade life-years for better health states. A second exclusion criterion was when respondents valued the worst possible health state higher than every other state. Finally, respondents who valued all states as worse than dead were excluded.
Results
CORE-6D
The sample contained responses from 225 respondents from South Yorkshire. The response rate was 45.7% for respondents answering their door at the time of the interview (after several attempts), with a TTO completion rate of 99.7% across all interviews. The sample is compared with the general populations of South Yorkshire and England in Table 5 (see Chapter 3). Overall, the study sample had a higher average age and a higher proportion of females, home owners and retired individuals, and a lower proportion of employed/self-employed individuals. All responses were included in the analysis, as no respondents met the exclusion criteria.
The results across all respondents are shown in Table 15. Each state was valued by between 74 and 76 respondents, except for state 222220, which was valued by all respondents. The mean TTO values for the emotional states shown in the first column are consistent with their severity: the best emotional state had a mean value of 0.95 (SD 0.15) down to the worst state at 0.14 (SD 0.48). The impact of adding the physical dimension is consistent for the best state (00000). The physical dimension has little impact at level 0 or level 1, but at level 2 it reduces the mean TTO value by 0.13. The paired t-tests found the impact of level 2 to be significant at the 5% level. For the other three emotional states, the impact of adding physical health follows a different pattern: physical problems at level 0 (i.e. saying there are no physical problems) result in an increase in TTO value of 0.07, 0.13 and 0.09 across the three states. Level 1 is also associated with increases, although they are smaller, at 0.05, 0.05 and 0.07. Only level 2 is associated with decreases of 0.07, 0.04 and 0.04. The ANOVA found the impact of physical health to have been statistically significant for the two milder states (i.e. 00000 and 11000).
Emotional component | No physical item | Response levels of physical item | ANOVA: F-statistic, p-value | ||
---|---|---|---|---|---|
0 | 1 | 2 | |||
00000 | 0.95 (0.15)a | 0.96 (0.13) | 0.93 (0.14) | 0.82 (0.32)a | 7.50, < 0.001 |
11000 | 0.81 (0.27)a | 0.88 (0.25) | 0.86 (0.27)a | 0.74 (0.31) | 4.91, 0.002 |
22110 | 0.44 (0.45)a | 0.57 (0.44)a | 0.49 (0.47) | 0.40 (0.49) | 1.937, 0.124 |
22222 | 0.14 (0.48)a | 0.23 (0.52)a | 0.21 (0.50) | 0.10 (0.53) | 1.821, 0.142 |
AQL-5D and AQL-6D
The response rate for all eligible respondents answering their door was 45.8%. The respondents were similar to those of South Yorkshire and the UK for age and gender, but tended to have a higher proportion of retired individuals, a lower proportion of employed individuals and a lower mean EQ-5D score (0.80 vs 0.86). There were no significant differences between the samples who valued the AQL-5D and AQL-6D. 89
Just two respondents out of the 184 successfully conducted interviews were excluded. This left 1455 TTO values elicited from 180 respondents, with 727 and 728 for the AQL-5D and the AQL-6D health states, respectively. Descriptive statistics across the health states are presented in Table 16. Three pairs of matched states were each valued between 60 and 62 times, the worst states (33333 and 333333) were valued 91 times each and the remaining states were valued between 29 and 31 times. Across the four matched states, mean values for the best (0.97 vs 0.98) and worst states (0.26 vs. 0.30) of AQL-5D and AQL-6D were not found to be significantly different (see Table 17). The mean value of 12132 from the AQL-5D (0.70) was significantly higher than 121323 from AQL-6D (0.56) (p-value = 0.061). By way of contrast, the mean value for the AQL-5D state 23131 (0.64) was lower than the AQL-6D state 231311 (0.78) (p-value = 0.034).
Measure | Health state | n | Mean (SD) | Median | IQR | Minimum | Maximum |
---|---|---|---|---|---|---|---|
AQL-5D | 11111a | 60 | 0.97 (0.14) | 1.00 | 1.00–1.00 | 0.03 | 1.00 |
12132b | 62 | 0.70 (0.41) | 0.78 | 0.64–1.00 | –0.98 | 1.00 | |
23131c | 60 | 0.64 (0.44) | 0.80 | 0.54–0.95 | –0.98 | 1.00 | |
33333d | 91 | 0.26 (0.53) | 0.33 | 0.00–0.63 | –0.98 | 1.00 | |
AQL-6D | 111111a | 61 | 0.98 (0.07) | 1.00 | 1.00–1.00 | 0.50 | 1.00 |
121323b | 60 | 0.56 (0.40) | 0.55 | 0.36–0.89 | –0.70 | 1.00 | |
231311c | 61 | 0.78 (0.24) | 0.90 | 0.54–1.00 | 0.10 | 1.00 | |
333333d | 91 | 0.30 (0.48) | 0.33 | 0.00–0.65 | –0.98 | 1.00 |
AQL-5D | AQL-6D | z-score | ||||
---|---|---|---|---|---|---|
(1) | (2) | (3) | (4) | (1) vs (3) | (2) vs (4) | |
Concern2 | 0.039 | 0.032 | 0.031 | 0.034 | 0.170 | –0.052 |
Concern3 | 0.035 | 0.041 | 0.046 | 0.047** | –0.210 | –0.165 |
Breath2 | 0.042 | 0.019 | –0.011 | –0.001 | 0.984 | 0.502 |
Breath3 | 0.200*** | 0.167*** | 0.054* | 0.047* | 2.853*** | 3.177 |
Weather2 | 0.069 | 0.024 | –0.015 | –0.016 | 1.599 | 1.035 |
Weather3 | 0.058 | 0.057** | 0.034 | 0.033 | 0.513 | 0.734 |
Sleep2 | –0.001 | 0.016 | 0.017 | –0.001 | –0.346 | 0.471 |
Sleep3 | 0.121*** | 0.106*** | 0.099*** | 0.091*** | 0.471 | 0.431 |
Activities2 | 0.080** | 0.074*** | 0.042 | 0.040* | 0.795 | 0.978 |
Activities3 | 0.290* | 0.307*** | 0.137*** | 0.150*** | 2.921*** | 4.213*** |
Pain2 | 0.071** | 0.071*** | ||||
Pain3 | 0.303*** | 0.301*** | ||||
Constant | 0.034 | 0.061 | 0.019 | 0.023 | 0.260 | 0.681 |
Observations | 727 | 727 | 728 | 728 | ||
No. of ID | 91 | 91 | ||||
R 2 | 0.223 | 0.280 | ||||
RMSE | 0.398 | 0.398 | 0.323 | 0.323 | ||
MAE (state level) | 0.031 | 0.038 | 0.027 | 0.030 |
Modelling of the preference data
For AQL-5D the coefficients across the five dimensions are consistent with the severity levels within each dimension, i.e. coefficients for level 3 > level 2 > level 1 (Table 17). The only exception is the sleep dimension, where the level 2 coefficient has the ‘wrong’ sign, although it is very small and non-significant. Level 3 of breath, weather and sleep are significant, as are levels 2 and 3 for activities. The RMSE at the individual level is quite high at 0.4, but the MAE at the state level is 0.038 and this compares very favourably with that achieved in the original model of 0.047. 21 The plot of observed and predicted mean health-state TTO values and residuals ordered by mean observed value suggests that there is no obvious pattern in the errors. 89 The N3 term was not significant in any model and so is not included in the model reported here.
For AQL-6D the pain/discomfort dimension had significant coefficients for levels 2 and 3 at the 5% level, with level 3 pain/discomfort having the largest coefficient (0.301) of any dimension in the AQL-6D for model (4). There were three inconsistencies, with levels 2 of breath (–0.001), weather (–0.016) and sleep (–0.001) being negative, but these are all < 0.02 and none was significant. Overall the model performed well in terms of MAE (0.030 vs 0.038 for AQL-5D) at the state level and again there is no obvious pattern in the errors. There was little change to the coefficients for concern and sleep compared with the AQL-5D model, but a noticeable reduction in the coefficient for level 3 of weather (which was significant in the AQL-5D model at the 5% level but non-significant in the AQL-6D model). However, there were substantial reductions in the coefficients for shortness of breath and activities, particularly for the level 3 coefficients of 0.167 compared with 0.047 and 0.307 compared with 0.150 for the AQL-5D and AQL-6D models, respectively. The results of the z-tests confirm that there were significant differences between the AQL-5D and AQL-6D models in the coefficients of level 3 for shortness of breath and activities at the 1% level. Further modelling is reported elsewhere. 89
Discussion
A major concern in the use of CSPBMs for making cross-programme comparisons arises from the potential impact of side effects and comorbidities. Side effects can have a direct impact on health-state values. Side effects, along with comorbidities, can also have an indirect impact. The presence of side effects and/or comorbidities also has implications for the dimensions included in the CSPBMs. Adding extra dimensions to a classification system might be thought to be neutral in the case of the highest level (i.e. indicating no problems) or to worsen the state for lower levels and hence result in a lower mean health-state value. These studies have shown that the addition of extra dimensions to the emotional component of CORE-6D and AQL-5D had a significant impact on mean health-state values, but not always in the expected direction. The addition of the extra dimension at its worst level reduced the health-state values, as would be expected. A comparison of matched pairs of states for the CORE-6D and AQL-5D, however, showed that the addition of a dimension at its best or intermediate level resulted in increases in health-state values in all except one case (and that was the best emotional state). The same result was found in a study that added sleep to the EQ-5D. 90 The AQL-5D/6D study went on to estimate the impact of the additional dimension for pain/discomfort on the coefficients of the other dimensions. The results are not consistent across dimensions and show that a simple additive adjustment would not adequately capture the effect of adding the pain/discomfort dimension. This provides evidence for a more complex interaction between the new dimension and the existing ones.
These apparently paradoxical results can be explained in a number of different ways. Respondents valuing the emotional states of the CORE-6D and the AQL-5D may assume that the state being valued involves physical problems and pain/discomfort, respectively. So when they are explicitly told the state does not involve these problems then this has the tendency to increase the health-state values. Even being told the state has intermediate physical problems resulted in higher values for the three states of emotional ill health. The magnitude of the unmentioned but imagined problems needs to be quite substantial to achieve such significant differences.
The addition of dimensions seems to have implications for the entire structure of the preference function for health. There was a shift in the size of the coefficients associated with the AQL-5D classification system. It could be that respondents focus on one dominant dimension as part of a heuristic to simplify the task. For AQL-5D this is breathlessness or activities and for AQL-6D this for many has become pain/discomfort. This is related to a focusing effect where respondents exaggerate the importance of asthma-related problems, but the addition of the pain/discomfort dimension with no problems helps put those asthma problems into perspective and so they become less important (as reflected in the lower weights). Addressing this type of explanation is better understood using more in-depth methods, such as cognitive de-briefing. Whatever the explanation, this raises serious concerns about missing dimensions from any health-state classification system.
It was only possible to design a study to estimate additive functional forms similar to those that already exist for the EQ-5D. It would have been desirable to have estimated more complex functional forms such as multiplicative or multilinear functional. 91 This was a consequence of funding limitations, but it was adequate for answering the primary question of whether adding a dimension to a classification system impacted on the size of the coefficients associated with other dimensions (including significant changes). These studies have been limited to two condition-specific instruments and this may limit the generalisability of the results to other CSPBMs or perhaps more importantly for policy-makers, to generic preference-based measures. CSPBMs by definition tend to exclude many common and important domains, and so the general issue addressed by these studies is relevant. Even generic measures exclude potentially important dimensions such as cognition and energy in the EQ-5D. However, whether other dimensions would have such a strong impact as pain/discomfort or the physical dimension requires further research.
Conclusions
The AQL-5D study has implications for the development of add-on dimensions to extend the coverage of generic measures, such as the EQ-5D. Studies have examined the impact of adding on dimensions for cognition92 and sleep,90 which in the case of the former was found to be significant in a student population and in the latter not significant in a sample of the general population using TTO. This study suggests that the extra dimensions for generic preference-based measures or CSPBMs can not be treated as simply an additive term, and so simply added to an existing tariff of values (such as the EQ-5D value sets). Although this was a reasonable simplification for the addition of a physical dimension to the emotional component of the CORE-6D across the three emotional ill health states, it did not work for the addition of pain and discomfort to the AQL-5D. Furthermore, this study has implications for using CSPBMs, particularly those with a very narrow focus. It calls into question the accuracy of utility values generated by narrow classification systems for patients who experience significant side effects or comorbidities. The implications for making cross-programme comparisons are addressed in Chapter 7.
Chapter 6 Performance of condition-specific preference-based measures in comparison with the original measure and generic preference-based measures
Introduction
The increased development and use of CSPBMs raises two important questions regarding the performance of these measures. First, how does the preference-based measure compare with the original non-preference-based measure used to derive it in terms of psychometric performance of validity and responsiveness to change? Second, do CSPBMs offer an improvement over existing generic preference-based measures in terms of these psychometric properties?
The first question arises because the derivation of a health-state classification system based on a small subset of items of the original measure inevitably involves a loss of information. Given that the original rationale for using a condition-specific measure is its expected greater relevance and sensitivity, it is important that this informational advantage is retained in the process of deriving a preference-based index from the existing measure. The existing non-preference-based measure has multiple items in order to maximise reliability and achieve better psychometric performance in terms of validity and responsiveness. Basing the health-state classification on a subset of items raises the question of whether the preference-based measure retains the reliability, responsiveness and sensitivity of the original measure. The extent of information loss can be examined using conventional psychometric analysis. 43 Any loss of information needs to be balanced against the ability of the preference-based measure to generate quality adjustment weights for QALYs.
The second question arises as condition-specific measures are often used in preference to generic measures because it is claimed that the condition-specific measures are more appropriate, valid and responsive. However, there is little published evidence that examines whether this is the case for preference-based measures. This is a development of the first question raised above and can be addressed by applying similar psychometric methods to data sets containing both generic and CSPBMs. If CSPBMs are used in preference to generic preference-based measures, it is important that these measures are valid and responsive. Their development also requires a large amount of time and resources, and it is important these resources are used effectively.
This raises the issue of how the CSPBMs compare with the generic preference-based measures, as this indicates the probable impact of using CSPBMs compared with generic measures to generate QALY values for use in economic evaluation. A large number of published studies compare the performance of EQ-5D with other generic preference-based measures, such as SF-6D and HUI2 (see Brazier et al. 5 for an overview), yet there is little evidence comparing the impact of using a CSPBMs with a generic preference-based measures.
This chapter assesses (1) the extent of any information loss from moving from the original measures to the preference-based measures derived from them for four condition-specific measures for asthma, common mental health problems, overactive bladder and cancer and (2) the performance of these CSPBMs in comparison with generic preference-based measures for the appropriate patient populations. Our analysis focuses on validity, responsiveness and correlation across the measures. Validity is examined in terms of ability to discriminate between patients with different levels of severity defined in terms of their specific condition. Responsiveness is examined in terms of sensitivity to change in trial data before and after treatment. It uses a number of data sets containing each condition-specific measure and one or more generic preference-based measures. The chapter concludes with a discussion of the implications of the results.
Methods
Generic measures
EQ-5D
The EQ-5D has five dimensions (mobility, self-care, usual activities, pain/discomfort, anxiety/depression), each with three levels of severity (no problems, moderate problems, extreme problems). 1 The health-state classification system describes 243 health states and modelled utility values for the UK general population range from 1 to –0.594. 43
SF-6D
The SF-6D has six dimensions (physical functioning, role limitation, social functioning, pain, mental health and vitality) each with between four and six levels of severity. 2 The health-state classification system generated using SF-36 data describes 18,000 health states and modelled utility values for the UK general population range from 1 to 0.301. 3 When derived from SF-12 data, the SF-6D includes three levels of response for physical functioning, four levels of response for role limitations, and five levels of response for each of the remaining dimensions, resulting in the formation of 7500 unique health states. In this version of SF-6D, utility values range from 1 to 0.345. 3
EQ-5D utility values mapped from SF-12 using published algorithms
When EQ-5D was not included in a study, EQ-5D utility values were mapped from SF-12 data using two published algorithms. Mapping enables EQ-5D utilities to be estimated for each patient at each time point using the collected SF-12 data. The Grey et al. 15 mapping algorithm is used here (referred to as Mapped EQ-5D). The algorithm used multinomial logit regression and Monte Carlo simulation methods to generate predictions of EQ-5D responses using individual question responses and summary scores from the SF-12 as explanatory variables. These EQ-5D predicted responses were then linked to utility values using the UK value set. 43 We assume that the errors associated with the mapping process are zero.
Condition-specific measures
Asthma: AQLQ and AQ-5D
The Asthma Quality of Life Questionnaire has 32 items covering four domains: symptoms (12 items), activity limitations (11 items), emotional function (five items) and environmental stimuli (four items). 93 Each item has seven severity levels. Domain scores are generated by summing all item scores within the domain, where high scores indicate good quality of life. 25 The mini-AQLQ is a shorter, standardised version of the AQLQ, covering the same domains (symptoms, activity limitations, emotional function, environmental stimuli). 24 The measure contains 15 items each with seven levels of severity, and the wording for some items differs slightly in wording from the AQLQ. The AQL-5D is a preference-based measure derived from the AQLQ and mini-AQLQ which has five domains (concern about asthma, shortness of breath, weather and pollution stimuli, sleep problems and activity limitation), each with five levels of severity. 79 The classification system derives 3125 health states with modelled utility values ranging from 1 to 0.45. 29
Common mental health problems: CORE-OM and CORE-6D
The CORE-OM has 34 self-report items across four domains [subjective well-being, problems (depression, anxiety, physical symptoms, and trauma), functioning (general functioning, close relationships, social relationships), and risk (risk to self and risk to others)]. Each item has five levels of severity. 70,71 The CORE-OM clinical score is calculated by adding all 34 item scores and multiplying by 10/34. The CORE-OM clinical score produces values between 0 and 40, where 10 is the cut-off point between clinical and non-clinical cases, 10 to < 15 indicates mild psychological distress, 15 to < 20 moderate distress, 20 to < 25 moderate to severe distress and 25–40 severe psychological distress. 71 A completed CORE-OM questionnaire can be considered ‘valid’ if at least 31 items have been completed. CORE-6D is a preference-based measure derived from the CORE-OM, which is specific to common mental health problems consisting of an emotional component with five domains (functioning – close relationships, symptoms – anxiety, risk/harm to self, functioning – general, functioning – social relationships) and a physical health item. Each of the six items has three levels of severity, which, combined, can produce 729 health states. 68 Modelled utility values range from 0.95 to 0.10 (see Chapter 3).
Cancer: EORTC QLC-C30 and EORTC-8D
The EORTC QLQ-C30 has 30 items that cover functioning (physical, role, social, emotional, cognitive) and common cancer symptoms (pain, fatigue, nausea, vomiting, dyspnoea, constipation and diarrhoea) plus global quality of life. The EORTC QLQ-C30 has 14 summary scales that each represents an aspect of functioning or a particular symptom with one additional global quality of life scale. Each summary scale ranges from 0 to 100. 94 The EORTC-8D is a preference-based measure derived from the EORTC QLQ-C30, which has eight dimensions (physical functioning, role functioning, pain, emotional functioning, social functioning, fatigue and sleep disturbance, nausea, constipation/diarrhoea) each with four or five levels of severity. The classification system describes 81,920 health states with modelled utility values ranging from 1 to 0.291. 34
Overactive bladder: OAB-q and OAB-5D
The OAB-q has 33 items separated into an eight-item symptom bother scale and a 25-item HRQoL scale with four subscales (coping, sleep, concern, social interaction). Each item has six levels of severity. Results can be reported using domain scores and overall scores. 95 The OAB-5D has five domains (urge to urinate, urine loss, sleep impact, coping strategy, concern with overactive bladder), each with five levels of severity. 31 The classification system defines 3125 states with modelled utility values ranging from 1 to 0.606. 30
Table 18 summarises the condition-specific measures and the preference-based measures derived from them. Each of the preference-based measures has a different range of potential utility values, with EQ-5D utility values having the largest possible range of 1.594 and OAB-5D having the smallest range of 0.394. The observed range of utility values in a patient data set often does not cover the full range, yet where the possible range is smaller it is probable that the observed range will be smaller.
Measure | Original measure | Domains | Scoring system | Preference-based measure | Dimensions | Scoring range |
---|---|---|---|---|---|---|
Generic | N/A | EQ-5D | Mobility; self-care; usual activities; pain/discomfort; anxiety/depression | 1 to –0.594 | ||
Generic | SF-36 | Physical functioning; role limitations – physical; role limitations – emotional, social functioning; bodily pain; mental health; vitality; general health | SF-6D | Physical functioning; role limitation; social functioning; pain; mental health; vitality | 1 to 0.301 | |
SF-12 | SF-6D | Physical functioning; role limitation; social functioning; pain; mental health; vitality | 1 to 0.345 | |||
Asthma | AQLQ, mini-AQLQ | Symptoms; activity limitations; emotional function; environmental stimuli | Four domain scores | AQL-5D | Concern about asthma; shortness of breath; weather and pollution stimuli; sleep problems; activity limitation | 1 to 0.45 |
Common mental health problems | CORE-OM | Subjective well-being; problems (depression; anxiety; physical symptoms; trauma); functioning (general functioning; close relationships; social relationships); risk (risk to self; risk to others) | One clinical score | CORE-6D | Emotional component with five domains (functioning – close relationships; symptoms – anxiety; risk/harm to self; functioning – general; functioning – social relationships); physical health item | 0.95 to 0.10 |
Cancer | EORTC QLQ-C30 | Functioning (physical; role; social; emotional; cognitive); common cancer symptoms (pain; fatigue; nausea; vomiting; dyspnoea; constipation; diarrhoea); two global items | Fourteen summary scales | EORTC-8D | Physical functioning; role functioning; pain; emotional functioning; social functioning; fatigue and sleep disturbance; nausea; constipation/diarrhoea | 1 to 0.291 |
Overactive bladder | OAB-q | Symptom bother; coping; sleep; concern; social interaction | Five domain summary scores plus HRQoL summary score | OAB-5D | Urge to urinate, urine loss, sleep impact, coping strategy, concern with overactive bladder | 1 to 0.606 |
Patient data sets
Asthma
Asthma Exacerbation Study
The Asthma Exacerbation Study was a prospective observational study examining the impact of asthma exacerbations on HRQoL in 112 patients with moderate to severe asthma (British Thoracic Society level 4 or 5) over 4 weeks. 96 The mean age of the full sample was 41.4 years (SD 12.2 years) and 37% were male.
COGENT study (Computerised Guidelines Evaluation in the North of England)
The COGENT study was a before and after, cluster randomised controlled trial evaluating the use of computerised decision support (Cochrane Database of Systematic Reviews) systems in implementing clinical guidelines for the primary care management of asthma in adults. 97 UK practices which used their computer systems intensively were eligible for the study. Asthma patients aged ≥ 18 years who were registered with the participating practices were identified from a computerised search. Questionnaires were administered before and approximately 1 year after the introduction of the computerised decision support system. The mean age of the full sample was 48.65 years (SD 17.71 years) and 40% were male. The Newcastle Asthma Symptom questionnaire (NASS) overall score was used to indicate severity, generated as the summed score of all 10 items included in the NASS (breathlessness during exercise, breathlessness during day when not exercising, wheezing during the day, coughing during the day, wheezing at night, breathlessness at night, coughing at night, disturbed sleep, fear because of asthma, feeling tightness in chest). 98
Common mental health problems
PMS data set
The PMS data set consisted of 553 adults selected from participants in a longitudinal study99 that followed the adult psychiatric morbidity survey conducted in the UK in 2000. 100 The 553 people in the data set belonged in a sample that had been randomly allocated to complete the CORE–OM (for further details see Connell et al. 101). All data in the PMS data set were collected at one time point; no follow-up data were available. Mean age of the full sample was 44.33 years (SD 14.35 years) and 43% were male. Severity was measured using responses to the Clinical Interview Schedule – Revised (CIS-R). 102
PHASE data set
The PHASE data set consisted of 112 adults participating in a randomised controlled trial evaluating self-help cognitive behavioural therapy (CBT) facilitated by practice nurses against ordinary general practitioner (GP) care (control group) for mild to moderate anxiety and/or depression. The trial was conducted in 17 general practices in north-east England. 103 Data were available at baseline, end of treatment (note: although there is no demarcated end of treatment for the control group, assessment occurred at the same time as end of treatment for the self-help CBT group to provide a ‘matched’ point of assessment), 1-month follow-up and 3-month follow-up. The mean age of the full sample was 39.25 years (SD 12.68 years) and 23.3% were male. Severity was measured using the CORE-OM clinical score. 71,72
Cancer
VISTA data set
The VISTA data were collected in a Phase III randomised open-label trial for patients newly diagnosed with multiple myeloma cancer (ClinicalTrials.gov no. NCT00111319). Patients were asked to complete both the EQ-5D and EORTC QLQ-C30 at their screening visit, on day 1 of each of the nine cycles of treatment, at their end of treatment visit and during the post-treatment phase (every 6 or 8 weeks) until disease progression. The mean age of the full sample was 71.82 years (SD 5.48 years) and 51% were male. Severity was measured using the Karnofsky Performance Scale, which classifies patients according to functional impairment typically using 10-point markers, where a score of 100 indicates that the patient is normal with no signs of disease and a score of ‘0’ is equivalent to death. 104
Vancouver Cancer Clinic Breast cancer data set
The Vancouver Cancer Clinic (VCC) Breast cancer data were collected at the VCC. Patients diagnosed with breast cancer attending an outpatient clinic were asked to complete EQ-5D and EORTC QLQ-C30. Mean age of the full sample was 67.92 years (SD 18.17 years) and all were female. Severity was measured using the stage of disease, from stage I (indicating that cancer is localised) to stage IV (indicating that cancer has metastasised or spread to other areas of the body).
Vancouver Cancer Clinic Lung cancer data set
As above, the data were collected at the VCC. Patients who had been diagnosed with lung cancer and were attending an outpatient clinic were asked to complete EQ-5D and EORTC QLQ-C30. The mean age of the full sample was 61.83 years (SD 21.13 years) and 48% were male. Severity was measured using the stage of disease.
Overactive bladder
Trial 023
Trial 023 was a clinical trial for overactive bladder patients. 105 OAB-q data were collected at baseline and follow-up but no generic data were collected. The mean age was 61.34 years (SD 14.74 years) and 29% were male.
Trial 037
Trial 037 was placebo-controlled trial for overactive bladder patients conducted in the USA. 106 Data were collected at baseline for OAB-q and SF-36, and OAB-q data were also collected at follow-up. The mean age was 58.8 years (SD 13.52 years) and 49% were male. Severity was measured using the number of urge incontinence episodes per 24 hours, grouped as 0 or > 0.
Analysis
Validity: discrimination across different severity groups
Construct validity is examined in terms of known group differences as indicated by ability to discriminate between patients with different levels of severity defined in terms of their specific condition. It is assumed that the severity groupings represent differences that are important to patients and the general population. This is examined partly by the statistical significance of differences using t-tests where there are only two groups and an overall F-test from an ANOVA, where there are more than two groups to explore the discriminative ability of the measures across different levels of severity. The sensitivity of the measures to the differences is also examined using the standardised effect size estimated as the difference in mean scores between two adjacent subgroups of study participants with different levels of severity divided by the SD of scores for the mildest of the two subgroups.
Responsiveness to change over time
Responsiveness is the sensitivity of the measure to known changes in health over time. In the data available to this study, this is examined in terms of sensitivity to change in trial data before and after treatment across all study arms. This is quite a crude test, as there may be some people who did not get better. The potential responsiveness to change over time was examined in terms of floor and ceiling effects and the statistical significance of differences across time periods. Floor and ceiling effects report the percentage of people in the sample in the most severe health state of the classification and in full health, respectively. Such effects suggest that the instrument is not well targeted to the study population, as it cannot measure the whole range of health; consequently the instrument is unable to capture either improvement or deterioration in health for those patients. Relative floor and ceiling effects across measures are most important here, as they indicate that one measure cannot distinguish, whereas the other can. These were reported using all responses where observations were available for every measure of interest.
The degree of responsiveness is also usually assessed in terms of the standardised response mean and effect size, where standardised response mean is the mean change score of a measure between two different time points divided by the SD of the change score. 107 Effect size in this case is the mean change score of a measure between two different time points divided by the SD of the score at baseline. Standardised response mean, effect size and t-tests are estimated using all responses for the periods of interest (e.g. baseline and end of treatment) for which observations are available for every measure of interest.
The potential impact of using different measures is also examined by looking at absolute values rather than the standardised ones. The mean utility values generated by CSPBMs and generic preference-based measures are therefore compared by severity group and compared before and after intervention. The statistical significance of any differences is examined by t-tests (as explained above and reported under responsiveness) where there are only two groups or ANOVA where there are more than two groups.
The preference-based measures are also compared using Pearson correlation coefficients, although this is a poor indicator of agreement, and using the intraclass correlation coefficient (ICC) as this assesses the consistency of the preference-based measures given that they are both measuring utility values on the same utility scale (0–1, dead to full health). Stata version 9 was used for all regression analysis and SPSS version 15 was used for the descriptive statistical analysis.
Results
Tables 19–21 report discrimination across severity groups, responsiveness to treatment and correlations, respectively.
Condition | Data set | Measure | Range of mean (SD) across groups | Range of effect sizes | ANOVA (or t-test values if only two groups) | No. of severity groups | Range of n per group |
---|---|---|---|---|---|---|---|
Asthma | COGENTa | AQLQ | 3.62 (1.09) to 6.27 (0.55) | 0.85 to 1.53 | < 0.001 | 4 | 1274 to 1692 |
AQL-5D | 0.75 (0.10) to 0.96 (0.04) | 0.83 to 1.50 | < 0.001 | 4 | |||
EQ-5D | 0.56 (0.31) to 0.87 (0.18) | 0.30 to 0.72 | < 0.001 | 4 | |||
SF-6D | 0.59 (0.11) to 0.75 (0.10) | 0.31 to 0.72 | < 0.001 | 4 | |||
Common mental health problems | PMSb | CORE-OM | 15.34 (5.94) to 3.60 (2.69) | –1.03 to –1.47 | < 0.001 | 4 | 11 to 428 |
CORE-6D | 0.70 (0.16) to 0.91 (0.05) | 0.77 to 1.00 | < 0.001 | 4 | |||
Mapped EQ-5D | 0.54 (0.31) to 0.89 (0.15) | 0.51 to 0.64 | < 0.001 | 4 | |||
SF-6D | 0.59 (0.11) to 0.83 (0.11) | 0.52 to 0.91 | < 0.001 | 4 | |||
PHASEc | CORE-OM | 27.60 (2.85) to 5.07 (2.93) | –2.63 to –3.94 | < 0.001 | 5 | 22 to 44 | |
CORE-6D | 0.40 (0.14) to 0.87 (0.07) | 0.96 to 1.59 | < 0.001 | 5 | |||
EQ-5D | 0.29 (0.27) to 0.82 (0.23) | 0.35 to 0.71 | < 0.001 | 5 | |||
Cancer | VISTAd | EORTC QLQ-C30 | 32.64 (19.76) to 68.95 (17.83) | 0.29 to 0.50 | < 0.001 | 6 | 36 to 1410 |
EORTC-8D | 0.56 (0.13) to 0.85 (0.12) | 0.35 to 0.65 | < 0.001 | 6 | |||
EQ-5D | 0.16 (0.36) to 0.81 (0.18) | 0.45 to 0.55 | < 0.001 | 6 | |||
VCC Breaste | EORTC QLQ-C30 | 60.98 (19.49) to 73.53 (13.25) | –0.07 to 0.71 | 0.015 | 4 | 14 to 41 | |
EORTC-8D | 0.73 (0.12) to 0.88 (0.10) | –0.18 to 0.85 | < 0.001 | 4 | |||
EQ-5D | 0.84 (0.12) to 0.70 (0.21) | –0.23 to 0.81 | 0.045 | 4 | |||
VCC Lungf | EORTC QLQ-C30 | 66.09 (23.03) to 59.95 (19.02) | 0.27 | 0.183 | 2 | 29 to 62 | |
EORTC-8D | 0.75 (0.12) to 0.81 (0.11) | 0.55 | 0.039 | 2 | |||
EQ-5D | 0.71 (0.21) to 0.79 (0.17) | 0.47 | 0.081 | 2 | |||
Overactive bladder | Trial 037g | OAB-q | 59.30 (20.62) to 68.91 (19.44) | 0.49 | < 0.001 | 2 | 307 to 451 |
OAB-5D | 0.82 (0.08) to 0.88 (0.08) | 0.74 | < 0.001 | 2 | |||
SF-6D | 0.72 (0.11) to 0.75 (0.10) | 0.26 | 0.001 | 2 |
Condition | Data set | Measure | Percentage at floor | Percentage at ceiling | Mean change (SD) | Standardised response mean | ES | t-test |
---|---|---|---|---|---|---|---|---|
Asthma | Asthma Exacerbationa | n = 201 | n = 90 | |||||
Mini-AQLQ | 0 | 0 | –0.153 (0.694) | –0.22 | –0.12 | 0.063 | ||
AQL-5D | 0.5 | 1.5 | –0.014 (0.079) | –0.18 | –0.11 | 0.096 | ||
EQ-5D | 0 | 44.8 | 0.007 (0.187) | 0.04 | 0.03 | 0.218 | ||
COGENT | n = 5884 | N/A | ||||||
AQLQ | 0 | 0.5 | N/A | |||||
AQL-5D | 0.3 | 10.8 | N/A | |||||
EQ-5D | 0 | 28.8 | N/A | |||||
SF-6D | 0.3 | 0 | N/A | |||||
Common mental health problems | PMS | n = 537 | N/A | |||||
CORE-OM | 0 | 1.3 | N/A | |||||
CORE-6D | 0 | 22.5 | N/A | |||||
Mapped EQ-5D | 0 | 36.1 | N/A | |||||
SF-6D | 0 | 1.5 | N/A | |||||
PHASEb | n = 185 | n = 39 | ||||||
CORE-OM | 0 | 0.5 | –6.26 (7.48) | –0.84 | –1.04 | < 0.001 | ||
CORE-6D | 0.5 | 4.3 | 0.087 (0.193) | 0.45 | 0.48 | 0.008 | ||
EQ-5D | 0 | 10.3 | 0.103 (0.268) | 0.38 | 0.35 | 0.021 | ||
Cancer | VISTAc | n = 5903 | n = 379 | |||||
EORTC QLQ-C30 | 1.7 | 2.7 | 9.081 (26.82) | 0.339 | 0.396 | < 0.001 | ||
EORTC-8D | 0.1 | 3.8 | 0.021 (0.154) | 0.134 | 0.138 | 0.010 | ||
EQ-5D | 0.2 | 12.6 | 0.095 (0.372) | 0.256 | 0.275 | < 0.001 | ||
VCC Breast | n = 100 | |||||||
EORTC QLQ-C30 | 0 | 5.0 | ||||||
EORTC-8D | 0 | 2.0 | ||||||
EQ-5D | 0 | 24.0 | ||||||
VCC Lung | n = 100 | |||||||
EORTC QLQ-C30 | 1.0 | 4.0 | ||||||
EORTC-8D | 0 | 0 | ||||||
EQ-5D | 0 | 17.0 | ||||||
Overactive bladder | Trial O23 | n = 1321 | n = 420 | |||||
OAB-q | 0.5 | 1.3 | –18.23 (21.08) | –0.865 | –0.781 | < 0.001 | ||
OAB-5D | 1.7 | 1.8 | –0.080 (0.093) | –0857 | –0.887 | < 0.001 | ||
Trial 037 | n = 758 | |||||||
OAB-q | 0 | 0.1 | N/A | |||||
OAB-5D | 0.4 | 3.4 | N/A | |||||
SF-6D | 0.1 | 0 | N/A |
Condition | Data set | Measures | Pearson correlation coefficient | ICC: mean (95% CI) | ICC: p-value |
---|---|---|---|---|---|
Asthma | Asthma Exacerbation | AQL-5D and EQ-5D | 0.64 | 0.538 (0.431 to 0.629) | < 0.001 |
COGENT | AQL-5D and EQ-5D | 0.53 | 0.316 (0.165 to 0.438) | < 0.001 | |
AQL-5D and SF-6D | 0.62 | 0.265 (–0.089 to 0.581) | < 0.001 | ||
EQ-5D and SF-6D | 0.75 | 0.536 (0.462 to 0.598) | < 0.001 | ||
Common mental health problems | PMS | CORE-6D and mapped EQ-5D | 0.63 | 0.459 (0.361 to 0.544) | < 0.001 |
CORE-6D and SF-6D | 0.65 | 0.475 (0.054 to 0.697) | < 0.001 | ||
Mapped EQ-5D and SF-6D | 0.81 | 0.712 (0.646 to 0.764) | < 0.001 | ||
PHASE | CORE-6D and EQ-5D | 0.58 | 0.474 (0.332 to 0.591) | < 0.001 | |
Cancer | VISTA | EQ-5D and EORTC-8D | 0.71 | 0.482 (0.264 to 0.624) | < 0.001 |
VCC Breast | EQ-5D and EORTC-8D | 0.63 | 0.563 (0.414 to 0.683) | < 0.001 | |
VCC Lung | EQ-5D and EORTC-8D | 0.61 | 0.527 (0.370 to 0.655) | < 0.001 | |
Overactive bladder | Trial 037 | OAB-5D and SF-6D | 0.37 | 0.203 (–0.056 to 0.420) | < 0.001 |
Asthma
Discrimination
Four severity groups were generated using the NASS for the COGENT data set (asthma exacerbation study data set did not have a suitable variable to capture severity). Table 19 indicates that AQLQ and AQL-5D had similar effect sizes, with larger effect sizes than both EQ-5D and SF-6D. The difference in scores across adjacent severity groups was statistically significant (at the 1% level) for all measures. The AQL-5D had a slightly smaller range of mean utility values across groups of 0.21 (ranging from 0.75 to 0.96) than EQ-5D of 0.31 (ranging from 0.56 to 0.87), and the SF-6D had the narrowest range of 0.16 (ranging from 0.59 to 0.75). Despite these differences, the smaller SD of AQL-5D resulted in effect sizes that were twice the size of those of EQ-5D.
Responsiveness
None of the measures suffered from floor effects. Across the COGENT data set the EQ-5D had the largest ceiling effects (28.8%), but it was also quite large for the AQL-5D (10.8%) in the COGENT data set but not in the asthma exacerbation study (1.5%). AQL-5D was the only measure that had observations across the full range of the measure. For the asthma exacerbation study, standardised response mean and effect size were similar for mini-AQLQ and AQL-5D. AQLQ and AQL-5D had mean change in the opposite direction to EQ-5D and the change was statistically significant (at the 10% level), suggesting that AQLQ and AQL-5D captured change in the right direction. EQ-5D had a very small change (0.007) that was not statistically significant and had smaller standardised response mean (0.04) and effect size (0.03) than AQL-5D.
Correlation
There were extremely high correlations of 0.92 between mini-AQLQ and AQL-5D for the asthma exacerbation study and 0.94 between AQLQ and AQL-5D for COGENT using the Pearson correlation coefficient. Pearson correlation coefficients between condition-specific mini-AQLQ, AQLQ and AQL-5D and generic SF-6D and EQ-5D across both data sets were much lower, ranging from 0.53 to 0.64. Pearson correlation coefficients between AQL-5D and EQ-5D were 0.64 for the asthma exacerbation study and 0.53 for the COGENT study, and the ICC were lower at 0.54 and 0.32, respectively, but both significant at the 1% level. The Pearson correlation coefficient between AQL-5D and SF-6D was 0.62 with lower ICC of 0.265 that is significant at the 1% level.
Common mental health problems
Discrimination
Severity groups were generated using the CIS-R for the PMS data set and the CORE-OM clinical score for the PHASE data set. CORE-OM had much larger effect sizes than CORE-6D, but both had larger effect sizes than EQ-5D in the PMS data set and mapped EQ-5D and SF-6D in the PHASE data set. The difference in scores was statistically significant for all measures in both data sets at the 1% level. The CORE-6D had a slightly smaller range of mean utility values across groups of 0.21 (0.70 to 0.91) in PMS and 0.47 (0.40 to 0.87) in PHASE compared with EQ-5D of 0.53 (0.29 to 0.82) in PHASE and mapped EQ-5D of 0.35 (range 0.54 to 0.89) in PMS, and the SF-6D had a narrow range of 0.24 (0.59 to 0.83). Effect sizes were larger for the CORE-6D than the SF-6D, and these in turn both had larger effect sizes than the EQ-5D owing to its SDs being larger.
Responsiveness
None of the measures suffered from floor effects. Across the PMS data set the mapped EQ-5D had the largest ceiling effects (36.1%), but the ceiling effect was also quite large for the CORE-6D (22.5%). The EQ-5D also had the largest ceiling effects (10.3%) in the PHASE data set, and ceiling effects were lower for CORE-6D (4.3%). For the PHASE data set, standardised response mean and effect size were larger for CORE-OM (range –0.84 to –1.04) than for CORE-6D (range 0.45 to 0.48). All measures report that health improves and that the change is statistically significant, but mean change and SD are higher for EQ-5D [0.103 (0.268)] than for CORE-6D [0.087 (0.193)]. Standardised response mean, effect size and statistical significance were better for CORE-6D than for EQ-5D.
Correlation
There were high Pearson correlation coefficients of –0.82 to –0.84 between CORE-OM and CORE-6D. Pearson correlation coefficients between condition-specific CORE-OM and CORE-6D and generic EQ-5D, mapped EQ-5D and SF-6D were much lower, ranging from –0.55 to –0.65. Pearson correlation coefficients between CORE-6D and EQ-5D or mapped EQ-5D were 0.58 and 0.63 and the ICCs were 0.474 and 0.459, and both were significant at the 1% level. The Pearson correlation coefficient between CORE-6D and SF-6D was 0.81 and the ICC of 0.712 was significant at the 1% level.
Cancer
Discrimination
Severity groups were generated using Karnofsky Performance Scale for VISTA and stage of disease for VCC Breast and VCC Lung data sets. EORTC-8D had higher effect sizes than EORTC QLQ-C30 across all data sets and similar effect sizes to EQ-5D. The difference in scores across adjacent severity groups was statistically significant at the 1% level for all measures in the VISTA data set, at the 5% level for all measures in the VCC Breast data set and at the 5% level for EORTC-8D in the VCC Lung data set. The CORE-8D had narrower range of 0.29 (0.56 to 0.85) than EQ-5D of 0.65 (0.16 to 0.81) in the VISTA data set but similar range in the other data sets. Despite these differences, the smaller SD of EORTC-8D resulted in generally larger effect sizes than those of EQ-5D.
Responsiveness
None of the measures suffered from floor effects, yet the EQ-5D suffered from ceiling effects from 12.6% to 24.0%. For the VISTA data set standardised response mean and effect sizes were largest for EORTC QLQ-C30 and lowest for EORTC-8D, suggesting some degree of information loss between the measures. Mean change and SD are larger for EQ-5D than EORTC-8D, and this leads to larger standardised response means and effect sizes. However, all measures captured a statistically significant change.
Correlation
There were moderate Pearson correlation coefficients of between 0.67 and 0.71 between EORTC QLQ-C30 and EORTC-8D. Pearson correlation coefficients between EORTC QLQ-C30 and EQ-5D were lower and differed by data set, ranging from 0.37 to 0.61. Pearson correlation coefficients between EORTC-8D and EQ-5D ranged from 0.61 to 0.71, and ICCs ranged from 0.482 to 0.563 and were significant at the 1% level.
Overactive bladder
Discrimination
Severity groups were generated using number of urge incontinence episodes per 24 hours (i.e. whether = 0 or > 0 for trial 037). OAB-5D had a larger effect size than both OAB-q and SF-6D. The difference in scores across the severity groups was statistically significant (at the 0.01% level) for all measures. OAB-5D mean difference across groups (0.06) was double that of SF-6D (0.03) with smaller SD.
Responsiveness
None of the measures suffered from floor or ceiling effects. For trial 023, standardised response mean and effect sizes were similar for both OAB-q and OAB-5D and both had significant changes (at the 1% level). There were no responsiveness data on the SF-6D.
Correlation
There were extremely high Pearson correlation coefficients ranging from 0.87 to 0.90 between OAB-q and OAB-5D. In trial 037 data set, the Pearson correlation coefficients between condition-specific OAB-q and OAB-5D and generic SF-6D were much lower, ranging from 0.36 to 0.37. The Pearson correlation coefficient between OAB-5D and SF-6D was relatively low at 0.37 and the ICC was 0.203, but this was significant at the 1% level.
Discussion
This chapter examines the extent of information loss arising from moving from the original condition-specific measures of AQLQ for asthma, CORE-OM for common mental health problems, EORTC QLQ-C30 for cancer and OAB-q for overactive bladder to CSPBMs derived from these: AQL-5D, CORE-6D, EORTC-8D and OAB-5D. The chapter also examines the performance of the original condition-specific measures and the CSPBMs in comparison with the widely used generic measures of EQ-5D and SF-6D and, where EQ-5D data are unavailable, uses estimated EQ-5D values produced using a published mapping function. It also provides evidence on the likely impact of using CSPBMs in place of generic preference-based measures. All results are briefly summarised in Table 22 and expanded below in more detail.
Condition | Data set | Condition-specific measure | Information loss from original measure | Discriminative validity | Responsiveness | Generic measure | Discriminative validity of EQ-5D | Responsiveness of EQ-5D |
---|---|---|---|---|---|---|---|---|
Asthma | Asthma Exacerbation | AQL-5D | No | Yes | Yes | EQ-5D | Yes | Yes |
COGENT | AQL-5D | No | Yes | N/A | EQ-5D | Yes | N/A | |
Common mental health problems | PMS | CORE-6D | No | Yes | N/A | Mapped EQ-5D | Yes | N/A |
SF-6D | Yes | N/A | ||||||
PHASE2 | CORE-6D | No | Yes | Yes | (Mapped EQ-5D) | Yes | ||
Cancer | VISTA3 | EORTC-8D | Some for responsiveness | Yes | Yes | EQ-5D | Yes | Yes |
VCC Breast | EORTC-8D | No | Yes | N/A | EQ-5D | Yes | N/A | |
VCC Lung | EORTC-8D | No | Yes | N/A | EQ-5D | Yes | N/A | |
Overactive bladder | Trial O23 | OAB-5D | No | Yes | N/A | N/A | N/A | N/A |
Trial 037 | OAB-5D | No | Yes | N/A | SF-6D | Yes | N/A |
Information loss
Asthma
There is no evidence of information loss in the move from asthma-specific AQLQ to the preference-based AQL-5D regarding discrimination across severity group and responsiveness. The AQL-5D suffered from a higher degree of ceiling effects than AQLQ but is otherwise similar.
Common mental health problems
There is evidence of little information loss in the move from mental health-specific CORE-OM to preference-based CORE-6D. Discrimination across severity groups had larger effect sizes for CORE-OM than CORE-6D, although for both measures the difference in scores was statistically significant. Responsiveness was similar across the two measures.
Cancer
There is evidence of little information loss between the cancer-specific EORTC QLQ-C30 global quality-of-life summary score to EORTC-8D. The analysis indicates that the EORTC-QLQ-C30 global quality-of-life summary score was more responsive than EORTC-8D to changes in HRQoL from screening to end of treatment using the VISTA data set, yet across all three data sets had smaller effect sizes when discriminating across different severity groups. The global EORTC QLQ-C30 quality-of-life summary score was generated using only two items from the EORTC QLQ-C30. This score was reported here as it is not recommended by the instrument developers that a summary score across items measuring different functionings and symptoms is generated. However, it cannot be concluded using the analyses reported here alone whether there is information loss when moving from the full EORTC QLQ-C30 measure to EORTC-8D. Further analysis on this data set indicated that the validity and responsiveness of EORTC-8D was similar to the EORTC-QLQ-C30 functioning and symptom summary scores. 108 Repeating this analysis using other data sets would indicate whether the findings are specific to this data set and patient population and research in this area is encouraged.
Overactive bladder
There is no evidence of information loss in the move from overactive bladder-specific OAB-q to OAB-5D regarding discrimination across severity group and responsiveness. In fact, the OAB-5D had a larger effect size than OAB-q.
Overall there is evidence for little loss of information in moving from the original measures to the preference-based measures. For the EORTC-8D there may be some loss of responsiveness but this was only able to be examined in one data set.
Performance in comparison with a generic preference-based measure
Asthma
The asthma-specific AQL-5D and generic EQ-5D were both able to discriminate across different severity groups. Mean difference and SD across groups was lower for AQL-5D than EQ-5D, but the effect sizes were larger for AQL-5D than both EQ-5D and SF-6D. EQ-5D was less responsive from baseline to 4 weeks than AQL-5D. Another study109 compared the discrimination of AQL-5D, AQLQ and three generic preference-based measures (EQ-5D, HUI3 and SF-6D) for asthma control status. The study also found that AQL-5D was superior to the generic measures regarding discrimination, and performed similarly to AQLQ.
Common mental health problems
The condition-specific CORE-6D performed better than the generic EQ-5D, SF-6D and mapped EQ-5D values regarding discrimination across severity groups. The CORE-6D and EQ-5D performed comparably for responsiveness, but the CORE-6D had higher standardised response mean and effect size despite lower mean change. The EQ-5D suffered from much higher ceiling effects than CORE-6D.
Cancer
The cancer-specific EORTC-8D performed better than the generic EQ-5D regarding discrimination across different severity groups using three data sets. The reverse was found for responsiveness, yet this was only measured using one data set. The EQ-5D had a higher proportion of ceiling effects than EORTC-8D.
Overactive bladder
The overactive bladder-specific OAB-5D performed better than SF-6D, but this was examined using only one data set. None of the available data sets was able to measure SF-6D responsiveness.
Overall discussion
Overall, the CSPBMs performed better than the generic measures in terms of discriminative validity measured using effect size. They had smaller mean differences than EQ-5D yet consistently had either similar or larger effect sizes. This may be important for the power of a study, as the CSPBMs may be able to detect significant differences with smaller sample size. Responsiveness for the CSPBMs and generic preference-based measures was only able to be examined in three data sets, but indicated that AQL-5D and CORE-6D had higher standardised response means and effect sizes than EQ-5D. The reverse was found for EORTC-8D, although it was still able to detect a statistically significant change. The CSPBMs were always able to detect a statistically significant change, yet EQ-5D was unable to detect a statistically significant change in the asthma exacerbation study data set. The larger effect size and standardised response mean for CSPBMs can reduce uncertainty, which can be important for the precision estimates and probabilistic sensitivity analysis in an economic evaluation. However this does not mean the CSPBMs are necessarily a more valid preference-based measure of health. One key limitation of this analysis is the lack of available data to examine all measures using multiple data sets for both discrimination and responsiveness and is limited by the range and representativeness of each sample for each population of interest. Further research using other data sets is encouraged.
The modestly better or comparable performance between CSPBMs and generic preference-based measures is reassuring given that they are all measures capturing HRQoL, but raises the question whether or not CSPBMs offer an advantage over the generic preference-based measures. The analysis suggests that one consistent advantage of using the CSPBMs rather than EQ-5D is greater refinement of values at the upper end of HRQoL and thus greater ability to discriminate between different severity groups. There was a high level of agreement between the generic preference-based measures and CSPBMs in terms of ICC and correlation. However, contrary to what may be expected, mean change in utility scores before and after treatment and mean differences across severity groups were often larger for the generic preference-based measures than the CSPBMs. One possible explanation for the larger mean change and differences is that generic measures also capture changes in HRQoL owing to comorbidities and side effects. Another possibility is that the EQ-5D value set used here has a wider range of potential values than all other preference-based measures used here. The large range of the UK EQ-5D value set is unique to that valuation study, as it has not been found in valuation studies of other measures or in valuation studies of the same classification in other countries (see, for example, Shaw et al. 110).
Conclusion
There is little evidence of information loss from moving from the original condition-specific measures to the preference-based measures derived from them for four condition-specific measures for asthma, common mental health problems, cancer and overactive bladder. The performance of the CSPBMs and generic preference-based measures was similar for responsiveness to capturing change following treatment yet CSPBMs performed better at discriminating between groups with different severity. Although the benefits of CSPBMs over generic preference-based measures may not be as marked as expected, their larger effect size is important for trials and for the reduced uncertainty in the values they generate. The larger effect sizes were due to smaller SDs, as mean change and differences were larger for EQ-5D than the CSPBMs. The large mean change and SD of EQ-5D may be due to the UK value set used here,43 and further research examining this is recommended. Ceiling effects were lower for the CSPBMs than generic EQ-5D, suggesting greater responsiveness for respondents at the upper end of HRQoL. The analysis conducted has been limited to nine data sets, the majority of which did not have multiple time points, and further research in this area is recommended.
These results suggest that the CSPBMs have better or similar performance to the generic preference-based measures regarding discriminative validity across severity groups and responsiveness to change following treatment. The performance of CSPBMs is similar to the measure they are derived from, suggesting that CSPBMs are only likely to offer an improvement on generic preference-based measures where the original condition-specific measure offers an improvement on the generic preference-based measures. The development of CSPBMs from existing measures should be limited to measures that are valid and responsive and that offer an improvement on generic preference-based measures, typically where the generic PBM is inappropriate.
Chapter 7 Discussion and conclusions
There has been a rapid proliferation in preference-based condition-specific measures and the methods for developing them (see Chapter 2). However, there remain some fundamental concerns whether they can be used to make comparisons between interventions for different conditions and programmes of care. 6,41,111 Even using generic preference-based measures does not ensure comparability, as significant differences between the different generic preference-based measures have been found. 5 It has been argued that the only way to achieve cross-programme comparability is to use the same generic preference-based measures. Using one instrument in all studies is the only way to ensure that different patient groups are being judged in terms of the same dimensions of health, using the same valuation methods and the values are obtained from the same sample. Comparability is very important to policy-making organisations such as NICE and is one reason why NICE has expressed a preference for the EQ-5D in its reference case set of methods for economic evaluations. 6
The argument against relying on one measure is that in many cases EQ-5D data (or whatever instrument is chosen) may not be available in the relevant studies (e.g. pivotal trials or other studies used to populate economic models) or may not be appropriate for the condition or patient group. Comparability between CSPBMs can be achieved to some extent by the use of a common numéraire, such as a year in full health or money. It has been argued that provided the values are obtained using the same valuation technique, with the same tightly controlled protocol (e.g. mode of administration, elicitation procedure, visual aids, wording of question, etc.), common anchors (full health and dead) and the same type of respondents (such as a representative sample of the general population), a common measuring stick is being used and so comparisons can be made between quality adjustment weights estimated using different classification systems. This argument would imply that there is no need to have a common classification system in order to achieve consistency in decision-making. It seems to have been an implicit assumption in the willingness-to-pay literature and the early QALY literature of the 1970s and 1980s that this is the case. Indeed there is no other area of applied economics in which the description of benefit has to be standardised across programmes. However, the extent to which this claim is likely to be true is revisited in this chapter in the light of the findings of the research reported in this report.
The development of preference-based condition-specific measures should not be seen as an alternative to generic preference-based measures but rather as a complement. The remainder of this chapter considers in more detail the potential role of CSPBMs in economic evaluations to inform resource allocation across health programmes and goes on to specify the conditions that need to be satisfied in order to justify the development of CSPBMs.
Problems in achieving cross-programme comparability
There are a number of obstacles to achieving comparability using different classification systems, including the exclusion of side effects, the problem of naming the condition, focusing effects, the lack of a common anchor and the potential impact of comorbidities.
Naming the condition
Many condition-specific measures name the condition in their items, and this is reflected in some of the health-state classifications, such as one for overactive bladder. 31 This is thought to improve its sensitivity. However, there are concerns that naming the condition in the health state may invite respondents valuing the state to consider their experience or preconceptions of the condition. These may be views that the condition is better or worse than being described. This would distort the values for a condition-specific health state. Another concern is the different nature of causal attribution in non-patients/hypothetical states and patients/real states. Take the statement ‘asthma interferes with getting a good night’s sleep’. If this is used in describing hypothetical states, we know that by definition that asthma is causing the sleep problem. But if this is used in patient self-report then how certain can we be that asthma is causing the sleep problem? We will not be asking for a purely factual statement, but for the patient’s guess as to what is causing the sleep problem. Maybe they have financial worries that are keeping them from sleeping well, and the worry and stress is also triggering asthma symptoms; does that count as asthma interfering with sleep?
Chapter 4 reported on a labelling study in which health states were valued by three groups of respondents that were identical, except that one group valued unlabelled states, one valued states labelled as cancer and the other valued states labelled as IBS. The inclusion of a cancer label was shown to significantly reduce the elicited health-state utility values and the impact differed depending on the severity of the state, whereas the inclusion of an IBS label had no impact on utility values. Further research is required to determine which other condition labels may potentially affect elicited utility values.
There are persuasive arguments that the difference in elicited values owing to a condition label represents greater accuracy in the value for that state. The condition label may provide more accuracy in the description of the health state that enables respondents to more accurately value the state. For example, respondents may place a different value on interference with social activities caused by needing to be near a toilet than as a result of undergoing chemotherapy. Furthermore, the condition label itself may affect the quality of life experienced in a particular health state. For example, a cancer health state may differ in its quality of life, although the health status is measured the same in terms of a classification system, such as the generic EQ-5D or cancer-specific EORTC-8D.
However, without qualitative research we cannot conclude that the differences in utility values owing to condition labels are not distortions arising from prior knowledge or preconceptions of the health state or factors irrelevant to valuing a health state. For example, the differences observed with the condition label could be caused by fear or dread associated with cancer that is not experienced by the patient (as reflected in the health-state description that will include emotional health), stigmatisation of patients with cancer or taking account of mortality. These factors would make the elicited TTO estimates biased. We therefore recommend the exclusion of condition labels from health-state descriptions, where practical, to ensure that utility values are not distorted by prior knowledge, experience or preconceptions of the condition. However, developing preference-based measures will be limited by the content of the original instrument and for many this includes the condition label. However, this may not matter for all conditions and further research is required to examine the extent of this problem. Qualitative research may demonstrate that the differences in values owing to a condition label represent greater accuracy rather than a distortion due to prior knowledge or preconceptions.
This raises the question of whether or not utility values used to inform resource allocation decisions should reflect this difference. We outlined in Chapter 4 that, as resource allocation distributes resources across different conditions and different patient groups, it is typically argued that there must be comparability in health-state descriptions irrespective of the underlying condition causing that health state. For example, the same health state experienced by a patient with cancer, IBS, heart disease, depression or diabetes should be given the same preference weighting in terms of its impact on utility. We therefore argue that health-state values used in economic evaluation should not reflect any difference in values due to a condition label. However, if qualitative research shows that differences in values due to a condition label are caused either by greater accuracy in the health-state description or the associated impact on the quality of life (rather than health per se) experienced in the condition, this implies that the utility value associated with a health state may legitimately vary by condition. These values could then be selected for use in economic evaluation on the grounds that they represent greater accuracy, and that comparability may be of secondary importance if comparability is met only by sacrificing accuracy. If it can be demonstrated using further research that the inclusion of a condition label in valuation studies enables greater accuracy in elicited utility values, this opens a new chapter in the debate of whether CSPBMs should be used in economic evaluation.
Side effects
An obvious limitation of condition-specific measures is that they may fail to pick up side effects of treatment. This could be dealt with by including additional dimensions to the condition-specific measure to cover known side effects, though this will miss any unknown side effects. This approach was examined in Chapter 5, in which a pain/discomfort dimension was added to the asthma-specific AQL-5D. Although these may not be common side effects of treatment, it provided an example of the impact of adding an extra dimension. The study found that the extra dimension had a significant impact on health-state values but that the impact was not additive. The net effect on a patient’s utility value depended on the severity of their state: the addition of pain/discomfort at level 1 (no pain/discomfort) or 2 (moderate pain/discomfort) significantly increased the mean health-state values in an asthma patient population, whereas level 3 pain/discomfort (extreme) reduced values. We also estimated a regression model of preference weights for the larger AQL-6D classification system and found that the additional dimension for pain/discomfort did impact on the condition-specific dimensions and that the impact was not consistent across dimensions. This implies that a CSPBMs would need to be completely revalued following the addition of a dimension to cover a side effect rather than simply adding the preference weights of an extra dimension to the existing value set.
Adding one or two dimensions would also reduce the practical advantage of basing a CSPBMs on an existing condition-specific measure. It would require extra data to be collected on the new dimension unless the extra dimension is based on another widely used measure (such as a generic measure).
Anchoring
Even for generic preference-based measures, the upper anchor used in the valuation task is the instrument-specific best state, and respondents may still imagine other health problems. So what is the respondent thinking about the dimensions that are not mentioned in the (condition-specific measure) health state they are being asked to value? For some dimensions they may not pay them any attention. Where they do think about them, respondents may simply assume that other dimensions are at their optimum level or that they are at the same level as their own health. However, anything other than ‘full health’ or ‘perfect health’ makes comparability between preference-based instruments problematic. Evidence from the add-on study suggests that respondents do not assume other dimensions are at full health. The addition of pain/discomfort at level 1 (no pain/discomfort) or 2 (moderate pain/discomfort) actually increased the mean health-state values in an asthma patient population; the addition of a physical health dimension at level 1 to the emotional component of the CORE-6D also increased their mean health-state value. This suggests that respondents were assuming there would be problems in other dimensions (rightly or wrongly). Our recommendation is to use a generic upper anchor in health-state valuation tasks to improve comparability between preference-based measures.
Focusing effects
Another possible concern with using CSPBMs is focusing effects. We tend to focus on those things that are placed in front of us. Respondents, therefore, will tend to focus on the problems described in the health state they are valuing rather than other aspects of their health or indeed other aspects of their life. This results in respondents exaggerating the importance of the problems associated with the condition being valued compared with other conditions. For any given health state, this suggests that CSPBMs may generate a larger decrement from any given dimension of health than a generic measure simply because the respondent is not being given the broader context. This also has important implications for comorbidities. Interestingly our research has suggested that respondents do think about other dimensions of health and so this may not be as important as initially suspected.
Comorbidities
Where there are comorbidities, the achievement of comparability between specific instruments requires the assumption that the impact of different dimensions on preferences is additive, whether or not they are included in the classification system. The impact of breathlessness on health-state values, for example, must be the same whether or not the patient has other health problems not covered by the classification system, such as joint pain. Otherwise, even in the scenario that the intervention only alters the dimensions covered in the specific instrument, the estimated change in health-state value may be incorrect due to preference dependence between dimensions included in the classification system of the specific measure and those dimensions not included. Having rheumatic pain, for example, may have an impact on the size of the health gain associated with the treatment of breathlessness.
It might have been expected that adding a comorbidity would reduce health-state values by a constant amount, regardless of the severity of the condition-specific state. The study in Chapter 5 has shown that the addition of an extra dimension at its worst level reduced the health-state values, as would be expected. However, it also showed that the addition of a dimension at the intermediate level or lowest level in most cases resulted in increases in health-state values. The AQL-5D/AQL-6D study went on to estimate the impact of the additional dimension for pain/discomfort on the coefficients of the other dimensions. The results were not consistent across dimensions and show that a simple additive adjustment would not adequately capture the effect of adding the pain/discomfort dimension to the classification system. The results from the CORE-6D study outlined in Chapter 5 were more consistent with a simple additive assumption.
Health-state utility values estimated from CSPBMs assume that the patient has only one condition. Where patients have comorbidities or where there are important side effects then our findings suggest that the values are not going to reflect the marginal impact of the condition. This is potentially a serious limitation for use within a condition as well for making cross-programme comparisons.
Conclusion
Respondents in health-state valuation studies struggle with many pieces of information at once and so there is a practical constraint on the size of classification systems designed for preference-based measures. Any classification system that is amenable to valuation will exclude some dimensions of health and so there will also be gaps in the coverage of generic measures such as EQ-5D. Generic measures were not developed to provide a complete picture of a person’s HRQoL. 112 The decision to use a CSPBMs in cross-programme comparisons is ultimately a trade-off. The advantage of a measure that is more relevant and sensitive to those things that matter to patients with the condition needs to be compared with the disadvantages from excluding side effects of treatment, distortions created by focusing effects and the potential loss of comparability from preference interactions with dimensions not covered by the narrower focus of the CSPBMs.
This trade-off will vary between condition-specific measures and CSPBMs. Some measures will be less prone to problems such as focusing effects and comorbidities, as they contain generic dimensions of HRQoL, such as the EORTC QLQ-C30 and preference-based EORTC-8D derived from it. Others will be more likely to suffer from these effects, such as those measures that focus on a narrow range of symptoms. More work is needed to explore the likely size of these effects and how they compare with any gain from using a particular measure. We believe that the priority for future research is to focus on how the focus, dimensionality and framing of the classification system impacts on health-state utility values and to provide recommendations for future studies developing preference-based measures.
Limitations
The limitations of each study are included in each chapter. There are some overall limitations of the project that are summarised here. First, it is often not possible to generalise across all CSPBMs; for example, some focus on symptoms and may be unable to capture side effects and comorbidities, whereas some focus more on HRQoL and it is realistic to expect they are able to capture side effects and comorbidities. Furthermore, for some conditions labelling may have an impact, while for others it may not. Likewise, some measures may include all important dimensions, whereas for others there may be important dimensions missing. Second, the lack of detail and clarity in the reporting of the development of CSPBMs makes comparisons across the development of measures difficult and provides significant challenges for the methodology in the derivation of the classification system to be understood and able to evolve to become more scientifically rigorous. Finally, it is important to examine the performance of CSPBMs in comparison with generic preference-based measures to determine whether they do have an advantage and to determine the impact on the results if these values are used in economic evaluation; however, there are few available data that can be used to examine this and the analysis conducted here has been constrained by this.
When should a condition-specific preference-based measure be developed and/or used?
There are two situations where it might be worth considering the development and use of a CSPBM to generate QALYs for use in economic evaluation; one is where generic data are not available and the other is where the generic measures are thought to be inappropriate.
Unavailability of generic data
The lack of generic data arises from the failure to use a generic preference-based measures in key clinical trials or other studies. However, the lack of data collected in specific trials may not demonstrate a lack of availability for populating an economic model. There could be other related studies or routine sources that provide the necessary evidence on the values for the key states used in the model that may be undertaken to support the submission, or the values might be identified by a systematic search of the literature. In some situations, an alternative approach to deriving health-state values will be to map the condition-specific measure (and other variables) on to the relevant generic measure (see Brazier and Tsuchiya113 for an overview of mapping). Where data sets containing both measures in a relevant patient sample are available then mapping may be a quicker and cheaper solution. Mapping has its own problems and its success varies between conditions and instruments, and current practice tends to ignore the uncertainty arising from the mapping function itself. 114 Where there are no means of obtaining relevant health-state utility values using generic measures, then a CSPBM will be the only solution.
Generic measures are not appropriate
Pharmacoeconomic guidelines often recommend the use of generic measures for economic evaluation, and in the case of NICE there is a strong preference for one generic measure (i.e. EQ-5D). 6 However, no agencies rule out the use of CSPBMs. In the case of NICE, alternative methods are permitted where the EQ-5D has been shown to be inappropriate. One approach is to consider the appropriateness of a generic preference-based measure for the patient group in terms of psychometric criteria, such as practicality, reliability, validity and responsiveness. 6 Given the problems with CSPBMs, this would seem to be a sensible approach and one we will adopt here. The assessment of the appropriateness of EQ-5D has recently been addressed in some detail in a NICE DSU Technical Support Document,115 so here we summarise the key concerns.
The practicality of an instrument depends on its acceptability to respondents. All of the generic measures are quite short and for most patients are quite modest in burden compared with other questionnaires and clinical assessments that they have to endure. However, there may be concerns in certain populations with whether it is possible for patients to meaningfully respond, such as when they are extremely ill or cognitively impaired (e.g. case of dementia). In these cases, proxy responses can be used and this problem would apply as much to CSPBMs as to generic measures.
Reliability is the ability of a measure to reproduce the same value on two separate administrations when there has been no change in health. This can be over time, between methods of administration or between raters. Evidence on the reliability of the generic instruments does indicate significant random variation between assessments, but again this is no more than would be found with CSPBMs. 5
The assessment of validity is far more problematic. The primary difficulty is the lack of a gold standard measure of health-state utilities. The challenge of assessing validity pervades the measurement of all psychological phenomena and has been met in the psychometric literature by the development of various tests that have been adapted for use with preference-based measures. 13,115 The validity of a preference-based measure is the product of the classification system and the methods of valuation. Assuming the methods of valuation are acceptable, then the issue of validity is concerned with the classification system that defines the coverage and sensitivity of the instrument [although most available evidence is concerned with the index (Papaioannou et al. 11)].
The validity of the content of a measure depends on the extent to which it covers the areas of quality of life that is likely to be altered by a condition and its treatment. This can be assessed using qualitative methods such as in-depth interviews and focus groups of patients, and this is the approach recommended by the US FDA12 for patient-reported outcome measures to support labelling claims. The face validity of a classification system can be assessed using cognitive interview techniques to establish whether patients, for example, understand the descriptions in the way they are intended to be understood. Such evidence will identify a potential problem but will not be definitive, as an absent dimension may be picked up by the other dimensions, particularly generic dimensions such as well-being and social activities.
An important quantitative test or series of tests comes under the term construct validity. This is a method for testing empirically the extent to which a measure agrees with other measures or indicators of the dimensions of HRQoL considered relevant to the patient group (such as those identified by qualitative work). There are two commonly used approaches in the psychometric literature to examining construct validity of a standardised classification system. One approach is to examine whether it is able to differentiate between groups thought to differ in terms of their health (i.e. known group differences) and the other is the extent to which it correlates with another measure of health (i.e. convergent validity). These tests provide evidence on the degree to which a measure is valid at measuring the concept being tested. A related test is responsiveness, which is the ability to respond to known changes in HRQoL.
There are two weaknesses in the use of such evidence. One is that the construct variable used to define ‘known group differences’ may not be valid. This will be particularly the case where clinical measures are used such as visual acuity, respiratory function or symptoms of schizophrenia that may have only a weak relationship to HRQoL in any case. For responsiveness, the alleged change in health can come from assumed changes before and after an intervention that may not have improved patient health. Second, validity is not dichotomous but a matter of degree, and deciding whether a measure is sufficiently valid is ultimately a matter of judgement. A CSPBM may achieve a larger difference or change as indicated by a standardised effect size [where the mean change in score is divided by either the SD at baseline or the SD of the change (Katz et al. 116)], for example, but effect sizes do not indicate the value or importance of a change.
Where the classification systems of generic measures seems to fail to pick up differences or changes suggested by the condition-specific measure, or at least suggests a much smaller difference, then there needs to be some other evidence that this is likely to be important to the general public. A study, for example, could be undertaken to establish whether a dimension of HRQoL excluded from the generic measures is important to members of the general public.
This careful assessment of the evidence provides the basis for deciding whether it is worth developing a full CSPBM. The next question is which condition-specific measure should be used.
Choice of condition-specific measure
The first issue is whether to work with an existing condition-specific measure or to develop a new preference-based condition-specific measure de novo. There may not be a suitable existing condition-specific measure and so it will be necessary to develop a new measure from scratch.
The advantages in working with an existing measure are that utility values can be generated for existing data sets, and this is likely to be a key practical advantage. Existing measures are more likely to be acceptable to the clinical and research communities at large. For many conditions, there are one or two established measures that are widely accepted and so would provide a useful vehicle for estimating health-state utility values. However, there are some conceptual and practical considerations. Our research suggests that measures focusing on a narrow range of dimensions are likely to be more prone to focusing and comorbidity effects. Conceptually, condition-specific measures that cover a broad range of dimensions are to be preferred over those that do not. It is also better to have HRQoL dimensions rather than symptoms, as these are more likely to be broader in coverage. Although it is not possible to entirely rule out symptoms (e.g. pain in EQ-5D), a measure that is entirely symptom-based is going to be most prone to the problems described above. Consideration also needs to be given to likely side effects. Some condition-specific measures, such as the EORTC QLQ-C30 in cancer, include dimensions designed to pick up known effects but this may miss those that are not predicted in advance.
At a practical level, some questionnaires are more difficult to convert into health-state classifications. One problem that we have addressed through the use of Rasch techniques is the high correlation between domains. Other practical difficulties may include items that use evaluative terms in the response choices such as ‘bother’ that actually already encapsulate values. Some condition-specific measure items and levels are too wordy or do not combine well to form health states that would be comprehensible to respondents undertaking a valuation task.
Before pursuing the expense of developing a CSPBM, it is advisable to compare the condition-specific measure with existing generic measures. Evidence here suggests a mixed picture, with the condition-specific measure performing better using the criteria of responsiveness and validity in some cases, but in other cases it does not seem to offer much advantage.
Valuation
To improve comparability between condition-specific measures, it is important that they are valued using the same methods. This is difficult to achieve, as there is no international co-ordination of this effort. However, when developing CSPBMs thought should be given to the likely use of the evidence and where it is going to be used.
Demonstrating the impact of using condition-specific preference-based measures
Given the problems with CSPBMs, it is important to demonstrate the advantages that any one measure has over existing generic measures. This would mean replicating the types of analyses performed in Chapter 6, in which we compared the performance of the CSPBMs with generic measures in terms of validity and responsiveness. The results in some cases suggested that the CSPBM was not better. In other cases, the CSPBMs did offer greater sensitivity and so policy-makers would need to decide whether this is worth the potential disadvantages in reduced comparability across programmes. This is a judgement that will be specific to the condition, the CSPBM and the advantages it appears to have over the preferred generic measures. However, it is worth noting that all CSPBMs produced similar results to EQ-5D, suggesting that the choice of measure may have minimal impact on results. In fact our results in Chapter 6 suggest that mean utility change and SD of the change may actually be smaller for the CSPBMs than EQ-5D for the measures examined in the report.
The add-on agenda
Research is under way looking at the potential role of adding dimensions to the EQ-5D to improve its relevance to specific conditions. 90 This would avoid or at least reduce some of the problems associated with CSPBMs. However, it requires the additional modules to be developed and valued and for the ‘EQ-5D-plus’ measure to be used in clinical studies. Furthermore, the number of extra dimensions that can be added at one time is limited by respondents’ ability to value large health states. There is important empirical work to be done to examine the scope for adding dimensions to common generic measures and to see whether this approach overcomes the limitations of generic measures without the need to use CSPBMs. This research suggests that it will not be possible to assume that the extra dimensions simply have an additive impact on existing EQ-5D value sets.
Conclusion
Respondents in health-state valuation studies struggle with too many pieces of information at once and so there is a practical constraint on the size of descriptive systems designed for preference-based measures. Any descriptive system that is amenable to valuation will exclude some dimensions of health and so there will be gaps in the coverage of generic measures such as EQ-5D. Generic measures were not developed to provide a complete picture of a person’s HRQoL (see Williams112). The decision to use a preference-based condition-specific measure in cross-programme comparisons is ultimately a trade-off between having a measure that is more relevant and sensitive to those things that matter to patients against less cross-programme comparability (due to from excluding side effects of treatment, distortions created by focusing effects and impact of comorbidities). Comparability is further reduced from using different valuation methods.
The development of CSPBMs has been increasing rapidly in recent years. Arguably, insufficient consideration has been given to the appropriateness of some of the CSPBMs that have been developed and whether or not they really do offer an improvement. This chapter has reviewed the important role of evidence on the validity and responsiveness of generic and condition-specific measures before deciding to use CSPBMs.
Chapter 8 Recommendations
This report provides a review of methods for developing CSPBMs, reports on a series of five studies that addressed various problems that have arisen in the field of CSPBMs, and examines the implications for using CSPBMs in economic evaluation. This chapter tries to bring together this research in the form of a series of recommendations about the development, testing and use of CSPBMs in economic evaluation and an agenda for future research.
When to develop and use condition-specific preference-based measures?
We have developed a simple flow chart of conditions that should be met before considering whether to develop a CSPBM for use in economic evaluation (Figure 3). This flow chart can be used to inform the decision of whether values from CSPBMs should be considered. CSPBMs have an important role where generic measures are inappropriate for a given condition. Inappropriateness is difficult to prove in this area in the absence of a gold standard, but we recommend that reviews are undertaken to inform any judgement about whether generic measures are not sensitive to potentially important differences. The decision to develop or use a CSPBM for economic evaluation is ultimately a judgement that involves a trade-off between any advantages in using a classification system more appropriate to the condition against potential reductions to comparability across conditions.
FIGURE 3.
Considerations in the development of a CSPBM for use in economic evaluation. CSM, condition-specific measure.
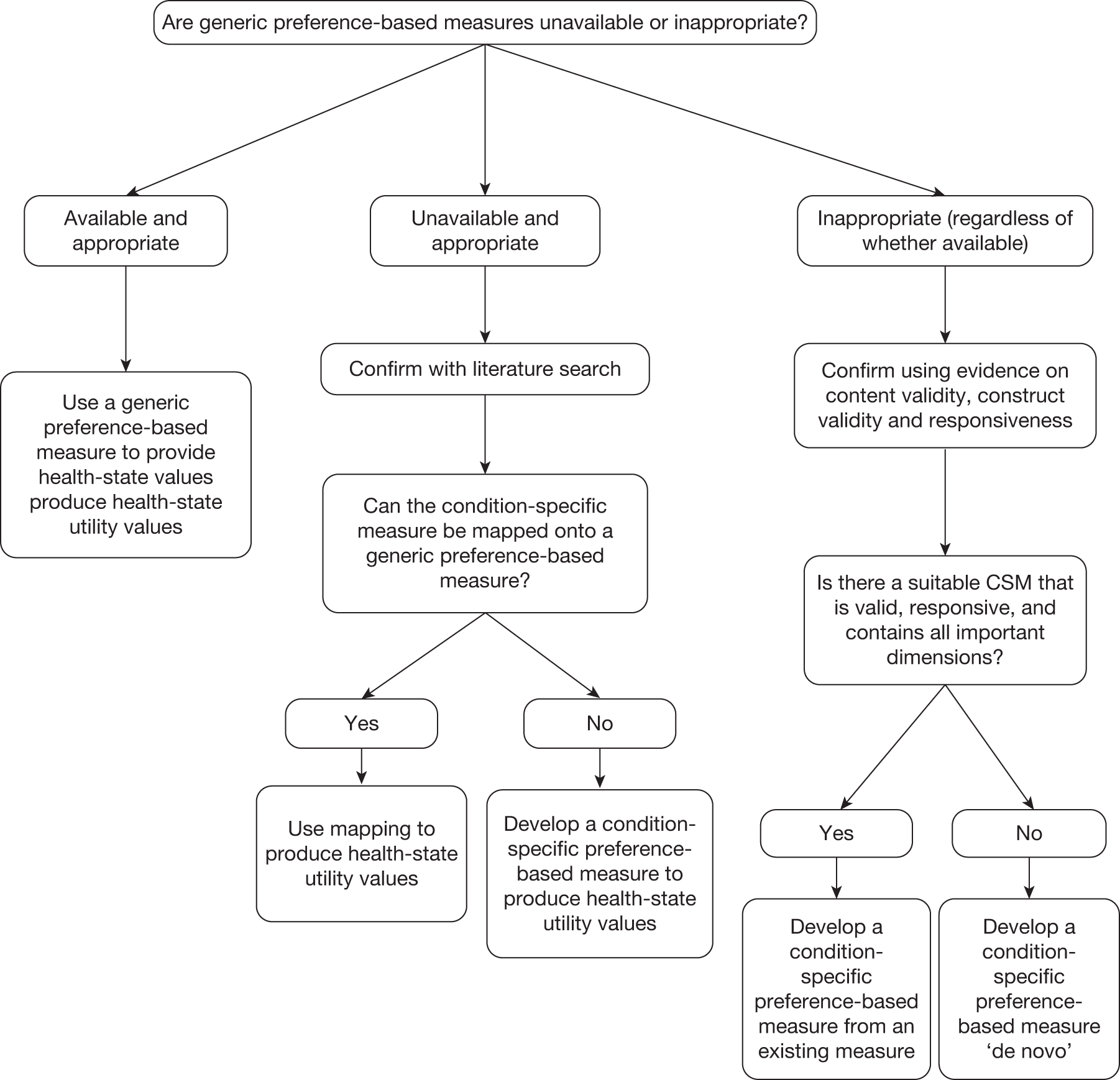
How to develop condition-specific preference-based measures
The first consideration is whether to develop a new condition-specific health-state classification or to develop one from an existing condition-specific measure. This decision should be based on considerations of the appropriateness of the condition-specific measure for the condition, including validity and responsiveness. It should also take into account the requirements of potential policy-makers, such as whether they require a measure of HRQoL or whether one based on symptoms would be acceptable.
This report describes the six stages to developing CSPBMs and the key recommendations are as follows:
Health-state classification
-
Use explicit criteria and methods for implementing those criteria in the development of a health-state classification system (stages I–III).
-
Not to use condition labels in the health-state classification system (where possible) to avoid any potential distortions at the valuation stage due to prior knowledge, preconceptions or irrelevant information about future prognosis.
-
Incorporate important side effects of treatment or comorbidities in the patient population into the health-state classification system to avoid potential inaccuracies at the valuation stage. This can be undertaken as add-ons for existing CSPBMs.
-
A health-state classification system developed from an existing measure should be validated on another data set (stage IV).
Valuation
-
To enhance comparability it is helpful for the CSPBMs to use the same valuation methods (stage V) as used for the generic and other CSPBMs with which it is likely to be compared.
-
The Rasch vignette approach rather than a conventional statistical design should be used to generate the states for valuation where the domains of an instrument are highly correlated and form a unidimensional scale (stage VI).
For further guidance on other aspects of the development of CSPBMs – including methods of eliciting values, the sources of values and the modelling of health-state values – readers should refer to the broader literature (see Brazier et al. 5 for an overview).
All methods should be fully reported.
Testing and comparison with generic measures
-
The degree of information loss of moving from the original condition-specific measure to the CSPBMs should be demonstrated in order to meet concerns of the wider community of clinical researchers.
-
The performance of the new CSPBMs should be compared with the generic preference-based measures in order to quantify any gains from its use.
-
The impact from using the CSPBMs compared with the generic measure should be examined in terms of the degree of agreement between the preference-based measures.
Research agenda
Condition-specific measures are going to continue to be an important source of data on the effectiveness of health-care interventions. CSPBMs have an important role to play in order to ensure that the benefits of health-care interventions are properly reflected in the QALY estimates for all patient groups. To meet this demand, there is future agenda of research to improve the development and usage of CSPBMs:
-
Further research is required into the appropriateness of generic preference-based measures compared with condition-specific measures of health including their psychometric performance and more qualitative work into the content and face validity of the measures.
-
On labelling, more quantitative work is required into the impact of naming different medical conditions to establish whether or not other condition labels impact on health-state values. For some CSPBMs it has not been possible to avoid any mention of the condition because it is part of the wording of the items from the original measure.
-
Qualitative research into labelling is also required to examine whether any impact (as was found for cancer) comes from a more accurate description of the state or from distortions caused by false preconceptions or irrelevant prognostic information (e.g. mortality).
-
For existing and future CSPBMs, research is recommended to incorporate major side effects and highly prevalent comorbidities in add-on studies to examine their likely impact and, where they are important, to increase their comparability with other preference-based measures.
-
CSPBMs need to be compared with generic preference-based measures in order to examine the extent of any advantages that they may have.
-
Research into the use of items in condition-specific measures as add-ons to the EQ-5D (as an alternative approach to developing full CSPBMs).
Acknowledgements
The authors would like to thank all the interviewees who took part in the valuation surveys for this project. The authors would also like to thank Angie Rees for undertaking the literature search for the review and Liz Metham for formatting the report.
Contribution of authors
John Brazier (Professor, Health Economics) managed the project and contributed to the methodology and interpretation of results at each stage.
Donna Rowen (Research Fellow, Health Economics) conducted the review of methodology of the development of CSPBMs and analysed the data for the labelling study, add-on studies and performance of measures.
Ifigeneia Mavranezouli (Senior Health Economist) conducted the development of the preference-based CORE-6D from CORE-OM and examined the performance of CORE-OM in comparison with other measures.
Aki Tsuchiya (Professor, Health Economics) contributed to the methodology and interpretation of results at each stage.
Tracey Young (Senior Research Fellow, Health Economics and Statistics) provided statistical input to the project.
Yaling Yang (Research Fellow, Health Economics) contributed to the review of methodology of CSPBMs, the AQL-6D add-on study and examined the performance of AQL-5D and OAB-5D in comparison with other measures.
Michael Barkham (Professor of Clinical Psychology) contributed to the development of the CORE-6D from the CORE-OM.
Rachel Ibbotson (Research Fellow, Data Management) managed the primary data collection for the labelling, add-on and CORE-6D valuation surveys.
John Brazier and Donna Rowen took responsibility for writing the report.
Disclaimers
The views expressed in this publication are those of the authors and not necessarily those of the HTA programme or the Department of Health.
References
- Brooks R. EuroQol: the current state of play. Health Policy 1996;37:53-72.
- Brazier J, Roberts J, Deverill M. The estimation of a preference-based measure of health from the SF-36. J Health Econ 2002;21:271-92.
- Brazier JE, Roberts J. The estimation of a preference-based measure of health from the SF-12. Med Care 2004;42:851-9.
- Feeny D, Furlong W, Torrance GW, Goldsmith CH, Zhu Z, Depauw S, et al. Multiattribute and single-attribute utility functions for the health utilities index mark 3 system. Med Care 2002;40:113-28.
- Brazier JE, Ratcliffe J, Solomon JA, Tsuchiya A. Measuring and valuing health for economic evaluation. Oxford: Oxford University Press; 2007.
- National Institute for Health and Clinical Excellence (NICE) . Guide to the Methods of Technology Appraisal 2008.
- Espallargues M, Czoski-Murray CJ, Bansback NJ, Carlton J, Lewis GM, Hughes LA, et al. The impact of age-related macular degeneration on health status utility values. Invest Ophthalmol Vis Sci 2005;46:4016-23.
- Barton GR, Bankart J, Davis AC, Summerfield QA. Comparing utility scores before and after hearing-aid provision : results according to the EQ-5D, HUI3 and SF-6D. Appl Health Econ Health Policy 2004;3:103-5.
- Walters SJ, Morrell CJ, Dixon S. Measuring health-related quality of life in patients with venous leg ulcers. Qual Life Res 1999;8:327-36.
- Haywood K, Garratt A, Lall R, Smith J, Lamb S. EuroQol EQ-5D and condition-specific measures of health outcome in women with urinary incontinence: reliability, validity and responsiveness. Qual Life Res 2008;17:475-83.
- Papaioannou D, Brazier J, Parry G. How valid and responsive are generic health status measures, such as the EQ-5D and SF-36, in schizophrenia? A systematic review. Value Health 2011;14:907-20.
- US Department of Health and Human Services Food and Drug Administration (FDA) . Guidance for Industry: Patient-Reported Outcome Measures: Use in Medical Product Development to Support Labeling Claims 2009.
- Brazier J, Deverill M. A checklist for judging preference-based measures of health related quality of life: learning from psychometrics. Health Econ 1999;8:41-5.
- Brazier JE, Yang Y, Tsuchiya A, Rowen DL. A review of studies mapping (or cross walking) non-preference based measures of health to generic preference-based measures. Eur J Health Econ 2010;11:215-25.
- Gray AM, Rivero-Arias O, Clarke PM. Estimating the association between SF-12 responses and EQ-5D utility values by response mapping. Med Decis Making 2006;26:18-29.
- Rowen D, Brazier J, Roberts J. Mapping SF-36 onto the EQ-5D index: how reliable is the relationship?. Health Qual Life Outcomes 2009;7.
- Torrance GW. Measurement of health state utilities for economic appraisal: a review. J Health Econ 1986;5:1-30.
- Tosh J, Longworth L, George E. Utility Values in NICE Technology Appraisals. Value Health 2010;14:102-9.
- Brazier J, Dixon S, Brazier J, Dixon S. The use of condition specific outcome measures in economic appraisal. Health Econ 1995;4:255-64.
- Revicki DA, Leidy NK, Brennan-Diemer F, Thompson C, Togias A. Development and preliminary validation of the multiattribute Rhinitis Symptom Utility Index. Qual Life Res 1998;7:693-702.
- Revicki DA, Leidy NK, Brennan-Diemer F, Sorenson S, Togias A. Integrating patient preferences into health outcomes assessment: the multiattribute asthma symptom utility index. Chest 1998;114:998-1007.
- Stolk EA, Busschbach J. Validity and feasibility of the use of condition-specific outcome measures in economic evaluation. Qual Life Res 2003;12:363-71.
- Brazier JE, Roberts J, Platts M, Zoellner YF. Estimating a preference-based index for a menopause specific health quality of life questionnaire. Health Qual Life Outcomes 2005;3.
- Juniper EF, Buist A, Cox F, Ferrie P, King D. Validation of a standardized version of the Asthma Quality of Life Questionnaire. Chest 1999;115:1265-70.
- Juniper EF, Guyatt GH, Ferrie P, Griffith L. Measuring quality of life in asthma. Am J Respir Dis 1993;147:832-8.
- Brazier JU. Deriving a preference-based single index from the UK SF-36 Health Survey. J Clin Epidemiol 1998;51:1115-28.
- Stevens KJ, Brazier J, McKenna SP, Doward LC, Cork MJ. The development of a preference-based measure of health in children with atopic dermatitis. Br J Dermatol 2005;153:372-7.
- Yang Y, Brazier J, Tsuchiya A, Young T. Estimating a preference-based index for a 5-dimensional health state classification for asthma derived from the Asthma Quality of Life Questionnaire. Med Decis Making 2011;31:281-91.
- Young T, Yang Y, Brazier J, Tsuchiya A. The use of Rasch analysis in reducing a large condition-specific instrument for preference valuation: the case of moving from AQLQ to AQL-5D. Med Decis Making 2011;31:195-210.
- Yang Y, Brazier J, Tsuchiya A, Coyne K. Estimating a preference-based single index from the overactive bladder questionnaire. Value Health 2009;12:159-66.
- Young T, Yang Y, Brazier JE, Tsuchiya A, Coyne K. The first stage of developing preference-based measures: constructing a health-state classification using Rasch analysis. Qual Life Res 2009;18:253-65.
- Brazier JE, Czoski-Murray C, Roberts J, Brown M, Symonds T, Kelleher C. Estimation of a preference-based index from a condition-specific measure: the King’s health questionnaire. Med Decis Making 2008;28:113-26.
- Ratcliffe J, Brazier J, Tsuchiya A, Symonds T, Brown M. Using DCE and ranking data to estimate cardinal values for health states for deriving a preference-based single index from the sexual quality of life questionnaire. Health Econ 2009;18:1261-76.
- Rowen D, Brazier J, Young T, Gaugris S, Craig BM, King MT, et al. Deriving a preference-based measure for cancer using the EORTC QLQ-C30. Value 2011;14:721-31.
- Young T, Yang Y, Brazier J, Tsuchiya A, Coyne K. Making Rasch decisions: the use of Rasch analysis in the construction of preference based health related quality of life instruments. Health Economics and Decision Science Discussion Paper 08/05. Sheffield: University of Sheffield; 2008.
- Rasch G. Probabilistic models for some intelligence and attainment tests. Chicago, IL: University of Chicago Press; 1960.
- Tesio L. Measuring behaviours and perceptions: Rasch analysis as a tool for rehabilitation research. Journal Rehabil Med 2003;35:105-15.
- Torrance GW, Boyle H, Horwood S. Application of Multi-Attribute Utility Theory to Measure Social Preferences for Health States. Oper Res 1982;30:1043-69.
- Torrance GW, Feeny DH, Furlong WJ, Barr RD, Zhang Y, Wang Q. Multiattribute utility function for a comprehensive health status classification system. Health Utilities Index Mark 2. Med Care 1996;34:702-22.
- Sugar CAS. Empirically defined health states for depression from the SF–12. Health Serv Res 1998;33:911-28.
- Dowie J. Decision validity should determine whether generic or condition-specific HRQOL measure is used in health care decisions. Health Econ 2002;11:1-8.
- Dolan P. Modeling valuations for EuroQol health states. Med Care 1997;35:1095-108.
- Fitzpatrick R, Davey C, Buxton MJ, Jones DR. Evaluating patient-based outcome measures for use in clinical trials. Health Technol Assess 1998;2.
- Beusterien K, Leigh N, Jackson C, Miller R, Mayo K, Revicki D, et al. Integrating preferences into health status assessment for amyotrophic lateral sclerosis: the ALS Utility Index. Amyotroph Lateral Scler 2005;6:169-76.
- Burr JM, Kilonzo M, Vale L, Ryan M. Developing a preference-based glaucoma utility index using a discrete choice experiment. Optom Vis Sci 2007;84:E797-809.
- Chiou C-FW. Measuring preference weights for american college of rheumatology response criteria for patients with rheumatoid arthritis. J Rheumatol 2005;32:2326-9.
- Goodey RD, Brickley MR, Armstrong RA, Shepherd JP, Goodey RD, Brickley MR, et al. The minor oral surgery outcome scale: a multi-attribute patient-derived outcome measure. J Oral Maxillofac Surg 2000;58:1096-101.
- Harwood RH, Rogers A, Dickinson E, Ebrahim S, Harwood RH, Rogers A, et al. Measuring handicap: the London Handicap Scale, a new outcome measure for chronic disease. Qual Health Care 1994;3:11-6.
- Hodder SC, Edwards MJ, Brickley MR, Shepherd JP, Hodder SC, Edwards MJ, et al. Multiattribute utility assessment of outcomes of treatment for head and neck cancer. Br J Cancer 1997;75:898-902.
- Kind P, Macran S. Eliciting social preference weights for functional assessment of cancer therapy-lung health states. Pharmacoeconomics 2005;23:1143-53.
- Lamers LM, Uyl-de Groot CA, Buijt I. The use of disease-specific outcome measures in cost-utility analysis: The development of Dutch societal preference weights for the FACT-L scale. Pharmacoeconomics 2007;25:591-603.
- McKenna SP, Ratcliffe J, Meads DM, Brazier JE. Development and validation of a preference based measure derived from the Cambridge Pulmonary Hypertension Outcome Review (CAMPHOR) for use in cost utility analyses. Health Qual Life Outcomes 2008;6.
- Misajon R, Hawthorne G, Richardson J, Barton J, Peacock S, Iezzi A, et al. Vision and quality of life: the development of a utility measure. Invest Ophthalmol Vis Sci 2005;46:4007-15.
- Peacock S, Misajon R, Iezzi A, Richardson J, Hawthorne G, Keeffe J. Vision and quality of life: Development of methods for the VisQoL vision-related utility instrument. Ophthalmic Epidemiol 2008;15:218-23.
- Palmer CS, Schmier J, Snyder E, Scott B. Patient preferences and utilities for ‘off-time’ outcomes in the treatment of Parkinson’s disease. Quality Life Res 2000;9:819-27.
- Poissant L, Mayo NE, Wood-Dauphinee S, Clarke AE, Poissant L, Mayo NE, et al. The development and preliminary validation of a Preference-Based Stroke Index (PBSI). Health Qual Life Outcomes 2003;1.
- Shaw RW, Brickley MR, Evans L, Edwards MJ, Shaw RW, Brickley MR, et al. Perceptions of women on the impact of menorrhagia on their health using multi-attribute utility assessment. Br J Obstet Gynaecol 1998;105:1155-9.
- Sundaram M, Smith MJ, Revicki DA, Elswick B, Miller LA. Rasch analysis informed the development of a classification system for a diabetes-specific preference-based measure of health. J Clin Epidemiol 2009;62:845-56.
- Sundaram M, Smith MJ, Revicki DA, Miller LA, Madhavan S, Hobbs G. Estimation of a valuation function for a diabetes mellitus-specific preference-based measure of health: the Diabetes Utility Index. Pharmacoeconomics 2010;28:201-16.
- Young T, Rowen D, Norquist J, Brazier J. Developing preference-based health measures: using Rasch analysis to generate health state values. Qual Life Res 2010;19:907-17.
- Babbie E. The practice of social research. Belmont, CA: Wadsworth Publishing; 1992.
- Brazier J, Rowen D. Alternatives to EQ-5D for generating health state utility values. London: NICE; 2011.
- Gold MR, Siegel JE, Russell LB, Weinstein MC. Cost-effectiveness in health and medicine. Oxford: Oxford University Press; 1996.
- Chisholm D, Healey A, Knapp M, Chisholm D, Healey A, Knapp M. QALYs and mental health care. Soc Psychiatry Psychiatr Epidemiol 1997;32:68-75.
- Knapp M, Mangalore R. ‘The trouble with QALYs. ’. Epidemiol Psichiatr Soc 2007;16:289-93.
- Brazier J. Measuring and valuing mental health for use in economic evaluation. J Health Serv Res Policy 2008;13:70-5.
- Kowalski JW, Rentz AM, Walt JG, Lloyd A, Lee J, Young T, et al. Rasch analysis in the development of a simplified version of the national eye institute visual-function questionnaire-25 for utility estimation. Qual Life Res 2011;21:323-34.
- Mavranezouli I, Brazier J, Young A, Barkham M. Using Rasch analysis to form plausible health states amenable to valuation: the development of the CORE-6D from a measure of common mental health problems (CORE-OM). Qual Life Res 2011;20:321-33.
- Mavranezouli I, Brazier J, Rowen D, Barkham M. Estimating a Preference-Based Index from the Clinical Outcomes in Routine Evaluation – Outcome Measure (CORE-OM): valuation of CORE-6D. Health Economics and Decision Science Discussion Paper. Sheffield: University of Sheffield; 2011.
- Barkham M, Margison F, Leach C, Lucock M, Mellor-Clark J, Evans C, et al. Service profiling and outcomes benchmarking using the CORE-OM: toward practice-based evidence in the psychological therapies. Clinical Outcomes in Routine Evaluation-Outcome Measures. J Consult Clin 2001;69:184-96.
- Barkham M, Mellor-Clark J, Connell J, Cahill J. A core approach to practice-based evidence: A brief history of the origins and applications of the CORE-OM and CORE System. Counsell Psychother Research J 2006;6:3-15.
- Evans C, Connell J, Barkham M, Margison F, McGrath G, Mellor-Clark J, et al. Towards a standardised brief outcome measure: psychometric properties and utility of the CORE-OM. Br J Psychiatry 2002;180:51-60.
- Smith AB, Rush R, Fallowfield LJ, Velikova G, Sharpe M. Rasch fit statistics and sample size considerations for polytomous data. BMC Med Res Methodol 2008;8.
- Smith EJ. Detecting and evaluating the impact of multidimensionality using item fit statistics and principal component analysis of residuals. J Appl Meas 2002;3:205-31.
- Tennant A, Conaghan P. The Rasch measurement model in rheumatology: What is it and why use it? When should it be applied, and what should one look for in a Rasch paper?. Arthritis Rheum 2007;57:1358-62.
- Tennant A, Pallant J. Unidimensionality matters! (A Tale of Two Smiths?). Rasch Meas Trans 2006;20:1048-51.
- Gudex C. Time trade-off user manual: props and self-completion methods. York: University of York, Centre for Health Economics; 1994.
- Kind P, Hardman G, Macran S. UK population norms for EQ-5D. Centre for Health Economics Discussion Paper Series. York: University of York; 1999.
- Young T, Yang Y, Brazier J, Tsuchiya A. The use of Rasch analysis in reducing a large condition-specific instrument for preference valuation: the case of moving from AQLQ to AQL-5D. Med Decis Making 2010;31:281-91.
- Gerard K, Dobson M, Hall J. Framing and labelling effects in health descriptions: quality adjusted life years for treatment of breast cancer. J Clin Epidemiol 1993;46:77-84.
- Llewellyn-Thomas H, Sutherland HJ, Tibshirani R, Ciampi A, Till JE, Boyd NF. Describing health states. Methodologic issues in obtaining values for health states. Med Care 1984;22:543-52.
- Rabin R, Rosser RM, Butler C. Impact of diagnosis on utilities assigned to states of illness. J R Soc Med 1993;86:444-8.
- Robinson S, Bryan S. ‘Naming and framing’: An Investigation of the Effect of Disease Labels on Health State Valuations n.d.
- Sackett DL, Torrance GW. The utility of different health states as perceived by the general public. J Chronic Dis 1978;31:697-704.
- Rowen D, Brazier J, Tsuchiya A, Young T, Ibbotson R. It’s all in the name, or is it? The impact of labelling on health state values. Med Decis Making 2012;32:31-40.
- McCabe C, Stevens K, Roberts J, Brazier J. Health state values for the HUI 2 descriptive system: results from a UK survey. Health Econ 2005;14:231-44.
- Goldstein H. Multilevel statistical methods. New York, NY: Halstead Press; 1995.
- Dolan P, Kahneman D. Interpretations of utilities and their implications for the valuation of health. Economic J 2008;118:215-34.
- Brazier J, Rowen D, Tsuchiya A, Yang Y, Young T. The impact of adding an extra dimension to a preference-based measure. Soc Sci Med 2011;73:245-53.
- Yang Y, Brazier J, Tsuchiya A. The Effect of Adding a Sleep Dimension to the EQ-5D n.d.
- Keeney RL, Raiffa H. Decisions with multiple objectives: preferences and value tradeoffs. New York, NY: Cambridge University Press; 1993.
- Krabbe PF, Stouthard ME, Essink-Bot ML, Bonsel GJ. The effect of adding a cognitive dimension to the EuroQol multiattribute health-status classification system. J Clin Epidemiol 1999;52:293-301.
- Juniper EF, Guyatt GH, Epstein R, Ferrie P, Jaeschke R, Hiller T. Evaluation of impairment of health related quality of life in asthma: development of a questionnaire for use in clinical trials. BMJ 1992;47.
- Aaronson NK, Ahmedzai S, Bregman B, Bullinger M, Cull A, Duez N, et al. The European Organization for Research and Treatment of Cancer QLQ-C30: a quality-of-life instrument for use in international clinical trials in oncology. J Natl Cancer Inst 1993;85:365-76.
- Coyne K, Revicki D, Hunt T, Corey R, Stewart W, Bentkover J, et al. Psychometric validation of an overactive bladder symptom and health related quality of life questionnaire: the OAB-q. Qual Life Res 2002;11:563-74.
- Lloyd A, Price D, Brown R. The impact of asthma exacerbations on health-related quality of life in moderate to severe asthma patients in the UK. Prim Care Respir J 2007;16.
- Eccles M, Grimshaw J, Steen N, Parkin D, Purves I, McColl E, et al. The design and analysis of an evaluation of computerised decision support: the COGENT Study. Fam Pract 2000;17.
- Steen N, Hutchinson A, McColl E, Eccles M, Hewison J, Meadows K, et al. Development of a symptom based outcome measure for asthma. BMJ 1994;309.
- Singleton N, Lewis G. Better or worse: a longitudinal study of the mental health of adults living in private households in Great Britain. London: The Stationery Office; 2003.
- Singleton N, Bumpstead R, O’Brien M, Lee A, Melttzer H. Psychiatric morbidity among adults living in private households, 2000. London: The Stationery Office; 2001.
- Connell J, Barkham M, Stiles WB, Twigg E, Singleton N, Evans O, et al. Distribution of CORE-OM scores in a general population, clinical cut-off points and comparison with the CIS-R. Br J Psychiatry 2007;190:69-74.
- Lewis G, Pelosi AJ, Araya R, Dunn G. Measuring psychiatric disorder in the community: a standardized assessment for use by lay interviewers. Psychol Med 1992;22:465-86.
- Richards A, Barkham M, Cahill J, Richards D, Williams C, Heywood P. PHASE: a randomised, controlled trial of supervised self-help cognitive behavioural therapy in primary care. Br J Gen Pract 2003;53:764-70.
- Karnofsky D, Burchenal J, MacLeod C. Evaluation of chemotherapeutic agents. New York, NY: Columbia University Press; 1949.
- Coyne K, Matza LS, Thompson CL. The responsiveness of the overactive bladder questionnaire (OAB-q). Qual Life Res 2005;14:849-55.
- Coyne K, Matza L, Thompson C. Psychometric properties and responsiveness of the OAB-q in patients with OAB and nocturia (study 583-uro-0084-037). Report prepared for Pfizer Inc; 2005.
- Cohen J. Statistical power analysis for the behavioural sciences. New York, NY: Academic Press; 1977.
- Rowen D, Young T, Brazier J, Gaugris S. Comparison of generic, condition-specific and mapped health state utility values. Sheffield: University of Sheffield; 2011.
- McTaggart-Cowan HM, Marra CA, Yang Y, Brazier JE, Kopec JA, FitzGerald JM, et al. The validity of generic and condition-specific preference-based instruments: the ability to discriminate asthma control status. Qual Life Res 2008;17:453-62.
- Shaw JW, Johnson JA, Coons SJ. US valuation of the EQ-5D health states: development and testing of the D1 valuation model. Med Care 2005;43:203-20.
- Gold M, Franks P, Erickson P, Gold M, Franks P, Erickson P. Assessing the health of the nation. The predictive validity of a preference-based measure and self-rated health. Med Care 1996;34:163-77.
- Williams A. The measurement and valuation of health: a chronicle. York: University of York; 1995.
- Brazier J, Tsuchiya A. Preference-based condition-specific measures of health: what happens to cross programme comparability?. Health Econ 2010;19:125-9.
- Longworth L, Rowen D. The use of mapping methods to estimate health state utility values. London: NICE; 2011.
- Brazier J, Longworth L. An introduction to the measurement and valuation of health for NICE submissions. London: NICE; 2011.
- Katz JN, Larson MG, Phillips CB, Fossel AH, Liang MH. Comparative measurement sensitivity of short and longer health status instruments. Med Care 1992;30:917-25.
- Tennant A, McKenna SP, Hagell P. Application of Rasch analysis in the development and application of quality of life instruments. Value Health 2004;7:S22-6.
- Masters GN. A Rasch model for partial credit scoring. Psychometrika 1982;47:149-74.
- Young T, Yang Y, Brazier JE, Tsuchiya A, Coyne K. The first stage of developing preference-based measures: Constructing a health-state classification using Rasch analysis. Qual Life Res 2009;18:253-65.
- Morrell CJ, Slade P, Warner R, Paley G, Dixon S, Walters SJ, et al. Clinical effectiveness of health visitor training in psychologically informed approaches for depression in postnatal women: pragmatic cluster randomised trial in primary care. BMJ 2009;338.
Appendix 1 Overview of Rasch analysis
Rasch analysis is a mathematical modelling technique36 that converts qualitative (categorical) responses to points on a continuous (unmeasured) latent scale using a logit model. In terms of HRQoL, Rasch analysis converts categorical items (i.e. questions) to a unidimensional continuous latent scale, which is conceived to be a continuous measure of HRQoL.
When applying Rasch analysis to HRQoL responses, each respondent’s position on the underlying latent (HRQoL) scale accounts for that person’s degree of health-related problems. To apply Rasch models to HRQoL instruments it is assumed that patients with more severe problems should indicate that they have difficulties with more items (representing tasks or facets of health) described in the instrument than patients with less severe problems. It is further assumed that the easier an item is to achieve the more likely it will be achieved. 117
There are several types of Rasch model; however, the one most commonly used when creating health-state classification systems is the Rasch Partial Credit model, which allows for multilevel item responses to all items and patient responses as variables that may be estimated independently,118 as is the case with the AQLQ.
From an economist’s perspective Rasch analysis helps to understand the relationship between items (and item levels) and HRQoL, but not the appropriate weighting for a health-state classification system. A Rasch model may indicate that respondents with different health problems have better (or worse) health, in comparison with one another, based on their responses to an item, but it does not indicate anything about the extent to which it would be preferred. This requires additional information on preferences as described in stages V and VI.
Appendix 2 Project protocol
Aims and objectives
Economic evaluation assesses health care interventions in terms of their cost per Quality Adjusted Life Year (QALY) gained. The most commonly used measure to put the ‘quality adjustment weight’ into the QALY is the EQ-5D, a generic preference-based measure of health. It has been claimed that generic preference-based measures are not applicable to all interventions and patient groups, and many clinicians and researchers prefer to use condition-specific measures. However, most condition-specific measures are not ‘preference-based’ and thus cannot be used to derive the ‘quality adjustment weight’ for use in QALYs.
This project will critically review, develop and test methods for deriving preference-based measures of health from condition specific non-preference-based measures of health (and other patient-based measures of outcome). The aim is to produce guidance on how to produce preference-based measures from existing non-preference-based measures and to identify areas for further research. The project will develop and test new or revised methods to compensate for the flaws of existing methods, derive a preference-based measure for mental health and test the preference-based condition-specific measures against the original instruments and generic preference-based measures.
It will build on previous work to develop condition specific measures from instruments in asthma (AQOL), overactive bladder (OAB-q), mental health (CORE-OM) and cancer (EORTC QLQ-C30).
The specific objectives of the project are as follows:
-
to identify and review the existing literature on current methods for deriving a preference-based measure of health from non-preference-based measures of health in order to develop a framework
-
to propose a set of conditions that need to be satisfied in order to justify the development and valuation of a condition-specific preference-based measure (CSM)
-
to examine and test a new method for generating health states, the Rasch-based vignette approach, from non-preference based measures using Rasch modelling
-
to examine the degree of information loss of moving from the original instrument to the preference-based index
-
to assess the impact of referring to the medical condition (or disease) in the descriptions
-
to assess the impact of attempting to capture side effects with CSMs
-
to assess the impact of co morbidities by testing the additivity assumption and the extent of any violation across two conditions (asthma and common mental health problems)
-
to compare preference-based measures derived from the CSMs with generic measures (including EQ-5D and SF-6D) in order to examine the degree of agreement and the extent of any gain in psychometric performance.
Description of the project methodology
Stage 1: To identify and critically review the current methods for developing preference-based measures of quality of life from condition specific measures
This stage will critically review published studies of theoretical and empirical work on the development of preference-based measures from CSMs and other non-preference-based measures of health. From the review, the following will be developed: a list of questions to address to decide whether it is worth deriving a preference-based CSM; a list of the methodological challenges (in addition to those identified above); methods for deriving health states descriptions amenable to valuation studies from CSMs; and a framework for testing resultant preference-based measures.
Stage 2: To develop and test new or revised methods to compensate for the flaws of existing methods
Objectives 1 and 2 will be addressed by the review of the literature in stage 1. The remaining six objectives will be addressed by three empirical phases of work.
Phase A: Derivation of health states from the CORE-OM
Exploration of a new approach, the Rasch-based vignette approach, that is being developed for instruments that do not contain a set of independent dimensions or in the case of the CORE-OM, where there is one large factor covering different sub-dimensions that are not independent. This problem is more likely to arise in CSMs since they have a narrower set of items.
Phase B: Valuation surveys – CORE-OM valuation; impact of labelling; and impact of side-effects and comorbidities
This is the component for which we are seeking ethical approval since it involves conducting interviews with 644 members of the general public. The objectives of the valuation study will be:
-
to generate values for the CORE-OM mental health states
-
to examine the impact of labelling of measures using EORTC-8D health states
-
to estimate the potential impact of side-effects and comorbidities on the valuation of the CORE-OM and AQL-5D in terms of: (a) The additive impact of the variable i.e. the size and significance of the coefficient on the additional dimension and (b) The impact of the additional dimensions on the size of the other dimensions.
There are three valuation studies to be undertaken to address these objectives. Only Phase B will involve the collection of data.
Phase C: Testing the preference-based CSMs against the original instruments and generic preference-based measures
The objectives of this phase of the research are: (1) to examine the extent of any information loss from moving from the original instruments to the health state classifications of the AQL-5D, OAB-5D and the health states derived from the CORE-OM and (2) to compare them with generic preference-based measures. This will be done by comparing their psychometric properties in terms of validity and responsiveness using available data sets.
Valuation surveys
A common format will be used for each of the three valuation surveys. The valuation surveys will all employ the time trade-off method (TTO), where respondents are asked how many years they would be willing to sacrifice in order to be in full health.
The representative sample of the general population will be asked firstly to complete the classification for their own health state for the relevant instrument and secondly to undertake a warm-up ranking task and eight TTO valuations of health states. For the CORE-OM valuation survey the warm-up ranking task will involve four cards which will then be valued using TTO, subsequently there will be a second ranking task involving four cards which will then be valued using TTO. The MVH group version of TTO will be used to allow comparison with the EQ-5D tariff. 43 This valuation protocol was also used in the AQL-5D, OAB-5D and EORTC QLQ-C30 valuation studies. Respondents will also be asked a number of background questions covering health, demographic and socio-economic characteristics. Similar interview schedules have been successfully used in a large scale general population survey undertaken in the UK43 and by ScHARR in a number of studies. Each interview is expected to take about 30 minutes. A small pilot study of 20 respondents will be undertaken prior to each valuation study to check respondents’ understanding of the TTO and to check that they are completing each task as expected.
The sample sizes for the three surveys are as follows:
-
This study has been designed to value 24 out of the possible 54 CORE-OM states (see above), to ensure an adequate mix of mental health states with and without mobility and pain problems. These states will be divided into four blocs of eight health states, one for each respondent to value. The valuation of these 24 states has two aims. One is to produce mean estimates for each health state. The second is to compare mean values between the states with and without the addition of mobility and pain using simple t-tests. Assuming a power of 0.8, significance level of 0.05, standard deviation of 0.3 and an expected difference of 0.1, then this requires a sample of 73 valuations for each state and a total of 220 completed interviews.
-
The EORTC-8D labelling study is concerned with testing the impact on health state values of removing the name of the cause of the problem. Mean values across eight states will be compared using simple t-tests and this requires a sample of 73 valuations for each state (see justification in 1). The previous EORTC-8D valuation study had approx. 30 values per state. So this requires an additional 43 valuations of the eight states with no label and 146 interviews for health states with two different labels (73 per label). A minimum of 219 completed interviews will be required.
-
The valuation of AQL-5D and the classification enhanced with a pain dimension requires 35 health states to be valued in total (see above). Each state will be valued 30 times producing a minimum of 132 completed interviews. This number has not been selected to enable comparisons across states, but to allow the estimation of a preference model.
Description of the outcome measures
There are four condition specific measures that will form the subject of this research.
Asthma Quality of Life Questionnaire (AQLQ) and Asthma Quality of Life-5 Dimensions (AQL-5D)
The AQLQ is a 32-item instrument designed to assess HRQL in patients with asthma. It has a set of items for self-completion covering four dimensions: symptoms (12 items), activity limitations (11 items), emotional function (five items) and environmental stimuli (four items), and each item has seven levels. 24,25 It has been shown to be reliable, valid and responsive in asthma populations and has been used in more than 170 papers (Medline). Based on the application of Rasch analysis and conventional psychometric tests, the AQLQ has been reduced to a five-dimension health state classification system called the AQL-5D29 and valued using time trade-off. 28 The dimensions are: concern about asthma, shortness of breath, weather and pollution stimuli, sleep impact and activity limitations. These dimensions were selected directly from the original AQLQ. Each dimension has five levels of severity with level 1 denoting no problem and level 5 indicating extreme problem. All patient data with complete AQLQ information can be mapped on to the AQL-5D.
Over Active Bladder Questionnaire (OAB-q) and Over Active Bladder Questionnaire-5 Dimensions (OAB-5D)
The OAB-q is a 33-item OAB-specific questionnaire that consists of an eight-item Symptom Bother scale and a 25-item health related quality of life (HRQL) scale that has four sub-scales: Coping, Concern, Sleep and Social Interaction. 95 Responses are based on a 6-point Likert scale. Amongst continent and incontinent OAB patients, the OAB-q has demonstrated good internal consistency, reliability, test-retest reliability, concurrent validity, discriminative validity, and responsiveness to treatment-related change. 95,105,106 A new five-dimension health state classification system named OAB-5D was constructed by selecting items directly from the OAB-q using Rasch analysis and conventional psychometric tests. 119 This resulted in five dimensions: urge to urinate, urine loss, sleep impact, coping strategy (“planning ‘escape route’ to rest room in public place”), and concern of OAB symptoms (‘Bladder symptoms cause you embarrassment’). A valuation survey was undertaken using the same methods as those for AQL-5D. 30
Clinical Outcomes in Routine Evaluation-Outcome Measure (CORE-OM)
The CORE-OM has been developed to assess the outcomes of therapeutic interventions for people with mental health problems seen in primary and secondary care settings. 70,71 It has 34 self-report items covering the domains of subjective well-being, symptoms, function and risk. Each item has five levels (‘not at all’ through to ‘most or all of the time’). It has been shown to be reliable, valid and sensitive to change in clinical samples. 72 It has become one of the most widely used mental health outcome measures in the NHS and is being used in a number of studies, see for example. 103,120
European Organisation for Research and Treatment of Cancer Quality of Life Questionnaire (EORTC QLQ-C30)
The EORTC QLQ-C30 is a widely-used patient report measure of symptoms in patients with cancer. It has 30 self-report items covering functionings (physical, role, social, emotional, cognitive), symptoms (fatigue, nausea and vomiting, pain, shortness of breath, sleep disturbance, appetite loss, constipation, diarrhoea, financial impact) and general health. 94 Each item has four levels (from ‘not at all’ to ‘very much’) with the exception of two general questions with seven levels (‘very poor’ to ‘excellent’). A preference-based instrument has been developed and the health state classification system has eight dimensions (physical functioning, role functioning, social functioning, pain, emotional functioning, fatigue, constipation and diarrhoea and nausea) each with four or five levels. A valuation study was undertaken using the time trade-off method. 34
Participants in valuation surveys
The research will be carried out across the UK. It will involve: (i) random selection using the PAF register of addresses in the UK of addresses within Yorkshire; (ii) Households will be approached in writing, introducing the study, the purpose of recruitment, asking for their participation and informing that an interviewer may call; and (iii) At each address the interviewer will identify themselves and request an interview, either with the person there, or with another person in the household if they are needed to fulfil the quota, or both. The interviewer will then obtain consent ensuring the member of the public has read the information form.
List of abbreviations
- CBT
- cognitive behavioural therapy
- CIS-R
- Clinical Interview Schedule – Revised
- CORE-OM
- Clinical Outcomes in Routine Evaluation – Outcome Measure
- CSPBM
- condition-specific preference-based measure
- DCE
- discrete choice experiment
- DIF
- differential item functioning
- EORTC QLQ-C30
- European Organisation for Research and Treatment of Cancer Quality of Life Questionnaire, version 30
- FDA
- Food and Drug Administration
- GLS
- generalised least squares
- HRQoL
- health-related quality of life
- IBS
- irritable bowel syndrome
- ICC
- intraclass correlation coefficient
- MAE
- mean absolute error
- MVH
- Measurement and Valuation of Health
- N/A
- not applicable
- NICE
- National Institute for Health and Clinical Excellence
- PROM
- patient-reported outcome measure
- QALY
- quality-adjusted life-year
- RMSE
- root-mean-square error
- SD
- standard deviation
- TTO
- time trade-off
- VAS
- visual analogue scale
- VCC
- Vancouver Cancer Clinic
All abbreviations that have been used in this report are listed here unless the abbreviation is well known (e.g. NHS), or it has been used only once, or it is a non-standard abbreviation used only in figures/tables/appendices, in which case the abbreviation is defined in the figure legend or in the notes at the end of the table.
Notes
Health Technology Assessment programme
-
Director, NIHR HTA programme, Professor of Clinical Pharmacology, Department of Pharmacology and Therapeutics, University of Liverpool
-
Professor of Dermato-Epidemiology, Centre of Evidence-Based Dermatology, University of Nottingham
Prioritisation Group
-
Director, NIHR HTA programme, Professor of Clinical Pharmacology, Department of Pharmacology and Therapeutics, University of Liverpool
-
Professor Imti Choonara, Professor in Child Health, Academic Division of Child Health, University of Nottingham
Chair – Pharmaceuticals Panel
-
Dr Bob Coates, Consultant Advisor – Disease Prevention Panel
-
Dr Andrew Cook, Consultant Advisor – Intervention Procedures Panel
-
Dr Peter Davidson, Director of NETSCC, Health Technology Assessment
-
Dr Nick Hicks, Consultant Adviser – Diagnostic Technologies and Screening Panel, Consultant Advisor–Psychological and Community Therapies Panel
-
Ms Susan Hird, Consultant Advisor, External Devices and Physical Therapies Panel
-
Professor Sallie Lamb, Director, Warwick Clinical Trials Unit, Warwick Medical School, University of Warwick
Chair – HTA Clinical Evaluation and Trials Board
-
Professor Jonathan Michaels, Professor of Vascular Surgery, Sheffield Vascular Institute, University of Sheffield
Chair – Interventional Procedures Panel
-
Professor Ruairidh Milne, Director – External Relations
-
Dr John Pounsford, Consultant Physician, Directorate of Medical Services, North Bristol NHS Trust
Chair – External Devices and Physical Therapies Panel
-
Dr Vaughan Thomas, Consultant Advisor – Pharmaceuticals Panel, Clinical
Lead – Clinical Evaluation Trials Prioritisation Group
-
Professor Margaret Thorogood, Professor of Epidemiology, Health Sciences Research Institute, University of Warwick
Chair – Disease Prevention Panel
-
Professor Lindsay Turnbull, Professor of Radiology, Centre for the MR Investigations, University of Hull
Chair – Diagnostic Technologies and Screening Panel
-
Professor Scott Weich, Professor of Psychiatry, Health Sciences Research Institute, University of Warwick
Chair – Psychological and Community Therapies Panel
-
Professor Hywel Williams, Director of Nottingham Clinical Trials Unit, Centre of Evidence-Based Dermatology, University of Nottingham
Chair – HTA Commissioning Board
Deputy HTA Programme Director
HTA Commissioning Board
-
Professor of Dermato-Epidemiology, Centre of Evidence-Based Dermatology, University of Nottingham
-
Department of Public Health and Epidemiology, University of Birmingham
-
Professor of Clinical Pharmacology, Director, NIHR HTA programme, Department of Pharmacology and Therapeutics, University of Liverpool
-
Professor Judith Bliss, Director of ICR-Clinical Trials and Statistics Unit, The Institute of Cancer Research
-
Professor David Fitzmaurice, Professor of Primary Care Research, Department of Primary Care Clinical Sciences, University of Birmingham
-
Professor John W Gregory, Professor in Paediatric Endocrinology, Department of Child Health, Wales School of Medicine, Cardiff University
-
Professor Steve Halligan, Professor of Gastrointestinal Radiology, Department of Specialist Radiology, University College Hospital, London
-
Professor Angela Harden, Professor of Community and Family Health, Institute for Health and Human Development, University of East London
-
Dr Martin J Landray, Reader in Epidemiology, Honorary Consultant Physician, Clinical Trial Service Unit, University of Oxford
-
Dr Joanne Lord, Reader, Health Economics Research Group, Brunel University
-
Professor Stephen Morris, Professor of Health Economics, University College London, Research Department of Epidemiology and Public Health, University College London
-
Professor Dion Morton, Professor of Surgery, Academic Department of Surgery, University of Birmingham
-
Professor Gail Mountain, Professor of Health Services Research, Rehabilitation and Assistive Technologies Group, University of Sheffield
-
Professor Irwin Nazareth, Professor of Primary Care and Head of Department, Department of Primary Care and Population Sciences, University College London
-
Professor E Andrea Nelson, Professor of Wound Healing and Director of Research, School of Healthcare, University of Leeds
-
Professor John David Norrie, Director, Centre for Healthcare Randomised Trials, Health Services Research Unit, University of Aberdeen
-
Dr Rafael Perera, Lecturer in Medical Statisitics, Department of Primary Health Care, University of Oxford
-
Professor Barney Reeves, Professorial Research Fellow in Health Services Research, Department of Clinical Science, University of Bristol
-
Professor Peter Tyrer, Professor of Community Psychiatry, Centre for Mental Health, Imperial College London
-
Professor Martin Underwood, Professor of Primary Care Research, Warwick Medical School, University of Warwick
-
Professor Caroline Watkins, Professor of Stroke and Older People’s Care, Chair of UK Forum for Stroke Training, Stroke Practice Research Unit, University of Central Lancashire
-
Dr Duncan Young, Senior Clinical Lecturer and Consultant, Nuffield Department of Anaesthetics, University of Oxford
-
Dr Tom Foulks, Medical Research Council
-
Dr Kay Pattison, Senior NIHR Programme Manager, Department of Health
HTA Clinical Evaluation and Trials Board
-
Director, Warwick Clinical Trials Unit, Warwick Medical School, University of Warwick and Professor of Rehabilitation, Nuffield Department of Orthopaedic, Rheumatology and Musculoskeletal Sciences, University of Oxford
-
Professor of the Psychology of Health Care, Leeds Institute of Health Sciences, University of Leeds
-
Director, NIHR HTA programme, Professor of Clinical Pharmacology, University of Liverpool
-
Professor Keith Abrams, Professor of Medical Statistics, Department of Health Sciences, University of Leicester
-
Professor Martin Bland, Professor of Health Statistics, Department of Health Sciences, University of York
-
Professor Jane Blazeby, Professor of Surgery and Consultant Upper GI Surgeon, Department of Social Medicine, University of Bristol
-
Professor Julia M Brown, Director, Clinical Trials Research Unit, University of Leeds
-
Professor Alistair Burns, Professor of Old Age Psychiatry, Psychiatry Research Group, School of Community-Based Medicine, The University of Manchester & National Clinical Director for Dementia, Department of Health
-
Dr Jennifer Burr, Director, Centre for Healthcare Randomised trials (CHART), University of Aberdeen
-
Professor Linda Davies, Professor of Health Economics, Health Sciences Research Group, University of Manchester
-
Professor Simon Gilbody, Prof of Psych Medicine and Health Services Research, Department of Health Sciences, University of York
-
Professor Steven Goodacre, Professor and Consultant in Emergency Medicine, School of Health and Related Research, University of Sheffield
-
Professor Dyfrig Hughes, Professor of Pharmacoeconomics, Centre for Economics and Policy in Health, Institute of Medical and Social Care Research, Bangor University
-
Professor Paul Jones, Professor of Respiratory Medicine, Department of Cardiac and Vascular Science, St George‘s Hospital Medical School, University of London
-
Professor Khalid Khan, Professor of Women’s Health and Clinical Epidemiology, Barts and the London School of Medicine, Queen Mary, University of London
-
Professor Richard J McManus, Professor of Primary Care Cardiovascular Research, Primary Care Clinical Sciences Building, University of Birmingham
-
Professor Helen Rodgers, Professor of Stroke Care, Institute for Ageing and Health, Newcastle University
-
Professor Ken Stein, Professor of Public Health, Peninsula Technology Assessment Group, Peninsula College of Medicine and Dentistry, Universities of Exeter and Plymouth
-
Professor Jonathan Sterne, Professor of Medical Statistics and Epidemiology, Department of Social Medicine, University of Bristol
-
Mr Andy Vail, Senior Lecturer, Health Sciences Research Group, University of Manchester
-
Professor Clare Wilkinson, Professor of General Practice and Director of Research North Wales Clinical School, Department of Primary Care and Public Health, Cardiff University
-
Dr Ian B Wilkinson, Senior Lecturer and Honorary Consultant, Clinical Pharmacology Unit, Department of Medicine, University of Cambridge
-
Ms Kate Law, Director of Clinical Trials, Cancer Research UK
-
Dr Morven Roberts, Clinical Trials Manager, Health Services and Public Health Services Board, Medical Research Council
Diagnostic Technologies and Screening Panel
-
Scientific Director of the Centre for Magnetic Resonance Investigations and YCR Professor of Radiology, Hull Royal Infirmary
-
Professor Judith E Adams, Consultant Radiologist, Manchester Royal Infirmary, Central Manchester & Manchester Children’s University Hospitals NHS Trust, and Professor of Diagnostic Radiology, University of Manchester
-
Mr Angus S Arunkalaivanan, Honorary Senior Lecturer, University of Birmingham and Consultant Urogynaecologist and Obstetrician, City Hospital, Birmingham
-
Dr Diana Baralle, Consultant and Senior Lecturer in Clinical Genetics, University of Southampton
-
Dr Stephanie Dancer, Consultant Microbiologist, Hairmyres Hospital, East Kilbride
-
Dr Diane Eccles, Professor of Cancer Genetics, Wessex Clinical Genetics Service, Princess Anne Hospital
-
Dr Trevor Friedman, Consultant Liason Psychiatrist, Brandon Unit, Leicester General Hospital
-
Dr Ron Gray, Consultant, National Perinatal Epidemiology Unit, Institute of Health Sciences, University of Oxford
-
Professor Paul D Griffiths, Professor of Radiology, Academic Unit of Radiology, University of Sheffield
-
Mr Martin Hooper, Public contributor
-
Professor Anthony Robert Kendrick, Associate Dean for Clinical Research and Professor of Primary Medical Care, University of Southampton
-
Dr Nicola Lennard, Senior Medical Officer, MHRA
-
Dr Anne Mackie, Director of Programmes, UK National Screening Committee, London
-
Mr David Mathew, Public contributor
-
Dr Michael Millar, Consultant Senior Lecturer in Microbiology, Department of Pathology & Microbiology, Barts and The London NHS Trust, Royal London Hospital
-
Mrs Una Rennard, Public contributor
-
Dr Stuart Smellie, Consultant in Clinical Pathology, Bishop Auckland General Hospital
-
Ms Jane Smith, Consultant Ultrasound Practitioner, Leeds Teaching Hospital NHS Trust, Leeds
-
Dr Allison Streetly, Programme Director, NHS Sickle Cell and Thalassaemia Screening Programme, King’s College School of Medicine
-
Dr Matthew Thompson, Senior Clinical Scientist and GP, Department of Primary Health Care, University of Oxford
-
Dr Alan J Williams, Consultant Physician, General and Respiratory Medicine, The Royal Bournemouth Hospital
-
Dr Tim Elliott, Team Leader, Cancer Screening, Department of Health
-
Dr Joanna Jenkinson, Board Secretary, Neurosciences and Mental Health Board (NMHB), Medical Research Council
-
Professor Julietta Patrick, Director, NHS Cancer Screening Programme, Sheffield
-
Dr Kay Pattison, Senior NIHR Programme Manager, Department of Health
-
Professor Tom Walley, CBE, Director, NIHR HTA programme, Professor of Clinical Pharmacology, University of Liverpool
-
Dr Ursula Wells, Principal Research Officer, Policy Research Programme, Department of Health
Disease Prevention Panel
-
Professor of Epidemiology, University of Warwick Medical School, Coventry
-
Dr Robert Cook, Clinical Programmes Director, Bazian Ltd, London
-
Dr Colin Greaves, Senior Research Fellow, Peninsula Medical School (Primary Care)
-
Mr Michael Head, Public contributor
-
Professor Cathy Jackson, Professor of Primary Care Medicine, Bute Medical School, University of St Andrews
-
Dr Russell Jago, Senior Lecturer in Exercise, Nutrition and Health, Centre for Sport, Exercise and Health, University of Bristol
-
Dr Julie Mytton, Consultant in Child Public Health, NHS Bristol
-
Professor Irwin Nazareth, Professor of Primary Care and Director, Department of Primary Care and Population Sciences, University College London
-
Dr Richard Richards, Assistant Director of Public Health, Derbyshire County Primary Care Trust
-
Professor Ian Roberts, Professor of Epidemiology and Public Health, London School of Hygiene & Tropical Medicine
-
Dr Kenneth Robertson, Consultant Paediatrician, Royal Hospital for Sick Children, Glasgow
-
Dr Catherine Swann, Associate Director, Centre for Public Health Excellence, NICE
-
Mrs Jean Thurston, Public contributor
-
Professor David Weller, Head, School of Clinical Science and Community Health, University of Edinburgh
-
Ms Christine McGuire, Research & Development, Department of Health
-
Dr Kay Pattison, Senior NIHR Programme Manager, Department of Health
-
Professor Tom Walley, CBE, Director, NIHR HTA programme, Professor of Clinical Pharmacology, University of Liverpool
External Devices and Physical Therapies Panel
-
Consultant Physician North Bristol NHS Trust
-
Reader in Wound Healing and Director of Research, University of Leeds
-
Professor Bipin Bhakta, Charterhouse Professor in Rehabilitation Medicine, University of Leeds
-
Mrs Penny Calder, Public contributor
-
Dr Dawn Carnes, Senior Research Fellow, Barts and the London School of Medicine and Dentistry
-
Dr Emma Clark, Clinician Scientist Fellow & Cons. Rheumatologist, University of Bristol
-
Mrs Anthea De Barton-Watson, Public contributor
-
Professor Nadine Foster, Professor of Musculoskeletal Health in Primary Care Arthritis Research, Keele University
-
Dr Shaheen Hamdy, Clinical Senior Lecturer and Consultant Physician, University of Manchester
-
Professor Christine Norton, Professor of Clinical Nursing Innovation, Bucks New University and Imperial College Healthcare NHS Trust
-
Dr Lorraine Pinnigton, Associate Professor in Rehabilitation, University of Nottingham
-
Dr Kate Radford, Senior Lecturer (Research), University of Central Lancashire
-
Mr Jim Reece, Public contributor
-
Professor Maria Stokes, Professor of Neuromusculoskeletal Rehabilitation, University of Southampton
-
Dr Pippa Tyrrell, Senior Lecturer/Consultant, Salford Royal Foundation Hospitals’ Trust and University of Manchester
-
Dr Nefyn Williams, Clinical Senior Lecturer, Cardiff University
-
Dr Kay Pattison, Senior NIHR Programme Manager, Department of Health
-
Dr Morven Roberts, Clinical Trials Manager, Health Services and Public Health Services Board, Medical Research Council
-
Professor Tom Walley, CBE, Director, NIHR HTA programme, Professor of Clinical Pharmacology, University of Liverpool
-
Dr Ursula Wells, Principal Research Officer, Policy Research Programme, Department of Health
Interventional Procedures Panel
-
Professor of Vascular Surgery, University of Sheffield
-
Consultant Colorectal Surgeon, Bristol Royal Infirmary
-
Mrs Isabel Boyer, Public contributor
-
Mr Sankaran Chandra Sekharan, Consultant Surgeon, Breast Surgery, Colchester Hospital University NHS Foundation Trust
-
Professor Nicholas Clarke, Consultant Orthopaedic Surgeon, Southampton University Hospitals NHS Trust
-
Ms Leonie Cooke, Public contributor
-
Mr Seumas Eckford, Consultant in Obstetrics & Gynaecology, North Devon District Hospital
-
Professor Sam Eljamel, Consultant Neurosurgeon, Ninewells Hospital and Medical School, Dundee
-
Dr Adele Fielding, Senior Lecturer and Honorary Consultant in Haematology, University College London Medical School
-
Dr Matthew Hatton, Consultant in Clinical Oncology, Sheffield Teaching Hospital Foundation Trust
-
Dr John Holden, General Practitioner, Garswood Surgery, Wigan
-
Dr Fiona Lecky, Senior Lecturer/Honorary Consultant in Emergency Medicine, University of Manchester/Salford Royal Hospitals NHS Foundation Trust
-
Dr Nadim Malik, Consultant Cardiologist/Honorary Lecturer, University of Manchester
-
Mr Hisham Mehanna, Consultant & Honorary Associate Professor, University Hospitals Coventry & Warwickshire NHS Trust
-
Dr Jane Montgomery, Consultant in Anaesthetics and Critical Care, South Devon Healthcare NHS Foundation Trust
-
Professor Jon Moss, Consultant Interventional Radiologist, North Glasgow Hospitals University NHS Trust
-
Dr Simon Padley, Consultant Radiologist, Chelsea & Westminster Hospital
-
Dr Ashish Paul, Medical Director, Bedfordshire PCT
-
Dr Sarah Purdy, Consultant Senior Lecturer, University of Bristol
-
Dr Matthew Wilson, Consultant Anaesthetist, Sheffield Teaching Hospitals NHS Foundation Trust
-
Professor Yit Chiun Yang, Consultant Ophthalmologist, Royal Wolverhampton Hospitals NHS Trust
-
Dr Kay Pattison, Senior NIHR Programme Manager, Department of Health
-
Dr Morven Roberts, Clinical Trials Manager, Health Services and Public Health Services Board, Medical Research Council
-
Professor Tom Walley, CBE, Director, NIHR HTA programme, Professor of Clinical Pharmacology, University of Liverpool
-
Dr Ursula Wells, Principal Research Officer, Policy Research Programme, Department of Health
Pharmaceuticals Panel
-
Professor in Child Health, University of Nottingham
-
Senior Lecturer in Clinical Pharmacology, University of East Anglia
-
Dr Martin Ashton-Key, Medical Advisor, National Commissioning Group, NHS London
-
Dr Peter Elton, Director of Public Health, Bury Primary Care Trust
-
Dr Ben Goldacre, Research Fellow, Division of Psychological Medicine and Psychiatry, King’s College London
-
Dr James Gray, Consultant Microbiologist, Department of Microbiology, Birmingham Children’s Hospital NHS Foundation Trust
-
Dr Jurjees Hasan, Consultant in Medical Oncology, The Christie, Manchester
-
Dr Carl Heneghan, Deputy Director Centre for Evidence-Based Medicine and Clinical Lecturer, Department of Primary Health Care, University of Oxford
-
Dr Dyfrig Hughes, Reader in Pharmacoeconomics and Deputy Director, Centre for Economics and Policy in Health, IMSCaR, Bangor University
-
Dr Maria Kouimtzi, Pharmacy and Informatics Director, Global Clinical Solutions, Wiley-Blackwell
-
Professor Femi Oyebode, Consultant Psychiatrist and Head of Department, University of Birmingham
-
Dr Andrew Prentice, Senior Lecturer and Consultant Obstetrician and Gynaecologist, The Rosie Hospital, University of Cambridge
-
Ms Amanda Roberts, Public contributor
-
Dr Gillian Shepherd, Director, Health and Clinical Excellence, Merck Serono Ltd
-
Mrs Katrina Simister, Assistant Director New Medicines, National Prescribing Centre, Liverpool
-
Professor Donald Singer, Professor of Clinical Pharmacology and Therapeutics, Clinical Sciences Research Institute, CSB, University of Warwick Medical School
-
Mr David Symes, Public contributor
-
Dr Arnold Zermansky, General Practitioner, Senior Research Fellow, Pharmacy Practice and Medicines Management Group, Leeds University
-
Dr Kay Pattison, Senior NIHR Programme Manager, Department of Health
-
Mr Simon Reeve, Head of Clinical and Cost-Effectiveness, Medicines, Pharmacy and Industry Group, Department of Health
-
Dr Heike Weber, Programme Manager, Medical Research Council
-
Professor Tom Walley, CBE, Director, NIHR HTA programme, Professor of Clinical Pharmacology, University of Liverpool
-
Dr Ursula Wells, Principal Research Officer, Policy Research Programme, Department of Health
Psychological and Community Therapies Panel
-
Professor of Psychiatry, University of Warwick, Coventry
-
Consultant & University Lecturer in Psychiatry, University of Cambridge
-
Professor Jane Barlow, Professor of Public Health in the Early Years, Health Sciences Research Institute, Warwick Medical School
-
Dr Sabyasachi Bhaumik, Consultant Psychiatrist, Leicestershire Partnership NHS Trust
-
Mrs Val Carlill, Public contributor
-
Dr Steve Cunningham, Consultant Respiratory Paediatrician, Lothian Health Board
-
Dr Anne Hesketh, Senior Clinical Lecturer in Speech and Language Therapy, University of Manchester
-
Dr Peter Langdon, Senior Clinical Lecturer, School of Medicine, Health Policy and Practice, University of East Anglia
-
Dr Yann Lefeuvre, GP Partner, Burrage Road Surgery, London
-
Dr Jeremy J Murphy, Consultant Physician and Cardiologist, County Durham and Darlington Foundation Trust
-
Dr Richard Neal, Clinical Senior Lecturer in General Practice, Cardiff University
-
Mr John Needham, Public contributor
-
Ms Mary Nettle, Mental Health User Consultant
-
Professor John Potter, Professor of Ageing and Stroke Medicine, University of East Anglia
-
Dr Greta Rait, Senior Clinical Lecturer and General Practitioner, University College London
-
Dr Paul Ramchandani, Senior Research Fellow/Cons. Child Psychiatrist, University of Oxford
-
Dr Karen Roberts, Nurse/Consultant, Dunston Hill Hospital, Tyne and Wear
-
Dr Karim Saad, Consultant in Old Age Psychiatry, Coventry and Warwickshire Partnership Trust
-
Dr Lesley Stockton, Lecturer, School of Health Sciences, University of Liverpool
-
Dr Simon Wright, GP Partner, Walkden Medical Centre, Manchester
-
Dr Kay Pattison, Senior NIHR Programme Manager, Department of Health
-
Dr Morven Roberts, Clinical Trials Manager, Health Services and Public Health Services Board, Medical Research Council
-
Professor Tom Walley, CBE, Director, NIHR HTA programme, Professor of Clinical Pharmacology, University of Liverpool
-
Dr Ursula Wells, Principal Research Officer, Policy Research Programme, Department of Health