Notes
Article history paragraph text
The research reported in this issue of the journal was funded by the HTA programme as project number 07/37/29. The contractual start date was in November 2008. The draft report began editorial review in March 2012 and was accepted for publication in July 2012. The authors have been wholly responsible for all data collection, analysis and interpretation, and for writing up their work. The HTA editors and publisher have tried to ensure the accuracy of the authors' report and would like to thank the reviewers for their constructive comments on the draft document. However, they do not accept liability for damages or losses arising from material published in this report.
Declared competing interests of authors
DKM is a paid consultant or member of a Data Monitoring Committee for Solvay Ltd, GlaxoSmithKline Ltd, Brainscope Ltd, Ornim Medical, Shire Medical and Neurovive Ltd. His institution receives payment for a registered patent for a new positron emission tomography ligand assessing mitochondrial function
Permissions
Copyright statement
© Queen's Printer and Controller of HMSO 2013. This work was produced by Harrison et al. under the terms of a commissioning contract issued by the Secretary of State for Health. This issue may be freely reproduced for the purposes of private research and study and extracts (or indeed, the full report) may be included in professional journals provided that suitable acknowledgement is made and the reproduction is not associated with any form of advertising. Applications for commercial reproduction should be addressed to: NIHR Journals Library, National Institute for Health Research, Evaluation, Trials and Studies Coordinating Centre, Alpha House, University of Southampton Science Park, Southampton SO16 7NS, UK.
Chapter 1 Introduction
Risk prediction models have been in established use in adult general critical care units for > 30 years, since the publication of the original Acute Physiology And Chronic Health Evaluation (APACHE) model in 1981. 1 In the UK, the first large-scale validation of a risk prediction model was the Intensive Care Society's APACHE II study in Britain and Ireland (1987–9). 2,3 This study produced recalibrated coefficients for the APACHE II model, and led, in 1994, to the formation of the Intensive Care National Audit and Research Centre (ICNARC) and the Case Mix Programme (CMP), the national clinical audit of adult general critical care units in England, Wales and Northern Ireland. ICNARC has continued to pioneer developments in risk prediction in the CMP, most recently through the validation and recalibration of a number of general risk prediction models4 and subsequent development of a new model – the ICNARC model. 5
Unlike adult general critical care, no data are routinely collected in the NHS for risk-adjusted comparison of outcomes from neurocritical care. Consequently, a number of dedicated neurocritical care units currently participate in the CMP. However, there are significant limitations to using models developed and validated for general critical care for patients receiving neurocritical care. Using a spectrum of measures for calibration and discrimination, risk prediction models – successfully developed and validated for adult admissions to general critical care units – showed significant departure from perfect calibration in admissions with head injuries to adult general and dedicated neurocritical care units. 6 The inclusion and handling within general risk models of variables of specific prognostic importance in acute traumatic brain injury (TBI) is often poor. 6 For example, the APACHE II model assumes that any patient who is sedated for the entire first 24 hours in the critical care unit is deemed neurologically normal, which is unlikely to be correct for TBI patients and has led to that the use of pre-sedation values of the Glasgow Coma Scale (GCS) for such patients. 7 The only general model to take any account of changes detected on computerised tomography (CT) scan is the Mortality Prediction Model (MPM) II, and the inclusion of CT information in this model is limited to the presence of an intracranial mass effect. Furthermore, all risk prediction models for adult general critical care use an outcome of mortality at discharge from acute hospital, which is not considered adequate for neurocritical care when longer-term (e.g. 6-month) mortality and, importantly, functional outcome are more valid. 8
Although a large number of risk prediction models for TBI exist, a systematic review found that most models are limited by being based on small samples of patients, having poor methodology, and rarely being validated on external populations. 9 Despite the more recent development of models based on larger, more representative data sources,10 these models require further prospective validation, and potentially recalibration, before they can be applied with confidence for research and audit in neurocritical care in the NHS.
In the NHS, adult patients with TBI are rarely managed by a single service. They are usually managed by a succession of services from first contact to definitive critical care and the latter is not always within in a dedicated neurocritical care unit within a neuroscience centre. Despite guidelines recommending that all patients with severe TBI be treated within a neuroscience centre,11 many (particularly those without surgically remedial lesions) are currently neither treated in nor transferred to one. A combination of geography, bed availability, local variation and clinical assessment of prognosis can often determine the location of definitive critical care for an adult patient with TBI. The Neurocritical Care Stakeholder Group, established to offer expert advice to Department of Health and Commissioners, indicated in its audit report that, within the NHS, only 67% of beds that are ring-fenced for neurocritical care were in dedicated neurocritical care units and that neurocritical care unit occupancy rates exceeded 90% (especially for Level 3 beds). 12 Most neurocritical care for adult patients with TBI was delivered either in dedicated neurocritical care units (42%) or in combined neuro/general critical care units within a neuroscience centre (35%). However, despite clear guidelines and the progressive regionalisation of neurosurgical care since 1948, 23% of patients with TBI were treated in general critical care units outside a neuroscience centre.
Local critical care consultant opinion indicated that at least 83% of these patients required transfer to a neuroscience centre. No data were available, or are routinely collected even in 2012, within the NHS for risk-adjusted comparisons.
Where adult patients with TBI should be optimally managed is an important question for the NHS, in terms of both outcomes and costs. Belief and limited evidence has underpinned the establishment, and continuing expansion, of dedicated, neurocritical care facilities in the UK13,14 but no formal evaluation has been undertaken. Increased centralisation has been hypothesised to improve outcomes through concentration of knowledge and expertise, higher volumes of patients and greater adherence to evidence-based protocols. Recent research has suggested benefit from managing severe head injury in specialist centres;15,16 however, the results are inconclusive owing to lack of adjustment for all known confounders, no data on costs of care, and having follow-up data only to hospital discharge. The existing research also does not address the issue of dedicated compared with combined critical care units within neuroscience centres. A key issue for policy-makers is whether the additional initial costs of more specialised care are justified by subsequent reductions in morbidity costs and/or improvements in patient outcomes. Although conventional randomised controlled trial (RCT) methodology may be impractical in this setting, the presence of variation in the way services are organised and delivered can allow them to be compared using observational methods. This is possible only if a valid, reliable, appropriate and accurate risk prediction model exists.
The Risk Adjustment In Neurocritical care (RAIN) study was originally conceived in 2001. At its inaugural meeting in February 2007, the newly formed Neurocritical Care Network (NCCNet), a network of units and staff providing neurocritical care to patients in both dedicated and general units, identified establishing a risk prediction model to investigate and evaluate the location and outcomes of care for adult patients with TBI as their first, and top, priority. It was recognised that this aim could only be achieved through validation of an accurate risk prediction model for adult patients with TBI and the RAIN study was therefore adopted by NCCNet.
The primary aims of the RAIN study were to validate risk prediction models for acute TBI in the setting of neurocritical care in the NHS, and to use these models to evaluate the optimum location and comparative costs of neurocritical care in the NHS. Specific, detailed objectives to achieve these aims were to:
-
identify, from the literature, the existing risk prediction models for acute TBI most likely to be applicable to a neurocritical care setting, and identify a full list of variables required in order to be able to calculate these models (see Chapter 2)
-
collect complete, valid and reliable data for the variables identified above for consecutive adult admissions with TBI to dedicated neurocritical care units within a neuroscience centre, combined neuro/general critical care units within a neuroscience centre and general critical care units outside a neuroscience centre within the NHS (see Chapter 3)
-
describe the case mix of these patients and their survival, neurological outcome and quality of life (QOL) at 6 months following the TBI (see Chapter 4)
-
undertake a prospective, external validation of existing models for adult patients with TBI admitted to critical care, to identify the strengths and weaknesses of each model, and, if possible, to identify the best model to use for risk adjustment in this setting (see Chapter 5)
-
describe and compare adjusted outcomes and cost-effectiveness of care for adult admissions with TBI between dedicated neurocritical care units within a neuroscience centre, combined neuro/general critical care units within a neuroscience centre and general critical care units outside a neuroscience centre within the NHS (see Chapter 6)
-
make recommendations for policy and practice within the NHS (see Chapter 7).
Chapter 2 Selection of risk prediction models for critically ill patients with acute traumatic brain injury
Introduction
This chapter reports the process that was undertaken to select the most appropriate risk prediction models for external validation in the RAIN study. The aim was to identify the models most likely to be applicable in a neurocritical care setting in the NHS.
Methods
Selection of candidate risk prediction models was conducted in two phases. First, a systematic review of the literature was conducted to identify existing risk prediction models for acute TBI that are most likely to be applicable to a critical care setting and to identify the variables required to be able to calculate these models. Second, eligible models identified by the systematic review were reviewed by the RAIN Study Steering Group (see Acknowledgements) to determine if there were any relevant models that had been missed and to select the most appropriate models for external validation in the RAIN study.
Perel et al. 9 previously conducted a systematic review to identify and assess existing risk prediction models for TBI. 9 The first stage of the systematic review therefore was to update the existing review to identify relevant risk prediction models that had been published since 2006. The second stage was to assess the eligibility of studies previously identified by Perel et al. 9 and by the updated searches for the RAIN study.
Search strategy
The electronic search strategy was based on that used by Perel et al. 9 (see Appendix 1) and was performed using EMBASE (incorporating MEDLINE) to identify eligible studies, published in English, from 2006 to 2008, which (1) gave an overall prognostic estimation by combining the predictive information from at least two variables – studies could develop new prognostic models (derivation studies) or evaluate previous ones (validation studies); (2) used variables collected before hospital discharge, which were therefore considered as predictors; (3) included patients of any age; (4) included patients with any type or severity of TBI; and (5) predicted any outcome, such as neurological impairment, disability, survival, etc. There was no time restriction for the evaluation of outcomes. Three search themes were combined: ‘traumatic brain injury’; ‘brain/coma/consciousness/craino/skull’; and ‘prognosis/predicts’. One reviewer (GPr) examined titles, abstracts and keywords of records identified by the electronic database searches for eligibility. The full text of all potentially eligible papers was obtained and independently assessed by two reviewers (GPr and DAH) for eligibility using the pre-defined inclusion criteria described above. Disagreement was resolved by a third reviewer (KMR). The reference lists of all full-text papers reviewed were checked for any additional potentially eligible studies.
The studies previously identified by Perel et al. 9 and by the updated searches were then independently assessed by two reviewers (GPr and DAH) for eligibility for the RAIN study. Studies were eligible for inclusion if they (1) were based on adult (> 16 years) populations; (2) had a sample size of greater than 500 patients in either the development or validation data set; (3) aimed to evaluate outcome regardless of care received during the hospital stay; and (4) were UK based or multicentre. Disagreement was resolved by a third reviewer (KMR).
Assessment of methodological quality
There is no gold standard tool for quality assessment of either RCTs or observational studies. Assessment of the methodological quality of studies eligible for the RAIN study was conducted using the same approach as Perel et al. 9 considering two domains: (1) internal validity, which refers to systematic error and is related to the study design and (2) external validity, which refers to the generalisability of the study and whether the results can be extrapolated to other populations and settings. Eighteen questions related to internal and external validity were considered for each of the studies, as follows:
Internal validity
-
Did the study have adequate follow-up?
-
Was a discussion included about the rationale to include predictors?
-
Were the predictive variables clearly defined?
-
Were the outcomes predicted valid?
-
Were missing data adequately managed?
-
Was an adequate strategy performed to build the multivariable model?
-
Were interactions between the variables examined?
-
Were the continuous variables handled appropriately?
-
Were there > 10 events per variable included?
External validity
-
Was a description of the sample population reported?
-
Was there a clear explanation on how to estimate the prognosis provided?
-
Were measures of discrimination reported?
-
Were measures of calibration reported?
-
Were confidence intervals (CIs) reported?
-
Was the model validated?
-
Was the model internally validated?
-
Was the model externally validated?
-
Was the effect of the model established?
Expert review
All studies eligible for the RAIN study were then reviewed by the RAIN Study Steering Group to identify any additional studies, either published or ongoing, of relevance, and to select the most appropriate models for validation in a UK critical care setting.
Results
The electronic database searches identified a total of 1832 citations. After screening of titles and abstracts, 23 potentially eligible papers were identified for full-text review. 17–39 In addition, the electronic database searches identified seven review articles,40–46 the references lists of which did not identify any further potentially eligible papers. Of the 23 full-text papers reviewed, 13 were excluded because either they were not studies that had developed or evaluated prognostic models for TBI or they were prognostic models that included predictors measured after discharge from hospital (Figure 1).
A total of 53 studies reporting 102 models were identified by Perel et al. 9 However, of these, the authors considered the models developed by Signorini et al. 47 and Hukkelhoven et al. 48 to be the most clinically useful for patients from high-income countries with moderate and severe TBI, as they fulfilled the majority of the methodological requirements and showed acceptable performance in the external validation. They were also considered to be available in a user-friendly way. A total of 12 potentially eligible studies were therefore identified from Perel et al. 9 and from the updated searches (see Figure 1).
Of the 12 potentially eligible studies, eight did not fulfil the RAIN study eligibility criteria because the models had been developed in paediatric populations, were based on samples of fewer than 500 patients, adjusted for care received within hospital or had been conducted in a single-centre, non-UK setting (Figure 2). This resulted in four eligible studies for review by the RAIN Study Steering Group.
FIGURE 2.
Stage 2 of the selection process: selection of models for external validation in the RAIN study. (a) Studies were excluded for more than one reason.
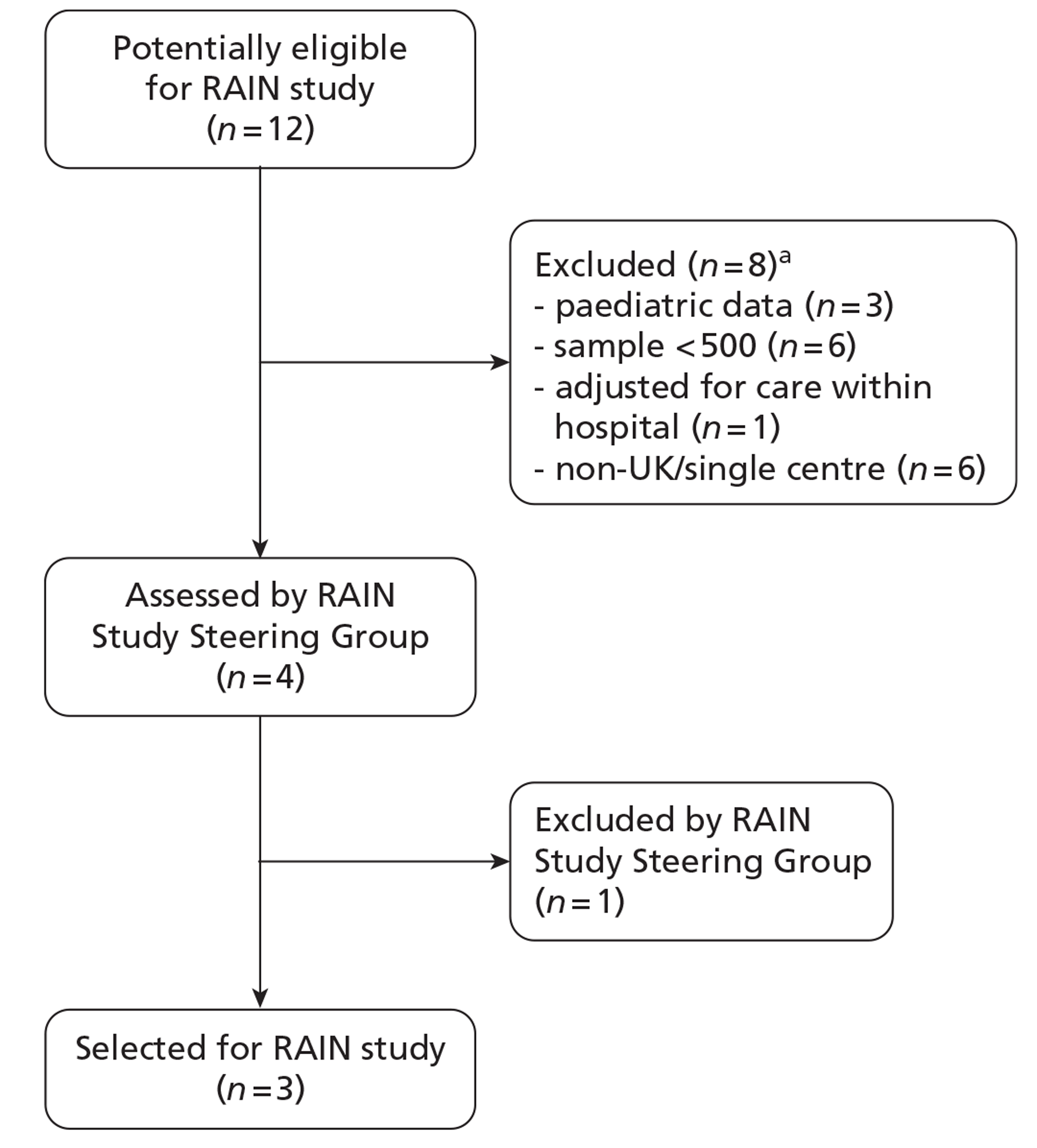
Description of eligible studies
Four studies,30,35,47,48 reporting a total of 17 risk prediction models, met the RAIN study eligibility criteria. A brief description of each is given below and a summary is provided in Table 1.
Model | Variables in model | Derivation sample | External validation | Outcome | Discrimination c-index (95% CI)a | Calibration, p-value from Hosmer-Lemeshow test | ||
---|---|---|---|---|---|---|---|---|
Signorini et al. (1999)47 | ||||||||
GCS score | Single-centre observational study (n = 372) | Single-centre observational study (n = 520) | Mortality at 1 year | 0.835 | < 0.001 | |||
Pupil reactivity | ||||||||
SS | ||||||||
Haematoma | ||||||||
Hukklehoven et al. (2005)48 | ||||||||
Model | Age | Two multicentre RCTs (n = 2,269) | EBIC (n = 796) | Mortality at 6 months | 0.87 (0.84 to 0.89) | 0.42 | ||
GCS motor score | TCDB (n = 746) | Mortality at 6 months | 0.89 (0.87 to 0.91) | < 0.001 | ||||
Pupil reactivity | ||||||||
Hypoxia | EBIC (n = 796) | Unfavourable outcome at 6 months | 0.83 (0.80 to 0.86) | 0.05 | ||||
Hypotension | ||||||||
CT classificationb | ||||||||
SAH | ||||||||
MRC CRASH trial collaborators (2008)30 – CRASH models | ||||||||
Basic model | Age | One multicentre RCT (n = 2185) | IMPACT database (n = 8509) | Unfavourable outcome at 6 months | 0.81 | 0.70 | ||
GCS score | ||||||||
Pupil reactivity | ||||||||
Major extracranial injuryc | ||||||||
CT model | As above for Basic model plus: | One multicentre RCT (n = 1955) | IMPACT database (n = 8509) | Unfavourable outcome at 6 months | 0.83 | 0.63 | ||
Petechial haemorrhagesc | ||||||||
Obliteration of the third ventricle or basal cisterns | ||||||||
SAH | ||||||||
Midline shift | ||||||||
Non-evacuated haematoma | ||||||||
Steyerberg et al. (2008)35 – IMPACT models | ||||||||
Core model | Age | IMPACT database (n = 8509) | CRASH tria (n = 6272) | Mortality at 6 months | 0.78 | – | ||
GCS motor score | Unfavourable outcome at 6 months | 0.78 | – | |||||
Pupil reactivity | ||||||||
Extended model | As above for core model plus: | IMPACT database (n = 6999) | CRASH tria (n = 6272) | Mortality at 6 months | 0.80 | – | ||
Hypoxiac | Unfavourable outcome at 6 months | 0.80 | – | |||||
Hypotensionc | ||||||||
CT classificationb | ||||||||
SAH | ||||||||
Extradural haemorrhagec | ||||||||
Laboratory model | As above for Core and Extended models plus: | IMPACT database (n = 3554) | Not externally validated | Mortality at 6 months | – | – | ||
Glucose | Unfavourable outcome at 6 months | – | – | |||||
Haemoglobin |
Signorini et al.
Signorini et al. 47 developed a risk prediction model in a cohort of consecutive patients admitted to a regional trauma centre with moderate or severe head injury (n = 372) between January 1989 and July 1991. The criteria for enrolment into the study were (1) age ≥ 14 years and (2) admission or last known GCS score of < 12, or of 13–15 with concomitant systemic injuries giving an injury severity score (ISS) of ≥ 16. The outcome assessed was mortality at 1 year. The variables included in the model are detailed in Table 1. The model was externally validated in a similar cohort of patients in the same centre accrued as part of an almost identical study between July 1991 and April 1996 (n = 520).
Hukkelhoven et al.
Hukkelhoven et al. 48 developed two risk prediction models using data from two multicentre RCTs: (1) the International Tirilazad trial (n = 1120) conducted in 40 centres in Europe, Israel and Australia from 1992 to 199449 and (2) the North America Tirilazad trial (n = 1149) conducted in 36 centres in the USA and Canada from 1991 to 1994. 50 Patients were included who (1) were aged ≤ 65 years; (2) had a total GCS score of < 9 or a total GCS score of 9–12 and an abnormal CT scan; (3) had a GCS motor score available; (4) had a CT scan available; and (5) had been admitted to hospital within 4 hours of the TBI. The outcomes assessed were mortality at 6 months and unfavourable outcome (death, vegetative state or severe disability) at 6 months, defined using the Glasgow Outcome Scale (GOS). The variables included in the models are detailed in Table 1. The model for mortality at 6 months was externally validated in two populations of patients: the core data survey conducted by the European Brain Injury Consortium (EBIC), which included 796 patients with severe or moderate TBI consecutively collected between February and April 1995 from 55 European centres in which the 6-month outcome assessment was routinely performed,51 and the Traumatic Coma Data Bank (TCDB), which contained data collected on 746 patients with non-penetrating severe TBI admitted to four centres in the USA between 1984 and 1988. 52 The model for unfavourable outcome at 6 months was externally validated in the EBIC data set only.
Medical Research Council CRASH trial collaborators
The Medical Research Council (MRC) Corticosteroid Randomisation After Significant Head injury (CRASH) trial collaborators30 developed eight risk prediction models using data from the MRC CRASH trial, a large international RCT which enrolled 10,008 patients between 1999 and 2004. 53–54 Risk models were developed for death at 14 days and unfavourable outcome at 6 months in patients with TBI in low-/middle- and high-income countries. The risk models that were eligible for the RAIN study were those developed using data from high-income countries (n = 2482). The outcome of interest for the RAIN study was unfavourable outcome at 6 months. Two models were developed: (1) the Basic model, which included only clinical and demographic variables, and (2) the CT model, which also included CT scan results. Patients were included who (1) were aged ≥ 16 years; (2) had a total GCS score of < 15; and (3) were within 8 hours of the TBI. The variables included in the model are detailed in Table 1. The models, with some modifications, were externally validated in a cohort of 8509 patients with moderate to severe TBI from the International Mission for Prognosis and Analysis of Clinical Trials in TBI [IMPACT (International Mission for Prognosis and Analysis of Clinical Trials in TBI)] database (described below). For validation of the Basic model, the ‘variable major extracranial injury’ was excluded, and for validation of the CT model, the ‘variable petechial haemorrhages’ was excluded, as these variables were not available in the validation sample.
Steyerberg et al.
Steyerberg et al. 35 developed three risk models using data from the IMPACT database,55 which includes data from eight RCTs and three observational studies conducted between 1984 and 1997. Patients were included who had a GCS score of < 13. The outcomes assessed were mortality and unfavourable outcome at 6 months. Three models were developed for each outcome: a Core model, an Extended model and a Laboratory model. The variables included in each of the models are detailed in Table 1. The Core model and a variant of the Extended model were validated using data from the CRASH trial (described above). For validation of the Extended model, the variables ‘hypoxia’, ‘hypotension’ and ‘extradural haemorrhage’ were excluded, as these were not available in the validation sample. It was not possible to externally validate the Laboratory model, as laboratory values were not recorded in the CRASH trial.
Assessment of methodological quality
The quality assessment of the four studies,30,35,47,48 using the criteria of Perel et al. ,9 is summarised in Table 2. Neither the MRC CRASH trial collaborators30 nor Steyerberg et al. 35 reported completeness of follow-up in their respective papers reporting development of the CRASH and IMPACT models. However, for the CRASH trial, a separate paper reporting the trial results indicated overall follow-up of 95% for GOS at 6 months,54 and a paper reporting the design of the IMPACT database indicated overall follow-up of 95% for GOS at 6 months (including last observation carried forward imputation of 18% of values from 3 months). 55
Quality criteria, Perel et al. (2006)9 | Signorini et al. (1999)47 | Hukkelhoven et al. (2005)48 | MRC CRASH trial collaborators (2008)30 | Steyerberg et al. (2008)35 | |
---|---|---|---|---|---|
Internal validity – study | |||||
1 | Did the study have adequate follow-up? | Yes (> 90%) | Yes (> 90%) | Yes (> 90%) | Yes (> 90%) |
Internal validity – variables | |||||
2 | Was a discussion included about rationale to include the predictors? | Yes | Yes | Yes | Yes |
3 | Were the predictive variables clearly defined? | No | No | No | No |
4 | Were the outcomes predicted valid? | Yes (mortality) | Yes (mortality/GOS) | Yes (GOS) | Yes (mortality/GOS) |
5 | Were missing data adequately managed? | No (complete case analysis) | Yes (regression imputation) | No (complete case analysis) | Yes (multiple imputation) |
Internal validity – analysis | |||||
6 | Was an adequate strategy performed to build the multivariable model? | Forward selection based on clinical criteria and completeness | Backward stepwise selection (p < 0.2) | p < 0.05 in full model | Partial R2 from previous analysis of same database |
7 | Were interactions between the variables examined? | Not reported | No | Noa | Yes |
8 | Were continuous variables handled appropriately? | Yes | Yes | Yes | Yes |
9 | Were > 10 events per variable included? | No | No | Yes | Yes |
External validity | |||||
10 | Was the description of the sample reported? | Yes | Yes | Yes | Yes |
11 | Was it clearly explained how to estimate the prognosis? | Yes (nomogram) | Yes (simple score) | Yes (web calculator) | Yes (simple score, web calculator and spreadsheet) |
12 | Were measures of discrimination reported? | Yes (with CI) | Yes | Yes | Yes |
13 | Were measures of calibration reported? | Yes | Yes | Yes | Yes |
14 | Were CIs presented? | Yes | Yes | Yes | Yes |
15 | Was the model validated? | Yes | Yes | Yes | Yes |
16 | Was the model internally validated? | No | Yes (bootstrap) | Yes (bootstrap) | Yes (cross-validation) |
17 | Was the model externally validated? | Yes | Yes | Yesb | Yes/noc |
18 | Was the effect of using the model established? | No | No | No | No |
All of the studies30,35,47,48 provided some justification for the predictors included in the models, reflecting a combination of an existing established relationship with outcome and ease of collection and use. However, none of the investigators reported clear definitions for the predictors. All of the studies30,35,47,48 used valid outcomes (mortality and/or GOS) in the models. Handling of missing data varied. In two studies,30,47 complete case analyses were used. The final number of patients included in the model of Signorini et al. 47 was not reported; however, 20% of patients were missing CT assessment alone and, therefore, a maximum of 80% of the original sample of 365 patients can have been used in fitting the final model. The MRC CRASH trial collaborators30 cited low levels of missing data as justification for their complete case approach; however, this approach resulted in only 88% of the original sample being included in the Basic model for high-risk countries and 79% in the CT model. In contrast, for the remaining two studies,35,48 statistical imputation methods were used, resulting in all patients being included in the final models. Hukkelhoven et al. 48 used regression imputation to impute the 4.8% of missing values, acknowledging that such an approach would slightly underestimate the true variability and Steyerberg et al. 35 used the gold standard method of multiple imputation.
In all four studies,30,35,47,48 risk prediction models were developed using logistic regression, although the approach to variable selection varied. Signorini et al. 47 used a form of forward stepwise selection but with the order of variables being added to the model based on a combination of data completeness and clinical criteria rather than statistical significance alone. Hukkelhoven et al. 48 fitted a full model and used backward stepwise selection to remove variables with p > 0.2. The MRC CRASH trial collaborators30 included variables in the final models if they were significant at the 5% level in a full multivariable model. Finally, Steyerberg et al. 35 based inclusion in their final models on partial Nagelkerke R2-values from a previous multivariable analysis of the same database. 57 The only authors who reported evaluating interactions between predictors were Steyerberg et al. ;35 however, it was unclear which, or how many, potential interactions were examined. All of the studies30,35,47,48 used continuous modelling for continuous variables, and all either included or considered some degree of non-linearity. The criterion of at least 10 events per predictor variable included in the modelling process was met by three of the four studies. 30,35,48 Signorini et al. 47 reported approximately eight events per predictor variable included in the model.
A description of the sample population, including important case mix variables, was reported for all four studies. 30,35,47,48 In addition, simple methods for calculating predictions, including a nomogram, simple clinical scores, web calculators and a spreadsheet calculator, were provided. Measures of discrimination were reported in all papers, although only Hukkelhoven et al. 48 included a CI on the c-index [area under the receiver operating characteristic (ROC) curve]. In all papers, calibration was summarised graphically and tested for perfect calibration with the Hosmer–Lemeshow test, with CIs reported on model estimates.
All models were validated either internally and/or externally as described above and summarised in Table 1. None of the models was evaluated for its effect in clinical practice.
Internal validation was performed by Hukkelhoven et al. 48 and the MRC CRASH trial collaborators30 using bootstrap methods, and by Steyerberg et al. 35 using cross-validation across the 11 separate study data sets comprising the IMPACT database. The model of Signorini et al. 47 was externally validated using data from a further 520 patients admitted to the same single centre. The models of Hukkelhoven et al. 48 were externally validated using data from approximately 1500 patients from observational registries. Modified versions of the CRASH models were validated using the IMPACT database. 30 The IMPACT Core models and modified versions of the IMPACT Extended models were validated in the CRASH trial data set; however, it was not possible to externally validate the IMPACT Lab models, as the CRASH trial did not record the required laboratory values. 35
Expert review
The RAIN Study Steering Group did not identify any further studies (either published or ongoing) that would be potentially eligible for the RAIN study. The four studies identified by the systematic review reported development and validation of 17 risk prediction models. Of these, 11 were potentially eligible for validation in the RAIN study; the models developed by the MRC CRASH trial collaborators30 for low-income countries and predicting mortality at 14 days were excluded. Following review by the RAIN Study Steering Group, 10 risk prediction models were selected for external validation in the RAIN study. The model developed by Signorini et al. 47 was excluded because the model was developed using data from a relatively small single-centre study with mortality at 1 year as the outcome. The remaining three studies were large multicentre studies, which considered functional outcome as well as mortality at 6 months. There was also concern about the data burden associated with the ISS included in the Signorini et al. model. 47
Discussion
Principal findings
The systematic review of the literature identified four studies30,35,47,48 reporting development and validation of 17 risk prediction models for TBI. Of the 17 models, 11 were eligible for the RAIN study. Following assessment of their methodological quality and review by the RAIN Study Steering Group, 10 models, developed and validated in three studies,30,35,48 were selected for external validation in the RAIN study. Four models were developed for mortality at 6 months35,48 and six models were developed for unfavourable outcome, using the GOS, at 6 months. 30,35,48
The variables included across the 10 selected models were age, GCS score, GCS motor score, pupil reactivity, presence of major extracranial injury, hypoxia, hypotension, glucose, haemoglobin, Marshall CT classification,56 presence of traumatic subarachnoid haemorrhage (SAH), presence of extradural haematoma, presence of petechial haemorrhages, obliteration of the third ventricle or basal cisterns, midline shift and non-evacuation of haematoma. The outcomes assessed were mortality and unfavourable outcome, using the GOS, at 6 months.
Strengths and weaknesses
A major strength of this systematic review was being built on a previous, rigorous systematic review. 9 One of the strengths of the Perel et al. 9 review is that there was no restriction on the types of patients, i.e. any age and any severity of TBI, or on the setting. Interestingly, although the burden of trauma is much greater in low-income countries, only 2% of the models identified included patients from these countries. The MRC CRASH trial collaborators30 identified significant interactions between the country's income level and several predictors and so developed separate models for low-/middle-income countries and for high-income countries. Given that the RAIN study was seeking to validate prognostic models for use in a UK NHS setting, models developed for low-/middle-income countries were excluded.
There are some limitations to the systematic review. The original literature search by Perel et al. 9 was restricted to 1990 onwards. However, this was on the basis that changes in management and diagnostic technology in recent years means that prognostic models developed before 1990 are unlikely to be relevant for the current medical care of patients with TBI. In addition, only studies that explicitly combined at least two predictors were included, which means that studies that used multivariable analysis to investigate individual predictors but did not report an overall estimation were excluded. Similarly, studies that assessed clinical prediction rules considering more than one variable were excluded if they did not combine them.
Methodological quality of the risk prediction models
The original systematic review by Perel et al. 9 and the recent update reveal that a large number of prognostic models for TBI have been published. However, their methodological quality is relatively poor. Limitations include, small sample sizes (fewer than 10 events per variable), loss to follow-up rates in excess of 10%, inappropriate handling of missing data (i.e. not using statistical imputation methods), and rarely being validated in external populations. Perel et al. 9 reported that of the 102 models (in 53 studies) identified, they considered only the three models developed by Signorini et al. 47 and Hukkelhoven et al. 48 to be clinically useful for patients from high-income countries. All three models fulfilled the eligibility criteria for inclusion in the RAIN study. The updated search identified an additional eight models (from two studies30,35) that were also eligible. In general, the methodological quality of these 11 models was good. However, eight models35,48 were developed using data from multiple sources and may therefore be limited by differences between data sets in eligibility criteria, definitions of variables and timings of measurements. Although all of the studies included discussion about the rationale for including specific predictors, none reported clear definitions for predictive variables. In addition, there was variation in how missing data were handled: two of the four studies used regression or multiple imputation and two used complete case analysis on the basis that there were few missing data; however, this meant that for at least one of the models, only 79% of the original sample was included. 30
Of the 11 eligible models, 10 risk prediction models were selected by the RAIN Study Steering Group for validation in the RAIN study. 30,35,48 All were developed using some or all data from RCTs, which may limit their external validity. Even in large pragmatic RCTs, such as the CRASH trial,30 external validity may be affected by self-selection of centres and patients to participate in the trial, as well as the potential for patients enrolled in a trial (in both the active and control arms) to receive better standard of care than in usual clinical practice. 58,59 To assess whether a model is generalisable to other populations, it is important to conduct external validation. Of the 10 models, all except two were validated on patients from different centres. However, for four of the remaining eight models, a limitation was that some variables had to be excluded from the models for validation as they were not available in the validation sample. Therefore, only four of the 10 models – those by Hukkelhoven et al. 48 and the Core models from Steyerberg et al. 35 – have been externally validated without undergoing any modifications.
Summary
In summary, three families of risk prediction models including 10 individual models were identified that are most likely to be applicable to a UK critical care setting. 30,35,48 These models require further prospective validation, and potentially recalibration, before they can be applied with confidence in neurocritical care in the NHS.
Chapter 3 The Risk Adjustment In Neurocritical care study
Introduction
The systematic review of the literature identified three families of risk prediction models for acute TBI that were likely to be applicable to a neurocritical care setting. In order to externally validate these models and to use them to evaluate the optimum location and comparative costs of neurocritical care in the NHS, a prospective cohort study was undertaken in dedicated neurocritical care units, combined neuro/general critical care units within a neuroscience centre and general critical care units outside a neuroscience centre. Data were collected on consecutive adult patients admitted to critical care with suspected acute TBI. This chapter reports the RAIN study set-up from research governance, through design and development of the data set, recruitment of sites and patients, data management, and 6-month follow-up of neurological outcomes and QOL.
Methods
Research governance
The RAIN study was sponsored by ICNARC. The contract for the study was signed in December 2008 and, once the study co-ordinator was appointed, the process of completing research governance approvals commenced. Owing to the inclusion of adults with incapacity, two separate applications were made to the NHS Research Ethics Committee (REC) for Wales, covering sites in England and Wales under the Mental Capacity Act 2005, and to the Scotland A REC, covering sites in Scotland under the Adults with Incapacity (Scotland) Act 2000. Favourable opinions were received on 13 March 2009 (ref. 09/MRE09/10) and 30 March 2009 (ref. 09/MRE00/15), respectively.
In response to the unique problems faced by patients with acute TBI in critical care, we delayed the request for consent until 6 months after the TBI at the point of follow-up. At hospital/critical care unit admission these patients are often unconscious and their level of consciousness continues to vary during their stay in the critical care unit. Generally, treatment needs to be started urgently, so there is little time for health-care staff to adequately explain research studies to patients or their families. This is, of course, a stressful and emotional time for families. In view of these difficulties in gaining informed consent, an application was made to the National Information Governance Board (NIGB) Ethics and Confidentiality Committee (ECC) for support under Section 251 of the NHS Act 2006 for permission to hold sufficient patient identifiable data, prior to patient consent, in order for us to contact the patient at 6 months post TBI and gain their consent. Section 251 support, covering sites in England and Wales, was obtained on 4 August 2009 [ref. ECC 2–06(d)/2009]. For the two sites in Scotland, falling outside the remit of the NHS Act 2006, approval was sought from the Caldicott Guardian and was granted on 10 November 2009 and 25 March 2010.
Central Research and Development (R&D) approval was gained on 4 August 2009. Site-specific information (SSI) forms were submitted for each for each NHS Trust, with the last form submitted on 3 November 2009 and the final approval gained on 28 January 2010.
The National Institute for Health Research (NIHR) Clinical Research Network (CRN) Portfolio details high-quality clinical research studies that are eligible for support from the NIHR CRN in England. The RAIN study was adopted on to the NIHR CRN Portfolio on 27 August 2009 (ref. 7349).
Changes to protocol
The initial RAIN study protocol submitted for approvals was Version 1.3 (21 January 2009). Amendments to the RAIN study follow-up documentation, not requiring changes to the study protocol, were submitted in March 2009, May 2009, July 2009 and November 2009, and approved by the RECs. A final non-substantial amendment to the Study Protocol (Version 1.4, 1 February 2011; see Appendix 2) was submitted in February 2011, clarifying explicitly that participating critical care units would be required to submit anonymised CT scans to the Wolfson Brain Imaging Centre (WBIC) at Addenbrooke's Hospital (University of Cambridge/Cambridge University Hospitals NHS Foundation Trust) for the purpose of the substudy on inter-rater reliability of CT scan reporting.
Design and development of data set
The initial RAIN study data set was developed and produced by the RAIN study team from the original model publications. Definitions for some of the fields were refined through discussion and consultation with the clinical experts on the RAIN Study Steering Group. There were five elements to the data set: (1) characteristics of the patients and their injury; (2) risk factors for the selected risk prediction models; (3) location of care details, to describe and cost the patient journey in order to investigate the effect of location of neurocritical care; (4) short-term outcomes at discharge from critical care and acute hospital; and (5) contact details, to provide the information required for the 6-month follow-up.
To avoid duplication of data collection, the RAIN study was piggybacked on to the CMP in England and Wales and linked with data provided by the Scottish Intensive Care Society Audit Group (SICSAG) in Scotland. Both the CMP and SICSAG databases have been independently assessed to be of high quality against 10 criteria for coverage and accuracy by the Directory of Clinical Databases at docdat.ic.nhs.uk. Critical care units in England and Wales that were not participating in the CMP recorded all required fields within the RAIN study data set.
The publications reporting each risk prediction model, other associated publications and, where available, original study documentation relating to the data sources were examined for definitions for each field to be included in the data set. The definitions and, in particular, the time points for data collection were often not clearly defined and/or varied both between risk models and between different data sources used for the development of the same risk model. Clinical experts from the RAIN Study Steering Group also identified a small number of additional fields that they felt to be important predictors of outcome following TBI that were not included in any of the risk prediction models, the collection of which was considered valuable for informing future work in this area.
The full RAIN study data set and definitions are provided in Appendix 3. A brief summary is given below.
Characteristics
Patients were characterised by their age, sex, residential postcode (permitting linkage to small area deprivation statistics), residence prior to hospital admission and prior dependency. The injury was characterised by timing, cause, intoxication at the time of injury and presence, and site(s) of major extracranial injury.
Risk factors
Pupil reactivity and GCS score data were collected both pre-hospital (prior to attendance at first hospital) and at admission to the first hospital (within 12 hours of attendance at the first hospital). GCS score was collected additionally as the last value prior to sedation, and pupil reactivity at the point of admission to the critical care unit. CT scan data were evaluated based on the first CT scan performed after the TBI.
Location of care details
The patient journey prior to admission to the critical care unit was recorded using the immediate prior location and, for patients who were admitted from a more transient location (e.g. theatre, imaging, emergency department), their location prior to this. Data for resource use was based on the total numbers of calendar days of both organ support and levels of care as defined for the Department of Health Critical Care Minimum Data Set (CCMDS).
Short-term outcomes
Survival status at discharge from the critical care unit was recorded in the RAIN study for all admissions. For survivors, subsequent information on location following discharge (including critical care transfers), and outcomes at final discharge from critical care and acute hospital were also collected.
Contact details
The patient's full name, address and any telephone number(s) were included to permit the follow-up of patients by postal (or telephone) questionnaire at 6 months following the TBI. The patient's NHS number was included to ensure accurate linkage to national death registration using the ‘list cleaning’ service of the Medical Research Information Service (MRIS) at the NHS Information Centre for Health and Social Care. Name and contact details for the patient's general practitioner (GP) were included to confirm from the GP that the patient was still alive prior to sending the questionnaire.
Sample size calculation
We performed a simulation study to assess the power to detect a difference in the c-index (area under the ROC curve) between two different risk prediction models applied to the same population. Simulations were based on the following assumptions: the rate of unfavourable outcome (death or severe disability) at 6 months in the population will be 40% (based on the observed rate of unfavourable outcomes in high-income countries in the CRASH trial30 and consistent with the results of a regional audit in East Anglia60); statistical tests will be based on a two-sided p-value of p = 0.05; and the ability to detect, with 80% power, a 10% relative difference in c-index from the value of 0.83 observed for the CRASH model in the development sample. 30 A total of 17,500 data sets were simulated at different sample sizes using a binormal model61 and the empirical power was assessed at each sample size as the proportion of data sets in which a statistically significant difference was detected (see Appendix 2).
Based on these simulations, a sample size of 3100 patients was required for model validation. To allow for 8% loss to follow-up [based on the observed follow-up rates from the CRASH62 and Randomised Evaluation of Surgery with Craniectomy for Uncontrollable Evaluation of Intra-Cranial Pressure (RESCUEicp) RCTs63], we aimed to recruit 3400 patients.
Using data from the CMP database, we anticipated the rate of admission of adult patients following acute TBI to be approximately eight per unit per month for dedicated neurocritical care units, six per unit per month for combined neuro/general critical care units, and 0.5 per unit per month for general critical care units outside a neuroscience centre. We therefore aimed to recruit at least 12 dedicated neurocritical care units, 13 combined neuro/general critical care units and 30 general critical care units outside neuroscience centres to complete recruitment within 18 months.
Recruitment of sites and patients
Recruitment of sites
All neurocritical care units in the UK and adult general critical care units participating in the CMP were invited to participate in the RAIN study. Standalone high-dependency units (HDUs) were not eligible for participation in the study. The RAIN study was publicised to critical care units via the CMP, NCCNet, the Intensive Care Society and the UK Critical Care Trials Forum (UKCCTF).
Maintenance and motivation of sites
Regular contact was maintained with all participating critical care units during the course of the RAIN study. Newsletters were sent on a monthly to quarterly basis, depending on the stage of the study, to maintain motivation and encourage involvement by keeping data collectors informed of study progress. Newsletters were also used as an opportunity to clarify any data issues and remind local collaborators to enrol all eligible patients. The study co-ordinator maintained close contact with all sites by telephone and e-mail throughout the study and was available to answer queries.
Updates on study progress were also given at meetings of the CMP, NCCNet, the Neuroanaesthesia Society of Great Britain and Ireland and the UKCCTF in order to maintain the profile of the RAIN study in the critical care community. Regular updates on study progress were also provided to the NIHR Comprehensive CRN Critical Care Specialty Group. Members of the RAIN Study Steering Group also publicised the study at relevant conferences.
Recruitment of patients
All adult patients (defined as aged ≥ 16 years) admitted to participating adult critical care units following an actual or suspected TBI, and with a GCS score of < 15, following resuscitation, were identified.
Data management
Start-up meetings/data set familiarisation courses
The start-up meetings/data set familiarisation courses were 1-day events, at which the background, aims and rationale for the RAIN study were discussed with the collaborating clinicians, research nurses and data clerks. This was followed by a detailed explanation of the definition for each field in the data set with opportunities for questions and examples. At least one member of staff from each site was required to attend a RAIN study start-up meeting/data set familiarisation course to ensure that they understood the aims of the RAIN study and the precise rules and definitions of the data set. Each delegate was given a RAIN study Data Collection Manual to take back with them to their site for reference purposes.
The Data Collection Manual contained precise, standardised definitions for each field (see Appendix 3), along with data collection forms and flows (see Appendix 4), to guide them through the data collection process. The Data Collection Manual was regularly reviewed and new versions released to ensure clarity and to answer common queries.
Data entry and monitoring of recruitment
From the data collection forms, data were entered by members of the research team at participating sites on to a dedicated, secure, web-based data entry system (‘web portal’) developed and hosted by ICNARC. A guide to using the web portal was produced and sent to research staff to assist in data entry. Data Collection Manuals, flows and forms, definitions and error checking were also available from the web portal, either for download or built into the design.
Data management was an ongoing process. Data were monitored throughout the data collection period in order to ensure that the database was as complete as possible and the rate of recruitment was as expected to minimise the time between the end of data collection and the start of data analysis. For each site, the number of patients entered on to the web portal and the date the last patient was entered was monitored. Neurocritical care units were contacted if no patients had been admitted in one calendar month. General critical care units outside a neuroscience centre received monthly e-mails to remind them to monitor for eligible patients. Quarterly CMP data submissions were reviewed to identify any admissions recorded in the CMP with a reason for admission to that critical care unit that potentially indicated a TBI that had not been entered on to the RAIN web portal.
Every patient initially thought to have TBI was entered on to the RAIN web portal to ensure completeness of recruitment. However, any patient that was subsequently found to have a different cause for their neurological impairment (e.g. cerebrovascular accident) was excluded from analyses.
Data validation reports
Two data validation reports (DVRs) were sent regularly to participating critical care units. The purpose of the first DVR was to ensure complete data entry of the fields required for patient follow-up. This DVR was sent on a weekly to fortnightly basis and checked all patients reaching 4 weeks post TBI to ensure that complete identifiers and contact details (full name and postal address, contact telephone number, NHS number, date of birth and GP details) were available. Data collectors were asked to enter data, where missing, or confirm with the RAIN study team that data were unavailable.
The second DVR checked data accuracy. These checks identified any incomplete data (missing values) and inconsistent data (unusual, although not impossible, data that must be confirmed as correct by the data collectors) both within individual fields and across fields. Following receipt of a DVR, data collectors either updated/corrected the data on the web portal or responded to the RAIN study team to confirm the data were correct in order to clear queries.
Data linkage with the Case Mix Programme
RAIN study data were linked with the corresponding CMP data using the CMP admission number and checked using date of birth, sex, NHS number, date and time of admission to the critical care unit and status on discharge from the critical care unit. Any discrepancies between the two databases were resolved with the respective data collectors. Data linkage between the RAIN study database and CMP database was performed regularly, to ensure outcome data required for the 6-month follow-up of patients were available.
Data linkage with the Scottish Intensive Care Society Audit Group
RAIN study data were linked with the corresponding SICSAG data using the SICSAG Key (unique identifier) and checked using the age in years, sex, and date and time of admission to the critical care unit. Data linkage between the RAIN study database and SICSAG database was performed once at the end of the study.
External validation against the Trauma Audit and Research Network database
The Trauma Audit and Research Network (TARN; www.tarn.ac.uk) is the trauma registry for England and Wales with coverage of approximately 70% of trauma-receiving hospitals. For hospital sites in TARN with a critical care unit participating in the RAIN study, TARN provided data on the number of admissions to critical care associated with TBI as an external data source to verify the completeness of recruitment to the RAIN study.
Data linkage with death registration
The follow-up of patients was carefully monitored to prevent any potential distress to those who care for the patient from receiving a letter addressed to a deceased relative or partner. In order to obtain an outcome for patients at 6 months after acute TBI, the follow-up process started at 4.5 months to allow for the administrative processes. On a weekly basis, the status of any patient that had reached 4.5 months post TBI was checked on the web portal. A list of patients who were not indicated as ‘dead’ was then sent to the MRIS to confirm the mortality status of patients. Patients indicated as having died were logged and the follow-up process ended; all other patients started the 6-month follow-up process to ascertain their neurological outcome and QOL. At the end of the study, a final file was sent to the MRIS to confirm the final survival status of all patients in the RAIN study.
Six-month follow-up of neurological outcome and quality of life
Patients identified as not having died at 4.5 months using data from the critical care unit and from MRIS followed the process shown in Figure 3.
FIGURE 3.
Patient follow-up process.
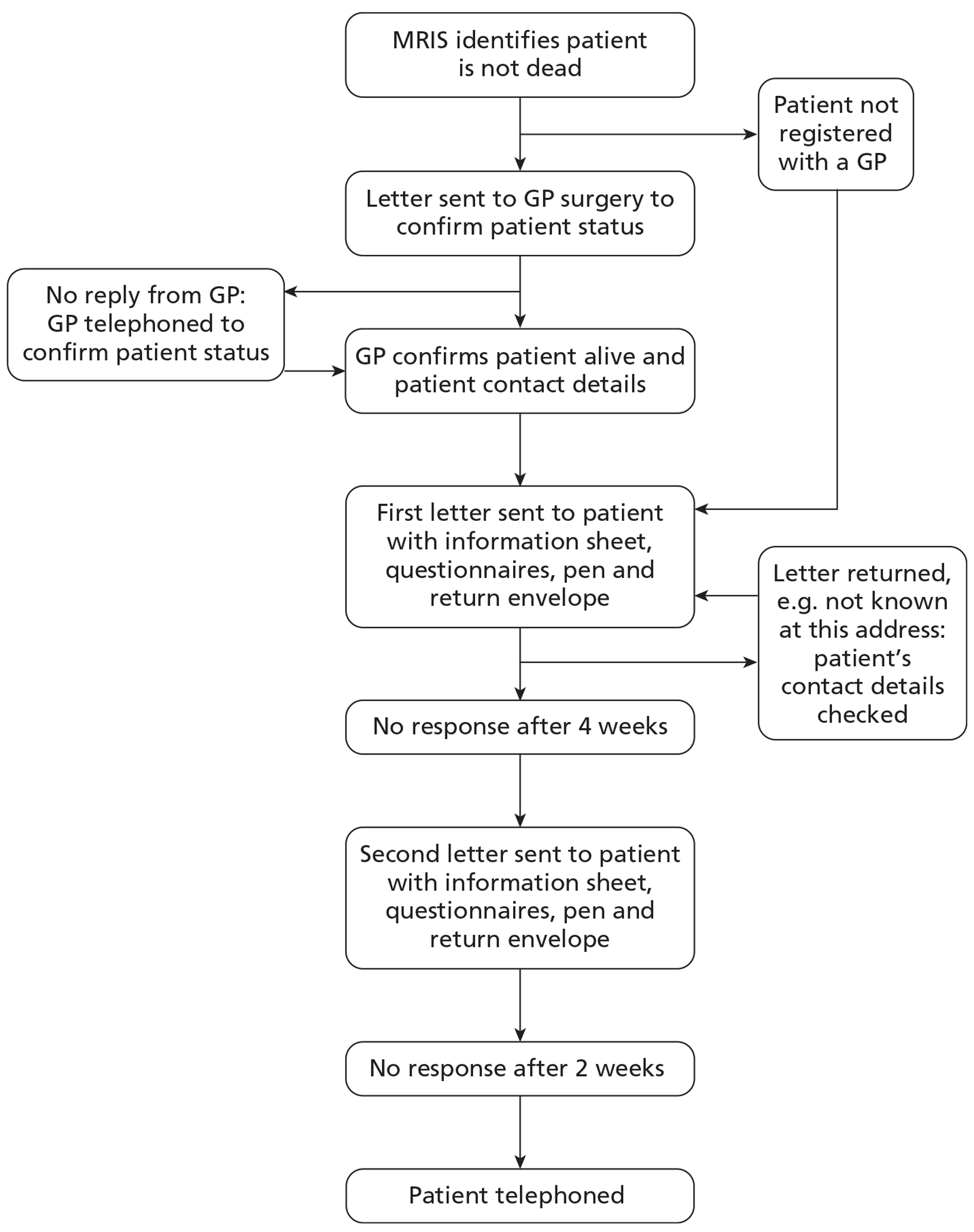
Patient outcomes were collected centrally by the RAIN study team at ICNARC, using methods based on those undertaken in previous research studies, including the CRASH62 and RESCUEicp RCTs. 63 For patients registered with a general practice, their GP was sent a letter explaining the RAIN study and a form to complete to confirm that the patient had not died and to verify the patient's address. GPs could confirm the patient's status and address in a variety of ways: by returning the form by post or fax, completing a secure on-line response form or telephoning the RAIN study team. If no response was received, a follow-up telephone call was made to ensure the original information had been received. In cases where patients were not registered with a GP, REC approval allowed for them to be contacted directly. When informed that a patient was no longer registered with the GP contacted, attempts were made to ascertain the patient's current GP.
Patients were then sent, by post, an introductory letter, information sheet, consent form, questionnaires, freepost return envelope and pen (see Appendix 5), following best evidence-based practice to maximise response. 64 In instances where the patient was unable to consent, a boxed section on the letter addressing their carer asked them to offer what they feel would be the presumed will of the patient. Two questionnaires were included: the Your Health Questionnaire and the Health Services Questionnaire. The Your Health Questionnaire included the required questions to evaluate the European Quality of life (EuroQol) 5-dimension, 3-level version (EQ-5D-3L)65 and the Glasgow Outcome Scale – Extended (GOSE)66 measures. The EQ-5D-3L was included to enable the calculation of quality-adjusted life-years (QALYs) as the best available global measure of health outcome. 67 The GOSE questionnaire is the most widely used measure of functional outcome following acute TBI,68 and has been used in most of the large, recent and ongoing RCTs. 54,63 Use of a postal questionnaire to collect the GOSE questionnaire responses has been found to have high reliability. 69 The Health Services Questionnaire included questions about the patients use of health services following discharge from acute hospital and was used to cost subsequent use of health services (see Chapter 6). Patients were asked to complete the consent form and questionnaires if they wished to take part in the RAIN study, or to return the questionnaires blank to indicate that they did not wish to take part. Non-responders were followed up with a second letter after 4 weeks, including the same enclosures as the original.
If no postal response was received after a further 2 weeks following the second letter, then patients were telephoned if contact details were available. A telephone interview template was used to explain the RAIN study and to ask for informed consent. In order not to overburden the interviewee, the telephone interview included only the GOSE questionnaire as the primary outcome for the RAIN study. Telephone calls were made at various times from Monday to Saturday between 0900 and 2030 hours to maximise the chances of contacting the patient.
Follow-up ended when a postal questionnaire was returned, either complete or blank, or when a telephone interview was completed or refusal obtained. When post was returned (e.g. not known at this address, no longer at this address, address inaccessible, etc.) the critical care unit and GP were contacted to check the address details and to elicit any updates to the patient information.
When patients were identified either as discharged to or subsequently moved to a care home, rehabilitation centre or another hospital, these institutions were contacted to establish the status of the patient and the most appropriate way to proceed with follow-up. If a patient had capacity to consent but required assistance in reading and/or completing the questionnaire then health-care professionals would often assist the patient. For those unable to consent, institutions advised on the most appropriate person to contact to gain consent. In cases in which a patient did not have the capacity to consent and either there was no next-of-kin or the next-of-kin was also unable to consent, where possible, an Independent Mental Capacity Advocate was identified.
When attempts to make contact by telephone were repeatedly unsuccessful, for example where telephone numbers would ring through to an answering service or a mobile telephone was continually switched off, and attempts had been made on various days and at various times of day over at least a month, and no alternative contact information was available, if an answering service was available, a message with information to contact the RAIN study team was left and if the call was not returned then the patient was considered lost to follow-up.
Data management of 6-month follow-up data
Two databases were set up for central data entry of questionnaire responses: one for the Your Health Questionnaire and one for the Health Services Questionnaire. Ambiguous responses (e.g. two boxes ticked, responses written instead of ticked, alterations made to questions, etc.) were initially left blank for subsequent review. Following data entry, all GOSE questionnaires with blank responses (except for those where responses were unnecessary for scoring, e.g. return to work for patients who were retired prior to the injury) were identified and manually reviewed to determine whether (1) the response was clearly indicated by the information available on the questionnaire (e.g. the word ‘yes’ or ‘very’ written next to a box rather than the box being ticked); (2) the response could be imputed with reasonable confidence from the other information on the questionnaire; or (3) there was insufficient information to assume a response. Changes to data owing to situations (1) or (2) were identified separately on the database, such that the data could be reanalysed with imputed GOSE responses excluded.
Glasgow Outcome Scale–Extended responses were used to assign each patient to a GOSE category based on their worst response using an algorithm supplied by the original developers of the postal questionnaire. Following guidelines for the application of the questionnaire,69,70 all of those assigned to the categories of upper or lower severe disability were reviewed if other responses appeared to contradict this categorisation (e.g. return to the same work as prior to the injury). In addition, all questionnaires for patients assigned to the category of pre-existing severe disability or severe disability not due to the injury were reviewed to confirm whether the questionnaire responses were consistent with this categorisation. When questionnaires were reviewed, the entire response to all questions, including the EQ-5D-3L when available, was used to assign the patient to the most appropriate GOSE category.
For a few patients, two separate responses were received (either two paper questionnaires or one paper questionnaire and one telephone interview). These were reviewed to determine the most appropriate questionnaire to use for the analysis, taking into account the respondent (patient preferred over family member preferred over carer), timing of the response (favouring responses closer to/after 6 months) and completeness of questionnaire (favouring more complete responses).
All questionnaire reviews were performed by two investigators with any areas of ambiguity or disagreement reviewed and discussed with a third.
Results
Recruitment of sites
Recruitment of sites took place between December 2008 and December 2009. In total, 74 critical care units expressed an interest in taking part in the study and were sent an SSI form to complete. Of these, one neurocritical care unit was unable to take part owing to a conflicting, ongoing research study and a further four general critical care units outside a neuroscience centre did not reach the R&D submission stage. Local R&D approval was sought and gained for 69 critical care units. R&D approvals took a median of 68 days [interquartile range (IQR) 32 to 237] from submission of SSI form. R&D approval to start of RAIN study data collection took a median of 27 days (IQR 13 to 119). Two neurocritical care units withdrew, as they were unable to meet the study start date giving a total of 67 critical care units. This exceeded the recruitment targets with 13 dedicated neurocritical care units, 14 combined neuro/general critical units, four additional critical care units within a neuroscience centre (admitting overflow patients from the neurocritical care unit) and 36 general critical care units outside a neuroscience centre participating in the RAIN study (Table 3). One neurocritical care unit (and an additional critical care unit within the same neuroscience centre) withdrew from the study in August 2010 owing to research staffing shortages. All other critical care units collected data until March 2011 (Figure 4).
Geographical region | Neuroscience centresa | Non-neuroscience centres | ||
---|---|---|---|---|
Number (%) in RAINb | Total number in region | Number (%) in RAIN | Total number in region | |
UK | 27 (84) | 32 | 36 (16) | 223 |
England | 23 (92) | 25 | 34 (19) | 180 |
East Midlands SHA | 1 (100) | 1 | 4 (33) | 12 |
East of England SHA | 1 (100) | 1 | 7 (41) | 17 |
London SHA | 6 (86) | 7 | 3 (11) | 28 |
North East SHA | 2 (100) | 2 | 2 (13) | 15 |
North West SHA | 2 (67) | 3 | 6 (24) | 25 |
South Central SHA | 2 (100) | 2 | 1 (9) | 11 |
South East Coast SHA | 1 (100) | 1 | 2 (11) | 18 |
South West SHA | 2 (100) | 2 | 3 (18) | 17 |
West Midlands SHA | 3 (100) | 3 | 3 (16) | 19 |
Yorkshire and the Humber SHA | 3 (100) | 3 | 3 (17) | 18 |
Wales | 2 (100) | 2 | 2 (14) | 14 |
Northern Ireland | 0 (0) | 1 | 0 (0) | 9 |
Scotland | 2 (50) | 4 | 0 (0) | 20 |
FIGURE 4.
Recruitment timeline.
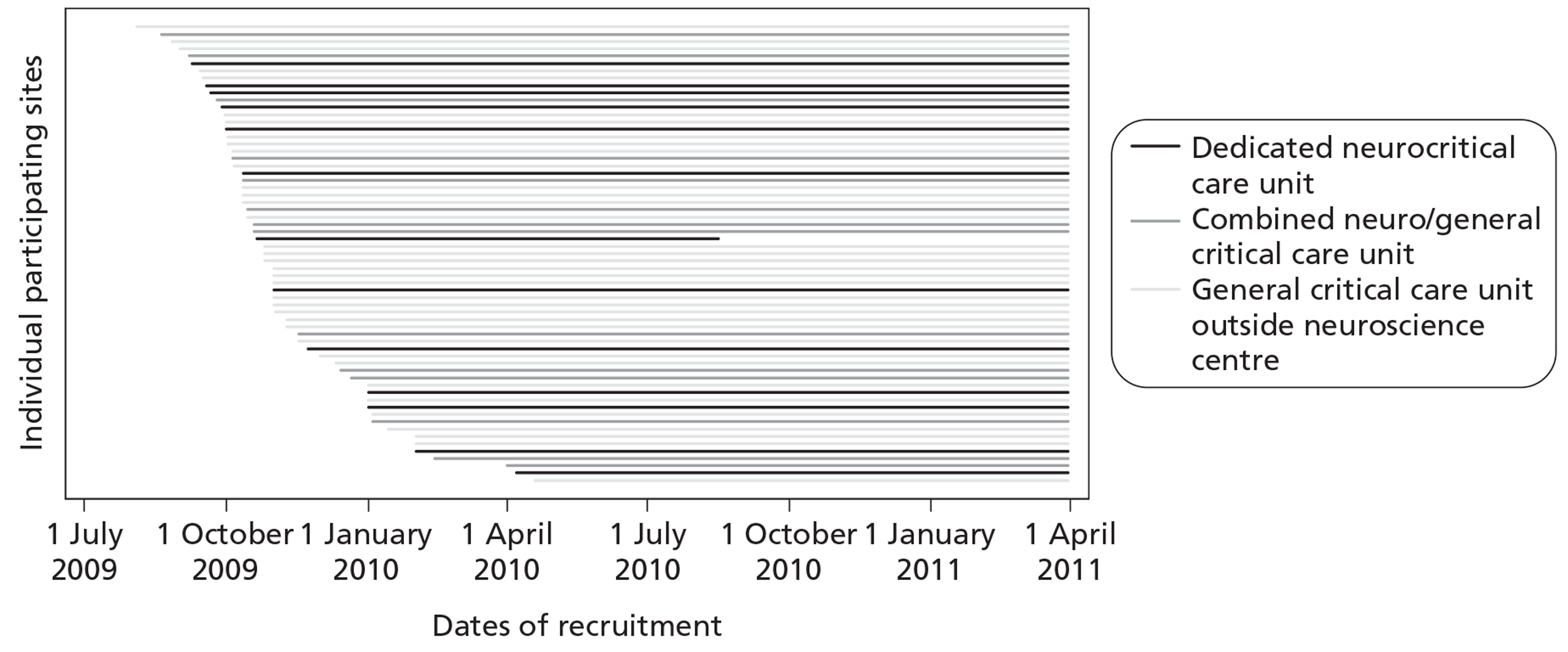
Each critical care unit was represented at a start-up meeting/data set familiarisation course. In total, seven start-up meetings/data set familiarisation courses were held between May 2009 and March 2010.
Recruitment of patients
The first patient was recruited to the RAIN study on 19 August 2009 and patient recruitment continued until 31 March 2011. The final RAIN study data set contained a total of 3626 critical care unit admissions. After excluding multiple admissions of the same patient and patients who did not prove finally to have a TBI, 3210 patients remained (Figure 5). Of these, 28 patients were homeless, 61 were non-UK residents and for 12 patients (military) the address details were withheld, resulting in a cohort of 3109 patients (97%) that were followed up for 6-month survival by data linkage with death registrations.
FIGURE 5.
Patient flow. a, Paper questionnaire included full Your Health Questionnaire (GOSE and EQ-5D-3L) and Health Services Questionnaire; telephone questionnaire included GOSE only.
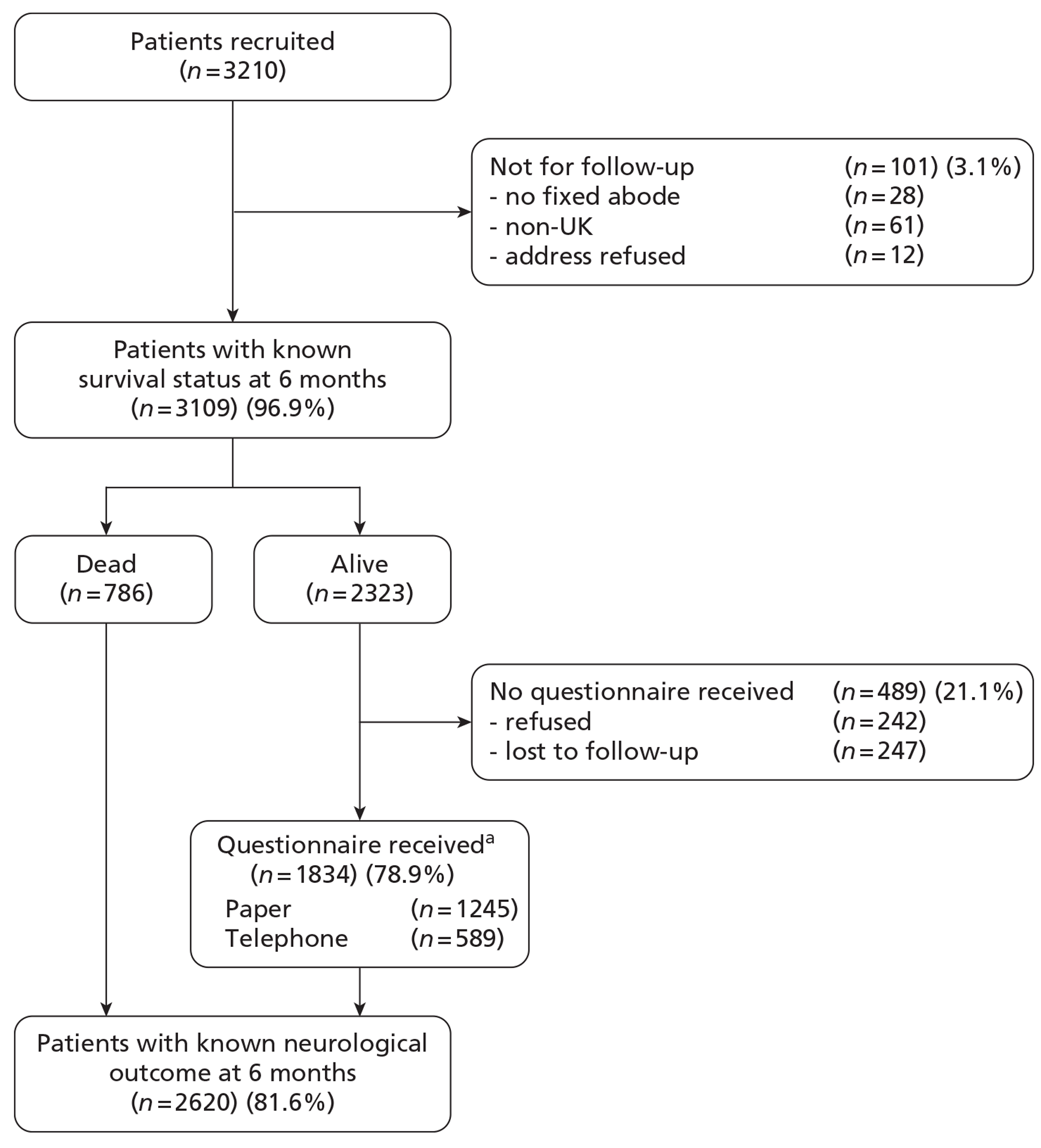
As a result of the regular feedback to critical care units participating in the CMP, four instances of a missed admission for TBI were identified after recruitment of patients had closed and these were, therefore, unable to be included in the study. There were also six admissions for which the research team at the unit was unable to confirm whether or not the patient had a TBI. Validation against figures from TARN indicated similar numbers of patients recruited to the RAIN study as reported through TARN. In some sites, the number of patients in the RAIN study exceeded the number reported to TARN by a small amount and, in other sites, the reverse was true, reflecting different definitions for TBI in the two projects.
Six-month follow-up of neurological outcome and quality of life
Of 2323 patients not reported as dead by MRIS, 242 (10%) refused follow-up and 247 (11%) were lost to follow-up (the first patient was recruited to the RAIN study on 19 August 2009 and patient recruitment continued until 31 March 2011).
A breakdown of the 242 patients for whom outcome data were refused is shown in Table 4. The majority of refusals (177, 73%) were by return of a blank questionnaire (from which no further information was available).
Method of/reason for refusal | Number of patients |
---|---|
Return of blank questionnaire | 177 |
During telephone follow-up | 65 |
By patient | 29 |
Patient refused before study could be explained | 2 |
Patient refused without giving reason | 23 |
Patient found questionnaire distressing or confusing | 4 |
By family member or carer | 36 |
Family member could not determine whether relative was able to consent | 7 |
Family member did not wish to answer on behalf of relative who was unable to consent | 9 |
Family member or friend blocked access to a patient who would have had capacity to consent | 5 |
Family member or carer informed us that patient did not wish to take part | 10 |
Carer informed us that family member did not wish the patient to take part | 5 |
A breakdown of the 247 patients lost to follow-up is shown in Table 5. The largest number of patients lost to follow-up (122, 49%) were those with whom we were unable to make any contact, despite exhausting all options available to us. On four occasions, contact to a patient was blocked by their GP because the patient had not consented in advance, despite NIGB and REC approval for the follow-up process used in the study. Eight requests were received from GPs not to contact the patient or family, either on compassionate grounds or because the GP had spoken to the patient or family and they did not wish to be contacted, and on six occasions family members did not want us to contact their relative. All such requests were respected.
Reason for loss to follow-up | Number of patients |
---|---|
Follow-up stopped by health-care professional | 20 |
GP blocked access to patient because the patient had not consented in advance | 4 |
GP requested we should not contact the patient or family | 8 |
Health-care professional informed us patient was unable to consent and no next-of-kin available | 8 |
Other reasons | 222 |
Patient died after 6 months while attempts to make contact were ongoing | 21 |
Family member informed us that patient had capacity to consent but did not want us to contact them | 6 |
Patient or family member informed us that a postal questionnaire had been returned but this was never received | 26 |
Patient or family member informed us that they had received a postal questionnaire but wished to consider the study and did not want to complete the questionnaire by telephone or be contacted again | 39 |
Adequate communication prevented by poor understanding of English | 4 |
Unable to contact the patient – prisoner | 4 |
Unable to contact the patient or family despite repeated attempts | 122 |
Processing errors | 5 |
Incorrect data entry on web portal – patient reported to have died | 5 |
Questionnaires were completed a median of 199 days (IQR 166 to 239 days) after the TBI (Figure 6). Two questionnaires were received very early, owing to data entry errors in the date of TBI that were not identified until follow-up had commenced. There was an initial peak at around 160 days corresponding to postal questionnaires returned following the initial posting, a second peak at around 200 days corresponding to postal questionnaires returned following the second posting, and a subsequent heavy tail of the patients followed up by telephone that were often difficult to contact. A total of 30 patients were eventually contacted for the 6-month follow-up more than 1 year after their TBI.
FIGURE 6.
Distribution of days from TBI to completion of questionnaire. Vertical line indicates 6 months post TBI.
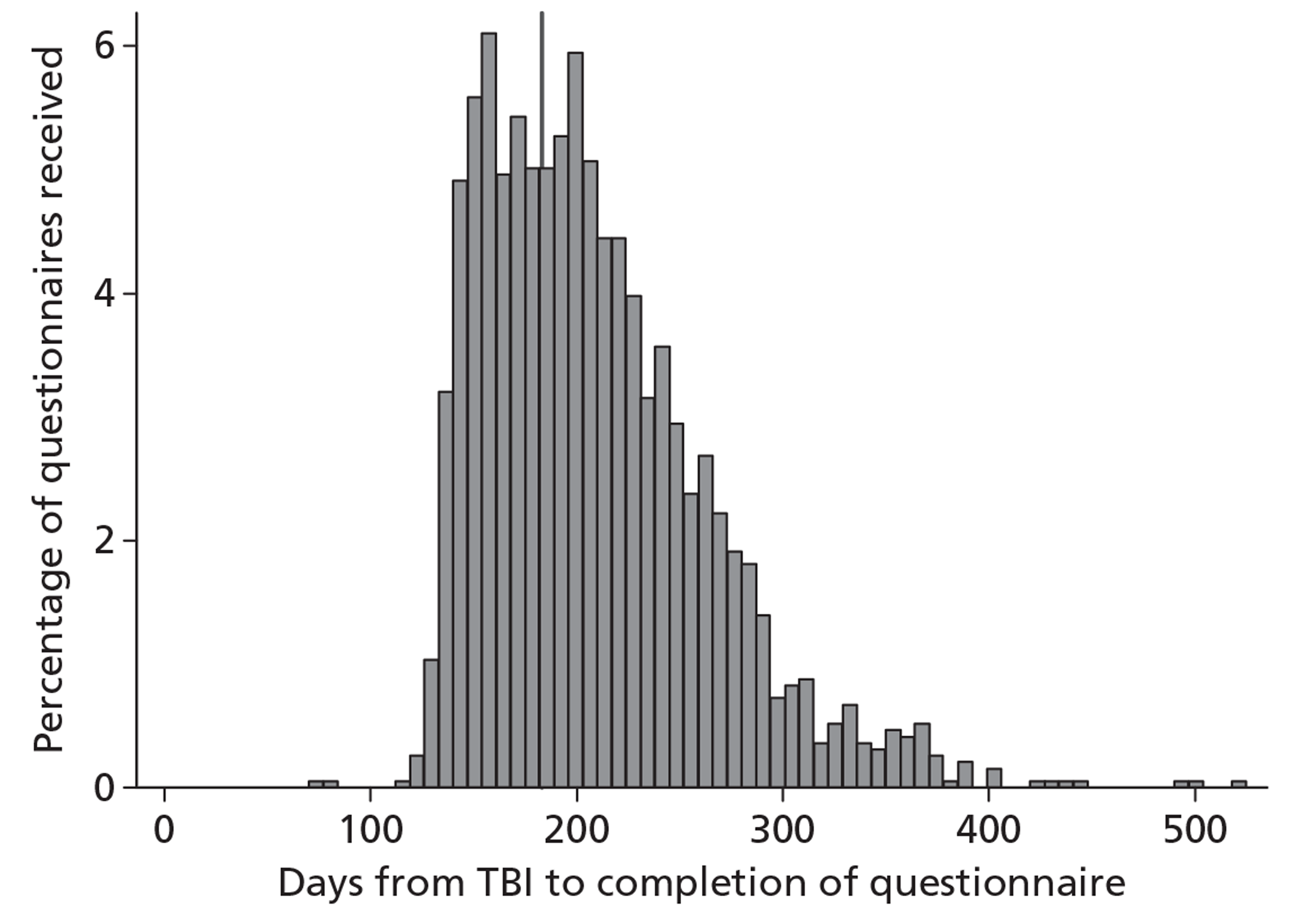
Discussion
Principal findings
The RAIN study has provided case mix data from the time of injury and presentation at hospital and outcomes data at 6 months following injury on a highly representative sample of patients receiving critical care following acute TBI in the UK.
Challenges in conducting the study
Patients with TBI present a challenging population for obtaining reliable, longer-term outcome data. The reasons for this are wide ranging and must be considered when discussing those who were lost to follow-up. Identifying the current location of the patient is not straightforward because of the frequent movement between hospitals and health-care institutions, entry into rehabilitation programmes and moving back to live with family and friends after TBI, as well as a relatively high proportion of homeless patients and those from overseas. Other challenges include ascertaining whether a patient has the capacity to consent and to accurately answer the questions. For example, in some instances, we were informed by health-care professionals or family that while the patient had the capacity to consent following the TBI they had tendencies to confabulate and that answers provided may not have been accurate.
Throughout the follow-up process, every effort was taken to avoid causing unnecessary distress to patients or their families. Following MRIS confirmation, for those registered with a GP, it was necessary to send a letter to confirm the patient's status. There were a small number of cases where the GP blocked contact with the patient or refused to provide the information because prior patient consent had not been obtained. This was despite GPs being fully informed, by letter and telephone, about the study and the governance and ethics approvals. There were also instances where the GP contacted the patient and the patient requested not to be contacted by the Study Team. This raises possible questions whether such patients were fully informed about the study. However, the benefits of contacting GPs do appear to outweigh these possible missed follow-ups. In 28 cases, patients were not reported as having died by MRIS but, on contacting the GP, were found to have died. Despite this being a relatively small number in comparison with the resources and time required to collect GP responses, the prevention of distress to these families by this intervention cannot be ignored. In cases where GPs requested that we did not contact the patient or family for compassionate reasons, this was respected. Despite the dual safeguards of checking for reported deaths both with MRIS and GPs, there were seven cases in which, on making contact with the family, we were informed the patient had recently died. It is inevitable, when following up a high-risk population, that such situations will occur, but it is the responsibility of the researcher to do everything possible to keep these to a minimum.
The use of postal questionnaires may have been a factor in our response rate. Twenty-six patient questionnaires were reported to have been posted by the patient or family but were never received and, once it appeared that undelivered mail could potentially be influencing response rates and fluctuations in the returns were noticed, Royal Mail was contacted. The registered freepost envelope presented a possible issue. Considering this, and research indicating that stamped addressed envelopes may improve response rates over reply-paid envelopes,64 we switched to using stamps during the course of the study. Additionally, when patients indicated during telephone follow-up that they had already returned a questionnaire by post, we requested that they also complete a telephone interview so that in the event the postal questionnaire was not received an outcome would still be available.
Chapter 4 Case mix and outcomes at 6 months for critically ill patients with acute traumatic brain injury
Introduction
This chapter describes the case mix of patients in the RAIN study admitted to critical care units following acute TBI and their survival, neurological outcome and QOL at 6 months following TBI.
Methods
Selection of patients
Despite a GCS score of < 15 being an inclusion criterion for the RAIN study, some patients with suspected TBI were recruited with no GCS score recorded, and others who had an initial GCS score of < 15, either pre-hospital or on presentation at hospital, subsequently improved prior to sedation. Patients from the RAIN study database were included in the analysis if their last GCS score prior to sedation/admission to critical care was < 15.
Statistical methods
Case mix, length of stay (LOS) and outcomes were summarised overall and for subgroups defined by the cause of TBI – road traffic accident (RTA), fall or assault. Patients with other or unknown causes of injury were included in the overall group but excluded from the subgroups. All analyses were descriptive and no statistical testing was undertaken.
Case mix
For presentation, case mix variables are categorised by causal factors; pre-injury status; date/time of TBI; hospital source of admission to critical care; age/sex; neurological dysfunction; and physiology.
Causal factors include the cause of TBI, categorised as above, with RTA details, height of fall (where relevant), presence and site(s) of major extracranial injury (defined as an injury that would require hospital admission in its own right) and presence of confirmed or suspected intoxication (with alcohol, drugs, etc.) at the time of injury.
Pre-injury status includes residence prior to admission to acute hospital, dependency prior to admission to acute hospital and deprivation status. Deprivation was assessed using the English Index of Multiple Deprivation (IMD) 201071 and the Welsh IMD 200872 for patients with a valid residential postcode. IMD quintiles of deprivation within Wales were assumed similar to the quintiles in England. Patients resident outside England and Wales were excluded from the assessment of deprivation, as sufficiently equivalent measures of deprivation were not available.
Date/time of TBI was categorised by the day of the week and hour of the day. Hospital source of admission to critical care was described by the location immediately prior to admission to the critical care unit. Age and sex were summarised both separately and by describing the overall age/sex distribution.
Neurological dysfunction includes the total GCS score, motor score component of the GCS score, pupil reactivity, Marshall CT classification56 and presence of traumatic SAH. GCS score, motor score and pupil reactivity were summarised for each time point at which they were recorded for all patients with an assessment available at that time point. Marshall CT classification and presence of traumatic SAH were assessed from the first CT scan following the injury.
Physiology includes the first recorded-at-hospital values of core physiology, arterial blood gases and laboratory parameters. Core physiological parameters recorded were temperature, systolic blood pressure, heart rate and oxygen saturation. Arterial blood gas parameters were partial pressure of oxygen (PaO2), fraction of inspired oxygen (FiO2), partial pressure of carbon dioxide (PaCO2) and pH. Laboratory parameters were serum glucose, haemoglobin and platelet count.
Length of stay
Length of stay in critical care was summarised by the median and IQR of the total stay in critical care, including transfers between critical care units, and stratified by the survival status at final discharge from critical care. Similarly, LOS in acute hospital was summarised by the median and IQR of the total stay in acute hospital, including transfers between acute hospitals, and stratified by the survival status at final discharge from acute hospital.
Outcomes
The outcomes reported were mortality, neurological outcome and QOL. Mortality was reported at final discharge from critical care, final discharge from acute hospital, and at 6 months following the TBI. Survival over time to 6 months following the TBI was displayed using Kaplan–Meier plots. Neurological outcome was summarised by the GOSE category at 6 months, and dichotomised as favourable (GOSE category of good recovery or moderate disability) or unfavourable (GOSE category of death or severe disability; note that the questionnaire used for collection of GOSE responses did not distinguish vegetative state from lower severe disability). Quality of life at 6 months was described by the responses to the five domains of the EQ-5D-3L and by the patients' self-rated current health on a visual analogue scale from 0 (worst imaginable) to 100 (best imaginable).
Results
Selection of patients
Of the 3210 patients in the RAIN study data set, 101 (3.1%) had no GCS score recorded, and 134 (4.2%) had a last pre-sedation GCS score of 15, resulting in a data set for analysis of 2975 patients.
Case mix
Causal factors
The most common causes of TBI were RTA (33%), fall (47%) and assault (12%), with 3% from other causes and 5% of unknown cause (Figure 7). RTA details are shown in Figure 8 and fall height in Figure 9.
FIGURE 7.
Cause of TBI.
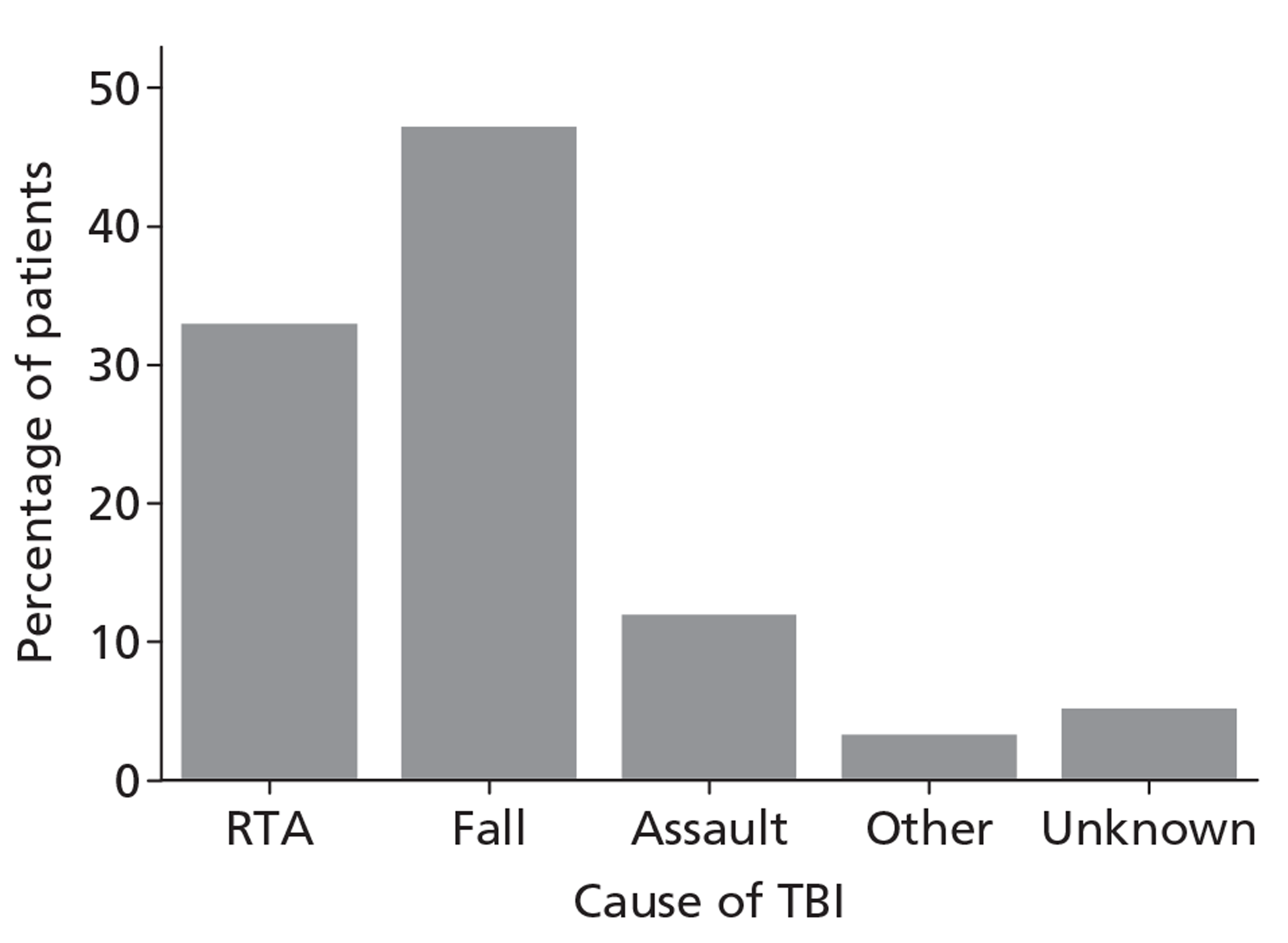
FIGURE 8.
Road traffic accident details.
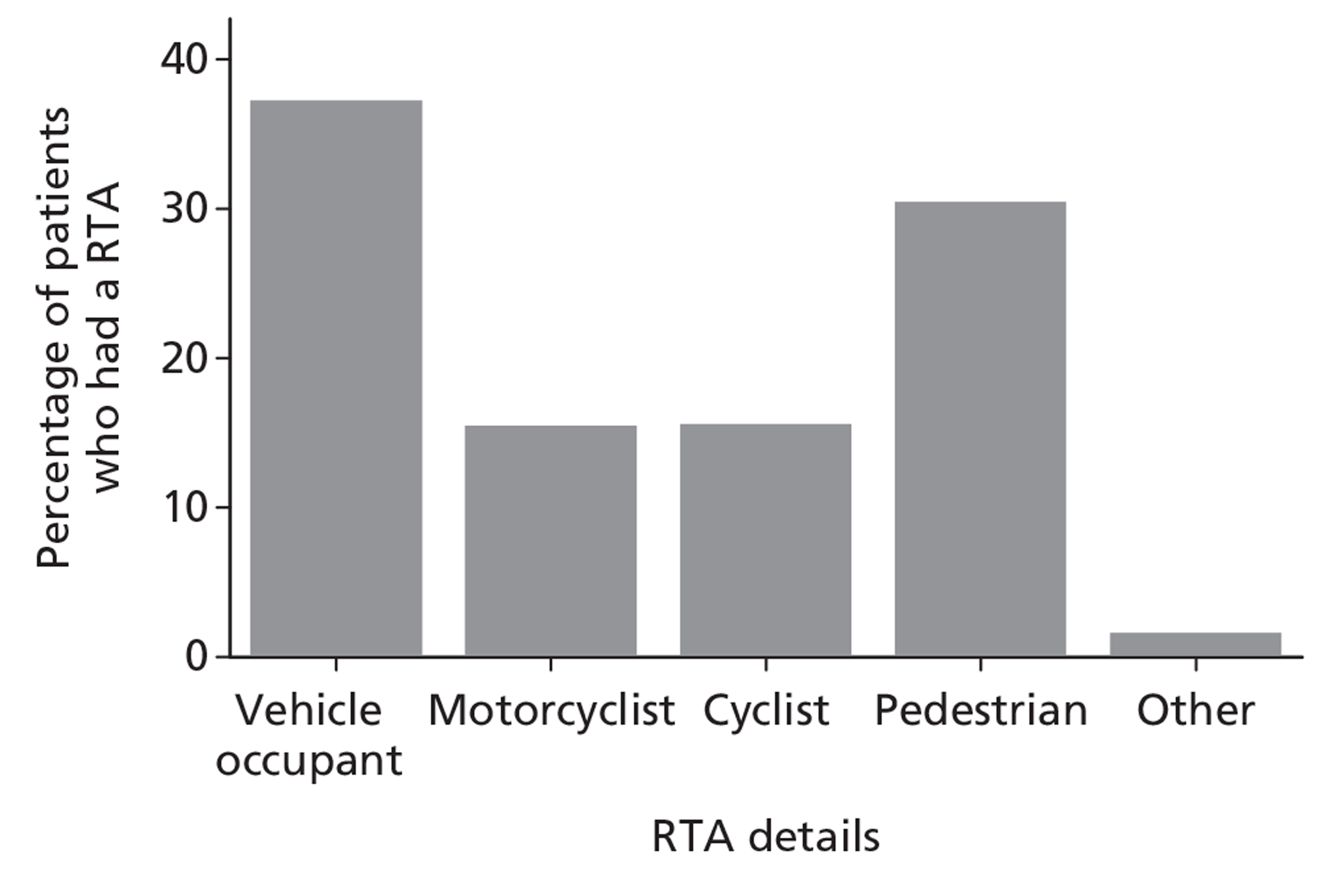
FIGURE 9.
Height of fall.
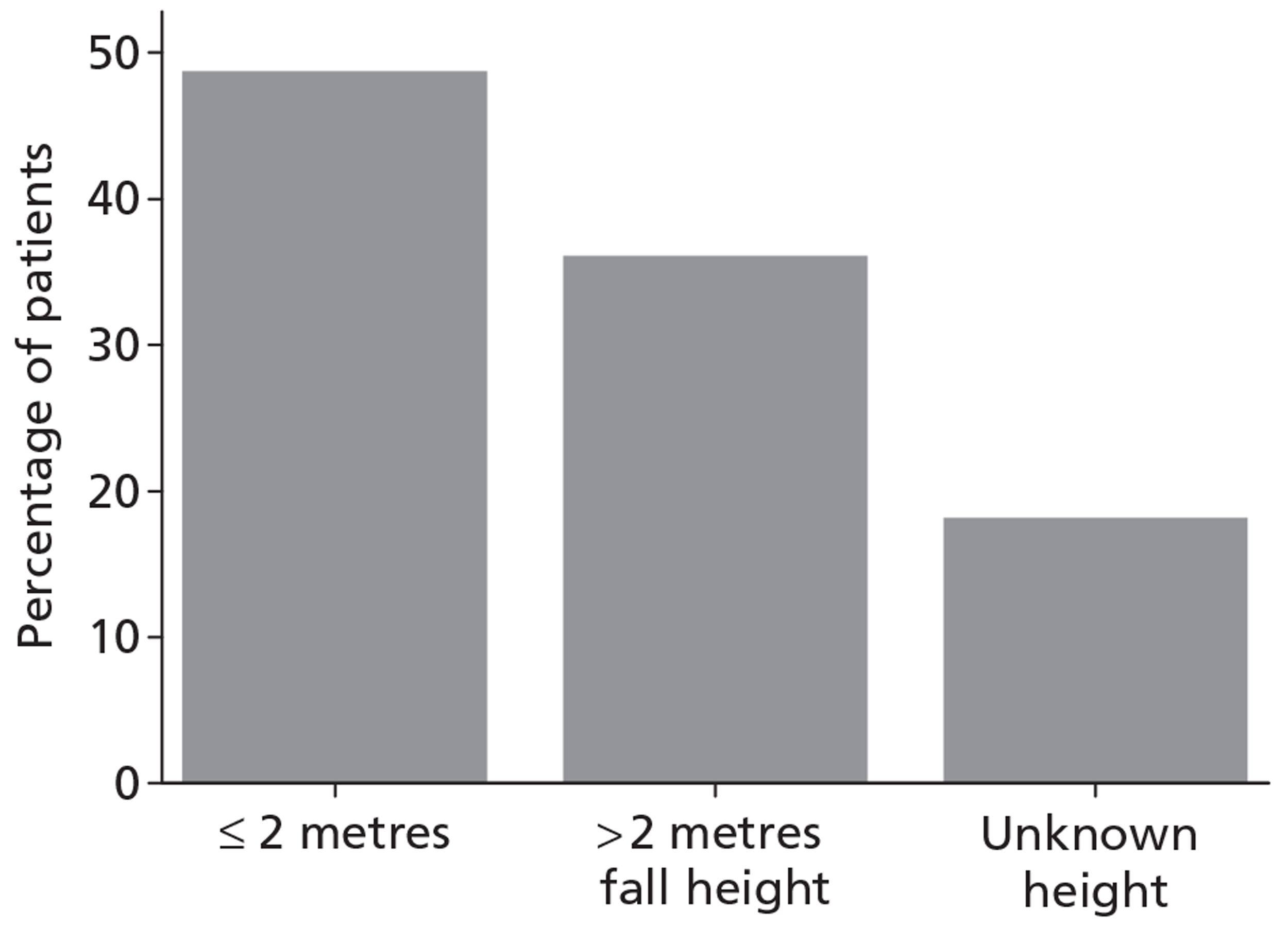
A major extracranial injury, sufficient to require hospital admission in its own right, was present in 41% and was particularly common for RTAs (70% compared with 28% for falls and 22% for assaults). Among assaults, major extracranial injuries were predominantly of the head and neck, whereas for falls and particularly for RTAs the sites of major extracranial injury were more widespread (Figure 10). Intoxication was either confirmed or suspected in almost half (45%), and varied by cause of TBI (26% for RTAs, 49% for falls and 74% for assaults; Figure 11).
FIGURE 10.
Major extracranial injury by site, overall and by cause of TBI. (a) Overall; (b) RTA; (c) fall; (d) assault.
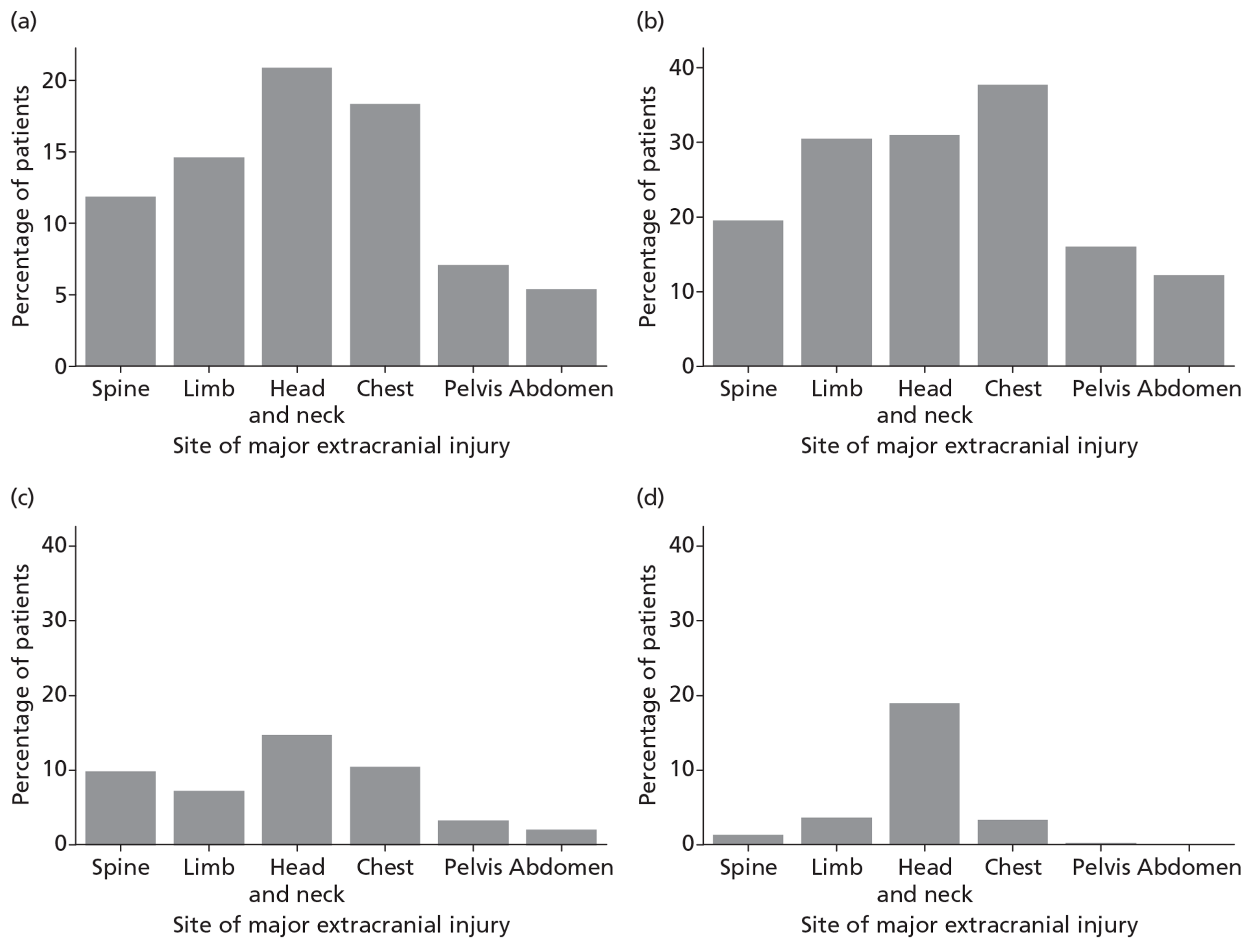
FIGURE 11.
Confirmed or suspected intoxication at time of TBI, overall and by cause of TBI. (a) Overall; (b) RTA; (c) fall; (d) assault.
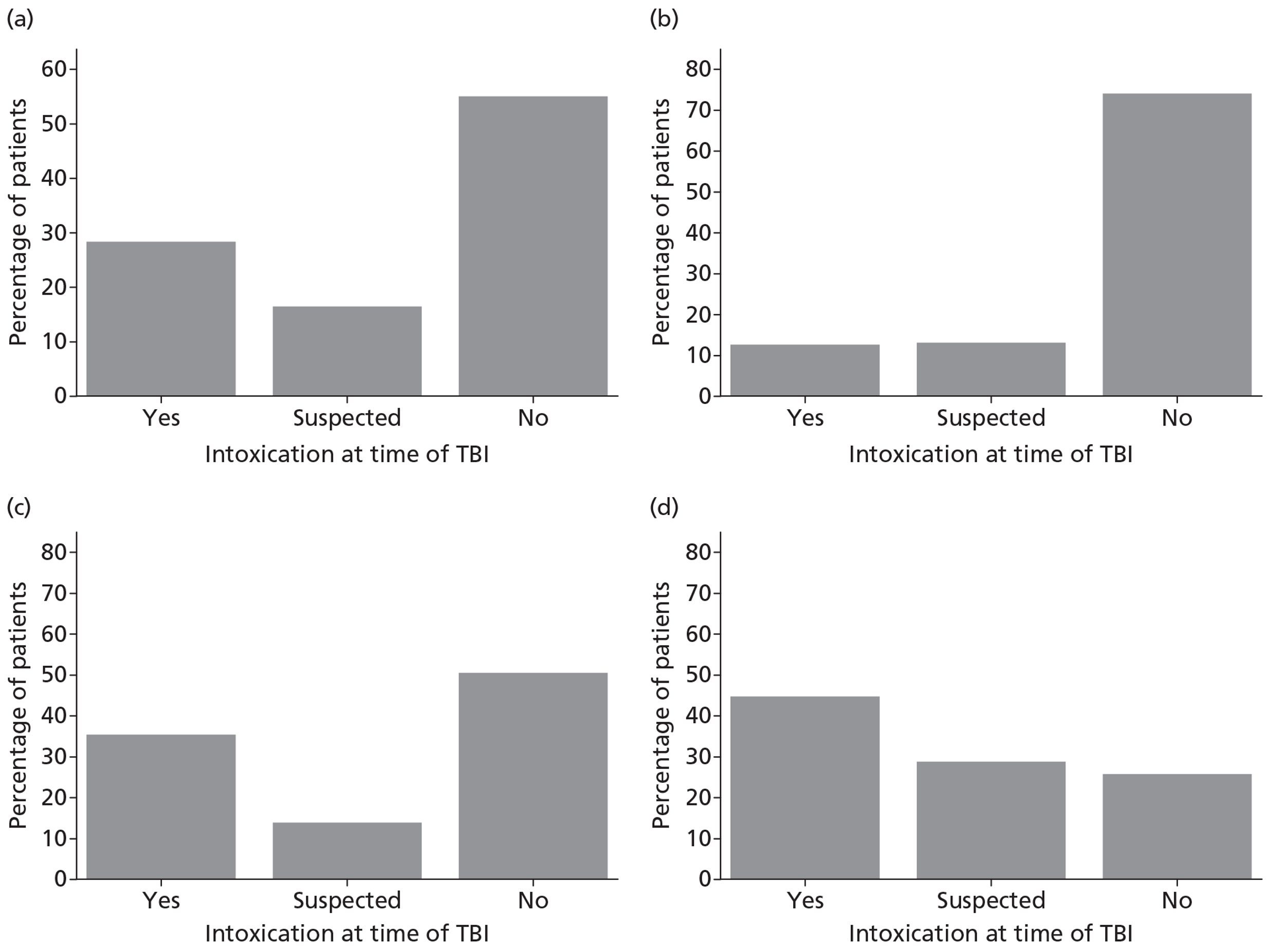
Pre-injury status
Table 6 reports the pre-injury status of patients, overall and by cause of TBI. The vast majority of patients were living independently prior to the TBI, indicated by 96% with a prior residence of ‘home’ and 95% able to live without assistance in activities of daily living. Figure 12 shows the distribution of deprivation by quintiles of IMD. There was a marked socioeconomic gradient that was strongest for assault (38% in the most deprived quintile vs 9% in the least deprived) and weakest for RTA (22% vs 19%).
Case mix factors | Overall (n = 2975a) | RTA (n = 976) | Fall (n = 1399) | Assault (n = 353) |
---|---|---|---|---|
Residence prior to admission, n (%) [N] | [2971] | [975] | [1398] | [351] |
Home | 2846 (95.8) | 948 (97.2) | 1345 (96.2) | 330 (94.0) |
No fixed abode or temporary abode | 57 (1.9) | 15 (1.5) | 20 (1.4) | 13 (3.7) |
Residential place of work/education | 27 (0.9) | 8 (0.8) | 3 (0.2) | 4 (1.1) |
Nursing home or equivalent | 18 (0.6) | 1 (0.1) | 16 (1.1) | 0 (0) |
Health-related institution | 13 (0.4) | 2 (0.2) | 10 (0.7) | 0 (0) |
Non-health-related institution | 10 (0.3) | 1 (0.1) | 4 (0.3) | 4 (1.1) |
Prior dependency, n (%) [N] | [2895] | [944] | [1376] | [337] |
Able to live without assistance in daily activities | 2742 (94.7) | 921 (97.6) | 1258 (91.4) | 333 (98.8) |
Minor assistance with some daily activities | 132 (4.6) | 19 (2.0) | 102 (7.4) | 3 (0.9) |
Major assistance with majority of/all daily activities | 19 (0.7) | 4 (0.4) | 15 (1.1) | 0 (0) |
Total assistance with all daily activities | 2 (0.1) | 0 (0) | 1 (0.1) | 1 (0.3) |
FIGURE 12.
Deprivation, overall and by cause of TBI. (a) Overall; (b) RTA; (c) fall; (d) assault.
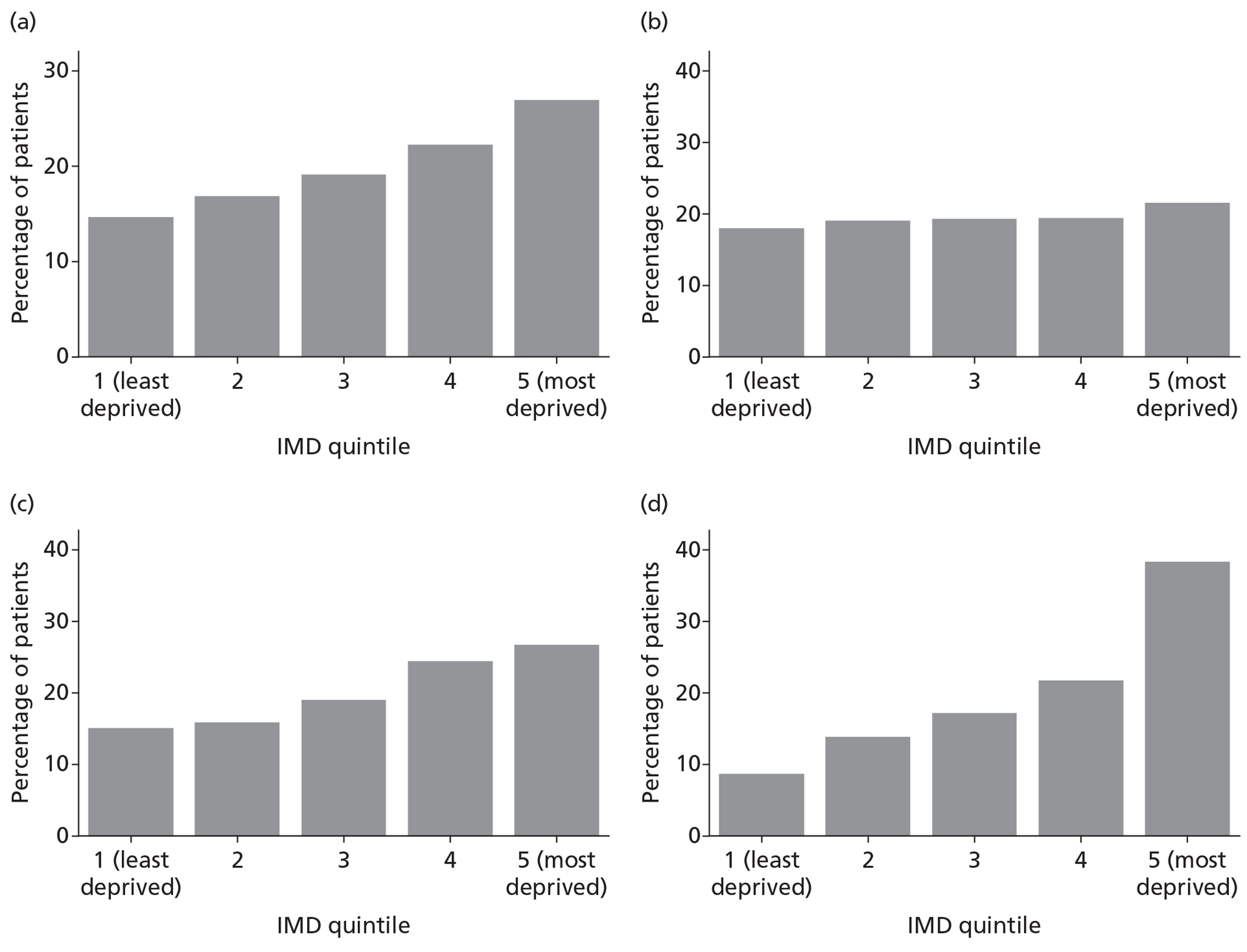
Date/time of traumatic brain injury
Figure 13 shows the day of TBI, overall and by cause. TBI was more common at the weekend (37% overall), particularly for assaults (50%). TBI occurred throughout the day with a distribution that varied considerably by cause of TBI (Figure 14). For RTA, there were peaks corresponding to the morning and evening rush hours and a further peak in the late evening. Falls occurred throughout the day, particularly during waking hours. Assaults were much more common in the late evening and early hours of the morning, with almost half of all assaults occurring between 2300 and 0300 hours.
FIGURE 13.
Day of TBI, overall and by cause. (a) Overall; (b) RTA; (c) fall; (d) assault.
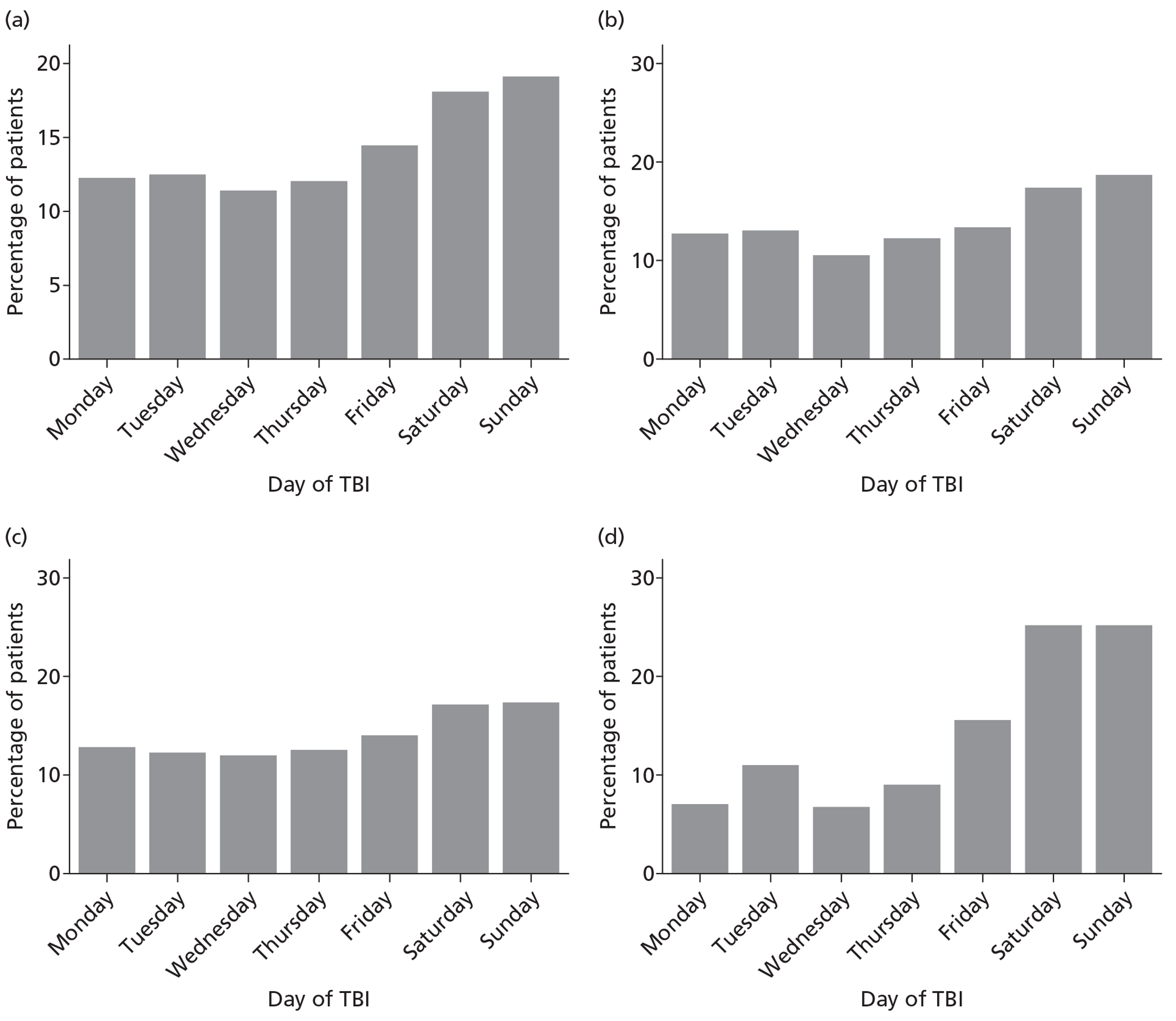
FIGURE 14.
Time of TBI, overall and by cause. (a) Overall; (b) RTA; (c) fall; (d) assault.
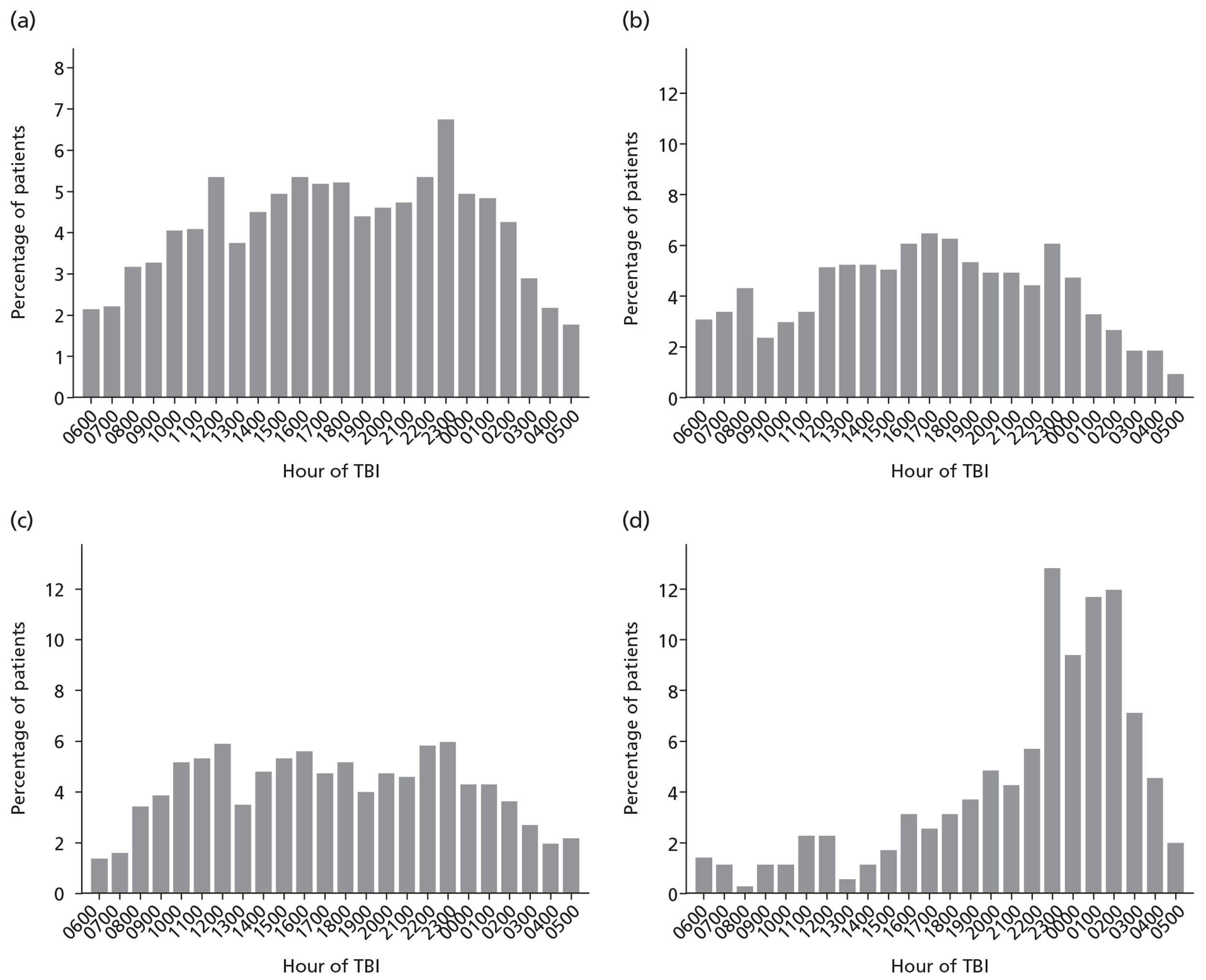
Hospital source of admission to critical care
The majority of patients in the RAIN study were admitted to the critical care unit either from an emergency department (56%) or from theatre and recovery (27%; Table 7). The distribution of source of admission was similar by cause of TBI, although more patients were admitted from theatre following a fall or assault than following an RTA. Overall, 9% of patients were transferred to the critical care unit directly from another critical care area; two-thirds of these were from a level 3 bed in another critical care unit (not participating in the RAIN study).
Case mix factors | Overall (n = 2975a) | RTA (n = 976) | Fall (n = 1399) | Assault (n = 353) |
---|---|---|---|---|
Hospital source of admission, n (%) [N] | [2972] | [975] | [1397] | [353] |
Emergency department | 1676 (56.4) | 605 (62.1) | 734 (52.5) | 215 (60.9) |
Theatre and recovery | 805 (27.1) | 222 (22.8) | 408 (29.2) | 100 (28.3) |
Recovery onlyb | 37 (1.2) | 9 (0.9) | 23 (1.7) | 4 (1.1) |
Level 3 bed in other ICU or ICU/HDU | 193 (6.5) | 74 (7.6) | 80 (5.7) | 17 (4.8) |
Level 2 bed in other ICU or ICU/HDU | 14 (0.5) | 3 (0.3) | 9 (0.6) | 0 (0) |
Paediatric ICU/HDU | 2 (0.1) | 1 (0.1) | 1 (0.1) | 0 (0) |
Adult HDU | 13 (0.4) | 0 (0) | 12 (0.9) | 0 (0) |
Intermediate care area | 53 (1.8) | 23 (2.4) | 16 (1.2) | 5 (1.4) |
Ward | 110 (3.7) | 13 (1.3) | 76 (5.4) | 8 (2.3) |
Imaging | 59 (2.0) | 20 (2.1) | 35 (2.5) | 3 (0.9) |
Specialist treatment area | 9 (0.3) | 5 (0.5) | 3 (0.2) | 0 (0) |
Not in hospital | 1 (< 0.1) | 0 (0) | 0 (0) | 1 (0.3) |
Age and sex
Patients were predominantly young (mean age 45 years overall) and male (76% overall; Table 8). The overall distribution of age was bimodal with peaks in the twenties and forties (Figure 15). This was, however, very much driven by different distributions by cause of TBI. For RTA, age of admissions peaked for males in their late teens and twenties, for falls the peak was among males in their forties, and for assaults, which were almost exclusively (95%) male, the peak was in the twenties.
Case mix factors | Overall (n = 2975a) | RTA (n = 976) | Fall (n = 1399) | Assault (n = 353) |
---|---|---|---|---|
Age [N] | [2975] | [976] | [1399] | [353] |
Mean (SD) | 44.7 (18.9) | 36.3 (17.6) | 53.4 (17.5) | 33.9 (12.6) |
Median (IQR) | 44 (28 to 59) | 31 (22 to 47) | 54 (42 to 67) | 30 (24 to 42) |
Sex, n (%) [N] | [2975] | [976] | [1399] | [353] |
Male | 2263 (76.1) | 727 (74.5) | 985 (70.4) | 334 (94.6) |
Female | 712 (23.9) | 249 (25.5) | 414 (29.6) | 19 (5.4) |
FIGURE 15.
Age and sex distribution, overall and by cause of TBI (a) Overall; (b) RTA; (c) fall; (d) assault.
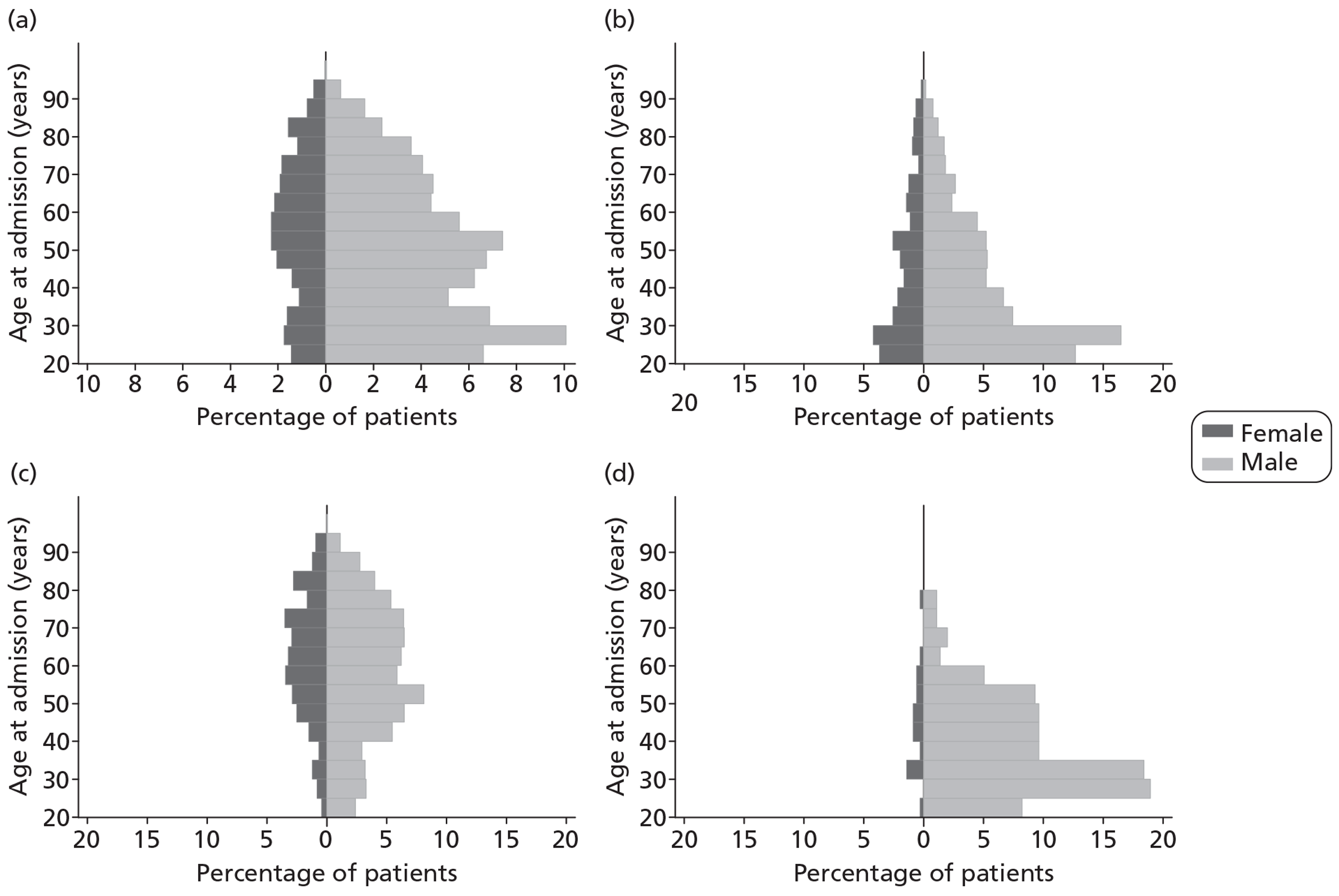
Neurological dysfunction
Glasgow Coma Scale score was assessed and recorded pre-hospital for 2252 (76%) patients, including 9% with a pre-hospital GCS score of 15 that subsequently deteriorated such that the last pre-sedation GCS score was < 15. For 576 patients (19%), the pre-hospital GCS score was their last pre-sedation GCS score. The remaining 2399 patients all had a first at hospital GCS score recorded (within 12 hours following initial presentation at hospital), including 7% with a first recorded-at-hospital GCS score of 15 that subsequently deteriorated such that the last pre-sedation GCS score was < 15. For 1275 patients (43%), the first recorded-at-hospital GCS score was their last pre-sedation GCS score and the remaining 1124 (38%) patients had a subsequent last pre-sedation GCS score documented. The severity of TBI, as assessed by the GCS score, was generally worst for RTA, followed by assault and then fall (Figure 16). The motor score component of GCS score showed a U-shaped distribution with the highest proportions observed at the most extreme (low and high) values (Figure 17). Pupil reactivity was assessed pre-hospital, within 12 hours of initial presentation at hospital and on admission to the critical care unit for 52%, 90% and 97% of patients, respectively. For 20 (0.7%), 11 (0.4%) and 8 (0.3%) patients at each time point, respectively, neither pupil was able to be assessed, whereas the majority of missing data were not documented. Patients admitted following an RTA were more likely to have unreactive pupils than those admitted following either a fall or an assault (Figure 18).
FIGURE 16.
Glasgow Coma Scale score, overall and by cause of TBI. (a) Overall; (b) RTA; (c) fall; (d) assault.
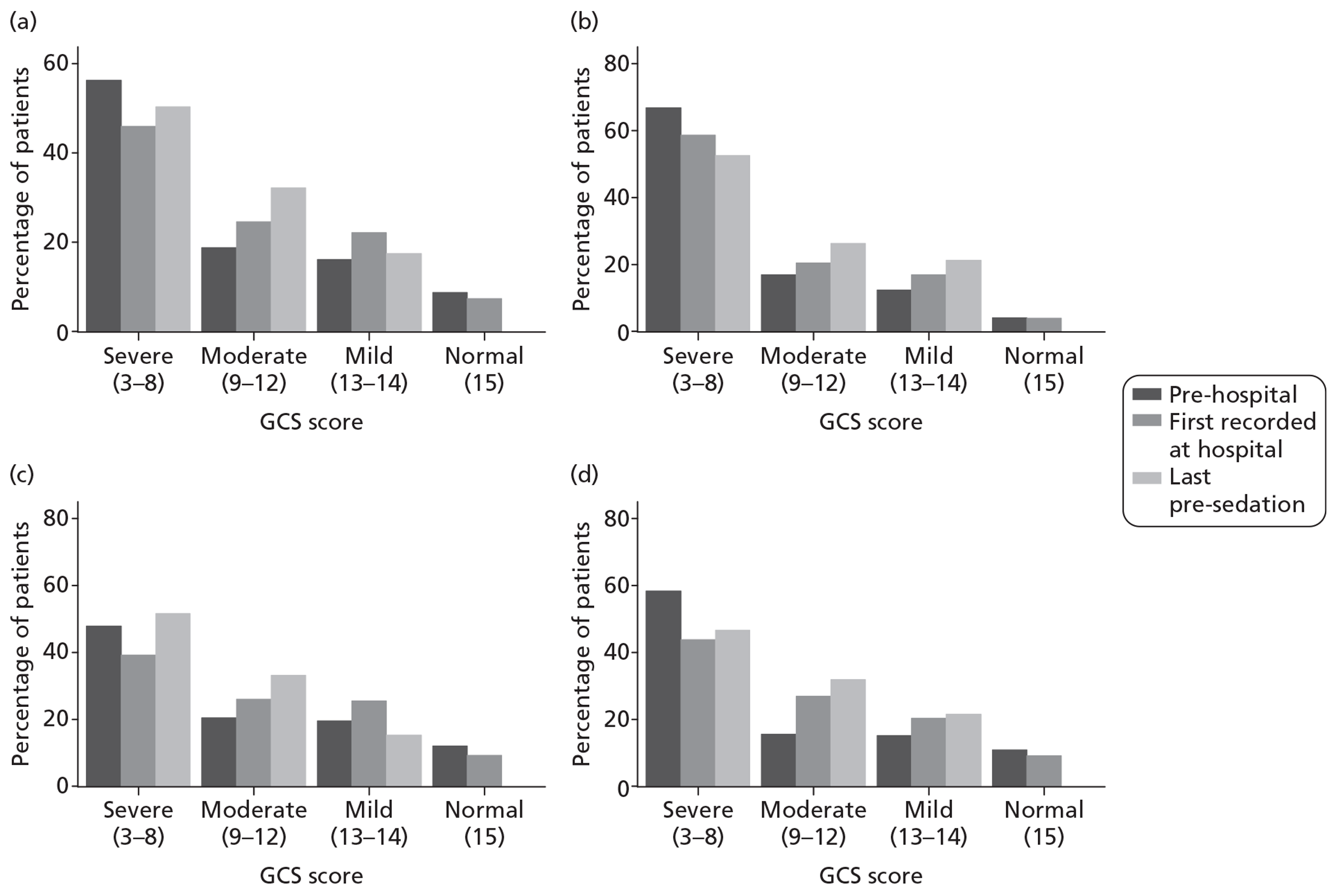
FIGURE 17.
Glasgow Coma Scale motor score component, overall and by cause of TBI. (a) Overall; (b) RTA; (c) fall; (d) assault.
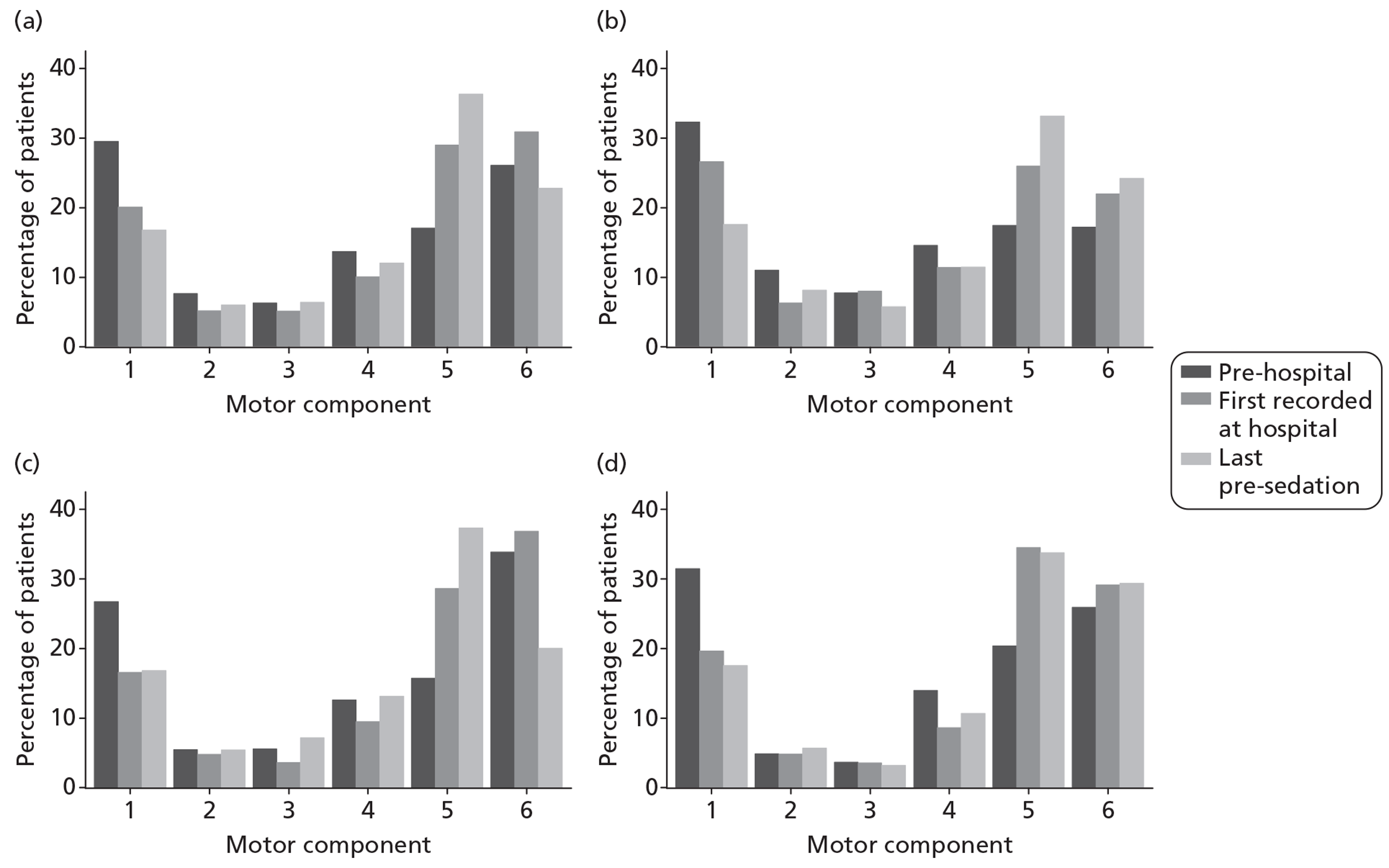
FIGURE 18.
Pupil reactivity, overall and by cause of TBI. (a) Overall; (b) RTA; (c) fall; (d) assault.
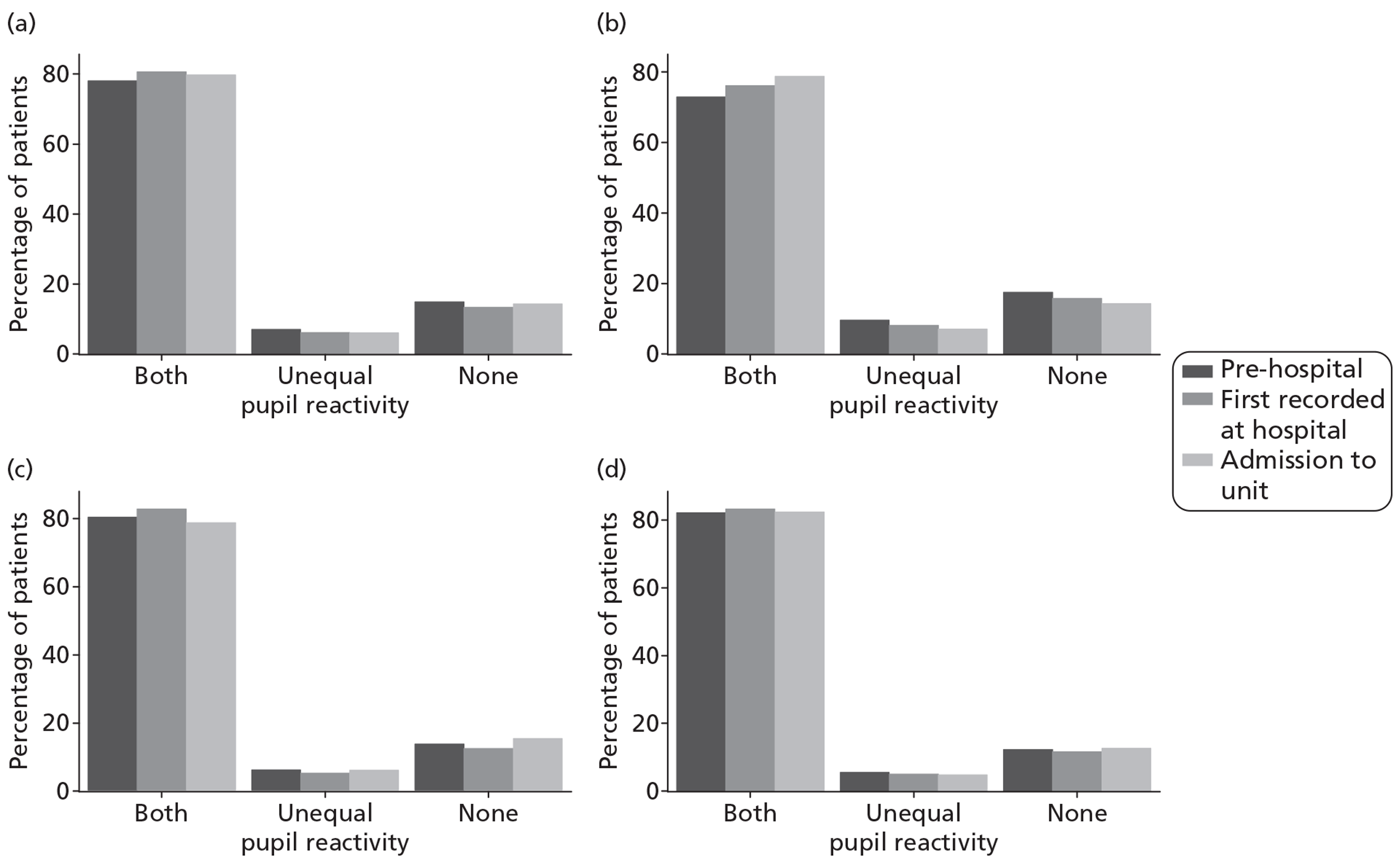
A first CT scan was available for 2817 (95%) patients and, of these, sufficient data were available to assign a Marshall CT classification for 2773 (98%; Figure 19). Very few patients (< 5%) had no abnormalities on the CT scan. The most common Marshall categories, each accounting for about one-third of patients, were diffuse injury II (basal cisterns present, midline shift 0–5 mm, no high- or mixed-density lesion > 25 ml) and evacuated mass lesion. Evacuation of a mass lesion was approximately twice as common among patients admitted following a fall (43%) or an assault (40%) than an RTA (20%). Overall, 56% of patients with a CT scan available had a traumatic SAH, and this was similar across the different causes of TBI (RTA 54%, fall 58%, assault 55%).
FIGURE 19.
Marshall CT classification, overall and by cause of TBI. (a) Overall; (b) RTA; (c) fall; (d) assault.
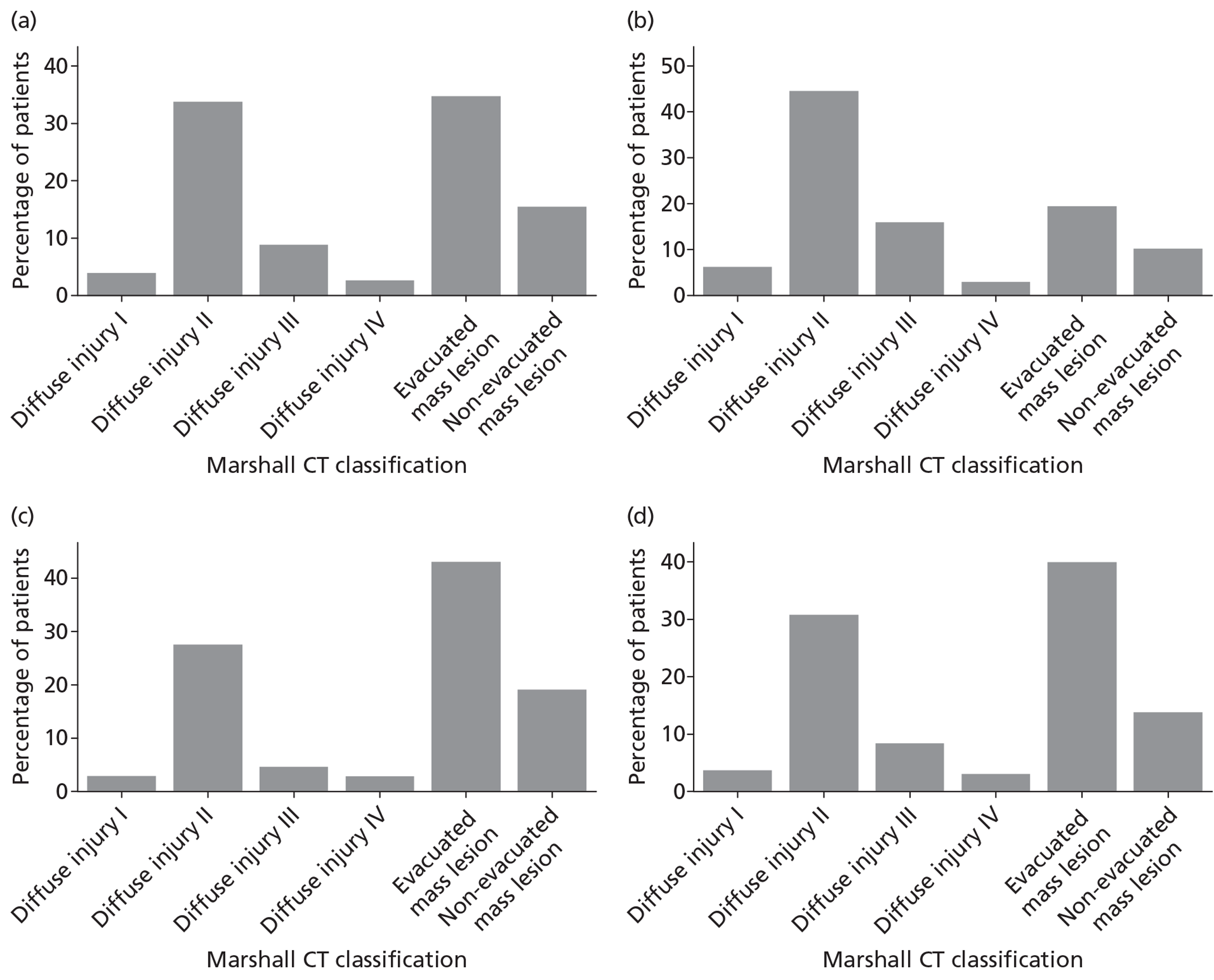
Physiology
Table 9 summarises the first recorded physiological parameters following presentation at hospital. Core physiological parameters were available for between 87% and 95% of patients, arterial blood gas parameters were available for between 72% and 80% of patients, and laboratory parameters were available for between 84% and 90% of patients. There was little difference in the degree of physiological derangement by cause of TBI.
Case mix factors | Overall (n = 2975a) | RTA (n = 976) | Fall (n = 1399) | Assault (n = 353) |
---|---|---|---|---|
Core physiology, median (IQR) [N] | ||||
Temperature (°C) | 36.0 (35.2 to 36.7) [2590] | 35.9 (35.0 to 36.5) [829] | 36.0 (35.3 to 36.8) [1235] | 36.0 (35.3 to 36.5) [311] |
Systolic blood pressure (mmHg) | 139 (121 to 157) [2829] | 136 (120 to 153) [933] | 142 (123 to 161) [1333] | 133 (120 to 149) [335] |
Heart rate (beats per minute) | 87 (72 to 105) [2830] | 93 (76 to 114) [934] | 85 (70 to 102) [1334] | 84 (70 to 100) [334] |
Oxygen saturation (%) | 99 (97 to 100) [2797] | 99 (97 to 100) [920] | 98 (96 to 100) [1322] | 99 (97 to 100) [333] |
Arterial blood gas parameters, median (IQR) [N] | ||||
PaO2 (kPa) | 28.7 (15.0 to 45.5) [2375] | 31.7 (15.3 to 50.5) [811] | 26.8 (14.2 to 41.4) [1106] | 31.7 (16.6 to 49.6) [271] |
FiO2 | 0.8 (0.5 to 1.0) [2131] | 1.0 (0.5 to 1.0) [729] | 0.7 (0.5 to 1.0) [992] | 0.6 (0.5 to 1.0) [245] |
PaCO2 (kPa) | 5.2 (4.5 to 6.1) [2378] | 5.3 (4.6 to 6.2) [812] | 5.2 (4.4 to 6.0) [1108] | 5.2 (4.6 to 6.0) [271] |
pH | 7.4 (7.3 to 7.4) [2360] | 7.4 (7.3 to 7.4) [806] | 7.4 (7.3 to 7.4) [1099] | 7.4 (7.3 to 7.4) [271] |
Laboratory parameters, median (IQR) [N] | ||||
Serum glucose (mmol/l) | 7.6 (6.3 to 9.5) [2496] | 7.7 (6.4 to 9.7) [817] | 7.6 (6.4 to 9.4) [1180] | 7.2 (6.1 to 8.8) [303] |
Haemoglobin (g/dl) | 13.4 (11.9 to 14.6) [2683] | 13.4 (11.7 to 14.6) [896] | 13.2 (11.8 to 14.5) [1259] | 14.0 (12.8 to 14.9) [315] |
Platelet count (× 109/l) | 219 (169 to 273) [2608] | 224 (178 to 274) [878] | 213 (157 to 271) [1216] | 222 (180 to 274) [307] |
Length of stay
Table 10 reports the LOS overall and by cause of TBI. The median total LOS in critical care was 7 days but this differed substantially between survivors (median 8 days) and non-survivors (median 3 days) of critical care. The critical care LOS distribution was similar for non-survivors across different causes of TBI, but for survivors was longer for patients admitted following an RTA (median 11 days) than for a fall or assault (median 7 and 6 days, respectively). The median total LOS in acute hospital was 30 days for survivors compared with 5 days for non-survivors. The LOS in acute hospital for survivors again varied substantially by cause of TBI, with the longest stay for patients admitted following an RTA (median 37 days), followed by for a fall (median 30 days), and for an assault (median 20 days).
Length of stay | Overall (n = 2975a) | RTA (n = 976) | Fall (n = 1,399) | Assault (n = 353) |
---|---|---|---|---|
LOS (days), median (IQR) [ N ] | ||||
Total LOS in critical care | ||||
Survivors | 8 (3 to 17) [2416] | 11 (4 to 20) [801] | 7 (2 to 16) [1099] | 6 (2 to 13) [304] |
Non-survivors | 3 (1 to 6) [540] | 3 (1 to 7) [169] | 2 (1 to 6) [291] | 3 (1 to 5) [49] |
All | 7 (2 to 15) [2956] | 9 (3 to 18) [970] | 6 (2 to 14) [1390] | 4 (1 to 12) [353] |
Total LOS in acute hospital | ||||
Survivors | 30 (14 to 59) [2163] | 37 (17 to 68) [741] | 30 (14 to 58) [941] | 20 (9 to 44) [290] |
Non-survivors | 5 (2 to 12) [696] | 5 (1 to 11) [195] | 5 (2 to 12) [403] | 3 (1 to 7) [55] |
All | 22 (8 to 50) [2859] | 28 (9 to 60) [936] | 20 (7 to 46) [1344] | 16 (6 to 39) [345] |
Mortality
Mortality at final discharge from critical care was 18% and was highest for falls (21%) followed by RTAs (18%) and assaults (14%) (Table 11). At final discharge from acute hospital, mortality had increased to 24%, with the differential outcomes by cause of TBI maintained. Of the patients who survived to discharge from acute hospital, very few died before 6 months following the TBI, with 6-month mortality of 26%. The Kaplan–Meier survival curve (Figure 20) indicates that the majority of deaths occurred within the first 30 days following the TBI, with a slow decline in survival thereafter. The same was true across each cause of TBI, with the differential mortality by cause established by 30 days and maintained to 6 months.
Outcomes | Overall (n = 2975a) | RTA (n = 976) | Fall (n = 1399) | Assault (n = 353) |
---|---|---|---|---|
Mortality, deaths (%) [N] | ||||
At final discharge from critical care | 546 (18.4) [2962] | 171 (17.6) [972] | 295 (21.2) [1394] | 49 (13.9) [353] |
At final discharge from acute hospital | 705 (24.5) [2883] | 197 (20.9) [944] | 410 (30.2) [1358] | 55 (15.9) [346] |
At 6 months | 748 (26.0) [2881] | 204 (21.5) [948] | 439 (32.0) [1370] | 56 (16.8) [333] |
Unfavourable outcome at 6 months,b n (%) [N] | 1481 (61.2) [2422] | 466 (57.3) [813] | 776 (66.7) [1164] | 137 (51.7) [265] |
FIGURE 20.
Kaplan–Meier survival curves to 6 months following TBI. (a) Overall; (b) by cause of TBI.
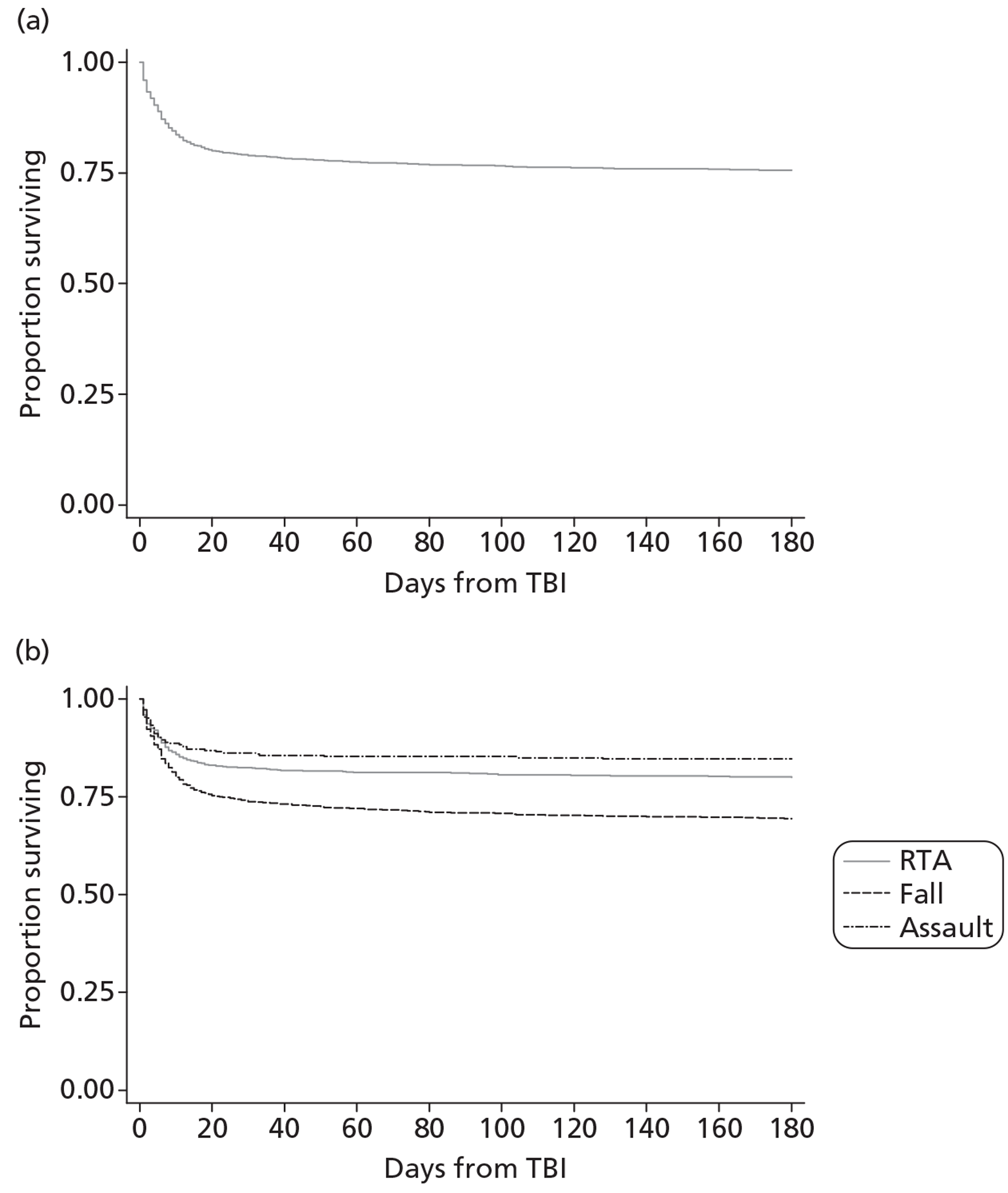
Neurological outcome at 6 months
There was a substantial burden of disability at 6 months, with only 26% of surviving patients with a known GOSE category reporting a good recovery compared with 44% reporting severe disability (Figure 21). In addition to the 26% of patients who had died by 6 months, a similar proportion was known to have severe disability (Figure 22). When presented as a percentage of those with a known GOSE category, this corresponds to a rate of unfavourable outcome (death or severe disability) at 6 months of 61% (see Table 11). Outcomes were worst for falls, then for RTAs and assaults, with unfavourable outcome rates of 67%, 57% and 52%, respectively. The GOSE categories were reported for all patients with known survival status at 6 months (n = 2881) to avoid biasing the proportions towards deaths. Unfavourable outcome at 6 months is reported only for those with a known GOSE category (n = 2422) and, therefore, the reported rate of 61% is likely to represent an overestimate of the actual percentage with unfavourable outcome at 6 months because deaths will be over-represented owing to more complete follow-up. This is addressed in the following chapter using multiple imputation.
FIGURE 21.
Glasgow Outcome Scale – Extended at 6 months following TBI as a percentage of survivors with known GOSE category, overall and by cause of TBI. (a) Overall; (b) RTA; (c) fall; (d) assault.
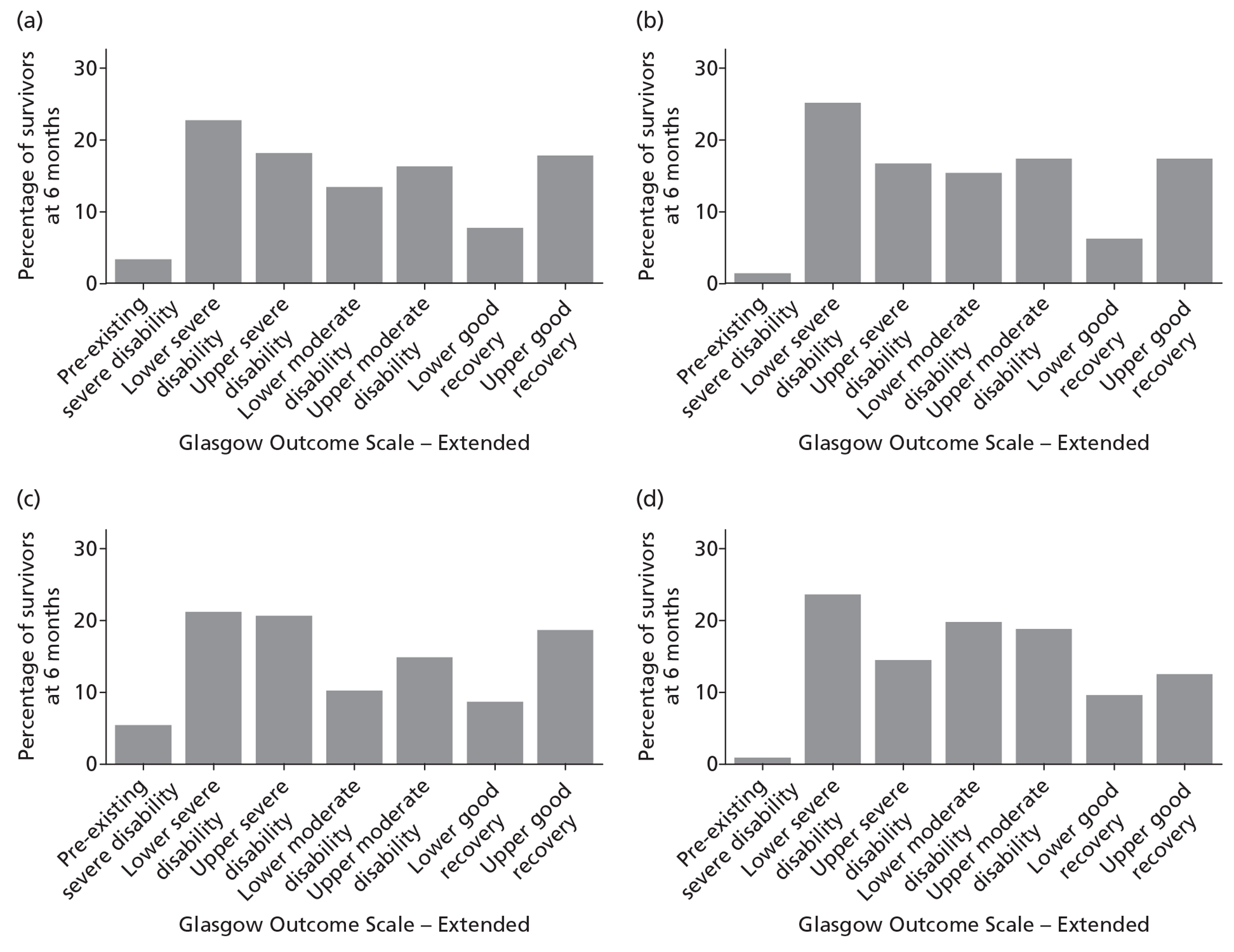
FIGURE 22.
Glasgow Outcome Scale – Extended at 6 months following TBI as a percentage of all patients with known survival status, overall and by cause of TBI. Denominator includes 483 (17%) patients who were alive at 6 months following TBI but with unknown GOSE category. (a) Overall; (b) RTA; (c) fall; (d) assault.
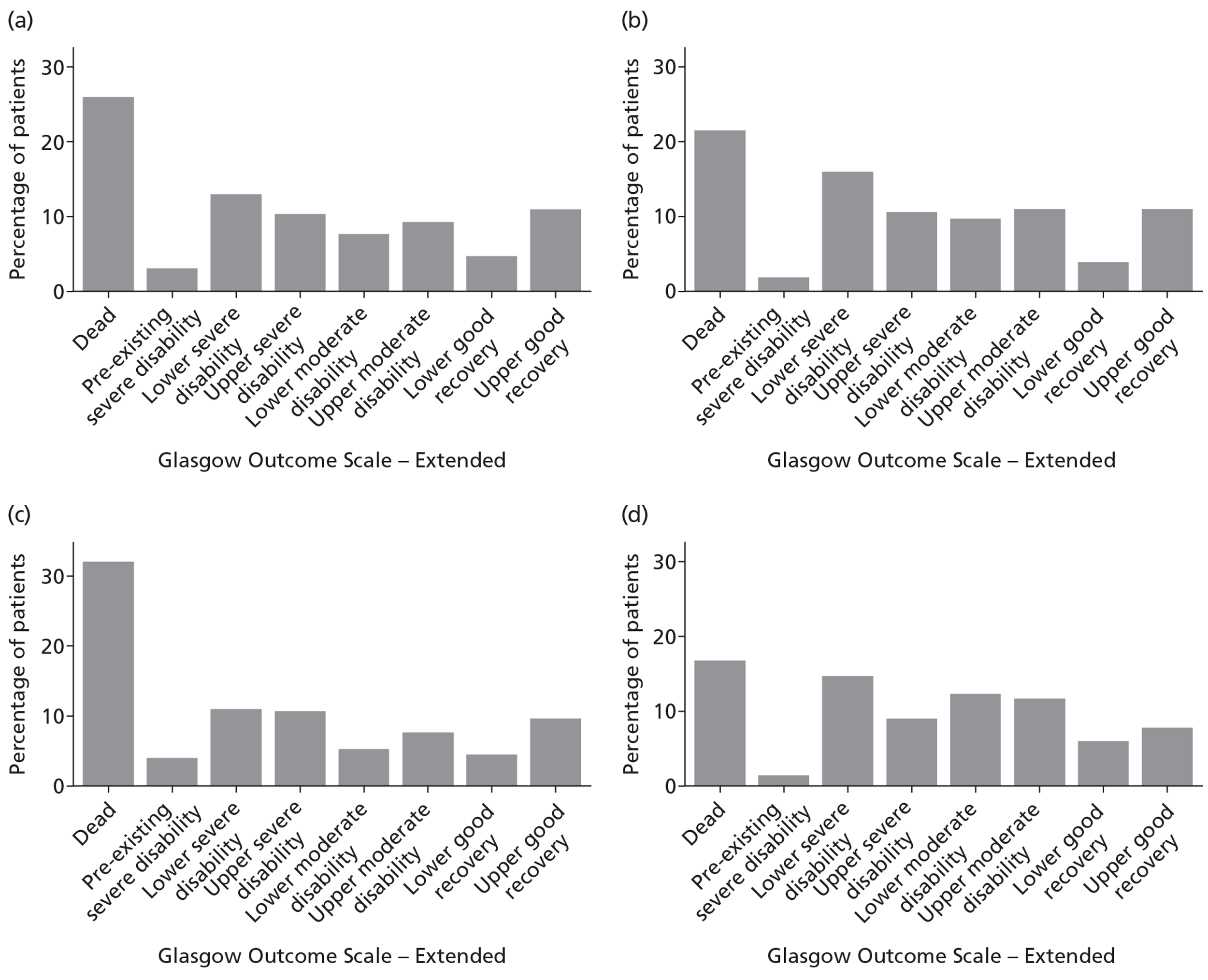
Quality of life at 6 months
European Quality of Life-5 Dimensions (3-level version) responses were available for 1132 patients (53% of those known to be alive at 6 months). Figure 23 summarises the EQ-5D-3L responses for each of the five domains. The EQ-5D-3L responses indicated substantial issues with QOL at 6 months, including 70% of patients reporting problems with performing usual activities, around 60% reporting problems with pain or discomfort and anxiety or depression, around 50% reporting problems with mobility and around 35% reporting problems with self care. There was little difference across the EQ-5D-3L domains by cause of TBI, although those admitted following an RTA reported slightly higher proportions in the worst categories for physical functioning (mobility, self care and usual activities), whereas patients admitted following assault reported slightly higher proportions in the worst categories for pain or discomfort, and anxiety or depression.
FIGURE 23.
European Quality of Life-5 Dimensions (3-level version) responses by domain, overall and by TBI cause (a) Mobility; (b) self-care; (c) usual activities; (d) pain/discomfort; (e) anxiety/depression.
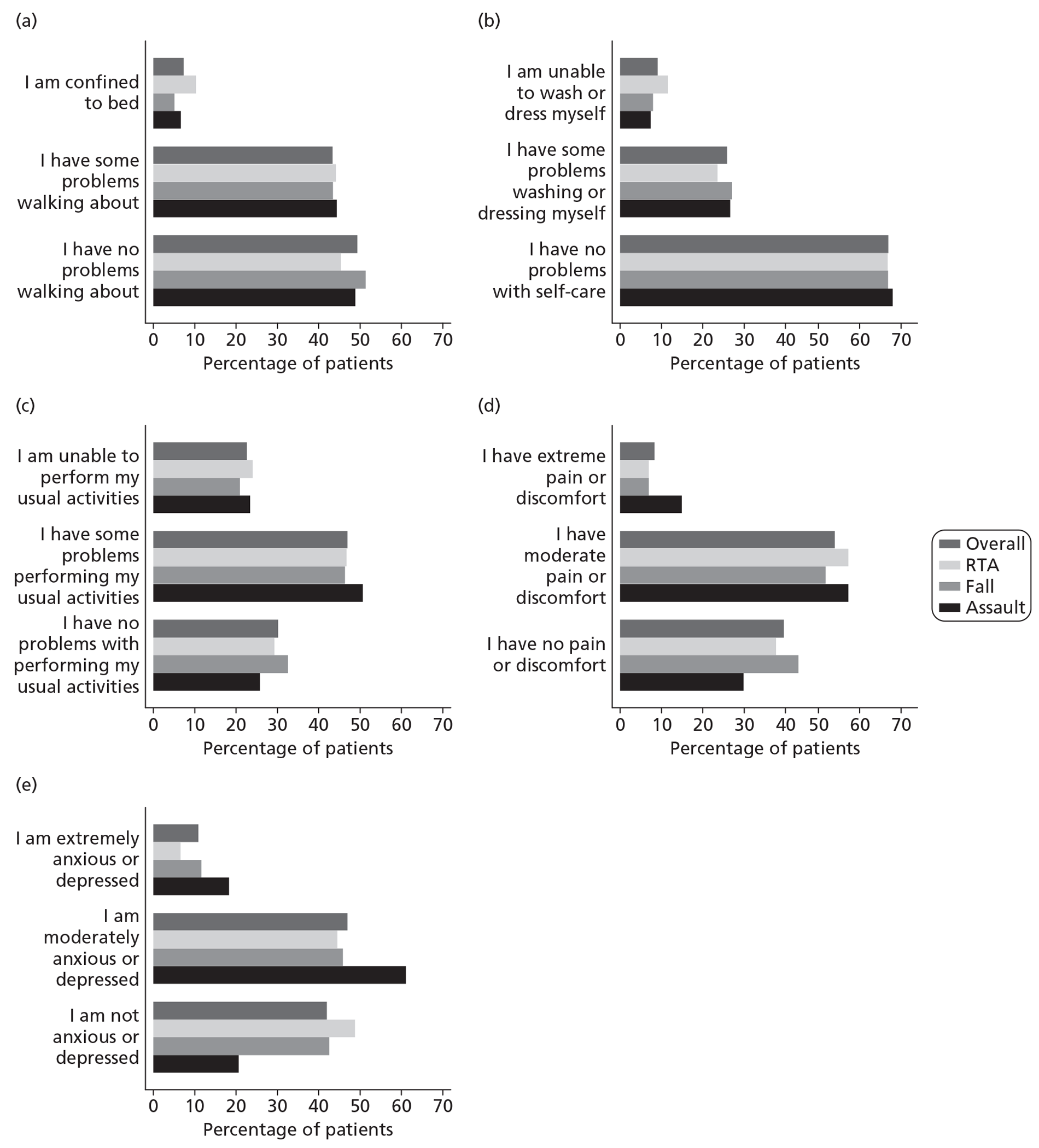
Figure 24 summarises the self-rated health from the visual analogue scale for the 1078 patients who completed this section of the questionnaire, overall and by cause of TBI. The overall median self-rated health was 70 (IQR 50 to 85). Self-rated health was lower for patients following assault (median 60, IQR 45 to 80) than for either RTA or fall (both 70, 50 to 85).
FIGURE 24.
Self-rated health at 6 months following TBI, overall and by cause (a) Overall; (b) RTA; (c) fall; (d) assault.
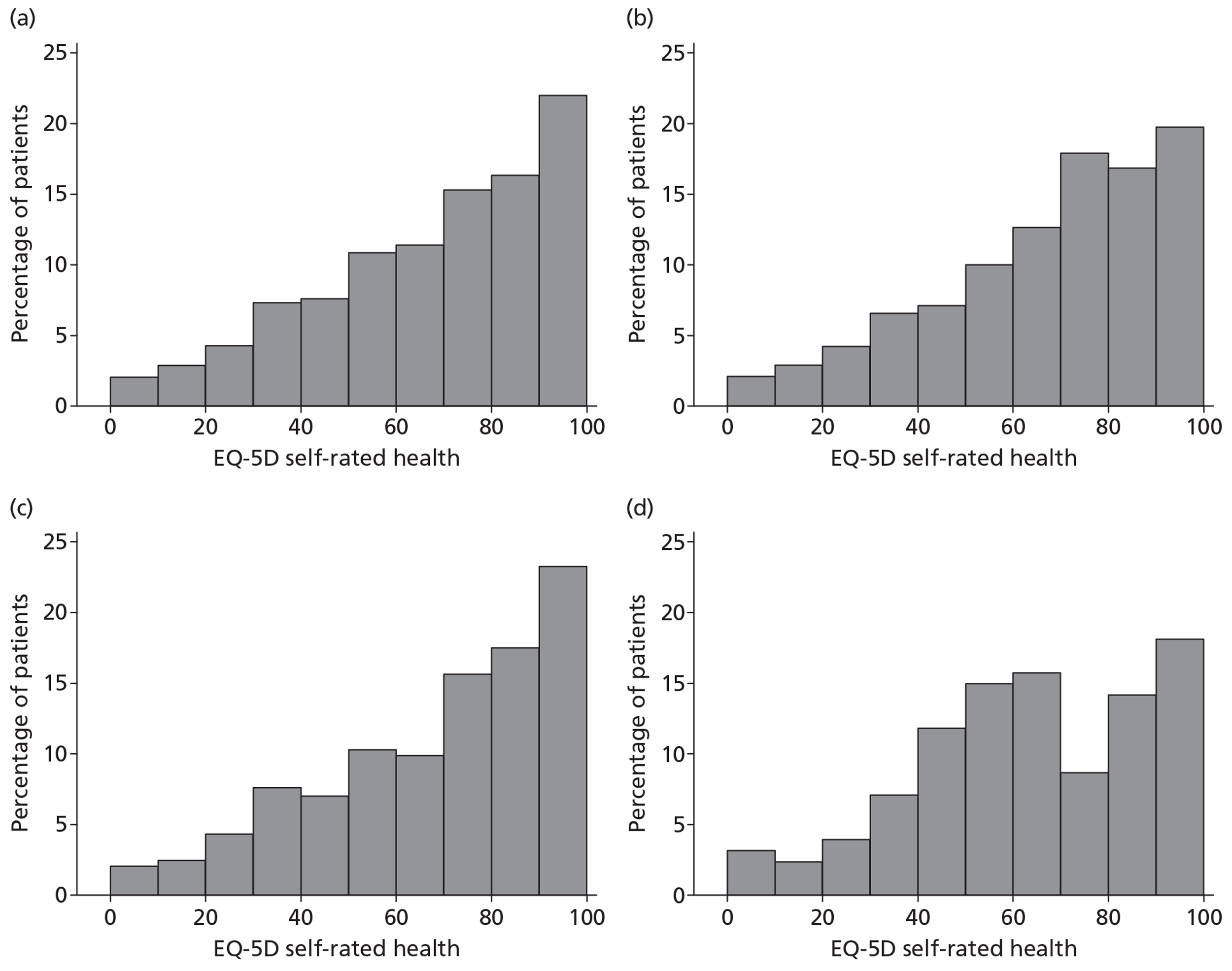
Discussion
Principal findings
Patients with acute TBI admitted to UK critical care units participating in the RAIN study were predominantly admitted following RTA (33%), fall (47%) or assault (12%). Characteristics and outcomes varied markedly by cause of TBI. The RAIN study demonstrated a substantial burden of poor neurological outcomes and QOL 6 months after TBI for adult patients admitted to critical care. Mortality at discharge from acute hospital was 16% for assault, 21% for RTA and 30% for falls, rising to 17%, 22% and 32%, respectively, at 6 months following the TBI. Of survivors at 6 months with known a known GOSE catgory, 44% had severe disability, 30% had moderate disability, and only 26% had made a good recovery. When combined with the 26% mortality at 6 months, 61% of patients with known outcome had an unfavourable outcome (death or severe disability) at 6 months. In addition, between 35% and 70% of survivors reported problems across the five domains of the EQ-5D-3L. Despite this, self-rated health was remarkably good, with an overall median of 70 out of 100 and 22% of respondents rating their current health as 90 out of 100 or higher.
Comparison with other studies
Little literature exists reporting specifically on multicentre cohorts of patients with acute TBI receiving critical care. A previous study from the CMP reported on over 11,000 admissions to 169 general critical care units (including combined neuro/general critical care units) and two dedicated neurocritical care units between 1995 and 2005 with a primary reason for admission to the critical care unit of ‘primary brain injury’, ‘extradural haematoma’ or ‘subdural haematoma’. 6 Three-quarters of admissions were male and the mean age was 44 years, corresponding very closely with the overall figures in the RAIN study. Patients were followed up to ultimate discharge from acute hospital, with mortality of 33.5% – considerably higher than the 24.5% acute hospital mortality observed in the RAIN study. A prospective study from Australia and New Zealand reported on 635 patients admitted to the critical care units of 16 major trauma centres following acute TBI. 73 The age and sex distribution was again similar to that of the RAIN study (mean age 42 years, 74% male). However, there was a different distribution of cause of TBI with 61% RTA, 25% falls, 8% assault and 8% other/unknown; 57% of TBIs were severe (GCS score 3–8) based on the first at hospital GCS score compared with 46% of those in the RAIN study with a first at hospital GCS score recorded. A remarkably high proportion of patients had no visible pathology on CT scan (24% overall including 23% of severe TBI) and of those with abnormal CT scans, diffuse injuries predominated (63%), consistent with the higher proportion of patients admitted following RTA. Mortality was similar to that in the RAIN study (15.1% vs 18.4% at discharge from the critical care unit and 24.4% vs 26.0% at 6 months following TBI).
The proportion of survivors with severe disability at 6 months in the RAIN study (44%) is high compared with previous studies, for example 26% for patients with severe TBI from a study in the Netherlands74 and 27% among critically ill patients following TBI in Australia and New Zealand.73 Some of these patients will still be on a trajectory of improving neurological outcome; the proportion with a good recovery increased from 38% at 6 months to 46% at 12 months in the study from the Netherlands,74 and from 27% to 42% in the study from Australia and New Zealand. 73 However, for many patients, these are likely to represent long-term disabilities, for example a long-term follow-up study in Glasgow found that of 96 patients with severe disability at 1 year following TBI, 44 (46%) had died, 30 (31%) still had severe disability, 15 (16%) had moderate disability and seven (7%) had made a good recovery when followed up 5–7 years following injury. 75
Chapter 5 External validation of risk prediction models for acute traumatic brain injury among critically ill patients
Introduction
This chapter reports the statistical validation of the risk prediction models identified following the updated systematic review (see Chapter 2) using the data collected for the RAIN study (see Chapters 3 and 4). In addition, this chapter reports a nested substudy testing the inter-rater reliability (reproducibility) of CT scan reporting in the RAIN study.
Methods
Summary of risk prediction models
Following the updated systematic review (see Chapter 2) and review by the RAIN Study Steering Group, three families of risk prediction models were selected for validation in the RAIN study – the models of Hukkelhoven et al. (Hukkelhoven models);48 the MRC CRASH trial collaborators (CRASH models);30 and Steyerberg et al. (IMPACT models). 35 A total of 10 models were included in the RAIN study validation (Figure 25): four predicting mortality at 6 months (one Hukkelhoven and three IMPACT) and six predicting unfavourable outcome at 6 months (one Hukkelhoven, two CRASH and three IMPACT). Details of the development and validation of these models were reported in Chapter 2. A brief summary of the development data sources, inclusion/exclusion criteria and included variables is presented for each model below.
FIGURE 25.
Overview of risk prediction models.
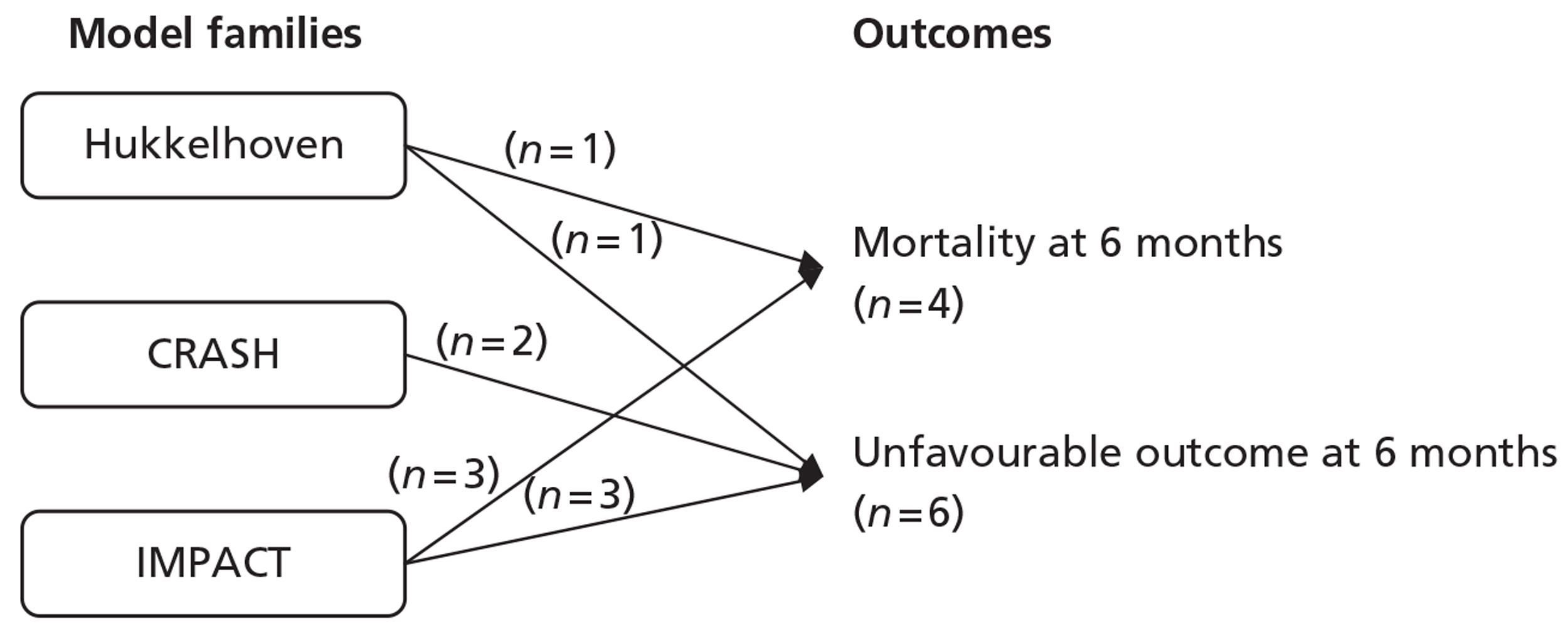
Hukkelhoven models
The Hukkelhoven models were developed using data from two multicentre RCTs, one from North America and one from Europe. 49,50 Inclusion criteria for both trials were patients aged 15–65 years with severe TBI (GCS score of < 9) regardless of CT findings or moderate TBI (GCS score of 9–12) with an abnormal CT scan. Patients with an absent motor score were excluded. Randomisation had to take place within 4 hours of injury. Two risk prediction models were developed: one for mortality at 6 months and one for unfavourable outcome at 6 months. 48 The variables included in the risk prediction models were: age (linear and quadratic terms); motor score; pupil reactivity; pre-hospital hypotension; pre-hospital hypoxia; Marshall CT classification; and traumatic SAH. Model coefficients were obtained from the published paper. 48
CRASH models
The CRASH models were developed using data from one multinational, multicentre RCT. 53,54,62 Inclusion criteria for the trial were patients aged ≥ 16 years with TBI and a GCS score of < 15 and no clear contraindication to receiving corticosteroids. Randomisation had to take place within 8 hours of injury. In total, eight risk prediction models were developed, for each combination of setting (low-/middle- and high-income countries), outcome (mortality at 14 days and unfavourable outcome at 6 months) and model complexity (‘Basic’ and ‘CT’ models). 30 Patients with no CT scan available were excluded from the development samples for the CT models. As the setting for the RAIN study was the UK NHS, and the importance of longer-term outcomes was identified at the outset of the study, the models of interest for the RAIN study were the two models based on data from high-income countries predicting unfavourable outcome at 6 months. The variables included in the Basic models were age (linear > 40 years); GCS score (linear); pupil reactivity; and major extracranial injury (requiring hospital admission independent of the TBI). The additional variables included in the CT models were one or more small petechial haemorrhages; obliteration of the third ventricle or basal cisterns; subarachnoid bleed (traumatic SAH); midline shift of > 5 mm; and non-evacuated haematoma. Model coefficients were obtained from the authors.
IMPACT models
The IMPACT models were developed using data from the IMPACT database, a pooled database of data from eight RCTs and three observational studies of patients with moderate or severe TBI (GCS score of < 13). 55 Individual data sets had different inclusion criteria. Six risk prediction models were developed for each combination of outcome (mortality at 6 months and unfavourable outcome at 6 months) and model complexity (‘Core’, ‘Extended’ and ‘Lab’ models). 35 Patients from one of the constituent data sets of the IMPACT database, which did not record hypoxia, hypotension or CT classification, were excluded from the development sample for the extended models and patients from a further six data sets, which did not record laboratory values, were excluded from the development sample for the Laboratory models. Missing data for individual patients in the development samples for each model were imputed. The variables included in the Core models were age (linear); motor score; and pupil reactivity. The additional variables included in the Extended models were Marshall CT classification; traumatic SAH; epidural (extradural) haematoma; pre-hospital hypoxia; and pre-hospital hypotension. The additional variables included in the Laboratory models were glucose (linear); and haemoglobin (linear). Model coefficients were obtained from the published paper. 35
Data sources and risk factors definitions
Patients were selected from the RAIN study database if their last pre-sedation GCS score was < 15. Definitions for fields in the RAIN study are provided in Appendix 3. Risk factor variables were calculated from the raw data as follows:
-
Age Age in whole years at the time of TBI, calculated from the date of birth and date of TBI.
-
GCS score The last recorded GCS score prior to sedation, which may have been either the pre-hospital GCS score, the first recorded-at-hospital GCS score or a subsequent measurement prior to sedation and admission to the critical care unit.
-
Motor score The motor score component of the last pre-sedation GCS score.
-
Pupil reactivity The first recorded-at-hospital pupil reactivity, if available, or the pre-hospital pupil reactivity if no first at hospital value was available. Pupil reactivity was recorded for the left and right eye separately and combined as either ‘both reactive’, ‘one reactive’ or ‘neither reactive’. If only one eye could be assessed, pupil reactivity was assigned as ‘both reactive’ if this eye was reactive, and ‘neither reactive’ if this eye was not reactive.
-
Major extracranial injury Recorded as a specific field in the RAIN study data set, defined as an injury requiring hospital admission in its own right.
-
Pre-hospital hypoxia Defined as either a pre-hospital oxygen saturation of < 90%, or pre-hospital hypoxia strongly suspected if there was clinical evidence of hypoxia (e.g. tension pneumothorax) but no pre-hospital oxygen saturation was recorded.
-
Pre-hospital hypotension Defined as either a pre-hospital systolic blood pressure of < 90 mmHg, or pre-hospital hypotension strongly suspected if there was clinical evidence of hypotension (e.g. multiple trauma with massive blood loss) but no pre-hospital systolic blood pressure was recorded.
-
Marshall CT classification Based on the first CT scan following the TBI, and defined as (1) diffuse injury I – no visible pathology seen on CT scan (first CT scan result normal); (2) diffuse injury II – basal cisterns present, midline shift of 0–5 mm, no high- or mixed-density lesion of > 25 ml; (3) diffuse injury III – basal cisterns compressed or absent, midline shift of 0–5 mm, no high- or mixed-density lesion of > 25 ml; (4) diffuse injury IV – midline shift of > 5 mm, no high- or mixed-density lesion > 25 ml; (5) evacuated mass lesion – any high- or mixed-density lesion of > 1 ml – surgically evacuated; (6) non-evacuated mass lesion of > 25 ml – high- or mixed-density lesion of > 25 ml not surgically evacuated. 56
-
Traumatic SAH Recorded as a specific field in the RAIN study data set.
-
One or more small petechial haemorrhages Recorded as a specific field in the RAIN study data set.
-
Obliteration of the third ventricle or basal cisterns Third ventricle recorded as ‘obliterated’ or basal cisterns recorded as ‘absent’.
-
Midline shift of > 5 mm Recorded as a specific field in the RAIN study data set.
-
Non-evacuated haematoma Any high- or mixed-density lesion of > 1 ml – not surgically evacuated.
-
Extradural haematoma Recorded as a specific field in the RAIN study data set.
-
First at hospital glucose Recorded as a specific field in the RAIN study data set.
-
First at hospital haemoglobin Recorded as a specific field in the RAIN study data set.
Handling of missing data
Missing data in the RAIN study data set were addressed with multiple imputation. 76,77 Multiple imputation aims to allow for the uncertainty about missing data by creating multiple copies of the data set with the missing values in each data set replaced by imputed values, sampled from their predicted distribution. 78 Analyses are conducted on each of the imputed data sets and combined together. As GOSE category is conditional on survival status, the imputation was conducted in two stages. In the first stage, imputation models were specified for risk factors and mortality at 6 months, according to observed covariates. In the second stage, for each of the previously imputed data sets, imputation models were specified for GOSE category for those patients who were either known to be alive at 6 months or were predicted to be alive by the first stage imputation model. Each multiple imputation model assumed that the data were ‘missing at random’, i.e. conditional on the variables included in the imputation models. 76 Each imputation model considered including, for example baseline characteristics (e.g. age) and resource use at 6 months (e.g. LOS in critical care). Five imputed data sets were generated for each stage of the imputation,78 giving 25 imputed data sets in total. All multiple imputation models were implemented in R (The R Foundation for Statistical Computing, Vienna, Austria: www.r-project.org) using Multivariate Imputation by Chained Equations (MICE). 79
Validation data sets
For the primary analyses, each model was validated in the multiply imputed data sets, using (1) all patients in the RAIN study with a last pre-sedation GCS score of < 15 and (2) only those patients meeting the original inclusion criteria for each model. As secondary analyses, and to reflect use of the models in real life settings, the validation was repeated in the original RAIN study data set prior to imputation using (3) all patients; (4) only those patients meeting the original inclusion criteria for each model; and (5) only those patients meeting the original inclusion criteria for each model and with complete data for all variables in the model. For analyses 3 and 4, missing values were assumed to be in the lowest risk category and laboratory data were singly imputed to a normal value.
As a sensitivity analysis, the analysis for models predicting unfavourable outcome in all patients in the RAIN study data set [data set (1) above] was repeated excluding those patients assigned to the GOSE category of severe disability that was either pre-existing or not due to the TBI.
Statistical analysis
The case mix and outcomes of patients reported for the original development sample for each family of models (Hukkelhoven, CRASH and IMPACT) was compared with those for the patients in the RAIN study data set that met the original inclusion criteria for the particular family of models, and with all patients in the RAIN study data set. The case mix factors included in these comparisons were those included in the papers reporting development of the models. Owing to the level of detail of reporting in the papers, it was possible to summarise the overall case mix and outcome for only each full development sample, and not specifically to the patients actually included in each development sample for each individual model.
The completeness of data for each risk factor included in each of the risk prediction models and the outcomes of mortality and unfavourable outcome at 6 months was assessed in the full RAIN study data set. Univariable analyses were conducted to assess the relationship between each risk factor and the outcomes.
Each risk prediction model was then validated in each of the five RAIN study validation data sets using measures of calibration, discrimination and overall fit, as described below. For the validation of risk prediction models for mortality at 6 months, the five data sets from the first stage imputation were used. For the validation of risk prediction models for unfavourable outcome at 6 months, the 25 data sets from the second stage imputation were used. In each case, measures of model performance were calculated in each imputed data set and combined using Rubin's rules,76 which recognise uncertainty both within and between imputations.
Discrimination describes the ability of the model to correctly separate the patients into different groups (survivors from non-survivors or favourable from unfavourable outcomes). Discrimination was assessed by the c-index,80 which is equivalent to the area under the ROC curve. 81 When c = 1 there is perfect discrimination between the groups (i.e. every patient that died has a higher predicted risk than every patient that survived, or every patient with an unfavourable outcome has a higher predicted risk than every patient with a favourable outcome) and when c = 0.5 the discrimination is no better than chance.
Calibration describes the degree of correspondence between the probability predicted by the model, and the observed proportion with the outcome. Calibration was assessed by graphical plots of observed against expected risk in 10 equal-sized groups by predicted risk. The Hosmer–Lemeshow test was used to test the hypothesis of perfect calibration. 82 However, the Hosmer–Lemeshow test does not provide a measure of the magnitude of miscalibration and is highly sensitive to sample size. 83 Therefore, the magnitude and direction of miscalibration was assessed using Cox's calibration regression. 84 Cox's calibration regression fits a logistic regression model to the predicted log odds from the risk prediction model to estimate the intercept, α, and slope, β. If the model is perfectly calibrated then α = 0 and β = 1. The value of α represents the calibration at a predicted risk of 0.5, and calibration more generally if β = 1. If 0 < β < 1, the predictions vary too much; if β > 1, the predictions ‘show the right general pattern of variation but do not vary enough’.
The overall fit (or accuracy) of the model describes how close the predictions are to the actual outcomes for individual patients. Overall fit was assessed using Brier's score, the mean squared error between the outcome and prediction. 85 Lower values of Brier's score represent better fit, with perfect predictions corresponding to B = 0 and a constant prediction of 0.5 for all patients corresponding to B = 0.25.
Inter-rater reliability of computerised tomography scan reporting
It is essential to know whether the data obtained from local reporting of CT scans are adequate for accurate risk adjustment, as this will have significant implications on the practicability of using any particular risk prediction model. Data collectors were therefore asked to record appropriate identifiers to allow access to CT scans for rereview at a later date, and were requested not to discard or destroy the films or digital imaging data for these patients until 5 years after entry into the RAIN study. Copies of the first CT scan following presentation at hospital were requested for a randomly selected sample of 10% of patients, weighted to include more patients from outside neuroscience centres, where patient throughput was lower, and including at least one patient from each site participating in the RAIN study (provided at least one patient had CT data available). Data collectors were requested to send anonymised CT scans to the WBIC. Images were transferred either electronically using the Image Exchange Portal or by recorded delivery or secure courier. The images were centrally viewed and assessed by four neurosurgical specialist registrars/research fellows experienced in assessing CT scans, working under the supervision of two of the clinical co-investigators (DKM, PJH). Each CT scan was assessed independently by two raters, working in two teams of two, and data were recorded using the same secure, web-based data entry system as for the original RAIN study data entry. Each rater was blinded to the site from which the CT scan originated, the original RAIN study data reported by that site, and the assessment of their co-rater.
Inter-rater reliability (reproducibility) was assessed by κ-statistics86 for each individual CT field in the RAIN study data set between:
-
each group of three ratings comprising the original RAIN study data and the two independent ratings from WBIC; and
-
each pair of ratings: RAIN compared with WBIC rater 1, RAIN compared with WBIC rater 2 and WBIC rater 1 compared with WBIC rater 2, separately for the two teams of two raters within WBIC.
Subgroup analyses were conducted to compare inter-rater reliability by the specialty and grade of assessor in the original RAIN study data, and by whether the scan was from a neuroscience centre or a non-neuroscience centre.
Confidence intervals for κ-statistics were calculated using the method of Donner and Eliasziw87 for two raters rating a binary outcome, the method of Zou and Donner88 for more than two raters rating a binary outcome, and using bootstrap resampling for more than two raters rating an outcome with more than two levels. 89
All statistical analyses were conducted using Stata/SE version 10.1 (StataCorp LP, College Station, TX, USA).
Results
Comparison of model development samples with the RAIN study data set
Tables 12–14 describe the case mix and outcomes of the development samples for the families of Hukkelhoven, CRASH and IMPACT models compared with patients from the RAIN study, both those meeting the original inclusion criteria for the respective model and all patients in the RAIN study data set. Compared with patients in the Hukkelhoven development sample (see Table 12), patients in the RAIN study were, on average, older (mean 45 years vs 33 years), more likely to have had a fall (47% vs 17%) and less likely to have had an RTA (33% vs 58%) as the cause of their TBI, had worse GCS motor scores (32% vs 13% with motor score 1 or 2) and were more likely to have had either an evacuated or non-evacuated mass lesion (Marshall class 5/6, 50% vs 32%). They were, however, less likely to have either pre-hospital hypoxia (13% vs 21%) or hypotension (7% vs 18%). These differences remained, but were generally reduced, after applying the Hukkelhoven inclusion criteria to the RAIN study data set, with the exception of the GCS motor score in which the difference became more extreme (42% vs 13% with motor score 1 or 2). Mortality at 6 months was slightly higher in the RAIN study than in the Hukkelhoven development sample (26% vs 22%) but was similar (23% vs 22%) after applying the inclusion criteria. Unfavourable outcome at 6 months was substantially higher in the RAIN study both before (61% vs 40%) and after (60% vs 40%) applying the inclusion criteria.
Case mix and outcomes | Hukkelhoven | RAIN (meeting Hukkelhoven inclusion) | RAIN (all) |
Number of admissions | 2269 | 1806 | 2975 |
Age (years), mean (SD) [N] | 33.2 (13.5) [2269] | 38.0 (14.4) [1806] | 44.7 (18.9) [2975] |
Male, n (%) [N] | 1757 (77.4) [2269] | 1414 (78.3) [1806] | 2263 (76.1) [2975] |
Cause of TBI, n (%) [N] | [2269] | [1806] | [2975] |
RTA | 1319 (58.1) | 708 (39.2) | 971 (32.6) |
Fall | 386 (17.0) | 699 (38.7) | 1399 (47.0) |
Othera | 564 (24.9) | 399 (22.1) | 605 (20.3) |
GCS motor score, n (%) [N] | [2269] | [1806] | [2907] |
5/6 (localises/obeys) | 933 (41.1) | 672 (37.2) | 1409 (48.5) |
4 (normal flexion) | 659 (29.0) | 242 (13.4) | 349 (12.0) |
3 (abnormal flexion) | 374 (16.5) | 140 (7.8) | 209 (7.2) |
1/2 (none/extension) | 303 (13.4) | 752 (41.6) | 940 (32.3) |
Pupil reactivity, n (%) [N] | [2269] | [1806] | [2975] |
Both reactive | 1401 (61.7) | 1275 (70.6) | 2215 (74.5) |
One reactive | 279 (12.3) | 122 (6.8) | 167 (5.6) |
Neither reactive | 308 (13.6) | 283 (15.7) | 378 (12.7) |
Unable/not assessed | 281 (12.4) | 126 (7.0) | 215 (7.2) |
Hypoxia, n (%) [N] | 429 (21.4) [2006] | 260 (15.2) [1709] | 354 (12.6) [2800] |
Hypotension, n (%) [N] | 395 (17.9) [2208] | 141 (8.3) [1703] | 190 (6.8) [2791] |
Marshall CT classification, n (%) [N] | [2249] | [1710] | [2773] |
1/2 (diffuse injury I/II) | 1006 (44.7) | 674 (39.4) | 1051 (37.9) |
3 (diffuse injury III) | 426 (18.9) | 193 (11.3) | 248 (8.9) |
4 (diffuse injury IV) | 88 (3.9) | 51 (3.0) | 78 (2.8) |
5/6 (evacuated mass lesion/non-evacuated mass lesion > 25 ml) | 729 (32.4) | 792 (46.3) | 1396 (50.3) |
Traumatic SAH, n (%) [N] | 1090 (49.0) [2223] | 1006 (58.4) [1724] | 1565 (56.0) [2797] |
Six-month outcome, n (%) [N]: | |||
Mortality | 500 (22.0) [2269] | 397 (22.7) [1746] | 748 (26.0) [2881] |
Unfavourable outcome | 862 (40.1) [2149] | 867 (60.0) [1444] | 1481 (61.3) [2420] |
Compared with patients in the CRASH development sample (see Table 13), patients in the RAIN study were, on average, slightly older (mean 45 vs 41 years), more likely to have had a fall (47% vs 20%) and less likely to have had an RTA (33% vs 50%) as the cause of their TBI, had worse GCS scores (59% vs 44% severe TBI, GCS score 3–8; 15% vs 33% mild TBI, GCS score 13–14), were more likely to have unreactive pupils (13% vs 9%) and major extracranial injury (41% vs 23%), and had higher rates of all risk factors on CT. The rate of unfavourable outcome at 6 months was substantially higher in the RAIN study (61% vs 39%). There was very little change after applying the CRASH inclusion criteria to the RAIN study data set, as the majority of patients in the RAIN study met CRASH inclusion.
Case mix and outcomes | CRASHa | RAIN (meeting CRASH inclusion) | RAIN (all) |
---|---|---|---|
Number of admissions | 2,482 | 2710 | 2975 |
Age (years), mean (SD) [N] | 40.6 (19.4) | 43.9 (18.8) [2710] | 44.7 (18.9) [2975] |
Male, n (%) [N] | (78.9) | 2069 (76.4) [2710] | 2263 (76.1) [2975] |
Cause of TBI, n (%) [N] | [2710] | [2975] | |
RTA | (50.2) | 949 (35.0) | 971 (32.6) |
Fall | (20.0) | 1218 (44.9) | 1399 (47.0) |
Otherb | (29.8) | 543 (20.0) | 605 (20.3) |
Hours since injury, mean (SD) [N] | 2.8 (2.0) | 0.4 (4.1) [2710] | 7.1 (46.6) [2975] |
GCS score, n (%) [N] | [2710] | [2975] | |
13–14 (mild TBI) | (32.6) | 398 (14.7) | 447 (15.0) |
9–12 (moderate TBI) | (23.6) | 677 (25.0) | 765 (25.7) |
3–8 (severe TBI) | (43.8) | 1635 (60.3) | 1763 (59.3) |
Pupil reactivity, n (%) [N] | [2710] | [2975] | |
Both reactive | (80.7) | 2016 (74.4) | 2215 (74.5) |
One reactive | (6.3) | 154 (5.7) | 167 (5.6) |
Neither reactive | (9.1) | 350 (12.9) | 378 (12.7) |
Unable/not assessed | (3.9) | 190 (7.0) | 215 (7.2) |
Major extracranial injury, n (%) [N] | (22.5) | 1163 (42.9) [2709] | 1213 (40.8) [2974] |
CT scan available, n (%) [N] | (88.0) | 2583 (95.3) [2710] | 2817 (94.7) [2975] |
Traumatic SAH, n (%) [N] | (26.4) | 1456 (56.8) [2563] | 1565 (56.0) [2797] |
Obliteration of third ventricle or basal cisterns, n (%) [N] | (9.6) | 641 (25.0) [2560] | 717 (25.7) [2793] |
Midline shift, n (%) [N] | (11.1) | 753 (29.4) [2563] | 880 (31.5) [2796] |
Haematoma, n (%) [N] | [2580] | [2813] | |
Evacuated | (7.9) | 831 (32.2) | 964 (34.3) |
Non-evacuated | (26.5) | 1341 (52.0) | 1432 (50.9) |
Six-month outcome, n (%) [N] | |||
Unfavourable outcome | (38.5) | 1340 (61.0) [2198] | 1481 (61.3) [2420] |
Compared with patients in the IMPACT development sample (see Table 14), patients in the RAIN study were, on average, older (median 44 vs 30 years), more likely to have GCS motor scores at the extremes of the range (24% vs 16% with motor score 1 and 47% vs 30% with motor score 6), and were less likely to have unreactive pupils (13% vs 21%), hypoxia (13% vs 21%) and hypotension (7% vs 18%). The distributions of CT findings and laboratory results were broadly similar. Mortality at 6 months was similar (26% vs 28%) but the rate of unfavourable outcome at 6 months was higher in the RAIN study (61% vs 48%). There was little change after applying the IMPACT inclusion criteria to the RAIN study data set.
Case mix and outcomes | IMPACT | RAIN (meeting IMPACT inclusion) | RAIN (all) |
---|---|---|---|
Number of admissions | 8509 | 2528 | 2975 |
Age (years), median (IQR) [N] | 30 (21 to 45) [8509] | 44 (28 to 59) [2528] | 44 (28 to 59) [2975] |
GCS motor score, n (%) [N] | [8509] | [2528] | [2975] |
5/6 (localises/obeys) | 2591 (30.5) | 974 (38.5) | 1409 (47.4) |
4 (normal flexion) | 1940 (22.8) | 345 (13.6) | 349 (11.7) |
3 (abnormal flexion) | 1085 (12.8) | 209 (8.3) | 209 (7.0) |
2 (extension) | 1042 (12.2) | 219 (8.7) | 219 (7.4) |
1 (none) | 1395 (16.4) | 721 (28.5) | 721 (24.2) |
Untestable/missing | 456 (5.4) | 60 (2.4) | 68 (2.3) |
Pupil reactivity, n (%) [N] | [8509] | [2528] | [2975] |
Both reactive | 4486 (52.7) | 1828 (72.3) | 2215 (74.5) |
One reactive | 886 (10.4) | 152 (6.0) | 167 (5.6) |
Neither reactive | 1754 (20.6) | 367 (14.5) | 378 (12.7) |
Unable/not assessed | 1383 (16.3) | 181 (7.2) | 215 (7.2) |
Hypoxia, n (%) [N] | 1116 (20.5) [5452] | 327 (13.8) [2379] | 354 (12.6) [2800] |
Hypotension, n (%) [N] | 1171 (18.2) [6420] | 169 (7.1) [2370] | 190 (6.8) [2791] |
Marshall CT classification, n (%) [N] | [5192] | [2355] | [2773] |
1 (diffuse injury I) | 360 (6.9) | 94 (4.0) | 113 (4.1) |
2 (diffuse injury II) | 1838 (35.4) | 770 (32.7) | 938 (33.8) |
3 (diffuse injury III) | 863 (16.6) | 214 (9.1) | 248 (8.9) |
4 (diffuse injury IV) | 187 (3.6) | 64 (2.7) | 78 (2.8) |
5 (evacuated mass lesion) | 1435 (27.6) | 834 (35.4) | 964 (34.8) |
6 (non-evacuated mass lesion > 25 ml) | 509 (9.8) | 379 (16.1) | 432 (15.6) |
Traumatic SAH, n (%) [N] | 3313 (44.8) [7393] | 1365 (57.5) [2375] | 1565 (56.0) [2797] |
Extradural haematoma(s), n (%) [N] | 999 (13.5) [7409] | 373 (15.6) [2392] | 469 (16.7) [2816] |
Glucose (mmol/l), median (IQR) [N] | 8.2 (6.7 to 10.4) [4830] | 7.7 (6.3 to 9.5) [2142] | 7.6 (6.3 to 9.5) [2496] |
Haemoglobin (g/dl), median (IQR) [N] | 12.7 (10.8 to 14.3) [4376] | 13.4 (11.8 to 14.6) [2280] | 13.4 (11.9 to 14.6) [2683] |
Six-month outcome, n (%) [N] | |||
Mortality | 2396 (28.2) [8509] | 685 (27.9) [2455] | 748 (26.0) [2881] |
Unfavourable outcome | 4082 (48.0) [8509] | 1323 (64.3) [2059] | 1481 (61.3) [2420] |
Data completeness
Table 15 reports the data completeness for risk factors and outcomes in the RAIN study data set. Age and GCS score were 100% complete (due to their requirement for meeting RAIN study inclusion criteria), and major extracranial injury was missing for only one patient (and assumed absent). All other risk factors and outcomes were included in the multiple imputation. The core risk factors were available for between 92.8% (pupil reactivity) and 97.7% (motor score) of patients. The first CT scan was available for 2817 patients (94.7%) and the majority of these had all CT fields recorded. Overall, 93.2% of CT scans could be assigned to a Marshall class; of the remaining 6.8%, 5.3% could not be assigned as no CT data were available and 1.5% were due to individual missing CT fields. Laboratory variables were the least complete with 83.9% completeness for glucose and 90.2% for haemoglobin. There was 96.8% follow-up for mortality at 6 months (being unknown only for patients who were homeless or a non-UK resident and could not be linked with the MRIS database; see Chapter 3. There was 81.4% follow-up overall for unfavourable outcome at 6 months (see Chapter 4). Following multiple imputation, the overall mortality at 6 months was estimated to be 25.7% and the rate of unfavourable outcomes 57.4% (note that this is lower than the observed rate owing to being more likely to observe outcomes for non-survivors).
Variable | Hukkelhoven | CRASH Basic | CRASH CT | IMPACT Core | IMPACT Extended | IMPACT Lab | Completeness, n (%) |
---|---|---|---|---|---|---|---|
Predictors | |||||||
Age | ✓ | ✓ | ✓ | ✓ | ✓ | ✓ | 2975 (100) |
GCS score | ✓ | ✓ | 2975 (100) | ||||
GCS motor score | ✓ | ✓ | ✓ | ✓ | 2907 (97.7) | ||
Pupil reactivity | ✓ | ✓ | ✓ | ✓ | ✓ | ✓ | 2760 (92.8) |
Major extracranial injury | ✓ | ✓ | 2974 (> 99.9) | ||||
Hypoxia | ✓ | ✓ | ✓ | ✓ | 2800 (94.1) | ||
Hypotension | ✓ | ✓ | ✓ | ✓ | 2791 (93.8) | ||
Marshall CT classification | ✓ | ✓ | ✓ | 2773 (93.2) | |||
Traumatic SAH | ✓ | ✓ | ✓ | ✓ | 2797 (94.0) | ||
Small petechial haemorrhages | ✓ | 2814 (94.6) | |||||
Obliteration of third ventricle/basal cisterns | ✓ | 2793 (93.9) | |||||
Midline shift | ✓ | 2796 (94.0) | |||||
Non-evacuated haematoma | ✓ | 2813 (94.6) | |||||
Extradural haematoma | ✓ | ✓ | 2816 (94.7) | ||||
Glucose | ✓ | 2496 (83.9) | |||||
Haemoglobin | ✓ | 2683 (90.2) | |||||
Outcomes | |||||||
Mortality at 6 months | ✓ | ✓ | ✓ | ✓ | 2881 (96.8) | ||
Unfavourable outcome at 6 months | ✓ | ✓ | ✓ | ✓ | ✓ | ✓ | 2422 (81.4) |
Univariable associations between risk factors and outcomes
Tables 16–18 report the univariable associations between risk factors and outcomes for core, CT and laboratory variables. The majority of core risk factors demonstrated similar patterns of associations with outcomes as in the risk prediction models (see Table 16). Mortality at 6 months was relatively flat below the age of 40 years and increased from the age of 40 years (reflecting the structure used in the CRASH models), whereas unfavourable outcome increased across the whole range of age (reflecting the structure used in the IMPACT models). The range of outcomes across categories of GCS motor score (16–40% for mortality and 45–75% for unfavourable outcome) was almost as wide as that seen across the total GCS score (15–43% and 44–78%), reflecting the consensus that the majority of prognostic information in the GCS score, for patients with TBI, comes from the motor score component. Non-reactive pupils and pre-hospital hypoxia and hypotension were associated with substantially worse outcomes. However, major extracranial injury was not associated with any increase in mortality or unfavourable outcome at 6 months.
Variable | Mortality at 6 months | Unfavourable outcome at 6 months | ||
---|---|---|---|---|
n/N | % (95% CI) | n/N | % (95% CI) | |
Age (years) | ||||
16–29 | 125/807 | 15.5 (13.2 to 18.1) | 303/669 | 45.3 (41.6 to 49.1) |
30–39 | 61/394 | 15.5 (12.2 to 19.4) | 181/325 | 55.7 (50.3 to 61.0) |
40–49 | 109/533 | 20.5 (17.2 to 24.1) | 269/444 | 60.6 (56.0 to 65.0) |
50–59 | 115/421 | 27.3 (23.3 to 31.8) | 228/343 | 66.5 (61.3 to 71.3) |
60–69 | 149/362 | 41.2 (36.2 to 46.3) | 232/315 | 73.7 (68.5 to 78.2) |
70–79 | 127/258 | 49.2 (43.2 to 55.3) | 186/229 | 81.2 (75.7 to 85.8) |
80+ | 62/106 | 58.5 (49.0 to 67.4) | 82/97 | 84.5 (76.0 to 90.4) |
GCS score | ||||
13–14 | 63/426 | 14.8 (11.7 to 18.5) | 158/362 | 43.6 (38.6 to 48.8) |
9–12 | 135/740 | 18.2 (15.6 to 21.2) | 308/613 | 50.2 (46.3 to 54.2) |
4–8 | 301/1137 | 26.4 (24.0 to 29.1) | 625/944 | 66.2 (63.1 to 69.2) |
3 | 249/578 | 43.1 (39.1 to 47.1) | 390/503 | 77.5 (73.7 to 81.0) |
GCS motor score | ||||
6 | 78/486 | 16.0 (13.1 to 19.6) | 189/416 | 45.4 (40.7 to 50.2) |
5 | 166/871 | 19.1 (16.6 to 21.8) | 377/713 | 52.9 (49.2 to 56.5) |
4 | 76/341 | 22.3 (18.2 to 27.0) | 173/283 | 61.1 (55.3 to 66.6) |
3 | 59/200 | 29.5 (23.6 to 36.2) | 112/166 | 67.5 (60.0 to 74.1) |
2 | 77/215 | 35.8 (29.7 to 42.4) | 141/176 | 80.1 (73.6 to 85.3) |
1 | 282/701 | 40.2 (36.7 to 43.9) | 453/608 | 74.5 (70.9 to 77.8) |
Pupil reactivity | ||||
Both reactive | 450/2146 | 21.0 (19.3 to 22.7) | 996/1785 | 55.8 (53.5 to 58.1) |
One reactive | 41/159 | 25.8 (19.6 to 33.1) | 92/135 | 68.1 (59.9 to 75.4) |
Neither reactive | 202/371 | 54.4 (49.4 to 59.4) | 282/328 | 86.0 (81.8 to 89.3) |
Major extracranial injury | ||||
No | 460/1714 | 26.8 (24.8 to 29.0) | 872/1429 | 61.0 (58.5 to 63.5) |
Yes | 288/1166 | 24.7 (22.3 to 27.3) | 608/992 | 61.3 (58.2 to 64.3) |
Pre-hospital hypoxia | ||||
No | 577/2361 | 24.4 (22.7 to 26.2) | 1184/1978 | 59.9 (57.7 to 62.0) |
Yes | 133/347 | 38.3 (33.4 to 43.5) | 209/297 | 70.4 (64.9 to 75.3) |
Pre-hospital hypotension | ||||
No | 631/2516 | 25.1 (23.4 to 26.8) | 1264/2105 | 60.0 (57.9 to 62.1) |
Yes | 76/184 | 41.3 (34.4 to 48.5) | 124/163 | 76.1 (69.0 to 82.0) |
For CT variables (see Table 17), mortality increased substantially across the four categories of diffuse injury in the Marshall CT classification, was lower for category 5 (evacuated mass lesion) and substantially higher for category 6 (non-evacuated mass lesion > 25 ml). This pattern does not appear to support the modelling approach taken in both the Hukkelhoven and IMPACT models of combining categories 5 and 6, although the relationship may be different once adjusted for other variables (e.g. the separate variable for extradural haematoma included in the IMPACT models). All other CT factors were associated with increased mortality and unfavourable outcome except small petechial haemorrhages and extradural haematoma. There was no univariable association between small petechial haemorrhages and outcomes. However, it should be noted that in the CRASH CT model, this variable was associated only with a small, and not statistically significant, increase in risk in high-income countries and was retained in the model because of the more substantial effect observed in low- and middle-income countries. Extradural haematoma was associated with a decreased risk of mortality and unfavourable outcome, consistent with the negative coefficient for this variable in the IMPACT Extended and IMPACT Lab models.
Variable | Mortality at 6 months | Unfavourable outcome at 6 months | ||
---|---|---|---|---|
n/N | % (95% CI) | n/N | % (95% CI) | |
Marshall CT classification | ||||
1 | 7/109 | 6.4 (3.1 to 12.7) | 36/85 | 42.4 (32.4 to 53.0) |
2 | 117/896 | 13.1 (11.0 to 15.4) | 337/727 | 46.4 (42.8 to 50.0) |
3 | 79/240 | 32.9 (27.3 to 39.1) | 136/211 | 64.5 (57.8 to 70.6) |
4 | 30/78 | 38.5 (28.4 to 49.6) | 50/74 | 67.6 (56.3 to 77.1) |
5 | 252/948 | 26.6 (23.9 to 29.5) | 523/797 | 65.6 (62.3 to 68.8) |
6 | 212/420 | 50.5 (45.7 to 55.2) | 299/372 | 80.4 (76.0 to 84.1) |
Traumatic SAH | ||||
No | 239/1193 | 20.0 (17.9 to 22.4) | 546/987 | 55.3 (52.2 to 58.4) |
Yes | 462/1522 | 30.4 (28.1 to 32.7) | 846/1298 | 65.2 (62.5 to 67.7) |
Small petechial haemorrhages | ||||
No | 422/1614 | 26.1 (24.1 to 28.3) | 834/1366 | 61.1 (58.4 to 63.6) |
Yes | 284/1118 | 25.4 (22.9 to 28.0) | 568/935 | 60.7 (57.6 to 63.8) |
Obliteration of third ventricle/basal cisterns | ||||
No | 383/1999 | 19.2 (17.5 to 20.9) | 906/1650 | 54.9 (52.5 to 57.3) |
Yes | 315/712 | 44.2 (40.6 to 47.9) | 483/631 | 76.5 (73.1 to 79.7) |
Midline shift of > 5 mm | ||||
No | 363/1843 | 19.7 (17.9 to 21.6) | 840/1529 | 54.9 (52.4 to 57.4) |
Yes | 338/871 | 38.8 (35.6 to 42.1) | 552/755 | 73.1 (69.8 to 76.2) |
Non-evacuated haematoma | ||||
No | 313/1350 | 23.2 (21.0 to 25.5) | 690/1133 | 60.9 (58.0 to 63.7) |
Yes | 394/1381 | 28.5 (26.2 to 31.0) | 714/1170 | 61.0 (58.2 to 63.8) |
Extradural haematoma | ||||
No | 612/2278 | 26.9 (25.1 to 28.7) | 1203/1926 | 62.5 (60.3 to 64.6) |
Yes | 94/456 | 20.6 (17.2 to 24.6) | 200/377 | 53.1 (48.0 to 58.0) |
For the laboratory variables (see Table 18), there was a substantial increasing risk of both mortality and unfavourable outcome at 6 months associated with both increasing glucose and decreasing haemoglobin, consistent with the relationships in the IMPACT Lab models.
Variable | Mortality at 6 months | Unfavourable outcome at 6 months | ||
---|---|---|---|---|
n/N | % (95% CI) | n/N | % (95% CI) | |
First at hospital glucose (mmol/l) | ||||
< 6 | 68/459 | 14.8 (11.9 to 18.4) | 178/377 | 47.2 (42.2 to 52.3) |
6–6.9 | 80/449 | 17.8 (14.6 to 21.6) | 190/362 | 52.5 (47.3 to 57.6) |
7–7.9 | 114/446 | 25.6 (21.7 to 29.8) | 225/373 | 60.3 (55.3 to 65.2) |
8–9.9 | 162/554 | 29.2 (25.6 to 33.2) | 303/473 | 64.1 (59.6 to 68.3) |
10+ | 203/509 | 39.9 (35.7 to 44.2) | 346/449 | 77.1 (73.0 to 80.7) |
First at hospital haemoglobin (g/dl) | ||||
< 10 | 100/235 | 42.6 (36.4 to 48.9) | 160/204 | 78.4 (72.3 to 83.5) |
10–11.9 | 146/429 | 34.0 (29.7 to 38.6) | 261/362 | 72.1 (67.3 to 76.5) |
12–12.9 | 101/390 | 25.9 (21.8 to 30.5) | 225/332 | 67.8 (62.6 to 72.6) |
13–13.9 | 123/539 | 22.8 (19.5 to 26.5) | 237/462 | 51.3 (46.7 to 55.8) |
14–14.9 | 122/488 | 25.0 (21.4 to 29.0) | 258/420 | 61.4 (56.7 to 66.0) |
15+ | 97/516 | 17.6 (14.6 to 21.2) | 202/408 | 49.5 (44.7 to 54.3) |
External validation of the risk prediction models
For the primary analysis, following multiple imputation, all 2975 patients were included in the validation of the risk models among all patients in the RAIN study data set and between 1868 (63%) and 2711 (91%) were included in the validation among patients eligible for each model (Table 19). Owing to multiple imputation of missing data, the sample sizes were the same for the validation data sets for mortality and for unfavourable outcome for each model, where relevant.
Risk model | All patients, validation data set (1) | Eligible for model, validation data set (2) |
---|---|---|
Number of admissions | ||
Hukkelhoven | 2975 | 1868 |
CRASH Basic | 2975 | 2711 |
CRASH CT | 2975 | 2584 |
IMPACT Core | 2975 | 2528 |
IMPACT Extended | 2975 | 2393 |
IMPACT Lab | 2975 | 2393 |
Mortality at 6 months (%) | ||
Hukkelhoven | 25.7 | 22.2 |
IMPACT Core | 25.7 | 27.6 |
IMPACT Extended | 25.7 | 27.6 |
IMPACT Lab | 25.7 | 27.6 |
Unfavourable outcome at 6 months (%) | ||
Hukkelhoven | 57.4 | 55.9 |
CRASH Basic | 57.4 | 57.0 |
CRASH CT | 57.4 | 56.9 |
IMPACT Core | 57.4 | 60.0 |
IMPACT Extended | 57.4 | 59.9 |
IMPACT Lab | 57.4 | 59.9 |
The discrimination (c-index) of the four models predicting mortality at 6 months is reported in Table 20. Discrimination was similar for the Hukkelhoven, IMPACT Extended and IMPACT Lab models (c-index 0.78) and higher than that for the IMPACT Core model (0.75). Inspection of the calibration plots (Figure 26) showed good calibration for the Hukkelhoven and IMPACT Lab models, whereas the IMPACT Core and Extended models tended to overestimate the risk of mortality at 6 months (i.e. observed mortality was lower than predicted). This visual interpretation is supported by the measures and tests of calibration (Table 21). Brier's score (Table 22), summarising the overall fit of the predictions, was best for the Hukkelhoven model and worst for the IMPACT Core model. There was little difference in any measures of model performance between assessment among all patients and among those eligible for the model.
Risk model | All patients | Eligible for model |
---|---|---|
c-index (95% CI) | ||
Hukkelhoven | 0.784 (0.764 to 0.805) | 0.773 (0.745 to 0.801) |
IMPACT Core | 0.754 (0.733 to 0.774) | 0.750 (0.728 to 0.772) |
IMPACT Extended | 0.780 (0.760 to 0.799) | 0.780 (0.760 to 0.801) |
IMPACT Lab | 0.777 (0.756 to 0.798) | 0.779 (0.758 to 0.800) |
FIGURE 26.
Calibration plots: mortality at 6 months. Each point represents the observed mortality (with 95% CI) plotted against the predicted mortality from the model for 10 equal-sized groups based on the predicted mortality. Bars at the foot of each figure (‘rug plots’) illustrate the distribution of predictions for survivors and non-survivors.
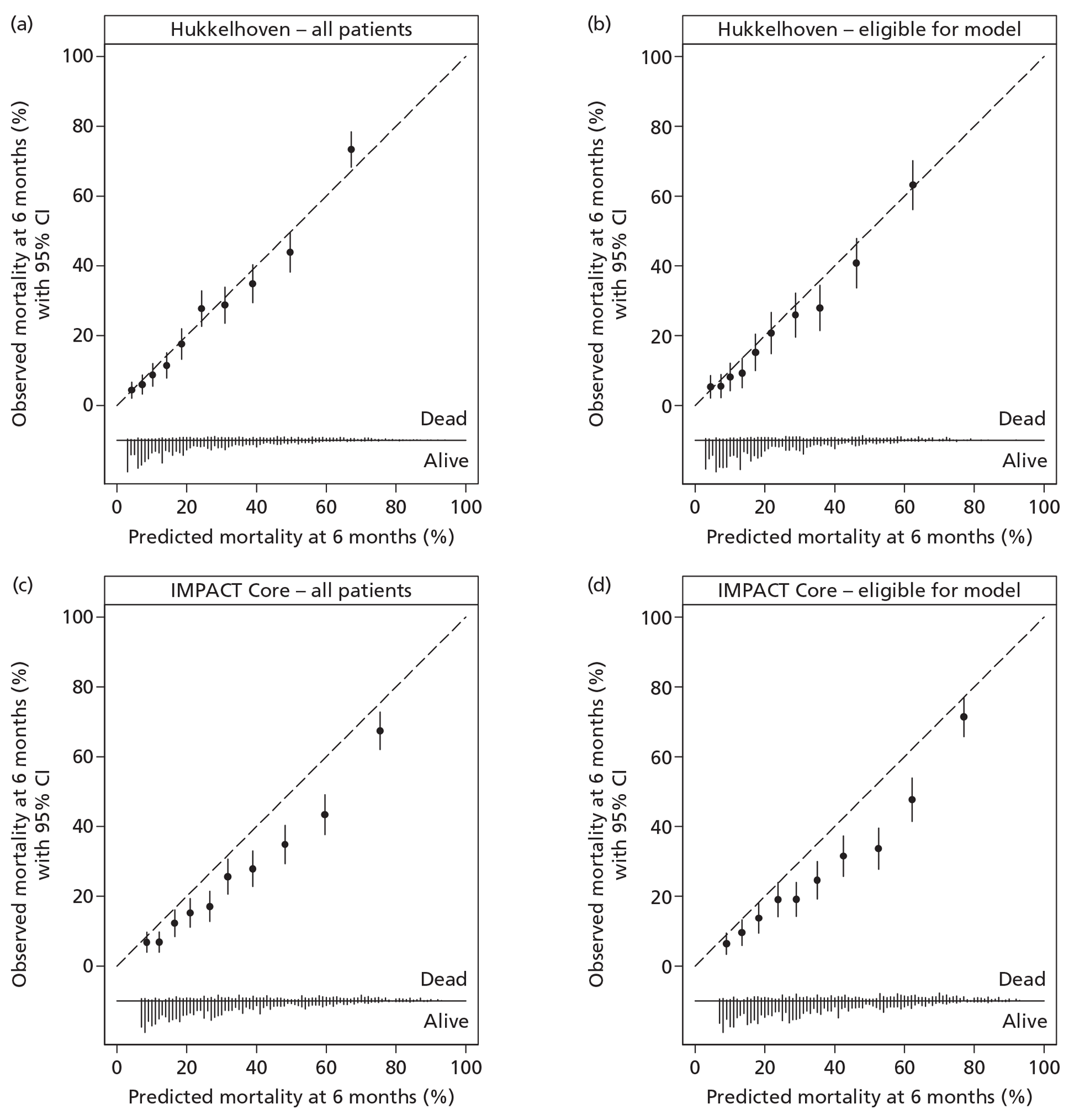
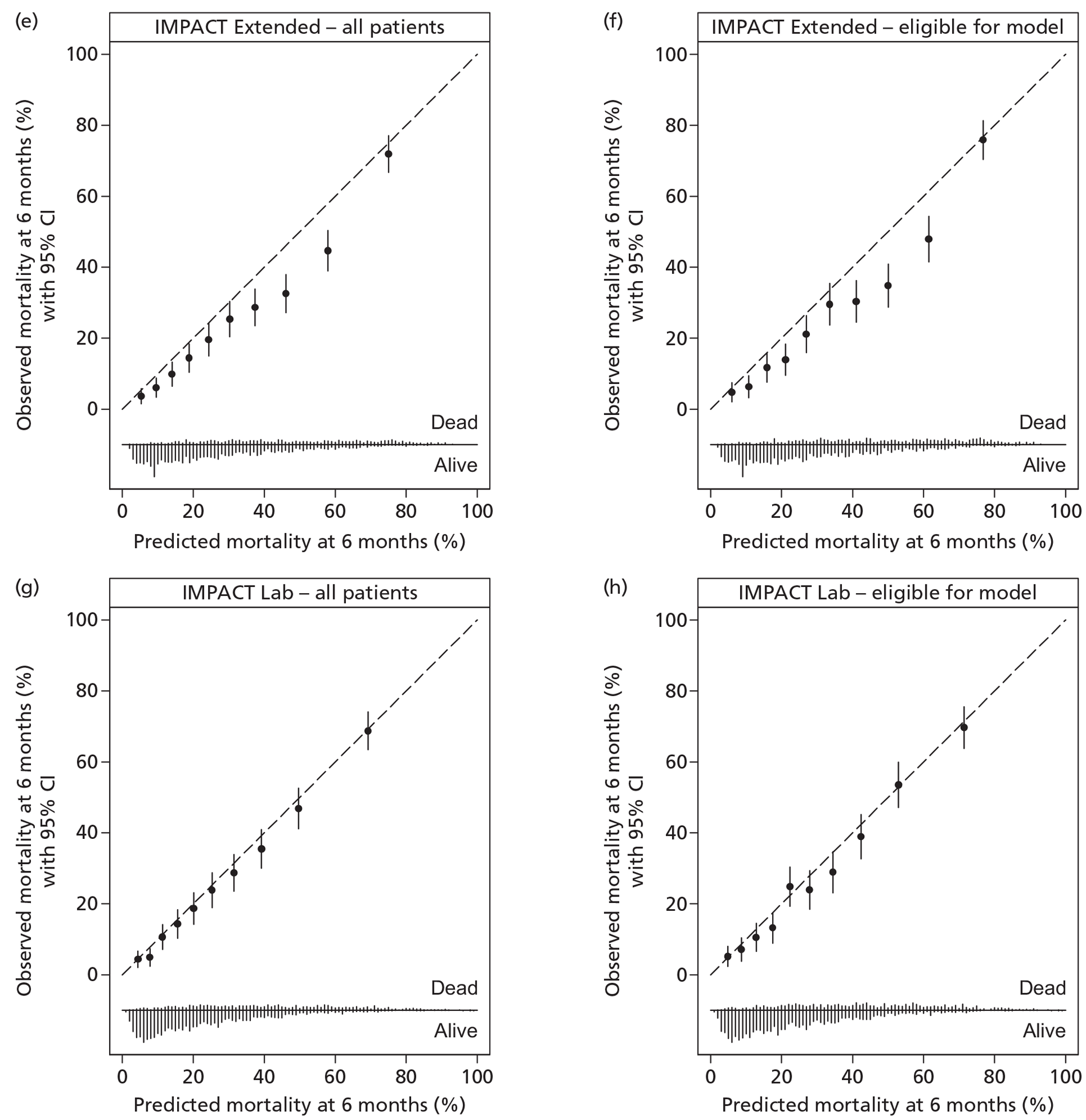
Risk model | All patients | Eligible for model |
---|---|---|
Hosmer–Lemeshow test, chi-squared statistic (p-value) | ||
Hukkelhoven | 18.8 [0.042] | 15.3 [0.12] |
IMPACT Core | 117 [< 0.001] | 112 [< 0.001] |
IMPACT Extended | 76.7[(< 0.001] | 74.8 [< 0.001] |
IMPACT Lab | 10.6 [0.39] | 14.0 [0.17] |
Cox calibration regression, α (95% CI) β (95% CI) [p-value] | ||
Hukkelhoven | −0.01 (−0.13 to 0.11) 1.06 (0.96 to 1.16) [0.22] | −0.13 (−0.29 to 0.04) 1.06 (0.92 to 1.20) [0.011] |
IMPACT Core | −0.50 (−0.60 to −0.40) 0.95 (0.86 to 1.05) [< 0.001] | −0.51 (−0.61 to −0.40) 0.94 (0.84 to 1.05) [< 0.001] |
IMPACT Extended | −0.37 (−0.48 to −0.27) 1.02 (0.92 to 1.11) [< 0.001] | −0.39 (−0.50 to −0.28) 1.03 (0.93 to 1.13) [< 0.001] |
IMPACT Lab | −0.11 (−0.23 to 0.01) 1.00 (0.90 to 1.11) [0.051] | −0.11 (−0.23 to 0.01) 1.01 (0.90 to 1.12) [0.059] |
Risk model | All patients | Eligible for model |
---|---|---|
Brier's score | ||
Hukkelhoven | 0.150 | 0.141 |
IMPACT Core | 0.166 | 0.175 |
IMPACT Extended | 0.156 | 0.163 |
IMPACT Lab | 0.153 | 0.159 |
Each point represents the observed mortality (with 95% CI) plotted against the predicted mortality from the model for 10 equal-sized groups based on the predicted mortality. Bars at the foot of each figure (‘rug plots’) illustrate the distribution of predictions for survivors and non-survivors.
The discrimination of the models for predicting unfavourable outcome at 6 months was worse than for mortality at 6 months, with c-index values ranging from 0.69 to 0.71 (Table 23). The IMPACT Lab model had slightly better discrimination (0.714), followed by the models making use of CT findings (Hukkelhoven, CRASH CT and IMPACT Extended; c-index 0.708) with the models using only core variables (CRASH Basic and IMPACT Core) having the worst discrimination (c-index 0.69–0.70). All models were poorly calibrated, substantially underestimating the risk of unfavourable outcome at 6 months, particularly at low predicted risk (Figure 27 and Table 24). The lack of calibration resulted in very poor values for Brier's score – little better than (and, in the case of the CRASH Basic model, worse than) the value of 0.25 that would be observed from predicting a constant risk of 0.5 for all patients (Table 25). The sensitivity analysis excluding patients with severe disability – either pre-existing or not due to the TBI – resulted in very small improvements in discrimination and no discernible change to calibration or overall fit (Table 26). Results were very similar when the analyses were repeated for the secondary analyses in the data sets prior to multiple imputation (see Appendix 6).
Risk model | All patients | Eligible for model |
---|---|---|
c-index (95% CI) | ||
Hukkelhoven | 0.708 (0.686 to 0.730) | 0.688 (0.660 to 0.716) |
CRASH Basic | 0.699 (0.677 to 0.721) | 0.697 (0.675 to 0.720) |
CRASH CT | 0.708 (0.686 to 0.729) | 0.710 (0.687 to 0.733) |
IMPACT Core | 0.693 (0.671 to 0.716) | 0.689 (0.665 to 0.713) |
IMPACT Extended | 0.708 (0.686 to 0.730) | 0.706 (0.683 to 0.730) |
IMPACT Lab | 0.714 (0.692 to 0.736) | 0.713 (0.689 to 0.737) |
FIGURE 27.
Calibration plots: unfavourable outcome at 6 months. Each point represents the observed proportion with unfavourable outcome (with 95% CI) plotted against the predicted risk of unfavourable outcome from the model for 10 equal-sized groups based on the predicted risk. Bars at the foot of each figure (‘rug plots’) illustrate the distribution of predictions for patients with favourable and unfavourable outcomes.
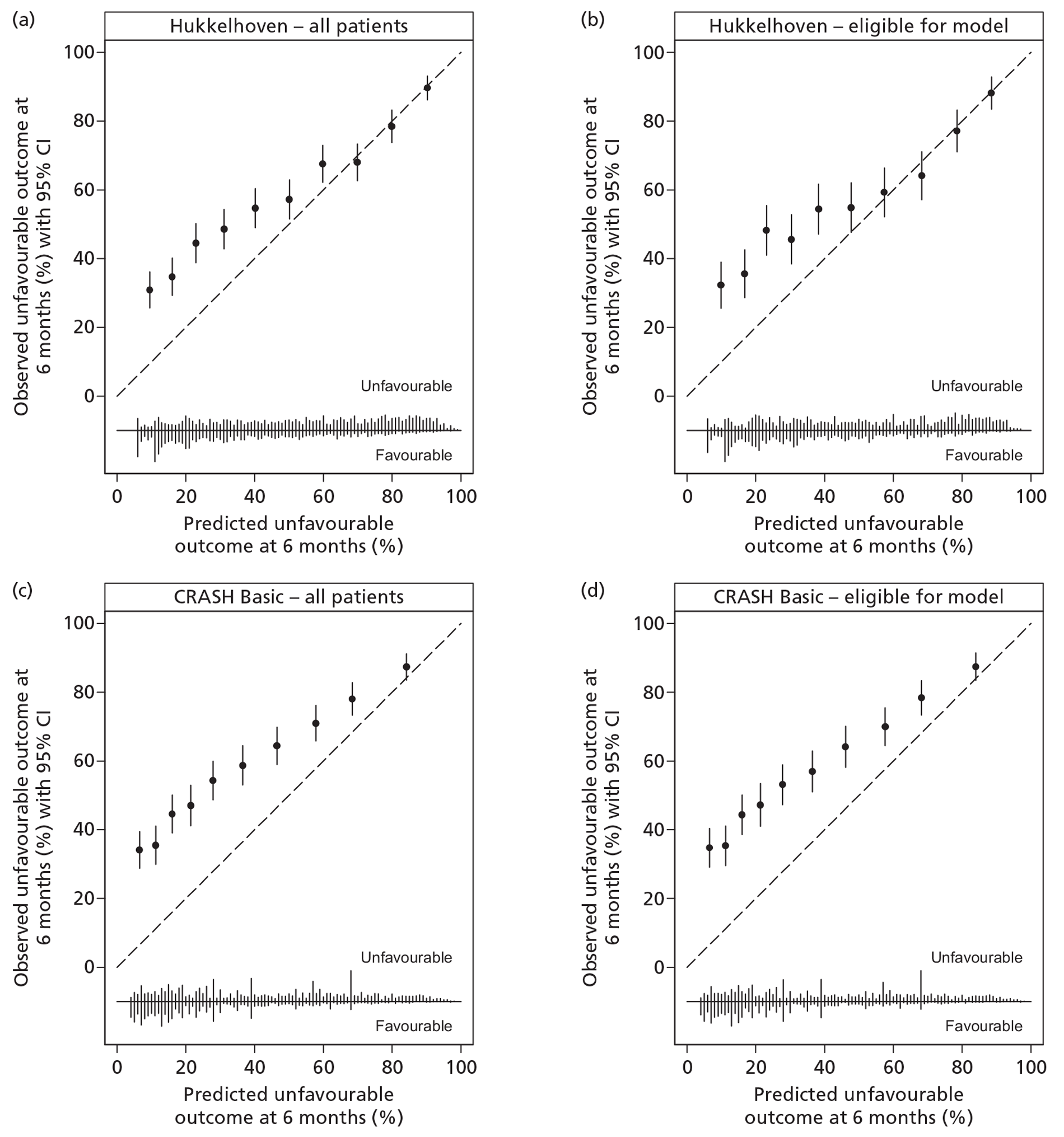
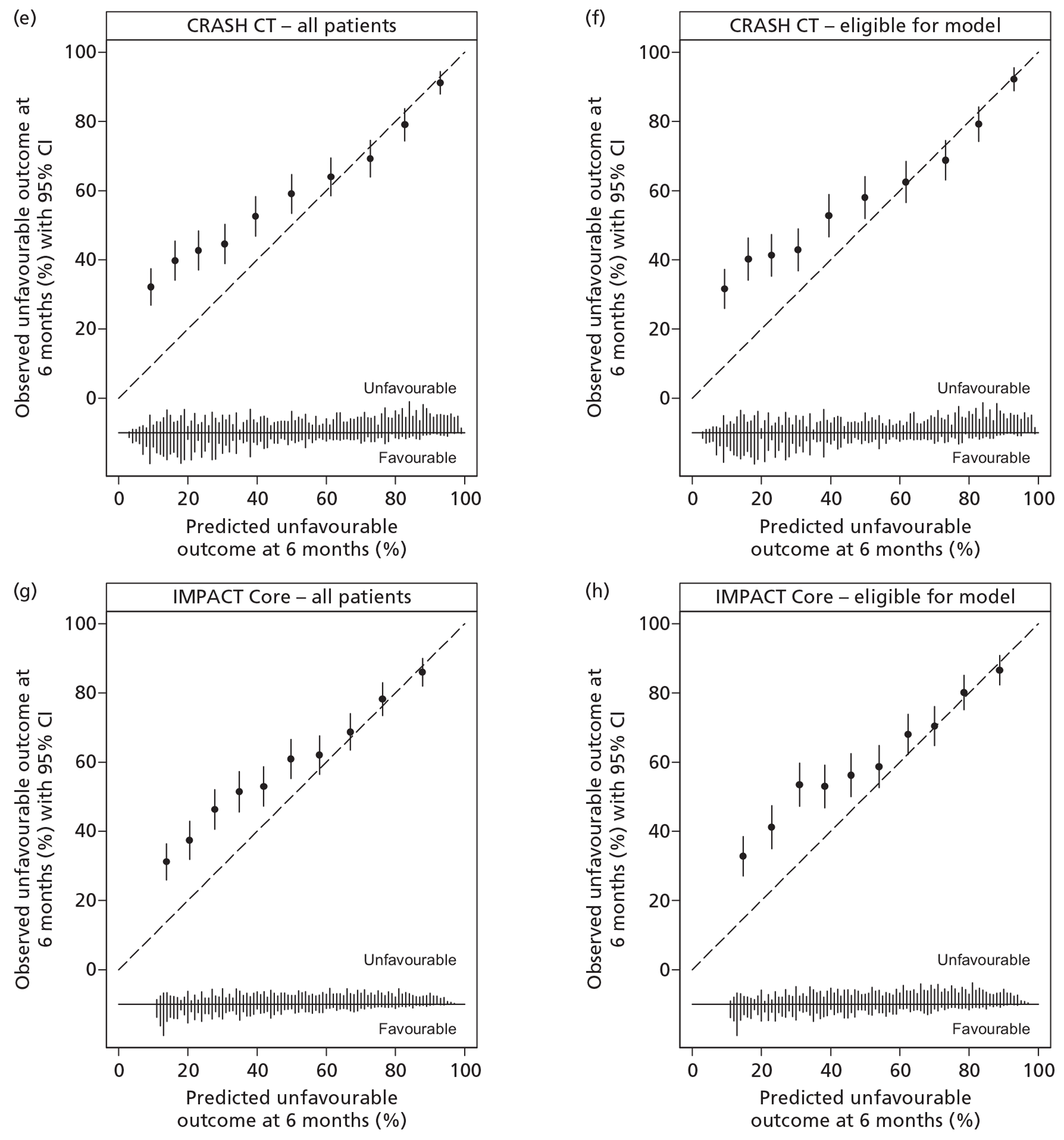
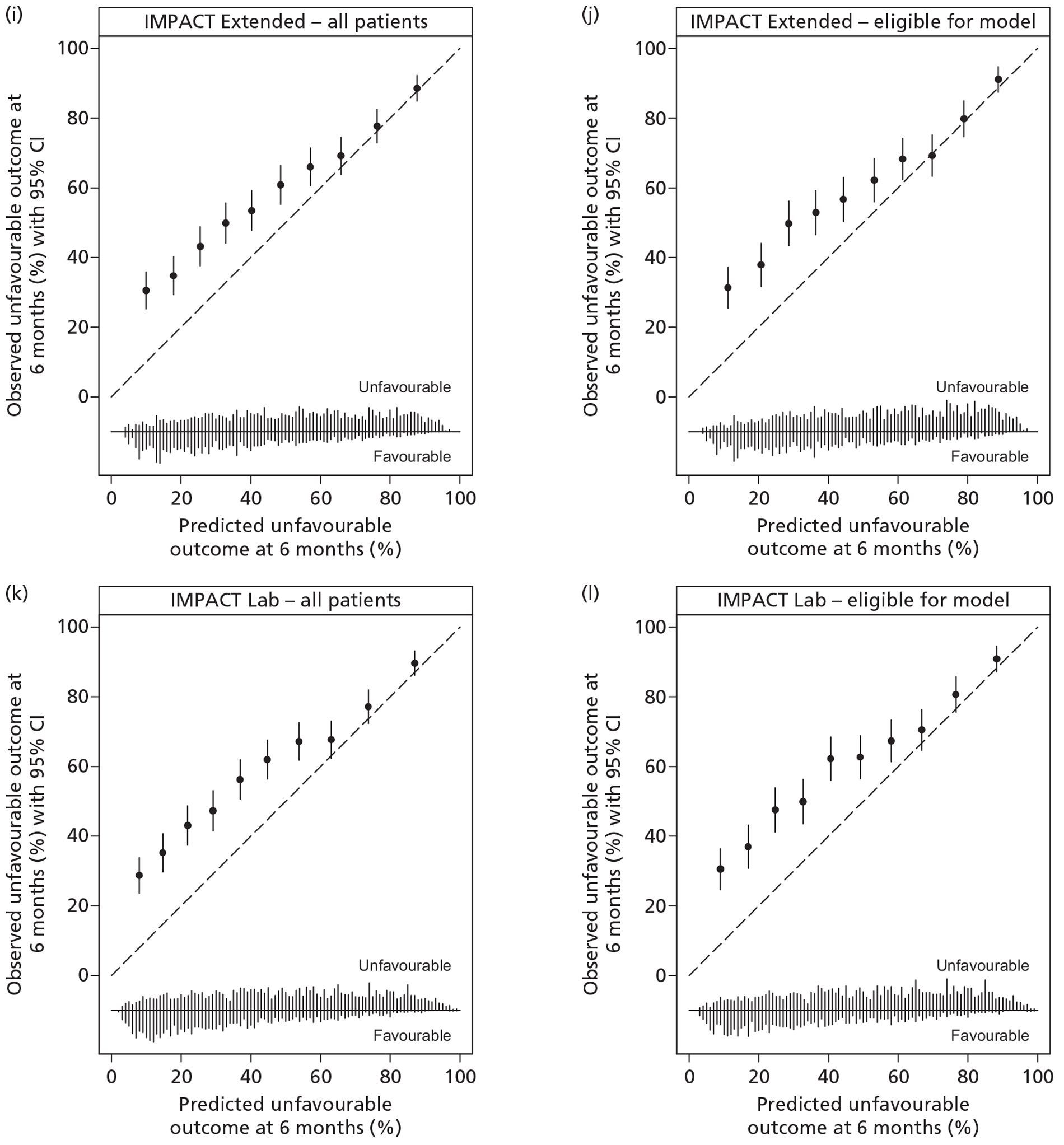
Risk model | All patients | Eligible for model |
---|---|---|
Hosmer–Lemeshow test, chi-squared statistic [p-value] | ||
Hukkelhoven | 401 [< 0.001] | 272 [< 0.001] |
CRASH Basic | 1102 [< 0.001] | 997 [< 0.001] |
CRASH CT | 437 [< 0.001] | 362 [< 0.001] |
IMPACT Core | 253 [< 0.001] | 219 [< 0.001] |
IMPACT Extended | 341 [< 0.001] | 252 [< 0.001] |
IMPACT Lab | 515 [< 0.001] | 384 [< 0.001] |
Cox calibration regression, α (95% CI) β (95% CI) [p-value] | ||
Hukkelhoven | 0.44 (0.35 to 0.53) 0.60 (0.52 to 0.68) [< 0.001] | 0.39 (0.28 to 0.50) 0.57 (0.47 to 0.66) [< 0.001] |
CRASH Basic | 0.74 (0.64 to 0.85) 0.59 (0.52 to 0.67) [< 0.001] | 0.73 (0.62 to 0.84) 0.60 (0.52 to 0.67) [< 0.001] |
CRASH CT | 0.40 (0.31 to 0.49) 0.56 (0.49 to 0.63) [< 0.001] | 0.38 (0.28 to 0.47) 0.57 (0.50 to 0.64) [< 0.001] |
IMPACT Core | 0.41 (0.32 to 0.50) 0.66 (0.58 to 0.75) [< 0.001] | 0.43 (0.34 to 0.52) 0.65 (0.55 to 0.74) [< 0.001] |
IMPACT Extended | 0.47 (0.38 to 0.56) 0.66 (0.58 to 0.75) [< 0.001] | 0.48 (0.38 to 0.58) 0.66 (0.57 to 0.75) [< 0.001] |
IMPACT Lab | 0.58 (0.48 to 0.67) 0.65 (0.57 to 0.73) [< 0.001] | 0.59 (0.49 to 0.74) 0.65 (0.56 to 0.74) [< 0.001] |
Risk model | All patients | Eligible for model |
---|---|---|
Brier's score | ||
Hukkelhoven | 0.232 | 0.239 |
CRASH Basic | 0.262 | 0.262 |
CRASH CT | 0.232 | 0.230 |
IMPACT Core | 0.232 | 0.230 |
IMPACT Extended | 0.230 | 0.227 |
IMPACT Lab | 0.236 | 0.233 |
Model | c-index (95% CI) | Hosmer–Lemeshow test, χ2 (p-value) | Cox calibration regression, α, β (p-value) | Brier's score |
---|---|---|---|---|
Hukkelhoven | 0.711 (0.689 to 0.733) | 353 (< 0.001) | 0.40 to 0.61 (< 0.001) | 0.230 |
CRASH Basic | 0.701 (0.679 to 0.722) | 1015 (< 0.001) | 0.71 to 0.60 (< 0.001) | 0.259 |
CRASH CT | 0.710 (0.689 to 0.732) | 394 (< 0.001) | 0.36 to 0.57 (< 0.001) | 0.230 |
IMPACT Core | 0.695 (0.672 to 0.717) | 224 (< 0.001) | 0.37 to 0.67 (< 0.001) | 0.231 |
IMPACT Extended | 0.711 (0.689 to 0.733) | 302 (< 0.001) | 0.44 to 0.67 (< 0.001) | 0.228 |
IMPACT Lab | 0.718 (0.696 to 0.740) | 454 (< 0.001) | 0.54 to 0.66 (< 0.001) | 0.233 |
Each point represents the observed proportion with unfavourable outcome (with 95% CI) plotted against the predicted risk of unfavourable outcome from the model for 10 equal-sized groups based on the predicted risk. Bars at the foot of each figure (‘rug plots’) illustrate the distribution of predictions for patients with favourable and unfavourable outcomes.
Inter-rater reliability of computerised tomography scan reporting
Computerised tomography scans were available for 312 (91%) of 342 patients randomly selected for inclusion in the substudy evaluating inter-rater reliability of CT scan reporting, including at least one scan from 64 of the 66 critical care units that recruited at least one patient to the RAIN study. The main reason cited for failure to provide a CT scan was the need to obtain the scan from a referring hospital. κ-Statistics ranged from 0.19 to 0.78 across the individual CT fields (Table 27). The majority of fields used in the risk model were found to have moderate to good inter-rater reliability (κ-statistic 0.4–0.8). The worst inter-rater reliability was found for the presence of small petechial haemorrhages, which may partly explain why no association was found between this factor and outcomes. Agreement between the WBIC assessors (WBIC1 vs WBIC2 and WBIC3 vs WBIC4; median κ-statistic 0.70, IQR 0.58 to 0.76) was generally better than between the original RAIN study data and the WBIC assessors (RAIN vs WBIC1, RAIN vs WBIC2, etc.; 0.52, 0.37–0.62). κ-Statistics for subgroup analyses are reported in Tables 28 and 29. There was little discernible variation in inter-rater reliability of CT scan assessments according to the specialty and grade of the assessor for the original RAIN study data (see Table 28). CT scans that originated from RAIN study critical care units within neuroscience centres had similar inter-rater reliability compared with CT scans that originated from non-neuroscience centres (see Table 29).
CT variable | Three-way comparison (n = 312) | WBIC team 1 (n = 156) | WBIC team 2 (n = 155) | ||||
---|---|---|---|---|---|---|---|
RAIN vs WBIC1 | RAIN vs WBIC2 | WBIC1 vs WBIC2 | RAIN vs WBIC3 | RAIN vs WBIC4 | WBIC3 vs WBIC4 | ||
CT scan result (normal/abnormal) | 0.59 (0.46 to 0.70) | 0.60 (0.40 to 0.75) | 0.62 (0.42 to 0.76) | 0.61 (0.41 to 0.77) | 0.46 (0.24 to 0.66) | 0.54 (0.31 to 0.71) | 0.73 (0.48 to 0.87) |
Traumatic SAH | 0.44 (0.36 to 0.52) | 0.34 (0.15 to 0.46) | 0.49 (0.33 to 0.61) | 0.54 (0.39 to 0.67) | 0.39 (0.21 to 0.50) | 0.37 (0.18 to 0.48) | 0.61 (0.46 to 0.73) |
Brainstem pathology | 0.20 (0.10 to 0.34) | 0.08 (−0.06 to 0.28) | 0.03 (−0.08 to 0.23) | −0.01 (−0.02 to 0.50) | 0.27 (0.06 to 0.42) | 0.24 (0.04 to 0.41) | 0.25 (0.08 to 0.42) |
Basal cisterns (absent/compressed/present) | 0.56 (0.52 to 0.64) | 0.50 (0.42 to 0.64) | 0.52 (0.39 to 0.67) | 0.68 (0.59 to 0.80) | 0.39 (0.30 to 0.51) | 0.52 (0.39 to 0.62) | 0.73 (0.62 to 0.83) |
Obliteration of third ventricle | 0.60 (0.49 to 0.69) | 0.61 (0.44 to 0.74) | 0.51 (0.32 to 0.66) | 0.75 (0.59 to 0.86) | 0.37 (0.17 to 0.56) | 0.54 (0.34 to 0.70) | 0.79 (0.61 to 0.90) |
Midline shift of > 5 mm | 0.78 (0.71 to 0.84) | 0.62 (0.47 to 0.74) | 0.69 (0.55 to 0.80) | 0.84 (0.71 to 0.92) | 0.87 (0.75 to 0.94) | 0.81 (0.67 to 0.90) | 0.91 (0.79 to 0.96) |
One or more small petechial haemorrhage(s) of ≤ 1 ml | 0.18 (0.11 to 0.26) | 0.14 (−0.06 to 0.26) | 0.37 (0.20 to 0.49) | 0.26 (−0.01 to 0.30) | 0.11 (−0.05 to 0.26) | 0.09 (−0.07 to 0.25) | 0.23 (0.07 to 0.38) |
High-/mixed-density lesion(s) of > 1 ml | 0.68 (0.60 to 0.74) | 0.68 (0.54 to 0.79) | 0.63 (0.48 to 0.74) | 0.74 (0.61 to 0.83) | 0.62 (0.47 to 0.74) | 0.63 (0.48 to 0.75) | 0.78 (0.64 to 0.87) |
Extradural haematoma | 0.60 (0.49 to 0.70) | 0.58 (0.37 to 0.74) | 0.64 (0.43 to 0.79) | 0.81 (0.62 to 0.91) | 0.43 (0.24 to 0.59) | 0.59 (0.41 to 0.73) | 0.64 (0.45 to 0.78) |
Subdural haematoma | 0.67 (0.60 to 0.73 | 0.64 (0.50 to 0.74) | 0.67 (0.53 to 0.77) | 0.84 (0.73 to 0.91) | 0.58 (0.43 to 0.69) | 0.59 (0.45 to 0.70) | 0.71 (0.58 to 0.81) |
Intracerebral haematoma/haemorrhage/contusion | 0.51 (0.44 to 0.58) | 0.52 (0.38 to 0.64) | 0.39 (0.20 to 0.50) | 0.58 (0.41 to 0.68) | 0.48 (0.33 to 0.60) | 0.47 (0.32 to 0.60) | 0.68 (0.54 to 0.78) |
Posterior fossa haematoma | 0.36 (0.17 to 0.56) | 0.53 (0.25 to 0.75) | 0.18 (0.005, 0.53) | 0.15 (−0.01 to 0.47) | 0.31 (0.06 to 0.62) | 0.21 (0.02 to 0.50) | 0.65 (0.36 to 0.83) |
Volume of largest high-/mixed-density lesion (> 25 ml/≤ 25 ml) | 0.65 (0.58 to 0.72) | 0.56 (0.40 to 0.67) | 0.60 (0.45 to 0.72) | 0.76 (0.64 to 0.85) | 0.65 (0.50 to 0.76) | 0.61 (0.46 to 0.73) | 0.71 (0.57 to 0.81) |
CT variable | Specialty and grade of assessor | |||||
---|---|---|---|---|---|---|
Critical care/anaesthesia consultant (n = 32) | Neurocritical care/neuroanaesthesia consultant (n = 71) | Neurosurgery/neuroradiology consultant (n = 28) | Neurosurgery/neuroradiology non-consultant (n = 25) | Radiology consultant (n = 87) | Radiology non-consultant (n = 49) | |
CT scan result (normal/abnormal) | 0.22 (0.00 to 0.74) | 0.62 (0.28 to 0.83) | N/A | N/A | 0.63 (0.45 to 0.77) | 0.40 (0.13 to 0.67) |
Traumatic SAH | 0.46 (0.19 to 0.69) | 0.48 (0.32 to 0.63) | 0.17 (−0.03 to 0.47) | 0.47 (0.18 to 0.72) | 0.50 (0.36 to 0.63) | 0.29 (0.10 to 0.49) |
Brainstem pathology | 0.37 (0.11 to 0.64) | 0.22 (0.05 to 0.45) | 0.14 (−0.01 to 0.67) | 0.46 (0.07 to 0.82) | 0.16 (0.01 to 0.48) | −0.05 (−0.03 to 0.39) |
Basal cisterns (absent/compressed/present) | 0.54 (0.39 to 0.81) | 0.55 (0.45 to 0.71) | 0.39 (0.19 to 0.59) | 0.49 (0.28 to 0.66) | 0.64 (0.51 to 0.78) | 0.50 (0.36 to 0.61) |
Obliteration of third ventricle | 0.59 (0.27 to 0.81) | 0.48 (0.26 to 0.68) | 0.46 (0.18 to 0.70) | 0.66 (0.35 to 0.85) | 0.68 (0.39 to 0.85) | 0.72 (0.42 to 0.88) |
Midline shift of > 5 mm | 0.84 (0.60 to 0.95) | 0.75 (0.59 to 0.86) | 0.57 (0.30 to 0.77) | 0.84 (0.60 to 0.94) | 0.90 (0.71 to 0.97) | 0.74 (0.48 to 0.88) |
One or more small petechial haemorrhage(s) of ≤ 1 ml | 0.17 (−0.02 to 0.41) | 0.16 (0.02 to 0.33) | 0.08 (−0.10 to 0.33) | 0.10 (−0.08 to 0.39) | 0.18 (0.05 to 0.33) | 0.28 (0.09 to 0.49) |
High-/mixed-density lesion(s) of > 1 ml | 0.33 (0.07 to 0.62) | 0.87 (0.70 to 0.94) | 0.63 (0.26 to 0.86) | N/A | 0.71 (0.58 to 0.81) | 0.59 (0.40 to 0.75) |
Extradural haematoma | 0.32 (0.06 to 0.63) | 0.70 (0.50 to 0.84) | 0.80 (0.50 to 0.93) | 0.52 (0.19 to 0.77) | 0.57 (0.23 to 0.81) | 0.56 (0.24 to 0.79) |
Subdural haematoma | 0.50 (0.26 to 0.69) | 0.73 (0.59 to 0.84) | 0.70 (0.45 to 0.86) | 0.60 (0.33 to 0.80) | 0.63 (0.49 to 0.75) | 0.78 (0.61 to 0.88) |
Intracerebral haematoma/haemorrhage/contusion | 0.24 (0.03 to 0.47) | 0.66 (0.51 to 0.77) | 0.22 (0.01 to 0.47) | 0.44 (0.17 to 0.67) | 0.57 (0.42 to 0.70) | 0.48 (0.28 to 0.65) |
Posterior fossa haematoma | 0.58 (0.11 to 0.89) | 0.38 (0.10 to 0.69) | N/A | 0.49 (0.12 to 0.80) | 0.31 (0.04 to 0.74) | N/A |
Volume of largest high-/mixed-density lesion (> 25 ml/≤ 25 ml) | 0.76 (0.53 to 0.90) | 0.76 (0.62 to 0.86) | 0.42 (0.17 to 0.64) | 0.39 (0.14 to 0.63) | 0.57 (0.38 to 0.72) | 0.63 (0.41 to 0.79) |
CT variable | Neuroscience centre | |
---|---|---|
Yes (n = 194) | No (n = 117) | |
CT scan result (normal/abnormal) | 0.57 (0.35 to 0.74) | 0.58 (0.41 to 0.72) |
Traumatic SAH | 0.40 (0.30 to 0.50) | 0.51 (0.38 to 0.62) |
Brainstem pathology | 0.26 (0.12 to 0.43) | 0.10 (−0.00 to 0.32) |
Basal cisterns (absent/compressed/present) | 0.53 (0.42 to 0.61) | 0.61 (0.50 to 0.71) |
Obliteration of third ventricle | 0.52 (0.38 to 0.64) | 0.75 (0.57 to 0.86) |
Midline shift of > 5 mm | 0.76 (0.67 to 0.83) | 0.82 (0.67 to 0.91) |
One or more small petechial haemorrhage(s) of ≤ 1 ml | 0.14 (0.05 to 0.24) | 0.25 (0.13 to 0.37) |
High/mixed density lesion(s) of > 1 ml | 0.68 (0.56 to 0.77) | 0.64 (0.52 to 0.74) |
Extradural haematoma | 0.61 (0.47 to 0.72) | 0.56 (0.31 to 0.75) |
Subdural haematoma | 0.67 (0.58 to 0.74) | 0.66 (0.54 to 0.76) |
Intracerebral haematoma/haemorrhage/contusion | 0.48 (0.39 to 0.57) | 0.54 (0.41 to 0.66) |
Posterior fossa haematoma | 0.38 (0.16 to 0.61) | 0.30 (0.06 to 0.65) |
Volume of largest high-/mixed-density lesion (> 25 ml/≤ 25 ml) | 0.65 (0.56 to 0.73) | 0.60 (0.45 to 0.72) |
Discussion
Principal findings
The RAIN study has demonstrated that the Hukkelhoven, CRASH and IMPACT risk prediction models for mortality and unfavourable outcome at 6 months have acceptable levels of discrimination when applied in a UK cohort of adult patients admitted to critical care units following acute TBI. Although the Hukkelhoven and IMPACT Lab models for mortality at 6 months had good calibration, the other IMPACT models for mortality at 6 months overpredicted risk, and all models for unfavourable outcome at 6 months underpredicted risk particularly at the lower end of the risk spectrum.
Strengths and weaknesses
The main strengths of the RAIN study are the large, representative sample with high levels of data completeness and the rigorous statistical methods. The RAIN study included 84% of all neuroscience centres in the UK plus representation from the important, but often overlooked, cohort of critically ill patients with acute TBI managed outside neuroscience centres. Although the completeness of follow-up for unfavourable outcome at 6 months (81%) was lower than ideal, this reflects the difficulties inherent in following up such a population (see Chapter 4). Furthermore, consent was obtained at the point of follow-up rather than prior to enrolment (as would be the case in an RCT), so patients who declined follow-up were still included within the initial study population. The low follow-up rate was addressed by implementing a two-stage multiple imputation process – first imputing risk factors and mortality, and subsequently, for those alive or imputed to be alive at 6 months, imputing the GOSE categories.
Strengths and weaknesses in comparison with other studies
The RAIN study is the first prospective external validation of these risk prediction models, with all previous validations having been undertaken using existing data sources from either RCTs or trauma registries. Consequently, although many previous validation studies have been restricted to validating modified and refitted versions of the models due to differences between the available data for validation and the data used for model development, the RAIN study was able to define variables in advance to accurately implement all risk models as originally reported.
The discrimination of the risk prediction models in the RAIN study was generally within the range observed in previous development and validation populations. The Hukkelhoven models had c-indices of 0.78 and 0.71 for mortality and unfavourable outcome at 6 months, respectively, in the RAIN study compared with 0.78 and 0.80 in the original development sample. 48 The Hukkelhoven models have been validated in four external existing data sources. The model development paper reported validation of the model for mortality at 6 months in observational data sets from the EBIC and the TCDB with a c-index of 0.87 and 0.89, respectively. 48 The model for unfavourable outcome at 6 months was validated in the EBIC data set only (as the timing of GOS reporting in the TCDB data set was variable) with a c-index of 0.83. Subsequently, Hukkelhoven et al. 90 presented validation data from one further data source, the Selfotel RCT, with a c-index of 0.74 for both mortality and unfavourable outcome at 6 month. A recently reported study from a 10-year cohort of patients admitted to a single UK neuroscience centre reported a c-index around 0.83 for both mortality and unfavourable outcome at 1 year. 91
The CRASH Basic model and CT model for unfavourable outcome at 6 months had c-indices of 0.70 and 0.71, respectively, in the RAIN study compared with 0.81 and 0.83 in the original development sample. 30 The model development paper reported validation of modified versions of both models (excluding major extracranial injury and small petechial haemorrhages) in the IMPACT database with a c-index of 0.77 for both models. The CRASH models have subsequently been validated in three further existing data sources from previous RCTs in a joint study by the IMPACT, CRASH and TARN groups. 92 These were, again, modified versions of the original models, refitted using only patients in the CRASH trial with GCS score of ≤ 8 and replacing total GCS with motor score and the CRASH CT variables with Marshall classification, resulting in a model structure substantially more similar to the Hukkelhoven and IMPACT models. The c-index for the modified CRASH Basic model in these three data sets was 0.68, 0.74 and 0.76, respectively; the c-index for the modified CRASH CT model was 0.71 in the only data set for which this could be calculated.
The IMPACT Core, Extended and Laboratory models for mortality at 6 months had c-indices of 0.75, 0.78 and 0.78, respectively, in the RAIN study compared with values of 0.66–0.84, 0.71–0.87 and 0.72–0.80 in cross-validation among the original data sets used for model development. 35 The corresponding figures for the models for unfavourable outcome at 6 months were 0.69, 0.71 and 0.71, respectively, in the RAIN study compared with 0.74–0.82, 0.73–0.84 and 0.75–0.82 in cross-validation among the original development data sets. The model development paper reported validation of the IMPACT Core model and a modified version of the Extended model (the ‘Core + CT’ model, excluding hypoxia, hypotension and extradural haematoma) in the CRASH trial data set with c-indices of 0.78 and 0.80, respectively, for mortality at 6 months, and the same results for unfavourable outcome at 6 months. The IMPACT models have subsequently been validated in six further data sets – a study from a single trauma centre in the USA,93 and the joint study from the IMPACT, CRASH and TARN groups using data from three RCTs (as for the validation of the CRASH models) and two observational studies. 92 The single-centre US study reported c-indices of 0.78, 0.83 and 0.83 for mortality at 6 months, and 0.76, 0.79 and 0.76 for unfavourable outcome at 6 months for the Core, Extended and Laboratory models, respectively. 93 The IMPACT/CRASH/TARN study reported a c-index for the IMPACT Core model ranging from 0.65–0.83 for mortality at 6 months across the five data sets, and 0.66–0.76 for unfavourable outcome at 6 months across four data sets in which this outcome was available. 92 The best discrimination for mortality was from the UK TARN data set; however, the outcome assessed in this data set was mortality at discharge from hospital not mortality at 6 months. The IMPACT Extended model had a c-index of 0.86 for hospital mortality in the TARN data set, and 0.71 for both mortality and unfavourable outcome at 6 months in the one RCT data set in which it could be applied. The IMPACT Lab model had c-index of 0.71 and 0.70 for mortality and unfavourable outcome at 6 months, respectively, in the same data set.
In the RAIN study, the presence of major extracranial injury was found not to be associated with an increased risk of either mortality or unfavourable outcome at 6 months and this is at variance with some previous studies. A recent meta-analysis on the effect of major extracranial injury on mortality in TBI found an inverse relationship between major extracranial injury and mortality according to the severity of the TBI with odds ratios of 2.14, 1.46 and 1.18 in mild (GCS score of 13–14), moderate (GCS score of 9–12) and severe (GCS score of 3–8) TBI, respectively. 94 Although there are patients with a range of severity of TBI within the RAIN study, approximately two-thirds had GCS scores of 3–8 and all patients were admitted to a critical care unit, which may also represent an additional marker of severity. One may therefore anticipate that the effect of major extracranial injury within the RAIN study would be small. It is also possible, however, that the lack of effect of major extracranial injury in the RAIN study may be due to a lack of consistent application of the definition, taken from the CRASH trial, which was somewhat subjective. Collection of more detailed injury severity scoring, although time consuming, would permit more detailed investigation of the effect of major extracranial injury both more consistently across sites and according to severity.
Advantages and disadvantages of the alternative risk prediction models
In terms of the statistical assessment of model performance, there was very little to choose between models of similar complexity from Hukkelhoven, CRASH and IMPACT. The best discrimination overall was from the IMPACT Lab model – the only one of the models to include laboratory parameters – however, the improvement in performance over the models of the next level of complexity (Hukkelhoven, CRASH CT, IMPACT Extended) was very small. There was a larger difference in performance between these models and the simplest models using core data only (CRASH Basic and IMPACT Core), suggesting that there is important prognostic information within the CT scan and the presence/absence of pre-hospital hypoxia/hypotension. The substudy on inter-rater reliability of CT scan reporting suggested that the CT findings included in the models could be assessed with acceptable reliability. The CRASH models included two variables – major extracranial injury and small petechial haemorrhages – that did not demonstrate any association with outcomes in the RAIN study. This may be seen as a reason to prefer the Hukkelhoven and IMPACT Extended models over the CRASH CT model; however, despite the inclusion of these apparently non-prognostic fields, the overall performance of the CRASH CT model was similar. For the subsequent analyses within the RAIN study, we therefore selected the IMPACT Lab model as the primary model for risk adjustment in the base-case analyses, with the CRASH CT model used for sensitivity analyses. The CRASH CT model was chosen over the Hukkelhoven model for sensitivity analyses as it included more substantially different predictor variables from the IMPACT Lab model.
Chapter 6 Evaluation of the costs, consequences and cost-effectiveness of alternative locations of care for critically ill patients with acute traumatic brain injury
Introduction
Acute TBI is a major cause of death and long-term disability. 75,95–99 The economic burden of TBI is considerable; studies in the USA have reported societal costs of approximately US$60B per year. 100 Care pathways for adult patients after acute TBI vary widely across regions within publicly funded health-care systems. 101,102 These clinical practice variations are not informed by evidence on the relative costs and outcomes following alternative care pathways. Instead, the location of critical care following TBI may reflect bed availability, local variation and clinical assessment of the patient's prognosis. Variations in the care pathway may be important determinants of mortality and morbidity following TBI. Studies have reported that management of adult TBI patients in specialist neuroscience centres is associated with improved outcomes compared with non-neuroscience centres. 15,16,103 Several case series have suggested that dedicated algorithms and protocols of care in neurocritical care settings can improve mortality and functional outcome following TBI. 14,104,105 Possible improvements from more specialised care for patients with TBI may reflect more concentrated knowledge among health professionals, and greater numbers of patients. 14,106 However, a common concern is that the evidence base on alterative locations of care following TBI is weak; in the absence of RCTs, confounding is a key concern, and previous studies have failed to undertake adequate risk-adjustment when comparing outcomes across settings. Further, few studies have compared the relative costs and cost-effectiveness of alternative locations of care following acute TBI. 107–109
Notwithstanding the lack of evidence, it has been recommended that, following a severe TBI, patients should be managed within a neuroscience centre. 11 Even within neuroscience centres, there are alternative models of critical care, with a recent report suggesting most TBI patients admitted to neuroscience centres are managed in neurocritical care units (about two-thirds of beds), or combined units that included neuro/general critical care beds (about one-third). 12
The distinction between these two models of care within neuroscience centres is potentially important. Recent expansion of dedicated neurocritical care facilities13,14 has been based on evidence of the potential benefits from managing severe head injury in specialist centres. 16 However, such evidence remains inconclusive, as these studies rely on risk prediction models that have not been validated, and fail to differentiate between any effects of specialist neurocritical care, per se, compared with rapid access to neurosurgical care. A key issue is whether any additional initial costs of dedicated compared with combined neuro/general critical care units, are justified by subsequent reductions in morbidity costs, mortality, or disability. Although there is some evidence that specific interventions for patients with TBI, such as aggressive intracranial pressure monitoring, can improve outcomes,14 these can be provided in either combined neuro/general critical care units or in dedicated neurocritical care units. The RAIN study compared the risk-adjusted costs, consequences and cost-effectiveness of dedicated neurocritical care units compared with combined neuro/general critical care units in neuroscience centres. In both settings, patients had access to other specialist resources, such as neurosurgery and neuroradiology.
A second key policy question is whether adult patients with TBI without an acute ‘neurosurgical’ lesion benefit from an ‘early’ decision to transfer to a neuroscience centre. The available evidence is inconclusive. 110–114 Although there is a consensus that those patients who have a space-occupying intracranial haematoma with worsening mass effect should be rapidly transferred to a neurosurgical centre,115 for other adult patients with TBI, there is little evidence on any relative gains from ‘early’ transfer. For critically ill TBI patients, in whom neurosurgery is not indicated, the risks from ‘early’ transfer and subsequent aggressive protocols of care may be substantial. 116,117 Indeed, for adult TBI patients the relatively aggressive approaches adopted in neurocritical care within neuroscience compared with non-neuroscience centres could lead to worse outcomes,118 higher costs and may not be cost-effective. 119 An alternative view is that an early decision to keep the patient within the non-neuroscience centre can lead to delayed transfers, for example if a critical lesion develops subsequently, with potentially higher risks. 120 Previous National Institute for Health and Care Excellence (NICE) guidelines for ‘non-neurosurgical’ patients with TBI recognised the importance of the issue of ‘early’ transfer by listing it as a key topic for future research. 11
A key challenge for such an evaluation is to choose an appropriate time point that reflects an ‘early’ decision to transfer. Here, an ‘early’ decision of whether or not to transfer the patient, is defined as one made immediately after the TBI has been diagnosed and the patient stabilised for transfer. Although limited NHS resources could delay transfer by several hours, transfer within 18 hours of hospital presentation still implies an early decision to transfer. A transfer more than 24 hours after hospital presentation implies a decision to delay transfer, rather than an intended early transfer delayed by logistics. In many cases the patient may not be transferred at all. 16
We therefore had two distinct research objectives that addressed separate decision problems of key policy relevance but were complementary in using risk prediction models to adjust for observed confounders when assessing the costs, consequences and cost-effectiveness of alternative care locations following TBI. These objectives were to compare the relative costs, consequences and cost-effectiveness of:
-
management in a dedicated neurocritical care unit compared with a combined neuro/general critical care unit for adult patients with TBI presenting at a neuroscience centre
-
‘early’ (within 18 hours of hospital presentation) transfer to a neuroscience centre compared with ‘no or late’ (after 24 hours) transfer, for adult patients with TBI who initially present at a non-neuroscience centre and do not require surgery for evacuation of a mass lesion.
These objectives are not easily addressed by RCTs but the variation in neurocritical care services across the NHS allowed us to undertake a rigorous non-randomised study. Such a non-randomised study requires valid, reliable and accurate risk prediction models. Earlier sections of this report suggest that pre-existing risk prediction models for patients with TBI can provide appropriate adjustment for patient factors at presentation.
Methods overview
This evaluation compared alternative care locations for adult patients admitted to critical care following TBI. The evaluation was undertaken in two phases. In the first phase, we compared the costs and consequences of the alternative care locations at 6 months following the TBI. In the second phase, we used estimates from these 6-month end points to project the lifetime cost-effectiveness of alternative care locations. The first phase cost–consequence study compared risk-adjusted 6-month health outcomes (mortality, GOSE and EQ-5D-3L) and costs across alternative care locations using data from the RAIN study. In the second phase, the cost-effectiveness analysis (CEA) followed NICE methods guidance and took a health and personal social perspective,67 and reported cost-effectiveness over the lifetime. Future costs and outcomes were discounted at the current recommended rates of 3.5%.
The subsequent sections motivate the alternative care pathways and comparators considered, report the methods and results of the cost–consequence analysis and then report the methods and results of the lifetime CEA. Finally, we discuss the findings from both phases of the evaluation.
Motivating the comparators chosen for the research objectives
Alternative care pathways in the NHS for adult patients with traumatic brain injury
There are many possible pathways for patients admitted to NHS critical care units following acute TBI, but the vast majority of patients can broadly be classified into one of four pathways (Figure 28). Patients may either present at a non-neuroscience centre (pathways A, B and C), or directly to a neuroscience centre (pathway D). Patients who present to a non-neuroscience centre may either receive all of their critical care within that centre (pathway A) or transfer to a neuroscience centre either directly from the emergency department (pathway B), or critical care within the non-neuroscience centre (pathway C). (Note that pathway C will include both patients for whom the initial decision is to transfer to a neuroscience centre but who receive a period of critical care within the non-neuroscience centre either for stabilisation prior to transfer or while a suitable bed is located, and also patients for whom the initial decision is to manage within the non-neuroscience centre but a subsequent decision is made to transfer, e.g. owing to deterioration.) Patients who present directly to a neuroscience centre are generally managed within a neurocritical care unit in that neuroscience centre (pathway D), which may be either a dedicated neurocritical care unit or a combined neuro/general critical care unit. (Occasionally, when beds are unavailable patients may be transferred to a general critical care unit in a neuroscience centre.)
FIGURE 28.
Simplified patient pathways.
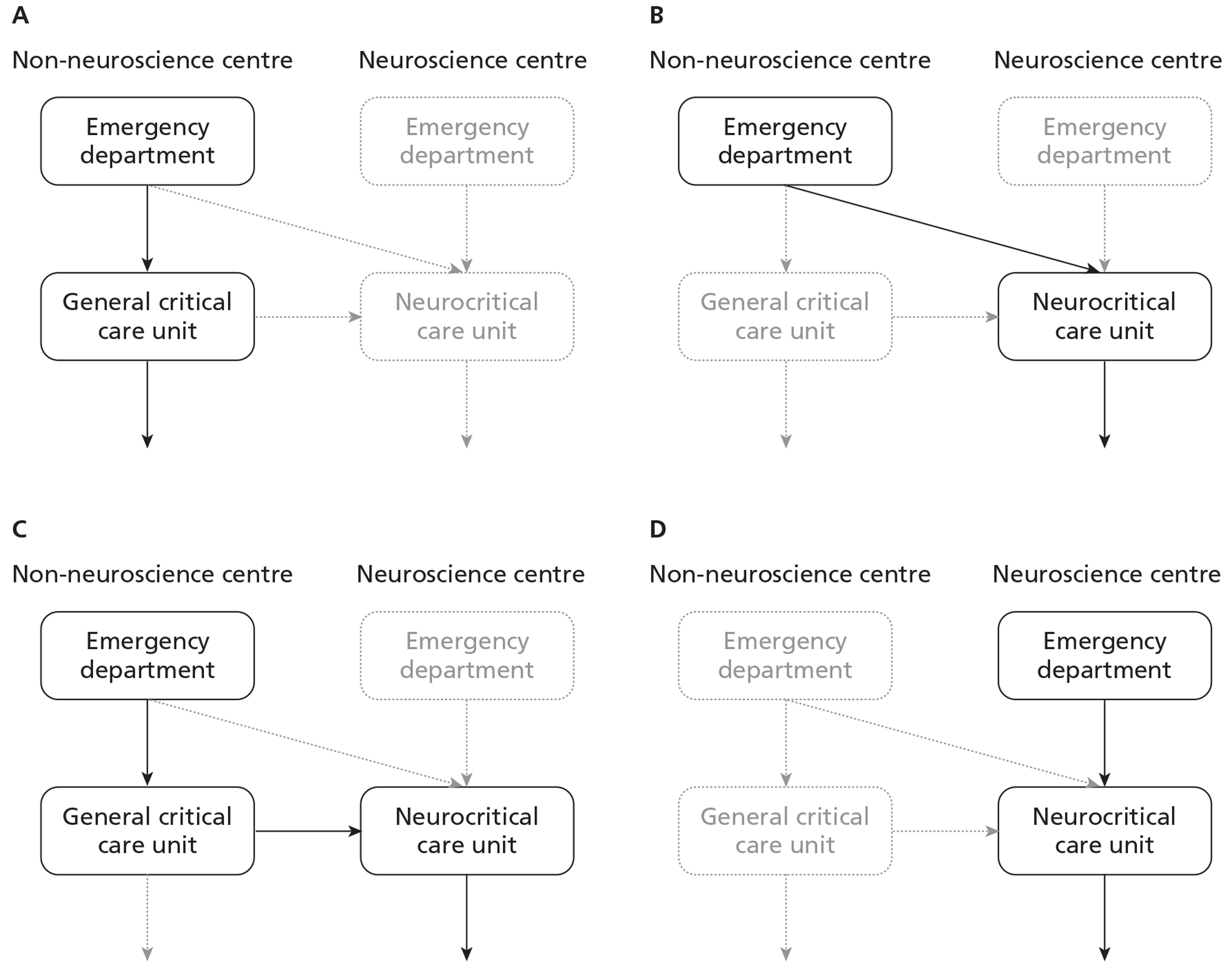
Comparators for addressing research objective 1
Research objective 1 considered alternative ways of organising and delivering neurocritical care services within a neuroscience centre for patients admitted following acute TBI (Figure 29). The population of interest was defined by all RAIN study patients who were admitted to critical care within a neuroscience centre, regardless of whether they initially presented at a neuroscience centre (pathway D) or were transferred from a non-neuroscience centre (pathways B or C). Patients who received all their critical care within a non-neuroscience centre were excluded (i.e. pathway A). The decision problem contrasted care in neuroscience centres with a dedicated neurocritical care unit (Figure 29a) compared with a combined neuro/general critical care unit (see Figure 29b). Hence, patients within each neuroscience centre were only included in one of the comparator groups, analogous to a cluster randomised trial in which individuals within a cluster are all randomised to the same ‘treatment’.
FIGURE 29.
Comparators for research objective 1: organisation of care within neuroscience centres. (a) Dedicated neuro unit; (b) combined neuro/general unit.
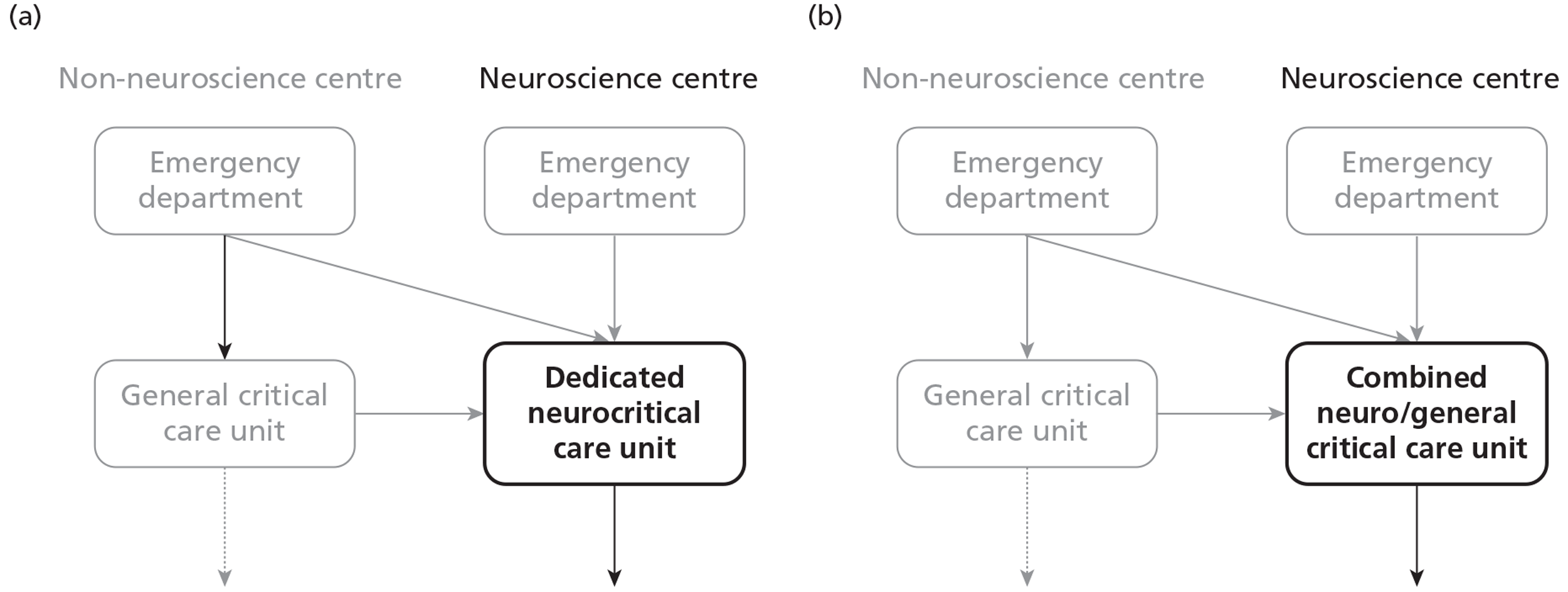
Comparators for addressing research objective 2
The second research objective considered whether, for those patients who presented at a non-neuroscience centre, the intention to undertake an early transfer to a neuroscience centre was cost-effective. This population was characterised by all RAIN study patients who presented at a non-neuroscience centre (i.e. pathways A, B and C) apart from those who underwent neurosurgery for evacuation of a mass lesion within 24 hours following presentation. These comparators were selected to contrast an initial decision to transfer the patient to a neuroscience centre (Figure 30a) compared with an initial decision to manage the patient within the non-neuroscience centre (see Figure 30b). The ‘early’ transfer group comprised patients transferred directly to a neuroscience centre from an emergency department in a non-neuroscience centre, and transfers from a critical care unit that were within 18 hours of initial hospital presentation. The ‘no or late’ transfer group comprised patients who received all their critical care within a non-neuroscience centre, and also patients from critical care units in non-neuroscience centres, who were transferred to a neuroscience centre more than 24 hours after initial hospital presentation. To ensure separation between the comparator arms, we excluded patients transferred between 18 and 24 hours following initial hospital presentation. The cut-off times were chosen by consensus among the clinical experts on the RAIN Study Steering Group. Patients within each participating critical care unit could be included within either comparator group.
FIGURE 30.
Comparators for research objective 2: ‘early’ vs ‘no or late’ transfer to a neuroscience centre (a) Early transfer; (b) no or late transfer.
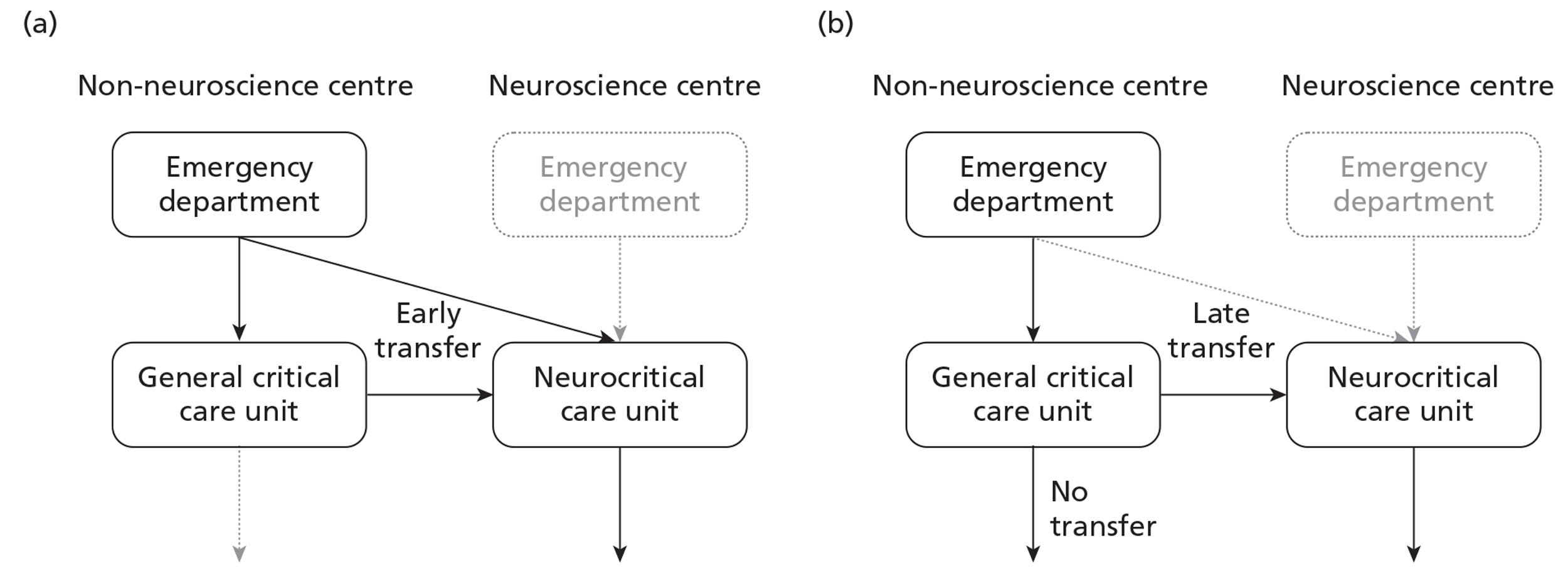
As part of research objective 2, three sets of prespecified subgroup analyses were undertaken: the first according to age, the second according to presence of major extracranial injury and the third by GCS score at baseline. The justification for reporting results by age group was that several recent studies have reported worse outcomes following TBI for older patients, defined as those above an age threshold ranging from 65 to 80 years. 121–124 This raises the hypothesis that ‘early’ transfer for an older subgroup may not be cost-effective. We categorised age groups according to a cut-off of 70 years, which is in the middle of the range of age thresholds used previously, and was anticipated to ensure sufficient sample sizes in each subgroup.
Major extracranial injury frequently accompanies TBI and can increase mortality. 16,94,125–127 However, it is unclear whether the presence of major extracranial injury will modify the effect of an early transfer to neuroscience centres on mortality, morbidity or cost. Hence, we reported the costs, consequences and cost-effectiveness of ‘early’ compared with ‘no or late’ transfer for subgroups with and without major extracranial injury, defined as an injury that would require hospital admission in its own right.
The third subgroup analysis was motivated by NICE guidance, which recommended transfer to a neuroscience centre for all patients with severe TBI, defined as a TBI with GCS score of ≤ 8, regardless of the need for neurosurgery. 11 We therefore reported the costs, consequences and cost-effectiveness of ‘early’ compared with ‘no or late’ transfer for subgroups with a last pre-sedation GCS scores of 3–8 and 9–14.
Methods for the cost–consequence analysis of alternative care locations at 6 months
Settings, inclusion criteria and measurement of case mix
Each critical care unit within the RAIN study was classified according to whether they were in a neuroscience centre and, if so, whether they were a dedicated neurocritical care unit or a combined neuro/general critical care unit (Table 30). (The one ‘major injuries unit’ participating in the RAIN study was classified as a dedicated neurocritical care unit due to the specialist nature of their case mix and the presence of a separate general critical care unit within the same hospital.) All dedicated neurocritical care units in the UK participated in the RAIN study, along with 74% of combined neuro/general critical care units and 16% of general critical care units in non-neuroscience centres. Dedicated neurocritical care units had a similar distribution of bed numbers to general critical care units in non-neuroscience centres, whereas combined neuro/general critical care units were larger. The throughput of TBI cases was similar for dedicated neurocritical care units and combined neuro/general critical care units, and substantially higher than for general critical care units in non-neuroscience centres.
Hospital type | Neuroscience centrea | Non-neuroscience centre | |
---|---|---|---|
Unit type | Dedicated neurocritical care unit | Combined neuro/general critical care unit | General critical care unit |
Number of units (% of all in UK) | 13 (100) | 14 (73.7) | 36 (16.1) |
Number of beds, n (%) | |||
5–9 | 5 (38.5) | 0 (0) | 17 (47.2) |
10–14 | 5 (38.5) | 1 (7.1) | 14 (38.9) |
15–19 | 3 (23.1) | 5 (35.7) | 4 (11.1) |
20+ | 0 (0) | 8 (57.1) | 1 (2.8) |
Number of admissions in RAIN study | 1565 | 1621 | 440 |
Admissions per unit per year (IQR across units) | 90 (60 to 103) | 84 (54 to 110) | 9 (6 to 11) |
Number of patients in analysis | 1324 | 1341 | 310 |
Data were extracted from the RAIN study data set for all patients with acute TBI and a last pre-sedation GCS score of < 15. For research objective 1, all patients with RAIN study data submitted from a neuroscience centre were included in the analysis. All patients who met the inclusion criteria within an eligible critical care unit were then assigned to either comparator group according to whether the neurocritical care unit met the criteria for a dedicated critical care or combined neuro/general critical care unit. 13,14
For research objective 2, all patients initially presenting at a non-neuroscience centre were included in the analysis. Patients included in this analysis came from RAIN study data submitted either from non-neuroscience centres or from neuroscience centres where the location prior to admission field indicated that the patient had been transferred from another acute hospital. Patients were assigned to either comparison group according to the time of transfer to a neuroscience centre. Patients transferred to a neuroscience centre between 18 and 24 hours following initial presentation at an acute hospital were excluded from the analysis.
Risk factor data for the IMPACT Lab35 and CRASH CT30 models were taken from the time of injury and initial presentation at acute hospital, and were defined in the same way as for the external validation of the risk prediction models (see Chapter 5).
Resource use before 6 months
For research objective 1, resource use was considered from the first admission to critical care within the neuroscience centre, i.e. any resource use before this critical care admission, was excluded. For the second research objective, resource use was considered from the first critical care admission following the TBI. For both research objectives, the RAIN study prospectively recorded the LOS in critical care for each admission, according to days in a dedicated neurocritical care unit within a neuroscience centre, days in a combined neuro/general critical care unit within a neuroscience centre, and days in a general critical care unit within a non-neuroscience centre. The RAIN study also recorded whether or not the patient had intracranial neurosurgery for evacuation of a mass lesion. We recorded the LOS following transfer to other wards, both within the same acute hospital or to other hospitals including rehabilitation centres (all termed LOS on general medical wards). Any readmissions to critical care within the RAIN study were recorded. We also extracted data on readmissions to critical care units that were not in the RAIN study via data linkage with the CMP database. Each day during critical care was assigned to the appropriate Healthcare Resource Group (HRG) using daily organ support data recorded for the CCMDS.
The 6-month follow-up in the RAIN study (see Chapter 3) included a Health Services Questionnaire to ascertain use of other hospital and personal social services up to 6 months following the TBI. These postal questionnaires asked patients or their carers to report any hospital and personal service use between discharge from hospital and 6 months. We adapted a questionnaire128 to report service use following a TBI. The items of service use included subsequent hospital readmissions (excluding critical care), visits to hospital outpatients, and community health services (contacts with the GP, health visitor, district nurse, physiotherapist, occupational therapist, psychologist and speech therapist) (see Appendix 5). The patients eligible for this questionnaire were those known to be alive, and discharged from hospital by the date of the 6-month follow-up.
Health outcomes at 6 months
Data on 6-month outcomes were collected centrally as detailed in Chapter 3. EQ-5D-3L profiles were combined with health-state preference values from the UK general population, to give an EQ-5D-3L utility index score on a scale anchored at 0 (death) and 1 (perfect health). 129 QALYs at 6 months were then reported for each patient by combining information on survival and utility score at 6 months. Patients who died before 6 months were assigned zero QALYs. For patients alive at 6 months who completed an EQ-5D-3L questionnaire, their utility score at 6 months was multiplied by 0.5 life-years (6/12 months) to give their 6-month QALY.
Unit costs
For all critical care admissions, each bed-day was costed with the corresponding unit cost per bed-day from the ‘Payment by Results’ database. 130 Each set of unit costs distinguished between the alternative care locations; for research objective 1, separate unit costs were extracted for neuroscience centres with dedicated neurocritical care units compared with neuroscience centres with combined neuro/general critical care units (Table 31). For research objective 2, separate unit costs were applied to critical care in non-neuroscience centres (general critical care) compared with neuroscience centres (dedicated neurocritical care or combined neuro/general critical care). The unit cost of transfer to a neuroscience centre was taken as the cost of an emergency transfer. 130 For those admissions that included an intracranial procedure, to recognise the additional costs of theatre time and consumables, we included an additional unit cost corresponding to neurosurgery for cases with complications or comorbidities. 130 Each set of unit costs was reported as an average across the centres in the RAIN study. Unit costs for general medical bed-days were taken across all categories of elective, excess bed-days, weighted for their relative prevalence. 131 Other unit costs of health and personal social services were taken from the literature (Table 32). 132 All unit costs were reported in 2010–11 prices.
Resource use | Unit costs (£) of inpatient care | ||
---|---|---|---|
Neuroscience centre with dedicated neurocritical care unit | Neuroscience centre with combined neuro/general critical care unit | Non-neuroscience centre with general critical care unit | |
Critical care bed-day | |||
One organ supported | 865 | 800 | 738 |
Two organs supported | 888 | 1269 | 899 |
Three organs supported | 1354 | 1181 | 1348 |
Four organs supported | 1369 | 1324 | 1553 |
Five organs supported | 1433 | 1360 | 1685 |
Six organs supported | 1481 | 1570 | 1895 |
Seven organs supported | 1448 | 1570 | 2204 |
General medical bed-daya | 252 | 252 | 252 |
Transfer to neuroscience centre | 253 | 253 | N/A |
Intracranial procedure | 5796 | 4570 | 6174b |
Community health service | Unit cost (£) |
---|---|
Hospital outpatient | 147 |
GP practice visit | 47 |
GP home visit | 99 |
GP practice nurse | 11 |
Hospital staff nurse | 11 |
Health visitor | 11 |
District nurse | 11 |
Psychologist | 16 |
Speech and language therapist | 8 |
Occupational therapist | 10 |
Physiotherapist | 8 |
Counsellor | 60 |
Hospital discharge co-ordinator | 8 |
Child psychologist | 16 |
Dietitian | 8 |
Mental health service | 27 |
Cognitive–behavioural therapist | 8 |
Social worker | 9 |
Art therapy | 10 |
Each item of resource use was combined with the appropriate unit cost to report a cost per patient for each cost category (inpatient, outpatient, community and total costs). Resource use was estimated at the individual patient-level for each specific location of care. The unit costs were average unit costs across the centres in the RAIN study specific to each location of care (see Table 32).
Analysis of 6-month costs and consequences
For both research objectives, we described baseline characteristics, unadjusted outcomes, resource use and costs for each comparator. For each end point we employed regression analysis to adjust for baseline differences between the comparators using the individual risk factor variables from the IMPACT Laboratory model. 35 For the mortality and unfavourable outcome end points, we report odds ratios with logistic regression. For research objective 1, where each patient within a critical care unit was by definition within the same comparison group, we specified multilevel models that included random effects for centre to recognise any between-centre differences. 133,134 For the EQ-5D-3L, QALY and cost end points, the multilevel model reported incremental effects of the alternative care locations, after adjusting for case mix. The multilevel models assumed that both individual and centre level residuals were drawn from normal distributions. For research objective 2, where patients within each critical care unit could be assigned to either comparison arm, we repeated the above analysis but with single-level regression models estimated by maximum likelihood. These models reported incremental effects of the alternative care locations, together with 95% CIs, assuming that individual level residuals followed normal distributions. For research objective 2, we undertook prespecified subgroup analyses to report: incremental effects according to age (aged ≤ 70 years or > 70 years), major extracranial injury (yes or no) and GCS score (3–8 or 9–14). All analyses were implemented in R using the General Linearized Models (GLM) package. 135
For those patients who did not complete the 6-month follow-up questionnaires, data were unavailable for GOSE (approximately 20%), EQ-5D-3L and health service use (both approximately 50%). For a minority of patients, information was missing for baseline characteristics (between 2% and 17%) and mortality (3%). We addressed missing data with multiple imputation following a similar approach to that applied in Chapter 5. 76,78,136 The EQ-5D-3L, GOSE and cost end points were conditional on survival status, so we conducted the imputation in two stages. In the first stage, we specified imputation models for baseline characteristics and mortality according to observed covariates. In the second stage, for each of the previously imputed data sets, we specified imputation models for GOSE, EQ-5D-3L and health service use for those patients who were either known to be alive at 6 months, or were predicted to be alive by the first stage imputation model. (Owing to the high number of health service use categories, we specified multiple imputation models only for three aggregated categories: inpatient, outpatient and ‘other’ service use. In order to present disaggregated descriptions of mean service use across all the patients sampled in each arm, we also undertook mean imputation. Here we replaced missing observations in each category with the unconditional mean resource use for each category by treatment arm, among those patients with the relevant resource use observed.)
Each multiple imputation model assumed that the data were ‘missing at random’, that is conditional on the variables included in each imputation model. 76 Each imputation model considered including baseline covariates (e.g. risk factor variables from the IMPACT Lab model), resource use (e.g. LOS in critical care), and end points (e.g. we used data for those patients who completed the GOSE questionnaire to predict missing EQ-5D-3L). For research objective 1, we developed multilevel imputation models to recognise the hierarchical structure of the data. 137 For research objective 2, we used standard multiple imputation. 77 As the missingness pattern may differ across treatment groups, we specified separate imputation models for each comparator, and five imputed data sets were generated for each stage of the imputation, giving a total of 25 imputed data sets for each arm. After imputation, we applied the analytical models to each imputed data set, and combined the resultant estimates with Rubin's rules,76 which recognise uncertainty both within and between imputations. All multiple imputation models were implemented in R using MICE. 79
Results of the cost–consequence analysis of alternative care locations at 6 months
Research objective 1: Dedicated neurocritical care unit compared with combined neuro/general critical care unit
The baseline characteristics of the patients were broadly similar between the dedicated neurocritical care units and combined neuro/general critical care units (Table 33). In particular, the median predicted risk of unfavourable outcome, according to the IMPACT Lab model, was 40% for those dedicated critical care units compared with 41% in the combined units.
Baseline covariates | Combined neuro/general critical care unit | Dedicated neurocritical care unit |
---|---|---|
Number of admissions | 1341 | 1324 |
Age (years) | ||
Mean (SD) | 44.4 (18.8) | 43.9 (18.7) |
Median (IQR) | 43 (28 to 58) | 43 (26 to 59) |
Male, n (%) | 1023 (76.3) | 1002 (75.7) |
Major extracranial injury, n (%) | 590 (44.0) | 488 (36.9) |
Pre-hospital hypoxia,a %b | 13.4 | 11.5 |
Pre-hospital hypotension,a %b | 8.1 | 4.8 |
Last pre-sedation GCS score, n (%) | ||
13–14 (mild TBI) | 166 (12.4) | 232 (17.5) |
9–12 (moderate TBI) | 352 (26.2) | 340 (25.7) |
3–8 (severe TBI) | 823 (61.4) | 752 (56.8) |
GCS motor score, %b | ||
6 (obeys) | 15.9 | 19.8 |
5 (localises) | 32.2 | 30.8 |
4 (normal flexion) | 12.3 | 12.0 |
3 (abnormal flexion) | 8.1 | 6.9 |
2 (extension) | 8.3 | 6.7 |
1 (none) | 23.2 | 23.7 |
Pupil reactivity,c %b | ||
Both reactive | 79.8 | 80.9 |
One reactive | 6.4 | 6.8 |
Neither reactive | 13.8 | 12.3 |
Marshall CT classification,d %b | ||
1 (diffuse injury I) | 2.7 | 4.3 |
2 (diffuse injury II) | 35.0 | 30.4 |
3 (diffuse injury III) | 8.4 | 9.6 |
4 (diffuse injury IV) | 2.5 | 3.3 |
5 (evacuated mass lesion) | 37.3 | 39.3 |
6 (non-evacuated mass lesion > 25 ml) | 14.1 | 13.1 |
Traumatic SAH,d %b | 57.1 | 56.6 |
Extradural haematoma,d %b | 16.7 | 17.5 |
Predicted risk (%) at 6 months, median (IQR)b | ||
Death (IMPACT Lab model) | 22.8 (11.5 to 39.6) | 22.5 (11.3 to 36.9)) |
Unfavourable outcome (IMPACT Lab model) | 41.0 (22.9 to 64.2) | 40.0 (21.1 to 60.7) |
Unfavourable outcome (CRASH CT model) | 44.2 (24.3 to 72.2) | 42.5 (22.4 to 70.3) |
Table 34 describes the main items of hospital resource use recorded from the RAIN study and the CMP database. Although the overall mean hospital LOS was similar between the groups, the dedicated neurocritical care unit group had a higher average LOS in critical care.
Resource | Combined neuro/general critical care unit (n = 1341) | Dedicated neurocritical care unit (n = 1324) |
---|---|---|
Index admission | ||
Mean (SD) days in critical care | 11.08 (10.83) | 13.19 (15.07) |
Mean (SD) days on general medical wards | 26.26 (36.03) | 24.69 (33.96) |
Mean (SD) total hospital days | 37.34 (40.49) | 37.89 (39.62) |
Neurosurgery,a n (%) | 477 (35.57) | 478 (36.10) |
Readmission | ||
n (%) readmission | 66 (4.92) | 100 (7.55) |
Mean (SD) days on critical care | 0.46 (3.27) | 0.59 (2.94) |
Mean (SD) days on general wards | 0.22 (3.28) | 0.22 (3.41) |
Mean (SD) total days | 0.68 (5.24) | 0.80 (5.05) |
Mean (SD) total hospital days up to 6 months | 38.02 (41.27) | 38.69 (40.49) |
Table 35 shows the flow of patients through the study. As the EQ-5D-3L and the Health Services Questionnaire were administered only by post, and not by telephone, the completion rates were lower for these questionnaires.
Stage of follow-up | Combined neuro/general critical care unit | Dedicated neurocritical care unit |
---|---|---|
Hospital presentation | 1341 | 1324 |
Total deaths before 6 months | 331 | 305 |
Missing data on death before 6 months | 42 | 45 |
Eligible for Your Health Questionnaire | 968 | 974 |
GOSE questionnaire completed | 750 (77%) | 796 (82%) |
EQ-5D-3L questionnaire completed | 471 (49%) | 508 (52%) |
Initial hospital episode of 6 months or more | 27 | 20 |
Eligible for Health Services Questionnaire | 941 | 954 |
Health Services Questionnaire completed | 495 (53%) | 529 (55%) |
Mortality at 6 months was similar between the groups (Table 36). The dedicated neurocritical care unit group had a higher mean EQ-5D-3L utility index score for survivors, a lower proportion of patients with unfavourable outcomes and higher mean QALYs, although none of these differences was statistically significant, after case mix adjustment.
Six-month outcomes | Combined neuro/general critical care unit (n = 1341) | Dedicated neurocritical care unit (n = 1324) | Odds ratio/incremental effecta (95% CI) | |
---|---|---|---|---|
Unadjusted | Adjusted | |||
Death before 6 months, n (%) | 341 (25%) | 312 (24%) | 0.982 (0.95 to 1.02) | 0.942 (0.77 to 1.16) |
Mean (SD) EQ-5D (survivors) | 0.43 (0.41) | 0.48 (0.41) | 0.049 (−0.001 to 0.098) | 0.048 (−0.002 to 0.099) |
GOSE category, n (%) | ||||
Upper good recovery | 155 (12%) | 169 (13%) | ||
Lower good recovery | 76 (6%) | 71 (5%) | ||
Upper moderate disability | 145 (11%) | 166 (13%) | ||
Lower moderate disability | 123 (9%) | 125 (9%) | ||
Upper severe disability | 181 (14%) | 165 (12%) | ||
Lower severe disability | 283 (21%) | 289 (22%) | ||
Pre-existing severe disability | 36 (3%) | 27 (2%) | ||
Dead | 341 (25%) | 312 (24%) | ||
Unfavourable outcome, n (%) | 841 (63%) | 793 (60%) | 0.888 (0.69 to 1.15) | 0.902 (0.74 to 1.10) |
Mean (SD) QALY | 0.16 (0.20) | 0.18 (0.21) | 0.023 (0.002 to 0.043) | 0.020 (−0.001 to 0.040) |
Table 37 reports the results from the Health Services Questionnaire for all patients sampled in each comparison group. The mean numbers of contacts were similar between the groups. For both groups, relatively low use, on average, was made of community health services.
Resource use | Combined neuro/general critical care unit (n = 1341) | Dedicated neurocritical care unit (n = 1324) |
---|---|---|
Inpatient days (general medical) | 8.3 (16.7) | 8.2 (17.6) |
Outpatient visits | 3.3 (4.6) | 2.7 (3.7) |
GP contacts | 2.1 (2.2) | 2.3 (2.4) |
Nurse contacts | 1.3 (2.4) | 1.2 (2.1) |
Occupational therapist contacts | 2.3 (3.6) | 2.5 (3.9) |
Health visitor contacts | 0.8 (2.2) | 0.8 (2.1) |
Clinical psychologist contacts | 1.3 (3.3) | 1.1 (3.0) |
Speech therapist contacts | 1.4 (3.5) | 1.5 (3.9) |
Physiotherapist contacts | 3.4 (3.9) | 3.4 (4.0) |
Mental health service contacts | 0.4 (2.0) | 0.4 (1.6) |
Cognitive–behavioural therapist contacts | 0.3 (1.3) | 0.2 (1.0) |
Table 38 reports the mean total costs at 6 months for each comparison arm. On average, critical care costs were higher for the dedicated neurocritical care units, and this led to a positive incremental cost compared with combined neuro/general critical care units.
Source of costs | Combined neuro/general critical care unit (n = 1341) | Dedicated neurocritical care unit (n = 1324) | Incremental cost (95% CI) |
---|---|---|---|
Index admissiona | |||
Critical care | 15,066 (15,190) | 18,225 (21,638) | |
General medical | 6619 (9081) | 6223 (8558) | |
Subsequent admissions | |||
Critical carea | 559 (3958) | 722 (3664) | |
General medicala | 56 (827) | 55 (860) | |
General medicalb | 757 (2468) | 782 (2503) | |
Outpatient careb | 445 (1405) | 407 (1322) | |
Community costsb | 332 (1274) | 364 (1375) | |
Grand totalab | 25,466 (21,468) | 28,855 (25,970) | 3694 (1899 to 5489) |
Research objective 2: ‘early’ compared with ‘no or late’ transfer to a neuroscience centre
There were substantial differences in case mix between patients in the ‘early’ transfer group and the ‘no or late’ transfer group (Table 39). The patients in the ‘early’ transfer group were on average younger but were generally of less severe case mix than the ‘no or late’ transfer group. The ‘no or late’ transfer group had a higher proportion of patients with a GCS score of 3–8, for whom neither pupil was reactive, and who had a Marshall CT classification of ‘non-evacuated mass lesion’. The net effect was that the median predicted risk of death at 6 months was higher for the ‘no or late’ transfer group than for the ‘early’ transfer group.
Baseline covariates | ‘No or late’ transfer to neuroscience centre | ‘Early’ transfer to neuroscience centre |
---|---|---|
Number of admissions | 263 | 584 |
Age (years) | ||
Mean (SD) | 40.0 (17.4) | 50.7 (20.1) |
Median (IQR) | 39 (24 to 53) | 49 (35 to 69) |
Male, n (%) | 207 (78.7) | 455 (77.9) |
Major extracranial injury, n (%) | 110 (41.8) | 209 (35.8) |
Pre-hospital hypoxia,a %b | 15.0 | 13.5 |
Pre-hospital hypotension,a %b | 9.7 | 5.2 |
Last pre-sedation GCS score, n (%) | ||
13–14 (mild TBI) | 41 (15.6) | 105 (18.0) |
9–12 (moderate TBI) | 62 (23.6) | 173 (29.6) |
3–8 (Severe TBI) | 160 (60.8) | 306 (52.4) |
Motor score from last pre-sedation GCS score, %b | ||
6 (obeys) | 14.1 | 20.8 |
5 (localises) | 26.5 | 35.4 |
4 (normal flexion) | 10.3 | 11.2 |
3 (abnormal flexion) | 4.9 | 4.9 |
2 (extension) | 7.3 | 5.9 |
1 (none) | 36.7 | 21.8 |
Pupil reactivity,c %b | ||
Both reactive | 77.0 | 83.8 |
One reactive | 1.4 | 6.1 |
Neither reactive | 21.6 | 10.0 |
Marshall CT classification,d %b | ||
1 (diffuse injury I) | 10.9 | 2.4 |
2 (diffuse injury II) | 45.6 | 42.4 |
3 (diffuse injury III) | 8.4 | 16.7 |
4 (diffuse injury IV) | 2.7 | 4.3 |
5 (evacuated mass lesion) | 0.6 | 9.6 |
6 (non-evacuated mass lesion > 25 ml) | 31.8 | 24.6 |
Traumatic SAH,d %b | 47.1 | 60.3 |
Extradural haematoma,d %b | 9.6 | 14.8 |
Predicted risk (%) at 6 months, median (IQR)b | ||
Death (IMPACT Lab model) | 24.6 (9.6 to 48.1) | 18.3 (9.4 to 32.7) |
Unfavourable outcome (IMPACT Lab model) | 47.8 (20.8 to 73.6) | 33.8 (17.7 to 54.3) |
Unfavourable outcome (CRASH CT model) | 56.4 (21.8 to 83.1) | 35.6 (19.1 to 58.4) |
Figure 31 presents a histogram of the time from initial presentation at hospital to transfer to a neuroscience centre, for all patients transferred. Each bin on the histogram represents a time period of 1 hour; the vertical line at 18 hours represent the maximum time to transfer for the ‘early’ group, and the line at 24 hours, the minimum transfer time for the ‘late’ group. In the ‘no or late’ transfer group, 94% of patients were not transferred to a neuroscience centre. The majority of transfers were between 3 and 10 hours from hospital presentation. For 77 patients transferred directly from an emergency department a time of transfer was not available; these patients were defined as in the ‘early’ transfer group.
FIGURE 31.
Distribution of time from initial presentation at hospital to transfer to a neuroscience centre. Note: 10 transfers beyond 48 hours are not shown; 77 transfers direct from the emergency department had missing transfer times and were assumed to be early.
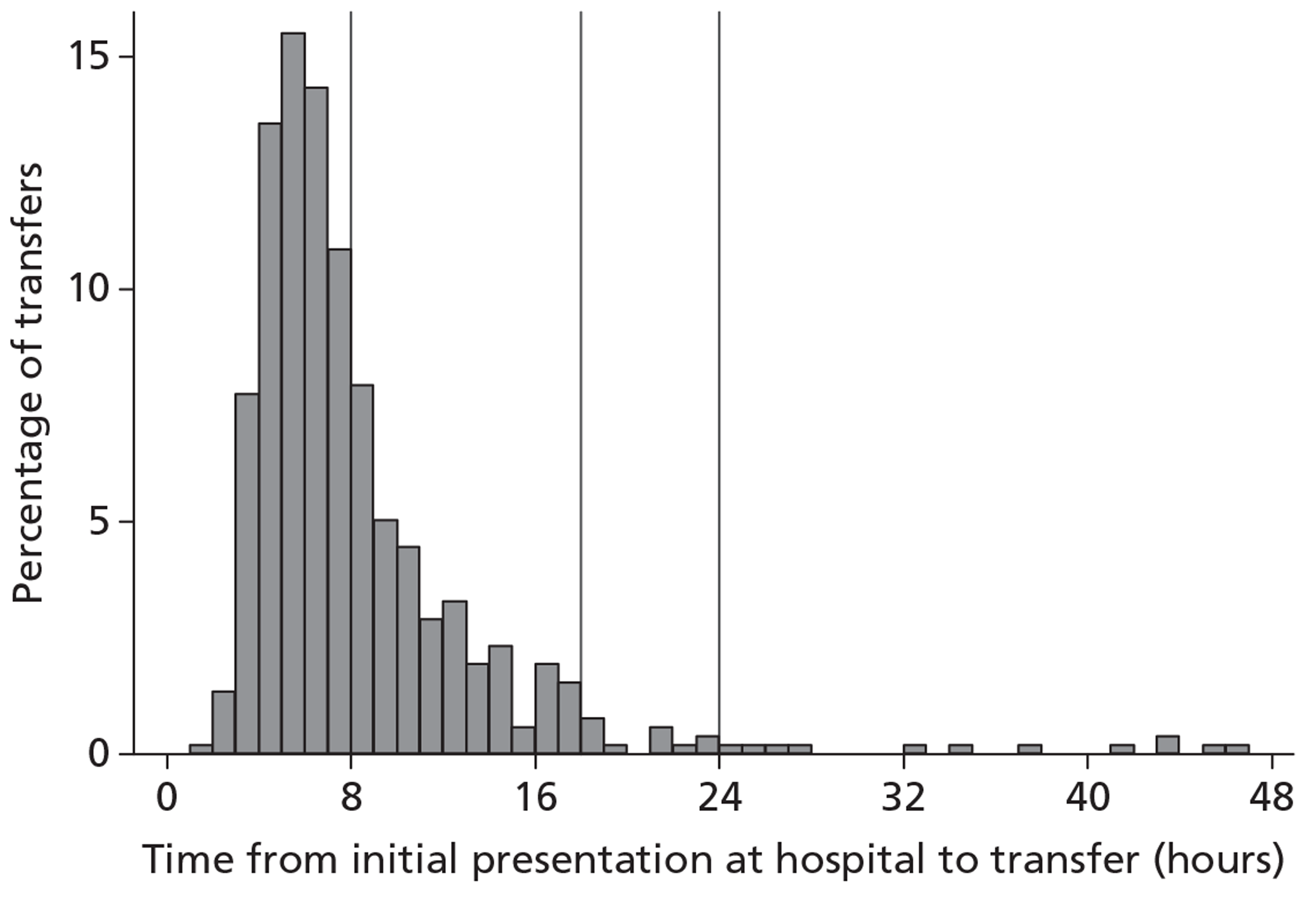
The ‘early’ transfer group had mean LOS in critical care, on general wards and in total that was approximately double that of the ‘no or late’ transfer group (Table 40). In addition, a higher proportion of patients in the ‘early’ transfer group had an intracranial procedure for evacuation of a mass lesion, at least 24 hours after initial presentation.
Resource use | ‘No or late’ transfer to neuroscience centre (n = 263) | ‘Early’ transfer to neuroscience centre (n = 584) |
---|---|---|
Index admission | ||
Mean (SD) days in critical care | 5.67 (6.66) | 13.93 (15.68) |
Mean (SD) days on general medical wards | 12.73 (23.94) | 24.31 (34.09) |
Mean (SD) total hospital days | 18.40 (26.98) | 38.24 (40.37) |
Neurosurgery,a n (%) | 1 (0.38) | 48 (8.22) |
Readmission | ||
n (%) readmission | 9 (3.42) | 38 (6.51) |
Mean (SD) days on critical care | 0.28 (2.03) | 0.57 (3.18) |
Mean (SD) days on general wards | 0.03 (0.31) | 0.13 (1.55) |
Mean (SD) total hospital days | 0.31 (2.24) | 0.69 (3.98) |
Mean (SD) total hospital days up to 6 months | 18.71 (27.02) | 38.94 (40.86) |
Table 41 shows the flow of patients through the study, and highlights that around half the eligible patients completed the postal questionnaires for the EQ-5D-3L and service use.
Stage of follow-up | ‘No or late’ transfer to neuroscience centre | ‘Early’ transfer to neuroscience centre |
---|---|---|
Hospital presentation | 263 | 584 |
Total deaths before 6 months | 104 | 106 |
Missing data on deaths by 6 months | 7 | 23 |
Eligible for Your Health Questionnaire | 152 | 455 |
GOSE questionnaire completed | 114 (75%) | 363 (80%) |
EQ-5D-3L questionnaire completed | 70 (46%) | 233 (51%) |
Initial hospital episode of 6 months or more | 2 | 7 |
Eligible for Health Services Questionnaire | 150 | 448 |
Health Services Questionnaire completed | 76 (51%) | 236 (53%) |
The proportion of patients who died before 6 months was substantially lower in the ‘early’ compared with the ‘no or late’ transfer group (odds ratio after case mix adjustment 0.52, 95% CI 0.34 to 0.80; Table 42). Although the CI on the odds ratio for unfavourable outcome included 1, the ‘early’ transfer group reported a higher mean EQ-5D-3L utility index score for survivors, and higher mean QALYs after case mix adjustment.
Six-month outcomes | ‘No or late’ transfer to neuroscience centre (n = 263) | ‘Early’ transfer to neuroscience centre (n = 584) | Odds ratio/incremental effecta (95% CI) | |
---|---|---|---|---|
Unadjusted | Adjusted | |||
Death before 6 months, n (%) | 107 (41%) | 109 (19%) | 0.334 (0.242 to 0.462) | 0.52 (0.34 to 0.80) |
Mean (SD) EQ-5D (survivors) | 0.44 (0.37) | 0.55 (0.41) | 0.108 (0.011 to 0.205) | 0.129 (0.032 to 0.225) |
GOSE category, n (%) | ||||
Upper good recovery | 40 (15%) | 80 (14%) | ||
Lower good recovery | 9 (3%) | 45 (8%) | ||
Upper moderate disability | 20 (7%) | 88 (15%) | ||
Lower moderate disability | 25 (9%) | 65 (11%) | ||
Upper severe disability | 19 (7%) | 74 (13%) | ||
Lower severe disability | 34 (13%) | 117 (20%) | ||
Pre-existing severe disability | 11 (4%) | 7 (1%) | ||
Dead | 107 (41%) | 109 (19%) | ||
Unfavourable outcome, n (%) | 169 (65%) | 307 (53%) | 0.61 (0.17 to 2.15) | 0.88 (0.28 to 2.79) |
Mean (SD) QALY | 0.13 (0.18) | 0.22 (0.21) | 0.093 (0.056 to 0.130) | 0.051 (0.015 to 0.086) |
Table 43 shows the hospital and community health service use reported by the patients at 6 months, averaged across the whole sample including those who died. The ‘early’ transfer group reported higher average LOS after readmissions to general medical wards, and had higher mean contacts with outpatient and community health services.
Resource use | ‘No or late’ transfer to neuroscience centre (n = 263) | ‘Early’ transfer to neuroscience centre (n = 584) |
---|---|---|
Inpatient days (general medical) | 6.4 (13.8) | 7.9 (15.5) |
Outpatient visits | 1.8 (2.3) | 2.4 (2.4) |
GP contacts | 2.2 (2.3) | 2.3 (2.0) |
Nurse contacts | 1.1 (2.6) | 1.1 (2.0) |
Occupational therapist contacts | 1.4 (3.3) | 2.5 (3.7) |
Health visitor contacts | 0.9 (2.1) | 0.7 (1.9) |
Clinical psychologist contacts | 0.6 (2.1) | 1.0 (2.5) |
Speech therapist contacts | 0.5 (1.6) | 1.1 (2.4) |
Physiotherapist contacts | 2.6 (3.2) | 2.8 (3.2) |
Mental health service contacts | 0.4 (1.4) | 0.2 (0.8) |
Cognitive–behavioural therapist contacts | 0.2 (1.1) | 0.2 (0.8) |
The higher proportion of patients surviving in the early transfer arm is reflected in the estimates of the total costs at 6 months (Table 44); the mean costs of critical care and the total costs were twice as high in the early transfer group, with a positive incremental cost of approximately £15,000, after case mix adjustment.
Source of costs | ‘No or late’ transfer to neuroscience centre (n = 263) | ‘Early’ transfer to neuroscience centre (n = 584) | Incremental cost (95% CI) |
---|---|---|---|
Index admissiona | |||
Critical care | 8330 (10,016) | 19,352 (22,874) | |
General medical | 3209 (6033) | 6126 (8589) | |
Subsequent admissions | |||
Critical carea | 395 (2939) | 720 (3957) | |
General medicala | 8 (79) | 33 (391) | |
General medicalb | 616 (2247) | 836 (2547) | |
Outpatient careb | 289 (1114) | 573 (1740) | |
Community costsb | 288 (1177) | 604 (1885) | |
Grand totala,b | 13,152 (14,563) | 28,525 (27,100) | 15,001 (11,123 to 18,880) |
Table 45 presents the results of the subgroup analyses for the 6-month end points, after case mix adjustment. Patients aged > 70 years had, on average, a higher odds of death in the ‘early’ compared with the ‘no or late’ transfer group, but the CIs around the odds ratios were wide and included 1. For older survivors, the mean odds ratio for death at 6 months was < 1 whether or not patients had a major extracranial injury but the subgroup with major extra cranial injury had very low odds of death in the ‘early’ transfer group.
Subgroups | Odds ratio for death (95% CI) | Incremental EQ-5D-3L (95% CI) | Incremental QALY (95% CI) | Incremental cost (95% CI) |
---|---|---|---|---|
Overall | 0.521 (0.34 to 0.80) | 0.129 (0.032 to 0.225) | 0.051 (0.015 to 0.086) | 15,001 (11,123 to 18,880) |
Age (years) | ||||
≤ 70 | 0.471 (0.289 to 0.766) | 0.132 (0.027 to 0.237) | 0.066 (0.014 to 0.118) | 15,770 (11,492 to 20,047) |
> 70 | 1.528 (0.441 to 5.299) | 0.074 (−0.334 to 0.483) | 0.037 (−0.167 to 0.241) | 5136 (−3604 to 13,876) |
Major extracranial injury | ||||
No | 0.797 (0.448 to 1.420) | 0.131 (−0.001 to 0.262) | 0.066 (−0.000 to 0.131) | 11,756 (6774 to 16,737) |
Yes | 0.215 (0.098 to 0.468) | 0.119 (−0.019 to 0.256) | 0.059 (−0.009 to 0.128) | 20,481 (14,374 to 26,588) |
GCS score | ||||
Mild or moderate TBI | 0.733 (0.358 to 1.498) | 0.127 (−0.004 to 0.259) | 0.064 (−0.002 to 0.130) | 9195 (4076 to 14,314) |
Severe TBI | 0.431 (0.237 to 0.783) | 0.135 (−0.005 to 0.275) | 0.067 (−0.003 to 0.137) | 18,293 (12,573 to 24,013) |
Methods for the lifetime cost-effectiveness analysis
Overview
For each research objective, we reported incremental cost-effectiveness of the alternative care locations over the lifetime. Such lifetime CEA requires information on long-term survival, QOL and cost of alternative interventions. Hence, it was necessary to make assumptions about the long-term prognosis of patients with TBI based on the 6-month data from the RAIN study, but also drawing on evidence from the literature. We tested whether the results were robust to alternative assumptions in extensive sensitivity analyses.
To calculate lifetime QALYs, long-term survival for each patient was calculated from observed survival for each patient within the first 6 months, and from their predicted survival after 6 months. Survival after 6 months was predicted for each patient by applying age-/sex-adjusted excess death rates for the RAIN study patients compared with those for the general population. This was undertaken in two steps. First, we calculated the excess death rates for RAIN study patients who survived after 6 months, compared with the age-/sex-matched UK general population. Second, these excess death rates were applied to predict life expectancy for each RAIN study patient alive at 6 months according to their age and sex.
Long-term survival
Patients in the RAIN study had their vital status followed up until September 2011, and dates of all cause death before that cut-off were provided by MRIS. For each patient the total survival time was calculated up to death or September 2011. We plotted Kaplan–Meier survival curves showing the number of patients who died over time until the last available time point, which was 800 days after the initial admission. For each comparator, the majority of deaths were within the first 30 days, as shown in Figure 32 for research objective 1.
FIGURE 32.
Kaplan–Meier survival curves for research objective 1.
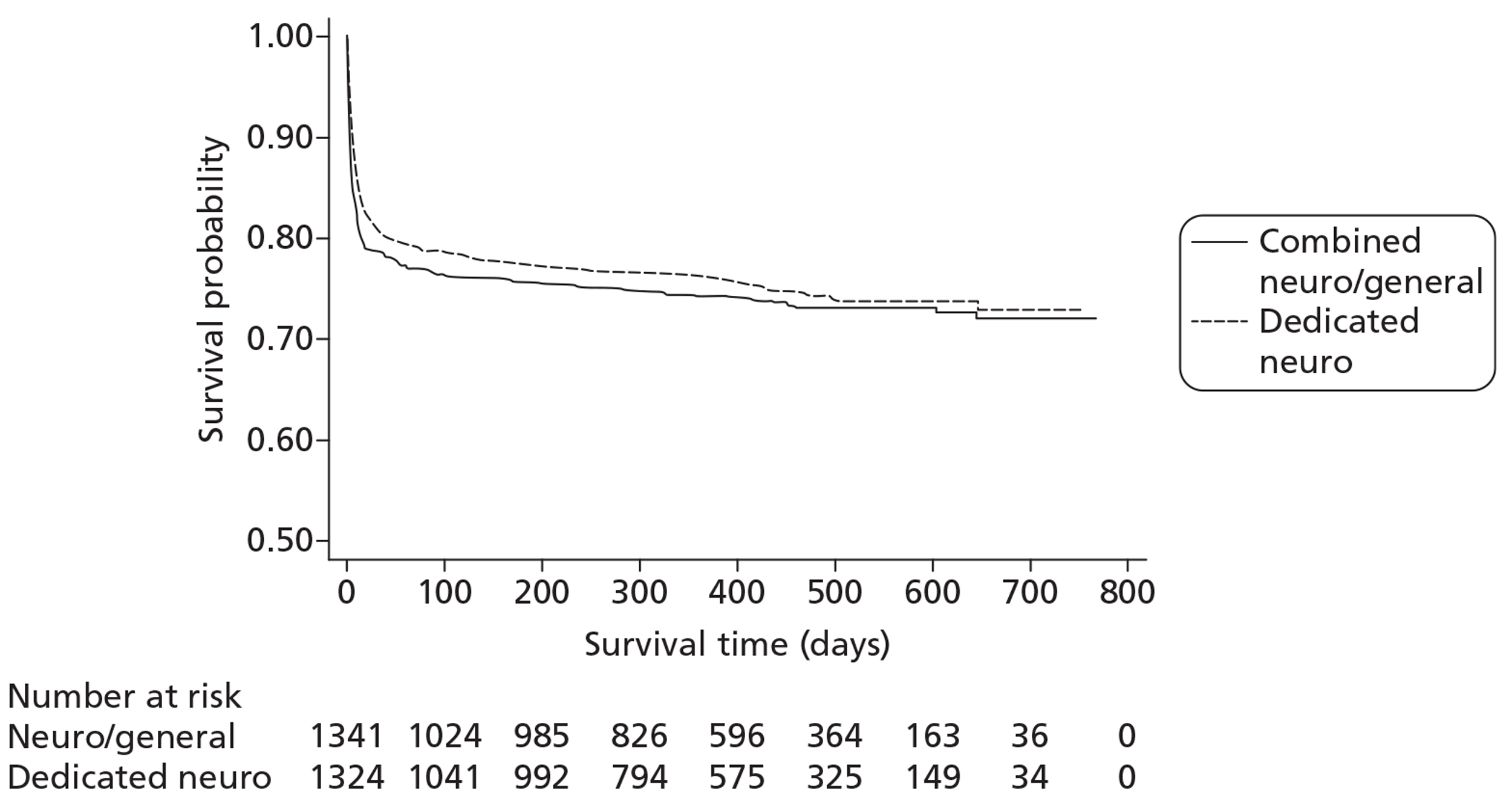
We followed methodological guidance and considered alternative parametric approaches for extrapolating the observed survival. 138 When considering alternative parametric specifications we excluded the first 30 days of follow-up, as during this during this time period, patients had high risks of death; it was judged inappropriate to use this portion of the data for predicting the long-term probability of death. Figure 33 shows alternative parametric extrapolations for research objective 1, for the two comparator arms combined.
FIGURE 33.
Comparison of alternative parametric extrapolations of survival for research objective 1.
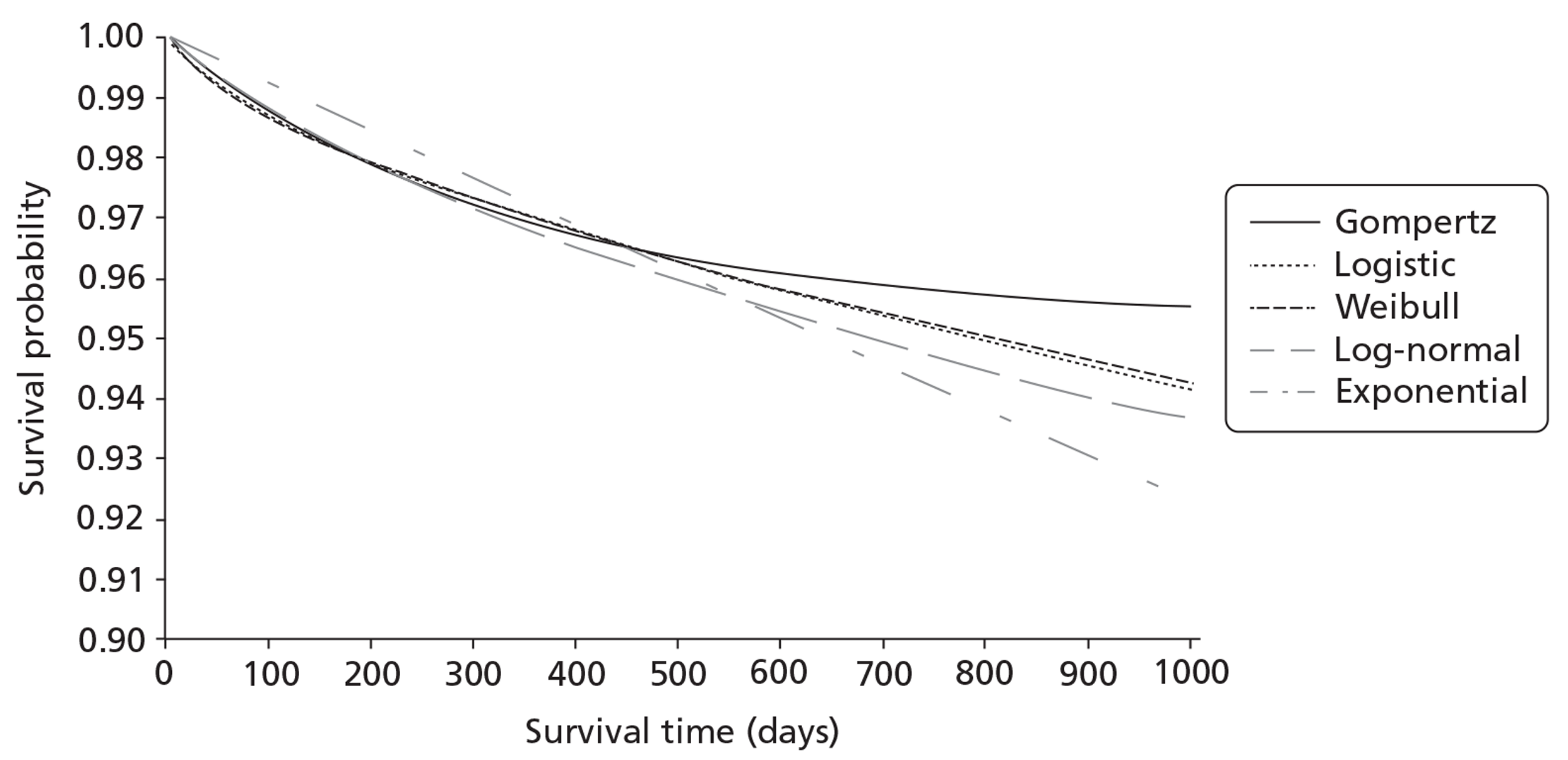
We compared the relative goodness of fit of the alternative parametric survival functions, for the remaining period of observed survival according to the Akaike information criterion (AIC) and Bayesian information criterion (BIC), the fit was similar with the logistic, log-normal or Weibull models (Table 46).
Distribution | AIC | BIC |
---|---|---|
Gompertz | 1302.839 | 1421.721 |
Log-normal | 1285.765 | 1404.647 |
Logistic | 1285.889 | 1404.771 |
Weibull | 1287.583 | 1406.465 |
Exponential | 1325.173 | 1438.394 |
We compared the survival predicted by the alternative extrapolation approaches at 6–12 months with that for the age-/sex-matched general population. 139 We used each parametric extrapolation to report the predicted probability of death at 6–12 months for all the 30-day survivors included in RAIN for research objective 1, and report the ratio of these probabilities of death compared with those for the age-/sex-matched general population, also over a 6-month period. Table 47 shows, for the older age group each of the parametric survival curves tended to predict lower mortality compared with the general population.
Age group (years) | Gompertz | Log-normal | Logistic | Weibull | Exponential |
---|---|---|---|---|---|
16–44 | 1.34 (1.26 to 1.43) | 1.24 (1.13 to 1.35) | 1.27 (1.18 to 1.36) | 1.29 (1.21 to 1.38) | 1.07 (1.00 to 1.14) |
45–59 | 1.15 (1.05 to 1.25) | 1.13 (1.02 to 1.23) | 1.09 (0.99 to 1.19) | 1.11 (1.01 to 1.21) | 0.91 (0.83 to 1.00) |
60+ | 0.70 (0.64 to 0.76) | 0.69 (0.64 to 0.74) | 0.69 (0.63 to 0.74) | 0.67 (0.61 to 0.73) | 0.58 (0.53 to 0.64) |
In the base case we therefore decided that the most plausible approach was to apply age-/sex-matched general population death rates after 6 months for each comparator. Hence, in each of the comparator arms for research objective 1, a similar proportion of patients were assumed to die each year. Of the alternative parametric functions, the Gompertz was judged the most plausible and so we applied this extrapolation as a sensitivity analysis.
For research objective 2, the Kaplan–Meier survival curves (Figure 34) highlight that differences in survival between the comparator groups were observed within the first 30 days, and were maintained over time. Here when the alternative parametric specifications were applied to the RAIN data (excluding the first 30 days after TBI), the fit was similar (Table 48). Each of the extrapolation approaches predicted death rates lower than those for the general population for 6–12 months for the younger age group (Table 49). In the base case, we again applied age-/sex-matched general population death rates after 6 months, and used the Gompertz extrapolation in a sensitivity analysis. For this decision problem, both approaches assumed that the differences between the comparison groups observed within the RAIN study were maintained over time.
FIGURE 34.
Kaplan–Meier survival curves for research objective 2.
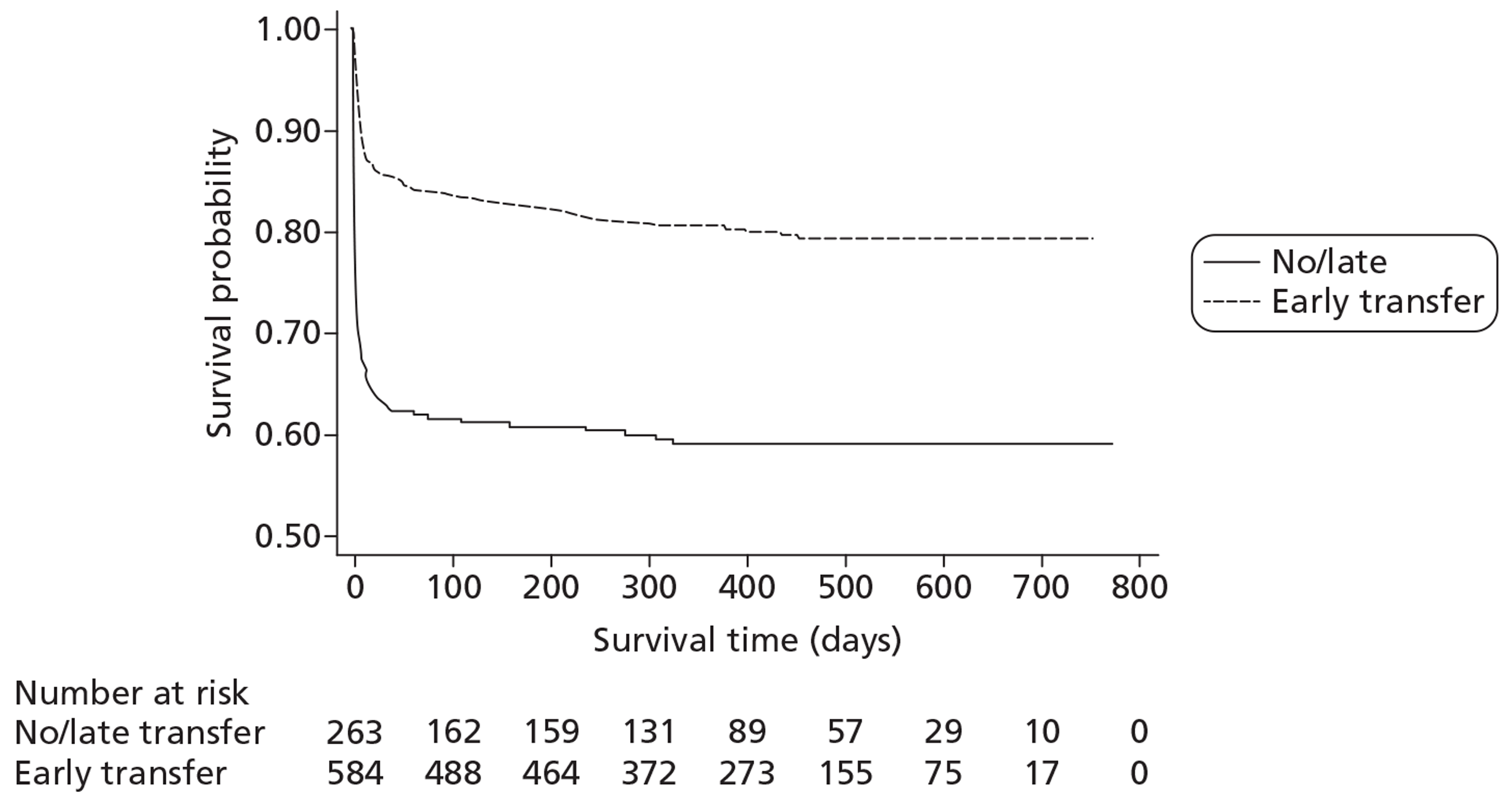
Distribution | AIC | BIC |
---|---|---|
Gompertz | 358.0628 | 448.0886 |
Log-normal | 363.7754 | 458.3024 |
Logistic | 366.0875 | 460.6145 |
Weibull | 368.5472 | 463.0743 |
Exponential | 374.2425 | 464.2683 |
Age group (years) | Gompertz | Log-normal | Logistic | Weibull | Exponential |
---|---|---|---|---|---|
16–44 | 0.61 (0.55 to 0.68) | 0.43 (0.35 to 0.50) | 0.52 (0.45 to 0.58) | 0.55 (0.49 to 0.61) | 0.47 (0.41 to 0.52) |
45–59 | 1.02 (0.80 to 1.24) | 0.83 (0.62 to 1.04) | 0.89 (0.66 to 1.11) | 0.92 (0.72 to 1.11) | 0.79 (0.61 to 0.96) |
60+ | 0.96 (0.78 to 1.13) | 0.93 (0.78 to 1.07) | 0.92 (0.76 to 1.08) | 0.89 (0.72 to 1.06) | 0.81 (0.64 to 0.98) |
Long-term quality of life
The lifetime CEA required estimates of QOL over time according to the initial locations of critical care. Ideally, CEAs are populated with longitudinal estimates of QOL reported using a generic, preference-based utility measure such as the EQ-5D-3L. We reviewed the literature to find appropriate evidence on QOL for this research objective. However, we did not find any studies that reported QOL over time with a preference-based measure. Our review did find two studies that reported QOL assessed with the Short Form questionnaire-36 items (SF-36) at approximately 10 years after TBI compared with the general population. Jacobsson et al. 140 collected data for 67 individuals in Sweden 10 years after acute TBI. This sample included 50% of patients defined as having had a mild TBI (GCS score of 13–15 at admission to the emergency department) and 50% who had either a moderate (GCS score of 9–12) or severe (GCS score of 3–8) TBI. However, the study did not find any differences in QOL according to initial severity. The main finding was that for the whole sample of patients with TBI, general health assessed by the SF-36 was approximately 15% lower than that for the age-/sex-matched general population (n = 1224). Andelic et al. 141 described QOL (SF-36) for a sample of 62 survivors 10 years after moderate (GCS score of 9–12; 52%) to severe (GCS score of 3–8; 48%) TBI in Norway. The main finding was that the study patients had systematically lower scores on each dimension of the SF-36 than the age-/sex-matched general population (n = 2323). The mean SF-36 score for general health was similar to that for a moderate to severe TBI sample in the USA (n = 228), and approximately 15% less than that of the general Norwegian population. A similar decrement to the general populations was reported for the moderate and the severe TBI groups. Other studies have considered disability over time for TBI survivors,75 and found that in patients admitted to hospital 5–7 years after a TBI, rates of disability were high (53%) and similar to those reported at 1 year (57%). Our review did not find any studies that estimated long-term QOL or disability for TBI patients managed in alternative locations of neurocritical care.
In light of the lack of evidence on long-term QOL following alternative locations of critical care, our estimates of QOL over time for each comparator were based on the 6-month data from the RAIN study. However, rather than assuming that the differences in QOL between the comparators at 6 months were maintained, we made the conservative assumption that the differences in QOL attenuated over time. For each comparator we predicted the mean QOL at 6 months for patients aged 40 years (approximately the median age of RAIN patients) with the same risk adjustment applied as in the cost–consequence analysis. We predict mean QOL for each treatment arm between year 1 and year 10 with a linear interpolation, such that after 10 years the mean for each treatment arm was 15% lower than that for the age-matched general population. After 10 years we assumed that for each comparator, the 15% decrement compared with the age-matched population was maintained. As Figure 35 shows, this implies that the relative gains in QOL at 6 months attenuate over time (see also Sensitivity analyses).
FIGURE 35.
Attenuation of relative QOL over time for (a) research objective 1 and (b) research objective 2.
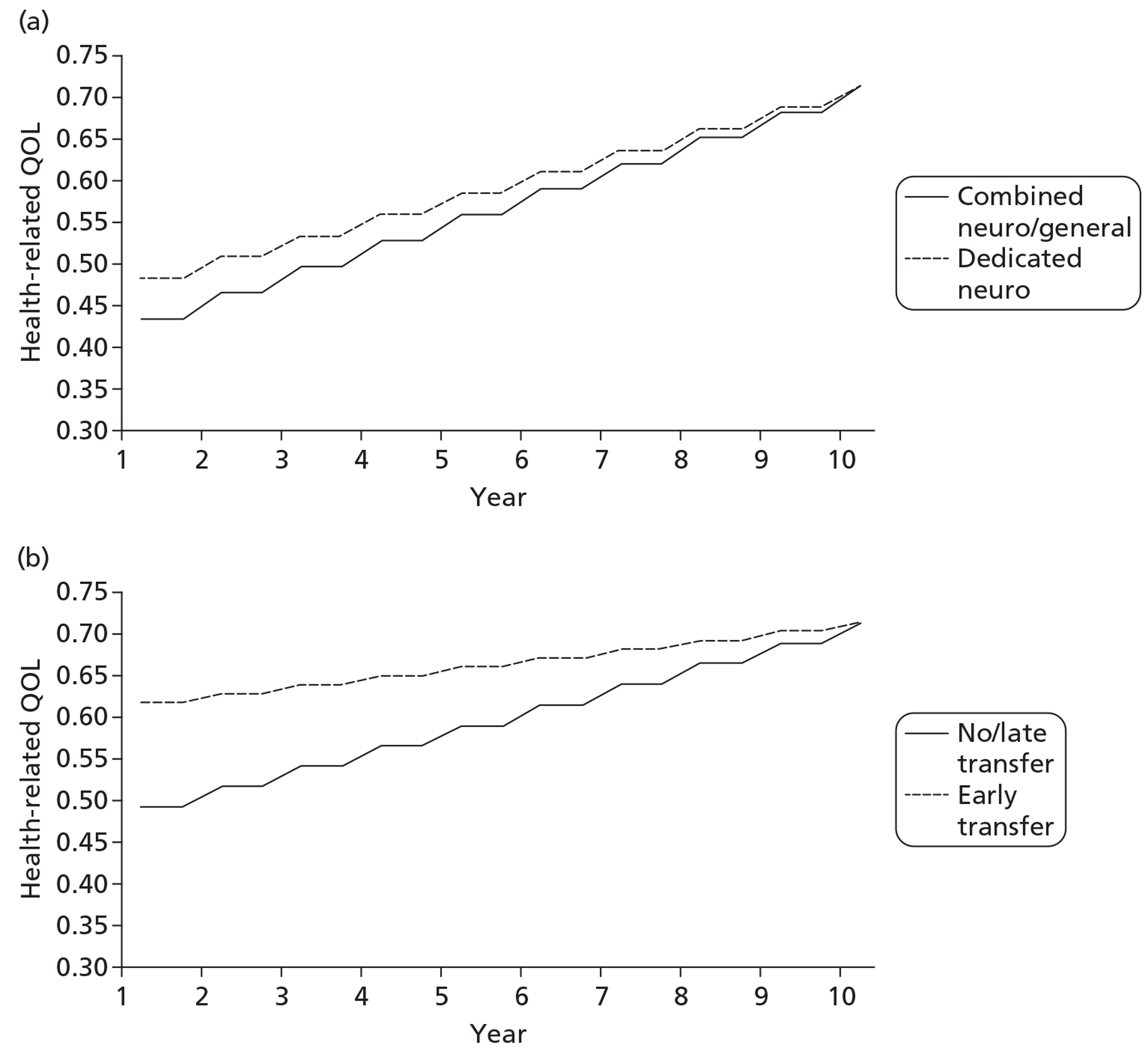
For each individual, we combined their predicted life expectancy (see Long-term survival), with their predicted QOL over time to give their projected lifetime QALY.
Long-term costs
The lifetime CEA also required costs to be projected for each individual for those surviving beyond 6 months. We reviewed the literature but did not find any relevant information on the relative costs of alternative care locations for patients who were alive 6 months after TBI. We therefore considered long-term costs based on the costs estimated in the RAIN study at 6 months. The costs considered fell into four categories: inpatient costs incurred after admission to critical care, inpatient costs on general wards, outpatient costs and community care costs.
We calculated inpatient costs incurred after critical care admission by recording ongoing admissions and readmissions to critical care units that participated in the RAIN study between 6 and 12 months after the TBI. We also considered admissions to critical care units that did not participate in the RAIN study but were included in the CMP, and to critical care units that participated in the CMP after patient recruitment to the RAIN study ended. The mean costs for each comparator group were then calculated for those patients who survived at least 6 months and were not censored between 6 and 12 months. These mean costs were used to impute mean costs between 6 and 12 months for the censored observations. For each comparator, these mean costs were relatively small (Tables 50 and 51). After 1 year it was assumed that there were no further readmissions to critical care that were attributable to the original TBI.
Source of costs | Measurement time frame | Combined neuro/general critical care unit | Dedicated neurocritical care unit | Assumptions: base case (sensitivity analysis) |
---|---|---|---|---|
Critical care admissionsa | 6–12 months | 207 (794) | 398 (1602) | 0 after 12 months |
General medical admissionsb | Discharge to 6 months | 1410 (4828) | 1478 (5093) | For 3 years (for 5 years) |
Outpatient visitsb | Discharge to 6 months | 337 (815) | 303 (653) | For 3 years (for 5/10 years) |
Community careb | Discharge to 6 months | 176 (382) | 188 (351) | For 3 years (for 5/10 years) |
Source of costs | Measurement time frame | ‘No or late’ transfer to neuroscience centre | ‘Early’ transfer to neuroscience centre | Assumptions: base case (sensitivity analysis) |
---|---|---|---|---|
Critical care admissionsa | 6–12 months | 77 (647) | 497 (2106) | 0 after 12 months |
General medical admissionsb | Discharge to 6 months | 1059 (3459) | 1809 (4925) | For 3 years (for 5 years) |
Outpatient visitsb | Discharge to 6 months | 211 (359) | 300 (472) | For 3 years (for 5/10 years) |
Community careb | Discharge to 6 months | 168 (361) | 221 (326) | For 3 years (for 5/10 years) |
For the other cost categories, we took each individual's inpatient, outpatient and community costs up to 6 months estimated from the Health Services Questionnaires. For each comparator arm we then reported the mean cost between initial hospital discharge and the 6-month time point but only for those surviving up to 6 months. We then applied these 6 monthly costs for each survivor to each subsequent 6-month period up to 3 years. After 3 years we assumed that there were no further costs attributable to the acute TBI (see Sensitivity analyses).
Base-case analysis
We reported mean lifetime costs (£) and QALYs per patient for each comparison group by predicting costs and outcomes for each individual as described above. Each incremental effect was reported using the risk factors from the IMPACT Lab risk prediction model to adjust for case mix. For research objective 1, incremental QALYs and costs were estimated with bivariate multilevel models to allow for clustering and any correlation between the end points. 133,134 For research objective 2, the correlation between the end points was recognised with Seemingly Unrelated Regression. 142
For each research objective, incremental net monetary benefits (INBs) were estimated by valuing incremental QALYs at a threshold of £20,000 per QALY and subtracting from this the incremental costs. For each lifetime end point (costs, QALYs and INBs) we used bivariate regression analysis to recognise correlation between individuals' costs and QALYs.77 Each of these regression models was applied to each of the 25 imputed data sets from the analysis of the 6-month end points (see Analysis of 6-month costs and consequences). We again combined the resultant estimates of the incremental effectiveness, costs and cost-effectiveness with Rubin's rules. Hence, CIs for incremental costs, QALYs and INBs again recognised the within- and between-imputation variation. Cost-effectiveness acceptability curves (CEACs) were calculated by reporting the probability that each alternative was the most cost-effective (i.e. had a positive INB) at different levels of willingness to pay for a QALY gain (£0−50,000 per QALY gained). For research objective 2, analyses were repeated for the previously defined subgroups using stratified analyses.
Sensitivity analyses
The base case made the following assumptions that, a priori, were judged to be potentially important: (a) costs attributable to the TBI were only incurred for up to 3 years; (b) the differences in unit costs across care locations represented genuine differences in resource inputs across settings; (c) QOL from the 6-month follow-up applied irrespective of the actual time of follow-up (which ranged from 4 to 10 months after initial presentation); (d) the QOL for survivors at 10 years post TBI was 15% of that of the general population; (e) transfers up to 18 hours following initial hospital presentation were categorised as ‘early’; (f) costs were normally distributed; (g) all-cause death rates applied from 6 months following hospital presentation; and (h) the IMPACT Lab risk prediction model was the most appropriate for case mix adjustment. The sensitivity analyses tested whether the base-case results were robust if the following alternative standpoints were taken:
-
Extending the period over which costs were attributable to the acute TBI To test whether the results were sensitive to the duration over which costs were attributable to the acute TBI, we repeated the analysis assuming that (i) inpatient costs in general medical wards, outpatient and community costs were incurred for 5 years, and (ii) outpatient and community costs continued for up to 10 years.
-
Using the same unit costs across all critical care locations We assumed that all critical care units in the RAIN study had the unit costs for combined neuro/general critical care units.
-
Limiting use of QOL data from the RAIN study to those patients whose QOL was measured at close to 6 months To assess the impact of only using QOL information from those patients in the RAIN study followed up between 150 and 220 days, we assumed that those patients who had QOL measured outside these time points had missing 6-month QOL and GOSE questionnaire data. We then applied a multiple imputation model to impute 6-month end points for these patients.
-
Assuming that the QOL decrement observed in RAIN was maintained Some studies have suggested that the level of residual disability reported at 12 months is maintained for 5–7 years. 75 Here we assumed that the decrement in QOL reported in the RAIN study at 6 months compared with the general population was maintained over the patients' lifetime.
-
Taking a stricter definition of ‘early’ transfer for research objective 2 To test whether or not the results for research objective 2 were robust to the arbitrary definition of ‘early’ transfer, we repeated the analyses defining an ‘early’ transfer as within 8 hours of hospital presentation and excluding patients transferred between 8 and 18 hours.
-
Assuming that individual patient costs were drawn from a gamma distribution compared with a normal distribution The assumption that costs are normally distributed may not be plausible,143 so here we allow costs to follow a gamma distribution. 144
-
Using a Gompertz survival function To test whether the results were robust to the choice of extrapolation approach we applied age-stratified death rates using a Gompertz survival function.
-
Undertaking risk adjustment with CRASH CT risk prediction model We reran the analytical models estimating incremental costs and QALYs using the variables from the CRASH CT model30 rather than the IMPACT Lab model35 to adjust for case mix.
Results of the lifetime cost-effectiveness analysis
Base-case results for research objective 1
The base-case lifetime cost-effectiveness results for the first research objective are shown in Table 52. Dedicated neurocritical care units had higher mean lifetime QALYs at small additional mean costs, with an incremental cost-effectiveness ratio (ICER) of approximately £14,000 per QALY. At a ceiling ratio of £20,000 per QALY the INB was positive (approximately £1300). The CEAC suggested that at ceiling ratios of £20,000 to £30,000 per QALY, the probability that dedicated compared with combined neurocritical care units are cost-effective for patients following acute TBI, is around 60% (Figure 36).
Parameter | Combined neuro/general critical care unit | Dedicated neurocritical care unit | Incremental effecta (95% CI) |
---|---|---|---|
Lifetime costs | 31,007 (22,471) | 34,909 (26,834) | 3167 (−464 to 6797) |
Lifetime QALYs | 9.49 (6.52) | 9.99 (6.56) | 0.224 (−0.332 to 0.780) |
Lifetime cost per QALY | 14,128 | ||
INBb | 1316 (−9857 to 12,489) |
FIGURE 36.
Probability that care following acute TBI is more cost-effective in a dedicated neurocritical care unit vs a combined neuro/general critical care unit at alternative levels of willingness to pay for a QALY gain.
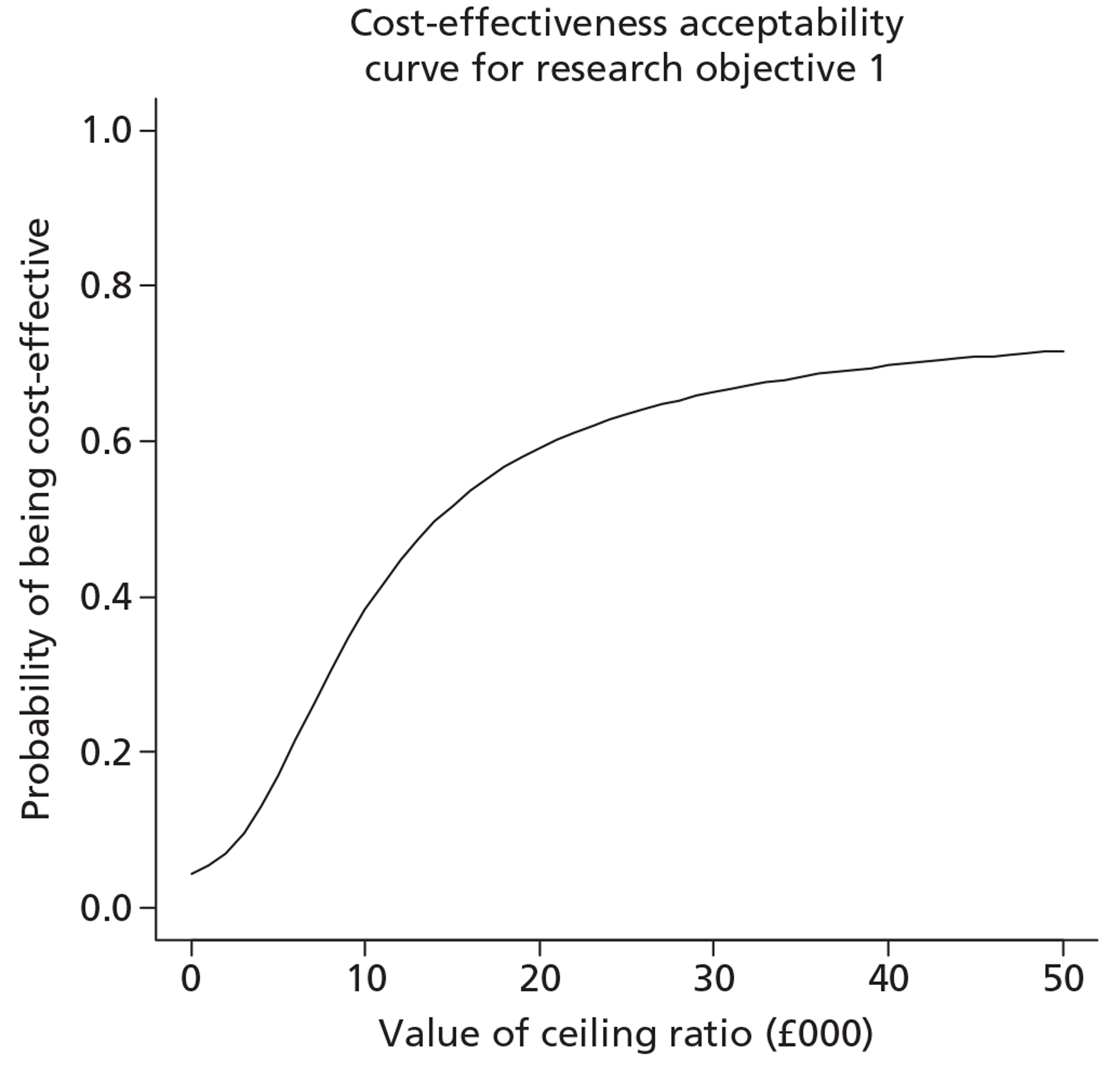
Sensitivity analysis results for research objective 1
The sensitivity analysis shows that the base-case finding, that dedicated critical care units were on average more cost-effective, is robust to the alternative approaches taken (Figure 37). Where the higher mean QOL for the dedicated neurocritical compared with the combined units observed in the RAIN study is maintained for the lifetime, the positive incremental QALY and INB in favour of the dedicated units increased. Here, sampling uncertainty is greater than in the base case as this approach recognises more fully individual-level variation in QOL across the sample. However, as for the other scenarios considered, the point estimate of the INB is consistent with that reported in the base case.
FIGURE 37.
Sensitivity analyses reporting mean (95% CI) INB comparing care for dedicated neurocritical care units vs combined neuro/general critical care units. Vertical dashed line indicates incremental net benefits in the base case analysis. Solid vertical line indicates no difference in net monetary benefits between comparator groups.
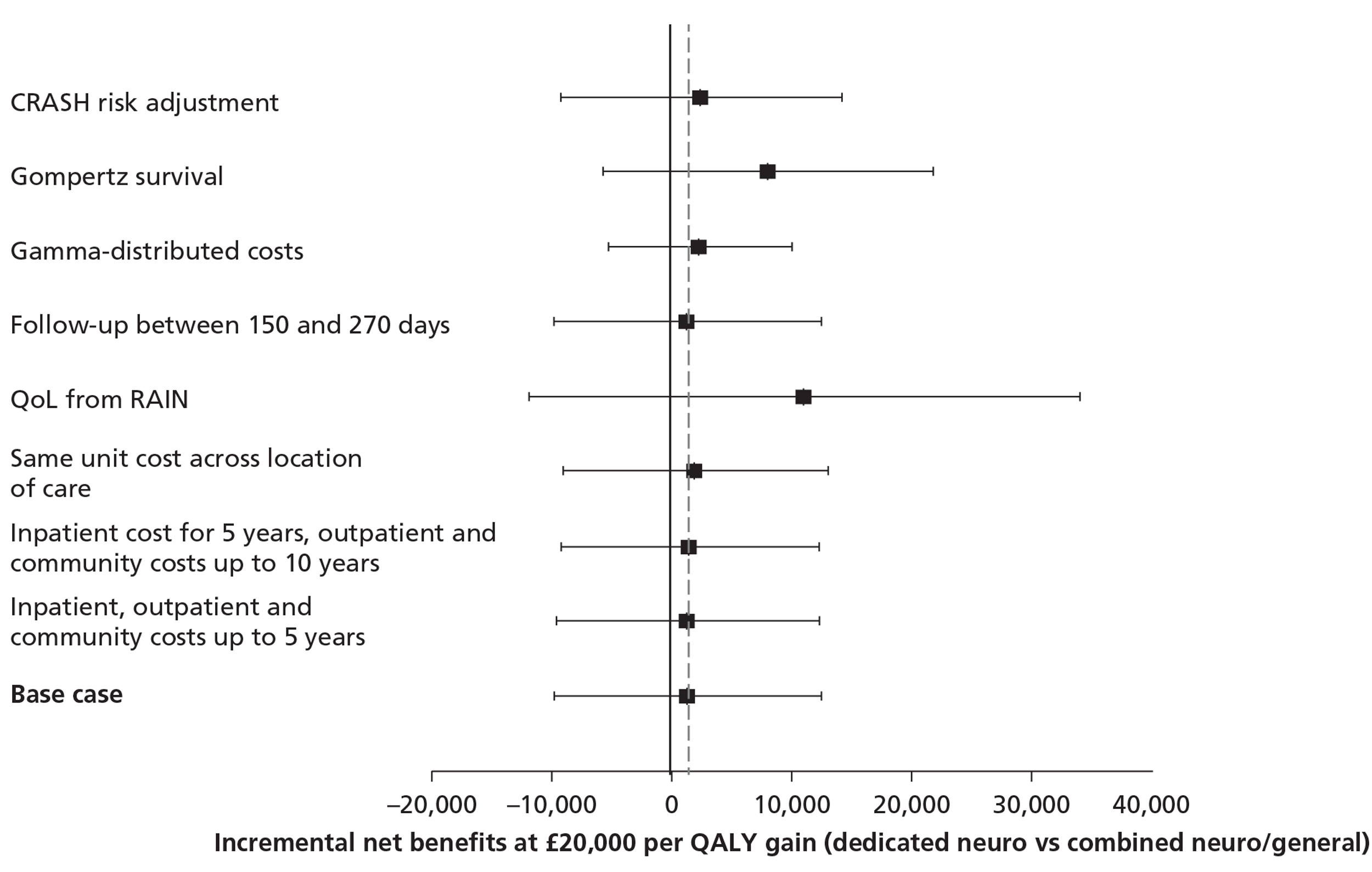
Base-case results for research objective 2
Table 53 reports the base-case results for the lifetime CEA comparing ‘early’ transfer compared with ‘no or late’ transfer to a neuroscience centre.
Parameter | ‘No or late’ transfer to neuroscience centre | ‘Early’ transfer to neuroscience centre | Incremental effecta (95% CI) |
---|---|---|---|
Lifetime costs | 16,105 (15,041) | 36,422 (28,030) | 19,209 (15,234 to 23,184) |
Lifetime QALYs | 7.19 (6.88) | 11.55 (6.43) | 1.795 (1.049 to 2.541) |
Lifetime cost per QALY | 10,704 | ||
INBb | 16,682 (2574 to 30,791) |
The results show that, after adjusting for differences in observed baseline characteristics the ‘early’ transfer group reported higher lifetime QALYs, at an additional cost, with an ICER of approximately £11,000 per QALY. The CEAC suggested that at the standard thresholds of £20,000–30,000 per QALY, the probability that early transfer was cost-effective is close to 100% (Figure 38).
FIGURE 38.
Probability that ‘early’ vs ‘no or late’ transfer is cost-effective at alternative levels of willingness to pay for a QALY gain.
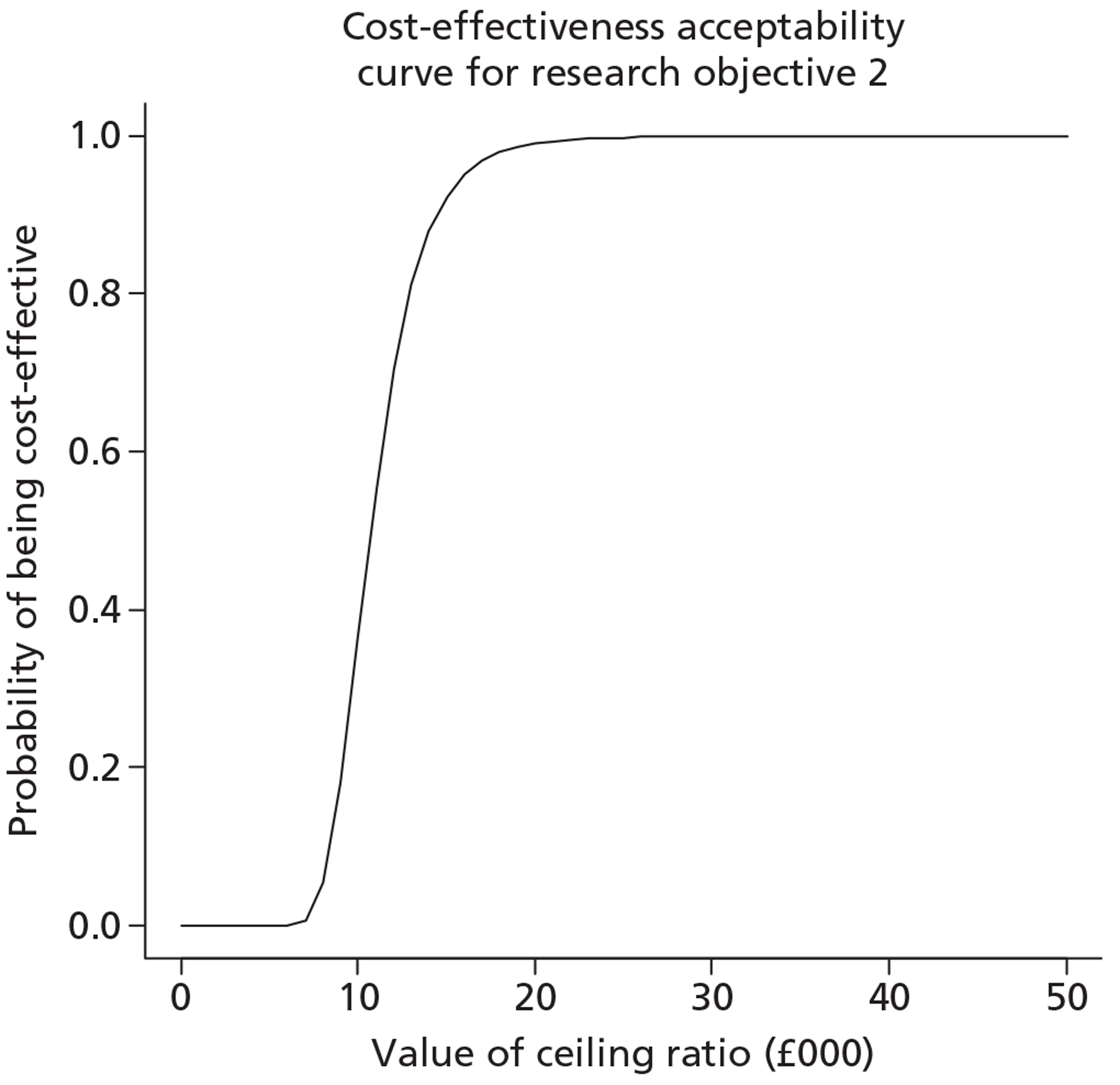
Sensitivity analysis results for research objective 2
The sensitivity analysis shows that the base-case cost-effectiveness results are relatively robust to the alternative methodological standpoints considered; the INB is broadly similar across scenarios (Figure 39). The additional scenario considered for this objective was to limit the sample for the ‘early’ transfer group to those patients who were transferred within 8 hours of presentation. Tightening the criteria for ‘early’ transfer led to slightly higher incremental QALYs and increased the cost-effectiveness of ‘early’ transfer.
FIGURE 39.
Sensitivity analyses reporting mean (95% CI) INB comparing care following TBI for ‘early’ vs ‘no or late’ transfer to a neuroscience centre. Vertical dashed line indicates incremental net benefits in the base case analysis. Solid vertical line indicates no difference in net monetary benefits between comparator groups.
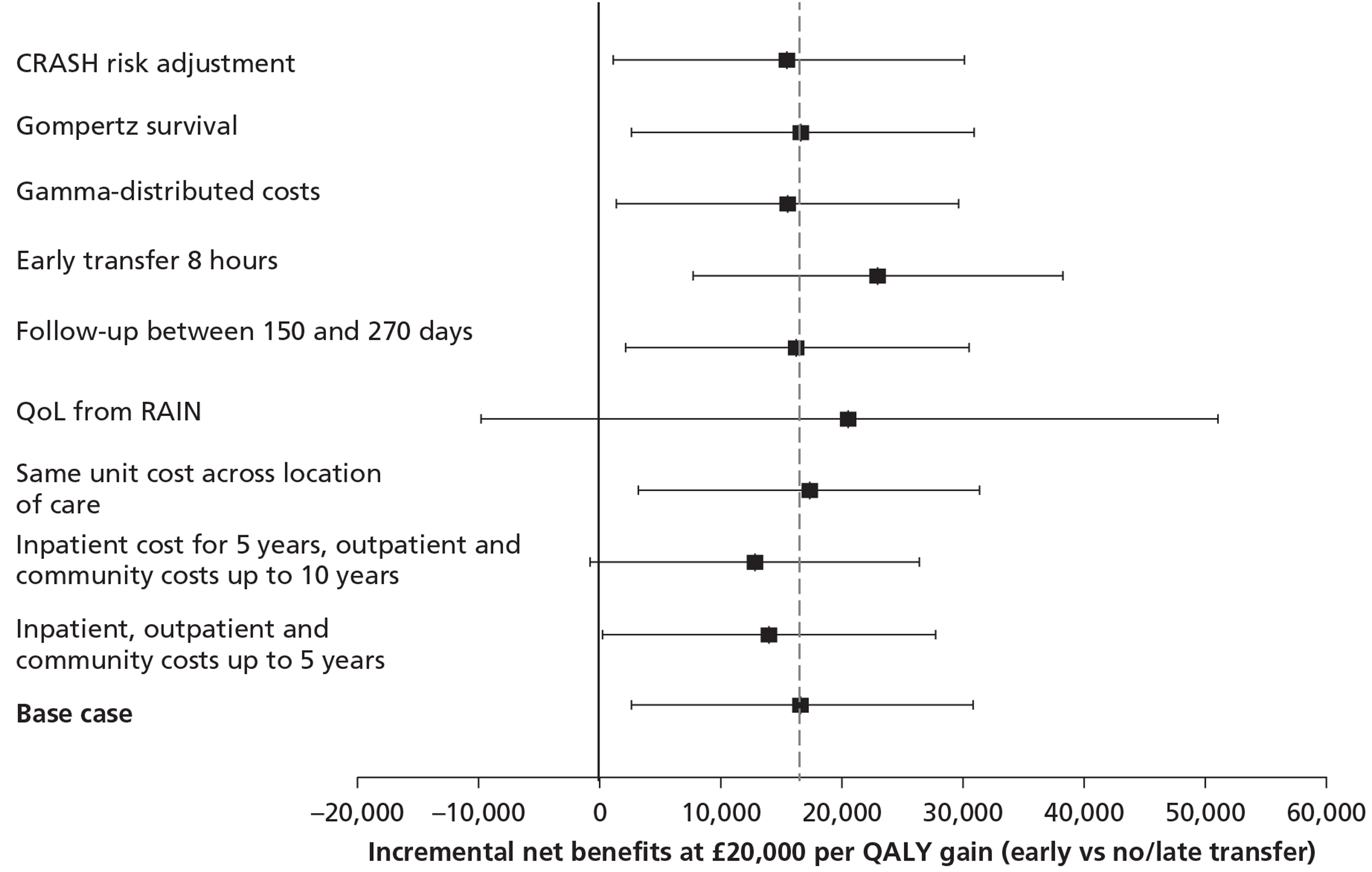
Subgroup analysis results for research objective 2
The results for the subgroup analysis according to age group suggest that ‘early’ transfer has a very low probability of being cost-effective for patients aged > 70 years (Figure 40).
FIGURE 40.
Probability that ‘early’ vs ‘no or late’ transfer is cost-effective, by age group, at alternative levels of willingness to pay for a QALY gain.
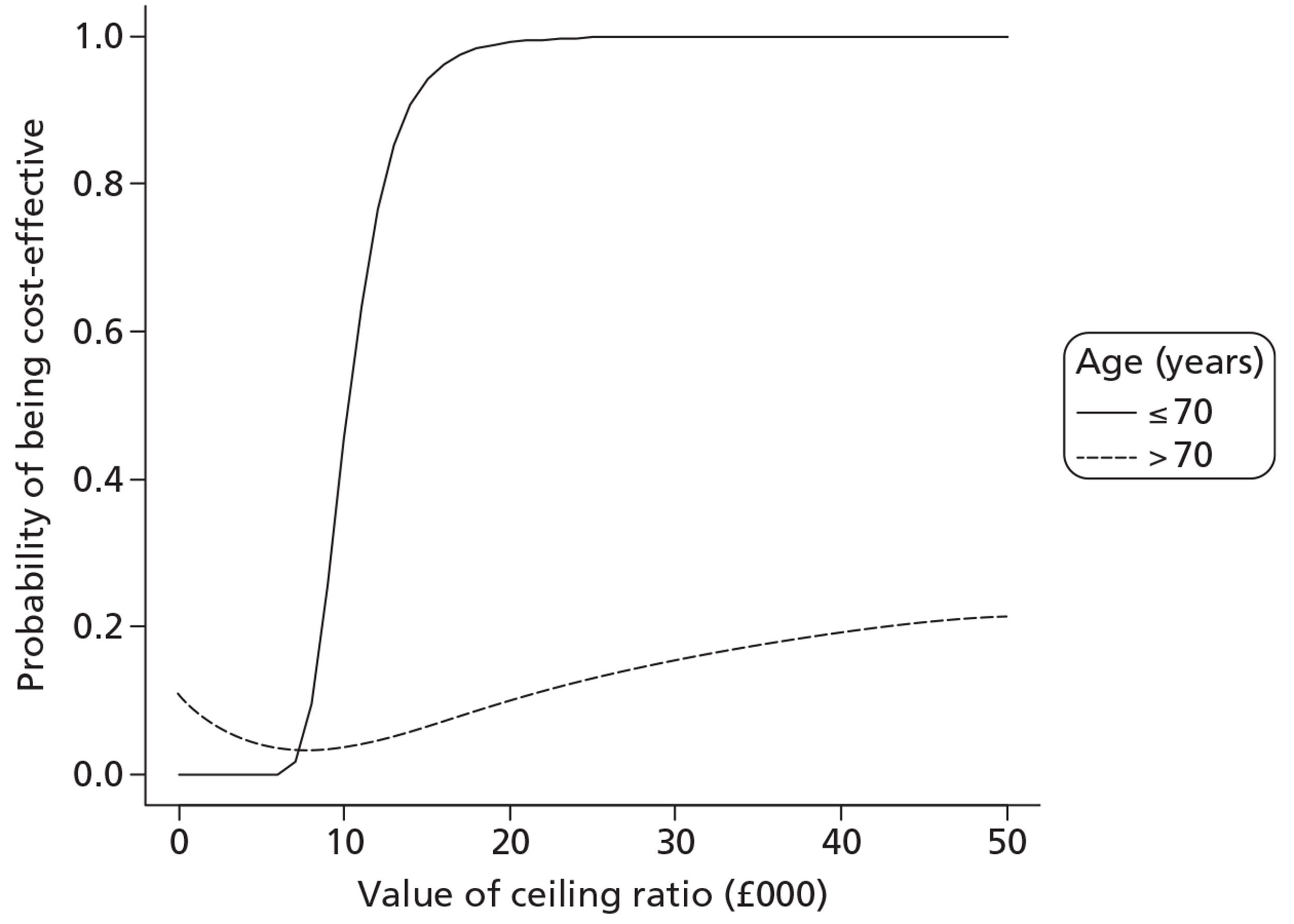
The probability that ‘early’ transfer is cost-effective is lower for the subgroup of patients defined as without major extracranial injury. Here the probability that ‘early’ transfer is cost-effective is around 60% compared with 100% for the subgroup defined as having a major extracranial injury (Figure 41).
FIGURE 41.
Probability that ‘early’ vs ‘no or late’ transfer is cost-effective, by presence or absence of major extracranial injury, at alternative levels of willingness to pay for a QALY gain.
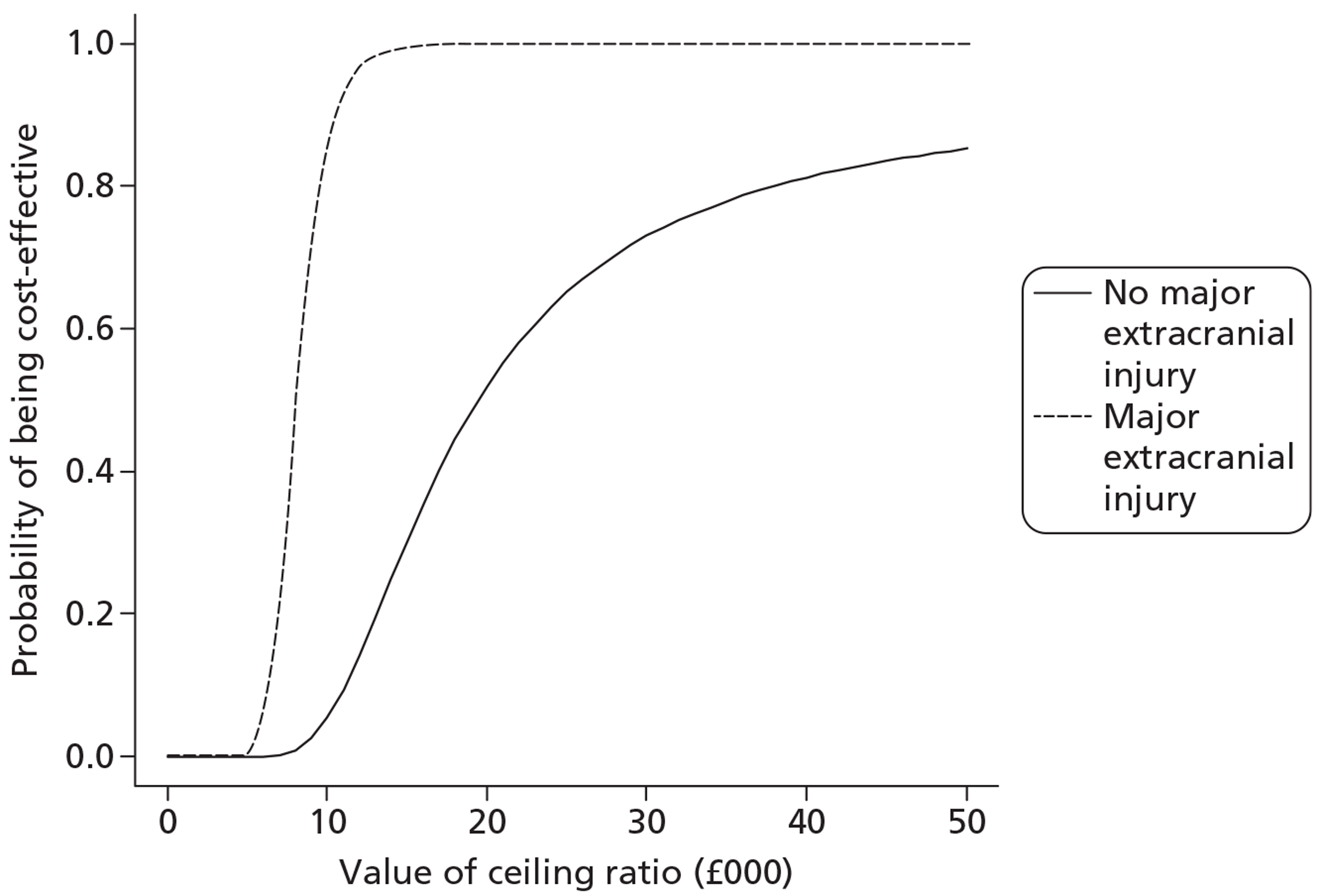
‘Early’ transfer appears most cost-effective for patients with severe TBI according to the baseline GCS score; the probability that ‘early’ transfer is relatively cost-effective is between 60% and 80% at ceiling ratios of between £20,000 and £30,000 per QALY (Figure 42).
FIGURE 42.
Probability that ‘early’ vs ‘no or late’ transfer is cost-effective, by baseline level of TBI severity, at alternative levels of willingness to pay for a QALY gain.
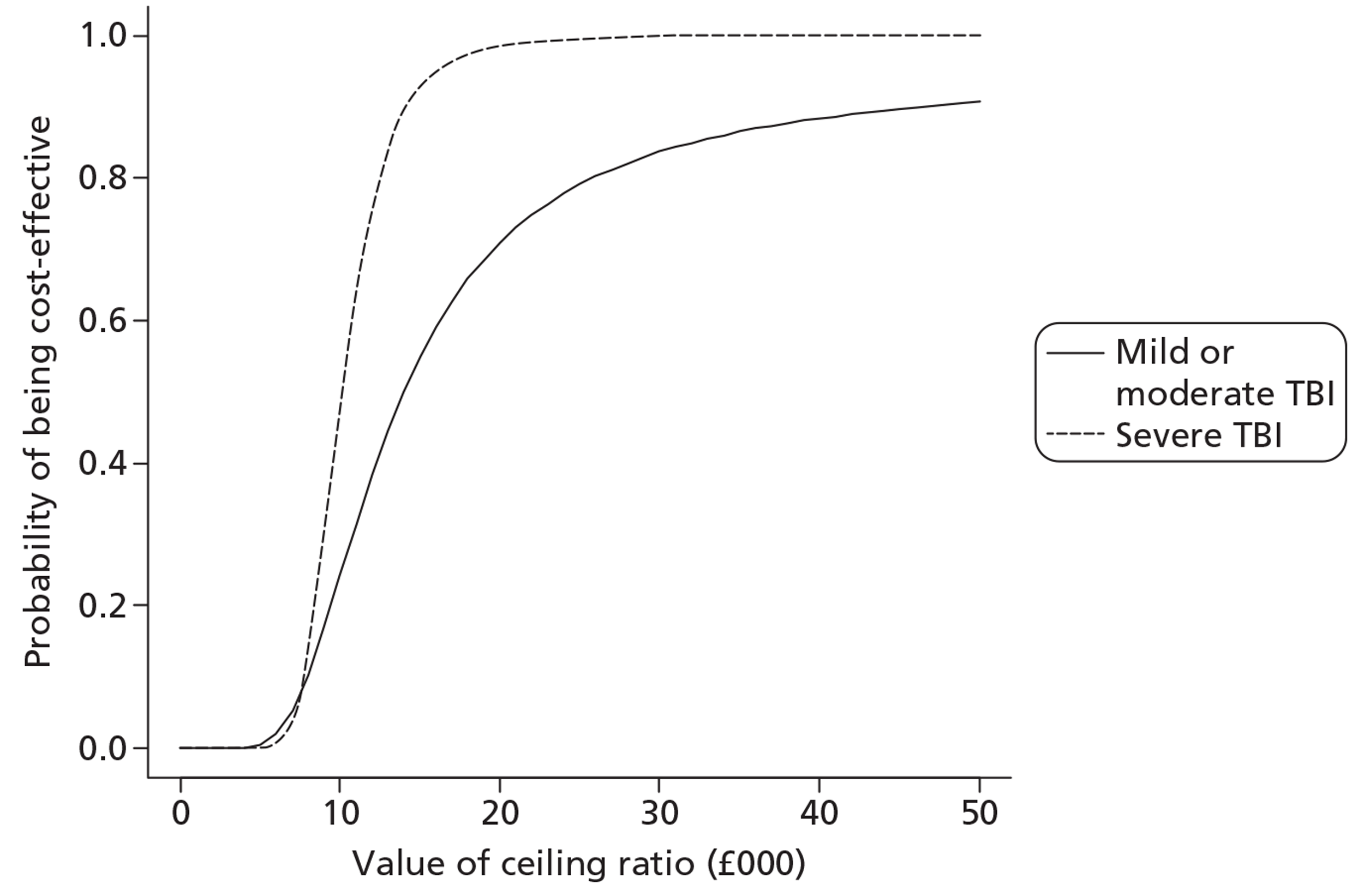
Discussion
Principal findings
This aspect of the study had two research objectives. The first of these was to compare the relative costs, consequences and cost-effectiveness of management in a dedicated neurocritical care unit compared with a combined neuro/general critical care unit for critically ill adult patients with acute TBI who present at, or are transferred to, a neuroscience centre. The second objective was to compare the relative costs, consequences and cost-effectiveness of ‘early’ (within 18 hours of hospital presentation) transfer compared with no transfer or ‘late’ (after 24 hours) transfer, for critically ill patients with acute TBI who initially present at a non-neuroscience centre and do not require surgery for evacuation of a mass lesion within 24 hours. The main findings are that, following case mix adjustment using the variables from the IMPACT Lab model,35 dedicated neurocritical care units lead to higher mean QOL than combined units but considerable statistical uncertainty surrounds this result; and dedicated units are associated with additional costs. The cost-effectiveness results suggest that it is highly uncertain that dedicated neurocritical care units are more cost-effective than combined units; the probability that dedicated units are more cost-effective is around 60%. For patients presenting at non-neuroscience centres who do not require immediate neurosurgery, we find that, after risk adjustment, early transfer is associated with gains in survival, and improvements in mean QOL for survivors. Although early transfer is also associated with higher costs, these appear to be justified by the relatively large QALY gains, with an incremental cost per QALY of around £11,000. Hence, at the £20,000 per QALY threshold typically used by NICE, the probability that ‘early’ transfer is cost-effective is close to 100%. Although this finding is robust to all the alternative assumptions considered, the potential role for unobserved confounding must be recognised.
Meaning of the study and comparison with previous studies
This study extends the previous literature on the relative costs and consequences of alternative locations of neurocritical care. 107–109 The finding that there is, on average, an improvement in risk-adjusted QOL if patients with acute TBI are managed in a dedicated neurocritical care unit rather than a combined neuro/general critical care unit has several possible explanations. First, a dedicated multidisciplinary team may provide more effective and immediate rehabilitation as part of acute care; they may also improve access to specialised neurorehabilitation after discharge from the unit, which can lead to improved outcomes. 145 Second, dedicated units may provide more aggressive monitoring and management, which have been suggested to reduce morbidity. 16 Although concerns have also been raised that more invasive approaches may increase mortality,116 the RAIN study did not find any differences in mortality at 6 months after TBI between patients managed in dedicated and combined units. A third explanation is that the differences in the mean QOL between the settings may simply reflect chance variation, and indeed the CIs include a difference of zero, and so the null hypothesis that there is no difference in QOL between the settings cannot be rejected. That said, we followed standard methods guidance for CEA, and the lifetime analysis incorporated the non-significant difference in the mean QOL observed at 6 months. We also made the conservative assumption that the gain in mean QOL for the dedicated neurocritical care units attenuated over time. Fourth, the improvement in average QOL for TBI patients managed in dedicated neurocritical care units rather than combined units could reflect unmeasured confounding between the settings. For this comparison, the baseline characteristics of the patients were similar between the groups. However, the mean improvement in QOL in favour of dedicated units is fairly small, and could be overturned if a factor associated with poor prognosis was somewhat more prevalent in the dedicated compared with combined units.
In this context, it is useful to consider the differences in patterns of care between specialist neurocritical care units and neuro/general critical care units that were perceived to exist at the study outset. Then, the published literature suggested there were between-unit differences in the ability to deliver protocol driven critical care according to expert guidelines. 13 However, since then, the dissemination of perceived best practice (e.g. through NCCNet) may have reduced clinical practice variation among neuroscience centres. This may explain why any differences observed in costs and consequences according to dedicated compared with combined neuro/general critical care are small.
The finding that early transfer to a neuroscience centre for TBI patients appears cost-effective is driven by the gains in survival and QOL observed in the RAIN study at 6 months after hospital presentation. Previous work has found that there are benefits from early transfer for patients who have a space-occupying haematoma with worsening mass effect,115 but this study extends the evidence base to other critically ill patients with TBI. Previous research raised conflicting hypotheses, with some studies suggesting that, for non-surgical patients, early transfer and subsequently more aggressive management may lead to increase risks and costs that outweigh any gains,119 whereas other studies suggested that, delayed transfer may lead to worse outcomes. 120 In the RAIN study, only a small proportion (6%) of the ‘no or late’ transfer group had a delayed transfer. Hence, any detrimental effects from delayed transfer would be unlikely to explain the findings, and the main contrast is between an intention to transfer the TBI patient to a neuroscience centre compared with continuing management at a non-neuroscience centre.
The definition of an ‘early’ transfer is clearly arbitrary, and may differ across contexts. For the RAIN study it was judged important to define the time point at which an ‘early’ transfer was made according to what was broadly appropriate for the NHS context. Hence, in advance of any analysis, the RAIN Study Steering Group defined an ‘early’ transfer as being within 18 hours of hospital presentation. This pragmatic choice of cut-off time recognised that there may be several alternative care pathways that patients may take from initial hospital presentation to transfer to a neuroscience centre. The chosen cut-off time also recognised that even if an early decision to transfer is made, there may be local logistical barriers to an immediate transfer. Hence, an 18 hours time point was chosen to be conservative and included all admissions where there was ‘an intention’ to made a rapid transfer. A potential concern is that the relative cost-effectiveness of an ‘early’ transfer policy could be highly sensitive to the cut-off point, and indeed previous evidence from trauma networks encourages a 4-hour time window, but for neurosurgical interventions. 101 Our sensitivity analysis suggested that applying an 8-hour rather than an 18 hours time window from hospital presentation, reduced the incremental cost per QALY for early transfer from £11,000 (base case) to £9000. Hence, the overall finding that ‘early’ transfer appears cost-effective is not sensitive to the choice of time threshold.
Our evaluation considered the relative costs and consequences of ‘early’ transfer according to alternative pre-defined subgroup analyses. The results suggest that ‘early’ transfer is relatively cost-effective, both for patients defined as having mild or moderate TBI (GCS score of 9–14) as well as for patients with severe TBI (GCS score of 3–8) at admission. Patients aged > 70 years have a higher odds ratio of death in the ‘early’ transfer group. For this subgroup the most cost-effective strategy appears to be to keep those patients presenting at a non-neuroscience centre within that setting. The effect of ‘early’ transfer on mortality appears to differ according to whether or not patients have a major extracranial injury. We observed large and significant gains from ‘early’ transfer in the subgroup of patients with major extracranial injury. However, for patients without a major extracranial injury, the improvements in mortality and QOL following ‘early’ transfer did not reach statistical significance, and we are, hence, unable to conclude that ‘early’ transfer of patients in this subgroup is cost-effective.
The relative gains from ‘early’ transfer in the subgroup with major extracranial injury are somewhat counterintuitive as it might be expected that the risks of physiological derangement associated with transfer would be greater in this subgroup. One possible explanation is that the presence of significant extracranial injury may represent a marker of a disease mechanism or injury type that predisposes patients to develop the pathophysiological derangements (such as severe intracranial hypertension) that most benefit from the specialist critical care available at a neuroscience centre. For example, high-speed RTAs may not only tend to cause more extracranial injury, but also may predispose to traumatic axonal injury and subdural haemorrhage, and enhance the benefits of specialist critical care. Another explanation is that the differential gains may reflect the broad definition of major extracranial injury adopted in the RAIN study. Specifically, patients who would not have met a more rigorous definition of major extracranial injury may have been misclassified as having significant extracranial injury. These patients may be at relatively low risk of death following transfer. This could have been coupled with the retention of unstable patients with truly significant extracranial injury in referring hospitals, which might be undetected by the RAIN study, as we had no metric of the severity of extracranial trauma. The net effect of such misclassification and inadequate severity characterisation may be to overstate the cost-effectiveness of early transfer in those defined as having major extracranial injury and understate the gains in the subgroup defined as not have major extracranial injury. A final explanation is that there may be residual confounding in the major extracranial injury stratum. This subgroup may have included patients who, according to unmeasured prognostic factors, were ‘too unstable for transfer’. Further research would ideally record such potential confounders and use them as a basis for excluding such patients from the decision problem. That is, the population of interest should only include patient groups where there is equipoise as to the costs and benefits of ‘early’ transfer.
Strengths and weaknesses
Lifetime CEAs requires assumptions to be made about the long-term mortality, cost and QOL following alternative interventions – in this case, care locations. As the RAIN study was only designed to measure survival and QOL for all eligible patients up to 6 months, we carefully considered alternative ways of extrapolating from these data to the lifetime. There is no consensus from the literature on the size or the duration of the impact that an acute TBI has on mortality, QOL or costs. More specifically, estimates from the RAIN study provided the strongest basis for projecting the relative effects of alternative care locations on mortality, QOL and health and personal social service costs. Hence, in the base case, information from the RAIN study was used to support assumptions about the long-term prognosis for patients following a TBI, according to the location of critical care.
A potential issue raised by the results of the RAIN study is that few deaths were reported between 30 days and 1 year after hospital presentation; in general, the death rates reported were either similar to or lower than the age-/sex-matched general population. Rather, than just choosing the extrapolation approach that appeared to best fit the observed data, we considered which extrapolation approach would be most plausible according to the previous literature. Other studies have reported excess deaths for patients at 5–7 years following a TBI,146–149 whereas a more relevant study that included patients admitted to critical care following TBI observed only one death between 6 and 12 months out of over 400 survivors at 6 months. 73 In the base-case analysis we judged that the most reasonable extrapolation approach was to apply all-cause death rates from 6 months onwards. Then, to examine whether the results would be robust to an alternative approach we ran sensitivity analyses in which we applied the most plausible and conservative of the parametric extrapolations (using the Gompertz function). This sensitivity analysis led to small differences in the ICER – for example, for research objective 2, the base-case ICER reduced marginally from £10,704 to £10,691. More, generally, the sensitivity analyses suggested that the findings did not appear to change substantively when alternative assumptions were made about the time period over which costs attributable to the TBI were applied, or whether the decrement in QOL observed at 6 months was maintained over the lifetime.
Although the RAIN study has added to the literature on alternative care pathways following TBI, it does have some limitations. First, the RAIN study only measured costs and outcomes for up to 6 months; however, the sensitivity analysis suggests that results appear robust to the choice of alternative assumptions made in extrapolating the data over the lifetime. Second, the study followed methodological guidance and took a health and personal social services perspective, and so excluded any broader societal costs, for example from productivity losses or ongoing costs of rehabilitation or nursing home care borne by the patient. Third, about half of patients eligible for follow-up at 6 months were missing data on health service use and QOL, and these data were not missing completely at random. We tackled this issue by using state-of-the-art approaches to handling missing data, in that we used multiple imputation models that respected the hierarchical structure of the data. That said, such approaches assume that the data are missing conditional on baseline factors and other end point and process measures that are observed, hence if missingness is driven by unobserved prognostic factors this could have led to biased estimates.
Finally, the major concern in any such non-randomised comparison is residual confounding. Although our sensitivity analysis that used variables from the CRASH risk prediction model suggested that the results were robust to the choice of risk adjustment method, unobserved confounders may explain the differences in costs and consequences between the comparator groups. Specifically, the comparison of observed baseline characteristics between the ‘early’ and ‘no or late’ transfer groups suggests that the patients transferred early were of much less severe case mix according to observed factors. This raises the concern that unobserved confounders associated with worse outcome may also be more prevalent in the ‘no or late’ transfer group. Further research is required to consider alternative approaches for handling the potential impact of unobserved confounders. Such approaches may include collecting data on additional baseline measures anticipated to be important, considering more flexible risk prediction and matching methods, formal sensitivity analyses that investigate whether the findings are robust to unobserved confounding, and alternative approaches as instrumental variable estimation that purport to handle unobserved confounding.
Summary
In summary, this evaluation finds that for critically ill patients with acute TBI who present at, or are transferred to, a neuroscience centre, management in a dedicated neurocritical care unit rather than a combined neuro/general critical care unit is associated with small gains in QALYs, at additional costs, but it remains highly uncertain whether dedicated units are more cost-effective. Overall, for adult patients with acute TBI who present at a non-neuroscience centre and do not require neurosurgery within 24 hours, ‘early’ transfer appears more cost-effective than ‘no or late’ transfer to a neuroscience centre, after risk adjustment. However, further research is required that considers alternative approaches for handling differences in baseline characteristics between settings. Such research could draw on the framework and data presented in the RAIN study to address alternative comparisons, for example contrasting management in high-volume settings with management in low-volume settings, or comparing settings with relatively high proportions of patients with TBI with those with relatively low proportions.
Chapter 7 Conclusions
Implications for health care
The risk prediction models evaluated in the RAIN study demonstrated sufficient statistical performance to support their use in research studies, for example as a basis for stratification in RCTs, but fell some way below the level that would be required to recommend their use to guide individual patient decision-making, particularly where this is likely to be of a life-or-death nature, for example withdrawal of life-sustaining therapy. The lack of calibration, particularly for models predicting unfavourable outcome at 6 months, also currently limits their utility as a tool for communication; however, once recalibrated or improved, and if used appropriately, there may be scope for the use of risk predictions at the individual patient level both to aid communication between health-care providers and in communicating risks to patients and their families.
The RAIN study provides the most robust evidence to date supporting the current NICE clinical guideline that all patients with severe TBI (GCS score of 3–8) would benefit from transfer to a neuroscience centre, regardless of their need for neurosurgery. 11 Indeed, the results of the RAIN study suggest that this guideline should potentially also extend to patients with mild or moderate TBI (GCS score of 9–14) requiring critical care. The only exception to this was in patients aged > 70 years, for whom transfer was associated with a (non-significant) increased risk of death, and the most cost-effective strategy was management within the hospital at which they originally presented. However, if such a strategy is to be implemented then it will be necessary to review neurocritical care capacity and resourcing at neuroscience centres to ensure sufficient capacity is available to meet the additional workload this would entail.
Although the results of the RAIN study suggest that, within a neuroscience centre, management in a dedicated neurocritical care unit may be cost-effective compared with management in a combined neuro/general critical care unit, there was considerable statistical uncertainty and these results are not sufficiently strong to warrant any major reconfiguration of existing neurocritical care services.
In terms of public health implications, the high proportion of critical care admissions of patients with TBI associated with confirmed or suspected intoxication reported in the RAIN study, and subsequent substantial costs of care, reinforce the importance of public health messages and interventions to attempt to reduce this health-care burden.
Pre-hospital data reported in the RAIN study, particularly regarding neurological dysfunction, were often of poor quality; GCS score was documented for around three-quarters of patients, and pupil reactivity for only half of patients. Better systems are required to improve the routine documentation of pre-hospital data and ensure these form part of the permanent patient record.
Recommendations for future research
Recommendation 1: Further research should be undertaken to explore the potential to improve on the current risk prediction models for acute traumatic brain injury
Although the existing risk prediction models demonstrated acceptable discrimination for mortality at 6 months and, less so, for unfavourable outcome at 6 months, there appears to be considerable scope for further improvement. As a minimum, the risk prediction models for unfavourable outcome all require recalibration for use in a UK critical care setting. However, additional areas that may be explored, either within the RAIN study data set, by data linkage (e.g. with the TARN database), and/or by new prospective data collection include: consideration of mechanism of injury; incorporation of pupil size in addition to reactivity; addressing the severity and/or site(s) of major extracranial injury; incorporating additional data on the physiological response to injury; and consideration of genetic factors in the host response to injury. Development of new risk prediction models may consider both classical statistical methods and machine learning approaches, such as artificial neural networks. The results of the RAIN study should be revisited in light of any improvements to risk modelling. A new risk prediction model may also be incorporated into a web-based prediction tool, as has been done for the CRASH and IMPACT models, to facilitate its use in practice.
Recommendation 2: Further research is required to consider alternative approaches for handling the potential impact of unobserved confounders on the RAIN study results
In addition to improving the risk prediction models used to underpin the non-randomised comparisons, alternative methodological approaches to complement risk adjustment include matching methods, such as propensity matching or GenMatch. 150 Such approaches would be complementary to the risk adjustment methods described, in that matching could be used to pre-process the RAIN study data before applying risk adjustment. 151 To consider unobserved confounding, future research could also be undertaken using instrumental variable estimation152 and formal sensitivity analyses that investigate whether the findings are robust to unobserved confounding. 153
Recommendation 3: Options should be explored for continuing to follow up the RAIN study cohort to obtain data on long-term mortality, functional outcomes and quality of life
The RAIN study identified substantial gaps and discordance in the literature regarding the long-term outcomes of patients with acute TBI. The RAIN study database provides a large, representative cohort of critically ill adult patients following acute TBI. Continuing follow-up of the RAIN study cohort would provide much valuable information, both to strengthen the lifetime CEA and to address additional issues, such as accelerated late cognitive decline. However, this would require changes to the current research ethics and governance approvals for the RAIN study and, therefore, these should be addressed urgently.
Recommendation 4: Further research is required to better understand the alternative pathways of care for patients following acute TBI and the impact of these on costs and outcomes
The RAIN study addressed certain specific aspects of the alternative pathways of care for critically ill adult patients following acute TBI and many other aspects remain unexplored. The simple comparison of dedicated neurocritical care units compared with combined neuro/general critical care units may be expanded to model a volume–outcome relationship with the absolute number of patients with TBI admitted or a dose–response relationship with the proportion of admissions that are patients with TBI to any given neurocritical care unit (or neurosciences patients more generally), or to give consideration to variation across centres in the use of treatment protocols or particular interventions. Further analyses for patients presenting outside neuroscience centres may consider the effect of time to transfer on costs and outcomes – both for patients who require neurosurgery (for whom current guidelines often recommend transfer within 4 hours101) and those who do not. An alternative model of service delivery, not currently implemented in most regions of the UK, is bypass of local hospitals to transport patients with significant TBI directly to a neuroscience centre. A feasibility study for a cluster RCT on this intervention – the Head Injury Transportation Straight to Neurosurgery (HITS-NS) trial (www.hta.ac.uk/project/2223.asp) – is currently ongoing.
Recommendation 5: Further research should explore equity of access to post-critical care support for patients following acute traumatic brain injury
Improved access to specialist neurorehabilitation services has been identified as a potential driver of better outcomes reported from neuroscience centres. 145 However, provision of and access to such services varies and questionnaire responses in the RAIN study indicated much dissatisfaction with the continuity of care and provision of rehabilitation and follow-up after discharge from critical care. Future research should identify the regional and local variation in provision of post-critical care support for patients following acute TBI and consider the impact of such provision on subsequent recovery.
Finally, the RAIN study should inform all future research studies in the neurocritical care of adult patients following acute TBI through provision of reliable data for sample size calculations and exploratory analyses, and informing the choice of risk adjustment methods and data set design.
Acknowledgements
We wish to thank all the participants for taking part in the study, GPs and health-care professionals for their assistance in patient follow-up, SICSAG for providing access to their database, and TARN for providing data for verification of recruitment. We wish to thank NCCNet for adopting and supporting the study.
We wish to acknowledge the following members of staff from Addenbrooke's Hospital for their contribution to the substudy on inter-rater reliability of CT scan reporting: Mr Matthew Guilfoyle; Mr Adel Helmy; Natalie Hoyles; Mr Ibrahim Jalloh; Mr Angelos Kolias; Anne Manktelow; and Glenda Virgo.
A thank you also to all the staff at ICNARC, with special thanks to Ruth Canter; Sarah Corlett; Andrew Fleming; Rahi Jahan; Andre Selmer; Petra Selmer; Suzy Taljard; Louise Ward-Bergeman; and Ana Weller.
Contribution of authors
Dr David A Harrison (Senior Statistician) designed the study, contributed to the analysis and interpretation of the data, and drafted and critically reviewed the manuscript.
Dr Gita Prabhu (Study Co-ordinator) conducted and analysed the data for the systematic review, contributed to acquisition of the data and drafted the manuscript.
Dr Richard Grieve (Reader in Health Economics) designed the economic evaluation, contributed to the analysis and interpretation of the data, and drafted and critically reviewed the manuscript.
Dr Sheila E Harvey (CTU Manager and Senior Research Fellow, Health Services Research) contributed to the acquisition and interpretation of the data, and drafted and critically reviewed the manuscript.
Dr M Zia Sadique (Research Fellow, Health Economics) contributed to the analysis and interpretation of the data and drafted the manuscript.
Dr Manuel Gomes (Research Assistant, Health Economics) contributed to the analysis and interpretation of the data and drafted the manuscript.
Kathryn A Griggs (Statistical Research Assistant) contributed to the analysis and interpretation of the data and drafted the manuscript.
Emma Walmsley (Research Assistant) contributed to the acquisition and interpretation of the data and drafted the manuscript.
Professor Martin Smith (Consultant and Honorary Professor in Neuroanaesthesia and Neurocritical Care) conceived and designed the study, contributed to the acquisition and interpretation of the data and critically reviewed the manuscript.
Dr Paddy Yeoman (Consultant, Critical Care Medicine and Anaesthesia) contributed to the design of the study, acquisition and interpretation of the data and critically reviewed the manuscript.
Professor Fiona E Lecky (Clinical Professor in Emergency Medicine and Research Director of TARN) contributed to the design of the study, interpretation of the data and critically reviewed the manuscript.
Mr Peter JA Hutchinson (Senior Surgical Scientist and Honorary Consultant Neurosurgeon) contributed to the design of the study, acquisition and interpretation of the data and critically reviewed the manuscript.
Professor David K Menon (Professor of Anaesthesia and Consultant, Neurosciences Critical Care, and Research Lead for NCCNet) conceived and designed the study, contributed to the acquisition and interpretation of the data, and drafted and critically reviewed the manuscript.
Professor Kathryn M Rowan (Director of ICNARC and Honorary Professor of Health Services Research) conceived and designed the study, contributed to acquisition, analysis and interpretation of the data and critically revised the manuscript.
Risk Adjustment In Neurocritical care study Management Team
Dr Gita Prabhu (Study Co-ordinator); Andrew Craven (previous Research Administrator); Kathryn Griggs (Statistical Research Assistant); Dr David Harrison (Chief Investigator); Dr Sheila Harvey (CTU Manager/Senior Research Fellow); Phil Restarick (previous Research Co-ordinator); Professor Kathy Rowan (Director); Andrew Stenson (previous Research Manager); and Emma Walmsley (Research Assistant).
Risk Adjustment In Neurocritical care Study Steering Group
Professor Monty Mythen (Independent chair); Julie Bridgewater (Independent); Dr Richard Grieve; Mr Peter Hutchinson; Mr Jonathan Hyam (Independent); Professor Fiona Lecky; Professor David Menon; Professor Martin Smith; Dr Ian Tweedie (Independent); and Dr Paddy Yeoman.
Research staff at participating sites
We acknowledge that there have been many other individuals who made a contribution within the participating hospitals including administrative staff, doctors, nurses, radiologists and neurosurgeons. It is impossible to thank everyone personally; however, we would like to thank the following research staff:
D Menon, L Moore and J Outtrim (Addenbrooke's Hospital); H Black, R Jacob and C Smalley (Arrowe Park Hospital); S Chau, A Bowry, C Denniss, D Raw and M Reid (Barnsley District Hospital); M Sun Wai, E Bilton and H Chitty (Basildon Hospital); J Cupitt and S Baddeley (Blackpool Victoria Hospital); R Meacher and M Templeton (Charing Cross Hospital); R Wroth, A Jarvis and J Toms (Chesterfield Royal Hospital); J Bleasdale, J Edwards and A McCann (City Hospital and Sandwell General Hospital); N Robin and H Jeffrey (Countess of Chester Hospital); V Prasad and P Wakefield (Darent Valley Hospital); E Thomas, H McMillan and T Quintrell (Derriford Hospital); A Asumang and M Wain (Diana, Princess of Wales Hospital); A Manara and S Grier (Frenchay Hospital); A Gratrix and N Smith (Hull Royal Infirmary); I Littlejohn, J Powell and G Spurling (Hurstwood Park Neurological Centre); H Madder, J Smith and J Titchell (John Radcliffe Hospital); P Hopkins, J Dawson and D Hadfield (King's College Hospital); D Holden, M Coggon and S Fleming (King's Mill Hospital); J Adams, Z Beardow and S Elliot (Leeds General Infirmary); N Flint, K Clarkson and A Smith (Leicester Royal Infirmary); D Simpson and N Pinto (Medway Maritime Hospital); H Jones (Morriston Hospital); M Smith, B Boyd, H Burgess and F Chong (National Hospital for Neurology and Neurosurgery); S Edwards and C Hughes (Nevill Hall Hospital); S Fletcher and M Rosbergen (Norfolk and Norwich University Hospital); A Walder and K Jones (North Devon District Hospital); J Cuesta and M Dlamini (North Middlesex University Hospital); J Wilkinson, R Marsh and K Brough (Northampton General Hospital); T Gallacher, M Lycett, T Matthews and T Thornhill (Queen Elizabeth Hospital Birmingham and Selly Oak Hospital); R Jain and K Reid (Queen's Hospital, Romford and Royal Berkshire Hospital); P Yeoman, A Jarvis, J Litchfield and H Wright (Queen's Medical Centre); S Thornton (Royal Bolton Hospital); J Paddle and K Burt (Royal Cornwall Hospital); J Andrzejowski, A Pritchard and K Bouakline (Royal Hallamshire Hospital); A Vincent, C Higham, P Laws and H McConnell (Royal Victoria Infirmary – previously Newcastle General Hospital); R Protheroe (Salford Royal Hospital); R Sharawi and A Day (Scunthorpe General Hospital); R Lightfoot, K Linford, J Mitchell, R Oram and S Tollerfield (Southampton General Hospital); D Higgins and S Andrews (Southend University Hospital); SP Young and L Stewart (Southern General Hospital); P Razis and V Frazer (St George's Hospital); M Watters, K Challis and K Mayell (The Great Western Hospital); R Lewis, S Bell and A Kong (The Ipswich Hospital); J Wright and K Hugill (The James Cook University Hospital); K Blenk and L Everett (The James Paget Hospital); D Dutta, C McClements and J Power (The Princess Alexandra Hospital); A Guha, J Nolan, A Walker and K Williams (The Royal Liverpool University Hospital); V Verma, K Maitland and G Marshall (The Royal London Hospital); D Watson, J Bradshaw, K Flahive, R Jackson and G Ward (University Hospital Coventry); M Mostert and V Todman (University Hospital Lewisham); V Gupta (University Hospital of Hartlepool and University Hospital of North Tees); S Krueper, R Ahern and D Cartlidge (University Hospital of North Staffordshire); G Scholey, S Fernandez, N Haskins, S Shah and A Williams (University Hospital of Wales); C Whitehead, J Cater, D Davies, C Owen and D Watling (Walton Centre for Neurology & Neurosurgery); J Cardy, S Humphreys and C Swanevelder (West Suffolk Hospital); P Andrews and E Grant (Western General Hospital); F Keane and I Bird (Whipps Cross University Hospital); C Jones, S Dowling and A McCairn (Whiston Hospital); and, D Southern, V Cunningham and C Hirst (Wrexham Maelor Hospital).
Disclaimer
This report presents independent research funded by the National Institute for Health Research (NIHR). The views and opinions expressed by authors in this publication are those of the authors and do not necessarily reflect those of the NHS, the NIHR, NETSCC, the HTA programme or the Department of Health.
References
- Knaus WA, Zimmerman JE, Wagner DP, Draper EA, Lawrence DE. APACHE-acute physiology and chronic health evaluation: a physiologically based classification system. Crit Care Med 1981;9:591-7. http://dx.doi.org/10.1097/00003246-198108000-00008.
- Rowan KM, Kerr JH, Major E, McPherson K, Short A, Vessey MP. Intensive Care Society's APACHE II study in Britain and Ireland – I: Variations in case mix of adult admissions to general intensive care units and impact on outcome. BMJ 1993;307:972-7. http://dx.doi.org/10.1136/bmj.307.6910.972.
- Rowan KM, Kerr JH, Major E, McPherson K, Short A, Vessey MP. Intensive Care Society's APACHE II study in Britain and Ireland – II: Outcome comparisons of intensive care units after adjustment for case mix by the American APACHE II method. BMJ 1993;307:977-81. http://dx.doi.org/10.1136/bmj.307.6910.977.
- Harrison DA, Brady AR, Parry GJ, Carpenter JR, Rowan K. Recalibration of risk prediction models in a large multicenter cohort of admissions to adult, general critical care units in the United Kingdom. Crit Care Med 2006;34:1378-88. http://dx.doi.org/10.1097/01.CCM.0000216702.94014.75.
- Harrison DA, Parry GJ, Carpenter JR, Short A, Rowan K. A new risk prediction model for critical care: the Intensive Care National Audit & Research Centre (ICNARC) model. Crit Care Med 2007;35:1091-8. http://dx.doi.org/10.1097/01.CCM.0000259468.24532.44.
- Hyam JA, Welch CA, Harrison DA, Menon DK. Case mix, outcomes and comparison of risk prediction models for admissions to adult, general and specialist critical care units for head injury: a secondary analysis of the ICNARC Case Mix Programme Database. Crit Care 2006;10. http://dx.doi.org/10.1186/cc5066.
- Livingston BM, Mackenzie SJ, MacKirdy FN, Howie JC. Should the pre-sedation Glasgow Coma Scale value be used when calculating Acute Physiology and Chronic Health Evaluation scores for sedated patients? Scottish Intensive Care Society Audit Group. Crit Care Med 2000;28:389-94. http://dx.doi.org/10.1097/00003246-200002000-00017.
- Hayes JA, Black NA, Jenkinson C, Young JD, Rowan KM, Daly K, et al. Outcome measures for adult critical care: a systematic review. Health Technol Assess 2000;4.
- Perel P, Edwards P, Wentz R, Roberts I. Systematic review of prognostic models in traumatic brain injury. BMC Med Inform Decis Making 2006;6. http://dx.doi.org/10.1186/1472-6947-6-38.
- Menon DK, Zahed C. Prediction of outcome in severe traumatic brain injury. Curr Opin Crit Care 2009;15:437-41. http://dx.doi.org/10.1097/MCC.0b013e3283307a26.
- Head injury: triage, assessment, investigation and early management of head injury in infants, children and adults. London: National Institute for Health and Care Excellence; 2007.
- Neurocritical Care Stakeholder Group . Neurocritical care capacity and demand. Neurocritical Care Stakeholder Group 2006. www.bann.org.uk/cms/resources/uploads/File/Neurocritical%20Care%20Snapshot%20Audit%20-%20Final.pdf (accessed 7 March 2013).
- Menon D. Neurocritical care: turf label, organizational construct, or clinical asset?. Curr Opin Crit Care 2004;10:91-3. http://dx.doi.org/10.1097/00075198-200404000-00002.
- Smith M. Neurocritical care: has it come of age?. Br J Anaesth 2004;93:753-5. http://dx.doi.org/10.1093/bja/aeh275.
- Fuller G, Bouamra O, Woodford M, Jenks T, Patel H, Coats TJ, et al. The effect of specialist neurosciences care on outcome in adult severe head injury: a cohort study. J Neurosurg Anesthesiol 2011;23:198-205.
- Patel HC, Bouamra O, Woodford M, King AT, Yates DW, Lecky FE. Trends in head injury outcome from 1989 to 2003 and the effect of neurosurgical care: an observational study. Lancet 2005;366:1538-44. http://dx.doi.org/10.1016/S0140-6736(05)67626-X.
- Catroppa C, Anderson VA, Morse SA, Haritou F, Rosenfeld JV. Outcome and predictors of functional recovery 5 years following pediatric traumatic brain injury (TBI). J Pediatr Psychol 2008;33:707-18. http://dx.doi.org/10.1093/jpepsy/jsn006.
- Colantonio A, Escobar MD, Chipman M, McLellan B, Austin PC, Mirabella G, et al. Predictors of postacute mortality following traumatic brain injury in a seriously injured population. J Trauma 2008;64:876-82. http://dx.doi.org/10.1097/TA.0b013e31804d493e.
- Corrigan JD, Selassie AW, Lineberry LA, Millis SR, Wood KD, Pickelsimer EE, et al. Comparison of the Traumatic Brain Injury (TBI) Model Systems national dataset to a population-based cohort of TBI hospitalizations. Arch Phys Med Rehabil 2007;88:418-26. http://dx.doi.org/10.1016/j.apmr.2007.01.010.
- Dawson DR, Schwartz ML, Winocur G, Stuss DT. Return to productivity following traumatic brain injury: cognitive, psychological, physical, spiritual, and environmental correlates. Disabil Rehabil 2007;29:301-13. http://dx.doi.org/10.1080/09638280600756687.
- Devitt R, Colantonio A, Dawson D, Teare G, Ratcliff G, Chase S. Prediction of long-term occupational performance outcomes for adults after moderate to severe traumatic brain injury. Disabil Rehabil 2006;28:547-59. http://dx.doi.org/10.1080/00222930500219258.
- Ducrocq SC, Meyer PG, Orliaguet GA, Blanot S, Laurent-Vannier A, Renier D, et al. Epidemiology and early predictive factors of mortality and outcome in children with traumatic severe brain injury: experience of a French pediatric trauma center. Pediatr Crit Care Med 2006;7:461-7. http://dx.doi.org/10.1097/01.PCC.0000235245.49129.27.
- Feiz-Erfan I, Horn EM, Theodore N, Zabramski JM, Klopfenstein JD, Lekovic GP, et al. Incidence and pattern of direct blunt neurovascular injury associated with trauma to the skull base. J Neurosurg 2007;107:364-9. http://dx.doi.org/10.3171/JNS-07/08/0364.
- Hesdorffer DC, Ghajar J. Marked improvement in adherence to traumatic brain injury guidelines in United States trauma centers. J Trauma 2007;63:841-7. http://dx.doi.org/10.1097/TA.0b013e318123fc21.
- Hsiao KY, Hsiao CT, Weng HH, Chen KH, Lin LJ, Huang YM. Factors predicting mortality in victims of blunt trauma brain injury in emergency department settings. Emerg Med J 2008;25:670-3. http://dx.doi.org/10.1136/emj.2007.053405.
- Jagannathan J, Okonkwo DO, Yeoh HK, Dumont AS, Saulle D, Haizlip J, et al. Long-term outcomes and prognostic factors in pediatric patients with severe traumatic brain injury and elevated intracranial pressure. J Neurosurg Pediatr 2008;2:240-9. http://dx.doi.org/10.3171/PED.2008.2.10.240.
- Johnson P, Thomas-Stonell N, Rumney P, Oddson B. Long-term outcomes of pediatric acquired brain injury. Brain Cogn 2006;60:205-6.
- Liao CC, Hsu YP, Chen CT, Tseng YY. Transarterial embolization for intractable oronasal hemorrhage associated with craniofacial trauma: evaluation of prognostic factors. J Trauma 2007;63:827-30. http://dx.doi.org/10.1097/TA.0b013e31814b9466.
- Mauritz W, Janciak I, Wilbacher I, Rusnak M. Severe traumatic brain injury in Austria IV: intensive care management. Wien Klin Wochenschr 2007;119:46-55. http://dx.doi.org/10.1007/s00508-006-0763-2.
- MRC CRASH trial collaborators . Predicting outcome after traumatic brain injury: practical prognostic models based on large cohort of international patients. BMJ 2008;336:425-9. http://dx.doi.org/10.1136/bmj.39461.643438.25.
- Oh HS, Seo WS, Lee S, Song H. Comparisons of the prognostic predictors of traumatic brain injury according to admission Glasgow Coma Scale scores-based on 1- and 6-month assessments. Taehan Kanho Hakhoe Chi 2006;36:621-9.
- Pang BC, Kuralmani V, Joshi R, Hongli Y, Lee KK, Ang BT, et al. Hybrid outcome prediction model for severe traumatic brain injury. J Neurotrauma 2007;24:136-46. http://dx.doi.org/10.1089/neu.2006.0113.
- Pape TL, Lundgren S, Heinemann AW, Guernon A, Giobbie-Hurder A, Wang J, et al. Establishing a prognosis for functional outcome during coma recovery. Brain Inj 2006;20:743-58. http://dx.doi.org/10.1080/02699050600676933.
- Salorio CF, Slomine BS, Guerguerian AM, Christensen JR, White JR, Natale JE, et al. Intensive care unit variables and outcome after pediatric traumatic brain injury: a retrospective study of survivors. Pediatr Crit Care Med 2008;9:47-53. http://dx.doi.org/10.1097/01.PCC.0000298638.66240.0E.
- Steyerberg EW, Mushkudiani N, Perel P, Butcher I, Lu J, McHugh GS, et al. Predicting outcome after traumatic brain injury: development and international validation of prognostic scores based on admission characteristics. PLoS Med 2008;5. http://dx.doi.org/10.1371/journal.pmed.0050165.
- Stulemeijer M, van der WS, Borm GF, Vos PE. Early prediction of favourable recovery 6 months after mild traumatic brain injury. J Neurol Neurosurg Psychiatry 2008;79:936-42. http://dx.doi.org/10.1136/jnnp.2007.131250.
- Thompson HJ, Rivara FP, Jurkovich GJ, Wang J, Nathens AB, MacKenzie EJ. Evaluation of the effect of intensity of care on mortality after traumatic brain injury. Crit Care Med 2008;36:282-90. http://dx.doi.org/10.1097/01.CCM.0000297884.86058.8A.
- Xu XY, Liu WG, Yang XF, Li LQ. Evaluation of models that predict short-term outcome after traumatic brain injury. Brain Inj 2007;21:575-82. http://dx.doi.org/10.1080/02699050701426881.
- Zheng WB, Liu GR, Li LP, Wu RH. Prediction of recovery from a post-traumatic coma state by diffusion-weighted imaging (DWI) in patients with diffuse axonal injury. Neuroradiology 2007;49:271-9. http://dx.doi.org/10.1007/s00234-006-0187-8.
- Hollingworth W, Vavilala MS, Jarvik JG, Chaudhry S, Johnston BD, Layman S, et al. The use of repeated head computed tomography in pediatric blunt head trauma: factors predicting new and worsening brain injury. Pediatr Crit Care Med 2007;8:348-56. http://dx.doi.org/10.1097/01.PCC.0000270837.66217.3B.
- Maas AI, Stocchetti N, Bullock R. Moderate and severe traumatic brain injury in adults. Lancet Neurol 2008;7:728-41. http://dx.doi.org/10.1016/S1474-4422(08)70164-9.
- Nampiaparampil DE. Prevalence of chronic pain after traumatic brain injury: a systematic review. JAMA 2008;300:711-19. http://dx.doi.org/10.1001/jama.300.6.711.
- Rocker GM, Cook DJ, Shemie SD. Brief review: Practice variation in end of life care in the ICU: implications for patients with severe brain injury. Can J Anaesth 2006;53:814-19. http://dx.doi.org/10.1007/BF03022799.
- Saatman KE, Duhaime AC, Bullock R, Maas AI, Valadka A, Manley GT. Classification of traumatic brain injury for targeted therapies. J Neurotrauma 2008;25:719-38. http://dx.doi.org/10.1089/neu.2008.0586.
- Tagliaferri F, Compagnone C, Korsic M, Servadei F, Kraus J. A systematic review of brain injury epidemiology in Europe. Acta Neurochir 2006;148:255-68. http://dx.doi.org/10.1007/s00701-005-0651-y.
- Willemse-van Son AH, Ribbers GM, Verhagen AP, Stam HJ. Prognostic factors of long-term functioning and productivity after traumatic brain injury: a systematic review of prospective cohort studies. Clin Rehabil 2007;21:1024-37. http://dx.doi.org/10.1177/0269215507077603.
- Signorini DF, Andrews PJ, Jones PA, Wardlaw JM, Miller JD. Predicting survival using simple clinical variables: a case study in traumatic brain injury. J Neurol Neurosurg Psychiatry 1999;66:20-5. http://dx.doi.org/10.1136/jnnp.66.1.20.
- Hukkelhoven CW, Steyerberg EW, Habbema JD, Farace E, Marmarou A, Murray GD, et al. Predicting outcome after traumatic brain injury: development and validation of a prognostic score based on admission characteristics. J Neurotrauma 2005;22:1025-39. http://dx.doi.org/10.1089/neu.2005.22.1025.
- Marshall LF, Maas AI, Marshall SB, Bricolo A, Fearnside M, Iannotti F, et al. A multicenter trial on the efficacy of using tirilazad mesylate in cases of head injury. J Neurosurg 1998;89:519-25. http://dx.doi.org/10.3171/jns.1998.89.4.0519.
- Hukkelhoven CW, Steyerberg EW, Farace E, Habbema JD, Marshall LF, Maas AI. Regional differences in patient characteristics, case management, and outcomes in traumatic brain injury: experience from the tirilazad trials. J Neurosurg 2002;97:549-57. http://dx.doi.org/10.3171/jns.2002.97.3.0549.
- Murray GD, Teasdale GM, Braakman R, Cohadon F, Dearden M, Iannotti F, et al. The European Brain Injury Consortium survey of head injuries. Acta Neurochir 1999;141:223-36. http://dx.doi.org/10.1007/s007010050292.
- Marshall LF, Becker DP, Bowers SA, Cayard C, Eisenberg H, Gross CR, et al. The National Traumatic Coma Data Bank. Part 1: Design, purpose, goals, and results. J Neurosurg 1983;59:276-84. http://dx.doi.org/10.3171/jns.1983.59.2.0276.
- Roberts I, Yates D, Sandercock P, Farrell B, Wasserberg J, Lomas G, et al. Effect of intravenous corticosteroids on death within 14 days in 10008 adults with clinically significant head injury (MRC CRASH trial): randomised placebo-controlled trial. Lancet 2004;364:1321-8. http://dx.doi.org/10.1016/S0140-6736(04)17188-2.
- Edwards P, Arango M, Balica L, Cottingham R, El Sayed H, Farrell B, et al. Final results of MRC CRASH, a randomised placebo-controlled trial of intravenous corticosteroid in adults with head injury-outcomes at 6 months. Lancet 2005;365:1957-9. http://dx.doi.org/10.1016/S0140-6736(05)66552-X.
- Marmarou A, Lu J, Butcher I, McHugh GS, Mushkudiani NA, Murray GD, et al. IMPACT database of traumatic brain injury: design and description. J Neurotrauma 2007;24:239-50. http://dx.doi.org/10.1089/neu.2006.0036.
- Marshall LF, Marshall SB, Klauber MR, Van Berkum CM, Eisenberg H, Jane JA, et al. The diagnosis of head injury requires a classification based on computed axial tomography. J Neurotrauma 1992;9:287-92.
- Murray GD, Butcher I, McHugh GS, Lu J, Mushkudiani NA, Maas AI, et al. Multivariable prognostic analysis in traumatic brain injury: results from the IMPACT study. J Neurotrauma 2007;24:329-37. http://dx.doi.org/10.1089/neu.2006.0035.
- Black N. Why we need observational studies to evaluate the effectiveness of health care. BMJ 1996;312:1215-18. http://dx.doi.org/10.1136/bmj.312.7040.1215.
- Menon D, Harrison D. Prognostic modelling in traumatic brain injury. BMJ 2008;336:397-8. http://dx.doi.org/10.1136/bmj.39461.616991.80.
- Patel HC, Menon DK, Tebbs S, Hawker R, Hutchinson PJ, Kirkpatrick PJ. Specialist neurocritical care and outcome from head injury. Intensive Care Med 2002;28:547-53. http://dx.doi.org/10.1007/s00134-002-1235-4.
- Hanley JA. The robustness of the ‘binormal’ assumptions used in fitting ROC curves. Med Decis Making 1988;8:197-203. http://dx.doi.org/10.1177/0272989X8800800308.
- The CRASH trial management group . The CRASH trial protocol (Corticosteroid randomisation after significant head injury). BMC Emerg Med 2001;1. http://dx.doi.org/10.1186/1471-227X-1-1.
- Hutchinson PJ, Corteen E, Czosnyka M, Mendelow AD, Menon DK, Mitchell P, et al. Decompressive craniectomy in traumatic brain injury: the randomized multicenter RESCUEicp study. Acta Neurochir Suppl 2006;96:17-20. http://dx.doi.org/10.1007/3-211-30714-1_4.
- Edwards P, Roberts I, Clarke M, DiGuiseppi C, Pratap S, Wentz R, et al. Increasing response rates to postal questionnaires: systematic review. BMJ 2002;324. http://dx.doi.org/10.1136/bmj.324.7347.1183.
- The EuroQol Group . EuroQol: a new facility for the measurement of health-related quality of life. Health Policy 1990;16:199-208. http://dx.doi.org/10.1016/0168-8510(90)90421-9.
- Teasdale GM, Pettigrew LE, Wilson JT, Murray G, Jennett B. Analyzing outcome of treatment of severe head injury: a review and update on advancing the use of the Glasgow Outcome Scale. J Neurotrauma 1998;15:587-97. http://dx.doi.org/10.1089/neu.1998.15.587.
- Guide to the methods of technology appraisal. London: NICE; 2008.
- Nichol AD, Higgins AM, Gabbe BJ, Murray LJ, Cooper DJ, Cameron PA. Measuring functional and quality of life outcomes following major head injury: common scales and checklists. Injury 2011;42:281-7. http://dx.doi.org/10.1016/j.injury.2010.11.047.
- Wilson JT, Edwards P, Fiddes H, Stewart E, Teasdale GM. Reliability of postal questionnaires for the Glasgow Outcome Scale. J Neurotrauma 2002;19:999-1005. http://dx.doi.org/10.1089/089771502760341910.
- Wilson JT, Pettigrew LE, Teasdale GM. Structured interviews for the Glasgow Outcome Scale and the extended Glasgow Outcome Scale: guidelines for their use. J Neurotrauma 1998;15:573-85. http://dx.doi.org/10.1089/neu.1998.15.573.
- The English Indices of Deprivation 2010. London: Department for Communities and Local Government; 2011.
- Welsh Index of Multiple Deprivation (WIMD) 2008: Summary Report. Cardiff: Welsh Assembly Government; 2011.
- Myburgh JA, Cooper DJ, Finfer SR, Venkatesh B, Jones D, Higgins A, et al. Epidemiology and 12-month outcomes from traumatic brain injury in Australia and New Zealand. J Trauma 2008;64:854-62. http://dx.doi.org/10.1097/TA.0b013e3180340e77.
- Andriessen TM, Horn J, Franschman G, van der NJ, Haitsma I, Jacobs B, et al. Epidemiology, severity classification, and outcome of moderate and severe traumatic brain injury: a prospective multicenter study. J Neurotrauma 2011;28:2019-31. http://dx.doi.org/10.1089/neu.2011.2034.
- Whitnall L, McMillan TM, Murray GD, Teasdale GM. Disability in young people and adults after head injury: 5–7 year follow up of a prospective cohort study. J Neurol Neurosurg Psychiatry 2006;77:640-5. http://dx.doi.org/10.1136/jnnp.2005.078246.
- Rubin DB. Multiple imputation for nonresponse in surveys. New York, NY: J Wiley & Sons; 1987.
- Schafer JL. Analysis of incomplete multivariate data. London: Chapman and Hall; 1997.
- Sterne JA, White IR, Carlin JB, Spratt M, Royston P, Kenward MG, et al. Multiple imputation for missing data in epidemiological and clinical research: potential and pitfalls. BMJ 2009;338. http://dx.doi.org/10.1136/bmj.b2393.
- van Buuren S, Groothuis-Oudshoorn K. MICE: Multivariate Imputation by Chained Equations in R. J Stat Software 2011;45:1-67.
- Harrell FE, Califf RM, Pryor DB, Lee KL, Rosati RA. Evaluating the yield of medical tests. JAMA 1982;247:2543-6. http://dx.doi.org/10.1001/jama.1982.03320430047030.
- Hanley JA, McNeil BJ. The meaning and use of the area under a receiver operating characteristic (ROC) curve. Radiology 1982;143:29-36.
- Hosmer DW, Lemeshow S. Goodness of fit tests for the multiple logistic regression model. Comm Stat 1980;A9:1043-69.
- Kramer AA, Zimmerman JE. Assessing the calibration of mortality benchmarks in critical care: The Hosmer–Lemeshow test revisited. Crit Care Med 2007;35:2052-6. http://dx.doi.org/10.1097/01.CCM.0000275267.64078.B0.
- Cox DR. Two further applications of a model for binary regression. Biometrika 1958;45:562-5.
- Brier GW. Verification of forecasts expressed in terms of probability. Mon Weather Rev 1950;75:1-3. http://dx.doi.org/10.1175/1520-0493(1950)078〈0001:VOFEIT〉2.0.CO;2.
- Landis JR, Koch GG. The measurement of observer agreement for categorical data. Biometrics 1977;33:159-74. http://dx.doi.org/10.2307/2529310.
- Donner A, Eliasziw M. A goodness-of-fit approach to inference procedures for the kappa statistic: confidence interval construction, significance-testing and sample size estimation. Stat Med 1992;11:1511-19. http://dx.doi.org/10.1002/sim.4780111109.
- Zou G, Donner A. Confidence interval estimation of the intraclass correlation coefficient for binary outcome data. Biometrics 2004;60:807-11. http://dx.doi.org/10.1111/j.0006-341X.2004.00232.x.
- Reichenheim ME. Confidence intervals for the kappa statistic. Stata J 2004;4:421-8.
- Hukkelhoven CW, Rampen AJ, Maas AI, Farace E, Habbema JD, Marmarou A, et al. Some prognostic models for traumatic brain injury were not valid. J Clin Epidemiol 2006;59:132-43. http://dx.doi.org/10.1016/j.jclinepi.2005.06.009.
- Yeoman P, Pattani H, Silcocks P, Owen V, Fuller G. Validation of the IMPACT outcome prediction score using the Nottingham Head Injury Register dataset. J Trauma 2011;71:387-92. http://dx.doi.org/10.1097/TA.0b013e31820ceadd.
- Roozenbeek B, Lingsma HF, Lecky FE, Lu J, Weir J, Butcher I, et al. Prediction of outcome after moderate and severe traumatic brain injury: external validation of the IMPACT and CRASH prognostic models. Crit Care Med 2012;40:1609-17. http://dx.doi.org/10.1097/CCM.0b013e31824519ce.
- Panczykowski DM, Puccio AM, Scruggs BJ, Bauer JS, Hricik AJ, Beers SR, et al. Prospective independent validation of IMPACT modeling as a prognostic tool in severe traumatic brain injury. J Neurotrauma 2012;29:47-52. http://dx.doi.org/10.1089/neu.2010.1482.
- van Leeuwen N, Lingsma HF, Perel P, Lecky F, Roozenbeek B, Lu J, et al. Prognostic value of major extracranial injury in traumatic brain injury: an individual patient data meta-analysis in 39,274 patients. Neurosurgery 2012;70:811-18. http://dx.doi.org/10.1227/NEU.0b013e318235d640.
- Fleminger S. Long-term psychiatric disorders after traumatic brain injury. Eur J Anaesthesiol Suppl 2008;42:123-30. http://dx.doi.org/10.1017/S0265021507003250.
- Harrison-Felix C, Whiteneck G, Devivo MJ, Hammond FM, Jha A. Causes of death following 1 year postinjury among individuals with traumatic brain injury. J Head Trauma Rehabil 2006;21:22-33. http://dx.doi.org/10.1097/00001199-200601000-00003.
- McMillan TM, Teasdale GM. Death rate is increased for at least 7 years after head injury: a prospective study. Brain 2007;130:2520-7. http://dx.doi.org/10.1093/brain/awm185.
- McMillan TM, Teasdale GM, Weir CJ, Stewart E. Death after head injury: the 13 year outcome of a case control study. J Neurol Neurosurg Psychiatry 2011;82:931-5. http://dx.doi.org/10.1136/jnnp.2010.222232.
- Selassie AW, Zaloshnja E, Langlois JA, Miller T, Jones P, Steiner C. Incidence of long-term disability following traumatic brain injury hospitalization, United States, 2003. J Head Trauma Rehabil 2008;23:123-31. http://dx.doi.org/10.1097/01.HTR.0000314531.30401.39.
- Traumatic Brain Injury in the United States. Emergency Department visits, hospitalizations and deaths 2002–2006. Washington, DC: US Department of Health and Human Services; 2010.
- Barratt H, Wilson M, Moore F, Raine R. The implications of the NICE guidelines on neurosurgical management for all severe head injuries: systematic review. Emerg Med J 2010;27:173-8. http://dx.doi.org/10.1136/emj.2009.075382.
- Gabbe BJ, Biostat GD, Lecky FE, Bouamra O, Woodford M, Jenks T, et al. The effect of an organized trauma system on mortality in major trauma involving serious head injury: a comparison of the United Kingdom and Victoria, Australia. Ann Surg 2011;253:138-43. http://dx.doi.org/10.1097/SLA.0b013e3181f6685b.
- Fuller G, Bouamra O, Woodford M, Jenks T, Patel H, Coats TJ, et al. Temporal trends in head injury outcomes from 2003 to 2009 in England and Wales. Br J Neurosurg 2011;25:414-21. http://dx.doi.org/10.3109/02688697.2011.570882.
- Bulger EM, Nathens AB, Rivara FP, Moore M, MacKenzie EJ, Jurkovich GJ. Management of severe head injury: institutional variations in care and effect on outcome. Crit Care Med 2002;30:1870-6. http://dx.doi.org/10.1097/00003246-200208000-00033.
- Elf K, Nilsson P, Enblad P. Outcome after traumatic brain injury improved by an organized secondary insult program and standardized neurointensive care. Crit Care Med 2002;30:2129-34. http://dx.doi.org/10.1097/00003246-200209000-00029.
- Kochanek PM, Snyder JV, Sirio CA, Saxena S, Bircher NG. Specialty neurointensive care: Is it just a name or a way of life?. Crit Care Med 2001;29:692-3. http://dx.doi.org/10.1097/00003246-200103000-00053.
- Faul M, Wald MM, Rutland-Brown W, Sullivent EE, Sattin RW. Using a cost-benefit analysis to estimate outcomes of a clinical treatment guideline: testing the Brain Trauma Foundation guidelines for the treatment of severe traumatic brain injury. J Trauma 2007;63:1271-8. http://dx.doi.org/10.1097/TA.0b013e3181493080.
- Ibrahim MI, Abdullah M, Naing L, Abdullah JM, Idris Z, Aljunid SM. Cost effectiveness analysis of using multiple neuromodalities in treating severe traumatic brain injury in a developing country like Malaysia. Asian J Surg 2007;30:261-6. http://dx.doi.org/10.1016/S1015-9584(08)60036-6.
- Park CA, McGwin G, Jr, Smith DR, May AK, Melton SM, Taylor AJ, et al. Trauma-specific intensive care units can be cost effective and contribute to reduced hospital length of stay. Am Surg 2001;67:665-70.
- Kim KH. Predictors for functional recovery and mortality of surgically treated traumatic acute subdural hematomas in 256 patients. J Korean Neurosurg Soc 2009;45:143-50. http://dx.doi.org/10.3340/jkns.2009.45.3.143.
- Koc RK, Akdemir H, Oktem IS, Meral M, Menku A. Acute subdural hematoma: outcome and outcome prediction. Neurosurg Rev 1997;20:239-44. http://dx.doi.org/10.1007/BF01105894.
- Seelig JM, Becker DP, Miller JD, Greenberg RP, Ward JD, Choi SC. Traumatic acute subdural hematoma: major mortality reduction in comatose patients treated within four hours. N Engl J Med 1981;304:1511-18. http://dx.doi.org/10.1056/NEJM198106183042503.
- Tian HL, Chen SW, Xu T, Hu J, Rong BY, Wang G, et al. Risk factors related to hospital mortality in patients with isolated traumatic acute subdural haematoma: analysis of 308 patients undergone surgery. Chin Med J 2008;121:1080-4.
- Wilberger JE, Jr, Harris M, Diamond DL. Acute subdural hematoma: morbidity, mortality, and operative timing. J Neurosurg 1991;74:212-18. http://dx.doi.org/10.3171/jns.1991.74.2.0212.
- Bullock MR, Chesnut R, Ghajar J, Gordon D, Hartl R, Newell DW, et al. Surgical management of acute epidural hematomas. Neurosurgery 2006;58:S7-15.
- Andrews PJ, Piper IR, Dearden NM, Miller JD. Secondary insults during intrahospital transport of head-injured patients. Lancet 1990;335:327-30. http://dx.doi.org/10.1016/0140-6736(90)90614-B.
- Gentleman D, Jennett B. Hazards of inter-hospital transfer of comatose head-injured patients. Lancet 1981;2:853-4. http://dx.doi.org/10.1016/S0140-6736(81)91115-6.
- Shafi S, Diaz-Arrastia R, Madden C, Gentilello L. Intracranial pressure monitoring in brain-injured patients is associated with worsening of survival. J Trauma 2008;64:335-40. http://dx.doi.org/10.1097/TA.0b013e31815dd017.
- Cremer OL, van Dijk GW, van Wensen E, Brekelmans GJ, Moons KG, Leenen LP, et al. Effect of intracranial pressure monitoring and targeted intensive care on functional outcome after severe head injury. Crit Care Med 2005;33:2207-13. http://dx.doi.org/10.1097/01.CCM.0000181300.99078.B5.
- Badjatia N, Carney N, Crocco TJ, Fallat ME, Hennes HM, Jagoda AS, et al. Guidelines for prehospital management of traumatic brain injury 2nd edition. Prehosp Emerg Care 2008;12:S1-2.
- Patel HC, Bouamra O, Woodford M, Yates DW, Lecky FE. Clinical article: mortality associated with severe head injury in the elderly. Acta Neurochir 2010;152:1353-7. http://dx.doi.org/10.1007/s00701-010-0666-x.
- Stocchetti N, Paterno R, Citerio G, Beretta L, Colombo A. Traumatic brain injury in an aging population. J Neurotrauma 2012;29:1119-25. http://dx.doi.org/10.1089/neu.2011.1995.
- Susman M, DiRusso SM, Sullivan T, Risucci D, Nealon P, Cuff S, et al. Traumatic brain injury in the elderly: increased mortality and worse functional outcome at discharge despite lower injury severity. J Trauma 2002;53:219-23. http://dx.doi.org/10.1097/00005373-200208000-00004.
- Tokutomi T, Miyagi T, Ogawa T, Ono J, Kawamata T, Sakamoto T, et al. Age-associated increases in poor outcomes after traumatic brain injury: a report from the Japan Neurotrauma Data Bank. J Neurotrauma 2008;25:1407-14. http://dx.doi.org/10.1089/neu.2008.0577.
- Ho KM, Burrell M, Rao S. Extracranial injuries are important in determining mortality of neurotrauma. Crit Care Med 2010;38:1562-8. http://dx.doi.org/10.1097/CCM.0b013e3181e2ccd8.
- Jacobs B, Beems T, Stulemeijer M, van Vugt AB, van der Vliet TM, Borm GF, et al. Outcome prediction in mild traumatic brain injury: age and clinical variables are stronger predictors than CT abnormalities. J Neurotrauma 2010;27:655-68. http://dx.doi.org/10.1089/neu.2009.1059.
- Lefering R, Paffrath T, Linker R, Bouillon B, Neugebauer EA. Head injury and outcome: what influence do concomitant injuries have?. J Trauma 2008;65:1036-43. http://dx.doi.org/10.1097/TA.0b013e318184ee48.
- Field D, Elbourne D, Truesdale A, Grieve R, Hardy P, Fenton AC, et al. Neonatal ventilation with inhaled nitric oxide versus ventilatory support without inhaled nitric oxide for preterm infants with severe respiratory failure: the INNOVO multicentre randomised controlled trial. Pediatrics 2005;115:926-36. http://dx.doi.org/10.1542/peds.2004-1209.
- Dolan P, Gudex C, Kind P, Williams A. CHE Discussion Paper 138. York: Centre for Health Economics, University of York; 1999.
- NHS reference costs 2010–11. London: DoH; 2011.
- Rowan K, Adam S, Ball C, Bray K, Baker-McLearn D, Carmel S, et al. Evaluation of outreach services in critical care: Project SDO/74/2004. London: NIHR Service Delivery and Organisation Programme; 2007.
- Curtis L. Unit Costs of Health & Social Care 2011 2011. www.pssru.ac.uk/archive/pdf/uc/uc2011/uc2011.pdf (accessed 1 May 2013).
- Gomes M, Ng ES, Grieve R, Nixon R, Carpenter J, Thompson SG. developing appropriate methods for cost-effectiveness analysis of cluster randomized trials. Med Decis Making 2012;32:350-61. http://dx.doi.org/10.1177/0272989X11418372.
- Grieve R, Nixon R, Thompson SG. Bayesian hierarchical models for cost-effectiveness analyses that use data from cluster randomized trials. Med Decis Making 2010;30:163-75. http://dx.doi.org/10.1177/0272989X09341752.
- Mccullagh P, Nelder JA. Generalized linear models. London: Chapman and Hall; 1989.
- Kenward MG, Carpenter J. Multiple imputation: current perspectives. Stat Methods Med Res 2007;16:199-218. http://dx.doi.org/10.1177/0962280206075304.
- Carpenter J, Goldstein H, Kenward M. REALCOM-IMPUTE software for multilevel multiple imputation with mixed response types. J Stat Software 2011;45:1-14.
- Latimer N. Survival analysis for economic evaluations alongside clinical trials: extrapolation with patient-level data. NICE DSU technical support documents: No. 14. Sheffield: University of Sheffield; 2011.
- Interim Life Tables, 2008–2010. Newport: ONS; 2011.
- Jacobsson LJ, Westerberg M, Lexell J. Health-related quality-of-life and life satisfaction 6–15 years after traumatic brain injuries in northern Sweden. Brain Inj 2010;24:1075-86. http://dx.doi.org/10.3109/02699052.2010.494590.
- Andelic N, Hammergren N, Bautz-Holter E, Sveen U, Brunborg C, Roe C. Functional outcome and health-related quality of life 10 years after moderate-to-severe traumatic brain injury. Acta Neurol Scand 2009;120:16-23. http://dx.doi.org/10.1111/j.1600-0404.2008.01116.x.
- Greene WH. Econometric analysis. Upper Saddle River, NJ: Prentice Hall; 2003.
- Thompson SG, Barber JA. How should cost data in pragmatic randomised trials be analysed?. BMJ 2000;320:1197-200. http://dx.doi.org/10.1136/bmj.320.7243.1197.
- Barber J, Thompson S. Multiple regression of cost data: use of generalised linear models. J Health Serv Res Policy 2004;9:197-204. http://dx.doi.org/10.1258/1355819042250249.
- Teig M, Smith M. Where should patients with severe traumatic brain injury be managed? All patient should be managed in a neurocritical care unit. J Neurosurg Anesthesiol 2010;22:357-9. http://dx.doi.org/10.1097/ANA.0b013e3181f0dada.
- Baguley IJ, Nott MT, Howle AA, Simpson GK, Browne S, King AC, et al. Late mortality after severe traumatic brain injury in New South Wales: a multicentre study. Med J Aust 2012;196:40-5. http://dx.doi.org/10.5694/mja11.10090.
- Cameron CM, Purdie DM, Kliewer EV, McClure RJ. Ten-year outcomes following traumatic brain injury: a population-based cohort. Brain Inj 2008;22:437-49. http://dx.doi.org/10.1080/02699050802060621.
- Harrison-Felix CL, Whiteneck GG, Jha A, Devivo MJ, Hammond FM, Hart DM. Mortality over four decades after traumatic brain injury rehabilitation: a retrospective cohort study. Arch Phys Med Rehabil 2009;90:1506-13. http://dx.doi.org/10.1016/j.apmr.2009.03.015.
- Ventura T, Harrison-Felix C, Carlson N, DiGuiseppi C, Gabella B, Brown A, et al. Mortality after discharge from acute care hospitalization with traumatic brain injury: a population-based study. Arch Phys Med Rehabil 2010;91:20-9. http://dx.doi.org/10.1016/j.apmr.2009.08.151.
- Noah MA, Peek GJ, Finney SJ, Griffiths MJ, Harrison DA, Grieve R, et al. Referral to an extracorporeal membrane oxygenation center and mortality among patients with severe 2009 influenza A(H1N1). JAMA 2011;306:1659-68. http://dx.doi.org/10.1001/jama.2011.1471.
- Abadie A, Imbens GW. Bias-corrected matching estimators for average treatment effects. J Bus Econ Stat 2011;29:1-11. http://dx.doi.org/10.1198/jbes.2009.07333.
- Pirracchio R, Sprung C, Payen D, Chevret S. Benefits of ICU admission in critically ill patients: whether instrumental variable methods or propensity scores should be used. BMC Med Res Methodol 2011;11. http://dx.doi.org/10.1186/1471-2288-11-132.
- Rosenbaum PR. Covariance adjustment in randomised experiments and observational studies. Stat Sci 2002;17:286-304. http://dx.doi.org/10.1214/ss/1042727942.
Appendix 1 Search strategy for updated systematic review of risk prediction models
Appendix 2 Risk Adjustment In Neurocritical care study protocol version 1.4
Appendix 3 Risk Adjustment In Neurocritical care study data definitions
Appendix 4 Risk Adjustment In Neurocritical care study data collection form and data set flow
Appendix 5 Risk Adjustment In Neurocritical care study follow-up materials
Appendix 6 Sensitivity analysis: external validation of risk prediction models in original (non-imputed) data sets
List of abbreviations
- AIC
- Akaike information criterion
- APACHE
- Acute Physiology And Chronic Health Evaluation
- BIC
- Bayesian information criterion
- CCMDS
- Critical Care Minimum Data Set
- CEA
- cost-effectiveness analysis
- CEAC
- cost-effectiveness acceptability curve
- CI
- confidence interval
- CMP
- Case Mix Programme
- CRASH
- Corticosteroid Randomisation After Significant Head injury
- CRN
- Clinical Research Network
- CT
- computerised tomography
- DVR
- data validation report
- EBIC
- European Brain Injury Consortium
- ECC
- Ethics and Confidentiality Committee
- EQ-5D-3L
- European Quality of Life-5 Dimensions, 3-level version
- FiO2
- fraction of inspired oxygen
- GCS
- Glasgow Coma Scale
- GOS
- Glasgow Outcome Scale
- GOSE
- Glasgow Outcome Scale – Extended
- GP
- general practitioner
- HDU
- high-dependency unit
- HRG
- Healthcare Resource Group
- ICER
- incremental cost-effectiveness ratio
- ICNARC
- Intensive Care National Audit and Research Centre
- ICU
- intensive-care unit
- IMD
- Index of Multiple Deprivation
- IMPACT
- International Mission for Prognosis and Analysis of Clinical Trials in TBI
- INB
- incremental net monetary benefit
- IQR
- interquartile range
- ISS
- injury severity score
- LOS
- length of stay
- MICE
- Multivariate Imputation by Chained Equations
- MRC
- Medical Research Council
- MRIS
- Medical Research Information Service
- NCCNet
- Neurocritical Care Network
- NICE
- National Institute for Health and Care Excellence
- NIGB
- National Information Governance Board
- NIHR
- National Institute for Health Research
- PaCO2
- partial pressure of carbon dioxide
- PaO2
- partial pressure of oxygen
- QALY
- quality-adjusted life-year
- QOL
- quality of life
- R&D
- research and development
- RAIN
- Risk Adjustment In Neurocritical care
- RCT
- randomised controlled trial
- REC
- Research Ethics Committee
- ROC
- receiver operating characteristic
- RTA
- road traffic accident
- SAH
- subarachnoid haemorrhage
- SF-36
- Short Form questionnaire-36 items
- SICSAG
- Scottish Intensive Care Society Audit Group
- SSI
- site-specific information
- TARN
- Trauma Audit and Research Network
- TBI
- traumatic brain injury
- TCDB
- Trauma Coma Data Bank
- UKCCTF
- UK Critical Care Trials Forum
- WBIC
- Wolfson Brain Imaging Centre
All abbreviations that have been used in this report are listed here unless the abbreviation is well known (e.g. NHS), or it has been used only once, or it is a non-standard abbreviation used only in figures/tables/appendices, in which case the abbreviation is defined in the figure legend or in the notes at the end of the table.