Notes
Article history
The MRC-NIHR Methodology Research Programme funded the PRET project (Preparatory study for the Re-valuation of the EQ-5D Tariff, MRC ref. G0901500), and the EuroQol Group funded the PRET-AS project (Preparatory study for the Re-valuation of the EQ-5D Tariff – Additional Sample) as an extension to the PRET project with formal agreement from the MRC.
To strengthen the evidence base for health research, the MRP oversees and implements the evolving strategy for high quality methodological research. In addition to the MRC and NIHR funding partners, the MRP takes into account the needs of other stakeholders including the devolved administrations, industry R&D, and regulatory/advisory agencies and other public bodies. The MRP funds investigator-led and needs-led research proposals from across the UK. In addition to the standard MRC and RCUK terms and conditions, projects commissioned/managed by the MRP are expected to provide a detailed report on the research findings and may publish the findings in the HTA journal, if supported by NIHR funds.
The authors have been wholly responsible for all data collection, analysis and interpretation, and for writing up their work. The HTA editors and publisher have tried to ensure the accuracy of the authors’ report and would like to thank the reviewers for their constructive comments on the draft document. However, they do not accept liability for damages or losses arising from material published in this report.
Declared competing interests of authors
The PRET-AS component of this project was funded by the EuroQol Group as an extension to the PRET project, with formal agreement from the Medical Research Council. The EQ-5D and EQ-5D-5L are intellectual property of the EuroQol Group. JB, ND, LL and AT are members of the EuroQol Group, and therefore could have a potential conflict of interest. The research reported here was carried out independently, and the views expressed in this report are not those of the EuroQol Group. NB’s institution has received financial support from Pfizer Canada as a postdoctoral funding award.
Permissions
Copyright statement
© Queen’s Printer and Controller of HMSO 2014. This work was produced by Mulhern et al. under the terms of a commissioning contract issued by the Secretary of State for Health. This issue may be freely reproduced for the purposes of private research and study and extracts (or indeed, the full report) may be included in professional journals provided that suitable acknowledgement is made and the reproduction is not associated with any form of advertising. Applications for commercial reproduction should be addressed to: NIHR Journals Library, National Institute for Health Research, Evaluation, Trials and Studies Coordinating Centre, Alpha House, University of Southampton Science Park, Southampton SO16 7NS, UK.
Chapter 1 Background to the Preparatory study for the Re-valuation of the EQ-5D Tariff project
Introduction
Measuring cost-effectiveness
Health-care resources are limited and need to be allocated efficiently. The National Institute for Health and Care Excellence (NICE) was set up to help make better health-care resource allocation decisions. NICE bases its recommendations on cost-effectiveness analyses, with the quality-adjusted life-years (QALYs) gained as the outcome measure. A QALY combines values for quality and quantity of life in a single figure, and allows the assessment of the effectiveness across interventions and treatments for different conditions using a common metric. This requires a value for the health-related quality of life (HRQL) or utility for a particular health state, which is then multiplied by the duration of the health state to calculate the number of QALYs. Utility values can be generated using generic preference-based measures of health, such as the EQ-5D.
EQ-5D
The EQ-5D1 is the preferred instrument to use to derive utility values to assess the HRQL impact of medical interventions. 2 The EQ-5D assesses HRQL across five dimensions (mobility, self care, usual activities, pain/discomfort and anxiety/depression) each with three response levels (no, some or extreme problems). Therefore, the entire descriptive system generates 243 health-state descriptions, each of which produces a utility value (known as the value set).
The current UK EQ-5D value set is based on the Measurement and Valuation of Health (MVH) study. This study used face-to-face interviews of a representative sample of the UK general population to value 45 hypothetical EQ-5D states using the preference elicitation method time trade-off (TTO). The results of the valuation study were modelled using regression to provide a utility score for all 243 health states (range of −0.594 to 1). 3 Utility scores are anchored on a 0–1 scale, where 1 is equivalent to full health, 0 to dead, and negative values to states worse than dead.
The UK EQ-5D value set is used for economic evaluations by a range of decision-makers and researchers. They are also used in a range of further applications, including population health surveys (e.g. the Health Survey for England); burden of disease studies; hospital inpatient surveys and the NHS Patient Reported Outcome Measures (PROMs) initiative. 4 However, there is the need for a new EQ-5D value set to be developed for the following reasons:
-
A five-level version of the EQ-5D, the EQ-5D-5L,5 has been developed (response categories: none, slight, moderate, severe, extreme/unable). This version generates a possible 3125 health states, and there is the need for a value set to be developed for the larger descriptive system so that the instrument can be used in the economic evaluation of new interventions and treatments.
-
It is possible that the preferences of the general population may have changed since the original MVH study was carried out in the 1990s.
-
Change in demography may mean that although individual preferences may not have changed, the composition of people across the country has changed, so that average preferences may have changed.
-
There has been recognition of the shortcomings of the MVH protocol used to generate the UK EQ-5D value set, in particular in regards to the valuation method used for states perceived by respondents as worse than dead.
-
There have been advances in health-state valuation methods, including the potential application of discrete choice experiments (DCEs) to derive utility values, by including duration as an attribute [discrete choice experiment incorporating duration (DCETTO)].
-
There have been advances in the administration modes available for valuation studies [e.g. using computer-assisted personal interviews (CAPIs) or online methods].
The ‘Preparatory study for the Re-valuation of the EQ-5D Tariff project’ and ‘Preparatory study for the Re-valuation of the EQ-5D Tariff project – Additional Sample’
The methods used for the generation of EQ-5D-5L population value sets needs to be up to date and informed by the latest understanding of the techniques used to value health states. The ‘Preparatory study for the Re-valuation of the EQ-5D Tariff project’ (PRET) is a methodological study that aims to contribute to the generation of EQ-5D-5L population value sets by exploring a range of methodological issues associated with a range of health-state valuation techniques, including TTO and DCETTO. The ‘PRET – Additional Sample’ (PRET-AS) study is an extension to PRET and allows further investigation into health-state valuation-related methods. This report will cover both projects.
The PRET study is a methodological study that has four stages. In stage 1, a large scale online survey is carried out to explore a series of methodological issues related to health-state valuation using binary choice questions. PRET-AS is an extension of stage 1, and involves a further online survey investigating two binary choice techniques that can be used to generate utility values. In stage 2, a segment of the PRET stage 1 online survey is carried out in a face-to-face environment using CAPI to test the equivalence of responses to the valuation questions across different modes of administration. Stage 3 uses CAPI to investigate the strategies and processes used to answer health-state valuation questions based around DCETTO and TTO. Stage 4 uses in-depth cognitive interviews to investigate the completion of TTO and DCETTO in more detail.
The remainder of this chapter is organised as follows. In the Methods of health-state valuation considered in PRET section below, the health-state valuation techniques TTO and DCETTO are introduced. In the Issues with the design of health-state valuation studies considered in PRET and PRET-AS section, the methodological issues that need to be considered in the design of any health-state valuation study are outlined, with reference to the issues investigated in this study.
Sections of this chapter are reported in an online discussion paper6 (accessible at www.shef.ac.uk/polopoly_fs/1.165490!/file/1116.pdf).
Methods of health-state valuation considered in PRET
TTO
Time trade-off7 is a widely used method for valuing health-states valuation, and the MVH TTO protocol8 that was used to derive the EQ-5D value set1,3 has also been used to generate utility scores for condition-specific preference-based measures of health. 9–13
Time trade-off is an iterative cardinal technique that elicits a health-state value by asking respondents to trade off time in full health to avoid living in a hypothetical health state described by the classification system. The value is derived at the point where respondents are indifferent between the scenarios. The MVH TTO protocol used face-to-face interviews, and the task follows a set procedure to derive each utility value. First, respondents are asked whether they would prefer to live in health state (H) for 10 years, or to die immediately, or whether they were indifferent between the options. This established whether the health state was perceived as better than, worse than, or equal to being dead.
For states perceived as better than dead, respondents choose between (1) living in H for T years (usually 10) or (2) living in full health for X years (where X ≤ 10). The duration of full health (X) is varied iteratively until respondents are indifferent between the options. The value for H is then calculated as X/10. Typically, zero time preference is assumed.
For health states that are ‘worse than dead’, respondents are asked to consider a choice between (1) H for W years followed by full health for X years (where W + X = 10) after which they will die or (2) immediate death. Both years in full health, X, and years in the health state, W = 10 − X, are varied until respondents are indifferent between the two options. However, this may result in extreme values. For example, if a respondent is indifferent between (1) H for 3 months followed by full health for 9 years 9 months after which they die and (2) immediate death, this would suggest that the value of health is −0.25/9.75 = −39 on a scale on which ‘1’ = full health and ‘0’ = being dead. Traditionally, this has been regarded as unacceptable and arbitrary transformations have been applied. 3,14–16 Under the established convention, H is calculated as −X/10.
Regardless of whether the state is better or worse than being dead, the iterative process is susceptible to bias. This is because when people are asked two (or more) consecutive questions, the later question is not independent of the earlier question. For example, the response given to a TTO question where X is 3 years will be affected by the value of X used in the preceding question (e.g. whether it was 1 year or 5 years). The issue of biases caused by iterative questioning is a key topic in the literature on the monetary valuation of health but is under-researched in the literature on health-state valuations.
To estimate utility values for each health state defined by a classification system, the results of the TTO study are modelled using multivariate regression. The disutility coefficient for each severity level of each dimension is calculated using level 1 (no problem) as the baseline. Therefore, full health is anchored at 1, and the utility value for each overall health state is calculated by subtracting the disutility value for each dimension from 1.
Lead time – time trade-off
A concern with the MVH TTO protocol above is the process used to value states worse than dead. 16–18 The following problems were identified with this procedure: (1) The method is not the same as the method for states better than dead; (2) time spent in health state H has become the decision variable, with the result that respondents are not valuing a specified time in the state (as is the case for states better than dead); and (3) the raw result is non-linear for the time spent in state H, such that as H approaches zero the index approaches negative infinity ( Figure 1 ) and, as is pointed out above, transformations used are arbitrary, and render the values incommensurable with those for states better than dead.
FIGURE 1.
Increase in health-state value as time in poor health decreases.
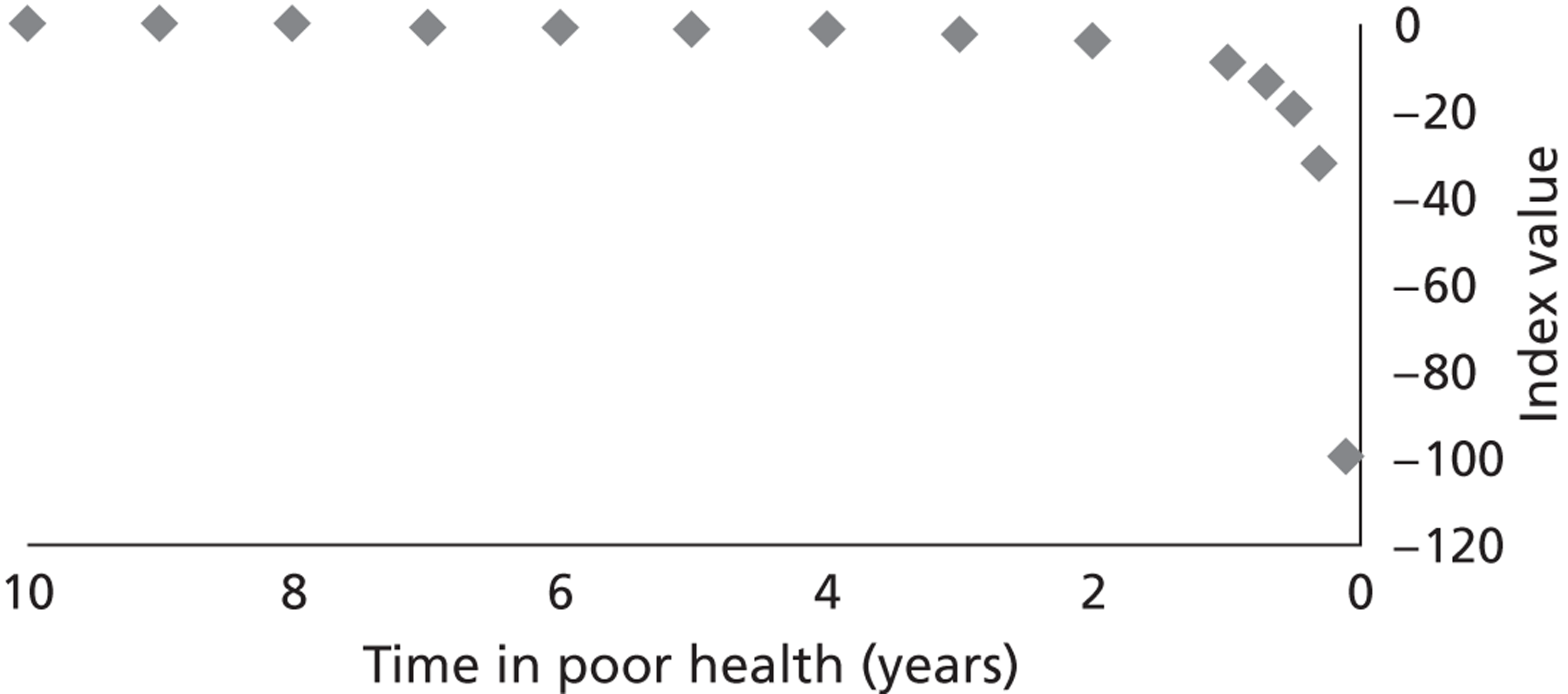
The lead time – time trade-off (LT-TTO) was devised to overcome these problems. In order to allow X to take negative values, LT-TTO appends a stretch of ‘lead time’ in full health before the usual TTO scenarios begin. For example, if lead time is 15 years, a LT-TTO question compares living in full health for 15 years followed by H for 10 years against living in full health for some duration between zero and (15 + X) years. If indifference is achieved at, say, 20 years in full health, then by removing the lead time this is the same as X = 5 in a TTO without lead time (20 − 15 = 5). If indifference is at 12 years in full health then this corresponds to a ‘negative duration’ (12 − 15 = −3). Note first that the value of living in H for 1 year is given by X/10, regardless of whether H is better or worse than dead, and, second, the calculation assumes that the size of T is unaffected by the addition of the lead time. 16
As can be seen, a lead time of 15 years against a duration of 10 years will allow LT-TTO to elicit values in the range [−1.5 to 1]. If the value of H is < −1.5 then a respondent will strongly prefer immediate death over the prospect of 15 years of lead time plus 10 years in state H. This is called ‘exhausting’ the lead time, and if the objective is to identify a point of indifference for all states for all respondents, it calls for a longer lead time (or a shorter duration). There have been a number of attempts to explore the optimal ratio of the lead time to the duration but a clear answer is yet to emerge. 19
DCEs
There is growing interest in using ordinal techniques such as DCE to generate health-state values. 20 DCEs generate ordinal preference data by asking respondents to indicate their preferred option from a set of health-state profiles (usually two profiles are presented), in which each profile is described in terms of attributes and levels. The results of the choice exercise are then modelled using regressions to generate a coefficient value for each level of each attribute, or dimension. DCE does not require the application of an iterative procedure, and therefore may be less cognitively demanding and avoid the bias associated with iterative procedures.
Discrete choice experiment assumes that preferences are measured on a ‘latent’ scale, and are directly unobservable, but can be modelled in terms of observed characteristic of each choice. As a result, the raw regression coefficients are also on a latent scale, with no direct meaning. Thus, to use DCE to generate utility values that can be used as the HRQL adjustment weights for the QALY, coefficients must be anchored on the full health–dead utility scale. This has typically been done using external values generated, for example, from a TTO exercise. 21 Recently, a method has been developed that avoids the need to use external values by incorporating duration as an attribute of the health-state profile, therefore interpreting DCE data as a TTO exercise (DCETTO). 22 To estimate utility values for health states, a regression model incorporating interaction terms between each level of the health-state dimensions and the duration attribute are estimated (see Chapter 8 , DCE TTO analysis, for more details). The approach has been tested using EQ-5D health states, and has been shown to be a feasible approach to deriving logical and consistent health-state values.
Issues with the design of health-state valuation studies considered in PRET and PRET-AS
In the design of health-state valuation studies, a range of key methodological issues that can impact on the validity and usability of the value sets derived need to be taken into account. These include:
-
Whose values to obtain?
-
What mode of administration to use?
-
What method of valuation to use?
-
How many, and which hypothetical health states to value?
-
The duration of each hypothetical health state?
Each is discussed in detail below, with reference to the issues investigated by PRET and PRET-AS.
Whose values?
The value sets for the three-level EQ-5D,3 and SF-6D,23,24 generic preference-based measures of health and a range of condition-specific measures9–13 are based on general population values, and this is recommended by NICE. 2 However, it is possible that the values given to hypothetical health states by the general population may differ from values given by patients, and there has been debate about whose values should be used. 25,26 Health state satisfaction and adaptation to the health state can be used as a proxy to test this issue as a recent study has demonstrated that if the general public can be informed about the extent to which patients are satisfied with their condition, the discrepancy in values may diminish. 27 PRET tests this further by incorporating a level of life satisfaction, health satisfaction, or adaptation into the health-state description. Alongside this, PRET also tests whether health-state values, which contain satisfaction levels, are influenced by the respondent’s level of satisfaction with their own health or life.
There is also a normative element to this debate, concerning whether general public values ought to be used over patient values. The use of general public values is typically justified with reference to the non-welfarist argument. This states that as the values are used for decision-making in a publicly funded health-care system, they should come from people as informed citizens, not from people as consumers. 28 The traditional approach to health-state valuation, and that used for the current EQ-5D MVH value set, has been to obtain valuations by asking respondents to imagine themselves in the health state. If an informed citizen perspective is taken then a different framing of the TTO question may be required to reflect that the respondent is valuing health states on behalf of other members of society. However, it is unclear what impact an alternative perspective will have on values. PRET investigates this by comparing responses using the standard individual perspective with two alternatives reflecting the citizen approach.
What mode of administration?
Face-to-face interviews with pen and paper questionnaires have been the most widely used method for collecting health-state valuation data using iterative techniques, and was the mode used for EQ-5D using TTO,3 and SF-6D using standard gamble (SG). 23,24 Advances in technology means that it is now also possible to carry out TTO studies using face-to-face CAPI, and this mode was used to derive EQ-5D population value sets for Australia29 and Denmark. 30 Health-state preferences have also been elicited using DCE in a face-to-face setting,21 and the feasibility of carrying out both TTO and DCETTO in an online setting has been investigated. 22
A comparison of the online and face-to-face delivery of TTO found that the responses differed by administration mode, with the online sample displaying more variation in response. 31 Tests of the person trade-off (PTO) valuation technique across online and CAPI administrations also found potential differences across modes. 32,33 Therefore, iterative health-state valuation tasks administered online may generate different results from face-to-face studies, but it is not clear whether the difference comes from the mode of administration or an interaction between the iterative task and the mode of administration. Furthermore, there are also concerns about potential differences in the characteristics of samples collected using face-to-face and online modes, and therefore the overall level of comparability.
The equivalence of responses to binary choice health-state valuation questions (which are amenable to online delivery) across administration modes has not been investigated. Therefore, one of the purposes of PRET was to carry out a head-to-head comparison of an online administration (in stage 1) and a CAPI administration (in stage 2) of an otherwise identical survey containing binary choice health-state valuation questions. A secondary aim is to investigate the similarities and differences of the samples recruited to each mode using the standard recruitment procedure utilised in studies of this kind.
What method of valuation?
As has been described above, there are a range of available techniques for the valuation of health states, and there is also the potential for new and innovative techniques to be developed in the future. The issues with each method need to be considered by those designing health-state valuation studies, as this may impact on the final value sets produced. Further work needs to be done to investigate a range of issues related to each technique described above (TTO, LT-TTO and DCETTO), and one of the aims of PRET is to further the knowledge base in this area, and therefore inform the choice of valuation technique for any health-state valuation exercise. This is described below.
As was described above, MVH TTO protocol used to value the EQ-5D has problems regarding the procedure used to value states worse than dead, and this lead to the development of LT-TTO. Further investigation of the LT-TTO technique is required to identify the optimal length of the lead time used, particularly in very poor health states for which a number of respondents may use up or ‘exhaust’ all of the lead time. One of the objectives of PRET was to provide evidence on this issue. Moreover, another concern is that if the value of a health state depends on its timing and on a preceding health state, then the addition of lead time may distort the TTO value. PRET compares the values produced using binary choice versions of the original and LT-TTO methodologies described in Chapter 2 .
The DCETTO has been shown to be a feasible method for producing values for EQ-5D. However, further testing using a larger descriptive system, such as that found in EQ-5D-5L, is required to investigate the validity of the technique further. PRET and PRET-AS investigate these issues further. Following on from the development of DCETTO, it is possible that other binary choice techniques could be used to produce utility values anchored on the full health–dead scale, and this includes versions of both TTO and LT-TTO, in which one of the choices includes full health. Little is known about the acceptability of both the traditional iterative and new binary choice methods for deriving utility values, and also the ways in which respondents perceive, process and complete the tasks. One of the objectives of PRET is to investigate these issues using both CAPI techniques and detailed qualitative interviews, and this may inform the choice of valuation technique used in future studies.
How many, and which hypothetical health states to value?
The original three-level EQ-5D has 243 possible states. The current MVH TTO value set is based on direct valuations of 45 of these. However, the introduction of EQ-5D-5L means that there are now 3125 possible health states to model. Findings from the DCETTO questions included in PRET, and the modelling approach used to select questions for the study, may be used as prior information to assist in the selection of states and design of the revaluation study.
One aspect that needs to be considered in the design of DCETTO studies is the number of choices each participant can be asked to make. In a recent review, De Bekker-Grob and colleagues20 found that the mean number of choice sets per respondent in health-related DCEs is 14 and it has been suggested that including 8–16 choice tasks is good practice. 34 Furthermore, limited formal work has been done to establish the sample size requirements for DCEs, and PRET and PRET-AS investigate these issues further.
How long should each hypothetical state last?
The current MVH TTO value set is based on participants being asked to imagine each health state lasting for a duration of 10 years. However, the MVH also estimated TTO tariffs for different durations because there was a concern that the tariff values may be a function of the duration of the health state. There are four related issues, all of which are also relevant to DCETTO. 35 One is whether or not constant proportional time trade-off (CP-TTO) holds so that the utility associated with a marginal survival in a given health state remains constant regardless of the health state or the duration. It has been argued that for very severe states there may be a ‘maximal endurable time’ limit, beyond which the marginal benefit of survival diminishes. 36 The second issue is whether or not respondents use a positive temporal discount rate when valuing hypothetical health scenarios. 37–39 The third is the impact of life stage concerns in health-state valuations. If the duration of the state is too long then the scenarios will not be credible for older respondents and vice versa. Furthermore, depending on the duration, people may be thinking about life stage events rather than about the trade-off between longevity and quality of life.
The final issue is whether or not 10 years is the most relevant duration for NICE decision-making. If the issues highlighted above mean that the value of a state is a function of its duration then the revaluation of the EQ-5D may not be based on scenarios with a 10-year duration. The PRET stage 1 online survey examines the impact of varying duration on health-state preferences.
Format of report
The aim of this report is to describe in detail the methods used across the project, and present the results of each stage. Chapter 2 gives a broad overview of the methods used for the PRET stage 1 and PRET-AS online surveys, and presents the demographic characteristics of the respondent samples overall and by each question type. Chapters 3 – 8 report the results of the PRET and PRET-AS online surveys with each of the chapters reporting the findings relating to one of the methodological issues addressed by the online surveys; Chapter 9 reports the methods and results of stage 2; Chapter 10 the methods and results of stage 3, and Chapter 11 the methods and results of stage 4. Finally, Chapter 12 provides a general discussion and recommendations for future research.
Chapter 2 General overview of the PRET stage 1 and PRET-AS online surveys
Introduction
The aim of this chapter is to briefly outline the methodological issues addressed by the online surveys carried out in stage 1 of the PRET project and PRET-AS, and to describe the format, recruitment process and administration of the online surveys. Stage 1 of the PRET project used a large online survey to investigate a range of methodological factors relating to health-state valuation, using binary choice questions. PRET-AS was a second online survey that investigated two binary choice health-state valuation techniques that can be used to produce population tariffs on the full health–dead utility scale. The study design and questions used to investigate each of the methodological issues is described in detail in the subsequent chapters reporting the results. In the rest of this chapter, the second section describes the overall aims and objectives of the studies, and provides an overview of the methodological issues tested; the third section reports the general format of the surveys, and the recruitment and administration procedures used; and the fourth section reports overall response rates for each online survey.
The first three sections of this chapter are reported in an online discussion paper6 (accessible at www.shef.ac.uk/polopoly_fs/1.165490!/file/1116.pdf).
Aims and objectives and the methodological issues tested
PRET stage 1
The aim of the PRET stage 1 online survey was to test a range of methodological assumptions and questions related to health-state valuation, using health states based on EQ-5D-5L. This was done using binary choice questions based on TTO and DCE. The questions tested were:
-
whether health-state preferences are independent of duration (see Chapter 3 )
-
whether health-state preferences are independent of person perspective used (see Chapter 4 )
-
investigation of LT-TTO (see Chapter 5 )
-
whether health-state preferences are independent of lead time
-
to what extent respondents exhaust lead time under very poor health
-
-
whether the preferences of others’ health is independent of when health events take place (see Chapter 6 )
-
whether health-state preferences are independent of satisfaction in the state (see Chapter 7 )
-
whether DCETTO is feasible for EQ-5D-5L, and if so which states should be valued (see Chapter 8 ).
Issues 1–5 are methodological, and therefore the questions used to investigate this are not designed to produce utility values anchored on the full health–dead scale for EQ-5D-5L. Issue 6 uses a method that is designed to elicit utility values.
PRET-AS
The aim of PRET-AS was to investigate the feasibility of two binary choice question types that can be used to derive utility values anchored on the full health–dead utility scale. This was done by conducting an additional online survey of similar size to that in stage 1.
-
The first question type in PRET-AS was also used in the PRET stage 1 survey to investigate issue (6) and presents a DCE with an associated duration level using EQ-5D-5L health states. The results are presented alongside the findings from PRET stage 1 (see Chapter 8 ).
-
The second question type presented a binary choice version of LT-TTO using whole three-level EQ-5D health states. The results are presented alongside the findings from the PRET stage 1 LT-TTO investigation (see Chapter 5 ).
The methods used for the surveys are briefly described in the next six sections, and the study design to test each methodological question is described in detail in the relevant chapter.
Basic question format
Binary choice questions were used to investigate the methodological issues highlighted above. A single response to a binary choice question cannot identify the level of HRQL that an individual feels is right for a given health state, and this was not the aim of the majority of the questions used for the PRET project. However, by examining the distribution of responses of multiple respondents across different binary choice questions incorporating different attributes included in valuation tasks, the methodological issues highlighted above can be tested.
The most ‘basic’ sort of binary choice question used for PRET stage 1 was as follows:
-
[Scenario A]: you will in health state H for 10 years and die
-
[Scenario B]: you will live in full health for (V × 10) years and die (where V is a value between 0 and 1)
-
Which of the two scenarios do you think is better?
The value of V corresponds to the level of HRQL and was varied across different versions of the questions included in the surveys. If we assume that there is an unobserved genuine value of the health state, say V*, then a respondent will, in effect, assess the duration in full health given in scenario B in light of this value. Thus (errors permitting), they will choose B when V* < V. Figure 2 displays an illustrative example of two hypothetical states: ‘severe’ (with a lower V*; but we do not know where it lies) and ‘mild’ (with a higher V*). Along the horizontal axis is the value of V with 0 for dead and 1 for full health. Along the vertical axis is the proportion of people choosing to live in full health (i.e. scenario B), given the task above with different values of V. The curve indicates that, as V increases, the proportion of people who think the given health state is no better than V (namely V* < V) will increase and therefore more will choose scenario B. Now, suppose V is at 0.6. If the state H in the example above is the severe state then, from the curve, around 90% of observations can be expected to be for scenario B, and be consistent with V* > 0.6, but if state H is the mild state then around 50% can be expected to be for scenario B. In other words, given a value of V in a binary choice scenario, the proportion of respondents choosing scenario B will be a function of the value V* that respondents give to the state H (and any further relevant factors, explained below).
FIGURE 2.
The value of V and the proportion of respondents choosing scenario B (full health).
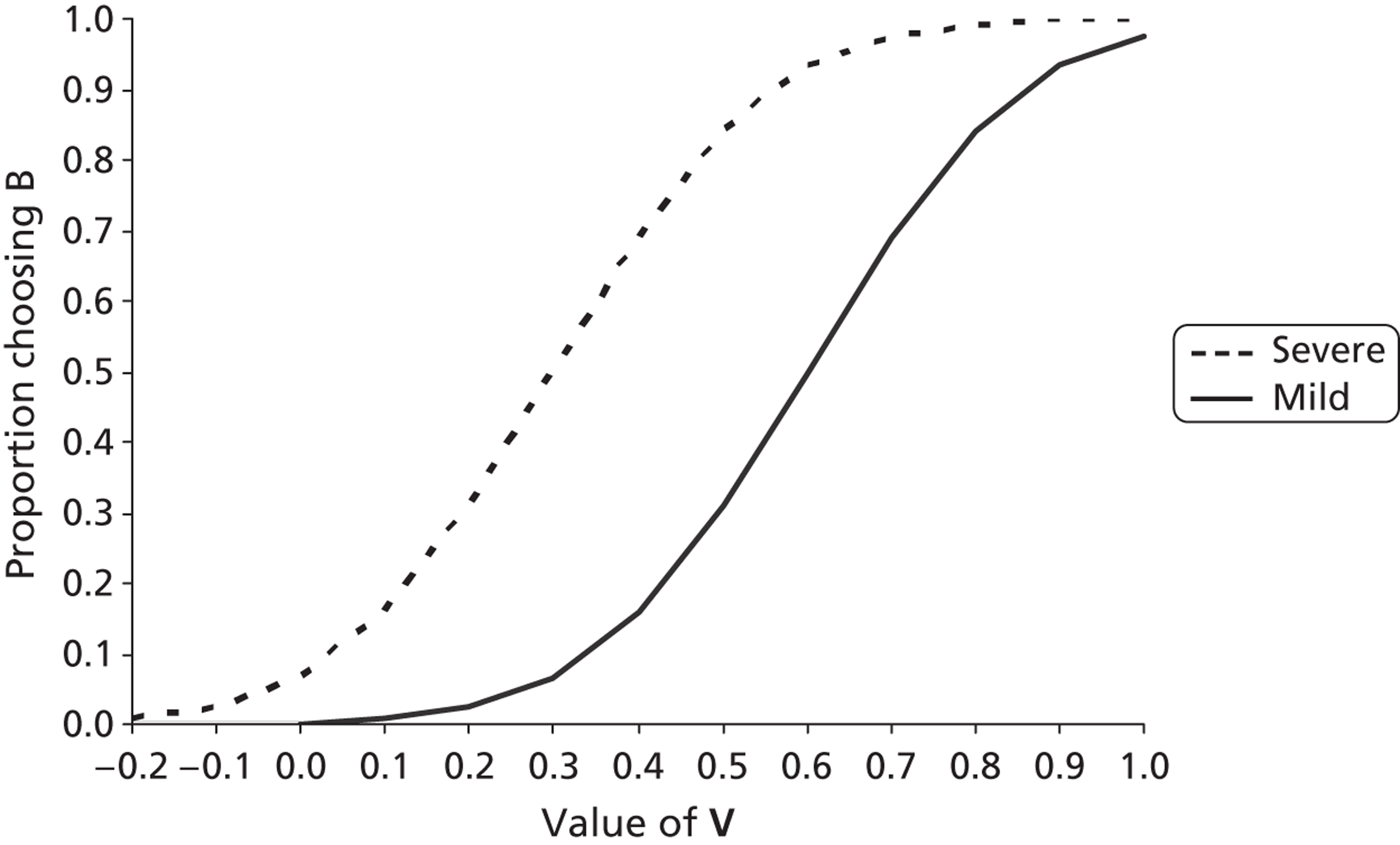
Thus the different scenarios used in the project were assessed in terms of the proportion of people choosing one scenario over the other. All binary choice scenarios included information about a health state, and the length of time lived in the state, followed by death.
Note that the binary questions used are a snapshot of one question from the conventional iterative TTO procedure. This is because the typical TTO exercise is a series of binary choice questions and involves changing V until the respondent is indifferent between the two scenarios. In fact, the procedure of TTO can be interpreted as a special case of DCE, in which scenario B always involves full health.
Question type summary
To investigate the methodological issues, eight types of binary choice questions were used, and these are summarised in Table 1 . PRET included question types I–VII, and PRET-AS included question types VII and VIII. The question types included one or more of the following parameters:
-
Single dimensions or full health states from EQ-5D-5L health states (H): see The hypothetical health states used.
-
Duration (T) lived in state H. PRET used durations of 10 weeks, 1 year, 5 years and 10 years.
-
Lead time stretches (L) in full health before (H) occurs, including 0, 10 weeks, 1 year, 5 years and 10 years.
-
Person perspective (P) that the hypothetical health states apply to. PRET used ‘you’, ‘somebody else like you’ and ‘somebody else’ perspectives.
-
Level of satisfaction with one’s own health or life (S). PRET used low health satisfaction, high health satisfaction, high life satisfaction, and ‘learnt to live with the health state’.
Question type parameter | I | II | III | IV | V | VI | VII | VII |
---|---|---|---|---|---|---|---|---|
State of health (H) | CS | CS | CS | CS | CS | 55555 | 5L | 3L |
Duration in full health (T) | ✓ | ✓ | ✓ | ✓ | ✓ | ✓ | n/m | ✓ |
Duration in H | ✓ | ✓ | ✓ | ✓ | ✓ | ✓ | ✓ | ✓ |
Lead time (L) | n/m | n/m | ✓ | ✓ | n/m | ✓ | n/m | ✓ |
Person/perspective (P) | You | Other | You | Other | You | You | You | You |
Satisfaction (S) | n/m | n/m | n/m | n/m | ✓ | n/m | n/m | n/m |
Each question type will be described in detail in the relevant chapter below. Each question type was used to investigate one (or more) of the PRET assumptions or PRET-AS methods. Question types I–VI were developed to assess methodological issues, and not to derive a utility tariff, whereas question types VII and VIII can be used for this purpose.
Question type I was used to investigate assumption 1 (health-state preferences are independent of duration, or an assessment of CP-TTO). It also provided a comparator question for question types II–V (which use the same question format but vary certain parameters to test the impact of the addition of these parameters); Question type II investigates question 2 (whether health-state preferences are independent of person perspective). Three question types are used for the investigation of LT-TTO: type III to investigate question 3a (whether health-state preferences are independent of lead time); type VI to investigate question 3b (the extent to which lead time is exhausted); and type VIII questions to investigate whether binary choice LT-TTO can be feasibly used to generate values on the utility scale (PRET-AS aim 2). Question type IV was used to investigate question 4 (whether the preferences for others’ health is independent of when health events take place, or an investigation of time preference); question type V investigated question 5 (whether health-state preferences are independent of satisfaction in the state); and question type VII was used to assess the feasibility of DCE with duration for producing utility values (PRET-AS aim 1).
The hypothetical health states used
Questions type I–V used the following five health dimensions adapted from EQ-5D-5L health states:
-
‘Slight problems walking about’ (level 2 of the mobility dimension from EQ-5D-5L state 21111).
-
‘Slight pain’ (level 2 of the pain dimension from EQ-5D-5L state 11121).
-
‘Unable to walk about’ (level 5 of the mobility dimension from EQ-5D-5L state 51111).
-
‘Extreme pain’ (level 5 of the pain/discomfort dimension using pain only from EQ-5D-5L state 11151).
-
‘Extreme depression’ (level 5 of the anxiety/depression using depression only from EQ-5D-5L state 11115).
For question types I–V, such corner states (CSs, with only one problem each) were chosen so that any variation across states could be linked to a single EQ-5D dimension, and to make the health scenarios easy to picture. The scenarios cover different aspects of health, and therefore enabled us to test the key methodological issues across different hypothetical health concerns. For the health states taken from the pain/discomfort and anxiety/depression dimensions, the questions only included either pain or depression. In order to reflect that the health states described had no problems (represented level 1) on the other EQ-5D dimensions, respondents were explicitly requested to assume that they have no other health problems other than those indicated. Two sets of V values were used: 0.8 and 0.9 for the two states involving level 2 (i.e. slight problems) and 0.4 and 0.6 for the three states involving level 5 (unable/extreme problems). These values were chosen in line with the MVH tariff values for the five comparable health states taken from the three-level version of EQ-5D. This was done to use V values near to the modelled indifference point from the MVH tariff to make the choices challenging. For the two mild states, the comparable MVH values were 0.850 (21111) and 0.796 (11121), and for the extreme states the comparable values were 0.213 (31111), 0.264 (11131) and 0.414 (11113).
Type VI questions used the worst possible state using EQ-5D-5L (state 55555), which is the most likely state to lead respondents to exhaust lead time.
For question type VII, whole EQ-5D-5L health states were used. This is because these question types investigate methods to produce values on the full health–dead utility scale for whole health-state descriptive systems, such as EQ-5D-5L.
For question type VIII, whole states from the three-level EQ-5D were used. The three-level version was used as we were assessing the feasibility of a new binary choice health-state valuation method, and the whole states used had also been used in previous research developing the LT-TTO method.
Survey completion process
Each survey began by providing a brief background explaining the purpose of the survey, and this was followed by a compulsory informed consent page. After consenting, respondents provided demographic information, including age, gender, marital status, employment status, whether they were educated past the minimum level, and whether they had a degree. Respondents answered questions about health status (on a five-point scale from ‘excellent’ to ‘poor’); health satisfaction and life satisfaction [measured on a 10-point scale from ‘completely satisfied’ to ‘completely dissatisfied’, and known as SWBH (own health satisfaction) and SWBL (own life satisfaction), respectively], and the EQ-5D-5L. For half of the respondents these questions were followed by the experimental question modules. However, the other half of the respondents completed the self-report questions after the experimental modules. On the final page there was a free text box to enable respondents to provide their opinions on the survey, or any other relevant information (see Appendix 1 for screenshots from version 15 of the online survey).
Allocation of questions to questionnaire versions
PRET
The seven different question types were presented across three experimental modules:
-
Module 1 Five type I questions.
-
Module 2 Five questions specific to the questionnaire version (using question types II–VI).
-
Module 3 Two type VII questions.
Each respondent completed 12 binary choice questions and there were 15 versions of the online survey overall ( Table 2 describes the question types included in each survey). The ordering of the questions within each module was randomised. For 14 of the versions, module 2 consisted of five binary choice questions from one of types II, III, IV, V or VI. Therefore, respondents who completed these versions faced three question types each. However, for version 15, module 2 included one question each of types II, III, IV, V or VI. Therefore, respondents allocated to version 15 completed all seven question types. This was done so that we could compare the results for all question types across different modes of administration at stage 2 of the project (see Chapter 4 ).
Group | Questions | No. of versions | Version names | Approximate n | ||
---|---|---|---|---|---|---|
Module 1 | Module 2 | Module 3 | ||||
1 | 5 × type I | 5 × type II | 2 × type VII | 3 (12 subversions) | V1/V2/V3 | 600 |
2 | 5 × type I | 5 × type III | 2 × type VII | 3 (12 subversions) | V4/V5/V6 | 600 |
3 | 5 × type I | 5 × type IV | 2 × type VII | 3 (12 subversions) | V7/V8/V9 | 600 |
4 | 5 × type I | 5 × type V | 2 × type VII | 2 (8 subversions) | V10/V11 | 400 |
5 | 5 × type I | 5 × type VI | 2 × type VII | 3 (12 subversions) | V12/V13/V14 | 600 |
6 | 5 × type I | 1 × type II/III/IV/V/VI | 2 × type VII | 1 (4 subversions) | V15 | 200 |
Furthermore, there were 60 subversions of the survey (each of the 15 versions has four subversions) for module 3. This enabled 120 DCETTO pairs to be allocated across the 60 subversions (i.e. two per subversion).
PRET-AS
The PRET-AS respondents completed either 15 type VII questions across three experimental modules of five questions or 10 type VIII questions across two experimental modules of five questions.
Recruitment and the sample
PRET
Respondents were recruited from an existing commercial internet panel. Overall, approximately 3000 respondents were recruited into stage 1 of PRET across the 60 subversions of the online survey, with each version completed by a minimum sample size of 50. Respondents were sourced from an existing internet panel following set quotas for age across five age groups (18–24, 25–34, 35–44, 45–54, and 55–65 years, although a handful of respondents reported that they were older than 65 years) and gender, in an attempt to recruit a sample that was representative of the UK general population in this age range. To recruit participants, invitations were sent out by e-mail. Respondents were screened out prior to starting the experimental questions if the relevant quota for age and gender was complete, or after completing the survey if they answered all of the survey questions in less than the minimum imposed time limit of 5 minutes. Those who successfully completed the survey received online points worth approximately £1.
The same recruitment procedures that were used for the PRET online survey was followed for PRET-AS, with approximately 1800 respondents across the 36 type VII question surveys and 1200 across the 27 type VIII surveys. We reduced the minimum completion times so that respondents were classified as non-completers if they completed the survey in < 3 minutes (and the time to complete the overall survey and each experimental question module was recorded).
Respondents entering the survey firstly completed the same demographic and self-reported health questions. They were then presented with information about the tasks. This included details about the EQ-5D-5L health dimensions, and instructions to imagine that they would experience each health state for the period shown without relief or treatment, that death would be very swift and completely painless, and that they would have no other health problems besides what was indicated. A practice task was then completed, followed by the valuation questions.
Respondent characteristics
Tables 3 and 4 present the characteristics of the respondents to the PRET and PRET-AS online surveys overall, and in comparison with the UK general population using census data. 40 Respondents who completed the PRET online survey were not invited to take part in the PRET-AS online survey. Following recommendations in Dolan and Metcalfe,41 we merge levels of SWBH and SWBL into the following categories: ‘low’ if 0–5; ‘medium’ if 6–7; ‘high’ if 8–9; and ‘very high’ if 10. This is because those scoring ‘10’ display different characteristics than those that might be expected – for example they tend to be older and less healthy.
Characteristic | General populationa | Invited | Non-responders | Respondersb | Responder, non-completerb | Completers | Type | ||||||
---|---|---|---|---|---|---|---|---|---|---|---|---|---|
I | II | III | IV | V | VI | VII | |||||||
n | 34,892 | 27,142 | 7750 | 4591 | 3159 | 3159 | 829 | 847 | 849 | 645 | 873 | 3159 | |
Age, years | |||||||||||||
Mean (SD) | 42.2 | 37.2 | 37 | 39.0 (13.6) | 37.4 (13.2) | 40.8 (13.8) | 40.8 (13.8) | 39.4 (13.7) | 41.0 (13.5) | 39.9 (13.9) | 42.5 (14.0) | 41.7 (13.8) | 40.8 (13.8) |
Range | 18–64 | 18–65 | 18–65 | 18–65 | 18–65 | 18–65 | 18–65 | 18–65 | 18–65 | 18–65 | 18–74 | 18–65 | 18–64 |
Age category, years (n, %) | |||||||||||||
18–24 | 14 | 5882 (16.9) | 17 | 1232 (18.2) | 744 (20.5) | 488 (15.4) | 488 (15.4) | 146 (17.6) | 123 (14.5) | 147 (17.3) | 90 (14) | 123 (14.1) | 488 (15.4) |
25–34 | 23 | 8377 (24.0) | 23 | 1849 (27.3) | 1064 (29.4) | 785 (24.9) | 785 (24.9) | 214 (25.8) | 209 (24.7) | 232 (27.3) | 147 (22.8) | 204 (23.4) | 785 (24.9) |
35–44 | 24 | 10,222 (29.3) | 32 | 1256 (18.5) | 698 (19.3) | 558 (17.7) | 558 (17.7) | 157 (18.9) | 166 (19.6) | 133 (15.7) | 109 (16.9) | 149 (17.0) | 558 (17.7) |
45–54 | 22 | 6682 (19.2) | 18 | 1391 (20.5) | 673 (18.6) | 718 (22.8) | 718 (22.8) | 188 (22.7) | 196 (23.2) | 194 (22.9) | 129 (20) | 213 (24.4) | 718 (22.8) |
55–65 | 17 | 3727 (10.7) | 18 | 1046 (15.4) | 442 (12.2) | 604 (19.2) | 604 (19.2) | 124 (15.0) | 153 (18.1) | 143 (16.8) | 169 (26.2) | 185 (21.2) | 604 (19.2) |
Male (n, %) | 47 | 22,452 (64.3) | 69 | 3273 (48.1) | 1833 (50.3) | 1440 (45.6) | 1440 (45.6) | 368 (44.4) | 372 (43.9) | 401 (47.2) | 278 (43.1) | 444 (49.2) | 1440 (45.6) |
Employment (n, %) | |||||||||||||
In employment | 62 | NA | NA | 3612 (53.1) | 1928 (52.9) | 1684 (53.3) | 1684 (53.3) | 444 (53.6) | 443 (52.3) | 467 (55.0) | 343 (53.3) | 468 (53.7) | 1684 (53.3) |
Student | 7 | NA | NA | 728 (10.7) | 446 (12.2) | 282 (8.9) | 282 (8.9) | 96 (11.6) | 78 (9.2) | 73 (8.6) | 52 (8.1) | 67 (7.7) | 282 (8.9) |
Not in employment | 31 | NA | NA | 1202 (17.7) | 614 (16.8) | 1193 (37.8) | 1193 (37.8) | 289 (34.9) | 326 (38.5) | 309 (36.4) | 249 (42.1) | 338 (38.7) | 1193 (37.8) |
Marital status (n, %) | |||||||||||||
Married/partner | 53 | NA | NA | 3613 (53.1) | 1858 (51.0) | 1755 (55.6) | 1755 (55.6) | 439 (52.9) | 486 (57.3) | 450 (53.0) | 366 (56.8) | 492 (56.3) | 1755 (55.6) |
Single | 47 | NA | NA | 3191 (46.9) | 1788 (49.0) | 1403 (44.4) | 1403 (44.4) | 390 (47.1) | 361 (42.7) | 399 (47.0) | 186 (28.88) | 381 (43.7) | 1403 (44.4) |
Education (n, %) | |||||||||||||
Education after minimum age | NA | NA | NA | 6115 (76.0) | 2796 (76.7) | 2273 (75.1) | 2273 (75.1) | 628 (75.7) | 634 (74.9) | 659 (77.6) | 482 (74.8) | 643 (73.7) | 2273 (75.1) |
Educated to degree level | 22 | NA | NA | 2702 (39.9) | 1491 (41.2) | 1211 (38.4) | 1211 (38.4) | 310 (37.4) | 321 (37.9) | 363 (42.7) | 250 (38.8) | 311 (35.6) | 1211 (38.4) |
Time taken to complete, minutes (mean, SD) | |||||||||||||
Overall | NA | NA | NA | NA | NA | 9.01 (4.6) | 9.01 (4.6) | 8.74 (5.0) | 8.69 (4.4) | 8.61 (3.9) | 8.6 (4.7) | 8.52 (3.9) | 9.01 (4.6) |
Module 1 | NA | NA | NA | NA | NA | 1.15 (1.1) | 1.15 (1.1) | NA | NA | NA | NA | NA | 1.15 (1.1) |
Module 2 | NA | NA | NA | NA | NA | 1.42 (1.4) | NA | 1.25 (1.1) | 1.48 (1.4) | 1.55 (1.6) | 1.4 (1.5) | 1.49 (1.4) | 1.42 (1.4) |
Module 3 | NA | NA | NA | NA | NA | 1.25 (1.2) | NA | NA | NA | NA | NA | NA | 1.25 (1.2) |
Health status (n, %) | |||||||||||||
Good | NA | NA | NA | 4306 (78.5) | 1942 (81.6) | 2364 (76.1) | 2364 (76.1) | 620 (74.8) | 647 (76.4) | 649 (76.4) | 497 (77.1) | 659 (75.5) | 2364 (76.1) |
Poor | NA | NA | NA | 1179 (21.5) | 438 (18.4) | 741 (23.9) | 741 (23.9) | 209 (25.2) | 200 (23.6) | 200 (23.6) | 148 (23) | 214 (24.5) | 741 (23.9) |
SWBH (n, %) | |||||||||||||
10 | NA | NA | NA | 537 (9.8) | 1470 (11.9) | 243 (7.8) | 243 (7.8) | 60 (7.2) | 59 (7.0) | 68 (8.03) | 64 (9.9) | 59 (6.8) | 243 (7.8) |
6–9 | NA | NA | NA | 3140 (57.3) | 1334 (56.0) | 1806 (58.2) | 1806 (58.2) | 489 (58.9) | 503 (59.4) | 498 (58.7) | 366 (56.7) | 503 (57.6) | 1806 (58.2) |
1–5 | NA | NA | NA | 1808 (33.0) | 752 (31.6) | 1056 (34.0) | 1056 (34.0) | 281 (33.9) | 285 (33.7) | 283 (33.3) | 215 (33.3) | 311 (35.6) | 1056 (34.0) |
SWBL (n, %) | |||||||||||||
10 | NA | NA | NA | 491 (9.0) | 282 (11.9) | 209 (6.7) | 209 (6.7) | 43 (5.2) | 54 (6.3) | 51 (6.0) | 60 (9.3) | 60 (6.9) | 209 (6.7) |
6–9 | NA | NA | NA | 3191 (58.2) | 1324 (55.6) | 1867 (60.1) | 1867 (60.1) | 495 (59.7) | 520 (61.4) | 531 (62.5) | 363 (56.3) | 528 (67.4) | 1867 (60.1) |
1–5 | NA | NA | NA | 1803 (32.9) | 774 (32.5) | 1029 (33.1) | 1029 (33.1) | 402 (35.1) | 273 (32.2) | 268 (31.5) | 222 (34.4) | 285 (32.7) | 1029 (33.1) |
Characteristic | General populationa | Invited | Non-responders | Respondersb | Responders, non-completersb | Completers |
---|---|---|---|---|---|---|
n | 5552 | 1039 | 4513 | 2714 | 1799 | |
Age, years | ||||||
Mean (SD) | 42.2 | 38.8 | 39.1 | 39.4 (13.2) | 37.9 (12.8) | 40.4 (13.3) |
Range | 18–64 | 18–65 | 18–65 | 18–65 | 18–65 | 18–65 |
Age category, years (n, %) | ||||||
18–24 | 14 | 735 (13.2) | 4.1 | 431 (14.7) | 178 (15.8) | 253 (14.1) |
25–34 | 23 | 1093 (19.7) | 8.0 | 752 (25.7) | 322 (28.5) | 430 (23.9) |
35–44 | 24 | 2261 (40.7) | 78.1 | 663 (22.6) | 283 (25.0) | 380 (21.1) |
45–54 | 22 | 852 (15.3) | 6.3 | 615 (21.0) | 212 (18.8) | 403 (22.4) |
55–65 | 17 | 607 (10.9) | 3.5 | 468 (15.9) | 135 (11.9) | 333 (18.5) |
Male (n, %) | 47 | 3425 (61.7) | 88.6 | 1499 (51.0) | 679 (59.6) | 820 (45.6) |
Employment (n, %) | ||||||
In employment | 62 | NA | NA | 1711 (70.3) | 669 (71.2) | 1042 (57.9) |
Student | 7 | NA | NA | 261 (10.9) | 108 (11.5) | 153 (8.5) |
Not in employment | 31 | NA | NA | 463 (19.0) | 163 (17.3) | 757 (42.1) |
Marital status (n, %) | ||||||
Married/partner | 53 | NA | NA | 1617 (55.9) | 598 (53.5) | 1019 (56.6) |
Single | 47 | NA | NA | 1274 (44.1) | 520 (46.5) | 780 (43.4) |
Education (n, %) | ||||||
Education after minimum age | NA | NA | NA | 3.835 (85.0) | 2239 (76.8) | 1404 (78.0) |
Educated to degree level | 22 | NA | NA | 1242 (42.6) | 1242 (55.5) | 762 (42.4) |
Time taken to complete, minutes (mean, SD) | ||||||
Overall | NA | NA | NA | NA | NA | 9.42 (5.4) |
Module 1 | NA | NA | NA | NA | NA | 2.00 (1.5) |
Module 2 | NA | NA | NA | NA | NA | 1.72 (1.7) |
Module 3 | NA | NA | NA | NA | NA | 1.55 (1.5) |
Health status (n, %) | ||||||
Good | NA | NA | NA | 1898 (78.4) | 516 (82.6) | 1384 (76.9) |
Poor | NA | NA | NA | 524 (21.6) | 109 (17.4) | 415 (23.1) |
SWBH (n, %) | ||||||
10 | NA | NA | NA | 196 (8.1) | 76 (12.2) | 120 (6.7) |
6–9 | NA | NA | NA | 1436 (59.3) | 359(57.4) | 1077 (59.9) |
1–5 | NA | NA | NA | 790 (32.6) | 190 (30.4) | 602 (33.5) |
SWBL (n, %) | ||||||
10 | NA | NA | NA | 174 (7.2) | 63 (10.1) | 111 (6.2) |
6–9 | NA | NA | NA | 1452 (60.0) | 370 (59.2) | 1082 (60.1) |
1–5 | NA | NA | NA | 796 (32.9) | 192 (30.7) | 606 (33.7) |
Response process
PRET
Overall, 34,892 panel members were invited to take part in the PRET stage 1 online survey, and 7750 (22.2%) clicked the link to access the survey. Of those who entered the survey, 668 (8.6%) did not start the questions, 2158 (27.8%) dropped out during the survey, 1765 (22.8%) either completed the survey in less than the minimum time of 5 minutes or did not click the final page so were classified as non-completers, and 3159 (40.8%) fully completed the survey. The available demographics for responder and non-responder samples overall and across the VII question types are reported in Table 3 .
PRET-AS type VII
Overall, 5552 respondents were invited to take part, and 4513 (81%) respondents accessed the survey. Of these, 1183 (26% of those accessing the survey) were turned away because their quota was full, leaving 3330 (74%) to enter the survey. Of these, 1020 (31%) dropped out before reaching the DCETTO questions. Of the remaining 2310 who entered the DCETTO questions, 23, 50 and 33 dropped out during the first, second, and third modules, respectively. A further nine completed all of the DCETTO questions but failed to formally sign out from the survey and to be counted. Finally, 396 respondents (17% of those who started the DCETTO questions) were excluded because they completed the survey in less than the minimum time limit of 3 minutes. Therefore, 1799 respondents (40% of those accessing the survey) fully completed the whole survey in > 3 minutes. This amounts to 40% of those accessed the survey, 54% of those who entered and 78% of those who started the DCETTO questions. The available demographics for responder and non-responder samples are reported in Table 4 .
PRET-AS type VIII
Overall, 4696 respondents were invited, and 3570 (76.0%) accessed the survey. Of those who accessed the survey, 1035 (29.0%) did not start the questions, 658 (18.4%) dropped out during the LT-TTO survey, 677 (19.0%) either fully completed the survey in < 3 minutes or completed but did not click the final link so were classified as non-completers, and 1200 (33.6%) fully completed the survey in > 3 minutes. The available demographics for responder and non-responder samples are reported in Table 5 .
Characteristic | General populationa | Invited | Non-respondersb | Responders | Responders, non-completers | Completers |
---|---|---|---|---|---|---|
n | 4696 | 1126 | 3570 | 2370 | 1200 | |
Age, years | ||||||
Mean (SD) | 42.2 | 38.6 | 39.2 | 38.9 (12.8) | 37.3 (12.4) | 40.4 (13.1) |
Range | 18–64 | 18–65 | 18–65 | 18–65 | 18–65 | 18–65 |
Age category, years (n, %) | ||||||
18–24 | 14 | 638 (13.6) | 36 (3.5) | 428 (17.6) | 245 (19.8) | 183 (15.3) |
25–34 | 23 | 874 (18.6) | 60 (5.8) | 612 (25.1) | 334 (26.9) | 278 (23.2) |
35–44 | 24 | 2025 (43.1) | 856 (83.2) | 593 (24.3) | 319 (25.7) | 274 (22.9) |
45–54 | 22 | 712 (15.2) | 46 (4.5) | 490 (20.1) | 225 (18.2) | 265 (22.1) |
55–64 | 17 | 447 (9.5) | 31 (3.0) | 314 (12.9) | 116 (9.4) | 198 (16.5) |
Male (n, %) | 47 | 2987 (63.6) | 945 (91.8) | 1297 (52.8) | 726 (57.9) | 571 (47.6) |
Employment (n, %) | ||||||
In employment | 62 | NA | NA | 1357 (55.7) | 748 (60.4) | 609 (50.8) |
Student | 7 | NA | NA | 227 (9.3) | 113 (9.1) | 114 (9.5) |
Not in employment | 31 | NA | NA | 854 (35.0) | 377 (30.5) | 591 (49.2) |
Marital status (n, %) | ||||||
Married/partner | 53 | NA | NA | 1350 (55.4) | 656 (53.0) | 694 (57.8) |
Single | 47 | NA | NA | 1088 (44.6) | 582 (47.0) | 506 (42.2) |
Education (n, %) | ||||||
Education after minimum age | NA | NA | NA | 1801 (73.9) | 913 (73.8) | 888 (74.0) |
Educated to degree level | 22 | NA | NA | 966 (39.7) | 526 (42.6) | 440 (36.7) |
Time taken to complete, minutes (mean, SD) | ||||||
Overall | NA | NA | NA | NA | NA | 7.41 (4.6) |
Module 1 | NA | NA | NA | NA | NA | 1.68 (1.4) |
Module 2 | NA | NA | NA | NA | NA | NA |
Module 3 | NA | NA | NA | NA | NA | NA |
Health status (n, %) | ||||||
Good | NA | NA | NA | 1617 (80.3) | 686 (84.1) | 932 (77.7) |
Poor | NA | NA | NA | 397 (19.7) | 129 (15.9) | 268 (22.3) |
SWBH (n, %) | ||||||
10 | NA | NA | NA | 155 (7.7) | 78 (9.5) | 77 (6.4) |
6–9 | NA | NA | NA | 1238 (61.4) | 509 (62.2) | 729 (60.8) |
1–5 | NA | NA | NA | 624 (31.0) | 231 (28.2) | 393 (32.8) |
SWBL (n, %) | ||||||
10 | NA | NA | NA | 78 (7.1) | 44 (9.0) | 75 (6.3) |
6–9 | NA | NA | NA | 661 (59.8) | 292 (59.5) | 712 (59.3) |
1–5 | NA | NA | NA | 366 (33.1) | 155 (31.6) | 413 (34.4) |
Summary
The development of the EQ-5D-5L and advances in the techniques used for health-state valuation means that there is the need to derive a new population value set for use in cost-effectiveness analysis. The PRET and PRET-AS projects investigate a range of methodological issues relating to the health-state valuations. The methodological issues are assessed using binary choice questions administered online. The aim of this chapter was to briefly describe the methodological issues addressed in the PRET and PRET-AS online surveys, and to outline the surveys used and recruitment procedure. More detailed descriptions of the methods, results and discussion of each stage are included in Chapter 3 .
Chapter 3 Are health-state preferences independent of duration (assessing CP-TTO using type I questions)?
Introduction
Constant proportional time trade-off is a key assumption underlying the use of TTO health-state values in the generation of QALYs. CP-TTO assumes that the health-state values produced by TTO are the same irrespective of the duration assigned to the health state. If the assumption does not hold, health states may be valued differently, dependent on their duration.
Evidence both for and against42,43 CP-TTO has been found, and the research reported in this chapter aimed to test the assumption using a binary choice question incorporating a range of duration values (and associated time in full health) and health-state dimensions. This was done using the most ‘basic’ binary choice question type I used in PRET stage 1. The objectives of the analysis of this question type were twofold:
-
To provide a baseline or reference point for the PRET binary choice question design in terms of the frequencies of respondents choosing scenario B (shorter time in full health) across different combinations of state H , value V and duration T The results of this baseline question can then be compared with question types II–V which incorporate the same health dimensions and duration along with information about additional attributes. We also assess the impact of respondent characteristics on the scenario choice, and examine the logical consistency of responses.
-
To test the CP-TTO assumption If health-state preferences are independent of duration then, for a given combination of state H and value V, the distribution of respondents between the two scenarios should not be affected by duration T. Therefore, if the duration (10 × V) years in the basic scenario above was replaced with (5 × V) years, the proportion of people choosing each scenario at a given V should not differ (i.e. are health-state preferences independent of duration or a test of CP-TTO).
Methods
Question format and study design
The type I binary choice questions used the following format (and an example of how the question was presented in the survey is shown in Appendix 2 ).
-
[Scenario A]: You will live in health state H for T years and then die.
-
[Scenario B]: You will live in full health for (VT) years and then die.
-
Which scenario do you think is better?
The health state H was a ‘CS’ and used the following five health dimensions adapted from EQ-5D-5L health dimensions. CSs were used so that variation could be linked to a single dimension, and also to make the health scenarios easy to imagine. Respondents were instructed to assume that they have no other health problems other than those indicated in the scenario.
-
‘Slight problems walking about’ (level 2 of the mobility dimension from EQ-5D-5L state 21111).
-
‘Slight pain’ (a segment of level 2 of the pain dimension using pain only from EQ-5D-5L state 11121).
-
‘Unable to walk about’ (level 5 of the mobility dimension from EQ-5D-5L state 51111).
-
‘Extreme pain’ (a segment of level 5 of the pain/discomfort dimension using pain only from EQ-5D-5L state 11151).
-
‘Extreme depression’ (a segment of level 5 of the anxiety/depression using depression only from EQ-5D-5L state 11115).
Duration T took one of four values: 10 weeks, 1 year, 5 years and 10 years. The values were chosen as follows: 10 years for comparability with the ‘standard’ MVH TTO protocol, 5 and 1 years as intermediate whole-year values, and 10 weeks to test the maximum endurable time of the more severe CSs.
Two sets of V values were used: 0.8 and 0.9 for the two states using level 2 (i.e. slight), and 0.4 and 0.6 for the three states using level 5 (unable/extreme). V values of 0.4 and 0.8 are described as ‘V(low)’, and 0.6 and 0.9 as ‘V(high)’.
All 15 versions of the online survey included five type I questions as the first module presented to respondents, meaning that there were 75 ‘slots’ for this question type overall. Combining the five health states H, four dimension levels T, and two values for V used for type I questions resulted in a total of 40 possible combinations. Therefore, 35 of the combinations appeared twice in different versions of the online survey, with five (one for each health state) appearing once. The allocation of the question combinations across the different survey versions are displayed in Appendix 3 .
In addition, each respondent was given a question similar to a type I question, but tests for logical consistency. In this question, scenario A was dominated by scenario B: scenario A was to live for a shorter duration in worse health and scenario B was to live for a longer duration in full health. Thus, the logical answer is to choose B. If respondents were choosing randomly between A and B then around half of them would choose A. In other words, double the proportion of those choosing A for the logical consistency test question may be interpreted to represent the proportion of respondents who were not fully engaged.
Analysis
For question type I, the outcome of interest is the proportion of respondents selecting scenario B, which means preferring less time in full health over more time in worse health, and thus represents the proportion of respondents for whom the value of V* of state H is lower than the value of V used in the scenario pair. The proportions of those choosing scenario B were analysed across the different scenario attribute combinations and background characteristics. For type I questions, the proportion of respondents who violated logical dominance was also assessed.
The findings were tested for the overall sample, and also by splitting the sample into two groups based on the median time taken to complete question module 1 (which included five type I questions). Group 1 included those who completed the question module in less than the median time taken to complete the module, and group 2 included those who completed the module in more than or equal to the time taken to complete the module.
Probit regression was used to explore the significant impacts on choosing scenario B across each set of scenario attributes and background characteristics. The equation used is as follows:
where Pr represents probability, the β is are the estimated parameters, D represents the background characteristics of respondents, SWB represents self-reported satisfaction levels (SWB H and SWB L), X represents the properties of the health state using health state (H), duration (T), lead time in full health (L), person perspective (P), and satisfaction level (S), and the function Φ(.) is the distribution function of the standard normal distribution. Marginal effects are reported where, for example, a marginal effect of −0.1 for female indicates that being female reduces the probability of choosing scenario B by 10%. Statistical significance levels of both < 0.05 and < 0.1 were used.
Results
Demographics
As all respondents were given type I questions, the sample here consists of 3159 full survey completers who each completed five type I questions (of which one was a test of logical consistency). Therefore, the responses to the four logical questions generated 12,636 type I observations. Each combination of H, V and T was completed by either (approximately) 200 or 400 respondents. The characteristics of the sample are displayed in Table 3 .
Objective 1: descriptive analysis
Of the 3159 respondents, 200 (6%) failed the test of logical consistency (i.e. responded that they would rather live for less time in one of the five health states than a longer time in full health). Using bivariate analysis, there is a disproportionate number of males (chi-squared test; p = 0.001) – those whose education continued after minimum school leaving age (p = 0.012) and those in poorer self-reported general health (p = 0.015) – who failed the logical consistency test. Age, having a degree, and time taken were not associated with failing the logical consistency test. When assessing the proportions of respondents failing the logical consistency test across the two groups defined by the time taken to complete question module 1 described above (see Analysis), it was found that the proportion of respondents failing the test did not differ significantly between group 1 (n = 85, 5.5%) and group 2 (n = 115, 7.7%) (p = 0.06).
Based on the remaining four type I questions in module 1, Figure 3 illustrates the proportion of respondents choosing scenario B, broken down by the health-state dimension, duration of the health state, and the value used to generate the associated time in full health in scenario B. In other words, this was the proportion of respondents for whom the value used in the scenario (V) was larger than the value they perceive (V*) for the state. It should be noted that the majority of bars are > 50%, some of them as high as 90%. This suggests that the values of V used in the design of the question types may have been set lower (this is discussed in relation to all of the question types in Chapter 12 , Weaknesses of the project).
FIGURE 3.
Proportion of sample choosing scenario B (type I questions).
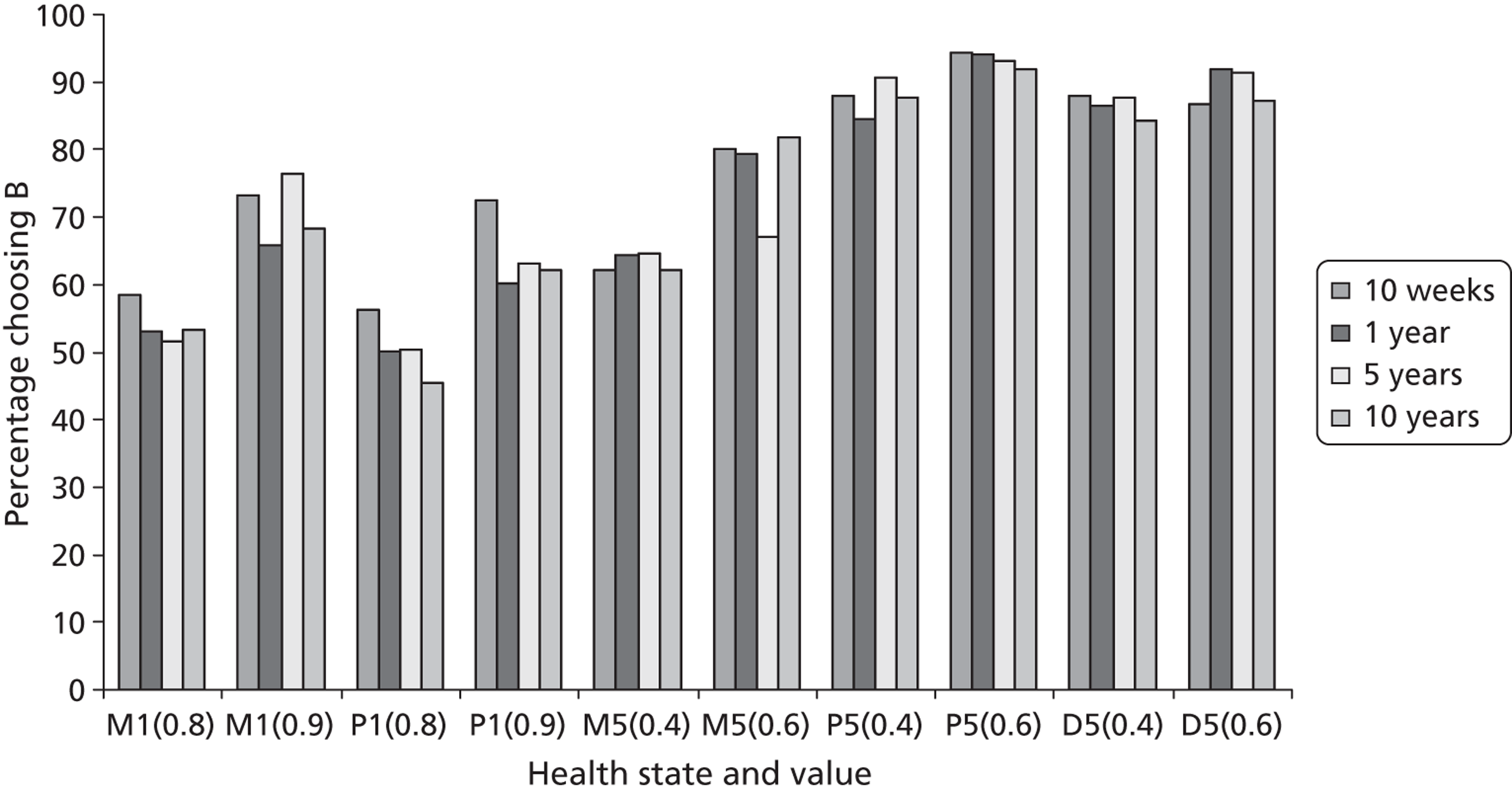
Within each health-state dimension, the proportion of respondents choosing B was higher when the value of V was larger. For example, the bars for ‘slight problems walking about’ with a high V value [M2(0.9)] are taller than the corresponding bars for ‘slight problems walking about’ with a lower V value [M2(0.8)] across the same duration of time spent in the health state. The exception (by a small margin) is for ‘extreme depression’ [D5(0.4)] and [D5(0.6)] with a duration of 10 weeks. Within each specific health problem (where a comparison is possible), the proportion choosing scenario B was always higher for the more severe state so that the bars for ‘unable to walk about’ (M5) are taller than corresponding bars for ‘slight problems walking about’ (M2), and the bars for ‘extreme pain’ (P5) are taller than the corresponding bars for ‘slight pain’ (P2). This demonstrates that respondents are more likely to choose the full health option when the state is severe. There does not seem to be a pattern to the proportions of respondents choosing scenario B across the four duration levels. For example, the bars for the 10-week duration scenarios tend to be taller than the corresponding bars for longer durations, but there are exceptions.
Table 6 summarises the results of a series of probit regressions explaining the propensity to choose scenario B (living in full health for a shorter period of time), without (models 1–5) and with (models 6–10) controlling for a series of covariates. As the distribution of data for own health in EQ-5D-5L is skewed, dummy variables indicating any problem in mobility, pain/discomfort or anxiety/depression were used. The models by state indicate that generally the higher the value of V, the higher the probability of choosing to live in full health for a shorter duration [although this is not significant for ‘extreme depression’ (D5)]. There were no covariates that affect the choice consistently across all states. Regarding the effect of respondents’ self-reported health in EQ-5D (models 6–10), the exercise finds that, controlling for duration and V value, having a mobility problem was associated with being less likely to choose scenario B (living for less time in full health) for the two mobility-based states (M2 and M5) and extreme pain (P5); having pain/discomfort was associated with being less likely to choose scenario B when the state is ‘slight problems in walking about’ (M2) and ‘slight pain’ (P2) but not ‘extreme pain’ (P5); and having anxiety/depression was associated with being less likely to choose scenario B when the state was ‘extreme depression’ (D5). This indicates that, to some extent, respondents who have experience of the health state they are valuing may be more likely to hypothetically associate it with a higher utility value.
Scenario attributes/background characteristics | (1) | (2) | (3) | (4) | (5) | (6) | (7) | (8) | (9) | (10) |
---|---|---|---|---|---|---|---|---|---|---|
M2 | P2 | M5 | P5 | D5 | M2 | P2 | M5 | P5 | D5 | |
V value (ref.: V low) | ||||||||||
V high | 0.452*** | 0.359*** | 0.407*** | 0.352*** | NS | 0.482*** | 0.358*** | 0.412*** | 0.359*** | NS |
Duration (ref.: 10 years) | ||||||||||
10 weeks | 0.154* | 0.273*** | NS | NS | NS | NS | 0.283*** | NS | NS | NS |
1 year | NS | NS | NS | NS | NS | NS | NS | NS | NS | NS |
5 years | NS | NS | NS | NS | NS | NS | NS | NS | NS | NS |
Marital status | NS | NS | NS | NS | NS | |||||
Employment status | NS | NS | NS | NS | −0.043** | |||||
Age category | −0.074*** | −0.055* | NS | 0.071* | NS | |||||
General health | NS | NS | NS | NS | NS | |||||
Health satisfaction | NS | NS | NS | 0.266** | NS | |||||
Report problems on EQ-5D-5L | ||||||||||
Mobility (score ≥ 2) | −0.261** | NS | −0.219** | −0.316** | NS | |||||
Pain (score ≥ 2) | −0.125* | −0.302*** | NS | NS | NS | |||||
Depression (score ≥ 2) | NS | NS | NS | NS | −0.275*** | |||||
Constant | NS | NS | 0.366*** | 1.132*** | 1.017*** | 0.442*** | 0.273* | 0.506*** | 0.944*** | 1.071*** |
n | 2533 | 2495 | 2550 | 2526 | 2532 | 2495 | 2461 | 2514 | 2493 | 2497 |
Log-likelihood | −1656.551 | −1682.460 | −1541.726 | −740.201 | −951.307 | −1600.001 | −1636.980 | −1501.139 | −702.528 | −911.776 |
All of the state dummies were significant when pooling across states ( Table 7 ; model 11 without covariates, and model 12 with covariates). It suggests that ‘slight problems walking about’ (M2) was perceived as being worse than ‘slight pain’ (P2), and ‘unable to walk about’ (M5) was perceived as worse than ‘extreme depression’ (D5), which, in turn, was worse than ‘extreme pain’ (P5). As the values are clustered by the severity groups, the state coefficients cannot be compared across the mild states and the severe states. All duration and value dummies were significant. The dummy for the V value 0.9 was omitted as there was collinearity in the design (this is because all scenarios with M2 or P2 that do not use the value of 0.8 use 0.9). Having problems on the EQ-5D-5L dimensions of Mobility, Pain/discomfort, and Anxiety/depression were all significant in the pooled model, indicating that having existing health concerns impacts on the propensity to choose full health (p < 0.001).
Scenario attributes/background characteristics | (11) | (12) |
---|---|---|
All states | All states | |
State (ref.: M2) | ||
P2 | −0.128*** | −0.124*** |
M5 | −0.131** | −0.128** |
P5 | 0.676*** | 0.688*** |
D5 | 0.513*** | 0.524*** |
V value (ref.: 0.4) | ||
0.6 | 0.311*** | 0.319*** |
0.8 | −0.392*** | −0.402*** |
0.9 | [Omitted] | [Omitted] |
Duration (ref.: 10 years) | ||
10 weeks | 0.109** | 0.107** |
1 year | NS | NS |
5 years | NS | NS |
Marital status | NS | |
Employment status | NS | |
Age category | −0.022* | |
General health | NS | |
SWBH | 0.104** | |
Report problems on EQ-5D-5L | ||
Mobility (score ≥ 2) | −0.163*** | |
Pain (score ≥ 2) | −0.124*** | |
Depression (score ≥ 2) | −0.081** | |
Constant | 0.467*** | 0.673*** |
n | 12,636 | 12,460 |
Log-likelihood | −6591.027 | −6415.893 |
Objective 2: assessing assumption 1 – CP-TTO
The coefficients of interest for assessing CP-TTO are those for duration spent in the health state. The 10-year duration value is used as the reference as this is the value used in the ‘standard’ MVH TTO protocol. For the two milder states of ‘slight problems walking about’ (M2) and ‘slight pain’ (P2), the 10-week duration had a significantly positive effect relative to 10 years. However, durations of 1 year and 5 years were not significant (see Table 6 , model 1 for M2 and model 2 for P2). For the states ‘unable to walk about’ (M5, model 3) and ‘extreme pain’ (P5, model 4), none of the duration coefficients was significant. For ‘extreme depression’ (D5, model 5), the 5-year duration is significant. The same pattern of significance was found when covariates were controlled for (see Table 7 , models 6–10). In the model pooling across states, only the 10-week coefficient was significant (see Table 8 , model 11 without and model 12 with controlling for covariates). The positive coefficients indicate that the shorter duration value was associated with having higher preferences for the health state presented.
The above analysis demonstrates that whether or not CP-TTO holds depends on the dimension and severity of the state. In the case of D5, the pattern was not monotonic. It is somewhat surprising that the extreme (level 5) states, where one may have expected maximal endurable time to apply, have resulted in no significant duration coefficients. There also seems to be no pattern of interactions between the state, associated duration, V value and respondent characteristics.
Discussion
The type I questions used in this chapter are a snapshot of one iteration of the TTO procedure, and, as such, provide a comparator for the other question types which incorporate various attributes to the standard question to allow for the testing of the methodological issues introduced in Chapter 2 . A small number of respondents failed the test of logical consistency, indicating that they may not be paying full attention to the online survey or answering truthfully, although we cannot investigate the reasons in more detail. However, failing the logical consistency test does not seem to be related to the time taken to complete the type I questions, as there was no difference between those completing the module quickly and those taking longer to complete (defined in terms of the median time taken).
The questions also allow us to test the assumption of CP-TTO. To the best of our knowledge, this is the first attempt to assess CP-TTO using binary choice questions that are a snapshot of the TTO procedure. The findings are inconclusive: there is no clear pattern to the coefficient values across each duration level, which suggests that the relationship between CP-TTO and the state description and duration value is complex and needs further investigation. We do not produce strong evidence for or against the CP-TTO assumption. Therefore, it is not clear whether the value of a state is a function of its duration, and we cannot give clear guidance on the best duration values to use in future valuation studies. Furthermore, there are limits to what can be implied from the binary choice questions as a small range of V values were used in this study. This is discussed more generally in terms of all question types in Chapter 12 (see Weaknesses of the project) of this report.
Chapter 4 Are health-state preferences independent of person perspective (using type II questions)?
Introduction
In the MVH TTO protocol, respondents are asked to imagine themselves living in the health state, and therefore provide preferences from their own perspective. However, it is unclear what impact using alternative perspectives may have on health-state preferences. The aim of the analysis reported in this chapter was to test whether preferences for health states are influenced by the perspective associated with the state. That is, if health-state preferences are independent of perspective then for a given combination of state H and value V, the distribution of respondents should not differ when person perspective P is changed. This was done by comparing type I questions (which use the ‘you’ perspective) and type II questions (which have matched health-state descriptions and duration values, but use two different perspectives reflecting the citizen’s approach).
Methods
Question format and study design
This analysis used type I and type II binary choice questions, which take the following format (see also Appendix 2 ).
Type I:
[Scenario A]: You will live in health state H for T years and then die.
[Scenario B]: You will live in full health for (VT) years and then die.
Which scenario do you think is better?
Type II:
[Scenario A]: [Person P] will live in health state H for T years and then die.
[Scenario B]: [Person P] will live in full health for (VT) years and then die.
Which scenario do you think is better?
The ‘corner’ health states and duration and V values used across both question types were the same as described in Chapter 3 (see Methods). For type II questions, the two perspectives used were ‘Somebody else’ (SE) and ‘Somebody else like you’ (SY). To assess the impact of perspective P, the health state H, duration T and V value combinations were matched across question types I and II, so the impact of varying only perspective could be assessed.
Question type II appeared on four survey versions, with 16 available ‘slots’ for questions across 80 possible combinations of health state H, duration T, perspective P, and V value. Eight slots were allocated to the perspective SE, with the same eight combinations allocated to SY. Four of the states across each perspective were allocated to the low V category, and four to the high V category. Two of the eight states across each perspective were allocated to each duration level. As described in Chapter 3 , type I questions appeared in all 15 versions of the survey, and the relevant state and duration combinations matched across the question types were extracted for the comparison.
Analysis
The effect of perspective was analysed using descriptive analysis, chi-squared tests and probit regression, which was used to explore the impact of different perspectives on choosing scenario B while controlling for background characteristics:
where Pr represents probability, the β is are the estimated parameters, D represents the background characteristics of respondents, SWB represents self-reported satisfaction levels (SWB H and SWB L), X represents the properties of the health state using health state (H), duration (T), lead time in full health (L), person perspective (P) and satisfaction level (S), and the function Φ(.) is the distribution function of the standard normal distribution. Equation 2 is the same as Equation 1 (see Equation 1 on p. 22) and, again, marginal effects are reported. A backwards stepwise approach was taken to the selection of explanatory sociodemographic variables included in the final models using a level of statistical significance of p < 0.1. Joint tests of statistical significance were used for categorical variables expressed as sets of dummy variables.
Results
Demographic characteristics
Overall, 829 respondents completed type II questions across four survey versions, and this sample was matched with those who completed the comparable type I questions. The characteristics of the samples are shown in Table 3 .
Descriptive analysis
Across all eight combinations between type I and II questions, 75% of respondents chose scenario B when perspective was SE, 73% chose scenario B when the perspective was phrased as SY, and 72% chose scenario B when the perspective was phrased as ‘you’. The proportions choosing scenario B for each question combination included are shown in Table 8 .
State | Duration in poor health (T) | Value (V) | Perspective (types I and II) (%) | p-value (chi-squared test) | ||
---|---|---|---|---|---|---|
SE | SY | You | ||||
M2 | 5 years | 0.8 | 57 | 54 | 52 | 0.475 |
P2 | 10 weeks | 0.9 | 63 | 66 | 72 | 0.118 |
P2 | 10 years | 0.8 | 53 | 45 | 45 | 0.174 |
M5 | 1 year | 0.6 | 70 | 79 | 79 | 0.030 |
P5 | 10 weeks | 0.4 | 91 | 90 | 88 | 0.565 |
P5 | 10 years | 0.6 | 93 | 89 | 92 | 0.282 |
D5 | 1 year | 0.4 | 84 | 81 | 86 | 0.215 |
D5 | 5 year | 0.6 | 87 | 83 | 91 | 0.035 |
Pearson’s chi-squared tests across the three perspectives established statistically significant differences in the proportion of respondents reporting a preference for scenario B for two of the states describing extreme problems (M5 and D5). More respondents favoured less time in full health (scenario B) than the extreme mobility state (M5) when the perspective for the scenario was phrased as ‘you’ or SY compared with when it was SE. Fewer respondents presented with the perspective SY preferred less time in full health when compared with one of the D5 states (T = 5 years, V = 0.6) when compared with the SE or ‘you’ perspective.
Regression analysis
Probit models were estimated for each question combination separately ( Tables 9 and 10 ). Four of the eight models found no impact of the different perspectives on the probability of choosing scenario B. Two models established that respondents were less likely to trade off full health for P2 and extreme mobility problems (M5) when the scenario perspective was SE compared with ‘you’ (p < 0.05). For D5, respondents were more likely to choose scenario B if the perspective was SY in comparison with ‘you’ (p < 0.05, where T = 5; p < 0.1, where T = 1). There is no clear pattern to the impact of demographic variables.
Scenario attributes/background characteristics | M2, T = 5 years, V = 0.8 | P2, T = 10 weeks, V = 0.9 | P2, T = 10 years, V = 0.8 | M5, T = 1 year, V = 0.6 | P5, T = 10 weeks, V = 0.4 | P5, T = 10 years, V = 0.6 | D5, T = 1 year, V = 0.4 | D5, T = 5 years, V = 0.6 |
---|---|---|---|---|---|---|---|---|
n | 852 | 610 | 774 | 830 | 615 | 622 | 848 | 612 |
Constant | 0.456** | −1.019*** | −0.356*** | 0.797*** | 1.393*** | 1.022*** | 0.448** | 1.102*** |
Perspective (ref.: you) | ||||||||
Someone elsea | NS | −0.284** | NS | −0.288** | NS | NS | NS | NS |
Someone like youa | NS | NS | NS | NS | NS | NS | −0.234* | −0.391** |
Age | −0.008** | NS | NS | NS | NS | NS | 0.009** | NS |
Gendera | NS | NS | NS | NS | NS | 0.365** | NS | 0.232* |
Marital statusa,b | NS | NS | NS | NS | NS | ** | NS | * |
Employmenta,b | NS | NS | NS | NS | NS | NS | NS | NS |
Educationa | NS | NS | NS | NS | NS | −0.553*** | −0.274** | −0.501*** |
Self-reported healtha,b | * | NS | NS | NS | ** | NS | NS | NS |
SWBH | NS | NS | 0.037** | 0.057** | NS | NS | 0.057** | NS |
SWBL | NS | −0.067*** | NS | −0.053** | NS | NS | NS | NS |
LR chi-squared | 13.57 | 12.72 | 7.78 | 11.86 | 7.97 | 32.43 | 18.57 | 36.71 |
Pseudo R 2 | 0.0115 | 0.0165 | 0.0073 | 0.0133 | 0.0196 | 0.0861 | 0.0254 | 0.0774 |
Log-likelihood | −581 | −380 | −531 | −440 | −199 | −172 | −355 | −218 |
Scenario attributes/background characteristics | Overall | M2 | P2 | M5 | P5 | D5 |
---|---|---|---|---|---|---|
Sample, no. of observations | 15,677 | 2931 | 3250 | 2903 | 3353 | 3334 |
Constant | −0.709*** | 0.555*** | −0.618*** | −0.306*** | 0.471*** | 0.560*** |
Health state (ref.: M2) | ||||||
P2 | −0.124*** | |||||
M5 | 0.893*** | |||||
P5 | 1.703*** | |||||
D5 | 1.500*** | |||||
Duration (ref.: 10 weeks) | ||||||
10 weeks | ||||||
1 year | −0.078** | −0.144** | −0.201*** | NS | NS | NS |
5 years | −0.090*** | NS | −0.168** | NS | NS | NS |
10 years | −0.116*** | −0.152** | −0.197*** | NS | −0.185** | NS |
V value (ref.: V low) | 0.326*** | 0.463*** | 0.313*** | 0.436*** | 0.314*** | NS |
Perspective (ref.: You) | ||||||
Someone elsea | NS | NS | NS | −0.268** | NS | −0.161* |
Someone like youa | −0.088** | NS | NS | NS | NS | −0.357*** |
Age | NS | −0.010*** | −0.005*** | NS | NS | 0.006** |
Gender a | NS | −0.114** | NS | NS | 0.211*** | 0.291*** |
Marital status a,b | NS | NS | NS | NS | NS | NS |
Employment a,b | *** | NS | NS | NS | ** | NS |
Education a | −0.097*** | NS | NS | NS | −0.227*** | −0.242*** |
Self-reported health a,b | *** | *** | NS | NS | NS | * |
SWBH | 0.027*** | NS | 0.036** | 0.057*** | 0.053*** | NS |
SWBL | −0.017*** | NS | −0.030*** | −0.024* | NS | NS |
LR chi-squared | 2059.39 | 133.22 | 95.40 | 99.26 | 92.42 | 102.05 |
Pseudo R 2 | 0.1131 | 0.0338 | 0.0214 | 0.0280 | 0.0457 | 0.0388 |
Log-likelihood | −8073 | −1903 | −2179 | −1721 | −964 | −1265 |
Analysing all of the type I and II responses together (see Table 10 ) showed that the reduction in the likelihood of choosing scenario B when referring to SY was modest but statistically significant. The coefficient for the dummy variable for SE was not statistically significant; however, a joint test for significance approached the 5% level (p = 0.059). No statistically significant interactions were found between perspective P and duration T, state H or value V.
Discussion
In a standard TTO exercise, the perspective generally used to frame the scenario is ‘you’, meaning that respondents should provide preferences based on imagining themselves in the health states presented to them. In the analysis reported in this section we have tested the impact using two different perspectives reflecting the citizen approach (SE and SY) in comparison with the standard ‘you’ perspective used for the MVH TTO protocol. We found no clear pattern to the impact of varying perspective across all states either in terms of comparing ‘you’ with SE and SY, or comparing SE and SY with each other. The conclusions that can be drawn from this analysis are limited by the combinations of health state, duration and V value that could be used to compare the perspectives. The results may also be limited by the alternative perspectives chosen, which could be perceived differently by different respondents.
There is some evidence for differences for the level 5 mobility (M5) and depression (D5) scenarios. Differences were found in the proportions of people preferring to choose full health than a shorter period of time with D5 (5 years), depending on how the perspective was phrased. A similar pattern of responses was seen for the other D5 (1 year) state, although the differences were not statistically significant. When looking at the overall picture for extreme depression, respondents are more likely to choose to live in full health when faced with the ‘you’ perspective in comparison with the SE and SY perspectives. After adjusting for potential confounding sociodemographic factors, the difference was larger for the perspective SY.
This indicates that, from a personal perspective, D5 is a health state to be avoided, irrespective of the duration spent in the health state and the corresponding time in full health. Respondents could have been more comfortable trading their own length of life than other people’s lives; however, if this were the case we would expect to see a more consistent pattern across all of the health states. That people were more willing to trade for the D5 state may suggest that respondents considered they would find that state worse than other people would. We did not include another depression health state, so cannot observe how varying the perspective would impact on other depression-related states, for example slight depression.
We can, however, compare the results for mobility levels 2 and 5. For M5, fewer people presented with the SE perspective chose scenario B than the other perspectives. This was not the case for the less severe mobility health state (M2). This may indicate that the impact of perspective is dependent upon the severity of the health state presented and the overall likelihood of choosing to trade less time in full health.
The two descriptions of the perspective relating to ‘someone else’ produced somewhat different results. In particular, there were some statistically significant differences for the ‘someone else’ perspective, for which problems were described on the pain and mobility dimensions, and statistically significant differences for the ‘someone else like you’ perspective, for which problems were described on the depression dimension. It should be noted that where any of the statistically significant differences were found, the coefficient for both ‘someone else’ perspectives were always in the same direction, and varied only in magnitude and significance. Even still, it is not clear why these differences should occur. Further work may attempt to investigate the impact of perspective using different descriptions based on the citizen perspective.
To focus more on the impact of the actual perspective used, it would be interesting to use whole EQ-5D-5L health states in a similar binary choice or iterative TTO task. Further investigation into the relationship between health-state severity and perspective would also be informative. There are many different perspectives that could be used to assess the impact of the framing of the question on preferences. This could include specifying different personal characteristics, such as age or gender, and this is an area for potential future research.
Chapter 5 Investigation of LT-TTO (using types III, VI and VIII questions)
Introduction
As discussed in detail in Chapter 1 , LT-TTO was developed to overcome the problems associated with the process used to value states worse than dead using the MVH TTO protocol (which involves a very different task to that used for states better than dead). To do this, LT-TTO adds a ‘lead time’ in full health before the usual TTO scenario. This allows states to be valued using the same procedure.
LT-TTO requires that the health-state values generated are independent of the addition of lead time. However, the impact of lead time (and different lengths of lead time) is currently unclear, and needs empirical examination. Further to this, a concern of the lead time approach is the extent to which respondents giving negative valuations use up all of the lead time available by choosing immediate death throughout the iterative exercise, or when the health state is particularly severe (so do not reach their actual value for the health state). This is called ‘exhausting’ the lead time, and there have been a number of attempts to explore the optimal ratio of lead time to duration to use in studies. 19 Earlier studies have found that there is a small proportion of respondents who, when faced with a very severe state, exhaust lead time even when the ratio between lead time and duration is very high. 16 It has been suggested that the respondent may become ‘locked in’ to choosing immediate death as a way of indicating qualitatively to the interviewer that the state is very severe. The implication is that such responses cannot be interpreted at face value. If this is the case, then we may expect to see fewer cases of exhaustion of lead time in binary choice LT-TTO conducted in an online environment. This is because in an online environment there is no interviewer to whom to demonstrate strong feelings and, as each binary choice question is independent of an iterative routing (i.e. the previous question presented), respondents cannot become locked into one response.
In the analysis reported here we use binary choice versions of LT-TTO for the following objectives:
-
To investigate whether health-state preferences are independent of lead time. If preferences are independent of lead time, then for a given combination of state H and value V, the distribution of respondents should not differ by the addition of lead time L.
-
To investigate the extent to which respondents exhaust lead time under very poor health.
-
To investigate the feasibility of eliciting health-state utility values using binary choice questions based on LT-TTO.
Methods
Question format and study design
Type I and III questions:
Type III questions were compared with type I questions to assess the extent to which the addition of lead time into the scenario impacts on health-state preferences. The two question types take the following format (see also Appendix 2 ).
Type I:
[Scenario A]: You will live in health state H for T years and then die.
[Scenario B]: You will live in full health for (VT) years and then die.
Which scenario do you think is better?
Type III:
[Scenario A]: You will live in full health for L followed by health state H for T years and then die.
[Scenario B]: You will live in full health for (L + VT) years and then die.
Which scenario do you think is better?
The health state H, duration T and value V combinations were matched across the question types so that the only difference was the addition of lead time. The same five CSs were used for type I and III questions, and the duration and lead time values were matched at 10 weeks, 1 year, 5 years and 10 years.
All 15 versions of the online survey included five type I questions. Question type III appeared on four survey versions, with 16 available slots for questions across the 160 possible combinations of health state H, duration T, lead time L and value V. Four slots were allocated to each lead time and health-state duration level, and each state was included in four scenarios. The full allocations for both question types are included in Appendix 3 .
Type VI questions
Type VI questions were used to investigate the extent to which the exhaustion of lead time when the state is severe is explained by different ratios of lead time to duration (the L : T ratio). This was done by mapping the proportion of respondents who exhaust lead time at various combinations of duration T and lead time L. Establishing this relationship will inform the choice of ratio of lead time L against duration T in future studies using LT-TTO. Type VI questions took the following format (where choosing scenario B means exhausting lead time – see also Appendix 2 ):
-
[Scenario A]: You will live L in full health followed by T in EQ-5D-5L state 55555 and then die.
-
[Scenario B]: You will die immediately.
By increasing the duration of lead time L against a set duration T, the chances of exhausting lead time should diminish. The ‘worst’ EQ-5D-5L state 55555 was used throughout (see Figure 6 for the health-state description). The duration and lead time values used were 10 weeks, 1 year, 5 years and 10 years.
Sixteen question slots were available across four versions of the online survey. Combinations of lead time L and duration T were selected, with the most frequently occurring L : T ratio being 1 : 1 (see Appendix 3 for the full allocation). It was judged that some very low ratios (e.g. 10 weeks’ lead time and 10 years’ duration, for which the majority of respondents would be expected to choose scenario B) and very high ratios (e.g. 10-year lead time and 10-week duration for which the majority would be expected to choose scenario A) were not meaningful and were therefore not included.
Type VIII questions
Type VIII questions, which can be used to derive a utility value anchored on the full health–dead scale, took the following form (see also Appendix 2 ):
-
[Scenario A]: You will live in full health for L followed by state H for duration T then die.
-
[Scenario B]: You will live in full health for (L + VT) then die (V < 1.0).
The challenge for a DCE of LT-TTO is the amount of information that is involved in each choice. A DCE of LT-TTO for EQ-5D will in effect have eight dimensions per scenario (full health, lead time, the five EQ-5D dimensions, and duration in the state), totalling 16 pieces of information to consider per binary choice. Furthermore, 14 of these will change randomly from one question to the next. Therefore, an alternative that is closer to the original TTO presentation is used for PRET-AS, in which scenario B always involves a shorter duration in full health. Scenario A has eight dimensions but scenario B has only two; of the 10 overall pieces of information, eight of them change from one question to the next. In this situation, V can take negative values, provided that L + VT is not negative. Similar designs have been used in a SG study. 44
As this was a feasibility study, full health states from the three-level EQ-5D were used, and the states were selected based on those used in previous LT-TTO research to reflect a combination of dimension and severity levels. 16,19 The five states used were 11211, 22121, 32211, 23232 and 33333.
In total, 27 versions of the online survey were administered. Each respondent was presented with 10 type VIII questions, with 270 ‘slots’ available overall. A range of combinations of state, lead time and V value were used, and Appendix 3 includes the combinations. The 10 questions were grouped into two modules of five. Half of the respondents in a version received the modules in one order and the other half in the reverse order. Within each module, the ordering of the five questions was randomised.
Analysis
Question types I, III and VI
The impact of lead time (types I and III), and the propensity to exhaust lead time across different L : T ratios (type VI) was analysed using descriptive analysis, chi-squared tests and probit regression, which was used to explore the impact of perspective on choosing scenario B whilst controlling for background characteristics:
where Pr represents probability, the β is are the estimated parameters, D represents the background characteristics of respondents, SWB represents self-reported satisfaction levels (SWB H and SWB L), X represents the properties of the health state using health state H, duration T, lead time in full health L, and the function Φ(.) is the distribution function of the standard normal distribution. Equation 3 is the same as Equation 1 (see Equation 1 on p. 22).
Question type VIII
For question type VIII, the data were analysed through a series of logit regressions. The first model regressed the propensity to choose scenario B on the V value used, pooling across all states. Model 2 controls for state by adding state dummies, and model 3 also controls for duration. Models 4 and 5 examine whether constant proportional time trade-off and zero temporal discounting (and additive separability) hold by controlling for duration T (and lead time L). Model 6 then controls for covariates. The choice of logit here (as opposed to the probit for types I, III and VI) was an entirely practical one, arising from the need to calculate the cumulative function.
Note that as the logit density function is symmetric, the mean and the median of the distribution coincide. The median value of a state is given as the value V* that has a predicted probability 50% of selecting scenario B. Assuming:
then, as
holds, for given duration T and lead time L the mean value for state H can be obtained by identifying the value V* that has a predicted probability of 50%. Predicted values of V* are reported for each state using regression coefficients from the logit models, under key assumptions regarding levels of duration T and lead time L, where relevant.
Results
Demographics
The number of respondents completing types I, III, VI and VIII varied from 847 (type III) to 3159 (type I). Approximately half were male and the average age was around 40 years. Tables 3 and 5 display the characteristics of each sample.
Objective 1: are health-state preferences independent of lead time?
Table 11 summarises the frequencies of respondents choosing in live in scenario B across question combinations with (type III) and without (type I) the addition of lead time. The correlation between the proportions of those choosing scenario B across the two question types was 0.93. Of the 16 matched scenarios, six resulted in statistically significant differences across question types I and III. The rows of Table 11 are ordered from the most significant to the least significant according to the chi-squared test for the null hypothesis that the proportion choosing scenario B in matched types I and III questions are the same. One may observe that severe problems tend to appear nearer the top of the table than the mild problems, or that the shortest duration or the higher ratios do not appear near the top. However, overall, there is no clear pattern across the dimension of health, the severity of health, duration or L : T ratio.
State | Duration in poor health | Type III | Type I | p-value (chi-squared test) | ||
---|---|---|---|---|---|---|
Lead time | L : T ratio | % B | % B | |||
D5 | 1 year | 10 weeks | 1 : 5 | 82.8 | 91.8 | 0.005 |
M5 | 10 years | 10 years | 1 : 1 | 67.9 | 81.9 | 0.001 |
D5 | 5 years | 5 years | 1 : 1 | 83.1 | 91.5 | 0.010 |
M2 | 5 years | 10 years | 2 : 1 | 60.5 | 51.7 | 0.034 |
P2 | 10 years | 10 years | 1 : 1 | 54.1 | 45.5 | 0.042 |
P5 | 1 year | 1 year | 1 : 1 | 89.9 | 94.2 | 0.051 |
P5 | 10 weeks | 10 weeks | 1 : 1 | 92.4 | 88.0 | 0.130 |
P2 | 1 year | 5 years | 5 : 1 | 56.0 | 50.2 | 0.241 |
D5 | 10 weeks | 1 year | 5 : 1 | 90.5 | 88.1 | 0.368 |
M5 | 5 years | 10 weeks | 2 : 1 | 61.4 | 64.7 | 0.417 |
M5 | 10 weeks | 5 years | 25 : 1 | 83.1 | 80.2 | 0.446 |
P5 | 10 years | 1 year | 1 : 10 | 89.5 | 87.8 | 0.529 |
P2 | 5 years | 1 year | 1 : 5 | 65.7 | 63.1 | 0.572 |
P2 | 10 weeks | 10 weeks | 1 : 1 | 71.0 | 72.4 | 0.754 |
M2 | 10 years | 5 years | 1 : 2 | 67.2 | 68.4 | 0.757 |
M2 | 1 year | 1 year | 1 : 1 | 65.1 | 65.7 | 0.887 |
Table 12 reports the results of probit regressions where the propensity to choose scenario B is explained in terms of the key scenario parameters and select covariates. The coefficients used for duration T and value V are significant, alongside state H, when the data are pooled across states. On the other hand, lead time L is not always significant, indicating that the inclusion of lead time L cannot be said to affect the propensity to choose scenario B in a systematic way. However, long lead times and the use of depression in the health scenario seem to make the introduction of lead time significant. A range of background variables are also significant.
Scenario attributes/background characteristics | Overall | M2 | P2 | M5 | P5 | D5 |
---|---|---|---|---|---|---|
n | 8192 | 1716 | 1875 | 1466 | 1668 | 1467 |
State (ref.: M2) | ||||||
P2 | −0.081* | |||||
M5 | NS | |||||
P5 | 0.745*** | |||||
D5 | 0.499*** | |||||
Duration (ref.: 10 years) | ||||||
5 years | NS | −0.434*** | 0.420*** | −0.522*** | ||
1 year | NS | NS | NS | 0.301*** | NS | |
10 weeks | 0.258*** | 0.707*** | NS | NS | −0.259* | |
V value | ||||||
0.4 | [Ref.] | [Ref.] | [Ref.] | [Ref.] | ||
0.6 | 0.254*** | [Omitted] | [Omitted] | [Omitted] | ||
0.8 | −0.354*** | [Ref.] | [Ref.] | |||
0.9 | [Omitted] | [Omitted] | [Omitted] | |||
Lead time (ref.: none) | ||||||
10 weeks | −0.12** | NS | NS | NS | −0.506*** | |
1 year | NS | NS | NS | NS | NS | |
5 years | NS | NS | NS | NS | −0.455*** | |
10 years | NS | 0.211** | 0.210* | −0.409*** | ||
Own health (ref.: excellent/very good) | ||||||
Good | NS | NS | NS | NS | 0.229* | NS |
Fair/Poor | 0.165*** | NS | NS | 0.235** | 0.378** | NS |
SWB H | 0.117** | 0.225** | NS | NS | 0.310** | NS |
SWB L | NS | NS | NS | NS | NS | NS |
Age | NS | −0.007*** | −0.004* | NS | 0.008** | NS |
Gender | 0.098*** | NS | NS | NS | 0.236*** | 0.338*** |
Education | NS | 0.170** | NS | NS | −0.339*** | −0.266*** |
Employment | NS | NS | NS | NS | NS | −0.241*** |
Marital status | 0.094*** | NS | 0.129* | NS | NS | NS |
Constant | NS | NS | NS | 0.795** | NS | 1.019** |
LR chi-squared | 1030.41 | 67.03 | 91.92 | 65.26 | 79.73 | 56.03 |
Pseudo R 2 | 0.1087 | 0.0295 | 0.0361 | 0.0376 | 0.0763 | 0.0522 |
Log-likelihood | −4223.75 | −1104.00 | −1228.11 | −835.24 | −482.78 | −508.19 |
Objective 2: to what extent do respondents exhaust lead time under very poor health?
Figure 4 illustrates the propensity to exhaust lead time. Figure 4a shows the data by L : T ratio, where the bars are ordered from high to low propensity. As can be seen, the bars are also ordered from the lowest ratio to the highest, except for the last two bars, where the ratio 10 : 1 achieves a lower incidence of exhaustion of lead time than 25 : 1. Figure 4b breaks this down by the actual length of lead time and duration. The bars continue to be bunched by the L : T ratio, suggesting that the within-ratio variation is relatively small. Each bar in Figure 4b represents roughly 200 or 400 respondents.
FIGURE 4.
Propensity to exhaust lead time (type VI questions). Bars are ordered from high to low propensity to exhaust lead time. Propensity to choose scenario B by (a) L : T ratio and (b) lead time and duration.
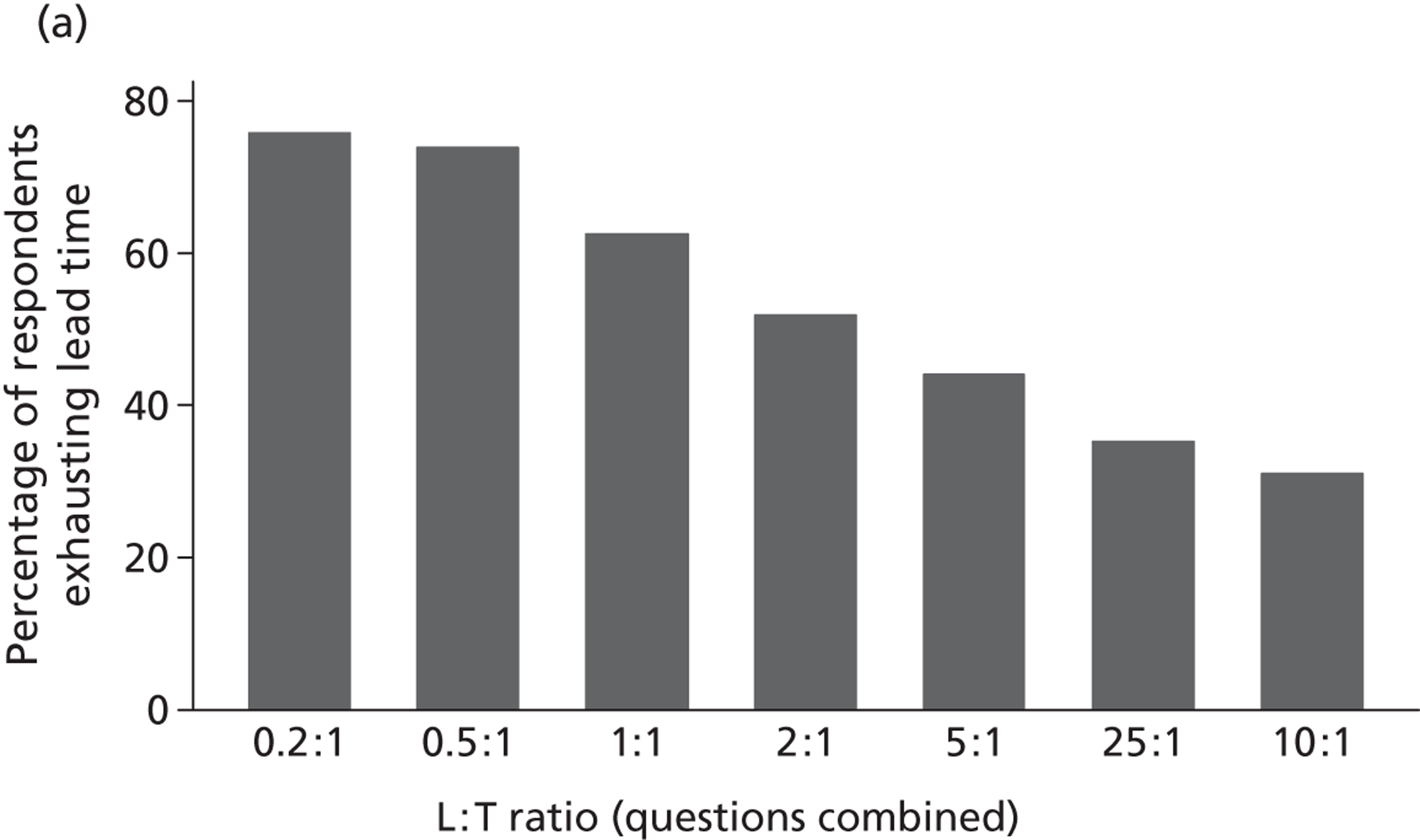
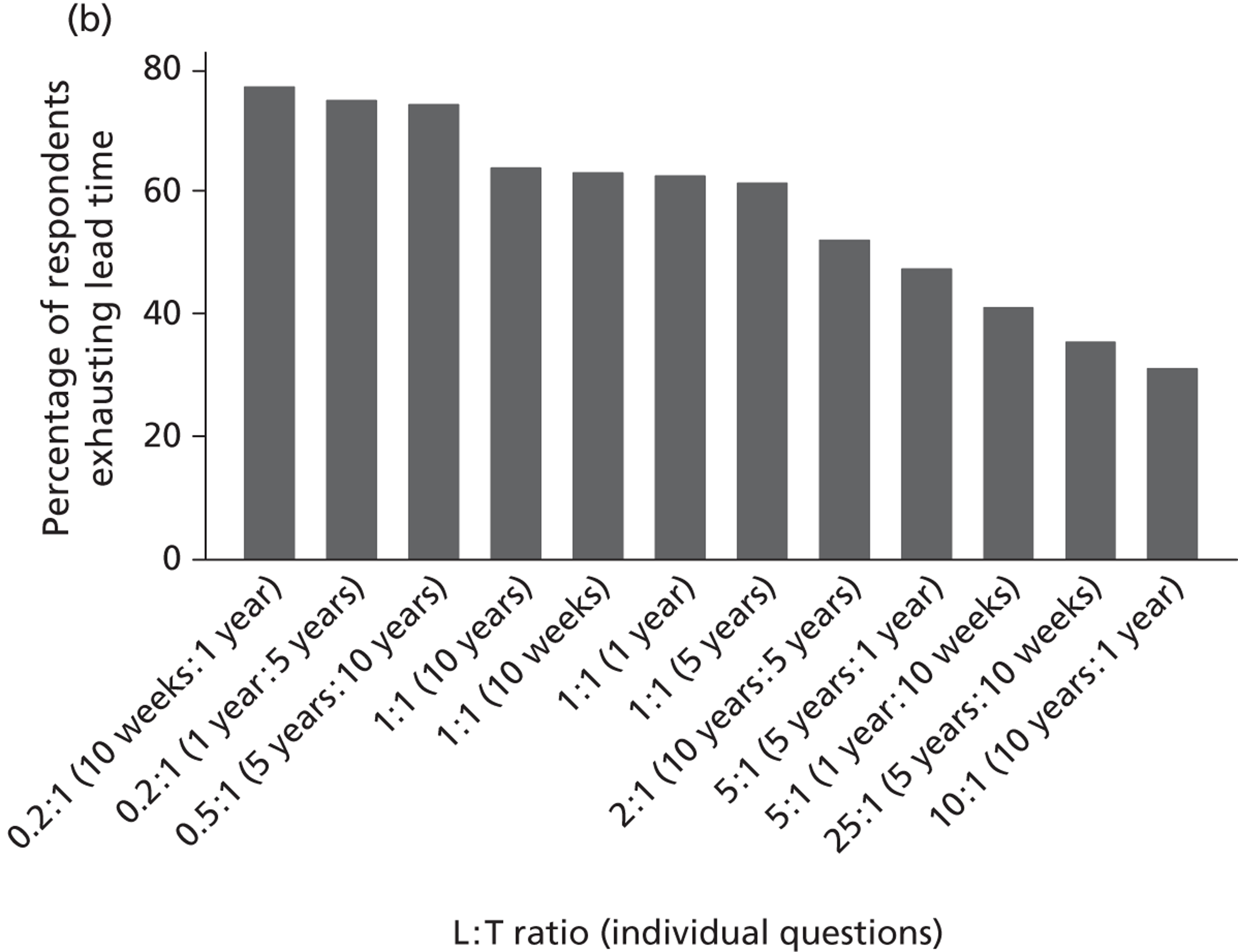
Regarding the actual incidence rate of exhaustion of lead time, it is only when the L : T ratio is as high as 5 : 1 that less than half of the respondents exhaust lead time. This is in contrast with previous work carried out using iterative LT-TTO in computer-assisted face-to-face interviews, where 20–25% of respondents exhausted lead time ( Table 13 , taken from earlier LT-TTO work19). Two of the L : T combinations were common across the two studies (10 years–5 years and 5 years–1 year) and it can be seen that, in either case, the incidence of exhaustion of lead time is much higher in the online administration of binary choice LT-TTO (see Figure 4 ) than in the face-to-face administration of iterative LT-TTO ( Table 14 ).
Health state in three-level EQ-5D | Variant (L : T ratio, years) | Valuation ≥ 0, % | Valuation < 0, % | Missing, % | ||
---|---|---|---|---|---|---|
Valued using lead time | Valued by ‘extension’ and/or ‘reduction’a | Not possible to achieve indifference | ||||
33333 | A (20 : 10) | 11 | 65 | 15 | 3 | 6 |
B (5 : 1) | 11 | 67 | 14 | 4 | 4 | |
C (10 : 5) | 14 | 67 | 12 | 5 | 2 |
Scenario attributes/background characteristics | Model 1 | Model 2 |
---|---|---|
Sample | All | Alla |
No. of observations | 3481 | 3471 |
constant | 0.327*** | NS |
Lead time (ref.: 10 weeks) | [Ref.] | [Ref.] |
1 yearb | −0.458*** | −0.461*** |
5 yearsb | −0.795*** | −0.801*** |
10 yearsb | −1.124*** | −1.141*** |
Duration (ref.: 10 weeks)b | [Ref.] | [Ref.] |
1 yearb | 0.401*** | 0.412*** |
5 yearsb | 0.786*** | 0.798*** |
10 yearsb | 1.135*** | 1.154*** |
Ageb , c | NS | |
Female | 0.277*** | |
Married/paired | NS | |
Employed | 0.128*** | |
Education beyond minimum age | 0.156*** | |
Good self-reported health | NS | |
SWBH | 0.173** | |
SWBL | −0.156*** | |
LR chi-squared | 242.42 | 319.13 |
Pseudo R 2 | 0.512 | 0.677 |
Log-likelihood | −2244 | −2198 |
Table 14 shows the results of regressing the propensity to choose scenario B (immediate death), and thus to exhaust lead time, on lead time L and duration T. The negative lead time coefficients indicate that, although controlling for duration, the longer the lead time the lower the incidence of exhaustion of lead time, which is as expected. The positive duration coefficients mean that, although controlling for lead time, the longer the duration the higher is the propensity to exhaust lead time, which is also expected. When background characteristics are controlled for, a number of them are significant but do not affect the sign or the magnitude of the main effects coefficients.
Is it feasible to elicit LT-TTO values using binary choice questions?
Figure 5 plots the proportion of those choosing scenario B along different values of V used, by state H: note that the horizontal axis is not continuous and the bars pool across the different combinations of duration T and lead time L. As expected, across all of the states, the proportion of those who choose scenario B increases as value of V used increases (the higher the value of V used then the longer the survival in full health in scenario B, thus the higher the proportion of those selecting scenario B over scenario A, other things the same). Except for state 33333, all of the bars start at < 50% and go beyond 50%. For state 33333, > 60% of respondents already choose scenario B when the value of V used is as low as −3.0.
FIGURE 5.
Raw plots of proportion choosing B against value V (type VIII questions). (a) Raw plots state 11211; (b) raw plots state 22121; (c) raw plots state 23232; (d) raw plots state 32211; and (e) raw plots state 33333.
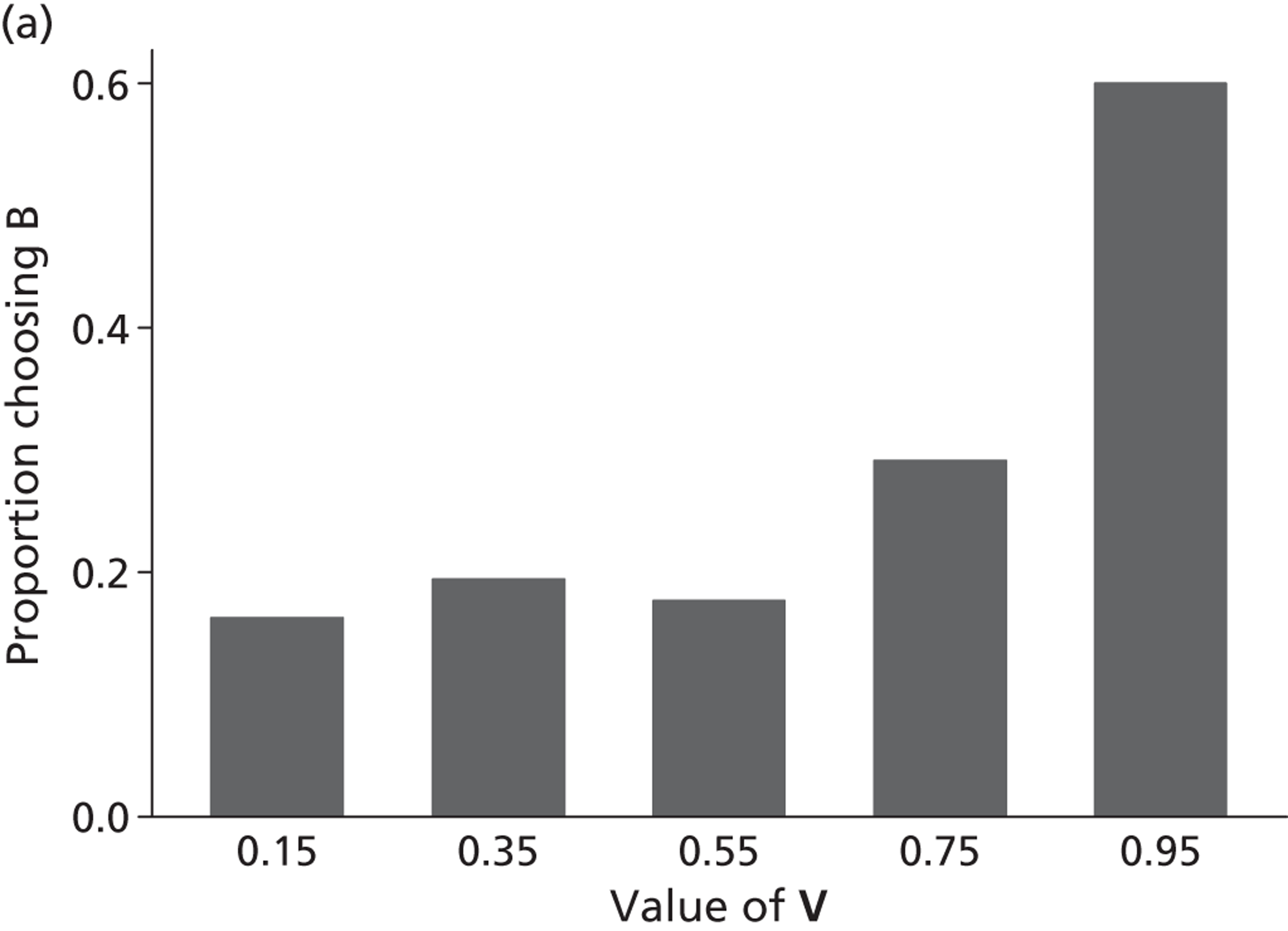
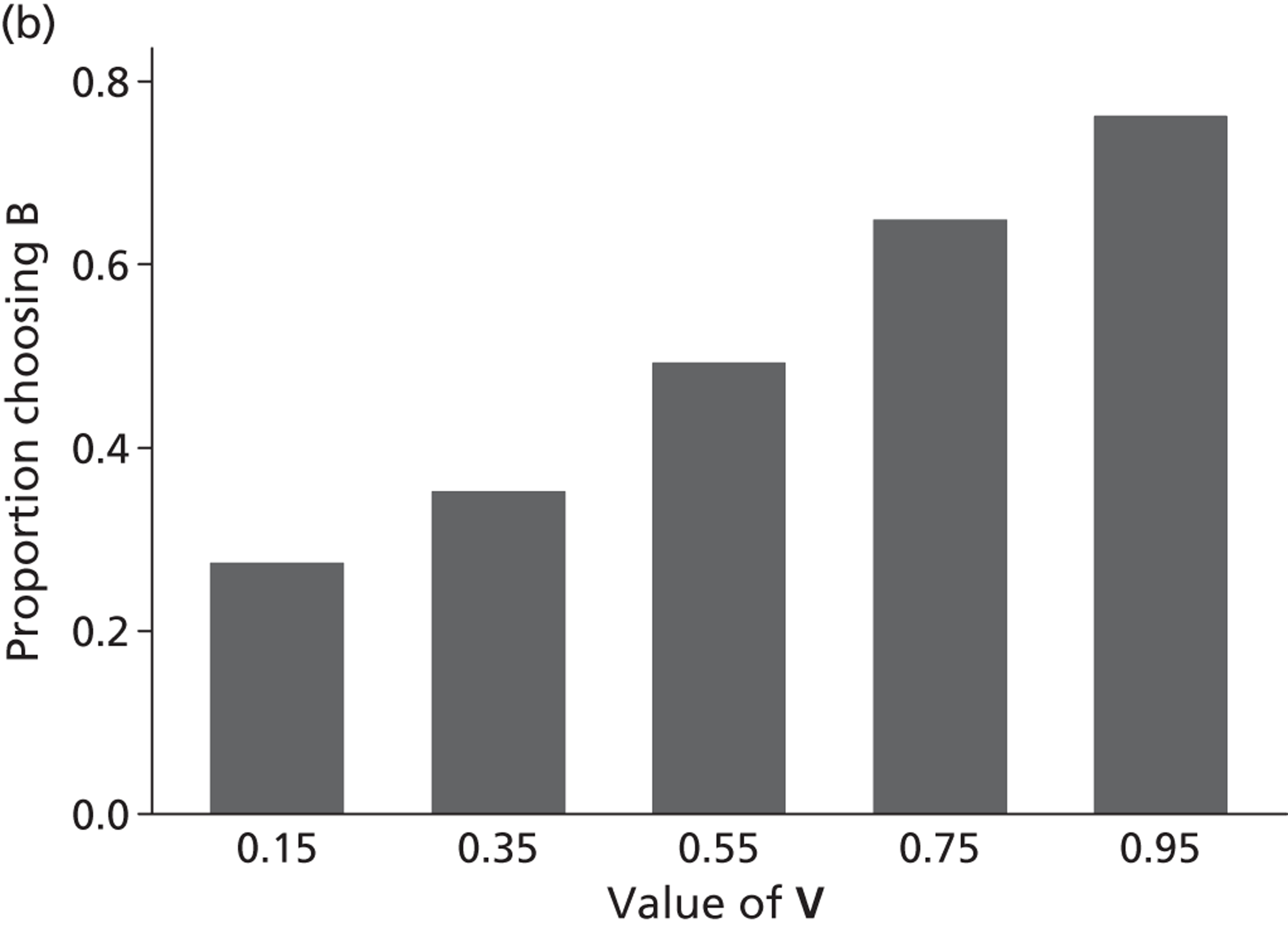
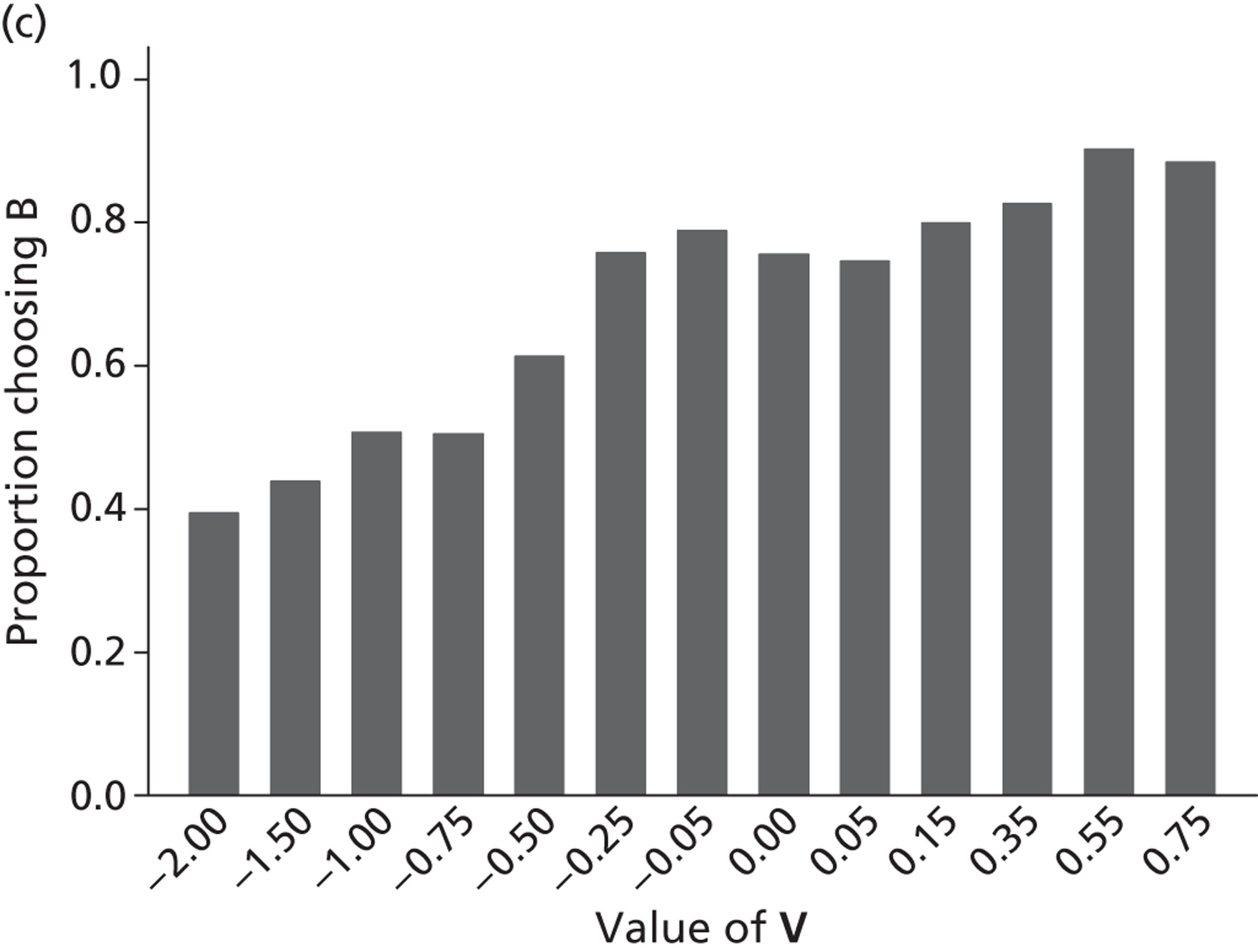
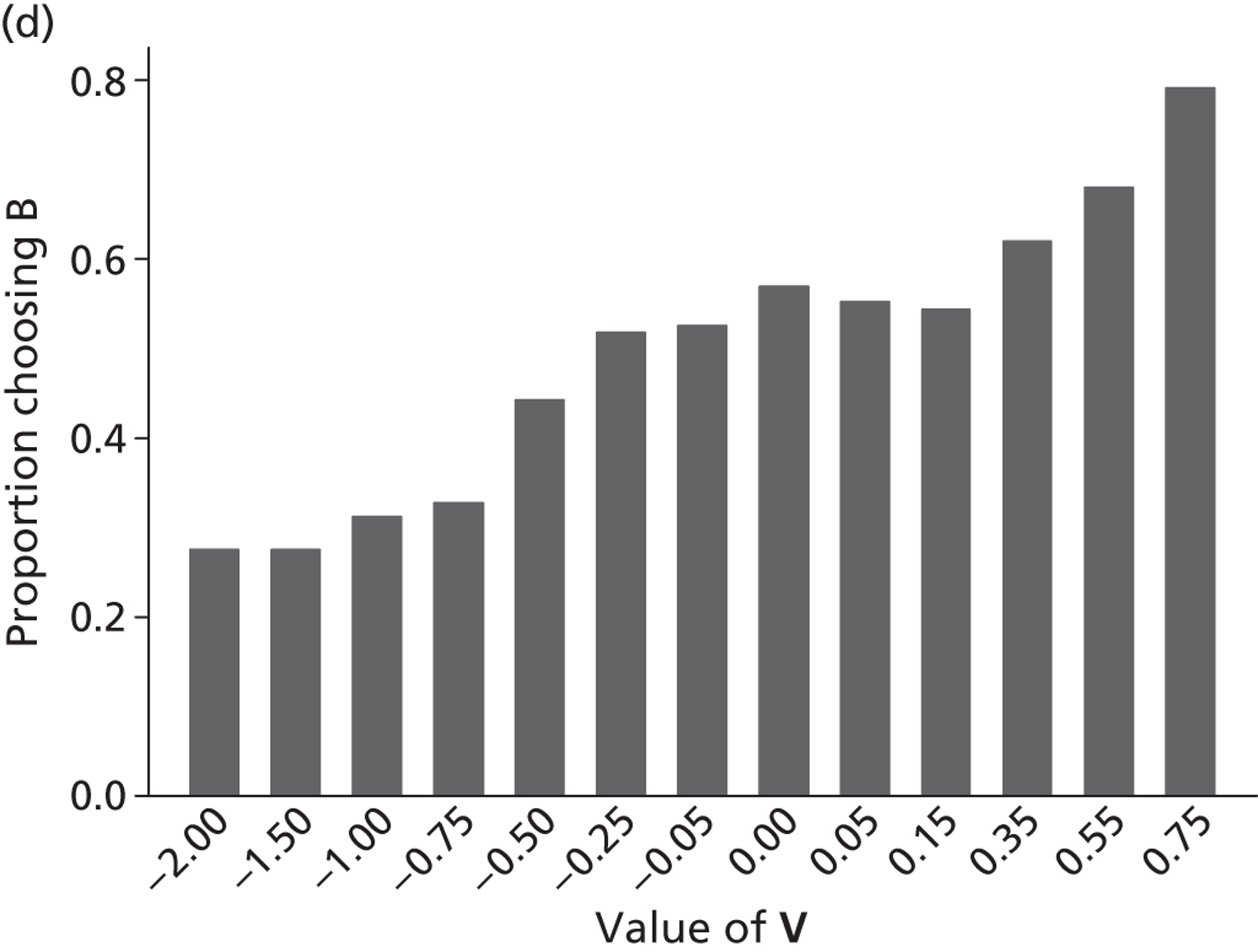
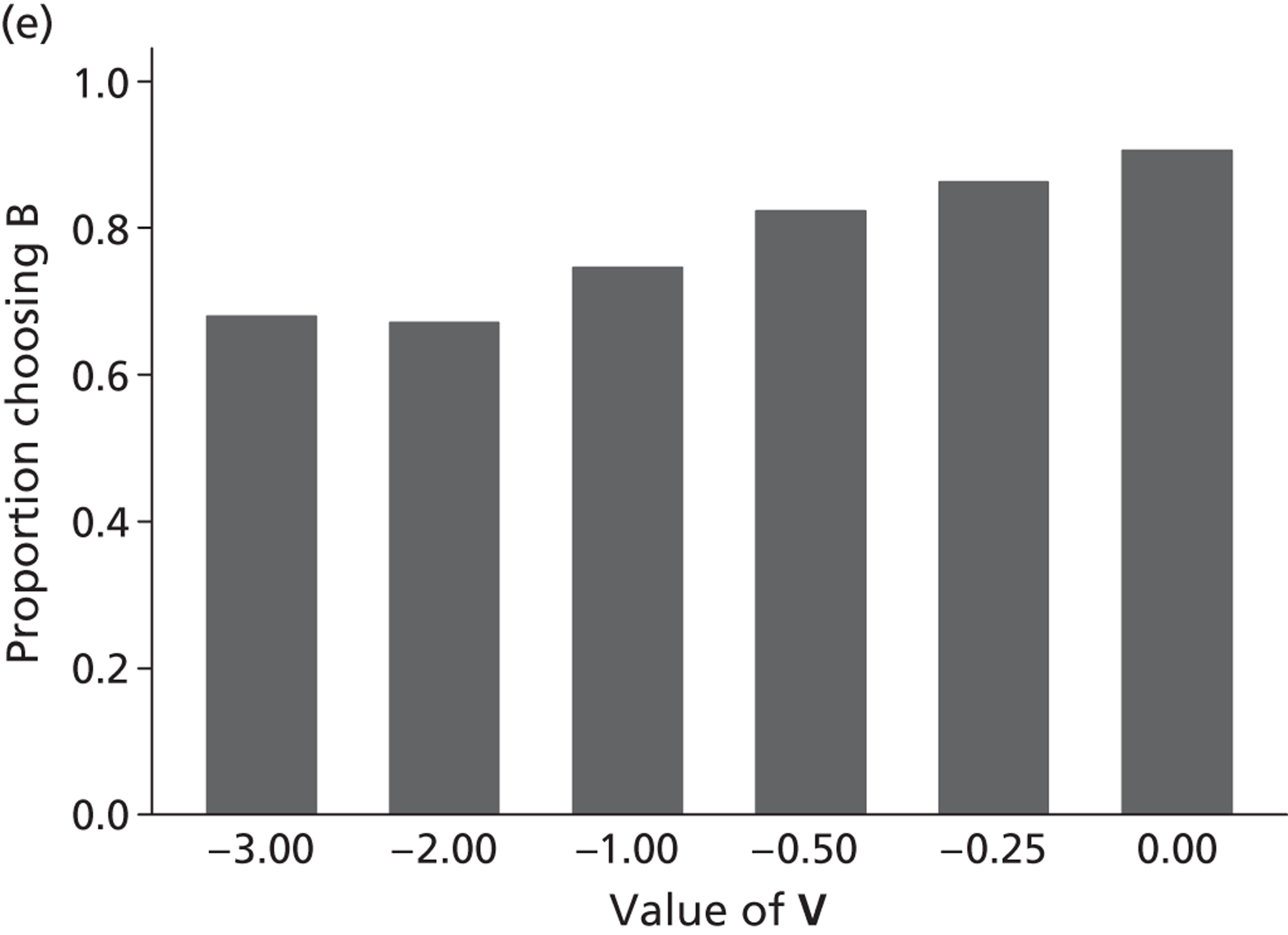
Table 15 summarises the regression results. All of the coefficients are highly significant. The propensity to choose scenario B is, as expected, a function of the value of V used (treated as continuous). The coefficients of the state dummies in model 2 onwards represent the severity of each state relative to 11211. Their magnitude is very stable across different specifications. Their positive sign indicates that the reference state 11211 is the best state. They also consistently imply that state 23232 is worse than state 32211. The regression results above from the type III data would suggest controlling the regressions for duration T and lead time L. In models 3–5, the duration coefficients are significant and negative, which is consistent with both positive time preference and maximal endurable time. When state-by-duration interactions are added to model 3, none of these is significant (results not shown), which may suggest that the negative coefficient for duration is not caused by maximal endurable time. However, when the same interactions are added to model 4, the coefficients for the worst two states are significant (for state 33333 at 1% and for state 23232 at 5%). The lead time coefficients in models 4 and 5 are significantly positive, indicating that the addition of lead time results in lower (non-discounted) values. Although a number of covariates are found to be significant in model 6, their inclusion does not affect the magnitude of the coefficients for the main effects.
Scenario attributes/background characteristics | Model | ||||
---|---|---|---|---|---|
1 | 2 | 3 | 4 | 5 | |
Sample | All | All | All | All | Alla |
No. of observations | 12,000 | 12,000 | 12,000 | 12,000 | 10,070 |
Constant | 0.529*** | −1.386*** | −1.296*** | −1.262*** | −1.414*** |
Value V | 0.159*** | 0.809*** | 0.812*** | 0.846*** | 0.840*** |
State (ref.: 11211b) | |||||
22121b | 0.955*** | 0.956*** | 0.960*** | 0.956*** | |
23232b | 2.511*** | 2.520*** | 2.513*** | 2.511*** | |
32211b | 1.596*** | 1.601*** | 1.588*** | 1.557*** | |
33333b | 3.627*** | 3.622*** | 3.511*** | 3.516*** | |
Duration | −0.023*** | −0.059*** | −0.060*** | ||
Lead time | 0.022*** | 0.023*** | |||
Ageb , c | *** | ||||
Marital statusb , c | NS | ||||
Employmentb , c | *** | ||||
Educationb | 0.198*** | ||||
Self-reported healthb , c | ** | ||||
LR chi-squared | 50.58 | 1919.69 | 1939.43 | 1966.89 | 1749 |
Pseudo R 2 | 0.0032 | 0.1203 | 0.1215 | 0.1233 | 0.1312 |
Log-likelihood | −7953 | −7019 | −7009 | −6995 | −5795 |
Table 16 reports the results of running models 2 and 4 by state H, in order to obtain predicted medians. Models by states are used because the pooled models in Table 16 do not give an intercept for the reference state. In Table 16 , each intercept represents the severity of the state (the worse the state, the smaller the intercept), and has the same relative ordering across states observed in Table 13 . The coefficients in Table 13 are used to calculate the value of V* that will result in a predicted propensity to choose scenario B of 50%, i.e. the median (and the mean) health-state value. Table 17 reports the predicted median values for each state. Model 2 does not have any parameter assumptions, but model 4 requires parameters on duration T and lead time L to be set exogenously. No scaling has been used. As can be seen, the values for the two mild states are very stable, whereas the same cannot be said of the remaining three states: they are affected widely by the model and by the parameter assumptions.
Sample | 11211 | 22121 | 23232 | 32211 | 33333 |
---|---|---|---|---|---|
No. of observations | 886 | 877 | 3757 | 3724 | 2756 |
Constant | −2.424*** | −1.497*** | 1.209*** | 0.216*** | 1.822*** |
V value | 2.532*** | 2.761*** | 1.051*** | 0.838*** | 0.475*** |
LR chi-squared | 82.38 | 117.12 | 374.56 | 265.95 | 96.27 |
Pseudo R 2 | 0.0781 | 0.0963 | 0.0827 | 0.0515 | 0.0338 |
Log-likelihood | −485 | −549 | −2078 | −2447 | −1377 |
Sample | 11211 | 22121 | 23232 | 32211 | 33333 |
---|---|---|---|---|---|
No. of observations | 886 | 877 | 3757 | 3724 | 2756 |
Constant | −2.385*** | −1.437*** | 1.404*** | 0.236*** | 2.014*** |
V value | 2.536*** | 2.764*** | 1.081*** | 0.860*** | 0.548*** |
Duration | −0.010*** | −0.015*** | −0.089*** | −0.047*** | −0.092*** |
Lead time | [Omitted] | [Omitted] | 0.031*** | 0.030*** | 0.020*** |
LR chi-squared | 82.63 | 117.78 | 400.92 | 271.98 | 119.45 |
Pseudo R 2 | 0.0784 | 0.0969 | 0.0885 | 0.0527 | 0.0419 |
Log-likelihood | −485 | −548 | −2064 | −2444 | −1366 |
Model | Assumptions | 11212 | 22121 | 23232 | 32211 | 33333 |
---|---|---|---|---|---|---|
Model 2 by state | 0.92 | 0.54 | −1.15 | −0.25 | −3.80 | |
Model 4 by state | T = 10 years; L = 0 years | 0.98 | 0.57 | −0.48 | 0.25 | −2.00 |
T = 10 years; L = 10 years | 0.98 | 0.58 | −0.75 | −0.10 | −2.35 | |
T = 5 years; L = 10 years | 0.96 | 0.55 | −1.18 | −0.35 | −3.20 | |
T = 1 year; L = 5 years | 0.95 | 0.53 | −1.35 | −0.40 | −3.70 |
Figure 6 plots the predicted probabilities of choosing scenario B at different values of V used in the range [−3 to 1]. The relative ordering of two of the states will depend on the value of V used.
FIGURE 6.
Predicted probabilities of choosing scenario B at different values of V used in the range [−3 to 1] by state (type VIII questions).
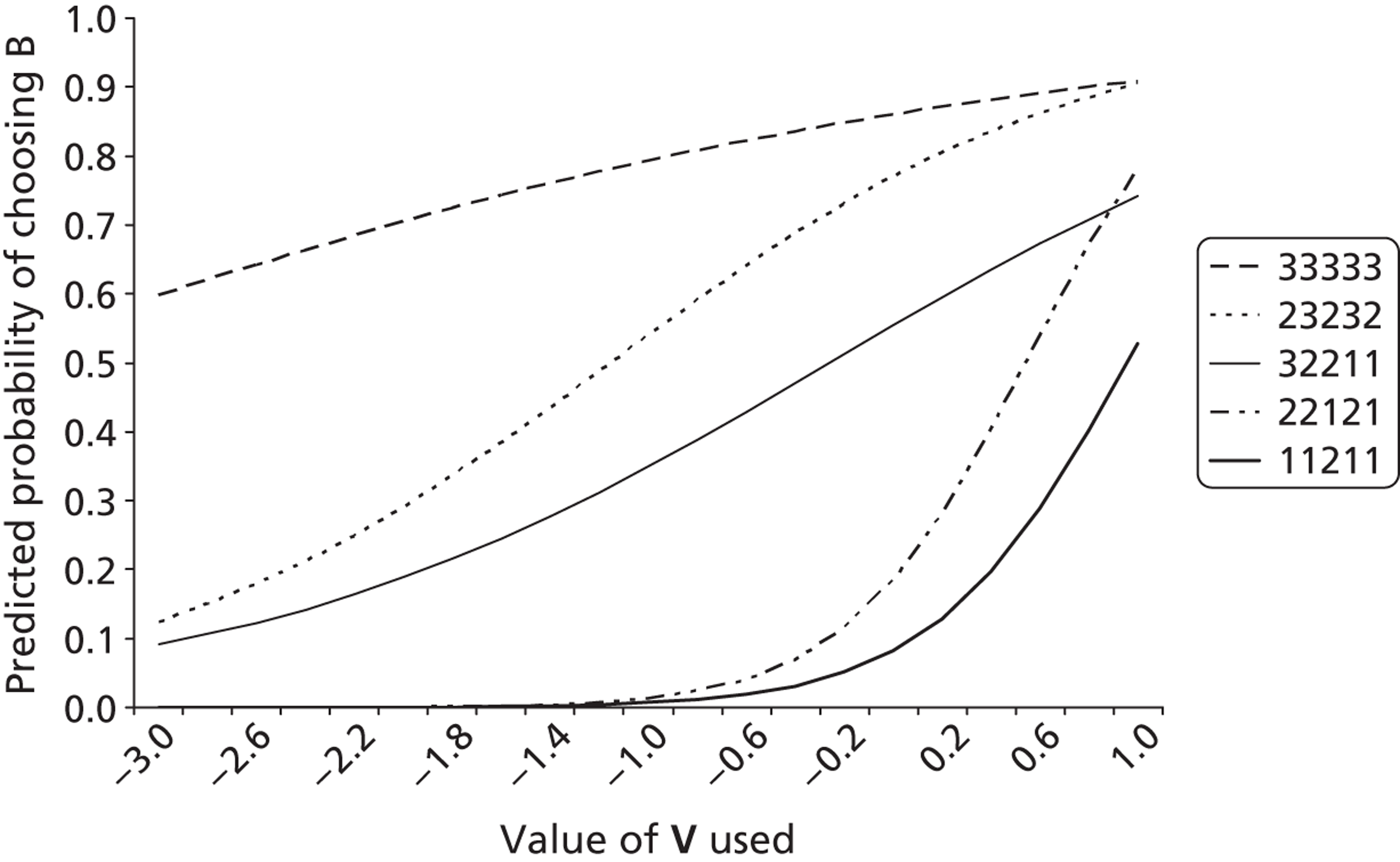
Discussion
The analysis of LT-TTO questions in the PRET and PRET-AS surveys has addressed three key issues regarding the administration of the Lead Time paradigm in an online environment. The first issue was whether the addition of a lead time to the TTO exercise had an impact on people’s health-state preferences. The results suggested that adding lead time impacts on the likelihood of choosing to live in full health, although there was no clear pattern to the results.
The second issue explored was the propensity of respondents to exhaust lead time when presented with an extremely poor health state. The results show that except for the highest L : T ratio (which was 25 : 1), the longer the lead time L relative to duration T, the lower the frequency of respondents exhausting lead time. The ratio 10 : 1 consisted of a lead time of 10 years and duration of 1 year, whereas the ratio 25 : 1 consisted of a lead time of 5 years and duration of 10 weeks. If respondents thought 10 weeks was too short to trade off survival from regardless of the state of health then this effect may cancel out the effect of the higher L : T ratio.
The online binary choice LT-TTO resulted in a substantially higher incidence of exhaustion of lead time than with an earlier face-to-face iterative LT-TTO, despite the inclusion of higher L : T ratios. We anticipated that the use of an online binary choice LT-TTO would result in a reduction of the proportions of respondents exhausting lead time, not in an increase. One possibility is that a substantial proportion of respondents either did not understand the question or were not fully engaged, and thus chose random answers. However, if this is the case, then this proportion would need to be very high (e.g. as high as 60% to cancel out the ‘excess’ incidence of around 30% observed).
The last topic addressed was the feasibility of conducting health-state valuation exercises using online binary choice LT-TTO (the design only used five EQ-5D states, and therefore does not allow the modelling of individual dimensions and levels of EQ-5D). The regression analysis indicated that the propensity to choose scenario B can be explained in terms of the value of V used in the scenarios. It also confirmed the findings from the analysis of type III data that duration T and lead time L affect this propensity. Coefficients from two regression models were used to predict the median (= mean) health-state value for the five EQ-5D health states used. The value for 11212 is just < 1.0, with no scaling. The implication is that almost half of the respondents require a V value of > 1.0 for them to select scenario B. At the opposite end, the predicted value for 33333 is between −2 and −3.8. This is consistent with the results of type VI questions, for which around half of the respondents exhausted lead time at L : T ratio of 2 : 1, and less than half exhausted lead time at 5 : 1, indicating that the median health-state value is between −2 and −5. However, the parameters in model 4 predict that when the value of V used is −10, the proportion of those exhausting lead time for state 33333 will be < 10%, which is not compatible with the results found for the type VI lead time exhaustion questions.
The next issue to consider is the assumption of zero time preference throughout. As the overall durations in some of the scenarios stretch beyond 10 years and the longest are 30 years, it would be useful to conduct further analysis incorporating reasonable levels of positive time preference. Another issue is the assumption of the logistic cumulative function and the use of logit regressions, especially given that the density distribution of health-state valuation data is asymmetric owing to truncation when the value of V used reaches 1.0.
To conclude, the analysis of the type VIII questions has shown that binary choice online LT-TTO could be used to elicit health-state values for EQ-5D states. However, a number of technical and normative issues remain unresolved.
Chapter 6 Are preferences for own and others’ health independent of when health events take place (assessing time preference)?
Introduction
Time preference in TTO is a potential source of bias that may impact on health-state values. TTO assumes that utility is constant across the life-years presented. However, there is growing evidence to suggest that when respondents complete a TTO task, future life-years are valued at a lower rate than current years.
The objective of the analysis reported in this section is to investigate respondents’ implied time preference rates. If health-state preferences are independent of when health events take place then for a given combination of state H, value V and perspective P, the distribution of respondents should not be affected by the timing of health events, represented by lead time L. This is tested by comparing three binary choice question types (type I, II and IV questions).
Methods
Question format and study design
The three binary choice questions have the following format (see also Appendix 2 ):
Type I:
-
[Scenario A]: You will live in health state H for T years and then die.
-
[Scenario B]: You will live in full health for (VT) years and then die.
-
Which scenario do you think is better?
Type II:
-
[Scenario A]: [Person P] will live in health state H for T years and then die.
-
[Scenario B]: [Person P] will live in full health for (VT) years and then die.
-
Which scenario do you think is better?
Type IV:
-
[Scenario A]: [Person P] will live in full health for L followed by health state H for T years and then die.
-
[Scenario B]: [Person P] will live in full health for (L + VT) years and then die.
-
Which scenario do you think is better?
The same CSs H, person perspectives P, duration T and lead time L values described in Chapters 3 – 5 were used. The combinations of person perspective, health state and duration were matched across question types II and IV so that the impact of the addition of lead time could be assessed in terms of time preference.
All 15 versions of the online survey included five type I questions. Question type II appeared on four survey versions, with 16 available ‘slots’ for questions across 80 possible combinations of health state H, duration T, perspective P and V value. Eight slots were allocated to the perspective SE, with the same eight combinations allocated to SY. Four of the states across each perspective were allocated to the low V category, and four to the high V category. Two of the eight states across each perspective were allocated to each duration level. There were also 16 available ‘slots’ for type IV questions across the 320 possible scenario combinations. Each lead time L, health state H, V value and duration T combination used was matched across each perspective P. L : T ratios of 1 : 1 were included for each duration level. Eight of the states used the low values for V, and eight used the high V value. Appendix 3 details the full allocation of attributes across the possible combinations.
Analysis
By making a number of assumptions the responses to question types I, II and IV can be used to derive information regarding the respondents’ time preference rates. One important assumption made in what follows is that the respondents’ time preferences are exponential rather than, say, hyperbolic. Notation is as introduced previously, with the addition that two values of V are distinguished in order to allow the value of the health state H (V 1) to differ from the approximate health-state value (V*) used to determine the time in full health offered in the choice. The conceptual representation of question types I, II and IV are displayed in Figure 7 . For all question types, the indifference on the part of the respondent between option A and option B implies that:
FIGURE 7.
Representation of question types I, II and IV for time preference analysis.
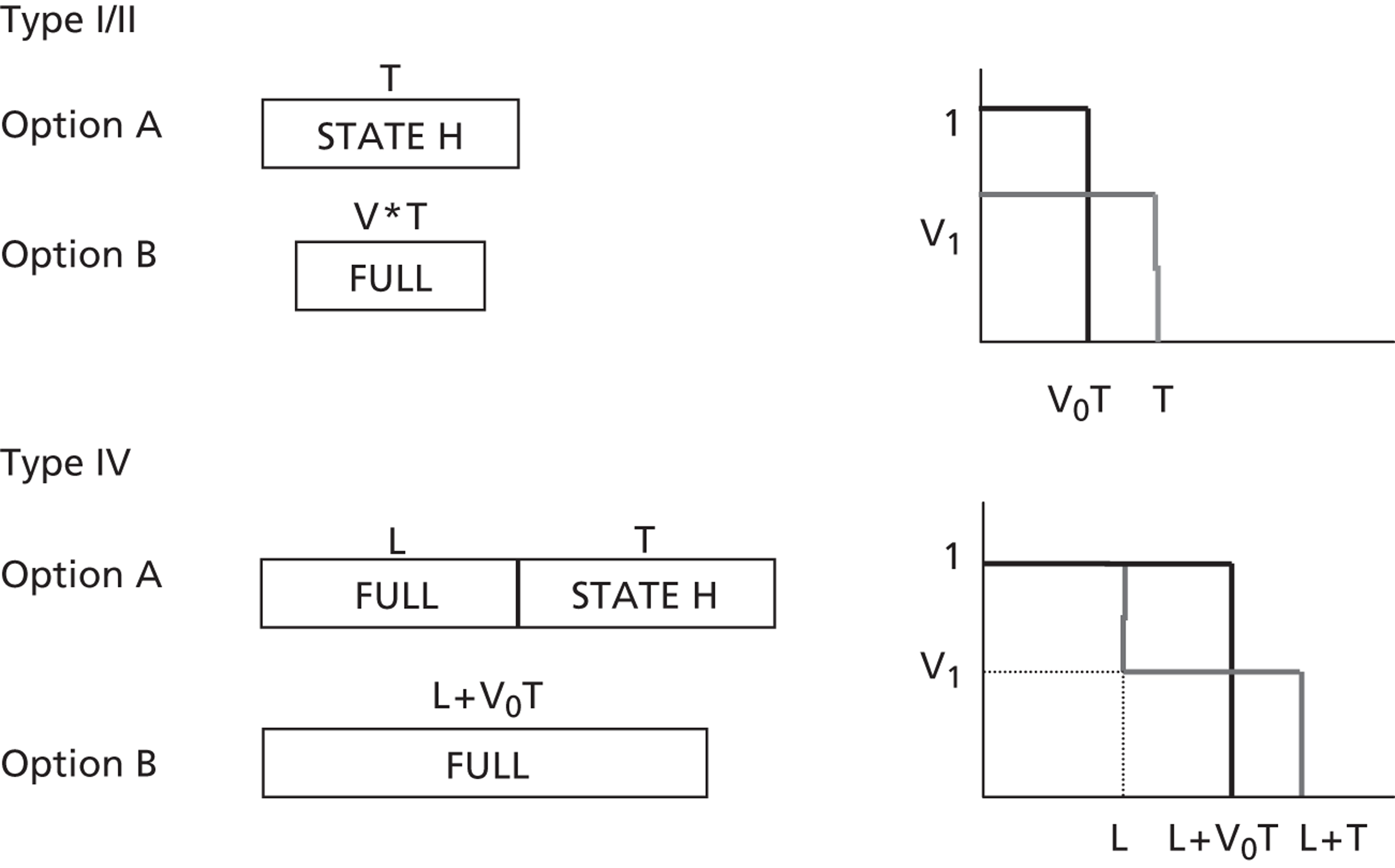
Given that V* and T are specified in the question, the value of r which solves Equation 6 is readily identified if V 1 is known or can be assumed. In the current case, V 1 for each individual is not known and thus it is necessary to assume a value. In the analysis that follows, V 1 is set equal to the DCETTO values generated in PRET-AS (see Chapter 8 ) that best describe the health state in the particular question.
Respondents choose option A or option B, and thus each choice they make implies that their time preference rate is above or below the value of r that solves the equation for that particular choice. More specifically, the proportion of respondents choosing B in response to a particular question (given the assumptions made above) indicates the proportion of respondents with a time preference rate above the equation-solving value of r.
Results
Demographics
The number of respondents completing types I, II and IV are 3159, 829 and 849, respectively. The demographic characteristics of the sample are reported in Table 3 .
Time preference analysis
The results for the type I questions (framed with a ‘you’ perspective) are reported in Table 18 . The value of r which would be implied by indifference between options A and B is generally positive (with few exceptions). This is simply a consequence of the choices offered and the health-state values assumed. The numbers presenting information regarding time preferences are the per cent of respondents choosing option B when confronted by a particular choice. For example, consider the choice between 10 years with the D5 state and four years in full health, given the assumed health-state value (0.522) the 64.4% of respondents choosing full health have an implied discount rate > 0.10.
Year A | Year B | Health state | Assumed value | Percentage choosing B | Implied r for those choosing B |
---|---|---|---|---|---|
0.192 | 0.077 | D5 | 0.522 | 88.1 | r > 5.18 |
0.077 | P5 | 0.504 | 88.0 | r > 4.41 | |
0.077 | M5 | 0.698 | 62.3 | r > 13.5 | |
0.115 | D5 | 0.522 | 86.7 | r > −3.30 | |
0.115 | P5 | 0.504 | 94.4 | r > −4.07 | |
0.115 | M5 | 0.698 | 80.2 | r > 4.50 | |
0.154 | P2 | 0.934 | 56.2 | r > 11.72 | |
0.173 | P2 | 0.934 | 73.7 | r > 5.28 | |
1 | 0.417 | D5 | 0.522 | 86.4 | r > 0.85 |
0.417 | P5 | 0.504 | 84.5 | r > 0.71 | |
0.417 | M5 | 0.698 | 64.3 | r > 2.41 | |
0.583 | D5 | 0.522 | 91.9 | r > −0.50 | |
0.583 | P5 | 0.504 | 94.2 | r > −0.64 | |
0.583 | M5 | 0.698 | 79.4 | r > 0.99 | |
0.833 | P2 | 0.934 | 50.2 | r > 1.89 | |
0.917 | P2 | 0.934 | 60.1 | r > 0.48 | |
5 | 2 | D5 | 0.522 | 87.7 | r > 0.20 |
2 | P5 | 0.504 | 90.5 | r > 0.17 | |
2 | M5 | 0.698 | 64.7 | r > 0.52 | |
3 | D5 | 0.522 | 91.5 | r > −0.13 | |
3 | P5 | 0.504 | 93.2 | r > −0.16 | |
3 | M5 | 0.698 | 67.0 | r > 0.17 | |
4 | P2 | 0.934 | 50.2 | r > 0.46 | |
4.5 | P2 | 0.934 | 63.1 | r > 0.17 | |
10 | 4 | D5 | 0.522 | 64.4 | r > 0.10 |
4 | P5 | 0.504 | 87.8 | r > 0.09 | |
4 | M5 | 0.698 | 62.2 | r > 0.26 | |
6 | D5 | 0.522 | 87.3 | r > −0.06 | |
6 | P5 | 0.504 | 91.9 | r > −0.08 | |
6 | M5 | 0.698 | 81.9 | r > 0.09 | |
8 | P2 | 0.934 | 45.5 | r > 0.23 | |
9 | P2 | 0.934 | 62.1 | r > 0.09 |
The data in Table 18 are slightly difficult to interpret as they comprise the percentage choosing B at different threshold values for r. If the time preference rates implied by the respondents’ choices belonged to the same distribution then we would expect that the lower the threshold value of r, the higher would be the percentage choosing B. But this is not observed. The main reason for this is that the implied discount rates are not independent of duration.
When the proportion choosing option B are considered for different durations of time in particular health states, there appears to be a tendency for the underlying distribution of time preferences to shift leftwards as duration increases, at least in the case of P5 and M5. In other words, the percentage choosing option B tends to hold up as the equilibrating rate falls with increasing duration. The overall picture is mixed, for example, in the case of P2, there is a tendency for the percentage choosing option B to fall slightly as duration increases.
The results for the lead-time choices reported in Table 19 also highlight that the implied time preference rates are influenced by time period over which discounting takes place. Consider the health state P5 (assumed value of 0.504), for which 90.2% of respondents have an implied discount rate of > 4.41 over 10 weeks and 93.4% have an implied discount rate of > 0.09 over 10 years. Similarly, for slight pain over 10 weeks 69.9% had an r > 5.28, whereas over 10 years 59.5% had an r > 0.23. Again these observations are consistent with leftward shifts of the distribution of time preferences as duration increases.
Lead time | Time in health state H | Perspective | Health state (assumed value) | No. (% choosing B) | Implied r for those choosing B |
---|---|---|---|---|---|
10 weeks | 10 weeks | SE | P5 (0.504) | 203 (88.2) | r > 4.41 |
10 weeks | SY | P5 (0.504) | 205 (92.2) | r > 4.41 | |
10 weeks | SE | P2 (0.934) | 203 (69.0) | r > 5.28 | |
10 weeks | SY | P2 (0.934) | 205 (70.7) | r > 5.28 | |
1 year | SE | D5 (0.522) | 205 (82.4) | r > 1.08 | |
1 year | SY | D5 (0.522) | 221 (81.9) | r > 1.08 | |
5 years | SY | D5 (0.522) | 220 (87.7) | r > −0.13 | |
1 year | 1 year | SE | M5 (0.698) | 203 (79.8) | r > 0.99 |
1 year | SY | M5 (0.698) | 220 (82.7) | r > 0.99 | |
5 years | 5 years | SE | D5 (0.522) | 203 (88.7) | r > −0.13 |
10 years | SE | P5 (0.504) | 220 (95.0) | r > 0.09 | |
10 years | SY | P5 (0.504) | 205 (91.7) | r > 0.09 | |
10 years | 10 years | SE | P2 (0.934) | 205 (63.4) | r > 0.23 |
10 years | SY | P2 (0.934) | 220 (55.9) | r > 0.23 |
Little can be said about the extent of negative time preferences because these are group data rather than individual data. There are, however, eight occasions when the equilibrating value of r is negative. The relatively low percentage choosing scenario A are indicating they have negative time preferences. As with the choices when the equilibrating rate was positive, there is clear evidence that time preferences are not independent of duration. For example, for choices concerning extreme pain, as duration increases and the equilibrating rate rises (−4.07, −0.64, −0.16, −0.08) the percentage choosing scenario B increases slightly. If time preferences were independent of duration one would expect the proportion choosing B to fall. The responses for the choices with a negative equilibrating r are consistent with a rightward shift in the distribution of implied discount rates as duration increases.
The impact of making an alternative assumption regarding the health-state value to apply to time spent in health state H is illustrated in Table 20 . Using the example of the health-state M5, the value of 0.698 (based on the values generated in PRET-AS) is replaced by 0.648 and 0.748. Higher (lower) health-state values increase (decrease) the value of r consistent with indifference between options A and B. The effect is greatest when considering shorter associated durations.
Years in health state H | Health-state value | ||
---|---|---|---|
0.648 | 0.698 | 0.748 | |
0.192 | r > 2.17 | r > 4.50 | r > 7.00 |
1 | r > 0.55 | r > 0.99 | r > 1.48 |
5 | r > 0.08 | r > 0.17 | r > 0.27 |
10 | r > 0.04 | r > 0.09 | r > 0.13 |
Table 21 compares the implied time preference rates by perspective. A higher proportion of respondents tended to choose option B when the perspective was SE rather than SY (but note the exception of the M5 state). The proportion choosing option B also tends to be higher for the ‘you’ rather SY perspective (but note there are two exceptions). However, the differences in proportions are not great and it would not be appropriate to suggest on these data that implied time preference rates are higher for SE compared to SY, or for ‘you’ compared to SY. A comparison of the SE and SY perspectives is also possible with the lead time choices and these data show no tendency for the proportion choosing B to be higher given a particular perspective.
Year A | Year B | Health state | Assumed value | Implied r for those choosing B | Perspective | Percentage who prefer B |
---|---|---|---|---|---|---|
0.192 | 0.077 | P5 | 0.504 | r > 4.41 | You | 88.0 |
SE | 91.0 | |||||
SY | 90.3 | |||||
1 | 0.417 | D5 | 0.522 | r > 0.85 | You | 86.4 |
SE | 84.1 | |||||
SY | 81.5 | |||||
1 | 0.583 | M5 | 0.698 | r > 0.99 | You | 79.4 |
SE | 70.4 | |||||
SY | 79.1 | |||||
5 | 3 | D5 | 0.522 | r > −0.13 | You | 91.5 |
SE | 86.6 | |||||
SY | 83.0 | |||||
5 | 4 | M2 | 1.000 | NA | You | 51.7 |
SE | 56.7 | |||||
SY | 54.2 | |||||
10 | 6 | P5 | 0.504 | r > −0.08 | You | 91.9 |
SE | 92.8 | |||||
SY | 88.6 | |||||
10 | 8 | P2 | 0.934 | r > 0.23 | You | 45.5 |
SE | 52.7 | |||||
SY | 44.7 |
Discussion
No data were collected in order to estimate the implied discount rates of individual respondents. Thus, the analysis of discounting is at the group level. This restricts the analysis that can be undertaken. The analysis requires an assumption that the health-state valuations of the respondents for the different health states in the choices can be approximated by the relevant values generated in PRET-AS. Thus it was assumed throughout that the group of respondents facing a particular choice had a median health-state value equal to the EQ-5D score assigned to that health state. If the true median were to be higher, the implied time preference rate would be higher. Thus, although it is possible that respondents may have different time preferences for different health states, any apparent differences could also result from differences in the median health-state values of the respondents and the EQ-5D score assigned to that health state.
The analysis also assumed the traditional (for economists) discounted utility model describes the respondents’ time preferences; however, as is generally found, time preference rates did not appear to be independent of duration. There was some evidence of the distribution of time preferences shifting leftwards (i.e. discount rates falling) as duration in health state H increases.
These data are not particularly well suited to test for differences with respect to time preferences by question perspective. An individual-level analysis would be required to explore such differences. Thus, although some tendencies were observed in these data, no firm conclusion should be drawn.
Chapter 7 Impact of satisfaction on preferences (using type V questions)
Introduction
Time trade-off requires respondents to forecast the impact of a change in health on their future selves. However, our preferences fail to predict adaptation processes. There is a strong case for incorporating information on adaptation in a preference elicitation task to allow respondents to weight it alongside the expected impact of different dimensions of health. 27,43 The analysis in this chapter seeks to elicit ‘better preferences’ obtained via a TTO that takes adaptation into consideration. We do this by incorporating a level of satisfaction with life or health into the way a health state is described.
It is important to see what effect including health satisfaction in the description of a health-state scenario has on the preferences people provide. The objective of the analysis reported in this chapter was to investigate the following two questions:
-
Are health-state preferences influenced by satisfaction levels in those states (i.e. is the preference for others’ health independent of satisfaction in the state?). If the assumption holds, then for a given combination of state H and value V, the distribution of respondents should not be affected by level of satisfaction S with the state.
-
Are preferences for health state that contain satisfaction levels influenced by the respondent’s satisfaction with their own health?
This was done by comparing question types I and V as described below.
Methods
Question format and study design
The binary choice questions took the following format (see also Appendix 2 ):
Type I:
-
[Scenario A]: You will live in health state H for T years and then die.
-
[Scenario B]: You will live in full health for (VT) years and then die.
-
Which scenario do you think is better?
Type V:
-
[Scenario A]: You will live in health state H for T years with satisfaction S and then die.
-
[Scenario B]: You will live in full health for (VT) years with satisfaction S and then die.
-
Which scenario do you think is better?
The health state H, duration T and value V were matched across both question types so that the impact of the addition of satisfaction could be assessed. The durations were fixed at 5 years for scenario A and 3 years for scenario B. Three of the CSs were used: M5, P5 and D5. These are combined with one of four satisfaction states: ‘high life satisfaction’ (High LS), ‘high health satisfaction’ (High HS), ‘low health satisfaction’ (Low HS), and a situation described as ‘learnt to live’ with the health condition (LL) – ‘high/low’ were chosen as there is no ambiguity in which of the two is better, and ‘learnt to live’ illustrates an adaptation to the state.
Type V questions appeared in three versions of the online survey, meaning 11 question ‘slots’ in total. Five slots were allocated to scenarios investigating HS, two slots to LS, and four slots to LL. Type I questions appeared on all 15 versions of the online survey, and the matched questions (in terms of health state and duration) from across the surveys were used in this analysis.
Analysis
The probability of respondent i choosing to live for 3 years in full health (scenario B) was estimated using probit regression:
where H × S denotes the combination of a health state H with a level of satisfaction S under scenario A. The subscript j represents the number of multiattribute health states. DEMO represents a set of demographic variables available for the respondent: these are gender, age, age squared, marital status, employment status and education level. HEALTH is a set of 15 dummy variables capturing respondents’ own state of health obtained from their completion of the EQ-5D-5L. 5 SWB represents a set of self-reported health or life satisfaction variables, each on a 0–10 scale, with ‘0’ denoting ‘not at all satisfied’ and ‘10’ denoting ‘completely satisfied’. The correlation between health and life satisfaction was 0.67, which may lead in multicollinearity. Therefore, the results control only for life satisfaction but the overall pattern of the results does not alter if health satisfaction is controlled for.
Equation 7 is estimated by pooling together all responses of the scenarios. As respondents in versions 1 and 2 of the survey face multiple scenarios of health state–satisfaction combinations, we estimate robust standard errors clustered at the individual level. Type I questions act as the reference category for each dimension to assess any change in preferences attributed to the introduction of satisfaction levels.
Results
Demographics
Overall, 645 respondents completed type V questions, 830 completed the matched type I questions, and the demographics of the sample are reported in Table 3 . Approximately 73%, 87% and 71% of the respondents did not have any problems with mobility, self-care, and performing usual activities, respectively, as measured by EQ-5D-5L. The corresponding proportions for pain/discomfort and anxiety/depression are about 45% and 56%, respectively. Average SWBH and SWBL of the sample were 6.4 and 6.3, respectively. The proportion of those reporting the best state in EQ-5D-5L (i.e. 11111) is 31.6%. Average own health satisfaction (SWBH) and life satisfaction (SWBL) are 6.4 and 6.3, respectively.
Impact of satisfaction
Pooling all 2128 responses, the proportion of respondents choosing the full-health scenario B is 70.1%. There is a significant difference between the proportion of respondents choosing scenario B between the low and high satisfaction groups for both M5 and P5 ( Table 22 ). The difference between High HS and LL for D5 is not significant.
State | Duration, years | Duration scenario B, years | Proportion across each satisfaction level (%) | Chi-squared test | |||
---|---|---|---|---|---|---|---|
Low HS | High HS | High LS | LL | ||||
M5 | 5 | 3 | 86.3 | 56.6 | 53.1 | 58.3 | 0.000 |
P5 | 5 | 3 | 90.1 | 73.0 | 71.1 | 71.8 | 0.000 |
D5 | 5 | 3 | NA | 70.9 | NA | 70.6 | 0.950 |
Table 23 reports marginal effects coefficients regressing experimental variables and demographic characteristics on to the propensity to choose scenario B (3 years in full health). The probability of choosing scenario B in reference to the base category (D5 and LL) with the health state increased significantly when the state was ‘M5 and Low HS’ (about 17%) and when it was ‘P5 and Low HS’ (about 20%). The remaining statistically significant states were associated with the health state M5; the High HS, High LS or LL descriptors significantly reduced the probability of choosing full health by approximately 17%, 19%, and 14%, respectively.
Health scenarios | Marginal effect |
---|---|
State and satisfaction level (ref.: D5 and LL) | |
M5 and Low HS | 0.168*** (0.027) |
M5 and High HS | −0.162*** (0.051) |
M5 and High LS | −0.187*** (0.053) |
M5 and LL | −0.138*** (0.035) |
P5 and Low HS | 0.203*** (0.032) |
P5 and High HS | NS |
P5 and High LS | NS |
P5 and LL | NS |
D5 and High HS | NS |
Employment (ref.: Employed) | |
Retired | 0.11** (0.055) |
Homemaker | −0.118** (0.053) |
EQ-5D-5L (ref.: Level 1) | |
Pain/discomfort level 3 | −0.131** (0.067) |
Anxiety/depression level 2 | −0.082** (0.041) |
Anxiety/depression level 3 | −0.204*** (0.062) |
Anxiety/depression level 4 | −0.205** (0.08) |
n | 2054 |
Pseudo R 2 | 0.107 |
Demographic variables were not statistically significant, except for respondents who were homemakers. Moderate pain/discomfort (level 3) and moderate to severe anxiety/depression (levels 3 and 4) on EQ-5D-5L also reduced the probability of choosing full health, and respondent’s SWBL level was not significant.
We also compared different combinations of states. Consider the scenario of M5 and Low HS, with a marginal effect of 0.168, and P5 and Low HS, with a marginal effect of 0.203. As the satisfaction level in both of these states was the same, any difference in marginal effects was arguably due to the health state. Therefore, P5 in comparison with M5 increased the likelihood of choosing 3 years in full health by 3.5% (= 0.203 − 0.168). A Wald test rejects the null hypothesis that these two coefficients are equal (χ2 (1) = 38.24, Prob = 0.000).
We also assessed matched health states that differ by satisfaction level. The scenario ‘M5 and Low HS’ had a marginal effect of 0.168, and ‘M5 and High HS’ had a marginal effect of −0.162. As the health state was the same, any difference may be due to the difference in the levels of satisfaction. Thus, facing ‘Low HS’ compared with ‘High HS’ increased the likelihood of choosing 3 years in full health by 33% (= 0.168 + 0.162).
Table 24 presents the marginal coefficients of estimations grouping scenarios based on EQ-5D dimensions. This compares type I and type V PRET questions [i.e. comparing health-state scenarios without any information on LS or HS included (type I) to comparable health-state scenarios but which contain information on satisfaction in the state (type V)]. For the health state M5, the probability of choosing full health significantly increased by 23% when associated with Low HS. However, when this state was combined with either High HS or LS, the probability of choosing the full health scenario decreased by about 12% and 14%, respectively. This indicates that respondents would prefer to cope with the given health dimension for a longer time period when they were more satisfied with their health or life. Learning to live with the condition as a proxy for adaptation had no statistically significant effect on preferences. For the health state P5, the addition of Low HS did not have a significant effect on preferences. In line with the mobility dimension (M5), the presence of High HS or LS reduced the probability of choosing the full health scenario by 29% and 32%, respectively. In contrast with M5, the addition of LL also reduced the probability of choosing the full health scenario by approximately 32%. For the health state D5, the effect of High HS and LL were similar to the corresponding effects for the M5 and P5 dimensions.
Scenario attributes/background characteristics | (1) ‘Unable to walk’ | (2) ‘Extreme pain’ | (3) ‘Extreme depression’ |
---|---|---|---|
Health scenarios | |||
M5 | [Ref.] | ||
M5 and Low HS | 0.23** (0.043) | ||
M5 and High HS | −0.121* (0.056) | ||
M5 and High LS | −0.144* (0.057) | ||
M5 and LL | NS | ||
P5 | [Ref.] | ||
P5 and Low HS | NS | ||
P5 and High HS | −0.292** (0.051) | ||
P5 and High LS | −0.316** (0.052) | ||
P5 and LL | −0.322** (0.052) | ||
D5 | [Ref.] | ||
D5 and High HS | −0.305** (0.051) | ||
D5 and LL | −0.301** (0.044) | ||
Demographics | |||
Age | 0.018* (0.007) | ||
Age squared | −0.0002* (0.0001) | ||
Employment (ref.: Employed) | |||
Retired | 0.076* (0.037) | ||
Long-term sick | 0.076* (0.037) | ||
Taking care of home | −0.15* (0.068) | ||
Education: below degree level | 0.06* (0.03) | 0.083* (0.041) | |
EQ-5D-5L (ref.: Level 1) | |||
Mobility level 5 | 0.126** (0.034) | ||
Usual activities level 2 | 0.104* (0.046) | ||
Pain/discomfort level 2 | −0.09* (0.045) | ||
Pain/discomfort level 3 | −0.134* (0.062) | ||
Anxiety/depression level 2 | −0.106* (0.051) | ||
Anxiety/depression level 3 | −0.14* (0.062) | −0.193** (0.059) | −0.162* (0.074) |
Anxiety/depression level 4 | −0.228** (0.087) | −0.26* (0.111) | |
n | 972 | 1211 | 613 |
Pseudo R 2 | 0.094 | 0.173 | 0.164 |
Table 25 demonstrates that the relative ordering between High LS and High HS was consistent, but the relative ordering of LL was not. This suggests that the meaning and importance of learning to live with a health condition depends heavily on the state. Across the dimensions, respondents’ SWBL did not have a statistically significant impact on preferences and there was no pattern to the impact of demographic variables.
Scenario attributes/background characteristics | (1) ‘Low HS’ | (2) ‘High HS’ | (3) ‘High LS’ | (4) ‘Learnt to live’ |
---|---|---|---|---|
Health scenarios | ||||
D5 and Low HS | [Ref.] | |||
P5 and Low HS | 0.047 (0.03) | |||
M5 and High HS | [Ref.] | |||
P5 and High HS | 0.163*** (0.045) | |||
D5 and High HS | 0.129*** (0.046) | |||
M5 and High LS | [Ref.] | |||
P5 and High LS | 0.181*** (0.051) | |||
M5 and LL | [Ref.] | |||
P5 and LL | 0.132*** (0.049) | |||
D5 and LL | 0.141*** (0.032) | |||
Demographics | ||||
Age | 0.015** (0.007) | |||
Age squared | −0.0002** (0.0001) | |||
Employment (ref.: Employed) | ||||
Retired | 0.101*** (0.021) | |||
Student | 0.089*** (0.024) | 0.198** (0.09) | ||
Taking care of home | −0.249*** (0.08) | |||
Unemployed | −0.188** (0.092) | |||
Education: below degree level | 0.114** (0.056) | |||
EQ-5D-5L (ref.: Level 1) | ||||
Mobility level 3 | −0.291** (0.147) | |||
Mobility level 5 | −0.681*** (0.019) | |||
Self-care level 3 | 0.261*** (0.069) | |||
Self-care level 5 | 0.346*** (0.02) | |||
Usual activities level 2 | 0.08** (0.031) | |||
Usual activities level 4 | 0.092*** (0.021) | 0.22** (0.109) | ||
Usual activities level 5 | −0.679*** (0.02) | |||
Pain/discomfort level 3 | −0.198** (0.095) | |||
Anxiety/depression level 2 | −0.133** (0.054) | −0.141** (0.067) | ||
Anxiety/depression level 3 | −0.212** (0.094) | −0.207*** (0.072) | −0.218** (0.087) | |
Anxiety/depression level 4 | −0.236** (0.109) | −0.326** (0.103) | ||
SWBL | ||||
LS Group (6–7) | −0.161** (0.073) | |||
n | 372 | 621 | 410 | 608 |
Pseudo R 2 | 0.191 | 0.086 | 0.08 | 0.118 |
The impact of the dimensions of the self-reported EQ-5D-5L on TTO preferences was also assessed. Respondents faced with the P5 and D5 tended to opt for scenario B if they self report extreme problems with mobility (level 5) and slight problems performing usual activities (level 2), respectively. Respondents who reported problems with pain and depression preferred living longer when facing the P5 and D5 scenarios.
Respondents facing ‘High HS’, ‘High LS’ or LL were significantly more likely to prefer the ‘poor’ health state (i.e. choosing scenario A) than those faced with Low HS. Therefore, we could also investigate preferences between health states for a given level of satisfaction in that state. Marginal effects probit coefficients based on the grouping of health states according to the associated satisfaction level are presented in Table 25 (with mobility as the reference category). For Low HS the health dimension included had no impact on the likelihood of choosing scenario A or B. This differs to the pooled model (see Table 23 ), in which a significant difference was found between ‘M5 and Low HS’ and ‘P5 and Low HS’.
With High HS, respondents facing either P5 or D5 in reference to M5 were more likely to prefer scenario B (full health). The difference in impact between P5 and D5 was not statistically significant (χ2 (1) = 0.54, Prob = 0.464). For scenarios including High LS, respondents were more likely to choose scenario B for P5 than M5. There was also some evidence that respondents with a medium level of SWBL had a stronger preference for choosing scenario A (living in the health state). The results for LL can be interpreted in a similar way, as extreme depression tended to have a stronger impact than extreme pain but the difference was not significant (χ2 (1) = 0.03, Prob = 0.852). When M5 and P5 are accompanied by Low HS (column 1), there was no significant difference between them. However, when these states were accompanied by High HS, High LS, or LL (columns 2–4) there was a significant difference, suggesting an interaction between the health state and the satisfaction level.
No demographic variables were significant across all four models. The age effect observed in Table 24 appears for only the Low HS model. Different employment status categories were significant across the different satisfaction levels. The demographic and own health variables suggest that high levels of health and life satisfaction were perceived differently by respondents.
Discussion
When health states in a TTO scenario are described in the ‘standard’ way (with a description of the health state and associated duration against a shorter period in full health), the preferences elicited for those states focus on the state of health. Considerations given to how the states will be experienced are not informed by the task, and are therefore incorporated into the scenarios by respondents (see Chapters 10 and 11 for a more detailed discussion). The analysis reported in this section investigates whether the addition of satisfaction into the standard TTO procedure influences preferences, and we find that this is indeed the case. In addition, we examine whether health-state preferences that contain satisfaction levels are influenced by the respondent’s own level of health or life satisfaction. No clear pattern was found.
We found that a scenario that contains Low HS can lead to a significant increase in the likelihood of preferring to live for a shorter duration in full health. However, this was not the case for the P5 health state, where the addition of Low HS does not impact preferences. This may be because respondents associate being in extreme pain with Low HS. Similarly, a scenario that contains either High HS or LS leads to an increase in the likelihood of preferring to live for a longer time in the associated health state rather than the shorter period in full health. However, respondent’s own satisfaction with health or life does not impact on preferences, and there is no pattern to the impact of background characteristics.
Respondents asked to give preferences for scenarios including states of P5 and D5 prefer living in those states when they self report problems on the pain and depression dimensions of the EQ-5D-5L. This may be because respondents believe that the health dimension that they are valuing cannot be much worse than their existing level of pain or depression, or they may believe that they could cope with the problem.
There is need for more research incorporating adaptation and the experience of living in poor health into valuation exercises. Future research could, for example, consider expanding the dimensions, levels of severity, and duration of scenarios studied here to offer more extensive evidence of accounting for adaptation in a preference-based setting. Research should also consider the impact on health-state preferences of different types and levels of information about the future consequences of those preferences (framed as satisfaction or adaptation). In this way, empirical data can illuminate the debate concerning the methods used and the information provided to value health.
Chapter 8 The feasibility of the DCETTO for deriving health-state values for EQ-5D-5L
Introduction
The PRET-AS component of this section has been adapted from papers presented to the Health Economist’s Study Group and the EuroQol Group. 45,46
Over the years there has been a focus on using DCE47,48 to derive utility values. DCE estimates values on an unobserved latent scale, and therefore studies have typically relied on external values (such as the value of the worst state derived by TTO)21 in order to anchor DCE values on the full health–dead utility scale.
Recently, a method was developed that avoids the need to use external values to generate a utility score by incorporating duration as an attribute of the health-state profile, therefore interpreting DCE data as a TTO exercise (DCETTO). 22 To estimate utility values for health states, a regression model incorporating interaction terms between each level of the health-state dimensions and the duration attribute are estimated.
The DCETTO development study was based on an internet survey in Canada, for which respondents were asked to value health states from the three-level EQ-5D. Although the preliminary results were encouraging, further investigation with a larger descriptive system, such as EQ-5D-5L, is necessary before the approach can be considered for use in future population-based health-state valuation surveys. The overall objective of this study was to use data from both the PRET and PRET-AS online surveys to elucidate some of the remaining questions.
The main objectives of the PRET analysis were:
-
To use PRET data to explore the feasibility of using DCETTO questions (type VII questions) to elicit health-state utility values for the EQ-5D-5L descriptive system.
The objectives of PRET-AS were to use a larger DCETTO study design to:
-
Further explore the feasibility of the DCETTO approach, and determine if the method can produce logically consistent values for EQ-5D-5L, with more detailed levels than EQ-5D. The hypothesis is that the coefficients will have the expected sign so that, on average, respondents preferred to live longer and in better health states.
-
Compare the consistency of the PRET-AS models for each dimension with those of three other studies: DCETTO results from the development study;22 DCETTO results from the PRET survey; and TTO results from the MVH survey. Of these, the development study and the MVH use the three-level EQ-5D. The null hypothesis is that the pattern of the corresponding coefficients from the PRET-AS study will not differ significantly from the others.
-
Explore the extent of agreement between individual ordinal preferences and aggregate cardinal values. The hypothesis is that there will be a high correlation between the proportion of respondents who select one health scenario over the other, and the difference in the number of QALYs for these two states predicted for the DCETTO scenario.
-
Explore the existence of learning or fatigue effects, i.e. whether respondents answer the choices at the beginning of the experiment less or more consistently than the choices towards the end.
-
Compare obtaining more DCETTO answers from a smaller sample and fewer DCETTO answers from a larger sample, holding the total number of DCETTO answers and the design constant. The null hypothesis is that when the design and the total volume of observations are held constant there is no difference in final results between (1) using one-third of the data from the whole sample; (2) using two-thirds of the data from half of the sample; and (3) using all of the data from one-third of the sample.
Methods
Question format
Type VII questions are based on the DCETTO design developed by Bansback and colleagues. 22 DCETTO questions present a whole EQ-5D-5L health state H with an associated attribute for duration T followed by death and take the following form (see also Appendix 2 ):
-
[Scenario A]: You live in EQ-5D-5L state H A for duration T A then die.
-
[Scenario B]: You live in EQ-5D-5L state H B for duration T B then die.
The DCETTO scenarios used in each pair consist of ‘you’ living in a particular EQ-5D-5L state for one of three-levels of duration T (where T = 1, 5 or 10 years) followed by death (see Figure 5 ). In contrast with question types I–V, whole EQ-5D-5L health states were used. We used a duration of 10 years to be commensurate with the standard time frame used for the MVH TTO protocol, 1 year was selected as it was also included in the development study (and it is the lowest possible whole year value), and 5 years was selected as an intermediate value. Respondents were asked which health scenario they think is better.
Type VII questions were used to first investigate the selection of health scenario pairs that could be used in a full DCETTO study, and, second, to investigate the feasibility of the DCETTO method with a larger health-state classification system. This was done by modelling the results to produce coefficients for each level of each EQ-5D-5L dimension, and comparing this with the results of the DCETTO development study. 22
The EQ-5D-5L has 3125 possible health states. Combining this with a duration attribute with three levels amounts to 9375 possible DCETTO scenarios. This means that 87.9 million DCETTO scenario pairs could be produced. The number of parameters for DCETTO of EQ-5D-5L with three duration levels is 62 [EQ-5D-5L main effects 5 × (5 − 1) = 20; duration main effects 3 − 1 = 2; and interactions 20 × 2 = 40]. This is the minimum number of pairs required to estimate coefficients for all of the parameters in the model. Confidence in the parameter estimates is improved with more pairs, and we therefore selected 120 based on the D-efficiency criterion using the modified Fedorov algorithm. 49,50 We produced 10 different designs based on different random starting points from the full factorial design, and assessed the resultant designs for efficiency level, and the number of pairs for which duration differed. We did not restrict the design to exclude potentially implausible states, as it is not clear what the criteria for implausible states in EQ-5D-5L should be. All 10 designs had similar efficiency levels, and, owing to the nature of the design algorithm included, potentially implausible dimension combinations. However, as it is difficult to establish sufficient evidence for a state to be determined implausible, it was decided not to restrict any design at this stage, and investigate the issue further during the qualitative phases of the study (see Chapters 10 and 11 ). For one design, duration differed between the scenarios on 18 (15%) of the pairs, which was higher than the other nine designs. Therefore, this design was used in PRET.
Study design
PRET
The 120 pairs were administered to participants across 60 subversions of the online survey (with four subversions of each of the 15 survey versions. Duration differed across 18 of the 120 pairs, and each main survey version included at least one of these pairs.
PRET-AS
The DCETTO pairs were presented in blocks of five across 36 survey versions. Each respondent completed 15 DCETTO scenario pairs across three experimental ‘modules’ made up of five pairs each. The survey had 36 ‘versions’, and therefore the 120 pairs selected by the D-optimal design were split into 36 ‘blocks’ of five pairs (where 60 of the pairs, including the 18 where duration differed across the scenarios, were repeated). A given block appeared in three different versions, each in a different module.
The 36 blocks were allocated across the 36 versions so that, where appropriate, the data could be analysed in three different ‘batches’. Table 26 gives a stylised representation of the design, with section A representing the entire data. Each row represents six of the 36 versions, or one-sixth of the whole sample, and the columns correspond to the 15 DCETTO task each respondent answers, grouped into three modules of five tasks. Assuming a sample size of 1800 (see below), each cell corresponds to 300 respondents answering one DCETTO task, whereas the whole grid represents 27,000 DCETTO tasks.
Module 1 | Module 2 | Module 3 | ||||||||||||
---|---|---|---|---|---|---|---|---|---|---|---|---|---|---|
1 | 2 | 3 | 4 | 5 | 6 | 7 | 8 | 9 | 10 | 11 | 12 | 13 | 14 | 15 |
Module 1 | Module 2 | Module 3 | ||||||||||||
---|---|---|---|---|---|---|---|---|---|---|---|---|---|---|
1 | 2 | 3 | 4 | 5 | 6 | 7 | 8 | 9 | 10 | 11 | 12 | 13 | 14 | 15 |
Module 1 (ALL) | Module 2 (ALL) | Module 3 (ALL) |
Module 1 | Module 2 | Module 3 | ||||||||||||
---|---|---|---|---|---|---|---|---|---|---|---|---|---|---|
1 | 2 | 3 | 4 | 5 | 6 | 7 | 8 | 9 | 10 | 11 | 12 | 13 | 14 | 15 |
Module 12(B1) | ||||||||||||||
Module 12(B2) | ||||||||||||||
Module 1 | Module 2 | Module 3 | ||||||||||||
---|---|---|---|---|---|---|---|---|---|---|---|---|---|---|
1 | 2 | 3 | 4 | 5 | 6 | 7 | 8 | 9 | 10 | 11 | 12 | 13 | 14 | 15 |
Module 123(B1) | ||||||||||||||
Module 123(B2) | ||||||||||||||
Module 123(B3) |
Module 1 | Module 2 | Module 3 | ||||||||||||
---|---|---|---|---|---|---|---|---|---|---|---|---|---|---|
1 | 2 | 3 | 4 | 5 | 6 | 7 | 8 | 9 | 10 | 11 | 12 | 13 | 14 | 15 |
ALL |
The first set of batches is ‘one-module batches’ and consists of five DCETTO tasks per respondent, based on one module across all respondents. Therefore, each batch would total 9000 DCETTO observations. These are called ‘module 1(All)’, ‘module 2(All)’ and ‘module 3(All)’, depending on which module the data come from. These one-module batches are illustrated in Table 26b .
The second set of batches is ‘two-module batches’ and comprises 10 DCETTO tasks per respondent, based on modules 1 and 2 of half of the respondents. Each batch has 900 respondents and includes 9000 observations. Two such batches are possible depending on which half of the sample, and these are called ‘module 12(B1)’ and ‘module 12(B2)’. These two-module batches are illustrated in Table 26c .
The third set of batches is ‘three-module batches’ and contains 15 DCETTO tasks per respondent, based on modules 1, 2 and 3 of one-third of the respondents. Each batch has 600 respondents providing 9000 observations. Three such batches are possible, referred to as ‘module 123(B1)’, ‘module 123(B2)’ and ‘module 123(B3)’. These three-module batches are illustrated in Table 26d . Note that the total number of DCETTO observations (9000) and the make-up of the scenario pairs (180) are kept constant across all of the above batches. Finally, the whole data set ‘ALL’ (n = 1800, 15 DCETTO tasks each, 27,000 observations) is shown in Table 26e .
DCETTO – analysis
Objectives 1 (PRET) and 2 (PRET-AS) To determine the coefficients for the DCETTO a conditional logit model was used as described by Bansback and colleagues. 22 Briefly, the utility function μ of each respondent i is defined to be a multiplicative between a vector of levels for each EQ-5D attribute x and life-years t in each scenario j so that:
Of these, the constant α can be included to examine level balance, but is expected to be equal to zero; β represents the value of living in full health for the specified duration and is expected to be positive; λ represents the disutility of living with the specified set of EQ-5D-5L health problems for the same duration and thus is expected to be negative; and ε ij is a random term which is assumed to be the independent and identically distributed extreme value. Duration is treated as continuous and conditional logit regression is used to estimate the coefficients, controlling for clustering of responses among respondents.
Bansback and colleagues22 show that the value for each health state anchored on the health utility scale (V) can be calculated from the estimated coefficients using the following formula:
Thus, the value of a health state is expressed in two arguments: the value of full health and the disutility determined by EQ-5D-5L. For the state of full health, λ ^ = 0 and so V = 1. If the absolute value of λ ^ is equivalent to β ^ then λ ^ / β ^ = 1 and V = 0. If the state is severe then the absolute value of λ ^ may exceed β ^ or in other words, the magnitude of the disutility associated with the state may be larger than the difference between full health and being dead. If so, this would result in a negative V, implying a state worse than being dead.
Note that the anchoring of the utility function for dead at 0 is achieved through the relative size of the two regression coefficients β and λ in Equation 8 above, and does not rely on the inclusion of the state of being dead in the DCETTO, or as a supplementary question. The anchoring of the utility function for full health at the value 1 is achieved through equation (4): as λ = 0 for full health, Equation 9 anchors full health at whatever value given in the first argument.
Three additional analyses were carried out to assess whether respondents trade time when presented with pairs where duration differs. First, time trading behaviour was explored by examining the frequencies of respondents who were willing to trade time when presented with a pair where duration differed between the scenarios. The proportions of respondents choosing the shorter duration was investigated irrespective of the EQ-5D-5L state presented. Second, the proportions of respondents who sometimes chose the longer and sometimes chose the shorter duration was examined, where the pairs presented allowed us to investigate this. Third, trading behaviour was assessed in relation to the utility value associated with each health scenario included in the pairs where duration differs.
Objective 3 To compare the results of PRET-AS data with the DCETTO development, and PRET studies, the size of the coefficients within each dimension used to generate utility values were compared. This is because a direct comparison across all three studies was not possible, as PRET and PRET-AS use EQ-5D-5L health states, and the DCETTO development and MVH studies3,22 used the three-level EQ-5D. The three DCETTO studies were compared graphically.
The same comparison was not possible for the MVH study coefficients owing to the use of the constant and the N3 term (a coefficient that is used when any EQ-5D dimension is at level 3) in the generation of utility values (i.e. the worst level). Therefore, to provide a broad overview of the potential feasibility of the values produced we compared the predicted values with the three-level EQ-5D tariff produced by the MVH study3 for the states where there is some level of comparability. The MVH TTO tariff is not used as a ‘gold standard’, but rather as a comparison tariff for the PRET-AS model. The comparison should not be interpreted to mean that DCETTO should reproduce, for EQ-5D-5L, the same range and distribution of values as the three-level EQ-5D. We matched level three of the EQ-5D-5L dimensions (i.e. moderate problems) with level 2 of the three-level EQ-5D (equivalent to some/moderate problems). Level 5 of EQ-5D-5L (extreme/unable) was matched with level 3 of the three-level instrument (with the caveat that the wording for the worst level of the mobility domain has changed significantly from ‘confined to bed’ to ‘unable to walk about’). We assessed the mean absolute difference between the predicted values, the relationship between the predictions across the utility scale, and also the comparability of states valued as worse than dead across the two models.
Objective 4 To explore the extent of agreement between individual ordinal preferences and aggregate cardinal values, first, the difference in the value of the health scenario in QALYs was calculated across the 120 health scenario pairs to represent the aggregate cardinal values. The value for each scenario was based on the predicted value of the EQ-5D-5L state multiplied by the specified duration. The differences in QALYs across scenario pairs were then compared with the proportion of respondents choosing each scenario. If the majority chooses the health-state scenario with the lower predicted QALYs then this would indicate a ‘disagreement’ between individual ordinal preferences and aggregate cardinal values.
Objective 5 We used two approaches to examine the existence of learning and fatigue effects. First, the predicted values obtained from models estimated on the module 1(All), module 2(All) and module 3(All) subsamples were compared against each other, and against the predicted values for the whole sample. As these batches represent the first, second and final modules that respondents answered, a divergence in the predictions can be interpreted as evidence of learning and fatigue effects. Second, along the lines of Swait and Louviere,51 we estimated a model on the full sample in which the scale of the error term is allowed to vary by batch. The scale of the first batch is normalised to one for identification purposes. As the scale is inversely proportional to the error variance, an increase in scale towards the end of the choice sequence can be interpreted as a learning effect, and vice versa. Furthermore, the LR statistic (see Equation 10) can be used to test the null hypothesis that the respondents’ preferences are stable throughout the choice sequence.
Here LL R is the log-likelihood of the model estimated on the full sample, which allows for scale differences but assumes that α, β and λ do not vary by batch. This restricted model is estimated using the Stata module clogithet (StataCorp LP, College Station, TX, USA). 52,53 LL U is the sum of the log-likelihoods of the three models estimated on the batch-specific subsamples. Together, these form the unrestricted model, which allows for variations in both scale and preferences by batch. Under the null hypothesis, the test statistic is chi-squared, distributed with 40 degrees of freedom. The number of degrees of freedom is given by the number of parameters in the unrestricted model minus the number of parameters in the restricted model.
Objective 6 To compare allocating the same number of DCETTO questions across different numbers of respondents holding the design constant, module 1(All), the two module 12 batches and the three module 123 batches are used. And, finally, to examine the effect of sample size holding the design constant, batches module 1(All), module 12(All) and All are used.
Results: PRET
Demographics
All 3159 PRET online survey respondents completed type VII questions. The demographic characteristics are displayed in Table 3 .
Objective 1: feasibility of DCETTO with EQ-5D-5L using PRET
Table 27 presents the ordered and unordered estimated coefficient values for each level of each dimension (model 1 with all levels of each attribute entered, and model 2 with unordered or similar levels combined). For model 1, most of the levels are logically ordered except Mobility level 3, which is positive. This is also the case for level 2 of the Pain/discomfort dimension. This indicates that an increase in severity according to the dimension would lead to an increase in utility value. The magnitude of the difference between 11 sets of dimension levels (out of 20) was not significant. These were: Mobility levels 1/2 and 4/5; Self-care levels 1/2, 2/3 and 4/5; Usual activities levels 1/2, 2/3 and 4/5; Pain/discomfort levels 1/2; and Anxiety/depression levels 2/3 and 4/5. Model 2 displays the coefficient values combined unordered or similar levels. Table 28 and Figure 8 display the anchored coefficient values that would be used to predict health-state utility values for EQ-5D-5L. The predicted range of utility scores is 1 (for state 11111) to −0.814 (for state 55555), and approximately one-third of values are of < 0 (equivalent to a state worse than dead). The general logical ordering of the coefficient values in the PRET data indicates that DCETTO may be a feasible method for generating utility values for EQ-5D-5L. At the same time, it also shows that two DCETTO observations each from 3000 respondents is not enough to produce a fully satisfactory value set for EQ-5D-5L.
Parameter | Model 1 | Model 2 | ||||
---|---|---|---|---|---|---|
Estimate | p-value | Robust standard error | Estimate | p-value | Robust standard error | |
MO2 × T | −0.0173 | 0.0102 | −0.0013 | 0.0079 | ||
MO3 × T | 0.0115 | 0.0093 | −0.0013 | 0.0079 | ||
MO4 × T | −0.0594 | *** | 0.0103 | −0.0582 | *** | 0.0081 |
MO5 × T | −0.0600 | *** | 0.0095 | −0.0582 | *** | 0.0081 |
SC2 × T | −0.0160 | 0.0101 | −0.0136 | 0.0093 | ||
SC3 × T | −0.0277 | ** | 0.0092 | −0.0279 | ** | 0.0091 |
SC4 × T | −0.0954 | *** | 0.0097 | −0.0932 | *** | 0.0093 |
SC5 × T | −0.1016 | *** | 0.0099 | −0.1029 | *** | 0.0092 |
UA2 × T | −0.0168 | 0.0105 | −0.0150 | * | 0.0083 | |
UA3 × T | −0.0138 | 0.0099 | −0.0150 | * | 0.0083 | |
UA4 × T | −0.0485 | *** | 0.0093 | −0.0474 | *** | 0.0091 |
UA5 × T | −0.0624 | *** | 0.0102 | −0.0638 | *** | 0.0093 |
PD2 × T | 0.0141 | 0.0098 | 0 | |||
PD3 × T | −0.0188 | * | 0.0097 | −0.0276 | *** | 0.0076 |
PD4 × T | −0.0812 | *** | 0.0108 | −0.0897 | *** | 0.0085 |
PD5 × T | −0.1138 | *** | 0.0106 | −0.1216 | *** | 0.0084 |
AD2 × T | −0.0343 | *** | 0.0091 | −0.0347 | *** | 0.0078 |
AD3 × T | −0.0300 | *** | 0.0097 | −0.0347 | *** | 0.0078 |
AD4 × T | −0.1129 | *** | 0.0103 | −0.1086 | *** | 0.0084 |
AD5 × T | −0.0978 | *** | 0.0108 | −0.1086 | *** | 0.0084 |
T | 0.2407*** | 0.0196 | 0.2509 | < 0.0001 | 0.0172 | |
Observations | 6318 | 6318 | ||||
Log-likelihood | −3971 | −3977 | ||||
AIC | 7984 | −7984 | ||||
McFadden’s R 2 | 0.0932 | 0.0918 |
Dimension parameters | Model 1 | Model 2 |
---|---|---|
MO2 | 0.072 | 0.005 |
MO3 | −0.048 | 0.005 |
MO4 | 0.247 | 0.232 |
MO5 | 0.249 | 0.232 |
SC2 | 0.066 | 0.054 |
SC3 | 0.115 | 0.111 |
SC4 | 0.396 | 0.371 |
SC5 | 0.422 | 0.410 |
UA2 | 0.070 | 0.060 |
UA3 | 0.057 | 0.060 |
UA4 | 0.201 | 0.189 |
UA5 | 0.259 | 0.254 |
PD2 | −0.059 | 0.110 |
PD3 | 0.078 | 0.110 |
PD4 | 0.337 | 0.358 |
PD5 | 0.473 | 0.485 |
AD2 | 0.143 | 0.138 |
AD3 | 0.125 | 0.138 |
AD4 | 0.469 | 0.433 |
AD5 | 0.406 | 0.433 |
FIGURE 8.
Values for each health state predicted by PRET data.
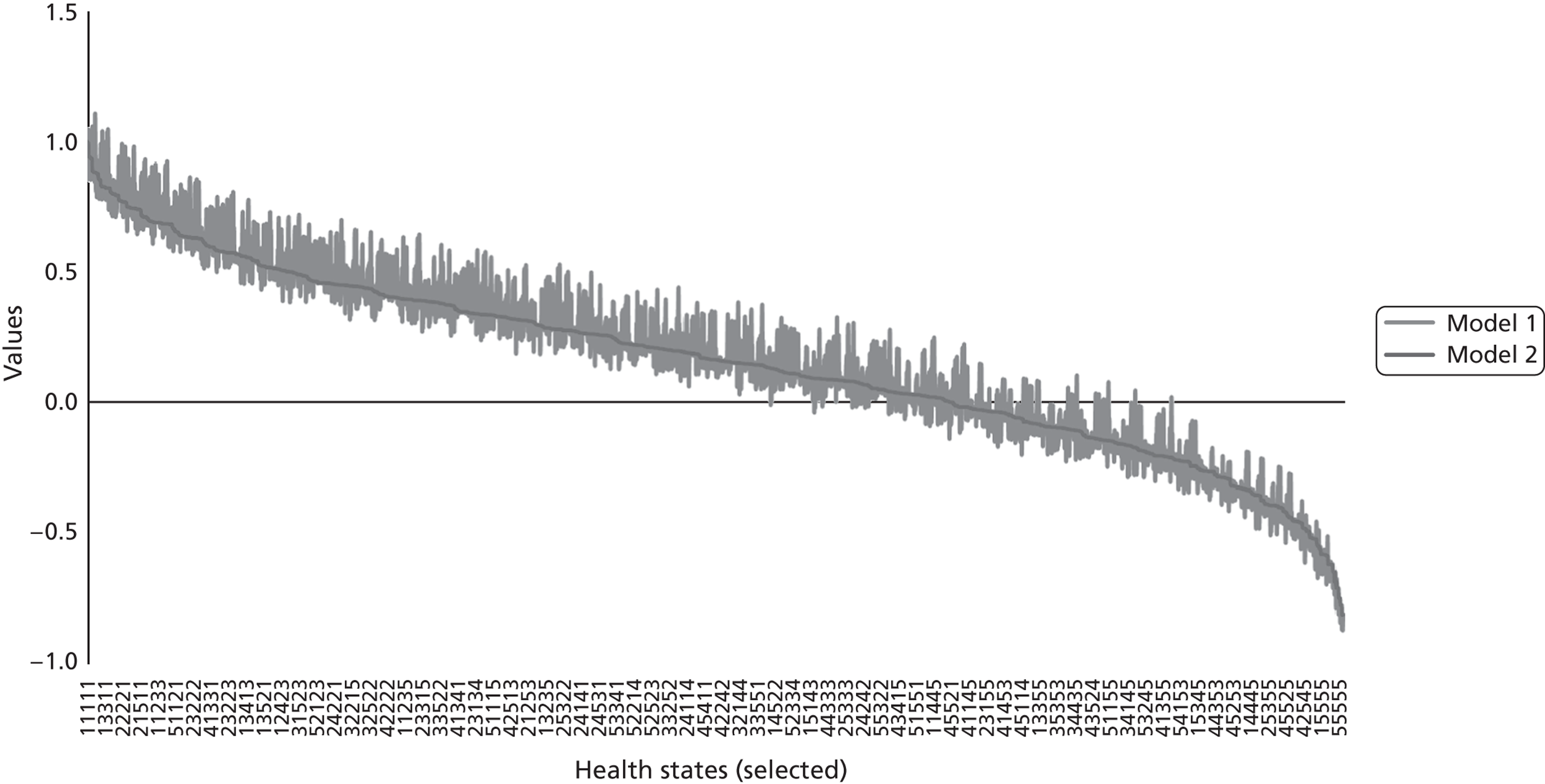
Results: PRET-AS
Demographics
Overall, 1799 respondents completed the survey (see Table 4 ). The background characteristics do not differ across batches or versions (not shown). The DCETTO pairs were presented in blocks of five across 36 survey versions. The number of respondents completing each of the 36 survey versions ranged from 43 to 52. The number of observations for each of the 120 pairs ranged from 145 to 309 (as a number of pairs were repeated in more than one block).
Objective 2: feasibility of DCETTO with EQ-5D-5L using PRET-AS
DCETTO coefficients
Table 29 reports the unanchored DCETTO regression coefficients. The coefficients reported are based on a model with no intercept. The model with an intercept results in a small but significantly positive intercept. The other coefficients change slightly but they only have a negligible effect on the anchored coefficients. The positive intercept suggests that there is a bias towards selecting the scenario presented on the left-hand side. The results of the model with the intercept are available on request. The coefficient for Mobility level 2 interacted with duration (M2 × T) did not have the expected sign but was not significant. All other coefficients were ordered as expected. The difference between three sets of dimension levels (out of 20) was not significant: Mobility levels 1/2; Self-care levels 4/5; Usual activities levels 4/5; and Anxiety/depression levels 4/5. Figure 9 displays the distribution of the predicted utility scores for all 3125 EQ-5D-5L health states produced from the anchored coefficients. The value predicted for the worst EQ-5D-5L state (55555) was −0.845, and 31.5% of the 3125 EQ-5D-5L health states had a negative value (i.e. are worse than dead).
Dimension × duration parameters | Whole sample | Unrestricted model | Restricted model | |||||||
---|---|---|---|---|---|---|---|---|---|---|
ALL | Module 1 | Module 2 | Module 3 | ALL | ||||||
No. of DCE | 15 | 5 | 5 | 5 | 15 | |||||
M2 × T | 0.006 | 0.014 | 0.001 | 0.002 | 0.006 | |||||
M3 × T | −0.021 | *** | −0.008 | −0.028 | *** | −0.027 | *** | −0.022 | *** | |
M4 × T | −0.096 | *** | −0.086 | *** | −0.095 | *** | −0.107 | *** | −0.102 | *** |
M5 × T | −0.116 | *** | −0.125 | *** | −0.117 | *** | −0.110 | *** | −0.125 | *** |
SC2 × T | −0.004 | 0.002 | 0.002 | −0.016 | * | −0.004 | ||||
SC3 × T | −0.022 | *** | −0.024 | ** | −0.016 | ** | −0.026 | *** | −0.024 | *** |
SC4 × T | −0.103 | *** | −0.114 | *** | −0.104 | *** | −0.095 | *** | −0.111 | *** |
SC5 × T | −0.133 | *** | −0.152 | *** | −0.123 | *** | −0.129 | *** | −0.144 | *** |
UA2 × T | −0.025 | *** | −0.037 | *** | −0.019 | ** | −0.021 | ** | −0.027 | *** |
UA3 × T | −0.048 | *** | −0.057 | *** | −0.049 | *** | −0.040 | *** | −0.052 | *** |
UA4 × T | −0.082 | *** | −0.088 | *** | −0.087 | *** | −0.074 | *** | −0.088 | *** |
UA5 × T | −0.092 | *** | −0.107 | *** | −0.095 | *** | −0.077 | *** | −0.099 | *** |
PD2 × T | −0.027 | *** | −0.040 | *** | −0.027 | *** | −0.016 | * | −0.029 | *** |
PD3 × T | −0.064 | *** | −0.081 | *** | −0.065 | *** | −0.049 | *** | −0.070 | *** |
PD4 × T | −0.155 | *** | −0.167 | *** | −0.153 | *** | −0.149 | *** | −0.167 | *** |
PD5 × T | −0.197 | *** | −0.216 | *** | −0.191 | *** | −0.188 | *** | −0.212 | *** |
AD2 × T | −0.033 | *** | −0.047 | *** | −0.025 | ** | −0.029 | *** | −0.036 | *** |
AD3 × T | −0.065 | *** | −0.081 | *** | −0.058 | *** | −0.059 | *** | −0.071 | *** |
AD4 × T | −0.169 | *** | −0.208 | *** | −0.156 | *** | −0.149 | *** | −0.184 | *** |
AD5 × T | −0.189 | *** | −0.207 | *** | −0.190 | *** | −0.174 | *** | −0.204 | *** |
T | 0.393 | *** | 0.431 | *** | 0.372 | *** | 0.384 | *** | 0.424 | *** |
Log of scale parameter: module 2 | −0.083 | *** | ||||||||
Log of scale parameter: module 3 | −0.144 | *** | ||||||||
LL statistic | −15,813.054 | −5162.8781 | −5281.012 | −5334.5721 | −15,806.85 | |||||
Observations | 26,985 | 8995 | 8995 | 8995 | 26,985 |
FIGURE 9.
Histogram of all 3125 EQ-5D-5L health-state values (PRET-AS data).
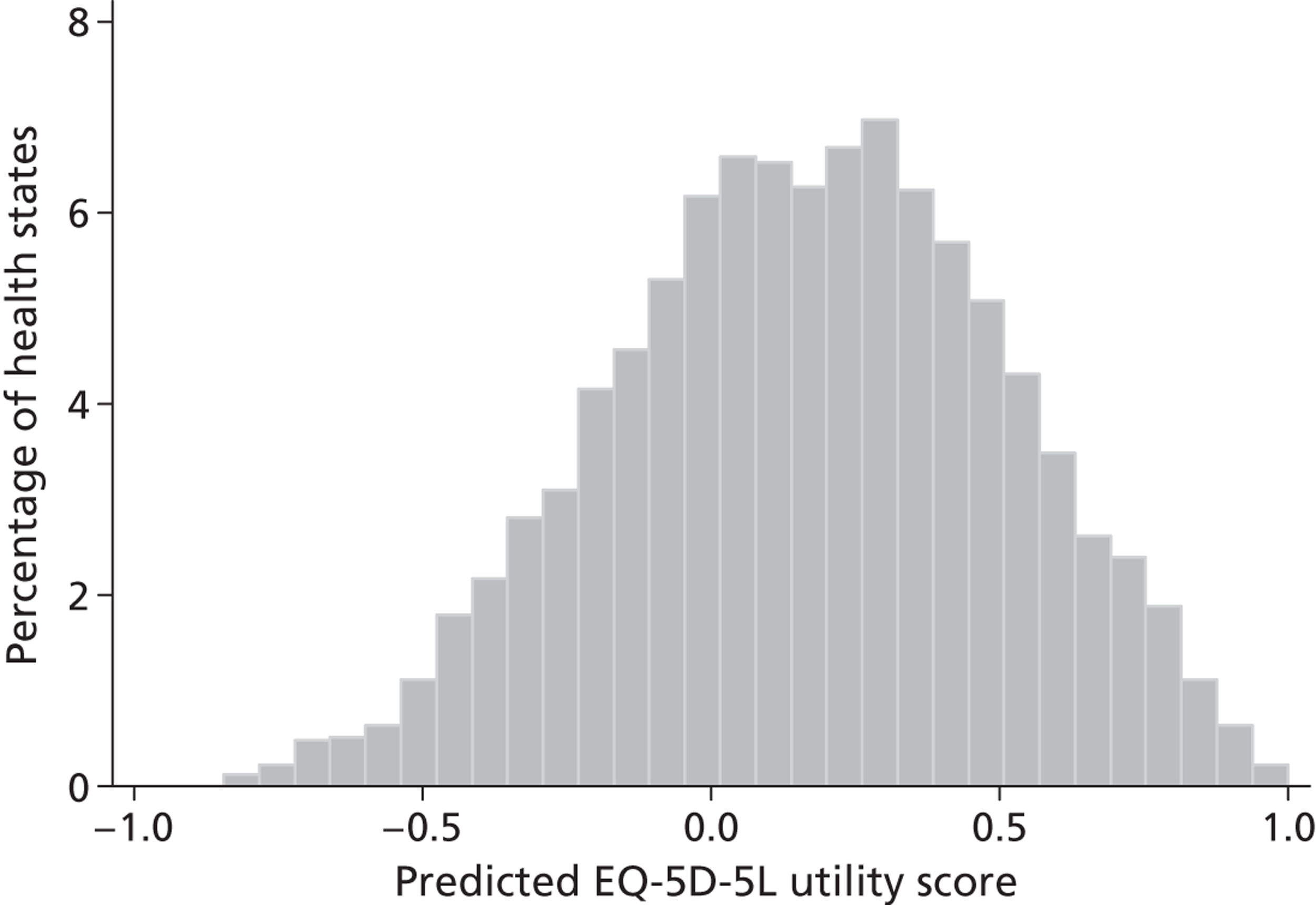
Examining time trading behaviour
Most of the respondents (1597; 88.8%) encountered at least one DCETTO task where duration differed across the scenario pair, and the time-trading behaviour of respondents by the number of such pairs they encountered is displayed in Table 30 . Overall, 266 (16.7%) did not trade time across any of the pairs that they completed (i.e. always selected the longer duration irrespective of the number of pairs completed where duration differed) and 160 (10.0%) traded every time (i.e. selected the shorter duration for every pair completed where duration varied). Therefore, 1171 (75.8% of those completing at least two pairs with different durations) displayed mixed trading behaviour (sometimes selecting the scenario with the longer duration and sometimes selecting the scenario with the shorter duration).
No. of pairs where duration differs | No. of survey versions | No. completing | No. (%) never trading | No. (%) always trading | No. (%) mixed trading |
---|---|---|---|---|---|
1 | 1 | 52 | 30 (57.7) | 22 (42.3) | NA |
2 | 1 | 50 | 8 (16.0) | 11 (22.0) | 31 (62.0) |
3 | 19 | 944 | 159 (16.8) | 104 (11.0) | 681 (72.1) |
4 | 6 | 299 | 32 (10.7) | 11 (3.7) | 256 (85.6) |
5 | 2 | 101 | 13 (12.9) | 7 (6.9) | 81 (80.2) |
6 | 3 | 151 | 24 (15.9) | 5 (3.3) | 122 (80.8) |
All | 31 | 1597 | 266 (16.7) | 160 (10.0) | 1171 (75.8a) |
Note that if the scenario with the longer duration has more QALYs then respondents are not expected to trade. Therefore, the 18 scenario pairs with different durations were ranked in terms of the gap in QALYs between the scenario with the longer duration and the scenario with the shorter duration: a negative gap indicates that the scenario with shorter duration has more QALYs. Table 31 presents this scenario ranking alongside the proportion of respondents selecting the scenario with the longer duration. The overall decreasing pattern observed is as expected: when the absolute difference in QALYs is large, a clear majority chooses the scenario with more QALYs; when the absolute difference is smaller, the margin becomes smaller. In three pairs, the majority fails to choose the scenario with more QALYs (rows 10, 12 and 13, shown in bold text). Roughly speaking, where the absolute difference in QALYs is similar, it does not seem the case that the split of responses is affected by whether the scenario with more QALYs has a shorter duration. So for example, scenario pairs in rows 1, 17 and 18 have an absolute QALY gap of 4.1–4.5 QALYs, and these pairs have a roughly 80% : 20% split of respondents in favour of the higher-QALY scenario, regardless of whether the scenario has longer or shorter duration. Similarly, rows 7 and 16 have an absolute QALY gap of 1.8, resulting in a 75% : 25% split of respondents, or rows 8 and 15 have a QALY gap of 1.3 and a respondent split of 63% : 37%. However, not all pairs follow this pattern.
Row ID | QALY difference | Percentage choosing larger QALY | Percentage choosing shorter duration | Scenario | ||||||||
---|---|---|---|---|---|---|---|---|---|---|---|---|
Longer duration | Shorter duration | |||||||||||
Observations | State | T | Tariff | QALY | State | T | Tariff | QALY | ||||
1 | 4.25 | 81.73 | 18.27 | 301 | 15212 | 10 | 0.516 | 5.159 | 42224 | 5 | 0.181 | 0.905 |
2 | 3.24 | 83.50 | 16.50 | 309 | 53332 | 10 | 0.277 | 2.773 | 42555 | 1 | −0.467 | −0.467 |
3 | 3.02 | 59.25 | 40.75 | 292 | 33251 | 10 | 0.323 | 3.232 | 25241 | 1 | 0.208 | 0.208 |
4 | 2.94 | 76.61 | 23.39 | 295 | 41523 | 10 | 0.288 | 2.884 | 51335 | 1 | −0.060 | −0.060 |
5 | 2.61 | 68.87 | 31.13 | 302 | 51141 | 10 | 0.307 | 3.070 | 33114 | 1 | 0.456 | 0.456 |
6 | 2.61 | 72.55 | 27.45 | 306 | 42124 | 10 | 0.245 | 2.454 | 23155 | 5 | −0.030 | −0.151 |
7 | 1.72 | 71.43 | 28.57 | 294 | 13314 | 5 | 0.393 | 1.967 | 42151 | 1 | 0.243 | 0.243 |
8 | 1.29 | 63.30 | 36.70 | 297 | 42531 | 5 | 0.348 | 1.739 | 21143 | 1 | 0.445 | 0.445 |
9 | 1.22 | 74.49 | 25.51 | 294 | 25141 | 5 | 0.272 | 1.360 | 45421 | 1 | 0.140 | 0.140 |
10 | 0.51 | 49.35 | 50.65 | 306 | 24551 | 10 | 0.009 | 0.094 | 23444 | 5 | −0.083 | −0.415 |
11 | 0.41 | 71.85 | 28.15 | 302 | 41515 | 5 | 0.041 | 0.207 | 54414 | 1 | −0.200 | −0.200 |
12 | 0.03 | 48.34 | 51.66 | 302 | 42324 | 10 | 0.123 | 1.231 | 35332 | 5 | 0.240 | 1.199 |
13 | −0.18 | 33.33 | 33.33 | 294 | 24144 | 5 | −0.080 | −0.402 | 54514 | 1 | −0.224 | −0.224 |
14 | −0.25 | 61.00 | 61.00 | 300 | 55223 | 5 | 0.066 | 0.332 | 13332 | 1 | 0.580 | 0.580 |
15 | −1.34 | 62.37 | 62.37 | 295 | 24153 | 10 | 0.078 | 0.776 | 14512 | 5 | 0.423 | 2.114 |
16 | −1.83 | 75.33 | 75.33 | 304 | 31455 | 5 | −0.240 | −1.202 | 32232 | 1 | 0.623 | 0.623 |
17 | −4.13 | 82.89 | 82.89 | 298 | 34435 | 10 | −0.165 | −1.652 | 31333 | 5 | 0.495 | 2.477 |
18 | −4.57 | 79.61 | 79.61 | 304 | 15445 | 10 | −0.413 | −4.128 | 14223 | 1 | 0.443 | 0.443 |
Objective 3: comparability of the PRET-AS model
DCETTO and PRET studies
Figure 10 depicts the anchored coefficients and confidence intervals from this study and those from the development and PRET studies. 22 The MVH coefficients could not be plotted on this figure owing to the use of the N3 term. The vertical axis shows the disutility associated with each level within each dimension. For the present study (the blue curves), it shows that for example, Mobility level 2 is not statistically significantly different from level 1, and has a positive value indicating that utility increases as health level decreases. Elsewhere, all of the curves are downward sloping, indicating that the level coefficients are logically ordered. It also shows that amongst the level 5 coefficients, pain/discomfort has the worst disutility, closely followed by anxiety/depression. These two dimensions demonstrate a wider gap between levels 3 and 4 than the other three dimensions. For the PRET data (represented by the green lines) there are a larger number of inconsistent coefficients.
FIGURE 10.
Comparing the anchored coefficients with the development study. L1–L5, levels 1 to 5. Note: level 3 is equivalent to the moderate level in EQ-5D used in development study and level 5 is equivalent to the extreme level in EQ-5D. Data on the development study taken from Bansback and colleagues. 22
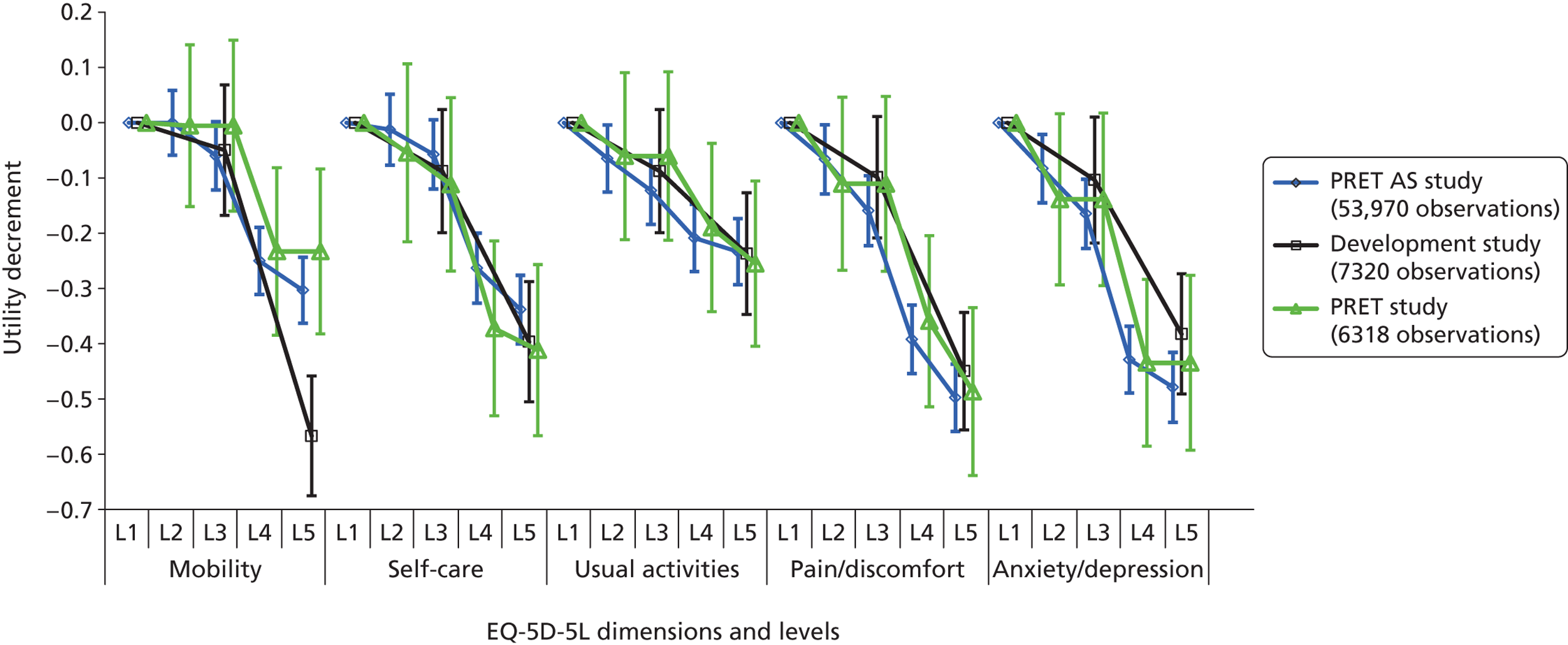
The anchored coefficients from the development study depicted by the black curves are based on the three-level EQ-5D, and so along the horizontal axis the middle level is placed with level 3 of EQ-5D-5L and the worst level is placed with level 5 of EQ-5D-5L. The major difference between the PRET and PRET-AS, and the development study coefficients is in the worst level of the Mobility attribute. The middle and worst levels for the Anxiety/depression dimension also fall outside the corresponding confidence intervals. Elsewhere, the level 3 and level 5 coefficients from the five-level model are similar to the level 2 and level 3 coefficients from the three-level model.
The coefficients produced for EQ-5D-5L from the larger PRET-AS study are more consistent than those produced for PRET. The predicted range of the utility values (using the ordered models) is similar, and both have similarities with the DCETTO development study. This indicates that the DCETTO approach may be a feasible method of producing utility values for large descriptive systems when a sufficient study design is used.
MVH study
Across the 243 comparable EQ-5D states, the mean absolute difference between the DCETTO and MVH TTO values was 0.11 (range 0–0.4). The absolute difference differed slightly across the utility scale. The mean difference for states valued by the MVH TTO tariff as equal to or worse than dead (i.e. a score of ≤ 0) was 0.09. This difference for states valued between 0.01 and 0.2 was 0.12, and for states valued between 0.21 and 1.00 was 0.11. The larger absolute difference in the middle of the utility scale is also reflected in Figure 11 , where the MVH TTO values are generally lower.
FIGURE 11.
Relationship between DCETTO and MVH TTO predicted values.
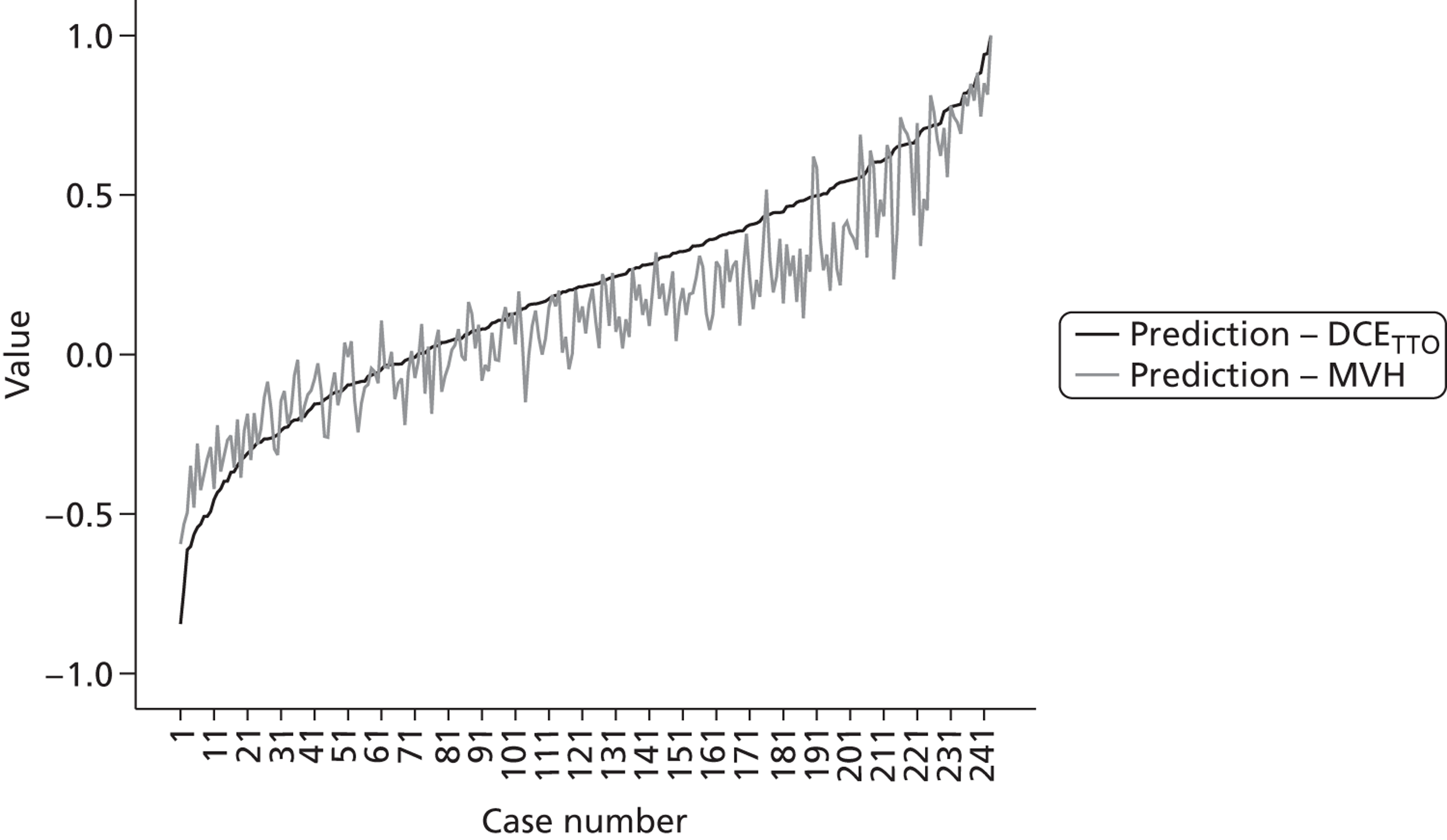
In terms of states worse than dead, 66 (27%) of states are valued at ≤ 0 across both predicted tariffs, 158 (65%) are valued as states better than dead, 14 (6%) are valued as worse than dead by just the MVH tariff, and five (2%) are valued as worse than dead by the DCETTO predicted tariff.
Objective 4: exploring the extent of agreement between individual ordinal preference and aggregate cardinal values
For each of the 120 pairs, we examined the difference in the percentage of respondents choosing the profile with more QALYs over less, so that a positive figure indicates that the majority of respondents chose the scenario with more QALYs. If all respondents facing the same pair choose the same scenario, this difference would be 100 − 0 = 100; if there is a 50% : 50% split then this difference would be 50 − 50 = 0. Figure 12 plots this difference along the vertical axis against the absolute difference in implied QALYs across the scenario pair along the horizontal axis. As can be seen, most plots are in the positive range, and there is a rough positive correlation so that the further apart in terms of QALYs the two scenarios are, the larger is the proportion of those who choose the scenario with the higher QALYs.
FIGURE 12.
Percentage of respondents choosing higher QALY scenario by the absolute difference in QALYs.
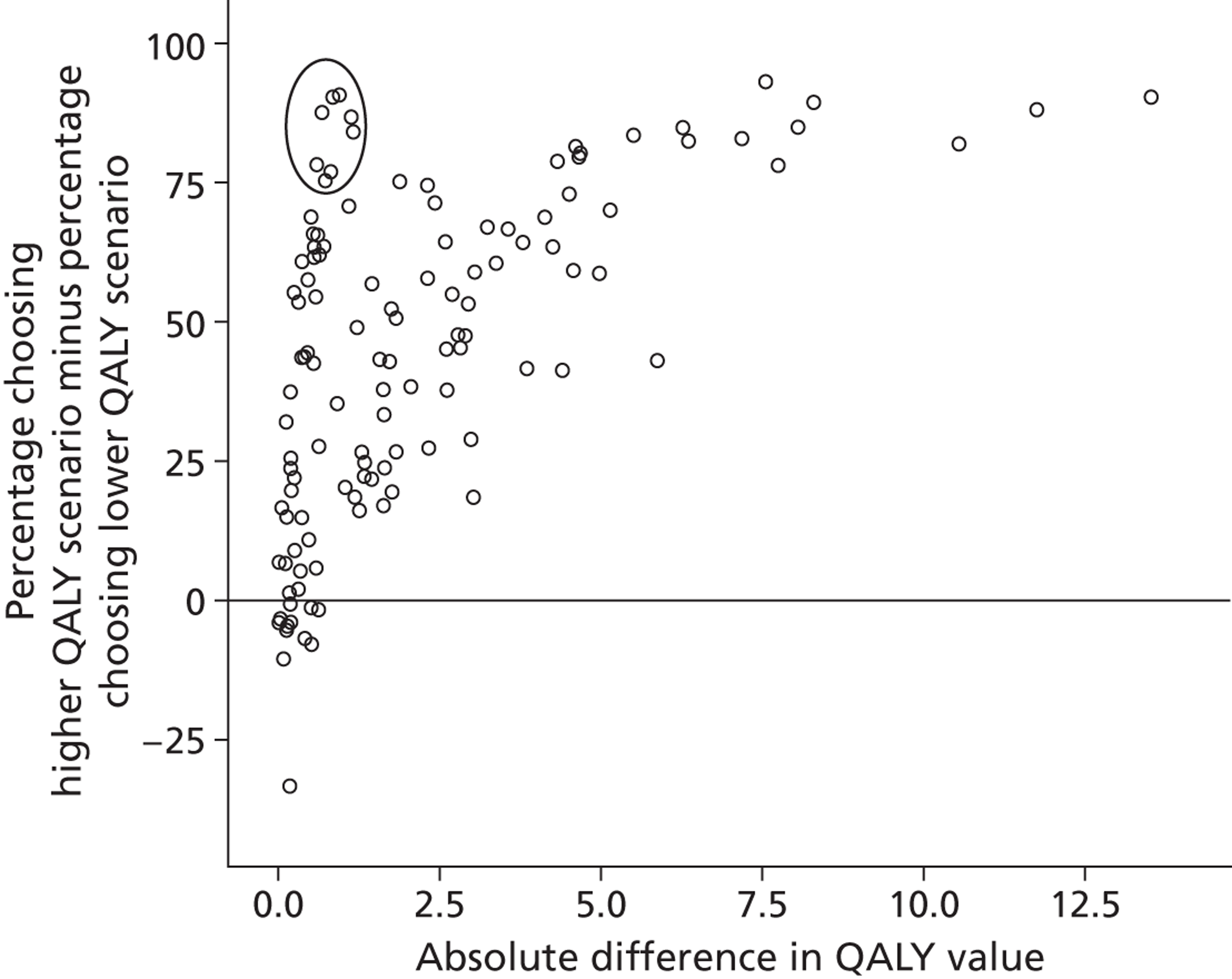
There is a group of pairs with very little difference in terms of QALYs, but a large difference in the response split across the pairs (see circled area on Figure 12 , which highlights eight pairs across which the difference in the response split is > 75% but the absolute difference in the value of the QALYs is minimal – < 1.2 QALYs). All eight of these pairs have a matched duration of 1 year and the difference in the health-state values across the scenarios is large (between 0.67 and 1.16) but this large difference is not reflected in the difference in QALYs across the scenarios because they are only 1 year long. In other words, if these eight pairs had the same EQ-5D-5L states combined with a 10-year duration then the difference in QALYs would have been much larger (6.7 to 11.6). Respondents consistently choose the less severe health state with the larger associated utility value.
The pairs in the negative range of Figure 9 indicate a disagreement between the ordinal preference and the implied cardinal values for the pairs. Of the 120 pairs, such a disagreement was observed in 12 pairs, all of them with very small difference in QALYs across the scenarios. For 11 of these, the difference between those choosing each scenario is ≤ 10%, indicating a low level of disagreement. For the one remaining pair, however, the difference is large (33%), indicating a higher level of disagreement. This pair consisted of scenario A with state 24144 for 5 years (−0.40 QALYs) compared with scenario B with state 54514 for 1 year (−0.22 QALYs). Of the five dimensions, A and B are the same in two (Self-care and Anxiety/depression); scenario A is better in two (Mobility and Usual activities); and scenario B is better in one (Pain/discomfort). In terms of QALYs, scenario B is better, but only a third of respondents agreed. The health-state values of the respective health states are −0.08 for scenario A and −0.22 for scenario B, suggesting that there may be a fair proportion of respondents who perceive that scenario A is not worse than dead, whereas a larger proportion would agree that scenario B is worse than dead. If a respondent believes that scenario A is worse than dead then 5 years of scenario A may be less preferable than 1 year of scenario B, so they may choose scenario B. However, if a respondent believes that scenario A is better than dead then 5 years of scenario A is more preferable than 1 year of scenario B, so they choose A. Thus, a small variation in individual perception around dead (namely slightly better than dead vs. slightly worse than dead) may lead to the opposite choice between the scenario pair.
Objective 5: examining learning and fatigue effects
The correlation coefficients of the predicted EQ-5D-5L values across the batches are very high. For example, all of the one-module batches have a correlation coefficient ranging from 0.985 for module 1(All) with module 3(All) to 0.998 for ALL with module 2(All). A scatterplot matrix is given in Figure 13a . The plots illustrate a very good direct correlation, with no bias by severity. This suggests that the three modules are each capturing similar preferences.
FIGURE 13.
Scatterplots of the 3125 predicted values, by batch. (a) By module with the whole sample; (b) two-module models using half of the sample; and (c) three-module models using one-third of the sample.
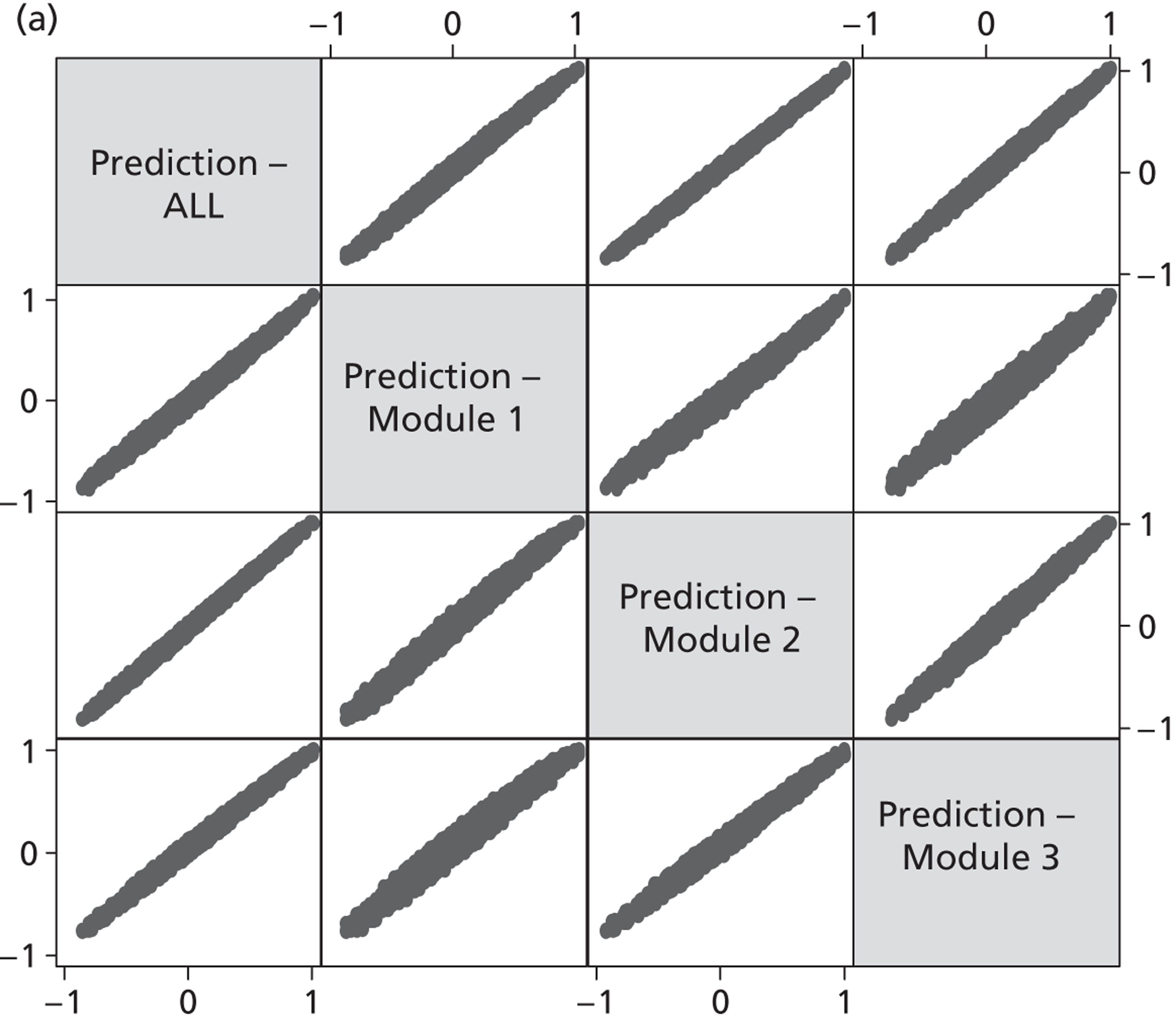
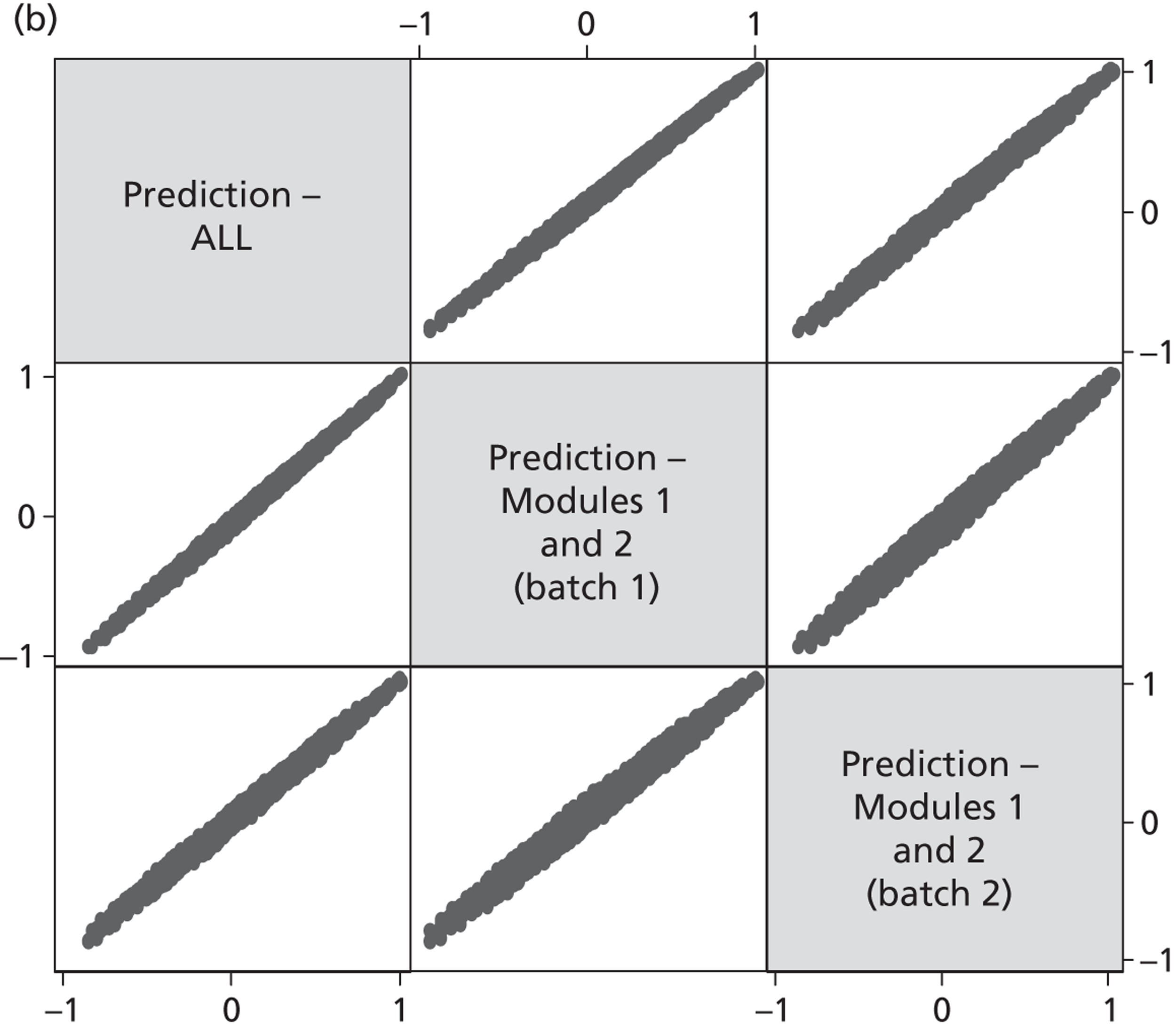
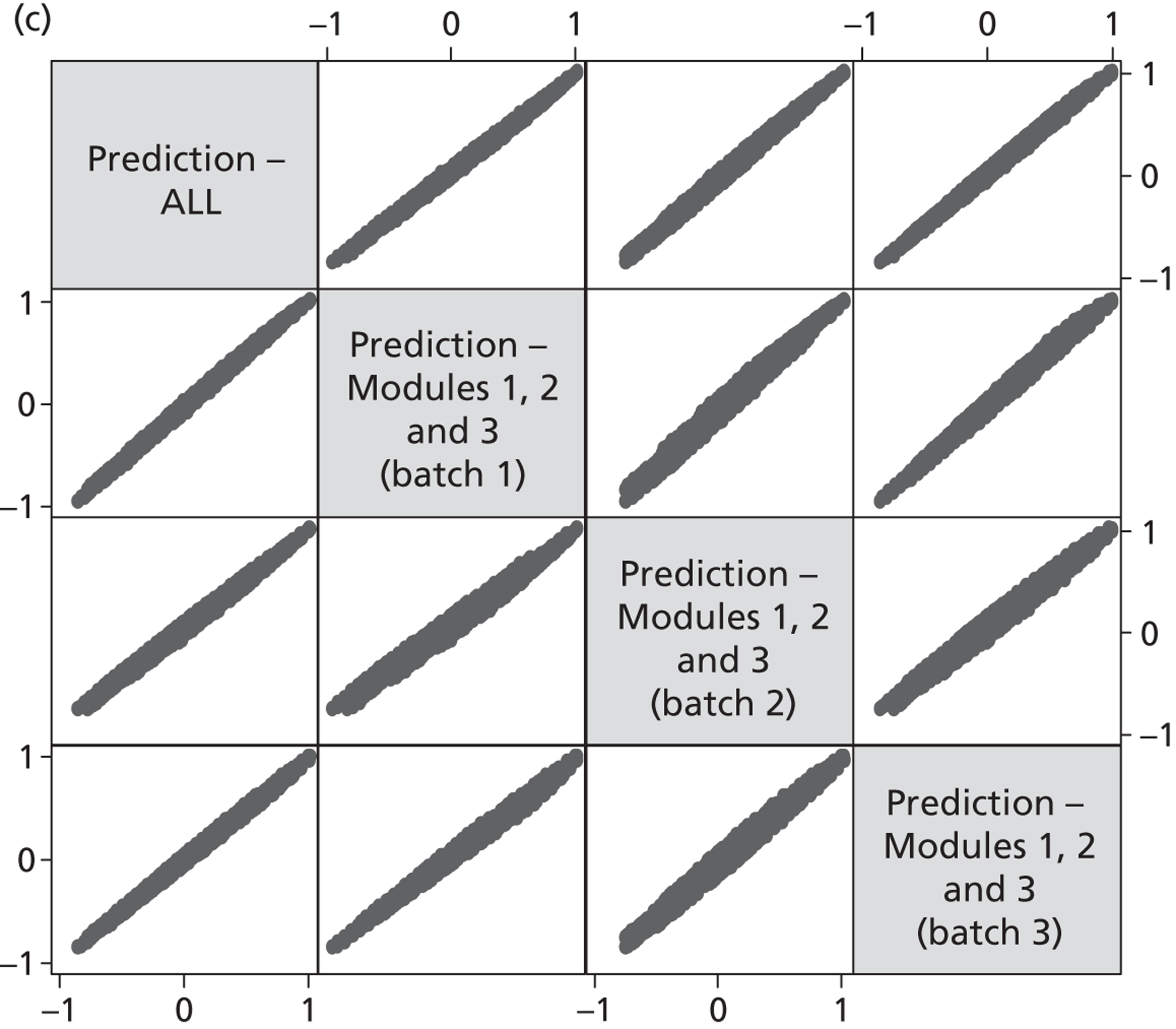
Table 29 presents the results for the restricted model, which allows for scale difference across batches. The size of estimated scale parameters increase negatively indicating that the error variance is increasing towards the end of the experiment. This suggests a fatigue effect. The LR statistic is 56.78, narrowly rejecting the null of preference homogeneity across the batches at the 5% significance level. The unrestricted models for each of the three modules are also presented.
Objective 6: comparing more DCETTO answers from a smaller sample and fewer DCETTO answers from a larger sample
From a visual inspection of the scatterplot matrices in Figure 13 , it can be seen that although there is little to choose between them, the two-module batches in Figure 13b achieve the highest concentration of the plots, followed by the three-module batches Figure 13c . The relatively less concentrated scatter for module 1(All) suggests that asking a large sample of respondents five DCETTO questions may not be the most efficient way of administering the tasks. The designs incorporating batches of 10 tasks with an intermediate-sized sample, and 15 tasks with a smaller sample provide stable results in comparison with the whole sample model.
Discussion
The analysis reported in this section demonstrates that the DCETTO is a feasible method for generating health-state utility values for larger descriptive systems such as those found in the EQ-5D-5L (for qualitative evidence about the approach, see Chapters 10 and 11 of this report). The PRET-AS DCETTO coefficients within each the health-state attribute are more consistent than the PRET coefficients, with only one coefficient (Mobility level 2) found to be non-significant (and disordered). Furthermore, a larger number of dimension level coefficients are significantly different from the adjacent coefficient in the PRET-AS data [and the non-significant dimension level sets are between levels 4 (severe problems) and 5 (extreme problems/unable to), which may imply that respondents find it challenging to tell the difference between these levels]. This issue is investigated further in Chapters 10 and 11 . The distribution of the predicted values for the 3125 health states is unimodal, and there is no statistically significant gap between the value for the best state (i.e. 11111), and the next best state (21111). In contrast, the three-level EQ-5D MVH value set based on TTO where the distribution of the 243 predicted values has a bimodal distribution, and a gap of 0.117 between the best (11111) and next best health state (11211). DCETTO allows values for states worse than dead to be predicted using the same methodology and modelling process as states better than dead, and with no arbitrary transformations. The utility values predicted in this study indicate that the worst state has a value of −0.845, and 31.5% of the states have a negative value.
The states used in the study were derived using a D-optimal algorithm, and the consistency of the coefficients in the PRET-AS study demonstrates that this method is a valid way of selecting states for use in future DCETTO studies when used alongside a sufficient sample size. One outstanding issue is implausible dimension combinations. The PRET-AS study produced feasible coefficients using DCETTO without restricting the design to exclude states that could be perceived as implausible. As it is not clear what the criteria for implausible states in EQ-5D-5L should be, one practical way ahead with DCETTO may be to not exclude any states from the study design. Furthermore, if states with certain combinations of levels were excluded, the design would be inefficient and/or biased. The model used to generate utility values is going to predict values for all states even if they are deemed implausible. Therefore, it may be argued that these states should not be excluded from the design.
Although we do not expect the PRET-AS, PRET and development studies to report identical coefficients, the consistency of the coefficients with the development study is encouraging. There are some similarities with the widely used MVH tariff in terms of the states predicted as worse than dead, but there is a mean absolute difference found between the tariff values across the utility scale. The only major change in comparable levels between the three- and the five-level versions of EQ-5D was in the worst level of mobility. As the wording has changed from ‘confined to bed’ (EQ-5D mobility level 3) to ‘unable to walk about’ (EQ-5D-5L mobility level 5), the fact that we found a difference gives some face validity to the method. Confidence intervals overlapped for all other comparable levels.
Analysis of data batches indicates that 10 or 15 DCETTO tasks may be better than five DCETTO tasks per respondent, and this is consistent with the study design recommendations put forward by other studies. 34 On the other hand, we found some evidence of a fatigue effect over 15 DCETTO tasks, so, although it does not take much time for an average respondent to complete them, it is probably prudent not to give respondents too many online DCETTO tasks. Our coefficients indicate that there may be a slight bias towards selecting the state on the left-hand side, and the position (i.e. either scenario A or scenario B) in which the states appeared was not randomised. Bias should be investigated further in future studies using the DCETTO technique by randomising the presentation of the health-state scenarios within a pair. The stability of the coefficients could also be assessed by altering the order in which the health-state dimensions are presented within each state.
To assess the impact of adding duration into the scenarios presented we investigated whether respondents are willing to trade time when completing DCETTO tasks (i.e. select the scenario with a shorter duration). Overall, we have shown that the majority of respondents are willing to select a scenario with a shorter time frame if the scenario with a shorter duration has a higher utility value. There is a limited number of pairs where this is not the case, and it may be important to systematically test trading behaviour using a wider range and number of duration values as outlined above.
Analysis of the level of agreement between ordinal preferences and aggregate values is important, as patients may think health scenario, or prospect, A is better than B, but the value set disagrees. Roberts and Dolan54 used the MVH tariff to assess agreement and found that for two-thirds of respondents to agree with the ordinal ranking between EQ-5D health states, the cardinal difference between the states had to be as large as 0.20. The results of our study show that for the majority of the pairs, there is agreement between the predicted value of the scenario in QALYs across the pairs and the health scenario chosen.
The analysis using Figure 12 attempted to explore the relationship between the gap in QALYs across a scenario pair and the split of responses. A fundamental assumption in DCE and random utility theory is that the split of responses across a scenario is explained by the difference in the latent value of the two scenarios. However, the plots have shown that some scenario pairs with little difference in QALYs across the two scenarios can still result in highly uneven splits of responses. This appears to be related to the fact that the health scenarios used are composed of a health state and its duration, with interactions between them. An innovative visual presentation of the relationship between the QALY gap and the responses split will be very useful to eyeball DCETTO data. Another anomaly was observed for a pair involving a state with a value close to 0.
The analysis presented here has treated duration as a continuous variable. However, the design allows for duration as a categorical variable. This further analysis will allow the examination of whether preferences are linear in duration, and indeed whether the QALY model holds.
There are a number of limitations with the study design used, which may impact on the findings presented. First, EQ-5D state 11111 is assumed to be equivalent to full health, so the issue of ‘upper end censoring’ is not addressed and this is a potential area for further work. Second, only 18 of the 120 pairs had differing duration levels between the health scenarios. To predict utility values, the attribute coefficients are divided by the duration coefficient so any bias in duration will bias the whole model. As can be seen, the confidence interval for the duration coefficient is large in comparison with the others, and it is possible that by increasing the number of pairs where duration varies, the size of the confidence interval could be reduced. At the same time, it should be borne in mind that the number of pairs in which duration may vary is limited by the fact that duration is interacted with the other attributes in the model. To identify the coefficients for these interactions, duration needs to be held constant within some pairs. Future developments of DCETTO should investigate the impact of increasing the number of pairs within which duration differs. This may be achieved by basing the D-optimality criterion on the covariance matrix of the anchored coefficients instead of the unanchored coefficients, as was done in the present study.
Chapter 9 Stage 2 online and CAPI comparison study methods and results
Objectives
This chapter has been adapted from papers presented at the Health Economist’s Study Group and the EuroQol Group. 55,56 The aim of stage 2 of PRET was to compare the online and face-to-face CAPI administration of binary choice health-state valuation questions. The first objective was to assess whether responses to the questions differed across modes. The second objective was to compare the background characteristics and self-reported health of the samples recruited for each study, and compared the sample characteristics with the general population. Version 15 of the stage 1 online survey (which included each of the seven question types outlined in Chapter 2 , Aims and objectives of the methodological issues tested) was repeated in a CAPI setting presenting identical questions in the same order. In order to achieve a comparison of the two modes of administration as they would happen in the real world, the two samples were recruited separately following procedures that would be used in typical online or face-to-face surveys. We also assessed potential differences between the demographic characteristics of the samples.
Methods
Recruitment and the sample
For the online survey, respondents were recruited from an internet panel as described in Chapter 2 (see PRET). Only those respondents completing version 15 of the online survey were considered in the stage 2 analysis.
For the CAPI interviews, 200 respondents were recruited following the same set quotas for age and gender based on the UK general population as those set for the online survey. Respondents were recruited by knocking on 1 in every 10 doors of selected postcodes in five areas of the UK, and those who participated were given a £5 gift voucher as an incentive. The interviewer explained the project and gained consent from an eligible member of the household (i.e. aged > 18 years and of an age and gender quota for which interviews were still required for the sample to be representative). The questions were presented to respondents on a laptop, with the interviewer reading out all of the content displayed on the screen, and recording the response. Interviews were conducted in a one-to-one setting, and participants were able to stop the interview at any time. As with the online survey, a minimum completion time of 5 minutes was imposed.
Survey format
The survey used to test for differences between the online and CAPI modes of administration was version 15 of the PRET stage 1 online survey. Table 32 outlines the questions and attribute combinations used in the matched survey.
Type | Scenario A | Scenario B | |||||
---|---|---|---|---|---|---|---|
H | T | L | P | S | H | T | |
I | Slight problems walking about | 10 years | n/m | You | n/m | Full health | 9 years |
Slight pain | 10 weeks | n/m | You | n/m | Full health | 8 weeks | |
Unable to walk about | 10 years | n/m | You | n/m | Full health | 8 years | |
Extreme pain | 2 years | n/m | You | n/m | Full health | 5 years | |
Extremely depressed | 1 year | n/m | You | n/m | Full health | 7 months | |
II | Extreme pain | 10 years | n/m | Somebody else | n/m | Full health | 6 years |
III | Slight pain | 10 weeks | 10 weeks | You | n/m | Full health | 19 weeks |
IV | Extremely depressed | 1 year | 10 weeks | Somebody else like you | n/m | Full health | 7 months |
V | Unable to walk about | 5 years | n/m | You | High | Full health | 3 years |
VIa | 55555 | 10 years | 10 years | You | n/m | Immediate death | NA |
VIIaa | 24144 | 5 years | n/m | You | n/m | 54514 | 1 year |
VIIba | 25555 | 1 year | n/m | You | n/m | 42424 | 1 year |
VIIca | 53543 | 10 years | n/m | You | n/m | 31354 | 10 years |
VIIda | 41234 | 1 year | n/m | You | n/m | 14112 | 1 year |
Analysis
Sociodemographics, health reported by the respondent, and time taken to complete each of the experimental question modules were compared across the two samples using chi-squared test and ANOVA analyses. The sociodemographic characteristics were also compared with the general population of England and Wales using statistics from the 2001 UK census for 18- to 64-year-olds. 45 As with stage 1, comparisons of the proportion of respondents who choose scenario B by administration mode and across the binary choice question types was carried out, with statistical significance indicated by p-values of < 0.05. Probit regressions were used to explore the variables significantly impacting on the likelihood of choosing scenario B for each question.
Two additional analyses were carried out to examine the effect of time taken to complete the tasks. First, the effect of time taken to complete individual modules was examined. Observations from those respondents who took 5 minutes or more to complete the whole survey were broken up into two groups within each module: Group 1 included those completing the module in less than the median time taken to complete the module; and Group 2 included those completing the module in more than or equal to the median time. For each module, the proportion of respondents choosing scenario B was compared across the two groups. Second, differences in the proportion of respondents choosing scenario B was assessed using a range of cut-off points in terms of the time taken to complete the whole survey (i.e. 5, 6, 7 and 8 minutes).
Results
Respondent characteristics
Overall, 422 respondents completed either the online or face-to-face CAPI version of the survey (see Table 34 for the full respondent characteristics). For the online version 2326 members of the UK general population were invited to take part. Of this group, 487 potential respondents (20.1%) clicked the link to access the survey, 266 (11% of those invited; 54% of those accessing the survey) were screened out as they belonged to a completed age and gender quota, left the survey during completion or completed the survey in < 5 minutes. This group were defined as non-completers. In total, 221 (9.5% of those invited, 46% of those accessing) fully completed the survey in ≥ 5 minutes. Age and gender did not significantly differ between those members of the online panel responding and those not responding.
The CAPI version of the survey was completed by 201 respondents. No respondents completed the survey in < 5 minutes, and no respondents dropped out during the completion of the questions. Information about the response rate for the survey is not available, as it was not recorded by the survey company.
In terms of the sample characteristics, age and gender did not significantly differ between the online and CAPI sample. However, differences were found across other background characteristics ( Table 33 ). A higher number of CAPI respondents were married, and more members of the online panel were educated to a higher level. The marital status of the online sample is more similar to the general population, as more of the CAPI sample are married or with a partner. In contrast, the CAPI sample education level is more similar to the general population.
Characteristic | General populationa | Overall | Online | CAPI | p-value | ||||
---|---|---|---|---|---|---|---|---|---|
Online, CAPI and GP | Online vs. CAPI | Overall vs. GP | Online vs. GP | CAPI vs. GP | |||||
N invited | NA | NA | 2326 | NA | |||||
n | NA | 422 | 221 (52.37) | 201 (47.63) | |||||
Age, years | |||||||||
Mean (SD) | 42.2 | 41.5 (13.96) | 41.6 (14.38) | 41.4 (13.52) | NA | p = 0.913 | NA | NA | NA |
Range | 18–64 | 18–65 | 18–65 | 18–65 | |||||
Age category (n, %) | p = 0.411 | p = 0.233 | p = 0.415 | p = 0.154 | p = 0.980 | ||||
18–24 | 14 | 64 (15.2) | 34 (15.4) | 30 (15.0) | |||||
25–34 | 23 | 97 (23.0) | 51 (23.1) | 46 (22.9) | |||||
35–44 | 24 | 85 (20.1) | 36 (16.29) | 49 (24.4) | |||||
45–54 | 22 | 86 (20.4) | 46 (21.8) | 40 (19.9) | |||||
55–64 | 17 | 90 (21.3) | 54 (24.4) | 36 (17.9) | |||||
Male (n, %) | 47 | 201 (47.6) | 102 (46.2) | 99 (49.3) | p = 0.836 | p = 0.524 | p = 0.945 | p = 0.703 | p = 0.765 |
Employment (n, %) | p < 0.001 | p = 0.223 | p = 0.713 | p = 0.589 | p < 0.845 | ||||
In employment | 62 | 245 (58.1) | 128 (57.9) | 117 (58.2) | |||||
Student | 7 | 36 (8.5) | 23 (10.4) | 13 (6.5) | |||||
Not in employment | 31 | 141 (33.4) | 70 (31.7) | 68 (33.8) | |||||
Marital status (n, %) | p = 0.047 | p = 0.013 | p = 0.297 | p = 0.705 | p = 0.044 | ||||
Married/partner | 53 | 236 (55.9) | 111 (50.7) | 125 (62.2) | |||||
Single | 47 | 184 (43.6) | 108 (49.3) | 76 (37.8) | |||||
Education after minimum age (n, %) | NA | 292 (69.2) | 174 (78.7) | 118 (58.7) | NA | p < 0.001 | NA | NA | NA |
Educated to degree level (n, %) | 22 | 136 (29.9) | 90 (40.7) | 46 (22.9) | p < 0.001 | p = 0.032 | p < 0.001 | p < 0.001 | p = 0.719 |
Time taken to complete, minutes (mean, SD) | |||||||||
Overall | NA | 9.88 (4.6) | 8.64 (3.84) | 11.26 (4.99) | NA | p < 0.001 | NA | NA | NA |
Module 1 | NA | 1.27 (0.76) | 1.07 (0.77) | 1.49 (0.70) | NA | p < 0.001 | NA | NA | NA |
Module 2 | NA | 1.92 (1.33) | 1.80 (1.63) | 2.06 (0.89) | NA | p = 0.045 | NA | NA | NA |
Module 3 | NA | 1.28 (0.99) | 1.20 (1.11) | 1.36 (0.84) | NA | p = 0.088 | NA | NA | NA |
Health status (n, %) | |||||||||
Good | NA | 340 (80.6) | 163 (73.8) | 177 (88.1) | |||||
Poor | NA | 82 (19.4) | 58 (26.2) | 24 (12.0) | |||||
SWBH (n, %) | |||||||||
10 | NA | 37 (8.8) | 15 (6.8) | 22 (11.0) | |||||
6–9 | NA | 279 (66.1) | 132 (59.7) | 147 (73.1) | |||||
1–5 | NA | 106 (25.1) | 74 (33.5) | 32 (15.9) | |||||
SWBL (n, %) | |||||||||
10 | NA | 44 (10.4) | 15 (6.8) | 29 (14.4) | |||||
6–9 | NA | 266 (63.0) | 131 (59.3) | 135 (67.2) | |||||
1–5 | NA | 112 (26.5) | 75 (33.9) | 37 (18.4) |
Time taken to complete the survey
The time taken to complete the overall survey was longer for the CAPI sample, whose participants also took longer to complete module 1 (five type I questions) and module 2 (one each of types II–VI questions). There were no differences for module 3 (two DCETTO questions). Across all modules the standard deviation of the time taken is bigger for the online group (see Table 33 ).
Self-reported health status
Responses to the self-report questions are displayed in Figure 14 . The CAPI sample self reports significantly better health (p = 0.002), and higher SWBH (p < 0.001) and SWBL (p < 0.001). The mean EQ-5D-5L index score for the online sample was 0.776 (SD 0.25) and for the CAPI group was 0.874 (SD 0.20). Index scores were generated using the interim mapping between the EQ-5D and EQ-5D-5L developed by van Hout and colleagues. 57 The difference between the mapped index scores was significant [F(1409) = 18.66, p < 0.001]. EQ-5D-5L dimension level response frequencies also differed significantly by mode of administration (with the exception of the mobility dimension). The CAPI sample self-reported less problems across the self care, usual activities, pain/discomfort and anxiety/depression dimensions.
FIGURE 14.
Self-reported health status, and satisfaction with health and life. (a) Self-reported health status; (b) SWBH; and (c) SWBL.
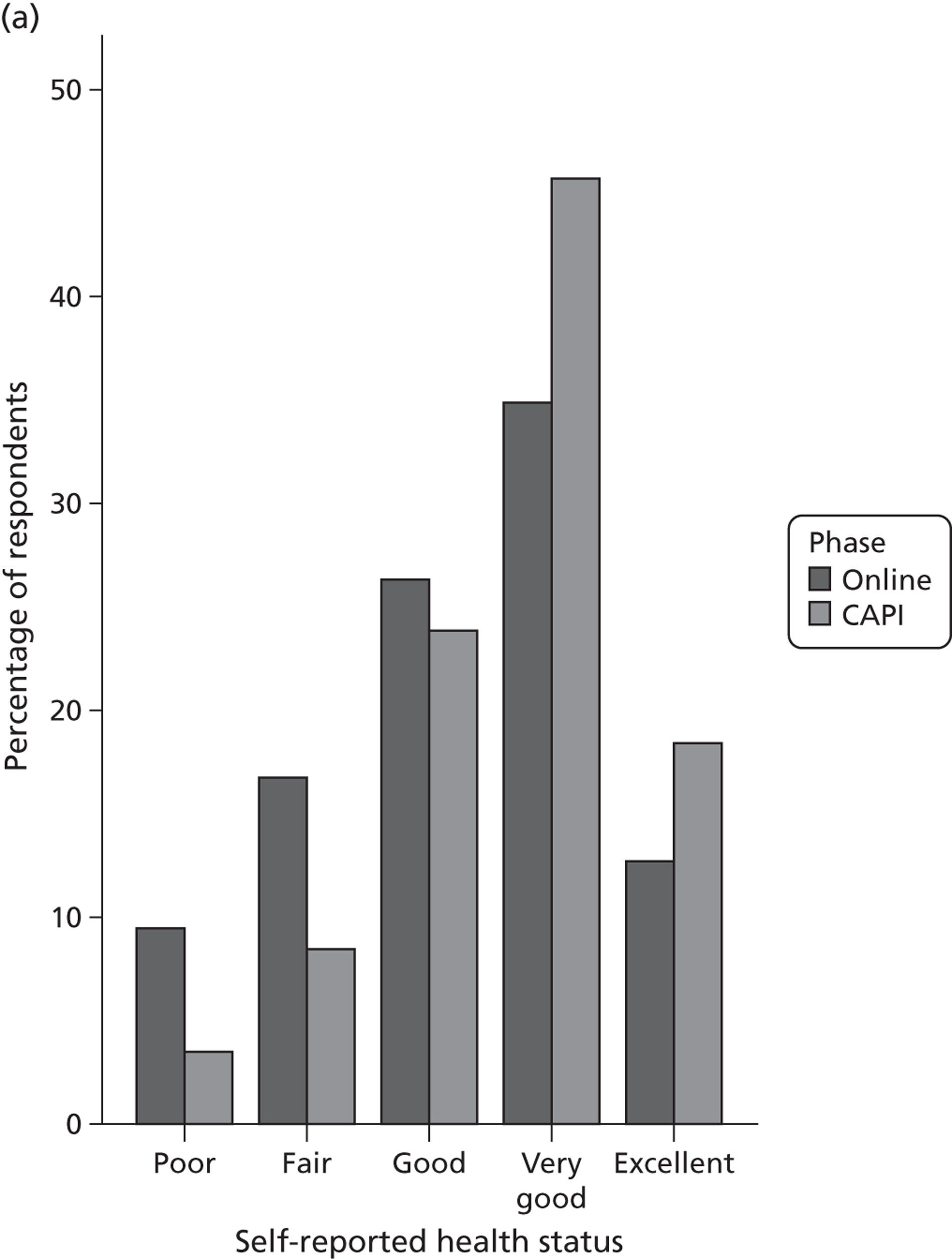
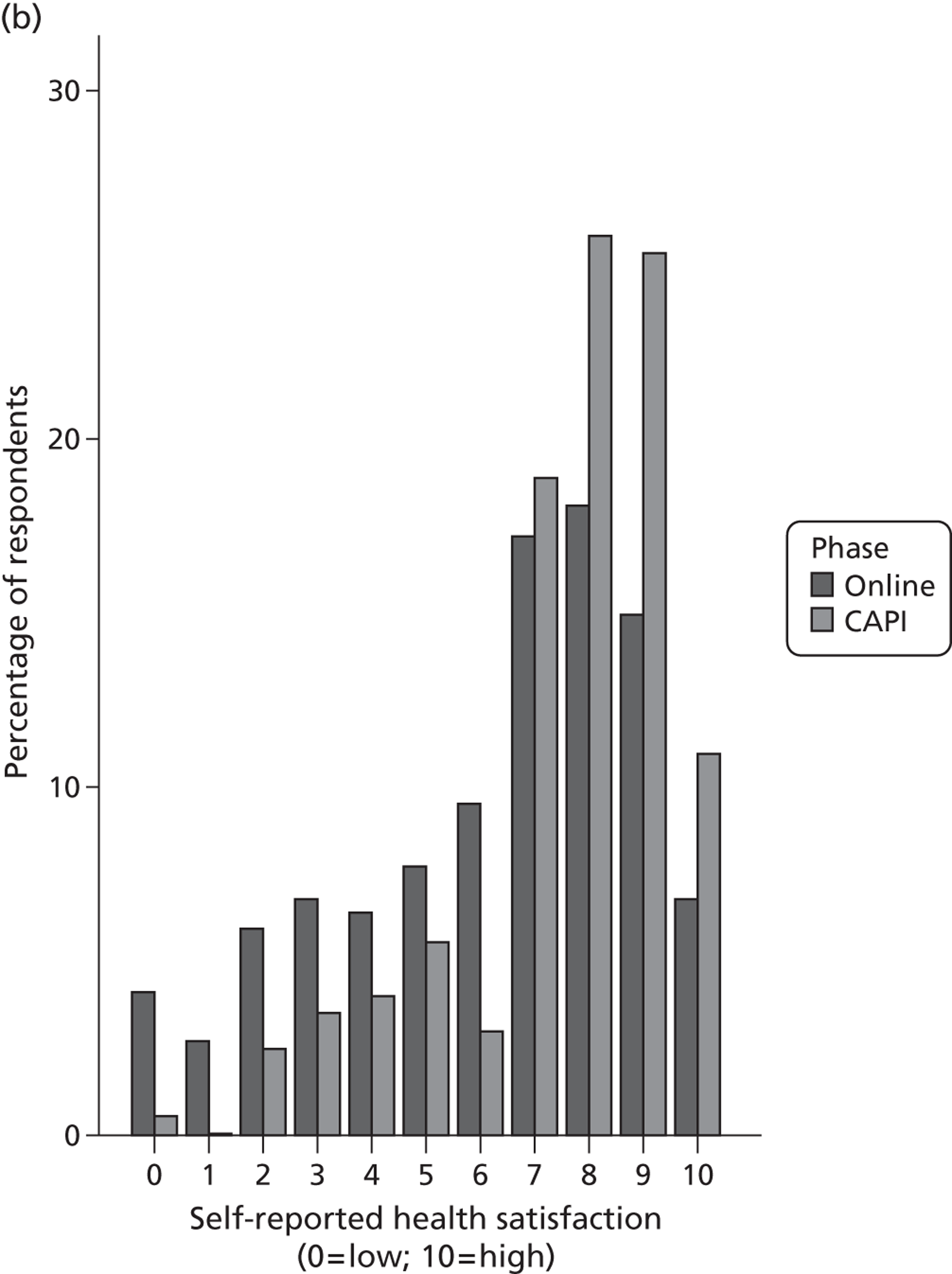
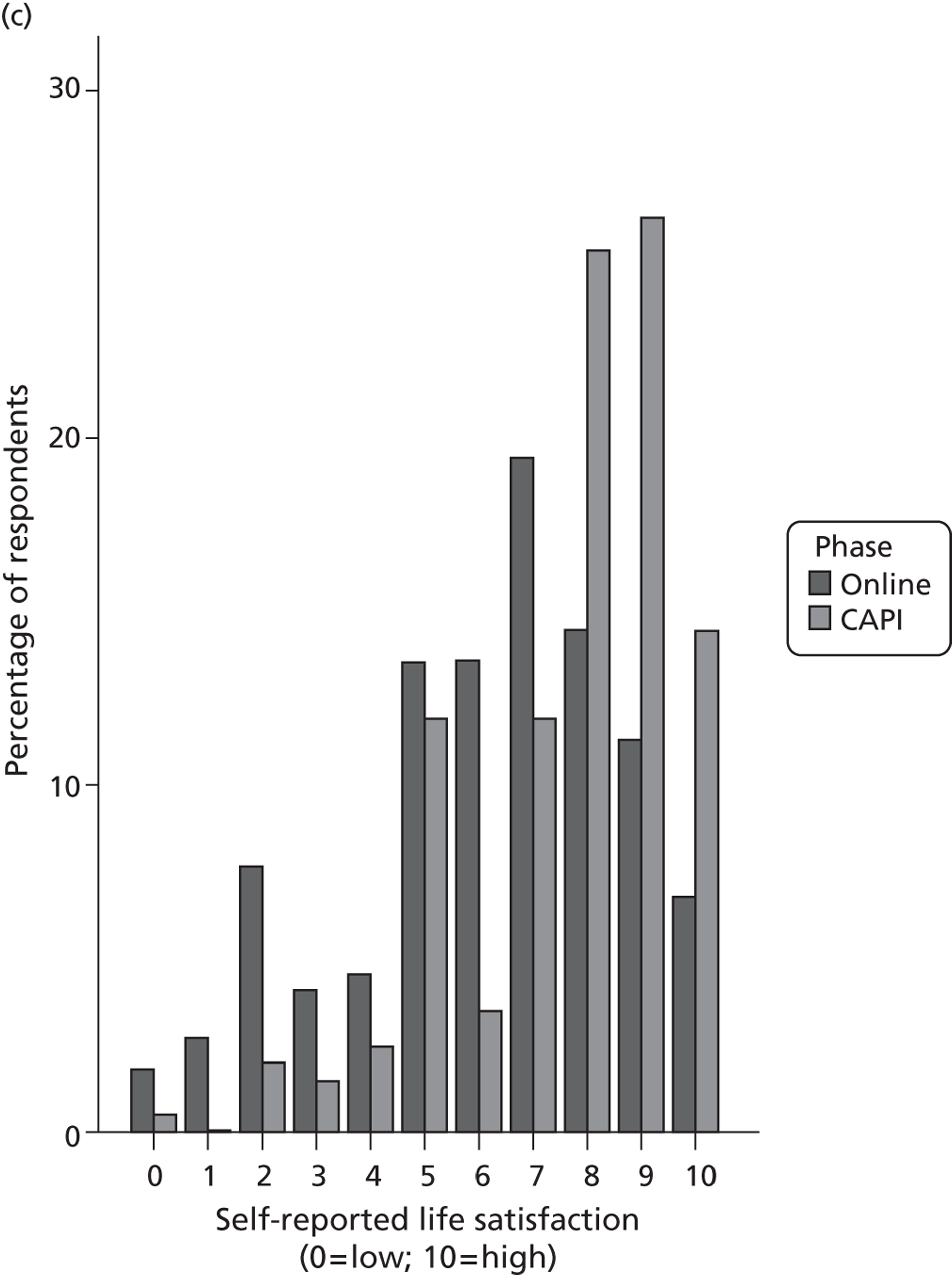
Comparison of responses to the binary choice valuation questions
The proportion of the sample choosing scenario B (which equated to choosing the shorter duration in full health; choosing immediate death or choosing the EQ-5D-5L health state and associated duration appearing as health scenario B in the DCETTO task) was not significantly different across the administration modes for any of the seven binary choice question types included in the study. This indicates that choices were consistent irrespective of the experimental attributes varied across the question types ( Table 34 ).
Type | Online (%) | CAPI (%) | p-value |
---|---|---|---|
I | 67.0 | 68.2 | 0.79 |
54.8 | 58.7 | 0.41 | |
81.9 | 81.6 | 0.94 | |
98.2 | 98.5 | 0.80 | |
91.9 | 91.5 | 0.91 | |
II | 92.8 | 94.0 | 0.60 |
III | 71.0 | 75.6 | 0.29 |
IV | 81.9 | 83.1 | 0.75 |
V | 56.6 | 60.7 | 0.39 |
VI | 65.6 | 64.6 | 0.84 |
VII | 49.1 | 49.8 | 0.91 |
77.8 | 76.6 | 0.82 |
This overall result was robust to different explorations by completion time. At the individual module level, there was no significant difference between those taking less than the median time. At the whole survey level, the results were not affected by varying the minimum time cut-off thresholds (detailed results available on request).
Probit regressions for each question reveal that a range of demographic and experimental attribute variables significantly predicts the likelihood of choosing scenario B for a number of the binary choice questions. However, the mode of administration, time taken to complete the questions, or the interaction between mode and completion time do not predict responses for any of the question types ( Table 35 ). For type I questions, response choice was significantly predicted by the health state and duration used in the question, where scenario B was more likely to be selected for the more severe health states or larger duration values. Question types II–VI include one health state and associated duration, so these results cannot be tested across these question types. For type II questions, females are 4% more likely and those with higher levels of SWBL are 1% more likely to choose to live in full health. For type IV questions, females are 8% more likely to choose scenario B, and for type V males are 10% more likely, and respondents who are retired are 19% more likely, to choose scenario B. Response to type VII questions is predicted by education level and SWBL but these results are difficult to interpret, as DCETTO questions include two whole EQ-5D-5L health states with associated duration. Response to question types III and VI was not predicted by any of the variables.
Variable | Question type | |||||||
---|---|---|---|---|---|---|---|---|
Type I | Type II | Type III | Type IV | Type V | Type VI | Type VIIa | Type VIIb | |
Health state | 0.09* | – | – | – | – | – | – | – |
V value | 0.05* | – | – | – | – | – | – | – |
Duration | 0.03* | – | – | – | – | – | – | – |
Administration mode | NS | NS | NS | NS | NS | NS | NS | NS |
Gender | NS | 0.04** | NS | 0.08* | −0.10* | NS | NS | NS |
Age | −0.01** | NS | NS | NS | NS | NS | NS | NS |
Education level | 0.03** | NS | NS | NS | NS | NS | NS | −0.10** |
Health status | NS | NS | NS | NS | NS | NS | NS | NS |
SWBH | 0.02* | NS | NS | NS | NS | NS | NS | NS |
SWBL | 0.01* | 0.01* | NS | NS | NS | NS | −0.03** | NS |
Employment level | ||||||||
Employed | NS | NS | NS | NS | NS | NS | NS | NS |
Retired | NS | NS | NS | NS | 0.19* | NS | NS | NS |
n | 2105 | 422 | 422 | 422 | 422 | 422 | 309 | 309 |
LR chi-squared | 348.39 | 10.26 | 6.34 | 16.74 | 9.02 | 10.37 | 18.23 | 8.01 |
Pseudo R 2 | 0.16 | 0.05 | 0.01 | 0.04 | 0.02 | 0.02 | 0.02 | 0.02 |
Log-likelihood | −899.61 | −97.88 | −242.03 | −187.55 | −281.83 | −267.60 | −210.16 | −163.32 |
Discussion
This chapter reports on a study comparing the administration of identical sets of binary choice questions designed to test issues related to health-state valuation conducted in online and face-to-face environments. The results demonstrate that there is no difference between the responses to the valuation tasks across the two administration modes. Sample characteristics of groups recruited following standard procedures were also investigated, and we found some differences. However, no differences between the responses to the valuation questions were found across the modes when differences in the sample characteristics were controlled. The results are also consistent across questions with both partial and whole EQ-5D health-state descriptions indicating that information burden may not impact on responses across different administration modes.
The findings demonstrate that when a health-state valuation task design is suited to online and CAPI administration, the null hypothesis that the mode of administration does not impact on the results cannot be rejected, as similar results are generated. The results reported here were established using samples recruited following the standard procedures for CAPI (i.e. achieving a representative sample in selected postcode areas following pre-established quotas) and online (i.e. using participant panels to achieve a representative sample following pre-established quotas). This demonstrates the potential applicability of our results for consideration in the design of health-state valuation studies using binary methods such as DCETTO (see Chapter 8 for more detail). However, it is unclear how these findings relate to other preference elicitation tasks, and previous work comparing an iterative valuation technique (TTO) found differences in responses between online and face-to-face administration, and concluded that this could be due to the iterative nature of the process. 32
It may be possible to extend our findings to other valuation methods, and further work should compare the results produce for both iterative and binary choice preference elicitation techniques across different administration modes.
The two samples in our study were recruited against age and gender quotas and therefore do not differ in terms of these characteristics. However, the two samples differ significantly in some observable characteristics and this raises the issue of representativeness with the UK general population. Compared with previous census data,40 the CAPI sample are more representative in terms of educational attainment. The online sample over-represents people educated to at least degree level, and this has also been found in other studies comparing online research groups to the general population. 58
The time taken was also assessed. The CAPI sample took longer to complete the overall survey, which is likely to be due to the presence of the interviewer. The shorter completion time for the online sample suggests that some respondents may not have been fully engaged with the task, and because of this we set a minimum time of 5 minutes. The results of the study are consistent using cut-off points of > 5 minutes and across different groupings by module completion time. The full applicability of the findings is limited, however, as we are unable to assess the stability of the results using cut-offs of < 5 minutes. Respondent engagement in online studies should be investigated further by analysing responses using a wide range of cut-off points, and examining the time taken to complete each task in comparison with other administration modes.
In terms of respondent health, it is possible that the online sample is genuinely less healthy than the CAPI sample. However, it has also been established that individuals may answer face-to-face surveys in a socially desirable way, particularly when answering questions about sensitive issues such as mental health. 59 This may vary according to whether responses were public or anonymous. 60 In the CAPI sample there may be a discrepancy between actual health and reported health status because of the presence of the interviewer. This did not, however, impact on responses to the health-state valuation questions.
We were not able to assess how mode of administration impact upon the responses of those aged > 65 years, as this group was not included in the sampling frame for the study. This potentially limits the applicability of our findings, and further comparisons of valuation tasks across different modes of administration should investigate responses among those aged > 65 years. This will establish the level of equivalence of health-state valuation exercises across different modes of administration for the overall adult population.
Even with highly selective screening, the samples may differ in terms of further unobserved characteristics. The CAPI sample characteristics are influenced by who is at home when the interviewer visits, who agrees to take part, and who completes the interview. The online sample using an internet panel has impact from who has access to the internet, who is a member of the online panel, who in the panel agrees to take part, and who of those agreeing to take part completes the survey. It is not clear how the different selection mechanisms impact on unobservable sample characteristics, and therefore on responses to health-state valuation questions. Furthermore, differences in the membership of online panels and the sorts of surveys administered by the panel company may also affect responses (e.g. some companies may complete more health surveys than others). An area for future research is to investigate the consistency of response rates and actual responses provided across different panels. Typically, characteristics of non-responders to interviews are not available, and one advantage of online surveys using existing internet panels is that certain characteristics of non-responders may be accessible. This allows for further insight into issues around non-response.
In summary, the two administrations have different advantages and disadvantages, and the similarities with the general population indicate that the standard sampling frames used for face-to-face and online research studies are valid. Responses to the main experimental binary choice questions were not significantly different across the modes, and mode of administration was not a significant factor explaining the responses. Therefore, both modes produce similar data, and both can be used to administer health-state valuation surveys including binary choice valuation questions such as DCETTO. The advantages and disadvantages of both modes must be considered when designing health-state valuation studies.
Chapter 10 PRET stage 3 CAPI investigation of health-state valuation task acceptability and completion
Stage 3 CAPI study summary
This chapter has been adapted from work presented to the Health Economist’s Study Group. 61 Little is known about how both personal subjective and task specific factors impact on the health-state valuations respondents provide to valuation exercises such as TTO and DCE. Stage 3 of the PRET project aimed to investigate the validity and acceptability of binary choice versions of TTO, LT-TTO and DCETTO using face-to-face CAPI with EQ-5D-5L health states. All three of the methods used in this chapter can be used to generate utility values on the full health–dead scale, and detailed quantitative work presenting two of the tasks in an online setting (and generating a utility tariff using one of the methods) is described in Chapters 5 and 8 of this report. The processes respondents use to complete health-state valuation tasks and the influence of a range of external factors and demographics on responses were also assessed. This included an investigation of the importance of the EQ-5D-5L dimensions in the decision-making process. Research investigating these issues will add to the literature about how health-state valuation tasks are completed, and why particular preferences are given.
Influences on responses to health-state valuation tasks
Health-state valuation tasks may be difficult for respondents to complete: for TTO this may be because (although the exercise is broken down step by step) it requires the identification of the point of indifference between life A and life B by trading between length of life and quality of life; and for DCE because (although only ordinal information is required of the respondent) it involves a choice between two options for which all of the attributes included in the options may differ from task to task. It is therefore important to understand the factors that may impact on the validity of responses, including the acceptability of the techniques to members of the general public. Research comparing DCE and TTO has found that both techniques have equivalent levels of respondent comprehension and completion. 62 However, this study did not test the DCETTO method, which may be more difficult than standard DCE owing to the addition of an attribute for duration.
The strategies and processes used by respondents to complete TTO and DCETTO tasks is also an important factor to understand, as this may influence the validity of responses, and therefore may inform the design of valuation studies. Robinson and colleagues63 found that respondents in a TTO study may use a ‘threshold of tolerability’ to establish whether a state is severe enough for them to trade any time. In qualitative work, it has been found that respondents to a DCE study introduced additional information and assumptions to help them answer the questions. 64 It has also been found that respondents may focus on key attributes, and may not attend to all attributes, both because the attribute is not relevant to the individual, and also to simplify the task. 65
The subjective importance to respondents of the actual health dimensions included in the hypothetical scenarios is also of interest, as this can cast light on the descriptive systems used. Values for both generic and condition-specific preference-based measures are mostly derived from the general population, and different descriptions of health dimensions across instruments differ in their level of importance to respondents. For example, a key health dimension may carry more weight, and it is important to understand the qualitative hierarchy of the importance of dimensions to respondents. Quantitative information about the importance of dimensions (and levels) is available from the regression analyses, but the dimensions with the most subjective importance may or may not be the same as those dimensions with the largest regression coefficients. Quantitative information about the importance of EQ-5D-5L dimensions is not currently available (as the valuation study has not yet been carried out). Furthermore, little is known about the qualitative importance of the EQ-5D dimensions and associated response levels, and how this might have an impact on health-state preferences.
External respondent related factors and background characteristics may also impact the results of health-state valuation studies. Dolan and Roberts66 found that age, gender and marital status influenced responses to TTO tasks, and respondents’ own health experiences have been found to impact on choices made in both TTO and DCE studies. 64,67 It has also been established that respondents who find valuation tasks complex are less likely to be educated to college level. 68
Iterative TTO and LT-TTO procedures can be conceptualised as multiple binary choice tasks following a similar format used to represent DCETTO scenarios (see Chapter 3 of this report). This means that the iterative task process can be simplified44 and direct comparisons with DCETTO can be carried out. Furthermore, the binary choice tasks are amenable to completion using a variety of media including CAPI and online, which produce similar results for binary choice questions (see Chapter 4 of this report). However, note that as individuals do not report their point of indifference, there is a fundamental shift in the focus of the analysis, from determining a mean over individual cardinal preference to modelling the cardinal preferences of groups using methods that do not rely on individual level cardinal data.
Methods
Valuation question format
In this study, question types VII and VIII were investigated alongside a new binary choice question (type IX) which was designed to represent TTO (see Figure 21 for the question format). DCETTO (type VII used in PRET and PRET-AS) presents an EQ-5D health state with an associated level for duration for both scenarios A and B (therefore 12 pieces of information in each question):
-
[Scenario A]: You live in state H A for duration T A then die.
-
[Scenario B]: You live in state H B for duration T B then die.
In binary choice LT-TTO (type VIII matched with those used in the PRET-AS survey), scenario A presents full health for a certain duration followed by an EQ-5D-5L health state for a certain duration, and scenario B presents full health for a specified duration (meaning 10 pieces of information in each question):
-
[Scenario A]: You will live in full health for L followed by state H for duration T then die.
-
[Scenario B]: You will live in full health for (L + VT) then die (V < 1.0).
Question type IX is based on TTO and takes the following form:
-
[Scenario A]: You live in state H for T years then die.
-
[Scenario B]: You live in full health for VT years then die (V < 1.0).
Here, scenario A includes an EQ-5D-5L health state with an associated duration level and scenario B presents full health for a shorter duration (therefore eight pieces of health state and duration information that are included in each question).
The format of the three types of binary choice questions used in this study is displayed below (see Figure 18 ).
Three tasks of each of the question types were set ( Table 36 ). For types VIII (LT-TTO) and XI (TTO), three EQ-5D-5L states – defined as mild, moderate and severe – were selected, and the same states were used across both question types. A duration level was selected to go with each state, and the full health duration was varied in accordance with the selected health-state duration level. For type VII (DCETTO), the same three states were presented as scenario A, with a state of similar severity presented as scenario B. Duration was fixed across the first of the three scenario pairs, but varied for the second and third pairs. Members of the research team selected the states and durations to provide a difficult choice for respondents that would enable us to investigate the strategies and processes used to answer the questions in more depth than if the choice was easier to make.
Question type | Scenario A | Percentage choosing A | Scenario B | Percentage choosing B | ||||
---|---|---|---|---|---|---|---|---|
EQ-5D-5L Health state | Lead time in full health | Duration, years | Health state | Duration, years | ||||
Type XI (TTO) | Example 1 | 12332 | n/a | 10 | 72.8 | Full health | 7.25 | 27.2 |
Example 2 | 34243 | n/a | 5 | 63.4 | Full health | 2.5 | 36.6 | |
Example 3 | 43554 | n/a | 1 | 69.8 | Full health | 10 weeks | 30.2 | |
Type VIII (LT-TTO) | Example 1 | 12332 | 10 | 10 | 44.6 | Full health | 17.25 | 55.4 |
Example 2 | 34243 | 10 | 5 | 25.5 | Full health | 12 | 74.5 | |
Example 3 | 43554 | 2 | 1 | 35.3 | Full health | 1.5 | 64.7 | |
Type VII (DCETTO) | Example 1 | 12332 | n/a | 10 | 50.5 | 21323 | 10 | 49.5 |
Example 2 | 34243 | n/a | 5 | 72.3 | 43344 | 10 | 27.7 | |
Example 3 | 43554 | n/a | 1 | 73.8 | 55355 | 5 | 26.2 |
Follow-up question format
After completing three tasks of a given question type, three kinds of follow-up probing questions were used to investigate the issues related to question acceptability and task completion (see Appendix 4 ). The first kind of the probing questions took the format of tick boxes, with a free text question available to allow for further issues to be raised by respondents if they wished. The tick box questions were developed through a series of pilot studies with a convenience sample of academic and non-academic university employees. The questions were conceptualised across four categories: task completion process and acceptability; potential difficulties answering the questions; importance of EQ-5D-5L dimensions; and external influences on response. The second kind of follow-up questions was sets of five type-specific follow-up questions, and these appeared after each type of binary choice questions. The third set of general follow-up questions was included to assess issues across types of valuation task.
Study design
To administer the health-state valuation and follow-up questions, CAPI interviews were used. Each respondent completed two of the three types of binary choice questions and associated type-specific follow-up questions. This was followed by the general feedback questions relating to both valuation methods. Each valuation task was presented as both the first and second of the two completed by respondents, and therefore there were six versions of the survey overall. Respondents also completed the same demographic and self-reported health questions that were included in the online surveys, with the addition of a question asking about whether they had children or dependants aged > 18 years. Following completion of the interview, interviewers completed three questions about the respondent’s understanding of the task, their level of concentration, and the environment in which the interview was conducted.
Recruitment and survey completion
The recruitment and survey completion process followed the same procedure as the CAPI study carried out at stage 2 of the project. Those who participated were given a £5 gift voucher as an incentive.
Analysis
Descriptive statistics including frequency and cross tab analyses were used to assess the results to the follow-up questions. Significance testing between demographic groups was carried out using chi-squared tests.
Results
Sample demographics, response and interview information
Interviewers visited 1783 houses to achieve 306 interviews (a response rate of 17.2%). Of those who did not participate, 789 (44.3%) were not at home or unavailable, 333 (18.6%) refused, and 355 (19.8%) were out of scope (i.e. if no one in the house fitted the age and gender quota groups that were still to be completed). The response rate for eligible contacts was 48%. Table 37 presents demographic information and data relating to the interview environment. Overall, the sample was generally representative of the UK general population and the majority self-reported good health and high levels of SWBH and SWBL. The majority of respondents displayed a good understanding of the task and concentrated on the questions. The majority of the interviews were conducted in a quiet environment with no distraction from other activities in the household.
Demographic | n (%) |
---|---|
Version no. | |
1 (type VII/type VIII) | 53 (17.3) |
2 (type VIII/type VII) | 51 (16.7) |
3 (type VII/type IX) | 50 (16.3) |
4 (type IX/type VII) | 52 (17.0) |
5 (type VIII/type IX) | 50 (16.3) |
6 (type IX/type VIII) | 50 (16.3) |
Male | 152 (49.7) |
Age, mean (SD) | 46.46 (17.88) |
Age range, years | |
18–24 | 47 (15.4) |
25–34 | 50 (16.3) |
35–44 | 56 (18.3) |
45–54 | 54 (17.6) |
55–64 | 42 (13.7) |
65+ | 57 (18.6) |
Marital status | |
Married/partner | 193 (63.1) |
Other | 113 (36.9) |
Employment status | |
Employed or self-employed | 168 (54.9) |
Student | 8 (2.6) |
Not working | 130 (42.5) |
Children aged < 18 years | 116 (37.9) |
Dependents aged > 18 years | 18 (5.9) |
Education | |
Beyond minimum age | 159 (52.0) |
Degree level | 66 (21.6) |
Self-reported health | |
EQ-5D | |
Index score, mean (SD) | 0.821 (0.29) |
In best health state (11111) | 145 (47.4) |
Health status | |
Good health | 268 (87.6) |
Poor health | 38 (12.4) |
Satisfied with health | |
Yes (6–10) | 254 (83.0) |
No (0–5) | 52 (17.0) |
Satisfied with life | |
Yes (6–10) | 265 (86.6) |
No (0–5) | 41 (13.4) |
Interviewer information | |
Understanding of task | |
Good | 241 (79.3) |
Moderate | 61 (20.1) |
Completion of task | |
Concentrated very hard | 232 (76.3) |
Concentrated fairly hard | 72 (23.7) |
Interview environment | |
Quiet with no distraction | 244 (80.3) |
Some background distraction | 47 (15.5) |
Disruptions and interruptions | 13 (4.3) |
Task comparison and acceptability
Overall, 52.3% of respondents reported that both of the question types that they completed were of equal difficulty. Of those who indicated a different level of difficulty across the questions, type VIII was perceived as the easiest followed by types XI and VII. Furthermore, 36% of those who completed both types VIII and VII, and 47% of those who completed both types IX and VII stated that the TTO (i.e. types VIII and IX) binary choice questions were easier to complete. This indicates that the binary choice conceptualisation of both TTO tasks may be more acceptable to respondents than DCETTO questions.
The majority of the sample (71.2%) reported that the layout of the questions meant that they could be answered easily. However, across all three question types, over half of the sample reported that they sometimes or always found it difficult to complete the task, with the most difficulties being reported by those who completed question type XI (TTO) first ( Figure 15 ). The difference in reported levels of difficulty between the groups is significant (p < 0.01). Of the overall group, 17% of respondents reported that DCETTO questions encouraged them to think about external influences the most when responding, and this is higher than the TTO (9%) and LT-TTO (10%) questions. However, the majority (64%) reported that the questions were equivalent in this regard.
FIGURE 15.
Proportions of the sample reporting difficulty completing the task.
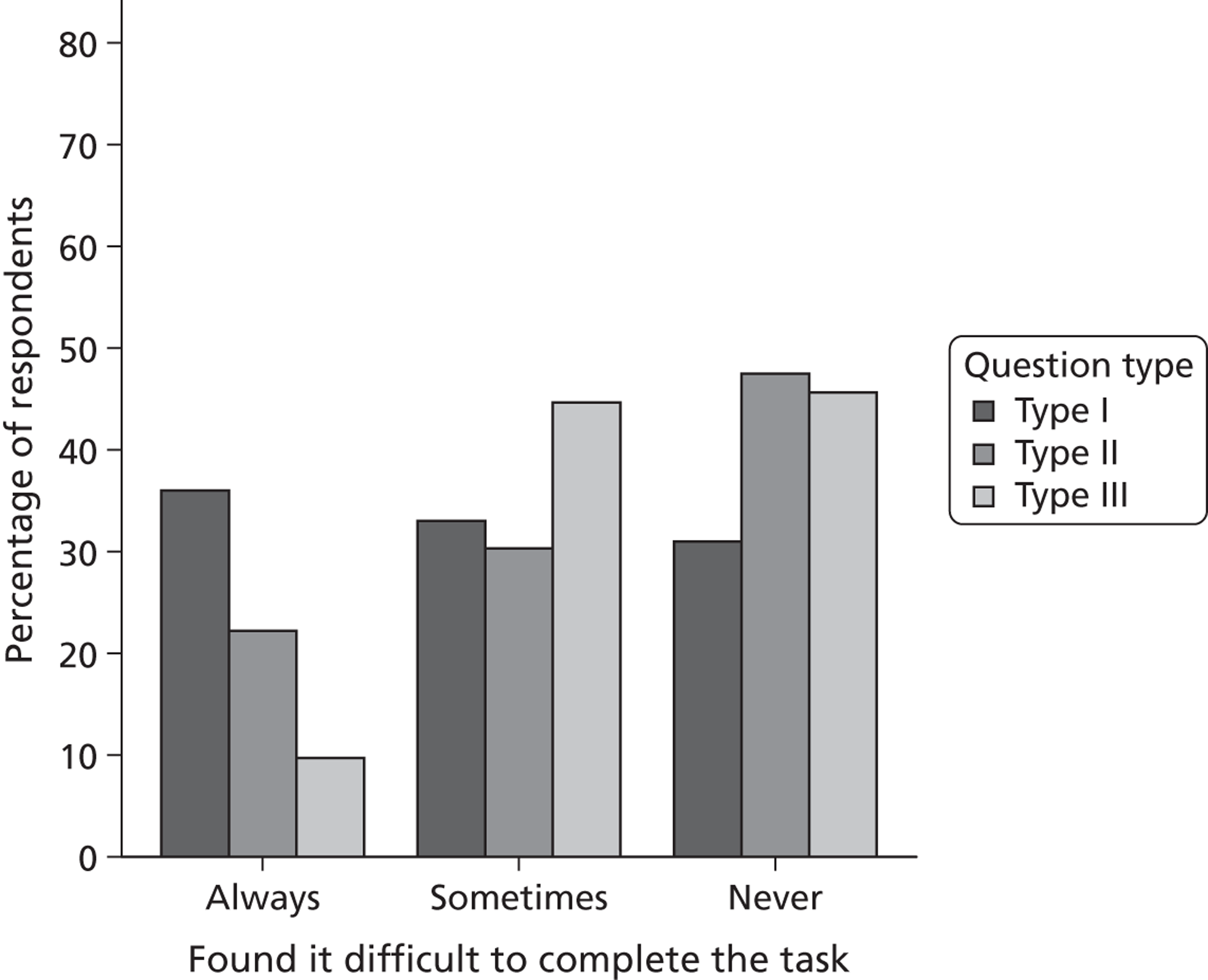
Attention to attributes
Overall, 43% of those completing question type XI, 33% of those completing type VIII and 24% of those completing type VII indicated that they always completed the task by only considering the most important attribute, and the difference in response between the tasks across the questions is not significant (p = 0.07). The majority of the sample agreed that they only consider the attributes that are subjectively important to them when completing the tasks, and this was generally consistent irrespective of which question the respondent found the easiest ( Figure 16 ). However, 35% of those who complete question type VII (DCETTO) indicated that they did not only consider the most important attribute, indicating that they are assessing a number of attributes when choosing between the options.
FIGURE 16.
Attendance to attributes across the question types. (a) Proportions of respondents who consider only the most important attribute; (b) proportions of respondents who do not consider all statements, only those that are subjectively important.
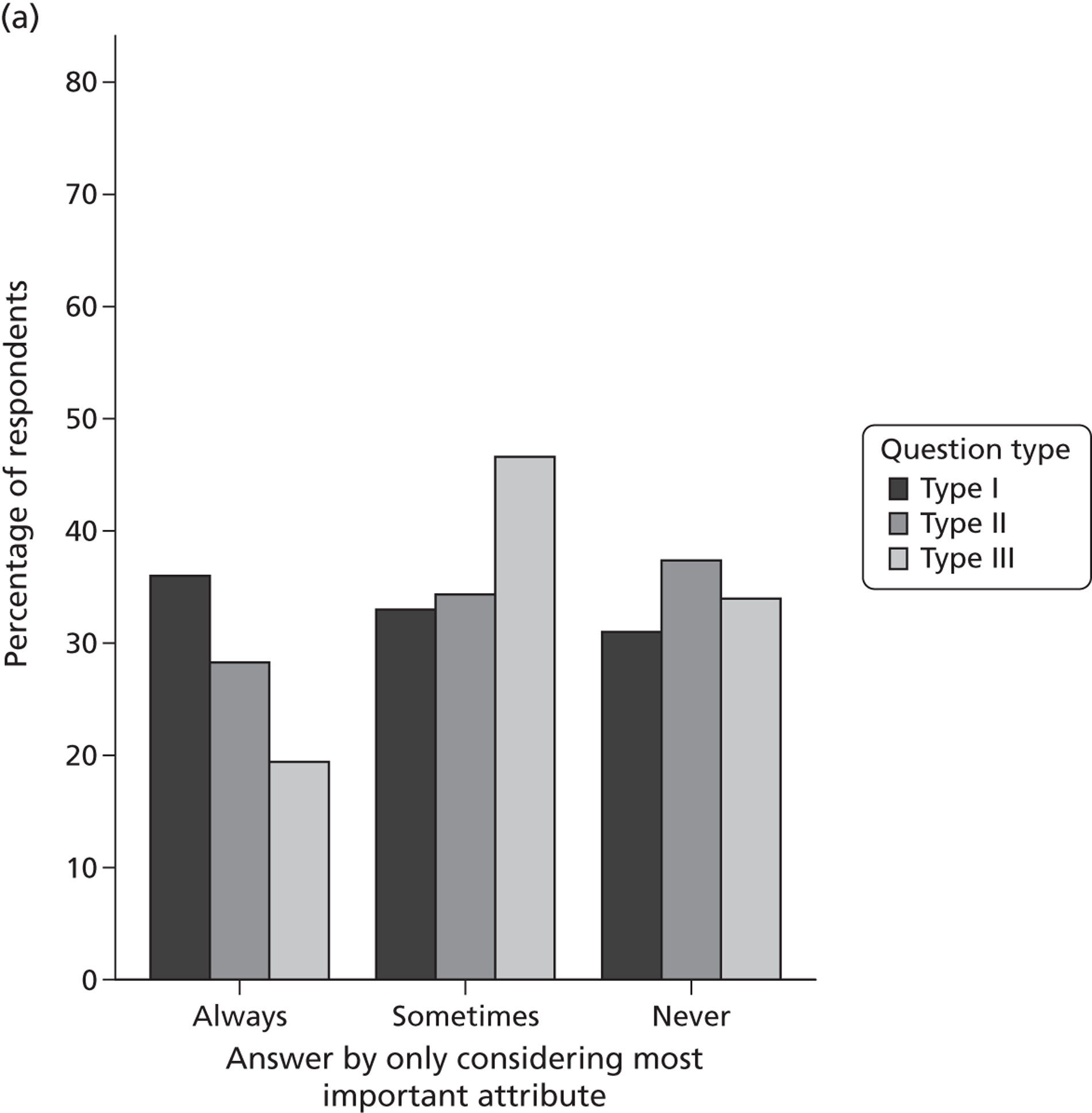
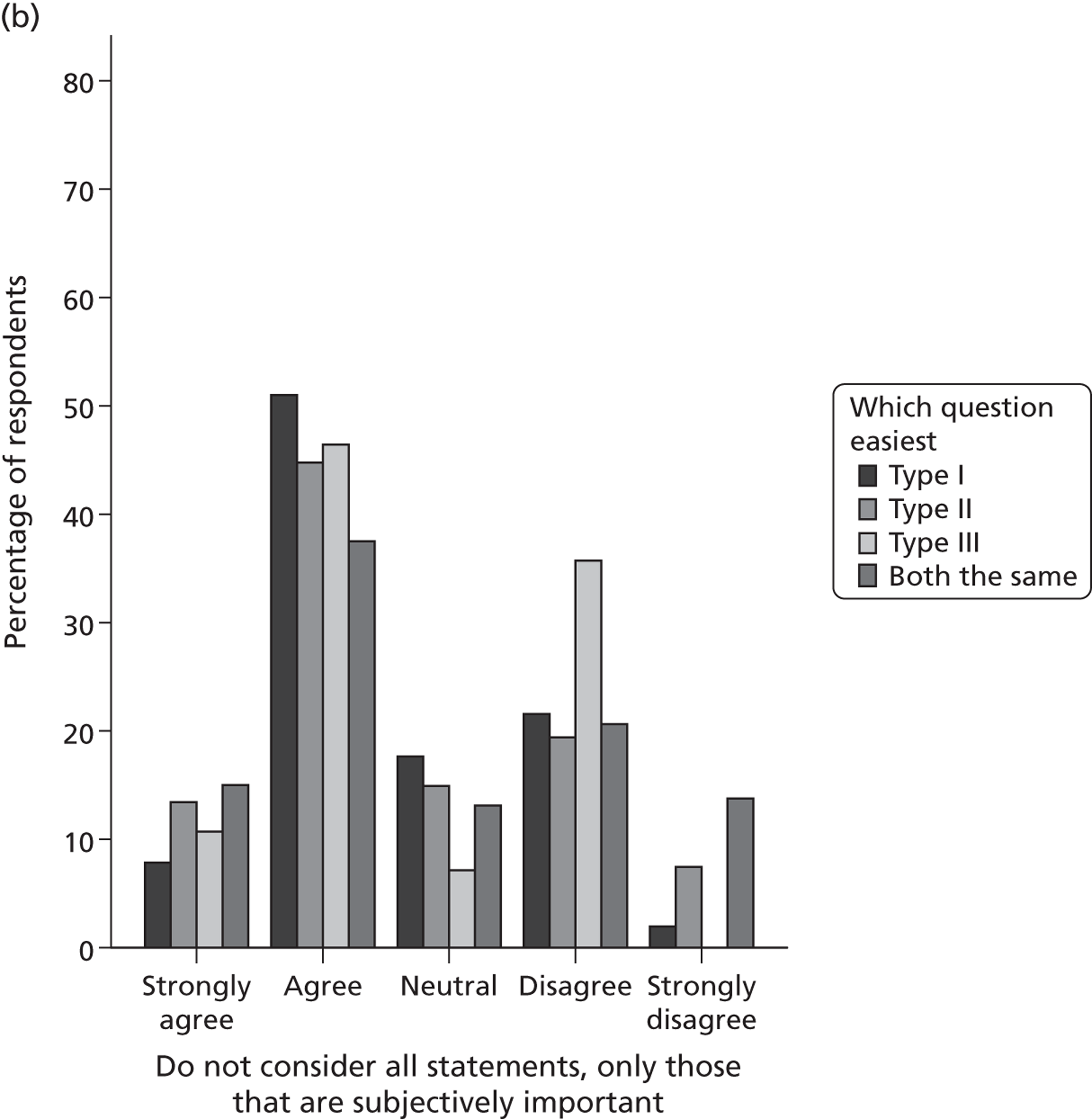
Importance of individual task attributes
Respondents were asked to indicate which single dimension included in the valuation task (i.e. EQ-5D dimensions and duration) was most important in the decision-making process ( Table 38 ). In types I and II, the duration spent in full health was consistently ranked as the most important attribute, and this was followed by the duration in the health state. When all task attributes were included, the EQ-5D dimension with the highest number of respondents, indicating that it was the most important in the decision-making process, was mobility. This was consistent across the question types.
Dimension | TTO (type XI) | LT-TTO (type VIII) | DCETTO (type VII) | |||
---|---|---|---|---|---|---|
n (%) | Rank | n (%) | Rank | n (%) | Rank | |
Duration | 44 (21.8) | 2 | 41 (26.6) | 2 | 77 (37.4) | 1 |
Duration in full health | 59 (29.2) | 1 | 44 (28.6) | 1 | NA | NA |
Mobility | 28 (13.9) | 3 | 15 (9.7) | 3 | 31 (15.0) | 2 |
Self-care | 28 (13.9) | 3 | 9 (5.8) | 6 | 26 (12.6) | 4 |
Usual activities | 16 (7.9) | 6 | 14 (9.1) | 4 | 17 (8.3) | 6 |
Pain/discomfort | 20 (9.9) | 5 | 21 (6.9) | 5 | 24 (11.7) | 5 |
Anxiety/depression | 5 (2.5) | 7 | 8 (2.6) | 7 | 31 (15.0) | 2 |
Importance of EQ-5D-5L dimensions
Respondents were asked to rank all five EQ-5D-5L dimensions (excluding the duration attributes) in order of importance in the decision-making process, and the results overall are displayed in Figure 17 . Mobility was ranked as most important by the largest number of respondents (29.4%), with Pain/discomfort ranked as the most important dimension by 24.5%. Anxiety/depression was ranked as the least important dimension in the decision-making process by the highest frequency of respondents (40.5%). The highest frequency across each of the rankings corresponds with the order the dimensions appear in the classification system. When the results were assessed by question type, a similar pattern was established: mobility was ranked as the most important dimension across all question types, with Anxiety/depression ranked as the least important. A large proportion of the sample reported that they were able to tell the difference between the EQ-5D-5L dimension response levels slight/moderate, moderate/severe and severe/extreme but 9.2% reported that they could never tell the difference between severe and extreme, and 20.5% reported that they could not tell the difference between severe and extreme in some situations.
FIGURE 17.
Importance of EQ-5D-5L dimensions overall (all question types pooled).
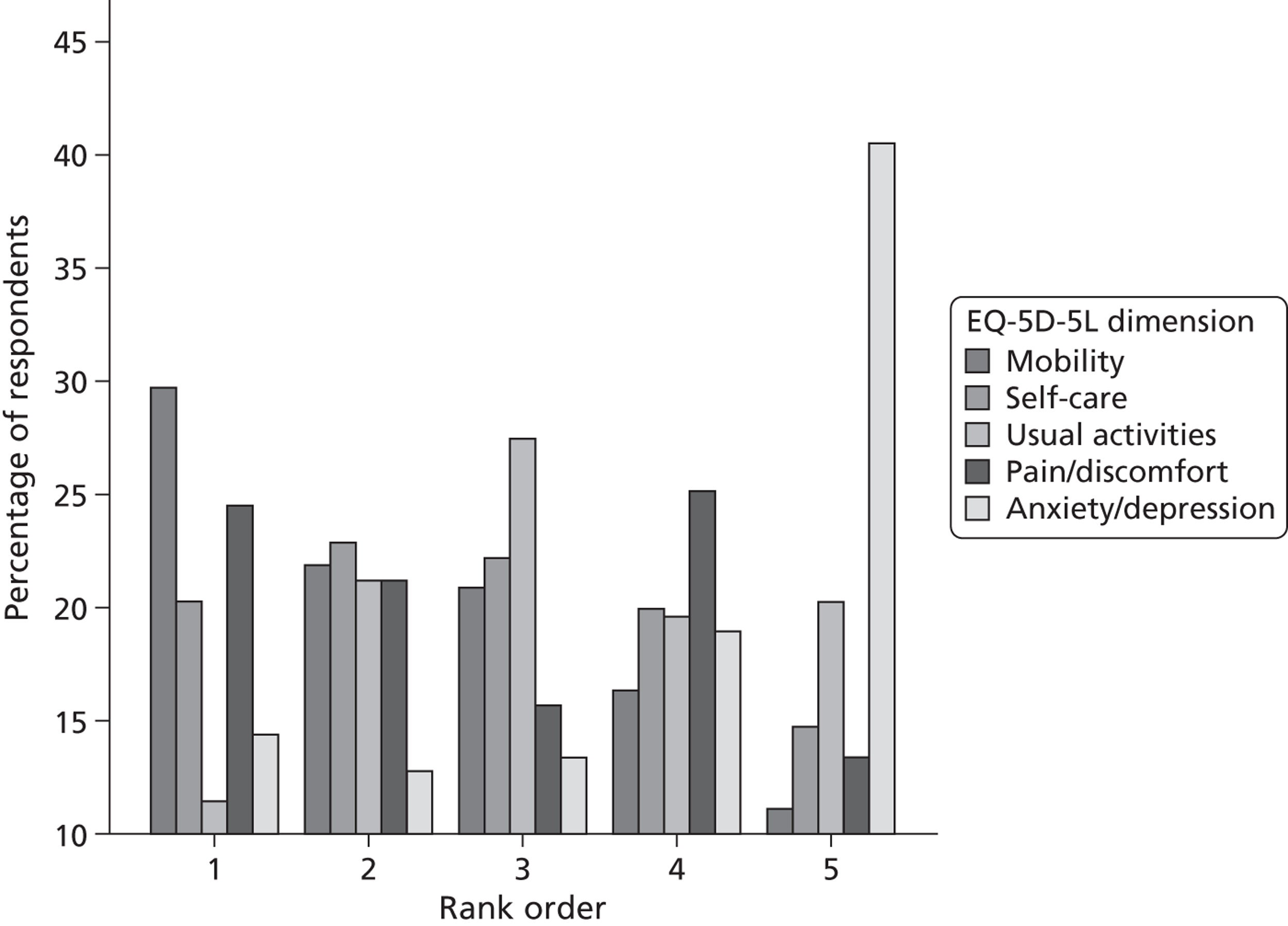
Influence of personal and subjective factors and background characteristics on response
Overall, 269 (87.9%) respondents reported that they imagined themselves living in the health state. However, 30.7% reported that their own health experiences influenced their response, 26.1% reported that other people’s experiences influenced their response, 31.4% reported that both groups influenced response and 11.8% reported that neither group influenced response. Of the 269 respondents reporting that they imagined themselves in the health states, 90 (33.4%) reported that their own health influenced their response, 66 (24.5%) reported that their response was influenced by other people with poor health, 79 (29.4%) said both and 34 (12.6%) said neither of these groups ( Figure 18 ). Table 39 reports the influence of a range of other personal factors.
FIGURE 18.
Impact of personal factors on response. (a) Proportions of respondents who imagined themselves or somebody else, or both or neither of these, living in the health state; and (b) proportions of respondents reporting that their responses were affected by their own health experiences, or those of other people they know who have had poor health.
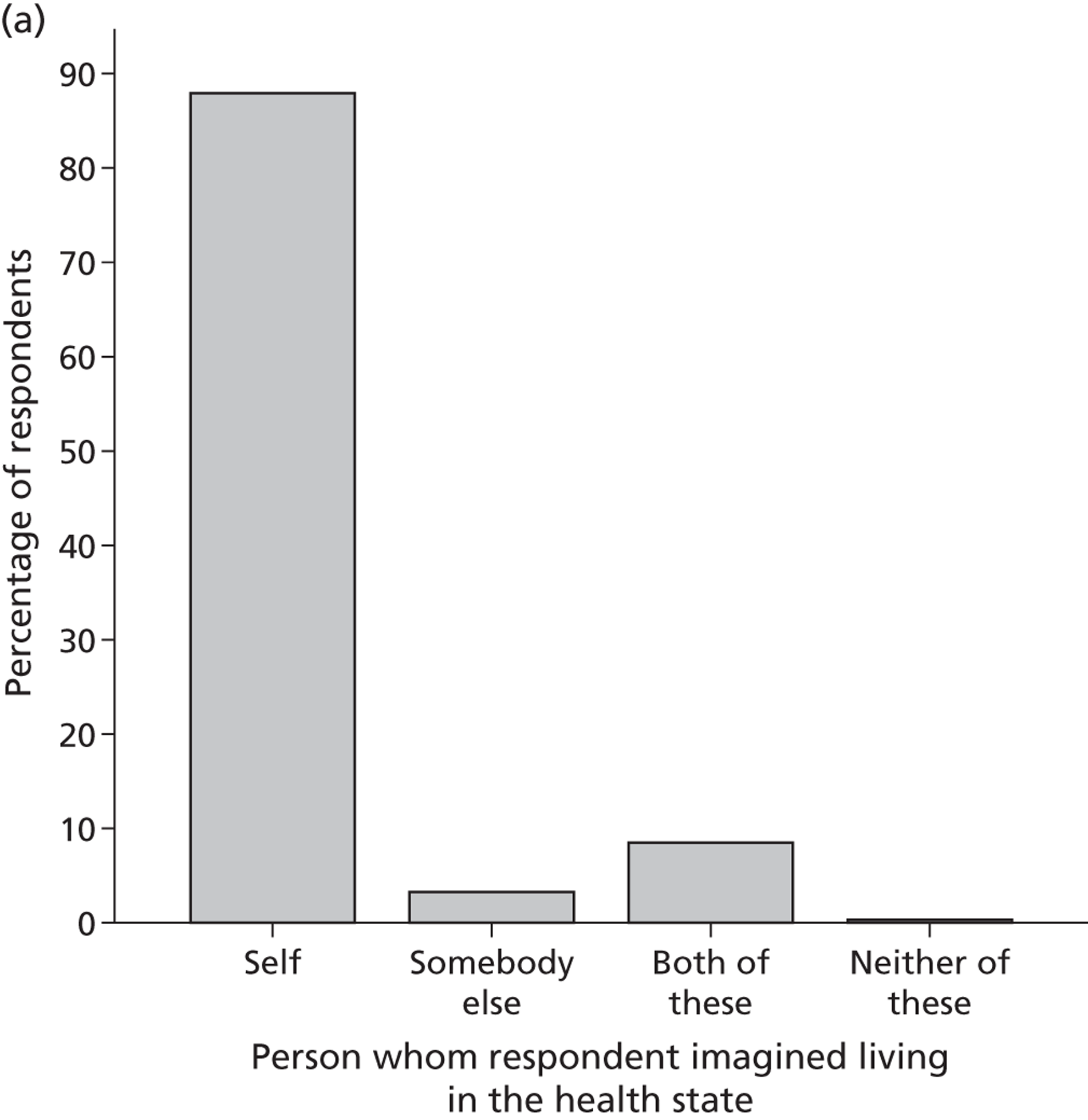
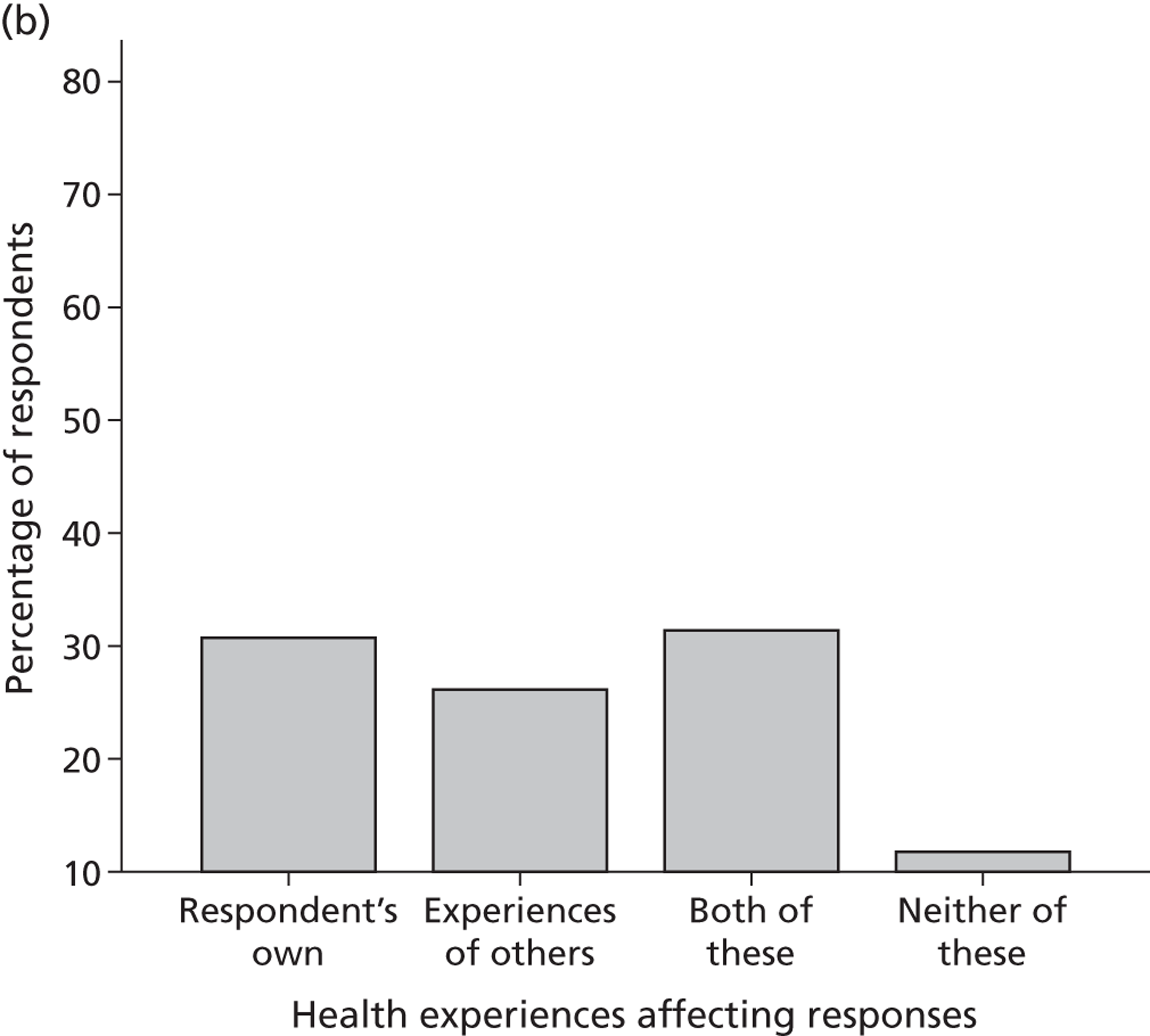
Personal factor | Response (n, %) | ||||
---|---|---|---|---|---|
Always | Often | Sometimes | Rarely | Never | |
Impact of feelings about health and life | 134 (43.8) | 106 (34.6) | 54 (17.6) | 4 (1.3) | 8 (2.6) |
Impact of health state on life and financial situation | 72 (23.5) | 75 (24.5) | 81 (26.5) | 27 (8.8) | 51 (16.7) |
Choose longer duration in order to spend more time with others | 59 (19.3) | 54 (17.6) | 71 (23.2) | 34 (11.1) | 88 (28.8) |
Overall, 78% of the sample indicated that they always or often considered how the health state would impact on their feeling about their health and life, 48% report that they would always or often consider the impact of the health state on their life and financial situation. Furthermore, 36% of the sample indicated that they would always or often choose a longer duration to spend time with others but 40% report that they rarely or never did this.
Figure 19 displays the impact of considerations about other people on response across a selection of background characteristics. Overall, 85.3% of the sample reported that their responses were influenced by considerations about how the health state would affect other people close to them either ‘sometimes’ or more often. Respondents who were married or with partner were significantly more likely to indicate that their answers were influenced by how the health state would affect those around them (p < 0.01). There is no overall difference in response for those with or without children aged < 18 years (p = 0.18) or those with dependants aged > 18 years (p = 0.58).
FIGURE 19.
Personal factors: impact on others. This shows the likelihood of respondents’ answers being influenced by how the health state would affect those around them, depending on whether they (a) are married/with a partner; (b) have children aged < 18 years; and (c) have dependants aged > 18 years (e.g. respondents who are carers).
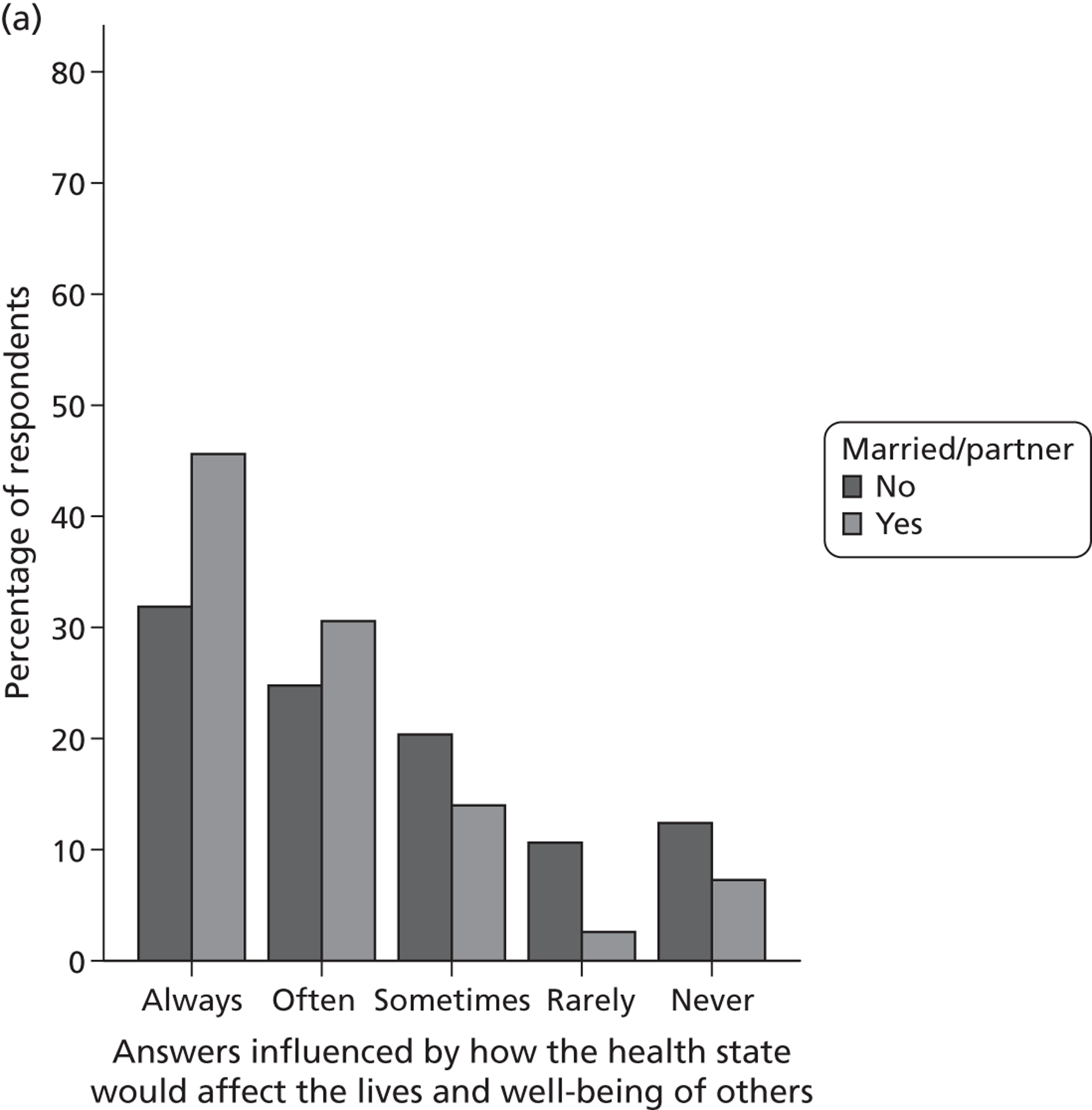
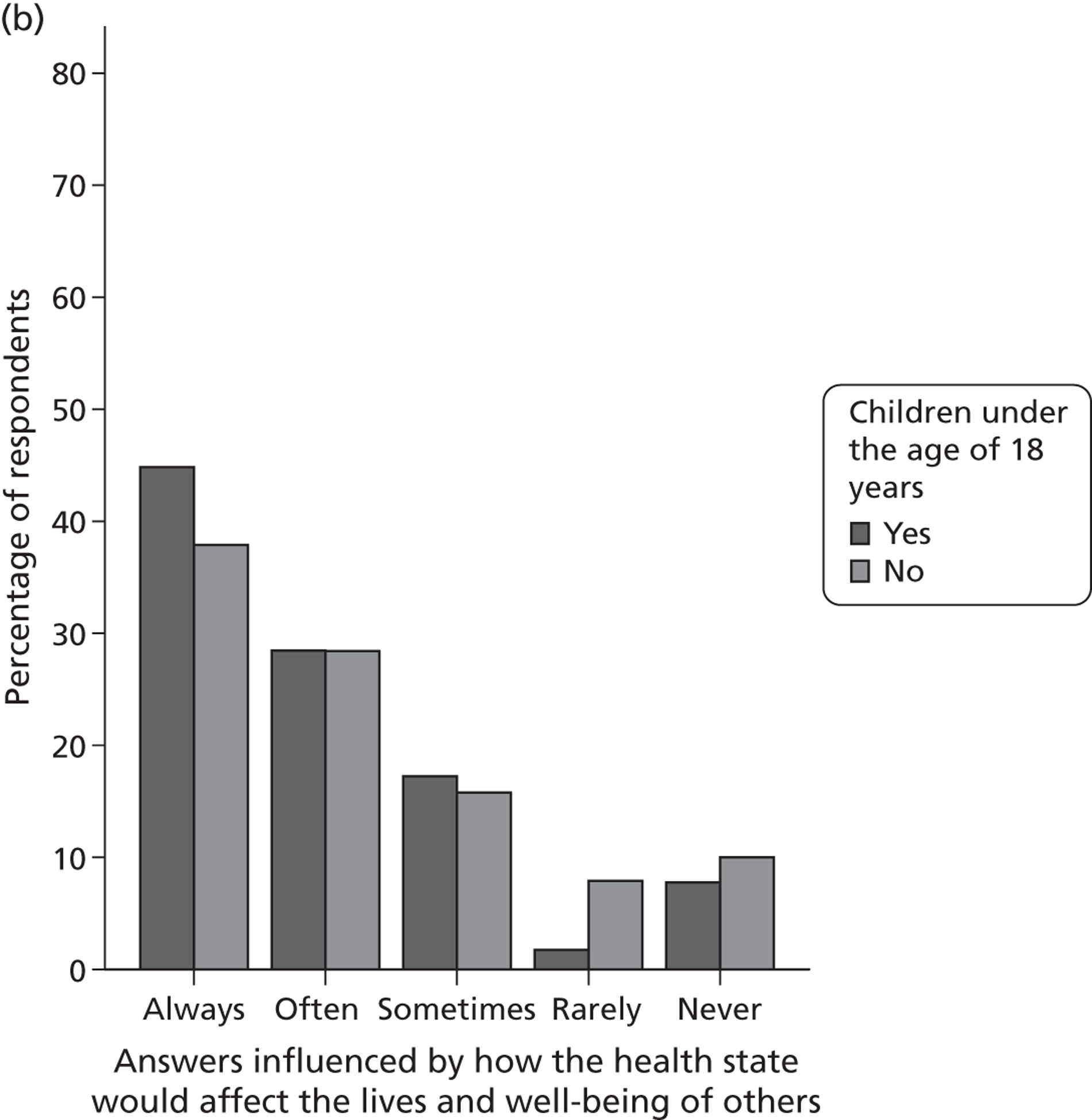
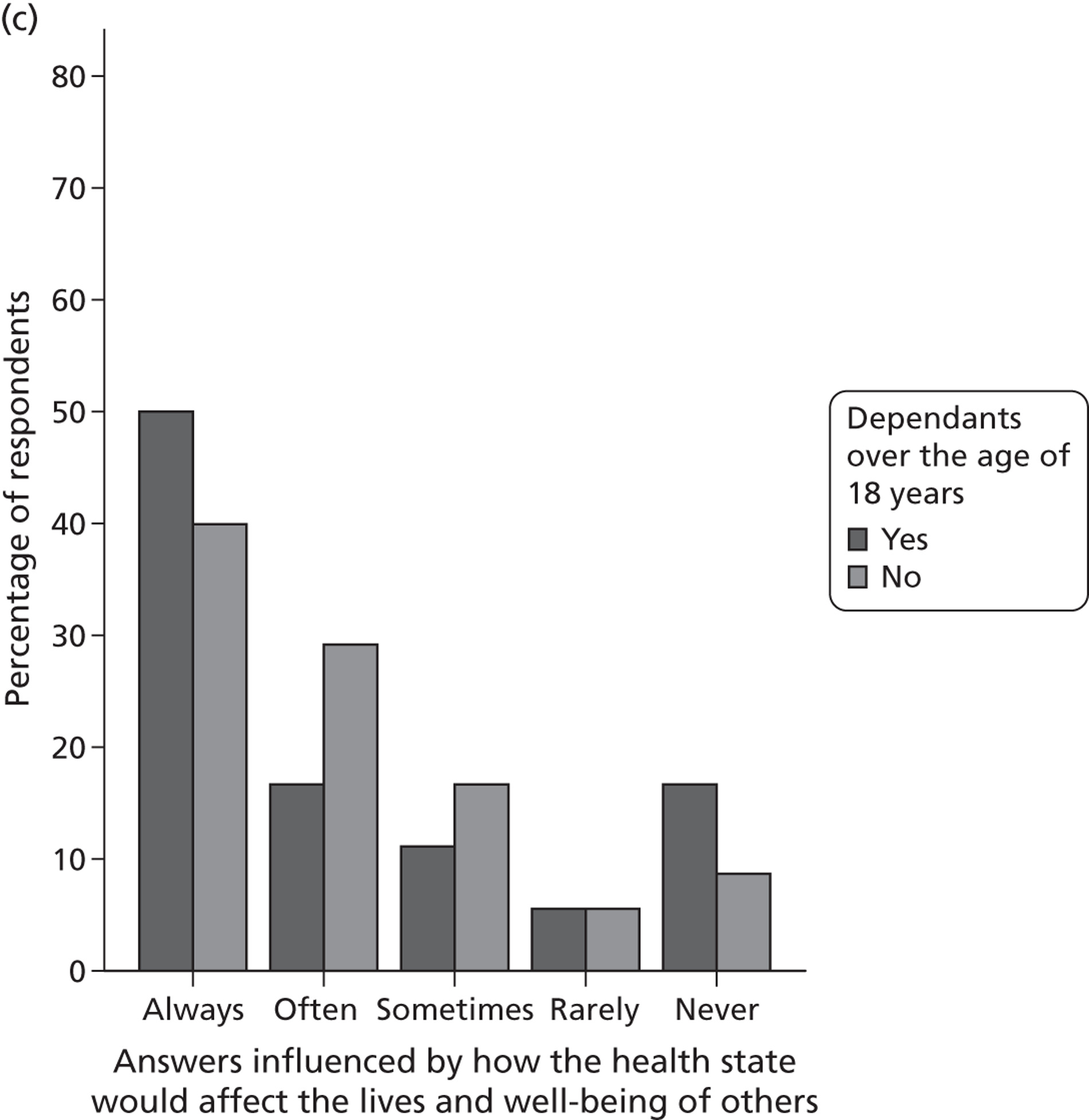
Figure 20 displays the impact of age and level of responsibility to others on responses across a number of key demographic variables. Overall, 79.1% of the sample report that their age and level of responsibility had an impact on their responses at least sometimes. There are no significant differences regarding how age and responsibilities impact on response by marital status (p = 0.11), having children (p = 0.54), having dependants aged > 18 years (p = 0.16), being employed (p = 0.51) or by age group (p = 0.51).
FIGURE 20.
Personal factors: age and level of responsibilities to others. The impact on responses of age and level of responsibility to others is shown across a number of key demographic variables. These include (a) marital status; (b) having children aged < 18 years; (c) having dependants aged > 18 years; and (d) employment status.
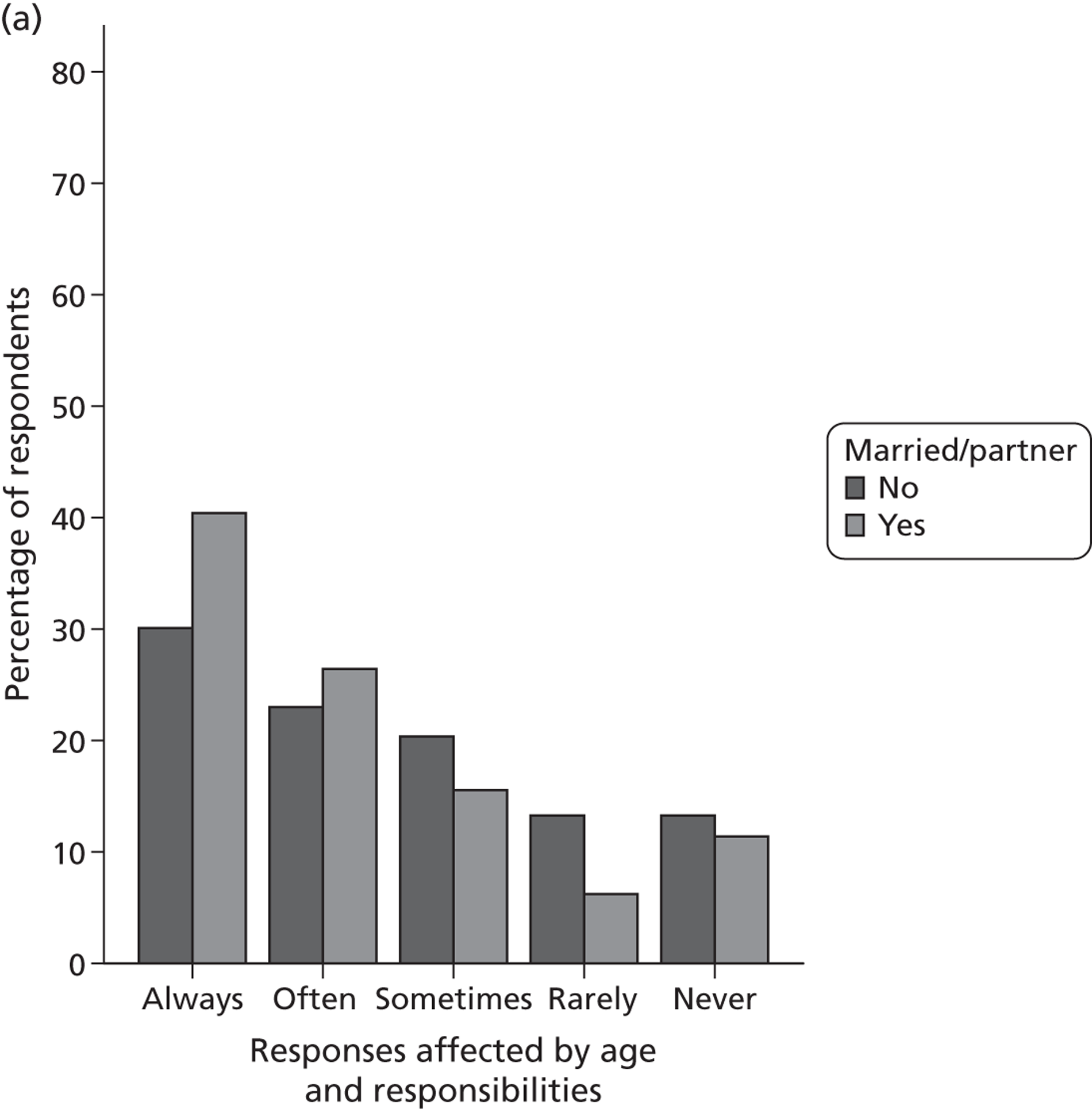
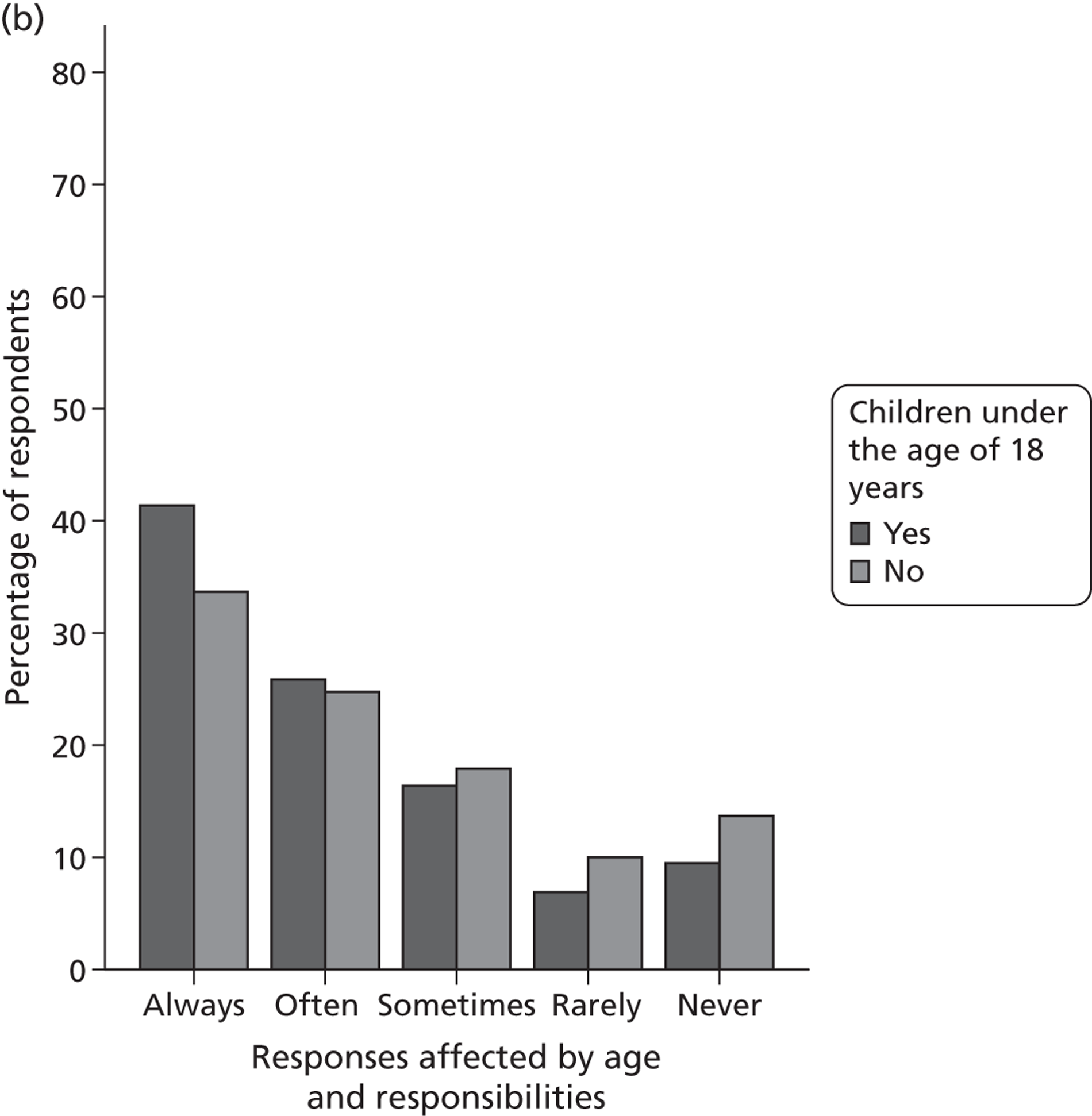
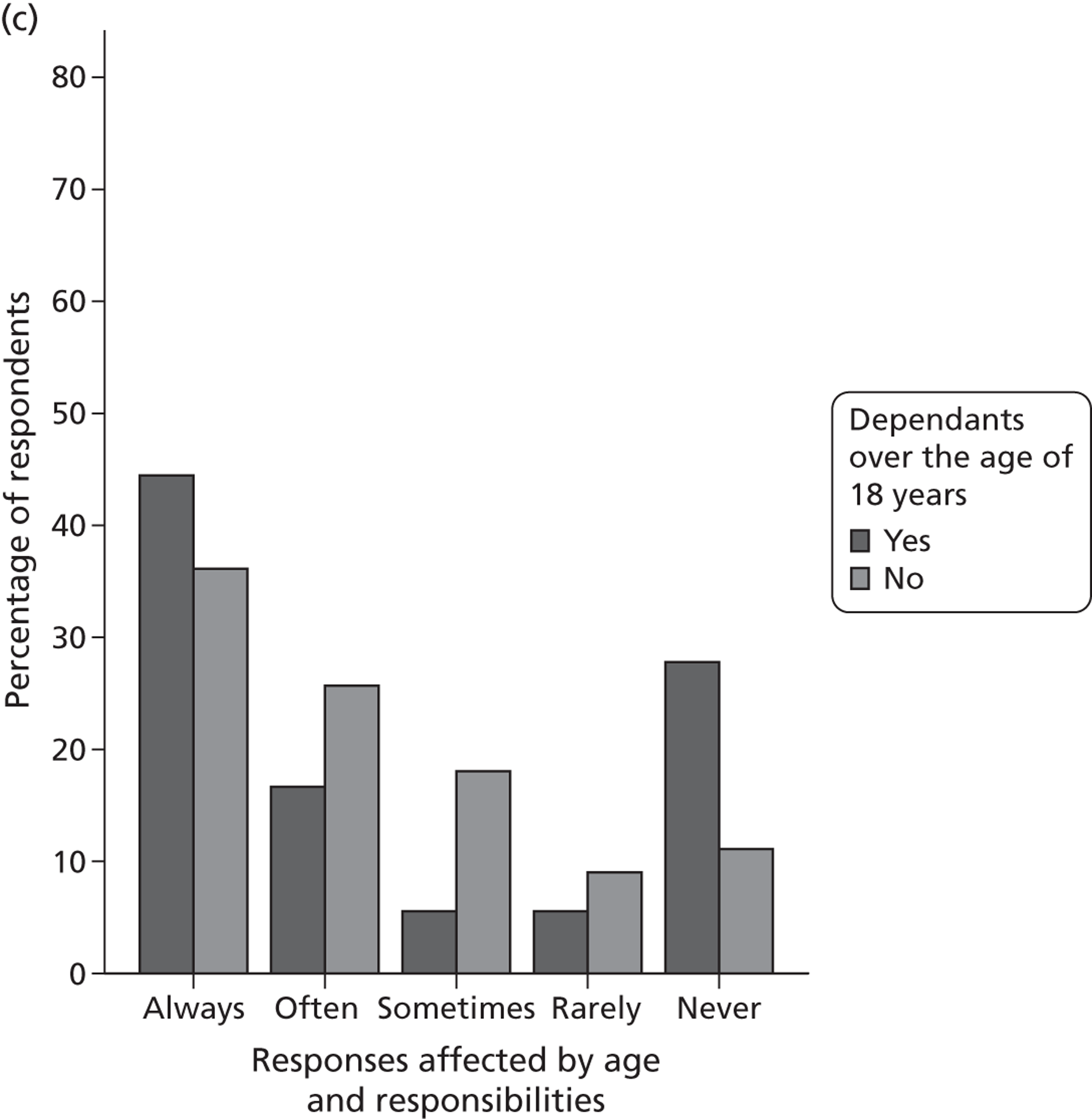
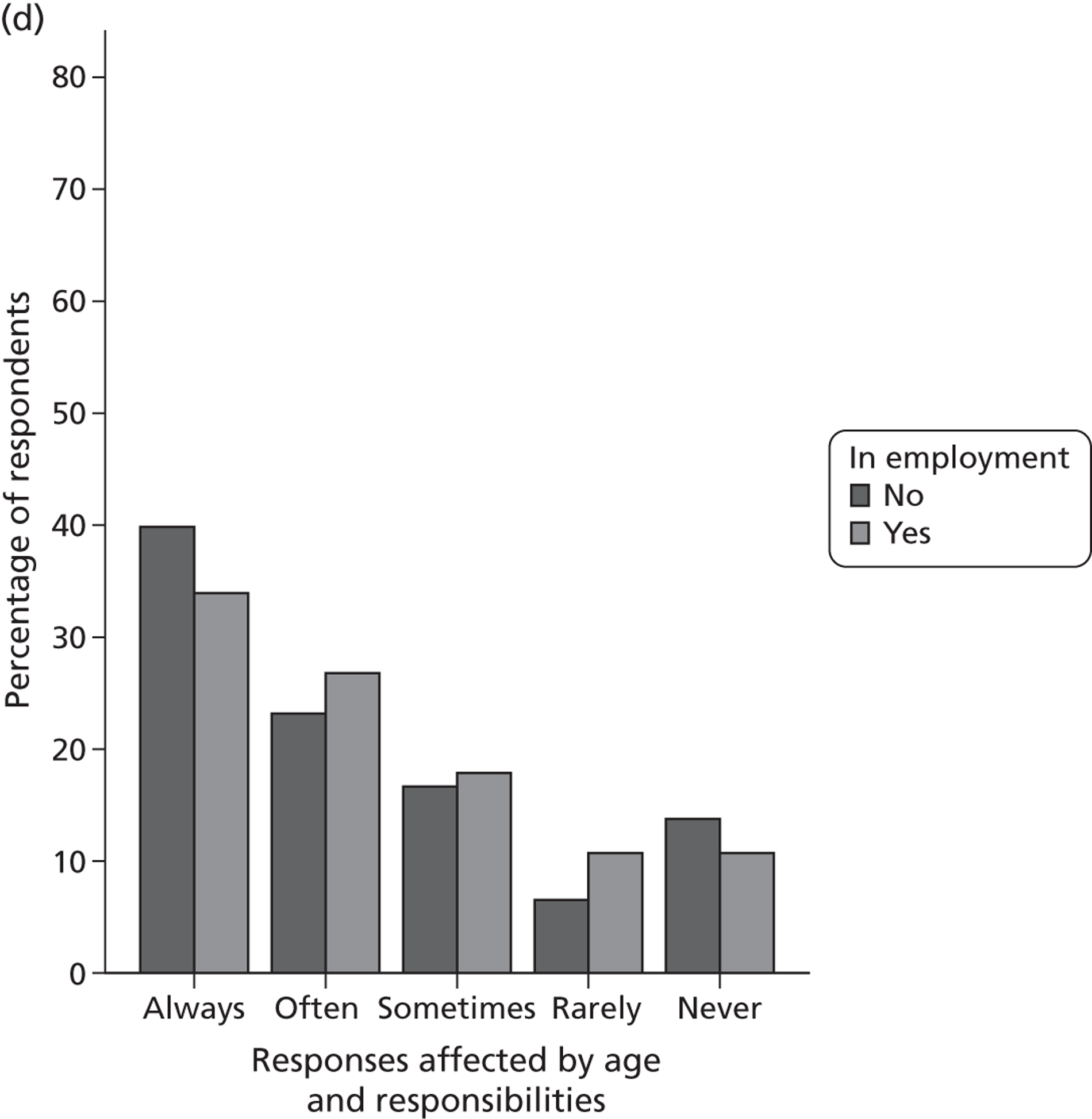
Discussion
Stage 3 of PRET used CAPI methods to investigate issues related to the completion of health-state valuation tasks using binary choice presentations of the methods. Presenting TTO and LT-TTO as binary choice questions enables a direct comparison with DCETTO. We found that the tasks were acceptable, and the TTO and LT-TTO tasks may be easier for respondents to complete than the DCETTO task. We also investigated the EQ-5D-5L descriptive system in terms of the importance of each dimension and whether respondents can differentiate between the five response levels. When respondents rank the order of importance of the EQ-5D-5L dimensions, there is some evidence of an effect of the order in which the dimensions are presented, so that they matched the ordering in the descriptive system (and, indeed, the ranking question).
In their conventional form, TTO and LT-TTO iterate until the point of indifference between the health state and full health is achieved, and this point is used to calculate the TTO value. This process is not followed when deriving utility values for health states using DCETTO, as only ordinal preferences are achieved for each task. However, by designing studies that incorporate many health-state pairs administered to a sufficient sample size, it is possible to model the ordinal results to derive a utility scale, and the feasibility of this has been demonstrated in Chapter 8 . It would also be possible to use the binary choice conceptualisations of TTO and LT-TTO to derive utility values as both include a duration attribute so can be anchored on the full health–dead scale as required. However, further work would be needed to produce a valid study design with a sufficient number of states, and also to establish the exact form that the regression model to estimate utility values would require.
Past work investigating how respondents complete DCE tasks has found that some introduce further assumptions and may not attend to all of the attributes presented. 64,65 To some extent this is supported by our study as a group of respondents reported that they answer by only considering the subjectively most important attribute, and this was found consistently across question types. This is an area that warrants further investigation to establish how many attributes it is reasonable to present in binary choice health-state valuation tasks. It may be possible to improve attribute attention by improving the study design and presentation of tasks. For example, participants could be asked to consider all of the attributes, or advances in computer technology could be used to develop innovative methods for presenting the health states.
This study also assessed the importance of EQ-5D-5L dimensions to general population respondents when presented in health-state valuation tasks. This relates to which dimensions respondents pay attention to (which may or may not be the same as the dimensions with the highest disutility). When assessing the overall ranking of EQ-5D dimensions, the results indicate that Mobility is the most important dimension followed by Pain/discomfort, Self-care, Usual activities, and Anxiety/depression. This suggests that when respondents are asked to rank the EQ-5D-5L dimensions, there is some evidence that the order of appearance of the dimensions may be important. However, firm conclusions cannot be drawn about this until further research has tested this by varying the order in which the dimensions are presented. In addition, we have found that some respondents cannot tell the difference between certain response levels, in particular level 4, ‘severe’, and level 5, ‘extreme’. These results, which have also been found elsewhere,69 may have implications for the sensitivity of the five-level descriptive system, as not all respondents may feel confident which level is worse, or perceive that there is a qualitative difference between the levels. If so, this will impact on the elicited preferences associated with the dimension.
When considering the overall importance of all attributes included in the binary choice tasks, duration, either in full health or in the selected health state, is the most important attribute. This suggests that a range of duration values should be administered in binary choice health-state valuation studies to test the importance of duration on responses. This can be done both quantitatively to assess the impact on utility values of varying duration, and qualitatively to investigate in detail why duration is the key attribute for respondents. Using a restricted set of durations means that the task used is deviating further from an iterative TTO. By having a richer set of durations we should be better able to model the group equivalent of the indifference point.
We found that a number of personal subjective factors and background characteristics may affect responses to the tasks. Marital status was an important factor, and this is in line with Dolan and Roberts. 66 At the beginning of valuation studies, respondents are not asked to consider how the state will impact on their lives beyond the health-state attribute included in the scenarios under consideration. However, these results indicate that the majority of respondents do not consider the health states in isolation. Therefore, certain background characteristics and personal factors are influential in the health-state valuation process. At the minimum it is important to collect a range of background characteristics, and it might also be possible to ask respondents what they considered when answering, and investigate the results excluding those completing the task the ‘wrong’ way. Further research should continue to consider the importance of a range of personal factors and how these might impact on choices made, and qualitative work into this has been carried out as part of stage 4 of the PRET project.
The CAPI study reported in this chapter has a number of limitations. We used follow-up probing questions to try to investigate reasons behind participants’ responses, and although they were designed using a pilot study, it is possible that important factors about the questions or response behaviour were not captured. We could not test in detail the reasons behind certain responses, for example why duration was consistently considered the most important attribute, as we did not have this capacity during the CAPI interview. To improve this aspect, stage 4 of the PRET project carried out a ‘think-aloud’ or cognitive interview study, with respondents completing both iterative TTO and LT-TTO, and DCETTO (see Chapter 6 ).
In summary, there is a growing interest in the use of binary choice questions to conduct health-state valuation exercises. However, little is understood about how respondents perceive the task and complete the exercise. We have tested three types of binary choice questions (TTO, LT-TTO and DCETTO) and found that the binary choice conceptualisation of both TTO tasks (i.e. those with less attributes that vary between tasks, and that only present time in full health as scenario B) may be more acceptable to respondents than DCETTO questions. There is also some evidence that certain attributes are more important than others that may be linked to an ordering effect. Furthermore, a range of external factors may impact on responses. These results may inform the design of binary choice question valuation studies, and the next stage of this work is to carry out detailed interviews testing the completion of both iterative (TTO and LT-TTO) and binary choice valuation tasks and to develop the methodology of designing and analysing a full valuation study for binary choice TTO and LT-TTO to produce utility weights.
Chapter 11 PRET stage 4: a qualitative investigation of the acceptability and completion of health-state valuation tasks
Introduction
This chapter has been adapted from work presented to the EuroQol Group. 70 Although both binary choice and iterative health-state valuation tasks are regularly used to derive preferences, there has not been widespread qualitative research into the ways in which respondents perceive and complete the tasks, and the personal and subjective factors used, and how this may impact the validity of responses and the subsequent utility values derived. Please see Chapter 10 (Influences on responses to health-state valuation tasks) for more information on the past work in this area.
The CAPI study reported in Chapter 10 allows the investigation of some of these issues using binary choice versions of TTO, LT-TTO and DCETTO. The acceptability of these tasks to respondents was high, but it was found that the majority of respondents do not attend to all of the health attributes when completing the tasks, which may have implications for the derived values. It was also found that there are a range of personal subjective factors that influence responses including experience of illness, how the health state would affect their lifestyle, and how they would cope with the state. Furthermore, when respondents ranked the order of importance of the EQ-5D-5L dimensions, there was some evidence of an ordering effect where they are matched with the ordering presented in the descriptive system (i.e. Mobility first, then Self Care . . .). There was also evidence that some respondents had difficulties distinguishing between the levels of EQ-5D-5L (in particular, level 4, ‘severe’, and level 5, ‘extreme’).
However, the stage 3 CAPI study was limited by the multiple choice probing questions used, which meant that the issues investigated were guided by the research team during the development of the survey, and could not be elaborated on extensively by the interviewee. Furthermore, we did not test the more conventional iterative versions of TTO and LT-TTO. Stage 4 attempts to deal with these limitations by carrying out an in-depth qualitative study investigating issues around the completion of both iterative (TTO and LT-TTO) and binary choice (DCE and DCETTO) health-state valuation exercises using EQ-5D-5L health states. This was carried out to help inform the use of iterative TTO, LT-TTO and DCE, which are the techniques to be used by the EuroQol Group in the ongoing worldwide valuations of EQ-5D-5L. 71 The think-aloud interview technique with follow-up questions was used to allow respondents rather than the interviewer to guide the discussion. We investigated respondent perception of the task, methods used to complete the task, the impact of task related factors on responses, the impact of personal and subjective factors on responses, and difficulties completing the tasks (including factors related to the EQ-5D-5L descriptive system).
Methods
Interview protocol
A ‘think-aloud’ interview protocol including semistructured follow-up questions was used to investigate how respondents completed health-state valuation exercises (see Appendix 5 ). Respondents were asked to complete each task while talking out loud about how they were answering the question, and any related thoughts or opinions about the health states or tasks in general. For each task the interviewer read out the health-state scenarios and asked the respondent to answer the question, but then did not interrupt until the answer was given, provided that the respondent was able to verbalise their thoughts. If the respondent could not successfully verbalise their thoughts they were asked the reasoning behind their decision after providing an answer. All respondents were then asked follow-up questions about a range of issues relating to each task, which were dependent on the thoughts verbalised while answering the question. This included questions about the difficulty of the task, the realism of the scenarios, personal subjective impacts and the influence of experiences of health on responses, and the EQ-5D-5L descriptive system. At the end of the study, respondents were given the chance to talk about any further issues that they wanted to discuss. A combination of think-aloud and semi structured questions was used to let respondents verbalise their thoughts without guidance whilst also investigating specific issues related to the health-state valuation tasks used.
Five types of valuation tasks were tested: DCE, DCETTO, iterative TTO with visual aid, iterative LT-TTO with visual aid and the ‘better or worse than dead’ screener question used at the beginning of a TTO exercise (see Appendix 6 for examples of each, and Table 40 for the states used). The ‘better or worse than dead’ screener question was used in isolation in an attempt to investigate respondent deliberations while deciding how severe a state is. This had three variants: duration or life A of 10 years with no lead time; lead time of 5 years followed by duration of 5 years; and no specified duration associated with the state. The EQ-5D-5L health states used were taken from past research, and hand selected to cover a range of severities and durations. All respondents completed DCE and DCETTO questions and at least one other valuation task. Half of the respondents completed TTO or LT-TTO, and the other half completed just the ‘better or worse than dead’ screener question. Following the EuroQol Group protocol for the forthcoming valuation studies,71 LT-TTO was completed if respondents indicated that the state presented was worse than dead. See Table 40 for a summary of the exercises, with the health states and durations used.
Task | State A | Duration, years | State B | Duration, years |
---|---|---|---|---|
DCE | 13321 | 22231 | ||
31223 | 21332 | |||
23232 | 32223 | |||
22123 | 13222 | |||
34454 | 43544 | |||
DCETTO | 34542 | 10 | 25443 | 10 |
23321 | 5 | 32231 | 7 | |
44333 | 8 | 53442 | 10 | |
23321 | 10 | 32231 | 8 | |
44333 | 5 | 53442 | 7 | |
22434 | 5 | 32325 | 5 | |
45434 | 4 | 54345 | 6 | |
23322 | 10 | 32231 | 8 | |
23322 | 10 | 32231 | 6 | |
45434 | 1 | 54345 | 2 | |
23322 | 5 | 32231 | 8 | |
22222 | 5 | 12212 | 3 | |
22434 | 5 | 32325 | 5 | |
Better worse | 33333 | 10 | Immediate death | |
55555 | 10 | Immediate death | ||
55555 | Immediate death | |||
55555 | 5 full health, 5 state | Full health | 5 | |
54423 | 10 | Immediate death | ||
54423 | Immediate death | |||
54423 | 5 full health, 5 state | Full health | 5 | |
44444 | Immediate death | |||
44444 | 10 | Immediate death | ||
31344 | 10 | Immediate death | ||
31344 | Immediate death | |||
53252 | 10 | Immediate death | ||
53252 | Immediate death | |||
53252 | 5 full health, 5 state | Full health | 5 | |
TTO | Full health | 0–10 | 33333 | 10 |
Full health | 0–10 | 55555 | 10 | |
Full health | 0–10 | 53252 | 10 | |
Full health | 0–10 | 54423 | 10 |
Procedure
A convenience sample of non-academic members of staff at the University of Sheffield was recruited using university e-mail lists and poster advertisements. Initially, participants read the project information and consented to take part in the study. They then completed the same demographic and self-reported health questions as at stage 3, with the addition of a question about experiences of illness. To introduce the think-aloud process, respondents completed two warm-up tasks. The first asked respondents to count the number of windows in the house or flat that they live in while thinking out loud, and the second presented a DCE question about a choice of holidays (an example used in a previous think-aloud study). 72 If respondents were happy with what was required, the recording was started and the respondents completed each of the valuation questions while thinking out loud, with follow-up questions asked after each task. When all of the questions were completed, interviewees were asked for any further comments about the tasks and study in general. All interviewees received a £5 voucher for participating.
Analysis
All interviews were recorded and transcribed verbatim. An initial coding frame based around how respondents completed the valuation exercises was developed from existing literature and the results of the CAPI interviews carried out at stage 3. Transcripts were read in detail and respondent statements were allocated to the initial coding frame. New categories were included in the coding frame to cover issues raised in the interviews that were not initially included. All transcripts were coded by a member of the project team (BM). A selection of transcripts was independently coded by an external researcher (JC), experienced in qualitative work to ensure reliability and consistency across the analysis.
Results
Sample
Descriptive statistics of the sample are reported in Table 41 . Two-thirds were female, with a mean age of 36 years. The sample was highly educated, and had good self-reported health levels.
Characteristic | n (%) |
---|---|
n | 29 |
Gender | |
Male | 10 (34.5) |
Female | 19 (65.5) |
Age (years) | |
Mean (SD) | 36.63 (10.17) |
Range | 24–57 |
Marital status | |
Married/partner | 15 (48.3) |
Single | 14 (51.7) |
In employment | 29 (100) |
Have children | 6 (20.7) |
Education post minimum | 28 (96.6) |
Educated to degree level | 23 (79.3) |
Experience of serious illness (asked from Interview 8) | 11 (50.0) |
EQ-5D index (mean, SD) | 0.907 (0.12) |
Health status | |
Excellent | 10 (34.5) |
Very good | 12 (41.4) |
Good | 6 (20.7) |
Fair | 0 (0) |
Poor | 1 (3.4) |
SWBH | |
10 | 1 (3.4) |
6–9 | 25 (82.6) |
1–5 | 3 (10.3) |
SWBL | |
10 | 2 (6.9) |
6–9 | 22 (75.9) |
1–5 | 5 (17.2) |
Interview results
The coding frame is outlined in Figure 21 . Transcripts were coded into five overall categories: ‘scenario/task-specific factors’, ‘personal and subjective factors’, ‘difficulties’, ‘opinions of task and task comparisons’, and ‘other’. The first three of these categories related to the task completion process, and each of these was split into four subcategories identifying the main themes (so, for example, ‘scenario/task-specific factors’ that the respondents used to complete the task included ‘comparing duration’, ‘comparing dimensions’, ‘comparing levels’ and ‘combination of these’).
FIGURE 21.
Analysis coding frame.
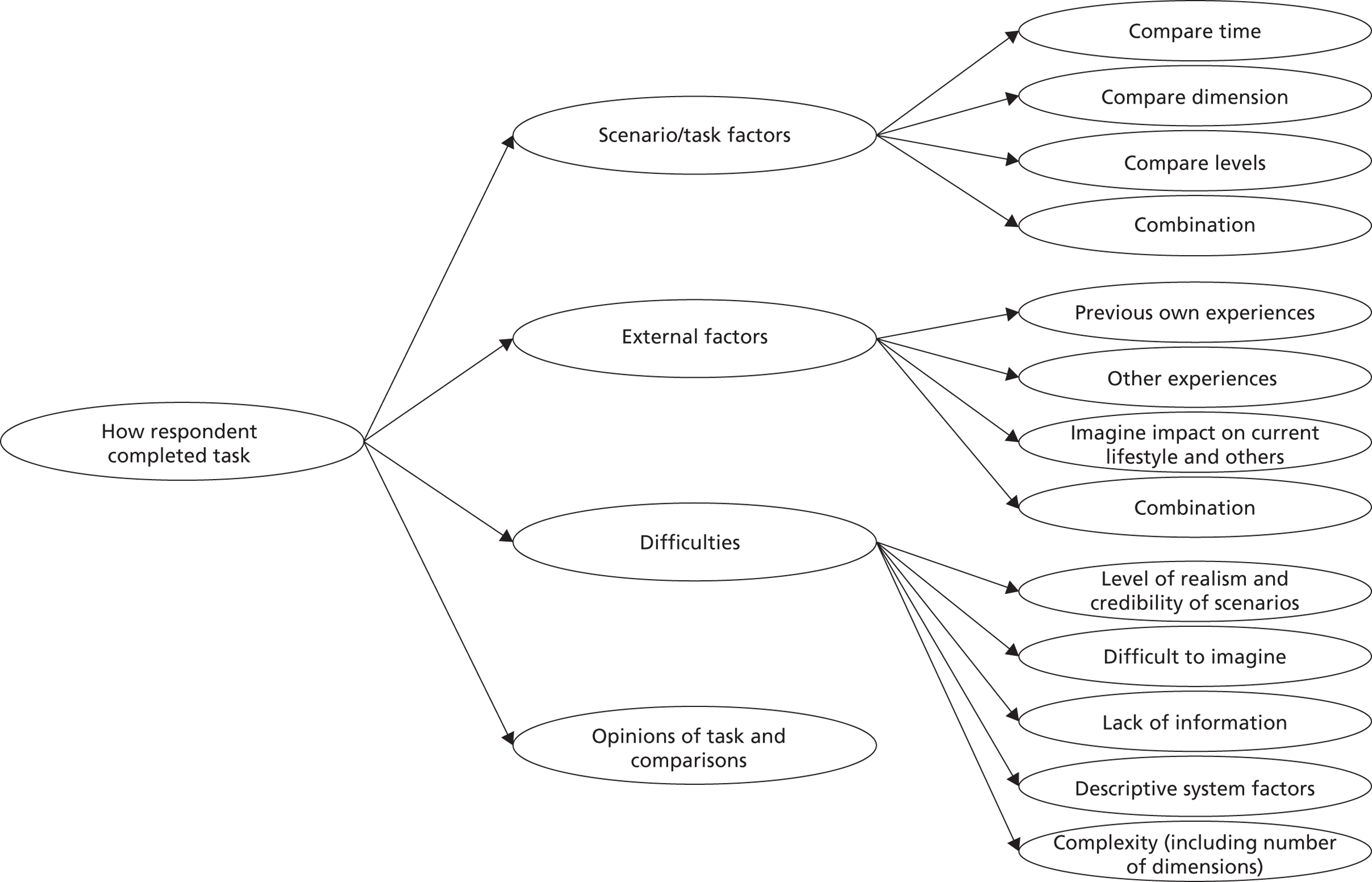
The results for each theoretical section of the coding frame with indicative quotes are outlined below.
How respondents complete the tasks: scenario/task factors
Comparing health-state dimensions and severity levels
Respondents reported considering the EQ-5D-5L classification system dimensions and severity levels in a variety of ways, and this influences the completion of the valuation tasks. For example, some respondents reported comparing every dimension and severity level to answer the question. However, other respondents did not consider all of the dimensions, and focused on those attributes most important to them (and therefore their answers were based on these dimensions only). Furthermore, some respondents focused on the severity levels rather than the actual health-state dimensions, and used systems to estimate the severity of the health states overall. The following quotes demonstrate some of the ways in which respondents perceived and completed the tasks:
. . . presuming actually it’s three slights and then a moderate in both so it’s actually the same . . .
Respondent 14
I was just comparing each bit of health and the scenarios and then just thinking is that one better than that one, and just doing that for each one.
Respondent 22
I looked at them overall and I looked at which state of health would be the worst for me . . .
Respondent 5
That’s the bit [level 2 vs. level 3 on the anxiety/depression dimension] that I zoomed into straight away. I just think you know erm if you feel really that bad in that way.
Respondent 7
. . . scenario B is better because I have no problems to walk about, washing or dressing myself is worse, third one [i.e. usual activities] is worse than A, fourth one [pain/discomfort] is the same as A, five [anxiety/depression] is better than A, it is difficult really . . . . I would say that is the same, slightly anxious and depressed, I think on the first side I think health scenario B looks better to me because it seems overall less issues.
Respondent 10
Okay, I suppose the trade off is between whether you have mobility problems like the walking about and washing and dressing yourself or whether you’re depressed and actually that’s kind of a mental/physical trade off.
Respondent 17
You know I do value some of these things [i.e. dimensions] obviously a little bit more than others . . .
Respondent 1
The impact of duration
For DCE without duration, some respondents reported hypothetically assigning a common duration for both health states and how this might impact on the way in which the state is valued, but some reported not considering duration in their response as the following quotes indicate.
I just presumed like neither of them [state 22123 vs. state 13222] seemed the sort of thing that would finish you off particularly quickly so I just imagined the rest of my normal life . . . . I was just sort of imagining normal life well not normal but normal length of life . . . . [for a different pair with states 34454 vs. 43544] if there was a limit on how long it was going to be life if it wasn’t particularly long then I might value the walking about.
Respondent 11
No it [duration] was raised as a question in my head but it wasn’t something that I made an assumption on.
Respondent 12
For TTO, DCE and DCETTO, some respondents reported that duration had an impact on the way they completed the question (e.g. by considering the overall state in more detail), and was an important component of the task but this was not consistent across all respondents (and may be influenced by the duration values used). Similarly, some respondents reported that duration became the most important attribute in the decision-making process (and therefore they were unlikely to trade any time), but other respondents did not consider duration to be an important factor, and were more concerned with quality of life over quantity of life. The following quotes provide examples of the impact of the addition of duration and comparisons between DCE and DCETTO:
I think this one is harder to compare because of the time difference erm so that makes me think about it a bit more.
Respondent 27
. . . you have kind of got to start weighing it up [when duration is added] and you have got to start thinking of everything then.
Respondent 8
So this means you die after 5 years and this one after three years well it goes without a doubt I’d go for health scenario A [with 5 years]. It gives me an extra two years to live and there is no price on life.
Respondent 18
I think if it had sort of said 5 years and 10 years I would have felt it was less of an issue because 5 years is still a decent bit of time. If you’re told you’ve got one year to live that’s sort of the actual timescale maybe not so much the one year virtually doubling but if it’s just one year I think . . .
Respondent 14
I would say to start with in between 10 years and eight years doesn’t make much of a difference to me I don’t think so erm I would probably take that out of consideration.
Respondent 10
I think if that quality of life during that duration is good for me it’s quality of life and I would take quality over quantity any day.
Respondent 21
Yes after looking at all the other things but it [duration] would come down on the lists on my priorities on the bottom.
Respondent 11
The severity of the health state interacts with duration, and some respondents traded time to avoid living in severe health states.
I feel that the worst scenario is B because although it is for a longer duration I think the quality of life would be very limited so I would choose A.
Respondent 16
If I’ve got a year of full health compared to 10 years of rubbish health I’d take the year.
Respondent 28
How respondents complete the tasks: personal and subjective factors impacting responses
When faced with health-state valuation exercises, respondents incorporated a range of personal factors that influenced their responses to the tasks. These were highly subjective and included personal experiences of illness, and the impact that the health state would have on their current lifestyle and the lives of those around them. Each section below describes the main factors discussed in the interviews.
Impact of own and others’ health experiences
Respondents reported answering the questions considering their own and other people’s health experiences, and this had an impact on the way they perceived the health states:
I was thinking about myself in them, having had past health problems myself, I can put myself into the situation.
Respondent 5
I’m kind of relating it to an experience that I had been going through with my dad and I just think you know to me that was more important [the anxiety/depression dimension of a DCE without duration].
Respondent 7
My own and one of my best friends as well who was severely depressed and I’ve got elderly family and friends who the idea of that for them is that kind of slipping away of their own independence and that sort of idea of not being able to wash or dress yourself feels like it’s more of an impact on my own independence which I value quite strongly so that’s where that came from.
Respondent 17
Imagine impact on current lifestyle and others
A key consideration of many respondents when presented with EQ-5D-5L health states was how they and others would cope with living in the state, both in terms of individual dimensions and in terms of interactions between the dimensions. Life stage issues were another consideration, including how the state would impact on others, and this informed their responses. The quotes below provide examples of how respondents imagined coping with the states:
I think I could probably come to live with moderate problems in walking about, there’s always TV.
Respondent 1
. . . if you have got a longer time to cope with it which then comes into effect the longer that you have to cope with it you could become more anxious and depressed about it.
Respondent 7
. . . I know that if I was feeling extremely anxious and depressed for 5 years then even slight pain would be very difficult for me to manage so it becomes kind of harder for me to pick it out.
Respondent 9
Well I think that is accurate for me when we were looking at the milder states I thought you know that would be unpleasant but I would still get by, whereas when I am looking at this I think this is so unpleasant that I would have to change everything about my life and the way I experience it in order to cope, erm which does make me view things differently.
Respondent 9
I think that I would go for the health scenario B because I would be less reliant on others . . .
Respondent 15
. . . from a personal point of view I am not the best person to suffer ill health anyway, so as well as suffering myself I think I would probably make things unbearable for everybody else . . .
Respondent 7
I would immediately go for health scenario A for the reasons that I have two young children and would want to live for longer.
Respondent 12
Although respondents were instructed at the beginning of the interview to ‘imagine that you will experience each health state for the period shown, even if receiving treatment for the health state’, some had difficulties considering the states without assuming that they would get relief for the problem. This had an impact on the decision-making process both for single dimensions and in comparisons across dimensions, and may mean that more weight is placed on dimensions which have wider lifestyle implications that cannot be medicated (such as self care).
. . . they’re all slight problems really except for pain which I suppose could be medicated . . .
Respondent 26
. . . you would only have moderate pain which you can relieve with tablets or whatever . . .
Respondent 5
How respondents complete the tasks: task complexities
Level of realism and credibility of scenarios
Some respondents reported that the EQ-5D-5L health states used were not always realistic in terms of the combination of the dimensions used. This has implications for the validity of the task and the design of valuation studies. However, this was not unanimous, and some respondents linked the same states to an actual condition. Furthermore, some respondents thought that some of the states they were presented with were realistic but others were not. See examples of these issues below concerning different EQ-5D-5L states:
I find it difficult to believe that somebody who had moderate problems washing and dressing themselves and had moderate problems doing their usual activities wouldn’t be at all anxious or depressed . . .
Respondent 27
I would have thought that in the general population there are plenty of people that would fit into both of those [states 23232 and 32223] . . . . I am not sure why you would be able to walk about with only slight problems if you had got extreme problems washing and dressing yourself [state 34542 (10y) and 25443 (10y)]
Respondent 6
. . . I do find it difficult to imagine that if somebody knew that they had eight years to live that they would not be anxious or depressed at any stage during those eight years.
Respondent 9
. . . but realistically you wouldn’t know that I’m going to live for 5 years and then I’m going to die. I mean if you did it would impact everything else.
Respondent 20
. . . yes I can [imagine], somebody with like multiple sclerosis or something like.
Respondent 13, discussing states 34454 and 43544
Task complexity
The tasks were generally perceived as complex, with DCETTO being more complex than DCE, and LT-TTO seen as more complex than TTO. The tasks were seen as complex due to the number of dimensions and considerations, and some respondents were concerned about the impact of the complexity on the way respondents go about answering the questions. Respondents reported that the tasks were complex, because of: the choices that were required; difficulties picturing the health scenarios and individual health dimensions; and the amount of information included in each scenario. In terms of lack of information, some respondents reported wanting more information about the task descriptive system (e.g. what a usual activity is) but this was not consistent:
I do yes [think DCE has a lot of information] ’cos I mean going down the list and stuff you know and having to think about that [scenario A] and then go to that [scenario B] and make the comparison and stuff.
Respondent 25
. . . there was quite a few factors that I’m having to sort of mentally weigh up and juggle a bit and maybe if I was to spend a bit more time on it I might there might be things I’d overlooked or underplayed but because it’s like an instantaneous reaction . . . . It does get a bit more confusing when there’s more [dimensions].
Respondent 23, comparing TTO/LT-TTO and DCE both with and without duration
They’re very similar and that makes it quite difficult . . .
Respondent 1
I think it’s a bit difficult to have a distinctive impression of what a usual activity is . . .
Respondent 29
I do think that does cover quite a few main issues of your living life, like your lifestyle what you do and what you enjoy, how you get about, at least you can put whatever things you like doing will fit into them boxes what you can do . . .
Respondent 7
Descriptive system factors
Some respondents had difficulty telling the difference between level 4, ‘severe’, and level 5, ‘extreme’, both at an abstract/linguistic level (i.e. unclear which is stronger: severe or extreme), and in the context of the particular problem (i.e. severe pain and extreme pain are indistinguishably bad). However, this was not consistent, and difficulties were also reported imagining the context of the self-care (between levels 2, ‘slight’, and 3, ‘moderate’) and mobility dimensions (between levels 3, ‘moderate’, and 4, ‘severe’), and the linguistic difference between levels 3, ‘moderate’, and 4, ‘severe’.
Is severely or extremely the higher ranking?
Respondent 9
I think severe sounds more than extreme I don’t know though for sure.
Respondent 19
If you were in severe pain you might well describe it as it being extremely bad, they are very similar.
Respondent 6
. . . at the other end of the spectrum when you are talking about severe and extreme, severe and extreme is quite easy to imagine the difference of, but the slightly and moderate is more difficult.
Respondent 28; talking about self-care
I am just trying to work out which, whether the washing and dressing is better to be severe or moderate.
Respondent 23
. . . imagining what it relates to in moderate problems in walking or severe problems in walking what does that mean?
Respondent 27, talking about mobility
‘Better or worse than dead’ screener question and the ‘immediate’ death option
When faced with the better or worse than dead screener question presented at the beginning of a TTO task using a severe EQ-5D-5L health state, respondents reported that the states used for this exercise (life A) and the concept of immediate death (life B) are both difficult to conceptualise, and therefore the question is difficult to answer. Again, respondents incorporated a range of personal and subjective factors to help them conceptualise the options.
I mean the idea that I’m sat here now and could walk over and keel over is quite different.
Respondent 17
I’m trying to sort of visualise it [the health state and immediate death], and erm and actually you know what when you are actually feeling healthy and good it is hard to visualise it, then I would say erm at the moment I wouldn’t want to say that I want to die immediately but that might be because I can’t believe or imagine it.
Respondent 10
I think this is a really, really personal choice I think it does completely depend on so many other circumstances around you. If you had fantastic support it might be that you want to stay and live and have the support there. If you’ve got no-one to support you through any of those things I can completely understand why you would want to die.
Respondent 14
. . . because I’m aware that there are a lot of older people in our population who probably are at this stage I’m less inclined to say I’d want to die immediately.
Respondent 15
Valuation task-specific issues and task comparisons
Respondents reported both positive and negative opinions about the tasks used, and the framing of the hypothetical scenarios. Overall, the tasks were acceptable to the majority of respondents, and differences in the complexity of the decision-making process between the TTO and DCE based exercises were reported. However, there were some concerns about the stability of preferences over time. The visual aspect of TTO (i.e. the props board) was seen as useful to explaining and visualising the task. Quotes regarding the comparison of DCE and DCETTO are discussed in the ‘impact of duration’ analysis section above.
. . . it [TTO] is definitely an easier decision to make it is a very different feeling answering those two questions types because basically this [TTO] is almost a bargain and this [DCETTO] feels less like a bargain.
Respondent 28
I think you would need some face-to-face stuff [alongside the possible use of online methods] erm possibly the opportunity to give people more chance to get more details about why, otherwise it could end up being quite abstract . . .
Respondent 6
I think that it asks some difficult questions and you know not everyone wants to imagine this kind of scenario, erm, however, it is a valid question and an important one that needs to be asked.
Respondent 26
. . . the positive of it is that it doesn’t say scenario A is cancer and scenario B is MS so you don’t have that kind of negative side, erm but I think that it is incredibly difficult to choose I mean, I am thankful that it is a choice that I have to make, having said that I feel so unsure about the choices that I am making.
Respondent 9
If you’d asked me these questions thirty years ago, forty years ago my answers would have been different because the thought of slight problems walking about would have been unthinkable. I think it makes it, I don’t know why it [the TTO board] makes it more real but it does erm kind of.
Respondent 13
. . . that [TTO] is quite good ’cos it is a visual thing, that [DCE] is just writing and it is kind of like you know you are constantly having to refer to it you know what I mean.
Respondent 25
Yeah they’re [TTO based exercises vs. DCE based exercises] both slightly different ways of looking at things and therefore I think they’re helpful in different ways.
Respondent 24
Discussion
This qualitative investigation of the completion of DCE, DCETTO, TTO and LT-TTO for health-state valuation has furthered the CAPI based work reported in Chapter 5 by carrying out more in-depth interviews that were not limited by the multiple choice follow-up questions. In addition, iterative versions of TTO and LT-TTO were used. Think-aloud interviewing was used, which allowed us to investigate in detail how respondents complete the tasks, and the personal and subjective factors and difficulties that influence responses. We have found that a range of strategies are used to complete the tasks. We have also found that respondents incorporate a range of personal factors that are linked to life and health experiences, and may impact on the responses provided.
Some respondents complete the exercises by fully attending to all attributes and associated severity levels. However, others focus on selected components of the task (that may be the health dimension or severity level that is most important to the respondent, or the first dimension attended to while completing the exercise). Attribute non-attendance in preference elicitation tasks has also been found in previous research assessing DCE66 and may have implications for the values derived for each attribute. To improve attribute attention it may be possible to design innovative ways of presenting the tasks to encourage respondents to consider each dimension. In this study, the TTO props board was seen as a useful visual aid, and it may be possible to visually represent DCE tasks, including DCETTO, used to value health states (visual representations are widely used in DCE studies other than health-state valuation).
The addition of duration to a DCE task (i.e. DCETTO) allows the derivation of utility values anchored on the full health–dead utility scale. In this study we have tested both DCE and DCETTO and the qualitative evidence suggests that when duration is added to the scenarios, it is considered as an important part of the choice exercise by many respondents, indicating some validity for the DCETTO approach. Some respondents report that it influences the way in which they respond to the task and is the most important consideration, but others report that the quality of life is more important than the quantity of life. Furthermore, some respondents suggested that the actual levels used for the DCETTO duration attribute, and the interaction of this with the health-state dimensions, influenced the perception and completion of the task. This demonstrates the importance of designing DCETTO studies so that the number of duration levels and the actual combinations of actual duration values used allow the impact of duration to be fully captured. Further qualitative and quantitative work into the impact of duration in DCETTO tasks would be useful.
We have shown that the health states presented in valuation tasks are considered by respondents in terms of personal and subjective factors such as experience of illness, how the state would affect their lifestyle, and how they would cope with the state. It is these considerations and experiences that are at the core of the values placed on different areas of health by different individuals. The way in which these factors interact with the valuation process, and the extent to which they are important to the preferences provided, varies from respondent to respondent, and also according to the severity of the state and the dimensions used. This study has attempted to outline some of the major personal themes that inform the perception of states. However, the themes reported here are not exhaustive, and further research beyond this exploratory study may carry out interviews with a wider sample to explore which subjective factors are important, and how these interact with, and therefore influence, the valuation process.
The importance of personal factors in the valuation process, and the influence that these may have on subsequent values, raises questions about whether any of the factors should be included in the actual valuation exercises. For example, should coping or satisfaction in the state be incorporated, how would this impact preferences, and how should this be done? In this study we also found that respondents often seem to require more information about the scenarios to make an informed choice. Examples of this included wanting information about how a usual activity should be perceived, and what each level of the descriptive system translates into practical terms for each dimension. It would be possible to provide some further information, but there is also a limit to what information can be provided so that studies do not become too complex and difficult to complete. Furthermore, the extent to which providing some types of information (e.g. a set of definitions) might affect the perception of the health states and therefore the valuation process, is unclear.
We found that some respondents have difficulties with some of the EQ-5D-5L health states. This lends support to the common practice in the design of valuation studies of restricting implausible states by hand, or checking the design for implausible states. Furthermore, we have found that a number of respondents cannot tell the difference between certain EQ-5D-5L levels, in particular level 4, ‘severe’, and level 5, ‘extreme’. These results may have implications for the sensitivity of the five-level descriptive system utility values, it may be possible to tackle this issue by informing respondents about which is the worst level (so that when respondents interpret the levels, the focus will be on whether or not they are different in terms of severity instead of which one is the worst). The issue can also be investigated when modelling forthcoming EQ-5D-5L valuation data in terms of the size of the difference between the levels.
This investigation is limited in a number of ways. First, we used a convenience sample of respondents, which was in no way representative of the general population who are the target population for most valuation studies. This is because our study was carried out with people employed in non-academic roles in a UK university, and therefore our sample is generally quite young, highly educated and healthy. To extend this work and investigate the issues in more detail it might be possible to interview a wider sample representative of the population in terms of age and health status, which might inform and extend the results described above. Second, we carried out a think-aloud study, which can be difficult, and may not fully replicate the thought processes of respondents completing the tasks (who may employ different thought processes to complete the tasks because of the need to think aloud). Furthermore, we do not know if respondents were fully verbalising all of their thoughts. To address this we also asked follow-up questions to attempt to investigate certain issues related to health-state valuation. However, insights gained from the follow-up questions are limited to those that the respondent can and is willing to reflect and recall. In either case (think aloud or follow-up), it is unlikely for respondents to indicate that, for example, their answers are at random or that they do not care.
Using think-aloud methods to test a range of valuation methods has raised a number of issues relating to the completion and acceptability of the tasks that need to be considered in the design of valuation studies. This includes the complexity of the tasks (which may mean that respondents should not be required to complete too many); the information provided to respondents; the attribute combinations used (which should be realistic and allow the impact of duration to be modelled); and whether other factors can and should be included in the valuation process.
Chapter 12 Discussion
This chapter presents a brief summary of the findings from the PRET (and PRET-AS) project, provides an outline of the EuroQol Group’s final protocol for the valuation of EQ-5D-5L, discusses a number of key issues, examines the weaknesses and areas for further research, and concludes.
Summary of findings
Stage 1: PRET
Of the topics examined, although the duration of the health state being valued affected the preference for the state, there was no clear pattern regarding the direction or the magnitude. In other words, there is no single answer to whether constant proportional TTO is violated; future research should focus on when it is violated. The perspective of the valuation exercise did not result in significant changes to health-state preferences across pooled data, although different patterns were observed across the severe states. Furthermore, exhaustion of lead time was affected by the length of the lead time relative to the duration of the health state in question. At the same time, exhaustion of lead time in online LT-TTO appeared to be much higher than that observed in face-to-face iterative LT-TTO. 19
Question type IV with lead time was used to examine time preference. The data allow the derivation of the minimum level of time preference that is consistent with a particular choice to be made, given the combinations of the relevant parameters. The implied minimum time preference rates were positive in most cases. In general, the rate was found to fluctuate by state and by duration. Some scenarios, in particular the ones with short durations, resulted in very high time preference rates (e.g. 500%). The implied time preferences were not affected by the different perspectives. On the other hand, the reference to the level of satisfaction in the health state in question had a significant impact on the preference for the state: higher satisfaction was associated with positive preference.
Stage 1: PRET-AS
The PRET-AS online survey indicated that DCETTO is a valid method for generating health-state utility values for EQ-5D-5L, and resulted in coefficients that are logically ordered within each dimension; it produced a unimodal set of predicted health-state values, ranging from −0.845 to 1.0, without relying on arbitrary transformation of negative values which has been shown to be problematic,14 or exogenous anchoring of the value of being dead. 21 In addition, it found that binary choice LT-TTO may be feasible to produce utility values, but further work is required to develop the optimal selection of the states to be used in the valuation, and for the modelling of results to generate predicted health-state values.
PRET stage 2
The online and CAPI methods were found to produce similar results for the seven binary choice tasks used in PRET. Although the two samples had some statistically significantly different demographic characteristics, controlling for these did not affect the overall outcome. It is noted that one of the main differences between the two samples was in terms of the respondents’ self-reported health: the online survey sample appeared to be significantly less healthy than the CAPI sample.
PRET stage 3
The three methods used (TTO, LT-TTO, DCETTO) were acceptable to respondents. Respondents typically found TTO and LT-TTO easier to complete than the DCETTO task. When respondents ranked the order of importance of the EQ-5D-5L dimensions, there was some evidence of an effect of the order in which the dimensions are presented. Some respondents were uncertain about the relative ordering of level 4, ‘severe’, and level 5, ‘extreme’, problems. A number of personal and/or subjective factors and background characteristics has an impact on responses to the tasks.
PRET stage 4
In addition to the three methods used in stage 3, a DCE with no duration was added, and TTO and LT-TTO were used in the full iterative administration. The think-aloud method and the follow-up questions revealed that respondents used a range of strategies to complete the various tasks. In line with stage 3, uncertainty regarding level 4, ‘severe’, and level 5, ‘extreme’, problems was observed. Furthermore, respondents incorporated a range of personal factors which were linked to their own life and health experiences.
EuroQol Group’s official protocol for the valuation of EQ-5D-5L
The PRET project has conducted research into the methodology of health-state valuation, and was set against the background of the revaluation of the newly developed EQ-5D-5L. Over the recent years, a number of methodological studies have been carried out around the world (some of which were funded by the EuroQol Group) to inform the development of a standard protocol for country-specific valuations of EQ-5D-5L. 71 This includes studies of different types of iterative LT-TTO, a ‘composite’ TTO using the MVH TTO for states better than dead and LT-TTO for states worse than dead, and DCE without duration. The PRET project forms part of this suite of methodological research activities, and the EuroQol Group has had access to the findings of PRET and PRET-AS projects as they developed.
Following this body of work, the EuroQol Group has developed its own official protocol for the valuation of EQ-5D-5L. Valuation studies will be CAPI-based face-to-face interviews involving 10 iterative TTO tasks and seven DCE tasks per respondent. The DCE will not include duration as an attribute. TTO is used to provide exact information about the utility of a small number of health states, and DCE offers censored data on a larger number of states that indicate whether the value of one state is higher than the value of another state, but not anchored on the full health–dead scale. The two kinds of data will then be modelled together, using both a likelihood and a Bayesian approach.
The immediate contribution from the PRET (and PRET-AS) project to this official EuroQol valuation protocol is from the last stage of the project (stage 4). The original aim of stage 4 in the PRET proposal was to develop and test a protocol for the revaluation of EQ-5D-5L (see proposal in Appendix 7 ). However, during the time frame of the project the EuroQol Group developed its own official protocol outlined in the paragraphs above. Therefore, stage 4 of PRET was adapted to use qualitative methods to test TTO, LT-TTO and DCE with and without duration. This work examines the ways in which respondents complete the valuation tasks used in the official protocol, and contributes towards the understanding of the reasons behind their responses. On the other hand, although PRET stages 1 to 3 and PRET-AS tested innovative non-iterative binary choice methods in an online environment for health-state valuation, these methods were regarded by the EuroQol Group as being still in development, and thus too risky to be appropriated as the central method for their official protocol. However, there is scope for additional tasks to be included alongside the official valuation method, and some countries are considering the use of DCETTO as an experimental method to further explore EQ-5D-5L values.
Key discussion points
Online surveys
Stage 1 of the project consisted of two large scale online surveys, using an existing commercial internet panel, and this may raise a number of concerns. The first is the nature of the sample. Commercial online panels enable the researcher to specify the sampling frame of the respondents out of a long list of criteria (e.g. age, gender, education level, employment status, marital status, disability status, ethnicity, housing status, residential area, number of children and their age, etc.), and thus achieve ‘representativeness’ in the attributes of their choice. Nevertheless, it can only achieve representativeness in terms of observed characteristics (assuming they are correctly self-reported), and the issue of self-selection into the panel will always remain a concern (the average 75-year-old panel member may not be an average 75-year-old in the wider population). Furthermore, by definition, internet panels cannot have people without access to online computer facilities. However, it should also be noted that a similar argument could be made of other modes of recruitment. An interview survey may suffer from selection into accepting an interviewer into one’s home – something not everybody has an equal propensity to accept.
The second concern is of legitimacy. Most commercial online panels offer some form of reward to participating members. In other words, panel members are people who have put themselves forward to be surveyed for various (mostly marketing research) purposes in exchange for a financial payback. There may be a concern over using the same facility for the purpose of academic research which may subsequently inform public policy. One may argue that democratic legitimacy would require that all citizens have an equal chance of being invited to take part in research that may form the basis of policy. There are two things to note. First, it is indeed the case that some panels appear to offer significant rewards, with no restrictions on the number of surveys a given member can complete; however, other panels offer ‘points’ to be converted into donations to charities of the members’ choice, and/or have restrictions on the number of surveys a given member can complete in a month. The researcher needs to be aware of such aspects of the panel and select them wisely. Second, it is of interest to note that the stage 3 CAPI survey found the majority (51%) of face-to-face interview respondents replying positive to the question ‘Do you think it is okay to base policy on the views of people who volunteer to answer internet surveys for a small reward?’
The third concern is with respect to the quality of the actual responses. Unlike interview-based surveys, but similar to postal surveys, there is no information about the environment in which the survey was taken, and whether the respondent was sufficiently focused on the tasks. Unlike postal surveys, information on time taken between individual clicks or data on the movement of cursors could be collected and analysed, but even they cannot distinguish between a respondent who is in deep contemplation and another who is distracted by other activities. This impact of poor respondent engagement is likely to affect different methods differently, depending on how the analyses treat error and noise. Methods such as iterative TTO, which elicit exact values from each individual for each state, are more likely to be vulnerable to poor data quality than methods such as DCETTO, which collect only ordinal preferences from individuals and models them, taking into account that data contain error and noise.
When the researcher makes a decision on the mode of administration, he/she needs to weigh up the pros and cons. The major advantages of online surveys are that a sample of thousands can be achieved within weeks, at relatively low cost. PRET (including PRET-AS) was an 18-month project, and the stage 1 survey of 6000 respondents was feasible only through using online technology.
Binary choice methods
With the exception of the iterative TTO and LT-TTO used in stage 4, all of the experimental questions used in PRET were of the binary choice kind. This means most of the data collected within this project do not allow the identification of a particular health-state value for a given individual respondent. However, the distribution of respondents across each binary choice allows the analysis to infer the violation or otherwise of different assumptions involved in health-state valuation. Arguably, it is particularly suited to studies such as stage 1 of PRET, in which a large number of methodological topics were tested out on a large number of respondents in a relatively short time frame.
Of interest, however, is whether binary choice methods can be used as the main method of a health-state valuation study, which aims to produce a value set of a health-state classification system. In this project, type VIII data using binary choice LT-TTO was found to be capable of generating health-state values. Unlike iterative LT-TTO, as it does not aim to identify the point of indifference for all states from all individuals, it has the major advantage of not being affected by exhaustion of lead time. 19 At the same time, further significant developments regarding the selection of the health scenarios and the health-state modelling are required before it can be used for actual health-state valuation studies. On the other hand, DCETTO is much better developed. This project has furthered the work carried out in the development study of DCETTO with the three-level EQ-5D,22 and shown that it is capable of valuing large descriptive systems such as the EQ-5D-5L.
One fundamental challenge for the use of binary choice methods for health-state valuation is the assumption from random utility theory that the difference in value across the paired scenarios will determine the distribution of responses between the two scenarios. It has no scope to distinguish between values and strength of preference; in other words, it does not allow a strong preference over a slight difference in value. Although random utility theory may not recognise such a preference, it is clear that they exist: indeed, the DCETTO value set estimated from PRET-AS implies that the difference in value between EQ-5D-5L 11111 and 21111 is negligible, although it is quite likely that most people in a binary choice would select 11111 for a fixed duration over 21111 for the same duration (if such a choice were given).
It is sometimes suggested that binary choice methods are easier for respondents than iterative methods because although in iterative methods respondents need to give an exact value for an answer, binary methods require respondents to give only an ordinal preference. However, it is rare that respondents of an iterative exercise are asked to come up with an exact value on their own. Most iterative health-state valuation methods are designed as a series of binary choice tasks, in which the parameter of the second task onwards is determined by the response to the preceding task. In this respect, answering, for example, eight independent binary choice questions where all the parameters of the scenarios change from question to question may well be more challenging than answering eight binary choice tasks from within a single iterative question. In the latter, only one parameter is likely to change from task to task. Results reported in stage 4 of this project support this view: respondents typically found iterative TTO and LT-TTO easier than binary choice DCE or DCETTO. However, earlier work comparing DCE and TTO found that both techniques could be understood equally well and had high completion rates. 62 On the other hand, the very factor that makes iterative tasks easier may introduce bias of its own. There is a literature on contingent valuation and willingness to pay in health economics, where it has been shown that iterative tasks may be susceptible to biases because respondents do not interpret the series of binary choices as independent. 73–75 In effect, the binary choice versions of TTO and LT-TTO can be interpreted as iterative (LT-)TTO surveys, but ones in which the individual tasks are presented at random order.
The use of DCETTO to value EQ-5D-5L
The move from the three-level EQ-5D (with 243 distinct health states) to the five-level EQ-5D-5L (with 3125 distinct health states) was to allow for improved sensitivity. An increased number of health states that can be described differently do not necessarily lead to improved sensitivity unless (1) patients recognise them as distinct states for self-reporting their own health and (2) the valuation studies result in distinct coefficients for the added levels within each dimension. In this respect, it is of interest that during stages 3 and 4 of the project at least some respondents expressed uncertainty (both unprompted during the think-aloud process, and prompted as part of a follow-up question) about the relative ordering between level 4, ‘severe’, and level 5, ‘extreme’, used in two dimensions of EQ-5D-5L (Anxiety/depression and Pain/discomfort).
However, the DCETTO data in PRET-AS found that at the aggregate, with one exception, all 20 anchored level dummies were ordered within each dimension, and statistically significantly different from level 1. The only exception – mobility level 2 – had the ‘incorrect’ sign, but this was not significant. It is highly unlikely that respondents had any conceptual uncertainty regarding the ordering of level 1 ‘no problems’ and level 2 ‘slight problems’; and therefore, this non-significant coefficient for mobility level 2 is likely to be caused by actual preferences rather than a cognitive challenge. In fact, compared with value sets based on TTO data, one attraction of a value set based on DCETTO is its apparent ability to model very small decrements from full health.
Furthermore, when a series of chi-squared tests were conducted to test the null hypothesis that adjacent level dummies (i.e. levels 2 vs. 3; 3 vs. 4; 4 vs. 5) within each dimension were no different from each other, all 15 resulted in rejecting the null (13 had p < 0.001; p = 0.029 for level 4 vs. 5 in usual activities; p = 0.009 for level 2 vs. 3 in self-care: details available from authors). The overall implication thus is that although there may be individual respondents who are uncertain about the relative ordering of levels, at the aggregate, a clear pattern exists across the levels within each dimension, so that EQ-5D-5L can be valued with an online survey using DCETTO.
Respondent characteristics
For stages 1–3 of PRET and PRET-AS, the characteristics of the samples used are generally representative of the UK population in terms of age and gender. Throughout the questions, there do not seem to be any covariates that are constantly significant or never significant. In stage 1, age, gender, own health and satisfaction (with own health or life) were often found to be significant, but not always. Furthermore, marital, employment and education status tend to be found significant for the severe states (as opposed to mild states or pooled models), but again, not all of the time. The results from stage 2 indicate that different covariates affect different question types differently.
Although we have the age and gender characteristics of those who did not respond to the online surveys, we do not know how this sample differs in other ways, and how this may have had an impact on results. This may be important as we have demonstrated at stages 3 and 4 that respondent characteristics (in terms of personal factors such as having children) or subjective factors (such as experience of coping with illness) have an impact on the way in which both iterative and binary choice tasks are perceived. We have outlined some of the personal and subjective considerations that are important in the valuation process. It is possible that these could be considered in the design of valuation studies, either by directly including questions about the factors, or including them in the valuation process.
Weaknesses of the project
First, the scenarios in question types I–V in stages 1 and 2 did not use whole EQ-5D-5L states. The five health states used focused on three of the five dimensions of the instrument, and then simply described the problem (e.g. ‘extreme pain’), without spelling out the other non-existent problems (i.e. no problems walking about; no problems with . . . ). This use of partial descriptions of CSs was chosen in light of the complexity of some of the binary choice questions, and enables the issues to be tested with a focus on varying the parameters within each question type using simple health dimension descriptions. The most complex type IV question took the following structure:
-
[Scenario A]: Person P lives L in full health followed by T in state H then dies.
-
[Scenario B]: Person P lives (L + VT) in full health then dies.
As the main purpose of the exercises was not to compare the results with actual health-state preferences, but rather investigate a wide range of issues using simple binary choice questions, it was felt unnecessary to burden the respondents further by using descriptions of state H that consisted of five pieces of information.
However, there are potential limitations with this approach, which may have some implications for the sensitivity and wider applicability of the findings related to the methodological issues tested by question types I–V. For example, although respondents were explicitly requested to assume that they have no other health problems other than those indicated, we do not know what assumptions respondents made when answering the questions. There is the possibility that, instead of imagining the intended corner health state, respondents used their own understanding of health and assumptions about related health and social problems to fill in the gaps (e.g. extreme pain is likely to involve other problems). This may impact on the findings, and has implications for the analysis on time preference as the derivation of the time preference range is based on an assumed value of V* used by the respondent. However, it should be noted that the qualitative work at stage 4 suggests that even when respondents are presented with whole EQ-5D-5L health states, many will use assumptions about how the state impacts on a wide range of other health-related areas. If partial descriptions of CSs are used in future methodological research it would be useful if qualitative work similar to that carried out for stage 4 could be used at the pilot stage to investigate this.
A related issue is the choice of the value of V used for question types I–V. The values (0.8 and 0.9 for the mild states and 0.4 and 0.6 for the severe states) were arrived at using the comparable health-state values from the MVH TTO tariff. The very high proportion of respondents choosing the full health option suggests that the values selected could have been lower, and it is possible that the sensitivity of the hypothesis testing has been impacted upon by this. We were also restricted in the number of V values that could be used owing to the range of questions included in the stage 1 online survey, However, it should be noted that the values of V were not used to produce cardinal health-state values, but rather, to examine a set of methodological issues and as such are useful to compare across the various question types.
Second, the respondents to stages 1–3 are limited to those aged < 65 years. This was a limitation arising from the use of an online panel in stage 1, and in order to keep in line with this, stages 2 and 3 did not recruit people aged ≥ 65 years. Although the inclusion of older respondents would have been more preferable, given the likely self-selection to online panels discussed above, it would have had its own problems.
Third, the design of the binary choice questions used in stage 1 was not the most efficient. There were a number of collinearities in the design, which resulted in a reduction in the overall number of combinations of scenarios that could be compared across types. The wording of the satisfaction levels, and their combination with health states were also potentially problematic (e.g. the combination of extreme problems with high satisfaction). The extent of the impact of these missed opportunities is not clear.
Fourth, we imposed minimum time limits to the online surveys of 5 minutes for PRET and 3 minutes for PRET-AS. The values were chosen following the pilot launch of the survey but were selected in a fairly arbitrary manner. We have been unable to assess how the results may have been impacted upon using overall time cut-off points below the minimum, and this limits the wider applicability of the findings. Future research may investigate responses in more detail, for example by using a wide range of time cut-off points, or recording the time taken to complete each task.
Fifth, owing to the limitation in funds and time, the topics addressed in stages 3 and 4 of the project are somewhat restricted compared with the breadth of topics explored in the earlier stages. Furthermore, although the in-depth think-aloud exploration of the thought processes that respondents use when answering health-state valuation exercises was highly informative, it was conducted using a somewhat skewed sample. Given the nature of the exercise, the aim was not to survey a representative sample, but there is the scope that recruiting from a wider range of respondents may have resulted in additional themes emerging.
Areas of possible future research
A number of areas can be identified for further research, and these are listed below.
-
Further research into DCE TTO The data from the PRET-AS study can be re-analysed by treating duration as categorical. This will enable the incorporation of duration dependent time preference, if deemed appropriate. Further developments requiring new DCETTO data include the use of more levels in duration, and the examination of the influence of different experimental designs, including the selection of more health scenario pairs with different durations. It is anticipated that DCETTO may be used in studies to value other generic and condition-specific classification systems, and, as such, will allow these issues to be tested further.
-
Further development of new binary choice methods Two new types of binary choice questions may merit further exploration. One is the binary choice LT-TTO, which avoids the problem of exhausting lead time, and the other is binary choice TTO, which is significantly simpler than DCETTO or LT-TTO but suffers from the inability to value states worse than dead. A hybrid binary choice design, which blends TTO and LT-TTO, may be of interest.
-
Online survey quality In terms of the quality of online surveys, empirical comparisons across different commercial internet panels, by replicating stage 2 of PRET, may be of interest. This would allow the exploration of whether specific methodological aspects of health-state valuation are more or less affected by the panel used.
-
Qualitative methods Stages 3 and 4 of the project can be improved upon in two ways. First is to widen the range of topics and issues addressed. The project focused on contrasting the valuation methods themselves, and did not pursue how factors such as satisfaction, perspective or timing are interpreted by respondents. Second is to widen the respondent base for the think-aloud study, which had a small and unrepresentative sample.
-
PRET online survey stage 1 Many of the stage 1 results are not clear-cut, because they depend on the state (and sometimes on duration). Regression results based on pooled data and controlling for state may mask the heterogeneity across the dimensions and levels of the health-state problem. Future research may look into the interactions between the health problem, its severity and the methodological topic.
Conclusions
The PRET and PRET-AS have conducted a series of empirical work surveying over 6500 respondents, across four stages. The overall project has examined a number of key topics associated with the valuation of hypothetical health states, in particular the EQ-5D-5L. The first stage had a very wide coverage, across eight topics, and these were explored using binary choice questions in large scale online surveys. The second stage compared a version of the online survey with a CAPI using identical questions. The third and fourth stages focused on more specific issues and explored them in increasing detail, using CAPIs and qualitative analysis. One theme that emerged from stage 1 was the relevance of health states themselves. The effects of duration, perspective, timing, and satisfaction were all somewhat different across different health states. Time preference also depended on duration. The other findings indicate that DCETTO is a promising approach, and that binary choice tasks are robust to an online administration. Binary choice LT-TTO has scope to be adapted for an online delivery, but the risk of increased exhaustion of lead time needs to be examined further.
Acknowledgements
The authors would like to thank the Medical Research Council (MRC)-National Institute for Health Research (NIHR) Methodology Research Programme for funding the PRET project (Preparatory study for the Re-valuation of the EQ-5D Tariff, MRC ref. G0901500), and the EuroQol Group for funding the PRET-AS project (Preparatory study for the Re-valuation of the EQ-5D Tariff – Additional Sample) as an extension to the PRET project with formal agreement from the MRC.
We are grateful to all respondents who took part. We would like to thank Dr Pelham Barton (type VII), Jill Carlton (stage 4), Sam England (PRET-AS type VII), Professor Jan van Busschbach (all stages) Professor Ben van Hout (all stages), Andy Jamieson (PRET-AS), Dr Clara Mukuria (PRET type V), Dr Tess Peasgood (PRET type V), Professor Julie Ratcliffe (stage 2), Professor Mandy Ryan (PRET-AS type VII), Professor Joshua Salomon (PRET-AS) and Liam Williamson (PRET type II) for their input.
Research ethics approval has been given by the ethics committee at the School of Health and Related Research in line with University of Sheffield research governance and ethics requirements.
Contributions of authors
Brendan Mulhern (Research Fellow in Health Economics) was involved in the design and analysis of all stages of PRET and PRET-AS; and was involved in the writing of each section of this report.
Dr Nick Bansback (Health Economist) contributed to the design of each stage of PRET and PRET-AS, and was jointly responsible for the analysis and reporting of the PRET-AS type VII DCETTO study.
Professor John Brazier (Professor of Health Economics) contributed to the conception of PRET and PRET-AS, and contributed to the reporting of stage 4.
Dr Ken Buckingham (Health Economist) contributed to the conception and design of PRET and PRET-AS type VIII, and was jointly responsible for the analysis of the question types linked to LT-TTO (types III, VI and VIII).
Professor John Cairns (Professor of Health Economics) was involved in the design of PRET, and the analysis and reporting of the time preference sections of the report.
Professor Nancy Devlin (Director of Research, Office of Health Economics) was involved in the conception and design of all stages of PRET and PRET-AS type VIII, was jointly responsible for the analysis relating to LT-TTO (types III, IV and VIII) and was involved in writing the LT-TTO, stage 2 and stage 3 sections of this report.
Professor Paul Dolan (Professor of Behavioural Science) was involved in the design of PRET stage 1, and was jointly responsible for the analysis and report writing for the satisfaction questions.
Dr Arne Risa Hole (Senior Lecturer in Economics) was involved in the design of PRET stage 1 and PRET-AS; and was jointly responsible for the analysis of the type VII DCETTO study.
Dr Georgios Kavetsos (Research Fellow in Social Policy) was jointly responsible for the analysis and reporting of the PRET type V satisfaction results.
Dr Louise Longworth (Reader in Health Economics) was involved in the design of PRET stage 1 and 2, was responsible for the analysis and reporting of the PRET type II perspective questions, and contributed to the reporting of stage 2.
Dr Donna Rowen (Research Fellow in Health Economics) contributed to the design of all stages of PRET and PRET-AS, and was involved in writing the report for stages 2, 3 and 4.
Professor Aki Tsuchiya (Professor of Health Economics) was the principal investigator of both PRET and PRET-AS; oversaw both projects, and contributed to the conception, design, analysis and reporting of every stage.
The main author of Chapter 3 was Professor Aki Tsuchiya.
The main author of Chapter 4 was Dr Louise Longworth.
The main authors of Chapter 5 were Dr Ken Buckingham, Professor Nancy Devlin, Brendan Mulhern and Professor Aki Tsuchiya.
The main author of Chapter 6 was Professor John Cairns.
The main authors of Chapter 7 were Dr Georgios Kavetsos and Professor Paul Dolan.
The main authors of Chapter 8 were Dr Nick Bansback, Dr Arne Risa Hole, Brendan Mulhern and Professor Aki Tsuchiya.
Disclaimers
This report presents independent research funded by the National Institute for Health Research (NIHR). The views and opinions expressed by authors in this publication are those of the authors and do not necessarily reflect those of the NHS, the NIHR, the MRC, NETSCC, the HTA programme or the Department of Health.
References
- Brooks R. EuroQol: The current state of play. Health Policy 1996;37:53-72. http://dx.doi.org/10.1016/0168-8510(96)00822-6.
- Guide to the Methods of Technology Appraisal. London: NICE; 2008.
- Dolan P. Modeling valuations for EuroQol health states. Med Care 1997;35:1095-108. http://dx.doi.org/10.1097/00005650-199711000-00002.
- Browne J, Jamieson L, Lawsey J, van der Meulen J, Black N, Cairns J, et al. Patient Reported Outcomes Measures (PROMs) in Elective Surgery. London: Department of Health; 2007.
- Herdman M, Gudex C, Lloyd A, Kind P, Parkin D, Bonsel G, et al. Development and preliminary testing of the new five-level version of EQ-5D (EQ-5D-5L). Qual Life Res 2011;20:1727-36. http://dx.doi.org/10.1007/s11136-011-9903-x.
- Tsuchiya A, Mulhern B. Preparation for the Re-valuation of the EQ-5D Tariff (PRET) project: overview of methods for project stages 1–3. HEDS Discussion Paper 2011;11/16.
- Torrance GW, Thomas W, Sackett D. A utility maximization model for evaluation of health care programmes. Health Serv Res 1972;7:118-33.
- Gudex C. Time Trade-Off User Manual: Props and Self-Completion Methods. York: Centre for Health Economics, University of York; 1994.
- Mulhern B, Rowen D, Jacoby A, . The development of a QALY measure for epilepsy: NEWQOL-6D. Epilepsy Behav 2012;24:36-43. http://dx.doi.org/10.1016/j.yebeh.2012.02.025.
- Rowen D, Brazier JE, Young T, Gaugris S, Craig BM, King MT, et al. Deriving a preference-based measure for cancer using the EORTC QLQ-C30. Value Health 2011;14:721-31. http://dx.doi.org/10.1016/j.jval.2011.01.004.
- Rowen D, Mulhern B, Banerjee S, van Hout B, Knapp M, Smith S, et al. Estimating preference based single index measures for dementia using DEMQOL and DEMQOL-Proxy. Value Health 2012;15:323-33. http://dx.doi.org/10.1016/j.jval.2011.10.016.
- Yang Y, Brazier J, Tsuchiya A, Coyne K. Estimating a preference-based index from the Overactive Bladder questionnaire. Value Health 2008;12:59-66.
- Yang Y, Brazier JE, Tsuchiya A, Young T. Estimating a preference based index for a 5-dimensional health state classification for asthma derived from the asthma quality of life questionnaire. Med Decis Making 2011;31:281-91. http://dx.doi.org/10.1177/0272989X10379646.
- Lamers L. The transformation of utilities for health states worse than dead: Consequences for the estimation of EQ-5D value sets. Med Care 2007;45:238-44.
- Craig BM, van Busschbach JJV. Towards a more universal approach in health state valuation. Health Econ 2011;20:864-75.
- Devlin N, Tsuchiya A, Buckingham K, Tilling C. A Uniform Time Trade Off Method for States Better and Worse than Dead: Feasibility Study of the ‘Lead Time’ Approach. Health Econ 2011;20:348-61. http://dx.doi.org/10.1002/hec.1596.
- Robinson A, Spencer A. Exploring challenges to TTO utilities: valuing states worse than dead. Health Econ 2006;15:393-402. http://dx.doi.org/10.1002/hec.1069.
- Tilling C, Devlin N, Tsuchiya A, Buckingham K. Protocols for time trade off valuations of health states worse than dead: a literature review. Med Decis Making 2010;30:610-19.
- Devlin N, Buckingham K, Shah K, Tsuchiya A, Tilling C, Wilkinson G, et al. A comparison of alternative variants of lead and lag time TTO. Health Econ 2013;22:517-32. http://dx.doi.org/10.1002/hec.2819.
- de Bekker-Grob EW, Ryan M, Gerard K. Discrete choice experiments in health economics: a review of the literature. Health Econ 2012;20:145-72. http://dx.doi.org/10.1002/hec.1697.
- Rowen D, Brazier J, van Hout B. A comparison of methods for converting DCE values onto the full health–dead QALY scale. HEDS Discussion Paper 2011;11/15.
- Bansback N, Brazier J, Tsuchiya A, Anis A. Using a discrete choice experiment to estimate societal health state utility values. Health Econ 2012;31:306-18.
- Brazier JE, Roberts J. Estimating a preference-based index from the SF-12. Med Care 2004;42:851-9.
- Brazier J, Roberts J, Deverill M. The estimation of a preference-based measure of health from the SF-36. J Health Econ 2002;21:271-92. http://dx.doi.org/10.1016/S0167-6296(01)00130-8.
- Brazier J, Akehurst R, Brennan A, Dolan P, Claxton K, McCabe C, et al. Should patients have a greater role in valuing health states?. Appl Health Econ Health Policy 2005;4:201-8. http://dx.doi.org/10.2165/00148365-200504040-00002.
- Krabbe PFM, Tromp N, Ruers TJM, van Riel PLCM. Are patients’ judgments of health status really different from the general population?. Health Qual Life Outcomes 2011;9. http://dx.doi.org/10.1186/1477-7525-9-31.
- McTaggart-Cowan H, Tsuchiya A, O’Cathain A, Brazier J. Understanding the effect of disease adaptation information on general population values for hypothetical health states. Soc Sci Med 2011;72:1904-12. http://dx.doi.org/10.1016/j.socscimed.2011.03.036.
- Tsuchiya A, Miyamoto J, Anand P, Puppe C, Pattanaik P. Handbook of Rational and Social Choice. Oxford: Oxford University Press; 2009.
- Viney R, Norman R, King MT, Cronin P, Street DJ, Knox S, et al. Time trade off EQ-5D weights for Australia. Value Health 2011;14:928-36. http://dx.doi.org/10.1016/j.jval.2011.04.009.
- Wittrup-Jensen KU, Lauridsen J, Gudex C, Pedersen KM. Generation of a Danish TTO value set for EQ-5D health states. Scand J Public Health 2009;37:459-66. http://dx.doi.org/10.1177/1403494809105287.
- Norman R, King MT, Clarke D, . Does mode of administration matter? Comparison of online and face-to-face administration of a time trade-off task. Qual Life Res 2010;19:499-508. http://dx.doi.org/10.1007/s11136-010-9609-5.
- Robinson A, Covey J, Jones-Lee M, Loomes G. Comparing the Results of Face-to-face and Web Based PTO Exercises. East Anglia, UK: Health Economics Study Group; 2008.
- Damschroder LJ, Baron J, Hershey JC, . The validity of person tradeoff measurements: randomized trial of computer elicitation versus face-to-face interview. Med Decis Making 2004;4:170-80. http://dx.doi.org/10.1177/0272989X04263160.
- Bridges JFP, Hauber AB, Marshall D, Lloyd A, Prosser LA, Regier DA, et al. Conjoint analysis applications in health – a checklist: a report of the ISPOR good research practices for conjoint analysis task force. Value Health 2011;4:403-13. http://dx.doi.org/10.1016/j.jval.2010.11.013.
- Tsuchiya A, Dolan P. The QALY model and individual preferences for health states and health profiles over time: a systematic review of the literature. Med Decis Making 2005;25:460-7. http://dx.doi.org/10.1177/0272989X05276854.
- Stalmeier PF, Lamers LM, Busschbach JJ, Krabbe PF. On the assessment of preferences for health and duration: maximal endurable time and better than dead preferences. Med Care 2007;45:835-41. http://dx.doi.org/10.1097/MLR.0b013e3180ca9ac5.
- van der Pol M, Cairns JA. Negative and zero time preference for health. Health Econ 2000;9:171-5. http://dx.doi.org/10.1002/(SICI)1099-1050(200003)9:2<171::AID-HEC492>3.3.CO;2-Q.
- Chapman GB, Elstein AS. Valuing the future: temporal discounting of health and money. Med Decis Making 1995;5:373-86. http://dx.doi.org/10.1177/0272989X9501500408.
- Cairns JA, Drummond MF, McGuire A. Theory and Practice of Economic Evaluation in Health Care. Oxford: Oxford University Press; 2001.
- Census 2001: General report for England and Wales. London: Office for National Statistics; 2005.
- Dolan P, Metcalfe R. Comparing Measures of Subjective Well-being and View About the Role They Should Play in Policy. London: Office for National Statistics; 2011.
- Dolan P, Stalmeier PFM. The validity of time trade-off values in calculating QALYs: constant proportional time trade-off versus the proportional heuristic. J Health Econ 2003;22:445-58. http://dx.doi.org/10.1016/S0167-6296(02)00120-0.
- Bleichrodt H, Pinto JL, Abellan-Perpinan JM. A consistency test of the time trade-off. J Health Econ 2003 n.d.;22:1037-52. http://dx.doi.org/10.1016/S0167-6296(03)00046-8.
- Bosch JL, Kammitt JK, Weinstein MC, Hunink MGM. Estimating general-population utilities using one binary-gamble question per respondent. Med Decis Mak 1998;18:381-90. http://dx.doi.org/10.1177/0272989X9801800405.
- Bansback N, Hole AR, Mulhern B, Tsuchiya A. Using a discrete choice experiment with duration to value health states: a feasibility study using EQ-5D-5L. Rotterdam, The Netherlands: EuroQol Group Plenary; 2012.
- Bansback N, Hole AR, Mulhern B, Tsuchiya A. Using a discrete choice experiment with duration to value health states: a feasibility study using EQ-5D-5L. Oxford, UK: Health Economics Study Group; 2012.
- Ryan M, Netten A, Skatun D, Smith P. Using discrete choice experiments to estimate a preference-based measure of outcome: an application to social care for older people. J Health Econ 2006;25:927-44. http://dx.doi.org/10.1016/j.jhealeco.2006.01.001.
- Ratcliffe J, Brazier J, Tsuchiya A, Symonds T, Brown M. Using DCE and ranking data to estimate cardinal values for health states for deriving a preference-based single index from the sexual quality of life questionnaire. Health Econ 2009;18:1261-76. http://dx.doi.org/10.1002/hec.1426.
- Carlsson F, Martinsson P. Design techniques for stated preference methods in health economics. Health Econ 2003;12:281-94. http://dx.doi.org/10.1002/hec.729.
- Kuhfeld WF. Marketing Research Methods in SAS. Cary, NC: SAS Institute Inc.; 2005.
- Swait J, Louviere J. The role of the scale parameter in the estimation and comparison of multinomial logit models. J Marketing Res 1993;30:305-14. http://dx.doi.org/10.2307/3172883.
- Hole AR. Statistical Software Components S456737. Boston, MA, USA: Boston College Department of Economics; 2006.
- Hole AR. Small-sample properties of tests for heteroscedasticity in the conditional logit model. Economics Bulletin 2006;3:1-14.
- Roberts J, Dolan P. To what extent do people prefer health states with higher values? A note on evidence from the EQ-5D valuation set. Health Econ 2004;13:733-7. http://dx.doi.org/10.1002/hec.875.
- Mulhern B, Tsuchiya A, Rowen D, Devlin N, Bansback N, Longworth L, et al. Health state valuation questions: head to head comparison on online and CAPI. Oxford, UK: EuroQol Group Plenary; 2011.
- Mulhern B, Tsuchiya A, Rowen D, Devlin N, Bansback N, Longworth L, et al. Health state valuation questions: does mode of administration matter?. Bangor, UK: Health Economics Study Group; 2011.
- van Hout B, Janssen MF, Feng YS, Kohlmann T, Busschbach J, Golicki D, et al. Interim Scoring for the EQ-5D-5L: Mapping the EQ-5D-5L to EQ-5D-3L Value Sets. Value Health 2012;15:708-15. http://dx.doi.org/10.1016/j.jval.2012.02.008.
- Liu H, Cella D, Gershon R, Shen J, Morales LS, Riley W, et al. Representativeness of the patient-reported outcomes measurement information system internet panel. J Clin Epidemiol 2010;63:1169-78. http://dx.doi.org/10.1016/j.jclinepi.2009.11.021.
- Robling MR, Ingledew DK, Greene G, Sayers A, Shaw C, Sander L, et al. Applying an extended theoretical framework for data collection mode to health services research. BMC Health Serv Res 2010;10. http://dx.doi.org/10.1186/1472-6963-10-180.
- Paulhus DL. Two-component models of socially desirable responding. J Pers Soc Psychol 1984;46:598-609. http://dx.doi.org/10.1037/0022-3514.46.3.598.
- Mulhern B, Tsuchiya A, Devlin N, Buckingham K, Rowen D, Brazier J. A comparison of three binary choice methods for health state valuation. Aix-en-Provence, France: Health Economics Study Group; 2012.
- Ratcliffe J, Couzner L, Flynn T, Sawyer M, Stevens K, Brazier J, et al. Valuing child health utility 9D health states with a Young Adolescent Sample: a feasibility study to compare best–worst scaling discrete choice experiment, standard gamble and time trade-off methods. Appl Health Econ Health Policy 2011;9:5-27. http://dx.doi.org/10.2165/11536960-000000000-00000.
- Robinson A, Dolan P, Williams A. Valuing health states using VAS and TTO: what lies behind the numbers?. Soc Science Med 1997;45:1289-97.
- San Miguel F, Ryan M, Amaya-Amaya M. ‘Irrational’ stated preferences: a quantitative and qualitative investigation. Health Econ 2005;14:307-22. http://dx.doi.org/10.1002/hec.912.
- Ryan M, Watson V, Entwistle V. Rationalising the ‘irrational’: a think aloud study of discrete choice experiment responses. Health Econ 2009;18:321-36. http://dx.doi.org/10.1002/hec.1369.
- Dolan P, Roberts J. To what extent can we explain time trade-off values from other information about respondents?. Soc Sci Med 2002;54:919-29. http://dx.doi.org/10.1016/S0277-9536(01)00066-1.
- Jansen SGT, Stigelbout AM, Wakker PP, Nooij MA, Noordijk EM, Kievit J. Unstable preferences: a shift in valuation or an effect of the elicitation procedure?. Med Decis Making 2002;20:62-71. http://dx.doi.org/10.1177/0272989X0002000108.
- Wittenburg E, Prosser LA. Ordering errors, objections and invariance in utility survey responses: A framework for understanding who, why and what to do. Appl Health Econ Health Policy 2011;9:225-41. http://dx.doi.org/10.2165/11590480-000000000-00000.
- Luo N, Li M, Chevalier J, Lloyd A, Herdman M. A cross-cultural study of the scale labels in the EQ-5D-3L and EQ-5D-5L descriptive systems. Qual Life Res 2012;20.
- Mulhern B, Tsuchiya A, Brazier J, Rowen D. How do respondents perceive health state valuation exercises? A ‘think aloud’ study investigating time trade off and discrete choice experiments. Rotterdam, The Netherlands: EuroQol Group Plenary; 2012.
- Krabbe P, Devlin N, de Charro F, van Hout B, Oppe M, Bakker G. Protocol to value the EQ-5D-5L. Rotterdam: The EuroQol Group; 2012.
- Cheraghi-Sohi S, Bower P, Mead N, McDonald R, Whalley D, Roland M. Making sense of patient priorities: applying discrete choice methods in primary care using ‘think aloud’ technique. Fam Pract 2007;24:276-82. http://dx.doi.org/10.1093/fampra/cmm007.
- Frew EJ, Wolstenholme JL, Whynes DK. Comparing willingness-to-pay: bidding game format versus open-ended and payment scale formats. Health Policy 2004;68:289-98. http://dx.doi.org/10.1016/j.healthpol.2003.10.003.
- McNamee P, Ternent L, Gbangou A, Newlands D. A game of two halves? Incentive incompatibility, starting point bias and the bidding game contingent valuation method. Health Econ 2010;19:75-87.
- Stalhammar NO. An empirical note on willingness to pay and starting-point bias. Med Decis Making 1996;16:242-7. http://dx.doi.org/10.1177/0272989X9601600308.
Appendix 1 PRET stage 1/CAPI online survey screenshots (version 15)
Page 1: Information page (part 1):
Page 2: Information (part 2):
Page 3: Respondent consent form:
Page 4: Demographic questions (part 1):
Please note that for stages 3 and 4, extra demographic questions about having children and dependants were added, along with a question about experiences of illness.
Page 5: Demographic questions (part 2)
Page 6: EQ-5D-5L
Page 7: Self-reported health and satisfaction with health and life questions
Page 8: Instruction page
The example question used at this stage for the PRET-AS online survey was either a type VII or a type VIII question. The example used for PRET stage 3 was a type VII, type VIII or type IX question, depending on the survey version completed.
Page 9: Question module 1 (type I questions)
Page 10: Question module 2
Page 11: Question module 3 (question type VII)
Page 12: Final page with free text response box
Appendix 2 Question format as presented in the online and CAPI surveys
Appendix 3 Allocation of attribute levels for each question type included in the surveys
Type I attribute combinations
T | 10 weeks | 1 year | 5 years | 10 years | ||||
---|---|---|---|---|---|---|---|---|
V | V(low) | V(high) | V(low) | V(high) | V(low) | V(high) | V(low) | V(high) |
Slight problems walking about | ✓✓ | ✓ | ✓✓ | ✓✓ | ✓✓ | ✓✓ | ✓✓ | ✓✓ |
Slight pain or discomfort | ✓✓ | ✓✓ | ✓ | ✓✓ | ✓✓ | ✓✓ | ✓✓ | ✓✓ |
Unable to walk about | ✓✓ | ✓✓ | ✓✓ | ✓✓ | ✓✓ | ✓ | ✓✓ | ✓✓ |
Extreme pain or discomfort | ✓✓ | ✓✓ | ✓✓ | ✓✓ | ✓✓ | ✓✓ | ✓✓ | ✓ |
Extremely anxious or depressed | ✓✓ | ✓✓ | ✓✓ | ✓✓ | ✓ | ✓✓ | ✓✓ | ✓✓ |
Type II attribute combinations
T | 10 weeks | 1 year | 5 years | 10 years | ||||||||||||
---|---|---|---|---|---|---|---|---|---|---|---|---|---|---|---|---|
V | V(low) | V(high) | V(low) | V(high) | V(low) | V(high) | V(low) | V(high) | ||||||||
Perspective | SY | SE | SY | SE | SY | SE | SY | SE | SY | SE | SY | SE | SY | SE | SY | SE |
Slight problems walking about | ✓ | ✓ | ||||||||||||||
Slight pain or discomfort | ✓ | ✓ | ✓ | ✓ | ||||||||||||
Unable to walk about | ✓ | ✓ | ||||||||||||||
Extreme pain or discomfort | ✓ | ✓ | ✓ | ✓ | ||||||||||||
Extremely anxious or depressed | ✓ | ✓ | ✓ | ✓ |
Type III attribute combinations
T | 10 weeks | 1 year | 5 years | 10 years | ||||||||||||
---|---|---|---|---|---|---|---|---|---|---|---|---|---|---|---|---|
L | 10 weeks | 1 year | 5 years | 10 weeks | 1 year | 5 years | 10 years | 10 weeks | 1 year | 5 years | 10 years | 1 year | 5 years | 10 years | ||
V | V(low) | V(high) | V(low) | V(high) | V(high) | V(high) | V(low) | V(high) | V(low) | V(high) | V(high) | V(low) | V(low) | V(high) | V(low) | V(high) |
Slight problems walking about | ✓ | ✓ | ✓ | |||||||||||||
Slight pain or discomfort | ✓ | ✓ | ✓ | ✓ | ||||||||||||
Unable to walk about | ✓ | ✓ | ✓ | |||||||||||||
Extreme pain or discomfort | ✓ | ✓ | ✓ | |||||||||||||
Extremely anxious or depressed | ✓ | ✓ | ✓ |
Type IV attribute combinations
T | 10 weeks | 1 year | 5 years | 10 years | ||||||||||||
---|---|---|---|---|---|---|---|---|---|---|---|---|---|---|---|---|
L | 10 weeks | 10 weeks | 1 year | 5 years | 10 years | 5 years | 10 years | |||||||||
P | SE | SY | SE | SY | SE | SY | SE | SY | SE | SY | V(low) | |||||
V | V(low) | V(high) | V(low) | V(high) | V(low) | V(low) | V(high) | V(high) | V(high) | V(high) | V(low) | V(low) | V(high) | V(high) | V(low) | |
Slight problems walking about | ✓ | ✓ | ||||||||||||||
Slight pain or discomfort | ✓ | ✓ | ✓ | ✓ | ||||||||||||
Unable to walk about | ✓ | ✓ | ||||||||||||||
Extreme pain or discomfort | ✓ | ✓ | ✓ | ✓ | ||||||||||||
Extremely anxious or depressed | ✓ | ✓ | ✓ | ✓ |
Type V attribute combinations
5 years | ||||
---|---|---|---|---|
HS | LS | LL | ||
Low | High | High | High | |
V(high) | V(high) | V(high) | V(high) | |
Slight problems walking about | ||||
Slight pain | ✓ | |||
Unable to walk about | ✓ | ✓ | ✓ | ✓ |
Extreme pain | ✓ | ✓ | ✓ | ✓ |
Extremely depressed | ✓ | ✓ |
Type VI attribute combinations
Lead time | Duration (T) | |||
---|---|---|---|---|
10 weeks | 1 year | 5 years | 10 years | |
10 weeks (0.2 years) | ✓✓ | ✓ | ||
1 year | ✓ | ✓✓ | ✓ | |
5 years | ✓ | ✓ | ✓✓ | ✓ |
10 years | ✓ | ✓ | ✓✓ |
Type VII attribute combinations
Version | Health scenario A | T | Health scenario B | T | Version | Health scenario A | T | Health scenario B | T | Version | Health scenario A | T | Health scenario B | T |
---|---|---|---|---|---|---|---|---|---|---|---|---|---|---|
V1a | 51241 | 5 | 33335 | 5 | V6a | 31455 | 5 | 32232 | 1 | V11a | 51552 | 10 | 45213 | 10 |
15212 | 10 | 42224 | 5 | 22112 | 10 | 35544 | 10 | 24253 | 1 | 45141 | 1 | |||
V1b | 12541 | 1 | 43252 | 1 | V6b | 11551 | 1 | 55215 | 1 | V11b | 12553 | 10 | 25432 | 10 |
44112 | 10 | 52434 | 10 | 14512 | 10 | 51223 | 10 | 25141 | 5 | 45421 | 1 | |||
V1c | 11131 | 10 | 52315 | 10 | V6c | 13441 | 10 | 54522 | 10 | V11c | 24153 | 10 | 14512 | 5 |
51453 | 5 | 14244 | 5 | 21424 | 1 | 12135 | 1 | 51123 | 5 | 34551 | 5 | |||
V1d | 44445 | 5 | 25214 | 5 | V6d | 43522 | 5 | 14314 | 5 | V11d | 54242 | 5 | 11124 | 5 |
54523 | 1 | 31412 | 1 | 25513 | 10 | 44321 | 10 | 12324 | 1 | 33133 | 1 | |||
V2a | 25415 | 5 | 13353 | 5 | V7a | 22255 | 5 | 31512 | 5 | V12a | 52134 | 5 | 25422 | 5 |
54241 | 1 | 32534 | 1 | 53525 | 1 | 44113 | 1 | 33452 | 1 | 44134 | 1 | |||
V2b | 23444 | 5 | 24551 | 10 | V7b | 45413 | 5 | 51232 | 5 | V12b | 32221 | 5 | 25132 | 5 |
43541 | 10 | 15133 | 10 | 42131 | 10 | 24245 | 10 | 13332 | 1 | 55223 | 5 | |||
V2c | 22345 | 1 | 15532 | 1 | V7c | 41341 | 5 | 54153 | 5 | V12c | 34131 | 5 | 12353 | 5 |
25321 | 10 | 33114 | 10 | 22555 | 10 | 53142 | 10 | 14331 | 10 | 45452 | 10 | |||
V2d | 54111 | 5 | 12432 | 5 | V7d | 25241 | 1 | 33251 | 10 | V12d | 11454 | 1 | 35321 | 1 |
51335 | 1 | 41523 | 10 | 34431 | 1 | 55553 | 1 | 43454 | 10 | 32145 | 10 | |||
V3a | 51411 | 10 | 23134 | 10 | V8a | 35323 | 5 | 11435 | 5 | V13a | 52325 | 5 | 43253 | 5 |
32143 | 5 | 13215 | 5 | 53215 | 10 | 22443 | 10 | 42413 | 1 | 34144 | 1 | |||
V3b | 41125 | 1 | 22231 | 1 | V8b | 53515 | 5 | 15431 | 5 | V13b | 15524 | 10 | 33353 | 10 |
42112 | 5 | 54424 | 5 | 42555 | 1 | 15431 | 5 | 35332 | 5 | 42324 | 10 | |||
V3c | 33114 | 1 | 51141 | 10 | V8c | 54451 | 1 | 22314 | 1 | V13c | 11315 | 1 | 42542 | 1 |
41233 | 10 | 23324 | 10 | 22413 | 10 | 45155 | 10 | 21342 | 10 | 54411 | 10 | |||
V3d | 45351 | 10 | 31224 | 10 | V8d | 55531 | 5 | 21142 | 5 | V13d | 15243 | 5 | 22351 | 5 |
42531 | 5 | 21143 | 1 | 13225 | 10 | 54144 | 10 | 41335 | 10 | 32221 | 10 | |||
V4a | 14423 | 10 | 21211 | 10 | V9a | 41354 | 5 | 12522 | 5 | V14a | 45254 | 1 | 31323 | 1 |
21512 | 1 | 35354 | 1 | 15443 | 1 | 52122 | 1 | 21533 | 5 | 33122 | 5 | |||
V4b | 15314 | 10 | 44435 | 10 | V9b | 52412 | 5 | 55334 | 1 | V14b | 14153 | 5 | 53444 | 5 |
45554 | 5 | 33413 | 5 | 12225 | 1 | 55334 | 1 | 12242 | 10 | 31414 | 10 | |||
V4c | 32445 | 10 | 53533 | 10 | V9c | 21552 | 5 | 45135 | 5 | V14c | 55352 | 5 | 22513 | 5 |
13342 | 1 | 31511 | 1 | 23155 | 5 | 42124 | 10 | 42151 | 1 | 13314 | 5 | |||
V4d | 41515 | 5 | 54414 | 1 | V9d | 11221 | 1 | 52443 | 1 | V14d | 44243 | 10 | 52155 | 10 |
44333 | 1 | 23144 | 1 | 44212 | 5 | 13541 | 5 | 52151 | 1 | 43542 | 1 | |||
V5a | 32454 | 5 | 23331 | 5 | V10a | 14223 | 1 | 15445 | 10 | V15a | 24144 | 5 | 54514 | 1 |
34352 | 1 | 13435 | 1 | 25132 | 1 | 33543 | 1 | 25555 | 1 | 42424 | 1 | |||
V5b | 44524 | 5 | 32211 | 5 | V10b | 51133 | 1 | 44515 | 1 | V15b | 31245 | 1 | 23311 | 1 |
34435 | 10 | 31333 | 5 | 32332 | 10 | 23125 | 10 | 11315 | 10 | 35252 | 10 | |||
V5c | 44421 | 5 | 35545 | 5 | V10c | 35323 | 10 | 42234 | 10 | V15c | 25455 | 1 | 53211 | 1 |
54254 | 10 | 33522 | 10 | 15213 | 1 | 24435 | 1 | 24333 | 5 | 15151 | 5 | |||
V5d | 33234 | 5 | 14345 | 5 | V10d | 35531 | 10 | 11452 | 10 | V15d | 53543 | 10 | 31354 | 10 |
51342 | 1 | 23123 | 1 | 35422 | 1 | 43351 | 1 | 41234 | 1 | 14112 | 1 |
Type VIII question allocation
State (H) | L : T ratio | L | T | Range of value V [no. of levels] |
---|---|---|---|---|
12211 | 1 : 1 | 10 weeks | 10 weeks | 0.15, 0.35, 0.55, 0.75, 0.95 [5] |
1 year | 1 year | |||
5 years | 5 years | |||
10 years | 10 years | |||
22121 | 1 : 1 | 10 weeks | 10 weeks | 0.15, 0.35, 0.55, 0.75, 0.95 [5] |
1 year | 1 year | |||
5 years | 5 years | |||
10 years | 10 years | |||
32211 | 1 : 1 | 10 weeks | 10 weeks | −1.0, −0.75, −0.5, −0.25, −0.05, 0.0, 0.05, 0.15, 0.35, 0.55, 0.75 [11] |
1 year | 1 year | |||
5 years | 5 years | |||
10 years | 10 years | |||
2 : 1 | 20 weeks | 10 weeks | −2.0, −1.5, −1.0, −0.5, −0.25, −0.05, 0.0, 0.15, 0.35, 0.55 [10] | |
2 years | 1 year | |||
10 years | 5 years | |||
20 years | 10 years | |||
23232 | 1 : 1 | 10 weeks | 10 weeks | −1.0, −0.75, −0.5, −0.25, −0.05, 0.0, 0.05, 0.15, 0.35, 0.55, 0.75 [11] |
1 year | 1 year | |||
5 years | 5 years | |||
10 years | 10 years | |||
2 : 1 | 20 weeks | 10 weeks | −2.0, −1.5, −1.0, −0.5, −0.25, −0.05, 0.0, 0.15, 0.35, 0.55 [10] | |
2 years | 1 year | |||
10 years | 5 years | |||
20 years | 10 years | |||
33333 | 1 : 1 | 10 weeks | 10 weeks | −1.0, −0.5, −0.25, 0.0 [4] |
1 year | 1 year | |||
5 years | 5 years | |||
10 years | 10 years | |||
2 : 1 | 20 weeks | 10 weeks | −2.0, −1.0, −0.5, 0.0 [4] | |
2 years | 1 year | |||
10 years | 5 years | |||
20 years | 10 years | |||
5 : 1 | 1 year | 10 weeks | −3.0, −2.0, −1.0, −0.5, 0.0 [5] | |
5 years | 1 year | |||
25 years | 5 years | |||
50 years | 10 years | |||
10 : 1 | 100 weeks | 10 weeks | −3.0, −2.0, −1.0, −0.5, 0.0 [5] | |
10 years | 1 year |
Appendix 4 Follow-up questions used
Probing questions:
1. When answering the questions, which part of the health state was most important to you?
-
length of time spent in the health state
-
problems walking about
-
problems washing or dressing yourself
-
problems with usual activities
-
level of pain or discomfort
-
level of anxiety or depression.
2.
Question | Yes | Sometimes | No |
---|---|---|---|
There is too much information included in these scenarios so I just look at the bit that is most important to me | |||
I found it difficult to answer these questions | |||
When answering these questions, I chose the scenario with the fewest number of severe health areas | |||
Unless the state is severe, the number of years that you live for is the most important part of the scenario | |||
It is not clear what full health means | |||
It is difficult to imagine changing from full health to a poor health state so suddenly | |||
It is not realistic that time in full health is always shorter |
3. Please rank, from 1–5, which areas of health were most important when answering the questions:
-
problems walking about
-
problems washing or dressing yourself
-
problems with usual activities
-
level of pain
-
level of anxiety/depression.
4. When answering the questions, who did you imagine living in the health state?
-
yourself
-
somebody else
-
both of the above
-
neither of the above.
5. Whose health experiences had an effect on your responses to the questions?
-
my own health experiences
-
people I know who have had poor health
-
both of the above
-
neither of the above.
6.
Which set of questions: | First set | Second set | Both the same |
---|---|---|---|
Did you find the easiest? | |||
Made you think the most about the effect of the health scenario on the other people around me (e.g. family)? |
7.
Question | Strongly agree | Agree | Neutral | Disagree | Strongly disagree |
---|---|---|---|---|---|
When answering, I do not consider all of the statements, just the ones that are important to me | |||||
The layout of the questions means that they can be answered easily | |||||
It is difficult to imagine what it would actually be like to live in the scenarios | |||||
The scenarios are not realistic | |||||
There is too much to think about to give a credible answer |
8.
Question | Yes | In some situations | No |
---|---|---|---|
I can tell the difference between slight and moderate problems for each health area | |||
I can tell the difference between moderate and severe problems for each health area | |||
I can tell the difference between severe and extreme problems for each health area |
9.
Question | Yes | In some situations | No |
---|---|---|---|
I can tell the difference between slight and moderate problems for each health area (VERSIONS 1 AND 4 ONLY) | |||
I can tell the difference between moderate and severe problems for each health area (VERSIONS 2 AND 5 ONLY) | |||
I can tell the difference between severe and extreme problems for each health area (VERSIONS 3 AND 6 ONLY) |
10.
Question | Yes | No |
---|---|---|
It is hard to believe that I would be left without relief or treatment by doctors and other health professionals | ||
It is possible that my answers would change if I was asked the same questions in a week’s time |
11.
Question | Always | Often | Sometimes | Rarely | Never |
---|---|---|---|---|---|
My answers were influenced by how the health state would affect the life and well-being of those around me (e.g. my children, parents or partner) | |||||
My age and my responsibilities to others had an effect on how I answered the questions | |||||
How severe the health scenario is does not matter, I would choose to live in the scenario with a longer duration to spend time with the people close to me | |||||
The impact that living in the each health state would have on my life and my financial situation was an important consideration | |||||
How I would feel about my health and life when living in the scenarios is an important consideration |
12.
Question | Definitely yes | Probably yes | Don’t mind | Probably not | Definitely not |
---|---|---|---|---|---|
Questions similar to the health scenario questions above may be asked to different groups of people to help decide health-care policy. ‘Do you think it is okay to base policy on the views of people who volunteer to answer internet surveys for a small reward?’ |
Appendix 5 Stage 4 interview protocol
1. Ask respondent to read information sheet.
2. When they have finished reading the information sheet ask them:
After reading the information sheet, do you have any questions about the interview or what we are asking you to do?
3. Hand the respondent a copy of the consent form and explain that they need to sign it before the interview can commence. Then ensure that the respondent knows that by signing the form they are consenting to being recorded.
4. Now collect demographic and self-reported health information using the answer booklet (the demographic questions are matched with those used at other stages of PRET and are included in Appendix 4 ). Say:
We would like you to complete some questions about you and questions about your health. We will not be recording this bit of the interview, and just to remind you that all of the answers you provide will be kept confidential and only seen by members of the project team.
5. When the respondent has completed the demographic questions the interview can commence. Start recording, and read out the following introduction (also available in the answer booklet):
In this interview we are interested in hearing your opinion of a range of health states. Knowing this help improve the methods that organisations such as the National Institute for Health and Clinical Excellence use to calculate how much health improvement new drugs will bring about.
We want you to take part in what is called a think-aloud interview. We will present you with a choice of health scenarios and ask you to tell me which one you would prefer to live in, or which one you think is better. The health states are imaginary, and we are interested in your opinions of the imaginary health state, not your own health state. When answering the questions we want you to think aloud, in other words talk about the thoughts that you are having whilst making your decision. Please just say everything that comes into your head when completing the tasks, and do not explain and plan what you are going to say. There is no right or wrong answer to any of the tasks, we are just interested in your opinions. The scenarios may include a number of areas of health, with or without an associated duration that you would spend living in the health state. The health areas include full health, mobility, self care, usual activities, pain/discomfort and anxiety/depression. The scenarios also include reference to death. When answering the questions please imagine that you will experience each health state for the period shown, without relief or treatment. Please imagine that death will be very swift and completely painless. Please also imagine that you will have no other health problems besides what is indicated. Do you have any questions at this point?
6. Complete example task 1. Say:
Firstly, and to get you used to completing think-aloud tasks we will complete a couple of examples. For the first example we would like you to answer a question whilst thinking aloud about the process that you are using to answer the question, and any thoughts that you are having.
7. Turn page in answer booklet to example 1. Say:
Please look at and answer the following question. The question is: ‘How many windows are in the house that you live in?’ When you are working out the answer to this question, please just say out loud whatever is going through your mind as you answer the questions even if it seems obvious.
8. RESPONDENT ANSWERS EXAMPLE 1
9. Complete example task 2. Say:
Thank you, now we have one more example question for you to complete where you will be presented with a choice of two options and asked to think aloud about the options and how you go about making a decision.
10. Turn page in answer booklet to example 2. Say:
The question presents a choice of two holidays each with specific attributes that may influence your decision. Please look at and answer the following question [read out full question]. When you are answering the question, please just say out loud whatever is going through your mind, even if it seems obvious. Remember that there are no right or wrong answers, we just want to hear how you think about these issues.
Holiday A | Holiday B | |
---|---|---|
7 nights | 10 nights | |
Good weather | Fair weather | |
3* hotel | 4* hotel | |
Beach holiday | Beach holiday | |
Which holiday would you choose? |
11. RESPONDENT ANSWERS EXAMPLE 2
-
If the respondent answers the question but does not think out loud:
-
Follow up by saying: Thank you for your answer but I am unclear about how you came to a decision as you did not really think aloud when answering the question. Can you tell me a little more about what you were thinking as you came up with that answer?
-
Follow the respondent’s answer with: Thank you. Do you think that you now understand what we are asking you to do and are happy to move on to the scenarios incorporating health states?
-
If yes:
-
Ask if they have any further questions and answer if necessary.
-
Then move on to the health-state questions.
-
-
If no:
-
Ask what part of the task they do not understand, and make sure that the process is clear before moving on to the health-state tasks.
-
-
-
If the respondent thinks out loud while answering the question, say:
-
Thank you. Do you think that you now understand what we are asking you to do and are happy to move on to the scenarios incorporating health states? Do you have any further questions?
-
Answer questions if any.
-
If they understand:
-
Move on to the health-state tasks.
-
-
If they do not understand:
-
Ask what part of the task they do not understand, and make sure that the process is clear before moving on to the health-state tasks.
-
-
12. Complete health-state task set that will be displayed in the answer booklet (see section D). For binary choice tasks, say:
Now we will move on to the tasks involving health. Please look at and answer the following question. When you are doing this, please just say out loud whatever is going through your mind as you answer the questions even if it seems obvious. There are no right or wrong answers, we just want to hear how you think about these issues.
-
Read through question and attributes and then remind respondent to think aloud.
-
If respondent is not thinking aloud use follow-up prompt: Can you tell me a little more about what you were thinking as you came up with that answer?
-
Then move on to probing questions. We will only ask questions if similar concepts have not been covered during the think-aloud process.
-
When task is iterative:
-
Ask them about the process following the better or worse than dead screen question, prompt during the iterative process if they are not thinking aloud (by saying: Please continue to think aloud whilst completing the task.) Ask them to expand at the end of the process if required by saying: Can you tell me a little more about what you were thinking as you came up with that answer? Then ask follow-up probe questions if required.
-
13. Repeat process for each valuation task included in the task set.
14. At the end of the valuation task set, ask the respondent if they have any further comments about the scenarios or study.
Appendix 6 Examples of stage 4 valuation tasks
DCE
DCETTO
Better or worse than dead screener question
TTO and LT-TTO tasks
TTO
Taken with permission from Gudex C, editor. Time Trade-Off User Manual: Props and Self-Completion Methods. CHE Occasional Paper 20. York: Centre for Health Economics, University of York; 1994.
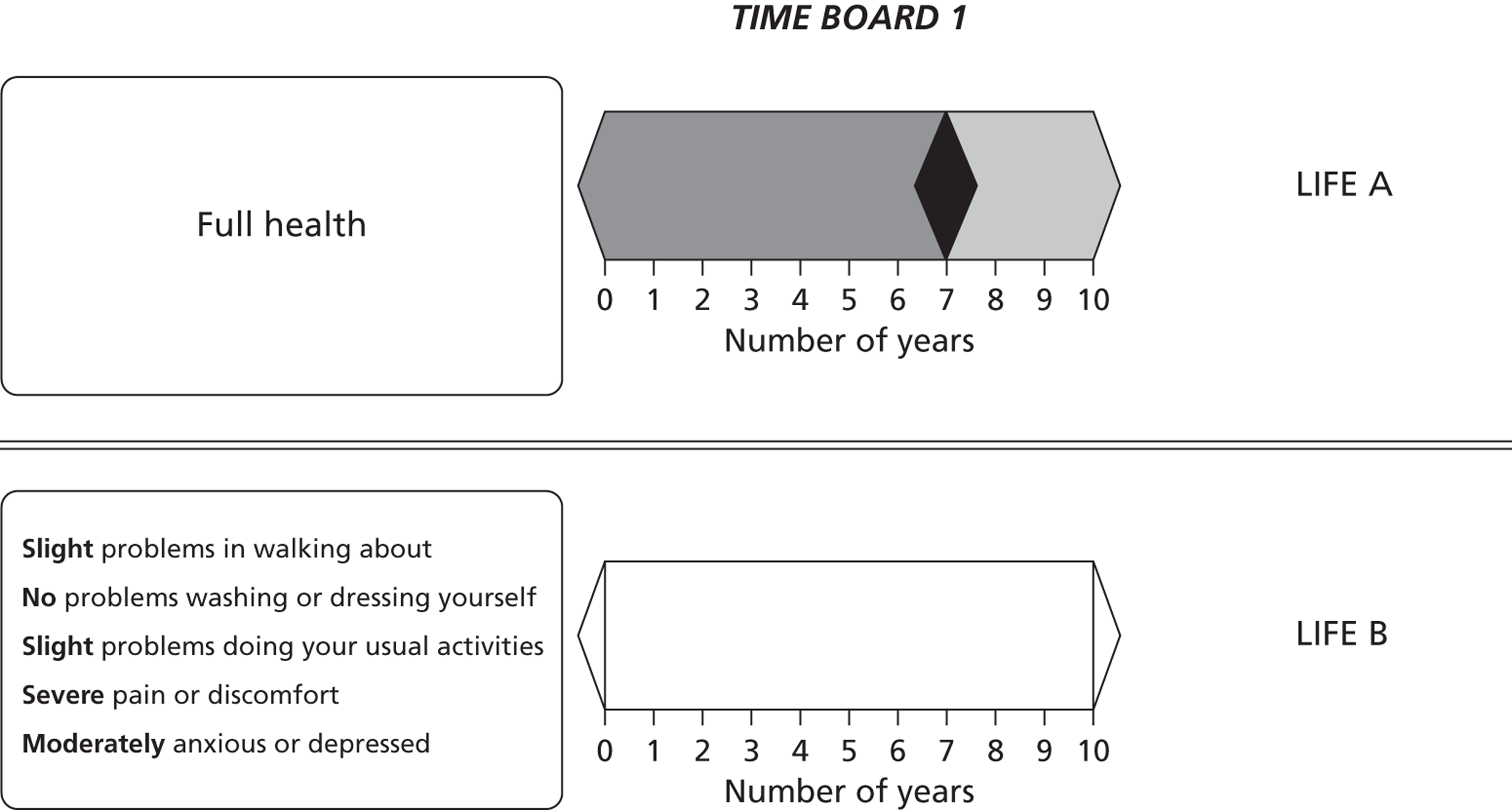
Appendix 7 PRET and PRET-AS proposals
PRET proposal
1. TITLE: Preparatory study for the Re-evaluation of the EQ-5D Tariff (PRET)
2. IMPORTANCE
Resources are limited and need to be allocated efficiently. The health care sector is no exception. The National Institute for Health and Clinical Excellence (NICE) was set up to help make better health care resource allocation decisions. NICE bases its recommendations on cost effectiveness analyses with the Quality Adjusted Life Year (QALY) as the outcome measure. The EQ-5D, with its population based preference indices, is the preferred instrument to use when quantifying the health related quality of life (HRQOL) impact of medical interventions (NICE, 2008).
Furthermore, the UK EQ-5D value sets are used not just by NICE, but are widely used as a basis for economic evaluation by other agencies both in the UK and elsewhere; and in a very wide range of applications, including population health surveys (e.g. the Health Survey for England); burden of disease studies; hospital inpatient surveys and, most recently, the NHS PROMs initiative (Browne et al. 2007).
The current EQ-5D ‘tariff’ is based on the Measurement and Valuation of Health (MVH) study, carried out in 1994 and published in 1997 (Dolan, 1997). The study used face to face interviews of a representative sample of the general public. A selection of hypothetical EQ-5D states was assessed using the time trade off (TTO) method. The results were modelled in terms of the EQ-5D descriptive system to provide a population value set, which in effect is a tariff of HRQOL weights given to all 243 EQ-5D states.
In the past 15 years, there have been developments that have lead to the need for a re- evaluation of the EQ-5D:
-
people may not have the same preferences as they did 15 years ago;
-
change in demography may mean that although individual preferences may not have changed, the composition of people across the country has changed, so that average preferences may have changed;
-
recognition of the shortcomings of the MVH TTO design, in particular in the context of observations worse than dead;
-
new advances in methods for valuing health states other than TTO, such as discrete choice
-
experiments (DCE);
-
new advances in the mode of valuation, other than face to face interviews; and
-
the development of a revised version of the EQ-5D, with 5 levels rather than 3.
In order for NICE to make the most appropriate decisions, the EQ-5D population value set needs to be one that is up to date, based on the latest understanding of health-state preferences.
The proposed study ‘Preparatory study for the Re-evaluation of the EQ-5D Tariff’ (PRET) is a methodological piece of work that will contribute to the re-evaluation of the EQ-5D population value set, by exploring a number of methodological issues, as identified in the Scoping Study (MRC, 2009). While there are no valuation or modelling components in the proposed project, the final product will include a protocol for an actual re-evaluation study to use.
The design of a valuation study of a health-state classification will need to take the following issues into account:
-
Whose values?
-
Which health-state classification system?
-
What mode of administration?
-
What method of valuation?
-
How many, and which hypothetical health states to value?
-
How long should each hypothetical state last?
Each is discussed in more detail below.
(1) Whose values?
The current MVH value set is based on general population values. There has been a debate on how general population values on hypothetical health states may differ from the way patients value hypothetical states or their own current state (Brazieret al. , 2005). PRET does not have the capacity actually to compare patient values with general public values, and will only survey members of the general public. However, a recent study has demonstrated that if non-patients can be informed about the extent to which it is possible for patients to still have a good life and be satisfied with their condition, the discrepancy in values may diminish (McTaggart-Cowanet al. , 2009). Therefore, PRET will examine this further by introducing an element of health satisfaction in the questions, so that the way in which patients feel about the state of health can be captured.
There is also a normative element to this debate, concerning whether general public values ought to be used over patient values. The use of general public values is typically justified with reference to the non-welfarist argument: viz. because the values are used in decision making in a publicly funded health care system, the values should come from people as informed citizens, not as people as consumers (see for example Tsuchiya, Miyamoto, 2009). The use of the well-being element in PRET will arguably contribute to making the non-patients better informed about how patients perceive their own health. In addition, background characteristics questions will examine the respondent’s illness experience and satisfaction with their own health.
(2) Which health-state classification system?
There are two issues here. The first is which version of EQ-5D to use. The version of EQ-5D that is most commonly in use has 3 levels across each of the 5 dimensions of health (Brooks, 1996), and this is the version of EQ-5D that the MVH population value set is for. However, the EuroQol Group has recently released the 5-level version of EQ-5D (Herdmanet al. 2008) and the next UK valuation study of the EQ-5D is likely to be around this new 5-level version. Therefore, PRET will, where relevant, use EQ-5D-5L.
A second issue is whether or not the 5 dimensions of EQ-5D cover all relevant aspects to be taken into account when health-state valuation exercises are conducted. (There is another proposal alongside this one, lead by Dr Longworth based also at the University of Sheffield that will explore the issue of preference based condition specific instruments, including EQ-5D add-ons.) There is a limit to the number of dimensions a preference-based instrument can have, so any further information to be incorporated needs to be fairly generic. As was explained above, PRET will look at the implications of introducing an element of health satisfaction alongside the standard EQ-5D health state descriptive system to contribute towards this topic.
(3) What mode of administration?
The current MVH TTO value set is based on face to face interviews. Whilst this is a method that results in high quality data, it is also a very expensive method of data generation. When the MVH study was carried out in the mid-90s, there were two more alternatives available: postal questionnaire and telephone interview (with or without a pre-posted questionnaire). While these two modes of administration are much less costly than face to face interviews, they are usually regarded as resulting in lower quality data.
However, over the past decade there have been major advances in communication technology, and one emerging, very attractive mode of survey administration is via the internet, using on-line panels. This is where market research companies have a pool of potential respondents with registered background characteristics. A survey instrument will be set up on the internet, and potential respondents will be invited via e-mail to access this and to complete it on-line. The advantages of such on-line surveys are: complex routing (or branching) of questionnaires are possible; question ordering can be easily randomised; the time taken for each question can be logged; there is no process of data entry and associated errors; background characteristics of non-respondents can be obtained; large samples can be achieved in a short time; and the sampling frame can be flexible. On the other hand, there are concerns, such as: the representativeness of the sample in terms of unobserved characteristics; the motive of participation; and whether respondents are genuinely engaged. A particular concern for iterative exercises on-line is that if respondents wished to get through the questions quickly, there is an incentive to accept the first trade off offered, without going through the iterative process to reach indifference. So any successful on-line version of the TTO is unlikely to be simple transplants of an existing interview-based iterative protocol to an on-line environment. PRET will carry out a head to head comparison of an on-line survey, and a face to face interview.
(4) What method of valuation?
The current MVH value set is based on TTO (Gudex, 1994). This TTO protocol is known to have a few problems, in particular, regarding the procedure used to value, and subsequently to transform, states worse than dead. It is not only different from but also incommensurable with the procedure used for states better than dead (Tilling et al. , 2009). While the average values for two thirds of states are positive, a large number of states have individual observations that are negative, which affect (or distort) the average values. Recently, funded by the EuroQol Group, an alternative TTO protocol, called the ‘lead time TTO’ has been devised (Devlinet al. , 2009). This processes all states in the same way, regardless of whether it is better or worse than dead, by adding a set number of years in full health preceding the time trade off exercise (and hence the name ‘lead time’). Further analysis is required to identify the optimal length of this lead time. Moreover, one concern is that if the value of a health state depends on a preceding health state, then the addition of lead time will distort the TTO value. PRET will include a comparison of the MVH TTO and the lead time TTO. Furthermore, it will identify any bias introduced by using the lead time TTO and recommend ways to correct for this in the re-evaluation study of EQ-5D.
In addition to TTO, there is a growing interest in the application of DCE in health-state valuation. One advantage of the DCE is that because individuals are not interrogated until they reach a point of indifference (as in TTO), but only asked to give ordinal preferences over pairwise choices, it is arguably less cognitively demanding than such methods. On the other hand, the well-known problem with the DCE has been that there has been no satisfactory method of combining the dimensions of health with survival and duration. However, a new method has recently been developed that interprets DCE data as a TTO exercise (Bansback,et al. , 2009). It includes duration as one of the DCE attributes, estimates a regression model with an interaction term between health state and duration, and then uses these regression coefficients to calculate the value of health states by solving the equivalence relationship for a binary choice situation between, on the one hand, living in a given health state for a specific duration of time and, on the other, living in full health for a shorter duration; viz. The indifference point in TTO. Not only does this potentially solve the problem of DCE, it also potentially solves the issue of observations worse than dead, identified in the context of TTO above, without recourse to the lead time structure. PRET will explore this novel approach further.
(5) Which hypothetical health state to value?
The current MVH TTO value set is based on direct valuations of 45 states. On the other hand, an orthogonal design of a 3-level 5-dimensional instrument will involve 16 states. Following the latest experimental design theory, and using the data collected within the project as prior information, PRET will make recommendations on the optimal selection of hypothetical EQ-5D-5L states for the re-valuation study, using TTO and DCE.
(6) How long should each hypothetical state last?
The current MVH TTO value set is based on participants being asked to imagine each health state lasting for a 10-year duration. However, the MVH also estimated TTO tariffs of different durations (based on VAS valuations of different durations). This was because there was a concern that the tariff values may be a function of the duration of the health state. There are four related issues, all of which are relevant to DCE as well. (See Tsuchiya, Dolan, 2005 for a review of these topics.) One is whether or not ‘constant proportional time trade off’ holds so that the utility associated with a marginal survival in a given health state remains constant regardless of the health state or the duration. Some people argue that for very severe states, there will come a ‘maximal endurable time’ limit, beyond which the marginal benefit of survival diminishes. The second issue is whether or not respondents use a positive temporal discount rate when valuing hypothetical health scenarios. Note that this is not the same as whether people have a positive time preference when they make decisions in the real world involving future health prospects. The third is the impact of life stage concerns in valuations involving long durations. If the duration of the state is too long, then the scenarios will not be credible for the older respondents. At the same time, shorter durations are not credible for the younger respondents. Furthermore, depending on the duration, people may be thinking about life stage events (e.g. pay off mortgage, child starting school) rather than about the trade off between longevity and quality of life).
The final issue is whether or not a 10-year duration is the most relevant duration of health states for NICE decision making. If the above issues mean that the value of a state is a function of its duration, and if most NICE decision making involve states that last for much shorter durations, then the re-evaluation of the EQ-5D should not be based on scenarios with a 10-year duration. Therefore, PRET will examine the impact of duration on health-state preferences and recommend the optimal combinations of durations to be used in the main re-evaluation of EQ-5D.
Thus, to summarise, there are a number of issues that need to be addressed before the re- evaluation of the EQ-5D population value set can take place. PRET will examine these and, based on the findings, recommend a study protocol. The project will take 18 months from the start to the production of the study protocol, with the aim of handing over to the UK re-evaluation study of the EQ-5D-5L as smoothly and promptly as possible. The recent launch of the EQ-5D-5L means that the opportunity for a re-evaluation study is ripe, and this should be preceded by a well planned intensive methodological study of the kind proposed here.
3. SCIENTIFIC POTENTIAL
3.1 People and track record
A team of health economists will be lead by Dr Tsuchiya. The PRET team covers expertise in the valuation of EQ-5D (in the UK, Japan, and Thailand), SF-6D, and several condition specific preference based measures. Furthermore, different members of the team have worked together on projects ranging from the development of lead time TTO; the use of TTO and DCE in an on-line environment; theoretical work on the QALY concept; EQ-5D in patient reported health outcomes; adaptation to chronic health; time preference for future health; and optimal experimental design. We have Professor van Busschbach (Erasmus University Rotterdam), who is a psychologist with research interests in health-state valuations, as an external advisor. Dr Tsuchiya, Dr Longworth, Professor Devlin, and Professor van Busschbach are members of the EuroQol Group. Professor Brazier has been invited to participate in Valuation Taskforce Meetings of the EuroQol Group.
3.2 Environment
PRET will be based at the School of Health and Related Research, University of Sheffield. University of Sheffield, School of Health and Related Research (ScHARR) is a multidisciplinary School within the Faculty of Medicine Dentistry and Health. It brings together a wide range of health related skills including: health economics, decision sciences, mixed methods, epidemiology, and medical statistics, and has strong ties with the NHS and NICE.
Health Economics and Decision Science (HEDS) is a section within ScHARR. It aims to promote excellence in national and international health care resource allocation decisions, through applied and theoretical research. HEDS makes major contributions in many areas, including valuation of health, analysis of health policy, welfare and equity, technology appraisal, trial-based economic evaluation, and econometrics. There has been a number of valuation studies carried out by HEDS, including the SF-6D, the HUI (UK value set), and a number of condition specific preference based measures. http://www.shef.ac.uk/scharr/research/health-economicsanddecisionscience
3.3 Research plans
3.3.1 Overview: aim of the project and objectives at each stage
PRET is a methodological study, and aims to produce a study protocol for the re-evaluation of the EQ-5D-5L. As was illustrated above, there are a number of relevant issues. The project will carry out a large scale on-line survey to identify the key issues that should be explored further in interview settings. The project consists of the following 4 stages over 18 months.
Stage 1: on-line survey: months 1–6
The objective of stage 1 is to examine a number of assumptions and lead time TTO and DCE design issues, with a view to inform the selection of issues to be examined in more detail in stage 3. The main component of stage 1 is a large scale on-line questionnaire.
Stage 2: parallel survey: months 3–7
The objective of stage 2 is to explore the strengths and weaknesses of the different modes of administration and to provide recommendations for the re-evaluation of the EQ-5D tariff. Stage 2 will consist of interview surveys that replicate one of the on-line survey versions in stage 1.
Stage 3: interview survey: months 8–13
The objective of stage 3 is to inform stage 4. Stage 3 will consist of face to face interviews to examine in more detail and depth the issues examined in stage 1 for further analyses.
Stage 4: developmental work: months 14–18
The objective of stage 4 is to produce and test out the final re-evaluation protocol, based on face to face interviews of a convenience sample. This will involve an iterative process of trial and error.
3.3.2 The survey question format and types of questions in stages 1 and 2
The survey questions in stages 1 and 2 will take the format of binary choice. A single response to a binary choice question cannot identify the level of HRQOL an individual feels is right for a health state. However, by examining the distribution of responses of multiple respondents across different binary choice questions, the relevance of key parameters can be identified.
For example, the most basic binary choice question used in PRET looks like this:
[Scenario A]: you will live in health state H for 10 years and die
[Scenario B]: you will live in full health for (V × 10) years and die
Which of the two options do you think is better?
The figure below is an illustrative example of two hypothetical states, ‘severe’ and ‘mild’. Along the horizontal axis is the value of V with 0 for dead and 1 for full health. Along the vertical axis is the proportion of people. The upward sloping curves indicate that, as V increases, the proportion of people who think the given health state is no better than V will increase. By definition, the curve for ‘severe’ lies to the left of a curve for ‘mild’. Now, suppose V is 0.6. If the state H in the example above is the severe state, then around 90% of people think it is no better than 0.6 and thus choose scenario B. But if state H is the mild state, then around 50% will think it is no better than 0.6 and thus choose scenario B.
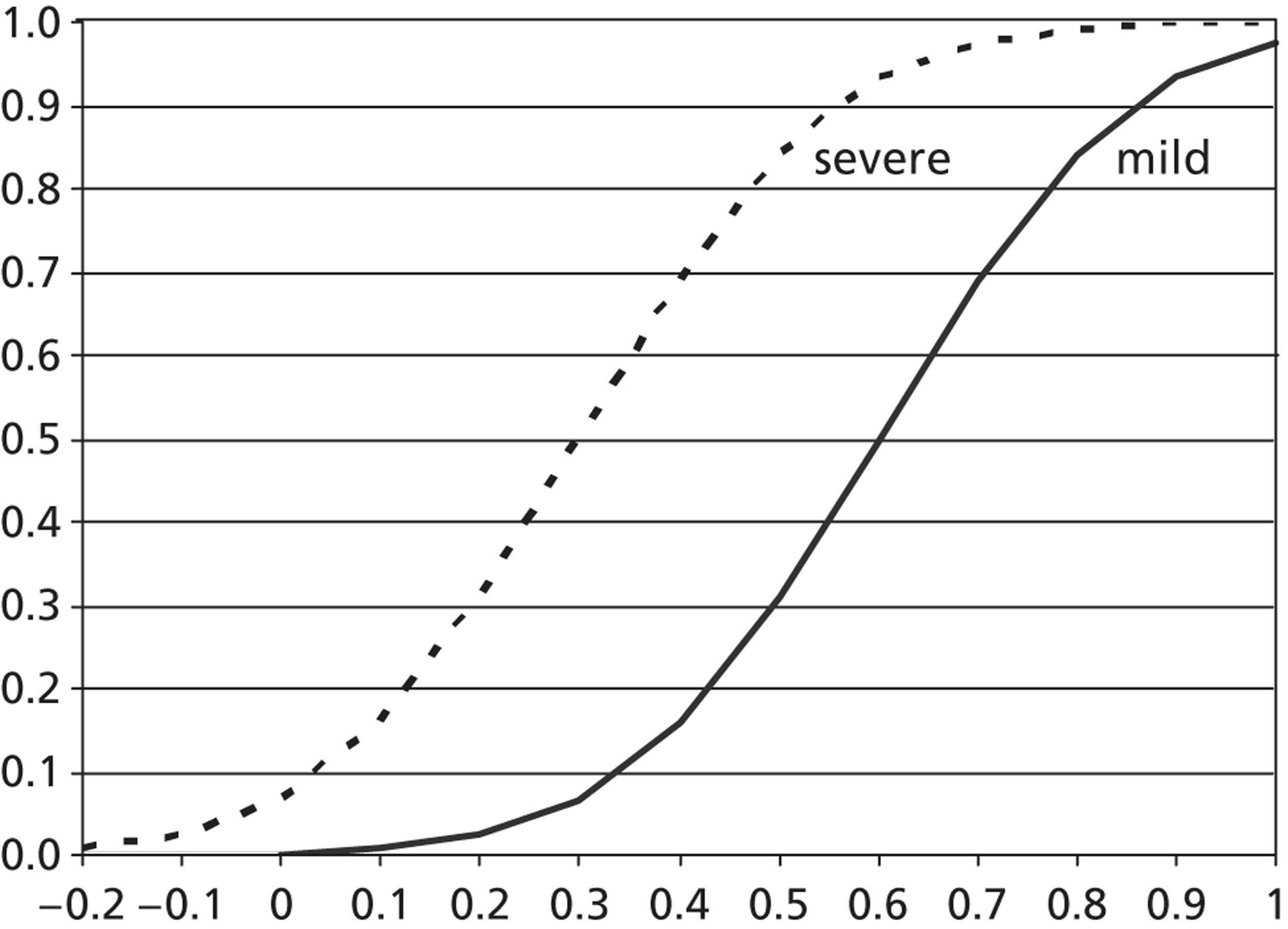
So the different health states are picked up in terms of the proportion of people choosing one scenario over the other. All binary choice scenarios will include information on a health state and the length of time lived in the state, followed by death. These binary questions could be part of a DCE design. At the same time, they are a snippet of a TTO procedure; the typical TTO exercise involves changing V until the respondent is indifferent between the two scenarios. In fact, TTO can be interpreted as a special case of DCE, where scenarioB always involves full health.
The final details including wording and presentation will be determined under the actual project, but in general terms, a scenario can include the following key parameters:
-
EQ-5D-5L states (H): see section 3.3.4 below
-
Duration in years (T) in state H: e.g. 1 year, 3 years, 10 years
-
Lead time stretches (L) in full health including zero: e.g. 0 years, 3 years, 5 years
-
Person perspective (P) that the TTO applies to: e.g. ‘you’, ‘somebody else like you’, ‘a group of people aged 30’
-
Levels of satisfaction with one’s own health (S): low / medium/ high level of satisfaction with one’s own health state.
There are 7 types of binary choice questions, and they are summarised below:
Question type | |||||||
---|---|---|---|---|---|---|---|
Parameter | I | II | III | IV | V | VI | VII |
EQ-5D health state (H) | ✓ | ✓ | ✓ | ✓ | ✓ | ✓ | ✓ |
Duration in EQ-5D health state | ✓ | ✓ | ✓ | ✓ | ✓ | ✓ | ✓ |
Time Trade Off (Lead time) | n/m (*) | n/m | ✓ | ✓ | n/m | ✓ | n/m |
Health state perspective | you | other | you | other | you | you | you |
Satisfaction with health | n/m | n/m | n/m | n/m | ✓ | n/m | n/m |
Thus, the above basic binary choice example is a type I question, and the MVH TTO is a type II question, because it uses no lead time, the ‘somebody like you’ perspective, and no satisfaction.
3.3.3 The assumptions tested using type I to type V questions
The first 5 question types are based on TTO, and present two possible scenarios:
[A]: Person P lives L in full health followed by T in state H with satisfaction S then dies
[B]: Person P lives (L + VT) in full health then dies
By comparing the responses to the first 5 question types, the following assumptions are explored.
Assumption 1: health-state values are independent of duration
Type I questions will be used. If the assumption holds, then for a given combination of state H and value V, the distribution of respondents between the two scenarios should not be affected by duration T. So, for example, if the basic example above was changed to 5 years for Scenario A and (V × 5) years for Scenario B, the cumulative curve for the state should remain in the same position, and the proportion of people choosing each Scenario at given V should be unaffected.
Assumption 2: health-state values are independent of persons perspectives
Types I and II questions are used. If the assumption holds, then for a given combination of state H and value V, the distribution of respondents should not be affected by person perspective P.
Assumption 3: health-state values are independent of lead time
Types I and III questions are used. If the assumption holds, then for a given combination of state H and value V, the distribution of respondents should not be affected by lead time L.
Assumption 4: the values of others’ health are independent of when health events take place
Types II and IV questions are matched so that they are identical except for the timing of health events. The exercise is not affected by life stage considerations that inevitably affect time preference exercises using the ‘you’ perspective. If the assumption holds, then for a given combination of state H, value V, and person perspective P, the distribution of respondents should not be affected by the timing of health events, represented by lead time L.
Assumption 5: the values of others’ health are independent of satisfaction in the state
Type V questions are used. If the assumption holds, then for a given combination of state and value V, the distribution of respondents should not be affected by satisfaction S.
These assumptions are analysed by comparing the proportions across question types. Further, probit regressions will be used to explain the propensity to choose scenario B in terms of the key parameter of interest, controlling for health state H. Throughout, pooled data across respondents are used, and separate regressions are used for each assumption. In addition, for each assumption, a probit regression per state will be used to explore whether the results may depend on the health state used, and thus, on the dimension of the health problem. Where relevant, duration T and lead time L will be entered as categorical variables to allow for non-linearity.
In stage 1, the effect of background characteristics on whether or not to respond to the survey and on what responses to give in the binary choice questions will be explored econometrically.
3.3.4 The hypothetical health states used in type 1 to type V questions
Questions type I to V will use the following 5 health states:
-
‘Mild problem walking about’ (EQ-5D-5L state 21111)
-
‘Mild pain’ (11112)
-
‘Severe difficulties walking about’ (51111)
-
‘Severe pain’ (11151)
-
‘Severe depression’ (11115)
Only one dimension in any health state has a problem, and therefore, these states are simple and easy to imagine. In addition, they cover different aspects of health, and thus enable the analysis to test the key assumptions by the different kinds of health problem. States will be presented verbally as in the above, and not with reference to all 5 dimensions of EQ-5D. Piloting will determine the level of V to be used, but it is envisaged that two values (e.g. 0.8 and 0.2) will be used throughout for the mild states and the severe states respectively.
3.3.5 Type VI questions to test the sufficiency of lead time under very poor health
Developmental work for lead time TTO has indicated that some respondents associate very poor states with extreme negative values that they will ‘use up’ or exhaust all their lead time (Devlin et al, 2009). When this happens, no TTO value can be inferred. While at least some of these may reflect a genuine quantitative preference, others may be a qualitative indication that the state is extremely poor. Type VI questions will use the worst possible state 55555 in Scenario A combined with a relatively short duration T and long lead time L; and set Scenario B to immediate death. The objective is to map the proportion of respondents who exhaust lead time at various combinations of duration T and lead time L to gauge the proportion of respondents who may be giving a qualitative preference for very poor states.
3.3.6 Type VII questions for informing the selection of states for DCE
Type VII questions are in effect a very small scale DCE study. Both scenarios here consist of ‘you’ living in a particular EQ-5D-5L state for a specified duration followed by death. These questions will use states with a good mix of problems across all 5 dimensions. The results will be used as prior information to guide the design of an efficient set of hypothetical health states to be used in the re-evaluation of EQ-5D-5L. The information will be used for both DCE and TTO design.
3.3.7 The allocation of questions to questionnaire versions
The 7 types of questions will be allocated across three modules:
-
Module 1: 5 questions of type 1
-
Module 2: one question each from types II, III, IV, V, and VI, totalling 5 questions
-
Module 3: 2 questions of type VII
Thus, each respondent will be presented with 12 binary choice questions across 3 modules, covering all 7 types of question. In order to avoid proportional heuristics, the 5 health states will be used only once each in module 1, and then once each in module 2, combined with a different duration T. There will be 15 versions of the on-line questionnaire. While all possible type I scenarios will appear in a few versions each, and all type VI scenarios will appear in at least one version, only select scenarios in types II to V will be used. One advantage of an on-line survey is the potential of achieving very large sample size and many different versions in relatively short time. This allows designs that examine cross-respondent differences, free from heuristics. Each version will aim for an achieved sample of 200 in the on-line survey. One of these versions will be used in the parallel survey interviews, which will also aim for an achieved sample of 200.
Furthermore, there will be 30 sub-versions (each of the 15 versions will have 2 sub-versions) for module 3. EQ-5D-5L has 3125 possible health states, and combining this with 3 durations amounts to 9375 possible DCE scenarios. Of these, 120 will be selected based on the latest experimental design theory (for example, Bliemer, Rose, 2006) and allocated to 60 binary choices, of which 2 will be allocated to each sub-version. Each sub-version will have an achieved sample of 100 in the on-line survey.
3.3.8 Stages 3 and 4
Stage 1 will identify the key assumptions that affect TTO and DCE. The objective of stage 3 is to examine these in interview settings that will allow more detailed feedback. For example, if stage 1 finds that the effect of duration T has a significant impact, then stage 3 interviews will explore how this comes about. Or, if a significant proportion of respondents exhausts lead time, then stage 3 will explore how to deal with these respondents. The interviews will consist of a relatively small number of TTO and DCE tasks, each combined with probing questions inviting the respondent to explain their choices, and thus to inform the development of a valuation protocol. A pilot study will generate the probing questions. A general population sample of 300 will be allocated across up to 4 versions. Stage 4 will test out an interview and on-line versions of the recommended protocol in small waves of up to 10 respondents each, and make further adjustments. A convenience sample of 30 will be used in interview settings.
4. ETHICS AND RESEARCH GOVERNANCE
The study will survey members of the public, and therefore will require research ethics approval. Appropriate research ethics approval will be obtained from the University of Sheffield Research Ethics Committee and the research will be carried out in line with the University policies on good research practice. All responses will be recorded under a code. Only aggregate level data will be published in scientific papers. (Further detail is available elsewhere in the application form.)
5. DATA PRESERVATION OR SHARING
Individual level coded data will be made available for use by other researchers once we have confirmed acceptances on any main papers arising from our work. This will consist of the stage 1 on-line survey (n = 3000), the stage 2 parallel survey (n = 200) and the stage 3 interview data (n = 300). These will be accompanied by appropriate documentation.
6. PUBLIC ENGAGEMENT
There will be a project webpage where interested members of the public, and in particular study participants, may find out about the progress and findings of the research.
7. EXPLOITATION AND DISSEMINATION
The central product of PRET is a study protocol for the re-evaluation of the EQ-5D population tariff. The protocol will include TTO and DCE for both face to face interview and on-line survey, with an indication of the preferred mode of administration, a recommended set of health states to use, and a guide to sample size calculation.
The scientific findings from PRET will be submitted to the UK Health Economists’ Study Group (HESG) meetings and the EuroQol Group Scientific Meeting for presentation. They will also be submitted for publication in international health economics journals.
The stage 3 interviews is not expected to fully exploit the outcomes of the stage 1 on-line survey, and the health economics research community will be welcome to use the outcomes of this to identify research topics for further examination. The study is not likely to generate commercially exploitable results.
REFERENCES
- Bansback N, Brazier J, Tsuchiya A, Anis A. A Comparison of a Discrete Choice Experiment to the Time Trade off to Value Health States for QALYs 2009.
- Bliemer MCJ, Rose JM. Designing Stated Choice Experiments: State-of-the-Art 2006.
- Brazier J, Akehurst R, Brennan A, Dolan P, Claxton K, McCabe C, et al. Should patients have a greater role in valuating health states?. Applied Health Economics and Health Policy 2005;4:201-8.
- Browne J, Jamieson L, Lawsey J, van der Meulen J, Black N, Cairns J, et al. Patient Reported Outcome Measures (PROMs) in Elective Surgery 2007.
- Brooks R. EuroQol: The current state of play. Health Policy 1996;37:53-72.
- Devlin N, Tsuchiya A, Buckingham K, Tilling C. A Uniform Time Trade Off Method for States Better and Worse Than Dead: Feasibility Study of the ‘Lead Time’ Approach 2009.
- Dolan P. Modelling valuations for EuroQol health states. Medical Care 1997;35:1095-108.
- Gudex C. Time Trade-Off User Manual: Props and Self-Completion Methods. Centre for Health Economics, University of York; 1994.
- Herdman M, Sanz L, Lloyd A, Badia X, Gudex C. Qualitative Testing of Two New 5-Level Versions of the EQ-5D in Spain: Preliminary Study Results 2008.
- McTaggart-Cowan H, O’Cathain A, Tsuchiya A, Brazier J. A Qualitative Study Exploring the General Population’s Perception of Rheumatoid Arthritis After Being Informed about Disease Adaptation 2009.
- Medical Research Council . NICE Methodology Scoping Project ‘Executive Summary’ 2009.
- ‘Priority Topics’ n.d. URL: www.mrc.ac.uk/Utilities/Documentrecord/index.htm?d = MRC006073 (accessed June 2009).
- Guide to the Methods of Technology Appraisal. 2008.
- Tilling C, Devlin N, Tsuchiya A, Buckingham K. Protocols for TTO Valuations of Health States Worse Than Dead: A Literature Review and Framework for Systematic Analysis 2008.
- Tsuchiya A, Dolan P. The QALY model and individual preferences for health states and health profiles over time: A systematic review of the literature. Medical Decision Making 2005;25:460-7.
- Tsuchiya A, Miyamoto J, Anand P, Puppe C, Pattanaik P. Handbook of Rational and Social Choice. Oxford University Press; 2009.
Abstract of research
Resources are limited and need to be allocated efficiently. The National Institute for Health and Clinical Excellence (NICE) was set up to help make better health care resource allocation decisions. NICE bases its recommendations on cost effectiveness, and the EQ-5D is the preferred instrument to use when quantifying the health related quality of life impact of medical interventions.
The current EQ-5D ‘tariff’ is based on a survey in 1994. The study asked members of the general public to value a selection of hypothetical EQ-5D states using the time trade off (TTO) method. However, in the past 15 years, there have been various developments that have lead to the need for a re-evaluation of the EQ-5D. In order for NICE to make the most appropriate decisions, the EQ-5D population value set needs to be one that is up to date, based on the latest understanding of health-state preferences.
The proposed preparatory study is a methodological piece of work that will contribute to the re- evaluation of the EQ-5D population value set, by exploring a number of methodological issues, as identified in the MRC Scoping Study. The aim is to produce a protocol for an actual re-evaluation study to use. This is done in 4 stages.
The objective of stage 1 is to examine a number of assumptions and design issues, with a view to inform the selection of issues to be examined in more detail in stage 3. Stage 1 will consist of a large scale on-line questionnaire.
The objective of stage 2 is to explore the strengths and weaknesses of the different modes of administration and to provide recommendations for the re-evaluation of the EQ-5D tariff. Stage 2 will consist of interview surveys that replicate one of the on-line survey versions in stage 1.
The objective of stage 3 is to inform stage 4. Stage 3 will consist of face to face interviews to examine in more detail and depth the issues examined in stage 1 for further analyses. The interviews will consist of TTO and discrete choice experiment tasks, each combined with probing questions inviting the respondent to explain their choices, and inform the development of a valuation protocol.
The objective of stage 4 is to produce and test out the final re-evaluation protocol, based on face to face interviews of a convenience sample. This will involve an iterative process of trial and error.
Summary of Health and Wealth Implications
The proposed project is a preparatory study towards the re-evaluation of the EQ-5D tariff. Therefore, the direct implications of the research will be on the methodology of health-state valuations. The health (and wealth) of a population is best supported by cost-effective allocation of limited resources. Where cost per Quality Adjusted Life Years is used as a method of assessing different health care interventions, it is crucial that the best available population value sets are used. The findings of the proposed project will contribute to a better design of the re-evaluation of the EQ-5D population value set in the UK, and thus to better quality decision making by NICE. In other words, there will be indirect implications to health and wealth from the proposed project. Furthermore, it will have implications for the design of similar evaluation studies internationally, using EQ-5D or other instruments.
Lay summary
Resources are limited and need to be allocated efficiently. The National Institute for Health and Clinical Excellence (NICE) was set up to help make better health care resource allocation decisions. NICE bases its recommendations on cost effectiveness, and the EQ-5D is the preferred instrument to use when quantifying the health related quality of life impact of medical interventions. EQ-5D is a tool that classifies 243 different health states with 5 different dimensions of health, each with an identified level of severity.
NICE uses an EQ-5D ‘tariff’. This is a set of numbers that indicate the level of health related quality of life of each EQ-5D health state, on a scale with 1 for full health and 0 for dead. By comparing the different EQ-5D states patients are in before and after treatment, analysts can calculate how effective the treatment is.
The current EQ-5D tariff is based on a survey in 1994. The study asked members of the general public to ‘value’ a selection of hypothetical EQ-5D states using a specially designed questionnaire. However, in the past 15 years, there have been various developments that have lead to the need for a ‘re-evaluation’ of the EQ-5D. In order for NICE to make the most appropriate decisions, the EQ-5D population value set needs to be one that is up to date, based on the latest understanding of how to value health states.
The proposed preparatory study is a methodological piece of work that will contribute to the re- evaluation of the EQ-5D tariff. The aim is to produce a protocol for an actual re-evaluation study to use. The project will look into different ways of surveying members of the public, for instance, by on-line surveys or by face to face interviews. The project will also look at different ways of designing and wording the questionnaire to value hypothetical EQ-5D states, or which EQ-5D states to use. It will start with a large scale on-line survey to gauge the relevant concerns, where a long list of issues can be explored by surveying a large number of people, but not in much detail. The study will then move on to a smaller scale interview study, where researchers can ask participants more detailed questions. The final stage of the project will be to test outa recommended study protocol for the actual re-evaluation study to use, and make any further fine tuning adjustments.
Does the research raise ethical issues?
If ‘Yes’, please state what these ethical issues are:
The study will survey members of the public, and thus will require research ethics approval. However, participants will not be recruited through the NHS. Appropriate research ethics approval will be obtained from the University of Sheffield Research Ethics Committee and the research will be carried out in line with the University policies on good research practice.
As participants will only take part in an on-line or an interview survey, the anticipated risks to participants are extremely low. The survey questions will be of two kinds: the first, and main set of questions are based on entirely hypothetical scenarios, and involves little sensitive or private material. The second kind is background characteristics questions, which will be of a fairly conventional nature.
For both the on-line survey and the general public interviews, market research agencies will be used. We will ensure that the agencies conform to standard market research governance regulations (e.g. Market Research Society Code of Conduct). Each participant will be given an ID code, and all responses will be recorded under the code. Members of the research team carrying out the analysis will only have access to coded responses. Only aggregate level data will be included in the project report or published in scientific papers.
PRET-AS proposal1
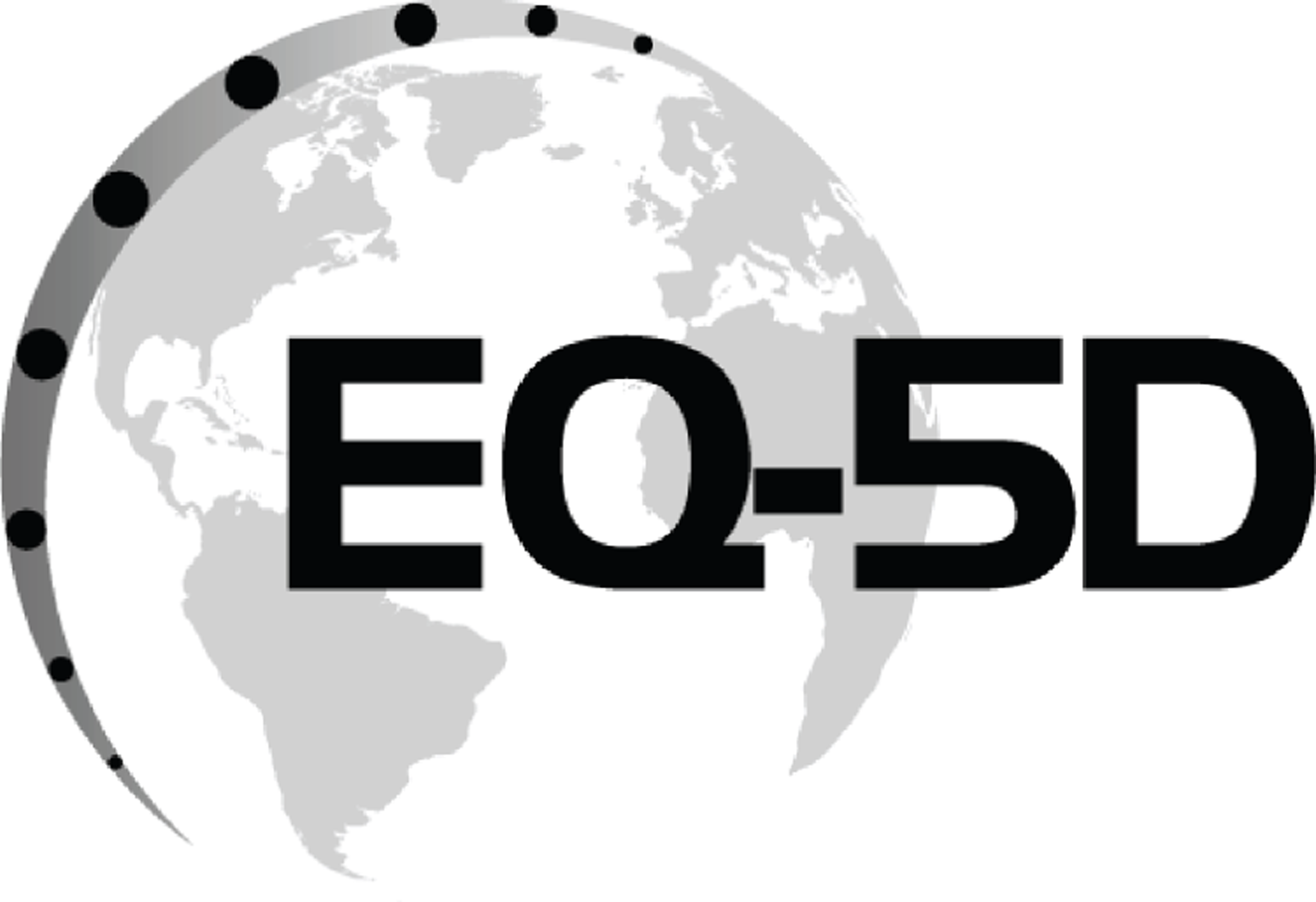
1. Background
The current EQ-5D population value set, or ‘tariff’, is based on a survey carried out in 1994. In the past 15 years, there have been developments that have lead to the need for a re-evaluation of the EQ-5D, for example:
-
recognition of the shortcomings of the MVH TTO design, in particular in the context of observations worse than dead;
-
new advances in methods for valuing health states, such as DCE;
-
new advances in the mode of valuation, other than face to face interviews; and
-
the development of a revised version of the EQ-5D, with 5 levels rather than 3.
PRET is a methodological project that will contribute to the re-evaluation of the EQ-5D population value set, by exploring a number of methodological issues. The deliverables will consist of recommendations for methods that future valuation studies could use.
When the grant was awarded, the UK Medical Research Council (MRC) made clear that there should be good communication and collaboration between PRET research team and the EuroQol Group. As is explained below, PRET includes a large scale on-line survey of members of the UK public. As the cost of one additional respondent in an on-line survey is relatively modest, this gives rise to an opportunity to explore any further methodological issues of common interest to the Group and to PRET, and hence this PRET-AS proposal to the EuroQol Group. The original PRET proposal has already been subject to peer review at the MRC, and we do not propose to make any changes to its design, only to add a number of survey versions to stage 1.
2. Brief outline of the PRET project
The project will carry out a large scale on-line survey to identify the key issues that should be explored further in subsequent smaller scale interview settings.
Stage 1: on-line survey: months 1–6
The objective of stage 1 is to examine a number of assumptions involved in the valuation of hypothetical health states, with a view to inform the selection of methodological issues to be examined in more detail in stage 3. Stage 1 will consist of a large scale on-line survey of members of the general public (main achieved sample n = 3000), using an existing internet panel. Each respondent will be presented with 12 binary choice questions designed to explore methodological issues including:
-
constant proportional time trade off
-
duration of lead time in lead time TTO
-
exhaustion of lead time for the worst possible state (EQ-5D-5L 55555)
-
timing of health states
-
selection of EQ-5D-5L states for DCE with duration as the 6th dimension
Stage 2: parallel survey: months 3–7
The objective of stage 2 is to explore the strengths and weaknesses of the different modes of administration. This stage will consist of face to face interviews of members of the general public (n = 200). This will replicate one of the on-line survey versions in stage 1.
Stage 3: interview survey: months 8–13
The objective of stage 3 is to examine in more detail and depth the issues examined in stage 1 for further analyses. This stage will consist of face to face interviews of the general public (n = 300).
Stage 4: developmental work: months 14–18
The objective of stage 4 is to produce and test out the final re-evaluation protocol. This stage will involve an iterative process of trial and error, and will be carried out through face to face interviews of a convenience sample (n = 30).
3. The PRET-AS proposal
3.1 Objectives
The aim of PRET-AS is to enhance the study design of PRET. The objectives of PRET-AS are twofold:
-
to increase the number of binary choice data to inform the optimal selection of EQ-5D-5L state pairs in DCE designs; and
-
to examine the number of binary choice questions that respondents can reasonably complete in an on-line environment.
3.2 Methods
Regarding the first objective, EQ-5D-3L has 243 health states, which leads to 29,403 different combinations of health-states pairs to select from. EQ-5D-5L has 3125 health states, with 4881,250 different combinations of health states to select from. Furthermore, in a DCE design with duration as the 6th attribute with 3 levels, there will in effect be 9375 different health scenarios, resulting in 43,940,625 scenario pairs to select from. PRET will contribute to inform this selection, but the number of pairs included in PRET is still miniscule compared to the number of all possible pairs. PRET- AS therefore proposes to cover up to a further 450 scenarios across 30 questionnaire versions, each version with between 10 to 20 binary choice questions.
Regarding the second objective, one challenge associated with on-line administration of valuation studies is that respondents may become bored and distracted with repetitious tasks, especially if very similar-looking questions are repeated across numerous tasks. Therefore, PRET-AS will propose to explore the number of binary choice questions that can be put to respondents in an on-line survey environment, and examine the deterioration in data quality by the number of questions presented.
PRET-AS proposes an additional achieved sample size of 3000 (the original sample of 3000 in PRET are unaffected by PRET-AS). The additional sample in PRET-AS will be broken down so that a third of them will be presented with 10 binary choice questions, another third of the sample will be presented with 15 binary choice questions, and the last third 20 questions.
All PRET-AS data will be analysed alongside the corresponding questions in PRET to address the first objective. Furthermore, in order to address the second objective above, drop out rates, time take to complete a binary choice question, and distribution of left/right choices, are examined as more binary choice questions are answered. Identical binary choice questions will be allocated at different points in the survey, allowing the comparison of data quality depending on at what point in the exercise they appear, and contribute towards the identification of the optimal number of binary choice questions to present to respondents.
3.3 Research ethics
Ethics approval for PRET-AS will be obtained alongside the ethics approval for PRET from the University of Sheffield Research Ethics Committee, and the research will be carried out in line with the University policies on good research practice. All responses will be recorded under a code. Only aggregate level data will be published in scientific papers.
3.4 Dissemination of results, acknowledgement of funding, and archiving of data
The scientific findings from PRET will be submitted to the UK Health Economists’ Study Group (HESG) meetings and the EuroQol Group Scientific Meeting for presentation. They will also be submitted for publication in international health economics journals. Wherever possible, data and outcomes following PRET-AS will be analysed, presented, and submitted for publication alongside the data and findings from PRET. If analysis of PRET-AS data are presented on its own, acknowledgement will be made to the EuroQol Group and MRC. (However, if analysis of PRET data are presented on its own, acknowledgement will be made to MRC alone.) PRET-AS data will be archived together with PRET data, where individual level coded data will be made available for use by other researchers once the research team has confirmed acceptances on any main papers arising from the work.
List of abbreviations
- CAPI
- computer-assisted personal interview
- CP-TTO
- constant proportional time trade-off
- CS
- corner state
- DCE
- discrete choice experiment
- DCETTO
- discrete choice experiment incorporating duration
- H
- health state (part of experimental question)
- HRQL
- health-related quality of life
- HS
- health satisfaction (part of experimental questions)
- L
- lead time in full health (part of experimental questions)
- LL
- learnt to live (part of experimental question)
- LLR
- log-likelihood of full sample
- LLU
- log-likelihood of individual blocks
- LR
- likelihood ratio
- LS
- life satisfaction (part of experimental question)
- LT-TTO
- lead time – time trade-off
- MRC
- Medical Research Council
- MVH
- Measurement and Valuation of Health study
- NA
- not available
- n/a
- not applicable
- NICE
- National Institute for Health and Care Excellence
- NIHR
- National Institute for Health Research
- n/m
- not mentioned in the scenario
- NS
- non-significant
- P
- perspective (part of experimental questions)
- PRET
- Preparatory study for the Re-valuation of the EQ-5D Tariff project
- PRET-AS
- Preparatory study for the Re-valuation of the EQ-5D Tariff project – Additional Sample
- PROMs
- Patient Reported Outcome Measures initiative
- PTO
- person trade-off
- QALY
- quality-adjusted life-year
- r
- implied rate of time preference
- S
- satisfaction in health state (part of experimental questions)
- SD
- standard deviation
- SE
- the ‘somebody else’ perspective (part of experimental questions)
- SG
- standard gamble
- SWBH
- own health satisfaction
- SWBL
- own life satisfaction
- SY
- the ‘somebody else like you’ perspective (part of experimental questions)
- T
- duration in health state (part of experimental questions)
- TTO
- time trade-off
- V
- value to calculate full health duration (part of experimental questions)
- V*
- unobserved ‘true’ value of the health state