Notes
Article history
The research reported in this issue of the journal was funded by the Health Technology Assessment programme as project number 11/46/07. The contractual start date was in August 2012. The report detailing the set up phase and initial outcomes began editorial review in October 2014 and was accepted for publication in December 2014. The authors have been wholly responsible for all data collection, analysis and interpretation, and for writing up their work. The Health Technology Assessment editors and production house have tried to ensure the accuracy of the authors’ report and would like to thank the reviewers for their constructive comments on the final report document. However, they do not accept liability for damages or losses arising from material published in this report. Should the study progress further, the full report will be published in the Health Technology Assessment journal.
Declared competing interests of authors
Steve Goodacre is deputy chairperson of the Health Technology Assessment Programme Clinical Evaluation and Trials Board, and was chairperson of the Pandemic Influenza Themed Call Board.
Permissions
Copyright statement
© Queen’s Printer and Controller of HMSO 2015. This work was produced by Goodacre et al. under the terms of a commissioning contract issued by the Secretary of State for Health. This issue may be freely reproduced for the purposes of private research and study and extracts (or indeed, the full report) may be included in professional journals provided that suitable acknowledgement is made and the reproduction is not associated with any form of advertising. Applications for commercial reproduction should be addressed to: NIHR Journals Library, National Institute for Health Research, Evaluation, Trials and Studies Coordinating Centre, Alpha House, University of Southampton Science Park, Southampton SO16 7NS, UK.
Chapter 1 Background
Description of the health problem
An influenza pandemic has the potential to place a huge strain upon health services, particularly the emergency care services, which may be exacerbated by staff sickness absence due to influenza. Prior to the 2009 H1N1 influenza pandemic, the UK influenza pandemic contingency plan predicted around 750,000 excess emergency department attendances and 82,500 excess hospitalisations during a pandemic. 1 A more recent estimate suggested that a pandemic could result in 50% of the population having some symptoms, of whom 30% would seek primary care and 1–4% would need hospital admission. 2 The Pandemic Influenza Advisory Committee subgroup on modelling have estimated a likely clinical attack rate of 3–35% (worst-case scenario 50%), with 10–25% of these to have complications and a peak demand in the worst-case scenario of 13% of the population being ill. 3 Pandemic planning needs to encompass a wide range of potential scenarios, but even projections at the less severe end of the spectrum could cause substantial problems of overcrowding at emergency departments that are already often working close to capacity. Methods of triage for patients presenting to the emergency department with suspected pandemic influenza are therefore required and need to be fair, robust and reproducible. 4
The term ‘triage’ is often used to describe a brief initial assessment in the emergency department to determine patient order of priority in the queue to be seen. In this proposal we use the term ‘triage’ more broadly to include the full process of emergency department assessment, potentially including investigations such as blood tests and X-rays, and apply it to decision-making regarding whether or not the patient should be admitted and whether or not they should be referred for high dependency or intensive care.
Emergency department triage methods need to accurately predict the individual patient’s risk of death or severe illness. The predicted risk can then guide decision-making. Patients with a low risk may be discharged home, those with a higher risk admitted to hospital, and those at highest risk referred for high dependency or intensive care. The level of risk used to trigger these decisions need not necessarily be fixed or determined in advance. Indeed, it is likely that decision-making thresholds could change during the course of a pandemic, as the balance between resource availability and demand changes. Triage methods that use a risk prediction score to determine the need for hospital care may therefore be more useful than a triage rule that classifies patients into admission and discharge categories.
Current triage methods for pandemic influenza
Health Protection Agency (HPA) guidance prior to the 2009 pandemic, supported by the British Thoracic Society and British Infection Society, recommended the use of the CURB-65 pneumonia score5 for adults with suspected influenza-related pneumonia. This score uses five variables [confusion, urea level, respiratory rate, blood pressure (BP) and age] to generate a score of between 0 and 5. Subsequent Department of Health guidelines on surge capacity in a pandemic also considered use of a physiological–social score [Pandemic Modified Early Warning Score (PMEWS)] for adults. 6 This score uses physiological variables, age, social factors, chronic disease and performance status to generate a score of between 0 and 7. National guidance specific to the 2009 H1N1 influenza pandemic included a swine flu hospital pathway for emergency department management of adults and children with seven criteria; any one of which predicts increased risk and the need for hospital assessment. 7
Existing evidence
We used the autumn/winter phase of the 2009 H1N1 influenza pandemic in Sheffield and Manchester to evaluate the discriminant value of three potential systems for triage of emergency department patients with pandemic influenza: CURB-65, PMEWS and the swine flu hospital pathway. 8,9 However, the pandemic in these areas was less severe than predicted and only five patients of the cohort of 481 met our predefined criteria for critical illness. Within this cohort, the discriminant value (c-statistic) of the three systems for predicting critical illness was moderate {CURB-65 0.78 [95% confidence interval (CI) 0.58 to 0.99], PMEWS 0.77 (95% CI 0.55 to 0.99) and the swine flu hospital pathway 0.70 (95% CI 0.45 to 0.96)}. Their performance in predicting hospital admission was worse: CURB-65 0.65 (95% CI 0.54 to 0.76), PMEWS 0.76 (95% CI 0.66 to 0.86) and the swine flu hospital pathway 0.62 (95% CI 0.51 to 0.72). These findings suggested that clinicians were not using the recommended triage methods when deciding whether to admit or discharge patients, and raised concerns about the accuracy of these methods for predicting adverse outcome.
Other research during the pandemic cast doubt on the utility of existing triage systems. The Swine Flu Triage (SwiFT) study of patients admitted to critical care with H1N1 influenza found that 68% scored 0 or 1 using CURB-65 (i.e. recommended for hospital discharge). 10 This is supported in evidence from a Canadian seasonal flu cohort, for which no triage system performed well in predicting intensive care admission [c-statistics PMEWS 0.63 (95% CI 0.57 to 0.69), CURB-65 0.58 (95% CI 0.52 to 0.64)]. 11 The best discriminator in this cohort was SMART-COP, a system specifically developed to predict intensive care admission in community-acquired pneumonia,12 which achieved a c-statistic of 0.73 (95% CI 0.67 to 0.79) but has not to our knowledge been examined in a pandemic cohort. The SwiFT study10 also developed a new score based on systolic BP, temperature, heart rate, respiratory rate, neurological status and inspired oxygen concentration to predict adverse outcome. The SMART-COP and SwiFT scores therefore offer alternative triage methods that require validation in a pandemic. Capelastegui et al. 13 developed a score including age, sex, comorbidities and clinical presentation, which had a c-statistic of 0.74 for predicting major complications, but this has also not been validated in any external cohort.
A number of cohort studies were undertaken during the 2009 H1N1 influenza pandemic and in subsequent flu seasons to identify risk factors for poor outcome in various groups. 14–57 We have systematically reviewed these studies and present the main findings in Appendix 1. The predominant predictors of adverse outcome were chronic comorbidities and obesity, with conflicting evidence regarding the risk of pregnancy. Acute physiological disturbances, particularly hypoxia, were also found to have prognostic value.
The existing research therefore suggests that, although there are a number of patient characteristics and clinical measures that can predict adverse outcome, the available data do not support the use of any specific triage methods in suspected pandemic influenza.
Chapter 2 Research questions
Further research is required to identify predictors of adverse outcome and develop triage methods for use in pandemic influenza. Research in seasonal influenza and other respiratory illnesses can help to inform triage methods for pandemic influenza, but differences in the clinical characteristics of different respiratory illnesses and influenza strains mean that any triage method developed outside a pandemic needs to be tested in a pandemic and, ideally, pandemic influenza triage methods should be developed or refined during a pandemic.
The PAINTED (PAndemic INfluenza Triage in the Emergency Department) study has been planned to allow rapid implementation of a research protocol in a pandemic affecting the UK NHS, which will identify the most accurate triage method for predicting severe illness among patients attending the emergency department with suspected pandemic influenza. Successful implementation of a research protocol in a pandemic will require all regulatory approvals to be secured in advance, piloting of data collection processes, regular updating of the protocol, and plans for protocol activation. This report describes the PAINTED protocol, the results of piloting the protocol, and the plans for protocol updating and activation in the event of a pandemic.
The aim of the PAINTED study is to identify the most accurate triage method for predicting severe illness among patients with suspected pandemic influenza who are attending the emergency department. The specific objectives are to:
-
determine the discriminant value of emergency department triage methods for predicting severe illness in patients presenting with suspected pandemic influenza
-
determine the discriminant value of presenting clinical characteristics and routine tests for identifying severe illness
-
determine the independent predictive value of presenting clinical characteristics and routine tests for severe illness
-
develop two new triage methods based upon (1) presenting clinical characteristics alone and (2) presenting clinical characteristics, electrocardiogram (ECG), chest X-ray (CXR), and routine blood test results.
The aim of the pilot study was to test the PAINTED study methods during a winter influenza season. The specific objectives were to:
-
develop and test the use of a standardised clinical assessment form (CAF) that could be used for both clinical record documentation and research data collection during a pandemic
-
develop and test a secure online database to allow efficient data management in a pandemic
-
analyse pilot data from patients with seasonal influenza to ensure that data are reasonably complete and within expected ranges
-
seek clinician views on the usability of the standardised CAF
-
obtain all regulatory approvals required for the main study so that it can be activated rapidly in the event of a pandemic.
Chapter 3 Methods
The PAINTED study will be a prospective observational cohort study of patients attending the emergency department with suspected pandemic influenza, which aims to develop and evaluate triage methods for identifying those at risk of serious adverse outcome. It will evaluate triage methods used to determine whether or not a patient with suspected pandemic influenza should be admitted to hospital or not, and whether or not they should be admitted to intensive or high dependency care. These will include the CURB-65 score, PMEWS, the swine flu hospital pathway, SMART-COP, the SwiFT score and any new methods developed before the next pandemic. It will also develop two new triage methods based upon presenting (1) clinical characteristics alone and (2) clinical characteristics, ECG, CXR and routine blood test results.
The first score will use only variables that are available at initial patient assessment, i.e. history and examination, including simple technologies, such as automated BP measurement and pulse oximetry. This triage method can be used to assess patients for the need for hospital investigation and identify patients who can be discharged without further assessment. It could potentially be used, with appropriate validation, to assess patients in the community.
The second triage method will be based upon all available emergency department data, including routine blood tests, ECG and CXR findings. This triage method can be used for two potential purposes: identification of (1) patients with a low risk of adverse outcome who can be discharged home after emergency department assessment; and (2) high-risk patients who are likely to need high dependency or intensive care.
The PAINTED study will evaluate the ability of each method to predict whether patients die or require respiratory, cardiac or renal support. We will not evaluate the impact of triage methods upon patient care. Intervention in the study will therefore consist of only data collection and follow-up. Patient management will continue according to whatever national guidance is in place at the time of the pandemic.
We will initially aim to develop triage methods that can be applied to the whole population of patients presenting to the emergency department. Age-dependent variables will be assessed and included in the triage method in relation to age-specific normal ranges. We will then explore whether different triage methods may be appropriate for different patients, particularly whether or not a different triage method may be appropriate for children.
Full details of the PAINTED study are provided in the PAINTED protocol (see Appendix 2). This chapter describes the pilot study methods.
The PAndemic INfluenza Triage in the Emergency Department pilot study
The PAINTED pilot study involved using the standardised CAF and online database to collect data from patients presenting to the emergency department with suspected seasonal influenza, thus testing processes planned for the PAINTED study in the event of a pandemic.
A standardised CAF was developed, which was intended to double as clinical notes and study data collection form. It was based on the form used in our 2009 pandemic influenza study,9 and was designed to be easy for clinical staff to complete and include the key information required for clinical record-keeping. It also included the elements of all currently available triage methods, variables identified in previous studies as being predictors of adverse outcome (see Appendix 1), any other potential predictors that are routinely recorded in the emergency department (comorbidities, physiological observations, routine blood tests, ECG and CXR), and details of any pre-presentation antiviral medication, antibiotics and immunisation status. The form is reproduced in Appendix 3.
The pilot study was undertaken during winter 2012–13 across six acute hospitals: the Northern General Hospital (Sheffield), Sheffield Children’s Hospital, Salford Royal Hospital (Greater Manchester), York Hospital, Scarborough Hospital and The Royal London Hospital. We included all adults and children presenting with suspected influenza at the emergency departments of the participating hospitals. Patients were eligible for inclusion if they met the current clinical diagnostic criteria of (1) fever (pyrexia ≥ 38 °C) or a history of fever and (2) influenza-like illness (two or more of cough, sore throat, rhinorrhoea, limb or joint pain, headache, vomiting or diarrhoea) or a severe and/or life-threatening illness that is suggestive of an infectious process. The assessing clinician determined eligibility and completed the standardised CAF if the patient was considered to have suspected influenza. Research nurses employed by each hospital then photocopied the form, secured the original form in the notes and took the copy away.
Patients were followed up until 30 days after attendance by hospital record review to identify adverse outcomes. Patients who died or required respiratory, cardiovascular or renal support were defined as having an adverse outcome. If they survived to 30 days without requiring respiratory, cardiovascular or renal support they were defined as having no adverse outcome. We also recorded whether or not they were treated with antiviral agents or antibiotics, and the length and location of any hospital stay.
Respiratory support was defined as any intervention used to protect the patient’s airway or assist their ventilation, including non-invasive ventilation or acute administration of continuous positive airway pressure. It did not include supplemental oxygen alone or nebulised bronchodilators. Cardiovascular support was defined as any intervention used to maintain organ perfusion, such as inotropic drugs, or invasively monitor cardiovascular status, such as central venous pressure or pulmonary artery pressure monitoring, or arterial BP monitoring. It did not include peripheral intravenous canulation and/or fluid administration. Renal support was defined as any intervention used to assist renal function, such as haemoperfusion, haemodialysis or peritoneal dialysis. It did not include intravenous fluid administration.
Outcome assessment was based primarily on research nurse review of hospital computer records and case notes. The hospital computer records were checked at least 30 days after presentation for death or hospital admissions. If the patient was alive at 30 days, was discharged home from the emergency department and did not reattend hospital they were recorded as having no adverse outcome. If they died, were admitted to hospital or reattended hospital within 30 days, their hospital notes were retrieved and reviewed by the research nurse. If there was no evidence in the hospital notes of an adverse outcome the patient was recorded as having no adverse outcome.
The research nurse recorded details of adverse events on a follow-up form. Once complete, the research nurse entered all non-personal data on to a secure online database provided by the Sheffield Clinical Trials Research Unit (CTRU). Personal details (date of birth, NHS number, hospital number and emergency department number) were accessed by the research nurses to allow linkage back to source data but were not accessible to research staff in Sheffield. A unique study number provided a common identifier for research nurses and CTRU researchers. The research nurses kept a record of any patient who withdrew from the project but did not communicate details to other staff.
We intended to collect data from around 400 cases for the pilot study, with each pilot site collecting data from 60 to 80 cases. We estimated that this would provide sufficient numbers to test data collection and management, and to explore missing data rates. The main purpose of the pilot phase was to test data completion rates and the processes for data abstraction and reporting, so only descriptive data analysis was undertaken.
The standardised CAF was evaluated for acceptability and usability through 12 semistructured interviews with emergency department doctors and nurse practitioners based at four of the pilot sites. Participants were purposively sampled on the basis of having had experience of using the form during the pilot phase. Those who had no experience of using the form were provided with a copy of the form and its guidance to review prior to the interview. The interviews were undertaken by a single researcher (DB) as part of an educational project. The researcher was not otherwise involved in the PAINTED project but was supervised by members of the research team (SG, AI, RW). The interview schedule is reproduced in Appendix 4. Data were analysed using a framework approach. 58
Chapter 4 Ethical and governance processes and pandemic study site set-up
An essential part of the pilot study involved identifying and addressing the ethical and governance processes that needed to be in place in advance of the main pandemic study. We intended to undertake the pilot study using the same protocol as the main pandemic study to ensure that ethical and governance issues were addressed in the pilot study, so that all necessary approvals were in place in advance of a pandemic.
Ethical issues
The pilot study did not alter patient management and neither will the pandemic study. Both simply involve collecting routinely available data at presentation and follow-up. No additional diagnostic tests are performed. The risks to patients involved in the study are therefore very low and principally relate to data protection and confidentiality.
In accordance with plans for the main study, posters were prominently displayed in all participating departments, advising patients of the project and providing contact details for further information. Leaflets that briefly described the nature and purpose of the study (see Appendix 5) were provided for staff to hand to patients with suspected influenza and to provide contact details for further information.
We did not seek patient consent to participate on the basis that the study was limited to collection of routinely available data and any delays in patient assessment would risk compromising patient care. The information leaflet provided a tear-off slip with contact details that patients could use to inform the hospital or research team if they wished to withdraw from the study. Patients who wished to withdraw from the study would have their study records deleted. Their decision to withdraw would not be communicated to clinical staff providing further care and would not influence their subsequent management.
In our previous pandemic study,9 we obtained approval from the National Information Governance Board (NIGB) to collect personal data without patient consent under Section 251 of the NHS Act 2006. 59 We intended to do the same in the pilot and pandemic phases of PAINTED. However, the Ethics and Confidentiality Committee (ECC) of the NIGB ruled that they were unable to (1) approve our use – during the pilot phase, in the absence of a pandemic – of certain items of identifiable information without informed consent and (2) give a decision concerning the main phase of the study, partly because of uncertainty regarding possible changes to the common law duty of confidentiality, and partly because of the imminent dissolution of the ECC itself. We felt that requesting consent would make the pilot study too different from the pandemic study for it to be a meaningful pilot of the processes involved. We therefore decided to remove all personal details from the data set during the pilot phase so that data could be collected without patient consent. Following this amendment, Research Ethics Committee (REC) approval was granted by the North West (Haydock) REC without the need for Section 251 approval.
Responsibility for Section 251 approval subsequently passed from the NIGB to the Confidentiality Advisory Group (CAG) of the Health Research Authority. We therefore submitted to the CAG an application for Section 251 approval for the pandemic phase of the project, together with a concomitant ‘notice of amendment’ to the REC. We were granted Section 251 conditional approval contingent upon satisfying certain conditions. REC approval for the pandemic study was granted, conditional upon Section 251 approval. Initially, correspondence with the NIGB questioned the need for an application at this point, advising that it may be best to wait until a pandemic situation arose. It was necessary to clarify that the nature of the funding award specified the expectation to seek regulatory approvals in readiness of a pandemic. Despite this, the NIGB maintained obvious concerns over the timing of a future pandemic and the legal and regulatory landscape that may exist at that time.
The main condition for approval was to satisfy (to the mandatory level) each of the 14 requirements of the Information Governance Toolkit assessment, including technical data security, standard operating procedures, information governance staff training and human resource contractual matters beyond the responsibility of the research team. Completing the Toolkit on a per-project basis appears to be a very cumbersome and inefficient way to operate, placing a significant burden on the resources of research teams. Both the initial application and yearly review represent a significant burden, which may damage the long-term viability of maintaining a pandemic study in long-term ‘hibernation’. The University of Sheffield [specifically the School of Health and Related Research (ScHARR)] has therefore appointed an information governance lead to develop an information governance policy that addresses the requirements of the Toolkit and will be maintained to demonstrate an ongoing institutional level commitment to adequate standards of information handling and security.
The study protocol now states that the only patient-identifiable information recorded on to the database and viewable by the research team will be NHS numbers. These will be used to cross-check the completeness of the data set by comparing NHS numbers with the Intensive Care National Audit and Research Centre (ICNARC) database and the Office for National Statistics (ONS). The cross-check with ICNARC will be to determine that our data set has captured all intensive care usage and associated adverse outcomes. Should there be cases in the ICNARC database that are not present in the PAINTED database, we would endeavour to retrieve the missing clinical data (linking the cases via the NHS number) to supplement the PAINTED data set. Capturing missing mortality data will be undertaken via a search organised by the ONS. Linking of records would again be made using the NHS number.
The qualitative interview study did not involve patients, so NHS REC approval was not sought. Instead, the study was approved by the University of Sheffield (ScHARR) REC.
Research governance
Sheffield Teaching Hospitals NHS Foundation Trust was the pilot study sponsor and will be sponsor for the main pandemic study. The pilot was managed by staff in the section of Health Services Research in ScHARR in the University of Sheffield: Steve Goodacre (Chief Investigator), Mike Campbell (Statistician), Andrew Lee (Public Health), Richard Wilson (Project Manager), Andy Irving (Research Associate), Kirsty Challen (Postgraduate Research Student) and Kathryn MacKellar (Administrator). Responsibility has now passed to the Sheffield CTRU (also based in ScHARR) but the Chief Investigator and project team remain essentially unchanged. The project management group met quarterly between March 2012 and September 2013, when the study was placed in hibernation. Yearly review meetings were scheduled from October 2014 to ensure that the study maintained its readiness in the event of a pandemic.
A Steering Committee was formed to oversee study progress. The committee included Timothy Coats (Chair, Professor of Emergency Medicine), Jonathan Van Tam (Professor of Health Protection), Paul Baxter (Senior Lecturer in Biostatistics), Stephen Morton (Regional Director of the HPA Yorkshire and the Humber), Lynn Winspear [Sheffield Emergency Care Forum (SECF), Patient and Public Representation], Enid Hirst (SECF), Steve Goodacre and Richard Wilson. Lynn Winspear, Enid Hirst and other members of SECF also provided specific input into the project’s lay summary and patient leaflets.
Regulatory approvals for the pandemic phase
The pandemic phase aims to collect data from 20,000 patients presenting with suspected pandemic influenza across 40 sites. We planned to identify sites and principal investigators, establish processes and obtain research governance approvals at these sites during the pilot phase. We aimed to recruit sites across the whole of the UK to ensure that we would not miss the early phases of a pandemic localised to a particular geographical area.
We secured approvals from 41 separate English trusts (49 separate sites), one Welsh site, one Northern Irish site and two Scottish sites. The sites are listed in Table 1, below.
No. | Hospital site | NHS trust |
---|---|---|
1 | Northern General Hospital | Sheffield Teaching Hospitals NHS Foundation Trust |
2 | Sheffield Children’s Hospital | Sheffield Children’s NHS Foundation Trust |
3 | Salford Royal Hospital | Salford Royal Hospital NHS Foundation Trust |
4 | York District Hospital | York Teaching Hospital NHS Foundation Trust |
5 | Scarborough General Hospital | York Teaching Hospital NHS Foundation Trust |
6 | The Royal London Hospital | Bart’s and The London NHS Trust |
7 | Wythenshawe Hospital | University Hospital of South Manchester NHS Foundation Trust |
8 | Barnsley Hospital | Barnsley Hospital NHS Foundation Trust |
9 | Poole Hospital | Poole Hospital NHS Foundation Trust |
10 | Northampton General Hospital | Northampton General Hospital NHS Trust |
11 | Hull Royal Infirmary | Hull and East Yorkshire Hospitals NHS Trust |
12 | Bristol Royal Infirmary | University Hospitals Bristol NHS Foundation Trust |
13 | Derriford Hospital | Plymouth Hospitals NHS Trust |
14 | Milton Keynes Hospital | Milton Keynes Hospital NHS Foundation Trust |
15 | Royal Berkshire Hospital | Royal Berkshire NHS Foundation Trust |
16 | Arrowe Park Hospital | Wirral University Teaching Hospital NHS Foundation Trust |
17 | Cumberland Infirmary | North Cumbria University Hospitals NHS Trust |
18 | West Cumberland Hospital | North Cumbria University Hospitals NHS Trust |
19 | Dorset County Hospital | Dorset County Hospital NHS Foundation Trust |
20 | Gloucestershire Royal Infirmary | Gloucestershire Hospitals NHS Foundation Trust |
21 | King’s Mill Hospital | Sherwood Forest Hospitals NHS Foundation Trust |
22 | New Cross Hospital | The Royal Wolverhampton Hospitals NHS Trust |
23 | The Princess Royal Hospital | Shrewsbury And Telford Hospital NHS Trust |
24 | Wansbeck Hospital | Northumbria Healthcare NHS Foundation Trust |
25 | Doncaster Royal Infirmary | Doncaster and Bassetlaw Hospitals NHS Foundation Trust |
26 | University Hospital North Staffordshire | University Hospital of North Staffordshire NHS Trust |
27 | Southend Hospital | Southend University Hospital NHS Foundation Trust |
28 | John Radcliffe Infirmary | Oxford Radcliffe Hospitals NHS Trust |
29 | Harrogate District General Hospital | Harrogate and District NHS Foundation Trust |
30 | Broomfield Hospital | Mid Essex Hospital Services NHS Trust |
31 | Royal Victoria Infirmary | The Newcastle Upon Tyne Hospitals NHS Foundation Trust |
32 | Manchester Royal Infirmary | Central Manchester University Hospitals NHS Foundation Trust |
33 | Royal Manchester Children’s Hospital | Central Manchester University Hospitals NHS Foundation Trust |
34 | Aintree University Hospital | Aintree University Hospitals NHS Foundation Trust |
35 | Coventry and Warwickshire University Hospital | University Hospitals Coventry and Warwickshire NHS Trust |
36 | Addenbrooke’s Hospital | Cambridge University Hospitals NHS Foundation Trust |
37 | Queen’s Medical Centre | Nottingham University Hospitals NHS Trust |
38 | Royal Lancaster Infirmary | University Hospitals of Morecambe Bay NHS Foundation Trust |
39 | Furness General Infirmary | University Hospitals of Morecambe Bay NHS Foundation Trust |
40 | Wexham Park Hospital | Heatherwood And Wexham Park Hospitals NHS Foundation Trust |
41 | Queen Elizabeth Hospital | The Queen Elizabeth Hospital King’s Lynn NHS Foundation Trust |
42 | Great Western Hospital | Great Western Hospitals NHS Foundation Trust |
43 | Royal United Hospital | Royal United Hospital Bath NHS Trust |
44 | King’s College Hospital | King’s College Hospital NHS Foundation Trust |
45 | Ashford and St Peter’s Hospital | Ashford and St Peter’s NHS Foundation Trust |
46 | Royal Gwent Hospital, Wales | Aneurin Bevan Health Board |
47 | Craigavon Area Hospital, Northern Ireland | Southern Health and Social Care Trust |
48 | Edinburgh Royal Infirmary, Scotland | NHS Lothian |
49 | Royal Alexandra Hospital, Scotland | NHS Greater Glasgow and Clyde |
Recruitment of Northern Irish and, in particular, Scottish sites involved a disproportionate amount of additional preparatory work. We briefly outline the issues here for the benefit of researchers planning similar studies across the devolved nations. Section 251 approval does not provide a lawful means to collect patient-identifiable information without consent in Northern Ireland or Scotland. Furthermore, research using data from incapacitated adults in Scotland requires informed consent to participate, either directly from the person concerned or indirectly via a relative or a welfare guardian. In Northern Ireland we had to provide an alternative assurance of good information handling, data protection systems and processes, which consisted of an 18-page ‘data access agreement’ to the trust Caldicott Guardian.
In Scotland, we were advised that PAINTED should be undertaken as an audit and, as such, we would be able to collect identifiable patient data from all categories of patient without any restrictions. This involved a new national governance application, development of a new bespoke local site contract process, and submission of a 48-page application for Privacy Advisory Committee (PAC) approval for the collection of patient identifiers, namely the date of birth and Community Health Index number (analogous to the NHS number). Like the Information Governance Toolkit assessment, the PAC application required extensive input from multiple stakeholders, including technical electronic data flow and data protection protocols, various standard operating procedures and information governance training.
In addition to approval from the PAC, we were advised that we required specific additional approvals to collect Community Health Index numbers, addressed via a Caldicott Guardian National Scrutiny Process. This National Caldicott Forum advised that we would need to retain the use of patient information sheets and study posters (as per protocol) to inform patients of the use of their data and provide means to withdraw their data if requested. However, we were informed by the National Records of Scotland that the use of patient data for audit is routine in Scottish hospitals, so the use of information sheets or posters would be inappropriate. We have established that, by directing each organisation to the other’s advice, authority lies with the National Caldicott Forum and we retain the use of these study documents in Scottish sites.
We created a Scottish-specific protocol to reflect implementation of PAINTED as an audit in Scotland, highlighting deviations in operating procedures from the rest of the UK. We sought advice from the English REC with regard to these matters and received favourable opinion to proceed as advised in Scotland.
In summary, we were able to set up sites across the whole of the UK in advance of the pandemic phase of PAINTED, but found that navigating divergent – and sometimes incongruent – ethical and governance frameworks resulted in a disproportionate amount of resources being committed to, including sites in the devolved nationals (particularly Scotland), which may have impaired our ability to include non-English sites.
Chapter 5 Pilot study results
The pilot study was undertaken across six hospitals in winter 2012–13. Table 2 shows the start and end dates for case identification at the participating hospitals. Recruitment at the Northern General Hospital was extended for 1 month in an attempt to identify more patients but without success.
Recruitment milestone | Sheffield Children’s Hospital | Salford Royal Hospital | Royal London Hospital | Northern General Hospital | York District General | Scarborough General Hospital |
---|---|---|---|---|---|---|
Recruitment starta | 14 November 2012 | 20 September 2012 | 13 November 2012 | 8 October 2012 | 5 November 2012 | 29 January 2013 |
Recruitment end | 31 March 2013 | 31 March 2013 | 31 March 2013 | 26 April 2013 | 31 March 2013 | 31 March 2013 |
Recruitment days | 137 | 191 | 138 | 198 | 146 | 62 |
Patients recruited | 36 | 50 | 64 | 2 | 11 | 2 |
There were low levels of seasonal influenza overall during winter 2012–13, with consultations for influenza-like illness peaking at 30.1 per 100,000. 60 As a result, we were unable to achieve our target of collecting data from 400 cases. Overall, 165 patients with suspected influenza were identified across the six participating hospitals and had CAFs completed. Ten patients subsequently withdrew their data from the study, leaving 155 (94%) available for analysis. Follow-up data were available from 129 of 155 patients at 30 days (83%). Of these, 50 of 129 (39%) were admitted to hospital and 3 of 129 (2%) suffered an adverse outcome. Figure 1 illustrates the flow of patients through the pilot study.
FIGURE 1.
Flow of patients through the pilot study.

Table 3 shows patient flow by hospital. Three of the hospitals identified 150 of 165 (91%) of the patients. This suggests that either seasonal influenza incidence was very low at the other three hospitals or these hospitals were failing to identify cases for the study. All 10 withdrawing patients were at the same hospital. Reasons for withdrawal were not requested from patients, so we are unable to draw any further conclusions regarding withdrawals. All three of the hospitals with meaningful patient numbers were unable to collect follow-up data from a proportion of cases, with proportions ranging from 8% to 31%. Admission rates varied substantially, even allowing for small numbers, from 4% to 85% across the hospitals reporting meaningful numbers of cases. All of the deaths were at one hospital. Although we should be careful about drawing conclusions from these small numbers, they suggest a substantial degree of variation between hospitals in rates of case identification, withdrawal, follow-up and hospital admission.
Patient nos. | Sheffield Children’s Hospital | Salford Royal Hospital | Royal London Hospital | Northern General Hospital | York District General | Scarborough General Hospital | All hospitals |
---|---|---|---|---|---|---|---|
Total | 36 | 50 | 64 | 2 | 11 | 2 | 165 |
Withdrawn | 0 | 0 | 10 (16%) | 0 | 0 | 0 | 10 (6%) |
Data available | 36 (100%) | 50 (100%) | 54 (84%) | 2 (100%) | 11 (100%) | 2 (100%) | 155 (94%) |
30-day follow-up completed | 25 (69%) | 46 (92%) | 45 (83%) | 0 | 11 (100%) | 2 (100%) | 129 (83%) |
Admitted | 1 (4%) | 39 (85%) | 7 (16%) | 0 | 2 (18%) | 1 (50%) | 50 (39%) |
Deaths | 0 | 3 (8%) | 0 | 0 | 0 | 0 | 3 (2%) |
Table 4 shows data completion for key variables on the case report form by hospital and across all hospitals. BP is usually measured in children only if they are severely ill. This explains why recording of BP is so low at Sheffield Children’s Hospital.
Variable | Sheffield Children’s Hospital | Salford Royal Hospital | Royal London Hospital | Northern General Hospital | York District General | Scarborough General Hospital | All hospitals |
---|---|---|---|---|---|---|---|
No. | 36 | 50 | 54 | 2 | 11 | 2 | 155 |
Age | 34 (94%) | 48 (96%) | 30 (56%) | 2 (100%) | 11 (100%) | 2 (100%) | 127 (82%) |
Sex | 36 (100%) | 48 (96%) | 44 (81%) | 2 (100%) | 11 (100%) | 2 (100%) | 143 (92%) |
Referral source | 34 (94%) | 43 (86%) | 51 (94%) | 2 (100%) | 11 (100%) | 2 (100%) | 143 (92%) |
Performance status | 16 (44%) | 43 (86%) | 45 (83%) | 2 (10%) | 10 (91%) | 1 (50%) | 117 (75%) |
Symptom duration | 33 (92%) | 39 (78%) | 47 (87%) | 1 (50%) | 10 (91%) | 2 (100%) | 132 (85%) |
Temperature | 34 (94%) | 47 (94%) | 52 (96%) | 2 (100%) | 11 (100%) | 2 (100%) | 148 (95%) |
Pulse rate | 35 (97%) | 45 (90%) | 51 (94%) | 2 (100%) | 10 (91%) | 1 (50%) | 144 (93%) |
Respiratory rate | 36 (100%) | 48 (96%) | 50 (93%) | 2 (100%) | 11 (100%) | 1 (50%) | 148 (95%) |
BP | 1 (3%) | 34 (68%) | 49 (91%) | 2 (100%) | 8 (73%) | 0 | 94 (61%) |
Oxygen saturation | 27 (75%) | 45 (90%) | 50 (93%) | 2 (100%) | 10 (91%) | 1 (50%) | 135 (87%) |
Inspired oxygen | 14 (39%) | 41 (82%) | 29 (54%) | 1 (50%) | 3 (27%) | 0 | 88 (57%) |
Capillary refill | 31 (86%) | 17 (34%) | 40 (74%) | 2 (100%) | 9 (82%) | 1 (50%) | 100 (64%) |
GCS | 11 (31%) | 30 (60%) | 39 (72%) | 2 (100%) | 7 (64%) | 0 | 89 (57%) |
AVPU | 25 (69%) | 44 (88%) | 35 (65%) | 2 (100%) | 2 (18%) | 1 (50%) | 109 (70%) |
CXR | 33 (92%) | 35 (70%) | 36 (67%) | 2 (100%) | 11 (100%) | 1 (50%) | 118 (76%) |
ECG | 33 (92%) | 27 (54%) | 38 (70%) | 1 (50%) | 11 (100%) | 1 (50%) | 111 (72%) |
The mean age of the cohort was 31 years (median 26.5 years, range 1–92 years), with 49 of 127 (39%) aged 0–16 years. There were 72 males and 71 females. The referral source among those with usable data for this variable was self-referral for 114 (80%), general practitioner (GP) for nine (6%), multiple for six (4%) and other for 14 (10%). Mean symptom duration was 5.6 days (median 3 days, range 1–56 days). Performance status among those with usable data for this variable was unrestricted/normal in 78 (67%), limited by strenuous activity in seven (6%), limited by non-strenuous activity in 25 (21%), limited by self-care in five (4%) and bed-/chair-bound in two (2%).
Table 5 reports descriptive statistics for physiological variables. Elevated temperature, heart rate and respiratory rate are all in keeping with acute febrile illness. Capillary refill was normal in 55 patients and not recorded in 100 patients. The modal values for eyes, Glasgow Coma Scale (GCS) verbal and GCS motor component scores were 4, 5 and 6, respectively. The alert, verbally responsive, responsive to pain or unconscious (AVPU) response scale was recorded as alert in all 109 cases in which it was recorded.
Variable | n | Mean (SD) | Range |
---|---|---|---|
Temperature (°C) | 148 | 37.8 (1.0) | 35.9–40.0 |
Pulse rate (per minute) | 144 | 108 (28) | 59–180 |
Respiratory rate (per minute) | 148 | 25 (10) | 13–60 |
Systolic BP (mmHg) | 94 | 124 (23) | 71–203 |
Diastolic BP (mmHg) | 94 | 71 (13) | 41–108 |
Oxygen saturation (%) | 135 | 96 (3) | 83–100 |
A CXR was normal in 28, abnormal in 23 and not undertaken in 67 of the 118 cases with details recorded. ECG was normal in 26 patients, abnormal in 14 and not undertaken in 71 of the 111 cases with ECG details recorded.
Social isolation and eight chronic diseases were recorded as being present by ticking a box, so it was not possible to determine whether no tick in the box indicated absence of the variable or missing data. Social isolation (defined as living alone or having no fixed abode) was reported by 27 patients (16%). Chronic diseases were recorded with the following frequencies: heart disease, 18; renal impairment, six; steroid therapy, one; asthma, 17; other chronic lung disease, 14; diabetes, nine; active malignancy, one; and immunosuppression, one.
Influenza was thought by the clinician to be the most likely diagnosis in 34 of 155 cases (22%). The most common alternatives were upper respiratory tract infection (17 cases), community-acquired pneumonia (11 cases) and lower respiratory tract infection (five cases).
Blood test results were available for 32 of 155 cases. Table 6 reports descriptive statistics for blood variables. Results were generally within normal ranges, with the exception of white cell count (WCC), which tended to be elevated in keeping with acute infective illness.
Variable | n | Mean (SD) | Range |
---|---|---|---|
Haemoglobin (g/dl) | 32 | 13.5 (1.9) | 9.5–16.0 |
WCC (× 109/l) | 10.1 (4.2) | 4–18 | |
Platelet count (× 109/l) | 234 (112) | 132–606 | |
Sodium (mmol/l) | 138 (4) | 125–145 | |
Potassium (mmol/l) | 3.9 (0.6) | 3.0–5.9 | |
Urea (mmol/l) | 6.2 (6.6) | 2.3–29.2 | |
Creatinine (µmol/l) | 87 (55) | 47–370 |
Follow-up data were available from 129 of 155 attendances. Table 7 shows the number of admissions across the centres with length of stay for the two hospitals with meaningful numbers of admissions. Overall, 50 of 129 patients were admitted with a mean length of stay of 3.9 days (median 2 days, range 0–22 days).
Parameter | Sheffield Children’s Hospital | Salford Royal Hospital | Royal London Hospital | Northern General Hospital | York District General | Scarborough General Hospital | All hospitals |
---|---|---|---|---|---|---|---|
No. of admissions | 1 | 39 | 7 | 0 | 2 | 1 | 50 |
LoS recorded | 1 | 38 | 7 | 0 | 2 | 1 | 49 |
Mean LoS | – | 4.2 | 3.3 | – | – | – | 3.9 |
Median LoS | – | 2 | 3 | – | – | – | 2 |
Range | – | 0–22 | 0–9 | – | – | – | 0–22 |
Three cases were recorded as having suffered an adverse outcome. All three died; two also received respiratory, cardiovascular and/or renal support. Details are as follows:
-
Female, aged 31 years, died after receiving extracorporeal membrane oxygenation, and respiratory, cardiovascular and renal support.
-
Male, aged 83 years, died after receiving respiratory and cardiovascular support.
-
Male, aged 87 years, died with no record of having received respiratory, cardiovascular or renal support.
Clinician views on the standardised clinical assessment form
Most participants believed that the content of the form was both appropriate and useful, although its adult-centric nature was noted by some. At the very least, they felt that it included the minimum level of information required in dealing with a case of pandemic flu, but elements of added value were noted, such as allowing for, and prompting, the documentation of a thorough history and examination. Some individuals noted that elements of the form were either unclear in terms of what they required or would benefit from greater detail, with suggestions for specific pointers in the ‘Clinical examination’ section and space to document details of abnormal findings.
The structure of the form was received well in terms of ease of use owing to its clarity, simplicity and concise nature. It was felt to be logical, with some participants commenting that it mirrored their own practice of taking notes. Despite positive reflections on the fact that the form fitted on to one A4 page, a greater number of those interviewed felt that it did not have enough space for them to write all of the free text that they would like.
A number of amendments to the form were suggested and are outlined in Table 8. There was little consistency across the participants in the amendments suggested, and the majority of suggestions were made by only single participants. Three suggestions were made independently by two participants. These were to reduce the size of the ‘Patient criteria’ and ‘Performance status’ sections, and to move the observations box to the top of the second page. Most participants favoured an electronic rather than paper version of the form, as they considered that it would be quicker, although it should be noted that the majority of those interviewed had experience of using the latter during the pilot phase.
Form content | |
---|---|
Include space to provide details about abnormal central capillary refill, CXR or ECG findings | |
Include specific pointers in the ‘Clinical examination’ section, detailing what clinicians should be looking for | |
Use of a separate paediatric form | Suggested by two participants, although acknowledged by one to be difficult to implement practically in a department seeing all ages |
Form structure | |
Reduce the size of the ‘Patient criteria’ and ‘Performance status’ sections | Suggested by two participants |
Move the observations box to the top of the second page | Suggested by two participants |
Relocate the title to the top of the form | |
Combine the ‘Past medical history’ and ‘Chronic disease’ sections | |
Reduce the size of the ‘Referral source’ section | |
Combine the ‘Previous’, ‘Antibiotic therapy?’ and ‘Symptom duration’ sections | |
Increase the size of the ‘Current medication’ section | |
Relocate the ‘Clinically obese?’ and ‘Pregnant?’ questions to somewhere more appropriate | |
With the observations box at the top of the page, move the ‘Investigations’ section after ‘Clinical examination’ (which should include the ‘Severe respiratory distress’ and ‘Respiratory exhaustion’ questions), followed by a renamed ‘Differential diagnosis including other clinical concerns’ | |
Split the ‘Clinical examination’ section to include specific respiratory examination information |
In terms of the purpose of the form, the majority of participants considered it to be a helpful clinical record and, almost without exception, felt that, putting aside their experience during the pilot, they would be confident using it in a future pandemic situation with the accompanying guidance. Indeed, one participant noted that his/her unit felt that it would be useful enough to continue using after the pilot had ended. However, some of those interviewed commented that it lacked use beyond data collection in terms of assisting in the patient management process, and one person commented that the condition-specific design of the form meant that it could direct health-care professionals towards a wrong diagnosis.
Chapter 6 Discussion
We developed a standardised CAF that was used for both clinical record documentation and research data collection. Also, we developed a secure online database and used it to collect usable data from participating hospitals. Interviews with clinicians revealed generally positive views on the standardised form and willingness to use the form in the event of a pandemic. A number of amendments were suggested but none was made by more than two participants. As a consequence, no substantial amendments were made to the form. All regulatory approvals were obtained for the main study to allow it to be activated rapidly in the event of a pandemic.
The pilot study was limited by the low incidence of influenza in the winter of 2012–13, which made it difficult to draw conclusions about case identification and generalise these conclusions to a pandemic. The variation in numbers of cases identified between the hospitals suggests that some hospitals were failing to identify eligible cases for the study. However, this may reflect the difficulties of achieving staff engagement when the incidence of cases is low and influenza is a low priority. Staff awareness and engagement is likely to be much higher during a pandemic, although other challenges are likely to arise in a pandemic that may compromise study progress in other unpredictable ways.
The pilot study also highlighted potential concerns about missing data, with data completeness varying between items and between hospitals. Data were relatively complete for heart rate, respiratory rate, temperature and peripheral oxygen saturation. They were lower for capillary refill, inspired oxygen, BP, GCS score and AVPU. This is probably because patients who are well do not routinely have capillary refill checked or receive supplementary oxygen, and children do not usually have their BP measured. The GCS score is probably assumed to be 15/15 and AVPU response = ‘alert’ and thus not recorded in patients who are well and able to provide a full history. When recorded, capillary refill was always normal, AVPU was always ‘alert’ and GCS score was mostly 15.
Chest X-rays were performed in 51 of 118 cases, with details recorded, and ECGs were performed in 40 of 111 cases, with details recorded, whereas blood tests were recorded for 32 of 155 patients. These low rates are unsurprising, as CXR, ECG and blood tests have a limited role in the assessment of patients with uncomplicated influenza. It would be reasonable to assume that those without investigations would have had normal investigations had they been performed, but the proportion of patients with no record of whether or not the investigation was performed highlights the importance of improving recording of this variable.
The primary analysis for the pandemic study will impute missing predictor variable data as being normal on the assumption that the most likely reason for it being missing is that the clinician did not feel that it was worth recording a variable or doing an investigation that was expected to be normal. However, this assumption may not hold in a pandemic if clinical resources are overstretched. We have therefore planned sensitivity analysis based on different imputation assumptions to test the assumption that missing values are normal.
The adverse event rate was 3 of 129 cases (2%), which is similar to that observed in our 2009 H1N1 influenza pandemic study9 and suggests that our assumption of a low adverse event rate in sample size calculation is appropriate.
Variation in missing data rates between hospitals is concerning, as it suggests that some hospitals were not recording potentially available data. Deliberately, we did not use the opportunity afforded by the pilot study to undertake rigorous chasing of missing data but instead used the light touch approach that is likely to be the only feasible approach in a pandemic. We are involved in ongoing efforts to identify why specific data items were missing at specific hospitals to determine whether or not processes can be improved prior to a pandemic. We are also exploring whether or not developments in routine data management, such as electronic emergency department records, can be harnessed to improve case identification and data completeness.
This is particularly important with regard to minimising the rate of missing outcome data. Some 26 of 155 patients (17%) did not have 30-day follow-up recorded and hence we were unable to verify whether or not they had an adverse outcome. It was not clear why this rate was so high, as 30-day follow-ups involved only record review. It may have reflected a low priority for research nurses, as the pilot might have lacked salience during a quiet influenza season, and recruitment to the pilot was not subject to any target or sanction (unlike studies involving patient consent). We are involved in ongoing efforts to ensure that research nurse support will be appropriate and sufficiently motivated in the event of a pandemic. For example, we have produced online screencast demonstrations of how to collect and manage the data.
It is also worth noting that data linkage methods that were not available for the pilot will be available in the pandemic study. The CAG approved the proposed use of the NHS number to link our data to ONS and ICNARC data in the event of a pandemic but was not able to approve this for the pilot study. This linkage can be used in a pandemic to identify those who have died or been admitted to critical care. If the risks of failed linkage or adverse events occurring outside critical care are accepted, and patients with no ONS or ICNARC event are assumed to have had no adverse event, then linkage methods could provide close to 100% follow-up. Developments in electronic data recording and linkage could facilitate efficient 30-day follow-up within hospitals and further reduce the risk of missing data. For these reasons we have decided not to revise the sample size calculation for the pandemic study to take missing outcome data into account.
In summary, the pilot has achieved the objectives of developing acceptable data collection methods, but only limited conclusions about case identification and data completeness to be drawn from a pilot in seasonal influenza and applied to a pandemic. On one hand, a pandemic is likely to be associated with overstretched resources that may further limit our ability to minimise missing data. On the other hand, PAINTED may achieve a higher profile and priority in a pandemic than is possible for a pilot phase that was undertaken during a relatively quiet influenza season.
Some changes to the protocol were required as a result of the pilot. As outlined above, we were unable to obtain Section 251 approval for the use of personal data and therefore undertook the pilot using only anonymised data. For the pandemic study, we obtained approval to use the NHS number to allow linkage to ONS and ICNARC data. This will provide a simple way of checking for missed adverse outcomes but will not allow us to contact GPs for additional follow-up data. The likelihood of additional adverse events being identified through GP contact is low and, in the event of a pandemic, unlikely to be feasible. We therefore intend to accept this small risk of patients being misclassified as not having an adverse outcome.
The process of addressing the requirements of the Information Governance Toolkit for CAG approval created a substantial and unanticipated additional burden. However, by developing the role of the Information Governance Lead in our institution, and developing an Information Governance Policy that can be maintained and applied to similar future projects, we have ensured that (hopefully) this issue will be easier to address in future projects.
The process of obtaining research governance approvals involved, as expected, varying degrees of delay. The process of obtaining approvals in hospitals in the devolved nations and, in particular, addressing different approaches to the use of confidential data, involved substantial additional work. We believe that for the PAINTED study this additional work is, on balance, worthwhile, given the importance of achieving coverage across the whole of the UK. Other projects that do not need to achieve such wide geographical coverage may regard the additional bureaucratic obstacles associated with undertaking a study across different regulatory regimes as a sufficient obstacle to warrant limiting to one area.
Maintenance of the project and activation in the event of a pandemic
The pilot phase is now completed and the project has been put on hold until a pandemic occurs. However, the protocol will need to be regularly reviewed and updated to ensure that it remains valid and relevant to the needs of the NHS. The following specific issues need to be regularly reviewed:
-
evidence for triage methods and predictors of adverse outcome in influenza
-
case identification, in particular the diagnostic criteria for suspected influenza and potential use of rapid diagnostic testing methods
-
national and international pandemic planning guidance
-
the ethical and regulatory framework for undertaking research, particularly in relation to using data without consent
-
data collection and management in emergency departments, particularly the use of electronic patient records.
Responsibility for PAINTED has now been passed to the Sheffield CTRU. Members of the project team remain engaged in the project but, with the exception of the Chief Investigator, all project roles and contact details are now role specific rather than person specific. This means that the project remains potentially active as long as the Chief Investigator is in post.
Detailed maintenance and activation plans have been drawn up to guide maintenance of the project until a pandemic and activation in the event of a pandemic. The maintenance plan involves an annual review of the project with the following key elements:
-
Update the literature review of predictors of adverse outcome in suspected influenza. This has been done for the first annual review in 2014 and is included in Appendix 1 of this report.
-
Review of international and national pandemic planning guidance and expert contact to identify development in pandemic planning, influenza diagnosis and treatment. The 2014 update has identified development in point-of-care testing for influenza,61 which may have implications for case identification in the future but has not yet been adopted into national guidance.
-
Contact with participating hospitals to ensure they remain willing and able to support the pandemic phase, and that regulatory approvals remain in place.
-
Review of expressions of interest from potential participating hospitals and, if appropriate, replacement of existing hospitals with new hospitals.
-
Contact with all members of the project team and steering committee to ensure that they remain willing to undertake their roles in the event of a pandemic. If appropriate, replacement of any members of the project team or steering committee who are unable to continue to fulfil their role.
-
Updating the protocol and review by the project team.
-
Informing the REC and CAG of any amendments to the protocol and, if appropriate, seeking approval for any substantial amendments.
-
Review of the maintenance and activation plans.
Recommendations for further research
The obvious recommendation for further research as a result of this pilot study is that the main study should be undertaken if and when a pandemic occurs. Regulatory approvals are in place, data collection methods have been developed and tested, and a process is in place to ensure that the protocol is regularly updated and readiness to undertake the research is maintained.
The pilot study has identified potential problems with missing data and these could be explored through further pilot work. However, given the difficulty of generalising conclusions from pilot work undertaken in a seasonal influenza to a full study undertaken in a pandemic, there is probably little value in simply repeating attempts to pilot the standardised assessment form in future influenza seasons. Instead, further research should involve using developments in routine data management and other technologies to develop the proposed pandemic research methods. For example, the increasing use of electronic data capture in emergency departments may provide the opportunity to develop an electronic CAF, thus reducing the risk of missing data by making data collection more efficient and integrated into routine care. Developments in linkage between administrative data sources could be used to simplify the collection of follow-up data and reduce the risk of missing outcome data. The development of rapid influenza testing could be integrated into hospital information systems to ensure that all cases receiving testing were identified and included in a pandemic study.
Conclusions
An observational cohort study to identify the most accurate triage method for predicting severe illness in emergency department attendees with suspected pandemic influenza is set up and ready to activate in a pandemic. Clinician views of the standardised CAF are generally positive. We were able to collect usable data using the standardised CAF, although problems of missing data were identified and the paucity of seasonal influenza cases limited our ability to fully test how case identification and data collection will proceed in pandemic.
Acknowledgements
Project co-applicants
Name | Affiliation | Role |
---|---|---|
Steve Goodacre | University of Sheffield | Chief Investigator |
Kirsty Challen | University of Sheffield | Expert in Pandemic Emergency Planning |
Andrew Bentley | University Hospital of South Manchester | Critical Care Expertise |
Darren Walter | University Hospital of South Manchester | Expert in Pandemic Emergency Planning |
Ian Maconochie | St Mary’s Hospital, London | Expert in Paediatric Emergency Medicine |
Chris Fitzsimmons | Sheffield Children’s Hospital | Expert in Paediatric Emergency Medicine |
Mike Campbell | University of Sheffield | Statistician |
Andrew Lee | University of Sheffield | Public Health Expertise |
Richard Wilson | University of Sheffield | Project Manager |
Project Management Group
Name | Affiliation | Role |
---|---|---|
Steve Goodacre | University of Sheffield | Chief Investigator |
Mike Campbell | University of Sheffield | Statistician |
Andrew Lee | University of Sheffield | Public Health Expertise |
Richard Wilson | University of Sheffield | Project Manager |
Andy Irving | University of Sheffield | Research Associate |
Kirsty Challen | University of Sheffield | Postgraduate Research Student |
Kathryn MacKellar | University of Sheffield | Administrator |
Project Steering Committee
Name | Position | Organisation |
---|---|---|
Tim Coats (Chair)a | Professor of Emergency Medicine | University of Leicester |
Jonathan Van Tama | Professor of Health Protection | University of Nottingham |
Paul Baxtera | Senior Lecturer in Biostatistics | University of Leeds |
Stephen Mortona | Regional Director, Yorkshire and the Humber | HPA |
Enid Hirsta | Public representative | SECF |
Lynn Winspeara | Public representative | SECF |
Steve Goodacre | Chief Investigator | University of Sheffield |
Richard Wilson | Project Manager | University of Sheffield |
Site leads and research nurses
Hospital | Principal Investigator | Research nurse/lead contact |
---|---|---|
Sheffield Teaching Hospital | Steve Goodacre | John Humphreys |
Sheffield Children’s Hospital | Chris Fitzsimmons | Julie Woodhead |
Salford Royal | Fiona Lecky | Kate Clayton |
York Teaching Hospital | Steven Crane | Rebecca Coop |
Scarborough General Hospital | Richard Smith | Simon Dyer |
The Royal London | Tim Harris | Jason Potts |
South Manchester | Darren Walter | Darren Walter |
Barnsley | James Griffiths | Sally Anne Pearson |
Poole | Deborah Mayne | Deborah Mayne |
Northampton | Tristan Dyer | Sue Brown |
Hull | Alasdair Pickering | Paul Williams |
Bristol Royal Infirmary/Bristol Royal Hospital for Children | Jonathan Benger | Georgina Elder |
Plymouth | Jason Smith | Rosalyn Squire |
Milton Keynes | Peter Thomas | Rowena Fletcher |
Reading | Liza Keating | Sarah Kempster |
Wirral | Andrea Wootten | Christopher Smalley |
North Cumbria | Olu Orugun | Leon Jonker |
Dorset | Tamsin Ribbons | Sarah Moreton |
Gloucestershire | Victoria Stacey | Gemma Race |
Sherwood Forest | Richard Clarkson | Lynne Allsop |
Wolverhampton | Andrew MacDuff | Claire Bailey |
Shrewsbury | Adrian Marsh | Charlotte Owen |
Northumbria | Mark Harrison | Maureen Armstrong |
Doncaster | Faisal Fasih | Rachel Codling |
North Staffordshire | Richard Hall | Ian Massey |
Southend | Bernard Hadebe | Sue Bowman |
Oxford | Melanie Darwent | Sally Beer |
Harrogate | Victoria Holloway | James Hughes |
Edinburgh (Scotland) | Alasdair Gray | Ola Gruszczynska |
Chelmsford | Steve Jenkins | Helena Walsh |
Newcastle | John Wright | Kelley Storey |
Paisley (Scotland) | Alasdair Corfield | Alasdair Corfield |
Central Manchester | Rick Body | Rick Body |
Aintree | Abdo Sattout | Gemma Richards |
Coventry | Magdy Sakr | Magdy Sakr |
Cambridge | Khurram Iftikhar | Katrina Gatley |
Nottingham | Frank Coffey | Philip Miller |
Craigavon (Northern Ireland) | Mike Smith | Sara Gilpin |
Lancaster | Samuel McBride | Jayne Craig |
Wexham Park | Sarah Wilson | Kate Eke |
Newport (Wales) | Ashkok Vaghela | Claire Price |
Swindon | Stephen Haig | Debbie Palmer |
King’s Lynn | Nam Tong | Kayleigh Shough |
Bath | David Watson | Paula Paterson |
King’s College Hospital (London) | Rob Pinate | Sinead Helyar |
Ashford and St Peter’s | Claire Atkinson | Martha Wrigley |
Journal outputs and associated publications
Goodacre S, Walter D. Research in an influenza pandemic [published online ahead of print August 27 2012]. Emerg Med J 2013;30:349. doi:10.1136/emermed-2012-201769
Challen K, Goodacre SW, Wilson R, Bentley A, Campbell M, Fitzsimmons C, et al. Evaluation of triage methods used to select patients with suspected pandemic influenza for hospital admission. Emerg Med J 2012;29:383–8.
Goodacre S, Challen K, Wilson R and Campbell M. Evaluation of triage methods used to select patients with suspected pandemic influenza for hospital admission: cohort study. Health Technol Assess 2010;14(46):173–263.
Disclaimers
This report presents independent research funded by the National Institute for Health Research (NIHR). The views and opinions expressed by authors in this publication are those of the authors and do not necessarily reflect those of the NHS, the NIHR, NETSCC, the HTA programme or the Department of Health. If there are verbatim quotations included in this publication the views and opinions expressed by the interviewees are those of the interviewees and do not necessarily reflect those of the authors, those of the NHS, the NIHR, NETSCC, the HTA programme or the Department of Health.
References
- Pandemic Flu: A National Framework for Responding to an Influenza Pandemic. London: DH; 2007.
- UK Influenza Pandemic Preparedness Strategy. London: DH; 2011.
- Subgroup on Modelling. Modelling Summary. London: DH; 2011.
- Challen K, Bentley A, Bright J, Walter D. Clinical review: mass casualty triage – pandemic influenza and critical care. Crit Care 2007;11:212-18. http://dx.doi.org/10.1186/cc5732.
- Lim W. Pandemic flu: clinical management of patients with an influenza-like illness during an influenza pandemic. Thorax 2007;62:1-46. http://dx.doi.org/10.1136/thx.2006.073080.
- Pandemic Influenza: Surge Capacity and Prioritisation in Health Services. London: DH; 2008.
- Swine Flu Clinical Package. London: DH; 2009.
- Challen K, Goodacre SW, Wilson R, Bentley A, Campbell M, Fitzsimmons C, et al. Evaluation of triage methods used to select patients with suspected pandemic influenza for hospital admission. Emerg Med J 2012;29:383-8. http://dx.doi.org/10.1136/emj.2010.104380.
- Goodacre S, Challen K, Wilson R, Campbell M. Evaluation of triage methods used to select patients with suspected pandemic influenza for hospital admission: cohort study. Health Technol Assess 2010;14:173-26. http://dx.doi.org/10.3310/hta14460-03.
- Rowan K, Harrison D, Walsh TS, McAuley DF, Perkins GD, Taylor BL, et al. The Swine Flu Triage (SwiFT) study: development and ongoing refinement of a triage tool to provide regular information to guide immediate policy and practice for the use of critical care services during the H1N1 swine influenza pandemic. Health Technol Assess 2010;14. http://dx.doi.org/10.3310/hta14550-05.
- Muller MP, McGeer AJ, Hassan K, Marshall J, Christian M. Toronto Invasive Bacterial Disease Network . Evaluation of pneumonia severity and acute physiology scores to predict ICU admission and mortality in patients hospitalized for influenza. PLOS One 2010;5. http://dx.doi.org/10.1371/journal.pone.0009563.
- Charles PG, Wolfe R, Whitby M, Fine MJ, Fuller AJ, Stirling R, et al. SMART-COP: a tool for predicting the need for intensive respiratory or vasopressor support in community-acquired pneumonia. Clin Infect Dis 2008;47:375-84. http://dx.doi.org/10.1086/589754.
- Capelastegui A, Quintana JM, Bilbao A, España PP, Garin O, Alonso J, et al. Score to identify the severity of adult patients with influenza A (H1N1) 2009 virus infection at hospital admission. Eur J Clin Microbiol Infect Dis 2012;31:2693-701. http://dx.doi.org/10.1007/s10096-012-1616-8.
- Miller RR, Markewitz BA, Rolfs RT, Brown SM, Dascomb KK, Grissom CK, et al. Clinical findings and demographic factors associated with ICU admission in Utah due to novel 2009 influenza A(H1N1) infection. Chest 2010;137:752-8. http://dx.doi.org/10.1378/chest.09-2517.
- Nguyen-Van-Tam J, Openshaw P, Hashim A, Gadd EM, Lim WS, Semple MG, et al. Risk factors for hospitalisation and poor outcome with pandemic A/H1N1 influenza: United Kingdom first wave (May–September 2009). Thorax 2010;65:645-51. http://dx.doi.org/10.1136/thx.2010.135210.
- ANZIC Influenza Investigators . Critical care services and 2009 H1N1 influenza in Australia and New Zealand [published online ahead of print October 8 2009]. N Engl J Med 2009;361:1925-34. http://dx.doi.org/10.1056/NEJMoa0908481.
- Harris PN, Dixit R, Francis F, Buettner PG, Leahy C, Burgher B, et al. Pandemic influenza H1N1 2009 in north Queensland: risk factors for admission in a region with a large indigenous population. Commun Dis Intell n.d.;34:102-9.
- Santa-Olalla Peralta P, Cortes-García M, Vicente-Herrero M, Castrillo-Villamandos C, Arias-Bohigas P, Pachon-del Amo I, et al. Risk factors for disease severity among hospitalised patients with 2009 pandemic influenza A (H1N1) in Spain, April–December 2009. Euro Surveill 2010;15.
- Cui W, Zhao H, Lu X, Wen Y, Zhou Y, Deng B, et al. Factors associated with death in hospitalized pneumonia patients with 2009 H1N1 influenza in Shenyang, China. BMC Infect Dis 2010;10. http://dx.doi.org/10.1186/1471-2334-10-145.
- Zimmerman O, Rogowski O, Aviram J, Misrahi M, Zeltser D, Justo D, et al. C-reactive protein serum levels as an early predictor of outcome in patients with pandemic H1N1 influenza A virus infection. BMC Infect Dis 2010;10. http://dx.doi.org/10.1186/1471-2334-10-288.
- Martin-Loeches I, Papiol E, Rodríguez A, Diaz E, Zaragoza R, Granada RM, et al. Acute kidney injury in critical ill patients affected by influenza A (H1N1) virus infection. Crit Care 2011;15. http://dx.doi.org/10.1186/cc10046.
- Echevarría-Zuno S, Mejía-Aranguré JM, Mar-Obeso AJ, Grajales-Muñiz C, Robles-Pérez E, González-León M, et al. Infection and death from influenza A H1N1 virus in Mexico: a retrospective analysis. Lancet 2009;374:2072-9. http://dx.doi.org/10.1016/S0140-6736(09)61638-X.
- Louie JK, Gavali S, Acosta M, Samuel MC, Winter K, Jean C, et al. Children hospitalized with 2009 novel influenza A (H1N1) in California. Arch Pediatr Adolesc Med 2010;164:1023-31. http://dx.doi.org/10.1001/archpediatrics.2010.203.
- Stein M, Tasher D, Glikman D, Shachor-Meyouhas Y, Barkai G, Yochai AB, et al. Hospitalization of children with influenza A (H1N1) virus in Israel during the 2009 outbreak in Israel. Arch Pediatr Adolesc Med 2010;164:1015-22. http://dx.doi.org/10.1001/archpediatrics.2010.195.
- Vasoo S, Singh K, Trenholme GM. Predicting need for hospitalization of patients with pandemic (H1N1) 2009, Chicago, Illinois, USA. Emerg Infect Dis 2010;16:1594-97. http://dx.doi.org/10.3201/eid1610.091889.
- Bagdure D, Curtis DJ, Dobyns E, Glode MP, Dominguez SR. Hospitalized children with 2009 pandemic influenza A (H1N1): comparison to seasonal influenza and risk factors for admission to the ICU. PLOS One 2010;5. http://dx.doi.org/10.1371/journal.pone.0015173.
- Fajardo-Dolci G, Gutiérrez-Vega R, Arbolaya-Casanova H, Villalobos A, Wilson KS, Garcia SG, et al. Clinical characteristics of fatalities due to influenza A (H1N1) virus in Mexico. Thorax 2010;65:505-9. http://dx.doi.org/10.1136/thx.2009.126953.
- Lee N, Choi K, Chan PK, Hui DS, Lui DC, Wong BC, et al. Outcomes of adults hospitalised with severe influenza. Thorax 2010;65:510-15. http://dx.doi.org/10.1136/thx.2009.130799.
- Libster R, Bugna J, Coviello S, Hijano Dr, Dunaiewsky M, Reynoso N, et al. Pediatric hospitalizations associated with 2009 pandemic influenza A (H1N1) in Argentina. N Engl J Med 2010;362:45-5. http://dx.doi.org/10.1056/NEJMoa0907673.
- Chien Y-S, Su C-P, Tsai H-T, Huang AS, Lien C-E, Hung M-N, et al. Predictors and outcomes of respiratory failure among hospitalized pneumonia patients with 2009 H1N1 influenza in Taiwan. J Infect 2010;60:168-74. http://dx.doi.org/10.1016/j.jinf.2009.12.012.
- Jain S, Kamimoto L, Bramley AM, Schmitz AM, Benoit SR, Louie J, et al. Hospitalized patients with 2009 H1N1 influenza in the United States, April–June 2009. N Engl J Med 2009;361:1935-44. http://dx.doi.org/10.1056/NEJMoa0906695.
- Tuite AR, Greer AL, Whelan M, Winter AL, Lee B, Yan P, et al. Estimated epidemiological parameters and morbidity associated with pandemic H1N1 influenza. CMAJ 2010;182:131-6. http://dx.doi.org/10.1503/cmaj.091807.
- Campbell A, Rodin R, Kropp R, Mao, Hong Z, Vachon J, . Risk of severe outcomes among patients admitted to hospital with pandemic (H1N1) influenza. CMAJ 2010;182:349-55. http://dx.doi.org/10.1503/cmaj.091823.
- Aviram G, Bar-Shai A, Sosna J, Rogowski O, Rosen G, Weinstein I, et al. H1N1 influenza: initial chest radiographic findings in helping predict patient outcome. Radiology 2010;255:252-9. http://dx.doi.org/10.1148/radiol.10092240.
- Bassetti M, Parisini A, Calzi A, Pallavicini FM, Cassola G, Artioli S, et al. Risk factors for severe complications of the novel influenza A (H1N1): analysis of patients hospitalized in Italy. Clin Micro Inf 2010;17:247-50. http://dx.doi.org/10.1111/j.1469-0691.2010.03275.x.
- Xi X, Xu Y, Jiang L, Li A, Duan J, Du B, et al. Hospitalized adult patient with 2009 influenza A (H1N1) in Beijing, China: risk factors for hospital mortality. BMC Infect Dis 2010;10. http://dx.doi.org/10.1186/1471-2334-10-256.
- Pebody R, McLean E, Zhao H, Cleary P, Bracebridge S, Foster K, et al. Pandemic influenza A (H1N1) 2009 and mortality in the United Kingdom: risk factors for death, April 2009 to March 2010. Euro Surveill 2010;15.
- Wilking H, Buda S, von der Lippe E, Altmann D, Krause G, Eckmanns T, et al. Mortality of 2009 pandemic influenza A(H1N1) in Germany. Euro Surveillance 2010;15.
- Martin-Loeches I, Díaz E, Vidaur L, Torres A, Laborda C, Granada R, et al. Pandemic and post-pandemic Influenza A (H1N1) infection in critically ill patients. Crit Care 2011;15. http://dx.doi.org/10.1186/cc10573.
- Pereira JM, Moreno RP, Matos R, Rhodes A, Martin-Loeches I, Cecconi M, et al. Severity assessment tools in ICU patients with 2009 Influenza A (H1N1) pneumonia. Clin Microbiol Infect 2012;18:1040-8. http://dx.doi.org/10.1111/j.1469-0691.2011.03736.x.
- Delgado-Rodrıguez M, Castilla J, Godoy P, Martın V, Soldevila N, Alonso J, et al. Prognosis of hospitalized patients with 2009 H1N1 influenza in Spain: influence of neuraminidase inhibitors. J Antimicrob Chemother 2012;67:1739-45. http://dx.doi.org/10.1093/jac/dks098.
- Bramley AM, Dasgupta S, Skarbinski J, Kamimoto L, Fry AM, Finelli L, et al. Intensive care unit patients with 2009 pandemic influenza A (H1N1pdm09) virus infection – United States, 2009. Influenza Other Respir Viruses 2012;6:e134-42. http://dx.doi.org/10.1111/j.1750-2659.2012.00385.x.
- Chen W-H, Lu C-Y, Shao P-L, Lee P-I, Kao C-L, Chung M-Y, et al. Risk factors of severe novel influenza A (H1N1) infections in hospitalized children. J Formos Med Assoc 2012;111:421-6. http://dx.doi.org/10.1016/j.jfma.2011.06.015.
- Chen K-F, Hsieh Y-H, Gaydos CA, Valsamakis A, Rothman RE. Derivation of a clinical prediction rule to predict hospitalization for influenza in EDs. Am J Emerg Med 2013;31:529-34. http://dx.doi.org/10.1016/j.ajem.2012.10.010.
- Kok J, Blyth CC, Foo H, Bailey MJ, Pilcher DV, Webb SA, et al. Viral pneumonitis is increased in obese patients during the first wave of pandemic A (H1N1) 2009 virus. PLOS One 2013;8. http://dx.doi.org/10.1371/journal.pone.0055631.
- Estella A. Usefulness of CURB-65 and pneumonia severity index for influenza A H1N1v pneumonia. Monaldi Arch Chest Dis 2012;77:118-21.
- Garnacho-Montero J, Gutierrez-Pizarraya A, Marquez JA, Zaragoza R, Granada R, Ruiz-Santana S, et al. Epidemiology, clinical features, and prognosis of elderly adults with severe forms of influenza A (H1N1). J Am Geriatr Soc 2013;61:350-6. http://dx.doi.org/10.1111/jgs.12152.
- Esterman EE, Lahra MM, Zurynski YA, Booy R, Elliott EJ. Influenza infection in infants aged < 6 months during the H1N1–09 pandemic: a hospital-based case series. J Paediatr Child Health 2013;49:635-40. http://dx.doi.org/10.1111/jpc.12266.
- Dalziel SR, Thompson JM, Macias CG, Fernandes RM, Johnson DW, Waisman Y, et al. Predictors of severe H1N1 infection in children presenting within Pediatric Emergency Research Networks (PERN): retrospective case–control study. BMJ 2013;347. http://dx.doi.org/10.1016/j.jpeds.2013.10.057.
- Lopez-Delgado JC, Rovira A, Esteve F, Rico N, Mendiluce RM, Noguera JB, et al. Thrombocytopenia as a mortality risk factor in acute respiratory failure in H1N1 influenza. Swiss Med Wkly 2013;143. http://dx.doi.org/10.4414/smw.2013.13788.
- Greenbaum A, Chaves SS, Perez A, Aragon D, Bandyopadhyay A, Bennett N, et al. Heavy alcohol use as a risk factor for severe outcomes among adults hospitalized with laboratory-confirmed influenza, 2005–2012. Infection 2014;42:165-70. http://dx.doi.org/10.1007/s15010-013-0534-8.
- Delgado-Rodriguez M, Castilla J, Godoy P, Martin V, Soldevila N, Alonso J, et al. Different prognosis in hospitalized patients with influenza one season after the pandemic H1N1 influenza of 2009–2010 in Spain. Influenza Other Respir Viruses 2013;7:1336-42. http://dx.doi.org/10.1111/irv.12119.
- Borse R, Kadam D, Sangle S, Basavraj A, Prasad H, Umarji P, et al. Clinicoradiologic correlation in adult patients diagnosed with novel influenza A (H1N1). J Assoc Physicians India 2013;61:600-7.
- Mortensen E, Louie J, Pertowski C, Cadwell BL, Weiss E, Acosta M, et al. Epidemiology and outcomes of adults with asthma who were hospitalized or died with 2009 pandemic influenza A (H1N1) – California, 2009. Influenza Other Respir Viruses 2013;7:1343-9. http://dx.doi.org/10.1111/irv.12120.
- Semple MG, Myles PR, Nicholson KG, Lim WS, Read RC, Taylor BL, et al. An evaluation of Community Assessment Tools (CATs) in predicting use of clinical interventions and severe outcomes during the A (H1N1)pdm09 pandemic. PLOS One 2013;8. http://dx.doi.org/10.1371/journal.pone.0075384.
- Kusznierz G, Uboldi A, Sosa G, Torales S, Colombo J, Moyano C, et al. Clinical features of the hospitalized patients with 2009 pandemic influenza A (H1N1) in Santa Fe, Argentina. Influenza Other Respir Viruses 2013;7:410-17. http://dx.doi.org/10.1111/j.1750-2659.2012.00405.x.
- Mertz D, Kim TH, Johnstone J, Lam P-P, Science M, Kuster SP, et al. Populations at risk for severe or complicated influenza illness: systematic review and meta-analysis. BMJ 2013;347. http://dx.doi.org/10.1136/bmj.f5061.
- Ritchie J, Spencer L, Bryman A, Burgess RG. Analyzing Qualitative Data. London: Routledge; 1994.
- National Health Service Act 2006. London: The Stationery Office; 2006.
- Surveillance of Influenza and Other Respiratory Viruses, Including Novel Respiratory Viruses, in the United Kingdom: Winter 2012/13. London: Public Health England; 2013.
- Bell J, Bonner A, Cohen DM, Birkhahn R, Ram Y, Triner W, et al. Multicenter clinical evaluation of the novel Alere Influenza A&B isothermal nucleic acid amplification test. J Clin Virol 2014;61:81-6. http://dx.doi.org/10.1016/j.jcv.2014.06.001.
Appendix 1 Studies evaluating clinical predictors of adverse outcome in pandemic influenza
Author | Site | Subjects | N | Outcome | Variable | Results |
---|---|---|---|---|---|---|
Rowan (ICNARC)10 | UK | ICU, suspected H1N1 (NB: Only 562 confirmed) | 1725 | Death | Current/recent pregnancy | HR 0.13 (95% CI 0.19 to 0.98); p = 0.048 |
Severe chronic organ dysfunction | HR 1.53 (95% Cl 1.16 to 2.02); p = 0.008 | |||||
Immunocompromised | HR 1.65 (95% Cl 1.16 to 2.33); p = 0.005 | |||||
SOFA score (per point) | HR 1.05 (95% Cl 1.02 to 1.08); p = 0.001 | |||||
Capelastegui13 | Spain | Hospitalised, > 18 years | 618 | Severe complication (death, IPPV, septic shock, ARDS, ‘resuscitation manoeuvres’ | Age | OR 2.6 (95% Cl 1.4 to 5) 46–65 years; 2.8 (95% Cl 1.3 to 6) > 65 years |
Male | OR 2.2 (95% Cl 1.3 to 3.8) | |||||
Smoker | OR 2.1 (95% Cl 1.1 to 3.9) yes; 2.2 (95% Cl 1.1 to 4.4) ex | |||||
No. of comorbidities | OR 2.9 (95% Cl 1.4 to 5.8) > 2 (ref. 0) | |||||
Multilobar/bilateral | OR 2.5 (95% Cl 1 to 5.9) | |||||
Pneumonia | OR 1.8 (95% Cl 1 to 3) | |||||
Confusion | OR 3.9 (95% Cl 1.8 to 8.5) | |||||
Fever | OR 0.4 (95% Cl 0.2 to 0.8) | |||||
Dyspnoea | OR 4.7 (95% Cl 2 to 11) | |||||
Score: 1 point for age > 45 years, male, > 2 comorbidities, pneumonia; 2 points for confusion, dyspnoea | AUROC 0.74 (95% Cl 0.68 to 0.8) | |||||
Miller14 | Utah | ICU admission, age > 15 years, PCR confirmation of H1N1 | 47 | ICU admission | Hispanic | 23% vs. 13% population; p = 0.01 |
Pacific/Hawaiian | 26% vs. 1% population; p < 0.001 | |||||
BMI 30–39 kg/m2 | 38% vs. 19% population; p < 0.001 | |||||
BMI > 39 kg/m2 | 36% vs. 3% population; p < 0.001 | |||||
Nguyen-Van-Tam (FLU-CIN)15 | UK | Hospitalised, confirmed H1N1 | 631 | Death/ICU/HDU | Chronic lung disease (not asthma/COPD) | OR 3.41 (95% CI 1.33 to 8.71); p = 0.010 |
Obesity | OR 6.96 (95% Cl 1.46 to 27.28); p = 0.008 | |||||
Altered consciousness | OR 1.11 (95% Cl 1.04 to 1.17); p = 0.001 | |||||
CXR pneumonia | OR 5.28 (95% Cl 2.95 to 9.47); p = 0.001 | |||||
CRP > 100 mg/dl | OR 4.41 (95% Cl 2.14 to 9.1); p = 0.001 | |||||
SaO2 < 94% on air | OR 3.6 (95% Cl 2.17 to 6.27); p = 0.001 | |||||
ANZIC16 | Australia/New Zealand | ICU-confirmed H1N1 | 722 | ICU admission | Pregnancy | 9.1% vs. 1% population |
BMI > 35 kg/m2 | 28.6% vs. 5.3% population | |||||
Chronic pulmonary disease | 32.7% vs. 13% population | |||||
Maori/Pacific islander | 25% vs. 13.6% population | |||||
Harris17 | Australia | H1N1 confirmed | 181 | Hospital admission | Aboriginal/Torres Strait | 37.7% vs. 60.3%; p = 0.004 |
Pregnant | 29% vs. 8.1%; p = 0.013 | |||||
DM | 24.6% vs. 4.2%; p < 0.001 | |||||
Renal disease | 18% vs. 3.3%; p = 0.001 | |||||
Cardiac disease | 26.2% vs. 8.3%; p = 0.001 | |||||
Obese | 28.3% vs. 10%; p = 0.002 | |||||
Santa-Olalla18 | Spain | Inpatients, H1N1 | 3025 | ICU/death | Asthma | 14.5% vs. 22.7%; p < 0.001 |
COPD | 11.5% vs. 16.9%; p < 0.001 | |||||
BMI > 40 kg/m2 | 19.3% vs. 11.1%; p < 0.001 | |||||
Diabetes | 13.8% vs. 9.4%; p < 0.001 | |||||
Other metabolic disease | 11.5% vs. 8.8%; p = 0.001 | |||||
Cardiovascular disease | 16.1% vs. 9.6%; p < 0.001 | |||||
Chronic hepatic disease | 9% vs. 6.1%; p = 0.025 | |||||
Seizures | 6.5% vs. 3.4%; p = 0.001 | |||||
Chronic renal insufficiency | 7.3% vs. 4.1%; p = 0.003 | |||||
Cui19 | China | Inpatient, H1N1 | 68 | Death | BMI > 27 kg/m2 | 8/10 death vs. 14/58 alive; p = 0.001 |
Zimmerman20 | Israel | Adults, CDC definition, PCR confirmation | 191 | ICU admission | SaO2 | Median 92% vs. 97%; p = 0.006 |
Examination lung findings | 71% vs. 31%; p = 0.002 | |||||
CRP | Median 123 vs. 40; p < 0.001 | |||||
Martin-Loeches21 | Spain | Adults, ICU admission for respiratory failure, no pre-existing CRF, microbiological confirmation | 661 | Acute kidney injury | Diabetes | 16.2% vs. 9.2%; p = 0.04 |
SOFA score | Mean 8.7 vs. 4.8; p < 0.001 | |||||
MODS | 92.4% vs. 54.7%; p < 0.001 | |||||
WCC | 8.3 vs. 6.8; p < 0.001 | |||||
CK | 290 vs. 170; p < 0.001 | |||||
CRP | 28 vs. 20; p < 0.001 | |||||
Echevarría-Zuno22 | Mexico | Confirmed H1N1 | 6945 | Death | Chronic disease | OR 6.1 (95% Cl 2.37 to 15.99) |
Tachypnoea | OR 4.26 (95% Cl 2.14 to 8.47) | |||||
Cyanosis | OR 3.46 (95% Cl 1.63 to 7.31) | |||||
Time of onset to admission (days) | OR 1.19 (95% Cl 1.11 to 1.28) | |||||
Louie23 | USA | Age < 18 years, hospitalised, H1N1 | 345 | Death/ICU | Hispanic (vs. white) | OR 0.4 (95% Cl 0.2 to 0.8) |
Pulmonary disease | OR 1.6 (95% Cl 1.0 to 2.6) | |||||
Cardiac disease | OR 4.3 (95% Cl 1.9 to 9.5) | |||||
Neurological disease | OR 2.8 (95% Cl 1.6 to 5.0) | |||||
Gastrointestinal disorder | OR 2.4 (95% Cl 1.3 to 4.5) | |||||
Acute altered mental status | 2% vs. 15%; p < 0.001 | |||||
Stein24 | Israel | Age < 18 years, hospitalised, H1N1 | 478 | ICU admission | Neurological disease | 19% vs. 7.6%; p = 0.02 |
Cardiovascular disease | 14.3% vs. 5.7%; p = 0.03 | |||||
Metabolic disease | 9.5% vs. 1.6%; p = 0.01 | |||||
Tachypnoea | 61.9% vs. 34.9%; p = 0.001 | |||||
Hypoxia | 57.1% vs. 21.8%; p < 0.001 | |||||
CXR effusion | 9.5% vs. 2.1%; p = 0.005 | |||||
CXR diffuse infiltrate | 33.3% vs. 8.1%; p < 0.001 | |||||
Vasoo25 | USA | ED presentations, H1N1 | 83 | Admission ICU | History of prematurity | 18.8% vs. 0; p = 0.002 |
Haemoglobinopathy | 12.5% vs. 0; p = 0.02 | |||||
Chronic neurological disease | OR 6.9 (95% Cl 1.3 to 35.5) | |||||
Malignancy | 9.4% vs. 0; p = 0.054 | |||||
Tachypnoea | OR 4.7 (95% Cl 1.7 to 13) | |||||
SaO2 < 92% | 31.3% vs. 0; p < 0.0001 | |||||
Acute renal failure | 15.6% vs. 0; p = 0.007 | |||||
CXR infiltrate | 37.9% vs. 0; p = 0.001 | |||||
Chronic pulmonary disease | OR 4.5 (95% Cl 1.4 to 14.0) | |||||
History of prematurity | OR 30 (95% Cl 3.2 to 281.8) | |||||
Chronic neurological disease | OR 4.1 (95% Cl 1 to 17.7) | |||||
Tachypnoea | OR 5.4 (95% Cl 1.7 to 17.5) | |||||
SaO2 < 92% | OR 84.9 (95% Cl 9.3 to 772) | |||||
Acute renal failure | OR 22.0 (95% Cl 2.3 to 214.2) | |||||
CXR infiltrate | 68.9% vs. 37.9 (inpatients); p < 0.0001 | |||||
Bagdure26 | USA | Paediatric admission, H1N1 | 307 | PICU | Neurological disorder | 38% vs. 19%; p = 0.002 |
Immunocompromised | 3% vs. 9%; p = 0.08 | |||||
Seizures (acute) | 15% vs. 3%; p < 0.001 | |||||
Mental status change | 20% vs. 2%; p < 0.001 | |||||
Hypoxia | 76% vs. 58%; p = 0.007 | |||||
Decreased breath sounds | 48% vs. 30%; p = 0.006 | |||||
WCC < 4 × 109/l | 13% vs. 26%; p = 0.04 | |||||
CRP > 1 mg/dl | 82% vs. 57%; p = 0.03 | |||||
pH < 7.35 | 75% vs. 27%; p = 0.002 | |||||
Fajardo-Dolci27 | Mexico | First 100 H1N1 confirmed deaths | 100 | Death | Cardiovascular disease | 20.9% vs. 4.1% population |
Metabolic syndrome | 39.5% vs. 14.5% population | |||||
DM | 19.8% vs. 7% population | |||||
Respiratory disease | 8.1% vs. 0.4% population | |||||
Hypertension | 19.8% vs. 15.4% population | |||||
Lee28 | Hong Kong | Adults, seasonal flu A/B | 754 | Death | Oseltamivir, Tamiflu® (Roche) | HR 0.27 (95% Cl 0.13 to 0.55); p < 0.001 |
Male | HR 3.92 (95% Cl 1.8 to 8.57); p = 0.001 | |||||
Major comorbidity | HR 2.27 (95% Cl 1.02 to 5.09); p = 0.045 | |||||
Libster29 | Argentina | Age < 18 years, confirmed H1N1 by PCR | 251 | ICU admission | Asthma | OR 4.92 (95% Cl 1.38 to 17.33); p = 0.002 |
Chien30 | Korea | H1N1 pneumonia | 96 | IPPV/NIV | Pregnancy | 2% vs. 9%; p = 0.05 |
Chronic renal insufficiency | 14% vs. 1%; p = 0.04 | |||||
SOFA | 4 vs. 1; p = 0.000 | |||||
Jain31 | USA | Confirmed H1N1 | 272 | ICU/death | Age (years) | Median 19 vs. 29 |
Neurocognitive disease | 5% vs. 13% | |||||
Neuromuscular disease | 5% vs. 13% | |||||
CXR pneumonia | 28% vs. 73% | |||||
Antivirals < 48 hours | 45% vs. 23% | |||||
Tuite32 | Canada | Confirmed H1N1 | 3152 | Death | Age > 50 years | OR 28.6 (95% Cl 7.3 to 111.2) |
Campbell33 | Canada | Hospital admission, H1N1 | 1479 | Death/ICU | Heart disease | RR 2.1 (95% Cl 1.6 to 2.7) |
DM | RR 2.2 (95% Cl 1.7 to 2.7) | |||||
Immunosuppression | RR 1.5 (95% Cl 1.1 to 2.0) | |||||
Aviram34 | Israel | ED H1N1, CXR in 24 hours | 97 | ICU/death | Bilateral opacities | 60% vs. 15%; p = 0.049 |
Multizonal opacities | 60% vs. 6%; p = 0.01 | |||||
Bassetti35 | Italy | Inpatients, confirmed H1N1 | 81 | ICU/death | Neurocognitive disease | 33.3% vs. 7%; p = 0.02 |
COPD/asthma | 19.7% vs. 50%; p = 0.03 | |||||
Pneumonia on admission | 100% vs. 44%; p = 0.0008 | |||||
Xi36 | China | Adult inpatients, H1N1 | 155 | Inpatient death | Hypertension | 37% vs. 19.5%; p = 0.048 |
Dyspnoea at presentation | 77.8% vs. 47.7%; p = 0.004 | |||||
Pebody37 | UK | UK national statistics (estimated case fatality rate) | 440 deaths | Death | Chronic renal disease | RR 36.3 (95% Cl 20.9 to 63.2) |
Heart disease | RR 15.2 (95% Cl 9.6 to 24.1) | |||||
Respiratory disease | RR 11.3 (95% Cl 7.9 to 16.1) | |||||
Liver disease | RR 63.3 (95% Cl 38.6 to 103.7) | |||||
DM | RR 9.2 (95% Cl 5.6 to 14.9) | |||||
Immunosuppression | RR 52.8 (95% Cl 36.3 to 76.6) | |||||
Stroke/TIA | RR 7.5 (95% Cl 2.3 to 23.7) | |||||
Chronic neurological disease | RR 115.3 (95% Cl 84.3 to 157.6) | |||||
Wilking38 | Germany | National statistics | 226,075 | Death | Age 15–34 years (ref. 35–60 years) | OR 0.18 (95% Cl 0.13 to 0.26) |
Age > 60 years | OR 5.4 (95% Cl 3.86 to 7.56) | |||||
Martin-Loeches39 | Spain | ICU admission, PCR-confirmed H1N1 (also assessed 2010–11 post pandemic) | 648 | Death | SOFA | Mean 4.9 vs. 8.4; p < 0.001 |
APACHE | Mean 12.53 vs. 19.69; p < 0.001 | |||||
Age (years) | Mean 43.7 vs. 48.4; p < 0.001 | |||||
Comorbidity | 69.6% vs. 79.4%; p = 0.02 | |||||
Heart failure | 6% vs. 11%; p = 0.03 | |||||
Chronic renal disease | 4% vs. 10%; p = 0.003 | |||||
Autoimmune disease | 2.6% vs. 5.7%; p = 0.06 | |||||
Haematological disease | 3.7% vs. 14.9%; p < 0.001 | |||||
Respiratory coinfection | 14.6% vs. 23.4%; p = 0.01 | |||||
Pereira40 | Multiple (ESICM) | ICU admission | 265 | Death | SAPS III | Mean 51 vs. 60; p < 0.001 |
APACHE II | Mean 25 vs. 20; p < 0.001 | |||||
Delgado-Rodriguez41 | Spain | Hospitalised | 813 | Death/ICU | Age 46–65 years (ref. < 19 years) | OR 2.21 (95% Cl 1.09 to 4.71) |
Age > 65 years (ref. < 19 years) | OR 2.44 (95% Cl 1.03 to 5.83) | |||||
Ex-smoker (NB: Current smoker not significant) | OR 1.97 (95% Cl 1.07 to 3.52) | |||||
COPD | OR 2.02 (95% Cl 1 to 3.87) | |||||
DM | OR 2.25 (95% Cl 1.21 to 4.02) | |||||
Corticosteroids | OR 3.05 (95% Cl 1.14 to 7.35) | |||||
H2 blockers | OR 2.08 (95% Cl 1.05 to 6.66) | |||||
Two to three comorbidities (ref. 0) | OR 2.21 (95% Cl 1.09 to 4.6) | |||||
More than three comorbidities (ref. 0) | OR 2.98 (95% Cl 1.47 to 6.24) | |||||
Bramley42 | USA | ICU admission | 108 adults + 46 children | Death | Illness to admission < 2 days | 10/37 deaths vs. 51/115; p = 0.06 |
Asthma | 4/11 death vs. 33/117; p = 0.05 | |||||
CXR pneumonia | 32/35 death vs. 69/107; p < 0.001 | |||||
Treatment < 2 days | 2/28 death vs. 34/97; p < 0.01 | |||||
Sepsis syndrome | 21/30 death vs. 15/100; p < 0.01 | |||||
Chen43 | Taiwan | Paediatric admission | 61 | Death/ICU | BMI > 25 kg/m2 | 3/11 vs. 0/37; p = 0.008 |
SOB | 8/14 vs. 8/47; p = 0.008 | |||||
CRP > 3 mg/dl | 6/12 vs. 5/46; p = 0.008 | |||||
Secondary bacterial infection | 4/14 vs. 2/47; p = 0.03 | |||||
Infiltration on CXR | 6/14 vs. 33/42; p = 0.03 | |||||
Pleural effusion on CXR | 3/14 vs. 0/42; p = 0.02 | |||||
Chen44 | Taiwan | ED presentations (NB: 2007–9, all flu) | 146 | Hospital admission | Underlying illness | 89% admission vs. 69% |
SOB | 13% admission vs. 6% | |||||
Headache | 0% admission vs. 5% | |||||
General ache | 2% admission vs. 8% | |||||
CXR positive finding | 29% admission vs. 15% | |||||
WCC | High 9% admission vs. 6%, low 25 vs. 19 | |||||
Neutrophil | High 25% admission vs. 12%, low 11 vs. 9 | |||||
Hb | Low 29% admission vs. 20% | |||||
Kok45 | Australia | ICU admission | 173 | Death (hospital) | Obesity | 6% in obese vs. 20% non-obese NB: Non-significant when corrected for severity of illness |
Estella46 | Spain | Hospital admission with viral pneumonia | 24 | ICU admission | SaO2 | 96.6 ± 2 ward vs. 87.7 ± 5 ICU |
Garnacho-Montero47 | Spain | ICU admission, H1N1 | 1120 | Death | Age > 65 years | 32% mortality vs. 22% |
Garnacho-Montero47 | Spain | ICU admission, H1N1, age > 65 years (subgroup of above) | 129 | Death | Haematological disease | OR 5.1 (95% Cl 1.7 to 14.7) |
Immunosuppression | OR 3.7 (95% Cl 1.5 to 8.7) | |||||
> 48 hours before oseltamivir | OR 2.7 (95% Cl 0.9 to 7.6) | |||||
Esterman48 | Australia | Admission < 6 months | 28 | Admission | Smoker in household | 36% vs. 20% population |
NICU/SCBU | 25% vs. 14.4% population | |||||
Preterm birth | 14% vs. 8.2% population | |||||
Median household size | 5 vs. 2.5 population | |||||
Dalziel49 | International (PERN) | Children, admission | 265 + 265 age matched | Severe outcome | Asthma | OR 2.7 (95% Cl 1.7 to 4.2) |
Chronic lung disease | OR 9.8 (95% Cl 4.2 to 22.8) | |||||
Heart disease | OR 6.0 (95% Cl 2.3 to 15.5) | |||||
Renal disease | OR 8.0 (95% Cl 1.0 to 64.0) | |||||
Cerebral palsy | OR 34.5 (95% Cl 8.5 to 141) | |||||
Preterm birth | OR 4.1 (95% Cl 2.0 to 8.5) | |||||
Dyspnoea | OR 9.9 (95% Cl 5.7 to 17.1) | |||||
Increase/purulent sputum | OR 11.0 (95% Cl 3.4 to 35.9) | |||||
Seizures (acute) | OR 5.6 (95% Cl 2.2 to 14.5) | |||||
Irritable/drowsy | OR 2.9 (95% Cl 1.7 to 5.1) | |||||
Wheeze (complaint) | OR 7.0 (95% Cl 3.5 to 14.10) | |||||
Respiratory rate | OR 0.15 (95% Cl 0.046 to 0.26) | |||||
Heart rate | OR –0.19 (95% Cl –0.3 to –0.086) | |||||
SaO2 < 93%/supplemental O2 | OR 39.7 (95% Cl 12.6 to 125) | |||||
Chest retraction | OR 18.5 (95% Cl 9 to 38) | |||||
Accessory muscle use | OR 25.2 (95% Cl 10.7 to 59.7) | |||||
Crepitations | OR 7.8 (95% Cl 4.1 to 14.8) | |||||
Wheeze on examination | OR 8.1 (95% Cl 4.6 to 14.4) | |||||
Prolonged CRT | OR 16.7 (95% Cl 5.2 to 53.4) | |||||
Altered mental status | OR 76.3 (95% Cl 10.3 to 564) | |||||
Signs of dehydration | OR 12.3 (95% Cl 4.5 to 33.6) | |||||
Abnormal CXR | OR 6.2 (95% Cl 3.1 to 12.5) | |||||
Lopez-Delgado50 | Spain | ICU with respiratory failure from H1N1 | 60 | Hospital mortality | BMI > 30 kg/m2 | 37% survivor vs. 0%; p = 0.021 |
Dyslipidaemia | 18% survivor vs. 8%; p = 0.049 | |||||
Creatinine | 108.4 ± 74 survivor vs. 186.4 ± 220; p = 0.043 | |||||
Hb | 13 ± 2 survivor vs. 11.4 ± 3.2; p = 0.033 | |||||
Platelets | 214 ± 101 survivor vs. 113 ± 82; p = 0.002 | |||||
pH | 7.4 ± 0.7 survivor vs. 7.28 ± 0.15; p < 0.001 | |||||
PCO2 (mmHg) | 41 ± 21 survivor vs. 58 ± 24; p = 0.04 | |||||
Bacterial coinfection | 10.4% survivor vs. 41.6%; p = 0.022 | |||||
Greenbaum51 | USA | Hospitalised, 18–65 years, with lab-confirmed flu (not all pandemic) | 9092 | Mortality or ICU admission | Heavy alcohol use | RR 1.34 (95% Cl 1.04 to 1.74) |
Chronic lung disease | RR 1.35 (95% Cl 1.23 to 1.48) | |||||
Asthma | RR 0.85 (95% Cl 0.77 to 0.93) | |||||
Cardiovascular disease | RR 1.12 (95% Cl 1.02 to 1.24) | |||||
Chronic metabolic disease | RR 1.29 (95% Cl 1.19 to 1.4) | |||||
Greenbaum51 | USA | Hospitalised, > 65 years, with lab-confirmed flu (not all pandemic) | 6584 | Mortality or ICU admission | Heavy alcohol use | RR 2.47 (95% Cl 1.69 to 3.6) |
Chronic lung disease | RR 1.51 (95% Cl 1.36 to 1.68) | |||||
Cardiovascular disease | RR 1.41 (95% CI 1.26 to 1.57) | |||||
Delgado-Rodriguez52 | Spain | Hospitalised with lab-confirmed flu | 1520 | Mortality or ICU admission | Respiratory failure | OR 2.14 (95% Cl 1.12 to 4.08) |
Cardiovascular disease | OR 3.10 (95% Cl 1.89 to 5.09) | |||||
Cancer | OR 2.61 (95% Cl 1.61 to 4.24) | |||||
Systemic steroids pre-admission | OR 4.69 (95% Cl 2.46 to 8.95) | |||||
Pneumonia at admission | OR 1.98 (95% Cl 1.33 to 9.5) | |||||
No. organ malfunction at admission (continuous) | OR 3.31 (95% Cl 2.62 to 4.2) | |||||
Alcohol > 80 g/day | OR 1.99 (95% Cl 1.09 to 3.64) | |||||
Borse53 | India | Adult ICU admission with lab-confirmed H1N1 | 100 | Hospital mortality | No significant clinical or radiological predictors | |
Mortensen54 | California | Hospitalised/died with influenza A and asthma | 170 | ICU admission/death | Renal disease | OR 3.87 (95% Cl 1.08 to 13.87) |
Infiltrates on CXR | OR 9.71 (95% Cl 3.93 to 23.99) | |||||
Semple55 | UK | Hospitalised (FLU-CIN), > 16 years | 1040 | HDU/ICU/death | Severe respiratory distress | OR 2.27 (95% Cl 1.63 to 3.16) |
Increased respiratory rate | OR 2.37 (95% Cl 1.69 to 3.31) | |||||
SaO2 < 93% | OR 6.42 (95% Cl 4.49 to 9.18) | |||||
Respiratory exhaustion | OR 6.13 (95% Cl 2.64 to 14.2) | |||||
Severe dehydration/shock | OR 2.89 (95% Cl 2.01 to 4.16) | |||||
Altered consciousness | OR 4.99 (95% Cl 2.82 to 8.81) | |||||
Other clinical concern | OR 2.19 (95% Cl 1.39 to 4.36) | |||||
Hospitalised, (FLU-CIN) < 16 years | 480 | Severe respiratory distress | OR 3.16 (95% Cl 1.91 to 5.22) | |||
SaO2 < 93% | OR 4.95 (95% Cl 2.97 to 8.25) | |||||
Severe dehydration/shock | OR 11 (95% Cl 1.98 to 61.1) | |||||
Altered consciousness | OR 6.44 (95% Cl 3.49 to 11.9) | |||||
Other clinical concern | OR 2.38 (95% Cl 1.16 to 4.9) | |||||
Kusznierz56 | Argentina | Hospitalised, lab-confirmed H1N1 | 242 | Death | Obesity | 4% survivor vs. 40%; p < 0.001 |
DM | 6% survivor vs. 19%; p = 0.002 | |||||
Heart disease | 6% survivor vs. 19%; p = 0.02 | |||||
Hypertension | 16% survivor vs. 38%; p = 0.03 | |||||
Renal disease | 4% survivor vs. 11%; p = 0.04 | |||||
CXR consolidation | 75% survivor vs. 38%; p < 0.001 | |||||
Secondary bacterial infection | 0.6% survivor vs. 7%; p = 0.002 | |||||
ARDS | 19% survivor vs. 72%; p < 0.001 | |||||
Sepsis/shock | 6% survivor vs. 54%; p < 0.001 | |||||
Tamiflu® < 48 hours | 27% survivor vs. 13%; p = 0.012 | |||||
Mertz57 | Multiple | Meta-analysis (seasonal flu) | 75,871 | Death | Obesity | OR 30.10 (95% Cl 1.17 to 773.12) |
Cardiovascular disease | OR 1.97 (95% Cl 1.06 to 3.9) | |||||
Immunocompromised | OR 3.81 (95% Cl 1.28 to 11.35) | |||||
Endocrine disease | OR 13.92 (95% Cl 3.71 to 52.13) | |||||
ICU admission | Chronic lung disease | OR 4.46 (95% Cl 1.34 to 14.79) | ||||
Meta-analysis (pandemic flu) | 534,911 | Death | < 4/52 post partum | OR 4.43 (95% Cl 1.24 to 15.81) | ||
Obesity | OR 2.74 (95% Cl 1.56 to 4.8) | |||||
Chronic lung disease | OR 1.71 (95% Cl 1.17 to 2.51) | |||||
Cardiovascular disease | OR 2.92 (95% Cl 1.76 to 4.82) | |||||
Immunocompromised | OR 3.67 (95% Cl 1.78 to 7.58) | |||||
Malignancy | OR 3.1 (95% Cl 2.35 to 4.1) | |||||
Neuromuscular disease | OR 2.68 (95% Cl 1.91 to 3.75) | |||||
Anaemia/haemoglobinopathy | OR 2.28 (95% Cl 1.35 to 3.84) | |||||
DM | OR 2.21 (95% Cl 1.37 to 3.57) | |||||
Liver disease | OR 2 (95% Cl 1.32 to 3.04) | |||||
Metabolic disease | OR 1.83 (95% Cl 1.19 to 2.79) | |||||
Renal disease | OR 3.11 (95% Cl 1.54 to 6.28) | |||||
ICU admission | Obesity | OR 1.81 (95% Cl 1.48 to 2.22) | ||||
Chronic lung disease | OR 1.48 (95% Cl 1.19 to 1.83) | |||||
Cardiovascular disease | OR 1.7 (95% Cl 1.39 to 2.08) | |||||
Neuromuscular disease | OR 2.63 (95% Cl 1.83 to 3.79) | |||||
DM | OR 1.6 (95% Cl 1.32 to 1.94) | |||||
Liver disease | OR 2.65 (95% Cl 1.44 to 4.88) |
Appendix 2 The PAndemic INfluenza Triage in the Emergency Department study protocol
Research objectives
We aim to identify the most accurate triage method for predicting severe illness among patients attending the emergency department with suspected pandemic influenza.
Our specific objectives are:
-
to determine the discriminant value of emergency department triage methods for predicting severe illness in patients presenting with suspected pandemic influenza
-
to determine the discriminant value of presenting clinical characteristics and routine tests for identifying severe illness
-
to determine the independent predictive value of presenting clinical characteristics and routine tests for severe illness
-
to develop two new triage methods based upon (a) presenting clinical characteristics alone and (b) presenting clinical characteristics, electrocardiogram (ECG), chest X-ray and routine blood test results.
Existing research
Prior to the 2009 H1N1 pandemic, the United Kingdom (UK) influenza pandemic contingency plan predicted around 750,000 excess emergency department attendances and 82,500 excess hospitalisations during a pandemic. 1 A recent consultation document suggested that a pandemic could result in 50% of the population having some symptoms, of whom 30% would seek primary care and 1–4% would need hospital admission. 2 The Pandemic Influenza Advisory Committee Subgroup on Modelling have estimated a likely clinical attack rate of 3–35% (worst case scenario 50%), with 10–25% of these to have complications and a peak demand in the worst case scenario of 13% of the population being ill. 3
Pandemic planning needs to encompass a wide range of potential scenarios, but even projections at the less severe end of the spectrum could cause substantial problems of overcrowding at emergency departments that are already often working close to capacity. Methods of triage for patients presenting to the emergency department with suspected pandemic influenza are therefore required and need to be fair, robust and reproducible. 4
The term triage is often used to describe a brief initial assessment in the emergency department to determine patient order of priority in the queue to be seen. In this proposal we use the term triage more broadly to include the full process of emergency department assessment, potentially including investigations such as blood tests and X-rays, and apply it to decision-making regarding whether the patient should be admitted and whether they should be referred for high dependency or intensive care.
Emergency department triage methods need to accurately predict the individual patient’s risk of death or severe illness. The predicted risk can then guide decision-making. Patients with a low risk may be discharged home, those with a high risk admitted to hospital, and those with a very high risk referred for high dependency or intensive care. The level of risk used to trigger these decisions need not necessarily be fixed or determined in advance. Indeed, it is likely that decision-making thresholds could change during the course of a pandemic as the balance between resource availability and demand changes. Triage methods that use a risk prediction score to determine the need for hospital care may therefore be more useful than a triage rule that classifies patients into admission and discharge categories.
Health Protection Agency (HPA) guidance prior to the 2009 pandemic, supported by the British Thoracic Society and British Infection Society, recommended the use of the CURB-65 pneumonia score5 for patients with suspected influenza-related pneumonia. This score uses five variables (confusion, urea level, respiratory rate, blood pressure and age) to generate a score between zero and five. Subsequent Department of Health guidelines on surge capacity in a pandemic also considered use of a physiological-social score [Pandemic Modified Early Warning Score (PMEWS)]. 6 This score uses physiological variables, age, social factors, chronic disease and performance status to generate a score between zero and seven. National guidance specific to the 2009 H1N1 pandemic included a swine flu hospital pathway for emergency department management with seven criteria, any one of which predicts increased risk and the need for hospital assessment. 7
We used the autumn/winter phase of the 2009 H1N1 pandemic in Sheffield and Manchester to evaluate the discriminant value of three potential systems for triage of pandemic influenza patients in the emergency department: CURB-65, PMEWS and the swine flu hospital pathway. 8,9 However, the pandemic in these areas was less severe than predicted and only five patients of the cohort of 481 met our predefined criteria for critical illness. Within this cohort the discriminant value (c-statistic) of the three systems for predicting critical illness was moderate [CURB-65 0.78, 95% confidence interval (CI) 0.58 to 0.99, PMEWS 0.77 (0.55 to 0.99) and the swine flu hospital pathway 0.70 (0.45 to 0.96)]. Their performance in predicting hospital admission was worse: CURB-65 0.65 (95% CI 0.54 to 0.76), PMEWS 0.76 (0.66 to 0.86) and the swine flu hospital pathway 0.62 (0.51 to 0.72). These findings suggested that clinicians were not using the recommended triage methods when deciding whether to admit or discharge patients, and raised concerns about the accuracy of these methods for predicting adverse outcome.
Other research during the pandemic cast doubt on the utility of existing triage systems. The SwiFT study of patients admitted to critical care with H1N1 found 68% scored 0 or 1 using CURB-65 (i.e. recommended for hospital discharge). 10 This is supported in evidence from a Canadian seasonal flu cohort, where no triage system performed well in predicting intensive care admission (c-statistics PMEWS 0.63 (0.57–0.69), CURB-65 0.58 (0.52–0.64). 11 The best discriminator in this cohort was SMART-COP, a system specifically developed to predict intensive care admission in community-acquired pneumonia12 which achieved a c-statistic of 0.73 (0.67–0.79) but has not to our knowledge been examined in a pandemic cohort. The SwiFT study10 also developed a new score based on systolic blood pressure, temperature, heart rate, respiratory rate, neurological status and inspired oxygen concentration to predict adverse outcome. The SMART-COP and SwiFT scores therefore offer alternative triage methods that require validation in a pandemic. We are not aware of any other new scores to emerge since the 2009 pandemic.
In addition to our study and SwiFT, a number of cohort studies were undertaken during the 2009 H1N1 pandemic to identify risk factors for poor outcome in various groups. We have systematically reviewed these studies and present the main findings in the appendix of this project description. The predominant predictors of adverse outcome were chronic comorbidities and obesity13–18 with conflicting evidence regarding the risk of pregnancy. 10,15 Acute physiological disturbances, particularly hypoxia, were also found to have prognostic value. 10,14,19–25
The existing research therefore suggests that, although there are a number of patient characteristics and clinical measures that can predict adverse outcome, the available data do not support the use of any specific triage methods in suspected pandemic influenza.
Research methods
We plan to undertake a prospective observational cohort study of patients attending the emergency department with suspected pandemic influenza to evaluate existing triage methods, identify clinical predictors of adverse outcome and develop new triage methods.
Predictor variable data collection
Emergency department staff will be provided with a standardised form for assessing patients with suspected influenza that will double as clinical notes and study data collection form. It will include the elements of all currently available triage methods, variables identified in previous studies as being predictors of adverse outcome (see appendix) and any other potential predictors that are routinely recorded in the emergency department (comorbidities, physiological observations, routine blood tests, ECG and chest X-ray). We will also record details of any pre-presentation anti-viral medication, antibiotics and immunisation status.
Planned interventions
We will evaluate triage methods used to determine whether a patient with suspected pandemic influenza should be admitted to hospital or not, and whether they should be admitted to intensive or high dependency care. These will include the CURB-65 score, PMEWS, the swine flu hospital pathway, SMART-COP, the SwiFT score and any new methods developed before the next pandemic. We will also develop two new triage methods based upon (a) presenting clinical characteristics alone and (b) presenting clinical characteristics, ECG, chest X-ray and routine blood test results.
The first score will only use variables available at initial patient assessment, i.e. history and examination, including simple technologies such as automated blood pressure measurement and pulse oximetry. This triage method can be used to assess patients for the need for hospital investigation and identify patients that can be discharged without further assessment. It could potentially be used, with appropriate validation, to assess patients in the community.
The second triage method will be based upon all available emergency department data, including routine blood tests, ECG and chest X-ray findings. This triage method can be used for two potential purposes: (1) identification of patients with a low risk of adverse outcome who can be discharged home after emergency department assessment; and (2) identification of high-risk patients who are likely to need high dependency or intensive care.
We will evaluate the ability of each method to predict whether patients die or require respiratory, cardiac or renal support. We will not evaluate the impact of triage methods upon patient care. Intervention in the study will therefore only consist of data collection and follow-up. Patient management will continue according to whatever Department of Health guidance is in place at the time of the pandemic.
We will initially aim to develop triage methods that can be applied to the whole population of patients presenting to the emergency department. Age dependent variables will be assessed and included in the triage method in relation to age specific normal ranges. We will then explore whether different triage methods may be appropriate for different patients, particularly whether a different triage method may be appropriate for children.
Planned inclusion/exclusion criteria
We will include all adults and children presenting the emergency department of the participating hospitals with suspected pandemic influenza during the peak of the pandemic. Patients will be eligible for inclusion if they meet the current clinical diagnostic criteria of (1) fever (pyrexia ≥ 38 °C) or a history of fever and (2) influenza-like illness (two or more of cough, sore throat, rhinorrhoea, limb or joint pain, headache, vomiting or diarrhoea) or severe and/or life-threatening illness suggestive of an infectious process; or if they meet any future clinical diagnostic criteria recommended by the Department of Health. The assessing clinician will determine eligibility and complete the data collection form if the patient is considered to have suspected pandemic influenza. We will not attempt to retrospectively apply the clinical diagnostic criteria and exclude patients who appear to have been inappropriately included. Patients will only be excluded if they request exclusion from the study.
Proposed outcome measures
Patients will then be followed up until 30 days after attendance by hospital record review. Patients who die or require respiratory, cardiovascular or renal support they will be defined as having an adverse outcome. If they survive to 30 days without requiring respiratory, cardiovascular or renal support they will be defined as having no adverse outcome. If a severe pandemic leads to hospital resources being overwhelmed we will categorise patients as having an adverse outcome if they were deemed to have needed respiratory, cardiovascular or renal support but were denied this due to lack of resources. We will also record whether they are treated with antiviral agents or antibiotics and the length and location of any hospital stay. At day 30 the data will be entered into the database.
Respiratory support is defined as any intervention to protect the patient’s airway or assist their ventilation, including non-invasive ventilation or acute administration of continuous positive airway pressure. It does not include supplemental oxygen alone or nebulised bronchodilators. Cardiovascular support is defined as any intervention to maintain organ perfusion, such as inotropic drugs, or invasively monitor cardiovascular status, such as central venous pressure or pulmonary artery pressure monitoring, or arterial blood pressure monitoring. It does not include peripheral intravenous canulation and/or fluid administration. Renal support is defined as any intervention to assist renal function, such as haemoperfusion, haemodialysis or peritoneal dialysis. It does not include intravenous fluid administration.
Outcome assessment will be based primarily on research nurse review of hospital computer records and case notes. The hospital computer records will be checked at least 30 days after presentation. If the patient is alive at 30 days, was discharged home from the emergency department and did not reattend hospital, they will be recorded as having no adverse outcome. If they died, were admitted to hospital or reattended hospital within 30 days, their hospital notes will be retrieved and reviewed by the research nurse. If there is no evidence in the hospital notes of an adverse outcome the patient will be recorded as having no adverse outcome. We intend to cross check the completeness of the dataset by comparing NHS numbers with the Intensive Care National Audit and Research Centre (ICNARC) database and the Office for National Statistics (ONS). The cross-check with ICNARC will be to determine that our dataset has captured all intensive care usage and associated adverse outcomes. Should there be cases in the ICNARC database that are not present in the PAINTED database we would endeavour to retrieve the missing clinical data (linking the cases via the NHS number) to supplement the PAINTED dataset. Capturing missing mortality data will be undertaken via a search organised by the ONS. Linking of records would again be made using the NHS number. If outcome still is uncertain (for example, if patient records are not obtainable) this will be recorded as no adverse outcome.
We have selected an outcome measure that has a relatively clear definition and unequivocally indicates a case in which hospital admission and high dependency care would be desirable. The disadvantage of this definition is that it excludes patients who might benefit from other aspects of hospitalisation, such as oxygen supplementation or intravenous fluids. However, oxygen and intravenous fluids are often administered to patients with little clinical need for these treatments, administration is often poorly recorded and administration may be based on the clinical variables being tested in this project rather than objective clinical need. Including these treatments in our definitions of respiratory or cardiovascular support would thus carry a substantial risk of over-estimating the prevalence of serious outcome and of over-estimating the association between predictor variables and outcome.
We will also not attempt to determine whether deaths were likely to be amenable to treatment and will thus not explore the issue of whether treatment would be futile. It is possible that a severe pandemic could result in a need to identify cases where treatment would be futile, but this is beyond the scope, and possibly incompatible with the aims, of this proposal.
Proposed sample size
The sample size will ultimately depend upon the size and severity of the pandemic. Our pragmatic data collection methods will ensure that we maximise any opportunity to evaluate emergency department triage methods in a pandemic.
Our experience in the 2009 pandemic has shown us that pre-pandemic estimates of case hospitalisation and case fatality rates can be very misleading and that sample size estimates must take into account considerable uncertainty in these estimates. Nevertheless, we have also shown that informative findings can be generated even in a pandemic with a very low rate of adverse outcome.
Given that most cases of suspected pandemic influenza (even in a severe pandemic) do not result in an adverse outcome, the key variable in determining study power is the number of cases with an adverse outcome. A single cohort including at least 150 cases with adverse outcome would allow us to estimate the c-statistic of a triage method, clinical variable or test with a standard error of 0.03 (assuming the true c-statistic was 0.8). The table below shows the standard error resulting from samples with smaller numbers of adverse outcomes.
N with adverse outcome | Standard error (assuming c-statistic was 0.8) |
---|---|
150 | 0.033 |
125 | 0.036 |
100 | 0.040 |
75 | 0.046 |
50 | 0.056 |
A sample with N = 150 adverse outcome would estimate the sensitivity of a dichotomised rule, variable or test with a standard error as outlined in the table below, depending on the sensitivity at the threshold used. Estimates of specificity would obviously be very precise given the anticipated low prevalence of adverse outcome.
Sensitivity | Lower limit of 95% CI |
---|---|
1.00 | 0.98 |
0.95 | 0.90 |
0.90 | 0.84 |
0.85 | 0.78 |
0.80 | 0.73 |
The same cohort could be used to identify independent predictors of outcome and develop new triage methods (objectives 3 and 4). The number of variables that could be tested as independent predictors of outcome in a multivariable model and for inclusion in a triage method would depend upon the sample size. Based on the rule of thumb of needing at least 10 events for each independent regression variable in a logistic regression, a cohort with 150 cases with adverse outcome would allow us to test up to 15 parameters. 26
These estimates assume that each triage method and predictor variable will be used and tested on the whole cohort. However, we plan to explore whether different patients require different triage methods, particularly whether a different triage method is required for children and adults. Data from the 2009 H1N1 pandemic suggest that around a quarter to a third of adverse outcomes may occur in children. 14,33 To increase the probability that we will have at least 50 cases with adverse outcome among children we will aim to recruit a total of 200 cases with adverse outcome rather than 150.
If we assume that the prevalence of adverse outcome is the same as our 2009 cohort (1%) then we would need to collect data from 20,000 cases to identify 200 with an adverse outcome. We have therefore used this estimate in planning, although it is likely to be a overestimate of the total numbers required given the mild nature of the 2009 pandemic. A more severe pandemic would allow more precise estimates to be made with no additional costs or would allow us to reduce the total number of cases required to identify 200 with an adverse outcome.
If we are able to develop a new triage method that appears to have superior discriminant value to existing methods then we would want to validate this method in a new cohort. A sample including 421 cases with adverse outcome would provide 80% power to compare an area under the ROC curve of 0.85 versus 0.90 at 5% significance, assuming a correlation of 0.6 between scores. We have not included validation of a new triage method in our objectives because this would require (a) successful development of a new method and (b) a much larger sample size, with associated costs and assumptions about pandemic severity. However, if the pandemic is severe (i.e. the prevalence of adverse outcome exceeds 3%, so the number with adverse outcome exceeds 450) we will split the cohort into two equal cohorts to allow testing of existing rules and derivation of new rules on one half and validation of new rules, with comparison to existing rules, on the other.
We plan to collect data across 40 hospitals and have based our sample size calculation on the assumption of receiving 500 completed forms, including an average of 5 adverse outcomes, per hospital over the course of the pandemic.
Statistical analysis
In all analyses only age will be treated as a continuous variable (with possible reparameterisation). All other continuous variables will be categorised on the basis of their use in existing risk scores or previous studies. This is because most continuous variables used in risk prediction have a non-linear association with adverse outcome, with increased risk at high and low values.
It is likely that a proportion of data for most predictor variables (especially blood results) will be missing. The most likely reason is that a measurement would not be made or test performed if it was expected to be normal. Missing data will therefore be handled in constructing scores and in multivariable analysis by assuming that all missing values are normal (i.e. score zero in the relevant risk score). A sensitivity analysis will be performed by imputing missing values and comparing results between the three scenarios of excluding cases with missing values, treating missing values as normal and using imputed values for missing values.
Existing triage methods will be assessed by calculating the area under the ROC curve (c-statistic) for discriminating between cases with and without an adverse outcome (defined as death or need for support of respiratory, cardiovascular or renal function) and sensitivity and specificity at key decision-making thresholds.
The discriminant value of each clinical variable or test for adverse outcome will be assessed by calculating the c-statistic and, for dichotomous variables, the sensitivity and specificity.
Independent predictors of outcome will be identified by entering all clinical variables with an association with outcome (p < 0.2) into a multivariate logistic regression model.
New triage methods will be developed by combining the independent predictors of outcome into two new triage scores: one based on clinical variables measured at initial assessment only and the other based on all clinical variables (including blood tests and X-rays) measured in the emergency department. Integer weights will be assigned to each category of predictor variable according to the coefficient derived from a multivariate model using categorised independent predictors. This will generate a composite clinical score in which risk of positive outcome increases with the total score.
To determine whether different clinical scores are required for adults and children we will derive separate scores for adults (age ≥ 16) and children. If any variables are included in one and not in the other we will compare c-statistics separately in each age group for models with and without the relevant variable. We will also test whether the weights attached to each variable differ sufficiently to affect prediction. The outcome may be that models with different predictors and/or different weights are required for adults and children.
If the pandemic is severe enough to allow the cohort to be split into derivation and validation cohorts with sufficient numbers of adverse outcome we will compare new triage methods developed during the project to existing triage methods by calculating c-statistics and sensitivity/specificity at key decision-making thresholds in the second cohort.
Data management
Data will be collected by the clinical staff caring for the patient using a standardised clinical assessment form that will double as routine clinical record and research data collection form. Research nurses employed by each hospital (and funded by the Comprehensive Local Research Network) will identify patients with suspected influenza for whom the standardised form was completed. Once 30 days have passed from attendance the research nurse will check the hospital computer system for deaths or hospital admissions. If death or hospital admission has occurred (estimated 15% of cases) the research nurse will retrieve hospital notes to record details of any adverse events. Once complete the research nurse will enter anonymised data into a secure online database provided by the Sheffield Clinical Trials Research Unit (CTRU). The only patient identifiable information recorded onto the database and viewable by the research team will be NHS numbers. We intend to use these to cross check the completeness of the dataset by comparing NHS numbers with the ICNARC database and the ONS. The cross-check with ICNARC will be to determine that our dataset has captured all intensive care usage and associated adverse outcomes. Should there be cases in the ICNARC database that are not present in the PAINTED database we would endeavour to retrieve the missing clinical data (linking the cases via the NHS number) to supplement the PAINTED dataset. Capturing missing mortality data will be undertaken via a search organised by the ONS. Linking of records would again be made using the NHS number.
Piloting the data collection form
We have piloted and developed a standardised clinical assessment form based on the 2009 pandemic. Staff at participating hospitals have used the form for routine assessment of patients with seasonal influenza during the winter of 2012–13. We have sought staff feedback to make the form as user-friendly as possible and to ensure that it serves dual needs of collecting relevant information for routine clinical records and the data required for our research. We will promote use of the form so that it becomes the routine clinical record for patients presenting to the participating hospitals with suspected influenza. Once the form is developed we will create a secure online database to ensure efficient data management.
We will ensure that the software supporting form production and the database is flexible so that the form can be amended and updated at short notice and with minimum inconvenience to clinical and research staff. During the pilot phase and at the point of activation of the full study (see below) we will update our literature review to identify any new triage methods or potentially useful predictors of adverse outcome.
Activation of the full study
The project will be activated if and when an influenza pandemic results in increased emergency department attendances with suspected influenza. Research staff will promote the use of the standardised data collection form, collect follow-up data and undertake data entry. We will update our literature review (as outlined above) and monitor reports from areas where the pandemic develops to identify any potentially new predictors of adverse outcome that may be unique to the emerging pandemic. If any potentially new predictors are identified we will cascade information to clinical staff and amend the clinical assessment form to ensure that they are systematically recorded.
Ethical arrangements
We have sought Research Ethics Committee (REC) approval prior to piloting and in advance of any pandemic. We have sought approval to activate the project in the event of a pandemic without a further REC review. Our previous similar project in the 2009 H1N1 pandemic was approved by the REC. The planned processes for informing patients of the project and managing data are very similar to those approved in our 2009 project. During the previous 2009 project patient identifiable information was taken to allow monitoring, data validation and GP contact. The National Information Governance Board (NIGB) gave section 251 approval to this use of identifiable patient data without consent. However the NIGB was unable to give approval to the use of patient identifiable information in the pilot phase of this project. We will therefore not be collecting identifiable details in the pilot phase, but will use the pilot phase to test whether the study can be undertaken without identifiable data.
We have assessed the potential limitations incurred by inability to use identifiable details we will pursue approval to use identifiable details during the pandemic phase from the appropriate competent body.
Risks and anticipated benefits for trial participants and society
The study will not alter patient management and will simply collect routinely available data at presentation and follow-up. No additional diagnostic tests will be performed. The risks to patients involved in the study are therefore very low and principally relate to data protection and confidentiality.
The standardised clinical assessment form will be used as both routine clinical record and data collection form to ensure that care is not delayed by unnecessary duplication of data recording. The pilot phase will be used to ensure that this form is fit for both purposes and acceptable to clinical staff. The research nurses will keep a record of all patients who withdraw from the project but will not communicate details to other staff. Only anonymous data will be entered into the database by the clinical staff. Other than the research team’s access to NHS numbers no one outside of the hospital will have access to patient identifiable information.
Patients involved in the study will potentially benefit from the use of the standardised clinical assessment form. This will ensure that important variables are recorded and communicated between staff providing care. The standardised form can also be used to remind staff of current guidance for management.
Future patients with suspected pandemic influenza and society in general will benefit from evaluation and development of accurate triage methods that have the potential to improve clinical decision-making and ensure that patients receive the right care and health service resources are optimally used.
Informing potential trial participants of possible benefits and known risks
Posters in all participating departments will be prominently displayed advising patients of the project and providing contact details for further information. Information leaflets will be provided for staff to hand to patients with suspected pandemic influenza that briefly describe the nature and purpose of the study and provides contact details for further information.
Obtaining informed consent from participants
We will not seek patient consent to participate on the basis that the study is limited to collection of routinely available data and any delays in patient assessment would risk compromising patient care. The information leaflet outlined above will provide a tear-off slip with contact details that patients can use to inform the hospital or research team if they wish to withdraw from the study. Patients who wish to withdraw from the study will have their study records deleted. Their decision to withdraw will not be communicated to clinical staff providing further care and will not influence their subsequent management.
Proposed time period for retention of relevant study documentation
The original data collection form will constitute the clinical notes and be kept in each hospital according to normal practice. The anonymised database will be maintained by the Clinical Trials Unit until ten years after the end of the project.
Proposed action to comply with ‘The Medicines for Human Use (Clinical Trials) Regulations 2004’
Not applicable – this is not a clinical trial.
Research governance
Sheffield Teaching Hospitals NHS Foundation Trust will be the study sponsor and the project will be managed by the School of Health and Related Research (ScHARR) in the University of Sheffield. The Hospital Trust and University share a joint research office in Sheffield to facilitate management of collaborative projects such as this. The Project Management Group (PMG), consisting of the co-applicants and any appointed research staff, will manage the study. The PMG will meet prior, during and after the pilot phase. After that meetings will be held annually until a pandemic emerges and the project is activated. During the pandemic the PMG will meet at least monthly, either in person or by teleconference. The Sheffield CTRU will manage data entry, data management and provide data ready for analysis by Professor Campbell.
A Steering Committee will be formed to oversee study progress. This will consist of an independent Chair and at least three independent members (including a relevant clinician, statistician and public/patient representative), the Chief Investigator and the Project Manager.
Project timetable and milestones
June 2012 to September 2012: REC submission, and seeking regulatory approvals from participating NHS Trusts.
October 2012 to January 2013: Piloting and development of clinical assessment form.
February 2013: Project put on hold until pandemic emerges (T0)
T0: Project activated
T0 to T0 + 3 months: Data collection from 20,000 cases, including 200 with an adverse outcome, across 40 hospitals (see sample size section for details)
T0 + 3 to T0 + 6 months: Analysis and reporting
Expertise
The research team combines experts on emergency management of suspected pandemic influenza (KC, DW and AB) with expertise in paediatric emergency medicine (IM), critical care (AB) and public health (AL), and the statistical expertise and research infrastructure of the Sheffield Clinical Trials Unit (SG, MC and RW).
The team collaborated on a similar previous project during the 2009 H1N1 pandemic (HTA09/84/66). This project was completed and reported despite difficulties caused by research governance procedures and the unexpectedly mild course of the pandemic.
Steve Goodacre was Chief Investigator for HTA09/84/66 and is lead applicant for this proposal. He has undertaken many major national evaluations in emergency care, including development of clinical prediction methods. His current projects provide the necessary infrastructure to rapidly undertake the proposed research. Richard Wilson managed the DAVROS study and has developed extensive expertise in data collection, management and protection in observation studies using routine data sources without patient consent. Mike Campbell is an experienced medical statistician with expertise in development and validation of clinical prediction rules. Andrew Lee is a Senior Clinical University Teacher in Public Health who has a research interest in emergency planning and is currently collaborating with SG, KC and DW on an NIHR Service Delivery and Organisation project involving scoping the emergency planning literature.
Kirsty Challen and Darren Walter are emergency physicians with research interests in pandemic influenza and emergency planning, and Andrew Bentley is an accredited critical care and respiratory physician. They have previously evaluated triage methods for pandemic influenza and are leading experts in this field. Ian Maconochie is a paediatric emergency physician who has evaluated paediatric early warning scores, the predictive value of clinical features in sick children and the management of febrile children.
Service users
Enid Hirst has agreed to be the patient/public representative for the project and has reviewed the proposal. She acted as patient and public representative for our project in the 2009 pandemic and was an independent member of the study Steering Committee.
Enid is a founder member of the Sheffield Emergency Care Forum. This is a patient and public representative group with a specific interest in emergency care research. The Forum has reviewed this proposal and provided feedback. Enid will continue to provide a link between the project and the Forum.
Enid previously spent eight years with Sheffield Community Health Council, was a lay member of the Steering Committee for NHS Direct Yorkshire and Humber, was a member of Unscheduled Care Network Board in Sheffield, spent three years with Sheffield Children’s Hospital Patient Forum, and has attended Trust Board meetings at Sheffield Children’s Hospital for many years as an observer for the Community Health Council and then the Patient Forum. She is now a member of Sheffield LINks (Local Involvement Network), a lay member of the Out of Hours Accreditation Group, is on the Dental Services Joint Planning Group for Sheffield, is a patient representative for the Group looking into Dentally Anxious Patients, and is a patient representative on the new Critical Care/Emergency Medicine Priority Group.
Her role will include the following:
-
reviewing the protocol and specifically advising on ethical issues and arrangements for data protection and confidentiality
-
reviewing the poster and information leaflet
-
patient/public representation on the Steering Committee
-
lay input into reporting and dissemination of findings
-
liaison between the project and the Sheffield Emergency Care Forum.
Protocol references
1. Department of Health. Pandemic Flu: A National Framework for Responding to an Influenza Pandemic. London: Department of Health; 2007.
2. Department of Health Pandemic Influenza Preparedness Team. UK Influenza Pandemic Preparedness Strategy. London: Department of Health; 2011.
3. Scientific Pandemic Influenza Advisory Committee (SPI). Subgroup on Modelling. Modelling Summary. London: Department of Health; 2011.
4. Challen K, Bentley A, Bright J, Walter D. Clinical review: mass casualty triage – pandemic influenza and critical care. Crit Care 2007;11:212.
5. Lim W. Pandemic flu: clinical management of patients with an influenza-like illness during an influenza pandemic. Thorax 2007;62(Suppl. 1):1–46.
6. Department of Health. Pandemic Influenza: Surge Capacity and Prioritisation in Health Services. London: Department of Health; 2008.
7. Department of Health. Swine Flu Clinical Package. London: Department of Health; 2009.
8. Challen K, Goodacre SW, Wilson R, Bentley A, Campbell M, Fitzsimmons C, et al. Evaluation of triage methods used to select patients with suspected pandemic influenza for hospital admission. Emerg Med J 2011; Published Online First: doi:10.1136/emj.2010.104380.
9. Goodacre S, Challen K, Wilson R, Campbell M. Evaluation of triage methods used to select patients with suspected pandemic influenza for hospital admission: cohort study. Health Technol Assess 2010;14(46):173–263.
10. Rowan K, Harrison D, et al. The Swine Flu Triage (SwiFT) study: development and ongoing refinement of a triage tool to provide regular information to guide immediate policy and practice for the use of critical care services during the H1N1 swine influenza pandemic. Health Technol Assess 2010;14(55).
11. Muller MP, McGeer AJ, et al. Evaluation of pneumonia severity and acute physiology scores to predict ICU admission and mortality in patients hospitalized for influenza. PLOS One 2010;5:e9563.
12. Charles PG, Wolfe R, et al. SMART-COP: a tool for predicting the need for intensive respiratory or vasopressor support in community-acquired pneumonia. Clin Infect Dis 2008;47:375–384.
13. Miller RR, Markewitz BA, et al. Clinical findings and demographic factors associated with ICU admission in Utah due to novel 2009 influenza A (H1N1) infection. Chest 2010;137:752–758.
14. Nguyen-Van-Tam J, Openshaw P, et al. Risk factors for hospitalisation and poor outcome with pandemic A/H1N1 influenza: United Kingdom first wave (May-September 2009). Thorax 2010;65:645–651.
15. ANZIC Influenza Investigators. Critical care services and 2009 H1N1 influenza in Australia and New Zealand. N Engl J Med 2009;361:10.1056/NEJMoa0908481.
16. Harris PN, Dixit R, et al. Pandemic influenza H1N1 2009 in north Queensland – risk factors for admission in a region with a large Indigenous population. Commun Dis Intell 201;34:102–109.
17. Santa-Olalla Peralta P, Cortes-García M, et al. Risk factors for disease severity among hospitalised patients with 2009 pandemic influenza A (H1N1) in Spain, April – December 2009. Euro Surveill 2010;15:19667.
18. Cui W, Zhao H, et al. Factors associated with death in hospitalized pneumonia patients with 2009 H1N1 influenza in Shenyang, China. BMC Infect Dis 2010;10:145.
19. Zimmerman O, Rogowski O, et al. C-reactive protein serum levels as an early predictor of outcome in patients with pandemic H1N1 influenza A virus infection. BMC Infect Dis 2010;10:288.
20. Martin-Loeches I, Papiol E, et al. Acute kidney injury in critical ill patients affected by influenza A (H1N1) virus infection. Crit Care 2011;15:R66.
21. Echevarría-Zuno S, Mejía-Aranguré JM, et al. Infection and death from influenza A H1N1 virus in Mexico: a retrospective analysis. Lancet 2009;374:2072–2079.
22. Louie JK, Gavali S, et al. Children hospitalized with 2009 novel influenza A(H1N1) in California. Arch Pediatr Adolesc Med 2010;164:1023–1031.
23. Stein M, Tasher D, et al. Hospitalization of children with influenza A(H1N1) virus in Israel during the 2009 outbreak in Israel.’ Arch Pediatr Adolesc Med 2010;164:1015–1022.
24. Vasoo S, Singh K, et al. Predicting need for hospitalization of patients with pandemic (H1N1) 2009, Chicago, Illinois, USA. Emerg Infect Dis 2010;16:1594–1597.
25. Bagdure D, Curtis DJ, et al. Hospitalized children with 2009 pandemic influenza A (H1N1): comparison to seasonal influenza and risk factors for admission to the ICU.’ PLOS One 2010;5:e15173.
26. Peduzzi P, Concato J, Kemper E, Holford TR, Feinstein AR. A simulation study of the number of events per variable in logistic regression analysis. J Clin Epidemiol 1996;49:1373–9.
27. Fajardo-Dolci G, Gutierrez-Vega R, et al. Clinical characteristics of fatalities due to influenza A (H1N1) virus in Mexico. Thorax 2010;65:505–9.
28. Lee N, Choi K, et al. Outcomes of adults hospitalised with severe influenza. Thorax 2010;65:510–5.
29. Libster R, Bugna J, et al. Pediatric hospitalizations associated with 2009 pandemic influenza A (H1N1) in Argentina. N Engl J Med 2010;362:45–55.
30. Chien Y-S, Su C-P, et al. Predictors and outcomes of respiratory failure among hospitalized pneumonia patients with 2009 H1N1 influenza in Taiwan. J Infect 2010;60:168–74.
31. Jain S, Kamimoto L, et al. Hospitalized patients with 2009 H1N1 influenza in the United States, April-June 2009. N Engl J Med 2009;361:1935–44.
32. Tuite AR, Greer AL, et al. Estimated epidemiological parameters and morbidity associated with pandemic H1N1 influenza. CMAJ 2010;182:131–6.
33. Campbell A, Rodin R, et al. Risk of severe outcomes among patients admitted to hospital with pandemic (H1N1) influenza. CMAJ 2010;182:349–55.
34. Aviram G, Bar-Shai A, et al. H1N1 influenza: initial chest radiographic findings in helping predict patient outcome. Radiology 2010;255:252–9.
35. Bassetti M, Parisini A, et al. Risk factors for severe complications of the novel influenza A (H1N1): analysis of patients hospitalized in Italy. Clin Microbiol Infect 2010;17:247–50.
36. Xi X, Xu Y, et al. Hospitalized adult patient with 2009 influenza A (H1N1) in Beijing, China: risk factors for hospital mortality. BMC Infect Dis 2010;10:256.
37. Pedbody R, McLean E, et al. Pandemic influenza A (H1N1) 2009 and mortality in the United Kingdom: risk factors for death, April 2009 to March 2010. Euro Surveill 2010;15:19571.
38. Wilking H, Buda S, et al. Mortality of 2009 pandemic influenza A (H1N1) in Germany. Euro Surveill 2010;15:19741.
Flow diagram
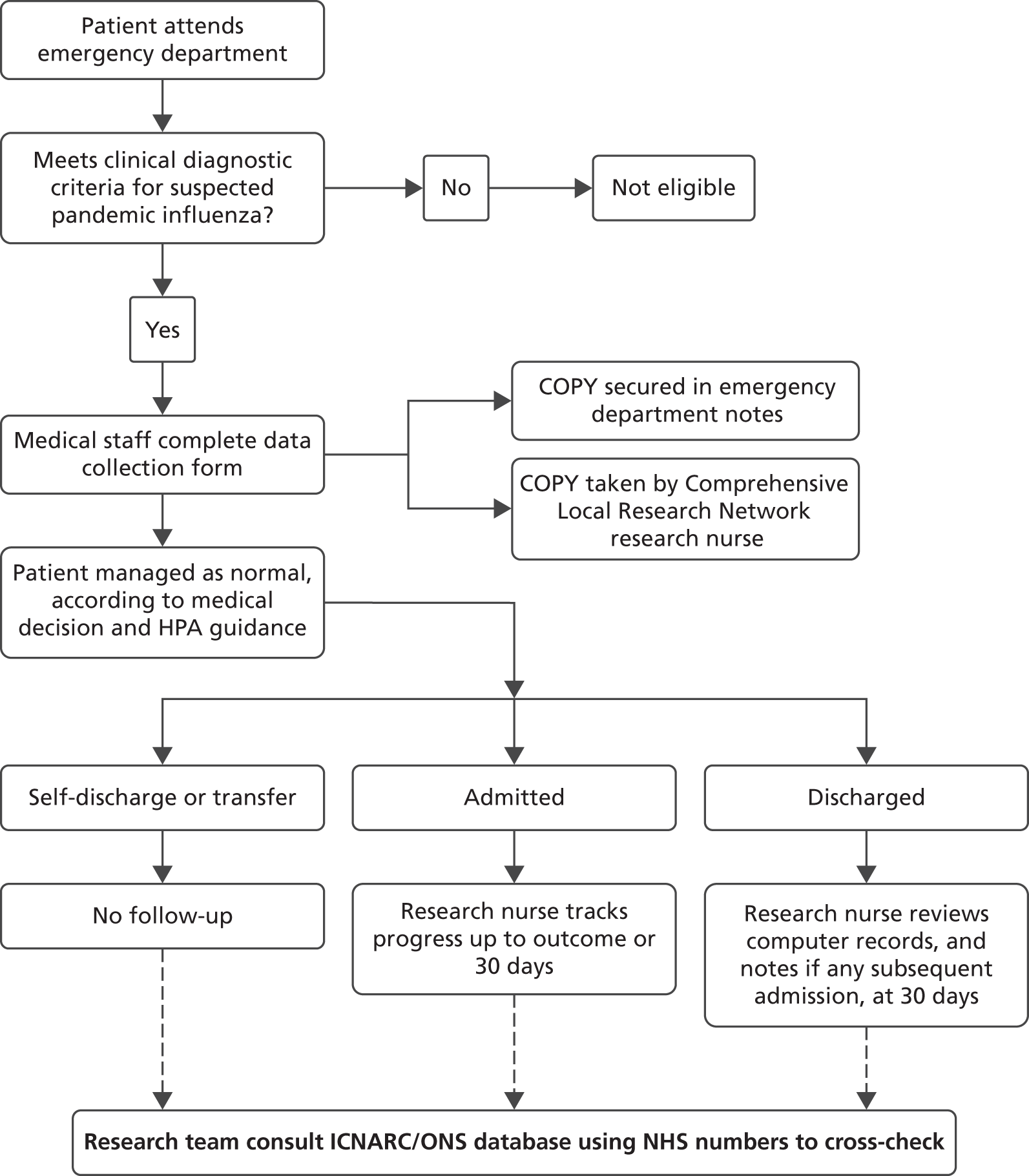
Appendix 3 Standardised clinical assessment form
Appendix 4 Schedule for the qualitative interviews
Pre-interview checks
-
Introductions.
-
Ensure that the participant is comfortable with the content of the information sheet and that any questions are answered.
-
Ensure that the participant is familiar with the PAINTED assessment form.
-
Ensure that consent form is signed and dated.
-
Ensure that interview details are completed.
Introduction
Briefly outline aims and objectives of the project and take participant details.
Participant information
Age:
Job title:
Length of employment at current site:
Length of employment within an emergency department setting:
Define clinical assessment form
General
Do you have experience of using clinical assessment forms in the emergency department?
-
Other than the PAINTED form?
-
Would you consider yourself to have significant experience of using such forms?
Would you consider yourself to have particularly strong views on clinical assessment forms?
-
[If so] Are these positive or negative?
What is your general opinion of such forms?
-
Could you explain why?
PAINTED Assessment Form
Thinking specifically about the PAINTED form . . .
Do you have experience of using the PAINTED assessment form?
-
How many forms have you completed?
-
Have you completed an electronic or paper-based version of the form, or both?
What is your general opinion of the form?
-
Could you explain why?
How would you assess the suitability of the form for its purpose [as a clinical record] (acceptability) ?
-
What about in comparison to existing alternatives (both internal and external)?
-
Could you explain why?
How easy do you think the form is to use (usability) ?
-
What about in comparison to other clinical assessment forms?
-
Would you feel confident in using the form in a pandemic situation (that may be many years away) with very little guidance?
-
Could you explain why?
What do you like about the form?
-
Could you explain why?
What do you dislike about the form?
-
Could you explain why?
If you could alter the form in any way, how would you do so?
-
Could you explain why?
Clinical assessment forms generally
Thinking about clinical assessment forms more generally . . .
How would you assess the suitability of the forms you have used in emergency department practice [as clinical records]?
-
Are there any specific examples you are thinking of?
-
Could you explain why?
What is your reflection on how easy these have been to use?
-
Are there any specific examples you are thinking of?
-
Could you explain why?
In your opinion, do you think that you complete such forms thoroughly and accurately?
-
Are there any factors that influence this?
-
Do you believe these to be specific to your setting?
If you were uncertain about how to complete a form, what would you do?
-
Could you explain why?
How important a part of your clinical practice do you consider assessment forms to be?
-
Could you explain why?
Electronic versus paper-based forms
Considering the use of electronic as well as paper-based forms . . .
Have you had experience of using both electronic and paper-based forms within the emergency department?
-
[If both] Would you consider yourself to have significant experience of using such forms?
How do you feel the two different types compare?
-
Could you explain why?
If you could choose to use one or the other, which would it be?
-
Could you explain why?
-
Would your decision be the same if considering the specific example of the PAINTED form? Why?
Considering the increased use of information technology within healthcare, how do you see the use of electronic forms developing within your emergency department?
-
Do you consider there to be any barriers to this?
-
Could you explain why?
Appendix 5 Patient information leaflet (adult)
Glossary
- Area under the receiver operator characteristic curve (c-statistic)
- A measure of the discriminant value of a risk prediction score.
- Community Assessment Application System Tool
- A decision pathway for determining which patients with suspected pandemic influenza require hospital assessment and admission; it forms the basis of the swine flu hospital pathway.
- CURB-65
- A risk prediction score for pneumonia, based on confusion, urea level, respiratory rate, blood pressure and age > 65 years.
- Ethics and Confidentiality Committee
- A subcommittee of the National Information Governance Board.
- Pandemic Modified Early Warning Score
- A risk score for pandemic influenza, based on physiological variables, age, social factors, chronic disease and performance status.
- SMART-COP
- A risk prediction score for pneumonia, based on systolic blood pressure, multiple lobes involvement on chest X-ray, albumin level, respiration, tachycardia, confusion, oxygenation and pH.
List of abbreviations
- AVPU
- alert, verbally responsive, responsive to pain or unconscious
- BP
- blood pressure
- CAF
- clinical assessment form
- CAG
- Confidentiality Advisory Group
- CI
- confidence interval
- CTRU
- Clinical Trials Research Unit
- CXR
- chest X-ray
- ECC
- Ethics and Confidentiality Committee
- ECG
- electrocardiogram
- GCS
- Glasgow Coma Scale
- GP
- general practitioner
- HPA
- Health Protection Agency
- ICNARC
- Intensive Care National Audit and Research Centre
- NIGB
- National Information Governance Board
- ONS
- Office for National Statistics
- PAC
- Privacy Advisory Committee
- PAINTED
- PAndemic INfluenza Triage in Emergency Department
- PMEWS
- Pandemic Modified Early Warning Score
- REC
- Research Ethics Committee
- ScHARR
- School of Health and Related Research
- SD
- standard deviation
- SECF
- Sheffield Emergency Care Forum
- SwiFT
- Swine Flu Triage
- WCC
- white cell count