Notes
Article history
The research reported in this issue of the journal was funded by the HTA programme as project number 10/97/01. The contractual start date was in January 2012. The draft report began editorial review in April 2014 and was accepted for publication in December 2014. The authors have been wholly responsible for all data collection, analysis and interpretation, and for writing up their work. The HTA editors and publisher have tried to ensure the accuracy of the authors’ report and would like to thank the reviewers for their constructive comments on the draft document. However, they do not accept liability for damages or losses arising from material published in this report.
Declared competing interests of authors
none
Permissions
Copyright statement
© Queen’s Printer and Controller of HMSO 2015. This work was produced by Perera et al. under the terms of a commissioning contract issued by the Secretary of State for Health. This issue may be freely reproduced for the purposes of private research and study and extracts (or indeed, the full report) may be included in professional journals provided that suitable acknowledgement is made and the reproduction is not associated with any form of advertising. Applications for commercial reproduction should be addressed to: NIHR Journals Library, National Institute for Health Research, Evaluation, Trials and Studies Coordinating Centre, Alpha House, University of Southampton Science Park, Southampton SO16 7NS, UK.
Chapter 1 Introduction and rationale
Cardiovascular disease: burden, causes and strategies for management
Cardiovascular disease (CVD) – comprising coronary heart disease (CHD), stroke and peripheral arterial disease – continues to be a major public health problem: it accounts for almost one-third of deaths in the UK, making it one of the leading causes of mortality,1–4 and it is responsible for one-fifth of all hospital admissions. 5 The cost implications of CVD are significant, with recent estimates of spending on cardiovascular care in the UK of approximately £12.5B: £9.6B on direct health-care cost, and £4.2B on informal care. 1
Importantly, a high proportion of CVD deaths are identified as premature (before the age of 75 years)1 and most may therefore be preventable. 4 Most CVD is caused by atheroma (or atherosclerotic plaques) in the arterial wall; these plaques develop progressively throughout life, and are often at an advanced stage before symptoms occur. 4,6 Three modifiable risk factors, smoking, blood pressure (BP) and cholesterol, particularly in combination, are thought to account for the majority of all premature CHD. 2,7
The number of deaths caused by CVD has gradually declined since reaching a peak in the 1970s. An estimated 60% of this decline is due to smoking cessation and reduction of other risk factors, with the other 40% resulting from improvements in treatment. 8 Strategies for CVD prevention therefore aim to address these risk factors. In the UK, there is currently a three-part strategy: a reduction in average levels of risk factors in the population; identifying those at a higher risk of CVD events; and reducing risk in people with established CVD through therapies to lower BP and lipid levels. 2,9,10
Lipid measurement and lipid-lowering treatments therefore play a vital role in CVD risk management: testing lipid levels provides a simple and safe way to identify those at increased risk of CVD, and enables the initiation and ongoing up-titration of therapies to lower lipid levels. Optimising the clinical strategy to identify dyslipidaemia for both primary and secondary CVD prevention is therefore of major importance; however, a number of key questions remain unanswered. There are several different cholesterol measures: total cholesterol (TC), low-density lipoprotein (LDL) cholesterol, high-density lipoprotein (HDL) cholesterol, the ratios of these three measures, non-HDL cholesterol and triglycerides (TGs), as well as more recent alternative measures such as apolipoprotein B (Apo B) and apolipoprotein A-I (Apo A-I). The utility of each of these measurements, including whether or not any meet the criteria for a screening test, is currently not known. Ideally, the selection of an optimal monitoring measure should take into account four different factors: (1) clinical validity, with the most useful tests being those strongly associated with the outcome and that give results early enough to allow action to be taken; (2) responsiveness to changes in therapy; (3) the ability to identify real changes from background measurement variability (short-term biological fluctuations and technical measurement error); and (4) practicalities such as affordability, accessibility, ease of testing and interpretation. 11
After an optimal monitoring test has been identified, translation to a monitoring strategy then requires further consideration of factors such as the frequency of testing, defining the clinical actions required if the target is out of range, evaluating the cost-effectiveness of the strategy, and ensuring that those implementing the strategy have adequate training and a quality assurance scheme is developed. 11 Cost-effectiveness is important because screening programmes need to produce a net benefit that should be achieved at a reasonable cost – accounting for savings from improved outcomes.
The factors for choosing an optimal monitoring measure and defining an optimal monitoring strategy have not been thoroughly addressed in the current guidelines that aim to help general practitioners (GPs) manage dyslipidaemia in primary and secondary populations with CVD. A recent systematic review12 examining the clarity and evidence base for such CVD prevention and treatment guidelines found that, of those with a section on lipids, only half recommended specific target levels or provided guidance on how to interpret initial measurements. The majority did not specify how or when to carry out subsequent monitoring, or provide management guidance when the target is out of range. Largely based upon consensus and expert opinion, the evidence base for an optimal monitoring interval is particularly weak.
This report forms part of a commissioned stream of work by the National Institute for Health Research (NIHR) Health Technology Assessment (HTA) programme, and aims to provide evidence for an effective strategy for monitoring dyslipidaemia in both primary and secondary prevention populations. It has involved the development and use of new methods to determine an effective strategy for screening and monitoring of lipid levels in relation to primary and secondary CVD prevention. It includes drawing together (1) a systematic review of the literature to identify the predictive values of different lipid measures and help determine the optimal disease parameter for monitoring; (2) a systematic review of the literature to determine the effectiveness of lipid modification therapies and establish the effect size for treatment in both primary and secondary prevention populations; (3) individual patient data (IPD) analyses to derive parameters from repeated measurements to identify biological variability, measurement error and variation in the rate of change in specific lipid measurements over time; (4) simulation modelling to estimate the likelihood of false decisions with respect to treatment under different monitoring strategies; (5) IPD analyses to examine the potential impact of different approaches to lipid measurement have on CVD risk scores; and (6) health economics modelling, which takes into account individual variability in lipid progression, as well as the poor precision of tests, to determine the cost-effectiveness of different monitoring strategies. This introductory chapter reviews the clinical background for this work, summarising current lipid management guidance, particularly in the UK, and highlighting the key gaps in the evidence base that this report aims to address.
Lipid monitoring and statin therapy
Cholesterol is a lipid, essential for normal bodily function; however, high levels are associated with several health problems, in particular CVD. Lipid measurement is important for accurately assessing an individual’s overall risk of CVD and CHD: high TC and LDL cholesterol, and low HDL cholesterol predict an increased risk. 13 There is a clear log-linear relationship between cholesterol and cardiovascular events, with each mmol/l rise in TC associated with a 72% risk of a major coronary event. 7,14 Management of dyslipidaemia therefore plays an important role in the prevention of both primary and secondary cardiovascular events.
Statins are a group of medicines that can reduce serum LDL cholesterol through the inhibition of 3-hydroxy-3-methylglutaryl coenzyme A (HMG-CoA) reductase, an enzyme involved in the biosynthesis of cholesterol, and the subsequent enhanced uptake of LDL from the bloodstream. 15,16 Since their introduction in the 1980s, they have made a dramatic difference in lipid management, with statin use reducing CHD mortality among those at both moderate and high risk of CHD. 17–25 Current guidelines in the UK2,9,10 propose their use for both primary and secondary prevention of CVD. Accordingly, UK statin expenditure has increased from approximately £20M in 199322 to £70M in 2004,26 and to > £800M in 2011. 27 Mirroring this treatment cost has been a large increase in lipid measurement. Lipid levels are measured both before and after the decision to initiate dietary or statin treatment. Our recent Oxfordshire-based study indicated a ≥ 15-fold rise in the overall number of lipid measurements over the past 20 years; although appropriate lipid testing has increased, and cholesterol levels appear to be lowered, there appears to be considerable, possibly unnecessary, repeat testing. 17
The objectives of lipid monitoring, which change over the course of treatment, can usefully be divided into the five phases described in Table 1. 11,28 Although individuals taking statins may require some monitoring of response to treatment or follow-up (phase 2: initial titration), most testing is likely to happen during screening (phase 1: pre-treatment) or long-term monitoring (phase 3: maintenance). This report focuses on the monitoring carried out in phases 1 and 3: screening and long-term monitoring.
Phase | Monitoring objectives | Optimal interval |
---|---|---|
1. Pre-treatment | Check need for treatment Establish a baseline for determining response and change |
Short – based on within person variability |
2. Initial titration | Assess individual response to treatment Assess immediate adverse effect Achieve control |
Medium – based on pharmacokinetics (e.g. drug half-life) and pharmacodynamics (physiological impact time) (wash-in) |
3. Maintenance | Detect drift from control limits Detect long-term harms |
Long – based on rate of random and systematic ‘drift’ |
4. Re-establish control | Bring level back within control limits | Medium – see (2) |
5. Cessation | Check safety of cessation | Medium – see (2) |
Screening and long-term monitoring of lipid levels requires interpretation of initial levels and also of the following sequential levels over time. 29 As part of this interpretation, it is necessary to consider both the short-term within-person variation (‘noise’) and the long-term variability among individuals in the population (‘signal’), illustrated in Figure 1. 30,31 At the time of writing, however, no published guidelines have considered either within-person or long-term variation in their re-screening strategies. 12 Recent evidence has suggested that, because of the weak signal–noise (SN) ratio of cholesterol level, frequent screening and/or monitoring – as part of long-term management – more often captures measurement error rather than true changes. 30–32 As clinical decisions on treatment are typically based upon these measurements, overly frequent monitoring may be detrimental to a patient’s health; therefore, less frequent testing of cholesterol, such as every 3–5 years, might be more appropriate.
FIGURE 1.
Variance of difference among individual cholesterol levels over 4 years.

Choice of lipid measure to monitor
Primary prevention
The seminal statin trials in the 1990s, such as 4S (Scandinavian simvastatin survival study),18 LIPID (The long-term intervention with pravastatin in ischaemic disease study),33 CARE (Cholesterol and current events trial)34 and WOSCOPS (West of Scotland coronary prevention study),19 focused on TC as the main lipid measurement for screening and monitoring. As a result, initial guidelines also focused on TC for screening, targets, and monitoring. 35,36 Although an important CVD risk factor, serum TC on its own is actually a relatively poor predictor of who will go on to have an event. 22,36 In practice, CVD can rarely be attributed to a single underlying risk factor; more commonly it is the additive and synergistic effect of several risk factors that lead to the atherosclerotic progression underlying most CVD. Thus, over time, there has been a shift towards assessment of absolute CVD risk, using CVD risk equations that include a combination of important risk factors. 37 For initial risk measurement, there is evidence from cohort studies38–40 and a meta-analysis41 to suggest that lipid ratios (TC/HDL cholesterol and LDL/HDL cholesterol) have greater independent predictive values for CHD than individual lipid levels. The better predictive ability, and ease of measuring TC compared with LDL, has led to the inclusion of TC/HDL cholesterol in many CVD risk equations,2,42 which, in turn, has meant a move to measuring and using a combination of TC and HDL for screening in primary prevention patients. Although TC and HDL, based upon cardiovascular risk, are the most commonly recommended lipids for screening, it is often suggested that LDL be either calculated using the Friedewald equation43 (requiring a fasting sample) or measured directly, even when guidelines do not specify what to do with the information. Additionally, most guidelines also recommend measuring TGs, although TGs play only a minor role in treatment choices.
Secondary prevention
For people with established CVD, statins reduce total mortality, cardiovascular mortality and morbidity, and are cost-effective, particularly for those with CHD. 16,44 However, substantial variation in the use of statins for secondary prevention combined with poor adherence to treatment, even in those who have experienced a CVD event,45–47 mean that in many instances serum cholesterol often remains at unacceptably high levels,48 and can be further improved with advice, support and treatment. Management of statins for the secondary prevention of CVD is usually based on controlling cholesterol levels to a target dose,2,49–51 but there is a lack of published evidence on the cost-effectiveness of treating to target compared with fixed doses of statins,2 and recommendations are based upon either economic simulation models or expert opinion.
When to monitor
Primary and secondary prevention
Information provided on the frequency of lipid monitoring in current CVD prevention guidelines differs greatly: some guidelines give vague references to testing lipids or assessing CVD risk at ‘regular intervals’, whereas others give specific intervals for specific groups of people, often with more frequent screening and measurement for those at higher risk or in secondary prevention groups. Most guidelines do not reference the evidence or level of evidence on which the recommendation was based, and, of those that do, most use only weak levels of evidence, consensus or expert opinion. 12
Screening and monitoring as part of primary cardiovascular prevention in the UK
The 2010 UK National Institute for Health and Care Excellence (NICE) lipid modification guidelines2 for the primary prevention of CVD recommend more active and systematic identification of those at high risk of CVD, but without full-scale (population wide) screening. 2 Based upon an economic analysis, the guidelines suggest the use of computerised GP records (with data on age, smoking, BP and, sometimes, serum lipids) to identify people aged between 40 and 74 years who are in need of a full CVD risk assessment. This full assessment would then include measurement of TC and HDL cholesterol (non-fasting samples would be adequate) and calculation of the patient’s 10-year risk of CVD using an appropriate CVD risk calculator, such as the QRisk2 equations from the QRESEARCH database,52 equations from the Framingham Heart Study,53 or the ASsessing cardiovascular risk using SIGN guidelines (ASSIGN) score. 54 Statin therapy is recommended as part of a management strategy for primary prevention people with a ≥ 20% 10-year risk of developing CVD. Although the economic analysis on which these guidelines were based allows for measurement error at a population level (through the incorporation of an error term based on published values for the coefficient of variation in TC and HDL cholesterol), individual variability in the progression of dyslipidaemia is not accounted for. Furthermore, only TC and HDL cholesterol measurement are considered, even although guidelines note that before offering lipid modification therapy, fasting levels of TC, HDL and TGs should be measured, and LDL should be calculated using the Friedewald equation. 43 The implication is that LDL cholesterol measurement is not required for risk assessment: complicated by the need for specialised assays to measure LDL cholesterol directly or a fasting sample to allow indirect calculation of LDL cholesterol.
Although guidelines note that a patient’s CVD risk should be reviewed on an ongoing basis, a specific interval is not provided. Target lipid levels are not recommended and once a patient has started taking statins, no further monitoring of lipids is considered necessary: review of drug therapy should be guided by clinical judgement and patient preference. 2 This differs to many guidelines that have suggested close monitoring of those on treatment, and was suggested because of the lack of clinical trial evidence for treating to targets, which target to use, and the lack of cost-effectiveness studies on lipid measurement for risk assessment. 2 Although initially proposed over 10 years ago,37 the ‘fire and forget’ approach has been criticised because of the possibility of failing to identify non-response to treatment, whether or not biological or through non-adherence. 55 Despite the known inaccuracy of lipid measurements, NICE guidelines2 note that multiple measurements are impractical and risk delay, and thus recommend that treatment should be generally based on only one or two measurements. Using an average of several repeated measurements is likely to improve the estimate of an individual’s ‘true’ underlying lipid level but is also likely to have an impact on CVD risk prediction; this has not yet been investigated.
Updated guidelines were published by NICE in 2014;56 Table 2 summarises key differences in monitoring strategies between the 2010 and the 2014 guidelines. Based upon economic analyses, the update recommends that, although TC and HDL will remain the lipid measurements for screening, the treatment eligibility threshold for people with no history of CVD will be lowered to a 10-year CVD risk of 10%, and that this should be calculated using the QRisk252 assessment tool. As QRisk252 has been validated for people aged up to 85 years of age, people aged 75–84 years will also become eligible for screening. Moreover, a fasting sample prior to initiating statin therapy is no longer needed; instead TC, HDL cholesterol, and TG concentrations should be measured and non-HDL cholesterol calculated (through the subtraction of HDL cholesterol from TC), when a non-fasting sample is considered sufficient. More detailed follow-up monitoring is suggested, with measurement of TC and HDL cholesterol, and calculation of non-HDL cholesterol at 3 months, with a target reduction of at least 40% in non-HDL cholesterol. This recommendation is based upon an evidence review of statin efficacy, and it is noted that there are wide confidence intervals (CIs) around this 40% estimated reduction. It is not clear whether or not further lipid measurements after another 3 months are recommended for those who did not meet these goals. As with previous economic analyses, individual variability is not accounted for.
Aspect of guideline | NICE Clinical Guideline | |
---|---|---|
2010: Clinical Guideline 672 | 2014: Clinical Guideline 18156 | |
Lipid measurements required | TC/HDL cholesterol measurements initially, followed by a full fasting panel pre treatment | Before starting lipid modification therapy for the primary prevention of CVD, take at least one lipid sample to measure a full lipid profile. This should include measurement of TC, HDL cholesterol, non-HDL cholesterol, and TG concentrations. A fasting sample is not needed |
CVD risk score(s) | QRisk252 Framingham 199153 ASSIGN54 |
QRisk252 |
CVD risk threshold for statin treatment | 20% | 10% |
Initial statin treatment recommendation | Simvastatin 40 mg | Atorvastatin 20 mg |
Follow-up recommendation | Once a person has been started on a statin . . . repeat lipid measurement is unnecessary. Clinical judgement and patient preference should guide the review of drug therapy and whether or not to review the lipid profile | Measure cholesterol, HDL cholesterol and non-HDL cholesterol in people who have been started on high-intensity statin treatment (atorvastatin, any dose) after 3 months of treatment and aim for a > 40% reduction in non-HDL cholesterol |
Follow-up action | Target for TC or LDL cholesterol is not recommended for people who are treated with a statin for primary prevention of CVD | If a > 40% reduction in non-HDL cholesterol is not achieved:
|
Monitoring as part of secondary cardiovascular prevention in the UK
In 2010, NICE’s lipid modification guidelines for the secondary prevention of CVD2 recommend initiation with simvastatin 40 mg (except if contraindicated), and that if TC levels of < 4 mmol/l or LDL cholesterol levels of < 2 mmol/l are not achieved on the initial dose then the dose can be increased to simvastatin 80 mg or a statin of similar potency and acquisition cost. These recommendations were based upon economic analyses, as a systematic literature search identified no studies on the cost-effectiveness of treating to target compared with a fixed dose of statin. 2 These analyses do not consider individual variability in lipid levels or the frequency at which to monitor. Since 2010, a drug safety update from the Medicines and Healthcare Products Regulatory Agency (MHRA),57 based upon results from the SEARCH study (Study of the effectiveness of additional reductions in cholesterol and homocysteine),58 recommended that simvastatin 80 mg should be considered only in patients with severe hypercholesterolaemia and a high risk of cardiovascular complications; furthermore, the UK patent for atorvastatin expired in 2012, and now that generic versions are available, this is considered a preferred choice of statin by some local authorities,59,60 and an updated analysis is clearly needed.
As noted above, these guidelines have been under review since 2011. 61,62 Updated recommendations from 201456 are compared with those from 20102 in Table 3, and suggest initiating therapy with a 80-mg dose of atorvastatin for people with established CVD62 and the replacement of general target levels for TC and LDL cholesterol with an individual target of a 40% reduction in non-HDL cholesterol after 3 months’ treatment. Potential actions for those not achieving this target include optimising adherence to statin therapy, diet and lifestyle measures and the timing of the dose (i.e. taken at night). In addition, people already taking a different statin/dose could be switched to atorvastatin 80 mg. These suggestions are, again, based upon economic analysis that does not account for individual variability in lipids or the frequency at which to monitor within a framework including both statins and the prevention of CVD.
Aspect of guideline | NICE Clinical Guideline | |
---|---|---|
2010: Clinical Guideline 672 | 2014: Clinical Guideline 18156 | |
Initial treatment recommendation | A drug with a low acquisition cost, such as simvastatin 40 mg | Atorvastatin 80 mg |
Lipids required for monitoring | TC and LDL cholesterol | Measure cholesterol, HDL cholesterol and non-HDL cholesterol in people who have been started on high-intensity statin treatment (atorvastatin any dose) after 3 months |
Lipid thresholds or follow-up recommendation | 4 mmol/l for TC or 2 mmol/l for LDL cholesterol | Aim for a > 40% reduction in non-HDL cholesterol at 3 months since statin initiation |
Follow-up action | Consider increasing to simvastatin 80 mg or a drug of similar efficacy and acquisition cost of TC of < 4 mmol/l or LDL of < 2 mmol/l is not attained An ‘audit’ level of TC of 5 mmol/l should be used to assess progress in populations or groups of people with CVD, in recognition that more than half of patients will not achieve a TC of < 4 mmol/l or LDL of < 2 mmol/l |
If a greater than 40% reduction in non-HDL cholesterol is not achieved:
|
No specific monitoring intervals are given and the frequency of the monitoring is not discussed. In contrast, until 2014, the Quality Outcomes Framework (QOF),63 a voluntary incentive-based scheme for GP practices in the UK, effectively recommends annual monitoring of TC levels in secondary prevention patients, as there was an indicator for the proportion of patients with CHD whose TC level, measured in the preceding 12 months, was ≤ 5 mmol/l. Updates for 2014–15 expect practices to continue to monitor TC/HDL cholesterol but targets have been removed,64 providing evidence to support that this decision may help to minimise the persistence of over-monitoring. Previous research has suggested that, as a result of measurement error, such frequent monitoring is just as likely to mislead when trying to decide whether or not changes in treatment are needed. 65
Aims and objectives
As described in this introductory chapter, screening and monitoring of lipid levels to aid treatment decisions provides a key method for reducing the risk of CVD; however, the optimal lipid measure, monitoring strategy and interval remain unclear. A large number of guidelines do not include clear information on what, when and how to monitor lipids, and, of those that do, the evidence base, for monitoring frequency in particular, is weak; moreover, within-person and long-term variation are not addressed in their re-screening strategies. 12 Therefore, the aim of the research reported in this monograph, and set out in the original protocol (see www.nets.nihr.ac.uk/projects/hta/109701), is to develop a clinical and economic model of dyslipidaemia and use this to determine an effective strategy for screening and monitoring of lipid levels in relation to primary and secondary CVD prevention, including current practice.
Our specific objectives are to:
-
identify the relative ability of different lipid measures (single or combination) to detect important changes in lipid status
-
estimate the incremental gains and costs of different strategies (lipid measurements and intervals) for risk assessment and monitoring of lipid levels in patients at risk of, or with, CVD
-
develop and populate an economic model of lipid monitoring
-
explore how the choice of lipid measure impacts on risk assessment of CVD compared with original risk scores
-
disseminate the impact of our findings on CVD risk assessment.
Modifications to the original protocol are listed in Appendix 2.
Overview of report structure
The scientific summary provides a brief overview of our main findings. The subsequent chapters (see Chapters 2–10) give full details of methods and comprehensive reporting of results. An outline of the content of each chapter and its relation to the objectives listed above is presented below. The report uses the following structure: systematic reviews, statistical modelling, health-economic modelling, and other chapters. Figure 2 shows the dependencies between chapters and the objectives.
FIGURE 2.
Dependencies between chapters and the objectives. PPI, patient and public involvement.

In Chapter 2, the predictive ability of different lipid measures for CVD events and all-cause mortality are compared in the systematic review of prognostic studies, addressing Objective 1 through secondary analyses of the literature.
In Chapter 3, we review the randomised controlled trial (RCT) evidence for atorvastatin, with particular emphasis on dose effects, because this is now the recommended statin in UK guidelines and as a prerequisite for Chapter 7.
In Chapter 4, we quantify the degree of variability (noise) in different lipid measures, and compare this to the rate of progression (SN ratio), as a measure of the ability of different lipid measures to detect real versus apparent changes in lipid status, addressing Objective 1 through analyses of patient-level data.
We address Objective 2 by extending the results of Chapter 4 into a computer model of lipid monitoring. In Chapter 5 this model is used to compare different intervals for lipid-monitoring strategies (Objective 2), and in Chapter 6 it is used to consider the use of different lipid measures in CVD risk estimation (Objective 4). In Chapter 7 the computer model is extended into an economic model (Objective 3) of lipid monitoring.
Chapter 8 describes a meeting with key researchers and stakeholders as part of our dissemination strategy (Objective 5), and Chapter 9 summarises a subsequent consultation with our patient and public involvement (PPI) representative. The concluding Chapter 10 contains the discussion of our overall findings.
Chapter 2 Systematic review of association between lipid measures and cardiovascular events and all-cause mortality
Background
As noted in Chapter 1, there are a number of different lipids that could be measured as part of a strategy for the prevention of primary or secondary CVD. However, at the time of writing, there is no consensus on which lipid is the optimal target for monitoring. One key criterion for a monitoring target is the ability to predict clinically significant events,11 preferably at a stage early enough to allow preventative action to be taken.
The Cholesterol Treatment Trialists’ Collaboration66 has extensively examined the safety and efficacy of statins for lowering LDL cholesterol but, to date, has not compared the predictive ability of different lipid measures for CVD events or mortality. A number of previous reviews and meta-analyses have compared the association of different lipid measures with CVD outcomes, all of which have examined subjects who are not taking statins separately to those who are. The reasons for this are not clear, and are potentially historical.
To date, five reviews41,67–71 have assessed studies with subjects not taking statins. Their key features and findings are summarised in Tables 4 and 5, respectively. Three of these studies obtained IPD,41,67,69,70 whereas the other two analysed trial-level data. 68,71 All support an association between lipid measures and the cardiovascular outcomes examined; however, as each focuses on a different subset of lipid measures and/or a different cardiovascular outcome, their conclusions for the lipid most strongly predictive of CVD varied, and comparisons are difficult.
Review name: number of studies – type | Review characteristics | Statistical methods and assumptions |
---|---|---|
APSC 2005:67 32 studies – IPD | No searches completed Studies included by invitation (subject to certain criteria) Studies included only if reported results for TC, HDL cholesterol and TGs at baseline Studies excluded if no events in follow-up Some subgroup analysis by gender and age and comments on heterogeneity of data because of regional differences, but essentially no quality assessment |
Prevention groups not stated Outcomes were analysed in equal quartiles (as assessed at baseline) and summarised through HRs Further analysis by use of likelihood ratio chi-squared statistic to compare models (results not shown) |
Thompson and Danesh 2006:68 23 studies – published data | Searches up to October 2005 Extensive search, but no strategy provided Studies only included if reported results for Apo B, Apo A-I, Apo B/Apo A-I at baseline No table of excluded studies No quality assessment of studies, although subgroup analysis by quality factors; however, no conclusions drawn from these results Grading system for adjustment of data; however, all date pooled whether unadjusted or adjusted Outcome included various definitions of CHD, subgroup analysis to examine this, but no conclusions drawn |
Mixed primary and secondary populations Point estimates extracted and standardised ORs, RRs and HRs were assumed to be an approximate measure for relative risk Results displayed as meta-analysis (bottom vs. top-third baseline values) |
PSC 2007:41 not stated – IPD | Search appears to be comprehensive but no search strategy available, no reference to language and search dates not published Screening not described No table of excluded studies Limited quality assessment Comprehensive analysis plan Results calculated only for participants with complete data for all four measures (TC, HDL cholesterol, non-HDL cholesterol, TC/HDL cholesterol) |
Primary prevention Age-specific HRs estimated by Cox regression (gender specific too in the original review) Results presented in this review as inverse of the published results to allow comparison Unreliable measurements excluded HRs are presented in unit measures approximately proportional to 1 SD (0.33 mmol/l HDL cholesterol, 1 mmol/l non-HDL cholesterol, 1.33 mmol/l TC/HDL cholesterol) |
ERFC 2009,69 2012:70 112 – IPD | Extended work by APSC and PSC Original search up to August 2007, subsequent search dates not given. No search strategy provided Full search completed, except databases searched not stated, nor language or publication restrictions No statement about screening process Studies only included if reported results for TC, HDL, TGs, non-HDL cholesterol and several conventional risk factors at baseline Quality assessment was completed through a variety of subgroup and sensitivity analyses |
Primary prevention HRs calculated using coproportional regression models stratified by gender Four matched nested case–control studies provided ORs which were considered to approximate HRs HRs adjusted for age, gender, systolic BP, smoking, BMI, history of diabetes |
Sniderman et al. 2011:71 107 – published data | Search based on previous reviews and PubMed search from 2005 until unstated date. No search strategy provided Previous reviews and experts contacted but no reference to publication or language restrictions Inclusion/exclusion criteria not stated Studies included only if reported relative risks for Apo B, non-HDL cholesterol and LDL cholesterol No statement about screening process No table of excluded studies Assessment of assay quality and degree of data adjustment, but no further quality assessment Outcome definition varied, sensitivity analysis completed to account for this |
Primary prevention RRR per 1 SD increment All ORs and HRs converted to RRRs Random-effects meta-analysis |
Review name [summary measure used] | Number of studies | Outcome | Lipid measure | Estimate (95% CI) |
---|---|---|---|---|
APSC 200567 [HR per 1 SD increase] Note: HDL cholesterol as 1 SD decrease |
17 | CVD mortality | TC | 1.09 (1.05 to 1.14) |
TG | 1.17 (log) (1.06 to 1.28) | |||
HDL cholesterol | 1.16 (1.04 to 1.30) | |||
Non-HDL cholesterol | 1.21 (1.11 to 1.32) | |||
TC/HDL cholesterol | 1.23 (log) (1.12 to 1.36) | |||
TG/HDL cholesterol | 1.21 (log) (1.09 to 1.34) | |||
CHD mortality | LDL cholesterol | 1.35 (1.13 to 1.61) | ||
Thompson and Danesh 200668 [RR] Note: Apo A-I reported as inverse |
21 | CHD | Apo A-I | 1.62 (1.43 to 1.83) |
Apo B | 1.99 (1.65 to 2.39) | |||
Apo B/Apo A-I | 1.86 (1.55 to 2.22) | |||
PSC 200741 [age-specific HR] | 61 | CHD mortality | TC 80–89 years | 1.18 (1.12 to 122) |
TC 70–79 years | 1.22 (1.18 to 1.25) | |||
TC 60–69 years | 1.39 (1.35 to 1.45) | |||
TC 50–59 years | 1.72 (1.64 to 1.76) | |||
TC 40–49 years | 2.27 (2.08 to 2.38) | |||
23 | HDL cholesterol 70–89 years | 0.74 (0.67 to 0.82) | ||
HDL cholesterol 60–69 years | 0.55 (0.49 to 0.61) | |||
HDL cholesterol 40–59 years | 0.61 (0.54 to 0.69) | |||
Non-HDL cholesterol 70–89 years | 1.37 (1.27 to 1.49) | |||
Non-HDL cholesterol 60–69 years | 1.52 (1.41 to 1.64) | |||
Non-HDL cholesterol 60–69 years | 1.75 (1.61 to 1.92) | |||
TC/HDL cholesterol 70–89 years | 1.45 (1.35 to 1.59) | |||
TC/HDL cholesterol 60–69 years | 1.67 (1.56 to 1.79) | |||
TC/HDL cholesterol 40–59 years | 1.79 (1.67 to 1.96) | |||
ERFC 2009,69 201270 [HR per 1 SD increase/decrease] | 68 (2009) | CHD and stroke | TG | 1.37 (1.31 to 1.42) |
HDL cholesterol | 0.71 (0.68 to 0.75) | |||
Non-HDL cholesterol | 1.56 (1.47 to 1.66) | |||
8 (2009) | CHD | LDL | 1.41 (1.11 to 1.81) | |
Non-HDL cholesterol | 1.49 (1.20 to 1.86) | |||
22 (2009) | CHD and stroke | Non-HDL cholesterol | 1.55 (1.34 to 1.80) | |
Apo B | 1.61 (1.44 to 1.80) | |||
HDL cholesterol | 0.71 (0.65 to 0.79) | |||
Apo A-I | 0.81 (0.74 to 0.88) | |||
Non-HDL cholesterol/HDL cholesterol | 1.52 (1.37 to 1.68) | |||
Apo B/Apo A-I | 1.51 (1.39 to 1.63) | |||
26 (2012) | CVD | Non-HDL cholesterol | 1.27 (1.22 to 1.33) | |
Apo B | 1.24 (1.19 to 1.29) | |||
Apo A-I | 0.87 (0.84 to 0.90) | |||
TC/HDL cholesterol | 1.32 (1.24 to 1.39) | |||
Apo B/Apo A-I | 1.3 (1.24 to 1.36) | |||
Sniderman et al. 201171 [geometric mean RRR] | 12 | CHD | LDL cholesterol | 1.25 (1.18 to 1.33) |
Non-HDL cholesterol | 1.34 (1.24 to 1.44) | |||
Apo B | 1.43 (1.35 to 1.51) |
Two recent systematic reviews have considered the predictive value of a limited selection of lipid measures in people taking statins. 72,73 Tables 6 and 7 summarise their characteristics and some key results; however, as each review used different methodology, and only one reported hazard ratios (HRs), it is hard to make a direct comparison. There was some indication that non-HDL cholesterol outperformed the other lipids examined; however, this was outcome dependent and differences were modest. Both reviews72,73 considered only RCTs for inclusion and, other than a sensitivity analyses in one review, examine primary and secondary prevention groups together, hence making the unverified assumption that lipid measures are similarly predictive of CVD events in populations both with, and without, a history of CVD.
Review name: number of studies – type | Review characteristics | Statistical methods and assumptions |
---|---|---|
Robinson 2012:73 32 studies – Published articles and data from Cholesterol Treatment Trialists’ Collaboration | Search from 1966 to 1 December 2010. No strategy published. Plus reference lists and previous reviews. No comment on publication or language restrictions Screening process thorough and clearly stated Clear inclusion/exclusion criteria and definitions provided Studies included only if reported results for Apo B, TC, LDL cholesterol, HDL cholesterol at baseline and one time point in follow-up No table of excluded studies No quality assessment of included studies |
Mixed primary and secondary populations Statin randomised control trials. Control arms either placebo or lower-dosage statin Changes in lipid measures were calculated as the difference between two treatment groups over the same time period Study specific crude relative risk and SE was estimated for each lipid measure for CVD Random-effects meta-analysis completed Results for Apo B presented. No further information on the impact of reduction of other lipid measures published |
Boekholdt et al. 2012:72 29 studies – IPD | Authors followed PRISMA Search up to December 2011. No search strategy provided. Search restricted to PubMed and English-language publications only. No comment on grey literature, reference lists, etc. Studies included only if reported results for TC, LDL cholesterol, HDL cholesterol, TGs, and Apo B, Apo A-I at baseline and follow-up over 2 years No table of excluded studies Full assessment of quality using Delphi score A Measurement Tool to Assess Systematic Reviews (AMSTAR) indicates a high-quality review, meeting all aspects of the assessment albeit some areas were not fully met |
Mixed primary and secondary populations HRs calculated using Cox proportional models Analyses were adjusted for gender, age, smoking, DM, systolic BP, and trial |
Review name [summary measure used] | Number of studies | Outcome | Lipid measure | Estimate (95% CI) |
---|---|---|---|---|
Robinson et al. 201273 [percentage relative risk decrease = 10-mg/dl decrease in lipid measure] | 12 | CVD | Apo B | 11.60 (3.5 to 20.5) |
Boekholdt et al. 201272 [HR per 1 SD increase] | 8 | CVD | LDL | 1.13 (1.10 to 1.17) |
Non-HDL cholesterol | 1.16 (1.12 to 1.19) | |||
Apo B | 1.14 (1.11 to 1.18) |
Thus, although a number of previous reviews have examined the predictive ability of different lipid measures for cardiovascular events, all have focused on a subset of lipids, a specific population or a specific cardiovascular outcome. In this chapter we therefore aim to evaluate the available evidence for the strength of association between each lipid measure and cardiovascular outcomes, including CVD events, CVD mortality and all-cause mortality, in both primary and secondary prevention populations.
Methods
Search methods for identification of studies
We reviewed all published systematic reviews since 2007 using the Montori systematic review filter74 for MEDLINE and the Wilczynki filter75 for EMBASE (both 75% sensitivity). Based on this overview, we opted to stratify results by statin use: populations taking statins and populations not taking statins. Consequently, two separate searches were carried out and two sets of results are presented, although the general methods remained the same for both groups. Dates for the searches were staggered, as the review was broken down into manageable tasks based on statin usage and existing reviews.
Searches were conducted in the Cochrane Central Register of Controlled Trials, MEDLINE, EMBASE, the Clinical Trials Register, the Current Controlled Trials register, and the Cumulative Index to Nursing and Allied Health Literature (CINAHL) to identify relevant studies.
All trials included in identified reviews were considered for inclusion, along with any trials identified by the work of the Cholesterol Treatment Trialists’ Collaboration. 66
Populations not taking statins A search was conducted (unrestricted for study design) from 1 September 2007 to 18 June 2013. This updated/extended the search carried out by the Emerging Risk Factors Collaboration (ERFC). 76 The search was restricted to English language only and did not include conference abstracts (see Appendix 3).
Populations taking statins Two strategies were used, based on study design type. For non-randomised controlled trials (non-RCTs) a search was completed for all publications up to 4 December 2012, with no restriction on language or publication type. For RCTs, all studies were included from previous reviews,72,73 plus a search was conducted to extend these review’s searches up to 9 April 2013. In line with these reviews, the strategy was for English language-only publications (both strategies are presented in Appendix 4). An additional search was conducted within the international Clinical Trials Registry and ClinicalTrials.gov for any studies in progress from 2010 to April 2013.
The title and abstract of each paper identified in the searches was reviewed by two reviewers to identify potentially relevant references. The full text of potentially relevant studies was then obtained, and two reviewers independently selected studies to be included in the review using predetermined inclusion criteria. In all cases, disagreements about study inclusion were resolved by consensus and a third reviewer was consulted if disagreements persisted. Flow charts were constructed to show the selection process.
Study selection criteria
We included all prognostic cohort studies of any design (univariable or multivariable) that measured lipids in humans. Studies from any setting regardless of statin treatment were included. RCTs with adequate follow-up were treated as cohorts providing evidence to a specific study group [e.g. a statin vs. placebo RCT would provide evidence for both populations taking statins (statin arm) and not taking statins (placebo arm) separately].
Consistent with previous reviews in this area, we included studies where at least 1000 relevant participants were recruited per cohort/intervention arm and a minimum 2-year average follow-up duration. 66,72 There was no restriction on gender. We included studies with only two or more lipid measures assessed at baseline/relevant time point, as the focus of the review was to compare predictive performance across different lipid measures.
Target group in PECO (Population, Exposure, Comparator, Outcome) format:
-
P Population with or without existing CVD.
-
E Measurement of lipid measures at baseline/relevant time point. Ten lipids measures were studied including TC, HDL cholesterol, LDL cholesterol, non-HDL cholesterol, TGs, Apo B, Apo A-I, plus combinations of these measures as ratios TC/HDL cholesterol, LDL/HDL cholesterol and Apo B/Apo A-I.
-
C Control or comparator may or may not be present.
-
O Outcomes included cardiovascular events, cardiovascular mortality and all-cause mortality. Strokes were excluded if they were the sole outcome (included if treated as part of composite outcome with other CVD events).
In addition, we considered all studies from previous reviews for inclusion irrespective of whether or not they met the 1000-participant, minimum two lipid measures at baseline or 2-year follow-up thresholds to maximise comparability of our results with those published in previous reviews.
Included studies provided quantitative results for one or more of the following outcomes:
-
cardiovascular events [CHD, non-fatal myocardial infarction (MI), stroke, angina attack, other CVD, coronary bypass]
-
cardiovascular mortality (fatal MI, sudden cardiac death, stroke, congestive heart failure, other fatal CVD)
-
all-cause mortality.
Outcome definition was based on that reported in the included studies.
Data extraction and management
For all included studies, two reviewers independently carried out data extraction. Details extracted included study name and year, cohort numbers, baseline age, gender, prevention group, statin usage and dose if applicable, follow-up period, blood sample details and lipid data. When several follow-up periods were reported then data for the longest follow-up were extracted. Disagreements were resolved by consensus with a third reviewer being consulted when required.
The methodological quality of included studies was assessed using the Quality in Prognostic Studies (QUIPS) assessment tool. 77 Quality of studies with populations taking statins was independently assessed by two reviewers and the level of agreement tested by the kappa statistic (κ). For studies of populations not taking statins, quality was assessed by one reviewer. Quality was assessed in six bias domains, with emphasis placed on prognostic factors and attrition:
-
Study participants No quality bias was anticipated in this area, as all populations were included in the review. Studies were categorised by prevention group, which provided inception cohorts.
-
Study attrition There is no clear guidance for the assessment of attrition rates in prognostic studies. Previous prognostic reviews have considered attrition rates of 10–20% as low risk of quality bias, but these have been based on small populations with relatively rare conditions and short follow-up periods. 78,79 As the new cohorts in this review have > 1000 participants with long follow-ups, we anticipated that dropout could be higher. Risk of study attrition bias was considered to be low – if it was documented – and < 25%, moderate if 25–40% or not reported, and high risk if > 40%.
-
Prognostic factor Lipid measures were considered to be at low risk of quality bias if all tests were processed by a central accredited laboratory and procedures for different measures were described. Risk was assessed as moderate if a central laboratory was used but no further information given, and high risk of bias when no information was provided.
-
Outcome measure This dimension was not considered to be significant, as it was a necessary requirement in the study entry criteria. However, outcome bias could have been introduced owing to clarity of outcome definitions; this was explicitly addressed in sensitivity analyses.
-
Study confounding This too was not considered to be a significant concern, as study confounders were specially accounted for in the data analysis of each study: studies were divided into unadjusted and adjusted data.
-
Statistical analysis As most of the included studies were not designed to report the outcome data required for this review, we did not assess the quality of the study in relation to how the study data were analysed; instead we addressed this issue separately by carrying out a sensitivity analysis considering the type of analysis used in the original study.
Data analysis
Data were sought from the included studies for predictive outcomes as follows:
-
Direct estimates for one standard deviation (SD) incremental change either as HRs, odds ratios (OR) or risk ratios (RRs) and their variances. These ratios were assumed to approximate the same measure of risk. This assumption was tested in a sample of studies with the highest event rates and the ratios showed little variation.
-
Data in an alternative format to incremental change by one SD were converted where possible.
-
Univariable and multivariable models (unadjusted and adjusted). Multivariable analyses included any studies with adjustment for one or more of the following covariates: age, gender, body mass index (BMI), smoking, etc. Where a study produced more than one multivariable model then the most advanced model was selected for data inclusion. However, we specifically excluded any model that adjusted for any other lipid measure.
-
IPD were not actively sought, but used when available.
-
When no direct estimates were reported, alternative indirect methods for estimation were used. 80,81 Additionally, two further alternative indirect methods for estimation were used based on (1) generalised least squares for trend82,83 and (2) simulation methods. A brief description of these can be found in Appendix 5.
The primary analysis estimated the predictive strength (HR) for each lipid measure by each of the three outcomes. Outcomes are reported separately and stratified by unadjusted/adjusted estimates. Analyses were subgrouped by prevention group (primary or secondary prevention). Studies were considered to be only primary or secondary prevention if > 95% of the participants fell in that category; otherwise studies were considered as mixed. Numbers of studies, participants and study characteristics are presented in tables.
For each lipid measure, random effect meta-analyses were carried out to obtain summary HRs (by one SD) using Review Manager (RevMan) 5.2 (The Cochrane Collaboration, The Nordic Cochrane Centre, Copenhagen, Denmark) and results were displayed as forest plots. A random-effects model was selected because high heterogeneity was anticipated in both populations. Any risk of small study bias should be reduced by the majority of included studies having > 1000 participants. Overall summary plots presenting pooled estimates for all lipid measures (HDL and Apo A-I as inverse associations to allow clear comparisons with other measures) by outcome and by unadjusted/adjusted models were produced. Although pooling of data for lipid measures with only three or fewer studies could be potentially misleading, the figures were included in the summary plots as an indication of potential trends had there been more study data.
Heterogeneity was summarised using the I2 and chi-squared statistics. Potential sources of any heterogeneity (clinical or statistical) were explored using subgroup and sensitivity analyses.
Subgroup analyses, where possible, were conducted by:
-
pre-existing condition (e.g. diabetes)
-
study type [prospective (including RCTs) or retrospective cohorts]
-
age (threshold of median 40 years at inception).
Sensitivity analyses were conducted to determine the impact of study quality and data extraction on outcome. Focus was placed on consistency of overall results with those obtained in better-quality studies. When sufficient studies were available, sensitivity analyses were carried out by omitting:
-
studies in which the predictive outcomes were defined by CHD, a surrogate measure of CVD
-
studies assessed to have a higher/moderate risk of quality bias (based on prognostic factors and attrition quality domains)
-
studies in which the majority of the lipid tests were not completed in a central laboratory (yes/no)
-
studies in which summary data were extracted indirectly
-
within the populations not taking statins, studies that confirmed the use of lipid modification therapy.
The search aimed to be as broad as possible to include as many studies as possible. However, it is acknowledged that there is considerable publication bias in prognostic studies that is difficult to characterise;84 therefore we did not carry an analysis for potential publication bias.
A post hoc analysis was conducted as recommended by the external peer reviewer with the aim of exploring sources of potential heterogeneity. A meta-regression was completed to examine the associations between variables considered in the subgroup and sensitivity analyses. Meta-regression was completed, where possible, for those outcomes and variables with sufficient study data.
Amendments to protocol
The original protocol was registered with International Prospective Register of Systematic Reviews (PROSPERO, 2013: CRD42013003727). A number of changes were made to the protocol. Originally, the search strategy specified that all studies would be considered for inclusion in this review. However, it proved necessary to modify the strategy to take into account three reviews published in 2012 and the scale of the review. All studies from the three existing reviews were included, and the new search strategy extended the search beyond the search date of these reviews. Exclusion criteria were introduced to reduce the number of smaller studies (minimum participant numbers, length of follow-up and baseline lipids).
The method for data extraction was amended once the data were examined. Two additional indirect methods for data extraction were used (see Appendix 5) to ensure that the maximum number of data could be obtained for analysis. In the analysis, all outcome data for each lipid measure were pooled; however, it was acknowledged that only those summary estimates including three or more sets of data would be used to draw conclusions. Quality assessment of included studies was amended from an adapted Quality Assessment of Diagnostic Accuracy Studies tool (QUADAS2) to a more recent tool77 specifically designed for prognostic reviews – QUIPS.
Results
We present the results grouped as studies based on populations (1) not taking statins and (2) taking statins.
All study names appear as abbreviations and the full study names are shown in Appendices 6 and 7.
Studies based on populations not taking statins
Figure 3 summarises the selection process; 3776 publications were screened from the search, with a further 165 potential studies identified from existing reviews and hand searching. Of these, 90 studies met the inclusion criteria and provided results (see Appendix 8), with 16 studies providing two or more sets of data; this resulted in a total of 110 sets of data. Twelve studies divided the data by gender [TLGS,88 Yao City,89 AMORIS,90 CB project,91 Copenhagen City,92 DUBBO,93 Framingham Offspring,40 IKNS (Yao),94 MONICA,95 Northwick Park I,96 Reykjavik,97 Rotterdam98]. Two studies divided the data by gender and then further by ethnicity (Charleston99) or presence of metabolic syndrome [European Prospective Investigation of Cancer (EPIC) – Norfolk study (EPIC-Norfolk)100] so providing four data sets each. Puerto Rico101 divided the data by rural and urban populations, and MRFIT102 by black and white men.
FIGURE 3.
Flow chart of study inclusion for populations not taking statins.
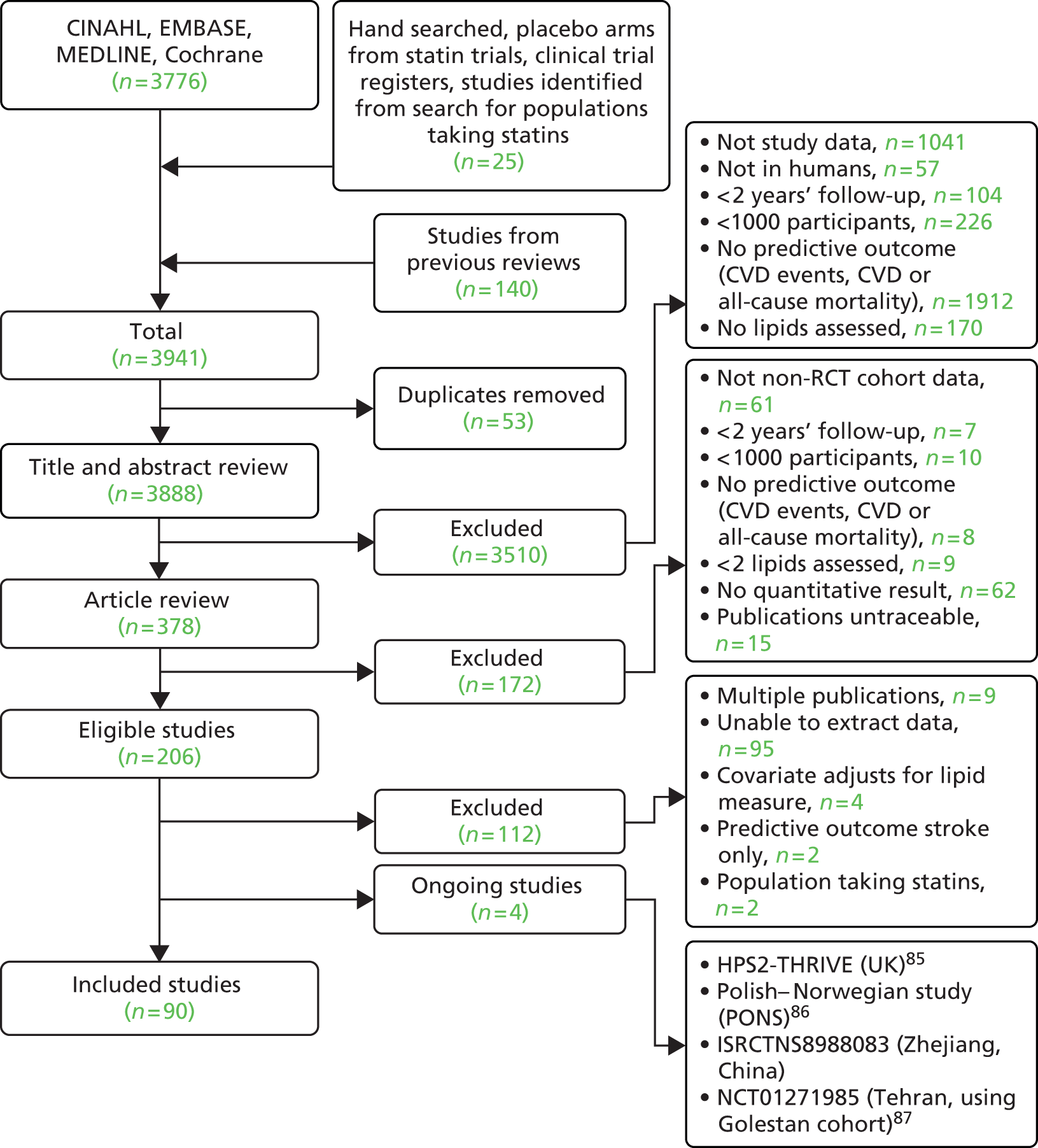
Full details of the characteristics of the 90 included studies are shown in Table 8. Of the included studies 73% were prospective cohorts and 17% were placebo arms of RCTs. The remaining nine studies were two retrospective cohorts (Swedish NDR;164 Juntendo, Japan134), three RCTs (of non-lipid modifying interventions) for which both arms were included in this review (SHEP,159 TREAT,167 Women’s Health172) and four nested case–control studies (BUPA,113 GLOSTRUP Population,124 Nurses’ Health,147 Physicians’ Health150). Studies provided data for between 1 and 10 lipid measures. Two studies provided data for all of the 10 lipid measures (FIELD,121 Women’s Health172). Data details for all studies are shown in Appendix 8. Table 9 shows the number of studies and participants by the 10 lipid measures. Numbers are also shown for three additional measures (Apo B/HDL cholesterol, TG/HDL cholesterol/HDL cholesterol/TC) that were revealed by the review; however, data were available from only one study for one outcome for each measure and therefore no pooled estimates were obtained.
Study name | Study type | Maximum number of participantsa | Age (years: mean unless indicated) | Gender (% female) | Underlying condition for total population | Primary prevention (%) | Lipid-lowering drugs (%) | Fasting assay | Central laboratory | Direct or estimated LDL (N/A if no LDL measure) | Follow-up (years; mean unless indicated) |
---|---|---|---|---|---|---|---|---|---|---|---|
7 Countries (Greece)103 | Prospective cohort | 1215 | 49 | 0 | NS | 100 | NS | NF | NS | N/A | 25 |
7 Countries (Italy)103 | Prospective cohort | 1712 | 49 | 0 | NS | 100 | NS | NF | NS | N/A | 25 |
7 Countries (Netherlands)104 | Prospective cohort | 885 | 71 | 0 | NS | 100 | 3 | NF | Y (accredited) | N/A | 25 |
7 Countries (Serbia)105 | Prospective cohort | 1563 | 40–59 range | 0 | NS | 99 | NS | NF | Y (accredited) | N/A | 25 |
AF/TexCAPS106 | RCT | 3301 | 58 | 15 | No | 100 | N | NS | Y (accredited) | Estimated (Friedewald) | 5.2 |
ALERT107 | RCT | 1052 | 50 | 35 | Renal or combined renal and pancreas transplants > 6 months and stable graft function | 97 | N | F | Y | NS | 5.1 |
AMORIS90 | Prospective cohort | 149,121 | 49+ | 44 | NS | 100 | NS | F/NF (fasting reported for 62% for Apos) | Y (accredited) | Estimated | 11.8 |
ANHF108 | Prospective cohort | 8662 | 43 | 52 | None | 100 | NS | NS | NS | N/A | 15 |
ASCOT-LLA109 | RCT | 1274 | 64 | 24 | Diabetes (II) | 100 | N | NF | Y | NS | 3.3 |
AURORA110 | RCT | 1384 | 64 | 37 | End-stage renal failure/haemodialysis for 3 months | 28.2 | N | F | Y (accredited) | NS | 3.2 |
BRHS111 | Prospective cohort | 7735 | 40–59 range | 0 | NS | 75 | NS | NF | NS | N/A | 7.5 |
Bruneck112 | Prospective cohort | 765 (previous review) | 61–70 mean range | 50 | NS | 90 | NS | F | NS | Estimated (Friedewald) | 10 |
BUPA113 | Nested case control | 1374 | 53 | 0 | NS | NS | NS | F | NS | Estimated (Friedewald) | 5+ |
Caerphilly114 | Prospective cohort | 2225 | 45–59 range | 0 | NS | 69 | NS | F | Y | Estimated (Friedewald) | 9 |
CARDS115 | RCT | 1410 | 62 | 32 | Diabetes (II) | 100 | N | F | Y (accredited) | Estimated (Friedewald) | 3.9 median |
CARE34 | RCT | 2078 | 59 | 14 | None | 0 | N | F | Y (accredited) | Estimated (Friedewald) | 5 median |
Casale Monferrato116 | Prospective cohort | 982 | 69 | 57 | Diabetes II | 76 | NS | F | Y | Estimated (Friedewald, except for 3%) | 11 |
CB project91 | Prospective cohort | 49,018 | 39 | 52 | NS | NS | NS | NF | Y (accredited) | N/A | 11.8 |
Charleston99 | Prospective cohort | 2181 | 50 | 55 | NS | NS | NS | F | NS | N/A | 30 |
Chinese veterans117 | Prospective cohort | 1268 | 55+ | 0 | NS | 70 | NS | NS | NS | N/A | up to 18 years |
CHS118 | Prospective cohort | 4885 | 73 | 60 | NS | 84 | ≤ 5.7 | F | Y (accredited) | Estimated (Friedewald) | 7.5 |
Copenhagen City92 | Prospective cohort | 9035 | 59 | 57 | NS | 99.50 | 0.5 | NF | NS | Estimated (Friedewald) | 14 |
CORONA119 | RCT | 2497 | 73 | 24 | None | 0 | N | NF | Y | NS | 2.7 median |
DAI120 | Prospective cohort | 2788 | 66–69 mean range | 43 | Diabetes (II) | 0 | 24–31.2 | F | N | NS | 4 |
DUBBO93 | Prospective cohort | 2805 | 70 | 56 | NS | 75 | NS | F | Y (accredited) | Estimated (Friedewald) | 5.2 median |
EPIC-Norfolk100 | Prospective cohort | 21,448 | 59 | 56 | NS | 100 | NS | NF | Y | Estimated (Friedewald) | 11 |
FIELD121 | RCT | 4900 | 62 | 37 | Diabetes (II) | 78 | N | F | Two central labs – both accredited | NS | 5 median |
FINRISK ‘92122 | Prospective cohort | 2378 | 54 | 52 | NS | 94 | NS | F | Y | N/A | 6.6 median |
Framingham Offspring40 | Prospective cohort | 3322 | 51 | 53 | NS | 100 | NS | F | NS | Estimated (Friedewald) | 14.8 |
GISSI123 | RCT (stopped early) | 2133 | 60 | 14 | No | 0 | N | F | Y (accredited) | NS | 2 |
GLOSTRUP Population124 | Nested case control | 264 | NS | 0 | NS | 100 | NS | F | Y (accredited) | NS | 8 |
GOH125 | Prospective cohort | 1745 | 53 | 0 | NS | 90 | NS | F | Y (accredited) | N/A | 18 |
GRIPS126 | Prospective cohort | 4368 | 40–59.9 range | 0 | NS | 100 | NS | F | NS | Direct | 10 |
Hamaguchi 2007127 | Prospective cohort | 1221 | 47–49 mean range | 39 | None | 100 | NS | NS | NS | NS | 5.8 |
Honolulu Heart128 | Prospective cohort | 3741 | 71–79 range | 0 | NS | 100 | NS | F | Y | N/A | 3–5 years |
Hoorn study129 | Prospective cohort | 1817 | 61 | 52 | NS | 90 | N | F | NS | N/A | 10 |
HPFS130 | Prospective cohort | 746 | 65 | 0 | Diabetes (II) | 100 | 9 | F (50%) | Y | Direct | 6 |
HPS24 | RCT | 10,267 | 64 | 18 | None | 20.8 | N | NF | Y | Direct | 5 |
IKNS (Yao)94 | Prospective cohort | 12,187 | 40–69 range | 66 | NS | 100 | NS | NF | Y (accredited) | N/A | 8.9 |
InCHIANTI131 | Prospective cohort | 1013 | 65+ | 56 | None | 95 | NS | F | NS | Estimated (Friedewald) | 6 |
Israeli Ischaemic Heart132 | Prospective cohort | 10,059 | 40+ | 0 | NS | NS | NS | NF | Y | N/A | 23 |
IwateKENCO133 | Prospective cohort | 24,566 | 60+ | 65 | NS | 100 | N | F/NF | Y (accredited) | Estimated (Friedewald) | 2.7 |
Juntendo, Japan134 | Retrospective cohort | 1074 | 59–60 mean range | 16 | All complete revascularisation/excluded those on haemodialysis | 0 | 7–10.5 | F | NS | Estimated (Friedewald) | 10.6 |
KIHD135 | Prospective cohort | 1931 | 42–60 range | 0 | NS | 100 | NS | F | NS | Direct | 3 |
Lan 2007136 | Prospective cohort | 2086 | 72 | 37 | None | 95 | NS | F | Y (accredited) | NS | 8.2 |
LIFE (subanalysis)137 | Prospective cohort | 8243 | 67 | 53 | NS | 75.3 | 7.1 in full LIFE trial | NS | Y | N/A | 4.8 |
LIPID138 | RCT | 4502 | 62 | 17 | No | 0 | N | F | Y | Estimated (Friedewald) | 6.1 |
Livermore139 | Prospective cohort | 40 | 0 | NS | 100 | NS | NF | NS | NS | 53.2 | |
LRC prevalence140 | Prospective cohort | 3678 | 35–74 range | 47 at 8-year follow-up | NS | 100 | N | F | NS | Estimated | 12.2 |
MEGA141 | RCT | 3966 | 58 | 69 | N | 100 | 23 use by study end | F | Y (accredited) | Estimated (Friedewald) | 5.3 |
MESA142 | Prospective cohort | 6693 | 62 | 53 | NS | 100 | 16.10 | F | Y | NS | 4.62 |
MHS143 | Prospective cohort | 11,060 | 62 | 44 | Diabetic | NS | 32.60 | NS | NS | NS | 4.62 |
MONICA95 | Prospective cohort | 2850 | 50 | 50 | NS | 100 | NS | NF | NS | N/A | 13 median |
MRFIT102 | Prospective cohort | 6257 | 46 | 0 | NS | 100 | NS | F | NS | NS | 7 |
NHANES III144 | Prospective cohort | 7594 | 45 | 50 | NS | NS | NS | F | Y (accredited) | Estimated (Friedewald) | 10.3 median |
NIPPON 80145 | Prospective cohort | 9216 | 50 | 56 | NS | 100 | NS | NF | Y (accredited) | N/A | 17.3 |
Northwick Park I96 | Prospective cohort | 3108 | 48 | 30 | NS | 97 | NS | NF | NS | N/A | 29 median |
Northwick Park II146 | Prospective cohort | 2508 | 56 | 0 | NS | 100 | NS | NF | NS | Estimated (Wallduis) | 6 |
Nurses’ Health147 | Nested case control | 683 (previous review) | 61 | 100 | NS | 100 | N | F | Y (accredited) | Direct | 8 |
Palma 2007148 | Prospective cohort | 2848 | 53 | 53 | Surgical patients (various) | NS | NS | F | Y | N/A | 6 |
PARIS149 | Prospective cohort | 6999 | 43–53 range | 0 | NS | 98 | NS | F | NS | N/A | 11.4 |
Physicians’ Health150 | Nested case control | 492 (previous review) | 59 | 0 | NS | 100 | NS | NF | Y (accredited) | N/A | 7 |
PreCIS151 | Prospective cohort | 3098 | 54–55 mean range | 43 | NS | 53 | 41 (statins) | F | NS | Estimated (Friedewald) | 7.6 |
PREVEND152 | Prospective cohort | 6948 | 48 | 52 | NS | 100 | NS | F | NS | Estimated (Friedewald) | 7.9 |
PROCAM153 | Prospective cohort | 5389 | 40–65 range | 0 | NS | 100 | NS | F | NS | Estimated (Friedewald) | 6 |
Progetto Cuore154 | Prospective cohort | 20,447 | 50 | 64 | NS | NS | NS | NS | NS | N/A | 10.4 |
PROSPER155 | RCT | 2913 | 75 | 52 | None | 30 | N | F | Y (accredited) | NS | 3.2 |
Puerto Rico101 | Prospective cohort | 6205 | 45–64 range | 0 | NS | 94 | NS | NF | Y | N/A | 12 |
Quebec Cardiovascular156 | Prospective cohort | 2177 | 57 | 0 | NS | 100 | NS | F | NS | Estimated (Friedewald) | 5 |
Rancho Bernardo157 | Prospective cohort | 2227 | 69 | 59 | NS | 100 | N | F | Y (accredited) | Estimated (Friedewald) | 9.6 |
Reykjavik97 | Prospective cohort | 18,569 | 53 | 48 | NS | 95 | NS | F | NS | N/A | 17.4 |
RIFLE158 | Prospective cohort | 20,386 | 30–69 range | 0 | NS | 100 | NS | F | N | N/A | 6 |
Rotterdam98 | Prospective cohort | 6006 | 55+ | 59 | NS | 96 | 2.90 | F/NF (both F and NF stated) | NS | N/A | 4.2 |
SHEP159 | Prospective cohort(both arms of RCT) | 4736 | ≥ 60 | 57 | NS | 93 | NS | F (60%) | Y | Estimated | 4.5 |
SHS160 | Prospective cohort | 2108 | 57 | 63 | Diabetes (II) | 100 | NS | F | Y | Direct | 9 |
SLVHS161 | Prospective cohort | 1682 | 52–55 mean range | 56 | no | 100 | 3 at baseline | F | NS | N/A | 9.3 |
Stanek 2007162 | Prospective cohort | 30,348 | 66 | 45 | NS | 65 | NS | NS | NS | NS | 2.25 |
Stockholm County163 | prospective cohort | 3502 | 60 | 54 | NS | 100 | NS | F | NS | Estimated (Friedewald) | 11 |
Swedish NDR164 | Retrospective cohort | 18,673 | 60 | 40 | Diabetes (II) | 86.6 | 42 | NS | N | Estimated (Friedewald) | 4.8 |
Thrombo165 | Prospective cohort | 1045 | 48 | 24 | None | 0 | 38 | F | NS | Estimated (Friedewald) | 26 |
Ting 2010166 | Prospective cohort | 4521 | 54+ median | 54 | Diabetic | 92 | 23 by study end | F | Y (accredited) | Estimated (Friedewald) | 4.9 |
TLGS88 | Prospective cohort | 6834 | 45+ | 56 | NS | 97 | ≤ 5 | F | Y | Estimated (Friedewald) | 9.3 |
TREAT167 | Prospective cohort (both arms of RCT) | 4038 | 68 | 57 | NS | 63 | 15% in 1000 subgroup | NS | NS | N/A | 2.4 median |
Turku Elderly168 | Prospective cohort | 1032 | 70 | 64 | NS | 0 | < 1% | F | Y | Estimated (Friedewald) | 12 |
ULSAM169 | Prospective cohort | 1108 | 50 | 0 | NS | 100 | NS | F | NS | Estimated (Friedewald) | 28.7 |
VA-HIT170 | RCT | 1267 | 64 | 0 | NS | 0 | N | F | Y (accredited) | Estimated (Friedewald) | 5.1 |
Whitehall I171 | Prospective cohort | 17,718 | 40–64 range | 0 | NS | NS | NS | NF | Y | N/A | 18 |
Women’s Health172 | Prospective cohort (both arms of RCT) | 15,632 | 54 | 100 | NS | NS | NS | NF | Y (accredited) | Direct | 10 |
WOSCOPS19 | RCT | 3293 | 55 | 0 | N | 100 | N | F | Y (accredited) | NS | 4.9 |
Yao City89 | Prospective cohort | 11,977 | 21–89 range | 32 | NS | 100 | NS | NF | Y (accredited) | N/A | 4.8 |
Lipid measure | Outcome | Number of primary prevention studies | Number of secondary prevention studies | Overall number of studies | Number of participants |
---|---|---|---|---|---|
LDL | CVD events | 15 | 7 | 28 | 110,021 |
CVD events (adjusted) | 17 | 1 | 22 | 286,038 | |
CVD mortality | 2 | 0 | 2 | 4691 | |
CVD mortality (adjusted) | 3 | 1 | 6 | 161,581 | |
All-cause mortality | 2 | 0 | 7 | 13,958 | |
All-cause mortality (adjusted) | 0 | 0 | 4 | 32,136 | |
TC | CVD events | 16 | 5 | 27 | 110,304 |
CVD events (adjusted) | 28 | 1 | 39 | 256,259 | |
CVD mortality | 6 | 0 | 14 | 36,514 | |
CVD mortality (adjusted) | 9 | 1 | 19 | 234,018 | |
All-cause mortality | 3 | 0 | 15 | 25,215 | |
All-cause mortality (adjusted) | 8 | 0 | 19 | 128,446 | |
HDL | CVD events | 18 | 7 | 33 | 129,205 |
CVD events (adjusted) | 29 | 1 | 39 | 323,111 | |
CVD mortality | 4 | 0 | 6 | 19,421 | |
CVD mortality (adjusted) | 5 | 1 | 10 | 179,359 | |
All-cause mortality | 2 | 0 | 9 | 62,497 | |
All-cause mortality (adjusted) | 1 | 0 | 5 | 29,372 | |
TGs | CVD events | 13 | 6 | 25 | 100,441 |
CVD events (adjusted) | 9 | 1 | 14 | 128,055 | |
CVD mortality | 4 | 0 | 5 | 11,837 | |
CVD mortality (adjusted) | 4 | 1 | 5 | 159,421 | |
All-cause mortality | 2 | 0 | 8 | 16,168 | |
All-cause mortality (adjusted) | 3 | 0 | 5 | 17,274 | |
Non-HDL | CVD events | 4 | 0 | 6 | 22,292 |
CVD events (adjusted) | 14 | 0 | 19 | 253,110 | |
CVD mortality | 0 | 0 | 1 | 8243 | |
CVD mortality (adjusted) | 3 | 1 | 4 | 152,422 | |
All-cause mortality | No data | ||||
All-cause mortality (adjusted) | No data | ||||
Apo B | CVD events | 7 | 2 | 10 | 26,528 |
CVD events (adjusted) | 17 | 1 | 21 | 207,994 | |
CVD mortality | 0 | 0 | 1 | 1145 | |
CVD mortality (adjusted) | 1 | 0 | 3 | 158,280 | |
All-cause mortality | No data | ||||
All-cause mortality (adjusted) | 0 | 0 | 1 | 7594 | |
Apo A | CVD events | 5 | 2 | 8 | 23,773 |
CVD events (adjusted) | 15 | 1 | 19 | 204,372 | |
CVD mortality | 0 | 0 | 1 | 1145 | |
CVD mortality (adjusted) | 1 | 0 | 3 | 158,280 | |
All-cause mortality | No data | ||||
All-cause mortality (adjusted) | 0 | 0 | 1 | 7594 | |
Apo B/Apo-I | CVD events | 3 | 1 | 4 | 16,192 |
CVD events (adjusted) | 14 | 0 | 16 | 195,445 | |
CVD mortality | No data | ||||
CVD mortality (adjusted) | 1 | 0 | 2 | 156,715 | |
All-cause mortality | No data | ||||
All-cause mortality (adjusted) | 0 | 0 | 1 | 7594 | |
TC/HDL cholesterol and non-HDL/HDL merged | CVD events | 5 | 1 | 7 | 17,659 |
CVD events (adjusted) | 17 | 1 | 23 | 258,011 | |
CVD mortality | 1 | 0 | 1 | 3678 | |
CVD mortality (adjusted) | 3 | 0 | 5 | 164,197 | |
All-cause mortality | No data | ||||
All-cause mortality (adjusted) | 0 | 0 | 2 | 12,849 | |
LDL/HDL cholesterol | CVD events | 3 | 0 | 3 | 8499 |
CVD events (adjusted) | 8 | 0 | 11 | 194,177 | |
CVD mortality | 1 | 0 | 1 | 3678 | |
CVD mortality (adjusted) | 1 | 1 | 3 | 157,789 | |
All-cause mortality | No data | ||||
All-cause mortality (adjusted) | 0 | 0 | 1 | 7594 | |
Additional lipid measures (ratios) revealed in included studies but not included in this review (insufficient data to analyse) | |||||
Apo B/HDL | CVD events | 1 | 0 | 1 | 683 |
CVD events (adjusted) | 0 | 0 | 1 | 15,632 | |
CVD mortality | No data | ||||
CVD mortality (adjusted) | No data | ||||
All-cause mortality | No data | ||||
All-cause mortality (adjusted) | No data | ||||
TG/HDL | CVD events | 1 | 0 | 1 | 3678 |
CVD events (adjusted) | No data | ||||
CVD mortality | No data | ||||
CVD mortality (adjusted) | No data | ||||
All-cause mortality | No data | ||||
All-cause mortality (adjusted) | No data | ||||
HDL/TC | CVD events | 1 | 0 | 1 | 710 |
CVD events (adjusted) | No data | ||||
CVD mortality | No data | ||||
CVD mortality (adjusted) | No data | ||||
All-cause mortality | No data | ||||
All-cause mortality (adjusted) | No data |
Outcome data were available for CVD events, CVD mortality and all-cause mortality. Data were further divided into unadjusted (CVD events, CVD mortality, all-cause mortality) and adjusted data [CVD events (adjusted), CVD mortality (adjusted), all-cause mortality (adjusted)] and then by lipid measure. Meta-analyses were not possible for all combinations of outcomes and lipid measures. Outcome data for all lipid measures were pooled for CVD events (except LDL/HDL cholesterol) and CVD events (adjusted). For CVD mortality, outcome data were available only for nine lipid measures (no studies examined the ratio Apo B/Apo A-I) and only HDL cholesterol, TGs and TC had sufficient (more than three studies) to pool data. Outcome data were available for all 10 lipid measures for CVD mortality (adjusted), but Apo A-I, LDL/HDL cholesterol, Apo B and Apo B/Apo A-I data could not be pooled due to an insufficient number of studies. Data were available for four lipid measures for all-cause mortality (LDL, HDL, TC, TGs), all of which had sufficient studies to pool data. Finally, for all lipid measures bar non-HDL cholesterol, outcome data for all-cause mortality (adjusted) were provided, although only HDL cholesterol, LDL cholesterol, TC and TGs could be pooled as a result of insufficient studies.
Low-density lipoprotein measurement
Of the 59 studies that provided data for LDL, seven measured it directly [HPS,24 GRIPS,126 Health Professionals Follow-up Study (HPFS),130 KIHD,135 Nurses’ Health,147 SHS,160 Women’s Health172]; 34 studies (59%) estimated LDL cholesterol, of which 26 used the Friedewald formula,43 three studies stated that the measure was estimated but with no further information (SHEP,159 AMORIS,90 LRC prevalence140) and Northwick Park II146 estimated LDL using the Wallduis formula;173 the remaining 17 studies did not report whether or not LDL was measured or estimated.
Heterogeneity
Heterogeneity was generally high across all prevention groups, outcome data and lipid measures with few exceptions. For CVD events, both adjusted and unadjusted, the overall heterogeneity was considerable (I2 > 73%) for all lipid measures except Apo A-I in the unadjusted data (56%) and LDL/HDL cholesterol (55%) in the adjusted data where it was moderate. When further examined, the unadjusted CVD event data for primary prevention groups is considerably heterogeneous (77–97%) for all lipid measures except Apo A-I (5% based on five studies) and Apo B/Apo A-I (59% based on three studies). For adjusted CVD events outcome data in the primary prevention populations the heterogeneity is large (92–98%) for all lipid measures except LDL/HDL cholesterol were it is low (27%); though this is based on eight data sets, these were obtained from only three studies. There were insufficient data to examine heterogeneity in any of the lipid measures in the secondary prevention populations for CVD events adjusted outcome data. However, for these populations, in the unadjusted CVD events outcome data the heterogeneity was measurable in five out of the nine lipid measures and varied: LDL cholesterol was considerable (76%), TC, HDL cholesterol and TGs were moderate (34%, 52% and 63%) and for Apo B and Apo A-I there was no heterogeneity (0%). This was for the same two studies each (LIPID,138 Thrombo165). Heterogeneity for CVD mortality outcome data (unadjusted and adjusted) was substantial for all pooled lipid measures (67–91%). There was no heterogeneity statistic for secondary prevention populations owing to insufficient data. For the primary prevention groups in these data the heterogeneity ranged from 40% to 89%. Few lipid measures provided sufficient data for all-cause mortality and therefore assessment of heterogeneity was limited. For those that did, LDL cholesterol, TC, HDL cholesterol, TGs, heterogeneity for the summary estimate was moderate to considerable (52–95%), there were insufficient data to assess secondary prevention groups. All-cause mortality unadjusted data for primary prevention groups varied between two measures (LDL cholesterol, TC) showing considerable heterogeneity (> 95%) and two measures (HDL cholesterol and TGs) showing no heterogeneity (0%). Data from only two studies were available for each of these last two measures: of the two studies reporting HDL data, one study had 44,457 participants (AMORIS90) and the other 1013 (InCHIANTI131); for TGs, the participant numbers were 1013 (InCHIANTI131) and 2086 (Lan 2007136). Forest plots of all of these results appear in Appendix 9.
Figures 4–9 show the summaries of the overall results for all lipid measures for CVD events, CVD events (adjusted), CVD mortality, CVD mortality (adjusted), all-cause mortality and all-cause mortality (adjusted), respectively.
FIGURE 4.
Summary results (pooled estimates) by all lipid measures as prognostic markers for cardiovascular events in populations not taking statins. All data sets include primary prevention data sets, secondary prevention data sets, and data sets with mixed or unreported prevention groups. Heterogeneity: For all measures, for all data sets, I2 > 87% except for Apo A-I (I2 = 56%). For all measures for primary prevention groups, I2 = 77–97% except Apo A-I (5%) and Apo B/Apo A-I (59%). For secondary prevention groups, I2 for limited measures as follows: LDL (76%), TC (34%), TGs (63%), HDL (52%), Apo B (0%), Apo A-I (0%). Full details are available in Appendix 9. IV, inverse variance.
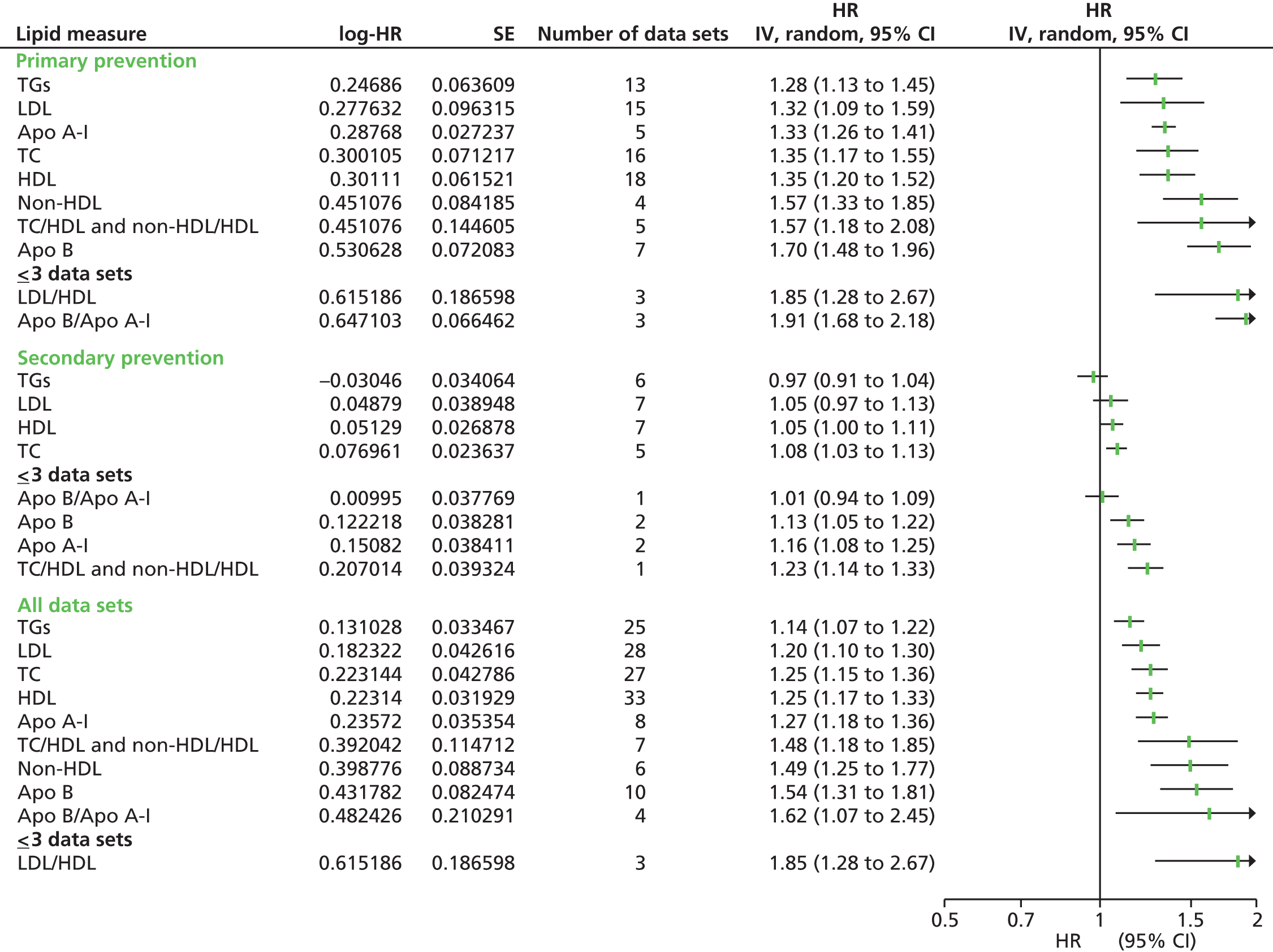
FIGURE 5.
Summary results (pooled estimates) by all lipid measures as prognostic markers for cardiovascular events (after adjustment) in populations not taking statins. All data sets include primary prevention data sets, secondary prevention data sets, and data sets with mixed or unreported prevention groups. Heterogeneity: For all measures, for all data sets, I2 = 73–98% except for LDL/HDL cholesterol (55%). For all measures for primary prevention groups, I2 = 92–98% except LDL/HDL cholesterol (27%). Unable to calculate heterogeneity for secondary prevention groups owing to insufficient data. Full details are available in Appendix 9. IV, inverse variance.

FIGURE 6.
Summary results (pooled estimates) by all lipid measures as prognostic markers for cardiovascular mortality in populations not taking statins. All data sets include primary prevention data sets, secondary prevention data sets, and data sets with mixed or unreported prevention groups. Heterogeneity: For limited measures, for all data sets, I2 as follows: TC (86%), HDL (67%), TGs (68%). For limited measures for primary prevention groups, I2 as follows: TC (85%), HDL (54%), TGs (40%). Unable to calculate heterogeneity for secondary prevention groups owing to lack of data. Full details are available in Appendix 9. IV, inverse variance.

FIGURE 7.
Summary results (pooled estimates) by all lipid measures, as prognostic markers for cardiovascular mortality (adjusted estimates) in populations not taking statins. All data sets include primary prevention data sets, secondary prevention data sets, and data sets with mixed or unreported prevention groups. Heterogeneity: For limited measures, for all data sets, I2 as follows: LDL (91%), TC (84%), HDL (71%), TGs (79%), non-HDL cholesterol (84%), TC/HDL (88%). For limited measures for primary prevention groups I2 as follows: LDL (84%), TC (70%), HDL (65%), TGs (84%), non-HDL cholesterol (89%), TC/HDL (52%). Unable to calculate heterogeneity for secondary prevention groups owing to insufficient data. Full details are available in Appendix 9. IV, inverse variance.
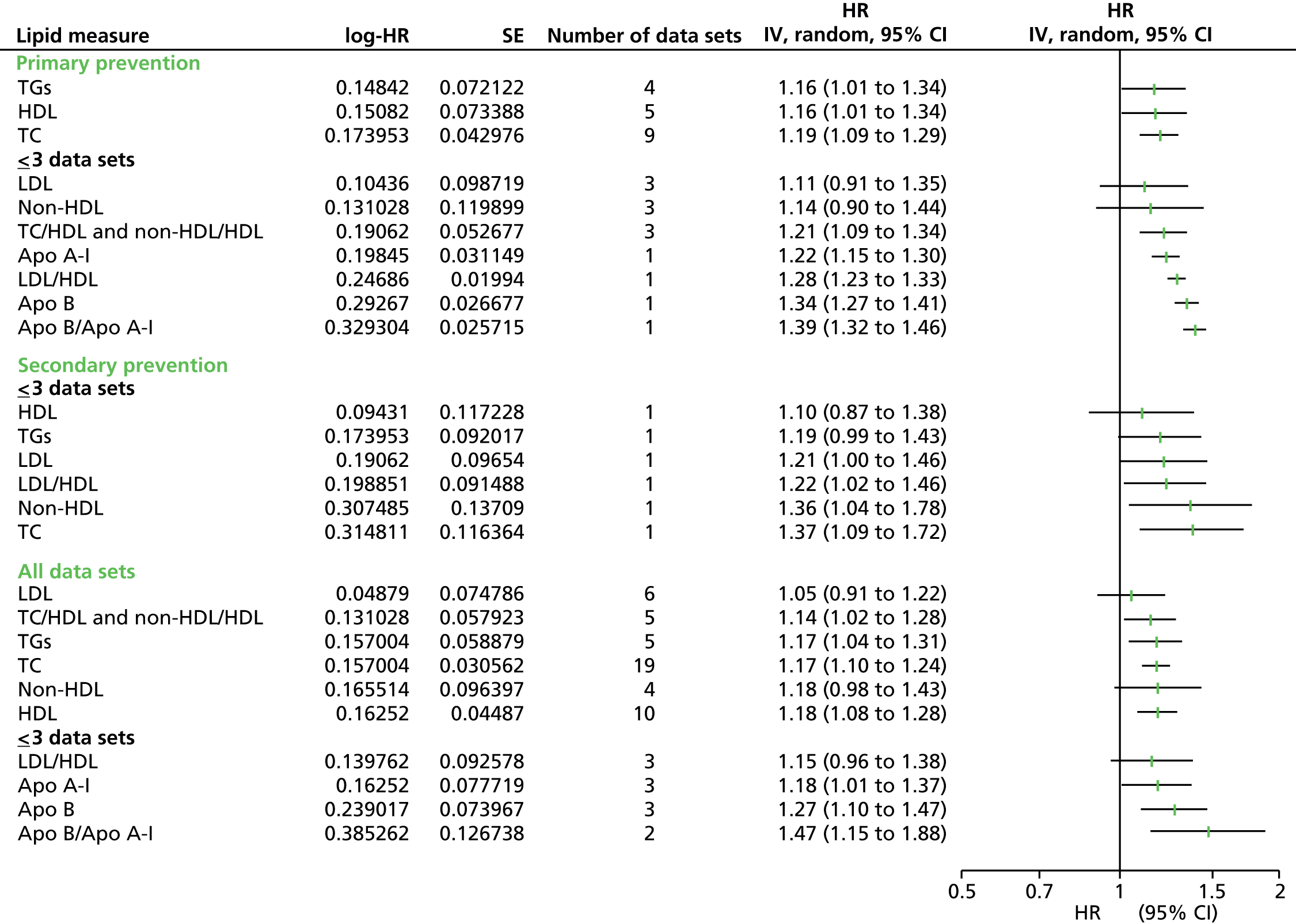
FIGURE 8.
Summary results (pooled estimates) by all lipid measures as prognostic markers for all-cause mortality in populations not taking statins. All data sets include primary prevention data sets, secondary prevention data sets, and data sets with mixed or unreported prevention groups. Heterogeneity: For limited measures, for all data sets, I2 as follows: LDL (92%), TC (95%), HDL (94%), TGs (52%). For limited measures for primary prevention groups I2 as follows: LDL (96%), TC (95%), HDL (0%), TGs (0%). Unable to calculate heterogeneity for secondary prevention groups owing to lack of data. Full details are available in Appendix 9. IV, inverse variance.

FIGURE 9.
Summary results (pooled estimates) by all lipid measures as prognostic markers for all-cause mortality (adjusted estimates) in populations not taking statins. All data sets include primary prevention data sets, secondary prevention data sets, and data sets with mixed or unreported prevention groups. Heterogeneity: For limited measures, for all data sets, I2 as follows: LDL (56%), TC (85%), HDL (53%), TGs (52%). For limited measures for primary prevention groups I2 as follows: TC (68%), TGs (56%). Unable to calculate heterogeneity for secondary prevention groups owing to lack of data. Full details are available in Appendix 9. IV, inverse variance.

For unadjusted estimates – CVD events:
-
When all of the data were combined for all of the lipid measures with more than three data sets, Apo B/Apo A-I (four studies: HR 1.62, 95% CI 1.07 to 2.45), followed by Apo B (10 studies: HR 1.54, 95% CI 1.31 to 1.81), non-HDL cholesterol (six studies: HR 1.49, 95% CI 1.25 to 1.77) and TC/HDL cholesterol (seven studies: HR 1.48, 95% CI 1.18 to 1.85) offer the highest predictive value.
-
For primary prevention populations, of the single measures Apo B (five studies: HR 1.70, 95% CI 1.48 to 1.96) and non-HDL cholesterol (four studies: HR 1.57, 95% CI 1.33 to 1.85), offer the strongest predictive value, whereas for the ratios, TC/HDL cholesterol (five studies: HR 1.57, 95% CI 1.18 to 2.08) offered the strongest value.
-
In the secondary prevention populations, only TC (five studies: HR 1.08, 95% CI 1.03 to 1.13) offers a significant association with CVD events.
For adjusted estimates – CVD events:
-
All lipid measures have data for this outcome. When all data are combined, Apo B/Apo A-I offers the strongest predictive value based on 16 sets of data (HR 1.35, 95% CI 1.22 to 1.50). The other two lipid ratios (TC/HDL cholesterol and LDL/HDL cholesterol), TC, Apo B and non-HDL cholesterol all have similar predictive values based on similar or more studies (HR range 1.22–1.28).
-
For primary prevention groups, the data follows the same pattern with Apo B/Apo A-I as the strongest predictor (HR 1.36, 95% CI 1.21 to 1.53). Of note in comparison with the unadjusted data, now Apo B/Apo A-I (14 studies) is the strongest predictor, followed by LDL/HDL cholesterol (eight studies: HR 1.29, 95% CI 1.27 to 1.32).
-
For secondary prevention there were insufficient study data to provide results.
For unadjusted estimates – CVD mortality:
-
The amount of evidence for CVD mortality is considerably smaller than for CVD events particularly in relation to lipid ratios.
-
Only three lipid measures had sufficient data to meta-analyse (HDL, TGs, TC).
-
HDL cholesterol offered the strongest predictive value for combined data (six studies: HR 1.28, 95% CI 1.11 to 1.48) with TC and TGs providing estimates of similar magnitude (combined: TC HR 1.14, 95% CI 1.14 to 1.42; five studies: TGs HR 1.24, 95% CI 1.08 to 1.42).
-
In the primary prevention group, TGs offered the strongest predictive value (four studies: HR 1.30, 95% CI 1.14 to 1.49), but, as with the combined data, TC and HDL cholesterol have estimates of a similar magnitude (six studies: TC HR 1.27, 95% CI 1.01 to 1.60; four studies: HDL cholesterol HR 1.27, 95% CI 1.06 to 1.51).
-
There were no data for secondary prevention populations.
For adjusted estimates – CVD mortality:
-
Similar to the unadjusted data, the amount of evidence for CVD mortality (adjusted) is considerably smaller than for CVD events particularly in relation to lipid ratios.
-
For all studies combined HDL (10 studies: HR 1.18, 95% CI 1.08 to 1.28), TC (19 studies: HR 1.17, 95% CI 1.10 to 1.24) and TGs (five studies: HR 1.17, 95% CI 1.04 to 1.31) offered the strongest predictive values.
-
For the primary prevention group only HDL (five studies: HR 1.16, 95% CI 1.01 to 1.34) and TGs (four studies: HR 1.16, 95% CI 1.01 to 1.34) showed an association with adjusted CVD mortality data.
-
Data were available for secondary prevention groups but with insufficient study numbers to obtain pooled results.
For unadjusted estimates – all-cause mortality:
-
There were data for only four measures (HDL cholesterol, TC, LDL cholesterol, TGs).
-
The associations followed a different pattern to that observed for CVD events and mortality (except for HDL cholesterol and TG).
-
For all studies combined, only HDL cholesterol (nine studies: HR 1.25, 95% CI 1.10 to 1.43) and TGs (eight studies: HR 1.09, 95% CI 1.04 to 1.15) showed an association with all-cause mortality.
-
For primary prevention populations there were insufficient data to provide results. There were no data for secondary prevention populations.
For adjusted estimates – all-cause mortality:
-
Lipid measure data were available for all measures, except for non-HDL cholesterol, but in a small number of studies.
-
TC (19 studies) was the only lipid measure reported in more than six studies.
-
For all studies combined, only TGs (five studies: HR 1.09, 95% CI 1.06 to 1.13) and TC (19 studies, HR 1.05, 95% CI 1.02 to 1.09) showed an association with all-cause mortality (adjusted).
-
For primary prevention groups there was no evidence of association or insufficient data.
-
There were no data for secondary prevention populations.
Subgroup analyses
Pre-existing conditions
Within the 90 included studies (see Appendix 8), 14 studies examined populations with pre-existing conditions at baseline. Ten studies were on diabetic populations: four of these providing outcome data for CVD events [CARDS (Collaborative atorvastatin diabetes study);115 ASCOT-LLA (Anglo Scandinavian cardiac outcomes trial – lipid-lowering arm);109 Ting 2010;166 DAI120)], five of these provided data for CVD events (adjusted) (HPFS;130 Ting 2010;166 FIELD;121 SHS;160 Swedish NDR164), Casale Monferrato116 provided data for CVD mortality (adjusted) and MHS143 contributed data to all-cause mortality (adjusted). Subgroup analyses were completed for CVD events (unadjusted and adjusted), but not for CVD mortality (adjusted) or all-cause mortality owing to insufficient data.
Subgroup analyses to examine the results for non-diabetic populations compared with diabetic populations are discussed and presented in Appendix 10. Although, in general, there were insufficient data in the diabetic population, for either prevention group, to compare results with the non-diabetic population, generally lipids were less predictive of CVD events in the diabetic populations than non-diabetic populations.
Two studies were either in the latter stages of renal disease (AURORA110) or post renal transplant (ALERT107); because of lack of study numbers, no subgroup analysis was completed for these baseline comorbidities.
Study design and age
Of the 90 studies included (see Appendix 8), only three studies133,161,163 were retrospective (Swedish NDR;164 Stanek 2007;162 Juntendo, Japan134), which meant that there were insufficient studies to compare study design, and there were insufficient studies with populations aged < 40 years to compare the effect of subgroup by age. All studies reported participants as > 40 years except for four studies (CB project,91 Yao City,89 LRC prevalence,140 RIFLE158).
Sensitivity analyses
A full discussion of the results of the sensitivity analyses and forest plots is presented in Appendix 10.
Surrogate measures for cardiovascular disease
A total of 61% of studies used CHD as a surrogate measure for CVD events (both adjusted and unadjusted data). Once these were removed, data were no longer sufficient to obtain pooled estimates for all lipid measures, and for those with sufficient data the results showed the same overall trends. Within the CVD mortality outcome data, 52% of the studies used a surrogate measure for cardiovascular risk. Similar to CVD events, this meant that, for most lipid measures, insufficient data were available to obtain pooled estimates and with little difference in the associations in those with enough data.
Study quality
Study quality was examined by placing emphasis on how the prognostic markers were measured and levels of attrition. No studies were assessed as being at high risk of attrition bias and 29% of studies were judged to be at moderate risk. With regard to bias due to prognostic marker measurement 38 studies were assessed to be at high risk, 21 at moderate risk and 31 at low risk of bias. Removal of all studies at moderate or high risk in either of these domains left 23 studies with fewer or no data available for pooled analysis and small changes to the associations observed in those that were obtained.
Laboratory methods
Ideally, blood samples would have been analysed in an accredited central laboratory for each study. In fact, 54% of studies completed the blood analysis in a central laboratory, with 57% of these reporting the laboratory as accredited; 37 studies did not report where the blood samples were analysed. Only three studies reported not using a central laboratory (DAI,120 Swedish NDR,164 RIFLE158). Sensitivity analyses were completed to remove all studies not reporting whether or not a central laboratory was used, and where a central laboratory was stated as not used. No major differences were observed with the primary analyses in the reduced lipid measures for which estimates were obtained.
Indirect data extraction
Sensitivity analysis was completed to examine whether or not the method of data extraction had any bearing on the results. Data were extracted directly from 53% of studies. For the remaining 42 studies, data were extracted indirectly using simulation or generalised least squares for trend methods. The only observed difference for the lipid measures for which pooled estimates were obtained was that for CVD events in the primary prevention group, and the overall group, LDL cholesterol now showed no association.
Use of lipid modification therapy
Finally, sensitivity analyses were completed to assess if contamination of the population by partial use of lipid-lowering medication would affect the results. Eighteen studies confirmed statin or lipid-lowering usage either at baseline or during the course of the studies. Usage ranged from 0.5% to 38% (seven studies < 5% usage and in Framingham Offspring53 the usage level was not confirmed). Removal of these studies made only marginal differences to the pooled estimates.
Studies based on populations taking statins
The study selection process is summarised in Figure 10. In total, 2260 publications from the non-RCT search and 667 publications from the RCT search were screened, reducing to 25 included studies, with 28 sets of data meeting the inclusion criteria and providing results. Three studies (J-LIT,174,175 Phase Z176 and SEARCH58) offered two sets of data; two studies had two intervention arms with different statin doses (Phase Z176 and SEARCH58). In addition, one study stratified results into separate primary and secondary prevention groups (J-LIT174,175).
FIGURE 10.
Flow chart of study inclusion for populations taking statins. CTT, Cholesterol Treatment Trialists’ Collaboration.
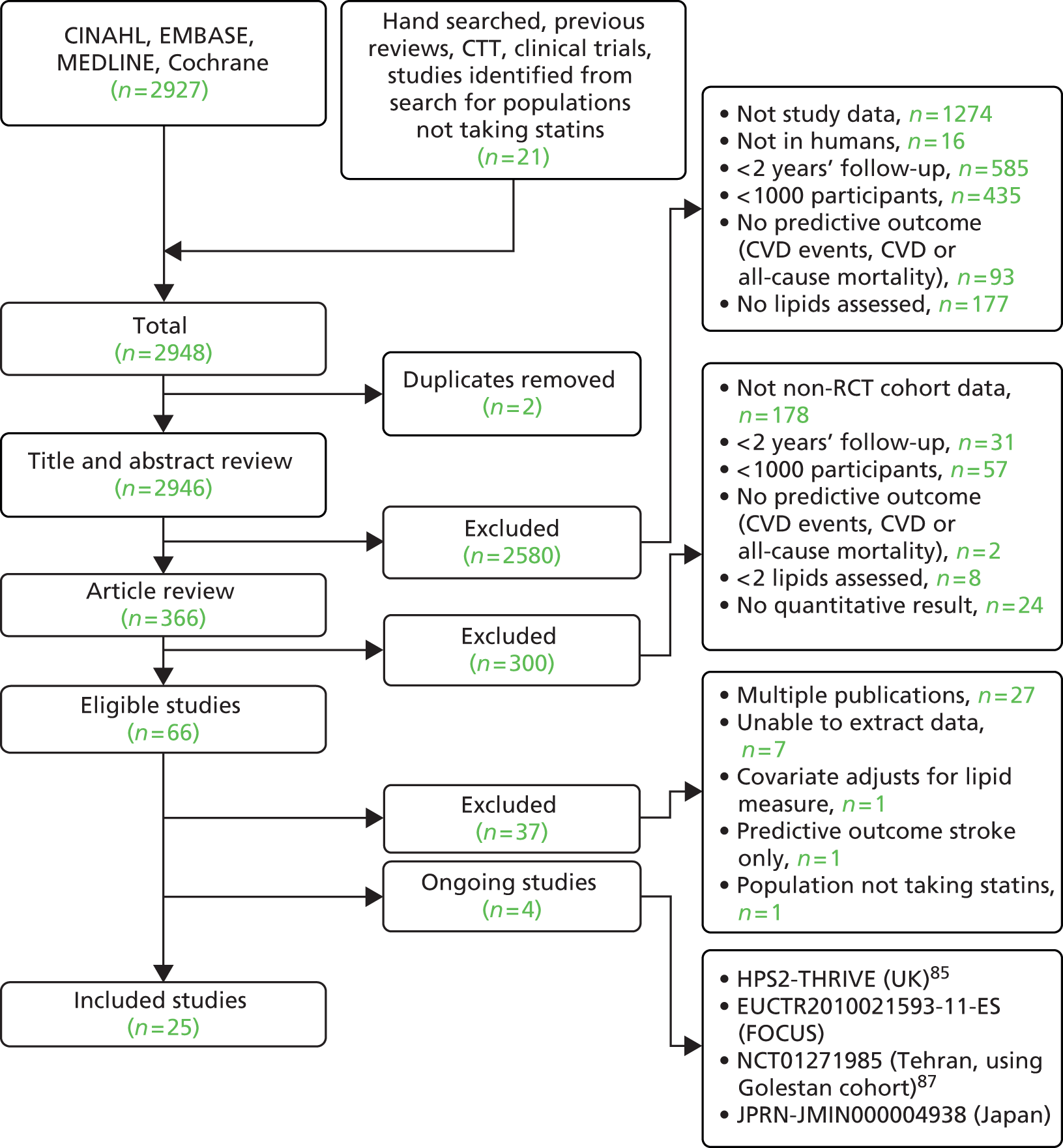
The characteristics of the included studies are shown in Table 10. Of the 25 studies, only three were non-RCTs (CLIP,177 LIVES Ex,182 J-LIT174,175), one was a nested case control from a RCT (TNT185) and the rest were RCTs. Studies provided data for between one and seven lipid measures, and no single study examined all markers. No data were available for LDL/HDL cholesterol. No results were available for CVD mortality or all-cause mortality. Full details of the data can be seen in Appendix 11. Table 11 shows the number of studies and participants by lipid measure. Outcome data per lipid measure were separated into unadjusted CVD events (CVD events) and adjusted CVD events [CVD events (adjusted)]. Only half of the reported combinations of lipid measures and outcomes could be pooled using meta-analysis. Individual forest plots for these measures are presented in Appendix 12. The remaining data had fewer studies than our threshold for meta-analysis; however, a pooled estimate was obtained for all lipid measure summaries, but meta-analyses have not been presented.
Study name | Study type | No. of participants | Mean age (years) | Gender (% female) | Underlying condition | Primary prevention (%) | Statin | Statin dose | Fasting assay | Central laboratory | Direct or estimated LDL (N/A if LDL not measured) | Mean follow-up (years) |
---|---|---|---|---|---|---|---|---|---|---|---|---|
4S18 | RCT | 2221 | 58.6a | 18 | NS | 0 | Simvastatin | 20–40 mg maximum | NS | Y | Estimated (Friedewald) | 5.4 |
AF/TexCAPS106 | RCT | 3304 | 58 | 18 | N | 100 | Lovastatin | 20–40 mg maximum | NS | Y (accredited) | Estimated (Friedewald) | 5.2 |
ALERT107 | RCT | 1050 | 50 | 33 | Renal or combined renal and pancreas transplants > 6 months and stable graft function | 97 | Fluvastatin | 40 mg | F | Y | NS | 5.1 |
ASCOT-LLA109 | RCT | 1258 | 64 | 23 | Diabetes (II) | 100 | Atorvastatin | 10 mg | NF | Y | NS | 3.3b |
AURORA110 | RCT | 1389 | 64 | 39 | End-stage renal failure/haemodialysis for 3 months | 40 | Pravastatin | 10 mg | F | Y (accredited) | NS | 3.2 |
CARDS115 | RCT | 1428 | 62 | 32 | Diabetes (II) | 100 | Atorvastatin | 10 mg | F | Y (accredited) | Estimated (Friedewald) | 4 |
CARE34 | RCT | 2081 | 59 | 14 | N | 0 | Pravastatin | 40 mg | F | Y (accredited) | Estimated (Friedewald) | 5b |
CLIP177 | Prospective cohort | 3249 | 59 | 69 | N | 100 | Pravastatin | 10–20 mg | F | N | Estimated (Friedewald) | 58 |
CORONA119 | RCT | 2514 | 73 | 24 | N | 0 | Rosuvastatin | 10 mg | NF | Y | NS | 2.73b |
dal-OUTCOMES178 | RCT | 7933 | 60 | 19 | N | 0 | Statin (NS) | NS | F | Y (accredited) | NS | 2.58 |
GISSI123 | RCT | 2138 | 58 | 13 | N | 0 | Pravastatin | 20 mg | F | Y (accredited) | NS | 2.1 |
HPS24 | RCT | 10,269 | 64a | 28 | N | 16.1 | Simvastatin | 40 mg | NF | Y | Direct | 4.8 |
IDEAL179 | RCT | 8888 | 61 | 19 | N | 0 | Atorvastatin and simvastatin | A = 80 mg, S = 20 mg | F | Y | Estimated (Friedewald) | 4.8b |
JELIS180 | RCT | 5806 | NS | NS | N | 100 | Pravastatin or simvastatin | P = 10 mg, S = 5 mg | F | Y | NS | 4.6 |
J-LIT Primary174 | Prospective cohort | 42,360 | 58 | 68 | N | 100 | Simvastatin | 5–10 mg | F | N (quality tested and no differences) | Estimated (Friedewald) | 5.39 |
J-LIT Secondary175 | Prospective cohort | 51,247 | 60 | 58 | N | 0 | Simvastatin | 5–10 mg | F | N (quality tested and no differences) | Estimated (Friedewald) | 5.39 |
JUPITER181 | RCT | 7832 | 66b | 38.5 | NS | 100 | Rosuvastatin | 20 mg | NS | Y | NS | 1.9b |
LIPID138 | RCT | 4512 | 62b | 17 | N | 0 | Pravastatin | 40 mg | F | Y | Estimated (Friedewald) | 6.1 |
LIVES Ex182 | Prospective cohort | 6582 | 64 | 69 | N | 89 | Pitavastatin | 1–4 mg | NS | NS | Estimated (Friedewald) | 5.31 |
Phase A-Z 20176 | RCT | 2265 | 61b | 24 | N | 0 | Simvastatin | 40 mg/day followed by 80 mg/day | NS | Y | NS | 1.98b |
Phase A-Z 40/80176 | RCT | 2232 | 61b | 25 | N | 0 | Simvastatin | Placebo followed by 20 mg/day | NS | Y | NS | 1.98b |
PROSPER155 | RCT | 2891 | 75 | 52 | N | 54 | Pravastatin | 40 mg | F | Y (accredited) | NS | 3.2 |
PROVE-IT183 | RCT | 3683 | 58 | 22 | N | 0 | Atorvastatin and pravastatin | A = 80 mg, P = 40 mg | NS | Y (accredited) | NS | 2 |
SEARCH 2058 | RCT | 6033 | 64 | Mixed | NS | 0 | Simvastatin | 20 mg | NS | Y | Direct | 6.7 |
SEARCH 8058 | RCT | 6031 | 64 | Mixed | NS | 0 | Simvastatin | 80 mg | NS | Y | Direct | 6.7 |
SPARCL184 | RCT | 2366 | 63 | 39 | NS | 0 | Atorvastatin | 80 mg | F | Y | NS | 4.9b |
TNT185 | Nested case–control (RCT) | 1527 | 61a | 17 | NS | 0 | Atorvastatin | 10 mg n = 810, 80 mg n = 717 | Y | NS | NS | 4.9 |
WOSCOPS19 | RCT | 3302 | 55 | 0 | N | 100 | Pravastatin | 40 mg | F | Central accredited | NS | 4.9 |
Lipid measure | Outcome | Number of primary prevention studies | Number of secondary prevention studies | Overall number of studies | Number of participants |
---|---|---|---|---|---|
LDL | CVD events | 7 | 14 | 25 | 96,978 |
CVD events (adjusted) | 2 | 2 | 5 | 110,507 | |
TC | CVD events | 4 | 6 | 11 | 41,620 |
CVD events (adjusted) | 2 | 2 | 4 | 103,925 | |
HDL | CVD events | 7 | 10 | 20 | 72,126 |
CVD events (adjusted) | 2 | 2 | 5 | 112,577 | |
TGs | CVD events | 5 | 5 | 11 | 33,328 |
CVD events (adjusted) | 1 | 2 | 3 | 98,119 | |
Non-HDL | CVD events | 1 | 4 | 5 | 24,990 |
CVD events (adjusted) | 1 | 0 | 1 | 5806 | |
Apo B | CVD events | 1 | 5 | 6 | 33,752 |
CVD events (adjusted) | 0 | 1 | 1 | 4512 | |
Apo A-I | CVD events | 0 | 1 | 1 | 4512 |
CVD events (adjusted) | 0 | 1 | 1 | 4512 | |
Apo B/Apo A-I | CVD events | 0 | 2 | 2 | 6197 |
CVD events (adjusted) | No data | ||||
TC/HDL cholesterol and non-HDL cholesterol/HDL cholesterol merged | CVD events | 0 | 2 | 2 | 8195 |
CVD events (adjusted) | 0 | 1 | 1 | 4512 |
Low-density lipoprotein measurement
Only two studies (SEARCH,58 HPS24) measured LDL directly; half of the remaining studies estimated LDL using the Friedewald equation43 and half did not report the method used.
Heterogeneity
Across both prevention groups, heterogeneity was substantial for all but one lipid measure (non-HDL cholesterol). Only LDL cholesterol (CVD events) showed moderate heterogeneity for primary prevention populations and non-HDL cholesterol (CVD events) for secondary prevention populations.
Figure 11 shows a summary of the overall results for all lipid measures for CVD events, whereas Figure 12 shows the same, for adjusted estimates.
FIGURE 11.
Summary results (pooled estimates) by all lipid measures as prognostic markers for cardiovascular events in populations taking statins. All data sets include primary prevention data sets, secondary prevention data sets, and data sets with mixed or unreported prevention groups. Heterogeneity: For limited measures for all data sets I2 as follows: TC (74%), LDL (84%), HDL (88%), TGs (82%), non-HDL cholesterol (34%), Apo B (83%). For limited measures for primary prevention groups I2 as follows: TC (64%), LDL (33%), HDL (88%), TGs (60%). For limited measures for secondary prevention groups I2 as follows: TC (81%), LDL (84%), HDL (80%), TGs (85%), non-HDL cholesterol (49%), Apo B (86%). Full details are available in Appendix 12. IV, inverse variance.
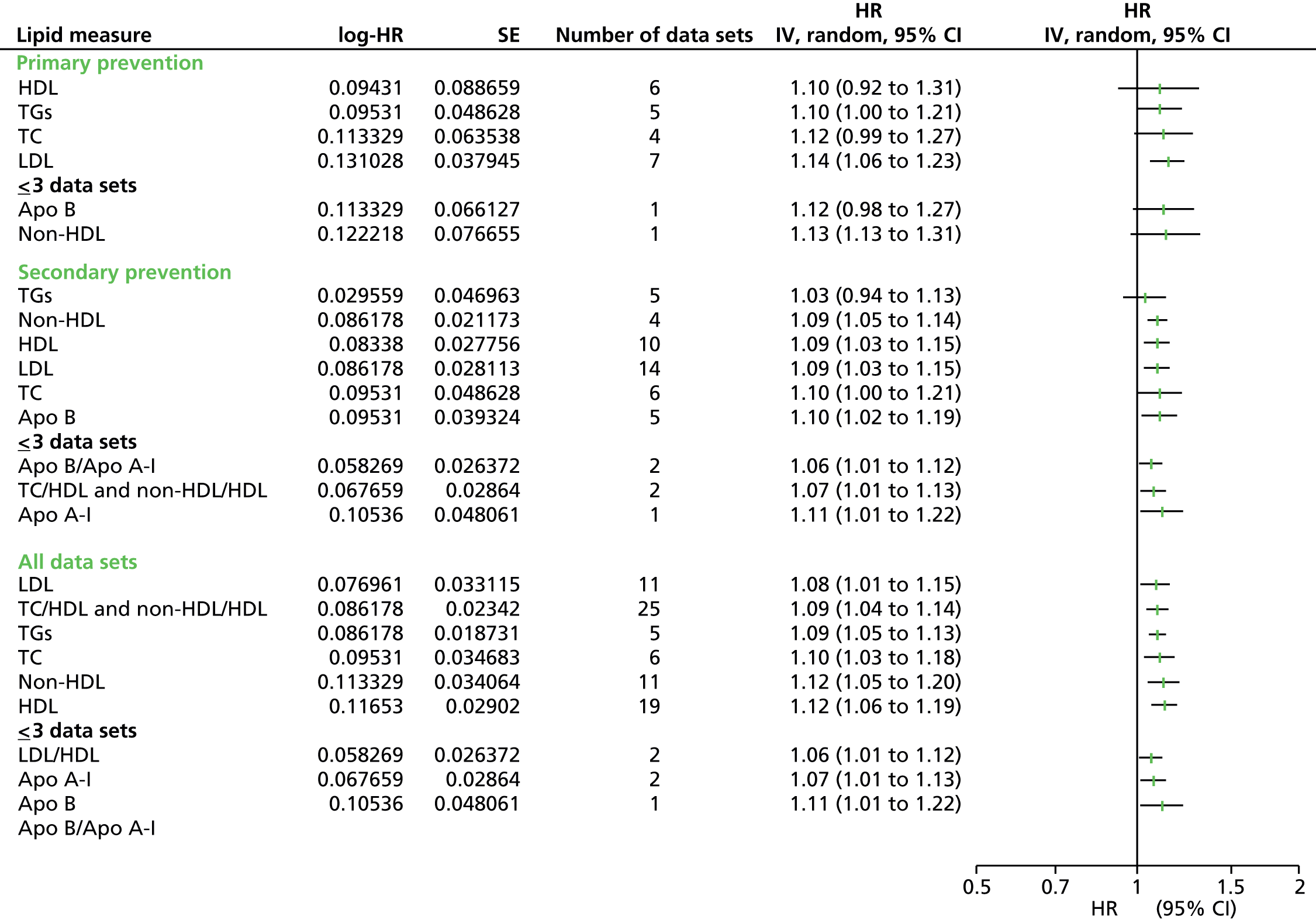
FIGURE 12.
Summary results (pooled estimates) by all lipid measures as prognostic markers for cardiovascular events (adjusted estimates) in populations taking statins. All data sets include primary prevention data sets, secondary prevention data sets, and data sets with mixed or unreported prevention groups. Heterogeneity: For limited measures for all data sets I2 as follows: TC (78%), LDL (94%), HDL (89%). For limited measures for primary prevention groups I2 as follows: TC (92%), LDL (95%), HDL (85%). For limited measures for secondary prevention groups I2 as follows: TC (0%), LDL (81%), HDL (84%). Full details are available in Appendix 12. IV, inverse variance.

For unadjusted estimates – CVD events:
-
Overall HDL (19 studies: HR 1.12, CI 1.06 to 1.19) and TC (11 studies: HR 1.12, CI 1.05 to 1.20) offered the strongest association with CVD events.
-
For primary prevention populations, LDL cholesterol (seven studies: HR 1.14, CI 1.06 to 1.23) offered the strongest predictive value. All other measures have a similar point estimate but are not significant.
-
For secondary prevention groups LDL, Apo B, non-HDL cholesterol and HDL offered a similar predictive value (HR range 1.09–1.10).
For adjusted estimates – CVD events:
-
Overall HDL (five studies: HR 1.61, CI 1.19 to 2.18) offered the strongest association with CVD events.
-
For both primary and secondary prevention groups there were insufficient data to form conclusions.
Subgroup analyses
Pre-existing conditions
There were four studies whose participants had underlying conditions at baseline: two diabetic populations (ASCOT-LLA,109 CARDS115) and two studies were either in the latter stages of renal disease (AURORA110) or post renal transplant (ALERT107). Owing to insufficient data, no subgroup analyses could be completed either for diabetic populations compared with non-diabetic populations or for renal disease populations compared with populations not suffering from renal disease. In a sensitivity analysis, removing these four studies from the analysis had no effect on the predictive value of each lipid measure for CVD events (unadjusted or adjusted).
Study design and age
No retrospective studies meant that no subgroup comparison was made for study design. All study populations were aged > 40 years at baseline.
Sensitivity analyses
Surrogate measures for cardiovascular disease
Definitions for cardiovascular events varied considerably; 7 out of 25 studies used CHD as a surrogate measure for predicting cardiovascular risk. Appendix 13 shows a sensitivity analysis comparing all of the pooled estimates for lipid measures removing the studies that used a surrogate measure. For the CVD events the summary measures followed the same trends as the main summaries, except for HDL which was no longer a strong predictor reducing from HR 1.12 to 1.01. There were no longer sufficient data to show results for the primary prevention group; in the secondary prevention group the results were the same. There were data for only HDL cholesterol and LDL cholesterol for CVD events (adjusted) and these measures had insufficient data.
Study quality
Studies were assessed for quality with emphasis placed on attrition and prognostic markers (lipid measures). Agreement between the two assessors was good (attrition: κ = 0.76) and very good (prognostic marker: κ = 0.84). Only two studies were assessed as high risk in either category (CLIP,177 PROVE-IT183). Removing these two studies from the analysis made no difference to the results. Removing all studies that were assessed as moderate or high risk in either category left five studies for consideration (CARDS,115 AURORA,110 CORONA,119 PROSPER,155 ALERT107). Again, this made no difference to the results, although the predictive value for LDL was no longer significant in the primary prevention group or overall.
Laboratory methods
All studies, bar four, completed the analysis of blood samples in a central laboratory. Two of these did not report whether or not a central laboratory was used (LIVES Ex,182 TNT183) and two studies did not use a central laboratory (CLIP,177 J-LIT174,175), although J-LIT174,175 did test for inequalities between laboratories and found no differences. No sensitivity analyses were completed owing to insufficient data.
Indirect data extraction
Finally, sensitivity analysis was conducted to examine whether or not the method of data extraction had any bearing on the results (see Appendix 13). Analysis was conducted on nine studies after removing all of the studies for which the data were extracted indirectly. For CVD events (unadjusted) in the secondary prevention groups and in all studies, only Apo B and LDL cholesterol continued to have sufficient data to provide results. The pooled estimate for Apo B was very similar but the estimate for LDL was increased (secondary prevention: HR increase from 1.09 to 1.17, all studies: HR 1.09 to 1.16). There were insufficient data to assess the impact of indirect data extraction on adjusted CVD events outcome data.
Excluded studies for both populations are shown in Appendix 14.
Post hoc meta-regression
Meta-regression was completed for CVD events (adjusted estimates) in populations not taking statins; this was the only outcome with data for all 10 lipid measures. However, none of the 35 studies contributed data for all lipid measures. Owing to the lack of data for each lipid measure, only three variables were examined in the meta-regression: diabetic population at baseline, use of a surrogate measure for CVD events and other baseline underlying conditions, as the interpretation of the effect of these variables could be of clinical relevance. Results of the meta-regression can be seen in Appendix 15.
Meta-regression was conducted for nine lipid measures as the LDL/HDL cholesterol ratio was reported in only eight data sets (three studies: AMORIS,90 EPIC-Norfolk100 and Framingham Offspring53). For LDL cholesterol and TGs, the underlying condition variable was excluded because of collinearity; for Apo A-I and Apo-B/Apo A-I, the diabetes variable was excluded for the same reason. The meta-regression for LDL cholesterol, HDL cholesterol, TGs, Apo B and Apo A-I found no effect for any of the three variables. Of the remaining measures, TC, non-HDL cholesterol, Apo B/Apo A-I, and TC/HDL cholesterol showed stronger associations with outcome in studies for which we extracted a surrogate, such as CHD events, rather than CVD events (p = 0.003, p = 0.007, p < 0.001 and p = 0.026, respectively).
Discussion
This is the first review designed to determine the predictive value of a panel of 10 lipid measures for cardiovascular events, cardiovascular mortality and all-cause mortality. It is the first review to include all populations taking, and not taking, statins, and to further divide that population into primary and secondary prevention groups.
Key finding for populations not taking statins
Results from the included studies in this population support Apo B/Apo A-I as the strongest predictor of CVD events (adjusted and unadjusted). Apo B, non-HDL cholesterol, LDL/HDL cholesterol and TC/HDL cholesterol offer similar predictive values and only slightly lower point estimates than Apo B/Apo A-I. However, all of the lipid measures offer positive associations with CVD events and there is considerable overlap in the CIs. This pattern is repeated when considering only primary prevention populations. There were insufficient data sets to pool data for Apo B/Apo A-I and LDL/HDL cholesterol for unadjusted data, but the limited data available do suggest these to be the strongest predictors, and this is reflected in the adjusted data. Although the number of studies were insufficient to pool data for Apo B/Apo A-I, the numbers of participants were comparable to those in the data for TC/HDL cholesterol and non-HDL cholesterol. Data for the secondary prevention group were very limited, but did appear to follow a similar trend, except for TGs, which appeared to have no association with CVD events.
The results from this review are in line with the latest research by the ERFC70 and the 2011 review by Sniderman et al. 71 The ERFC70 examined the association among non-HDL cholesterol, Apo B, Apo A-I, TC/HDL cholesterol and Apo B/Apo A-I and CVD (adjusted outcome data) in 26 studies (primary and secondary prevention groups). The results in this review are almost identical for non-HDL cholesterol, Apo B, and Apo A-I, but the CIs are slightly narrower. Both reviews have found that the ratios TC/HDL cholesterol and Apo B/Apo A-I were more strongly associated with CVD events than other lipid measures. The ERFC’s final conclusion was that TC/HDL cholesterol offers a stronger association with CVD events than the individual measures Apo B and Apo A-I; our review agrees with these findings. However, the ERFC70 did not include LDL/HDL cholesterol in their assessment and our review suggests that this is almost as predictive as Apo B/Apo A-I. Sniderman et al. 71 only considered three lipid measures and their association with CHD. Although our outcome definition differs, results were similar, suggesting that Apo B is superior to non-HDL cholesterol and non-HDL cholesterol is superior to LDL in predicting events. Examination of diabetic populations not taking statins was limited by the lack of data. However, there is some indication that for CVD events (unadjusted) the lipid measures examined provide lower predictive values or no association for this population. Within the adjusted data, more lipid measures were represented, but trends were not clear: most, but not all, lipids were less predictive of CVD events in the diabetic populations than non-diabetic populations.
For CVD mortality (unadjusted and adjusted), HDL offered the strongest association with CVD mortality, but the CIs overlapped with those for TC and TGs. In the unadjusted primary prevention group the same three lipid measures showed an association, with overlapping CIs, but here TGs was the strongest, whereas in the adjusted primary prevention group TC no longer had an association, and HDL and TGs had exactly the same results. However, in the adjusted data, five other lipid measures (TC/HDL cholesterol, LDL/HDL cholesterol, LDL cholesterol, Apo A-I, Apo B) offered an indication that they could provide a similar, or stronger, predictive value in primary prevention groups and for all participants, if more study data were available.
The Asia Pacific Studies Collaboration (APSC)67 assessed 17 studies, and examined the association between seven lipid measures (TC, TGs, HDL cholesterol, non-HDL cholesterol, TC/HDL cholesterol, TG/HDL cholesterol) and CVD mortality, and the association between LDL cholesterol and CHD. For the lipid measures for which we were able to pool data in our review (TC, TG, HDL), the HRs were only slightly greater than the APSC results,67 and, similar to our review, the CIs in their data all overlapped. So, although the strength of our associations was slightly larger, there was similarity in the pattern of results. Results for TG, TC/HDL cholesterol and TG/HDL cholesterol were presented as log results, and therefore were not comparable, and we had no results for non-HDL cholesterol and CVD mortality (unadjusted data) in our review.
Post hoc meta-regression showed an association only between CVD events (adjusted) and using a surrogate measure for CVD events for four lipid measures (TC, non-HDL cholesterol, Apo B/Apo A-I, TC/HDL cholesterol). The analysis suggests that including the surrogate measure of CHD could potentially lead to an overestimation of the HR for CVD events (adjusted) by approximately 20%.
From the limited outcome data available for all-cause mortality (unadjusted and adjusted), only two lipid measures had an association. For unadjusted data, TGs had a stronger association, whereas for adjusted data, HDL cholesterol was more strongly associated. Although there were insufficient studies to analyse Apo B, Apo A-I and their ratio, these could potentially offer a stronger predictive value. Unexpectedly, higher levels of some lipid measures (LDL cholesterol, TC, LDL/HDL cholesterol) were associated with a lower risk of all-cause mortality. The level of comorbidities in these populations might account for this result. The IKNS study in Yao City94 concluded that the decreased association with mortality was stronger in heavy drinkers and more significant in follow-up over 5 years. There is no existing review to compare results for all-cause mortality.
Key finding for populations taking statins
For all included studies, HDL and TC offered the strongest association with CVD events (unadjusted) and HDL cholesterol with adjusted data. For unadjusted data, only LDL cholesterol offered an association for primary preventions groups, whereas for secondary preventions groups Apo B, TC, LDL cholesterol, non-HDL cholesterol and HDL cholesterol all had a similar association. For adjusted data, although there were insufficient studies to produce combined results, there was some indication that HDL cholesterol for primary prevention groups and TC for secondary prevention groups could offer strong associations with CVD events.
Boekholdt et al. 72 completed an IPD systematic review using adjusted data from eight studies to assess the association between LDL cholesterol, non-HDL cholesterol, and Apo B and CVD events. In comparison, although 25 studies contributed data to this review, the maximum numbers of studies contributing data to any single measure in our comparable analysis was five. Comparison with the work of Boekholdt et al. 72 was only partially possible. This review’s LDL cholesterol point estimate was higher than the estimate of Boekholdt et al. 72 and not significant; for both Apo B and HDL cholesterol our point estimates were higher, with large CIs and based on only one included study. Boekholdt et al. 72 concluded that there was a stronger association for non-HDL cholesterol with CVD events than LDL and Apo B; this review suggests agreement with this finding but does not have sufficient evidence to confirm it. However, Boekholdt et al. 72 did not assess HDL cholesterol in his research, and this review would suggest that HDL cholesterol has a higher predictive value than non-HDL cholesterol, LDL cholesterol or Apo B for CVD events in populations taking statins.
No outcome data for CVD mortality or all-cause mortality were available for this group.
The lipid measures with the higher associations with CVD events and CVD mortality are consistent with current NICE recommendations for monitoring. 2,62 Our results suggest that alternative measures such as Apo B or the ratios LDL/HDL cholesterol and Apo B/Apo A-I, could be given further consideration in the future based on their clinical validity; however, in 2014 these were unlikely to be affordable or practical.
Strengths and limitations
The main strength of this review is its extensive coverage of all study designs, populations taking and not taking statins, and the wide panel of 10 lipid measures. The review has subgrouped populations into primary and prevention groups to seek to understand whether or not the predictive ability of various lipids varies according to these populations. Previous reviews in this area often look at only a subset of lipid measures, which complicates comparisons and the development of clinical recommendations. There is a tendency for reviews to exclude studies if they do not have data for all of the lipids being assessed in the review. In this review we have included study data even if they contributed to only some of the lipid measures or outcomes under consideration. We have extracted data from publications using indirect methods, as well as direct and converted data.
To complete a review of the scale and complexity of this one, it is required to make decisions about the scope and focus that impact on study identification and selection. Although other lipid-lowering drug groups could have been included in this review (such as fibrates), together with the advisory board we decided to focus only on statins. It is possible that by searching from the dates of previous reviews and modifying the search strategy, studies may have been missed; however, as we have aimed to include all studies from previous reviews and larger trials of > 1000 participants, we believe it is unlikely that large relevant studies have been overlooked. From the existing reviews we were unable to trace 15 potential studies, accounting for 10% of our final sample. This could have been because studies were mentioned as joining collaborations, but the studies never completed or the findings were not published. Despite the large number of included studies in this review, the number of studies reporting data for each pooled estimate is much smaller. This is typical of prognostic reviews in the area; for example, the ERFC used only 68 out of a potential 112 studies. 69
Many included studies did not disclose details such as whether or not the participants had pre-existing CVD or whether or not the participants were taking statins. Assumptions have been made in the data analysis to accommodate these studies, and sensitivity analyses were conducted to account for these assumptions.
No outcome data for CVD mortality or for all-cause mortality were available in populations taking statins, and data for these outcomes were provided only in a smaller proportion of studies of populations not taking statins, compared with CVD events. As expected, heterogeneity was high for most analyses, and contributed to our decision to use a random-effects model for the pooled estimates. The populations included in the studies were hugely varied, with no specific inclusion criteria relating to baseline characteristics. Furthermore, it was expected that there would be significant variation in the way the lipid measures were tested. The strength of predictive value of the lipid measures was largely based on the point estimates, but it should be noted that the CIs were often overlapping and the degree of difference between lipid measures was on the whole small. In meta-regression we did not find any measures that considerably reduced heterogeneity.
All estimates were obtained independently for each lipid measure or ratio. Therefore, comparisons between these measures are all indirect comparisons. We did not explore the alternative approach based around network meta-analysis (NMA) of a subset of studies to make direct comparisons, because this approach would use fewer studies and the methods for NMA are better developed for treatment comparisons than for prognostic reviews.
Assessment of methodological quality of the individual studies was difficult because included studies were not designed to address the same objective as this review and, therefore, the information required for this review to assess quality might not have been a priority for the study authors. Statistical analysis was rarely in a form that was appropriate for our outcomes. Assessment of attrition quality bias had no precedent in previous reviews, and setting boundaries for levels of low, moderate and high attrition bias were proven, subsequently, to be too generous, as no studies had high attrition.
There were a number of changes from the original protocol. The search methods were modified to accommodate the scale of the review and recognise the publication of three reviews in 2012. Similarly, by introducing the minimum number of participants, a 2-year follow-up and a minimum two lipid measures at baseline it was anticipated this would reduce the inclusions of smaller, more-prone-to-bias studies. This also removed the need for sensitivity analysis for smaller studies. In the analysis, all outcome data for each lipid measure was pooled. Although acknowledging that conclusions drawn from three or fewer sets of data could be misleading, it was important to enable an overall comparison between lipid measures and their trends, and view potentially useful lipid measures for future research. The indirect methods for data extraction were changed once included studies were examined; the best methods were used to extract the maximum amount of data. Quality assessment of included studies was instead based on a more recent tool77 that was specifically designed for prognostic reviews: QUIPS.
This review did not use IPD. It is well acknowledged that this is the ideal data source for these analyses and this means that a number of the limitations above would have been reduced or removed. Future research should look to utilise this approach if possible.
Prognostic systematic reviews are still in the early stages of methodological development. Search strategies, data extraction techniques, quality assessment tools and analysis software are not well established compared with other types of systematic reviews. This made each stage of the review challenging; as this area of research develops, it will provide a clearer path for undertaking this type of review.
Chapter 3 Meta-analysis of the effect of atorvastatin on serum lipid levels
Background
As discussed in Chapter 1, the value of a clinical monitoring programme partly depends on the existence of clinical actions to improve or maintain patient health that can be guided by monitoring. 11 Monitoring cholesterol is potentially worthwhile because there is an effective clinical response available to health practitioners if monitored levels are high: the prescription of a statin. It follows that in order to understand the value of different approaches to monitoring cholesterol or other available lipid measures, the benefits of the treatment must first be understood.
Of the statins available in the UK, simvastatin was the most widely prescribed when this project began in 2011. It has been available in generic form since 2003 and is therefore relatively cheap; for example, in 2014 a 28-tab pack cost either just over or just under £1 per pack, depending on dose. 186 In contrast, atorvastatin remained on patent until 2012; while on patent, the price of atorvastatin (Lipitor) was > £28 per pack (28 × 40- or 80-mg tablets)16 compared with prices of < £2 for 10-, 20- and 40-mg doses and just over £3 for a 80-mg dose of the generic form available since the patent expired. 186
Previous studies of the cost-effectiveness of atorvastatin concluded that, given the high cost, there was insufficient evidence of adequately large benefits to justify switching the majority of patients to atorvastatin. However, these studies were conducted while atorvastatin was still on patent, and, given the dramatic change in cost since the patent expired, the question needs to be re-examined. This chapter focuses on estimating the effect (or benefits) of atorvastatin on lipid measures, compared with the effect of other statins. The reason for this is twofold: first, trials of atorvastatin have not, in general, been large enough to use heart disease (heart attack and stroke) or death as their primary outcome measure, and, second, we are particularly interested in the effect of atorvastatin on the different lipid measures to inform the economic models presented in Chapter 7.
Typically, this would require a systematic search of the literature for RCTs comparing atorvastatin with placebo or any other statin. However, a number of other systematic reviews addressing this specific research question were published recently; of particular relevance are a Cochrane review examining the dose-related effect of atorvastatin on lipid levels in 254 trials187 and a systematic review and network meta-analysis evaluating the dose-related effects of different statins on lipid levels in 181 RCTs. 188 Both of these reviews could provide the information required to inform economic analyses of the effect of atorvastatin in lipid monitoring; however, a number of included trials were very small and of short duration, and, if these were excluded, results may differ. On the advice of the project advisory board, this chapter presents a re-analysis based on the studies identified in the most recent network meta-analysis, focusing on trials of atorvastatin with a minimum of 1000 individuals and at least 12-month follow-up, and therefore deemed to have a lower chance of bias.
Methods
Selection of studies
The 181 RCTs reviewed by Naci et al. 188 were considered for inclusion, including trials of both primary and secondary prevention populations. Two authors independently identified relevant publications and extracted data. Disagreements were resolved by consensus with a third author. Included trials met the following criteria: (1) at least 1000 individuals were randomised in total; (2) the average follow-up was at least 1 year (52 weeks); and (3) at least one of the groups was randomised to receive atorvastatin (‘Atorva’), in any dose (fixed or titrated). Risk of bias was assessed independently by two authors using the risk of bias tool provided in the Cochrane Handbook,189 which ascribes subjective ‘low’, ‘high’ or ‘unclear’ risk of bias to seven domains (two domains relating to blinding were combined into a single category).
Outcome measures and data extraction
Seven lipid measures and ratios (LDL cholesterol, HDL cholesterol, TC, TGs, non-HDL cholesterol, TC/HDL cholesterol and LDL/HDL cholesterol) were included as outcomes. Results from studies that reported data for longer periods of follow-up suggest that the most marked change in lipid measures occurs within the first 12 months, remaining relatively constant or with a moderate drift towards baseline values thereafter. 188 For this reason, lipid measure data were extracted at baseline and at the time point closest to 12 months (52 weeks) for which data were reported.
Data analysis
For each study, effect sizes [differences between the means in the atorvastatin treatment group and the comparator group, with standard errors (SEs)] were estimated based on the means, SDs and sample sizes reported at follow-up. As differences between groups at baseline were small, no adjustment was made for baseline values in the estimation of the effect size at follow-up. Units for all lipid measures were converted to millimoles per litre. For studies that did not report numerical data in the form required to make comparisons between the studies, or to directly estimate the effect size, the following steps were used, as required:
-
use the group-specific baseline SD and sample size for the equivalent quantities at follow-up, in the absence of additional information
-
use the median as an estimate of the mean
-
estimate within-group SD as SE multiplied by the square root of the sample size
-
use 0.75 times the interquartile range as an estimate of the SD.
Network meta-analysis was then used to investigate whether or not it was possible to combine the estimates of the effect size of atorvastatin in lipid measures across all included studies, taking into account differences in treatments and doses used in different studies. 190 For studies that used dose titration, the average dose across the study period was recorded as an estimate of the dose used in the study. Analyses were carried out using Stata 12.1 (StataCorp LP, College Station, TX, USA) and RevMan 5.2.
Amendments to protocol
Our protocol initially specified the estimation of effects of all statins on lipid measures based on all available trials. In August 2013, a comprehensive network meta-analysis and systematic review including all available statins was published. 188 Given this evidence combined with the particular interest in atorvastatin, reflecting current UK guidelines, we suggested a protocol modification to extract data only from RCTs that evaluated atorvastatin and that were large enough (> 1000 participants) with a minimum follow-up of 52 weeks. The study advisory board acting on behalf of the HTA approved this modification. We have tested through sensitivity analyses if our choice of threshold for the minimum number of participants had an impact on our findings.
Results
Based on the screening criteria, 171 trials were excluded; 10 studies are therefore included in this review115,179,184,191–197 (Figure 13). Three of the lipid measures (non-HDL cholesterol, TC/HDL cholesterol and LDL/HDL cholesterol) were either not reported or reported only in a single study, so only the other four measures (LDL cholesterol, HDL cholesterol, TC and TGs) are reported here. Nine of the studies115,179,184,191–196 reported each of these four measures at one or more follow-up times. The other study, PROVE IT-TIMI,195 reported LDL and HDL at both baseline and follow-up, but reported TC and TGs only at baseline.
FIGURE 13.
Flow chart showing selection of studies for inclusion.

Table 12 shows the characteristics of the 10 included studies. 113,179,184,191–197 The total number of participants randomised ranged from 1049 to 10,305, and the dose of atorvastatin used ranged from 10 to 80 mg/day. The studies113,179,184,191–195 showed wide variation in both their length of follow-up (with average length of follow-up ranging between 1 and 5 years) and the follow-up times at which outcomes measures were reported, even though the follow-up time closest to 12 months for which data were reported was used for data extraction.
Study | Treatment | Mean dose (mg/day) | Participants (by arm) | Study follow-up | Follow-up for data extraction |
---|---|---|---|---|---|
ALLIANCE194 | Atorvastatin | Median 40.5 | 1217 | Median 54.3 months | Mean 51.5 months |
Usual care | – | 1225 | |||
ASCOT-LLA192 | Atorvastatin | 10 | 5168 | Median 3.3 years | 1 years |
Placebo | – | 5137 | |||
aASG-I197 | Atorvastatin | 11 | 789 | 52W | 52 weeks |
Lovastatin | 24 | 260 | |||
ASPEN191 | Atorvastatin | 10 | 1211 | Median 4 years | 4 years |
Placebo | – | 1199 | |||
CARDS115 | Atorvastatin | 10 | 1428 | Median 3.9 years | 1 years |
Placebo | – | 1410 | |||
GREACE196 | Atorvastatin | 24 | 800 | 3 years | Mean over whole |
Usual care | – | 800 | Follow-up | ||
IDEAL179 | Atorvastatin | 80 | 4439 | 5 years | 1 years |
Simvastatin | 20 | 4449 | |||
PROVE IT-TIMI195 | Atorvastatin | 80 | 2009 | Mean 24 months | 12 months |
Pravastatin | 40 | 2063 | |||
SPARCL184 | Atorvastatin | 80 | 2365 | Median 4.9 years | Mean over whole |
Placebo | – | 2366 | Follow-up | ||
TNT193 | Atorvastatin | 80 | 4995 | Median 4.9 years | 12 months |
Atorvastatin | 10 | 5006 |
Figure 14 shows the pattern of treatments and doses in network form. A line between two treatments indicates that a direct comparison of those treatments was made in the named study or studies. In this figure, doses of atorvastatin of < 40 mg/day have been combined into a single category (‘Atorva 10–20’), and ‘Placebo’ and ‘Usual care’ groups are also treated as equivalent. Four trials provide a direct comparison of ‘Atorva 10–20’ against ‘Placebo/Usual care’. None of the other direct comparisons of treatments was reported in more than a single study.
FIGURE 14.
Network representation of the treatments and doses compared in the included studies.
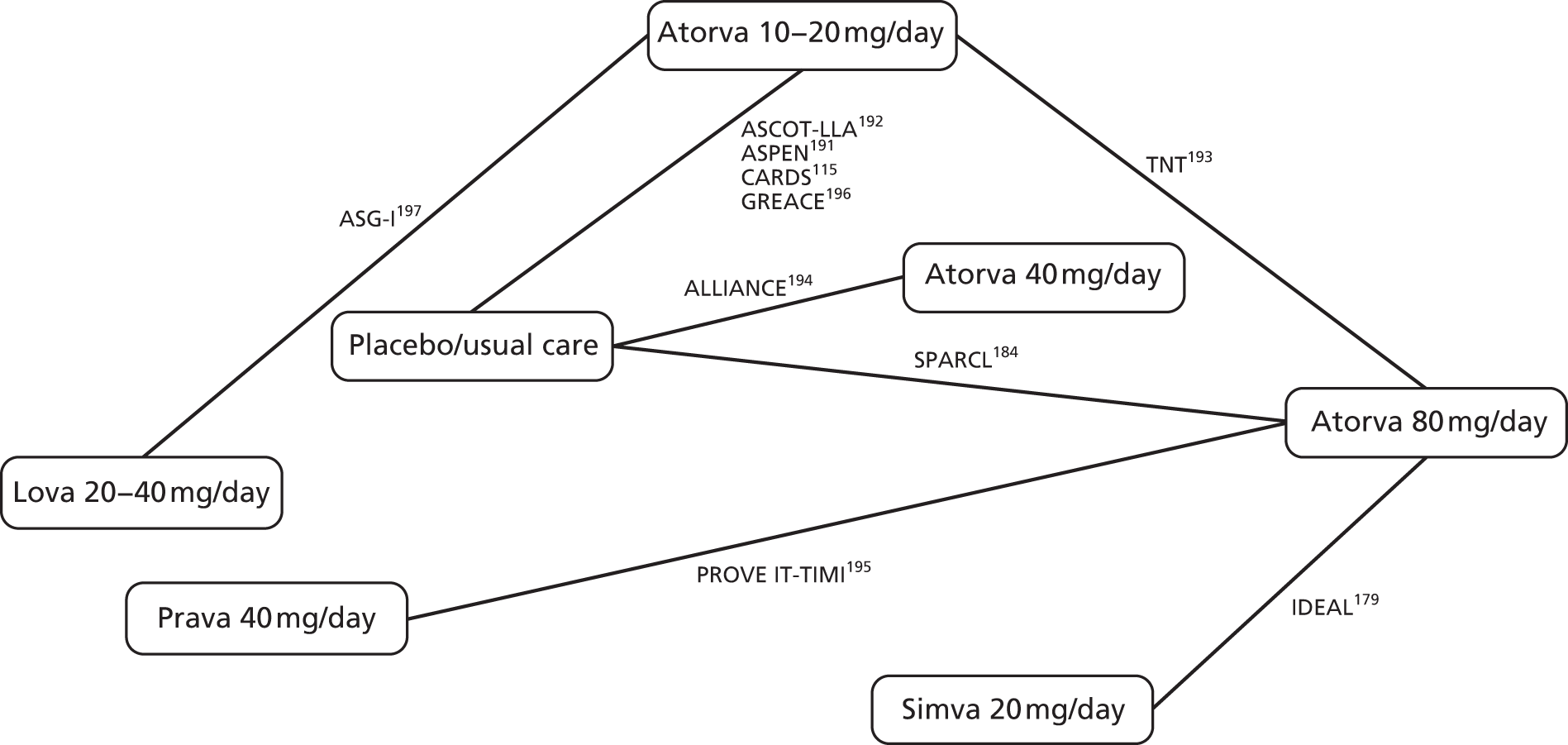
Table 13 summarises the baseline characteristics of the participants in each study. The average age in all studies was approximately 60 years, and the percentage of female participants ranged from 17% to 43%. Some studies targeted individuals with a history of MI (e.g. IDEAL179), CVD (e.g. ALLIANCE194) or diabetes (e.g. ASPEN191), whereas others (e.g. SPARCL184) specifically excluded individuals with one or more of these conditions.
Study | Treatment | Age, years: mean (SD) | Female % | MI % | CHD % | DM % | BMI (kg/m2): mean (SD) | Smoking % |
---|---|---|---|---|---|---|---|---|
ALLIANCE194 | Atorva | 61.1 (9.0) | 17.8 | 60.1 | 100 | 23.2 | C19.6 | |
Usual care | 61.3 (8.6) | 17.7 | 55.5 | 100 | 21.2 | C19.3 | ||
ASCOT-LLA192 | Atorva | 63.1 (8.5) | 18.9 | 24.3 | 28.6 (4.7) | C33.2 | ||
Placebo | 63.2 (8.6) | 18.7 | 24.8 | 28.7 (4.6) | C32.2 | |||
ASG-I197 | Atorva | 57 (11) | 41 | 15 | 27 (2.8) | |||
Lova | 58 (11) | 43 | 16 | 27 (3.2) | ||||
ASPEN191 | Atorva | 61.1 (8.1) | 34 | 17 | 100 | 28.9 (3.7) | C12 | |
Placebo | 61.0 (8.2) | 33 | 16 | 100 | 28.8 (3.8) | C13 | ||
CARDS115 | Atorva | 61.5 (8.3) | 32 | 0 | 100 | 28.7 (3.6) | C22, F44 | |
Placebo | 61.8 (8.0) | 32 | 0 | 100 | 28.8 (3.5) | C23, F43 | ||
GREACE196 | Atorva | 58 (12) | 22 | 82 | 100 | 20 | 24.2 (0.8) | |
Usual care | 59 (14) | 21 | 80 | 100 | 19 | 23.9 (0.6) | ||
IDEAL179 | Atorva | 61.8 (9.5) | 19.1 | 100 | 12 | 27.3 (3.9) | C20.1, F58.1 | |
Simva | 61.6 (9.5) | 19.1 | 100 | 12.1 | 27.3 (3.8) | C21.2, F58.8 | ||
PROVE IT-TIMI195 | Atorva | 58.1 (11.2) | 22.2 | 17.8 | C36.5 | |||
Prava | 58.3 (11.3) | 21.6 | 17.5 | C37.1 | ||||
SPARCL184 | Atorva | 63 | 39.7 | 0 | 16.7 | 27.5 | C19.1, F40.7 | |
Placebo | 62.5 | 41 | 0 | 16.9 | 27.4 | C19.3, F38.8 | ||
TNT193 | Atorva 80 | 61.2 (8.8) | 18.8 | 59 | 100 | 28.4 (4.5) | C13.4, F63.2 | |
Atorva 10 | 60.9 (8.8) | 19.2 | 57.7 | 100 | 28.6 (4.7) | C13.4, F63.3 |
Table 14 shows the risk of bias scores assigned to the studies by the two authors. The majority of the trials were rated as low risk of bias for most criteria, and there was a high level of agreement between the authors. Reasons for classifications of high risk of bias included a lack of blinding: one trial used an ‘open-label’ design (IDEAL179) and two used ‘Usual care’ as the comparator, making blinding infeasible (ALLIANCE194 and GREACE197) – and giving concerns about an unusually high level of dropout of withdrawal of consent (ALLIANCE194 and ASG-I197).
Study | Random sequence generation | Allocation concealment | Blinding | Incomplete outcome data | Selective reporting | Other sources of bias |
---|---|---|---|---|---|---|
ALLIANCE194 | L/L | U/H | H/H | H/H | H/L | L/L |
ASCOT-LLA192 | L/L | L/L | L/L | L/L | L/L | L/L |
ASG-I197 | L/L | U/L | L/L | H/H | L/U | L/L |
ASPEN191 | L/U | U/U | L/L | U/L | L/L | L/L |
CARDS115 | L/L | L/L | L/L | L/L | L/L | L/L |
GREACE196 | L/L | U/U | H/H | L/U | L/L | L/L |
IDEAL179 | L/L | U/U | H/H | L/L | L/L | L/L |
PROVE IT-TIMI195 | L/U | L/U | U/L | L/L | L/L | L/L |
SPARCL184 | L/L | L/L | L/L | L/L | L/U | L/L |
TNT193 | L/L | L/L | L/L | L/L | L/L | L/L |
Table 15 shows average lipid measures for each study at baseline and follow-up. There are noticeable disparities between studies at baseline: for example, average LDL cholesterol in different studies at baseline ranges from 2.74 mmol/l (TNT193) to 4.97 mmol/l (ASG-I197).
Study | Treatment | LDL cholesterol | HDL cholesterol | TC | TGs | ||||
---|---|---|---|---|---|---|---|---|---|
Baseline | Follow-up | Baseline | Follow-up | Baseline | Follow-up | Baseline | Follow-up | ||
ALLIANCE194 | Atorva | 3.80 (0.70) | 2.46 (0.70) | 1.03 (0.26) | 1.09 (0.26) | 5.84 (0.88) | 4.40 (0.96) | 2.22 (1.22) | 1.92 (1.53) |
Usual care | 3.78 (0.62) | 2.84 (0.57) | 1.06 (0.28) | 1.11 (0.34) | 5.82 (0.83) | 4.89 (0.98) | 2.24 (1.28) | 2.07 (1.37) | |
ASCOT-LLA192 | Atorva | 3.44 (0.72) | 2.25 (0.69) | 1.31 (0.37) | 1.30 (0.37) | 5.48 (0.78) | 4.16 (0.82) | 1.66 (0.92) | 1.37 (0.85) |
Placebo | 3.44 (0.72) | 3.45 (0.76) | 1.31 (0.36) | 1.28 (0.35) | 5.48 (0.78) | 5.45 (0.85) | 1.65 (0.87) | 1.65 (0.99) | |
ASG-I197 | Atorva | 4.97 (0.56) | 3.13 | 1.27 (0.28) | 1.36 | 7.14 (0.84) | 5.21 | 2.02 (0.84) | 1.70 |
Lova | 4.89 (0.64) | 3.47 | 1.27 (0.32) | 1.36 | 7.11 (0.81) | 5.62 | 2.07 (0.81) | 1.90 | |
ASPEN191 | Atorva | 2.92 (0.65) | 2.04 | 1.22 (0.36) | 1.24 | 5.02 (0.80) | 4.03 | 1.66 (0.91) | 1.59 |
Placebo | 2.95 (0.67) | 2.92 | 1.22 (0.34) | 1.22 | 5.02 (0.80) | 4.94 | 1.64 (0.94) | 1.80 | |
CARDS115 | Atorva | 3.04 (0.72) | 1.86 (0.69) | 1.39 (0.32) | 1.37 (0.33) | 5.36 (0.83) | 3.97 (0.81) | 1.95 (1.08) | 1.58 (0.88) |
Placebo | 3.02 (0.70) | 3.10 (0.80) | 1.42 (0.34) | 1.37 (0.37) | 5.35 (0.82) | 5.42 (0.90) | 1.93 (1.09) | 1.96 (1.23) | |
GREACE196 | Atorva | 4.65 (0.70) | 2.51 (0.10) | 1.01 (0.18) | 1.09 (0.18) | 6.65 (1.01) | 4.27 (0.26) | 2.08 (0.87) | 1.44 (0.35) |
Usual care | 4.63 (0.72) | 4.37 (0.83) | 1.01 (0.18) | 1.03 (0.18) | 6.59 (0.96) | 6.34 (1.06) | 2.01 (0.75) | 1.94 (0.72) | |
IDEAL179 | Atorva | 3.14 (0.86) | 2.05 (0.67) | 1.19 (0.34) | 1.18 (0.34) | 5.09 (1.03) | 3.81 (0.84) | 1.71 (0.90) | 1.31 (0.73) |
Simva | 3.24 (0.86) | 2.64 (0.68) | 1.19 (0.34) | 1.22 (0.34) | 5.07 (1.03) | 4.55 (0.85) | 1.66 (0.83) | 1.58 (0.96) | |
PROVE IT-TIMI195 | Atorva | 2.74 (0.76) | 1.66 (0.56) | 0.98 (0.27) | 1.05 | 4.68 (0.87) | 1.79 (0.81) | ||
Prava | 2.74 (0.78) | 2.51 (0.66) | 1.01 (0.25) | 1.09 | 4.65 (0.85) | 1.74 (0.78) | |||
SPARCL184 | Atorva | 3.43 (0.63) | 1.89 | 1.29 (0.38) | 1.35 | 5.47 (0.76) | 3.81 | 1.63 (1.04) | 1.26 |
Placebo | 3.46 (0.63) | 3.32 | 1.29 (0.38) | 1.32 | 5.49 (0.76) | 5.39 | 1.62 (0.77) | 1.64 | |
TNT193 | Atorva 80 | 2.51 (0.47) | 1.91 | 1.22 (0.28) | 1.19 | 4.53 (0.62) | 3.78 | 1.71 (0.79) | 1.49 |
Atorva 10 | 2.53 (0.47) | 2.56 | 1.22 (0.28) | 1.19 | 4.53 (0.62) | 4.60 | 1.71 (0.82) | 1.79 |
Table 16 shows the effect sizes for each lipid measure in each study. Figures 15–18 display this information in network form, separately for each measure. In these figures, each estimate refers to the effect of the treatment to the right of the line to which it is attached compared with the treatment to the left of the line. For example, the results of ALLIANCE194 show a reduction in LDL cholesterol at follow-up of 0.38 mmol/l for the atorvastatin treatment group compared with the usual care group.
Study | Comparison | LDL cholesterol | HDL cholesterol | TC | TGs |
---|---|---|---|---|---|
ALLIANCE194 | A40.5 – U | –0.38 (0.031) | –0.03 (0.014) | –0.49 (0.046) | –0.15 (0.070) |
ASCOT-LLA192 | A10 – U | –1.20 (0.015) | 0.02 (0.007) | –1.29 (0.017) | –0.28 (0.019) |
ASG-I197 | A11 – L24 | –0.34 (0.042) | 0 (0.021) | –0.40 (0.060) | –0.21 (0.060) |
ASPEN191 | A10 – U | –0.88 (0.027) | 0.03 (0.014) | –0.92 (0.033) | –0.21 (0.038) |
CARDS115 | A10 – U | –1.24 (0.029) | 0 (0.014) | –1.45 (0.033) | –0.38 (0.042) |
GREACE196 | A24 – U | –1.86 (0.029) | 0.05 (0.009) | –2.07 (0.039) | –0.50 (0.029) |
IDEAL179 | A80 – S20 | –0.59 (0.015) | –0.04 (0.007) | –0.74 (0.018) | –0.26 (0.019) |
PROVE IT-TIMI195 | A20 – P40 | –0.85 (0.019) | –0.04 (0.008) | ||
SPARCL184 | A80 – U | –1.44 (0.018) | 0.03 (0.011) | –1.58 (0.022) | –0.38 (0.021) |
TNT193 | A80 – A10 | –0.65 (0.009) | 0 (0.006) | –0.83 (0.012) | –0.29 (0.016) |
FIGURE 15.
Network representation of effect sizes (with SEs) for LDL at follow-up in each study. In the ‘Atorva 10–20’ vs. ‘Placebo/Usual care’ comparison, the four studies are presented in the same order as in Figure 14. Each estimate refers to the effect of the treatment to the right of line to which it is attached, relative to the treatment to the left of the line (e.g. the mean LDL for CARDS115 at 1 year was 1.24 mmol/l lower in the Atorva 10-mg group than in the ‘Usual care’ group).
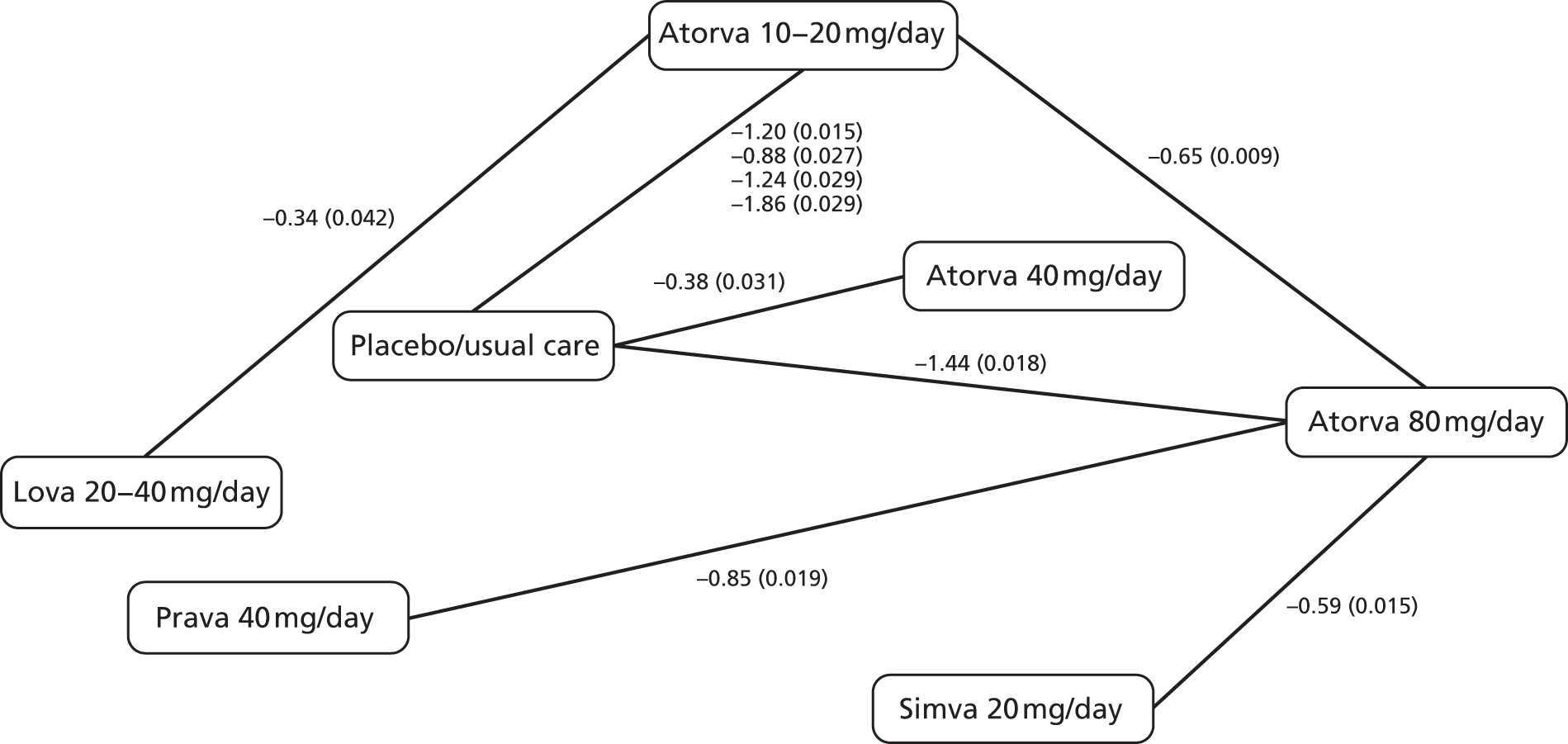
FIGURE 16.
Network representation of effect sizes (with SEs) for HDL at follow-up in each study.

FIGURE 17.
Network representation of effect sizes (with SEs) for TC at follow-up in each study.

FIGURE 18.
Network representation of effect sizes (with SEs) for TGs at follow-up in each study.

The results of the four studies that report a comparison of ‘Atorva 10–20’ against ‘Placebo/Usual care’ (ASCOT-LLA,192 ASPEN,191 CARDS115 and GREACE196) at follow-up are also reported in a forest plot in Figure 19. There is notably large heterogeneity between the studies (I2 > 99%), which is amplified by the small width of the CIs, a consequence of ascribing a minimum sample size as an inclusion criterion. For this reason we do not provide a pooled estimate of the effect across the four studies. 113,189,192,196
FIGURE 19.
Forest plot to show results from four studies that compare ‘Atorva 10–20’ with ‘Placebo/Usual care’. IV, inverse variance.

The balance of evidence shows a clear reduction in LDL cholesterol, TC and TGs at all doses of atorvastatin compared with placebo. There appears to be some evidence of a dose-related effect (see Figures 15, 17 and 18; Table 15), but this is difficult to assess definitively given the limited number of studies in this review that used one of the larger atorvastatin doses (≥ 40 mg/day). The studies that used other statins as treatment arms [ASG-I, which used lovastatin at an average dose of 24 mg/day; IDEAL,179 which used simvastatin 20 mg/day; and PROVE IT-TIMI,195 which used pravastatin 40 mg/day] also demonstrated greater reductions in LDL, TC and TGs (where reported) with atorvastatin relative to its comparator. None of the 10 studies113,179,184,191–197 showed more than a small change in HDL cholesterol between treatments. There was also consistently little change in HDL cholesterol between baseline and follow-up, irrespective of the length of the follow-up period. These results were consistent to our choice of a minimum number of participants in a study (see sensitivity analysis in Appendix 16).
Fitting the network meta-analysis model was computationally feasible despite the sparsity of the network. However, we considered it inappropriate to report combined summary estimates because of the extremely high heterogeneity observed in the ‘Atorva 10–20’ and ‘Placebo/Usual care’ treatment comparison. This was the only pairwise comparison for which there was more than one contributing study. For pairs of treatment for which there was a single contributing study, the point estimate of the effect size from the network meta-analysis was similar to the effect size observed in the only study that directly compared the two treatments. However, we present results from this analysis in Appendix 16.
The Cochrane systematic review examining the lipid-lowering efficacy of atorvastatin187 estimated effects for different doses and modelled the dose–effect relationship. A comparison between these estimates and the ones obtained in the present chapter can be seen in Figure 20 for TC and LDL cholesterol.
FIGURE 20.
Comparison of treatment effects in Cochrane systematic reviews and Chapter 3. (a) Total cholesterol; (b) LDL cholesterol.
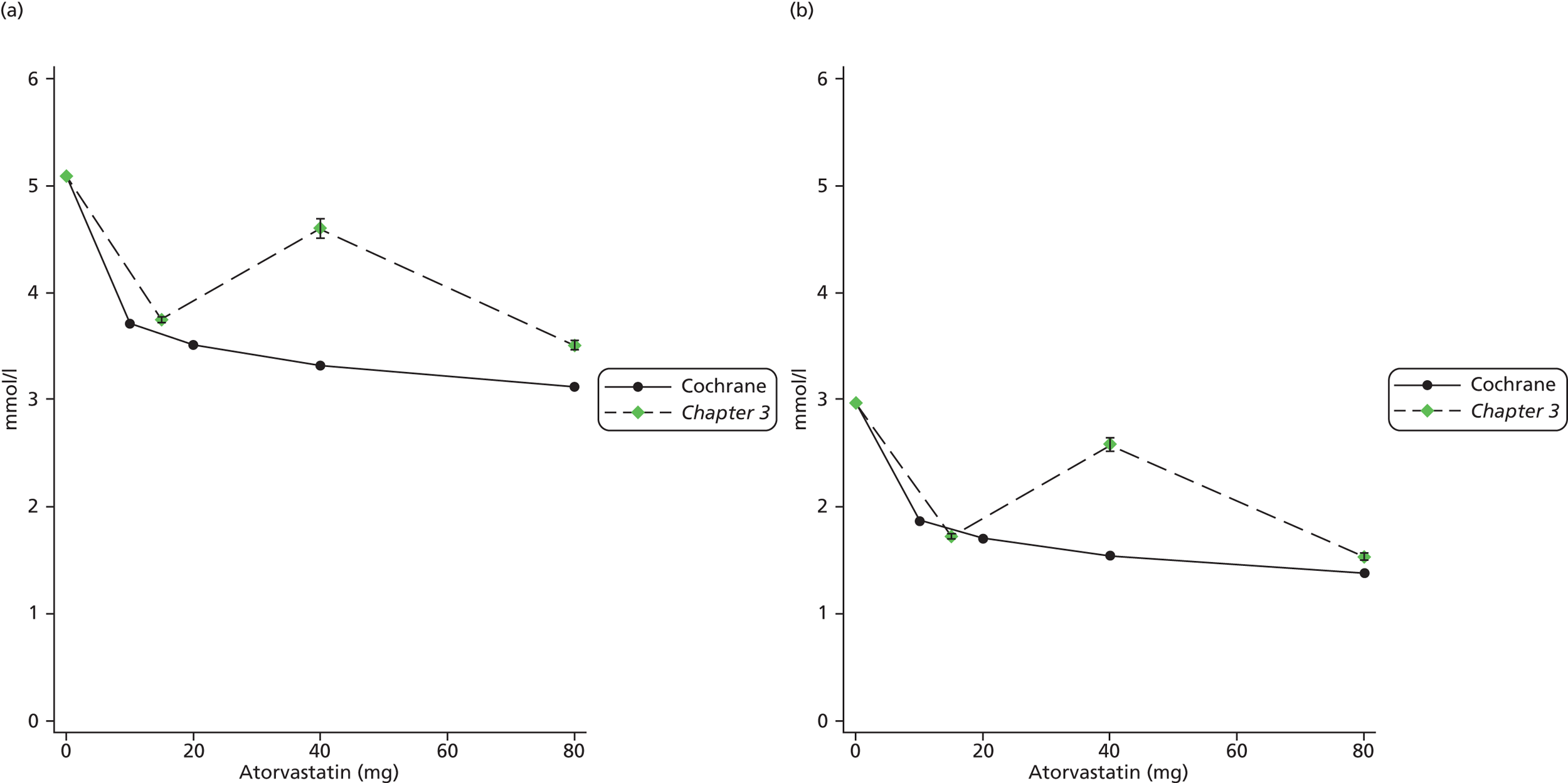
Discussion
Based on this review of large randomised trials with long periods of follow-up, we have found that, compared with other statins, placebo or usual care, atorvastatin substantially lowers (improves) TC, LDL cholesterol and TGs but does not affect HDL cholesterol. This finding was consistently observed across all included studies, and suggests that atorvastatin may have a greater effect than other statins in reducing cardiovascular risk.
The number of large studies that have evaluated the effect of atorvastatin at doses of > 20 mg/day is very limited. This raises issues about the scale of the true dose–effect association. There was only one eligible study (TNT193) that directly compared a higher dose (80 mg/day) of atorvastatin with a lower dose (10 mg/day). In that study, there was a clear improvement in TC, LDL cholesterol and TGs in the higher-dose arm, although the magnitude of the reduction in the two cholesterol measures was somewhat smaller than that observed in the four studies (ASCOT-LLA,192 ASPEN,191 CARDS115 and GREACE196) that compared the lowest atorvastatin dose to placebo or usual care. However, on the basis of the limited information available regarding the effect of higher doses of atorvastatin, our results are broadly similar with the findings of the Cochrane systematic review published in 2012. 187 Having confirmed the Cochrane estimates, we therefore used them for economic modelling (see Chapter 7).
This study has some limitations. We did not perform a full database search to identify studies to be included. Instead, the search was based on a list of studies already identified in a wide-ranging review paper, which was published as recently as 2013. 188 As none of the 10 studies115,179,184,191–197 that we included reported lipid measures as primary outcome measures, relevant data were in many cases limited. For this reason, we were not able to assess three of the intended lipid measures and ratios. In addition, extremely high heterogeneity between studies made it inappropriate to pool numerical results.
Although we attempted to use a 12-month period of follow-up in the primary comparison, several studies did not report lipid measures at this time point, and so in several cases other follow-up periods were used as an approximation to the effect after 1 year. Furthermore, summary statistics were not reported in a consistent way across studies, which required several assumptions to be made regarding effect size estimates and their SEs. We do not believe that these assumptions are likely to have a large impact on our overall conclusions: the studies included are large, with total sample size of at least 1000 individuals, and so SEs are likely to be extremely small, even if there is error in the estimate of the SD on which the calculation is based.
Chapter 4 Modelling progression of lipid levels
Background
Screening and long-term monitoring of cholesterol levels requires interpretation of initial levels and also of sequential levels over time. 29 When such quantities are measured, we can think of the observed value as a product of the true underlying value and its variability. Interpretation of observed levels must therefore consider the short-term within-person variability and the long-term variability among individuals in the population. 11 Previous research examining variation in cholesterol levels in an Australian population and a Japanese population used statistical models to quantify within-individual changes over time and variations between individuals, both in the level of cholesterol and in the rate of change. 30,31 This methodology has since been extended to allow for uncertainty in the initial measurement as well as subsequent monitoring tests. 32 In this chapter we model the progression of lipid measures over time using data from individual patients; we fit models that allow for variation between patients at baseline, change over time, variation between patients in rate of change (potentially including patients with no change), and combined assay and biological variability in any given measurement. Parameter estimates from these models form the basis for examining the impact that different monitoring strategies have on clinical decision-making on eligibility for statin use, as discussed in Chapter 5, and inform the development of health economics models for the progression of dyslipidaemia, presented in Chapter 7 of this report.
Methods
We limit our analysis to patients aged ≥ 40 years for consistency with the earliest age at which cholesterol monitoring starts in programmes such as the NHS Health Check programme. 198,199 Because cholesterol levels in women change throughout the transition from premenopause to perimenopause to post menopause,200,201 we further restrict our analyses in women to those aged ≥ 55 years. 202
Data sources
Parameters for progression and variability of cholesterol were estimated using repeated measures data from two cohorts of patients: the St Luke’s cohort from Tokyo, Japan, described previously,31 and the UK general practice-based Clinical Practice Research Datalink (CPRD; formerly General Practice Research Database). 203 The derivation of the analysis cohorts and relevant variables is described below. The rationale for using the Japanese data set was to take advantage of the rigorous data collection at St Luke’s, with almost 100% complete data collection at regular intervals. Although some values (e.g. population mean of TC, HDL cholesterol and LDL cholesterol) would be expected to vary between Japanese and UK populations, it was hoped that some parameters, in particular, the within-person short-term variability of each lipid might be unaffected by setting and ethnic group. If so, we could take advantage of the St Luke’s cohort data to obtain a highly precise estimate of short-term variability. Noting that this variability is the sum of laboratory or assay variability, and day-to-day variability within individuals, it seemed likely that at least the first component would be transferable to a UK setting.
St Luke’s cohort, Japan
Variation in cholesterol levels in the St Luke’s cohort has previously been examined using other statistical methods. 31 For comparison with these previous results, we initially fitted models to a closed cohort of patients with four measurements at regular intervals. We then also fitted models to an open cohort with varying numbers of measurements per patient at intervals as available, for greater potential relevance to routine practice. As the proportion of patients using statins and/or with previous CVD in the St Luke’s cohort is small, we restricted analyses to primary prevention patients not using statins. Lipid measures available included TC, HDL and TGs; from these, we also calculated LDL using the Friedewald equation,43 non-HDL cholesterol, and the ratios of TC/HDL cholesterol and LDL/HDL cholesterol.
The closed cohort consisted of men aged ≥ 40 years and women aged ≥ 55 years, whose first health check was in 2005, and who then had at least one health check per year in 2006, 2007 and 2008. Only the first measurement was used if patients had more than one measurement per year, so all patients contributed four measurements at approximately annual intervals to the model. Patients who reported a CVD event or the use of cholesterol-lowering medication before their first health check or during follow-up were excluded.
The open cohort consisted of men aged ≥ 40 years and women aged ≥ 55 years, who attended the centre for a health check at any point after 1 January 2005. Patients who reported a CVD event or cholesterol-lowering drug use before their first health check were excluded. If a patient reported either a CVD event or statin use after their first health check, we included lipid readings up to the age they reported the CVD event, or the health check at which they reported statin use; i.e. we ‘censored’ the data at the last lipid reading before the change in medication or the CVD event. The latest date of follow-up available was 30 December 2010. Patients contributed between 1 and 12 lipid measures over a period of up to 6 years.
The following variables were available and used in analyses in St Luke’s data:
-
Baseline characteristics Participant identifier, gender, reported age at first health check, dates of first lipid measurement after 1 January 2005 and lipid readings at this date, reported age of first reported CVD event and reported statin use at each health check.
-
Repeated measures Lipid readings and dates of all lipid measurements between start of follow-up and 30 December 2010.
Analyses were stratified by gender, and each lipid type was considered separately.
Clinical Practice Research Datalink
Patients aged ≥ 40 years (men) or ≥ 55 years (women) with cholesterol measurement(s) on at least one occasion after 1 January 2005 were identified from the CPRD. As an objective of this research is the comparison of different lipid measures, patients were required to have had at least three types of lipid measurement – from TC, HDL cholesterol, LDL cholesterol and TGs – recorded on at least one occasion. In addition to these four types of lipid measurement, we studied the calculated measurements non-HDL cholesterol, TC/HDL cholesterol and LDL/HDL cholesterol. Analyses were stratified by gender and by CVD prevention group, defined as follows.
Primary prevention patients not taking statins were defined as those who had not had a CVD event and were not taking statin medication at the time of the lipid measurement. For each lipid type, the start of follow-up for these patients (in the target age groups) was the date of their first measurement of that lipid. All subsequent lipid measurements were included until the end of follow-up, defined as the earliest date of CVD event, statin prescription or end of eligible data (death, patient transferred out of the practice, end of patient registration or end of follow-up on 21 September 2012).
Primary prevention patients taking statins were defined as those who had not had a CVD event and were taking statin medication at the time of the lipid measurement, and had prescription records for that type of statin for a minimum of 6 months. The start of follow-up was defined as the date of the first lipid measurement that was at least 12 weeks after the date the statin was prescribed. Follow-up ended at the earliest date of CVD event or end of eligible data (as above).
Secondary prevention patients taking statins were defined as those who had had a CVD event prior to the time of the lipid measurement and who were taking statin medication at this time and had prescription records for that type of statin for a minimum of 6 months. The start of follow-up was defined as the date of first lipid measurement that was at least 12 weeks after the date of the CVD event and the date of statin prescription. Follow-up ended at the end of eligible data (as above). We did not study a group of secondary prevention patients not taking statins due to small numbers.
The following variables were defined for use in these analyses:
-
Baseline characteristics Participant identifier, gender, year of birth, date of first lipid measurement after 1 January 2005 and lipid readings at this date, earliest date of CVD event, and earliest date of statin prescription of at least 6 months’ duration.
-
Repeated measures Lipid readings and dates of all lipid measurements between start and end of follow-up.
Statistical methods
We study four components of variability and change in lipid measurements:
-
the tendency for lipid levels to differ systematically between patients, described as the between-subject variation in baseline lipid levels
-
the average rate of change over time in the lipid measure for the whole group
-
the variation in the rate of change between individuals
-
the short-term variability in any single measurement, which is a combination of the analytic variability in the assay and the short-term biological variability.
From a monitoring perspective, the short-term variability equates to the measurement error or ‘noise’ (component 4 above), whereas the true rate of change in individuals is the ‘signal’.
As both the average baseline lipid levels and trends over time may vary, separate models were fitted for each lipid measure, for men and for women, and for each prevention group as defined above. Thus in CPRD data, six separate models (two gender subgroups in each of three prevention groups) were fitted for each lipid measure, whereas the St Luke’s cohort’s two models (two gender subgroups in a single prevention group, primary prevention not taking statins) were fitted per lipid measure.
We use the framework introduced by Glasziou et al. 30 to represent the components of variability and change listed above. Age-adjusted linear random-effects models were fitted to each gender subgroup of each prevention group as follows:
[Note: αi ∼ N(α, σa2), βi ∼ N(β, σb2), with covariance (αi, βi) = σab; εij ∼ N(0, σw2)]
where Yij is the jth observed lipid measurement in the ith person in the cohort and Ai is the age of the ith person at baseline. The rationale, interpretation and properties of this model have been discussed in detail previously:32,204 briefly, α is the average initial value (component 1 above), β is the average annual rate of change in the cohort (component 2) and σb, the SD of the individual rates of change, represents the variation of the rate of change between individuals (component 3). The SD σw represents the short-term variability of a single measurement (component 4 above). Model assumptions used here include independent normal distributions (denoted N) for α, β and ε. Normal distribution assumptions and model fit were checked graphically through comparison of the empirical and estimated distributions of the residuals. Model assumptions were not met for TGs without transformation and, because of the subsequent difficulties in interpretation, are not presented.
Parameter estimates are presented as mean and SD for each lipid, with 95% CIs. We also report SN ratios for each lipid, calculated as follows:
Recalibration of Japanese parameters to a UK perspective
The progression of each lipid was modelled through fitting a linear random-effects model, as described above, to data from primary prevention subjects not taking statins in the CPRD, except that the noise variable σw2 was constrained to that estimated from the equivalent St Luke’s data set. This was done using the runmlwin package in Stata version 12.1. 205 Assumptions of normality and model fit were checked graphically. As above, results are presented as mean and SD for each lipid (in millimoles per litre for single measures or no units for the ratios), with 95% CIs, and as SN ratios.
All models were fitted in Stata version 12.1.
Amendments to protocol
Our protocol initially specified that we would also model lipid progression in a secondary prevention population taking statins using data from the Long-Term Intervention with Pravastatin in Ischaemic Disease (LIPID) trial. 33 We were unable to gain direct access to the IPD from this trial; however, we did obtain summary estimates which corroborate that parameter estimates obtained from the CPRD for secondary prevention patients taking statins (presented in this chapter) are consistent with those found in the LIPID33 data.
Results
St Luke’s cohort
Baseline characteristics of participants in the St Luke’s closed and open cohorts are shown in Table 17. From a total of 42,557 subjects of the correct age range in the St Luke’s data, 7694 and 38,388 were eligible for inclusion in the closed and open cohort analyses, respectively, with some subjects included in both analyses. The inclusion criteria (at least 40 years of age in men or 55 years in women) are reflected in a high male–female ratio, and higher average age in women. The mean number of visits and length of follow-up were similar in men and women in each cohort.
Variable | Mean (SD) | |||
---|---|---|---|---|
St Luke’s closed cohort, N = 7694 | St Luke’s open cohort, N = 38,388 | |||
Men | Women | Men | Women | |
n | 5774 | 1920 | 28,670 | 9718 |
No. of years’ follow-up | 3.04 (0.21) | 3.08 (0.21) | 2.04 (1.92) | 1.99 (1.98) |
No. of visits | 4.00 (0.00) | 4.00 (0.00) | 2.90 (1.85) | 2.83 (1.89) |
Age at baseline, years | 54 (10) | 62 (6) | 52 (10) | 62 (6) |
TC, mmol/l | 5.25 (0.79) | 5.81 (0.75) | 5.30 (0.83) | 5.88 (0.84) |
HDL cholesterol, mmol/l | 1.45 (0.35) | 1.80 (0.39) | 1.44 (0.35) | 1.77 (0.41) |
LDL cholesterol, mmol/l | 3.53 (0.75) | 3.82 (0.72) | 3.18 (0.79) | 3.62 (0.78) |
TGs, mmol/l | 1.38 (0.94) | 0.98 (0.53) | 1.47 (1.13) | 1.05 (0.59) |
Non-HDL, mmol/l | 3.81 (0.81) | 4.02 (0.76) | 3.86 (0.86) | 4.10 (0.86) |
TC/HDL cholesterol | 3.82 (0.99) | 3.38 (0.82) | 3.87 (1.05) | 3.47 (0.88) |
LDL/HDL cholesterol | 2.28 (0.77) | 1.98 (0.66) | 2.34 (0.80) | 2.07 (0.72) |
Table 18 shows estimated variability and change parameters in the closed St Luke’s cohort for each lipid measurement. For TC, the average value at baseline, adjusted to age zero, was 5.22 mmol/l (95% CI 5.12 to 5.33 mmol/l) in men and 5.99 mmol/l (95% CI 5.68 to 6.29 mmol/l) in women. Average change per year was 0.00 mmol/l (95% CI –0.01 to 0.00 mmol/l) in both men and women. However, the variation around this average was comparatively large, with SD of 0.09 mmol/l per year in both men and women: this corresponds to an estimate that for 95% of men and women, the rate of change lies between a decrease of 0.18 mmol/l and an increase of 0.18 mmol/l. The estimated SD of the within-measurement variability was 0.35 (95% CI 0.35 to 0.36) in men and 0.37 (95% CI 0.37 to 0.38) in women. The estimated SN ratio at 1 year is therefore 0.092/0.352 = 0.06 in men and 0.092/0.372 = 0.06 in women. The estimated SN ratios over longer time periods are shown in Table 19. The SN ratio does not appear to exceed one until 4–5 years. The SN ratios for other lipids showed similar trends, although a stronger SN ratio was seen for the two lipid ratios (TC/HDL cholesterol and LDL/HDL cholesterol) and for LDL cholesterol.
Interpretation | α | β | γ | σw | σa | σb | σab |
---|---|---|---|---|---|---|---|
Mean lipid level at first measurement, at age zero (mmol/l or unitless) | Mean annual change in lipid measurement | Effect of age at first measurement on mean lipid measurement | SD of the within-measurement variability of lipid | SD of lipid | SD of annual change in lipid | Covariance between actual value of lipid and rate of change | |
Men | |||||||
TC | 5.22 (5.12 to 5.33) | –0.00 (–0.01 to 0.00) | 0.00 (–0.00 to 0.00) | 0.35 (0.35 to 0.36) | 0.71 | 0.09 | –0.01 |
HDL cholesterol | 1.32 (1.27 to 1.37) | 0.01 (0.01 to 0.01) | 0.00 (0.00 to 0.00) | 0.12 (0.12 to 0.12) | 0.33 | 0.03 | 0.00 |
LDL cholesterol | 3.52 (3.42 to 3.62) | –0.01 (–0.01 to –0.01) | 0.00 (–0.00 to 0.00) | 0.32 (0.31 to 0.32) | 0.69 | 0.08 | –0.01 |
Non-HDL cholesterol | 3.90 (3.80 to 4.01) | –0.01 (–0.02 to –0.01) | –0.00 (–0.00 to 0.00) | 0.33 (0.33 to 0.34) | 0.74 | 0.09 | –0.01 |
TC/HDL cholesterol | 4.09 (3.96 to 4.22) | –0.03 (–0.03 to –0.02) | –0.01 (–0.01 to –0.00) | 0.34 (0.33 to 0.34) | 0.92 | 0.11 | –0.02 |
LDL/HDL cholesterol | 2.53 (2.43 to 2.64) | –0.00 (–0.01 to –0.00) | –0.00 (–0.01 to –0.00) | 0.26 (0.26 to 0.27) | 0.72 | 0.08 | –0.01 |
Women | |||||||
TC | 5.99 (5.68 to 6.29) | –0.00 (–0.01 to 0.00) | –0.00 (–0.01 to 0.00) | 0.37 (0.37 to 0.38) | 0.65 | 0.09 | –0.01 |
HDL cholesterol | 2.05 (1.88 to 2.22) | –0.00 (–0.00 to 0.00) | –0.00 (–0.01 to –0.00) | 0.14 (0.13 to 0.14) | 0.37 | 0.03 | –0.00 |
LDL cholesterol | 3.82 (3.52 to 4.12) | –0.00 (–0.01 to 0.00) | –0.00 (–0.00 to 0.00) | 0.33 (0.32 to 0.34) | 0.66 | 0.09 | –0.01 |
Non-HDL cholesterol | 3.95 (3.63 to 4.27) | –0.00 (–0.01 to 0.01) | 0.00 (–0.00 to 0.01) | 0.34 (0.33 to 0.34) | 0.70 | 0.09 | –0.01 |
TC/HDL cholesterol | 3.06 (2.71 to 3.41) | –0.00 (–0.01 to 0.01) | 0.00 (–0.00 to 0.01) | 0.26 (0.25 to 0.26) | 0.78 | 0.09 | –0.01 |
LDL/HDL cholesterol | 1.79 (1.50 to 2.07) | 0.01 (0.01 to 0.02) | 0.00 (–0.00 to 0.01) | 0.21 (0.21 to 0.22) | 0.63 | 0.08 | –0.01 |
Lipid measure | SN ratio at different periods after baseline (years) | |||||||||
---|---|---|---|---|---|---|---|---|---|---|
1 | 2 | 3 | 4 | 5 | 6 | 7 | 8 | 9 | 10 | |
Men | ||||||||||
TC | 0.06 | 0.24 | 0.54 | 0.95 | 1.49 | 2.15 | 2.92 | 3.82 | 4.83 | 5.96 |
HDL cholesterol | 0.06 | 0.24 | 0.54 | 0.96 | 1.50 | 2.16 | 2.94 | 3.84 | 4.86 | 6.00 |
LDL cholesterol | 0.07 | 0.27 | 0.61 | 1.08 | 1.69 | 2.43 | 3.31 | 4.33 | 5.48 | 6.76 |
Non-HDL cholesterol | 0.08 | 0.32 | 0.71 | 1.26 | 1.97 | 2.84 | 3.86 | 5.04 | 6.38 | 7.88 |
TC/HDL cholesterol | 0.10 | 0.39 | 0.88 | 1.56 | 2.45 | 3.52 | 4.79 | 6.26 | 7.92 | 9.78 |
LDL/HDL cholesterol | 0.09 | 0.37 | 0.84 | 1.49 | 2.32 | 3.34 | 4.55 | 5.94 | 7.52 | 9.29 |
Women | ||||||||||
TC | 0.06 | 0.24 | 0.54 | 0.95 | 1.49 | 2.14 | 2.92 | 3.81 | 4.82 | 5.96 |
HDL cholesterol | 0.05 | 0.20 | 0.46 | 0.81 | 1.27 | 1.82 | 2.48 | 3.24 | 4.10 | 5.07 |
LDL cholesterol | 0.08 | 0.30 | 0.68 | 1.21 | 1.89 | 2.72 | 3.70 | 4.83 | 6.11 | 7.55 |
Non-HDL cholesterol | 0.08 | 0.31 | 0.69 | 1.23 | 1.93 | 2.77 | 3.77 | 4.93 | 6.24 | 7.70 |
TC/HDL cholesterol | 0.11 | 0.45 | 1.02 | 1.81 | 2.84 | 4.08 | 5.56 | 7.26 | 9.19 | 11.34 |
LDL/HDL cholesterol | 0.13 | 0.53 | 1.18 | 2.10 | 3.28 | 4.73 | 6.44 | 8.41 | 10.64 | 13.13 |
Parameter estimates and SN ratios from the open St Luke’s cohort are shown in Tables 20 and 21. Mean age-adjusted lipid levels at first measurement were similar in both the closed and open Japanese cohorts. The within-measurement variation (noise) for each lipid was greater in the open cohort, and the variance in the annual change in lipid (signal) was smaller, so that the SN ratio did not exceed one until 6 years (e.g. lipid ratios) or 8 years (e.g. TC).
Interpretation | α | β | γ | σw | σa | σb | σab |
---|---|---|---|---|---|---|---|
Mean lipid level at first measurement, at age zero | Mean annual change in lipid measurement | Effect of age at first measurement on mean lipid measurement | SD of the within-measurement variability of lipid | SD of lipid | SD of annual change in lipid | Covariance between actual value of lipid and rate of change | |
Men | |||||||
TC | 5.24 (5.19 to 5.30) | –0.00 (–0.01 to –0.00) | 0.00 (–0.00 to 0.00) | 0.41 (0.41 to 0.42) | 0.71 | 0.06 | –0.01 |
HDL cholesterol | 1.37 (1.34 to 1.39) | 0.01 (0.00 to 0.01) | 0.00 (0.00 to 0.00) | 0.16 (0.16 to 0.16) | 0.32 | 0.02 | –0.00 |
LDL cholesterol | 3.02 (2.97 to 3.07) | –0.00 (–0.00 to 0.00) | 0.00 (0.00 to 0.00) | 0.39 (0.38 to 0.39) | 0.65 | 0.05 | –0.01 |
Non-HDL cholesterol | 3.88 (3.82 to 3.94) | –0.01 (–0.01 to –0.01) | –0.00 (–0.00 to 0.00) | 0.40 (0.40 to 0.40) | 0.75 | 0.06 | –0.01 |
TC/HDL cholesterol | 4.00 (3.93 to 4.07) | –0.01 (–0.02 to –0.01) | –0.00 (–0.00 to –0.00) | 0.43 (0.43 to 0.44) | 0.93 | 0.07 | –0.02 |
LDL/HDL cholesterol | 2.48 (2.42 to 2.54) | –0.01 (–0.01 to –0.00) | –0.00 (–0.00 to –0.00) | 0.34 (0.33 to 0.34) | 0.72 | 0.06 | –0.01 |
Women | |||||||
TC | 6.11 (5.93 to 6.29) | –0.02 (–0.02 to –0.01) | –0.00 (–0.01 to –0.00) | 0.44 (0.44 to 0.45) | 0.69 | 0.07 | –0.01 |
HDL cholesterol | 2.01 (1.91 to 2.10) | –0.01 (–0.01 to –0.01) | –0.00 (–0.00 to –0.00) | 0.17 (0.17 to 0.17) | 0.37 | 0.02 | –0.00 |
LDL cholesterol | 3.73 (3.56 to 3.90) | –0.01 (–0.01 to –0.00) | –0.00 (–0.00 to 0.00) | 0.39 (0.39 to 0.40) | 0.65 | 0.06 | –0.01 |
Non-HDL cholesterol | 4.12 (3.93 to 4.30) | –0.01 (–0.01 to –0.01) | –0.00 (–0.00 to 0.00) | 0.41 (0.40 to 0.41) | 0.73 | 0.07 | –0.02 |
TC/HDL cholesterol | 3.16 (2.96 to 3.37) | 0.01 (0.00 to 0.01) | 0.00 (0.00 to 0.01) | 0.36 (0.35 to 0.36) | 0.79 | 0.06 | –0.01 |
LDL/HDL cholesterol | 1.89 (1.73 to 2.06) | 0.01 (0.01 to 0.01) | 0.00 (–0.00 to 0.00) | 0.30 (0.29 to 0.30) | 0.64 | 0.05 | –0.00 |
Lipid measure | SN ratio at different periods after baseline (years) | |||||||||
---|---|---|---|---|---|---|---|---|---|---|
1 | 2 | 3 | 4 | 5 | 6 | 7 | 8 | 9 | 10 | |
Men | ||||||||||
TC | 0.02 | 0.08 | 0.18 | 0.32 | 0.49 | 0.71 | 0.97 | 1.27 | 1.60 | 1.98 |
HDL cholesterol | 0.02 | 0.08 | 0.18 | 0.32 | 0.51 | 0.73 | 0.99 | 1.29 | 1.64 | 2.02 |
LDL cholesterol | 0.02 | 0.07 | 0.16 | 0.29 | 0.45 | 0.65 | 0.88 | 1.15 | 1.46 | 1.80 |
Non-HDL cholesterol | 0.02 | 0.10 | 0.22 | 0.40 | 0.62 | 0.90 | 1.22 | 1.60 | 2.02 | 2.50 |
TC/HDL cholesterol | 0.03 | 0.11 | 0.25 | 0.45 | 0.70 | 1.01 | 1.38 | 1.80 | 2.28 | 2.82 |
LDL/HDL cholesterol | 0.03 | 0.12 | 0.27 | 0.49 | 0.76 | 1.10 | 1.49 | 1.95 | 2.47 | 3.05 |
Women | ||||||||||
TC | 0.02 | 0.09 | 0.20 | 0.36 | 0.56 | 0.80 | 1.09 | 1.42 | 1.80 | 2.22 |
HDL cholesterol | 0.02 | 0.08 | 0.18 | 0.32 | 0.49 | 0.71 | 0.97 | 1.26 | 1.60 | 1.98 |
LDL cholesterol | 0.02 | 0.10 | 0.22 | 0.39 | 0.61 | 0.88 | 1.20 | 1.57 | 1.99 | 2.46 |
Non-HDL cholesterol | 0.03 | 0.11 | 0.25 | 0.45 | 0.71 | 1.02 | 1.39 | 1.81 | 2.29 | 2.83 |
TC/HDL cholesterol | 0.03 | 0.11 | 0.25 | 0.44 | 0.68 | 0.99 | 1.34 | 1.75 | 2.22 | 2.74 |
LDL/HDL cholesterol | 0.03 | 0.11 | 0.24 | 0.43 | 0.67 | 0.97 | 1.32 | 1.72 | 2.18 | 2.69 |
Clinical Practice Research Datalink
Baseline characteristics for men and women in each analysis group in CPRD are presented in Table 22. On average, primary prevention patients not taking statins were younger than primary prevention patients taking statins, who were younger than secondary prevention patients taking statins. Secondary prevention patients taking statins had a longer follow-up period and more lipid tests than both primary prevention groups. On average, lipid levels were lower in those taking statin therapy.
Variable | Men | Women | ||||||||||
---|---|---|---|---|---|---|---|---|---|---|---|---|
Primary prevention not taking statins, N = 46,785 | Primary prevention taking statins, N = 18,593 | Secondary prevention taking statins, N = 13,197 | Primary prevention not taking statins, N = 37,563 | Primary prevention taking statins, N = 15,846 | Secondary prevention taking statins, N = 8797 | |||||||
n | Mean (SD) | n | Mean (SD) | n | Mean (SD) | n | Mean (SD) | n | Mean (SD) | n | Mean (SD) | |
No. of years’ follow-up | 46,785 | 2.05 (2.29) | 18,593 | 3.55 (2.39) | 13,197 | 4.34 (2.45) | 37,563 | 2.19 (2.32) | 15,846 | 3.66 (2.40) | 8797 | 4.09 (2.46) |
No. of visits | 46,785 | 2.59 (2.04) | 18,593 | 5.33 (3.70) | 13,197 | 6.48 (3.77) | 37,563 | 2.81 (2.17) | 15,846 | 5.50 (3.72) | 8797 | 6.18 (3.77) |
Age at baseline, years | 46,785 | 59 (11) | 18,593 | 63 (10) | 13,197 | 69 (10) | 37,563 | 66 (9) | 15,846 | 68 (9) | 8797 | 74 (9) |
TC, mmol/l | 46,785 | 5.57 (1.06) | 18,593 | 4.57 (1.10) | 13,197 | 4.18 (0.94) | 37,563 | 6.01 (1.08) | 15,846 | 4.89 (1.11) | 8797 | 4.59 (1.05) |
HDL cholesterol, mmol/l | 45,168 | 1.33 (0.37) | 17,788 | 1.29 (0.40) | 12,707 | 1.26 (0.35) | 36,184 | 1.66 (0.45) | 15,186 | 1.57 (0.45) | 8446 | 1.55 (0.43) |
LDL cholesterol, mmol/l | 44,031 | 3.45 (0.93) | 16,965 | 2.48 (0.95) | 12,279 | 2.22 (0.80) | 35,177 | 3.66 (0.96) | 14,520 | 2.62 (1.00) | 8077 | 2.35 (0.92) |
TGs, mmol/l | 44,523 | 1.72 (1.27) | 17,211 | 1.79 (1.27) | 12,406 | 1.56 (1.00) | 35,546 | 1.47 (0.83) | 14,694 | 1.60 (0.87) | 8154 | 1.53 (0.83) |
Non-HDL cholesterol, mmol/l | 45,151 | 4.23 (1.06) | 17,780 | 3.28 (1.08) | 12,705 | 2.92 (0.91) | 36,168 | 4.33 (1.08) | 15,181 | 3.34 (1.11) | 8443 | 3.05 (1.04) |
TC/HDL cholesterol | 45,151 | 4.45 (1.34) | 17,782 | 3.76 (1.24) | 12,706 | 3.50 (1.09) | 36,168 | 3.83 (1.15) | 15,183 | 3.32 (1.10) | 8443 | 3.15 (1.03) |
LDL/HDL cholesterol | 44,003 | 2.77 (1.02) | 16,960 | 2.05 (0.91) | 12,275 | 1.87 (0.82) | 35,164 | 2.37 (0.91) | 14,517 | 1.79 (0.84) | 8076 | 1.64 (0.78) |
Tables 23 and 24 show the parameters estimates for men and women for each lipid measure in each prevention group, and Tables 25 and 26 show the SN ratios over follow-up. The mean age-adjusted lipid levels were higher in the UK patients than in the Japanese patients, although average age at baseline was higher in the UK (59 and 66 years in UK men and women, respectively, and 52 and 62 years in Japanese men and women, respectively). Similar trends were seen across all lipid measures, all prevention groups and in both men and women in the UK data. On average, there was only a small average change per year in the lipid measure but the variation around this was comparatively large. The estimated within-measurement variability was large for all lipid measures. The SN ratio exceeds 1 earlier in the secondary prevention patients taking statins – at approximately 5 years for all lipid measures in both men and women. For primary prevention patients taking statins, it was between 5 and 6 years after first measurement before the SN ratio was > 1, and for primary prevention patients not taking statins the time taken for the signal to exceed the noise was longer, at between 6 and 10 years depending on the lipid measure. The lipid ratios and HDL cholesterol appeared to have a stronger SN ratio.
Interpretation | α | β | γ | σw | σa | σb | σab |
---|---|---|---|---|---|---|---|
Mean lipid level at first measurement, at age zero | Mean annual change in lipid measurement | Effect of age at first measurement on mean lipid measurement | SD of the within-measurement variability of lipid | SD of lipid | SD of annual change in lipid | Covariance between actual value of lipid and rate of change | |
Primary prevention not taking statins | |||||||
TC | 6.27 (6.22 to 6.32) | –0.017 (–0.019 to –0.015) | –0.012 (–0.013 to –0.012) | 0.52 (0.52 to 0.52) | 0.90 | 0.06 | –0.012 |
HDL cholesterol | 1.12 (1.10 to 1.14) | –0.003 (–0.004 to –0.002) | 0.004 (0.003 to 0.004) | 0.18 (0.18 to 0.18) | 0.33 | 0.03 | –0.002 |
LDL cholesterol | 4.11 (4.06 to 4.15) | –0.013 (–0.016 to –0.011) | –0.010 (–0.011 to –0.010) | 0.47 (0.46 to 0.47) | 0.80 | 0.05 | –0.011 |
Non-HDL cholesterol | 5.15 (5.10 to 5.19) | –0.015 (–0.017 to –0.013) | –0.016 (–0.017 to –0.015) | 0.50 (0.50 to 0.50) | 0.91 | 0.06 | –0.015 |
TC/HDL cholesterol | 5.69 (5.62 to 5.75) | –0.001 (–0.004 to 0.002) | –0.021 (–0.022 to –0.020) | 0.62 (0.61 to 0.62) | 1.17 | 0.10 | –0.020 |
LDL/HDL cholesterol | 3.73 (3.68 to 3.78) | –0.002 (–0.005 to 0.000) | –0.015 (–0.016 to –0.014) | 0.50 (0.49 to 0.50) | 0.92 | 0.07 | –0.016 |
Primary prevention taking statins | |||||||
TC | 5.85 (5.77 to 5.93) | –0.021 (–0.024 to –0.018) | –0.021 (–0.022 to –0.020) | 0.63 (0.63 to 0.63) | 0.85 | 0.12 | –0.034 |
HDL cholesterol | 0.95 (0.91 to 0.98) | –0.002 (–0.003 to –0.001) | 0.005 (0.005 to 0.006) | 0.16 (0.16 to 0.16) | 0.35 | 0.04 | –0.004 |
LDL cholesterol | 3.61 (3.53 to 3.69) | –0.016 (–0.019 to –0.012) | –0.017 (–0.018 to –0.016) | 0.55 (0.55 to 0.56) | 0.74 | 0.11 | –0.024 |
Non-HDL cholesterol | 4.90 (4.82 to 4.98) | –0.021 (–0.024 to –0.017) | –0.026 (–0.027 to –0.025) | 0.61 (0.61 to 0.62) | 0.82 | 0.13 | –0.034 |
TC/HDL cholesterol | 5.82 (5.73 to 5.92) | –0.011 (–0.015 to –0.007) | –0.033 (–0.034 to –0.031) | 0.66 (0.66 to 0.67) | 0.98 | 0.14 | –0.044 |
LDL/HDL cholesterol | 3.50 (3.43 to 3.58) | –0.010 (–0.013 to –0.006) | –0.022 (–0.023 to –0.021) | 0.54 (0.54 to 0.55) | 0.76 | 0.11 | –0.027 |
Secondary prevention taking statins | |||||||
TC | 5.25 (5.16 to 5.34) | –0.018 (–0.021 to –0.015) | –0.016 (–0.017 to –0.015) | 0.55 (0.54 to 0.55) | 0.76 | 0.11 | –0.031 |
HDL cholesterol | 0.91 (0.87 to 0.95) | –0.004 (–0.005 to –0.003) | 0.005 (0.004 to 0.006) | 0.15 (0.15 to 0.15) | 0.32 | 0.03 | –0.002 |
LDL cholesterol | 3.10 (3.02 to 3.18) | –0.014 (–0.017 to –0.010) | –0.012 (–0.014 to –0.011) | 0.48 (0.47 to 0.48) | 0.65 | 0.10 | –0.023 |
Non-HDL cholesterol | 4.30 (4.22 to 4.39) | –0.015 (–0.018 to –0.012) | –0.020 (–0.022 to –0.019) | 0.52 (0.52 to 0.53) | 0.72 | 0.11 | –0.029 |
TC/HDL cholesterol | 5.37 (5.26 to 5.48) | –0.002 (–0.005 to 0.002) | –0.027 (–0.029 to –0.026) | 0.58 (0.58 to 0.59) | 0.91 | 0.12 | –0.033 |
LDL/HDL cholesterol | 3.16 (3.07 to 3.24) | –0.004 (–0.007 to –0.001) | –0.018 (–0.019 to –0.017) | 0.48 (0.48 to 0.49) | 0.70 | 0.10 | –0.023 |
Interpretation | α | β | γ | σw | σa | σb | σab |
---|---|---|---|---|---|---|---|
Mean lipid level at first measurement, at age 0 | Mean annual change in lipid measurement | Effect of age at first measurement on mean lipid measurement | SD of the within-measurement variability of lipid | SD of lipid | SD of annual change in lipid | Covariance between actual value of lipid and rate of change | |
Primary prevention not taking statins | |||||||
TC | 6.36 (6.29 to 6.43) | –0.011 (–0.014 to –0.009) | –0.006 (–0.007 to –0.005) | 0.55 (0.54 to 0.55) | 0.92 | 0.06 | –0.013 |
HDL cholesterol | 1.56 (1.53 to 1.60) | –0.003 (–0.004 to –0.002) | 0.001 (0.001 to 0.002) | 0.18 (0.18 to 0.18) | 0.41 | 0.04 | –0.003 |
LDL cholesterol | 4.17 (4.10 to 4.24) | –0.010 (–0.012 to –0.007) | –0.007 (–0.008 to –0.006) | 0.48 (0.47 to 0.48) | 0.83 | 0.06 | –0.015 |
Non-HDL cholesterol | 4.83 (4.76 to 4.91) | –0.009 (–0.011 to –0.006) | –0.008 (–0.009 to –0.007) | 0.52 (0.52 to 0.52) | 0.94 | 0.06 | –0.017 |
TC/HDL cholesterol | 4.35 (4.27 to 4.44) | 0.002 (–0.001 to 0.005) | –0.008 (–0.009 to –0.007) | 0.48 (0.48 to 0.48) | 1.04 | 0.09 | –0.021 |
LDL/HDL cholesterol | 2.87 (2.80 to 2.93) | –0.001 (–0.003 to 0.002) | –0.007 (–0.008 to –0.006) | 0.40 (0.40 to 0.40) | 0.84 | 0.07 | –0.016 |
Primary prevention taking statins | |||||||
TC | 5.56 (5.44 to 5.67) | –0.000 (–0.004 to 0.004) | –0.010 (–0.012 to –0.009) | 0.67 (0.66 to 0.67) | 0.87 | 0.13 | –0.026 |
HDL cholesterol | 1.11 (1.05 to 1.16) | –0.001 (–0.002 to 0.000) | 0.007 (0.006 to 0.007) | 0.17 (0.17 to 0.17) | 0.41 | 0.04 | –0.003 |
LDL cholesterol | 3.50 (3.39 to 3.61) | –0.003 (–0.007 to 0.001) | –0.013 (–0.014 to –0.011) | 0.60 (0.60 to 0.61) | 0.78 | 0.12 | –0.026 |
Non-HDL cholesterol | 4.47 (4.35 to 4.59) | –0.002 (–0.006 to 0.001) | –0.017 (–0.019 to –0.015) | 0.65 (0.65 to 0.65) | 0.86 | 0.13 | –0.030 |
TC/HDL cholesterol | 4.79 (4.66 to 4.91) | 0.001 (–0.002 to 0.005) | –0.022 (–0.023 to –0.020) | 0.56 (0.55 to 0.56) | 0.91 | 0.12 | –0.029 |
LDL/HDL cholesterol | 2.91 (2.81 to 3.01) | 0.000 (–0.003 to 0.003) | –0.016 (–0.017 to –0.015) | 0.48 (0.48 to 0.49) | 0.70 | 0.10 | –0.017 |
Secondary prevention taking statins | |||||||
TC | 5.09 (4.95 to 5.24) | –0.006 (–0.010 to –0.001) | –0.007 (–0.009 to –0.005) | 0.63 (0.62 to 0.63) | 0.81 | 0.12 | –0.025 |
HDL cholesterol | 1.17 (1.11 to 1.24) | –0.005 (–0.007 to –0.004) | 0.005 (0.004 to 0.006) | 0.18 (0.18 to 0.18) | 0.40 | 0.04 | –0.003 |
LDL cholesterol | 2.89 (2.75 to 3.03) | –0.002 (–0.007 to 0.002) | –0.007 (–0.009 to –0.005) | 0.56 (0.56 to 0.57) | 0.73 | 0.11 | –0.025 |
Non-HDL cholesterol | 3.90 (3.75 to 4.04) | –0.002 (–0.006 to 0.003) | –0.012 (–0.014 to –0.010) | 0.61 (0.60 to 0.61) | 0.81 | 0.12 | –0.030 |
TC/HDL cholesterol | 4.33 (4.17 to 4.48) | 0.007 (0.003 to 0.011) | –0.016 (–0.018 to –0.014) | 0.55 (0.55 to 0.55) | 0.87 | 0.11 | –0.024 |
LDL/HDL cholesterol | 2.44 (2.32 to 2.57) | 0.004 (0.000 to 0.008) | –0.011 (–0.012 to –0.009) | 0.45 (0.45 to 0.46) | 0.67 | 0.09 | –0.018 |
Lipid measure | SN ratio at different periods after baseline (years) | |||||||||
---|---|---|---|---|---|---|---|---|---|---|
1 | 2 | 3 | 4 | 5 | 6 | 7 | 8 | 9 | 10 | |
Primary prevention not taking statins | ||||||||||
TC | 0.01 | 0.05 | 0.12 | 0.21 | 0.32 | 0.46 | 0.63 | 0.82 | 1.04 | 1.28 |
HDL cholesterol | 0.03 | 0.12 | 0.27 | 0.47 | 0.74 | 1.07 | 1.45 | 1.90 | 2.40 | 2.97 |
LDL cholesterol | 0.01 | 0.05 | 0.11 | 0.19 | 0.30 | 0.43 | 0.58 | 0.76 | 0.96 | 1.19 |
Non-HDL cholesterol | 0.01 | 0.06 | 0.13 | 0.24 | 0.37 | 0.53 | 0.73 | 0.95 | 1.20 | 1.48 |
TC/HDL cholesterol | 0.02 | 0.10 | 0.22 | 0.39 | 0.61 | 0.88 | 1.20 | 1.56 | 1.98 | 2.44 |
LDL/HDL cholesterol | 0.02 | 0.09 | 0.20 | 0.35 | 0.55 | 0.79 | 1.08 | 1.41 | 1.78 | 2.20 |
Primary prevention taking statins | ||||||||||
TC | 0.04 | 0.15 | 0.35 | 0.62 | 0.96 | 1.39 | 1.89 | 2.47 | 3.12 | 3.85 |
HDL cholesterol | 0.06 | 0.24 | 0.54 | 0.96 | 1.50 | 2.16 | 2.94 | 3.84 | 4.85 | 5.99 |
LDL cholesterol | 0.04 | 0.15 | 0.34 | 0.60 | 0.94 | 1.35 | 1.84 | 2.41 | 3.04 | 3.76 |
Non-HDL cholesterol | 0.04 | 0.17 | 0.37 | 0.67 | 1.04 | 1.50 | 2.04 | 2.66 | 3.37 | 4.16 |
TC/HDL cholesterol | 0.04 | 0.17 | 0.39 | 0.70 | 1.09 | 1.57 | 2.13 | 2.79 | 3.53 | 4.35 |
LDL/HDL cholesterol | 0.04 | 0.16 | 0.37 | 0.65 | 1.02 | 1.47 | 2.00 | 2.61 | 3.30 | 4.08 |
Secondary prevention taking statins | ||||||||||
TC | 0.04 | 0.15 | 0.35 | 0.62 | 0.96 | 1.39 | 1.89 | 2.47 | 3.13 | 3.86 |
HDL cholesterol | 0.05 | 0.20 | 0.46 | 0.81 | 1.27 | 1.82 | 2.48 | 3.24 | 4.10 | 5.06 |
LDL cholesterol | 0.04 | 0.16 | 0.36 | 0.64 | 1.00 | 1.44 | 1.96 | 2.56 | 3.24 | 4.00 |
Non-HDL cholesterol | 0.04 | 0.16 | 0.37 | 0.65 | 1.02 | 1.46 | 1.99 | 2.60 | 3.30 | 4.07 |
TC/HDL cholesterol | 0.05 | 0.18 | 0.41 | 0.73 | 1.13 | 1.63 | 2.22 | 2.90 | 3.67 | 4.53 |
LDL/HDL cholesterol | 0.04 | 0.17 | 0.38 | 0.68 | 1.06 | 1.52 | 2.07 | 2.70 | 3.42 | 4.22 |
Lipid measure | SN ratio at different periods after baseline (years) | |||||||||
---|---|---|---|---|---|---|---|---|---|---|
1 | 2 | 3 | 4 | 5 | 6 | 7 | 8 | 9 | 10 | |
Primary prevention not taking statins | ||||||||||
TC | 0.01 | 0.05 | 0.11 | 0.19 | 0.30 | 0.44 | 0.59 | 0.78 | 0.98 | 1.21 |
HDL cholesterol | 0.04 | 0.16 | 0.35 | 0.63 | 0.98 | 1.41 | 1.92 | 2.51 | 3.18 | 3.92 |
LDL cholesterol | 0.02 | 0.06 | 0.14 | 0.24 | 0.38 | 0.55 | 0.75 | 0.97 | 1.23 | 1.52 |
Non-HDL cholesterol | 0.01 | 0.06 | 0.13 | 0.23 | 0.35 | 0.51 | 0.69 | 0.91 | 1.15 | 1.42 |
TC/HDL cholesterol | 0.03 | 0.14 | 0.31 | 0.56 | 0.87 | 1.26 | 1.71 | 2.23 | 2.83 | 3.49 |
LDL/HDL cholesterol | 0.03 | 0.13 | 0.29 | 0.51 | 0.80 | 1.15 | 1.57 | 2.04 | 2.59 | 3.20 |
Primary prevention taking statins | ||||||||||
TC | 0.04 | 0.15 | 0.34 | 0.60 | 0.94 | 1.35 | 1.84 | 2.40 | 3.04 | 3.76 |
HDL cholesterol | 0.05 | 0.21 | 0.47 | 0.84 | 1.31 | 1.88 | 2.56 | 3.34 | 4.23 | 5.22 |
LDL cholesterol | 0.04 | 0.15 | 0.34 | 0.60 | 0.94 | 1.36 | 1.85 | 2.41 | 3.05 | 3.77 |
Non-HDL cholesterol | 0.04 | 0.15 | 0.34 | 0.61 | 0.96 | 1.38 | 1.88 | 2.45 | 3.10 | 3.83 |
TC/HDL cholesterol | 0.05 | 0.18 | 0.41 | 0.73 | 1.14 | 1.65 | 2.24 | 2.93 | 3.70 | 4.57 |
LDL/HDL cholesterol | 0.04 | 0.16 | 0.36 | 0.63 | 0.99 | 1.42 | 1.94 | 2.53 | 3.20 | 3.95 |
Secondary prevention taking statins | ||||||||||
TC | 0.04 | 0.16 | 0.35 | 0.63 | 0.98 | 1.41 | 1.92 | 2.50 | 3.17 | 3.91 |
HDL cholesterol | 0.04 | 0.18 | 0.40 | 0.71 | 1.10 | 1.59 | 2.16 | 2.82 | 3.57 | 4.41 |
LDL cholesterol | 0.04 | 0.16 | 0.35 | 0.62 | 0.97 | 1.40 | 1.90 | 2.49 | 3.15 | 3.89 |
Non-HDL cholesterol | 0.04 | 0.16 | 0.36 | 0.64 | 1.01 | 1.45 | 1.97 | 2.58 | 3.26 | 4.02 |
TC/HDL cholesterol | 0.04 | 0.17 | 0.38 | 0.67 | 1.04 | 1.50 | 2.04 | 2.67 | 3.38 | 4.17 |
LDL/HDL cholesterol | 0.04 | 0.17 | 0.39 | 0.69 | 1.08 | 1.56 | 2.12 | 2.77 | 3.51 | 4.33 |
St Luke’s cohort recalibrated to the UK
Table 27 shows estimated variability and change parameters for men and women. The estimated within-measurement variability from the Japanese data set was smaller than that seen in the UK data: for example, TC/HDL cholesterol parameter estimates in men were 0.43 (0.43 to 0.44) in the Japanese data, and 0.62 (0.61 to 0.62) in the UK data. The estimates of the variation in the lipid value and annual rate of change were correspondingly larger, leading to a stronger SN ratio, as shown in Table 28.
Interpretation | α | β | γ | σw | σa | σb | σab |
---|---|---|---|---|---|---|---|
Mean lipid level at first measurement, at age 0 | Mean annual change in lipid measurement | Effect of age at first measurement on mean lipid measurement | SD of the within-measurement variability of lipid from the open St Luke’s cohort, Tokyo, Japan | SD of lipid | SD of annual change in lipid | Covariance between actual value of lipid and rate of change | |
Men | |||||||
TC | 6.25 (5.95 to 6.56) | –0.02 (–0.08 to 0.05) | –0.01 (–0.05 to 0.03) | 0.41 | 0.94 | 0.10 | –0.028 |
HDL cholesterol | 1.12 (0.93 to 1.31) | –0.00 (–0.04 to 0.04) | 0.00 (–0.02 to 0.03) | 0.16 | 0.34 | 0.04 | –0.003 |
LDL cholesterol | 4.10 (3.80 to 4.39) | –0.01 (–0.08 to 0.05) | –0.01 (–0.05 to 0.03) | 0.37 | 0.84 | 0.09 | –0.025 |
Non-HDL cholesterol | 5.13 (4.82 to 5.44) | –0.01 (–0.08 to 0.05) | –0.02 (–0.06 to 0.02) | 0.40 | 0.95 | 0.10 | –0.029 |
TC/HDL cholesterol | 5.67 (5.31 to 6.02) | 0.00 (–0.08 to 0.08) | –0.02 (–0.07 to 0.02) | 0.43 | 1.23 | 0.18 | –0.053 |
LDL/HDL cholesterol | 3.71 (3.40 to 4.03) | –0.00 (–0.08 to 0.07) | –0.02 (–0.06 to 0.03) | 0.34 | 0.98 | 0.15 | –0.043 |
Women | |||||||
TC | 6.35 (5.97 to 6.73) | –0.01 (–0.08 to 0.06) | –0.01 (–0.05 to 0.04) | 0.44 | 0.96 | 0.10 | –0.027 |
HDL cholesterol | 1.56 (1.31 to 1.82) | –0.00 (–0.05 to 0.04) | 0.00 (–0.03 to 0.03) | 0.17 | 0.42 | 0.04 | –0.003 |
LDL cholesterol | 4.16 (3.80 to 4.53) | –0.01 (–0.08 to 0.06) | –0.01 (–0.05 to 0.04) | 0.38 | 0.87 | 0.09 | –0.027 |
Non-HDL cholesterol | 4.82 (4.44 to 5.20) | –0.01 (–0.08 to 0.06) | –0.01 (–0.05 to 0.04) | 0.41 | 0.98 | 0.10 | –0.032 |
TC/HDL cholesterol | 4.34 (3.94 to 4.74) | 0.00 (–0.07 to 0.08) | –0.01 (–0.06 to 0.04) | 0.36 | 1.08 | 0.13 | –0.037 |
LDL/HDL cholesterol | 2.85 (2.49 to 3.22) | 0.00 (–0.07 to 0.07) | –0.01 (–0.05 to 0.04) | 0.30 | 0.87 | 0.11 | –0.029 |
Lipid measure | SN ratio at different periods after baseline (years) | |||||||||
---|---|---|---|---|---|---|---|---|---|---|
1 | 2 | 3 | 4 | 5 | 6 | 7 | 8 | 9 | 10 | |
Men | ||||||||||
TC | 0.06 | 0.24 | 0.54 | 0.95 | 1.49 | 2.14 | 2.92 | 3.81 | 4.82 | 5.95 |
HDL cholesterol | 0.06 | 0.25 | 0.56 | 0.99 | 1.55 | 2.24 | 3.04 | 3.98 | 5.03 | 6.21 |
LDL cholesterol | 0.07 | 0.27 | 0.61 | 1.08 | 1.69 | 2.43 | 3.30 | 4.32 | 5.46 | 6.74 |
Non-HDL cholesterol | 0.06 | 0.25 | 0.57 | 1.02 | 1.59 | 2.29 | 3.11 | 4.06 | 5.14 | 6.35 |
TC/HDL cholesterol | 0.17 | 0.67 | 1.51 | 2.68 | 4.19 | 6.03 | 8.21 | 10.72 | 13.56 | 16.74 |
LDL/HDL cholesterol | 0.19 | 0.78 | 1.75 | 3.12 | 4.87 | 7.01 | 9.54 | 12.46 | 15.77 | 19.47 |
Women | ||||||||||
TC | 0.05 | 0.20 | 0.45 | 0.80 | 1.24 | 1.79 | 2.44 | 3.19 | 4.03 | 4.98 |
HDL cholesterol | 0.05 | 0.22 | 0.49 | 0.86 | 1.35 | 1.94 | 2.65 | 3.46 | 4.38 | 5.40 |
LDL cholesterol | 0.06 | 0.25 | 0.55 | 0.99 | 1.54 | 2.22 | 3.02 | 3.95 | 4.99 | 6.17 |
Non-HDL cholesterol | 0.06 | 0.25 | 0.56 | 1.00 | 1.56 | 2.25 | 3.06 | 4.00 | 5.06 | 6.25 |
TC/HDL cholesterol | 0.14 | 0.57 | 1.28 | 2.27 | 3.55 | 5.11 | 6.95 | 9.08 | 11.49 | 14.18 |
LDL/HDL cholesterol | 0.15 | 0.58 | 1.32 | 2.34 | 3.65 | 5.26 | 7.16 | 9.36 | 11.84 | 14.62 |
Discussion
Recent research has noted that the variability of measures used for monitoring chronic conditions, such as lipid levels, may limit their usefulness. 30,32,206,207 These analyses are consistent with such previous research, showing that frequent measurement of lipid levels is more likely to identify change owing to biological variability and inherent measurement error than true changes in an individual’s health status. As clinical decisions on treatment are based upon these measurements, this may be detrimental to a patient’s health. Chapter 5 explores the impact of this variability on clinical decisions on statin use under different monitoring schedules.
The protocol initially specified that the models fitted in the St Luke’s cohort, and then recalibrated using the UK population using CPRD data, would be used to examine clinical decisions on treatment. However, the estimates of the within-person short-term variability were unexpectedly smaller in the St Luke’s cohort than in the UK cohort. Hypotheses to explain this included the following: the CPRD estimate was so inflated by the nature of routine clinical data (missed appointments varying laboratory methods, etc.) that the St Luke’s estimate was indeed an improved estimate but to a greater extent than anticipated; or that it is unsafe to make the modelling assumption that this short-term variability would be transportable between ethnic groups and settings. Under the former hypothesis it would be highly advisable to combine parameter estimates from the St Luke’s cohort and CPRD, as specified in the protocol. Under the latter hypothesis it would be highly inappropriate to do so. Therefore, we sought external data from the literature to inform the choice between these two interpretations and hence determine whether or not the methods in the protocol should be modified. The expected amount of short-term biological variability might be described as either a SD that is directly comparable to estimates from this chapter (σw), or as regression dilution ratios that can be calculated from estimates from this chapter. More detail is given in Appendix 17. Sparse literature on SD was found but multiple large studies reported regression dilution ratios of 0.69–0.78 in the UK for TC (see Appendix 17). For comparison, our models estimate the ratio to be 0.84 from St Luke’s recalibrated to the UK, or 0.75 from the CPRD. Similar results were found for other cholesterol subfractions. Thus the regression dilution ratio results provide support for estimating variability from CPRD alone, and models in Chapters 5 and 7 are therefore based upon parameters estimated directly to the CPRD data, rather than to St Luke’s data with recalibration to the UK. For completeness, the latter analyses (methods and results) are presented in Appendix 18.
We have used established methods to draw conclusions about the variability in the progression of dyslipidaemia in two strong data sets. Although these models are subject to a number of assumptions and limitations, such as the difficulty of external validation, the literature comparison of individual parameter estimates (see Appendix 17) show that individual parts of the model are consistent with other populations. Both sources of data have strengths and limitations. Data collection in the St Luke’s cohort is extremely rigorous and all tests were analysed in the same laboratory, limiting assay variation. However, findings may not be generalisable: the majority of patients have not had a CVD event, are not taking statin therapy, and are from only one institution in Tokyo. CPRD data are collected during routine practice rather than for research purposes, and may therefore be more prone to inaccuracies and bias; however, the data used for these analyses is based upon information such as test results and test dates that are largely uploaded to the computer system electronically. Moreover, these data are clearly representative of routine general practice in the UK.
Chapter 5 Simulation models for primary and secondary prevention in a UK population
Background
This chapter consists of results from modelling the impact different monitoring strategies might have on clinical decisions on statin use. Current UK guidelines recommend that primary prevention patients at high risk of CVD should be prescribed statins. 2,56 At the time of writing the project protocol, high CVD risk was defined as a 10-year CVD risk score of ≥ 20%, and the recommended risk scores, such as QRisk2,52 ASSIGN54 and Framingham 199153 all include the TC/HDL cholesterol ratio as a factor. In 2014, guidelines56 changed the definition of high risk to a 10-year CVD risk score of ≥ 10%, calculated using QRisk2. 52 Statin therapy is recommended for all secondary prevention patients; guidelines published in 2014 recommend that patients are prescribed 80 mg of atorvastatin, whereas earlier guidelines recommended a lower initiating dose, and that an increased dose should be considered when TC levels of > 4 mmol/l and/or LDL cholesterol levels of > 2 mmol/l are measured. 2 Thus, UK recommendations for changes to statin treatment are, at least partially, based upon measured lipid levels. However, as illustrated in Chapter 4, the estimated variation within each lipid measurement is greater than the likely change over 1 year, thus, monitoring at short intervals promotes unnecessary pharmacological treatment with high likelihood. Possible implications are labelling people with ‘normal’ or lower lipid levels as ‘sick’, complacency with regards to lifestyle changes and self-care, and lower adherence to medication later in life when increased age places patients at higher CVD risk and medication adherence may be more beneficial. The key concern of false-negative tests is that patients are receiving lower doses than those recommended, potentially leading to a raised risk of CVD. We applied established methods206,207 to data from the CPRD to estimate the rates of true and false test results, and examine the optimal interval for monitoring dyslipidaemia in primary and secondary prevention patients.
Methods
Model structure
We characterised a population of 100,000 men and 100,000 women for each prevention group (primary, primary on statins, and secondary on statins) through sampling with replacement from the CPRD. The populations were generated with the same distribution of observed lipid measures and baseline cardiovascular risk factors as patients in that prevention group in our CPRD data. Risk factors included those used to calculate 10-year CVD risk using QRisk252 and Framingham 1991,53 and were based on individuals without a history of familial hypercholesterolaemia who had any one lipid measurement (TC, HDL cholesterol, LDL cholesterol or TGs) recorded in 2006, the most recent complete year of data in our sample, for which the minimum age was 40 years for men and 55 years for women. This patient group was selected to represent those attending lipid monitoring. Prevention groups were defined as described in Chapter 4. CVD risk factors were defined in reference to the baseline date, which was the date of the first lipid measurement in 2006. Lipid measurements recorded on this date were used as the baseline observed readings. Age at baseline was defined from year of birth and the date of first lipid measurement. Each condition from diabetes, atrial fibrillation, chronic kidney disease, rheumatoid arthritis, use of BP medication, a family history of angina or heart attack in a first-degree relative of < 60 years of age or left-ventricular hypertrophy, was assumed to be present if there was a medical code relating to the condition in patient medical records up to 1 year after the baseline date. The first measurement recorded up to 1 year after baseline was used for baseline smoking status, BMI and systolic BP. Missing data for TC, HDL, LDL, TGs, BMI, systolic BP and smoking status were imputed using the multivariate chained equations method; lipid values and BMI were log transformed prior to imputing. 208 Models assumed that non-lipid CVD risk factors remain constant over time. Separate simulation analyses were run for each lipid, prevention group and gender.
Estimates of lipid variability and the between- and within person trends, derived in UK data in Chapter 4, were used to simulate lipid progression over time (see Tables 23 and 24). The ‘true’ lipid reading for each patient at baseline adjusted for age at measurement, assumed to be unobservable to the patient or clinician, was simulated conditional on the initial observed value, and then an individual annual rate of change was simulated (see Appendix 19). The simulated true lipid reading for each patient at each year of follow-up is then calculated using Equation 1 (see Chapter 4). The observed lipid value in any given sample is simulated from their true value at that time and the model estimate of the within-measurement variability of the lipid (see Equation 2, Chapter 4). For primary prevention patients, the true and observed 10-year CVD risk scores at each time point were also calculated.
The proportion of recommended treatment changes at each time point that are attributable to dyslipidaemia (true-positive results) can be estimated through calculating the proportion of changes for which both the simulated true and observed lipid values in secondary prevention patients, or both true and observed 10-year CVD risk scores in primary prevention patients, were above threshold; likewise the proportion attributable to within-person variability (false-positive results) can be estimated from the proportion for which the simulated observed lipid value/10-year CVD risk on which the decision was based was above threshold, while the true lipid level/10-year CVD risk was below threshold. Similarly, we can estimate the proportions of patients who correctly (true-negative) or incorrectly (false-negative) tested negative. The calculation of these proportions is illustrated in Table 29. For CIs, we repeated the simulations changing the model parameters to plausible values given the data, obtained through non-parametric bootstrapping. Random samples of 100,000 patients were drawn with replacement, 100 times from the simulated data set described above; for each, the simulation was then run 50 times and the average proportions of true-positive, false-positive, true-negative and false-negative treatment decisions were calculated. The SD across the 100 averages is used to estimate the SE and calculate CIs.
True lipid level or true 10-year CVD risk above treatment threshold: need treatment | Proportions of false treatment decisions | |||
No | Yes | |||
Observed lipid level or observed 10-year CVD risk above treatment threshold: Prescribed treatment | No | True negative [TN] | False negative [FN] | False negative = FN/(FN + TN) |
Yes | False positive [FP] | True positive [TP] | False positive = FP/(FP + TP) |
For each patient group, each lipid and both genders, we carried out simulation modelling for annual monitoring, biennial monitoring (every 2 years), triennial monitoring (every 3 years) and quinquennial monitoring (every 5 years). We first consider the proportions of false-positive and false-negative treatment decisions for strategies based on guidelines current, at the time of analysis,2 for which primary prevention treatment guidelines use CVD risk thresholds calculated using QRisk252 and secondary prevention uses lipid thresholds; we then consider how rates might be affected by monitoring using different lipids, different CVD risk scores and thresholds, and different patterns of measurement frequency.
Scenarios based on current guidelines
Primary prevention patients not taking statins
Our simulation assumed that monitoring would follow NICE guidance2 as follows:
-
Lipid screening for primary prevention patients begins at 40 years for men or 55 years for women.
-
A 10-year CVD risk score for the observed TC/HDL cholesterol ratio is calculated using the QRisk252 algorithm.
-
An observed risk of ≥ 20% classes a patient as high risk.
-
Patients not taking statins are eligible for a statin prescription if high risk.
-
Patients not eligible for a treatment change continue to be monitored and can hence be eligible for a statin prescription in subsequent years.
Therefore, a male aged ≥ 40 years and a female aged ≥ 55 years in the simulated primary prevention population are assumed to be eligible for a statin prescription if their 10-year CVD risk calculated from their observed lipid value is above the 20% threshold. We then classify a treatment change as a ‘true-positive’ if the calculated 10-year CVD risk based on the true lipid reading is above threshold, or a ‘false-positive’ if the calculated CVD risk based on true lipid reading is below threshold (as illustrated in Table 29). Similarly, when a patient is not eligible for a statin prescription because the observed lipid gave a 10-year CVD risk below threshold, we describe this as a ‘true-negative’ if their CVD risk calculated from their true lipid value is below threshold, and ‘false-negative’ if the true CVD risk is above threshold. Patients who are classed as a false-positive can later become true-positives if their ‘true’ CVD risk goes over the threshold. Patients who are true-positives remain classed as true-positives.
Primary prevention patients taking statins
Although NICE guidance suggests that monitoring in primary prevention patients already taking statins is not necessary,2 we examined the consequences of monitoring this group as described above, except that patients whose observed 10-year CVD risk was over threshold were eligible to be prescribed an intensified dose of statin.
Secondary prevention patients taking statins
Total and LDL cholesterol levels rather than CVD risk are used to guide treatment for secondary prevention patients. 2 The progression of TC and LDL were considered separately. Our simulation assumed that patients:
-
were already taking a statin
-
are eligible for treatment intensification if the modelled lipid reading was over recommended thresholds (4 mmol/l for TC, 2 mmol/l for LDL)
-
not eligible for an up-titration continue to be monitored and can hence be eligible to be prescribed a higher dose in subsequent years.
Therefore, a patient (male aged ≥ 40 years or female aged ≥ 55 years) in the simulated population is assumed to be eligible to be prescribed an intensified dose if the simulated observed lipid (either TC or LDL) was above threshold, (4 or 2 mmol/l, respectively). The proportions of true and false treatment decisions were calculated as previously described using the true and observed lipid values.
Scenarios based on alternative strategies for monitoring lipids
Framingham 1991 as an alternative 10-year cardiovascular risk score
Until 2014, NICE guidelines2 did not name a specific risk score for calculating 10-year CVD risk, allowing health-care practitioners to use a method best suited to their requirements, such as QRisk2,52 Framingham 199153 or ASSIGN,54 all of which are based upon TC and HDL. Therefore, we carried out simulation modelling for primary prevention men and women not taking statins using the Framingham 1991 risks score,53 on which the Joint British Societies risk charts10 are based, to guide treatment decisions.
Lowering the cardiovascular risk threshold for primary prevention patients
As noted in Chapter 1, NICE guidelines were being reviewed throughout the duration of this project,62 and it was thought that the risk threshold for primary prevention patients would be lowered. We therefore carried out simulation modelling using both 15% and 10% CVD risk thresholds, calculated using QRisk2. 52 In July 2014, revised guidelines lowered the treatment threshold to 10%.
Alternative lipid measures
We considered how results would vary if different lipid measures were used in monitoring. Based on the results from Chapter 2, we focused on TC, LDL and HDL only. For primary prevention patients, QRisk252 was edited, to use TC, LDL or LDL/HDL cholesterol instead of TC/HDL cholesterol to calculate a 10-year CVD risk. Simulation models were then run as described above for each lipid type, using a treatment eligibility threshold of 20%.
For secondary prevention patients we also examined monitoring using the ratios of TC/HDL cholesterol and LDL/HDL cholesterol. Thresholds for these lipid measures were selected from existing guidelines as far as possible: Table 30 summarises the rationale for each selected threshold.
Lipid measure | Threshold | Source of threshold |
---|---|---|
TC | 4 mmol/l | 2010 NICE guidance2 |
LDL | 2 mmol/l | 2010 NICE guidance2 |
TC/HDL cholesterol | 4 | 2010 NICE guidance for TC level,2 and a recommended goal of > 1 mmol/l for HDL209,210 |
LDL/HDL cholesterol | 2 | 2010 NICE guidance for LDL level,2 and a recommended value of > 1 mmol/l for HDL209,210 |
Alternative strategies for lipid measurement
Further simulation modelling was carried out, in which treatment eligibility decisions were based upon the average of repeated lipid measurements taken over a short time period; this strategy aimed to improve the accuracy of the lipid measurement on which treatment was based. Such repeated measurements could either be taken for all patients or limited to those whose first test is ‘near’ the NICE thresholds, for example ± x mmol/l from threshold; however, given the high variability in lipid levels, as described in Chapter 4, it was decided to model this repetition for all secondary prevention patients taking statins and primary prevention patients taking statins. The proportions of true- and false-positive treatment eligibility decisions under three possible alternative strategies were compared:
-
five repeat tests are used to calculate an average lipid level every 5 years
-
three repeat tests are averaged every 3 years, and
-
three repeat tests are averaged every 5 years.
Repeat tests were modelled at 1-month intervals and the mean observed lipid level was calculated. Proportions of true and false treatment decisions were then calculated as described above.
A further alternative monitoring strategy might be to update a subject’s risk score annually using a previous lipid measurement. We therefore also carried out additional simulations in the primary prevention population not taking statins, where the lipid reading used to calculate the 10-year CVD risk score was measured (1) once every 3 years, and (2) once every 5 years, but the risk score was calculated annually using the most recent lipid measure, risk factor data from baseline, and current – i.e. updated – age.
Amendments to protocol
The protocol initially specified that the parameters obtained from models fitted in the St Luke’s cohort and then recalibrated to the UK population using CPRD data would be used to examine clinical decisions on eligibility for treatment. However, as explained in Chapter 4 (see Discussion) and Appendix 17, estimates of the within-person short-term variability were unexpectedly smaller in the St Luke’s cohort than in the UK cohort, and, after comparison with external data from the literature, we proposed to the advisory board a protocol modification to use the direct CPRD estimates in our simulation models, as this adjustment meant that our models were more consistent with those reported in previous literature. This chapter therefore presents results based upon parameters estimated from the CPRD data; however, for completeness, Appendix 18 presents methods and results according to the original protocol.
Our protocol specified that calculations of 10-year CVD risk would be based upon the Framingham 1991 risk score;53 however; QRisk252 has been increasingly favoured in CVD prevention guidelines as the risk score of choice. The annual update to QRisk252 in 2012 allowed calculation of risk over periods of 1–10 years, thus facilitating its incorporation into health-economic models (presented in Chapter 7). Therefore, with the approval of our advisory board, QRisk252 was used as the reference CVD risk score in Chapters 5 and 7, and in Appendix 18 we also present analyses modelling treatment decisions based upon the Framingham risk score as originally specified.
Results
The baseline characteristics for the simulated data based on CPRD patients are shown for each gender/prevention group in Table 31.
Variable | Men | Women | ||||
---|---|---|---|---|---|---|
Primary, not taking statins | Primary, taking statins | Secondary, taking statins | Primary, not taking statins | Primary, taking statins | Secondary, taking statins | |
Mean (SD) | ||||||
Age, years | 60 (11) | 64 (10) | 69 (9) | 67 (8) | 69 (7) | 73 (7) |
TC, mmol/l | 5.45 (1.06) | 4.51 (1.04) | 4.18 (0.88) | 5.93 (1.08) | 4.84 (1.07) | 4.55 (0.97) |
HDL, mmol/l | 1.33 (0.37) | 1.30 (0.41) | 1.26 (0.35) | 1.65 (0.45) | 1.56 (0.45) | 1.54 (0.42) |
LDL, mmol/l | 3.41 (0.95) | 2.47 (0.89) | 2.25 (0.76) | 3.62 (0.97) | 2.59 (0.93) | 2.35 (0.84) |
TGs, mmol/l | 1.72 (1.18) | 1.83 (1.22) | 1.57 (0.94) | 1.51 (0.90) | 1.63 (0.90) | 1.56 (0.82) |
TC/HDL cholesterol, mmol/l | 4.35 (1.29) | 3.68 (1.16) | 3.50 (1.05) | 3.80 (1.12) | 3.29 (1.02) | 3.12 (0.93) |
LDL/HDL cholesterol, mmol/l | 2.75 (1.06) | 2.04 (0.93) | 1.92 (0.84) | 2.36 (0.92) | 1.78 (0.82) | 1.64 (0.75) |
Non-HDL cholesterol, mmol/l | 4.12 (1.05) | 3.21 (1.02) | 2.92 (0.85) | 4.27 (1.08) | 3.28 (1.04) | 3.01 (0.94) |
Systolic BP, mmHg | 139 (18) | 139 (17) | 135 (17) | 141 (19) | 141 (17) | 138 (19) |
BMI, kg/m2 | 28.31 (5.15) | 29.09 (5.06) | 28.21 (4.67) | 27.87 (5.67) | 29.01 (5.86) | 28.07 (5.74) |
% (n) | ||||||
Smoking status | ||||||
Non-smoker | 35.82 (35,823) | 26.04 (26,039) | 18.26 (18,257) | 49.43 (49,429) | 42.85 (42,854) | 36.68 (36,677) |
Ex-smoker | 44.50 (44,501) | 54.32 (54,322) | 65.29 (65,291) | 36.67 (36,667) | 42.58 (42,578) | 48.61 (48,606) |
Light smoker (< 10 cigarettes/day) | 5.35 (5352) | 5.75 (5752) | 6.57 (6572) | 4.78 (4784) | 5.85 (5850) | 5.37 (5366) |
Moderate smoker (10–19 cigarettes/day) | 7.38 (7383) | 7.43 (7433) | 5.94 (5938) | 5.29 (5291) | 5.30 (5297) | 5.77 (5769) |
Heavy smoker (≥ 20 cigarettes/day) | 6.94 (6941) | 6.45 (6454) | 3.94 (3942) | 3.83 (3829) | 3.42 (3421) | 3.58 (3582) |
History of diabetesa | 13.05 (13,045) | 44.68 (44,683) | 24.42 (24,417) | 9.30 (9297) | 38.02 (38,021) | 25.27 (25,273) |
History of atrial fibrillationa | 3.71 (3714) | 5.41 (5414) | 11.98 (11,979) | 3.30 (3301) | 4.62 (4623) | 11.20 (11,195) |
History of rheumatoid arthritisa | 1.13 (1125) | 1.08 (1081) | 1.92 (1919) | 2.76 (2756) | 2.54 (2542) | 3.48 (3475) |
History of chronic kidney diseasea | 0.66 (655) | 1.92 (1917) | 2.38 (2378) | 0.47 (466) | 1.49 (1490) | 2.26 (2257) |
Angina or heart attack in a first-degree relativea | 5.02 (5019) | 6.05 (6052) | 7.88 (7875) | 4.90 (4904) | 6.69 (6689) | 9.47 (9467) |
On BP treatmenta | 42.07 (42,065) | 61.98 (61,979) | 54.64 (54,638) | 54.55 (54,554) | 69.67 (69,674) | 65.77 (65,772) |
History of left ventricular hypertrophyb | 0.60 (601) | 1.00 (1005) | 1.15 (1152) | 0.56 (560) | 0.93 (926) | 1.14 (1142) |
Primary prevention not taking statins
Results of modelling the rate of eligibility for statin prescriptions for males and females in this group are shown in Figure 21 and Tables 32 and 33. Figure 21 shows the percentage of tests that are false-positives (left) and false-negatives (right) in men and women, for annual, biennial, triennial and quinquennial monitoring strategies over 15 years of follow-up. More frequent monitoring resulted in a higher proportion of patients incorrectly recommended for a statin prescription (left). The proportion of patients incorrectly recommended for a statin prescription decreased over time as patients moved from being classed as false-positive to true-positive (as their underlying CVD risk increased). The proportion of patients incorrectly not recommended for a statin was higher when monitoring occurred at longer intervals. Over follow-up, there was a gradual increase in the proportion of false-negative tests for each monitoring interval. As all non-lipid risk factors other than age were held constant and, on average, the change in lipid levels was approximately zero (see Tables 23 and 24), over time, an increasing proportion of the population is classed as at high CVD risk as a result of population ageing, and therefore an increasing proportion of patients who should be eligible for treatment are missed.
FIGURE 21.
Men and women, primary prevention not taking statins, QRisk252 (TC/HDL cholesterol) using ≥ 20% as threshold. Model estimates for the percentage of changes in treatment that are attributable to the within-measurement variability (false-positive) rather than true changes in lipid value; and the percentage of those not prescribed a change in treatment who are incorrectly classified (false-negative), reported at intervals following a first test using screening intervals of 1, 2, 3 and 5 years.

Years since first test | (1) Cumulative number of positive treatment changes per 1000 (95% CI) | (2) Percentage of positive treatment changes that are false (95% CI) | (3) Percentage of negative treatment changes that are false (95% CI) | |||||||||
---|---|---|---|---|---|---|---|---|---|---|---|---|
Every year | Every 2 years | Every 3 years | Every 5 years | Every year | Every 2 years | Every 3 years | Every 5 years | Every year | Every 2 years | Every 3 years | Every 5 years | |
0 | 422 (422 to 422) | 422 (422 to 422) | 422 (422 to 422) | 422 (422 to 422) | 3.5 (3.4 to 3.6) | 3.5 (3.4 to 3.6) | 3.5 (3.4 to 3.6) | 3.5 (3.4 to 3.6) | 3.0 (2.9 to 3.1) | 3.0 (2.9 to 3.1) | 3.0 (2.9 to 3.1) | 3.0 (2.9 to 3.1) |
1 | 464 (463 to 464) | 5.1 (5.0 to 5.3) | 1.7 (1.7 to 1.8) | |||||||||
2 | 496 (495 to 497) | 486 (485 to 487) | 5.5 (5.3 to 5.7) | 4.3 (4.1 to 4.5) | 1.5 (1.5 to 1.5) | 2.6 (2.5 to 2.6) | ||||||
3 | 525 (524 to 527) | 512 (511 to 512) | 5.3 (5.1 to 5.6) | 3.7 (3.5 to 3.8) | 1.4 (1.4 to 1.5) | 3.2 (3.1 to 3.2) | ||||||
4 | 554 (553 to 556) | 544 (543 to 545) | 5.1 (4.9 to 5.3) | 4.0 (3.8 to 4.2) | 1.5 (1.5 to 1.5) | 2.7 (2.7 to 2.8) | ||||||
5 | 583 (581 to 584) | 566 (565 to 567) | 4.8 (4.6 to 5.0) | 3.0 (2.9 to 3.1) | 1.6 (1.6 to 1.6) | 4.0 (3.8 to 4.1) | ||||||
6 | 610 (609 to 612) | 601 (599 to 602) | 597 (596 to 598) | 4.5 (4.3 to 4.7) | 3.5 (3.3 to 3.6) | 3.0 (2.9 to 3.2) | 1.6 (1.6 to 1.7) | 3.0 (2.9 to 3.1) | 3.7 (3.6 to 3.8) | |||
. . . | ||||||||||||
10 | 713 (712 to 715) | 705 (703 to 706) | 699 (697 to 701) | 3.4 (3.3 to 3.6) | 2.7 (2.6 to 2.8) | 2.1 (2.0 to 2.2) | 2.0 (1.9 to 2.0) | 3.6 (3.5 to 3.7) | 5.0 (4.8 to 5.2) | |||
12 | 760 (758 to 762) | 752 (750 to 754) | 749 (747 to 750) | 3.0 (2.9 to 3.1) | 2.3 (2.2 to 2.4) | 2.1 (2.0 to 2.1) | 2.2 (2.1 to 2.2) | 4.0 (3.8 to 4.1) | 4.8 (4.7 to 5.0) | |||
15 | 823 (821 to 825) | 813 (811 to 815) | 811 (809 to 813) | 2.5 (2.3 to 2.6) | 1.7 (1.6 to 1.7) | 1.5 (1.4 to 1.5) | 2.6 (2.5 to 2.6) | 5.7 (5.5 to 5.9) | 6.4 (6.2 to 6.7) |
Years since first test | (1) Cumulative number of positive treatment changes per 1000 (95% CI) | (2) Percentage of positive treatment changes that are false (95% CI) | (3) Percentage of negative treatment changes that are false (95% CI) | |||||||||
---|---|---|---|---|---|---|---|---|---|---|---|---|
Every year | Every 2 years | Every 3 years | Every 5 years | Every year | Every 2 years | Every 3 years | Every 5 years | Every year | Every 2 years | Every 3 years | Every 5 years | |
0 | 385 (385 to 385) | 385 (385 to 385) | 385 (385 to 385) | 385 (385 to 385) | 2.8 (2.7 to 2.9) | 2.8 (2.7 to 2.9) | 2.8 (2.7 to 2.9) | 2.8 (2.7 to 2.9) | 1.9 (1.9 to 2.0) | 1.9 (1.9 to 2.0) | 1.9 (1.9 to 2.0) | 1.9 (1.9 to 2.0) |
1 | 422 (422 to 422) | 3.7 (3.5 to 3.8) | 1.4 (1.3 to 1.4) | |||||||||
2 | 454 (454 to 455) | 449 (449 to 449) | 3.6 (3.5 to 3.8) | 2.9 (2.8 to 3.0) | 1.3 (1.3 to 1.3) | 1.9 (1.9 to 2.0) | ||||||
3 | 486 (485 to 486) | 479 (479 to 480) | 3.5 (3.3 to 3.6) | 2.5 (2.5 to 2.6) | 1.4 (1.4 to 1.4) | 2.2 (2.1 to 2.3) | ||||||
4 | 518 (517 to 518) | 513 (512 to 513) | 3.3 (3.2 to 3.5) | 2.6 (2.5 to 2.8) | 1.5 (1.5 to 1.5) | 2.2 (2.1 to 2.2) | ||||||
5 | 551 (550 to 552) | 544 (543 to 545) | 3.2 (3.1 to 3.3) | 2.3 (2.2 to 2.4) | 1.7 (1.6 to 1.7) | 2.7 (2.6 to 2.8) | ||||||
6 | 585 (584 to 586) | 580 (579 to 581) | 579 (578 to 580) | 3.1 (2.9 to 3.2) | 2.5 (2.4 to 2.6) | 2.3 (2.2 to 2.4) | 1.9 (1.8 to 1.9) | 2.7 (2.6 to 2.8) | 2.9 (2.8 to 3.0) | |||
. . . | ||||||||||||
10 | 731 (729 to 732) | 725 (724 to 727) | 724 (722 to 726) | 2.5 (2.4 to 2.6) | 2.0 (1.9 to 2.0) | 1.8 (1.7 to 1.8) | 3.0 (3.0 to 3.1) | 4.3 (4.2 to 4.4) | 4.8 (4.6 to 4.9) | |||
12 | 802 (800 to 804) | 797 (795 to 799) | 796 (794 to 798) | 2.1 (2.0 to 2.1) | 1.6 (1.6 to 1.7) | 1.5 (1.5 to 1.6) | 3.9 (3.8 to 4.0) | 5.5 (5.4 to 5.6) | 5.9 (5.7 to 6.1) | |||
15 | 894 (892 to 896) | 890 (888 to 892) | 890 (888 to 891) | 1.4 (1.3 to 1.4) | 1.0 (1.0 to 1.0) | 1.0 (0.9 to 1.0) | 5.7 (5.5 to 5.9) | 8.6 (8.2 to 9.0) | 8.8 (8.4 to 9.1) |
Tables 32 and 33 show the cumulative number eligible for statin prescriptions, the percentage of those that are false-positives, and the percentage of those repeatedly testing negative that are false-negative. Each outcome is shown for annual, biennial, triennial and quinquennial monitoring strategies. Column 1 of Table 32 shows the modelled rate of eligibility for statin prescriptions per 1000 men in the first year after monitoring begins, and the cumulative rate of eligibility for statin prescriptions over the subsequent years assuming 1-, 2-, 3- and 5-yearly monitoring. For example, over the first 6 years of follow-up, annual monitoring would result in 610 per 1000 men being eligible for a statin prescription, whereas biennial and triennial monitoring would result in 601 per 1000 men and 597 per 1000 men eligible for prescriptions, respectively. Over 15 years, annual, triennial and quinquennial monitoring would result in 823, 813 and 811 per 1000 men eligible for prescriptions. Column 2 of Table 32 shows the estimated proportion of individuals who are eligible for statin prescriptions that are incorrectly treated (false-positives), as a percentage of the total number of men who are eligible for a statin prescription, if monitoring is conducted every 1, 2, 3 and 5 years. The percentage of true-positives can be obtained by subtracting the false-positives from 100%. At the first monitoring test, 3.5% of recommended treatment changes can be attributed to variability in the TC/HDL cholesterol measurements (false-positive), whereas 96.5% of recommended statin prescriptions would be attributable to dyslipidaemia (true-positives). This corresponds to about 15 per 1000 men (422 per 1000 prescriptions × 3.5% false) recommended for treatment unnecessarily. At 6 years after first measurement, we estimate that the proportion of false-positive prescriptions under annual, biennial and triennial monitoring would be 4.5%, 3.5% and 3.0%, respectively (corresponding to about 27, 21 and 18 per 1000 men being over-treated), and by 15 years, under annual, triennial and quinquennial monitoring, 2.5%, 1.7% and 1.5% respectively (corresponding to about 21, 14 and 12 men per 1000). The estimated proportion of patients who were incorrectly not recommended for a statin (false-negatives) over follow-up under annual, biennial, triennial and quinquennial monitoring is shown in column 3. At first test 3% of men are incorrectly not recommended for a statin, corresponding to 17 men per 1000 (578 men are not treated × 3.0% false). At 6 years, the proportions of men incorrectly not recommended for a statin are 1.6%, 3.0% or 3.7% when monitoring intervals of 1, 2 or 3 years, respectively. These proportions correspond to 6, 12 or 15 men per 1000. Similar results for women are shown in Tables 33.
Primary prevention taking statins
Corresponding estimates for modelling the rate of eligibility for an intensified dose of statin under different monitoring schedules in primary prevention patients taking statins are shown in Figure 22 and Tables 34 (men) and 35 (women). Similar trends were seen to primary prevention patients not taking statins.
FIGURE 22.
Men and women, primary prevention taking statins, QRisk252 (TC/HDL cholesterol) using ≥ 20% as threshold. Model estimates for the percentage of changes in treatment that are attributable to the within-measurement variability (false-positive) rather than true changes in lipid value, and the percentage of those not prescribed a change in treatment who are incorrectly classified (false-negative), reported at intervals following a first test using screening intervals of 1, 2, 3 and 5 years.

Years since first test | (1) Cumulative number of positive treatment changes per 1000 (95% CI) | (2) Percentage of positive treatment changes that are false (95% CI) | (3) Percentage of negative treatment changes that are false (95% CI) | |||||||||
---|---|---|---|---|---|---|---|---|---|---|---|---|
Every year | Every 2 years | Every 3 years | Every 5 years | Every year | Every 2 years | Every 3 years | Every 5 years | Every year | Every 2 years | Every 3 years | Every 5 years | |
0 | 664 (664 to 664) | 664 (664 to 664) | 664 (664 to 664) | 664 (664 to 664) | 2.9 (2.8 to 2.9) | 2.9 (2.8 to 2.9) | 2.9 (2.8 to 2.9) | 2.9 (2.8 to 2.9) | 6.0 (5.8 to 6.1) | 6.0 (5.8 to 6.1) | 6.0 (5.8 to 6.1) | 6.0 (5.8 to 6.2) |
1 | 708 (707 to 709) | 3.9 (3.8 to 4.0) | 3.4 (3.3 to 3.5) | |||||||||
2 | 739 (738 to 740) | 728 (727 to 729) | 4.0 (3.9 to 4.1) | 3.2 (3.1 to 3.2) | 2.7 (2.7 to 2.8) | 4.9 (4.8 to 5.0) | ||||||
3 | 765 (764 to 766) | 750 (749 to 751) | 3.8 (3.7 to 3.9) | 2.6 (2.5 to 2.7) | 2.6 (2.5 to 2.6) | 6.0 (5.9 to 6.1) | ||||||
4 | 789 (788 to 790) | 779 (778 to 780) | 3.6 (3.5 to 3.7) | 2.8 (2.7 to 2.9) | 2.6 (2.6 to 2.6) | 4.9 (4.9 to 5.0) | ||||||
5 | 812 (810 to 813) | 795 (794 to 795) | 3.3 (3.2 to 3.4) | 2.0 (1.9 to 2.0) | 2.7 (2.7 to 2.7) | 7.6 (7.4 to 7.7) | ||||||
6 | 832 (831 to 834) | 823 (822 to 824) | 819 (818 to 820) | 3.0 (2.9 to 3.1) | 2.4 (2.3 to 2.4) | 2.1 (2.0 to 2.1) | 2.8 (2.8 to 2.9) | 5.4 (5.3 to 5.4) | 6.7 (6.6 to 6.9) | |||
. . . | ||||||||||||
10 | 897 (896 to 899) | 891 (890 to 892) | 886 (884 to 887) | 2.0 (1.9 to 2.1) | 1.6 (1.5 to 1.6) | 1.2 (1.1 to 1.2) | 3.0 (2.9 to 3.1) | 5.8 (5.7 to 6.0) | 8.7 (8.5 to 9.0) | |||
12 | 921 (920 to 922) | 916 (914 to 917) | 913 (912 to 914) | 1.6 (1.5 to 1.6) | 1.2 (1.2 to 1.3) | 1.0 (1.0 to 1.1) | 3.0 (2.9 to 3.1) | 5.9 (5.7 to 6.0) | 7.5 (7.2 to 7.7) | |||
15 | 947 (946, 948) | 942 (941 to 943) | 941 (940 to 942) | 1.1 (1.0 to 1.1) | 0.7 (0.7 to 0.7) | 0.6 (0.6 to 0.6) | 3.0 (2.9 to 3.1) | 7.5 (7.2 to 7.8) | 9.0 (8.6 to 9.3) |
Years since first test | (1) Cumulative number of positive treatment changes per 1000 (95% CI) | (2) Percentage of positive treatment changes that are false (95% CI) | (3) Percentage of negative treatment changes that are false (95% CI) | |||||||||
---|---|---|---|---|---|---|---|---|---|---|---|---|
Every year | Every 2 years | Every 3 years | Every 5 years | Every year | Every 2 years | Every 3 years | Every 5 years | Every year | Every 2 years | Every 3 years | Every 5 years | |
0 | 568 (568 to 568) | 568 (568 to 568) | 568 (568 to 568) | 568 (568 to 568) | 2.5 (2.4 to 2.6) | 2.5 (2.5 to 2.6) | 2.5 (2.5 to 2.6) | 2.5 (2.5 to 2.6) | 4.2 (4.1 to 4.3) | 4.2 (4.1 to 4.3) | 4.2 (4.1 to 4.3) | 4.2 (4.1 to 4.3) |
1 | 615 (615 to 616) | 3.5 (3.4 to 3.6) | 2.7 (2.6 to 2.7) | |||||||||
2 | 653 (652 to 654) | 645 (645 to 646) | 3.6 (3.5 to 3.7) | 2.8 (2.7 to 2.9) | 2.4 (2.4 to 2.5) | 3.8 (3.7 to 3.9) | ||||||
3 | 688 (687 to 689) | 678 (677 to 679) | 3.5 (3.4 to 3.6) | 2.4 (2.4 to 2.5) | 2.6 (2.6 to 2.6) | 4.6 (4.5 to 4.7) | ||||||
4 | 723 (721 to 724) | 715 (714 to 716) | 3.2 (3.1 to 3.3) | 2.5 (2.4 to 2.6) | 2.8 (2.8 to 2.9) | 4.4 (4.4 to 4.5) | ||||||
5 | 755 (754 to 756) | 745 (743 to 746) | 2.9 (2.8 to 3.0) | 1.9 (1.8 to 1.9) | 3.1 (3.0 to 3.1) | 5.8 (5.6 to 5.9) | ||||||
6 | 786 (784 to 787) | 779 (778 to 780) | 777 (776 to 778) | 2.5 (2.4 to 2.6) | 2.0 (1.9 to 2.1) | 1.8 (1.7 to 1.8) | 3.3 (3.2 to 3.3) | 5.1 (5.0 to 5.2) | 5.8 (5.7 to 5.9) | |||
. . . | ||||||||||||
10 | 885 (884 to 887) | 881 (880 to 882) | 879 (878 to 880) | 1.5 (1.4 to 1.5) | 1.1 (1.1 to 1.2) | 1.0 (0.9 to 1.0) | 4.2 (4.1 to 4.3) | 6.4 (6.3 to 6.6) | 7.6 (7.4 to 7.8) | |||
12 | 922 (921 to 924) | 919 (918 to 920) | 918 (917 to 919) | 1.1 (1.0 to 1.1) | 0.8 (0.8 to 0.8) | 0.7 (0.7 to 0.8) | 4.7 (4.6 to 4.8) | 7.2 (7.0 to 7.4) | 8.1 (7.8 to 8.3) | |||
15 | 962 (961 to 963) | 960 (959 to 961) | 960 (959 to 960) | 0.6 (0.5 to 0.6) | 0.4 (0.4 to 0.4) | 0.4 (0.4 to 0.4) | 5.6 (5.4 to 5.9) | 9.5 (9.1 to 9.9) | 9.7 (9.3 to 10.1) |
Secondary prevention taking statins
Figure 23 and Tables 36 and 37 show corresponding estimates in secondary prevention patients taking statins when eligibility for treatment change to an intensified dose of statin was based upon a TC threshold. Estimates based upon LDL measurements are presented in Figure 24 and Tables 38 and 39. The proportions of both false-positive and false-negative decisions with regards to eligibility for treatment were relatively large. The proportion of patients recommended for up-titration unnecessarily (false-positives) was greater when the monitoring interval was shorter. After 3 years of follow-up, 27.1% of men recommended for a higher statin dose were up-titrated unnecessarily when monitoring every year using a TC threshold of 4 mmol/l compared with 21.9% when monitoring at 3-year intervals. This corresponds to 224 per 1000 men and 157 per 1000 men, respectively (825 per 1000 up-titrations × 27.1% false and 718 per 1000 men × 21.9% false). In women, using a TC threshold of 4 mmol/l, the equivalent proportions recommended for unnecessary up-titration were 14.6% and 12.2%, corresponding to 136 per 1000 women and 105 per 1000 women recommended for unnecessary treatment under annual and triennial monitoring, respectively. In contrast, more frequent monitoring resulted in a lower proportion of patients eligible for treatment being missed. At 3 years since first test, the proportion of under-treated men was 3.7% under annual monitoring using a TC threshold of 4 mmol/l, and 12.7% under triennial monitoring, corresponding to 6 per 1000 men and 36 per 1000 men, respectively. The equivalent proportions in women were 7.9% and 24.0% or 5 per 1000 women and 33 per 1000 women under annual and triennial monitoring, respectively. When each of TC and LDL levels in secondary prevention patients were first measured, approximately one-quarter to one-third of men and almost half of women were estimated to be recommended too low a dose of statin; the proportion of patients recommended too low a treatment dose decreased over follow-up. However, the high numbers of patients who test over threshold, and are therefore eligible for an up-titration, mean this corresponds to between 111 per 1000 (men, TC 4 mmol/l) and 134 per 1000 (women, LDL 2 mmol/l) patients being under-treated at first test, dropping to between 35 to 36 per 1000 (men, TC 4 mmol/l) and 38 and 42 per 1000 (women, LDL 2 mmol/l) at second test (depending on the monitoring interval).
FIGURE 23.
Men and women, secondary prevention taking statins, using TC ≥ 4 mmol/l as threshold. Model estimates for the percentage of changes in treatment that are attributable to the within-measurement variability (false-positive) rather than true changes in lipid value; and the percentage of those not prescribed a change in treatment who are incorrectly classified (false-negative), reported at intervals following a first test using screening intervals of 1, 2, 3 and 5 years.
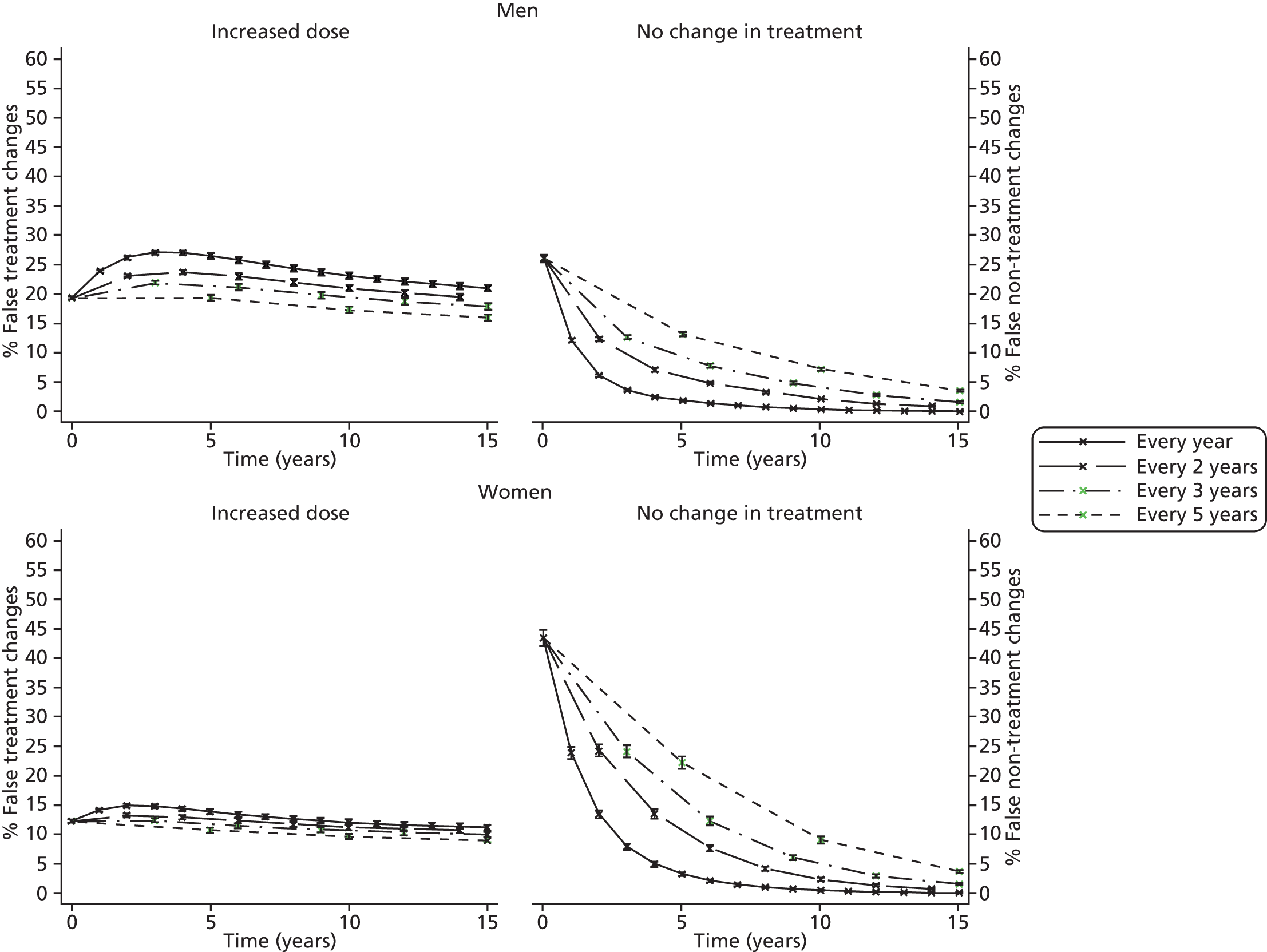
Years since first test | (1) Cumulative number of positive treatment changes per 1000 (95% CI) | (2) Percentage of positive treatment changes that are false (95% CI) | (3) Percentage of negative treatment changes that are false (95% CI) | |||||||||
---|---|---|---|---|---|---|---|---|---|---|---|---|
Every year | Every 2 years | Every 3 years | Every 5 years | Every year | Every 2 years | Every 3 years | Every 5 years | Every year | Every 2 years | Every 3 years | Every 5 years | |
0 | 573 (573 to 573) | 573 (573 to 573) | 573 (573 to 573) | 573 (573 to 573) | 19.4 (19.1 to 19.6) | 19.4 (19.1 to 19.6) | 19.4 (19.1 to 19.7) | 19.4 (19.1 to 19.6) | 26.1 (25.5 to 26.7) | 26.1 (25.5 to 26.7) | 26.1 (25.5 to 26.7) | 26.1 (25.4 to 26.7) |
1 | 714 (712 to 716) | 24.0 (23.8 to 24.2) | 12.2 (11.8 to 12.5) | |||||||||
2 | 783 (780 to 786) | 715 (713 to 718) | 26.3 (26.0 to 26.5) | 23.1 (22.8 to 23.3) | 6.2 (6.0 to 6.4) | 12.4 (12.0 to 12.8) | ||||||
3 | 825 (821 to 828) | 718 (716 to 721) | 27.1 (26.7 to 27.4) | 21.9 (21.6 to 22.3) | 3.7 (3.5 to 3.9) | 12.7 (12.3 to 13.1) | ||||||
4 | 853 (850 to 857) | 787 (784 to 791) | 27.0 (26.6 to 27.4) | 23.7 (23.4 to 24.1) | 2.5 (2.4 to 2.7) | 7.2 (6.9 to 7.5) | ||||||
5 | 873 (870 to 877) | 727 (723 to 730) | 26.5 (26.0 to 26.9) | 19.4 (19.0 to 19.8) | 1.9 (1.8 to 2.1) | 13.2 (12.7 to 13.7) | ||||||
6 | 888 (884 to 892) | 829 (826 to 833) | 791 (788 to 795) | 25.8 (25.3 to 26.3) | 23.0 (22.5 to 23.5) | 21.2 (20.7 to 21.7) | 1.5 (1.4 to 1.6) | 4.9 (4.6 to 5.2) | 7.8 (7.5 to 8.2) | |||
. . . | ||||||||||||
10 | 918 (914 to 922) | 872 (868 to 876) | 798 (793 to 802) | 23.1 (22.5 to 23.7) | 21.0 (20.4 to 21.5) | 17.3 (16.8 to 17.8) | 0.5 (0.4 to 0.5) | 2.2 (2.1 to 2.3) | 7.3 (7.0 to 7.6) | |||
12 | 925 (921 to 929) | 882 (878 to 887) | 852 (848 to 857) | 22.1 (21.6 to 22.7) | 20.2 (19.6 to 20.7) | 18.7 (18.2 to 19.3) | 0.3 (0.2 to 0.3) | 1.4 (1.3 to 1.5) | 2.9 (2.7 to 3.0) | |||
15 | 931 (927 to 935) | 865 (860 to 869) | 828 (824 to 833) | 21.0 (20.5 to 21.6) | 17.9 (17.4 to 18.4) | 16.0 (15.5 to 16.5) | 0.1 (0.1 to 0.1) | 1.7 (1.6 to 1.8) | 3.7 (3.5 to 3.8) |
Years since first test | (1) Cumulative number of positive treatment changes per 1000 (95% CI) | (2) Percentage of positive treatment changes that are false (95% CI) | (3) Percentage of negative treatment changes that are false (95% CI) | |||||||||
---|---|---|---|---|---|---|---|---|---|---|---|---|
Every year | Every 2 years | Every 3 years | Every 5 years | Every year | Every 2 years | Every 3 years | Every 5 years | Every year | Every 2 years | Every 3 years | Every 5 years | |
0 | 737 (737 to 737) | 737 (737 to 737) | 737 (737 to 737) | 737 (737 to 737) | 12.1 (11.8 to 12.3) | 12.1 (11.8 to 12.3) | 12.1 (11.8 to 12.4) | 12.1 (11.8 to 12.3) | 43.3 (41.9 to 44.7) | 43.3 (42.0 to 44.7) | 43.3 (41.9 to 44.6) | 43.3 (41.9 to 44.6) |
1 | 859 (856 to 861) | 14.0 (13.8 to 14.2) | 23.8 (22.8 to 24.8) | |||||||||
2 | 907 (904 to 910) | 861 (859 to 863) | 14.7 (14.4 to 14.9) | 13.1 (12.8 to 13.3) | 13.3 (12.6 to 14.1) | 24.2 (23.2 to 25.2) | ||||||
3 | 932 (930 to 935) | 863 (861 to 866) | 14.6 (14.3 to 14.9) | 12.2 (11.9 to 12.5) | 7.9 (7.3 to 8.4) | 24.0 (23.0 to 25.1) | ||||||
4 | 947 (944 to 950) | 910 (907 to 912) | 14.2 (13.8 to 14.6) | 12.7 (12.4 to 13.1) | 4.9 (4.5 to 5.3) | 13.5 (12.7 to 14.3) | ||||||
5 | 956 (954 to 959) | 868 (865 to 871) | 13.7 (13.3 to 14.1) | 10.6 (10.2 to 10.9) | 3.2 (2.9 to 3.5) | 22.1 (21.1 to 23.2) | ||||||
6 | 962 (960 to 965) | 932 (930 to 935) | 910 (907 to 913) | 13.3 (12.8 to 13.7) | 12.1 (11.7 to 12.5) | 11.3 (10.9 to 11.7) | 2.1 (1.9 to 2.3) | 7.6 (7.0 to 8.1) | 12.2 (11.5 to 13.0) | |||
. . . | ||||||||||||
10 | 973 (971 to 975) | 951 (948 to 954) | 908 (905 to 912) | 11.9 (11.4 to 12.4) | 11.1 (10.6 to 11.5) | 9.5 (9.1 to 9.9) | 0.4 (0.4 to 0.5) | 2.3 (2.1 to 2.5) | 9.0 (8.4 to 9.6) | |||
12 | 975 (973 to 977) | 955 (952 to 957) | 939 (936 to 942) | 11.4 (11.0 to 11.9) | 10.7 (10.3 to 11.2) | 10.1 (9.7 to 10.6) | 0.2 (0.2 to 0.2) | 1.3 (1.2 to 1.4) | 3.0 (2.7 to 3.2) | |||
15 | 976 (974 to 979) | 944 (940 to 947) | 922 (919 to 926) | 11.0 (10.5 to 11.5) | 9.8 (9.4 to 10.2) | 8.9 (8.5 to 9.3) | 0.1 (0.0 to 0.1) | 1.5 (1.4 to 1.6) | 3.6 (3.4 to 3.9) |
FIGURE 24.
Men and women, secondary prevention taking statins, using LDL ≥ 2 mmol/l as threshold. Model estimates for the percentage of changes in treatment that are attributable to the within-measurement variability (false-positive) rather than true changes in lipid value; and the percentage of those not prescribed a change in treatment who are incorrectly classified (false-negative), reported at intervals following a first test using screening intervals of 1, 2, 3 and 5 years.

Years since first test | (1) Cumulative number of positive treatment changes per 1000 (95% CI) | (2) Percentage of positive treatment changes that are false (95% CI) | (3) Percentage of negative treatment changes that are false (95% CI) | |||||||||
---|---|---|---|---|---|---|---|---|---|---|---|---|
Every year | Every 2 years | Every 3 years | Every 5 years | Every year | Every 2 years | Every 3 years | Every 5 years | Every year | Every 2 years | Every 3 years | Every 5 years | |
0 | 623 (623 to 623) | 623 (623 to 623) | 623 (623 to 623) | 623 (623 to 623) | 15.9 (15.5 to 16.2) | 15.8 (15.5 to 16.1) | 15.9 (15.6 to 16.2) | 15.9 (15.5 to 16.2) | 32.0 (31.3 to 32.7) | 32.0 (31.3 to 32.7) | 32.0 (31.3 to 32.8) | 32.0 (31.2 to 32.7) |
1 | 766 (764 to 769) | 19.8 (19.5 to 20.0) | 16.0 (15.4 to 16.5) | |||||||||
2 | 832 (829 to 835) | 769 (766 to 771) | 21.6 (21.3 to 21.9) | 18.8 (18.5 to 19.1) | 8.5 (8.2 to 8.9) | 16.4 (15.8 to 17.0) | ||||||
3 | 870 (867 to 873) | 772 (770 to 775) | 22.0 (21.7 to 22.4) | 17.7 (17.4 to 18.1) | 5.1 (4.8 to 5.4) | 16.6 (16.0 to 17.3) | ||||||
4 | 894 (891 to 898) | 836 (833 to 840) | 21.8 (21.4 to 22.2) | 19.1 (18.8 to 19.5) | 3.5 (3.2 to 3.7) | 9.6 (9.1 to 10.1) | ||||||
5 | 911 (907 to 914) | 780 (776 to 784) | 21.2 (20.8 to 21.7) | 15.4 (15.1 to 15.8) | 2.5 (2.3 to 2.8) | 16.8 (16.1 to 17.5) | ||||||
6 | 923 (919 to 926) | 874 (870 to 877) | 840 (836 to 844) | 20.6 (20.1 to 21.0) | 18.4 (18.0 to 18.8) | 17.0 (16.5 to 17.4) | 1.9 (1.7 to 2.0) | 6.2 (5.8 to 6.6) | 9.8 (9.3 to 10.3) | |||
. . . | ||||||||||||
10 | 945 (941 to 948) | 907 (903 to 912) | 843 (838 to 847) | 18.3 (17.8 to 18.8) | 16.7 (16.3 to 17.2) | 13.8 (13.4 to 14.3) | 0.5 (0.5 to 0.6) | 2.4 (2.2 to 2.6) | 8.3 (7.8 to 8.7) | |||
12 | 949 (946 to 953) | 915 (911 to 919) | 890 (886 to 894) | 17.5 (17.0 to 18.0) | 16.1 (15.6 to 16.6) | 15.0 (14.6 to 15.5) | 0.2 (0.2 to 0.3) | 1.4 (1.3 to 1.6) | 3.0 (2.8 to 3.3) | |||
15 | 953 (950 to 957) | 899 (895 to 904) | 867 (863 to 872) | 16.7 (16.2 to 17.2) | 14.4 (14.0 to 14.9) | 12.9 (12.4 to 13.3) | 0.1 (0.1 to 0.1) | 1.7 (1.6 to 1.8) | 3.8 (3.6 to 4.0) |
Years since first test | (1) Cumulative number of positive treatment changes per 1000 (95% CI) | (2) Percentage of positive treatment changes that are false (95% CI) | (3) Percentage of negative treatment changes that are false (95% CI) | |||||||||
---|---|---|---|---|---|---|---|---|---|---|---|---|
Every year | Every 2 years | Every 3 years | Every 5 years | Every year | Every 2 years | Every 3 years | Every 5 years | Every year | Every 2 years | Every 3 years | Every 5 years | |
0 | 658 (658 to 658) | 658 (658 to 658) | 658 (658 to 658) | 658 (658 to 658) | 14.9 (14.6 to 15.2) | 14.9 (14.6 to 15.2) | 14.9 (14.6 to 15.2) | 14.9 (14.6, 15.2) | 39.2 (38.0 to 40.4) | 39.2 (38.1 to 40.4) | 39.2 (38.0 to 40.4) | 39.2 (38.0 to 40.4) |
1 | 808 (805 to 811) | 17.6 (17.4 to 17.9) | 21.4 (20.4 to 22.3) | |||||||||
2 | 871 (868 to 875) | 812 (809 to 815) | 18.7 (18.3 to 19.1) | 16.5 (16.1 to 16.8) | 12.1 (11.4 to 12.9) | 22.2 (21.2 to 23.2) | ||||||
3 | 905 (902 to 909) | 816 (812 to 819) | 18.7 (18.2 to 19.2) | 15.3 (14.9 to 15.8) | 7.5 (6.9 to 8.0) | 22.5 (21.4 to 23.6) | ||||||
4 | 926 (922 to 930) | 876 (872 to 880) | 18.2 (17.6 to 18.8) | 16.2 (15.6 to 16.7) | 4.9 (4.4 to 5.4) | 13.1 (12.2 to 14.0) | ||||||
5 | 939 (936 to 943) | 824 (819 to 828) | 17.5 (16.9 to 18.2) | 13.1 (12.6 to 13.7) | 3.3 (2.9 to 3.7) | 21.6 (20.4 to 22.8) | ||||||
6 | 948 (944 to 952) | 908 (904 to 913) | 879 (874 to 884) | 16.8 (16.2 to 17.5) | 15.3 (14.7 to 15.9) | 14.2 (13.6 to 14.8) | 2.3 (2.0 to 2.6) | 7.7 (7.0 to 8.5) | 12.4 (11.4 to 13.3) | |||
. . . | ||||||||||||
10 | 964 (960 to 967) | 935 (930 to 939) | 879 (874 to 885) | 14.9 (14.2 to 15.6) | 13.8 (13.1 to 14.5) | 11.6 (11.0 to 12.2) | 0.5 (0.4, 0.6) | 2.6 (2.3 to 2.9) | 9.5 (8.7 to 10.3) | |||
12 | 967 (963 to 970) | 940 (936 to 945) | 920 (914 to 925) | 14.3 (13.5 to 15.0) | 13.3 (12.6 to 14.0) | 12.5 (11.8 to 13.1) | 0.2 (0.2 to 0.3) | 1.5 (1.3 to 1.7) | 3.3 (2.9 to 3.6) | |||
15 | 969 (965 to 973) | 926 (921 to 932) | 899 (893 to 905) | 13.6 (12.9 to 14.3) | 12.0 (11.4 to 12.6) | 10.9 (10.3 to 11.4) | 0.1 (0.1 to 0.1) | 1.7 (1.5 to 1.9) | 4.0 (3.6 to 4.4) |
Alternative strategies for monitoring
Lowering the CVD risk threshold for primary prevention patients
We evaluated the impact of a reduction on the CVD risk threshold to 15% and 10%. Prescription eligibility rates and estimates of the proportion of these that are unnecessary (false-positives) for monitoring in primary prevention men and women not currently taking statins and based on a CVD risk threshold of 15% and 10% are shown in Figures 25 and 26. Trends were similar to those seen with a 20% CVD risk threshold, with higher proportion of false-positive eligibility decisions and lower proportions of false-negative eligibility decisions when monitoring is more frequent, although the proportion of false-positive eligibility decisions decreased as the threshold lowered, whereas the proportion of false-negative treatment eligibility decisions was higher.
FIGURE 25.
Men and women, primary prevention not taking statins, QRisk252 (TC/HDL cholesterol) using ≥ 15% as threshold. Model estimates for the percentage of changes in treatment that are attributable to the within-measurement variability (false-positive) rather than true changes in lipid value; and the percentage of those not prescribed a change in treatment who are incorrectly classified (false-negative), reported at intervals following a first test using screening intervals of 1, 2, 3 and 5 years.
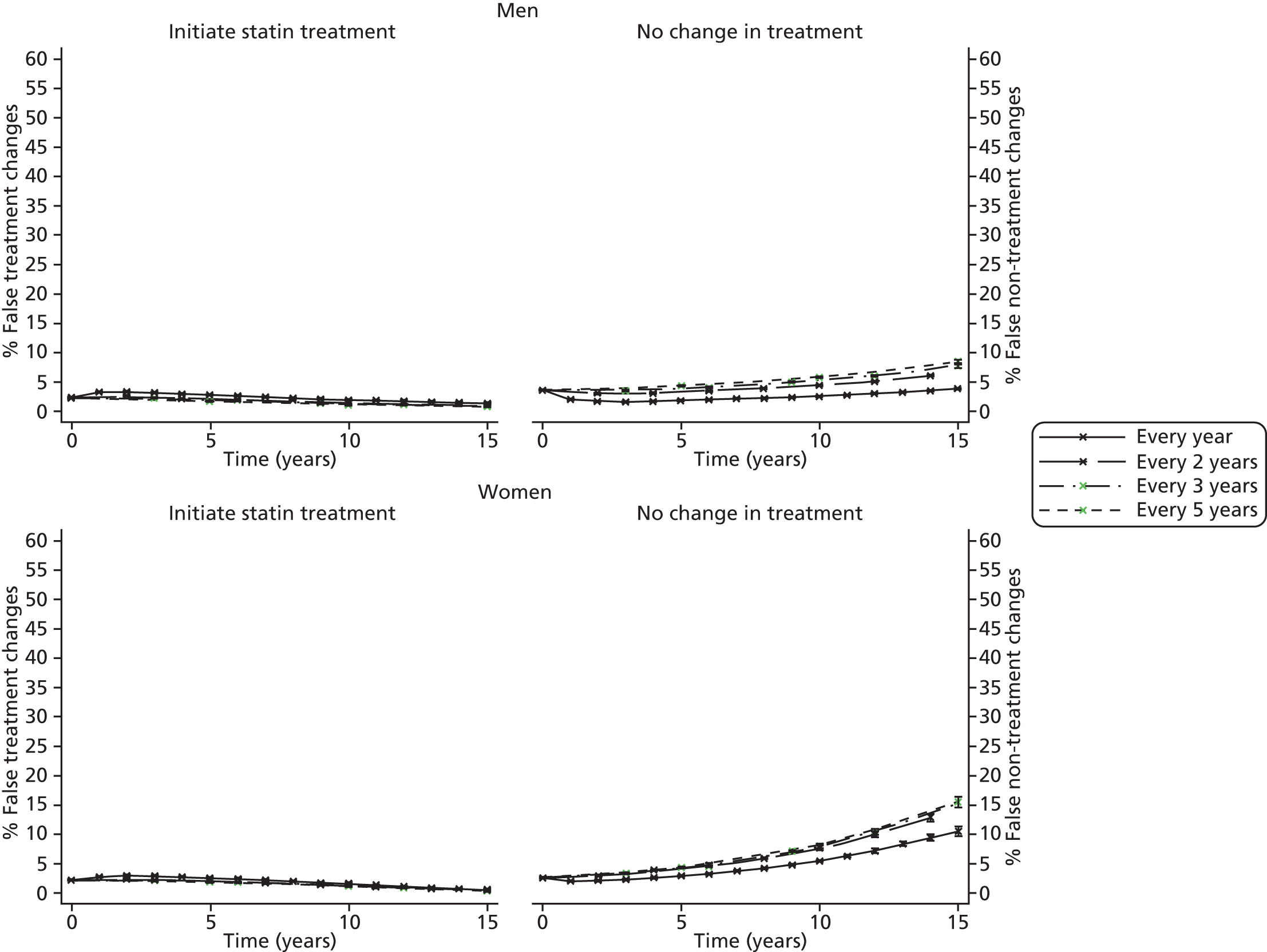
FIGURE 26.
Men and women, primary prevention not taking statins, QRisk252 (TC/HDL cholesterol) using ≥ 10% as threshold. Model estimates for the percentage of changes in treatment that are attributable to the within-measurement variability (false-positive) rather than true changes in lipid value; and the percentage of those not prescribed a change in treatment who are incorrectly classified (false-negative), reported at intervals following a first test using screening intervals of 1, 2, 3 and 5 years.

Alternative cardiovascular risk measures
Corresponding figures for the following alternative monitoring strategies listed below are presented in Appendix 20:
-
Primary prevention not taking statins using Framingham 1991 as risk score.
-
Primary prevention not taking statins using modified QRisk252 based on TC, LDL and LDL/HDL cholesterol instead of TC/HDL cholesterol.
-
Secondary prevention using the ratio of TC/HDL cholesterol instead of TC, and LDL/HDL cholesterol instead of LDL.
Similar trends as described above were seen in all the alternative scenarios.
Alternative strategies for lipid measurement: updating on single or multiple measures
In our primary analysis (above) we assumed that, in primary prevention, if lipids are measured less than annually then CVD risk would also be estimated less than annually (at each lipid measurement). For completeness we now consider strategies in which lipids are measured every 3 or 5 years, but CVD risk is nonetheless recalculated annually, using the most recent available lipid measurement and current age. Figures 27 and 28 compare these strategies with our primary analysis (CVD risk estimated only when lipid is measured). Periodic measurement with annual calculation of CVD risk has led to a non-monotonic trend in estimates of the patients both over- and under-treated, although this is likely an artefact of the simulation, as in practice subjects would not be measured at the same time point. Proportions of false-positives (unnecessary changes to treatment) were higher when the alternative strategy guided treatment than when monitoring occurred at longer intervals but were lower than under annual monitoring. Higher proportions of false-negative tests (undertreatment) were seen when monitoring at longer intervals than when using the alternative strategy; annual monitoring gave the lowest proportion of false-negatives.
FIGURE 27.
Men and women, primary prevention not taking statins, QRisk252 (TC/HDL cholesterol) using ≥ 20% as threshold, Recalculating QRisk2 yearly based on measures every 3 years. Model estimates for the percentage of changes in treatment that are attributable to the within-measurement variability (false-positive) rather than true changes in lipid value; and the percentage of those not prescribed a change in treatment who are incorrectly classified (false-negative), reported at intervals following a first test.

FIGURE 28.
Men and women, primary prevention not taking statins, QRisk252 (TC/HDL cholesterol) using ≥ 20% as threshold, recalculating QRisk252 yearly based on measures every 5 years. Model estimates for the percentage of changes in treatment that are attributable to the within-measurement variability (false-positive) rather than true changes in lipid value; and the percentage of those not prescribed a change in treatment who are incorrectly classified (false-negative), reported at intervals following a first test.

We explored how using the average of multiple lipid measures could impact on the proportions of incorrect eligibility decisions amongst both primary and secondary prevention populations taking statins. Estimates comparing this alternative monitoring strategy with annual measurement are presented in Figures 29–34. Similar trends were seen in both prevention groups. The proportion of incorrect treatment eligibility decisions was smaller when decisions were based upon the average of a larger number of repeat measurements, with a lower proportion of both false-positive and false-negative decisions when the average of five repeated measurements was used to guide treatment, although annual monitoring with only one measurement still gave a lower proportion of under-treated patients. When strategies with assumed similar total cost were compared (see Appendix 20, Figures 135–137) for primary and secondary patients on statins, respectively, more repeated measurements with a longer interval gave a lower proportion of false-positive eligibility decisions, and estimates of the proportion of false-negative eligibility decisions were similar.
FIGURE 29.
Men and women, primary prevention taking statins, QRisk252 (TC/HDL cholesterol) using ≥ 20% as threshold, impact of using the average of three measurements. Model estimates for the percentage of changes in treatment that are attributable to the within-measurement variability (false-positive) rather than true changes in lipid value, and the percentage of those not prescribed a change in treatment who are incorrectly classified (false-negative), reported at intervals following a first test.

FIGURE 30.
Men and women, primary prevention taking statins, QRisk252 (TC/HDL cholesterol) using ≥ 20% as threshold, impact of using the average of five measurements. Model estimates for the percentage of changes in treatment that are attributable to the within-measurement variability (false-positive) rather than true changes in lipid value, and the percentage of those not prescribed a change in treatment who are incorrectly classified (false-negative), reported at intervals following a first test.

FIGURE 31.
Men and women, secondary prevention taking statins using TC ≥ 4 mmol/l as threshold, impact of using the average of three measurements. Model estimates for the percentage of changes in treatment that are attributable to the within-measurement variability (false-positive) rather than true changes in lipid value, and the percentage of those not prescribed a change in treatment who are incorrectly classified (false-negative), reported at intervals following a first test.

FIGURE 32.
Men and women, secondary prevention taking statins using TC ≥ 4 mmol/l as threshold, impact of using the average of five measurements. Model estimates for the percentage of changes in treatment that are attributable to the within-measurement variability (false-positive) rather than true changes in lipid value, and the percentage of those not prescribed a change in treatment who are incorrectly classified (false-negative), reported at intervals following a first test.

FIGURE 33.
Men and women, secondary prevention taking statins using LDL ≥ 2 mmol/l as threshold, impact of using the average of three measurements. Model estimates for the percentage of changes in treatment that are attributable to the within-measurement variability (false-positive) rather than true changes in lipid value, and the percentage of those not prescribed a change in treatment who are incorrectly classified (false-negative), reported at intervals following a first test.

FIGURE 34.
Men and women, secondary prevention taking using LDL ≥ 2 mmol/l as threshold, impact of using the average of five measurements. Model estimates for the percentage of changes in treatment that are attributable to the within-measurement variability (false-positive) rather than true changes in lipid value, and the percentage of those not prescribed a change in treatment who are incorrectly classified (false-negative), reported at intervals following a first test.

Discussion
Using parameters presented in Chapter 4, we modelled the rate of eligibility for statin prescriptions, quantifying the proportions of incorrect treatment eligibility decisions when monitoring at intervals of 1, 2, 3 and 5 years. The high variability in each lipid measurement resulted in recommendations for unnecessary initiation or up-titration (false-positive ) and undertreatment (false-negative) in all patient groups and under all scenarios considered. Consistent with previous research on long-term chronic conditions such as hypertension, renal function and hypercholesterolaemia,30,204,206,207 we found that more frequent monitoring resulted in a higher proportion of false-positive tests, which, in these analyses, corresponds to a higher proportion of patients incorrectly recommended for a statin prescription or an up-titration. This is attributable to the low true rate of change over time. Conversely, the proportion of patients incorrectly not recommended for a statin or an increased dose (false-negative results) was lower when monitoring at shorter intervals. However, given the comparatively high numbers of patients over threshold and hence recommended for treatment (in both primary and secondary prevention groups), fewer absolute numbers of patients were undertreated as opposed to unnecessarily recommended for an up-titration.
Given that such lipid measurements guide clinical decisions, this may be detrimental to a patient’s health, and alternative monitoring strategies that aim to estimate the underlying lipid levels more accurately may be preferable. Previous research examining optimal screening intervals for cholesterol has not examined the proportion of under-treated patients. 30,31 As statin treatment has been shown to reduce CVD risk, even when patients have low lipid levels, whether or not potential overtreatment in these patients is detrimental requires a full health economics analysis that can account for the potential harms of over and undertreatment; this is the focus of Chapter 7.
Updated guidelines recommended lowering the CVD risk threshold for initiation of statin treatment in primary prevention patients to 10%. As we lower the treatment threshold, a greater proportion of the population will have a true CVD risk that is over the threshold, and this means that more negative test results are likely to be false. However, as the proportion of patients testing positive will increase, fewer patients will therefore test negative. For example, when monitoring every year or 3 years, a threshold of 20% led to 1.6% or 3.7% of men incorrectly not recommended for treatment at 6 years since first test, whereas a threshold of 10% led to 3.1% and 5.4%, respectively. These proportions correspond to 6 per 1000 and 15 per 1000 men incorrectly not recommended for treatment with a 20% threshold, and 5 per 1000 and 8 per 1000 men at a 10% threshold. The proportion of patients incorrectly not recommended for treatment increases with age under both thresholds, however as the proportion of patients recommended for treatment also increases with age, when the threshold is lowered, the absolute number of patients affected is lower.
An alternative to reduce the measurement variability would be the use of the average of a number of measures (e.g. three TC measures taken over time). However, QRisk252 was developed based upon risk factors measured in a primary care database (QResearch), where single measurements of TC and HDL were used to estimate CVD risk. Any change in the variability of a factor included in a risk score (such as systolic BP or TC) would impact on the performance of the score. Therefore, a limitation of these additional analyses is that we did not modify the regression coefficients in the risk equation to account for the potential regression dilution effect. In Chapter 6 we discuss the expected impact of these monitoring alternatives on QRisk2. 52
We have used modelling techniques rather than primary data (such as that obtained from a randomised clinical trial design); however, the feasibility of collecting data in the scale required for an experimental design testing diagnostic or monitoring strategies is unlikely, and therefore we have used established methods to quantify the long-term implications of monitoring in data representative of routine general practice in the UK.
One limitation of these analyses is the (unlikely) assumption that CVD risk factors other than age and lipid level remain constant over time; however, this project focuses on lipid levels as a risk factor for CVD, and accurately modelling the changes in each risk factor in QRisk252 was beyond the scope of this project (requiring alternative modelling as seen in Chapter 4 for each of the factors included in the risk score).
In May 2012, the UK patent for atorvastatin expired and this may affect statin prescribing. We found that a high proportion of secondary prevention patients who should have been in the control phase of monitoring (i.e. they should already have been recommended for an up-titration to a therapeutic dose) were prescribed too low a dose of statin according to current guidelines. One possible explanation for this might be availability of appropriate statins. These simulations are based upon patient characteristics from 2006. In 2006, guidance for these patients recommended initial treatment with simvastatin 40 mg, and the higher-intensity statin choices were limited to simvastatin 80 mg,2 a dose of simvastatin that further guidance57,211 suggests limiting to only those patients who have severe hypercholesterolaemia and a high risk of cardiovascular complications. Updated local guidelines59 recommend initial use of atorvastatin 40 mg, with the option of potential up-titration to atorvastatin 80 mg. Thus, patients whose lipid levels are not controlled by simvastatin 40 mg might now be prescribed atorvastatin 40 mg, which is associated with a higher percentage reduction in LDL. 59 We were not able to investigate the effects recent potential changes to statin prescribing might have on the proportions of false-positive and false-negative treatment eligibility decisions with the available data. CPRD data was available for the period of May to 21 September 2012; however, the proportion of patients prescribed atorvastatin and simvastatin among secondary prevention patients who were prescribed statins, was similar to that seen in 2006 (atorvastatin: 30% in 2012, 34% in 2006) and it may take time before prescription patterns change.
The threshold for guiding treatment in primary prevention patients was lowered from 20% to 10% in July 2014;56 sensitivity analyses using thresholds of both 15% and 10% showed similar patterns, although the estimated proportion of under-treated patients increased as the threshold decreased. In addition, there is some variation in the interpretation of the 2010 NICE guidelines for secondary prevention; specifically whether both or only one of TC and LDL is over threshold for up-titration to be considered. 2,59 Our models do not enable us to examine the progression of both TC and LDL simultaneously so we have instead examined monitoring schedules for each lipid individually. A joint model to guide treatment changes could be part of future research, although the updated guidelines recommend that secondary prevention patients are prescribed 80 mg of atorvastatin,56 and, as such, there is no higher dose for which subjects would need to be monitored for up-titration.
Analyses are restricted to a population attending monitoring. We have not attempted to model the complexity of individual treatment decision-making; however, the analyses presented here aim to form the basis for evaluation of national monitoring strategies. Neither have we attempted to quantify the relative harms of false-positive and false-negative treatment eligibility decisions; this is addressed in Chapter 7, where we extend the work presented here to a full health-economic model that estimates the relative cost-effectiveness of different monitoring strategies.
Chapter 6 Impact on risk scores of different approaches to lipid measurement
Background
The risk of CVD can be assessed using tools such as QRisk2,52 ASSIGN54 and Framingham 1991,53 all of which include the TC/HDL cholesterol ratio. As noted previously (see Chapters 1 and 5), the latest NICE guidance on lipid monitoring for primary prevention populations2 recommends using such risk scores to estimate 10-year risk of developing CVD to identify patients at ‘high risk’ and hence guide treatment decisions, such as the prescription of statins. The 2010 high-risk threshold was a 10-year risk of ≥ 20%, with the choice of risk calculator left to the GP. Updated guidance in 2014 recommended lowering the treatment threshold to 10% and the use of QRisk252 to calculate risk. 56 The methods in this chapter therefore focus on 10-year CVD risk calculation using QRisk2,52 and include both 10% and 20% risk thresholds.
Although findings in Chapter 2 suggest that there is unlikely to be much improvement in risk calculators if other common lipids (TC, HDL, LDL cholesterol as single measurements or ratios) were used in place of TC/HDL cholesterol, there was some indication that alternative measures such as non-HDL cholesterol or Apo B may be more predictive in some population subgroups. If risk scores were recalculated to use different lipid measurements, such as non-HDL cholesterol or Apo B, some patients, currently classified as low risk, would be reclassified as high CVD risk, and vice versa; we examine the effect that modifying QRisk252 in this way would have on the proportions of patients in different risk categories, and whether or not reclassified subjects are predominantly those close to the risk threshold. As Apo B is not widely available in general practice, analyses presented in this chapter therefore focus on modification of risk scores to use non-HDL cholesterol, which can be calculated from TC and HDL measurements and does not need fasting blood samples.
There is also potential to refine cardiovascular risk estimation within a lipid monitoring programme by taking the average of lipid measurements on two or more separate occasions; as shown in Chapter 4, the high level of within-person variability means that using the average of a number of measurements, taken over a short time period, might better represent the true underlying lipid level for that patient at that time point. This would reduce the within-measurement variability (see Table 23, column 4) of the resulting estimate. Regression dilution theory shows that coefficients in a generalised linear model, such as a risk score, are affected by within-person variability. 212 Specifically, they are attenuated (closer to 0) with increased variability. It follows that if the variability of the measure is to be reduced, for example by taking an average, the coefficient in the risk score must be increased. 213 Here we use the results of Chapter 2 to estimate the modifications necessary to use CVD risk calculators with the mean of three and five lipid measurements.
Methods
Open source C code to calculate QRisk252 is available online,214 and version QRisk2–2012 (Q68_QRisk2_2012, created on 3 January 2012) was downloaded. QRisk252 is updated approximately annually; we did not update to the QRisk2–2013, as the latest date in CPRD data available in this project was 21 September 2012. The C code was compiled for use with both Windows and Linux machines using Microsoft Visual C++ 2010 Express (Microsoft Corporation, Redmond, WA, USA) and the GNU Compiler Collection (GCC) compiler, respectively. A plug-in was then created using Stata, version 12.1, to calculate QRisk252 directly from the C code. 215
Alternative lipid measures: non-HDL cholesterol
Risk estimates calculated using a single measurement of TC/HDL cholesterol in the original QRisk252 equation were compared with those calculated using a modified risk equation utilising a single measurement of non-HDL cholesterol. The modified equation was created by substituting – for the centring constant and beta coefficients (log-HRs) for TC/HDL cholesterol in the original C code – values relevant to non-HDL cholesterol, derived as follows.
For each gender, new centring values (means) were taken from the CPRD primary prevention subjects not taking statins, having excluded individuals with measurements outside the allowed ranges for the QRisk252 variables. 214 New beta coefficients were taken from the EPIC-Norfolk study,216 as this was the largest study in our systematic review (see Chapter 2) to investigate the prognostic significance of non-HDL cholesterol in a UK primary prevention population who were not taking statins. This study reports HRs per 1 SD increase in non-HDL cholesterol separately for men and women, and for both genders combined, and reported the SD for non-HDL cholesterol by gender and the occurrence of a CVD event. The SDs across the CVD and non-CVD event groups for men and women can be combined to obtain the overall SD of non-HDL cholesterol for men and women, as shown in Appendix 21.
A sensitivity analysis was carried out using the overall HR per SD increase in non-HDL cholesterol for the primary prevention group not taking statins calculated in the systematic review (see Chapter 2). To convert to a HR per millimole/litre increase, we raised the overall HR per SD to the power of 1/SD, with the SD calculated from the primary prevention off-statin group in the CPRD data (having excluded individuals with measurements outside the allowed ranges for the QRisk252 variables and different lipid measures). Centring values were also taken from this data set, as in the primary analysis.
Predicted risk using non-HDL cholesterol in the modified score was compared with predicted risk using TC/HDL cholesterol ratio in the original QRisk252 score, in primary prevention patients in CPRD. Results are presented as scatterplots and Bland–Altman plots. 217 We also grouped CVD risk into 10-year risk categories in 5% increments from 0% to ≥ 25%, and used tables to compare the numbers of subjects reclassified.
Repeated lipid measures
We estimate the regression dilution ratios for a single measure of any lipid fraction as:
where σw2 and σa2 are the within-measurement and between-measurement variability. The values of σw2 and σa2 for TC and the TC/HDL cholesterol have been estimated in Chapter 4.
The regression dilution ratio for the mean of k measures is:
and hence the coefficient of a single measurement can be converted to the coefficient of the mean by multiplying by:
as has been reported previously. 213 The regression dilution ratio method is exact for linear models and has been shown to apply approximately to other generalised linear models. 212 It can be shown that it applies approximately in the case of univariate survival models with censoring. 218 We conducted further simulations to confirm the approximation also holds for multivariate (multiple covariate) survival models (details available on request).
For consistency with the simulation models examining alternative lipid-monitoring strategies, presented in Chapter 5, we initially compare risk estimates from the original QRisk252 equation (that uses a single measurement of TC/HDL cholesterol) with estimates calculated using the mean of three and the mean of five TC/HDL cholesterol measurements in the original QRisk252 equation. We then compare estimates from the original QRisk252 equation, using one measurement of TC/HDL cholesterol with the equations modified to adjust for regression dilution using the mean of three and the mean of five repeated TC/HDL cholesterol measurements. Analyses were carried out using Stata 12.1 and R 3.0.1 (The R Foundation for Statistical Computing, Vienna, Austria). 219
Results
Alternative lipid measure: non-HDL cholesterol
Table 40 presents frequency counts of subjects in each of six categories of risk estimated using the original QRisk252 score, calculated using a single measure of TC/HDL cholesterol, compared with risk estimated using QRisk252 modified for use with a single measurement of non-HDL cholesterol, using estimates from EPIC-Norfolk. 216 A large proportion of patients have very high risk (> 25%) according to the original QRisk252 score. These high-risk individuals are primarily aged ≥ 70 years and have at least one of the following conditions: atrial fibrillation, diabetes, treated hypertension. Figures 35 and 36 show scatterplots comparing the two QRisk252 scores, with the number of subjects reclassified at 20% and 10% CVD risk thresholds, respectively. Approximately 5% of subjects are reclassified from low to high risk, and 5% from high to low risk using a threshold of 20%; smaller proportions are reclassified when the threshold is lowered to 10%. Figure 37 shows a Bland–Altman plot comparing the QRisk252 estimates using TC/HDL cholesterol and non-HDL cholesterol; absolute differences in the risk estimates are less than approximately 10% for 95% of subjects. Absolute differences of > 10% were generally observed in people over the 20% risk threshold. Of the 1757 people with risk differences outside the normal range, 80.9% had risk estimates of > 20% according to both the original and modified equations. Similar results were obtained when the systematic review results from Chapter 2 were used to modify the risk equation (Table 41 and Figures 38–40).
QRisk2 estimate of 10-year CVD risk calculated using single TC/HDL cholesterol measure (%) | QRisk2 estimate of 10-year CVD risk calculated using single non-HDL cholesterol measure (%) | Total | |||||
---|---|---|---|---|---|---|---|
< 5 | 5–10 | 10–15 | 15–20 | 20–25 | 25+ | ||
< 5 | 2055 | 365 | 4 | 1 | 0 | 0 | 2425 |
5–10 | 640 | 3,756 | 958 | 76 | 8 | 4 | 5442 |
10–15 | 0 | 1025 | 2659 | 1066 | 214 | 52 | 5016 |
15–20 | 0 | 63 | 1012 | 1719 | 911 | 391 | 4096 |
20–25 | 0 | 6 | 157 | 886 | 1123 | 1015 | 3187 |
25+ | 0 | 0 | 18 | 287 | 1022 | 6892 | 8219 |
Total | 2695 | 5215 | 4808 | 4035 | 3278 | 8354 | 28,385 |
FIGURE 35.
Comparison of estimates of 10-year cardiovascular risk calculated using TC/HDL cholesterol in the original QRisk252 equation or using non-HDL cholesterol in a modified equation, with the low-/high-risk threshold set at 20%.

FIGURE 36.
Comparison of estimates of 10-year cardiovascular risk calculated using TC/HDL cholesterol in the original QRisk252 equation or using non-HDL cholesterol in a modified equation, with the low-/high-risk threshold set at 10%.
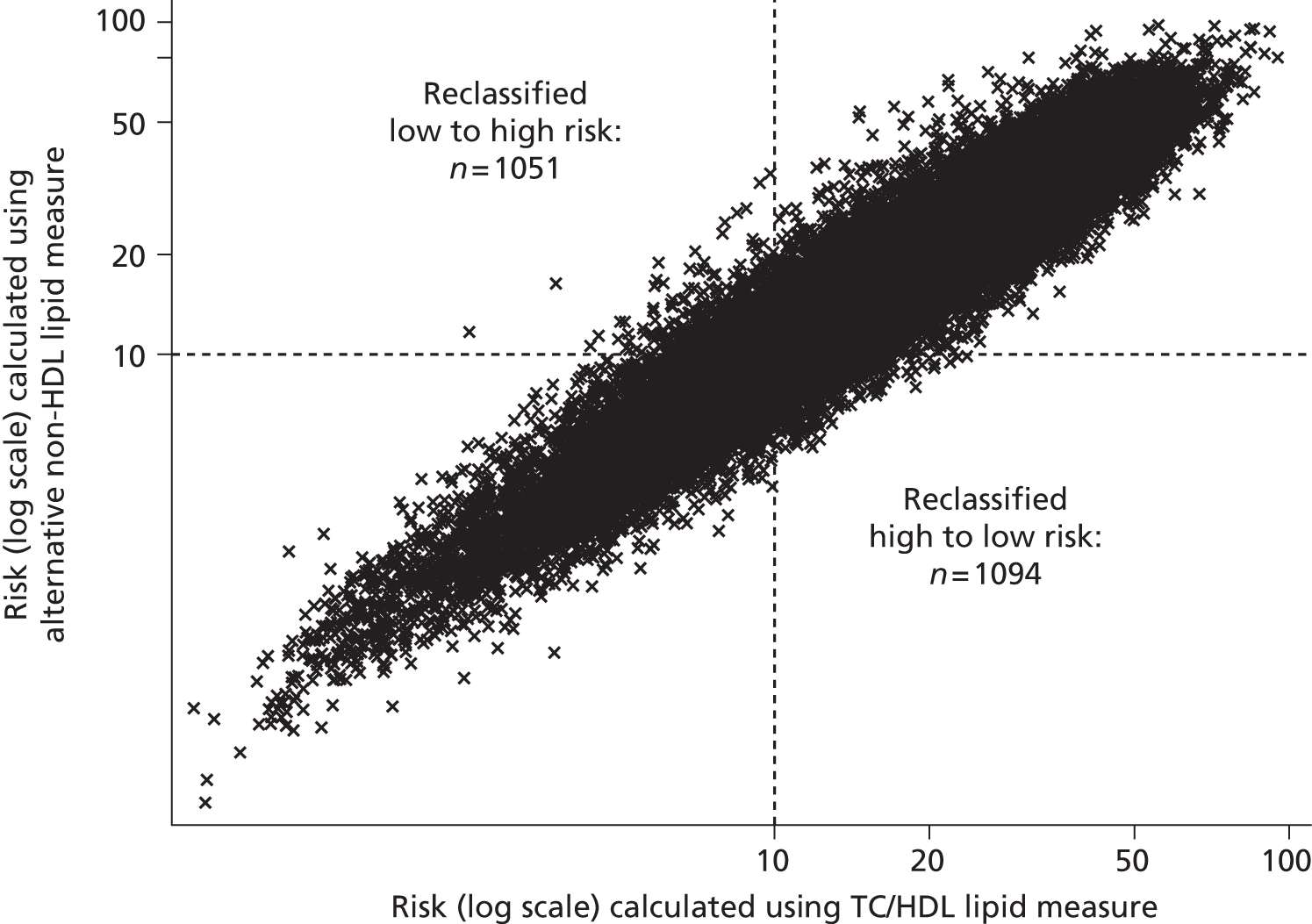
FIGURE 37.
Bland–Altman plot of estimates of 10-year cardiovascular risk calculated using TC/HDL cholesterol in the original QRisk252 equation or using non-HDL cholesterol in a modified equation.

QRisk2 estimate of 10-year CVD risk calculated using single TC/HDL cholesterol measure (%) | QRisk2 estimate of 10-year CVD risk calculated using single non-HDL cholesterol measure (%) | Total | |||||
---|---|---|---|---|---|---|---|
< 5 | 5–10 | 10–15 | 15–20 | 20–25 | 25+ | ||
< 5 | 2148 | 276 | 1 | 0 | 0 | 0 | 2425 |
5–10 | 316 | 4446 | 670 | 9 | 1 | 0 | 5442 |
10–15 | 0 | 611 | 3520 | 833 | 48 | 4 | 5016 |
15–20 | 0 | 3 | 720 | 2417 | 853 | 103 | 4096 |
20–25 | 0 | 0 | 24 | 745 | 1659 | 759 | 3187 |
25+ | 0 | 0 | 0 | 41 | 755 | 7423 | 8219 |
Total | 2464 | 5336 | 4935 | 4045 | 3316 | 8289 | 28,385 |
FIGURE 38.
Comparison of estimates of 10-year cardiovascular risk calculated using TC/HDL cholesterol in the original QRisk252 equation or using non-HDL cholesterol in a modified equation (sensitivity analysis), with the low-/high-risk threshold set at 20%.

FIGURE 39.
Comparison of estimates of 10-year cardiovascular risk calculated using TC/HDL cholesterol in the original QRisk252 equation or using non-HDL cholesterol in a modified equation (sensitivity analysis), with the low-/high-risk threshold set at 10%.
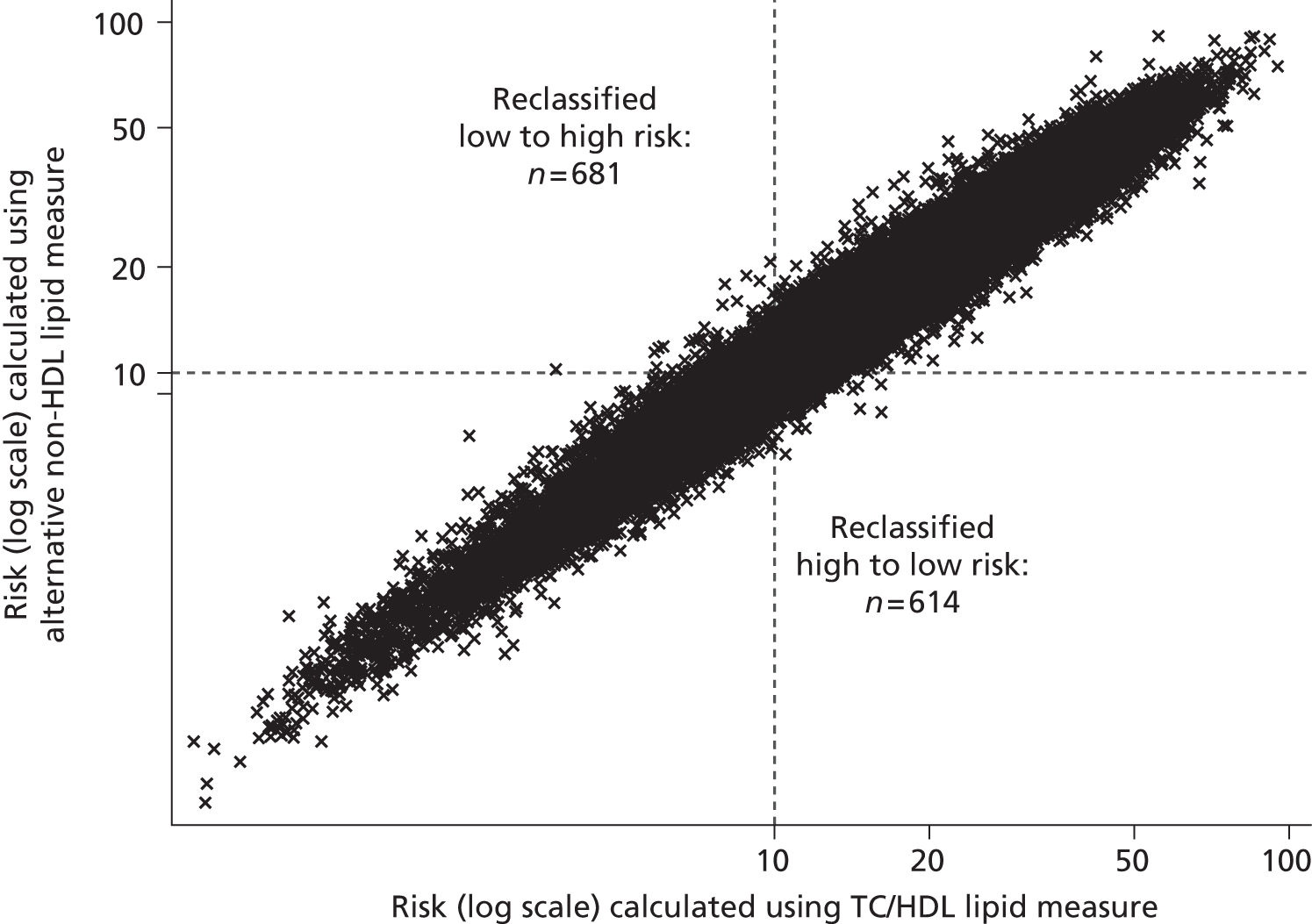
FIGURE 40.
Bland–Altman plot of estimates of 10-year cardiovascular risk calculated using TC/HDL cholesterol in the original QRisk252 equation or using non-HDL cholesterol in a modified equation (sensitivity analysis).
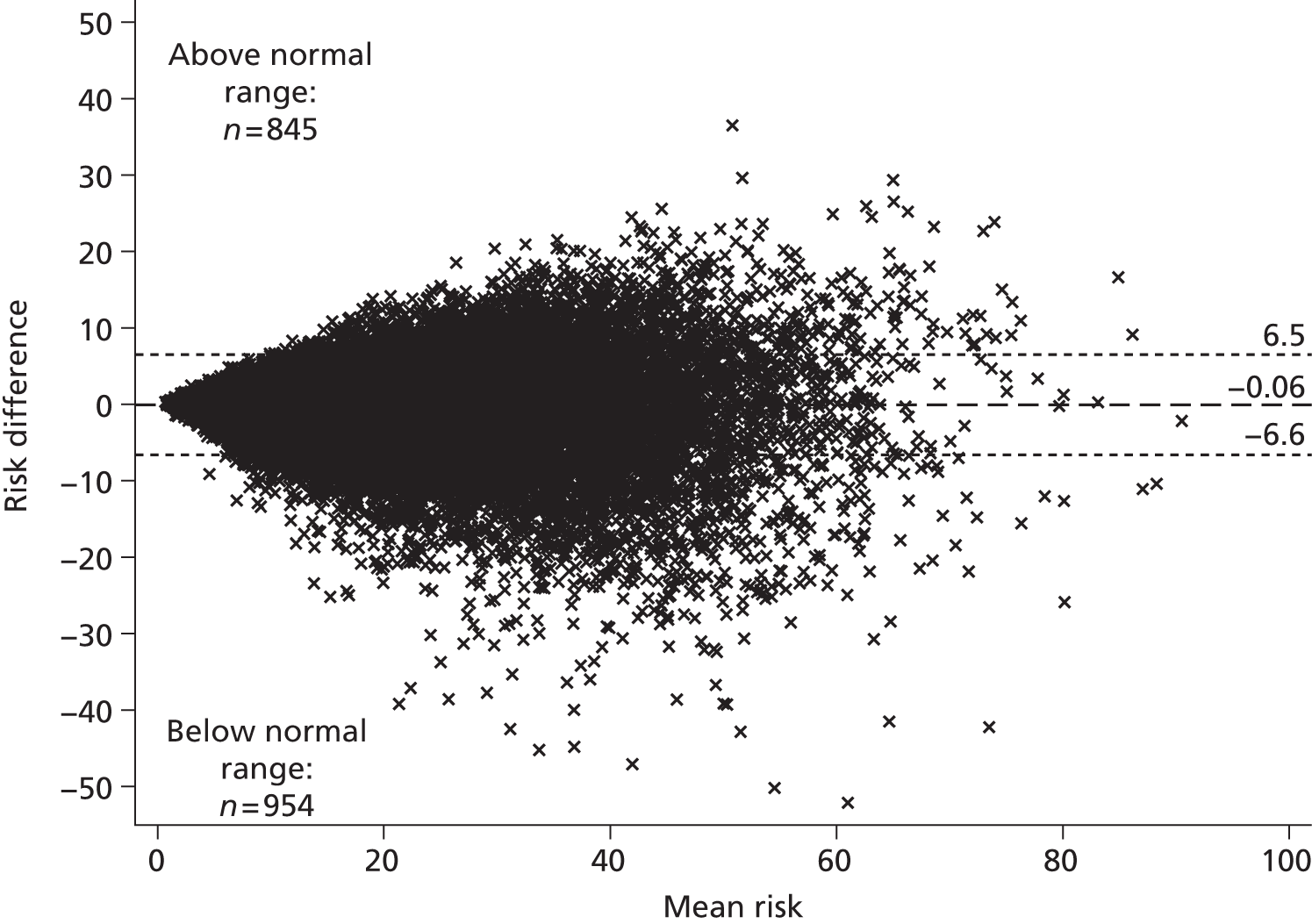
Repeated lipid measures
Before adjusting for regression dilution
Table 42 and Figures 41 and 42 show the numbers of subjects reclassified between risk categories when CVD risk is calculated from one measurement or the mean of three measurements using the original QRisk252 equation, with no adjustment for regression dilution. Only a very small number of subjects are reclassified between risk categories, and for 95% of subjects any absolute change in calculated risk is < 3% (Figure 43). Similar results were seen when CVD risk estimated using one measurement was compared with that calculated using the mean of five measurements in the original QRisk252 equation without adjusting for regression dilution; corresponding estimates are shown in Table 43 and Figures 44–46.
QRisk2 estimate of 10-year CVD risk calculated using single TC/HDL cholesterol measure (%) | QRisk2 estimate of 10-year CVD risk calculated using mean of three TC/HDL cholesterol measures (%) | Total | |||||
---|---|---|---|---|---|---|---|
< 5 | 5–10 | 10–15 | 15–20 | 20–25 | 25+ | ||
< 5 | 1284 | 87 | 0 | 0 | 0 | 0 | 1371 |
5–10 | 53 | 3397 | 164 | 0 | 0 | 0 | 3614 |
10–15 | 0 | 125 | 3108 | 189 | 0 | 0 | 3422 |
15–20 | 0 | 0 | 160 | 2423 | 186 | 2 | 2771 |
20–25 | 0 | 0 | 1 | 169 | 1726 | 152 | 2048 |
25+ | 0 | 0 | 0 | 1 | 165 | 4751 | 4917 |
Total | 1337 | 3609 | 3433 | 2782 | 2077 | 4905 | 18,143 |
FIGURE 41.
Comparison of estimates of 10-year cardiovascular risk calculated using a single TC/HDL cholesterol measure or the mean of three TC/HDL cholesterol measures in the original QRisk252 equation, with the low-/high-risk threshold set at 20%.
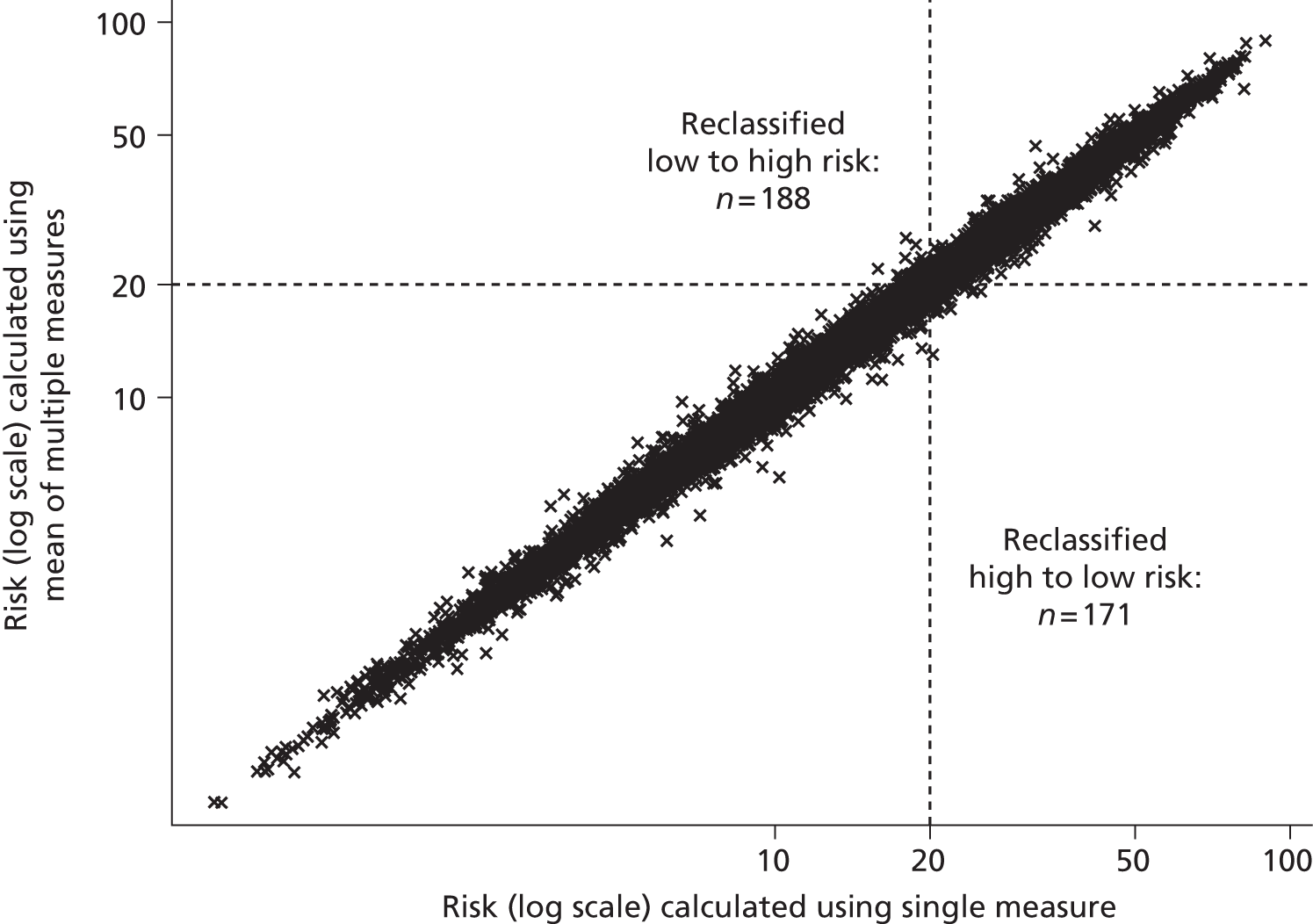
FIGURE 42.
Comparison of estimates of 10-year cardiovascular risk calculated using a single TC/HDL cholesterol measure or the mean of three TC/HDL cholesterol measures in the original QRisk252 equation, with the low-/high-risk threshold set at 10%.

FIGURE 43.
Bland–Altman plot of estimates of 10-year cardiovascular risk calculated using a single TC/HDL cholesterol measure or the mean of three TC/HDL cholesterol measures in the original QRisk252 equation.

QRisk2 estimate of 10-year CVD risk calculated using single TC/HDL cholesterol measure (%) | QRisk2 estimate of 10-year CVD risk calculated using mean of five TC/HDL cholesterol measures (%) | Total | |||||
---|---|---|---|---|---|---|---|
< 5 | 5–10 | 10–15 | 15–20 | 20–25 | 25+ | ||
< 5 | 1282 | 89 | 0 | 0 | 0 | 0 | 1371 |
5–10 | 57 | 3,383 | 174 | 0 | 0 | 0 | 3614 |
10–15 | 0 | 132 | 3091 | 199 | 0 | 0 | 3422 |
15–20 | 0 | 0 | 168 | 2391 | 210 | 2 | 2771 |
20–25 | 0 | 0 | 1 | 184 | 1690 | 173 | 2048 |
25+ | 0 | 0 | 0 | 1 | 181 | 4735 | 4917 |
Total | 1339 | 3604 | 3434 | 2775 | 2081 | 4910 | 18,143 |
FIGURE 44.
Comparison of estimates of 10-year cardiovascular risk calculated using a single TC/HDL cholesterol measure or the mean of five TC/HDL cholesterol measures in the original QRisk252 equation, with the low-/high-risk threshold set at 20%.

FIGURE 45.
Comparison of estimates of 10-year cardiovascular risk calculated using a single TC/HDL cholesterol measure or the mean of five TC/HDL cholesterol measures in the original QRisk252 equation, with the low-/high-risk threshold set at 10%.

FIGURE 46.
Bland–Altman plot of estimates of 10-year cardiovascular risk calculated using a single TC/HDL cholesterol measure or the mean of five TC/HDL cholesterol measures in the original QRisk252 equation.
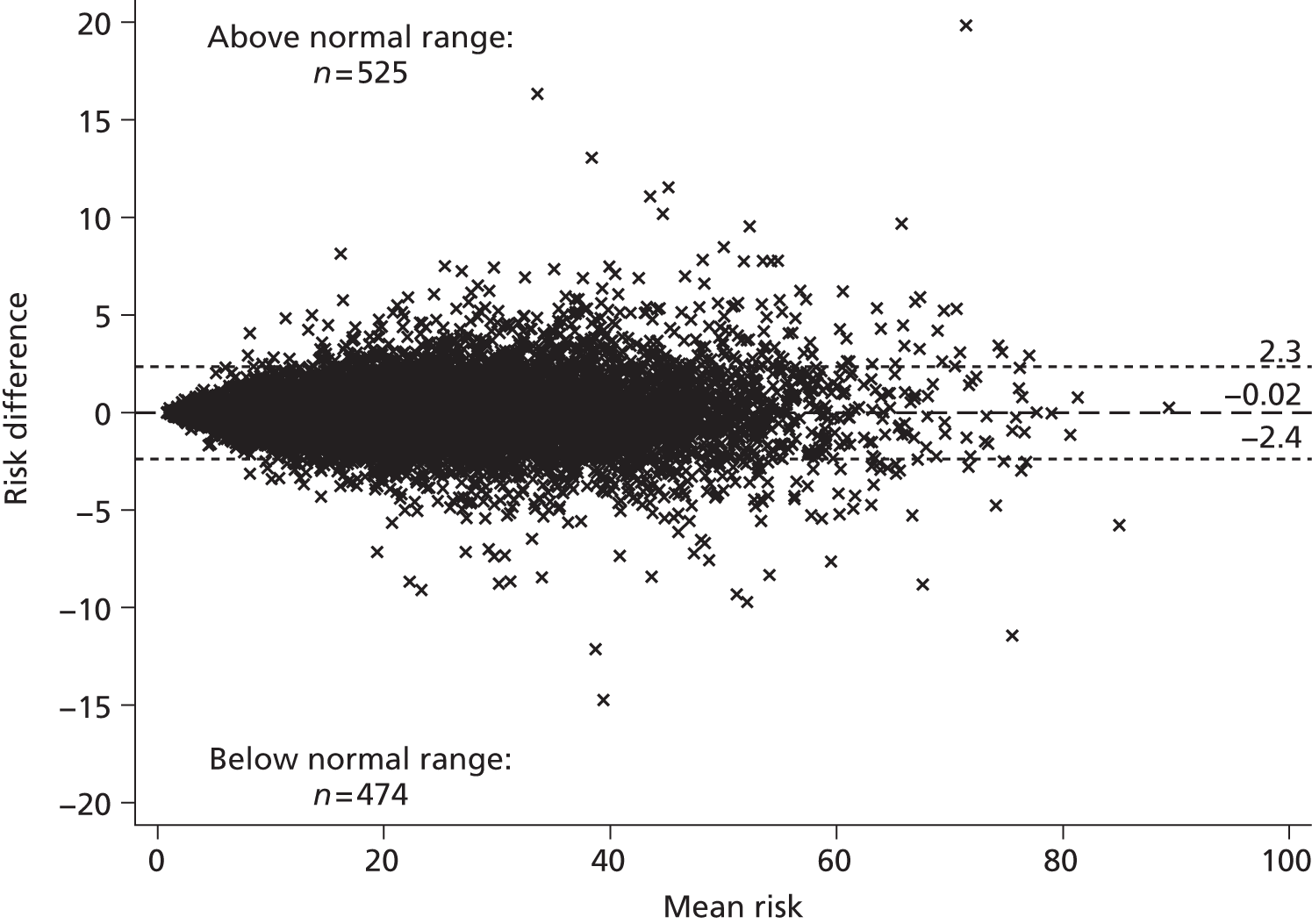
After adjusting for regression dilution
The estimates of parameters σw and σa in Table 23 give a regression dilution ratio of 1.281 for the TC/HDL cholesterol ratio in men. Coefficients for TC/HDL cholesterol should be therefore multiplied by 1.281/(1 + 0.281/3) = 1.171 for the mean of three measurements, and by 1.213 for the mean of five measurements. We therefore increased the log-HR in the QRisk252 equation from 0.17 to 0.199 (corresponding to HR 1.22) for three measurements and to 0.206 (HR 1.23) for five measurements. In women, the regression dilution ratio from Table 24 is 1.213 and the QRisk252 log-HR is 0.16, giving rise to log-HRs (and HRs) of 0.181 (1.20) for the mean of three measurements and 0.186 (1.20 to two decimal places) for the mean of five measurements.
Table 44 and Figures 47–49, and Table 45 and Figures 50–52, show the effect of these changes on risk classification. Differences in the estimated risk were small, and few subjects were reclassified between risk categories.
QRisk2 estimate of 10-year CVD risk calculated using single TC/HDL cholesterol measure (%) | QRisk2 estimate of 10-year CVD risk calculated using LIPID measures (%) | Total | |||||
---|---|---|---|---|---|---|---|
< 5 | 5–10 | 10–15 | 15–20 | 20–25 | 25+ | ||
< 5 | 1280 | 91 | 0 | 0 | 0 | 0 | 1371 |
5–10 | 59 | 3374 | 181 | 0 | 0 | 0 | 3614 |
10–15 | 0 | 132 | 3084 | 206 | 0 | 0 | 3422 |
15–20 | 0 | 0 | 188 | 2365 | 217 | 1 | 2771 |
20–25 | 0 | 0 | 1 | 189 | 1,685 | 173 | 2048 |
25+ | 0 | 0 | 0 | 1 | 192 | 4724 | 4917 |
Total | 1339 | 3597 | 3454 | 2761 | 2094 | 4898 | 18,143 |
FIGURE 47.
Comparison of estimates of 10-year cardiovascular risk calculated using a single TC/HDL cholesterol measure in the original QRisk252 equation or the mean of three TC/HDL cholesterol measures in a modified equation, with the low-/high-risk threshold set at 20%.

FIGURE 48.
Comparison of estimates of 10-year cardiovascular risk calculated using a single TC/HDL cholesterol measure in the original QRisk252 equation or the mean of three TC/HDL cholesterol measures in a modified equation, with the low-/high-risk threshold set at 10%.

FIGURE 49.
Bland–Altman plot of estimates of 10-year cardiovascular risk calculated using a single TC/HDL cholesterol measure in the original QRisk252 equation or the mean of three TC/HDL cholesterol measures in a modified equation.

QRisk2 estimate of 10-year CVD risk calculated using single TC/HDL cholesterol measure (%) | QRisk2 estimate of 10-year CVD risk calculated using LIPID measures (%) | Total | |||||
---|---|---|---|---|---|---|---|
< 5 | 5–10 | 10–15 | 15–20 | 20–25 | 25+ | ||
< 5 | 1282 | 89 | 0 | 0 | 0 | 0 | 1371 |
5–10 | 68 | 3354 | 192 | 0 | 0 | 0 | 3614 |
10–15 | 0 | 145 | 3050 | 227 | 0 | 0 | 3422 |
15–20 | 0 | 0 | 201 | 2324 | 243 | 3 | 2771 |
20–25 | 0 | 0 | 1 | 210 | 1641 | 196 | 2048 |
25+ | 0 | 0 | 0 | 1 | 222 | 4694 | 4917 |
Total | 1350 | 3588 | 3444 | 2762 | 2106 | 4893 | 18,143 |
FIGURE 50.
Comparison of estimates of 10-year cardiovascular risk calculated using a single TC/HDL cholesterol measure in the original QRisk252 equation or the mean of five TC/HDL cholesterol measures in a modified equation, with the low-/high-risk threshold set at 20%.
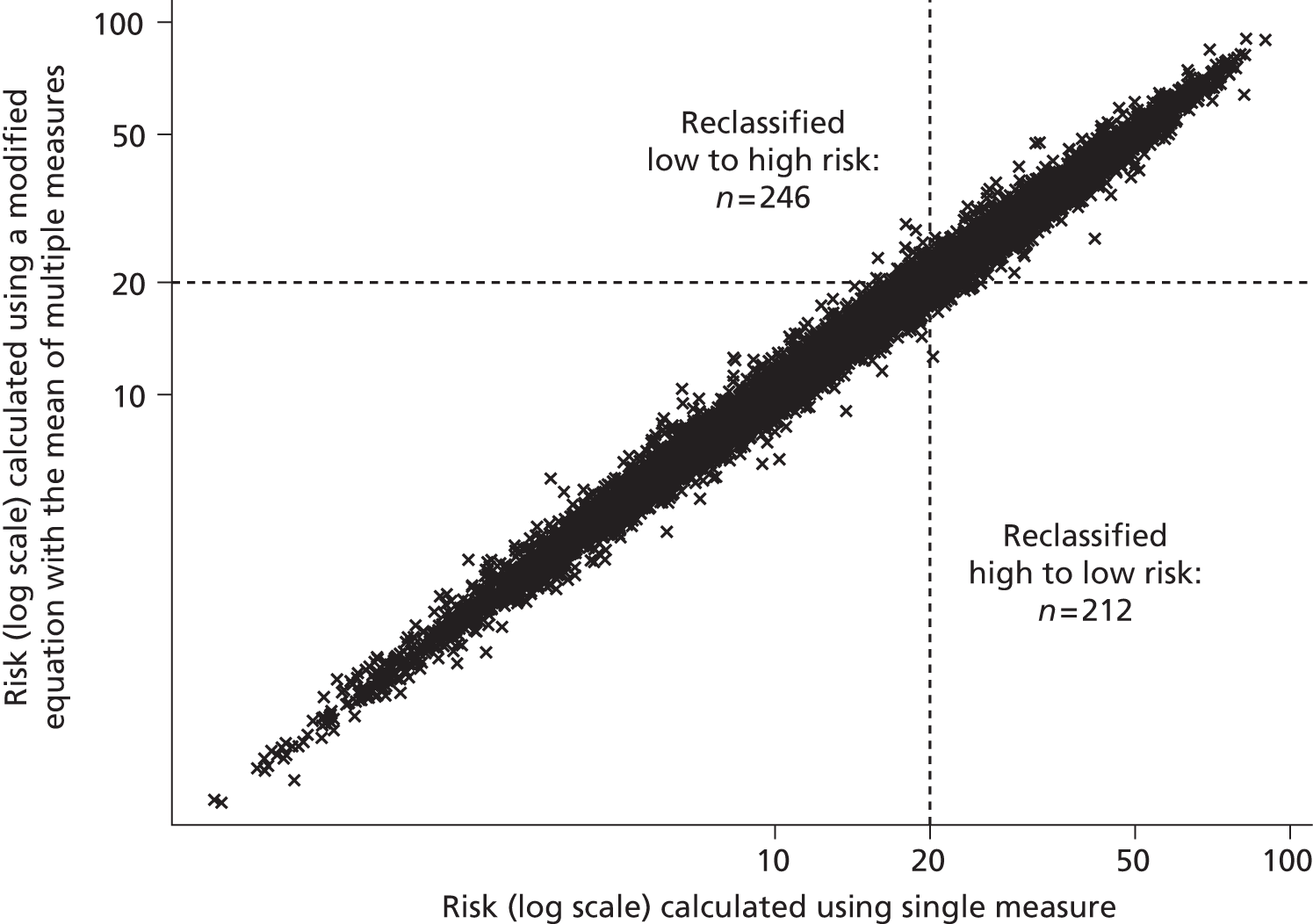
FIGURE 51.
Comparison of estimates of 10-year cardiovascular risk calculated using a single TC/HDL cholesterol measure in the original QRisk252 equation or the mean of five TC/HDL cholesterol measures in a modified equation, with the low-/high-risk threshold set at 10%.
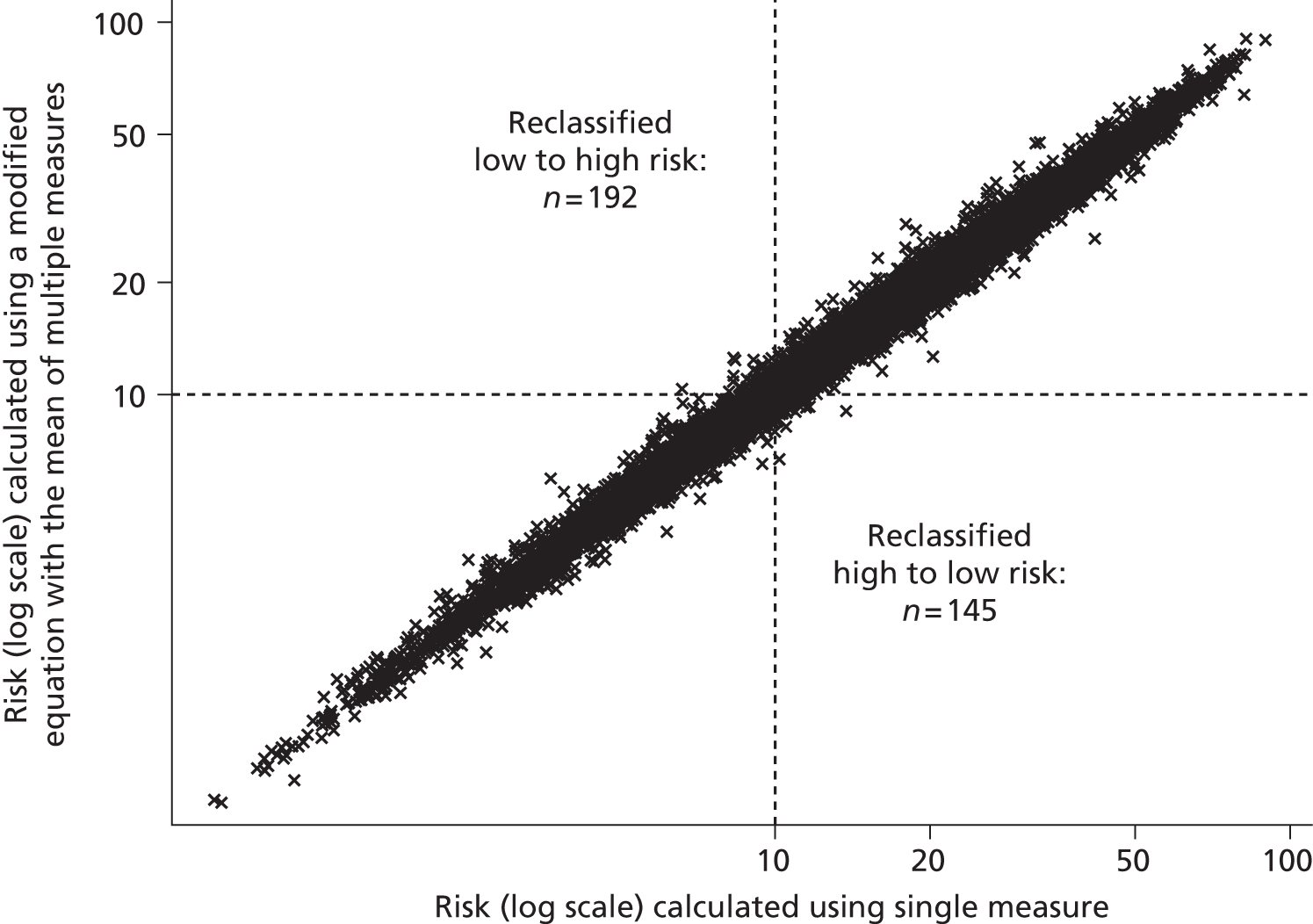
FIGURE 52.
Bland–Altman plot of estimates of 10-year cardiovascular risk calculated using a single TC/HDL cholesterol measure in the original QRisk252 equation or the mean of five TC/HDL cholesterol measures in a modified equation.

Discussion
We have considered two possible strategies for improving the lipid measurement within a single cardiovascular risk estimate. We first considered whether or not risk estimates could be improved by an alternative lipid measurement. Motivated by Chapter 2, we posited a risk equation based on non-HDL cholesterol, and found potential for about 1 person in 10 to be classified differently (high vs. low risk and vice versa) compared with a risk calculator based on TC/HDL cholesterol ratio. These results are only illustrative but at least demonstrate the potential for a change. This contrasts with our second analysis, in which we considered whether or not risk estimates could be improved by taking multiple blood samples and using the average of a lipid measure. This appears to make negligible difference to risk estimation, probably because the within-measurement variability of TC/HDL cholesterol, although not negligible, is not large compared with other sources of variation.
We did not have primary data on which to build a risk equation with non-HDL cholesterol. A further, and major, limitation of these analyses is that we can estimate the degree of reclassification but not the extent to which our modifications represent an improvement to risk estimation. To quantify this would require a large cohort with cardiovascular risk factors including both lipid measurements, hard outcome measures and sufficient follow-up, and without treatment changes during follow-up. 220,221 Databases of routine clinical data, such as the CPRD,222 can provide cohorts of sufficient size, coverage and follow-up; however, they will be subject to a large amount of treatment initiation or change during follow-up, especially in those, or those estimated to be, at high risk of CVD. Particularly problematic for a comparison of TC/HDL cholesterol to another lipid is that under current practice much of such treatment change will have been triggered by high risk according to TC/HDL cholesterol. Therefore, we can estimate that using non-HDL cholesterol could produce appreciably different risk estimates but cannot determine whether or not these would be more predictive of future CVD. Other limitations of our analyses include the use of an approximate risk equation for non-HDL cholesterol, and an approximate though well-established method for adjusting for regression dilution. 218,223,224
Taking multiple blood samples in order to average a lipid measurement such as TC/HDL cholesterol ratio would increase financial and practical costs but is unlikely to appreciably improve cardiovascular risk estimation. Improvements to cardiovascular risk estimates, within a lipid monitoring programme, could instead be sought through alternative choices of lipid measure. Our illustrative results, using non-HDL cholesterol in a modified cardiovascular risk equation, show that this has potential to appreciably alter risk estimates. However, at present the data do not exist to definitively determine whether or not the estimates based on non-HDL cholesterol or the estimates based on the ratio are more useful in clinical practice.
Chapter 7 Health-economic simulation model and cost-effectiveness analysis for monitoring lipids in the general population in the UK
Background
Chapter 5 showed the variability in lipid measurements leading to ‘false-positive’ and ‘false-negative’ monitoring tests, but did not attempt to weigh the costs of unnecessary treatment changes arising from the former against the health and other costs resulting from the missed treatment opportunities of the latter. Here we extend the models of Chapter 5 to full patient-level simulation models incorporating modelling assumptions about cost and quality of life.
Patient-level simulation models are a valuable tool to model the progression of clinical outcomes for an individual patient over a long period of time. The inherent difficulties and expense of collecting data in the scale required to evaluate a monitoring strategy using an experimental design means that these simulation models are increasingly used to estimate the lifetime costs and benefits of different disease management strategies. Outcomes are usually quantified in terms of mean life expectancy or mean quality-adjusted life expectancy. 225
Importantly, the economic simulations explicitly account for the high levels of variability in lipids (see Chapter 4), which is of particular relevance for an economic evaluation of optimal lipid-monitoring strategies. Moreover, as economic analysis can be sensitive to changes in the price of treatments, the recent expiry of the patent for atorvastatin and the subsequent dramatic price reduction, mean that an updated economic analysis is clearly required. This chapter therefore outlines the methods, underlying assumptions and subsequent economic evaluation of a patient-level simulation model for CVD, which considers variability in lipid levels and the frequency at which to monitor. This model was developed to integrate the information reported in the preceding chapters and hence examine optimal lipid-monitoring strategies in the UK.
Methods
Simulation models
Model structure
We developed a simulation model to predict the occurrence of cardiovascular events (grouped into MI, stroke or other CVD) in order to estimate lifetime outcomes and quality-adjusted life expectancy. For primary prevention patients we explicitly model the first occurrence of an event, whereas for secondary prevention patients we model the first event subsequent to joining the monitoring programme. The model is built around a series of key health states through which patients can progress over their lifetime. Figure 53 illustrates the sequence of steps within annual cycles of the model. Patients enter the model in one of the three prevention groups described in Chapter 4:
-
primary prevention patients not taking statins
-
primary prevention patients taking statins
-
secondary prevention patients taking statins.
FIGURE 53.
Pathway of the simulation model and risk equations involved. QALY, quality-adjusted life-year.
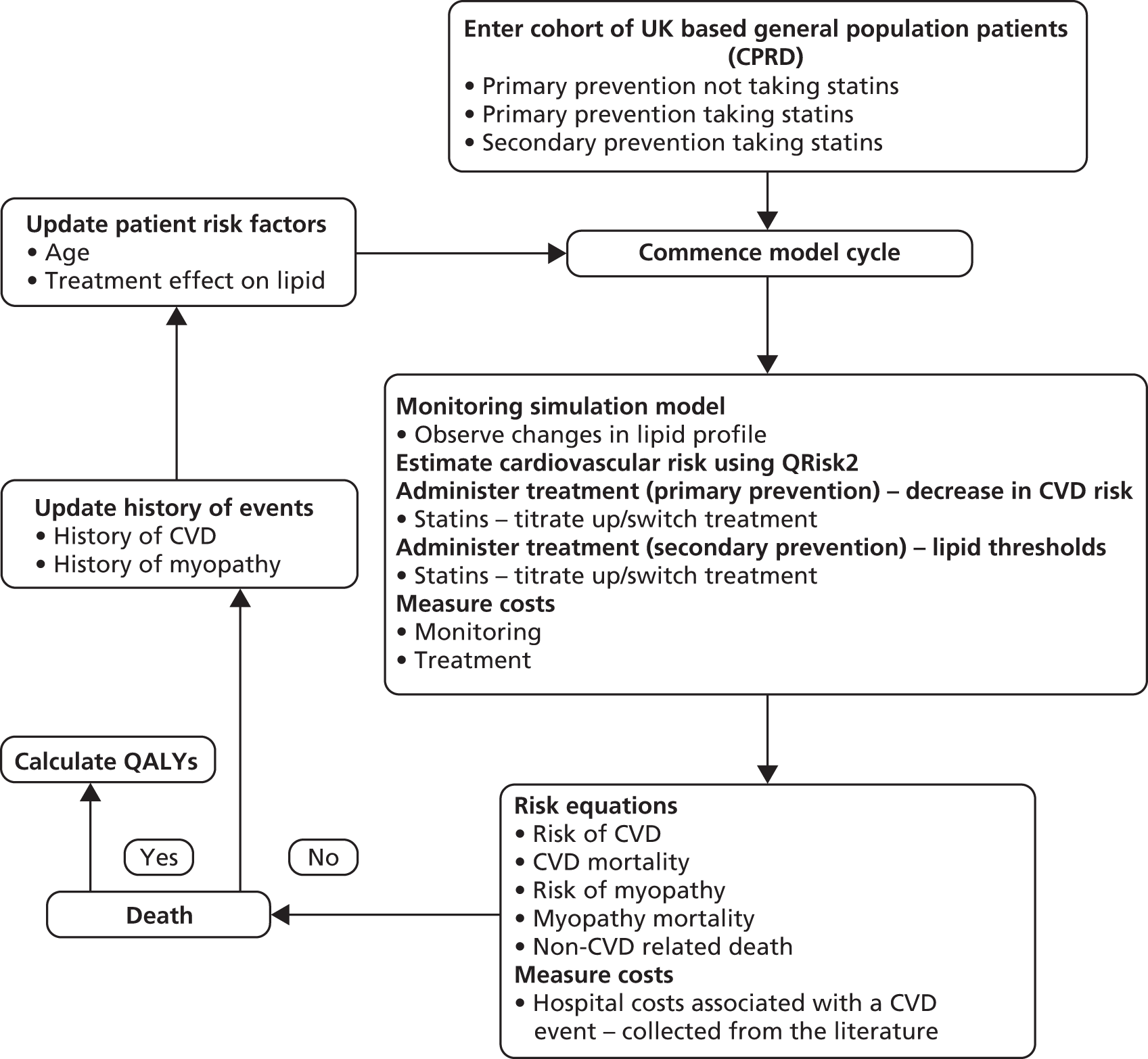
As in Chapters 4 and 5, we assume monitoring begins at 40 years for men and 55 years for women. The model is described in detail below: briefly, it runs in annual cycles and calculates cardiovascular risk using QRisk252 (for people in the primary prevention group) and monitors patients’ lipid levels (for patients in the secondary prevention group). Changes in treatment are dependent on these two things and are explained in detail further below. Different lipid monitoring scenarios (annual, 3-yearly, 5-yearly, etc.) are modelled and compared to determine the most optimal lipid monitoring interval that reduces costs and maximises benefits. The model is applied to a simulated population of men and women with baseline characteristics as presented in Table 46.
Variable | Male | Female | ||||
---|---|---|---|---|---|---|
Primary | Primary on statins | Secondary | Primary | Primary on statins | Secondary | |
n | 17,434 | 7714 | 8803 | 14,438 | 7016 | 5660 |
Age (years): mean (SD) | 60.3 (11.8) | 64.1 (10.3) | 69.7 (9.9) | 68.5 (9.5) | 69.9 (8.2) | 74.7 (8.6) |
History of diabetes (%) | 13.4 | 44.7 | 24.1 | 9.8 | 38.4 | 24.6 |
History of atrial fibrillation (%) | 4.1 | 5.7 | 12.7 | 4.1 | 5.1 | 12.8 |
History of chronic kidney disease (%) | 0.7 | 2 | 2.5 | 0.6 | 1.5 | 2.5 |
Systolic BP (mmHg): mean (SD) | 139.3 (17.7) | 139.4 (16.7) | 134.9 (17.5) | 141.5 (18.9) | 140.7 (17.5) | 138.3 (19.2) |
BMI (kg/m2): mean (SD) | 28.2 (5.1) | 29.0 (5.1) | 28.0 (4.7) | 27.7 (5.6) | 28.8 (5.8) | 27.7 (5.6) |
TC (mmol/l): mean (SD) | 5.4 (1.1) | 4.5 (1.0) | 4.2 (0.9) | 5.9 (1.1) | 4.8 (1.1) | 4.5 (0.9) |
HDL (mmol/l): mean (SD) | 1.3 (0.4) | 1.2 (0.4) | 1.2 (0.3) | 1.7 (0.4) | 1.6 (0.4) | 1.5 (0.4) |
LDL (mmol/l): mean (SD) | 3.4 (0.9) | 2.5 (0.9) | 2.2 (0.8) | 3.6 (0.9) | 2.6 (0.9) | 2.3 (0.8) |
TGs (mmol/l): mean (SD) | 1.7 (1.2) | 1.8 (1.2) | 1.5 (0.9) | 1.5 (0.8) | 1.6 (0.9) | 1.5 (0.8) |
Data sources used in model construction and parametric equations
Lipid progression and treatment thresholds
In the UK, guidelines for prescribing statins are based upon estimated CVD risk (primary) or lipid levels (secondary), as described earlier (see Chapters 1 and 5). The model used to simulate the progression of lipids over time, described in Chapter 5, has therefore been integrated into this simulation model. The progression of each lipid measure is considered separately, so separate simulation modelling was carried out for TC and for LDL. As in Chapter 5, the simulation model presented in this chapter assumes that decisions in primary prevention are guided by CVD risk estimated by QRisk252 and that decisions in secondary prevention populations are guided by lipid targets of 4 mmol/l for TC and 2 mmol/l for LDL. For primary prevention, in our primary analysis we assumed a 10-year CVD risk threshold of 20%, based on guidelines current at time of analysis. In sensitivity analyses we consider how our conclusions would differ under risk thresholds of 15% or 10%.
Statin dose and type
As noted in Chapter 1, the atorvastatin patent expired in 2010. 59,62 We therefore assumed that monitoring would be based upon strategies recommended in the NICE guidelines that were current at time of the analysis,226 but that atorvastatin, instead of simvastatin, would be recommended for both primary and secondary prevention populations. We assumed a recommended initial dose of 10 mg (for comparability of LDL-lowering effect with the then-recommended simvastatin 40 mg) in primary prevention and 40 mg in secondary prevention populations. As illustrated in Figure 54, we assumed that treatment regimens would be changed as follows:
Primary prevention not taking statins
-
Patients would be prescribed 10 mg of atorvastatin if their estimated 10-year risk of CVD was above threshold.
Primary prevention taking statins
-
Patients taking statins other than atorvastatin would be prescribed 10 mg of atorvastatin if their 10-year CVD risk was above threshold.
-
Patients taking 10 mg of atorvastatin would be prescribed 40 mg of atorvastatin if their 10-year CVD risk was above threshold.
-
Patients taking 40 mg of atorvastatin would be prescribed atorvastatin 80 mg, the highest treatment available, if their 10-year risk of CVD was above threshold.
Secondary prevention taking statins
-
Patients taking statins other than atorvastatin would be prescribed 40 mg of atorvastatin if their measured lipid level was above threshold.
-
Secondary prevention patients taking atorvastatin 40 mg would be up-titrated to 80 mg of atorvastatin if their measured lipid level was above threshold.
FIGURE 54.
Dosages of statins and methods of titration assumed in simulation model.

Treatment effects of statins
As reported in Chapter 3, few RCTs have examined the lipid-lowering effects of atorvastatin with doses higher than 20 mg/day. However, the findings in Chapter 3 are broadly consistent with the dose–response equations reported in the 2012 Cochrane Systematic Review. 187 We therefore model the statin effects in our model on these (see equations 7–9, below), obtaining the percentage reductions in TC and LDL shown in Table 47.
where y is percentage, x is the statin dosage in mg and log is logarithm to base 10.
Current treatment (mg) | Reason for change | Treatment change (mg) | TC (%) | LDL (%) |
---|---|---|---|---|
No treatment | QRisk252 estimate > threshold | Atorvastatin 10 | 73 | 63 |
Atorvastatin 10 | QRisk252 estimate > threshold | Atorvastatin 40 | 8 | 11 |
No treatment | CVD event but were not on statins originally | Atorvastatin 40 | 65 | 52 |
Atorvastatin 40 | QRisk252 estimate > threshold Lipid > threshold |
Atorvastatin 80 | 4 | 5 |
In our main analyses we assumed 80% adherence (20% non-adherence) to statin medication when calculating costs and effects. Non-adherence was modelled as statin cessation in the first year of commencing treatment.
Cardiovascular disease incidence and cardiovascular disease-related mortality
Our model simulates the first CVD event after model entry, but takes into account subsequent costs, mortality and impact on quality of life associated with this event over the patients’ remaining lifetime. We used QRisk2,52 as described in Chapter 6, to estimate the incidence of CVD. Chapter 6 also describes the methods used to modify the risk equation to use different lipids instead of TC/HDL cholesterol or to use the mean value of a number of repeated measures. The treatment effect of statins in reducing lipids will be reflected in a reduced CVD incidence through using the modified QRisk252 equations in Chapter 6.
Gender-specific mortality rates following the occurrence of CVD events were estimated from CPRD data. To estimate mortality in the first month following a CVD event, we used a logistic regression, and for mortality > 1 month post CVD event we used a Gompertz proportional hazards model. Coefficients of age and gender were included in both models. Parameter estimates are shown in Table 48. The probability that a CVD event was a MI, stroke or other type of event was also estimated using the CPRD data: patients experiencing an event were assigned a 36% probability that the event was a MI, 20% that it was a stroke, and 44% it was another CVD event.
Parameter or model structure | Equation 1 | Equation 2 | Equation 3 | Equation 4 |
---|---|---|---|---|
Model section | CVD death 1 month post event (males) | CVD death 1 month post event (females) | History of CVD (males) | History of CVD (females) |
Functional form coefficients | Logistic | Logistic | Gompertz | Gompertz |
γ/ρ (shape parameter of model) | 0.00032 (0.00030 to 0.00034) | 0.0003 (0.0002 to 0.0004) | ||
Constant | –7.72 (–7.16 to –8.28) | –9.19 (–9.88 to –8.49) | –12.84 (–13.16 to –12.58) | –11.35 (–11.59 to –11.12) |
Age at event | 0.07 (0.06 to 0.08) | 0.09 (0.08 to 0.10) | 0.058 (0.054 to 0.062) | 0.04 (0.038 to 0.044) |
Stroke | –0.59 (–0.78 to –0.41) | –0.38 (–0.49 to –0.27) | 0.23 (0.17 to 0.28) | 0.17 (0.11 to 0.23) |
Other CVD | –1.39 (–1.61 to –1.16) | –1.89 (–2.15 to –1.64) |
Mortality from other causes
Age-specific risk of non-CVD-related death was estimated from life tables by subtracting CVD cause-specific mortality rates from all-cause mortality rates. In the absence of cause-specific life tables for the UK, we used US cause-specific life tables for the general population. 227
Quality of life
Estimates of the utilities associated with different health states (No complications, MI, Stroke, Other CVD, Statin medication) were drawn from previous studies, predominantly using time trade-off methods, listed in Table 49. Utility values are expressed as quality-adjusted life-years (QALYs). This measure captures both increases in life expectancy, and improved quality of life resulting from prevention of complications, providing a composite outcome measure of fatal and non-fatal events that permits comparisons between a wide range of interventions. A utility value of ‘1’ on the QALY scale is equivalent to full health and ‘0’ is equivalent to death. Patients were assumed to have a utility of ‘1’ before CVD. MI patients were assumed to have a utility of 0.88 in the first year post MI, and 0.9 in subsequent years. 229 Stroke and other CVD patients were assumed to have utilities of 0.58 and 0.73, respectively. Patients with non-fatal myopathy had a utility of 0.97.
Model component | Source | Description | Annual costs (£) |
---|---|---|---|
Monitoring | HTA commissioned report (2004)a | First year of monitoringb | 195.80 |
Subsequent yearsc | 52.77 | ||
Treatment | Category M prices second quarter July 2013228 | Atorvastatin 10 mg | 10.80 |
Atorvastatin 20 mg | 15.48 | ||
Atorvastatin 40 mg | 22.68 | ||
Atorvastatin 80 mg | 37.56 | ||
Hospital costs from a cardiovascular event | UKPDS hospital costs (2003)a | Fatal MI | 1819 (1486 to 2204) |
Fatal stroke | 5342 (3055 to 8576) | ||
Non-fatal MI | 6427 (5653 to 7456) | ||
Non-fatal stroke | 3737 (2525 to 5170) | ||
Utilities | Greving et al. 2011229 | Post MI first year | 0.88 (0.80 to 0.96) |
Subsequent years post MI | 0.90 (0.80 to 0.95) | ||
Post stroke | 0.50 (0.00 to 0.75) | ||
Pignone et al. 2006230 | Myopathy | 0.97 (0.94 to 1.00) |
Outcome measure
Our prespecified main outcome measure was the cost per QALY gained. For each lipid-monitoring strategy that we examined, the incremental cost per QALY gained was estimated by calculating the difference between the cost of the strategy of interest and the cost of another strategy, which was then divided by the differences in QALYs between each strategy. In comparisons in which one scenario is both cost-saving and more effective than another, the ratio measure cost per QALY is uninterpretable, and we report instead that one scenario ‘dominates’ the other.
Model testing and validation
Validity of the model estimates were tested by comparing life expectancy estimates of the model to the UK life table 2009–11. 231
Cost-effectiveness analyses
Economic evaluation
Incremental cost-effectiveness analyses were carried out for several monitoring strategies outlined below and in line with those presented in Chapter 5. The perspective of the economic evaluation was of the health-care provider and so only the following direct health service costs were included in the analysis: costs of monitoring; costs of treatment; and costs of hospitalised cardiovascular events. Both costs and effects were discounted at 3.5% per year for the first 30 years, and, subsequently, 3% in line with current guidelines. 232
Results are presented as mean values and SDs, or mean differences, and as ‘cost-to-clinical-effectiveness’ ratios. The effects of uncertainty surrounding costs and the utility values used in the study were examined using sensitivity analyses.
Model simulations
Using the methods outlined above, simulations were undertaken at the patient level. The results presented are the mean of 1000 simulations, to stabilise the expectation of different individual Monte Carlo simulations.
Resource data and costs
We use annual monitoring as our reference strategy except where otherwise indicated. All costs included were obtained from published sources and the values and sources of cost data are described in Table 49. Costs are expressed in 2013 pounds sterling, and data from previous years have been adjusted to 2013 values using the Hospital and Community Health Services (HCHS) pay and price index. 233
The cost of lipid monitoring in primary care is estimated at £195.80 for the first year of monitoring, and £52.77 for each subsequent year, according to the HTA report in 2004234 and adjusted for inflation.
Annual costs for treatment of atorvastatin 10, 20 and 40 and 80 mg are listed as £10.80, £15.48, £22.68 and £37.56, respectively, according to the Category M prices in the second quarter of July 2013. 228 Similarly, we used hospital costs for a CVD event from the UK Prospective Diabetes Study (UKPDS)235 hospital costs of 2003 and inflated up to 2013 prices.
Sensitivity analyses
Sensitivity analyses were conducted on major assumptions. We considered how comparisons between monitoring strategies might vary under alternative risk thresholds in primary prevention (15% or 10%, instead of 20%); if using alternative lipid measures (in a modified QRisk252 score in primary prevention or directly in secondary prevention); and the effect of averaging lipid measurements over values from multiple blood tests. Sensitivity analyses to address increased incidence of diabetes in statin users and a quality-of-life decrement in diabetes were carried out by increasing these parameters and re-running the model until a value was found at which annual monitoring was no longer cost-effective compared with less frequent monitoring. For these analyses we took ‘less frequent’ monitoring to be biennial lipid monitoring. We examined sensitivity to assumptions about medication adherence similarly.
We used a sensitivity analysis approach to consider whether or not our results are robust against hypothetical, currently unreported or unquantified adverse effects of statins. That is, we posited a hypothetical utility decrement associated with statin usage itself, and re-ran the model gradually increasing this from zero until a utility decrement was found at which annual monitoring was no longer cost-effective. We used bootstrapping methods to draw 1000 samples of the same size as the original sample for the primary prevention group with replacement to account for sampling variability. Point estimates of mean total costs and mean total QALYs were reported comparing annual monitoring with triennial monitoring in the primary prevention group when the risk threshold was lowered to 10%, for both males and females, using TC/HDL cholesterol as the lipid being monitored.
Scenarios
In the main analyses, we compare monitoring intervals of between 1 and 5 years. Incorporating the primary analysis, and the sensitivity analyses listed above, the following scenarios were considered:
-
Current guidelines:
-
primary – TC/HDL cholesterol ratio in QRisk2 – threshold of 20%
-
primary on statins – TC/HDL cholesterol ratio in QRisk2 – threshold of 20%
-
secondary on statins – TC – threshold of 4 mmol/l
-
secondary on statins – LDL – threshold of 2 mmol/l
-
-
Alternative lipid measures:
-
primary – LDL/HDL cholesterol ratio in modified QRisk2 score – 20%
-
primary on statins – LDL/HDL cholesterol ratio in modified QRisk2 score – 20%
-
secondary on statins – TC/HDL cholesterol ratio – threshold of 4 mmol/l
-
secondary on statins – LDL/HDL cholesterol ratio – threshold of 2 mmol/l
-
primary – LDL – 20%
-
primary – TC – 20%
-
primary on statins – LDL – 20%
-
primary on statins – TC – 20%
-
-
Sensitivity analysis of current guidelines and alternative lipid measures:
-
lower CVD threshold to 15%
-
adjust treatment effects to upper and lower 95% CIs
-
adjusting the incidence rate of diabetes from statin usage
-
a quality of life decrement for males and females who have diabetes
-
a quality of life decrement for males and females who use statins
-
adjusting the adherence rate for people on statins
-
-
Primary prevention taking statins – TC/HDL cholesterol – threshold of 20%:
-
average of five repeat tests, then monitor at 5-year intervals
-
average of three repeat tests, then monitor at 3-year intervals
-
average of three repeat tests, then monitor at 5-year intervals
-
-
Secondary prevention taking statins – TC – threshold 4 mmol/l:
-
average of five repeat tests, then monitor at 5-year intervals
-
average of three repeat tests, then monitor at 3-year intervals
-
average of five repeat tests, then monitor at 3-year intervals
-
-
Primary prevention (including primary prevention not taking statins and primary prevention taking statins) – TC/HDL cholesterol:
-
10% CVD risk threshold (in the context of the new NICE guidelines56 for CVD prevention)
-
the effect of implementing a utility decrement for patients who have diabetes
-
the effect of implementing a utility decrement for patients on statins
-
the effect of an increased incidence of diabetes for patients on statins
-
the effect of reduced adherence for patients on statins
-
cost-effectiveness of lowering the risk threshold from 20% to 15%.
-
Major amendments to protocol
Our main analyses assumed that a 10-year CVD risk threshold of 20% would be used to guide treatment decisions in primary prevention, based on guidelines at time of protocol development. In sensitivity analyses described above, we also considered the use of a 15% risk threshold. Subsequent to completion of the analyses, new NICE guidelines56 were published recommending that treatment decisions should be based on a 10% risk threshold. We therefore conducted a further, post hoc, sensitivity analysis comparing annual to triennial monitoring under a 10% risk threshold.
The original protocol specified that, in anticipation of difficulties using QRisk252 algorithms, we would use Framingham53 for CVD risk estimation, recalibrated to QRisk252 if possible. In the event, we were able to obtain open-source software implementing the QRisk252 algorithm from the developers and have therefore used QRisk252 throughout. This change is in line with the most recent UK guidelines, which give emphasis to QRisk252 over Framingham methods53 for risk estimation.
The protocol specified that in addition to the above scenarios we would evaluate a strategy mentioned in the 2008 NICE guideline2 in which ‘once a person has been started on a statin for primary therapy, repeat lipid measurement is unnecessary’. Because of constraints of computational time we were not able to include this analysis.
In the event of substantial uncertainty in model results, the protocol specified that we would evaluate the costs and benefits to the NHS of further research to reduce this uncertainty. Following the results of our main and sensitivity analyses, in which one monitoring strategy dominated, there was not sufficient uncertainty to justify such an analysis.
Results
Monitoring a primary prevention population not taking statins
Table 50 compares annual monitoring to triennial and quinquennial monitoring of lipids. Under 2010 guidelines using a 20% CVD risk threshold, annual monitoring appears to be less costly and more effective than less frequent levels of monitoring with all lipid measures: annual intervals dominate over less frequent intervals of monitoring. Under a CVD risk threshold of 15%, again annual monitoring appears to be less costly and more effective than less frequent levels of monitoring.
Gender | Treatment group | Risk threshold | 3 year vs. 1 year | 5 year vs. 3 year | 10 year vs. 5 year |
---|---|---|---|---|---|
Female | Primary not taking statins (TC/HDL cholesterol) | 20% | Annual monitoring dominates | Annual monitoring dominates | Annual monitoring dominates |
Primary taking statins (TC/HDL cholesterol) | 20% | Annual monitoring dominates | Annual monitoring dominates | Annual monitoring dominates | |
Secondary taking statins (TC) | TC 4 mmol/l | Annual monitoring dominates | Annual monitoring dominates | Annual monitoring dominates | |
Secondary taking statins (LDL) | LDL 2 mmol/l | Annual monitoring dominates | Annual monitoring dominates | Annual monitoring dominates | |
Male | Primary not taking statins (TC/HDL cholesterol) | 20% | Annual monitoring dominates | Annual monitoring dominates | Annual monitoring dominates |
Primary taking statins (TC/HDL cholesterol) | 20% | Annual monitoring dominates | Annual monitoring dominates | Annual monitoring dominates | |
Secondary taking statins (TC) | TC 4 mmol/l | Annual monitoring dominates | Annual monitoring dominates | Annual monitoring dominates | |
Secondary taking statins (LDL) | LDL 2 mmol/l | Annual monitoring dominates | Annual monitoring dominates | Annual monitoring dominates |
Figures 55 and 56 report a post hoc sensitivity analysis, comparing annual monitoring with triennial monitoring under a CVD risk threshold of 10%, as bootstrapped estimates for mean costs and QALYs on a cost-effectiveness plane. For males, the discounted cost of annual monitoring was, on average, £465 (95% CI –£124 to –£806) less than triennial monitoring, with a discounted incremental QALY gain of 0.31 (95% CI 0.11 to 0.51). For females, the discounted cost of annual monitoring was, on average, £387 (95% CI –£8 to –£766) less than triennial monitoring, with a discounted incremental QALY gain of 0.29 (95% CI 0.12 to 0.47). Annual monitoring dominates (achieving lower incremental costs and higher incremental QALYs) triennial monitoring in 98% and 99% of all replications for males and females, respectively.
FIGURE 55.
Cost-effectiveness plane graphing differences between annual vs. triennial monitoring of TC/HDL cholesterol for males after 1000 bootstrap replications when considering the new treatment threshold guidelines of 10% in the primary prevention group. Vertical axis shows differences in mean total costs, horizontal axis shows differences in mean QALYs. Annual monitoring generated lower costs and more QALYs than the control treatment in 98% of all replications.
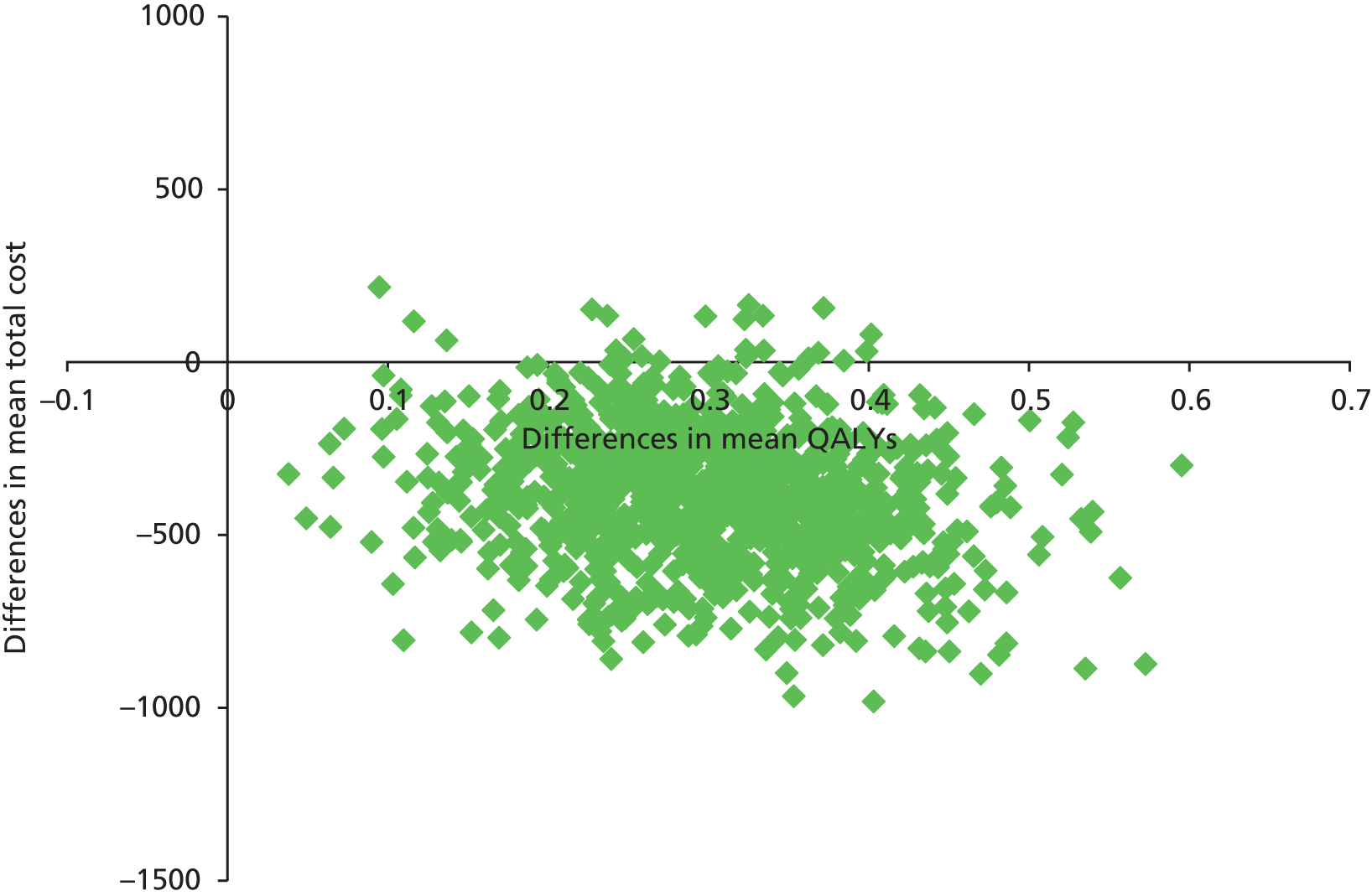
FIGURE 56.
Cost-effectiveness plane showing differences between annual vs. triennial monitoring of TC/HDL cholesterol for females after 1000 bootstrap replications when considering the new treatment threshold guidelines of 10% in the primary prevention group. Vertical axis shows differences in mean total costs, horizontal axis shows differences in mean QALYs. Annual monitoring generated lower costs and more QALYs than the control treatment in 99% of all replications.

Monitoring a primary prevention population taking statins
Results in a primary prevention population taking statins based on current guidelines (see Table 50) are similar, in that annual monitoring appears to be less costly and more effective than less frequent levels of monitoring with all lipid measures. This applies under both 20% and 15% risk thresholds.
Monitoring a secondary prevention population taking statins
Results for a secondary prevention population based on current guidelines are shown above (see Table 50). In this population who have already experienced a CVD event, changes in costs and QALYs with different monitoring intervals are smaller than in primary prevention, but annual monitoring still appears to be less costly and more effective than less frequent levels of monitoring with all lipid measures.
Sensitivity analyses
A summary of analyses based on alternative lipid measures, alternative treatment thresholds, and other sensitivity analyses conducted can be found in Tables 51–56.
Gender | Treatment group | Risk threshold | 3 year vs. 1 year | 5 year vs. 3 year | 10 year vs. 5 year |
---|---|---|---|---|---|
Female | Primary not taking statins (LDL/HDL cholesterol) | 20% | Annual monitoring dominates | Annual monitoring dominates | Annual monitoring dominates |
Primary taking statins (LDL/HDL cholesterol) | 20% | Annual monitoring dominates | Annual monitoring dominates | Annual monitoring dominates | |
Secondary taking statins (TC/HDL cholesterol) | TC/HDL cholesterol 4 mmol/l | Annual monitoring dominates | Annual monitoring dominates | Annual monitoring dominates | |
Secondary taking statins (LDL/HDL cholesterol) | LDL/HDL cholesterol 2 mmol/l | Annual monitoring dominates | Annual monitoring dominates | Annual monitoring dominates | |
Primary not taking statins (LDL) | 20% | Annual monitoring dominates | Annual monitoring dominates | Annual monitoring dominates | |
Primary not taking statins (TC) | 20% | Annual monitoring dominates | Annual monitoring dominates | Annual monitoring dominates | |
Primary taking statins (LDL) | 20% | Annual monitoring dominates | Annual monitoring dominates | Annual monitoring dominates | |
Primary taking statins (TC) | 20% | Annual monitoring dominates | Annual monitoring dominates | Annual monitoring dominates | |
Male | Primary not taking statins (LDL/HDL cholesterol) | 20% | Annual monitoring dominates | Annual monitoring dominates | Annual monitoring dominates |
Primary taking statins (LDL/HDL cholesterol) | 20% | Annual monitoring dominates | Annual monitoring dominates | Annual monitoring dominates | |
Secondary taking statins (TC/HDL cholesterol) | TC/HDL cholesterol 4 | Annual monitoring dominates | Annual monitoring dominates | Annual monitoring dominates | |
Secondary taking statins (LDL/HDL cholesterol) | LDL/HDL cholesterol 2 | Annual monitoring dominates | Annual monitoring dominates | Annual monitoring dominates | |
Primary not taking statins (LDL) | 20% | Annual monitoring dominates | Annual monitoring dominates | Annual monitoring dominates | |
Primary not taking statins (TC) | 20% | Annual monitoring dominates | Annual monitoring dominates | Annual monitoring dominates | |
Primary taking statins (LDL) | 20% | Annual monitoring dominates | Annual monitoring dominates | Annual monitoring dominates | |
Primary taking statins (TC) | 20% | Annual monitoring dominates | Annual monitoring dominates | Annual monitoring dominates |
Gender | Treatment group | Risk threshold | 3 year vs. 1 year | 5 year vs. 3 year | 10 year vs. 5 year |
---|---|---|---|---|---|
Female | Primary not taking statins (TC/HDL cholesterol) | 15% | Annual monitoring dominates | Annual monitoring dominates | Annual monitoring dominates |
Primary not taking statins (LDL/HDL cholesterol) | 15% | Annual monitoring dominates | Annual monitoring dominates | Annual monitoring dominates | |
Primary not taking statins (TC) | 15% | Annual monitoring dominates | Annual monitoring dominates | Annual monitoring dominates | |
Primary taking statins (TC/HDL cholesterol) | 15% | Annual monitoring dominates | Annual monitoring dominates | Annual monitoring dominates | |
Primary taking statins (LDL/HDL cholesterol) | 15% | Annual monitoring dominates | Annual monitoring dominates | Annual monitoring dominates | |
Primary taking statins (TC) | 15% | Annual monitoring dominates | Annual monitoring dominates | Annual monitoring dominates | |
Male | Primary not taking statins (TC/HDL cholesterol) | 15% | Annual monitoring dominates | Annual monitoring dominates | Annual monitoring dominates |
Primary not taking statins (LDL/HDL cholesterol) | 15% | Annual monitoring dominates | Annual monitoring dominates | Annual monitoring dominates | |
Primary not taking statins (TC) | 15% | Annual monitoring dominates | Annual monitoring dominates | Annual monitoring dominates | |
Primary taking statins (TC/HDL cholesterol) | 15% | Annual monitoring dominates | Annual monitoring dominates | Annual monitoring dominates | |
Primary taking statins (LDL/HDL cholesterol) | 15% | Annual monitoring dominates | Annual monitoring dominates | Annual monitoring dominates | |
Primary taking statins (TC) | 15% | Annual monitoring dominates | Annual monitoring dominates | Annual monitoring dominates |
TC/HDL cholesterol | |||
---|---|---|---|
Gender | Treatment group | Risk threshold | 3 year vs. 1 year |
Female | Primary not taking statins | 10% | Annual monitoring dominates |
Male |
Sensitivity analysis (20% CVD risk threshold) | Results |
---|---|
Incidence of diabetes per year for patients while on statins for males | 14% (10–18%) |
Incidence of diabetes per year for patients while on statins for females | 12% (7–17%) |
A utility decrement associated for patients with diabetes for males | 0.03 (0.02–0.04) |
A utility decrement associated for patients with diabetes for females | 0.02 (0.01–0.03) |
A utility decrement associated with statins for females | 0.11 (0.09–0.13) |
A utility decrement associated with statins for males | 0.08 (0.07–0.09) |
Adherence to statins for females | 50% (39–61%) |
Adherence to statins for males | 65% (56–74%) |
Sensitivity analysis (20% CVD risk threshold) | Results |
---|---|
Incidence of diabetes per year for patients while on statins for males | 8% (4–12%) |
Incidence of diabetes per year for patients while on statins for females | 6% (4–8%) |
A utility decrement associated for patients with diabetes for males | 0.02 (0.01–0.03) |
A utility decrement associated for patients with diabetes for females | 0.01 (0.005–0.015) |
A utility decrement associated with statins for females | 0.06 (0.03–0.09) |
A utility decrement associated with statins for males | 0.04 (0.02–0.06) |
Adherence to statins for females | 70% (55–85%) |
Adherence to statins for males | 83% (72–94%) |
Gender | Treatment group | Threshold | Five repeat tests, monitor 5-year intervals vs. three repeat tests, 3-year intervals | Three repeat tests, monitor 5-year intervals vs. three repeat tests, 3-year intervals |
---|---|---|---|---|
Female | Primary taking statins (TC/HDL cholesterol) | QRisk252 > 20% | Three repeat tests, 3-year intervals dominates | Three repeat tests, 3-year intervals dominates |
Male | Primary taking statins (TC/HDL cholesterol) | QRisk252 > 20% | Three repeat tests, 3-year intervals dominates | Three repeat tests, 3-year intervals dominates |
Female | Secondary taking statins | TC 4 mmol/l | Three repeat tests, 3-year intervals dominates | Three repeat tests, 3-year intervals dominates |
Male | Secondary taking statins | TC 4 mmol/l | 7.6 –1.92 £0.24M three repeat tests, 3-year intervals dominates | 5.3–1.56 £0.29M three repeat tests, 3-year intervals dominates |
Diabetes
In sensitivity analyses applying a utility decrement for patients with diabetes, in primary prevention using a 20% CVD risk threshold, for any decrement up to 0.03 for females and 0.02 for males, annual monitoring remains cost-effective compared with less frequent monitoring. Similar results, 0.02 and 0.01 for females and males, respectively, were obtained in secondary prevention. For reference, a decrement of 0.05 is equivalent to a patient with type 2 diabetes who has experienced a stroke (transient ischaemic attack) compared with the overall study population in a study that measured health–utility scores from a multicentre, prospective observational study of 7327 patients with type 2 diabetes. 236 In sensitivity analyses exploring the effect of increased incidence of diabetes in patients taking statins, for any incidence rate up to 14% per year in males and 12% per year in females, annual monitoring remained cost-effective compared with less frequent monitoring in primary prevention. Results of 8% and 6% for females and males, respectively, were obtained for secondary prevention.
Other adverse effects
In sensitivity analyses applying a hypothetical utility (QALY) decrement to all patients on statins, it was found that, for any utility decrement up to 0.11 for females and 0.08 for males, annual monitoring in primary prevention remains cost-effective compared with less frequent monitoring. Results of 0.06 and 0.04 for females and males, respectively, were obtained for secondary prevention.
Adherence
In sensitivity analyses exploring the effect of reduced adherence for patients on statins, for any adherence rate > 35% (non-adherence up to 65%) in male patients and for any adherence rate > 50% (non-adherence up to 50%) in female patients, annual monitoring remained cost-effective compared with less frequent monitoring. The corresponding lower limits of adherence (upper limits of non-adherence) are 17% (83%) in males and 30% (70%) in females in secondary prevention.
Comparison of risk thresholds
A comparison of the cost-effectiveness of annual monitoring with a 10-year cardiovascular risk threshold of 20% or 15% in primary prevention is presented in Table 57. The additional costs of treating more patients, when using a threshold of 15%, are offset by the decrease in CVD events, resulting in higher utilities and lower costs for all lipid groups in the primary prevention group and primary prevention with statins group.
Lipid | Prevention group | Gender | Costs (£) | Utilities | ICERS |
---|---|---|---|---|---|
TC/HDL cholesterol | Primary not taking statins | Female | –3,200,000 | 430.85 | 15% threshold dominates |
TC/HDL cholesterol | Primary not taking statins | Male | –6,200,000 | 930.54 | |
LDL/HDL cholesterol | Primary not taking statins | Female | –4,700,000 | 522.92 | |
LDL/HDL cholesterol | Primary not taking statins | Male | –7,200,000 | 835.61 | |
TC | Primary not taking statins | Female | –16,300,000 | 1706.93 | |
TC | Primary not taking statins | Male | –14,000,000 | 1753.13 | |
LDL | Primary not taking statins | Female | –13,500,000 | 1409.04 | |
LDL | Primary not taking statins | Male | –11,100,000 | 1388.72 | |
TC/HDL cholesterol | Primary taking statins | Female | –4,300,000 | 450.16 | |
TC/HDL cholesterol | Primary taking statins | Male | –7,200,000 | 710.29 | |
LDL/HDL cholesterol | Primary taking statins | Female | –5,800,000 | 517.78 | |
LDL/HDL cholesterol | Primary taking statins | Male | –6,900,000 | 878.92 | |
TC | Primary taking statins | Female | –21,100,000 | 2171.44 | |
TC | Primary taking statins | Male | –16,100,000 | 1956.33 | |
LDL | Primary taking statins | Female | –16,100,000 | 1693.78 | |
LDL | Primary taking statins | Male | –12,700,000 | 1661.96 |
Discussion and interpretation of results
Following patent expiration in the UK, statins at different dosages have now become very affordable. The low costs associated with lipid monitoring and assessing cardiovascular risk mean that monitoring lipid levels more frequently would identify patients who are at high cardiovascular risk (or above lipid threshold) who would then need to be given statin treatment. The increased costs of more frequent monitoring and treatment are offset by the number of potential cardiovascular events prevented. As the hospital costs associated with a cardiovascular event are quite large in comparison with the cost of statins, this means that more frequent monitoring is more cost-effective. This was found whether or not cardiovascular risk thresholds of 20%, 15% or 10% are assumed to guide primary prevention treatment decisions.
Although there are associated negative effects of taking statins (fibromyalgia, fatigue, muscle soreness), our sensitivity analysis model results have shown that the negative effects need to be substantially greater (i.e. a utility decrement for all patients associated with the usage of statins) than recorded in the literature in order to change the decision to more frequently monitor lipids at current cardiovascular risk thresholds. This is consistent with the latest published research. 237,238
Another issue that we considered was adherence to statin medication. Uptake and adherence of statin medication in real clinical practice may not be the same as in the RCTs from which our effect estimates derive. Chowdhury et al. ’s 2013 meta-analysis239 of 12 prospective studies found that the prevalence of good adherence to statins was suboptimal at 60% (95% CI 52% to 68%). We have not attempted to model a distinction between non-adherence and discontinuation: none of the studies in the Chowdhury review was > 10 years, and we do not know how discontinuation rates may behave in the longer term. In sensitivity analyses we found that annual monitoring remained cost-effective compared with less frequent monitoring, even if adherence is substantially lower than in trials. As in Chapter 5, our simulations are based on a CPRD population with at least one lipid measurement present in the year before baseline. Our results therefore will not apply to patients who decline invitations to attend lipid screening. Such patients will accrue no costs and receive neither harms nor benefits from lipid screening.
The 15% risk threshold for treatment decisions in monitoring primary prevention patients dominates the 20% risk threshold, which suggests that the risk threshold could be further reduced and still be cost-effective according to NICE’s £30,000 per QALY threshold. Since this work was conducted, NICE has published new guidelines for cardiovascular risk assessment in the UK, suggesting the lowering of the 10-year cardiovascular risk from 20% to 10%. 56 Recently, the American Heart Association (AHA) also lowered the suggested threshold for 10-year cardiovascular risk to 7.5%,240 again suggesting an increase in uptake of statins. These new guidelines would substantially increase the number of patients undertaking statin medication. Our analyses, carried out before the guidance was published, do not directly compare the 10% to the 15% or 20% threshold. However, we did compare monitoring intervals at various thresholds including, in a sensitivity analysis, 10%: we found that annual monitoring is more cost-effective than less frequent monitoring at all of the risk thresholds that we considered.
Comparison with previous literature
Previous HTA-commissioned research234 in 2007 conducted a systematic review and meta-analysis of economic evaluations of statins for the primary prevention of coronary events. The study234 concluded that statin therapy in secondary prevention was cost-effective compared with other treatments; the cost-effectiveness ratio results were mixed in the primary prevention group. However, the analyses did not take into account costs of identifying and screening the relevant population at risk, which this current study does. A key difference between the current study and previous studies is the expiration of patents on statins in the UK. The previous HTA report suggested that ‘the analyses are sensitive to the cost of statins . . . the cost-effectiveness results will need to be reviewed in the light of any significant changes in the price of statins’. The changes in the price of statins has resulted in a major shift towards the cost-effectiveness of more frequent monitoring of lipids and encouraging an increase in the uptake of statins.
The 2013 Cochrane review of primary prevention with statins concluded that statins reduce all-cause mortality and cardiovascular events without increasing the risk of adverse events among people at low risk of CVD (< 10% over 10 years). 237 Previous reviews of RCTs have investigated adverse effects of statins. The Cholesterol Treatment Trialists collaboration found no increased risk of cancer associated with statin use. 241 Sattar et al. 242 found a 9% increase in the odds of developing diabetes in those randomised to statins and further this is most apparent at high doses. 243 Increased risk of intra cerebral haemorrhage has been reported but was not confirmed, in populations without previous stroke, by two systematic reviews of RCTs. 244,245 A Cochrane review did not find increased risk of rhabdomyolysis in statin users. 237 Recent systematic reviews238,246 found no increase in muscle problems in statin groups compared with placebo groups across randomised trials; however, two trials not included in those reviews did find such effects. 247,248 A recent overview commented that there were other possible effects of statins that did not have strong RCT evidence, such as the possible increase of cataracts. 249
An extreme scenario to consider would be to suggest universal treatment at highest tolerated dose, as the associated costs of overtreatment are very small in relation to the costs of undertreatment. However, it is difficult to draw firm policy conclusions regarding the merits of universal treatment because of the limited number of studies reporting continued long-term statin usage. Therefore, it is necessary to collect more evidence surrounding the benefits and adherence for patients with statins in a much longer time period. This should be examined in future work before universal treatment can be considered a viable policy option.
Strengths and limitations
Our results were robust when challenged in sensitivity analyses (especially for females, in whom monitoring is assumed to begin later in life). There are a number of limitations that require acknowledgement in our model. Multiple CVD events are not explicitly included in the model as the QRisk252 equation, but the impact of these events on costs and mortality is taken into account for those with a history of the event. Second, our analysis is over a lifetime, whereas the data we have from the CPRD is limited to only 7 years and so we have had to make a ‘life table’ assumption that the current risk in the UK population can represent risks over a lifetime. When accounting for uncertainty in our model, we assumed independence between (1) variables in the same risk equations and (2) different risk equations. The level of uncertainty could potentially be impacted upon if the covariance between parameters is significant.
The choice of starting dose of atorvastatin at 10 mg was based on available information at the time the protocol was written, being similar in LDL reduction to the simvastatin dose (40 mg) in recommendations at that time. 2,59 After the analyses were carried out, the 2014 NICE guidance recommended atorvastatin 20 mg as the starting dose in primary prevention. 56 Given the small difference between 10 mg and 20 mg found in the review conducted in Chapter 3, this discrepancy would have only modest impact on specific cost–utility estimates and will not affect our overall conclusion that annual monitoring dominates.
Our model captures the cardiovascular benefits, potential increase in myopathy, and, in a sensitivity analysis, increased incidence of diabetes, of statins, but, as discussed above, there are other potential effects of statins for which definitive RCT evidence is not currently available. To address this, a sensitivity analysis explored the potential impact of any currently unquantified harms of statin prescription, and found that unless such harms had QALY decrements as high as 0.04 to 0.11 then our overall conclusions, in favour of annual monitoring, remain justified. This analysis addresses concerns about both clinical adverse effects of statins and psychological effects of labelling such as those reported for hypertension. 250
Conclusion
We estimate that annual monitoring is not only more effective, but also cost-saving (dominates) compared with less frequent monitoring strategies. This finding appears robust under a variety of assumptions, including varying risk thresholds used to guide decision-making in primary prevention.
Chapter 8 Early dissemination meeting of findings
Format of meeting and outputs
As part of our dissemination strategy, and in order to be able to incorporate comments from key researchers and stakeholders in the scientific community, we held an early meeting at which provisional results were presented, followed by a group discussion. The meeting was held on Wednesday 18 September 2013 from 13.00 to 17.00 at St Hugh’s College, Oxford, with lunch provided beforehand to allow the group to network and get to know each other.
In total, 57 guests were invited, made up from the 18 people involved in the project, seven internal staff members from the Nuffield Department of Primary Care Health Sciences (NDPCHS) and 32 external academics who have published key papers on lipids or CVD in relevant fields. A personal letter of invitation was sent to all of those invited, via post and through e-mail 3 months prior to the meeting on behalf of Professor Richard Hobbs, Director of NDPCHS and Dr Rafael Perera (Principal Investigator), Director of Medical Statistics, NDPCHS. In total, 33 people responded, wishing to attend the meeting, and 19 responded to say that they had prior arrangements.
Those who attended the meeting were considered to be largely representative of those invited, and included both UK and international opinion leads in the field of lipid and cardiovascular research, a representative from NICE, and various relevant researchers who have collaborated with members of the NDPCHS.
The meeting began with Professor Richard Hobbs welcoming the group to Oxford and an overview of the NDPCHS. Dr Rafael Perera then gave the group an overview of lipid monitoring and a brief summary of the main aims of the project. This was followed by three separate presentations, one from each of the section leads, which focused on the choice of lipid measurements for monitoring and possible monitoring strategies for both primary and secondary prevention population groups. The presentations were as follows:
-
Julie McLellan gave an overview of findings from Chapter 2 on the predictive value of different lipid measures for CVD and mortality in primary and secondary populations. Ms Julie McLellan explained the background of this work, summarised the methods used, and presented early results.
-
Dr Emily McFadden gave a presentation on the work completed for the statistical modelling section, presented in this report in Chapters 4 and 5. Dr Emily McFadden summarised the data sources and statistical methods used, before describing results on lipid variability and the corresponding estimates of false treatment decisions under different monitoring scenarios.
-
Dr Philip Clarke and Thomas Lung then presented an overview and explained provisional results from the economic model used to estimate the cost-effectiveness of different monitoring scenarios seen in Chapter 7.
A question and answer session followed, which developed into a lively debate on the clinical implications of the work presented and some suggestions for further analyses for potential inclusion into this report (HTA monograph).
The whole of the dissemination meeting, including both presentations and the discussion, were filmed to enable us to disseminate the findings from the meeting as quickly as possible. Two films have now been produced:
-
Optimal Lipid Monitoring dissemination meeting: study findings
-
The role of early dissemination meetings in the research process
The first film included interviews from Dr Rafael Perera, Professor Richard Hobbs, Dr Emily McFadden, Dr Richard Stevens, Dr Rubin Minhas, Professor Carl Heneghan and Dr Gautam Deshpande, and lasts 7 minutes 29 seconds. This film gives a general overview on how a long-term condition might impact on people’s health, and concentrates on the main results at the time of the meeting, with information about the models used and how the findings would impact on the general public.
The second film included interviews with Dr Rafael Perera, Professor Richard Hobbs, Dr Richard Stevens, Dr Rubin Minhas and Dr Gautam Deshpande, and lasts 2 minutes 35 seconds. This film concentrates on the outcome of the meeting, and gives the different groups a chance to discuss how this project will have an impact on people’s health. The dissemination meeting helped us to identify opinions from different experts in the field, and enabled debate on potential monitoring strategies and their clinical impact. It was a successful way to disseminate our provisional findings quickly, enabling us to incorporate valuable feedback into the final report, and will help us to deliver the evidence as fast as possible into the general public.
These films will be available at the NDPCHS, University of Oxford website: http://youtu.be/Hhv87ocvH6Q.
Chapter 9 Patient and public involvement representative comment on universal treatment
Preface
The results of Chapter 7 show that annual monitoring is more cost-effective than less frequent monitoring strategies, even though (see Chapter 5) annual monitoring carries the highest rate of statin prescriptions that are ‘unnecessary’: that is, prescription of statins to people whose underlying cholesterol value (or cardiovascular risk) is below a treatment threshold, but whose cholesterol value (and hence apparent cardiovascular risk) measures high on a particular occasion due to within-measurement variability (see Chapter 4).
This cost-effectiveness, despite over-prescription, arises because statins are cheap, so that the main deterrent to their wide use is possible harms, and, because they are well-tolerated, with few side-effects compared to their cardiovascular benefits, in almost all populations the cardiovascular benefits outweigh the few known health risks. Further, as a result of the expiry of atorvastatin patent status in 2012, the costs associated with prescribing statins are lower than the cost of a monitoring programme to allocate (‘ration’) them. Thus, frequent monitoring programmes, with a relatively high false-positive rate and relatively low false-negative rate (see Chapters 5 and 7) are more cost-effective than less frequent monitoring programmes with a lower false-positive rate but higher false-negative rate.
At the early dissemination meeting (see Chapter 7) this interpretation of the cost-effectiveness results was discussed. It was observed that, taken to the extreme, this line of argument would advocate universal statin treatment (in some age-defined population) rather than a monitoring programme. Provisional results from the simulation model of Chapter 5 were presented showing the modelled cardiovascular benefit of universal treatment compared with treatment based on a monitoring programme (Figure 57).
FIGURE 57.
Modelled cardiovascular event rates under the assumption of universal treatment with 80 mg of atorvastatin, universal treatment with 40 mg of atorvastatin, or treatment allocated according to current UK guidelines, in a primary prevention population.

The PPI member of the project steering committee was therefore invited to consider, from a patient perspective, the potential implications of replacing cholesterol monitoring with universal treatment in an age-defined population. The PPI member reviewed drafts of Chapters 2–7, met with the project team for discussion, and prepared a written commentary, which follows below.
To monitor or not to monitor? A patient and public involvement view
Summary As the PPI representative, I was asked to consider the proposition that universal prescription of statins could sensibly be adopted for everyone over a certain age without prior measuring or subsequent monitoring of cholesterol or lipids. My personal conclusion, which I would guess would be the view of many patients and members of the public, is that such a change of practice might turn out, on closer inspection, to be a very finely balanced question and one to be treated with great caution. I suggest a list of factors that should be considered and, if possible, quantified. And – if the balance of advantage still appears to lie with universal prescription – some thoughts on a cautious step-by-step introduction.
The question
-
Now that atorvastatin has come off patent it would cost less to prescribe statins to everyone over a given age than to conduct tests. The known side effects are thought to be slight and the benefits great. We had to consider whether or not this was a safe option with great benefits to health as well as to expenditure. Speaking personally, I find it exciting when a new insight rearranges all the furniture and one knows that the room will never look the same again. But new insights generally arrive with the beauty of simplicity, uncluttered by caveats and conditions. They need to be interrogated.
-
In the present case the insight hangs on a direct comparison of the net costs of two options. In discussion we came to call these ‘measure and monitor’ compared with ‘fire and forget’. The former included the costs of initial measurement and subsequent monitoring, selective prescription and known side effects, and the benefits of reduced cardiovascular incidents. The latter included the costs of universal prescription and known side effects, and the benefits of reduced cardiovascular incidents.
-
As a patient on statins and a lay member of the public, I wanted to know whether or not those costs and benefits painted the full picture. If there were additional costs or drawbacks to ‘fire and forget’ or potential savings and improvements in ‘measure and monitor’ then the gap between the two options might close or the balance of advantage reverse. I suggested a list of potential costs and savings that patients or members of the public might wish to be considered (many of these having been already identified in team discussion).
Potential extra costs with ‘fire and forget’
-
Unknown side effects These might arise either as (a) a result of new medical research or (b) new evidence accruing with the passage of years (to date it has not been possible to study the side effects of taking statins over long periods of time but a universal prescription could place hundreds of thousands of people on statins for 30, 40 or 50 years).
-
Over- or under-prescription Statins prescribed without measuring the patient’s condition would necessarily mean a standard dosage. A low dose would fail to achieve the protection needed by high-risk people, with consequent costs from cardiovascular incidents. A high dose would imply additional costs from side effects, both known and (if any) unknown.
-
Non-adherence I learnt that occasional lapses by a patient in taking statins are generally unimportant. But there are those who discontinue their treatment for long periods or completely, for a variety of personal reasons ranging from anxiety about side effects to over-optimism about their health. Non-adherence brings costs to health. We should expect non-adherence to be greater once statins are prescribed without any prior measurement because many people would doubt that they have any need for them.
-
Labelling Would being told that they need statins lead to more people labelling themselves as having a medical condition to worry about? This might increase costs due to (a) more visits to their GP (see discussion of GP costs below) and (b) an increase in sick leave from work.
Fear of side effects
-
People vary. Some are keen to take prescriptions, alternative medicines, dietary supplements, etc. Others are reluctant to muck around with their body or are even fearful of side effects. With a universal, unmonitored ‘fire and forget’ policy, this latter group might become significant. One person talks to another. Resistance to taking the prescription would probably spread, non-adherence rates would rise among those who start the regime, and the costs from unprevented cardiovascular incidents would rise.
Media scares
-
Investigative journalism and marketing managers, in newspapers and TV alike, love a good scare story about health. Media stories are magnified by Twitter and YouTube. People do not trust scientists, pharmaceutical companies or governments. There must be a risk of a scare about the side effects of long-term use of statins by people who do not need them. Perhaps the probability of a scare is no higher than moderate, but the consequences could be highly costly: potentially not only a collapse of faith in universal prescription, but also of growing distrust of statins themselves, leading to more cardiovascular incidents in the population than at present. And perhaps a good scare about statins would smooth the path to a new scare about some other science-based proposal.
Potential new savings for ‘measure and monitor’
-
GPs’ time The main costs of measurement are in staff time, and the GPs’ time is the most costly. Visits to the doctor are triggered by the patients themselves. I question whether or not GPs’ time might be overstated in the present analysis of ‘measure and monitor’: (1) some of the present GP visits should be discounted from the equation (because the patient wants a visit for other reasons); (2) general practices could promote widespread cholesterol monitoring without a prior consultation with the GP – just going straight to blood sampling; and (3) as a minor point, I wonder why the first-year monitoring costs in the model are assumed to be three to four times greater than those for subsequent years – is this dependent on assumptions about GP time?
-
Lower cost measures The study is looking at the effectiveness of different measures in predicting health problems. If (without choosing less effective tools) measures can be adopted that would require fewer GP consultations or fewer visits to the clinic for blood sampling then costs would reduce.
-
Longer intervals between monitoring Given the observation that statins are so cheap and relatively harmless that you might as well do away with measurement altogether, there is at least an argument for extending the periods between taking measurements in a regime of monitoring. Ten-year intervals, rather than five-year intervals, would halve the monitoring costs.
Intermediate positions
-
If, after exploring these possible costs and savings, the balance of advantage still appears to lie with a ‘fire and forget’ regime then it might be prudent to consider partial or incremental implementation.
-
For example, as a first step, universal prescription without measurement might be brought in for those aged > 70 years. The threshold might be reduced progressively to ≤ 60 years. This would seem particularly attractive if the balance of advantage seemed to favour ‘fire and forget’ on those costs that are readily quantifiable, but there were to remain great doubts about the hard-to-quantify factors. These might include, especially, widespread public doubts about the long-term side effects of taking statins and the risk of a media scare story. A cautious first step to those aged > 70 years old would allow time for the public to accept a new approach, time for the public reaction to be assessed, and time for medical research into long-term effects of statins.
Conclusion
-
I hope these thoughts are helpful. I cannot judge how far these factors are amenable to quantification, or even how far the arguments are valid. They are a lay person’s perception, but I think it is reasonable to emphasise that I suspect ‘fire and forget’ would be very exposed to repercussions from public doubts and anxieties, and vulnerable to media scare.
John Stevens
Chapter 10 Discussion and conclusions
This work extends a body of knowledge that has identified that many observed changes in lipid measurements, in the absence of a lipid-lowering treatment change, are due to short-term variability rather than true change. We have reviewed the literature on predictive power of lipids and effects of atorvastatin, estimated variability of lipids, and modelled the consequences, including costs and health effects, of a variety of lipid-monitoring strategies.
Summary of findings
Our systematic review results point to apolipoproteins and composite lipid measures, such as ratios and non-HDL cholesterol, as the strongest predictors of CVD risk and mortality. In particular, the ratio of Apo B to Apo A-I shows a strong association with future CVD events, although few studies evaluated its association with all-cause mortality. In general, associations with all-cause mortality were weaker than associations with CVD events and CVD mortality. Our re-analysis of RCTs of atorvastatin confirmed its effectiveness for lowering LDL and TC at low and high doses. Our analyses of large databases from the UK and Japan show that biological variability and measurement error make up about one-quarter of the total variation in lipid measures. As a result, we estimate that many observed changes in lipid monitoring are due to measurement error and biological variability instead of true change; findings were consistent for all studied lipid measures, single or composite. The relative frequency of incorrectly detecting individuals as above threshold when they are below, and vice versa, is dependent on the interval between measurements in a monitoring strategy. In general, more frequent measurements will lead to an increase in the number of both true-positives and false-positives above threshold classifications. Cost-effectiveness modelling allowed us to incorporate the costs and health consequences of monitoring, treatment and long-term health outcomes in a lipid monitoring programme. Based on these models, we find that any regime that places more individuals on treatment is likely to be cost-effective compared with one that treats less. These results held for both primary and secondary prevention of CVD, and whether or not a 10-year CVD risk threshold of 20%, 15% or 10% is used to guide primary prevention.
Strengths and limitations
We have carried out the most complete review to date of prospective studies reporting on the predictive value of single and composite lipid measures for CVD events, CVD mortality and all-cause mortality. Limitations, such as the relatively small number of studies addressing all of the lipid measures of interest and the use of indirect comparisons, are discussed in Chapter 2. Despite large statistical heterogeneity, strengths include the consistency of many findings across different outcome measures, populations and treatment groups.
Our meta-analysis to determine the efficacy of atorvastatin in reducing lipid levels (increasing in the case of HDL) was based on studies identified in a recently published systematic review of lipid-lowering across statins. 188 We did not carry out a new search for trials to avoid duplication of effort. We independently filtered the studies, extracted data, and quality assessed the 10 included studies115,179,184,191–197 in order to produce estimates of lipid-lowering effects of atorvastatin at different doses. Our estimates were consistent with dose–response equations reported in a Cochrane review,187 except at doses of 40 mg, for which we had only one study. 194
Our statistical analyses of variability and long-term change in lipids were based on data from high-quality data sources. In particular, data from the CPRD are likely to be representative of contemporary clinical practice in the UK and therefore not include those not accepting invitation to participate in CVD risk assessment or taking over-the-counter statin treatment – currently less than 1% of the eligible population. 251 We were also able to show that our estimates are broadly consistent with published data on lipid variability in similar populations (see Appendix 17). Our estimates of rates of false-positive and false-negative tests for lipid or CVD risk above treatment threshold are based on statistical modelling and not a trial comparing different monitoring strategies. Full randomised trials of diagnostic or monitoring strategies require, in general, unfeasibly large sample sizes. 252 Given the complexity and number of monitoring strategies that we have studied, it is hard to conceive of a feasible trial addressing the same research questions.
Our simulations in Chapters 5 and 7 necessarily make simplifying assumptions: for example, that risk factors besides lipid levels and age remain constant for the period under study. BP may remain constant in the short term204 but in the longer term both systolic and diastolic BP are likely to rise over time,253 and even weight is unlikely to remain constant over a 10-year period. 254 However, modelling changes in all risk factors is outside the remit of this report, which focuses specifically on lipid measures in the management of CVD risk. Another necessary simplifying assumption is that treatment decisions follow guidelines, whereas in practice treatment decisions are multifactorial. However, for the purposes of evaluating monitoring strategies we consider it reasonable to model TC and LDL in secondary prevention, and CVD risk in primary prevention, as the driving factors in statin prescribing decisions. Our results were robust in sensitivity analyses to alternate treatment pathways including the use of a 10% threshold of CVD risk for decisions in primary prevention, as recently proposed. 62 Our analyses were also robust under a variety of assumptions about adherence to medication and about known and unknown adverse effects.
The value of lipid monitoring and our conclusions that annual monitoring is cost-effective are dependent on the value of lipid-lowering therapies, including cost, benefits and harms. We have considered only statins, and in particular atorvastatin (as currently recommended by NICE guidelines56) at low, medium and high dose; other lipid-lowering agents, such as proprotein convertase subtilisin-kexin type 9 (PCSK9) inhibitors,255 are not currently recommended by guidelines. In Chapter 3 we reviewed the dose-dependent effects of atorvastatin to complement the many previous reviews of statin benefits and harms. Our cost-effectiveness analyses incorporate cardiovascular benefits of statins and, as discussed in Chapter 7, known and (in sensitivity analyses) potential unknown harms of statins. The latter could include psychological and other effects of ‘labelling’ or medicalisation of those monitored. Nor have we included potential additional benefits of statins, such as possible anti-inflammatory effects256 and non-cardiovascular benefits;249 in this respect, our estimates of benefits of monitoring are likely to be conservative. A further limitation is that we have not considered how risk of adverse events may vary with other patient characteristics.
We used an early dissemination meeting to give stakeholders and experts in the field the opportunity to comment and critique our primary results, their interpretation and implications, and invited our PPI representative (involved since the project was designed) to comment on the discussion. These inputs informed and improved the content of this final report as well as its tone and focus.
Interpretation
The falling costs of statins combined with the current estimates of efficacy and safety indicate that any regime that places more individuals under treatment is likely to be more cost-effective compared with one that treats less. An example of this would be using a 10% threshold of 10-year CVD risk to guide primary prevention, as recommended in the 2014 revisions to NICE clinical guidelines for CVD prevention. 56 Another example in these revised guidelines56 is the move to wider use of maximum dose in secondary prevention. Composites of total and HDL cholesterol are also emphasised, rather than measurement or estimation of LDL cholesterol. Our review of prognostic studies supports this. Although apolipoprotein measurements may have still greater prognostic power, at present they are not generally available in primary care.
Strategies with more frequent lipid monitoring reduce the number of undertreated individuals at the cost of increased treatment in people who do not require it under current guidelines. However, the health and economic costs of undertreatment are much higher than those of unnecessary treatment. As the consequences of failing to treat individuals who later experience a CVD event are overwhelmingly more severe than those of unnecessary treatment, any strategy that treats more individuals is cost-effective. In the extreme, this leads to an argument for universal treatment with statins to the highest tolerated dose, or at least support for a move towards population-based rather than risk-based treatment. 257,258 Some of the concerns about universal or population-based strategies for treatment with lipid-lowering drugs have been alluded to in Chapter 9; full evaluation of such strategies is beyond the scope of this report but could be a priority for future research.
Future research
Gaps in the existing literature that we encountered include (1) confirming the strength of the associations between lipid measures and CVD events, CVD mortality and all-cause mortality; (2) long-term (surveillance) studies to quantify safety profiles of atorvastatin; and (3) better estimation of the benefits and harms of atorvastatin at high doses. Of these, our research would most benefit from further information on effects, and especially safety profiles, of statins at high doses and over the longer term.
Further areas of research could include the potential for other markers in monitoring, beginning with (1) obtaining estimates of short- and long-term variability for apolipoproteins and (2) the role of other associated markers on CVD risk estimation potentially including genetic markers.
Beyond the scope of this project, future research should address whether or not (1) monitoring has an alternative role to improve compliance/adherence and uptake of treatment; and (2) there are interventions that can improve uptake of lipid monitoring.
Gaps in the literature
The evidence we found of association was strongest for CVD events and most sparse for all-cause mortality. Recent systematic reviews of trials237,238,259 have confirmed the perceived safety profile of statins. However, none of these studies provides evidence of long-term adherence or of safety after long-term exposure to these treatments. With individuals likely to be on these medications for longer than 40 years, this evidence is therefore needed.
We found most of the trial evidence about the effects of atorvastatin was for 10 or 20 mg. Given that current recommended treatment strategies emphasise higher doses, further evidence from RCTs of medium (40 mg) or high (80 mg) dose are required. Related to this, as atorvastatin (or any other statin) does not impact on HDL levels, alternative treatment regimes that might increase HDL could be of benefit.
Further areas
Most lipid measures show similar estimates of association with CVD events. Alternative markers such as Apo B and Apo A-I and in particular their ratio (Apo B/Apo A-I) might be better predictors, although not widely available at present. It is plausible that data already exist (as part of international IPD collaborations) to get better estimates of association. If studies confirm Apo B and Apo A-I (or their ratio) as the most relevant markers for CVD risk, evaluation of short-term variability (noise) and average change over time would be required to study monitoring with these markers. We did not have access to data sets with repeated measures of apolipoproteins.
We identified more potential to improve CVD risk scores through use of alternative lipid measurements than through averaging the measures currently used. Whether or not the reclassifications seen are genuine improvements in risk prediction should be assessed in a cohort with follow-up for CVD outcomes and a design or analysis that addresses treatment change. We were unable to assess how inflammatory or genetic markers might further improve risk estimation.
Beyond our scope
Although the focus of this report is on numerical quantification of predictive association, efficacy, rates of change, variability, and costs; we have not evaluated the role of monitoring as a tool to increase compliance or adherence to treatment. Hypercholesterolaemia is asymptomatic, and it is possible that regular visits to a GP or nurse practitioner could serve as reminders to carry on taking medications. Qualitative and quantitative studies exploring and quantifying the role of monitoring in adherence would help in determining its potential benefits.
Not everyone invited to lipid monitoring will take part. Given the potential benefit of monitoring, research into interventions demonstrating improved uptake would be highly relevant.
An extreme scenario to consider would be to suggest universal treatment at highest tolerated dose, as the associated costs of overtreatment are very small in relation to the costs of undertreatment. However, it is difficult to draw firm policy conclusions regarding the merits of universal treatment because of the limited number of studies reporting continued long-term statin usage. Therefore, it is necessary to collect more evidence surrounding the benefits and adherence for patients with statins in a much longer time period. This should be examined in future work before universal treatment can be considered a viable policy option.
Conclusion
We find that any monitoring strategy that places more individuals under treatment is likely to be more cost-effective compared with one that treats fewer. Our findings provide support for the proposal – currently under consideration by NICE62 – that the CVD risk threshold for primary prevention with statins be lowered from 20% to 10%. In all comparisons considered in this report, annual monitoring was cost saving and effective. The implications of universal treatment without monitoring require further investigation: until then, regular lipid monitoring in those with and without CVD is likely to be beneficial to patients and to the health service.
Acknowledgements
In addition to the authors, we wish to thank: Nicola Pidduck, Nia Roberts, Mary Selwood, Daniela Coelho Goncalves, Haruka Sakamoto, Alice Fuller, Jason Oke, and Maria Vazquez-Montes for their help collating the documents and administrative support throughout the project, screening studies, data management and statistical advice. We would also like to thank Richard Hobbs, Head of the NDPCHS, for his support throughout the project and, in particular, during the dissemination meeting. We would like to give special thanks to Professor Andrew Neil, Professor Oliver Rivero-Arias and Dr Derrick Bennet for contributions as members of the project advisory board.
The authors would like to acknowledge the use of the University of Oxford Advanced Research Computing (ARC) facility in carrying out this work.
Contribution of authors
Rafael Perera (Professor in Medical Statistics) led the programme of work; contributed to the design of all of the studies; wrote the first draft of the last chapter, the abstract and the scientific summary; contributed to all of the work packages and the drafting of all the sections; and edited the final report.
Emily McFadden (Senior Epidemiologist/Medical Statistician) led the statistical component (analysis and data management) of the programme of work; wrote the first draft of the first, fourth, fifth and sixth chapters; contributed to health economics and the drafting of all the sections; and edited the final report.
Julie McLellan (Systematic Reviewer) led the systematic review (screening, data extraction and data analysis) component (see Chapter 2) of the programme of work, and wrote the first draft of the second chapter.
Tom Lung (Research Fellow) developed the cardiovascular simulation model; produced the cost-effectiveness results; and drafted, edited and finalised Chapter 7.
Philip Clarke (Professor and Health Economist) contributed to the design of the study; the development of the cardiovascular simulation model; and the drafting and editing of Chapter 7.
Teresa Pérez (Associate Professor in Statistics) contributed to the screening of full-text papers (see Chapter 2); developed codes for indirect methods of extraction; performed data extraction; and contributed and revised the draft of Chapter 2.
Thomas Fanshawe (Medical Statistician) carried out the data extraction and meta-analysis; prepared the first draft of Chapter 3; and commented and contributed to drafts of all of the other chapters.
Andrew Dalton (Senior Researcher) carried out the data extraction and meta-analysis; helped with the first draft of Chapter 3; and commented on Chapters 1 and 10.
Andrew Farmer (Professor of General Practice) contributed to the design of the studies; provided clinical feedback for the simulation models; and commented on drafts.
Paul Glasziou (Professor in Evidence-Based Medicine) contributed to the design of the studies, and provided feedback on results and drafts.
Osamu Takahashi (Director of the Centre for Clinical Epidemiology) contributed to the design of the programme of work and the analysis of the Japanese data.
John Stevens (PPI representative) advised on the conception of the programme of work; wrote Chapter 9; and commented on drafts for all chapters.
Les Irwig (Professor) contributed to discussion about the concepts and data underpinning the initiation of the work, and commented on selected chapters.
Jennifer Hirst (Senior Systematic Reviewer and Research Officer) contributed to designing the searches, reviewing papers and data extraction; the editing of drafts of Chapter 2; and commented on the drafts of Chapter 3.
Sarah Stevens (Medical Statistician) contributed to the analysis contained within Chapter 6 and its drafting.
Asuka Leslie (Public Health specialty registrar) contributed to the draft protocol and the screening and data extraction for the systematic review in Chapter 2, and also commented on drafts of Chapters 1, 2 and 10.
Sachiko Ohde (Senior Researcher) carried out the data extraction and analysis of the Japanese cohort, as well as contributing to the design of Chapter 4.
Gautam Deshpande (Senior Researcher) contributed clinical expertise to the analysis and discussion of the Japanese cohort, as well as critical comments to Chapters 1, 4 and 10.
Kevin Urayama (Senior Researcher) contributed to the design and analysis of data from the Japanese cohort and commented on the draft of Chapter 4.
Brian Shine (Consultant Clinical Pathologist) contributed to the design of the programme of work, commenting on it as it progressed, and commented on drafts.
Richard Stevens (Associate Professor in Medical Statistics) contributed to the conception of the programme of work, advised on design, analysis and interpretation of all studies, and contributed to the drafting of Chapters 4–6, as well as revisions of the final report.
Data sharing statement
The data in the systematic reviews reported here can be obtained from the corresponding author on request.
Disclaimers
This report presents independent research funded by the National Institute for Health Research (NIHR). The views and opinions expressed by authors in this publication are those of the authors and do not necessarily reflect those of the NHS, the NIHR, NETSCC, the HTA programme or the Department of Health. If there are verbatim quotations included in this publication the views and opinions expressed by the interviewees are those of the interviewees and do not necessarily reflect those of the authors, those of the NHS, the NIHR, NETSCC, the HTA programme or the Department of Health.
References
- Townsend N, Wickramasinghe K, Bhatnager P, Smolina K, Nicols M, Leal J. Coronary Heart Disease Statistics. London: British Heart Foundation; 2012.
- Cooper A, Nherera L, Calvert N, O’Flynn N, Turnbull N, Robson J, et al. Clinical Guidelines and Evidence Review for Lipid Modification: Cardiovascular Risk Assessment and the Primary and Secondary Prevention of Cardiovascular Disease. London: National Collaborating Centre for Primary Care and Royal College of General Practitioners; 2010.
- Cardiovascular Disease – NHS Choices. London: Department of Health; 2014.
- PH25 Prevention of Cardiovascular Disease: Guidance. London: NICE; 2010.
- Putting Prevention First – Vascular Checks: Risk Assessment and Management. London: Department of Health; 2008.
- Perk J, De Backer G, Gohlke H, Graham I, Reiner Z, Verschuren M, et al. European Guidelines on cardiovascular disease prevention in clinical practice (version 2012). The Fifth Joint Task Force of the European Society of Cardiology and Other Societies on cardiovascular disease prevention in clinical practice. Eur Heart J 2012;33:1635-701. http://dx.doi.org/10.1093/eurheartj/ehs092.
- Emberson JR, Whincup PH, Morris RW, Walker M. Re-assessing the contribution of serum total cholesterol, blood pressure and cigarette smoking to the aetiology of coronary heart disease: impact of regression dilution bias. Eur Heart J 2003;24:1719-26. http://dx.doi.org/10.1016/S0195-668X(03)00471-8.
- Unal B, Critchley JA, Capewell S. Explaining the decline in coronary heart disease mortality in England and Wales between 1981 and 2000. Circulation 2004;109:1101-7. http://dx.doi.org/10.1161/01.CIR.0000118498.35499.B2.
- Risk Estimation and the Prevention of Cardiovascular Disease (Guideline 97). Edinburgh: SIGN, NHS Scotland; 2007.
- Stroke Association . JBS 2: Joint British Societies’ guidelines on prevention of cardiovascular disease in clinical practice. Heart 2005;91:v1-52. http://dx.doi.org/10.1136/hrt.2005.079988.
- Glasziou PP, Irwig L, Aronson J. Evidence-Based Medical Monitoring: From Principles to Practice. Oxford: BMJ Books, Blackwell Publishing; 2008.
- Moschetti I, Brandt D, Perera R, Clarke M, Heneghan C. Adequacy of reporting monitoring regimens of risk factors for cardiovascular disease in clinical guidelines: systematic review. BMJ 2011;342. http://dx.doi.org/10.1136/bmj.d1289.
- Wilson PWF, D’Agostino RB, Levy D, Belanger AM, Silbershatz H, Kannel WB. Prediction of coronary heart disease using risk factor categories. Circulation 1998;97:1837-47. http://dx.doi.org/10.1161/01.CIR.97.18.1837.
- Shai I, Rimm EB, Hankinson SE, Curhan G, Manson JE, Rifai N, et al. Multivariate assessment of lipid parameters as predictors of coronary heart disease among postmenopausal women: potential implications for clinical guidelines. Circulation 2004;110:2824-30. http://dx.doi.org/10.1161/01.CIR.0000146339.57154.9B.
- Smith M, Lee NJ, Haney E, Carson S. Drug Class Review: HMG-CoA Reductase Inhibitors (Statins) n.d. www.ncbi.nlm.nih.gov/books/NBK47273/pdf/Bookshelf_NBK47273.pdf (accessed 14 August 2015).
- NICE Technical Appraisal 94. Statins for the Prevention of Cardiovascular Events. London: NICE; 2006.
- Doll H, Shine B, Kay J, James T, Glasziou P. The rise of cholesterol testing: how much is unnecessary. Br J Gen Pract 2011;61:e81-8. http://dx.doi.org/10.3399/bjgp11X556245.
- Scandinavian Simvastatin Survival Study Group . Randomised trial of cholesterol lowering in 4444 patients with coronary heart disease: the Scandinavian Simvastatin Survival Study (4S). Lancet 1994;344:1383-9.
- Shepherd J, Cobbe SM, Ford I, Isles CG, Lorimer AR, MacFarlane PW, et al. Prevention of coronary heart disease with pravastatin in men with hypercholesterolemia. West of Scotland Coronary Prevention Study Group. N Engl J Med 1995;333:1301-7. http://dx.doi.org/10.1056/NEJM199511163332001.
- The Long-Term Intervention with Pravastatin in Ischaemic Disease (LIPID) Study Group . Prevention of cardiovascular events and death with pravastatin in patients with coronary heart disease and a broad range of initial cholesterol levels. N Engl J Med 1998;339:1349-57. http://dx.doi.org/10.1056/NEJM199811053391902.
- Pedersen TR, Kjekshus J, Pyörälä K, Olsson AG, Cook TJ, Musliner TA, et al. Effect of simvastatin on ischemic signs and symptoms in the Scandinavian Simvastatin Survival Study (4S). Am J Cardiol 1998;81:333-5. http://dx.doi.org/10.1016/S0002-9149(97)00904-1.
- NHS Centre for Reviews and Dissemination University of York . Cholesterol and coronary heart disease: screening and treatment. Eff Heal Care 1998;4.
- National Service Framework for Coronary Heart Disease. London: DH; 2000.
- Heart Protection Study Collaborative Group . MRC/BHF Heart Protection Study of cholesterol-lowering with simvastatin in 20,536 high-risk individuals: a randomised placebo-controlled trial. Lancet 2002;360:7-22. http://dx.doi.org/10.1016/S0140-6736(02)09327-3.
- Heart Protection Study Collaborative Group . MRC/BHF Heart Protection Study of cholesterol-lowering with simvastatin in 5963 people with diabetes: a randomised placebo-controlled trial. Lancet 2003;361:2005-16. http://dx.doi.org/10.1016/S0140-6736(03)13636-7.
- The Lancet, Editorial . OTC statins: a bad decision for public health. Lancet 2004;363. http://dx.doi.org/10.1016/S0140-6736(04)16284-3.
- Trusler D. Statin prescriptions in UK now total a million each week. BMJ 2011;343. http://dx.doi.org/10.1136/bmj.d4350.
- Glasziou P, Irwig L, Mant D. Monitoring in chronic disease: a rational approach. BMJ 2005;330:644-8. http://dx.doi.org/10.1136/bmj.330.7492.644.
- Del Mar C, Doust J, Glasziou P. Clinical Thinking: Evidence, Communication and Decision-Making. Malden, MA: Blackwell Publishing Ltd; 2006.
- Glasziou PP. Monitoring cholesterol levels: measurement error or true change?. Ann Intern Med 2008;148. http://dx.doi.org/10.7326/0003-4819-148-9-200805060-00005.
- Takahashi O, Glasziou PP, Perera R, Shimbo T, Suwa J, Hiramatsu S, et al. Lipid re-screening: what is the best measure and interval?. Heart 2010;96:448-52. http://dx.doi.org/10.1136/hrt.2009.172619.
- Stevens RJ, Oke J, Perera R. Statistical models for the control phase of clinical monitoring. Stat Methods Med Res 2010;19:394-41. http://dx.doi.org/10.1177/0962280209359886.
- The LIPID Study Group . Design features and baseline characteristics of the LIPID (long-term intervention with pravastatin in ischemic disease) study: a randomized trial in patients with previous acute myocardial infarction and/or unstable angina pectoris. Am J Cardiol 1995;76:474-9. http://dx.doi.org/10.1016/S0002-9149(99)80133-7.
- Sacks FM, Pfeffer MA, Moye LA, Rouleau JL, Rutherford JD, Cole TG, et al. The effect of pravastatin on coronary events after myocardial infarction in patients with average cholesterol levels. Cholesterol and Recurrent Events Trial investigators. N Engl J Med 1996;335:1001-9. http://dx.doi.org/10.1056/NEJM199610033351401.
- Carleton RA, Dwyer J, Finberg L, Flora J, Goodman DS, Grundy SM, et al. Report of the Expert Panel on Population Strategies for Blood Cholesterol Reduction. A statement from the National Cholesterol Education Program, National Heart, Lung, and Blood Institute, National Institutes of Health. Circulation 1991;83:2154-232. http://dx.doi.org/10.1161/01.CIR.83.6.2154.
- Shaper AG, Pocock SJ, Phillips AN, Walker M. A scoring system to identify men at high risk of a heart attack. Health Trends 1987;19:37-9.
- Shepherd J. Resource management in prevention of coronary heart disease: optimising prescription of lipid-lowering drugs. Lancet 2002;359:2271-3. http://dx.doi.org/10.1016/S0140-6736(02)09299-1.
- Kinosian B, Glick H, Garland G. Cholesterol and coronary heart disease: predicting risks by levels and ratios. Ann Intern Med 1994;121:641-7. http://dx.doi.org/10.7326/0003-4819-121-9-199411010-00002.
- Ridker PM, Rifai N, Cook NR, Bradwin G, Buring JE. Non-HDL cholesterol, apolipoproteins A-I and B100, standard lipid measures, lipid ratios, and CRP as risk factors for cardiovascular disease in women. JAMA 2005;294:326-33. http://dx.doi.org/10.1001/jama.294.3.326.
- Ingelsson E, Schaefer EJ, Contois JH, McNamara JR, Sullivan L, Keyes MJ, et al. Clinical utility of different lipid measures for prediction of coronary heart disease in men and women. JAMA 2007;298:776-85. http://dx.doi.org/10.1001/jama.298.7.776.
- Lewington S, Whitlock G, Clarke R, Sherliker P, Emberson J, . Prospective Studies Collaboration (PSC) . Blood cholesterol and vascular mortality by age, sex, and blood pressure: a meta-analysis of individual data from 61 prospective studies with 55,000 vascular deaths. Lancet 2007;370:1829-39. http://dx.doi.org/10.1016/S0140-6736(07)61778-4.
- Grover SA. Identifying adults at increased risk of coronary disease. JAMA 1995;274. http://dx.doi.org/10.1001/jama.1995.03530100041032.
- Friedewald WT, Levy RI, Fredrickson DS. Estimation of the concentration of low-density lipoprotein cholesterol in plasma, without use of the preparative ultracentrifuge. Clin Chem 1972;18:499-502.
- Baigent C, Keech A, Kearney PM, Blackwell L, Buck G, Pollicino C, et al. Efficacy and safety of cholesterol-lowering treatment: prospective meta-analysis of data from 90,056 participants in 14 randomised trials of statins. Lancet 2005;366:1267-78. http://dx.doi.org/10.1016/S0140-6736(05)67394-1.
- Howell N, Trotter R, Mottram DR, Rowe D. Compliance with statins in primary. Pharm J 2004;272:23-6.
- Rasmussen JN, Chong A, Alter DA. Relationship between adherence to evidence-based pharmacotherapy and long-term mortality after acute myocardial infarction. JAMA 2007;297:177-86. http://dx.doi.org/10.1001/jama.297.2.177.
- Wei L, Ebrahim S, Bartlett C, Davey PD, Sullivan FM, MacDonald TM. Statin use in the secondary prevention of coronary heart disease in primary care: cohort study and comparison of inclusion and outcome with patients in randomised trials. BMJ 2005;330. http://dx.doi.org/10.1136/bmj.38398.408032.8F.
- Capewell S, Unal B, Critchley JA, McMurray JJ V. Over 20,000 avoidable coronary deaths in England and Wales in 2000: the failure to give effective treatments to many eligible patients. Heart 2006;92:521-3. http://dx.doi.org/10.1136/hrt.2004.053645.
- United States Preventive Services Task Force . Screening for Lipid Disorders in Adults: U.S. Preventive Services Task Force Recommendation Statement n.d. www.uspreventiveservicestaskforce.org/uspstf08/lipid/lipidrs.htm (accessed 3 March 2014).
- Detection, Evaluation, and Treatment of High Blood Cholesterol in Adults (ATP III Final Report). Bethesda, MD: National Institutes of Health; 2002.
- Reducing Risk in Heart Disease: an Expert Guide to Clinical Practice for Secondary Prevention of Coronary Heart Disease. Melbourne, VI: Heart Foundation and Cardiac Society of Australia and New Zealand; 2012.
- Hippisley-Cox J, Coupland C, Vinogradova Y, Robson J, Minhas R, Sheikh A, et al. Predicting cardiovascular risk in England and Wales: prospective derivation and validation of QRISK2. BMJ 2008;336:1475-82. http://dx.doi.org/10.1136/bmj.39609.449676.25.
- Anderson KM, Odell PM, Wilson PWF, Kannel WB. Cardiovascular disease risk profiles. Am Heart J 1991;121:293-8. http://dx.doi.org/10.1016/0002-8703(91)90861-B.
- Woodward M, Brindle P, Tunstall-Pedoe H. Adding social deprivation and family history to cardiovascular risk assessment: the ASSIGN score from the Scottish Heart Health Extended Cohort (SHHEC). Heart 2007;93:172-6. http://dx.doi.org/10.1136/hrt.2006.108167.
- Karpe F, Holman R. Fire-and-forget in prevention of coronary heart disease. Lancet 2002;360. http://dx.doi.org/10.1016/S0140-6736(02)11890-3.
- NICE Clinical Guideline 181. Lipid Modification: Cardiovascular Risk Assessment and the Modification of Blood Lipids for the Primary and Secondary Prevention of Cardiovascular Disease. London: NICE; 2014.
- Medicines and Healthcare products Regulatory Agency (MHRA) . Simvastatin: Increased Risk of Myopathy at High Dose (80 mg) n.d. www.gov.uk/drug-safety-update/simvastatin-increased-risk-of-myopathy-at-high-dose-80-mg (accessed 14 August 2015).
- Armitage J, Bowman L, Wallendszus K, Bulbulia R, Rahimi K, . Study of the Effectiveness of Additional Reductions in Cholesterol and Homocysteine (SEARCH) Collaborative Group . Intensive lowering of LDL cholesterol with 80 mg versus 20 mg simvastatin daily in 12,064 survivors of myocardial infarction: a double-blind randomised trial. Lancet 2010;376:1658-69. http://dx.doi.org/10.1016/S0140-6736(10)60310-8.
- Tully L, Buchanan K. Oxfordshire CCG Lipid Management Guidance Updated April 2013. Oxford: Oxfordshire CCG; 2013.
- Lipid Management Guidelines – 2013. South West Yorkshire: NHS; 2013.
- Summary of the Development Clinical Guideline on Lipid Modification (Update). London: National Clinical Guideline Centre; 2014.
- Lipid Modification (Update): Guideline Consultation. London: National Clinical Guideline Centre; 2014.
- QOF Business Rules v27.1. Data and Business Rules – Secondary Prevention of Coronary Heart Disease (CHD) Indicator Set. London: HSCIC, NHS England; 2013.
- NHS Employers . 2014/2015/General/Medical/Services/(GMS)/Contract./Quality/and/Outcomes/Framework/(QOF)./Guidance/for/GMS/Contract/2014/2015 n.d. http://bma.org.uk/support-at-work/contracts/gp-contracts-and-funding/independent-contractors/qof-guidance-7th-revision (accessed 12 November 2015).
- Irwig L. Estimating an individual’s true cholesterol level and response to intervention. JAMA 1991;266. http://dx.doi.org/10.1001/jama.1991.03470120080037.
- CTSV . CTT (Cholesterol Treatment Trialists’ Collaboration) – CTSU n.d. www.ctsu.ox.ac.uk/research/meta-trials/ctt/ctt-website (accessed 26 March 2014).
- Barzi F, Patel A, Woodward M, Lawes CM, Ohkubo T, Gu D, et al. A comparison of lipid variables as predictors of cardiovascular disease in the Asia Pacific region. Ann Epidemiol 2005;15:405-13. http://dx.doi.org/10.1016/j.annepidem.2005.01.005.
- Thompson A, Danesh J. Associations between apolipoprotein B, apolipoprotein AI, the apolipoprotein B/AI ratio and coronary heart disease: a literature-based meta-analysis of prospective studies. J Intern Med 2006;259:481-92. http://dx.doi.org/10.1111/j.1365-2796.2006.01644.x.
- Di Angelantonio E, Sarwar N, Perry P, Kaptoge S, Ray KK, . Emerging Risk Factors Collaboration . Major lipids, apolipoproteins, and risk of vascular disease. JAMA 2009;302:1993-2000. http://dx.doi.org/10.1001/jama.2009.1619.
- Di Angelantonio E, Gao P, Pennells L, Kaptoge S, Caslake M, . Emerging Risk Factors Collaboration . Lipid-related markers and cardiovascular disease prediction. JAMA 2012;307:2499-506. http://dx.doi.org/10.1001/jama.2012.6571.
- Sniderman AD, Williams K, Contois JH, Monroe HM, McQueen MJ, Graaf J, et al. A meta-analysis of low-density lipoprotein cholesterol, non-high-density lipoprotein cholesterol, and apolipoprotein B as markers of cardiovascular risk. Circulation Cardiovasc Qual Outcomes 2011;4:337-45. http://dx.doi.org/10.1161/CIRCOUTCOMES.110.959247.
- Boekholdt SM, Arsenault BJ, Mora S, Pedersen TR, LaRosa JC, Nestel PJ, et al. Association of LDL cholesterol, non-HDL cholesterol, and apolipoprotein B levels with risk of cardiovascular events among patients treated with statins: a meta-analysis. JAMA 2012;307:1302-9. http://dx.doi.org/10.1001/jama.2012.366.
- Robinson JG, Wang S, Jacobson TA. Meta-analysis of comparison of effectiveness of lowering apolipoprotein B versus low-density lipoprotein cholesterol and non-high-density lipoprotein cholesterol for cardiovascular risk reduction in randomized trials. Am J Cardiol 2012;110:1468-76. http://dx.doi.org/10.1016/j.amjcard.2012.07.007.
- Montori VM, Wilczynski NL, Morgan D, Haynes RB. Optimal search strategies for retrieving systematic reviews from MEDLINE: analytical survey. BMJ 2005;330. http://dx.doi.org/10.1136/bmj.38336.804167.47.
- Wilczynski NL, Haynes RB. EMBASE search strategies achieved high sensitivity and specificity for retrieving methodologically sound systematic reviews. J Clin Epidemiol 2007;60:29-33. http://dx.doi.org/10.1016/j.jclinepi.2006.04.001.
- Danesh J, Erqou S, Walker M, Thompson SG, Tipping R, . Emerging Risk Factors Collaboration . The Emerging Risk Factors Collaboration: analysis of individual data on lipid, inflammatory and other markers in over 1.1 million participants in 104 prospective studies of cardiovascular diseases. Eur J Epidemiol 2007;22:839-69. http://dx.doi.org/10.1007/s10654-007-9165-7.
- Hayden JA, Cote P, Bombardier C. Evaluation of the quality of prognosis studies in systematic reviews. Ann Intern Med 2006;144:427-37. http://dx.doi.org/10.7326/0003-4819-144-6-200603210-00010.
- Zandbergen EG, de Haan RJ, Hijdra A. Systematic review of prediction of poor outcome in anoxic-ischaemic coma with biochemical markers of brain damage. Intensive Care Med 2001;27:1661-7. http://dx.doi.org/10.1007/s001340101076.
- Perel P, Edwards P, Wentz R, Roberts I. Systematic review of prognostic models in traumatic brain injury. BMC Med Inf Decis Mak 2006;6. http://dx.doi.org/10.1186/1472-6947-6-38.
- Parmar MK, Torri V, Stewart L. Extracting summary statistics to perform meta-analyses of the published literature for survival endpoints. Stat Med 1998;17:2815-34. http://dx.doi.org/10.1002/(SICI)1097-0258(19981230)17:24<2815::AID-SIM110>3.0.CO;2-8.
- Riley RD, Abrams KR, Sutton AJ, Lambert PC, Jones DR, Heney D, et al. Reporting of prognostic markers: current problems and development of guidelines for evidence-based practice in the future. Br J Cancer 2003;88:1191-8. http://dx.doi.org/10.1038/sj.bjc.6600886.
- Greenland S, Longnecker MP. Methods for trend estimation from summarized dose-response data, with applications to meta-analysis. Am J Epidemiol 1992;135:1301-9.
- Orsini N, Bellocco R, Greenland S. Generalized least squares for trend estimation of summarized dose–response data. Stata J 2006;6:40-57.
- Kyzas PA, Loizou KT, Ioannidis JP. Selective reporting biases in cancer prognostic factor studies. J Natl Cancer Inst 2005;97:1043-55. http://dx.doi.org/10.1093/jnci/dji184.
- HPS2-THRIVE . HPS2-THRIVE (Treatment of HDL to Reduce the Incidence of Vascular Events) n.d. www.thrivestudy.org (accessed 11 November 2015).
- Zatonski W, Mańczuk M, Kieke PONS. POlish-Norwegian Study (PONS): research on chronic non-communicable diseases in European high risk countries – study design. Ann Agri Environ Med 2011;18:203-6.
- Tehran University of Medical Sciences . Prevention of Cardiovascular Disease in Middle-Aged and Elderly Iranians Using a Single PolyPill (PolyIran) n.d. https://clinicaltrials.gov/ct2/show/record/NCT01271985 (accessed 11 November 2015).
- Tohidi M, Hatami M, Hadaegh F, Safarkhani M, Harati H, Azizi F. Lipid measures for prediction of incident cardiovascular disease in diabetic and non-diabetic adults: results of the 8.6 years follow-up of a population based cohort study. Lipids Health Dis 2010;9.
- Sato S, Nakamura M, Iida M, Naito Y, Kitamura A, Okamura T, et al. Plasma fibrinogen and coronary heart disease in urban Japanese. Am J Epidemiol 2000;152:420-3. http://dx.doi.org/10.1093/aje/152.5.420.
- Holme I, Aastvelt AH, Jungner I, Walldius G, . Relationships between lipoprotein components and risk of myocardial infarction: age, gender and short vs. longer follow-up periods in the Apolipoprotein MOrtality RISk study (AMORIS). J Intern Med 2008;264:30-8. http://dx.doi.org/10.1111/j.1365-2796.2008.01925.x.
- Verschuren WMM, Kromhout D. Total cholesterol concentration and mortality at a relatively young age: do men and women differ?. BMJ 1995;311:779-83. http://dx.doi.org/10.1136/bmj.311.7008.779.
- Langsted A, Freiberg JJ, Nordestgaard BG. Fasting and nonfasting lipid levels: influence of normal food intake on lipids, lipoproteins, apolipoproteins, and cardiovascular risk prediction. Circulation 2008;118:2047-56. http://dx.doi.org/10.1161/CIRCULATIONAHA.108.804146.
- Simons LA. Predictors of mortality in the prospective Dubbo study of Australian elderly. Aust N Z J Med 1996;26:40-8. http://dx.doi.org/10.1111/j.1445-5994.1996.tb02905.x.
- Iso H, Naito Y, Kitamura A, Sato S, Kiyama M, Takayama Y, et al. Serum total cholesterol and mortality in a Japanese population. J Clin Epidemiol 1994;47:961-9. http://dx.doi.org/10.1016/0895-4356(94)90110-4.
- Meisinger C, Loewel H, Mraz W, Koenig W. Prognostic value of apolipoprotein B and A-I in the prediction of myocardial infarction in middle-aged men and women: results from the MONICA/KORA Augsburg cohort study. Eur Heart J 2005;26:271-8. http://dx.doi.org/10.1093/eurheartj/ehi003.
- De Stavola BL, Meade TW. Long-term effects of hemostatic variables on fatal coronary heart disease: 30-year results from the first prospective Northwick Park Heart Study (NPHS-I). J Thromb Haemost 2007;5:461-71. http://dx.doi.org/10.1111/j.1538-7836.2007.02330.x.
- Jonsdóttir LS, Sigfussen N, Gudnason V, Sigvaldason H, Thorgeirsson G. Do lipids, blood pressure, diabetes, and smoking confer equal risk of myocardial infarction in women as in men? The Reykjavik Study. Eur J Cardiovasc Risk 2002;9:67-76. http://dx.doi.org/10.1097/00043798-200204000-00001.
- Houterman S, Verschuren WM, Hofman A, Witteman JC. Serum cholesterol is a risk factor for myocardial infarction in elderly men and women: the Rotterdam study. J Intern Med 1999;246:25-33. http://dx.doi.org/10.1046/j.1365-2796.1999.00525.x.
- Keil JE, Sutherland SE, Knapp RG, Lackland DT, Gazes PC, Tyroler HA. Mortality rates and risk factors for coronary disease in black as compared with white men and women. N Engl J Med 1993;329:73-8. http://dx.doi.org/10.1056/NEJM199307083290201.
- Arsenault BJ, Despres JP, Stoes ES, Wareham NJ, Kastelein JJ, Khaw KT, et al. European Prospective Investigation into Cancer and Nutrition-Norfolk study . Lipid assessment, metabolic syndrome and coronary heart disease risk. Eur J Clin Invest 2010;40:1081-93.
- Garcia-Palmieri MR, Costas R. Progress in Cardiology. Philadelphia: Lea and Febiger; 1986.
- Watkins LO, Neaton JD, Kuler LH. Racial differences in high-density lipoprotein cholesterol and coronary heart disease incidence in the Usual-Care Group of the Multiple Risk Factor Intervention Trial. Am J Cardiol 1966;57:536-45.
- Dontas AS, Menotti A, Aravanns C, Ioannidis P, Seccarecchia F. Comparative total mortality in 25 years in Italian and Greek middle aged rural men. J Epidemiol Community Health 1998;52:638-44. http://dx.doi.org/10.1136/jech.52.10.638.
- Weijenberg MP, Feskins EJM, Kromhout D. Total and high density lipoprotein cholesterol as risk factors for coronary heart disease in elderly men during 5 years of follow-up. The Zutphen Elderly Study. Am J Epidemiol 1996;143:151-8. http://dx.doi.org/10.1093/oxfordjournals.aje.a008724.
- Nedeljkovic S, Ostojic MC, Grujic MZ, Josipovic V, Keys A, Menotti A, et al. Coronary heart disease in 25 years. The experience in the three Serbian cohorts of the Seven Countries Study. Acta Cardiol 1993;1:11-24.
- Downs JR, Clearfield M, Weiss S, Whitney E, Shapiro DR, Beere PA, et al. Primary prevention of acute coronary events with lovastatin in men and women with average cholesterol levels. JAMA 1998;279:1615-22. http://dx.doi.org/10.1001/jama.279.20.1615.
- Jardine AG, Holdass H, Felsstrom B, Cole E, Nyberg G, Gronhagen-Riska C, et al. Fluvastatin prevents cardiac death and myocardial infraction in renal transplant recipients: post-hoc subgroup analyses of the ALERT study. Am J Transplant 2004;4:988-95. http://dx.doi.org/10.1111/j.1600-6143.2004.00445.x.
- Dhaliwal SS, Welborn TA. Central obesity and cigarette smoking are key determinants of CVD deaths in Australia: a public health perspective. Prev Med 2009;49:153-7. http://dx.doi.org/10.1016/j.ypmed.2009.07.019.
- Sever PS, Poulter NR, Dahlöf B, Wedel H, Collins R, Beevers G, et al. Reduction in cardiovascular events with atorvastatin in 2,532 patients with type 2 diabetes: Anglo-Scandinavian Cardiac Outcomes Trial – Lipid-Lowering Arm (ASCOT-LLA). Diabetes Care 2005;28:1151-7.
- Fellstrom BC, Jardine AG, Schmieder RE, Holdass H, Bannister K, Beutler J, et al. Rosuvastatin and cardiovascular events in patients undergoing hemodialysis. N Engl J Med 2009;360:1395-407. http://dx.doi.org/10.1056/NEJMoa0810177.
- Pocock J, Shaper AG, Phillips AN. Concentrations of high density lipoprotein cholesterol, triglycerides, and total cholesterol in ischaemic heart disease. BMJ 1989;298:998-1002. http://dx.doi.org/10.1136/bmj.298.6679.998.
- Kiechl S, Willeit J, Mayr MA, Viehweider B, Oberhollenzer M, Hronenberg F, et al. Oxidized phospholipids, lipoprotein(a), lipoprotein-associated phospholipase A2 activity, and 10-year cardiovascular outcome: prospective results from the Bruneck study. Arterioscler Thromb Vasc Biol 2007;27:1788-95. http://dx.doi.org/10.1161/ATVBAHA.107.145805.
- Wald NJ, Law M, Watt HC, Wu T, Bailey A, Johnson AM, et al. Apolipoproteins and ischaemic heart disease: implications for screening. Lancet 1994;343:75-9.
- Sweetnam PM, Bolton CH, Downs LG, Durrington PN, MacKness MI, Elwood PC, et al. Apolipoproteins A-I, A-II and B, lipoprotein(a) and the risk of ischaemic heart disease: the Caerphilly study. Eur J Clin Invest 2000;30:947-56.
- Colhoun HM, Betteridge DJ, Durrington PN, Hitman GA, Neil HAW, Livingstone SJ, et al. Primary prevention of cardiovascular disease with atorvastatin in type 2 diabetes in the Collaborative Atorvastatin Diabetes Study (CARDS): multicentre randomised placebo-controlled trial. Lancet 2004;364:685-96. http://dx.doi.org/10.1016/S0140-6736(04)16895-5.
- Bruno G, Merlett F, Biggeri A, Bargero G, Prina-Cerai S, Pagano G, et al. Effect of age on the association of non-high-density-lipoprotein cholesterol and apolipoprotein B with cardiovascular mortality in a Mediterranean population with type 2 diabetes: the Casale Monferrato study. Diabetologia 2006;49:937-44. http://dx.doi.org/10.1007/s00125-006-0195-6.
- Sai X, He Y, Men K, Wang B, Huang JY, Shi QL, et al. All-cause mortality and risk factors in a cohort of retired military male veterans, Xi’an, China: an 18-year follow up study. BMC Public Health 2007;7. http://dx.doi.org/10.1186/1471-2458-7-290.
- Psaty BM, Anderson M, Krinmal RA, Tracy RP, Orchard T, Fied LP, et al. The association between lipid levels and the risks of incident myocardial infarction, stroke, and total mortality: The Cardiovascular Health Study. J Am Geriatr Soc 2004;52:1639-47. http://dx.doi.org/10.1111/j.1532-5415.2004.52455.x.
- Kjekshus J, Apetrei E, Barrios V, Bohm N, Cleland JG, Cornel JH, et al. Rosuvastatin in older patients with systolic heart failure. N Engl J Med 2007;357:2248-61. http://dx.doi.org/10.1056/NEJMoa0706201.
- Giorda CB, Avogaro A, Maggini M, Lombardo F, Mannucci E, Turco S, et al. Recurrence of cardiovascular events in patients with type 2 diabetes. Diabetes Care 2008;31:2154-9. http://dx.doi.org/10.2337/dc08-1013.
- Taskinen MR, Barter PJ, Ehnholm C, Sullivan DR, Mann K, Simes J, et al. Ability of traditional lipid ratios and apolipoprotein ratios to predict cardiovascular risk in people with type 2 diabetes. Diabetologia 2010;53:1846-55. http://dx.doi.org/10.1007/s00125-010-1806-9.
- Salomaa V, Rasi V, Kulathinal S, Vahtera E, Jauhiainen M, Ehnholm C, et al. Hemostatic factors as predictors of coronary events and total mortality: The FINRISK ‘92 Hemostasis Study. Arterioscler Thromb Vasc Biol 2002;22:353-8. http://dx.doi.org/10.1161/hq0202.104078.
- GISSI Prevenzione Investigators . Results of the low-dose (20 mg) pravastatin GISSI Prevenzione trial in 4271 patients with recent myocardial infarction: do stopped trials contribute to overall knowledge?. Ital Heart J 2000;1:810-20.
- Klausen IC, Sjol A, Hansen PS, Geredes LU, Moller L, Leming L, et al. Apolipoprotein(a) isoforms and coronary heart disease in men: a nested case–control study. Atherosclerosis 1997;132:77-84. http://dx.doi.org/10.1016/S0021-9150(97)00071-3.
- Gerber Y, Dankner R, Chetrit A, Novikov I, Goldbourt U. The role of risk factor time trends in the steep decline of CHD mortality between two Israeli cohort studies. Prev Med 2005;41:85-91. http://dx.doi.org/10.1016/j.ypmed.2004.10.020.
- Cremer P, Nagel D, Mann H, Labrot B, Muller-Berninger R, Elster H, et al. Ten-year follow-up results from the Goettingen Risk, Incidence and Prevalence Study (GRIPS). I. Risk factors for myocardial infarction in a cohort of 5790 men. Atherosclerosis 1997;129:221-30. http://dx.doi.org/10.1016/S0021-9150(96)06030-3.
- Hamaguchi M, Kojima T, Takeda M, Sarui H, Kawahito Y, Yoshida N, et al. Non-alcoholic fatty liver disease is a novel predictor of CVD. World J Gastroenterol 2007;13:1579-84. http://dx.doi.org/10.3748/wjg.v13.i10.1579.
- Schatz IJ, Masaki K, Yano K, Chen R, Rodriguez BL, Curb JD. Cholesterol and all-cause mortality in elderly people from the Honolulu Heart Program: a cohort study. Lancet 2001;358:351-5. http://dx.doi.org/10.1016/S0140-6736(01)05553-2.
- Bos G, Dekker JM, Nijpels D, de Vegt F, Diamant M, Stehouwer CD, et al. A combination of high concentrations of serum triglyceride and non-high-density-lipoprotein-cholesterol is a risk factor for CVD in subjects with abnormal glucose metabolism: The Hoorn Study. Diabetologia 2003;46:910-16. http://dx.doi.org/10.1007/s00125-003-1141-5.
- Jiang R, Schultz MB, Li T, Rifai N, Stampfer MJ, Rimm EB, et al. Non-HDL cholesterol and apolipoprotein B predict cardiovascular disease events among men with type 2 diabetes. Diabetes Care 2004;27:1991-7. http://dx.doi.org/10.2337/diacare.27.8.1991.
- Semba RD, Bandinelli S, Sun K, Gurainik JM, Ferrucc L, . Plasma carboxymethyl-lysine, an advanced glycation end product, and all-cause and cardiovascular disease mortality in older community-dwelling adults. J Am Geriatr Soc 2009;57:1874-80. http://dx.doi.org/10.1111/j.1532-5415.2009.02438.x.
- Goldbourt U, Yaari S, Medalie JH. Factors predictive of long-term coronary heart disease mortality among 10,059 male Israeli civil servants and municipal employees. Cardiology 1993;8:100-21. http://dx.doi.org/10.1159/000175862.
- Yokokawa H, Yasamura S, Tanno K, Ohsawa M, Onoda T, Itai K, et al. Serum low-density lipoprotein to high density lipoprotein ratio as a predictor of future acute myocardial infarction among men in a 2.7-year cohort study of a Japanese northern rural population. J Atheroscler Thromb 2011;18:89-98. http://dx.doi.org/10.5551/jat.5215.
- Fukushima Y, Ohmura H, Mokuno H, Kajimoto K, Kasai T, Hirayama S, et al. Non-high-density lipoprotein cholesterol is a practical predictor of long-term cardiac death after coronary artery bypass grafting. Atherosclerosis 2012;221:206-11. http://dx.doi.org/10.1016/j.atherosclerosis.2011.12.012.
- Lakka H, Lakka TA, Tuomilehto J, Sivenius J, Salonoen JT. Hyperinsulinemia and the risk of cardiovascular death and acute coronary and cerebrovascular events in men. Arch Intern Med 2000;160:1160-8. http://dx.doi.org/10.1001/archinte.160.8.1160.
- Lan TY, Chiu HC, Chang HY, Chang WC, Chen HY, Tai TY. Clinical and laboratory predictors of all-cause mortality in older population. Arch Gerontol Geriatr 2007;45:327-34. http://dx.doi.org/10.1016/j.archger.2007.02.001.
- De Simone G, Olsen MH, Wachtel K, Hille DA, Dahlof B, Ibsen H, et al. Clusters of metabolic risk factors predict cardiovascular events in hypertension with target-organ damage: the LIFE study. J Hum Hypertens 2007;21:625-32. http://dx.doi.org/10.1038/sj.jhh.1002203.
- Simes RJ, Marschner IC, Hunt D, Colquhoun D, Sullivan D, Stewart RA, et al. Relationship between lipid levels and clinical outcomes in the Long-term Intervention with Pravastatin in Ischemic Disease (LIPID) Trial: to what extent is the reduction in coronary events with pravastatin explained by on-study lipid levels?. Circulation 2002;105:1162-9.
- Williams PT. Low high-density lipoprotein 3 reduces the odds of men surviving to age 85 during 53-year follow-up. JAGS 2012;60:430-6. http://dx.doi.org/10.1111/j.1532-5415.2011.03851.x.
- Grover SA, Coupal L, Hu X. Identifying adults at increased risk of coronary disease. JAMA 1995;274:801-6. http://dx.doi.org/10.1001/jama.1995.03530100041032.
- Mizuno K, Nakaya N, Teramoto T, Yokoyama S, Ohashi Y, Ueki A, et al. Usefulness of LDL-C-related parameters to predict cardiovascular risk and effect of pravastatin in mild-to-moderate hypercholesterolemia. J Atheroscler Thromb 2012;19:176-85. http://dx.doi.org/10.5551/jat.9183.
- Manickam P, Rathod A, Panaich S, Hari P, Veeranna V, Badheka A, et al. Comparative prognostic utility of conventional and novel lipid parameters for CVD risk prediction: do novel lipid parameters offer an advantage?. J Clin Lipidol 2011;5:82-90. http://dx.doi.org/10.1016/j.jacl.2010.12.001.
- Shalev V, Chodick B, Bialik M, Green MS, Heymann AD. In a population-based cohort of diabetes patients, men and women had similar risks for all-cause mortality. J Clin Epidemiol 2007;60:86-93. http://dx.doi.org/10.1016/j.jclinepi.2006.04.010.
- Sierra-Johnson J, Fisher RM, Romero-Corral A, Somers VK, Lopez-Jiminez F, Chrvik J, et al. Concentration of apolipoprotein B is comparable with the apolipoprotein B/apolipoprotein A-I ratio and better than routine clinical lipid measurements in predicting coronary heart disease mortality: findings from a multi-ethnic US population. Eur Heart J 2009;30:710-17. http://dx.doi.org/10.1093/eurheartj/ehn347.
- Okamura T, Tanaka H, Miyamatsu N, Hayakawa T, Kadowaki T, Kita Y, et al. The relationship between serum total cholesterol and all-cause or cause-specific mortality in a 17.3-year study of a Japanese cohort. Atherosclerosis 2007;190:216-23. http://dx.doi.org/10.1016/j.atherosclerosis.2006.01.024.
- Cooper JA, Miller GJ, Bauer KA, Morrissey JH, Meade TW, Howarth DJ, et al. Comparison of novel hemostatic factors and conventional risk factors for prediction of coronary heart disease. Circulation 2000;102:2816-22. http://dx.doi.org/10.1161/01.CIR.102.23.2816.
- Shai I, Rimm EB, Hankinson SE, Curhan G, Manson JE, Rifal N, et al. Multivariate assessment of lipid parameters as predictors of coronary heart disease among postmenopausal women: potential implications for clinical guidelines. Circulation 2004;110:2824-30. http://dx.doi.org/10.1161/01.CIR.0000146339.57154.9B.
- Palma S, Cosano A, Mariscal M, Martinez-Gallego CG, Medina-Cuadros M, Delgado-Rogriguez M. Cholesterol and serum albumin as risk factors for death in patients undergoing general surgery. Br J Surg 2007;94:369-75. http://dx.doi.org/10.1002/bjs.5626.
- Cambien F, Jacqueson A, Richard JL, Warnet JM, Ducimetiere P, Claude JR. Is the level of serum triglyceride a significant predictor of coronary death in ‘normocholesterolemic’ subjects?. Am J Epidemiol 1986;124:624-32.
- Stampfer MJ, Sacks FM, Salvini S, Willett WC, Hennekens CH. A prospective study of cholesterol, apolipoproteins, and the risk of myocardial infraction. N Engl J Med 1991;325:373-81. http://dx.doi.org/10.1056/NEJM199108083250601.
- Ioachimescu AG, Brennan DM, Hoar BM, Hazen SL, Hoogwerf BJ. Preventive Cardiology Information System Database Cohort Study . Serum uric acid is an independent predictor of all-cause mortality in patients at high risk of cardiovascular disease. Arthritis Rheum 2008;58:623-30. http://dx.doi.org/10.1002/art.23121.
- Kappelle PJ, Gansevoort RT, Hillege JL, Wolffenbuttel BH, Dullaart RP. PREVEND study group . Apolipoprotein B/A-I and total cholesterol/high-density lipoprotein cholesterol ratios both predict cardiovascular events in the general population independently of nonlipid risk factors, albuminuria and C-reactive protein. J Intern Med 2011;269:232-42. http://dx.doi.org/10.1111/j.1365-2796.2010.02323.x.
- Assmann G, Cullen P, Schulte Helmut. Simple scoring scheme for calculating the risk of acute coronary events based on the 10-year follow-up of the Prospective Cardiovascular Münster (PROCAM) Study. Circulation 2002;105:310-15. http://dx.doi.org/10.1161/hc0302.102575.
- Palmieri L, Donfrancesco G, Giampaoli S, Torjani M, Panico S, Vanuzzo D, et al. Favorable cardiovascular risk profile and 10-year coronary heart disease incidence in women and men: results from the Progetto Cuore. Eur J Cardiovasc Prevent Rehabil 2006;13. http://dx.doi.org/10.1097/01.hjr.0000221866.27039.4b.
- Shepherd J, Blauw GJ, Murphy MB, Bollen EL, Buckley BM, Cobbe SM, et al. Pravastatin in elderly individuals at risk of vascular disease (PROSPER): a randomised controlled trial. Lancet 2002;360:1623-30. http://dx.doi.org/10.1016/S0140-6736(02)11600-X.
- St-Pierre AC, Cantin B, Dagenais GR, Mauriege P, Bernard PM, Despres JP, et al. Low-density lipoprotein subfractions and the long-term risk of ischemic heart disease in men: 13-year follow-up data from the Québec Cardiovascular Study. Arterioscler Thromb Vasc Biol 2005;25:553-9. http://dx.doi.org/10.1161/01.ATV.0000154144.73236.f4.
- Von Mühlen D, Langer RD, Barrett-Connor E. Rancho Bernardo Study . Sex and time differences in the associations of non-high-density lipoprotein cholesterol versus other lipid and lipoprotein factors in the prediction of cardiovascular death (The Rancho Bernardo Study). Am J Cardiol 2003;91:1311-15. http://dx.doi.org/10.1016/S0002-9149(03)00319-9.
- Menotti A, Farchi G, Seccareccia F. The prediction of coronary heart disease mortality as a function of major risk factors in over 30,000 men in the Italian RIFLE pooling project. A comparison with the MRFIT primary screening. J Cardiovasc Risk 1994;1:263-70.
- Frost PH, Davis BR, Burlanddo AJ, Curb JD, Guthrie JP, Isaacsohn JL, et al. Serum lipids and incidence of coronary heart disease. Circulation 1996;94:2381-8. http://dx.doi.org/10.1161/01.CIR.94.10.2381.
- Lu W, Resnick HE, Jablonski KA, Jones KL, Jain AK, Howard WJ, et al. Non-HDL cholesterol as a predictor of CVD in type 2 diabetes. Diabetes Care 2003;26:16-23. http://dx.doi.org/10.2337/diacare.26.1.16.
- Abdel-Maksoud MF, Eckel RH, Hamman RF, Hokanson JE, . Risk of coronary heart disease is associated with triglycerides and high-density lipoprotein cholesterol in women and non-high-density lipoprotein cholesterol in men. J Clin Lipidol 2012;6:374-81. http://dx.doi.org/10.1016/j.jacl.2012.02.011.
- Stanek EJ, Eckel RH, Hamman RF, Hokanson JE. Risk of cardiovascular events in patients at optimal values for combined lipid parameters. Curr Med Res Opin 2007;23:553-63. http://dx.doi.org/10.1185/030079906X167660.
- Gigante B, Leaner K, Vikstrom M, Frumento P, Carlsson AC, Bottai M, et al. Elevated Apo B serum levels strongly predict early cardiovascular events. Heart 2012;98:1242-5. http://dx.doi.org/10.1136/heartjnl-2012-301852.
- Eliasson B, Cederholm J, Eeg-Olofsson K, Svensson AM, Zethellus B, Gudbjornsdottir S, et al. Clinical usefulness of different lipid measures for prediction of coronary heart disease in type 2 diabetes. Diabetes Care 2011;34:2095-100. http://dx.doi.org/10.2337/dc11-0209.
- Moss AJ, Goldstein RE, Marder VJ, Sparks CE, Oakes D, Greenberg H, et al. Thrombogenic factors and recurrent coronary events. Circulation 1999;99:2517-22. http://dx.doi.org/10.1161/01.CIR.99.19.2517.
- Ting RZ, Yang X, Yu LW, Luk AO, Kong AP, Tong PC, et al. Lipid control and use of lipid-regulating drugs for prevention of cardiovascular events in Chinese type 2 diabetic patients: a prospective cohort study. Cardiovasc Diabetol 2010;9. http://dx.doi.org/10.1186/1475-2840-9-77.
- McMurray JJV, Uno H, Jarolim P, Desai AS, de Zeeuw D, Eckhardt KU, et al. Predictors of fatal and non-fatal cardiovascular events in patients with type 2 diabetes mellitus, chronic kidney disease, and anemia: an analysis of the Trial to Reduce cardiovascular Events with Aranesp (darbepoetin-alfa) Therapy (TREAT). Am Heart J 2011;162:748-55. http://dx.doi.org/10.1016/j.ahj.2011.07.016.
- Upmeier E, Lavonius S, Leytonen A, Viltanen M, Isoaho H, Arve S. Serum lipids and their association with mortality in the elderly: a prospective cohort study. Aging Clin Exp Res 2009;21:424-30. http://dx.doi.org/10.1007/BF03327441.
- Dunder K, Lind L, Zethelius B, Berglund L, Lithell H. Evaluation of a scoring scheme, including proinsulin and the apolipoprotein B/apolipoprotein A1 ratio, for the risk of acute coronary events in middle-aged men: Uppsala Longitudinal Study of Adult Men (ULSAM). Am Heart J 2004;148:596-601. http://dx.doi.org/10.1016/j.ahj.2004.03.021.
- Bloomfield RH, Robins SJ, Collins D, Fye CL, Anderson JW, Elam MB, et al. Gemfibrozil for the secondary prevention of coronary heart disease in men with low levels of high-density lipoprotein cholesterol. N Engl J Med 1999;341:410-18. http://dx.doi.org/10.1056/NEJM199908053410604.
- Davey Smith G, Shipley MJ, Marmot MG, Rose G. Plasma cholesterol concentration and mortality. JAMA 1992;267:70-6. http://dx.doi.org/10.1001/jama.1992.03480010078028.
- Ridker PM, Rifal N, Cook NR, Bradwin G, Buring JE. Non-HDL cholesterol, apolipoproteins A-I and B100, standard lipid measures, lipid ratios, and CRP as risk factors for CVD in women. JAMA 2005;294:326-33. http://dx.doi.org/10.1001/jama.294.3.326.
- Cooper JA, Miller GJ, Bauer KA, Morrissey JH, Meade TW, Howarth DJ, et al. Comparison of novel hemostatic factors and conventional risk factors for prediction of coronary heart disease. Circulation 2000;102:2816-22. http://dx.doi.org/10.1161/01.CIR.102.23.2816.
- Matsuzaki M, Kita T, Mabuchi H, Matsuzawa Y, Nakaya N, Oikawa S, et al. Primary Prevention Cohort Study of the Japan Lipid Intervention Trial . Large scale cohort study of the relationship between serum cholesterol concentration and coronary events with low-dose simvastatin therapy in Japanese patients with hypercholesterolemia. Circ J 2002;66:1087-95. http://dx.doi.org/10.1253/circj.66.1087.
- Mabuchi H, Kita T, Matsuzaki M, Matsuzawa Y, Nakaya N, Oikawa S, et al. Secondary Prevention Cohort Study of the Japan Lipid Intervention Trial . Large scale cohort study of the relationship between serum cholesterol concentration and coronary events with low-dose simvastatin therapy in Japanese patients with hypercholesterolemia and coronary heart disease. Circ J 2002;66:1096-100. http://dx.doi.org/10.1253/circj.66.1096.
- de Lemos JA, Blazing MA, Wiviott SD, Lewis EF, Fox KA, White HD, et al. Early intensive vs a delayed conservative simvastatin strategy in patients with acute coronary syndromes. JAMA 2004;292:1307-16. http://dx.doi.org/10.1001/jama.292.11.1307.
- Saito Y, Shirai K, Sasaki N, Shinomya M, Yoshida S. Prognosis of hypercholesterolemic patients taking pravastatin for 5 years: the Chiba lipid intervention program (CLIP) study. J Atheroscler Thromb 2002;9:99-108. http://dx.doi.org/10.5551/jat.9.99.
- Schwartz GG, Olsson AG, Abt M, Ballantyne CM, Barter PJ, Brumm J, et al. Effects of dalcetrapib in patients with recent acute coronary syndrome. N Engl J Med 2012;367:2089-99. http://dx.doi.org/10.1056/NEJMoa1206797.
- Pedersen TR, Faergeman O, Kastelein JJP, Olsson AG, Tikkanen MJ, Holme I, et al. High-dose atorvastatin vs usual-dose simvastatin for secondary prevention after myocardial infarction: the IDEAL study – a randomized controlled trial. JAMA 2005;294:2437-45. http://dx.doi.org/10.1001/jama.294.19.2437.
- Sasaki J, Yokoyama M, Matsuzaki M, Saito Y, Origasa H, Ishikawa Y, et al. Relationship between coronary artery disease and non-HDL-C and effect of highly purified EPA on the risk of coronary artery disease in hypercholesterolemic patients treated with statins: sub-analysis of the Japan EPA lipid intervention study (JELIS). J Atheroscler Thromb 2012;19:194-20. http://dx.doi.org/10.5551/jat.8326.
- Ridker PM, Danielson E, Fonseca FA, Genest J, Gotto AM, Kastelein JJ, et al. Rosuvastatin to prevent vascular events in men and women with elevated C-Reactive Protein. N Engl J Med 2008;359:2195-207. http://dx.doi.org/10.1056/NEJMoa0807646.
- Teramoto T, Urashima M, Shimano H. A large-scale survey on cardio-cerebrovascular events during pitavastatin (LIVALO tablet) therapy in Japanese patients with hypercholesterolemia – LIVALO effectiveness and safety study extension. Jpn Pharmacol Ther 2011;39:789-803.
- Ray KK, Cannon CP, Cairns R, Morrow DA, Ridker PM, Braunwaald E. Prognostic utility of Apo B/AI, total cholesterol/HDL, non-HDL cholesterol, or hs-CRP as predictors of clinical risk in patients receiving statin therapy after acute coronary syndromes: results from PROVE IT-TIMI 22. Arterioscler Thromb Vasc Biol 2009;29:424-30. http://dx.doi.org/10.1161/ATVBAHA.108.181735.
- Amarenco P, Bogousslavsky J, Callahan A, Gold LB, Hennerici M, . The Stroke Prevention by Aggressive Reduction in Cholesterol Levels (SPARCL) Investigators . High-dose atorvastatin after stroke or transient ischemic attack. N Engl J Med 2006;355:549-59. http://dx.doi.org/10.1056/NEJMoa061894.
- Arsenault BJ, Barter P, DeMicco DA, Bao W, Preston GM, LaRosa JC, et al. Prediction of cardiovascular events in statin-treated stable coronary patients of the treating to new targets randomized controlled trial by lipid and non-lipid biomarkers. PLOS ONE 2014;9.
- British National Formulary. London: BMJ Group and Pharmaceutical Press; 2014.
- Adams S, Tsang M, Wright JM. Lipid lowering efficacy of atorvastatin. Cochrane Database Syst Rev 2012;12. http://dx.doi.org/10.1002/14651858.cd008226.pub2.
- Naci H, Brugts JJ, Fleurence R, Ades A. Dose-comparative effects of different statins on serum lipid levels: a network meta-analysis of 256,827 individuals in 181 randomized controlled trials. Eur J Prev Cardiol 2013;20:658-70. http://dx.doi.org/10.1177/2047487313483600.
- Higgins J, Green S. Cochrane Handbook for Systematic Reviews of Interventions Version 5.1.0. Chichester: Wiley-Blackwell; 2011.
- White IR. Multivariate random-effects meta-analysis. Stata J 2009;9:40-56.
- Knopp RH, d’Emden M, Smilde JG, Pocock SJ. Efficacy and safety of atorvastatin in the prevention of cardiovascular end points in subjects with type 2 diabetes: the Atorvastatin Study for Prevention of Coronary Heart Disease Endpoints in non-insulin-dependent diabetes mellitus (ASPEN). Diabetes Care 2006;29:1478-85. http://dx.doi.org/10.2337/dc05-2415.
- Sever PS, Dahlöf B, Poulter NR, Wedel H, Beevers G, Caulfield M, et al. Prevention of coronary and stroke events with atorvastatin in hypertensive patients who have average or lower-than-average cholesterol concentrations, in the Anglo-Scandinavian Cardiac Outcomes Trial – Lipid Lowering Arm (ASCOT-LLA): a multicentre randomised controlled trial. Lancet 2003;361:1149-58. http://dx.doi.org/10.1016/S0140-6736(03)12948-0.
- LaRosa JC, Grundy SM, Waters DD, Shear C, Barter P, Fruchart J-C, et al. Intensive lipid lowering with atorvastatin in patients with stable coronary disease. N Engl J Med 2005;352:1425-35. http://dx.doi.org/10.1056/NEJMoa050461.
- Koren MJ, Hunninghake DB. Clinical outcomes in managed-care patients with coronary heart disease treated aggressively in lipid-lowering disease management clinics: the Alliance Study. J Am Coll Cardiol 2004;44:1772-9. http://dx.doi.org/10.1016/j.jacc.2004.07.053.
- Davidson M, Mckenney J, Stein E, Schrott H, Bakker-Arkema R, Fayyad R. Comparison of one-year efficacy and safety of atorvastatin versus lovastatin in primary hypercholesterolemia. Am J Cardiol 1997;79:1475-81. http://dx.doi.org/10.1016/S0002-9149(97)00174-4.
- Athyros VG, Papageorgiou AA, Mercouris BR, Athyrou V V, Symeonidis AN, Basayannis EO, et al. Treatment with atorvastatin to the National Cholesterol Educational Program Goal versus ‘usual’ care in secondary coronary heart disease prevention. Curr Med Res Opin 2002;18:220-8. http://dx.doi.org/10.1185/030079902125000787.
- Cannon CP, Braunwald E, McCabe CH, Rader DJ, Rouleau JL, Belder R, et al. Intensive versus moderate lipid lowering with statins after acute coronary syndromes. N Engl J Med 2004;350:1495-504. http://dx.doi.org/10.1056/NEJMoa040583.
- NHS Choices . The History of the NHS 2010. www.nhs.uk/Tools/Documents/ThehistoryoftheNHS.htm (accessed 14 August 2015).
- NHS Choices . NHS Health Check n.d. www.nhs.uk/planners/nhshealthcheck/pages/nhshealthcheck.aspx (accessed 14 August 2015).
- Matthews KA, Crawford SL, Chae CU, Everson-Rose SA, Sowers MF, Sternfeld B, et al. Are changes in cardiovascular disease risk factors in midlife women due to chronological aging or to the menopausal transition?. J Am Coll Cardiol 2009;54:2366-73. http://dx.doi.org/10.1016/j.jacc.2009.10.009.
- Bittner V. Menopause, age, and cardiovascular risk: a complex relationship. J Am Coll Cardiol 2009;54:2374-5. http://dx.doi.org/10.1016/j.jacc.2009.10.008.
- Dratva J, Gómez Real F, Schindler C, Ackermann-Liebrich U, Gerbase MW, Probst-Hensch NM, et al. Is age at menopause increasing across Europe? Results on age at menopause and determinants from two population-based studies. Menopause 2009;16:385-94. http://dx.doi.org/10.1097/gme.0b013e31818aefef.
- Clinical Practice Research Datalink (CPRD) . Clinical Practice Research Datalink n.d. www.cprd.com/home/ (accessed 31 July 2013).
- Keenan K, Hayen A, Neal BC, Irwig L. Long term monitoring in patients receiving treatment to lower blood pressure: analysis of data from placebo controlled randomised controlled trial. BMJ 2009;338. http://dx.doi.org/10.1136/bmj.b1492.
- Leckie G, Charlton C. runmlwin: A Program to Run the MLwiN Multilevel Modeling Software from within Stata. J Stat Softw 2013;52:1-40.
- Oke JL, Stevens RJ, Gaitskell K, Farmer AJ. Establishing an evidence base for frequency of monitoring glycated haemoglobin levels in patients with Type 2 diabetes: projections of effectiveness from a regression model. Diabet Med 2012;29:266-71. http://dx.doi.org/10.1111/j.1464-5491.2011.03412.x.
- Oke J, Farmer A, Neil A, Dalton RN, Dunger D, Stevens R. Monitoring young people with type 1 diabetes for diabetic nephropathy: potential errors of annual ACR testing. Diabetes Res Clin Pract 2013;99:307-14. http://dx.doi.org/10.1016/j.diabres.2012.12.010.
- Morris TP, White IR, Royston P, Seaman SR, Wood AM. Multiple imputation for an incomplete covariate that is a ratio. Stat Med 2014;33:88-104. http://dx.doi.org/10.1002/sim.5935.
- Reducing your Blood Cholesterol. Birmingham: BHF; 2011.
- NHS Choices . High Cholesterol – Diagnosis 2013. www.nhs.uk/Conditions/Cholesterol/Pages/Diagnosis.aspx (accessed 23 January 2014).
- Bowman L, Armitage J, Bulbulia R, Parish S, Collins R. Study of the effectiveness of additional reductions in cholesterol and homocysteine (SEARCH): characteristics of a randomized trial among 12064 myocardial infarction survivors. Am Heart J 2007;154:815-23.
- Frost C, Thompson SG. Correcting for regression dilution bias: comparison of methods for a single predictor variable. J R Stat Soc Ser A 2000;163:173-89. http://dx.doi.org/10.1111/1467-985X.00164.
- Stevens RJ, Kothari V, Adler AI, Stratton IM. The UKPDS risk engine: a model for the risk of coronary heart disease in Type II diabetes (UKPDS 56). Clin Sci 2001;101:671-9. http://dx.doi.org/10.1042/cs1010671.
- ClinRisk Subversion Repository n.d. http://svn.clinrisk.co.uk/opensource/qrisk2 (accessed 6 March 2013).
- StataCorp . Creating and Using Stata Plugins n.d. www.stata.com/plugins/ (accessed 3 February 2014).
- Arsenault BJ, Rana JS, Stroes ESG, Després J-P, Shah PK, Kastelein JJP, et al. Beyond low-density lipoprotein cholesterol: respective contributions of non-high-density lipoprotein cholesterol levels, triglycerides, and the total cholesterol/high-density lipoprotein cholesterol ratio to coronary heart disease risk in apparently healthy men and women. J Am Coll Cardiol 2009;55:35-41.
- Bland JM, Altman DG. Statistical methods for assessing agreement between two methods of clinical measurement. Lancet 1986;1:307-10. http://dx.doi.org/10.1016/S0140-6736(86)90837-8.
- Hughes MD. Regression dilution in the proportional hazards model. Biometrics 1993;49:1056-66. http://dx.doi.org/10.2307/2532247.
- R: A Language and Environment for Statistical Computing. Vienna: R Foundation for Statistical Computing; 2013.
- Altman DG, Royston P. What do we mean by validating a prognostic model?. Stat Med 2000;19:453-73. http://dx.doi.org/10.1002/(SICI)1097-0258(20000229)19:4<453::AID-SIM350>3.0.CO;2-5.
- Reilly BM, Evans AT. Translating clinical research into clinical practice: impact of using prediction rules to make decisions. Ann Intern Med 2006;144:201-9. http://dx.doi.org/10.7326/0003-4819-144-3-200602070-00009.
- Walley T, Mantgani A. The UK General Practice Research Database. Lancet 1997;350:1097-9. http://dx.doi.org/10.1016/S0140-6736(97)04248-7.
- Prentice RL. Covariate measurement errors and parameter estimation in a failure time regression model. Biometrika 1982;69:331-42. http://dx.doi.org/10.1093/biomet/69.2.331.
- Hu P, Tsiatis AA, Davidian M. Estimating the parameters in the Cox model when covariate variables are measured with error. Biometrics 1998;54:1407-19. http://dx.doi.org/10.2307/2533667.
- Clarke PM, Gray AM, Briggs A, Farmer AJ, Fenn P, Stevens RJ, et al. A model to estimate the lifetime health outcomes of patients with type 2 diabetes: the United Kingdom Prospective Diabetes Study (UKPDS) Outcomes Model (UKPDS no. 68). Diabetologia 2004;47:1747-59. http://dx.doi.org/10.1007/s00125-004-1527-z.
- NICE Care Guideline 67. Lipid Modification: Full Guideline. NICE; 2010.
- Arias E, Heron M, Tejada-Vera B. United States Life Tables Eliminating Certain Causes of Death, 1999–2001. Hyattsville, MD: National Center for Health Statistics; 2013.
- Category M Prices – Quarter 2 July 2013. London: NHSBSA; 2013.
- Greving JP, Visseren FLJ, de Wit GA, Algra A. Statin treatment for primary prevention of vascular disease: whom to treat? Cost-effectiveness analysis. BMJ 2011;342. http://dx.doi.org/10.1136/bmj.d1672.
- Pignone M. Aspirin, statins, or both drugs for the primary prevention of coronary heart disease events in men: a cost–utility analysis. Ann Intern Med 2006;144. http://dx.doi.org/10.7326/0003-4819-144-5-200603070-00007.
- Interim Life Tables, England and Wales, 2009–2011. London: ONS; 2013.
- The Green Book: Appraisal and Evaluation in Central Government. London: TSO; 2011.
- Curtis L. Unit Costs of Health and Social Care 2010. Canterbury: PSSRU, University of Kent; 2010.
- Ward S, Lloyd Jones M, Pandor A, Holmes M, Ara R, Ryan A, et al. A systematic review and economic evaluation of statins for the prevention of coronary events. Health Technol Assess 2007;11. http://dx.doi.org/10.3310/hta11140.
- Clarke P, Gray A, Legood R, Briggs A, Holman R. The impact of diabetes-related complications on healthcare costs: results from the United Kingdom Prospective Diabetes Study (UKPDS Study No. 65). Diabet Med 2003;20:442-50. http://dx.doi.org/10.1046/j.1464-5491.2003.00972.x.
- Zhang P, Brown MB, Bilik D, Ackermann RT, Li R, Herman WH. Health utility scores for people with type 2 diabetes in U.S. managed care health plans: results from Translating Research Into Action for Diabetes (TRIAD). Diabetes Care 2012;35:2250-6. http://dx.doi.org/10.2337/dc11-2478.
- Taylor F, Huffman MD, Macedo AF, Moore THM, Burke M, Davey Smith G, et al. Statins for the primary prevention of cardiovascular disease. Cochrane Database Syst Rev 2013;1. http://dx.doi.org/10.1002/14651858.cd004816.pub5.
- Finegold JA, Manisty CH, Goldacre B, Barron AJ, Francis DP. What proportion of symptomatic side effects in patients taking statins are genuinely caused by the drug? Systematic review of randomized placebo-controlled trials to aid individual patient choice. Eur J Prev Cardiol 2014;21:464-74. http://dx.doi.org/10.1177/2047487314525531.
- Chowdhury R, Khan H, Heydon E, Shroufi A, Fahimi S, Moore C, et al. Adherence to cardiovascular therapy: a meta-analysis of prevalence and clinical consequences. Eur Heart J 2013;34:2940-8.
- Stone NJ, Robinson JG, Lichtenstein AH, Goff DC, Lloyd-Jones DM, Smith SC, et al. Treatment of Blood Cholesterol to Reduce Atherosclerotic Cardiovascular Disease Risk in Adults: Synopsis of the 2013 ACC/AHA Cholesterol Guideline. Ann Intern Med. Am Coll Phys 2014;160:339-43.
- Mihaylova B, Emberson J, Blackwell L, Keech A, Simes J, Barnes EH, et al. The effects of lowering LDL cholesterol with statin therapy in people at low risk of vascular disease: meta-analysis of individual data from 27 randomised trials. Lancet 2012;380:581-90. http://dx.doi.org/10.1016/S0140-6736(12)60367-5.
- Sattar N, Preiss D, Murray HM, Welsh P, Buckley BM, de Craen AJM, et al. Statins and risk of incident diabetes: a collaborative meta-analysis of randomised statin trials. Lancet 2010;375:735-42. http://dx.doi.org/10.1016/S0140-6736(09)61965-6.
- Preiss D, Seshasai SRK, Welsh P, Murphy SA, Ho JE, Waters DD, et al. Risk of incident diabetes with intensive-dose compared with moderate-dose statin therapy: a meta-analysis. JAMA 2011;305:2556-64. http://dx.doi.org/10.1001/jama.2011.860.
- Hackam DG, Woodward M, Newby LK, Bhatt DL, Shao M, Smith EE, et al. Statins and intracerebral hemorrhage: collaborative systematic review and meta-analysis. Circulation 2011;124:2233-42. http://dx.doi.org/10.1161/CIRCULATIONAHA.111.055269.
- McKinney JS, Kostis WJ. Statin therapy and the risk of intracerebral hemorrhage: a meta-analysis of 31 randomized controlled trials. Stroke 2012;43:2149-56. http://dx.doi.org/10.1161/STROKEAHA.112.655894.
- Ganga HV, Slim HB, Thompson PD. A systematic review of statin-induced muscle problems in clinical trials. Am Heart J 2014;168:6-15. http://dx.doi.org/10.1016/j.ahj.2014.03.019.
- Golomb BA, Evans MA, Dimsdale JE, White HL. Effects of statins on energy and fatigue with exertion: results from a randomized controlled trial. Arch Intern Med 2012;172:1180-2. http://dx.doi.org/10.1001/archinternmed.2012.2171.
- Parker BA, Capizzi JA, Grimaldi AS, Clarkson PM, Cole SM, Keadle J, et al. Effect of statins on skeletal muscle function. Circulation 2013;127:96-103. http://dx.doi.org/10.1161/CIRCULATIONAHA.112.136101.
- Desai CS, Martin SS, Blumenthal RS. Non-cardiovascular effects associated with statins. BMJ 2014;349. http://dx.doi.org/10.1136/bmj.g3743.
- Hamer M, Batty GD, Stamatakis E, Kivimaki M. Hypertension awareness and psychological distress. Hypertension 2010;56:547-50. http://dx.doi.org/10.1161/HYPERTENSIONAHA.110.153775.
- Mainous AG, Baker R, Everett CJ, King DE. Impact of a policy allowing for over-the-counter statins. Qual Prim Care 2010;18:301-6.
- Bossuyt PM, Lijmer JG, Mol BW. Randomised comparisons of medical tests: sometimes invalid, not always efficient. Lancet 2000;356:1844-7. http://dx.doi.org/10.1016/S0140-6736(00)03246-3.
- Takahashi O, Glasziou PP, Perera R, Shimbo T, Fukui T. Blood pressure re-screening for healthy adults: what is the best measure and interval?. J Hum Hypertens 2012;26:540-6. http://dx.doi.org/10.1038/jhh.2011.72.
- Peeters A, Magliano DJ, Backholer K, Zimmet P, Shaw JE. Changes in the rates of weight and waist circumference gain in Australian adults over time: a longitudinal cohort study. BMJ Open 2014;4. http://dx.doi.org/10.1136/bmjopen-2013-003667.
- Stein EA. Low-density lipoprotein cholesterol reduction by inhibition of PCSK9. Curr Opin Lipidol 2013;24:510-17. http://dx.doi.org/10.1097/MOL.0000000000000021.
- Balk EM. Effects of statins on nonlipid serum markers associated with cardiovascular disease. Ann Intern Med 2003;139. http://dx.doi.org/10.7326/0003-4819-139-8-200310210-00011.
- Rose G. Strategy of prevention: lessons from cardiovascular disease. Br Med J 1981;282:1847-51. http://dx.doi.org/10.1136/bmj.282.6279.1847.
- Strachan D, Rose G. Strategies of prevention revisited: effects of imprecise measurement of risk factors on the evaluation of ‘high-risk’ and ‘population-based’ approaches to prevention of cardiovascular disease. J Clin Epidemiol 1991;44:1187-96. http://dx.doi.org/10.1016/0895-4356(91)90151-X.
- Naci H, Brugts J, Ades T. Comparative tolerability and harms of individual statins: a study-level network meta-analysis of 246 955 participants from 135 randomized, controlled trials. Circ Cardiovasc Qual Outcomes 2013;6:390-9. http://dx.doi.org/10.1161/CIRCOUTCOMES.111.000071.
- Taylor AJ, Kent SM, Flaherty PJ, Coyle LC, Markwood TT, Vernalis MN. ARBITER: Arterial biology for the investigation of the treatment effects of reducing cholesterol: a randomized trial comparing the effects of atorvastatin and pravastatin on carotid intima medial thickness. Circulation 2002;106:2055-60. http://dx.doi.org/10.1161/01.CIR.0000034508.55617.65.
- Smilde T, van Wissen S, Awollersheim H, Trip M, Kastelein J, Stalenhoef A. Effect of aggressive versus conventional lipid lowering on atherosclerosis progression in familial hypercholesterolemia (ASAP): a prospective, randomised, double-blind trial. Lancet 2001;357:577-81. http://dx.doi.org/10.1016/S0140-6736(00)04053-8.
- Raggi P, Davidson M, Callister TQ, Welty FK, Bachmann GA, Hecht H, et al. Aggressive versus moderate lipid-lowering therapy in hypercholesterolemic postmenopausal women: Beyond Endorsed Lipid Lowering with EBT Scanning (BELLES). Circulation 2005;112:563-71. http://dx.doi.org/10.1161/CIRCULATIONAHA.104.512681.
- Bertolini S, Bittolo Bon G, Campbell LM, Farnier M, Langan J, Mahla G, et al. Efficacy and safety of atorvastatin compared to pravastatin in patients with hypercholesterolemia. Atherosclerosis 1997;130:191-7. http://dx.doi.org/10.1016/S0021-9150(96)06052-2.
- Brown AS, Bakker-Arkema RG, Yellen L, Henley RW, Guthrie R, Campbell CF, et al. Treating patients with documented atherosclerosis to national cholesterol education program-recommended low-density-lipoprotein cholesterol goals with atorvastatin, fluvastatin, lovastatin and simvastatin. J Am Coll Cardiol 1998;32:665-72. http://dx.doi.org/10.1016/S0735-1097(98)00300-3.
- Colivicchi F, Tubaro M, Mocini D, Genovesi Ebert A, Strano S, Melina G, et al. Full-dose atorvastatin versus conventional medical therapy after non-ST-elevation acute myocardial infarction in patients with advanced non-revascularisable coronary artery disease. Curr Med Res Opin 2010;26:1277-84. http://dx.doi.org/10.1185/03007991003751496.
- Kadoglou NPE, Sailer N, Moumtzouoglou A, Kapelouzou A, Gerasimidis T, Liapis CD. Aggressive lipid-lowering is more effective than moderate lipid-lowering treatment in carotid plaque stabilization. J Vasc Surg 2010;51:114-21. http://dx.doi.org/10.1016/j.jvs.2009.07.119.
- Mohler ER, Hiatt WR, Creager MA. Cholesterol reduction with atorvastatin improves walking distance in patients with peripheral arterial disease. Circulation 2003;108:1481-6. http://dx.doi.org/10.1161/01.CIR.0000090686.57897.F5.
- Nissen SE, Tuzcu EM, Schoenhagen P, Brown BG, Ganz P, Vogel RA, et al. Effect of intensive compared with moderate lipid-lowering therapy on progression of coronary atherosclerosis: a randomized controlled trial. JAMA 2004;291:1071-80. http://dx.doi.org/10.1001/jama.291.9.1071.
- Deedwania P, Stone PH, Bairey Merz CN, Cosin-Aguilar J, Koylan N, Luo D, et al. Effects of intensive versus moderate lipid-lowering therapy on myocardial ischemia in older patients with coronary heart disease: results of the Study Assessing Goals in the Elderly (SAGE). Circulation 2007;115:700-7. http://dx.doi.org/10.1161/CIRCULATIONAHA.106.654756.
- Schmermund A, Achenbach S, Budde T, Buziashvili Y, Förster A, Friedrich G, et al. Effect of intensive versus standard lipid-lowering treatment with atorvastatin on the progression of calcified coronary atherosclerosis over 12 months: a multicenter, randomized, double-blind trial. Circulation 2006;113:427-37. http://dx.doi.org/10.1161/CIRCULATIONAHA.105.568147.
- Sola S, Mir MQS, Lerakis S, Tandon N, Khan BV. Atorvastatin improves left ventricular systolic function and serum markers of inflammation in nonischemic heart failure. J Am Coll Cardiol 2006;47:332-7. http://dx.doi.org/10.1016/j.jacc.2005.06.088.
- Stone PH, Lloyd-Jones DM, Kinlay S, Frei B, Carlson W, Rubenstein J, et al. Effect of intensive lipid lowering, with or without antioxidant vitamins, compared with moderate lipid lowering on myocardial ischemia in patients with stable coronary artery disease: the Vascular Basis for the Treatment of Myocardial Ischemia Study. Circulation 2005;111:1747-55. http://dx.doi.org/10.1161/01.CIR.0000160866.90148.76.
- Higgins JPT, Jackson D, Barrett JK, Lu G, Ades AE, White IR. Consistency and inconsistency in network meta-analysis: concepts and models for multi-arm studies. Res Synth Methods 2012;3:98-110. http://dx.doi.org/10.1002/jrsm.1044.
- White IR, Barrett JK, Jackson D, Higgins JPT. Consistency and inconsistency in network meta-analysis: model estimation using multivariate meta-regression. Res Synth Methods 2012;3:111-25. http://dx.doi.org/10.1002/jrsm.1045.
- Clarke R, Lewington S, Youngman L, Sherliker P, Peto R, Collins R. Underestimation of the importance of blood pressure and cholesterol for coronary heart disease mortality in old age. Eur Heart J 2002;23:286-93. http://dx.doi.org/10.1053/euhj.2001.2781.
- Law MR, Wald NJ, Wu T, Hackshaw A, Bailey A. Systematic underestimation of association between serum cholesterol concentration and ischaemic heart disease in observational studies: data from the BUPA study. BMJ 1994;308:363-6. http://dx.doi.org/10.1136/bmj.308.6925.363.
- Parish S, Peto R, Palmer A, Clarke R, Lewington S, Offer A, et al. The joint effects of apolipoprotein B, apolipoprotein A1, LDL cholesterol, and HDL cholesterol on risk: 3510 cases of acute myocardial infarction and 9805 controls. Eur Heart J 2009;30:2137-46. http://dx.doi.org/10.1093/eurheartj/ehp221.
- Yarnell JWG, Patterson CC, Sweetnam PM, Lowe GDO. Haemostatic/inflammatory markers predict 10-year risk of IHD at least as well as lipids: the Caerphilly collaborative studies. Eur Heart J 2004;25:1049-56. http://dx.doi.org/10.1016/j.ehj.2004.04.011.
- Qizilbash N, Duffy SW, Rohan TE. Repeat measurement of case-control data: correcting risk estimates for misclassification owing to regression dilution of lipids in transient ischaemic attacks and minor ischaemic strokes. Am J Epidemiol 1991;133:832-8.
- Demacker PN, Schade RW, Jansen RT, Van’t Laar A. Intra-individual variation of serum cholesterol, triglycerides and high density lipoprotein cholesterol in normal humans. Atherosclerosis 1982;45:259-66. http://dx.doi.org/10.1016/0021-9150(82)90227-1.
- Nazir DJ, Roberts RS, Hill SA, McQueen MJ. Monthly intra-individual variation in lipids over a 1-year period in 22 normal subjects. Clin Biochem 1999;32:381-9. http://dx.doi.org/10.1016/S0009-9120(99)00030-2.
- Smith SJ, Cooper GR, Myers GL, Sampson EJ. Biological variability in concentrations of serum lipids: sources of variation among results from published studies and composite predicted values. Clin Chem 1993;39:1012-22.
- Ortolá J, Castiñeiras MJ, Fuentes-Arderiu X. Biological variation data applied to the selection of serum lipid ratios used as risk markers of coronary heart disease. Clin Chem 1992;38:56-9.
- Jeffs JA, Godsland IF, Johnston DG. Less than 50% of variation in HDL cholesterol between and within individuals, is explained by established predictors. Atherosclerosis 2006;184:178-87.
Appendix 1 Excluded references
Alaupovic P, Fesmire JD, Hunnighake D, Domanski M, Forman S, Knatterud GL, et al. The effect of aggressive and moderate lowering of LDL-cholesterol and low dose anticoagulation on plasma lipids, apolipoproteins and lipoprotein families in post coronary artery bypass graft trial. Atherosclerosis 1999;146:369–79.
Athyros VG, Papageorgiou AA, Mercouris BR, Athyrou VV, Symeonidis AN, Basayannis EO, et al. Treatment with atorvastatin to the National Cholesterol Educational Program goal versus’ usual’care in secondary coronary heart disease prevention. Curr Med Res Opin 2002;18:220–8.
Bae J-M, Yang Y-J, Li Z-M, Ahn Y-O. Low cholesterol is associated with mortality from cardiovascular diseases: a dynamic cohort study in Korean adults. J Korean Med Sci 2012;27:58–63.
Ballesteros-Pomar M, Rubio-Herrera M, Gutiérrez-Fuentes J, Gómez-Gerique J, Gómez-De-La-Cámara A, Pascual O, et al. Dietary habits and cardiovascular risk in the Spanish population: the DRECE study (I). Ann Nutr Metab 2000;44:108–14.
Beishuizen ED, Jukema JW, Tamsma JT, Van De Ree MA, Van Der Vijver JCM, Putter H, et al. No effect of statin therapy on silent myocardial ischemia in patients with type 2 diabetes without manifest cardiovascular disease. Diabetes Care 2005;28:1675–9.
Boden WE, Probstfield JL, Anderson T, Chaitman BR, Desvignes-Nickens P, Koprowicz KM, et al. Niacin in patients with low HDL cholesterol levels receiving intensive statin therapy. NEJM 2011;365:2255–67.
Bonaa K, Thelle D. Association between blood pressure and serum lipids in a population: the Tromsø study. Circulation 1991;83:1305–14.
Brunner E, Marmot M, White I, O’Brien J, Etherington M, Slavin B, et al. Gender and employment grade differences in blood cholesterol, apolipoproteins and haemostatic factors in the Whitehall II study. Atherosclerosis 1993;102:195–207.
Buring J, O’Connor G, Goldhaber S, Rosner B, Herbert P, Blum C, et al. Decreased HDL2 and HDL3 cholesterol, Apo AI and Apo A-II, and increased risk of myocardial infarction. Circulation 1992;85:22–9.
Carlson LA, Rosenhamer G. Reduction of mortality in the Stockholm Ischaemic Heart Disease Secondary Prevention Study by combined treatment with clofibrate and nicotinic acid. Acta Med Scand 1988;223:405–18.
Casiglia E, Palatini P. Cardiovascular risk factors in the elderly. J Hum Hypertens 1998;12:575–81.
Chen Z, Peto R, Collins R, MacMahon S, Lu J, Li W. Serum cholesterol concentration and coronary heart disease in population with low cholesterol concentrations. BMJ 1991;303:276–82.
Chien K-L, Hsu H-C, Su T-C, Chen M-F, Lee Y-T, Hu FB. Apolipoprotein B and non-high density lipoprotein cholesterol and the risk of coronary heart disease in Chinese. J Lipid Res 2007;48:2499–505.
Coleman M, Key T, Wang D, Hermon C, Fentiman I, Allen D, et al. A prospective study of obesity, lipids, apolipoproteins and ischaemic heart disease in women. Atherosclerosis 1992;92:177–85.
Cox CS, Mussolino ME, Rothwell ST, Lane MA, Golden CD, Madans JH, et al. Plan and operation of the NHANES I Epidemiologic Follow-up Study, 1992. Vital Health Stat Ser 1 1997;35:1–231.
Cui R, Iso H, Toyoshima H, Date C, Yamamoto A, Kikuchi S, et al. Serum total cholesterol levels and risk of mortality from stroke and coronary heart disease in Japanese: the JACC study. Atherosclerosis 2007;194:415–20.
Davis WA, Chin E, Jee A, Martins J, Bruce DG, Beilby J, et al. Apolipoprotein E genotype and mortality in Southern European and Anglo-Celt patients with type 2 diabetes: the Fremantle Diabetes Study. Eur J Endocrinol 2010;163:559–64.
De Backer G. Regional differences in dietary habits coronary risk factors and mortality rates in Belgium. 1. Design and methodology. Acta Cardiol 1984;39:285–92.
Deeg DJ, van Tilburg T, Smit JH, de Leeuw ED. Attrition in the Longitudinal Aging Study Amsterdam. The effect of differential inclusion in side studies. J Clin Epidemiol 2002;55:319–28.
Doney AS, Dannfald J, Kimber CH, Donnelly LA, Pearson E, Morris AD, et al. The FTO gene is associated with an atherogenic lipid profile and myocardial infarction in patients with type 2 diabetes. A Genetics of Diabetes Audit and Research Study in Tayside Scotland (Go-DARTS) Study. Circulation 2009;2:255–9.
Dorr AE, Gundersen K, Schneider JC Jr, Spencer TW, Martin WB. Colestipol hydrochloride in hypercholesterolemic patients: effect on serum cholesterol and mortality. J Chronic Dis 1978;31:5–14.
Elley C, Kenealy T, Robinson E, Drury P. Glycated haemoglobin and cardiovascular outcomes in people with Type 2 diabetes: a large prospective cohort study. Diabetic Med 2008;25:1295–301.
Engström G, Stavenow L, Hedblad B, Lind P, Eriksson K-F, Janzon L, et al. Inflammation-sensitive plasma proteins, diabetes, and mortality and incidence of myocardial infarction and stroke. A population-based study. Diabetes 2003;52:442–7.
Ericsson C-G, Nilsson J, Grip L, Svane B, Hamsten A. Effect of bezafibrate treatment over five years on coronary plaques causing 20% to 50% diameter narrowing (The Bezafibrate Coronary Atherosclerosis Intervention Trial [BECAIT]). Am J Cardiol 1997;80:1125–9.
Fang X-H, Kronmal RA, Li S-C, Longstreth W, Cheng X-M, Wang W-Z, et al. Prevention of stroke in urban China. A community-based intervention trial. Stroke 1999;30:495–501.
Fowkes F, Housley E, Cawood E, MacIntyre C, Ruckley C, Prescott R. Edinburgh Artery Study: prevalence of asymptomatic and symptomatic peripheral arterial disease in the general population. Int J Epidemiol 1991;20:384–92.
The Medical Research Council’s General Practice Research Framework. Thrombosis prevention trial: randomised trial of low-intensity oral anticoagulation with warfarin and low-dose aspirin in the primary prevention of ischaemic heart disease in men at increased risk. The Medical Research Council’s General Practice Research Framework. Lancet 1998;351:233–41.
Furberg CD, Adams H, Applegate WB, Byington RP, Espeland MA, Hartwell T, et al. Effect of lovastatin on early carotid atherosclerosis and cardiovascular events. Asymptomatic Carotid Artery Progression Study (ACAPS) Research Group. Circulation 1994;90:1679–87.
Ginsberg HN, Elam MB, Lovato LC, Crouse J, III, Leiter LA, Linz P, et al. Effects of combination lipid therapy in type 2 diabetes mellitus. N Engl J Med 2010;362:1563–74.
Goldenberg I, Boyko V, Tennenbaum A, Tanne D, Behar S, Guetta V. Long-term benefit of high-density lipoprotein cholesterol-raising therapy with bezafibrate: 16-year mortality follow-up of the Bezafibrate Infarction Prevention Trial. Arch Intern Med 2009;169:508–14.
Look AHEAD Research Group, Wing RR. Long-term effects of a lifestyle intervention on weight and cardiovascular risk factors in individuals with type 2 diabetes mellitus: four-year results of the Look AHEAD trial. Arch Intern Med 2010;170:1566–75.
Haheim LL, Holme I, Hjermann I, Leren P. Nonfasting serum glucose and the risk of fatal stroke in diabetic and nondiabetic subjects. 18-year follow-up of the Oslo Study. Stroke 1995;26:774–7.
Hamer M, Stamatakis E. Physical activity and risk of cardiovascular disease events: inflammatory and metabolic mechanisms. Med Sci Sports Exerc 2009;41:1206–11.
Harris J, Giles L, Finucane P, Andrews G. Prevalence of coronary heart disease and cardiovascular risk factors in a sample of older Australians: Australian Longitudinal Study of Ageing. Australas J Ageing 2004;23:25–32.
Hayashi T, Araki A, Kawashima S, Sone H, Watanabe H, Ohrui T, et al. Metabolic predictors of ischemic heart disease and cerebrovascular attack in elderly diabetic individuals: difference in risk by age. Cardiovasc Diabetol 2013;12:10.
He Y, Lam TH, Li LS, Li LS, He SF, Liang BQ. Triglyceride and coronary heart disease mortality in a 24-year follow-up study in Xi’an, China. Ann Epidemiol 2004;14:1–7.
Held C, Hjemdahl P, Rehnqvist N, Björkander I, Forslund L, Brodin U, et al. Cardiovascular prognosis in relation to apolipoproteins and other lipid parameters in patients with stable angina pectoris treated with verapamil or metoprolol: results from the Angina Prognosis Study in Stockholm (APSIS). Atherosclerosis 1997;135:109–18.
Heyman A, Karp HR, Heyden S, Bartel A, Cassel JC, Tyroler HA, et al. Cerebrovascular disease in the bi-racial population of Evans County, Georgia. Stroke 1971;2:509–18.
Higgins M, Keller JB. Cholesterol, coronary heart disease, and total mortality in middle-aged and elderly men and women in Tecumseh. Ann Epidemiol 1992;2:69–76.
Hildrum B, Mykletun A, Dahl A, Midthjell K. Metabolic syndrome and risk of mortality in middle-aged versus elderly individuals: the Nord-Trøndelag Health Study (HUNT). Diabetologia 2009;52:583–90.
Ho SC, Woo J, Sham A, Chan SG, Yu AL. A 3-year follow-up study of social, lifestyle and health predictors of cognitive impairment in a Chinese older cohort. Int J Epidemiol 2001;30:1389–96.
Howard BV, Roman MJ, Devereux RB, Fleg JL, Galloway JM, Henderson JA, et al. Effect of lower targets for blood pressure and LDL cholesterol on atherosclerosis in diabetes: the SANDS randomized trial. JAMA 2008;299:1678–89.
Howard BV, Van Horn L, Hsia J, Manson JE, Stefanick ML, Wassertheil-Smoller S, et al. Low-fat dietary pattern and risk of cardiovascular disease: the Women’s Health Initiative Randomized Controlled Dietary Modification Trial. JAMA 2006;295:655–66.
Igland J, Vollset SE, Nygård OK, Gjessing HK, Ueland PM, Tell GS. Relative importance of risk factors for coronary heart disease. The Hordaland Homocysteine Study. Scand Cardiovasc J 2012;46:316–23.
Imai K, Matsuyama S, Miyake S, Suga K, Nakachi K. Natural cytotoxic activity of peripheral-blood lymphocytes and cancer incidence: an 11-year follow-up study of a general population. Lancet 2000;356:1795–9.
Isles CG, Hole DJ, Gillis CR, Hawthorne VM, Lever AF. Plasma cholesterol, coronary heart disease, and cancer in the Renfrew and Paisley survey. BMJ 1989;298:920.
Itakura H, Nakaya N, Kusunoki T, Shimizu N, Hirai S, Mochizuki S, et al. Long-term event monitoring study of fluvastatin in Japanese patients with hypercholesterolemia: efficacy and incidence of cardiac and other events in elderly patients (≥ 65 years old). J Cardiol 2011;57:77–88.
Iwashita M, Matsushita Y, Sasaki J, Arakawa K, Kono S, Group KLIS. Relation of serum total cholesterol and other risk factors to risk of coronary events in middle-aged and elderly Japanese men with hypercholesterolemia: the Kyushu Lipid Intervention Study. Circulation 2004;68:405.
Jansen A, Aalst-Cohen E, Tanck M, Trip M, Lansberg P, Liem A, et al. The contribution of classical risk factors to cardiovascular disease in familial hypercholesterolaemia: data in 2400 patients. J Intern Med 2004;256:482–90.
Kadota A, Miura K, Okamura T, Hozawa A, Murakami Y, Fujiyoshi A, et al. Relationship of moderate metabolic risk factor clustering to cardiovascular disease mortality in non-lean Japanese: a 15-year follow-up of NIPPON DATA90. Atherosclerosis 2011;215:209–13.
Kappelle PJ, Zwang L, Huisman MV, Banga JD, Sluiter WJ, Dallinga-Thie GM, et al. Atorvastatin affects low density lipoprotein and non-high density lipoprotein cholesterol relations with apolipoprotein B in type 2 diabetes mellitus: modification by triglycerides and cholesteryl ester transfer protein. Expert Opin Ther Targets 2009;13:743–51.
Katsuki S. Epidemiological and clinicopathological study on cerebrovascular disease in Japan. Prog Brain Res 1966;21:64–89.
Kawano Y, Ogihara T, Saruta T, Goto Y, Ishii M. Association of blood pressure control and metabolic syndrome with cardiovascular risk in elderly Japanese: JATOS Study. Am J Hypertens 2011;24:1250–6.
Kitamura A, Iso H, Naito Y, Iida M, Konishi M, Folsom AR, et al. High-density lipoprotein cholesterol and premature coronary heart disease in urban Japanese men. Circulation 1994;89:2533–9.
Klungel OH, Heckbert SR, de Boer A, Leufkens HG, Sullivan SD, Fishman PA, et al. Lipid-lowering drug use and cardiovascular events after myocardial infarction. Ann Pharmacother 2002;36:751–7.
Knopp RH, d’Emden M, Smilde JG, Pocock SJ. Efficacy and safety of atorvastatin in the prevention of cardiovascular end points in subjects with type 2 diabetes. The Atorvastatin Study for Prevention of Coronary Heart Disease Endpoints in Non-Insulin-Dependent Diabetes Mellitus (ASPEN). Diabetes Care 2006;29:1478–85.
Knuiman MW, Vu HT, Bartholomew HC. Multivariate risk estimation for coronary heart disease: the Busselton Health Study. Aus N Z J Public Health 1998;22:747–53.
Kojima S, Sakamoto T, Ogawa H, Kitagawa A, Matsui K, Shimomura H, et al. Standard-dose statin therapy provides incremental clinical benefits in normocholesterolemic diabetic patients. Circulation 2010;74:779–85.
Koren MJ, Hunninghake DB. Clinical outcomes in managed-care patients with coronary heart disease treated aggressively in lipid-lowering disease management clinics. The ALLIANCE Study. J Am Coll Cardiol 2004;44:1772–9.
Lee J, Heng D, Chia KS, Chew SK, Tan BY, Hughes K. Risk factors and incident coronary heart disease in Chinese, Malay and Asian Indian males: the Singapore Cardiovascular Cohort Study. Int J Epidemiol 2001;30:983–8.
Li J-J, Lu Z-L, Kou W-R, Bolli R, Chen Z, Wu Y-F, et al. Impact of long-term Xuezhikang therapy on cardiovascular events in high-risk patients with nonspecific, pre-existing abnormal liver tests: a post-hoc analysis from Chinese Coronary Secondary Prevention Study (CCSPS). Int J Cardiol 2012;154:362–5.
Lichtenstein KA, Armon C, Buchacz K, Chmiel JS, Buckner K, Tedaldi E, et al. Provider compliance with guidelines for management of cardiovascular risk in HIV-infected patients. Prev Chronic Dis 2013;10:E10.
Lindroos M, Kupari M, Heikkila J, Tilvis R. Prevalence of aortic valve abnormalities in the elderly: an echocardiographic study of a random population sample. J Am Coll Cardiol 1993;21:1220–5.
Lopez FL, Agarwal SK, MacLehose RF, Soliman EZ, Sharrett AR, Huxley RR, et al. Blood lipid levels, lipid-lowering medications, and the incidence of atrial fibrillation: the Atherosclerosis Risk in Communities Study. Circulation 2012;5:155–62.
Lowe LP, Liu K, Greenland P, Metzger BE, Dyer AR, Stamler J. Diabetes, asymptomatic hyperglycemia, and 22-year mortality in black and white men: the Chicago Heart Association Detection Project in Industry Study. Diabetes Care 1997;20:163–9.
Luc G, Bard JM, Ferrieres J, Evans A, Amouyel P, Arveiler D, et al. Value of HDL cholesterol, apolipoprotein A-I, lipoprotein A-I, and lipoprotein A-I/A-II in prediction of coronary heart disease: the PRIME Study. Prospective Epidemiological Study of Myocardial Infarction. Arterioscler Thromb Vasc Biol 2002;22:1155–61.
Luepker RV, Rästam L, Hannan PJ, Murray DM, Gray C, Baker WL, et al. Community education for cardiovascular disease prevention morbidity and mortality results from the Minnesota Heart Health Program. Am J Epidemiol 1996;144:351–62.
MacMahon S, Norton R, Jackson R, Mackie M, Cheng A, Vander Hoorn S, et al. Fletcher Challenge-University of Auckland Heart & Health Study: design and baseline findings. N Z Med J 1995;108:499–502.
Manninen V, Tenkanen L, Koskinen P, Huttunen J, Mänttäri M, Heinonen O, et al. Joint effects of serum triglyceride and LDL cholesterol and HDL cholesterol concentrations on coronary heart disease risk in the Helsinki Heart Study. Implications for treatment. Circulation 1992;85:37–45.
Marín A, Medrano MJ, González J, Pintado H, Compaired V, Bárcena M, et al. Risk of ischaemic heart disease and acute myocardial infarction in a Spanish population: observational prospective study in a primary-care setting. BMC Public Health 2006;6:38.
Mast BT, Miles T, Penninx BW, Yaffe K, Rosano C, Satterfield S, et al. Vascular disease and future risk of depressive symptomatology in older adults: findings from the Health, Aging, and Body Composition study. Biol Psychiatry 2008;64:320–6.
May M, Lawlor DA, Brindle P, Patel R, Ebrahim S. Cardiovascular disease risk assessment in older women: can we improve on Framingham? British Women’s Heart and Health prospective cohort study. Heart 2006;92:1396–401.
McGovern PG, Jacobs DR, Shahar E, Arnett DK, Folsom AR, Blackburn H, et al. Trends in acute coronary heart disease mortality, morbidity, and medical care from 1985 through 1997 the Minnesota Heart Survey. Circulation 2001;104:19–24.
McQueen MJ, Hawken S, Wang X, Ounpuu S, Sniderman A, Probstfield J, et al. Lipids, lipoproteins, and apolipoproteins as risk markers of myocardial infarction in 52 countries (the INTERHEART study): a case-control study. Lancet 2008;372:224–33.
Meade T, Zuhrie R, Cook C, Cooper J. Bezafibrate in men with lower extremity arterial disease: randomised controlled trial. BMJ 2002;325:1139.
Menotti A, Kromhout D, Nissinen A, Giampaoli S, Seccareccia F, Feskens E, et al. Short-term all-cause mortality and its determinants in elderly male populations in Finland, the Netherlands, and Italy: The FINE Study. Prev Med 1996;25:319–26.
Monami M, Luzzi C, Lamanna C, Chiasserini V, Addante F, Desideri CM, et al. Three-year mortality in diabetic patients treated with different combinations of insulin secretagogues and metformin. Diabetes Metabol Res Rev 2006;22:477–82.
Morrell J, Zeymer U, Baumgartner I, Limbourg T, Röther J, Bhatt DL, et al. Differences in management and outcomes between male and female patients with atherothrombotic disease: results from the REACH Registry in Europe. Eur J Cardiovasc Prevent Rehabil 2011;18:270–7.
Nakayama T, Date C, Yokoyama T, Yoshiike N, Yamaguchi M, Tanaka H. A 15.5-year follow-up study of stroke in a Japanese provincial city. The Shibata Study. Stroke 1997;28:45–52.
Navas-Nacher EL, Colangelo L, Beam C, Greenland P. Risk factors for coronary heart disease in men 18 to 39 years of age. Ann Intern Med 2001;134:433–9.
Australian Institute of Health and Welfare (AIHW) and National Heart Foundation of Australia (NHF). Risk Factor Prevalence Study: survey no. 3 1989 cities analysis: authoritative information and statistics to promote better health and wellbeing. Cat. no. NHF 220. Canberra: AIHW; 1990.
Njolstad I, Arnesen E, Lund-Larsen PG. Smoking, serum lipids, blood pressure, and sex differences in myocardial infarction. A 12-year follow-up of the Finnmark Study. Circulation 1996;93:450–6.
Nordestgaard BG, Benn M, Schnohr P, Tybjærg-Hansen A. Nonfasting triglycerides and risk of myocardial infarction, ischemic heart disease, and death in men and women. JAMA 2007;298:299–308.
Obara F, Saitoh S, Takagi S, Shimamoto K. Influence of hypertension on the incidence of cardiovascular disease in two rural communities in Japan: the Tanno-Sobetsu [corrected] study. Hypertens Res 2007;30:677–82.
Okada H, Horibe H, Yoshiyuki O, Hayakawa N, Aoki N. A prospective study of cerebrovascular disease in Japanese rural communities, Akabane and Asahi. Part 1: evaluation of risk factors in the occurrence of cerebral hemorrhage and thrombosis. Stroke 1976;7:599–607.
Onat A, Ozhan H, Can G, Hergenc G, Karabulut A, Albayrak S. [Serum apolipoprotein B is superior to LDL-cholesterol level in predicting incident coronary disease among Turks.] Anadolu Kardiyol Derg 2007;7:128–33.
Onat A, Yazici M, Can G, Sniderman A. Evidence for a complex risk profile in obese postmenopausal Turkish women with hypertriglyceridaemia and elevated apolipoprotein B. Clin Sci 2004;107:97–104.
Parish S, Peto R, Palmer A, Clarke R, Lewington S, Offer A, et al. The joint effects of apolipoprotein B, apolipoprotein A1, LDL cholesterol, and HDL cholesterol on risk: 3510 cases of acute myocardial infarction and 9805 controls. Eur Heart J 2009;30:2137–46.
Peters S, Palmer M, Grobbee D, Crouse J, O’Leary D, Raichlen J, et al. C-reactive protein lowering with rosuvastatin in the METEOR study. J Intern Med 2010;268:155–61.
Raum E, Lietzau S, Stegmaier C, Brenner H, Rothenbacher D. For the majority of patients with diabetes blood pressure and lipid management is not in line with recommendations. Results from a large population-based cohort in Germany. Pharmacoepidemiol Drug Safety 2008;17:485–94.
Ren J, Grundy SM, Liu J, Wang W, Wang M, Sun J, et al. Long-term coronary heart disease risk associated with very-low-density lipoprotein cholesterol in Chinese: the results of a 15-Year Chinese Multi-Provincial Cohort Study (CMCS). Atherosclerosis 2010;211:327–32.
Rodeghiero F, Tosetto A. The VITA Project: population-based distributions of protein C, antithrombin III, heparin-cofactor II and plasminogen: relationship with physiological variables and establishment of reference ranges. Thrombosis Haemostasis 1996;76:226–33.
Rose G, Tunstall-Pedoe H, Heller R. UK heart disease prevention project: incidence and mortality results. Lancet 1983;321:1062–6.
Rosengren A, Dotevall A, Eriksson H, Wilhelmsen L. Optimal risk factors in the population: prognosis, prevalence, and secular trends. Data from Göteborg population studies. Eur Heart J 2001;22:136–44.
Rosengren A, Wilhelmsen L, Welin L, Tsipogianni A, Teger-Nilsson A-C, Wedel H. Social influences and cardiovascular risk factors as determinants of plasma fibrinogen concentration in a general population sample of middle aged men. BMJ 1990;300:634–8.
Rossebø AB, Pedersen TR, Boman K, Brudi P, Chambers JB, Egstrup K, et al. Intensive lipid lowering with simvastatin and ezetimibe in aortic stenosis. N Engl J Med 2008;359:1343–56.
Rossouw JE, Cushman M, Greenland P, Lloyd-Jones DM, Bray P, Kooperberg C, et al. Inflammatory, lipid, thrombotic, and genetic markers of coronary heart disease risk in the women’s health initiative trials of hormone therapy. Arch Intern Med 2008;168:2245–53.
Rudnichi A, Safar M, Asmar R, Guize L, Benetos A. Prevalence of cardiovascular risk factors in a French population. J Hypertens 1998;16:S85–90.
Satoh H, Tomita K, Fujii S, Kishi R, Tsutsui H. Lower high-density lipoprotein cholesterol is a significant and independent risk for coronary artery disease in Japanese men. J Atherosclerosis Thrombosis 2008;16:792–8.
Schwartz GG, Olsson AG, Ezekowitz MD, Ganz P, Oliver MF, Waters D, et al. Effects of atorvastatin on early recurrent ischemic events in acute coronary syndromes: the MIRACL study: a randomized controlled trial. JAMA 2001;285:1711–18.
Serruys PW, de Feyter P, Macaya C, Kokott N, Puel J, Vrolix M, et al. Fluvastatin for prevention of cardiac events following successful first percutaneous coronary intervention: a randomized controlled trial. JAMA 2002;287:3215–22.
Shimamoto T, Komachi Y, Inada H, Doi M, Iso H, Sato S, et al. Trends for coronary heart disease and stroke and their risk factors in Japan. Circulation 1989;79:503–15.
Shinozaki T, Matsuyama Y, Iimuro S, Umegaki H, Sakurai T, Araki A, et al. Effective prevention of cardiovascular disease and diabetes-related events with atorvastatin in Japanese elderly patients with type 2 diabetes mellitus: adjusting for treatment changes using a marginal structural proportional hazards model and a rank-preserving structural failure time model. Geriatr Gerontol Int 2012;12:88–102.
Simon A, Chironi G, Gariepy J, Pino MD, Levenson J. Differences between markers of atherogenic lipoproteins in predicting high cardiovascular risk and subclinical atherosclerosis in asymptomatic men. Atherosclerosis 2005;179:339–44.
Smith GD, Hart C, Hole D, MacKinnon P, Gillis C, Watt G, et al. Education and occupational social class: which is the more important indicator of mortality risk? J Epidemiol Community Health 1998;52:153–60.
Sone H, Tanaka S, Tanaka S, Iimuro S, Oida K, Yamasaki Y, et al. Serum level of triglycerides is a potent risk factor comparable to LDL cholesterol for coronary heart disease in Japanese patients with type 2 diabetes: subanalysis of the Japan Diabetes Complications Study (JDCS). J Clin Endocrinol Metab 2011;96:3448–56.
Soyama Y, Miura K, Morikawa Y, Nishijo M, Nakanishi Y, Naruse Y, et al. High-density lipoprotein cholesterol and risk of stroke in Japanese men and women: The Oyabe Study. Stroke 2003;34:863–8.
Staessen JA, Wang J-G, Brand E, Barlassina C, Birkenhäger WH, Herrmann S-M, et al. Effects of three candidate genes on prevalence and incidence of hypertension in a Caucasian population. J Hypertens 2001;19:1349–58.
Straczek C, Tafflet M, Barberger-Gateau P, Bertrand M, Dupuy A, Ducimetière P, et al. Do lipids and apolipoproteins predict coronary heart disease under statin and fibrate therapy in the primary prevention setting in community-dwelling elderly subjects? The 3C Study. Atherosclerosis 2011;214:426–31.
Suadicani P, Hein HO, Gyntelberg F. Occupational noise exposure, social class, and risk of ischemic heart disease and all-cause mortality: a 16-year follow-up in the Copenhagen Male Study. Scand J Work Environ Health 2012;38:19–26.
Suh I, Jee SH, Kim HC, Nam CM, Kim IS, Appel LJ. Low serum cholesterol and haemorrhagic stroke in men: Korea Medical Insurance Corporation Study. Lancet 2001;357:922–5.
Ten Kate G-JR, Neefjes LA, Dedic A, Nieman K, Langendonk JG, Galema-Boers AJ, et al. The effect of LDLR-negative genotype on CT coronary atherosclerosis in asymptomatic statin treated patients with heterozygous familial hypercholesterolemia. Atherosclerosis 2013;227:334–41.
Teramoto T, Kitagawa Y, Daida H. APPROACH-J study: design, rationale, and baseline data of the affirmation primary prevention with pravastatin in reduction of occlusive atherosclerotic complications in hypercholesterolemia: Japan study. J Atheroscler Thromb 2010;18:1054–61.
Tocharoenvanich P, Yipintsoi T, Choomalee K, Boonwanno P, Rodklai A. Risk factors for a five-year death in the InterASIA-South cohort. Med J Med Assoc Thailand 2008;91:471.
Törnberg SA, Holm L-E, Carstensen JM, Eklund GA. Risks of cancer of the colon and rectum in relation to serum cholesterol and beta-lipoprotein. N Engl J Med 1986;315:1629–33.
Tsuji I, Imai Y, Nagai K, Ohkubo T, Watanabe N, Minami N, et al. Proposal of reference values for home blood pressure measurement: prognostic criteria based on a prospective observation of the general population in Ohasama, Japan. Am J Hypertens 1997;10:409–18.
Tunstall-Pedoe H, Woodward M, Tavendale R, A’Brook R, McCluskey MK. Comparison of the prediction by 27 different factors of coronary heart disease and death in men and women of the Scottish Heart Health Study: cohort study. BMJ 1997;315:722–9.
Ulmer H, Kelleher C, Diem G, Concin H. Long-term tracking of cardiovascular risk factors among men and women in a large population-based health system: the Vorarlberg Health Monitoring & Promotion Programme. Eur Heart J 2003;24:1004–13.
Van Lennep JER, Westerveld HT, van Lennep HWR, Zwinderman AH, Erkelens DW, van der Wall EE. Apolipoprotein concentrations during treatment and recurrent coronary artery disease events. Arterioscler Thromb Vasc Biol 2000;20:2408–13.
Wanner C, Krane V, März W, Olschewski M, Mann JF, Ruf G, et al. Atorvastatin in patients with type 2 diabetes mellitus undergoing hemodialysis. N Engl J Med 2005;353:238–48.
Wilhelmsen L, Johansson S, Rosengren A, Wallin I, Dotevall A, Lappas G. Risk factors for cardiovascular disease during the period 1985–1995 in Göteborg, Sweden. The GOT-MONICA Project. J Intern Med 1997;242:199–211.
Willey JZ, Xu Q, Boden-Albala B, Paik MC, Moon YP, Sacco RL, et al. Lipid profile components and risk of ischemic stroke: the Northern Manhattan Study (NOMAS). Arch Neurol 2009;66:1400–6.
Worm SW, Sabin CA, Reiss P, El-Sadr W, Monforte ADA, Pradier C, et al. Presence of the metabolic syndrome is not a better predictor of cardiovascular disease than the sum of its components in HIV-infected individuals. Data Collection on adverse events of anti-HIV Drugs (D:A:D) study. Diabetes Care 2009;32:474–80.
Xiaomei L, Yaguang F, Jiang Y, Xiang J, Jixian W, Zhijuan S, et al. A cohort study on risk factors of lung cancer in Yunnan tin miners. Chin J Lung Cancer 2013;16:184–90.
Zylberstein DE, Bengtsson C, Björkelund C, Landaas S, Sundh V, Thelle D, et al. Serum homocysteine in relation to mortality and morbidity from coronary heart disease a 24-year follow-up of the population study of women in Gothenburg. Circulation 2004;109:601–6.
Appendix 2 Modifications to original protocol
This monograph was commissioned by the NIHR Health Technology Programme to provide an evidence base for decisions about the best measure and optimal re-testing interval for lipid levels in patients at risk of, or with, CVD. The research proposal submitted to the HTA was converted into a basic protocol registered in PROSPERO (PROSPERO 2011: CRD42011001753). A more detailed protocol (also submitted to PROSPERO) was created for the systematic review to determine the predictive value of lipid measures for CVD (PROSPERO 2013: CRD42013003727). This appendix presents the original proposal submitted to the HTA, as well as the protocol submitted to Prospero for reference. During the course of the work a number of amendments or modifications to these protocols were proposed and agreed by the advisory group.
Amendments and modifications included
Minor amendments to the systematic review of predictive value of lipid measures (see Chapter 2). The search methods were modified to accommodate the scale of the review and recognise the publication of three reviews in 2012. All outcome data for each lipid measure were pooled regardless of level of heterogeneity, as the primary objective was to enable an overall comparison between lipid measures, their trends and view potentially useful lipid measures for future research. The indirect methods for data extraction were changed once included studies were examined to allow maximum amount of data to be included. Quality assessment of included studies was based on a more recent tool specifically designed for prognostic reviews called QUIPS.
Changes to the focus of the Systematic Review of Efficacy into a Meta-analysis of identified studies (see Chapter 3). In our protocol we set to carry out a systematic review to identify RCTs of lipid-lowering therapy. However, a number of other systematic reviews addressing this specific research question were published recently. These reviews could provide the information required to inform economic analyses of the effect of atorvastatin in lipid monitoring; however, a number of included trials were very small and of short duration, and, if these were excluded, results may differ. On the advice of the project advisory board, Chapter 3 was modified to a re-analysis based on the studies identified in the most recent network meta-analysis, focusing on trials of atorvastatin with a minimum of 1000 individuals and at least 12-month follow-up, and therefore deemed to have a lower chance of bias.
Statistical and economic simulation models based on estimates of noise from CPRD rather than St Luke’s cohort (see Chapters 5 and 7, full discussion in Appendix 17). The protocol initially specified the use of estimates of noise from the St Luke’s cohort adjusted to the UK population (CPRD) to examine impact on clinical decisions for treatment. However, these estimates were considerably smaller when compared with the UK cohort. Based on external data from the literature, we proposed to the advisory board a protocol modification to use the direct CPRD estimates in our simulation models, as this adjustment meant that our models were more consistent with those reported in previous literature.
Lack of IPD analysis for LIPID trial33 data. We were unable to gain direct access to the IPD for the LIPID trial33 data. We did obtain summary estimates that corroborated that those obtained from CPRD for secondary prevention were consistent with those found in LIPID. 33 As we had decided to use only CPRD estimates for the models used for primary prevention, a similar strategy was used for secondary prevention.
Use of QRisk253 as reference CVD risk score instead of Framingham (see Chapters 4–7). Since the protocol was written in 2010, QRisk253 has been increasingly favoured in CVD prevention guidelines as the risk score of choice. The annual update to QRisk253 in 2012 allowed calculation of risk over periods of 1–10 years, thus facilitating its incorporation into health-economic models. Therefore, with the approval of our advisory board, QRisk253 was used as the reference CVD risk score in the statistical modelling and the health economics modelling chapters.
Specific changes to protocol are further described within each chapter.
Appendix 3 Search strategies for populations not taking statins
Search report
The search was conducted on 18 June 2013. The subject search terms were developed through an iterative process testing against known studies. Methodological filters for RCTs were applied; studies were limited to papers published in English from 2007 onwards.
Search numbers
Database | Interface | Observational studies | RCTs |
---|---|---|---|
CENTRAL | The Cochrane Library, Wiley [Issue 3, 2013] | 699 | |
CINAHL | EBSCOhost [1980 – present] | 322 | 133 |
EMBASE | OvidSP [1974 – present] | 1422 | 1513 |
MEDLINE | OvidSP [1946 – present, In process] | 688 | 1692 |
Total: | 2432 | 4037 | |
Duplicates removed: | 2162 | ||
Total: | 1604 | 2703 | |
Duplicates from ON STATIN removed | |||
Final total: | 1410 | 2366 |
Search strategies
CINAHL
S83 | S82 NOT S78 |
S82 | S70 AND S81 |
S81 | S79 OR S80 |
S80 | (MH ‘Treatment Outcomes+’) |
S79 | TI (random* OR experimental studies) OR AB (random* OR experimental studies) OR MW (random* OR experimental studies) |
S78 | (S70 AND S77) |
S77 | S71 OR S72 OR S73 OR S74 OR S75 OR S76 |
S76 | TI ((observational n1 (study or studies))) OR AB ((observational n1 (study or studies))) |
S75 | TI ((cohort n1 (study or studies))) OR AB ((cohort n1 (study or studies))) |
S74 | (MH ‘Nonconcurrent Prospective Studies’) |
S73 | (MH ‘Correlational Studies’) |
S72 | (MH ‘Case Control Studies+’) |
S71 | (MH ‘Prospective Studies’) |
S70 | S29 AND S69 |
S69 | S60 OR S61 OR S62 OR S63 OR S64 OR S65 OR S66 OR S67 OR S68 |
S68 | TI (vascular and (mortality or death#)) |
S67 | TI (stroke and (mortality or death#)) |
S66 | TI ((coronary or heart or cardiac or myocardial or chd) and (mortality or death#)) |
S65 | TI ((cardiovascular or cardio-vascular or cvd) and (mortality or death#)) |
S64 | (MH ‘Stroke/MO/PR’) |
S63 | (MH ‘Vascular Diseases/MO/PR’) |
S62 | (MH ‘Myocardial Ischemia/MO/PR’) OR (MH ‘Coronary Disease+/MO/PR’) OR (MH ‘Myocardial Infarction+/MO/PR’) |
S61 | (MH ‘Cardiovascular Diseases/MO/PR’) |
S60 | S51 AND S59 |
S59 | S52 OR S53 OR S54 OR S55 OR S56 OR S57 OR S58 |
S58 | TI life expectancy OR AB life expectancy |
S57 | TI ((mortality or death# or survival)) OR AB ((mortality or death# or survival)) |
S56 | TI (prognosis or prognostic) OR AB (prognosis or prognostic) |
S55 | TI ((predict or predictor# or prediction#)) OR AB ((predict or predictor# or prediction#)) |
S54 | (MH ‘Survival’) OR (MH ‘Survival Analysis’) |
S53 | (MH ‘Cause of Death’) |
S52 | (MH ‘Prognosis’) |
S51 | S30 OR S31 OR S32 OR S33 OR S34 OR S35 OR S36 OR S37 OR S38 OR S39 OR S40 OR S41 OR S42 OR S43 OR S44 OR S45 OR S46 OR S47 OR S48 OR S49 OR S50 |
S50 | TI (((all cause or total) n3 (mortality or death#))) OR AB (((all cause or total) n3 (mortality or death#))) |
S49 | TI mortality or death# or life expectancy |
S48 | TI Stroke OR AB stroke |
S47 | TI atherosclerosis OR AB atherosclerosis |
S46 | TI ((vascular n3 (event# or outcome#))) OR AB ((vascular n3 (event# or outcome#))) |
S45 | TI (vascular n3 disease#) OR AB (vascular n3 disease#) |
S44 | TI myocardial infarct* OR AB myocardial infarct* |
S43 | TI (((ischaemic or ischemic or ischaemia or ischemia) n3 (event# or outcome#))) OR AB (((ischaemic or ischemic or ischaemia or ischemia) n3 (event# or outcome#))) |
S42 | TI (((ischaemic or ischemic or ischaemia or ischemia) n3 disease#)) OR AB (((ischaemic or ischemic or ischaemia or ischemia) n3 disease#)) |
S41 | TI ischaemic or ischemic or ischaemia or ischemia |
S40 | TI (((coronary or heart) n3 (event# or outcome#))) OR AB (((coronary or heart) n3 (event# or outcome#))) |
S39 | TI (((coronary or heart) n3 disease#)) OR AB (((coronary or heart) n3 disease#)) |
S38 | TI coronary or heart or chd |
S37 | TI (((cardiovascular or cardio-vascular) n3 (event# or outcome#))) OR AB (((cardiovascular or cardio-vascular) n3 (event# or outcome#))) |
S36 | TI (((cardiovascular or cardio-vascular) n3 disease#)) OR AB (((cardiovascular or cardio-vascular) n3 disease#)) |
S35 | TI cardiovascular or cardio-vascular or cvd |
S34 | (MM ‘Mortality’) |
S33 | (MM ‘Stroke’) |
S32 | (MM ‘Peripheral Vascular Diseases’) |
S31 | (MM ‘Myocardial Ischemia’) OR (MM ‘Coronary Disease+’) OR (MM ‘Myocardial Infarction+’) |
S30 | (MM ‘Cardiovascular Diseases’) |
S29 | S28 NOT S11 |
S28 | S12 OR S13 OR S14 OR S15 OR S16 OR S17 OR S18 OR S19 OR S20 OR S21 OR S22 OR S23 OR S24 OR S25 OR S26 OR S27 |
S27 | TI ((cholesterol n2 (profile# or ratio#))) OR AB ((cholesterol n2 (profile# or ratio#))) |
S26 | TI ((lipid# n2 (profile# or ratio#))) OR AB ((lipid# n2 (profile# or ratio#))) |
S25 | TI triglyceride* OR AB triglyceride* |
S24 | TI (apoa or Apo B or apo-a or apo-b) OR AB (apoa or Apo B or apo-a or apo-b) |
S23 | TI apolipoprotein* OR AB apolipoprotein* |
S22 | TI ldlc OR AB ldlc |
S21 | TI ldl-c OR AB ldl-c |
S20 | TI hdlc OR AB hdlc |
S19 | TI hdl-c OR AB hdl-c |
S18 | TI ((ldl or low density lipoprotein#) n1 cholesterol) OR AB ((ldl or low density lipoprotein#) n1 cholesterol) |
S17 | TI (((hdl or high density lipoprotein#) n1 cholesterol)) OR AB (((hdl or high density lipoprotein#) n1 cholesterol)) |
S16 | TI ((total n1 cholesterol)) OR AB ((total n1 cholesterol)) |
S15 | TI ((total n1 lipid#)) OR AB ((total n1 lipid#)) |
S14 | (MM ‘Triglycerides’) |
S13 | (MM ‘Apolipoproteins’) |
S12 | (MM ‘Lipoproteins, HDL Cholesterol’) OR (MM ‘Lipoproteins, LDL Cholesterol’) |
S11 | S1 OR S2 OR S3 OR S4 OR S5 OR S6 OR S7 OR S8 OR S9 OR S10 |
S10 | TI (simvastatin or Zocor or Lipex) OR AB (simvastatin or Zocor or Lipex) |
S9 | TI (rosuvastatin or Crestor) OR AB (rosuvastatin or Crestor) |
S8 | TI (pravastatin or Pravachol or Selektine or Lipostat) OR AB (pravastatin or Pravachol or Selektine or Lipostat) |
S7 | TI (pitavastatin or Livalo or Pitava) OR AB (pitavastatin or Livalo or Pitava) |
S6 | TI (lovastatin or Mevacor or Altocor or Altoprev) OR AB (lovastatin or Mevacor or Altocor or Altoprev) |
S5 | TI (fluvastatin or Lescol) OR AB (fluvastatin or Lescol) |
S4 | TI (atorvastatin or Lipitor or Torvast) OR AB (atorvastatin or Lipitor or Torvast) |
S3 | TI ((HMG-CoA reductase inhibitor# or 3-hydroxy-3-methyl-glutaryl-CoA reductase inhibitor# or 3-hydroxy-3-methylglutaryl coenzyme A reductase inhibitor# or HMGCR inhibitor# or Hydroxymethylglutaryl-CoA Reductase Inhibitor#)) OR AB ((HMG-CoA reductase inhibitor# or 3-hydroxy-3-methyl-glutaryl-CoA reductase inhibitor# or 3-hydroxy-3-methylglutaryl coenzyme A reductase inhibitor# or HMGCR inhibitor# or Hydroxymethylglutaryl-CoA Reductase Inhibitor#)) |
S2 | TI statin# OR AB statin# |
S1 | (MH ‘Statins+’) |
Cochrane Central Register of Controlled Trials
#1 MeSH descriptor: [Hydroxymethylglutaryl-CoA Reductase Inhibitors] explode all trees
#2 statin*:ti,ab,kw (Word variations have been searched)
#3 HMG-CoA reductase inhibitor* or 3-hydroxy-3-methyl-glutaryl-CoA reductase inhibitor* or 3-hydroxy-3-methylglutaryl coenzyme A reductase inhibitor* or HMGCR inhibitor* or Hydroxymethylglutaryl-CoA Reductase Inhibitor*:ti,ab,kw (Word variations have been searched)
#4 atorvastatin or Lipitor or Torvast:ti,ab,kw (Word variations have been searched)
#5 fluvastatin or Lescol:ti,ab,kw (Word variations have been searched)
#6 lovastatin or Mevacor or Altocor or Altoprev:ti,ab,kw (Word variations have been searched)
#7 pitavastatin or Livalo or Pitava:ti,ab,kw (Word variations have been searched)
#8 pravastatin or Pravachol or Selektine or Lipostat:ti,ab,kw (Word variations have been searched)
#9 rosuvastatin or Crestor:ti,ab,kw (Word variations have been searched)
#10 simvastatin or Zocor or Lipex:ti,ab,kw (Word variations have been searched)
#11 #1 or #2 or #3 or #4 or #5 or #6 or #7 or #8 or #9 or #10
#12 MeSH descriptor: [Lipoproteins, HDL] explode all trees
#13 MeSH descriptor: [Lipoproteins, LDL] explode all trees
#14 MeSH descriptor: [Apolipoproteins] explode all trees
#15 MeSH descriptor: [Triglycerides] explode all trees
#16 ((lipid* or cholesterol) near (profile* or ratio*)):ti,ab,kw (Word variations have been searched)
#17 (total next cholesterol):ti,ab,kw (Word variations have been searched)
#18 ((hdl or high density lipoprotein*) next cholesterol):ti,ab,kw (Word variations have been searched)
#19 ((ldl or low density lipoprotein*) next cholesterol):ti,ab,kw (Word variations have been searched)
#20 apolipoprotein* or apoa or Apo B or apo-a or apo-b:ti,ab,kw (Word variations have been searched)
#21 triglyceride*:ti,ab,kw (Word variations have been searched)
#22 #12 or #13 or #14 or #15 or #16 or #17 or #18 or #19 or #20 or #21
#23 #22 not #11
#24 cardiovascular or cardio-vascular or cvd or coronary or heart or ischaem* or ischem* or myocardial or angina or chd or vascular or stroke or atheroclero* or arteriosclero*:ti,ab,kw from 2007 to 2013 (Word variations have been searched)
#25 #23 and #24
EMBASE: observational
-
statin?.ti.
-
(HMG-CoA reductase inhibitor? or 3-hydroxy-3-methyl-glutaryl-CoA reductase inhibitor? or 3-hydroxy-3-methylglutaryl coenzyme A reductase inhibitor? or HMGCR inhibitor? or Hydroxymethylglutaryl-CoA Reductase Inhibitor?).ti.
-
(atorvastatin or Lipitor or Torvast).ti.
-
(fluvastatin or Lescol).ti.
-
(lovastatin or Mevacor or Altocor or Altoprev).ti.
-
(pitavastatin or Livalo or Pitava).ti.
-
(pravastatin or Pravachol or Selektine or Lipostat).ti.
-
(rosuvastatin or Crestor).ti.
-
(simvastatin or Zocor or Lipex).ti.
-
exp *hydroxymethylglutaryl coenzyme A reductase inhibitor/
-
1 or 2 or 3 or 4 or 5 or 6 or 7 or 8 or 9 or 10
-
*high density lipoprotein/
-
*low density lipoprotein/
-
low density lipoprotein cholesterol/
-
*apolipoprotein/or *apolipoprotein a/or *apolipoprotein a1/or *apolipoprotein b/
-
*triacylglycerol/or *triacylglycerol blood level/
-
((lipid? or cholesterol?) adj2 (profile? or ratio?)).ti,ab.
-
(total adj2 (lipid? or cholesterol)).ti,ab.
-
((hdl or high density lipoprotein?) adj cholesterol).ti,ab.
-
((ldl or low density lipoprotein?) adj cholesterol).ti,ab.
-
hdl-c.ti,ab.
-
hdlc.ti,ab.
-
ldl-c.ti,ab.
-
ldlc.ti,ab.
-
apolipoprotein*.ti,ab.
-
(apoa or Apo B or apo-a or apo-b).ti,ab.
-
triglyceride*.ti,ab.
-
12 or 13 or 14 or 15 or 16 or 17 or 18 or 19 or 20 or 21 or 22 or 23 or 24 or 25 or 26 or 27
-
*cardiovascular disease/
-
*coronary artery disease/
-
*ischemic heart disease/
-
*heart muscle ischemia/
-
*heart infarction/
-
exp *angina pectoris/
-
*sudden death/
-
*vascular disease/
-
*Stroke/
-
*atherosclerosis/or *arteriosclerosis/
-
*Mortality/
-
(cardiovascular or cardio-vascular or cvd).ti.
-
((cardiovascular or cardio-vascular) adj3 disease?).ti,ab.
-
((cardiovascular or cardio-vascular) adj3 (event? or outcome?)).ti,ab.
-
(coronary or heart or chd).ti.
-
((coronary or heart) adj3 disease?).ti,ab.
-
((coronary or heart) adj3 (event? or outcome?)).ti,ab.
-
(ischaemic or ischemic or ischaemia or ischemia).ti.
-
((ischaemic or ischemic or ischaemia or ischemia) adj3 disease?).ti,ab.
-
((ischaemic or ischemic or ischaemia or ischemia) adj3 (event? or outcome?)).ti,ab.
-
myocardial infarct*.ti,ab.
-
(vascular adj3 disease?).ti,ab.
-
(vascular adj3 (event? or outcome?)).ti,ab.
-
atherosclerosis.ti,ab.
-
stroke.ti,ab.
-
(mortality or death? or life expectancy).ti.
-
((all cause or total) adj3 (mortality or death?)).ti,ab.
-
or/29–55
-
*Prognosis/
-
*’Cause of Death’/
-
*survival/or *survival rate/or *life expectancy/
-
proportional hazards model/
-
*Mortality/
-
(predict or predictor? or prediction?).ti,ab.
-
(prognosis or prognostic).ti,ab.
-
(mortality or death? or survival).ti,ab.
-
life expectancy.ti,ab.
-
57 or 58 or 59 or 60 or 61 or 62 or 63 or 64 or 65
-
28 and 56 and 66
-
((cardiovascular or cardio-vascular or cvd) and (risk? or mortality or death?)).ti.
-
((coronary or heart or cardiac or myocardial or chd) and (risk? or mortality or death?)).ti.
-
(stroke and (risk? or mortality or death?)).ti.
-
(vascular and (risk? or mortality or death?)).ti.
-
68 or 69 or 70 or 71
-
28 and 72
-
67 or 73
-
74 not 11
-
Clinical study/
-
Family study/
-
Longitudinal study/
-
(Cohort adj (study or studies)).mp.
-
(Case control adj (study or studies)).tw.
-
(follow up adj (study or studies)).tw.
-
(observational adj (study or studies)).tw.
-
(epidemiologic$adj (study or studies)).tw.
-
76 or 77 or 78 or 79 or 80 or 81 or 82 or 83
-
75 and 84
-
limit 85 to (conference abstract or conference paper or conference proceeding or ‘conference review’)
-
85 not 86
EMBASE: RCT
-
statin?.ti.
-
(HMG-CoA reductase inhibitor? or 3-hydroxy-3-methyl-glutaryl-CoA reductase inhibitor? or 3-hydroxy-3-methylglutaryl coenzyme A reductase inhibitor? or HMGCR inhibitor? or Hydroxymethylglutaryl-CoA Reductase Inhibitor?).ti.
-
(atorvastatin or Lipitor or Torvast).ti.
-
(fluvastatin or Lescol).ti.
-
(lovastatin or Mevacor or Altocor or Altoprev).ti.
-
(pitavastatin or Livalo or Pitava).ti.
-
(pravastatin or Pravachol or Selektine or Lipostat).ti.
-
(rosuvastatin or Crestor).ti.
-
(simvastatin or Zocor or Lipex).ti.
-
exp *hydroxymethylglutaryl coenzyme A reductase inhibitor/
-
1 or 2 or 3 or 4 or 5 or 6 or 7 or 8 or 9 or 10
-
*high density lipoprotein/
-
*low density lipoprotein/
-
low density lipoprotein cholesterol/
-
*apolipoprotein/or *apolipoprotein a/or *apolipoprotein a1/or *apolipoprotein b/
-
*triacylglycerol/or *triacylglycerol blood level/
-
((lipid? or cholesterol?) adj2 (profile? or ratio?)).ti,ab.
-
(total adj2 (lipid? or cholesterol)).ti,ab.
-
((hdl or high density lipoprotein?) adj cholesterol).ti,ab.
-
((ldl or low density lipoprotein?) adj cholesterol).ti,ab.
-
hdl-c.ti,ab.
-
hdlc.ti,ab.
-
ldl-c.ti,ab.
-
ldlc.ti,ab.
-
apolipoprotein*.ti,ab.
-
(apoa or Apo B or apo-a or apo-b).ti,ab.
-
triglyceride*.ti,ab.
-
12 or 13 or 14 or 15 or 16 or 17 or 18 or 19 or 20 or 21 or 22 or 23 or 24 or 25 or 26 or 27
-
*cardiovascular disease/
-
*coronary artery disease/
-
*ischemic heart disease/
-
*heart muscle ischemia/
-
*heart infarction/
-
exp *angina pectoris/
-
*sudden death/
-
*vascular disease/
-
*Stroke/
-
*atherosclerosis/or *arteriosclerosis/
-
*Mortality/
-
(cardiovascular or cardio-vascular or cvd).ti.
-
((cardiovascular or cardio-vascular) adj3 disease?).ti,ab.
-
((cardiovascular or cardio-vascular) adj3 (event? or outcome?)).ti,ab.
-
(coronary or heart or chd).ti.
-
((coronary or heart) adj3 disease?).ti,ab.
-
((coronary or heart) adj3 (event? or outcome?)).ti,ab.
-
(ischaemic or ischemic or ischaemia or ischemia).ti.
-
((ischaemic or ischemic or ischaemia or ischemia) adj3 disease?).ti,ab.
-
((ischaemic or ischemic or ischaemia or ischemia) adj3 (event? or outcome?)).ti,ab.
-
myocardial infarct*.ti,ab.
-
(vascular adj3 disease?).ti,ab.
-
(vascular adj3 (event? or outcome?)).ti,ab.
-
atherosclerosis.ti,ab.
-
stroke.ti,ab.
-
(mortality or death? or life expectancy).ti.
-
((all cause or total) adj3 (mortality or death?)).ti,ab.
-
or/29–55
-
*Prognosis/
-
*’Cause of Death’/
-
*survival/or *survival rate/or *life expectancy/
-
proportional hazards model/
-
*Mortality/
-
(predict or predictor? or prediction?).ti,ab.
-
(prognosis or prognostic).ti,ab.
-
(mortality or death? or survival).ti,ab.
-
life expectancy.ti,ab.
-
57 or 58 or 59 or 60 or 61 or 62 or 63 or 64 or 65
-
28 and 56 and 66
-
((cardiovascular or cardio-vascular or cvd) and (risk? or mortality or death?)).ti.
-
((coronary or heart or cardiac or myocardial or chd) and (risk? or mortality or death?)).ti.
-
(stroke and (risk? or mortality or death?)).ti.
-
(vascular and (risk? or mortality or death?)).ti.
-
68 or 69 or 70 or 71
-
28 and 72
-
67 or 73
-
74 not 11
-
random*.tw. or placebo*.mp. or double blind*.tw.
-
75 and 76
-
limit 77 to (conference abstract or conference paper or conference proceeding or ‘conference review’)
-
77 not 78
MEDLINE: observational
-
exp Hydroxymethylglutaryl-CoA Reductase Inhibitors/
-
statin?.ti.
-
(HMG-CoA reductase inhibitor? or 3-hydroxy-3-methyl-glutaryl-CoA reductase inhibitor? or 3-hydroxy-3-methylglutaryl coenzyme A reductase inhibitor? or HMGCR inhibitor? or Hydroxymethylglutaryl-CoA Reductase Inhibitor?).ti.
-
(atorvastatin or Lipitor or Torvast).ti.
-
(fluvastatin or Lescol).ti.
-
(lovastatin or Mevacor or Altocor or Altoprev).ti.
-
(pitavastatin or Livalo or Pitava).ti.
-
(pravastatin or Pravachol or Selektine or Lipostat).ti.
-
(rosuvastatin or Crestor).ti.
-
(simvastatin or Zocor or Lipex).ti.
-
1 or 2 or 3 or 4 or 5 or 6 or 7 or 8 or 9 or 10
-
exp Lipoproteins, LDL/an, bl [Analysis, Blood]
-
exp Lipoproteins, HDL/an, bl [Analysis, Blood]
-
exp Apolipoproteins/an, bl [Analysis, Blood]
-
Triglycerides/an, bl [Analysis, Blood]
-
exp *Lipoproteins, LDL/
-
exp *Lipoproteins, HDL/
-
exp *Apolipoproteins/
-
*Triglycerides/
-
(total adj2 (lipid* or cholesterol)).ti,ab.
-
((lipid? or cholesterol*) adj2 (profile? or ratio?)).ti,ab.
-
((hdl or high density lipoprotein?) adj cholesterol).ti,ab.
-
((ldl or low density lipoprotein?) adj cholesterol).ti,ab.
-
hdl-c.ti,ab.
-
hdlc.ti,ab.
-
ldl-c.ti,ab.
-
ldlc.ti,ab.
-
apolipoprotein*.ti,ab.
-
(apoa or Apo B or apo-a or apo-b).ti,ab.
-
triglyceride*.ti,ab.
-
12 or 13 or 14 or 15 or 16 or 17 or 18 or 19 or 20 or 21 or 22 or 23 or 24 or 25 or 26 or 27 or 28 or 29 or 30
-
*Cardiovascular Diseases/
-
*Coronary Disease/
-
*Myocardial Ischemia/
-
exp *Myocardial Infarction/
-
exp *Angina Pectoris/
-
*Coronary Artery Disease/
-
*Death, Sudden, Cardiac/
-
*Vascular Diseases/
-
*Stroke/
-
*Arteriosclerosis/
-
*Mortality/
-
(cardiovascular or cardio-vascular or cvd).ti.
-
((cardiovascular or cardio-vascular) adj3 disease?).ti,ab.
-
((cardiovascular or cardio-vascular) adj3 (event? or outcome?)).ti,ab.
-
(coronary or heart or chd).ti.
-
((coronary or heart) adj3 disease?).ti,ab.
-
((coronary or heart) adj3 (event? or outcome?)).ti,ab.
-
(ischaemic or ischemic or ischaemia or ischemia).ti.
-
((ischaemic or ischemic or ischaemia or ischemia) adj3 disease?).ti,ab.
-
((ischaemic or ischemic or ischaemia or ischemia) adj3 (event? or outcome?)).ti,ab.
-
myocardial infarct*.ti,ab.
-
(vascular adj3 disease?).ti,ab.
-
(vascular adj3 (event? or outcome?)).ti,ab.
-
atherosclerosis.ti,ab.
-
stroke.ti,ab.
-
(mortality or death? or life expectancy).ti.
-
((all cause or total) adj3 (mortality or death?)).ti,ab.
-
or/32–58
-
Prognosis/
-
‘Cause of Death’/
-
Survival Analysis/or Survival Rate/or Life Expectancy/
-
proportional hazards models/
-
Mortality/
-
(predict or predictor? or prediction?).ti,ab.
-
(prognosis or prognostic).ti,ab.
-
(mortality or death? or survival).ti,ab.
-
life expectancy.ti,ab.
-
60 or 61 or 62 or 63 or 64 or 65 or 66 or 67 or 68
-
31 and 59 and 69
-
Cardiovascular Diseases/mo [Mortality]
-
Coronary Disease/mo [Mortality]
-
Myocardial Ischemia/mo [Mortality]
-
exp Myocardial Infarction/mo [Mortality]
-
Angina Pectoris/mo [Mortality]
-
Coronary Artery Disease/mo [Mortality]
-
Death, Sudden, Cardiac/mo [Mortality]
-
Vascular Diseases/mo [Mortality]
-
Arteriosclerosis/mo [Mortality]
-
Stroke/mo [Mortality]
-
((cardiovascular or cardio-vascular or cvd) and (mortality or death?)).ti.
-
((coronary or heart or cardiac or myocardial or chd) and (mortality or death?)).ti.
-
(stroke and (mortality or death?)).ti.
-
(vascular and (mortality or death?)).ti.
-
71 or 72 or 73 or 74 or 75 or 76 or 77 or 78 or 80 or 81 or 82 or 83 or 84
-
31 and 85
-
70 or 86
-
87 not 11
-
Epidemiologic studies/
-
Case control.tw.
-
(cohort adj (study or studies)).tw.
-
Cohort analy$.tw.
-
(Follow up adj (study or studies)).tw.
-
(observational adj (study or studies)).tw.
-
89 or 90 or 91 or 92 or 93 or 94
-
88 and 95
MEDLINE: randomised controlled trial
-
exp Hydroxymethylglutaryl-CoA Reductase Inhibitors/
-
statin?.ti.
-
(HMG-CoA reductase inhibitor? or 3-hydroxy-3-methyl-glutaryl-CoA reductase inhibitor? or 3-hydroxy-3-methylglutaryl coenzyme A reductase inhibitor? or HMGCR inhibitor? or Hydroxymethylglutaryl-CoA Reductase Inhibitor?).ti.
-
(atorvastatin or Lipitor or Torvast).ti.
-
(fluvastatin or Lescol).ti.
-
(lovastatin or Mevacor or Altocor or Altoprev).ti.
-
(pitavastatin or Livalo or Pitava).ti.
-
(pravastatin or Pravachol or Selektine or Lipostat).ti.
-
(rosuvastatin or Crestor).ti.
-
(simvastatin or Zocor or Lipex).ti.
-
1 or 2 or 3 or 4 or 5 or 6 or 7 or 8 or 9 or 10
-
exp Lipoproteins, LDL/an, bl [Analysis, Blood]
-
exp Lipoproteins, HDL/an, bl [Analysis, Blood]
-
exp Apolipoproteins/an, bl [Analysis, Blood]
-
Triglycerides/an, bl [Analysis, Blood]
-
exp *Lipoproteins, LDL/
-
exp *Lipoproteins, HDL/
-
exp *Apolipoproteins/
-
*Triglycerides/
-
(total adj2 (lipid* or cholesterol)).ti,ab.
-
((lipid? or cholesterol*) adj2 (profile? or ratio?)).ti,ab.
-
((hdl or high density lipoprotein?) adj cholesterol).ti,ab.
-
((ldl or low density lipoprotein?) adj cholesterol).ti,ab.
-
hdl-c.ti,ab.
-
hdlc.ti,ab.
-
ldl-c.ti,ab.
-
ldlc.ti,ab.
-
apolipoprotein*.ti,ab.
-
(apoa or Apo B or apo-a or apo-b).ti,ab.
-
triglyceride*.ti,ab.
-
12 or 13 or 14 or 15 or 16 or 17 or 18 or 19 or 20 or 21 or 22 or 23 or 24 or 25 or 26 or 27 or 28 or 29 or 30
-
*Cardiovascular Diseases/
-
*Coronary Disease/
-
*Myocardial Ischemia/
-
exp *Myocardial Infarction/
-
exp *Angina Pectoris/
-
*Coronary Artery Disease/
-
*Death, Sudden, Cardiac/
-
*Vascular Diseases/
-
*Stroke/
-
*Arteriosclerosis/
-
*Mortality/
-
(cardiovascular or cardio-vascular or cvd).ti.
-
((cardiovascular or cardio-vascular) adj3 disease?).ti,ab.
-
((cardiovascular or cardio-vascular) adj3 (event? or outcome?)).ti,ab.
-
(coronary or heart or chd).ti.
-
((coronary or heart) adj3 disease?).ti,ab.
-
((coronary or heart) adj3 (event? or outcome?)).ti,ab.
-
(ischaemic or ischemic or ischaemia or ischemia).ti.
-
((ischaemic or ischemic or ischaemia or ischemia) adj3 disease?).ti,ab.
-
((ischaemic or ischemic or ischaemia or ischemia) adj3 (event? or outcome?)).ti,ab.
-
myocardial infarct*.ti,ab.
-
(vascular adj3 disease?).ti,ab.
-
(vascular adj3 (event? or outcome?)).ti,ab.
-
atherosclerosis.ti,ab.
-
stroke.ti,ab.
-
(mortality or death? or life expectancy).ti.
-
((all cause or total) adj3 (mortality or death?)).ti,ab.
-
or/32–58
-
Prognosis/
-
‘Cause of Death’/
-
Survival Analysis/or Survival Rate/or Life Expectancy/
-
proportional hazards models/
-
Mortality/
-
(predict or predictor? or prediction?).ti,ab.
-
(prognosis or prognostic).ti,ab.
-
(mortality or death? or survival).ti,ab.
-
life expectancy.ti,ab.
-
60 or 61 or 62 or 63 or 64 or 65 or 66 or 67 or 68
-
31 and 59 and 69
-
Cardiovascular Diseases/mo [Mortality]
-
Coronary Disease/mo [Mortality]
-
Myocardial Ischemia/mo [Mortality]
-
exp Myocardial Infarction/mo [Mortality]
-
Angina Pectoris/mo [Mortality]
-
Coronary Artery Disease/mo [Mortality]
-
Death, Sudden, Cardiac/mo [Mortality]
-
Vascular Diseases/mo [Mortality]
-
Arteriosclerosis/mo [Mortality]
-
Stroke/mo [Mortality]
-
((cardiovascular or cardio-vascular or cvd) and (mortality or death?)).ti.
-
((coronary or heart or cardiac or myocardial or chd) and (mortality or death?)).ti.
-
(stroke and (mortality or death?)).ti.
-
(vascular and (mortality or death?)).ti.
-
71 or 72 or 73 or 74 or 75 or 76 or 77 or 78 or 80 or 81 or 82 or 83 or 84
-
31 and 85
-
70 or 86
-
87 not 11
-
randomized controlled trial.pt.
-
controlled clinical trial.pt.
-
randomized.ab.
-
placebo.ab.
-
drug therapy.fs.
-
randomly.ab.
-
trial.ab.
-
groups.ab.
-
89 or 90 or 91 or 92 or 93 or 94 or 95 or 96
-
exp animals/not humans.sh.
-
97 not 98
-
88 and 99
Appendix 4 Search strategies for populations taking statins studies
Search report: non-randomised control trials
The search was conducted on 4 December 2012. The subject search terms were developed through an iterative process testing against known studies. The search filter for observational studies was adapted from that presented on the SIGN website: www.sign.ac.uk/methodology/filters.html#obs.
Search numbers
Database | Interface | Results |
---|---|---|
CINAHL | EBSCOhost [1980 – present] | 204 |
EMBASE | OvidSP [1974 – present] | 1438 |
MEDLINE | OvidSP [1946 – present, In process] | 1431 |
Total | 3073 | |
Duplicates removed | 813 | |
Final total | 2260 |
Search strategies
CINAHL: (EBSCOhost) [1980 – present]
S100 | S92 AND S99 | (204) |
S99 | S93 OR S94 OR S95 OR S96 OR S97 OR S98 | (182,420) |
S98 | TI ((observational n1 (study or studies))) OR AB ((observational n1 (study or studies))) | (9500) |
S97 | TI ((cohort n1 (study or studies)))OR AB ((cohort n1 (study or studies))) | (18,393) |
S96 | (MH ‘Nonconcurrent Prospective Studies’) | (115) |
S95 | (MH ‘Correlational Studies’) | (13,984) |
S94 | (MH ‘Case Control Studies+’) | (27,507) |
S93 | (MH ‘Prospective Studies’) | (137,519) |
S92 | S80 OR S91 | (1214) |
S91 | S11 AND S40 AND S90 | (807) |
S90 | S81 OR S82 OR S83 OR S84 OR S85 OR S86 OR S87 OR S88 OR S89 | (41,254) |
S89 | TI (vascular and (risk# or mortality or death#)) | (599) |
S88 | TI (stroke and (risk# or mortality or death#)) | (2383) |
S87 | TI ((coronary or heart or cardiac or myocardial or chd) and (risk# or mortality or death#)) | (8755) |
S86 | TI ((cardiovascular or cardio-vascular or cvd) and (risk# or mortality or death#)) | (5558) |
S85 | (MH ‘Stroke/EP/ET/MO/RF/PR’) | (6930) |
S84 | (MH ‘Vascular Diseases/EP/ET/MO/PR/RF’) | (482) |
S83 | (MH ‘Myocardial Ischemia/EP/ET/MO/PR/RF’) OR (MH ‘Coronary Disease+/EP/ET/MO/PR/RF’) OR (MH ‘Myocardial Infarction+/EP/ET/MO/PR/RF’) | (13,088) |
S82 | (MH ‘Cardiovascular Diseases/EP/ET/RF/MO/PR’) | (7030) |
S81 | (MH ‘Cardiovascular Risk Factors’) | (10,752) |
S80 | S11 AND S40 AND S62 AND S79 | (899) |
S79 | S63 OR S64 OR S65 OR S66 OR S67 OR S68 OR S69 OR S70 OR S71 OR S72 OR S73 OR S74 OR S75 OR S76 OR S77 OR S78 | (358,882) |
S78 | TI life expectancy OR AB life expectancy | (2536) |
S77 | TI ((mortality or death# or survival)) OR AB ((mortality or death# or survival)) | (113,181) |
S76 | TI (prognosis or prognostic) OR AB (prognosis or prognostic) | (20,299) |
S75 | TI prevalence OR AB prevalence | (46,120) |
S74 | TI incidence OR AB incidence | (43,281) |
S73 | TI ((predict or predictor# or prediction#)) OR AB ((predict or predictor# or prediction#)) | (57,994) |
S72 | TI (risk n1 scor*) OR AB (risk n1 scor*) | (1662) |
S71 | TI (risk* n1 marker#) OR AB (risk* n1 marker#) | (646) |
S70 | TI (risk* n1 assessment) OR AB (risk* n1 assessment) | (5322) |
S69 | TI (risk* n1 factor#) OR AB (risk* n1 factor#) | (47,311) |
S68 | TI risk* | (71,245) |
S67 | (MH ‘Survival’) OR (MH ‘Survival Analysis’) | (25,261) |
S66 | (MH ‘Cause of Death’) | (4529) |
S65 | (MH ‘Prognosis’) | (17,288) |
S64 | (MH ‘Incidence’) OR (MH ‘Prevalence’) | (41,706) |
S63 | (MH ‘Risk Factors’) | (54,554) |
S62 | S41 OR S42 OR S43 OR S44 OR S45 OR S46 OR S47 OR S48 OR S49 OR S50 OR S51 OR S52 OR S53 OR S54 OR S55 OR S56 OR S57 OR S58 OR S59 OR S60 OR S61 | (157,684) |
S61 | TI (((all cause or total) n3 (mortality or death#))) OR AB (((all cause or total) n3 (mortality or death#))) | (4490) |
S60 | TI mortality or death# or life expectancy | (30,739) |
S59 | TI Stroke OR AB stroke | (29,634) |
S58 | TI atherosclerosis OR AB atherosclerosis | (4890) |
S57 | TI ((vascular n3 (event# or outcome#))) OR AB ((vascular n3 (event# or outcome#))) | (752) |
S56 | TI (vascular n3 disease#) OR AB (vascular n3 disease#) | (3335) |
S55 | TI myocardial infarct* OR AB myocardial infarct* | (14,093) |
S54 | TI (((ischaemic or ischemic or ischaemia or ischemia) n3 (event# or outcome#))) OR AB (((ischaemic or ischemic or ischaemia or ischemia) n3 (event# or outcome#))) | (1217) |
S53 | TI (((ischaemic or ischemic or ischaemia or ischemia) n3 disease#)) OR AB (((ischaemic or ischemic or ischaemia or ischemia) n3 disease#)) | (2416) |
S52 | TI ischaemic or ischemic or ischaemia or ischemia | (8018) |
S51 | TI (((coronary or heart) n3 (event# or outcome#))) OR AB (((coronary or heart) n3 (event# or outcome#))) | (2921) |
S50 | TI (((coronary or heart) n3 disease#)) OR AB (((coronary or heart) n3 disease#)) | (22,302) |
S49 | TI coronary or heart or chd | (45,444) |
S48 | TI (((cardiovascular or cardio-vascular) n3 (event# or outcome#))) OR AB (((cardiovascular or cardio-vascular) n3 (event# or outcome#))) | (4266) |
S47 | TI (((cardiovascular or cardio-vascular) n3 disease#)) OR AB (((cardiovascular or cardio-vascular) n3 disease#)) | (14,076) |
S46 | TI cardiovascular or cardio-vascular or cvd | (14,758) |
S45 | (MM ‘Mortality’) | (4750) |
S44 | (MM ‘Stroke’) | (20,130) |
S43 | (MM ‘Peripheral Vascular Diseases’) | (1511) |
S42 | (MM ‘Myocardial Ischemia’) OR (MM ‘Coronary Disease+’) OR (MM ‘Myocardial Infarction+’) | (32,434) |
S41 | (MM ‘Cardiovascular Diseases’) | (11,744) |
S40 | S12 OR S13 OR S14 OR S15 OR S16 OR S17 OR S18 OR S19 OR S20 OR S21 OR S22 OR S23 OR S24 OR S25 OR S26 OR S27 OR S28 OR S29 OR S30 OR S31 OR S32 OR S33 OR S34 OR S35 OR S36 OR S37 OR S38 OR S39 | (22,753) |
S39 | TI triglyceride* OR AB triglyceride* | (4211) |
S38 | TI (apoa or Apo B or apo-a or apo-b) OR AB (apoa or Apo B or apo-a or apo-b) | (404) |
S37 | TI apolipoprotein* OR AB apolipoprotein* | (1581) |
S36 | TI ldlc OR AB ldlc | (30) |
S35 | TI ldl-c OR AB ldl-c | (820) |
S34 | TI hdlc OR AB hdlc | (43) |
S33 | TI hdl-c OR AB hdl-c | (741) |
S32 | TI ((lipoprotein# n2 (factor# or marker))) OR AB ((lipoprotein# n2 (factor# or marker))) | (44) |
S31 | TI lipoprotein# | (1778) |
S30 | TI ((ldl or low density lipoprotein#) n1 cholesterol) OR AB ((ldl or low density lipoprotein#) n1 cholesterol) | (3638) |
S29 | TI (((hdl or high density lipoprotein#) n1 cholesterol)) OR AB (((hdl or high density lipoprotein#) n1 cholesterol)) | (3766) |
S28 | TI (((serum or blood or total) n1 cholesterol)) OR AB (((serum or blood or total) n1 cholesterol)) | (4240) |
S27 | TI ((cholesterol n2 (marker# or parameter# or profile# or measure* or level# or management or ratio#))) OR AB ((cholesterol n2 (marker# or parameter# or profile# or measure* or level# or management or ratio#))) | (3417) |
S26 | TI cholesterol | (3745) |
S25 | TI (((serum or blood or total) n1 lipid#)) OR AB (((serum or blood or total) n1 lipid#)) | (1997) |
S24 | TI ((lipid# n2 (marker# or parameter# or profile# or measure* or level# or management or ratio#))) OR AB ((lipid# n2 (marker# or parameter# or profile# or measure* or level# or management or ratio#))) | (3546) |
S23 | TI lipid# | (3676) |
S22 | (MM ‘Triglycerides’) | (876) |
S21 | (MM ‘Apolipoproteins’) | (897) |
S20 | (MM ‘Lipoproteins, HDL Cholesterol’) OR (MM ‘Lipoproteins, LDL Cholesterol’) | (1468) |
S19 | (MM ‘Cholesterol’) | (2055) |
S18 | (MM ‘Lipids’) | (1724) |
S17 | (MH ‘Triglycerides/AN/BL’) | (3146) |
S16 | (MH ‘Apolipoproteins/AN/BL’) | (676) |
S15 | (MH ‘Lipoproteins, LDL+/BL/AN’) | (3618) |
S14 | (MH ‘Lipoproteins, HDL+/BL/AN’) | (2935) |
S13 | (MH ‘Cholesterol/AN/BL’) | (4853) |
S12 | (MH ‘Lipids/AN/BL’) | (2578) |
S11 | S1 OR S2 OR S3 OR S4 OR S5 OR S6 OR S7 OR S8 OR S9 OR S10 | (8108) |
S10 | TI (simvastatin or Zocor or Lipex) OR AB (simvastatin or Zocor or Lipex) | (733) |
S9 | TI (rosuvastatin or Crestor) OR AB (rosuvastatin or Crestor) | (359) |
S8 | TI (pravastatin or Pravachol or Selektine or Lipostat) OR AB (pravastatin or Pravachol or Selektine or Lipostat) | (328) |
S7 | TI (pitavastatin or Livalo or Pitava) OR AB (pitavastatin or Livalo or Pitava) | (32) |
S6 | TI (lovastatin or Mevacor or Altocor or Altoprev) OR AB (lovastatin or Mevacor or Altocor or Altoprev) | (159) |
S5 | TI (fluvastatin or Lescol) OR AB (fluvastatin or Lescol) | (128) |
S4 | TI (atorvastatin or Lipitor or Torvast) OR AB (atorvastatin or Lipitor or Torvast) | (759) |
S3 | TI ((HMG-CoA reductase inhibitor# or 3-hydroxy-3-methyl-glutaryl-CoA reductase inhibitor# or 3-hydroxy-3-methylglutaryl coenzyme A reductase inhibitor# or HMGCR inhibitor# or Hydroxymethylglutaryl-CoA Reductase Inhibitor#)) OR AB ((HMG-CoA reductase inhibitor# or 3-hydroxy-3-methyl-glutaryl-CoA reductase inhibitor# or 3-hydroxy-3-methylglutaryl coenzyme A reductase inhibitor# or HMGCR inhibitor# or Hydroxymethylglutaryl-CoA Reductase Inhibitor#)) | (392) |
S2 | TI statin# OR AB statin# | (4416) |
S1 | (MH ‘Statins+’) | (5689) |
EMBASE: (OvidSP) [1974 – present]
1 | statin?.ti,ab. | 33,754 |
2 | (HMG-CoA reductase inhibitor? or 3-hydroxy-3-methyl-glutaryl-CoA reductase inhibitor? or 3-hydroxy-3-methylglutaryl coenzyme A reductase inhibitor? or HMGCR inhibitor? or Hydroxymethylglutaryl-CoA Reductase Inhibitor?).ti,ab. | 5712 |
3 | (atorvastatin or Lipitor or Torvast).ti,ab. | 7356 |
4 | (fluvastatin or Lescol).ti,ab. | 1960 |
5 | (lovastatin or Mevacor or Altocor or Altoprev).ti,ab. | 3777 |
6 | (pitavastatin or Livalo or Pitava).ti,ab. | 745 |
7 | (pravastatin or Pravachol or Selektine or Lipostat).ti,ab. | 4342 |
8 | (rosuvastatin or Crestor).ti,ab. | 2566 |
9 | (simvastatin or Zocor or Lipex).ti,ab. | 8543 |
10 | exp hydroxymethylglutaryl coenzyme A reductase inhibitor/ | 82,256 |
11 | 1 or 2 or 3 or 4 or 5 or 6 or 7 or 8 or 9 or 10 | 91,408 |
12 | *lipid/ | 50,861 |
13 | *cholesterol/ | 52,784 |
14 | *lipid blood level/ | 7515 |
15 | *cholesterol blood level/ | 8372 |
16 | *lipoprotein blood level/ | 2957 |
17 | *high density lipoprotein/ | 11,159 |
18 | *Lipids/ | 50,861 |
19 | *Cholesterol/ | 52,784 |
20 | *low density lipoprotein/ | 16,012 |
21 | low density lipoprotein cholesterol/ | 49,163 |
22 | *apolipoprotein/or *apolipoprotein a/or *apolipoprotein a1/or *apolipoprotein b/ | 13,420 |
23 | *triacylglycerol/or *triacylglycerol blood level/ | 22,138 |
24 | lipid?.ti. | 118,213 |
25 | (lipid? adj2 (marker? or parameter? or profile? or measure* or level? or management or ratio?)).ti,ab. | 52,686 |
26 | ((serum or blood or total) adj lipid?).ti,ab. | 32,371 |
27 | cholesterol.ti. | 52,808 |
28 | (cholesterol adj2 (marker? or parameter? or profile? or measure* or level? or management or ratio?)).ti,ab. | 43,275 |
29 | ((serum or blood or total) adj cholesterol).ti,ab. | 60,269 |
30 | ((hdl or high density lipoprotein?) adj cholesterol).ti,ab. | 44,423 |
31 | ((ldl or low density lipoprotein?) adj cholesterol).ti,ab. | 37,563 |
32 | lipoprotein?.ti. | 47,631 |
33 | (lipoprotein? adj2 (factor? or marker)).ti,ab. | 492 |
34 | hdl-c.ti,ab. | 13,054 |
35 | hdlc.ti,ab. | 1136 |
36 | ldl-c.ti,ab. | 12,035 |
37 | ldlc.ti,ab. | 845 |
38 | apolipoprotein*.ti,ab. | 41,079 |
39 | (apoa or Apo B or apo-a or apo-b).ti,ab. | 18,448 |
40 | triglyceride*.ti,ab. | 93,821 |
41 | 12 or 13 or 14 or 15 or 16 or 17 or 18 or 19 or 20 or 21 or 22 or 23 or 24 or 25 or 26 or 27 or 28 or 29 or 30 or 31 or 32 or 33 or 34 or 35 or 36 or 37 or 38 or 39 or 40 | 397,436 |
42 | *cardiovascular disease/ | 67,011 |
43 | *coronary artery disease/ | 80,847 |
44 | *ischaemic heart disease/ | 46,497 |
45 | *heart muscle ischaemia/ | 38,628 |
46 | *heart infarction/ | 103,076 |
47 | exp *angina pectoris/ | 36,292 |
48 | *sudden death/ | 15,059 |
49 | *vascular disease/ | 18,884 |
50 | *Stroke/ | 60,507 |
51 | *atherosclerosis/or *arteriosclerosis/ | 70,685 |
52 | *Mortality/ | 45,556 |
53 | (cardiovascular or cardio-vascular or cvd).ti. | 103,060 |
54 | ((cardiovascular or cardio-vascular) adj3 disease?).ti,ab. | 120,912 |
55 | ((cardiovascular or cardio-vascular) adj3 (event? or outcome?)).ti,ab. | 32,483 |
56 | (coronary or heart or chd).ti. | 432,889 |
57 | ((coronary or heart) adj3 disease?).ti,ab. | 238,264 |
58 | ((coronary or heart) adj3 (event? or outcome?)).ti,ab. | 18,145 |
59 | (ischaemic or ischaemic or ischaemia or ischaemia).ti. | 135,324 |
60 | ((ischaemic or ischaemic or ischaemia or ischaemia) adj3 disease?).ti,ab. | 43,343 |
61 | ((ischaemic or ischaemic or ischaemia or ischaemia) adj3 (event? or outcome?)).ti,ab. | 11,894 |
62 | myocardial infarct*.ti,ab. | 178,477 |
63 | (vascular adj3 disease?).ti,ab. | 46,062 |
64 | (vascular adj3 (event? or outcome?)).ti,ab. | 6668 |
65 | atherosclerosis.ti,ab. | 97,460 |
66 | stroke.ti,ab. | 176,865 |
67 | (mortality or death? or life expectancy).ti. | 190,936 |
68 | ((all cause or total) adj3 (mortality or death?)).ti,ab. | 30,910 |
69 | or/42–68 | 1,423,865 |
70 | *risk factor/ | 21,991 |
71 | *risk/ | 22,848 |
72 | *risk assessment/ | 23,481 |
73 | *Incidence/ | 2966 |
74 | *Prevalence/ | 13,500 |
75 | *Prognosis/ | 18,310 |
76 | *’Cause of Death’/ | 7616 |
77 | *survival/or *survival rate/or *life expectancy/ | 23,542 |
78 | proportional hazards model/ | 30,921 |
79 | *Mortality/ | 45,556 |
80 | risk*.ti. | 333,931 |
81 | (risk* adj factor?).ti,ab. | 400,378 |
82 | (risk* adj assessment).ti,ab. | 35,216 |
83 | (risk* adj marker?).ti,ab. | 4207 |
84 | (risk adj scor*).ti,ab. | 9918 |
85 | (predict or predictor? or prediction?).ti,ab. | 568,686 |
86 | incidence.ti,ab. | 599,364 |
87 | prevalence.ti,ab. | 424,983 |
88 | (prognosis or prognostic).ti,ab. | 435,850 |
89 | (mortality or death? or survival).ti,ab. | 1,528,922 |
90 | life expectancy.ti,ab. | 21,753 |
91 | 70 or 71 or 72 or 73 or 74 or 75 or 76 or 77 or 78 or 79 or 80 or 81 or 82 or 83 or 84 or 85 or 86 or 87 or 88 or 89 or 90 | 3,346,042 |
92 | 41 and 69 and 91 | 54,828 |
93 | cardiovascular disease/ep, et [Epidemiology, Etiology] | 33,679 |
94 | *coronary artery disease/ep, et [Epidemiology, Etiology] | 13,871 |
95 | *ischemic heart disease/ep, et [Epidemiology, Etiology] | 7547 |
96 | *heart muscle ischemia/ep, et [Epidemiology, Etiology] | 4539 |
97 | *heart infarction/ep, et [Epidemiology, Etiology] | 9955 |
98 | exp *angina pectoris/ep, et [Epidemiology, Etiology] | 3741 |
99 | *stroke/ep, et [Epidemiology, Etiology] | 9113 |
100 | *sudden death/ep, et [Epidemiology, Etiology] | 2512 |
101 | *atherosclerosis/ep, et [Epidemiology, Etiology] | 12,183 |
102 | *arteriosclerosis/ep, et [Epidemiology, Etiology] | 4638 |
103 | ((cardiovascular or cardio-vascular or cvd) and (risk? or mortality or death?)).ti. | 27,267 |
104 | ((coronary or heart or cardiac or myocardial or chd) and (risk? or mortality or death?)).ti. | 46,583 |
105 | (stroke and (risk? or mortality or death?)).ti. | 7837 |
106 | (vascular and (risk? or mortality or death?)).ti. | 3923 |
107 | 93 or 94 or 95 or 96 or 97 or 98 or 99 or 100 or 102 or 103 or 104 or 105 or 106 | 148,565 |
108 | 41 and 107 | 260,76 |
109 | 92 or 108 | 59,534 |
110 | 11 and 109 | 9938 |
111 | Clinical study/ | 88,187 |
112 | Case control study/ | 72,450 |
113 | Family study/ | 9792 |
114 | Longitudinal study/ | 56,995 |
115 | Retrospective study/ | 300,771 |
116 | Prospective study/ | 220,347 |
117 | Randomized controlled trials/ | 23,300 |
118 | 116 not 117 | 219,709 |
119 | Cohort analysis/ | 135,849 |
120 | (Cohort adj (study or studies)).mp. | 91,599 |
121 | (Case control adj (study or studies)).tw. | 65,387 |
122 | (follow up adj (study or studies)).tw. | 43,276 |
123 | (observational adj (study or studies)).tw. | 49,482 |
124 | (epidemiologic$adj (study or studies)).tw. | 69,401 |
125 | 111 or 112 or 113 or 114 or 115 or 118 or 119 or 120 or 121 or 122 or 123 or 124 | 988,392 |
126 | 110 and 125 | 1438 |
MEDLINE: (OvidSP) [1946 – present, In process]
1 | exp Hydroxymethylglutaryl-CoA Reductase Inhibitors/ | 24,278 |
2 | statin?.ti,ab. | 23,004 |
3 | (HMG-CoA reductase inhibitor? or 3-hydroxy-3-methyl-glutaryl-CoA reductase inhibitor? or 3-hydroxy-3-methylglutaryl coenzyme A reductase inhibitor? or HMGCR inhibitor? or Hydroxymethylglutaryl-CoA Reductase Inhibitor?).ti,ab. | 4436 |
4 | (atorvastatin or Lipitor or Torvast).ti,ab. | 4830 |
5 | (fluvastatin or Lescol).ti,ab. | 1429 |
6 | (lovastatin or Mevacor or Altocor or Altoprev).ti,ab. | 3030 |
7 | (pitavastatin or Livalo or Pitava).ti,ab. | 437 |
8 | (pravastatin or Pravachol or Selektine or Lipostat).ti,ab. | 3222 |
9 | (rosuvastatin or Crestor).ti,ab. | 1561 |
10 | (simvastatin or Zocor or Lipex).ti,ab. | 5937 |
11 | 1 or 2 or 3 or 4 or 5 or 6 or 7 or 8 or 9 or 10 | 37,929 |
12 | Lipids/an, bl [Analysis, Blood] | 57,106 |
13 | Cholesterol/an, bl [Analysis, Blood] | 63,056 |
14 | exp Lipoproteins, LDL/an, bl [Analysis, Blood] | 26,465 |
15 | exp Lipoproteins, HDL/an, bl [Analysis, Blood] | 26,534 |
16 | exp Apolipoproteins/an, bl [Analysis, Blood] | 12,093 |
17 | Triglycerides/an, bl [Analysis, Blood] | 42,674 |
18 | *Lipids/ | 40,555 |
19 | *Cholesterol/ | 39,731 |
20 | exp *Lipoproteins, LDL/ | 16,587 |
21 | exp *Lipoproteins, HDL/ | 12,054 |
22 | exp *Apolipoproteins/ | 21,958 |
23 | *Triglycerides/ | 13,599 |
24 | lipid?.ti. | 98,748 |
25 | (lipid? adj2 (marker? or parameter? or profile? or measure* or level? or management or ratio?)).ti,ab. | 39,597 |
26 | ((serum or blood or total) adj lipid?).ti,ab. | 25,730 |
27 | cholesterol.ti. | 44,602 |
28 | (cholesterol adj2 (marker? or parameter? or profile? or measure* or level? or management or ratio?)).ti,ab. | 34,267 |
29 | ((serum or blood or total) adj cholesterol).ti,ab. | 46,159 |
30 | ((hdl or high density lipoprotein?) adj cholesterol).ti,ab. | 35,055 |
31 | ((ldl or low density lipoprotein?) adj cholesterol).ti,ab. | 29,173 |
32 | lipoprotein?.ti. | 41,003 |
33 | (lipoprotein? adj2 (factor? or marker)).ti,ab. | 423 |
34 | hdl-c.ti,ab. | 8820 |
35 | hdlc.ti,ab. | 759 |
36 | ldl-c.ti,ab. | 7857 |
37 | ldlc.ti,ab. | 499 |
38 | apolipoprotein*.ti,ab. | 34,858 |
39 | (apoa or Apo B or apo-a or apo-b).ti,ab. | 15,088 |
40 | triglyceride*.ti,ab. | 72,013 |
41 | 12 or 13 or 14 or 15 or 16 or 17 or 18 or 19 or 20 or 21 or 22 or 23 or 24 or 25 or 26 or 27 or 28 or 29 or 30 or 31 or 32 or 33 or 34 or 35 or 36 or 37 or 38 or 39 or 40 | 345,025 |
42 | *Cardiovascular Diseases/ | 60,067 |
43 | *Coronary Disease/ | 91,567 |
44 | *Myocardial Ischemia/ | 21,803 |
45 | exp *Myocardial Infarction/ | 102,612 |
46 | exp *Angina Pectoris/ | 29,049 |
47 | *Coronary Artery Disease/ | 26,315 |
48 | *Death, Sudden, Cardiac/ | 5967 |
49 | *Vascular Diseases/ | 16,392 |
50 | *Stroke/ | 38,506 |
51 | *Arteriosclerosis/ | 35,801 |
52 | *Mortality/ | 16,781 |
53 | (cardiovascular or cardio-vascular or cvd).ti. | 80,244 |
54 | ((cardiovascular or cardio-vascular) adj3 disease?).ti,ab. | 91,447 |
55 | ((cardiovascular or cardio-vascular) adj3 (event? or outcome?)).ti,ab. | 22,346 |
56 | (coronary or heart or chd).ti. | 346,977 |
57 | ((coronary or heart) adj3 disease?).ti,ab. | 181,015 |
58 | ((coronary or heart) adj3 (event? or outcome?)).ti,ab. | 12,806 |
59 | (ischaemic or ischemic or ischaemia or ischemia).ti. | 105,797 |
60 | ((ischaemic or ischemic or ischaemia or ischemia) adj3 disease?).ti,ab. | 33,058 |
61 | ((ischaemic or ischemic or ischaemia or ischemia) adj3 (event? or outcome?)).ti,ab. | 8691 |
62 | myocardial infarct*.ti,ab. | 135,442 |
63 | (vascular adj3 disease?).ti,ab. | 35,913 |
64 | (vascular adj3 (event? or outcome?)).ti,ab. | 4810 |
65 | atherosclerosis.ti,ab. | 75,237 |
66 | stroke.ti,ab. | 128,143 |
67 | (mortality or death? or life expectancy).ti. | 163,977 |
68 | ((all cause or total) adj3 (mortality or death?)).ti,ab. | 22,550 |
69 | or/42–68 | 1,114,257 |
70 | risk factors/ | 509,984 |
71 | risk/ | 91,663 |
72 | risk assessment/ | 157,422 |
73 | Incidence/ | 159,745 |
74 | Prevalence/ | 168,888 |
75 | Prognosis/ | 332,051 |
76 | ‘Cause of Death’/ | 32,936 |
77 | Survival Analysis/or Survival Rate/or Life Expectancy/ | 214,774 |
78 | proportional hazards models/ | 37,408 |
79 | Mortality/ | 32,876 |
80 | risk*.ti. | 260,605 |
81 | (risk* adj factor?).ti,ab. | 306,925 |
82 | (risk* adj assessment).ti,ab. | 27,441 |
83 | (risk* adj marker?).ti,ab. | 3098 |
84 | (risk adj scor*).ti,ab. | 6483 |
85 | (predict or predictor? or prediction?).ti,ab. | 458,060 |
86 | incidence.ti,ab. | 469,242 |
87 | prevalence.ti,ab. | 336,308 |
88 | (prognosis or prognostic).ti,ab. | 322,645 |
89 | (mortality or death? or survival).ti,ab. | 1,216,008 |
90 | life expectancy.ti,ab. | 17,244 |
91 | 70 or 71 or 72 or 73 or 74 or 75 or 76 or 77 or 78 or 79 or 80 or 81 or 82 or 83 or 84 or 85 or 86 or 87 or 88 or 89 or 90 | 3,114,367 |
92 | 41 and 69 and 91 | 46,530 |
93 | Cardiovascular Diseases/ep, et, mo [Epidemiology, Etiology, Mortality] | 44,498 |
94 | Coronary Disease/ep, et, mo [Epidemiology, Etiology, Mortality] | 32,798 |
95 | Myocardial Ischemia/ep, et, mo [Epidemiology, Etiology, Mortality] | 7049 |
96 | exp Myocardial Infarction/ep, et, mo [Epidemiology, Etiology, Mortality] | 38,747 |
97 | Angina Pectoris/ep, et, mo [Epidemiology, Etiology, Mortality] | 6036 |
98 | Coronary Artery Disease/ep, et, mo [Epidemiology, Etiology, Mortality] | 9256 |
99 | Death, Sudden, Cardiac/ep, et [Epidemiology, Etiology] | 6470 |
100 | Vascular Diseases/ep, et, mo [Epidemiology, Etiology, Mortality] | 6261 |
101 | Arteriosclerosis/ep, et, mo [Epidemiology, Etiology, Mortality] | 14,000 |
102 | Stroke/ep, et, mo [Epidemiology, Etiology, Mortality] | 19,782 |
103 | ((cardiovascular or cardio-vascular or cvd) and (risk? or mortality or death?)).ti. | 20,145 |
104 | ((coronary or heart or cardiac or myocardial or chd) and (risk? or mortality or death?)).ti. | 35,276 |
105 | (stroke and (risk? or mortality or death?)).ti. | 5592 |
106 | (vascular and (risk? or mortality or death?)).ti. | 2961 |
107 | 93 or 94 or 95 or 96 or 97 or 98 or 99 or 100 or 102 or 103 or 104 or 105 or 106 | 182,179 |
108 | 41 and 107 | 26,904 |
109 | 92 or 108 | 50,683 |
110 | 11 and 109 | 5835 |
111 | Epidemiologic studies/ | 5579 |
112 | exp case control studies/ | 586,246 |
113 | exp cohort studies/ | 1,234,178 |
114 | Case control.tw. | 67,718 |
115 | (cohort adj (study or studies)).tw. | 70,719 |
116 | Cohort analy$.tw. | 3087 |
117 | (Follow up adj (study or studies)).tw. | 35,103 |
118 | (observational adj (study or studies)).tw. | 36,618 |
119 | Longitudinal.tw. | 124,262 |
120 | Retrospective.tw. | 238,637 |
121 | 111 or 112 or 113 or 114 or 115 or 116 or 117 or 118 or 119 or 120 | 1,542,206 |
122 | 110 and 121 | 1431 |
Search report: randomised control trials
The search was conducted on 9 April 2013. The subject search terms were developed through an iterative process testing against known studies. Methodological filters for RCTs were applied; studies were limited to papers published in English from 2012 onwards.
Search numbers
Database | Interface | 2011 onwards |
---|---|---|
Cochrane Central Register of Controlled Trials | The Cochrane Library, Wiley [Issue 3, 2013] | 36 |
CINAHL | EBSCOhost [1980 – present] | 64 |
EMBASE | OvidSP [1974 – present] | 354 |
MEDLINE | OvidSP [1946 – present, In process] | 429 |
Total | 883 | |
Duplicates removed | 216 | |
Final total | 667 |
Search strategies
CENTRAL
-
#1 MeSH descriptor: [Hydroxymethylglutaryl-CoA Reductase Inhibitors] explode all trees
-
#2 statin*:ti,ab,kw
-
#3 HMG-CoA reductase inhibitor* or 3-hydroxy-3-methyl-glutaryl-CoA reductase inhibitor* or 3-hydroxy-3-methylglutaryl coenzyme A reductase inhibitor* or HMGCR inhibitor* or Hydroxymethylglutaryl-CoA Reductase Inhibitor*:ti,ab,kw (Word variations have been searched)
-
#4 atorvastatin or Lipitor or Torvast:ti,ab,kw (Word variations have been searched)
-
#5 fluvastatin or Lescol:ti,ab,kw (Word variations have been searched)
-
#6 lovastatin or Mevacor or Altocor or Altoprev:ti,ab,kw (Word variations have been searched)
-
#7 pitavastatin or Livalo or Pitava:ti,ab,kw (Word variations have been searched)
-
#8 pravastatin or Pravachol or Selektine or Lipostat:ti,ab,kw (Word variations have been searched)
-
#9 rosuvastatin or Crestor:ti,ab,kw (Word variations have been searched)
-
#10 simvastatin or Zocor or Lipex:ti,ab,kw (Word variations have been searched)
-
#11 #1 or #2 or #3 or #4 or #5 or #6 or #7 or #8 or #9 or #10
-
#12 MeSH descriptor: [Lipids] explode all trees and with qualifiers: [Analysis – AN, Blood – BL]
-
#13 MeSH descriptor: [Cholesterol] explode all trees and with qualifiers: [Analysis – AN, Blood – BL]
-
#14 MeSH descriptor: [Lipoproteins, LDL] explode all trees
-
#15 MeSH descriptor: [Lipoproteins, HDL] explode all trees
-
#16 MeSH descriptor: [Apolipoproteins] explode all trees
-
#17 MeSH descriptor: [Triglycerides] explode all trees
-
#18 lipid*:ti (Word variations have been searched)
-
#19 (lipid* near (marker* or parameter* or profile* or measure* or level* or management or ratio*)):ti,ab,kw (Word variations have been searched)
-
#20 ((serum or blood or total) next lipid*):ti,ab,kw (Word variations have been searched)
-
#21 cholesterol:ti (Word variations have been searched)
-
#22 (cholesterol near (marker* or parameter* or profile* or measure* or level* or management or ratio*)):ti,ab,kw (Word variations have been searched)
-
#23 ((serum or blood or total) next cholesterol):ti,ab,kw (Word variations have been searched)
-
#24 ((hdl or high density lipoprotein*) next cholesterol):ti,ab,kw (Word variations have been searched)
-
#25 ((ldl or low density lipoprotein*) next cholesterol):ti,ab,kw (Word variations have been searched)
-
#26 lipoprotein*:ti (Word variations have been searched)
-
#27 (lipoprotein* near (factor* or marker*)):ti,ab,kw (Word variations have been searched)
-
#28 hdl-c or hdlc or ldl-c or ldlc:ti,ab,kw (Word variations have been searched)
-
#29 apolipoprotein* or apoa or Apo B or apo-a or apo-b:ti,ab,kw (Word variations have been searched)
-
#30 triglyceride*:ti,ab,kw (Word variations have been searched)
-
#31 #12 or #13 or #14 or #15 or #16 or #17 or #18 or #19 or #20 or #21 or #22 or #23 or #24 or #25 or #26 or #27 or #28 or #29 or #30
-
#32 #11 and #31
CINAHL: (EBSCOhost) [1980 – present]
S96 | (S92 AND S95) Published date from: 20120101–20130431; English language | (36) |
S95 | S93 OR S94 | (229,883) |
S94 | (MH ‘Treatment Outcomes+’) | (108,846) |
S93 | TI (random* OR experimental studies) OR AB (random* OR experimental studies) OR MW (random* OR experimental studies) | (145,214) |
S92 | S80 OR S91 | (1240) |
S91 | S11 AND S40 AND S90 | (829) |
S90 | S81 OR S82 OR S83 OR S84 OR S85 OR S86 OR S87 OR S88 OR S89 | (42,613) |
S89 | TI (vascular and (risk# or mortality or death#)) | (609) |
S88 | TI (stroke and (risk# or mortality or death#)) | (2457) |
S87 | TI ((coronary or heart or cardiac or myocardial or chd) and (risk# or mortality or death#)) | (9000) |
S86 | TI ((cardiovascular or cardio-vascular or cvd) and (risk# or mortality or death#)) | (5756) |
S85 | (MH ‘Stroke/EP/ET/MO/RF/PR’) | (7292) |
S84 | (MH ‘Vascular Diseases/EP/ET/MO/PR/RF’) | (493) |
S83 | (MH ‘Myocardial Ischemia/EP/ET/MO/PR/RF’) OR (MH ‘Coronary Disease+/EP/ET/MO/PR/RF’) OR (MH ‘Myocardial Infarction+/EP/ET/MO/PR/RF’) | (13,465) |
S82 | (MH ‘Cardiovascular Diseases/EP/ET/RF/MO/PR’) | (7276) |
S81 | (MH ‘Cardiovascular Risk Factors’) | (10,997) |
S80 | S11 AND S40 AND S62 AND S79 | (917) |
S79 | S63 OR S64 OR S65 OR S66 OR S67 OR S68 OR S69 OR S70 OR S71 OR S72 OR S73 OR S74 OR S75 OR S76 OR S77 OR S78 | (369,748) |
S78 | TI life expectancy OR AB life expectancy | (2599) |
S77 | TI ((mortality or death# or survival)) OR AB ((mortality or death# or survival)) | (116,627) |
S76 | TI (prognosis or prognostic) OR AB (prognosis or prognostic) | (21,070) |
S75 | TI prevalence OR AB prevalence | (47,512) |
S74 | TI incidence OR AB incidence | (44,421) |
S73 | TI ((predict or predictor# or prediction#)) OR AB ((predict or predictor# or prediction#)) | (59,834) |
S72 | TI (risk n1 scor*) OR AB (risk n1 scor*) | (1758) |
S71 | TI (risk* n1 marker#) OR AB (risk* n1 marker#) | (672) |
S70 | TI (risk* n1 assessment) OR AB (risk* n1 assessment) | (5462) |
S69 | TI (risk* n1 factor#) OR AB (risk* n1 factor#) | (48,604) |
S68 | TI risk* | (73,568) |
S67 | (MH ‘Survival’) OR (MH ‘Survival Analysis’) | (26,178) |
S66 | (MH ‘Cause of Death’) | (4734) |
S65 | (MH ‘Prognosis’) | (18,064) |
S64 | (MH ‘Incidence’) OR (MH ‘Prevalence’) | (43,388) |
S63 | (MH ‘Risk Factors’) | (56,113) |
S62 | S41 OR S42 OR S43 OR S44 OR S45 OR S46 OR S47 OR S48 OR S49 OR S50 OR S51 OR S52 OR S53 OR S54 OR S55 OR S56 OR S57 OR S58 OR S59 OR S60 OR S61 | (161,926) |
S61 | TI (((all cause or total) n3 (mortality or death#))) OR AB (((all cause or total) n3 (mortality or death#))) | (4688) |
S60 | TI mortality or death# or life expectancy | (31,676) |
S59 | TI Stroke OR AB stroke | (30,475) |
S58 | TI atherosclerosis OR AB atherosclerosis | (5018) |
S57 | TI ((vascular n3 (event# or outcome#))) OR AB ((vascular n3 (event# or outcome#))) | (777) |
S56 | TI (vascular n3 disease#) OR AB (vascular n3 disease#) | (3420) |
S55 | TI myocardial infarct* OR AB myocardial infarct* | (14,418) |
S54 | TI (((ischaemic or ischemic or ischaemia or ischemia) n3 (event# or outcome#))) OR AB (((ischaemic or ischemic or ischaemia or ischemia) n3 (event# or outcome#))) | (1245) |
S53 | TI (((ischaemic or ischemic or ischaemia or ischemia) n3 disease#)) OR AB (((ischaemic or ischemic or ischaemia or ischemia) n3 disease#)) | (2486) |
S52 | TI ischaemic or ischemic or ischaemia or ischemia | (8282) |
S51 | TI (((coronary or heart) n3 (event# or outcome#))) OR AB (((coronary or heart) n3 (event# or outcome#))) | (2992) |
S50 | TI (((coronary or heart) n3 disease#)) OR AB (((coronary or heart) n3 disease#)) | (22,761) |
S49 | TI coronary or heart or chd | (46,572) |
S48 | TI (((cardiovascular or cardio-vascular) n3 (event# or outcome#))) OR AB (((cardiovascular or cardio-vascular) n3 (event# or outcome#))) | (4403) |
S47 | TI (((cardiovascular or cardio-vascular) n3 disease#)) OR AB (((cardiovascular or cardio-vascular) n3 disease#)) | (14,481) |
S46 | TI cardiovascular or cardio-vascular or cvd | (15,189) |
S45 | (MM ‘Mortality’) | (4894) |
S44 | (MM ‘Stroke’) | (21,024) |
S43 | (MM ‘Peripheral Vascular Diseases’) | (1554) |
S42 | (MM ‘Myocardial Ischemia’) OR (MM ‘Coronary Disease+’) OR (MM ‘Myocardial Infarction+’) | (33,158) |
S41 | (MM ‘Cardiovascular Diseases’) | (12,144) |
S40 | S12 OR S13 OR S14 OR S15 OR S16 OR S17 OR S18 OR S19 OR S20 OR S21 OR S22 OR S23 OR S24 OR S25 OR S26 OR S27 OR S28 OR S29 OR S30 OR S31 OR S32 OR S33 OR S34 OR S35 OR S36 OR S37 OR S38 OR S39 | (23,307) |
S39 | TI triglyceride* OR AB triglyceride* | (4330) |
S38 | TI (apoa or Apo B or apo-a or apo-b) OR AB (apoa or Apo B or apo-a or apo-b) | (417) |
S37 | TI apolipoprotein* OR AB apolipoprotein* | (1615) |
S36 | TI ldlc OR AB ldlc | (30) |
S35 | TI ldl-c OR AB ldl-c | (837) |
S34 | TI hdlc OR AB hdlc | (43) |
S33 | TI hdl-c OR AB hdl-c | (761) |
S32 | TI ((lipoprotein# n2 (factor# or marker))) OR AB ((lipoprotein# n2 (factor# or marker))) | (44) |
S31 | TI lipoprotein# | (1808) |
S30 | TI ((ldl or low density lipoprotein#) n1 cholesterol) OR AB ((ldl or low density lipoprotein#) n1 cholesterol) | (3694) |
S29 | TI (((hdl or high density lipoprotein#) n1 cholesterol)) OR AB (((hdl or high density lipoprotein#) n1 cholesterol)) | (3849) |
S28 | TI (((serum or blood or total) n1 cholesterol)) OR AB (((serum or blood or total) n1 cholesterol)) | (4314) |
S27 | TI ((cholesterol n2 (marker# or parameter# or profile# or measure* or level# or management or ratio#))) OR AB ((cholesterol n2 (marker# or parameter# or profile# or measure* or level# or management or ratio#))) | (3469) |
S26 | TI cholesterol | (3791) |
S25 | TI (((serum or blood or total) n1 lipid#)) OR AB (((serum or blood or total) n1 lipid#)) | (2033) |
S24 | TI ((lipid# n2 (marker# or parameter# or profile# or measure* or level# or management or ratio#))) OR AB ((lipid# n2 (marker# or parameter# or profile# or measure* or level# or management or ratio#))) | (3644) |
S23 | TI lipid# | (3761) |
S22 | (MM ‘Triglycerides’) | (905) |
S21 | (MM ‘Apolipoproteins’) | (916) |
S20 | (MM ‘Lipoproteins, HDL Cholesterol’) OR (MM ‘Lipoproteins, LDL Cholesterol’) | (1516) |
S19 | (MM ‘Cholesterol’) | (2080) |
S18 | (MM ‘Lipids’) | (1787) |
S17 | (MH ‘Triglycerides/AN/BL’) | (3238) |
S16 | (MH ‘Apolipoproteins/AN/BL’) | (689) |
S15 | (MH ‘Lipoproteins, LDL+/BL/AN’) | (3711) |
S14 | (MH ‘Lipoproteins, HDL+/BL/AN’) | (3009) |
S13 | (MH ‘Cholesterol/AN/BL’) | (4945) |
S12 | (MH ‘Lipids/AN/BL’) | (2666) |
S11 | S1 OR S2 OR S3 OR S4 OR S5 OR S6 OR S7 OR S8 OR S9 OR S10 | (8296) |
S10 | TI (simvastatin or Zocor or Lipex) OR AB (simvastatin or Zocor or Lipex) | (740) |
S9 | TI (rosuvastatin or Crestor) OR AB (rosuvastatin or Crestor) | (364) |
S8 | TI (pravastatin or Pravachol or Selektine or Lipostat) OR AB (pravastatin or Pravachol or Selektine or Lipostat) | (329) |
S7 | TI (pitavastatin or Livalo or Pitava) OR AB (pitavastatin or Livalo or Pitava) | (34) |
S6 | TI (lovastatin or Mevacor or Altocor or Altoprev) OR AB (lovastatin or Mevacor or Altocor or Altoprev) | (163) |
S5 | TI (fluvastatin or Lescol) OR AB (fluvastatin or Lescol) | (129) |
S4 | TI (atorvastatin or Lipitor or Torvast) OR AB (atorvastatin or Lipitor or Torvast) | (771) |
S3 | TI ((HMG-CoA reductase inhibitor# or 3-hydroxy-3-methyl-glutaryl-CoA reductase inhibitor# or 3-hydroxy-3-methylglutaryl coenzyme A reductase inhibitor# or HMGCR inhibitor# or Hydroxymethylglutaryl-CoA Reductase Inhibitor#)) OR AB ((HMG-CoA reductase inhibitor# or 3-hydroxy-3-methyl-glutaryl-CoA reductase inhibitor# or 3-hydroxy-3-methylglutaryl coenzyme A reductase inhibitor# or HMGCR inhibitor# or Hydroxymethylglutaryl-CoA Reductase Inhibitor#)) | (396) |
S2 | TI statin# OR AB statin# | (4549) |
S1 | (MH ‘Statins+’) | (5771) |
EMBASE: (OvidSP) [1974 – present]
1 | statin?.ti,ab. | 35,110 |
2 | (HMG-CoA reductase inhibitor? or 3-hydroxy-3-methyl-glutaryl-CoA reductase inhibitor? or 3-hydroxy-3-methylglutaryl coenzyme A reductase inhibitor? or HMGCR inhibitor? or Hydroxymethylglutaryl-CoA Reductase Inhibitor?).ti,ab. | 5779 |
3 | (atorvastatin or Lipitor or Torvast).ti,ab. | 7638 |
4 | (fluvastatin or Lescol).ti,ab. | 1988 |
5 | (lovastatin or Mevacor or Altocor or Altoprev).ti,ab. | 3834 |
6 | (pitavastatin or Livalo or Pitava).ti,ab. | 783 |
7 | (pravastatin or Pravachol or Selektine or Lipostat).ti,ab. | 4424 |
8 | (rosuvastatin or Crestor).ti,ab. | 2722 |
9 | (simvastatin or Zocor or Lipex).ti,ab. | 8816 |
10 | exp hydroxymethylglutaryl coenzyme A reductase inhibitor/ | 84,991 |
11 | 1 or 2 or 3 or 4 or 5 or 6 or 7 or 8 or 9 or 10 | 94,445 |
12 | *lipid/ | 51,572 |
13 | *cholesterol/ | 53,094 |
14 | *lipid blood level/ | 7590 |
15 | *cholesterol blood level/ | 8428 |
16 | *lipoprotein blood level/ | 2975 |
17 | *high density lipoprotein/ | 11,339 |
18 | *Lipids/ | 51,572 |
19 | *Cholesterol/ | 53,094 |
20 | *low density lipoprotein/ | 16,187 |
21 | low density lipoprotein cholesterol/ | 50,952 |
22 | *apolipoprotein/or *apolipoprotein a/or *apolipoprotein a1/or *apolipoprotein b/ | 13,564 |
23 | *triacylglycerol/or *triacylglycerol blood level/ | 22,349 |
24 | lipid?.ti. | 119,981 |
25 | (lipid? adj2 (marker? or parameter? or profile? or measure* or level? or management or ratio?)).ti,ab. | 54,454 |
26 | ((serum or blood or total) adj lipid?).ti,ab. | 32,964 |
27 | cholesterol.ti. | 53,397 |
28 | (cholesterol adj2 (marker? or parameter? or profile? or measure* or level? or management or ratio?)).ti,ab. | 44,200 |
29 | ((serum or blood or total) adj cholesterol).ti,ab. | 61,697 |
30 | ((hdl or high density lipoprotein?) adj cholesterol).ti,ab. | 45,565 |
31 | ((ldl or low density lipoprotein?) adj cholesterol).ti,ab. | 38,567 |
32 | lipoprotein?.ti. | 48,091 |
33 | (lipoprotein? adj2 (factor? or marker)).ti,ab. | 499 |
34 | hdl-c.ti,ab. | 13,597 |
35 | hdlc.ti,ab. | 1171 |
36 | ldl-c.ti,ab. | 12,555 |
37 | ldlc.ti,ab. | 866 |
38 | apolipoprotein*.ti,ab. | 41,864 |
39 | (apoa or Apo B or apo-a or apo-b).ti,ab. | 18,789 |
40 | triglyceride*.ti,ab. | 962,65 |
41 | 12 or 13 or 14 or 15 or 16 or 17 or 18 or 19 or 20 or 21 or 22 or 23 or 24 or 25 or 26 or 27 or 28 or 29 or 30 or 31 or 32 or 33 or 34 or 35 or 36 or 37 or 38 or 39 or 40 | 405,351 |
42 | *cardiovascular disease/ | 68,577 |
43 | *coronary artery disease/ | 82,044 |
44 | *ischemic heart disease/ | 47,091 |
45 | *heart muscle ischemia/ | 38,965 |
46 | *heart infarction/ | 104,156 |
47 | exp *angina pectoris/ | 36,503 |
48 | *sudden death/ | 15,399 |
49 | *vascular disease/ | 19,219 |
50 | *Stroke/ | 60,494 |
51 | *atherosclerosis/or *arteriosclerosis/ | 71,618 |
52 | *Mortality/ | 47,119 |
53 | (cardiovascular or cardio-vascular or cvd).ti. | 105,762 |
54 | ((cardiovascular or cardio-vascular) adj3 disease?).ti,ab. | 125,607 |
55 | ((cardiovascular or cardio-vascular) adj3 (event? or outcome?)).ti,ab. | 34,144 |
56 | (coronary or heart or chd).ti. | 440,855 |
57 | ((coronary or heart) adj3 disease?).ti,ab. | 244,310 |
58 | ((coronary or heart) adj3 (event? or outcome?)).ti,ab. | 18,791 |
59 | (ischaemic or ischemic or ischaemia or ischemia).ti. | 138,122 |
60 | ((ischaemic or ischemic or ischaemia or ischemia) adj3 disease?).ti,ab. | 44,242 |
61 | ((ischaemic or ischemic or ischaemia or ischemia) adj3 (event? or outcome?)).ti,ab. | 12,312 |
62 | myocardial infarct*.ti,ab. | 182,681 |
63 | (vascular adj3 disease?).ti,ab. | 47,150 |
64 | (vascular adj3 (event? or outcome?)).ti,ab. | 6902 |
65 | atherosclerosis.ti,ab. | 100,304 |
66 | stroke.ti,ab. | 182,779 |
67 | (mortality or death? or life expectancy).ti. | 195,213 |
68 | ((all cause or total) adj3 (mortality or death?)).ti,ab. | 32,462 |
69 | or/42–68 | 1,455,239 |
70 | *risk factor/ | 23,278 |
71 | *risk/ | 25,190 |
72 | *risk assessment/ | 24,094 |
73 | *Incidence/ | 3043 |
74 | *Prevalence/ | 14,523 |
75 | *Prognosis/ | 19,239 |
76 | *’Cause of Death’/ | 7746 |
77 | *survival/or *survival rate/or *life expectancy/ | 24,935 |
78 | proportional hazards model/ | 33,386 |
79 | *Mortality/ | 47,119 |
80 | risk*.ti. | 344,746 |
81 | (risk* adj factor?).ti,ab. | 414,844 |
82 | (risk* adj assessment).ti,ab. | 36,425 |
83 | (risk* adj marker?).ti,ab. | 4391 |
84 | (risk adj scor*).ti,ab. | 10,650 |
85 | (predict or predictor? or prediction?).ti,ab. | 590,407 |
86 | incidence.ti,ab. | 615,050 |
87 | prevalence.ti,ab. | 439,526 |
88 | (prognosis or prognostic).ti,ab. | 451,161 |
89 | (mortality or death? or survival).ti,ab. | 1,578,413 |
90 | life expectancy.ti,ab. | 22,482 |
91 | 70 or 71 or 72 or 73 or 74 or 75 or 76 or 77 or 78 or 79 or 80 or 81 or 82 or 83 or 84 or 85 or 86 or 87 or 88 or 89 or 90 | 3,447,985 |
92 | 41 and 69 and 91 | 56,517 |
93 | cardiovascular disease/ep, et [Epidemiology, Etiology] | 34,030 |
94 | *coronary artery disease/ep, et [Epidemiology, Etiology] | 13,941 |
95 | *ischaemic heart disease/ep, et [Epidemiology, Etiology] | 7583 |
96 | *heart muscle ischaemia/ep, et [Epidemiology, Etiology] | 4560 |
97 | *heart infarction/ep, et [Epidemiology, Etiology] | 10,011 |
98 | exp *angina pectoris/ep, et [Epidemiology, Etiology] | 3752 |
99 | *stroke/ep, et [Epidemiology, Etiology] | 9112 |
100 | *sudden death/ep, et [Epidemiology, Etiology] | 2530 |
101 | *atherosclerosis/ep, et [Epidemiology, Etiology] | 12,290 |
102 | *arteriosclerosis/ep, et [Epidemiology, Aetiology] | 4643 |
103 | ((cardiovascular or cardio-vascular or cvd) and (risk? or mortality or death?)).ti. | 28,367 |
104 | ((coronary or heart or cardiac or myocardial or chd) and (risk? or mortality or death?)).ti. | 48,008 |
105 | (stroke and (risk? or mortality or death?)).ti. | 8114 |
106 | (vascular and (risk? or mortality or death?)).ti. | 4052 |
107 | 93 or 94 or 95 or 96 or 97 or 98 or 99 or 100 or 102 or 103 or 104 or 105 or 106 | 151,699 |
108 | 41 and 107 | 26,633 |
109 | 92 or 108 | 61,246 |
110 | 11 and 109 | 10,232 |
111 | random*.tw. or placebo*.mp. or double-blind*.mp. | 1,024,177 |
112 | 110 and 111 | 3324 |
113 | (2012* or 2013*).dp,em,yr. | 1,633,588 |
114 | 112 and 113 | 364 |
115 | limit 114 to english language | 354 |
MEDLINE: (OvidSP) [1946 – present, In process]
1 | exp Hydroxymethylglutaryl-CoA Reductase Inhibitors/ | 24,343 |
2 | statin?.ti,ab. | 23,244 |
3 | (HMG-CoA reductase inhibitor? or 3-hydroxy-3-methyl-glutaryl-CoA reductase inhibitor? or 3-hydroxy-3-methylglutaryl coenzyme A reductase inhibitor? or HMGCR inhibitor? or Hydroxymethylglutaryl-CoA Reductase Inhibitor?).ti,ab. | 4409 |
4 | (atorvastatin or Lipitor or Torvast).ti,ab. | 4890 |
5 | (fluvastatin or Lescol).ti,ab. | 1431 |
6 | (lovastatin or Mevacor or Altocor or Altoprev).ti,ab. | 3033 |
7 | (pitavastatin or Livalo or Pitava).ti,ab. | 442 |
8 | (pravastatin or Pravachol or Selektine or Lipostat).ti,ab. | 3203 |
9 | (rosuvastatin or Crestor).ti,ab. | 1604 |
10 | (simvastatin or Zocor or Lipex).ti,ab. | 6004 |
11 | 1 or 2 or 3 or 4 or 5 or 6 or 7 or 8 or 9 or 10 | 38,247 |
12 | Lipids/an, bl [Analysis, Blood] | 57,228 |
13 | Cholesterol/an, bl [Analysis, Blood] | 63,013 |
14 | exp Lipoproteins, LDL/an, bl [Analysis, Blood] | 26,654 |
15 | exp Lipoproteins, HDL/an, bl [Analysis, Blood] | 26,703 |
16 | exp Apolipoproteins/an, bl [Analysis, Blood] | 12,146 |
17 | Triglycerides/an, bl [Analysis, Blood] | 42,791 |
18 | *Lipids/ | 40,728 |
19 | *Cholesterol/ | 39,607 |
20 | exp *Lipoproteins, LDL/ | 16,603 |
21 | exp *Lipoproteins, HDL/ | 12,117 |
22 | exp *Apolipoproteins/ | 22,002 |
23 | *Triglycerides/ | 13,640 |
24 | lipid?.ti. | 99,201 |
25 | (lipid? adj2 (marker? or parameter? or profile? or measure* or level? or management or ratio?)).ti,ab. | 40,360 |
26 | ((serum or blood or total) adj lipid?).ti,ab. | 25,973 |
27 | cholesterol.ti. | 44,546 |
28 | (cholesterol adj2 (marker? or parameter? or profile? or measure* or level? or management or ratio?)).ti,ab. | 34,466 |
29 | ((serum or blood or total) adj cholesterol).ti,ab. | 46,672 |
30 | ((hdl or high density lipoprotein?) adj cholesterol).ti,ab. | 35,492 |
31 | ((ldl or low density lipoprotein?) adj cholesterol).ti,ab. | 29,598 |
32 | lipoprotein?.ti. | 41,066 |
33 | (lipoprotein? adj2 (factor? or marker)).ti,ab. | 429 |
34 | hdl-c.ti,ab. | 9038 |
35 | hdlc.ti,ab. | 768 |
36 | ldl-c.ti,ab. | 8087 |
37 | ldlc.ti,ab. | 504 |
38 | apolipoprotein*.ti,ab. | 35,086 |
39 | (apoa or Apo B or apo-a or apo-b).ti,ab. | 15,162 |
40 | triglyceride*.ti,ab. | 72,822 |
41 | 12 or 13 or 14 or 15 or 16 or 17 or 18 or 19 or 20 or 21 or 22 or 23 or 24 or 25 or 26 or 27 or 28 or 29 or 30 or 31 or 32 or 33 or 34 or 35 or 36 or 37 or 38 or 39 or 40 | 346,985 |
42 | *Cardiovascular Diseases/ | 60,721 |
43 | *Coronary Disease/ | 91,391 |
44 | *Myocardial Ischemia/ | 21,903 |
45 | exp *Myocardial Infarction/ | 102,883 |
46 | exp *Angina Pectoris/ | 29,260 |
47 | *Coronary Artery Disease/ | 26,769 |
48 | *Death, Sudden, Cardiac/ | 6067 |
49 | *Vascular Diseases/ | 16,191 |
50 | *Stroke/ | 39,462 |
51 | *Arteriosclerosis/ | 35,324 |
52 | *Mortality/ | 16,834 |
53 | (cardiovascular or cardio-vascular or cvd).ti. | 80,998 |
54 | ((cardiovascular or cardio-vascular) adj3 disease?).ti,ab. | 93,108 |
55 | ((cardiovascular or cardio-vascular) adj3 (event? or outcome?)).ti,ab. | 22,838 |
56 | (coronary or heart or chd).ti. | 348,302 |
57 | ((coronary or heart) adj3 disease?).ti,ab. | 182,181 |
58 | ((coronary or heart) adj3 (event? or outcome?)).ti,ab. | 12967 |
59 | (ischaemic or ischemic or ischaemia or ischemia).ti. | 105,964 |
60 | ((ischaemic or ischemic or ischaemia or ischemia) adj3 disease?).ti,ab. | 33,230 |
61 | ((ischaemic or ischemic or ischaemia or ischemia) adj3 (event? or outcome?)).ti,ab. | 8773 |
62 | myocardial infarct*.ti,ab. | 136,018 |
63 | (vascular adj3 disease?).ti,ab. | 35,712 |
64 | (vascular adj3 (event? or outcome?)).ti,ab. | 4792 |
65 | atherosclerosis.ti,ab. | 75,403 |
66 | stroke.ti,ab. | 129,881 |
67 | (mortality or death? or life expectancy).ti. | 164,583 |
68 | ((all cause or total) adj3 (mortality or death?)).ti,ab. | 22,991 |
69 | or/42–68 | 1,119,270 |
70 | risk factors/ | 514,238 |
71 | risk/ | 92,327 |
72 | risk assessment/ | 158,812 |
73 | Incidence/ | 161,604 |
74 | Prevalence/ | 172,295 |
75 | Prognosis/ | 334,751 |
76 | ‘Cause of Death’/ | 33,236 |
77 | Survival Analysis/or Survival Rate/or Life Expectancy/ | 216,536 |
78 | proportional hazards models/ | 38,202 |
79 | Mortality/ | 32,916 |
80 | risk*.ti. | 265,235 |
81 | (risk* adj factor?).ti,ab. | 312,588 |
82 | (risk* adj assessment).ti,ab. | 28,150 |
83 | (risk* adj marker?).ti,ab. | 3199 |
84 | (risk adj scor*).ti,ab. | 6759 |
85 | (predict or predictor? or prediction?).ti,ab. | 468,529 |
86 | incidence.ti,ab. | 473,279 |
87 | prevalence.ti,ab. | 343,423 |
88 | (prognosis or prognostic).ti,ab. | 327,990 |
89 | (mortality or death? or survival).ti,ab. | 1,226,745 |
90 | life expectancy.ti,ab. | 17,457 |
91 | 70 or 71 or 72 or 73 or 74 or 75 or 76 or 77 or 78 or 79 or 80 or 81 or 82 or 83 or 84 or 85 or 86 or 87 or 88 or 89 or 90 | 3,149,540 |
92 | 41 and 69 and 91 | 47,011 |
93 | Cardiovascular Diseases/ep, et, mo [Epidemiology, Etiology, Mortality] | 45,011 |
94 | Coronary Disease/ep, et, mo [Epidemiology, Etiology, Mortality] | 32,734 |
95 | Myocardial Ischaemia/ep, et, mo [Epidemiology, Etiology, Mortality] | 7100 |
96 | exp Myocardial Infarction/ep, et, mo [Epidemiology, Etiology, Mortality] | 38,794 |
97 | Angina Pectoris/ep, et, mo [Epidemiology, Etiology, Mortality] | 6018 |
98 | Coronary Artery Disease/ep, et, mo [Epidemiology, Aetiology, Mortality] | 9415 |
99 | Death, Sudden, Cardiac/ep, et [Epidemiology, Etiology] | 6561 |
100 | Vascular Diseases/ep, et, mo [Epidemiology, Etiology, Mortality] | 6204 |
101 | Arteriosclerosis/ep, et, mo [Epidemiology, Etiology, Mortality] | 13,850 |
102 | Stroke/ep, et, mo [Epidemiology, Aetiology, Mortality] | 20,132 |
103 | ((cardiovascular or cardio-vascular or cvd) and (risk? or mortality or death?)).ti. | 20,619 |
104 | ((coronary or heart or cardiac or myocardial or chd) and (risk? or mortality or death?)).ti. | 35,680 |
105 | (stroke and (risk? or mortality or death?)).ti. | 5689 |
106 | (vascular and (risk? or mortality or death?)).ti. | 2959 |
107 | 93 or 94 or 95 or 96 or 97 or 98 or 99 or 100 or 102 or 103 or 104 or 105 or 106 | 183,700 |
108 | 41 and 107 | 27,139 |
109 | 92 or 108 | 51,158 |
110 | 11 and 109 | 5899 |
111 | randomized controlled trial.pt. | 346,302 |
112 | controlled clinical trial.pt. | 85,685 |
113 | randomized.ab. | 264,313 |
114 | placebo.ab. | 143,132 |
115 | drug therapy.fs. | 1,598,414 |
116 | randomly.ab. | 192,458 |
117 | trial.ab. | 272,911 |
118 | groups.ab. | 1,242,034 |
119 | 111 or 112 or 113 or 114 or 115 or 116 or 117 or 118 | 3,096,302 |
120 | exp animals/not humans.sh. | 3,798,097 |
121 | 119 not 120 | 2,648,242 |
122 | 110 and 121 | 4288 |
123 | (2012* or 2013*).dp,ed,yr. | 1,685,294 |
124 | 122 and 123 | 451 |
125 | limit 124 to english language | 429 |
Appendix 5 Methods for indirect data extraction
Meta-analysis was performed for 10 different lipid measurements (TC, HDL, LDL, non-HDL cholesterol, triglycerides and Apo B, Apo A-I, plus combinations of these measures and ratios TC/HDL cholesterol, LDL/HDL cholesterol and Apo B/Apo A-I).
For the meta-analysis of prognostic data, estimates of the natural log of the HR (log(HR)) and its variance were sought. When direct estimates were not reported, indirect estimations were used to obtain unadjusted or adjusted estimates. 80,81
Summary measures sought included:
-
HR and 95% CI
-
loge HR and p-value
-
HR and p-value
-
HR, group numbers and total events
-
C2, group numbers and total events
-
p-value from chi-square test, group numbers and total events.
If none of these was available, relative risk or ORs with CIs were sought.
Two additional alternatives of data extraction were considered.
Method 1
In 16% of the studies, instead of global size effect, the information provided was the risk of event across different levels of the lipid measurement, with one category serving as the reference group. In these cases, generalised least squares for trend estimation were used. 83
Method 2
In 32% of the studies, data were obtained by simulating IPD. We distinguished two different cases:
First case. Baseline parameters, within each group (mean and SD for patients with event and patients without event) for each lipid measurement were extracted. Then an algorithm for computing the risk of event for a one-unit increase was followed (Table 58).
Step | Procedure |
---|---|
1 | Simulate two random samples of sizes n1 = number of patients with event and n2 = number of patients without event, from normal distributions, using baseline parameters |
2 | Join both samples in a new variable |
3 | Create an indicator variable that takes on the value ‘1’ for data from the first sample and the value ‘0’ other case |
4 | Fit a logistic regression model considering variables obtained on step 2 and step 3, keeping the corresponding OR |
5 | Repeat steps (1)–(4), 103 times |
6 | Calculate the average of the ORs: this value is the estimate of the OR and the 2.5th and 97.5th percentiles are the estimates of the lower and upper confidence limits, respectively |
Second case. In some studies, baseline parameters for each lipid measurement were available for only the whole sample and not by groups (events/non-events). In these cases, simulation methods could be applied when the number of events and no events by levels of the lipid measurements were provided, i.e. lipid measurement was divided into two or three categories and the number of events/non-events, of each category, were reported. A different algorithm for computing the risk of an event was used (Table 59). This method was used to estimate ORs by treatment for all randomised studies involving simulated IPD.
Step | Procedure |
---|---|
1 | Simulate a random sample of size n = number total of patients, from a normal distribution, considering the overall baseline mean and SD as the parameters of the global distribution |
2 | Sort this sample according to predetermined categories |
3 | Determinate the observed frequency in the sample that belongs to each category |
4 | Calculate the expected number of events of each category so that the probability of event matches with information reported in the article |
5 | Define a dummy variable (‘1’ means event, ‘0’ means no event) that accurately represents the information obtained in step 4 |
6 | Fit a logistic regression model considering variables obtained on step 2 and step 5, keeping the corresponding OR |
7 | Repeat steps (1)–(5), 103 times |
8 | Calculate the average of the ORs: this value is the estimate of the OR and the 2.5th and 97.5th percentiles are the estimates of the lower and upper confidence limits, respectively |
Appendix 6 Populations not taking statins: study names and references
Abbreviated study name | Full study name | Publication author | Title | Reference |
---|---|---|---|---|
7 Countries (Italy and Greece)103 | Seven Countries study (Italy and Greece) | Dontas AS et al. | Comparative total mortality in 25 years in Italian and Greek middle aged rural men | J Epidemiol Community Health 1998;52:638–44 |
7 Countries (Netherlands)104 | Seven Countries study (Netherlands); also known as Zutphen Elderly study | Weijenberg MP et al. | Total and high density lipoprotein cholesterol as risk factors for coronary heart disease in elderly men during 5 years of follow-up. The Zutphen Elderly Study | Am J Epidemiol 1996;143:151–8 |
7 Countries (Serbia)105 | Seven Countries study (Serbia) | Nedeljkovic S et al. | Coronary heart disease in 25 years. The experience in the three Serbian cohorts of the Seven Countries Study | Acta Cardiol 1993;1:11–24 |
AF/TexCAPS106 | Air Force/Texas Coronary Atherosclerosis Prevention Study | Downs JR et al. | Primary prevention of acute coronary events with lovastatin in men and women with average cholesterol levels | JAMA 1998;279:1615–22 |
ALERT107 | Assessment of LEscol in Renal Transplantation trial | Jardine AG et al. | Fluvastatin prevents cardiac death and myocardial infraction in renal transplant recipients: post-hoc subgroup analyses of the ALERT study | Am J Transplant 2004;4:988–95 |
AMORIS90 | Apolipoprotein Mortality Risk study | Holme I et al. | Relationships between lipoprotein components and risk of myocardial infarction: age, gender and short vs. longer follow-up periods in the Apolipoprotein MOrtality RISk study (AMORIS) | J Intern Med 2008;264:30–8 |
ANHF108 | Australian National Heart Foundation | Dhaliwal SS, Welborn TA | Central obesity and cigarette smoking are key determinants of CVD deaths in Australia: a public health perspective | Prev Med 2009;49:153–7 |
ASCOT-LLA109 | Anglo Scandinavian Cardiac Outcomes Trial – Lipid-Lowering Arm | Sever PS et al. | Reduction in cardiovascular events with atorvastatin in 2,532 patients with type 2 diabetes | Diabetes Care 29;28:1151–7 |
AURORA110 | A study to evaluate the use of rosuvastatin in subjects on regular haemodialysis: an assessment of survival and cardiovascular events | Fellstrom BC et al. | Rosuvastatin and cardiovascular events in patients undergoing hemodialysis | N Engl J Med 2009;360:1395–407 |
BRHS111 | British Regional Heart Study | Pocock J et al. | Concentrations of high density lipoprotein cholesterol, triglycerides, and total cholesterol in ischaemic heart disease | BMJ 1989;298:998–1002 |
Bruneck112 | Bruneck Study | Kiechl S et al. | Oxidized phospholipids, lipoprotein(a), lipoprotein-associated phospholipase A2 activity, and 10-year cardiovascular outcome: prospective results from the Bruneck study | Arterioscler Thromb Vasc Biol 2007;27:1788–95 |
BUPA113 | British United Provident Association study | Wald NJ et al. | Apolipoproteins and ischaemic heart disease: implications for screening | Lancet 1994;343:75–9 |
Caerphilly114 | Caerphilly Study | Sweetman P et al. | Apolipoproteins A±I, A±II and B, lipoprotein(a) and the risk of ischaemic heart disease: the Caerphilly Study | Eur J Clin Invest 2000;30:947–56 |
CARDS115 | Collaborative Atorvastatin Diabetes Study | Colhoun HM et al. | Primary prevention of CVD with atorvastatin in type 2 diabetes in the Collaborative Atorvastatin Diabetes Study (CARDS): multicentre randomised placebo-controlled trial | Lancet 2004;364:685–96 |
CARE34 | Cholesterol and Recurrent Events Trial | Sacks FM et al. | The effect of pravastatin on coronary events after myocardial infarction in patients with average cholesterol levels | N Engl J Med 1996;335:1001–9 |
Casale Monferrato116 | Casale Monferrato Study | Bruno G et al. | Effect of age on the association of non-high-density-lipoprotein cholesterol and apolipoprotein B with cardiovascular mortality in a Mediterranean population with type 2 diabetes: the Casale Monferrato study | Diabetologia 2006;49:937–44 |
CB project91 | Consultation Bureau Project | Verschuren WMM, Kromhout D | Total cholesterol concentration and mortality at a relatively young age: do men and women differ? | BMJ 1995;311:779–83 |
Charleston99 | Charleston Heart Study | Keil JE et al. | Mortality rates and risk factors for coronary disease in black as compared with white men and women | N Engl J Med 1993;329:73–8 |
Chinese veterans117 | N/A | Sai X et al. | All-cause mortality and risk factors in a cohort of retired military male veterans, Xi’an, China: an 18-year follow up study | BMC Public Health 2007;7:290 |
CHS118 | Cardiovascular Health Study | Psaty BM et al. | The association between lipid levels and the risks of incident myocardial infarction, stroke, and total mortality: The Cardiovascular Health Study | J Am Geriatr Soc 2004;52:1639–47 |
Copenhagen City92 | Copenhagen City Heart Study | Langsted A et al. | Fasting and nonfasting lipid levels: influence of normal food intake on lipids, lipoproteins, apolipoproteins, and cardiovascular risk prediction | Circulation 2008;118:2047–56 |
CORONA119 | Controlled Rosuvastatin Multinational Trial in Heart Failure | Kjekshus J et al. | Rosuvastatin in older patients with systolic heart failure | N Engl J Med 2007;357:2248–61 |
DAI120 | Diabetes and Informatics Study | Giorda CB et al. | Recurrence of cardiovascular events in patients with type 2 diabetes | Diabetes Care 2008;31:2154–9 |
DUBBO93 | Dubbo Study | Simons LA | Predictors of mortality in the prospective Dubbo study of Australian elderly | Aust N Z J Med 1996;26:40–8 |
EPIC-Norfolk100 | European Prospective Investigation into Cancer and Nutrition-Norfolk study | Arsenault BJ et al. | Lipid assessment, metabolic syndrome and coronary heart disease risk | Eur J Clin Invest 2010;40:1081–93 |
FIELD121 | Fenofibrate Intervention and Event Lowering in Diabetes study | Taskinen MR et al. | Ability of traditional lipid ratios and apolipoprotein ratios to predict cardiovascular risk in people with type 2 diabetes | Diabetologia 2010;53:1846–55 |
FINRISK ‘92122 | FINRISK ‘92 Hemostasis Study | Salomaa V et al. | Hemostatic factors as predictors of coronary events and total mortality: The FINRISK ‘92 Hemostasis Study | Arterioscler Thromb Vasc Biol 2002;22:353–8 |
Framingham Offspring53 | Framingham Offspring Study | Ingelsson E et al. | Clinical utility of different lipid measures for prediction of coronary heart disease in men and women | JAMA 2007;298:776–85 |
GISSI123 | GISSI Prevenzione Trial | GISSI Prevenzione Investigators | Results of the low-dose (20 mg) pravastatin GISSI Prevenzione trial in 4271 patients with recent myocardial infarction: do stopped trials contribute to overall knowledge? | Ital Heart J 2000;1:810–20 |
Glostrup Population124 | The Glostrup Population Studies | Klausen IC et al. | Apolipoprotein(a) isoforms and coronary heart disease in men A nested case–control study | Atherosclerosis 1997;132:77–84 |
GOH125 | Glucose Intolerance, Obesity and Hypertension Study | Gerber Y et al. | The role of risk factor time trends in the steep decline of CHD mortality between two Israeli cohort studies | Prev Med 2005;41:85–91 |
GRIPS126 | Goettingen Risk, Incidence and Prevalence Study | Cremer P et al. | Ten-year follow-up results from the Goettingen Risk, Incidence and Prevalence Study (GRIPS). I. Risk factors for myocardial infarction in a cohort of 5790 men | Atherosclerosis 1997;129:221–30 |
Hamaguchi 2007127 | N/A | Hamaguchi M et al. | Non-alcoholic fatty liver disease is a novel predictor of CVD | World J Gastroenterol 2007;13:1579–84 |
Honolulu Heart128 | Honolulu Hear Program | Schatz IJ et al. | Cholesterol and all-cause mortality in elderly people from the Honolulu Heart Program: a cohort study | Lancet 2001;358:351–5 |
Hoorn129 | Hoorn Study | Bos G et al. | A combination of high concentrations of serum triglyceride and non-high-density-lipoprotein-cholesterol is a risk factor for CVD in subjects with abnormal glucose metabolism: The Hoorn Study | Diabetologia 2003;46:910–16 |
HPFS130 | Health Professionals Follow-up Study | Jiang R et al. | Non-HDL cholesterol and apolipoprotein B predict cardiovascular disease events among men with type 2 diabetes | Diabetes Care 2004;27:1991–7 |
HPS24 | Heart Protection Study | Heart Protection Study Collaborative Group | MRC/BHF Heart protection study of cholesterol lowering with simvastatin in 20,536 high-risk individuals: a randomised placebo-controlled trial | Lancet 2002;360:7–22 |
IKNS (Yao)94 | Ikawa, Kyowa and Noichi and Yao Study | Iso H et al. | Serum total cholesterol and mortality in a Japanese population | J Clin Epidemiol 1994;47:961–9 |
InCHIANTI131 | Aging in Chianti Area Study | Semba RD et al. | Plasma carboxymethyl-lysine, an advanced glycation end product, and all-cause and cardiovascular disease mortality in older community-dwelling adults | J Am Geriatr Soc 2009;57:1874–80 |
Israeli Ischaemic Heart132 | Israeli Ischaemic Heart Disease Study | Goldbourt U et al. | Factors predictive of long-term coronary heart disease mortality among 10,059 male Israeli civil servants and municipal employees | Cardiology 1993;8:100–21 |
IwateKENCO133 | Iwate Kenpoku Cohort Study | Yokokawa H et al. | Serum low-density lipoprotein to high density lipoprotein ratio as a predictor of future acute myocardial infarction among men in a 2.7-year cohort study of a Japanese northern rural population | J Atheroscler Thromb 2011;18:89–98 |
Juntendo, Japan134 | N/A | Fukushima Y et al. | Non-high-density lipoprotein cholesterol is a practical predictor of long-term cardiac death after coronary artery bypass grafting | Atherosclerosis 2012;221:206–11 |
KIHD135 | Kuopio Ischaemic Heart Disease Risk Factor Study | Lakka H et al. | Hyperinsulinemia and the risk of cardiovascular death and acute coronary and cerebrovascular events in men | Arch Intern Med 2000;160:1160–8 |
Lan 2007136 | N/A | Lan TY et al. | Clinical and laboratory predictors of all-cause mortality in older population | Arch Gerontol Geriatr 2007;45:327–34 |
LIFE (subanalysis)137 | Losartan Intervention For Endpoint Reduction in Hypertension Study | De Simone G et al. | Clusters of metabolic risk factors predict cardiovascular events in hypertension with target-organ damage: the LIFE study | J Hum Hypertens 2007;21:625–32 |
LIPID138 | The Long-term Intervention with Pravastatin in Ischaemic Disease Study | Simes RJ et al. | Relationship between lipid levels and clinical outcomes in the long-term intervention with pravastatin in ischemic disease (LIPID) trial | Circulation 2002;105:1162–9 |
Livermore139 | N/A | Williams PT | Low high-density lipoprotein 3 reduces the odds of men surviving to age 85 during 53-year follow-up | JAGS 2012;60:430–6 |
LRC prevalence140 | Lipid Research Clinic Prevalence and Follow-up Studies | Grover SA et al. | Identifying adults at increased risk of coronary disease | JAMA 1995;274:801–6 |
MEGA141 | Management of Elevated Cholesterol in the Primary Prevention Group of Adult Japanese Study | Mizuno K et al. | Usefulness of LDL-C-related parameters to predict cardiovascular risk and effect of pravastatin in mild-to-moderate hypercholesterolemia | J Atheroscler Thromb 2012;19:176–185 |
MESA142 | Multi-ethnic Study of Atherosclerosis | Manickam P et al. | Comparative prognostic utility of conventional and novel lipid parameters for CVD risk prediction: do novel lipid parameters offer an advantage? | J Clin Lipidol 2011;5:82–90 |
MHS143 | Maccabi Healthcare Services | Shalev V et al. | In a population-based cohort of diabetes patients, men and women had similar risks for all-cause mortality | J Clin Epidemiol 2007;60:86–93 |
MONICA95 | Monitoring of Trends and Determinants in Cardiovascular Disease Augsburg Project | Meisinger C et al. | Prognostic value of apolipoprotein B and A-I in the prediction of myocardial infarction in middle-aged men and women: results from the MONICA/KORA Augsburg cohort study | Eur Heart J 2005;26:271–8 |
MRFIT102 | Multiple Risk Factor Intervention Trial | Watkins LO et al. | Racial differences in high-density lipoprotein cholesterol and coronary heart disease incidence in the Usual-Care Group of the Multiple Risk Factor Intervention Trial | Am J Cardiol 1966;57:536–45 |
NHANES III144 | Third National Health and Nutrition Examination Survey | Sierra-Johnson J et al. | Concentration of apolipoprotein B is comparable with the apolipoprotein B/apolipoprotein A-I ratio and better than routine clinical lipid measurements in predicting coronary heart disease mortality: findings from a multi-ethnic US population | Eur Heart J 2009;30:710–17 |
NIPPON 80145 | Nippon 80 Data Research Group | Okamura T et al. | The relationship between serum total cholesterol and all-cause or cause-specific mortality in a 17.3-year study of a Japanese cohort | Atherosclerosis 2007;190:216–23 |
Northwick Park I96 | Northwick Park Heart Study I | De Stavola BL, Meade TW | Long-term effects of hemostatic variables on fatal coronary heart disease: 30-year results from the first prospective Northwick Park Heart Study (NPHS-I) | J Thromb Haemost 2007;5:461–71 |
Northwick Park II146 | Northwick Park Heart Study II | Cooper JA et al. | Comparison of novel hemostatic factors and conventional risk factors for prediction of coronary heart disease | Circulation 2000;102:2816–22 |
Nurses’ Health147 | Nurses’ Health Study | Shai I et al. | Multivariate assessment of lipid parameters as predictors of coronary heart disease among postmenopausal women: potential implications for clinical guidelines | Circulation 2004;110:2824–30 |
Palma 2007148 | N/A | Palma S et al. | Cholesterol and serum albumin as risk factors for death in patients undergoing general surgery | Br J Surg 2007;94:369–75 |
PARIS149 | Paris Prospective Study | Cambien F et al. | Is the level of serum triglyceride a significant predictor of coronary death in ‘normocholesterolemic’ subjects? | Am J Epidemiol 1986;124:624–32 |
Physicians’ health190 | Physicians Health Study | Stampfer MJ et al. | A prospective study of cholesterol, apolipoproteins, and the risk of myocardial infraction | N Engl J Med 1991;325:373–81 |
PreCIS151 | Preventive Cardiology Information System Database Cohort Study | Ioachimescu AG et al. | Serum uric acid is an independent predictor of all-cause mortality in patients at high risk of cardiovascular disease | Arthritis Rheum 2008;58:623–30 |
PREVEND152 | Prevention of Renal and Vascular End-stage Disease cohort | Kappelle PJWH et al. | Apolipoprotein B A-I and total cholesterolâ high-density lipoprotein cholesterol ratios both predict cardiovascular events in the general population independently of nonlipid risk factors, albuminuria and C-reactive protein | J Intern Med 2011;269:232–42 |
PROCAM153 | Prospective Cardiovascular Münster Study | Assmann G, Cullen P, Schulte Helmut | Simple scoring scheme for calculating the risk of acute coronary events based on the 10-year follow-up of the Prospective Cardiovascular Münster (PROCAM) Study | Circulation 2002;105:310–15 |
Progetto Cuore154 | Progetto Cuore | Palmieri L et al. | Favorable cardiovascular risk profile and 10-year coronary heart disease incidence in women and men: results from the Progetto Cuore | Eur J Cardiovasc Prevent Rehabil 2006;13:562 |
PROSPER155 | Prospective Study of Pravastatin in the Elderly at Risk | Shepherd J et al. | Pravastatin in elderly individuals at risk of vascular disease (PROSPER): a randomised controlled trial | Lancet 2002;360:1623–30 |
Puerto Rico101 | Puerto Rico Heart Health Program | Garcia-Palmieri MR, Costas R | Risk factors of coronary heart disease: a prospective epidemiologic study in Puerto Rico | Progress in Cardiology. Philadelphia: Lea and Febiger; 1986. pp. 101–90 |
Quebec Cardiovascular156 | Québec Cardiovascular Study | St-Pierre AC et al. | Low-density lipoprotein subfractions and the long-term risk of ischemic heart disease in men: 13-year follow-up data from the Québec Cardiovascular Study | Arterioscler Thromb Vasc Biol 2005;25:553–9 |
Rancho Bernardo157 | Rancho Bernardo Study | Von Mühlen D et al. | Sex and time differences in the associations of non-high-density lipoprotein cholesterol versus other lipid and lipoprotein factors in the prediction of cardiovascular death (The Rancho Bernardo Study) | Am J Cardiol 2003;91:1311–15 |
Reykjavik97 | Reykjavik Study | Jonsdóttir LS et al. | Do lipids, blood pressure, diabetes, and smoking confer equal risk of myocardial infarction in women as in men? The Reykjavik Study | Eur J Cardiovasc Risk 2002;9:67–76 |
RIFLE158 | Risk Factors and Life Expectancy Project | Menotti A et al. | The prediction of coronary heart disease mortality as a function of major risk factors in over 30,000 men in the Italian RIFLE pooling project. A comparison with the MRFIT primary screening | J Cardiovasc Risk 1994;1:263–70 |
Rotterdam98 | Rotterdam Study | Houterman S et al. | Serum cholesterol is a risk factor for myocardial infarction in elderly men and women: the Rotterdam study | J Intern Med 1999;246:25–33 |
SHEP159 | Systolic Hypertension in the Elderly Program | Frost PH et al. | Serum lipids and incidence of coronary heart disease | Circulation 1996;94:2381–8 |
SHS160 | Strong Heart Study | Lu W et al. | Non-HDL cholesterol as a predictor of CVD in type 2 diabetes | Diabetes Care 2003;26:16–23 |
SLVHS161 | San Luis Valley Health Study | Abdel-Maksoud MF et al. | Risk of coronary heart disease is associated with triglycerides and high-density lipoprotein cholesterol in women and non-high-density lipoprotein cholesterol in men | J Clin Lipidol 2012;6:374–81 |
Stanek 2007162 | N/A | Stanek EJ et al. | Risk of cardiovascular events in patients at optimal values for combined lipid parameters | Curr Med Res Opin 2007;23:553–63 |
Stockholm County163 | N/A | Gigante B et al. | Elevated Apo B serum levels strongly predict early cardiovascular events | Heart 2012;98:1242–5 |
Swedish NDR164 | Swedish National Diabetes Register | Eliasson B et al. | Clinical usefulness of different lipid measures for prediction of coronary heart disease in type 2 diabetes | Diabetes Care 2011;34:2095–100 |
Thrombo165 | Thrombogenic Factors and Recurrent Coronary Events | Moss AJ et al. | Thrombogenic factors and recurrent coronary events | Circulation 1999;99:2517–22 |
Ting 2010166 | N/A | Ting R et al. | Lipid control and use of lipid-regulating drugs for prevention of cardiovascular events in Chinese type 2 diabetic patients: a prospective cohort study | Cardiovasc Diabetol 2010;9:77 |
TLGS88 | Tehran Lipid and Glucose Study | Tohidi M et al. | Lipid measures for prediction of incident CVD in diabetic and nondiabetic adults: results of the 8.6 years follow-up of a population-based cohort study | Lipids Health Dis 2010;9:6 |
TREAT167 | Trial to Reduce Cardiovascular Events with Aranesp Therapy | McMurray JJV et al. | Predictors of fatal and non-fatal cardiovascular events in patients with type 2 diabetes mellitus, chronic kidney disease, and anemia: an analysis of the Trial to Reduce cardiovascular Events with Aranesp (darbepoetin-alfa) Therapy (TREAT) | Am Heart J 2011;162:748–55 |
Turku Elderly168 | Turku Elderly Study | Upmeier E et al. | Serum lipids and their association with mortality in the elderly: a prospective cohort study | Aging Clin Exp Res 2009;21:424–30 |
ULSAM169 | Uppsala Longitudinal Study of Adult Men | Dunder K et al. | Evaluation of a scoring scheme, including proinsulin and the apolipoprotein B/apolipoprotein A1 ratio, for the risk of acute coronary events in middle-aged men: Uppsala Longitudinal Study of Adult Men (ULSAM) | Am Heart J 2004;148:596–601 |
VA-HIT170 | Veterans Affairs Cooperative Studies Program High-density Lipoprotein Cholesterol Intervention Trial | Bloomfield Rubins H et al. | Gemfibrozil for the secondary prevention of coronary heart disease in men with low levels of high-density lipoprotein cholesterol | N Engl J Med 1999;341:410–18 |
Whitehall I171 | The Whitehall Study I | Davey Smith G et al. | Plasma cholesterol concentration and mortality | JAMA 1992;267:70–6 |
Women’s Health172 | Women’s Health Study | Ridker PM et al. | Non-HDL cholesterol, apolipoproteins A-I and B100, standard lipid measures, lipid ratios, and CRP as risk factors for CVD in women | JAMA 2005;294:326–33 |
WOSCOPS19 | West of Scotland Coronary Prevention Study | Shepherd J et al. | Prevention of coronary heart disease with Pravastatin in men with hypercholestolemia | N Engl J Med 1995;333:1301–7 |
Yao City89 | N/A | Sato S et al. | Plasma fibrinogen and coronary heart disease in urban Japanese | Am J Epidemiol 2000;152:420–3 |
Appendix 7 Populations taking statins: study names and references
Abbreviated study name | Full study name | Publication author | Title | Reference |
---|---|---|---|---|
4S18 | Scandinavian Simvastatin Survival Study | Scandinavian Simvastatin Survival Study group | Randomised trial of cholesterol lowering in 4444 patients with coronary heart disease: the Scandinavian simvastatin survival study (4S) | Lancet 1994;344:1383–89 |
AF/TexCAPS106 | Air Force/Texas Coronary Atherosclerosis Prevention Study | Downs JR et al. | Primary prevention of acute coronary events with lovastatin in men and women with average cholesterol levels | JAMA 1998;279:1615–22 |
ALERT107 | Assessment of LEscol in Renal Transplantation Trial | Jardine AG et al. | Fluvastatin prevents cardiac death and myocardial infraction in renal transplant recipients: post-hoc subgroup analyses of the ALERT study | Am J Transplant 2004;4:988–95 |
ASCOT-LLA109 | Anglo Scandinavian Cardiac Outcomes Trial – Lipid-Lowering Arm | Sever PS et al. | Reduction in cardiovascular events with atorvastatin in 2,532 patients with type 2 diabetes | Diabetes Care 2005;28:1151–7 |
AURORA110 | A Study to Evaluate the Use of Rosuvastatin in Subjects on Regular Haemodialysis: an Assessment of Survival and Cardiovascular Events | Fellstrom BC et al. | Rosuvastatin and cardiovascular events in patients undergoing hemodialysis | N Engl J Med 2009;360:1395–407 |
CARDS115 | Collaborative Atorvastatin Diabetes Study | Colhoun HM et al. | Primary prevention of CVD with atorvastatin in type 2 diabetes in the collaboratie atorvastatin diabetes study (CARDS): multicentre randomised placebo-controlled trial | Lancet 2004;364:685–96 |
CARE34 | Cholesterol and Recurrent Events trial | Sacks FM et al. | The effect of pravastatin on coronary events after myocardial infarction in patients with average cholesterol levels | N Engl J Med 1996;335:1001–9 |
CLIP177 | Chiba Lipid Intervention Program Study | Saito Y et al. | Prognosis of hypercholesterolemic patients taking pravastatin for 5 years: the Chiba lipid intervention program (CLIP) study | J Atheroscler Thromb 2002;9:99–108 |
CORONA119 | Controlled Rosuvastatin multinational Trial in Heart Failure | Kjekshus J et al. | Rosuvastatin in older patients with systolic heart failure | N Engl J Med 2007;357:2248–61 |
dal-OUTCOMES178 | dal-OUTCOMES | Schwartz GG et al. | Effects of dalcetrapib in patients with recent acute coronary syndrome | N Engl J Med 2012;367:2089–99 |
GISSI123 | GISSI Prevenzione trial | GISSI Prevenzione Investigators | Results of the low-dose (20 mg) pravastatin GISSI Prevenzione trial in 4271 patients with recent myocardial infarction: do stopped trials contribute to overall knowledge? | Ital Heart J 2000;1:810–20 |
HPS24 | Heart Protection Study | Heart Protection Study Collaborative Group | MRC/BHF Heart protection study of cholesterol lowering with simvastatin in 20,536 high-risk individuals: a randomised placebo-controlled trial | Lancet 2002;360:7–22 |
IDEAL179 | Incremental Decrease in End Points Through Aggressive Lipid Lowering Study | Pedersen TR et al. | High-dose atorvastatin vs usual-dose simvastatin for secondary prevention of myocardial infarction | JAMA 2005;294:2437–45 |
JELIS180 | Japan EPA Lipid Intervention Study | Sasaki J et al. | Relationship between coronary artery disease and non-HDL-C and effect of highly purified EPA on the risk of coronary artery disease in hypercholesterolemic patients treated with statins: sub-analysis of the Japan EPA lipid intervention study (JELIS) | J Atheroscler Thromb 2012;19:194–204 |
J-LIT Primary174 | Primary Prevention Cohort Study of the Japan Lipid Intervention Trial | Matsuzaki M et al. | Large scale cohort study of the relationship between serum cholesterol concentration and coronary events with low-dose simvastatin therapy in Japanese patients with hypercholesterolemia | Circ J 2002;66:1087–95 |
J-LIT Secondary175 | Secondary Prevention Cohort Study of the Japan Lipid Intervention Trial | Mabuchi H et al. | Large scale cohort study of the relationship between serum cholesterol concentration and coronary events with low-dose simvastatin therapy in Japanese patients with hypercholesterolemia and coronary heart disease | Circ J 2002;66:1096–100 |
JUPITER181 | Justification for the Use of Statins in Prevention: an Intervention Trial Evaluating Rosuvastatin | Ridker PM et al. | Rosuvastatin to prevent vascular events in men and women with elevated C-Reactive Protein | N Engl J Med 2008;359:2195–207 |
LIPID138 | The Long-term Intervention with Pravastatin in Ischaemic Disease Study | Simes RJ et al. | Relationship between lipid levels and clinical outcomes in the long-term intervention with pravastatin in ischemic disease (LIPID) trial | Circulation 2002;105:1162–9 |
LIVES Ex182 | LIVALO Effectiveness and Safety Study Extension | Teramoto T et al. | A large-scale survey on cardio-cerebrovascular events during pitavastatin (LIVALO tablet) therapy in Japanese patients with hypercholesterolemia – LIVALO effectiveness and safety study extension | Jpn Pharmacol Ther 2011;39:789–803 |
Phase Z (20 & 40/80)176 | A to Z Trial | de Lemos JA et al. | Early intensive vs a delayed conservative simvastatin strategy in patients with acute coronary syndromes | JAMA 2004;292:1307–16 |
PROSPER155 | Prospective Study of Pravastatin in the Elderly at Risk | Shepherd J et al. | Pravastatin in elderly individuals at risk of vascular disease (PROSPER): a randomised controlled trial | Lancet 2002;360:1623–30 |
PROVE-IT183 | Pravastatin or Atorvastatin Evaluation and Infection Therapy-thrombolysis in Myocardial Infarction 22 Trial | Ray KK et al. | Prognostic utility of Apo B/AI, total cholesterol/HDL, non-HDL cholesterol, or hs-CRP as predictors of clinical risk in patients receiving statin therapy after acute coronary syndromes: results from PROVE IT-TIMI 22 | Arterioscler Thromb Vasc Biol 2009;29:424–30 |
SEARCH (80 & 20)58 | Study of the Effectiveness of Additional Reductions in Cholesterol and Homocysteine | Study of the effectiveness of additional reductions in cholesterol and homocysteine (SEARCH) collaborative group | Intensive lowering of LDL cholesterol with 80 mg vs. 20 mg simvastatin daily in 12,064 survivors of myocardial infarction: a double-blind randomised trial | Lancet 2010;376:1658–69 |
SPARCL184 | Stroke Prevention by Aggressive Reduction in Cholesterol Levels Trial | Stroke prevention by aggressive reduction in cholesterol levels (SPARCL) investigators | High-dose atorvastatin after stroke or transient ischemic attack | N Engl J Med 2006;355:549–59 |
TNT185 | Treating to New Targets | Arsenault BJ et al. | Prediction of cardiovascular events in statin-treated stable coronary patients by lipid and non-lipid biomarkers | J Am Coll Cardiol 2011;57:63–9 |
WOSCOPS19 | West of Scotland Coronary Prevention Study | Shepherd J et al. | Prevention of coronary heart disease with Pravastatin in men with hypercholestolemia | N Engl J Med 1995;333:1301–7 |
Appendix 8 Extracted data from studies for populations not taking statins
Study name | Data extraction method | Outcome | Surrogate outcome | Effect measure | No. of participants | Lipid measure units | Measure per 1 SD change | Lower limit | Upper limit | Log-HR | SE | Adjusted | Covariates if adjusted |
---|---|---|---|---|---|---|---|---|---|---|---|---|---|
7 Countries (Greece)103 | Direct | All-cause mortality | N/A | HR | 1215 | TC | 0.91289 | 0.81903 | 1.017508 | –0.0911401 | 0.0553559 | Y | Age, systolic BP, smoking, arm circumference, BMI, vital capacity, forced expiratory volume |
7 Countries (Italy)103 | Direct | CVD event | Y | HR | 1712 | TC | 1.2744 | 1.13482 | 1.431194 | 0.2424723 | 0.0591922 | N | N/A |
Direct | All-cause mortality | N/A | HR | 1712 | TC | 1.13159 | 1.0507 | 1.218693 | 0.1236202 | 0.0378386 | Y | Age, systolic BP, smoking, arm circumference, BMI, vital capacity, forced expiratory volume | |
7 Countries (Netherlands)104 | Direct | CVD event | Y | HR | 710 | TC | 1.03 | 0.81 | 1.32 | 0.0295588 | 0.1245798 | N | N/A |
TC | 1.17 | 0.9 | 1.52 | 0.1570037 | 0.1336915 | Y | Age, BMI, smoking, systolic BP, alcohol | ||||||
HDL | 0.78 | 0.59 | 1.03 | –0.2484614 | 0.1421407 | N | N/A | ||||||
HDL | 0.8 | 0.6 | 1.08 | –0.2231436 | 0.1499456 | Y | Age, BMI, smoking, Systolic BP, alcohol | ||||||
CVD mortality | Y | HR | 885 | TC | 1.25 | 0.96 | 1.59 | 0.2231436 | 0.1287133 | N | N/A | ||
TC | 1.4 | 1.07 | 1.83 | 0.3364722 | 0.1369024 | Y | Age, BMI, smoking, systolic BP, alcohol | ||||||
HDL | 1 | 0.78 | 1.28 | 0 | 0.1263575 | N | N/A | ||||||
HDL | 1.01 | 0.76 | 1.34 | 0.0099503 | 0.14467 | Y | Age, BMI, smoking, Systolic BP, alcohol | ||||||
7 Countries (Serbia)105 | Direct | CHD mortality | Y | HR | 1563 | TC | 1.13907 | 0.97276 | 1.3337736 | 0.1302079 | 0.0805184 | Y | Age, BMI, smoking, systolic BP |
AF/TexCAPS106 | Orsini (2) | CVE event | Y | RR | 3301 | LDL | 1.54066 | 1.04654 | 2.268087 | 0.4322122 | 0.1973085 | N | N/A |
HDL | 0.60066 | 0.40464 | 0.8916439 | –0.5097249 | 0.2015492 | ||||||||
ALERT107 | Simulation (2) | Cardiac mortality or definite non-fatal MI | Y | OR | 1052 | LDL | 1.29577 | 1.13682 | 1.486613 | 0.2591074 | 0.0684348 | N | N/A |
TC | 1.36301 | 1.20354 | 1.562351 | 0.3096926 | 0.0665622 | ||||||||
HDL | 0.84434 | 0.74828 | 0.9470393 | –0.1691991 | 0.0600934 | ||||||||
TGs | 1.175 | 1.03573 | 1.324315 | 0.1612707 | 0.0627017 | ||||||||
AMORIS90 | Direct | CVD event | N | HR | 65050 | TC | 1.18 | 1.16 | 1.21 | 0.1655144 | 0.0107654 | Y | Age, gender, hospital recorded hypertension, diabetes |
TGS | 1.11 | 1.1 | 1.12 | 0.10436 | 0.0045966 | ||||||||
CVD event | Y | HR | 83954 | Apo A-I | 0.8 | 0.78 | 0.83 | –0.2231436 | 0.0158499 | Y | Age | ||
65167 | Apo A-I | 0.8 | 0.76 | 0.83 | –0.2231436 | 0.0224763 | |||||||
83954 | Apo B | 1.46 | 1.42 | 1.5 | 0.3784364 | 0.0139817 | |||||||
65167 | Apo B | 1.4 | 1.35 | 1.46 | 0.3364722 | 0.0199826 | |||||||
83954 | LDL | 1.4 | 1.37 | 1.43 | 0.3364722 | 0.0109346 | |||||||
65167 | LDL | 1.34 | 1.29 | 1.39 | 0.2926696 | 0.0190463 | |||||||
83954 | HDL | 0.75 | 0.73 | 0.77 | –0.2876821 | 0.0136087 | |||||||
65167 | HDL | 0.72 | 0.69 | 0.76 | –0.3285041 | 0.0246497 | |||||||
83954 | Non-HDL cholesterol | 1.46 | 1.43 | 1.5 | 0.3784364 | 0.0121915 | |||||||
65167 | Non-HDL cholesterol | 1.43 | 1.37 | 1.48 | 0.3576744 | 0.0197019 | |||||||
83954 | Apo B/Apo A-I | 1.5 | 1.46 | 1.53 | 0.4054651 | 0.0119468 | |||||||
65167 | Apo B/Apo A-I | 1.43 | 1.38 | 1.48 | 0.3576744 | 0.0178466 | |||||||
83954 | LDL/HDL cholesterol | 1.3 | 1.28 | 1.32 | 0.2623643 | 0.0078499 | |||||||
65167 | LDL/HDL cholesterol | 1.28 | 1.26 | 1.31 | 0.2468601 | 0.0099274 | |||||||
83954 | TC/HDL cholesterol | 1.29 | 1.27 | 1.31 | 0.2546422 | 0.0079108 | |||||||
65167 | TC/HDL cholesterol | 1.28 | 1.26 | 1.31 | 0.2468601 | 0.0099274 | |||||||
CVD mortality | Y | HR | 149121 | TC | 1.24 | 1.17 | 1.3 | 0.2151114 | 0.0268777 | Y | Age and gender | ||
TG | 1.34 | 1.28 | 1.41 | 0.2926696 | 0.0246759 | ||||||||
Apo A-I | 0.82 | 0.77 | 0.87 | –0.1984509 | 0.0311486 | ||||||||
Apo B | 1.34 | 1.27 | 1.41 | 0.2926696 | 0.0266767 | ||||||||
LDL | 1.26 | 1.2 | 1.33 | 0.2311117 | 0.0262391 | ||||||||
HDL | 0.74 | 0.69 | 0.78 | –0.3011051 | 0.0312761 | ||||||||
Non-HDL cholesterol | 1.35 | 1.28 | 1.42 | 0.3001046 | 0.0264788 | ||||||||
Apo B/Apo A-I | 1.39 | 1.32 | 1.46 | 0.3293037 | 0.0257155 | ||||||||
LDL/HDL cholesterol | 1.28 | 1.23 | 1.33 | 0.2468601 | 0.01994 | ||||||||
TC/HDL cholesterol | 1.28 | 1.24 | 1.33 | 0.2468601 | 0.0178744 | ||||||||
Simulation (1) | All-cause mortality | N/A | OR | 44,457 | HDL | 0.83 | 0.8 | 0.85 | –0.1863296 | 0.0154655 | N | N/A | |
ANHF108 | Direct | CVD mortality | N | OR | 8662 | TC | 1.17734 | 0.94513 | 1.47067 | 0.1632577 | 0.1127936 | N | N/A |
HDL | 0.75175 | 0.57434 | 0.99195 | –0.2853515 | 0.1394008 | ||||||||
ASCOT-LLA109 | Simulation (2) | CVD event | N | OR | 1274 | LDL | 1.26723 | 1.1626 | 1.404007 | 0.2368358 | 0.0481305 | N | N/A |
HDL | 0.93255 | 0.85343 | 1.051044 | –0.0698336 | 0.0531307 | ||||||||
TGs | 0.91138 | 0.82493 | 1.00828 | –0.0927953 | 0.0511997 | ||||||||
AURORA110 | Simulation (2) | CVD event | N | OR | 1384 | LDL | 1.0286 | 0.93953 | 1.11867 | 0.0281987 | 0.0445194 | N | N/A |
BRHS111 | Simulation (1) | CVD event | Y | OR | 7735 | TC | 1.62296 | 1.46773 | 1.774085 | 0.4842492 | 0.0483595 | N | N/A |
TGs | 1.13359 | 0.84993 | 1.5013471 | 0.1253862 | 0.1451439 | ||||||||
HDL | 0.84112 | 0.67406 | 1.084117 | –0.1730209 | 0.1212261 | ||||||||
Bruneck112 | Simulation (1) | CVD event | N | OR | 765 | TC | 1.3445 | 1.10379 | 1.659849 | 0.2960207 | 0.1040753 | N | N/A |
TGs | 1.13193 | 0.8989 | 1.4158174 | 0.1239202 | 0.1158908 | ||||||||
HDL | 0.8786 | 0.72518 | 1.103316 | –0.1294296 | 0.1070556 | ||||||||
LDL | 1.37326 | 1.13522 | 1.872485 | 0.3171882 | 0.1276644 | ||||||||
Apo A | 0.94659 | 0.78294 | 1.1741719 | –0.0548873 | 0.103384 | ||||||||
Apo B | 1.38737 | 1.17967 | 1.766929 | 0.327407 | 0.1030629 | ||||||||
BUPA113 | Simulation (1) | CVD mortality | Y | OR | 1374 | TC | 1.53782 | 1.29932 | 1.731425 | 0.4303626 | 0.0732415 | N | N/A |
HDL | 0.60428 | 0.44593 | 0.7294944 | –0.5037148 | 0.1255594 | ||||||||
Apo A-I | 0.74469 | 0.65936 | 0.8314919 | –0.2947859 | 0.0591716 | ||||||||
Apo B | 1.89716 | 1.60975 | 2.234868 | 0.6403586 | 0.0837004 | ||||||||
Caerphilly114 | Direct | CVD event | Y | OR | 2225 | TC | 1.26 | 1.1 | 1.44 | 0.2311117 | 0.0687074 | Y | Age + non-lipid risk factors (diastolic BP, smoking habit, BMI, and evidence of ischaemia at baseline) |
Apo B | 1.2 | 1.05 | 1.37 | 0.1823216 | 0.0678624 | ||||||||
HDL | 0.787 | 0.6802 | 0.917 | –0.239527 | 0.0762042 | ||||||||
Apo A-I | 0.847 | 0.7299 | 0.9708 | –0.1660546 | 0.0727584 | ||||||||
CARDS115 | Simulation (2) | CVD event | N | OR | 1410 | LDL | 1.0479 | 0.9439 | 1.1733 | 0.0467882 | 0.0554988 | N | N/A |
TC | 1.1112 | 1.001 | 1.2386 | 0.1054405 | 0.0543322 | ||||||||
HDL | 0.9484 | 0.8495 | 1.0615 | –0.0529789 | 0.0568343 | ||||||||
TGs | 0.8438 | 0.5929 | 1.2109 | –0.1698398 | 0.1821667 | ||||||||
CARE34 | Simulation (2) | CVD event | Y | OR | 2078 | LDL | 1.0194 | 0.95714 | 1.0830078 | 0.0192177 | 0.0315165 | N | N/A |
TC | 1.06292 | 0.99873 | 1.1270821 | 0.0610224 | 0.0308423 | ||||||||
HDL | 0.99359 | 0.90507 | 1.0930539 | –0.0064301 | 0.0481437 | ||||||||
TGs | 0.98042 | 0.92488 | 1.0362048 | –0.0197733 | 0.0289929 | ||||||||
Casale Monferrato116 | Orsini (1) | CVD mortality | N | OR | 982 | TC | 0.94377 | 0.88764 | 1.003443 | –0.0578771 | 0.0312827 | Y | Age, gender, hypertension, smoking, CHD, fibrinogen, cumulative average individual HbA1c, and referring physician |
LDL | 0.95418 | 0.87845 | 1.036446 | –0.0468979 | 0.0421914 | ||||||||
HDL | 0.8975 | 0.8309 | 0.9694457 | –0.1081374 | 0.0393401 | ||||||||
Apo A-I | 0.9567 | 0.89177 | 1.026368 | –0.044263 | 0.0358619 | ||||||||
Apo B | 1.14058 | 1.03028 | 1.262697 | 0.1315378 | 0.0518937 | ||||||||
CB project91 | Orsini (1) | All-cause mortality | N/A | HR | 23389 | TC | 1.25507 | 1.17833 | 1.336815 | 0.2271929 | 0.0321924 | Y | Age, smoking, systolic BP, BMI |
25629 | TC | 1.17838 | 1.05012 | 1.322308 | 0.1641415 | 0.0587945 | |||||||
Charleston99 | Direct | CVD mortality | Y | HR | 653 existing review | TC | 1.19 | 1 | 1.43 | 0.1739533 | 0.0912435 | N | N/A |
333 | TC | 1 | 0.76 | 1.33 | 0 | 0.1427591 | |||||||
741 | TC | 1.61 | 1.4 | 1.86 | 0.4762342 | 0.0724756 | |||||||
454 | TC | 1.43 | 1.15 | 1.78 | 0.3576744 | 0.1114417 | |||||||
653 | TC | 1.18 | 0.98 | 1.43 | 0.1655144 | 0.0963972 | Y | Systolic BP, BMI, years of education, smoking status, diabetes status | |||||
333 | TC | 1.15 | 0.83 | 1.58 | 0.1397619 | 0.1642231 | |||||||
741 | TC | 1.28 | 1.07 | 1.54 | 0.2468601 | 0.0928887 | |||||||
454 | TC | 1.29 | 0.99 | 1.67 | 0.2546422 | 0.1333862 | |||||||
All-cause mortality | N/A | 653 | TC | 1.09 | 0.98 | 1.22 | 0.0861777 | 0.055881 | N | N/A | |||
333 | TC | 0.93 | 0.8 | 1.08 | –0.0725707 | 0.0765573 | |||||||
741 | TC | 1.42 | 1.29 | 1.55 | 0.3506569 | 0.04684 | |||||||
454 | TC | 1.19 | 1.03 | 1.37 | 0.1739533 | 0.0727684 | |||||||
653 | TC | 1.05 | 0.94 | 1.18 | 0.0487902 | 0.0580076 | Y | Systolic BP, BMI, years of education, smoking status, diabetes status | |||||
333 | TC | 0.99 | 0.83 | 1.17 | –0.0100503 | 0.087585 | |||||||
741 | TC | 1.11 | 0.99 | 1.24 | 0.10436 | 0.0574392 | |||||||
454 | TC | 1.19 | 1.03 | 1.37 | 0.1739533 | 0.0727684 | |||||||
Chinese veterans117 | Direct | All-cause mortality | N/A | HR | 1268 | TGs | 1.14324 | 1.06926 | 1.222257 | 0.1338663 | 0.0341154 | N | N/A |
TGs | 1.14324 | 1.06926 | 1.222257 | 0.1338663 | 0.0341154 | Y | Age, systolic BP, cigarettes per day, duration of smoking, age of starting smoking, exercise, BMI, negative affairs, family history of diseases, existing baseline diseases | ||||||
CHS118 | Direct | CVD event | Y | HR | 4885 | TC | 0.95 | 0.86 | 1.04 | –0.0512933 | 0.0484805 | N | N/A |
LDL | 1.02 | 0.93 | 1.12 | 0.0198026 | 0.0474233 | ||||||||
HDL | 0.71 | 0.64 | 0.79 | –0.3424903 | 0.0537155 | ||||||||
TGs | 1.11 | 1.03 | 1.2 | 0.10436 | 0.0389701 | ||||||||
TC | 1.09 | 0.98 | 1.2 | 0.0861777 | 0.0516644 | Y | Age, gender, diabetes, smoking status, CVD and systolic BP | ||||||
LDL | 1.1 | 1 | 1.21 | 0.0953102 | 0.0486276 | ||||||||
HDL | 0.85 | 0.76 | 0.96 | –0.1625189 | 0.0595956 | ||||||||
TGs | 1.07 | 0.99 | 1.16 | 0.0676586 | 0.0404261 | ||||||||
All-cause mortality | N/A | TC | 0.86 | 0.81 | 0.91 | –0.1508229 | 0.0296965 | N | N/A | ||||
LDL | 0.89 | 0.84 | 0.95 | –0.1165338 | 0.0313929 | ||||||||
HDL | 0.86 | 0.81 | 0.92 | –0.1508229 | 0.0324845 | ||||||||
TGs | 1.05 | 0.99 | 1.11 | 0.0487902 | 0.0291863 | ||||||||
TC | 0.98 | 0.92 | 1.04 | –0.0202027 | 0.0312761 | Y | Age, gender, diabetes, smoking status, CVD and systolic BP | ||||||
LDL | 0.97 | 0.92 | 1.04 | –0.0304592 | 0.0312761 | ||||||||
HDL | 0.99 | 0.92 | 1.06 | –0.0100503 | 0.0361353 | ||||||||
TGs | 1.05 | 0.99 | 1.11 | 0.0487902 | 0.0291863 | ||||||||
Copenhagen City92 | Orsini (2) | CVD event | N | HR | 5181 | TC | 1.08077 | 1.021 | 1.144 | 0.0776738 | 0.0290174 | Y | Age, BP, smoking, use of lipid-lowering drugs and use of hormone replacement therapy, diabetes plus body mass plus high-sensitivity C-reactive protein |
Non-HDL cholesterol | 1.1254 | 1.0516 | 1.2043 | 0.1181385 | 0.0345882 | ||||||||
LDL | 1.12185 | 1.0538 | 1.1942 | 0.1149791 | 0.0319066 | ||||||||
HDL | 0.9805 | 0.926 | 1.0383 | –0.0196926 | 0.0292005 | ||||||||
Apo A-I | 0.961 | 0.888 | 1.0402 | –0.0397809 | 0.0403563 | ||||||||
Apo B | 1.082 | 1.03852 | 1.1273 | 0.0788112 | 0.0209257 | ||||||||
TGs | 1.03277 | 1.00034 | 1.0662 | 0.0322445 | 0.0162656 | ||||||||
TC/HDL cholesterol | 1.3855 | 1.22524 | 1.5669 | 0.3260611 | 0.0627455 | ||||||||
Apo B/Apo A-I | 1.1675 | 1.09704 | 1.2424 | 0.1548647 | 0.0317422 | ||||||||
3854 | TC | 1.0678 | 1.01616 | 1.122074 | 0.0656023 | 0.0252938 | |||||||
Non-HDL cholesterol | 1.14095 | 1.07077 | 1.215717 | 0.1318569 | 0.032386 | ||||||||
LDL | 1.13014 | 1.0409 | 1.227026 | 0.1223371 | 0.0419672 | ||||||||
HDL | 0.8377 | 0.7298 | 0.9616 | –0.1770952 | 0.0703643 | ||||||||
Apo A-I | 0.9698 | 0.9045 | 1.0398 | –0.0306654 | 0.0355616 | ||||||||
Apo B | 1.0998 | 1.04928 | 1.152757 | 0.0951283 | 0.0239939 | ||||||||
TGs | 1.02357 | 1.00296 | 1.044613 | 0.0232985 | 0.0103816 | ||||||||
TC/HDL cholesterol | 1.08393 | 1.05288 | 1.115885 | 0.0805887 | 0.0148262 | ||||||||
Apo B/Apo A-I | 1.05082 | 1.03206 | 1.069921 | 0.049568 | 0.0091921 | ||||||||
All-cause mortality | N/A | 7587 | TGs | 1.12 | 1.09 | 1.15 | 0.1133287 | 0.0136695 | Y | Age | |||
6394 | TGs | 1.08 | 1.05 | 1.11 | 0.076961 | 0.014176 | |||||||
CORONA119 | Simulation (2) | CVD event | N | OR | 2497 | LDL | 0.88613 | 0.82453 | 0.962596 | –0.1208939 | 0.0394943 | N | N/A |
Apo B/Apo A-I | 1.01317 | 0.95069 | 1.09422 | 0.0130811 | 0.0358688 | ||||||||
HDL | 0.94347 | 0.86926 | 1.03011 | –0.0581865 | 0.0433097 | ||||||||
TGs | 0.8688 | 0.81125 | 0.930863 | –0.1406412 | 0.0350863 | ||||||||
DAI120 | Simulation (1) | CVD event | N | OR | 2788 | TC | 1.01275 | 0.93174 | 1.1005407 | 0.0126697 | 0.0424751 | N | N/A |
HDL | 0.97052 | 0.89393 | 1.0472745 | –0.0299201 | 0.0403866 | ||||||||
LDL | 1.112 | 0.88805 | 1.3917753 | 0.1061571 | 0.114618 | ||||||||
DUBBO93 | Direct | All-cause mortality | N/A | OR | 1236 | TC | 0.96504 | 0.85106 | 1.0977102 | –0.0355845 | 0.0649224 | N | N/A |
LDL | 0.93101 | 0.79588 | 1.1238549 | –0.0714824 | 0.0880277 | ||||||||
TGs | 1.0784 | 0.92136 | 1.2206902 | 0.0754817 | 0.0717654 | ||||||||
HDL | 0.92655 | 0.81489 | 1.0519367 | –0.0762881 | 0.0651375 | ||||||||
1569 | TC | 0.86241 | 0.7386 | 1.0021556 | –0.148019 | 0.0778465 | |||||||
LDL | 0.82014 | 0.69329 | 0.9732195 | –0.1982791 | 0.086521 | ||||||||
TGs | 1.34915 | 1.09185 | 1.57112 | 0.299474 | 0.0928353 | ||||||||
HDL | 0.79893 | 0.69391 | 0.8923803 | –0.2244813 | 0.0641694 | ||||||||
EPIC-Norfolk100 | Direct | CVD event | Y | HR | 21448 | LDL | 1.22 | 1.17 | 1.27 | 0.1988509 | 0.0209217 | Y | Age, gender, smoking, waist circumference, physical activity, systolic BP, and hormone replacement therapy (women) |
Non-HDL cholesterol | 1.54 | 1.35 | 1.74 | 0.4317824 | 0.0647399 | ||||||||
TC/HDL cholesterol | 1.19 | 1.14 | 1.24 | 0.1739533 | 0.0214498 | ||||||||
610 | TC | 1.34 | 0.99 | 1.81 | 0.2926696 | 0.1539228 | Y | Age, smoking, physical activity | |||||
HDL | 0.68 | 0.48 | 0.95 | –0.3856625 | 0.174152 | ||||||||
Apo B | 1.46 | 1.04 | 2.06 | 0.3784364 | 0.1743585 | ||||||||
Apo A-I | 0.75 | 0.55 | 0.99 | –0.2876821 | 0.1499456 | ||||||||
Apo B/Apo A-I | 1.99 | 1.3 | 3.05 | 0.6881346 | 0.2175452 | ||||||||
LDL/HDL cholesterol | 2.27 | 1.39 | 3.69 | 0.8197798 | 0.2490619 | ||||||||
983 | TC | 1.25 | 1.07 | 1.45 | 0.2231436 | 0.0775268 | |||||||
HDL | 0.96 | 0.78 | 1.17 | –0.040822 | 0.103435 | ||||||||
Apo B | 1.32 | 1.12 | 1.56 | 0.2776317 | 0.0845299 | ||||||||
Apo A-I | 0.98 | 0.81 | 1.17 | –0.0202027 | 0.0938073 | ||||||||
Apo B/Apo A-I | 1.33 | 1.12 | 1.59 | 0.2851789 | 0.0893891 | ||||||||
LDL/HDL cholesterol | 1.25 | 1.05 | 1.47 | 0.2231436 | 0.0858348 | ||||||||
406 | TC | 1.37 | 0.88 | 2.12 | 0.3148107 | 0.2242983 | |||||||
HDL | 1.13 | 0.78 | 1.66 | 0.1222176 | 0.1926732 | ||||||||
Apo B | 1.38 | 0.85 | 2.23 | 0.3220835 | 0.2460512 | ||||||||
Apo A-I | 0.99 | 0.68 | 1.42 | –0.0100503 | 0.1878366 | ||||||||
Apo B/Apo A-I | 1.36 | 0.82 | 2.28 | 0.3074847 | 0.2608741 | ||||||||
LDL/HDL cholesterol | 1.99 | 1.05 | 3.76 | 0.6881346 | 0.3254155 | ||||||||
530 | TC | 1.03 | 0.8 | 1.32 | 0.0295588 | 0.1277488 | |||||||
HDL | 0.74 | 0.55 | 0.98 | –0.3011051 | 0.1473557 | ||||||||
Apo B | 1.02 | 0.81 | 1.3 | 0.0198026 | 0.120685 | ||||||||
Apo A-I | 0.71 | 0.57 | 0.91 | –0.3424903 | 0.1193388 | ||||||||
Apo B/Apo A-I | 1.32 | 1.02 | 1.7 | 0.2776317 | 0.1303127 | ||||||||
LDL/HDL cholesterol | 1.21 | 0.96 | 1.52 | 0.1906204 | 0.1172276 | ||||||||
FIELD121 | Direct | CVD event | N | HR | 4900 | TC/HDL cholesterol | 1.21 | 1.12 | 1.31 | 0.1906204 | 0.0399741 | Y | Gender, age, prior MI, prior coronary artery bypass grafting, smoking, creatinine concentration, insulin use, HbA1c, country of residence |
LDL/HDL cholesterol | 1.17 | 1.09 | 1.27 | 0.1570037 | 0.0389896 | ||||||||
HDL | 0.84 | 0.76 | 0.91 | –0.1743534 | 0.0459506 | ||||||||
Non-HDL cholesterol | 1.13 | 1.04 | 1.22 | 0.1222176 | 0.040722 | ||||||||
TGs | 1.07 | 1 | 1.15 | 0.0676586 | 0.0356536 | ||||||||
TC | 1.06 | 0.98 | 1.15 | 0.0582689 | 0.0408073 | ||||||||
LDL | 1.05 | 0.98 | 1.14 | 0.0487902 | 0.0385793 | ||||||||
Apo B/Apo A-I | 1.2 | 1.12 | 1.3 | 0.1823216 | 0.0380193 | ||||||||
Apo A-I | 0.85 | 0.78 | 0.93 | –0.1625189 | 0.0448701 | ||||||||
Apo B | 1.14 | 1.05 | 1.23 | 0.1310283 | 0.0403633 | ||||||||
FINRISK ‘92122 | Simulation (1) | CVD event | Y | OR | 2378 | TC | 1.51 | 1.128 | 1.987 | 0.4121097 | 0.1444336 | N | N/A |
HDL | 0.574 | 0.459 | 0.704 | –0.5551259 | 0.1091143 | ||||||||
All-cause mortality | N/A | TC | 1.032 | 0.851 | 1.246 | 0.0314987 | 0.0972657 | ||||||
HDL | 0.778 | 0.647 | 0.907 | –0.2510288 | 0.0861725 | ||||||||
Framingham Offspring40 | Direct | CVD event | N | HR | 3066 | LDL | 1.11 | 1.01 | 1.22 | 0.10436 | 0.0481889 | Y | Age, gender, antihypertensive treatment, smoking, systolic and diastolic BP, lipid medication use |
Non-HDL cholesterol | 1.21 | 1.1 | 1.33 | 0.1906204 | 0.0484359 | ||||||||
CVD event | Y | 1562 | TC | 1.12 | 0.97 | 1.28 | 0.1133287 | 0.0707447 | Y | Age, antihypertensive treatment, diabetes, smoking and systolic BP | |||
3322 | HDL | 0.71 | 0.6 | 0.83 | –0.3424903 | 0.0827796 | |||||||
Apo A-I | 0.83 | 0.72 | 0.96 | –0.1863296 | 0.0733883 | ||||||||
Apo B | 1.37 | 1.2 | 1.57 | 0.3148107 | 0.0685597 | ||||||||
TC/HDL cholesterol | 1.39 | 1.22 | 1.58 | 0.3293037 | 0.0659628 | ||||||||
LDL/HDL cholesterol | 1.35 | 1.18 | 1.54 | 0.3001046 | 0.0679255 | ||||||||
Apo B/Apo A-I | 1.39 | 1.23 | 1.58 | 0.3293037 | 0.0638803 | ||||||||
1760 | TC | 1.18 | 0.96 | 1.44 | 0.1655144 | 0.103435 | |||||||
3322 | HDL | 0.72 | 0.57 | 0.92 | –0.3285041 | 0.1221269 | |||||||
Apo A-I | 0.85 | 0.68 | 1.07 | –0.1625189 | 0.1156431 | ||||||||
Apo B | 1.38 | 1.15 | 1.67 | 0.3220835 | 0.0951688 | ||||||||
TC/HDL cholesterol | 1.39 | 1.17 | 1.66 | 0.3293037 | 0.0892382 | ||||||||
LDL/HDL cholesterol | 1.36 | 1.14 | 1.63 | 0.3074847 | 0.0912122 | ||||||||
Apo B/Apo A-I | 1.4 | 1.16 | 1.67 | 0.3364722 | 0.0929601 | ||||||||
GISSI123 | Simulation (2) | CVD event | N | OR | 2133 | LDL | 1.11802 | 0.9794 | 1.26884 | 0.1115593 | 0.0660506 | N | N/A |
TC | 1.16389 | 1.0748 | 1.26652 | 0.1517678 | 0.041872 | ||||||||
HDL | 1.05374 | 0.94298 | 1.18484 | 0.0523467 | 0.0582444 | ||||||||
TGs | 0.99153 | 0.8952 | 1.10744 | –0.0085111 | 0.0542753 | ||||||||
Glostrup population124 | Simulation(1) | CVD event | Y | OR | 264 | TC | 1.83217 | 1.35662 | 2.606839 | 0.6054983 | 0.1666188 | N | N/A |
TGs | 2.71015 | 1.86844 | 4.112419 | 0.9970055 | 0.2012514 | ||||||||
Apo A-I | 0.76439 | 0.57708 | 1.0513916 | –0.2686812 | 0.153035 | ||||||||
Apo B | 1.71308 | 1.27742 | 2.3691566 | 0.5382958 | 0.1575752 | ||||||||
GOH125 | Direct | CVD mortality | Y | HR | 1745 | TC | 1.15 | 0.95 | 1.38 | 0.1397619 | 0.0952492 | N | N/A |
All-cause mortality | N/A | TC | 1.05 | 0.96 | 1.14 | 0.0487902 | 0.0438394 | ||||||
GRIPS126 | Simulation (1) | CVD event | Y | OR | 4368 | TC | 2.28996 | 2.03968 | 2.540059 | 0.8285348 | 0.0559681 | N | N/A |
TGs | 1.42192 | 1.28897 | 1.595354 | 0.3520053 | 0.0544021 | ||||||||
LDL | 2.69414 | 2.37036 | 3.020998 | 0.9910783 | 0.0618739 | ||||||||
HDL | 0.7239 | 0.64232 | 0.7926559 | –0.3231022 | 0.0536468 | ||||||||
Apo B | 2.29347 | 2.02958 | 2.551539 | 0.8300664 | 0.058385 | ||||||||
Apo A-I | 0.7739 | 0.69308 | 0.8458674 | –0.2563188 | 0.0508225 | ||||||||
LDL/HDL cholesterol | 2.58044 | 2.24957 | 2.927679 | 0.947958 | 0.0672125 | ||||||||
TC/HDL cholesterol | 2.36319 | 2.10311 | 2.671609 | 0.8600116 | 0.0610363 | ||||||||
Apo B/Apo A-I | 2.10583 | 1.79 | 2.347714 | 0.7447078 | 0.0691906 | ||||||||
Hamaguchi127 | Direct | CVD event | N | OR | 1221 | LDL | 1.36839 | 0.91099 | 2.0546984 | 0.3136337 | 0.2074888 | N | N/A |
HDL | 0.57708 | 0.34925 | 0.9490384 | –0.5497744 | 0.2550156 | ||||||||
TGs | 1.46373 | 1.08707 | 1.960606 | 0.3809873 | 0.1504505 | ||||||||
Honolulu Heart128 | Orsini (1) | All-cause mortality | N/A | HR | 3741 | TC | 0.9934 | 0.9833 | 1.0036 | –0.0066219 | 0.0052129 | Y | Age, cardiovascular risk factors, frailty measures and chronic diseases |
Hoorn129 | Direct | CVD event | N | HR | 1817 | Non-HDL cholesterol | 1.41248 | 1.28896 | 1.550295 | 0.3453499 | 0.0470953 | Y | Age and gender |
HPFS130 | Orsini (1) | CVD event | N | HR | 746 | TC | 1.17791 | 1.02711 | 1.350921 | 0.1637442 | 0.069907 | Y | Age, BMI, family history of MI, physical activity, smoking, alcohol, fasting status, history of hypertension, aspirin use, HbA1c |
LDL | 1.16947 | 1.02279 | 1.337187 | 0.1565472 | 0.0683773 | ||||||||
HDL | 0.88047 | 0.77305 | 1.0028116 | –0.1273017 | 0.0663824 | ||||||||
NonHDL | 1.21781 | 1.06135 | 1.397374 | 0.1970501 | 0.0701678 | ||||||||
Apo B | 1.19488 | 1.03737 | 1.37626 | 0.1780416 | 0.0721118 | ||||||||
TGs | 1.1171 | 1.00823 | 1.237722 | 0.1107387 | 0.0523146 | ||||||||
TC/HDL cholesterol | 1.35399 | 1.18192 | 1.551099 | 0.3030536 | 0.0693421 | ||||||||
HPS24 | Orsini (2) | CVD event | N | RR | 10,267 | TC | 1.16793 | 1.06794 | 1.27729 | 0.1552338 | 0.0456668 | N | N/A |
LDL | 1.37032 | 1.20874 | 1.553494 | 0.3150414 | 0.0640127 | ||||||||
HDL | 0.5533 | 0.48561 | 0.6304192 | –0.5918636 | 0.0665783 | ||||||||
TGs | 1.14361 | 1.0642 | 1.228957 | 0.1341925 | 0.036721 | ||||||||
IKNS (Yao)94 | Direct | All-cause mortality | N/A | RR | 4087 | TC | 1.21 | 1.04 | 1.42 | 0.1906204 | 0.079448 | Y | Age, job, hypertensive status, alcohol intake, smoking Relative weight index |
8100 | TC | 1.02 | 0.87 | 1.17 | 0.0198026 | 0.075578 | |||||||
InCHIANTI131 | Simulation (1) | CVD mortality | N | OR | 1013 | TC | 0.75377 | 0.57888 | 0.9812759 | –0.2826653 | 0.1346303 | N | N/A |
LDL | 0.86559 | 0.71799 | 1.0496138 | –0.1443401 | 0.0968688 | ||||||||
HDL | 0.79941 | 0.64935 | 0.9708074 | –0.2238773 | 0.1025893 | ||||||||
TGs | 1.05079 | 0.83308 | 1.3150491 | 0.0495395 | 0.116455 | ||||||||
All-cause mortality | N/A | TC | 0.60039 | 0.51937 | 0.6926233 | –0.5101788 | 0.0734347 | ||||||
LDL | 0.67972 | 0.58184 | 0.8034778 | –0.3860811 | 0.0823357 | ||||||||
HDL | 0.78408 | 0.67755 | 0.9132076 | –0.2432447 | 0.0761445 | ||||||||
TGs | 1.03156 | 0.88566 | 1.191815 | 0.0310722 | 0.0757388 | ||||||||
Israeli Ischaemic Heart132 | Direct | CVD mortality | Y | HR | 10,059 | TC | 1.29 | 1.2 | 1.39 | 0.2546422 | 0.0374955 | N | N/A |
HDL | 0.735 | 0.671 | 0.8 | –0.3078848 | 0.0448578 | N | N/A | ||||||
All-cause mortality | N/A | TC | 1.07 | 1.03 | 1.12 | 0.0676586 | 0.0213699 | Y | Initial malignant disease | ||||
HDL | 0.917 | 0.877 | 0.961 | –0.0866478 | 0.0233335 | ||||||||
IwateKENCO133 | Orsini (1) | CVD event | Y | HR | 8714 | TC | 1.08872 | 0.96947 | 1.2226629 | 0.085007 | 0.0591942 | Y | Age, current smoking, systolic BP, BMI, uric acid, HbA1c |
LDL | 1.13676 | 0.95701 | 1.3502649 | 0.1281862 | 0.0878156 | ||||||||
HDL | 0.77186 | 0.64276 | 0.926891 | –0.2589489 | 0.0933822 | ||||||||
15,852 | TC | 1.00401 | 0.90839 | 1.1096611 | 0.0039998 | 0.0510564 | |||||||
LDL | 0.94318 | 0.81805 | 1.087448 | –0.0585027 | 0.0726201 | ||||||||
HDL | 0.92973 | 0.81348 | 1.062614 | –0.0728561 | 0.0681553 | ||||||||
Juntendo, Japan134 | Direct | CVD mortality | N | HR | 1074 | TC | 1.3727 | 1.076 | 1.691 | 0.3167796 | 0.1153239 | Y | Gender, age, current smoker, hypertension, and DM |
TGs | 1.1862 | 1.009 | 1.4451 | 0.1707549 | 0.0916374 | ||||||||
HDL | 0.909 | 0.7214 | 1.1395 | –0.0954102 | 0.1166202 | ||||||||
LDL | 1.2102 | 0.9967 | 1.4489 | 0.1907856 | 0.0954363 | ||||||||
LDL/HDL cholesterol | 1.2171 | 1.0105 | 1.4515 | 0.196471 | 0.0923858 | ||||||||
Non-HDL cholesterol | 1.361 | 1 | 1.7091 | 0.3082197 | 0.1367263 | ||||||||
KIHD135 | Direct | CVD event | Y | HR | 1931 | LDL | 1.22 | 0.94 | 1.59 | 0.1988509 | 0.134084 | Y | Age and baseline examination year |
CVD event | N | HR | 1521 | TGs | 1.143 | 0.984 | 1.317 | 0.1336564 | 0.0743586 | ||||
Apo B | 1.212 | 1.052 | 1.398 | 0.1922719 | 0.0725381 | ||||||||
HDL | 0.886 | 0.753 | 1.045 | –0.1210383 | 0.0835987 | ||||||||
Lan 2007136 | Direct | All-cause mortality | N/A | HR | 2086 | TC | 0.90595 | 0.82074 | 1 | –0.0987737 | 0.0503959 | N | N/A |
TGs | 1.00999 | 0.9201 | 1.1098426 | 0.0099385 | 0.047828 | ||||||||
TC | 0.95305 | 0.85868 | 1.0561473 | –0.0480871 | 0.0528031 | Y | Age, gender, hypertension, diabetes, heart disease, lung disease | ||||||
TGs | 1.03995 | 0.94008 | 1.1497831 | 0.0391699 | 0.0513696 | ||||||||
LIFE (subanalysis)137 | Direct | CVD event | N | HR | 8243 | Non-HDL cholesterol | 1 | 1 | 1 | 0 | 0 | N | N/A |
CVD mortality | N | Non-HDL cholesterol | 1 | 1 | 1 | 0 | 0 | ||||||
LIPID138 | Direct | CVD event | Y | HR | 4502 | LDL | 1.1136 | 1.0306 | 1.202 | 0.107598 | 0.0392463 | N | N/A |
TC | 1.0973 | 1.0163 | 1.185 | 0.0928526 | 0.0391771 | ||||||||
HDL | 0.8532 | 0.7799 | 0.9274 | –0.1587613 | 0.0441886 | ||||||||
TGs | 1.0577 | 0.9916 | 1.5229 | 0.0560967 | 0.109452 | ||||||||
TC/HDL cholesterol | 1.23 | 1.1293 | 1.3163 | 0.2070142 | 0.0390885 | ||||||||
Apo A-I | 0.8508 | 0.7887 | 0.9245 | –0.1615782 | 0.0405273 | ||||||||
Apo B | 1.1316 | 1.0488 | 1.2192 | 0.1236326 | 0.0384052 | ||||||||
LDL | 1.2093 | 1.0761 | 1.3382 | 0.1900417 | 0.0556077 | Y | Age, gender, smoking status, history of hypertension, diabetes, stroke or transient ischaemic attack, peripheral vascular disease, previous coronary revascularisation, stable angina and qualifying event | ||||||
TC | 1.1929 | 1.0651 | 1.3485 | 0.1763873 | 0.0601847 | ||||||||
HDL | 0.8491 | 0.7579 | 0.9515 | –0.1635783 | 0.0580327 | ||||||||
TGs | 1.0659 | 0.975 | 1.1714 | 0.0638195 | 0.0468157 | ||||||||
TC/HDL cholesterol | 1.2815 | 1.1458 | 1.4227 | 0.2480313 | 0.0552177 | ||||||||
Apo A-I | 0.8218 | 0.7305 | 0.9216 | –0.1962582 | 0.0592811 | ||||||||
Apo B | 1.1994 | 1.0718 | 1.3395 | 0.1818214 | 0.0568768 | ||||||||
Livermore139 | Simulation (1) | All-cause mortality | N/A | OR | 1144 | TC | 1.16465 | 1.02288 | 1.370263 | 0.1524206 | 0.0745869 | N | N/A |
LDL | 1.18376 | 1.01739 | 1.333615 | 0.1686992 | 0.0690451 | ||||||||
LRC prevalence140 | Simulation (1) | CVD mortality | Y | OR | 3678 | TC | 1.85378 | 1.52876 | 2.218434 | 0.6172268 | 0.0949867 | N | N/A |
HDL | 0.62517 | 0.48832 | 0.8406527 | –0.4697261 | 0.1385743 | ||||||||
LDL | 2.22558 | 1.81245 | 2.664015 | 0.8000189 | 0.0982532 | ||||||||
TGs | 1.34821 | 1.14333 | 1.648718 | 0.2987741 | 0.0933811 | ||||||||
TC/HDL cholesterol | 1.9442 | 1.56834 | 2.400281 | 0.6648511 | 0.1085636 | ||||||||
LDL/HDL cholesterol | 2.0877 | 1.68009 | 2.600024 | 0.7360625 | 0.1113961 | ||||||||
MEGA141 | Orsini (1) | CVD event | N | HR | 3966 | TC | 1.01465 | 0.99584 | 1.0338298 | 0.0145469 | 0.0095519 | Y | Gender, age, diabetes, hypertension, BMI, cigarette smoking |
LDL | 1.0442 | 1.00152 | 1.088698 | 0.0432549 | 0.021293 | ||||||||
HDL | 1.25136 | 1.06097 | 1.475879 | 0.224227 | 0.0842023 | ||||||||
MESA142 | Direct | CVD event | Y | HR | 6693 | TC | 1.34392 | 1.144 | 1.520757 | 0.2955892 | 0.0726224 | Y | Age, gender, cigarette smoking, DM, systolic BP, diastolic BP, antihypertensive use, lipid-lowering therapy, family history of CHD, creatinine, waist–hip ratio |
TGs | 1.27779 | 1.13175 | 1.439334 | 0.2451312 | 0.0613305 | ||||||||
HDL | 0.85652 | 0.72035 | 1.0295373 | –0.1548794 | 0.0911046 | ||||||||
LDL | 1.30624 | 1.12929 | 1.460642 | 0.2671505 | 0.0656356 | ||||||||
Non-HDL cholesterol | 1.39198 | 1.22408 | 1.575648 | 0.3307272 | 0.0644066 | ||||||||
MHS143 | Direct | All-cause mortality | N/A | HR | 11,060 | LDL | 0.9699 | 0.9115 | 1.0307 | –0.0305623 | 0.0313525 | Y | Age |
8597 | LDL | 1.0649 | 0.9685 | 1.1328 | 0.0628809 | 0.0399743 | |||||||
MONICA95 | Direct | CVD event | Y | HR | 1414 | TC/HDL cholesterol | 1.48 | 1.22 | 1.78 | 0.3920421 | 0.096368 | Y | Diabetes, smoking, BMI, alcohol, hypertensive status, age |
TC | 1.45 | 1.22 | 1.73 | 0.3715636 | 0.0890996 | ||||||||
HDL | 0.78 | 0.62 | 0.98 | –0.2484614 | 0.1167942 | ||||||||
Non-HDL cholesterol | 1.49 | 1.26 | 1.75 | 0.3987761 | 0.0838021 | ||||||||
Apo A-I | 0.91 | 0.75 | 1.12 | –0.0943107 | 0.1022987 | ||||||||
Apo B | 1.49 | 1.25 | 1.78 | 0.3987761 | 0.0901709 | ||||||||
Apo B/Apo A-I | 1.42 | 1.2 | 1.68 | 0.3506569 | 0.0858348 | ||||||||
1436 | TC/HDL cholesterol | 1.69 | 1.22 | 2.35 | 0.5247285 | 0.1672358 | |||||||
TC | 1.71 | 1.33 | 2.18 | 0.5364934 | 0.1260576 | ||||||||
HDL | 0.74 | 0.48 | 1.12 | –0.3011051 | 0.2161474 | ||||||||
Non-HDL cholesterol | 1.79 | 1.4 | 2.3 | 0.5822156 | 0.1266421 | ||||||||
Apo A-I | 0.91 | 0.62 | 1.32 | –0.0943107 | 0.1927723 | ||||||||
Apo B | 1.73 | 1.32 | 2.27 | 0.5481214 | 0.1383031 | ||||||||
Apo B/Apo A-I | 1.55 | 1.15 | 2.11 | 0.4382549 | 0.1548281 | ||||||||
MRFIT102 | Direct | CVD event | Y | HR | 5792 | HDL | 0.8027 | 0.71683 | 0.8988412 | –0.2197803 | 0.05772 | N | N/A |
LDL | 0.68876 | 0.62363 | 0.7606923 | –0.3728586 | 0.0506802 | ||||||||
465 | HDL | 0.96478 | 0.52915 | 1.759023 | –0.0358604 | 0.3064393 | |||||||
LDL | 0.52956 | 0.3125 | 0.897388 | –0.6357013 | 0.2690998 | ||||||||
NHANES III144 | Direct | All-cause mortality | OR | 7594 | TC | 1 | 0.93 | 1.07 | 0 | 0.0357728 | Y | Age, race, gender | |
HDL | 0.87 | 0.72 | 1.04 | –0.1392621 | 0.0938073 | ||||||||
LDL | 0.9 | 0.81 | 1.01 | –0.1053605 | 0.0562937 | ||||||||
Apo B | 1.17 | 0.83 | 1.64 | 0.1570037 | 0.1737311 | ||||||||
Apo A-I | 0.84 | 0.65 | 1.09 | –0.1743534 | 0.1318777 | ||||||||
LDL/HDL cholesterol | 0.98 | 0.88 | 1.08 | –0.0202027 | 0.0522435 | ||||||||
TC/HDL cholesterol | 1.04 | 0.98 | 1.08 | 0.0392207 | 0.0247867 | ||||||||
Apo B/Apo A-I | 1.35 | 0.92 | 1.98 | 0.3001046 | 0.1955302 | ||||||||
CVD mortality | TC | 1.08 | 0.97 | 1.21 | 0.076961 | 0.0563978 | |||||||
HDL | 0.76 | 0.56 | 1.05 | –0.2744368 | 0.1603594 | ||||||||
LDL | 1.03 | 0.87 | 1.21 | 0.0295588 | 0.0841537 | ||||||||
Apo B | 1.63 | 1.02 | 2.6 | 0.48858 | 0.2387012 | ||||||||
Apo A-I | 0.61 | 0.42 | 0.9 | –0.4942963 | 0.1944235 | ||||||||
LDL/HDL cholesterol | 0.95 | 0.81 | 1.12 | –0.0512933 | 0.0826657 | ||||||||
TC/HDL cholesterol | 1.08 | 1.02 | 1.14 | 0.076961 | 0.0283739 | ||||||||
Apo B/Apo A-I | 1.94 | 1.14 | 3.27 | 0.662688 | 0.2688168 | ||||||||
NIPPON data 80145 | Orsini (1) | All-cause mortality | HR | 9216 | TC | 0.98477 | 0.93333 | 1.039053 | –0.0153425 | 0.0273737 | Y | Age, serum albumin, BMI, hypertension, diabetes, cigarette smoking category, alcohol intake category, gender | |
CVD event | TC | 1.09522 | 1.00538 | 1.193083 | 0.0909516 | 0.0436676 | |||||||
Northwick Park I96 | Direct | CVD mortality | Y | RR | 2167 | TC | 1.48 | 1.27 | 1.73 | 0.3920421 | 0.0788532 | N | N/A |
TGs | 1.36 | 1.17 | 1.58 | 0.3074847 | 0.076638 | ||||||||
941 | TC | 1.32 | 0.93 | 1.88 | 0.2776317 | 0.1795517 | |||||||
TGs | 1.55 | 1.12 | 2.13 | 0.4382549 | 0.1639779 | ||||||||
Northwick Park II146 | Direct | CVD event | Y | OR | 1153 | TC | 1.44 | 1.14 | 1.82 | 0.3646431 | 0.1193388 | N | N/A |
TGs | 1.09 | 0.87 | 1.34 | 0.0861777 | 0.1101867 | ||||||||
Apo A-I | 0.74 | 0.62 | 0.88 | –0.3011051 | 0.0893374 | Y | Age and medical practice recruitment centre | ||||||
HR | 2508 | Apo B | 1.42 | 1.19 | 1.7 | 0.3506569 | 0.0909885 | ||||||
HDL | 0.78 | 0.68 | 0.94 | –0.2484614 | 0.0825987 | ||||||||
LDL | 1.31 | 1.12 | 1.52 | 0.2700271 | 0.0779035 | ||||||||
OR | 1153 | TC | 1.52 | 1.23 | 1.89 | 0.4187103 | 0.1095823 | ||||||
Nurses’ Health147 | Direct | CVD event | Y | OR | 683 | LDL | 1.4 | 1.2 | 1.6 | 0.3364722 | 0.0733883 | N | N/A |
Apo B | 1.8 | 1.5 | 2.2 | 0.5877867 | 0.0977021 | ||||||||
HDL | 0.5 | 0.4 | 0.7 | –0.6931472 | 0.1427591 | ||||||||
TGs | 1.5 | 1.2 | 1.7 | 0.4054651 | 0.0888537 | ||||||||
TC | 1.3 | 1.1 | 1.5 | 0.2623643 | 0.0791212 | ||||||||
TC/HDL cholesterol | 1.8 | 1.5 | 2.1 | 0.5877867 | 0.0858348 | ||||||||
LDL/HDL cholesterol | 1.7 | 1.5 | 2.1 | 0.5306283 | 0.0858348 | ||||||||
Apo B/HDL | 1.9 | 1.6 | 2.3 | 0.6418539 | 0.0925779 | ||||||||
Non-HDL cholesterol | 1.6 | 1.3 | 1.9 | 0.4700036 | 0.0968086 | ||||||||
Palma 2007148 | Orsinis (1) | All-cause mortality | N/A | RR | 2848 | TC | 1.10832 | 1.01913 | 1.2053269 | 0.1028442 | 0.0428073 | N | N/A |
HDL | 1.07252 | 0.96218 | 1.195504 | 0.0700066 | 0.0553881 | ||||||||
PARIS149 | Direct | CVD mortality | Y | HR | 6999 | TC | 1.38397 | 1.22134 | 1.56827 | 0.3249526 | 0.0637813 | Y | Age, BMI, cigarette, systolic BP, diabetes |
TGs | 1.08317 | 0.97029 | 1.2092747 | 0.0798904 | 0.056168 | ||||||||
Physicians’ Health150 | Direct | CVD event | Y | OR | 492 | TGs | 1.43 | 1.22 | 1.68 | 0.3576744 | 0.0816181 | Y | Age and smoking |
HDL | 0.87 | 0.76 | 0.98 | –0.1392621 | 0.0648556 | ||||||||
TC | 1.65 | 1.37 | 1.99 | 0.5007753 | 0.0952357 | ||||||||
TC/HDL cholesterol | 1.35 | 1.19 | 1.53 | 0.3001046 | 0.0641108 | ||||||||
PreCIS151 | Simulation (2) | All-cause mortality | N/A | OR | 3098 | TC | 0.99778 | 0.87646 | 1.1380555 | –0.0022261 | 0.0666301 | N | N/A |
LDL | 0.98417 | 0.83913 | 1.1522271 | –0.0159607 | 0.0808884 | ||||||||
HDL | 0.98277 | 0.82725 | 1.1625821 | –0.0173771 | 0.0868098 | ||||||||
TGs | 1.01505 | 0.87358 | 1.1920616 | 0.0149394 | 0.0792967 | ||||||||
PREVEND152 | Simulation (1) | CVD event | N | OR | 6948 | TC | 1.65718 | 1.49707 | 1.82376 | 0.5051186 | 0.0503544 | N | N/A |
LDL | 1.73994 | 1.55099 | 1.911797 | 0.5538478 | 0.0533541 | ||||||||
Non-HDL cholesterol | 1.84983 | 1.64308 | 2.026045 | 0.6150943 | 0.0534471 | ||||||||
HDL | 0.67444 | 0.61071 | 0.7422967 | –0.3938712 | 0.0497771 | ||||||||
Apo B | 1.76772 | 1.56905 | 1.957507 | 0.5696883 | 0.0564288 | ||||||||
Apo A-I | 0.77846 | 0.72136 | 0.8415349 | –0.2504392 | 0.0393102 | ||||||||
Apo A-II | 0.87281 | 0.75626 | 0.9489064 | –0.1360386 | 0.0578875 | ||||||||
TC/HDL cholesterol | 1.97133 | 1.78832 | 2.249869 | 0.6787095 | 0.0585705 | ||||||||
Apo B/Apo A-I | 1.96161 | 1.67431 | 2.209359 | 0.6737666 | 0.0707398 | ||||||||
TGs | 1.61824 | 1.43578 | 1.797426 | 0.4813416 | 0.0573073 | ||||||||
Direct | HR | TC | 1.27 | 1.15 | 1.41 | 0.2390169 | 0.0519969 | Y | Age and gender | ||||
LDL | 1.3 | 1.17 | 1.45 | 0.2623643 | 0.0547346 | ||||||||
Non-HDL cholesterol | 1.36 | 1.23 | 1.5 | 0.3074847 | 0.0506252 | ||||||||
HDL | 0.69 | 0.6 | 0.79 | –0.3710637 | 0.0701794 | ||||||||
Apo B | 1.24 | 1.13 | 1.35 | 0.2151114 | 0.0453793 | ||||||||
Apo A-I | 0.78 | 0.7 | 0.88 | –0.2484614 | 0.058378 | ||||||||
Apo A-II | 0.88 | 0.78 | 0.98 | –0.1278334 | 0.0582292 | ||||||||
TC/HDL cholesterol | 1.19 | 1.11 | 1.29 | 0.1739533 | 0.0383373 | Y | Age, gender, hypertension, diabetes, obesity, smoking, urinary albumin excretion, high-sensitivity C-reactive proteins | ||||||
Apo B/Apo A-I | 1.24 | 1.12 | 1.38 | 0.2151114 | 0.0532538 | ||||||||
PROCAM153 | Simulation (1) | CVD event | Y | OR | 5389 | TC | 2.00907 | 1.75066 | 2.359544 | 0.6976724 | 0.0761424 | N | N/A |
HDL | 0.6178 | 0.5387 | 0.7142927 | –0.4815949 | 0.0719718 | ||||||||
LDL | 2.2513 | 1.95188 | 2.681919 | 0.8115087 | 0.081056 | ||||||||
Progetto Cuore154 | Direct | CVD event | Y | HR | 20,447 | TC | 1.4 | 1.31 | 1.5 | 0.3364722 | 0.0345505 | Y | Age and gender |
HDL | 1.35 | 1.23 | 1.48 | 0.3001046 | 0.047201 | ||||||||
TGs | 1.11 | 1.05 | 1.18 | 0.10436 | 0.0297766 | ||||||||
PROSPER155 | Simulation (2) | CVD event | Y | OR | 2913 | LDL | 0.97281 | 0.92993 | 1.0154191 | –0.0275624 | 0.022436 | N | N/A |
HDL | 0.80445 | 0.77566 | 0.8379954 | –0.2175952 | 0.0197197 | ||||||||
Puerto Rico101 | Direct | CVD event | Y | OR | 5766 | TC | 1.2866 | 1.15229 | 1.436558 | 0.252 | 0.0562499 | Y | Age, physical activity, systolic BP, relative weight, smoking, heart rate |
2389 | TC | 1.12075 | 0.90592 | 1.386524 | 0.1139999 | 0.1085714 | |||||||
CVD mortality | Y | 6205 | TC | 1.17941 | 1.08143 | 1.286234 | 0.165016 | 0.0442439 | Y | Age, physical activity, systolic BP, relative weight, smoking, heart rate, urban or rural residence, abnormal blood glucose, education, alcohol, vital capacity, haematocrit | |||
Quebec Cardiovascular156 | Direct | CVD event | Y | HR | 2155 | TC | 1.45208 | 1.22444 | 1.7220578 | 0.373 | 0.087 | Y | Age, systolic BP, DM, smoking habits, and medication |
TGs | 2.00571 | 1.79019 | 2.2471888 | 0.696 | 0.058 | ||||||||
HDL | 0.43605 | 0.2095 | 0.9075923 | –0.83 | 0.374 | ||||||||
TC/HDL cholesterol | 1.18057 | 1.09584 | 1.2718595 | 0.166 | 0.038 | ||||||||
Apo B | 1.01207 | 1.00614 | 1.0180408 | 0.012 | 0.003 | ||||||||
Apo A-I | 0.99203 | 0.98236 | 1.0018016 | –0.008 | 0.005 | ||||||||
Simulation (1) | OR | 2072 | TC | 1.31614 | 1.12107 | 1.493042 | 0.2747009 | 0.0730961 | N | N/A | |||
LDL | 1.4073 | 1.18806 | 1.654956 | 0.3416708 | 0.0845536 | ||||||||
HDL | 0.85616 | 0.75531 | 1.0037664 | –0.1553017 | 0.0725486 | ||||||||
TC/HDL cholesterol | 1.40443 | 1.15449 | 1.673964 | 0.3396322 | 0.0947789 | ||||||||
Apo B | 1.44155 | 1.26764 | 1.690916 | 0.3657217 | 0.0734975 | ||||||||
TGs | 0.95344 | 0.85602 | 1.0770886 | –0.0476776 | 0.0586039 | ||||||||
2177 | LDL/HDL cholesterol | 1.4288 | 1.19647 | 1.654185 | 0.3568377 | 0.082637 | |||||||
Rancho Bernardo157 | Direct | CVD mortality | N | HR | 922 | TC | 1.13 | 0.95 | 1.35 | 0.1222176 | 0.0896423 | Y | Age, smoking pack-years, systolic BP, fasting plasma glucose, BMI, exercise, current use of oestrogen for women |
TC/HDL cholesterol | 1.17 | 0.99 | 1.39 | 0.1570037 | 0.0865699 | ||||||||
HDL | 0.87 | 0.69 | 1.09 | –0.1392621 | 0.1166432 | ||||||||
Non-HDL cholesterol | 1.17 | 0.98 | 1.39 | 0.1570037 | 0.0891598 | ||||||||
LDL | 1.14 | 0.96 | 1.36 | 0.1310283 | 0.0888537 | ||||||||
TGs | 1.13 | 0.96 | 1.29 | 0.1222176 | 0.0753735 | ||||||||
1305 | TC | 0.87 | 0.72 | 1.05 | –0.1392621 | 0.0962485 | |||||||
TC/HDL cholesterol | 1.07 | 0.88 | 1.29 | 0.0676586 | 0.0975703 | ||||||||
HDL | 0.92 | 0.75 | 1.13 | –0.0833816 | 0.1045663 | ||||||||
Non-HDL cholesterol | 0.91 | 0.76 | 1.09 | –0.0943107 | 0.0919935 | ||||||||
LDL | 0.9 | 0.75 | 1.09 | –0.1053605 | 0.0953724 | ||||||||
TGs | 1.05 | 0.86 | 1.29 | 0.0487902 | 0.103435 | ||||||||
Reykjavik study97 | Direct | CVD event | Y | HR | 9681 | TC | 1.34478 | 1.25702 | 1.433509 | 0.2962319 | 0.0335155 | Y | Age |
8888 | TC | 1.36848 | 1.31199 | 1.436535 | 0.313697 | 0.0231347 | |||||||
RIFLE158 | Direct | CVD mortality | Y | OR | 20,386 | TC | 1.36412 | 1.16387 | 1.598842 | 0.3105066 | 0.0810014 | Y | Age, distolic BP, mean number of cigarettes per day |
Rotterdam98 | Direct | CVD event | Y | HR | 2453 | TC | 1.23552 | 1.03488 | 1.489684 | 0.2114927 | 0.0929275 | Y | Age, systolic BP, BMI, smoking, alcohol |
HDL | 0.97027 | 0.95288 | 0.993556 | –0.0301799 | 0.0106631 | ||||||||
Non-HDL/HDL | 1.27181 | 1.08225 | 1.491695 | 0.2404403 | 0.0818553 | ||||||||
3553 | TC | 1.5025 | 1.24684 | 1.832971 | 0.4071317 | 0.0982982 | |||||||
HDL | 0.95892 | 0.93512 | 0.9854115 | –0.0419528 | 0.0133629 | ||||||||
Non-HDL/HDL | 1.43227 | 1.26992 | 1.640581 | 0.3592613 | 0.0653301 | ||||||||
CVD mortality | Y | 5255 | TC/HDL cholesterol | 0.984 | 0.7137 | 1.3387 | –0.0161294 | 0.160457 | Y | Age | |||
All-cause mortality | N/A | TC/HDL cholesterol | 0.8903 | 0.6858 | 1.1647 | –0.1161968 | 0.1351104 | ||||||
SHEP159 | Direct | CVD event | Y | HR | 4736 | TC | 1.14621 | 1.03355 | 1.260026 | 0.1364565 | 0.0505434 | N | N/A |
HDL | 0.74574 | 0.65722 | 0.8437637 | –0.2933844 | 0.0637384 | ||||||||
Non-HDL cholesterol | 1.2575 | 1.13364 | 1.393968 | 0.2291224 | 0.0527353 | ||||||||
TC/HDL cholesterol | 1.33619 | 1.21612 | 1.459418 | 0.28982 | 0.0465231 | ||||||||
TC | 1.24859 | 1.12358 | 1.374931 | 0.2220181 | 0.0515014 | Y | Age, race, gender, history of CHD, diastolic BP, smoking, diabetes, alcohol use, Rose angina questionnaire, presence of carotid bruit, and uric acid | ||||||
HDL | 0.90243 | 0.78499 | 1.0389546 | –0.1026589 | 0.0715065 | ||||||||
Non-HDL cholesterol | 1.29149 | 1.15607 | 1.451222 | 0.2557935 | 0.0580061 | ||||||||
TC/HDL cholesterol | 1.23095 | 1.09935 | 1.366703 | 0.2077879 | 0.0555305 | ||||||||
SHS160 | Orsini (2) | CVD event | N | HR | 2108 | Non-HDL cholesterol | 1.1491 | 1.082 | 1.2204 | 0.138979 | 0.030706 | Y | Age, gender, BMI, smoking status, study centre, SBP, HbA1c, fibrinogen, insulin, albumin to creatinine ratio |
HDL | 0.9507 | 0.9048 | 0.9989 | –0.0505567 | 0.02524 | ||||||||
LDL | 1.1241 | 1.0582 | 1.1941 | 0.1169827 | 0.0308223 | ||||||||
TGs | 1.03519 | 1.01334 | 1.0575 | 0.0345821 | 0.0108806 | ||||||||
TC/HDL cholesterol | 1.22874 | 1.12542 | 1.341547 | 0.2059917 | 0.0448124 | ||||||||
SLVHS161 | Simulation (2) | CVD event | Y | OR | 1682 | TC | 0.9541 | 0.82293 | 1.1079933 | –0.046982 | 0.0758774 | N | N/A |
HDL | 0.74258 | 0.66179 | 0.8269951 | –0.2976204 | 0.0568499 | ||||||||
HR | 936 | Non-HDL cholesterol | 1.5236 | 1.26416 | 1.835 | 0.421076 | 0.0950604 | ||||||
746 | Non-HDL cholesterol | 1.2933 | 1.0897 | 1.5339 | 0.2571971 | 0.0872222 | |||||||
Stanek 2007162 | Simulation (1) | CVD event | N | OR | 30,348 | LDL | 1.02605 | 0.99445 | 1.0580013 | 0.0257155 | 0.0158019 | N | N/A |
HDL | 0.93708 | 0.90589 | 0.9667735 | –0.064987 | 0.0165939 | ||||||||
TGs | 1.01895 | 0.99196 | 1.0504162 | 0.0187696 | 0.0146066 | ||||||||
TC | 1.00839 | 0.97479 | 1.037563 | 0.008359 | 0.0159201 | ||||||||
Stockholm County163 | Simulation (1) | CVD event | N | OR | 3502 | TC | 1.11827 | 0.99938 | 1.2482277 | 0.1117855 | 0.0567194 | N | N/A |
LDL | 1.21089 | 1.09725 | 1.351346 | 0.1913548 | 0.0531372 | ||||||||
HDL | 0.65242 | 0.59352 | 0.7131401 | –0.4270698 | 0.0468387 | ||||||||
TGs | 1.46591 | 1.25004 | 1.779722 | 0.3824769 | 0.0901227 | ||||||||
Apo B | 1.49395 | 1.35008 | 1.649826 | 0.4014236 | 0.0511502 | ||||||||
Apo A-I | 0.67937 | 0.60199 | 0.7735397 | –0.3865913 | 0.0639641 | ||||||||
Swedish NDR164 | Direct | CVD event | Y | HR | 18,673 | Non-HDL cholesterol | 1.2 | 1.14 | 1.27 | 0.1823216 | 0.0275481 | Y | Age, gender, diabetes duration, type of hypoglycaemic treatment, HbA1c, systolic BP, smoking, BMI, albuminuria, and a history of CVD |
LDL | 1.17 | 1.1 | 1.24 | 0.1570037 | 0.0305615 | ||||||||
HDL | 0.85 | 0.79 | 0.91 | –0.1625189 | 0.0360744 | ||||||||
TC | 1.14 | 1.08 | 1.21 | 0.1310283 | 0.0289947 | ||||||||
TC/HDL cholesterol | 1.23 | 1.17 | 1.3 | 0.2070142 | 0.0268777 | ||||||||
LDL/HDL cholesterol | 1.22 | 1.15 | 1.28 | 0.1988509 | 0.027321 | ||||||||
Thrombo165 | Simulation (1) | CVD event | Y | OR | 1045 | Apo A-I | 0.92685 | 0.74502 | 1.1611849 | –0.0759613 | 0.1132102 | N | N/A |
Apo B | 1.13324 | 0.85321 | 1.5439409 | 0.1250778 | 0.1512985 | ||||||||
TC | 1.03533 | 0.81983 | 1.2710019 | 0.0347215 | 0.1118545 | ||||||||
HDL | 1.07837 | 0.80226 | 1.5243062 | 0.0754505 | 0.1637395 | ||||||||
LDL | 1.08819 | 0.8915 | 1.4692173 | 0.0845191 | 0.1274437 | ||||||||
TGs | 1.00974 | 0.79287 | 1.228994 | 0.0096958 | 0.1118092 | ||||||||
Ting 2010166 | Direct | CVD event | N | HR | 4521 | TGs | 1.0586 | 0.97771 | 1.1514927 | 0.0569512 | 0.0417344 | N | N/A |
HDL | 0.8402 | 0.75106 | 0.9366095 | –0.1741188 | 0.0563231 | Y | Age, smoking status, duration of diabetes, HbA1c, natural log (urine albumin–creatinine ratio + 1), use of statins, fibrates, gliclazide and rosiglitazone during FU, years of enrolment (selected by the stepwise algorithm with p = 0.10 for inclusion and removal) | ||||||
TGs | 1.01476 | 0.90974 | 1.1232011 | 0.0146539 | 0.0537703 | ||||||||
HDL | 0.84949 | 0.76835 | 0.9440873 | –0.1631178 | 0.0525436 | N | N/A | ||||||
TLGS88 | Direct | CVD event | N | HR | 2317 | TC | 0.76 | 0.73 | 0.8 | –0.2744368 | 0.023359 | Y | Age, waist–hip ratio, diastolic blood pressure, smoking status, anti-depressive drug use, family history of premature cardiovascular disease |
LDL | 0.77 | 0.74 | 0.8 | –0.2613648 | 0.0198881 | ||||||||
HDL | 0.77 | 0.73 | 0.8 | –0.2613648 | 0.023359 | ||||||||
Non-HDL cholesterol | 0.77 | 0.74 | 0.8 | –0.2613648 | 0.0198881 | ||||||||
TC/HDL cholesterol | 0.77 | 0.74 | 0.8 | –0.2613648 | 0.0198881 | ||||||||
2993 | TC | 1.22 | 1.01 | 1.48 | 0.1988509 | 0.0974724 | |||||||
LDL | 1.22 | 0.99 | 1.49 | 0.1988509 | 0.1042925 | ||||||||
HDL | 1 | 0.81 | 1.24 | 0 | 0.1086307 | ||||||||
Non-HDL cholesterol | 1.22 | 1.01 | 1.48 | 0.1988509 | 0.0974724 | ||||||||
TC/HDL cholesterol | 1.17 | 0.97 | 1.41 | 0.1570037 | 0.0954206 | ||||||||
CVD mortality | N | 3004 | TC | 1.126 | 0.943 | 1.345 | 0.1186715 | 0.0905824 | Y | Age | |||
HDL | 0.991 | 0.827 | 1.187 | –0.0090407 | 0.0921887 | ||||||||
3830 | TC | 1.133 | 0.886 | 1.446 | 0.124869 | 0.124959 | |||||||
HDL | 0.939 | 0.723 | 1.218 | –0.0629398 | 0.1330501 | ||||||||
All-cause mortality | N/A | 3004 | TC | 0.8923 | 0.778 | 1.022 | –0.1139529 | 0.0695893 | |||||
HDL | 1.06 | 0.936 | 1.201 | 0.0582689 | 0.0635955 | ||||||||
3830 | TC | 0.921 | 0.771 | 1.101 | –0.0822952 | 0.0908892 | |||||||
HDL | 1.043 | 0.88 | 1.237 | 0.0421012 | 0.086868 | ||||||||
TREAT167 | Simulation (1) | CVD mortality | N | OR | 4038 | TC | 1.01095 | 0.93796 | 1.087578 | 0.0108943 | 0.0377559 | N | N/A |
HDL | 0.86975 | 0.80148 | 0.9204163 | –0.1395548 | 0.0352963 | ||||||||
TGs | 1.08711 | 0.99863 | 1.1893585 | 0.0835222 | 0.0445879 | ||||||||
Turku Elderly168 | Simulation (2) | All-cause mortality | N/A | OR | 1032 | TC | 0.71885 | 0.67948 | 0.7531239 | –0.3301059 | 0.0262513 | N | N/A |
LDL | 0.70642 | 0.66572 | 0.7493017 | –0.3475462 | 0.0301726 | ||||||||
HDL | 0.36388 | 0.31074 | 0.422555 | –1.0109309 | 0.0784053 | ||||||||
TGs | 1.14738 | 1.06299 | 1.23794 | 0.1374837 | 0.0388691 | ||||||||
ULSAM169 | Direct | CVD event | Y | HR | 1108 | Apo B | 1.5 | 1.27 | 1.77 | 0.4054651 | 0.0846843 | N | N/A |
Apo A-I | 0.7 | 0.58 | 0.84 | –0.3566749 | 0.0944831 | ||||||||
Apo B/Apo A-I | 1.65 | 1.39 | 1.96 | 0.5007753 | 0.0876635 | ||||||||
TC | 1.27 | 1.08 | 1.5 | 0.2390169 | 0.0838021 | ||||||||
LDL | 1.38 | 1.17 | 1.61 | 0.3220835 | 0.0814363 | ||||||||
HDL | 0.56 | 0.46 | 0.69 | –0.5798185 | 0.103435 | ||||||||
TGs | 1.42 | 1.21 | 1.66 | 0.3506569 | 0.0806626 | ||||||||
Apo B/Apo A-I | 1.46 | 1.24 | 1.71 | 0.3784364 | 0.0819852 | Y | Intact proinsulin, systolic BP, family history of MI, smoking | ||||||
VA-HIT170 | Simulation (2) | CVD event | N | OR | 1267 | HDL | 0.90063 | 0.84345 | 0.976114 | –0.1046563 | 0.0372662 | N | N/A |
TGs | 1.03635 | 0.96193 | 1.1215739 | 0.0357011 | 0.0391711 | ||||||||
LDL | 1.09639 | 1.03455 | 1.151628 | 0.092023 | 0.027349 | ||||||||
Whitehall I171 | Direct | All-cause mortality | N/A | HR | 17,718 | TC | 1.0752 | 1.0526 | 1.1111 | 0.0725067 | 0.0137978 | Y | Age |
CVD mortality | N | TC | 1.2048 | 1.1627 | 1.25 | 0.1863136 | 0.018469 | ||||||
Women’s Health172 | Orsini (1) | CVD event | N | HR | 15,632 | TC | 1.1137 | 1.05361 | 1.177214 | 0.1076869 | 0.0282978 | Y | Age, BP (Framingham categories), BMI, diabetes, smoking |
LDL | 1.08869 | 1.02196 | 1.159787 | 0.0849779 | 0.0322745 | ||||||||
HDL | 0.86379 | 0.81525 | 0.91522 | –0.1464272 | 0.0295084 | ||||||||
Non-HDL cholesterol | 1.20253 | 1.11155 | 1.300949 | 0.184426 | 0.0401369 | ||||||||
Apo A-I | 0.93969 | 0.91278 | 0.9673951 | –0.062206 | 0.0148254 | ||||||||
Apo B | 1.18758 | 1.09539 | 1.287522 | 0.1719159 | 0.0412263 | ||||||||
TC/HDL cholesterol | 1.41397 | 1.2708 | 1.573282 | 0.3464042 | 0.0544693 | ||||||||
LDL/HDL cholesterol | 1.41144 | 1.25807 | 1.583513 | 0.3446105 | 0.0586916 | ||||||||
Apo B/Apo A-I | 1.31969 | 1.19193 | 1.46114 | 0.2773946 | 0.05195 | ||||||||
WOSCOPS19 | Simulation (2) | CVD event | Y | OR | 3293 | LDL | 1.03552 | 0.95972 | 1.115381 | 0.0348989 | 0.0383438 | N | N/A |
HDL | 1.20456 | 1.12058 | 1.290148 | 0.1861168 | 0.0359459 | ||||||||
TC | 0.98056 | 0.91338 | 1.05561 | –0.0196335 | 0.0369197 | ||||||||
TGs | 1.1652 | 1.07397 | 1.253741 | 0.1528962 | 0.0394819 | ||||||||
Yao City89 | Simulation (1) | CVD event | Y | OR | 8129 | TC | 1.646 | 1.13673 | 2.325978 | 0.4983463 | 0.1826497 | Y | Age and gender |
HDL | 0.62401 | 0.46981 | 0.8419168 | –0.471587 | 0.1488153 | ||||||||
3848 | TC | 1.93286 | 1.35981 | 2.739954 | 0.6590018 | 0.1787231 | |||||||
HDL | 0.75962 | 0.34308 | 2.0820889 | –0.2749337 | 0.4599921 |
Appendix 9 Forest plots for each lipid measure as prognostic markers in populations not taking statins
(Plots presented only when more than three data sets per prognostic marker.)
FIGURE 58.
Low-density lipoprotein as prognostic marker for cardiovascular events in populations not taking statins. IV, inverse variance.

FIGURE 59.
Low-density lipoprotein as prognostic marker for cardiovascular events (adjusted estimates) in populations not taking statins. IV, inverse variance.

FIGURE 60.
Low-density lipoprotein as prognostic marker for cardiovascular mortality (adjusted estimates) in populations not taking statins. IV, inverse variance.

FIGURE 61.
Low-density lipoprotein as prognostic marker for all-cause mortality in populations not taking statins. IV, inverse variance.

FIGURE 62.
Low-density lipoprotein as prognostic marker for all-cause mortality (adjusted estimates) in populations not taking statins. IV, inverse variance.

FIGURE 63.
Total cholesterol as prognostic marker for cardiovascular events in populations not taking statins. IV, inverse variance.

FIGURE 64.
Total cholesterol as prognostic marker for cardiovascular events (adjusted estimates) in populations not taking statins. IV, inverse variance.

FIGURE 65.
Total cholesterol as prognostic marker for cardiovascular mortality in populations on statins. IV, inverse variance.
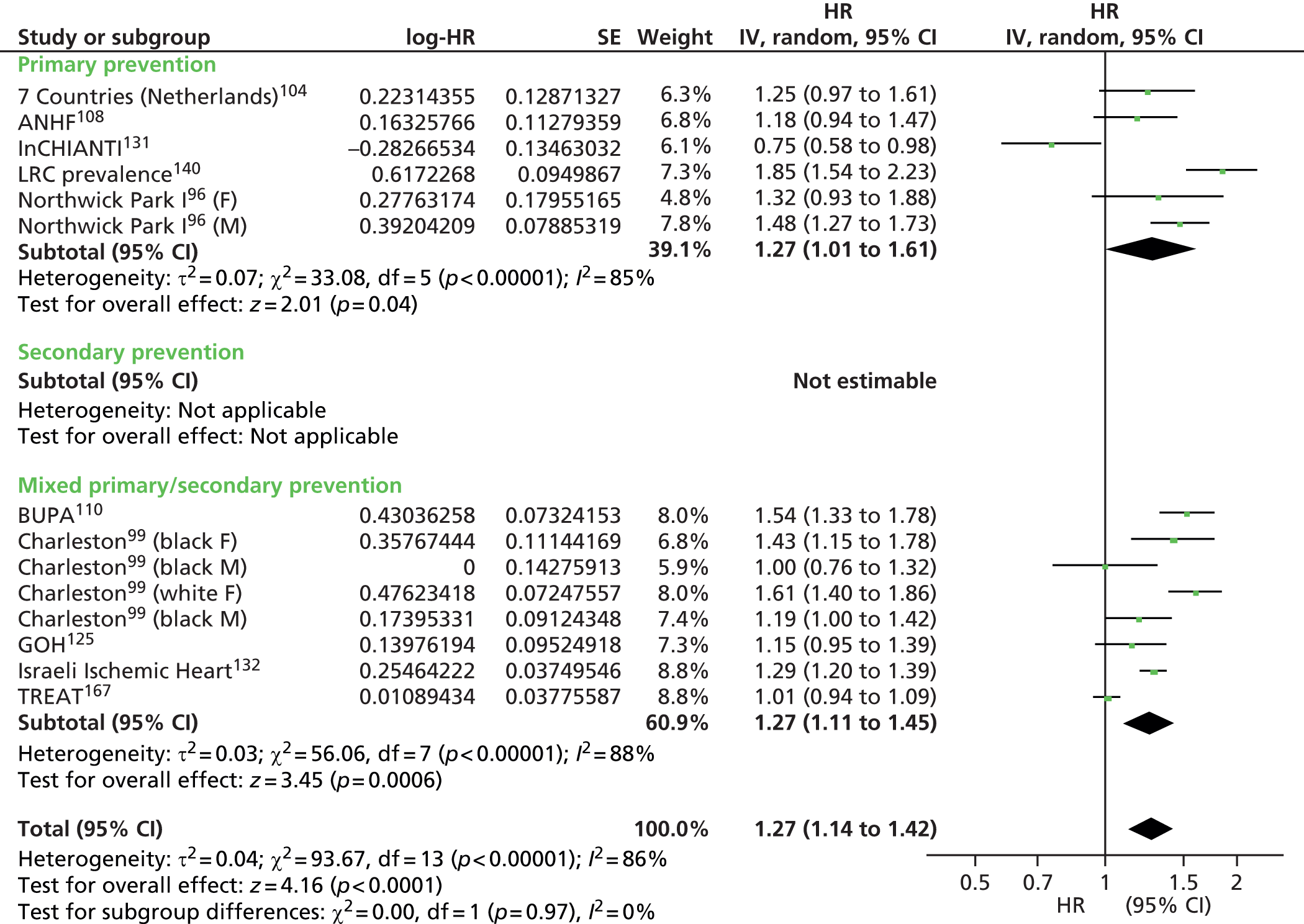
FIGURE 66.
Total cholesterol as prognostic marker for cardiovascular mortality (adjusted estimates) in populations not taking statins. IV, inverse variance.

FIGURE 67.
Total cholesterol as prognostic marker for all-cause mortality in populations not taking statins. IV, inverse variance.
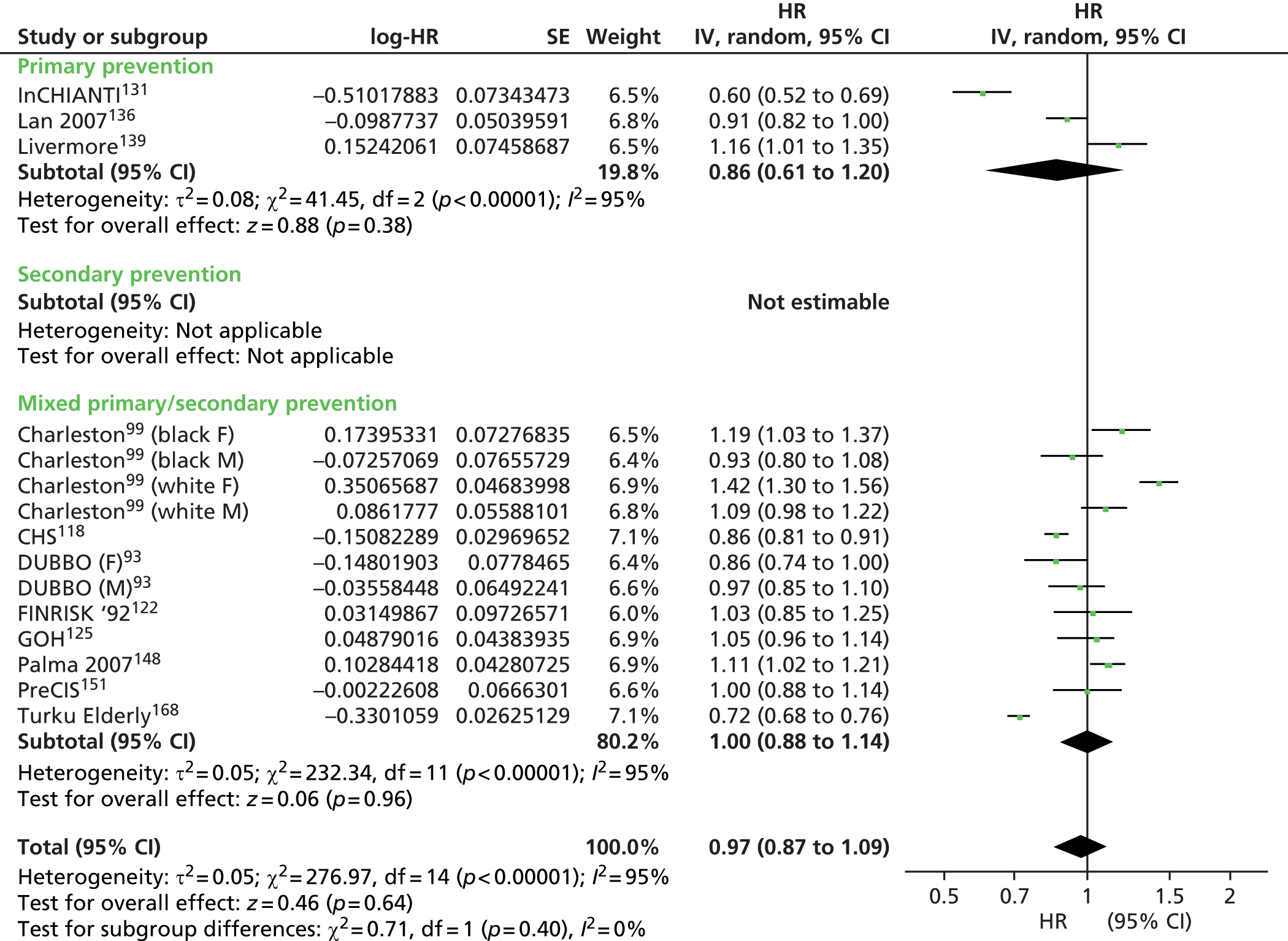
FIGURE 68.
Total cholesterol as prognostic marker for all-cause mortality (adjusted estimates) in populations not taking statins. IV, inverse variance.

FIGURE 69.
High-density lipoprotein cholesterol as prognostic marker for cardiovascular events in populations not taking statins. IV, inverse variance.
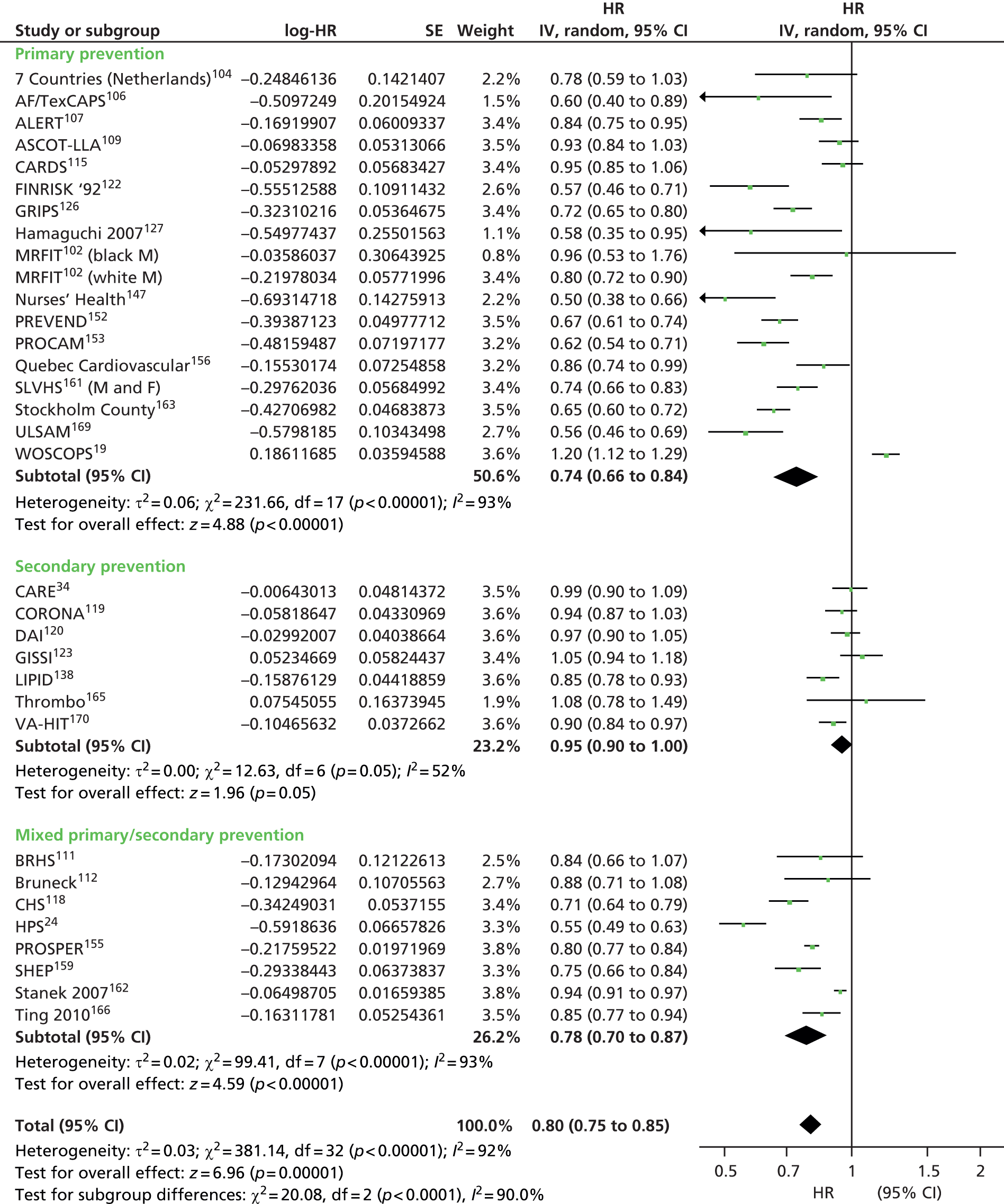
FIGURE 70.
High-density lipoprotein cholesterol as prognostic marker for cardiovascular events (adjusted estimates) in populations not taking statins. IV, inverse variance.

FIGURE 71.
High-density lipoprotein cholesterol as prognostic marker for cardiovascular mortality in populations not taking statins. IV, inverse variance.

FIGURE 72.
High-density lipoprotein cholesterol as prognostic marker for cardiovascular mortality (adjusted estimates) in populations not taking statins. IV, inverse variance.

FIGURE 73.
High-density lipoprotein cholesterol as prognostic marker for all-cause mortality in populations not taking statins. IV, inverse variance.

FIGURE 74.
High-density lipoprotein cholesterol as prognostic marker for all-cause mortality (adjusted estimates) in populations not taking statins. IV, inverse variance.

FIGURE 75.
Triglycerides as prognostic marker for cardiovascular events in populations not taking statins. IV, inverse variance.
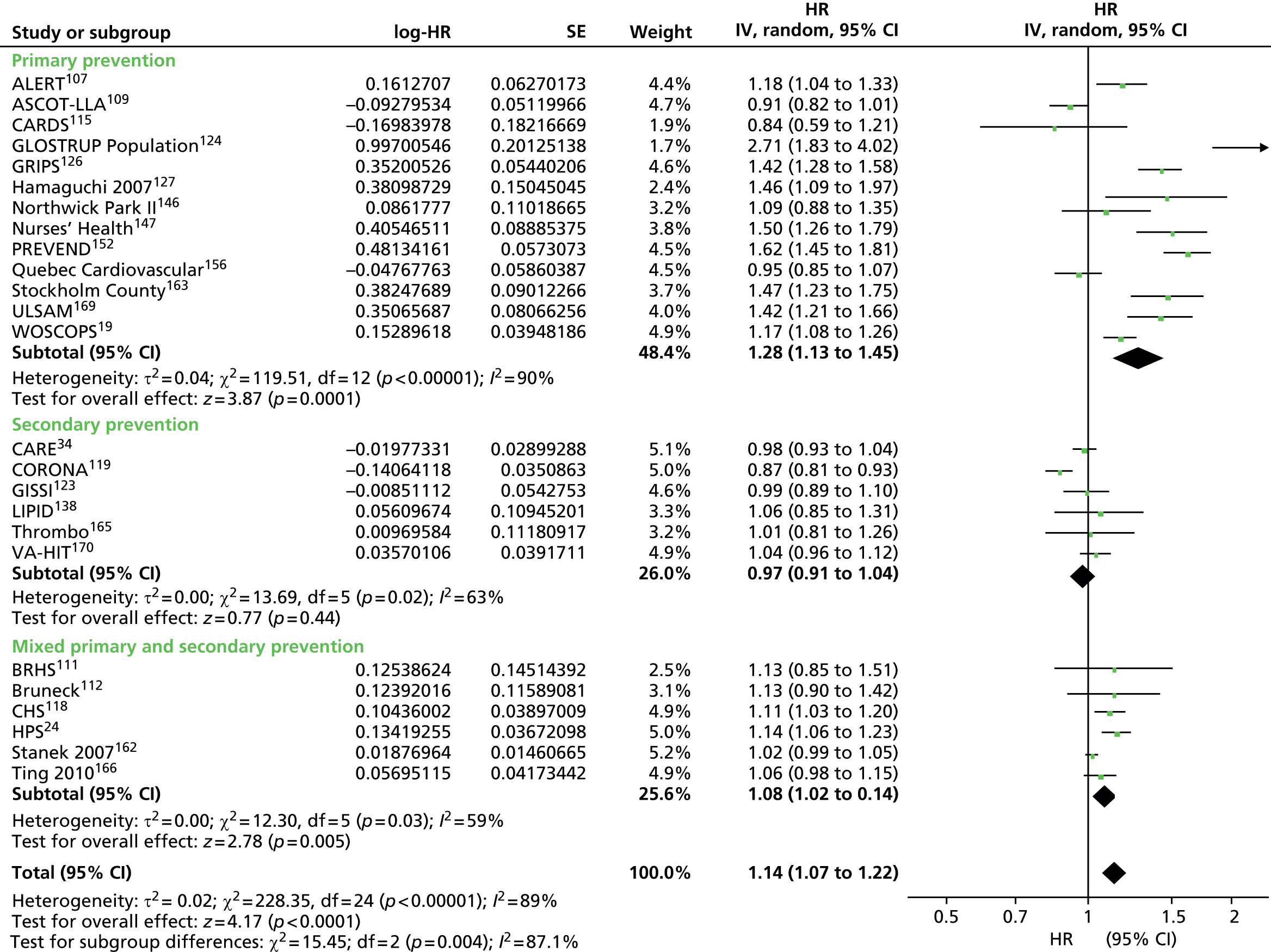
FIGURE 76.
Triglycerides as prognostic marker for cardiovascular events (adjusted estimates) in populations not taking statins. IV, inverse variance.

FIGURE 77.
Triglycerides as prognostic marker for cardiovascular mortality in populations not taking statins. IV, inverse variance.

FIGURE 78.
Triglycerides as prognostic marker for cardiovascular mortality (adjusted estimates) in populations not taking statins. IV, inverse variance.
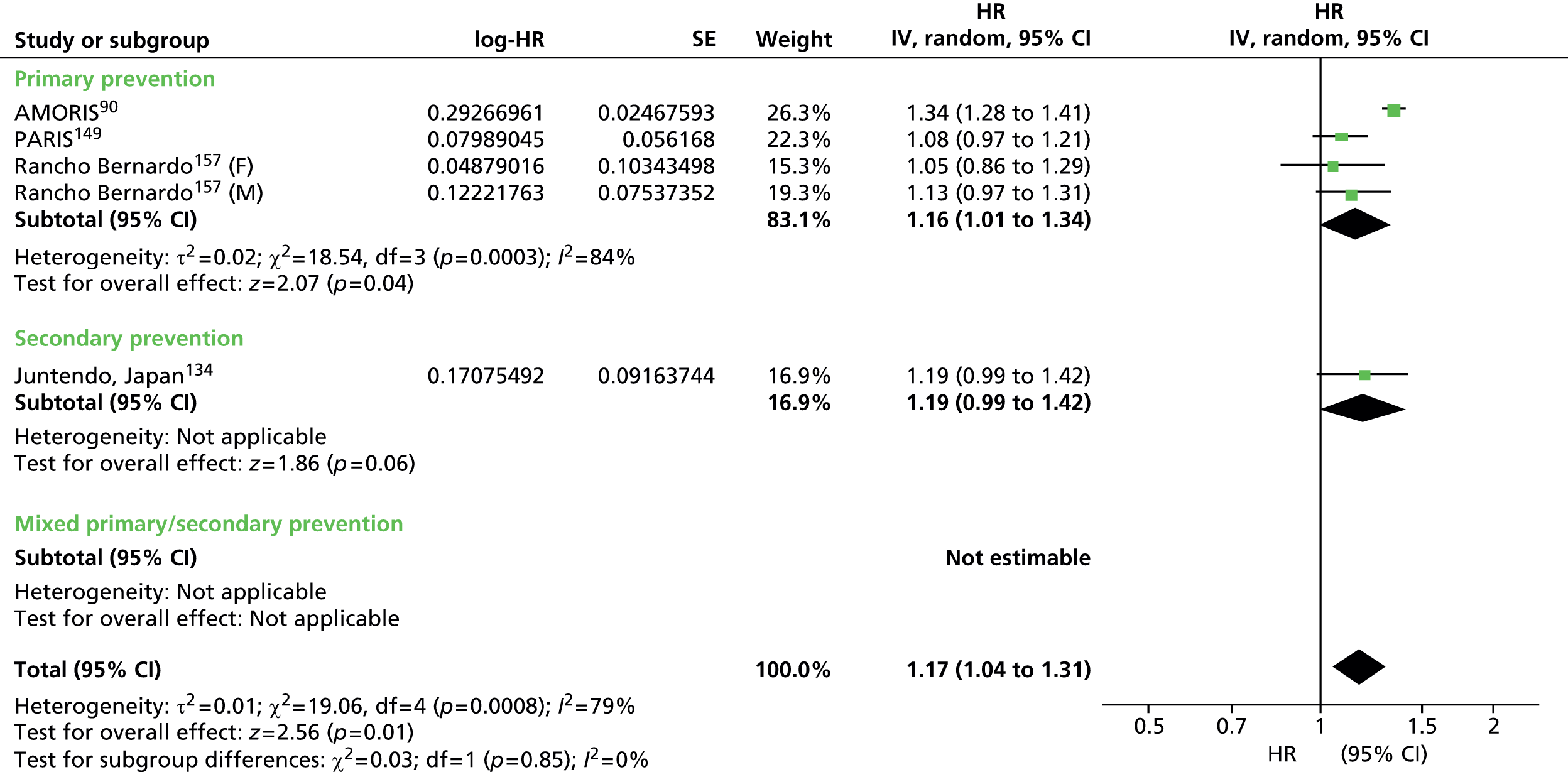
FIGURE 79.
Triglycerides as prognostic marker for all-cause mortality in populations not taking statins. IV, inverse variance.

FIGURE 80.
Triglycerides as prognostic marker for all-cause mortality (adjusted estimates) in populations not taking statins. IV, inverse variance.
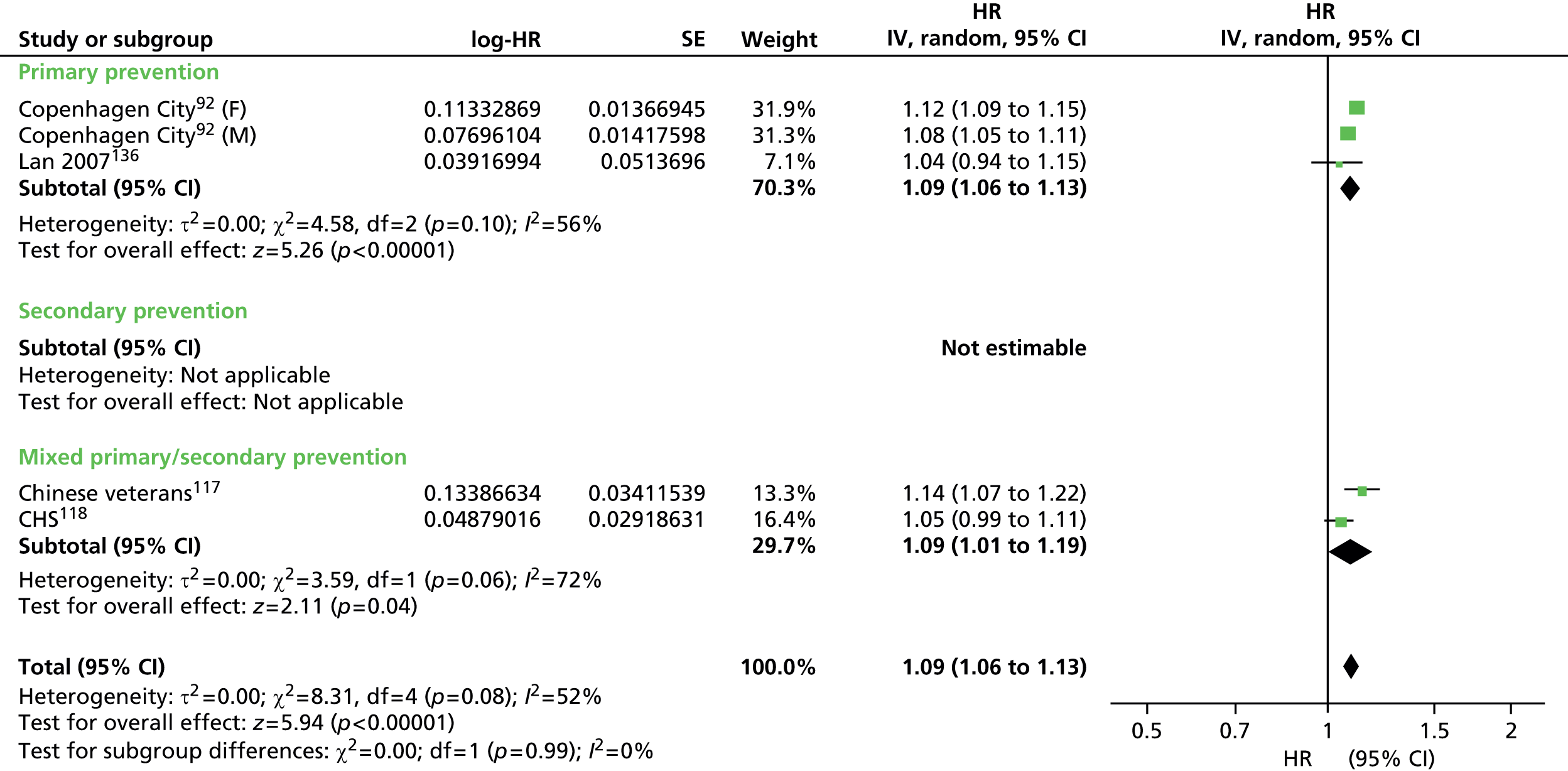
FIGURE 81.
Non-HDL cholesterol as prognostic marker for cardiovascular events in populations not taking statins. IV, inverse variance.

FIGURE 82.
Non-HDL cholesterol as prognostic marker for cardiovascular events (adjusted estimates) in populations not taking statins. IV, inverse variance.
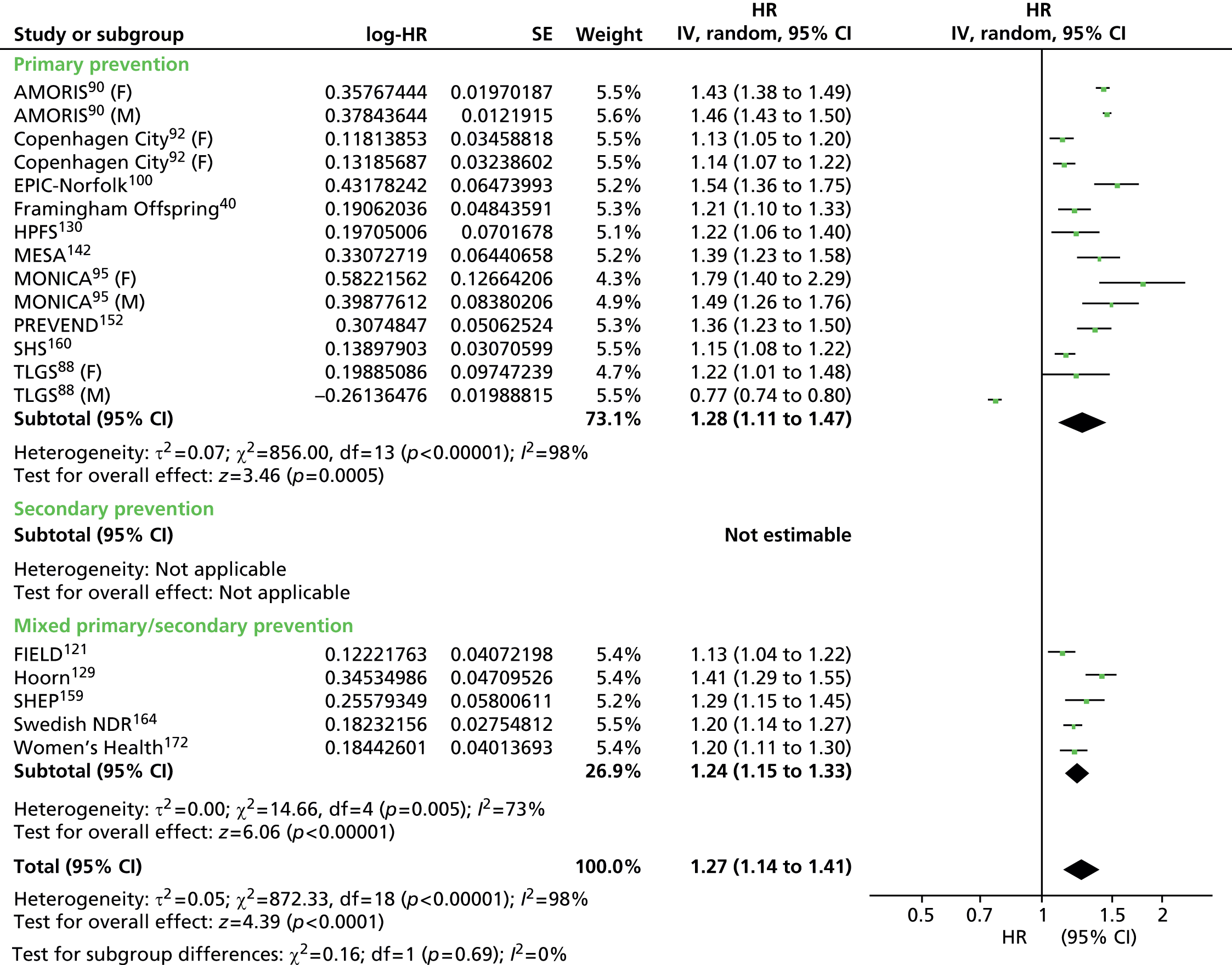
FIGURE 83.
Non-HDL cholesterol as prognostic marker for cardiovascular mortality (adjusted estimates) in populations not taking statins. IV, inverse variance.

FIGURE 84.
Apolipoprotein B as prognostic marker for cardiovascular events in populations not taking statins. IV, inverse variance.

FIGURE 85.
Apolipoprotein B as prognostic marker for cardiovascular events (adjusted estimates) in populations not taking statins. IV, inverse variance.

FIGURE 86.
Apolipoprotein A-I as prognostic marker for cardiovascular events in populations not taking statins. IV, inverse variance.
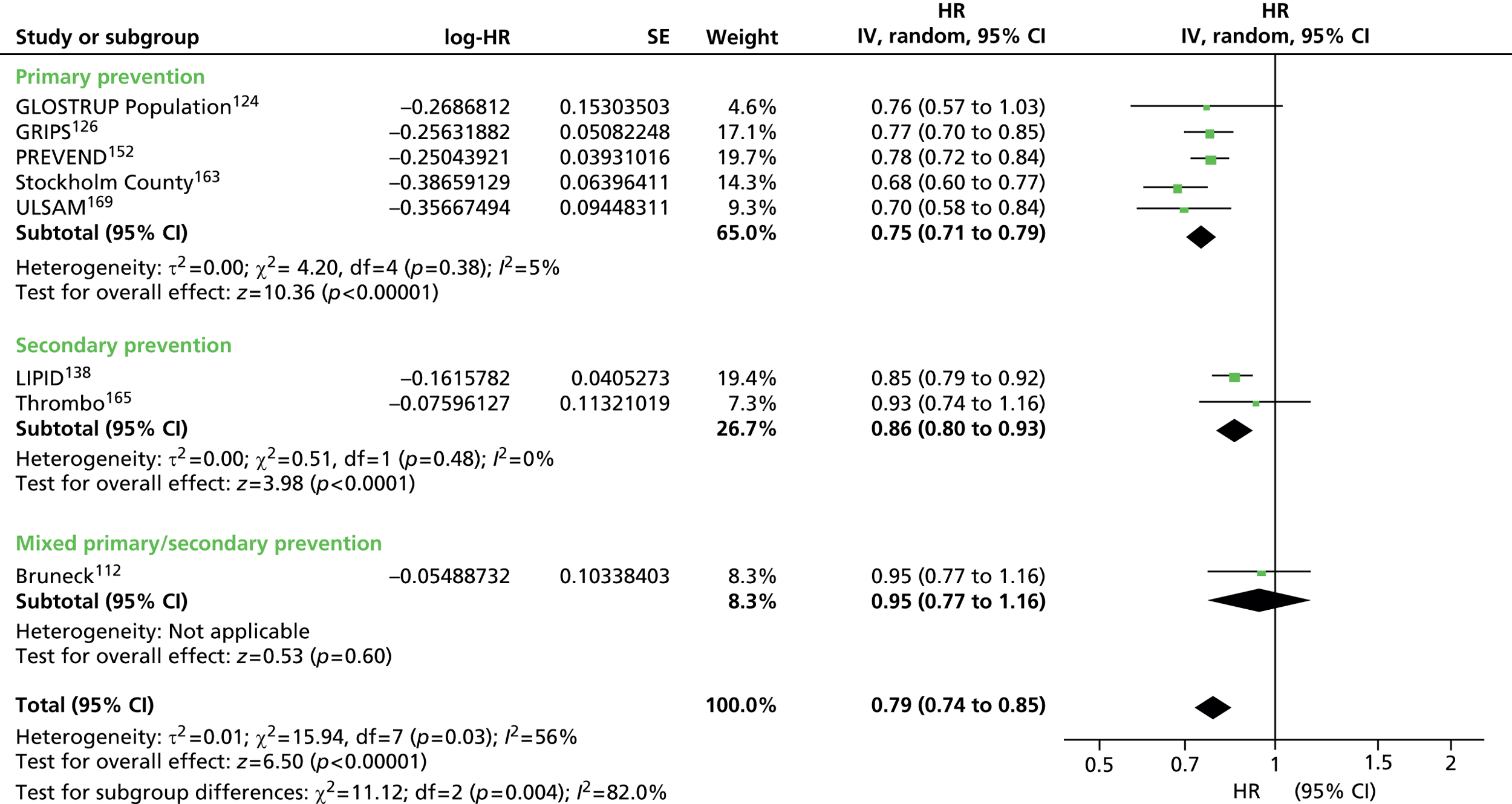
FIGURE 87.
Apolipoprotein A-I as prognostic marker for cardiovascular events (adjusted estimates) in populations not taking statins. IV, inverse variance.
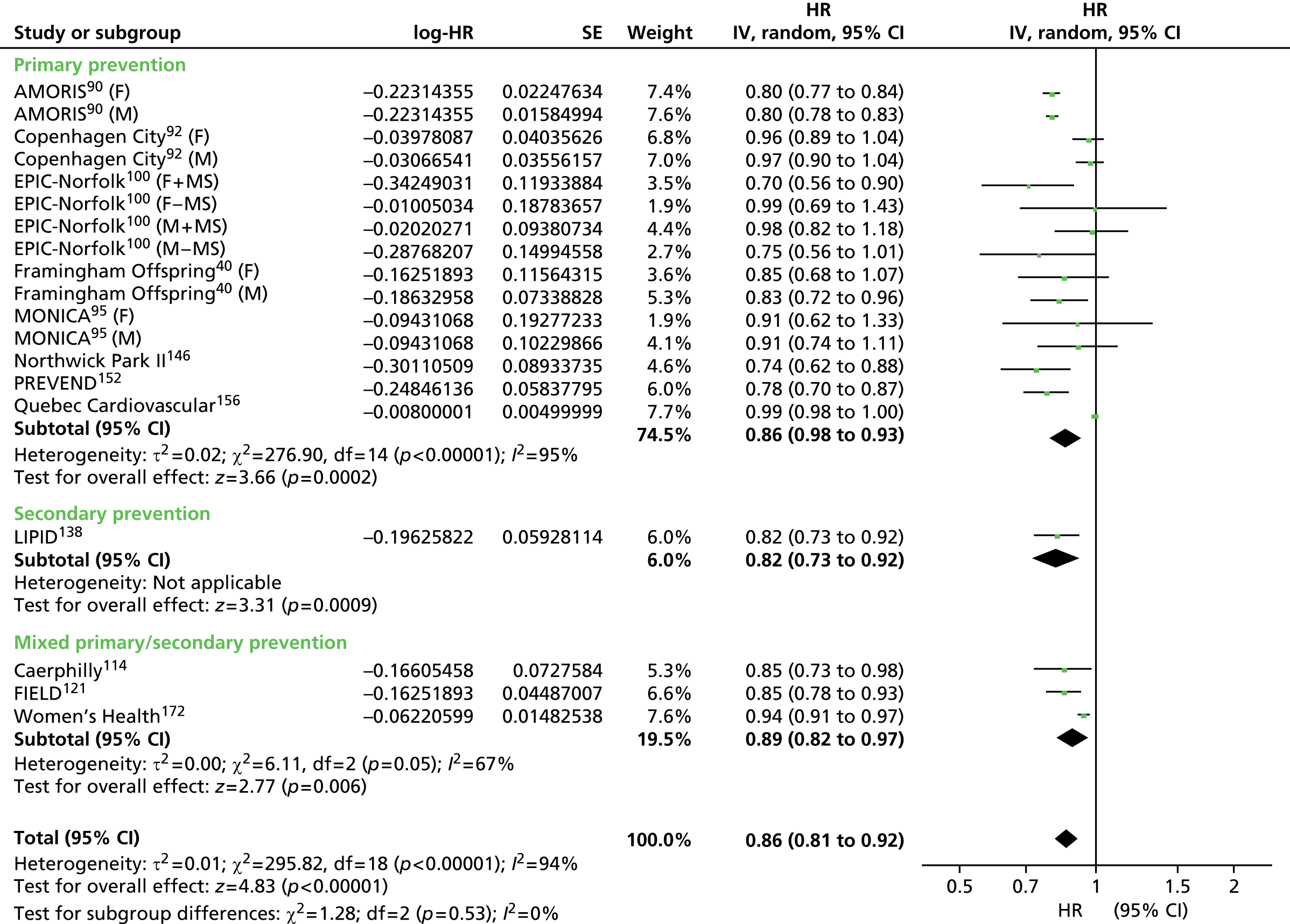
FIGURE 88.
Apolipoprotein B/Apo A-I as prognostic marker for cardiovascular events in populations not taking statins. IV, inverse variance.
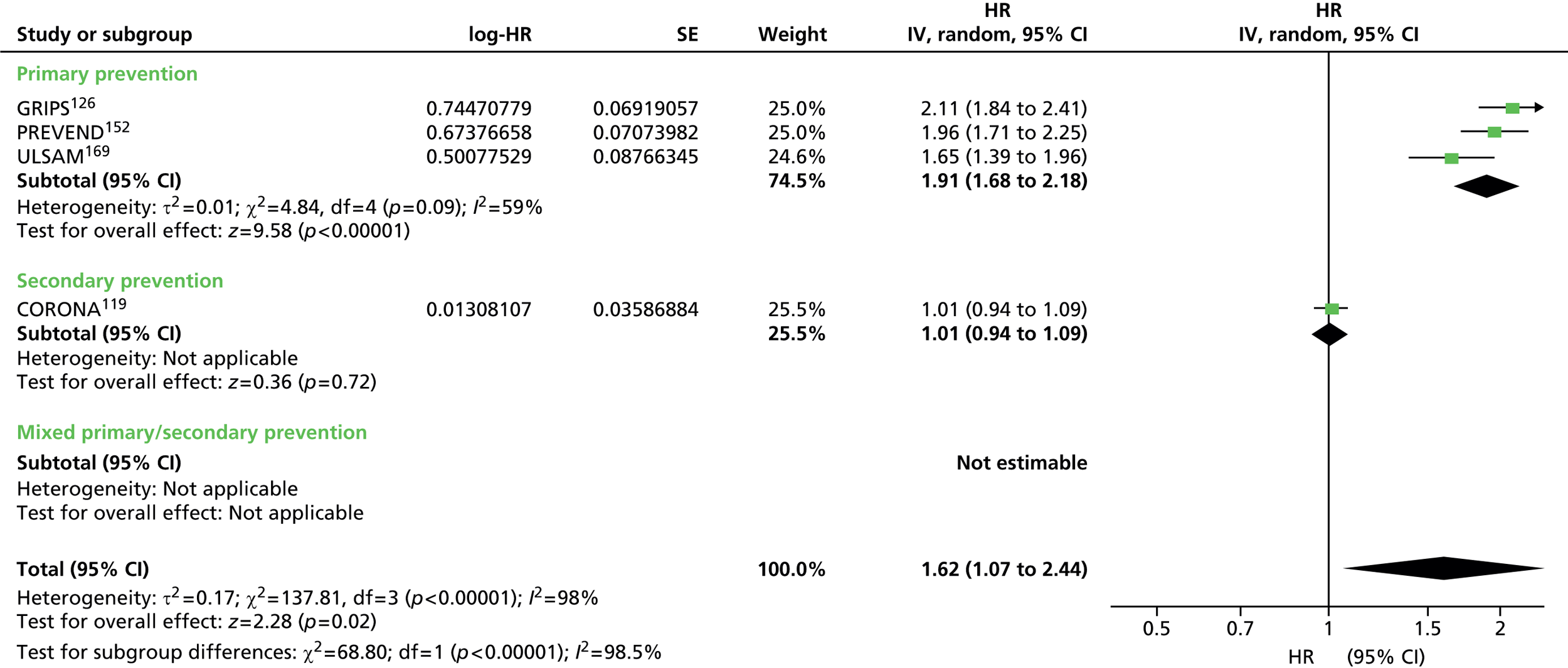
FIGURE 89.
Apolipoprotein B /Apo A-I as prognostic marker for cardiovascular events (adjusted estimates) in populations not taking statins. IV, inverse variance.
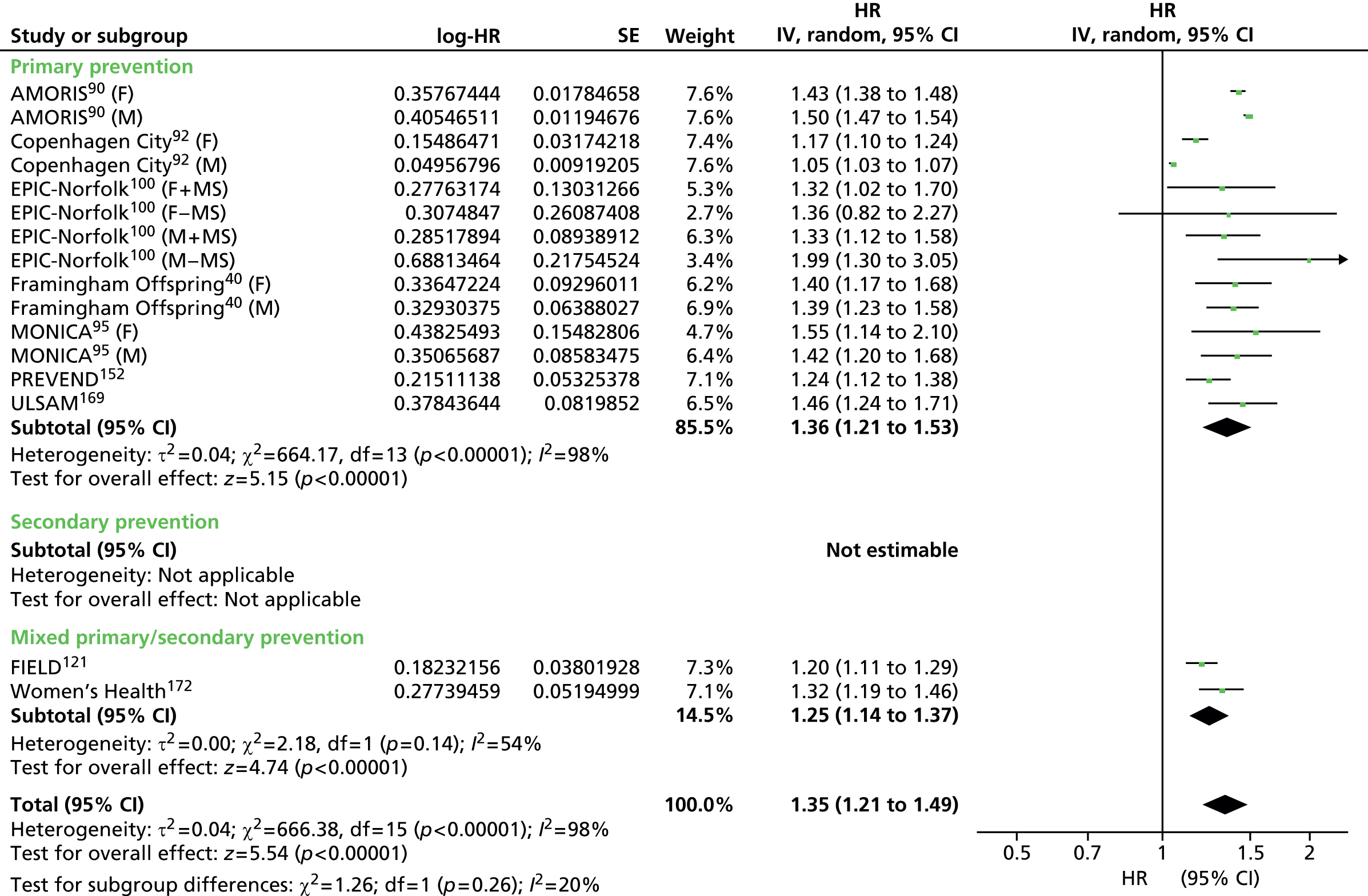
FIGURE 90.
Total cholesterol/HDL cholesterol as prognostic marker for cardiovascular events in populations not taking statins. IV, inverse variance.

FIGURE 91.
Total cholesterol/HDL cholesterol as prognostic marker for cardiovascular events (adjusted estimates) in populations not taking statins. IV, inverse variance.

FIGURE 92.
Total cholesterol/HDL cholesterol as prognostic marker for cardiovascular mortality (adjusted estimates) in populations not taking statins. IV, inverse variance.

FIGURE 93.
Low-density lipoprotein/HDL cholesterol as prognostic marker for cardiovascular events (adjusted estimates) in populations not taking statins. IV, inverse variance.

Appendix 10 Subgroup and sensitivity analyses in populations not taking statins
Subgroup analyses
Pre-existing conditions
Within the 90 included studies (see Appendix 8), 14 examined populations with pre-existing conditions at baseline. Ten studies were on diabetic populations: four of these providing outcome data for CVD events (CARDS,113 ASCOT-LLA,109 Ting 2010,166 DAI120), five of these provided data for CVD events (adjusted) (HPFS,130 Ting 2010,166 FIELD,121 SHS,160 Swedish NDR164), Casale Monferrato116 provided data for CVD mortality (adjusted) and MHS143 contributed data to all-cause mortality (adjusted). Subgroup analyses were completed for CVD events (unadjusted and adjusted), but not for CVD mortality (adjusted) or all-cause mortality as a result of insufficient data.
Forest plots of the subgroup analyses to examine the results for non-diabetic populations compared with diabetic populations are shown below. For CVD events, only four lipid measures had data on diabetic populations (LDL, TC, TGs and HDL). For these four measures the estimates for the non-diabetic population showed only a small increase in comparison with the main analysis (HR range 1.17–1.28 compared with 1.14–1.25), but still with overlapping CIs. In the diabetic population, only HDL had sufficient data to compare with the non-diabetic populations and the predictive value was much reduced (diabetic: HR 1.08, 95% CI 1.02 to 1.13; non-diabetic: HR 1.28, 95% CI 1.20 to 1.38). For primary and secondary prevention groups, the non-diabetic population varied little from the main analysis except that in the primary prevention group TGs increased in predictive value to HR 1.38 (95% CI 1.21 to 1.58). There were insufficient data in the diabetic population for either prevention group to compare results to the non-diabetic population. Within the CVD events (adjusted) data there were diabetic populations providing data for all lipid measures. Overall, in the non-diabetic population the pooled estimates were very similar to the main analysis and followed the same pattern. Only five lipid measures within the diabetic populations had sufficient data sets to compare to non-diabetic populations. These were slightly lower in the diabetic population for TC/HDL cholesterol and HDL, but reduced from HR 1.29 to HR 1.17 for non-HDL cholesterol and further still for TGs and LDL. For primary and secondary prevention groups the non-diabetic populations were similar to the main analysis; no comparisons with diabetic populations could be made as there were insufficient or no data for these populations.
Two studies were either in the latter stages of renal disease (AURORA110) or post renal transplant (ALERT107), and affected the CVD events (unadjusted) outcome data. EPIC-Norfolk100 included populations suffering from metabolic syndrome and contributed data to CVD events (adjusted) outcome data. Finally, Palma 2007148 comprised a population of post-surgical patients, who provided results to all-cause mortality outcome data. Owing to lack of study numbers no subgroup analysis was completed for these baseline comorbidities.
FIGURE 94.
Summary results (pooled estimates) for cardiovascular events subgrouped by non-diabetic and diabetic populations for selected lipid measures. All data sets include primary prevention data sets, secondary prevention data sets, and data sets with mixed or unreported prevention groups. IV, inverse variance.

FIGURE 95.
Summary results (pooled estimates) for cardiovascular events by all lipid measures (adjusted estimates) subgrouped by non-diabetic and diabetic populations. All data sets include primary prevention data sets, secondary prevention data sets, and data sets with mixed or unreported prevention groups. IV, inverse variance.

Sensitivity analyses
Surrogate measures for cardiovascular disease
Definitions for cardiovascular events varied considerably. Forest plots of sensitivity analyses [CVD events, CVD events (adjusted), CVD mortality, CVD mortality (adjusted)] comparing all of the pooled estimates for lipid measures, removing the studies that used a surrogate measure, are presented below. In total, 61% of studies used CHD as a surrogate measure for predicting cardiovascular risk (both adjusted and unadjusted data). For unadjusted data, once the surrogate measure data were removed, the results show the same overall trends, although the data were no longer available for all lipid measures, in particular the apolipoproteins and the lipid ratios. For adjusted CVD event data, the primary prevention group and all data sets show a similar trend, although for a number of lipid measures (all studies – TC, LDL; primary prevention – TC, LDL, non-HDL cholesterol, TC/HDL cholesterol) the associations were no longer significant. Within the CVD mortality outcome data, 52% of the studies used a surrogate measure for cardiovascular risk. For the unadjusted CVD mortality data, removal of these studies meant that data for only four lipid measures remained (TC, LDL, HDL, TGs) and all of these had three or fewer studies, meaning that analysis was not possible. For adjusted CVD mortality data, there were now insufficient studies in the prevention groups to warrant analysis. For all studies, only three lipid measures (LDL, TC, HDL) had sufficient study numbers to make analysis meaningful. There is little difference in pattern from the main analysis, except that the strength of associations is reduced and TC is no longer significant.
Study quality
Study quality was examined by placing emphasis on how the prognostic markers were measured and levels of attrition. No studies were assessed as high risk of attrition bias and 29% of studies were judged to be at moderate risk. With regard to bias due to prognostic marker measurement, 38 studies were assessed to be high risk, 21 moderate risk, and 31 low risk. Removal of all studies at moderate or high risk in either of these domains left 23 studies. For CVD events, only four measures had sufficient studies left to draw any conclusions (LDL, TC, HDL, TGs). The trends remained the same as the main analyses for these measures, but for LDL and TC there was a small reduction in the estimates, although data were no longer available from secondary populations. For HDL and TGs there was a small increase in all point estimates except for HDL in the secondary prevention groups. In the remaining studies within the CVD events (adjusted), no data were available from secondary prevention populations. Small reductions or small increases in the summary measures were seen in the primary prevention populations and overall, but no differences to the overall trends compared with the main analyses. CVD mortality (unadjusted and adjusted) data no longer had sufficient studies to justify obtaining pooled estimates, except for LDL, TC and HDL in the adjusted data, which showed very small reductions in the point estimates for primary prevention and overall populations. Again, data were no longer available from secondary prevention populations. For all-cause mortality data, not enough studies were available to make any comparisons with the main analyses except for TC, which showed a very small reduction in the overall summary measure for both adjusted and unadjusted data.
Laboratory methods
Ideally, blood samples would have been analysed in an accredited central laboratory for each study: 54% of studies completed the blood analysis in a central laboratory, with 57% of these reporting the laboratory as accredited. FIELD121 analysed samples in two laboratories, both of which were accredited. Thirty-seven studies did not report where the blood samples were analysed. Only three studies reported not using a central laboratory (DAI,120 Swedish NDR,164 RIFLE158). Sensitivity analysis was completed to remove all studies not reporting whether or not a central laboratory was used and when a central laboratory was stated as not used. For CVD events, only LDL, TC, HDL, TGs had sufficient number of studies to examine if removing these studies had any effect. There was a small reduction in the summary estimates for the primary prevention populations and the overall estimates, but no change to the conclusions drawn in the main analyses. A similar effect was seen for all lipid measures in the adjusted CVD events data. For CVD mortality (unadjusted and adjusted) there were either no differences from the main analyses or insufficient studies left to make a comment; except for TC, which for both outcomes showed a small reduction in the estimates for the primary prevention group and overall. No results could be shown for all-cause mortality (unadjusted and adjusted) as few results were left.
FIGURE 96.
Summary results (pooled estimates) for cardiovascular events by all lipid measures based on removing studies with surrogate definitions of CVD. All data sets include primary prevention data sets, secondary prevention data sets, and data sets with mixed or unreported prevention groups. IV, inverse variance.

FIGURE 97.
Summary results (pooled estimates) for cardiovascular events by all lipid measures (adjusted estimates) based on removing studies with surrogate definitions of CVD. All data sets include primary prevention data sets, secondary prevention data sets, and data sets with mixed or unreported prevention groups. IV, inverse variance.
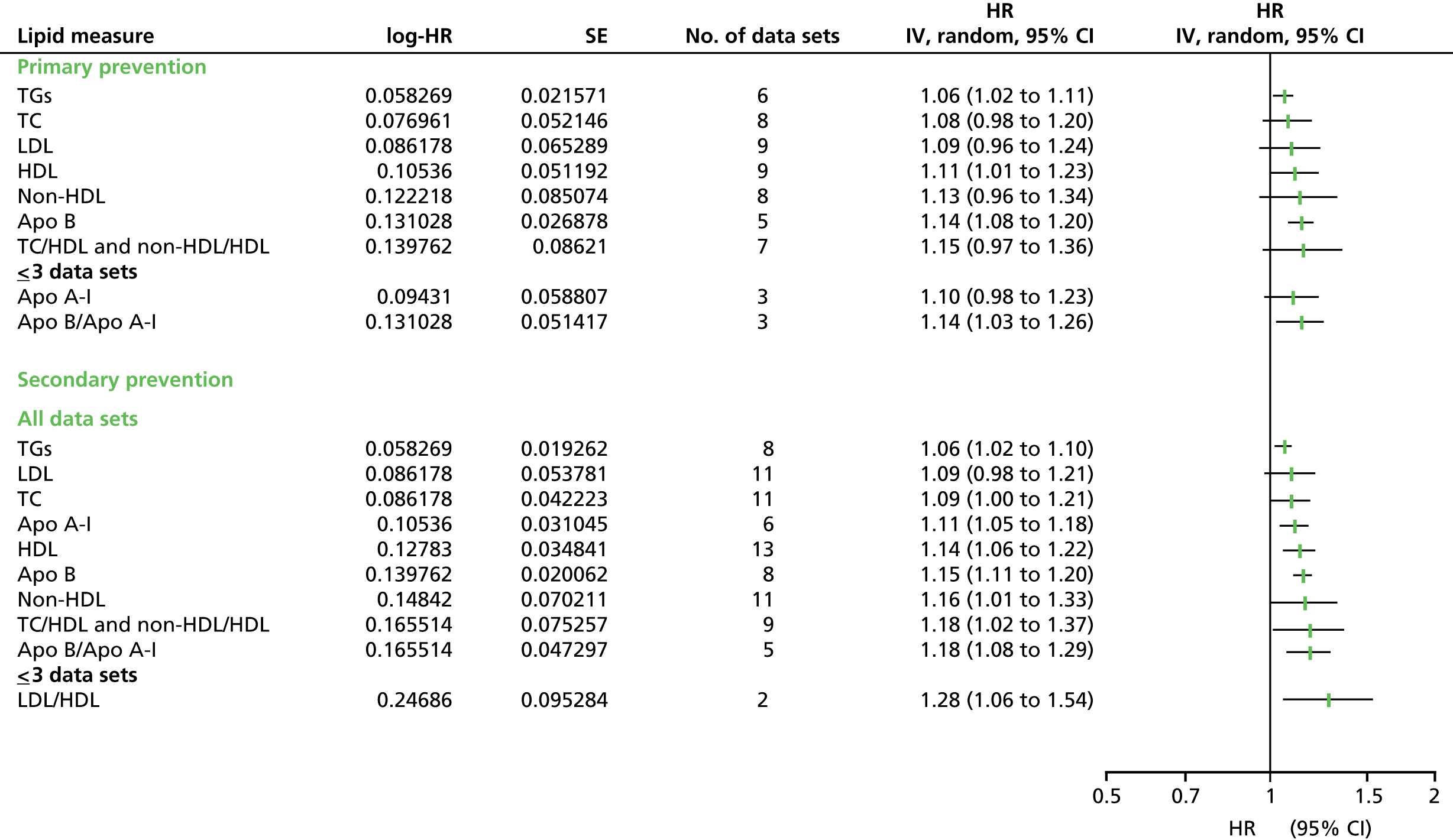
FIGURE 98.
Summary results (pooled estimates) for cardiovascular mortality by all lipid measures based on removing studies with surrogate definitions of CVD. All data sets include primary prevention data sets, secondary prevention data sets, and data sets with mixed or unreported prevention groups. IV, inverse variance.
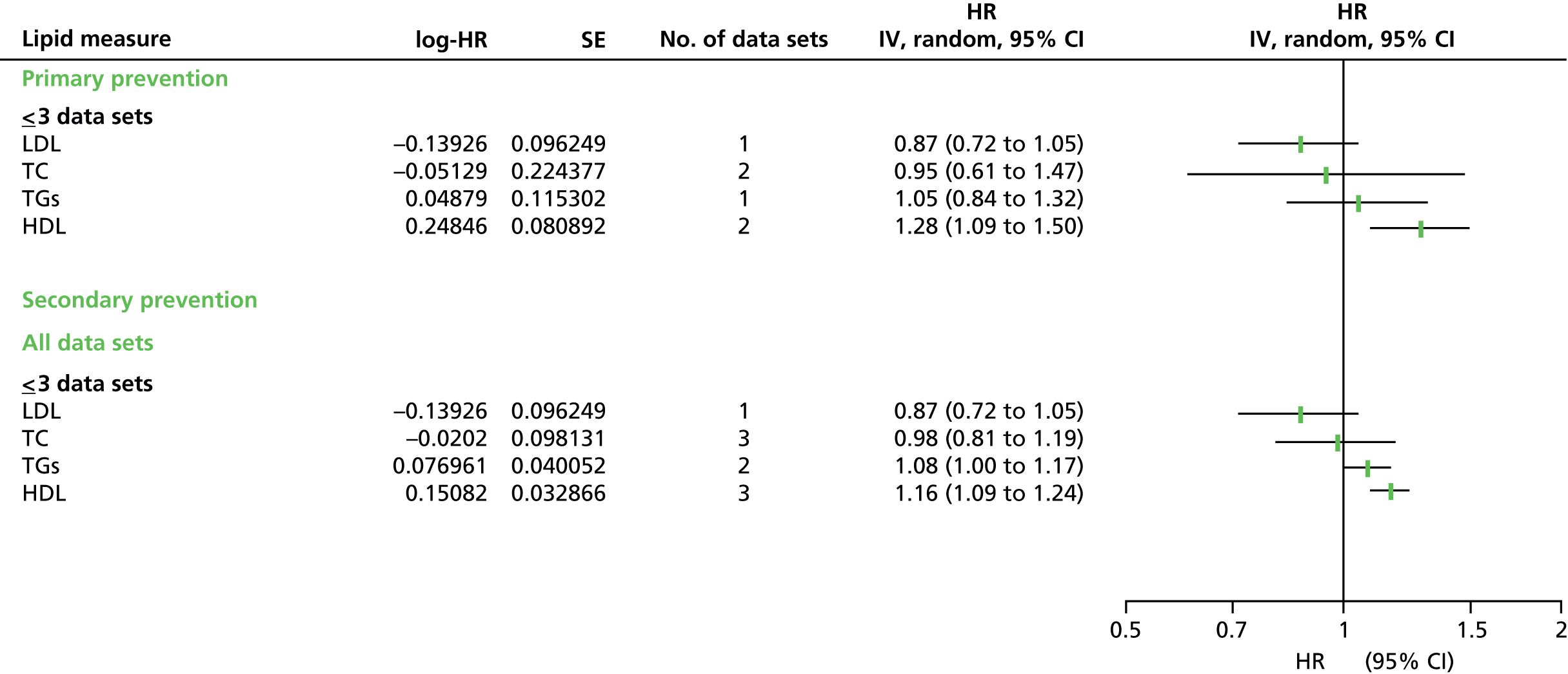
FIGURE 99.
Summary results (pooled estimates) for cardiovascular mortality by all lipid measures (adjusted estimates) based on removing studies with surrogate definitions of CVD. All data sets include primary prevention data sets, secondary prevention data sets, and data sets with mixed or unreported prevention groups. IV, inverse variance.
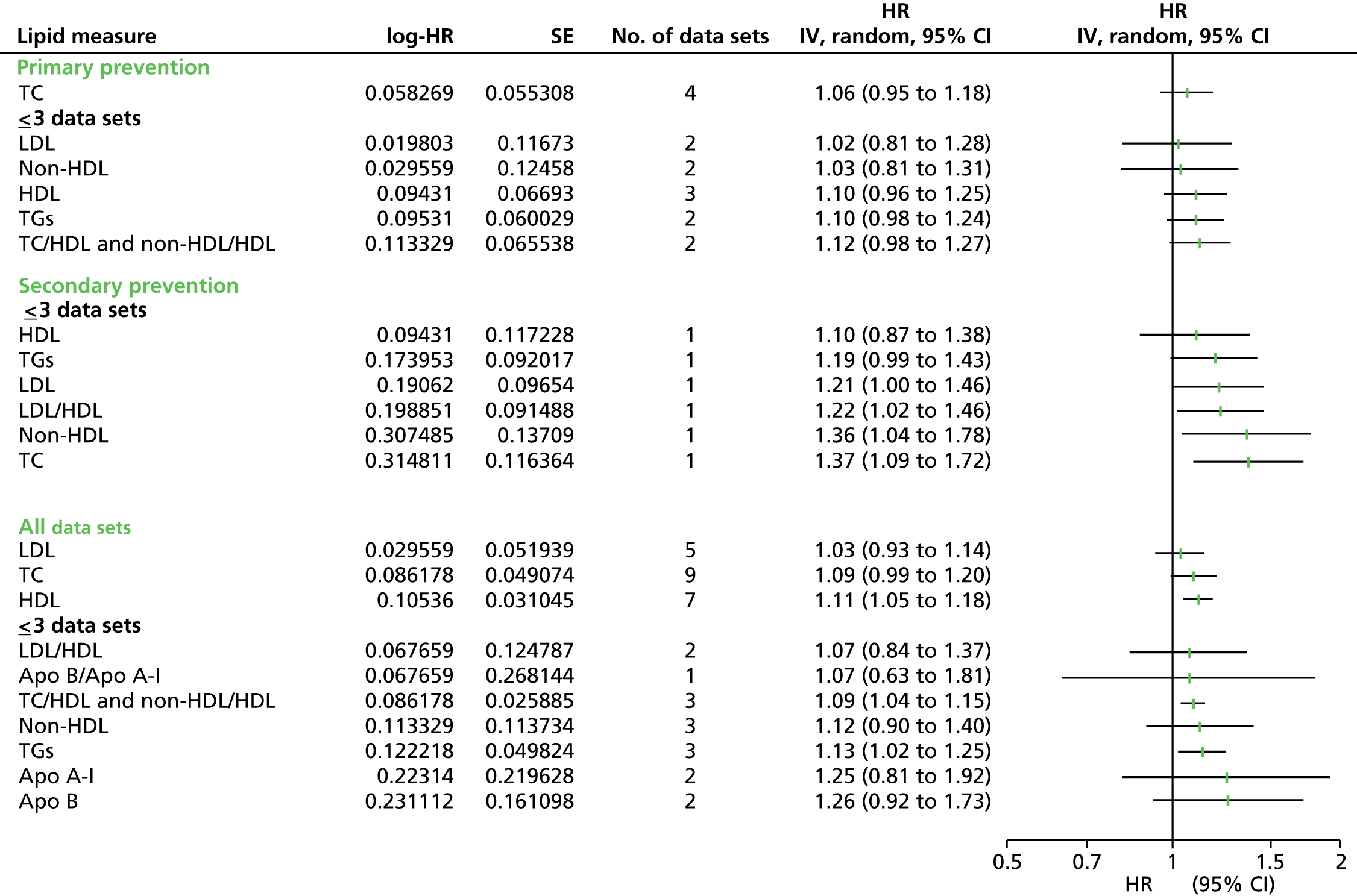
Indirect data extraction
Sensitivity analysis was completed to examine whether or not the method of data extraction had any bearing on the results. Data were extracted directly from 53% of studies. For the remaining 42 studies, data were extracted indirectly using simulation or generalised least squares for trend methods. Two studies provided both direct and simulated data, but for different outcomes (Quebec cardiovascular,156 PREVEND152). Studies with indirect data were omitted to see if this affected the results (see the forest plots below). For CVD events, removal of these studies meant that meta-analysis estimates for a number of the lipid measures were no longer viable, although they followed the same basic trend with reduced strengths of association. However, in the primary prevention group and for all data sets LDL now showed no association. Results for adjusted CVD event results followed a similar pattern to the main analysis. For CVD mortality directly extracted data only TC now had sufficient data for analysis, which now provided an association in the primary prevention group. There was no difference in the results from the adjusted CVD mortality data. For unadjusted and adjusted all-cause mortality data there was little difference from the main analyses except that sufficient data were no longer available for LDL (unadjusted) and TGs (adjusted).
FIGURE 100.
Summary results (pooled estimates) for cardiovascular events by all lipid measures based on removing studies with indirectly extracted data. All data sets include primary prevention data sets, secondary prevention data sets, and data sets with mixed or unreported prevention groups. IV, inverse variance.

FIGURE 101.
Summary results (pooled estimates) for cardiovascular events by all lipid measures (adjusted estimates) based on removing studies with indirectly extracted data. All data sets include primary prevention data sets, secondary prevention data sets, and data sets with mixed or unreported prevention groups. IV, inverse variance.
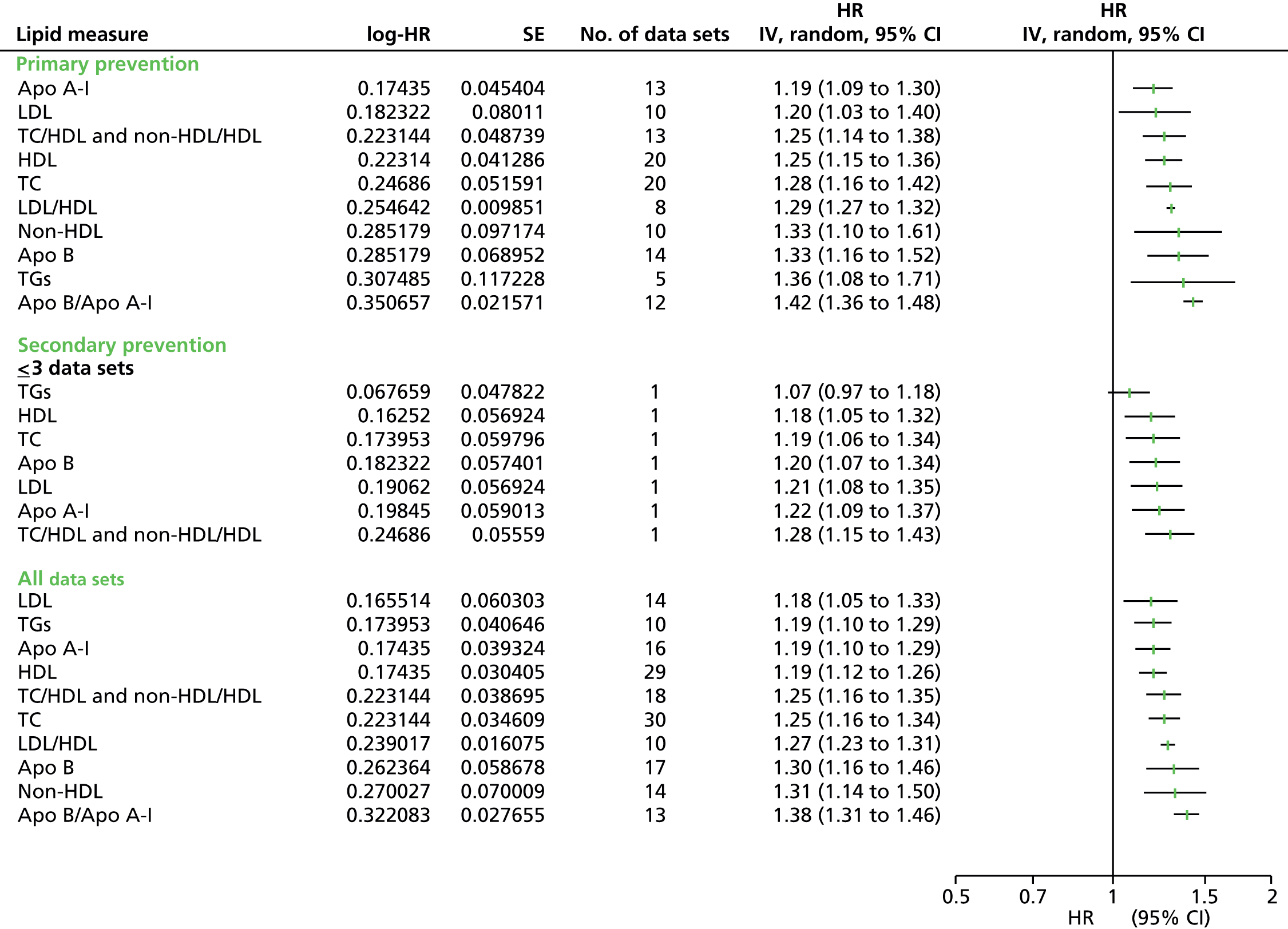
FIGURE 102.
Summary results (pooled estimates) for cardiovascular mortality by all lipid measures based on removing studies with indirectly extracted data. All data sets include primary prevention data sets, secondary prevention data sets, and data sets with mixed or unreported prevention groups. IV, inverse variance.

FIGURE 103.
Summary results (pooled estimates) for cardiovascular mortality by all lipid measures (adjusted estimates) based on removing studies with indirectly extracted data. All data sets include primary prevention data sets, secondary prevention data sets, and data sets with mixed or unreported prevention groups. IV, inverse variance.

FIGURE 104.
Summary results (pooled estimates) for all-cause mortality by all lipid measures based on removing studies with indirectly extracted data. All data sets include primary prevention data sets, secondary prevention data sets, and data sets with mixed or unreported prevention groups. IV, inverse variance.

FIGURE 105.
Summary results (pooled estimates) for all-cause mortality by all lipid measures (adjusted estimates) based on removing studies with indirectly extracted data. All data sets include primary prevention data sets, secondary prevention data sets, and data sets with mixed or unreported prevention groups. IV, inverse variance.

FIGURE 106.
Summary results (pooled estimates) for cardiovascular events by all lipid measures based on removing studies with reported use of lipid-lowering medication. All data sets include primary prevention data sets, secondary prevention data sets, and data sets with mixed or unreported prevention groups. IV, inverse variance.
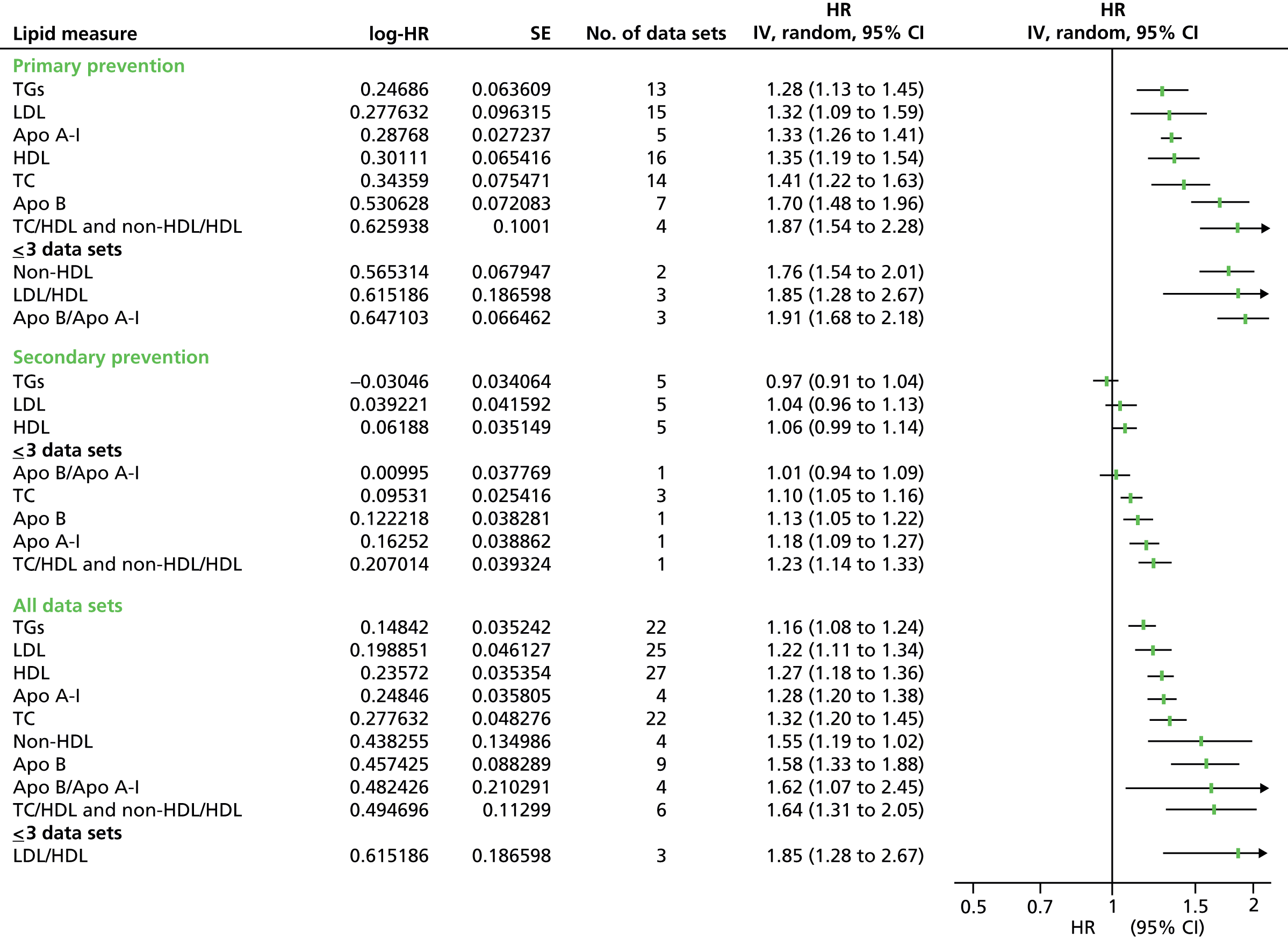
FIGURE 107.
Summary results (pooled estimates) for cardiovascular events by all lipid measures (adjusted estimates) based on removing studies with reported use of lipid-lowering medication. All data sets include primary prevention data sets, secondary prevention data sets, and data sets with mixed or unreported prevention groups. IV, inverse variance.

FIGURE 108.
Summary results (pooled estimates) for cardiovascular mortality by all lipid measures based on removing studies with reported use of lipid-lowering medication. All data sets include primary prevention data sets, secondary prevention data sets, and data sets with mixed or unreported prevention groups. IV, inverse variance.

FIGURE 109.
Summary results (pooled estimates) for cardiovascular mortality by all lipid measures (adjusted estimates) based on removing studies with reported use of lipid-lowering medication. All data sets include primary prevention data sets, secondary prevention data sets, and data sets with mixed or unreported prevention groups. IV, inverse variance.
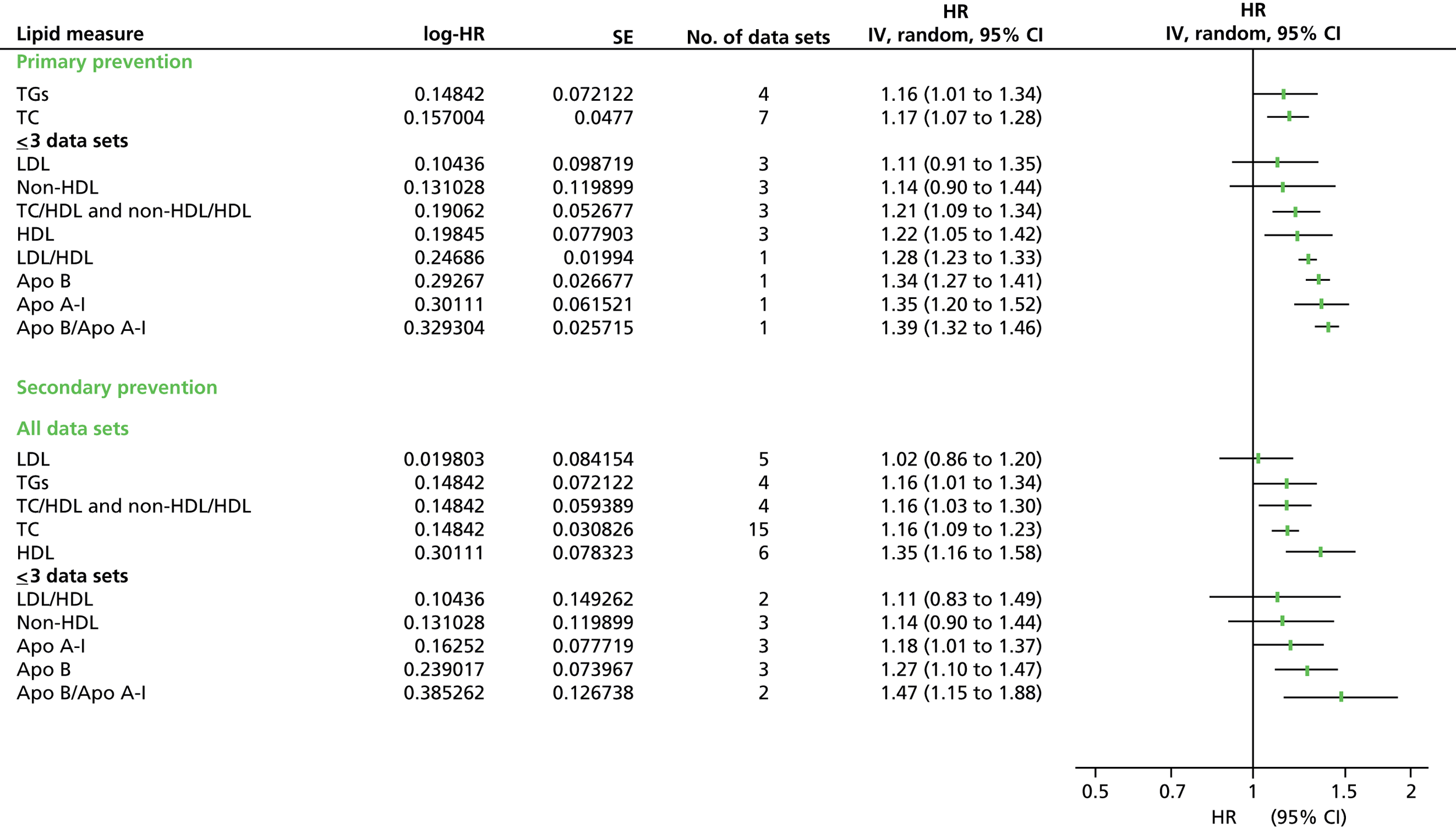
FIGURE 110.
Summary results (pooled estimates) for all-cause mortality by all lipid measures based on removing studies with reported use of lipid-lowering medication. All data sets include primary prevention data sets, secondary prevention data sets, and data sets with mixed or unreported prevention groups. IV, inverse variance.
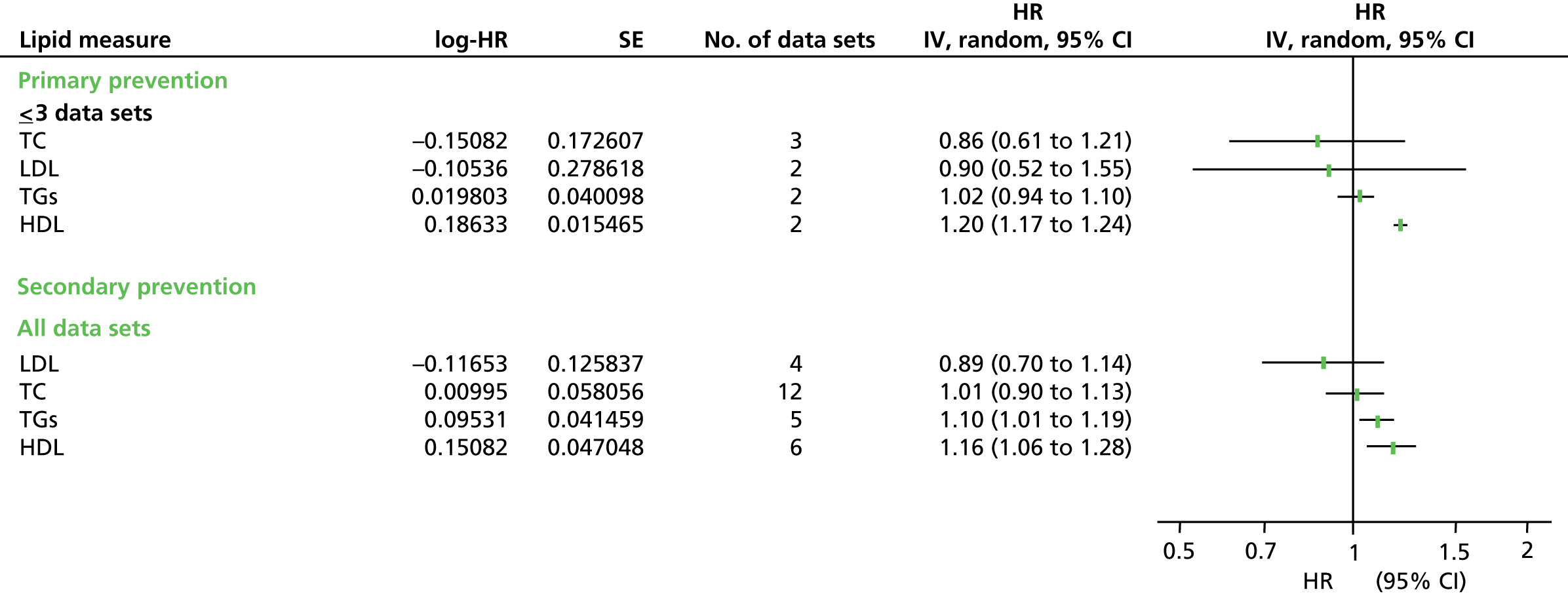
FIGURE 111.
Summary results (pooled estimates) for all-cause mortality by all lipid measures (adjusted estimates) based on removing studies with reported use of lipid-lowering medication. All data sets include primary prevention data sets, secondary prevention data sets, and data sets with mixed or unreported prevention groups. IV, inverse variance.

Use of lipid modification therapy
Finally, sensitivity analysis was competed to assess if contamination of the population by partial use of lipid-lowering medication would affect the results. Eighteen studies confirmed statin or lipid-lowering usage either at baseline or during the course of the studies. Usage ranged from 0.5% to 38% (seven studies with < 5% usage and Framingham Offspring40 usage level not confirmed). Two studies (Ting 2010,166 Framingham Offspring40) included unadjusted and adjusted outcome data, and for the adjusted data one of the adjustments was for lipid-lowering medication; therefore analysis for adjusted data did not include these two studies. For both unadjusted and adjusted CVD events data the removal of studies with contaminated populations made marginal difference to the pooled estimates, and the same four lipid measures had the strongest predictive values. There were very small differences between the CVD mortality data (unadjusted and adjusted) with contaminated populations removed and the main analyses. For unadjusted and adjusted data there were no longer sufficient data for HDL in the primary prevention groups, nor for non-HDL cholesterol in all data sets for the adjusted data. For all of the data, the point estimates were similar except for HDL, which was stronger in the adjusted data (HR 1.35, CI 1.16, 1.58). There was no difference in the results from all-cause mortality data. For all-cause adjusted data, only TC retained sufficient data to be analysed; the result for this was no different.
Appendix 11 Extracted data from studies for populations taking statins
Study name | Data extraction method | Outcome | Surrogate outcome | Effect measure | No. of participants | Lipid measure | Measure per 1 SD change | Lower limit | Upper limit | log-HR | SE | Adjusted | Covariates |
---|---|---|---|---|---|---|---|---|---|---|---|---|---|
4S18 | Direct | CVD event | N | HR | 2221 | LDL | 1.2 | 1.07 | 1.34 | 0.1823216 | 0.0574008 | N | N/A |
Non-HDL cholesterol | 1.06 | 1.19 | 1.33 | 0.0582689 | 0.0283739 | ||||||||
Apo B | 1.14 | 1 | 1.31 | 0.1310283 | 0.0688845 | ||||||||
AF/TexCAPS106 | Orsini (2) | CVD event | Y | RR | 3304 | LDL | 1.307 | 0.8048327 | 2.122489 | 0.2677344 | 0.2473751 | N | N/A |
HDL | 0.8536102 | 0.5261637 | 1.384836 | –0.1582806 | 0.2468685 | ||||||||
ALERT107 | Simulation (2) | CVD event | Y | OR | 1050 | LDL | 1.226397 | 1.059242 | 1.413322 | 0.2040806 | 0.0735687 | N | N/A |
TC | 1.305899 | 1.130212 | 1.501814 | 0.2668917 | 0.0725175 | ||||||||
HDL | 0.839768 | 0.726805 | 0.9678052 | –0.1746296 | 0.0730542 | ||||||||
TGs | 1.237197 | 1.074949 | 1.430299 | 0.2128483 | 0.0728598 | ||||||||
ASCOT-LLA109 | Simulation (2) | CVD event | N | OR | 1258 | LDL | 1.10402 | 0.986971 | 1.243201 | 0.0989581 | 0.0588786 | N | N/A |
HDL | 1.004978 | 0.894415 | 1.115344 | 0.0049657 | 0.0563133 | ||||||||
TGs | 1.03913 | 0.93403 | 1.16704 | 0.0383838 | 0.0568157 | ||||||||
AURORA110 | Simulation (2) | CVD event | N | OR | 1389 | LDL | 0.92354 | 0.84968 | 1.0013 | –0.0795412 | 0.0418864 | N | N/A |
CARDS115 | Simulation (2) | CVD event | N | OR | 1428 | LDL | 1.0339 | 0.9063 | 1.1867 | 0.0333381 | 0.0687656 | N | N/A |
TC | 1.06743 | 0.9274 | 1.22078 | 0.0652539 | 0.0701174 | ||||||||
HDL | 0.9156 | 0.7978 | 1.0477 | –0.0881757 | 0.0695139 | ||||||||
TGs | 0.9598 | 0.8417 | 1.1058 | –0.0410303 | 0.0696175 | ||||||||
CARE34 | Simulation (2) | CVD event | Y | OR | 2081 | LDL | 1.025084 | 0.95892 | 1.0939094 | 0.0247746 | 0.0335983 | N | N/A |
TC | 1.0253383 | 0.9589505 | 1.0936867 | 0.0250226 | 0.0335383 | ||||||||
HDL | 0.9780343 | 0.9631657 | 0.9919854 | –0.0222105 | 0.0075212 | ||||||||
TGs | 1.0736066 | 1.0010375 | 1.1464495 | 0.0710236 | 0.0346002 | ||||||||
CLIP177 | Simulation (1) | CVD event | N | OR | 3249 | LDL | 1.1613089 | 0.8605814 | 1.6223296 | 0.1495477 | 0.1617373 | N | N/A |
TC | 1.1778323 | 0.7190525 | 1.3861625 | 0.1636757 | 0.1674388 | ||||||||
HDL | 0.6297541 | 0.4892235 | 0.8073103 | –0.4624259 | 0.1277777 | ||||||||
TGs | 1.1383781 | 0.8783289 | 1.6780671 | 0.1296045 | 0.1651471 | ||||||||
CORONA119 | Orsini (1) | CVD event | N | OR | 2514 | LDL | 0.914595 | 0.842691 | 0.994476 | –0.0892739 | 0.0422489 | N | N/A |
Apo B/Apo A-I | 1.039328 | 0.970691 | 1.104575 | 0.0385744 | 0.0329612 | ||||||||
HDL | 0.910742 | 0.8507389 | 0.9597831 | –0.0934956 | 0.0307658 | ||||||||
TGs | 0.913886 | 0.8631722 | 0.9670051 | –0.0900494 | 0.0289769 | ||||||||
dal-OUTCOMES178 | Direct | CVD event | N | RR | 7933 | HDL | 1.04151 | 0.964433 | 1.125176 | 0.0406716 | 0.0393251 | N | N/A |
LDL | 1.223514 | 1.140305 | 1.313406 | 0.201727 | 0.0360531 | ||||||||
Apo B | 1.248155 | 1.162074 | 1.342833 | 0.2216665 | 0.0368814 | ||||||||
GISSI123 | Simulation (2) | CVD event | N | OR | 2138 | LDL | 1.020846 | 0.90409 | 1.15793 | 0.0206317 | 0.0631276 | N | N/A |
TC | 1.339714 | 1.22196 | 1.4683 | 0.2924562 | 0.0468493 | ||||||||
HDL | 0.926379 | 0.82573 | 1.03691 | –0.0764718 | 0.058095 | ||||||||
TGs | 0.963861 | 0.86301 | 1.08628 | –0.0368082 | 0.0586959 | ||||||||
HPS24 | Orsini (2) | CVD event | N | RR | 10,269 | TC | 1.236506 | 1.11351 | 1.373089 | 0.2122897 | 0.0534556 | N | N/A |
LDL | 1.429138 | 1.234838 | 1.654012 | 0.3570715 | 0.0745572 | ||||||||
HDL | 0.6251777 | 0.5372922 | 0.7274386 | –0.4697193 | 0.0772927 | ||||||||
TGs | 1.208799 | 1.113494 | 1.312261 | 0.1896273 | 0.0419002 | ||||||||
IDEAL179 | Direct | CVD event | N | HR | 8888 | LDL | 1.16 | 1.08 | 1.25 | 0.14842 | 0.0372915 | N | N/A |
Non-HDL cholesterol | 1.1 | 1.19 | 1.27 | 0.0953102 | 0.0165979 | ||||||||
Apo B | 1.08 | 1.14 | 1.2 | 0.076961 | 0.013085 | ||||||||
JELIS180 | Direct | CVD event | Y | HR | 5806 | TC | 1.01 | 0.82 | 1.24 | 0.0099503 | 0.1055006 | Y | Gender, age, hypertension, diabetes, smoking |
LDL | 1.19 | 1 | 1.42 | 0.1739533 | 0.0894533 | ||||||||
HDL | 0.6 | 0.47 | 0.76 | –0.5108256 | 0.1225984 | ||||||||
Non-HDL cholesterol | 1.35 | 1.11 | 1.66 | 0.3001046 | 0.1026678 | ||||||||
J-LIT Primary174 | Orsini (1) | CVD event | Y | HR | 42,360 | TC | 1.644333 | 1.37865 | 1.96133 | 0.4973348 | 0.0899281 | Y | Gender, age at baseline (a continuous variable), hypertension, DM, and smoking habit |
LDL | 2.104233 | 1.771323 | 2.500364 | 0.743951 | 0.0879361 | ||||||||
HDL | 0.3558607 | 0.2602924 | 0.4865178 | –1.0332159 | 0.1595581 | ||||||||
TGs | 1.327338 | 1.096823 | 1.606496 | 0.2831754 | 0.0973565 | ||||||||
J-LIT Secondary175 | Orsini (1) | CVD event | Y | HR | 51,247 | TC | 1.361851 | 1.027201 | 1.805564 | 0.3088448 | 0.1438866 | Y | Gender, age at baseline (a continuous variable), hypertension, DM, and smoking habit |
LDL | 1.745394 | 1.26287 | 2.41228 | 0.5569803 | 0.1650983 | ||||||||
HDL | 0.5221532 | 0.3466503 | 0.7865106 | –0.6497942 | 0.2090025 | ||||||||
TGs | 0.9248072 | 0.5396509 | 1.5848582 | –0.07817 | 0.2748285 | ||||||||
JUPITER181 | Direct | CVD event | N | HR | 7832 | LDL | 1.56 | 1.17 | 2.05 | 0.4446858 | 0.1430704 | N | N/A |
Non-HDL cholesterol | 1.13 | 1.47 | 1.98 | 0.1222176 | 0.0759782 | ||||||||
Apo B | 1.12 | 1.43 | 1.85 | 0.1133287 | 0.0656916 | ||||||||
LIPID138 | Direct | CVD event | Y | HR | 4512 | LDL | 1.061 | 0.9534 | 1.1728 | 0.0592119 | 0.0528354 | N | N/A |
TC | 1.0893 | 0.9917 | 1.1929 | 0.0855353 | 0.0471229 | ||||||||
HDL | 0.9243 | 0.8366 | 1.0241 | –0.0787186 | 0.0515876 | ||||||||
TGs | 1.0904 | 1.0082 | 1.1714 | 0.0865446 | 0.0382737 | ||||||||
TC/HDL cholesterol | 1.0478 | 1 | 1.0964 | 0.0466927 | 0.0234776 | ||||||||
Apo A-I | 0.8969 | 0.8128 | 0.9841 | –0.1088109 | 0.0487863 | ||||||||
Apo B | 1.10483 | 1.0049 | 1.2137 | 0.0996915 | 0.0481596 | ||||||||
LDL | 1.1507 | 1 | 1.3312 | 0.1403705 | 0.0729798 | Y | Age, gender, smoking status, history of hypertension, diabetes, stroke or transient ischaemic attack, peripheral vascular disease, previous coronary revascularisation, stable angina and qualifying event | ||||||
TC | 1.2007 | 1.0408 | 1.3715 | 0.1829047 | 0.0703866 | ||||||||
HDL | 0.9114 | 0.7952 | 1.0378 | –0.0927734 | 0.0679247 | ||||||||
TGs | 1.1148 | 1 | 1.2432 | 0.108675 | 0.0555328 | ||||||||
TC/HDL cholesterol | 1.0801 | 1.0158 | 1.1458 | 0.0770536 | 0.0307211 | ||||||||
Apo A-I | 0.8547 | 0.7435 | 0.9818 | –0.1570047 | 0.0709232 | ||||||||
Apo B | 1.2038 | 1.0488 | 1.3812 | 0.1854832 | 0.0702311 | ||||||||
LIVES Ex182 | Direct | CVD event | N | HR | 6582 | LDL | 0.932486 | 0.807277 | 1.145343 | –0.0699011 | 0.0892328 | N | N/A |
HDL | 0.597566 | 0.491383 | 0.712232 | –0.5148905 | 0.0946887 | ||||||||
LDL | 0.807277 | 0.6945119 | 0.9658245 | –0.2140884 | 0.0841257 | Y | Age, gender, hypertension, diabetes, smoking status, history of ischaemic heart disease | ||||||
HDL | 0.788093 | 0.6538556 | 0.9492477 | –0.2381392 | 0.0950978 | ||||||||
Phase A-Z 20176 | Simulation (2) | CVD event | N | OR | 2265 | LDL | 1.002124 | 0.947646 | 1.052307 | 0.0021217 | 0.0267243 | N | N/A |
HDL | 0.972594 | 0.904927 | 1.044719 | –0.0277886 | 0.0366451 | ||||||||
Phase A-Z 40/80176 | Simulation (2) | CVD event | N | OR | 2232 | LDL | 0.944812 | 0.897938 | 0.994047 | –0.0567693 | 0.0259397 | N | N/A |
HDL | 0.955006 | 0.890001 | 1.018952 | –0.0460377 | 0.0345172 | ||||||||
PROSPER155 | Simulation (2) | CVD event | N | OR | 2891 | LDL | 0.944586 | 0.904055 | 0.980223 | –0.0570085 | 0.0206352 | N | N/A |
HDL | 0.985297 | 0.942636 | 1.049381 | –0.0148122 | 0.0273662 | ||||||||
PROVE-IT183 | Direct | CVD event | Y | HR | 3683 | LDL | 1.2 | 1.07 | 1.35 | 0.1823216 | 0.0592974 | N | N/A |
Apo B/A-I | 1.1 | 1.01 | 1.2 | 0.0953102 | 0.0439723 | ||||||||
TC/HDL cholesterol | 1.12 | 1.01 | 1.24 | 0.1133287 | 0.052337 | ||||||||
Non-HDL cholesterol | 1.2 | 1.07 | 1.35 | 0.1823216 | 0.0592974 | ||||||||
SEARCH 2058 | Orsini (2) | CVD event | N | RR | 6033 | TC | 0.9885257 | 0.8490197 | 1.150954 | –0.0115406 | 0.0776184 | N | N/A |
LDL | 1.107274 | 0.9707363 | 1.263015 | 0.1019011 | 0.0671434 | ||||||||
HDL | 0.7779744 | 0.6271996 | 0.9649946 | –0.2510617 | 0.1099127 | ||||||||
SEARCH 8058 | Orsini (2) | CVD event | N | RR | 6031 | TC | 1.00286 | 0.8643467 | 1.16357 | 0.0028559 | 0.0758353 | N | N/A |
LDL | 1.104946 | 0.9737662 | 1.253798 | 0.0997965 | 0.0644799 | ||||||||
HDL | 0.7036491 | 0.5681171 | 0.871514 | –0.3514755 | 0.1091593 | ||||||||
SPARCL184 | Direct | CVD event | N | HR | 2366 | LDL | 1.13 | 0.99 | 1.33 | 0.1222176 | 0.0753136 | N | N/A |
Non-HDL cholesterol | 1.03 | 1.16 | 1.36 | 0.0295588 | 0.0405777 | ||||||||
Apo B | 0.97 | 1.1 | 1.24 | –0.0304592 | 0.0305615 | ||||||||
TNT185 | Simulation (1) | CVD event | N | OR | 1527 | LDL | 1.266617 | 1.137362 | 1.409606 | 0.2363496 | 0.0547446 | N | N/A |
TC | 1.137976 | 1.021825 | 1.266721 | 0.1292512 | 0.0548065 | ||||||||
HDL | 0.7458936 | 0.6681091 | 0.8318255 | –0.2931723 | 0.055911 | ||||||||
TGs | 1.152997 | 1.038202 | 1.270762 | 0.1423646 | 0.0515628 | ||||||||
WOSCOPS19 | Simulation (2) | CVD event | N | OR | 3302 | LDL | 1.094023 | 0.9963 | 1.197026 | 0.0898617 | 0.0468232 | N | N/A |
HDL | 1.212498 | 1.109733 | 1.310267 | 0.1926827 | 0.0423754 | ||||||||
TC | 1.0171593 | 0.9098123 | 1.107519 | 0.0170137 | 0.0501631 | ||||||||
TGs | 1.181543 | 1.083324 | 1.284759 | 0.1668212 | 0.0435044 |
Appendix 12 Forest plots for each lipid measure as prognostic markers in populations taking statins
(Plots presented only when more than three data sets per prognostic marker.)
FIGURE 112.
Total cholesterol as prognostic marker for cardiovascular events in populations on statins. IV, inverse variance.
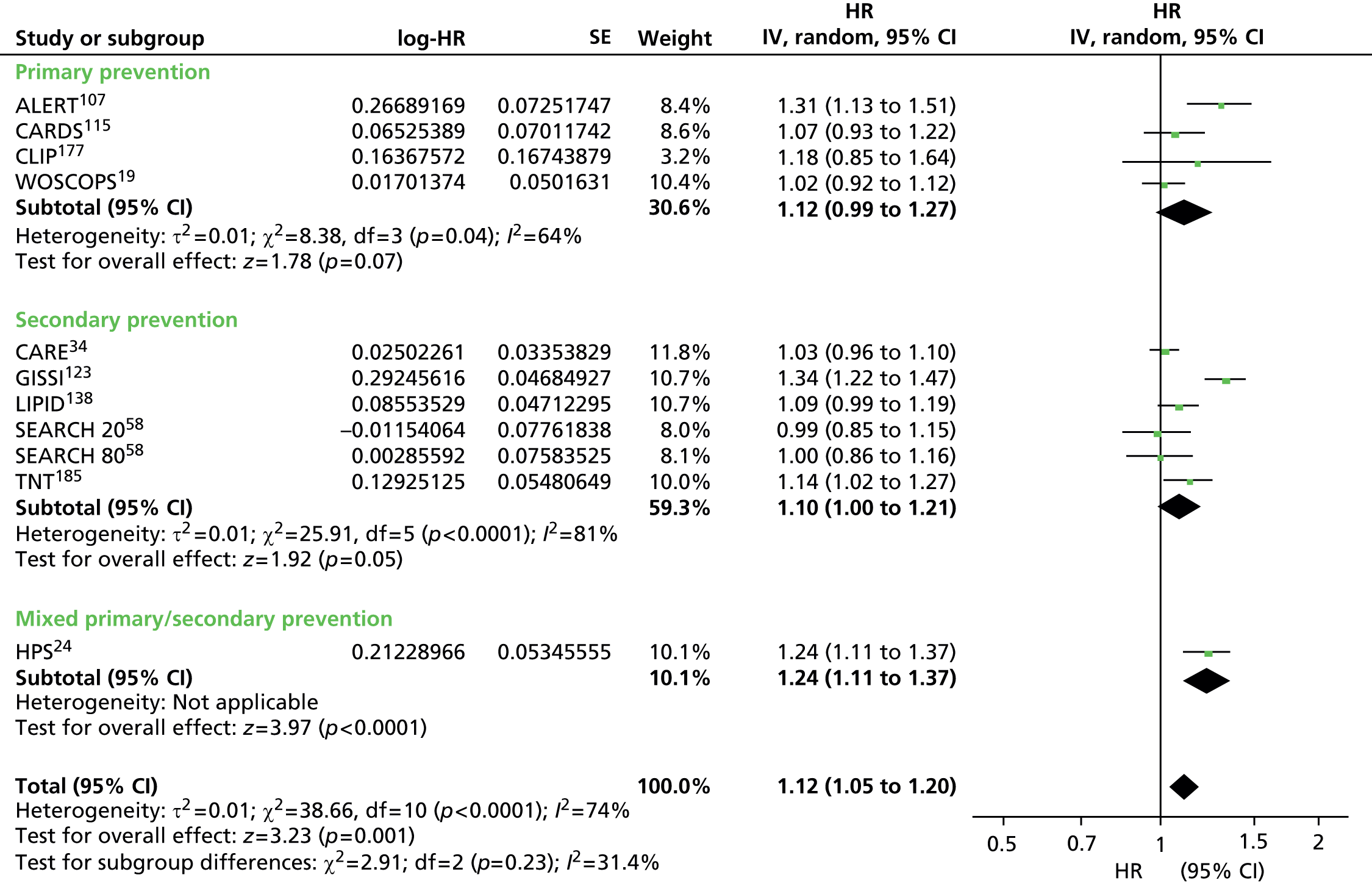
FIGURE 113.
Total cholesterol as prognostic marker for cardiovascular events (adjusted estimates) in populations on statins. IV, inverse variance.
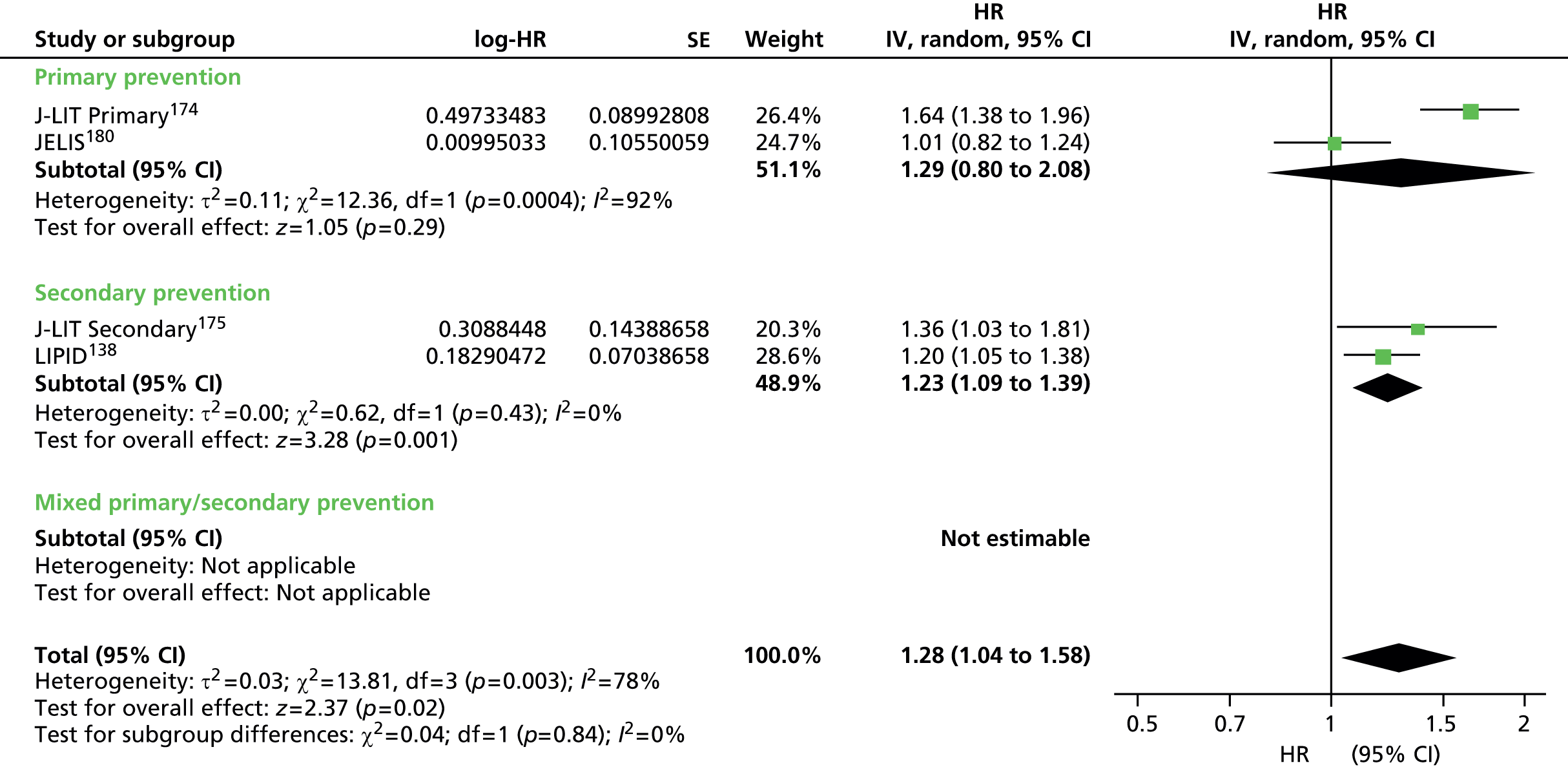
FIGURE 114.
Low-density lipoprotein as prognostic marker for cardiovascular events in populations on statins. IV, inverse variance.
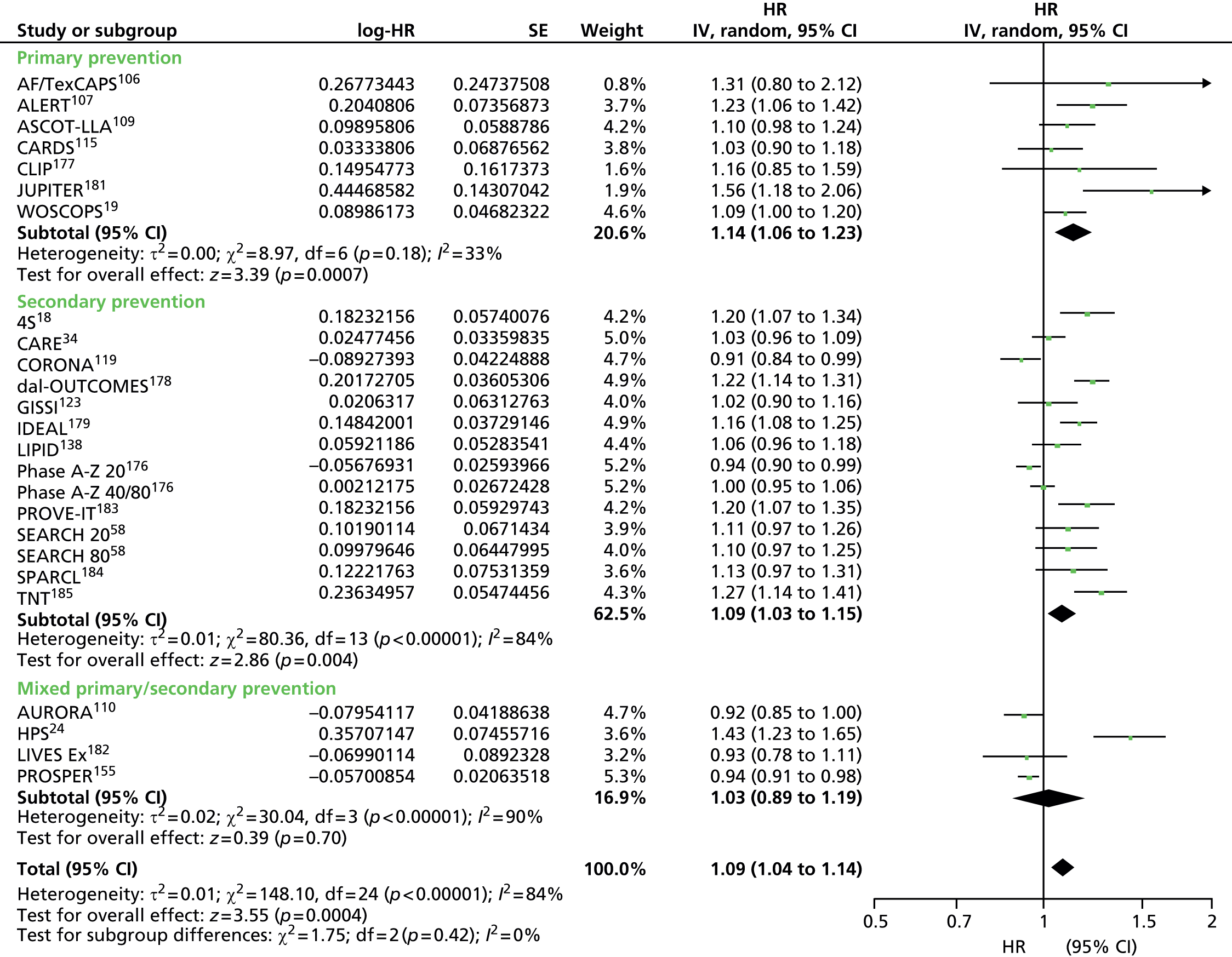
FIGURE 115.
Low-density lipoprotein as prognostic marker for cardiovascular events (adjusted estimates) in populations on statins. IV, inverse variance.

FIGURE 116.
High-density lipoprotein as prognostic marker for cardiovascular events in populations on statins. IV, inverse variance.
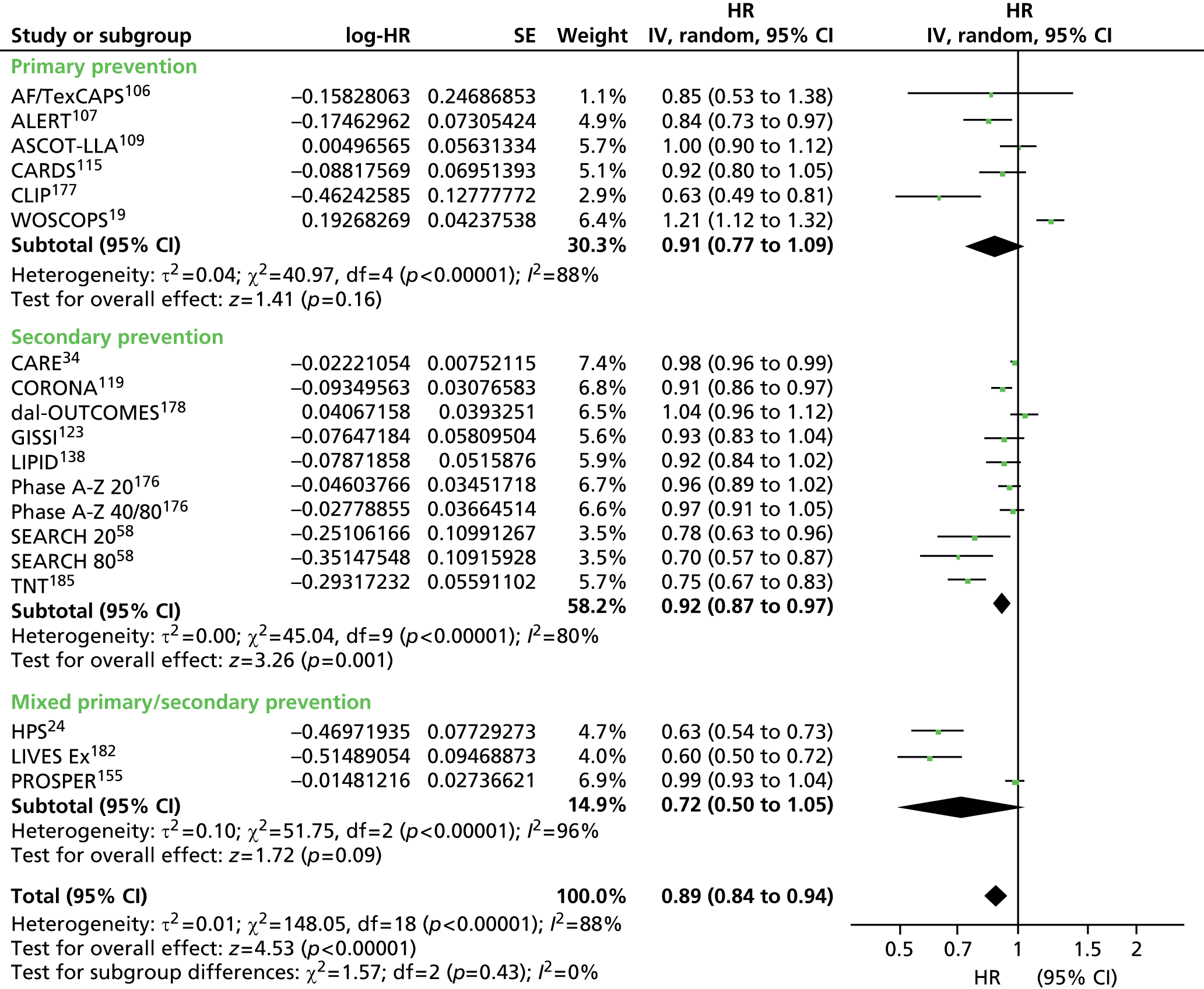
FIGURE 117.
High-density lipoprotein as prognostic marker for cardiovascular events (adjusted estimates) in populations on statins. IV, inverse variance.

FIGURE 118.
Triglycerides as prognostic marker for cardiovascular events in populations on statins. IV, inverse variance.

FIGURE 119.
Non-HDL as prognostic marker for cardiovascular events in populations on statins. IV, inverse variance.

FIGURE 120.
Apolipoprotein B as prognostic marker for cardiovascular events in populations on statins. IV, inverse variance.

Appendix 13 Sensitivity analyses in populations taking statins
FIGURE 121.
Summary results (pooled estimates) for cardiovascular events by all lipid measures based on removing studies with surrogate definitions of CVD. All data sets include primary prevention data sets, secondary prevention data sets, and studies with mixed or unreported prevention data sets. IV, inverse variance.

FIGURE 122.
Summary results (pooled estimates) for cardiovascular events by all lipid measures based on removing studies with indirectly extracted data. All data sets include primary prevention data sets, secondary prevention data sets, and studies with mixed or unreported prevention data sets. IV, inverse variance.
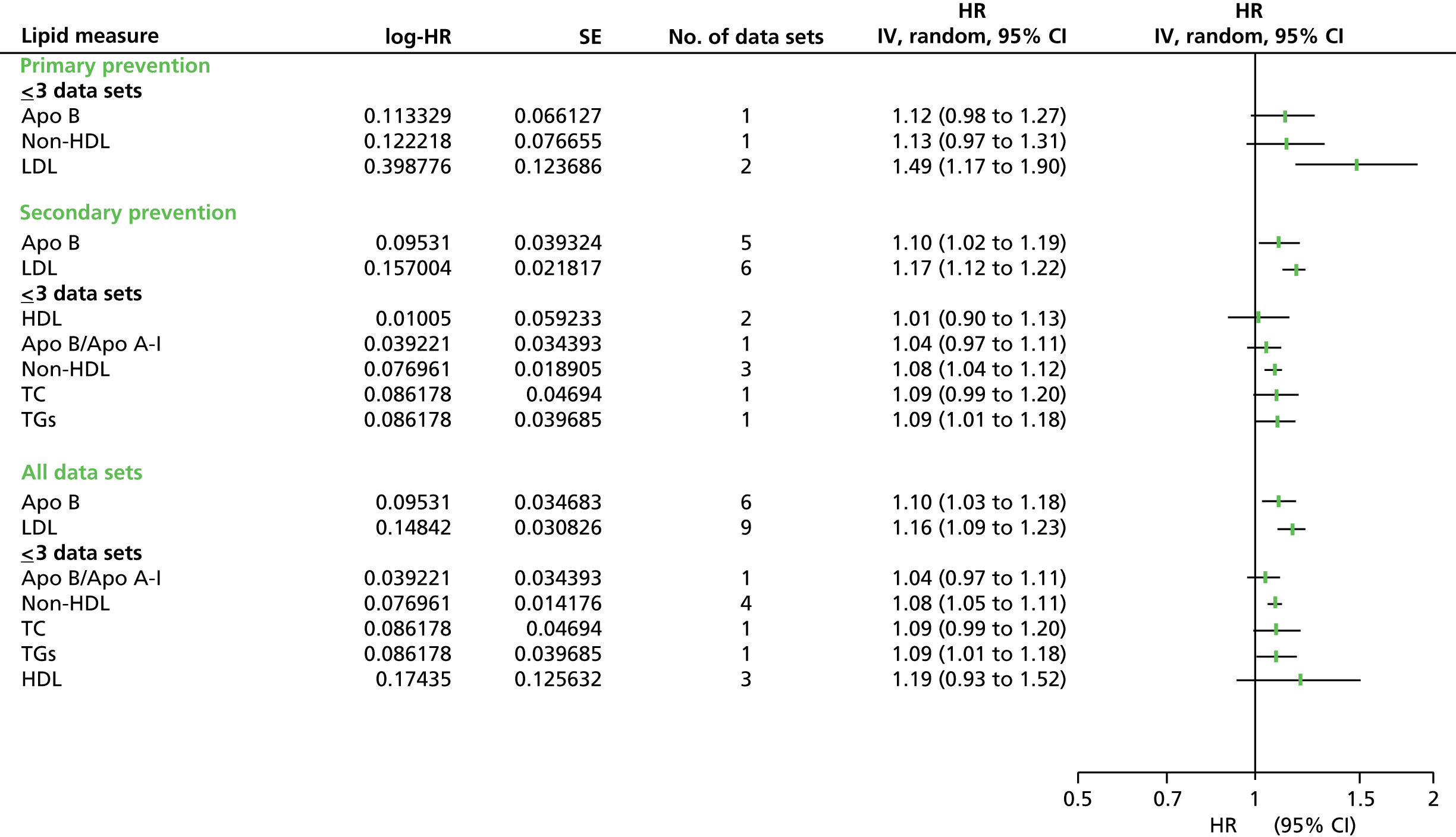
FIGURE 123.
Summary results (pooled estimates) for cardiovascular events by all lipid measures (adjusted estimates) based on removing studies with indirectly extracted data. All data sets include primary prevention data sets, secondary prevention data sets, and studies with mixed or unreported prevention data sets. IV, inverse variance.

Appendix 14 Excluded studies
Author (lead) | Study name | Reason for exclusion |
---|---|---|
AIHW and NHF | Risk Factors Prevalence Study | Unable to extract data |
Alaupovic P | Post-CABG | Unable to extract data |
Athyros V | GREACE | < 1000 participants per cohort |
Bae JM | Dynamic Cohort Study in Korean Adults | < 1 lipid measure assessed |
Ballesteros-Pomar MD | The DRECE study | Unable to extract data |
Beishuizen ED | Cerdia | < 1000 participants per cohort |
Boden W | AIM-HIGH | Unable to extract data |
Bonaa KH | The Tromso Study | Unable to extract data |
Brunner EJ | Whitehall II Study | Unable to extract data |
Buring JE | Boston Area Health Study | < 1000 participants per cohort |
Carlson LA | Stockholm Ischaemic Heart Disease Secondary Prevention Study (PMS) | < 1000 participants per cohort |
Casiglia E | Cardiovascular Study in the Elderly (CASTEL) | |
Chen Z | Unable to extract data | |
Chien KL | Chin-Shan Community Cardiovascular Cohort Study | Unable to extract data |
Coleman MP | < 1000 participants per cohort | |
Cox CS | The NHANES I Epidemiologic Follow-up Study | Unable to extract data |
Cui R | JACC study | Unable to extract data |
Davis WA | Fremantle Diabetes Study FDS | Unable to extract data |
De Backer G | Belgium Interuniversity Research on Nutrition and Health (BIRNH) | Unable to extract data |
Deeg DJH | Longitudinal Aging Study Amsterdam | Unable to extract data |
Doney A | Go-DARTS | No lipid data |
Dorr AE | < 1000 | |
Elley CR | Unable to extract data | |
Engstrom G | Malmo | Unable to extract data |
Ericsson MD | BECAIT | < 1000 participants per cohort |
Fang XH | Seven Cities China | Unable to extract data |
Fowkes FGR | Edinburgh Artery Study | Unable to extract data |
Furberg C | ACAPS | < 1000 participants per cohort |
Ginsberg H | ACCORD | Unable to extract data |
Goldenberg I | Bezafibrate Infarction Prevention Study | Unable to extract data |
Haheim L | Oslo Study | Unable to extract data |
Hamer M | Scottish Health Survey | Unable to extract data |
Harris J | Australian Longitudinal Study of Ageing | Unable to extract data |
Hayashi T | Japan Cholesterol and Diabetes Mellitus Study | Unable to extract data |
He Y | Xian | Lipids as covariates |
Held C | APSIS | < 1000 participants per cohort |
Heyman A | Evans County | Unable to extract data |
Higgins M | Tecumseh Community Health Study | Unable to extract data |
Hildrum B | HUNT | Unable to extract data |
Ho SC | unable to extract data | |
Howard BV | Women’s Health Initiative Randomized Controlled Dietary Modification Trial | Unable to extract data |
Howard BV | SANDS | No outcome data |
Igland J | Hordaland Homocysteine Study | Unable to extract data |
Imai K | SAITAMA | Unable to extract data |
Isles CG | Renfrew and Paisley | Unable to extract data |
Itakura H | LEM | No outcome data by lipid measure |
Iwashita M | Kyushi Lipid Intervention Study | Lipids as covariates |
Jansen ACM | Follow-up < 2 years | |
Kadota A | NIPPON 90 | Unable to extract data |
Kappelle P | < 1000 participants per cohort | |
Katsuki S | Hisayama Study | Unable to extract data |
Kawano Y | JATOS Study | Unable to extract data |
Kitamura A | Osaka 1979–86 | Stroke only |
Klungel O | Unable to extract data | |
Knopp RH | ASPEN | Unable to extract data |
Knuiman MW | Busselton Health Study | Unable to extract data |
Kojima S | < 1000 participants per cohort | |
Koren M | ALLIANCE | Unable to extract data |
Lee J | Singapore Cardiovascular Cohort Study | Unable to extract data |
Li JJ | China Coronary Secondary Prevention Study (CCSPS) | No outcome data by lipid measure |
Lichtenstein KA | HIV Outpatient Study (HOPS) | Unable to extract data |
Lindroos M | The Helsinki Ageing study | Unable to extract data |
Liu X | Yunnan Tin Minor Cohort study | Untraceable |
Look AHEAD Research group | Look AHEAD | Unable to extract data |
Lopez F | ARIC | Lipids as covariates |
Lowe LP | Chicago Heart Association | No outcome by lipid measure |
Luc G | The Prime Study | Unable to extract data |
Luepker R | The Minnesota Heart Health Program | Unable to extract data |
MacMahon S | Fletcher Challenge | Unable to extract data |
Manninen V | Helsinki Heart Study | Unable to extract data |
Marin A | Zaragoza | Unable to extract data |
Mast BT | Health, Aging and Body Composition Study | No outcome data |
May M | British Women’s Heart & Health Study | Unable to extract data |
McGovern PG | The Minnesota Heart Survey | Unable to extract data |
McQueen M | The Interheart Study | No follow-up |
Meade T | LEADER | Unable to extract data |
Menotti A | FINE | Lipids as covariates |
Monami M | No outcome by lipid measure | |
Morrell J | REACH Registry in Europe | Unable to extract data |
MRC General Practice Research Framework | Thrombosis Prevention Trial | Unable to extract data |
Nakayama T | The Shibata Study | Stroke only |
Navas-Nacher E | CHADP | Only 1 lipid measure |
Njolstad I | Norwegian Counties | Unable to extract data |
Nordestgaard B | Copenhagen General Population Study | Unable to extract data |
Obara F | The Tanno-Soubetsu Study | Unable to extract data |
Okada H | Akabane and Asahi Study | Unable to extract data |
Onat A | Turkish Adult Risk Factor Study | Unable to extract data |
Onat A | TEKHARF | Unable to extract data |
Parish S | International Studies of Infarct Survival (ISIS) | No follow-up |
Peters SAE | Meteor | No outcome data |
Raum E | Esther study | Unable to extract data |
Ren J | Chinese Multi Provincial Cohort Study (CMCS) | Lipids as covariates |
Rodeghiero F | The VITA project | Unable to extract data |
Rose G | UK Heart Disease Prevention Project | Unable to extract data |
Rosengren A | Goteborg Population studies | Unable to extract data |
Rosengren A | Gothenburg 1933 | Unable to extract data |
Rossebo A | SEAS | < 1000 participants per cohort |
Rossouw JF | Women’s Health Initiative Trials | < 1000 participants per cohort |
Rudnichi A | IPC Paris | Unable to extract data |
Satoh H | Lipids as covariates | |
Schwartz G | MIRACL | Follow-up < 2 years |
Serruys P | LIPS | < 1000 participants per cohort |
Shimamoto T | Ikawa | Unable to extract data |
Shinozaki T | J-EDIT | Unable to extract data |
Simon A | < 1000 participants per cohort | |
Smith GD | 27 Workplaces, Scotland | Unable to extract data |
Sone H | Japan Diabetes Complications Study | Lipids as covariates |
Soyama Y | The Oyabe Study | Stroke only |
Staessen JA | FLEMENGHO | Unable to extract data |
Straczek C | 3C | lipids as covariates |
Suadicani P | Copenhagen Male Study | unable to extract data |
Suh II | Korea Medical Insurance Corporation Study | Stroke only |
ten Kate G | < 1000 participants per cohort | |
Teramoto T | APPROACH J | No lipid data |
Tocharoenvanich P | InterASIA-south | Unable to extract data |
Tornberg S | VARMLAND | Unable to extract data |
Tsuji I | Ohasama, Japan | Unable to extract data |
Tunstall-Pedoe H | Scottish Heart Health Study | Unable to extract data |
Ulmer H | The Vorarlberg Health Monitoring & Promotion Program | Unable to extract data |
Van Lennep J | Leiden Heart Study | < 1000 participants per cohort |
Wanner C | 4D | < 1000 participants per cohort |
Wilhelmsen L | The GOT-MONICA Project | Unable to extract data |
Willey J | NOMAS | Stroke only |
Worm SW | D:A:D | Unable to extract data |
Zylberstein DE | Population Study of Women in Gothenburg | Unable to extract data |
Appendix 15 Meta-regressions: Stata output tables for selected lipid measures analysing three variables* for cardiovascular events (adjusted estimates) in populations not taking statins
*Presence of diabetes or underlying condition in baseline populations, use of surrogate measure of cardiovascular events.
Meta-regression | No. of observations = 17 | ||||
Restricted maximum likelihood estimate of between-study variance | tau2 = 0.01854 | ||||
% residual variation due to heterogeneity | I-squared_res = 95.10 | ||||
Proportion of between-study variance explained | Adjusted R-squared = 12.18% | ||||
Joint test for all covariates | Model F(2,14) = 1.67 | ||||
With Knapp–Hartung modification | Probability > F = 0.2237 | ||||
Underlying condition dropped because of collinearity | |||||
ln_LDLesti∼e | Coefficient | SE | t | p >|t| | 95% CI |
---|---|---|---|---|---|
Diabetes | 0.0622571 | 0.1165698 | 0.53 | 0.602 | –0.1877602 to 0.3122743 |
Surrogate | 0.1395165 | 0.0764276 | 1.83 | 0.089 | –0.0244044 to 0.3034374 |
_cons | 0.0727824 | 0.0547029 | 1.33 | 0.205 | –0.0445437 to 0.1901085 |
Meta-regression | No. of observations | 28 | |||
Restricted maximum likelihood estimate of between-study variance | tau2 | 0.02196 | |||
% residual variation due to heterogeneity | I-squared_res | 94.15 | |||
Proportion of between-study variance explained | Adjusted R-squared | 27.55% | |||
Joint test for all covariates | Model F(2,14) | 3.78 | |||
With Knapp–Hartung modification | Probability > F | 0.0236 | |||
ln_LDLesti∼e | Coefficient | SE | t | p >|t| | 95% CI |
---|---|---|---|---|---|
Diabetes | 0.2603132 | 0.2195192 | 1.19 | 0.247 | –0.1927521 to 0.7133785 |
Surrogate | 0.2379818 | 0.0719744 | 3.31 | 0.003 | 0.0894339 to 0.3865298 |
Condition | –0.1584406 | 0.1340066 | –1.18 | 0.249 | –0.4350165 to 0.1181354 |
_cons | 0.0618716 | 0.0581716 | 1.06 | 0.298 | –0.0581887 to 0.1819318 |
Meta-regression | No. of observations | 29 | |||
Restricted maximum likelihood estimate of between-study variance | tau2 | 0.01635 | |||
% residual variation due to heterogeneity | I-squared_res | 91.78 | |||
Proportion of between-study variance explained | Adjusted R-squared | –0.24% | |||
Joint test for all covariates | Model F(2,14) | 1.23 | |||
With Knapp–Hartung modification | Probability > F | 0.3198 | |||
ln_LDLesti∼e | Coefficient | SE | t | p >|t| | 95% CI |
---|---|---|---|---|---|
Diabetes | –0.0481637 | 0.1734864 | –0.28 | 0.784 | –0.4054657 to 0.3091383 |
Surrogate | –0.1120133 | 0.0703374 | –1.59 | 0.124 | –0.256876 to 0.0328493 |
Condition | 0.0763977 | 0.1315423 | 0.58 | 0.567 | –0.1945187 to 0.3473141 |
_cons | –0.1133303 | 0.0587723 | –1.93 | 0.065 | –0.234374 to 0.0077135 |
Meta-regression | No. of observations | 8 | |||
Restricted maximum likelihood estimate of between-study variance | tau2 | 0.03094 | |||
% residual variation due to heterogeneity | I-squared_res | 95.48 | |||
Proportion of between-study variance explained | Adjusted R-squared | 33.37% | |||
Joint test for all covariates | Model F(2,14) | 2.41 | |||
With Knapp–Hartung modification | Probability > F | 0.1849 | |||
Underlying condition dropped because of collinearity | |||||
ln_TGesti∼e | Coefficient | SE | t | p >|t| | 95% CI |
---|---|---|---|---|---|
Diabetes | 0.0177365 | 0.1675231 | 0.11 | 0.920 | –0.4128953 to 0.4483683 |
Surrogate | 0.3098726 | 0.1528325 | 2.03 | 0.098 | –0.0829958 to 0.702741 |
_cons | 0.0533839 | 0.1047012 | 0.51 | 0.632 | –0.215759 to 0.3225269 |
Meta-regression | No. of observations | 14 | |||
Restricted maximum likelihood estimate of between-study variance | tau2 | 0.02028 | |||
% residual variation due to heterogeneity | I-squared_res | 95.67 | |||
Proportion of between-study variance explained | Adjusted R-squared | 44.35% | |||
Joint test for all covariates | Model F(2,14) | 5.78 | |||
With Knapp–Hartung modification | Probability > F | 0.0193 | |||
Underlying condition dropped because of collinearity | |||||
ln_NHDLesti∼e | Coefficient | SE | t | p >|t| | 95% CI |
---|---|---|---|---|---|
Diabetes | 0.0613456 | 0.1239055 | 0.50 | 0.630 | –0.2113685 to 0.3340598 |
Surrogate | 0.2958856 | 0.088926 | 3.33 | 0.007 | 0.1001608 to 0.4916104 |
_cons | 0.1053788 | 0.0616339 | 1.71 | 0.115 | –0.0302764 to 0.2410341 |
Meta-regression | No. of observations | 17 | |||
Restricted maximum likelihood estimate of between-study variance | tau2 | 0.01494 | |||
% residual variation due to heterogeneity | I-squared_res | 98.67 | |||
Proportion of between-study variance explained | Adjusted R-squared | 8.40% | |||
Joint test for all covariates | Model F(2,14) | 1.61 | |||
With Knapp–Hartung modification | Probability > F | 0.2349 | |||
ln_ApoBesti∼e | Coefficient | SE | t | p >|t| | 95% CI |
---|---|---|---|---|---|
Diabetes | 0.1846332 | 0.2002893 | 0.92 | 0.373 | –0.2480655 to 0.6173319 |
Surrogate | 0.1738488 | 0.0857072 | 2.03 | 0.064 | –0.0113104 to 0.359008 |
condition | –0.1335675 | 0.1211023 | –1.10 | 0.290 | –0.395193 to 0.128058 |
_cons | 0.1269758 | 0.0728726 | 1.74 | 0.105 | –0.0304559 to 0.2844075 |
Meta-regression | No. of observations | 15 | |||
Restricted maximum likelihood estimate of between-study variance | tau2 | 0.009801 | |||
% residual variation due to heterogeneity | I-squared_res | 95.61 | |||
Proportion of between-study variance explained | Adjusted R-squared | –9.05% | |||
Joint test for all covariates | Model F(2,14) | 0.39 | |||
With Knapp–Hartung modification | Probability > F | 0.6827 | |||
Diabetes dropped because of collinearity | |||||
ln_ApoBesti∼e | Coefficient | SE | t | p >| t | | 95% CI |
---|---|---|---|---|---|
Surrogate | –0.06577 | 0.075142 | –0.88 | 0.399 | –0.2294904 to 0.0979505 |
condition | 0.0038242 | 0.110407 | 0.03 | 0.973 | –0.2367319 to 0.2443802 |
_cons | –0.0988367 | 0.0627986 | –1.57 | 0.142 | –0.2356631 to 0.0379898 |
Meta-regression | No. of observations | 14 | |||
Restricted maximum likelihood estimate of between-study variance | tau2 | 0.002205 | |||
% residual variation due to heterogeneity | I-squared_res | 59.46 | |||
Proportion of between-study variance explained | Adjusted R-squared | 83.45% | |||
Joint test for all covariates | Model F(2,14) | 18.42 | |||
With Knapp–Hartung modification | Probability > F | 0.0003 | |||
Diabetes dropped because of collinearity | |||||
ln_ApoB_Aesti∼e | Coefficient | SE | t | p >|t| | 95% CI |
---|---|---|---|---|---|
Surrogate | 0.256769 | 0.0423127 | 6.07 | 0.000 | 0.1636393 to 0.3498988 |
condition | 0.0038242 | 0.110407 | 0.03 | 0.973 | –0.2367319 to 0.2443802 |
_cons | –0.0988367 | 0.0627986 | –1.57 | 0.142 | –0.2356631 to 0.0379898 |
Meta-regression | No. of observations | 17 | |||
Restricted maximum likelihood estimate of between-study variance | tau2 | 0.01964 | |||
% residual variation due to heterogeneity | I-squared_res | 95.00 | |||
Proportion of between-study variance explained | Adjusted R-squared | 23.46% | |||
Joint test for all covariates | Model F(2,14) | 3.09 | |||
With Knapp–Hartung modification | Probability > F | 0.0775 | |||
Underlying condition dropped because of collinearity | |||||
ln_TC_HDLesti∼e | Coefficient | SE | t | p >|t| | 95% CI |
---|---|---|---|---|---|
Diabetes | 0.12351 | 0.1615461 | 0.76 | 0.457 | –0.2229719 to 0.469992 |
Surrogate | 0.2056153 | 0.0827753 | 2.48 | 0.026 | 0.02808 to 0.3831506 |
_cons | 0.0824817 | 0.0666923 | 1.24 | 0.237 | –0.0605592 to 0.2255225 |
Appendix 16 Meta-analysis of the effect of atorvastatin on serum lipid levels: sensitivity analysis and results from the network meta-analysis
This appendix presents results from a sensitivity analysis of the impact our choice of a minimum sample size of 1000 participants had on estimates of the effect of atorvastatin on serum lipid levels. It also presents full results from two network meta-analyses, based on (1) the studies included in Chapter 3 and (2) the extended network (including studies with < 1000 participants).
The effect of relaxing the minimum sample size inclusion criterion
Re-searching the 181 trials cited in Naci et al. ,188 with no minimum sample size constraint, provided 13 additional studies for inclusion. Data were extracted from these studies independently by two researchers (TF and JM), as previously (Tables 74–77).
Study | Treatment | Mean dose (mg/day) | Participants (by arm) | Study follow-up | Follow-up for data extractiona |
---|---|---|---|---|---|
ARBITER260 | Atorva | 80 | 79 | 12 months | 12 months |
Prava | 40 | 82 | |||
ASAP261 | Atorva | 80 | 160 | 2 years | 2 years |
Simva | 40 | 165 | |||
BELLES262 | Atorva | 80 | 305 | 12 months | 12 months |
Prava | 40 | 310 | |||
Bertolini263 | Atorva | 11.8 | 227 | 12 months | 12 months |
Prava | 29.4 | 78 | |||
Brown264 | Atorva | 20 | 80 | 54 weeks | 54 weeks |
Fluva | 40 | 80 | |||
Lova | 80 | 81 | |||
Simva | 40 | 77 | |||
Colivicchi265 | Atorva | 80 | 144 | 12 months | 12 months |
Atorva | 24.8 | 146 | |||
Kadoglou266 | Atorva | 80 | 70 | 12 months | 12 months |
Atorva | 10–20 | 70 | |||
Mohler267 | Atorva | 80 | 120 | 12 months | 12 months |
Atorva | 10 | 120 | |||
Placebo | – | 114 | |||
REVERSAL268 | Atorva | 80 | 328 | 18 months | 18 months |
Prava | 40 | 329 | |||
SAGE269 | Atorva | 80 | 446 | 12 months | 12 months |
Prava | 40 | 445 | |||
Schmermund270 | Atorva | 80 | 235 | 12 months | 12 months |
Atorva | 10 | 236 | |||
Sola271 | Atorva | 20 | 54 | 12 months | 12 months |
Placebo | – | 54 | |||
Stone272 | Atorva | Median 80 | 96 | 12 months | 12 months |
Atorva + V | Median 80 | 101 | |||
Lova | Median 5 | 103 |
Study | Treatment | Age mean, years (SD) | Female % | MI % | CHD % | DM % | BMI mean, kg/m2 (SD) | Smoking % |
---|---|---|---|---|---|---|---|---|
ARBITER260 | Atorva | 58 (11) | 31.6 | 49.4 | 14.3 | C13.9 | ||
Prava | 61 (12) | 25.6 | 42.7 | 10.4 | C7.3 | |||
ASAP261 | Atorva | 59 | ||||||
Simva | 62 | |||||||
BELLES262 | Atorva | 64.2 (6.5) | 100 | 3.3 | 13.8 | 28.5 (5.4) | C20.7 | |
Prava | 64.5 (6.0) | 100 | 2.3 | 14.2 | 29.0 (5.5) | C16.5 | ||
Bertolini263 | Atorva | 56 (10.5) | 53 | 0 | 26 (3.0) | |||
Prava | 57 (10.6) | 59 | 0 | 25 (2.6) | ||||
Brown264 | Atorva | 62 (9.8) | 38 | |||||
Fluva | 62 (8.9) | 23 | ||||||
Lova | 64 (9.8) | 37 | ||||||
Simva | 63 (9.8) | 31 | ||||||
Colivicchi265 | Atorva 80 | 73.9 (9.4) | 47.9 | 100 | 100 | 72.2 | ||
Atorva 25 | 75.2 (9.9) | 49.3 | 100 | 100 | 69.8 | |||
Kadoglou266 | Atorva 80 | 63.2 (6.8) | 55 | 0 | 33 | 28.3 (4.0) | ||
Atorva 10 | 64.8 (7.3) | 54 | 0 | 38 | 29.1 (4.9) | |||
Mohler267 | Atorva 80 | 68 | 21 | 18 | C35 | |||
Atorva 10 | 69 | 25 | 19 | C41 | ||||
Placebo | 67 | 23 | 15 | C46 | ||||
REVERSAL268 | Atorva | 55.8 (9.8) | 28.9 | 20 | 30.5 (6.5) | |||
Prava | 56.6 (9.2) | 26.9 | 18 | 30.5 (5.6) | ||||
SAGE269 | Atorva | 72.4 (5.1) | 31.2 | 45.5 | 100 | 22.4 | 27.4 (4.0) | C5.4, F51.8 |
Prava | 72.6 (5.2) | 29.9 | 46.3 | 100 | 24.0 | 26.8 (3.5) | C7.0, F54.6 | |
Schmermund270 | Atorva 80 | 62 (8) | 25 | 0 | 13 | 28 (4) | C or F 74 | |
Atorva 10 | 61 (8) | 26 | 0 | 13 | 27 (4) | C or F 73 | ||
Sola271 | Atorva | 53.3 (6.2) | 37 | 100 | 24.1 (4.0) | |||
Placebo | 54.1 (6.9) | 39 | 100 | 24.4 (3.8) | ||||
Stone272 | Atorva | 14 | 42 | 17 | C or F 70 | |||
Atorva+V | 16 | 40 | 15 | C or F 56 | ||||
Lova | 12 | 36 | 16 | C or F 69 |
Study | Treatment | LDL | HDL | TC | TGs | ||||
---|---|---|---|---|---|---|---|---|---|
Baseline | Follow-up | Baseline | Follow-up | Baseline | Follow-up | Baseline | Follow-up | ||
ARBITER260 | Atorva | 3.83 (0.83) | 1.97 (0.59) | 1.27 (0.41) | 1.29 (0.47) | 5.92 (1.09) | 3.78 (0.93) | 2.38 (1.53) | 1.51 (0.87) |
Prava | 4.01 (0.88) | 2.84 (0.78) | 1.27 (0.36) | 1.34 (0.39) | 6.05 (1.09) | 4.91 (1.03) | 2.29 (1.21) | 2.09 (1.19) | |
ASAP261 | Atorva | 8.00 (1.83) | 3.88 (1.21) | 1.18 (0.32) | 1.32 (0.39) | 9.99 (1.87) | 5.73 (1.31) | 1.87 (1.09) | 1.23 (0.76) |
Simva | 8.33 (2.03) | 4.81 (1.38) | 1.16 (0.28) | 1.30 (0.36) | 10.27 (2.10) | 6.71 (1.38) | 1.85 (1.34) | 1.41 (0.96) | |
BELLES262 | Atorva | 4.53 (0.84) | 2.38 (0.67) | 1.46 (0.36) | 1.47 (0.35) | 6.95 (0.91) | 4.56 (1.08) | 2.09 (0.97) | 1.53 (0.73) |
Prava | 4.48 (0.92) | 3.34 (0.80) | 1.52 (0.37) | 1.56 (0.38) | 6.92 (1.04) | 5.68 (0.92) | 1.99 (1.03) | 1.70 (0.84) | |
Bertolini263 | Atorva | 5.02 (0.60) | 3.26 | 1.37 (0.30) | 1.47 | 7.16 (0.75) | 5.37 | 1.65 (0.75) | 1.41 |
Prava | 5.07 (0.53) | 3.90 | 1.27 (0.26) | 1.40 | 7.19 (0.62) | 6.04 | 1.80 (0.79) | 1.75 | |
Brown264 | Atorva | 4.47 (0.93) | 2.64 | 1.06 (0.28) | 1.13 | 6.57 (1.02) | 4.60 | 2.29 (0.88) | 1.85 |
Fluva | 4.40 (0.72) | 3.08 | 1.06 (0.28) | 1.13 | 6.46 (0.93) | 5.17 | 2.16 (0.71) | 2.12 | |
Lova | 4.53 (0.77) | 2.67 | 1.03 (0.28) | 1.19 | 6.67 (0.86) | 4.74 | 2.42 (0.95) | 2.08 | |
Simva | 4.45 (0.77) | 2.80 | 1.06 (0.32) | 1.18 | 6.52 (0.95) | 4.82 | 2.18 (0.77) | 1.85 | |
Colivicchi265 | Atorva 80 | 3.18 (0.72) | 1.6 (0.13) | 1.03 (0.31) | 5.77 (1.06) | 1.91 (0.47) | |||
Atorva 25 | 3.26 (0.70) | 2.4 (0.10) | 1.01 (0.26) | 5.64 (0.96) | 1.87 (0.44) | ||||
Kadoglou266 | Atorva 80 | 4.32 (1.03) | 1.98 (0.45) | 1.09 (0.34) | 1.21 (0.29) | 5.87 (1.41) | 3.81 (1.26) | 1.73 (0.61) | 1.34 (0.48) |
Atorva 10 | 4.19 (0.87) | 2.71 (0.53) | 1.20 (0.37) | 1.26 (0.27) | 6.30 (1.15) | 4.73 (0.88) | 1.98 (0.88) | 1.67 (0.86) | |
Mohler267 | Atorva 80 | 3.29 (1.60) | 1.66 (1.09) | 1.16 (0.39) | 1.22 (0.39) | 5.51 (0.57) | 3.62 (0.54) | 2.16 (1.70) | 1.46 (0.96) |
Atorva 10 | 3.23 (0.54) | 2.07 (1.09) | 1.19 (0.39) | 1.24 (0.26) | 5.46 (0.57) | 4.16 (1.11) | 2.34 (1.45) | 1.85 (1.21) | |
Placebo | 3.23 (0.52) | 3.23 (0.52) | 1.22 (0.39) | 1.19 (0.39) | 5.40 (1.09) | 5.40 (1.09) | 2.08 (1.41) | 2.14 (1.41) | |
REVERSAL268 | Atorva | 3.88 (0.72) | 2.04 (0.78) | 1.09 (0.26) | 1.11 (0.29) | 5.99 (0.88) | 3.91 (1.01) | 2.23 (1.08) | 1.68 (1.07) |
Prava | 3.88 (0.67) | 2.85 (0.67) | 1.11 (0.29) | 1.15 (0.29) | 6.02 (0.88) | 4.85 (0.83) | 1.13 (1.19) | 1.87 (1.04) | |
SAGE269 | Atorva | 3.81 (0.78) | 1.70 | 1.18 (0.30) | 1.24 | 5.84 (0.93) | 3.53 | 1.86 (0.81) | 1.37 |
Prava | 3.72 (0.81) | 2.51 | 1.20 (0.29) | 1.29 | 5.74 (0.93) | 4.52 | 1.78 (0.88) | 1.66 | |
Schmermund270 | Atorva 80 | 2.74 (0.57) | 2.25 (0.85) | 1.32 (0.31) | 1.37 (0.31) | 4.53 (0.62) | 4.09 (0.93) | 1.77 (0.89) | 1.55 (0.80) |
Atorva 10 | 2.79 (0.59) | 2.82 (0.72) | 1.34 (0.34) | 1.40 (0.36) | 4.58 (0.57) | 4.73 (0.72) | 1.59 (0.78) | 1.71 (0.85) | |
Sola271 | Atorva | 3.05 (0.39) | 2.40a | 1.14 (0.18) | 1.19 (0.21) | 1.68 (0.21) | 1.56 (0.23) | ||
Placebo | 3.21 (0.52) | (0.44)a | 1.09 (0.21) | 1.11 (0.21) | 1.63 (0.26) | 1.68 (0.25) | |||
Stone272 | Atorva | 3.85 (0.85) | 2.09 (0.41) | 1.14 (0.28) | 1.16 (0.26) | 5.90 (0.88) | 3.80 (0.54) | 2.00 (0.90) | 1.21 (0.67) |
Atorva+V | 3.65 (0.70) | 2.22 (0.59) | 1.27 (0.54) | 1.11 (0.28) | 5.77 (0.72) | 3.88 (0.75) | 1.90 (1.22) | 1.41 (1.23) | |
Lova | 3.90 (0.70) | 3.10 (0.57) | 1.11 (0.26) | 1.24 (0.34) | 5.95 (0.75) | 5.09 (0.80) | 1.93 (0.97) | 1.75 (1.19) |
Study | Comparison | LDL | HDL | TC | TGs |
---|---|---|---|---|---|
ARBITER260 | A80 – P40 | –0.87 (0.118) | –0.05 (0.073) | –1.13 (0.167) | –0.58 (0.178) |
ASAP261 | A80 – S40 | –0.93 (0.155) | 0.02 (0.045) | –0.98 (0.161) | –0.18 (0.103) |
BELLES262 | A80 – P40 | –0.96 (0.068) | –0.09 (0.034) | –1.12 (0.092) | –0.17 (0.073) |
Bertolini263 | A12 – P29 | –0.64 (0.079) | –0.07 (0.039) | –0.67 (0.097) | –0.15 (0.103) |
Brown264 | A20 – F40 | –0.44 (0.134) | 0 (0.045) | –0.57 (0.157) | –0.27 (0.129) |
A20 – L80 | –0.03 (0.137) | –0.06 (0.045) | –0.14 (0.151) | –0.23 (0.147) | |
A20 – S40 | –0.16 (0.138) | –0.05 (0.048) | –0.22 (0.159) | 0 (0.133) | |
Colivicchi265 | A80 – A25 | –0.80 (0.089) | |||
Kadoglou266 | A80 – A10 | –0.73 (0.086) | –0.05 (0.049) | –0.92 (0.190) | –0.33 (0.121) |
Mohler267 | A80 – A10 | –0.41 (0.141) | –0.02 (0.043) | –0.54 (0.113) | –0.39 (0.141) |
A80 – U | –1.57 (0.113) | 0.03 (0.051) | –1.78 (0.112) | –0.68 (0.157) | |
A10 – U | –1.16 (0.113) | 0.05 (0.043) | –1.24 (0.144) | –0.29 (0.171) | |
REVERSAL268 | A80 – P40 | –0.81 (0.065) | –0.04 (0.026) | –0.94 (0.083) | –0.19 (0.094) |
SAGE269 | A80 – P40 | –0.81 (0.058) | –0.05 (0.022) | –0.99 (0.068) | –0.29 (0.062) |
Schmermund270 | A80 – A10 | –0.57 (0.082) | –0.03 (0.035) | –0.64 (0.087) | –0.16 (0.086) |
Sola271 | A20 – U | a | 0.08 (0.045) | –0.12 (0.051) | |
Stone272 | A80 – A80 +V | –0.13 (0.078) | 0.05 (0.042) | –0.08 (0.101) | –0.20 (0.154) |
A80 – L5 | –1.01 (0.077) | –0.08 (0.047) | –1.29 (0.105) | –0.54 (0.150) | |
A80 +V – L5 | –0.88 (0.088) | –0.13 (0.047) | –1.21 (0.118) | –0.34 (0.183) |
Ten of these studies were two-arm trials. Of the remaining three, one272 was a three-arm trial in which one of the arms was to atorvastatin plus vitamin supplementation. For the purposes of analysis, this arm was ignored and so the trial was treated as a two-arm trial. One study267 compared two different doses of atorvastatin with placebo, and one study264 was a four-arm trial of four different statins. For these trials, all of the data were retained for the purpose of network meta-analysis.
Some studies did not report follow-up data for all of the outcome measures, and these studies are excluded from the analysis where necessary. Figures 124–128 show, in network form, the effect sizes from all contributing studies for LDL cholesterol, HDL cholesterol, TC and TGs, respectively.
FIGURE 124.
Extended network (23 studies): trials of the interventions evaluated. Bold text indicates the 10 studies included in the original review. Coloured text indicates comparisons obtained from the same study.
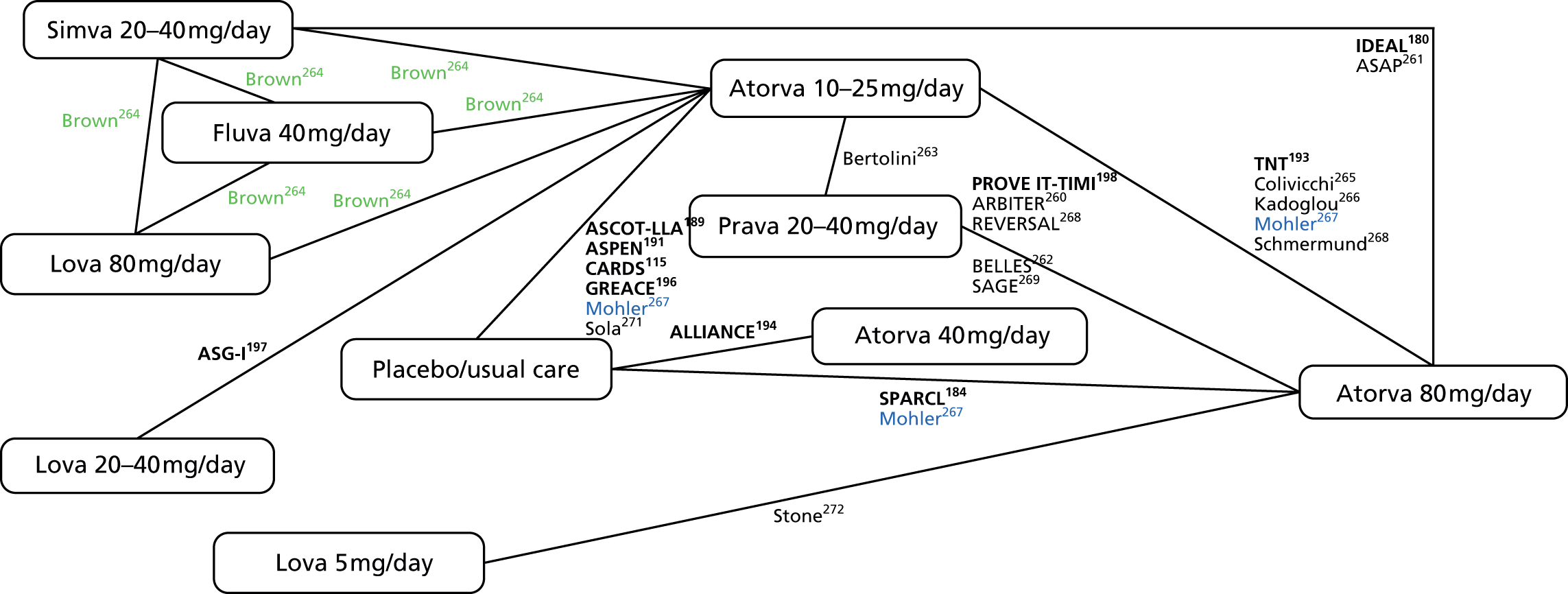
FIGURE 125.
Extended network (23 studies): LDL differences reported in trials of the interventions evaluated. Bold text indicates the 10 studies included in the original review. Coloured text indicates comparisons obtained from the same study.
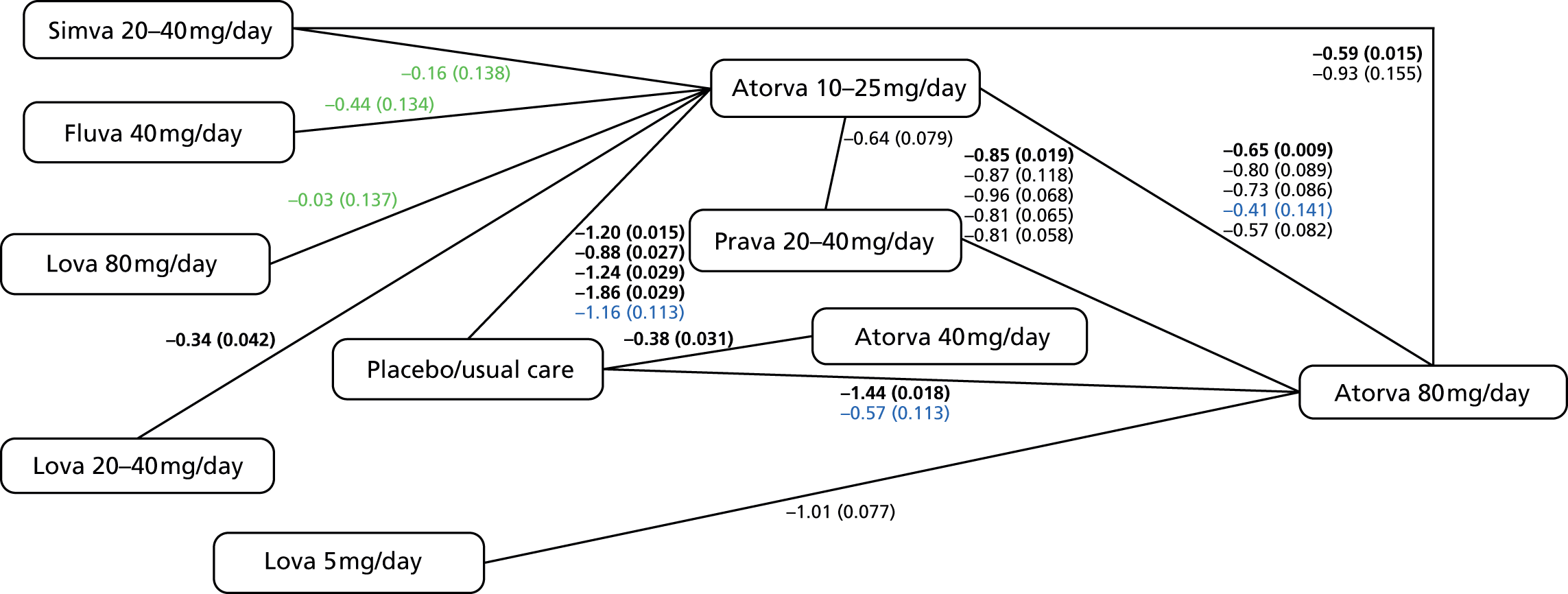
FIGURE 126.
Extended network (23 studies): HDL differences reported in trials of the interventions evaluated. Bold text indicates the 10 studies included in the original review. Coloured text indicates comparisons obtained from the same study.

FIGURE 127.
Extended network (23 studies): TC difference reported in trials of the interventions evaluated. Bold text indicates the 10 studies included in the original review. Coloured text indicates comparisons obtained from the same study.

FIGURE 128.
Extended network (23 studies): TGs differences reported in trials of the interventions evaluated. Bold text indicates the 10 studies included in the original review. Coloured text indicates comparisons obtained from the same study.

Network meta-analysis, original network
The results of the network meta-analysis are shown in Table 78. The model used was random-effects meta-analysis, with assumed equal between-study SD among all pairwise treatment comparisons. As there was, at most, only one contributing study for most pairwise comparisons (see Figures 125–128) it was impractical to fit more general models with varying between-study SD. The random-effects model was chosen because of extremely high heterogeneity between studies.
Statin (mg) | Lipid measure (mmol/l) | |||
---|---|---|---|---|
LDL | HDL | TC | Triglycerides | |
Atorvastatin 10–25 | –1.23 (0.18) | 0.027 (0.010) | –1.30 (0.22) | –0.31 (0.066) |
Atorvastatin 40 | –0.38 (0.39) | –0.020 (0.024) | –0.49 (0.47) | –0.15 (0.15) |
Atorvastatin 80 | –1.64 (0.29) | 0.028 (0.016) | –1.79 (0.34) | –0.49 (0.10) |
Lovastatin 20 | –0.88 (0.43) | 0.027 (0.031) | –0.83 (0.52) | –0.11 (0.16) |
Pravastatin 40a | –0.79 (0.49) | 0.068 (0.026) | – | – |
Simvastatin 20 | –1.04 (0.49) | 0.068 (0.026) | –1.01 (0.57) | –0.22 (0.17) |
sdb | 0.39 | 0.019 | 0.47 | 0.14 |
Following Higgins et al. 273 and White et al. ,274 we also fitted the full treatment-by-design interaction to examine inconsistency in the network, treating inconsistency parameters as fixed effects. For the meta-analysis consisting of the 10 studies originally identified, there is only one loop (between the Atorvastatin 10–25, Atorvastatin 80 and Placebo arms; see Figure 14) and therefore only one identifiable inconsistency parameter. This is a loop inconsistency parameter that can be chosen to contrast any two of the treatments within the loop. This choice is arbitrary, as different choices are simply different parameterisations of the same model.
The statistical significance of this loop inconsistency parameter can be tested using a Wald test (White et al. ,274 p. 115). Unexpectedly, when different parameterisations were used in models for the same network, this test statistic varied slightly, possibly because of instability in the estimate of this term or its SE (given the sparsity of the network and the presence of the heterogeneity random effect term, this is, perhaps, not surprising). However, for each of the four lipid measures, the loop inconsistency parameter was not statistically significant, irrespective of the parameterisation chosen. The conclusion is that there is no evidence for inconsistency in the network for any of the lipid measures.
The point estimates in Table 78 relate to the effect of each treatment relative to the reference category, Placebo/Usual Care. The results can be compared with those in the network diagram (see Figures 15–18) presented in Chapter 3.
For the treatment comparisons for which there are contributing studies that include a Placebo/Usual Care arm, the pooled effects in Table 78 are similar to those in the contributing studies (or, in the case of atorvastatin 10–25 vs. Placebo/Usual Care, close to the average effect observed in the contributing studies). The discrepancy in the estimate for atorvastatin 80 versus Placebo/Usual Care (from –1.44 mmol/l in the SPARCL trial184 to –1.64 mmol/l in Table 78) is a consequence of making the assumption of consistency, as this contrast forms part of the ‘atorvastatin 80 – atorvastatin 10–25 – Placebo/Usual Care’ loop (see Figure 15); as discussed above, this change is not statistically significant. The estimate of the heterogeneity random effect SD is driven by the multiplicity of studies comparing atorvastatin 10–25 with Placebo/Usual Care. The estimate (0.39 mmol/l) appears reasonable, given the range of point estimates in these four studies.
Interpretation for HDL cholesterol, TC and TGs is similar. For HDL cholesterol, the estimated heterogeneity SD is very small, as no study found more than a very small difference between groups in HDL. For all lipid measures, results for atorvastatin 40 appear a little unusual; however, these estimates are based on a single study (ALLIANCE194) that was rated as having high risk of bias on several domains (see Table 14).
Network meta-analysis, extended network
Repeating similar steps to those above (see Network meta-analysis, original network) to the extended network of 23 studies yields the results in Table 79.
Statin (mg) | Lipid measure (mmol/l) | |||
---|---|---|---|---|
LDL | HDL | TC | Triglycerides | |
Atorvastatin 10–25 | –1.18 (0.10) | 0.033 (0.0098) | –1.31 (0.12) | –0.29 (0.050) |
Atorvastatin 40 | –0.38 (0.25) | –0.020 (0.024) | –0.49 (0.29) | –0.15 (0.14) |
Atorvastatin 80 | –1.70 (0.12) | 0.018 (0.014) | –1.89 (0.15) | –0.49 (0.067) |
Fluvastatin 40 | –0.72 (0.27) | 0.020 (0.045) | –0.72 (0.32) | –0.0042 (0.16) |
Lovastatin 5 | –0.69 (0.28) | 0.098 (0.052) | –0.59 (0.34) | 0.047 (0.20) |
Lovastatin 20 | –0.84 (0.27) | 0.033 (0.031) | –0.91 (0.31) | –0.087 (0.14) |
Lovastatin 80 | –1.13 (0.27) | 0.080 (0.045) | –1.15 (0.31) | –0.044 (0.18) |
Pravastatin 40 | –0.79 (0.15) | 0.056 (0.018) | –0.80 (0.19) | –0.18 (0.091) |
Simvastatin 20 | –0.98 (0.19) | 0.053 (0.022) | –1.05 (0.22) | –0.26 (0.10) |
sda | 0.24 | 0.019 | 0.28 | 0.12 |
In this larger network, there were five available degrees of freedom (d.f.) for inconsistency parameters: there is one four-arm design, one three-arm design and nine two-arm designs (14 d.f.), and 10 treatments (9 d.f.), and therefore the number of additional degrees of freedom available for the inconsistency model is 5 (14 – 9). Under one parameterisation, these might be viewed as four loop inconsistency parameters, corresponding, respectively, to the loops:
‘Atorvastatin 80 – Atorvastatin 10–25 – Placebo/Usual Care’
‘Atorvastatin 80 – Atorvastatin 10–25 – Pravastatin 20–40’
‘Atorvastatin 80 – Simvastatin 20–40 – Atorvastatin 10’
‘Atorvastatin 80 – Simvastatin 20–40 – Atorvastatin 10–25 – Placebo/Usual Care’
and one design inconsistency parameter, induced by the three-arm trial,267 which has Atorvastatin 80, Atorvastatin 10 and Placebo/Usual Care arms. The single four-arm trial264 includes three arms that are not shared by any of the other 22 studies, and so does not provide scope for further loop inconsistency, because consistency of contrasts within a single trial is guaranteed.
As above (see Network meta-analysis, original network), there was no evidence of inconsistency in the network for any of the four lipid measures. This was true of both the global Wald test and from examining the inconsistency parameters individually. Therefore the results presented in Table 79 are those obtained from the consistency model.
The point estimates of effect size in Tables 78 and 79 are broadly comparable, indicating that the inclusion of the additional 13 studies in the extended network has not changed the conclusions in Chapter 3.
The final rows of each table reveal a reduction of around 40% in the estimate of the heterogeneity SD parameter for both LDL cholesterol and TC, with a concomitant reduction in the SEs. This appears to be due primarily to the multiplicity of studies in the ‘Atorvastatin 80 – Pravastatin 20–40’ contrast: previously there was only one such study, whereas in the extended network there are five, and these five have remarkably similar point estimates. The estimate of the heterogeneity SD parameter in the original network was driven solely by the studies in the ‘Atorvastatin 10–25 – Placebo/Usual Care’ contrast, for which there appears to be substantially greater heterogeneity.
The model assumes common heterogeneity in all treatment contrasts. The inclusion of different levels of heterogeneity in the model would create the major further problem of deciding a suitable level of heterogeneity for treatment contrasts for which there is only one contributing study (such as ‘Atorvastatin 40 – Placebo/Usual Care’), hence this approach was not explored.
Comparing the results of Table 79 to those in figure 3 of Naci et al. ,188 we can see that the estimates of effect size are extremely similar for two of the atorvastatin doses (10–25 and 80). The corresponding effect sizes in Naci et al. 188 are approximately –1.16 mmol/l for atorvastatin ≤ 10, –1.29 for atorvastatin 10–20 and –1.68 for atorvastatin > 40. For the intermediate dose (effect size –1.55 in Naci et al. 188), there is some discrepancy, although, as discussed above, our network provides little reliable information about this contrast.
Conclusions
We investigated inconsistency in the network presented in Chapter 3, and in an enlarged network in which the sample size inclusion criterion was relaxed. In no case was there evidence for inconsistency, and the addition of 13 extra studies to the network did not have a large effect on pooled point estimates of effect size.
Appendix 17 Rationale for modelling protocol change
Background
Our protocol specified that the models of Chapters 5 and 7 would be based on parameters estimated from data from St Luke’s Hospital, Tokyo, and recalibrated to the UK using CPRD data. During early analyses of both St Luke’s data and CPRD data it became apparent that this variability was substantially lower when estimated from St Luke’s data than from CPRD data by a factor close to two. Hypotheses to explain this included that the CPRD estimate was so inflated by the nature of routine clinical data (missed appointments, varying laboratory methods, etc.) that the St Luke’s estimate was indeed an improved estimate but to a much greater extent than anticipated, or, that it is unsafe to make the modelling assumption that this short-term variability would be transportable between ethnic groups and settings. Under the former hypothesis it would be highly advisable to combine parameter estimates from St Luke’s and CPRD, as specified in the protocol. Under the latter hypothesis it would be highly inappropriate to do so. Therefore, we sought external data from the literature to inform the choice between these two interpretations and hence determine whether or not the methods in the protocol should be modified.
Methods
PubMed was searched with the terms (‘regression dilution’[Title/Abstract] OR ((‘biological’ OR ‘measurement’) AND (variation OR variability’) AND (‘cholesterol OR ‘LDL’ OR ‘HDL’ OR ‘lipid’)) [Title] and results were reviewed for UK studies reporting either the SD of within-person short-term variability, or the regression dilution ratio, for any of total, HDL, LDL or non-HDL cholesterol, and their ratios. Within-person coefficients of variation (CVs) were converted to estimated SDs whenever possible.
Reported SDs of within-person short-term variability were compared with the corresponding estimates sw from the models of Chapter 4 applied to the St Luke’s data set and the CPRD data set. For comparison with reported regression dilution ratios, we calculated the modelled regression dilution ratio from the models of Chapter 4 using the formula sa2/(sa2+sw2) where sa2 is the between-person variance in true cholesterol, sw2 is the within-person variance of short-term biological and assay variability in observed cholesterol, and hence sa2+sw2 is the total between-person variance of observed cholesterol. Calculations were repeated for the models fitted to the CPRD (sa and sw estimated from CPRD), to the St Luke’s data sa and sw estimated from St Luke’s), and to the St Luke’s data with recalibration to CPRD sa estimated from St Luke’s but sa2+sw2 estimated from CPRD).
Results
The PubMed search returned 64 hits of which 41 matched ‘regression dilution’ in the title or abstract and 23 matched other terms. Of the 41 matching ‘regression dilution’, six were UK studies reporting regression dilution ratios for cholesterol or its subfractions and ratios. Table 80 shows the reported regression dilution ratios for cholesterol or other lipid measures, and the estimated regression dilution ratio from the model of Chapter 4 when fitted to data from the CPRD, when fitted to St Luke’s Hospital data, or when fitted to St Luke’s Hospital data and recalibrated to population values from the CPRD.
Lipid measure | Gender | CPRD | St Luke’s, Tokyo | St Luke’s recalibrated to CPRD | Whitehall study275 | BRHS7 | BUPA study276 | ISIS277 | Caerphilly278 | Oxford279 |
---|---|---|---|---|---|---|---|---|---|---|
TC | Male | 0.75 (0.75 to 0.75) | 0.75 (0.74 to 0.75) | 0.84 (0.84 to 0.84) | 0.77 | 0.72 | 0.71 | 0.76 | 0.69 | 0.78 |
Female | 0.74 (0.74 to 0.74) | 0.71 (0.70 to 0.72) | 0.83 (0.82 to 0.83) | |||||||
HDL | Male | 0.77 (0.77 to 0.78) | 0.80 (0.80 to 0.81) | 0.82 (0.82 to 0.82) | – | – | – | 0.77 | 0.56 | 0.58 |
Female | 0.84 (0.84 to 0.84) | 0.83 (0.82 to 0.83) | 0.86 (0.85 to 0.86) | |||||||
LDL | Male | 0.74 (0.74 to 0.75) | 0.76 (0.76 to 0.76) | 0.84 (0.84 to 0.84) | – | – | – | 0.73 | – | 0.72 |
Female | 0.75 (0.75 to 0.76) | 0.74 (0.73 to 0.75) | 0.84 (0.83 to 0.84) | |||||||
Non-HDL | Male | 0.77 (0.77 to 0.77) | 0.78 (0.77 to 0.78) | 0.85 (0.85 to 0.85) | – | – | – | 0.77 | – | – |
Female | 0.77 (0.76 to 0.77) | 0.76 (0.76 to 0.77) | 0.85 (0.85 to 0.86) | |||||||
Total/HDL | Male | 0.78 (0.78 to 0.78) | 0.82 (0.82 to 0.82) | 0.89 (0.89 to 0.89) | – | – | – | 0.72 | – | – |
Female | 0.83 (0.82 to 0.83) | 0.83 (0.82 to 0.83) | 0.90 (0.90 to 0.90) | |||||||
LDL/HDL cholesterol | Male | 0.77 (0.77 to 0.78) | 0.82 (0.82 to 0.82) | 0.89 (0.89 to 0.90) | – | – | – | 0.74 | – | – |
Female | 0.81 (0.81 to 0.82) | 0.82 (0.82 to 0.83) | 0.90 (0.89 to 0.90) |
Of the 23 hits matching other search terms, one study280 was found reporting SD for the total short-term within-person variability, and one study281 was found reporting both SD for short-term biological variability and SD for total assay variability. Other studies reported only one or other component, or defined short-term variability in ways not comparable with our models, or reported CVs without sufficient information to reliably estimate SD. Forward and backward citation searching yielded additional similar studies but no further usable studies.
In 22 healthy adults, using enzymatic assays and calculating LDL by the Friedwald formula, Nazir et al. 281 found that the biological variability of TC was substantially greater than the assay variability, with ratio of SDs (SD biological/SD analytical) 6.8. The equivalent ratio for TGs, HDL cholesterol and LDL cholesterol was 33.1, 4.2, and 6.2, respectively.
In 53 adults, using enzymatic assays, Demacker et al. 280 reported that the SD of intra-individual variation for cholesterol was 0.23 mmol/l (assay variability 0.14 mmol/l, biological variability 0.19 mmol/l); and for HDL cholesterol 0.10 mol/l (assay 0.06 mmol/l, biological 0.08 mmol/l).
Discussion
The regression dilution ratio results provide support for estimating variability from CPRD alone. Regression dilution ratios estimated from the CPRD data alone (see Table 80, column 3) appear comparable with the regression dilution ratios reported in the literature (see Table 80, columns 6–11). The ratio estimated from St Luke’s recalibrated to the CPRD appears too high (closer to 1) than any value reported in the literature.
The large size and consistency of the results of the studies reporting regression dilution ratios are an encouraging basis for decision-making. By contrast, few studies reporting variability in other ways were small (most n < 50 and all n < 100) compared with the regression dilution studies, typically used older (enzymatic) assay methods, and were difficult to compare with within-person SD as defined in Chapter 4. This was, in part, attributable to preferences for reporting CV (often without the corresponding mean) over SD, and for studying assay variability alone, but also many other reporting idiosyncrasies – for example, reporting only biological variation after subtracting an unreported quantity of assay variation (Smith et al. 282), reporting CV with median rather than mean (Ortolá et al. 283), reporting factors explaining variability without reporting total variability (Jeffs et al. 284), and more.
We therefore based our decision on the studies reporting regression dilution ratios. A limitation of this approach is that these studies are historical and have also used a variety of assay methods. However, we found at least one paper281 showing that the within-person variability is dominated by biological rather than assay variability, even when using older (enzymatic) assay methods.
It is interesting that the regression dilution ratio estimated solely within a Japanese data set (see Table 80, column 4) is similar to estimates from a UK population: smaller within-person variability relative to the UK appears to be offset by smaller between-person variability. However, this result from a single modelling study should not be over-interpreted and does not provide a basis for our decisions about our modelling of a UK cohort. The purpose of this review was to determine whether or not variability would be better modelled by recalibration from St Luke’s to CPRD or estimation from CPRD alone, and Table 80 (columns 5 and 3, respectively) provides a strong signal in favour of the latter. We therefore modified our protocol to estimate the model within CPRD rather than by recalibration from St Luke’s.
Appendix 18 Methods for recalibrating St Luke’s cohort to Clinical Practice Research Datalink
Lipid progression
The progression of each lipid was modelled through fitting a linear random-effects model, as described in Chapter 4, to data from primary prevention subjects not taking statins in the CPRD, except that the noise variable σw2 was constrained to that estimated from the equivalent St Luke’s data set (see Table 20). This was done using runmlwin in Stata version 12.1. 205 Assumptions of normality were checked graphically. Results are presented as mean and SD for each lipid (in millimoles per litre for single measures or no units for the ratios), with 95% CIs. We also present SN ratios as defined in Chapter 4.
Clinical decisions on statin use
Simulation modelling, as described in Chapter 5, was used to examine the proportion of patients recommended a treatment change (new prescription or prescription of an increased dosage of statin) attributable to a true change in lipid level or within measurement variability. Likewise, the proportions of patients that were correctly and incorrectly not allocated to treatment change were estimated. We assume that treatment recommendations would follow NICE guidance,2,56 as described in Chapter 5.
Results
The estimated within-measurement variability from the Japanese data set was smaller than that seen in the UK data: for example, TC/HDL cholesterol parameter estimates in men were 0.43 (95% CI 0.43 to 0.44) in the Japanese data (see Table 20) and 0.62 (95% CI 0.61 to 0.62) in the UK data (see Table 23). Table 81 shows estimated variability and change parameters for men and women when the noise variable was constrained to that estimated from the St Luke’s data. The estimates of the variation in the lipid value and annual rate of change were correspondingly larger, leading to a stronger SN ratio, as shown in Table 82.
Interpretation | α | β | γ | σw | σa | σb | σab |
---|---|---|---|---|---|---|---|
Mean lipid level at first measurement, at age 0 | Mean annual change in lipid measurement | Effect of age at first measurement on mean lipid measurement | SD of the within-measurement variability of lipid from the open St Luke’s cohort, Tokyo, Japan | SD of lipid | SD of annual change in lipid | Covariance between actual value of lipid and rate of change | |
Men | |||||||
TC | 6.25 (5.95 to 6.56) | –0.02 (–0.08 to 0.05) | –0.01 (–0.05 to 0.03) | 0.41 | 0.94 | 0.10 | –0.028 |
HDL | 1.12 (0.93 to 1.31) | –0.00 (–0.04 to 0.04) | 0.00 (–0.02 to 0.03) | 0.16 | 0.34 | 0.04 | –0.003 |
LDL | 4.10 (3.80 to 4.39) | –0.01 (–0.08 to 0.05) | –0.01 (–0.05 to 0.03) | 0.37 | 0.84 | 0.09 | –0.025 |
Non-HDL cholesterol | 5.13 (4.82 to 5.44) | –0.01 (–0.08 to 0.05) | –0.02 (–0.06 to 0.02) | 0.40 | 0.95 | 0.10 | –0.029 |
TC/HDL cholesterol | 5.67 (5.31 to 6.02) | 0.00 (–0.08 to 0.08) | –0.02 (–0.07 to 0.02) | 0.43 | 1.23 | 0.18 | –0.053 |
LDL/HDL cholesterol | 3.71 (3.40 to 4.03) | –0.00 (–0.08 to 0.07) | –0.02 (–0.06 to 0.03) | 0.34 | 0.98 | 0.15 | –0.043 |
Women | |||||||
TC | 6.35 (5.97 to 6.73) | –0.01 (–0.08 to 0.06) | –0.01 (–0.05 to 0.04) | 0.44 | 0.96 | 0.10 | –0.027 |
HDL | 1.56 (1.31 to 1.82) | –0.00 (–0.05 to 0.04) | 0.00 (–0.03 to 0.03) | 0.17 | 0.42 | 0.04 | –0.003 |
LDL | 4.16 (3.80 to 4.53) | –0.01 (–0.08 to 0.06) | –0.01 (–0.05 to 0.04) | 0.38 | 0.87 | 0.09 | –0.027 |
Non-HDL cholesterol | 4.82 (4.44 to 5.20) | –0.01 (–0.08 to 0.06) | –0.01 (–0.05 to 0.04) | 0.41 | 0.98 | 0.10 | –0.032 |
TC/HDL cholesterol | 4.34 (3.94 to 4.74) | 0.00 (–0.07 to 0.08) | –0.01 (–0.06 to 0.04) | 0.36 | 1.08 | 0.13 | –0.037 |
LDL/HDL cholesterol | 2.85 (2.49 to 3.22) | 0.00 (–0.07 to 0.07) | –0.01 (–0.05 to 0.04) | 0.30 | 0.87 | 0.11 | –0.029 |
Lipid measure | SN ratio at different periods after baseline (years) | |||||||||
---|---|---|---|---|---|---|---|---|---|---|
1 | 2 | 3 | 4 | 5 | 6 | 7 | 8 | 9 | 10 | |
Men | ||||||||||
TC | 0.06 | 0.24 | 0.54 | 0.95 | 1.49 | 2.14 | 2.92 | 3.81 | 4.82 | 5.95 |
HDL | 0.06 | 0.25 | 0.56 | 0.99 | 1.55 | 2.24 | 3.04 | 3.98 | 5.03 | 6.21 |
LDL | 0.07 | 0.27 | 0.61 | 1.08 | 1.69 | 2.43 | 3.30 | 4.32 | 5.46 | 6.74 |
Non-HDL cholesterol | 0.06 | 0.25 | 0.57 | 1.02 | 1.59 | 2.29 | 3.11 | 4.06 | 5.14 | 6.35 |
TC/HDL cholesterol | 0.17 | 0.67 | 1.51 | 2.68 | 4.19 | 6.03 | 8.21 | 10.72 | 13.56 | 16.74 |
LDL/HDL cholesterol | 0.19 | 0.78 | 1.75 | 3.12 | 4.87 | 7.01 | 9.54 | 12.46 | 15.77 | 19.47 |
Women | ||||||||||
TC | 0.05 | 0.20 | 0.45 | 0.80 | 1.24 | 1.79 | 2.44 | 3.19 | 4.03 | 4.98 |
HDL | 0.05 | 0.22 | 0.49 | 0.86 | 1.35 | 1.94 | 2.65 | 3.46 | 4.38 | 5.40 |
LDL | 0.06 | 0.25 | 0.55 | 0.99 | 1.54 | 2.22 | 3.02 | 3.95 | 4.99 | 6.17 |
Non-HDL cholesterol | 0.06 | 0.25 | 0.56 | 1.00 | 1.56 | 2.25 | 3.06 | 4.00 | 5.06 | 6.25 |
TC/HDL cholesterol | 0.14 | 0.57 | 1.28 | 2.27 | 3.55 | 5.11 | 6.95 | 9.08 | 11.49 | 14.18 |
LDL/HDL cholesterol | 0.15 | 0.58 | 1.32 | 2.34 | 3.65 | 5.26 | 7.16 | 9.36 | 11.84 | 14.62 |
Results of modelling the rate of eligibility for statin prescriptions for primary prevention men and women according to current practice at the time of analysis (QRisk252 with a threshold for treatment of 20%) are shown in Tables 83 and 84, respectively. As in Chapter 5, the three sections of Tables 83 and 84 show the cumulative number eligible for statin prescriptions, the percentage of those that are false-positives and the percentage of those repeatedly testing negative that are false-negative; each outcome is shown for monitoring strategies with annual, 2-yearly, 3-yearly and 5-yearly testing. Results are similar to those presented in Chapter 5, using parameters from models fitted to UK data.
Years since first test | (1) Cumulative number of positive treatment changes per 1000 (95% CI) | (2) Percentage of positive treatment changes that are false (95% CI) | (3) Percentage of negative treatment changes that are false (95% CI) | |||||||||
---|---|---|---|---|---|---|---|---|---|---|---|---|
Every year | Every 2 years | Every 3 years | Every 5 years | Every year | Every 2 years | Every 3 years | Every 5 years | Every year | Every 2 years | Every 3 years | Every 5 years | |
0 | 422 | 422 | 422 | 422 | 2.5 | 2.5 | 2.5 | 2.5 | 2.1 | 2.2 | 2.1 | 2.1 |
(422 to 422) | (422 to 422) | (422 to 422) | (422 to 422) | (2.4 to 2.5) | (2.5 to 2.5) | (2.5 to 2.5) | (2.5 to 2.5) | (2.1 to 2.1) | (2.2 to 2.2) | (2.1 to 2.1) | (2.1 to 2.1) | |
1 | 458 | 3.5 | 1.4 | |||||||||
(458 to 459) | (3.5 to 3.5) | (1.4 to 1.4) | ||||||||||
2 | 489 | 484 | 3.6 | 2.9 | 1.3 | 2.0 | ||||||
(489 to 490) | (484 to 485) | (3.6 to 3.6) | (2.9 to 2.9) | (1.3 to 1.3) | (2.0 to 2.0) | |||||||
3 | 518 | 511 | 3.5 | 2.5 | 1.3 | 2.3 | ||||||
(518 to 519) | (510 to 512) | (3.5 to 3.5) | (2.5 to 2.5) | (1.3 to 1.4) | (2.3 to 2.3) | |||||||
4 | 547 | 542 | 3.3 | 2.7 | 1.4 | 2.1 | ||||||
(547 to 548) | (542 to 543) | (3.3 to 3.3) | (2.7 to 2.7) | (1.4 to 1.4) | (2.0 to 2.1) | |||||||
5 | 576 | 567 | 3.1 | 2.1 | 1.4 | 2.7 | ||||||
(575 to 577) | (566 to 568) | (3.1 to 3.1) | (2.1 to 2.1) | (1.4 to 1.5) | (2.6 to 2.7) | |||||||
6 | 603 | 598 | 596 | 2.9 | 2.3 | 2.1 | 1.5 | 2.3 | 2.6 | |||
(602 to 604) | (597 to 599) | (595 to 597) | (2.9 to 3.0) | (2.3 to 2.4) | (2.1 to 2.1) | (1.5 to 1.5) | (2.2 to 2.3) | (2.5 to 2.6) | ||||
. . . | ||||||||||||
10 | 704 | 698 | 697 | 2.3 | 1.8 | 1.5 | 1.7 | 2.6 | 3.2 | |||
(703 to 706) | (697 to 700) | (695 to 698) | (2.2 to 2.3) | (1.7 to 1.8) | (1.5 to 1.5) | (1.7 to 1.8) | (2.6 to 2.7) | (3.1 to 3.3) | ||||
12 | 750 | 744 | 744 | 1.9 | 1.5 | 1.4 | 1.8 | 2.8 | 3.2 | |||
(749 to 751) | (743 to 746) | (743 to 745) | (1.9 to 2.0) | (1.5 to 1.6) | (1.3 to 1.4) | (1.8 to 1.9) | (2.7 to 2.9) | (3.1 to 3.3) | ||||
15 | 812 | 807 | 806 | 1.5 | 1.0 | 0.9 | 2.0 | 3.5 | 3.8 | |||
(810 to 813) | (805 to 808) | (805 to 808) | (1.4 to 1.5) | (1.0 to 1.1) | (0.9 to 1.0) | (2.0 to 2.1) | (3.3 to 3.7) | (3.7 to 3.9) |
Years since first test | (1) Cumulative number of positive treatment changes per 1000 (95% CI) | (2) Percentage of positive treatment changes that are false (95% CI) | (3) Percentage of negative treatment changes that are false (95% CI) | |||||||||
---|---|---|---|---|---|---|---|---|---|---|---|---|
Every year | Every 2 years | Every 3 years | Every 5 years | Every year | Every 2 years | Every 3 years | Every 5 years | Every year | Every 2 years | Every 3 years | Every 5 years | |
0 | 385 | 385 | 385 | 385 | 2.1 | 2.1 | 2.1 | 2.1 | 1.5 | 1.5 | 1.5 | 1.5 |
(385 to 385) | (385 to 385) | (385 to 385) | (385 to 385) | (2.1 to 2.1) | (2.1 to 2.1) | (2.1 to 2.1) | (2.1 to 2.1) | (1.5 to 1.5) | (1.5 to 1.5) | (1.5 to 1.5) | (1.5 to 1.5) | |
1 | 420 | 2.6 | 1.2 | |||||||||
(419 to 420) | (2.6 to 2.6) | (1.2 to 1.2) | ||||||||||
2 | 451 | 449 | 2.5 | 2.1 | 1.2 | 1.5 | ||||||
(451 to 451) | (448 to 449) | (2.5 to 2.5) | (2.0 to 2.1) | (1.1 to 1.2) | (1.5 to 1.5) | |||||||
3 | 483 | 479 | 2.4 | 1.9 | 1.2 | 1.7 | ||||||
(482 to 483) | (479 to 480) | (2.4 to 2.4) | (1.9 to 1.9) | (1.2 to 1.2) | (1.7 to 1.7) | |||||||
4 | 515 | 512 | 2.3 | 1.9 | 1.3 | 1.7 | ||||||
(514 to 515) | (511 to 513) | (2.3 to 2.3) | (1.9 to 1.9) | (1.3 to 1.4) | (1.7 to 1.8) | |||||||
5 | 548 | 545 | 2.2 | 1.7 | 1.5 | 2.0 | ||||||
(547 to 549) | (544 to 545) | (2.2 to 2.2) | (1.7 to 1.7) | (1.5 to 1.5) | (2.0 to 2.0) | |||||||
6 | 582 | 580 | 579 | 2.1 | 1.7 | 1.7 | 1.6 | 2.1 | 2.2 | |||
(581 to 583) | (579 to 581) | (578 to 580) | (2.1 to 2.1) | (1.7 to 1.8) | (1.7 to 1.7) | (1.6 to 1.7) | (2.1 to 2.1) | (2.2 to 2.2) | ||||
. . . | ||||||||||||
10 | 727 | 725 | 724 | 1.6 | 1.4 | 1.3 | 2.6 | 3.3 | 3.4 | |||
(726 to 729) | (723 to 726) | (723 to 725) | (1.6 to 1.7) | (1.3 to 1.4) | (1.3 to 1.3) | (2.5 to 2.7) | (3.2 to 3.3) | (3.3 to 3.5) | ||||
12 | 797 | 795 | 795 | 1.3 | 1.1 | 1.1 | 3.2 | 4.0 | 4.1 | |||
(796 to 799) | (794 to 797) | (794 to 796) | (1.3 to 1.4) | (1.1 to 1.1) | (1.0 to 1.1) | (3.1 to 3.3) | (3.9 to 4.1) | (4.0 to 4.2) | ||||
15 | 887 | 885 | 885 | 0.9 | 0.7 | 0.7 | 4.1 | 5.4 | 5.4 | |||
(885 to 888) | (883 to 886) | (883 to 886) | (0.8 to 0.9) | (0.7 to 0.7) | (0.7 to 0.7) | (3.9 to 4.3) | (5.1 to 5.7) | (5.2 to 5.7) |
Discussion
More frequent monitoring resulted in a slightly higher proportion of patients being incorrectly recommended for a statin prescription (false-positives). The cumulative proportion of false-positives decreased over time as some patients moved from false-positive to true-positive status, as their underlying CVD risk increased. The proportion of patients incorrectly not recommended for a statin was higher when monitoring occurred at longer intervals. Over follow-up, there was a gradual increase in the proportion of false-negative tests for each monitoring interval. As all non-lipid risk factors other than age were held constant and, on average, the change in lipid levels was approximately zero (see Table 81), over time, an increasing proportion of the population is classed as at high CVD risk as a result of population ageing, and therefore an increasing proportion of patients who should be eligible for treatment are missed.
Parameter estimates were similar to those modelled using σw2 from CPRD, shown in Tables 23 (men) and 24 (women) in Chapter 4. The smaller estimated within-measurement variability, led to larger parameter estimates for the variability in each baseline lipid measurement and in the annual rate of change. Trends were similar in men and women and for each lipid type.
Appendix 19 Simulating the unobserved and the slope conditional on the observed
Appendix 20 Figures for alternative monitoring strategies
This appendix presents figures for the following alternative monitoring strategies:
-
Primary prevention not on statins using Framingham 1991 as risk score (Figure 129).
-
Primary prevention not on statins using QRisk2 based on TC instead of TC/HDL cholesterol (Figure 130).
-
Primary prevention not on statins using QRisk2 based on LDL instead of TC/HDL cholesterol (Figure 131).
-
Primary prevention not on statins using QRisk2 based on LDL/HDL cholesterol instead of TC/HDL cholesterol (Figure 132).
-
Secondary prevention using the ratio of TC/HDL cholesterol instead of TC (Figure 133).
-
Secondary prevention using the ratio of LDL/HDL cholesterol instead of LDL (Figure 134).
-
Primary prevention on statins using QRisk2 based on average number of TC/HDL cholesterol readings and comparable costs (Figure 135).
-
Secondary prevention using TC ≥ 4 mmol/l as threshold based on average number of TC readings and comparable costs (Figure 136).
-
Secondary prevention using LDL ≥ 2 mmol/l as threshold based on average number of LDL readings and comparable costs (Figure 137).
FIGURE 129.
Men and women, primary prevention not taking statins, Framingham 1991 using ≥ 20% as threshold.

FIGURE 130.
Men and women, primary prevention not taking statins, modified QRisk252 (TC) using ≥ 20% as threshold.

FIGURE 131.
Men and women, primary prevention not taking statins, modified QRisk252 (LDL) using ≥ 20% as threshold.

FIGURE 132.
Men and women, primary prevention not taking statins, modified QRisk252 (LDL/HDL cholesterol) using ≥ 20% as threshold.

FIGURE 133.
Men and women, secondary prevention taking statins, using TC/HDL cholesterol ≥ 4 as threshold.

FIGURE 134.
Men and women, secondary prevention taking statins, using LDL/HDL cholesterol ≥ 2 as threshold.
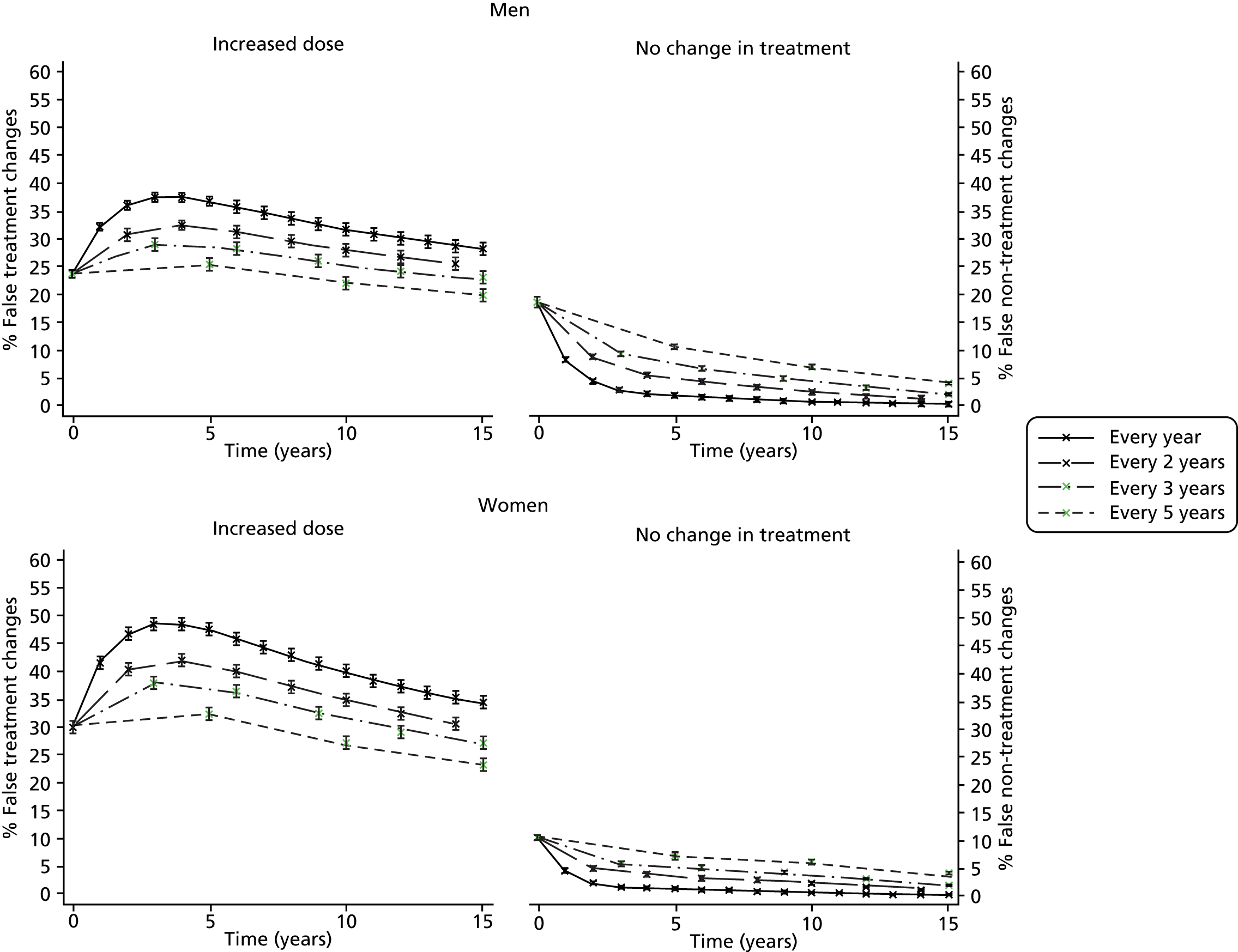
FIGURE 135.
Men and women, primary prevention taking statins, QRisk252 (TC/HDL cholesterol) using ≥ 20% as threshold based on average of several measures using equivalent costs.

FIGURE 136.
Men and women, secondary prevention taking statins, using TC ≥ 4 mmol/l as threshold based on average of several measures using equivalent costs.

FIGURE 137.
Men and women, secondary prevention taking statins, using LDL ≥ 2 mmol/l as threshold based on average of several measures using equivalent costs.
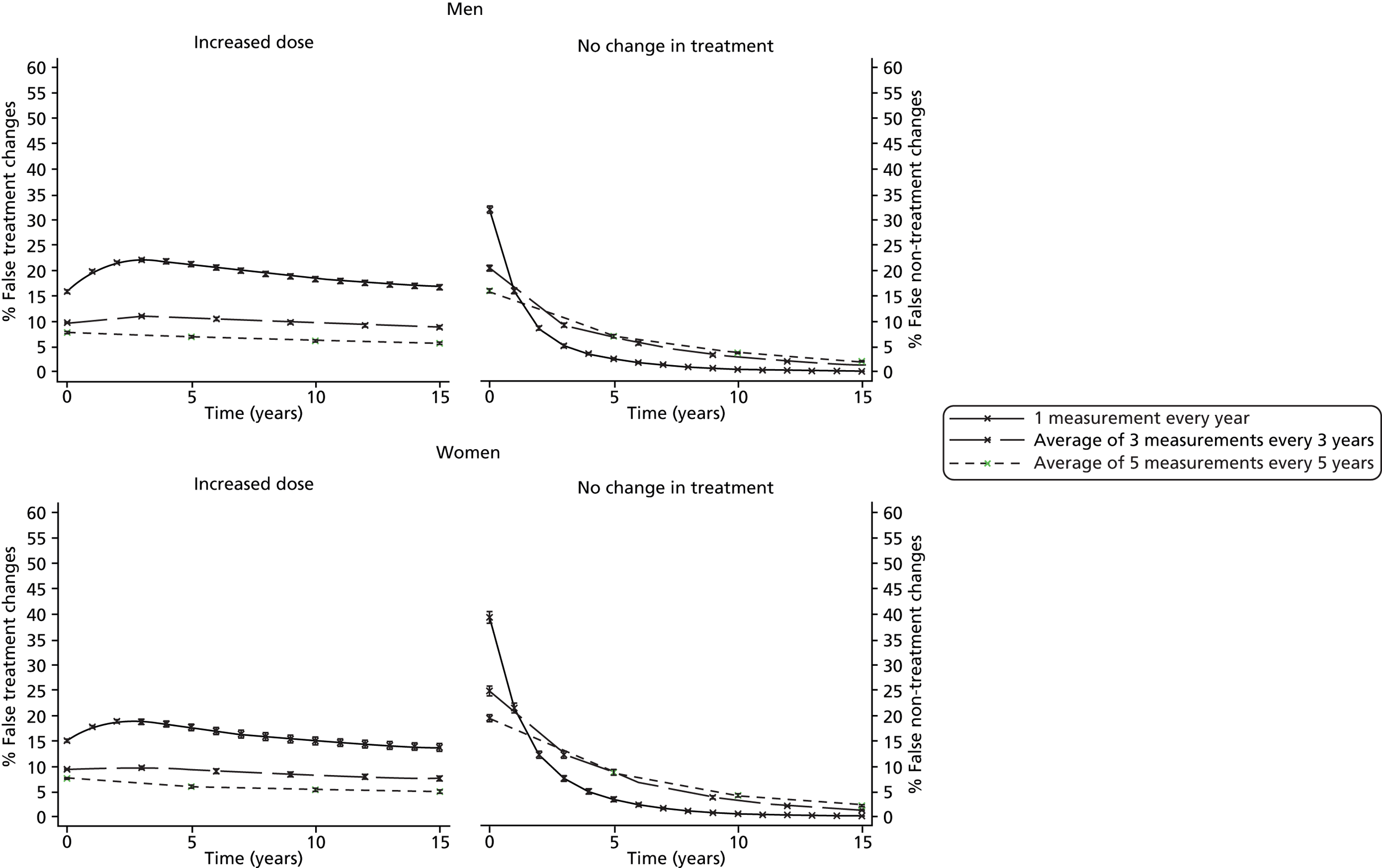
Appendix 21 Methods for the conversion of reported hazard ratios to log-hazard ratios per millimole per litre
Aim
To convert HRs reported in the EPIC-Norfolk analysis100 to the coefficient required to modify QRisk252 equation (log-HR per mmol/l).
Let ‘X’ equal the group of subjects with no CHD events and ‘Y’ equal the group with CHD events. Using the equations below and the parameters summarised in Table 85, we calculate that for men the log-HR per mmol/l is 0.339 (to three significant figures) and for women it is 0.376.
Parameter | Men | Women |
---|---|---|
Subjects without CHD events | ||
Nx, number of subjects | 8038 | 11,324 |
µx, mean non-HDL cholesterol in mg/dl | 181.9 | 179.5 |
σx, SD for non-HDL cholesterol in mg/dl | 41.7 | 47.1 |
Subjects with CHD events | ||
Ny, number of subjects | 1310 | 776 |
µy, mean non-HDL cholesterol in mg/dl | 196.1 | 205.0 |
σy, SD for non-HDL cholesterol in mg/dl | 49.0 | 48.3 |
All subjects (with and without CHD event) | ||
σxy, SD for non-HDL cholesterol in mg/dl | 43.1 mg/dl | 47.6 mg/dl |
σxy, SD for non-HDL cholesterol in mmol/l | 1.12 mmol/l | 1.23 mmol/l |
HR per SD increase in non-HDL cholesterol | 1.46 | 1.59 |
Log HR per SD increase in non-HDL cholesterol | 0.37 | 0.46 |
Log HR per mmol/l | 0.339 | 0.376 |
List of abbreviations
- Apo A-I
- apolipoprotein A-I
- Apo B
- apolipoprotein B
- BMI
- body mass index
- BP
- blood pressure
- CHD
- coronary heart disease
- CI
- confidence interval
- CPRD
- Clinical Practice Research Datalink
- CV
- coefficient of variation
- CVD
- cardiovascular disease
- ERFC
- Emerging Risk Factors Collaboration
- GP
- general practitioner
- HCHS
- Hospital and Community Health Services
- HDL
- high-density lipoprotein
- HMG-CoA
- 3-hydroxy-3-methylglutaryl coenzyme A
- HR
- hazard ratio
- HTA
- Health Technology Assessment
- IPD
- individual patient data
- LDL
- low-density lipoprotein
- MI
- myocardial infarction
- NDPCHS
- Nuffield Department of Primary Care Health Sciences
- NICE
- National Institute for Health and Care Excellence
- NIHR
- National Institute for Health Research
- NMA
- network meta-analysis
- OR
- odds ratio
- PPI
- patient and public involvement
- QALY
- quality-adjusted life-year
- QOF
- Quality Outcomes Framework
- RCT
- randomised controlled trial
- RR
- risk ratio
- SD
- standard deviation
- SE
- standard error
- SN
- signal–noise
- TC
- total cholesterol
- TG
- triglyceride
- UKPDS
- UK Prospective Diabetes Study