Notes
Article history paragraph text
This issue of the Health Technology Assessment journal series contains a project commissioned/managed by the Methodology research programme (MRP). The Medical Research Council (MRC) is working with NIHR to deliver the single joint health strategy and the MRP was launched in 2008 as part of the delivery model. MRC is lead funding partner for MRP and part of this programme is the joint MRC–NIHR funding panel ‘The Methodology Research Programme Panel’.
To strengthen the evidence base for health research, the MRP oversees and implements the evolving strategy for high quality methodological research. In addition to the MRC and NIHR funding partners, the MRP takes into account the needs of other stakeholders including the devolved administrations, industry R&D, and regulatory/advisory agencies and other public bodies. The MRP funds investigator-led and needs-led research proposals from across the UK. In addition to the standard MRC and RCUK terms and conditions, projects commissioned/managed by the MRP are expected to provide a detailed report on the research findings and may publish the findings in the HTA journal, if supported by NIHR funds.
The authors have been wholly responsible for all data collection, analysis and interpretation, and for writing up their work. The HTA editors and publisher have tried to ensure the accuracy of the authors’ report and would like to thank the reviewers for their constructive comments on the draft document. However, they do not accept liability for damages or losses arising from material published in this report.
Declared competing interests of authors
Karl Claxton, Nigel Rice, Mark Sculpher, Nancy Devlin, Marta Soares and Eldon Spackman have undertaken consultancy/research for pharmaceutical manufacturers and/or other commercial life sciences companies that may have an interest in this research. Peter C Smith is a member of the NHS Co-operation and Competition Panel, and the policy board of the Office of Health Economics. Nancy Devlin is employed by the Office of Health Economics, which receives funding from the Association of the British Pharmaceutical Industry.
Permissions
Copyright statement
© Queen’s Printer and Controller of HMSO 2015. This work was produced by Claxton et al. under the terms of a commissioning contract issued by the Secretary of State for Health. This issue may be freely reproduced for the purposes of private research and study and extracts (or indeed, the full report) may be included in professional journals provided that suitable acknowledgement is made and the reproduction is not associated with any form of advertising. Applications for commercial reproduction should be addressed to: NIHR Journals Library, National Institute for Health Research, Evaluation, Trials and Studies Coordinating Centre, Alpha House, University of Southampton Science Park, Southampton SO16 7NS, UK.
Chapter 1 Introduction
Policy context
A comparison of the incremental cost-effectiveness ratio (ICER) of a new technology with a cost-effectiveness threshold is not the only consideration when the National Institute for Health and Care Excellence (NICE) and its advisory committees issues guidance. However, it is an important one as it allows an assessment of whether or not the health expected to be gained from the use of a technology exceeds the health expected to be forgone elsewhere as other NHS activities are displaced. For this reason a comparison of the ICER of a technology to a threshold range is a critical part of the reference case in the NICE Guide to the Methods of Technology Appraisal3 and is often taken to be the starting point for deliberations about other considerations including judgements of social value. Therefore, the value of the threshold is critical to the assessment of whether or not technologies can be regarded as cost-effective. This is also true for other NHS resource allocation decisions which potentially impose additional costs on local NHS commissioners.
Estimating the cost-effectiveness threshold
A key part of NICE’s remit is to make decisions which are consistent with the efficient use of NHS resources. In the context of the NHS budget constraint, a consideration of efficiency has to reflect the implications of imposing additional costs on the system which will displace existing services thus leading to health decrements for patients other than those benefiting from the new technology being appraised. The cost-effectiveness threshold is an estimate of health forgone as other NHS activities are displaced to accommodate the additional costs of new technologies. A national decision-making body such as NICE needs an estimate of what is likely to be forgone across the NHS as we currently find it. 1 Of course, this will change as circumstances and the NHS change; tending to rise with increases in budget and health-care costs but tending to fall with increases in the productivity of health technologies and the efficiency of the NHS in general (including better local commissioning decisions). 2 A body such as NICE cannot and does not necessarily need to know what specific services and treatments will be displaced in particular localities or who will actually forgo health.
What is required, therefore, is an accountable and empirically-based assessment of the health that is likely to be forgone on average across the NHS. Currently NICE uses a threshold range of £20,000–30,000 per quality-adjusted life-year (QALY) gained, where additional considerations are required towards the upper bound. 3 The empirical basis of this range of values is very limited and there have been calls for further research in this area. 4 Explicit scientific methods are required which will provide accountability so that estimates can be scrutinised by a range of stakeholders. As estimates of the threshold will need to be periodically revised, methods which make best use of routinely available NHS data are needed. As well as accountability, this will provide more predictability in likely changes to the threshold for the investment decisions of technology manufacturers.
Aims and objectives
The aim of this research is to develop and to demonstrate methods to estimate the cost-effectiveness threshold for the NHS which makes best use of routinely available data. Methods are required which can capture the impact of a change in expenditure on length and quality-of-life (QoL), indicate how estimates of the threshold have changed over time, reflect uncertainty in any estimates and assess its implications, and indicate the impact of increases or decreases in spending. The project also aims to discuss options for developing data sources in the UK to estimate the threshold more precisely over time.
The research has four main objectives:
-
informed by relevant literature, to provide a conceptual framework to define the threshold and the basis of its estimation
-
using programme budgeting (PB) data for the English NHS, to estimate the cost per years of life gained (YLG) on average across the NHS, for marginal changes in budget
-
to extend the measure of the health effects of changes in expenditure by estimating the QoL associated with additional years of life and the direct impact of health services on QoL
-
to synthesise this work to bring evidence on life-years and QALYs together, to present the best estimate of the cost-effectiveness threshold given existing data, to show the implications of the uncertainty in the current evidence and to provide recommendations for future data collection and analysis.
Report structure
The main report is set out as a series of chapters, most of which are linked to more detailed analysis in separate appendices. Chapter 2 provides a policy context for the research and a conceptual framework for the subsequent empirical work. Chapter 3 outlines a simple theoretical model and associated econometric analysis of PB data to estimate the link between changes in overall NHS expenditure and mortality. Chapter 4 considers a range of analyses to extend the measure of health effect from mortality to YLGs and to QALYs. Chapter 5 draws out the main conclusions and insights from the research.
Chapter 2 Policy context and conceptual framework
Introduction
The purpose of this chapter is to provide the foundation for the empirical chapters that follow. It addresses a series of questions regarding the nature of the cost-effectiveness threshold that NICE use to guide its decisions, and the principles of how it should be estimated.
The chapter is informed by the results of a systematic literature search relating to these questions. Details of the methods and results of that search, together with a summary of the papers identified, are provided in Appendix 1. In brief, the search uses a ‘pearl growing’ method to identify relevant papers. This identifies a number of initial key articles (‘pearls’) on the basis of expert advice, and ‘grows’ these pearls in a series of steps: extraction of citations and references from the initial pearls; identification of further pearls from cited and referenced papers; repetition of citation and reference searches; and manual search of references. This process is repeated until no further papers of relevance are identified. On this basis, 76 relevant papers were identified and are referred to, when relevant, in this chapter.
This chapter is organised as follows. The next section considers, at a conceptual level, what the cost-effectiveness threshold to inform NHS decisions, such as those made by NICE’s advisory committees, should represent. Estimating the threshold considers alternative routes to generating an empirical estimate of such a threshold. The final section provides a brief overview of the methods used in the study.
What should the National Institute for Health and Care Excellence threshold represent?
The threshold as a measure of opportunity cost
The National Institute for Health and Care Excellence uses cost-effectiveness analysis (CEA) to inform the decisions underlying most types of guidance that it publishes. The use of CEA is most prominent in appraisals relating to new medicines,3 but is also a key input into diagnostics appraisals as well as clinical guidelines and public health guidance. 3,5 For those interventions and programmes which impose additional costs on the NHS budget, their ICERs indicate the incremental cost per additional QALY achieved relative to appropriate comparators. Although the ICER is one of a number of evidential inputs into NICE committees’ decisions, is has been shown to be the most important, at least for technology appraisals. 6
Interpreting whether or not a given ICER is acceptable requires the use of a cost-effectiveness threshold. Given that NICE has no influence on the level of the NHS budget, its decisions need to consider that budget as a fixed constraint. 1 Therefore, the threshold should reflect the opportunity costs, in terms of health forgone, resulting from the imposition of additional costs on the NHS. When NICE issues positive guidance for a new intervention which imposes additional costs on the system, the resources required to deliver it must be found by disinvesting from other interventions and services elsewhere. 7 This displacement of existing services will result in health decrements for other types of individual. 8 Thus, the threshold represents the additional cost that has to be imposed on the system to forgo 1 QALY’s worth of health through displacement.
Resource allocation decisions based on comparing an ICER with a cost-effectiveness threshold uses some simplifying assumptions, including those of constant returns to scale and perfect divisibility of programmes. 9 Some have suggested that this makes these methods unreliable,10 although it has also been argued that they provide useful approximations to guide decisions. 11 This report takes NICE’s use of these methods as a starting point, and does not review the literature relating to this debate in any depth.
As Figure 1 illustrates, CEA effectively becomes an analysis of net health benefits (NHBs): does the health gain from the new intervention outweigh the health decrements associated with the displacement of existing services necessary to fund it? Figure 1 shows the incremental costs and QALYs associated with a new intervention relative to a comparator (the latter being shown at the origin). The new intervention generates 2 additional QALYs per patient and, at price P1, imposes an additional £20,000 per patient; the ICER is, therefore, £10,000 per QALY gained. At a threshold of £20,000 per QALY, the additional cost of £20,000 per patient translates into a decrement of 1 QALY (the distance between the y-axis and the threshold). This is because the threshold indicates the additional cost that needs to be imposed on the NHS budget in order to displace services that result in 1 QALY being forgone. Therefore, at that price, there is a net health gain of 1 QALY per patient (2 gained from the new intervention and 1 forgone through displacement). At a price of P2, the additional cost per patient of the new intervention is £40,000 and the net health gain is 0: the 2 additional QALYs from the new intervention are the same as the QALYs forgone through displacement. At the highest price of P3, the adoption of the new intervention would actually result in a net health decrement of 1 QALY as it generates fewer QALYs (2) than are forgone (3).
FIGURE 1.
Graph showing illustration of the NICE threshold as a basis for assessing NHB. Reproduced from Value based pricing for NHS drugs: an opportunity not to be missed?, Claxton K, et al. , vol. 336, pp. 251–4, 2008 with permission from BMJ Publishing Group Ltd.
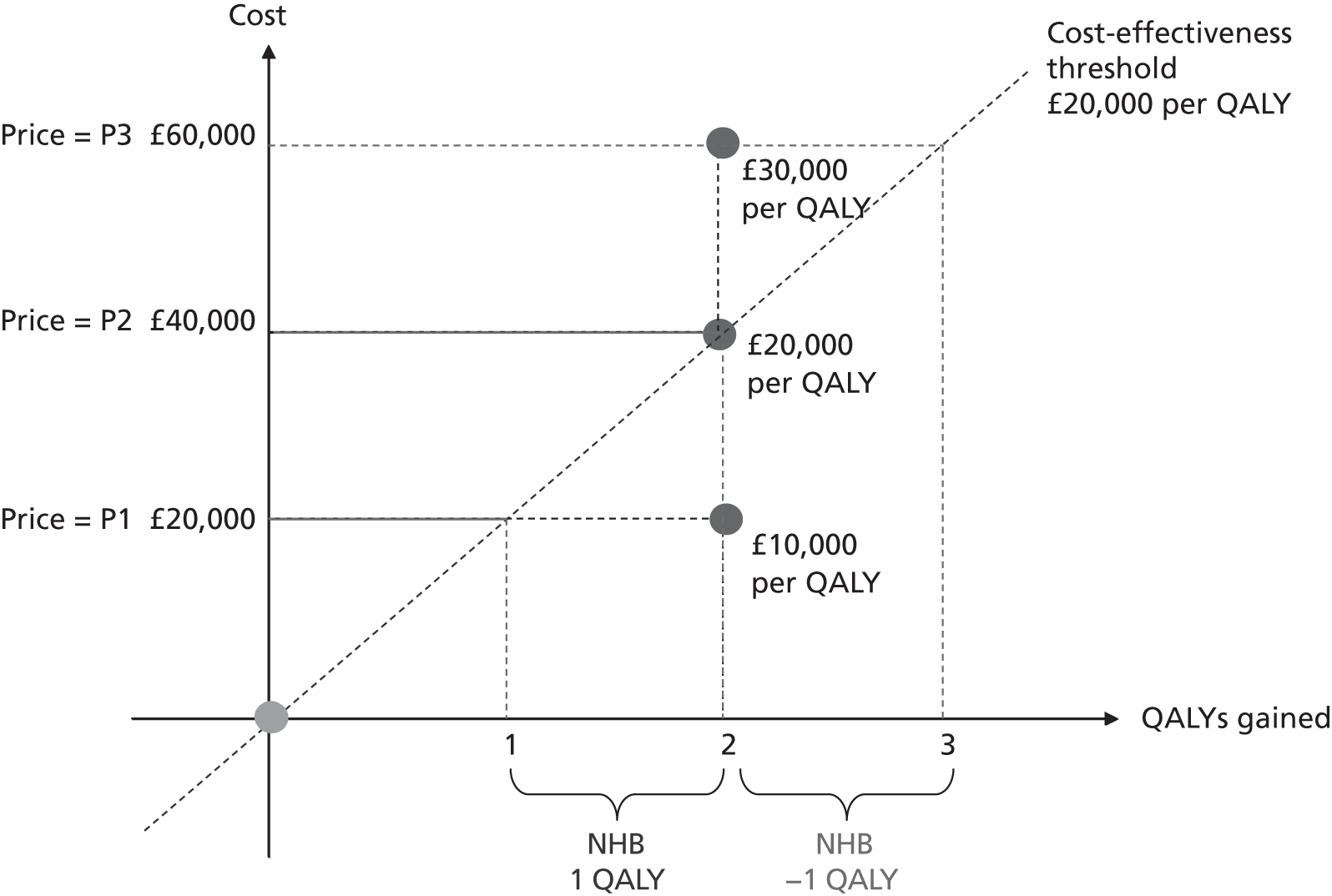
The use of the threshold to facilitate this NHB analysis can be expressed as in Equation 1:
where Δh is the change in health generated by the new intervention, ΔCh is the additional health-care cost imposed on the NHS, and k is the cost-effectiveness threshold. The net health gain from adopting the new intervention is therefore the health gained, Δh, minus the health forgone, ΔChk.
Understanding the NICE cost-effectiveness threshold as representing opportunity costs in terms of health is explicit in NICE documentation (e.g. the Guide to the Methods for Technology Appraisal3). It is also clear in reports published by the Department of Health, such as the consultation report on value-based pricing. 4,12,13 This conceptualisation of the principles of the NICE threshold is also described in the broader literature. 1,2 Formally, the threshold can be seen as the shadow price of the budget constraint. 1,2,8,14–16 Although this project focused on the use and estimation of a cost-effectiveness threshold for NICE decisions, the methods and estimates relate to any resource allocation decision within the NHS where the opportunity cost could fall anywhere in the system. Hence it could apply, for example, to Department of Health targets or to NHS England directives, as well as NICE guidance.
The threshold as the consumption value of health
Another view of what the threshold used in CEA should represent exists in the literature; however, in general terms this is based on the rate at which individuals are willing to forgo other forms of consumption to achieve health improvement [sometimes referred to as ‘willingness to pay’ (WTP)]. 17–36 Although this consumption value of health can provide information on the value of health improvement and may guide decisions such as the level of the overall NHS budget, it does not inform decisions regarding how to allocate a fixed budget within the health-care system.
The reason for this is that the consumption value of health applies equally to health gained as well as to health forgone. This is shown in Equation 2 where the consumption value of health, v, is added to the definition of NHB in Equation 1. This simply involves valuing both health gained and health forgone by the same consumption value of a unit of health, v. Therefore, the use of the consumption value is irrelevant: a treatment considered cost-effective in Equation 1 (i.e. to have a positive NHB) will inevitably be considered cost-effective in Equation 2, and an intervention with negative NHB (i.e. not cost-effective) will remain as such in Equation 2. a Therefore, the magnitude of the threshold, k, is not a value judgment but an empirical question which can, in principle, be estimated.
Estimating the threshold
The National Institute for Health and Care Excellence’s threshold range
The National Institute for Health and Care Excellence has been reluctant to specify a single cost-effectiveness threshold used in its decision-making. 6 It has also consistently emphasised that factors other than CEA are taken into consideration by the various advisory committees. 3,5,6,38–40 Therefore, it has preferred to indicate the range within which its threshold value lies (i.e. £20,000–30,000 per QALY gained). 3,5 Alongside this, it has provided an indication of the role other factors play in determining which point of threshold range is relevant. The latest guide3 suggests that an ICER < £20,000 is likely to lead to recommendation unless the evidence is considered highly uncertain; an ICER between £20,000 and £30,000 will lead to recommendation if the committee is also happy with the levels of uncertainty in the evidence and/or the QALY does not capture all aspects of benefit; and an ICER > £30,000 would be recommended only if issues related to levels of evidential uncertainty and a failure to capture all benefits in the QALY are particularly compelling.
In 2009, NICE issued further supplementary guidance relating to the appraisal of interventions for patients with short life expectancy (LE), although this can be considered to relate more to the measure of benefit than factors to be considered outside of cost-effectiveness. 41 In 2012 NICE issued a draft update of its methods guide which added that, if a new technology has an ICER > £20,000 per QALY, the committee’s deliberations would also consider ‘aspects that relate to non-health objectives of the NHS’ (e.g. wider social considerations and/or costs that fall outside the NHS budget). 42
Although NICE has carefully argued the case for why its decisions are not driven entirely by a comparison of the ICER with its threshold range, it has not provided any empirical evidence for why the threshold range takes the value it does. Indeed it has been widely argued than an empirical basis for these values should be generated. 4,43–47 For example, the House of Commons Health Select Committee in 2008 argued:
The affordability of NICE guidance and the threshold it uses to decide whether a treatment is cost-effective is of serious concern. The threshold is not based on empirical research and is not directly related to the budget, it seems to be higher than the threshold used by [primary care trusts] PCTs for treatments not assessed by NICE. Some witnesses, including patient organisations and pharmaceutical companies, thought that NICE should be more generous in the cost per QALY threshold it uses, and should approve more products. On the other hand, some PCTs struggle to implement NICE guidance at the current threshold and other witnesses argued that a lower threshold should be used. We recommend that the threshold used by NICE in its full assessments be reviewed; further research comparing thresholds used by PCTs and those used by NICE should be undertaken . . .
p. 64
The basis for empirical work
Although there is acceptance of the need for empirical work on the NICE cost-effectiveness threshold, a set of issues exists regarding the starting point for such analysis. One aspect of this is the view that the nature of the services that are displaced in response to additional costs being imposed by NICE guidance, and hence the magnitude of the health forgone for other patients, will depend on the productivity of the NHS and its overall (inflation-adjusted) budget, both of which have increased since NICE initially defined its threshold range. 48,49 In principle an increase in the (real) NHS budget would allow it to introduce interventions which were previously not cost-effective which might be expected to increase the threshold if these interventions were the marginal ones displaced in response to the budget impacts of NICE recommendations. However, any increase in the NHS budget may be allocated to non-discretionary expenditure. This would include, for example, expenditure relating to national initiatives such as new contracts for consultants and activities to meet waiting list targets as well as, of course, the implementation of NICE guidance. The non-discretionary nature of such expenditure means that these types of activities cannot easily be disinvested from given a need to release resources to fund NICE guidance. Therefore, if an increase in the NHS budget is largely devoted to these types of non-discretionary expenditure, there will be a limited impact on the threshold.
Gains in productivity may come through doing worthwhile activities more cost-effectively, including for those marginal interventions displaced by NICE recommendations, suggesting a reduction in the threshold. Alternatively, productivity gains might come through discontinuing activities which are not worth doing (i.e. that produce no health improvement), freeing resources for additional cost-effective interventions which may be the marginal services displaced by NICE guidance – this can have the result of increasing the threshold.
The net effect of these changes on the threshold could not be determined a priori and would depend on how any additional (real) budgets were allocated and how the gains in productivity where achieved. This does emphasise the fact that the threshold may change over time in response to these and other broader developments, and this would have to be considered as part of any regular updating of the empirical analysis of the threshold.
A second issue to be considered relates to how decisions are taken locally about any displacement following NICE guidance. The principles of CEA suggest that such displacement should relate to interventions which are the least cost-effective of those currently covered by the budget. 14 The basis for how local commissioners and providers make their disinvestment decisions is not clear, however, and there have been calls for greater transparency and guidance in this area. 48 It would be entirely unrealistic to assume that displacement only takes place in those existing services which are the least cost-effective. The reality is that numerous criteria are likely to be used by commissioners in implementing disinvestment, and that significant variation will exist between local decision-makers. 8 Such criteria might include, for example, equity concerns about a particular disadvantaged group locally or capacity constraints regarding particular services. Therefore NICE needs to know what is likely to happen on average across the NHS given the reality of local decisions. If local decision-making changes over time – for example, if local commissioners become more focussed on displacing services which are the least cost-effective, in terms of population health – this may affect the estimate of the threshold.
Studying displacement locally
A reasonable conclusion from a consideration of these issues is, therefore, that local decisions about disinvestment are likely to be an important determinant of the NICE threshold. 50–55 Appleby et al. 56 sought to assess whether or not it was possible to study local decisions about service investment and disinvestment to infer the cost-effectiveness thresholds being used (implicitly) locally and to draw conclusions about the appropriate level of the NICE threshold. They identified six PCTs and undertook structured interviews with each of the directors of public health. They also administrated questionnaires to an opportunistic sample of finance directors from NHS trusts. On this basis they developed a list of new services as well as those that had been deferred or discontinued. An attempt was made to estimate the implicit local ICER relating to these decisions by using any cost-effectiveness evidence used to inform the decisions together with relevant evidence on cost-effectiveness from the published literature.
The study found it quite straightforward to identify specific services that had been introduced, discontinued or deferred, but concluded that these decisions were typically based on clinical and other non-economic factors. A number of ‘decisions at the margin’ were identified but none of these were based on CEA. Instead, the basis for changes in services was a ‘business case’, or overall cost impact. It was possible to impute cost-effectiveness for most of the services affected, but the study concluded that, even with a larger sample of commissioners and providers, it would be very difficult to estimate an implied cost-effectiveness threshold locally. This would be because, first, most PCT decisions were service reconfigurations including demand management and waiting list initiatives. By their nature, teasing out the incremental cost and health effects, potentially across numerous types of patients, would be an enormous challenge. Second, there would be difficulty in identifying all local decisions as many options for investment, deferment or discontinuation are rejected before they are made more explicit in documentation. A third problem would be the finding that a range of criteria is used to make local decisions, with relatively little concern for cost-effectiveness, making a local threshold estimated in this way hard to interpret. A final challenge would be that it would be very difficult to establish a causal link between a change in local NHS budget and specific local investment and disinvestment decisions. The Appleby et al. 56 study highlights the problems that exist in deriving a cost-effectiveness threshold from a bespoke study of specific local resource allocation decisions.
What evidence is needed?
Given the challenges of studying local decisions as a means of establishing the NICE threshold, and keeping in mind NICE’s remit, it is possible to suggest a series of important characteristics that estimation methods should have from the perspective of principle and practice:
-
They should reflect the effect of NICE guidance on the average of the displacement decisions taken across the NHS, with less consideration on which types of patients and interventions are affected and why the decisions are taken. NICE cannot be expected to reflect what is likely to be marked variation between local commissioners and providers in how they react to an effective reduction in their budget as a result of positive guidance. Given NICE’s remit, it is the expected health effects (in terms of length and QoL) of the average displacement within the current NHS (given existing budgets, productivity and the quality of local decisions) that is relevant to the estimate of the threshold.
-
The methods used should not be a ‘once and for all’ effort but should facilitate regular updates to reflect changes in the broader NHS context such as changes in the overall real budget and productivity. This requires the use of data sources that are currently routinely available, are expected to become so in the future or could be made available at reasonable cost. It may be possible to glean some idea of how the threshold may change in the future by studying how it has changed in the past, which would require routine data sources to extend back over a period of time. Periodic updating using explicit scientific methods would encourage accountability through scrutiny of estimates by relevant stakeholders. It would also provide more predictability in likely changes to the threshold for the investment decisions of technology manufacturers.
-
The nature of the displacement of existing services (and hence the magnitude of the health forgone) will depend on the scale of the budget impact coming through NICE guidance. Therefore, the methods used to estimate the threshold should ideally be able to reflect this budget impact.
-
The methods should recognise the inevitable uncertainty relating to the evidence currently available for threshold estimation and translate this into an expression of the uncertainty in the estimate of the threshold. As well as providing information with which NICE can determine the appropriate implications for its choice of a threshold value, this consideration of uncertainty can help to prioritise further research or the collection of routine data.
An introduction to study methods
The current study has sought to develop methods consistent with these desired characteristics. This section provides a summary of the methods used. Further details are provided in each of the later chapters relating to the various components of work, and in the associated appendices. The general approach taken is to use routinely available data to look at the relationship between overall NHS expenditure and patients’ health outcomes. By exploiting differences between PCTs in expenditure and outcomes, it is possible to infer the costs of generating health improvement from NHS services at the margin. In principle, this is what is needed as the basis of the NICE cost-effectiveness threshold as it provides an indication of the health forgone through the services displaced by the additional budget effect of the Institute’s guidance.
Past work
The study was able to build on some key existing research relating to the relationship between NHS expenditure and mortality. 57–59 Since 2003 data on expenditure on health care across 23 programme budget categories (PBCs) of care have been available for each PCT in the NHS in England. These PB data seek to allocate, to broad areas of illness according to the primary diagnosis [using International Classification of Diseases, Tenth Edition (ICD-10) codes], all items of NHS expenditure, including expenditure on inpatient care, outpatient care, community care, primary care, and pharmaceuticals and devices.
For the purposes of this study, the merit of these data is that they open up the possibility of examining the relationship between differences in local spending and associated disease-specific mortality outcomes routinely available from the National Centre for Health Outcomes Development. In each programme, the elasticity of outcome with respect to changes in expenditure was estimated controlling for differences between PCTs in need. Changes in mortality were then transformed into YLG using assumptions regarding LE without the change in expenditure. This provides estimates of the marginal cost per YLG on average across the NHS by PBC.
This work focused largely on spending and outcomes in two of the largest programmes: circulatory disease and cancer,60 but has also informed the link across other programme categories. 58,61 Estimates of the cost per YLG for 2006/7 were £15,387 for cancer, £9974 for circulation problems, £5425 for respiratory problems, £21,538 for gastrointestinal problems and £26,428 for diabetes. These estimates were based on a straightforward, though carefully constructed, theoretical model of health production which informs the specification and estimation of a set of equations. These dealt with the challenge of there being alternative plausible directions of causation (e.g. between expenditure and health outcomes within a programme). This problem of endogeneity was addressed by identifying and testing suitable instrumental variables (IVs). In doing so, they accounted for variation in the clinical needs of the local population relevant to each programme together with broader local environmental factors relevant to the costs of care and outcomes.
This earlier work provides a strong foundation for the current study through its consideration of the average marginal elasticity of outcome with respect to programme expenditure. However, to estimate the threshold suitable for NICE decision-making, a number of further elements of research are necessary, and these are described below.
Further econometric analysis
This further econometric research is covered in Chapter 3, with full details in Appendix 2. The earlier work estimated the cost per YLG for the major programme areas. The NICE threshold needs to relate to the whole NHS and will, therefore, depend on all the programmes of care where disinvestment takes place. Given that each programme of care has been estimated separately, it is not clear how expenditure on particular programmes changes with the overall budget. For example, does disinvestment tend to fall on respiratory care or diabetes following a budget impact from NICE guidance? Therefore, the current study has further developed the econometric analysis to reflect the need for PCTs to operate within a fixed overall budget. This provides an estimate of the ‘budget elasticity of expenditure’ in each PBC, and facilities estimates of the impact of marginal increases (or decreases) in overall PCT budgets on spending in each PBC.
As well as indicating budgetary influences on programme spending, these elasticities have then been linked to changes in mortality outcomes by programme. These changes are used to estimate years of life lost (YLL) taking account of the fact that some of the observed deaths would have occurred anyway (had the same population not been at risk in the particular PBC); that is, taking account of unobserved counterfactual deaths. This takes into account how such budgetary changes (such as those imposed by NICE guidance) translate through local decisions into changes in expenditure on programmes of care and then to health outcomes.
Changes in budgets are in practice incremental rather than marginal, and it may be the case that the outcome elasticities of programme expenditure in times of budgetary increase (when new initiatives are introduced) are not the same as in times of budgetary decrease (when the focus is on disinvestment). The possible effects of non-marginal changes have therefore been explored. The project has also sought to explore how both expenditure and outcome elasticities, and hence the threshold, vary over time, and this has been assessed by generating relevant estimates for three sets of data.
A development from earlier work has been to relate expenditure in period t to mortality in periods t, t + 1 and t + 2. Although the data used are largely cross-sectional, mortality data are linked so as to follow expenditures. Given the inevitable uncertainty relating to assumptions in the analysis, extensive sensitivity analysis is undertaken to consider the implications for the estimates.
Moving from life-years to quality-adjusted life-years gained
A key element of the research has been to take the results of the econometric work linking NHS spending and mortality, and to translate this into effects on life-years and QALYs. The methods planned for the study included a consideration of local data, collected routinely by PCTs, on the types of interventions in which local decision-makers were investing and disinvesting. The aim was to inform the link between the effects of expenditure changes on mortality and impacts on broader health in terms of QALYs. These data may have indicated the types of interventions and services, within a given PBC, on which investment and disinvestment were taking place. Using targeted literature reviews, estimates of QoL for those activities may have been identified. However, it was established that there were limited data available at a local level to facilitate this type of analysis, so other data sources were used for this purpose (see Addendum 2: the role of data on local NHS decisions in Appendix 3).
It has therefore been necessary to consider alternative data and approaches. This is tackled using three sequential steps:
-
translate the estimated effects on mortality from the econometrics work into life-years by exploring the limitations of the mortality data available at PCT level and the published YLL figures used in the econometric analysis, and by considering how to improve the estimates using additional data and analysis
-
consider how estimates of life-year effects can be adjusted for the QoL in which they are lived, taking account of the gender and the age at which life-years are gained or lost as well as the QoL implications of particular diseases
-
explore ways to take account of those effects on health not directly associated with mortality and life-year affects (i.e. the ‘pure’ QoL effects) to estimate an overall cost per QALY threshold.
This aspect of the analysis is described in Chapter 4 with further details provided in Appendix 3.
The central or ‘best’ estimate is based on two assumptions relating to the health effects associated with expenditure, one conservative and the other more optimistic. The first assumption is that the health effects of changes in 1 year of expenditure are restricted to 1 year. This is implicit in the estimates of outcome elasticities estimated in the econometric analysis. This is likely to underestimate effects on mortality as expenditure that reduces mortality risk for an individual in 1 year may well also reduce their risk over subsequent years, and expenditure may also prevent disease in future patient populations. Therefore, total health effects will be underestimated and the cost per life-year or QALY threshold will be overestimated. Although undoubtedly conservative, it may be offset to some extent by the more optimistic assumption. It is assumed that any death averted by expenditure in 1 year will return the individual to the mortality risk of the general population, that is the years of life gained (YLG) associated with each death averted are based on what would have been their LE taking account of their of age and gender (using life tables for the general population).
The extreme upper and lower bounds for cost per life-year and cost per QALY thresholds are based on making both of these assumptions either optimistic (providing the lower bound for the threshold) or conservative (an upper bound for the threshold). The lower bound is based on assuming that health effects are not restricted to 1 year but apply to the remaining disease duration for the population at risk during the expenditure year. The upper bound is based on the combination of assuming that health effects are restricted to 1 year and that any death averted is only averted for the minimum duration consistent with the mortality data used to estimate the outcome. It is very important to note that the lower and upper bounds are very much extreme values with limited plausibility.
Conclusions
A cost-effectiveness threshold is needed to inform decisions by NICE, the NHS more generally or the Department of Health which reflects the fact that opportunity costs fall on services and population health at a local level. Given that it is (and will continue to be) unfeasible to know precisely which services are displaced across all localities within the NHS, the threshold should reflect the average implications on health of actual local decisions about marginal changes in local service caused by changes in expenditure. The absence of an empirical estimate of the threshold which reflects these principles lies behind the project. Using data routinely collected in the NHS or available data that could be routinely updated, the study is organised into two major parts. The first updates earlier analysis to estimate the relationship between NHS expenditure and mortality, and the second seeks to translate these mortality effects into the more general measure of health – the QALY.
Chapter 3 The link between NHS spending, mortality and the cost of a life-year
Introduction
This section presents an overview of the econometric work undertaken to estimate the link between NHS spending and mortality and how this is used to calculate the cost of a life-year. As well as providing the analytical foundations for estimates of cost per QALY threshold presented in Chapters 4 and 5, this work contributes to the ongoing debate about the extent to which additional health-care expenditure yields improved patient health outcomes.
The work presented in this report takes advantage of the availability of two new data sets to examine the relationship between NHS expenditure and mortality rates for various disease categories. One data set contains mortality rates for various disease categories at the level of geographically defined local health authorities, PCTs. The other data set presents NHS expenditure by PCT on 23 broad programmes of care (these programmes are listed in Table 1). This data set embraces most items of publicly funded expenditure, including inpatient, outpatient and community care, and pharmaceutical prescriptions. NHS revenue derives almost entirely from national taxation, and access to the system is generally free to the patient. The system is organised geographically, with responsibility for the local administration of the NHS devolved to PCTs. a PCTs are allocated fixed annual budgets by the Department of Health, within which they are expected to manage the health care in the locality.
PBC | Spend (£) per head | Spend (£) per head | Spend (£) per head | Spend (£) per head | Spend (£) per head | Spend (£) per head | Growth (%) | Growth (%) | Growth (%) | Growth (%) | Growth (%) | Share of total spend (%) | Share of total spend (%) | |
---|---|---|---|---|---|---|---|---|---|---|---|---|---|---|
2003/4 | 2004/5 | 2005/6 | 2006/7 | 2007/8 | 2008/9 | 2004/5 | 2005/6 | 2006/7 | 2007/8 | 2008/9 | 2004/5 | 2008/9 | ||
1 | Infectious diseases | 17.95 | 20.22 | 23.61 | 20.88 | 22.08 | 23.46 | 13 | 17 | –12 | 6 | 6 | 1.7 | 1.5 |
2 | Cancers and tumours | 64.95 | 75.54 | 83.24 | 81.67 | 90.21 | 94.55 | 16 | 10 | –2 | 10 | 5 | 6.3 | 6.2 |
3 | Blood disorders | 14.08 | 17.00 | 17.48 | 16.58 | 19.44 | 19.50 | 21 | 3 | –5 | 17 | 0 | 1.4 | 1.3 |
4 | Endocrine, nutritional | 28.96 | 31.86 | 37.26 | 36.70 | 39.39 | 43.38 | 10 | 17 | –1 | 7 | 10 | 2.7 | 2.8 |
5 | Mental health | 133.31 | 146.83 | 158.95 | 166.53 | 180.90 | 191.21 | 10 | 8 | 5 | 9 | 6 | 12.2 | 12.5 |
6 | Learning disability | 37.93 | 43.37 | 46.54 | 48.36 | 54.20 | 56.11 | 14 | 7 | 4 | 12 | 4 | 3.6 | 3.7 |
7 | Neurological | 29.83 | 35.09 | 41.06 | 55.27 | 62.43 | 67.64 | 18 | 17 | 35 | 13 | 8 | 2.9 | 4.4 |
8 | Vision problems | 24.61 | 27.65 | 28.24 | 26.97 | 30.69 | 32.95 | 12 | 2 | –4 | 14 | 7 | 2.3 | 2.2 |
9 | Hearing problems | 5.73 | 6.32 | 6.27 | 6.21 | 8.07 | 8.16 | 10 | –1 | –1 | 30 | 1 | 0.5 | 0.5 |
10 | Circulatory disease | 110.12 | 122.37 | 124.28 | 122.06 | 124.77 | 129.94 | 11 | 2 | –2 | 2 | 4 | 10.2 | 8.5 |
11 | Respiratory system | 54.60 | 62.71 | 69.56 | 65.07 | 67.68 | 77.97 | 15 | 11 | –6 | 4 | 15 | 5.2 | 5.1 |
12 | Dental problems | 10.78 | 13.55 | 24.91 | 51.93 | 59.45 | 62.44 | 26 | 84 | 108 | 14 | 5 | 1.1 | 4.1 |
13 | Gastrointestinal system | 63.56 | 73.22 | 81.30 | 73.30 | 75.05 | 77.89 | 15 | 11 | –10 | 2 | 4 | 6.1 | 5.1 |
14 | Skin problems | 20.98 | 24.90 | 26.84 | 28.31 | 30.41 | 32.34 | 19 | 8 | 5 | 7 | 6 | 2.1 | 2.1 |
15 | Musculoskeletal system | 61.36 | 71.72 | 74.74 | 66.75 | 75.91 | 79.68 | 17 | 4 | –11 | 14 | 5 | 6.0 | 5.2 |
16 | Trauma and injuries | 62.31 | 72.13 | 76.41 | 57.29 | 57.56 | 63.54 | 16 | 6 | –25 | 0 | 10 | 6.0 | 4.2 |
17 | Genitourinary system | 55.32 | 62.38 | 67.38 | 68.98 | 67.83 | 73.78 | 13 | 8 | 2 | –2 | 9 | 5.2 | 4.8 |
18 | Maternity | 52.28 | 55.04 | 60.42 | 57.64 | 57.09 | 60.44 | 5 | 10 | –5 | –1 | 6 | 4.6 | 3.9 |
19 | Neonate conditions | 11.72 | 13.93 | 13.42 | 13.17 | 15.15 | 17.23 | 19 | –4 | –2 | 15 | 14 | 1.2 | 1.1 |
20 | Poisoning | 9.68 | 12.32 | 14.25 | 14.59 | 15.84 | 18.31 | 27 | 16 | 2 | 9 | 16 | 1.0 | 1.2 |
21 | Healthy individuals | 20.29 | 22.77 | 26.18 | 26.85 | 31.44 | 35.74 | 12 | 15 | 3 | 17 | 14 | 1.9 | 2.3 |
22 | Social care needs | 24.81 | 30.93 | 33.59 | 30.29 | 35.29 | 36.58 | 25 | 9 | –10 | 17 | 4 | 2.6 | 2.4 |
23 | Other (includes GMS/PMS) | 136.94 | 157.75 | 171.82 | 209.70 | 232.02 | 227.71 | 15 | 9 | 22 | 11 | –2 | 13.2 | 14.9 |
1–23 | All PBCs | 1052.12 | 1199.60 | 1307.76 | 1345.10 | 1452.91 | 1530.59 | 14 | 9 | 3 | 8 | 5 |
We employ a model that assumes that each PCT receives an annual financial lump sum budget and allocates its resources across the 23 programmes of care to maximize the health benefits associated with that expenditure. Estimation of this model using the expenditure and mortality data facilitates two related studies: first, a study of how changes in the NHS budget impact on expenditure in each care programme; and second, a study of the link between expenditure in a programme and the health outcomes achieved, notably in the form of disease-specific mortality rates. The latter also permits the calculation of the cost of an additional life-year for individual programmes of expenditure.
The work presented here draws heavily on previous studies using these data57,59,60,62,63 and innovates in four major ways: (1) we relate expenditure in time period t to outcomes in periods t, t + 1, and t + 2 combined;b (2) we present plausible outcome models for a large number of budgeting categories – previous studies have tended to focus on the four largest care programmes; (3) we present estimates of the cost of a life-year for the enlarged number of programmes and, importantly, with the aid of assumptions about the productivity of programmes without a meaningful mortality-based outcome indicator, we extend our individual programme estimates to incorporate expenditure across all programmes of care; and (4) although the models we present appear well specified according to appropriate statistical tests, we subject our results to a substantial sensitivity analysis.
The next section presents a brief review of the relevant literature on which the study builds. This is followed by a summary overview of our approach to estimating the cost per life-year across the various programmes of care and the results obtained using PB data provided by the Department of Health. Further details of all aspects of the modelling approach, description of the data, the results we derive and calculation of costs per life-year are set out in Appendix 2. This section is intended to be supported by the information contained within Appendix 2.
Previous studies
One of the most fundamental yet unresolved issues in health policy is the extent to which additional health-care expenditure yields patient benefits, in the form of improved health outcomes. The work of health technology agencies such as NICE has greatly improved our understanding at the micro level of the costs and benefits of individual technologies. However, there remains a dearth of reliable evidence at the macro level on the benefits of increased health system expenditure.
The empirical problems of estimating the link between spending and health outcomes are manifest. If one relies on a time series of health outcome data for an individual health system it is difficult to disentangle the impact of expenditure from a wide range of other temporal influences on health, such as technological advances, epidemiological changes, and variations in broader economic circumstances. Similar methodological difficulties arise if one attempts a cross-sectional comparison of different health systems. In particular, when seeking to draw inferences from international comparisons, researchers might have failed to adjust for all the potential external influences on health outcomes and this might account in part for their findings. For example, in an early cross-sectional study of 18 developed countries, Cochrane et al. 64 use regression analysis to examine the statistical relationship between mortality rates on the one hand and per capita gross national product (GNP) and per capita consumption of inputs such as health-care provision on the other. They found that the indicators of health-care provision were generally not associated with outcomes in the form of mortality rates. Thereafter, the failure to identify strong and consistent relationships between health-care expenditure and health outcomes (after controlling for other factors) has become a consistent theme in the literature, whereas, in contrast, socioeconomic factors are often found to be good determinants of health outcomes. 65–67
There is furthermore the possibility that indicators of health system inputs, such as expenditure, are endogenous, in the sense that they have to some extent been influenced by the levels of health outcome achieved. In addition, the difficulty of satisfactorily estimating the impact of health system inputs on outcomes is compounded by the great heterogeneity of health care, the multiple influences on outcomes, and the rather general nature of the outcome mortality measure traditionally used. Consequently, the failure to detect a significant positive relationship between expenditure and health outcome might reflect the difficulties associated with any such study rather than the absence of such a relationship. For example, Gravelle and Backhouse68 examine some of the methodological difficulties associated with empirical investigation of the determinants of mortality rates. These include simultaneous equation bias and the associated endogeneity problem (that the level of health-care input might reflect the level of health outcome achieved in the past), and that a lag may occur between expenditure and outcomes (studies typically assume that expenditure has an immediate effect on mortality).
To avoid the difficulties imposed by data heterogeneity inherent in international analyses, the study by Cremieux et al. 69 examines the relationship between expenditure and outcomes across 10 Canadian provinces over the 15-year period 1978–92. They find that lower health-care spending is associated with a significant increase in infant mortality and a decrease in LE. Although challenging the received empirical wisdom, one difficulty with the Cremieux et al. 69 study is that the estimated regression equation consists of a mixture of potentially endogenous variables (such as the number of physicians, health spending, alcohol and tobacco consumption, expenditure on meat and fat) and exogenous variables (such as income and population density). The authors’ chosen estimation technique (generalised least squared; GLS) does not allow for this endogeneity and consequently the coefficients on the endogenous variables may be biased. 68 Similarly, Nixon and Ulmann’s study,70 which uses three health outcome measures and various explanatory variables (such as per capita health expenditure) for 15 European Union (EU) countries over the period 1980–95, does not allow for the possibility that some of the explanatory variables may be endogenous.
More recently, studies have started to address the endogeneity issue. 71,72 Bokhari et al. 71 estimate a cross-section model for 127 countries using data for 2000. They employ two health outcome indicators (the under-5 mortality rate and the maternal mortality rate). Bokhari et al. 71 allow for the endogeneity of health expenditure via the use of IV techniques, and they estimate the elasticity of these indicators with respect to total government health expenditure conditional on the level of education and basic infrastructure (such as road transport and sanitation). They find that health expenditure has a statistically significant negative impact on both under-five mortality and maternal mortality. The authors do note, however, that their focus on child and maternal mortality implicitly assumes that these outcome indicators are in some way representative of outcomes across all activities financed by government health-care expenditure. Data permitting, it would be preferable to relate health-care expenditure on under-fives to under-five mortality, and expenditure on maternal care to maternal mortality.
In this study we relate expenditure in a specific disease area to mortality associated with those diseases. We also address the endogeneity issue through the use of IVs and, unlike previous studies; we examine the sensitivity of our results to questions of instrument validity. Moreover, although previous empirical work has been loosely based on the notion of a health production function, it has rarely been informed by an explicit theoretical model. This is understandable, as the processes giving rise to the observed health outcome are likely to be very complex, and any theoretical model might become rather unwieldy. However, this absence of atheoretical model has sometimes led to atheoretical search for measures of health inputs demonstrating a statistically ‘significant’ association with health outcomes. In contrast, in this study we inform our empirical modelling with a theoretical framework. We believe that this may lead to a more convincing and better specified model of health outcomes than that used in many previous studies, and this model is outlined in the next section.
Modelling framework
In the literature on the relationship between health expenditure and health outcomes, the statistical model estimated often contains a mixture of exogenous variables (such as income and population density) and endogenous variables (such as health spending, the number of doctors, and spending on cigarettes and alcohol). In such circumstances, the application of ordinary least squares will lead to biased coefficients on the endogenous variables. To avoid this problem, Gravelle and Backhouse68 recommend that analysts model, even if only informally, the decision-making process which generates the observed data set.
To avoid the problem of simultaneous equation bias we have constructed a very basic model of the budgeting and outcomes data generation processes. In places, the model makes some heroic assumptions (which we hope to relax in future work) but the framework reveals some of the more salient features of the data generation processes.
We assume – quite realistically – that each PCT, i, receives an annual financial lump sum allocation, yi, from the Department of Health and that total within year expenditure for each PCT cannot exceed this amount. We also assume – less realistically – that this lump sum is allocated across the J programmes of care (J = 23) by a single decision-maker (although we know that in practice the programme budget data will in part reflect the myriad of individual clinical decisions that health-care professionals take every day and that these are decisions over which PCTs exercise little control).
We assume that each PCT adheres to a social welfare function, W(.), that incorporates the health outcome (h) across all 23 programmes of care so that for each PCT:
Health outcomes might be measured in a variety of ways, but the most obvious is to consider some measure of improvement in LE, possibly adjusted for QoL, in the form of a QALY.
We assume that, for each PCT and for each programme of care, there is a ‘health production function’ that indicates the link between local spending on programme j (xj) and health outcomes in the same programme (hj). Two such production functions are illustrated in Figure 2. We assume that increased expenditure yields improvements in health outcomes, as expressed, for example, in local mortality rates, but at a diminishing rate. Clearly the shape of the curve might depend on the health needs of the local population (such as epidemiological conditions) and other local circumstances, such as socioeconomic conditions and local service input prices. Note that in Figure 2 the cost of securing a given level of health outcome is – for whatever reason – higher in PCTa than PCTb.
FIGURE 2.
The health production function for programme j in two PCTs.

In algebraic form, each PCT seeks to maximise total welfare across all J programmes of care (J = 23) subject to the health production function for each programme of care of the form:
where nj is the need for health care in programme j, xj is PCT expenditure on programme j, and zj represents environmental variables affecting the production of health outcomes in programme j [which might include private (non-PCT) health-care expenditure in the disease area]. Each PCT’s problem is to select an expenditure level for each programme ((xj*)), so as to maximise the utility function in Equation 3 subject to the health production functions in Equation 4 and the budget constraint that total expenditure on all programmes should not exceed PCT income (y).
Algebraically, the budget constraint is:
Solving this maximisation problem yields the result that the optimal level of PCT expenditure in each category, (xj*), is a function of the need for health care in each category (n1, n2, . . ., n23), environmental variables affecting the production of health outcomes in each category, (z1, z2, . . ., z23) and PCT income (y). Thus:
These results imply that each PCT will allocate expenditure across the 23 programmes of care so that the marginal utility of the last pound spent in each programme of care is the same. Of course, this does not mean that each programme receives the same amount of cash; financial allocations will depend on both the relationship between utility and outcomes, and on the relationship between outcomes and expenditure for each programme of care. If we assume that one extra unit of health outcome improves managerial utility by the same amount irrespective of the programme of care, then the decision-maker simply allocates expenditure across all programmes to maximise total health outcomes. This is achieved by ensuring that the marginal health outcome benefit (measured perhaps in QALYs) is the same for the last pound spent across all programmes of care.
Thus, for each programme of care, there exists an expenditure equation (see Equation 6) explaining the expenditure choice of PCTs and a health outcome equation (see Equation 4) which models the associated health outcomes achieved. As presented, our basic model is static in the sense that the health production function (see Equation 4) assumes that all health benefits occur contemporaneously with expenditure. We acknowledge that for some programmes of care benefits might occur ≥ 1 year after expenditure has occurred. This is particularly likely to be the case for those programmes aimed at encouraging healthy lifestyles, where some benefits may occur decades after the actual programme expenditure. For other programmes, such as maternity/reproductive conditions and neonate conditions, benefits may be largely contemporaneous with expenditure. However, although our data are largely cross-sectional in nature, we are able to link mortality data in such a way that this follows expenditures. Accordingly, for our empirical modelling we estimate models using expenditure for period t with mortality data for periods t, t + 1, and t + 2 combined. Appendix 2 presents a number of sensitivity checks on these assumptions including models where mortality data precedes expenditure datac and shows that these results are fairly consistent with the results presented here.
Data
Programme budgeting in England
Prior to October 2006, there were 303 PCTs in England with an average population of about 160,000 people. In October 2006 the 303 PCTs became 152 PCTs. Some PCT boundaries remained unchanged while other PCTs were merged with one or more neighbours to form a new, larger, PCT. In a few cases the geographic area covered by an existing PCT was split between two or more new PCTs. These 152 PCTs have an average population of about 330,000 people. PCTs are allocated fixed annual budgets within which they are expected to meet expenditure on most aspects of health care, including inpatient, outpatient and community care, primary care and pharmaceutical prescriptions.
Programme budgeting data collection was initiated by the Department of Health in April 2003 when each PCT was required to prepare expenditure data disaggregated according to 23 programmes of health care. These programmes are defined by reference to ICD-10 codes at the four digit level, and most PBCs reflect ICD-10 chapter headings (e.g. cancer and tumours, circulation problems, renal problems, neonates, problems associated with the skin, problems associated with vision, problems associated with hearing, etc.). In some cases the 23 categories are broken down into further subareas to achieve a closer match with the various National Service Frameworks (NSFs); for example, the large mental health category is broken down into ‘substance abuse’, ‘dementia’, and ‘other’.
Programme budgeting seeks to allocate all types of PCT expenditure to the various PBCs, including secondary care, community care and prescribing. However, the system acknowledges that a medical model of care may not always be appropriate, and two specific non-clinical groups – ‘healthy individuals’ and ‘social care needs’ – have been created. These are intended to capture the costs of disease-prevention programmes and the costs of services that support individuals with social rather than health-care needs. In addition, in some cases it is not possible to assign activity by medical condition, preventative activity, or social care need and, in these cases, expenditure is assigned to a residual category (PBC 23) entitled ‘other’. The most important element of this residual programme is expenditure on general practitioner (GP) services (PBC 23a). In principle it should be possible to allocate each GP consultation to a particular care programme. However, at the moment the available data information systems do not permit such an allocation and so all primary care expenditure is allocated to this residual programme. The use of this residual category ensures that all expenditure is assigned to a programme of care. 73
The aim of the programme budget classifications is to identify the entire volume of health-care resources assigned to broad areas of illness according to the primary diagnosis associated with an intervention. It serves a number of purposes, most notably to assist in the local planning of health care. However, for this study its crucial merit is that it opens up the possibility of examining the statistical relationship between local programme spending and the associated disease-specific outcome. Various forms of data collection and analysis are required to map PCT expenditure on acute, community and other services to the 23 PBCs. From the PCT perspective, however, the construction of each PCT’s return largely involves collating information provided by other bodies and drawing on other information already in the PCT’s own annual accounts. Details of how expenditure is assigned to programmes of care can be found in Appendix 2, The collection of programme budgeting data.
Table 1 shows the expenditure per head and the growth in this expenditure for each PBC for 2003/4–2008/9. d Year-on-year comparisons of expenditure in each group are complicated by the fact that the algorithms used to allocate activity to PBCs are regularly revised. e However, by 2008/9 total PCT expenditure per person had increased to £1531 (up 28% from 2004/5). The residual ‘other’ category (PBC 23) still accounted for the largest share of expenditure (14.9%) with per capita expenditure of almost £228, of which £145 was accounted for by primary care expenditure. Mental health (PBC 5) accounted for just over 12% of expenditure, but the expenditure share recorded by circulation problems (PBC 10) had fallen from 10.2% to 8.5%. Other categories recording a fall in budget share of more than a half of 1 percentage point included: the gastrointestinal system (down from 6.1% to 5.1%), the musculoskeletal system (down from 6.0% to 5.2%), trauma and injuries (down from 6.0% to 4.2%), and maternity (down from 4.6% to 3.9%). Categories recording an increase in budget share of more than a half of 1 percentage point included neurological problems (up from 2.9% to 4.4%) and dental problems (up from 1.1% to 4.1%).
Some of these changes will partly reflect revisions to the algorithms used to allocate expenditure to particular PBCs. For example in 2006/7 expenditure per person on musculoskeletal problems fell by 11% and expenditure on trauma and injuries fell by 25%. In the same year, expenditure on neurological problems increased by 35%. This suggests that some types of activity, which were previously allocated to musculoskeletal problems and/or trauma and injuries, were reallocated to neurological problems.
Similarly, up to and including 2006/7 expenditure that was not directly attributable to a particular programme category was apportioned using admitted patient care percentages. f In other words, if x% of total admitted patient care expenditure was allocated to PBC 1, then x% of all expenditure that was not directly attributable to a particular programme category was also allocated to PBC 1. With effect from 2007/8, however, NHS organisations were asked to select an appropriate basis for the apportionment of this non-programme-specific expenditure and that, where no reasonable basis existed, such expenditure was to be allocated to the ‘other – miscellaneous’ (PBC 23X) category. These two changes to the algorithm used to allocate expenditure to particular PBCs illustrate that year-on-year comparisons of expenditure need to be interpreted with care.
Expenditure per head on any given programme varies from one PCT to another and Table 2 presents some statistics that indicate the degree of variation in expenditure levels across PCTs by PBC. Columns 3–6 of Table 2 present descriptive statistics for PCT expenditure per person. These reveal that, for example, PCT per capita expenditure in the cancer programme averaged £96.30 across all PCTs, with the minimum spend being £62.90 and the maximum being £155.70.
PBC | Spend per head (unadjusted) (£) | Spend per head (cost adjusted) (£) | Spend per head (cost and need adjusted) (£) | ||||||||||
---|---|---|---|---|---|---|---|---|---|---|---|---|---|
Mean | SD | Min. | Max. | Mean | SD | Min. | Max. | Mean | SD | Min. | Max. | ||
1 | Infectious diseases | 26.5 | 24.6 | 8.6 | 151.8 | 25.7 | 21.7 | 8.6 | 136.7 | 25.0 | 21.4 | 9.5 | 139.5 |
2 | Cancers and tumours | 96.3 | 16.9 | 62.9 | 155.7 | 96.7 | 19.7 | 59.1 | 163.1 | 94.2 | 15.3 | 55.2 | 154.0 |
3 | Blood disorders | 20.3 | 7.0 | 7.7 | 49.4 | 20.2 | 6.5 | 8.0 | 49.1 | 19.7 | 6.0 | 8.2 | 44.2 |
4 | Endocrine, nutritional | 44.6 | 8.8 | 28.9 | 74.8 | 44.7 | 9.5 | 27.4 | 77.0 | 43.3 | 6.1 | 29.9 | 61.5 |
5 | Mental health | 201.4 | 60.0 | 118.9 | 474.1 | 200.3 | 54.0 | 122.8 | 422.8 | 194.0 | 41.9 | 132.3 | 362.0 |
6 | Learning disability | 56.8 | 18.8 | 7.7 | 125.9 | 57.0 | 19.4 | 6.8 | 123.6 | 55.7 | 18.8 | 6.7 | 136.6 |
7 | Neurological | 68.5 | 13.8 | 41.1 | 133.8 | 68.8 | 15.6 | 38.4 | 137.5 | 66.9 | 12.1 | 41.5 | 125.2 |
8 | Vision problems | 33.2 | 6.7 | 16.7 | 57.7 | 33.4 | 7.5 | 14.8 | 59.2 | 32.5 | 6.1 | 15.6 | 48.3 |
9 | Hearing problems | 8.6 | 3.7 | 0.9 | 24.0 | 8.7 | 3.9 | 0.9 | 25.5 | 8.3 | 3.3 | 0.8 | 22.0 |
10 | Circulatory disease | 131.6 | 26.7 | 88.0 | 317.3 | 132.2 | 30.5 | 78.2 | 327.6 | 128.5 | 24.4 | 75.7 | 326.9 |
11 | Respiratory system | 80.5 | 17.4 | 48.0 | 141.2 | 80.9 | 19.8 | 42.7 | 145.3 | 78.1 | 12.4 | 48.2 | 126.0 |
12 | Dental problems | 64.8 | 13.4 | 28.0 | 111.9 | 64.9 | 14.1 | 24.9 | 115.8 | 63.0 | 10.7 | 28.1 | 97.1 |
13 | Gastrointestinal system | 80.0 | 14.5 | 46.7 | 119.6 | 80.4 | 16.8 | 41.5 | 124.6 | 78.0 | 11.3 | 41.6 | 114.4 |
14 | Skin problems | 33.1 | 8.0 | 18.1 | 66.4 | 33.3 | 8.6 | 16.5 | 69.1 | 32.2 | 6.3 | 16.0 | 57.7 |
15 | Musculoskeletal system | 79.9 | 17.6 | 43.3 | 127.3 | 80.4 | 19.9 | 39.6 | 132.5 | 78.2 | 16.6 | 41.0 | 116.4 |
16 | Trauma and injuries | 63.2 | 16.7 | 12.5 | 139.3 | 63.4 | 17.4 | 11.5 | 125.0 | 61.8 | 15.6 | 10.4 | 103.6 |
17 | Genitourinary system | 75.7 | 13.7 | 49.9 | 112.3 | 75.6 | 13.6 | 48.4 | 108.9 | 73.7 | 10.1 | 50.6 | 105.5 |
18 | Maternity | 63.3 | 16.7 | 24.6 | 124.4 | 63.1 | 15.8 | 21.9 | 117.9 | 61.4 | 12.8 | 24.4 | 96.5 |
19 | Neonate conditions | 18.4 | 7.3 | 6.4 | 46.4 | 18.2 | 6.8 | 6.6 | 43.7 | 17.8 | 6.6 | 5.8 | 47.8 |
20 | Poisoning | 18.6 | 4.2 | 10.8 | 31.2 | 18.7 | 4.7 | 9.6 | 32.3 | 18.2 | 3.9 | 10.1 | 33.1 |
21 | Healthy individuals | 38.4 | 18.1 | 9.7 | 125.0 | 38.4 | 17.8 | 8.9 | 115.6 | 36.7 | 14.5 | 9.4 | 104.5 |
22 | Social care needs | 40.8 | 56.6 | 0.1 | 415.2 | 41.2 | 59.2 | 0.1 | 432.9 | 39.7 | 55.0 | 0.0 | 411.5 |
23 | Other (includes GMS/PMS) | 230.8 | 44.5 | 138.2 | 396.1 | 230.2 | 42.4 | 140.7 | 356.5 | 226.8 | 45.8 | 134.1 | 346.0 |
All | All PBCs | 1575.6 | 196.7 | 1225.7 | 2079.9 | 1576.3 | 217.3 | 1183.0 | 2173.1 | 1534.0 | 86.2 | 1390.1 | 1987.0 |
Some PCTs will be spending more than other PCTs simply because they face higher input costs. Columns 7–10 in Table 2 present descriptive statistics for PCT per capita expenditure that has been adjusted for the unavoidable geographical variation in costs (input prices) faced by PCTs. g However, if anything, this adjustment appears to increase the variation in expenditure across PCTs; for example, the range of per capita expenditure on cancer increases from between £62.90 and £155.70 (unadjusted) to between £59.10 and £163.10 (adjusted for local health-care input prices).
Another cause of the variation in expenditure levels is the fact that the need for health care varies from one PCT to another. For example, areas with a relatively large proportion of elderly residents, or PCTs operating in relatively deprived locations, can be expected to experience relatively high levels of spending. The Department of Health has a well-developed methodology for estimating the relative health-care needs, which it uses as the basis for allocating health-care funds to PCTs. 75
Columns 11–14 in Table 2 present descriptive statistics for PCT per capita expenditure that has been adjusted for both the unavoidable geographical variation in costs and the local need for health care faced by PCTs. h For virtually every PBC, this adjustment reduces the variation in expenditure across PCTs; for example, the standard deviation of PCT per capita expenditure falls from £19.70 to £15.30 for the cancer programme. Although this adjustment reduces the variation in expenditure levels across PCTs, this decline is quite modest and there are still substantial differences in expenditure even after allowing for differences in local cost and need. For example, expenditure per head in the circulation problems category varies between £78 and £328 using cost-adjusted expenditure data, but falls between £76 and £327 using cost- and need-adjusted population data.
The variation in expenditure across PCTs has led some commentators to question the reliability of the PB data. The National Audit Office (NAO)77 undertook a survey of trusts, PCTs and Strategic Health Authorities (SHAs) to assess the quality of the data. They concluded that although the processes for collecting the budgeting data were well defined in most areas, there remained scope for improvements to the robustness of some of the data (e.g. non-admitted patient care). Appleby et al. 78 also considered the issue of data reliability in variations in spending on cancer services and noted some large year-on-year changes. However, the authors point out that it is difficult to define what might be either an implausible level of expenditure or an implausibly large change in expenditure. This is complicated by the fact that the Department of Health makes regular improvements to the way in which activity is matched to programme categories.
As with most data sets, there are likely to be recording and other errors associated with the PB data. However, although we note that the allocation of PB data might not be perfect there is no systematic evidence of this. Accordingly, for each disease category, we observe that PCT expenditure per person varies considerably and this variation – holding constant input prices and the need for health care – offers the opportunity to examine whether or not PCTs that spend more on health care achieve a better outcome and, if so, at what cost. Empirical estimates of the strength of this relationship for several programmes of care are presented in this report.
Health outcome data
Most studies of the relationship between expenditure and outcome have used some measure of mortality as an indicator of the latter. We also employ mortality as an outcome measure. First, it is a relevant (albeit not comprehensive) measure of the outcome of health-care expenditure; and second, it is available for more disease areas than any other outcome measure at PCT level.
Although mortality is available (by PCT) for several disease areas, it is not available for just over a half of all programmes not least because it is simply not relevant for these programmes (e.g. for learning disabilities, vision problems, hearing problems, dental problems and skin problems). Moreover, even where a mortality measure is available, the ICD-10 coverage of the mortality data often falls short of the coverage of the expenditure data. For some programmes, therefore, we have combined the published mortality rates for two or more disease areas in an attempt to match the ICD-10 coverage of the mortality data with that of the expenditure data.
Table 37 (see Appendix 2) shows how we have attempted to marry the mortality data (column C) and the expenditure data (column A). ICD-10 coverage of the component mortality rates for some PBCs falls short of the expenditure data and the extent of this shortfall is illustrated by the ratio reported in the final column of Table 3. For example, the cancers and tumours programme covers all expenditure associated with ICD-10 codes C00–C97 and D00–D49 but the PCT-based mortality data only relates to ICD-10 codes C00–C97. At the national (all England) level, figures are available which show that, in 2008, there were 62,072 deaths of those aged < 75 years from codes C00–C97 and that there were 63,076 deaths from codes C00–C97 and D00–D49 combined. In other words, the PCT-level mortality data reflect 98.4% of all deaths associated with the expenditure codes. We adjust our cost of life (year) estimates for this mismatch.
Description | Observations | Mean | SD | Min. | Max. |
---|---|---|---|---|---|
Proportion of residents born outside the EU | 151 | 0.0794 | 0.0876 | 0.0088 | 0.3817 |
Proportion of population in white ethnic group | 151 | 0.8927 | 0.1299 | 0.3942 | 0.9926 |
Proportion of population of working age (16–74 years) with LLT | 151 | 0.1182 | 0.0250 | 0.0709 | 0.1798 |
Proportion of population providing unpaid care | 151 | 0.0990 | 0.0118 | 0.0662 | 0.1221 |
Proportion of population providing unpaid care (< 20 hours per week) | 151 | 0.0667 | 0.0079 | 0.0461 | 0.0817 |
Proportion of population providing unpaid care (20–49 hours per week) | 151 | 0.0113 | 0.0025 | 0.0065 | 0.0195 |
Proportion of population providing unpaid care (> 50 hours per week) | 151 | 0.0210 | 0.0051 | 0.0093 | 0.0353 |
Proportion of population aged 16–74 years with no qualifications | 151 | 0.2960 | 0.0642 | 0.1301 | 0.4555 |
Proportion of population aged 16–74 tears that are full-time students | 151 | 0.0720 | 0.0270 | 0.0425 | 0.1626 |
Proportion of households without a car | 151 | 0.2932 | 0.1046 | 0.1325 | 0.5761 |
Proportion of owner occupied households | 151 | 0.6692 | 0.1128 | 0.2891 | 0.8205 |
Proportion of households in rented social (LA/HA) housing | 151 | 0.2071 | 0.0918 | 0.0817 | 0.5356 |
Proportion of households in rented private housing | 151 | 0.0924 | 0.0449 | 0.0349 | 0.2961 |
Proportion of lone pensioner households | 151 | 0.1434 | 0.0184 | 0.0979 | 0.1942 |
Proportion of one parent households | 151 | 0.0684 | 0.0180 | 0.0401 | 0.1207 |
Proportion of population aged 16–74 years that are permanently sick | 151 | 0.0574 | 0.0213 | 0.0242 | 0.1215 |
Proportion of population aged 16–74 years that are long-term unemployed | 151 | 0.0113 | 0.0052 | 0.0036 | 0.0287 |
Proportion of population aged 16–74 years in employment that are in agriculture | 151 | 0.0117 | 0.0119 | 0.0016 | 0.0668 |
Proportion of those aged 16–74 years that are in professional occupations | 151 | 0.2672 | 0.0688 | 0.1470 | 0.4958 |
IMD2007 | 151 | 23.8098 | 9.1168 | 8.0857 | 48.2627 |
Need index (incorporates CARAN formula) | 151 | 1.0253 | 0.1334 | 0.7311 | 1.3479 |
MFF index for HCHS and prescribing | 151 | 1.0021 | 0.0559 | 0.9410 | 1.1243 |
Diabetes prevalence rate 2007/8 (%, over 17 years) | 151 | 5.4872 | 0.7982 | 3.2200 | 8.5100 |
Epilepsy prevalence rate 2007/8 (%, over 18 years) | 151 | 0.7884 | 0.1489 | 0.4100 | 1.0900 |
HIV need index | 151 | 1.1848 | 1.4984 | 0.1648 | 8.3332 |
Chronic kidney disease 2007/8 (%, over 18 years) | 151 | 4.1687 | 1.2711 | 1.3500 | 8.4100 |
Maternity need index | 151 | 1.0345 | 0.2106 | 0.6845 | 1.8129 |
Raw (unadjusted) population 2007/8 | 151 | 335,735 | 196,501 | 90,142 | 1,264,298 |
We acknowledge that mortality is a more relevant outcome indicator for some programmes (e.g. for circulatory problems) than for others (e.g. for epilepsy) and, for this reason, we would expect better results in some programmes than others. We also acknowledge that this focus on mortality ignores the impact of expenditure aimed at chronic care and at palliative care. Nevertheless, our focus on mortality is purely practical: it is both a widely available measure and clearly a relevant outcome indicator. i
The mortality data provide us with a number of possible outcome indicators including the < 75 years of age standardised mortality rate (SMR) and the (< 75 years) standardised years of life lost rate (SYLLR). The SMR gives equal weight to all deaths irrespective of the age at which they occur but the SYLLR gives greater weight to deaths that occur at earlier ages. For our purposes we focus on a measure of the avoidable YLL. j This is calculated by summing over ages 1–74 years the number of deaths at each age multiplied by the number of years of life remaining up to age 75 years. The crude YLL rate is simply the number of YLL divided by the resident population aged < 75 years. Like conventional mortality rates, the crude YLL rate can be age standardised to eliminate the effects of differences in population age structures between areas, and this (age) standardised YLL rate is the health outcome variable generally employed in this study. 79
Other variables
We employ an IV estimation technique to our empirical models of the outcome and expenditure equations as described in the next section. This is due to (i) own programme expenditure is likely to be endogenous in the outcome equation and (ii) other programme need is likely to be endogenous in the own programme expenditure equation. Endogeneity of programme expenditure results from expenditure levels being responsive to levels of outcomes and/or unobserved need rendering expenditure correlated with the residuals in an ordinary least squared (OLS) regression of outcomes on expenditure. Due to limitations in the data available, need in the expenditure equation in the ‘other’ programmes is proxied by death rates (minus that due to the programme under investigation). This will be influenced by expenditure decisions, including expenditure in other programmes and is treated as endogenous in the expenditure model.
Instrumental variable estimation basically involves replacing the endogenous variable in the equation of interest with its predicted value from an OLS regression which regresses the endogenous variable on a set of IVs. These instruments should be good predictors of the endogenous variable (i.e. they should be relevant and strong predictors) but should be appropriately excluded from the equation of interest (i.e. they should be valid instruments).
We have a number of potential instruments available, mostly derived from the 2001 Population Census. 80 In our earlier studies we found that a small subset of these instruments proved sufficient to generate plausible results. These included the proportion of the population providing unpaid care; the proportion of households that are one pensioner households; index of multiple deprivation; and proportion of the population in the white ethnic group.
We also had available a further set of potential instruments and, where our more limited set of instruments failed to generate plausible results, we extended our instrument search to include this wider set of variables. This extended set of instruments is shown in Table 3. k
Our instruments reflect factors, such as socioeconomic deprivation and the availability of informal care in the community, which might indirectly impact on mortality rates and/or health-care expenditure levels. As we shall see, although our instruments ‘pass’ the appropriate statistical tests, some commentators claim that such tests may have ‘low power’ to detect the presence of invalid instruments. Consequently, in Appendix 2, The sensitivity of the outcome elasticity to the validity of the instrument exclusion restrictions we examine how sensitive our results are to the presence of invalid instruments.
Table 3 reports descriptive statistics for the socioeconomic and needs variables used in the study (these statistics are for the variables in absolute form). For example, on average, lone pensioner households comprise 14% of all households, the ‘white ethnic’ group accounts for 89% of the population and 10% of the population provide unpaid care.
In addition to the IVs, Table 3 also reports descriptive statistics for the Department of Health’s ‘need for health care’ index,l its need for human immunodeficiency virus (HIV) services index, and its need for maternity services index. The latter two indices are used to either supplement or replace the all service measure of need when estimating our models. The ‘need for health care’ index averages about 1 but varies substantially, with some PCTs having a needs index more than 25% below the national average and others facing a need for health care more than 30% above the national average. Table 3 also reports descriptive statistics for some disease prevalence rates (e.g. for diabetes and for epilepsy) and, again, these are used to either supplement or replace the all service measure of need when estimating our models. Finally, the MFF index shows that input prices in the most expensive PCT are almost 20% above those in the least expensive PCT.
Approach to model estimation
The theoretical framework suggests the specification and estimation of a system of equations, with an expenditure and health outcome equation for each of the 23 programmes of care. However, this approach makes infeasible data demands, requiring variables to identify expenditure, need, environmental factors and health outcomes in each of the 23 programmes of care. Moreover, mortality rates are available for less than half of the 23 programmes. Rather than estimate a system of equations, we proceed on a programme-by-programme basis, estimating health outcome and expenditure equations for those programmes for which mortality data are available.
In line with the theoretical framework presented above, we specify the following expenditure (see Equation 7) and health outcome (see Equation 8) models for each of the 23 programmes of care. Accordingly, for the j-th programme of care we have:
where xi is expenditure; ni is the own programme need for care; mi is the need for care in other programmes; yi is the total budget and hi is the health gain in PCT i.
Ideally we should employ a programme-specific indicator of the level of need for each care programme (nij) but these are not readily available. When estimating both the outcome and expenditure models we therefore proxy the own programme health-care need using the ‘needs’ component of the Department of Health’s resource allocation formula. m This needs element is specifically designed to adjust PCT allocations for local health-care needs and accordingly, ceteris paribus, we would expect a positive relationship between expenditure and need for each programme of care. We would also expect a positive relationship between need and adverse health outcomes. n
The expenditure model includes both the own programme health-care need (which is proxied using the ‘needs’ component of the Department of Health’s resource allocation formula) and the need for health care in all other programmes. In the absence of programme-specific measures of need, we use the ‘all-cause mortality rate excluding the mortality rate in the programme of interest’, mi, as the proxy for need in other programmes of care.
All variables have been log-transformed so that parameter estimates can be interpreted as elasticities. In other words, a regression coefficient of 0.5 implies that a 1% increase in the regressor is associated with a 0.5% increase in the dependent variable.
Instrumental variable estimation
Other programme need, mi, in the expenditure Equation 7 and expenditure, xi, in the outcome Equation 8 are both likely to be endogenous rendering OLS both biased and inconsistent. Endogeneity of programme expenditure results from expenditure levels being responsive to levels of outcomes and/or unobserved need. Other programme need in the expenditure equation is proxied by death rates which is influenced by expenditure decisions and hence is treated as endogenous. To deal with this endogeneity we employ IVs estimation and implement two-stage least squares (2SLS). Unlike OLS, IV is a consistent estimator in the presence of an endogenous regressor and, although in finite samples the IV estimator will be biased, with the bias (providing certain assumptions are met) being less than that associated with OLS.
For the health outcome equation, IV estimation can be viewed as finding variables (instruments) that are good predictors of programme expenditure but which are appropriately excluded from the outcome equation of interest (that is, from Equation 8) because they are not predictive of outcome. The assumption is that these instruments impact on health outcome through their impact on expenditure only, and that they do not have a direct effect on the outcome. o
Similarly, for the expenditure equation, IV estimation can be viewed as finding variables (instruments) that are good predictors of the proxy for other programme need (mi) but which do not belong in the expenditure equation of interest (that is, Equation 7). The assumption is that these predictors impact on own programme expenditure only through their impact on other programme need and that they do not have a direct effect on own programme expenditure.
The outcome and expenditure equations for any given programme may contain different IVs because these instruments are trying to predict different variables (own programme expenditure and other programme mortality respectively). In addition, the instrument set for, say, the expenditure equation may vary across programmes because the other programme need variable will reflect need in a different basket of programmes for each expenditure equation.
We have a number of potential instruments available, mostly derived from the 2001 Population Census. 80 In previous studies, we have often found that a small subset (four) of these instruments often proved sufficient to generate plausible results. However, if plausible results were not obtainable with some combination of these four instruments, we employed an extended instrument set. Further details of the identification of suitable instruments for each model can be found in Appendix 2, Re-estimation of poorly performing models with an extended instrument set.
The available instruments reflect factors, such as socioeconomic deprivation and the availability of informal care in the community, which might indirectly impact on mortality rates and/or health-care expenditure levels. The set of instruments associated with each estimated equation was selected on both technical and pragmatic grounds. From a pragmatic point of view, we require a parsimonious set of instruments that satisfy the necessary technical criteria. These are, first, that they have face validity, that is, that they are plausible determinants of the endogenous variable being instrumented, and second, that the instruments are both relevant and valid. The relevance of an instrument set refers to its ability to predict the endogenous variable of concern, whereas validity refers to the requirement that instruments should be uncorrelated with the error term in the equation of interest.
Should the instrument set be strong, relevant and valid, 2SLS will produce consistent estimates of the parameters of the reduced form models. We subject the instrument sets to tests for validity using the Sargan–Hansen test of over identifying restrictions. The joint null hypothesis is that the instruments are valid instruments (i.e. they are uncorrelated with the error term), and that the instruments are correctly excluded from the outcome equation of interest. A rejection of the null hypothesis casts doubt on the validity of the instruments. We test for instrument relevance using Shea’s81 partial R2 measure; this reflects the correlation between the excluded instruments and the endogenous regressor. However, even where valid and relevant, a non-zero but small correlation between the set of instruments and the endogenous regressors can lead to the problem of weak instruments, again rendering IV estimation biased. We test for the presence of weak instruments using the procedures set out in Stock and Yogo82 and the Kleibergen–Paap Lagrange multiplier (LM) statistic. A general test of model specification is provided through the use of Ramsey’s83 reset test for OLS and an adapted version of the test for IVs. 84
Finally, we check that the presumed endogenous variable is in fact endogenous using the test proposed by Durbin. 85 If the null hypothesis of exogeneity cannot be rejected, then we revert to using OLS. Although, in general, our instruments ‘pass’ the appropriate statistical tests, some commentators claim that such tests may have ‘low power’ to, and hence may fail, to reject the validity of the instruments when this is false in small samples. Consequently, in Appendix 2, The sensitivity of the outcome elasticity to the validity of the instrument exclusion restrictions we examine how sensitive our results are to the relaxation of the assumption that the instruments are valid.
Further details of our approach to IV estimation are set out in Appendix 2.
Results
The work presented here builds on previous studies of the link between expenditure and health outcomes. Martin et al. 60 reported outcome elasticities for two programmes (cancer and circulatory disease) using expenditure data for 2004/5 and pooled mortality data for 2002, 2003 and 2004. p This work was extended in a subsequent study63 to include several other programmes and updated expenditure data (2005/6). However, the authors struggled to obtain sensible outcome models for some programmes of care. Attempts to improve model estimates by considering alternating measures of the population need for health careq and an extended set of potential IVs are presented in Appendix 2, Analysis of programme budgeting expenditure for 2005/6 and mortality data for 2002/3/4. This work forms the basis for the set of key results from the empirical modelling of health-care expenditures and outcomes using more contemporaneous data presented in the following sections. Details of all results presented are set out in Appendix 2.
2006/7 expenditure data and mortality data for 2006/7/8
This section presents results that relate expenditure in 2006 to mortality in the same year and in the 2 following years (i.e. in 2006, 2007 and 2008). Throughout our measure of the need for health care is derived from the Department of Health’s resource allocation model based on the CARAN needs formula. 74 This represents a more up-to-date needs adjustment than the AREA based model76 that has been applied in previous studies,60,63 and is directly applicable to the 152 PCTs in existence in the 2006/7 expenditure year. Expenditure data has been adjusted for differences in input prices using the MFFs for HCHS and prescribing. r The outcome and expenditure results for the big four programmes are shown in Table 4 with the relevant outcome and expenditure elasticities highlighted.
Equation variable | (1) PBC 2, cancer | (2) PBC 2, cancer | (3) PBC 10, circulation | (4) PBC 10, circulation | (5) PBC 11, respiratory | (6) PBC 11, respiratory | (7) PBC 13, gastrointestinal | (8) PBC 13, gastrointestinal |
---|---|---|---|---|---|---|---|---|
Outcome model | Spend model | Outcome model | Spend model | Outcome model | Spend model | Outcome model | Spend model | |
Own programme spend per head | –0.342*** | –1.434*** | –2.029*** | –1.536*** | ||||
[0.099] | [0.218] | [0.636] | [0.468] | |||||
Need (CARAN per head) | 0.995*** | 1.626*** | 2.860*** | 2.306*** | 2.696*** | 1.449*** | 4.160*** | 2.040*** |
[0.106] | [0.343] | [0.252] | [0.372] | [1.044] | [0.331] | [0.577] | [0.378] | |
Need (CARAN per head squared) | 1.163*** | 2.451 | ||||||
[0.348] | [1.561] | |||||||
SYLLR (all deaths excluding cancer) | –0.855*** | |||||||
[0.191] | ||||||||
PCT budget per head | 0.465 | 0.540* | 0.679*** | 0.446* | ||||
[0.300] | [0.299] | [0.251] | [0.263] | |||||
SYLLR (all deaths excluding circulatory) | –1.666*** | |||||||
[0.295] | ||||||||
Permanently sick | 0.759** | |||||||
[0.367] | ||||||||
SYLLR (all deaths excluding respiratory) | –0.672** | |||||||
[0.305] | ||||||||
SYLLR (all deaths excluding gastrointestinal) | –1.206*** | |||||||
[0.314] | ||||||||
Constant | 6.501*** | 5.913*** | 11.413*** | 10.696*** | 13.756*** | 3.346 | 9.719*** | 8.370*** |
[0.436] | [2.815] | [1.046] | [2.379] | [3.279] | [2.075] | [2.009] | [2.299] | |
Endogeneity test statistic | 13.695 | 19.421 | 42.548 | 24.461 | 17.687 | 8.439 | 16.373 | 15.211 |
Endogeneity p-value | 0.000215 | 1.05e-05 | 6.90e-11 | 7.58e-07 | 2.60e-05 | 0.00367 | 5.20e-05 | 9.61e-05 |
Hansen–Sargan test statistic | 0.685 | 0.021 | 0.949 | 1.262 | 1.462 | 0.302 | 2.761 | 0.0164 |
Hansen–Sargan p-value | 0.408 | 0.084 | 0.814 | 0.261 | 0.227 | 0.583 | 0.0966 | 0.0898 |
Shea’s partial R2 | 0.164 | 0.445 | 0.300 | 0.296 | 0.0785 | 0.327 | 0.140 | 0.356 |
Kleibergen–Paap LM test statistic | 17.85 | 41.88 | 32.37 | 32.02 | 10.02 | 34.98 | 14.86 | 35.72 |
Kleibergen–Paap p-value | 0.000133 | 8.04e-10 | 1.61e-06 | 1.11e-07 | 0.00666 | 2.54e-08 | 0.000592 | 1.75e-08 |
Kleibergen–Paap F-statistic | 13.28 | 56.69 | 17.14 | 31.84 | 7.022 | 20.94 | 11.63 | 22.40 |
Pesaran–Taylor reset statistic | 0.00537 | 0.18 | 0.136 | 0.00349 | 0.0120 | 1.497 | 1.669 | 0.007 |
Pesaran–Taylor p-value | 0.942 | 0.668 | 0.712 | 0.953 | 0.913 | 0.221 | 0.196 | 0.935 |
In all four outcome models expenditure has a significant negative effect on mortality and the all service measure of need has a significant positive effect. The squared value of the measure of need is also positive and significant in the cancer outcome equation. In the respiratory outcome model, there is an additional indicator of need – the proportion of the population that are permanently sick – and this is both positive and statistically significant. The diagnostic statistics suggest that, in all four cases, own programme expenditure is endogenous and that the instruments are valid. They also suggest that the instruments are relevant. There is no evidence that the instruments are weak in three of the four outcome results. The Pesaran–Taylor test suggests that there is no evidence of model misspecification.
However, the Kleibergen–Paap F-statistic for the respiratory disease outcome model is 7.022 and this is less than the ‘critical’ target of 10.0. This indicates that the instruments may be weak and not good predictors of the programme expenditure. However, if we re-estimate this model having dropped the least significant instrument, the coefficient on own programme expenditure becomes −2.622 and is significant at the 1% level. Moreover, there is now no evidence of weak instruments (the Kleibergen–Paap F-statistic is 11.025) and it is this coefficient that we use for the respiratory outcome model in the cost of a life-year calculations below.
In three of the four expenditure models both the need and budget variables have a positive and significant effect on own programme expenditure. In addition, the proxy for need in other programmes is negative and significant in all four cases. The diagnostic statistics suggest that, for all four expenditure models, expenditure is endogenous and the instruments are valid. They also suggest that the instruments are relevant and there is no evidence that the instruments are weak. The Pesaran–Taylor test suggests that there is no evidence of model misspecification.
Cost of a life-year
The outcome and expenditure elasticities presented in Table 4 can be used to calculate the cost of a life-year in each programme. These calculations – for both the big four programmes as well as for the other six programmes with mortality based outcome indicators – are shown in Table 5. The cost of a life (year) estimates presented in Table 5 assume a 1% increase in each PCT’s budget and are calculated as:
the cost of an additional life in a particular programme
= the change in expenditure in that programme/the change in mortality in that programme
= (annual spend × expenditure elasticity)/(annual mortality × outcome elasticity × expenditure elasticity)
and
the cost of an additional life-year in a particular programme
= the change in expenditure in that programme/the change in life-years lost in that programme
= (annual spend × expenditure elasticity)/(annual life-years lost × outcome elasticity × expenditure elasticity).
PBC | Expenditure 2006/7, outcome 2006/7/8 | Expenditure 2007/8, outcome 2007/8/9 | Expenditure 2008/9, outcome 2008/9/10 | |||||||||
---|---|---|---|---|---|---|---|---|---|---|---|---|
Spend (£M) 2006/7 | Total life-years lost, < 75 years, 2006/7/8 | Cost per YLG (£) | Cost per YLG adjusted for YLL coverage (£) | Spend (£M) 2007/8 | Total life-years lost, < 75 years, 2007/8/9 | Cost per YLG (£) | Cost per YLG adjusted for YLL coverage (£) | Spend (£M) 2008/9 | Total life-years lost, < 75 years, 2008/9/10 | Cost per YLG (£) | Cost per YLG adjusted for YLL coverage (£) | |
Cancer | 4122 | 2,207,021 | 16,383 | 16,121 | 4573 | 2,189,685 | 17,165 | 16,891 | 4843 | 2,170,660 | 21,802 | 21,454 |
Circulatory problems | 6161 | 1,361,634 | 9466 | 9390 | 6325 | 1,313,223 | 11,315 | 11,224 | 6655 | 1,285,026 | 11,779 | 11,685 |
Respiratory problems | 3285 | 324,223 | 11,593 | 8961 | 3431 | 315,457 | 14,798 | 11,439 | 3994 | 311,034 | 21,307 | 16,470 |
Gastrointestinal problems | 3700 | 345,908 | 20,892 | 11,929 | 3805 | 343,355 | 25,034 | 14,295 | 3989 | 341,884 | 25,662 | 14,653 |
Big four programmes summary | 17,268 | 4,238,786 | 12,333 | 10,604 | 18,134 | 4,161,720 | 16,345 | 13,830 | 19,481 | 4,108,604 | 16,688 | 14,650 |
Infectious diseases | 1053 | 106,552 | 630,798 | 630,798 | 1119 | 106,092 | 57,742 | 57,742 | 1201 | 100,078 | 71,432 | 71,432 |
Endocrine problems | 1852 | 57,672 | 114,416 | 72,539 | 1997 | 55,492 | 190,745 | 120,932 | 2222 | 54,779 | 104,008 | 65,941 |
Neurological problems | 2790 | 66,137 | 1,129,960 | 153,675 | 3165 | 64,873 | 431,749 | 58,718 | 3466 | 64,222 | 388,267 | 52,804 |
Genitourinary problems | 3482 | 10,030 | 20,421,090 | 3,512,427 | 3439 | 8,529 | 652,096 | 112,160 | 3779 | 8004 | 877,038 | 150,851 |
Trauma and injuries | 2892 | 30,000 | N/A | N/A | 2918 | 21,273 | 1,115,197 | 195,159 | 3255 | 6881 | N/A | N/A |
Maternity and neonates | 3574 | 492,600 | 45,158 | 30,662 | 3662 | 489,170 | 204,168 | 138,630 | 3978 | 479,905 | 198,939 | 135,080 |
Other six programmes summary | 15,643 | 762,991 | 258,046 | 146,108 | 16,300 | 745,429 | 274,309 | 99,428 | 17,901 | 713,869 | 254,794 | 112,674 |
All 10 programmes summary | 32,911 | 5,001,777 | £23,780 | 19,965 | 34,434 | 4,907,149 | 38,110 | 28,983 | 37,382 | 4,822,473 | 38,328 | 30,883 |
Other 13 programmes summary | 34,985 | 39,223 | 41,016 | |||||||||
All 23 programmes | 67,896 | 87,494 | 73,457 | 73,657 | 108,829 | 82,765 | 78,398 | 105,460 | 84,974 |
To illustrate this calculation let us calculate the cost of a life-year for, say, the cancer programme. The annual spend on cancer in 2006/7 is £4122M and the expenditure elasticity for the programme is 0.465 so that the change in expenditure associated with a 1% increase in each PCT’s budget is £19.1673M (= 1% × £4122M × 0.465). The total number of life-years lost to cancer for 2006/7/8 totals 2,207,021 life-years and so the average annual loss is 735,674 life-years. The outcome elasticity for the cancer programme is 0.342 and the expenditure elasticity is 0.465 so the reduction in the number of life-years lost associated with a 1% increase in each PCT’s budget is 1170 (= 1% × 735,674 life-years × 0.342 × 0.465). The cost of an additional life-year is therefore £19.1673M (the change in expenditure in the programme) divided by 1170 (the reduction in the number of life-years lost), and this equals £16,383.
An integral part of the calculation of the cost of a life-year is the annual mortality (life-years lost) figure associated with a particular programme. Ideally, the ICD-10 coverage of the expenditure data should coincide with that of the mortality data. However, as shown in Table 37 of Appendix 2, the ICD-10 coverage of the mortality data typically falls short of that for the expenditure data. Unless we adjust the annual mortality figure so that its ICD-10 coverage approximates that of the expenditure data, our cost of life (year) estimates will be too large because they will underestimate the mortality gain.
Table 5 reports cost of a life-year estimates both with and without this adjustment for ICD-10 coverage. Having incorporated this adjustment, the results show that the cost of a life-year for the big four PBCs is estimated as £10,604 and, for all 10 programmes with a mortality outcome measure, the estimate is £19,965. For all programmes, assuming a zero gain for the 13 PBCs without an outcome indicator, the corresponding estimate is £73,457.
If we assume that PBC 23 (largely primary care) generates a zero health gain (because the gains from primary care are already reflected in the mortality rates for disease-specific programmes) and that the gain attributable to the remaining 12 programmes is, on average, the same as that attributable to those with a mortality outcome measure, then the cost of a life-year across all programmes is £22,565. s
Non-primary care trust Department of Health funded expenditure
Primary care trust expenditure accounts for a large proportion of Department of Health expenditure but PCTs do not account for the Department’s entire budget. In 2006/7 the Department of Health’s gross expenditure totalled £83.5B. Charges raised £3.4B so net expenditure totalled £80.1B. Of this net expenditure, PCTs accounted for £67.3B (that is, 84%) and various other bodies accounted for the remaining £12.8B. A breakdown of this gross and net expenditure by major body is shown in Table 70 of Appendix 2. The Department of Health has allocated net non-PCT expenditure across the 23 PBCs. Of the additional £12B of net expenditure, £11.2B (93%) has been allocated to PBC 23. This largely reflects (a) the allocation of almost all SHA expenditure to either PBC 23B (‘other: SHAs including workforce development committees’) or PBC 23X (‘other: miscellaneous’); and (b) the allocation of almost two-thirds of Department of Health expenditure to PBC 23X (‘other: miscellaneous’). The remaining £0.8B of additional net expenditure is spread across all PBCs according to various allocation rules and although this approach avoids allocating expenditure to the ‘other: miscellaneous’ category, this allocation of expenditure does not necessarily reflect actual expenditure.
The cost of a life (year) estimates presented above are based on the impact of a 1% exogenous change in total net PCT spend. All of our outcome and expenditure models have been estimated using net PCT expenditure, and all of our elasticities relate to this expenditure. Implicitly we assume that any budgetary shock only affects PCT funding and that it leaves non-PCT funding unchanged. Suppose instead we assume a 1% exogenous change in the Departmental budget. We have no information on how this Departmental budgetary shock is likely to be split between PCT and non-PCTs budgets. One might assume that the non-PCT budget is as responsive to a Departmental budgetary shock as is the PCT budget. If this was the case then it would add 17.7% to our cost of a life-year estimate for 2006/7. However, in the absence of any information about the responsiveness of the non-PCT budget, it is difficult to come to any firm conclusion about the impact of non-PCT expenditure on our cost of a life-year estimates.
2007/8 expenditure data and mortality data for 2007/8/9
Outcome and expenditure models were estimated using updated data for expenditure (from 2006/7 to 2007/8) and updated mortality data (from 2006/7/8 to 2007/8/9). Appendix 2, Analysis of programme budgeting expenditure for 2007/8 and mortality data for 2007/8/9 presents detailed discussion of the findings including tables of results.
Outcome models
As before, we model outcome as a function of own programme expenditure and a measure of health-care need, where the latter is proxied by the measure of need as employed by the Department of Health for resource allocation purposes. t There are, however, a few exceptions. For the respiratory programme we further included the square of the measure of need to improve model fit. In some other PBCs we found that the all service measure of need performed poorly and we replaced or supplemented this measure with either a more programme-specific measure (e.g. the epilepsy prevalence rate for neurological mortality) or with a better performing proxy for need (e.g. the percentage of residents born outside the EU for maternity/neonate mortality). These amendments improved model specification. u Full results for all programmes are presented in Appendix 2, Table 81; below is a summary of the findings.
Two sets of models were estimated for three of the big four programmes (cancer, respiratory problems and gastrointestinal problems). One of the two models used two instruments and so we report the instrument validity test statistic. In all three cases we failed to reject the null hypothesis of instrument validity. However, there is some evidence of weak instruments (at least in the respiratory and gastrointestinal programmes) and if we dropped one instrument and re-estimated the model, evidence of instrument weakness disappeared. The removal of one instrument has little impact on the coefficient on expenditure and it is this coefficient that we use below in our cost of a life-year calculations reported in Table 5.
For the big four programmes the need variable has a positive and significant effect on mortality, and expenditure has the anticipated negative effect. The diagnostic statistics reveal that, in all four PBCs, own programme expenditure is endogenous and that the instruments are valid. They also suggest that the instruments are relevant and there is no evidence that they are weak in the models with one excluded instrument. The Pesaran–Taylor test reveals no evidence of model misspecification.
The outcome results for the other programmes are similar to but more diverse than those for the big four programmes. This is to be anticipated because mortality is a much rarer outcome in these programmes than it is in the big four programmes. Own programme expenditure is not endogenous in four of these programmes, but we retain the IV estimator for three of these four because this yields more plausible results than the OLS estimator (the results are more plausible in the sense that the signs on the coefficients are more in line with our prior expectations). v
Expenditure has the anticipated negative effect on mortality in the endocrine problems programme but this is not statistically significant. The all service measure of need is not relevant for this PBC; instead, we find that the diabetes prevalence rate is positively associated with mortality, as is a measure of deprivation (the IMD2007).
Mortality from epilepsy is negatively and significantly associated with expenditure in the neurological programme. Both the all service need for health care and the epilepsy prevalence rate are positively and significantly associated with mortality in this programme.
Expenditure has a negative and statistically significant effect on mortality (from renal problems) in the genitourinary problems programme. The prevalence of lone parent households is positively associated with mortality.
Expenditure has the anticipated negative effect on mortality in the infectious disease programme and this is statistically significant. The all service measure of need is not relevant for this PBC; instead, we find that a measure of need associated with HIV is positively associated with mortality, as is a measure of deprivation (the IMD2007).
Expenditure has the anticipated negative effect on mortality in the maternity and neonates programme but the estimated coefficient is not statistically significant. In this PBC the generic all service measure of need has been replaced with two other indicators of deprivation – the proportion of residents born outside the EU and the proportion of those aged 16–74 years without any qualifications – both of these are positively associated with mortality.
Finally, expenditure and need have the anticipated effects on mortality in the trauma and injuries programme. In addition, the proportion of households without access to a car is negatively associated with mortality from fractures (perhaps access to a car facilitates involvement in serious road traffic accidents), and the proportion of residents that are students is positively associated with mortality from fractures.
The relevant statistical test suggests that expenditure is endogenous in 6 of the 10 programmes but we have retained the IV estimates for three of the other four programmes because they provide plausible results. The Hansen–Sargen test suggests that the selected instruments are valid, and the Kleibergen–Paap LM statistic suggests that they are relevant (i.e. correlated with the endogenous regressor). With the possible exception of the trauma and injuries programme, the Kleibergen–Paap F-statistic suggests that we do not have a problem with weak instruments. w Finally, the Pesaran–Taylor reset test statistics and the Ramsey reset F-statistics reveal no evidence of misspecification.
Expenditure models
The majority of the expenditure models contain the three variables: the PCT budget, a proxy for the own programme need for health care, and a proxy for the need for health care in other programmes. The budget term is positive in all 11 models and it is statistically significant in 8 of these 11 models.
The usual proxy for the own programme need for health care (i.e. the all service measure of need) is present in six of the models and it is significant in five of them. Its presence is supplemented with the addition of its squared value to improve model fit in the respiratory problems programme. In some programmes (e.g. the endocrine, metabolic and nutritional, and neurological),x we have replaced and/or supplemented the all service measure of need with a more programme specific measure (e.g. the diabetes prevalence rate and the epilepsy prevalence rate) and these measures of need have the anticipated positive impact on expenditure.
In addition, in a couple of other programmes we have used alternative proxies for the own programme need (e.g. with the use of the Department of Health’s measure of maternity need in the maternity/neonates expenditure equation). Full results for all programmes are presented in Appendix 2, Table 82; below is a summary of the findings.
For 8 of the 11 programmes we have used the all-cause mortality rate less own programme mortality rate as the proxy for the need for health care in other programmes, and the coefficient on this term is negative in seven programmes and statistically significant in six of the seven. In three programmes – maternity/neonates, GMS/PMS and trauma and injuries – we have used the all-cause mortality rate as the proxy for the need for health care in other programmes due to difficulties associated with the measurement of the own programme mortality rate. The coefficient on this term is not significant in any of the three models.
The relevant statistical test suggests that expenditure is endogenous in 6 of the 11 programmes but we have retained the IV estimates for two other programmes (GMS/PMS and trauma and injuries) because the IV estimator provides more plausible results. In the other three programmes we report OLS results.
The Hansen–Sargen test suggests that the selected instruments are valid, and the Kleibergen–Paap LM statistic suggests that they are relevant (i.e. correlated with the endogenous regressor). The Kleibergen–Paap F-statistic suggests that we do not have a problem with weak instruments. Finally, the Pesaran–Taylor reset test statistics and the Ramsey reset F-statistics reveal no evidence of model misspecification.
Calculation of the cost of a life and life-year
Expenditure and outcome elasticities for preferred models are used to calculate the cost of a life-year, both for individual programmes and for all programmes collectively. The relevant figures are summarised in Table 5. y The cost per YLG is £13,830 for the big four programmes and £28,983 for all 10 programmes with a mortality-based outcome indicator. These represent 30% and 45% increases on the respective costs for the previous year (i.e. using expenditure data for 2006/7 and mortality data for 2006/7/8).
If we assume that the other 13 programmes (all without a mortality-based outcome indicator) offer no health gain, then the cost per life-year across all PCT expenditure is £82,765. This is up from £73,457 using data for the previous year (an increase of 13%).
In addition, if we assume that PBC 23 generates a zero health gain and that the gain attributable to the remaining 12 programmes is, on average, the same as that attributable to those with a mortality outcome measure, then the cost of a life-year across all programmes is £31,846 (it was £22,565 using data for the previous year).
The next section presents outcome and expenditure models using programme budget data for 2008/9 and mortality data for 2008/9/10, and it explores the reasons for the increase in the cost of an additional life-year identified in this section.
2008/9 expenditure data and mortality data for 2008/9/10
Outcome and expenditure models were estimated using updated data for expenditure (from 2007/8 to 2008/9) and updated mortality data (from 2007/8/9 to 2008/9/10). Detailed results for the outcome model and expenditure model are shown in Appendix 2, Tables 85 and 86 respectively. First-stage regressions for these IV models can be found in Tables 99 and 100 in the Annex to Appendix 2.
Outcome models
The majority of the outcome models contain the two variables: own programme expenditure and a measure of the need for health care (the measure of need as employed by the Department of Health for resource allocation purposesz). For the respiratory disease programme we have added the square of the need measure to improve the model fit. In other PBCs (e.g. for the endocrine, metabolic and nutritional), we found that the all service measure of need performed poorly and we have replaced it with a more programme specific measure (e.g. the diabetes prevalence rate) or with a better performing proxy for need (e.g. the percentage of residents born outside the EU for maternity/neonate mortality). aa
The relevant statistical test suggests that expenditure is endogenous in 6 of the 10 programmes but we have retained the IV estimates for the other four because they provide plausible results. The Hansen–Sargen test suggests that the selected instruments are valid, and the Kleibergen–Paap LM statistic suggests that they are relevant (i.e. correlated with the endogenous regressor). The Kleibergen–Paap F-statistic suggests that we do not have a problem with weak instruments. Finally, the Pesaran–Taylor reset test statistics reveal no evidence of misspecification.
In all of the big four programmes the need for health-care variable has a positive and significant effect on mortality, and expenditure has the anticipated negative effect. As we have noted before, the outcome results for the other programmes are similar to, but more diverse than, those for the big four programmes. This is to be anticipated because mortality is a much rarer outcome in these programmes than it is in the big four programmes.
Expenditure has the anticipated negative effect on mortality in the endocrine problems programme and this is statistically significant. The all service measure of need is not relevant for this PBC; instead, we find that the diabetes prevalence rate is positively associated with mortality, as is a measure of deprivation (the IMD2007).
Expenditure has a negative but statistically insignificant impact on mortality from epilepsy in the neurological programme, and the all service indicator of the need for health care is positively and significantly associated with mortality in this programme.
Expenditure also has a negative but not statistically significant effect on mortality (from renal problems) in the genitourinary problems programme. The prevalence of lone parent households is positively associated with mortality.
Expenditure has the anticipated negative effect on mortality in the infectious disease programme and this is statistically significant. The all service measure of need is not relevant for this PBC; instead, we find that a measure of need associated with HIV is positively associated with mortality, as is a measure of deprivation (the IMD2007).
Expenditure has the anticipated negative effect on mortality in the maternity and neonates programme. In this PBC the coefficient on the generic all service measure of need is positive but not significant. It has been supplemented with two other indicators of deprivation – the proportion of residents born outside the EU and the proportion of those aged 16–74 years without any qualifications – and both of these are positively associated with mortality.
Finally, we were unable to develop a plausible outcome model for the trauma and injuries programme.
Expenditure models
The majority of expenditure models contain the three variables: the PCT budget, a proxy for the own programme need for health care, and a proxy for the need for health care in other programmes.
The budget term is positive and statistically significant in 10 of the 11 models.
The usual proxy for the own programme need for health care (i.e. the all service measure of need) is positive and significant in 5 of the 11 results. In a couple of programmes (respiratory disease and endocrine problems) we have added the squared value of need to improve the model fit and in both cases this term is positive and significant. In some programmes (e.g. the endocrine PBC and the neurological PBC), we have replaced and/or supplemented the all service measure of need with a more programme-specific measure (e.g. the diabetes and the epilepsy prevalence rates) and these usually have a positive and significant impact on expenditure. In addition, in a couple of programmes we have used alternative proxies for own programme need (e.g. with the use of the Department of Health’s measure of maternity need in the maternity/neonates expenditure equation and the use of HIV need in the infectious diseases programme). ab
For 8 of the 11 programmes we have used the all-cause mortality rate less the own programme mortality rate as the proxy for the need for health care in other programmes, and the coefficient on this term is negative in seven programmes and statistically significant in six of the seven. In three programmes – maternity/neonates, GMS/PMS and trauma and injuries – we have used the all-cause mortality rate as the proxy for the need for health care in other programmes due to difficulties associated with the measurement of the own programme mortality rate. The coefficient on this term is negative but not significant in these three models.
The relevant statistical test suggests that expenditure is endogenous in 5 of the 11 programmes but we have retained the IV estimates for two further programmes (endocrine problems and maternity/neonates) because the IV estimator provides more plausible results than the OLS estimator. In the other four programmes we report OLS results.
The Hansen–Sargen test suggests that the selected instruments are valid, and the Kleibergen–Paap LM statistic suggests that they are relevant (i.e. correlated with the endogenous regressor). The Kleibergen–Paap F-statistic suggests that we do not have a problem with weak instruments. Finally, the Pesaran–Taylor reset test statistics and the Ramsey reset F-statistics reveal no evidence of model misspecification.
Calculation of the cost of a life and life-year
Expenditure and outcome elasticities for our preferred models are used to calculate the cost of a life-year, both for individual programmes and for all programmes collectively. This results in the cost per YLG having increased slightly compared with using the previous expenditure and mortality data set (i.e. for 2007 and 2007/8/9 respectively): increasing from £13,830 to £14,650 for the big four programmes and from £28,983 to £30,883 for all 10 programmes with a mortality-based outcome indicator. If we assume that the other 13 programmes offer no health gain, then the cost per life-year across all PCT expenditure has increased from £82,765 in 2007/8 to £84,974 in 2008/9.
In addition, if we assume that PBC 23 generates a zero health gain and that the gain attributable to the remaining 12 programmes is, on average, the same as that attributable to those with a mortality outcome measure, then the cost of a life-year across all programmes in 2008/9 is £33,333. This is a 5% increase on the figure (£31,846) for the previous year.
Comparing the cost of life-year estimates associated with different data sets
Table 6 presents expenditure and outcome elasticities for the five combinations of expenditure and outcome data that have been used to estimate our model. It also reports the corresponding unadjusted cost of life-year estimates (i.e. estimates that are unadjusted for the mismatch in the ICD-10 coverage of the expenditure and mortality data). It is clear from this Table 6 (see row 13) that the (unadjusted) cost of a life-year for the 10 programmes with a mortality-based outcome indicator fluctuated around £22,000 for the first three sets of estimations (see columns M–O). However, using the two most recent sets of expenditure data (i.e. for 2007/8 and then for 2008/9), the figures in the table suggest that this cost has increased to about £38,000.
A | B | C | D | E | F | G | H | I | J | K | L | M | N | O | P | Q |
---|---|---|---|---|---|---|---|---|---|---|---|---|---|---|---|---|
PBC | Spend elasticities | Outcome elasticities | Cost of an additional life-year (£) (unadjusted for YLL coverage) | |||||||||||||
Using spend for 2005 and mortality for 2002/3/4 | Using spend for 2006 and mortality for 2004/5/6 | Using spend for 2006 and mortality for 2006/7/8 | Using spend for 2007 and mortality for 2007/8/9 | Using spend for 2008 and mortality for 2008/9/10 | Using spend for 2005 and mortality for 2002/3/4 | Using spend for 2006 and mortality for 2004/5/6 | Using spend for 2006 and mortality for 2006/7/8 | Using spend for 2007 and mortality for 2007/8/9 | Using spend for 2008 and mortality for 2008/9/10 | Using spend for 2005 and mortality for 2002/3/4 | Using spend for 2006 and mortality for 2004/5/6 | Using spend for 2006 and mortality for 2006/7/8 | Using spend for 2007 and mortality for 2007/8/9 | Using spend for 2008 and mortality for 2008/9/10 | ||
1 | Cancer | 0.968 | 0.548 | 0.465 | 0.890 | 0.525 | −0.394 | −0.337 | −0.342 | −0.365 | −0.307 | 13,741 | 16,518 | 16,383 | 17,165 | 21,802 |
2 | Circulatory problems | 0.682 | 0.701 | 0.540 | 0.293 | 0.648 | −1.370 | −1.447 | −1.434 | −1.277 | −1.319 | 8328 | 8725 | 9466 | 11,315 | 11,779 |
3 | Respiratory problems | 0.849 | 0.718 | 0.679 | 0.536 | 0.652 | −1.574 | −3.507 | −2.622 | −2.205 | −1.808 | 20,601 | 8747 | 11,593 | 14,798 | 21,307 |
4 | Gastrointestinal problems | 0.772 | 0.667 | 0.446 | 0.622 | 0.456 | −2.018 | −2.137 | −1.536 | −1.328 | −1.364 | 18,303 | 15,795 | 20,892 | 25,034 | 25,662 |
5 | All big four PBCs | 0.801 | 0.660 | 0.528 | 0.559 | 0.579 | −0.941 | −1.083 | −0.965 | −0.872 | −0.825 | 12,855 | 10,783 | 12,333 | 16,345 | 16,688 |
6 | Infectious diseases | 0.742 | 0.731 | 0.792 | 1.436 | 1.545 | −0.152 | −0.030 | −0.047 | −0.548 | −0.504 | 215,054 | 1,036,377 | 630,798 | 57,742 | 71,432 |
7 | Endocrine problems | 0.425 | 0.966 | 0.953 | 0.264 | 0.484 | −0.244 | −0.812 | −0.842 | −0.566 | −1.170 | 371,601 | 112,882 | 114,416 | 190,745 | 104,008 |
8 | Neurological problems | 1.111 | 0.648 | 0.616 | 1.035 | 0.98 | −0.182 | −0.098 | −0.112 | −0.339 | −0.417 | 503,201 | 1,241,253 | 1,129,960 | 431,749 | 388,267 |
9 | Genitourinary problems | 1.041 | 0.837 | 0.912 | 1.004 | 0.697 | −0.034 | −0.073 | −0.051 | −1.855 | −1.615 | 29,144,918 | 12,384,965 | 20,421,090 | 652,096 | 877,038 |
10 | Trauma and injuries | 0.627 | 0.617 | 0.358 | 1.686 | 1.344 | −1.332 | −0.527 | 0 | −0.369 | 0 | 282,132 | 548,767 | N/A | 1,115,197 | N/A |
11 | Maternity and neonates | 0.388 | 0.601 | 0.224 | 0.514 | 0.975 | −0.237 | −0.035 | −0.482 | −0.110 | −0.125 | 17,490 | 631,700 | 45,158 | 204,168 | 198,939 |
12 | All small six PBCs | 0.780 | 0.717 | 0.596 | 0.961 | 0.962 | −0.262 | −0.122 | −0.392 | −0.254 | −0.300 | 295,074 | 449,706 | 258,046 | 274,309 | 254,794 |
13 | All 10 PBCs with mortality | 0.792 | 0.687 | 0.561 | 0.749 | 0.762 | −0.844 | −0.940 | −0.877 | −0.778 | −0.747 | 21,256 | 20,893 | 23,780 | 38,110 | 38,328 |
14 | All 23 PBCs assuming zero gain in PBCs without mortality indicator | 56,799 | 62,718 | 87,494 | 108,829 | 105,460 | ||||||||||
15 | GMS/PMS | 0.926 | 0.759 | 0.739 | 0.563 | 0.494 | N/A | N/A | N/A | N/A | N/A | N/A | N/A | N/A | N/A | N/A |
16 | All 23 PBCs assuming zero gain in PBC 23 but average gain in other PBCs without a mortality indicator | 24,200 | 23,697 | 26,876 | 41,875 | 41,369 |
What are the proximate causes of this increase? Recall that the cost of a life-year is calculated as:
For 2006/7 (using mortality data for 2006/7/8) and for the 10 programmes with a mortality-based outcome indicator, the change in expenditure associated with a 1% budget increase is £184.53M and the change in the number of life-years lost associated with this increase is 7760 (see Appendix 2, Table 67 for the calculation of these figures). Thus the cost of a life-year is £23,780 (= £184.53M/7760).
For 2007/8 (using mortality data for 2007/8/9) and for the 10 programmes with a mortality-based outcome indicator, the change in expenditure associated with a 1% budget increase is £257.94M and the change in the number of life-years lost associated with this increase is 6768 (see Appendix 2, Table 83 for the calculation of these figures). Thus the cost of a life-year is £38,110 (= £257.94M/6768).
It is clear that the 60% increase in the cost of a life-year between 2006/7 and 2007/8 is largely attributable to (a) the 40% increase in the additional expenditure (up from £184.53M to £257.94M) directed towards these 10 programmes following a 1% budget increase; and (b) the 12% decline in the number of life-years saved by this increase in expenditure (down from 7760 to 6768 life-years).
The rise in the share of the budget increase directed towards these programmes can be attributed to the increase in the implied expenditure elasticity associated with these 10 programmes (up from 0.561 to 0.749). The decrease in the number of years of life saved appears to be due to (a) an overall reduction in the (absolute) size of the outcome elasticities; and (b) a shift in the additional expenditure towards those programmes with a relatively high cost of a life-year. For example, the cost of a life-year for the ‘small six’ programmes is much larger than for the ‘big four PBCs’. However, in 2007/8 the spend elasticity for the small six increases from 0.561 to 0.961 (71%) whereas the expenditure elasticity for the big four rises from 0.528 to 0.559 (6%). A similar pattern – of additional expenditure shifting away from the low cost PBCs – can be seen within the big four programmes. However, it is not clear why such rather dramatic changes should have taken place.
If we correct the cost of life-year estimates adjusting for the mismatch in the ICD-10 coverage of the expenditure and mortality data, these reveal similar increases in the cost of a life-year between 2006/7 on the one hand and 2007/8 and 2008/9 on the other. The cost of a life-year increased from £19,965 in 2006/7 to £28,983 in 2007/8 for the 10 programmes with mortality rate, an increase of 45%; and it increased from £22,565 to £31,846 for all programmes if we assume a zero health gain in PBC 23 and the same gain in the other 12 programmes as in the 10 with a mortality rate (an increase of 41%).
A potential reason for this apparent step change in the cost of a life-year is the adjustment that was made to the methodology for the collection of the 2007/8 PB data. In previous years expenditure that was not directly attributable to a particular programme category was apportioned using admitted patient care percentages. ac In other words, if x% of total admitted patient care expenditure was allocated to PBC 1, then x% of all expenditure that was not directly attributable to a particular programme category was also allocated to PBC 1. With effect from 2007/8, however, NHS organisations were asked to select an appropriate basis for the apportionment of this non-programme-specific expenditure and that, where no reasonable basis existed, such expenditure was to be allocated to the ‘other – miscellaneous’ (PBC 23X) category.
The Department of Health estimates that this allocation rule change increased the amount of expenditure attributed to PBC 23X by £700M. It will also, of course, have reduced expenditure across other programmes by the same amount in total. However, not all programmes will have been equally affected; PBCs that are more heavily inpatient based would have ‘lost’ expenditure whereas others, such as learning disabilities, social care and mental health, will have ‘lost’ considerably less. In addition, not all PCTs will have been equally affected because each will have employed different apportionment rules for the non-programme-specific expenditure (Bryn Shorney, Department of Health, 2012, personal communication).
Although this allocation rule change has considerably increased the estimated cost of a life-year, we believe that this rule change has led to a more accurate allocation of expenditure across PBCs, and that the more recent estimates of the cost of a life-year (for 2007/8 and 2008/9) are more accurate than those for the earlier years (for 2005/6 and 2006/7).
Adjusting the cost of a life-year estimates to constant prices
The estimates of the cost of a life-year presented above are all at current prices. To put them on a constant price basis, we need an index of pay and price inflation for the labour and goods/services purchased by the NHS. Curtis87 reports a pay and prices index for HCHS and this implies an inflation rate of 3.7% in 2006/7, 2.9% in 2007/8 and 3.9% in 2008/9. ad If we assume that similar inflation rates also apply to the purchase of pharmaceuticals and the provision of primary care (items that are excluded from the HCHS index), then we can use these figures to put the estimates of the cost of a life-year on a constant price basis.
For example, if we assume that PBC 23 generates a zero health gain and that the gain attributable to the 12 programmes without a mortality indicator is, on average, the same as that attributable to those with a mortality outcome measure, then the cost of a life-year across all programmes in 2008/9 is £33,333 at current (2008/9) prices. The cost for 2007/8 is £31,846 at current (2007/8) prices or £33,088 at constant (2008/9) prices, and the figure for 2006/7 is £22,565 at current (2006/7) prices or £24,125 at constant (2008/9) prices. The conversion of the costs from a current to constant price basis has relatively little impact because the inflation rate over the relevant period is quite small.
Summary and concluding remarks
The findings presented in this report build on four previous studies. These studies and the results presented here draw on the availability of two new data sets to obtain empirical estimates of the relationship between mortality and expenditure across all English local health authorities.
In this research we have extended the previous studies in several ways. First, we have derived plausible outcome and expenditure models for a larger number of programmes (10) than previous studies.
Second, we relate expenditure in time period t to mortality in that period (t) and in the next two periods (t + 1 and t + 2). In other words, we assume that the health benefits associated with expenditure occur either in the same period as the expenditure or in the next two periods. This is an improvement on past practice where data constraints forced researchers to relate expenditure to the current and two previous periods. ae When we re-estimated our models using expenditure data for 2006/7 and mortality data for 2006/7/8, we found that the cost of a life-year across the 10 programmes with a mortality-based outcome indicator is £23,780 (up from £20,893 when expenditure data for 2006/7 is combined with mortality data for 2004/5/6; an increase of 14%).
Third, we have noted the mismatch in the ICD-10 coverage of the expenditure and mortality data. If we adjust the calculation of the cost of a life-year for 2006/7 for this mismatch then the cost of a life-year across the 10 programmes with a mortality-based outcome indicator declines from £23,780 to £19,965 (a decrease of 16%).
Fourth, previous estimates of the cost of a life-year have been for individual programmes of care. In this report we have presented estimates of the cost of a life-year for an enlarged number of programmes and, with the aid of assumptions about the productivity (health gain) of programmes without a meaningful mortality-based outcome indicator, we have extended our individual programme estimates to incorporate expenditure across all programmes of care. Thus for 2006/7, the cost of a life-year for those PBCs with a mortality-based outcome indicator is £19,965. If we assume (a) that the health gains associated with PBC23, which includes primary care and workforce training expenditure, are reflected in the mortality rates for disease-specific programmes and (b) that the average health gain across the other programmes without a mortality-based outcome indicator is the same as that for those PBCs with a mortality-based outcome indicator, then the cost of life-year across all programmes is £22,565.
Fifth, we have extended our cost of life-year estimates beyond 2006/7. Re-estimation of our model using budgeting expenditure for 2007/8 generates an all programme cost of a life-year estimate of £31,846, and re-estimation of our model using budgeting expenditure for 2008/9 generates a similar cost of a life-year estimate (£33,333). Together, the last two estimates suggest that there has been step change in the cost of a life-year, and that this appears to have occurred between 2006/7 and 2007/8. The cost of a life-year estimates are very similar up to and including 2006/7, and they are very similar for 2007/8 and 2008/9. However, there is a substantial difference between the figures for 2004/5, 2005/6 and 2006/7 on the one hand (at about £22,000), and for 2007/8 and 2008/9 on the other (at about £33,000). The reason for this step change is not obvious but it might be due to changes in the algorithm used by the Department of Health to allocate non-admitted patient care activity to budget categories. Although this allocation rule change has considerably increased the estimated cost of a life-year, we believe that this rule change has led to a more accurate allocation of expenditure across PBCs, and that the more recent estimates of the cost of a life-year (for 2007/8 and 2008/9) are more accurate than those for the earlier years (for 2005/6 and 2006/7). A summary of the estimates of the cost of a life-year adjusted for the mismatch between ICD-10 chapters for expenditure and mortality are provided in Table 7.
A | B | C | D | E |
---|---|---|---|---|
PBC | Cost per life-year (£) (adjusted for ICD-10 coverage of spend and mortality data) | |||
2006/7 | 2007/8 | 2008/9 | ||
1 | Cancer | 16,121 | 16,891 | 21,454 |
2 | Circulatory disease | 9390 | 11,224 | 11,685 |
3 | Respiratory problems | 8961 | 11,439 | 16,470 |
4 | Gastrointestinal problems | 11,929 | 14,295 | 14,653 |
5 | All big four programmes | 10,604 | 13,830 | 14,650 |
6 | Other six programmes with a mortality rate | 146,108 | 99,428 | 112,674 |
7 | All 10 PBCs with a mortality rate | 19,965 | 28,983 | 30,883 |
(a) If we assume a zero health gain in those PBCs without a mortality rate . . . | ||||
8 | All 23 programmes | 73,457 | 82,765 | 84,974 |
. . . or (b) if we assume a zero gain in PBC 23 and that the average gain from the | ||||
the 10 PBCs with a mortality rate is applied to the remaining programmes | ||||
9 | All 23 programmes | 22,565 | 31,846 | 33,333 |
Virtually all of the cost of a life-year estimates presented in this report are calculated at current prices. However, it is possible to put them on a constant price basis using the HCHS pay and prices index. 87 For 2006/7, 2007/8 and 2008/9 this index recorded an annual rate of inflation of about 3.5% and so the impact of this constant price adjustment is fairly minimal. For example, if we assume that PBC 23 generates a zero health gain and that the gain attributable to the 12 programmes without a mortality indicator is, on average, the same as that attributable to those with a mortality outcome measure, then the cost of a life-year across all programmes at constant 2008/9 prices is £33,333 for 2008/9, £33,088 for 2007/8, and £24,125 for 2006/7.
Finally, although previous results and our current models ‘pass’ the appropriate statistical tests and, in particular, the Hansen–Sargen test for valid instruments, we are aware that this test might be unable to detect the presence of invalid instruments in some circumstances and that the validity of IVs is often open to question. Responding to this, several studies88,89 have suggested that researchers using IV techniques should subject the estimated coefficient on the endogenous variable to a sensitivity analysis. We undertake a comprehensive sensitivity analysis for the outcome equation for each of the big four models. This sensitivity analysis reveals that uncertainty associated with instrument validity has little effect on our estimate of the cost of a life-year but it does increase the degree of uncertainty associated with this estimate.
We recognise that this study has a number of limitations. The estimates of the cost of an additional life-year for programmes with a mortality-based outcome indicator are unadjusted for the QoL during the additional year. Accordingly, the quoted costs will be an underestimate of the QALY cost of a life-year to the extent that additional life-years are not in perfect health. In previous studies we have noted that a rudimentary adjustment for this issue using Health Outcomes Data Repository (HODaR) data increased the cost of a life-year by about 50–60%. 60,63
At the same time, however, the estimated costs will exaggerate the cost of an additional QALY for those programmes with a mortality-based outcome indicator because they ignore any health benefits that are not associated with a reduction in mortality. In other words, expenditure that improves the QoL (e.g. cancer palliative care) but which does not extend the length of life is implicitly given a zero health gain value.
In addition, the expenditure data relate to expenditure on all patients whereas the mortality data are based on a LE of 75 years. Thus implicitly our calculations attribute a zero health gain to all expenditure on those aged over 75 years. To illustrate the magnitude of the potential health gain ignored by this restriction, note that in a recent study of costs associated with all inpatient and outpatient activity (excluding mental health), those aged over 75 years accounted for 25% of all costs in 2007/8. 86
The results presented in this study are all from the estimation of the relationship between expenditure and mortality using data for a single time period. With the availability of several years of data for both expenditure and mortality, we wanted to estimate a panel data model because a panel can offer advantages over a one period model (e.g. it is better able to handle any unobserved heterogeneity across PCTs). However, most of the instruments employed here are based on the 2001 Census80 and, thus, time invariant, rendering them of little use in panel data modelling.
Chapter 4 Translating mortality effects into life-years and quality-adjusted life-years
Introduction
This chapter presents an overview of how the results of the econometric work undertaken to estimate the link between NHS spending and mortality, which was summarised in the previous chapter and detailed in Appendix 2, can be translated into effects on life-years and QALYs.
In this chapter we present three sequential steps of analysis which lead to estimates of the overall cost per QALY threshold for the NHS:
-
In From mortality to life-years we reconsider how the estimated effects on mortality from the econometrics work conducted in Chapter 3 might better translate in to life-years by exploring the limitations of mortality data available at PCT level and the published YLL figures presented. We explore how these estimates might be improved using additional data and analysis.
-
In Adjusting life-years for quality-of-life we consider how these estimates of life-year effects might be adjusted for the QoL in which they are lived, taking account of the gender and the age at which life-years are gained or lost as well as the disutility associated with particular diseases.
-
In Including quality-of-life effects during disease we explore ways to also take account of those effects on health not directly associated with mortality and life-year affects (i.e. the ‘pure’ QoL effects) to estimate an overall cost per QALY threshold.
This sequence of analysis is set out and explained based on the analysis of 2006 expenditure and mortality data from 2006 to 2008. In Chapter 5, Re-estimating the cost per quality-adjusted life-year threshold using more recent data we present estimates for 2008 expenditure and 2008–10 mortality data using the same methods and discuss the uncertainties associated with these estimates. As in the previous chapter much of the detail of data and analysis that supports this overview is presented in an appendix (see Appendix 3). At the end of each section we present a summary which includes a central ‘best’ estimate as well as extreme lower and upper bounds for the cost per life-year and cost per QALY threshold.
The core assumptions which underpin these three values are common across the above mentioned sections. The central or ‘best’ estimate is based on two assumptions: one conservative and the other more optimistic with respect to the health effects associated with expenditure. The first is that the health effects of changes in 1 year of expenditure are restricted to 1 year. This is implicit in the estimates of outcome elasticities presented in the previous chapter. a This is likely to underestimate effects on mortality as expenditure that reduces mortality risk for an individual in 1 year may well also reduce their risk over subsequent years; possibly over the whole of their remaining disease duration. Expenditure may also prevent disease in future patient populations. Therefore, total health effects will be underestimated and the cost per life-year or QALY threshold will be overestimated. Although undoubtedly conservative, it may be offset to some extent by the more optimistic assumption used to translate mortality effects into life-years. Any death averted by expenditure in 1 year is assumed to return the individual to the mortality risk of the general population, i.e. the YLG associated with each death averted are based on what would have been their LE taking account of their of age and gender (using life tables for the general population).
The extreme upper and lower bounds for cost per life-year and cost per QALY thresholds are based on making both assumptions either optimistic (providing the lower bound for the threshold) or both conservative (an upper bound for the threshold). The lower bound is based on assuming that health effects are not restricted to 1 year but apply to the remaining disease duration for the population at risk during the expenditure year (although this still does not account for the effects of expenditure on preventing disease). The upper bound is based on the combination of assuming that health effects are restricted to 1 year and that any death averted is only averted for the minimum duration consistent with the mortality data used to estimate the outcome elasticities in Chapter 3 (see Summary of cost per life-year estimates for a more detailed discussion). It is very important to note that the lower and upper bounds represent extreme values rather than alternative but plausible views that could reasonably be taken. We discuss this in more detail in Chapter 5, Future research and improving estimates and explain why establishing narrower bounds, which might retain some plausibility, has not been possible given the data available and therefore the analysis that has been feasible.
From mortality to life-years
In this section we summarise our examination of a number of issues associated with available PCT-based mortality data and the associated published estimates of YLL. We then examine how, given the limited information available about the population at risk in each PBC, we might take proper account of the fact that some of the observed deaths would have occurred anyway (had the same population not been at risk in the particular PBC) when estimating YLL (i.e. taking account of unobserved counterfactual deaths). This allows us to estimate the YLL that better reflects the effect of expenditure on the mortality observed in each PBC, and infer the excess deaths associated with each PBC. Finally we present cost per death averted and cost per life-year which accounts for the issues raised in this section.
Mortality and years of life lost coverage
The mortality data that is available at PCT level does not offer full coverage of all deaths across all the ICD-10 codes that make up each PBC (see Table 37 in Appendix 2 for how three-digit ICD-10 codes are mapped to PBCs). However, national (English) data are available that covers all deaths associated with all the ICD-10 codes that make up each PBC. Therefore, it is possible to adjust the incomplete reporting of mortality at PCT level (see Chapter 3, Previous studies) before applying the estimated outcome elasticities to calculate the deaths averted due to expenditure. b Applying published estimates of YLL per death to all the deaths averted provides the estimate of the cost per life-year reported in Chapter 3.
The published estimates of YLL (National Health Service Information Centre; NHS IC) used in Chapter 3 only include deaths below the age of 75 years (but exclude deaths below age 1 year) and are based on the difference between age 75 years and the age of each death below 75 years. These estimates have the same limited coverage as PCT-level mortality data so are not available for all the ICD-10 codes that make up each PBC. Therefore, applying the available estimates of YLL per death to the estimated number of deaths averted requires an assumption that the YLL per death is similar for those groups of ICD-10 codes covered and not covered by the published YLL figures.
This can be examined by using national Office for National Statistics (ONS) data to calculate YLL in the same way as NHS IC, but with full coverage of all the ICD-10 codes that make up each PBC. c Although ONS data provides complete coverage and reports gender, age at death is only reported in 5-year ranges (these data are not available at PCT level so could not be used when estimating outcome elasticities in Chapter 3). Therefore, using ONS data to estimate YLL requires taking the mid-point of each range as the age of death, i.e. assuming reported deaths are equally likely over the range in which they are reported. For this reason it is not possible to precisely recover the published YLL figures using ONS data for those ICD groupings that can be precisely matched to the NHS IC coverage. However, the differences are small (see Appendix 3, Table 102), suggesting that taking the mid-point of each range as the age of death may be a reasonable approximation.
The differences between estimates of YLL based on ONS and NHS IC data are, however, much more significant and are reported in Table 8. These reflect differences in the distribution of ages at death between those groups of ICD-10 codes covered and not covered in the NHS IC figures. For example, NHS IC figures available at PCT level for PBC 7 (neurological problems) have low coverage of all deaths in this PBC [0.14 in column (1)]. The deaths that are reported in NHS IC are associated with epilepsy and the YLL [22,046 in column (2)] reflects the generally younger age at death in this group. When adjusted for full coverage [22,046/0.14 = 162,100 in column (3)] the estimated YLL is much greater than the YLL based directly on all deaths by age group reported in ONS. This difference in YLL reflects the fact that the deaths in PBC 7 which are not covered by NHS IC figures tend to be in older age groups so generate fewer YLL.
PBC | (1) Coverage of mortality data relative to spend data | (2) YLL < 75 years (NHS IC) | (3) YLL < 75 years, adjusted (NHS IC) | (4) YLL < 75 years, no adjustment needed (ONS) | (5) Difference from adjusted NHS IC to ONS (%) | |
---|---|---|---|---|---|---|
1 | Infectious diseases | 1.00 | 35,517 | 35,517 | 40,928 | 15 |
2 | Cancer | 0.98 | 735,674 | 747,636 | 758,804 | 1 |
4 | Endocrine problems | 0.63 | 19,224 | 30,322 | 41,548 | 37 |
7 | Neurological problems | 0.14 | 22,046 | 162,100 | 93,755 | −42 |
10 | Circulatory | 0.99 | 453,878 | 457,538 | 481,246 | 5 |
11 | Respiratory | 0.77 | 108,074 | 139,812 | 147,465 | 6 |
13 | Gastrointestinal | 0.57 | 115,303 | 201,931 | 177,532 | −12 |
17 | Genitourinary | 0.17 | 3343 | 19,438 | 17,380 | −11 |
18 + 19 | Maternity and neonates | 0.68 | 164,200 | 241,826 | 15,409 | −94 |
Using ONS data also allows deaths under the age of 1 year to be appropriately assigned to PBCs via the ICD-10 code in which they occurred (NHS IC YLL figures exclude deaths under 1 year), rather than assigning them all to PBC 18 + 19 as in the previous chapter. d This explains the large reduction in YLL for PBC 18 + 19 (maternity and neonates) as much of the mortality is reassigned to ICD-10 codes which contribute to other PBCs. As most of the deaths that are reassigned are allocated to PBC 1 (infectious diseases) the YLL for this PBC increases despite complete reporting of deaths at PCT level and full coverage by NHS IC figures (see also Table 104 in Appendix 3).
Life expectancy and years of life lost
As noted above, the NHS IC estimates of YLL only include deaths below age 75 years and are based on the difference between age 75 years and the age of each death below 75 years. Implicitly this treats 75 years as the appropriate normal LE for males and females for the population at risk in each PBC. However, with the exception of maternity and neonates most deaths in PBCs occur above the age of 75 years and LEs are significantly > 75 years. For example, based on 2006–8 data, LE for the general population is 80.7 years for males and 84.4 years for females (considering age distribution) and even LE at birth is > 75 years (77.74 years for males and 81.88 years for females). 90
Based on ONS data YLL can be recalculated using gender-specific LE for the general population. e When increasing LE two effects occur, both of which tend to increase estimates of YLL. First, more deaths are included in the YLL calculation (those that occur between age 75 years and LE) and second, each death previously counted below 75 years will generate 5.7 or 9.4 more YLL for males and females respectively. The effect on the number of deaths and the YLL for each PBC of using the LE of the general population is reported in Table 9 [see columns (1), (2) and (3)].
PBC | (1) Deaths < 75 years (ONS) | (2) Deaths < LE (ONS) | (3) Difference in deaths due to increased LE (%) | (4) YLL < 75 years (ONS) | (5) YLL < LE (ONS) | (6) Difference in YLL due to increased LE (%) | |
---|---|---|---|---|---|---|---|
1 | Infectious diseases | 2050 | 3710 | 81 | 40,928 | 62,051 | 52 |
2 | Cancer | 62,944 | 95,212 | 51 | 758,804 | 1,345,013 | 77 |
4 | Endocrine | 2367 | 4000 | 69 | 41,548 | 65,015 | 56 |
7 | Neurological | 5095 | 8975 | 76 | 93,755 | 145,526 | 55 |
10 | Circulatory | 41,487 | 82,098 | 98 | 481,246 | 916,170 | 90 |
11 | Respiratory | 14,000 | 30,500 | 118 | 147,465 | 310,326 | 110 |
13 | Gastrointestinal | 10,611 | 15,827 | 49 | 177,532 | 273,303 | 54 |
17 | Genitourinary | 1588 | 4197 | 164 | 17,380 | 39,098 | 125 |
18 + 19 | Maternity and neonates | 226 | 226 | 0 | 15,409 | 17,167 | 11 |
The number of deaths counted below LE increases for every PBC except for maternity and neonates because, as expected, all deaths are below age 75 years in PBC 18 + 19. However, YLL increases for all PBCs reflecting the additional years otherwise expected to be lived to an older LE. Of course including more of the deaths observed in each PBC and the greater YLL associated with them will generate more deaths averted and more YLGs when applying the same proportionate effects from the outcome elasticities estimated in Chapter 3. Therefore, the cost per death averted and cost per life-year threshold are lower using these figures than those reported in Chapter 3 (see Table 13 and Table 107 in Appendix 3 for a summary of the effects on the thresholds). However, there are good reasons why YLL figures calculated as the difference between age of death and LE are likely to be overestimated. This is dealt with in the next section (see Years of life lost and accounting for counterfactual deaths). In Inferring excess deaths we take account of the fact that some of the deaths observed in a PBC would have occurred anyway in a similar ‘normal’ population (i.e. the counterfactual population not at risk through membership of the PBC) so not all observed deaths are ‘excess’ and generate YLL.
Years of life lost and accounting for counterfactual deaths
The estimates of YLL based on ONS data overcome many of the limitations of the published NHS IC figures. However, the YLLs reported in Tables 8 and 9, are calculated in the same way as the NHS IC figures, by taking the difference between a fixed LE and the age at death of deaths observed below that LE. This will tend to overestimate the YLL for two reasons: (1) it does not account for the fact that not all deaths observed below LE are ‘excess’ deaths in the sense that some deaths would have occurred (at the same age) in a similar population not at risk in the PBC; and (2) some of the deaths observed above LE may be ‘excess’ deaths that would not otherwise have occurred at that age. The overall effect on YLL, and the cost per life-year, will depend on the number of deaths above and below LE that are excess. Therefore, estimates of YLL are required which take account of the ‘counterfactual’ deaths that would have occurred even if the population in the PBC was not at risk through membership of the ICD-10 codes that make it up, but faced the same mortality risks as the general population, accounting for the age and gender distribution of the PBC population.
Ideally, with reliable information about the size of the population at risk in each PBC and its age and gender distribution it would be possible to estimate the number of deaths that would be expected to occur had this population not been at risk, based on mortality data for the general population. The difference between deaths observed across all ages and the deaths expected to have occurred in this matched ‘normal’ population would provide the number of ‘excess’ deaths by age and gender. f The YLL associated with each of these excess deaths is the LE conditional on gender and on surviving to the age at which the excess death occurred. The total YLL for the at risk population is simply the sum of these YLLs over all excess deaths, which could occur at any age. This YLL is equivalent to the area between the survival curve for the population at risk in a PBC and the counterfactual survival curve for the same population but not at risk from membership of the PBC. The difficultly is that routinely available data do not provide any information about the size of the population at risk or its age and gender distribution. All that is routinely available are observed deaths (by age and gender). Therefore, it is not possible to directly estimate excess deaths or compare survival curves.
Even if the size of the at risk population is unknown we can still use information that might be available about its age and gender distribution (or make reasonable assumptions) to estimate a matched ‘normal’ LE using life tables for the general population (such a LE summarises the area under the counterfactual survival curve). Unfortunately, it is not possible to also calculate the LE for the population at risk in the PBC (or represent the survival curve) without information about the size of the at risk population (if it was possible the difference between these life expectancies would approximate the YLL per patient at risk in a PBC).
Fortunately, we can still recover a consistent estimate of YLL using observed deaths and a LE that represents the normal LE of a matched population that is not at risk. This requires all observed deaths, both those that occur below and those that occur above this LE to be taken into account. Those deaths occurring below LE generate YLL – compared with the average of a matched population not at risk. However, we must also account for those deaths that occur at ages above LE. These deaths generate YLGs compared with the average of a matched population not at risk. Therefore, the appropriate estimate is a net YLL (i.e. YLL – YLG). In effect, by subtracting YLG from YLL we take account of the fact that not all deaths below LE are excess deaths but some deaths above LE are (see Appendix 3 for more formal explanation of the equivalence of these ways of calculating YLL). g
Using the life expectancy of the general population
Routinely available data provides the age and gender of observed deaths but no information about the age and gender distribution of the at risk population itself. Using observed age and gender at death as an indication of the distribution of the at risk population will significantly overestimate the LE of a normal matched population insofar as a disease may be chronic (not all PBC mortality occurs on entry into the at risk population), and that PBC-related mortality risk may increase with age (see Appendix 3, Table 114). h
In the absence of additional external information the net YLL could be based on the LE of the general population, reflecting its current age and gender distribution. These are reported in Table 10 and illustrate the impact of accounting for counterfactual deaths in the way described above. The YLL reported in column (5) of Table 10 are calculated the same way and are the same as the figures previously reported [column (5) of Table 9]. That is, they do not account for deaths that would have otherwise occurred below LE or the very many deaths that occur above LE. With the exception of PBC 18 + 19 many deaths occur above the LE of the general population [see column (4) in Table 10] in all PBCs. As a consequence there are YLG associated with all other PBCs [see Table 10, column (6)] so the net YLL in column (7) are lower than YLL based on the same LE. Therefore, failure to account for counterfactual deaths would lead to an overestimate of the YLL associated with a PBC and the effects of expenditure on YLL. Consequently, the cost per life-year threshold would be underestimated (see Table 13).
PBC | (1) LE of males (years) | (2) LE of females (years) | Average 2006–8 | |||||
---|---|---|---|---|---|---|---|---|
(3) Deaths < LE | (4) Deaths > LE | (5) YLL | (6) YLG | (7) Net YLL | ||||
1 | Infectious diseases | 80.7 | 84.4 | 3710 | 3248 | 62,052 | 18,796 | 43,256 |
2 | Cancer | 80.7 | 84.4 | 95,213 | 35,597 | 1,345,038 | 175,350 | 1,169,689 |
4 | Endocrine | 80.7 | 84.4 | 4000 | 2764 | 65,016 | 15,864 | 49,152 |
7 | Neurological | 80.7 | 84.4 | 8975 | 6378 | 145,529 | 34,621 | 110,908 |
10 | Circulatory | 80.7 | 84.4 | 82,099 | 77,752 | 916,192 | 444,694 | 471,498 |
11 | Respiratory | 80.7 | 84.4 | 30,500 | 34,945 | 310,334 | 215,829 | 94,505 |
13 | Gastrointestinal | 80.7 | 84.4 | 15,827 | 8320 | 273,308 | 45,295 | 228,012 |
17 | Genitourinary | 80.7 | 84.4 | 4198 | 6427 | 39,099 | 40,530 | –1431 |
18 + 19 | Maternity and neonates | 80.7 | 84.4 | 226 | 0 | 17,167 | 0 | 17,167 |
However, these figures are only correct insofar as the distribution of age and gender in each PBC is similar to the general population. For example, if the at risk population tends to be younger the correct LE for the PBC will be lower and the net YLL will also tend to be lower. Similarly, if the at risk population tends to be older than the general population the correct LE will be higher and net YLL will also tend to be higher. i This explains the apparent net gain in YLL (negative net YLL) for PBC 17 (genitourinary) where most deaths occur at ages greater than the LE of the general population so that YLG exceeds YLL. As we are able to show later (see Table 11) this is because the age distribution in this PBC tends to be older than the general population, that is the LE for a matched normal population should be higher with fewer deaths above and more below this LE.
Using additional information about age and gender distribution
It is evident that estimates of YLL require some account to be taken of counterfactual deaths. In the absence of routinely available information this requires examination of alternative sources of information which might provide a basis for more credible assumptions about the age and gender distribution of the PBC population than either, the distribution of observed deaths or the general population. j The World Health Organization (WHO) Global Burden of Disease (GBD) study, updated in 2008 using 2004 data (see Addendum 1: data sources in Appendix 3 for more details),k provides a range of summary health indicators for the UK, which are, in part, based on estimates of the incidence of sequelae associated with different types of disease by age and gender. l Therefore, the type of information used by WHO in the GBD study to generate summary estimates for the UK can also be used to improve the assumptions required about the age and gender distribution of the PBC populations. Importantly, at this stage, we do not need to rely on estimates of the absolute size of the at risk population, but only the relative ‘share’ by age and gender.
The Global Burden of Disease study classifies diseases by U-codes, which are groups of three digit ICD-10 codes (see Addendum 1: data sources in Appendix 3 for details of how U-codes map to ICD-10 codes). m As we know which ICD-10 codes contribute to each PBC we can map information from U-codes to PBCs via the ICD-10 codes that contribute to each. The resulting average age and LE for each PBC is reported in columns (3) and (4) of Table 11 using the information available from GBD in combination with life tables for the general population.
PBC | Sex | (1) Average age of general population (years) | (2) LE of general population (years) | (3) Average age in PBC (years) (GBD) | (4) LE of at risk population (years) (GBD) | |
---|---|---|---|---|---|---|
1 | Infectious diseases | M | 38.5 | 80.7 | 28.6 | 79.6 |
F | 40.8 | 84.4 | 30.2 | 83.6 | ||
2 | Cancer | M | 38.5 | 80.7 | 61.3 | 83.0 |
F | 40.8 | 84.4 | 52.3 | 84.7 | ||
4 | Endocrine | M | 38.5 | 80.7 | 44.2 | 81.0 |
F | 40.8 | 84.4 | 50.8 | 84.7 | ||
7 | Neurological | M | 38.5 | 80.7 | 24.8 | 79.6 |
F | 40.8 | 84.4 | 23.5 | 83.3 | ||
10 | Circulatory | M | 38.5 | 80.7 | 55.4 | 83.0 |
F | 40.8 | 84.4 | 57.9 | 86.5 | ||
11 | Respiratory | M | 38.5 | 80.7 | 32.1 | 80.3 |
F | 40.8 | 84.4 | 33.7 | 84.0 | ||
13 | Gastrointestinal | M | 38.5 | 80.7 | 35.8 | 80.6 |
F | 40.8 | 84.4 | 41.9 | 84.5 | ||
17 | Genitourinary | M | 38.5 | 80.7 | 63.2 | 83.5 |
F | 40.8 | 84.4 | 47.3 | 85.6 | ||
18 + 19 | Maternity and neonates | M | 38.5 | 80.7 | 3.0 | 78.7 |
F | 40.8 | 84.4 | 24.1 | 83.1 |
These summary estimates suggest that some of the PBC populations may on average be older than the general population (e.g. cancer, circulatory and genitourinary) or younger (e.g. maternity and neonates, infectious diseases and neurological). However, when trying to interpret these summaries it should be noted that the average age reported in Table 11 is the average over the ages at which sequelae occur within the ICD-10 codes contributing to the PBC. Therefore, a similar average age can reflect very different age distributions. Some reflect a markedly bimodal distribution (e.g. respiratory, where there is high incidence at very young and older ages), or very different age distributions across the type of diseases that contribute to the PBC. For example PBC 7 (neurological) includes dementia which accounts for the vast majority of the PBC population older than 70 years. However, a greater proportion of the population is in much younger age groups with other conditions, especially migraine (see Appendix 3, Addendum 1: data sources). When interpreting these summary estimates it should also be noted that the reported life expectancies are not the life expectancies at the average ages reported in column (3), but the average over the life expectancies for each age group within the contributing ICD-10 codes weighted by the age distribution of sequelae from GBD U-codes.
The implications for net YLL of using these PBC-specific estimates of ‘normal’ LE are reported in Table 12. As expected, the net YLL for those PBC with a LE greater than the general population are higher than those reported in column (5) in Table 10 (e.g. PBC 10 circulatory and PBC 17 genitourinary, which now has positive net YLL). Similarly, those PBCs with a LE less than the general population have lower net YLL than reported in column (5) in Table 10 (e.g. PBC 1 infectious diseases and PBC 18 + 19 maternity and neonates, where the effect of a lower LE is more modest as there are no deaths above either of the estimates of LE).
PBC | (1) LE of males (years) | (2) LE of females (years) | Average 2006–8 | (7) Net YLL | ||||
---|---|---|---|---|---|---|---|---|
(3) Death < LE | (4) Death > LE | (5) YLL | (6) YLG | |||||
1 | Infectious diseases | 79.6 | 83.6 | 3498 | 3460 | 58,686 | 21,724 | 36,962 |
2 | Cancer | 83.0 | 84.7 | 101,203 | 29,607 | 1,473,733 | 126,549 | 1,347,184 |
4 | Endocrine | 81.0 | 84.7 | 4068 | 2696 | 66,283 | 15,058 | 51,225 |
7 | Neurological | 79.6 | 83.3 | 8370 | 6983 | 135,686 | 41,770 | 93,917 |
10 | Circulatory | 83.0 | 86.5 | 96,694 | 63,157 | 1,102,020 | 278,251 | 823,768 |
11 | Respiratory | 80.3 | 84.0 | 29,549 | 35,897 | 298,343 | 230,313 | 68,030 |
13 | Gastrointestinal | 80.6 | 84.5 | 15,824 | 8323 | 273,117 | 45,414 | 227,703 |
17 | Genitourinary | 83.5 | 85.6 | 4969 | 5655 | 47,229 | 29,101 | 18,127 |
18 + 19 | Maternity and neonates | 78.7 | 83.1 | 226 | 0 | 16,801 | 0 | 16,801 |
The impact on the cost per life-year threshold of the issues discussed in the Introduction, From mortality to life-years and Adjusting life-years for quality-of-life are summarised in Table 13 (see Table 116 in Appendix 3 for detailed breakdown of changes in spend and YLLs across PBCs).
PBC grouping | Using cut-off in estimating YLL (ONS) (£) | Using net YLL estimates (£) | ||
---|---|---|---|---|
(1) Cut-off of 75 years | (2) Cut-off of LE of the general population | (3) Using LE of the general population | (4) Using LE of the PBC population (GBD) | |
All big four programmes | 10,398 | 5487 | 10,421 | 8080 |
11 PBCs (with mortality) | 20,031 | 10,660 | 19,928 | 15,628 |
All 23 PBCs (zero health effects for remaining 12 PBCs) | 73,697 | 39,218 | 73,317 | 57,497 |
All 23 PBCs (non-zero health effects for remaining 12 PBCs, except GMS)a | 22,639 | 12,048 | 22,523 | 17,663 |
Using ONS data to calculate YLL in the same way as the published NHS IC figures, but overcoming some of the issues associated with the reporting of mortality at PCT level and the coverage of published estimates of YLL (see Mortality and years of life lost coverage), generates similar estimates of a cost per life-year threshold [see Table 13, column (1)] to those reported in Chapter 3. Calculating YLL in the same way, but based on the LE of the general population significantly overestimates YLL for the reasons set out in Life expectancy and years of life lost so underestimates the cost per life-year threshold [see Table 13, column (2)]. Taking account of counterfactual deaths by calculating net YLL based on the LE of the general population [see Table 13, column (3)] provides similar estimates to those reported in Chapter 3. Assuming that PBC populations have the same age and gender distribution as the general population when the, albeit limited, information that is available suggests otherwise, seems inappropriate. Therefore, our preferred central estimate of the cost per life-year threshold is reported in Table 13, column (4). These are lower than those based on the general population, reflecting the impact on net YLL of evidence that the population at risk in some key PBCs (especially PBCs 2 and 10) tend to be older than the general population. In Summary of cost per life-year estimates we consider extreme upper and lower bounds that might be placed on this central estimate.
Inferring excess deaths
We have been able to establish a measure of net YLL, which takes account of deaths that would have occurred anyway below a normal LE for the PBC population (i.e. not all deaths observed in a PBC are excess), and that some deaths observed above this LE would not otherwise have occurred at that age (i.e. some of these deaths are excess). As explained in Years of life lost and accounting for counterfactual deaths, net YLL calculated in this way is equivalent to first establishing the number of excess deaths at each age, then calculating YLL for each excess death (based on the LE conditional on the age at which each excess death occurred) and then summing these YLL across all excess deaths (i.e. across all ages). In other words, the estimates of net YLL imply a number of excess deaths required to generate them in each PBC. Therefore, it is possible to solve for the total number of excess deaths based on the net YLL and the average YLL per observed death. n The net YLL divided by the average YLL per death provides the number of excess deaths required, which on average will generate the estimated net YLL. o
The implied excess deaths associated with net YLL based on the LE of the PBCs [see Table 12, column (7)] are reported in Table 14. With the exception of PBC 18 + 19, excess deaths are some proportion of total observed deaths in each PBC. The proportion of excess deaths differs by PBC reflecting the distribution of deaths relative to the LE of the PBC. p For example, in those PBCs where a large proportion of deaths occur below LE [see Table 12, columns (3) and (4)], excess deaths tend to be a greater proportion of the total deaths (e.g. PBC 2, 13 and 10). Where most deaths occur above LE, excess deaths as a proportion of the total deaths tend to be lower (e.g. PBC 1, 11 and 17).
PBC | (1) Net YLL | (2) YLL per observed death | (3) Excess deaths | (4) Total deaths | (5) % excess deaths | |
---|---|---|---|---|---|---|
1 | Infectious diseases | 36,962 | 13.4 | 2797 | 6958 | 40 |
2 | Cancer | 1,347,184 | 14.1 | 95,715 | 130,810 | 73 |
4 | Endocrine | 51,225 | 13.7 | 3769 | 6764 | 56 |
7 | Neurological | 93,917 | 13.7 | 6909 | 15,353 | 45 |
10 | Circulatory | 823,768 | 10.5 | 79,218 | 159,851 | 50 |
11 | Respiratory | 68,030 | 9.2 | 7386 | 65,445 | 11 |
13 | Gastrointestinal | 227,703 | 15.2 | 15,199 | 24,147 | 63 |
17 | Genitourinary | 18,127 | 8.3 | 2172 | 10,625 | 20 |
18 + 19 | Maternity and neonates | 16,801 | 73.9 | 226 | 226 | 100 |
Estimates of net YLL and changes in life-years due to expenditure (see Tables 12 and 13) have already accounted for the fact that not all deaths are excess and don’t generate YLL. Nevertheless, solving for the number of implied excess deaths associated with these net YLL estimates allows a comparison of the cost per excess and observed PBC death avoided and an examination of the interpretation that can be placed on the life-years expected to be gained from an excess or observed death averted. As only deaths observed in the PBC can be used to estimate the effects of expenditure (excess deaths are not directly observed as they rely on an unobserved counterfactual population and would occur outside the PBC), the outcome elasticities can be interpreted as the proportionate change in observed PBC mortality due to a proportionate change in PBC expenditure. Equally, however, they can also be interpreted as the proportionate effect on excess death due to a proportionate change in expenditure so can be applied to either total observed or total excess deaths. q
The cost per excess death and the cost per PBC death averted are reported in Table 15 (see Table 119 in Appendix 3 for a detailed breakdown of changes in spend and excess or PBC deaths across PBCs). The cost per PBC death averted is, of course, significantly lower than the cost per excess death as excess deaths are only a proportion of total deaths (see Table 14). Also the cost per PBC death averted is substantially lower than those reported in Chapter 3 (see Tables 68 and 69 in Appendix 2), as these estimates do not restrict the effects of expenditure to PBC deaths under the age of 75 years. r The cost per PBC or excess death averted (or life saved) should not be over interpreted as they are of little direct policy interest because lives are never saved (death is only delayed), and the significance of a death averted depends critically on how long it is averted for (the YLGs, see Table 13) and the QoL in which additional years are lived (see Adjusting life-years for quality-of-life).
PBC grouping | (1) Cost per excess death averted (£) | (2) Cost per PBC death averted (£) |
---|---|---|
All big four programmes | 91,129 | 32,864 |
11 PBCs (with mortality) | 177,692 | 64,774 |
All 23 PBCs (zero health effects for remaining 12 PBCs) | 653,748 | 238,310 |
All 23 PBCs (non-zero health effects for remaining 12 PBCs, except GMS)a | 200,829 | 73,208 |
However, establishing the number of excess and PBC deaths averted which are associated with net YLL is useful because it enables an assessment of the number of YLGs associated with each death averted. On average, across all 11 PBCs, each excess death averted is associated with 11.4 YLGs. These are reported for each PBC in Table 121 in Appendix 3, and range from 74.3 years per excess death for PBC 18 + 19 maternity and neonates to 8.3 years for PBC 17 genitourinary. However, clinicians or the evaluative literature cannot distinguish whether or not an observed death is excess. What can be observed is whether or not groups of similar patients with and without access to a treatment survive and for how long. Therefore, it is the life-years associated with each observed death that provide a context that can be interpreted based on experience and evidence of how effective those interventions that could be invested or disinvested intend to be. The average life-years expected to be gained associated with each observed PBC death averted takes account of the fact that some deaths that are avoided in the PBC are not delayed for very long but quickly occurs elsewhere and do not generate YLGs (i.e. they were not excess deaths). These are also reported for each PBC in Table 121 in Appendix 3 and range from 74.3 years per observed death for PBC 18 + 19 maternity and neonatest to 1.0 year for PBC 11 respiratory problems (i.e. the YLL per PBC death are much lower for those PBCs where a small proportion of observed deaths are excess). Each PBC death averted is associated with 4.1 YLGs on average across all 11 PBCs.
Summary of cost per life-year estimates
The sequence of analysis set out above has enabled an examination of the impact of the limitations associated with the incomplete reporting of mortality data at PCT level and incomplete coverage of published YLL estimates. We have also been able to consider effects above the age of 75 years while taking account of that fact that many deaths would have occurred anyway, despite the limited information available about the population at risk within a PBC. The GBD study does provide some information about the age and gender distribution of the population at risk in a PBC so offers some improvement over the other assumptions that would otherwise be required (i.e. that the distribution of age and gender is the same as the general population or follows the distribution of observed deaths). For this reason the cost per life-year threshold in column (4) of Table 13 and repeated in lines (1)–(4) in Table 16 are regarded as the central or best estimates given the evidence available and the credibility of alternative assumptions that could be made. As explained in the Introduction of this chapter, these are based on the conservative assumption that any health effects of changes in expenditure are restricted to 1 year, which, to some extent, may be offset by the more optimistic assumption any death averted returns the individual to the mortality risk faced by the general population, matched for age and gender.
PBC grouping | Cost per life-year threshold |
---|---|
Best estimate | |
Effect of expenditure on mortality | 1 year |
YLL per PBC death averted | ∼ 4.1a |
(1) All big four programmes | £8080 |
(2) 11 PBCs (with mortality) | £15,628 |
(3) All 23 PBCs (zero health effects for remaining 12 PBCs) | £57,497 |
(4) All 23 PBCs (non-zero health effects for remaining 12 PBCs, except GMS)b | £17,663 |
Lower bound | |
Effect of expenditure on mortality | Remainder of disease |
YLL per PBC death averted | ∼ 4.1a |
(5) All big four programmes | £3846 |
(6) 11 PBCs (with mortality) | £6106 |
(7) All 23 PBCs (zero health effects for remaining 12 PBCs) | £22,463 |
(8) All 23 PBCs (non-zero health effects for remaining 12 PBCs, except GMS)b | £6901 |
Upper bound | |
Effect of expenditure on mortality | 1 year |
YLL per PBC death averted | 2 |
(9) All big four programmes | £16,432 |
(10) 11 PBCs (with mortality) | £32,387 |
(11) All 23 PBCs (zero health effects for remaining 12 PBCs) | £119,155 |
(12) All 23 PBCs (non-zero health effects for remaining 12 PBCs, except GMS)b | £36,604 |
It does not seem credible to imagine that NHS expenditure has no health effects in the 12 PBCs which do not have sufficient mortality reported at PCT level to estimate outcome elasticities – what is implied by the estimate reported in Table 16, line (3). Therefore, it is the estimates reported in Table 16, lines (2) and (4) that are of policy interest. The estimate of £15,628 per life-year [see Table 16, line (2)] is restricted to the effects of changes in expenditure in the 11 PBCs where outcome elasticities can be estimated. The threshold of £17,663 per life-year uses the estimated health effects of expenditure in these PBC as a surrogate for health effects in the others, that is assuming that the effects that can be observed will be similar to those that cannot. However, no health effects are assigned to PBC 23 (GMS) on the basis that any health effects of this expenditure would be recorded in the other PBCs. u
The extreme upper and lower bounds for the cost per life-year thresholds in Table 16 are based on making the necessary assumptions about duration of health effects and how long a death might be averted optimistic (providing the lower bound for the threshold) or conservative (an upper bound for the threshold). The lower bound [see Table 16, lines (5)–(8)] is based on assuming that health effects are not restricted to 1 year but apply to the whole of the remaining disease duration of the population at risk in PBCs during the expenditure year. v Although this combines optimistic assumptions, it is possible, indeed likely, that at least some expenditure may have effects on the health outcomes of future patients who are not currently part of the population at risk in a PBC (e.g. investments or disinvestment in prevention will have an impact on populations that are incident to PBCs in the future). Such effects are not captured in any of the estimates presented in this chapter so all are conservative with respect to this type of health effect of changes in expenditure.
The upper bound [see Table 16, lines (9)–(12)] is based on the combination of assuming that health effects are restricted to 1 year for the population currently at risk and that any death averted is only averted for the minimum duration consistent with the mortality data. The econometrics work used the average of 3 years of mortality (2006–8), so the estimated outcome elasticities are based on differences in mortality that remain after averaging over 3 years. Therefore, the estimated effects are based on differences in observed PBC deaths that must have been sustained, on average, for more than a minimum of 2 years. w
Adjusting life-years for quality-of-life
The central or best estimates of the cost per life-year threshold, which were presented in Table 16, lines (2) and (4), take no account of the health-related QoL in which years of life, expected to be gained or lost through changes in expenditure, are likely to be lived. Even if attention is restricted to the direct health consequences of changes in mortality, estimates of the cost per life-year will tend to overestimate the effects of changes in expenditure (i.e. underestimate the threshold) compared with a more complete measure of health that accounts for the quality in which the years of life are expected to be lived. In this section we examine the ways in which the life-years reported in From mortality to life-years can be adjusted for quality, taking account of information that is available about (i) how QoL differs by age and gender (see Quality of life based on the general population); and (ii) how the quality-of-life-years associated with mortality changes might be affected by the types of diseases that make up each PBC (see Adjusting age-related quality-of-life for disease decrements). Throughout, we continue to take account of counterfactual deaths in the way described in Years of life lost and accounting for counterfactual deaths by making the adjustment for quality to the life-years associated with every observed death before calculating a quality-adjusted net YLL. The implications for a cost per QALY threshold that only accounts for the health effects of mortality changes are presented in Summary of the cost per quality-adjusted life-year threshold based only on mortality effects. In Including quality-of-life effects during disease we explore the ways in which the likely direct effects of expenditure on QoL (other than through mortality) might also be taken into account.
Quality of life based on the general population
The most commonly used metric of health-related QoL in the UK is European Quality of Life-5 Dimensions (EQ-5D),91 which is specified in the NICE reference case for methods of technology appraisal. 3 This metric has five dimensions of quality each with three possible levels. Each of these 243 possible health states is valued relative to a score of one, which represents full or best imaginable health (the best score across all five dimensions), and a score of zero, which represents death, based on a representative sample of the UK population. 92 Therefore, insofar as the years of life expected to be gained (or lost) through changes in expenditure would be lived in this state of full health, the cost per life-year thresholds reported in Table 16 would also be the cost per QALY thresholds, albeit ones that only account for the health effects of mortality changes. However, unsurprisingly, there is good evidence that, on average, the general population is not in this state of full health. Therefore, the QoL score associated with the health states experienced by the general population are less than 1, decline with age and differ by gender. These QoL ‘norms’ for the general population by age and gender are illustrated in Figure 3 based on an analysis of data from the Health Survey for England (HSE). x
FIGURE 3.
Quality of life for the general population by age and gender. Pooled QoL estimates provided by personal communication with Dr Anju Keetharuth, University of Sheffield, 2013.
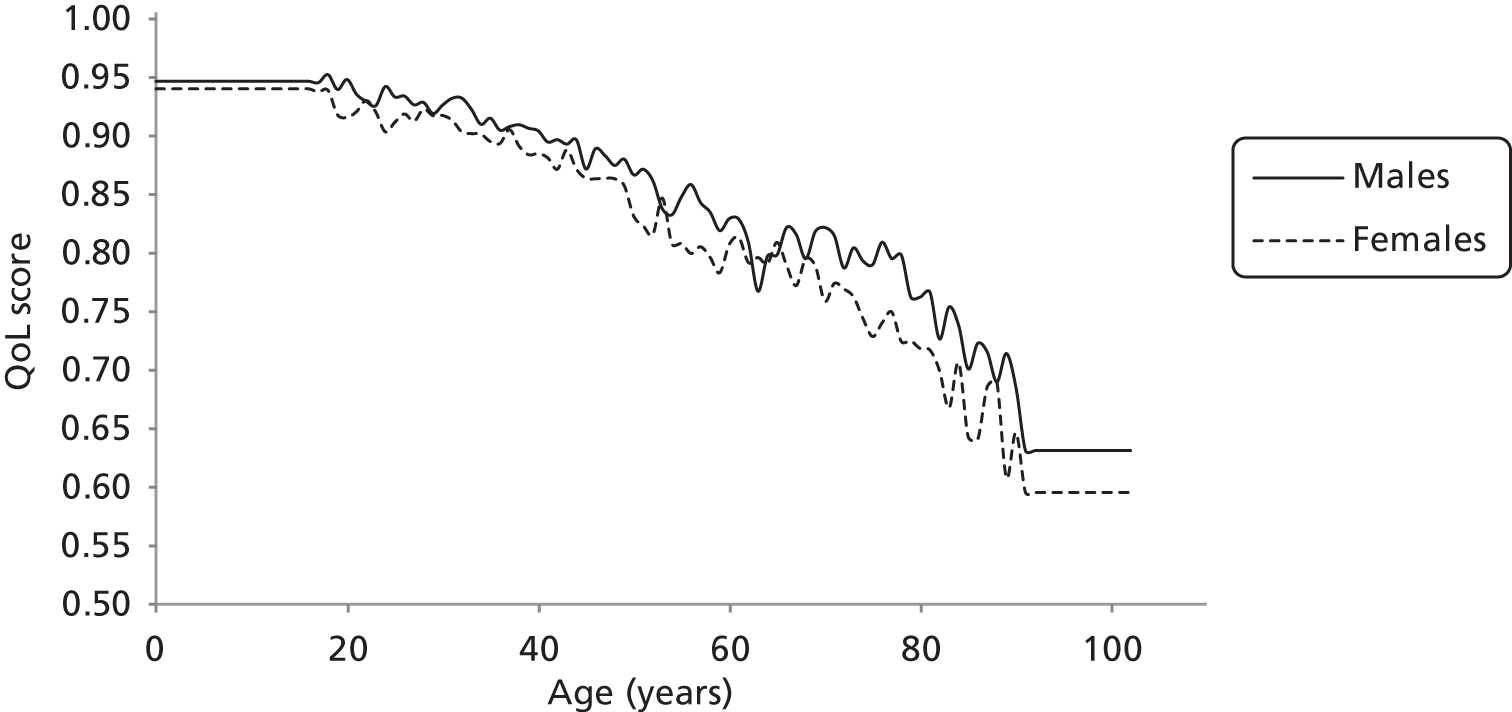
These QoL norms can be applied to the YLL associated with all observed deaths in each PBC, taking account of gender and age at death. The results are reported in columns (4)–(6) of Table 17. There are two effects of adjusting life-years for quality: (i) as QoL norms are always < 1 the adjusted YLL and YLG are always lower than the unadjusted values in columns (1) and (2) (previously reported in Table 12); and (ii) deaths above LE are necessarily at older ages with poorer QoL norms than those below, so the difference between adjusted and unadjusted values is greater for YLG than YLL. The overall effect of quality adjustment on net YLL is the balance of these two effects. The overall effect of quality adjustment is to reduce the net YLL [compare Table 17, columns (3) and (6)]. y
PBC | Unadjusted life-years | QALYs | |||||
---|---|---|---|---|---|---|---|
(1) YLL | (2) YLG | (3) Net YLL | (4) YLL | (5) YLG | (6) Net YLL | ||
1 | Infectious diseases | 58,686 | 21,724 | 36,962 | 47,481 | 14,618 | 32,864 |
2 | Cancer | 1,473,733 | 126,549 | 1,347,184 | 1,143,445 | 84,036 | 1,059,409 |
4 | Endocrine | 66,283 | 15,058 | 51,225 | 52,856 | 9973 | 42,883 |
7 | Neurological | 135,686 | 41,770 | 93,917 | 109,349 | 28,262 | 81,087 |
10 | Circulatory | 1,102,020 | 278,251 | 823,768 | 848,046 | 183,330 | 664,717 |
11 | Respiratory | 298,343 | 230,313 | 68,030 | 231,578 | 154,743 | 76,835 |
13 | Gastrointestinal | 273,117 | 45,414 | 227,703 | 216,256 | 30,277 | 185,979 |
17 | Genitourinary | 47,229 | 29,101 | 18,127 | 35,929 | 18,947 | 16,982 |
18 + 19 | Maternity and neonates | 16,801 | 0 | 16,801 | 14,568 | 0 | 14,568 |
The quality adjusted net YLL figures in Table 17, column (6) suggests that the health effects of mortality are lower than when relying only on unadjusted life-years in From mortality to life-years. Therefore the health effects of changes in expenditure on this more complete measure of health are lower. The implications of these adjustments on a cost per QALY threshold that only accounts for the direct health effects of mortality are reported in Table 18. As expected the cost per QALY threshold based on adjusting the YLGs or lost [see Table 18, column (2)] is higher than a threshold based on unadjusted life-years [see Table 18, column (1) and previously reported in Table 16].
PBC grouping | (1) Cost per life-year threshold (£) | (2) Cost per QALY threshold (population norms) (£) |
---|---|---|
All big four programmes | 8080 | 9631 |
11 PBCs (with mortality) | 15,628 | 18,622 |
All 23 PBCsa | 17,663 | 21,047 |
Adjusting age-related quality-of-life for disease decrements
Adjusting life-years for age- and gender-related QoL norms assumes that any YLG through a change in expenditure would be lived in a similar QoL to the general population. It is possible, however, that patients benefiting from reduced mortality may, nevertheless, continue to be affected by the type of diseases that make up each PBC and experience the QoL associated with the original disease.
The HODaR93 provides over 30,000 observations of EQ-5D measures of QoL by ICD-10 code and the age and gender of the patients in the sample (see Appendix 3, Addendum 1: data sources). Although this is a rich UK data set, there were a limited number of observations for some of the less common ICD-10 codes. For this reason HODaR was supplemented with information from the Medical Expenditure Panel Survey (MEPS)94 which also provides EQ-5D by ICD-10 and reports the average age of respondents (see Appendix 3, Addendum 1: data sources). These data provided a means of estimating the QoL associated with each ICD-10 code at the average age of respondents in the pooled sample. z The QoL associated with each PBC can be expressed as an average of the QoL associated with its component ICD-10 codes. aa The QoL effects of being in each PBC can then be expressed as a disease-related decrement compared with the population norms at the same age (see Table 129 in Appendix 3). This is illustrated for PBC 1 (infectious disease) in Figure 4, where the weighted average of QoL scores across the component ICD-10 codes was 0.667, at an average age average age of 54 years for male respondents. As the QoL norms for males age 54 years is 0.859 this suggests a decrement associated with membership of PBC 1 of 0.192, which can then be applied to QoL norms by age. ab
FIGURE 4.
Quality of life for males in PBC 1 (infectious disease) and the general population by age.

Quality-of-life norms adjusted for disease-related decrements can be applied to the YLL associated with observed deaths in each PBC, taking account of gender and age at death in the same way as in Quality of life based on the general population. ac The results are reported in columns (4)–(6) of Table 19. The overall effect of quality adjustment that also applies a disease-related decrement is to reduce the net YLL to a greater extent than adjustment with population norms alone [compare column (6) in Table 19 with column (6) in Table 17].
PBC | Unadjusted life-years | QALYs | |||||
---|---|---|---|---|---|---|---|
(1) YLL | (2) YLG | (3) Net YLL | (4) YLL | (5) YLG | (6) Net YLL | ||
1 | Infectious diseases | 58,686 | 21,724 | 36,962 | 37,055 | 10,793 | 26,262 |
2 | Cancer | 1,473,733 | 126,549 | 1,347,184 | 955,690 | 67,930 | 887,760 |
4 | Endocrine | 66,283 | 15,058 | 51,225 | 43,394 | 7844 | 35,550 |
7 | Neurological | 135,686 | 41,770 | 93,917 | 68,893 | 15,842 | 53,050 |
10 | Circulatory | 1,102,020 | 278,251 | 823,768 | 656,145 | 135,241 | 520,905 |
11 | Respiratory | 298,343 | 230,313 | 68,030 | 169,269 | 106,505 | 62,764 |
13 | Gastrointestinal | 273,117 | 45,414 | 227,703 | 163,593 | 21,677 | 141,916 |
17 | Genitourinary | 47,229 | 29,101 | 18,127 | 29,749 | 15,152 | 14,598 |
18 + 19 | Maternity and neonates | 16,801 | 0 | 16,801 | 13,662 | 0 | 13,662 |
It should be noted that combining QoL adjustments for both population norms and disease-related decrements assumes that any YLGs due to a reduction in mortality will be lived in the diseased state until LE (i.e. that all diseases are not just chronic but disease duration is lifelong). Inevitably this assumption means that the health effects of changes in mortality will be reduced. Consequently, the cost per QALY threshold reported in Table 20, column (2) will be higher than adjusting YLGs for population norms in Table 18.
PBC grouping | (1) Cost per life-year threshold (£) | (2) Cost per QALY threshold (disease-related decrements) (£) |
---|---|---|
All big four programmes | 8080 | 12,109 |
11 PBCs (with mortality) | 15,628 | 23,395 |
All 23 PBCsa | 17,663 | 26,441 |
Summary of the cost per quality-adjusted life-year threshold based only on mortality effects
The analysis to this point is summarised in Table 21. The three estimates of a cost per QALY threshold are based on assuming that each YLG is either lived in full health [see Table 21, column (1), equal to the cost per life-year estimates in Table 16]; lived in a QoL that reflects age and gender norms of the general population [see Table 21, column (2)]; or lived in a QoL that reflects the original disease state [see Table 21, column (3)].
PBC grouping | (1) QoL score = 1 | (2) QoL norm | (3) QoL diseased |
---|---|---|---|
Best estimate | |||
Effect of expenditure on mortality | 1 year | 1 year | 1 year |
YLL per PBC death averted | ∼ 4.1a | ∼ 4.1a | ∼ 4.1a |
QALYs per death averted | ∼ 4.1 | ∼ 3.5 | ∼ 2.8 |
(1) All big four programmes | £8080 | £9631 | £12,109 |
(2) 11 PBCs (with mortality) | £15,628 | £18,622 | £23,395 |
(3) All 23 PBCsb | £17,663 | £21,047 | £26,441 |
Lower bound | |||
Effect of expenditure on mortality | Remainder of disease | Remainder of disease | Remainder of disease |
YLL per PBC death averted | ∼ 4.1a | ∼ 4.1a | ∼ 4.1a |
QALYs per death averted | ∼ 4.1 | ∼ 3.5 | ∼ 2.8 |
(4) All big four programmes | £3846 | £4252 | £5319 |
(5) 11 PBCs (with mortality) | £6106 | £6852 | £8568 |
(6) All 23 PBCsb | £6901 | £7744 | £9683 |
Upper bound | |||
Effect of expenditure on mortality | 1 year | 1 year | 1 year |
YLL per PBC death averted | 2 | 2 | 2 |
QALYs per death averted | 2 | ∼ 1.9 | ∼ 1.5 |
(7) All big four programmes | £16,432 | £17,456 | £21,747 |
(8) 11 PBCs (with mortality) | £32,387 | £34,492 | £42,967 |
(9) All 23 PBCsb | £36,604 | £38,983 | £48,561 |
Assuming that YLGs are lived in full health is not credible and should be regarded as an underestimate of the threshold, given what is known about QoL norms for the general population (see Figure 3). Equally, assuming that all YLGs are lived in the QoL of the original disease state does not seem credible either and is likely to overestimate the threshold as it assumes that all disease is not only chronic but lifelong and all life-years would be lived in the diseased state until death. ad Although adjusting YLGs for the QoL of the general population, taking account of age and gender [see Table 21, column (2)], is likely to underestimate a cost per QALY threshold based only on mortality effects, it probably represents the ‘best’ of the three alternative estimates available at this stage of the analysis (see Using estimates of the QALY burden of disease for how analysis based on measures of QALY burden allows this assumption to be relaxed). ae The lower and upper bounds are based on combining optimistic and pessimistic assumptions about the duration of health effects and how long a death might be averted as described in Summary of cost per life-year estimates.
However, it should be noted that these cost per QALY thresholds only account for the direct health effects of changes in mortality due to changes in expenditure. Insofar as much, or at least some, of NHS activity and expenditure is intended to improve QoL, not just mortality, then these estimates will underestimate total health effects and overestimate a cost per QALY threshold based on a more complete measure of possible health effects. In Including quality-of-life effects during disease we explore the ways in which the likely effects of expenditure on QoL (other than through mortality) might also be taken into account.
Including quality-of-life effects during disease
The cost per QALY thresholds presented in Adjusting life-years for quality-of-life only account for the health (QALY) effects of changes in mortality due to changes in expenditure. It does not seem credible to suppose that all NHS activity and expenditure only influences mortality with no effect on the QoL while alive and experiencing a disease. Insofar as changes in NHS expenditure will also affect QoL as well as mortality then total health effects will be underestimated and the thresholds presented in Table 21 will overestimate the cost per QALY threshold. In this section we explore ways to also take account of those effects on health not directly associated with mortality and life-year effects (i.e. the ‘pure’ QoL effects) to estimate an overall cost per QALY threshold.
The routine reporting of QoL outcomes is increasingly available at PCT level (see Addendum 1: data sources in Appendix 3 for a description of these data). In principle, the variation in such measures of outcome across PCTs could be used to estimate outcome elasticities for QoL rather than mortality effects using similar econometric methods to those described in Chapter 3 (see Application of method to other non-mortality-based outcome indicators in Appendix 2 for the results of an exploratory econometric analysis of these data). However, the currently limited coverage of routine reporting of these outcomes means that it is not feasible to estimate QoL effects across all the PBCs using these data. In Chapter 5, Future research and improving estimates of the threshold we discuss how these data might be used to improve estimates of the threshold as the coverage and routine reporting of QoL outcomes improves, and how the analysis presented in Chapter 5, Which programme budget categories matter most?, might help prioritise reporting in particular areas (i.e. those PBCs and ICD-10 codes that have the greatest influence on estimates of the threshold).
Here we explore how estimates of effects of expenditure that can be observed (i.e. on mortality) can be used to infer the likely effects on what cannot be directly observed (QoL), rather than making extreme assumptions that are not credible (e.g. assuming that changes in expenditure will have no effects on QoL outcomes).
In Using ratios of quality-adjusted life-years to years of life lost we use three alternative estimates of the ratio of QALYs to life-years lost due to different types of disease as a means of inferring the change in QALYs that is likely to be associated with the estimated change in YLL (i.e. applying the total QALYs lost associated with each YLL with disease). This is consistent with regarding the estimates of the mortality and life-year effects as a surrogate for a more complete measure of the health effects of a change in expenditure.
However, these ratios of QALYs lost to life-years lost due to disease in those PBCs where outcome elasticities could not be estimated cannot inform estimates of the threshold (there are no estimated life-year effects with which to apply the ratios). Nonetheless, the sources of information on which ratios are based also provides much of the information required to calculate the QALY burden of disease in these areas, which can be used to inform estimates of the threshold. Therefore, in Using estimates of the quality-adjusted life-years burden of disease we use estimates of the QALY burden of disease, infer a proportionate effect on burden from the estimated effects on life-years, and then apply this proportionate effect to the measures of QALY burden for all the other PBCs. In this way we can use all the information available about the mortality and QoL effects of the different types of disease that make up each PBC, including those where mortality-based outcome elasticities are not available.
Using ratios of quality-adjusted life-years to years of life lost
The ratio of the total QALYs to YLL due to a disease indicates the number of QALYs associated with each YLL. Therefore, any change in YLL is likely to generate a number of QALYs indicated by the ratio – if it is reasonable to interpret the estimated effects on mortality and life-years as a surrogate for a more complete measure of total health effects. For example, a disease with a ratio > 1 suggests that each YLL across the at risk population is associated with more than 1 QALY (i.e. where there are significant QoL effects while experiencing the disease). af Therefore, a change in expenditure that leads to 1 YLG in this type of disease may be expected to generate > 1 QALY and a greater QALY effect than the same life-year effects in a disease where this ratio is < 1 (i.e. where most of the effect of disease is on mortality rather than QoL). Therefore, information which allows these ratios to be estimated for the diseases that make up each PBC provides a means of accounting for the likely effect on QoL other than through effects on mortality.
To understand the differences between the three ratios presented below it is useful to regard the total QALY lost to YLL ratio (R) for a particular disease as the sum of two ratios: (i) the QALYs lost due to premature death to YLL ratio (Rdeath);ag and (ii) the QALYs lost during disease (while alive) to YLL ratio (Ralive) (see Using ratios of quality-adjusted life-year to years of life lost in Appendix 3 for more detailed explanation).
Disability-adjusted life-year to years of life lost ratios
The WHO GBD study provides UK-specific estimates of the years of life lived with disability (YLD) and the YLL due to different types of disease (classified by U-codes that can be mapped to ICD-10, see From mortality to life-years and Addendum 1: data sources in Appendix 3). The GBD study uses disability-adjusted life-years (DALYs) as a measure of the burden of disease. This DALY measure has two components: (i) the YLD, which incorporates weights (between 0 and 1) to reflect the scale of disability experienced each year and the number of YLDs over the durations of disease; and (ii) the YLL. The total DALY associated with a disease is simply YLL + YLD. Therefore, the DALY to YLL ratio is (YLL + YLD)/YLL or equivalently YLL/YLL + YLD/YLL. As the first term (YLL/YLL = Rdeath) must equal 1 and the second (Ralive = YLD/YLL) must be ≥ 0, a ratio based on DALYs must necessarily be bounded below by 1. This is illustrated in Table 22 for four different types of diseases (classified by U-codes) which reflect diseases where mortality is the major component (e.g. U016) and where the impact of disease on the QoL while alive is the major component (e.g. U141).
U-code | DALY ratios | (Rdeath + Ralive) |
---|---|---|
U037 (other infectious diseases) | 1.23 | (1 + 0.23) |
U016 (tetanus) | 1.00 | (1 + 0)a |
U061 (mouth and oropharynx cancers) | 1.05 | (1 + 0.05) |
U141 (spina bifida) | 2.34 | (1 + 1.34)b |
Adjusting disability-adjusted life-years for quality-of-life norms
The use of DALY ratios bounded below by 1 essentially assumes that YLL would have otherwise been lived in a state of full health. As was discussed in Quality of life based on the general population this is not credible given information available about the QoL in the general population (see Figure 3). It would lead to over estimating the QALYs associated with mortality and life-year effects and underestimating the cost per QALY threshold. Therefore, it is important to adjust these DALY ratios for the QoL norms by age and gender in the same way as described in Quality of life based on the general population. The effect of this adjustmentah is illustrated in Table 23. Now those types of disease where mortality rather than QoL with the disease is the major component can have ratios < 1. Indeed, the first term of these ratios (Rdeath) is consistent with, and is implied by, the analysis in Quality of life based on the general population where the ratio of quality-adjusted net YLL to unadjusted net YLL represents this ratio on average for each PBC.
U-code | Modified DALY ratios | (Rdeath + Ralive) |
---|---|---|
U037 (other infectious diseases) | 1.01 | (0.78 + 0.23) |
U016 (tetanus) | 0.78 | (0.78 + 0) |
U061 (mouth and oropharynx cancers) | 0.83 | (0.78 + 0.05) |
U141 (spina bifida) | 2.18 | (0.85 + 1.34) |
Using quality-of-life estimates (based on Health Outcomes Data Repository and Medical Expenditure Panel Survey data)
The disability weights used in the DALY measure are not based on the same description of health states as the EQ-5D measure, nor are the weights based on a representative sample of the UK population responding to choice-based elicitation questions. EQ-5D-based QoL decrements (adjustments to age-related QoL norms) associated with different types of disease can be estimated from HODaR and MEPS data (previously described in Adjusting age-related quality-of-life for disease decrements). ai These disease-related QoL decrements be can be calculated for each U-code (based on the contributing ICD-10 codes) so can be used to replace the DALY disability weights in Ralive reported in Tables 22 and 23. aj This final adjustment is illustrated in Table 24 and turns, what were originally, DALY ratios into EQ-5D QALY ratios. ak For these reasons we regard the QALY to YLL ratios rather than DALY or modified DALY ratios as the preferred basis of estimating a cost per QALY threshold that provides a more complete picture of the likely health effects of changes in expenditure.
U-code | QALY ratios (HODaR and MEPS) | (Rdeath + Ralive) |
---|---|---|
U037 (other infectious diseases) | 1.37 | (0.78 + 0.60) |
U016 (tetanus) | 0.78 | (0.78 + 0) |
U061 (mouth and oropharynx cancers) | 0.80 | (0.78 + 0.02) |
U141 (spina bifida) | 1.88 | (0.85 + 1.03) |
Allocating effects at programme budget category level to International Classification of Diseases codes
Tables 22–24 illustrate how QALY ratios can be calculated for and differ by U-code. al Unsurprisingly, these ratios differ across the type of diseases that make up each PBC (see Table 147 in Appendix 3). When using this information to estimate a cost per QALY threshold the mortality and life-year effects observed at PBC level must be allocated in some way to the component ICD-10 codes before ratios are applied to life-year effects and the resulting QALY effects are summed across all the contributing ICD-10 codes. am For this reason it is important to consider how other information might inform the different ways in which the effects observed at PBC level might be generated by the distribution of impacts at ICD-10 level (i.e. where investment or disinvestment is likely to occur within the PBC and, therefore, which ICD-10 codes are likely to contribute most to overall health effects).
An important and complementary element to the econometric analysis of routinely reported information at PBC level was to investigate whether or not other information, commonly available at a local level within the NHS, might provide a useful indication of where, within a PBC, investment or disinvestment is more likely across the NHS. The details of this investigation are reported in Addendum 2: the role of data on local NHS decisions in Appendix 3. The review of local data sources suggested that there are very few routinely collected data on investment and disinvestment by local NHS organisations beyond the high-level aggregate data on spending by PBC which are used in the econometric analysis. Although more disaggregated data on spending decisions about specific services relevant to particular ICD-10 codes could in principle be acquired through additional primary research (surveys or freedom of information requests), this would be costly and with a risk that information acquired in this way may not be complete, consistent or representative.
In the absence of useful information at a local level it is possible to assume that a change in PBC expenditure will be allocated equally (on a per-patient basis) across the component ICD-10 codes (i.e. any investment or disinvestment is equally likely across the population at risk within the PBC). Hospital Episode Statistics (HES) (see Addendum 1: data sources in Appendix 3) provides information about the costs associated with each ICD-10 code by PCT so it is possible to establish which ICD-10 codes contribute most to the variability in HES costs within a PBC across PCTs. Those that contribute most to this variance might be expected to be more likely to have been subject to differential investment or disinvestment across PCTs. an
There are differences in relative weight assigned to ICD-10 code based on the size of the population or its contribution to variance in HES costs. If investment or disinvestment within a PBC tends to focus on ICD-10 codes representing areas of marginal value, the health effects of a change in PBC expenditure may be overestimated and a cost per QALY threshold underestimated when allocating effects equally across the population at risk within each PBC. However, weighting ICD-10 codes based on HES data is likely to favour those ICD-10 codes which represent more severe disease requiring more hospital care. This may over represent ICD-10 codes with lower QALY to YLL ratios if mortality effects tend to be a major component of these types of disease and may be conservative with respect to the health effects of changes in expenditure.
The implications for a cost per QALY threshold that uses the estimated mortality and life-year effects as a surrogate for a more complete measure of the likely health effects (i.e. that includes QoL as well as QALY effects) is summarised in Table 25. These results use the contribution to variance in HES costs to ‘weight’ the different ICD-10 codes within a PBC (when allocating the life-year effects), before applying the QALY ratios associated with each ICD-10 code (see Table 143 in Appendix 3).
PBC grouping | (1) DALY ratios (£) | (2) Modified DALY ratios (£) | (3) QALY ratios (HODaR and MEPS) (£) |
---|---|---|---|
All big four programmes | 5402 | 6419 | 5990 |
11 PBCs (with mortality) | 9958 | 11,718 | 10,297 |
All 23 PBCs | 11,254 | 13,244 | 11,638a |
The QALY to YLL ratio implied by this analysis for all 11 PBCs with outcome elasticities is 1.52, which suggests that every (unadjusted) life-year is associated with 1.52 QALYs on average across these PBCs. However, this implied QALY ratio differs across these PBCs, ranging from 0.79 in PBC 2 to 15.05 in PBC 18 + 19 (see Table 145 in Appendix 3). As all the analysis in this section seeks to use the estimated mortality and life-year effects as a surrogate for a more complete measure of likely health effects, it is the cost per QALY threshold for all 23 PBCs that is most relevant. As expected, this threshold (£11,638) is lower than a cost per QALY threshold based only the QoL adjusted YLL effects (£21,047 in Table 21 that assumes no effects of NHS expenditure on QoL itself). This difference gives some indication of the relative importance of QALY effects due to avoidance of premature death and the QALY effects of avoiding disability during disease.
Table 26 reports how the estimated QALY effects for each PBC can be decomposed into that part associated with life-year effects and that part associated with ‘pure’ QoL effects. These results appear credible for the first 11 PBCs, where those for which mortality is the major concern have a much greater share of total QALY effects associated with avoidance of premature death (e.g. PBC 2 and PBC 10) compared with those where QoL is the major concern (e.g. PBC 7). ao
PBC | QALY change (total) | QALY change (death) | % QALY gained | ||
---|---|---|---|---|---|
Due to avoidance of premature death | Due to avoidance of disability while alive | ||||
2 | Cancer | 1699 | 1641 | 97 | 3 |
10 | Circulatory | 6713 | 4856 | 72 | 28 |
11 | Respiratory | 3215 | 923 | 29 | 71 |
13 | Gastrointestinal | 3605 | 1193 | 33 | 67 |
1 | Infectious diseases | 27 | 11 | 40 | 60 |
4 | Endocrine | 2036 | 323 | 16 | 84 |
7 | Neurological | 342 | 52 | 15 | 85 |
17 | Genitourinary | 12 | 6 | 52 | 48 |
16 | Trauma and injuries | 0 | 0 | N/A | N/A |
18 + 19 | Maternity and neonates | 273 | 15 | 6 | 94 |
3 | Disorders of blood | 1087 | 547 | 50 | 50 |
5 | Mental health | 19,828 | 9979 | 50 | 50 |
6 | Learning disability | 2990 | 1505 | 50 | 50 |
8 | Problems of vision | 2348 | 1181 | 50 | 50 |
9 | Problems of hearing | 621 | 313 | 50 | 50 |
12 | Dental problems | 2282 | 1148 | 50 | 50 |
14 | Skin | 1021 | 514 | 50 | 50 |
15 | Musculoskeletal | 1469 | 739 | 50 | 50 |
20 | Poisoning and adverse events | 426 | 215 | 50 | 50 |
21 | Healthy individuals | 1781 | 896 | 50 | 50 |
22 | Social care needs | 6566 | 3304 | 50 | 50 |
23 | Other | 0 | 0 | N/A | N/A |
The ratios of QALYs to YLL due to disease in those PBCs where outcome elasticities could not be estimated cannot be used to inform estimates of the threshold because there are no estimated life-year effects with which to apply the ratios. Therefore, as in previous sections, the estimated effect of expenditure on health for the 11 PBCs with outcome elasticities is applied to the estimated changes in PBC expenditure for the other 12 PBCs (excluding GMS for the reasons given in From mortality to life-years), i.e. assuming that the health effects that can be observed from a change in expenditure will be similar to those that cannot. However, the use of QALY ratios also implies that the share of total health effects between QALY effects and that part associated with ‘pure’ QoL effects are also similar to those PBC with estimated outcome elasticities. Summing the different types of health effects across these 11 PBCs suggests that 50% is due to avoidance of premature death and 50% is due to avoidance of disability. This is clearly not credible when applied to the other PBCs. For example, to mental health, vision and hearing are likely to have a much greater share of total health effects associated with QoL effects and very little associated with premature mortality.
The problem is that using QALY to YLL ratios means that much of the information that is available about the other 12 PBCs cannot be used to inform the estimates of the cost per QALY threshold. Fortunately, the sources of information on which ratios are based also provide much of the information required to calculate the QALY burden of disease in these areas. Using estimates of the quality-adjusted life-year burden of disease explores how measures of burden can be used to estimate a cost per QALY threshold that captures the likely effects of a change in expenditure on all aspects of health while using all the information that is available about all the PBCs.
Using estimates of the quality-adjusted life-year burden of disease
In this section we use estimates of the QALY burden of disease to infer QALY effects in those PBCs where the mortality effects of changes in expenditure can be observed and then extrapolate the estimated proportionate effects to those PBCs where the health effects of changes in expenditure cannot be observed. The estimated proportionate effect of change in expenditure on the life-year burden of disease in the 11 PBCs where mortality-based outcome elasticities could be estimated are applied to measures of QALY burden in each of these PBCs (i.e. effects on the mortality burden of disease are used as a surrogate for effects on QALY burden). The proportionate effect on burden of disease due to the change in expenditure across these PBCs can then be applied to measures of QALY burden in the other 11 PBCs where mortality effects could not be estimated (i.e. the observed effects of changes in expenditure on burden of disease is extrapolated to the other PBCs where health effects cannot be observed). In this way we can use all the information available about the mortality and QoL effects of the different types of disease that make up each PBC, particularly those where mortality-based outcome elasticities are not available. Applying a proportionate effect to measures of QALY burden of disease is equivalent to assuming that any effects on life-years are lived at a QoL that reflects a proportionate improvement to the QoL with disease. ap It also allows QoL effects of changes in expenditure to be included; also based on proportionate improvement in the QoL with disease.
Previously in Chapter 3 and From mortality to life-years, Adjusting life-years for quality-of-life and Using ratios of quality-adjusted life-years to years of life lost, expenditure elasticities were not estimated for the other 11 PBCs where outcome elasticities could not be estimated because the same health effect of changes in expenditure was assumed (i.e. it did not matter how changes in expenditure was allocated between them). However, in this section it does matter how the remaining change in expenditure is allocated between the other 11 PBCs as they have different QALY burdens so different implied health effects of expenditure. Therefore, expenditure elasticities are estimated for all 23 PBCs (see column 2 of Table 108 in Appendix 3). However, it is not possible to estimate expenditure equations for all 23 PBCs simultaneously (see Chapter 5, Future research and improving estimates of the threshold), so the 23 independently-estimated expenditure elasticities do not account for all of the change in overall spend (i.e. the sum of changes in PBC expenditure based on the estimated PBC expenditure elasticities accounts for a < 1% change in total spend). This remaining change in total spend is allocated between all 23 PBCs reflecting their relative share of changes in expenditure based on their estimated expenditure elasticities [see column (4) of Table 108 in Appendix 3]. aq
The total QALY burden of disease for the population with disease in a particular year includes (i) the quality-adjusted YLL due to all the disease-related mortality that could occur in this population over their remaining duration of disease; and (ii) the reduction in QoL while alive also for their remaining disease duration. However, applying the estimated proportionate effects on mortality and life-years to such a measure of total burden would provide an estimate of the effects of a change in expenditure, not just in 1 year, but in all the remaining years of disease for the population at risk in that year. Recall from From mortality to life-years that we have adopted the conservative assumption that changes in expenditure will only have health effects in 1 year for the population with disease in that year. Therefore it is not a measure of total burden that is required, but a measure of the QALY burden of disease during 1 year for the population with disease (prevalent and incident) in that year. The estimated outcome elasticities can then be appropriately applied to this measure of burden. ar
The information from GBD used to derive QALY ratios in Using ratios of quality-adjusted life-years to years of life lost includes information about the YLL and duration of disease for those incident to a U-code [i.e. the measure of QALY burden from the information included in the ratios is a measure of the total burden of the disease, but only for the population that is incident (rather than the total population with disease) in 1 year]. Assuming that incidence is stable over the disease duration, this is also equivalent to the QALY burden of disease during 1 year for the population with disease (i.e. those that are incident and prevalent) in that year. as
However, in moving from ratios to absolute measures of burden it becomes more important to examine and then adjust for any inconsistency between information about YLL and size of the incident population from GBD (which is available by U-codes and can be mapped to ICD-10 codes), and the information about net YLL and observed deaths for each PBC based on ONS data as described in Inferring excess deaths (see Table 146 in Appendix 3). at
The implications for the cost per QALY threshold of using information about the QALY burden of disease for all PBCs rather than QALY ratios for those where an outcome elasticity can be estimated are reported in Table 27. The QALY effects of a change in PBC expenditure are a weighted average of the QALY effects within each of the ICD-10 codes that contribute to the PBC. The figures reported in Table 27, column (2) are based on weighing the effects at ICD-10 level by the proportion of the total PBC population within each contributing ICD-10 code, rather than the contribution to variance in HES costs. au
PBC grouping | Cost per QALY gained (£)a | |
---|---|---|
(1) QALY ratios (HODaR and MEPS) | (2) QALY burden (HODaR and MEPS) | |
All big four programmes | 5990 | 3036 |
11 PBCs (with mortality) | 10,297 | 5128 |
All 23 PBCs | 11,638 | 10,187a |
The cost per QALY threshold for the 11 PBCs with outcome elasticities is lower using a measure of QALY burden (£5128) rather than the QALY ratios (£10,297) described in Mortality and years of life lost. This is in part because GBD calculates YLL in the same way as published NHS IC figures and so will tend to overestimate a net YLL which accounts for counterfactual deaths (see Years of life lost and accounting for counterfactual deaths). This will make little difference to the first term in the QALY ratio (Rdeath) used in Mortality and years of life lost coverage as an overestimate of YLL affects both denominator and numerator of the ratio. However, the second term (Ralive) is likely to be underestimated. Therefore the ratios used in Using ratios of quality-adjusted life-years to years of life lost will tend to underestimate the QALY effects of expenditure and overestimate the cost per QALY threshold (see Table 27). We are able to adjust the GBD-based measure of QALY burden for this overestimation of net YLL in calculating the QALY threshold reported in Table 27, column (2). av
As the purpose of this section is to use the estimated mortality and life-year effects as a surrogate for a more complete measure of likely health effects, it is the cost per QALY threshold for all 23 PBCs that is of most relevance. The cost per QALY threshold for all 23 PBCs is based on applying the proportionate effects on the QALY burden of disease, based on the observed effects of changes in expenditure on mortality in the 11 PBCs with outcome elasticities,aw to the QALY burden of disease in the other PBCs. This generates a higher cost per QALY threshold (£10,187) than the one based only on the 11 PBCs with outcome elasticities (£5128). The reason is that the QALY burden of disease in the other PBCs is, in general, lower than the QALY burden of disease across those PBCs where outcome elasticities can be estimated (see Table 147 in Appendix 3). Therefore, applying the same proportionate effects to a lower QALY burden generates a smaller health effect of a change in expenditure. ax
In essence the difference between the estimates in Table 27 is that in column (1) the absolute effect on health associated with an absolute change in expenditure is extrapolated to the other PBCs, whereas in column (2) it is the relative effect on health of an absolute change in expenditure that is extrapolated. As we know that QALY burden differs between (and within) PBCs and especially between the groups of PBCs with and without estimated outcome elasticities (see Table 147 in Appendix 3),ay it is the values based on QALY burden in column (2) that are regarded as most credible and represent our central or best estimate.
A detailed breakdown of changes in expenditure and changes in QALYs across all PBCs is provided in Table 150 in Appendix 3, both when the analysis is based on QALY ratios and when it is based on QALY burden of disease. A comparison of these values suggests that QALY effects for the other PBCs are generally lower and therefore the cost per QALY for each of these PBCs are, in general, higher when based on a proportionate effect on QALY burden. Of course, we have not directly observed QoL effects in these PBCs but inferred them from the proportionate effects that we can observe. Insofar as investment and disinvestment opportunities in these PBCs might have been more valuable (offered greater improvement in QoL)az than suggested by the implied PBC thresholds, then overall QALY effects will tend to be underestimated and the cost per QALY threshold overestimated. For the reasons discussed in previous sections, we regard all the costs per QALY threshold reported in column (2) of Table 27 as on balance conservative with respect to overall health effects of a change in expenditure. However, the estimate of £10,187 may be conservative with respect to health effects (i.e. overestimated), as it is based on an extrapolation of the proportionate effects to measures of burden on these PBCs, rather than observations of the direct impact of changes in expenditure on QoL in these types of disease. This is especially important in PBC 5 (mental health disorders), which accounts for a large proportion of the change in overall expenditure (22%) and where a review of the evidence suggests that the investment and disinvestment opportunities in this PBC may have been more valuable than the implied PBC cost per QALY of £13,876 (see Table 155, Appendix 3 and Addendum 3: characterisation of the investment and disinvestment decisions in mental health – depression and schizophrenia). az The lower cost per QALY threshold for the 11 PBCs with outcome elasticities (£5128) might be regarded as more secure in this respect but they only account for a proportion (38%) of any change in overall expenditure (see Table 155 in Appendix 3).
Table 28 reports how the estimated QALY effects based on measures of QALY burden for each PBC can be decomposed into that part associated with life-year effects adjusted for quality and that part associated with ‘pure’ QoL effects. These results are similar to those reported in Table 26 which were based on QALY ratios for the 11 PBCs with an estimated outcome elasticity. Those PBCs for which mortality is the major concern have a much greater share of total QALY effects associated with avoidance of premature death (e.g. PBC 2 and PBC 10) compared with those where QoL is the major concern (e.g. PBC 7). The differences tend to favour QALYs gained though avoidance of disability, which reflects the underestimation of the effects on ‘pure’ QoL when using QALY ratios based on estimates of YLL from GBD (see the discussion above). aaa The QALY to YLL ratios that are implied by this analysis are reported in Appendix 3, Table 152. As expected the implied QALY ratio across all 11 PBCs with outcome elasticities is higher (3.05aab) than reported in Using ratios of quality-adjusted life-years to years of life lost because the previous bias against QoL effects by using QALY ratios based on unadjusted GBD information has been removed.
PBC | (1) QALY change (total) | (2) QALY change (death) | % QALY gained | ||
---|---|---|---|---|---|
(3) For premature death | (4) For disability while alive | ||||
2 | Cancer | 2121 | 1968 | 93 | 7 |
10 | Circulatory | 8347 | 5727 | 69 | 31 |
11 | Respiratory | 28,072 | 1072 | 4 | 96 |
13 | Gastrointestinal | 3922 | 1446 | 37 | 63 |
1 | Infectious diseases | 74 | 13 | 18 | 82 |
4 | Endocrine | 6905 | 380 | 5 | 95 |
7 | Neurological | 1361 | 60 | 4 | 96 |
17 | Genitourinary | 34 | 8 | 22 | 78 |
16 | Trauma and injuries | 0 | 0 | N/A | N/A |
18 + 19 | Maternity and neonates | 14 | 10 | 69 | 31 |
3 | Disorders of blood | 1215 | 62 | 5 | 95 |
5 | Mental health | 10,878 | 949 | 9 | 91 |
6 | Learning disability | 207 | 41 | 20 | 80 |
8 | Problems of vision | 561 | 22 | 4 | 96 |
9 | Problems of hearing | 1168 | 9 | 1 | 99 |
12 | Dental problems | 578 | 1 | 0 | 100 |
14 | Skin | 103 | 38 | 37 | 63 |
15 | Musculoskeletal | 1005 | 50 | 5 | 95 |
20 | Poisoning and adverse events | 42 | 7 | 16 | 84 |
21 | Healthy individuals | 40 | 6 | 16 | 84 |
22 | Social care needs | 0 | 0 | N/A | N/A |
23 | Other | 0 | 0 | N/A | N/A |
In Using ratios of quality-adjusted life-years to years of life lost the ratios of QALYs to YLL due to disease in those PBCs where outcome elasticities could not be estimated could not be used to inform estimates of the threshold or indicate how any total health effects in these other PBCs are likely to be ‘shared’ between life-year effects adjusted for quality and that part associated with ‘pure’ QoL effects (see Table 26). By applying the observed proportionate effects of changes in expenditure to measures of QALY burden of disease in these other PBCs, the likely share of any effects on QALYs between avoidance of premature mortality and avoidance of disability more closely reflect the nature of these types of diseases (see Table 28). As expected, a much greater proportion of QALY effects are associated with QoL during the disease compared with the 11 PBCs where mortality-based outcome elasticities could be estimated. The share of effects in particular PBCs are also much more credible. For example, in PBC 5 (mental health disorders) the overwhelming share of QALY effects are associated with QoL itself and for others, such as PBC 12 (dental problems), PBC 9 (problems of hearing) and PBC 8 (problems of vision); almost all effects are associated with QoL rather than mortality and life-years. For this, and the other reasons discussed above, the analysis based on measures of QALY burden are regarded as the best estimate of a cost per QALY ratio that reflects a more complete picture of the likely health effects of changes in overall expenditure.
Summary of the cost per quality-adjusted life-year threshold
The results of the three sequential steps of analysis described in this chapter are summarised in Table 29. In From mortality to life-years we explored ways in which the estimated effects on mortality from the econometrics work in Chapter 3 might be better translated in to life-year effects by overcoming some of the limitations of mortality data available at PCT level and taking account of counterfactual deaths. The results of this analysis were reported in Table 28 and are repeated in column (1) of Table 29. aac In Adjusting life-years for quality-of-life we considered how the estimated life-year effects might be adjusted for the QoL in which they are likely to be lived, taking account of the gender and the age at which life-years are gained or lost (see Table 21). The results of this analysis are repeated in column (2) of Table 29. Finally in Including quality-of-life effects during disease we explored ways to also take account of the likely effects of changes in expenditure on QoL during disease as well as the effects associated with mortality and life-years [see Table 29, column (3)]. These estimates provide our central estimate of a cost per QALY threshold, because they make best use of available information while the assumptions required, which on balance are likely to be conservative with respect to health effects, appear more reasonable than the other alternatives available. aad
PBC grouping | (1) From mortality to life-years analysis | (2) Adjusting life-years for quality-of-life analysis | (3) Including quality-of-life effects during disease analysis |
---|---|---|---|
QoL associated with life extension | 1 | Norm | |
QoL during disease | 0 | 0 | Based on burden |
Best estimate | |||
Effect of expenditure on mortality | 1 year | 1 year | 1 year |
YLL per death averted | ∼ 4.1 | ∼ 4.1 | ∼ 4.1 |
QALYs per death averted | ∼ 4.1 | ∼ 3.5 | ∼ 14.9 |
(1) All big four programmes | £8080 | £9631 | £3036 |
(2) 11 PBCs (with mortality) | £15,628 | £18,622 | £5128 |
(3) All 23 PBCs | £17,663 | £21,047 | £10,187 |
Lower bound | |||
Effect of expenditure on mortality | Remainder of disease duration | Remainder of disease duration | Remainder of disease duration |
YLL per death averted | ∼ 4.1 | ∼ 4.1 | ∼ 4.1 |
QALYs per death averted | ∼ 4.1 | ∼ 3.5 | ∼ 14.9 |
(4) All big four programmes | £3846 | £4252 | £674 |
(5) 11 PBCs (with mortality) | £6106 | £6852 | £860 |
(6) All 23 PBCs | £6901 | £7744 | £1843 |
Upper bound | |||
Effect of expenditure on mortality | 1 year | 1 year | 1 year |
YLL per death averted | 2 | 2 | 2 |
QALYs per death averted | ∼ 2 | ∼ 1.9 | ∼ 7.2 |
(7) All big four programmes | £16,432 | £17,456 | £6292 |
(8) 11 PBCs (with mortality) | £32,387 | £34,492 | £10,626 |
(9) All 23 PBCs | £36,604 | £38,983 | £21,111 |
The estimate of £5128 per QALY [see Table 29, line (2)] is restricted to the effects of changes in expenditure in the 11 PBCs where outcome elasticities can be estimated. Although this might be regarded as more secure, these PBCs only account for a proportion of a change in overall expenditure [approximately 38%, see column (6) in Table 108 in Appendix 3]. The threshold of £10,187 uses the estimated proportionate effects of expenditure on the QALY burden of disease in these PBCs as a surrogate for proportionate effects in the others (i.e. assuming that the effects that can be observed will be similar to those that cannot). As discussed in Using estimates of the quality-adjusted life-years burden of disease there are reasons to suspect that this may underestimate health effects in these PBCs which have most influence on the overall threshold. As in previous sections, no health effects are assigned to PBC 23 (GMS) on the basis that any health effects of this expenditure would be recorded in the other PBCs. aae Therefore, the best or central estimate of cost per QALY threshold is £10,187 [see Table 29, column (3), line (3)]. However, this estimate reflects changes in undiscounted QALYs associated with changes in expenditure. Although all the health effects of a change in expenditure are restricted to 1 year (so no discounting is necessary) some of the QALY effects of a change in mortality in that year will occur in future years, so in principle should be discounted. However, discounting these life-year effects, even at the higher rate of 3.5% recommended by NICE, only increases the cost per QALY threshold to £10,333 (see Table 154 in Appendix 3 for discounted values).
As in previous sections of this chapter, the upper and lower bounds for the cost per QALY thresholds in Table 29, column (3) are based on making the necessary assumptions about duration of health effects and how long a death might be averted optimistic (providing the lower bound for the threshold) or conservative (providing an upper bound for the threshold). The lower bound [see Table 29, lines (4)–(6)] is based on assuming that health effects are not restricted to 1 year but apply to the whole of the remaining disease duration of the population at risk in PBCs during 1 year. Although this combines optimistic assumptions, it is possible that at least some part of a change in expenditure may prevent disease so will have an impact on populations that are incident to PBCs in the future. Such effects are not captured in any of the estimates presented in this chapter so all are conservative with respect to this type of health effects of expenditure. The upper bound [see Table 29, lines (7)–(9)] is based on the combination of assuming that health effects are restricted to 1 year for the population currently at risk and that any death averted is only averted for 2 years (see Summary of cost per life-year estimates).
Chapter 5 Implications for a policy threshold
Introduction
The three sequential steps of analysis, which provide a cost per life-year threshold (see Chapter 4, From mortality to life-years) through a cost per life-year adjusted for quality (see Chapter 4, Adjusting life-years for quality-of-life) to a cost per QALY threshold (see Chapter 4, Including quality-of-life effects during disease), have been explained in Chapter 4 using the analysis of 2006 expenditure and mortality data from 2006 to 2008 (see Instrumental variable estimation in Chapter 3 and Model estimation using 2006/7 expenditure data and mortality data for 2006/7/8: CARAN need and two market forces factors in Appendix 2) to illustrate the implications for the threshold estimates. At each step we explored the different ways that routinely available data could be used and how additional information could improve our estimates. In doing so we identified a preferred analysis at each stage based on which made the best use of available information, whether or not the necessary assumptions appeared more reasonable than the alternatives available, and which provided a more complete picture of the likely health effects of a change in expenditure. Although other assumptions and judgements are possible that retain some level of plausibility, they do not necessarily favour a higher threshold. Indeed, when considered together, they suggest that on balance the central or best estimate presented in Chapter 4 and in Table 30 is, if anything, likely to be an overestimate (see How uncertain are the estimates and what are the implications? for a more detailed discussion and summary). In Future research and improving estimates of the threshold we discuss how some of these remaining uncertainties might be resolved through access to additional and better data and the type of analysis that would then be possible.
PBC grouping | (1) From mortality to life-years analysis | (2) Adjusting life-years for quality-of-life analysis | (3) Including quality- of-life effects during disease analysis |
---|---|---|---|
QoL associated with life extension | 1 | Norm | |
QoL during disease | 0 | 0 | Based on burden |
Best estimate | |||
Effect of expenditure on mortality | 1 year | 1 year | 1 year |
YLL per death averted | ∼ 4.5 | ∼ 4.5 | ∼ 4.5 |
QALYs per death averted | ∼ 4.5 | ∼ 3.8 | ∼ 15.0 |
(1) All big four programmes | £10,220 | £12,338 | £4872 |
(2) 11 PBCs (with mortality) | £23,360 | £28,045 | £8308 |
(3) All 23 PBCs | £25,214 | £30,270 | £12,936 |
Lower bound | |||
Effect of expenditure on mortality | Remainder of disease duration | Remainder of disease duration | Remainder of disease duration |
YLL per death averted | ∼ 4.5 | ∼ 4.5 | ∼ 4.5 |
QALYs per death averted | ∼ 4.5 | ∼ 3.8 | ∼ 15.0 |
(4) All big four programmes | £5083 | £5811 | £1194 |
(5) 11 PBCs (with mortality) | £8579 | £9861 | £1175 |
(6) All 23 PBCs | £9260 | £10,644 | £2018 |
Upper bound | |||
Effect of expenditure on mortality | 1 year | 1 year | 1 year |
YLL per death averted | 2 | 2 | 2 |
QALYs per death averted | ∼ 2 | ∼ 1.4 | ∼ 6.6 |
(7) All big four programmes | £23,346 | £26,138 | £11,040 |
(8) 11 PBCs (with mortality) | £52,936 | £59,151 | £18,827 |
(9) All 23 PBCs | £57,136 | £63,844 | £29,314 |
Re-estimating the cost per quality-adjusted life-year threshold using more recent data
The same methods of analysis can be applied to the econometric analysis of the 2008 expenditure and 2008 to 2010 mortality data (see 2008/9 expenditure data and mortality for 2008/9/10 in Chapter 3 and The correlation between the outcome and expenditure elasticities in Appendix 2). The differences between the 2006 analysis reported in Chapter 4 and the analysis of expenditure in 2008 reported below are the (i) total PBC expenditure; (ii) estimated expenditure elasticities; (iii) estimated outcome elasticities; (iv) observed PBC deaths by age and gender; and (v) LE by age and gender. The other information about QoL norms (see Chapter 4, Quality of life based on the general population), disease-related decrements in QoL (see Chapter 4, Adjusting age-related quality-of-life for disease decrements) and the information from GBD about incidence and duration of disease remain unchanged between 2006 and 2008 (we discuss how these estimates might be improved through access to more recent and better data in Future research and improving estimates of the threshold).
It should be noted that important improvements were made to the classification and collection of PBC expenditure data that took place after the 2006 data were collected. Therefore, the differences in threshold estimates between 2006 and 2008 partly reflect this (see Comparing the cost of life-year estimates associated with different data sets in Chapter 3 and Comparing the cost of life-year estimates associated with different data sets in Appendix 2) so should not be overinterpreted. The results of the analysis of 2007 and 2008 expenditure are comparable in this respect, providing insights into how the threshold might change over time and with changes in the overall budget. The implications of this analysis on the need for periodic reassessment are discussed in How does the threshold change with overall expenditure? For the purposes of this methodological research the 2008 expenditure and 2008–10 mortality data were the latest to be analysed. As it is the analysis of the most recent data that is of most policy relevance, our discussion throughout this section is based on analysis of 2008 expenditure, although the same sensitivity analysis (see Which programme budget categories matter most?) and analysis of uncertainty (see How uncertain are the estimates and what are the implications?) is available for 2006 and 2007 expenditure (see How uncertain are the estimates? in Appendix 3).
It is unnecessary to repeat all the analysis presented in Chapter 4, From mortality to life-years, Adjusting life-years for quality-of-life and Including quality-of-life effects during disease (the details of each stage of the analysis of 2008 data can be found in Appendix 3). Instead the results of the three sequential steps of analysis are summarised in Table 30. They include (i) the cost per life-year [see Table 30, column (1)],a based on the methods of analysis outlined in Chapter 4, From mortality to life-years; (ii) the cost per life-year adjusted for QoL [see Table 30, column (2)],b based on the methods of analysis outlined in Chapter 4, Adjusting life-years for quality-of-life; and (iii) the cost per QALY [see Table 30, column (3)], based on the methods of analysis outlined in Chapter 4, Using estimates of the quality-adjusted life-year burden of disease. These estimates, in Table 30, column (3), take account of the likely effects of changes in expenditure on QoL during disease as well as the effects associated with mortality and life-years; making best use of available information, while the assumptions required appear more reasonable than the other alternatives available. For this reason these estimates remain our central or best estimates for all the waves of expenditure and mortality data.
The estimate of £8308 per QALY [see Table 30, column (3), line (2)] is restricted to the effects of changes in expenditure in the 11 PBCs where outcome elasticities can be estimated. However, these PBCs only account for a proportion of the change in overall expenditure (approximately 50%, Table 31). As was explained in Chapter 4, Using estimates of the quality-adjusted life-year burden of disease and Summary of the cost per quality-adjusted life-year threshold, the QALY threshold of £12,936 [see Table 30, column (3), line (3)] uses the estimated proportionate effects of expenditure on the QALY burden of disease in the 11 PBCs as a surrogate for proportionate effects in the others (i.e. assuming that the effects that can be observed will be similar to those that cannot), and represents our central or best estimate. As in previous sections, no health effects are assigned to PBC 23 or 22 (GMS and social care) on the basis that any health effects of this expenditure would be recorded in the other PBCs. c Although this estimate of £12,936 reflects changes in undiscounted QALYs associated with changes in expenditure, discounting the QALY effects only increases the cost per QALY threshold to £13,141. d
PBC | (1) % Share of change in overall expenditure | (2) % Share of total health effects (QALY) | (3) Elasticity of the thresholda | (4) PBC cost per QALY (£) | |
---|---|---|---|---|---|
2 | Cancer | 4.47 | 3.41 | 0.34 | 16,997 |
10 | Circulatory | 7.59 | 13.95 | 1.40 | 7038 |
11 | Respiratory | 4.58 | 29.67 | 2.97 | 1998 |
13 | Gastrointestinal | 3.20 | 5.68 | 0.57 | 7293 |
1 | Infectious diseases | 3.27 | 2.03 | 0.20 | 20,829 |
4 | Endocrine | 1.89 | 7.84 | 0.78 | 3124 |
7 | Neurological | 5.98 | 14.11 | 1.41 | 5480 |
17 | Genitourinary | 4.64 | 1.37 | 0.14 | 43,813 |
16 | Trauma and injuries | 7.70 | 0 | 0 | N/A |
18 + 19 | Maternity and neonates | 6.83 | 0.03 | < 0.01 | 2,969,208 |
3 | Disorders of blood | 2.06 | 2.82 | 0.28 | 9419 |
5 | Mental health | 17.86 | 12.32 | 1.23 | 18,744 |
6 | Learning disability | 1.04 | 0.09 | 0.01 | 149,883 |
8 | Problems of vision | 1.94 | 0.55 | 0.05 | 45,788 |
9 | Problems of hearing | 0.87 | 1.81 | 0.18 | 6239 |
12 | Dental problems | 2.89 | 0.88 | 0.09 | 42,472 |
14 | Skin | 1.97 | 0.25 | 0.03 | 101,042 |
15 | Musculoskeletal | 3.63 | 3.00 | 0.30 | 15,628 |
20 | Poisoning and adverse events | 0.93 | 0.11 | 0.01 | 113,546 |
21 | Healthy individuals | 3.53 | 0.09 | 0.01 | 526,771 |
22 | Social care needs | 3.00 | 0 | 0 | N/A |
23 | Other | 10.14 | 0 | 0 | N/A |
The upper and lower bounds for the cost per QALY thresholds in column (3) in Table 30 are based on making the necessary assumptions about duration of health effects of expenditure and how long a death might be averted optimistic (providing the lower bound for the threshold) or conservative (providing an upper bound for the threshold). The lower bound [see Table 30, lines (4)–(6)] is based on assuming that the health effects of expenditure are not restricted to 1 year but apply to the whole of the remaining disease duration of the population at risk in PBCs during 1 year. Although this combines optimistic assumptions, it is possible that at least some part of a change in expenditure may prevent disease so will have an impact on populations that are incident to PBCs in the future. Such effects are not captured in any of the estimates presented in this report so all estimates are conservative in this respect (the possibility of a longer and more complex lag structure for the effects of expenditure are discussed in Future research and improving estimates of the threshold). The upper bound [see Table 30, lines (7)–(9)] is based on the combination of assuming that health effects are restricted to 1 year for the population currently at risk and that any death averted is only averted for 2 years (see Chapter 4, Summary of cost per life-year estimates).
The estimated QALY effects associated with each PBC can be decomposed into that part due to life-year effects adjusted for quality and that part associated with effects on QoL during disease. The proportionate share of these different aspects of the total health effects are the same as reported in Table 28; where those PBCs for which mortality is the major concern have a much greater share of total QALY effects associated with avoidance of premature death (e.g. PBC 2 and PBC 10) than those where QoL is the major concern (e.g. PBC 7).
Which programme budget categories matter most?
Which PBCs have the greatest influence on the overall threshold depends, to a large extent, on how a change in overall expenditure is allocated to the different PBCs [see column (1) in Table 31],e i.e. those that account for a greater share of the change in expenditure will tend to have the greater influence. However, it also depends on the proportionate effect of a change in PBC expenditure on the QALY burden associated with the PBCf and the scale of the QALY burden (for the population at risk) associated with the type of diseases that make up each PBC. g These determine the cost per QALY associated with each PBC [see Table 31, column (4) and Table 180 in Appendix 3]. The share, attributable to each PBC, of the total health effects of a change in overall expenditure [see column (2) of Table 31] is the combined effect of all of these. The proportionate impact on the overall cost per QALY threshold of a 10% change in PBC health effects in Table 31, column (3) gives an indication of how sensitive the overall threshold is to the estimate of health effects associated with each PBC. It starts to suggest where further efforts to improve estimates of the overall threshold might be most usefully directed.
Although the 11 PBCs where outcome elasticities could be estimated only account for 50% of the change in overall expenditure, they account for 78% of the overall health effects. Within this group some PBCs contribute more than others. For example, PBC 11 (respiratory) accounts for a greater share of total health effects and has a higher elasticity (2.97%) than PBC 10 (circulatory) even though the latter accounts for a greater part of a change in overall expenditure. The reason is that the cost per QALY associated with changes in expenditure in PBC 11 is lower than PBC 10 and much lower than the overall threshold (so generates more health effects for the same, or even smaller, change in expenditure). h The elasticities in Table 31, column (3), are instructive, for example the elasticity for PBC 11 suggests that even if the health effects of a change in expenditure in this PBC were overestimated by 30% the overall threshold would increase by 8.90% to £14,089. All other PBCs have much less influence in this respect. Nonetheless, PBC 10 is important compared with others as it does contribute a large share of total health effects and has one of the highest elasticities (1.40%). i Also PBC 7 (neurological), although accounting for a smaller share of a change in overall expenditure, does contribute a large share of total health effects with an elasticity of 1.41% and a relatively low cost per QALY associated with changes in PBC expenditure. j
The other 11 PBCs, where outcome elasticities could not be estimated (excluding PBC 23, GMS) account for a large part of a change in overall expenditure (40%) but only 22% of the overall health effects (i.e. the cost per QALYs associated with a change in expenditure in these PBCs is, in general, higher). Of course, we have not directly observed QoL effects in these PBCs but inferred them from the proportionate effects that we can observe. Insofar as investment and disinvestment opportunities in these PBCs might have been more valuable (i.e. offered greater improvement in QoL) than suggested by the implied PBC thresholds in Table 31, column (4), the overall QALY effects will tend to be underestimated and the overall cost per QALY threshold will be overestimated.
Programme budget category 5 (mental health disorders) accounts for a large proportion of the change in overall expenditure (18%), contributes most to the overall health effects (12%) and has the highest elasticity (1.23%) compared with these other PBCs. The cost per QALY associated with this PBC (£18,744) is based on an extrapolation of estimated proportionate effects to a population-based measure of QALY burden in this PBC, rather than observations of the direct impact of changes in expenditure on QoL in the types of diseases that make up the PBC. Evidence that is available suggests that the investment and disinvestment opportunities in this PBC may have been more valuable than this implied cost per QALY. A review of the evidence of the cost-effectiveness of the investment and disinvestment opportunities that have been available in mental health during this period is reported in Appendix 3, Addendum 3: characterisation of the investment and disinvestment decisions in mental health – depression and schizophrenia. A search for evidence about interventions in those ICD-10 codes that contribute most to the PBC (based on prevalence or the contribution to the variance in PBC costs), suggests that pharmacological, psychological and social interventions for depression are all more cost-effective (in general much < £10,000 per QALY) than the overall threshold, and significantly more valuable than the implied QALY threshold for this PBC. Based on the contribution that each ICD-10 code makes to variance in HES costs across PCTs, it is schizophrenia that contributes most. k Although interventions that may have been invested or disinvested in in schizophrenia are, in general, less cost-effective (in general < £24,000 per QALY) than those available for depression, they do not appear any less valuable than the implied cost per QALY of this PBC in Table 31. l
It is very important not to misinterpret the cost per QALY associated with each PBC in column (4) of Table 31. These are not cost-effectiveness thresholds. That is, they do not represent the QALYs likely to be forgone due to costs imposed (e.g. by the approval of a new and more costly technology by NICE) in a particular PBC because NHS expenditure is not devolved and constrained to PBC specific budgets. Rather, the overall expenditure is constrained through government decisions about public expenditure, but within the NHS resources (at the margin at least) can be reallocated in anything other than the very short run across different activities and disease areas. For example, the additional net NHS costs of approving a new but more costly technology in PBC 10 (circulatory) will not be restricted to the circulatory PBC [7.6% will, see column (1) in Table 31], but are likely to be reallocated in the same way as an equivalent reduction in overall expenditure [i.e. the shares of a change in overall expenditure in Table 31, column (1)]. m Therefore, the relevant cost per QALY threshold for a technology in the circulatory PBC is not £7038 but the overall threshold of £12,936.
The primary purpose of Table 31 is to identify which PBCs have greatest influence on the estimate of the overall threshold and examine whether the implied values for the other PBCs are likely to lead to under- or overestimation. There are differences in the implied cost per QALY ratio between PBCs, including some with very high implied cost per QALY (e.g. PBCs 18 + 19, 20 and 21 reflecting small estimated health effects in the denominator), although they have limited influence on the overall estimate of the threshold. These differences in the implied cost per QALY across PBCs should not be overinterpreted. For example, these differences could be interpreted as evidence of a misallocation of resources (e.g. reallocating expenditure from PBCs with higher to lower cost per QALY would improve health) if the purpose of the NHS and PCTs is to maximise unweighted QALYs. However, rather than a misallocation, these differences (between the first 11 PBCs) might indicate that the actual QoL effects of expenditure are proportionally greater (lower) than mortality effects in those with a higher (lower) cost per QALY, or that the health effects in these PBCs are more socially valuable with a greater implicit weight attached to QALYs gained or lost in these areas (e.g. maternity and neonates). The higher cost per QALY for the remaining PBCs may reflect that the actual QoL effects of changes in expenditure may be more than proportional to QALY burden (e.g. evidence from mental health PBC suggests that investment and disinvestment opportunities may have been more valuable than the implied PBC cost per QALY of £18,744). Additionally, it was not possible to estimate the health effects of changes in PBC expenditure simultaneously across PBCs. Consequently, the effects of changes in expenditure in one PBC may be recorded in ICD-10 codes relevant to other PBCs, so it is possible that PBCs with a higher implied cost per QALY may be contributing health effects to other (recipient) PBCs. n
Whether or not these differences are regarded as evidence of a misallocation, however, is unimportant for an estimate of a cost per QALY threshold that reflects the health effects of how changes in overall expenditure are currently expected to be allocated. Whether or not PCTs do or should maximise QALYs has no influence on the current estimate of the threshold, given that NICE currently uses an unweighted QALY threshold. o In addition, insofar as local objectives do change or national policy does reallocate expenditure, the impact of these and other changes that will take place over time will be reflected in estimates of the threshold in subsequent periods once these changes have taken place (see How does the threshold change with overall expenditure?).
How uncertain are the estimates and what are the implications?
There are a number of sources of uncertainty which contribute to an assessment of how uncertain a central or best estimate of the cost per QALY threshold might be. There are three reasons why uncertainty in the estimate of the threshold might be of policy interest: (i) the uncertainty in the parameters that determine the threshold might influence the mean or expected value of the threshold if they have a non-linear relationship to the threshold or when they have a multilinear relationship but are correlated with each other; (ii) the consequences of over- or underestimating the threshold differ so the uncertainty may have an influence on the extent to which a policy threshold (a single value that can be compared to the ICER of a new technology) should differ from the mean or expected value of the central or best estimate; and (iii) in conjunction with other methods of analysisp,95 it can indicate the potential value of gathering more information to improve these estimates in the future. Of course, hypothesis testing and the traditional rules of inference associated with it, such as statistical significance, p-values and confidence intervals, have no relevance when making unavoidable decisions about policy relevant quantities based on information currently available and the best use thereof. 96
An assessment of parameter uncertainty
Two sets of parameters are critical to the threshold, the expenditure elasticities estimated for each of the 23 PBCs, and the outcome elasticities estimated for 11 of these. These parameters are estimated with uncertainty, indicated by the standard errors on the relevant coefficients in the econometric analysis outlined in Chapter 3 and detailed in Appendix 2. As these statistical models estimate coefficients using normality on the relevant scale, normal distributions can be assigned to each of these estimated coefficients, each with a mean and standard deviation based on the results of the econometric analysis. q These distributions, represent the uncertainty in the mean estimate of each of the parameters and can be propagated through the various calculations required to estimate an overall cost per QALY threshold (i.e. through the sequence of analysis detailed in Chapter 4, From mortality to life-years, Adjusting life-years for quality-of-life and Including quality-of-life effects during disease) using Monte Carlo simulation which randomly samples from the assigned distributions. The results of each random sample represent one possible realisation of the overall threshold, given the uncertainty in estimates of the mean parameter values that determine it. By repeatedly sampling, a distribution of potential values that the overall threshold might take can be revealed. The results of this simulation are illustrated in Figure 5 which shows the cumulative probability density function for a cost per QALY threshold based only on the 11 PBCs with estimated outcome elasticities and for all 23 PBCs. It represents the probability (on the y-axis) that the threshold lies below a particular value.
FIGURE 5.
Cumulative probability density function for the cost per QALY threshold.

It has already been noted that restricting attention only to changes in expenditure in those 11 PBCs where an outcome elasticity can be estimated results in a much lower estimate of the threshold than considering all changes in expenditure across all PBCs This lower estimate of £8308 per QALY is much less uncertain but these PBCs only account for 50% of a change in overall expenditure, so it is the higher estimate, for all 23 PBCs, that is of most relevance for policy (see Re-estimating the cost per quality-adjusted life-year threshold using more recent data and Chapter 4, Summary of the cost per quality-adjusted life-year threshold). The fact that this estimate is more uncertain simply reflects the quality and quantity of data currently available. As useful analysis should endeavour to faithfully characterise uncertainty in policy relevant quantities, rather than select those quantities or questions for which precise estimates are possible, it is the more uncertain estimate for all 23 PBCs that should be of primary interest. The values that are used to generate Figure 5 are available in Table 183 in Appendix 3. They indicate that the probability that the overall threshold is < £20,000 per QALY is 0.89 and the probability that it is < £30,000 is 0.97.
The implications of uncertainty
Integrating this parameter uncertainty into the estimates of the overall threshold does not change the mean or expected value of the cost per QALY threshold. r This is to be expected as the expenditure and outcome elasticities have a multilinear relationship to the overall threshold and the analysis sampled independently from the distributions assigned to estimated coefficients. We did investigate the potential correlation between the expenditure and outcome elasticities by repeatedly re-estimating both based on randomly sampling with replacement the 152 PCTs – creating bootstrapped data sets where the original PCTs could appear more than once or not at all in the re-sampled data. This analysis indicated a small positive correlation between outcome and expenditure elasticities in four PBCs using 2006 expenditure data (see Analysis of programme budgeting expenditure for 2007/8 and mortality data for 2007/8/9 in Appendix 2). Such levels of correlation will tend to have a modest but positive influence on the mean value of the cost per QALY threshold. s
Uncertainty in the estimate of the overall threshold means that a policy threshold set at its mean or expected value may be inappropriate. Insofar as the consequences (to the NHS) of under- or overestimation are symmetrical, then the expected or mean value would be the appropriate policy threshold irrespective of the scale of uncertainty. However, the consequences of overestimating the threshold are more serious than underestimating it. This is illustrated in Figure 6 which is similar to Figure 1 presented in Chapter 2.
FIGURE 6.
Consequences of over- and underestimating the overall threshold.

Figure 6 shows the impact on NHB if the central estimate of £20,000 is in fact an overestimate and the threshold should be £10,000 per QALY. In these circumstances the technology should not have been approved at price P2. This overestimation leads to a loss of NHB of 2 QALYs as a consequence. Alternatively, the central estimate of £20,000 may be an underestimate and the threshold should be £30,000 per QALY. In these circumstances the technology could just as easily have been rejected or approved based on the central estimate and price P2. However, if the threshold is £30,000 per QALY rather than £20,000 it should be approved. If it was rejected, this underestimation leads to a loss of NHB of two-thirds of a QALY as a consequence (i.e. less than the loss associated with the same scale of overestimation). If the scale of under- or overestimation of the central estimate is equally likely (the distribution of possible values of the threshold is symmetrical) then using the mean or expected value as a policy threshold (one that can be compared with the ICER of a new technology) will lead to a loss of net benefit. t A policy threshold that represents the maximum the NHS can afford to pay for QALY gains offered by a technology will be lower than the mean of the cost per QALY threshold (i.e. < £12,936) to compensate for the more serious consequences of overestimating the ‘true’ value. u Importantly, this remains the case even if effects are expressed in terms of their equivalent consumption value (net monetary benefit based on WTP) rather than a measure of NHB. v
How much lower a policy threshold should be set below the mean or expected value depends on three considerations: (i) the scale of uncertainty in the estimate of the threshold (greater uncertainty implies a lower policy threshold); (ii) the scale of the incremental costs relative to incremental health benefits offered by the technology (policy threshold should only be equal to mean estimate if there are no additional NHS costs associated with the technology); and (iii) the skewness of the distribution of cost per QALY threshold (a negative skew tends to offset these effects – see Figure 52 in Appendix 3). The overall scale of the impact on a policy threshold will be specific to the additional NHS costs associated with a technology as well as the other sources of uncertainty, discussed below, and possible correlations between expenditure and outcome elasticities, discussed above. We have not quantitatively integrated all these considerations into an analysis of an appropriate policy threshold, although this may be possible in future research.
Structural uncertainty
The uncertainty associated with the parameters estimated in the econometric models is only one, and not necessarily the most important, source of uncertainty associated with the cost per QALY threshold. The parameter uncertainty presented above is conditional on the econometric model being ‘correct’. In particular, that the instruments used to identify the causal effect on health of changes in expenditure are valid. Although all the models passed the relevant tests of validity, there remains some uncertainty about the validity of the instruments used, i.e. there remains structural or model uncertainty (see Chapter 3 for an overview). 97 For this reason we undertook an analysis of how sensitive estimates of outcome elasticities might be to instrumental validity (see The identification of values to be imposed on the coefficients on the excluded instruments in Appendix 2). We were also able to specify a distribution for the measure of instrumental validity used in this sensitivity analysis, i.e. how ‘likely’ each value might be (see Obtaining the outcome elasticities associated with sampled coefficients on the excluded instruments in Appendix 2). Therefore, there are two ‘levels’ of uncertainty: (i) the parameter uncertainty (uncertainty in estimated coefficients given a particular ‘level’ of instrumental validity); and (ii) the structural uncertainty in the level of instrumental validity. Both sources of uncertainty were integrated by randomly sampling the distribution of measures of instrumental validity and then, conditional on this sampled value, re-estimating outcome equations and sampling the estimated coefficients. This analysis in Obtaining the outcome elasticities associated with sampled coefficients on the excluded instruments of Appendix 2 shows that model or structural uncertainty constitutes a greater part of the overall uncertainty associated with the outcome elasticities, so fully integrating this source of uncertainty is likely to have a significant impact on the extent to which a policy threshold should be lower than the mean or expected value of the cost per QALY threshold. Importantly, this additional structural uncertainty has little effect on the point estimates of the outcome elasticities, i.e. the central estimate of the cost per QALY threshold is robust to uncertainty in instrumental validity in the econometric models. w
Other sources of uncertainty
Of course the parameter and structural uncertainty associated with the econometrics work outlined in Chapter 3 is itself only one source of uncertainty associated with the estimated cost per QALY threshold. Each of the steps of analysis in Chapter 4, From mortality to life-years, Adjusting life-years for quality-of-life and Including quality-of-life effects during disease explored the different ways routinely available data could be used and how additional information could improve the estimates. We identified a preferred analysis (or scenario) at each stage based on which made the best use of available information, whether or not the assumptions required appeared more reasonable than the other alternatives available, and which provided a more complete picture of the likely health effects of a change in expenditure. Insofar as the preferred analysis is the only plausible scenario, there would be no other sources of uncertainty. However, other assumptions and judgements are possible, which although they may be judged less credible might, nonetheless, have some probability of being the most credible (given the evidence currently available). Therefore, there will be uncertainty between these alternative ‘scenarios’ as well as within each (the parameter and model uncertainty described above). 95 Although in principle this can be integrated into the analysis even in the absence of data to test alternative views,98 we do not do so here as assigning probabilities to alternative scenarios would be somewhat speculative and inevitably disputed. Instead we offer a summary of the qualitative considerations. Of course any increase in the uncertainty associated with the central estimate of the cost per QALY will impact on the extent to which a policy threshold should be lower than the mean. However, a critical issue is whether or not consideration of other ‘scenarios’ might change this central estimate (e.g. if scenarios that lead to a lower estimate are judged more credible than those that lead to higher ones). In other words the question is whether on balance the central or best estimate of £12,936 in Table 30 is likely to be an under- or overestimate of the cost per QALY threshold.
Most of the considerations have been discussed in detail throughout Chapter 4 so are only briefly summarised here. The key assumptions made in Chapter 4 that underpin the central estimate of the cost per QALY threshold reported in Table 30 are briefly summarised in Table 32, including a brief indication why such an assumption was required, the likely qualitative effect that each is likely to have on estimates for the health effects of changes in expenditure and where these are introduced in Chapter 4.
Assumption | Justification | Likely impact on estimates | Reference |
---|---|---|---|
1. Deaths averted by a change in expenditure returns an individual to the mortality risk of the general population (matched for age and gender) | No data to directly estimate the effects on survival. Appears more credible than restricting life-year effects of changes in mortality to the period of observed variation in mortality outcomes | Overestimate health effects | Chapter 4, Introduction and Summary of cost per life-year estimates Chapter 4, notes b and w, and Chapter 5, note ak |
2. Expenditure and outcome elasticities are uncorrelated | Expenditure and outcome equations were estimated separately | If the small but positive correlation between outcome and expenditure elasticises found in four PBCs was applied to all PBCs it is likely to have a modest but positive impact on the expected value of the threshold | How does the threshold change with overall expenditure? Chapter 5, note u |
3. Mortality effects of changes in expenditure (reported at PCT level) can be applied to all mortality recorded in a PBC | Assuming no health effects of expenditure in areas of disease where mortality is not recorded at PCT level or in over 75-years age groups appears arbitrary and less plausible than basing estimates of effects that cannot be observed on what can | Although including persons aged over 75 years mortality may overestimate the effects on observed PBC deaths (if mortality in older ages groups is less sensitive to changes in expenditure) it has a much more limited impact on life-year effects (i.e. including mortality above LE reduces net YLL) | Chapter 4, Mortality and years of life lost coverage, Life expectancy and years of life lost, Years of life lost and accounting for counterfactual deaths and Inferring excess deaths Chapter 4, notes b, q and s |
4. The PBC QALY effects are a weighted average of effects within each of the ICD-10 codes that contribute to the PBC based on the proportion of the total PBC population within each contributing ICD-10 code | PBC costs are not available at ICD-10 level across PCTs. Although costs from HES data are available at ICD-10 level they are only a small component of total PBC costs and contribute very little to the variability in PBC costs across PCTs, especially when considering PBCs where mortality effects could not be estimated | There is no information about how changes in PBC expenditure are allocated to particular ICD-10 codes so the effect is unclear. However, it may overestimate health effects if investment within a PBC is focused on ICD-10 codes where expenditure has greater health effects and disinvestment focuses on ICD-10 codes with less health effects | Chapter 4, Using ratios of quality-adjusted life-years to years of life lost and Using estimates of the quality-adjusted life-year burden of disease Chapter 4, notes an and au, and Chapter 5, note ai |
5. Health effects of changes in expenditure are restricted to the population at risk during 1 year | It was not possible to estimate a longer and more complex lag structure. Assuming that estimated health effects could be applied to the whole remaining duration of disease for the population at risk appears less plausible | Underestimate health effects | Chapter 4, Introduction, Summary of cost per life-year estimates, Summary of the cost per quality-adjusted life-year threshold and Future research and improving estimates of the threshold Chapter 4, note a, and Chapter 5, notes aj and ao |
6. Health effects restricted to the PBC in which expenditure changes. No health effects associated with changes in GMS expenditure (or PBC 22, social care) | It was not possible to estimate outcome equations for PBCs simultaneously so estimated outcome elasticities do not account for health effects due to changes in expenditure in other PBCs | Likely to underestimate health effects because effects of changes in expenditure in ‘contributory’ PBCs will not be reflected in estimates of health effects in other (recipient) PBCs unless they happen to be correlated with changes in expenditure in these PBCs | Chapter 4, Years of life lost and accounting for counterfactual deaths and Summary of cost per life-year estimates, Which programme budget categories matter most? and Future research and improving estimates of the threshold Chapter 4, notes c, m and u, Chapter 5, notes b, e, p, an and ar |
7. Same proportional effect on QALY burden of disease as the estimated proportional effect on the life-year burden of disease | Estimates of effects on mortality and life-years are used as a surrogate for effects on QoL. Appears more plausible than assuming no effects of NHS expenditure on QoL outcomes | May underestimate the QoL effects of changes in expenditure in these PBCs if effects are more than proportional to mortality and life-year effects or overestimate them if they are less than proportional | Chapter 4, Summary of the cost per quality-adjusted life-year threshold based only on mortality effects and Using estimates of the quality-adjusted life-year burden of disease Chapter 4, note ad |
8. Life-year effects are lived at a QoL that reflects a proportionate improvement to the QoL with disease | Consistent with using estimated mortality and life-year effects as a surrogate for a more complete measure of health outcome (QALYs). Appears more plausible than assigning QoL norms or a QoL with disease to life-year effects | This assumption is more conservative than assigning QoL norms to life-years (assuming that all disease is acute), but less conservative than assigning QoL with disease (assuming that all life-years would be lived in the diseased state until death) | Chapter 4, Summary of the cost per quality-adjusted life-year threshold based only on mortality effects and Using estimates of the quality-adjusted life-year burden of disease Chapter 4, notes ad and ap |
9. Proportional effect on QALY burden of disease in PBCs where mortality effects could not be estimated is assumed to be the same as the overall proportional effect on the life-year burden of disease across those PBCs where mortality effects could be estimated | Consistent with using estimated mortality and life-year effects as a surrogate for health effects (QALYs) where mortality effects cannot be directly estimated. Appears more plausible than assuming no health effects of NHS expenditure in these PBCs | May underestimate the QALY effects of changes in expenditure in these PBCs if effects are more than proportional to QALY burden of disease. Other evidence suggests that the effect of this assumption may be to underestimate health effects in key PBCs (mental health) | Chapter 4, Using estimates of the quality-adjusted life-year burden of disease, Summary of the cost per quality-adjusted life-year threshold and Which programme budget categories matter most? Chapter 4, notes aw and ax, Chapter 5, notes g, al, am |
On the one hand, there are some reasons why the health effects might be overestimated and the central estimate of the QALY threshold underestimated (e.g. see assumptions 1–4 in Table 32). Calculating the life-years lost that account for deaths that would have otherwise occurred as described in Chapter 4, Years of life lost and accounting for counterfactual deaths and Inferring excess deaths is equivalent to assuming that those deaths averted by a change in expenditure returns the individuals to the mortality risk of the general population (matched for age and gender). Although this appears more credible than the alternative assumptions that could be made (e.g. restricting life-year effects of changes in mortality to the period of observed variation in mortality outcomes), it is likely to be optimistic with respect to the life-year effects of changes in mortality, tending to underestimate the cost per QALY threshold.
On the other hand there are a number of reasons why the central estimate might be overestimated (e.g. see assumptions 5 and 6 in Table 32). The health effects of a change in expenditure are restricted to the population at risk during 1 year. This is undoubtedly pessimistic in three respects: (i) it means that effects on QoL during disease only occur for 1 year (the effect of investment that might have long-term effects on QoL, e.g. hip replacement are excluded); (ii) mortality effects are also restricted to 1 year, so the full effect of investments that reduce mortality for patients throughout their disease duration, not just in the first year, will not be captured; and (iii) changes in expenditure that reduce incidence in the at risk population in the future (i.e. prevention of disease) will not be captured either. A more formal and longer lag structure in the estimation of outcome elasticities would be likely to capture more health effects of a change in expenditure.
The effect of other assumptions that have been necessary are more ambiguous although some evidence suggests their net effect may be conservative with respect to health effects of changes in expenditure (e.g. see assumptions 7–9 in Table 32). The observed effects of a change in expenditure on mortality and life-years in the 11 PBCs where outcome elasticities could be estimated was used as a surrogate for health effects in the other 12 PBCs (excluding GMS), i.e. the estimated effects of a change in expenditure that could be observed were used to inform those effects that currently, at least, cannot. This approach is not necessarily optimistic with respect to overall health effects. In fact, there are reasons to believe it may underestimate them (overestimate the threshold). As discussed previously in Chapter 4, Summary of the cost per quality-adjusted life-year threshold and Re-estimating the cost per quality-adjusted life-year threshold using more recent data, if this means of extrapolating from observed to unobserved effects is rejected then threshold estimates could be based only on the health effects of changes in expenditure in those PBCs where outcome elasticities can be estimated. This generates a much lower cost per QALY threshold (£8308) even if that portion of GMS expenditure was allocated to these 11 PBCs (see Chapter 4, Summary of cost per life-year estimates). Alternatively, taking account of the large proportion of the change in expenditure allocated to the other 11 PBCs but assuming that there are no health effects of expenditure in all these other PBCs is not plausible. The evidence that is available about the value of investment and disinvestment opportunities in the most important of these other PBCs (PBC 5 mental health disorders), suggests that the health effects of changes in expenditure in this PBC might be underestimated and the central estimate of the threshold overestimated (see Which programme budget categories matter most? and Addendum 3: characterisation of the investment and disinvestment decisions in mental health – depression and schizophrenia in Appendix 3).
In addition, we have also shown that the uncertainty associated with our central estimate (from all sources) means that an appropriate policy threshold is likely to be below its mean or expected value. Finally, in Impact of investment, disinvestment and non-marginal effects we explore how the threshold is likely to differ when considering opportunities to make investments (i.e. an increase in overall expenditure, or cost saving accruing to the NHS), and when disinvestment is required (a reduction in overall expenditure or costs imposed on the NHS). This analysis shows that a cost per QALY threshold relevant to technologies which impose costs on the NHS is likely to be less than our central estimate of £12,936. Therefore, although other assumptions and judgements are possible that retain some level of plausibility, they do not all favour a higher threshold. Indeed, when considered together, they suggest that on balance the central or best estimate of £12,936 presented in Table 30 is, if anything, likely to be an overestimate. In Future research and improving estimates of the threshold we discuss how some of these remaining uncertainties might be resolved through access to additional and better data and the type of analysis that would then be possible.
Impact of investment, disinvestment and non-marginal effects
The central estimate of the cost per QALY threshold in Table 30 is based on estimates of the health effects of changes in expenditure across all 152 PCTs, some of which will be making investments (where expenditure is increasing) and others making disinvestments (where expenditure is reduced or growing more slowly). The cost per QALY threshold, however, is likely to differ across these different types of PCTs. This is illustrated in Figure 7 where the total observed variation in expenditure includes the impact of disinvestment (–ΔE) (e.g. where costs are imposed on the NHS by the approval of a more costly technology) and investment (ΔE) (e.g. where cost savings are accruing to the NHS). The central estimate of the cost per QALY threshold is the health effect of a change in expenditure across this variation in expenditure (k1). x One would expect that, other things being equal, more expenditure (expanding the budget from B1) would increase health but at a diminishing rate. Therefore, the amount of health displaced by disinvestment, or a reduction in expenditure, would be expected to be greater, i.e. the threshold associated with –ΔE (k1–) will be lower than the central estimate, k1. Equally, the health gained from investments, or an increase in expenditure, would be expected to be lower, that is the threshold associated with +ΔE (k1+) will be higher than k1.
FIGURE 7.
Investment, disinvestment and budget impact. –ΔE, disinvestment; +ΔE, investment; B1, current budget; H1, current level of health; k1, cost per QALY threshold; k1–, QALY threshold associated with disinvestment; k1+, QALY threshold associated with investment.

We have been able to examine this by re-estimating the outcome and expenditure elasticities separately for those PCTs where their actual budget is under the target allocation from the Department of Health resource allocation formula (i.e. those under greater financial pressure and more likely to be disinvesting than investing), and those that are over target (under less financial pressure and more likely to be investing than disinvesting). The detail of this analysis (based on 2006 expenditure and restricted to the ‘big four′ PBCs) are reported in Comparing outcome models for ‘high’ spending and ‘low’ spending primary care trusts in Appendix 2. The results confirm what would be expected given Figure 7 and the discussion above – the outcome elasticities are smaller (in absolute terms) for all four PBCs in the group of PCTs above their target allocation and larger for all four PBCs below their target allocation. Therefore, the health effects of changes in expenditure are greater in all these PBCs when PCTs are under more financial pressure and are more likely to be disinvesting then investing. The cost per life-year estimates for these PBCs are reported in Appendix 2: £10,604 for all PCTs combined (k1); £8441 for those PCTs under their target allocation (i.e. k1– associated with –ΔE); and £14,083 for PCTs over their target allocation (i.e. k1+ associated with +ΔE). Although these cost per life-year estimates are not based on the same calculations as Chapter 4, From mortality to life-years, they do start to indicate the scale of the effect on a threshold that is most relevant for new technologies that impose net costs on the NHS.
Expenditure elasticities for these PBCs also differ between these groups of PCTs – they are higher for those under their target allocation. These PBCs together consistently offer the greatest value in terms of cost per death averted, life-year or QALY (see Tables 30 and 31). This suggests that budget impact not only displaces more valuable activities within each PBC (outcome elasticities are larger), but that overall expenditure tends to be reallocated to more valuable PBCs. The effect of this reallocation on the overall threshold is not captured in the cost per life-year estimates reported above, which are restricted to these four PBCs. Therefore, extending this type of analysis to all PBCs in future research is likely to show that the effect on the cost per QALY threshold of both the sign and scale of changes in overall expenditure will be greater. Subsequent work might enable a quantitative assessment of how the relevant threshold should be adjusted for the scale of the budget impact of technologies appraised by NICE.
Although further work is needed to fully specify the quantitative effect of the scale of the non-marginal impact of new technologies on an appropriate threshold, the qualitative impact seems clear. First, the central estimate of the threshold is likely to be an overestimate for all technologies which impose net costs on the NHS (almost all technologies appraised by NICE have positive incremental NHS costs). 12,99,100 Second, the appropriate threshold to apply should be lower for technologies which have a greater impact on NHS costs.
How does the threshold change with overall expenditure?
The same methods of analysis can be applied to the econometric analysis of the 2007 expenditure and 2007–9 mortality data (see Analysis of programme budgeting expenditure for 2007/8 and mortality data for 2007/8/9 in Appendix 2). This provides an opportunity to consider how the cost per QALY threshold is likely to have changed from 2007 to 2008 as overall expenditure has increased. This can provide some insights into how the threshold might be expected to change over time, for example, overall expenditure changes and productivity in the NHS might be expected to rise with innovation in health technologies, clinical practice and service delivery. This has implications for a judgement about the appropriate frequency of periodic reassessment of the cost per QALY threshold.
It is not necessarily the case that the threshold will rise with overall expenditure or even with NHS prices. This is illustrated in Figure 8 where the threshold at budget B1 is represented by k1. If overall expenditure increases to B2 then, other things being equal, the threshold would also be expected to increase (i.e. k1 now overestimates the health effects of a change in expenditure at B2). y Increasing overall expenditure from B1 to B2 is equivalent to eliminating the same amount of waste in Figure 8, i.e. by reallocating resources devoted to activities unproductive to health. Again, other things being equal, the threshold would be expected to increase (k1 now overestimates the health effects of a change in expenditure at B1) once the waste has been eliminated. However, insofar as the productivity of those activities that are valuable to the NHS also improve through innovation in health technologies, clinical practice and service delivery, the threshold will tend to fall. Figure 8 illustrates a situation where the effects of eliminating waste (NHS stopping doing things it should not be doing) and, at the same time, improving productivity (NHS getting better at doing things it should do) means that the overall threshold is unchanged.
FIGURE 8.
Impact of changes in budget and productivity. B1, current budget; B2, increased budget; H1, current level of health; k1, cost per QALY threshold.

In making an assessment of whether or not the threshold is likely to increase with the NHS budget it is also necessary to consider whether or not there is discretion over how additional resources can be spent. For example, if any growth in the overall budget is spent on national initiatives or other activities that cannot easily be disinvested, then the additional costs of technologies approved by NICE must be accommodated by displacing other activities elsewhere. Therefore, it is growth in expenditure on more ‘discretionary’ parts of NHS expenditure and changes in the productivity and input prices of those health care activities which are more likely to be displaced, which are most relevant.
Over recent years much of the real budget growth in the NHS has been devoted to national initiatives that are not easily displaced (e.g. new contracts for GPs and consultants, national waiting time targets, information technology initiatives, etc.). 101 It also includes technology appraisal guidance issued by NICE itself, which has a funding mandate. Therefore, any real growth in what remains may have been more modest, so it is more likely to have been offset by any growth in the productivity of displaceable activities (e.g. drugs, devices, procedures and other services). Similarly, although there has been a general rise in input prices for the NHS, much of this inflation has been driven by staff as well as capital and overhead costs, some of which cannot be easily displaced. What are more relevant are the prices of inputs which could be displaced, an important element of which is drug prices. Although branded drug prices have tended to rise, at the same time there has been generic entry on patent expiry with dramatic reductions in prices for important classes of drugs. 102 Therefore, it is not self-evident that the threshold has grown over recent years, despite real increases in the NHS budget.
The central estimates of the cost per QALY threshold for 2007 and 2008 expenditure years are reported in Table 33. In comparing these estimates of the QALY threshold it should be noted that important improvements were made to the classification and collection of PBC expenditure data that took place after the 2006 data were collected. Therefore, the differences in threshold estimates for 2006 and 2007 partly reflect this (see Comparing the cost of life-year estimates associated with different data sets in Chapter 3 and Comparing the cost of life-year estimates associated with different data sets in Appendix 2) so should not be overinterpreted. The results of the analysis of 2007 and 2008 expenditure are comparable in this respect.
PBC grouping | (1) Cost per QALY threshold (2007) (£) | (2) Cost per QALY threshold (2008) (£) | (3) Nominal growth (%) | (4) Cost per QALY threshold (2008), 2007 NHS prices (£) | (5) Real growth (%) |
---|---|---|---|---|---|
All big four programmes | 4549 | 4872 | 7 | 4689 | 3 |
11 PBCs (with mortality) | 8513 | 8308 | –2 | 7996 | –6 |
All 23 PBCs | 13,554 | 12,936 | –5 | 12,450 | –8 |
Although overall expenditure increased by 6% between 2007 and 2008 which represented real growth of 2% in 2007 prices,z the overall threshold for all 23 PBCs fell by 5% in nominal terms and by 8% in real terms.
The reasons are complex but reflect changes in productivity, which differs across PBCs (changes in outcome elasticities), but also a general reallocation of a change in overall expenditure (changes in expenditure elasticities) towards those PBCs that appear more valuable in 2008. aa Given the sources of uncertainty described above, subtle differences between 2007 and 2008 should not be overinterpreted. However, this analysis does suggest that the overall threshold will not necessarily increase with growth in the real or even nominal NHS budget. In conjunction with the results of the analysis described in How uncertain are the estimates and what are the implications? it does suggest that the threshold is more likely to fall at a time when real budget growth is flat or falling and PCTs find themselves under increasing financial pressure.
Within the NICE technology appraisal process, the future incremental costs of a technology are expressed in real terms (at current prices) prior to discounting. Therefore, the estimates that are relevant to NICE decisions are: (i) the nominal threshold in the current year;ab and (ii) some assessment of the real growth in the threshold over the time horizon where incremental NHS costs are incurred. If there is an expectation of real growth (or fall) in the threshold over time then one way to incorporate this is through a higher (lower) discount rate applied to future cost. 103 Indeed, an expectation of changes in the real threshold over time also suggests something about the social rate of time preference for health, as revealed by budget allocations decisions. 104 However, incorporating an expected growth or decline in the threshold over time by adjusting discount rates is likely to be problematic once it is recognised that the expected incremental costs imposed by a technology are rarely uniform over time.
This discussion and the results reported in Table 33 suggest that there is little empirical support for an assumption that there will have been growth in the nominal threshold between 2008 and 2012. ab Growth in the nominal or real threshold seems much less likely in the future with the prospect of reduced budget growth, increased pressures to improve productivity and downward pressure on input prices. As how the nominal or real threshold is likely to change over time cannot be assumed to follow prices or overall expenditure nor empirical estimates or theoretical predictions of a growth in the private consumption value of health (WTP), it becomes especially important to be able to regularly update estimates of the cost per QALY threshold based on routinely available data (see Future research and improving estimates of the threshold).
What type of health is forgone by approval of a new technology?
The methods of analysis described in Chapters 3 and 4 and discussed in this chapter can identify, not only how many QALYs are likely to be forgone across the NHS as a consequence of approving a technology which imposes incremental costs on the NHS, it can also indicate where those QALYs are likely to be forgone and how they are made up, i.e. the additional deaths, life-years lost (unadjusted and adjusted for QoL) and the QoL impacts on those with disease.
For example, in 2011, NICE considered whether ranibizumab (Lucentis®, Roche) for the treatment of diabetic macular oedema should be approved for widespread use in the NHS (TA237). 105 Initially this technology was rejected by NICE on the grounds that, at its current price, it would be unlikely to be cost-effective. In 2012, however, a rapid review of TA237 approved ranibizumab if use was restricted to the most cost-effective subgroup (those with central retinal thickness ≥ 400 micrometres) and after a Patient Access Scheme (PAS) for this subgroup of patients was offered (details of the PAS which provides a discount to the NHS is commercial in confidence). 106
The appraisal and guidance documents105–109,ac provide the information required to estimate the additional NHS costs of treating this subgroup of patients each year (see Appendix 3, Addendum 4: what type of health is forgone by the approval of a new technology? for details of this example). Up to 44,000 NHS patients would be eligible for treatment with ranibizumab each year based on its licensed indication. 107 However, the subgroup of patients where ranibizumab was ultimately approved is likely to be 23,000 each year. This suggests that the approval of ranibizumab in this subgroup at the original appraisal price set in 2011 (i.e. without a PAS) would impose just over £80M of additional NHS costs for treating the eligible population each year.
Based on the 2008 central estimate of the cost per QALY threshold (£12,936 in Table 30) the approval of ranibizumab without a PAS would have been likely to displace 6184 QALYs elsewhere in the NHS. However, the analysis which underpins the threshold estimate can also be used to identify where the additional NHS cost of £80M are likely to impact and where and what type of health effects are likely to be forgone. This is illustrated in Table 34. For example, the estimated expenditure elasticities and total PBC expenditure indicates how these costs will tend to affect spending in each of the 23 PBCs [see Table 34, column (1)]. The estimated outcome elasticities allow this change in spending in each PBC to be translated into a change in deaths and life-year effects for the 11 PBCs where mortality effects could be estimated [see Table 34, columns (2) and (3)]. Applying the estimated proportional effect on the mortality burden of disease to measures of QALY (including the other PBCs) provides an estimate of the total QALY effect of the change in spend in each PBC [see Table 34, column (4)]. ad The comparison of life-year and total QALY effects allows the distinction to be made between QALY effects due the life-year effects of additional deaths and QALY effects due only to QoL [see Table 34, columns (5) and (6)].
PBC | (1) Change in spend (£M) | (2) Additional deaths | (3) Life-years forgone | QALYs forgone | |||
---|---|---|---|---|---|---|---|
(4) Total QALYs forgone | (5) Due to premature death | (6) QoL effects | |||||
2 | Cancer | 3.58 | 30 | 300 | 211 | 195 | 16 |
10 | Circulatory problems | 6.07 | 182 | 928 | 863 | 590 | 273 |
11 | Respiratory problems | 3.67 | 107 | 129 | 1,835 | 80 | 1754 |
13 | Gastrointestinal | 2.56 | 21 | 197 | 351 | 129 | 222 |
All big four programmes | 16 | 340 | 1554 | 3259 | 995 | 2265 | |
1 | Infectious diseases | 2.61 | 6 | 43 | 125 | 29 | 97 |
4 | Endocrine problems | 1.51 | 5 | 40 | 485 | 26 | 459 |
7 | Neurological problems | 4.78 | 10 | 52 | 873 | 34 | 838 |
17 | Genitourinary problems | 3.71 | 18 | 26 | 85 | 17 | 68 |
16 | Trauma and injuries | 6.16 | 0 | 0 | 0 | 0 | 0 |
18 + 19 | Maternity and neonates | 5.46 | 0 | 3 | 2 | 1 | 1 |
11 PBCs | 40 | 389 | 1717 | 4828 | 1101 | 3727 | |
3 | Disorders of blood | 1.65 | 3 | 13 | 175 | 9 | 166 |
5 | Mental health disorders | 14.29 | 23 | 103 | 762 | 67 | 696 |
6 | Learning disability | 0.83 | 0 | 2 | 6 | 1 | 4 |
8 | Problems of vision | 1.55 | 0 | 2 | 34 | 1 | 33 |
9 | Problems of hearing | 0.70 | 0 | 1 | 112 | 1 | 111 |
12 | Dental problems | 2.31 | 0 | 0 | 54 | 0 | 54 |
14 | Skin | 1.57 | 2 | 9 | 16 | 6 | 10 |
15 | Musculoskeletal system | 2.90 | 3 | 14 | 186 | 9 | 176 |
20 | Poisoning and adverse events | 0.74 | 0 | 2 | 7 | 1 | 5 |
21 | Healthy individuals | 2.83 | 0 | 1 | 5 | 1 | 5 |
22 | Social care needs | 2.40 | 0 | 0 | 0 | 0 | 0 |
23 | Other | 8.11 | 0 | 0 | 0 | 0 | 0 |
All 23 PBCs | 80 | 411 | 1864 | 6184 | 1197 | 4987 |
The results reported in Table 34 suggests that approval is likely to result in 411 additional deaths [most of which are likely to occur in PBCs circulatory, respiratory and cancer see Table 34, column (2)], and 1864 life-years forgone [most of which are likely to occur in PBCs circulatory, cancer and gastrointestinal – see Table 34, column (3)]. ae However, the impact of approval of this technology on QALYs forgone due to premature death [see Table 34, column (5)] only accounts for a proportion of the total QALY effects [see Table 34, column (4)]. Most (4987) are associated with QoL forgone during disease [see Table 34, column (6)]. These QoL impacts are most likely to occur in PBCs respiratory, neurological and mental health. The PBC level effects in Table 34 can also be examined at ICD-10 code level, although recognising the caveats discussed in Chapter 4, Adjusting life-years for quality of life and Including quality-of-life effects during disease. af For example, in the respiratory PBC it appears to be chronic lower respiratory diseases (J40–J47) where most additional deaths, life-years and QoL are forgone. In the mental health PBC the additional deaths appear to be associated with disorders due to psychoactive substance use (F10–F19) and mood (affective) disorders (F30–F39) (see Addendum 4: what type of health is forgone by the approval of a new technology? in Appendix 3). However, it should be recognised that these effects, which are based on the central estimate in Table 30, are likely to underestimate the health forgone given the discussion in How uncertain are the estimates and what are the implications? and especially in Impact of investment, disinvestment and non-marginal effects.
The impact of a reduction in the price of this technology, through either value-based pricing or the PAS that was offered during the rapid review,106 can also be examined in the same way. The PAS was commercial in confidence but we will consider a scenario where a 30% reduction in NHS costs was applied for this subgroup of patients. Such a discount would be expected to save 1855 QALYs including 126 deaths averted, 559 life-years (359 when adjusted for quality) and QoL effects during disease equivalent to 1496 QALYs, when compared with approval of the technology at the original price (see Addendum 4: what type of health is forgone by the approval of a new technology? in Appendix 3 for more details on this scenario analysis).
In many respects this starts to make ‘real’ the previously abstract notion that additional NHS costs are the health and opportunities of other unknown NHS patients. The methods of analysis presented in this report go some way to providing an empirically based and explicit quantification of the scale of opportunity costs the NHS faces when considering whether or not the health benefits associated with new technologies are expected to offset the health that is likely to be forgone elsewhere in the NHS. It also starts to make the other NHS patients, who ultimately bear the opportunity costs of such decisions, less abstract and more ‘known’ in social decisions. As who happens to be known or unknown is only a matter of perspective, time and ignorance,110 ethical and coherent social decisions require that both should be treated in the same way. The methods of analysis discussed in this chapter have contributed to removing some of the ‘ignorance’ and making the unknown more real.
Future research and improving estimates of the threshold
There are a number of ways in which this research could be usefully extended based on existing data and the information currently available, most of which have been discussed in previous sections of this chapter. Here we consider the scale of the evaluation problem in this context, examining what, in principle, would be required to resolve some of the key uncertainties discussed in How uncertain are the estimates and what are the implications?, before a more detailed examination of how additional routine data and greater access to existing data or data that are likely to become available, might improve estimates of the cost per QALY threshold in the future.
Two important questions remain when attempting to translate the estimated proportionate effects on mortality due to a change in expenditure into a more complete measure of the health effects (see Chapter 4, Introduction and How uncertain are the estimates and what are the implications?). These are (i) whether the health effects of a change in expenditure in 1 year should be restricted to 1 year or extend over a longer period; and (ii) the extent to which any death averted by expenditure in 1 year returns an individual to the mortality risk of the general population matched for age and gender. The central or ‘best’ estimates presented in Chapters 4 and 5 are based on combining the conservative assumption that the health effects of changes in 1 year of expenditure are restricted to 1 year,ag with the more optimistic assumption that any death averted by expenditure in 1 year returns the individual to the mortality risk of the general population. ah The combination of assumptions that underpin the central estimates appear to be on balance conservative (see Table 32 and discussion in How uncertain are the estimates and what are the implications?) and are certainly more credible than the implausibly pessimistic or optimistic assumptions that underpin the upper and lower bounds for the threshold that are also reported in Chapters 4 and 5. Key questions remain, however. Why can routine data not resolve some of these remaining uncertainties? What would be required to found a central estimate of the cost per life-year or cost per QALY threshold only on econometric estimates rather than, in part at least, resting on judgements about the credibility of these alternative assumptions?ai
A brief reiteration of the scale of this evaluation problem and the approaches to estimation that might be taken illustrates the quite profound difficulties and, therefore, the unavoidable need for explicit and accountable judgement and assumptions. aj
A longer and more complex lag structure
Of course, a longer and more complex lag structure exploiting the PBC panel data set (i.e. both cross-section and time series observations) that is becoming available over time, could in principle at least, identify the effect of a change in expenditure taking place in year t on health in years t, t + 1 , . . . , t + n. However, the health effects in subsequent years would need to be isolated from the effects of change in expenditure also occurring in subsequent years (which would also have both immediate and lagged health effects). The health effects of changes in expenditure in year t would need to be isolated from the lagged effects of changes in expenditure in previous years. Depending on the length of time series data available it may be possible to specify and estimate a richer empirical model to account for the lagged health effects of past expenditure and of lagged expenditure effects of past health outcomes. ak
Although this is not a problem in principle it does pose difficulties as there are very real limits to the current time series because (i) there are a limited number of observations in the cross-section (152 PCTs); (ii) the definition (and boundaries) of PCTs has changed and has recently changed again with the formation of CCGs; (iii) there are a limited number of years of observation in the time series (especially if lags are long); and (iv) as noted in Chapters 3 and 5, the quality of PBC reporting has changed over time (recall that estimates from 2006 and 2007 PBC expenditure were not as comparable as 2007 and 2008). Nonetheless, as the panel data evolves over time there will be more opportunities to explore whether judgements about the duration of effects on mortality can be informed using the type of analysis presented in the report. Insofar as there are later lagged health effects this will tend to reduce the estimate of the cost per death averted, cost per life-year and cost per QALY threshold. al
In many respects the problem of duration of mortality effects is a relatively straightforward one compared with the second issue of how changes in mortality (whether immediate or lagged) translate into life-years. In principle, estimating the effect of change in expenditure on life-years is really estimating the effect of changes in expenditure on the survival curves of the population at risk through membership of ICD-10 codes that contribute to each PBC. Even if the issue of lags is set aside, and attention is restricted to mortality effects in the expenditure year, translating these effects into life-years would require observations on the entire survival curve of the at risk population. This poses two profound difficulties: (i) we would need detailed information about the members of the at risk population (patient identifiers); and (ii) sufficient time to follow up the entire cohort from expenditure change to death (also accounting for other changes that are likely to take place during that time). Even if these data were available and such heroic estimation was possible, any estimate would be so historic that it would be of limited policy relevance. This is not a problem unique to this research but remains a problem for all estimates of the YLL due to disease. It may be possible to use external, non-routine, historic data sources where patient identifiers are available to inform a judgement about whether or not changes in mortality in critical ICD-10 codes (e.g. respiratory) tend to return patients to mortality risks similar to those of the general population. If historic evidence suggests that they remain at higher mortality risk it might indicate the likely scale of overestimation if life-year effects are based on the mortality risk of the general population. However, this would not be without major problems of distinguishing causality from selection effects.
The evolving panel data do have another advantage that could be exploited in the future. Currently it is only cross-sectional variation (i.e. between PCTs) that contributes to the estimates of outcome elasticities. This means that changes in expenditure that all PCTs tend to make together, that might have very large health effects (they all tend to invest in obviously valuable activities at the same time) or limited health effects (they all disinvest in some activities that are not valuable at the same time), may not be fully reflected in the current estimates. am However, using variation in expenditure and outcome in both cross-section and time series could more confidently pick up the full effects of simultaneous investment and disinvestment. The likely net effect on the overall threshold is unclear and will depend on whether PCTs tend to be more co-ordinated when investing in valuable activities (tending to reduce the threshold) or when disinvesting in ineffective ones (tending to increase the threshold). an
Simultaneous estimation across programme budget categories
Although expenditure equations are estimated for all 23 PBCs and outcome equations for the 11 PBCs where there are sufficient mortality data, these are estimated separately; each accounting for other PBC expenditure and other PBC need (see Chapter 3, Modelling framework). The correlations between expenditure and outcome elasticities within each PBC were also estimated by repeatedly resampling the data set and re-estimating expenditure and outcome elasticities (see Appendix 2, The correlation between the outcome and expenditure elasticities). Although the estimate of the overall threshold accounts for changes in expenditure across all 23 PBCs with health effects estimated in 11 and inferred in the others, it is possible that changes in expenditure in one PBC may have health effects in others. Although total deaths across all 23 PBCs are accounted for, unless the possible ‘external’ mortality effects in other PBCs happen to be associated with variation in expenditure in those PBCs then these health effects will not be reflected in the estimated outcome elasticities. This seems likely to underestimate the total health effects of changes in expenditure unless positive health effects are thought to be offset by expenditure in one PBC damaging health outcome in others (e.g. adverse events associated with treatment or other iatrogenic effects). ao To account properly for these possible effects would require estimating the interaction of changes in expenditure in each PBC on all the others while still accounting for possible endogeneity. Unfortunately, with only 152 observations in the cross-section (PCTs), this type of simultaneous estimation is currently not feasible.
Throughout Chapters 3–5 we have not imputed health effects for PBC 23 (GMS) or procedural ICD-10 codes on the grounds that the health effects of this type of expenditure will appear in ICD-10 codes that contribute to other PBCs. However, the health effects of this type of expenditure (PBCs 22 and 23) will only be reflected in the estimated outcome elasticities insofar as the variation in outcomes reported in other PBCs, due to variation in GMS expenditure, happens to be associated with variation in expenditure in those other PBCs. Therefore our approach to GMS (and social care, PBC 22) expenditure is likely to be conservative with respect to overall health effects; tending to overestimate the cost per life-year and cost per QALY threshold.
Exogenous shocks and quasi-experiments
One response to these difficulties would be to look for exogenous budgetary shocks to the whole health-care system and then estimate the health effects of the shock at a macro level. In principle this is very attractive as it would avoid all the difficulties of endogeneity and identifying valid instruments, exploring sensitivity and structural uncertainty. If a complete measure of health outcome was available at a health system level it would also avoid much of the complexity of working at a PBC and ultimately at ICD-10 code level.
Unfortunately, there are a number of difficulties. Although the NHS budget is set each year through an essentially political process (so each year’s change in budget might be regarded as an exogenous shock), insofar as public expenditure decisions are to some extent influenced by public sector performance, then these apparent ‘shocks’ are endogenous in a very similar way to PCT expenditure decisions about particular PBCs, but just at a higher level of aggregation. However, even if some arbitrary exogenous change to overall expenditure could be identified there are other serious difficulties. There is no comprehensive measure of outcome relevant to all NHS activities currently reported. This has two implications: (i) the mortality data that are available are only relevant to approximately 36% of a change in overall expenditure (see Which programme budget categories matter most?); and (ii) how mortality translates into life-years and QALYs depends critically on where those effects occur (the ICD-10 codes that contribute to each PBC). In addition, there are very good reasons why one would expect covariates (especially measures on need) and instruments to differ between different programmes of care. For all these reasons this research has focused on using routinely available data at its lowest level of aggregation.
By doing so we not only provide an estimate of a threshold based on a more complete measure of health effects, we are also able to indicate what type of health is affected and where they are most likely to occur. This provides a means to update estimates of the threshold should other aspects of social value be applied to measures of health or other aspects of social value be included in the future (e.g. consumption and other public expenditure effects). For example, any ‘weights’ that might be assigned to different types of QALY gains or consumption and other public expenditure effects associated with health effects and the patient characteristics associated with ICD-10 codes (e.g. QALY burden, YLL or other patient characteristics, such as age and gender) can be included in the current framework and a threshold re-estimated for ‘weighted’ QALYs or, give an estimate of the consumption value of a QALY, a threshold benefit–cost ratio that includes consumption as well as health effects.
Evolving programme budget category data
Each year offers another wave of PBC expenditure data which means that a potentially useful panel data set is developing. This offers some useful opportunities that have been described above. However, with only 152 PCTs in the cross-section, there is a limit to how much of the remaining uncertainty might be resolved. The utility of this evolving panel will also be limited by the formation of CCGs rather than PCTs as an important locus of expenditure decisions. Changes in PCT boundaries and the formation of CCGs will make the time series problematic unless CCGs can be mapped to previous PCT boundaries. However, updating expenditure and outcome elasticities based on variation in expenditure and outcomes across CCGs would be possible (it would provide more observations in the cross-section) so long as PBC expenditure and mortality outcomes are reported at CCG level.
Of course it would also be useful to be able to observe PBC expenditure at a lower level of aggregation (ideally at ICD-10 code) as this would avoid the assumption necessary to allocate PBC level effects to ICD-10 codes based on either estimates of the size of the at risk population or the crude (unadjusted for covariates) contribution to variance in PBC expenditure. As the only expenditure data that are available by ICD-10 code (and therefore PBC) for each PCT are HES-based estimates of cost, the relevance of measures of contribution to variance in PBC expenditure depends on what proportion of PBC costs are accounted for by HES. However, HES costs are only a small component of total PBC expenditure and contribute very little to the variability in PBC expenditure across PCTs especially when considering PBCs where mortality effects could not be estimated (see Chapter 4, Including quality-of-life effects during disease and Chapter 4, notes an and au). Greater disaggregation within PBCs would be particularly useful as the examination of information routinely collected by PCTs was not particularly helpful in identifying what investment and disinvestments within a PBC explain the variation in expenditure across PCTs (see Addendum 2: the role of data on local NHS decisions in Appendix 3).
Extending measures of health outcome
Currently the only routinely collected health outcome data that can be matched to expenditure by PBC at PCT level is mortality. For this reason outcome equations could only be estimated for 11 of the 23 PBCs. As discussed in Chapter 4, Adjusting life-years for quality-of-life and Including quality-of-life effects during disease, this represents only one aspect of health outcome and is not particularly relevant to many disease categories and much of the care that the NHS offers, when the primary purpose is to improve health experience and QoL rather than to extend survival. Therefore, the estimated proportionate effects of expenditure on the QALY burden of disease in these 11 PBCs were used as a surrogate for proportionate effects in the others, i.e. assuming that the proportionate effects that can be observed will be similar to those that cannot (see Re-estimating the cost per quality-adjusted life-year threshold using more recent data).
Of course, with access to a more complete measure of health outcome, which is routinely reported at PCT level and that can also be associated with PBC expenditure, it would be possible to use the same econometric methods to estimate the health effects of a change in expenditure across all PBCs, rather than imputing them in those PBCs where mortality is not the most relevant measure of health outcome.
The English NHS PROMs programme was introduced in 2009 and routinely collects self-reported health status of patients receiving surgery for four elective procedures: knee replacement, hip replacement, groin hernia repair and varicose vein surgery. The data that are collected include both condition-specific questions (the Oxford Hip Score, Oxford Knee Score and the Aberdeen Varicose Vein score; no condition-specific instrument is available for hernia) as well as the generic instrument, the EQ-5D (both the EQ-5D profile, and the patient’s global assessment of their health, the European Quality of Life-visual analogue scale (EQ-VAS). Patient-level data from the PROMs programme are freely available and can be linked to the HES database which provides a potential link to PBCs. Standardised reports on the PROMs data, including the average (case-mix adjusted) performance of providers, are regularly published by the NHS IC, currently on a quarterly basis. Although currently offering very limited coverage for our purposes, there are plans to extend the PROMs programme in the future, with work under way or being planned around the potential use of PROMs in a wide range of long-term conditions, primary care, in cancer survivorship, cardiovascular services, musculoskeletal and cosmetic surgery.
In Appendix 2, Application of method to other non-mortality-based outcome indicators we demonstrate how the econometric methods set out in Chapter 3 can be extended to these other non-mortality-based outcome measures. EQ-5D utility scores (pre and post an operative procedure) from the PROMs programme are used to generate a non-mortality-based outcome measure, which we use to estimate our outcome model. Although the Department of Health does not report the number of patients undergoing an eligible operation by commissioner (PCT) it was possible to use the HES data set to obtain this information. Routine reporting of procedure or intervention by commissioner in the PROMs data set would seem a simple but important and valuable extension, especially as data are extended to primary care where HES cannot be used to substitute for this omission.
With data for both the average health gain per operation and the number of operations, we were able to estimate ‘the health gain per head of population’ for hip and knee replacements as defined above. This estimated outcome elasticity can then be used as an outcome measure for changes in expenditure in the ‘problems of the musculoskeletal system’ programme (i.e. PBC 15). ap However, translating the short-term impact of an intervention on QoL, which can be estimated from PROMs data, into an estimate of the longer-term effects on QoL remains problematic.
Table 72 in Appendix 2 reports the estimated outcome equation for PBC 15 (musculoskeletal system) using the PROMs-based outcome measure. The result is intuitively plausible; an increase in expenditure improves health outcomes but, for a given spend, more need reduces the gain. The diagnostic statistics suggest that expenditure is endogenous and that the instruments are valid. They also suggest that the instruments are relevant and there is no evidence that the instruments are weak. Therefore, it is feasible to extend our modelling approach beyond those programmes with mortality outcomes should PROMs be extended more widely. Insofar as PROMs can contribute to a more secure estimate of the overall cost per QALY threshold in the future, the sensitivity analysis discussed in Which programme budget categories matter most? starts to indicate where this type of information might be most useful.
Musculoskeletal is an important PBC, accounting for over 5% of a change on overall expenditure and almost 5% of the change in health outcomes. However, of those PBCs without mortality outcomes, it is PBC 5 (mental health) that is most critical (see Table 31 in Which programme budget categories matter most?).
Measures of anxiety and depression are already routinely collected before, during and at the end of interventions as part of Improving Access to Psychological Therapies (IAPT), which is an NHS programme rolling out services across England offering interventions approved by NICE for the treatment of depression and anxiety disorders. By March 2011 IAPT services were offered in 142 of 151 PCTs. A requirement of the programme is to complete the Patient Health Questionnaire-9 (a measure of depression)110 and Generalised Anxiety Disorder Assessment-7 (a measure of anxiety). 111 Both of these disease-specific measures can be linked to Short Form questionnaire-20 items and further work could, in principle, link these scores to EQ-5D. This is a rich, valuable and evolving data set which potentially provides much of the information required to extend the econometric modelling to the mental health PBC. The experience with PROMs data suggests that this would be feasible, and the analysis in Which programme budget categories matter most? indicates that this could make a significant contribution to strengthening the assessment of the overall threshold. It would also contribute to an assessment of the cost-effectiveness of this programme both nationally and by PCT, which would be of value in its own right. Unfortunately, despite the collection of these data for every patient encounter for a number of years, unlike PROMs, these data have not yet been made publicly available. aq Of course, the services offered by the IAPT programme do not account for all the variation in expenditure in the mental health PBC. Nevertheless, access to data that have been and continues to be collected by practitioners and NHS patients, could provide estimates of changes in mental health outcomes due to changes in some types of mental health expenditure, which would be a significant advance. ar
Incidence and duration of disease
Chapter 4, From mortality to life-years sets out the series of steps required to translate mortality effects into life-years while taking account of competing risks or counterfactual deaths. This analysis used ONS data on deaths by age and gender in the ICD-10 codes that contribute to each PBC, as well as LEs by age and gender for the general population. Some information was also required about the age and gender distribution of the population at risk in the ICD-10 codes that contribute to each PBC (see Tables 11 and 12). In Chapter 4, Years of life lost and accounting for counterfactual deaths this was based on age and gender distribution of estimates of incidence from the WHO GBD study. The same information was also used in Chapter 4, Quality of life based on the general population to adjust life-years for the QoL norms of the general population by age and gender. In Chapter 4, Using estimates of the quality-adjusted life-year burden of disease the measures of QALY burden of disease also used information about the duration as well as incidence of disease from the same GBD study. These estimates, published in 2008, were based on 2004 UK data and proved to be the best available source of this type of information given the resources available for this research. However, the GBD study has recently been updated with the findings first publicly presented in December 2012. 113 The methodology of the new study as well as sources of information used have been much improved and any subsequent research on the threshold could integrate these new and improved estimates.
However, the GBD study is not the only potential source of information about estimates of incidence of disease by age and gender and disease duration across all the ICD-10 codes that contribute to the 23 PBCs. For example, the Clinical Practice Research Datalink (CPRD) [previously named General Practice Research Database (GPRD)] contains over 3 million active patient records drawn from approximately 400 primary care practices in the UK. CPRD is jointly funded by the NHS National Institute for Health Research (NIHR) and the Medicines and Healthcare products Regulatory Agency. The database has clinical and prescription data and can provide information to support pharmacovigilance (indication, utilisation, and risk/benefit profiles of drugs) and formal pharmacoepidemiological studies, including information on demographics, medical symptoms, therapy (medicines, vaccines, devices) and treatment outcomes.
Although this research was not funded to purchase access to CPRD data, we were able to examine a sample which comprised 22,313,086 rows/patient–ICD-10 events (three digit) representing 4,229,910 patients with data on diagnosis of diseases observed between 1 January 2006 and 24 June 2011 (see Addendum 1: data sources in Appendix 3). Although CPRD data could, in principle, provide the type of information required, the difficulties faced and the interpretation of the sample of data in the form available to us meant that it was not directly useful. The particular problems faced included: (i) read rather than ICD-10 codes reported in the data set, although mapping is and was possible; (ii) being able to identify when an episode of disease ended; (iii) estimating duration of disease from the sample of data when observations were censored by the limited years of data available to us; and (iv) confidently identifying incident patients in diseases of longer duration despite 2 years of washout prior to extracting the sample. CPRD is quite clearly a rich and valuable data set. However, our experience suggests that, to make best use of these data, specialist knowledge and experience of these data is really needed as well as access to a much larger sample than we were able to acquire with the limited resources available. Therefore, although CPRD could well help to improve estimates of incidence by age, gender and duration of disease, it would require additional well-resourced research including excess to specialist expertise and experience with this particular data set.
Recommendations for research
The priorities for further research that may be feasible based on data which are, or will become, available can be summarised as follows:
-
Any growth in the nominal or real threshold cannot be assumed (see Impact of investment, disinvestment and non-marginal effects and How does the threshold change with overall expenditure?), so it will be important to update estimates of the threshold with more recent and future waves of expenditure and mortality data.
-
If other aspects of social value are applied to health benefits of a new technology they must also be attached to the type of health that is likely to be forgone due to additional NHS costs. The methods developed in this research can be extended to allow the same weights to be also attached to the type of health that is forgone and estimate the wider social benefits that are likely to be lost when the NHS must accommodate the additional costs of new drugs.
-
We have demonstrated that these methods of analysis can be applied to QoL data collected as part of PROMs. This type of analysis could be applied to these data in key PBCs as PROMs is rolled out providing some evidence about the QoL effects of changes in PBC expenditure.
-
A key PBC is mental health. Currently, outcomes data that could be linked to measures of QoL are routinely collected in primary care. In principle, the same methods of analysis can be applied to these data once they are made available providing some evidence about the QoL effects of changes in mental health expenditure.
-
Improved and more recent estimates of incidence (by age and gender) and duration of disease will soon be available from the recently published updated GBD study. These data could be used when the threshold is re-estimated for later waves of expenditure data. Alternatively, estimates could be based on CPRD data. However, our experience suggests that utilising CPRD data would need research that is well resourced with access to specialist expertise and experience with this particular data set.
-
Estimating a more complex lag structure based on the evolving panel data would provide valuable evidence about the duration of the health effects of changes in expenditure. The recent release of census data for 2011 may allow a panel model to be estimated allowing better control for unobserved heterogeneity across PCTs as well as exploiting variation in outcomes, expenditure and other covariates over time. There are, however, significant challenges including the formation of CCGs in 2013, which will make the time series problematic for waves of expenditure and outcomes after 2012.
-
If PBC expenditure and outcome data are available at CCG level (as well as covariates and suitable instruments), it might become possible to estimate outcome and expenditure equations simultaneously across PBCs. This would enable more of the likely health effects of changes in expenditure to be reflected in the analysis.
Conclusions and implications for practice
The methods of analysis presented here go some way to providing an empirically-based and explicit quantification of the scale of opportunity costs the NHS faces when considering whether or not the health benefits associated with new technologies are expected to offset the health that is likely to be forgone elsewhere in the NHS. As such, it provides a basis for determining the appropriate threshold for NICE decisions as well as those made centrally by the NHS and Department of Health more generally.
Since 2004, NICE has used a threshold range of £20,000–30,000 per QALY. It has been widely recognised for many years that this range is not based on evidence. The central estimate of the cost per QALY threshold (£12,936 per QALY based on 2008 expenditure) suggests that the upper bound to this range is almost certainly too high and the lower bound is also likely to be an overestimate (see Re-estimating the cost per quality-adjusted life-year threshold using more recent data). For example, the analysis of the uncertainty associated with the estimated expenditure and outcome elasticities indicates that the chance the threshold is < £20,000 per QALY is 89% and the chance that it is < £30,000 is 97% (see How uncertain are the estimates and what are the implications?).
The central estimate is based on identifying a preferred analysis at each stage based on the analysis that made the best use of available information, whether or not the assumptions required appeared more reasonable than the other alternatives available, and which provided a more complete picture of the likely health effects of a change in expenditure. Although other assumptions and judgements are possible that retain some level of plausibility, they do not all favour a higher threshold. Indeed, when considered together, they suggest that on balance the central estimate of £12,936 is, if anything, likely to be an overestimate (see How uncertain are the estimates and what are the implications?).
Although there is substantial uncertainty associated with the estimate of the overall threshold (including parameter, structural and other sources of uncertainty), a policy threshold set at its mean or expected value may be inappropriate because the consequences for the NHS of overestimating the threshold are more serious than underestimating it (see How uncertain are the estimates and what are the implications?). In principle, a policy threshold (a single value that can be compared to an ICER) should be set below its mean value to take account of the non-linear relationship between the threshold and the additional NHB offered by a technology.
The analysis of PCTs that are under more or less financial pressure (above or below their target resource allocation) starts to indicate the quantitative effect of the scale of the non-marginal impact of new technologies on an appropriate threshold (see Impact of investment, disinvestment and non-marginal effects). It suggests that the central estimate of the threshold is likely to be an overestimate for all technologies which impose net costs on the NHS (almost all technologies appraised by NICE have positive incremental NHS costs), and that the threshold might be lower for technologies which have a greater impact on NHS costs.
The research found no evidence that the threshold had increased with real growth in the NHS budget or with NHS prices (2007–8) (see How does the threshold change with overall expenditure?). There is little empirical support for an assumption that there will have been growth in the nominal threshold between 2008 and 2012. As how the nominal or real threshold is likely to change over time cannot be assumed to follow prices or overall expenditure, nor empirical estimates or theoretical predictions of a growth in the private consumption value of health (WTP), it becomes especially important to be able to regularly update estimates of the cost per QALY threshold based on routinely available data (see Future research and improving estimates of the threshold).
The methods of analysis can not only identify how many QALYs are likely to be forgone across the NHS as a consequence of approving a technology which imposes incremental costs on the NHS, they can also indicate where those QALYs are likely to be forgone and how they are made up, that is the additional deaths, life-years lost (unadjusted and adjusted for QoL) and the QoL impacts on those with disease (see What type of health is forgone by approval of a new technology?). In doing so the study starts to make the other NHS patients, who ultimately bear the opportunity costs of such decisions, less abstract and more ‘known’ in social decisions. As who happens to be known or unknown is only a matter of perspective, time and ignorance, ethical and coherent social decisions require that both should be treated in the same way. These methods contribute to removing some of the ‘ignorance’ and making the unknown more real.
These methods also allow other aspects of health outcome to be incorporated in the estimate of the threshold. This has implications should a system of value-based pricing for new prescription pharmaceuticals be introduced, which may include some additional weight for health benefits in diseases which impose a large health burden and/or where there are wider social benefits for patients, their carers and the wider economy. The methods developed in this research will allow the same weights to be also attached to the type of health that is lost and estimate the wider social benefits that are likely to be lost when the NHS must accommodate the additional costs of new drugs.
The methods of analysis can be used as a framework for further empirical work as additional and more appropriate data emerge in the NHS (see Future research and improving estimates of the threshold). They also offer a basis for threshold estimation in other health-care systems which face constraints on the growth of health-care expenditure and use CEA to inform resource allocation decisions.
Acknowledgements
The authors would like to acknowledge funding received from MRC/NIHR through its Methodology Research Programme (award G0901498/1).
We acknowledge the contribution of the following individuals who participated in various aspects of this research: Ling-Hsiang Chuang, Craig Currie, Jamie Garside, Simon Gilbody, Charlotte Haylock, Sarah Jenkins-Jones, Tim Kendall, David Parkin and John Parkinson.
We are also grateful to those colleagues who attended our research workshop in May 2010 and offered comments on our preliminary methods. We would especially like to thank Peter Littlejohns for his support in advocating this topic to the Methodology Research Programme.
All views expressed here, and any errors, are entirely the responsibility of the authors.
Contributions of authors
All of the named authors below contributed to the development of the research questions, study design and implementation (including membership of the study management group), analysis and/or interpretation of data and submission of the final report. Contributions to particular elements of the study are described below.
Karl Claxton (Professor of Economics, University of York) was a co-applicant, and led the overall design of the study, interpretation of results and writing of the report.
Steve Martin (Researcher, University of York) was a co-applicant and undertook and wrote up the econometric analyses detailed in Chapter 3 and Appendix 2.
Marta Soares (Research Fellow, University of York) undertook the analyses extending the econometric analysis on mortality changes to broader health effects on QALYs (see Chapter 4 and Appendix 3).
Nigel Rice (Professor of Health Economics, University of York) was a co-applicant and contributed to the design, interpretation and write-up of the econometric analysis.
Eldon Spackman (Research Fellow, University of York) designed and undertook the systematic review (see Appendix 1) and contributed to analyses linking mortality changes to broader health effects on QALYs.
Sebastian Hinde (Research Fellow, University of York) designed, undertook and wrote up the systematic review (see Appendix 1), and contributed to analyses linking mortality changes to broader health effects on QALYs.
Nancy Devlin (Director of Research, Office of Health Economics) was a co-applicant and led the design and write-up of the review of local data availability.
Peter C Smith (Professor of Health Policy, Imperial College London) was a co-applicant, and contributed to the design and interpretation of all aspects of the analysis.
Mark Sculpher (Professor of Health Economics, University of York) was the principal applicant, chaired the study management group and contributed to the design, interpretation and write-up of all aspects of the analysis.
Disclaimers
This report presents independent research funded by the National Institute for Health Research (NIHR). The views and opinions expressed by authors in this publication are those of the authors and do not necessarily reflect those of the NHS, the NIHR, the MRC, NETSCC, the HTA programme or the Department of Health. If there are verbatim quotations included in this publication the views and opinions expressed by the interviewees are those of the interviewees and do not necessarily reflect those of the authors, those of the NHS, the NIHR, the MRC, NETSCC, the HTA programme or the Department of Health.
Notes
Chapter 2
Chapter 3
Chapter 4
Chapter 5
- n.d. URL: www.hscic.gov.uk/iapt.
Appendix 1
This is the aim of a value-based pricing approach previously considered by the Department of Health. 2
Appendix 2
Appendix 3
- n.d. URL: www.connectingforhealth.nhs.uk/systemsandservices/data/clinicalcoding/crossmap.
References
- Culyer A, McCabe C, Briggs A, Claxton K, Buxton M, Akehurst R, et al. Searching for a threshold, not setting one: the role of the National Institute for Health and Care Excellence. J Health Serv Res Policy 2007;12:56-8. http://dx.doi.org/10.1258/135581907779497567.
- McCabe C, Claxton K, Culyer AJ. The NICE cost-effectiveness threshold – what it is and what that means. Pharmacoeconomics 2008;26:733-44. http://dx.doi.org/10.2165/00019053-200826090-00004.
- Guide to the Methods of Technological Appraisal. London: NICE; 2008.
- NICE: First report of the Health Committee 2007–2008. London: The Stationery Office; 2008.
- Guide to the Methods of Technological Appraisal. London: NICE; 2004.
- Devlin N, Parkin D. Does NICE have a cost-effectiveness threshold and what other factors influence its decisions? A binary choice analysis. Health Econ 2004;13:437-52. http://dx.doi.org/10.1002/hec.864.
- Gafni A, Birch S. Guidelines for the adoption of new technologies – a prescription for uncontrolled growth in expenditures and how to avoid the problem. CMAJ 1993;148:913-17.
- Williams A. What Could be Nicer than NICE? OHE Annual Lecture. London: Office of Health Economics; 2004.
- Drummond M, Torrance G, O’Brien BJ, Stoddard G. Methods for the Economic Evaluation of Health Care Programmes. Oxford University Press; 2005.
- Birch S, Gafni A. Changing the problem to fit the solution: Johannesson and Weinstein’s (mis) application of economics to real world problems. J Health Econ 1993;12:469-76. http://dx.doi.org/10.1016/0167-6296(93)90006-Z.
- Johannesson M, Weinstein MC. On the decision rules of cost-effectiveness analysis. J Health Econ 1993;12:459-67. http://dx.doi.org/10.1016/0167-6296(93)90005-Y.
- A New Value-Based Approach to the Pricing of Branded Medicines – a Consultation. London: Department of Health; 2010.
- First Report of the Health Committee 2007–2008. London: The Stationery Office; 2008.
- Weinstein M, Zeckhauser R. Critical ratios and efficient allocation. J Public Econ 1973;2:147-57. http://dx.doi.org/10.1016/0047-2727(73)90002-9.
- Stinnett AA, Paltiel AD. Mathematical programming for the efficient allocation of health care resources. J Health Econ 1996;15:641-53. http://dx.doi.org/10.1016/S0167-6296(96)00493-6.
- Epstein D, Chalabi Z, Claxton K, Sculpher MJ. Efficiency, equity and budgetary policies: informing decisions using mathematical programming. Med Decis Making 2007;27:128-37. http://dx.doi.org/10.1177/0272989X06297396.
- Abelson P. The value of life and health for public policy. Econ Record 2003;79:S2-13. http://dx.doi.org/10.1111/1475-4932.00087.
- Bobinac A, van Exel NJ, Rutten FF, Brouwer WB. Willingness to pay for a quality-adjusted life-year: the individual perspective. Value Health 2010;13:1046-55. http://dx.doi.org/10.1111/j.1524-4733.2010.00781.x.
- Byrne MM, O’Malley K, Suarez-Almazor ME. Willingness to pay per quality-adjusted life-year in a study of knee osteoarthritis. Med Decis Making 2005;25:655-66. http://dx.doi.org/10.1177/0272989X05282638.
- Dolan P, Shaw R, Tsuchiya A, Williams A. QALY maximisation and people’s preferences: a methodological review of the literature. Health Econ 2004;14:197-208. http://dx.doi.org/10.1002/hec.924.
- Green C, Gerard K. Exploring the social value of health-care interventions: a stated preference discrete choice experiment. Health Econ 2009;18:951-76. http://dx.doi.org/10.1002/hec.1414.
- Groot W, van den Brink HM. The value of health. BMC Health Serv Res 2008;8.
- Gyrd-Hansen D. Willingness to pay for a QALY. Health Econ 2003;12:1049-60. http://dx.doi.org/10.1002/hec.799.
- Gyrd-Hansen D. Willingness to pay for a QALY – theoretical and methodological issues. Pharmacoeconomics 2005;23:423-32. http://dx.doi.org/10.2165/00019053-200523050-00002.
- Johnson FR, Backhouse M. Eliciting stated preferences for health-technology adoption criteria using paired comparisons and recommendation judgments. Value Health 2006;9:303-11. http://dx.doi.org/10.1111/j.1524-4733.2006.00119.x.
- King JT, Tsevat J, Lave JR, Roberst MS. Willingness to pay for a quality-adjusted life-year: Implications for societal health care resource allocation. Med Decis Making 2005;25:667-77. http://dx.doi.org/10.1177/0272989X05282640.
- Lieu TA, Ray GT, Ortega-Sanchez IR, Kleinman K, Rusinak D, Prosser LA. Willingness to pay for a QALY based on community member and patient preferences for temporary health states associated with Herpes Zoster. Pharmacoeconomics 2009;27:1005-16. http://dx.doi.org/10.2165/11314000-000000000-00000.
- Mason H, Jones-Lee M, Donaldson C. Modelling the monetary value of a qaly: a new approach based on UK data. Health Econ 2009;18:933-50. http://dx.doi.org/10.1002/hec.1416.
- Pinto-Prades JL, Loomes G, Brey R. Trying to estimate a monetary value for the QALY. J Health Econ 2009;28:553-62. http://dx.doi.org/10.1016/j.jhealeco.2009.02.003.
- Shiroiwa T, Sung YK, Fukuda T, Lang HC, Bae SC, Tsutani K. International survey on willingness-to-pay (wtp) for one additional qaly gained: what is the threshold of cost effectiveness?. Health Econ 2010;19:422-37. http://dx.doi.org/10.1002/hec.1481.
- Yaesoubi R, Roberts SD. A game-theoretic framework for estimating a health purchaser’s willingness-to-pay for health and for expansion. Health Care Manag Sci 2010;13:358-77. http://dx.doi.org/10.1007/s10729-010-9135-6.
- Polsky D. Does willingness to pay per quality-adjusted life-year bring us closer to a useful decision rule for cost-effectiveness analysis?. Med Decis Making 2005;25:605-6. http://dx.doi.org/10.1177/0272989X05283136.
- Smith RD, Richardson J. Can we estimate the ‘social’ value of a QALY? Four core issues to resolve. Health Policy 2005;74:77-84. http://dx.doi.org/10.1016/j.healthpol.2004.12.009.
- Hirth RA, Chernew ME, Miller E, Fendrick AM, Weissert WG. Willingness to pay for a quality-adjusted life-year: in search of a standard. Med Decis Making 2000;20:332-42. http://dx.doi.org/10.1177/0272989X0002000310.
- Haninger K, Hammitt J. Willingness to Pay for Quality-Adjusted Life Years: Empirical Inconsistency Between Cost-effectiveness Analysis and Economic Welfare Theory. France: Organisation for Economic Co-operation and Development; 2006.
- Baker R, Bateman I, Donaldson C, Jones-Lee M, Lancsar E, Loomes G, et al. Weighting and valuing quality-adjusted life-years using stated preference methods: preliminary results from the Social Value of a QALY Project. Health Technol Assess 2010;14.
- Claxton K, Walker S, Sculpher MJ, Palmer S. Appropriate Perspectives for Heath Care Decisions. CHE Research Paper 54. York: Centre for Health Economics, University of York; 2010.
- Rawlins MD, Culyer AJ. National Institute for Clinical Excellence and its value judgments. BMJ 2004;329:224-7. http://dx.doi.org/10.1136/bmj.329.7459.224.
- Rawlins MD, Barnett D, Stevens A. Pharmacoeconomics: NICE’s approach to decision-making. Br J Clin Pharmacol 2010;70:346-9. http://dx.doi.org/10.1111/j.1365-2125.2009.03589.x.
- Tappenden P, Brazier J, Ratcliffe J, Chilcott J. A stated preference binary choice experiment to explore NICE decision making. Pharmacoeconomics 2007;25:685-93. http://dx.doi.org/10.2165/00019053-200725080-00006.
- Appraising Life Extending End-of-Life Treatments. London: NICE; 2009.
- Draft Guide to the Methods of Technology Appraisal. London: NICE; 2012.
- NICE Response to the First Report of Session 2007–2008. London: The Stationery Office; 2008.
- The Government’s Response to the Health Select Committee’s First Report of Session 2007–08 on the National Institute for Health and Care Excellence. London: The Stationery Office; 2008.
- Braithwaite R, Roberts M. $50,000 Per QALY: Inertia, Indifference, or Irrationality? n.d.
- Birch S, Gafni A. The biggest bang for the buck or bigger bucks for the bang: the fallacy of the cost-effectiveness threshold. J Health Serv Res Policy 2006;11:46-51. http://dx.doi.org/10.1258/135581906775094235.
- Collier J. Parliamentary review asks NICE to do better still. BMJ 2008;336:53-8. http://dx.doi.org/10.1136/bmj.39454.496748.80.
- Towse A. Should NICE’s threshold range for cost per QALY be raised? Yes. BMJ 2009;338:268-9. http://dx.doi.org/10.1136/bmj.b181.
- Appleby J, Devlin N, Parkin D, Buxton M, Chalkidou K. Searching for cost effectiveness thresholds in the NHS. Health Policy 2009;91:239-45. http://dx.doi.org/10.1016/j.healthpol.2008.12.010.
- Hughes DA, Ferner RE. New drugs for old: disinvestment and NICE. BMJ 2010;340:690-2. http://dx.doi.org/10.1136/bmj.c572.
- Buxton M. How much are health-care systems prepared to pay to produce a QALY?. Eur J Health Econ 2005;6:285-7. http://dx.doi.org/10.1007/s10198-005-0325-y.
- Elshaug A, Moss JR, Littlejohns P, Karnon J, Merlin TL, Hiller JE. Identifying existing health care services that do not provide value for money. Med J Aust 2009;190:269-73.
- Laupacis A, Feeny D, Detsky AS, Tugwell PX. How attractive does a new technology have to be to warrant adoption and utilization – tentative guidelines for using clinical and economic evaluations. CMAJ 1992;146:473-81.
- Birch S, Gafni A. Cost-effectiveness ratios – in a league of their own. Health Policy 1994;28:133-41. http://dx.doi.org/10.1016/0168-8510(94)90031-0.
- Drummond M, Torrance G, Mason J. Cost-effectiveness league tables – more harm than good. Soc Sci Med 1993;37:33-40. http://dx.doi.org/10.1016/0277-9536(93)90315-U.
- Appleby J, Devlin N, Parkin D. NICE’s cost effectiveness threshold – how high should it be?. BMJ 2007;335:358-9. http://dx.doi.org/10.1136/bmj.39308.560069.BE.
- Martin S, Rice N, Smith P. The Link Between Health Care Spending and Health Outcomes: Evidence from English Programme Budgeting Data. CHE Research Paper 24. York: Centre for Health Economics; 2007.
- Martin S, Rice N, Smith P. Further Evidence on the Link Between Health Care Spending and Health Outcomes in England. CHE Research Paper 32. York: Centre for Health Economics; 2007.
- Martin S, Rice N, Smith P. The Link Between Health Care Spending and Health Outcomes for the New English Primary Care Trusts. CHE Research Paper 42. York: Centre for Health Economics; 2008.
- Martin S, Rice N, Smith P. Does health care spending improve health outcomes?. J Health Econ 2008;27:826-42. http://dx.doi.org/10.1016/j.jhealeco.2007.12.002.
- Martin S, Smith P. How Good at Commissioning Health are English Primary Care Trusts? A Preliminary Statistical Analysis. Report to the Health Foundation. London: The Health Foundation; 2009.
- Martin S, Rice N, Smith P. Panel Data Estimates of the Link Between Health Care Spending and Health Outcomes for English Primary Care Trusts. York: Centre for Health Economics; 2010.
- Martin S, Rice N, Smith P. Comparing costs and outcomes across programmes of health care. Health Econ 2012;21:316-37. http://dx.doi.org/10.1002/hec.1716.
- Cochrane AL, St. Leger AS, Moore F. Health service ‘input’ and mortality ‘output’ in developed countries. J Epidemiol Community Health 1997;51:344-8. http://dx.doi.org/10.1136/jech.51.4.344.
- Young FW. An explanation of the persistent doctor–mortality association. J Epidemiol Community Health 2001;55:80-4. http://dx.doi.org/10.1136/jech.55.2.80.
- St Leger S. The anomaly that finally went away?. J Epidemiol Community Health 2001;55. http://dx.doi.org/10.1136/jech.55.2.79.
- Nolte E, McKee M. Does Health Care Save Lives. London: The Nuffield Trust; 2004.
- Gravelle HSE, Backhouse ME. International cross-section analysis of the determination of mortality. Soc Sci Med 1987;25:427-41. http://dx.doi.org/10.1016/0277-9536(87)90167-5.
- Cremieux PY, Ouellette P, Pilon C. Health care spending as determinants of health outcomes. Health Econ 1999;8:627-39. http://dx.doi.org/10.1002/(SICI)1099-1050(199911)8:7<627::AID-HEC474>3.0.CO;2-8.
- Nixon J, Ulmann P. The relationship between health care expenditure and health outcomes. Evidence and caveats for a causal link. Eur J Health Econ 2006;7:7-18. http://dx.doi.org/10.1007/s10198-005-0336-8.
- Bokhari FA, Gai Y, Gottret P. Government health expenditures and health outcomes. Health Econ 2007;16:257-73. http://dx.doi.org/10.1002/hec.1157.
- Moreno-Serra R, Smith PC. The Effects of Health Coverage on Population Outcomes: A Country-Level Panel Data analysis. Washington DC: Results for Development Institute; 2011.
- Department of Health . NHS Finance Manual n.d. www.info.doh.gov.uk/doh/finman.nsf (accessed 12 January 2012).
- PCT Recurrent Revenue Allocations Exposition Book: 2009/10 and 2010/11. London: Department of Health; 2009.
- Smith PC, Rice N, Carr-Hill R. Capitation funding in the public sector. J R Stat Soc Series A 2001;164:217-41. http://dx.doi.org/10.1111/1467-985X.00200.
- Recurrent Resource Allocations: 2006/07, 2007/08. London: Department of Health; 2005.
- Good Governance Report: Review of Programme Budgeting. London: NAO; 2008.
- Appleby J, Harrison T, Foot C, Smith A, Gilmour S. Explaining Variations in Primary Care Trusts’ Spending on Cancer Services. London: The King’s Fund; 2011.
- Lakhani A, Olearnik H, Eayres DE. Compendium of Clinical and Health Indicators: Data Definitions and User Guide for Computer Files. London: National Centre for Health Outcomes Development; 2006.
- Census 2001: General Report for England and Wales. London: Office for National Statistics; 2005.
- Shea J. Instrumental relevance in multivariate linear models: a simple measure. Rev Econ Stat 1997;79:348-52. http://dx.doi.org/10.1162/rest.1997.79.2.348.
- Stock JH, Yogo M. Testing for Weak Instruments in Linear IV Regression. NBER Technical Working Paper 284. New York, NY: Cambridge University Press; 2002.
- Ramsey JB. Tests for specification errors in classical linear least-squares regression analysis. J R Stat Soc Series B 1969;31.
- Pesaran MH, Taylor LW. Diagnostics for IV regressions. Oxford Bull Econ Stat 1999;61. http://dx.doi.org/10.1111/1468-0084.00128.
- Durbin J. Errors in variables. Rev Int Stat Inst 1954;22:23-32. http://dx.doi.org/10.2307/1401917.
- Dixon J, Smith P, Gravelle H, Martin S, Bardsley M, Rice N, et al. A person based formula for allocating commissioning funds to general practices in England: development of a statistical model. BMJ 2011;343.
- Curtis L. Unit Costs of Health and Social Care 2011. Canterbury: PSSRU, University of Kent; 2011.
- Conley TG, Hansen CB, Rossi PE. Plausibly exogenous. Rev Econ Stat 2012;94:260-72. http://dx.doi.org/10.1162/REST_a_00139.
- Small DS. Sensitivity analysis for instrumental variables regression with overidentifying restrictions. J Am Stat Assoc 2007;102:1049-58. http://dx.doi.org/10.1198/016214507000000608.
- Office for National Statistics . Figures for England. National Life Tables 2006–2008 n.d. www.ons.gov.uk/ons/rel/subnational-health4/life-expec-at-birth-age-65/2004-06-to-2008-10/statistical-bulletin.html#tab-National-life-expectancy (accessed 12 January 2013).
- Wailoo AD, Tosh SJ. The Incorporation of Health Benefits in Cost Utility Analysis Using the EQ-5D: Report by the Decision Support Unit. Sheffield: School of Health and Related Research, University of Sheffield; 2010.
- Dolan P, Gudex C, Kind P, Williams A. A Social Tariff for EuroQol: Results from a UK General Population Survey. York: University of York; 1995.
- Currie CJ, McEwan P, Peters JR, Patel TC, Dixon S. The routine collation of health outcomes data from hospital treated subjects in the Health Outcomes Data Repository (HODaR): descriptive analysis from the first 20,000 subjects. Value Health 2005;8:581-90. http://dx.doi.org/10.1111/j.1524-4733.2005.00046.x.
- Cohen JW, Monheit AC, Beauregard KM, Cohen SB, Lefkowitz DC, Potter DE, et al. The Medical Expenditure Panel Survey: a national health information resource 1996;33:373-89.
- Claxton K, Palmer S, Longworth L, Bojke L, Griffin S, McKenna C, et al. Informing a decision framework for when NICE should recommend the use of health technologies only in the context of an appropriately designed programme of evidence development. Health Technol Assess 2012;16.
- Claxton K. The irrelevance of inference: a decision-making approach to the stochastic evaluation of health care technologies. J Health Econ 1999;18:341-64. http://dx.doi.org/10.1016/S0167-6296(98)00039-3.
- Jackson C, Bojke L, Thompson S, Claxton K, Sharples L. A framework for addressing structural uncertainty in decision models. Med Decis Making 2011;31:662-74. http://dx.doi.org/10.1177/0272989X11406986.
- Soares MO, Bojke L, Dumville J, Iglesias C, Cullum N, Claxton K. Methods to elicit experts’ beliefs over uncertain quantities: application to a cost effectiveness transition model of negative pressure wound therapy for severe pressure ulceration. Stat Med 2011;30:2363-80. http://dx.doi.org/10.1002/sim.4288.
- Claxton K, Lindsay AB, Buxton MJ, Culyer AJ, McCabe C, Walker S, et al. Value based pricing for NHS drugs: an opportunity not to be missed?. BMJ 2008;336:251-4. http://dx.doi.org/10.1136/bmj.39434.500185.25.
- Claxton K, Sculpher MJ, Carroll S. Value-based Pricing for Pharmaceuticals: Its Role, Specification and Prospects in a Newly Devolved NHS. CHE Research Paper 60. York: Centre for Health Economics, University of York; 2011.
- Maynard A, Street A. Seven years of feast, seven years of famine: boom to bust in the NHS?. BMJ 2006;332:906-8. http://dx.doi.org/10.1136/bmj.332.7546.906.
- The Pharmaceutical Price Regulation Scheme. An OFT Market Study. VBP Report 2007. London: Office of Fair Trading; 2007.
- Claxton K, Paulden M, Gravelle HSE, Brouwer W, Culyer AJ. Discounting and decision making in the economic evaluation of health care technologies. Health Econ 2011;20:2-15. http://dx.doi.org/10.1002/hec.1612.
- Paulden M, Claxton K. Budget allocation and the revealed social rate of time preference for health. Health Econ 2011;24:612-18.
- TA237: Ranibizumab for the Treatment of Diabetic Macular Oedema. London: NICE; 2011.
- Macular Oedema (Diabetic) – Ranibizumab (Rapid Review of TA237): Appraisal Consultation Document. London: NICE; 2012.
- Single Technology Appraisal (STA) Manufacturer Submission: Lucentis® (Ranibizumab) for the Treatment of Visual Impairment due to Diabetic Macular Oedema (DMO). Chamberley: Novartis; 2010.
- National Institute for Health and Care Excellence (NICE) . Technology Appraisal TA237: Ranibizumab for the Treatment of Diabetic Macular Oedema n.d. http://guidance.nice.org.uk/TA237 (accessed 12 January 2013).
- National Institute for Health and Care Excellence (NICE) . Rapid Review of TA237: Ranibizumab for the Treatment of Diabetic Macular Oedema n.d. http://guidance.nice.org.uk/TA/Wave23/41 (accessed 12 January 2013).
- Broome J. Trying to value a life. J Public Econ 1978;9:91-100. http://dx.doi.org/10.1016/0047-2727(78)90029-4.
- Kroenke K, Spitzer RL, Williams JB. The PHQ-9: validity of a brief depression severity measure. J Gen Intern Med 2001;16:606-13. http://dx.doi.org/10.1046/j.1525-1497.2001.016009606.x.
- Spitzer RL, Kroenke K, Williams JB, Lowe B. A brief measure for assessing generalized anxiety disorder: the GAD-7. Arch Intern Med 2006;166:1092-7. http://dx.doi.org/10.1001/archinte.166.10.1092.
- World Health Organization (WHO) . Global Burden of Disease Study 2010. Lancet 2012.
- Barrett A, Roques T, Small M, Smith R. How much will Herceptin really cost?. BMJ 2006;333:1118-20. http://dx.doi.org/10.1136/bmj.39008.624051.BE.
- Brock DW. How much is more life worth?. Hastings Cent Rep 2006;36:17-9. http://dx.doi.org/10.1353/hcr.2006.0036.
- Brouwer W, van Exel J, Baker R, Donaldson C. The new myth – the social value of the QALY. Pharmacoeconomics 2008;26:1-4. http://dx.doi.org/10.2165/00019053-200826010-00001.
- Chambers JD, Neumann PJ, Buxton MJ. Does medicare have an implicit cost-effectiveness threshold?. Med Decis Making 2010;30:E14-27. http://dx.doi.org/10.1177/0272989X10371134.
- Cohen J, Looney W. Re: how much is life worth: cetuximab, non-small cell lung cancer, and the $440 billion question. J Ntl Cancer Inst 2010;102:1044-8. http://dx.doi.org/10.1093/jnci/djq246.
- Eichler HG, Kong SX, Gerth WC, Mavros P, Jonsson B. Use of cost-effectiveness analysis in health-care resource allocation decision-making: How are cost-effectiveness thresholds expected to emerge?. Value Health 2004;7:518-28. http://dx.doi.org/10.1111/j.1524-4733.2004.75003.x.
- Gafni A, Birch S. Incremental cost-effectiveness ratios (ICERs): the silence of the lambda. Soc Sci Med 2006;62:2091-100. http://dx.doi.org/10.1016/j.socscimed.2005.10.023.
- Garber A, Phelps C. Economic foundations of cost-effectiveness analysis. J Health Econ 1997;16:1-31. http://dx.doi.org/10.1016/S0167-6296(96)00506-1.
- Grosse SD. Assessing cost-effectiveness in healthcare: history of the $50,000 per QALY threshold. Expert Rev Pharmacoecon Outcomes Res 2008;8:165-78. http://dx.doi.org/10.1586/14737167.8.2.165.
- Johannesson M, Meltzer D. Some reflections on cost-effectiveness analysis. Health Econ 1998;7:1-7. http://dx.doi.org/10.1002/(SICI)1099-1050(199802)7:1<1::AID-HEC327>3.0.CO;2-U.
- Johnson FR. Einstein on willingness to pay per QALY: is there a better way?. Med Decis Making 2005;25:607-8. http://dx.doi.org/10.1177/0272989X05283084.
- Kaplan R, Bush J. Health-related quality-of-life measurement for evaluation research and policy analysis. Health Psychol 1982;1:61-80. http://dx.doi.org/10.1037/0278-6133.1.1.61.
- Laufer F. Thresholds in cost-effectiveness analysis – more of the story. Value Health 2005;8:86-7. http://dx.doi.org/10.1111/j.1524-4733.2005.08103.x.
- Mauskopf J, Rutten F, Schonfeld W. Cost-effectiveness league tables – valuable guidance for decision makers?. Pharmacoeconomics 2003;21:991-1000. http://dx.doi.org/10.2165/00019053-200321140-00001.
- Maynard A, Bloor K. The future role of NICE. BMJ 2010;341:1006-7. http://dx.doi.org/10.1136/bmj.c6286.
- Rascati KL. The $64,000 question – what is a quality-adjusted life-year worth?. Clin Ther 2006;28:1042-3. http://dx.doi.org/10.1016/j.clinthera.2006.07.002.
- Towse A, Pritchard C, Devlin N. Cost-Effectiveness Thresholds: Economic and Ethical Issues. London: Office of Health Economics, The King’s Fund; 2002.
- O’Brien BJ, Gertsen K, Willan AR, Faulkner LA. Is there a kink in consumers’ threshold value for cost-effectiveness in health care?. Health Econ 2002;11:175-80. http://dx.doi.org/10.1002/hec.655.
- Speight J, Reaney M. Wouldn’t it be NICE to consider patients’ views when rationing health care?. BMJ 2009;338. http://dx.doi.org/10.1136/bmj.b85.
- Raftery J. Should NICE’s threshold range for cost per QALY be raised? No. BMJ 2009;338:268-9. http://dx.doi.org/10.1136/bmj.b185.
- Bridges JFP, Onukwugha E, Mullins CD. Healthcare rationing by proxy cost-effectiveness analysis and the misuse of the $50 000 threshold in the US. Pharmacoeconomics 2010;28:175-84. http://dx.doi.org/10.2165/11530650-000000000-00000.
- Mason AR, Drummond MF. Public funding of new cancer drugs: is NICE getting nastier?. Eur J Cancer 2009;45:1188-92. http://dx.doi.org/10.1016/j.ejca.2008.11.040.
- Ubel PA, Hirth RA, Chernew ME, Fendrick AM. What is the price of life and why doesn’t it increase at the rate of inflation?. Arch Intern Med 2003;163:1637-41. http://dx.doi.org/10.1001/archinte.163.14.1637.
- Vernon JA, Goldberg R, Golec J. Economic evaluation and cost-effectiveness thresholds signals to firms and implications for R&D investment and innovation. Pharmacoeconomics 2009;27:797-806. http://dx.doi.org/10.2165/11313750-000000000-00000.
- The World Health Report: Reducing Risks, Promoting Healthy Life. Geneva: WHO; 2002.
- Lee CP, Chertow GM, Zenios SA. An empiric estimate of the value of life: updating the renal dialysis cost-effectiveness standard. Value Health 2009;12:80-7. http://dx.doi.org/10.1111/j.1524-4733.2008.00401.x.
- Gerdtham U, Jonsson B, Culyer A, Newhouse J. Handbook of Health Economics. Amsterdam: Elsevier; 2000.
- Fisher ES, Welch HG. Avoiding the unintended consequences of growth in medical care – how might more be worse?. JAMA 1999;281:446-53. http://dx.doi.org/10.1001/jama.281.5.446.
- Or Z. Exploring the Effects of Health Care on Mortality Across OECD Countries. Paris: OECD; 2001.
- Bound J, Jaeger DA, Baker RM. Problems with instrumental variables estimation when the correlation between the instruments and the endogenous explanatory variable is weak. JAMA 1995;90:443-50.
- Staiger D, Stock JH. Instrumental variables regression with weak instruments. Econometrica 1997;65:557-86.
- Cragg JG, Donald SG. Testing identifiability and specification in instrumental variable models. Econometric Theory 1993;9:222-40. http://dx.doi.org/10.1017/S0266466600007519.
- Wooldridge J. Econometric Analysis of Cross Section and Panel Data. Cambridge: The MIT Press; 2002.
- Payment by Results: Tariff Information. London: Department of Health; 2007.
- Unified Exposition Book: 2003/04, 2004/05 and 2005/06 PCT Revenue Resource Limits. London: Department of Health; 2005.
- Dixon JY, Smith P, Gravelle M, Martin S, Bardsley M, Rice N, et al. A person based formula for allocating commissioning funds to general practices in England: development of a statistical model. BMJ 2011;343. http://dx.doi.org/10.1136/bmj.d6608.
- Kovandic T, Schaffer M, Kleck G. Estimating the Causal Effect of Gun Prevalence on Homicide Rates: A Local Average Treatment Effect Approach. Bonn: IZA; 2008.
- Health and Social Information Centre . Provisional Monthly Patient Reported Outcome Measures (PROMs) in England: A Guide to PROMs Methodology 2011.
- Office for National Statistics . Life Expectance at Birth and at Age 65 By Local Areas in the United Kingdom, 2004-06 to 2008-10 n.d. www.ons.gov.uk/ons/rel/subnational-health4/life-expec-at-birth-age-65/2004-06-to-2008-10/statistical-bulletin.html#tab-National-life-expectancy (accessed 17 April 2014).
- Guide to the Methods of Technological Appraisal. London: NICE; 2012.
- Murray C, Lopez AE. The Global Burden of Disease. Geneva: World Health Organization, Harvard School of Public Health, World Bank; 1996.
- The Global Burden of Disease: 2004 Update. Geneva: WHO; 2008.
- World Health Organization . The National Burden of Disease (NBD) Toolkit n.d. www.who.int/healthinfo/global_burden_disease/tools_nbd_toolkit/en/index.html (accessed 29 April 2014).
- Mathers C, Bernard C, Iburg K, Inoue M, Ma Fat D, Shibuya K, et al. Global Burden of Disease in 2002: Data Sources, Methods and Results. Geneva: World Health Organization; 2003.
- Health and Social Care Information Centre . Health Surveys for England n.d. www.hscic.gov.uk/article/3659/Health-Survey-for-England (accessed 29 April 2014).
- Appleby J, Devlin N. Getting the Most Out of PROMs: Putting Health Outcomes at the Heart of NHS Decision-Making. London: The King’s Fund; 2010.
- Gutacker N, Bojke C, Daidone S, Devlin N, Parkin D, Street A. Truly inefficient or providing better quality of care? Analysing the relationship between risk-adjusted hospital costs and patients’ health outcomes. Health Econ 2013;22:931-47.
- Feng Y, Parkin D, Devlin N. Assessing the Performance of the EQ-VAS in the NHS PROMs Programme. London: Office of Health Economics, The King’s Fund; 2012.
- Provisional Monthly Patient Reported Outcome Measures (PROMs) in England. Leeds: NHS Information Centre; 2012.
- Public Health England . PCT CCG Spend and Outcome Factsheets and Tools (SPOT) n.d. www.yhpho.org.uk/resource/view.aspx?RID=49488 (accessed 29 April 2014).
- Public Health England . NHS Atlas of Variation in Healthcare Services n.d. www.rightcare.nhs.uk/atlas/index.html (accessed 29 April 2014).
- NHS Choices n.d. www.nhs.uk/Pages/HomePage.aspx (accessed 10 October 2011).
- CG82: Core Interventions in the Treatment and Management of Schizophrenia in Primary and Secondary Care. London: NICE; 2009.
- Knapp M, Windmeijer F, Brown J, Kontodimas S, Tzivelekis S, Haro JM, et al. Cost-utility analysis of treatment with Olanzapine compared with other antipsychotic treatments in patients with schizophrenia in the Pan-European SOHO study. Pharmacoeconomics 2008;26:341-58. http://dx.doi.org/10.2165/00019053-200826040-00006.
- Davies LM, Barnes LM, Jones PB, Lewis S, Gaughran F, Hayhurst K, et al. A randomized controlled trial of the cost-utility of second-generation antipsychotics in people with psychosis and eligible for clozapine. Value Health 2008;11:549-62. http://dx.doi.org/10.1111/j.1524-4733.2007.00280.x.
- Bagnall AM, Jones L, Ginnelly L, Lewis R, Glanville J, Gilbody S, et al. A systematic review of atypical antipsychotic drugs in schizophrenia. Health Technol Assess 2003;7.
- Barton GR, Hodgekins J, Mugford M, Jones PBB, Croudace T, Fowler D. Cognitive behaviour therapy for improving social recovery in psychosis: cost-effectiveness analysis. Schizophr Res 2009;112:158-63. http://dx.doi.org/10.1016/j.schres.2009.03.041.
- CG90: Depression the Treatment and Management of Depression in Adults. London: NICE; 2009.
- Lenox-Smith A, Greenstreet L, Burslem K, Knight C. Cost effectiveness of venlafaxine compared with generic fluoxetine or generic amitriptyline in major depressive disorder in the UK. Clin Drug Invest 2009;29:173-84. http://dx.doi.org/10.2165/00044011-200929030-00004.
- Kendrick T, Peveler R, Longworth L, Baldwin D, Moore M, Chatwin J, et al. Cost-effectiveness and cost-utility of tricyclic antidepressants, selective serotonin reuptake inhibitors and lofepramine: randomised controlled trial. Br J Psychiatry 2006;188:337-45. http://dx.doi.org/10.1192/bjp.188.4.337.
- Hatziandreu EJ, Brown RE, Revicki DA, Turner R, Martindale J, Levine S, et al. Cost utility of maintenance treatment of recurrent depression with sertraline versus episodic treatment with dothiepin. Pharmacoeconomics 1994;5:249-68. http://dx.doi.org/10.2165/00019053-199405030-00008.
- Peveler R, Kendrick T, Buxton M, Longworth L, Baldwin D, Moore M, et al. A randomised controlled trial to compare the cost-effectiveness of tricyclic antidepressants, selective serotonin reuptake inhibitors and lofepramine. Health Technol Assess 2005;9.
- Kendrick T, Chatwin J, Dowrick C, Tylee A, Morriss R, Peveler R, et al. Randomised controlled trial to determine the clinical effectiveness and cost-effectiveness of selective serotonin reuptake inhibitors plus supportive care, versus supportive care alone, for mild to moderate depression with somatic symptoms in primary care: the THREAD (THREshold for AntiDepressant response) study. Health Technol Assess 2009;13.
- Simon J, Pilling S, Burbeck R, Goldberg D. Treatment options in moderate and severe depression: decision analysis supporting a clinical guideline. Br J Psychiatry 2006;189:494-501. http://dx.doi.org/10.1192/bjp.bp.105.014571.
- Kaltenthaler E, Shackley P, Stevens K, Beverley C, Parry G, Chilcott J. A systematic review and economic evaluation of computerised cognitive behaviour therapy for depression and anxiety. Health Technol Assess 2002;6.
- Kaltenthaler E, Brazier J, De Nigris E, Tumur I, Ferriter M, Beverley C, et al. Computerised cognitive behaviour therapy for depression and anxiety update: a systematic review and economic evaluation. Health Technol Assess 2006;10.
- Hollinghurst S, Peters TJ, Kaur S, Wiles N, Lewis G, Kessler D. Cost-effectiveness of therapist-delivered online cognitive-behavioural therapy for depression: randomised controlled trial. Br J Psychiatry 2010;197:297-304. http://dx.doi.org/10.1192/bjp.bp.109.073080.
- Mears A, Kendall T, Strathdee G, Sinfield R, Aldridge I. Progress on NICE guideline implementation in mental health trusts: meta-analyses. Psychiatrist 2008;32:383-7.
- Rosenheck RA, Leslie DL, Sindelar J, Miller EA, Lin H, Stroup TS, et al. Cost-effectiveness of second-generation antipsychotics and perphenazine in a randomized trial of treatment for chronic schizophrenia. Am J Psychiatry 2006;163:2080-9. http://dx.doi.org/10.1176/appi.ajp.163.12.2080.
- Lambert MT, Copeland LA, Sampson N, Duffy SA. New-onset type-2 diabetes associated with atypical antipsychotic medications. Prog Neuro-Psychopharmacol Biol Psychiatry 2006;30:919-23. http://dx.doi.org/10.1016/j.pnpbp.2006.02.007.
- Dispensing Doctors Association . Patent Expiry 2010–11 n.d. www.dispensingdoctor.org/content.php?id=1335 (accessed 3 May 2012).
- Haas SJ, Hill R, Krum H, Liew D, Tonkin A, Demos L, et al. Clozapine-associated myocarditis: a review of 116 cases of suspected myocarditis associated with the use of clozapine in Australia during 1993–2003. Drug Saf 2007;30:47-5. http://dx.doi.org/10.2165/00002018-200730010-00005.
- Alvir JM, Lieberman JA, Safferman AZ, Schwimmer JL, Schaaf JA. Clozapine-induced agranulocytosis. Incidence and risk factors in the United States. N Engl J Med 1993;329:162-7. http://dx.doi.org/10.1056/NEJM199307153290303.
- Crawford MJ, Killaspy H, Barnes TRE, Barrett B, Byford S, Clayton K, et al. Group art therapy as an adjunctive treatment for people with schizophrenia: multicentre pragmatic randomised trial. BMJ 2012;344.
- Kendall T. Treating negative symptoms of schizophrenia. BMJ 2012;344.
- Tarrier N, Barrowclough C, Porceddu K, Fitzpatrick E. The Salford Family Intervention Project: relapse rates of schizophrenia at five and eight years. Br J Psychiatry 1994;165:829-32. http://dx.doi.org/10.1192/bjp.165.6.829.
- Jarbrink K, Kreif N, Benedict A, Locklear J. Quality of life and drug costs associated with switching antipsychotic medication to once-daily extended release quetiapine fumarate in patients with schizophrenia. Curr Med Res Opin 2009;25:709-16. http://dx.doi.org/10.1185/03007990902738810.
- Davies LM, Lewis S, Jones PB, Barnes TR, Gaughran F, Hayhurst K, et al. Cost-effectiveness of first- v. second-generation antipsychotic drugs: results from a randomised controlled trial in schizophrenia responding poorly to previous therapy. Br J Psychiatry 2007;191:14-22. http://dx.doi.org/10.1192/bjp.bp.106.028654.
- CG82: Schizophrenia (Update). London: NICE; 2009.
- CG90: Depression in Adults: The Treatment and Management of Depression in Adults. London: NICE; 2007.
- A New Value-Based Approach to the Pricing of Branded Medicines: Government Response to Consultation. London: Department of Health; 2011.
Appendix 1 Systematic review of the literature on the cost-effectiveness threshold
Systematic review approach
Introduction
The aim of the systematic review was to inform the development of the conceptual framework, as well as the design, implementation and interpretation of the empirical analyses. Rather than define a set of very specific questions to answer through the review, the objective was to characterise the existing literature in terms of the questions addressed and approaches taken. However, it was hoped that insights would be provided on topics including:
-
general conceptualisation of the cost-effectiveness threshold
-
how NICE’s cost-effectiveness threshold should be defined, characterised and operationalised
-
approaches to estimating cost-effectiveness thresholds in general and the NICE threshold in particular.
In the initial stages of this systematic review it became clear that the ‘traditional’ method of conducting systematic searches of existing literature on the topic of the cost-effectiveness threshold would be insufficient to deal with the requirements of this particular study. Here we refer to the ‘traditional’ method as the practice of finding key terms and medical subject headings (MeSHs) that most accurately capture the range of literature relevant to the topic, while attempting to include as few irrelevant studies as possible (making use of programs such as MEDLINE).
The main weaknesses of using such an approach for a systematic review of this topic is that it requires a pre-existing knowledge of the terms used and topics covered in the current literature. This process has always required a degree of expertise (and luck) as to the strategy taken, including both knowledge of the literature to find likely search terms and skill in the construction of the strategies. The implications of excluding a single key term are potentially equivalent to ignoring vast areas of the literature. In addition, the traditional approach relies on key terms existing that suitably encapsulate the relevant literature. Finding common terms used in literature with potential relevance to the cost-effectiveness threshold was found to be a significant problem as many relevant topics were not specifically aimed at issues relating to the NICE cost-effectiveness threshold (e.g. the Martin et al. publications57–59 which provide a precursor to this project). In addition, due to the wide range of coverage of topics such a ‘threshold’ and ‘cost-effective’, any attempts at a systematic review would be either excessively large or result in a clearly limited snap-shot of the existing literature.
As a result a pragmatic approach was taken to the identification of relevant papers, one of ‘pearl growing’ which can be defined here as the use of existing collections of studies to identify additional relevant parts of the literature. The approach uses a pool of ‘initial pearls’ to grow the literature both through references and citations until all relevant papers have been discovered. This approach therefore relies on the expertise of the authors of the existing literature to populate the pool of studies rather than the searcher’s potentially limited knowledge.
Although this approach of ‘pearl growing’ was significantly limited by the existing software available and has a time consuming element, it represents an approach that corrects for many of the failings of traditional searches for topics that share the characteristics of the cost-effectiveness threshold.
Systematic review methods
The ‘pearl growing’ method of systematic review can be characterised into five steps for the identification of relevant papers.
-
Identification and extraction of ‘initial pearls’.
-
‘Initial pearls’ were identified through consultation with researchers with experience of the cost-effectiveness threshold literature. Fourteen initial pearls were identified through this process. These publications were chosen for their wide-ranging coverage of the topic as well as their anticipated significance.
-
-
Extraction of citations and references from ‘initial pearls’.
-
Citations: Web of Knowledge was selected to perform the citation searches. The reason for this selection was in part due to expert advice from an information specialist as well as brief and non-systematic investigations of citation results from a range of alternative software packages.
-
References: Web of Knowledge was also used for the collection of papers’ references.
-
Both citations and references were exported into an EndNote library (EndNote X6, Thomson Reuters, CA, USA) for the purpose of collection and further analysis (exclusion of repeats, title searching and review of the abstracts).
-
-
Identification of further ‘pearls’ from cited and referenced papers.
-
Once citations and references of the ‘initial pearls’ had been collected, they were subjected to a set of investigations to identify further ‘pearls’.
-
Papers were excluded based on whether or not the titles or abstracts suggested the paper contained information on five topics of interest. These topics had been previously identified given the objectives of the project and from a review of the ‘initial pearls’ and included papers were classified by whether or not they could inform:
-
introduction to the cost-effectiveness threshold topic and policy context
-
discussion and debate around the current value use of the threshold
-
potential methods suggested to find a suitable threshold value
-
specific values proposed
-
the use of individual and societal valuations of health gains to inform the value of the threshold.
-
-
-
Repetition of citation and reference searches.
-
The process was then repeated for the ‘pearls’ identified in step 3.
-
This process was repeated until no new ‘pearls’ were discovered by additional iterations.
-
-
Manual search of references.
-
To ensure as complete a search had been conducted as possible a retrospective manual search of all of the ‘pearls’ references was conducted. Any potentially relevant references not discovered previously (most likely due to a mix of user error and limitations with the software used) were added to the analysis at the relevant step and further pearl growing methods applied to them to ensure completeness of results.
-
Systematic review results
The ‘pearl growing’ method of systematic review revealed 76 papers deemed relevant. The results from each stage of the process are reported in Figure 9. The figure highlights that after four iterations no new relevant papers were identified by the systematic process.
FIGURE 9.
Graph showing process results from pearl growing systematic review.
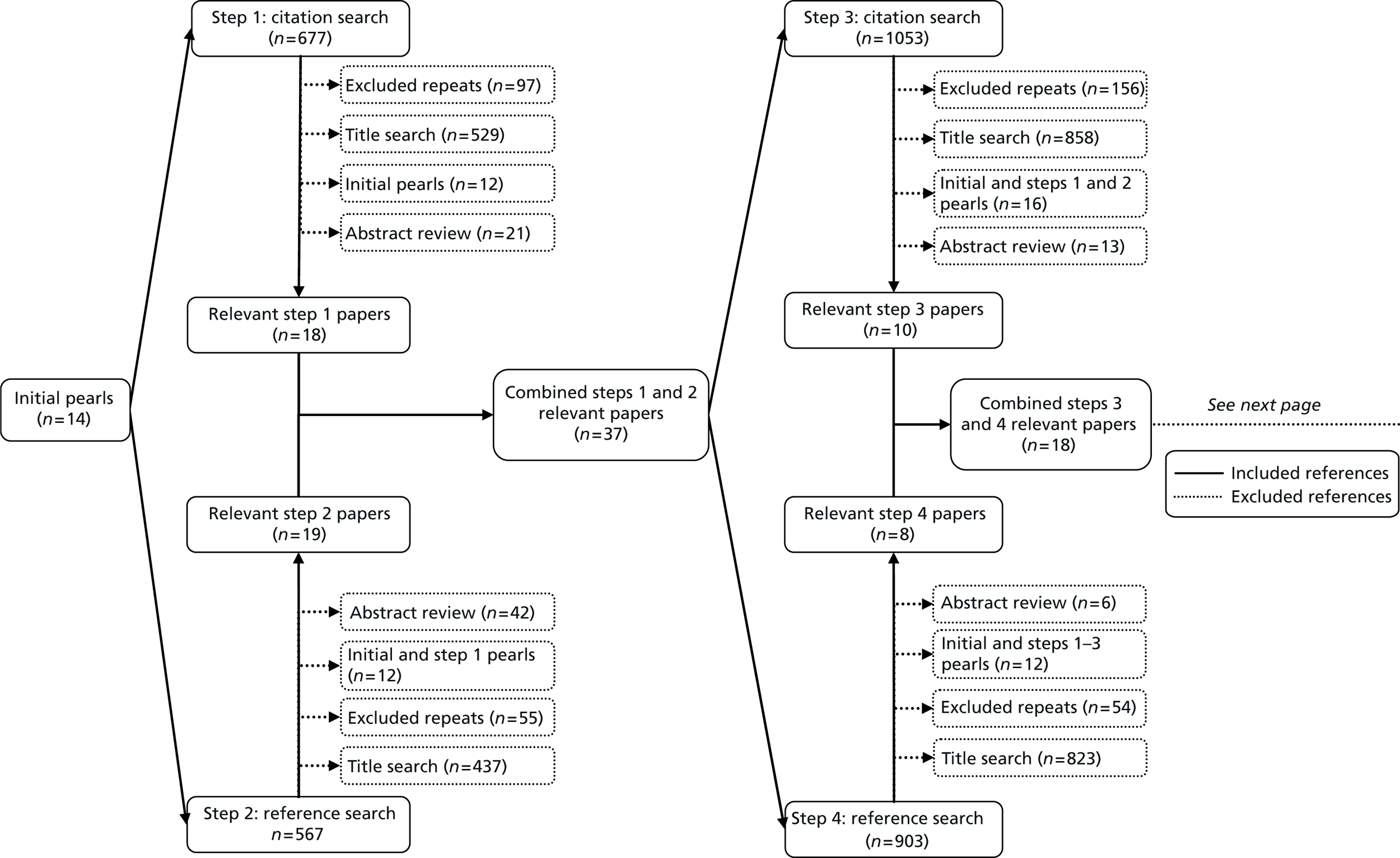

Review of the literature
Introduction and policy context
Due to the broad range of context which the relevant literature covers it is necessary to break down the literature review into several topics, these will be discussed independently. The 76 papers (see Papers discovered by the literature review for all of these papers) identified by the systematic review were defined into three different categories:
-
literature covering the introduction to the cost-effectiveness threshold topic and policy context
-
discussion and debate around the current value use of the threshold
-
potential methods suggested to find a suitable threshold value.
These categories were chosen to reflect the broad range of relevant topics and areas of discussion covered by the cost-effectiveness threshold literature. It should be noted that the majority of the literature identified by the literature review fell into the first and last categories, with very few covering multiple categories sufficiently to be discussed in more than one section. The final category will only be discussed briefly as it can be seen as a separate, unrelated approach to the threshold required for purposes of decision-making by NICE.
The majority of papers (34 of the 76 papers discovered) identified in the literature review could be characterised as introducing the idea of a cost-effectiveness threshold (these consist of the very early literature pre-dating NICE) or discussing the policy context through the years. 1–3,5,7,8,15,33,40,46,47,50,52–55,114–130 This section will characterise the main areas of discussion in the literature and briefly describe the key parts of the literature development.
Definition of the cost-effectiveness threshold
An important place to start is the consideration of how the literature has defined the cost-effectiveness threshold. This is important to analyse in the review as not only is it worth ensuring that a good definition has been presented, but it also allows us to assess whether or not the existing literature uses a definition that is both consistent and accurate.
One of the earliest definitions of something resembling the modern interpretation of the cost-effectiveness threshold comes from Weinstein and Zeckhauser. 15 Their paper identifies a ‘critical ratio’ between monetary costs and a measure of health gains. This critical ratio was argued to represent ‘a cut-off point for allocation’ of an activity in a budget-constrained public sector entity (p. 1). 15
A similar, more recent approach to define the threshold is that taken by Towse et al. 130 where the author considered a hypothetical budget-constrained health-care sector, with a perfectly informed decision-maker who only considers the cost per QALY of health technologies. Assuming perfect information, the decision-maker is able to rank all of the potential health-care activities based on their cost per QALY. A decision-maker will implement as many of the relatively low cost per QALY activities as possible until the budget is used up. Eventually a point will be reached where society is not willing to pay for a further marginal increase in QALYs and would rather the funding be used on other consumption. The cost per QALY at which this cut-off occurs can be described as the cost-effectiveness threshold as it represents the switching point between an activity being funded and not. As the budget is assumed to be fully responsive, any new technologies with a cost per QALY below this threshold will be funded in the future.
National Institute for Health and Care Excellence and the cost-effectiveness threshold
The use and valuation of a cost-effectiveness threshold by NICE has been controversial. Williams8 highlighted three events that may be argued to have particularly muddied the water. First, NICE did not set a threshold value by the government at the time of its inception in 1999. This meant that NICE was obliged to come up with a de novo estimate fairly rapidly. Through his set of discussions with NICE, Williams stated that at the point of inception NICE came up with a value of ‘roughly £30,000 per QALY, plus or minus £5000 depending on the specific circumstances’ (p. 7)8
The second event which Williams refers to was NICE’s initial resistance to acknowledging that any form of threshold value existed. Following analyses such as Towse et al. 130 and Devlin and Parkin6 investigating previous NICE decisions and inferring an implicit threshold, NICE began to publish details of its approach to an ICER threshold. The major step was the 2004 Guide to the Methods of Technological Appraisal5 that provided these details, although the definition of the £20,000–30,000 threshold range may be considered loose and open to interpretation. Although the 2004 guide was one of the first official references to the threshold, Sir Michael Rawlins did state at the 2001 NICE Annual General Meeting that the Institute would ‘need to be very clear in its reasons for supporting technologies with cost-effectiveness ratios higher than £30,000 per QALY’. 130
Williams’ final event is the often quoted £20,000–30,000 threshold range having never been scientifically justified. Authors such as Rawlins and Culyer38 have argued that there has never been an empirical basis for the values or any definitive meaning behind the range. They therefore argued that the threshold should not be the only tool for NICE to draw conclusions about new technologies.
The threshold as a range
The idea of such a threshold range has been part of the literature for some time. Kaplan and Bush125 considered the idea of a less abrupt approach than that suggested by Weinstein and Zeckhauser. 17 Kaplan and Bush125 investigated a set of early Medicare adoption decisions and presented broad criteria of acceptance based on a set of threshold ranges in terms of cost per additional well-year. These were defined as < $20,000/well-year (cost-effective), $20,000–100,000 (possibly controversial but justifiable), > $100,000 (questionable when compared with other expenditure). However, the authors noted that a$100,000 cut-off was not relevant to the policy decisions at the time and that all results would need significant future investigation. Similarly, Laupacis et al. 53 presented five ‘grades of recommendation’ for decisions about technological reimbursement in Canada.
The conclusions of both of these papers can be represented graphically by Figure 10, which is also described or presented in much of the literature (see Rawlins and Culyer,38 Littlejohn in Towse et al. ,130 McCabe et al. 2 and Devlin and Parkin6). This graph represents the probability of rejection of a new technology as a function of the technology’s ICER. The graph clearly shows two points of inflection (A and B in Figure 10), these two points represent an interpretation of the lower and upper bounds of a cost-effectiveness threshold range.
FIGURE 10.
Probability of rejection with a ‘soft’ cost-effectiveness threshold. A and B represent the two points of infection.
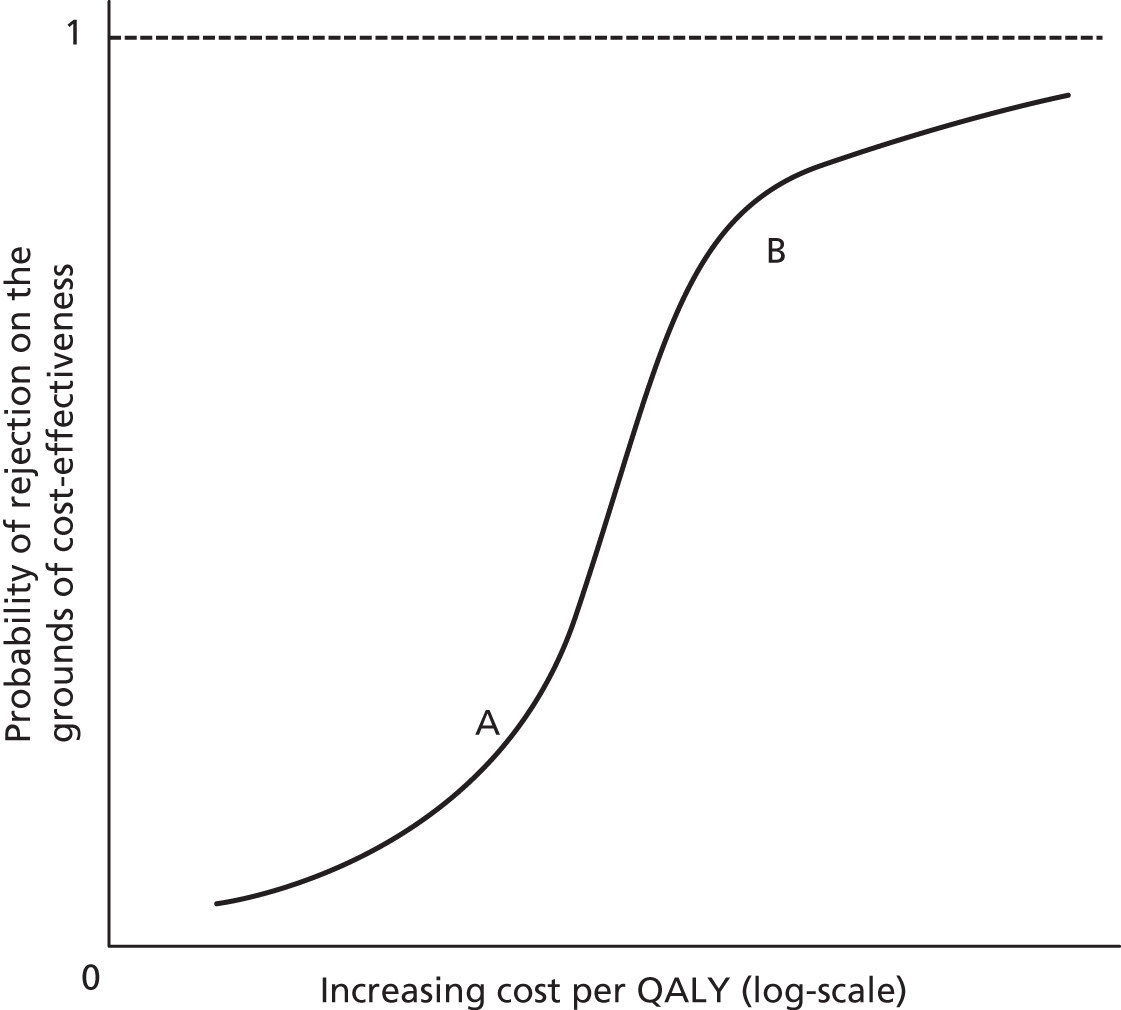
The literature often makes use of the terms ‘soft’ and ‘hard’ when referring to the threshold. The term ‘soft’ is often used in a similar way to the threshold ‘range’ (alternatively Akehurst’s ‘smudge’130). Although the underlying idea is the same, a ‘soft’ threshold has also been used to refer to a single threshold. For example, McCabe et al. 2 argued that it is both feasible and probably desirable to use a single threshold rather than a range, as the threshold should represent the point beyond which factors other than cost-effectiveness are considered. This approach would suggest that all new technologies with an ICER below the threshold should receive funding (regardless of their impact on other factors such as equity of health). It is, however, unclear from this paper what the implications are for technologies with an ICER beyond the single threshold value.
In contrast, a ‘hard’ threshold represents the situation where the ICER valuation is the sole relevant variable in an adoption decision, as demonstrated in Figure 11. 119 It is an important point that if a ‘hard’ threshold is set, no other factors can be considered in the decision-maker’s consideration of a new technology. The difference between a ‘hard’ and a ‘soft’ threshold is therefore largely based on whether or not the ICER reflects all considerations. Assuming the decision-maker is optimising health, a hard threshold should represent the most effective allocation of a health-care budget, but cannot account for any equity concerns (such as the severity of the condition, unmet need and orphan diseases) that are not included in the calculation of the ICER. Authors such as Dolan et al. 21 have demonstrated that a ‘hard’ threshold may not be able to suitably reflect the non-linearity of social or political values of QALYs to factors such as quality and length-of-life and for those with worse health prospects or dependents.
FIGURE 11.
Graph showing a ‘hard’ cost-effectiveness threshold.

What does the threshold represent?
Two broad lines of thought have developed on what the threshold represents, social WTP and shadow pricing. 1,2,8,12,16,17 The key difference between the two is the budget that should be considered by those accepting or rejecting health technologies. The social WTP approach (usually implicitly) assumes that the budget of the health-care sector is flexible to the value of health gains determined by society. So in this case it is the value society places on the health benefits (e.g. in QALYs) generated by new health-care programmes and technologies is estimated first, and then the health-care budget is the sum of society’s WTP for all treatments. In other words, the threshold is set exogenously with no reference to a budget constraint.
In contrast, the shadow pricing approach takes the budget as given (at least beyond the control of those who determine the cost-effectiveness threshold). 1,2 The threshold is, therefore, endogenously based on the services currently provided within the system. When a new programme or technology is accepted into the system and imposes an additional cost onto the budget, the only way to meet those costs is to remove or down-scale existing services which will incur opportunity costs in terms of population health. Hence the threshold represents the ICER of the least cost-effective existing service covered by the budget. In principle, it is this service which is removed to fund a new programme or technology. In practice, a range of criteria is likely to be used to identify appropriate services for displacement to make room in the budget for new interventions.
In the UK, the main source of debate about which of these concepts of the threshold is the correct one lies in NICE’s remit. Authors such as Culyer et al. 1 have discussed NICE’s position as a ‘searcher’ or a ‘setter’ of the threshold. The distinction between these two roles is that a threshold ‘searcher’ does not set a threshold with the motivation of maximising social welfare under the assumption of a flexible NHS budget, but instead investigates the threshold value that is appropriate given current NHS activities and the fixed budget as set down by Parliament.
Much of the literature on this topic is founded in the discussion of the correct constitutional role of NICE, the potential negative implications of setting a threshold and the feasibility of identifying displaced activities. In 2007, Culyer et al. 1 argued that it is not appropriate for NICE to be characterised as a threshold setter. The authors argued that the setting of a threshold would effectively imply that NICE sets the NHS budget. The setting of the NHS budget, they highlight, is the constitutional responsibility of Parliament, not NICE. Hence the paper argues that NICE should concern themselves with being threshold ‘searchers’, seeking to identify ‘an optimal threshold ICER, at the ruling rate of expenditure, that is consistent with the aim of the health service to maximise population health’ (p. 4). 1
In a similar vein Appleby et al. 56 concluded that the threshold used by NICE should be consistent with the decisions made by local commissioners within the NHS. This is important given that NICE provides little guidance to the NHS regarding interventions suitable for disinvestment to release the funding necessary to cover the new technologies it recommends. If the threshold is set too high NICE may well accept new technologies which are less cost-effective than the services which local commissioners displace to fund those technologies. Conversely, if the threshold is set too low, NICE is likely to reject services that are cost-effective relative to existing services delivered from the NHS budget. The authors conclude that, in the short term, NICE have to act as a threshold ‘searcher’ to ensure continuity in the NHS.
Alternative arguments have been put forward which reject the idea of NICE as a threshold ‘searcher’. First, some authors (such as Gafni and Birch7,120) have made the case that an implicit threshold has the potential to lead to spiralling inflation if new cost-effective technologies are funded without sufficient disinvestment. However, McCabe et al. 2 argued that Culyer’s characterisation of the NICE threshold could overcome this challenge if it were regularly reviewed so as to be flexible over time to changes in the NHS budget and the productivity of the sector, and if the threshold for new activities with a non-marginal budget impact was greater than those with a marginal impact. The issue of the inflationary pressure of a threshold is discussed further below.
Another concern raised about Culyer et al. ’s1 characterisation of the NICE threshold is that of Towse. 48 They argue that a lack of knowledge of the true opportunity cost of new activities makes us unable to identify the value of those activities being displaced and, therefore, it is impossible for NICE to ‘search’ for a threshold relating to activities displaced at the margin. The issue of the difficulty of identifying current activities at the margin in terms of cost-effectiveness will be dealt with later in this chapter.
Factors considered by the National Institute for Health and Care Excellence other than the comparison of the incremental cost-effectiveness ratio and threshold
As was discussed in the section The threshold as a range, the suitable threshold approach is dependent on the policy context around it, specifically if the comparison of the ICER with the threshold represents the only relevant piece of information that informs an adoption decision (a ‘hard’ threshold) or if it is simply one of many factors considered (‘soft’ threshold). In the case of the UK, NICE has openly stated the ICER of a technology is not the sole consideration of the committee in its adoption decisions. 5
Both NICE and a number of other authors have provided overviews of the other factors that are considered by NICE in the adoption decision, these are provided in Table 35.
NICE3,5 | Rawlins et al.39 | Tappenden et al.40 | Devlin and Parkin6 |
---|---|---|---|
Uncertainty of variables | Severity of illness | Uncertainty of the ICER | Uncertainty of the ICER |
Availability of comparators | End-of-life treatment | Availability of comparators | Burden of disease |
Clinical priorities (as set by Secretary of State) | Stakeholder opinion | Severity of illness | |
Clinical need | Innovation | ||
Availability of resources | Population characteristics (disadvantaged and children) | ||
Innovation | |||
Disease characteristics and population size | |||
Wider social costs and benefits | |||
Length of benefit |
Table 35 suggests that the threshold is only one consideration to decision-makers at NICE. However, in principle, these other types of benefits could be added to health benefits and compared with potential treatments for displacement which also have wider social benefits. In other words, this wider set of considerations relating to the benefits of new technologies should arguably also be reflected in the threshold. a
Multiple thresholds
Similarly, some have argued for using different thresholds for different situations. 2,17 The two main cases for using different thresholds are the size of the budgetary impact, or depending on whether the decision represents an investment in additional activities or a disinvestment in current activities.
The topic of different thresholds for different budgetary impacts of a proposed technology has received very little analytical attention from the literature. McCabe et al. 2 argue that technologies with a large budgetary impact should be evaluated against a lower threshold than those with a relatively small impact. The reason for this is a large budgetary impact will require a greater displacement of current activities (assuming a fixed overall budget); this may result in displacement of non-marginal activities which may be associated with a lower ICER than those at the margin.
Several authors have suggested the use of different threshold values depending on whether the decision represents an investment in additional activities or a disinvestment in current activities. O’Brien et al. ’s 2002 paper131 considers the difference in willingness to accept monetary compensation to forgo a health-care programme and WTP for the same benefit and link it to the cost-effectiveness threshold. This paper came from the perspective of the threshold representing social preferences rather than the shadow price of a fixed budget constraint and highlights that from a traditional ‘welfarist’ economics standpoint; a greater threshold value for disinvestment may be welfare maximising. Similarly, both Devlin and Parkin6 and Speight and Reaney132 have suggested a threshold for disinvestment of currently performed activities could be lower than for new activities, however, neither present any methodology for calculating the weight of a disinvested activity.
This is in contrast with the view that CEA guides the decisions of health systems with the objective of maximising some measure of health benefit subject to a budget constraint. Hughes and Ferner50 argued that differential thresholds with respect to investment and disinvestment would result in suboptimal levels of population health. This is because a new technology that would improve health may be rejected under a policy of having different thresholds for investment and disinvestment but not if the threshold values were the same. The authors argue that this failure to maximise population health represents an avoidable inefficiency not related to the aim of the health-care sector to maximise health and thus making the case for a single threshold value for disinvestment and investment. This point can be seen as a further case for the shadow price approach as opposed to the social WTP perspective as it highlights that, given a fixed NHS budget, the social WTP approach will not lead to a maximisation of health.
The need for an independent threshold panel
Related to the discussion over the correct role of NICE in determining a suitable cost-effectiveness threshold for the NHS is the literature on the potential for an independent threshold panel. Such a panel has been characterised in a similar manner to the Monetary Policy Committee (the setters of the Bank of England’s interest rate who act independently of the Government of the UK), as an independent committee responsible for the setting and updating of the cost-effectiveness threshold used by NICE.
The papers covering this topic are consistent in their call for an independent threshold panel, with no papers identified arguing against it. The main case provided in the literature for an independent setter is the removal of political influence; Claxton et al. 99 argue that political influence may drive the threshold up as politicians seek to use the threshold as a means to encourage investment by pharmaceutical companies. Williams8 suggests that NICE is biased in the setting of a threshold, as its political connections mean a higher threshold makes it more popular with the ‘sellers’ (the author defines sellers as not only the pharmaceutical industry but health-care professionals and patient groups) by allowing more technologies to be approved. Similarly, papers by Appleby et al. 56 and Raftery133 call for the creation of an independent threshold setter. The 2008 Health Select Committee14 recommended that a body independent of NICE should be established to set and review the threshold. However, it is unclear if such a body would also be independent of political influence or just of the NICE structure.
Arguments against the use of a cost-effectiveness threshold
A number of authors have argued against the use of a threshold. As mentioned earlier authors such as Gafni and Birch7,120 have suggested that the threshold approach risks leading to spiralling increases in inflationary pressures on health-care spending, and present an alternative approach based on the use of league tables of cost-effectiveness. The reason, they argue, is that there is no guarantee that the activities displaced are less cost-effective than those new technologies imposing costs on the health system budget. This observation is coupled with the expectation of authors such as Cohen and Looney118 that pharmaceutical firms will inevitably price their drugs so as to ensure the ICER of their proposed new technology is sufficiently close to the threshold to ensure adoption and thereby gain maximum producer surplus. This observation implies that providers such as the NHS may be forced to pay above market costs of new technologies by revealing their maximum WTP, in the form of the threshold. In addition the point raised in McCabe et al. 2 that the threshold should be adjusted regularly over time to ensure its efficiency seeks to address both of these arguments.
Other authors such as Eichler et al. 52 have raised and debated the issues around the theoretical base for the cost-effectiveness threshold, namely the assumption of perfect divisibility of health-care programmes, constant returns to scale and constant marginal opportunity costs.
Bridges et al. 119 argues that a unique threshold value imposes impractical assumptions in the case of the US health-care sector, and fails to account for supply and demand side variations in the market. As an alternative the authors propose a series of thresholds that reflect regional, dynamic budgeting and general methodological differences. They conclude that the case for abandoning a fixed threshold outweighs those for keeping one in the USA and that any threshold should vary across payer, over time, in the true budget impact of interventions and in the measurement of the effectiveness of interventions. This argument has clear links to the argument for shadow pricing of the threshold rather than the social WTP approach, as the shadow price approach is based on the view that the threshold is determined by budget and current efficiency which can be seen to differ over time and across payers. The unresolved issue here is the degree to which different subgroups (e.g. by region or budget) require different threshold values.
Identification of activities under the threshold
An important part of the literature is the discussion around the identification of activities with an ICER greater than the proposed threshold. The importance of this discussion stems from the requirement of new activities to displace current activities that are at the margin of what is cost-effective. If it is not possible to identify these activities separately from others then threshold analysis is methodologically flawed, as the funding of a new activity may impact on an activity with an ICER above the proposed threshold.
Most literature on this topic focuses on the importance of identifying activities to be displaced rather than the process and feasibility of doing so. For example, Hughes and Ferner46 and McCabe et al. 2 highlight the implications of inconsistent displacement on geographic variations in health-care provision and that the lack of consistency in the displacement process undercuts the use of a single cost-effectiveness threshold for the evaluation of new technologies. Similarly, Buxton51 suggests that, in order to fully appreciate the opportunity cost of the implementation of a new technology, we must have a clear knowledge of those activities displaced at the cost-effectiveness margin.
Few authors have sought to develop methods to identify the activities that should be displaced to free-up budget for new more cost-effective activities. Elshaug et al. 52 outlines a set of criteria for the identification of existing, potentially non-cost-effective practices which could then be further assessed to determine their cost-effectiveness using health technology assessment. The criteria suggested include factors such as new evidence on safety; efficacy or cost-effectiveness; geographic variation that have become apparent since technology adoption; heterogeneity in the clinical procedure; and technological development.
The current value of the threshold
As it became evident that decision-making bodies such as NICE are using (more or less explicit) cost-effectiveness thresholds, there has been a significant level of debate over its appropriate value. 6,8,38,39,45,48,51,56,130,134–137 In this section we will present three areas of the debate:
-
the lack of empirical basis to the current value
-
arguments over the value being generally too high or too low
-
if and how the threshold should change over time.
Lack of empirical basis to the current value
Since NICE made it clear that it uses an explicit threshold5 there has been little hiding the lack of evidential justification behind the £20,000–30,000 range. Indeed the Health Select Committee14 heard (during their enquiry into NICE in 2008) that the NICE threshold has no basis in hard science. Similarly, Appleby et al. 56 noted that ‘the uncomfortable truth is that NICE’s threshold has no basis in either theory or evidence’.
Similarly, the US value of $50,000 per QALY, which is often cited as the cost-effectiveness threshold relevant to resource allocation decisions in that country, is often attacked for its lack of empirical founding. 33,45,122,124 Some have suggested that the US figure is rooted in the cost-effectiveness of hospital renal dialysis,122 although why this makes it suitable for use more generally is unclear.
The threshold changing over time
Another concern of current NICE practice is the apparent lack of change in the threshold value used since the body’s inception. Many authors have argued that factors such as the NHS budget, price inflation, technological developments in the NHS and the discount rate applied to economic evaluations35,122,125,126 have all changed since the first use of the cost-effectiveness threshold. As such, the threshold should have changed to reflect this fact. Braithwaite and Roberts45 sought to demonstrate the impact of budget and technological growth on the optimal threshold. By creating a computer simulation of the US Medicare system, the authors were able to demonstrate the impact of these factors. Although there is no doubt in the literature that the NICE threshold should potentially change over time,a no papers have been identified which model the impact of any changes on the threshold.
Both Ubel et al. 136 and Raftery133 discuss the principles behind the directional change the threshold should take over time. Ubel et al. 136 have argued that the optimal threshold value needs to fall over time assuming medical innovation continues at roughly its current rate. Raftery133 has noted that, in real terms, the threshold has been falling since 1999 as, in order to stay constant in real terms, it should have increased given inflation (up 40% in the time period) and increased NHS spending (up 90%). The authors argue that this decline in the threshold should have been observed in the value used by NICE in decision-making. They describe the suggestion of a rise in the threshold being linked to the observed growth of the NHS budget over the last decade as ‘audacious’. 133 It is unclear to what extent the authors disagree with this interpretation of NHS efficiency as a relevant factor affecting the optimal threshold.
Threshold value generally too high or low
The majority of the debate over the current use of the threshold in the UK (and elsewhere) has been centred on whether the current value is too low or too high. The papers that will be discussed in this section focus on the general discussion of necessary directional change in the value rather than the presentation of a specific value; the latter is discussed in more detail in the following section on the proposed values of the threshold in the literature.
Vernon et al. 137 presented an analysis of the implications of the threshold being above or below its optimum value in terms of signals to the companies involved in research and development of new medical products. The authors concluded that if the threshold is set too low (below the economic value of the health benefit) it will result in research and development investment levels that are too low relative to their economic value (at the margin). The reason for this lies in a lack of returns to investments for the pharmaceutical companies. However, in the isolated case of the threshold relevant to the NHS (a small proportion of the world pharmaceutical market), the impact of changes to the threshold on the international pharmaceutical market equilibrium is unknown but likely to be small.
Similarly, thresholds set too high (above the economic value of the health benefit) will result in inefficiently high levels of research and development spending, such that the health-care provider is funding projects that do not have a sufficient impact on social welfare.
The literature that argued the threshold is too high in the UK can be broadly characterised into three key papers. Williams8 made the case that, intuitively, the threshold should not be significantly greater than the gross domestic product (GDP) per capita (roughly £18,000 in the UK in 2004). He made the case that, although it may be possible to provide a lot of the population with health care when the threshold is above the GDP per capita, it is not possible to provide health care for much of the population without imposing great hardship on those expected to foot the bill (the tax payer or government debt).
Second, Raftery133 argued that, although the UK threshold has been historically too high, it does not need reducing as the real value has decreased since 1999 due to inflationary pressure and increases in the NHS budget. He also suggests that recent policies implemented by NICE, such as greater weight being given to the benefits of treatment accruing to patients at the end of their life, need to be offset by reductions in the threshold for all other treatments for expenditure to remain within the NHS budget. Finally, Raftery cites the opportunity cost analysis of trastuzumab (Herceptin®, Roche)114 which showed that more cost-effective oncology services were being sacrificed to fund trastuzumab in breast cancer. This result suggests directly that, in some cases at least, the threshold value is too high.
Work by Martin et al. 57–59 investigated the cost per life-year saved in a selection of the 23 PBCs used in the NHS; these results are presented in Table 36. It is important to note that these results are presented as the cost per YLG rather than the cost per QALY of the least cost-effective current activity. The authors and others have used these results to argue that the threshold used by NICE may be too high. 128 Similarly, Collier’s47 report of the Health Select Committee suggests that the threshold used by NICE is higher than that used by PCTs.
PBC | Cost per YLG (£) | |
---|---|---|
2005/6 data | 2004/5 data | |
Cancer | 13,137 | 13,931 |
Circulation problems | 8426 | 7979 |
Respiratory problems | 7397 | N/A |
Gastrointestinal problems | 18,999 | N/A |
Diabetes | 26,453 | N/A |
In contrast, a range of authors have argued that the current NICE threshold is too low. Both Speight and Reany132 and Towse48 argued that the inclusion of wider social costs/benefits and full consideration of social WTP for additional health gains show that the threshold should be significantly larger. Both cite recent NICE work by Mason et al. 29,135 which suggested the threshold should be between £30,000 and £75,000 per QALY based on attempts to model a WTP-based value of a QALY based on observations of the value of avoiding a statistical fatality. Similarly, in the USA, Ubel et al. 136 have argued that, if inflation and WTP valuations are taken into account, the relevant threshold in the US should be closer to $200,000 per QALY than the regularly cited $50,000.
Those analyses which conclude the UK and US thresholds should be significantly higher have, at the core of their argument, the assumption that the respective health-care budget is fully capable of responding to society’s WTP for additional health gains.
Potential methods for threshold estimation
There are broadly three approaches that can be taken to determine the threshold value:51,56 social WTP, non-analytical approaches. Such as expert elicitation and shadow pricing of the budget constraint. This project is concerned with the latter approach to estimating the cost-effectiveness threshold. This is entirely consistent with the remit of the NHS in general and NICE in particular – they do not set the NHS budget but have to allocate those finite resources appropriately.
Papers seeking to elicit social willingness to pay and non-analytical approaches
The majority of the literature that has presented a proposed value for the threshold (in the UK, USA and elsewhere) has done so using valuation methods based on WTP for an additional health benefit. 18–32,34–37 However, other approaches have been suggested. For example, the WHO’s 2002 report138 suggested that interventions costing less than three times GDP per capita for each DALY averted represent good value for money.
Lee et al. 139 sought to update the US ‘dialysis standard’ often claimed to be the base of the US Medicare threshold. 122 The authors present a valuation of $129,090 per QALY based on current dialysis practice in the USA. Finally, in an appendix to their edited book, Towse et al. 130 provide an interesting set of results drawn from a set of participants to the associated workshop (the majority of which were health economists). The participants were asked to anonymously record their view on what threshold NICE should apply. Eighteen responses were recorded with the average of all responses being £29,000 per QALY.
Papers considering the shadow price of the budget constraint
The systematic review only identified four different papers by three different authors that suitably fell into the category of shadow pricing of the budget constraint.
Williams8 suggested investigating the cost-effectiveness of NHS interventions that represent the majority of the budget (he speculated that some 300 interventions accounted for about 90% of the cost incurred by the NHS). The purpose of this would be to identify current NHS activities that might not be cost-effective. He acknowledged the implausibility of conducting full technological appraisals on such a large number of interventions (estimating this would take 10 years, at which point it would be necessary to re-evaluate the initial appraisals), and thus suggested relying on expert opinion and existing patient data to speed up the process.
While Williams’ recommendations related to identifying current interventions with a high cost per QALY as the basis for disinvestment, there is the potential to take this approach further and use it for a method to determine the cost-effectiveness threshold even down to the level of a local decision-maker. This was attempted by Appleby et al. 49 who conducted a feasibility experiment into the estimation of the appropriate NHS threshold by examining decision-making in the NHS at a local level. The authors propose a structured model considering the new technology’s cost per weighted QALY gain in a table of all existing services. In an attempt to test the feasibility of this model they conducted interviews with senior NHS staff as well as investigating information on public health to construct a list of health-care services introduced or discontinued in 2006/7. The authors found that it was feasible to identify decisions and to make the important step of estimating their cost-effectiveness; however, they noted that any attempts to fully evaluate sufficient decisions as to estimate a threshold would require a detailed understanding of the decision structure at a local level as well as a significant number of observations.
The other key papers seeking to develop and implement methods for estimating the NHS threshold were those of Martin et al. 57–59 They aimed to establish a link between health-care spending and health outcomes in the NHS after having adjusted for the need of the patient population. They made use of data around the observed mortality at PCT level in the NHS alongside expenditure data on health care across 23 programmes of care based on ICD-10 disease categories. As has been mentioned earlier in this chapter these papers present the cost per life-year for a range of PBCs; however, the key result of these papers is that it is possible to make use of existing data to determine such valuations for current NHS interventions. The authors concluded that although their results are highly limited and do not present a single cost per QALY estimate for the optimal threshold they can ‘inform the decisions of NICE on whether their current threshold for accepting new technologies is set at an appropriate level’ (p. 37). These studies are the precursor of analyses presented in this report, and further details can be found in Appendix 2 and in Chapter 3 of the main report.
In the area of the efficient allocation of health care it is also important to note the contribution of the earlier mathematical papers such as Stinnett and Paltiel16 who outlined mathematical techniques to approach the problem through the use of a mixed integer programming approach. Although their approach differs from the interpretation of the threshold as used in this study it represented an important step in the evaluation of the methodology of seeking to solve the optimisation problem apparent in health care.
Conclusion
This systematic review of the literature surrounding the cost-effectiveness threshold has highlighted the significant range and diversity of the literature. Despite the international and mature nature of the literature there are significant differences in the suggested methods to represent a cost-effectiveness threshold. The main areas of debate relevant to this report have revolved around the role of NICE as a ‘searcher’ or ‘setter’ of the threshold. 1,2 Although some authors have implicitly argued for NICE to fulfil the role of a threshold ‘setter’ by suggesting methods of elicitation of social WTP valuations of a QALY, death or life-year,18–32,34–37 the literature of most relevance to this research has sought to consider estimation methods consistent with its role as a ‘searcher’. 56–59
Papers discovered by the literature review
Note: not all of these papers are referenced in this appendix and some references used were not discovered through the systematic review.
Initial pearls
Appleby J, Devlin N, Parkin D. NICE’s cost effectiveness threshold – how high should it be? BMJ 2007;335:358–9.
Appleby J, Devlin N, Parkin D, Buxton M, Chalkidou K. Searching for cost effectiveness thresholds in the NHS. Health Policy 2009;91:239–45.
Bridges JFP, Onukwugha E, Mullins CD. Healthcare rationing by proxy cost-effectiveness analysis and the misuse of the $50 000 threshold in the US. Pharmacoeconomics 2010;28:175–84.
Culyer A, McCabe C, Briggs A, Claxton K, Buxton M, Akehurst R, et al. Searching for a threshold, not setting one: the role of the National Institute for Health and Care Excellence. J Health Serv Res Policy 2007;12:56–8.
Devlin N, Parkin D. Does NICE have a cost-effectiveness threshold and what other factors influence its decisions? A binary choice analysis. Health Econ 2004;13:437–52.
Gafni A, Birch S. Incremental cost-effectiveness ratios (ICERs): the silence of the lambda. Soc Sci Med 2006;62:2091–100.
McCabe C, Claxton K, Culyer AJ. The NICE cost-effectiveness threshold – what it is and what that means. Pharmacoeconomics 2008;26:733–44.
Raftery J. Should NICE’s threshold range for cost per QALY be raised? No. BMJ 2009;338:268–9.
Towse A. Should NICE’s threshold range for cost per QALY be raised? Yes. BMJ 2009;338:268–9.
Braithwaite RS, Meltzer DO, King JT Jr, Leslie D, Roberts MS. What does the value of modern medicine say about the $50,000 per quality-adjusted life-year decision rule? Med Care 2008;46:349–56.
Grosse S. Assessing cost-effectiveness in healthcare: history of the $50,000 per QALY threshold. Exp Rev Pharmacoecon Outcomes Res 2008;8:165–78.
Rawlins MD, Culyer AJ. National Institute for Clinical Excellence and its value judgments. BMJ 2004;329:224–7.
Chambers JD, Neumann PJ, Buxton MJ. Does Medicare have an implicit cost-effectiveness threshold? Med Decis Making 2010;30:E14–27.
Step 1 results
Brouwer W, can Exel J, Baker R, Donaldson C. The new myth – the social value of the QALY. Pharmacoeconomics 2008;26:1–4.
Claxton K, Lindsay AB, Buxton MJ, Culyer AJ, McCabe C, Walker S, et al. Value based pricing for NHS drugs: an opportunity not to be missed? BMJ 2008;336:251–4.
Cohen J, Looney W. Re: How much Is life worth: cetuximab, non-small cell lung cancer, and the $440 billion question. J Natl Cancer Inst 2010;102:1044–8.
Eichler HG, Kong SX, Gerth WC, Mavros P, Jonsson B. Use of cost-effectiveness analysis in health-care resource allocation decision-making: how are cost-effectiveness thresholds expected to emerge? Value Health 2004;7:518–28.
Green C, Gerard K. Exploring the social value of health-care interventions: a stated preference discrete choice experiment. Health Econ 2009;18:951–76.
Groot W, van den Brink HM. The value of health. BMC Health Serv Res 2008;8:136.
Hughes DA, Ferner RE. New drugs for old: disinvestment and NICE. BMJ 2010;340:690–2.
Lieu TA, Ray G, Ortega-Sanchez I, Kleinman K, Rusinak D, Prosser L. Willingness to pay for a QALY based on community member and patient preferences for temporary health states associated with herpes zoster. Pharmacoeconomics 2009;27:1005–16.
Mason AR, Drummond MF. Public funding of new cancer drugs: is NICE getting nastier? Eur J Cancer 2009;45:1188–92.
Mason H, Jones-Lee M, Donaldson C. Modelling the monetary value of a qaly: a new approach based on UK data. Health Econ 2009;18:933–50.
Maynard A, Bloor K. The future role of NICE. BMJ 2010;341:c6286.
Rascati KL. The $64,000 question – what is a quality-adjusted life-year worth? Clin Ther 2006;28:1042–3.
Rawlins M, Barnett D, Stevens A. Pharmacoeconomics: NICE’s approach to decision-making. Br J Clin Pharmacol 2010;70:346–9.
Shiroiwa T, Sung YK, Fukuda T, Lang HC, Bae SC, Tsutani K. International survey on willingness-to-pay (WTP) for one additional QALY gained: what is the threshold of cost effectiveness? Health Econ 2010;19:422–37.
Speight J, Reaney M. Wouldn’t it be NICE to consider patients’ views when rationing health care? BMJ 2009;338:297.
Tappenden P, Brazier J, Ratcliffe J, Chilcott J. A stated preference binary choice experiment to explore NICE decision making. Pharmacoeconomics 2007;25:685–93.
Weinstein MC. How much are Americans willing to pay for a quality-adjusted life-year? Med Care 2008;46:343–45.
Yaesoubi R, Roberts SD. A game-theoretic framework for estimating a health purchaser’s willingness-to-pay for health and for expansion. Health Care Manag Sci 2010;13:358–77.
Step 2 results
Appleby J, Devlin N, Parkin D, Buxton M, Chalkidou K. Searching for Local NHS Cost Effectiveness Thresholds: A Feasibility Study. NICE Conference Manchester. 5–6 December 2007. URL: www.nice2007.co.uk/ApplebyDevlin.pdf (accessed 12 January 2012).
Birch S, Gafni A. The biggest bang for the buck or bigger bucks for the bang: the fallacy of the cost-effectiveness threshold. J Health Serv Res Policy 2006;11:46–51.
Braithwaite RS, Roberts MS. $50,000 per QALY: Inertia, Indifference, or Irrationality? Presented at the Annual Meeting of the Society for Medical Decision Making. Atlanta, GA, USA, 17–20 October, 2004.
Drummond M, Torrance G, Mason J. Cost-effectiveness league tables: more harm than good? Soc Sci Med 1993;37:33–40.
Gerard K, Mooney G. QALY league tables: handle with care. Health Econ 1993;2:59–64.
Gyrd-Hansen D. Willingness to pay for a QALY: theoretical and methodological issues. Pharmacoeconomics 2005;23:423–32.
Hammitt JK. The $64,000 question: what are we willing to pay for a QALY. ISPOR Connect 2005;11:7–9.
Hirth RA, Chernew ME, Miller E, Fenderick AM, Weissert WG. Willingness to pay for a quality-adjusted life-year: in search of a standard. Med Decis Making 2000;20:332–42.
King JT Jr, Tsevat J, Lave JR, Roberts MS. Willingness to pay for a quality-adjusted life-year: implications for societal health care resource allocation. Med Decis Making 2005;25:667–77.
Lee C, Chertow G, Zenios S. An empiric estimate of the value of life: updating the renal dialysis cost-effectiveness standard. Value Health 2009;12:80–7
Martin S, Rice N, Smith P. Further Evidence on the Link Between Health Care Spending and Health Outcomes in England [CHE 28. National Institute for Health and Care Excellence. NICE discussion paper 32]. York: University of York; 2007.
Martin S, Rice N, Smith PC. The Link Between Health Care Spending and Health Outcomes for the New English Primary Care Trusts. CHE Research Paper 42. York: University of York; 2008.
Mauskopf J, Rutten F, Schonfeld W. Cost-effectiveness league tables: valuable guidance for decision makers? Pharmacoeconomics 2003;21:991–1000.
Smith RD, Richardson J. Can we estimate the ‘social’ value of a QALY? Four core issues to resolve. Health Policy 2005;74:77–84.
Stinnett AA, Paltiel AD. Mathematical programming for the efficient allocation of health care resources. J Health Econ 1996;15:641–53.
Towse A, Pritchard C, Devlin N, eds. Cost Effectiveness Thresholds: Economic and Ethical issues. London: Office of Health Economics, The King’s Fund; 2002.
Ubel PA, Hirth RA, Chernew ME, Fendrick AM. What is the price of life and why doesn’t it increase at the rate of inflation? Arch Int Med 2002;163:1637–41.
Williams A. What Could Be Nicer Than NICE? London: Office for Health Economics; 2004.
Winkelymayer WC, Weinstein MC, Mittelman MA, Glynn RJ, Pliskin JS. Health economic evaluations: the special case of end-stage renal disease treatment. Med Decis Making 2002;22:417–30.
Step 3 results
Baker R, Bateman I, Donaldson C, Jones-Lee M, Lancsar E, Loomes G, et al. Weighting and valuing quality-adjusted life-years using stated preference methods: preliminary results from the Social Value of a QALY Project. Health Technol Assess 2010:14(27).
Bobinac A, van Exel N, Rutten FFN, Werner B. Willingness to pay for a quality-adjusted life-year: the individual perspective. Value Health 2010;13:1046–55.
Brock DW. How much is more life worth? Hastings Center Rep 2006;36:17–19.
Byrne MM, O’Malley K, Suarez-Almazor ME. Willingness to pay per quality-adjusted life-year in a study of knee osteoarthritis. Med Decis Making 2005;25:655–66.
Griffin S, Claxton K, Sculpher M. Decision analysis for resource allocation in health care. J Health Serv Res Policy 2008;13:23–30.
Gyrd-Hansen D. Willingness to pay for a QALY. Health Econ 2003;12:1049–60.
Harrison S. A policy agenda for health-care rationing. Br Med Bull 1995;51:885–99.
Laufer F. Thresholds in cost-effectiveness analysis – more of the story. Value Health 2005;8:86–7.
Pinto-Prades JL, Loomes G, Brey R. Trying to estimate a monetary value for the QALY. J Health Econ 2009;28:553–62.
Vernon JA, Goldberg R, Golec J. Economic evaluation and cost-effectiveness thresholds signals to firms and implications for R&D investment and innovation. Pharmacoeconomics 2009;27:797–806.
Step 4 results
Abelson P. The value of life and health for public policy. Econ Record 2003;79:S2–13.
Fryback DG, Lawrence WF. Dollars may not buy as many QALYs as we think: a problem with defining quality-of-life adjustments. Med Decis Making 1997;17:276–84.
Gafni A, Birch S. Guidelines for the adoption of new technologies – a prescription for uncontrolled growth in expenditures and how to avoid the problem. CMAJ 1993;148:913–17.
Johnson FR. Einstein on willingness to pay per QALY: is there a better way? Med Decis Making 2005;25:607–8.
Laupacis A, Feeny D, Detsky A, Tugwell P. How attractive does a new technology have to be to warrant adoption and utilization – tentative guidelines for using clinical and economic evaluations. CMAJ 1992;146:473–81.
Polsky D. Does willingness to pay per quality-adjusted life-year bring us closer to a useful decision rule for cost-effectiveness analysis? Med Decis Making 2005;25:605–6.
Martin S, Rice N, Smith P. The Link Between Health Care Spending and Health Outcomes: Evidence from English Programme Budgeting Data. CHE Research Paper 24. York: Centre for Health Economics; 2007.
Chambers JD, Neumann PJ, Buxton MJ. Does Medicare have an implicit cost-effectiveness threshold? Med Decis Making 2010;30:E14–27.
Step 5 results
Birch S, Gafni A. Cost-effectiveness ratios – in a league of their own. Health Policy 1994;28:133–41.
Johnson FR, Backhouse M. Eliciting stated preferences for health-technology adoption criteria using paired comparisons and recommendation judgments. Value Health 2006;9:303–11.
Step 6 results
Dolan P, Shaw R, Tsuchiya A, Williams A. QALY maximisation and people’s preferences: a methodological review of the literature. Health Econ 2004;14:197–208.
Baker R, Bateman I, Donaldson C, Jones-Lee M, Lancsar E, Loomes G, et al. Weighting and valuing quality-adjusted life-years using stated preference methods: preliminary results from the Social Value of a QALY Project. Health Technol Assess 2010;14(27).
O’Brien BJ, Gertsen K, Willan A, Faulkner L. Is there a kink in consumers’ threshold value for cost-effectiveness in health care? Health Econ 2002;11:175–80.
Buxton M. How much are health-care systems prepared to pay to produce a QALY? Eur J Health Econ 2005;6:285–7.
Mason AR, Drummond MF. Public funding of new cancer drugs: is NICE getting nastier? Eur J Cancer 2009;45:1188–92.
Appendix 2 The link between NHS spending and mortality: estimating the cost of a life-year in Englanda
Prologue
This report presents, in a linear fashion, details of the econometric work undertaken to estimate the link between NHS spending and mortality. It also presents details of how the econometric work is used to calculate the cost of a life-year. This report is designed to serve as a reference document in support of the main project report, which highlights the major findings from the project. As a supporting document this report provides far more detail than most interested parties will require. Nevertheless, those who seek more detail than that contained in the main project report may find the material here useful.
Background, model, data, and estimation approach
Introduction
In a recent White Paper the new British Conservative government emphasised the importance of clinical outcomes. It notes that, in future, success will be measured, not through the achievement of process targets, such as short waiting times, but against outcomes such as cancer and stroke survival rates. 12 Although the NHS budget is ring-fenced against the ongoing public sector deficit reduction programme, its budget is still likely to be under considerable pressure, and attention is likely to focus on the extent to which any additional health-care expenditure yields genuine patient benefits in the form of improved health outcomes.
However, one of the most fundamental yet unresolved issues in health policy is the extent to which additional health-care expenditure yields patient benefits, in the form of improved health outcomes. The work of health technology agencies, such as NICE, has greatly improved our understanding at the micro-level of the costs and benefits of individual therapeutic technologies. However, there remains a dearth of evidence at the macro-level on the benefits of increased health system expenditure.
Recently a series of studies has taken advantage of the availability of two new data sets to examine the relationship between NHS expenditure and mortality rates for various disease categories. 59,60,62,63 One data set contains mortality rates for various disease categories at the level of geographically defined local health authorities, known as PCTs. The other data set presents NHS expenditure by PCT on 23 broad programmes of care. This data set embraces most items of publicly-funded expenditure, including inpatient, outpatient and community care, and pharmaceutical prescriptions.
Like previous studies, we employ a model that assumes that each PCT receives an annual financial lump sum budget from the national ministry and allocates its resources across the 23 programmes of care to maximize the health benefits associated with that expenditure. Estimation of this model using the expenditure and mortality data facilitates two related studies: first, a study of how changes in the NHS budget impact on expenditure in each care programme; and second, a study of the link between expenditure in a programme and the health outcomes achieved, notably in the form of disease-specific mortality rates. The latter study also permits the calculation of the cost of an additional life-year for individual programmes of expenditure.
The work presented here draws heavily on previous studies. These were constrained in a number of ways and, in this analysis, we build on and improve these previous studies in four major ways:
-
First, due to data limitations previous studies related expenditure in time period t to mortality in periods t, t – 1, and t – 2 combined. In doing this, such studies assumed that PCTs had reached some sort of equilibrium in the expenditure choices they make and the outcomes they secure. This is probably not an unreasonable assumption given the relatively slow pace at which both types of variable change but, with more recent mortality data now available, here we relate expenditure in time period t to mortality in periods t, t + 1, and t + 2 combined (see Model estimation using 2006/7 expenditure data and mortality data for 2006/7/8: CARAN need and two market forces factors).
-
Second, previous studies have tended to focus on a very limited number of care programmes (e.g. cancer, circulatory disease, gastrointestinal problems and respiratory problems). Here we present plausible outcome models for a larger number of budgeting categories.
-
Third, previous estimates of the cost of a life-year have been for individual programmes of care. Here we present estimates of the cost of a life-year for an enlarged number of programmes and, importantly, with the aid of assumptions about the productivity of programmes without a meaningful mortality-based outcome indicator, we extend our individual programme estimates to incorporate expenditure across all programmes of care.
-
Finally, although previous results and our current models ‘pass’ the appropriate statistical tests, we subject our latest results to a substantial sensitivity analysis.
The structure of this report is as follows. Previous studies presents a brief review of previous empirical studies in this domain, which have often yielded conflicting results. A straightforward theoretical model of the budgetary problem faced by a PCT manager seeking to allocate limited funds between competing programmes of care is presented in Theoretical model. The PB and health outcome (mortality) data are described in NHS programme budgeting in England and Health outcome and other data respectively. Estimation issues and strategy outlines our estimation methods and some of the issues surrounding them.
In Analysis of programme budgeting expenditure for 2005/6 and mortality data for 2002/3/4 we commence our empirical work by estimating well-specified econometric models that outline (a) the budgetary expenditure choices and (b) the health outcomes achieved by PCTs using expenditure data for 2005/6 and mortality data for 2002/3/4. Analysis of programme budgeting expenditure for 2006/7 presents results using expenditure data for 2006/7 and mortality data for 2004/5/6. It also presents results using the same expenditure data but updating the mortality data to 2006/7/8. Several pieces of sensitivity analysis are also included in Analysis of programme budgeting expenditure for 2006/7, but the major piece of sensitivity analysis – examining the impact of relaxing the instrument validity restriction – is reported in The sensitivity of the outcome elasticity to the validity of the instrument exclusion restrictions.
In Analysis of programme budgeting expenditure for 2007/8 and mortality data for 2007/8/9 we re-estimate our model using updated expenditure and mortality data. In particular, we use the PB expenditure for 2007/8 and mortality data for 2007/8/9 to re-estimate our outcome and expenditure equations. In Analysis of programme budgeting expenditure for 2008/9 and mortality data for 2008/9/10 we update the data set again, and this time we employ PB expenditure data for 2008/9 and mortality data for 2008/9/10. We also compare the elasticities and cost of a life-year estimates that we have obtained using expenditure and mortality data for different years.
Finally, Summary and concluding remarks presents a summary of our findings and some concluding remarks.
Previous studies
There is a large body of literature on the determinants of international variations in health-care spending in which income levels often play a central role. 140 However, whether or not more expenditure generates better outcomes – for example, in terms of reduced mortality – remains a matter of debate. For example, Fisher and Welch141 note various ways in which more health care might harm patients and they cite various studies supporting their arguments. In a comprehensive review, Nolte and McKee67 discuss many studies that examine the impact of health care and other explanatory variables on some measure of health-care outcome. Nolte and McKee67 point out that researchers usually combine a production function approach with the application of regression analysis. For example, in an early cross-sectional study of 18 developed countries, Cochrane et al. 64 use regression analysis to examine the statistical relationship between mortality rates on the one hand and per capita GNP and per capita consumption of inputs such as health-care provision on the other. They find that the indicators of health-care provision were generally not associated with the outcomes in the form of mortality rates. Thereafter, the failure to identify strong and consistent relationships between health-care expenditure and health outcomes (after controlling for other factors) has become a consistent theme in the literature, while, in contrast, socioeconomic factors are often found to be good determinants of health outcomes. 65–67
This failure to detect a significant positive relationship between expenditure and health outcome might reflect the difficulties associated with any such study rather than the absence of such a relationship. For example, Gravelle and Backhouse68 examine some of the methodological difficulties associated with empirical investigation of the determinants of mortality rates. These include simultaneous equation bias and the associated endogeneity problem (that the level of health-care input might reflect the level of health outcome achieved in the past), and that a lag may occur between expenditure and outcomes (studies typically assume that expenditure has an immediate effect on mortality). To avoid the difficulties imposed by data heterogeneity inherent in international analyses, the study by Cremieux et al. 69 examines the relationship between expenditure and outcomes across 10 Canadian provinces over the 15-year period 1978–92. They find that lower health-care spending is associated with a significant increase in infant mortality and a decrease in LE.
Although challenging the received empirical wisdom, one difficulty with the Cremieux et al. 69 study is that the estimated regression equation consists of a mixture of potentially endogenous variables (such as the number of physicians, health spending, alcohol and tobacco consumption, and expenditure on meat and fat) and exogenous variables (such as income and population density). The authors’ chosen estimation technique (GLS) does not allow for this endogeneity and consequently the coefficients on the endogenous variables may be biased. 68 Or’s142 study of the determinants of variations in mortality rates across 21 Organisation for Economic Co-operation and Development countries between 1970 and 1995 may suffer from the same weakness. She finds that the contribution of the number of doctors to reducing mortality in Organisation for Economic Co-operation and Development countries is substantial but her estimation technique assumes that the number of doctors is exogenous to the health system.
Nixon and Ulmann70 provide a detailed review of 16 studies that have examined the relationship between health-care inputs and health outcomes, using macro-level data. They also undertake their own study using data for 15 EU countries over the period 1980–95. They employ three health outcome measures – LE at birth for males and females, and the infant mortality rate – and a dozen or more explanatory variables including per capita health expenditure; number of physicians (per 10,000 head of population); number of hospital beds (per 1000 head of population); the average length of stay in hospital; the inpatient admission rate; alcohol and tobacco consumption; nutritional characteristics; and environmental pollution indicators. Nixon and Ulmann70 conclude that although health expenditure and the number of physicians have made a significant contribution to improvements in infant mortality, ‘. . . health care expenditure has made a relatively marginal contribution to the improvements in LE in the EU countries over the period of the analysis’. Again, however, the study does not allow for the possibility that some of the explanatory variables may be endogenous.
Although loosely based on the notion of a health production function, the traditional empirical study described above has rarely been informed by an explicit theoretical model. This is understandable, as the processes giving rise to the observed health outcomes are likely to be very complex, and any theoretical model might become rather unwieldy. However, this absence of a model has usually led to a theoretical search for measures of health inputs demonstrating a statistically ‘significant’ association with health outcomes. In contrast, in this study we inform our empirical modelling with a theoretical framework. We believe that this may lead to a more convincing and better specified model of health outcomes than that used in many previous studies, and this model is outlined in the next section.
Theoretical model
Our modelling framework assumes that each PCT i receives an annual financial lump sum budget yi from the national ministry, and that annual total expenditure cannot exceed this amount. The PCT must then decide how to allocate its budget across the J programmes of care (J = 23 in this case). For each programme of care there is a ‘health production function’ fi(.) that indicates the link between local spending xij on programme j and health outcomes in that programme hij. Health outcomes might be measured in a variety of ways, but the most obvious is to consider some measure of improvement in LE, possibly adjusted for QoL, in the form of a QALY.
The nature of the specific health production function confronted by a PCT will depend on two types of local factors: the clinical needs of the local population relevant to the programme of care (which we denote nij); and broader local environmental factors zij relevant to delivering the programme of care (such as input prices, geographical factors, or other uncontrollable influences on outcomes). Both clinical and environmental factors may be multidimensional in nature. Increased expenditure then yields improvements in health outcomes, as expressed, for example, in improved local mortality rates, but at a diminishing rate. That is:
We assume there is a PCT social welfare function W(.) that embodies health outcomes across the J programmes of care. Assuming no interaction between programmes of care, each PCT allocates its budget so as to maximise total welfare, subject to the local budget constraint and the health production function for each programme of care:
It can of course quite plausibly be argued that decision-makers do not discriminate between health outcomes in different programmes of care, and that W(.) is merely the sum of such outcomes. However, there is no need for that assumption in our formulation.
Each PCT allocates expenditure across the 23 programmes of care so that the marginal benefit of the last pound spent in each programme of care is the same. This is represented diagrammatically in Figure 12, which illustrates the trade-off between just two programmes of care. The top left-hand quadrant indicates the health production function for programme 1, whereas the bottom right-hand quadrant indicates the health production function for programme 2, albeit in transposed form. The bottom left-hand quadrant indicates the budget constraint; the expenditure choice must lie on the budget line. This means that for each feasible pair of expenditure choices (points on the budget constraint line), a pair of health outcomes in the two programmes emerges, which is traced out as the health production possibility frontier in the top-right quadrant. The PCT will choose the point on this frontier that maximizes welfare. In this example, we have indicated a simple health maximizing approach (the maximum health summing across the two programmes), leading to optimal health outcomes (H1*, H2*) and expenditure (X1*, X2*).
FIGURE 12.
Graph showing optimal trade-off between two programmes of care.

Solving the constrained maximisation problem yields the result that the optimal level of expenditure in each category, xij*, is a function of the need for health care in each category (ni1, ni2, . . . , niJ), environmental variables affecting the production of health outcomes in each category (zi1, zi2, . . ., ziJ), and PCT income (yi). Thus:
Thus, for each programme of care there exists an expenditure equation (see Equation 11) explaining expenditure choice of PCTs and a health outcome equation (see Equation 9) that models the associated health outcomes achieved.
Our model is static in the sense that the health production function (see Equation 9) assumes that all health benefits occur contemporaneously with expenditure. We acknowledge that for some programmes of care benefits might occur ≥ 1 year after expenditure has occurred. This is particularly likely to be the case for those programmes aimed at encouraging healthy lifestyles, where some benefits may occur decades after the actual programme expenditure. For other programmes, such as maternity/reproductive conditions and neonate conditions, benefits may be largely contemporaneous with expenditure. Furthermore, we do not model the decision-maker’s time preferences.
For our empirical modelling, however, we are constrained by the data we have available, which are largely cross-sectional in nature. Owing to data limitations, previous studies have had to relate expenditure in period t to mortality data in periods t, t – 1, and t – 2 combined so that the mortality data precedes the expenditure data. This is not ideal. Implicitly previous studies have had to assume that the data represent a quasi long-run equilibrium position, and that relative expenditure levels and health outcomes within each PCT have been reasonably stable over a period of time. As we shall see, this appears to be a reasonable assumption because we obtain similar results when we estimate our models using expenditure for period t with either mortality data for periods t, t – 1, and t – 2 combined (see Model estimation using 2006/7 expenditure data and mortality data for 2004/5/6: CARAN need and two market forces factors) or with mortality data for periods t, t + 1, and t + 2 combined (see Health outcome and other data).
Having outlined our model, in the next section we discuss the data sets used to estimate this model.
NHS programme budgeting in England
The English NHS is the archetypal centrally-planned and publicly-funded health-care system. Its revenue derives almost entirely from national taxation, and access to the system is generally free to the patient. Primary care is an important element of the system, and GPs act as gatekeepers to secondary care and pharmaceuticals. The system is organised geographically, with responsibility for the local administration of the NHS devolved to local health authorities known as PCTs. b For the purposes of this study, there were 303 PCTs with an average population of about 160,000 people until October 2006. In October 2006 the 303 PCTs became 152 PCTs. Some PCT boundaries remained unchanged whereas other PCTs were merged with one or more neighbours to form a new larger PCT. In a few cases the geographic area covered by an existing PCT was split between two or more new PCTs. These 152 PCTs have an average population of about 330,000 people. c PCTs are allocated fixed annual budgets by the national ministry, within which they are expected to meet expenditure on most aspects of health care, including inpatient, outpatient and community care, primary care and pharmaceutical prescriptions.
The rationale behind the construction of programme budget data
Traditionally, PCTs and their predecessors have reported expenditure on the basis of inputs (e.g. total expenditure on pay and non-pay items). However, NHS policy-makers have for some time realised that this approach does not create clinically meaningful financial data or help in the design and evaluation of programmes of patient care. The Department of Health therefore initiated a ‘Programme Budgeting’ project. This has sought to create an accounting system that is more aligned with the distinct outputs and health outcomes of the health-care system. Since April 2003, in addition to its conventional accounting data, each PCT has prepared expenditure data disaggregated according to 23 programmes of health care. These programmes are defined by reference to the ICD-10 codes at the four-digit level, and most PBCs reflect ICD-10 chapter headings (e.g. cancer and tumours, circulation problems, renal problems, neonates, problems associated with the skin, problems associated with vision, problems associated with hearing, etc.). In some cases, the 23 categories are broken down into further subareas to achieve a closer match with the various NSFs; for example, the large mental health category is broken down into ‘substance abuse’, ‘dementia’, and ‘other’.
Programme budgeting seeks to allocate all types of PCT expenditure to the various PBCs, including secondary care, community care and prescribing. However, the system acknowledges that a medical model of care may not always be appropriate, and two specific non-clinical groups – ‘healthy individuals’ and ‘social care needs’ – have been created. These are intended to capture the costs of disease-prevention programmes and the costs of services that support individuals with social rather than health-care needs. In addition, in some cases it is not possible to assign activity by medical condition, preventative activity, or social care need and, in these cases, expenditure is assigned to a residual category (PBC 23) entitled ‘other’. The most important element of this residual programme is expenditure on GP services (PBC 23a). In principle, it should be possible to allocate each GP consultation to a particular care programme. However, at the moment the available data information systems do not permit such an allocation and so all primary care expenditure is allocated to this residual programme. The use of this residual category ensures that all expenditure is assigned to a programme of care. 73
The aim of the programme budget classifications is to identify the entire volume of health-care resources assigned to broad areas of illness according to the primary diagnosis associated with an intervention. It serves a number of purposes, most notably to assist in the local planning of health care. However, for this study its crucial merit is that it opens up the possibility of examining the statistical relationship between local programme spending and the associated disease-specific outcome.
The collection of programme budgeting data
Programme budgeting information is collected centrally by the Department of Health as part of the annual accounts process. Each PCT is required to submit an annual PB return to the Department which shows how their total expenditure is allocated across the 23 PBCs.
Various forms of data collection and analysis are required to map PCT expenditure onto acute, community and other services to the 23 PBCs. From the PCT perspective, however, the construction of each PCT’s return largely involves collating information provided by other bodies and drawing on other information already in the PCT’s own annual accounts. Thus GMS/PMS expenditure, which is already reported in PCT accounts, relates to direct primary care and is mapped in its entirety to PBC 23a (other: GMS/PMS); general ophthalmic service expenditure (again from PCT accounts) maps directly to PBC 8 (eye/vision problems); and general dental service expenditure maps directly to PBC 12 (dental problems). Prescribing and pharmaceutical services expenditure is allocated to PBCs on the basis of an annual apportionment report provided by the prescription pricing authority for each PCT as part of the annual accounts process. This apportionment report allocates each PCT’s annual family health service prescribing expenditure across the 23 PBCs. The balance of any primary health care purchased by the PCT is allocated/apportioned across the 23 PBCs on the basis of local records, with any remaining expenditure allocated/apportioned in line with the distributions already made across the budget categories.
It is the responsibility of all NHS providers – which includes PCTs, NHS trusts, and foundation hospitals – to allocate admitted patient care expenditure across the PBCs, specific to each PCT that utilises its services. These allocations are constructed using ‘finished consultant episodes’ (FCEs) (from the mandatory administrative HES data set returned by each provider) each of which is assigned to a Healthcare Resource Group (HRG), an English version of Diagnosis-Related Groups. National grouping software automatically assigns each HRG to 1 of the 23 PBCs and attaches the provider’s average reference cost for the relevant HRG to each record. For each PCT this information generates a split of inpatient care expenditure by PBC for each of its secondary health-care providers.
There are numerous difficulties faced when attempting to allocate non-admitted patient care activity (that is, outpatients, community services, direct access, A&E, etc.) to PBCs. The difficulties are primarily due to the absence of clear diagnostic codes. The ‘primary reason for care’ (equivalent to a diagnosis code) is not information that is routinely collected for community patients. Because of this, the approach prescribed is for service providers to produce a generic allocation analysis/report, for all PCTs making use of their services, for all non-admitted patient care costs across the 23 PBCs. Once derived, this generic allocation analysis/report is made available to PCTs at the same time as the unique (PCT-specific) inpatient care information described above. Unlike the first apportionment report relating to admitted patient care, the non-admitted patient care apportionment report will not be unique to the PCT, but will represent the provider’s overall experience. PCTs are expected to use these data to inform the apportionment of their own spend on non-admitted patient care across the 23 PBCs.
The Department of Health recognises that this approach – the provision of a PCT-specific breakdown of admitted patient care costs and a generic allocation of all PCTs non-admitted patient care spend by providers – is likely to generate a crude method for apportioning non-admitted patient care costs. PCTs and their providers are therefore encouraged to put in place other arrangements that allow a more sophisticated analysis of non-admitted patient care expenditure. Such arrangements may well rely on an activity sampling approach. 73
Mental health providers may not need to complete and forward detailed admitted and non-admitted patient care apportionment reports to PCTs. The nature of the services they provide may be such that the entire spend with them relates exclusively to the mental health programme (PBC 5). Ambulance trusts are required to provide non-admitted patient care information to those PCTs for whom they provide services. Where it is not possible to split the activity by PCT, a generic non-admitted patient care report is produced for all purchasers. 73
The Department of Health has been criticised for the rather simplistic way in which it has apportioned certain costs among categories, and there are obvious issues with the allocation of costs associated with patients who have multiple disorders. However, the Programme Budgeting project is very much work-in-progress and the Department is investigating ways to improve the accuracy with which costs are allocated across programmes (e.g. the Department is investigating the possibility of allocating training expenditures to specific programmes rather than to the generic medical training programme PBC 23b). d
Programme budgeting expenditure, 2003/4–2008/9
National (all PCT) expenditure per head and the growth in this expenditure are shown for each PBC for 2003/4–2008/9 in Table 1. Comparable data for each programme budget subcategory are shown in Table 91 in the Annex. Year-on-year comparisons of expenditure in each group are complicated by the fact that the algorithms used to allocate activity to PBCs are regularly revised. For example, for 2006/7 two major changes were made to the methods employed to construct the PB data. First, expert medical opinion was employed to re-evaluate the existing mapping from inpatient diagnosis codes to PBC. This led to the reassignment of just over 10% of all diagnosis (ICD-10) codes from one PBC to another. e,f Second, activity to be costed used the newly introduced version 4 of the HRG software which, among other things, changed the methodology for calculating non-admitted patient care costs. HRG4 reflected advances in clinical practice and was designed to generate a much more accurate costing of complex cases. Other developments, such as the transfer of responsibility for dental funding from local dental boards to PCTs, also complicate the interpretation of comparisons through time (e.g. per capita dental expenditure by PCTs increased from £13.55 in 2004/5 to £51.93 in 2006/7).
The expenditure figures for the first year (2003/4) are calculated on a slightly different basis to those for the other years (2004/5–2008/9). In particular, the figures for 2003/4 are on a ‘net expenditure’ basis whereas the figures for 2004/5–2008/9 are on an ‘own population’ basis. The ‘own population’ figure starts with net expenditure, it adds any expenditure funded from sources outside of the NHS, and then deducts any expenditure on other PCTs’ populations incurred through lead/host commissioning arrangements. In 2006/7 and across all PBCs, expenditure per head on an own population basis was 2.3% greater than expenditure on a net population basis.
In 2004/5 total PCT expenditure per person was £1200. The category attracting the most expenditure was the ‘other’ category (PBC 23) with per capita expenditure of almost £158 (13.2%). This category included primary care expenditure, workforce training expenditure, and a range of other miscellaneous expenditure items. Of these components, primary care expenditure was by far the largest element at £127 per head.
In 2004/5 there were two other categories with a budget share of over 10%: mental health (PBC 5) attracted 12.2% of expenditure (£147 per person), and circulation problems (PBC 10) recorded 10.2% of expenditure (£122 per person). Seven PBCs – cancers and tumours (£76), gastrointestinal problems (£73), trauma and injuries (£72), musculoskeletal problems (£72), respiratory problems (£63), genitourinary problems (£62) and maternity and reproductive conditions (£55) – had expenditure shares of between 4.6% and 6.3%. Finally, the 13 remaining PBCs – from hearing problems (£6) to learning disability (£43) – each account for between 0.5% and 3.6% of total expenditure.
By 2008/9 total PCT expenditure per person had increased to £1531 (up 28% from 2004/5). The residual ‘other’ category (PBC 23) still accounted for the largest share of expenditure (14.9%) with per capita expenditure of almost £228, of which £145 was accounted for by primary care expenditure. Mental health (PBC 5) still accounted for just over 12% of expenditure, but the expenditure share recorded by circulation problems (PBC 10) had fallen from 10.2% to 8.5%. Other categories recording a fall in budget share of more than one half of one percentage point included the gastrointestinal system (down from 6.1% to 5.1%), the musculoskeletal system (down from 6% to 5.2%), trauma and injuries (down from 6% to 4.2%) and maternity (down from 4.6% to 3.9%).
Categories recording an increase in budget share of more than one half of one percentage point included neurological problems (up from 2.9% to 4.4%) and dental problems (up from 1.1% to 4.1%).
Some of these changes will partly reflect revisions to the algorithms used to allocate expenditure to particular PBCs. For example in 2006/7 expenditure per person on musculoskeletal problems fell by 11% and expenditure on trauma and injuries fell by 25%. In the same year, expenditure on neurological problems increased by 35%. This suggests that some types of activity, which were previously allocated to musculoskeletal problems and/or trauma and injuries, were reallocated to neurological problems.
Similarly, up to and including 2006/7, expenditure that was not directly attributable to a particular programme category was apportioned using admitted patient care percentages. g In other words, if x% of total admitted patient care expenditure was allocated to PBC 1, then x% of all expenditure that was not directly attributable to a particular programme category was also allocated to PBC 1. With effect from 2007/8, however, NHS organisations were asked to select an appropriate basis for the apportionment of this non-programme-specific expenditure and that, where no reasonable basis existed, such expenditure was to be allocated to the ‘other – miscellaneous’ (PBC 23X) category.
These two changes to the algorithm used to allocate expenditure to particular PBCs illustrate that year-on-year comparisons of expenditure need to be interpreted with care.
Obviously, expenditure per head on any given programme varies from one PCT to another and Table 2 presents some statistics that indicate the degree of variation in expenditure levels across PCTs by PBC. Columns (2)–(5) of Table 2 present descriptive statistics for PCT expenditure per person by PBC. These reveal that, for example, PCT per capita expenditure in the cancer programme averaged £96.30 across all PCTs, with the minimum spend being £62.90 and the maximum being £155.70.
Some PCTs will be spending more than other PCTs simply because they face higher input costs. Columns (6)–(9) in Table 2 present descriptive statistics for PCT per capita expenditure that has been adjusted for the unavoidable geographical variation in costs (input prices) faced by PCTs. h However, if anything this adjustment appears to increase the variation in expenditure across PCTs; for example, the range of per capita expenditure on cancer increases from between £62.90 and £155.70 (unadjusted) to between £59.10 and £163.10 (adjusted for local health-care input prices).
Another cause of the variation in expenditure levels will be the fact that the need for health care will vary from one PCT to another. For example, areas with a relatively large proportion of elderly residents, or PCTs operating in relatively deprived locations, can be expected to experience relatively high levels of spending. The Department of Health has a well-developed methodology for estimating the relative health-care needs of PCTs, which it uses as the basis for allocating health-care funds to PCTs. 74 Recent ‘needs’ formulae have been derived from an adjustment for the demographic profile of the PCT and a series of econometric analyses of the link between health-care expenditure and other socioeconomic factors at a small area level within England. 74
Columns (10)–(13) in Table 2 present descriptive statistics for PCT per capita expenditure that has been adjusted for both the unavoidable geographical variation in costs and the local need for health care faced by PCTs. i For virtually every PBC this adjustment reduces the variation in expenditure across PCTs (e.g. the standard deviation of PCT per capita expenditure, falls from £19.70 to £15.30 for the cancer programme). Although this adjustment reduces the variation in expenditure levels across PCTs, this decline is quite modest and there are still substantial differences in expenditure even after allowing for differences in local cost and need. For example, expenditure per head in the circulation problems category varies between £78 and £328 using cost-adjusted expenditure data, but falls between £76 and £327 using cost- and need-adjusted population data.
This variation in expenditure across PCTs has led some commentators to question the reliability of the PB data. In a good governance report, the NAO75 sought to ‘ . . . examine the quality, timeliness and suitability of PB data to support [their] audit of the Department of Health Resource Account and determine whether the systems and processes in place to provide the data are accurate’. The NAO undertook a survey of trusts, PCTs and SHAs. The NAO noted that a number of PCTs expressed concern about the accuracy of data supplied to them by their service providers and noted that this was believed to be because most trusts did not use or find the data they supply to PCTs of any use to themselves. Overall, the NAO’s main conclusion was that although the processes for collecting the budgeting data were well defined in most areas, there remained scope for improvement to the robustness of some of the data (such as the non-admitted patient care data).
Appleby et al. 76 also considered the issue of data reliability in their study of variations in PCT spending on cancer services. They noted a rather dramatic variation in spending across PCTs for any given year, and that a relatively large number of PCTs report relatively large year-on-year changes in cancer expenditure. However, and as the authors point out, it is difficult to define what might be either an implausible level of expenditure or an implausibly large change in expenditure. Moreover, the interpretation of a large change in expenditure is complicated by the fact that the Department of Health makes regular changes (improvements) to the algorithm used to allocated activity to PBCs (as detailed above).
As a case study of the reliability of the PB data, Appleby et al. 76 report the results of West Kent PCT’s use of an alternative approach to producing the PB data for cancer and tumours. This alternative approach identified similar levels of expenditure to the traditional method at the aggregate level, but there were differences between the two approaches at the subprogramme level (that is, for expenditure on specific cancer sites and in the residual ‘other cancers’ category).
As with any data set, there are likely to be recording and other errors associated with the PB data. However, there is no evidence on the magnitude of such errors and we have no reason to believe that such errors are likely to bias our estimates in one particular direction (e.g. we have no reason to believe that measurement errors are systematically related to other relevant factors such as mortality rates). In this study, our focus is on whole programme expenditure and thus we avoid the data reliability issues inherent in any analysis of the subprogramme expenditure data. j Moreover, although we present estimates of the cost of a life-year for individual programmes, our primary focus is on the cost of a life-year across all programmes combined. The advantage of this is that the impact of a PCT reporting, for example, too little expenditure in one category might be offset by reporting too much expenditure in another.
Although we note that the allocation of expenditure might not be consistent across PCTs there is no systematic evidence that the magnitude of any inconsistency is sufficiently large to cause concern. Accordingly, for each disease category, the observed variation in expenditure per person – holding constant input prices and the need for health care – offers the opportunity to examine whether or not PCTs that spend more on health care achieve a better outcome and, if so, at what cost. Empirical estimates of the strength of this relationship for both individual and all programmes of care are presented later in this report.
Health outcome and other data
Health outcome data
Most studies of the relationship between expenditure and outcome have used some measure of mortality as an indicator of the latter. We too employ mortality as our outcome measure for two reasons: first, it is a relevant (but admittedly not comprehensive) measure of the outcome of health-care expenditure; and second, it is available for more disease areas than any other outcome measure at PCT level.
Although mortality is available (by PCT) for several disease areas, it is not available for just over a half of all programmes not least because it is simply not relevant for these programmes (e.g. for learning disabilities, vision problems, hearing problems, dental problems and skin problems). Moreover, even where a mortality measure is available, the ICD-10 coverage of the mortality data often falls short of the coverage of the expenditure data. For some programmes, therefore, we have combined the published mortality rates for two or more disease areas in an attempt to match the ICD-10 coverage of the mortality data with that of the expenditure data.
Table 37 shows how we have attempted to marry the mortality data [see Table 37, column (4)] and the expenditure data [see Table 37, column (2)]. However, and as Table 37 shows, the ICD-10 coverage of the component mortality rates for some PBCs still falls short that of the expenditure data and the extent of this shortfall is illustrated by the ratio reported in the final column of Table 37. For example, the cancers and tumours programme covers all expenditure associated with ICD-10 codes C00–C97 and D00–D49 but the PCT-based mortality data only relates to ICD-10 codes C00–C97. At the national (all England) level, figures are available which show that, in 2008, there were 62,072 deaths of those aged under 75 years from codes C00–C97 and that there were 63,076 deaths from codes C00–C97 and D00–D49 combined. In other words, the PCT-level mortality data reflects 98.4% of all deaths associated with the expenditure codes. Initially, we did not adjust our cost of life (year) estimates for this mismatch but, as we will see in Adjusting the cost of life (year) estimates for the mismatch in the ICD-10 coverage of the expenditure and the mortality data, an adjustment has been made for this mismatch in the final calculation of the cost of a life (year) associated with expenditure for 2006/7. The same adjustment has also been applied to the cost of a life (year) estimates associated with expenditure for 2007/8 and for 2008/9.
(1) PBC | (2) ICD-10 coverage of PBC | (3) Number of deaths, < 75 years, 2008, England (ONS, VS3) corresponding to column (2) ICD-10 codes | (4) ICD-10 coverage of best match PCT-based mortality rate(s) | (5) Number of deaths, < 75 years, 2008, England (ONS, VS3) corresponding to column (4) ICD-10 codes | (6) Ratio [column (5)/column (3)] |
---|---|---|---|---|---|
1 | Infectious diseases (large parts of A00–B99) | 1968 | Infectious diseases (A00–B99) | 1968 | 1 |
2 | Cancers and tumours (C00–C97, D00–D49) | 63,076 | All cancers (C00–C97) | 62,072 | 0.984 |
3 | Blood disorders (D500–D899) | 393 | No relevant mortality rate by PCT available | N/A | N/A |
4 | Endocrine, nutritional and metabolic problems (E000–E899) | 2368 | Diabetes (E10–E14) | 1501 | 0.634 |
5 | Mental health (F00–F69, Z55, Z56) | N/A | No relevant mortality rate available | N/A | N/A |
6 | Learning disability (F700–F739, F780–F849, F88–F90, Q90, Q91) | N/A | No relevant mortality rate available | N/A | N/A |
7 | Neurological system (G000–G999, Q000–Q079, R200–R999) | 5238 | Epilepsy (G40–G41) | 713 | 0.136 |
8 | Eye and vision problems (H000–H599, Q100–Q159) | N/A | No relevant mortality rate available | N/A | N/A |
9 | Hearing problems (H600–H999, Q160–Q179) | N/A | No relevant mortality rate available | N/A | N/A |
10 | Circulation problems (I00–I99, Q20–Q28) | 39,923 | Circulatory diseases (I00–I99) | 39,590 | 0.992 |
11 | Respiratory problems (A150–A169, A190–A199, J000–J989, Q300–Q349, R000–R099) | 14,417 | Asthma (J45–J46); bronchitis, emphysema, other COPD (J40–J44); pneumonia (J12–J18) | 11,147 | 0.773 |
12 | Dental problems (K000–K099) | N/A | No relevant mortality rate available | N/A | N/A |
13 | Gastrointestinal problems (I840–I859, K091–K929, Q380–Q459, R100–R198) | 10,656 | Liver disease (K70, K73–K74); ulcers (K25–K27) | 6082 | 0.571 |
14 | Skin problems (L000–L999, Q351–Q379, Q800–Q859) | 367 | No relevant mortality rate available | N/A | N/A |
15 | Musculoskeletal problems (M00–M99, Q18, Q650–Q799) | 933 | No relevant mortality rate available | N/A | N/A |
16 | Trauma, burns and injuries (S000–S999, T000–T357, T79, T90–T98) | 5809 | Skull, cranial injury (S02, S06, T90); fracture of thighbone (S72) | 1014 | 0.175 |
17 | Genitourinary problems (A50–A64, N00–N99, Q500–Q649, R30–R39, R86–R87) | 1565 | Chronic renal failure (N18) | 269 | 0.172 |
18 | Maternity and reproductive problems (N96–N98, O000–O999, Z300–Z391) | 41 | No relevant mortality rate available | N/A | 8.213, but see note below |
19 | Neonate conditions (P000–P299, P350–P399, P500–P619, P700–P839, P900–P969) | 226 | Infant mortality rate per 1000 live births, aged < 28 days (all ICD-10 codes) | 4345 | 8.213, but see note below |
20 | Poisoning (Q86, R78, R82, T360–T888) | N/A | No relevant mortality rate available | N/A | N/A |
21 | Healthy individuals | N/A | No relevant mortality rate available | N/A | N/A |
22 | Social care needs | N/A | No relevant mortality rate available | N/A | N/A |
Of course, we acknowledge that mortality is a more relevant outcome indicator for some programmes (e.g. for circulatory problems) than for others (e.g. for epilepsy) and, for this reason, we would expect better results in some programmes than others. We also acknowledge that this focus on mortality ignores the impact of expenditure aimed at chronic care and at palliative care. Nevertheless, our focus on mortality is purely practical: it is both a widely available measure and it is clearly a relevant outcome indicator. Moreover, the approach adopted here is extendable in principle to other non-mortality-based outcome indicators. We illustrate such an application in Application of method to other non-mortality-based outcome indicator where we use EQ-5D utility scores pre and post an operative procedure from the PROMs programme to generate a non-mortality-based outcome indicator, and we use this indicator to estimate our outcome model.
Previous studies using the PB data have employed two alternative mortality-based outcome indicators: the under 75 years of age SMR and the under 75 years SYLLR. The SMR gives equal weight to all deaths irrespective of the age at which they occur but the SYLLR gives greater weight to deaths that occur at earlier ages.
We employed both the SMR and the SYLLR when undertaking some preliminary sens7itivity analysis (i.e. in Estimation issues associated with the use of 2006/7 expenditure data when considering, for example, which measure of need to use), but elsewhere we have focussed solely on a measure of the avoidable YLL. k This is calculated by summing over ages 1–74 years the number of deaths at each age multiplied by the number of years of life remaining up to age 75 years. The crude YLL rate is simply the number of YLL divided by the resident population aged < 75 years. Like conventional mortality rates, the crude YLL rate can be age standardised to eliminate the effects of differences in population age structures between areas, and this (age) standardised YLL rate is the health outcome variable generally employed in this study (Lakhani et al. , p. 379). 77
Descriptive statistics for the SYLLRs employed in this study are shown in Table 38. For example, for all deaths over the 3-year period from 2006 to 2008, the annual SYLLR across all PCTs for those aged < 75 years averaged 467 YLL per 10,000 population, but this rate varied considerably across PCTs, ranging between 288 and 749 YLL per 10,000 population. Similarly, large variations in the mortality rate across PCTs are evident for other disease groups. l
Variable | Observations | Mean | SD | Min. | Max. |
---|---|---|---|---|---|
All causes of death, SYLLR, 2002/3/4 | 303 | 489.2 | 94.2 | 320.3 | 889.5 |
All causes of death, SYLLR, 2004/5/6 | 152 | 483.4 | 83.9 | 318.1 | 742.5 |
All causes of death, SYLLR, 2006/7/8 | 152 | 467.3 | 83.7 | 287.8 | 748.9 |
All causes of death, SYLLR, 2007/8/9 | 151 | 457.1 | 81.8 | 297.2 | 731.6 |
All causes of death, SYLLR, 2008/9/10 | 151 | 446.4 | 78.6 | 290.8 | 736.9 |
Cancer, SYLLR, 2002/3/4 | 303 | 161.9 | 20.8 | 115.6 | 263.4 |
Cancer, SYLLR, 2004/5/6 | 152 | 158.4 | 18.3 | 103.4 | 218.8 |
Cancer, SYLLR, 2006/7/8 | 152 | 154.2 | 19.0 | 90.5 | 212.2 |
Cancer, SYLLR, 2007/8/9 | 151 | 151.0 | 18.5 | 98.3 | 201.9 |
Cancer, SYLLR, 2008/9/10 | 151 | 147.9 | 17.5 | 100.2 | 193.9 |
Circulatory disease, SYLLR, 2002/3/4 | 303 | 114.4 | 31.3 | 57.7 | 225.7 |
Circulatory disease, SYLLR, 2004/5/6 | 152 | 108.6 | 25.2 | 65.2 | 177.8 |
Circulatory disease, SYLLR, 2006/7/8 | 152 | 99.0 | 23.7 | 54.4 | 156.7 |
Circulatory disease, SYLLR, 2007/8/9 | 151 | 94.4 | 22.6 | 51.4 | 149.9 |
Circulatory disease, SYLLR, 2008/9/10 | 151 | 91.1 | 21.7 | 50.9 | 154.8 |
Asthma, SYLLR, 2002/3/4 | 303 | 2.7 | 2.0 | 0.0 | 12.2 |
Asthma, SYLLR, 2004/5/6 | 152 | 2.4 | 1.3 | 0.1 | 6.3 |
Asthma, SYLLR, 2006/7/8 | 152 | 2.0 | 1.1 | 0.0 | 5.0 |
Asthma, SYLLR, 2007/8/9 | 151 | 1.9 | 1.1 | 0.0 | 5.7 |
Asthma, SYLLR, 2008/9/10 | 151 | 1.7 | 1.1 | 0.0 | 4.6 |
Bronchitis, emphysema and other COPD, SYLLR, 2002/3/4 | 303 | 12.5 | 5.7 | 2.6 | 35.5 |
Bronchitis, emphysema and other COPD, SYLLR, 2004/5/6 | 152 | 12.0 | 4.8 | 3.7 | 26.1 |
Bronchitis, emphysema and other COPD, SYLLR, 2006/7/8 | 152 | 12.0 | 4.8 | 4.0 | 24.4 |
Bronchitis, emphysema and other COPD, SYLLR, 2007/8/9 | 151 | 11.8 | 4.7 | 4.1 | 24.8 |
Bronchitis, emphysema and other COPD, SYLLR, 2008/9/10 | 151 | 11.6 | 4.9 | 4.2 | 26.6 |
Pneumonia, SYLLR, 2002/3/4 | 303 | 9.1 | 4.1 | 1.4 | 24.6 |
Pneumonia, SYLLR, 2004/5/6 | 152 | 9.7 | 3.7 | 3.6 | 21.9 |
Pneumonia, SYLLR, 2006/7/8 | 152 | 9.7 | 3.9 | 3.6 | 32.4 |
Pneumonia, SYLLR, 2007/8/9 | 151 | 9.8 | 4.0 | 3.9 | 34.4 |
Pneumonia, SYLLR, 2008/9/10 | 151 | 9.3 | 4.0 | 2.8 | 36.1 |
Tuberculosis, SYLLR, 2002/3/4 | N/A | ||||
Tuberculosis, SYLLR, 2004/5/6 | 152 | 0.8 | 1.1 | 0.0 | 5.2 |
Tuberculosis, SYLLR, 2006/7/8 | 152 | 0.8 | 1.0 | 0.0 | 7.6 |
Tuberculosis, SYLLR, 2007/8/9 | N/A | ||||
Tuberculosis, SYLLR, 2008/9/10 | N/A | ||||
Respiratory problems, SYLLR, 2002/3/4 (excluding TB) | 303 | 24.3 | 9.7 | 5.4 | 64.2 |
Respiratory problems, SYLLR, 2004/5/6 (including TB) | 152 | 24.9 | 8.9 | 9.7 | 51.7 |
Respiratory problems, SYLLR, 2006/7/8 (including TB) | 152 | 24.6 | 8.5 | 11.3 | 56.4 |
Respiratory problems, SYLLR, 2007/8/9 (excluding TB) | 151 | 23.4 | 8.1 | 8.5 | 57.4 |
Respiratory problems, SYLLR, 2008/9/10 (excluding TB) | 151 | 22.6 | 8.5 | 8.5 | 65.0 |
Liver disease, SYLLR, 2002/3/4 | 303 | 20.1 | 10.0 | 3.6 | 70.9 |
Liver disease, SYLLR, 2004/5/6 | 152 | 22.9 | 9.9 | 8.2 | 75.0 |
Liver disease, SYLLR, 2006/7/8 | 152 | 23.9 | 10.8 | 7.0 | 81.7 |
Liver disease, SYLLR, 2007/8/9 | 151 | 23.7 | 10.6 | 9.4 | 81.1 |
Liver disease, SYLLR, 2008/9/10 | 151 | 23.5 | 9.9 | 8.4 | 77.4 |
Gastric, duodenal and peptic ulcers, SYLLR, 2002/3/4 | 303 | 2.6 | 1.6 | 0.0 | 10.2 |
Gastric, duodenal and peptic ulcers, SYLLR, 2004/5/6 | 152 | 2.7 | 1.5 | 0.1 | 11.6 |
Gastric, duodenal and peptic ulcers, SYLLR, 2006/7/8 | 152 | 2.4 | 1.3 | 0.5 | 8.5 |
Gastric, duodenal and peptic ulcers, SYLLR, 2007/8/9 | 151 | 2.4 | 1.3 | 0.4 | 7.0 |
Gastric, duodenal and peptic ulcers, SYLLR, 2008/9/10 | 151 | 2.3 | 1.4 | 0.4 | 7.6 |
Gastrointestinal problems, SYLLR, 2002/3/4 | 303 | 22.7 | 11.0 | 4.7 | 77.8 |
Gastrointestinal problems, SYLLR, 2004/5/6 | 152 | 25.6 | 10.7 | 9.3 | 80.3 |
Gastrointestinal problems, SYLLR, 2006/7/8 | 152 | 26.3 | 11.5 | 8.1 | 87.6 |
Gastrointestinal problems, SYLLR, 2007/8/9 | 151 | 26.1 | 11.1 | 10.7 | 86.3 |
Gastrointestinal problems, SYLLR, 2008/9/10 | 151 | 25.8 | 10.5 | 9.2 | 82.5 |
Infectious diseases, SYLLR, 2002/3/4 | 303 | 7.0 | 4.2 | 0.1 | 28.1 |
Infectious diseases, SYLLR, 2004/5/6 | 152 | 8.1 | 4.3 | 2.4 | 24.9 |
Infectious diseases, SYLLR, 2006/7/8 | 152 | 8.3 | 4.4 | 0.6 | 26.1 |
Infectious diseases, SYLLR, 2007/8/9 | 151 | 8.2 | 4.2 | 2.1 | 25.1 |
Infectious diseases, SYLLR, 2008/9/10 | 151 | 7.7 | 4.0 | 1.6 | 22.6 |
Diabetes, SYLLR, 2002/3/4 | 303 | 4.7 | 2.3 | 0.0 | 13.4 |
Diabetes, SYLLR, 2004/5/6 | 152 | 4.5 | 2.1 | 1.3 | 15.3 |
Diabetes, SYLLR, 2006/7/8 | 152 | 4.3 | 2.0 | 0.5 | 14.6 |
Diabetes, SYLLR, 2007/8/9 | 151 | 4.0 | 1.8 | 0.3 | 11.2 |
Diabetes, SYLLR, 2008/9/10 | 151 | 4.0 | 1.7 | 0.4 | 10.0 |
Epilepsy, SYLLR, 2002/3/4 | 303 | 5.2 | 2.7 | 0.3 | 16.1 |
Epilepsy, SYLLR, 2004/5/6 | 152 | 5.3 | 2.1 | 0.5 | 13.1 |
Epilepsy, SYLLR, 2006/7/8 | 152 | 5.1 | 2.1 | 0.9 | 12.7 |
Epilepsy, SYLLR, 2007/8/9 | 151 | 4.9 | 1.9 | 1.3 | 14.5 |
Epilepsy, SYLLR, 2008/9/10 | 151 | 4.8 | 2.0 | 1.1 | 13.7 |
Renal failure, SYLLR, 2002/3/4 | 303 | 0.9 | 0.9 | 0.0 | 6.0 |
Renal failure, SYLLR, 2004/5/6 | 152 | 0.9 | 0.7 | 0.0 | 4.0 |
Renal failure, SYLLR, 2006/7/8 | 152 | 0.8 | 0.7 | 0.0 | 5.5 |
Renal failure, SYLLR, 2007/8/9 | 151 | 0.7 | 0.6 | 0.0 | 4.3 |
Renal failure, SYLLR, 2008/9/10 | 151 | 0.6 | 0.6 | 0.0 | 3.0 |
Fracture of femur (S72), SMR, 2002/3/4 (ages 65–84 years) | 303 | 8.9 | 6.9 | 0.0 | 39.3 |
Fracture of femur (S72), SMR, 2004/5/6 (ages 65–84 years) | 152 | 10.1 | 6.6 | 0.0 | 30.6 |
Fracture of femur (S72), SMR, 2006/7/8 (ages < 75 years) | 152 | 0.4 | 0.3 | 0.0 | 1.4 |
Fracture of femur (S72), SYLLR, 2007/8/9 (ages < 75 years) | 151 | 0.3 | 0.3 | 0.0 | 1.7 |
Fracture of femur (S72), SYLLR, 2008/9/10 (ages < 75 years) | 151 | 0.3 | 0.3 | 0.0 | 2.1 |
Skull fracture/injury, SMR, 2002/3/4 (ages < 75 years) | 303 | 2.8 | 1.2 | 0.4 | 7.6 |
Skull fracture/injury, SMR, 2004/5/6 (ages < 75 years) | 152 | 1.9 | 0.8 | 0.4 | 4.4 |
Skull fracture/injury, SMR, 2006/7/8 (ages < 75 years) | 152 | 1.8 | 0.7 | 0.5 | 4.2 |
Skull fracture/injury, SYLLR, 2007/8/9 (ages < 75 years) | 151 | 1.7 | 0.7 | 0.2 | 4.2 |
Skull fracture/injury, SYLLR, 2008/9/10 (ages < 75 years) | 151 | 1.6 | 0.6 | 0.1 | 3.0 |
Trauma, SMR, 2002/3/4 (weighted average of femur and skull fractures) | 303 | 4.8 | 2.4 | 0.3 | 15.3 |
Trauma, SMR, 2004/5/6 (sum of femur and skull fracture rates) | 152 | 12.0 | 6.8 | 1.9 | 32.8 |
Trauma, SMR, 2006/7/8 (sum of femur and skull fracture rates) | 152 | 2.1 | 0.8 | 0.6 | 4.7 |
Trauma, SMR, 2007/8/9 (sum of femur and skull fracture rates) | 151 | 2.1 | 0.8 | 0.2 | 4.6 |
Trauma, SMR, 2008/9/10 (sum of femur and skull fracture rates) | 151 | 1.9 | 0.8 | 0.1 | 4.4 |
Infant mortality rate, < 28 days per 1000 live births, 2002/3/4 | 303 | 3.4 | 1.3 | 0.9 | 7.8 |
Infant mortality rate, < 28 days per 1000 live births, 2004/5/6 | 130 | 3.4 | 0.9 | 1.2 | 6.2 |
Infant mortality rate, < 28 days per 1000 live births, 2006/7/8 | 152 | 3.3 | 1.0 | 1.4 | 6.4 |
Infant mortality rate, < 28 days per 1000 live births, 2007/8/9 | 151 | 3.2 | 1.0 | 1.2 | 6.9 |
Infant mortality rate, < 28 days per 1000 live births, 2008/9/10 | 151 | 3.2 | 1.0 | 1.2 | 6.9 |
Other variables
We employ an IV estimation technique to estimate our outcome and expenditure equations because (i) own programme expenditure is likely to be endogenous in the outcome equation and (ii) other programme need is likely to be endogenous in the own programme expenditure equation.
Instrumental variable estimation is described in Instrumental variable estimation, below, but basically it involves replacing the endogenous variable in the equation of interest with its predicted value from an OLS regression which regresses the endogenous variable on a set of IVs. These instruments should be good predictors of the endogenous variable (i.e. they should be relevant and strong predictors) but should be appropriately excluded from the equation of interest (i.e. they should be valid instruments).
We have a number of potential instruments available, mostly derived from 2001 Population Census. 80 In our earlier studies we found that a small subset of these instruments proved sufficient to generate plausible results and these included:
-
the proportion of the population providing unpaid care
-
the proportion of households that are one-pensioner households
-
the index of multiple deprivation
-
the proportion of the population in the white ethnic group.
We also had available a further set of potential instruments and, where our more limited set of instruments failed to generate plausible results, we extended our instrument search to include this wider set of variables. This extended set of instruments included:
-
the proportion of residents born outside the EU
-
the proportion of the population of working age (16–74 years) with a limiting long-term illness (LLT)
-
the proportion of the population aged 16–74 years with no qualifications
-
the proportion of the population aged 16–74 years who are full-time students
-
the proportion of households without a car
-
the proportion of households that are owner occupied
-
the proportion of households that are rented from a local association (LA) or housing association (HA)
-
the proportion of households that are rented from private landlords
-
the proportion of households that are lone-parent households with dependent children
-
the proportion of the population aged 16–74 years who are permanently sick
-
the proportion of those aged 16–74 years who are long-term unemployed
-
the proportion of those aged 16–74 years in employment who are working in agriculture
-
the proportion of those aged 16–74 years in managerial and professional occupations.
Details of the construction of all instruments are shown in Table 92 in the Annex.
Our instruments reflect factors, such as socioeconomic deprivation and the availability of informal care in the community, which might indirectly impact on mortality rates and/or health-care expenditure levels. As we shall see, although our instruments ‘pass’ the appropriate statistical tests, some commentators claim that such tests may have ‘low power’ to detect the presence of invalid instruments. Consequently, in The sensitivity of the outcome elasticity to the validity of the instrument exclusion restrictions we examine how sensitive our results are to the presence of invalid instruments.
Table 3 reports descriptive statistics for the socioeconomic and needs variables as available for the regression analysis of PB expenditure data for 2007/8 and for 2008/9 (these statistics are for the variables in absolute form). For example, on average, lone-pensioner households comprise 14% of all households, the ‘white ethnic’ group accounts for 89% of the population, and 10% of the population provide unpaid care.
In addition to the IVs, Table 3 also report descriptive statistics for various other variables available for the regression analysis including the of Department of Health’s ‘need for health care’ index (this incorporates the CARAN formula for HCHS and reflects need across all health-care services), its need for HIV services index, and its need for maternity services index. The latter two indices are used to either supplement or replace the all service measure of need when estimating our models. The ‘need for health care’ index averages about 1 but varies substantially, with some PCTs having a needs index more than 25% below the national average and others facing a need for health care more than 30% above the national average.
Table 3 also reports descriptive statistics for some disease prevalence rates (e.g. for diabetes and for epilepsy) and, again, these are used to either supplement or replace the all service measure of need when estimating our models.
Finally, the MFF index shows that input prices in the most expensive PCT are almost 20% above those in the least expensive PCT.
Estimation issues and strategy
Introduction
The theoretical framework suggests the specification and estimation of a system of equations, with an expenditure and health outcome equation for each of the 23 programmes of care. However, this approach makes infeasible data demands, requiring variables to identify expenditure, need, environmental factors and health outcomes in each of the 23 programmes of care. Moreover, mortality rates are available for less than half of the 23 programmes. Rather than estimate a system of equations, we proceed on a programme-by-programme basis, estimating health outcome and expenditure equations for those programmes for which mortality data is available.
In line with the theoretical framework presented in Theoretical model, we specify the following expenditure (see Equation 12) and health outcome (see Equation 13) models for each of the J programmes of care (J = 23):
where:
-
xi is the expenditure in PCTi in the selected programme
-
nij is the need for care in PCTi in programme j
-
yi is the total budget for PCTi
-
hi is the health gain in PCTi in the selected programme
-
ni is the need for care in PCTi in the selected programme.
Ideally we should employ a programme-specific indicator of the level of need for each care programme but these are not readily available. When estimating both the outcome and expenditure models we therefore proxy the own programme health-care need using the ‘needs’ component of the Department of Health’s resource allocation formula. m This needs element is specifically designed to adjust PCT allocations for local health-care needs and accordingly, ceteris paribus, we would expect a positive relationship between expenditure xi and need ni for each programme of care. We would also expect a positive relationship between need ni and adverse health outcomes hi. n
The expenditure model includes both the own programme health-care need (which is proxied using the ‘needs’ component of the Department of Health’s resource allocation formula) and the need for health care in all other programmes. When estimating the expenditure model previous studies have proxied the need for health care in other (competing) programmes using the mortality rate in those other programmes. The precise definition of the programmes included in the ‘other programme’ mortality rate has varied a little, but here all of our preferred results from 2006/7 onwards use the ‘all-cause mortality rate excluding the mortality rate in the programme of interest’ as the proxy for need in other programmes. o
Instrumental variable estimation
We do not use OLS to estimate Equations 12 and 13 because both are likely to contain an endogenous regressor. Expenditure in the outcome equation (see Equation 13) and other programme need in the expenditure equation (see Equation 12) are both likely to be endogenous and, in the presence of an endogenous regressor, OLS is both a biased and an inconsistent estimator. Instead, we use IV estimation and implement 2SLS using the -ivreg2- routine in Stata v11. Unlike OLS, IV is a consistent estimator in the presence of an endogenous regressor and, although in finite samples the IV estimator will be biased, the belief is that (providing certain assumptions are met) this bias will be less than that associated with OLS.
For the health outcome equation, IV estimation can be viewed as finding variables (instruments) that are good predictors of programme expenditure but which are appropriately excluded from the equation of interest (that is, from Equation 13). The assumption is that the instruments and exogenous variables from the equation of interest impact on the health outcome through their impact on expenditure only, and that they do not have a direct effect on the outcome. p If, on the other hand, an instrument reflects unobserved factors that affect both expenditure and mortality directly, then the IV estimator becomes both biased and inconsistent. Such an instrument is said to be ‘invalid’ because it belongs in the equation of interest in its own right.
We have a number of potential instruments available, mostly derived from the 2001 Population Census,80 and these are described in Other variables. In our earlier studies we found that a small subset (four) of these instruments often proved sufficient to generate plausible results and we commenced our empirical work with these. If plausible results were not obtainable with some combination of these four instruments, we employed an extended instrument set. Further details of the identification of suitable instruments for each model can be found in Re-estimation of poorly performing models with an extended instrument set.
The available instruments reflect factors, such as socioeconomic deprivation and the availability of informal care in the community, which might indirectly impact on mortality rates and/or health-care expenditure levels. The set of instruments associated with each estimated equation was selected on both technical and pragmatic grounds. From a pragmatic point of view, we require a parsimonious set of instruments that satisfy the necessary technical criteria. These are, first, that they have face validity, that is, that they are plausible determinants of the endogenous variable being instrumented, and second, that the instruments are both relevant and valid. The relevance of an instrument set refers to its ability to predict the endogenous variable of concern, whereas validity refers to the requirement that instruments should be uncorrelated with the error term in the equation of interest. The set of instruments was modified if, for example, the Hansen–Sargan test suggested that the set under test was not valid.
Should the instrument set be strong, relevant and valid, 2SLS will produce consistent estimates of the parameters of the reduced form models. We subject the instrument sets to tests for validity using the Sargan–Hansen test of overidentifying restrictions. The joint null hypothesis is that the instruments are valid instruments (i.e. they are uncorrelated with the error term), and that the excluded instruments are correctly excluded from the estimated equation. A rejection of the null hypothesis casts doubt on the validity of the instruments. We test for instrument relevance using Shea’s79 partial R2 measure; this reflects the correlation between the excluded instruments and the endogenous regressor. However, even if valid and relevant, non-zero but small correlations between the instruments and the endogenous regressors can lead to the problem of weak instruments. This can be the case even where correlations are shown to be significant at conventional levels of testing and sample sizes are large. 143 The IV estimator becomes a biased estimator if the instruments are weakly correlated with the endogenous regressors, and the extent of the bias can be specified relative to the bias of the OLS estimator.
For the case of a single regressor, Staiger and Stock144 suggest applying the criterion that if the first-stage F-statistic, testing the null hypothesis that the instrument set does not significantly predict the endogenous regressor, is less than 10 then the instruments can be thought to be weak. Stock and Yogo80 extend these ideas to the case where there can be multiple endogenous regressors and propose a test for the null hypothesis that the instruments are weak and provide appropriate critical values. This is an extension of the Cragg and Donald145 test for instrument relevance. For the case of a single endogenous regressor, the Cragg–Donald statistic is simply the F-statistic of the test of the hypothesis that the instruments do not enter the first-stage regression. Stock and Yogo80 provide critical values of the F-statistic (and the Cragg–Donald statistic for multiple endogenous regressors) that tabulates the ratio of 2SLS bias to the bias of OLS. The weakness or otherwise of the instruments can then be assessed by the relative bias exceeding a given threshold (e.g. 2SLS bias exceeding 5% of OLS bias). q
To ensure the robustness of our estimates to arbitrary heteroskedasticity, we estimate our models with Stata’s -robust- option. The Cragg–Donald statistics are not valid in the presence of heteroskedasticity. We therefore report the Kleibergen–Paap LM statistic (testing instrument relevance) and the Kleibergen–Paap F-statistic (testing for weak instruments) which are valid in the presence of heteroskedasticity.
A general test of model specification is provided through the use of Ramsey’s81 reset test for OLS and an adapted version of the test for IVs. r,81 The tests are more properly thought of as tests of a linearity assumption in the mean function or a test of functional form restrictions and omitted variables146 and can be useful as a general check of model specification.
Finally, we check that the presumed endogenous variable is in fact endogenous using the test proposed by Durbin. 83 If the null hypothesis of exogeneity cannot be rejected, then we also use the OLS estimator. In addition, although our instruments ‘pass’ the appropriate statistical tests, some commentators claim that such tests may have ‘low power’ to detect the presence of invalid instruments. Consequently, in The sensitivity of the outcome elasticity to the validity of the instrument exclusion restrictions we examine how sensitive our results are to the relaxation of the assumption that the instruments are valid.
Other estimation issues
In this research we build on previous studies that have used the PB data to estimate the outcome and expenditure models described in Introduction. This previous research was undertaken over a period of years and a number of changes were made between these studies (these were sometimes forced on the researchers by, for example, data availability considerations). Here we persevere with the previous approach used to analyse the 2005/6 PB data,63 but we make some changes to the way in which the 2006/7 (and subsequent) PB data are analysed.
In the next section we start by revisiting the results obtained by Martin et al. 63 who used the 2005/6 PB data. In 2005/6 there were 303 PCTs but a series of mergers reduced this total to 152 in 2006/7. These mergers exacerbated greatly the difference in size between the PCTs and so from 2006/7 it makes less sense to give each PCT equal weight in any regression. This is discussed further in Estimation issues associated with the use of 2006/7 expenditure data when we come to estimate our model using 2006/7 PB data.
Different PCTs face different costs when buying health-care inputs. For example, some health economy input prices are up to 40% higher in London and the south east of England than elsewhere. In a previous study,57 we used the MFF index that feeds into the payment by results tariffs for 2007/8 to adjust PB expenditure in 2006/7 for local input prices. 147 This index only reflects costs associated with the purchase of HCHS services but this was the only index available for the new (post-October 2006) set of PCTs at the time of that study. Since then, a more comprehensive set of MFF indices for the 152 PCTs has been published. 74 In Estimation issues associated with the use of 2006/7 expenditure data we investigate the use of alternative weighted averages of the HCHS, prescribing, and GMS/PMS MFF indices with weights reflecting the national share of expenditure across these three categories (these weights are 76.3%, 12.4% and 11.3% respectively). s For 2005/6, however, we persevere with the MFF employed in the original Martin et al. study,63 namely the HCHS MFF. 148
Estimation of the expenditure equation for any individual programme requires a proxy for the need for health care across all other programmes. Previous studies of PB expenditure in 2004/5, 2005/6 and 2006/7 have used the circulatory disease mortality rate as a proxy for the need for health care in other programmes in the cancer expenditure equation, and the cancer mortality rate as the proxy for need in other programmes in the circulatory disease expenditure equation. 59,60,63 As these are both programmes that attract considerable expenditure and record considerable mortality, it is not implausible that mortality and expenditure in one of the programmes will impact on expenditure in the other. For other programmes (e.g. respiratory problems and gastrointestinal problems) Martin et al. 59,63 used the all-cause mortality rate as a proxy for the ‘need in other programmes’ variable when analysing expenditure in both 2005/6 and 2006/7. Here, however, we persevere with the previous approach when using 2005/6 PB data but, from 2006/7, in all programmes we proxy the need for health care in other (competing) programmes using the mortality rate in those other programmes (i.e. the all-cause mortality rate minus the own programme mortality rate).
Finally, one data transformation that has been applied in all previous studies and is applied here too is to log-transform all variables so that parameter estimates can be interpreted as elasticities. In other words, a regression coefficient of 0.5 implies that a 1% increase in the regressor is associated with a 0.5% increase in the dependent variable.
Empirical results
Analysis of programme budgeting expenditure for 2005/6 and mortality data for 2002/3/4
This work builds on previous studies. Martin et al. 60 reported outcome elasticities for two programmes (cancer and circulatory disease) using expenditure data for 2004/5 and pooled mortality data for 2002, 2003 and 2004. Martin et al. 63 extended their preliminary analysis to include several other programmes and, in this extension, they used updated expenditure data (for 2005/6). However, the authors found it difficult to obtain sensible outcome models for some programmes of care. Here we commence our empirical work with an attempt to obtain plausible outcome models for those programmes that defeated Martin et al. in their study. 63
Construction of an alternative measure of need
Our preferred measure of the need for health care is calculated from the Department of Health’s PB data set. This data set includes PB expenditure for each care programme as well as the raw population and the ‘unified weighted’ population for each PCT. The unified weighted population incorporates adjustments to the raw population for both the need for health care as well unavoidable variations in local input costs. The latter are captured via an index which is known as the MFF. By removing the raw population and MFF adjustment from the unified weighted population we are left with the implied level of need, and this is the measure of need that was initially used in the estimation of the model. 63
The Department of Health PB measure of need associated with expenditure for 2005/6 incorporates the AREA resource allocation formula. This has since been replaced with the CARAN formula and recent work by colleagues at York and the Nuffield Trust has investigated the possibility of constructing a person-based resource allocation (PBRA) measure of need. 149 We therefore decided to investigate the possibility of applying PBRA methods to the construction of an alternative measure of need.
The construction of all of these measures of need involves two steps. The first step requires the estimation of the econometric relationship between the previous utilisation of services and the characteristics of the local areas as existed at the time of the utilisation (e.g. their demographic profile and other indicators of service need such as socioeconomic measures of deprivation). The second step involves the use of this relationship to predict future health-care use given predictions about future demographic characteristics and socioeconomic measures of deprivation.
The major difference between the AREA and CARAN formulae and the PBRA formula is that the former largely use small area-based indicators of socioeconomic characteristics as indicators of the need for health care, whereas the latter largely obviates the requirement for these through the extensive use of individual-based indicators of need. In particular, the PBRA formula employed here is based on an analysis of inpatient and outpatient cost data for 2007/8 for 10% of the entire population of England. 149 As regressors the PBRA utilisation model includes:
-
38 age/gender dummies
-
150 ICD-10 morbidity markers for each patient reflecting their use of inpatient services in the previous 2 years (that is, in 2005/6 and 2006/7 combined)
-
four hospital encounter variables for each patient reflecting the intensity of their use of both outpatient and inpatient services in the previous 2 years (that is, in 2005/6 and 2006/7 combined)
-
10 small area-based indicators of either local deprivation or health-care supply characteristics; and
-
151 PCT dummies (reflecting variations in health-care supply).
The coefficients from this modelling procedure are applied to patient registration data as at 1 April of the year for which the measure of need is required. Here we are studying expenditure in 2005/6 and so we applied the results of the modelling to patient registration data as at 1 April 2005. This requires the construction of a data set containing the patient registration details of all 50 million patients registered with an English practice at this date. To this we added the patient’s age and gender as at 1 April 2005. We also added each patient’s ICD-10 morbidity markers and their encounter variables for 2003/4 and 2004/5 combined. Each patient’s address [lower super output area (LSOA)] is also added to the data set and this is used to attach the small number of indicators reflecting the LSOA’s socioeconomic and health-care supply characteristics.
Given this data set, the calculation of PCT need (given supply) proceeds as follows. First, calculate the national average supply effect. This is the sum of the products of the national average values of the supply variables for the population as at 1 April 2005 and the relevant regression coefficients.
Second, ignore supply and calculate PCT need. This involves calculating the PCT average values of the needs variables by age and gender group for the population as at 1 April 2005. Next, for each PCT, calculate need by age and gender as the sum of the products of the mean values of the needs variables and their respective regression coefficients. Then total PCT need is the sum of need in each age/gender group multiplied by the number of patients in that age/gender group.
Finally, need given supply is calculated as total PCT need plus the number of patients multiplied by the national average supply effect. PCT need per person is simply total PCT need divided by the PCT population. Further details of how to use the results of the PBRA modelling to derive PCT weighted needs indices are presented in Dixon et al. 149
Re-estimation of models using a new measure of need
We re-estimated the outcome and expenditure models for the big four programmes as reported by Martin et al. 63 using the new (PBRA-based) measure of need. t In summary, the results for the cancer programme were acceptable but not quite as good as previously obtained, and the results for circulation problems, gastrointestinal problems and respiratory problems were poor (e.g. the signs on the expenditure and need variables in the outcome equation were counterintuitive). These were unanticipated results and we were curious to know why our alternative measure of need performed less well than the more established measure.
We undertook a brief comparison of the two measures of need. Figure 13 provides a scatter plot of the PB and PBRA measures of need. There is a clear positive correlation between the two measures (correlation coefficient = 0.6146), and the summary statistics in Table 39 suggest that they have similar ranges.
FIGURE 13.
Graph showing scatter plot of PB measure of need and PBRA measure of need.

Variable | Number of PCTs | Mean | SD | Min. | Max. |
---|---|---|---|---|---|
PB need | 295 | 1.0062 | 0.1511 | 0.6883 | 1.4889 |
PBRA need | 303 | 1.0146 | 0.1448 | 0.6884 | 1.4554 |
Table 40 reports values for the PB- and PBRA-based measures of need for selected types of PCTs. These figures suggest that:
-
the PBRA measure attributes more need to the least needy areas as defined by the PB measure [see Table 40, section (a)]
-
the PBRA measure attributes more need to the coastal/retirement areas than does the PB measure [see Table 40, section (b)]; and
-
the PBRA measure attributes far less need to inner city areas than does the PB measure [see Table 40, section (c)].
Area | PB need | PBRA need |
---|---|---|
(a) Examples of more affluent areas | ||
Wokingham PCT | 0.6883 | 0.7703 |
Blackwater Valley and Hart PCT | 0.7376 | 0.8395 |
Bracknell Forest PCT | 0.7410 | 0.8262 |
Royston, Buntingford and Bishop’s Stortford PCT | 0.7426 | 0.8484 |
Windsor, Ascot and Maidenhead PCT | 0.7460 | 0.8404 |
Woking PCT | 0.7499 | 0.8097 |
Chiltern and South Bucks PCT | 0.7515 | 0.8530 |
Uttlesford PCT | 0.7583 | 0.8861 |
North East Oxfordshire PCT | 0.7588 | 0.8372 |
South Cambridgeshire PCT | 0.7619 | 0.9647 |
(b) Examples of coastal/retirement areas | ||
Suffolk Coastal PCT | 0.9159 | 1.0815 |
Western Sussex PCT | 0.9613 | 1.3248 |
North Somerset PCT | 0.9651 | 1.1746 |
Poole PCT | 0.9860 | 1.2045 |
South and East Dorset PCT | 1.0079 | 1.2439 |
Fylde PCT | 1.0304 | 1.2157 |
Southport and Formby PCT | 1.0657 | 1.2141 |
North Norfolk PCT | 1.0658 | 1.3684 |
Adur, Arun and Worthing PCT | 1.0716 | 1.2641 |
East Devon PCT | 1.0870 | 1.3325 |
(c) Examples of inner city areas | ||
Brent PCT | 0.9848 | 0.6991 |
Lambeth PCT | 1.0454 | 0.7512 |
Islington PCT | 1.1222 | 0.9014 |
Southwark PCT | 1.1412 | 0.8163 |
Newham PCT | 1.1746 | 0.7897 |
City and Hackney PCT | 1.1849 | 0.8472 |
Bradford City PCT | 1.2131 | 0.8757 |
Tower Hamlets PCT | 1.2192 | 0.9299 |
Heart of Birmingham Teaching PCT | 1.2466 | 0.9052 |
Central Manchester PCT | 1.2965 | 0.9262 |
Central Liverpool PCT | 1.4065 | 1.0948 |
Although these differences are at first perplexing, they become more understandable when it is noted that the PB and PBRA measures record the level of need across different baskets of services. The PB measure of need refers to all health-care activity, that is, HCHS, prescribing and GMS/PMS (primary care), but the PBRA model only incorporates hospital activity (and it excludes mental health and maternity from this).
The need for hospital-based services is less related to deprivation than are other health-care services. Hence the PBRA measure of need – because it only relates to hospital services – redistributes need away from the more deprived PCTs and towards the more affluent ones. Moreover, expenditure on cancer services is largely hospital based and hence a measure of need based on HCHS spend alone will be reasonably satisfactory for cancer (as indeed we found). However, such a measure of need will perform less well for other programmes (e.g. circulatory disease), where more of the expenditure is on prescribing and/or primary care.
To test these hypotheses we need to compare our PBRA measure of need with a PB measure of need that only relates to acute services (i.e. that excludes maternity and mental health, and all prescribing and GMS/PMS). The Department of Health’s measure of need used for the 2005/6 allocations employs the AREA formula for HCHS. This formula does not permit a separation of acute and maternity need and so we cannot compare the PBRA measure of need for 2005/6 with the PB measure for 2005/6 for the same group of specialties (i.e. for acute services excluding maternity and mental health).
However, the CARAN formula, first implemented for the 2009/10 allocations, does distinguish between acute and maternity. But this formula has only been applied to the new (post-October 2006, n = 152) PCTs whereas our PBRA-based measure is for the old (pre-October 2006, n = 303) PCTs because we are modelling PB expenditure in 2005/6. However, not all of the old PCTs were involved in mergers in October 2006. Thus for about half of all PCTs, we can compare our PBRA-based measure of need for 2005/6 with the CARAN-based measure of need for 2009/10 for the same set of HCHS services (i.e. for acute services excluding maternity and mental health).
The correlation between PBRA need and CARAN acute services need is much higher (correlation coefficient = 0.8722) than that between the PBRA and PB need measures. In addition, an inspection of the values taken by the various need indices (e.g. for acute, maternity, and mental health) for the inner city PCTs (where the PBRA and PB measures of need diverge the most) supports the hypothesis that it is the different service coverage of the PBRA and PB measures of need that explains why they are so poorly correlated (Table 41).
PCT | PB need (all services) | PBRA need (acute) | CARAN need | ||
---|---|---|---|---|---|
Acute | Maternity | Mental health | |||
City and Hackney PCT | 1.1849 | 0.8472 | 0.8751 | 1.6783 | 1.5340 |
Tower Hamlets PCT | 1.2192 | 0.9299 | 0.8451 | 1.4988 | 1.6663 |
Newham PCT | 1.1746 | 0.7897 | 0.8683 | 1.8130 | 1.4486 |
Haringey PCT | 1.0448 | 0.8347 | 0.8471 | 1.4023 | 1.2886 |
Brent PCT | 0.9848 | 0.6991 | 0.8558 | 1.3420 | 1.2608 |
Camden PCT | 1.0336 | 0.8402 | 0.7667 | 0.9163 | 1.3209 |
Islington PCT | 1.1222 | 0.9014 | 0.8842 | 1.1399 | 1.4516 |
Lambeth PCT | 1.0454 | 0.7512 | 0.8111 | 1.3916 | 1.3349 |
Southwark PCT | 1.1412 | 0.8163 | 0.8445 | 1.3755 | 1.3905 |
Lewisham PCT | 1.0402 | 0.7793 | 0.8549 | 1.4253 | 1.2236 |
Heart of Birmingham PCT | 1.2466 | 0.9052 | 0.9078 | 1.5976 | 1.5621 |
For example, the PB index suggests that per capita need in the Newham PCT is 17% above the national average, but the PBRA index suggests that it is 21% below the national average. We believe that this difference is due to the fact that the PB index relates to all services whereas the PBRA index only relates to acute services. The separate figures for acute, maternity and mental health need from the CARAN formula confirm this hypothesis: CARAN acute need, like PBRA acute need, is well below the national average, but maternity and mental health need are well above it.
Re-estimation of poorly performing models with an extended instrument set
Martin et al. 63 found it difficult to obtain sensible outcome models for some programmes of care. As we were unable to find an improved measure of need, we sought to improve the outcome and expenditure models reported in Martin et al. 63 through the use of an extended set of regressors/instruments. Martin et al. 63 had focussed on the use of four instruments but here we extend the modelling to include an additional 13 regressors/instruments [born outside EU, LLT, no qualifications, full-time students, no car households, owner occupiers, privately rented, socially rented, lone parents, permanently sick, long-term unemployed, work in agriculture, work in professional occupation]. Further details about these variables can be found in The threshold changing over time and precise details about how they were constructed can be found in Table 92 in the Annex.
For each PBC, our modelling strategy with these additional regressors/instruments was the same:
-
First, estimate an IV model using our preferred set of regressors (with need, budget, and other programme need for the own programme spend model, and with need and spend for the outcome model) and preferred set of instruments (proportion of households that are lone pensioner households, per cent of the population providing unpaid care, the IMD2000, and the per cent of the population in the white ethnic group). Then adjust this set of instruments if necessary (e.g. remove from the instrument set or add an instrument to the regressor set if the Hansen–Sargan test indicates that this is appropriate). Estimate an OLS version of the IV model if the theoretically endogenous regressor is exogenous according to the relevant statistical test.
-
Second, if (a) fails to generate a reasonable model, add the same additional variables to both the regressor and instrument sets. Then eliminate insignificant regressors (least significant first, but always retaining, for example, the budget and other need variables in the expenditure model, and own programme spend in the outcome model). Then eliminate insignificant instruments until a reasonable model is obtained. Again, estimate an OLS version of the IV model if the theoretically endogenous regressor is exogenous according to the relevant statistical test.
Instrumental variable estimates of outcome and expenditure models
The above approach generates preferred outcome and expenditure models for each of the programmes with a mortality-based outcome indicator. Outcome models are shown in Table 42 with expenditure models in Table 43. The corresponding first-stage regression results can be found in Tables 93 and 94, respectively, in the Annex.
Variable | 2005/6 outcome model, instrument spend, unweighted, second stage | 2005/6, outcome model, spend exogenous, unweighted, OLS | 2005/6, outcome model, instrument spend, unweighted, second stage | 2005/6, outcome model, spend exogenous, unweighted, OLS | ||||||
---|---|---|---|---|---|---|---|---|---|---|
(1) PBC 2 cancer | (2) PBC 10 circulation | (3) PBC 11 respiratory | (4) PBC 13 gastrointestinal | (5) PBC 1 infectious disease | (6) PBC 7 neurological | (7) PBC 16 trauma | (8) PBC 19 neonates | (9) PBC 17 genitourinary | (10) PBC 4 endocrine | |
Own programme spend per head | –0.394*** | –1.370*** | –1.574*** | –2.018*** | –0.152 | –0.182 | –1.332*** | –0.237* | –0.034 | –0.244* |
[0.100] | [0.156] | [0.483] | [0.364] | [0.117] | [0.143] | [0.469] | [0.127] | [0.220] | [0.129] | |
Need per head | 0.905*** | 2.628*** | 4.076*** | 4.254*** | 1.157*** | 1.588*** | ||||
[0.083] | [0.163] | [0.562] | [0.412] | [0.252] | [0.445] | |||||
Lone pensioner households | –0.930*** | |||||||||
[0.158] | ||||||||||
Born outside EU | 0.111* | |||||||||
[0.063] | ||||||||||
No car households | 0.701*** | |||||||||
[0.114] | ||||||||||
HIV need per head | 0.212** | |||||||||
[0.082] | ||||||||||
Unpaid carers | 1.164*** | |||||||||
[0.392] | ||||||||||
Low birth weight births | 0.919*** | |||||||||
[0.223] | ||||||||||
Lone parents households | 0.549*** | 1.035*** | ||||||||
[0.121] | [0.211] | |||||||||
White ethic group | –1.246*** | |||||||||
[0.329] | ||||||||||
Population weighted IMD2000 | 0.421*** | |||||||||
[0.076] | ||||||||||
Diabetes prevalence rate 2004/5 | 14.236*** | |||||||||
[5.195] | ||||||||||
Diabetes prevalence rate squared | 2.026*** | |||||||||
[0.759] | ||||||||||
Constant | 4.101*** | 1.849*** | –2.892** | –2.052** | 2.654*** | 0.917** | 0.689 | 1.621*** | 2.188*** | 24.258*** |
[0.248] | [0.324] | [1.250] | [0.916] | [0.443] | [0.459] | [1.462] | [0.455] | [0.681] | [8.859] | |
Observations | 295 | 295 | 295 | 295 | 295 | 294 | 295 | 294 | 267 | 294 |
R 2 | 0.328 | 0.068 | 0.169 | 0.203 | ||||||
Endogeneity test statistic | 29.216 | 65.024 | 12.630 | 39.106 | 3.542 | 4.071 | ||||
Endogeneity p-value | 6.47e-08 | 0 | 0.000380 | 4.01e-10 | 0.0598 | 0.0436 | ||||
Hansen–Sargan test statistic | 0.786 | 7.209 | 1.877 | 2.468 | 1.200 | 5.976 | ||||
Hansen–Sargan p-value | 0.375 | 0.0655 | 0.171 | 0.291 | 0.273 | 0.0504 | ||||
Shea’s partial R2 | 0.133 | 0.311 | 0.0376 | 0.173 | 0.112 | 0.0735 | ||||
Kleibergen–Paap LM test statistic | 26.59 | 42.31 | 20.56 | 34.83 | 26.97 | 19.04 | ||||
Kleibergen–Paap p-value | 1.68e-06 | 1.44e-08 | 3.44e-05 | 1.32e-07 | 1.39e-06 | 0.000268 | ||||
Kleibergen–Paap F-statistic | 16.94 | 29.51 | 10.29 | 23.32 | 17.76 | 11.49 | ||||
Pesaran–Taylor reset statistic | 0.0347 | 0.162 | 0.0929 | 2.196 | 0.756 | 1.388 | ||||
Pesaran–Taylor p-value | 0.852 | 0.688 | 0.761 | 0.138 | 0.385 | 0.239 | ||||
Ramsey reset F-statistic | 2.089 | 0.665 | 1.075 | 1.118 | ||||||
Probability > F | 0.102 | 0.574 | 0.360 | 0.342 |
Variable | 2005/6 spend model, instrument other programme need, unweighted, second stage | 2005/6 spend model, other programme need exogenous, unweighted, OLS | 2005/6 spend model, instrument other programme need, unweighted, second stage | 2005/6 spend model, other programme need exogenous, unweighted, OLS | 2005/6 spend model, instrument other programme need, unweighted, second stage | ||||||
---|---|---|---|---|---|---|---|---|---|---|---|
(1) PBC 2 cancer | (2) PBC 10 circulation | (3) PBC 11 respiratory | (4) PBC 13 gastrointestinal | (5) PBC 1 infectious disease | (6) PBC 7 neurological | (7) PBC 16 trauma | (8) PBC 19 neonates | (9) PBC 17 genitourinary | (10) PBC 4 endocrine | (11) PBC 23 GMS/PMS | |
SYLLR cancer | –0.954*** | ||||||||||
[0.249] | |||||||||||
PCT budget per head | 0.968*** | 0.682*** | 0.849*** | 0.772*** | 0.742*** | 1.111*** | 0.627*** | 0.388 | 1.041*** | 0.425** | 0.926*** |
[0.191] | [0.161] | [0.223] | [0.166] | [0.180] | [0.244] | [0.173] | [0.391] | [0.141] | [0.175] | [0.199] | |
Need per head | 0.703*** | 0.885*** | 2.226*** | 1.115*** | 0.773*** | 1.720*** | 0.570*** | ||||
[0.248] | [0.261] | [0.436] | [0.230] | [0.298] | [0.401] | [0.207] | |||||
White ethnic group | 0.198*** | –0.739*** | |||||||||
[0.066] | [0.181] | ||||||||||
Provision of unpaid care | 0.364*** | –0.339* | |||||||||
[0.136] | [0.190] | ||||||||||
SYLLR circulatory disease | –0.577*** | ||||||||||
[0.107] | |||||||||||
Lone pensioners | –0.612*** | –0.257** | |||||||||
[0.165] | [0.101] | ||||||||||
SYLLR all deaths | –1.367*** | –0.639*** | –0.437*** | –0.899*** | –1.157*** | 0.121 | 0.035 | –0.158 | –1.003*** | ||
[0.328] | [0.149] | [0.157] | [0.182] | [0.274] | [0.307] | [0.099] | [0.116] | [0.276] | |||
Born outside EU | 0.069** | ||||||||||
[0.029] | |||||||||||
Full-time students | –0.165*** | 0.127*** | |||||||||
[0.053] | [0.031] | ||||||||||
No car households | 0.444*** | ||||||||||
[0.099] | |||||||||||
HIV need per head | 0.142*** | ||||||||||
[0.034] | |||||||||||
London boroughs dummy | 0.942*** | ||||||||||
[0.106] | |||||||||||
LA/HA rented housing | 0.377*** | ||||||||||
[0.126] | |||||||||||
No qualifications | 0.521*** | ||||||||||
[0.140] | |||||||||||
Private rented housing | 0.102** | ||||||||||
[0.041] | |||||||||||
Work in agriculture | –0.058*** | ||||||||||
[0.022] | |||||||||||
Constant | –0.020 | 3.440*** | 4.368** | 1.241 | –0.969 | 2.066* | 3.631** | –4.760** | –2.854*** | –2.435*** | 4.320** |
[0.517] | [1.111] | [1.757] | [0.930] | [1.037] | [1.131] | [1.414] | [1.920] | [0.604] | [0.726] | [1.703] | |
Observations | 295 | 295 | 295 | 295 | 295 | 295 | 295 | 295 | 295 | 295 | 295 |
Endogeneity test statistic | 6.465 | 12.921 | 19.325 | 7.218 | 13.865 | 12.690 | 5.273 | ||||
Endogeneity p-value | 0.0110 | 0.000325 | 1.10e-05 | 0.00722 | 0.000196 | 0.000368 | 0.0217 | ||||
Hansen–Sargan test statistic | 0.416 | 1.925 | 0.00232 | 2.441 | 0.826 | 3.577 | 3.213 | ||||
Hansen–Sargan p-value | 0.519 | 0.165 | 0.962 | 0.118 | 0.662 | 0.0586 | 0.201 | ||||
Shea’s partial R2 | 0.450 | 0.141 | 0.168 | 0.416 | 0.450 | 0.239 | 0.290 | ||||
Kleibergen–Paap LM test statistic | 63.99 | 31.27 | 33.11 | 57.16 | 64.15 | 39.80 | 47.98 | ||||
Kleibergen–Paap p-value | 0 | 1.62e-07 | 6.47e-08 | 0 | 0 | 2.28e-09 | 2.15e-10 | ||||
Kleibergen–Paap F-statistic | 109.7 | 21.74 | 19.08 | 98.29 | 70.14 | 40.01 | 43.10 | ||||
Pesaran–Taylor reset statistic | 2.679 | 0.231 | 0.848 | 0.987 | 0.0184 | 0.912 | 0.668 | ||||
Pesaran–Taylor p-value | 0.102 | 0.631 | 0.357 | 0.320 | 0.892 | 0.340 | 0.414 | ||||
R 2 | 0.709 | 0.177 | 0.399 | 0.267 | |||||||
Ramsey reset F-statistic | 1.572 | 0.250 | 1.358 | 0.765 | |||||||
Probability > F | 0.196 | 0.861 | 0.256 | 0.514 |
The first four results in Table 42 show the outcome model for the big four programmes (i.e. for cancer, circulatory disease, respiratory problems and gastrointestinal problems). In all four programmes the need variable has a positive and significant effect on mortality, and expenditure has the anticipated negative effect. The diagnostic statistics reveal that, in all four cases, own programme expenditure is endogenous and that the instruments are valid. They also suggest that the instruments are relevant and there is no evidence that the instruments are weak. The Pesaran–Taylor test suggests that there is no evidence of model misspecification.
The results for the other programmes are similar to but more diverse than those for the big four programmes. This is to be anticipated because mortality is a much rarer outcome in these programmes than it is in, say, the cancer programme. Own programme expenditure is not endogenous in the next two programmes (infectious diseases and neurological problems) and we revert to the use of the OLS estimator. Expenditure has the anticipated negative effect on mortality in the infectious disease programme, but this is not statistically significant. The all service measure of need is not relevant for this PBC; instead, we find that a measure of need associated with HIV is positively associated with mortality, as is a measure of deprivation (households with no car). Mortality from epilepsy is negatively associated with expenditure in the neurological programme. The need for health-care variables has a positive and significant effect on mortality.
Expenditure and need have the anticipated effects on mortality in the trauma and injuries programme. In addition, the provision of unpaid care appears to be associated with an increase in mortality from fractures. This might be because the availability of care allows the elderly to continue to live in their own home and that they are more likely to fall and die from a fall at home than they are in alternative accommodation (such as in a residential home or sheltered housing).
Expenditure has the anticipated negative effect on mortality in the neonates programme where the generic all service measure of need has been replaced with two more programme-specific indicators of need (the proportion of births that are low birth weight births, and the proportion of households that are lone parent households).
The final two results both employ the OLS estimator. Expenditure in the genitourinary programme has a small negative effect on mortality (from renal problems). The prevalence of one parent households and non-white residents both seem to be positively associated with mortality.
Finally, expenditure has the anticipated negative effect on mortality in the endocrine problems programme where the generic all service measure of need has again been replaced with a more programme-specific indicator of need (the diabetes prevalence rate). Mortality in this programme is also positively associated with the IMD2000.
The first four results in Table 43 show the expenditure model for the big four programmes (i.e. for cancer, circulatory disease, respiratory problems and gastrointestinal problems). In all four programmes both the need and budget variables have a positive and significant effect on own programme expenditure. In addition, the proxy for need in other programmes is negative and significant in all four cases. In the circulatory expenditure programme the provision of unpaid care is associated with more expenditure (patients may buy care in more affluent areas), as is the proportion of residents in the white ethnic group (there might be some unmet need associated with circulatory problems in the non-white ethnic groups).
The PCT budget variable is positive in all of the remaining seven programmes and this variable is significant in six of the seven. The proxy for other programme need (SYLLR all deaths) has the anticipated negative sign in five of the seven programmes and, where it is positive, it is never statistically significant.
The all service proxy for own programme need is positive and significant in three programmes. In the other four programmes, however, it has been replaced by various other socioeconomic indicators of need (in the trauma programme, for example, the provision of unpaid care is associated with a reduction in NHS expenditure and, in the neonates programme, the proportion of residents in the white ethnic group is negatively associated with expenditure).
The diagnostic statistics reveal that, for all seven IV models, expenditure is endogenous and the instruments are valid. They also suggest that the instruments are relevant and there is no evidence that the instruments are weak. The Pesaran–Taylor test suggests that there is no evidence of model misspecification.
Instrumental variable estimates of outcome and expenditure models: the first-stage equations
For the health outcome equation, IV estimation involves finding variables (instruments) that are good predictors of programme expenditure but which are appropriately excluded from the equation of interest (that is, from the outcome equation). The assumption is that the instruments impact on the health outcome through their impact on expenditure only, and that they do not have a direct effect on the outcome. If, on the other hand, an instrument reflects unobserved factors that affect both expenditure and mortality directly, then the IV estimator becomes both biased and inconsistent. Such an instrument is said to be ‘invalid’ because it belongs in the equation of interest in its own right.
In our outcome model we typically employ two instruments (call these z1 and z2) for expenditure. IV estimation assumes that these instruments do not belong in the outcome equation. In other words, IV estimation assumes that the coefficients γ1 and γ2 in the outcome model:
are identically zero (where y is mortality, x is expenditure, and n is a measure of the own programme need for health care and all variables relate to a particular programme of care). Such exclusion restrictions can be debatable and researchers who employ IV techniques often devote considerable effort towards convincing the reader that their assumed exclusion restrictions are a good approximation. 88,89 These efforts usually take two forms: first, researchers often offer a strong theoretical economic argument why their instruments do not belong in the equation of interest; and second, statistical tests for the validity of the exclusion restrictions (Sargan 2SLS, Hansen J-test generalised method of moments) are routinely reported as part of the results for any study that employs IV techniques.
It is difficult for us to identify clear theoretical reasons why our instruments (such as the proportion of lone pensioner households, the provision of unpaid care and an index of multiple deprivation) do not belong in the equation of interest (that is, that they will not directly affect mortality). Of necessity, therefore, we must be guided by the available statistical tests for the validity of the exclusion restrictions. However, although our outcome models ‘pass’ the relevant statistical test, some commentators have argued that the Sargan–Hansen test may have weak power and may fail to reject the null hypothesis of instrument validity even when an exclusion restriction is not valid. As we shall see in The sensitivity of the outcome elasticity to the validity of the instrument exclusion restrictions, this is likely to be the case when the induced biases in the estimates of β1 (the coefficient on the endogenous variable) are the same across all instruments. The Hansen–Sargan J-test statistic will be small when the null hypothesis of valid instruments is correct; but it will also be small if the biases induced in β1^ by invalid instruments all coincide (i.e. the instruments all identify the same wrong parameter). 150 In other words, for the Hansen–Sargan J test to have low power the use of any subset of instruments should generate the same asymptotic bias in β1^.
Our approach, implemented below, is to identify theoretical reasons why our instruments might belong in the first-stage expenditure equation but not in the second-stage outcome equation. Even if our arguments are thought unconvincing, a critic would also have to argue that any subset of our selected instruments will each induce the same bias in the coefficient on the endogenous variable. This is because it is only in these circumstances that the Hansen–Sargan test will be unable to reject the null hypothesis of instrument validity even when an exclusion restriction is not valid.
The first-stage regressions associated with the IV outcome results in Table 42 can be found in Table 93 in the Annex. A brief summary of the first-stage regressions is provided below.
Cancer programme of care
The instrument set for the cancer programme of care [see column (1) in Table 93] includes the proportion of households that are lone pensioner households and the proportion of the population providing unpaid care. These instruments have intuitive appeal. The first-stage regression of cancer expenditure on the instruments and the need for health care (as an exogenous regressor in the 2SLS model) reveals a positive and significant coefficient on lone pensioners and a negative but non-significant coefficient on the proportion of unpaid carers. The proportion of lone pensioners is likely to reflect an additional adjustment for health-care need specific to an elderly and needy population. The omission of this variable from the second-stage regression is plausible as the dependent variable relates to mortality under 75 years of age and some of the lone pensioners will be aged over 75 years, and members of this group are, by definition, relatively healthy individuals. Unpaid care might act as a substitute for the provision of health-care services and, in these circumstances, a negative relationship with expenditure is to be expected. There is no obvious relationship between the provision of unpaid care and mortality.
Circulatory disease programme of care
The two instruments used for cancer were also employed to predict expenditure in the circulatory disease programme and they were augmented with the addition of the population weighted index of multiple deprivation (IMD2000). The relevance of the latter variable is theoretically plausible as circulatory disease is more related to disadvantage than is cancer. In addition, we also employed the proportion of residents in the white ethnic group as an additional instrument for expenditure, but its coefficient is very small and it is not statistically significant.
Increased expenditure on circulatory disease in the first-stage regression is associated with a greater proportion of pensioners living alone and a greater proportion of unpaid carers. The latter may reflect an increased awareness and compliance with medical intervention, particularly preventative measures, brought about by carers, but this will not affect our outcome model if the impact of this additional support is largely on the mortality of those aged > 75 years. Expenditure on circulatory problems is also negatively associated with the IMD2000. As the IMD incorporates an access to medical services domain, this negative association might reflect some unmet need which largely affects mortality in those aged > 75 years.
Respiratory problems programme of care
The IMD2000 is negatively associated with expenditure on respiratory problems. As the IMD incorporates an access to medical services domain, this negative association might reflect some unmet need which largely affects mortality in those aged > 75 years. The proportion of the population aged 16–74 years that is permanently sick has a positive association with expenditure but might not affect mortality in those aged < 75 years if expenditure is largely directed towards managing chronic disease.
Gastrointestinal problems programme of care
Increased expenditure on gastrointestinal problems in the first-stage regression is positively associated with the proportion of residents providing unpaid care. This may reflect an increased awareness and compliance with medical intervention, particularly preventative measures, brought about by carers, but this will not affect our outcome model if the impact of this additional support is largely on the mortality of those aged > 75 years.
Trauma, burns and injuries programme of care
Increased expenditure on trauma, burns and injuries in the first-stage regression is positively associated with the proportion of pensioners living alone. This may reflect longer stays in hospital and an increased need for community care. However, the proportion of pensioners living alone will have little effect on our mortality measure if most of this expenditure is associated with patients > 75 years of age.
Neonate programme of care
The percentage of those aged 16–74 years that are long-term unemployed and the proportion of households that are in social rented housing are both positively associated with expenditure on neonate care. These are both indicators of socioeconomic deprivation and might be associated with the presence of larger families (i.e. more children per family). This would affect expenditure per head of population but not necessarily mortality per 1000 live births. The negative coefficient on the proportion of those aged 16–74 years with no qualifications might reflect the ‘emigration’ of young adults from those areas that are particularly deprived. This would reduce expenditure per head of population but would have no impact on the mortality measure.
The first-stage regressions associated with the IV expenditure results in Table 43 can be found in Table 94 in the Annex.
Cancer programme of care
The first-stage equation for the cancer expenditure model includes two instruments – lone pensioners and unpaid carers – that are excluded as regressors from the second stage of estimation. In this model the first-stage regression of other programme need (as proxied here by the circulatory disease mortality rate) on the instrument set generates a negative coefficient on both instruments excluded from the second-stage regression. A greater proportion of unpaid carers might reflect an increased level of care (and perhaps increased compliance with care programmes and drug regimes) resulting in a decrease in other programme deaths. The availability of unpaid care in the community might not have a direct effect on cancer expenditure if such care supplements rather than substitutes for NHS-funded care. Conditional on need and the total PCT budget, the negative coefficient on the proportion of lone pensioners may be indicative of the presence of increased networks of social support. If this additional support reduces other programme mortality but does not substitute for NHS care, then the lone pensioner variable will not belong in the expenditure equation.
Circulatory disease programme of care
In the circulatory disease expenditure model, the first-stage regression of other programme need (as proxied here by the cancer mortality rate) on the instrument set results in a negative coefficient on one instrument (lone pensioners) and a positive coefficient on the other (the IMD2000). As noted above, the negative coefficient on the proportion of lone pensioners may be indicative of areas with increased networks of social support. If this additional support does not substitute for NHS care then the lone pensioner variable will not belong in the expenditure equation. It is plausible that the IMD2000 should have a positive effect on other programme need but not belong in the expenditure equation if, for example, there is some unmet need in another (but not the circulatory disease) care programme.
Respiratory problems programme of care
In the respiratory disease expenditure model, the first-stage regression of other programme need (as proxied here by the all cause SYLLR) on the instrument set results in a negative coefficient on one instrument (unpaid care) and a positive coefficient on another (the IMD2000). A greater proportion of unpaid carers might reflect an increased level of care (and perhaps increased compliance with care programmes and drug regimes) resulting in a decrease in other programme deaths. The availability of unpaid care might not have a direct effect on own programme expenditure if such care does not substitute for NHS-funded care. It is plausible that the IMD2000 should have a positive effect on other programme need but not belong in the expenditure equation if, for example, there is some unmet need in another (but not the respiratory disease) care programme.
Gastrointestinal problems programme of care
In the gastrointestinal problems expenditure model, the first-stage regression of other programme need (as proxied here by the all cause SYLLR) on the instrument set (including need and total budget) results in a negative coefficient on one instrument (lone pensioners) and a positive coefficient on the other (IMD2000). As noted above, the negative coefficient on the proportion of lone pensioners may be indicative of areas with increased networks of social support. If this additional support does not substitute for NHS care then the lone pensioner variable will not belong in the expenditure equation. It is plausible that the IMD2000 should have a positive effect on other programme need but not belong in the expenditure equation if, for example, there is some unmet need in another (but not the gastrointestinal) care programme.
Neurological problems programme of care
The first-stage equation for the neurological expenditure model includes three instruments – lone pensioners, unpaid carers and IMD2000 – that are excluded as regressors from the second stage of estimation. Explanations for the signs on these variables have been outlined above when discussing the other first-stage regressions.
Trauma and injuries programme of care
The first-stage equation for the trauma expenditure model includes two instruments – lone pensioners and the IMD2000 – that are excluded as regressors from the second stage of estimation. Explanations for the signs on these variables have been outlined above when discussing the other first-stage regressions.
General medical services/primary medical services programme of care
The first-stage equation for the GMS/PMS expenditure model includes three instruments – households with no car, lone parents and permanently sick – that are excluded as regressors from the second stage of estimation. All three are plausibly positively associated with other programme need (as proxied here by the all cause SYLLR) but do not occur as regressors in the second-stage GMS/PMS expenditure model. The latter includes at least one measure of deprivation – the proportion of people aged 16–74 years without any qualifications – and the Hansen–Sargan test suggests that the three excluded instruments offer no additional explanatory power for observed variations in GMS/PMS expenditure.
We appreciate that not everyone will be convinced by our arguments about the validity of our instruments and so in The sensitivity of the outcome elasticity to the validity of the instrument exclusion restrictions we undertake a sensitivity analysis that examines the impact of weakening the instrument exclusion restriction.
Calculation of the cost of a life and life-year
The preferred models identified in Tables 42 and 43 indicate the responsiveness of mortality to changes in expenditure, and of own programme expenditure to changes in budget, using expenditure data for 2005/6. Together with information about programme expenditure and mortality, the coefficients on the own programme expenditure and PCT budget variables listed in Tables 42 and 43 can be used to calculate the cost of an additional life-year for the 10 programmes for which outcome and expenditure models are available. u For a relatively small budget change:
and
Table 44 presents the necessary information to calculate the cost of an additional life (or life-year) for each of these 10 programmes. There is an assumed small (1%) increase in the national budget and it is also assumed that this increase is applied to each PCT’s budget. The total additional spend in each programme associated with this injection [see Table 44, column (D)] is determined by the initial level of expenditure in the programme [see Table 44, column (B)] and the programme’s expenditure elasticity [see Table 44, column (C)]. In addition, this additional spend, in conjunction with the outcome elasticity (column F) and the number of deaths in the programme [see Table 44, column (E)], determine the number of lives saved that is associated with the additional expenditure. If we divide the change in programme expenditure [see Table 44, column (D)] by the change in the number of lives lost [see Table 44, column (G)] we obtain the cost per life gained [see Table 44, column (H)].
PBC scenario | (A) PBC description | (B) Spend (£M) 2005/6 | (C) Spend elasticity | (D)(= 0.01 × B × C) change in spend (£M) | (E) Annual mortality, < 75 years, 2002/3/4 | (F) Outcome elasticity | (G) (= 0.01 × C × E × F) change in annual mortality | (H)(= D/G) cost per life gained (£) | (I) Total life-years lost, < 75 years, 2002/3/4 | (J) (= 0.01 × C × F × I/3) change in annual life-years lost | (K) (= D/J) cost per YLG (£) |
---|---|---|---|---|---|---|---|---|---|---|---|
1 | Cancer | 4094 | 0.968 | 39.63 | 62,259 | 0.394 | 237.45 | 166,897 | 2,268,541 | 2884 | 13,741 |
2 | Circulatory problems | 6112 | 0.682 | 41.68 | 45,504 | 1.370 | 425.16 | 98,042 | 1,607,171 | 5005 | 8328 |
3 | Respiratory problems | 3421 | 0.849 | 29.04 | 11,601 | 1.574 | 155.03 | 187,350 | 316,506 | 1410 | 20,601 |
4 | Gastrointestinal problems | 3998 | 0.772 | 30.86 | 5926 | 2.018 | 92.32 | 334,318 | 324,735 | 1686 | 18,303 |
5 | Big four programmes | 17,625 | 141.22 | 125,290 | 909.96 | 155,196 | 4,516,953 | 10,986 | 12,855 | ||
6 | Infectious diseases | 1161 | 0.742 | 8.61 | 2050 | 0.152 | 2.31 | 3,725,931 | 106,552 | 40 | 215,054 |
7 | Endocrine problems | 1832 | 0.425 | 7.79 | 1690 | 0.244 | 1.75 | 4,442,720 | 60,615 | 21 | 371,601 |
8 | Neurological problems | 2019 | 1.111 | 22.43 | 729 | 0.182 | 1.47 | 15,217,293 | 66,137 | 45 | 503,201 |
9 | Genitourinary problems | 3313 | 1.041 | 34.49 | 294 | 0.034 | 0.10 | 331,432,573 | 10,030 | 1 | 29,144,918 |
10 | Trauma and injuries | 3758 | 0.627 | 23.56 | 1037 | 1.332 | 8.66 | 2,720,657 | 30,000 | 84 | 282,132 |
11 | Neonate conditions | 660 | 0.388 | 2.56 | 2123 | 0.237 | 1.95 | 1,311,733 | 477,675 | 146 | 17,490 |
12 | All 10 programmes | 30,368 | 0.792 | 240.67 | 133,213 | 926.22 | 259,838 | 5,267,962 | 11,322 | 21,256 |
Alternatively, we can apply the outcome elasticity [see Table 44, column (F)] to the annual number of life-years lost in the programme [see Table 44, column (E)] to determine the number of life-years saved that is associated with the additional expenditure. If we divide the change in programme expenditure [see Table 44, column (D)] by the change in the number of life-years lost [see Table 44, column (J)] we obtain the cost per YLG [see Table 44, column (K)]. Note that none of these figures are QALY adjusted and that all costs are at current (2005/6) prices.
The cost per life-year associated with the cancer programme is £13,741 and this is almost identical to that calculated using expenditure data for 2004/5, but with the same mortality data as that employed here. 60 Similarly, the cost per life-year associated with the circulatory disease programme is £8328 and this is also almost identical to that calculated using expenditure data for 2004/5 but with the same mortality data as that employed here. 60 The cost per life-year for the respiratory programme (£20,601) and for the gastrointestinal programme (£18,303) are a little larger than these figures but are still of the same order of magnitude. Taken together, the cost per life-year for these ‘big four PBCs’ is £12,855. v,w
Table 44 also contains cost per life-year estimates for the six other programmes for which a mortality-based outcome indicator is available. These cost estimates are much larger than those for the big four programmes. This is to be expected as mortality is a less relevant outcome indicator for these PBCs than for the big four programmes. The cost per life-year across all 10 programmes for which a mortality-based outcome indicator is available is £21,256.
Although we have an estimate of the cost per life-year for 10 programmes, it is unclear how we should adjust this estimate for the expenditure associated with the other 13 programmes. We attempted to estimate an outcome and expenditure model for expenditure and mortality in all 13 of these programmes combined. x However, this was not successful with, for example, counterintuitive signs on some variables. y
Instead, we decided to make some assumptions about the cost per life-year associated with the other 13 programmes. We examined two possibilities. First, we assumed that the other 13 programmes generate no mortality gain at all. This is clearly unrealistic but it does provide an upper bound for the cost per life-year across all programmes of care. Table 45 is similar to Table 44 but it incorporates this zero gain assumption for the 13 other programmes. z It shows that the cost per life-year across all 23 programmes – assuming a zero mortality gain in the 13 programmes without a mortality based indicator – is £56,799.
PBC scenario | (A) PBC description | (B) Spend (£M) 2005/6 | (C) Spend elasticity | (D) (= 0.01 × B × C) change in spend (£M) | (E) Annual mortality, < 75 years, 2002/3/4 | (F) Outcome elasticity | (G) (= 0.01 × C × E × F) change in annual mortality | (H) (= D/G) cost per life gained (£) | (I) Total life-years lost, < 75 years, 2002/3/4 | (J) (= 0.01 × C × F × I/3) change in annual life-years lost | (K) (= D/J) cost per YLG (£) |
---|---|---|---|---|---|---|---|---|---|---|---|
1 | Cancer | 4094 | 0.968 | 39.63 | 62,259 | 0.394 | 237.45 | 166,897 | 2,268,541 | 2884 | 13,741 |
2 | Circulatory problems | 6112 | 0.682 | 41.68 | 45,504 | 1.370 | 425.16 | 98,042 | 1,607,171 | 5005 | 8328 |
3 | Respiratory problems | 3421 | 0.849 | 29.04 | 11,601 | 1.574 | 155.03 | 187,350 | 316,506 | 1410 | 20,601 |
4 | Gastrointestinal problems | 3998 | 0.772 | 30.86 | 5926 | 2.018 | 92.32 | 334,318 | 324,735 | 1686 | 18,303 |
5 | Big four programmes | 17,625 | 141.22 | 125,290 | 909.96 | 155,196 | 4,516,953 | 10,986 | 12,855 | ||
6 | Infectious diseases | 1161 | 0.742 | 8.61 | 2050 | 0.152 | 2.31 | 3,725,931 | 106,552 | 40 | 215,054 |
7 | Endocrine problems | 1832 | 0.425 | 7.79 | 1690 | 0.244 | 1.75 | 4,442,720 | 60,615 | 21 | 371,601 |
8 | Neurological problems | 2019 | 1.111 | 22.43 | 729 | 0.182 | 1.47 | 15,217,293 | 66,137 | 45 | 503,201 |
9 | Genitourinary problems | 3313 | 1.041 | 34.49 | 294 | 0.034 | 0.10 | 331,432,573 | 10,030 | 1 | 29,144,918 |
10 | Trauma and injuries | 3758 | 0.627 | 23.56 | 1037 | 1.332 | 8.66 | 2,720,657 | 30,000 | 84 | 282,132 |
11 | Neonate conditions | 660 | 0.388 | 2.56 | 2123 | 0.237 | 1.95 | 1,311,733 | 477,675 | 146 | 17,490 |
12 | All 10 programmes | 30,368 | 0.792 | 240.67 | 133,213 | 926.22 | 259,838 | 5,267,962 | 11,322 | 21,256 | |
13 | Assume no health gain | 33,942 | 402.43 | 0.00 | 0 | ||||||
14 | All 23 programmes | 64,310 | 643.10 | 926.22 | 694,330 | 11,322 | 56,799 |
Second, the zero mortality gain assumption is an extreme one but possibly relevant for the residual programme (PBC 23) – where about two-thirds of the expenditure is attributable to primary care – if we assume that any mortality gain associated with primary care expenditure is reflected in mortality rates associated with other, more disease-specific, programmes (e.g. cancer, circulatory disease, etc.). However, if we assume a zero mortality gain in PBC 23, what assumption should we make about the mortality gain associated with the remaining 12 programmes?
One possibility is to assume that the cost per life (year) in the remaining 12 programmes is on average the same as that associated with the 10 programmes for which a mortality-based outcome indicator is available. At first this may sound strange as we have already noted that mortality is not regularly associated with these programmes whereas it is a normal outcome for the 10 programmes for which a mortality-based outcome indicator is available (and this is of course why mortality data at PCT level is available for these 10 PBCs). However, if we broaden our interpretation of health gain to include non-mortality effects (such as those on the QoL), then this assumption – that the cost per life (year) in the remaining 12 programmes is on average the same as that associated with the 10 programmes for which a mortality-based outcome indicator is available – becomes far more plausible.
Thus, Table 46 is similar to Table 45 but incorporates (a) a zero gain assumption for the residual (including primary care) programme (PBC 23); and (b) an average gain assumption for the remaining 12 programmes for which no mortality-based outcome indicator is available. Table 46 shows that the cost per life-year across all 23 programmes [see Table 46, row (15)] is £24,200. This is, of course, slightly greater than the cost of a life-year for the 10 programmes for which a mortality-based outcome indicator is available (£21,256) because a small proportion of expenditure (that on primary care) is assumed to have no health benefit beyond that captured by the more disease-specific programmes (e.g. in cancer, circulatory disease, etc.).
PBC scenario | (A) PBC description | (B) Spend (£M) 2005/6 | (C) Spend elasticity | (D) (= 0.01 × B × C) change in spend (£M) | (E) Annual mortality, < 75 years, 2002/3/4 | (F) Outcome elasticity | (G) (= 0.01 × C × E × F) change in annual mortality | (H) (= D/G) cost per life gained (£) | (I) Total life-years lost, < 75 years, 2002/3/4 | (J) (= 0.01 × C × F × I/3) change in annual life-years lost | (K) (= D/J) cost per YLG (£) |
---|---|---|---|---|---|---|---|---|---|---|---|
1 | Cancer | 4094 | 0.968 | 39.63 | 62,259 | 0.394 | 237.45 | 166,897 | 2,268,541 | 2884 | 13,741 |
2 | Circulatory problems | 6112 | 0.682 | 41.68 | 45,504 | 1.370 | 425.16 | 98,042 | 1,607,171 | 5005 | 8328 |
3 | Respiratory problems | 3421 | 0.849 | 29.04 | 11,601 | 1.574 | 155.03 | 187,350 | 316,506 | 1410 | 20,601 |
4 | Gastrointestinal problems | 3998 | 0.772 | 30.86 | 5926 | 2.018 | 92.32 | 334,318 | 324,735 | 1686 | 18,303 |
5 | Big four programmes | 17,625 | 141.22 | 125,290 | 909.96 | 155,196 | 4,516,953 | 10,986 | 12,855 | ||
6 | Infectious diseases | 1161 | 0.742 | 8.61 | 2050 | 0.152 | 2.31 | 3,725,931 | 106,552 | 40 | 215,054 |
7 | Endocrine problems | 1832 | 0.425 | 7.79 | 1690 | 0.244 | 1.75 | 4,442,720 | 60,615 | 21 | 371,601 |
8 | Neurological problems | 2019 | 1.111 | 22.43 | 729 | 0.182 | 1.47 | 15,217,293 | 66,137 | 45 | 503,201 |
9 | Genitourinary problems | 3313 | 1.041 | 34.49 | 294 | 0.034 | 0.10 | 331,432,573 | 10,030 | 1 | 29,144,918 |
10 | Trauma and injuries | 3758 | 0.627 | 23.56 | 1037 | 1.332 | 8.66 | 2,720,657 | 30,000 | 84 | 282,132 |
11 | Neonate conditions | 660 | 0.388 | 2.56 | 2123 | 0.237 | 1.95 | 1,311,733 | 477,675 | 146 | 17,490 |
12 | All 10 programmes | 30,368 | 0.792 | 240.67 | 133,213 | 926.22 | 259,838 | 5,267,962 | 11,322 | 21,256 | |
13 | (a) Assume no health gain for GMS/PMS | 8449 | 0.926 | 78.24 | 0.00 | 0 | |||||
14 | (b) Assume average gain in the other 12 PBCs | 25,493 | 1.272 | 324.20 | 1247.69 | 259,838 | 15,252 | 21,256 | |||
15 | All 23 programmes | 64,310 | 643.10 | 2173.90 | 295,827 | 26,575 | 24,200 |
The costs quoted in Tables 44–46 make no QALY adjustment but such an adjustment would add between 50% and 66% to the costs quoted. 100
Summary and conclusion
In this section we have extended the results reported by Martin et al. 63 by obtaining plausible outcome and expenditure models for all 10 programmes of care with a mortality-based outcome indicator. In addition, we have, for the first time, calculated the cost of a life-year across the big four programmes combined (£12,855) and across all 10 programmes (£21,256). Moreover, with the aid of an assumption about the productivity (health gain) of programmes without a meaningful mortality-based outcome indicator, we have extended our individual programme estimates to incorporate expenditure across all programmes of care.
If we assume that the other 13 programmes without a mortality-based outcome indicator generate no health gain then the cost of an additional life-year across all expenditure for 2005/6 is £56,799.
Alternatively, if we assume that any health-care gain associated with primary care expenditure is reflected in mortality rates associated with other, more disease-specific programmes, and that the health gain associated with the remaining 12 programmes is, on average, the same as that recorded by the PBCs with a mortality-based indicator, then the cost per life-year across all expenditure for 2005/6 is £24,200.
This concludes our analysis of the 2005/6 PB data. In the next section we apply our model to the 2006/7 PB data.
Analysis of programme budgeting expenditure for 2006/7
Construction of an alternative measure of need
The analysis of the 2005/6 PB data employed a measure of the need for health care that incorporated the AREA resource allocation formula for acute services. As was described in Instrumental variable estimation, we attempted to construct a better measure of need using a recently developed person-based approach. 149 However, we were unable to construct a viable alternative PBRA-based measure of need for use with the PB data for 2005/6 because the PBRA formula only relates to acute services yet the PB data incorporates elements for acute, maternity, mental health, prescribing and primary care, and we were unable to separate these component parts.
The construction of an alternative measure of need is, however, possible for use with the 2006/7 PB data. Spend and mortality data are available for the new (152) PCTs, and the Department of Health’s resource allocation exposition book for 2009/10 (which employs the CARAN model) provides separate measures of need for acute, maternity, mental health, prescribing and GMS/PMS services. We can therefore replace the (CARAN-based) measure of acute need for the 2009/10 allocation with our own PBRA-based measure of acute need (albeit for 2006/7) to calculate an alternative to the AREA-based measure of need across all health-care services.
The PBRA model was applied to all patients on practice lists as at 1 April 2006 to generate a PCT-level measure of acute need (see Construction of an alternative measure of need for a description of this approach as applied to patients on practice lists as at 1 April 2005). The resulting PBRA measure of acute need can be compared with the CARAN-based measure of acute need as reported in the Department of Health’s resource allocation exposition book for 2009/10. The correlation coefficient for these two measures is 0.8514 and descriptive statistics for the two measures are shown below in Table 47.
Variable | Number of PCTs | Mean | SD | Min. | Max. |
---|---|---|---|---|---|
CARAN_acute need | 152 | 1.0033 | 0.1113 | 0.7659 | 1.2153 |
PBRA_acute need | 152 | 1.0037 | 0.1218 | 0.7606 | 1.3420 |
The all service measure of need (which is a weighted average of the acute, maternity, mental health, prescribing and GMS/PMS measures) as reported in the Department of Health’s resource allocation exposition book for 2009/10 can be recalculated by replacing the CARAN-based acute measure with the PBRA-based acute measure of need. The correlation coefficient for these two all service measures of need is 0.9714 and Figure 14 shows a scatter plot of these two measures. Descriptive statistics for these two all service measures of need along with the (AREA-based) PB measure of need are shown in Table 48.
FIGURE 14.
Graph showing scatter plot of all service measures of need: incorporating CARAN- or PBRA-based measures of acute need.

Variable | Observations | Mean | SD | Min. | Max. |
---|---|---|---|---|---|
PCTneed_CARAN | 152 | 1.0240 | 0.1339 | 0.7311 | 1.3479 |
PCTneed_PBRA | 152 | 1.0242 | 0.1395 | 0.7287 | 1.3769 |
PCTneed_AREA | 152 | 1.0293 | 0.1380 | 0.7165 | 1.4006 |
The correlation coefficients for the three measures are shown in Table 49.
Variable | PCTneed_AREA | PCTneed_PBRA | PCTneed_CARAN |
---|---|---|---|
PCTneed_AREA | 1 | ||
PCTneed_PBRA | 0.9583 | 1 | |
PCTneed_CARAN | 0.9839 | 0.9714 | 1 |
Estimation issues associated with the use of 2006/7 expenditure data
As well as having to select a preferred measure of need from the three available, the estimation of our model using PB data for 2006/7 requires the resolution of several other issues.
Estimation issue 1: ‘net spend’ or ‘own population’ spend?
The Department of Health reports two sets of PB spend data: the first is on a ‘net spend’ basis and the second is on an ‘own population’ basis. The ‘own population’ data starts with the ‘net spend’ figure, adds any expenditure funded from non-NHS sources, and adjusts for expenditure made under PCT lead/host commissioning arrangements. These adjustments are usually very small. For 2005/6 we used the net spend data (because only net spend data was produced in the first year and we were hoping to build a panel) but given the now regular production of own population data this would seem to be the more appropriate data set to use as, for example, it includes all expenditure irrespective of its funding source.
Estimation issue 2: to weight or not to weight?
Ordinary least squares and IV estimation implicitly gives the same weight to each PCT when estimating our expenditure and outcome models. With the reorganisation of PCTs in October 2006, the number of such organisations was reduced from 303 to 152. However, far from making them more similar in terms of size (as measured by their population), this reorganisation actually increased the disparity in size between the largest and the smallest PCTs, with the largest PCT now being 14 times the size of the smallest. Unless we explicitly weight each observation (PCT) by its size, we will be giving the same weight (influence) to PCTs that are much smaller than other PCTs.
Estimation issue 3: which market forces factors?
This study builds on previous work using PB data. Martin et al. 59 report the results of the estimation of our model using PB data for 2006/7. One essential step in this estimation is the removal of the impact of unavoidable variations in local costs from the reported measure of the ‘unified weighted’ population. At the time of the earlier study the authors only had access to a MFF based on HCHS for the new 152 PCTs. Now, however, a more broadly-based MFF is available, that is, one based on a weighted average of MFFs for HCHS, prescribing, and GMS/PMS. Should we use a MFF for HCHS only, or one that incorporates HCHS and prescribing, or one that incorporates HCHS, prescribing and GMS/PMS?
Estimation issue 4: standardised mortality rates or standardised years of life lost rates, and which proxy for the other programme need variable?
Previous studies have reported results using both SMRs and SYLLRs but the sheer number of models being estimated requires that we focus on one measure only. Various proxies for other programme need have been employed in previous studies (see section Other estimation issues for further discussion). In this subsection we persevere with this variety but consistency demands that we focus in on a preferred proxy for other programme need.
This study builds on previous work using PB data. Martin et al. 59 report the results of the estimation of our model using PB data for 2006/7. With several alternative measures of need and MFF available, we undertook a preliminary empirical analysis of the 2006/7 PB data using the outcome and expenditure models for the big four programmes as reported in Martin et al. 59 as our starting point. These models incorporated the AREA-based measure of need and a MFF based on HCHS only.
We first re-estimated the outcome and expenditure models by replacing the AREA-based measure of need with one incorporating the PBRA formula. Then we re-estimated these models again with a measure of need incorporating the CARAN model. The results suggest that (a) for the outcome models, the use of the PBRA measure of need generates a smaller coefficient on expenditure than does the AREA measure of need; and (b) that for the spend models, the use of the PBRA measure of need generates a larger coefficient on PCT budget than does the AREA measure of need. For both the outcome and expenditure models, the use of the CARAN measure of need generates outcome and expenditure elasticities that lie between those generated by the AREA and PBRA measures.
Next, the results reported by Martin et al. 59 employ a MFF based on HCHS only to remove unavoidable variations in local costs from the reported measure of the (unified weighted) need for health-care services. This was the only MFF available for the new PCTs at the time of that study. Now, however, a more broadly-based MFF is available (that is, one based on a weighted average of MFFs for HCHS, prescribing, and GMS/PMS).
To examine the consequences of using the CARAN MFF (i.e. a weighted average of the HCHS, prescribing, and GMS/PMS MFFs), this MFF was used to calculate the implied level of need given the unified weighted populations for 2006/7 which are reported alongside the PB spend data by the Department of Health. aa We found that the use of an extended set of MFFs can sometimes affect the coefficient on the variable of interest.
Models were also estimated using a weighted average of the CARAN MFFs for HCHS and prescribing only. The latter results were very similar to those using all three of the CARAN MFFs (i.e. a weighted average of the HCHS, prescribing, and GMS/PMS MFFs).
We also tried re-estimating the outcome and expenditure models from Martin et al. 59 using the ‘own population’ expenditure data rather than the ‘net spend’ data but this adjustment had very little effect on the results. In addition, the impact of ‘weighting’ each observation by PCT size was usually rather modest.
Because of the sheer number of variations possible, we decided to estimate 13 particular variants of our model and details of these variants are summarised in Table 50. These variants were estimated for each of the big four programmes using both the outcome and expenditure equations. The results are presented in Tables 51–58.
Variant | PCTs weighted? | MFF indicator | Indicator of need | Mortality indicator |
---|---|---|---|---|
1 | No weights | HCHS | AREA-based UWP/HCHS MFF | SMR |
2 | No weights | HCHS | PBRA model applied to patients on list at 1 April 2006 | SMR |
3 | No weights | HCHS | CARAN model used for allocations in 2009/10 | SMR |
4 | No weights | HCHS | AREA-based UWP/HCHS MFF | SYLLR |
5 | No weights | HCHS | PBRA model applied to patients on list at 1 April 2006 | SYLLR |
6 | No weights | HCHS | CARAN model used for allocations in 2009/10 | SYLLR |
7 | Yes | HCHS | AREA-based UWP/HCHS MFF | SMR |
8 | No weights | HCHS, prescribing and GMS/PMS | AREA-based UWP/(HCHS, prescribing and GMS/PMS) MFF | SMR |
9 | Yes | HCHS, prescribing and GMS/PMS | AREA-based UWP/(HCHS, prescribing and GMS/PMS) MFF | SMR |
10 | No weights | HCHS and prescribing | AREA-based UWP/(HCHS and prescribing) MFF | SMR |
11 | Yes | HCHS and prescribing | AREA-based UWP/(HCHS and prescribing) MFF | SMR |
12 | No weights | HCHS, prescribing and GMS | CARAN model used for allocations in 2009/10 | SMR |
13 | Yes | HCHS, prescribing and GMS | CARAN model used for allocations in 2009/10 | SMR |
Variable | PBC 2 cancer, 2006/7, spend model | ||||||||||||
---|---|---|---|---|---|---|---|---|---|---|---|---|---|
(1) Uses SMR, no weighting, second stage, iAREA need 1, HCHS MFF | (2) Uses SMR, no weighting, second stage, PBRA need, HCHS MFF | (3) Uses SMR, no weighting, second stage, CARAN need, HCHS MFF | (4) Uses SYLLR, no weighting, second stage, iAREA need, HCHS MFF | (5) Uses SYLLR, no weighting, second stage, PBRA need, HCHS MFF | (6) Uses SYLLR, no weighting, second stage, CARAN need, HCHS MFF | (7) Uses SMR, weighted, second stage, iAREA need 1, HCHS MFF | (8) Uses SMR, no weighting, second stage, iAREA need 2, CARAN 3 MFFs | (9) Uses SMR, weighted, second stage, iAREA need 2, CARAN 3 MFFs | (10) Uses SMR, no weighting, second stage, iAREA need 3, CARAN 2 MFFs | (11) Uses SMR, weighted, second stage, iAREA need 3, CARAN 2 MFFs | (12) Uses SMR, no weighting, second stage, CARAN need, CARAN 3 MFFs | (13) Uses SMR, weighted, second stage, CARAN need, CARAN 3 MFFs | |
PCT budget per head | 0.353 | 0.681*** | 0.572** | 0.388 | 0.752*** | 0.618** | 0.326 | 0.250 | 0.245 | 0.246 | 0.241 | 0.552** | 0.544* |
[0.273] | [0.235] | [0.247] | [0.272] | [0.238] | [0.247] | [0.362] | [0.284] | [0.357] | [0.284] | [0.357] | [0.239] | [0.308] | |
NeedAREA1 | 1.513*** | 1.557*** | 1.351*** | ||||||||||
[0.288] | [0.284] | [0.367] | |||||||||||
Other programme need 1 | −0.654*** | −0.771*** | −0.733*** | −0.604*** | −0.749*** | −0.661*** | −0.749*** | −0.661*** | −0.680*** | −0.616*** | |||
[0.124] | [0.125] | [0.124] | [0.131] | [0.152] | [0.154] | [0.151] | [0.154] | [0.126] | [0.139] | ||||
NeedPBRA | 1.320*** | 1.352*** | |||||||||||
[0.261] | [0.265] | ||||||||||||
NeedCARAN | 1.431*** | 1.477*** | 1.347*** | 1.160*** | |||||||||
[0.287] | [0.289] | [0.246] | [0.294] | ||||||||||
Other programme need 2 | −0.649*** | −0.773*** | −0.728*** | ||||||||||
[0.119] | [0.124] | [0.119] | |||||||||||
NeedAREA2 | 1.778*** | 1.554*** | |||||||||||
[0.329] | [0.389] | ||||||||||||
NeedAREA3 | 1.765*** | 1.545*** | |||||||||||
[0.328] | [0.388] | ||||||||||||
Constant | 0.271 | 0.707 | 0.569 | 0.356 | 0.835 | 0.665 | 0.067 | 5.901*** | 5.559** | 5.932*** | 5.582** | 3.430** | 3.220 |
[0.544] | [0.551] | [0.554] | [0.542] | [0.567] | [0.554] | [0.572] | [2.062] | [2.550] | [2.063] | [2.550] | [1.645] | [2.087] | |
Observations | 152 | 152 | 152 | 152 | 152 | 152 | 152 | 152 | 152 | 152 | 152 | 152 | 152 |
Endogeneity test statistic | 13.112 | 18.683 | 18.420 | 13.313 | 18.736 | 18.716 | 11.940 | 13.098 | 11.708 | 13.017 | 11.504 | 16.985 | 13.460 |
Endogeneity p-value | 0.000293 | 1.54e-05 | 1.77e-05 | 0.000264 | 1.50e-05 | 1.52e-05 | 0.000549 | 0.000296 | 0.000622 | 0.000309 | 0.000695 | 3.77e-05 | 0.000244 |
Hansen–Sargan test statistic | 0.870 | 1.139 | 0.560 | 0.504 | 0.748 | 0.281 | 1.089 | 1.730 | 1.875 | 1.711 | 1.857 | 0.381 | 0.321 |
Hansen–Sargan p-value | 0.351 | 0.286 | 0.454 | 0.478 | 0.387 | 0.596 | 0.297 | 0.188 | 0.171 | 0.191 | 0.173 | 0.537 | 0.571 |
Shea’s partial R2 | 0.607 | 0.526 | 0.586 | 0.570 | 0.482 | 0.548 | 0.612 | 0.511 | 0.537 | 0.510 | 0.536 | 0.572 | 0.583 |
Kleibergen–Paap LM test statistic | 40.55 | 38.73 | 40.45 | 40.49 | 38.17 | 41.14 | 38.91 | 38.41 | 37.13 | 38.38 | 37.11 | 43.82 | 41.36 |
Kleibergen–Paap p-value | 1.57e-09 | 3.88e-09 | 1.64e-09 | 1.61e-09 | 5.14e-09 | 1.16e-09 | 3.55e-09 | 4.56e-09 | 8.66e-09 | 4.63e-09 | 8.75e-09 | 3.06e-10 | 1.05e-09 |
Kleibergen–Paap F-statistic | 73.17 | 63.72 | 68.50 | 67.14 | 51.14 | 60.78 | 72.14 | 57.74 | 61.44 | 58.09 | 61.58 | 68.29 | 66.96 |
Pesaran–Taylor reset statistic | 0.233 | 0.299 | 0.0271 | 0.198 | 0.211 | 0.00518 | 0.000324 | 0.00529 | 0.0391 | 0.0345 | 0.00971 | 9.41e-07 | 0.0158 |
Pesaran–Taylor p-value | 0.629 | 0.585 | 0.869 | 0.656 | 0.646 | 0.943 | 0.986 | 0.942 | 0.843 | 0.853 | 0.922 | 0.999 | 0.900 |
Variable | PBC 10 circulation, 2006/7, spend model | ||||||||||||
---|---|---|---|---|---|---|---|---|---|---|---|---|---|
(1) Uses SMR, no weighting, second stage, iAREA need 1, HCHS MFF | (2) Uses SMR, no weighting, second stage, PBRA need, HCHS MFF | (3) Uses SMR, no weighting, second stage, CARAN need, HCHS MFF | (4) Uses SYLLR, no weighting, second stage, iAREA need 1, HCHS MFF | (5) Uses SYLLR, no weighting, second stage, PBRA need, HCHS MFF | (6) Uses SYLLR, no weighting, second stage, CARAN need, HCHS MFF | (7) Uses SMR, weighted, second stage, iAREA need 1, HCHS MFF | (8) Uses SMR, no weighting, second stage, iAREA need 2, CARAN 3 MFFs | (9) Uses SMR, weighted, second stage, iAREA need 2, CARAN 3 MFFs | (10) Uses SMR, no weighting, second stage, iAREA need 3, CARAN 2 MFFs | (11) Uses SMR, weighted, second stage, iAREA need 3, CARAN 2 MFFs | (12) Uses SMR, no weighting, second stage, CARAN need, CARAN 3 MFFs | (13) Uses SMR, weighted, second stage, CARAN need, CARAN 3 MFFs | |
Other programme need 1 | −0.766** | −0.736** | −0.811** | −0.939*** | −0.776** | −0.927*** | −0.781** | −0.935*** | −0.831** | −1.112*** | |||
[0.298] | [0.299] | [0.370] | [0.305] | [0.315] | [0.324] | [0.316] | [0.326] | [0.367] | [0.392] | ||||
PCT budget per head | 0.861*** | 1.162*** | 1.035*** | 0.836*** | 1.191*** | 0.998*** | 0.719*** | 0.832*** | 0.661** | 0.829*** | 0.657** | 0.983*** | 0.914*** |
[0.240] | [0.218] | [0.219] | [0.229] | [0.220] | [0.210] | [0.259] | [0.242] | [0.264] | [0.242] | [0.264] | [0.213] | [0.231] | |
NeedAREA1 | 0.624* | 0.732* | 0.967** | ||||||||||
[0.355] | [0.389] | [0.378] | |||||||||||
White ethnic group | 0.215*** | 0.187** | 0.207** | 0.232*** | 0.199** | 0.225** | 0.278*** | 0.219** | 0.284*** | 0.219** | 0.284*** | 0.209** | 0.286*** |
[0.079] | [0.080] | [0.086] | [0.083] | [0.083] | [0.095] | [0.084] | [0.085] | [0.091] | [0.085] | [0.091] | [0.086] | [0.098] | |
Provision of unpaid care | 0.457** | 0.554*** | 0.488** | 0.437** | 0.549*** | 0.466* | 0.239 | 0.527*** | 0.336 | 0.528*** | 0.335 | 0.477** | 0.200 |
[0.205] | [0.186] | [0.227] | [0.212] | [0.183] | [0.247] | [0.227] | [0.190] | [0.210] | [0.190] | [0.211] | [0.227] | [0.268] | |
NeedPBRA | 0.250 | 0.295 | |||||||||||
[0.275] | [0.280] | ||||||||||||
NeedCARAN | 0.480 | 0.610 | 0.546 | 0.925** | |||||||||
[0.401] | [0.479] | [0.369] | [0.399] | ||||||||||
Other programme need 2 | −0.904** | −0.871** | −0.956* | ||||||||||
[0.365] | [0.347] | [0.491] | |||||||||||
NeedAREA2 | 0.655* | 1.020** | |||||||||||
[0.383] | [0.424] | ||||||||||||
NeedAREA3 | 0.652* | 1.018** | |||||||||||
[0.381] | [0.422] | ||||||||||||
Constant | 2.380** | 2.377* | 2.621* | 3.246** | 3.244** | 3.533* | 2.744** | 3.763* | 5.275** | 3.803* | 5.337** | 2.827 | 4.028** |
[1.212] | [1.251] | [1.469] | [1.572] | [1.553] | [2.088] | [1.222] | [2.133] | [2.306] | [2.143] | [2.319] | [1.863] | [1.995] | |
Observations | 152 | 152 | 152 | 152 | 152 | 152 | 152 | 152 | 152 | 152 | 152 | 152 | 152 |
Endogeneity test statistic | 8.506 | 10.727 | 8.136 | 6.475 | 8.793 | 5.743 | 9.019 | 8.939 | 8.654 | 9.036 | 8.745 | 8.315 | 9.729 |
Endogeneity p-value | 0.00354 | 0.00106 | 0.00434 | 0.0109 | 0.00302 | 0.0166 | 0.00267 | 0.00279 | 0.00326 | 0.00265 | 0.00310 | 0.00393 | 0.00181 |
Hansen–Sargan test statistic | 2.454 | 0.640 | 1.841 | 2.364 | 0.423 | 2.166 | 2.993 | 1.770 | 2.225 | 1.792 | 2.237 | 1.777 | 2.030 |
Hansen–Sargan p-value | 0.117 | 0.424 | 0.175 | 0.124 | 0.515 | 0.141 | 0.0836 | 0.183 | 0.136 | 0.181 | 0.135 | 0.183 | 0.154 |
Shea’s partial R2 | 0.235 | 0.205 | 0.184 | 0.207 | 0.184 | 0.148 | 0.238 | 0.225 | 0.230 | 0.224 | 0.228 | 0.183 | 0.183 |
Kleibergen–Paap LM test statistic | 22.99 | 24.15 | 20.59 | 21.32 | 25.01 | 17.46 | 26.79 | 23.47 | 27.59 | 23.63 | 27.59 | 20.91 | 24.11 |
Kleibergen–Paap p-value | 1.02e-05 | 5.70e-06 | 3.39e-05 | 2.35e-05 | 3.70e-06 | 0.000161 | 1.52e-06 | 8.02e-06 | 1.02e-06 | 7.41e-06 | 1.02e-06 | 2.88e-05 | 5.80e-06 |
Kleibergen–Paap F-statistic | 23.14 | 22.53 | 18.64 | 17.28 | 20.44 | 12.62 | 22.37 | 21.47 | 22.07 | 21.27 | 21.63 | 19.10 | 18.93 |
Pesaran–Taylor reset statistic | 0.00329 | 0.156 | 0.102 | 0.0384 | 0.333 | 0.288 | 0.0270 | 0.123 | 0.152 | 0.189 | 0.235 | 0.0165 | 0.190 |
Pesaran–Taylor p-value | 0.954 | 0.693 | 0.750 | 0.845 | 0.564 | 0.592 | 0.869 | 0.726 | 0.696 | 0.664 | 0.628 | 0.898 | 0.663 |
Variable | PBC 11 respiratory, 2006/7, spend model | ||||||||||||
---|---|---|---|---|---|---|---|---|---|---|---|---|---|
(1) Uses SMR, no weighting, second stage, iAREA need 1, HCHS MFF | (2) Uses SMR, no weighting, second stage, PBRA need, HCHS MFF | (3) Uses SMR, no weighting, second stage, CARAN need, HCHS MFF | (4) Uses SYLLR, no weighting, second stage, iAREA need 1, HCHS MFF | (5) Uses SYLLR, no weighting, second stage, PBRA need, HCHS MFF | (6) Uses SYLLR, no weighting, second stage, CARAN need, HCHS MFF | (7) Uses SMR, weighted, second stage, iAREA need 1, HCHS MFF | (8) Uses SMR, no weighting, second stage, iAREA need 2, CARAN 3 MFFs | (9) Uses SMR, weighted, second stage, iAREA need 2, CARAN 3 MFFs | (10) Uses SMR, no weighting, second stage, iAREA need 3, CARAN 2 MFFs | (11) Uses SMR, weighted, second stage, iAREA need 3, CARAN 2 MFFs | (12) Uses SMR, no weighting, second stage, CARAN need, CARAN 3 MFFs | (13) Uses SMR, weighted, second stage, CARAN need, CARAN 3 MFFs | |
PCT budget per head | 0.781** | 0.992*** | 0.957*** | 1.045*** | 1.315*** | 1.204*** | 0.808** | 0.592** | 0.591* | 0.588** | 0.585* | 0.865*** | 0.958*** |
[0.318] | [0.330] | [0.363] | [0.370] | [0.409] | [0.432] | [0.334] | [0.282] | [0.310] | [0.283] | [0.310] | [0.287] | [0.329] | |
NeedAREA1 | 1.714*** | 1.741*** | 1.813*** | ||||||||||
[0.597] | [0.497] | [0.563] | |||||||||||
Lone pensioner households | −0.497 | −0.243 | −0.483 | −0.419* | −0.240 | −0.380 | −0.595* | −0.078 | −0.304 | −0.089 | −0.320 | −0.447 | −0.556 |
[0.346] | [0.243] | [0.356] | [0.252] | [0.210] | [0.271] | [0.346] | [0.285] | [0.378] | [0.286] | [0.380] | [0.337] | [0.344] | |
Other programme need 1 | −0.803** | −0.602** | −0.890** | −0.866** | −0.391 | −0.664 | −0.407 | −0.687 | −0.834* | −0.931** | |||
[0.397] | [0.294] | [0.439] | [0.392] | [0.364] | [0.478] | [0.364] | [0.481] | [0.428] | [0.437] | ||||
NeedPBRA | 1.176*** | 1.226*** | |||||||||||
[0.391] | [0.366] | ||||||||||||
NeedCARAN | 1.686*** | 1.720*** | 1.680*** | 1.782*** | |||||||||
[0.627] | [0.536] | [0.609] | [0.561] | ||||||||||
Other programme need 2 | −1.109** | −0.955** | −1.197** | ||||||||||
[0.455] | [0.406] | [0.552] | |||||||||||
NeedAREA2 | 1.312** | 1.770** | |||||||||||
[0.667] | [0.803] | ||||||||||||
NeedAREA3 | 1.325** | 1.788** | |||||||||||
[0.661] | [0.800] | ||||||||||||
Constant | −0.143 | −0.649 | 0.258 | 2.954 | 2.293 | 3.531 | −0.046 | 1.605 | 2.457 | 1.686 | 2.578 | 1.022 | 0.595 |
[1.250] | [0.961] | [1.415] | [2.335] | [2.094] | [2.856] | [1.220] | [2.372] | [2.547] | [2.389] | [2.574] | [1.980] | [2.082] | |
Observations | 152 | 152 | 152 | 152 | 152 | 152 | 152 | 152 | 152 | 152 | 152 | 152 | 152 |
Endogeneity test statistic | 7.821 | 8.431 | 9.089 | 9.157 | 10.215 | 9.242 | 6.984 | 4.679 | 4.326 | 4.853 | 4.475 | 9.016 | 6.863 |
Endogeneity p-value | 0.00516 | 0.00369 | 0.00257 | 0.00248 | 0.00139 | 0.00236 | 0.00822 | 0.0305 | 0.0375 | 0.0276 | 0.0344 | 0.00268 | 0.00880 |
Hansen–Sargan test statistic | 1.655 | 3.108 | 0.983 | 0.214 | 1.502 | 0.0135 | 0.615 | 4.704 | 2.922 | 4.621 | 2.855 | 0.866 | 0.156 |
Hansen-Sargan p-value | 0.198 | 0.0779 | 0.321 | 0.644 | 0.220 | 0.908 | 0.433 | 0.0301 | 0.0874 | 0.0316 | 0.0911 | 0.352 | 0.693 |
Shea’s partial R2 | 0.164 | 0.211 | 0.149 | 0.183 | 0.203 | 0.172 | 0.167 | 0.161 | 0.131 | 0.161 | 0.131 | 0.142 | 0.146 |
Kleibergen–Paap LM test statistic | 18.58 | 21.08 | 18.17 | 22.04 | 17.51 | 18.27 | 18.78 | 20.00 | 13.94 | 20.14 | 13.90 | 17.12 | 16.62 |
Kleibergen–Paap p-value | 9.22e-05 | 2.64e-05 | 0.000113 | 1.64e-05 | 0.000158 | 0.000108 | 8.34e-05 | 4.55e-05 | 0.000940 | 4.23e-05 | 0.000960 | 0.000192 | 0.000246 |
Kleibergen–Paap F-statistic | 8.163 | 14.31 | 7.772 | 8.729 | 8.513 | 6.885 | 8.383 | 13.56 | 7.241 | 13.77 | 7.297 | 7.776 | 7.220 |
Pesaran–Taylor reset statistic | 0.238 | 0.0135 | 0.141 | 0.0704 | 0.0139 | 0.0164 | 1.083 | 2.231 | 2.206 | 2.311 | 2.283 | 3.699 | 4.984 |
Pesaran–Taylor p-value | 0.625 | 0.907 | 0.707 | 0.791 | 0.906 | 0.898 | 0.298 | 0.135 | 0.138 | 0.128 | 0.131 | 0.0545 | 0.0256 |
Variable | PBC 13 gastrointestinal, 2006/7, spend model | ||||||||||||
---|---|---|---|---|---|---|---|---|---|---|---|---|---|
(1) Uses SMR, no weighting, second stage, iAREA need 1, HCHS MFF | (2) Uses SMR, no weighting, second stage, PBRA need, HCHS MFF | (3) Uses SMR, no weighting, second stage, CARAN need, HCHS MFF | (4) Uses SYLLR, no weighting, second stage, iAREA need 1, HCHS MFF | (5) Uses SYLLR, no weighting, second stage, PBRA need, HCHS MFF | (6) Uses SYLLR, no weighting, second stage, CARAN need, HCHS MFF | (7) Uses SMR, weighted, second stage, iAREA need 1, HCHS MFF | (8) Uses SMR, no weighting, second stage, iAREA need 2, CARAN 3 MFFs | (9) Uses SMR, weighted, second stage, iAREA need 2, CARAN 3 MFFs | (10) Uses SMR, no weighting, second stage, iAREA need 3, CARAN 2 MFFs | (11) Uses SMR, weighted, second stage, iAREA need 3, CARAN 2 MFFs | (12) Uses SMR, no weighting, second stage, CARAN need, CARAN 3 MFFs | (13) Uses SMR, weighted, second stage, CARAN need, CARAN 3 MFFs | |
PCT budget per head | 0.538 | 0.876** | 0.862** | 1.058** | 1.461*** | 1.396*** | 0.627* | 0.240 | 0.271 | 0.237 | 0.265 | 0.509* | 0.692** |
[0.355] | [0.371] | [0.414] | [0.446] | [0.513] | [0.533] | [0.371] | [0.301] | [0.334] | [0.301] | [0.334] | [0.305] | [0.340] | |
NeedAREA1 | 2.627*** | 2.840*** | 2.775*** | ||||||||||
[0.851] | [0.758] | [0.755] | |||||||||||
Lone pensioner households | −0.838* | −0.269 | −0.740 | −0.793** | −0.362 | −0.736* | −1.080** | 0.122 | −0.262 | 0.121 | −0.270 | −0.612 | −0.901** |
[0.492] | [0.347] | [0.520] | [0.385] | [0.314] | [0.422] | [0.460] | [0.290] | [0.375] | [0.287] | [0.374] | [0.453] | [0.445] | |
Other programme need 1 | −1.386** | −0.820** | −1.416** | −1.572*** | −0.279 | −0.751 | −0.281 | −0.763 | −1.216** | −1.510*** | |||
[0.566] | [0.402] | [0.634] | [0.520] | [0.366] | [0.476] | [0.363] | [0.475] | [0.562] | [0.552] | ||||
NeedPBRA | 1.422*** | 1.627*** | |||||||||||
[0.488] | [0.478] | ||||||||||||
NeedCARAN | 2.369*** | 2.740*** | 2.375*** | 2.619*** | |||||||||
[0.889] | [0.839] | [0.836] | [0.748] | ||||||||||
Other programme need 2 | −2.093*** | −1.524*** | −2.250*** | ||||||||||
[0.663] | [0.588] | [0.817] | |||||||||||
NeedAREA2 | 1.267* | 1.916** | |||||||||||
[0.662] | [0.794] | ||||||||||||
NeedAREA3 | 1.264* | 1.920** | |||||||||||
[0.650] | [0.784] | ||||||||||||
Constant | 2.133 | 0.486 | 2.386 | 8.379** | 5.626* | 9.374** | 2.512 | 4.107 | 5.367* | 4.129 | 5.450* | 5.180** | 4.691** |
[1.763] | [1.237] | [1.998] | [3.338] | [2.958] | [4.164] | [1.605] | [2.520] | [2.774] | [2.524] | [2.787] | [2.437] | [2.310] | |
Observations | 152 | 152 | 152 | 152 | 152 | 152 | 152 | 152 | 152 | 152 | 152 | 152 | 152 |
Endogeneity test statistic | 3.530 | 1.329 | 4.118 | 11.390 | 5.366 | 9.900 | 7.202 | 0.041 | 1.085 | 0.052 | 1.144 | 4.849 | 8.194 |
Endogeneity p-value | 0.0603 | 0.249 | 0.0424 | 0.000738 | 0.0205 | 0.00165 | 0.00728 | 0.839 | 0.298 | 0.820 | 0.285 | 0.0277 | 0.00420 |
Hansen–Sargan test statistic | 7.192 | 9.154 | 4.655 | 2.282 | 6.867 | 1.169 | 5.058 | 12.98 | 11.45 | 12.91 | 11.31 | 4.276 | 2.414 |
Hansen–Sargan p-value | 0.00732 | 0.00248 | 0.0310 | 0.131 | 0.00878 | 0.280 | 0.0245 | 0.000316 | 0.000715 | 0.000327 | 0.000771 | 0.0387 | 0.120 |
Shea’s partial R2 | 0.164 | 0.211 | 0.149 | 0.183 | 0.203 | 0.172 | 0.167 | 0.161 | 0.131 | 0.161 | 0.131 | 0.142 | 0.146 |
Kleibergen–Paap LM test statistic | 18.58 | 21.08 | 18.17 | 22.04 | 17.51 | 18.27 | 18.78 | 20.00 | 13.94 | 20.14 | 13.90 | 17.12 | 16.62 |
Kleibergen–Paap p-value | 9.22e-05 | 2.64e-05 | 0.000113 | 1.64e-05 | 0.000158 | 0.000108 | 8.34e-05 | 4.55e-05 | 0.000940 | 4.23e-05 | 0.000960 | 0.000192 | 0.000246 |
Kleibergen–Paap F-statistic | 8.163 | 14.31 | 7.772 | 8.729 | 8.513 | 6.885 | 8.383 | 13.56 | 7.241 | 13.77 | 7.297 | 7.776 | 7.220 |
Pesaran–Taylor reset statistic | 0.00544 | 0.107 | 0.00251 | 0.0613 | 0.0576 | 0.000667 | 0.167 | 1.735 | 2.598 | 1.752 | 2.633 | 2.450 | 3.579 |
Pesaran–Taylor p-value | 0.941 | 0.743 | 0.960 | 0.804 | 0.810 | 0.979 | 0.683 | 0.188 | 0.107 | 0.186 | 0.105 | 0.118 | 0.0585 |
Variable | PBC 2 cancer, 2006/7, outcome model | ||||||||||||
---|---|---|---|---|---|---|---|---|---|---|---|---|---|
(1) Uses SMR, no weighting, second stage, iAREA need 1, HCHS MFF | (2) Uses SMR, no weighting, second stage, PBRA need, HCHS MFF | (3) Uses SMR, no weighting, second stage, CARAN need, HCHS MFF | (4) Uses SYLLR, no weighting, second stage, iAREA need 1, HCHS MFF | (5) Uses SYLLR, no weighting, second stage, PBRA need, HCHS MFF | (6) Uses SYLLR, no weighting, second stage, CARAN need, HCHS MFF | (7) Uses SMR, weighted, second stage, iAREA need 1, HCHS MFF | (8) Uses SMR, no weighting, second stage, iAREA need 2, CARAN 3 MFFs | (9) Uses SMR, weighted, second stage, iAREA need 2, CARAN 3 MFFs | (10) Uses SMR, no weighting, second stage, iAREA need 3, CARAN 2 MFFs | (11) Uses SMR, weighted, second stage, iAREA need 3, CARAN 2 MFFs | (12) Uses SMR, no weighting, second stage, CARAN need, CARAN 3 MFFs | (13) Uses SMR, weighted, second stage, CARAN need, CARAN 3 MFFs | |
NeedAREA1 | 1.142*** | 1.048*** | 1.121*** | ||||||||||
[0.161] | [0.143] | [0.169] | |||||||||||
Cancer spend per head | −0.426*** | −0.287*** | −0.351*** | −0.356*** | −0.223*** | −0.291*** | −0.487*** | −0.284*** | −0.367*** | −0.284*** | −0.366*** | −0.421*** | −0.494*** |
[0.125] | [0.080] | [0.098] | [0.110] | [0.068] | [0.082] | [0.148] | [0.109] | [0.133] | [0.109] | [0.133] | [0.126] | [0.156] | |
NeedPBRA | 0.972*** | 0.881*** | |||||||||||
[0.104] | [0.090] | ||||||||||||
NeedCARAN | 1.087*** | 1.005*** | 1.126*** | 1.112*** | |||||||||
[0.130] | [0.110] | [0.153] | [0.167] | ||||||||||
NeedAREA2 | 1.048*** | 1.070*** | |||||||||||
[0.128] | [0.143] | ||||||||||||
NeedAREA3 | 1.035*** | 1.058*** | |||||||||||
[0.128] | [0.142] | ||||||||||||
Constant | 3.689*** | 4.049*** | 3.884*** | 4.139*** | 4.482*** | 4.309*** | 3.536*** | 6.012*** | 6.375*** | 6.009*** | 6.373*** | 6.614*** | 6.938*** |
[0.318] | [0.202] | [0.249] | [0.278] | [0.172] | [0.207] | [0.372] | [0.476] | [0.583] | [0.476] | [0.583] | [0.552] | [0.684] | |
Observations | 152 | 152 | 152 | 152 | 152 | 152 | 152 | 152 | 152 | 152 | 152 | 152 | 152 |
Endogeneity test statistic | 18.518 | 16.063 | 19.086 | 15.982 | 12.454 | 17.096 | 18.965 | 11.026 | 13.552 | 10.985 | 13.490 | 19.697 | 19.500 |
Endogeneity p-value | 1.68e-05 | 6.13e-05 | 1.25e-05 | 6.40e-05 | 0.000417 | 3.55e-05 | 1.33e-05 | 0.000898 | 0.000232 | 0.000918 | 0.000240 | 9.07e-06 | 1.01e-05 |
Hansen–Sargan test statistic | 0.248 | 0.239 | 0.0431 | 0.163 | 0.161 | 0.00820 | 0.192 | 0.860 | 0.690 | 0.857 | 0.686 | 0.000632 | 0.0933 |
Hansen–Sargan p-value | 0.619 | 0.625 | 0.835 | 0.686 | 0.688 | 0.928 | 0.661 | 0.354 | 0.406 | 0.355 | 0.407 | 0.980 | 0.760 |
Shea’s partial R2 | 0.200 | 0.246 | 0.226 | 0.200 | 0.246 | 0.226 | 0.176 | 0.202 | 0.167 | 0.202 | 0.166 | 0.169 | 0.142 |
Kleibergen–Paap LM test statistic | 18.49 | 23.43 | 21.27 | 18.49 | 23.43 | 21.27 | 16.89 | 19.40 | 17.25 | 19.39 | 17.20 | 18.35 | 15.71 |
Kleibergen–Paap p-value | 9.66e-05 | 8.17e-06 | 2.41e-05 | 9.66e-05 | 8.17e-06 | 2.41e-05 | 0.000215 | 6.12e-05 | 0.000179 | 6.17e-05 | 0.000184 | 0.000104 | 0.000389 |
Kleibergen–Paap F-statistic | 19.15 | 28.08 | 24.39 | 19.15 | 28.08 | 24.39 | 15.16 | 19.90 | 14.69 | 19.81 | 14.58 | 16.81 | 12.05 |
Pesaran–Taylor reset statistic | 2.789 | 5.422 | 3.218 | 3.506 | 5.986 | 3.796 | 3.838 | 4.129 | 5.271 | 4.234 | 5.259 | 4.399 | 5.890 |
Pesaran–Taylor p-value | 0.0949 | 0.0199 | 0.0728 | 0.0611 | 0.0144 | 0.0514 | 0.0501 | 0.0422 | 0.0217 | 0.0396 | 0.0218 | 0.0360 | 0.0152 |
Variable | PBC 10 circulation, 2006/7, outcome model | ||||||||||||
---|---|---|---|---|---|---|---|---|---|---|---|---|---|
(1) Uses SMR, no weighting, second stage, iAREA need 1, HCHS MFF | (2) Uses SMR, no weighting, second stage, PBRA need, HCHS MFF | (3) Uses SMR, no weighting, second stage, CARAN need, HCHS MFF | (4) Uses SYLLR, no weighting, second stage, iAREA need 1, HCHS MFF | (5) Uses SYLLR, no weighting, second stage, PBRA need, HCHS MFF | (6) Uses SYLLR, no weighting, second stage, CARAN need, HCHS MFF | (7) Uses SMR, weighted, second stage, iAREA need 1, HCHS MFF | (8) Uses SMR, no weighting, second stage, iAREA need 2, CARAN 3 MFFs | (9) Uses SMR, weighted, second stage, iAREA need 2, CARAN 3 MFFs | (10) Uses SMR, no weighting, second stage, iAREA need 3, CARAN 2 MFFs | (11) Uses SMR, weighted, second stage, iAREA need 3, CARAN 2 MFFs | (12) Uses SMR, no weighting, second stage, CARAN need, CARAN 3 MFFs | (13) Uses SMR, weighted, second stage, CARAN need, CARAN 3 MFFs | |
NeedAREA1 | 2.442*** | 2.657*** | 2.554*** | ||||||||||
[0.239] | [0.256] | [0.251] | |||||||||||
Circulation spend per head | −1.166*** | −0.945*** | −1.080*** | −1.245*** | −0.983*** | −1.138*** | −1.258*** | −0.968*** | −1.077*** | −0.966*** | −1.075*** | −1.285*** | −1.379*** |
[0.203] | [0.180] | [0.195] | [0.215] | [0.190] | [0.205] | [0.207] | [0.191] | [0.194] | [0.191] | [0.194] | [0.243] | [0.238] | |
NeedPBRA | 2.104*** | 2.262*** | |||||||||||
[0.208] | [0.220] | ||||||||||||
NeedCARAN | 2.394*** | 2.587*** | 2.508*** | 2.624*** | |||||||||
[0.242] | [0.257] | [0.278] | [0.282] | ||||||||||
NeedAREA2 | 2.303*** | 2.452*** | |||||||||||
[0.218] | [0.233] | ||||||||||||
NeedAREA3 | 2.281*** | 2.426*** | |||||||||||
[0.217] | [0.231] | ||||||||||||
Constant | 1.971*** | 2.456*** | 2.165*** | 1.983*** | 2.555*** | 2.222*** | 1.771*** | 9.078*** | 9.596*** | 9.073*** | 9.584*** | 10.605*** | 11.050*** |
[0.429] | [0.379] | [0.411] | [0.454] | [0.400] | [0.432] | [0.438] | [0.916] | [0.931] | [0.914] | [0.928] | [1.168] | [1.145] | |
Observations | 152 | 152 | 152 | 152 | 152 | 152 | 152 | 152 | 152 | 152 | 152 | 152 | 152 |
Endogeneity test statistic | 32.774 | 30.750 | 39.253 | 38.776 | 28.130 | 42.881 | 28.691 | 28.410 | 28.030 | 28.471 | 27.939 | 40.272 | 38.934 |
Endogeneity p-value | 1.04e-08 | 2.94e-08 | 3.72e-10 | 4.75e-10 | 1.13e-07 | 5.82e-11 | 8.49e-08 | 9.82e-08 | 1.19e-07 | 9.51e-08 | 1.25e-07 | 2.21e-10 | 4.38e-10 |
Hansen–Sargan test statistic | 7.315 | 11.76 | 3.706 | 5.337 | 12.53 | 3.288 | 5.937 | 10.43 | 7.965 | 10.24 | 7.888 | 2.449 | 1.678 |
Hansen–Sargan p-value | 0.0625 | 0.00827 | 0.295 | 0.149 | 0.00576 | 0.349 | 0.115 | 0.0153 | 0.0467 | 0.0166 | 0.0484 | 0.484 | 0.642 |
Shea’s partial R2 | 0.368 | 0.383 | 0.376 | 0.368 | 0.383 | 0.376 | 0.349 | 0.370 | 0.346 | 0.371 | 0.347 | 0.305 | 0.291 |
Kleibergen–Paap LM test statistic | 29.32 | 26.93 | 31.11 | 29.32 | 26.93 | 31.11 | 32.68 | 31.06 | 34.79 | 31.25 | 34.98 | 28.69 | 31.43 |
Kleibergen–Paap p-value | 6.73e-06 | 2.05e-05 | 2.90e-06 | 6.73e-06 | 2.05e-05 | 2.90e-06 | 1.39e-06 | 2.97e-06 | 5.14e-07 | 2.72e-06 | 4.70e-07 | 9.03e-06 | 2.50e-06 |
Kleibergen–Paap F-statistic | 20.24 | 19.21 | 20.32 | 20.24 | 19.21 | 20.32 | 19.89 | 20.09 | 19.66 | 20.15 | 19.70 | 15.50 | 16.33 |
Pesaran–Taylor reset statistic | 0.0847 | 0.0884 | 2.19e-07 | 0.257 | 0.0261 | 0.0107 | 0.0185 | 0.0138 | 0.00282 | 0.0143 | 0.00315 | 2.369 | 0.196 |
Pesaran–Taylor p-value | 0.771 | 0.766 | 1.000 | 0.612 | 0.872 | 0.918 | 0.892 | 0.906 | 0.958 | 0.905 | 0.955 | 0.124 | 0.658 |
Variable | PBC 11 respiratory, 2006/7, outcome model | ||||||||||||
---|---|---|---|---|---|---|---|---|---|---|---|---|---|
(1) Uses SMR, instrument spend, no weighting, second stage, iAREA need 1, HCHS MFF | (2) Uses SMR, instrument spend, no weighting, second stage, PBRA need, HCHS MFF | (3) Uses SMR, instrument spend, no weighting, second stage, CARAN need, HCHS MFF | (4) Uses SYLLR, instrument spend, no weighting, second stage, iAREA need 1, HCHS MFF | (5) Uses SYLLR, instrument spend, no weighting, second stage, PBRA need, HCHS MFF | (6) Uses SYLLR, instrument spend, no weighting, second stage, CARAN need, HCHS MFF | (7) Uses SMR, instrument spend, weighted, second stage, iAREA need 1, HCHS MFF | (8) Uses SMR, instrument spend, no weighting, second stage, iAREA need 2, CARAN 3 MFFs | (9) Uses SMR, instrument spend, weighted, second stage, iAREA need 2, CARAN 3 MFFs | (10) Uses SMR, instrument spend, no weighting second stage, iAREA need 3, CARAN 2 MFFs | (11) Uses SMR, instrument spend, weighted, second stage, iAREA need 3, CARAN 2 MFFs | (12) Uses SMR, instrument spend, no weighting, second stage, CARAN need, CARAN 3 MFFs | (13) Uses SMR, instrument spend, weighted, second stage, CARAN need, CARAN 3 MFFs | |
NeedAREA1 | 8.008*** | 9.158*** | 8.647*** | ||||||||||
[2.969] | [3.298] | [3.317] | |||||||||||
Respiratory spend per head | −4.845** | −3.364*** | −4.149** | −5.568** | −3.894*** | −4.808** | −5.182** | −3.535** | −3.773** | −3.536** | −3.764** | −6.738* | −6.640* |
[2.147] | [1.225] | [1.734] | [2.388] | [1.359] | [1.945] | [2.352] | [1.412] | [1.503] | [1.400] | [1.479] | [3.799] | [3.464] | |
NeedPBRA | 5.941*** | 6.789*** | |||||||||||
[1.706] | [1.887] | ||||||||||||
NeedCARAN | 7.238*** | 8.306*** | 10.184** | 10.352** | |||||||||
[2.492] | [2.788] | [5.006] | [4.635] | ||||||||||
NeedAREA2 | 6.501*** | 7.025*** | |||||||||||
[1.985] | [2.176] | ||||||||||||
NeedAREA3 | 6.460*** | 6.965*** | |||||||||||
[1.959] | [2.130] | ||||||||||||
Constant | −10.277* | −6.163* | −8.328* | −12.218* | −7.567** | −10.087* | −11.234* | 17.749*** | 18.712*** | 17.755*** | 18.675*** | 31.101** | 30.667** |
[5.898] | [3.355] | [4.749] | [6.563] | [3.723] | [5.328] | [6.465] | [5.877] | [6.252] | [5.824] | [6.151] | [15.828] | [14.436] | |
Observations | 152 | 152 | 152 | 152 | 152 | 152 | 152 | 152 | 152 | 152 | 152 | 152 | 152 |
Endogeneity test statistic | 51.569 | 49.552 | 54.608 | 55.731 | 52.069 | 58.974 | 48.137 | 42.431 | 44.464 | 42.671 | 44.683 | 57.889 | 53.094 |
Endogeneity p-value | 0 | 0 | 0 | 0 | 0 | 0 | 0 | 7.32e-11 | 0 | 6.48e-11 | 0 | 0 | 0 |
Hansen–Sargan test statistic | 0.302 | 1.253 | 0.123 | 0.305 | 1.828 | 0.211 | 0.179 | 0.785 | 0.354 | 0.700 | 0.303 | 0.00383 | 0.0915 |
Hansen–Sargan p-value | 0.582 | 0.263 | 0.726 | 0.581 | 0.176 | 0.646 | 0.673 | 0.376 | 0.552 | 0.403 | 0.582 | 0.951 | 0.762 |
Shea’s partial R2 | 0.0491 | 0.0791 | 0.0593 | 0.0491 | 0.0791 | 0.0593 | 0.0462 | 0.0654 | 0.0624 | 0.0661 | 0.0633 | 0.0235 | 0.0246 |
Kleibergen–Paap LM test statistic | 5.660 | 8.303 | 7.499 | 5.660 | 8.303 | 7.499 | 5.437 | 7.311 | 6.973 | 7.461 | 7.117 | 3.866 | 3.772 |
Kleibergen–Paap p-value | 0.0590 | 0.0157 | 0.0235 | 0.0590 | 0.0157 | 0.0235 | 0.0660 | 0.0258 | 0.0306 | 0.0240 | 0.0285 | 0.145 | 0.152 |
Kleibergen–Paap F-statistic | 3.344 | 5.857 | 4.507 | 3.344 | 5.857 | 4.507 | 2.959 | 4.328 | 3.804 | 4.402 | 3.875 | 2.030 | 1.859 |
Pesaran–Taylor reset statistic | 0.791 | 4.049 | 0.000560 | 1.490 | 5.225 | 0.00355 | 0.327 | 0.0202 | 0.00861 | 0.0218 | 0.0116 | 3.788 | 1.716 |
Pesaran–Taylor p-value | 0.374 | 0.0442 | 0.981 | 0.222 | 0.0223 | 0.952 | 0.568 | 0.887 | 0.926 | 0.883 | 0.914 | 0.0516 | 0.190 |
Variable | PBC 13 gastrointestinal, 2006/7, outcome model | ||||||||||||
---|---|---|---|---|---|---|---|---|---|---|---|---|---|
(1) Uses SMR, instrument spend, no weighting, second stage, iAREA need 1, HCHS MFF | (2) Uses SMR, instrument spend, no weighting, second stage, PBRA need, HCHS MFF | (3) Uses SMR, instrument spend, no weighting, second stage, CARAN need, HCHS MFF | (4) Uses SYLLR, instrument spend, no weighting, second stage, iAREA need 1, HCHS MFF | (5) Uses SYLLR, instrument spend, no weighting, second stage, PBRA need, HCHS MFF | (6) Uses SYLLR, instrument spend, no weighting, second stage, CARAN need, HCHS MFF | (7) Uses SMR, instrument spend, weighted, second stage, iAREA need 1, HCHS MFF | (8) Uses SMR, instrument spend, no weighting, second stage, iAREA need 2, CARAN 3 MFFs | (9) Uses SMR, instrument spend, weighted, second stage, iAREA need 2, CARAN 3 MFFs | (10) Uses SMR, instrument spend, no weighting, second stage, iAREA need 3, CARAN 2 MFFs | (11) Uses SMR, instrument spend, weighted, second stage, iAREA need 3, CARAN 2 MFFs | (12) Uses SMR, instrument spend, no weighting, second stage, CARAN need, CARAN 3 MFFs | (13) Uses SMR, instrument spend, weighted, second stage, CARAN need, CARAN 3 MFFs | |
NeedAREA1 | 3.853*** | 3.966*** | 3.779*** | ||||||||||
[0.551] | [0.558] | [0.499] | |||||||||||
Gastrointestinal spend per head | −1.755*** | −1.420*** | −1.641*** | −1.544*** | −1.180*** | −1.404*** | −1.750*** | −1.275*** | −1.317*** | −1.275*** | −1.315*** | −2.056*** | −2.192*** |
[0.397] | [0.353] | [0.427] | [0.399] | [0.358] | [0.429] | [0.385] | [0.335] | [0.326] | [0.335] | [0.325] | [0.589] | [0.574] | |
NeedPBRA | 3.342*** | 3.413*** | |||||||||||
[0.486] | [0.498] | ||||||||||||
NeedCARAN | 3.794*** | 3.887*** | 4.140*** | 4.250*** | |||||||||
[0.612] | [0.621] | [0.768] | [0.710] | ||||||||||
NeedAREA2 | 3.426*** | 3.479*** | |||||||||||
[0.466] | [0.419] | ||||||||||||
NeedAREA3 | 3.393*** | 3.443*** | |||||||||||
[0.462] | [0.415] | ||||||||||||
Constant | −2.155** | −1.251 | −1.838 | −0.954 | 0.028 | −0.566 | −2.166** | 7.919*** | 8.073*** | 7.916*** | 8.064*** | 11.273*** | 11.835*** |
[1.047] | [0.928] | [1.121] | [1.054] | [0.943] | [1.127] | [1.016] | [1.431] | [1.391] | [1.430] | [1.387] | [2.524] | [2.460] | |
Observations | 152 | 152 | 152 | 152 | 152 | 152 | 152 | 152 | 152 | 152 | 152 | 152 | 152 |
Endogeneity test statistic | 23.347 | 18.985 | 25.405 | 17.048 | 11.389 | 17.857 | 16.834 | 16.638 | 11.980 | 16.689 | 11.942 | 25.632 | 22.341 |
Endogeneity p-value | 1.35e-06 | 1.32e-05 | 4.65e-07 | 3.65e-05 | 0.000739 | 2.38e-05 | 4.08e-05 | 4.52e-05 | 0.000538 | 4.40e-05 | 0.000549 | 4.13e-07 | 2.28e-06 |
Hansen–Sargan test statistic | 3.067 | 4.604 | 1.555 | 4.936 | 7.575 | 2.637 | 7.476 | 5.029 | 8.762 | 4.907 | 8.714 | 1.284 | 3.554 |
Hansen–Sargan p-value | 0.216 | 0.100 | 0.459 | 0.0847 | 0.0227 | 0.268 | 0.0238 | 0.0809 | 0.0125 | 0.0860 | 0.0128 | 0.526 | 0.169 |
Shea’s partial R2 | 0.193 | 0.231 | 0.200 | 0.193 | 0.231 | 0.200 | 0.191 | 0.208 | 0.198 | 0.208 | 0.198 | 0.139 | 0.135 |
Kleibergen–Paap LM test statistic | 16.47 | 17.51 | 16.32 | 16.47 | 17.51 | 16.32 | 17.68 | 17.09 | 18.26 | 17.14 | 18.34 | 13.39 | 13.98 |
Kleibergen–Paap p-value | 0.000910 | 0.000556 | 0.000974 | 0.000910 | 0.000556 | 0.000974 | 0.000511 | 0.000679 | 0.000389 | 0.000661 | 0.000375 | 0.00386 | 0.00293 |
Kleibergen–Paap F-statistic | 12.12 | 13.24 | 10.79 | 12.12 | 13.24 | 10.79 | 11.96 | 13.23 | 12.98 | 13.24 | 13.00 | 7.550 | 7.248 |
Pesaran–Taylor reset statistic | 0.233 | 0.0427 | 0.0897 | 1.246 | 1.121 | 0.258 | 0.170 | 0.0935 | 0.443 | 0.0893 | 0.411 | 0.00841 | 0.117 |
Pesaran–Taylor p-value | 0.629 | 0.836 | 0.765 | 0.264 | 0.290 | 0.611 | 0.680 | 0.760 | 0.506 | 0.765 | 0.521 | 0.927 | 0.732 |
The assimilation of the impact of alternative measures of need, weights and MFFs proved overwhelming. Instead, we approached the selection of the appropriate need ∼ weighting ∼ MFF combination from an a priori perspective. The AREA-based need formula has been replaced by the CARAN formula for the purposes of resource allocation and therefore it must be believed to be a better indicator of relative health-care need. The PBRA approach is relatively new and has not been implemented yet. We therefore decided to use the CARAN-based measure as our indicator of the level of need.
With some PCTs several times larger than others, it is difficult to justify giving them all the same weighting. It was therefore decided to weight all of our models by PCT size (where size is measured by the PCT’s population).
We also decided to use the ‘own population’ expenditure data on the grounds that all NHS expenditure, irrespective of its funding source, should be included in the analysis (although there is the issue about how this income is split between PBCs).
Finally, it was decided to focus on the use of the SYLLR as the outcome indicator, and to proxy ‘other programme need’ in the expenditure equation using the all-cause SYLLR minus the own programme SYLLR.
Model estimation using 2006/7 expenditure data and mortality data for 2004/5/6: CARAN need and three market forces factors
Initially, acceptable models were obtained by using the CARAN measure of need and adjusting expenditure for local input prices using a weighted average of the MFFs for all three services (HCHS, prescribing and GMS/PMS). The outcome and expenditure results for the big four programmes are shown in Table 59 with the relevant outcome and expenditure elasticities highlighted.
Variable | PBC 2 cancer, 2006/7 | PBC 10 circulation, 2006/7 | PBC 11 respiratory, 2006/7 | PBC 13 gastrointestinal, 2006/7 | ||||
---|---|---|---|---|---|---|---|---|
(1) | (2) | (3) | (4) | (5) | (6) | (7) | (8) | |
Regressors | Outcome model | Spend model | Outcome model | Spend model | Outcome model | Spend model | Outcome model | Spend model |
All-cause SYLLR excluding cancer | −0.952*** | |||||||
[0.179] | ||||||||
Budget per head (HPG MFF) | 0.542** | 0.694** | 0.712*** | 0.650** | ||||
[0.242] | [0.292] | [0.252] | [0.289] | |||||
Need CARAN | 0.958*** | 1.765*** | 2.830*** | 2.185*** | 1.764 | 1.371*** | 4.609*** | 2.696*** |
[0.129] | [0.286] | [0.252] | [0.355] | [1.192] | [0.297] | [0.700] | [0.679] | |
Own programme spend per head | −0.351*** | −1.441*** | −2.830*** | −2.125*** | ||||
[0.117] | [0.219] | [0.767] | [0.563] | |||||
All-cause SYLLR excluding circulatory problems | −1.782*** | |||||||
[0.336] | ||||||||
Permanently sick | 1.371*** | |||||||
[0.405] | ||||||||
All-cause SYLLR excluding respiratory problems | −0.670** | |||||||
[0.288] | ||||||||
All-cause SYLLR excluding gastrointestinal problems | −1.856*** | |||||||
[0.612] | ||||||||
Lone pensioner households | −0.593** | |||||||
[0.297] | ||||||||
Constant | 6.588*** | 5.937*** | 11.538*** | 10.299*** | 18.965*** | 3.117 | 12.208*** | 9.752*** |
[0.515] | [1.775] | [1.050] | [2.384] | [3.853] | [1.976] | [2.416] | [3.053] | |
Observations | 152 | 152 | 152 | 152 | 152 | 152 | 152 | 152 |
Endogeneity test statistic | 14.496 | 20.274 | 43.352 | 25.784 | 27.923 | 7.922 | 21.862 | 13.531 |
Endogeneity p-value | 0.000140 | 6.71e-06 | 0 | 3.82e-07 | 1.26e-07 | 0.00488 | 2.93e-06 | 0.000235 |
Hansen–Sargan test statistic | 0.208 | 0.293 | 1.507 | 0.542 | 1.879 | 0.356 | 1.006 | 0.0267 |
Hansen–Sargan p-value | 0.649 | 0.588 | 0.681 | 0.462 | 0.170 | 0.550 | 0.316 | 0.870 |
Shea’s partial R2 | 0.163 | 0.445 | 0.303 | 0.296 | 0.0802 | 0.366 | 0.142 | 0.206 |
Kleibergen–Paap LM test statistic | 16.97 | 42.38 | 32.53 | 32.70 | 10.51 | 36.33 | 15.00 | 19.07 |
Kleibergen–Paap p-value | 0.000207 | 6.28e-10 | 1.49e-06 | 7.93e-08 | 0.00523 | 1.29e-08 | 0.000553 | 7.22e-05 |
Kleibergen–Paap F-statistic | 12.47 | 48.32 | 17.31 | 25.71 | 7.482 | 24.32 | 11.80 | 8.660 |
Pesaran–Taylor reset statistic | 5.471 | 0.00111 | 0.0912 | 0.0183 | 3.090 | 1.915 | 0.267 | 0.0880 |
Pesaran–Taylor p-value | 0.0193 | 0.973 | 0.763 | 0.892 | 0.0788 | 0.166 | 0.605 | 0.767 |
In all four outcome models expenditure has a significant negative effect on mortality and, in three of these, the all service measure of need has a significant positive effect. In the respiratory outcome model, where the all service need term is not significant, there is another indicator of need – the proportion of the population that are permanently sick – and this is both positive and statistically significant. The diagnostic statistics suggest that, in all four cases, own programme expenditure is endogenous and that the instruments are valid. They also suggest that the instruments are relevant. There is some evidence that the instruments are slightly weak in one of the four outcome results (the respiratory model). ab The Pesaran–Taylor test suggests that there is no evidence of model misspecification.
In all four expenditure models both the need and budget variables have a positive and significant effect on own programme expenditure. In addition, the proxy for need in other programmes is negative and significant in all four cases. In the gastrointestinal expenditure programme the prevalence of lone pensioners households is associated with less NHS expenditure; there might be some unmet need here or perhaps this is a self-selecting group.
The diagnostic statistics suggest that, for all four expenditure models, the proxy for other programme need is endogenous and that the instruments are valid. They also suggest that the instruments are relevant and, with the possible exception of the gastrointestinal expenditure result, there is no evidence that the instruments are weak. The Pesaran–Taylor test suggests that there is no evidence of model misspecification.
The elasticities shown in Table 59 can be used to calculate the cost of a life-year in each programme and these calculations – for both these four programmes as well as for the other six programmes with a mortality-based outcome indicator – are shown in Tables 60 and 61 (the full outcome and expenditure models for the other six programmes with a mortality-based outcome indicator are not shown here).
(A) PBC scenario | (B) PBC description | (C) Spend (£M) 2006/7 | (D) Spend elasticity | (E) (= 0.01 × C × D) change in spend (£M) | (F) Average annual mortality, < 75 years, 2004/5/6 | (G) Outcome elasticity | (H) (= 0.01 × D × F × G) change in annual mortality | (I) (= E/H) cost per life gained (£) | (J) Total life-years lost, < 75 years, 2004/5/6 | (K) (= 0.01 × D × G × J/3) change in annual life-years lost | (L) (= E/K) cost per YLG (£) |
---|---|---|---|---|---|---|---|---|---|---|---|
1 | Cancer | 4122 | 0.542 | 22.34 | 62,259 | 0.351 | 118.44 | 188,625 | 2,221,530 | 1409 | 15,859 |
2 | Circulatory problems | 6161 | 0.694 | 42.76 | 45,504 | 1.441 | 455.06 | 93,959 | 1,463,912 | 4880 | 8762 |
3 | Respiratory problems | 3285 | 0.712 | 23.39 | 11,601 | 2.83 | 233.76 | 100,058 | 321,264 | 2158 | 10,839 |
4 | Gastrointestinal problems | 3700 | 0.65 | 24.05 | 5926 | 2.125 | 81.85 | 293,820 | 328,853 | 1514 | 15,884 |
5 | Big four programmes | 17,268 | 112.54 | 125,290 | 889.12 | 126,573 | 4,335,559 | 9961 | 11,298 | ||
6 | Big four programmes 2005/6 | 17,625 | 141.22 | 125,290 | 909.96 | 155,196 | 4,516,953 | 10,986 | 12,855 | ||
7 | Infectious diseases | 1053 | 0.725 | 7.63 | 2050 | 0.03 | 0.45 | 17,121,951 | 101604 | 7 | 1,036,377 |
8 | Endocrine problems | 1852 | 0.954 | 17.67 | 1690 | 0.965 | 15.56 | 1,135,604 | 60,615 | 186 | 94,985 |
9 | Neurological problems | 2790 | 0.64 | 17.86 | 729 | 0.1 | 0.47 | 38,271,605 | 68,808 | 15 | 1,216,428 |
10 | Genitourinary problems | 3482 | 0.799 | 27.82 | 294 | 0.074 | 0.17 | 160,047,803 | 11,554 | 2 | 12,217,601 |
11 | Trauma and injuries | 2892 | 0.609 | 17.61 | 1037 | 0.527 | 3.33 | 5,291,867 | 30,000 | 32 | 548,767 |
12 | Maternity and neonates | 3574 | 0.601 | 21.48 | 2123 | 0.036 | 0.46 | 46,762,966 | 484,950 | 35 | 614,153 |
13 | Other six programmes | 15,643 | 110.07 | 7923 | 20.43 | 5,387,190 | 757,531 | 277 | 396,796 | ||
14 | Other six programmes 2005/6 | 12,743 | 99.44 | 7923 | 16.26 | 6,115,621 | 751,009 | 337 | 295,074 | ||
15 | All 10 programmes | 32,911 | 0.676 | 222.61 | 133,213 | 909.55 | 244,747 | 5,093,090 | 10,238 | 21,743 | |
16 | All 10 programmes 2005/6 | 30,368 | 0.792 | 240.67 | 133,213 | 926.22 | 259,838 | 5,267,962 | 11,322 | 21,256 | |
Assume zero health gain in the other 13 programmes | |||||||||||
18 | Other 13 programmes | 34,985 | 1.304 | 456.35 | 0.00 | 0 | |||||
19 | Other 13 programmes 2005/6 | 33,942 | 1.186 | 402.43 | 0.00 | 0 | |||||
20 | All 23 programmes | 67,896 | 678.96 | 909.55 | 746,481 | 10,238 | 66,318 | ||||
21 | All 23 programmes 2005/6 | 64,310 | 643.10 | 926.22 | 694,330 | 11,322 | 56,799 |
(A) PBC scenario | (B) PBC description | (C) Spend (£M) 2006/7 | (D) Spend elasticity | (E) (= 0.01 × C × D) change in spend (£M) | (F) Average annual mortality, < 75 years, 2004/5/6 | (G) Outcome elasticity | (H) (= 0.01 × D × F × G) change in annual mortality | (I) (= E/H) cost per life gained (£) | (J) Total life-years lost, < 75 years, 2004/5/6 | (K) (= 0.01 × D × G × J/3) change in annual life-years lost | (L) (= E/K) cost per YLG (£) |
---|---|---|---|---|---|---|---|---|---|---|---|
1 | Cancer | 4122 | 0.542 | 22.34 | 62,259 | 0.351 | 118.44 | 188,625 | 2,221,530 | 1409 | 15,859 |
2 | Circulatory problems | 6161 | 0.694 | 42.76 | 45,504 | 1.441 | 455.06 | 93,959 | 1,463,912 | 4880 | 8762 |
3 | Respiratory problems | 3285 | 0.712 | 23.39 | 11,601 | 2.83 | 233.76 | 100,058 | 321,264 | 2158 | 10,839 |
4 | Gastrointestinal disease | 3700 | 0.65 | 24.05 | 5926 | 2.125 | 81.85 | 293,820 | 328,853 | 1514 | 15,884 |
5 | Big four programmes | 17,268 | 112.54 | 125,290 | 889.12 | 126,573 | 4,335,559 | 9961 | 11,298 | ||
6 | Big four programmes 2005/6 | 17,625 | 141.22 | 125,290 | 909.96 | 155,196 | 4,516,953 | 10,986 | 12,855 | ||
7 | Infectious diseases | 1053 | 0.725 | 7.63 | 2050 | 0.03 | 0.45 | 17,121,951 | 101604 | 7 | 1,036,377 |
8 | Endocrine problems | 1852 | 0.954 | 17.67 | 1690 | 0.965 | 15.56 | 1,135,604 | 60,615 | 186 | 94,985 |
9 | Neurological problems | 2790 | 0.64 | 17.86 | 729 | 0.1 | 0.47 | 38,271,605 | 68,808 | 15 | 1,216,428 |
10 | Genitourinary problems | 3482 | 0.799 | 27.82 | 294 | 0.074 | 0.17 | 160,047,803 | 11,554 | 2 | 12,217,601 |
11 | Trauma and injuries | 2892 | 0.609 | 17.61 | 1037 | 0.527 | 3.33 | 5,291,867 | 30,000 | 32 | 548,767 |
12 | Maternity and neonates | 3574 | 0.601 | 21.48 | 2123 | 0.036 | 0.46 | 46,762,966 | 484,950 | 35 | 614,153 |
13 | Other six programmes | 15,643 | 110.07 | 7923 | 20.43 | 5,387,190 | 757,531 | 277 | 396,796 | ||
14 | Other six programmes 2005/6 | 12,743 | 99.44 | 7923 | 16.26 | 6,115,621 | 751,009 | 337 | 295,074 | ||
15 | All 10 programmes | 32,911 | 0.676 | 222.61 | 133,213 | 909.55 | 244,747 | 5,093,090 | 10,238 | 21,743 | |
16 | All 10 programmes 2005/6 | 30,368 | 0.792 | 240.67 | 133,213 | 926.22 | 259,838 | 5,267,962 | 11,322 | 21,256 | |
Assume zero health gain in PBC 23, and gain in 10 PBCs applies to other 12 PBCs | |||||||||||
17 | PBC 23 | 10,585 | 0.844 | 89.34 | 0.00 | 0.00 | |||||
18 | PBC 23 2005/6 | 8449 | 0.926 | 78.24 | 0.00 | 0.00 | |||||
19 | Other 12 programmes | 24,400 | 367.01 | 1499.56 | 244,747 | 16,880 | 21,743 | ||||
20 | Other 12 programmes 2005/6 | 25,493 | 324.20 | 1247.69 | 259,838 | 15,252 | 21,256 | ||||
21 | All 23 programmes | 67,896 | 678.96 | 2409.11 | 281,830 | 27,118 | 25,038 | ||||
22 | All 23 programmes 2005/6 | 64,310 | 643.10 | 2173.90 | 295,827 | 26,575 | 24,200 |
Table 60 reveals that the cost of a life-year for the big four programmes combined is £11,298. This is remarkably close to the figure obtained using expenditure data for 2005/6, an AREA-based measure of need, and a HCHS MFF (£12,855). The cost of a life-year for all 10 programmes with a mortality-based measure of need is £21,743, which is even closer to the figure obtained using 2005/6 expenditure data (£21,256). If we assume a zero gain in the 13 programmes without a mortality-based indicator then the cost per life-year across all 23 programmes is £66,318 (it is £56,799 for 2005/6 data).
Alternatively, if we assume that PBC 23 generates a zero health gain and that the gain attributable to the remaining 12 programmes is, on average, the same as that attributable to those with a mortality outcome measure, then Table 61 shows that the cost of a life-year across all programmes is £25,038 (it is £24,200 for 2005/6 data).
Model estimation using 2006/7 expenditure data and mortality data for 2004/5/6: CARAN need and two market forces factors
Further discussion by the project team noted that the PB data incorporates all PCT expenditure and that, as there is a separate category for GMS/PMS expenditure (PBC 23a), it seems appropriate that the GMS/PMS MFF should be applied to this category. However, other categories of expenditure exclude GMS/PMS expenditure but incorporate both HCHS and prescribing expenditure. It therefore seems appropriate that a weighted averaged of the HCHS and prescribing MFFs should be applied to these other (non-GMS/PMS) categories of expenditure.
We therefore re-estimated the outcome and expenditure models for those programmes with a mortality-based outcome indicator using the CARAN measure of need and adjusting expenditure for local input prices using the MFFs for HCHS and prescribing services. The outcome and expenditure results for the big four programmes are shown in Table 62 with the relevant outcome and expenditure elasticities again highlighted (the first-stage regressions associated with these results can be found in Table 95 in the Annex).
PBC scenario | PBC 2, cancer, 2006/7 | PBC 10, circulation, 2006/7 | PBC 11, respiratory, 2006/7 | PBC 13, gastrointestinal, 2006/7 | ||||
---|---|---|---|---|---|---|---|---|
(1) Outcome model | (2) Spend model | (3) Outcome model | (4) Spend model | (5) Outcome model | (6) Spend model | (7) Outcome model | (8) Spend model | |
Own programme spend per head | −0.337*** | −1.447*** | −2.839*** | −2.137*** | ||||
[0.104] | [0.220] | [0.772] | [0.569] | |||||
Need CARAN per head | 0.974*** | 1.772*** | 2.860*** | 2.191*** | 1.782 | 1.375*** | 4.657*** | 2.697*** |
[0.110] | [0.287] | [0.257] | [0.355] | [1.198] | [0.297] | [0.716] | [0.676] | |
NeedCARAN per head squared | 1.314*** | |||||||
[0.352] | ||||||||
All-cause SYLLR excluding cancer | −0.951*** | |||||||
[0.180] | ||||||||
PCT budget per head | 0.548** | 0.701** | 0.718*** | 0.655** | ||||
[0.242] | [0.292] | [0.253] | [0.289] | |||||
All-cause SYLLR excluding circulatory disease | −1.778*** | |||||||
[0.336] | ||||||||
Permanently sick aged 16–74 years | 1.385*** | |||||||
[0.405] | ||||||||
All-cause SYLLR excluding respiratory problems | −0.663** | |||||||
[0.288] | ||||||||
All-cause SYLLR excluding gastrointestinal problems | −1.847*** | |||||||
[0.609] | ||||||||
Lone pensioner households | −0.590** | |||||||
[0.295] | ||||||||
Constant | 6.506*** | 5.881*** | 11.567*** | 10.227*** | 19.047*** | 3.032 | 12.260*** | 9.664*** |
[0.455] | [1.778] | [1.058] | [2.387] | [3.877] | [1.977] | [2.441] | [3.046] | |
Endogeneity test statistic | 15.173 | 20.248 | 43.405 | 25.854 | 27.876 | 7.863 | 21.853 | 13.607 |
Endogeneity p-value | 9.81e-05 | 6.80e-06 | 0 | 3.68e-07 | 1.29e-07 | 0.00505 | 2.94e-06 | 0.000225 |
Hansen–Sargan test statistic | 0.00201 | 0.306 | 1.440 | 0.530 | 1.912 | 0.344 | 1.011 | 0.0294 |
Hansen–Sargan p-value | 0.964 | 0.580 | 0.696 | 0.467 | 0.167 | 0.557 | 0.315 | 0.864 |
Shea’s partial R2 | 0.164 | 0.445 | 0.300 | 0.296 | 0.0793 | 0.366 | 0.140 | 0.206 |
Kleibergen–Paap LM test statistic | 17.85 | 42.38 | 32.37 | 32.70 | 10.42 | 36.33 | 14.86 | 19.07 |
Kleibergen–Paap p-value | 0.000133 | 6.28e-10 | 1.61e-06 | 7.93e-08 | 0.00545 | 1.29e-08 | 0.000592 | 7.22e-05 |
Kleibergen–Paap F-statistic | 13.28 | 48.32 | 17.14 | 25.71 | 7.390 | 24.32 | 11.63 | 8.660 |
Pesaran–Taylor reset statistic | 0.00226 | 0.00178 | 0.0945 | 0.0215 | 3.139 | 1.908 | 0.266 | 0.0605 |
Pesaran–Taylor p-value | 0.962 | 0.966 | 0.759 | 0.883 | 0.0764 | 0.167 | 0.606 | 0.806 |
In all four outcome models expenditure has a significant negative effect on mortality and, in three of these, the all service measure of need has a significant positive effect. In the respiratory outcome model, where the all service need term is not significant, there is another indicator of need – the proportion of the population that are permanently sick – and this is both positive and statistically significant. The all service measure of need squared is also positive and significant in the cancer outcome equation. The diagnostic statistics suggest that, in all four cases, own programme expenditure is endogenous and that the instruments are valid. They also suggest that the instruments are relevant. There is a little evidence that the instruments are weak in one of the four outcome results, namely the respiratory model. Re-estimation of the latter model but without the least significant instrument generates a coefficient of −3.507 on expenditure and the Kleibergen–Paap F-statistic now exceeds 10 (it is 11.799). The Pesaran–Taylor test suggests that there is no evidence of model misspecification in any of the outcome models.
In all four expenditure models both the need and budget variables have a positive and significant effect on own programme expenditure. In addition, the proxy for need in other programmes is negative and significant in all four cases. In the gastrointestinal expenditure programme the prevalence of lone pensioners households is associated with less NHS expenditure; there might be some unmet need here or perhaps this is self-selecting group.
The diagnostic statistics suggest that, for all four expenditure models, expenditure is endogenous and the instruments are valid. They also suggest that the instruments are relevant and, with the possible exception of the gastrointestinal expenditure result, there is no evidence that the instruments are weak. Re-estimation of the gastrointestinal expenditure model without the least significant instrument generates a coefficient of 0.667 on the budget variable and the Kleibergen–Paap F-statistic now exceeds 10 (it is 16.871). The Pesaran–Taylor test suggests that there is no evidence of model misspecification.
The outcome and expenditure elasticities are little changed from those presented in Table 59 and, like those, these new elasticities can be used to calculate the cost of a life-year in each programme. These calculations – for both these four programmes as well as for the other six programmes with a mortality-based outcome indicator – are shown in Tables 63 and 64 (the full outcome and expenditure models for the other six programmes with a mortality-based outcome indicator are not shown here).
(A) PBC scenario | (B) PBC description | (C) Spend (£M) 2006/7 | (D) Spend elasticity | (E) (= 0.01 × C × D) change in spend (£M) | (F) Average annual mortality, < 75 years, 2004/5/6 | (G) Outcome elasticity | (H) (= 0.01 × D × F × G) change in annual mortality | (I) (= E/H) cost per life gained (£) | (J) Total life-years lost, < 75 years, 2004/5/6 | (K) (= 0.01 × D × G × J/3) change in annual life-years lost | (L) (= E/K) cost per YLG (£) |
---|---|---|---|---|---|---|---|---|---|---|---|
1 | Cancer | 4122 | 0.548 | 22.59 | 62,259 | 0.337 | 114.98 | 196,461 | 2,221,530 | 1368 | 16,518 |
2 | Circulatory problems | 6161 | 0.701 | 43.19 | 45,504 | 1.447 | 461.57 | 93,569 | 1,463,912 | 4950 | 8725 |
3 | Respiratory problems | 3285 | 0.718 | 23.59 | 11,601 | 3.507 | 292.12 | 80,743 | 321,264 | 2697 | 8747 |
4 | Gastrointestinal problems | 3700 | 0.667 | 24.68 | 5926 | 2.137 | 84.47 | 292,170 | 328,853 | 1562 | 15,795 |
5 | Big four programmes | 17,268 | 114.04 | 125,290 | 953.13 | 119,650 | 4,335,559 | 10,576 | 10,783 | ||
6 | Big four programmes 2005/6 | 17,625 | 141.22 | 125,290 | 909.96 | 155,196 | 4,516,953 | 10,986 | 12,855 | ||
7 | Infectious diseases | 1053 | 0.731 | 7.70 | 2050 | 0.03 | 0.45 | 17,121,951 | 101,604 | 7 | 1,036,377 |
8 | Endocrine problems | 1852 | 0.966 | 17.89 | 1690 | 0.812 | 13.26 | 1,349,579 | 60,615 | 158 | 112,882 |
9 | Neurological problems | 2790 | 0.648 | 18.08 | 729 | 0.098 | 0.46 | 39,052,658 | 68,808 | 15 | 1,241,253 |
10 | Genitourinary problems | 3482 | 0.837 | 29.14 | 294 | 0.073 | 0.18 | 162,240,239 | 11,554 | 2 | 12,384,965 |
11 | Trauma and injuries | 2892 | 0.617 | 17.84 | 1037 | 0.527 | 3.37 | 5,291,867 | 30,000 | 33 | 548,767 |
12 | Maternity and neonates | 3574 | 0.601 | 21.48 | 2123 | 0.035 | 0.45 | 48,099,051 | 484,950 | 34 | 631,700 |
13 | Other six programmes | 15,643 | 112.13 | 7923 | 18.17 | 6,172,491 | 757,531 | 249 | 449,706 | ||
14 | Other six programmes 2005/6 | 12,743 | 99.44 | 7923 | 16.26 | 6,115,621 | 751,009 | 337 | 295,074 | ||
15 | All 10 programmes | 32,911 | 0.687 | 226.18 | 133,213 | 971.30 | 232,861 | 5,093,090 | 10,826 | 20,893 | |
16 | All 10 programmes 2005/6 | 30,368 | 0.792 | 240.67 | 133,213 | 926.22 | 259,838 | 5,267,962 | 11,322 | 21,256 | |
Assume zero health gain in the other 13 programmes | |||||||||||
18 | Other 13 programmes | 34,985 | 1.294 | 452.78 | 0.00 | 0 | |||||
19 | Other 13 programmes 2005/6 | 33,942 | 1.186 | 402.43 | 0.00 | 0 | |||||
20 | All 23 programmes | 67,896 | 678.96 | 971.30 | 699,024 | 10,826 | 62,718 | ||||
21 | All 23 programmes 2005/6 | 64,310 | 643.10 | 926.22 | 694,330 | 11,322 | 56,799 |
(A) PBC scenario | (B) PBC description | (C) Spend (£M) 2006/7 | (D) Spend elasticity | (E) (= 0.01 × C × D) change in spend (£M) | (F) Average annual mortality, < 75 years, 2004/5/6 | (G) Outcome elasticity | (H) (= 0.01 × D × F × G) change in annual mortality | (I) (= E/H) cost per life gained (£) | (J) Total life-years lost, < 75 years, 2004/5/6 | (K) (= 0.01 × D × G × J/3) change in annual life-years lost | (L) (= E/K) cost per YLG (£) |
---|---|---|---|---|---|---|---|---|---|---|---|
1 | Cancer | 4122 | 0.548 | 22.59 | 62,259 | 0.337 | 114.98 | 196,461 | 2,221,530 | 1368 | 16,518 |
2 | Circulatory problems | 6161 | 0.701 | 43.19 | 45,504 | 1.447 | 461.57 | 93,569 | 1,463,912 | 4950 | 8725 |
3 | Respiratory problems | 3285 | 0.718 | 23.59 | 11,601 | 3.507 | 292.12 | 80,743 | 321,264 | 2697 | 8747 |
4 | Gastrointestinal problems | 3700 | 0.667 | 24.68 | 5926 | 2.137 | 84.47 | 292,170 | 328,853 | 1562 | 15,795 |
5 | Big four programmes | 17,268 | 114.04 | 125,290 | 953.13 | 119,650 | 4,335,559 | 10,576 | 10,783 | ||
6 | Big four programmes 2005/6 | 17,625 | 141.22 | 125,290 | 909.96 | 155,196 | 4,516,953 | 10,986 | 12,855 | ||
7 | Infectious diseases | 1053 | 0.731 | 7.70 | 2050 | 0.03 | 0.45 | 17,121,951 | 101,604 | 7 | 1,036,377 |
8 | Endocrine problems | 1852 | 0.966 | 17.89 | 1690 | 0.812 | 13.26 | 1,349,579 | 60,615 | 158 | 112,882 |
9 | Neurological problems | 2790 | 0.648 | 18.08 | 729 | 0.098 | 0.46 | 39,052,658 | 68,808 | 15 | 1,241,253 |
10 | Genitourinary problems | 3482 | 0.837 | 29.14 | 294 | 0.073 | 0.18 | 162,240,239 | 11,554 | 2 | 12,384,965 |
11 | Trauma and injuries | 2892 | 0.617 | 17.84 | 1037 | 0.527 | 3.37 | 5,291,867 | 30,000 | 33 | 548,767 |
12 | Maternity and neonates | 3574 | 0.601 | 21.48 | 2123 | 0.035 | 0.45 | 48,099,051 | 484,950 | 34 | 631,700 |
13 | Other six programmes | 15,643 | 112..13 | 7923 | 18.17 | 6,172,491 | 757,531 | 249 | 449,706 | ||
14 | Other six programmes 2005/6 | 12,743 | 99.44 | 7923 | 16.26 | 6,115,621 | 751,009 | 337 | 295,074 | ||
15 | All 10 programmes | 32,911 | 0.687 | 226.18 | 133,213 | 971.30 | 232,861 | 5,093,090 | 10,826 | 20,893 | |
16 | All 10 programmes 2005/6 | 30,368 | 0.792 | 240.67 | 133,213 | 926.22 | 259,838 | 5,267,962 | 11,322 | 21,256 | |
Assume zero health gain in PBC 23, and gain in 10 PBCs applies to other 12 PBCs | |||||||||||
17 | PBC 23 | 10,585 | 0.759 | 80.34 | 0.00 | 0.00 | |||||
18 | PBC 23 2005/6 | 8449 | 0.926 | 78.24 | 0.00 | 0.00 | |||||
19 | Other 12 programmes | 24,400 | 372.44 | 1,599.42 | 232,861 | 17,826 | 20,893 | ||||
20 | Other 12 programmes 2005/6 | 25,493 | 324.20 | 1,247.69 | 259,838 | 15,252 | 21,256 | ||||
21 | All 23 programmes | 67,896 | 678.96 | 2,570.72 | 264,113 | 28,652 | 23,697 | ||||
22 | All 23 programmes 2005/6 | 64,310 | 643.10 | 2,173.90 | 295,827 | 26,575 | 24,200 |
The figures for 2006/7 in Table 63 (which incorporate two MFFs) can be compared with those for 2006/7 in Table 60 (which incorporate three MFFs). Table 63 reveals that the use of a different MFF has little impact on the cost of a life-year for the big four PBCs (it was £11,298, it is now £10,783) as well as on the cost of a life-year for all programmes with a mortality outcome measure (it was £21,743, it is now £20,893).
In addition, Table 64 shows that if we assume that PBC 23 generates a zero health gain and that the gain attributable to the remaining 12 programmes is, on average, the same as that attributable to those with a mortality outcome measure, then the cost of a life-year across all programmes is now £23,697 (it was £25,038 for 2006/7 in Table 61).
The figures in Table 64 also reveal that the cost of a life-year in 2006/7 for all programmes (£23,697) is little changed from the comparable figure for 2005/6 (£24,200).
Model estimation using 2006/7 expenditure data and mortality data for 2006/7/8: CARAN need and two market forces factors
One shortcoming with the models presented above is that they relate expenditure in 2006/7 to mortality in the same period and in the two previous periods (i.e. in 2004, 2005 and 2006). The difficulty with this is that one would expect expenditure in year t to affect mortality in year t and possibly subsequent years (t + 1, t + 2, etc.) but not mortality in previous years (t – 1, t – 2, etc.). However, if we assume that PCTs have reached some sort of equilibrium in the expenditure choices they make and the outcomes they secure, so that expenditure levels change relatively little from one year to the next, then mortality over the 3-year period t, t – 1 and t – 2 might be a good proxy for mortality in t, t + 1 and t + 2. Indeed, this is probably not an unreasonable assumption given the relatively slow pace at which both types of variable change.
Although this assumption of equilibrium is not an unreasonable one, it is one that ideally we would like to be able to drop. Fortunately, with the recent availability of more up-to-date mortality data, we have the opportunity to relate expenditure in 2006 to mortality in the same year and in the two following years (i.e. in 2006, 2007 and 2008). ac Thus, the models reported in Table 62 were re-estimated replacing the mortality rate for 2004/5/6 with that for 2006/7/8. The outcome and expenditure results for the big four programmes are shown in Table 65 with the relevant outcome and expenditure elasticities again highlighted (the first-stage regressions associated with these results can be found in Table 96 in the Annex). These elasticities are similar to those presented previously in Table 62 but there are some changes (e.g. the outcome elasticity in the respiratory outcome equation falls from −2.839 to −2.029).
Variable | PBC 2 cancer | PBC 10 circulation | PBC 11 respiratory | PBC 13 gastrointestinal | ||||
---|---|---|---|---|---|---|---|---|
(1) Outcome model | (2) Spend model | (3) Outcome model | (4) Spend model | (5) Outcome model | (6) Spend model | (7) Outcome model | (8) Spend model | |
Own programme spend per head | −0.342*** | −1.434*** | −2.029*** | −1.536*** | ||||
[0.099] | [0.218] | [0.636] | [0.468] | |||||
Need CARAN per head | 0.995*** | 1.626*** | 2.860*** | 2.306*** | 2.696*** | 1.449*** | 4.160*** | 2.040*** |
[0.106] | [0.343] | [0.252] | [0.372] | [1.044] | [0.331] | [0.577] | [0.378] | |
Need CARAN per head squared | 1.163*** | 2.451 | ||||||
[0.348] | [1.561] | |||||||
SYLLR all deaths excluding cancer | −0.855*** | |||||||
[0.191] | ||||||||
PCT budget per head | 0.465 | 0.540* | 0.679*** | 0.446* | ||||
[0.300] | [0.299] | [0.251] | [0.263] | |||||
SYLLR all deaths excluding circulatory | −1.666*** | |||||||
[0.295] | ||||||||
Permanently sick | 0.759** | |||||||
[0.367] | ||||||||
SYLLR all deaths excluding respiratory | −0.672** | |||||||
[0.305] | ||||||||
SYLLR all deaths excluding gastrointestinal | −1.206*** | |||||||
[0.314] | ||||||||
Lone pensioner households | ||||||||
Constant | 6.501*** | 5.913*** | 11.413*** | 10.696*** | 13.756*** | 3.346 | 9.719*** | 8.370*** |
[0.436] | [2.815] | [1.046] | [2.379] | [3.279] | [2.075] | [2.009] | [2.299] | |
Endogeneity test statistic | 13.695 | 19.421 | 42.548 | 24.461 | 17.687 | 8.439 | 16.373 | 15.211 |
Endogeneity p-value | 0.000215 | 1.05e-05 | 6.90e-11 | 7.58e-07 | 2.60e-05 | 0.00367 | 5.20e-05 | 9.61e-05 |
Hansen–Sargan test statistic | 0.685 | 0.021 | 0.949 | 1.262 | 1.462 | 0.302 | 2.761 | 0.0164 |
Hansen–Sargan p-value | 0.408 | 0.084 | 0.814 | 0.261 | 0.227 | 0.583 | 0.0966 | 0.0898 |
Shea’s partial R2 | 0.164 | 0.445 | 0.300 | 0.296 | 0.0785 | 0.327 | 0.140 | 0.356 |
Kleibergen–Paap LM test statistic | 17.85 | 41.88 | 32.37 | 32.02 | 10.02 | 34.98 | 14.86 | 35.72 |
Kleibergen–Paap p-value | 0.000133 | 8.04e-10 | 1.61e-06 | 1.11e-07 | 0.00666 | 2.54e-08 | 0.000592 | 1.75e-08 |
Kleibergen–Paap F-statistic | 13.28 | 56.69 | 17.14 | 31.84 | 7.022 | 20.94 | 11.63 | 22.40 |
Pesaran–Taylor reset statistic | 0.00537 | 0.18 | 0.136 | 0.00349 | 0.0120 | 1.497 | 1.669 | 0.007 |
Pesaran–Taylor p-value | 0.942 | 0.668 | 0.712 | 0.953 | 0.913 | 0.221 | 0.196 | 0.935 |
In all four outcome models expenditure has a significant negative effect on mortality and the all service measure of need has a significant positive effect. The all service measure of need squared is also positive and significant in the cancer outcome equation. In the respiratory outcome model, there is an additional indicator of need – the proportion of the population that are permanently sick – and this is both positive and statistically significant. The diagnostic statistics suggest that, in all four cases, own programme expenditure is endogenous and that the instruments are valid. They also suggest that the instruments are relevant. There is no evidence that the instruments are weak in three of the four outcome results. The Pesaran–Taylor test suggests that there is no evidence of model misspecification.
However, the Kleibergen–Paap F-statistic for the respiratory disease outcome model is 7.022 and this is less than the ‘critical’ target of 10.0. This indicates that the instruments may be weak. However, if we re-estimate this model having dropped the least significant instrument, the coefficient on own programme expenditure is now −2.622 and this is significant at the 1% level. Moreover, there is now no evidence of weak instruments (the Kleibergen–Paap F-statistic is 11.025) and it is this coefficient that we use for the respiratory outcome model in the cost of a life-year calculations below.
In three of the four expenditure models both the need and budget variables have a positive and significant effect on own programme expenditure. In addition, the proxy for need in other programmes is negative and significant in all four cases. The diagnostic statistics suggest that, for all four expenditure models, expenditure is endogenous and the instruments are valid. They also suggest that the instruments are relevant and there is no evidence that the instruments are weak. The Pesaran–Taylor test suggests that there is no evidence of model misspecification.
The outcome and expenditure elasticities presented in Table 65 can be used to calculate the cost of a life-year in each programme. These calculations – for both the big four programmes as well as for the other six programmes with mortality-based outcome indicators – are shown in Table 66. They show that the use of a more appropriate measure of mortality (i.e. for 2006/7/8 rather than for 2004/5/6) slightly increases the cost of a life-year for the big four PBCs (from £10,783 to £12,333) as well as for all 10 programmes with a mortality outcome measure (from £20,893 to £23,780).
(A) PBC scenario | (B) PBC description | (C) Spend (£M) 2006/7 | (D) Spend elasticity | (E) (= 0.01 × C × D) change in spend (£M) | (F) Annual mortality, < 75 years, 2006/7/8 | (G) Outcome elasticity (without negative sign) | (H) (= 0.01 × D × F × G) change in annual mortality | (I) (= E/H) cost per life gained (£) | (J) Total life-years lost, < 75 years, 2006/7/8 | (K) (= 0.01 × D × G × J/3) change in annual life-years lost | (L) (= E/K) cost per YLG (£) |
---|---|---|---|---|---|---|---|---|---|---|---|
1 | Cancer | 4122 | 0.465 | 19.17 | 61,961 | 0.342 | 98.54 | 194,520 | 2,207,021 | 1170 | 16,383 |
2 | Circulatory problems | 6161 | 0.540 | 33.27 | 41,106 | 1.434 | 318.31 | 104,519 | 1,361,634 | 3515 | 9466 |
3 | Respiratory problems | 3285 | 0.679 | 22.31 | 11,574 | 2.622 | 206.06 | 108,248 | 324,223 | 1924 | 11,593 |
4 | Gastrointestinal problems | 3700 | 0.446 | 16.50 | 6160 | 1.536 | 42.20 | 391,048 | 345,908 | 790 | 20,892 |
Big four programmes summary | |||||||||||
5 | Spend 2006 and mortality 2006/7/8 | 17,268 | 91.24 | 120,801 | 665.10 | 137,188 | 4,238,786 | 7399 | 12,333 | ||
6a | Spend 2006 and mortality 2004/5/6 | 17,268 | 114.04 | 125,290 | 953.13 | 119,650 | 4,335,559 | 10,576 | 10,783 | ||
7b | Spend 2005 and mortality 2002/3/4 | 17,625 | 141.22 | 125,290 | 909.96 | 155,196 | 4,516,953 | 10,986 | 12,855 | ||
8 | Infectious diseases | 1053 | 0.792 | 8.34 | 2050 | 0.047 | 0.76 | 10,928,905 | 106,552 | 13 | 630,798 |
9 | Endocrine problems | 1852 | 0.953 | 17.65 | 1542 | 0.842 | 12.37 | 1,426,410 | 57,672 | 154 | 114,416 |
10 | Neurological problems | 2790 | 0.616 | 17.19 | 727 | 0.112 | 0.50 | 34,265,082 | 66,137 | 15 | 1,129,960 |
11 | Genitourinary problems | 3482 | 0.912 | 31.76 | 294 | 0.051 | 0.14 | 232,226,224 | 10,030 | 2 | 20,421,090 |
12 | Trauma and injuries | 2892 | 0.358 | 10.35 | 1037 | 0 | 0.00 | N/A | 30,000 | 0 | N/A |
13 | Maternity and neonates | 3574 | 0.224 | 8.01 | 2189 | 0.482 | 2.36 | 3,387,363 | 492,600 | 177 | 45,158 |
Other six programmes summary | |||||||||||
14 | Spend 2006 and mortality 2006/7/8 | 15,643 | 93.29 | 7839 | 16.14 | 5,780,723 | 762,991 | 362 | 258,046 | ||
15a | Spend 2006 and mortality 2004/5/6 | 15,643 | 112.13 | 7923 | 18.17 | 6,172,491 | 757,531 | 249 | 449,706 | ||
16b | Spend 2005 and mortality 2002/3/4 | 12,743 | 99.44 | 7923 | 16.26 | 6,115,621 | 751,009 | 337 | 295,074 | ||
All 10 programmes summary | |||||||||||
17 | Spend 2006 and mortality 2006/7/8 | 32,911 | 0.561 | 184.53 | 128,640 | 681.24 | 270,881 | 5,001,777 | 7760 | 23,780 | |
18a | Spend 2006 and mortality 2004/5/6 | 32,911 | 0.687 | 226.18 | 133,213 | 971.30 | 232,861 | 5,093,090 | 10,826 | 20,893 | |
19b | Spend 2005 and mortality 2002/3/4 | 30,368 | 0.792 | 240.67 | 133,213 | 926.22 | 259,838 | 5,267,962 | 11,322 | 21,256 | |
Assume zero health gain in the other 13 programmes: other 13 programmes summary | |||||||||||
20 | Spend 2006 and mortality 2006/7/8 | 34,985 | 1.413 | 494.43 | 0.00 | 0 | |||||
21a | Spend 2006 and mortality 2004/5/6 | 34,985 | 1.294 | 452.78 | 0.00 | 0 | |||||
22b | Spend 2005 and mortality 2002/3/4 | 33,942 | 1.186 | 402.43 | 0.00 | 0 | |||||
All 23 programmes | |||||||||||
23 | Spend 2006 and mortality 2006/7/8 | 67,896 | 678.96 | 681.24 | 996,655 | 7760 | 87,494 | ||||
24a | Spend 2006 and mortality 2004/5/6 | 67,896 | 678.96 | 971.30 | 699,024 | 10,826 | 62,718 | ||||
25b | Spend 2005 and mortality 2002/3/4 | 64,310 | 643.10 | 926.22 | 694,330 | 11,322 | 56,799 |
In addition, Table 67 shows that if we assume that PBC 23 generates a zero health gain and that the gain attributable to the remaining 12 programmes is, on average, the same as that attributable to those with a mortality outcome measure, then the cost of a life-year across all programmes is now £26,876 (it was £23,697 using mortality for 2004/5/6).
(A) PBC scenario | (B) PBC description | (C) Spend (£M) 2006/7 | (D) Spend elasticity | (E) (= 0.01 × C × D) change in spend (£M) | (F) Annual mortality, < 75 years, 2006/7/8 | (G) Outcome elasticity (without negative sign) | (H) (= 0.01 × D × F × G) change in annual mortality | (I) (= E/H) cost per life gained (£) | (J) Total life-years lost, < 75 years, 2006/7/8 | (K) (= 0.01 × D× G × J/3) change in annual life-years lost | (L) (= E/K) cost per YLG (£) |
---|---|---|---|---|---|---|---|---|---|---|---|
1 | Cancer | 4122 | 0.465 | 19.17 | 61,961 | 0.342 | 98.54 | 194,520 | 2,207,021 | 1170 | 16,383 |
2 | Circulatory problems | 6161 | 0.540 | 33.27 | 41,106 | 1.434 | 318.31 | 104,519 | 1,361,634 | 3515 | 9466 |
3 | Respiratory problems | 3285 | 0.679 | 22.31 | 11,574 | 2.622 | 206.06 | 108,248 | 324,223 | 1924 | 11,593 |
4 | Gastrointestinal problems | 3700 | 0.446 | 16.50 | 6160 | 1.536 | 42.20 | 391,048 | 345,908 | 790 | 20,892 |
Big four programmes summary | |||||||||||
5 | Spend 2006 and mortality 2006/7/8 | 17,268 | 91.24 | 120,801 | 665.10 | 137,188 | 4,238,786 | 7399 | 12,333 | ||
6a | Spend 2006 and mortality 2004/5/6 | 17,268 | 114.04 | 125,290 | 953.13 | 119,650 | 4,335,559 | 10,576 | 10,783 | ||
7b | Spend 2005 and mortality 2002/3/4 | 17,625 | 141.22 | 125,290 | 909.96 | 155,196 | 4,516,953 | 10,986 | 12,855 | ||
8 | Infectious diseases | 1053 | 0.792 | 8.34 | 2050 | 0.047 | 0.76 | 10,928,905 | 106,552 | 13 | 630,798 |
9 | Endocrine problems | 1852 | 0.953 | 17.65 | 1542 | 0.842 | 12.37 | 1,426,410 | 57,672 | 154 | 114,416 |
10 | Neurological problems | 2790 | 0.616 | 17.19 | 727 | 0.112 | 0.50 | 34,265,082 | 66,137 | 15 | 1,129,960 |
11 | Genitourinary problems | 3482 | 0.912 | 31.76 | 294 | 0.051 | 0.14 | 232,226,224 | 10,030 | 2 | 20,421,090 |
12 | Trauma and injuries | 2892 | 0.358 | 10.35 | 1037 | 0 | 0.00 | N/A | 30,000 | 0 | N/A |
13 | Maternity and neonates | 3574 | 0.224 | 8.01 | 2189 | 0.482 | 2.36 | 3,387,363 | 492,600 | 177 | 45,158 |
Other six programmes summary | |||||||||||
14 | Spend 2006 and mortality 2006/7/8 | 15,643 | 93.29 | 7839 | 16.14 | 5,780,723 | 762,991 | 362 | 258,046 | ||
15a | Spend 2006 and mortality 2004/5/6 | 15,643 | 112.13 | 7923 | 18.17 | 6,172,491 | 757,531 | 249 | 449,706 | ||
16b | Spend 2005 and mortality 2002/3/4 | 12,743 | 99.44 | 7923 | 16.26 | 6,115,621 | 751,009 | 337 | 295,074 | ||
All 10 programmes summary | |||||||||||
17 | Spend 2006 and mortality 2006/7/8 | 32,911 | 0.561 | 184.53 | 128,640 | 681.24 | 270,881 | 5,001,777 | 7760 | 23,780 | |
18a | Spend 2006 and mortality 2004/5/6 | 32,911 | 0.687 | 226.18 | 133,213 | 971.30 | 232,861 | 5,093,090 | 10,826 | 20,893 | |
19b | Spend 2005 and mortality 2002/3/4 | 30,368 | 0.792 | 240.67 | 133,213 | 926.22 | 259,838 | 5,267,962 | 11,322 | 21,256 | |
Other 13 PBCs? Assume zero health gain in PBC 23 . . . | |||||||||||
20 | PBC 23: spend 2006 and mortality 2006/7/8 | 10,585 | 0.739 | 78.22 | 0.00 | 0.00 | |||||
21 | PBC 23: spend 2006 and mortality 2004/5/6 | 10,585 | 0.759 | 80.34 | 0.00 | 0.00 | |||||
22 | PBC 23: spend 2005 and mortality 2002/3/4 | 8449 | 0.926 | 78.24 | 0.00 | 0.00 | |||||
. . . and that the gain in 10 PBCs (see above) applies to the remaining 12 PBCs | |||||||||||
23 | 12 PBCs: spend 2006 and mortality 2006/7/8 | 24,400 | 416.20 | 1536.48 | 270,881 | 17,502 | 23,780 | ||||
24a | 12 PBCs: spend 2006 and mortality 2004/5/6 | 24,400 | 372.44 | 1599.42 | 232,861 | 17,826 | 20,893 | ||||
25b | 12 PBCs: spend 2005 and mortality 2002/3/4 | 25,493 | 324.20 | 1247.69 | 259,838 | 15,252 | 21,256 | ||||
All 23 programmes | |||||||||||
26 | 23 PBCs: spend 2006 and mortality 2006/7/8 | 67,896 | 678.96 | 2217.72 | 306,153 | 25,262 | 26,876 | ||||
27a | All 23 PBCs: spend 2006 and mortality 2004/5/6 | 67,896 | 678.96 | 2570.72 | 264,113 | 28,652 | 23,697 | ||||
28b | All 23 PBCs: spend 2005 and mortality 2002/3/4 | 64,310 | 643.10 | 2173.90 | 295,827 | 26,575 | 24,200 |
Adjusting the cost of life (year) estimates for the mismatch in the ICD-10 coverage of the expenditure and the mortality data
The cost of a life (year) estimates presented in Tables 66 and 67 assume a 1% increase in each PCT’s budget and are calculated as:
and
Thus, an integral part of the calculation of the cost of a life (year) is the annual mortality (life-years lost) figure associated with a particular programme. Ideally, the ICD-10 coverage of the expenditure data should coincide with that of the mortality data but, as we know from Table 37, the ICD-10 coverage of the mortality data typically falls short of that for the expenditure data. Unless we adjust the annual mortality figure so that its ICD-10 coverage approximates that of the expenditure data, our cost of life (year) estimates will usually be too large because they will usually underestimate the mortality gain.
Table 68 reproduces Table 66 but incorporates this ICD-10 coverage adjustment [see columns (J) and (O) in Table 68]. This adjustment reduces the cost of a life-year:
-
for the big four programmes from £12,333 to £10,604
-
for the 10 programmes with a mortality-based outcome indicator from £23,780 to £19,965
-
for all programmes assuming a zero gain for the 13 PBCs without an outcome indicator from £87,494 to £73,457.
(A) PBC scenario | (B) PBC description | (C) Spend (£M) 2006/7 | (D) Spend elasticity | (E) (= 0.01 × C × D) change in spend (£M) | (F)Annual mortality, < 75 years, 2006/7/8 | (G) Outcome elasticity (without negative sign) | (H) (= 0.01 × D × F × G) change in annual mortality | (I) (= E/H) cost per life gained (£) | (J) Coverage of mortality data relative to spend data | (K) (= H/J) change in annual mortality adjusted for coverage | (L) (= E/K) cost per life gained (£) adjusted for coverage | (M) Total life-years lost, < 75 years, 2006/7/8 | (N) (= 0.01 × D × G × M/3) change in annual life-years lost | (O) Coverage of mortality data relative to spend data | (P) (= N/O) change in annual life-years lost adjusted for YLL | (Q) (= E/N) cost per YLG (£) | (R) (= E/P)Cost per YLG adjusted for YLL coverage (£) |
---|---|---|---|---|---|---|---|---|---|---|---|---|---|---|---|---|---|
1 | Cancer | 4122 | 0.465 | 19.17 | 61,961 | 0.342 | 98.54 | 194,520 | 0.984 | 100.14 | 191,407 | 2,207,021 | 1170 | 0.984 | 1189 | 16,383 | 16,121 |
2 | Circulatory problems | 6161 | 0.540 | 33.27 | 41,106 | 1.434 | 318.31 | 104,519 | 0.992 | 320.88 | 103,683 | 1,361,634 | 3515 | 0.992 | 3543 | 9466 | 9390 |
3 | Respiratory problems | 3285 | 0.679 | 22.31 | 11,574 | 2.622 | 206.06 | 108,248 | 0.773 | 262.57 | 83,676 | 324,223 | 1924 | 0.773 | 2489 | 11,593 | 8961 |
4 | Gastrointestinal problems | 3700 | 0.446 | 16.50 | 6160 | 1.536 | 42.20 | 391,048 | 0.571 | 73.90 | 223,288 | 345,908 | 790 | 0.571 | 1383 | 20,892 | 11,929 |
Big four programmes summary | |||||||||||||||||
5 | Spend 2006 and mortality 2006/7/8 | 17,268 | 91.24 | 120,801 | 665.10 | 137,188 | 761.49 | 119,823 | 4,238,786 | 7399 | 8604 | 12,333 | 10,604 | ||||
6 | Spend 2006 and mortality 2004/5/6 | 17,268 | 114.04 | 125,290 | 953.13 | 119,650 | 4,335,559 | 10,576 | 10,783 | ||||||||
7 | Spend 2005 and mortality 2002/3/4 | 17,625 | 141.22 | 125,290 | 909.96 | 155,196 | 4,516,953 | 10,986 | 12,855 | ||||||||
8 | Infectious diseases | 1053 | 0.792 | 8.34 | 2050 | 0.047 | 0.76 | 10,928,905 | 1.000 | 0.76 | 10,928,905 | 106,552 | 13 | 1.000 | 13 | 630,798 | 630,798 |
9 | Endocrine problems | 1852 | 0.953 | 17.65 | 1542 | 0.842 | 12.37 | 1,426,410 | 0.634 | 19.52 | 904,344 | 57,672 | 154 | 0.634 | 243 | 114,416 | 72,539 |
10 | Neurological problems | 2790 | 0.616 | 17.19 | 727 | 0.112 | 0.50 | 34,265,082 | 0.136 | 3.69 | 4,660,051 | 66,137 | 15 | 0.136 | 112 | 1,129,960 | 153,675 |
11 | Genitourinary problems | 3482 | 0.912 | 31.76 | 294 | 0.051 | 0.14 | 232,226,224 | 0.172 | 0.80 | 39,942,910 | 10,030 | 2 | 0.172 | 9 | 20,421,090 | 3,512,427 |
12 | Trauma and injuries | 2892 | 0.358 | 10.35 | 1037 | 0 | 0.00 | N/A | 0.175 | 0.00 | N/A | 30,000 | 0 | 0.175 | 0 | N/A | N/A |
13 | Maternity and neonates | 3574 | 0.224 | 8.01 | 2189 | 0.482 | 2.36 | 3,387,363 | 8.213 | 0.29 | 27,820,413 | 492,600 | 177 | 0.679 | 261 | 45,158 | 30,662 |
Other six programmes summary | |||||||||||||||||
14 | Spend 2006 and mortality 2006/7/8 | 15,643 | 93.29 | 7839 | 16.14 | 5,780,723 | 25.05 | 3,724,129 | 762,991 | 362 | 639 | 258,046 | 146,108 | ||||
15 | Spend 2006 and mortality 2004/5/6 | 15,643 | 112.13 | 7923 | 18.17 | 6,172,491 | 757,531 | 249 | 449,706 | ||||||||
16 | Spend 2005 and mortality 2002/3/4 | 12,743 | 99.44 | 7923 | 16.26 | 6,115,621 | 751,009 | 337 | 295,074 | ||||||||
All 10 programmes summary | |||||||||||||||||
17 | Spend 2006 and mortality 2006/7/8 | 32,911 | 0.561 | 184.53 | 128,640 | 681.24 | 270,881 | 786.54 | 234,617 | 5,001,777 | 7760 | 9243 | 23,780 | 19,965 | |||
18 | Spend 2006 and mortality 2004/5/6 | 32,911 | 0.687 | 226.18 | 133,213 | 971.30 | 232,861 | 5,093,090 | 10,826 | 20,893 | |||||||
19 | Spend 2005 and mortality 2002/3/4 | 30,368 | 0.792 | 240.67 | 133,213 | 926.22 | 259,838 | 5,267,962 | 11,322 | 21,256 | |||||||
Assume zero health gain in the other 13 programmes: other 13 programmes summary | |||||||||||||||||
20 | Spend 2006 and mortality 2006/7/8 | 34,985 | 1.413 | 494.43 | 0.00 | 0 | 0 | ||||||||||
21 | Spend 2006 and mortality 2004/5/6 | 34,985 | 1.294 | 452.78 | 0.00 | 0 | |||||||||||
22 | Spend 2005 and mortality 2002/3/4 | 33,942 | 1.186 | 402.43 | 0.00 | 0 | |||||||||||
All 23 programmes | |||||||||||||||||
23 | Spend 2006 and mortality 2006/7/8 | 67,896 | 678.96 | 681.24 | 996,655 | 786.54 | 863,228 | 7760 | 9243 | 87,494 | 73,457 | ||||||
24 | Spend 2006 and mortality 2004/5/6 | 67,896 | 678.96 | 971.30 | 699,024 | 10,826 | 62,718 | ||||||||||
25 | Spend 2005 and mortality 2002/3/4 | 64,310 | 643.10 | 926.22 | 694,330 | 11,322 | 56,799 |
Similarly, Table 69 reproduces Table 67 but incorporates this ICD-10 coverage adjustment [see columns (J) and (O) in Table 69]. If we assume a zero health gain in PBC 23 and an average gain in the other 12 PBCs without a mortality-based outcome indicator, then this adjustment reduces the cost of a life-year for all programmes from £26,876 to £22,565.
(A) PBC scenario | (B) PBC description | (C) Spend (£M) 2006/7 | (D) Spend elasticity | (E) (= 0.01 × C × D) change in spend (£M) | (F) Annual mortality, < 75 years, 2006/7/8 | (G) Outcome elasticity (without negative sign) | (H) (= 0.01 × D × F × G) change in annual mortality | (I) (= E/H) cost per life gained (£) | (J) Coverage of mortality data relative to spend data | (K) (= H/J) change in annual mortality adjusted for coverage | (L) (= E/K) cost per life gained (£) adjusted for coverage | (M) Total life-years lost, < 75 years, 2006/7/8 | (N) (= 0.01 × D × G × M/3) change in annual life-years lost | (O) Coverage of mortality data relative to spend data | (P) (= N/O) change in annual life-years lost adjusted for coverage | (Q) (= E/N) cost per YLG (£) | (R) (= E/P) cost per YLG adjusted for YLL coverage (£) |
---|---|---|---|---|---|---|---|---|---|---|---|---|---|---|---|---|---|
1 | Cancer | 4122 | 0.465 | 19.17 | 61,961 | 0.342 | 98.54 | 194,520 | 0.984 | 100.14 | 191,407 | 2,207,021 | 1170 | 0.984 | 1189 | 16,383 | 16,121 |
2 | Circulatory problems | 6161 | 0.540 | 33.27 | 41,106 | 1.434 | 318.31 | 104,519 | 0.992 | 320.88 | 103,683 | 1,361,634 | 3515 | 0.992 | 3543 | 9466 | 9390 |
3 | Respiratory problems | 3285 | 0.679 | 22.31 | 11,574 | 2.622 | 206.06 | 108,248 | 0.773 | 262.57 | 83,676 | 324,223 | 1924 | 0.773 | 2489 | 11,593 | 8961 |
4 | Gastrointestinal problems | 3700 | 0.446 | 16.50 | 6160 | 1.536 | 42.20 | 391,048 | 0.571 | 73.90 | 223,288 | 345,908 | 790 | 0.571 | 1,83 | 20,892 | 11,929 |
Big four programmes summary | |||||||||||||||||
5 | Spend 2006 and mortality 2006/7/8 | 17,268 | 91.24 | 120,801 | 665.10 | 137,188 | 761.49 | 119,823 | 4,238,786 | 7399 | 8604 | 12,333 | 10,604 | ||||
6 | Spend 2006 and mortality 2004/5/6 | 17,268 | 114.04 | 125,290 | 953.13 | 119,650 | 4,335,559 | 10,576 | 10,783 | ||||||||
7 | Spend 2005 and mortality 2002/3/4 | 17,625 | 141.22 | 125,290 | 909.96 | 155,196 | 4,516,953 | 10,986 | 12,855 | ||||||||
8 | Infectious diseases | 1053 | 0.792 | 8.34 | 2050 | 0.047 | 0.76 | 10,928,905 | 1.000 | 0.76 | 10,928,905 | 106,552 | 13 | 1.000 | 13 | 630,798 | 630,798 |
9 | Endocrine problems | 1852 | 0.953 | 17.65 | 1542 | 0.842 | 12.37 | 1,426,410 | 0.634 | 19.52 | 904,344 | 57,672 | 154 | 0.634 | 243 | 114,416 | 72,539 |
10 | Neurological problems | 2790 | 0.616 | 17.19 | 727 | 0.112 | 0.50 | 34,265,082 | 0.136 | 3.69 | 4,660,051 | 66,137 | 15 | 0.136 | 112 | 1,129,960 | 153,675 |
11 | Genitourinary problems | 3482 | 0.912 | 31.76 | 294 | 0.051 | 0.14 | 232,226,224 | 0.172 | 0.80 | 39,942,910 | 10,030 | 2 | 0.172 | 9 | 20,421,090 | 3,512,427 |
12 | Trauma and injuries | 2892 | 0.358 | 10.35 | 1037 | 0 | 0.00 | N/A | 0.175 | 0.00 | N/A | 30,000 | 0 | 0.175 | 0 | N/A | N/A |
13 | Maternity and neonates | 3574 | 0.224 | 8.01 | 2189 | 0.482 | 2.36 | 3,387,363 | 8.213 | 0.29 | 27,820,413 | 492,600 | 177 | 0.679 | 261 | 45,158 | 30,662 |
Other six programmes summary | |||||||||||||||||
14 | Spend 2006 and mortality 2006/7/8 | 15,643 | 93.29 | 7839 | 16.14 | 5,780,723 | 25.05 | 3,724,129 | 762,991 | 362 | 639 | 258,046 | 146,108 | ||||
15 | Spend 2006 and mortality 2004/5/6 | 15,643 | 112.13 | 7923 | 18.17 | 6,172,491 | 757,531 | 249 | 449,706 | ||||||||
16 | Spend 2005 and mortality 2002/3/4 | 12,743 | 99.44 | 7923 | 16.26 | 6,115,621 | 751,009 | 337 | 295,074 | ||||||||
All 10 programmes summary | |||||||||||||||||
17 | Spend 2006 and mortality 2006/7/8 | 32,911 | 0.561 | 184.53 | 128,640 | 681.24 | 270,881 | 786.54 | 234,617 | 5,001,777 | 7760 | 9243 | 23,780 | 19,965 | |||
18 | Spend 2006 and mortality 2004/5/6 | 32,911 | 0.687 | 226.18 | 133,213 | 971.30 | 232,861 | 5,093,090 | 10,826 | 20,893 | |||||||
19 | Spend 2005 and mortality 2002/3/4 | 30,368 | 0.792 | 240.67 | 133,213 | 926.22 | 259,838 | 5,267,962 | 11,322 | 21,256 | |||||||
Other 13 PBCs? Assume zero health gain in PBC 23 . . . | |||||||||||||||||
20 | PBC 23 spend 2006 and mortality 2006/7/8 | 10,585 | 0.739 | 78.22 | 0.00 | 0.00 | 0.00 | ||||||||||
21 | PBC 23 spend 2006 and mortality 2004/5/6 | 10,585 | 0.759 | 80.34 | 0.00 | 0.00 | |||||||||||
22 | PBC 23 spend 2005 and mortality 2002/3/4 | 8449 | 0.926 | 78.24 | 0.00 | 0.00 | |||||||||||
. . . and that the gain in 10 PBCs (see row 17) applies to the remaining 12 PBCs | |||||||||||||||||
23 | 12 PBCs spend 2006 and mortality 2006/7/8 | 24,400 | 416.20 | 1536.48 | 270,881 | 1773.97 | 234,617 | 17,502 | 20,847 | 23,780 | 19,965 | ||||||
24 | 12 PBCs spend 2006 and mortality 2004/5/6 | 24,400 | 372.44 | 1599.42 | 232,861 | 17,826 | 20,893 | ||||||||||
25 | 12 PBCs spend 2005 and mortality 2002/3/4 | 25,493 | 324.20 | 1247.69 | 259,838 | 15,252 | 21,256 | ||||||||||
26 | 23 PBCs spend 2006 and mortality 2006/7/8 | 67,896 | 678.96 | 2217.72 | 306,153 | 2560.50 | 265,167 | 25,262 | 30,090 | 26,876 | 22,565 | ||||||
27 | 23 PBCs spend 2006 and mortality 2004/5/6 | 67,896 | 678.96 | 2570.72 | 264,113 | 28,652 | 23,697 | ||||||||||
28 | 23 PBCs spend 2005 and mortality 2002/3/4 | 64,310 | 643.10 | 2173.90 | 295,827 | 26,575 | 24,200 |
Adjusting the cost of life (year) estimates for Department of Health-funded expenditure that is not undertaken by primary care trusts
Primary care trust expenditure accounts for a large proportion of Department of Health expenditure but PCTs do not account for all of the Department of Health’s budget. In 2006/7 the Department of Health’s gross expenditure totalled £83.5B. Charges raised £3.4B so net expenditure totalled £80.1B. Of this net expenditure, PCTs accounted for £67.3B (that is, 84%) and various other bodies accounted for the remaining £12.8B. A breakdown of this gross and net expenditure by major body is shown in Table 70.
Body | Gross spend, £B | Income, £B | Net spend, £B |
---|---|---|---|
PCTs | 69.8 | 2.5 | 67.3 |
SHAs | 3.8 | 0.0 | 3.8 |
Special Health Authoritiesa | 2.8 | 1.3 | 1.5 |
Department of Health own costs (e.g. PSS grants, grants to LAs) | 7.1 | −0.4 | 7.5 |
Total Department of Health | 83.5 | 3.4 | 80.1 |
The Department of Health has allocated net non-PCT expenditure across the 23 PBCs and the impact of this allocation on total spend by PBC is shown in Table 71. No geographic breakdown (e.g. by PCT) of this expenditure is available.
PBC | Net spend, £B, 2006/7 | (D) Others’ spend as % of PCT spend | |||
---|---|---|---|---|---|
(A) All PCTs | (B) Others | (C) All Department of Health | |||
1 | Infectious diseases | 1.1 | 0.1 | 1.2 | 13.3 |
2 | Cancers and tumours | 4.1 | 0.0 | 4.2 | 0.8 |
3 | Disorders of blood | 0.8 | 0.1 | 0.9 | 12.0 |
4 | Endocrine, nutritional and metabolic issues | 1.9 | 0.1 | 2.0 | 5.4 |
5 | Mental health disorders | 8.4 | 0.3 | 8.7 | 3.2 |
6 | Problems of learning disability | 2.4 | −0.1 | 2.4 | −2.5 |
7 | Neurological | 2.8 | 0.0 | 2.8 | 1.3 |
8 | Problems of vision | 1.4 | 0.0 | 1.3 | −1.8 |
9 | Problems of hearing | 0.3 | 0.0 | 0.3 | 0.5 |
10 | Problems of circulation | 6.2 | 0.2 | 6.4 | 4.0 |
11 | Problems of the respiratory system | 3.3 | 0.1 | 3.3 | 1.6 |
12 | Dental problems | 2.6 | −0.2 | 2.4 | −7.0 |
13 | Problems of the gastrointestinal system | 3.7 | 0.0 | 3.7 | −0.5 |
14 | Problems of the skin | 1.4 | 0.0 | 1.5 | 2.2 |
15 | Problems of the musculoskeletal system | 3.4 | 0.0 | 3.4 | −0.3 |
16 | Problems due to trauma and injuries | 2.9 | 0.0 | 2.9 | −0.5 |
17 | Problems of the genitourinary system | 3.5 | 0.1 | 3.6 | 2.1 |
18 | Maternity and reproductive health | 2.9 | −0.1 | 2.8 | −2.2 |
19 | Conditions of neonates | 0.7 | 0.1 | 0.7 | 10.3 |
20 | Adverse effects and poisoning | 0.7 | 0.0 | 0.7 | −0.8 |
21 | Healthy individuals | 1.4 | 0.0 | 1.4 | 0.8 |
22 | Social care needs | 1.5 | 0.0 | 1.5 | 0.9 |
23 | Other areas of spend/conditions | 10.6 | 11.2 | 21.8 | 106.1 |
All categories | 67.9 | 12.0 | 79.9 | 17.7 |
Of the additional £12B of net expenditure, £11.2B (93%) has been allocated to PBC 23. This largely reflects (a) the allocation of almost all SHA expenditure to either PBC 23B (‘other: SHAs including workforce development committees’) or PBC 23X (‘other: miscellaneous’); and (b) the allocation of almost two-thirds of Department of Health expenditure to PBC 23X (‘other: miscellaneous’).
The remaining £0.8B of additional net expenditure is spread across all PBCs according to various allocation rules. For example, the majority of expenditure on Special Health Authorities is apportioned across programme categories on the basis of the PCT and SHA expenditure breakdown. The exception is the NHS Business Services Authority expenditure which is apportioned on the basis of primary care prescribing expenditure splits. Although this approach avoids allocating expenditure to the ‘other: miscellaneous’ category, this allocation of expenditure does not necessarily reflect actual expenditure. For example, NHS Litigation Authority expenditure may not be incurred in the same areas as overall PCT expenditure. 34
It is clear that most of the non-PCT expenditure is not specific to any disease area and that, to avoid putting all of it into a residual category, the Department of Health has identified what are reasonable, but largely arbitrary, rules to spread what is a relatively small proportion of this non-PCT expenditure across all PBCs.
The cost of a life (year) estimates presented above are based on the impact of a 1% exogenous change in total net PCT spend. All of our outcome and expenditure models have been estimated using net PCT expenditure, and all of our elasticities relate to this expenditure. Implicitly we assume that any budgetary shock only affects PCT funding and that it leaves non-PCT funding unchanged.
Suppose instead we assume a 1% exogenous change in the Departmental budget. How might this budgetary shock be split between PCT and non-PCT expenditure? There are two obvious options to consider. We could assume either (a) that all of this change is applied to PCT budgets and that there is no change in the non-PCT budget (as we do implicitly at the moment); or (b) that the budgetary shock affects both PCT and non-PCT budgets.
If the non-PCT budget is wholly unresponsive to the exogenous shock then our cost of a life-year estimates will be unchanged because this expenditure category attracts none of the budgetary change (although this expenditure will clearly contribute to a measure of average productivity).
If the non-PCT budget is to some degree responsive to the exogenous shock then it will affect our cost of a life-year estimates. To calculate the size of this impact we would need to know:
-
how responsive the non-PCT budget is to a total Departmental budgetary shock
-
how the responsive part of the non-PCT budget is allocated across PBCs; and
-
the size of the health effects associated with changes in the non-PCT budget at PBC level.
We have no evidence on how responsive the non-PCT budget is likely to be to a total budgetary shock. However, from Table 71 and the discussion about the rather arbitrary (but understandable) rules employed by the Department of Health to allocate non-PCT expenditure to PBCs, it would seem reasonable to assume that any change in the non-PCT budget should all be allocated to PBC 23. This ‘solves’ the problem of identifying the health gains associated with this change in the non-PCT budget because, in our cost of a life-year calculations, we assume that expenditure in this category attracts no health gains.
Thus, although we have no evidence on how responsive the non-PCT budget is likely to be to a total budgetary shock, we can present two scenarios. In the first scenario, the non-PCT budget is wholly unresponsive to a budgetary shock and any budgetary change is fully implemented via PCT expenditure. In this case, there is no impact on the cost of a life-year.
In the second scenario, one might assume that the non-PCT budget is as responsive to Departmental budgetary changes as is the PCT budget. In this case a 1% change in the Departmental budget is translated into a 1% change in both the total PCT and total non-PCT budgets, and this will increase the cost of a life-year by 17.7% for 2006/7, that is, from £22,565 to £26,553. This percentage increase is, of course, the same figure as total non-PCT expenditure expressed as a percentage of PCT expenditure. This is because all of the additional non-PCT expenditure is allocated to PBC 23 and the assumption is that all expenditure in this category offers no health gain.
We have no information on how any Departmental budgetary shock is likely to be split between PCT and non-PCTs budgets. Our cost of a life-year estimates implicitly assume that the non-PCT budget is wholly unresponsive to any budgetary shock. This is clearly a possibility. Alternatively, one might assume that the non-PCT budget is as responsive to a Departmental budgetary shock as is the PCT budget. If this was the case then it would add 17.7% to our cost of a life-year estimate for 2006/7. However, in the absence of any information about the responsiveness of the non-PCT budget, it is difficult to come to any firm conclusion about the impact of non-PCT expenditure on our cost of a life-year estimates. We therefore persevere with the assumption that the non-PCT budget is wholly unresponsive to Departmental budgetary shocks.
Application of method to other non-mortality-based outcome indicators
Not all health-care expenditure will be directed towards the reduction of mortality but it is relatively easy to envisage how our methods might be applied to other, non-mortality-based, outcome indicators. To illustrate how our approach might be applied to other such indicators we note that PROMs (health gain) data for various operations is available from the HES online website. For each PCT this data set reports the average health gain for those survey respondents who have had a specific operation (e.g. for hip replacement, for knee replacement, for varicose veins, and for groin hernia) over the survey period.
As a starting point, and to illustrate the principles involved, we focus on hip and knee replacements. As our outcome indicator for these procedures, we calculate:
for each PCT (this ignores age standardisation). This health gain measure is broadly comparable with our usual mortality measure, which is a ‘YLL’ rate per 10,000 of population (again, ignoring age standardisation).
Ideally the expenditure, number of operations and PROMs data should all relate to the same time period, but here the PROMs data covers operations undertaken between April 2009 and October 2010 yet the expenditure and number of operations data relate to 2006/7. Implicitly, we are assuming that the average gain per operation in 2006/7 is the same as over the PROMs survey period (although this is not particularly important as we are only illustrating principles here).
Unfortunately, the Department of Health does not report the number of patients undergoing an eligible operation by commissioner (PCT) so we use the HES data set for 2006/7 to obtain this information. Eligible hip and knee operations are defined in annex 1 of the Guide to PROMs methodology (on the HES website)151 and we use these definitions (of eligible operation codes) to obtain a count of eligible hip and knee FCEs by PCT for 2006/7.
With data for both the average health gain per operation and the number of operations, we are now in a position to calculate ‘the health gain per head of population’ for hip and knee replacements as defined above. We can then use this as an outcome indicator for expenditure in the ‘problems of the musculoskeletal system’ programme (i.e. PBC 15) because the vast majority of hip and knee replacements are for osteoarthritis and this diagnosis is included in PBC 15.
Table 72 reports the estimated outcome equation for PBC 15 using the PROMs-based outcome indicator. The result is intuitively plausible. More expenditure boosts the health gain but, for a given spend, more need reduces the gain. Of course we should remember that the health gain data relates to operations undertaken between April 2009 and October 2010 yet the expenditure and number of operations data (FCEs) relate to 2006/7. However, one might assume that the gain associated with each operation in 2009/10 is the same as the gain associated with each operation in 2006/7.
Regressors | Coefficient (standard error) |
---|---|
Expenditure per person in PBC 15 | 1.9068*** |
(0.4289) | |
Need CARAN per person | –1.6807*** |
(0.4533) | |
Constant | –6.3486*** |
(1.794) | |
Number of PCTs | 143 |
Diagnostic test statistics | |
Endogeneity test statistic | 24.677 |
Endogeneity p-value | 0.0000 |
Hansen-Sargan test statistic | 1.136 |
Hansen-Sargan p-value | 0.2865 |
Kleibergen–Paap LM test statistic | 14.702 |
Kleibergen–Paap p-value | 0.0006 |
Kleibergen–Paap F-statistic | 10.367 |
Pesaran–Taylor reset statistic | 0.03 |
Pesaran–Taylor p-value | 0.8588 |
The diagnostic statistics suggest that expenditure is endogenous and that the instruments are valid. They also suggest that the instruments are relevant and there is no evidence that the instruments are weak. The Pesaran–Taylor test suggests that there is no evidence of model misspecification.
This brief example illustrates the principles involved in extending our modelling approach beyond those programmes with a mortality indicator.
Comparing outcome models for ‘high’ spending and ‘low’ spending primary care trusts
As we have already noted, not all PCTs spend the same amount in each programme of care. Even after allowing for differences in local circumstances (such as input prices and need), some PCTs spend more than others, and it is this variation in expenditure that facilitates the estimation of our outcome and expenditure models.
Figure 15 illustrates the familiar health gain production function; as expenditure increases so too does health output but it increases at a diminishing rate. If all PCTs face the same production function (having controlled for input prices and need), and all PCTs are wholly efficient, then we would expect those PCTs that spend more (e.g. at point B) to experience a lower outcome elasticity than those that spend less (e.g. at point A), simply because they are further along the production function and are experiencing greater diminishing marginal returns.
FIGURE 15.
Graph showing health gain production function.

To test this hypothesis we used the expenditure model for each of the big four programmes to divide the 152 PCTs into two groups: those whose predicted spend is greater than the average predicted spend in that programme (ceteris paribus), and those whose predicted spend is smaller than the average predicted spend (ceteris paribus). We then re-estimated our outcome model for each of these two groups of PCTs and the results of this re-estimation are shown in Table 73. ad
IV regression result | All PCTs | ‘High spend’ PCTs | ‘Low spend’ PCTs |
---|---|---|---|
Cancer outcome equation | |||
1 Constant | 6.500*** | 7.132*** | 5.352*** |
2 Need for health care | 0.995*** | 1.265*** | 0.848*** |
3 Need for health care squared | 1.162*** | 0.588 | 0.842 |
4 Cancer expenditure per person | −0.342*** | −0.488*** | −0.074 |
Number of observations | 152 | 76 | 76 |
Endogeneity test statistic | 13.695*** | 7.165*** | 0.501 |
Instrument validity: Hansen J statistic | 0.685 | 0.734 | 1.587 |
Instrument relevance: Kleibergen–Paap LM statistic | 17.847*** | 7.102** | 13.617*** |
Weak instrument: Kleibergen–Paap F-statistic | 13.279 | 6.722 | 7.436 |
Reset test | 0.01 | 0.68 | 1.95 |
Circulatory disease outcome equation | |||
1 Constant | 11.413*** | 11.254*** | 9.356*** |
2 Need for health care | 2.859*** | 2.741*** | 2.636*** |
3 Circulatory expenditure per person | −1.434*** | −1.403*** | −0.995*** |
Number of observations | 152 | 76 | 76 |
Endogeneity test statistic | 42.548*** | 9.424*** | 20.489*** |
Instrument validity: Hansen J statistic | 0.949 | 4.782 | 0.366 |
Instrument relevance: Kleibergen–Paap LM statistic | 32.372*** | 12.658** | 15.123*** |
Weak instrument: Kleibergen–Paap F-statistic | 17.143 | 6.275 | 12.421 |
Reset test | 0.14 | 0 | 1.29 |
Respiratory problems outcome equation | |||
1 Constant | 17.023*** | 22.617** | 11.695*** |
2 Need for health care | 2.683** | 2.512 | 3.095** |
3 Need for health care squared | 3.08 | 5.537 | 8.097*** |
4 Permanently sick | 1.031** | 1.401 | 0.844 |
5 Respiratory expenditure per person | −2.622*** | −3.697* | −1.461* |
Number of observations | 152 | 76 | 76 |
Endogeneity test statistic | 20.860*** | 10.254*** | 5.380** |
Instrument validity: Hansen J statistic | N/A | N/A | N/A |
Instrument relevance: Kleibergen–Paap LM statistic | 9.091*** | 3.591 | 5.108** |
Weak instrument: Kleibergen–Paap F-statistic | 11.025 | 4.568 | 6.227 |
Reset test | 0 | 0.08 | 0.21 |
Gastrointestinal outcome equation | |||
1 Constant | 9.718*** | 9.306*** | 6.675*** |
2 Need for health care | 4.159*** | 5.156*** | 3.236*** |
3 Gastrointestinal spend per person | −1.536*** | −1.471*** | −0.819 |
Number of observations | 152 | 76 | 76 |
Endogeneity test statistic | 16.373*** | 7.781*** | 3.700* |
Instrument validity: Hansen J statistic | 2.761 | 1.529 | 3.824* |
Instrument relevance: Kleibergen–Paap LM statistic | 14.865*** | 10.094*** | 7.956** |
Weak instrument: Kleibergen–Paap F-statistic | 11.629 | 10.607 | 7.985 |
Reset test | 1.67 | 0.15 | 0.56 |
In Table 73 the first column of the IV regression results presents the outcome model for all PCTs; the second column reports the results for the ‘high spend’ PCTs; and the third column reports the results for the ‘low spend’ PCTs. For all four programmes, the coefficient on the expenditure variable is larger (in an absolute sense) for the ‘high spend’ PCTs than for the ‘low spend’ PCTs. This result contradicts our hypothesis that ‘high spenders’ will have a lower elasticity than ‘low spenders’.
However, if we drop the assumption that all PCTs are equally efficient – so that that some lie within the frontier defined by the production function – then it is clearly possible for ‘high’ spending PCTs to experience a larger outcome elasticity than a ‘low’ spending one. In addition, it is rather difficult to defend the assumption that all PCTs are equally efficient.
We can use the outcome elasticities reported in Table 73 to calculate the cost of a life-year for ‘high’ and ‘low’ spenders in each of the big four programmes. These calculations are shown in Table 74. ae As is to be anticipated, they reveal that the cost of a life-year is much smaller in ‘high spend’ PCTs than it is in ‘low spend’ PCTs. For example, the cost of a life-year in the cancer programme is £16,383 across all PCTs, but for ‘high spenders’ it is much less than this (£11,350) and for ‘low spenders’ it is much greater than this (£76,620). Presumably ‘high spending’ PCTs are high spenders because the cost of a life-year is relatively low and additional health gains in a particular programme can be had relatively cheaply. Similarly, ‘low spending’ PCTs are low spenders because the cost of a life-year is relatively high and additional health gains are relatively expensive.
Scenario | (A) PBC description | (B) Type of PCT | (C) Spend (£M) financial year 2006/7 | (D) 1% of spend (£M) financial year 2006/7 | (E) Outcome elasticity (without negative sign) | (F) Total life-years lost, < 75 years, 2006/7/8 | (G) Annual average life-years lost (= F/3) | (H) Change in annual life-years lost associated with 1% increase in spend (= E × G)/100 | (I) Cost (£) per YLG (= D/H) unadjusted for ICD-10 coverage |
---|---|---|---|---|---|---|---|---|---|
Split PCTs according to whether they are ‘high spenders’ (n = 76) or ‘low spenders’ (n = 76) | |||||||||
1 | Cancers | All | 4122 | 41.22 | 0.342 | 2,207,021 | 735,674 | 2516 | 16,383 |
2 | Cancers | High spend | 2080 | 20.80 | 0.488 | 1,126,580 | 375,527 | 1833 | 11,350 |
3 | Cancers | Low spend | 2042 | 20.42 | 0.074 | 1,080,442 | 360,147 | 267 | 76,620 |
4 | Circulatory problems | All | 6161 | 61.61 | 1.434 | 1,361,634 | 453,878 | 6509 | 9466 |
5 | Circulatory problems | High spend | 3148 | 31.48 | 1.403 | 695,890 | 231,963 | 3254 | 9673 |
6 | Circulatory problems | Low spend | 3012 | 30.12 | 0.995 | 665,744 | 221,915 | 2208 | 13,641 |
7 | Respiratory problems | All | 3285 | 32.85 | 2.622 | 324,223 | 108,074 | 2834 | 11,593 |
8 | Respiratory problems | High spend | 1645 | 16.45 | 3.697 | 174,639 | 58,213 | 2152 | 7644 |
9 | Respiratory problems | Low spend | 1640 | 16.4 | 1.461 | 149,584 | 49,861 | 728 | 22,513 |
10 | Gastrointestinal problems | All | 37.00 | 37.00 | 1.536 | 345,908 | 115,303 | 1771 | 20,892 |
11 | Gastrointestinal problems | High spend | 1868 | 18.68 | 1.471 | 190,231 | 63,410 | 933 | 20,026 |
12 | Gastrointestinal problems | Low spend | 1832 | 18.32 | 0.819 | 155,676 | 51,892 | 425 | 43,106 |
Comparing outcome models for over target and under target primary care trusts
The Department of Health has a well-developed resource allocation formula that determines the size of each PCTs ‘target’ budget given local conditions (such as population size and the need for health care). Every few years an improved resource allocation formula is developed and this generates a new ‘target’ budget for each PCT. The new target might be quite different from the old target and the immediate implementation of the new formula might lead to a large change in the budget for some PCTs. To avoid the difficulties that sudden large budgetary changes might bring, actual annual financial allocations are gradually moved towards the latest target budget. This means that in any year some PCTs receive an actual allocation which is greater than their target allocation, and that others receive an actual allocation which is less than their target allocation.
To examine whether or not being over or under the target allocation has any impact on the results, we split the 152 PCTs into two groups: those that received a budget over their target allocation in 2006/7 and those that received a budget under their target allocation in 2006/7. The outcome elasticities from the estimation of these models are shown in column (E) of Table 75, and these elasticities are used to calculate the cost of a life-year for each of these two groups of PCTs for each of the big four programmes [see column (I) of Table 75].
Scenario | (A) PBC description | (B) Type of PCT | (C) Spend (£M) financial year 2006/7 | (D) 1% of spend (£M) financial year 2006/7 | (E) Outcome elasticity (without negative sign) | (F) Total life-years lost, < 75 years, 2006/7/8 | (G) Annual average life-years lost (= F/3) | (H) Change in annual life-years lost associated with 1% increase in spend (= E × G)/100 | (I) Cost (£) per YLG (= D/H) |
---|---|---|---|---|---|---|---|---|---|
Split PCTs according to whether they are over target allocation (n = 67) or under target allocation (n = 85) | |||||||||
1 | Cancers | All | 4122 | 41.22 | 0.342 | 2,207,021 | 735,674 | 2516 | 16,383 |
2 | Cancers | Over target | 1733 | 17.33 | 0.179 | 897,403 | 299,134 | 535 | 32,365 |
3 | Cancers | Under target | 2390 | 23.90 | 0.476 | 1,309,618 | 436,539 | 2078 | 11,502 |
4 | Circulatory problems | All | 6161 | 61.61 | 1.434 | 1,361,634 | 453,878 | 6509 | 9466 |
5 | Circulatory problems | Over target | 2587 | 25.87 | 1.115 | 544,326 | 181,442 | 2023 | 12,787 |
6 | Circulatory problems | Under target | 3574 | 35.74 | 1.947 | 817,308 | 272,436 | 5304 | 6738 |
7 | Respiratory problems | All | 3285 | 32.85 | 2.622 | 324,223 | 108,074 | 2834 | 11,593 |
8 | Respiratory problems | Over target | 1357 | 13.57 | 2.637 | 127,810 | 42,603 | 1123 | 12,079 |
9 | Respiratory problems | Under target | 1928 | 19.28 | 2.674 | 196,413 | 65,471 | 1751 | 11,013 |
10 | Gastrointestinal problems | All | 3700 | 37.00 | 1.536 | 345,908 | 115,303 | 1771 | 20,892 |
11 | Gastrointestinal problems | Over target | 1566 | 15.66 | 0.569 | 142,281 | 47,427 | 270 | 58,030 |
12 | Gastrointestinal problems | Under target | 2134 | 21.34 | 1.869 | 203,626 | 67,875 | 1269 | 16,822 |
The results are consistent for each programme: PCTs whose budget is beyond their target allocation record a smaller outcome elasticity and a larger cost of a life-year than PCTs whose budget is less than their target allocation. For example, in the cancer programme and across all PCTs the outcome elasticity is −0.342 and the cost of a life-year is £16,383 (unadjusted for the ICD-10 coverage of the mortality data). For PCTs with a budget that exceeds their target allocation, the outcome elasticity is smaller (−0.179) and the cost of a life-year is larger (£32,365) than for all PCTs combined. However, for PCTs with a budget that falls short of their target allocation, the outcome elasticity is larger (−0.476) and the cost of a life-year is smaller (£11,502) than for all PCTs combined.
One explanation for this result is that PCTs whose budget is beyond their target allocation are under less financial pressure than other PCTs, and that one consequence of this is that there is less pressure on them to behave in the most efficient manner possible. There is some evidence in the literature to support the hypothesis that the degree of PCT inefficiency is positively related to the amount by which a PCTs is over its target allocation. 61
If we also re-estimate the expenditure models for both groups of PCTs we can calculate the cost of a life-year for the big four programmes combined. The relevant expenditure elasticities are shown in column (D) of Table 76. These expenditure elasticities are far larger for under target PCTs than they are for over target PCTs. One reason for this might be that the big four programmes are priority (‘hard’) programmes. Over target PCTs are able to devote sufficient resources to the big four programmes so that any additional budget is directed towards other (‘softer’) programmes which are less well funded than the priority programmes. In contrast, under target PCTs are struggling to devote sufficient resources to the priority programmes so that, when further funding does become available, this is directed towards the priority programmes.
(A) PBC scenario | (B) PBC description | (C) Spend (£M) 2006/7 | (D) Spend elasticity | (E) (= 0.01 × C × D) change in spend (£M) | (F) Annual mortality < 75 years, 2006/7/8 | (G) Coverage of mortality data relative to spend data | (H) Outcome elasticity (without negative sign) | (I) (= 0.01 × D × F × H/G) change in annual mortality, adjusted for coverage | (J) (= E/I) cost per life gained adjusted for coverage (£) | (K) Total life-years lost, < 75 years, 2006/7/8 | (L) (= 0.01 × D × H × K/3) change in annual life-years lost | (M) Coverage of mortality data relative to spend data | (N) (= L/M) change in annual life-years lost, adjusted for coverage | (O) (= E/L) cost per YLG (£) | (P) (= E/N) cost per YLG adjusted for YLL coverage (£) |
---|---|---|---|---|---|---|---|---|---|---|---|---|---|---|---|
All PCTs together | |||||||||||||||
1 | Cancer | 4122 | 0.465 | 19.17 | 61,961 | 0.984 | 0.342 | 100.14 | 191,407 | 2,207,021 | 1170 | 0.984 | 1189 | 16,383 | 16,121 |
2 | Circulatory problems | 6161 | 0.540 | 33.27 | 41,106 | 0.992 | 1.434 | 320.88 | 103,683 | 1,361,634 | 3515 | 1.000 | 3543 | 9466 | 9390 |
3 | Respiratory problems | 3285 | 0.679 | 22.31 | 11,574 | 0.773 | 2.622 | 266.57 | 83,676 | 324,223 | 1924 | 0.773 | 2489 | 11,593 | 8961 |
4 | Gastrointestinal problems | 3700 | 0.446 | 16.50 | 6160 | 0.571 | 1.536 | 73.90 | 223,228 | 345,908 | 790 | 0.650 | 1383 | 20,892 | 11,929 |
Big four programmes summary | |||||||||||||||
5 | Spend 2006 and mortality 2006/7/8 | 17,268 | 91.24 | 761.49 | 119,823 | 7399 | 8604 | 12,333 | 10,604 | ||||||
For over target PCTs only (n = 67) | |||||||||||||||
6 | Cancer | 1733 | 0.193 | 3.34 | 24,918 | 0.984 | 0.179 | 8.75 | 382,320 | 897,403 | 103 | 0.984 | 105 | 32,365 | 31,847 |
7 | Circulatory problems | 2587 | 0.150 | 3.88 | 16,346 | 0.992 | 1.115 | 27.56 | 140,806 | 544,326 | 303 | 1.000 | 303 | 12,787 | 12,685 |
8 | Respiratory problems | 1357 | 0.326 | 4.42 | 4588 | 0.773 | 2.637 | 51.02 | 86,701 | 127,810 | 366 | 0.773 | 474 | 12,079 | 9337 |
9 | Gastrointestinal problems | 1566 | 0.090 | 1.41 | 2525 | 0.571 | 0.569 | 2.26 | 622,378 | 142,281 | 24 | 0.650 | 43 | 58,030 | 33,135 |
Big four programmes summary | |||||||||||||||
10 | Spend 2006 and mortality 2006/7/8 | 7243 | 13.06 | 89.60 | 145,748 | 797 | 927 | 16,378 | 14,083 | ||||||
For under target PCTs only (n = 85) | |||||||||||||||
11 | Cancer | 2390 | 0.785 | 18.76 | 37,043 | 0.984 | 0.476 | 140.67 | 133,377 | 1,309,618 | 1631 | 0.984 | 1658 | 11,502 | 11,318 |
12 | Circulatory problems | 3574 | 0.748 | 26.73 | 24,760 | 0.992 | 1.947 | 363.50 | 73,544 | 817,308 | 3968 | 1.000 | 4000 | 6738 | 6684 |
13 | Respiratory problems | 1982 | 1.035 | 20.51 | 6986 | 0.773 | 2.674 | 250.12 | 82,015 | 196,413 | 1812 | 0.773 | 2344 | 11,321 | 8751 |
14 | Gastrointestinal problems | 2134 | 0.592 | 12.63 | 3602 | 0.571 | 1.869 | 69.80 | 181,000 | 203,626 | 751 | 0.650 | 1315 | 16,822 | 9605 |
Big four programmes summary | |||||||||||||||
15 | Spend 2006 and mortality 2006/7/8 | 10,080 | 78.64 | 824.09 | 95,429 | 8162 | 9317 | 9635 | 8441 |
These expenditure and outcome elasticities in Table 76 can be used to calculate the cost of a life-year for the big four programmes combined (adjusted for the ICD-10 coverage of the mortality data). This cost is:
-
£10,604 for all PCTs combined
-
£14,083 for PCTs whose budget is beyond its target allocation
-
£8441 for PCTs whose budget falls short of its target allocation.
Again, the cost of a life-year is much smaller for PCTs whose budget falls short of its target allocation.
The correlation between the outcome and expenditure elasticities
To investigate the correlation between the outcome and expenditure elasticities for any given programme, a random sample (with replacement) of 152 PCTs was drawn from the population of 152 PCTs. In this random drawing, some of the original observations will appear once, some more than once, and some not at all. Using this resampled data set, outcome and expenditure models for the selected programme were estimated (as per Table 65) and the outcome and expenditure elasticities saved. This step was repeated 500 times and the correlation coefficient for the outcome and expenditure elasticities was calculated. Table 77 shows these correlation coefficients for each of the big four programmes.
Programme of care | Correlation coefficient between the outcome and expenditure elasticities |
---|---|
Cancers and tumours | 0.1542 |
Circulatory disease | 0.1968 |
Respiratory problems | 0.0368 |
Gastrointestinal problems | 0.0611 |
Summary and conclusion
In this section we have undertaken several tasks. First, we have identified and resolved several estimation issues relating to the appropriate measure of need, the appropriate price index to be used to adjust PCT expenditure for local variations in input prices, and the fact that PCTs vary in size.
Second, we have derived plausible outcome and expenditure models for 10 care programmes using expenditure data for 2006/7 and mortality data for 2004/5/6. The cost of a life-year across these 10 programmes is £20,893 (it was £21,256 using expenditure data for 2005/6).
Third, we have re-estimated the outcome and expenditure models using the same expenditure data but replacing the mortality data for 2004/5/6 with data for 2006/7/8. The advantage of this is that it assumes that the health benefits associated with expenditure occur either in the same period as the expenditure or in the next two periods. This is an improvement on past practice where data constraints forced researchers to relate expenditure to the current and two previous periods. This re-estimation increased the cost of a life-year across all 10 programmes by 14%, from £20,893 to £23,780.
Fourth, we have adjusted the cost of a life-year calculations for the mismatch in the ICD-10 coverage of the expenditure and mortality data. This reduces the cost of a life-year for 2006/7 for those 10 PBCs with a mortality indicator from £23,780 to £19,965 (a decrease of 16%).
Fifth, we have noted that our cost of a life-year estimates are based on the assumption that any Departmental budgetary change falls entirely on PCTs. Although PCTs account for most of the Department of Health’s budget, non-PCTs still accounted for 15% of the budget in 2006/7. As we have no information on how any budgetary change would be split between PCTs and non-PCTs, our estimates implicitly assume that any Departmental budgetary change falls entirely on PCTs. If, on the other hand, the non-PCT budget is responsive to changes in the Department’s budget then our cost of a life-year estimates will be slightly too low [e.g. if the non-PCT budget is as responsive as the PCT budget, then our cost of a life-year estimate for 2006/7 will be increased by 17.7% (that is, from £22,565 to £26,553)].
We have also illustrated how our modelling framework can be applied to other non-mortality-based outcome indicators, and the cost of a life-year estimates that are obtained if PCTs are split into different groups (e.g. those that are under and those that are over their target budget allocations). In the next section we examine the impact of relaxing the instrument validity restriction on our results.
The sensitivity of the outcome elasticity to the validity of the instrument exclusion restrictions
Introduction
One of the crucial elements in the calculation of the cost of a life-year for any programme of care is the coefficient on the expenditure variable in the outcome equation. This coefficient indicates the amount by which mortality changes following a (small) change in expenditure in that care programme. It is to be expected that this coefficient will have a negative sign so that as expenditure increases, for example, mortality will decline. If this coefficient is small (in an absolute sense) then it implies that any change in expenditure will have little effect on mortality and so the cost of a life-year will be relatively large (ceteris paribus). Alternatively, if this coefficient is large (in an absolute sense) then any change in expenditure will have a large effect on mortality and so the cost of a life-year will be relatively small (ceteris paribus). For this reason it is important that we correctly identify the magnitude of this ‘treatment parameter’.
Our basic outcome model for each programme of care is:
where y is mortality, x is expenditure, and n is a measure of the need for health care (with all variables relating to a particular programme of care). We are particularly interested in the size of the coefficient on expenditure (β1). We do not use OLS to estimate this outcome model because expenditure (x) is endogenous and, in the presence of an endogenous regressor, OLS will provide both a biased and an inconsistent estimator of β1. Instead, we use IV techniques. Unlike OLS, IV will provide a consistent estimator of β1 and, although in finite samples the IV estimator will be biased, the belief is that (providing certain assumptions are met) this bias will be less than that associated with OLS.
Instrumental variable estimation involves finding variables (instruments) that are good predictors of expenditure (x), but which are appropriately excluded from the equation of interest (that is, Equation 15). The assumption is that the instruments impact on mortality (y) through their impact on expenditure (x) only, and that they do not have a direct effect on mortality (y). If, on the other hand, an instrument reflects unobserved factors that affect both expenditure and mortality directly, the use of this instrument will lead to a biased and inconsistent estimate of the coefficient on expenditure. Such an instrument is said to be ‘invalid’ because it belongs in the equation of interest in its own right.
In our outcome models we typically employ two instruments (call these z1 and z2) for expenditure. IV assumes that these instruments do not belong in the outcome (see Equation 15). In other words, IV assumes that the coefficients γ1 and γ2 in the outcome model:
are identically zero. Such exclusion restrictions can be debatable and researchers who employ IV techniques often devote considerable effort towards convincing the reader that their assumed exclusion restrictions are a good approximation. 88,89 These efforts usually take two forms: first, researchers often offer a strong theoretical economic argument why their instruments do not belong in the equation of interest; and, second, statistical tests for the validity of the exclusion restrictions (Sargan 2SLS, Hansen J-test GMM) are routinely reported as part of the results for any study that employs IV techniques.
It is difficult for us to identify clear theoretical reasons why our instruments (such as the proportion of lone pensioner households, the provision of unpaid care and an index of multiple deprivation) do not belong in the equation of interest (that is, that they will not directly affect mortality). Of necessity, therefore, we must be guided by the available statistical tests for the validity of the exclusion restrictions. However, although our outcome models ‘pass’ the relevant statistical test, some commentators have argued that the Sargan/Hansen test may have weak power and may fail to reject the null hypothesis of instrument validity even when an exclusion restriction is not valid. Given our reliance on this test, it is important that we examine the circumstances in which this test may have weak power.
The Hansen–Sargan test of overidentifying restrictions: when will it have low power?
As we have one endogenous variable (expenditure) in our outcome model and more than one instrument available for health-care expenditure, our estimating equation is said to be ‘overidentified’. With more instruments than endogenous regressors, there is more than one way of using the instruments to estimate the parameter β1 on the endogenous variable. The Hansen–Sargan J test of overidentifying restrictions calculates whether different instruments or different combinations of instruments generate significantly different values for the coefficient (β1) on the endogenous variable in the equation of interest. If significant differences are detected then the test will reject the null hypothesis that all instruments are jointly valid. Of course, the test does not reveal which instrument(s) is(are) invalid; instead, the test uses the fact that different instruments (or combinations thereof) generate different estimates of β1 to infer that something is wrong with the set of instruments. Even if all of the instruments are invalid in the sense that they are all correlated with the error term in the equation of interest (and thus belong in the outcome equation as regressors), the test can detect this failure if the induced biases in the estimates of β1 differ across instruments. This ‘vector-of-contrasts’ interpretation of the Hansen–Sargan test makes it clear when the J test will lack power to reject the null hypothesis when it is false. The J statistic will be small when the null hypothesis of valid instruments is correct; but it will also be small if the biases induced in β1^ by invalid instruments all coincide (i.e. the instruments all identify the same wrong parameter). 150
Most of our estimated models involve the use of two instruments. Kovandic et al. 150 point that when there are only two instruments:
. . . the J test statistic is numerically identical to a Hausman test statistic that contrasts the estimator using both instruments with an estimator using just one instrument. The intuition [behind this result] is . . . straightforward: a Hausman test will reject the null hypothesis that the two estimators being contrasted are both consistent so long as the estimators converge to different values. It is not a requirement for one of the two estimators to be consistent for the Hausman test (and therefore the J test) to have power to reject the null.
One implication of this observation is that misspecification, in the conditional mean of the model, need not necessarily cause the Hansen–Sargan test to fail.
Kovandic et al. point out that these arguments suggest:
‘. . . that the more unrelated the instruments are to each other, the more credible is a failure to reject the null that the instruments are exogenous, since a failure to reject would require that two unrelated instruments generate the same asymptotic bias in β1^
p. 19150
Schaffer argues that:
[d]ifferent sets of instruments are likely to have more or less power depending on where they come from. If all the instruments are minor variations on the same variable – e.g. they are the same variable but lagged a few different periods – then they are all likely to identify the same psuedo-parameter. The critique of low power is going to be fairly convincing here.
Professor Mark Schaffer, Heriot-Watt University, 2011, personal communication
On the other hand, if the instruments are very different and, even better, there are ex ante reasons for thinking that if they are invalid, they are invalid in ‘different ways’, the J test will have more power. For example, suppose two instruments are available and it is thought that, if one is invalid, it will bias the estimated parameter upwards but, if the other instrument is invalid, it will bias the estimated parameter downwards. If the Hansen–Sargan J test fails to reject in this setting, it is a convincing result (Professor Mark Schaffer, personal communication).
In this study we typically use any two from three available instruments when estimating our outcome equations. These three instruments are:
-
the proportion of households that are lone pensioner households (from the 2001 Census80)
-
the proportion of residents providing more than 1 hour of unpaid care per week (from the 2001 Census80); and
-
the IMD2007.
For the Hansen–Sargan J test to have low power the use of any two of these instruments should generate the same asymptotic bias in β1^. However, it is far from obvious that this will be the case, particularly given that our outcome equation already includes a measure of the need for health care.
Nevertheless, we must admit that it is possible that our instruments are correlated with both expenditure and some unobserved factor which is directly influencing the mortality rate, and that the induced bias in β1^ is the same for both instruments. In the next section we therefore examine the sensitivity of the estimated outcome elasticity to the validity of the exclusion restrictions.
In summary:
-
The Hansen–Sargan J test of overidentifying restrictions calculates whether different instruments or different combinations of instruments generate significantly different values for the coefficient (β1) on the endogenous variable in the equation of interest. If significant differences are detected then the test will reject the null hypothesis that all instruments are jointly valid.
-
The J test uses the fact that different instruments (or combinations thereof) generate different estimates of β1 to infer that something is wrong with the chosen set of instruments.
-
Even if all of the instruments are invalid in the sense that they are all correlated with the error term in the equation of interest, the test can detect this failure if the induced biases in the estimates of β1 differ across instruments.
-
This ‘vector-of-contrasts’ interpretation of the Hansen–Sargan test also makes it clear when the J test will lack power to reject the null hypothesis when it is false. The J statistic will be small when the null hypothesis of valid instruments is correct; but it will also be small if the biases induced in β1^ by invalid instruments all coincide (i.e. the instruments all identify the same wrong parameter).
-
Most of our outcome models use two from the following three instruments: lone pensioners, multiple deprivation and unpaid carers. Thus, our Hansen–Sargan test statistics are likely to have low power if our selected pair of instruments are both inducing the same bias in β1^. It is far from obvious that these instruments will induce the same bias in the coefficient on expenditure.
-
However, in case our instruments are imparting the same bias to β1^, the next section examines the sensitivity of the estimated outcome elasticity to the validity of the exclusion restrictions.
The value selection problem
Given that the Hansen–Sargan J test might be unable to detect the presence of invalid instruments in some (rather restrictive) circumstances, several studies have suggested that researchers using IV techniques should subject the estimated coefficient on the endogenous variable to a sensitivity analysis (e.g. Conley et al. ;88 Small89). Recall that IV estimation involves the assumption that the instruments do not belong in the equation of interest (i.e. in the outcome equation). In other words, the assumption is that the coefficients γ1 and γ2 on the instruments z1 and z2 in the outcome model:
are identically zero (where y is mortality, x is expenditure, and n is a measure of the need for health care). One suggestion is that investigators should relax the assumption that γ1 and γ2 are identically zero and examine the impact of this relaxation on the estimated value for β1. This proposal, however, raises the issue of which non-zero values should be imposed on γ1 and γ2.
Proponents of this approach suggest that prior information about the extent of deviations from the exact exclusion restriction might be drawn from other research studies or from subject matter experts. 88,89 In the present context, however, we have no prior beliefs about the likely values for, or even the signs on, γ1 and γ2.
As a starting point we re-estimated the outcome model for the 2006/7 cancer programme 420 times, assuming a uniform distribution between −1 and 1 for both γ1 and γ2. af,agTable 78 shows the estimated coefficients on expenditure (β1^) in our cancer outcome equation associated with the various pairs of values imposed on γ1 and γ2. The coefficients in Table 78 indicate that the outcome elasticity is rather sensitive to the precise values assigned to γ1 and γ2. However, in the absence of any guidance from other research studies or from subject matter experts, we require a method that will identify a plausible range of values for both γ1 and γ2, and which we can use as the basis for our sensitivity analysis.
Coefficients on expenditure (β1^) | Imposed coefficient on IMD variable (γ2) | |||||||||||||||||||||
---|---|---|---|---|---|---|---|---|---|---|---|---|---|---|---|---|---|---|---|---|---|---|
−1 | −0.9 | −0.8 | −0.7 | −0.6 | −0.5 | −0.4 | −0.3 | −0.2 | −0.1 | 0.0 | 0.1 | 0.2 | 0.3 | 0.4 | 0.5 | 0.6 | 0.7 | 0.8 | 0.9 | 1.0 | ||
Imposed coefficient on lone pensioner households variable (γ1) | 1.00 | −4.47 | −4.22 | −3.96 | −3.71 | −3.45 | −3.20 | −2.95 | −2.70 | −2.45 | −2.20 | −1.96 | −1.71 | −1.47 | −1.23 | −0.99 | −0.75 | −0.50 | −0.24 | 0.03 | 0.30 | 0.57 |
0.90 | −4.31 | −4.06 | −3.80 | −3.54 | −3.29 | −3.04 | −2.79 | −2.53 | −2.29 | −2.04 | −1.80 | −1.55 | −1.31 | −1.07 | −0.83 | −0.59 | −0.33 | −0.07 | 0.20 | 0.47 | 0.74 | |
0.80 | −4.15 | −3.90 | −3.64 | −3.38 | −3.13 | −2.88 | −2.62 | −2.37 | −2.13 | −1.88 | −1.64 | −1.40 | −1.16 | −0.92 | −0.67 | −0.42 | −0.16 | 0.10 | 0.37 | 0.65 | 0.92 | |
0.70 | −3.99 | −3.74 | −3.48 | −3.22 | −2.97 | −2.72 | −2.46 | −2.21 | −1.96 | −1.72 | −1.48 | −1.24 | −1.00 | −0.76 | −0.51 | −0.26 | 0.01 | 0.28 | 0.55 | 0.82 | 1.10 | |
0.60 | −3.83 | −3.58 | −3.32 | −3.06 | −2.81 | −2.55 | −2.30 | −2.05 | −1.80 | −1.56 | −1.32 | −1.08 | −0.84 | −0.60 | −0.35 | −0.09 | 0.18 | 0.45 | 0.73 | 1.00 | 1.28 | |
0.50 | −3.67 | −3.41 | −3.16 | −2.90 | −2.65 | −2.39 | −2.14 | −1.89 | −1.64 | −1.40 | −1.16 | −0.92 | −0.68 | −0.44 | −0.18 | 0.08 | 0.35 | 0.63 | 0.91 | 1.18 | 1.45 | |
0.40 | −3.51 | −3.25 | −3.00 | −2.74 | −2.49 | −2.23 | −1.98 | −1.73 | −1.48 | −1.23 | −0.99 | −0.76 | −0.52 | −0.27 | −0.01 | 0.26 | 0.53 | 0.81 | 1.09 | 1.36 | 1.63 | |
0.30 | −3.35 | −3.09 | −2.84 | −2.58 | −2.32 | −2.07 | −1.82 | −1.57 | −1.32 | −1.07 | −0.83 | −0.60 | −0.35 | −0.10 | 0.16 | 0.44 | 0.71 | 0.99 | 1.26 | 1.53 | 1.80 | |
0.20 | −3.19 | −2.93 | −2.68 | −2.42 | −2.16 | −1.91 | −1.65 | −1.40 | −1.15 | −0.91 | −0.67 | −0.43 | −0.19 | 0.07 | 0.34 | 0.62 | 0.90 | 1.17 | 1.44 | 1.71 | 1.97 | |
0.10 | −3.03 | −2.77 | −2.51 | −2.26 | −2.00 | −1.75 | −1.49 | −1.24 | −0.99 | −0.75 | −0.51 | −0.27 | −0.02 | 0.25 | 0.52 | 0.80 | 1.07 | 1.34 | 1.61 | 1.88 | 2.14 | |
0.00 | −2.87 | −2.61 | −2.35 | −2.10 | −1.84 | −1.58 | −1.33 | −1.07 | −0.83 | −0.58 | −0.34 | −0.10 | 0.15 | 0.43 | 0.70 | 0.98 | 1.25 | 1.52 | 1.78 | 2.04 | 2.31 | |
−0.10 | −2.71 | −2.45 | −2.19 | −1.93 | −1.68 | −1.42 | −1.16 | −0.91 | −0.66 | −0.42 | −0.18 | 0.07 | 0.33 | 0.61 | 0.88 | 1.15 | 1.42 | 1.68 | 1.95 | 2.21 | 2.47 | |
−0.20 | −2.55 | −2.29 | −2.03 | −1.77 | −1.51 | −1.26 | −1.00 | −0.75 | −0.50 | −0.25 | −0.01 | 0.24 | 0.51 | 0.79 | 1.06 | 1.32 | 1.59 | 1.85 | 2.11 | 2.37 | 2.63 | |
−0.30 | −2.39 | −2.13 | −1.87 | −1.61 | −1.35 | −1.10 | −0.84 | −0.58 | −0.33 | −0.09 | 0.15 | 0.41 | 0.68 | 0.95 | 1.22 | 1.48 | 1.75 | 2.01 | 2.27 | 2.53 | 2.79 | |
−0.40 | −2.23 | −1.97 | −1.71 | −1.45 | −1.19 | −0.93 | −0.68 | −0.42 | −0.17 | 0.06 | 0.30 | 0.57 | 0.84 | 1.11 | 1.38 | 1.64 | 1.91 | 2.17 | 2.43 | 2.69 | 2.95 | |
−0.50 | −2.07 | −1.81 | −1.55 | −1.29 | −1.03 | −0.78 | −0.52 | −0.27 | −0.03 | 0.20 | 0.44 | 0.72 | 1.00 | 1.27 | 1.54 | 1.80 | 2.06 | 2.32 | 2.58 | 2.84 | 3.10 | |
−0.60 | −1.91 | −1.65 | −1.39 | −1.13 | −0.87 | −0.62 | −0.37 | −0.13 | 0.10 | 0.32 | 0.58 | 0.87 | 1.15 | 1.42 | 1.69 | 1.95 | 2.22 | 2.48 | 2.74 | 3.00 | 3.26 | |
−0.70 | −1.75 | −1.49 | −1.23 | −0.97 | −0.72 | −0.47 | −0.22 | 0.01 | 0.22 | 0.46 | 0.73 | 1.02 | 1.30 | 1.57 | 1.84 | 2.11 | 2.37 | 2.63 | 2.89 | 3.16 | 3.42 | |
−0.80 | −1.59 | −1.33 | −1.07 | −0.82 | −0.57 | −0.32 | −0.09 | 0.14 | 0.35 | 0.60 | 0.88 | 1.17 | 1.45 | 1.72 | 1.99 | 2.26 | 2.52 | 2.79 | 3.05 | 3.31 | 3.57 | |
−0.90 | −1.43 | −1.17 | −0.92 | −0.67 | −0.42 | −0.18 | 0.05 | 0.27 | 0.50 | 0.76 | 1.04 | 1.32 | 1.60 | 1.88 | 2.15 | 2.41 | 2.68 | 2.94 | 3.20 | 3.47 | 3.73 | |
−1.00 | −1.27 | −1.02 | −0.76 | −0.52 | −0.27 | −0.04 | 0.18 | 0.41 | 0.65 | 0.92 | 1.20 | 1.48 | 1.76 | 2.03 | 2.30 | 2.57 | 2.83 | 3.09 | 3.36 | 3.62 | 3.88 |
The identification of values to be imposed on the coefficients on the excluded instruments
Our outcome equations typically involve two instruments and one endogenous regressor. With this structure we can re-estimate our outcome model twice, each time including one of the previously excluded instruments to the equation of interest. In particular, we can estimate:
and then
with the same set of (included and excluded) instruments (n, z1, and z2) being used to instrument × 1 in both cases. This provides us with coefficient and variance estimates for γ1 and γ2 and we can sample from these point estimates and their distributions to examine the impact of different (non-zero) values for γ1 and γ2 on the outcome elasticity (β1^).
The sampling procedure is straightforward. We sample from these estimates and their distributions by drawing two random numbers from a standard normal distribution and we form the product of these numbers and the standard errors associated with our estimates of γ1 and γ2. Our sampled pair of values of γ1 and γ2 (call these sampled values γ˜1 and γ˜2) are then the sum of these products and the respective coefficient estimates of γ1 and γ2. Table 79 shows the relevant coefficient and variance estimates for γ1 and γ2 that are used as part of this sampling procedure.
Programme | Instrument | Coefficient | Standard error | Variance | Covariance |
---|---|---|---|---|---|
Cancer | z1: lone pensioner households | −0.2074942 | 0.1773647 | 0.0314582 | 0.00494454 |
z2: IMD2007 | −0.0827677 | 0.1141054 | 0.0130200 | ||
Circulatory disease | z1: lone pensioner households | −0.2606290 | 0.2441101 | 0.059590 | 0.01122591 |
z2: IMD2007 | −0.2105334 | 0.2879230 | 0.082900 | ||
Respiratory problems | z1: long-term unemployment rate | 0.2642582 | 0.13273061 | 0.0176174 | −0.02136305 |
z2: LLT rate | −1.739808 | 1.611403 | 2.5966196 | ||
Gastrointestinal problems | z1: unpaid carers | 1.812286 | 2.347459 | 5.510564 | 0.08016639 |
z2: IMD2007 | 0.5567431 | 0.2066839 | 0.0427822 |
The estimation of Equations 18 and 19 does not generate estimates of γ1 and γ2 as part of the same model and so the sampling procedure outlined above implicitly assumes a zero covariance between these estimates. If we want to incorporate a covariance term into the sampling procedure this must be obtained from elsewhere. In the absence of an obviously better approach, we obtain a covariance term from the OLS estimation of our outcome model with the previously excluded instruments both included in the regression equation. Thus we estimate:
where y, x, n, z1 and z2 have their usual meaning, and where β1 is constrained to be equal to its value from the IV estimation of Equation 15. The value for the covariance term between the estimates of γ1 and γ2 for each of the big four programmes is shown in the final column of Table 79.
The sampling procedure from our estimates of γ1 and γ2 with a non-zero covariance is essentially the same as that outlined above but it incorporates the presence of a covariance term for the estimates of γ1 and γ2. We can illustrate this procedure using data from the cancer outcome model. First, we form the implied variance-covariance matrix for the estimates of γ1 and γ2:
lone_pensioners | IMD2007 | |
lone_pensioners | 0.031458 | 0.004944 |
IMD2007 | 0.004944 | 0.013020 |
Second, we form the product of a pair of random numbers from the standard normal distribution (r1, r2) with the Cholesky decomposition matrix from the variance-covariance matrix for the estimates of γ1 and γ2. The latter is given by:
lone_pensioners | IMD2007 | |
lone_pensioners | 0.177364 | 0 |
IMD2007 | 0.027877 | 0.110647 |
Finally, we add this pair of products to the respective coefficient estimates of γ1 and γ2 to obtain our sampled pair of values of γ1 and γ2 (call these γ˜1 and γ˜2). In other words, for each pair of random numbers (r1, r2), we calculate the sampled values γ˜1 and γ˜2 where:
This sampling procedure is undertaken 1000 times, both with a zero covariance between γ^1 and γ^2, and again with a non-zero covariance.
These procedures generate two sets of 1000 pairs of values for γ^1 and γ^2 (one set assumes a zero covariance between γ^1 and γ^2, and the other does not). These sets of values for γ^1 and γ^2 can be used to examine the sensitivity of the estimated outcome elasticity to alternative non-zero values for the coefficients on the excluded instruments.
Obtaining the outcome elasticities associated with sampled coefficients on the excluded instruments
For each pair of sampled values of γ1 and γ2 (γ^1 and γ^2), we can use IV techniques to estimate the model:
with the usual instrument set (x2, z1 and z2) used to instrument the endogenous variable × 1 (expenditure). For each pair of sampled values γ˜1 and γ˜2, we obtain a different outcome elasticity (β1^) and these different values can be plotted in a histogram. Such a plot illustrates the uncertainty associated with our point estimate of the outcome elasticity due to doubts about the validity of our instruments; we call this type of uncertainty ‘level 2’ uncertainty. This ‘level 2’ uncertainty is in addition to what we label ‘level 1’ uncertainty, that is, the uncertainty about the value of the outcome elasticity assuming the validity of our exclusion restrictions (remember that our estimated outcome elasticity is only a point estimate and that it has a distribution attached to it). To illustrate this ‘level 1’ uncertainty, we can sample from the distribution of the point estimate for the outcome elasticity from our basic IV model (where γ˜1 and γ˜2 are zero in Equation 21) and plot the sampled values (Figures 16–43).
Plots illustrating the degree of level 1 uncertainty for each of the big four programmes are shown as Figures 16 (cancer), 23 (circulatory disease), 30 (respiratory problems) and 37 (gastrointestinal problems). These level 1 uncertainty plots can be compared with plots of β1^ from the estimation of Equation 21. The latter plots illustrate the degree of level 2 uncertainty, that is, the uncertainty associated with our point estimate of the outcome elasticity due to doubts about the validity of the instruments. Figures 17 (cancer), 24 (circulatory disease), 31 (respiratory problems) and 38 (gastrointestinal problems) show plots of the outcome elasticity (β1 from Equation 21) assuming a zero covariance between γ^1 and γ^2 in Equations 18 and 19. Figures 18 (cancer), 25 (circulatory disease), 32 (respiratory problems) and 39 (gastrointestinal problems) show plots of the outcome elasticity (β1^ from Equation 21) assuming a non-zero covariance between γ^1 and γ^2 in Equations 18 and 19.
FIGURE 16.
Sampling 1000 values from the distribution of the point estimate for the cancer outcome elasticity. Note: the mean value of these 1000 sampled outcome elasticities is −0.338. The outcome elasticity for cancer expenditure in the basic IV model is −0.342.

FIGURE 17.
Sensitivity of the outcome elasticity for cancer expenditure to 1000 alternative pairs of coefficients on the excluded instruments with a zero covariance between these coefficients. Note: the mean value of the 1000 outcome elasticities is −0.209 (mean SE = 0.109). The outcome elasticity for cancer expenditure in the basic IV model is −0.342 (SE = 0.099). SE, standard error.

FIGURE 18.
Sensitivity of the outcome elasticity for cancer expenditure to 1000 alternative pairs of coefficients on the excluded instruments with a non-zero covariance between the coefficients. Note: the mean value of the 1000 outcome elasticities is −0.209 (mean SE = 0.105). The outcome elasticity for cancer expenditure in the basic IV model is −0.342 (SE = 0.099). SE, standard error.

FIGURE 19.
Sampling from the 1000 outcome elasticities for cancer expenditure (that were generated using 1000 alternative pairs of coefficients on the excluded instruments with a zero covariance between these coefficients). Note: the mean value of these 1000 sampled outcome elasticities is −0.220. The outcome elasticity for cancer expenditure in the basic IV model is −0.342.
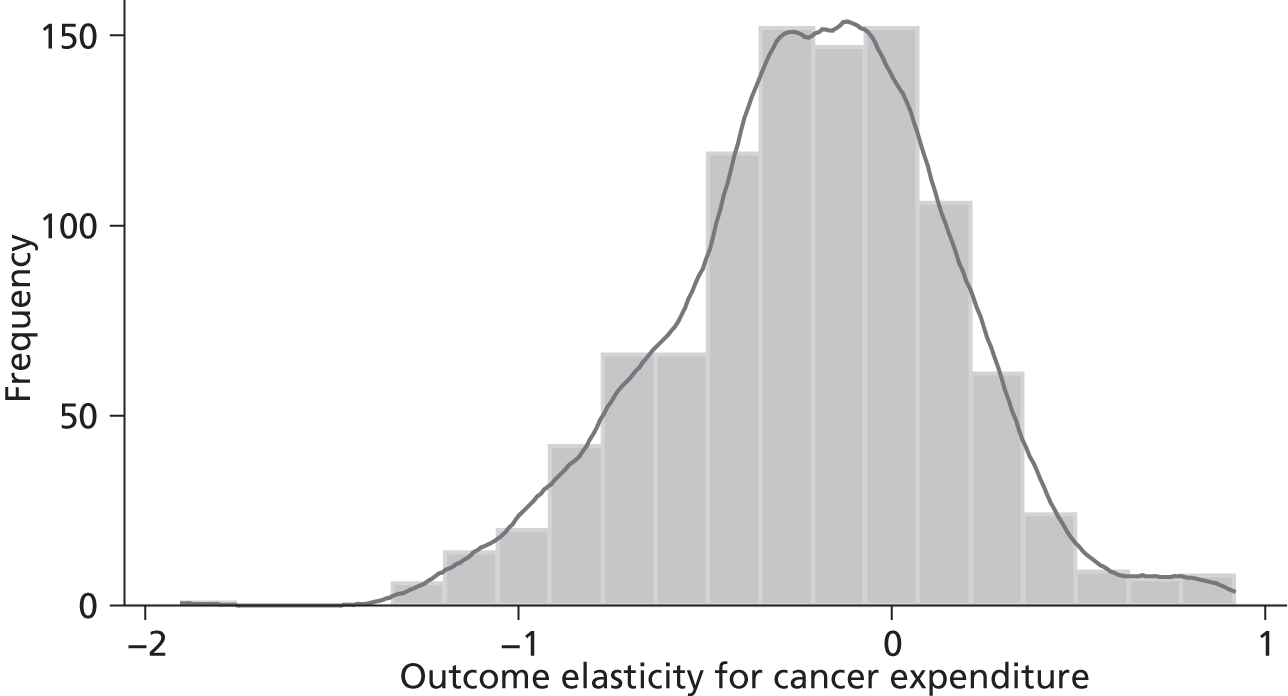
FIGURE 20.
Sampling from the 1000 outcome elasticities for cancer expenditure (that were generated using 1000 alternative pairs of coefficients on the excluded instruments with a non-zero covariance between these coefficients). Note: the mean value of these 1000 sampled outcome elasticities is −0.218. The outcome elasticity for cancer expenditure in the basic IV model is −0.342.

FIGURE 21.
Kernel density plots from Figures 16, 17 and 19: illustrating the uncertainty associated with the point estimate for the cancer outcome. Note: the mean value of the level 1/level 2/level 3 elasticities is −0.338/−0.209/−0.220. The outcome elasticity for cancer expenditure in the basic IV model is −0.342.

FIGURE 22.
Kernel density plots from Figures 16, 18 and 20: illustrating the uncertainty associated with the point estimate for the cancer outcome. Note: the mean value of the level 1/level 2/level 3 elasticities is −0.338/−0.209/−0.218. The outcome elasticity for cancer expenditure in the basic IV model is −0.342.

FIGURE 23.
Sampling 1000 values from the distribution of the point estimate for the circulatory disease outcome elasticity. Note: the mean value of these 1000 sampled outcome elasticities is −1.418. The outcome elasticity for circulatory disease expenditure in the comparable IV model is −1.427.

FIGURE 24.
Sensitivity of the outcome elasticity for circulatory disease expenditure to 1000 alternative pairs of coefficients on the excluded instruments with a zero covariance between these coefficients. Note: the mean value of the 1000 outcome elasticities is −1.697 (mean SE = 0.269). The outcome elasticity for circulatory expenditure in the comparable IV model is −1.427 (SE = 0.228). SE, standard error.
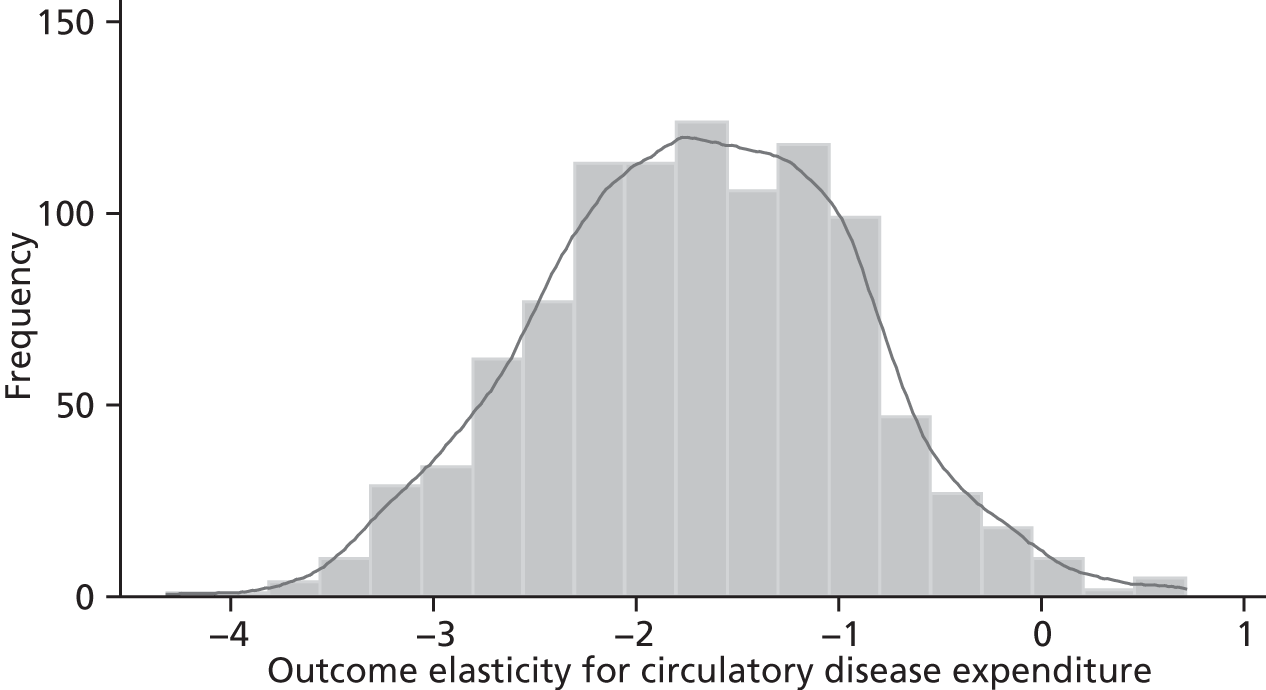
FIGURE 25.
Sensitivity of the outcome elasticity for circulatory disease expenditure to 1000 alternative pairs of coefficients on the excluded instruments with non-zero covariance between the coefficients. Note: the mean value of the 1000 outcome elasticities is −1.700 (mean SE = 0.269). The outcome elasticity for circulatory expenditure in the comparable IV model is −1.427 (SE = 0.228). SE, standard error.

FIGURE 26.
Sampling from the 1000 outcome elasticities for circulatory disease expenditure (that were generated using 1000 alternative pairs of coefficients on the excluded instruments with a zero covariance between these coefficients). Note: the mean value of these 1000 sampled outcome elasticities is −1.717. The outcome elasticity for circulatory expenditure in the comparable IV model is −1.427.
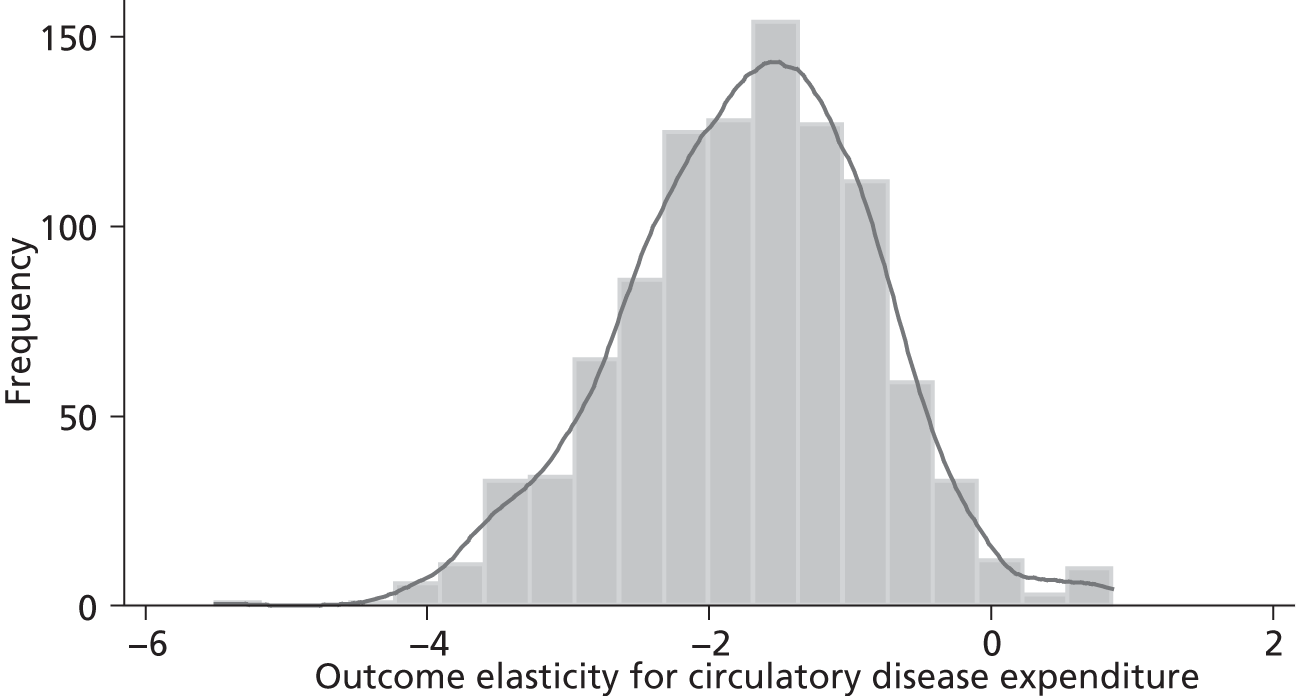
FIGURE 27.
Sampling from the 1000 outcome elasticities for circulatory disease expenditure (that were generated using 1000 alternative pairs of coefficients on the excluded instruments with a non-zero covariance between these coefficients). Note: the mean value of these 1000 sampled outcome elasticities is −1.718. The outcome elasticity for circulatory expenditure in the comparable IV model is −1.427.
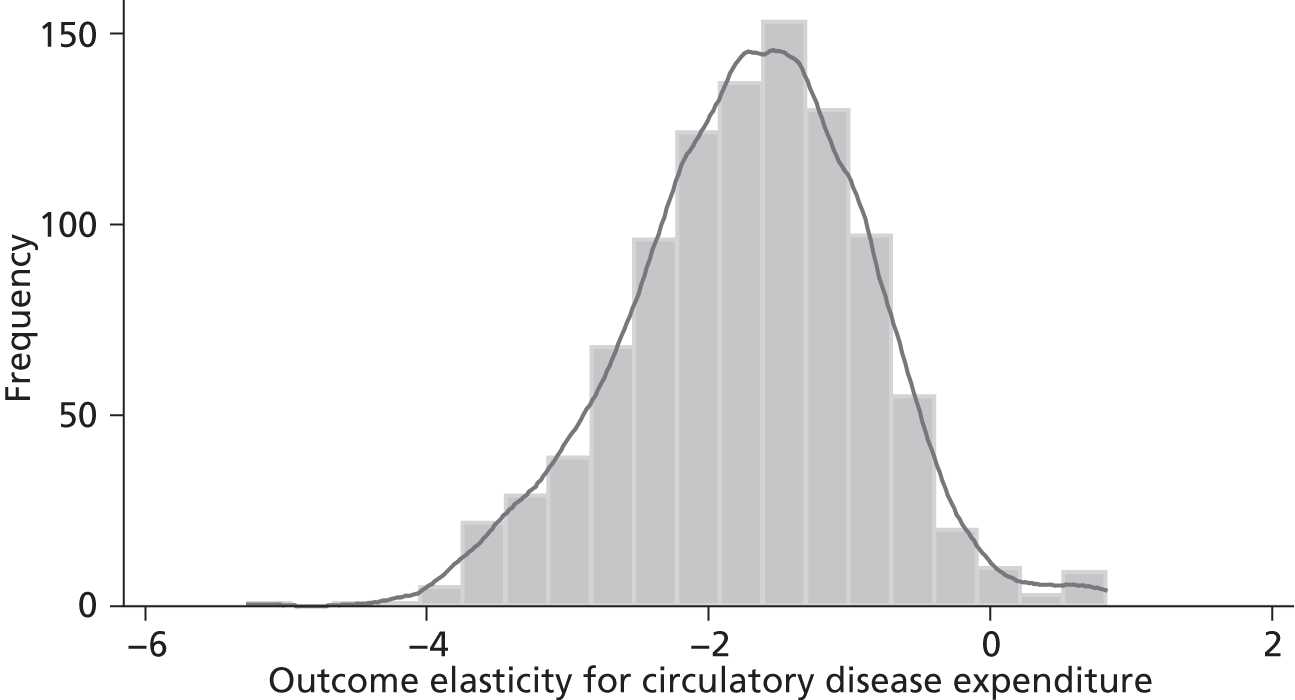
Finally, the point estimates β1^ from the estimation of Equation 21 also have a standard error and we can sample from these distributions. These sampled values illustrate what we term ‘level 3’ uncertainty, that is, the uncertainty associated with the value of the outcome elasticity due to both level 1 (sampling) and level 2 (instrument invalidity) effects.
Plots illustrating the degree of level 3 uncertainty, assuming a zero covariance between γ^1 and γ^2 in Equations 18 and 19, are shown as Figures 19 (cancer), 26 (circulatory disease), 33 (respiratory problems) and 40 (gastrointestinal problems). Plots illustrating the degree of level 3 uncertainty, assuming a non-zero covariance between γ^1 and γ^2 in Equations 18 and 19, are shown as Figures 20 (cancer), 27 (circulatory disease), 34 (respiratory problems) and 41 (gastrointestinal problems).
FIGURE 28.
Kernel density plots from Figures 23, 24 and 26: illustrating the uncertainty associated with the point estimate for the circulatory disease outcome elasticity. Note: the mean value of the level 1/level 2/level 3 elasticities is –1.418/–1.697/–1.717. The outcome elasticity for circulatory disease expenditure in the comparable IV model is −1.427.
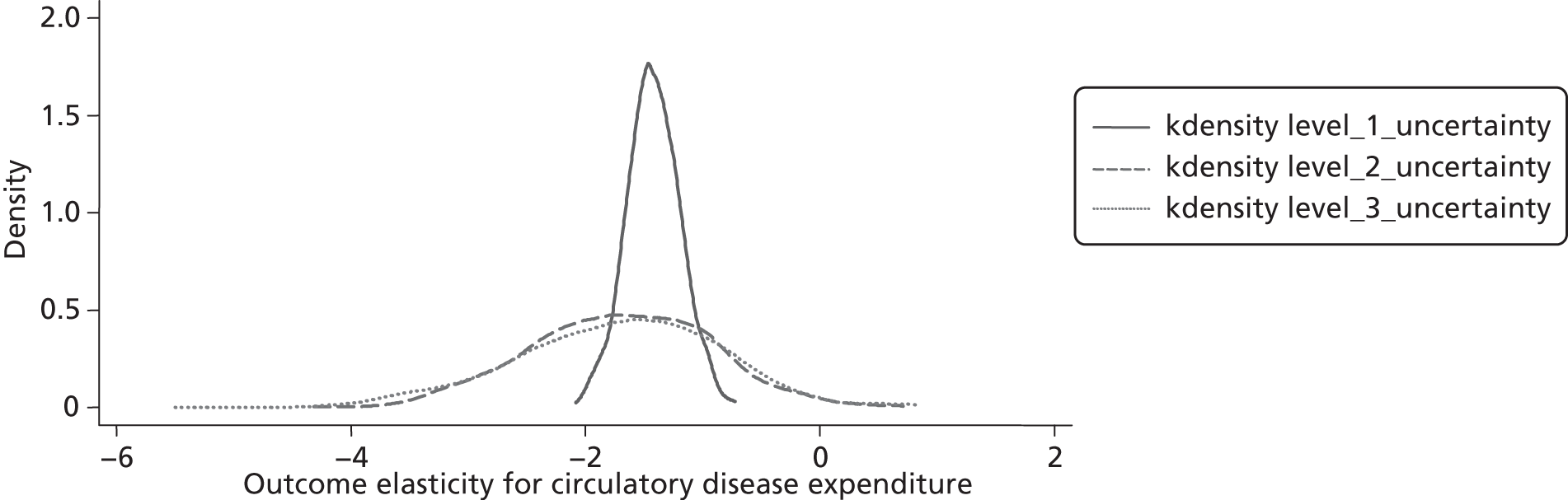
FIGURE 29.
Kernel density plots from Figures 23, 25 and 27: illustrating the uncertainty associated with the point estimate for the circulatory disease outcome elasticity. Note: the mean value of the level 1/level 2/level 3 elasticities is –1.418/–1.700/–1.718. The outcome elasticity for circulatory disease expenditure in the comparable IV model is −1.427.
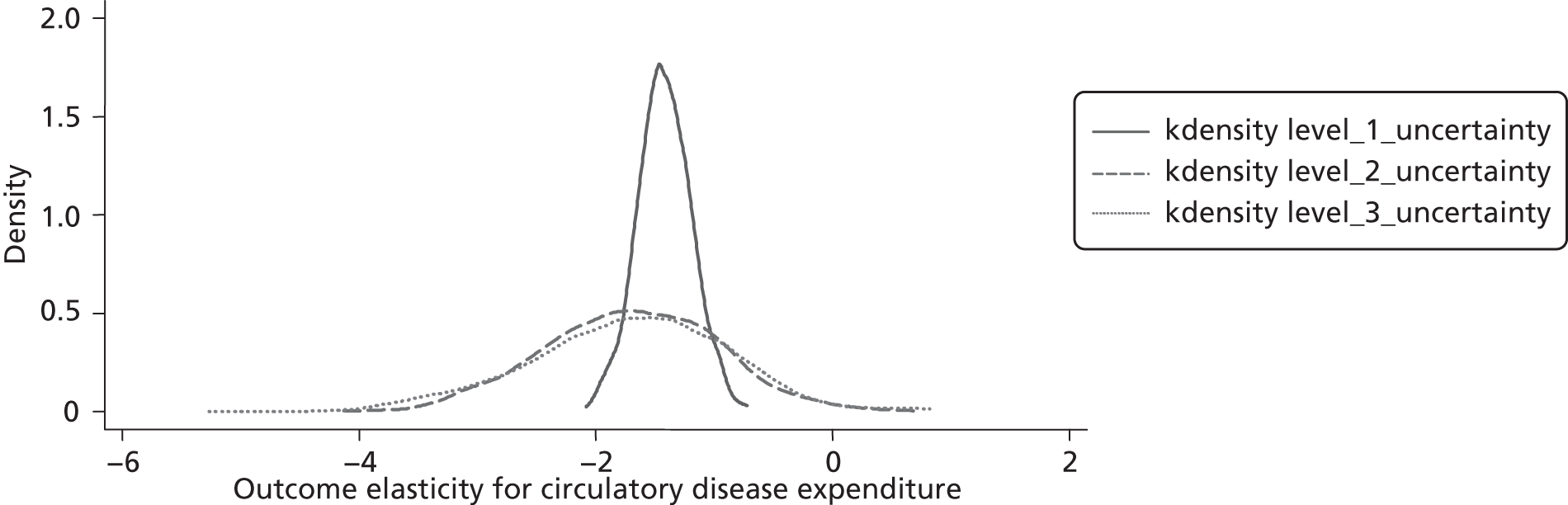
FIGURE 30.
Sampling 1000 values from the distribution of the point estimate for the respiratory disease outcome elasticity. Note: The mean value of these 1000 sampled outcome elasticities is –2.004. The outcome elasticity for respiratory expenditure in the comparable IV model is –2.029.

FIGURE 31.
Sensitivity of the outcome elasticity for respiratory disease expenditure to 1000 alternative pairs of coefficients on the excluded instruments with a zero covariance between these coefficients. Note: the mean value of the 1000 outcome elasticities is –1.145 (mean SE = 0.489). The outcome elasticity for respiratory expenditure in the comparable IV model is –2.029 (SE = 0.636). SE, standard error.

FIGURE 32.
Sensitivity of the outcome elasticity for respiratory disease expenditure to 1000 alternative pairs of coefficients on the excluded instruments with a non−zero covariance between the coefficients. Note: the mean value of the 1000 outcome elasticities is –1.149 (mean SE = 0.485). The outcome elasticity for respiratory expenditure in the comparable IV model is –2.029 (SE = 0.636). SE, standard error.

FIGURE 33.
Sampling from the 1000 outcome elasticities for respiratory disease expenditure (that were generated using 1000 alternative pairs of coefficients on the excluded instruments with a zero covariance between these coefficients). Note: the mean value of these 1000 sampled outcome elasticities is −1.146. The outcome elasticity for respiratory expenditure in the comparable IV model is −2.029.

FIGURE 34.
Sampling from the 1000 outcome elasticities for respiratory disease expenditure (that were generated using 1000 alternative pairs of coefficients on the excluded instruments with a non-zero covariance between these coefficients). Note: the mean value of these 1000 sampled outcome elasticities is −1.151. The outcome elasticity for respiratory expenditure in the comparable IV model is −2.029.

FIGURE 35.
Kernel density plots from Figures 30, 31 and 33: illustrating the uncertainty associated with the point estimate for the respiratory disease outcome elasticity. Note: the mean value of the level 1/level 2/level 3 elasticities is –2.004/–1.145/–1.146. The outcome elasticity for respiratory disease expenditure in the comparable IV model is –2.029.

Uncertainty and the value of the cancer outcome elasticity
Figure 16 plots 1000 values from the distribution of the point estimate for the cancer outcome elasticity. The mean value of these sampled values is −0.338 (the outcome elasticity in the basic IV model is −0.342 and its standard error is 0.099) and virtually all of them lie between 0 and −0.6.
The histogram in Figure 17 provides a plot of 1000 point estimates for the cancer outcome elasticity if we drop the assumption that the coefficients on the excluded instruments are exactly zero (and we also assume a zero covariance between γ^1 and γ^2 in Equations 18 and 19). The mean value of these 1000 outcome elasticities is −0.209 and this is about one-third lower than the elasticity in the basic IV model (−0.342). In addition, the mean value of the standard errors associated with these 1000 elasticities (0.109) is slightly greater than the standard error in the basic IV model (0.099) so that about one-quarter of the outcome elasticities in Figure 17 take a non-negative value.
The histogram in Figure 18 provides a similar plot to that in Figure 17 but this time we assume a non-zero covariance between γ^1 and γ^2 in Equations 18 and 19. There is very little difference between the zero (see Figure 17) and non-zero (see Figure 18) covariance plots, with both the mean elasticity and mean standard error virtually identical in both plots.
The histograms in Figures 17 and 18 provide plots of the point estimate for the cancer outcome elasticity if we drop the assumption that the coefficients on the excluded instruments are exactly zero. Each point estimate also has a standard error and we can sample from these estimates and their distributions to obtain the histograms shown in Figures 19 and 20. With the exception of a slight lengthening in the tail on the left hand side, these plots are very similar to the plots in Figures 17 and 18.
Figure 21 reproduces the three kernel density plots shown in Figures 16, 17 and 19 (remember that Figures 17 and 19 assume a zero covariance between γ^1 and γ^2 in Equations 18 and 19). Together these plots illustrate the impact of all three levels of uncertainty on our estimate of the cancer outcome elasticity. It is clear that the uncertainty induced by the instrument validity issue considerably increases the uncertainty associated with our estimate of the outcome elasticity (compare, for example, the density plot for level 1 uncertainty with those for both level 2 and level 3 uncertainty).
FIGURE 36.
Kernel density plots from Figures 30, 32 and 34: illustrating the uncertainty associated with the point estimate for the respiratory disease outcome elasticity. Note: the mean value of the level 1/level 2/level 3 elasticities is –2.004/–1.149/–1.151. The outcome elasticity for respiratory disease expenditure in the comparable IV model is –2.029.
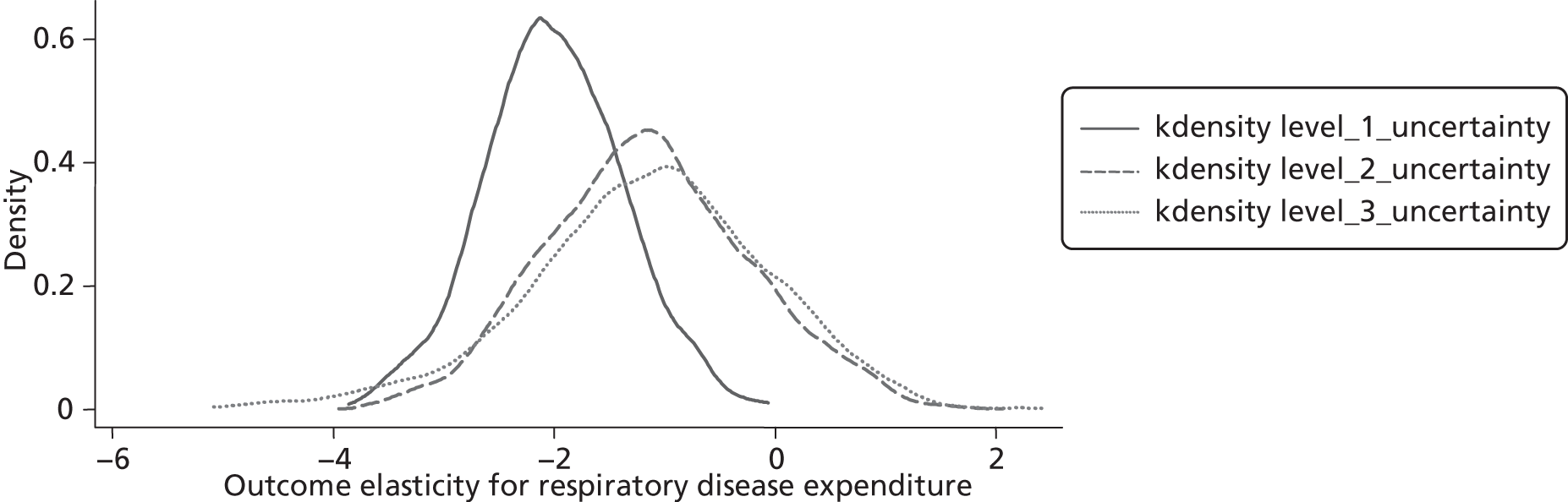
Figure 22 reproduces the three kernel density plots shown in Figures 16, 18 and 20 (remember that Figures 18 and 20 assume a non-zero covariance between γ^1 and γ^2 in Equations 18 and 19). As is the case for Figure 21, these plots illustrate the impact of all three levels of uncertainty on our estimate of the cancer outcome elasticity and, again, it is clear that it is the uncertainty induced by the instrument validity issue that considerably increases the uncertainty associated with our estimate of the outcome elasticity. For example, the standard deviation associated with the level 1 uncertainty density plot is 0.099 but the standard deviation for the level 2 (0.338) and level 3 (0.379) uncertainty density plots are both considerably larger than this.
FIGURE 37.
Sampling 1000 values from the distribution of the point estimate for the gastrointestinal disease outcome elasticity. Note: the mean value of these 1000 sampled outcome elasticities is –1.518. The outcome elasticity for gastrointestinal expenditure in the comparable IV model is –1.536.

Uncertainty and the value of the circulatory disease outcome elasticity
Figure 23 plots 1000 values from the distribution of the point estimate for the circulatory disease outcome elasticity. The mean value of these sampled values is −1.418 and virtually all of these values lie between −2.0 and −0.75. The outcome elasticity in the comparable IV model is −1.427. ah
The histogram in Figure 24 provides a plot of 1000 point estimates for the circulatory disease outcome elasticity if we drop the assumption that the coefficients on the excluded instruments are exactly zero (and we also assume a zero covariance between γ^1 and γ^2 in Equations 18 and 19). The mean value of these 1000 outcome elasticities is −1.697 and this is about one-fifth larger than the elasticity in the comparable IV model (−1.427). Similarly, the mean value of the standard errors associated with these 1000 elasticities (0.269) is also about one-fifth larger than the standard error in the comparable basic IV model (0.228). Virtually all of the point estimates values lie between −4.0 and 0.0, and there are very few non-negative values.
The histogram in Figure 25 provides a similar plot to that in Figure 24 but this time we assume a non-zero covariance between γ^1 and γ^2 in Equations 18 and 19. There is very little difference between the zero (see Figure 24) and non-zero (see Figure 25) covariance plots, with both the mean elasticity and mean standard error virtually identical in both plots.
The histograms in Figures 24 and 25 provide plots of the point estimate for the circulatory disease outcome elasticity if we drop the assumption that the coefficients on the excluded instruments are exactly zero. Each point estimate also has a standard error and we can sample (with replacement) from these estimates and their distributions to obtain the histograms shown in Figures 26 and 27. With the exception of a slight lengthening in the tail on the left hand side (as was also observed for the cancer programme), these plots are very similar to the plots in Figures 24 and 25.
Figure 28 reproduces the three kernel density plots shown in Figures 23, 24 and 26 (remember that Figures 24 and 26 assume a zero covariance between γ^1 and γ^2 in Equations 18 and 19). Together these plots illustrate the impact of all three levels of uncertainty on our estimate of the circulatory disease outcome elasticity. It is clear that the uncertainty induced by the instrument validity issue considerably increases the uncertainty associated with our estimate of the outcome elasticity (note that the range of values increases dramatically from the density plot illustrating level 1 uncertainty to that illustrating level 2 uncertainty).
FIGURE 38.
Sensitivity of the outcome elasticity for gastrointestinal disease expenditure to 1000 alternative pairs of coefficients on the excluded instruments with a zero covariance between these coefficients. Note: the mean value of the 1000 outcome elasticities is –2.365 (mean SE = 0.853). The outcome elasticity for gastrointestinal expenditure in the basic IV model is –1.536 (SE = 0.468). SE, standard error.
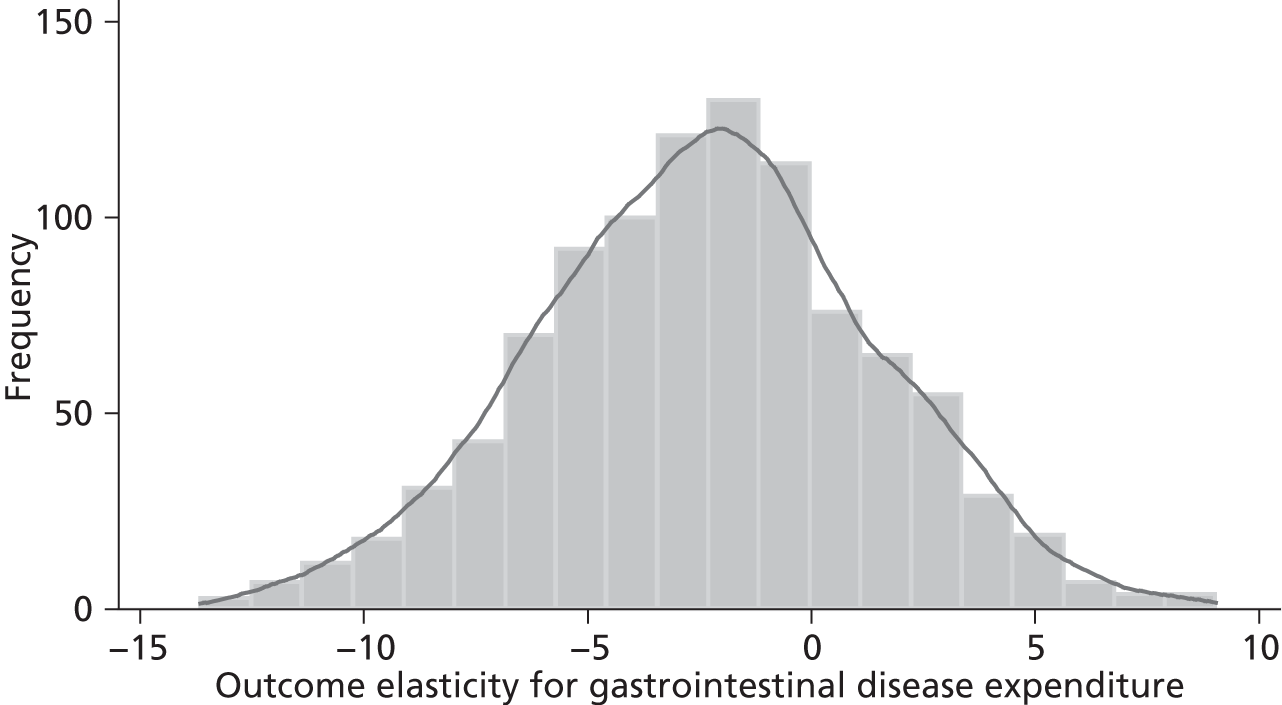
Figure 29 reproduces the three kernel density plots from Figures 23, 25 and 27 (remember that Figures 25 and 27 assume a non-zero covariance between γ^1 and γ^2 in Equations 18 and 19). As is the case for Figure 28, these plots illustrate the impact of all three levels of uncertainty on our estimate on the circulatory disease outcome elasticity and, again, it is clear that it is the uncertainty induced by the instrument validity issue that considerably increases the uncertainty associated with our estimate of the outcome elasticity. For example, the standard deviation associated with the level 1 uncertainty density plot is 0.228 but the standard deviation for the level 2 (0.735) and level 3 (0.843) uncertainty density plots are both considerably larger than this.
FIGURE 39.
Sensitivity of the outcome elasticity for gastrointestinal disease expenditure to 1000 alternative pairs of coefficients on the excluded instruments with non-zero covariance between the coefficients. Note: the mean value of the 1000 outcome elasticities is –2.360 (mean SE = 0.839). The outcome elasticity for gastrointestinal expenditure in the basic IV model is –1.536 (SE = 0.468). SE, standard error.
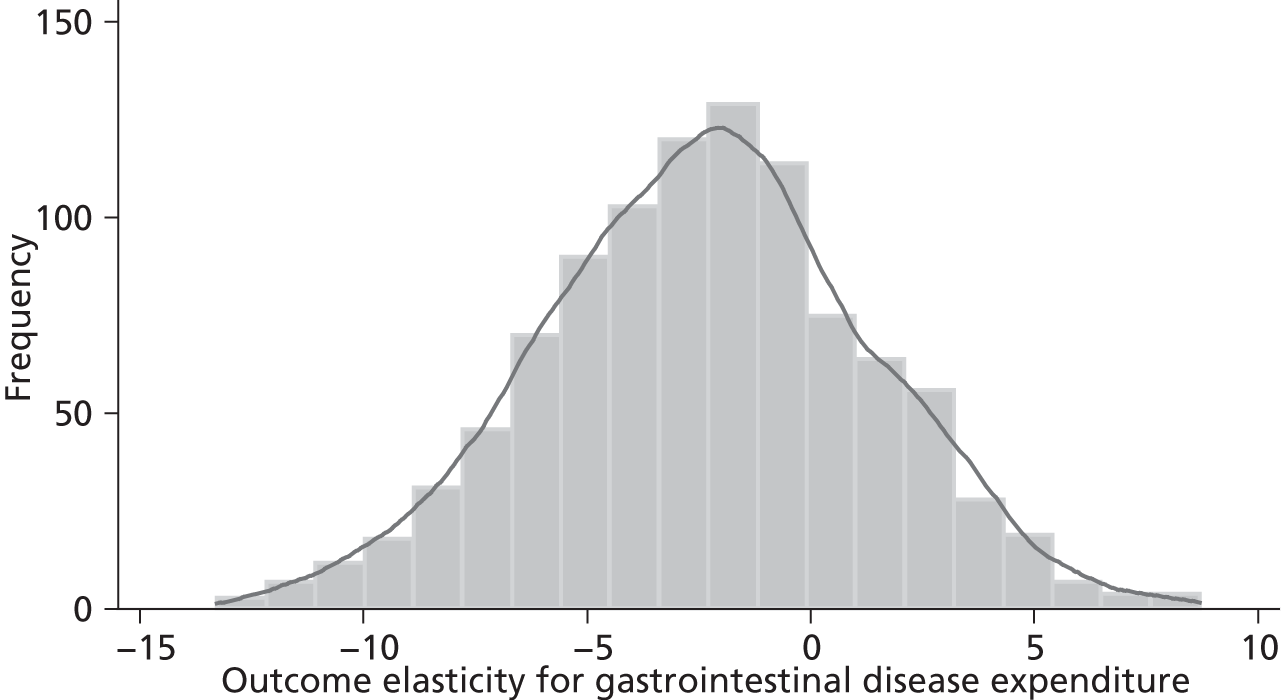
Uncertainty and the value of the respiratory disease outcome elasticity
Figure 30 plots 1000 values from the distribution of the point estimate for the respiratory disease outcome elasticity [see column (5) of Table 65]. The mean value of these sampled values is −2.004 (the outcome elasticity in the comparable IV model is −2.029) and all of these values lie between −4.0 and 0.
The histogram in Figure 31 provides a plot of 1000 point estimates for the respiratory disease outcome elasticity if we drop the assumption that the coefficients on the excluded instruments are exactly zero (and we also assume a zero covariance between γ^1 and γ^2 in Equations 18 and 19). The mean value of these 1000 outcome elasticities from the respiratory disease outcome model (−1.145) is almost one-half of the size of the elasticity in the comparable basic IV model (−2.029). And the mean value of the standard errors associated with these 1000 elasticities (0.489) is about one-quarter less than the standard error in the comparable basic IV model (0.636).
The histogram in Figure 32 provides a similar plot to that in Figure 31 but this time we assume a non-zero covariance between γ^1 and γ^2 in Equations 18 and 19. However, there is very little difference between the zero (see Figure 31) and non-zero (see Figure 32) covariance plots, with both the mean elasticity and mean standard error virtually identical in these two plots.
The histograms in Figures 31 and 32 provide plots of the point estimate for the respiratory disease outcome elasticity if we drop the assumption that the coefficients on the excluded instruments are exactly zero. Each point estimate also has a standard error and we can sample from these estimates and their distributions to obtain the histograms shown in Figures 33 and 34. With the exception of a slight lengthening of the tail on the left-hand side, these plots are similar to those in Figures 31 and 32 so the sampling procedure would appear to have little impact on the distribution of the point elasticities.
Figure 35 reproduces the three kernel density plots shown in Figures 30, 31 and 33 (remember that Figures 31 and 33 assume a zero covariance between γ^1 and γ^2 in Equations 18 and 19). Together these plots illustrate the impact of all three levels of uncertainty on our estimate of the respiratory disease outcome elasticity. It is clear that the uncertainty induced by the instrument validity issue both shifts the density plot to the right and increases the uncertainty associated with our estimate of the outcome elasticity (e.g. the range of values increases from −4.0 to 0.0 at level 1 to −5.0 to 2.5 at level 3).
FIGURE 40.
Sampling from the 1000 outcome elasticities for gastrointestinal disease expenditure (that were generated using 1000 alternative pairs of coefficients on the excluded instruments with a zero covariance between these coefficients). Note: the mean value of these 1000 sampled outcome elasticities is –2.442. The outcome elasticity for gastrointestinal expenditure in the comparable IV model is –1.536.
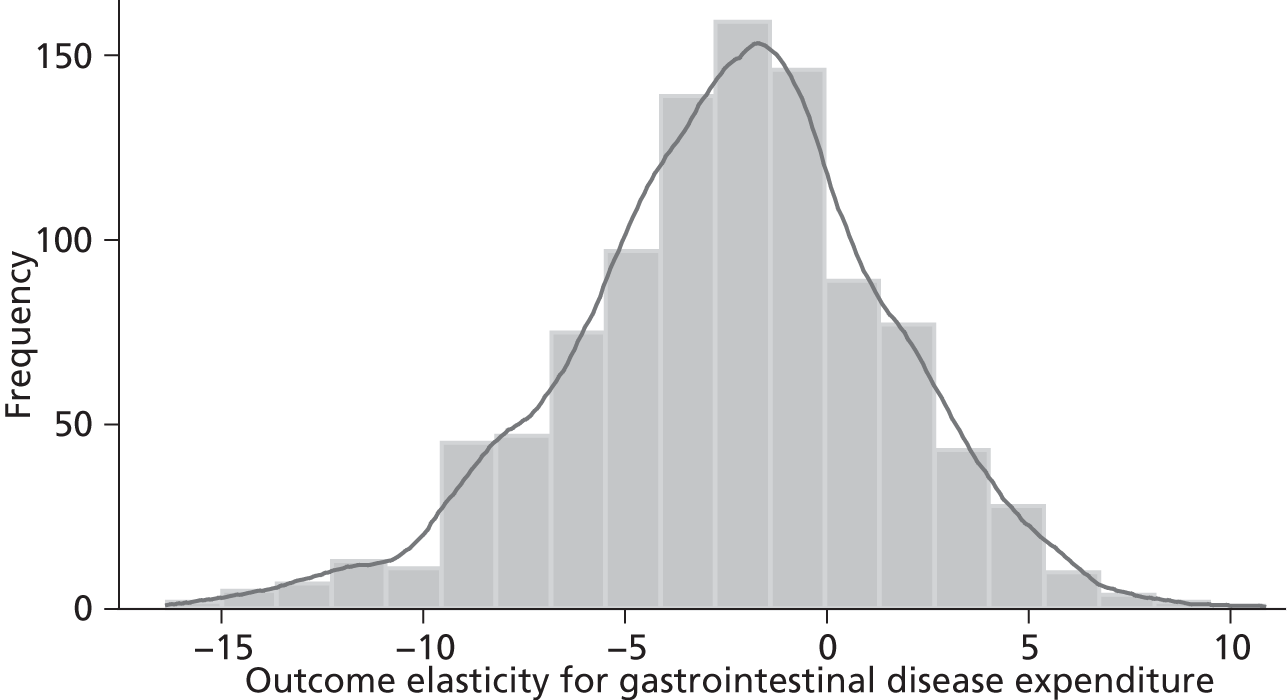
Figure 36 reproduces the three kernel density plots shown in Figures 30, 32 and 34 (remember that Figures 32 and 34 assume a non-zero covariance between γ^1 and γ^2 in Equations 18 and 19). As is the case for Figure 35, these plots illustrate the impact of all three levels of uncertainty on our estimate of the respiratory disease outcome elasticity and, again, it is clear that the uncertainty induced by the instrument validity issue both shifts the density plot to the right and considerably increases the uncertainty associated with our estimate of the outcome elasticity. More precisely, the standard deviation associated with the level 1 uncertainty density plot is 0.636 but the standard deviation for the level 2 (0.919) and level 3 (1.098) uncertainty density plots are both considerably larger than this.
FIGURE 41.
Sampling from the 1000 outcome elasticities for gastrointestinal disease expenditure (that were generated using 1000 alternative pairs of coefficients on the excluded instruments with a non-zero covariance between these coefficients). Note: the mean value of these 1000 sampled outcome elasticities is –2.434. The outcome elasticity for gastrointestinal expenditure in the comparable IV model is –1.536.

Uncertainty and the value of the gastrointestinal disease outcome elasticity
Figure 37 plots 1000 values from the distribution of the point estimate for the gastrointestinal disease outcome elasticity [see column (7) of Table 65]. The mean of these sampled values is −1.518 (the outcome elasticity in the comparable IV model is −1.536) and all of these values lie between −3.0 and 0.0.
The histogram in Figure 38 provides a plot of 1000 point estimates for the gastrointestinal disease outcome elasticity if we drop the assumption that the coefficients on the excluded instruments are exactly zero (and we also assume a zero covariance between γ^1 and γ^2 in Equations 18 and 19). The mean value of these 1000 outcome elasticities (−2.365) is 50% larger than the size of the elasticity in the comparable IV model (−1.536). And the mean value of the standard errors associated with these 1000 elasticities (0.853) is about 80% larger than the standard error in the basic IV model (0.468).
The histogram in Figure 39 provides a similar plot to that in Figure 38, but this time we assume a non-zero covariance between γ^1 and γ^2 in Equations 18 and 19. However, there is very little difference between the zero (see Figure 38) and non-zero (see Figure 39) covariance plots, with both the mean elasticity and mean standard error virtually identical in these plots.
The histograms in Figures 38 and 39 provide plots of point estimates for the gastrointestinal disease outcome elasticity if we drop the assumption that the coefficients on the excluded instruments are exactly zero. Each point estimate also has a standard error and we can sample from these estimates and their distributions to obtain the histograms shown in Figures 40 and 41. With the exception of a slight extension to both tails, these plots are similar to the plots in Figures 38 and 39.
Figure 42 reproduces the three kernel density plots shown in Figures 37, 38 and 40 (remember that Figures 38 and 40 assume a zero covariance between γ^1 and γ^2 in Equations 18 and 19). These plots illustrate the impact of all three levels of uncertainty on our estimate of the gastrointestinal disease outcome elasticity. It is clear that the uncertainty induced by the instrument validity issue both shifts the density plot to the left slightly and dramatically increases the uncertainty associated with our estimate of the outcome elasticity (e.g. the range of values increases from −3 to 0 at level 1, from −13 to 9 at level 2, and then further from −16 to 11 at level 3).
FIGURE 42.
Kernel density plots from Figures 37, 38 and 40: illustrating the uncertainty associated with the point estimate for the gastrointestinal disease outcome elasticity. Note: the mean value of the level 1/level 2/level 3 elasticities is −1.518/−2.365/−2.442. The outcome elasticity for gastrointestinal expenditure in the comparable IV model is −1.536.
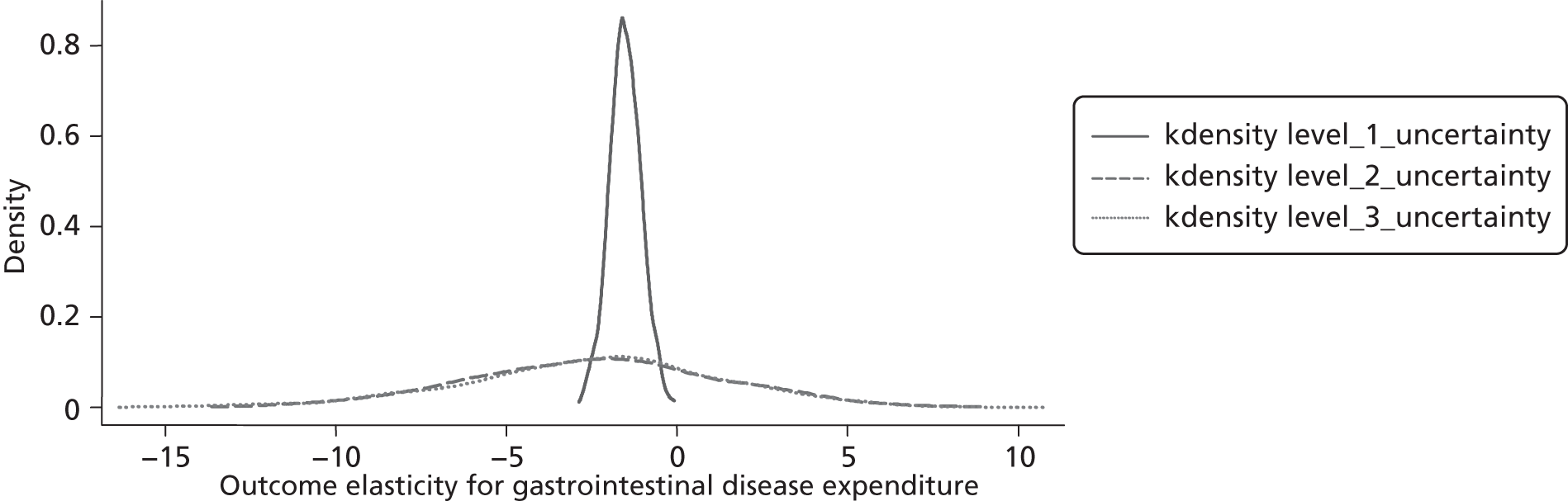
Figure 43 reproduces the three kernel density plots shown in Figures 37, 39 and 41 (remember that Figures 39 and 41 assume a non-zero covariance between γ^1 and γ^2 in Equations 18 and 19). As is the case for Figure 42, these plots illustrate the impact of all three levels of uncertainty on our estimate of the gastrointestinal disease outcome elasticity. And again, it is clear that the uncertainty induced by the instrument validity issue both shifts the density plot to the left slightly and considerably increases the uncertainty (range) associated with our estimate of the outcome elasticity. More precisely, the standard deviation associated with the level 1 uncertainty density plot is 0.468 but the standard deviation for the level 2 (3.658) and level 3 (3.834) uncertainty density plots are both eight times larger than this.
FIGURE 43.
Kernel density plots from Figures 37, 39 and 41: illustrating the uncertainty associated with the point estimate for the gastrointestinal disease outcome elasticity. Note: the mean value of the level 1/level 2/level 3 elasticities is −1.518/−2.360/−2.434. The outcome elasticity for gastrointestinal expenditure in the comparable IV model is −1.536.
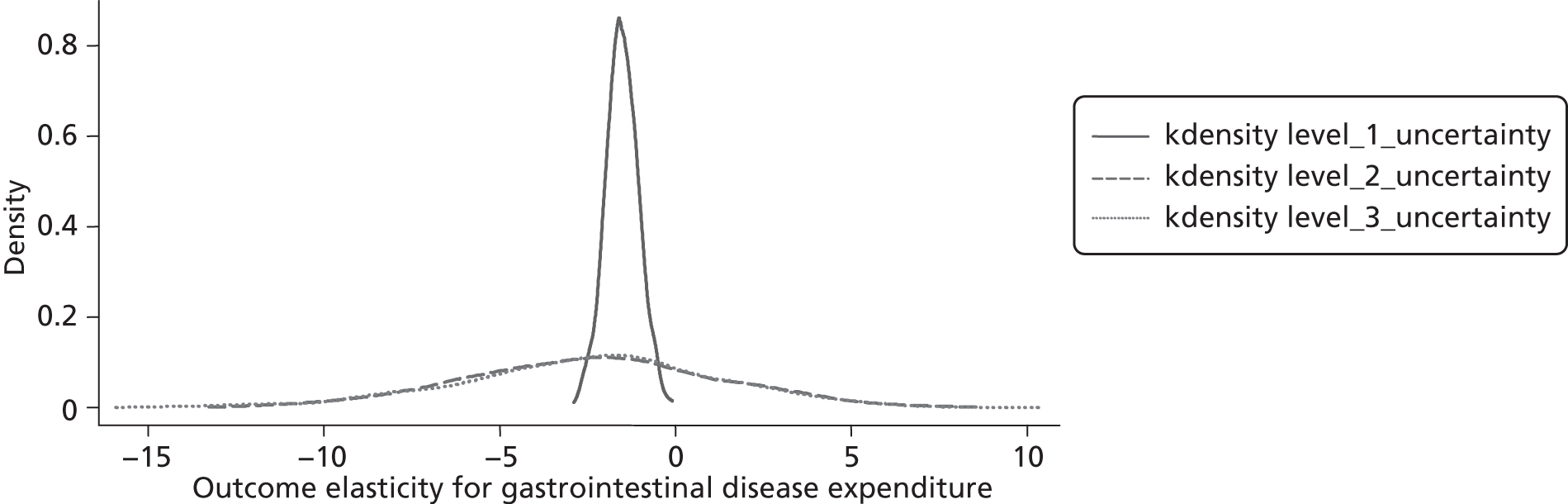
Implications of uncertainty for the estimate of the cost of a life-year
In the previous subsection, we have evaluated the outcome equation elasticities when uncertainty over the validity of instrument variables is considered (‘level 3’ uncertainty), in contrast to assuming the instruments are valid (‘level 1’ uncertainty). This analysis showed that including level 3 uncertainty affects the central value of the outcome elasticities; however, it is difficult to predict its effect on the expectation of the threshold given the impact of expenditure on mortality appears reduced in some programmes but increased in others. In Table 80, the mean estimates of the outcome elasticities under level 3 uncertainty were used to calculate the threshold for the big four programmes of health. The results show that relaxing the assumption of validity of the instruments has little impact on the expectation of the threshold for the big four PBCs [the cost per YLG threshold changed from £10,604 (see Table 68) to £11,009 (see Table 80)].
PBC description | Spend (£M) 2006/7 | Spend elasticity | Change in spend (£M) | Annual mortality, < 75 years, 2006/7/8 | Outcome elasticity (without negative sign) | Coverage of mortality data relative to spend data | Change in annual mortality adjusted for coverage | Cost per life gained (£) adjusted for coverage | Total life-years lost, < 75 years, 2006/7/8 | Coverage of mortality data relative to spend data | Change in annual life-years lost adjusted for YLL | Cost per YLG (£) | Cost per YLG adjusted for YLL coverage (£) | |
---|---|---|---|---|---|---|---|---|---|---|---|---|---|---|
1 | Cancer | 4122 | 0.465 | 19.17 | 61,961 | 0.218 | 0.984 | 63.90 | 299,975 | 2,207,021 | 0.984 | 759 | 16,383 | 25,265 |
2 | Circulatory problems | 6161 | 0.540 | 33.27 | 41,106 | 1.718 | 0.992 | 384.42 | 86,544 | 1,361,634 | 0.992 | 4245 | 9466 | 7838 |
3 | Respiratory problems | 3285 | 0.679 | 22.31 | 11,574 | 1.151 | 0.773 | 116.99 | 190,666 | 324,223 | 0.773 | 1092 | 11,593 | 20,419 |
4 | Gastrointestinal problems | 3700 | 0.446 | 16.50 | 6160 | 2.434 | 0.571 | 117.11 | 140,906 | 345,908 | 0.571 | 2192 | 20,892 | 7528 |
5 | Spend 2006 and mortality 2006/7/8 | 17,268 | 91.24 | 120,801 | 682.42 | 133,707 | 4,238,786 | 8288 | 12,333 | 11,009 |
The assumption of validity of instruments is expected to affect significantly the level of uncertainty over the cost-effectiveness threshold estimate. Illustrations of this source of uncertainty were presented in the previous section (see Obtaining the outcome elasticities associated with sampled coefficients on the excluded instruments) using empirical distributions derived from the sampling procedure implemented; these illustrations represent the uncertainty in the mean estimate for each of the elasticities. To characterise the effect of levels 1 and 3 uncertainty on the overall threshold we used the sets of simulated elasticities (one for each of the four programmes of care) to compute a threshold value; in doing so for all simulated sets, a sample of threshold values was obtained. In this way, uncertainty was propagated from the outcome elasticities to the threshold estimates, and an empirical distribution describing uncertainty over threshold estimates obtained. The cumulative density function can be used to display such uncertainty; this plots the probability (y-axis) of the threshold being below certain values (x-axis) in the simulated sample (this corresponds to a Bayesian interpretation of uncertainty). Figure 44 plots the cumulative density curve for the cost per life gained threshold when level 1 and level 3 uncertainty are considered in turn, and Figure 45 for the cost per YLG threshold.
FIGURE 44.
Cumulative density plot for the cost per life gained threshold for the big four PBCs (considers covariance between the coefficients on the excluded instruments). In drawing the cumulative density function, negative threshold values were dealt with by evaluating whether it was the health component or the cost component that was negative. For simulations where health change was negative (0% were observed for both levels 1 and 3), the threshold was left as a negative value. Simulations showing a negative change in spend were assigned a very high positive threshold value – in these an asymptote is generated in the plot (respectively 0% and 5.6% were observed for levels 1 and 3).

FIGURE 45.
Cumulative density plot for the cost per YLG threshold for the big four PBCs (considers covariance between the coefficients on the excluded instruments). In drawing the cumulative density function, negative threshold values were dealt with by evaluating whether it was the health component or the cost component that was negative. For simulations where health change was negative (0% were observed for both levels 1 and 3), the threshold was left as a negative value. Simulations showing a negative change in spend were assigned a very high positive threshold value – in these an asymptote is generated in the plot (respectively 0.04% and 7.7% were observed for levels 1 and 3).

The probability that the overall threshold is < £7500 per life-year is around 0.2 when uncertainty over the validity of instruments is considered (level 3), whereas when the instruments are assumed valid (level 1) this probability is 0. Under level 1 uncertainty, we would be confident that the threshold is < £30,000 (probability of 1), but when considering level 3 uncertainty there is some chance that the threshold is > £30,000 (probability of 0.2). These plots show that uncertainty on the validity of the instruments generates significant uncertainty over the threshold value.
Summary and conclusion
One of the crucial elements in the calculation of the cost of a life-year for any care programme is the coefficient on the expenditure variable in the outcome equation. The endogenous nature of expenditure in our model means that OLS estimation is inappropriate and that instead IV techniques must be used. The application of these techniques requires the identification of variables that are good predictors of the endogenous variable (expenditure) but which do not have a direct effect on the dependent variable (mortality).
It is difficult to provide theoretical arguments why our selected instruments will not affect mortality directly. Instead, we rely on the widely used Hansen–Sargen test of instrument validity. Although our models ‘pass’ this test, some commentators have argued that this test has weak power and may fail to reject the null hypothesis of instrument validity even when an exclusion restriction is not valid. Given our reliance on this test, we noted that this test will only lack power if the biases induced in the coefficient on the endogenous variable by invalid instruments all coincide (i.e. the instruments all identify the same wrong parameter). However, it is far from obvious that this will be so in this case, particularly given that our outcome equation already includes a measure of the need for health care.
Nevertheless, it is possible that our instruments are correlated with both expenditure and some unobserved factor which is directly influencing the mortality rate, and that the induced bias in β1^ is the same for both instruments.
We therefore undertook an extensive sensitivity analysis of the estimated outcome elasticity to the validity of the exclusion restrictions. In summary, we found that both the central value and distribution of the outcome elasticity may change if we drop the assumption that the coefficients on the excluded instruments are identically zero.
This change in the central value of the outcome elasticity reduces the impact of expenditure on mortality in some programmes (e.g. for cancer the ‘average’ outcome elasticity falls from −0.338 to −0.210, and for respiratory disease it falls from −2.004 to −1.151). However, in other programmes this change in the central value increases the impact of expenditure on mortality (e.g. for circulatory disease the ‘average’ outcome elasticity increases from −1.418 to −1.718, and for gastrointestinal problems it increases from −1.518 to −2.434).
However, in all four programmes the standard deviation associated with the distribution of the value for the outcome elasticity increased: for cancer it increased from 0.099 to 0.379; for circulatory disease it increased from 0.228 to 0.843; for respiratory disease it increased from 0.636 to 1.098; and for gastrointestinal disease it increased from 0.468 to 3.834.
Analysis of programme budgeting expenditure for 2007/8 and mortality data for 2007/8/9
Outcome and expenditure models were estimated using updated data for expenditure (from 2006/7 to 2007/8) and updated mortality data (from 2006/7/8 to 2007/8/9). Results for the outcome model are shown in Table 81 and results for the expenditure model are in Table 82. First-stage regressions for these IV models can be found in Tables 97 and 98 in the Annex.
Variable | PBC 2, cancer, 2007/8, outcome model, instrument spend, weighted, second stage | PBC 10, circulation, 2007/8, outcome model, instrument spend, weighted, second stage | PBC 11, respiratory, 2007/8, outcome model, instrument spend, weighted, second stage | PBC 13, gastrointestinal, 2007/8, outcome model, instrument spend, weighted, second stage | PBC 4, endocrine, 2007/8, outcome model, instrument spend, weighted, second stage | PBC 7, neurological, 2007/8, outcome model, other programme need exogenous, weighted, OLS | PBC 17, genitourinary, 2007/8, outcome model, instrument spend, weighted, second stage | PBC 1, infectious diseases, 2007/8, outcome model, instrument spend, weighted, second stage | PBC 18 + 19, maternity and neonates, 2007/8, outcome model, instrument spend, weighted, second stage | PBC 16, trauma and injuries, 2007/8, outcome model, instrument spend, weighted, second stage | |||
---|---|---|---|---|---|---|---|---|---|---|---|---|---|
(1) | (2) | (3) | (4) | (5) | (6) | (7) | (8) | (9) | (10) | (11) | (12) | (13) | |
Own programme spend per head | −0.365*** | −0.365*** | −1.277*** | −2.205*** | −2.211*** | −1.292*** | −1.328** | −0.566 | −0.339** | −1.898** | −0.546* | −0.110 | −0.369 |
[0.106] | [0.107] | [0.206] | [0.705] | [0.739] | [0.497] | [0.519] | [0.550] | [0.144] | [0.921] | [0.300] | [0.139] | [0.353] | |
Need CARAN per head | 0.984*** | 0.985*** | 2.818*** | 5.119*** | 5.113*** | 3.908*** | 3.947*** | 0.853** | 3.029*** | ||||
[0.108] | [0.110] | [0.256] | [1.052] | [1.105] | [0.633] | [0.658] | [0.344] | [0.717] | |||||
Need CARAN per head squared | 4.085** | 3.982** | |||||||||||
[1.721] | [1.774] | ||||||||||||
IMD2007 | 0.517*** | 0.481*** | |||||||||||
[0.109] | [0.098] | ||||||||||||
Diabetes prevalence rate 2007/8 | 0.820** | ||||||||||||
[0.359] | |||||||||||||
Epilepsy prevalence rate 2007/8 | 0.652*** | ||||||||||||
[0.231] | |||||||||||||
Lone parent households | 1.767*** | ||||||||||||
[0.430] | |||||||||||||
HIV need per head squared | 0.143** | ||||||||||||
[0.064] | |||||||||||||
HIV need per head | 0.487*** | ||||||||||||
[0.120] | |||||||||||||
Born outside the EU | 0.152*** | ||||||||||||
[0.028] | |||||||||||||
No qualifications aged 16–74 years | 0.990*** | ||||||||||||
[0.115] | |||||||||||||
No car households | −0.658*** | ||||||||||||
[0.221] | |||||||||||||
Full-time students | 0.528*** | ||||||||||||
[0.128] | |||||||||||||
Constant | 6.635*** | 6.637*** | 10.643*** | 12.244*** | 12.269*** | 8.688*** | 8.845*** | 0.512 | 3.072*** | 12.110** | 2.176*** | 3.303*** | 2.654** |
[0.480] | [0.483] | [0.996] | [2.947] | [3.090] | [2.142] | [2.237] | [1.349] | [0.614] | [4.852] | [0.675] | [0.762] | [1.346] | |
Observations | 151 | 151 | 151 | 151 | 151 | 151 | 151 | 151 | 151 | 147 | 151 | 151 | 151 |
Endogeneity test statistic | 17.288 | 16.323 | 39.948 | 21.368 | 28.333 | 18.871 | 17.769 | 1.293 | 3.916 | 3.603 | 0.551 | 1.375 | |
Endogeneity p-value | 3.21e-05 | 5.34e-05 | 1.42e-05 | 3.79e-06 | 1.02e-07 | 1.40e-05 | 2.49e-05 | 0.255 | 0.0478 | 0.0577 | 0.458 | 0.241 | |
Hansen–Sargan test statistic | 0.00124 | n/a | 0.056 | n/a | 0.163 | 0.120 | n/a | n/a | 6.710 | 0.583 | 0.675 | 5.001 | |
Hansen-Sargan p-value | 0.972 | 0.814 | 0.686 | 0.729 | 0.0349 | 0.747 | 0.411 | 0.0820 | |||||
Shea’s partial R2 | 0.162 | 0.162 | 0.323 | 0.0832 | 0.0977 | 0.126 | 0.112 | 0.133 | 0.160 | 0.104 | 0.201 | 0.137 | |
Kleibergen–Paap LM test statistic | 19.52 | 19.44 | 20.71 | 8.807 | 8.840 | 10.76 | 10.53 | 20.71 | 20.01 | 16.45 | 30.58 | 16.82 | |
Kleibergen–Paap p-value | 5.76e-05 | 1.04e-05 | 0.0000 | 0.00300 | 0.0120 | 0.00462 | 0.00117 | 5.36e-06 | 0.000169 | 0.000917 | 2.29e-07 | 0.000770 | |
Kleibergen–Paap F-statistic | 14.50 | 29.13 | 34.54 | 12.26 | 6.533 | 7.809 | 14.70 | 25.56 | 9.624 | 9.688 | 23.31 | 7.835 | |
Pesaran–Taylor/Ramsey test statistic | 0.00606 | 0.0115 | 2.06 | 2.839 | 2.850 | 0.418 | 0.106 | 0.00725 | 0.469 | 0.393 | 2.251 | 0.00684 | 0.0128 |
Pesaran–Taylor/Ramsey p-value | 0.938 | 0.915 | 0.1515 | 0.0920 | 0.0914 | 0.518 | 0.744 | 0.932 | 0.704 | 0.531 | 0.134 | 0.934 | 0.910 |
Variable | (1) PBC 2, cancer, 2007/8, spend model, second stage | (2) PBC 10, circulation, 2007/8, spend model, second stage | (3) PBC 11, respiratory, 2007/8, spend, model, second stage | (4) PBC 13, gastrointestinal, 2007/8, spend model, second stage | (5) PBC 1, infectious disease, 2007/8, spend model, OLS | (6) PBC 4, endocrine, 2007/8, spend model, second stage | (7) PBC 7, neurological, 2007/8, spend model, second stage | (8) PBC 17, genitourinary, 2007/8, spend model, OLS | (9) PBC 18 + 19, maternity and neonates, 2007/8, spend model, OLS | (10) PBC 23, GMS/PMS, etc., 2007/8, spend model, second stage | (11) PBC 16, trauma and injuries, 2007/8, spend model, second stage |
---|---|---|---|---|---|---|---|---|---|---|---|
All-cause SYLLR excluding cancer | −1.227*** | ||||||||||
[0.220] | |||||||||||
PCT budget per head | 0.890** | 0.293 | 0.536* | 0.622* | 1.435*** | 0.264 | 1.036*** | 1.004*** | 0.514* | 0.563 | 1.686*** |
[0.431] | [0.350] | [0.298] | [0.321] | [0.258] | [0.206] | [0.307] | [0.356] | [0.264] | [0.344] | [0.384] | |
Need CARAN per head | 1.659*** | 3.117*** | 1.786*** | 1.982*** | 0.925*** | 0.029 | |||||
[0.430] | [0.535] | [0.334] | [0.422] | [0.305] | [0.371] | ||||||
All-cause SYLLR excluding circulatory | −2.115*** | ||||||||||
[0.397] | |||||||||||
All-cause SYLLR excluding respiratory | −0.781*** | ||||||||||
[0.236] | |||||||||||
Need CARAN per head squared | 1.687*** | ||||||||||
[0.446] | |||||||||||
All-cause SYLLR excluding gastrointestinal | −1.279*** | ||||||||||
[0.333] | |||||||||||
HIV need per head | 0.440*** | ||||||||||
[0.025] | |||||||||||
All-cause SYLLR excluding infectious diseases | −0.543** | ||||||||||
[0.249] | |||||||||||
HIV need per head squared | 0.183*** | ||||||||||
[0.021] | |||||||||||
All-cause SYLLR excluding diabetes | −0.384* | ||||||||||
[0.218] | |||||||||||
Diabetes prevalence rate 2007/8 | 0.332*** | ||||||||||
[0.123] | |||||||||||
All-cause SYLLR excluding epilepsy | −0.259 | ||||||||||
[0.223] | |||||||||||
Epilepsy prevalence rate 2007/8 | 0.571*** | ||||||||||
[0.072] | |||||||||||
All-cause SYLLR excluding renal | −0.072 | ||||||||||
[0.168] | |||||||||||
Maternity need per head | 0.582*** | ||||||||||
[0.098] | |||||||||||
All-cause SYLLR | 0.286 | −0.169 | −0.277 | ||||||||
[0.193] | [0.290] | [0.363] | |||||||||
Lone pensioner households | −0.480*** | ||||||||||
[0.182] | |||||||||||
Population working in agriculture | 0.132*** | ||||||||||
[0.022] | |||||||||||
Constant | 4.973 | 15.081*** | 4.986** | 7.488*** | −4.212*** | 3.555* | −1.684 | −2.675 | −1.222 | 1.413 | −5.960*** |
[3.047] | [3.303] | [2.342] | [2.786] | [1.034] | [1.817] | [1.130] | [2.562] | [1.388] | [1.373] | [1.104] | |
Observations | 151 | 151 | 151 | 151 | 151 | 151 | 151 | 151 | 151 | 151 | 151 |
Endogeneity test statistic | 20.985 | 19.454 | 11.612 | 15.477 | 2.846 | 4.958 | 0.060 | 1.769 | |||
Endogeneity p-value | 4.63e-06 | 1.03e-05 | 0.000655 | 8.35e-05 | 0.0916 | 0.0260 | 0.807 | 0.183 | |||
Hansen–Sargan test statistic | 0.411 | 0.003 | 1.369 | 0.0201 | 0.510 | 2.748 | 1.091 | 1.121 | |||
Hansen–Sargan p-value | 0.522 | 0.959 | 0.504 | 0.887 | 0.775 | 0.0974 | 0.296 | 0.571 | |||
Shea’s partial R2 | 0.384 | 0.253 | 0.398 | 0.325 | 0.402 | 0.518 | 0.416 | 0.364 | |||
Kleibergen–Paap LM test statistic | 40.04 | 28.14 | 39.41 | 33.23 | 40.29 | 31.53 | 16.51 | 27.19 | |||
Kleibergen–Paap p-value | 2.02e-09 | 7.76e-07 | 1.42e-08 | 6.09e-08 | 9.26e-09 | 1.42e-07 | 0.000260 | 5.37e-06 | |||
Kleibergen–Paap F-statistic | 51.44 | 29.097 | 40.69 | 20.04 | 37.14 | 73.21 | 26.60 | 32.54 | |||
Pesaran–Taylor/Ramsey test statistic | 2.262 | 0.0002 | 0.0236 | 0.0341 | 0.721 | 2.351 | 0.619 | 1.297 | 1.018 | 1.757 | 0.193 |
Pesaran–Taylor/Ramsey p-value | 0.133 | 0.988 | 0.878 | 0.854 | 0.541 | 0.125 | 0.432 | 0.278 | 0.387 | 0.185 | 0.660 |
Outcome models
Some of the outcome models in Table 81 contain just two variables: own programme expenditure and a measure of the need for health care. The latter is usually the measure of need as employed by the Department of Health for resource allocation purposes and this incorporates the CARAN formula for acute services. For the respiratory programme we have added the square of this need measure to improve the model fit. In other PBCs we found that the all service measure of need performed poorly and we have replaced or supplemented it with either a more programme-specific measure (e.g. the epilepsy prevalence rate for neurological mortality) or with a better performing proxy for need (e.g. the percentage of residents born outside the EU for maternity/neonate mortality).
Two results are reported for three of the big four programmes. One of these two results uses two instruments and so we report the instrument validity test statistic. We cannot reject the null hypothesis of instrument validity in all three cases. However, there is some evidence of weak instruments (at least in the respiratory and gastrointestinal programmes) but, if we drop one instrument and re-estimate the model, the evidence of instrument weakness disappears (but of course there is no instrument validity test statistic with this re-estimation). The removal of one instrument has little impact on the coefficient on expenditure and it is this coefficient from this one instrument model that we use below in our cost of a life-year calculations.
The first seven results in Table 81 show the outcome model for the big four programmes (i.e. for cancer, circulatory disease, respiratory problems and gastrointestinal problems). In all four programmes the need variable has a positive and significant effect on mortality, and expenditure has the anticipated negative effect. The diagnostic statistics reveal that, in all four PBCs, own programme expenditure is endogenous and that the instruments are valid. They also suggest that the instruments are relevant and there is no evidence that the instruments are weak in the models with one excluded instrument. The Pesaran–Taylor test suggests that there is no evidence of model misspecification.
The outcome results for the other programmes [see columns (8)–(13) in Table 81] are similar to but more diverse than those for the big four programmes. This is to be anticipated because mortality is a much rarer outcome in these programmes than it is in the big four programmes. Own programme expenditure is not endogenous in four of these programmes but we retain the IV estimator for three of these four because this yields more plausible results than the OLS estimator (the results are more plausible in the sense that the signs on the coefficients are more in line with our prior expectations).
Expenditure has the anticipated negative effect on mortality in the endocrine problems programme but this is not statistically significant. The all service measure of need is not relevant for this PBC; instead, we find that the diabetes prevalence rate is positively associated with mortality, as is a measure of deprivation (the IMD2007).
Mortality from epilepsy is negatively and significantly associated with expenditure in the neurological programme. Both the all service need for health care and the epilepsy prevalence rate are positively and significantly associated with mortality in this programme.
Expenditure has a negative and statistically significant effect on mortality (from renal problems) in the genitourinary problems programme. The prevalence of lone parent households is positively associated with mortality.
Expenditure has the anticipated negative effect on mortality in the infectious disease programme and this is statistically significant. The all service measure of need is not relevant for this PBC; instead, we find that a measure of need associated with HIV is positively associated with mortality, as is a measure of deprivation (the IMD2007).
Expenditure has the anticipated negative effect on mortality in the maternity and neonates programme, but the estimated coefficient is not statistically significant. In this PBC the generic all service measure of need has been replaced with two other indicators of deprivation – the proportion of residents born outside the EU and the proportion of those aged 16−74 years without any qualifications – and both of these are positively associated with mortality.
Finally, expenditure and need have the anticipated effects on mortality in the trauma and injuries programme. In addition, the proportion of households without access to a car is negatively associated with mortality from fractures (perhaps access to a car facilitates involvement in serious road traffic accidents), and the proportion of residents that are students is positively associated with mortality from fractures.
The relevant statistical test suggests that expenditure is endogenous in 6 of the 10 programmes but we have retained the IV estimates for three of the other four programmes because they provide plausible results. The Hansen–Sargen test suggests that the selected instruments are valid, and the Kleibergen–Paap LM statistic suggests that they are relevant (i.e. correlated with the endogenous regressor). With the possible exception of the trauma and injuries programme, the Kleibergen–Paap F-statistic suggests that we do not have a problem with weak instruments. ai Finally, the Pesaran–Taylor/Ramsey reset test statistics reveal no evidence of misspecification.
Expenditure models
Most of the expenditure models in Table 82 contain just three variables: the PCT budget, a proxy for the own programme need for health care, and a proxy for the need for health care in other programmes.
The budget term is positive in all 11 models and it is statistically significant in 8 of these 11 models.
The usual proxy for the own programme need for health care (i.e. the all service measure of need) is present in six of the models and it is significant in five of them. Its presence is supplemented with the addition of its squared value to improve model fit in the respiratory problems programme.
In some programmes (e.g. endocrine, metabolic and nutritional, and neurological), we have replaced and/or supplemented the all service measure of need with a more programme-specific measure (e.g. the diabetes prevalence rate and the epilepsy prevalence rate) and these measures of need have the anticipated positive impact on expenditure.
In addition, in a couple of other programmes we have used alternative proxies for the own programme need (e.g. with the use of the Department of Health’s measure of maternity need in the maternity and neonates expenditure equation).
For 8 of the 11 programmes we have used the all-cause mortality rate less the own programme mortality rate as the proxy for the need for health care in other programmes, and the coefficient on this term is negative in seven programmes and statistically significant in six of the seven. In three programmes – maternity and neonates, GMS/PMS, and trauma and injuries programmes – we have used the all-cause mortality rate as the proxy for the need for health care in other programmes due to difficulties associated with the measurement of the own programme mortality rate. The coefficient on this term is not significant in any of the three models.
The relevant statistical test suggests that expenditure is endogenous in 6 of the 11 programmes, but we have retained the IV estimates for two other programmes (GMS/PMS, and trauma and injuries) because the IV estimator provides more plausible results. In the other three programmes we report OLS results.
The Hansen–Sargen test suggests that the selected instruments are valid, and the Kleibergen–Paap LM statistic suggests that they are relevant (i.e. correlated with the endogenous regressor). The Kleibergen–Paap F-statistic suggests that we do not have a problem with weak instruments. Finally, the Pesaran–Taylor reset test statistics and the Ramsey reset F-statistics reveal no evidence of model misspecification.
Calculation of the cost of a life and life-year
Expenditure and outcome elasticities for our preferred models are shown in Table 83 [see columns (D) and (G)] and these are used to calculate the cost of a life and the cost of a life-year, both for individual programmes and for all programmes collectively.
(A) PBC scenario | (B) PBC description | (C) Spend (£M) 2007/8 | (D) Spend elasticity | (E) (= 0.01 × C × D) change in spend (£M) | (F) Annual mortality, < 75 years, 2007/8/9 | (G) Outcome elasticity (without negative sign) | (H) (= 0.01 × D × F × G) change in annual mortality | (I) (= E/H) cost per life gained (£) | (J) Coverage of mortality data relative to spend data | (K) (= H/J) change in annual mortality adjusted for coverage | (L) (= E/K) cost per life gained (£) adjusted for coverage | (M) Total life-years lost, < 75 years, 2007/09 | (N) (= 0.01 × D × G × M/3) change in annual life-years lost | (O) Coverage of mortality data relative to spend data | (P) (= N/O) change in annual life-years lost adjusted for coverage | (Q) (= E/N) cost per YLG (£) | (R) (= E/P) cost per YLG adjusted for coverage (£) |
---|---|---|---|---|---|---|---|---|---|---|---|---|---|---|---|---|---|
1 | Cancer | 4573 | 0.890 | 40.70 | 61,960 | 0.365 | 201.28 | 202,207 | 0.984 | 204.55 | 198,972 | 2,189,685 | 2371 | 0.984 | 2410 | 17,165 | 16,891 |
2 | Circulatory problems | 6325 | 0.293 | 18.53 | 39,304 | 1.277 | 147.06 | 126,018 | 0.992 | 148.25 | 125,010 | 1,313,223 | 1638 | 0.992 | 1651 | 11,315 | 11,224 |
3 | Respiratory problems | 3431 | 0.536 | 18.39 | 10,764 | 2.205 | 127.22 | 144,557 | 0.773 | 164.58 | 111,742 | 315,457 | 1243 | 0.773 | 1608 | 14,798 | 11,439 |
4 | Gastrointestinal problems | 3805 | 0.622 | 23.67 | 6031 | 1.328 | 49.82 | 475,081 | 0.571 | 87.25 | 271,271 | 343,355 | 945 | 0.571 | 1656 | 25,034 | 14,295 |
Big four programmes summary | |||||||||||||||||
5 | Spend 2007 and mortality 2007/8/9 | 18,134 | 101.29 | 118,059 | 525.37 | 192,795 | 604.62 | 167,526 | 4,161,720 | 6197 | 7324 | 16,345 | 13,830 | ||||
6 | Spend 2006 and mortality 2006/7/8 | 17,268 | 91.24 | 120,801 | 665.10 | 137,188 | 761.49 | 119,823 | 4,238,786 | 7399 | 8604 | 12,333 | 10,604 | ||||
7 | Spend 2006 and mortality 2004/5/6 | 17,268 | 114.04 | 125,290 | 953.13 | 119,650 | 4,335,559 | 10,576 | 10,783 | ||||||||
8 | Spend 2005 and mortality 2002/3/4 | 17,625 | 141.22 | 125,290 | 909.96 | 155,196 | 4,516,953 | 10,986 | 12,855 | ||||||||
9 | Infectious diseases | 1119 | 1.436 | 16.07 | 1977 | 0.548 | 15.56 | 1,032,863 | 1.000 | 15.56 | 1,032,863 | 106,092 | 278 | 1.000 | 278 | 57,742 | 57,742 |
10 | Endocrine problems | 1997 | 0.264 | 5.27 | 1471 | 0.566 | 2.20 | 2,398,551 | 0.634 | 3.47 | 1,520,681 | 55,492 | 28 | 0.634 | 44 | 190,745 | 120,932 |
11 | Neurological problems | 3165 | 1.035 | 32.76 | 718 | 0.339 | 2.52 | 13,003,180 | 0.136 | 18.52 | 1,768,432 | 64,873 | 76 | 0.136 | 558 | 431,749 | 58,718 |
12 | Genitourinary problems | 3439 | 1.004 | 34.53 | 270 | 1.855 | 5.03 | 6,866,327 | 0.172 | 29.24 | 1,181,008 | 8529 | 53 | 0.172 | 308 | 652,096 | 112,160 |
13 | Trauma and injuries | 2918 | 1.686 | 49.20 | 1013 | 0.369 | 6.30 | 7,806,376 | 0.175 | 36.01 | 1,366,116 | 21,273 | 44 | 0.175 | 252 | 1,115,197 | 195,159 |
14 | Maternity and neonates | 3662 | 0.514 | 18.82 | 2199 | 0.110 | 1.24 | 15,139,113 | 8.213 | 0.15 | 124,337,534 | 489,170 | 92 | 0.679 | 136 | 204,168 | 138,630 |
Other six programmes summary | |||||||||||||||||
15 | Spend 2007 and mortality 2007/8/9 | 16,300 | 156.65 | 7648 | 32.85 | 4,768,699 | 102.95 | 1,521,610 | 745,429 | 571 | 1575 | 274,309 | 99,428 | ||||
16 | Spend 2006 and mortality 2006/7/8 | 15,643 | 93.29 | 7839 | 16.14 | 5,780,723 | 25.05 | 3,724,129 | 762,991 | 362 | 639 | 258,046 | 146,108 | ||||
17 | Spend 2006 and mortality 2004/5/6 | 15,643 | 112.13 | 7923 | 18.17 | 6,172,491 | 757,531 | 249 | 449,706 | ||||||||
18 | Spend 2005 and mortality 2002/3/4 | 12,743 | 99.44 | 7923 | 16.26 | 6,115,621 | 751,009 | 337 | 295,074 | ||||||||
All 10 programmes summary | |||||||||||||||||
19 | Spend 2007 and mortality 2007/8/9 | 34,434 | 257.94 | 125,707 | 558.22 | 462,067 | 707.57 | 364,540 | 4,907,149 | 6768 | 8900 | 38,110 | 28,983 | ||||
20 | Spend 2006 and mortality 2006/7/8 | 32,911 | 184.53 | 128,640 | 681.24 | 270,881 | 786.54 | 234,617 | 5,001,777 | 7760 | 9243 | 23,780 | 19,965 | ||||
21 | Spend 2006 and mortality 2004/5/6 | 32,911 | 226.18 | 133,213 | 971.30 | 232,861 | 5,093,090 | 10,826 | 20,893 | ||||||||
22 | Spend 2005 and mortality 2002/3/4 | 30,368 | 240.67 | 133,213 | 926.22 | 259,838 | 5,267,962 | 11,322 | 21,256 | ||||||||
Assume zero health gain in the other 13 programmes: other 13 programmes summary | |||||||||||||||||
23 | Spend 2007 and mortality 2007/8/9 | 39,223 | 478.63 | 0.00 | 0.00 | 0.00 | 0.00 | ||||||||||
24 | Spend 2006 and mortality 2006/7/8 | 34,985 | 494.43 | 0.00 | 0.00 | 0.00 | |||||||||||
25 | Spend 2006 and mortality 2004/5/6 | 34,985 | 452.78 | 0.00 | 0.00 | ||||||||||||
26 | Spend 2005 and mortality 2002/3/4 | 33,942 | 402.43 | 0.00 | 0.00 | ||||||||||||
All 23 programmes | |||||||||||||||||
27 | Spend 2007 and mortality 2007/8/9 | 73,657 | 736.57 | 558.22 | 1,319,496 | 707.57 | 1,040,992 | 6768 | 8900 | 108,829 | 82,765 | ||||||
28 | Spend 2006 and mortality 2006/7/8 | 67,896 | 678.96 | 681.24 | 996,655 | 786.54 | 863,228 | 7760 | 9243 | 87,494 | 73,457 | ||||||
29 | Spend 2006 and mortality 2004/5/6 | 67,896 | 678.96 | 971.30 | 699,024 | 10,826 | 62,718 | ||||||||||
30 | Spend 2005 and mortality 2002/3/4 | 64,310 | 643.10 | 926.22 | 694,330 | 11,322 | 56,799 |
Column (L) (see Table 83) reports the cost per life gained and column (R) (see Table 83) reports the cost per YLG. From the latter we can see that the cost per YLG is £13,830 for the big four programmes and £28,983 for all 10 programmes with a mortality-based outcome indicator. These represent 30% and 45% increases on the respective costs for the previous year (i.e. using expenditure data for 2006/7 and mortality data for 2006/7/8).
If we assume that the other 13 programmes (all without a mortality-based outcome indicator) offer no health gain, then the cost per life-year across all PCT expenditure is £82,765. This is up from £73,457 using data for the previous year (an increase of 13%).
In addition, Table 84 shows that if we assume that PBC 23 generates a zero health gain and that the gain attributable to the remaining 12 programmes is, on average, the same as that attributable to those with a mortality outcome measure, then the cost of a life-year across all programmes is £31,846 (it was £22,565 using data for the previous year).
(A) PBC scenario | (B) PBC description | (C) Spend (£M) 2007/8 | (D) Spend elasticity | (E) (= 0.01 × C × D) change in spend (£M) | (F) Annual mortality, < 75 years, 2007/8/9 | (G) Outcome elasticity (without negative sign) | (H) (= 0.01 × D × F × G) change in annual mortality | (I) (= E/H) cost per life gained (£) | (J) Coverage of mortality data relative to spend data | (K) (= H/J) change in annual mortality adjusted for coverage | (L) (= E/K) cost per life gained (£) adjusted for coverage | (M) Total life-years lost, < 75 years, 2007/8/9 | (N) (= 0.01 × D × G × M/3) change in annual life-years lost | (O) Coverage of mortality data relative to spend data | (P) (= N/O) change in annual life-years lost adjusted for coverage | (Q) (= E/N) cost per YLG (£) | (R) (= E/P) cost per YLG adjusted for coverage (£) |
---|---|---|---|---|---|---|---|---|---|---|---|---|---|---|---|---|---|
1 | Cancer | 4573 | 0.890 | 40.70 | 61,960 | 0.365 | 201.28 | 202,207 | 0.984 | 204.55 | 198,972 | 2,189,685 | 2371 | 0.984 | 2410 | 17,165 | 16,891 |
2 | Circulatory problems | 6325 | 0.293 | 18.53 | 39,304 | 1.277 | 147.06 | 126,018 | 0.992 | 148.25 | 125,010 | 1,313,223 | 1638 | 0.992 | 1651 | 11,315 | 11,224 |
3 | Respiratory problems | 3431 | 0.536 | 18.39 | 10,764 | 2.205 | 127.22 | 144,557 | 0.773 | 164.58 | 111,742 | 315,457 | 1243 | 0.773 | 1608 | 14,798 | 11,439 |
4 | Gastrointestinal problems | 3805 | 0.622 | 23.67 | 6031 | 1.328 | 49.82 | 475,081 | 0.571 | 87.25 | 271,271 | 343,355 | 945 | 0.571 | 1656 | 25,034 | 14,295 |
Big four programmes summary | |||||||||||||||||
5 | Spend 2007 and mortality 2007/8/9 | 18,134 | 101.29 | 118,059 | 525.37 | 192,795 | 604.62 | 167,526 | 4,161,720 | 6197 | 7324 | 16,345 | 13,830 | ||||
6 | Spend 2006 and mortality 2006/7/8 | 17,268 | 91.24 | 120,801 | 665.10 | 137,188 | 761.49 | 119,823 | 4,238,786 | 7399 | 8604 | 12,333 | 10,604 | ||||
7 | Spend 2006 and mortality 2004/5/6 | 17,268 | 114.04 | 125,290 | 953.13 | 119,650 | 4,335,559 | 10,576 | 10,783 | ||||||||
8 | Spend 2005 and mortality 2002/3/4 | 17,625 | 141.22 | 125,290 | 909.96 | 155,196 | 4,516,953 | 10,986 | 12,855 | ||||||||
9 | Infectious diseases | 1119 | 1.436 | 16.07 | 1977 | 0.548 | 15.56 | 1,032,863 | 1.000 | 15.56 | 1,032,863 | 106,092 | 278 | 1.000 | 278 | 57,742 | 57,742 |
10 | Endocrine problems | 1997 | 0.264 | 5.27 | 1471 | 0.566 | 2.20 | 2,398,551 | 0.634 | 3.47 | 1,520,681 | 55,492 | 28 | 0.634 | 44 | 190,745 | 120,932 |
11 | Neurological problems | 3165 | 1.035 | 32.76 | 718 | 0.339 | 2.52 | 13,003,180 | 0.136 | 18.52 | 1,768,432 | 64,873 | 76 | 0.136 | 558 | 431,749 | 58,718 |
12 | Genitourinary problems | 3439 | 1.004 | 34.53 | 270 | 1.855 | 5.03 | 6,866,327 | 0.172 | 29.24 | 1,181,008 | 8529 | 53 | 0.172 | 308 | 652,096 | 112,160 |
13 | Trauma and injuries | 2918 | 1.686 | 49.20 | 1013 | 0.369 | 6.30 | 7,806,376 | 0.175 | 36.01 | 1,366,116 | 21,273 | 44 | 0.175 | 252 | 1,115,197 | 195,159 |
14 | Maternity and neonates | 3662 | 0.514 | 18.82 | 2199 | 0.110 | 1.24 | 15,139,113 | 8.213 | 0.15 | 124,337,534 | 489,170 | 92 | 0.679 | 136 | 204,168 | 138,630 |
Other six programmes summary | |||||||||||||||||
15 | Spend 2007 and mortality 2007/8/9 | 16,300 | 156.65 | 7648 | 32.85 | 4,768,699 | 102.95 | 1,521,610 | 745,429 | 571 | 1575 | 274,309 | 99,428 | ||||
16 | Spend 2006 and mortality 2006/7/8 | 15,643 | 93.29 | 7839 | 16.14 | 5,780,723 | 25.05 | 3,724,129 | 762,991 | 362 | 639 | 258,046 | 146,108 | ||||
17 | Spend 2006 and mortality 2004/5/6 | 15,643 | 112.13 | 7923 | 18.17 | 6,172,491 | 757,531 | 249 | 449,706 | ||||||||
18 | Spend 2005 and mortality 2002/3/4 | 12,743 | 99.44 | 7923 | 16.26 | 6,115,621 | 751,009 | 337 | 295,074 | ||||||||
All 10 programmes | |||||||||||||||||
19 | Spend 2007 and mortality 2007/8/9 | 34,434 | 257.94 | 125,707 | 558.22 | 462,067 | 707.57 | 364,540 | 4,907,149 | 6768 | 8900 | 38,110 | 28,983 | ||||
20 | Spend 2006 and mortality 2006/7/8 | 32,911 | 184.53 | 128,640 | 681.24 | 270,881 | 786.54 | 234,617 | 5,001,777 | 7760 | 9243 | 23,780 | 19,965 | ||||
21 | Spend 2006 and mortality 2004/5/6 | 32,911 | 226.18 | 133,213 | 971.30 | 232,861 | 5,093,090 | 10,826 | 20,893 | ||||||||
22 | Spend 2005 and mortality 2002/3/4 | 30,368 | 240.67 | 133,213 | 926.22 | 259,838 | 5,267,962 | 11,322 | 21,256 | ||||||||
Other 13 PBCs? Assume zero health gain in PBC 23 . . . | |||||||||||||||||
23 | PBC 23 spend 2007 and mortality 2007/8/9 | 11,763 | 0.563 | 66.23 | 0.00 | 0.00 | 0.00 | 0.00 | |||||||||
24 | PBC 23 spend 2006 and mortality 2006/7/8 | 10,585 | 0.739 | 78.22 | 0.00 | 0.00 | 0.00 | ||||||||||
25 | PBC 23 spend 2006 and mortality 2004/5/6 | 10,585 | 0.759 | 80.34 | 0.00 | 0.00 | |||||||||||
26 | PBC 23 spend 2005 and mortality 2002/3/4 | 8449 | 0.926 | 78.24 | 0.00 | 0.00 | |||||||||||
. . . and that the gain in 10 PBCs (see row 19) applies to the remaining 12 PBCs | |||||||||||||||||
27 | 12 PBCs spend 2007 and mortality 2007/8/9 | 27,460 | 412.41 | 892.53 | 462,067 | 1131.31 | 364,540 | 10,821 | 14,229 | 38,110 | 28,983 | ||||||
28 | 12 PBCs spend 2006 and mortality 2006/7/8 | 24,400 | 416.20 | 1536.48 | 270,881 | 1773.97 | 234,617 | 17,502 | 20,847 | 23,780 | 19,965 | ||||||
29 | 12 PBCs spend 2006 and mortality 2004/5/6 | 24,400 | 372.44 | 1599.42 | 232,861 | 17,826 | 20,893 | ||||||||||
30 | 12 PBCs spend 2005 and mortality 2002/3/4 | 25,493 | 324.20 | 1247.69 | 259,838 | 15,252 | 21,256 | ||||||||||
All 23 programmes | |||||||||||||||||
31 | 23 PBCs spend 2007 and mortality 2007/8/9 | 73,657 | 736.57 | 1450.75 | 507,717 | 1838.88 | 400,554 | 17,590 | 23,129 | 41,875 | 31,846 | ||||||
32 | 23 PBCs spend 2006 and mortality 2006/7/8 | 67,896 | 678.96 | 2217.72 | 306,153 | 2560.50 | 265,167 | 25,262 | 30,090 | 26,876 | 22,565 | ||||||
33 | 23 PBCs spend 2006 and mortality 2004/5/6 | 67,896 | 678.96 | 2570.72 | 264,113 | 28,652 | 23,697 | ||||||||||
34 | 23 PBCs spend 2005 and mortality 2002/3/4 | 64,310 | 643.10 | 2173.90 | 295,827 | 26,575 | 24,200 |
Summary and conclusion
In this section we have estimated outcome and expenditure models using PB data for 2007/8 and mortality data for 2007/8/9. The cost of an additional life-year for all 10 programmes with a mortality-based outcome is £28,983. This is a 45% increase on the cost (£19,965) for the previous year (i.e. using expenditure data for 2006/7 and mortality data for 2006/7/8). The next section presents outcome and expenditure models using PB data for 2008/9 and mortality data for 2008/9/10, and it explores the reasons for the increase in the cost of an additional life-year identified in this section.
Analysis of programme budgeting expenditure for 2008/9 and mortality data for 2008/9/10
Outcome and expenditure models were estimated using updated data for expenditure (from 2007/8 to 2008/9) and updated mortality data (from 2007/8/9 to 2008/9/10). Results for the outcome model are shown in Table 85 and results for the expenditure model are in Table 86. First-stage regressions for these IV models can be found in Tables 99 and 100 in the Annex.
Variable | 2008/9, outcome model, instrument spend, weighted, second stage | |||||||||
---|---|---|---|---|---|---|---|---|---|---|
(1) PBC 2, cancer | (2) PBC 10, circulation | (3) PBC 11, respiratory | (4) PBC 13, gastrointestinal | (5) PBC 13, gastrointestinal | (6) PBC 4, endocrine | (7) PBC 7, neurological | (8) PBC 17, genitourinary | (9) PBC 1, infectious disease | (10) PBC 18 + 19, maternity and neonates | |
Own programme spend per head | −0.307*** | −1.319*** | −1.808*** | −1.287*** | −1.364** | −1.170*** | −0.417 | −1.615 | −0.504** | −0.125 |
[0.084] | [0.186] | [0.488] | [0.478] | [0.549] | [0.431] | [0.473] | [1.608] | [0.223] | [0.188] | |
Need CARAN | 0.954*** | 2.840*** | 4.811*** | 3.907*** | 3.993*** | 1.280** | 0.405 | |||
[0.095] | [0.247] | [0.760] | [0.625] | [0.700] | [0.579] | [0.288] | ||||
Need CARAN2 | 3.016** | |||||||||
[1.284] | ||||||||||
Diabetes prevalence rate | 0.903** | |||||||||
[0.371] | ||||||||||
IMD2007 | 0.711*** | 0.528*** | ||||||||
[0.108] | [0.091] | |||||||||
Lone parent households | 1.820*** | |||||||||
[0.659] | ||||||||||
HIV need per head | 0.468*** | |||||||||
[0.093] | ||||||||||
HIV need per head squared | 0.163*** | |||||||||
[0.046] | ||||||||||
Born outside EU | 0.169*** | |||||||||
[0.031] | ||||||||||
Population with no qualifications | 0.752*** | |||||||||
[0.129] | ||||||||||
Constant | 6.372*** | 10.861*** | 10.818*** | 8.715*** | 9.048*** | 2.107** | 3.233 | 11.065 | 1.844*** | 3.097*** |
[0.381] | [0.908] | [2.111] | [2.076] | [2.386] | [1.022] | [1.987] | [8.588] | [0.500] | [0.949] | |
Observations | 151 | 151 | 151 | 151 | 151 | 151 | 151 | 148 | 151 | 151 |
Endogeneity test statistic | 11.547 | 25.007 | 30.177 | 14.839 | 11.963 | 6.209 | 2.251 | 0.530 | 2.952 | 0.340 |
Endogeneity p-value | 0.000679 | 5.71e-07 | 3.94e-08 | 0.000117 | 0.000543 | 0.0127 | 0.133 | 0.467 | 0.0858 | 0.560 |
Hansen–Sargan test statistic | 0.843 | 0.801 | 0.00285 | 0.101 | 0.558 | 4.446 | 3.513 | 4.412 | 0.225 | |
Hansen–Sargan p-value | 0.358 | 0.371 | 0.957 | 0.751 | 0.757 | 0.108 | 0.0609 | 0.220 | 0.635 | |
Shea’s partial R2 | 0.245 | 0.282 | 0.176 | 0.192 | 0.150 | 0.193 | 0.155 | 0.103 | 0.191 | 0.263 |
Kleibergen–Paap LM test statistic | 23.51 | 24.85 | 13.79 | 13.60 | 11.64 | 25.23 | 21.85 | 12.51 | 20.29 | 22.02 |
Kleibergen–Paap p-value | 7.85e-06 | 4.02e-06 | 0.00101 | 0.00111 | 0.000644 | 1.38e-05 | 7.02e-05 | 0.00192 | 0.000437 | 1.65e-05 |
Kleibergen–Paap F-statistic | 21.14 | 47.87 | 15.10 | 11.93 | 16.51 | 13.56 | 20.13 | 9.000 | 9.306 | 16.92 |
Pesaran–Taylor reset statistic | 0.416 | 0.405 | 0.104 | 0.483 | 0.0584 | 1.211 | 0.838 | 1.681 | 0.0456 | 0.107 |
Pesaran–Taylor p-value | 0.519 | 0.524 | 0.747 | 0.487 | 0.809 | 0.271 | 0.360 | 0.195 | 0.831 | 0.744 |
Variable | (1) PBC 2, cancer, 2008/9, spend model, instrument other programme need, weighted, second stage | (2) PBC 10, circulatory, 2008/9, spend model, instrument other programme need, weighted, second stage | (3) PBC 11, respiratory, 2008/9, spend model, instrument other programme need, weighted, second stage | (4) PBC 13, gastrointestinal, 2008/9, spend model, instrument other programme need, weighted, second stage | (5) PBC 1, infectious disease, 2008/9, spend model, other programme need exogenous, weighted, OLS | (6) PBC 4, endocrine, 2008/9, spend model, instrument other programme need, weighted, second stage | (7) PBC 7, neurological, 2008/9, spend model, instrument other programme need, weighted, second stage | (8) PBC 17, genitourinary, 2008/9, spend model, other programme need exogenous, weighted, OLS | (9) PBC 18 + 19, maternity and neonates, 2008/9, spend model, instrument other programme need, weighted, second stage | (10) PBC 23a, GMS/PMS, 2008/9, spend model, other programme need exogenous, weighted, OLS | (11) PBC 16, trauma and injuries, 2008/9, spend model, other programme need exogenous, weighted, OLS |
---|---|---|---|---|---|---|---|---|---|---|---|
All-cause SYLLR excluding cancer | −1.216*** | ||||||||||
[0.186] | |||||||||||
PCT budget per head | 0.525* | 0.648 | 0.652* | 0.456* | 1.546*** | 0.484** | 0.980*** | 0.697*** | 0.975*** | 0.494*** | 1.344*** |
[0.296] | [0.552] | [0.337] | [0.254] | [0.265] | [0.240] | [0.220] | [0.209] | [0.303] | [0.140] | [0.236] | |
Need CARAN per head | 2.081*** | 2.606*** | 2.036*** | 2.095*** | 0.553 | 0.295 | 0.724** | ||||
[0.389] | [0.623] | [0.377] | [0.411] | [0.369] | [0.310] | [0.334] | |||||
All-cause SYLLR excluding circulatory | −1.987*** | ||||||||||
[0.351] | |||||||||||
All-cause SYLLR excluding respiratory | −1.081*** | ||||||||||
[0.264] | |||||||||||
Need CARAN per head squared | 1.336*** | 1.602*** | |||||||||
[0.501] | [0.495] | ||||||||||
All-cause SYLLR excluding gastrointestinal | −1.256*** | ||||||||||
[0.317] | |||||||||||
HIV need per head | 0.456*** | ||||||||||
[0.027] | |||||||||||
All-cause SYLLR excluding infectious disease | −0.472** | ||||||||||
[0.227] | |||||||||||
HIV need per head squared | 0.178*** | ||||||||||
[0.023] | |||||||||||
All-cause SYLLR excluding diabetes | −0.164 | ||||||||||
[0.197] | |||||||||||
Diabetes prevalence rate | 0.439*** | ||||||||||
[0.112] | |||||||||||
All-cause SYLLR excluding epilepsy | −0.257* | ||||||||||
[0.153] | |||||||||||
Epilepsy prevalence rate | 0.414*** | ||||||||||
[0.063] | |||||||||||
Born outside EU | 0.039*** | ||||||||||
[0.014] | |||||||||||
All-cause SYLLR excluding renal | −0.029 | ||||||||||
[0.139] | |||||||||||
All-cause SYLLR | −0.348 | −0.106 | −0.269 | ||||||||
[0.302] | [0.104] | [0.195] | |||||||||
Maternity need per head | 0.846*** | ||||||||||
[0.120] | |||||||||||
Lone pensioner households | −0.166** | ||||||||||
[0.079] | |||||||||||
Permanently sick aged 16–74 years | −0.310*** | ||||||||||
[0.092] | |||||||||||
Professional occupations | −0.124* | ||||||||||
[0.064] | |||||||||||
Working in agriculture | 0.107*** | ||||||||||
[0.022] | |||||||||||
Constant | 7.556*** | 11.702*** | 6.044** | 8.551*** | −5.471*** | 0.488 | −1.315 | −0.521 | −0.696 | 0.586 | −3.605*** |
[2.406] | [4.445] | [2.651] | [2.592] | [1.096] | [2.282] | [1.005] | [1.857] | [0.800] | [1.133] | [1.027] | |
Observations | 151 | 151 | 151 | 151 | 151 | 151 | 151 | 151 | 151 | 150 | 151 |
R 2 | 0.776 | 0.497 | 0.278 | 0.339 | |||||||
Endogeneity test statistic | 17.101 | 22.697 | 17.212 | 12.023 | 1.803 | 7.163 | 3.243 | ||||
Endogeneity p-value | 3.54e-05 | 1.90e-06 | 3.34e-05 | 0.000525 | 0.179 | 0.00744 | 0.0717 | ||||
Hansen–Sargan test statistic | 0.0538 | 0.332 | 0.858 | 0.420 | 0.138 | 0.594 | 1.349 | ||||
Hansen–Sargan p-value | 0.817 | 0.565 | 0.354 | 0.517 | 0.710 | 0.441 | 0.509 | ||||
Shea’s partial R2 | 0.379 | 0.265 | 0.389 | 0.331 | 0.399 | 0.500 | 0.257 | ||||
Kleibergen–Paap LM statistic | 39.01 | 29.71 | 37.32 | 33.84 | 38.45 | 35.08 | 22.81 | ||||
Kleibergen–Paap p-value | 3.38e-09 | 3.54e-07 | 7.87e-09 | 4.48e-08 | 4.48e-09 | 2.41e-08 | 4.43e-05 | ||||
Kleibergen–Paap F-statistic | 39.97 | 26.93 | 44.98 | 20.13 | 47.20 | 75.67 | 16.35 | ||||
Pesaran–Taylor reset statistic | 1.129 | 0.0810 | 0.000203 | 0.557 | 0.354 | 0.366 | 0.00412 | ||||
Pesaran–Taylor p-value | 0.288 | 0.776 | 0.989 | 0.456 | 0.552 | 0.545 | 0.949 | ||||
Ramsey reset F-statistic | 1.723 | 1.431 | 0.072 | 1.044 | |||||||
Probability > F | 0.165 | 0.236 | 0.975 | 0.375 |
Outcome models
Most of the outcome models in Table 85 contain just two variables: own programme expenditure and a measure of the need for health care. The latter is usually the measure of need as employed by the Department of Health for resource allocation purposes and this incorporates the CARAN formula for acute services. For the respiratory disease programme we have added the square of the need measure to improve the model fit. In other PBCs (e.g. for the endocrine, metabolic and nutritional programmes), we found that the all service measure of need performed poorly and we have replaced it with a more programme-specific measure (e.g. the diabetes prevalence rate) or with a better performing proxy for need (e.g. the percentage of residents born outside the EU for maternity/neonate mortality).
The relevant statistical test suggests that expenditure is endogenous in 6 of the 10 programmes but we have retained the IV estimates for the other four because they provide plausible results. The Hansen–Sargen test suggests that the selected instruments are valid, and the Kleibergen–Paap LM statistic suggests that they are relevant (i.e. correlated with the endogenous regressor). The Kleibergen–Paap F-statistic suggests that we do not have a problem with weak instruments (although the F-statistic is marginally less than the conventional target value of 10 in the genitourinary and infectious disease programmes). Finally, the Pesaran–Taylor reset test statistics reveal no evidence of misspecification.
Results for the big four programmes are shown in the first five columns of Table 85. Two results are reported for the gastrointestinal programme. The first of these [see Table 85, column (4)] uses two instruments and so we report the instrument validity test statistic. However, one of these instruments is insignificant in the first-stage regression and, if we drop this instrument and re-estimate the model, we obtain the result in Table 85, column (5) (but of course there is no instrument validity test statistic with this re-estimation). The removal of one instrument has little impact on the coefficient on expenditure but the Kleibergen–Paap F-statistic is now much greater than 10.
In all of the big four programmes the need variable has a positive and significant effect on mortality, and expenditure has the anticipated negative effect. As we have noted before, the outcome results for the other programmes [see Table 85, columns (6)–(10)] are similar to but more diverse than those for the big four programmes. This is to be anticipated because mortality is a much rarer outcome in these programmes than it is in the big four programmes.
Expenditure has the anticipated negative effect on mortality in the endocrine problems programme and this is statistically significant. The all service measure of need is not relevant for this PBC; instead, we find that the diabetes prevalence rate is positively associated with mortality, as is a measure of deprivation (the IMD2007).
Expenditure has a negative but statistically insignificant impact on mortality from epilepsy in the neurological programme, and the all service indicator of the need for health care is positively and significantly associated with mortality in this programme.
Expenditure also has a negative but not statistically significant effect on mortality (from renal problems) in the genitourinary problems programme. The prevalence of lone parent households is positively associated with mortality.
Expenditure has the anticipated negative effect on mortality in the infectious disease programme and this is statistically significant. The all service measure of need is not relevant for this PBC; instead, we find that a measure of need associated with HIV is positively associated with mortality, as is a measure of deprivation (the IMD2007).
Expenditure has the anticipated negative effect on mortality in the maternity and neonates programme. In this PBC the coefficient on the generic all service measure of need is positive but not significant. It has been supplemented with two other indicators of deprivation – the proportion of residents born outside the EU and the proportion of those aged 16−74 years without any qualifications – and both of these are positively associated with mortality.
Finally, we were unable to develop a plausible outcome model for the trauma and injuries programme.
Expenditure models
Most of the expenditure models in Table 86 contain just three variables: the PCT budget, a proxy for the own programme need for health care, and a proxy for the need for health care in other programmes.
The budget term is positive and statistically significant in 10 of the 11 models.
The usual proxy for the own programme need for health care (i.e. the all service measure of need) is positive and significant in 5 of the 11 results. In a couple of programmes (respiratory disease and endocrine problems) we have added the squared value of need to improve the model fit and in both cases this term is positive and significant.
In some programmes (e.g. endocrine and neurological), we have replaced and/or supplemented the all service measure of need with a more programme-specific measure (e.g. the diabetes and the epilepsy prevalence rates) and these usually have a positive and significant impact on expenditure.
In addition, in a couple of programmes we have used alternative proxies for own programme need (e.g. with the use of the Department of Health’s measure of maternity need in the maternity/neonates expenditure equation and the use of HIV need in the infectious diseases programme).
For 8 of the 11 programmes we have used the all-cause mortality rate less the own programme mortality rate as the proxy for the need for health care in other programmes, and the coefficient on this term is negative in seven programmes and statistically significant in six of the seven. In three programmes – maternity and neonates, GMS/PMS, and trauma and injuries programmes – we have used the all-cause mortality rate as the proxy for the need for health care in other programmes due to difficulties associated with the measurement of the own programme mortality rate. The coefficient on this term is negative but not significant in these three models.
The relevant statistical test suggests that expenditure is endogenous in 5 of the 11 programmes but we have retained the IV estimates for two further programmes (endocrine problems, and maternity and neonates) because the IV estimator provides more plausible results than the OLS estimator. In the other four programmes we report OLS results.
The Hansen–Sargen test suggests that the selected instruments are valid, and the Kleibergen–Paap LM statistic suggests that they are relevant (i.e. correlated with the endogenous regressor). The Kleibergen–Paap F-statistic suggests that we do not have a problem with weak instruments. Finally, the Pesaran–Taylor reset test statistics and the Ramsey reset F-statistics reveal no evidence of model misspecification.
Calculation of the cost of a life and life-year
Expenditure and outcome elasticities for our preferred models are shown in Table 87 [see columns (D) and (G)] and these are used to calculate the cost of a life and the cost of a life-year, both for individual programmes and for all programmes collectively.
(A) PBC scenario | (B) PBC description | (C) Spend (£M) 2008/9 | (D) Spend elasticity | (E) (= 0.01 × C × D) change in spend (£M) | (F) Annual mortality, < 75 years, 2008/9/10 | (G) Outcome elasticity (without negative sign) | (H) (= 0.01 × D × F × G) change in annual mortality | (I) (= E/H) cost per life gained (£) | (J) Coverage of mortality data relative to spend data | (K) (= H/J) change in annual mortality adjusted for coverage | (L) (= E/K) cost per life gained (£) adjusted for coverage | (M) Total life-years lost, < 75 years, 2008/9/10 | (N) (= 0.01 × D × G × M/3) change in annual life-years lost | (O) Coverage of mortality data relative to spend data | (P) (= N/O) change in annual life-years lost adjusted for coverage | (Q) (= E/N) cost per YLG (£) | (R) (= E/P) cost per YLG adjusted for coverage (£) | |
---|---|---|---|---|---|---|---|---|---|---|---|---|---|---|---|---|---|---|
1 | Cancer | 4843 | 0.525 | 25.43 | 61,899 | 0.307 | 99.77 | 254,855 | 0.984 | 101.39 | 250,777 | 2,170,660 | 1166 | 0.984 | 1185 | 21,802 | 21,454 | |
2 | Circulatory problems | 6655 | 0.648 | 43.12 | 38,075 | 1.319 | 325.43 | 132,514 | 0.992 | 328.06 | 131,454 | 1,285,026 | 3661 | 0.992 | 3691 | 11,779 | 11,685 | |
3 | Respiratory problems | 3994 | 0.652 | 26.04 | 10,660 | 1.808 | 125.66 | 207,230 | 0.773 | 162.56 | 160,189 | 311,034 | 1222 | 0.773 | 1581 | 21,307 | 16,470 | |
4 | Gastrointestinal problems | 3989 | 0.456 | 18.19 | 6015 | 1.364 | 37.41 | 486,199 | 0.571 | 65.52 | 277,620 | 341,884 | 709 | 0.571 | 1241 | 25,662 | 14,653 | |
Big four programmes summary | ||||||||||||||||||
5 | Spend 2008 and mortality 2008/9/10 | 19,481 | 112.78 | 116,649 | 588.27 | 191,716 | 657.53 | 171,552 | 4,108,604 | 6758 | 7698 | 16,688 | 14,650 | |||||
6 | Spend 2007 and mortality 2007/8/9 | 18,134 | 101.29 | 118,059 | 525.37 | 192,795 | 604.62 | 167,526 | 4,161,720 | 6197 | 7324 | 16,345 | 13,830 | |||||
7 | Spend 2006 and mortality 2006/7/8 | 17,268 | 91.24 | 120,801 | 665.10 | 137,188 | 761.49 | 119,823 | 4,238,786 | 7399 | 8604 | 12,333 | 10,604 | |||||
8 | Spend 2006 and mortality 2004/5/6 | 17,268 | 114.04 | 125,290 | 953.13 | 119,650 | 4,335,559 | 10,576 | 10,783 | |||||||||
9 | Spend 2005 and mortality 2002/3/4 | 17,625 | 141.22 | 125,290 | 909.96 | 155,196 | 4,516,953 | 10,986 | 12,855 | |||||||||
10 | Infectious diseases | 1201 | 1.545 | 18.56 | 1828 | 0.504 | 14.23 | 1,303,576 | 1.000 | 14.23 | 1,303,576 | 100,078 | 260 | 1.000 | 260 | 71,432 | 71,432 | |
11 | Endocrine problems | 2222 | 0.484 | 10.75 | 1398 | 1.170 | 7.92 | 1,358,473 | 0.634 | 12.49 | 861,272 | 54,779 | 103 | 0.634 | 163 | 104,008 | 65,941 | |
12 | Neurological problems | 3466 | 0.980 | 33.97 | 711 | 0.417 | 2.91 | 11,690,226 | 0.136 | 21.36 | 1,589,871 | 64,222 | 87 | 0.136 | 643 | 388,267 | 52,804 | |
13 | Genitourinary problems | 3779 | 0.697 | 26.34 | 240 | 1.615 | 2.70 | 9,749,742 | 0.172 | 15.71 | 1,676,956 | 8004 | 30 | 0.172 | 175 | 877,038 | 150,851 | |
14 | Trauma and injuries | 3255 | 1.344 | 43.75 | 983 | 0.000 | 0.00 | N/A | 0.175 | 0.00 | N/A | 6881 | 0 | 0.175 | 0 | N/A | N/A | |
15 | Maternity and neonates | 3978 | 0.975 | 38.79 | 2156 | 0.125 | 2.63 | 14,760,668 | 8.213 | 0.32 | 121,229,365 | 479,905 | 195 | 0.679 | 287 | 198,939 | 135,080 | |
Other six programmes summary | ||||||||||||||||||
16 | Spend 2008 and mortality 2008/9/10 | 17,901 | 172.15 | 7316 | 30.39 | 5,665,475 | 64.11 | 2,685,119 | 713,869 | 676 | 1528 | 254,794 | 112,674 | |||||
17 | Spend 2007 and mortality 2007/8/9 | 16,300 | 156.65 | 7648 | 32.85 | 4,768,699 | 102.95 | 1,521,610 | 745,429 | 571 | 1575 | 274,309 | 99,428 | |||||
18 | Spend 2006 and mortality 2006/7/8 | 15,643 | 93.29 | 7839 | 16.14 | 5,780,723 | 25.05 | 3,724,129 | 762,991 | 362 | 639 | 258,046 | 146,108 | |||||
19 | Spend 2006 and mortality 2004/5/6 | 15,643 | 112.13 | 7923 | 18.17 | 6,172,491 | 757,531 | 249 | 449,706 | |||||||||
20 | Spend 2005 and mortality 2002/3/4 | 12,743 | 99.44 | 7923 | 16.26 | 6,115,621 | 751,009 | 337 | 295,074 | |||||||||
All 10 programmes summary | ||||||||||||||||||
21 | Spend 2008 and mortality 2008/9/10 | 37,382 | 284.93 | 123,965 | 618.66 | 460,562 | 721.64 | 394,836 | 4,822,473 | 7434 | 9226 | 38,328 | 30,883 | |||||
22 | Spend 2007 and mortality 2007/8/9 | 34,434 | 257.94 | 125,707 | 558.22 | 462,067 | 707.57 | 364,540 | 4,907,149 | 6768 | 8900 | 38,110 | 28,983 | |||||
23 | Spend 2006 and mortality 2006/7/8 | 32,911 | 184.53 | 128,640 | 681.24 | 270,881 | 786.54 | 234,617 | 5,001,777 | 7760 | 9243 | 23,780 | 19,965 | |||||
24 | Spend 2006 and mortality 2004/5/6 | 32,911 | 226.18 | 133,213 | 971.30 | 232,861 | 5,093,090 | 10,826 | 20,893 | |||||||||
25 | Spend 2005 and mortality 2002/3/4 | 30,368 | 240.67 | 133,213 | 926.22 | 259,838 | 5,267,962 | 11,322 | 21,256 | |||||||||
Assume zero health gain in the other 13 programmes: other 13 programmes summary | ||||||||||||||||||
26 | Spend 2008 and mortality 2008/9/10 | 41,016 | 499.05 | 0.00 | 0.00 | 0 | 0 | |||||||||||
27 | Spend 2007 and mortality 2007/8/9 | 39,223 | 478.63 | 0.00 | 0.00 | 0 | 0 | |||||||||||
28 | Spend 2006 and mortality 2006/7/8 | 34,985 | 494.43 | 0.00 | 0 | 0 | ||||||||||||
29 | Spend 2006 and mortality 2004/5/6 | 34,985 | 452.78 | 0.00 | 0 | |||||||||||||
30 | Spend 2005 and mortality 2002/3/4 | 33,942 | 402.43 | 0.00 | 0 | |||||||||||||
All 23 programmes | ||||||||||||||||||
31 | Spend 2008 and mortality 2008/9/10 | 78,398 | 783.98 | 618.66 | 1,267,229 | 721.64 | 1,086,385 | 7434 | 9226 | 105,460 | 84,974 | |||||||
32 | Spend 2007 and mortality 2007/8/9 | 73,657 | 736.57 | 558.22 | 1,319,496 | 707.57 | 1,040,992 | 6768 | 8900 | 108,829 | 82,765 | |||||||
33 | Spend 2006 and mortality 2006/7/8 | 67,896 | 678.96 | 681.24 | 996,655 | 786.54 | 863,228 | 7760 | 9243 | 87,494 | 73,457 | |||||||
34 | Spend 2006 and mortality 2004/5/6 | 67,896 | 678.96 | 971.30 | 699,024 | 10,826 | 62,718 | |||||||||||
35 | Spend 2005 and mortality 2002/3/4 | 64,310 | 643.10 | 926.22 | 694,330 | 11,322 | 56,799 |
Again, Table 87, column (L) reports the cost per life gained and column (R) reports the cost per YLG. From the latter we can see that the cost per YLG has increased slightly compared with that using the previous expenditure and mortality data set (i.e. for 2007 and 2007/8/9, respectively): it has increased from £13,830 to £14,650 for the big four programmes and from £28,983 to £30,883 for all 10 programmes with a mortality-based outcome indicator.
If we assume that the other 13 programmes offer no health gain, then the cost per life-year across all PCT expenditure has increased from £82,765 in 2007/8 to £84,974 in 2008/9.
In addition, Table 88 shows that if we assume that PBC 23 generates a zero health gain and that the gain attributable to the remaining 12 programmes is, on average, the same as that attributable to those with a mortality outcome measure, then the cost of a life-year across all programmes in 2008/9 is £33,333. This is a 5% increase on the figure (£31,846) for the previous year.
(A) PBC scenario | (B) PBC description | (C) Spend (£M) 2008/9 | (D) Spend elasticity | (E) (= 0.01 × C × D) change in spend (£M) | (F) Annual mortality, < 75 years, 2008/9/10 | (G) Outcome elasticity (without negative sign) | (H) (= 0.01 × D × F × G) change in annual mortality | (I) (= E/H) cost per life gained (£) | (J) Coverage of mortality data relative to spend data | (K) (= H/J) change in annual mortality adjusted for coverage | (L) (= E/K) cost per life gained (£) adjusted for coverage | (M) Total life-years lost, < 75 years, 2008/9/10 | (N) (= 0.01 × D × G × M/3) change in annual life-years lost | (O) Coverage of mortality data relative to spend data | (P) (= N/O) change in annual life-years lost adjusted for coverage | (Q) (= E/N) cost per YLG (£) | (R) (= E/P) cost per YLG adjusted for coverage (£) |
---|---|---|---|---|---|---|---|---|---|---|---|---|---|---|---|---|---|
1 | Cancer | 4843 | 0.525 | 25.43 | 61,899 | 0.307 | 99.77 | 254,855 | 0.984 | 101.39 | 250,777 | 2,170,660 | 1166 | 0.984 | 1185 | 21,802 | 21,454 |
2 | Circulatory problems | 6655 | 0.648 | 43.12 | 38,075 | 1.319 | 325.43 | 132,514 | 0.992 | 328.06 | 131,454 | 1,285,026 | 3661 | 0.992 | 3691 | 11,779 | 11,685 |
3 | Respiratory problems | 3994 | 0.652 | 26.04 | 10,660 | 1.808 | 125.66 | 207,230 | 0.773 | 162.56 | 160,189 | 311,034 | 1222 | 0.773 | 1581 | 21,307 | 16,470 |
4 | Gastrointestinal problems | 3989 | 0.456 | 18.19 | 6015 | 1.364 | 37.41 | 486,199 | 0.571 | 65.52 | 277,620 | 341,884 | 709 | 0.571 | 1241 | 25,662 | 14,653 |
Big four programmes summary | |||||||||||||||||
5 | Spend 2008 and mortality 2008/9/10 | 19,481 | 112.78 | 116,649 | 588.27 | 191,716 | 657.53 | 171,552 | 4,108,604 | 6758 | 7698 | 16,688 | 14,650 | ||||
6 | Spend 2007 and mortality 2007/8/9 | 18,134 | 101.29 | 118,059 | 525.37 | 192,795 | 604.62 | 167,526 | 4,161,720 | 6197 | 7324 | 16,345 | 13,830 | ||||
7 | Spend 2006 and mortality 2006/7/8 | 17,268 | 91.24 | 120,801 | 665.10 | 137,188 | 761.49 | 119,823 | 4,238,786 | 7399 | 8604 | 12,333 | 10,604 | ||||
8 | Spend 2006 and mortality 2004/5/6 | 17,268 | 114.04 | 125,290 | 953.13 | 119,650 | 4,335,559 | 10,576 | 10,783 | ||||||||
9 | Spend 2005 and mortality 2002/3/4 | 17,625 | 141.22 | 125,290 | 909.96 | 155,196 | 4,516,953 | 10,986 | 12,855 | ||||||||
10 | Infectious diseases | 1201 | 1.545 | 18.56 | 1828 | 0.504 | 14.23 | 1,303,576 | 1.000 | 14.23 | 1,303,576 | 100,078 | 260 | 1.000 | 260 | 71,432 | 71,432 |
11 | Endocrine problems | 2222 | 0.484 | 10.75 | 1398 | 1.17 | 7.92 | 1,358,473 | 0.634 | 12.49 | 861,272 | 54,779 | 103 | 0.634 | 163 | 104,008 | 65,941 |
12 | Neurological problems | 3466 | 0.980 | 33.97 | 711 | 0.417 | 2.91 | 11,690,226 | 0.136 | 21.36 | 1,589,871 | 64,222 | 87 | 0.136 | 643 | 388,267 | 52,804 |
13 | Genitourinary problems | 3779 | 0.697 | 26.34 | 240 | 1.615 | 2.70 | 9,749,742 | 0.172 | 15.71 | 1,676,956 | 8004 | 30 | 0.172 | 175 | 877,038 | 150,851 |
14 | Trauma and injuries | 3255 | 1.344 | 43.75 | 983 | 0.000 | 0.00 | N/A | 0.175 | 0.00 | N/A | 6881 | 0 | 0.175 | 0 | N/A | N/A |
15 | Maternity and neonates | 3978 | 0.975 | 38.79 | 2156 | 0.125 | 2.63 | 14,760,668 | 8.213 | 0.32 | 121,229,365 | 479,905 | 195 | 0.679 | 287 | 198,939 | 135,080 |
Other six programmes summary | |||||||||||||||||
16 | Spend 2008 and mortality 2008/9/10 | 17,901 | 172.15 | 7316 | 30.39 | 5,665,475 | 64.11 | 2,685,119 | 713,869 | 676 | 1528 | 254,794 | 112,674 | ||||
17 | Spend 2007 and mortality 2007/8/9 | 16,300 | 156.65 | 7648 | 32.85 | 4,768,699 | 102.95 | 1,521,610 | 745,429 | 571 | 1575 | 274,309 | 99,428 | ||||
18 | Spend 2006 and mortality 2006/7/8 | 15,643 | 93.29 | 7839 | 16.14 | 5,780,723 | 25.05 | 3,724,129 | 762,991 | 362 | 639 | 258,046 | 146,108 | ||||
19 | Spend 2006 and mortality 2004/5/6 | 15,643 | 112.13 | 7923 | 18.17 | 6,172,491 | 757,531 | 249 | 449,706 | ||||||||
20 | Spend 2005 and mortality 2002/3/4 | 12,743 | 99.44 | 7923 | 16.26 | 6,115,621 | 751,009 | 337 | 295,074 | ||||||||
All 10 programmes summary | |||||||||||||||||
21 | Spend 2008 and mortality 2008/9/10 | 37,382 | 284.93 | 123,965 | 618.66 | 460,562 | 721.64 | 394,836 | 4,822,473 | 7434 | 9226 | 38,328 | 30,883 | ||||
22 | Spend 2007 and mortality 2007/8/9 | 34,434 | 257.94 | 125,707 | 558.22 | 462,067 | 707.57 | 364,540 | 4,907,149 | 6768 | 8900 | 38,110 | 28,983 | ||||
23 | Spend 2006 and mortality 2006/7/8 | 32,911 | 184.53 | 128,640 | 681.24 | 270,881 | 786.54 | 234,617 | 5,001,777 | 7760 | 9243 | 23,780 | 19,965 | ||||
24 | Spend 2006 and mortality 2004/5/6 | 32,911 | 226.18 | 133,213 | 971.30 | 232,861 | 5,093,090 | 10,826 | 20,893 | ||||||||
25 | Spend 2005 and mortality 2002/3/4 | 30,368 | 240.67 | 133,213 | 926.22 | 259,838 | 5,267,962 | 11,322 | 21,256 | ||||||||
Other 13 PBCs? Assume zero health gain in PBC 23 . . . | |||||||||||||||||
26 | PBC 23 spend 2008 and mortality 2008/9/10 | 11,663 | 0.494 | 57.62 | 0.00 | 0.00 | 0.00 | 0.00 | |||||||||
27 | PBC 23 spend 2007 and mortality 2007/8/9 | 11,763 | 0.563 | 66.23 | 0.00 | 0.00 | 0.00 | 0.00 | |||||||||
28 | PBC 23 spend 2006 and mortality 2006/7/8 | 10,585 | 0.739 | 78.22 | 0.00 | 0.00 | 0.00 | ||||||||||
29 | PBC 23 spend 2006 and mortality 2004/5/6 | 10,585 | 0.759 | 80.34 | 0.00 | 0.00 | |||||||||||
30 | PBC 23 spend 2005 and mortality 2002/3/4 | 8449 | 0.926 | 78.24 | 0.00 | 0.00 | |||||||||||
. . . and that the gain in 10 PBCs (see row 21) applies to the remaining 12 PBCs | |||||||||||||||||
31 | 12 PBCs spend 2008 and mortality 2008/9/10 | 29,353 | 441.43 | 958.47 | 460,562 | 1118.02 | 394,836 | 11,517 | 14,294 | 38,328 | 30,883 | ||||||
32 | 12 PBCs spend 2007 and mortality 2007/8/9 | 27,460 | 412.41 | 892.53 | 462,067 | 1131.31 | 364,540 | 10,821 | 14,229 | 38,110 | 28,983 | ||||||
33 | 12 PBCs spend 2006 and mortality 2006/7/8 | 24,400 | 416.20 | 1536.48 | 270,881 | 1773.97 | 234,617 | 17,502 | 20,847 | 23,780 | 19,965 | ||||||
34 | 12 PBCs spend 2006 and mortality 2004/5/6 | 24,400 | 372.44 | 1599.42 | 232,861 | 17,826 | 20,893 | ||||||||||
35 | 12 PBCs spend 2005 and mortality 2002/3/4 | 25,493 | 324.20 | 1247.69 | 259,838 | 15,252 | 21,256 | ||||||||||
All 23 programmes | |||||||||||||||||
36 | 23 PBCs spend 2008 and mortality 2008/9/10 | 78,398 | 783.98 | 1577.13 | 497,094 | 1839.66 | 426,155 | 18,951 | 23,520 | 41,369 | 33,333 | ||||||
37 | 23 PBCs spend 2007 and mortality 2007/8/9 | 73,657 | 736.57 | 1450.75 | 507,717 | 1838.88 | 400,554 | 17,590 | 23,129 | 41,875 | 31,846 | ||||||
38 | 23 PBCs spend 2006 and mortality 2006/7/8 | 67,896 | 678.96 | 2217.72 | 306,153 | 2560.50 | 265,167 | 25,262 | 30,090 | 26,876 | 22,565 | ||||||
39 | 23 PBCs spend 2006 and mortality 2004/5/6 | 67,896 | 678.96 | 2570.72 | 264,113 | 28,652 | 23,697 | ||||||||||
40 | 23 PBCs spend 2005 and mortality 2002/3/4 | 64,310 | 643.10 | 2173.90 | 295,827 | 26,575 | 24,200 |
Comparing the cost of life-year estimates associated with different data sets
Table 89 presents expenditure and outcome elasticities for the five combinations of expenditure and outcome data that have been used to estimate our model. It also reports the corresponding unadjusted cost of life-year estimates (i.e. estimates that are unadjusted for the mismatch in the ICD-10 coverage of the expenditure and mortality data). It is clear from Table 89 [see row (13)] that the (unadjusted) cost of a life-year for the 10 programmes with a mortality-based outcome indicator fluctuated around £22,000 for the first three sets of estimations [see columns Table 89, (M)–(O)]. However, using the two most recent sets of expenditure data (i.e. for 2007/8 and then for 2008/9), the figures in the table suggest that this cost has increased to about £38,000.
(A) | (B) PBC description | Spend elasticities | Outcome elasticities | Cost of an additional life-year (£) (unadjusted for YLL coverage) | |||||||||||||||
---|---|---|---|---|---|---|---|---|---|---|---|---|---|---|---|---|---|---|---|
(C) Using spend for 2005 and mortality for 2002/3/4 | (D) Using spend for 2006 and mortality for 2004/5/6 | (E) Using spend for 2006 and mortality for 2006/7/8 | (F) Using spend for 2007 and mortality for 2007/8/9 | (G) Using spend for 2008 and mortality for 2008/9/10 | (H) Using spend for 2005 and mortality for 2002/3/4 | (I) Using spend for 2006 and mortality for 2004/5/6 | (J) Using spend for 2006 and mortality for 2006/7/8 | (K) Using spend for 2007 and mortality for 2007/8/9 | (L) Using spend for 2008 and mortality for 2008/9/10 | (M) Using spend for 2005 and mortality for 2002/3/4 | (N) Using spend for 2006 and mortality for 2004/5/6 | (O) Using spend for 2006 and mortality for 2006/7/8 | (P) Using spend for 2007 and mortality for 2007/8/9 | (Q) Using spend for 2008 and mortality for 2008/9/10 | |||||
1 | Cancer | 0.968 | 0.548 | 0.465 | 0.890 | 0.525 | −0.394 | −0.337 | −0.342 | −0.365 | −0.307 | 13,741 | 16,518 | 16,383 | 17,165 | 21,802 | |||
2 | Circulatory problems | 0.682 | 0.701 | 0.540 | 0.293 | 0.648 | –1.370 | −1.447 | −1.434 | −1.277 | −1.319 | 8328 | 8725 | 9466 | 11,315 | 11,779 | |||
3 | Respiratory problems | 0.849 | 0.718 | 0.679 | 0.536 | 0.652 | –1.574 | −3.507 | −2.622 | −2.205 | −1.808 | 20,601 | 8747 | 11,593 | 14,798 | 21,307 | |||
4 | Gastrointestinal problems | 0.772 | 0.667 | 0.446 | 0.622 | 0.456 | –2.018 | −2.137 | −1.536 | −1.328 | −1.364 | 18,303 | 15,795 | 20,892 | 25,034 | 25,662 | |||
5 | All big four PBCs | 0.801 | 0.660 | 0.528 | 0.559 | 0.579 | –0.941 | −1.083 | −0.965 | −0.872 | −0.825 | 12,855 | 10,783 | 12,333 | 16,345 | 16,688 | |||
6 | Infectious diseases | 0.742 | 0.731 | 0.792 | 1.436 | 1.545 | –0.152 | −0.030 | −0.047 | −0.548 | −0.504 | 215,054 | 1,036,377 | 630,798 | 57,742 | 71,432 | |||
7 | Endocrine problems | 0.425 | 0.966 | 0.953 | 0.264 | 0.484 | –0.244 | −0.812 | −0.842 | −0.566 | −1.170 | 371,601 | 112,882 | 114,416 | 190,745 | 104,008 | |||
8 | Neurological problems | 1.111 | 0.648 | 0.616 | 1.035 | 0.980 | –0.182 | −0.098 | −0.112 | −0.339 | −0.417 | 503,201 | 1,241,253 | 1,129,960 | 431,749 | 388,267 | |||
9 | Genitourinary problems | 1.041 | 0.837 | 0.912 | 1.004 | 0.697 | –0.034 | −0.073 | −0.051 | −1.855 | −1.615 | 29,144,918 | 12,384,965 | 20,421,090 | 652,096 | 877,038 | |||
10 | Trauma and injuries | 0.627 | 0.617 | 0.358 | 1.686 | 1.344 | –1.332 | −0.527 | 0.000 | −0.369 | 0.000 | 282,132 | 548,767 | N/A | 1,115,197 | N/A | |||
11 | Maternity and neonates | 0.388 | 0.601 | 0.224 | 0.514 | 0.975 | –0.237 | −0.035 | −0.482 | −0.110 | −0.125 | 17,490 | 631,700 | 45,158 | 204,168 | 198,939 | |||
12 | All small six PBCs | 0.780 | 0.717 | 0.596 | 0.961 | 0.962 | –0.262 | −0.122 | −0.392 | −0.254 | −0.300 | 295,074 | 449,706 | 258,046 | 274,309 | 254,794 | |||
13 | All 10 PBCs with mortality indicator | 0.792 | 0.687 | 0.561 | 0.749 | 0.762 | –0.844 | −0.940 | −0.877 | −0.778 | −0.747 | 21,256 | 20,893 | 23,780 | 38,110 | 38,328 | |||
14 | All 23 PBCs assuming zero gain in PBCs without mortality indicator | N/A | N/A | N/A | N/A | N/A | N/A | N/A | N/A | N/A | N/A | 56,799 | 62,718 | 87,494 | 108,829 | 105,460 | |||
15 | GMS/PMS | 0.926 | 0.759 | 0.739 | 0.563 | 0.494 | N/A | N/A | N/A | N/A | N/A | N/A | N/A | N/A | N/A | N/A | |||
16 | All 23 PBCs assuming zero gain in PBC 23 but average gain in other PBCs without a mortality indicator | N/A | N/A | N/A | N/A | N/A | N/A | N/A | N/A | N/A | N/A | 24,200 | 23,697 | 26,876 | 41,875 | 41,369 |
What are the proximate causes of this increase? Recall that the cost of a life-year is calculated as:
For 2006/7 (using mortality data for 2006/7/8) and for the 10 programmes with a mortality-based outcome indicator, the change in expenditure associated with a 1% budget increase is £184.53M and the change in the number of life-years lost associated with this increase is 7760 (see Table 67 for the calculation of these figures). Thus, the cost of a life-year is £23,780 (= £184.53M/7760).
For 2007/8 (using mortality data for 2007/8/9) and for the 10 programmes with a mortality-based outcome indicator, the change in expenditure associated with a 1% budget increase is £257.94M and the change in the number of life-years lost associated with this increase is 6768 (see Table 83 in the appendix for the calculation of these figures). Thus, the cost of a life-year is £38,110 (= £257.94M/6768).
It is clear that the 60% increase in the cost of a life-year between 2006/7 and 2007/8 is largely attributable to (a) the 40% increase in the additional expenditure (up from £184.53M to £257.94M) directed towards these 10 programmes following a 1% budget increase; and (b) the 12% decline in the number of YLGs associated with this increase in expenditure (down from 7760 to 6768 life-years).
The rise in the share of the budget increase directed towards these programmes can be attributed to the increase in the implied expenditure elasticity associated with these 10 programmes (up from 0.561 to 0.749). The decrease in the number of YLG appears to be due to (a) an overall reduction in the (absolute) size of the outcome elasticities; and (b) a shift in the additional expenditure towards those programmes with a relatively high cost of a life-year. For example, the cost of a life-year for the ‘small six’ programmes is much larger than for the ‘big four PBCs’. However, in 2007/8 the spend elasticity for the small six increases from 0.561 to 0.961 (71%), whereas the expenditure elasticity for the big four rises from 0.528 to 0.559 (6%). A similar pattern – of additional expenditure shifting away from the low cost PBCs – can be seen within the big four programmes. However, it is not clear why such rather dramatic changes should have taken place.
Table 90 presents cost of life-year estimates (adjusted for the mismatch in the ICD-10 coverage of the expenditure and mortality data) for various combinations of programmes. These reveal similar increases in the cost of a life-year between 2006/7 on the one hand and 2007/8 and 2008/9 on the other. The cost of a life-year increased from £19,965 in 2006/7 to £28,983 in 2007/8 for the 10 programmes with mortality rate, an increase of 45%; it increased from £22,565 to £31,846 for all programmes if we assume a zero health gain in PBC 23 and the same gain in the other 12 programmes as in the 10 with a mortality rate (an increase of 41%).
(A) | (B) PBC description | Cost per life-year (£) (adjusted for ICD-10 coverage of spend and mortality data) | ||
---|---|---|---|---|
(C) 2006/7 | (D) 2007/8 | (E) 2008/9 | ||
1 | Cancer | 16,121 | 16,891 | 21,454 |
2 | Circulatory disease | 9390 | 11,224 | 11,685 |
3 | Respiratory problems | 8961 | 11,439 | 16,470 |
4 | Gastrointestinal problems | 11,929 | 14,295 | 14,653 |
5 | All big four programmes | 10,604 | 13,830 | 14,650 |
6 | Other six programmes with a mortality rate | 146,108 | 99,428 | 112,674 |
7 | All 10 PBCs with a mortality rate | 19,965 | 28,983 | 30,883 |
(a) if we assume a zero health gain in those PBCs without a mortality rate . . . | ||||
8 | All 23 programmes | 73,457 | 82,765 | 84,974 |
. . . or (b) if we assume a zero gain in PBC 23 and that the average gain from the 10 PBCs with a mortality rate is applied to the remaining programmes | ||||
9 | All 23 programmes | 22,565 | 31,846 | 33,333 |
One reason for this apparent step change in the cost of a life-year might be the adjustment that was made to the methodology for the collection of the 2007/8 PB data. In previous years, expenditure that was not directly attributable to a particular programme category was apportioned using admitted patient care percentages. aj In other words, if x% of total admitted patient care expenditure was allocated to PBC 1, then x% of all expenditure that was not directly attributable to a particular programme category was also allocated to PBC 1. With effect from 2007/8, however, NHS organisations were asked to select an appropriate basis for the apportionment of this non-programme-specific expenditure and that, where no reasonable basis existed, such expenditure was to be allocated to the ‘other – miscellaneous’ (PBC 23X) category.
The Department of Health estimates that this allocation rule change increased the amount of expenditure attributed to PBC 23X by £700M. It will also, of course, have reduced expenditure across other programmes by the same amount in total. However, not all programmes will have been equally affected; PBCs that are more heavily inpatient based would have ‘lost’ expenditure whereas others, such as learning disabilities, social care and mental health, will have ‘lost’ considerably less. In addition, not all PCTs will have been equally affected because each will have employed different apportionment rules for the non-programme-specific expenditure (Bryn Shorney, personal communication).
Although this allocation rule change has considerably increased the estimated cost of a life-year, we believe that this rule change has led to a more accurate allocation of expenditure across PBCs, and that the more recent estimates of the cost of a life-year (for 2007/8 and 2008/9) are more accurate than those for the earlier years (for 2005/6 and 2006/7).
Adjusting the cost of a life-year estimates to constant prices
The cost of a life-year estimates presented above are all at current prices. To put them on a constant price basis, we need an index of pay and price inflation for the labour and goods/services purchased by the NHS. Curtis87 reports a pay and prices index for HCHSs and this implies an inflation rate of 3.7% in 2006/7, 2.9% in 2007/8 and 3.9% in 2008/9. ak If we assume that similar inflation rates also apply to the purchase of pharmaceuticals and the provision of primary care (items that are excluded from the HCHS index), then we can use these figures to put the cost of a life-year estimates on a constant price basis.
For example, if we assume that PBC 23 generates a zero health gain and that the gain attributable to the 12 programmes without a mortality indicator is, on average, the same as that attributable to those with a mortality outcome measure, then the cost of a life-year across all programmes in 2008/9 is £33,333 at current (2008/9) prices. The cost for 2007/8 is £31,846 at current (2007/8) prices or £33,088 at constant (2008/9) prices, and the figure for 2006/7 is £22,565 at current (2006/7) prices or £24,125 at constant (2008/9) prices. The conversion of the costs from a current to constant price basis has relatively little impact because the inflation rate over the relevant period is quite small.
Summary and conclusions
In this section we have estimated outcome and expenditure models using PB data for 2008/9 and mortality data for 2008/9/10. The cost of an additional life-year for all 10 programmes with a mortality-based outcome is £30,883. This is similar to the comparable figure (£28,983) for the previous year (i.e. using expenditure data for 2007/8 and mortality data for 2007/8/9). If we assume that PBC 23 generates a zero health gain and that the gain attributable to the 12 programmes without a mortality indicator is, on average, the same as that attributable to those with a mortality outcome measure, then the cost of a life-year across all programmes in 2008/9 is £33,333 and this, too, is similar to the figure for the previous year (£31,846).
We have also identified a pay and prices index that can be used to put the estimated costs on a constant price basis. This index has recorded an annual inflation rate of about 3.5% since 2005/6.
There appears to have been a step change in the cost of an additional life-year. The cost of a life-year estimates are very similar up to and including 2006/7, and they are very similar for 2007/8 and 2008/9. However, there is a substantial difference between the figures for 2004/5, 2005/6 and 2006/7 on the one hand, and for 2007/8 and 2008/9 on the other. The reason for this step change is not obvious but it might be due to changes in the algorithm used by the Department of Health to allocate non-admitted patient care activity to budget categories. Although this allocation rule change has considerably increased the estimated cost of a life-year, we believe that this rule change has led to a more accurate allocation of expenditure across PBCs, and that the more recent estimates of the cost of a life-year (for 2007/8 and 2008/9) are more accurate than those for the earlier years (for 2005/6 and 2006/7).
Summary and concluding remarks
The findings presented in this report build on four previous studies. 59,60,62,63 These studies and the results presented here draw on the availability of two new data sets to obtain empirical estimates of the relationship between mortality and expenditure across all English local health authorities.
In this research we have extended the previous studies in several ways. First, we have derived plausible outcome and expenditure models for a larger number of programmes (n = 10) than previous studies. The cost of a life-year across all 10 programmes with a mortality-based outcome indicator using expenditure data for 2006/7 and mortality data for 2004/5/6 is £20,893.
Second, we relate expenditure in time period t to mortality in that period (t) and in the next two periods (t + 1 and t + 2). In other words, we assume that the health benefits associated with expenditure occur either in the same period as the expenditure or in the next two periods. This is an improvement on past practice where data constraints forced researchers to relate expenditure to the current and two previous periods. When we re-estimated our models having replaced mortality data for 2004/5/6 with that of 2006/7/8, we found that the cost of a life-year across the 10 programmes with a mortality-based outcome indicator using expenditure data for 2006/7 is £23,780 (up from £20,893, an increase of 14%).
Third, we have noted the mismatch in the ICD-10 coverage of the expenditure and mortality data. If we adjust the calculation of the cost of a life-year for 2006/7 for this mismatch then the cost of a life-year across the 10 programmes with a mortality-based outcome indicator declines from £23,780 to £19,965 (a decrease of 16%).
Fourth, previous estimates of the cost of a life-year have been for individual programmes of care. In this report we have presented estimates of the cost of a life-year for an enlarged number of programmes and, with the aid of assumptions about the productivity (health gain) of programmes without a meaningful mortality-based outcome indicator, we have extended our individual programme estimates to incorporate expenditure across all programmes of care. Thus, for 2006/7 the cost of a life-year for those PBCs with a mortality-based outcome indicator is £19,965. If we assume that (a) that the health gains associated with PBC 23, which includes primary care and workforce training expenditure, are reflected in the mortality rates for disease-specific programmes and (b) that the average health gain across the other programmes without a mortality-based outcome indicator is the same as that for those PBCs with a mortality-based outcome indicator, then the cost of life-year across all programmes is £22,565.
Fifth, we have extended our cost of life-year estimates beyond 2006/7. Re-estimation of our model using budgeting expenditure for 2007/8 generates an all programme cost of a life-year estimate of £31,846, and re-estimation of our model using budgeting expenditure for 2008/9 generates a similar cost of a life-year estimate (£33,333). Together, the last two estimates suggest that there has been a step change in the cost of a life-year, and that this appears to have occurred between 2006/7 and 2007/8. The cost of a life-year estimates are very similar up to and including 2006/7, and they are very similar for 2007/8 and 2008/9. However, there is a substantial difference between the figures for 2004/5, 2005/6 and 2006/7 on the one hand (at about £22,000), and for 2007/8 and 2008/9 on the other (at about £33,000). The reason for this step change is not obvious but it might be due to changes in the algorithm used by the Department of Health to allocate non-admitted patient care activity to budget categories. Although this allocation rule change has considerably increased the estimated cost of a life-year, we believe that this rule change has led to a more accurate allocation of expenditure across PBCs, and that the more recent estimates of the cost of a life-year (for 2007/8 and 2008/9) are more accurate than those for the earlier years (for 2005/6 and 2006/7).
Virtually all of the cost of a life-year estimates presented in this report are at current prices. However, it is possible to put them on a constant price basis using the HCHSs pay and prices index. 87 For 2006/7, 2007/8 and 2008/9 this index recorded an annual rate of inflation of about 3.5% and so the impact of this constant price adjustment is fairly minimal. For example, if we assume that PBC 23 generates a zero health gain and that the gain attributable to the 12 programmes without a mortality indicator is, on average, the same as that attributable to those with a mortality outcome measure, then the cost of a life-year across all programmes at constant 2008/9 prices is £33,333 for 2008/9, £33,088 for 2007/8, and £24,125 for 2006/7.
Finally, although previous results and our current models ‘pass’ the appropriate statistical tests and, in particular, the Hansen–Sargen test for valid instruments, we are aware that this test might be unable to detect the presence of invalid instruments in some (albeit rather restrictive) circumstances. Responding to this, several studies have suggested that researchers using IV techniques should subject the estimated coefficient on the endogenous variable to a sensitivity analysis. We do precisely this for the outcome equation for each of the big four models. This sensitivity analysis reveals that uncertainty associated with instrument validity has little effect on our estimate of the cost of a life-year, but it does increase the degree of uncertainty associated with this estimate.
We recognise that this study has a number of limitations. The cost of an additional life-year estimates for those programmes with a mortality-based outcome indicator are unadjusted for the QoL during the additional year; the quoted costs will be an underestimate of the QALY-adjusted cost of a life-year to the extent that additional life-years are not in perfect health. In previous studies we have noted that a rudimentary adjustment for this issue using HODaR data increased the cost of a life-year by about 50–60%. 12,100
At the same time, however, the estimated costs will exaggerate the cost of an additional QALY-adjusted year for those programmes with a mortality-based outcome indicator because they ignore any health benefits that are not associated with a reduction in mortality. In other words, expenditure that improves the QoL (e.g. cancer palliative care) but which does not extend the length of life is implicitly given a zero health gain value.
In addition, the expenditure data relates to expenditure on all patients whereas the mortality data is based on a LE of 75 years. Thus, implicitly our calculations attribute a zero health gain to all expenditure on those aged > 75 years. To illustrate the magnitude of the potential health gain ignored by this restriction, note that in a recent study of costs associated with all inpatient and outpatient activity (excluding mental health), those aged > 75 years accounted for 25% of all costs in 2007/8. 147
Moreover, our cost of a life-year estimates are based on the assumption that any Departmental budgetary change falls entirely on PCTs. Although PCTs account for most of the Department of Health’s budget, non-PCTs still accounted for 15% of the budget in 2006/7. As we have no information on how any budgetary change would be split between PCTs and non-PCTs, we have assumed that that any Departmental budgetary change falls entirely on PCTs. If the non-PCT budget is responsive to changes in the Department’s budget then our cost of a life-year estimates will be too low. If the non-PCT budget is as responsive as the PCT budget, then our cost of a life-year estimate for 2006/7 will be increased by 17.7% (that is, from £22,565 to £26,553).
The results presented in this study are all from the estimation of the relationship between expenditure and mortality using data for a single time period. With the availability of several years of data for both expenditure and mortality, we wanted to estimate a panel data model because a panel can offer advantages over a one-period model (e.g. it is better able to handle any unobserved heterogeneity across PCTs). However, most of the instruments employed here are based on the 2001 Census80 and thus estimation of a panel model will not be possible until these instruments become time variant; this should occur later this year with release of the 2011 Census data at PCT level. This is one piece of work that we intend to pursue in the near future.
Annex
PBC description | Spend (£) per head 2003/4 | Spend (£) per head 2004/5 | Spend (£) per head 2005/6 | Spend (£) per head 2006/7 | Spend (£) per head 2007/8 | Growth (%) 2007/8 | Spend (£) per head 2008/9 | Growth (%) 2008/9 | |
---|---|---|---|---|---|---|---|---|---|
1 | Infectious diseases | 17.95 | 20.22 | 23.61 | 20.88 | 22.08 | 6 | 23.46 | 6 |
1A | HIV and AIDS | 7.39 | 8.54 | 16 | 10.36 | 21 | |||
1X | Infectious diseases (other) | 13.49 | 13.54 | 0 | 13.10 | –3 | |||
2 | Cancers and tumours | 64.95 | 75.54 | 83.24 | 81.67 | 90.21 | 10 | 94.55 | 5 |
2A | Cancer, head and neck | 2.83 | 2.65 | –6 | 2.72 | 3 | |||
2B | Cancer, upper GI | 4.05 | 4.38 | 8 | 4.73 | 8 | |||
2C | Cancer, lower GI | 6.46 | 6.71 | 4 | 7.47 | 11 | |||
2D | Cancer, lung | 3.89 | 4.28 | 10 | 4.48 | 5 | |||
2E | Cancer, skin | 1.88 | 2.05 | 9 | 2.05 | 0 | |||
2F | Cancer, breast | 7.39 | 8.35 | 13 | 9.34 | 12 | |||
2G | Cancer, gynaecological | 2.97 | 2.93 | –1 | 3.05 | 4 | |||
2H | Cancer, urological | 7.76 | 7.84 | 1 | 8.17 | 4 | |||
2I | Cancer, haematological | 8.40 | 9.22 | 10 | 9.47 | 3 | |||
2X | Cancers and tumours (other) | 36.04 | 41.79 | 16 | 43.07 | 3 | |||
3 | Disorders of the blood | 14.08 | 17.00 | 17.48 | 16.58 | 19.44 | 17 | 19.50 | 0 |
4 | Endocrine, nutritional and metabolic | 28.96 | 31.86 | 37.26 | 36.70 | 39.39 | 7 | 43.38 | 10 |
4A | Diabetes | 17.76 | 19.44 | 9 | 21.73 | 12 | |||
4B | Endocrine, nutritional and metabolic | 6.95 | 7.47 | 8 | 7.96 | 6 | |||
4X | Other endocrine, nutritional, metabolic | 11.99 | 12.48 | 4 | 13.69 | 10 | |||
5 | Mental health disorders | 133.31 | 146.83 | 158.95 | 166.53 | 180.90 | 9 | 191.21 | 6 |
5A | Substance misuse | 13.81 | 15.76 | 14 | 17.81 | 13 | |||
5B | Organic mental disorders | 14.24 | 14.83 | 4 | 17.39 | 17 | |||
5C | Psychotic disorders | 23.84 | 31.19 | 31 | 33.69 | 8 | |||
5D | Child and adolescent mental health | 12.13 | 12.15 | 0 | 13.33 | 10 | |||
5X | Other mental health disorders | 102.51 | 106.97 | 4 | 108.99 | 2 | |||
6 | Problems of learning disability | 37.93 | 43.37 | 46.54 | 48.36 | 54.20 | 12 | 56.11 | 4 |
7 | Neurological | 29.83 | 35.09 | 41.06 | 55.27 | 62.43 | 13 | 67.64 | 8 |
7A | Chronic pain | 19.31 | 22.12 | 15 | 22.79 | 3 | |||
7X | Neurological (other) | 35.96 | 40.31 | 12 | 44.85 | 11 | |||
8 | Problems of vision | 24.61 | 27.65 | 28.24 | 26.97 | 30.69 | 14 | 32.95 | 7 |
9 | Problems of hearing | 5.73 | 6.32 | 6.27 | 6.21 | 8.07 | 30 | 8.16 | 1 |
10 | Problems of circulation | 110.12 | 122.37 | 124.28 | 122.06 | 124.77 | 2 | 129.94 | 4 |
10A | Coronary heart disease | 38.91 | 40.32 | 4 | 41.20 | 2 | |||
10B | Cerebrovascular disease | 16.05 | 17.30 | 8 | 19.35 | 12 | |||
10C | Problems of rhythm | 7.22 | 8.21 | 14 | 8.43 | 3 | |||
10X | Problems of circulation (other) | 59.88 | 58.95 | –2 | 60.96 | 3 | |||
11 | Problems of the respiratory system | 54.60 | 62.71 | 69.56 | 65.07 | 67.68 | 4 | 77.97 | 15 |
11A | Obstructive airways disease | 10.64 | 10.64 | 0 | 12.70 | 19 | |||
11B | Asthma | 14.04 | 15.73 | 12 | 16.99 | 8 | |||
11X | Problems of the respiratory system, other | 40.40 | 41.31 | 2 | 48.27 | 17 | |||
12 | Dental problems | 10.78 | 13.55 | 24.91 | 51.93 | 59.45 | 14 | 62.44 | 5 |
13 | Problems of the gastrointestinal system | 63.56 | 73.22 | 81.30 | 73.30 | 75.05 | 2 | 77.89 | 4 |
13A | Upper GI | 19.88 | 19.51 | –2 | 19.89 | 2 | |||
13B | Lower GI | 20.46 | 21.92 | 7 | 22.63 | 3 | |||
13C | Hepatobiliary | 11.26 | 12.23 | 9 | 12.90 | 5 | |||
13X | Problems of the gastrointestinal system, other | 21.69 | 21.39 | –1 | 22.46 | 5 | |||
14 | Problems of the skin | 20.98 | 24.90 | 26.84 | 28.31 | 30.41 | 7 | 32.34 | 6 |
14A | Burns | 1.08 | 1.56 | 44 | 1.02 | –34 | |||
14X | Problems of the skin, other | 27.23 | 28.86 | 6 | 31.32 | 9 | |||
15 | Problems of the musculoskeletal system | 61.36 | 71.72 | 74.74 | 66.75 | 75.91 | 14 | 79.68 | 5 |
16 | Problems due to trauma and injuries | 62.31 | 72.13 | 76.41 | 57.29 | 57.56 | 0 | 63.54 | 10 |
17 | Problems of the genitourinary system | 55.32 | 62.38 | 67.38 | 68.98 | 67.83 | –2 | 73.78 | 9 |
17A | Genital tract problems | 19.33 | 18.80 | –3 | 19.36 | 3 | |||
17B | Renal problems | 21.54 | 19.74 | –8 | 22.29 | 13 | |||
17C | STDs | 4.26 | 4.71 | 10 | 5.43 | 15 | |||
17X | Problems of the genitourinary system, other | 23.85 | 24.58 | 3 | 26.69 | 9 | |||
18 | Maternity and Reproductive health | 52.28 | 55.04 | 60.42 | 57.64 | 57.09 | –1 | 60.44 | 6 |
19 | Conditions of neonates | 11.72 | 13.93 | 13.42 | 13.17 | 15.15 | 15 | 17.23 | 14 |
20 | Adverse effects and poisoning | 9.68 | 12.32 | 14.25 | 14.59 | 15.84 | 9 | 18.31 | 16 |
20A | Unintended consequences of treatment | 10.54 | 12.14 | 15 | 12.96 | 7 | |||
20B | Poisoning | 2.13 | 2.44 | 15 | 2.91 | 19 | |||
20C | Violence | 0.47 | 0.49 | 3 | 1.75 | 258 | |||
20X | Adverse effects and poisoning, other | 1.45 | 0.77 | –47 | 0.70 | –9 | |||
21 | Healthy individuals | 20.29 | 22.77 | 26.18 | 26.85 | 31.44 | 17 | 35.74 | 14 |
21A | NSF prevention programme | 2.30 | 3.75 | 63 | 4.82 | 29 | |||
21B | NSF mental health prevention | 0.17 | 0.47 | 176 | 0.46 | –2 | |||
21X | Healthy individuals (other) | 24.38 | 27.22 | 12 | 30.46 | 12 | |||
22 | Social care needs | 24.81 | 30.93 | 33.59 | 30.29 | 35.29 | 17 | 36.58 | 4 |
23 | Other | 136.94 | 157.75 | 171.82 | 209.70 | 232.02 | 11 | 227.71 | –2 |
23A | GMS/PMS | 141.42 | 147.53 | 4 | 145.26 | –2 | |||
23B | Training (WDCs) | 0.60 | 0.30 | –49 | 0.24 | –21 | |||
23X | Miscellaneous | 67.67 | 84.19 | 24 | 82.20 | –2 | |||
1 to 23 | All PBCs | 1052.12 | 1199.60 | 1307.76 | 1345.10 | 1452.91 | 8 | 1530.59 | 5 |
Indicator name | Short description | Long description |
---|---|---|
BORNEXEU | Residents born outside the EU | Residents born outside the EU divided by all residents (census cell definition: KS005008/KS005001) |
WHITEEG | Population in white ethnic group | Population in white ethnic group divided by total population (KS006002 + KS006003 + KS006004)/KS006001 |
PCWALLTI | Population of working age with illness | Proportion of population of working age with LLT aged 16–74 years (KS008003/KS09A001) |
POPPUCAR | Unpaid care providers in population | Proportion of population providing unpaid care (KS008007/KS008001) |
POPPUCA1 | Unpaid care (< 20 hours/week) in population | Proportion of population providing unpaid care for 1–19 hours a week (KS008008/KS008001) |
POPPUCA2 | Unpaid care (20–49 hours/week) in population | Proportion of population providing unpaid care for 20–49 hours per week (KS008009/KS008001) |
POPPUCA3 | Unpaid care (> 50 hours/week) in population | Proportion of population providing unpaid care for > 50 hours a week (KS008007/KS008001) |
NQUAL1674 | Proportion aged 16–74 years with no qualifications | Proportion of population aged 16–74 years with no qualifications (KS013002/KS013001 |
FTSTUDEN | Proportion aged 16–74 years full-time students | Proportion of population aged 16–74 years that are full-time students (KS013008 + KS013009)/KS013001 |
HHNOCAR | Households without a car | Proportion of households without a car (KS017002/KS017001) |
OWNOCC | Owner occupied households | Proportion of households that are owner occupied (KS018002 + KS018003 + KS018004)/KS018001) |
LAHARENT | Rented social housing | Proportion of households that are rented from LA or HA (KS018005 + KS018006)/KS018001 |
PRIVRENT | Rented private housing | Proportion of households that are rented from private landlords (KS018007/KS018001) |
LONEPENH | Lone pensioner households | Proportion of households that are one pensioner households (KS020002/KS020001) |
LONEPARH | Lone parent households | Proportion of households that are lone parent households with dependent children (KS020011/KS020001) |
PERMSICK | Permanently sick of those aged 16–74 years | Proportion of population aged 16–74 years that are permanently sick (KS09A010/KS09A001) |
PC74LTUN | Long-term unemployed of those aged 16–74 years | Proportion of those aged 16–74 years that are long-term unemployed (KS09A015/KS09A001) |
WORKAGRI | Employed in agriculture | Proportion of those aged 16–74 years in employment that are working agriculture (KS11A002/KS11A001) |
PROFOCCU | People in professional occupations | Proportion of those aged 16–74 years in managerial and professional occupations (KS14A002 + KS14A003 + KS14A004)/KS14A001 |
Variable | 2005/6, outcome model, instrument spend, unweighted, first stage | |||||
---|---|---|---|---|---|---|
(1) PBC 2 cancer | (2) PBC 10 circulation | (3) PBC 11 respiratory | (4) PBC 13 gastrointestinal | (5) PBC 16 trauma and injuries | (6) PBC 19 neonates | |
Need per head | 0.406*** | 1.173*** | 1.533*** | 0.970*** | 0.727** | |
[0.097] | [0.235] | [0.401] | [0.243] | [0.289] | ||
Lone pensioner households | 0.593*** | 0.229*** | −0.118 | 0.045 | 0.561*** | |
[0.109] | [0.084] | [0.112] | [0.093] | [0.108] | ||
Provision of unpaid care | −0.013 | 0.374*** | 0.574*** | −0.148 | ||
[0.135] | [0.115] | [0.089] | [0.132] | |||
IMD2000 | −0.152*** | −0.247*** | −0.047 | −0.016 | ||
[0.056] | [0.069] | [0.060] | [0.074] | |||
White ethnic group | −0.007 | |||||
[0.067] | ||||||
Permanently sick | 0.192** | |||||
[0.085] | ||||||
Low birth weight births | 0.393 | |||||
[0.308] | ||||||
Lone parent households | 0.034 | |||||
[0.209] | ||||||
No qualifications | −0.599*** | |||||
[0.148] | ||||||
Long-term unemployed | 0.394*** | |||||
[0.122] | ||||||
LA/HA rented accommodation | 0.283** | |||||
[0.127] | ||||||
Constant | −1.373*** | −0.311 | −1.562*** | −0.959*** | −1.780*** | −2.797*** |
[0.304] | [0.244] | [0.344] | [0.192] | [0.260] | [0.433] | |
Observations | 295 | 295 | 295 | 295 | 295 | 294 |
R 2 | 0.297 | 0.629 | 0.434 | 0.571 | 0.396 | 0.197 |
Variable | 2005/6, spend model, instrument other programme need, unweighted, first stage | ||||||
---|---|---|---|---|---|---|---|
(1) PBC 2 cancer | (2) PBC 10 circulation | (3) PBC 11 respiratory | (4) PBC 13 gastrointestinal | (5) PBC 7 neurological | (6) PBC 16 trauma and injuries | (7) PBC 23 GMS/PMS | |
No qualifications | 0.240*** | ||||||
[0.038] | |||||||
Lone pensioner households | −0.686*** | −0.244*** | −0.234*** | −0.266*** | −0.234*** | −0.234*** | −0.129*** |
[0.067] | [0.052] | [0.049] | [0.049] | [0.049] | [0.049] | [0.038] | |
Private rented housing | 0.072*** | ||||||
[0.017] | |||||||
Work in agriculture | −0.006 | ||||||
[0.008] | |||||||
PCT budget per head | −0.146 | −0.003 | −0.077 | −0.022 | −0.077 | −0.077 | 0.043 |
[0.117] | [0.074] | [0.070] | [0.070] | [0.070] | [0.070] | [0.069] | |
No car households | 0.092** | ||||||
[0.039] | |||||||
Lone parent households | 0.171*** | ||||||
[0.035] | |||||||
Permanently sick | 0.125*** | ||||||
[0.027] | |||||||
Need per head | 1.933*** | 0.651*** | 0.875*** | 0.597*** | 0.875*** | 0.875*** | |
[0.110] | [0.157] | [0.175] | [0.157] | [0.175] | [0.175] | ||
White ethnic group | 0.197*** | ||||||
[0.038] | |||||||
Provision of unpaid care | −0.371*** | −0.153** | −0.217*** | −0.217*** | −0.217*** | ||
[0.071] | [0.065] | [0.055] | [0.055] | [0.055] | |||
IMD2000 | 0.056* | 0.128*** | 0.179*** | 0.128*** | 0.128*** | ||
[0.033] | [0.038] | [0.035] | [0.038] | [0.038] | |||
Constant | 2.562*** | 4.103*** | 4.851*** | 5.114*** | 4.851*** | 4.851*** | 7.361*** |
[0.159] | [0.140] | [0.109] | [0.082] | [0.109] | [0.109] | [0.139] | |
Observations | 295 | 295 | 295 | 295 | 295 | 295 | 295 |
R 2 | 0.804 | 0.680 | 0.858 | 0.849 | 0.858 | 0.858 | 0.881 |
Variable | (1) PBC 2 cancer, 2006/7, outcome model, uses SYLLR, instrument spend, weighted, first stage, CARAN need, two MFFs | (2) PBC 2, cancer, 2006/7, spend model, uses SYLLR, instrument other programme need, weighted, first stage, CARAN need, two MFFs | (3) PBC 10 circulation, 2006/7, outcome model, uses SYLLR, instrument spend, weighted, first stage, CARAN need, two MFFs | (4) PBC 10 circulation, 2006/7, spend model, uses SYLLR, instrument other programme need, weighted, first stage, CARAN need, two MFFs | (5) PBC 11 respiratory, 2006/7, outcome model, uses SYLLR, instrument spend, weighted, first stage, CARAN need, two MFFs | (6) PBC 11 respiratory, 2006/7, spend model, uses SYLLR, instrument other programme need, weighted, first stage, CARAN need, two MFFs | (7) PBC 13 gastrointestinal, 2006/7, outcome model, uses SYLLR, instrument spend, weighted, first stage, CARAN need, two MFFs | (8) PBC 13 gastrointestinal, 2006/7, spend model, uses SYLLR, instrument other programme need, weighted, first stage, CARAN need, two MFFs |
---|---|---|---|---|---|---|---|---|
Need CARAN per head | 1.162*** | 1.602*** | 1.539*** | 0.606*** | 1.026*** | 0.836*** | 1.292*** | 0.938*** |
[0.250] | [0.126] | [0.323] | [0.141] | [0.368] | [0.175] | [0.358] | [0.167] | |
Need CARAN per head squared | 0.912 | |||||||
[0.666] | ||||||||
Lone pensioner households | 0.383*** | −0.431*** | 0.321*** | −0.221*** | ||||
[0.134] | [0.073] | [0.111] | [0.067] | |||||
IMD2007 | −0.153** | −0.247*** | 0.117*** | 0.104** | −0.115 | 0.107*** | ||
[0.074] | [0.087] | [0.037] | [0.043] | [0.094] | [0.041] | |||
PCT budget per head | 0.120 | 0.183* | 0.077 | −0.020 | ||||
[0.124] | [0.094] | [0.084] | [0.093] | |||||
Provision of unpaid care | −0.410*** | 0.097 | −0.309*** | 0.373* | −0.325*** | |||
[0.088] | [0.197] | [0.090] | [0.215] | [0.078] | ||||
White ethnic group | −0.060 | |||||||
[0.082] | ||||||||
Permanently sick | 0.681** | |||||||
[0.269] | ||||||||
Long-term unemployed | −0.123*** | |||||||
[0.035] | ||||||||
LLT | −0.785* | |||||||
[0.449] | ||||||||
Constant | 5.586*** | 3.074*** | 6.387*** | 3.790*** | 3.906*** | 4.501*** | 5.496*** | 5.119*** |
[0.235] | [0.887] | [0.363] | [0.703] | [0.474] | [0.595] | [0.314] | [0.657] | |
Observations | 152 | 152 | 152 | 152 | 152 | 152 | 152 | 152 |
R 2 | 0.438 | 0.846 | 0.623 | 0.814 | 0.623 | 0.840 | 0.554 | 0.827 |
Variable | (1) PBC 2 cancer, 2006/7, outcome model, SYLLR 2006/7/8, instrument spend, weighted, first stage, two MFFs | (2) PBC 2 cancer, 2006/7, spend model, SYLLR 2006/7/8, instrument other programme need, weighted, first stage, two MFFs | (3) PBC 10 circulation, 2006/7, outcome model, SYLLR 2006/7/8, instrument spend, weighted, first stage, two MFFs | (4) PBC 10 circulation, 2006/7, spend model, SYLLR 2006/7/8, instrument other programme need, weighted, first stage, two MFFs | (5) PBC 11 respiratory, 2006/7, outcome model, SYLLR 2006/7/8, instrument spend, weighted, first stage, two MFFs | (6) PBC 11 respiratory, 2006/7, spend model, SYLLR 2006/7/8, instrument other programme need, weighted, first stage, two MFFs | (7) PBC 13 gastrointestinal, 2006/7, outcome model, SYLLR 2006/7/8, instrument spend, weighted, first stage, two MFFs | (8) PBC 13 gastrointestinal, 2006/7, spend model, SYLLR 2006/7/8, instrument other programme need, weighted, first stage, two MFFs |
---|---|---|---|---|---|---|---|---|
Need CARAN per head | 1.162*** | 1.574*** | 1.539*** | 0.791*** | 1.061*** | 0.909*** | 1.292*** | 1.059*** |
[0.250] | [0.138] | [0.323] | [0.157] | [0.386] | [0.167] | [0.358] | [0.166] | |
Need CARAN per head squared | 0.912 | 0.455 | ||||||
[0.666] | [0.599] | |||||||
Lone pensioner household | 0.383*** | −0.375*** | 0.321*** | −0.269*** | −0.313*** | |||
[0.134] | [0.079] | [0.111] | [0.067] | [0.072] | ||||
IMD2007 | −0.153** | −0.247*** | 0.097** | 0.107*** | −0.115 | 0.066 | ||
[0.074] | [0.087] | [0.039] | [0.041] | [0.094] | [0.040] | |||
PCT budget per head | 0.126 | 0.128 | 0.020 | 0.040 | ||||
[0.136] | [0.101] | [0.090] | [0.091] | |||||
Provision of unpaid care | −0.386*** | 0.097 | −0.289*** | 0.373* | −0.203** | |||
[0.097] | [0.197] | [0.080] | [0.215] | [0.088] | ||||
White ethnic group | −0.060 | |||||||
[0.082] | ||||||||
Permanently sick | 0.677** | |||||||
[0.272] | ||||||||
Long-term unemployed | −0.121*** | |||||||
[0.035] | ||||||||
LLT | −0.798* | |||||||
[0.454] | ||||||||
Constant | 5.586*** | 3.160*** | 6.387*** | 4.132*** | 3.864*** | 4.916*** | 5.496*** | 4.481*** |
[0.235] | [0.963] | [0.363] | [0.729] | [0.493] | [0.637] | [0.314] | [0.654] | |
Observations | 152 | 152 | 152 | 152 | 152 | 152 | 152 | 152 |
R 2 | 0.438 | 0.821 | 0.623 | 0.823 | 0.624 | 0.831 | 0.554 | 0.857 |
Variable | (1) PBC 2 cancer, 2007/8, instrument spend, weighted, first stage | (2) PBC 2 cancer, 2007/8, instrument spend, weighted, first stage | (3) PBC 10 circulation, 2007/8, instrument spend, weighted, first stage | (4) PBC 11 respiratory, 2007/8, instrument spend, weighted, first stage | (5) PBC 11 respiratory, 2007/8, instrument spend, weighted, first stage | (6) PBC 13 gastrointestinal, 2007/8, instrument spend, weighted, first stage | (7) PBC 13 gastrointestinal, 2007/8, instrument spend, weighted, first stage | (8) PBC 4 endocrine, 2007/8, instrument spend, weighted, first stage | (9) PBC 17 genitourinary, 2007/8, instrument spend, weighted, first stage | (10) PBC 1 infectious disease, 2007/8, instrument spend, weighted, first stage | (11) PBC 18 + 19 maternity and neonates, 2007/8, instrument spend, weighted, first stage | (12) PBC 16 trauma and injuries, 2007/8, instrument spend, weighted, first stage |
---|---|---|---|---|---|---|---|---|---|---|---|---|
Need CARAN per head | 0.582** | 0.545*** | 0.724*** | 1.251*** | 1.196*** | 0.999*** | 1.047*** | 1.111*** | 0.856*** | |||
[0.284] | [0.105] | [0.168] | [0.094] | [0.113] | [0.105] | [0.099] | [0.259] | [0.282] | ||||
No car households | 0.512* | 0.288** | ||||||||||
[0.267] | [0.137] | |||||||||||
Lone pensioner households | 0.632*** | 0.644*** | 0.468*** | 0.360*** | 0.269*** | 0.199 | ||||||
[0.148] | [0.119] | [0.100] | [0.103] | [0.100] | [0.133] | |||||||
IMD2007 | −0.012 | −0.067 | 0.325 | |||||||||
[0.088] | [0.053] | [0.224] | ||||||||||
Need CARAN per head squared | 1.332*** | 1.338*** | ||||||||||
[0.428] | [0.425] | |||||||||||
Provision of unpaid care | 0.441** | 0.200 | ||||||||||
[0.174] | [0.160] | |||||||||||
Born outside EU | −0.054*** | −0.067*** | 0.004 | −0.079** | ||||||||
[0.018] | [0.017] | [0.041] | [0.039] | |||||||||
Diabetes prevalence rate 2007/8 | 0.358*** | |||||||||||
[0.123] | ||||||||||||
Permanently sick | 0.307*** | |||||||||||
[0.061] | ||||||||||||
Lone parent households | 0.029 | |||||||||||
[0.124] | ||||||||||||
Chronic kidney disease prevalence rate 2007/8 | 0.123** | |||||||||||
[0.061] | ||||||||||||
Long-term unemployed | 0.146** | |||||||||||
[0.061] | ||||||||||||
LLT | 0.207 | |||||||||||
[0.134] | ||||||||||||
HIV need per head squared | 0.128*** | |||||||||||
[0.031] | ||||||||||||
HIV need per head | 0.300*** | |||||||||||
[0.044] | ||||||||||||
Work in agriculture | 0.152** | 0.126*** | ||||||||||
[0.064] | [0.033] | |||||||||||
Work in professional occupation | 0.647*** | |||||||||||
[0.172] | ||||||||||||
No qualifications | −0.214 | |||||||||||
[0.160] | ||||||||||||
Maternity need per head | 0.647*** | |||||||||||
[0.159] | ||||||||||||
Full-time students | 0.126 | |||||||||||
[0.095] | ||||||||||||
LA/HA accommodation | −0.197* | |||||||||||
[0.104] | ||||||||||||
Constant | 5.759*** | 5.747*** | 6.754*** | 4.886*** | 5.172*** | 4.531*** | 4.104*** | 4.224*** | 5.269*** | 4.226*** | 4.004*** | 4.766*** |
[0.252] | [0.234] | [0.322] | [0.201] | [0.350] | [0.281] | [0.057] | [0.390] | [0.209] | [1.007] | [0.297] | [0.339] | |
Observations | 151 | 151 | 151 | 151 | 151 | 151 | 151 | 151 | 147 | 151 | 151 | 151 |
R 2 | 0.369 | 0.369 | 0.653 | 0.659 | 0.664 | 0.531 | 0.524 | 0.436 | 0.296 | 0.724 | 0.407 | 0.361 |
Variable | (1) PBC 2 cancer, 2007/8, spend model, instrument other programme need, weighted, first stage | (2) PBC 10 circulation, 2007/8, spend model, instrument other programme need, weighted, first stage | (3) PBC 11 respiratory, 2007/8, spend model, instrument other programme need, weighted, first stage | (4) PBC 13 gastrointestinal, 2007/8, spend model, instrument other programme need weighted, first stage | (5) PBC 4 endocrine, 2007/8, spend model, instrument other programme need, weighted, first stage | (6) PBC 7 neurological, 2007/8, spend model, instrument other programme need, weighted, first stage | (7) PBC 23 GMS/PMS, etc., 2007/8, spend model, instrument other programme need, weighted, first stage | (8) PBC 16 trauma and injuries, 2007/8, spend model, instrument other programme need, weighted, first stage |
---|---|---|---|---|---|---|---|---|
PCT budget per head 2007/8 | 0.071 | 0.066 | 0.039 | −0.034 | 0.057 | 0.170 | 0.360** | 0.366*** |
[0.137] | [0.123] | [0.105] | [0.105] | [0.121] | [0.130] | [0.144] | [0.136] | |
Need CARAN per head | 1.613*** | 1.201*** | 1.050*** | 0.971*** | 1.090*** | 1.148*** | ||
[0.149] | [0.136] | [0.191] | [0.188] | [0.198] | [0.137] | |||
Need CARAN per head squared | 0.343 | |||||||
[0.266] | ||||||||
Lone pensioner households | −0.357*** | −0.220*** | −0.261*** | −0.274*** | −0.444*** | −0.255*** | −0.229*** | |
[0.067] | [0.060] | [0.063] | [0.063] | [0.055] | [0.061] | [0.072] | ||
Provision of unpaid care | −0.362*** | −0.215** | −0.156* | −0.296*** | −0.181* | 0.195** | ||
[0.094] | [0.090] | [0.093] | [0.086] | [0.100] | [0.092] | |||
IMD2007 | 0.070 | 0.099** | 0.067 | 0.309*** | 0.276*** | |||
[0.042] | [0.043] | [0.045] | [0.043] | [0.039] | ||||
Diabetes prevalence rate 2007/8 | 0.008 | |||||||
[0.069] | ||||||||
Epilepsy prevalence rate 2007/8 | 0.020 | |||||||
[0.049] | ||||||||
White ethnic group | 0.221*** | |||||||
[0.060] | ||||||||
Work in agriculture | 0.009 | |||||||
[0.011] | ||||||||
Constant | 3.624*** | 4.454*** | 4.667*** | 5.298*** | 4.496*** | 3.974*** | 2.054** | 2.630*** |
[0.969] | [0.848] | [0.735] | [0.724] | [0.920] | [0.954] | [0.994] | [0.978] | |
Observations | 151 | 151 | 151 | 151 | 151 | 151 | 151 | 151 |
R 2 | 0.847 | 0.828 | 0.861 | 0.834 | 0.860 | 0.839 | 0.830 | 0.824 |
Variable | (1) PBC 2 cancer, 2008/9, outcome model, instrument spend, weighted, first stage | (2) PBC 10 circulation, 2008/9, outcome model, instrument spend, weighted, first stage | (3) PBC 11 respiratory, 2008/9, outcome model, instrument spend, weighted, first stage | (4) PBC 13 gastrointestinal, 2008/9, outcome model, instrument spend, weighted, first stage | (5) PBC 13 gastrointestinal, 2008/9, outcome model, instrument spend, weighted, first stage | (6) PBC 4 endocrine, 2008/9, outcome model, instrument spend, weighted, first stage | (7) PBC 7 neurological, 2008/9, outcome model, instrument spend, weighted, first stage | (8) PBC 17 genitourinary, 2008/9, outcome model, instrument spend, weighted, first stage | (9) PBC 1 infectious disease, 2008/9, outcome model, instrument spend, weighted, first stage | (10) PBC 18 + 19 maternity and neonates, 2008/9, outcome model, instrument spend, weighted, first stage |
---|---|---|---|---|---|---|---|---|---|---|
Need CARAN per head | 1.122*** | 1.274*** | 1.228*** | 0.989*** | 1.056*** | 0.373** | 0.659*** | |||
[0.198] | [0.146] | [0.085] | [0.088] | [0.087] | [0.175] | [0.236] | ||||
Lone pensioner households | 0.490*** | 0.426*** | 0.252** | 0.272** | 0.287*** | |||||
[0.127] | [0.090] | [0.110] | [0.109] | [0.109] | ||||||
IMD2007 | −0.145** | −0.082 | 0.236 | |||||||
[0.060] | [0.091] | [0.214] | ||||||||
No car households | −0.188*** | 0.502** | ||||||||
[0.053] | [0.227] | |||||||||
Need CARAN per head squared | 1.071** | |||||||||
[0.426] | ||||||||||
Provision of unpaid care | 0.339*** | 0.539** | 1.393*** | |||||||
[0.117] | [0.232] | [0.340] | ||||||||
Born outside EU | −0.042*** | −0.060*** | 0.080*** | −0.031 | ||||||
[0.016] | [0.015] | [0.026] | [0.032] | |||||||
Diabetes prevalence rate 2007/8 | 0.167 | |||||||||
[0.132] | ||||||||||
Permanently sick | 0.380*** | |||||||||
[0.104] | ||||||||||
Epilepsy prevalence rate 2007/8 | 0.486*** | |||||||||
[0.121] | ||||||||||
Owner occupied households | −0.235** | |||||||||
[0.113] | ||||||||||
Lone parent households | 0.175** | 0.013 | ||||||||
[0.080] | [0.106] | |||||||||
Chronic kidney disease prevalence rate 2007/8 | 0.089*** | |||||||||
[0.033] | ||||||||||
Long-term unemployed | 0.148*** | |||||||||
[0.045] | ||||||||||
HIV need per head | 0.471*** | |||||||||
[0.050] | ||||||||||
HIV need per head squared | 0.146*** | |||||||||
[0.027] | ||||||||||
No qualifications | −0.751*** | −0.092 | ||||||||
[0.189] | [0.113] | |||||||||
Work in agriculture | 0.150*** | |||||||||
[0.051] | ||||||||||
Maternity need per head | 0.834*** | |||||||||
[0.162] | ||||||||||
Constant | 5.937*** | 5.435*** | 5.610*** | 4.752*** | 4.167*** | 6.379*** | 4.808*** | 5.363*** | 6.010*** | 4.171*** |
[0.221] | [0.230] | [0.238] | [0.236] | [0.048] | [0.768] | [0.193] | [0.121] | [1.513] | [0.375] | |
Observations | 151 | 151 | 151 | 151 | 151 | 151 | 151 | 148 | 151 | 151 |
R 2 | 0.521 | 0.612 | 0.746 | 0.665 | 0.648 | 0.559 | 0.477 | 0.378 | 0.791 | 0.614 |
Variable | (1) PBC 2 cancer, 2008/9, spend model, instrument other programme need, weighted, first stage | (2) PBC 10 circulatory, 2008/9, spend model, instrument other programme need, weighted, first stage | (3) PBC 11 respiratory, 2008/9, spend model, instrument other programme need, weighted, first stage | (4) PBC 13 gastrointestinal, 2008/9, spend model, instrument other programme need, weighted, first stage | (5) PBC 4 endocrine, 2008/9, spend model, instrument other programme need, weighted, first stage | (6) PBC 7 neurological, 2008/9, spend model, instrument other programme need, weighted, first stage | (7) PBC 18 + 19 maternity and neonates, 2008/9, spend model, instrument other programme need, weighted, first stage |
---|---|---|---|---|---|---|---|
PCT budget per head 2008/9 | 0.090 | 0.049 | 0.020 | –0.115 | 0.055 | 0.180 | 0.452*** |
[0.155] | [0.118] | [0.113] | [0.106] | [0.122] | [0.132] | [0.128] | |
Need CARAN per head | 1.589*** | 1.215*** | 1.305*** | 1.042*** | 1.112*** | 1.119*** | |
[0.168] | [0.133] | [0.129] | [0.207] | [0.211] | [0.141] | ||
Need CARAN per head squared | 0.221 | ||||||
[0.270] | |||||||
Lone pensioner households | –0.371*** | –0.209*** | –0.286*** | –0.256*** | –0.453*** | –0.106* | |
[0.071] | [0.060] | [0.057] | [0.062] | [0.053] | [0.059] | ||
Provision of unpaid care | –0.349*** | –0.236** | –0.266*** | –0.302*** | –0.239** | ||
[0.105] | [0.092] | [0.088] | [0.099] | [0.119] | |||
IMD2007 | 0.099** | 0.051 | 0.223*** | ||||
[0.045] | [0.048] | [0.044] | |||||
Diabetes prevalence rate 2007/8 | 0.067 | ||||||
[0.064] | |||||||
Epilepsy prevalence rate 2007/8 | 0.036 | ||||||
[0.044] | |||||||
Maternity need per head | 0.266*** | ||||||
[0.078] | |||||||
White ethnic group | 0.292*** | ||||||
[0.065] | |||||||
Constant | 3.457*** | 4.528*** | 4.687*** | 5.851*** | 4.340*** | 3.858*** | 1.901** |
[1.131] | [0.849] | [0.814] | [0.753] | [0.940] | [0.979] | [0.864] | |
Observations | 151 | 151 | 151 | 151 | 151 | 151 | 151 |
R 2 | 0.840 | 0.828 | 0.854 | 0.830 | 0.857 | 0.837 | 0.843 |
Appendix 3 Translating mortality effects into life-years and quality-adjusted life-years
Introduction
This appendix describes how the results of the econometric work undertaken to estimate the link between NHS spending and mortality, which was detailed in Appendix 2, can be translated in to effects on life-years and QALYs. This appendix presents much of the detail of data and analyses that support Chapter 4 of the main report.
We present three sequential steps of analysis which lead to estimates of the overall cost per QALY threshold for the NHS:
-
In From mortality to life-years we reconsider how the estimated effects on mortality from the econometrics work might better translate into life-years by exploring the limitations of mortality data available at PCT level and the published YLL figures presented in the previous chapter. We explore how these estimates might be improved using additional data and analysis.
-
In Adjusting life-years for quality-of-life we consider how these estimates of life-year effects might be adjusted for the QoL in which they are lived, taking account of the gender and the age at which life-years are gained or lost as well as the disutility associated with particular diseases.
-
In Including quality-of-life effects during disease we explore ways to also take account of those effects on health not directly associated with mortality and life-year effects (i.e. the ‘pure’ QoL effects) to estimate an overall cost per QALY threshold.
This sequence of analysis is set out and explained based on the analysis of 2006/7 expenditure and mortality data from 2006 to 2008. At the end of each section, we present a summary which includes a central ‘best’ estimate as well as extreme lower and upper bounds for the cost per life-year and cost per QALY threshold. The core assumptions which underpin these three values are common across sections From mortality to life-years, Adjusting life-years for quality-of-life, Including quality-of-life effects during disease and Which programme budget categories matter most?. The central or ‘best’ estimate is based on two assumptions one conservative and the other more optimistic with respect to the health effects associated with expenditure. The first is that the health effects of changes in 1 year of expenditure are restricted to 1 year. Analyses in Appendix 2 uses 3 years of mortality data, but these are averaged to an annual value prior to estimating outcome elasticities. Therefore, the estimated outcome elasticities represent the proportionate effect on mortality in 1 year due to a proportionate change in expenditure. This is likely to underestimate effects on mortality as expenditure that reduces mortality risk for an individual in 1 year may well also reduce their risk over subsequent years; possibly over the whole of their remaining disease duration. Expenditure may also prevent disease in future patient populations. Therefore, total health effects will be underestimated and the cost per life-year or QALY threshold will be overestimated. Although undoubtedly conservative, it may be offset to some extent by the more optimistic assumption used to translate mortality effects into life-years. In common with YLL figures published by NHS IC and the WHO GBD study it is assumed that any death averted by expenditure in 1 year will return the individual to the mortality risk of the general population, i.e. the YLG associated with each death averted are based on what would have been their LE taking account of their of age and gender (using life tables for the general population).
The extreme upper and lower bounds for cost per life-year and cost per QALY thresholds are based on making both assumptions either optimistic (providing the lower bound for the threshold) or conservative (an upper bound for the threshold). The lower bound is based on assuming that health effects are not restricted to 1 year but apply to the remaining disease duration for the population at risk during the expenditure year (although this still does not account for the effects of expenditure on preventing disease). The upper bound is based on the combination of assuming that health effects are restricted to 1 year and that any death averted is only averted for the minimum duration consistent with the mortality data used to estimate the outcome elasticities in Appendix 2. It is very important to note that the lower and upper bounds represent extreme values rather than alternative but plausible views that could reasonably be taken.
The three sequential steps of analysis, which provide a cost per life-year threshold, through a cost per life-year adjusted for quality to a cost per QALY threshold, are explained and detailed in From mortality to life-years, Adjusting life-years for quality of life and Including quality of life effects during disease, using the analysis of 2006 expenditure and mortality data from 2006 to 2008. In Which programme budget categories matter most?, further analysis using these data highlight which PBCs have the greatest influence on the overall threshold. An exploration of the impact of the uncertainty over the outcome and spend elasticities in estimates of the threshold is also presented in How uncertain are the estimates?. The sequence of analyses is then applied to 2008/9 expenditure and 2008/9/10 mortality data; results of the cost per QALY threshold for the most recent years of analysis are presented in Re-estimating the cost per quality-adjusted life-year threshold using 2008 expenditure data. In Re-estimating the cost per quality-adjusted life-year threshold using 2007 expenditure data we present our best estimate of the threshold cost per QALY based on the analysis of 2007/8 expenditure and mortality data from 2007 to 2009.
Analysis of 2006/7 expenditure and 2006/7/8 mortality data
From mortality to life-years
In this section we summarise our examination of a number of issues associated with available PCT-based mortality data and the associated published estimates of YLL. We then examine how, given the limited information available about the population at risk in each PBC, we might take proper account of the fact that some of the observed deaths would have occurred anyway (had the same population not been at risk in the particular PBC) when estimating YLL, i.e. taking account of unobserved counterfactual deaths. This allows us to estimate the YLL that better reflects the effect of expenditure on the mortality observed in each PBC, and infer the excess deaths associated with each PBC. Finally, we present the cost per death averted and cost per life-year which accounts for the issues raised in this section.
Mortality and years of life lost coverage
The mortality data that is available at PCT level does not offer full coverage of all deaths across all the ICD-10 codes that make up each PBC. Table 101 illustrates, using a few PBCs as examples, the mapping of three-digit ICD-10 codes to PBCs [see Table 101, column (1)] and the incomplete coverage of these ICD-10 codes in mortality data [see Table 101, column (2)]. A more detailed account of the extent of coverage is presented in Table 37 in Appendix 2.
PBC | (1) ICD-10 codes covered by the spend data | (2) ICD-10 codes covered by the mortality data (NHS IC) | (3) Coverage of mortality data relative to spend data (2008) | |
---|---|---|---|---|
1 | Infectious diseases | Large parts of A00–B99 | A00–B99 | 1.000 |
2 | Cancer | C00–C97, D00–D49 | C00–C97 | 0.984 |
4 | Endocrine | E000–E899 | E10–E14 | 0.634 |
10 | Circulatory | I00–I99, Q20–Q28 | I00–I99 | 0.992 |
11 | Respiratory | A150–A169, A190–A199, J000–J989, Q300–Q349, R000–R099 | J12–J18, J40–J44, J45–J46 | 0.773 |
National (English) data are, however, available that cover all deaths associated with all the ICD-10 codes that make up each PBC. Therefore, it is possible to adjust the incomplete reporting of mortality at PCT level (see Health outcome data in Appendix 2) before applying the estimated outcome elasticities to calculate the deaths averted due to expenditure. Applying published estimates of YLL per death to all the deaths averted using coverage adjustment factors [as illustrated in column (3) of Table 101] provides the estimate of the cost per life-year reported in Appendix 2. Note that the proportionate effects on mortality (due to changes in expenditure) are therefore assumed to be similar for mortality that is and is not recorded at PCT level. This seems more reasonable than assuming no effect of expenditure on mortality that happens not to be recorded at PCT level.
The published estimates of YLL (NHS IC) used in Chapter 3 only include deaths that occurred before the age of 75 years (but exclude deaths before age 1 year) and are based on the difference between age 75 years and the age of each death before 75 years. These estimates have the same limited coverage as PCT-level mortality data, so are not available for all the ICD-10 codes that make up each PBC. Therefore, applying the available estimates of YLL per death to the estimated number of deaths averted requires an assumption that the YLL per death is similar for those groups of ICD-10 codes covered and not covered by the published YLL figures.
This can be examined by using national ONS data to calculate YLL in the same way as NHS IC, but with full coverage of all the ICD-10 codes that make up each PBC. Although ONS data provides complete coverage and reports gender, age at death is only reported in 5-year ranges (these data are not available at PCT level so could not be used when estimating outcome elasticities in Chapter 3). Therefore, using ONS data to estimate YLL requires taking the mid-pointa of each range as the age of death (i.e. assuming reported deaths are equally likely over the range in which they are reported). For this reason it is not possible to precisely recover the published YLL figures using ONS data for those ICD-10 code groupings that can be precisely matched to the NHS IC coverage. However, the differences are small (ranging from −1% to 2% as shown in Table 102), suggesting that taking the mid-point of each range as the age of death is a reasonable approximation.
PBC | (1) YLL< 75 (NHS IC)a | (2) YLL< 75 (ONS)b | (3) Difference in YLL (%) | |
---|---|---|---|---|
1 | Infectious diseases | 35,517 | 35,688 | 0.5 |
2 | Cancer | 735,674 | 744,240 | 1 |
4 | Endocrine problems | 19,224 | 19,445 | 1 |
10 | Circulatory | 453,878 | 461,062 | 2 |
18 + 19 | Maternity and neonates | 164,200 | 163,105 | –1 |
Published estimates of YLL are available from the NHS IC for PBC 16 (trauma and injuries), but ONS does not provide the information required to calculate YLL for this PBC. The estimated outcome elasticity for PBC 16 (trauma and injuries) was zero for 2006 and could not be estimated for 2008 expenditure. Therefore, this PBC does not contribute any changes in health outcomes, although the changes in this expenditure are included in subsequent estimates of cost per life-year and QALY thresholds. However, there was very limited coverage of mortality data recorded at PCT level and the expenditure data for this PBC. In addition, the mortality data that was available (ICD-10 codes S72, S02, S06 and T90) was less likely to be associated with changes expenditure in this PBC and more likely to be associated with changes in expenditure in others. Consequently, the health effects of changes in expenditure in PBC 16 may be underestimated. Therefore, this PBC does not contribute any changes in health outcomes due to changes in expenditure in subsequent estimates of cost per life-year and QALY thresholds.
The differences between estimates of YLL based on ONS and NHS IC data are, however, much more significant and are reported in Table 103. These reflect differences in the distribution of ages at death between those groups of ICD-10 codes covered and not covered in the NHS IC figures. For example, NHS IC figures available at PCT level for PBC 7 (neurological problems) have low coverage of all deaths in this PBC [0.136 in Table 103, column (1)]. The deaths that are reported in NHS IC are associated with epilepsy and the YLL [22,046 in Table 103, column (2)] reflects the generally younger age at death in this group. When adjusted for full coverage [22,046/0.136 = 162,100 in Table 103, column (3)], the estimated YLL is much greater than the YLL based directly on all deaths by age group reported for the PBC in ONS. This difference in YLL reflects the fact that the deaths in PBC 7 which are not covered by NHS IC figures tend to be in older age groups so generate fewer YLL.
PBC | (1) Coverage of mortality data relative to spend data | (2) YLL< 75 (NHS IC) | (3) YLL< 75 adjusted (NHS IC) | (4) YLL< 75 no adjustment needed (ONS) | (5) Difference from adjusted NHS IC to ONS (%) | |
---|---|---|---|---|---|---|
1 | Infectious diseases | 1.000 | 35,517 | 35,517 | 40,928 | 15 |
2 | Cancer | 0.984 | 735,674 | 747,636 | 758,804 | 1 |
4 | Endocrine problems | 0.634 | 19,224 | 30,322 | 41,548 | 37 |
7 | Neurological problems | 0.136 | 22,046 | 162,100 | 93,755 | –42 |
10 | Circulatory | 0.992 | 453,878 | 457,538 | 481,246 | 5 |
11 | Respiratory | 0.773 | 108,074 | 139,812 | 147,465 | 6 |
13 | Gastrointestinal | 0.571 | 115,303 | 201,931 | 177,532 | –12 |
17 | Genitourinary | 0.172 | 3343 | 19,438 | 17,380 | –11 |
18 + 19 | Maternity and neonates | 0.679 | 164,200 | 241,826 | 15,409 | –94 |
Using ONS data also allows deaths under the age of 1 year to be appropriately assigned to PBCs via the ICD-10 code in which they occurred (NHS IC YLL figures exclude deaths under 1 year), rather than assigning them all to PBC 18 + 19 as in Appendix 2. b This explains the large reduction in YLL for PBC 18 + 19 (maternity and neonates) as much of the mortality is reassigned to ICD-10 codes which contribute to other PBCs. As most of the deaths that are reassigned are allocated to PBC 1 (infectious diseases) the YLL for this PBC increases despite complete reporting of deaths at PCT level and full coverage by NHS IC figures (Table 104).
PBC | (1) YLL< 75 (NHS IC) | (2) YLL< 75 (ONS)a | (3) Difference in YLL (%) | |
---|---|---|---|---|
1 | Infectious diseases | 35,517 | 40,928 | 15 |
2 | Cancer | 735,674 | 744,960 | 1 |
4 | Endocrine | 19,224 | 19,445 | 1 |
10 | Circulatory | 453,878 | 464,763 | 2 |
18 + 19 | Maternity and neonates | 164,200 | 15,409b | –91 |
Using ONS data to calculate YLL in the same way as the published NHS IC figures, but overcoming some of the issues associated with the reporting of mortality at PCT level and the coverage of published estimates of YLL, generates similar estimates of a cost per life-year threshold [see column (2) in Table 106] to those reported in Appendix 2.
Life expectancy and years of life lost
As noted above, the NHS IC estimates of YLL only include deaths below 75 years and are based on the difference between age 75 years and the age of each death below 75 years. Implicitly, this treats 75 years as the appropriate normal LE for males and females for the population at risk in each PBC. However, with the exception of maternity and neonates, most deaths in PBCs occur above the age of 75 years and LEs are significantly greater than 75 years. Based on 2006–8 data, LE at birth is greater than 75 years (77.74 years for males and 81.88 years for females). c Given the need to reflect the normal LE for the at risk population, it is more appropriate to use the age distribution of the general population, and calculate LE conditional on age averaged over the general population’s age distribution. General population LEs are estimated to be 80.7 years for males and 84.4 years for females. These LE estimates will always be higher than LEs at birth.
Based on ONS data, YLL can be recalculated using the above estimates of gender-specific LE for the general population. When increasing LE two effects occur, both of which tend to increase estimates of YLL. First, more deaths are included in the YLL calculation (those that occur between age 75 years and LE) and second, each death previously counted below 75 years will generate 5.7 or 9.4 more YLL for males and females respectively. The effect on the number of deaths and the YLL for each PBC using the LE of the general population is reported in Table 106.
PBC | (1) Deaths< 75 (ONS) | (2) Deaths< LE (ONS) | (3) Difference in deaths due to increased LE (%) | (4) YLL< 75 (ONS) | (5) YLL< LE (ONS) | (6) Difference in YLL due to increased LE (%) | |
---|---|---|---|---|---|---|---|
1 | Infectious diseases | 2050 | 3710 | 81 | 40,928 | 62,051 | 52 |
2 | Cancer | 62,944 | 95,212 | 51 | 758,804 | 1,345,013 | 77 |
4 | Endocrine | 2367 | 4000 | 69 | 41,548 | 65,015 | 56 |
7 | Neurological | 5095 | 8975 | 76 | 93,755 | 145,526 | 55 |
10 | Circulatory | 41,487 | 82,098 | 98 | 481,246 | 916,170 | 90 |
11 | Respiratory | 14,000 | 30,500 | 118 | 147,465 | 310,326 | 110 |
13 | Gastrointestinal | 10,611 | 15,827 | 49 | 177,532 | 273,303 | 54 |
17 | Genitourinary | 1588 | 4197 | 164 | 17,380 | 39,098 | 125 |
18 + 19 | Maternity and neonates | 226 | 226 | 0 | 15,409 | 17,167 | 11 |
The number of deaths counted below LE increases for every PBC except for maternity and neonates because, as expected, all deaths are below age 75 years in PBC 18 + 19. However, YLL increases for all PBCs reflecting the additional years otherwise expected to be lived to an older LE. Of course including more of the deaths observed in each PBC and the greater YLL associated with them will generate more deaths averted and more YLGs when applying the same proportionate effects from the outcome elasticities estimated in Appendix 2. Therefore, the cost per death averted and cost per life-year thresholds are expected to be lower using these figures than those reported in Appendix 2.
The impact on the cost per life-year and cost per death averted thresholds is summarised in Table 105. A detailed breakdown of the changes in spend and YLL across PBCs is presented in Table 107. A listing of the spend and outcome elasticities used in threshold calculations throughout this section is in Table 108 [see columns (2) and (5)]. Note that in the analyses in Appendix 2 and sections in this appendix up to Using ratios of quality-adjusted life-years to years of life lost (corresponding to Chapter 3 and Chapter 4, From mortality to life-years, Adjusting life-years for quality-of-life and Using ratios of quality-adjusted life-years to years of life lost in main report), expenditure elasticities were not estimated for the other 11 PBCs where outcome elasticities could not be estimated because the same health effect of changes in expenditure was assumed, i.e. it did not matter how changes in expenditure were allocated between them. However, in this section it does matter how the remaining change in expenditure is allocated.
PBC scenario | Using 75 years as the cut-off (ONS) | Using LE as the cut-off (ONS) | ||
---|---|---|---|---|
(1) Cost per death averted (£) | (2) Cost per LY gained (£) | (3) Cost per death averted (£) | (4) Cost per YLG (£) | |
All big four programmes | 122,756 | 10,398 | 63,426 | 5487 |
11 PBCs (with mortality) | 240,433 | 20,031 | 124,655 | 10,660 |
All 23 PBCs (zero health effects for remaining 12 PBCs) | 884,579 | 73,697 | 458,620 | 39,218 |
All 23 PBCs (non-zero health effects for remaining 12 PBCs, except GMS)a | 271,739 | 22,639 | 140,886 | 12,048 |
PBC | Change in spend (£M) | Using 75 years as the cut-off (ONS) | Using LE as the cut-off (ONS) | |||||||||||
---|---|---|---|---|---|---|---|---|---|---|---|---|---|---|
Deaths | Change in death | Cost per death averted (£) | YLL | Change in YLL | Cost per YLG (£) | Deaths | Change in deaths | Cost per death averted (£) | YLL | Change in YLL | Cost per YLG (£) | |||
2 | Cancer | 19 | 62,944 | 100.10 | 191,500 | 758,804 | 1207 | 15,885 | 95,212 | 151.42 | 126,599 | 1,345,013 | 2139 | 8962 |
10 | Circulatory | 33 | 41,487 | 321.26 | 103,560 | 481,246 | 3727 | 8928 | 82,098 | 635.73 | 52,333 | 916,170 | 7094 | 4690 |
11 | Respiratory | 22 | 14,000 | 249.25 | 89,482 | 147,465 | 2625 | 8495 | 30,500 | 543.00 | 41,074 | 310,326 | 5525 | 4037 |
13 | Gastrointestinal | 17 | 10,611 | 72.69 | 227,013 | 177,532 | 1216 | 13,568 | 15,827 | 108.42 | 152,198 | 273,303 | 1872 | 8814 |
All big four programmes | 122,756 | 10,398 | 63,426 | 5487 | ||||||||||
1 | Infectious diseases | 8 | 2050 | 0.76 | 10,936,680 | 40,928 | 15 | 547,796 | 3710 | 1.38 | 6,043,179 | 62,051 | 23 | 361,319 |
4 | Endocrine | 18 | 2367 | 18.99 | 929,559 | 41,548 | 333 | 52,957 | 4000 | 32.10 | 550,066 | 65,015 | 522 | 33,842 |
7 | Neurological | 17 | 5095 | 3.52 | 4,889,114 | 93,755 | 65 | 265,693 | 8975 | 6.19 | 2,775,491 | 145,526 | 100 | 171,172 |
17 | Genitourinary | 32 | 1588 | 0.74 | 42,993,075 | 17,380 | 8 | 3,928,251 | 4197 | 1.95 | 16,267,096 | 39,098 | 18 | 1,746,202 |
16 | Trauma and injuries | 10 | N/A | 0.00 | N/A | N/A | 0 | N/A | N/A | 0 | N/A | N/A | 0 | N/A |
18 + 19 | Maternity and neonates | 8 | 226 | 0.24 | 32,813,038 | 15,409 | 17 | 481,261 | 226 | 0.24 | 32,813,038 | 17,167 | 19 | 431,977 |
First 11 PBCs | 240,433 | 20,031 | 124,655 | 10,660 | ||||||||||
3 | Disorders of blood | 11 | 46.57 | 240,433 | 559 | 20,031 | 89.83 | 124,655 | 1050 | 10,660 | ||||
5 | Mental health | 204 | 849.17 | 240,433 | 10,193 | 20,031 | 1637.87 | 124,655 | 19,153 | 10,660 | ||||
6 | Learning disability | 31 | 128.05 | 240,433 | 1537 | 20,031 | 246.98 | 124,655 | 2888 | 10,660 | ||||
8 | Vision | 24 | 100.54 | 240,433 | 1207 | 20,031 | 193.92 | 124,655 | 2268 | 10,660 | ||||
9 | Hearing | 6 | 26.60 | 240,433 | 319 | 20,031 | 51.30 | 124,655 | 600 | 10,660 | ||||
12 | Dental | 23 | 97.72 | 240,433 | 1173 | 20,031 | 188.48 | 124,655 | 2204 | 10,660 | ||||
14 | Skin | 11 | 43.72 | 240,433 | 525 | 20,031 | 84.34 | 124,655 | 986 | 10,660 | ||||
15 | Musculoskeletal | 15 | 62.93 | 240,433 | 755 | 20,031 | 121.38 | 124,655 | 1419 | 10,660 | ||||
20 | Poisoning and adverse events | 4 | 18.27 | 240,433 | 219 | 20,031 | 35.23 | 124,655 | 412 | 10,660 | ||||
21 | Healthy individuals | 18 | 76.27 | 240,433 | 915 | 20,031 | 147.10 | 124,655 | 1720 | 10,660 | ||||
22 | Social care needs | 68 | 281.19 | 240,433 | 3375 | 20,031 | 542.35 | 124,655 | 6342 | 10,660 | ||||
23 | Other | 78 | 0 | N/A | N/A | N/A | N/A | |||||||
All (23 PBCs) | 271,739 | 22,639 | 140,886 | 12,048 |
PBC | (1) Total spend 2006/7 (£M) | Spend elasticities | Change in spend, £M (% share) | (7) Outcome elasticitiesb | ||||
---|---|---|---|---|---|---|---|---|
(2) Unadjusteda | (3) Analysis up to Chapter 4, Using ratios of quality-adjusted life-years to years of life lost (and Appendix 3) | (4) Analysis in Chapter 4, Using estimates of the quality-adjusted life-year burden of disease (and Appendix 3) | (5) Analysis up to Chapter 4, Using ratios of quality-adjusted life-years to years of life lost (and Appendix 3) | (6) Analysis in Chapter 4, Using estimates of the quality-adjusted life-year burden of disease (and Appendix 3) | ||||
2 | Cancer | 4122 | 0.465 | 0.465 | 0.657 | 19 (2.8) | 27 (4) | 0.342 |
10 | Circulatory | 6161 | 0.540 | 0.540 | 0.763 | 33 (4.9) | 47 (6.9) | 1.434 |
11 | Respiratory | 3285 | 0.679 | 0.679 | 0.959 | 22 (3.3) | 32 (4.6) | 2.622 |
13 | Gastrointestinal | 3700 | 0.446 | 0.446 | 0.630 | 17 (2.4) | 23 (3.4) | 1.536 |
All big four programmes | 17,268 | 91 (13.4) | 129 (19) | |||||
1 | Infectious diseases | 1054 | 0.792 | 0.792 | 1.119 | 8 (1.2) | 12 (1.7) | 0.047 |
4 | Endocrine | 1853 | 0.953 | 0.953 | 1.346 | 18 (2.6) | 25 (3.7) | 0.842 |
7 | Neurological | 2790 | 0.616 | 0.616 | 0.870 | 17 (2.5) | 24 (3.6) | 0.112 |
17 | Genitourinary | 3482 | 0.912 | 0.912 | 1.289 | 32 (4.7) | 45 (6.6) | 0.051 |
16 | Trauma and injuries | 2892 | 0.358 | 0.358 | 0.506 | 10 (1.5) | 15 (2.2) | – |
18 + 19 | Maternity and neonates | 3574 | 0.224 | 0.224 | 0.316 | 8 (1.2) | 11 (1.7) | 0.482 |
First 11 PBCs | 32,912 | 185 (27.2) | 261 (38.4) | |||||
3 | Blood | 837 | 0.700 | 1.338 | 0.989 | 11 (1.6) | 8 (1.2) | – |
5 | Mental health | 8406 | 1.271 | 2.429 | 1.796 | 204 (30.1) | 151 (22.2) | – |
6 | Learning disability | 2441 | 0.660 | 1.261 | 0.933 | 31 (4.5) | 23 (3.4) | – |
8 | Vision | 1362 | 0.929 | 1.775 | 1.313 | 24 (3.6) | 18 (2.6) | – |
9 | Hearing | 314 | 1.067 | 2.039 | 1.508 | 6 (0.9) | 5 (0.7) | – |
12 | Dental | 2621 | 0.469 | 0.896 | 0.663 | 23 (3.5) | 17 (2.6) | – |
14 | Skin | 1429 | 0.385 | 0.736 | 0.544 | 11 (1.5) | 8 (1.1) | – |
15 | Musculoskeletal | 3369 | 0.235 | 0.449 | 0.332 | 15 (2.2) | 11 (1.6) | – |
20 | Poisoning and adverse events | 737 | 0.312 | 0.596 | 0.441 | 4 (0.6) | 3 (0.5) | – |
21 | Healthy individuals | 1355 | 0.708 | 1.353 | 1.000 | 18 (2.7) | 14 (2.0) | – |
22 | Social care needs | 1529 | 2.314 | 4.422 | 3.269 | 68 (10.0) | 50 (7.4) | – |
23 | Other | 10,585 | 0.739 | 0.739 | 1.044 | 78 (11.5) | 111 (16.3) | – |
All 23 PBCs | 67,896 | 679 (100) | 679 (100) |
The cost per death averted (or life saved) threshold should not be overinterpreted because this is of little direct policy interest as lives are never saved (death is only delayed), and the significance of a death averted depends critically on how long it is averted and the QoL in which additional years are lived (see Adjusting life-years for quality-of-life). However, establishing the number of deaths averted which are associated with net YLL is useful because it enables an assessment of the number of YLGs associated with each death averted. Table 109 presents the YLL saved for each death averted implied by the assumptions underlying calculations of the cost per life-year threshold in Table 107. For the 11 PBCs with a mortality signal, each death averted is assumed to be associated with a gain of 11.7 YLL [when LE is used, see Table 109, column (2)]. This value is smaller than when using 75 years old as a cut-off [see Table 109, column (1)] because a higher proportion of deaths closer to the cut-off age are being considered (i.e. with lower YLL associated).
PBC | (1) Implied YLL per death averted (< 75 years) | (2) Implied YLL per death averted (< LE) | |
---|---|---|---|
2 | Cancer | 12.1 | 14.1 |
10 | Circulatory problems | 11.6 | 11.2 |
11 | Respiratory problems | 10.5 | 10.2 |
13 | Gastrointestinal problems | 16.7 | 17.3 |
All big four programmes | 11.8 | 11.6 | |
1 | Infectious diseases | 20.0 | 16.7 |
4 | Endocrine problems | 17.6 | 16.3 |
7 | Neurological problems | 18.4 | 16.2 |
17 | Genitourinary problems | 10.9 | 9.3 |
16 | Trauma and injuries | N/A | N/A |
18 + 19 | Maternity and neonates | 68.2 | 76.0 |
First 11 PBCs | 12.0 | 11.7 |
There are good reasons why YLL figures calculated as the difference between age of death and LE are likely to be overestimated. This is dealt with in the next section (see Years of life lost and accounting for counterfactual deaths). In Inferring excess deaths we take account of the fact that some of the deaths observed in a PBC would have occurred anyway in a similar ‘normal’ population (i.e. the counterfactual population not at risk through membership of the PBC), so not all observed deaths are ‘excess’ and generate YLL.
Years of life lost and accounting for counterfactual deaths
The estimates of YLL based on ONS data overcome many of the limitations of the published NHS IC figures. However, the YLL reported in Table 106 are calculated in the same way as the NHS IC figures, by taking the difference between a fixed LE and the age at death for deaths observed below that LE. Simply taking the difference between a fixed LE and the age at death of deaths that occur below LE and ignoring those deaths that occur above LE, is only an accurate representation of the YLL if it is reasonable to assume that no deaths would have otherwise occurred prior to LE (so all ‘normal’ deaths must occur at LE) and that there are no deaths (survivors) beyond LE in the at risk population (i.e. all deaths below LE are excess deaths and there are no excess deaths above LE). The estimate of YLL in the previous section may thus be biased for two reasons: (i) it does not account for the fact that not all deaths observed below LE are ‘excess’ deaths in the sense that some deaths would have occurred (at the same age) in a similar population not at risk in the PBC; and (ii) some of the deaths observed above LE may be ‘excess’ deaths that would not otherwise have occurred at that age (see breakdown of deaths below and above LE in Table 110).
PBC | (1) < LE 2006 | (2) > LE 2006 | (3) < LE 2007 | (4) > LE 2007 | (5) < LE 2008 | (6) > LE 2008 | (7) Annual deaths < LE | (8) Annual deaths > LE | |
---|---|---|---|---|---|---|---|---|---|
1 | Infectious diseases | 3824 | 3420 | 3902 | 3735 | 3403 | 2589 | 3710 | 3248 |
2 | Cancer | 95,549 | 34,192 | 95,331 | 35,455 | 94,758 | 37,144 | 95,213 | 35,597 |
4 | Endocrine | 4006 | 2661 | 3967 | 2750 | 4028 | 2882 | 4000 | 2764 |
7 | Neurological | 8454 | 5762 | 8845 | 6501 | 9626 | 6871 | 8975 | 6378 |
10 | Circulatory | 84,909 | 78,369 | 80,610 | 78,481 | 80,779 | 76,407 | 82,099 | 77,752 |
11 | Respiratory | 29,925 | 34,549 | 29,540 | 35,060 | 32,036 | 35,227 | 30,500 | 34,945 |
13 | Gastrointestinal | 15,893 | 8311 | 15,658 | 8376 | 15,930 | 8274 | 15,827 | 8320 |
17 | Genitourinary | 4056 | 6049 | 4072 | 6558 | 4465 | 6673 | 4198 | 6427 |
18 + 19 | Maternity and neonates | 195 | 0 | 216 | 0 | 267 | 0 | 226 | 0 |
The overall effect on YLL, and on the cost per life-year, will depend on the number of deaths above and below LE that are excess. However, it is more likely that deaths below LE are ‘excess’. Estimates of YLL are required which take account of the ‘counterfactual’ deaths that would have occurred even if the population in the PBC was not at risk through membership of the ICD-10 codes that make it up, but faced the same mortality risks as the general population, accounting for the age and gender distribution of the PBC population.
Ideally, with reliable information about the size of the population at risk in each PBC, and its age and gender distribution, it would be possible to estimate the number of deaths that would be expected to occur had this population not been at risk, based on mortality data for the general population. The difference between deaths observed across all ages in the PBC and the deaths expected to have occurred in this matched ‘normal’ population would provide the number of ‘excess’ deaths by age and gender. These ‘counterfactual’ deaths will occur in the other PBCs insofar as all deaths are recorded in an ICD-10 code, taking account of the unavoidable fact that everyone must die of something at some time. For example, even if all observed cancer mortality was avoidable and could in principle be eliminated with sufficient expenditure, lives would not be ‘saved’ but deaths delayed and reallocated to other causes. d
The YLL associated with each of these excess deaths is the LE conditional on gender and on surviving to the age at which the excess death occurred. The total YLL for the at risk population is simply the sum of these YLL over all excess deaths, which could occur at any age. We do not (and will never) know the counterfactual expected age of death for each individual patient. However, two perfectly matched populations of individuals, one at risk and another not at risk in the PBC can be compared in terms of their survival curves (Figure 46). The area below each survival curve reflects the LE and the area between the two survival curves returns the YLL. This is equivalent to comparing the average age of death across patients in the population at risk in the PBC (N patients), with the average age of death in the matched, not at risk, population (for simplicity assumed to be equally sized). Equation 22 describes the YLL per patient as the difference in the average age of death, agedeath, observed for each individual, i (out of N individuals), in each population. The YLL for the population is simply the per patient YLL multiplied by the size of the population N.
FIGURE 46.
Survival curve of a population at risk in a PBC and of a matched ‘normal’ population.
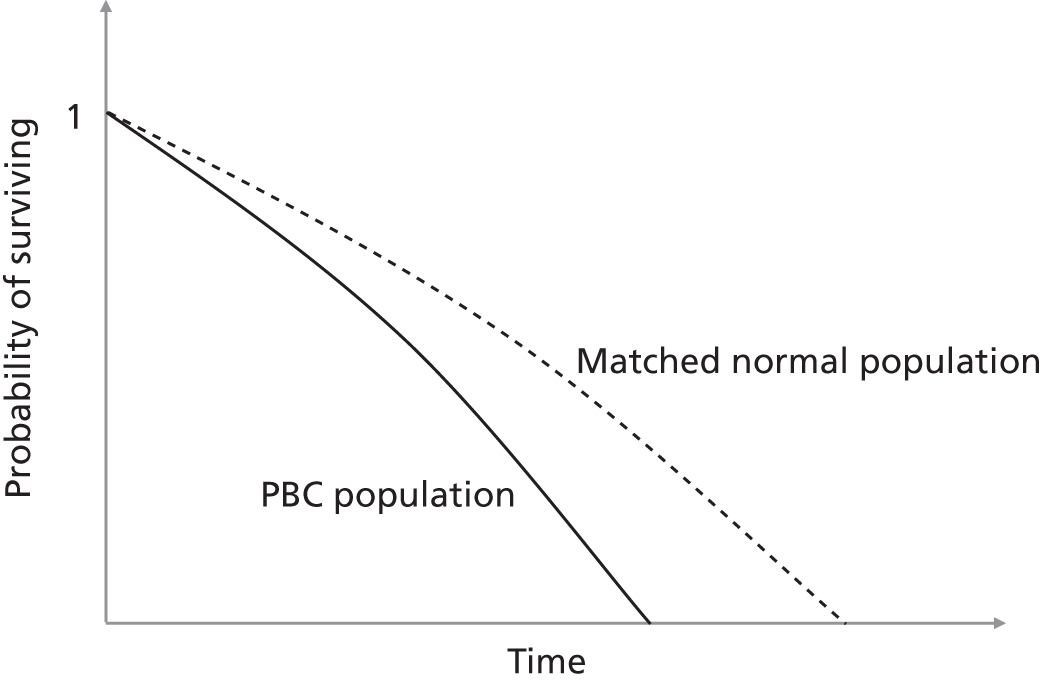
The difficultly is that routinely available data do not provide any information about the size of the population at risk or its age and gender distribution (matching criteria). Thus, a matched population cannot be generated, and the area between the two curves cannot be evaluated. Therefore, it is not possible to directly estimate excess deaths or compare survival curves. Even if the size of the at risk population is unknown we can still use information that might be available about its age and gender distribution (or make reasonable assumptions) to estimate a matched ‘normal’ LE using life tables for the general population – such a LE summarises the area under the counterfactual survival curve (LEnorm=1N∑i=1Nagedeath,inorm in Equation 22). Unfortunately, it is not possible to also calculate the LE for the population at risk in the PBC (or represent the survival curve) without information about the size of the at risk population – if it was possible, the difference between these life expectancies would approximate the YLL per patient at risk in a PBC.
Fortunately, we can still recover a consistent estimate of YLL using the normal LE of a matched population that is not at risk (a summary of the counterfactual average age of death), alongside the death data available for the PBC population. Equation 23 shows that population YLL can be approximated by subtracting the age at which each observed death in a PBC has occurred to the normal LE.
The data on the PBC observed deaths available expresses the ages at which deaths occurred in age groups (k out of K groups). Following from Equation 23, the population YLL can be evaluated considering the number of patients dying in each of the age groups, Ndie,k, as depicted in Equation 24. This is equivalent to comparing survival curves where age is discretised into intervals and the mid-point of the intervals used as age of death – this is illustrated in Figure 47.
FIGURE 47.
Area between the survival curves, discretised.

The calculations (in Equations 23 and 24) require all observed deaths – both those that occur below and those that occur above this LE – to be taken into account. Those deaths occurring below LE generate YLL – compared with the average of a matched population not at risk. However, we must also account for those deaths that occur at ages above LE. These deaths generate life-years ‘gained’ compared with the average of a matched population not at risk. Therefore, the appropriate estimate is a net YLL (i.e. YLL – YLG). In effect, by subtracting YLG from YLL we take account of the fact that not all deaths below LE are excess deaths but some deaths above LE are. Insofar as deaths above LE have been observed in a specific PBC, the net YLL estimate will always be lower than the estimate of YLL. Consequently, the estimates in Years of life lost and accounting for counterfactual deaths overestimate YLL and, hence, underestimate the cost per life-year threshold.
Using the life expectancy of the general population
Routinely available data provides the age and gender of observed deaths but no information about the age and gender distribution of the at risk population itself. Using observed age and gender at death [Table 111, column (3)] as an indication of the distribution of the at risk population will significantly overestimate the LE of a normal matched population insofar as a disease may be chronic (not all PBC mortality occurs on entry into the at risk population). If mortality risk increases over the disease duration, more deaths would be observed in groups that have been prevalent for some time (i.e. are older) than those that are incident. Older age groups will thus be over-represented in observed deaths compared with a matched normal population. For these reasons LE and YLL would be overestimated using age at death as a proxy for the age distribution of the at risk population, and the cost per life-year would be underestimated.
PBC | Sex | (1) Average age of general population | (2) LE of general population | (3) Age at death | (4) LE at age of death | |
---|---|---|---|---|---|---|
1 | Infectious diseases | M | 38.5 | 80.7 | 72.8 | 87.5 |
F | 40.8 | 84.4 | 79.3 | 91.1 | ||
2 | Cancer | M | 38.5 | 80.7 | 73.3 | 86.5 |
F | 40.8 | 84.4 | 73.8 | 88.8 | ||
4 | Endocrine | M | 38.5 | 80.7 | 72.5 | 87.1 |
F | 40.8 | 84.4 | 77.9 | 90.6 | ||
7 | Neurological | M | 38.5 | 80.7 | 72.8 | 87.2 |
F | 40.8 | 84.4 | 77.7 | 90.5 | ||
10 | Circulatory | M | 38.5 | 80.7 | 76.4 | 87.9 |
F | 40.8 | 84.4 | 82.7 | 91.7 | ||
11 | Respiratory | M | 38.5 | 80.7 | 79.4 | 89.0 |
F | 40.8 | 84.4 | 82.9 | 91.8 | ||
13 | Gastrointestinal | M | 38.5 | 80.7 | 68.9 | 85.7 |
F | 40.8 | 84.4 | 77.1 | 90.1 | ||
17 | Genitourinary | M | 38.5 | 80.7 | 81.6 | 90.1 |
F | 40.8 | 84.4 | 84.0 | 92.3 | ||
18 + 19 | Maternity and neonates | M | 38.5 | 80.7 | 1.1 | 78.3 |
F | 40.8 | 84.4 | 11.4 | 82.7 |
In the absence of additional external information the net YLL could be based on the LE of the general population, reflecting its current age and gender distribution. Such net YLL estimates are reported in Table 112, and illustrate the impact of accounting for counterfactual deaths in the way described above. The YLL reported in column 5 of Table 112 are calculated the same way and are the same as the figures previously reported [see column (5) of Table 106]. That is, they do not account for deaths that would have otherwise occurred below LE or the very many deaths that occur above LE. With the exception of PBC 18 + 19, many deaths occur above the LE of the general population [see column (4) in Table 112] in all PBCs. As a consequence, there are YLG associated with all other PBCs [see column (6)] so the net YLL in column (7) are lower than YLL based on the same LE. Therefore, failure to account for counterfactual deaths would lead to an overestimate of the YLL associated with a PBC and the effects of expenditure on YLL. The cost per life-year threshold would be underestimated (see Table 115).
PBC | (1) LE of males (years) | (2) LE of females (years) | Average 2006–8 | |||||
---|---|---|---|---|---|---|---|---|
(3) Deaths < LE | (4) Deaths > LE | (5) YLL | (6) YLG | (7) Net YLL | ||||
1 | Infectious diseases | 80.7 | 84.4 | 3710 | 3248 | 62,052 | 18,796 | 43,256 |
2 | Cancer | 80.7 | 84.4 | 95,213 | 35,597 | 1,345,038 | 175,350 | 1,169,689 |
4 | Endocrine | 80.7 | 84.4 | 4000 | 2764 | 65,016 | 15,864 | 49,152 |
7 | Neurological | 80.7 | 84.4 | 8975 | 6378 | 145,529 | 34,621 | 110,908 |
10 | Circulatory | 80.7 | 84.4 | 82,099 | 77,752 | 916,192 | 444,694 | 471,498 |
11 | Respiratory | 80.7 | 84.4 | 30,500 | 34,945 | 310,334 | 215,829 | 94,505 |
13 | Gastrointestinal | 80.7 | 84.4 | 15,827 | 8320 | 273,308 | 45,295 | 228,012 |
17 | Genitourinary | 80.7 | 84.4 | 4198 | 6427 | 39,099 | 40,530 | –1431 |
18 + 19 | Maternity and neonates | 80.7 | 84.4 | 226 | 0 | 17,167 | 0 | 17,167 |
However, these figures are only correct insofar as the distribution of age and gender in each PBC is similar to the general population. For example, if the at risk population tends to be younger the correct LE for the PBC will be lower. A lower LE will mean that there are less deaths below LE each generating fewer YLL, and more deaths above LE each generating more YLG. The net YLL will thus tend to be lower. Similarly, if the at risk population tends to be older than the general population, the correct LE will be higher and net YLL will also tend to be higher.
This explains the apparent net gain in YLL (negative net YLL) for PBC 17 (genitourinary) where most deaths occur at ages greater than the LE of the general population so that YLG exceeds YLL. As we are able to show later (see Table 114), this is because the age distribution in this PBC tends to be older than the general population (i.e. the LE for a matched normal population should be higher with fewer deaths above and more below this LE).
Using additional information about age and gender distribution
It is evident that estimates of YLL require some account to be taken of counterfactual deaths. In the absence of routinely available information this requires examination of alternative sources of information which might provide a basis for more credible assumptions about the age and gender distribution of the at risk population in each PBC than either the distribution of observed deaths or of the general population. e The WHO GBD study, updated in 2008 using 2004 data (see Addendum 1: data sources for more details)f provides a range of summary health indicators for the UK, which are, in part, based on estimates of the incidence and duration of sequelae associated with different types of disease by age and gender. Therefore, the type of information used by the WHO in the GBD study to generate summary estimates for the UK can also be used to improve the assumptions required about the age and gender distribution of the PBC populations. Importantly, at this stage, we do not need to rely on estimates of the absolute size of the at risk population, but only the relative ‘share’ by age and gender.
Specifically, the information reported by the GBD study (estimates specific to the UK provided in the National Burden of Disease toolkit) reported the incidence and duration of sequelae associated with different types of disease by age and gender. As it is possible that a patient may experience more than one of the types of sequelae reported in the GBD study we use the gender and age distribution of the sequelae with the highest prevalence, i.e. the minimum estimate of prevalence consistent with these figures (see Addendum 1: data sources), to evaluate the age and gender distribution within each disease.
Global Burden of Disease classifies diseases by U-codes, which are groups of three-digit ICD-10 codes (see Addendum 1: data sources for details of how U-codes map to ICD-10 codes). g As we know which ICD-10 codes contribute to each PBC we can map information from U-codes to PBCs via the ICD-10 codes that contribute to each. The resulting average age and LE for each PBC is reported in columns (1) and (2) of Table 113 using the information available from the GBD study in combination with life tables for the general population.
PBC | Sex | (1) Average age of general population (years) | (2) LE of general population (years) | (3) Proportion of males in PBC (GBD) (%) | (4) Average age in PBC (GBD) (years) | (5) Normal LE of PBC population (GBD) (years) | |
---|---|---|---|---|---|---|---|
1 | Infectious diseases | M | 38.5 | 80.7 | 54.1 | 28.6 | 79.6 |
F | 40.8 | 84.4 | 45.9 | 30.2 | 83.6 | ||
2 | Cancer | M | 38.5 | 80.7 | 28.0 | 61.3 | 83.0 |
F | 40.8 | 84.4 | 72.0 | 52.3 | 84.7 | ||
4 | Endocrine | M | 38.5 | 80.7 | 38.4 | 44.2 | 81.0 |
F | 40.8 | 84.4 | 61.6 | 50.8 | 84.7 | ||
7 | Neurological | M | 38.5 | 80.7 | 28.1 | 24.8 | 79.6 |
F | 40.8 | 84.4 | 71.9 | 23.5 | 83.3 | ||
10 | Circulatory | M | 38.5 | 80.7 | 51.6 | 55.4 | 83.0 |
F | 40.8 | 84.4 | 48.4 | 57.9 | 86.5 | ||
11 | Respiratory | M | 38.5 | 80.7 | 48.0 | 32.1 | 80.3 |
F | 40.8 | 84.4 | 52.0 | 33.7 | 84.0 | ||
13 | Gastrointestinal | M | 38.5 | 80.7 | 42.9 | 35.8 | 80.6 |
F | 40.8 | 84.4 | 57.1 | 41.9 | 84.5 | ||
17 | Genitourinary | M | 38.5 | 80.7 | 85.9 | 63.2 | 83.5 |
F | 40.8 | 84.4 | 14.1 | 47.3 | 85.6 | ||
18 + 19 | Maternity and neonates | M | 38.5 | 80.7 | 16.3 | 3.0 | 78.7 |
F | 40.8 | 84.4 | 83.7 | 24.1 | 83.1 |
These summary estimates suggest that some of the PBC populations may be on average older than the general population (e.g. cancer, circulatory and genitourinary) or younger (e.g. maternity and neonates, infectious diseases and neurological). However, when trying to interpret these summaries it should be noted that the average age reported in Table 113 is the average over the ages at which sequelae occur within the ICD-10 codes contributing to the PBC. Therefore, a similar average age can reflect very different age distributions. Some reflect a markedly bimodal distribution (e.g. PBC 11 respiratory), where there is high incidence at very young and older ages (Figure 48), or very different age distributions across the type of diseases that contribute to the PBC. For example, PBC 7 (neurological) includes dementia which accounts for the vast majority of the PBC population older than 70 years. However, a greater proportion of the population is in much younger age groups with other conditions, especially migraine (see Addendum 1: data sources for a detailed description of age and gender distributions in all PBCs). When interpreting these summary estimates it should also be noted that the reported LEs are not the LEs at the average ages reported in column (2) (see Table 113), but the average over the life expectancies for each age group within the contributing ICD-10 codes weighted by the age distribution of the sequelae with maximum prevalence from GBD U-codes.
FIGURE 48.
Distribution of PBC 11 prevalence by age, gender and contributing ICD-10 codes, alongside proportion of prevalent patients in the PBC and contribution to variance of each ICD-10 code.

The implications for net YLL of using these PBC specific estimates of ‘normal’ LE are reported in Table 114. As expected, the net YLL for those PBCs with a LE greater than the general population are higher than those reported in column (7) in Table 112 (e.g. PBC 10 circulatory and PBC 17 genitourinary, which now has positive net YLL). Similarly, those PBCs with a LE less than the general population have lower net YLL than reported in column (7) in Table 112 (e.g. PBC 1 infectious diseases and PBC 18 + 19 maternity and neonates, where the effect of a lower LE is more modest as there are no deaths above either of the estimates of LE).
PBC | (1) LE of males | (2) LE of females | Average 2006–8 | (7) Net YLL | ||||
---|---|---|---|---|---|---|---|---|
Deaths | (5) YLL | (6) YLG | ||||||
(3) < LE | (4) > LE | |||||||
1 | Infectious diseases | 79.6 | 83.6 | 3498 | 3460 | 58,686 | 21,724 | 36,962 |
2 | Cancer | 83.0 | 84.7 | 101,203 | 29,607 | 1,473,733 | 126,549 | 1,347,184 |
4 | Endocrine | 81.0 | 84.7 | 4068 | 2696 | 66,283 | 15,058 | 51,225 |
7 | Neurological | 79.6 | 83.3 | 8370 | 6983 | 135,686 | 41,770 | 93,917 |
10 | Circulatory | 83.0 | 86.5 | 96,694 | 63,157 | 1,102,020 | 278,251 | 823,768 |
11 | Respiratory | 80.3 | 84.0 | 29,549 | 35,897 | 298,343 | 230,313 | 68,030 |
13 | Gastrointestinal | 80.6 | 84.5 | 15,824 | 8323 | 273,117 | 45,414 | 227,703 |
17 | Genitourinary | 83.5 | 85.6 | 4969 | 5655 | 47,229 | 29,101 | 18,127 |
18 + 19 | Maternity and neonates | 78.7 | 83.1 | 226 | 0 | 16,801 | 0 | 16,801 |
The impact on the cost per life-year threshold of the issues discussed in this section is summarised in columns (3) and (4) of Table 115.
PBC scenario | Using cut-off in estimating YLL (ONS) (£) | Using net YLL estimates (£) | ||
---|---|---|---|---|
(1) Cut-off of 75 years | (2) Cut-off of LE of the general population | (3) Using LE of the general population | (4) Using LE of the PBC population (GBD) | |
All big four programmes | 10,398 | 5487 | 10,421 | 8080 |
11 PBCs (with mortality) | 20,031 | 10,660 | 19,928 | 15,628 |
All 23 PBCs (zero health effects for remaining 12 PBCs) | 73,697 | 39,218 | 73,317 | 57,497 |
All 23 PBCs (non-zero health effects for remaining 12 PBCs, except GMS)a | 22,639 | 12,048 | 22,523 | 17,663 |
Taking account of counterfactual deaths by calculating net YLL based on the LE of the general population [see Table 115, column (3)] provides similar estimates to those reported in Appendix 2. Assuming that PBC populations have the same age and gender distribution as the general population when the, albeit limited, information that is available suggests otherwise, seems inappropriate. Therefore, our preferred central estimate of the cost per life-year threshold is reported in column (4) (see Table 115). These are lower than those based on the general population, reflecting the impact on net YLL of evidence that the population at risk in some key PBCs (especially PBCs 2 and 10) tend to be older than the general population. A detailed breakdown of the changes in spend and YLL across PBCs that originate this central estimate are presented in columns (5)–(7) of Table 116. In Summary of cost per life-year estimates we consider extreme upper and lower bounds that might be placed on this central estimate.
PBC | (1) Change in spend (£M) | Using LE of the general population | Using LE of the PBC population | |||||
---|---|---|---|---|---|---|---|---|
(2) Net YLL | (3) Change in Net YLL | (4) Cost per YLG (£) | (5) Net YLL | (6) Change in Net YLL | (7) Cost per YLG (£) | |||
2 | Cancer | 19 | 1,169,689 | 1860 | 10,305 | 1,347,184 | 2142 | 8947 |
10 | Circulatory problems | 33 | 471,498 | 3651 | 9112 | 823,768 | 6379 | 5216 |
11 | Respiratory problems | 22 | 94,505 | 1683 | 13,256 | 68,030 | 1211 | 18,415 |
13 | Gastrointestinal problems | 17 | 228,012 | 1562 | 10,564 | 227,703 | 1560 | 10,579 |
All big four programmes | 10,421 | 8080 | ||||||
1 | Infectious diseases | 8 | 43,256 | 16 | 518,314 | 36,962 | 14 | 606,574 |
4 | Endocrine problems | 18 | 49,152 | 394 | 44,765 | 51,225 | 411 | 42,953 |
7 | Neurological problems | 17 | 110,908 | 77 | 224,601 | 93,917 | 65 | 265,235 |
17 | Genitourinary problems | 32 | –1431 | –1 | –47,709,995 | 18,127 | 8 | 3,766,371 |
16 | Trauma and injuries | 10 | N/A | 0 | N/A | N/A | 0 | N/A |
18 + 19 | Maternity and neonates | 8 | 17,167 | 19 | 431,977 | 16,801 | 18 | 441,387 |
First 11 PBCs | 19,928 | 15,628 | ||||||
3 | Disorders of blood | 11 | 562 | 19,928 | 716 | 15,628 | ||
5 | Mental health disorders | 204 | 10,245 | 19,928 | 13,064 | 15,628 | ||
6 | Learning disability | 31 | 1545 | 19,928 | 1970 | 15,628 | ||
8 | Problems of vision | 24 | 1213 | 19,928 | 1547 | 15,628 | ||
9 | Problems of hearing | 6 | 321 | 19,928 | 409 | 15,628 | ||
12 | Dental problems | 23 | 1179 | 19,928 | 1503 | 15,628 | ||
14 | Skin | 11 | 528 | 19,928 | 673 | 15,628 | ||
15 | Musculoskeletal system | 15 | 759 | 19,928 | 968 | 15,628 | ||
20 | Poisoning and adverse events | 4 | 220 | 19,928 | 281 | 15,628 | ||
21 | Healthy individuals | 18 | 920 | 19,928 | 1173 | 15,628 | ||
22 | Social care needs | 68 | 3393 | 19,928 | 4326 | 15,628 | ||
23 | Other | 78 | 0 | N/A | 0 | N/A | ||
All (23 PBCs) | 22,523 | 17,663 |
Inferring excess deaths
We have been able to establish a measure of net YLL which takes account of deaths that would have occurred anyway below a normal LE for the PBC population (i.e. not all deaths observed in a PBC are excess), and that some deaths observed above this LE would not otherwise have occurred at that age (i.e. some of these deaths are excess). As explained in Years of life lost and accounting for counterfactual deaths, net YLL calculated in this way is equivalent to first establishing the number of excess deaths at each age, then calculating YLL for each excess death (based on the LE conditional on the age at which each excess death occurred) and then summing these YLL across all excess deaths (i.e. across all ages). In other words, the estimates of net YLL imply a number of excess deaths required to generate them in each PBC. Therefore, it is possible to solve for the total number of excess deaths based on the net YLL and the average YLL per observed death (the average of the sum of the YLL for every observed death where the YLL for each observed death is the difference between age at death and LE conditional on age of death). The net YLL divided by the average YLL per death provides the number of excess deaths required, which on average will generate the estimated net YLL.
In the absence of information about the age distribution of excess deaths, calculations assume that the average YLL associated with observed and excess deaths are similar. Insofar as excess deaths are thought likely to generate more YLL than observed deaths the number of excess deaths will tend to be overestimated. This would tend to underestimate the cost per excess death averted. However, the cost per life-year estimates remain unchanged and do not require such an assumption.
The implied excess deaths associated with net YLL based on the LE of the PBCs [see Table 114, column (7)] are reported in Table 117. With the exception of PBC 18 + 19, excess deaths are some proportion of total observed deaths in each PBC. The proportion of excess deaths differs by PBC reflecting the distribution of deaths relative to the LE of the PBC. For example, in those PBCs where a large proportion of deaths occur below LE [see Table 117, columns (3) and (4)], excess deaths tend to be a greater proportion of total deaths (e.g. PBCs 2, 13 and 10). Where most deaths occur above LE, excess deaths as a proportion of total deaths tend to be lower (e.g. PBCs 11, 17 and 1). Nevertheless, the impact of the age distribution of deaths and the age distribution of the at risk population (summarised as LE) on the calculation of excess deaths is not always obvious as both will affect the numerator (net YLL) as well the denominator (average YLL per death) in this calculation.
PBC | (1) Net YLL | (2) YLL per observed death | (3) Excess deaths | (4) Total deaths | (5) % excess deaths | |
---|---|---|---|---|---|---|
1 | Infectious diseases | 36,962 | 13.4 | 2797 | 6958 | 40 |
2 | Cancer | 1,347,184 | 14.1 | 95,715 | 130,810 | 73 |
4 | Endocrine | 51,225 | 13.7 | 3769 | 6764 | 56 |
7 | Neurological | 93,917 | 13.7 | 6909 | 15,353 | 45 |
10 | Circulatory | 823,768 | 10.5 | 79,218 | 159,851 | 50 |
11 | Respiratory | 68,030 | 9.2 | 7386 | 65,445 | 11 |
13 | Gastrointestinal | 227,703 | 15.2 | 15,199 | 24,147 | 63 |
17 | Genitourinary | 18,127 | 8.3 | 2172 | 10,625 | 20 |
18 + 19 | Maternity and neonatesa | 16,801 | 73.9 | 226 | 226 | 100 |
Estimates of net YLL and changes in life-years due to expenditure (see Tables 114 and 115) have already accounted for the fact that not all deaths are excess and do not generate YLL. Nevertheless, solving for the number of implied excess deaths associated with these net YLL estimates allows a comparison of the cost per excess and observed PBC death avoided, and an examination of the interpretation that can be placed of the life-years expected to be gained from an excess or observed death averted.
As only deaths observed in the PBC can be used to estimate the effects of expenditure (excess deaths are not directly observed as they rely on an unobserved counterfactual population and would occur outside the PBC), the outcome elasticities can be interpreted as the proportionate change in observed PBC mortality due to a proportionate change in PBC expenditure. Equally, however, they can also be interpreted as the proportionate effect on excess death due to a proportionate change in expenditure so can be applied to either total observed or total excess deaths. Observed PBC mortality that is sensitive to changes in expenditure can be regarded as ‘avoidable’ and it is only this mortality that contributes to the estimates of outcome elasticities (not all observed mortality is necessarily avoidable and sensitive to expenditure – such mortality will not contribute to the estimates). Not all observed mortality is excess when compared with the counterfactual population, but this is unrelated to the question of how sensitive it is to expenditure (i.e. observed mortality will be just as sensitive to expenditure whether or not it is regarded as excess). Therefore, the estimated outcome elasticities can be applied to either observed PBC deaths or excess PBC deaths.
The cost per excess death and the cost per PBC death averted are reported in Table 118, and a detailed breakdown of changes in spend and excess or total deaths across PBCs is shown in Table 119. The cost per PBC death averted is, of course, significantly lower than the cost per excess death as excess deaths are only a proportion of total deaths (see Table 117). Also, the costs per PBC death averted are substantially lower than those reported in Appendix 2 (see Tables 68 and 69), as these estimates do not restrict the effects of expenditure to PBC deaths under 75 years of age.
PBC scenario | (1) Cost per excess death averted (£) | (2) Cost per PBC death averted (£) |
---|---|---|
All big four programmes | 91,129 | 32,864 |
11 PBCs (with mortality) | 177,692 | 64,774 |
All 23 PBCs (zero health effects for remaining 12 PBCs) | 653,748 | 238,310 |
All 23 PBCs (non-zero health effects for remaining 12 PBCs, except GMS)a | 200,829 | 73,208 |
PBC | (1) Change in spend (£M) | PBC deaths | Excess deaths | |||||
---|---|---|---|---|---|---|---|---|
(2) Total PBC deaths | (3) Change in PBC deaths | (4) Cost per PBC death averted (£) | (5) Excess deaths | (6) Change in excess deaths | (7) Cost per excess death averted (£) | |||
2 | Cancer | 19 | 130,809 | 208.03 | 92,147 | 95,715 | 152.22 | 125,934 |
10 | Circulatory problems | 33 | 159,851 | 1237.82 | 26,878 | 79,218 | 613.43 | 54,235 |
11 | Respiratory problems | 22 | 65,446 | 1165.14 | 19,142 | 7386 | 131.49 | 169,616 |
13 | Gastrointestinal problems | 17 | 24,148 | 165.42 | 99,757 | 15,199 | 104.12 | 158,488 |
All big four programmes | 32,864 | 0 | 91,129 | |||||
1 | Infectious diseases | 8 | 6958 | 2.59 | 3,222,218 | 2797 | 1.04 | 8,014,595 |
4 | Endocrine problems | 18 | 6765 | 54.28 | 325,291 | 3769 | 30.24 | 583,830 |
7 | Neurological problems | 17 | 15,353 | 10.59 | 1,622,486 | 6909 | 4.77 | 3,605,579 |
17 | Genitourinary problems | 32 | 10,625 | 4.94 | 6,425,694 | 2172 | 1.01 | 31,430,287 |
16 | Trauma and injuries | 10 | N/A | 0.00 | N/A | N/A | 0.00 | N/A |
18 + 19 | Maternity and neonates | 8 | 226 | 0.24 | 32,813,038 | 226 | 0.24 | 32,813,038 |
First 11 PBCs | 64,774 | 177,691 | ||||||
3 | Disorders of blood | 11 | 172.87 | 64,774 | 63.01 | 177,692 | ||
5 | Mental health disorders | 204 | 3152.02 | 64,774 | 1149.00 | 177,692 | ||
6 | Learning disability | 31 | 475.30 | 64,774 | 173.26 | 177,692 | ||
8 | Problems of vision | 24 | 373.19 | 64,774 | 136.04 | 177,692 | ||
9 | Problems of hearing | 6 | 98.72 | 64,774 | 35.99 | 177,692 | ||
12 | Dental problems | 23 | 362.72 | 64,774 | 132.22 | 177,692 | ||
14 | Skin | 11 | 162.30 | 64,774 | 59.16 | 177,692 | ||
15 | Musculoskeletal system | 15 | 233.59 | 64,774 | 85.15 | 177,692 | ||
20 | Poisoning and adverse events | 4 | 67.80 | 64,774 | 24.71 | 177,692 | ||
21 | Healthy individuals | 18 | 283.09 | 64,774 | 103.19 | 177,692 | ||
22 | Social care needs | 68 | 1043.74 | 64,774 | 380.47 | 177,692 | ||
23 | Other | 78 | 0.00 | 0.00 | 0.00 | N/A | ||
All 23 PBCs | 73,208 | 200,828 |
Recall from Appendix 2 that the measure of mortality that is available at PCT level and used to estimate the outcome elasticities is restricted to deaths under 75 years of age, as are the published estimates of YLL associated with them (see Mortality and years of life lost). However, to restrict effects only to those aged under 75 years would imply that there is no excess mortality above 75 years of age or equivalently that there are no health effects of PBC expenditure above 75 years of age. Rather than assume no effects of NHS activity in older populations, we apply the effects that can be observed to the whole PBC but account for deaths that would have otherwise occurred in our estimate of net YLL in Years of life lost and accounting for counterfactual deaths. Table 120 illustrates the number deaths averted for a 1% change in budget implicit in the alternative calculations of the cost per death averted threshold.
PBC scenario | Using deaths < 75 years (see Appendix 2, Table 67) | Using excess deaths (see Table 118) | Using PBC deaths (see Table 118) | |||
---|---|---|---|---|---|---|
(1) Cost per death averted (£) | (2) Number of deaths averted for a 1% change in budget | (3) Cost per excess death averted (£) | (4) Number of excess deaths averted for a 1% change in budget | (5) Cost per PBC death averted (£) | (6) Number of PBC deaths averted for a 1% change in budget | |
All big four programmes | 137,188 | 665 | 91,129 | 1001 | 32,864 | 2776 |
11 PBCs (with mortality) | 270,881 | 681 | 177,692 | 1039 | 64,774 | 2849 |
All 23 PBCs (zero health effects for remaining 12 PBCs) | 996,655 | 681 | 653,748 | 1039 | 238,310 | 2849 |
All 23 PBCs (non-zero health effects for remaining 12 PBCs, except GMS)a | 306,153 | 2218 | 200, 828 | 3381 | 73,208 | 5191 |
In many respects, whether or not PBC deaths at older ages are as sensitive to changes in expenditure is not critical, as any observed deaths that might be averted at older ages are less likely to generate YLGs because they are more likely to have occurred anyway in that year (i.e. are excess so generate zero YLGs anyway). Therefore, they will have very limited impact on cost per life-year or, subsequently, on cost per QALY estimates (in Adjusting life-years for quality-of-life and Including quality-of-life effects during disease). For this reason, it is the cost per life-year rather than cost per death averted, whether excess or observed, that is of primary interest. The cost per PBC or excess death averted (or life saved) should thus not be overinterpreted, as lives are never saved (death is only delayed). However, establishing the number of excess and PBC deaths averted which are associated with net YLL is useful because it enables an assessment of the number of YLGs associated with each death averted. These are reported for each PBC in Table 121 and range from 74.3 years per excess death for PBC 18 + 19 maternity and neonates to 8.3 years per excess death for PBC 17 genitourinary. On average, across all 11 PBCs each excess death averted is associated with 11.4 YLGs.
PBC | (1) Implied YLL per excess death averted | (2) Implied YLL per PBC death averted | |
---|---|---|---|
2 | Cancer | 14.07 | 10.30 |
10 | Circulatory problems | 10.40 | 5.15 |
11 | Respiratory problems | 9.21 | 1.04 |
13 | Gastrointestinal problems | 14.98 | 9.43 |
All big four programmes | 11.28 | 4.07 | |
1 | Infectious diseases | 13.21 | 5.31 |
4 | Endocrine problems | 13.59 | 7.57 |
7 | Neurological problems | 13.59 | 6.12 |
17 | Genitourinary problems | 8.34 | 1.71 |
16 | Trauma and injuries | N/A | N/A |
18 + 19 | Maternity and neonates | 74.34 | 74.34 |
First 11 PBCs | 11.37 | 4.14 |
However, clinicians or the evaluative literature cannot distinguish whether or not an observed death is excess. What can be observed is if groups of similar patients with and without access to a treatment survive and for how long. Therefore, it is the life-years associated with each observed death that provides a context that can be interpreted based on experience and evidence of how effective those interventions that could be invested or disinvested tend to be. The average life-years expected to be gained associated with each observed PBC death averted takes account of the fact that some deaths that are avoided in the PBC are not delayed for very long but quickly occur elsewhere and do not generate YLG (i.e. they were not excess deaths). The portion of observed deaths that are regarded as excess depend on how time is discretised. The data available report deaths in annual intervals so in this context ‘quickly’ means within 1 year. If deaths were reported in narrower time intervals then a greater proportion of observed deaths would be regarded as excess and, in the limit, with continuous time all observed deaths would be excess. Of course, the average YLL associated with them would be smaller and is approximated by the net YLL per observed death reported (the effect of approximation is likely to be small but unavoidable as it is due to deaths being reported in annual intervals).
The data available report deaths in annual intervals so in this context ‘quickly’ means within 1 year. If deaths were reported in narrower time intervals then a greater proportion of observed deaths would be regarded as excess and in the limit with continuous time all observed deaths would be excess. Of course, the average YLL associated with them would be smaller and is approximated by the net YLLs reported in Table 12 per observed death (the effects of approximation is likely to be small but unavoidable as it is due to deaths being reported in annual intervals). These are also reported for each PBC in Table 121 and range from 74.3 years per observed death for PBC 18 + 19 maternity and neonatesh to 1.0 for PBC 11 respiratory problems, i.e. the YLL per PBC death are much lower for those PBCs where a small proportion of observed deaths are excess. On average across all 11 PBCs each PBC death averted is associated with 4.1 YLGs.
Summary of cost per life-year estimates
The sequence of analysis set out above has enabled an examination of the impact of the limitations associated with the incomplete reporting of mortality data at PCT level and incomplete coverage of published YLL estimates. We have also been able to consider effects above age 75 years while taking account of the fact that many deaths would have occurred anyway, despite the limited information available about the population at risk within a PBC. The GBD study does provide some information about the age and gender distribution of the population at risk in a PBC so offers some improvement over the other assumptions that would otherwise be required (i.e. that the distribution of age and gender is the same as the general population or follows the distribution of observed deaths). For this reason, the cost per life-year threshold in column (4) of Table 115 and repeated in lines (1)–(4) in Table 122 are regarded as the central or best estimates given the evidence available and the credibility of alternative assumptions that could be made. As explained in the Introduction, these are based on the conservative assumptions that any health effects of changes in expenditure are restricted to 1 year, which, to some extent, may be offset by the more optimistic assumption that any death averted returns the individual to the mortality risk faced by the general population, matched for age and gender.
PBC grouping | Cost per life-year threshold |
---|---|
Best estimate | |
Effect of expenditure on mortality | 1 year |
YLL per PBC death averted | ∼ 4.1 |
(1) All big four programmes | £8080 |
(2) 11 PBCs (with mortality) | £15,628 |
(3) All 23 PBCs (zero health effects for remaining 12 PBCs) | £57,497 |
(4) All 23 PBCs (non-zero health effects for remaining 12 PBCs, except GMS)a | £17,663 |
Lower bound | |
Effect of expenditure on mortality | Remainder of disease |
YLL per PBC death averted | ∼ 4.1 |
(5) All big four programmes | £386 |
(6) 11 PBCs (with mortality) | £6106 |
(7) All 23 PBCs (zero health effects for remaining 12 PBCs) | £22,463 |
(8) All 23 PBCs (non-zero health effects for remaining 12 PBCs, except GMS)a | £6901 |
Upper bound | |
Effect of expenditure on mortality | 1 year |
YLL per PBC death averted | 2 |
(9) All big four programmes | £16,432 |
(10) 11 PBCs (with mortality) | £32,387 |
(11) All 23 PBCs (zero health effects for remaining 12 PBCs) | £119,155 |
(12) All 23 PBCs (non-zero health effects for remaining 12 PBCs, except GMS)a | £36,604 |
It does not seem credible to imagine that NHS expenditure has no health effects in the 12 PBCs which do not have sufficient mortality reported at PCT level to estimate outcome elasticities – what is implied by the estimate reported in Table 122, line (3). Therefore, it is the estimates reported in lines (2) and (4) that are of policy interest. The estimate of £15,628 per life-year [see line (2)] is restricted to the effects of changes in expenditure in the 11 PBCs where outcome elasticities can be estimated. The threshold of £17,663 per life-year uses the estimated health effects of expenditure in these PBCs as a surrogate for health effects in the others, that is assuming that the effects that can be observed will be similar to those that cannot. However, no health effects are assigned to PBC 23 (GMS) on the basis that any health effects of this expenditure would be recorded in the other PBCs.
It would be inappropriate to assign all the change in GMS expenditure to the estimate of cost per life-year based only on the 11 PBCs with outcome elasticities as it would imply that GMS only contributes to these PBCs. Restricting attention to the 11 PBCs with outcome elasticities but allocating part of the change in GMS expenditure to them based on their proportional share of changes in overall expenditure would yield the same cost per life-year as reported in line (4). i
The extreme upper and lower bounds for the cost per life-year thresholds in Table 122 are based on making the necessary assumptions about duration of health effects and how long a death might be averted optimistic (providing the lower bound for the threshold) or conservative (an upper bound for the threshold). The lower bound [see lines (5)–(8)] is based on assuming that health effects are not restricted to 1 year but apply to the whole of the remaining disease duration of the population at risk in PBCs during the expenditure year. Estimates of the average disease durations across the PBCs used in this calculation are depicted in Table 123 [see column (2)]. j These were obtained from the GBD study (see Addendum 1: data sources). Although this lower bound for the threshold combines optimistic assumptions, it is possible, indeed likely, that at least some expenditure may have effects on the health outcomes of future patients who are not currently part of the population at risk in a PBC, e.g. investments or disinvestment in prevention will have an impact on populations that are incident to PBCs in the future. Such effects are not captured in any of the estimates presented in this chapter so all are conservative with respect to this type of health effect of changes in expenditure.
PBC | (1) Duration of disease for an incident patient (years) (GBD) | (2) Remaining duration of disease for at risk population (years) (GBD) |
---|---|---|
1 | 6.21 | 3.11 |
2 | 1.19 | 0.59 |
3 | 1.07 | 0.53 |
4 | 24.83 | 12.42 |
5 | 7.41 | 3.70 |
6 | 3.46 | 1.73 |
7 | 30.91 | 15.45 |
8 | 13.96 | 6.98 |
9 | 16.40 | 8.20 |
10 | 3.21 | 1.61 |
11 | 11.24 | 5.62 |
12 | 0.33 | 0.17 |
13 | 0.27 | 0.13 |
14 | 1.01 | 0.50 |
15 | 9.56 | 4.78 |
16 | 3.74 | 1.87 |
17 | 1.11 | 0.56 |
18 | 0.58 | 0.29 |
19 | 9.71 | 4.86 |
20 | 0.93 | 0.47 |
21 | 1.07 | 0.53 |
22 | 3.74 | 1.87 |
23 | 3.74 | 1.87 |
The upper bound [see lines (9)–(12) in Table 122] is based on the combination of assuming that health effects are restricted to 1 year for the population currently at risk and that any death averted is only averted for the minimum duration consistent with the mortality data. The econometrics work used the average of 3 years of mortality (2006–8), so the estimated outcome elasticities are based on differences in mortality that remain after averaging over 3 years. Therefore, the estimated effects are based on differences in observed PBC deaths that must have been sustained, on average, for more than a minimum of 2 years. This is because variation in mortality in the first year of data will only contribute to estimates if differences are sustained for a minimum of 3 years, variation in mortality in the second year will only contribute if it is sustained for a minimum of 2 years, and in the third year only if sustained for 1 year. If differences in mortality are similar each year (the 3 years contribute equally to the estimates), then estimated effects must have been sustained, on average, for a minimum of 2 years. k These estimates can be interpreted as an upper bound given the data available and therefore the analysis that has been feasible.
Adjusting life-years for quality of life
The central or best estimates of the cost per life-year threshold, which were presented in Table 122 [see lines (2) and (4)], take no account of the health-related QoL in which years of life, expected to be gained or lost through changes in expenditure, are likely to be lived. Even if attention is restricted to the direct health consequences of changes in mortality, estimates of the cost per life-year will tend to overestimate the effects of changes in expenditure (underestimate the threshold) compared with a more complete measure of health that accounts for the quality in which the years of life are expected to be lived. In this section we examine the ways in which the life-years reported in From mortality to life-years can be adjusted for quality, taking account of information that is available about (i) how QoL differs by age and gender (see From mortality to life-years); and (ii) how the quality of life-years associated with mortality changes might be affected by the types of diseases that make up each PBC (see Adjusting life-years for quality of life). Throughout we continue to account for counterfactual deaths in the way described in Years of life lost and accounting for counterfactual deaths, by making the adjustment for quality to the life-years associated with every observed death, before calculating a quality-adjusted net YLL. The implications for a cost per QALY threshold that only accounts for the health effects of mortality changes are presented in Using estimates of the quality-adjusted life-year burden of disease. In Including quality-of-life effects during disease we explore the ways in which the likely direct effects of expenditure on QoL (other than through mortality) might also be taken into account.
Quality of life based on the general population
The most commonly used metric of health-related QoL in the UK is EQ-5D,91 which is specified in the NICE reference case for methods of technology appraisal. 153 This metric has five dimensions of quality, each with three possible levels. Each of these 243 possible health states is valued relative to a score of 1, which represents full or best imaginable health (the best score across all five dimensions), and a score of 0, which represents death, based on a representative sample of the UK population. 92 Therefore, insofar as the years of life expected to be gained or lost through changes in expenditure would be lived in this state of full health, the cost per life-year thresholds reported in Table 122 would also be the cost per QALY thresholds, albeit ones that only account for the health effects of mortality changes.
However, unsurprisingly, there is good evidence that, on average, the general population is not in this state of full health. Therefore, the QoL score associated with the health states experienced by the general population are less than 1, and are expected to decline with age and to differ by gender. These QoL ‘norms’ for the general population by age and gender are illustrated in Figure 49 based on an analysis of data from the HSE (see Addendum 1: data sources for a description of HSE data and the analysis of QoL norms illustrated in Figure 49).
FIGURE 49.
Quality of life for the general population by age and gender.

These QoL norms can be applied to the YLL associated with all observed deaths in each PBC, taking account of gender and age at death. The results are reported in columns (4)–(6) of Table 124.
PBC | Unadjusted life-years | QALYs | |||||
---|---|---|---|---|---|---|---|
(1) YLL | (2) YLG | (3) Net YLL | (4) YLL | (5) YLG | (6) Net YLL | ||
1 | Infectious diseases | 58,686 | 21,724 | 36,962 | 47,481 | 14,618 | 32,864 |
2 | Cancer | 1,473,733 | 126,549 | 1,347,184 | 1,143,445 | 84,036 | 1,059,409 |
4 | Endocrine | 66,283 | 15,058 | 51,225 | 52,856 | 9973 | 42,883 |
7 | Neurological | 135,686 | 41,770 | 93,917 | 109,349 | 28,262 | 81,087 |
10 | Circulatory | 1,102,020 | 278,251 | 823,768 | 848,046 | 183,330 | 664,717 |
11 | Respiratory | 298,343 | 230,313 | 68,030 | 231,578 | 154,743 | 76,835 |
13 | Gastrointestinal | 273,117 | 45,414 | 227,703 | 216,256 | 30,277 | 185,979 |
17 | Genitourinary | 47,229 | 29,101 | 18,127 | 35,929 | 18,947 | 16,982 |
18 + 19 | Maternity and neonates | 16,801 | 0 | 16,801 | 14,568 | 0 | 14,568 |
There are two effects of adjusting life-years for quality: (i) since QoL norms are always less than 1 the adjusted YLL and YLG are always lower than the unadjusted values in Table 124, columns (1) and (2) (previously reported in Table 106); and (ii) deaths above LE are necessarily at older ages with poorer QoL norms than those below, so the difference between adjusted and unadjusted values is greater for YLG than YLL (Table 125 illustrates these effects by showing the implied QoL scores applied to YLL and YLG). The overall effect of quality adjustment on net YLL is the balance of these two effects, and tends to reduce the net YLL [compare columns (3) and (6) in Table 124]. The only exception is PBC 11 (respiratory) which has a large proportion of deaths occurring above the LE of the PBC population (see Table 114).
PBC | (1) QoL score for YLL | (2) QoL score for YLG | |
---|---|---|---|
1 | Infectious diseases | 0.81 | 0.67 |
2 | Cancer | 0.78 | 0.66 |
4 | Endocrine | 0.80 | 0.66 |
7 | Neurological | 0.81 | 0.68 |
10 | Circulatory | 0.77 | 0.66 |
11 | Respiratory | 0.78 | 0.67 |
13 | Gastrointestinal | 0.79 | 0.67 |
17 | Genitourinary | 0.76 | 0.65 |
18 + 19 | Maternity and neonates | 0.87 | N/A |
The quality-adjusted net YLL figures in Table 124, column (6) suggest that the health effects of mortality are lower than when relying only on unadjusted life-years in Years of life lost and accounting for counterfactual deaths. Therefore, the health effects of changes in expenditure on this more complete measure of health will also be lower. The implications of these adjustments to a cost per QALY threshold that only accounts for the direct health effects of mortality are summarised in Table 126, and detailed in Table 127. As expected, the cost per QALY threshold based on adjusting the YLGs or lost [see Table 126, column (2)] is higher than a threshold based on unadjusted life-years [see Table 126, column (1), these results were previously reported in Tables 115 and 122].
PBC grouping | (1) Cost per life-year threshold (£) | (2) Cost per QALY threshold (population norms) (£) |
---|---|---|
All big four programmes | 8080 | 9631 |
11 PBCs (with mortality) | 15,628 | 18,622 |
All 23 PBCsa | 17,663 | 21,047 |
PBC | (1) Change in spend (£M) | (2) Change in QALY | (3) Cost per QALY gained (£) | |
---|---|---|---|---|
2 | Cancer | 19 | 1685 | 11,378 |
10 | Circulatory problems | 33 | 5147 | 6464 |
11 | Respiratory problems | 22 | 1368 | 16,304 |
13 | Gastrointestinal problems | 17 | 1274 | 12,952 |
All big four programmes | 9631 | |||
1 | Infectious diseases | 8 | 12 | 682,211 |
4 | Endocrine problems | 18 | 344 | 51,309 |
7 | Neurological problems | 17 | 56 | 307,201 |
17 | Genitourinary problems | 32 | 8 | 4,020,316 |
16 | Trauma and injuries | 10 | 0 | N/A |
18 + 19 | Maternity and neonates | 8 | 16 | 509,044 |
First 11 PBCs | 18,622 | |||
3 | Disorders of blood | 11 | 601 | 18,622 |
5 | Mental health disorders | 204 | 10,964 | 18,622 |
6 | Learning disability | 31 | 1653 | 18,622 |
8 | Problems of vision | 24 | 1298 | 18,622 |
9 | Problems of hearing | 6 | 343 | 18,622 |
12 | Dental problems | 23 | 1262 | 18,622 |
14 | Skin | 11 | 565 | 18,622 |
15 | Musculoskeletal system | 15 | 812 | 18,622 |
20 | Poisoning and adverse effects | 4 | 236 | 18,622 |
21 | Healthy individuals | 18 | 985 | 18,622 |
22 | Social care needs | 68 | 3630 | 18,622 |
23 | Other | 78 | 0 | N/A |
All 23 PBCs | 21,047 |
Table 128 depicts the judgements over life-years, QoL weights and total QALYs implicit in calculations of the threshold cost per QALY in Table 126. Specifically, columns (1) and (2) of Table 128 report the number of life-years associated with each death averted for each PBC; as expected, the values are equal to those in Table 121 as estimates rely on the net YLL evaluated in Years of life lost and accounting for counterfactual deaths. In columns (3) and (4), the number of QALYs gained associated with each death averted are presented. These ranged from 64.46 QALYs gained per PBC death averted for PBCs 18 and 19 (maternity and neonates) to 1.17 QALYs per PBC death averted for PBC 11 (respiratory), see column (4). In general, these values are expected to be smaller than the unadjusted YLL per PBC death averted in column (2). The exception is PBC 11 (respiratory) – in this PBC, the number of YLL and YLG are more similar than in other PBCs [respectively, columns (1) and (2) of Table 124], and given that YLG are weighted more heavily (with lower QoL scores) than YLL, the netting of adjusted estimates returns a higher number than the netting of unadjusted estimates. On average, across all 11 PBCs each PBC death averted is associated with 3.5 QALYs gained.
PBC | (1) Implied YLL per excess death averted | (2) Implied YLL per PBC death averted | (3) Implied QALYs gained per excess death averted | (4) Implied QALYs gained per PBC death averted | |
---|---|---|---|---|---|
2 | Cancer | 14.07 | 10.30 | 11.07 | 8.10 |
10 | Circulatory | 10.40 | 5.15 | 8.39 | 4.16 |
11 | Respiratory | 9.21 | 1.04 | 10.40 | 1.17 |
13 | Gastrointestinal | 14.98 | 9.43 | 12.24 | 7.70 |
All big four programmes | 11.28 | 4.07 | 9.46 | 3.41 | |
1 | Infectious diseases | 13.21 | 5.31 | 11.75 | 4.72 |
4 | Endocrine | 13.59 | 7.57 | 11.38 | 6.34 |
7 | Neurological | 13.59 | 6.12 | 11.74 | 5.28 |
17 | Genitourinary | 8.34 | 1.71 | 7.82 | 1.60 |
16 | Trauma and injuries | N/A | N/A | N/A | N/A |
18 + 19 | Maternity and neonates | 74.34 | 74.34 | 64.46 | 64.46 |
First 11 PBCs | 11.37 | 4.14 | 9.54 | 3.48 |
Adjusting age-related quality-of-life for disease decrements
Adjusting life-years for age- and gender-related QoL norms assumes that any YLG through a change in expenditure would be lived in a similar QoL to the general population. It is possible, however, that patients benefiting from reduced mortality may, nevertheless, continue to be affected by the type of diseases that make up each PBC and experience the QoL associated with the original disease.
The HODaR93 provides over 30,000 observations of EQ-5D measures of QoL by ICD-10 code and the age and gender of the patients in the sample (see Addendum 1: data sources). Although this is a rich UK data set, there were a limited number of observations for some of the less common ICD-10 codes. For this reason HODaR was supplemented with information from the MEPS94 which also provides EQ-5D by ICD and reports the average age of respondents (see Addendum 1: data sources). These data provided a means of estimating the QoL associated with each ICD-10 code at the average age of respondents in the pooled sample (ICD-10 code estimates of the QoL score and age were pooled across data sets by considering the number of patients from each data set contributing to estimates, i.e. a weighted average). The QoL associated with each PBC was then expressed as the average of the QoL associated with its component ICD-10 codes. The average QoL scores across ICD-10 codes which contribute to each PBC and the average age and gender of respondents were used to calculate a PBC disease-related decrement (disutility) based on QoL norms from the general population – it is important to note that by expressing the QoL effects of different diseases as age-related decrements we do not require the HODaR and MEPS samples to necessarily be representative of the age distribution of the population at risk in the PBCs.
Table 129 summarises the data from HODaR and MEPS and the QoL decrements used in further calculations of the threshold, namely the number of patients for which QoL scores were available [see column (1)]; the average age of these patients by gender [see columns (2) and (3)]; the average QoL scores across PBCs [see column (4)]; the QoL scores for the population norms by gender [see columns (5) and (6)]; and the calculated disease-related decrements [see columns (7) and (8)].
PBC | HODaR/MEPS | Population norms | Disease-related decrement compared with population norms | |||||
---|---|---|---|---|---|---|---|---|
(1) n | Average age | (4) QoL score for diseased | ||||||
(2) Male | (3) Female | (5) Male | (6) Female | (7) Male | (8) Female | |||
1 | 263 | 54.0 | 47.1 | 0.667 | 0.859 | 0.830 | 0.192 | 0.163 |
2 | 13,324 | 64.3 | 59.8 | 0.692 | 0.809 | 0.830 | 0.117 | 0.138 |
3 | 2464 | 58.6 | 58.1 | 0.656 | 0.859 | 0.830 | 0.203 | 0.174 |
4 | 7128 | 57.3 | 56.5 | 0.701 | 0.859 | 0.830 | 0.157 | 0.128 |
5 | 12,733 | 47.8 | 47.9 | 0.557 | 0.859 | 0.830 | 0.301 | 0.272 |
6 | 301 | 25.8 | 25.3 | 0.671 | 0.937 | 0.924 | 0.266 | 0.253 |
7 | 10,296 | 55.8 | 53.8 | 0.546 | 0.859 | 0.830 | 0.312 | 0.283 |
8 | 11,536 | 63.8 | 64.5 | 0.719 | 0.809 | 0.796 | 0.089 | 0.077 |
9 | 1023 | 61.7 | 59.8 | 0.778 | 0.809 | 0.830 | 0.031 | 0.051 |
10 | 33,854 | 64.4 | 64.1 | 0.629 | 0.809 | 0.796 | 0.179 | 0.167 |
11 | 19,646 | 48.4 | 47.2 | 0.634 | 0.859 | 0.830 | 0.224 | 0.195 |
12 | 1811 | 40.9 | 40.0 | 0.781 | 0.910 | 0.894 | 0.129 | 0.113 |
13 | 23,138 | 57.3 | 55.5 | 0.653 | 0.859 | 0.830 | 0.206 | 0.177 |
14 | 5659 | 54.8 | 54.0 | 0.695 | 0.859 | 0.830 | 0.164 | 0.134 |
15 | 34,590 | 56.4 | 56.6 | 0.578 | 0.859 | 0.830 | 0.280 | 0.251 |
16 | 2652 | 46.0 | 58.3 | 0.652 | 0.859 | 0.830 | 0.207 | 0.178 |
17 | 13,651 | 57.5 | 53.0 | 0.711 | 0.859 | 0.830 | 0.147 | 0.118 |
18 + 19 | 1566 | 37.8 | 31.7 | 0.848 | 0.910 | 0.894 | 0.063 | 0.047 |
20 | 1569 | 59.1 | 52.3 | 0.584 | 0.859 | 0.830 | 0.275 | 0.246 |
21 | 7488 | 60.6 | 60.3 | 0.661 | 0.809 | 0.796 | 0.147 | 0.135 |
22 | 25 | 78.4 | 81.4 | 0.156 | 0.798 | 0.636 | 0.642 | 0.480 |
23 | 1002 | 62.6 | 60.8 | 0.639 | 0.809 | 0.796 | 0.170 | 0.158 |
Figure 50 illustrates the use of the decrement to QoL norms for PBC 1 (infectious disease) across a range of ages. For PBC 1, the QoL score, evaluated across the component ICD-10 codes, was found to be 0.667 in HODaR and MEPS, at an average age of 54 years for male respondents. As the QoL norms for males aged 54 years is 0.859, this suggests a decrement associated with membership of PBC 1 of 0.192, which can then be applied to QoL norms by age as illustrated in Figure 50.
FIGURE 50.
Quality of life for males in PBC 1 (infectious disease) and the general population by age.
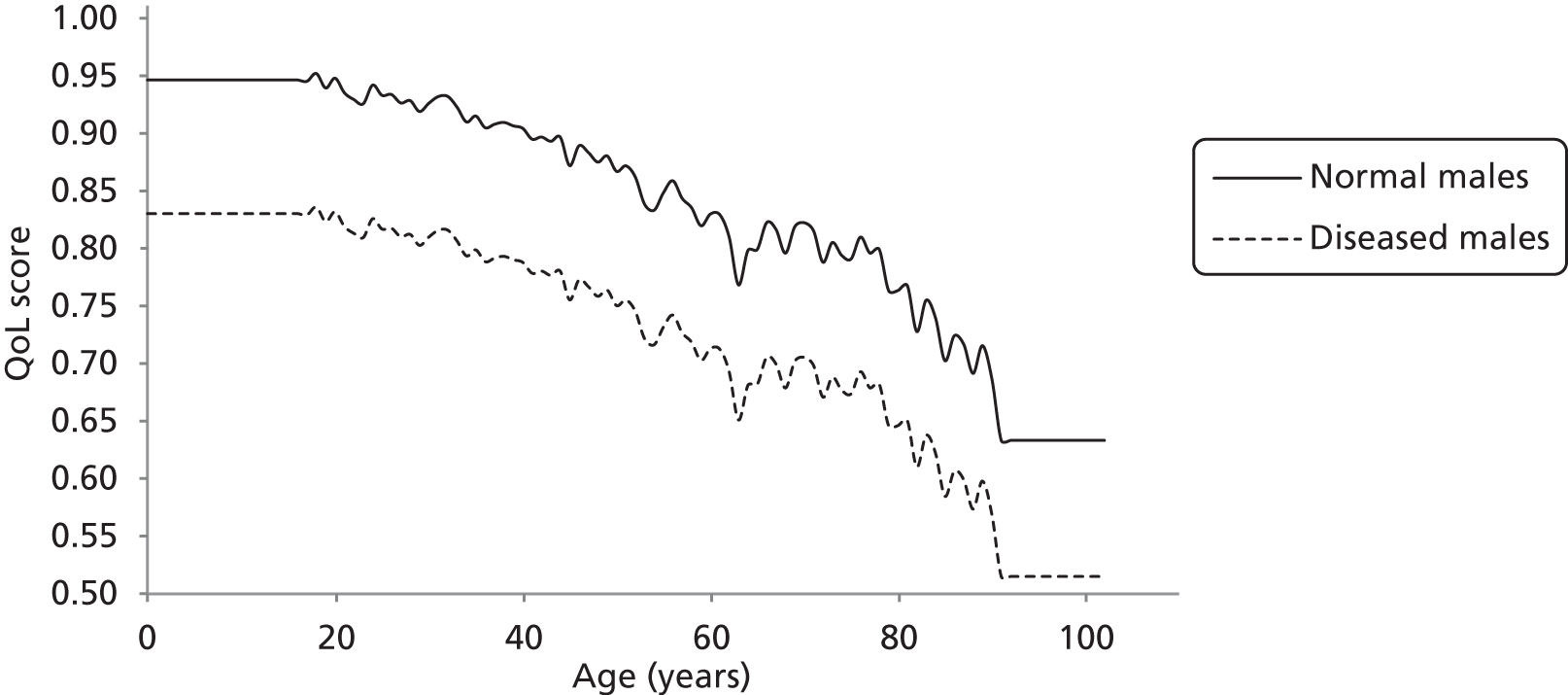
In principle, it would be possible to estimate disease-related disutility by age rather than assume a fixed additive decrement. HODaR does provide age for each reported QoL score, but MEPS only provides average age of respondents in published summaries. However, even with access to ‘raw’ scores and the age and gender of each, it is very unlikely that there would be sufficient data to estimate age-related decrements in each of the component ICD-10 codes. It would, however, be possible to assume a proportionate rather than fixed decrement by age. However, the average age of respondents in the pooled HODaR and MEPS sample [see columns (2) and (3) of Table 129] tends to be older than the age distribution of the PBC populations [see columns (3) and (4) of Table 113]. Given that older individuals are expected to have a lower QoL (norm), relative decrements can overestimate the decrements observed in younger patients. By applying overestimated decrements, the quality-adjusted net YLL would be underestimated and the cost per QALY threshold increased compared with the fixed decrement applied here.
Quality-of-life norms adjusted for disease-related decrements can be applied to the YLL associated with observed deaths in each PBC, taking account of gender and age at death in the same way as From mortality to life-years. To do so, the ‘PBC decrements’ calculated from HODaR and MEPS were applied to each observed death and the age at which each life-year was gained or lost (from ONS). The results are reported in columns (4)–(6) of Table 130. The overall effect of quality adjustment that also applies a disease-related decrement is to reduce the net YLL to a greater extent than adjustment with population norms alone [compare column (6) in Table 130 with column (6) in Table 124].
PBC | Unadjusted life-years | QALYs | |||||
---|---|---|---|---|---|---|---|
(1) YLL | (2) YLG | (3) Net YLL | (4) YLG | (5) YLL | (6) Net YLG | ||
1 | Infectious diseases | 58,686 | 21,724 | 36,962 | 37,055 | 10,793 | 26,262 |
2 | Cancer | 1,473,733 | 126,549 | 1,347,184 | 955,690 | 67,930 | 887,760 |
4 | Endocrine | 66,283 | 15,058 | 51,225 | 43,394 | 7844 | 35,550 |
7 | Neurological | 135,686 | 41,770 | 93,917 | 68,893 | 15,842 | 53,050 |
10 | Circulatory | 1,102,020 | 278,251 | 823,768 | 656,145 | 135,241 | 520,905 |
11 | Respiratory | 298,343 | 230,313 | 68,030 | 169,269 | 106,505 | 62,764 |
13 | Gastrointestinal | 273,117 | 45,414 | 227,703 | 163,593 | 21,677 | 141,916 |
17 | Genitourinary | 47,229 | 29,101 | 18,127 | 29,749 | 15,152 | 14,598 |
18 + 19 | Maternity and neonates | 16,801 | 0 | 16,801 | 13,662 | 0 | 13,662 |
The implied QoL weights (considering the disease-related decrements) for YLL and YLG are shown in Table 131. Note that, as expected, the weights assume a lower value than in Table 125.
PBC | (1) QoL weights for YLL | (2) QoL weights for YLG | |
---|---|---|---|
1 | Infectious diseases | 0.63 | 0.50 |
2 | Cancer | 0.65 | 0.54 |
4 | Endocrine | 0.65 | 0.52 |
7 | Neurological | 0.51 | 0.38 |
10 | Circulatory | 0.60 | 0.49 |
11 | Respiratory | 0.57 | 0.46 |
13 | Gastrointestinal | 0.60 | 0.48 |
17 | Genitourinary | 0.63 | 0.52 |
18 + 19 | Maternity and neonates | 0.81 | N/A |
Combining QoL adjustments for both population norms and disease-related decrements assumes that any YLGs due to a reduction in mortality will be lived in the diseased state until LE (i.e. that all diseases are not just chronic but disease duration is lifelong). Inevitably, this assumption means that the health effects of changes in mortality will be reduced. Consequently, the cost per QALY threshold reported in Table 132 [see column (2)] will be higher than adjusting YLGs for population norms in Table 126 [see column (2)]. A detailed breakdown of the cost per QALY threshold based on disease-related disability and mortality effects is shown is Table 133.
PBC scenario | (1) Cost per life-year threshold (£) | (2) Cost per QALY threshold (£) disease-related disutility |
---|---|---|
All big four programmes | 8080 | 12,109 |
11 PBCs (with mortality) | 15,628 | 23,395 |
All 23 PBCs (zero health effects for remaining 12 PBCs) | 57,497 | 86,072 |
All 23 PBCs (non-zero health effects for remaining 12 PBCs, except GMS)a | 17,663 | 26,441 |
PBC | (1) Change in spend (£M) | (2) Change in QALY | (3) Cost per QALY gained (£) | |
---|---|---|---|---|
2 | Cancer | 19 | 1412 | 13,578 |
10 | Circulatory problems | 33 | 4034 | 8248 |
11 | Respiratory problems | 22 | 1117 | 19,960 |
13 | Gastrointestinal problems | 17 | 972 | 16,974 |
All big four programmes | 0 | 12,109 | ||
1 | Infectious diseases | 8 | 10 | 853,712 |
4 | Endocrine problems | 18 | 285 | 61,892 |
7 | Neurological problems | 17 | 37 | 469,558 |
17 | Genitourinary problems | 32 | 7 | 4,676,874 |
16 | Trauma and injuries | 10 | 0 | N/A |
18 + 19 | Maternity and neonates | 8 | 15 | 542,801 |
First 11 PBCs | 0 | 23,395 | ||
3 | Disorders of blood | 11 | 479 | 23,395 |
5 | Mental health disorders | 204 | 8727 | 23,395 |
6 | Learning disability | 31 | 1316 | 23,395 |
8 | Problems of vision | 24 | 1033 | 23,395 |
9 | Problems of hearing | 6 | 273 | 23,395 |
12 | Dental problems | 23 | 1004 | 23,395 |
14 | Skin | 11 | 449 | 23,395 |
15 | Musculoskeletal system | 15 | 647 | 23,395 |
20 | Poisoning and adverse effects | 4 | 188 | 23,395 |
21 | Healthy individuals | 18 | 784 | 23,395 |
22 | Social care needs | 68 | 2890 | 23,395 |
23 | Other | 78 | 0 | N/A |
All 23 PBCs | 26,441 |
The number of YLGs associated with each death averted [columns (1) and (2) in Table 134] is, again, consistent with previous estimates (see Tables 121 and 128). The average number of QALYs gained across all 11 PBCs is 2.8 QALYs per death averted [see column (4) in Table 134]. As expected this value is lower than in the previous section [see column (4) in Table 128].
PBC | (1) Implied YLL per excess death averted | (2) Implied YLL per PBC death averted | (3) Implied QALYs gained per excess death averted | (4) Implied QALYs gained per PBC death averted | |
---|---|---|---|---|---|
2 | Cancer | 14.07 | 10.30 | 9.28 | 6.79 |
10 | Circulatory | 10.40 | 5.15 | 6.58 | 3.26 |
11 | Respiratory | 9.21 | 1.04 | 8.50 | 0.96 |
13 | Gastrointestinal | 14.98 | 9.43 | 9.34 | 5.88 |
All big four programmes | 11.28 | 4.07 | 7.53 | 2.71 | |
1 | Infectious diseases | 13.21 | 5.31 | 9.39 | 3.77 |
4 | Endocrine | 13.59 | 7.57 | 9.43 | 5.26 |
7 | Neurological | 13.59 | 6.12 | 7.68 | 3.46 |
17 | Genitourinary | 8.34 | 1.71 | 6.72 | 1.37 |
16 | Trauma and injuries | N/A | N/A | N/A | N/A |
18 + 19 | Maternity and neonates | 74.34 | 74.34 | 60.45 | 60.45 |
First 11 PBCs | 11.37 | 4.14 | 7.60 | 2.77 |
Summary of the cost per quality-adjusted life-year threshold based only on mortality effects
The analysis to this point is summarised in Table 135. The three estimates of a cost per QALY threshold are based on assuming that each YLG is either lived in full health [see column (1), equal to the cost per life-year estimates in Table 122]; lived in a QoL that reflects age and gender norms of the general population [see column (2)]; or lived in a QoL that reflects the original disease state [see column (3)].
PBC grouping | (1) QoL score = 1 | (2) QoL norm | (3) QoL diseased |
---|---|---|---|
Best estimate | |||
Effect of expenditure on mortality | 1 year | 1 year | 1 year |
YLL per death averted | ∼ 4.1 YLLa | ∼ 4.1 YLLa | ∼ 4.1 YLLa |
QALYs per death averted | ∼ 4.1 QALYs | ∼ 3.5 QALYs | ∼ 2.8 QALYs |
(1) All big four programmes | £8080 | £9631 | £12,109 |
(2) 11 PBCs (with mortality) | £15,628 | £18,622 | £23,395 |
(3) All 23 PBCsa | £17,663 | £21,047 | £26,441 |
Lower bound | |||
Effect of expenditure on mortality | Remainder of disease | Remainder of disease | Remainder of disease |
YLL per PBC death averted | ∼ 4.1 YLL | ∼ 4.1 YLL | ∼ 4.1 YLL |
QALYs per death averted | ∼ 4.1 QALYs | ∼ 3.5 QALYs | ∼ 2.8 QALYs |
(4) All big four programmes | £3846 | £4252 | £5319 |
(5) 11 PBCs (with mortality) | £6106 | £6852 | £8568 |
(6) All 23 PBCsa | £6901 | £7744 | £9683 |
Upper bound | |||
Effect of expenditure on mortality | 1 year | 1 year | 1 year |
YLL per PBC death averted | 2 YLL | 2 YLL | 2 YLL |
QALYs per death averted | 2 QALYs | ∼ 1.9 QALYs | ∼ 1.5 QALYs |
(7) All big four programmes | £16,432 | £17,456 | £21,747 |
(8) 11 PBCs (with mortality) | £32,387 | £34,492 | £42,967 |
(9) All 23 PBCsa | £36,604 | £38,983 | £48,561 |
The weights reflecting the quality in which the years of life saved are lived in each of these three estimates is shown in Table 136.
PBC | (1) Full health | (2) Population norms | (3) Disease-related disutility | |
---|---|---|---|---|
2 | Cancer | 1 | 0.79 | 0.66 |
10 | Circulatory | 1 | 0.81 | 0.63 |
11 | Respiratory | 1 | 1.13 | 0.92 |
13 | Gastrointestinal | 1 | 0.82 | 0.62 |
All big four programmes | 1 | 0.84 | 0.67 | |
1 | Infectious diseases | 1 | 0.89 | 0.71 |
4 | Endocrine | 1 | 0.84 | 0.69 |
7 | Neurological | 1 | 0.86 | 0.56 |
17 | Genitourinary | 1 | 0.94 | 0.81 |
16 | Trauma and injuries | N/A | N/A | N/A |
18 + 19 | Maternity and neonates | 1 | 0.87 | 0.81 |
First 11 PBC’s | 1 | 0.84 | 0.67 |
Assuming that YLGs are lived in full health is not credible and should be regarded as an underestimate of the threshold given what is known about QoL norms for the general population (see Figure 49). Equally, assuming that all YLGs are lived in the QoL of the original disease state does not seem credible either, and is likely to overestimate the threshold as it assumes that all disease is not only chronic but lifelong and all life-years would be lived in the diseased state until death. The information that is available about disease duration suggests that many types of disease that comprise the PBCs are not chronic and certainty not lifelong (see Table 123). Although adjusting YLGs for the QoL of the general population, taking account of age and gender [see column 2 in Table 135), is likely to underestimate the cost per QALY threshold based only on mortality effects, it probably represents the ‘best’ of the three alternative estimates available at this stage of the analysis. The lower and upper bounds are based on combining optimistic and pessimistic assumptions about the duration of health effects and how long a death might be averted as described in Summary of cost per life-year estimates.
However, it should be noted that these cost per QALY thresholds only account for the direct health effects of changes in mortality due to changes in expenditure. Insofar as much, or at least some part, of NHS activity and expenditure is intended to improve QoL, not just mortality, then these estimates will underestimate total health effects and overestimate a cost per QALY threshold based on a more complete measure of possible health effects. In Including quality-of-life effects during disease we explore the ways in which the likely effects of expenditure on QoL (other than through mortality) might also be taken into account.
Including quality-of-life effects during disease
The cost per QALY thresholds presented in Adjusting life-years for quality-of-life only account for the health (QALY) effects of changes in mortality due to changes in expenditure. It does not seem credible to suppose that all NHS activity and expenditure only influences mortality with no effect on the QoL while alive and experiencing a disease. Insofar as changes in NHS expenditure will also affect QoL, as well as mortality, then total health effects will be underestimated and the thresholds presented in Table 135 will overestimate the cost per QALY threshold. In this section we explore ways to also take account of those effects on health not directly associated with mortality and life-year effects (i.e. the ‘pure’ QoL effects) to estimate an overall cost per QALY threshold.
The routine reporting of QoL outcomes are increasingly available at PCT level (see Addendum 1: data sources for a description of these data). In principle, the variation in such measures of outcome across PCTs could be used to estimate outcome elasticities for QoL rather than mortality effects using similar econometric methods to those described in Appendix 2 (see Application of method to other non-mortality-based outcome indicators for the results of an exploratory econometric analysis of these data). However, the currently limited coverage of routine reporting of these outcomes means that it is not feasible to estimate QoL effects across all the PBCs using these data. Here we explore how estimates of effects of expenditure that can be observed (i.e. on mortality) can be used to infer the likely effects on what cannot be directly observed (QoL), rather than making extreme assumptions that are not credible (e.g. assuming that changes in expenditure will have no effects on QoL outcomes).
In Using ratios of quality-adjusted life-years to years of life lost we use three alternative estimates of the ratio of QALYs to life-years lost due to different types of disease as a means of inferring the change in QALYs that is likely to be associated with the estimated change in YLL (i.e. applying the total QALYs lost associated with each YLL with disease). This is consistent with regarding the estimates of the mortality and life-year effects as a surrogate for a more complete measure of the health effects of a change in expenditure.
However, these ratios of QALYs lost to life-years lost due to disease in those PBCs where outcome elasticities could not be estimated cannot inform estimates of the threshold (there are no estimated life-year effects with which to apply the ratios). Nonetheless, the sources of information on which ratios are based also provide much of the information required to calculate the QALY burden of disease in these areas, which can be used to inform estimates of the threshold. Therefore, in Using estimates of the quality-adjusted life-year burden of disease we use an estimate of the QALY burden of disease, infer a proportionate effect on burden from the observed effects on life-years, and then apply this proportionate effect to the measures of QALY burden for all the other PBCs. In this way we can use all the information available about the mortality and QoL effects of the different types of disease that make up each PBC, including those where mortality-based outcome elasticities are not available.
Using ratios of quality-adjusted life-years to years of life lost
The ratio of the total QALYs to YLL due to a disease indicates the number of QALYs associated with each year of life lost. Therefore, any change in YLL is expected to generate a number of QALYs indicated by the ratio – in this way, the estimated effects on mortality and life-years are interpreted as a surrogate for a more complete measure of total health effects, which is reasonable. For example, a disease with a ratio > 1 suggests that each year of life lost across the at risk population is associated with more than one QALY (i.e. there are significantly greater QoL effects while experiencing the disease). Therefore, a change in expenditure that leads to 1 YLG in this type of disease may generate a greater QALY effect than the same life-year effects in a disease where this ratio is < 1 (i.e. where most of the effect of disease is on mortality rather than QoL). Therefore, using these ratios provides a means of accounting for the likely effect on QoL other than through effects on mortality.
To understand the differences between the three ratios presented below, it is useful to regard the total QALYs lost to YLL ratio (R) for a particular disease as the sum of two ratios: (i) the QALYs lost due to premature death to YLL ratio (Rdeath); and (ii) the QALYs lost during disease (while alive) to YLL ratio (Ralive), as depicted in Equation 25.
Insofar as YLL would not have been lived in full health, the QoL effects captured in Rdeath are estimated to be lower than 1. Note that the analyses in Adjusting life-years for quality-of-life already imply a Rdeath ratio at PBC level. The second component of the ratio, Ralive, represents QALYs lost during disease for the at risk population as a proportion of the YLL observed in the same population – in diseases for which QoL during disease is compromised but LE is not changed significantly Ralive may thus assume high values. The ratios do not represent the balance of QALY gains due to mortality and morbidity in a single patient, but rather in the population. Where Rdeath is < 1, only when the pure QALY effects offset the less than full QoL of the YLL is the ratio > 1. Therefore, ratios < 1 are possible even when disease has measurable QoL effects for those experiencing it.
Disability-adjusted life-year to years of life lost ratios
The WHO GBD study provides UK-specific estimates of the YLD and the YLL due to different types of disease. Diseases in GBD are classified using U-codes that can then be mapped to ICD-10 codes, as illustrated in Table 137 (Addendum 1: data sources provides more details on the mapping procedure). GBD uses DALYs as a measure of the burden of disease. This DALY measure has two components: (i) the YLD, which evaluates the number of years lived with disability over the durations of disease, and incorporates weights (between zero and one) to reflect the scale of disability experienced in each year; and (ii) the YLL.
U-code | ICD-10 codes |
---|---|
U037 (other infectious diseases) | A02, A05, A20–A28, A31, A32, A38, A40–A49, A65–A70, A74–A79, A81, A82, A83.1–A83.9, A84–A89, A92–A99, B00–B04, B06–B15, B25–B49, B58–B60, B64, B66–B72, B74.3–B74.9, B75, B82–B89, B92–B99, G04 |
U016 (tetanus) | A33–A35 |
U061 (mouth and oropharynx cancers) | C00–C14 |
U057 (iron-deficiency anaemia) | D50, D64.9 |
The total DALYs associated with a disease is simply YLL + YLD. Therefore, the DALYs to YLL ratio is (YLL + YLD)/YLL or equivalently YLL/YLL + YLD/YLL. As the first term (YLL/YLL = Rdeath) must equal 1 and the second (Ralive = YLD/YLL) must be ≥ 0, a ratio based on DALYs must necessarily be bounded by one.
This is illustrated in Table 138 for the four different diseases (classified by U-codes) introduced in Table 137 which reflect diseases where mortality is the major component (e.g. U016) and where the impact of disease on the QoL while alive is the major component (e.g. U141).
U-code | DALY ratios | (Rdeath + Ralive) |
---|---|---|
U037 (other infectious diseases) | 1.23 | (1 + 0.23) |
U016 (tetanus) | 1.00 | (1 + 0)a |
U061 (mouth and oropharynx cancers) | 1.05 | (1 + 0.05) |
U141 (spina bifida) | 2.34 | (1 + 1.34)b |
Note that the estimates of GBD YLL used here are derived using UK data on mortality (relating to the year 2004) by age and gender groups – we assume these data to be from ONS and, thus, consistent with the data used in this work. However, the calculation of YLL in GBD differs from both the approach adopted by the NHS IC and the approach adopted here of using net YLL. For each death observed in the data, GBD evaluates YLL by considering the LE at the age at which the death occurred (and gender). 154 This is expected to overestimate net YLL (which accounts for counterfactual deaths, as detailed in Summary of the cost per quality-adjusted life-year threshold based only on mortality effects). This will make no difference to the first term in the QALY ratio (Rdeath) as an overestimate of YLL affects both denominator and numerator of the ratio. However, the second term (Ralive) is likely to be underestimated. Therefore, the ratios will tend to underestimate the QALY effects of expenditure and overestimate the cost per QALY threshold. This will be adjusted for in Using estimates of the quality-adjusted life-year burden of disease, where our preferred analysis based on burden of disease is presented.
Adjusting disability-adjusted life-years for quality-of-life norms
The use of DALY ratios bounded below by 1 essentially assumes that YLL would have otherwise been lived in a state of full health. As was discussed in Using ratios of quality-adjusted life-years to years of life lost, this is not credible given information available about the QoL in the general population (see Figure 49). It would lead to overestimating the QALYs associated with mortality and life-year effects and underestimating the cost per QALY threshold. Therefore, it is important to adjust these DALY ratios for the QoL norms by age and gender in the same way as described in Using ratios of quality-adjusted life-years to years of life lost. Equation 27 shows how the adjusted ratio is formulated when YLL are adjusted by the QoL in the general population, un. This is a simplified representation of the adjustment as despite gender and age having been considered in calculations these are not shown in the notation below.
The effect of this adjustment (within each U-code, see Addendum 1: data sources) is illustrated in Table 139. Now those types of disease where mortality rather than QoL with the disease is the major component can have ratios < 1. Indeed, the first term of these ratios (Rdeath) is consistent with (but not equivalent to) the analysis in Using ratios of quality-adjusted life-years to years of life lost, where the ratio of quality adjusted net YLL to unadjusted net YLL represents this ratio on average for each PBC.
U-code | Adjusted DALY ratios | (Rdeath + Ralive) |
---|---|---|
U037 (other infectious diseases) | 1.01 | (0.78 + 0.23) |
U016 (tetanus) | 0.78 | (0.78 + 0) |
U061 (mouth and oropharynx cancers) | 0.83 | (0.78 + 0.05) |
U141 (spina bifida) | 2.18 | (0.85 + 1.34) |
Using quality-of-life estimates (based on the Health Outcomes Data Repository and Medical Expenditure Panel Survey)
The disability weights used in the DALY measure (in Ralive) are not based on the same description of health states as the EQ-5D measure, nor are the weights based on a representative sample of the UK population responding to choice-based elicitation questions. EQ-5D based QoL decrements (in relation to age adjusted QoL norms) associated with different types of disease can be estimated from HODaR and MEPS data for the groups of ICD-10 codes that make up each U-code. The calculations of the QoL decrements from HODaR were conducted as previously described in Adjusting age-related quality-of-life for disease decrements. In summary, the average QoL scores across the ICD-10 codes which contribute to each U-code (based on the contributing ICD-10 codes, see Table 137 and Addendum 1: data sources for how ICD-10 codes map to U-codes) and the average age and gender of respondents from HODaR and MEPS were used to calculate a disease decrement for each U-code, based on QoL norms from the general population. Note that, by expressing the QoL effects of different diseases as age-related decrements (see Figure 50), we do not require the HODaR and MEPS samples to necessarily be representative of the age distribution of the population at risk.
The disease-related QoL decrements can then be used to replace the DALY disability weights in Ralive reported in Tables 138 and 139. This final adjustment is illustrated in Table 140; for example, the evidence about QoL from HODaR and MEPS suggests that the impact of U037 on QoL is greater than indicated by DALY disability weights. The QoL effects of U141, although still very significant, are lower than indicated by DALY disability weights.
U-code | QALY ratios (HODaR and MEPS) | (Rdeath + Ralive) |
---|---|---|
U037 (other infectious diseases) | 1.37 | (0.78 + 0.60) |
U016 (tetanus) | 0.78 | (0.78 + 0) |
U061 (mouth and oropharynx cancers) | 0.80 | (0.78 + 0.02) |
U141 (spina bifida) | 1.88 | (0.85 + 1.03) |
By turning what were originally DALY ratios into EQ-5D QALY ratios, we regard the QALY to YLL ratios rather than DALY or modified DALY ratios as the preferred basis of estimating a cost per QALY threshold. We consider these estimates to provide a more complete picture of the likely health effects of changes in expenditure.
U-code quality-adjusted life-year ratios to International Classification of Disease quality-adjusted life-year ratios
Information about the size and age and gender distribution is only available at U-code level. Therefore U-code ratios are applied to all the ICD-10 codes that contribute to a particular U-code. Note that, unlike ICD-10 codes, U-codes do not map directly to PBCs so some ICD-10 codes in different PBCs may belong to the same U-code and therefore have the same U-code ratio. Some ICD-10 codes are not included in the U-code classification of disease. Some of these are procedural codes (84 out of 1562) where mortality and QALY effects were not assigned mortality or QALY effects anyway (any health effects would be evident in other ICD-10 codes) so it was not necessary to impute ratios for them. Of the others, some were associated with PBC 16 (186 out of 1562) with a zero outcome elasticity so did not require imputation either. Imputation based on the median ratio across the ICD-10 codes within the PBC was required for the remaining (482 out of 1562). Eighty eight of these are not mapped into U-codes – these include three big categories of ICD-10 codes: symptoms and signs (R00–R69); abnormal clinical and laboratory findings, not elsewhere classified (R70–R99); and ill-defined and unknown causes of mortality (R95–R99). The remaining 394 were associated with U-codes where the ratio was undefined because the denominator (YLL) was zero. In both these cases, values were also imputed based on the median ratio across the ICD-10 codes within the PBC. As the distribution of ratios within a PBC tend to be highly positively skewed, imputation based on the median is likely to be conservative with respect to health effects and especially in the latter case where mortality effects appear to be a much less important aspect of the disease.
Table 141 illustrates the variation observed in the ratios (imputed) across ICD-10 codes within the same PBC.
PBC | Percentiles of the adjusted DALY ratios | |||||||
---|---|---|---|---|---|---|---|---|
5% | 15% | 25% | 50% | 75% | 85% | 95% | ||
2 | Cancer | 0.76 | 0.76 | 0.76 | 0.81 | 0.85 | 0.85 | 0.91 |
10 | Circulatory problems | 0.86 | 0.86 | 0.86 | 0.86 | 0.96 | 1.00 | 2.65 |
11 | Respiratory problems | 0.22 | 0.73 | 1.00 | 1.67 | 1.67 | 1.96 | 2.67 |
13 | Gastrointestinal problems | 0.86 | 0.96 | 1.01 | 1.63 | 1.63 | 1.78 | 2.73 |
1 | Infectious diseases | 0.00 | 0.83 | 1.01 | 1.01 | 1.01 | 1.01 | 2.64 |
4 | Endocrine problems | 0.77 | 1.37 | 1.43 | 2.97 | 2.97 | 2.97 | 2.97 |
7 | Neurological problems | 0.86 | 1.01 | 1.01 | 2.01 | 2.01 | 2.01 | 2.30 |
17 | Genitourinary problems | 0.74 | 0.77 | 0.77 | 1.10 | 1.10 | 1.10 | 12.41 |
18 | Maternity | 0.00 | 0.79 | 0.81 | 20.39 | 20.39 | 20.39 | 20.39 |
19 | Neonates | 1.17 | 1.17 | 1.17 | 1.17 | 2.29 | 2.29 | 2.29 |
Percentiles of the QALY ratios (HODaR and MEPS) | ||||||||
5% | 15% | 25% | 50% | 75% | 85% | 95% | ||
2 | Cancer | 0.76 | 0.76 | 0.76 | 0.79 | 0.80 | 0.80 | 0.83 |
10 | Circulatory problems | 0.83 | 0.83 | 0.83 | 0.83 | 0.94 | 1.01 | 1.83 |
11 | Respiratory problems | 0.73 | 0.86 | 1.37 | 2.09 | 2.09 | 2.24 | 2.80 |
13 | Gastrointestinal problems | 0.84 | 1.01 | 1.37 | 1.70 | 1.70 | 2.17 | 7.10 |
1 | Infectious diseases | 0.83 | 1.37 | 1.37 | 1.37 | 1.37 | 1.37 | 3.26 |
4 | Endocrine problems | 0.77 | 2.37 | 2.55 | 5.12 | 5.12 | 5.12 | 10.15 |
7 | Neurological problems | 0.84 | 0.90 | 1.37 | 5.90 | 5.90 | 5.90 | 5.90 |
17 | Genitourinary problems | 0.74 | 0.78 | 0.78 | 0.99 | 0.99 | 0.99 | 9.80 |
18 | Maternity | 0.81 | 0.81 | 0.83 | 49.30 | 49.30 | 49.30 | 49.30 |
19 | Neonates | 0.87 | 0.87 | 0.87 | 0.88 | 0.88 | 0.88 | 0.88 |
Allocating effects at programme budget category level to International Classification of Disease codes
Tables 138–140 illustrate how QALY ratios can be calculated for and differ by U-code. Unsurprisingly, these ratios differ across the type of diseases that make up each PBC (see Table 141). When using this information to estimate a cost per QALY threshold the mortality and life-year effects observed at PBC level must be allocated in some way to the component ICD-10 codes before ratios are applied to life-year effects and the resulting QALY effects are summed across all the contributing ICD-10 codes.
Alternatively, one could calculate an average of the ratios within a PBC and then apply this ‘average ratio’ to life-year effects at PBC level, rather than calculate QALY effects at ICD-code level by applying the relevant ratio. This would be inappropriate for two reasons. First, ratios should not be averaged; instead, the total QALYs lost and YLL should be summed across ICD-10 codes and the ratio of these sums used to represent a PBC level estimate (i.e. a ratio of averages). Second, even if the appropriate estimate of the QALY to YLL ratio is calculated at the PBC level, this estimate would assume ICD-10 codes to be equally representative of the PBC – i.e. that expenditure would be equally likely to affect any of the ICD-10 codes that compose a particular PBC. This is unlikely to be true not only due to the inherent differences in the disease described by the ICD-10 coding, but also as ICD-10 codes are likely to differ significantly in what concerns the size of the at risk population they represent.
It is important to consider explicitly how other information might inform the different ways in which the effects observed at PBC level might be generated by the distribution of impacts at ICD-10 code level, i.e. where investment or disinvestment is likely to occur within the PBC and therefore which ICD-10 codes are likely to contribute most to overall health effects. An important and complementary element to the econometric analysis of routinely reported information at PBC level, was to investigate this by looking at local level information available within the NHS. The details of this investigation are reported in Addendum 2: the role of data on local NHS decisions. The review of local data sources suggested that there is very little routinely collected data on investment and disinvestment by local NHS organisations beyond the high-level aggregate data on spending by PBCs which are used in the econometric analysis. Although more disaggregated data on spending decisions about specific services relevant to particular ICD-10 codes could in principle be acquired through additional primary research (surveys or freedom of information requests) this would be costly and with a risk that information acquired in this way may not be complete, consistent or representative.
In the absence of useful information at a local level, it is possible to assume that a change in PBC expenditure will be allocated equally (on a per patient basis) across the component ICD-10 codes, i.e. any investment or disinvestment is equally likely across the population at risk within the PBC. HES (see Addendum 1: data sources) provides information about the costs associated with each ICD-10 code by PCT. The variation in per patient costs between PCTs (where total costs allocated to individual ICD-10 codes were divided by the number of patients using services in the PCT) was analysed to establish which ICD-10 codes contribute most to the variability in HES costs within a PBC, across PCTs. The ICD-10 codes that contribute most to this variance might be expected to be more likely to have been subject to differential investment or disinvestment across PCTs. Unfortunately, total PBC costs are not available at ICD-10 code level across PCTs so could not be used for this purpose. Costs from HES data are only a component of total PBC costs (41% of total PBC costs for the 11 PBCs where mortality effects can be estimated) and contribute less to the variability in PBC costs across PCTs (HES contribute only 23% of the variability for the 11 PBCs where mortality effects can be estimated).
There are differences in relative weight assigned to ICD-10 codes based solely on the size of the population or its contribution to variance in HES costs. If investment or disinvestment within a PBC tends to focus on ICD-10 codes representing areas of marginal value the health effects of a change in PBC expenditure may be overestimated and a cost per QALY threshold underestimated when allocating effects equally across the population at risk within each PBC. However, weighting ICD-10 codes based on HES data is likely to favour those ICD-10 codes which represent more severe disease requiring more hospital care. This may over represent ICD-10 codes with lower QALY to YLL ratios if mortality effects tend to be a major component of these types of disease and may be conservative with respect to the health effects of changes in expenditure.
The implications for a cost per QALY threshold that uses the estimated mortality and life-year effects as a surrogate for a more complete measure of the likely heath effects (i.e. that includes QoL as well as quality adjusted life-year effects) is summarised in Table 142, and detailed in Table 143. These results use the contribution to variance in HES costs to ‘weight’ the different ICD-10 codes within a PBC (when allocating the life-year effects), before applying the QALY ratios associated with each ICD-10 code (see footnote l reporting results using weights based on the size of the population).
PBC scenario | Cost per QALY threshold (£) | ||
---|---|---|---|
(1) DALY ratios | (2) Adjusted DALY ratios | (3) QALY ratios (HODaR and MEPS) | |
All big four programmes | 5402 | 6419 | 5990 |
11 PBCs (with mortality) | 9958 | 11,718 | 10,297 |
All 23 PBCs | 11,254 | 13,244 | 11,638a |
PBC | (1) Change in spend (£M) | Adjusted DALY ratios | QALY ratios (HODaR and MEPS) | |||
---|---|---|---|---|---|---|
(2) Change in QALY | (3) Cost per QALY gained (£) | (4) Change in QALY | (5) Cost per QALY gained (£) | |||
2 | Cancer | 19 | 1763 | 10,871 | 1699 | 11,283 |
10 | Circulatory problems | 33 | 7677 | 4334 | 6713 | 4956 |
11 | Respiratory problems | 22 | 2379 | 9375 | 3215 | 6937 |
13 | Gastrointestinal problems | 17 | 2396 | 6886 | 3605 | 4577 |
All big four programmes | 6419 | 5990 | ||||
1 | Infectious diseases | 8 | 21 | 388,430 | 27 | 305,724 |
4 | Endocrine problems | 18 | 1077 | 16,396 | 2036 | 8673 |
7 | Neurological problems | 17 | 296 | 58,158 | 342 | 50,295 |
17 | Genitourinary problems | 32 | 15 | 2,158,296 | 12 | 2,623,379 |
16 | Trauma and injuries | 10 | 0 | N/A | 0 | N/A |
18 + 19 | Maternity and neonates | 8 | 125 | 64,173 | 273 | 29,327 |
First 11 PBCs | 11,718 | 10,297 | ||||
3 | Disorders of blood | 11 | 956 | 11,718 | 1087 | 10,297 |
5 | Mental health disorders | 204 | 17,423 | 11,718 | 19,828 | 10,297 |
6 | Learning disability | 31 | 2627 | 11,718 | 2990 | 10,297 |
8 | Problems of vision | 24 | 2063 | 11,718 | 2348 | 10,297 |
9 | Problems of hearing | 6 | 546 | 11,718 | 621 | 10,297 |
12 | Dental problems | 23 | 2005 | 11,718 | 2282 | 10,297 |
14 | Skin | 11 | 897 | 11,718 | 1021 | 10,297 |
15 | Musculoskeletal system | 15 | 1291 | 11,718 | 1469 | 10,297 |
20 | Poisoning and adverse effects | 4 | 375 | 11,718 | 426 | 10,297 |
21 | Healthy individuals | 18 | 1565 | 11,718 | 1781 | 10,297 |
22 | Social care needs | 68 | 5769 | 11,718 | 6566 | 10,297 |
23 | Other | 78 | 0 | N/A | 0 | N/A |
All 23 PBCs | 13,244 | 11,638 |
As all the analysis in this section seeks to use the estimated mortality and life-year effects as a surrogate for a more complete measure of likely health effects, it is the cost per QALY threshold for all 23 PBCs that is most relevant. As expected, this threshold (£11,638), is lower than a cost per QALY threshold based only the QALY effects (£21,047 and £26,441 in Table 135 that assumes no effects of NHS expenditure on QoL itself). This difference gives some indication of the relative importance of QALY effects due to avoidance of premature death and the QALY effects of avoiding disability during disease. Table 144 reports how the estimated QALY effects for each PBC can be decomposed into that part associated with QALY effects and that part associated with ‘pure’ QoL effects. These results appear credible for the first 11 PBCs, where those for which mortality is the major concern have a much greater share of total QALY effects associated with avoidance of premature death (e.g. PBCs 2 and 10) compared with those where QoL is the major concern (e.g. PBC 7).
PBC | QALY change (total) | QALY change (death) | % QALY gained | ||
---|---|---|---|---|---|
Due to avoidance of premature death | Due to avoidance of disability while alive | ||||
2 | Cancer | 1699 | 1641 | 97 | 3 |
10 | Circulatory | 6713 | 4856 | 72 | 28 |
11 | Respiratory | 3215 | 923 | 29 | 71 |
13 | Gastrointestinal | 3605 | 1193 | 33 | 67 |
1 | Infectious diseases | 27 | 11 | 40 | 60 |
4 | Endocrine | 2036 | 323 | 16 | 84 |
7 | Neurological | 342 | 52 | 15 | 85 |
17 | Genitourinary | 12 | 6 | 52 | 48 |
16 | Trauma and injuries | 0 | 0 | N/A | N/A |
18 + 19 | Maternity and neonates | 273 | 15 | 6 | 94 |
3 | Disorders of blood | 1087 | 547 | 50 | 50 |
5 | Mental health | 19,828 | 9979 | 50 | 50 |
6 | Learning disability | 2990 | 1505 | 50 | 50 |
8 | Problems of vision | 2348 | 1181 | 50 | 50 |
9 | Problems of hearing | 621 | 313 | 50 | 50 |
12 | Dental problems | 2282 | 1148 | 50 | 50 |
14 | Skin | 1021 | 514 | 50 | 50 |
15 | Musculoskeletal | 1469 | 739 | 50 | 50 |
20 | Poisoning and adverse events | 426 | 215 | 50 | 50 |
21 | Healthy individuals | 1781 | 896 | 50 | 50 |
22 | Social care needs | 6566 | 3304 | 50 | 50 |
23 | Other | 0 | 0 | N/A | N/A |
The ratios of QALYs to YLL due to disease in those PBCs where outcome elasticities could not be estimated cannot be used to inform estimates of the threshold because there are no estimated life-year effects with which to apply the ratios. Therefore, as in previous sections, the estimated effect of expenditure on health for the 11 PBCs with outcome elasticities is applied to the estimated changes in PBC expenditure for the other 12 PBCs (excluding GMS for the reasons given in Summary of cost per life-year estimates), i.e. assuming that the health effects that can be observed from a change in expenditure will be similar to those that cannot. However, the use of QALY ratios also implies that the share of total health effects between QALY effects and that part associated with ‘pure’ QoL effects are also similar to those PBCs with estimated outcome elasticities. Summing the different types of health effects across these 11 PBCs suggests that 50% is due to avoidance of premature death and 50% due to avoidance of disability. This is clearly not credible when applied to the other PBCs (e.g. mental health, vision and hearing are likely have a much greater share of total health effects associated with QoL effects and very little associated with premature mortality).
By comparing the change in QALYs in each PBC [that originates cost per QALY threshold estimates, column (2) in Table 145], with the corresponding change in YLL [see column (6) in Table 116], we can infer the implied QALY to YLL ratio in each of the PBCs with a mortality signal. These are shown in Table 145. The QALY to YLL ratio implied by the analysis using QALY ratios for all 11 PBCs with outcome elasticities is 1.52, which suggests that every life-year is associated with 1.52 QALYs on average across these PBCs. However, this implied QALY ratio differs across these PBCs, ranging from 0.79 in PBC 2 to 15.05 in PBC 18 + 19 [see column (4) of Table 145]. It should be noted that the implied QALY ratio of 1.33 for the 11 PBCs with outcome elasticities is a ratio of QALYs to unadjusted YLL. The proportion of total QALY effects due to premature deaths for the same PBCs (50% in Table 144) also implies a ratio – equal to two. However, this is a ratio of total QALY effects to quality-adjusted YLL. The difference between these two ratios is the denominator (i.e. quality-adjusted YLL are lower than unadjusted YLL).
PBC | Adjusted DALY ratios | QALY ratios (HODaR and MEPS) | |||||
---|---|---|---|---|---|---|---|
(1) Implied QALY per YLG | (2) Implied QALY per excess death averted | (3) Implied QALY per PBC death averted | (4) Implied QALY per YLG | (5) Implied QALY per excess death averted | (6) Implied QALY per PBC death averted | ||
2 | Cancer | 0.82 | 11.58 | 8.48 | 0.79 | 11.16 | 8.17 |
10 | Circulatory problems | 1.20 | 12.51 | 6.20 | 1.05 | 10.94 | 5.42 |
11 | Respiratory problems | 1.96 | 18.09 | 2.04 | 2.65 | 24.45 | 2.76 |
13 | Gastrointestinal problems | 1.54 | 23.02 | 14.49 | 2.31 | 34.63 | 21.80 |
All big four programmes | 1.26 | 14.20 | 5.12 | 1.35 | 15.21 | 5.49 | |
1 | Infectious diseases | 1.56 | 20.64 | 8.30 | 1.98 | 26.22 | 10.54 |
4 | Endocrine problems | 2.62 | 35.61 | 19.84 | 4.95 | 67.31 | 37.51 |
7 | Neurological problems | 4.56 | 61.99 | 27.90 | 5.27 | 71.69 | 32.26 |
17 | Genitourinary problems | 1.75 | 14.56 | 2.98 | 1.44 | 11.98 | 2.45 |
16 | Trauma and injuries | N/A | N/A | N/A | N/A | N/A | N/A |
18 + 19 | Maternity and neonates | 6.88 | 511.33 | 511.33 | 15.05 | 1118.85 | 1118.85 |
First 11 PBCs | 1.33 | 15.16 | 5.53 | 1.52 | 17.26 | 6.29 |
The problem is that using QALY to YLL ratios means that much of the information that is available about the other 12 PBCs cannot be used to inform the estimates of the cost per QALY threshold. Fortunately, the sources of information on which the ratios are based also provide much of the information required to calculate the QALY burden of disease in these areas. Using estimates of the quality-adjusted life-year burden of disease explores how measures of burden can be used to estimate a cost per QALY threshold that captures the likely effects of a change in expenditure on all aspects of health while using all the information that is available about all the PBCs.
Using estimates of the quality-adjusted life-year burden of disease
In this section we use estimates of the QALY burden of disease to infer QALY effects in those PBCs where the mortality effects of changes in expenditure can be observed, and extrapolate the estimated proportionate effects to those PBCs where the health effects of changes in expenditure cannot be observed. The estimated proportionate effect of change in expenditure on the life-year burden of disease in the 11 PBCs where mortality-based outcome elasticities could be estimated are applied to measures of QALY burden in each of these PBCs (i.e. effects on the mortality burden of disease are used as a surrogate for effects on QALY burden). The proportionate effect on burden of disease due to the change in expenditure across these PBCs can then be applied to measures of QALY burden in the other 11 PBCs where mortality effects could not be estimated (i.e. the observed effects of changes in expenditure on burden of disease is extrapolated to the other PBCs where health effects cannot be observed). In this way, we can use all the information available about the mortality and QoL effects of the different types of disease that make up each PBC, particularly those where mortality-based outcome elasticities are not available. Applying a proportionate effect to measures of QALY burden of disease is equivalent to assuming that any effects on life-years are lived at QoL that reflects a proportionate improvement to the QoL with disease. m It also allows QoL effects of changes in expenditure to be included; also based on proportionate improvement in the QoL with disease.
In Adjusting life-years for quality-of-life, each YLG could be assumed to be lived in full health, lived in a QoL that reflects age and gender norms of the general population or lived in a QoL that reflects the original disease state. Applying an estimated proportionate effect on the life-year burden of disease to measures of QALY burden of disease implies a proportionate improvement in the QoL with disease applied to any life-year effects. Therefore, basing estimates on measures of QALY burden provides a more conservative estimate of the QALY effects of changes in mortality than the best estimate reported in Adjusting life-years for quality-of-life, which was based on QoL norms.
Previously, in sections up to Using ratios of quality-adjusted life-years to years of life lost (corresponding to Chapter 3 and Chapter 4, From mortality to life-years, Adjusting life-years for quality-of-life and Using ratios of quality-adjusted life-years to years of life lost of main report), expenditure elasticities were not estimated for the other 11 PBCs where outcome elasticities could not be estimated because the same health effect of changes in expenditure was assumed (i.e. it did not matter how changes in expenditure were allocated between them). Given expenditure elasticities were only estimated for PBC 23 and the 11 PBCs where outcome elasticities could be estimated, in analyses up to Using ratios of quality-adjusted life-years to years of life lost the remaining change in total spend was assigned to the other 11 PBCs. As a consequence, proportionally more of the share of a change in total spend was allocated to these other PBCs in previous sections [see column (3) of Table 108]. However, in this section it does matter how the remaining change in expenditure is allocated between the other 11 PBCs as they have different QALY burdens so different implied health effects of expenditure. Therefore, expenditure elasticities are estimated for all 23 PBCs [see column (2) of Table 108]. However, it is not possible to estimate expenditure equations for all 23 PBCs simultaneously, so the 23 independently estimated expenditure elasticities do not account for all of the change in overall spend (i.e. the sum of changes in PBC expenditure based on the estimated PBC expenditure elasticities accounts for less than a 1% change in total spend). This remaining change in total spend is allocated between all 23 PBCs reflecting their relative share of changes in expenditure based on their estimated expenditure elasticities [see column (4) of Table 108].
The total QALY burden of disease for the population with disease in a particular year includes (i) the quality-adjusted YLL due to all the disease-related mortality that could occur in this population over their remaining duration of disease; and (ii) the reduction in QoL while alive also for their remaining disease duration. These components of burden represent, respectively, the QALYs lost due to premature death (QALYldeath) and the QALYs lost while alive (QALYlalive) as a consequence of disease.
However, applying the estimated proportionate effects on mortality and life-years to such a measure of total burden would provide an estimate of the effects of a change in expenditure, not just in 1 year, but in all the remaining years of disease for the population at risk in that year. Recall from From mortality to life-years that we have adopted the conservative assumption that changes in expenditure will only have health effects in 1 year for the population with disease in that year. Therefore, it is not a measure of total burden that is required, but a measure of the QALY burden of disease during 1 year for the population with disease (prevalent and incident) in that year. The estimated outcome elasticities can then be appropriately (and directly) applied to this measure of burden. Of course, it would be possible to solve for a lower outcome elasticity that could be applied to total burden which would return the required estimate of total QALY effects restricted to 1 year.
The information from GBD used to derive QALY ratios in Using estimates of the quality-adjusted life-year burden of disease includes information about the YLL and duration of disease for those incident to a U-code, i.e. the measure of QALY burden from the information included in the ratios is a measure of the total burden of the disease but only for the population that is incident (rather than the total population with disease) in 1 year. Assuming that incidence is stable over the disease duration this is also equivalent to the QALY burden of disease during 1 year for the population with disease (i.e. those that are incident and prevalent) in that year. This is valid as long as estimates of the QoL decrement of disease from HODaR and MEPS are assumed representative of average effects across those earlier (incident) and later (prevalent) in their disease duration.
However, in moving from ratios to absolute measures of burden it becomes more important to examine and then adjust for any inconsistency between information about YLL and size of the incident population from GBD (which is available by U-codes and can be mapped to ICD-10 codes), and the information about net YLL and observed deaths for each PBC based on ONS data as described in Summary of the cost per quality-adjusted life-year threshold based only on mortality effects (Table 146).
PBC | (1) Excess deaths ONS | Deaths | YLL | |||||
---|---|---|---|---|---|---|---|---|
(2) All deaths ONS | (3) All deaths GBD | (4) Adjustment factor (deaths) | (5) Net estimates ONS | (6) Total YLL GBD | (7) Adjustment factor (YLL) | |||
1 | Infectious diseases | 2797 | 6958 | 1408 | 4.94 | 36,962 | 25,142 | 1.47 |
2 | Cancer | 95,715 | 130,810 | 140,124 | 0.93 | 1,347,184 | 1,932,637 | 0.70 |
4 | Endocrine | 3769 | 6765 | 7509 | 0.90 | 51,225 | 95,401 | 0.54 |
7 | Neurological | 6909 | 15,353 | 12,854 | 1.19 | 93,917 | 164,796 | 0.57 |
10 | Circulatory | 79,218 | 159,852 | 178,454 | 0.90 | 823,768 | 1,750,608 | 0.47 |
11 | Respiratory | 7386 | 65,446 | 67,441 | 0.97 | 68,030 | 594,529 | 0.11 |
13 | Gastrointestinal | 15,199 | 24,147 | 28,329 | 0.85 | 227,703 | 396,829 | 0.57 |
17 | Genitourinary | 2172 | 10,625 | 8606 | 1.23 | 18,127 | 77,338 | 0.23 |
18 + 19 | Maternity and neonates | 226 | 226 | 2211 | 0.10 | 16,801 | 149,868 | 0.11 |
Total | 213,391 | 420,182 | 446,936 | 0.94 | 2,683,717 | 5,187,148 | 0.52 |
There are a number of reasons for the potential inconsistencies: (i) GBD is based on earlier years of mortality data; (ii) the imprecision of mapping from U-codes to PBC via ICD-10 codes; and (iii) the YLL reported in GBD are based on LE at the age of death (see Adjusting life-years for quality-of-life and Including quality-of-life effects during disease) and will overestimate the net YLL. The YLL by U-code, reported in GBD, that are mapped to ICD-10 codes are adjusted by these proportionate differences [see column (7) of Table 146] to ensure that the YLL associated with all contributing ICD-10 codes are consistent with (and do not overestimate) the net YLL for the PBC as a whole. The variation across ICD-10 codes in the adjusted QALY burden associated with mortality gains (for the population with disease in a particular year) is depicted in column (2) of Table 147.
PBC | Median [5th to 95th percentile] | |||
---|---|---|---|---|
(1) Burden while alive | (2) Burden due to premature death | (3) Burden | ||
1 | Infectious diseases | 0.47 [0.00 to 0.82] | 0.25 [0.11 to 42.87] | 0.72 [0.72 to 42.87] |
2 | Cancers and tumours | 0.09 [0.00 to 0.10] | 2.82 [0.51 to 5.11] | 2.92 [0.58 to 5.11] |
3 | Disorders of blood | 0.05 [0.05 to 0.07] | 0.01 [0.01 to 0.03] | 0.06 [0.06 to 0.09] |
4 | Endocrine | 0.10 [0.00 to 0.17] | 0.01 [0.01 to 4.82] | 0.11 [0.11 to 4.82] |
5 | Mental health | 0.10 [0.07 to 0.22] | 0.02 [0.00 to 0.04] | 0.12 [0.07 to 0.26] |
6 | Learning disability | 0.10 [0.06 to 0.10] | 0.02 [0.00 to 5.34] | 0.12 [0.10 to 5.41] |
7 | Neurological | 0.27 [0.00 to 0.37] | 0.02 [0.02 to 22.79] | 0.29 [0.25 to 22.79] |
8 | Vision | 0.05 [0.00 to 0.06] | 0.00 [0.00 to 20.99] | 0.05 [0.03 to 20.99] |
9 | Hearing | 0.05 [0.00 to 0.05] | 0.00 [0.00 to 20.99] | 0.05 [0.00 to 20.99] |
10 | Circulation | 0.09 [0.09 to 0.19] | 0.37 [0.06 to 0.39] | 0.48 [0.18 to 0.56] |
11 | Respiratory system | 0.14 [0.00 to 0.21] | 0.01 [0.00 to 5.17] | 0.15 [0.00 to 5.18] |
12 | Dental | 0.03 [0.01 to 0.03] | 0.01 [0.00 to 0.01] | 0.04 [0.01 to 0.04] |
13 | Gastrointestinal system | 0.10 [0.00 to 0.18] | 0.05 [0.00 to 23.67] | 0.15 [0.00 to 23.67] |
14 | Skin | 0.06 [0.00 to 0.06] | 0.02 [0.02 to 20.99] | 0.08 [0.08 to 20.99] |
15 | Musculoskeletal system | 0.10 [0.00 to 0.10] | 0.02 [0.00 to 20.99] | 0.12 [0.06 to 20.99] |
16 | Trauma and injury | N/A | N/A | N/A |
17 | Genitourinary system | 0.11 [0.00 to 0.13] | 0.04 [0.00 to 8.90] | 0.15 [0.05 to 8.90] |
18 | Maternity | 0.01 [0.00 to 0.01] | 0.00 [0.00 to 4.68] | 0.01 [0.00 to 4.68] |
19 | Conditions of neonates | 0.00 [0.00 to 0.00] | 0.03 [0.02 to 0.03] | 0.03 [0.02 to 0.03] |
20 | Poisoning and adverse events | 0.03 [0.00 to 0.06] | 0.00 [0.00 to 18.63] | 0.03 [0.02 to 18.63] |
21 | Healthy individuals | 0.05 [0.05 to 0.05] | 0.01 [0.01 to 0.01] | 0.06 [0.06 to 0.06] |
It is QALY burden per patient with disease in a particular year that is reported in Table 147, including the median and range across the ICD-10 codes contributing to each PBC. Such measure of burden considers QoL burden while alive in 1 year and mortality burden for the same time period. Note that mortality effects of disease in 1 year can lead to the loss of more than 1 life-year, and, for this reason, burden due to premature death (and consequently overall burden) may assume values bigger than 1. Burden values in Table 147 reflect variation across ICD-10 codes and should not be misinterpreted as the ‘average’ QALY burden for the PBC, as this depends on how PBC effects are allocated to ICD-10 codes and the ‘average’ burden for groups of PBCs depends on how a change in overall expenditure is shared between them (i.e. the expenditure elasticities estimated for each PBC in Appendix 2).
Due to the earlier years of data and imprecision in mapping from U-codes to ICD-10 codes there might also be some inconsistency in estimates of the total incidence of disease for a PBC. Insofar as disease-related mortality risk is stable, the same number of deaths should be observed in GBD and ONS data for the same at risk population. The PBC deaths recorded in GBD and those observed in ONS data [see columns (2) and (3) in Table 146] are similar but nonetheless the proportionate difference is used to adjust the scale of QoL burden while alive based on GBD information (equivalent to adjusting estimates of incidence). Notable exceptions are PBC 1 and PBC 18 + 19 where the discrepancies are likely to be due to imperfect mapping from U-code to PBC via ICD-10 codes. Summaries of the ICD-10-specific values of the adjusted burden of disease while alive are depicted in column (1) of Table 147. Total burden (for the population with disease in a particular year) is the sum of the two components of burden (Table 148 presents a few examples for illustration).
U-code | QALY burden | (QALY lostdeath + QALY lostalive) |
---|---|---|
U037 (other infectious diseases)a | 0.20 | (0.09 + 0.11) |
U016 (tetanus) | 2.73 | (2.73 + 0.00) |
U061 (mouth and oropharynx cancers) | 2.97 | (2.87 + 0.10) |
U141 (spina bifida) | 0.65 | (0.18 + 0.46) |
The implications for the cost per QALY threshold of using information about the QALY burden of disease for all PBCs, rather than QALY ratios for those where an outcome elasticity can be estimated, are reported summarily in Table 149 and in detail in Table 150. The QALY effects of a change in PBC expenditure are a weighted average of the QALY effects within each of the ICD-10 codes that contribute to the PBC. The figures reported in column 2 (Table 149) are based on weighing the effects at ICD-code level by the proportion of the total PBC population within each contributing ICD-10 code, rather than the contribution to variance in HES costs. n
PBC grouping | Cost per QALY gained (£)a | |
---|---|---|
(1) QALY ratios (HODaR and MEPS) | (2) QALY burden (HODaR and MEPS) | |
All big four programmes | 5990 | 3036 |
11 PBCs (with mortality) | 10,297 | 5128 |
All 23 PBCs | 11,638 | 10,187a |
PBC | (1) Change in spend (£M) | QALY ratios (HODaR and MEPS) | QALY burden (HODaR and MEPS) | |||
---|---|---|---|---|---|---|
(2) Change in QALY | (3) Cost per QALY gained (£) | (4) Change in QALY | (5) Cost per QALY gained (£) | |||
2 | Cancer | 19 | 1699 | 11,283 | 2121 | 12,772 |
10 | Circulatory problems | 33 | 6713 | 4956 | 8347 | 5631 |
11 | Respiratory problems | 22 | 3215 | 6937 | 28,072 | 1123 |
13 | Gastrointestinal problems | 17 | 3605 | 4577 | 3922 | 5944 |
All big four programmes | 5990 | 3036 | ||||
1 | Infectious diseases | 8 | 27 | 305,724 | 74 | 158,349 |
4 | Endocrine problems | 18 | 2036 | 8673 | 6905 | 3613 |
7 | Neurological problems | 17 | 342 | 50,295 | 1361 | 17,844 |
17 | Genitourinary problems | 32 | 12 | 2,623,379 | 34 | 1,320,516 |
16 | Trauma and injuries | 10 | 0 | N/A | 0 | N/A |
18 + 19 | Maternity and neonates | 8 | 273 | 29,327 | 14 | 813,578 |
First 11 PBCs | 10,297 | 5128 | ||||
3 | Disorders of blood | 11 | 1087 | 10,297 | 1215 | 6814 |
5 | Mental health disorders | 204 | 19,828 | 10,297 | 10,878 | 13,876 |
6 | Learning disability | 31 | 2990 | 10,297 | 207 | 109,806 |
8 | Problems of vision | 24 | 2348 | 10,297 | 561 | 31,858 |
9 | Problems of hearing | 6 | 621 | 10,297 | 1168 | 4047 |
12 | Dental problems | 23 | 2282 | 10,297 | 578 | 30,030 |
14 | Skin | 11 | 1021 | 10,297 | 103 | 75,158 |
15 | Musculoskeletal system | 15 | 1469 | 10,297 | 1005 | 11,129 |
20 | Poisoning and adverse effects | 4 | 426 | 10,297 | 42 | 76,909 |
21 | Healthy individuals | 18 | 1781 | 10,297 | 40 | 336,325 |
22 | Social care needs | 68 | 6566 | 10,297 | 0 | N/A |
23 | Other | 78 | 0 | N/A | 0 | N/A |
All 23 PBCs | 11,638 | 10,187 |
The cost per QALY threshold for the 11PBCs with outcome elasticities is a little lower using a measure of QALY burden (£5128) rather than the QALY ratios (£10,297) described in Using ratios of quality-adjusted life-years to years of life lost. This is in part because the way GBD calculates YLL overestimates net YLL (which accounts for counterfactual deaths, as detailed in Summary of the cost per quality-adjusted life-year threshold based only on mortality effects). This will make no difference to the first term in the QALY ratio (Rdeath) used in Using ratios of quality-adjusted life-years to years of life lost as an overestimate of YLL affects both denominator and numerator of the ratio. However, the second term (Ralive) is likely to be underestimated. Therefore, the ratios will tend to underestimate the QALY effects of expenditure and overestimate the cost per QALY threshold (see Table 149). We are able to adjust the GBD-based measure of QALY burden for this overestimation in calculating the QALY threshold reported in column (2) of Table 149.
As the purpose of this section is to use the estimated mortality and life-year effects as a surrogate for a more complete measure of likely health effects, it is the cost per QALY threshold for all 23 PBCs that is of most relevance. The cost per QALY threshold for all 23 PBCs is based on applying the proportionate effects on the QALY burden of disease, based on the observed effects of changes in expenditure on mortality in the 11 PBCs with outcome elasticities,o to the QALY burden of disease in the other PBCs. This generates a higher cost per QALY threshold (£10,187) than the one based only on the 11 PBCs with outcome elasticities (£11,638). The reason is that the QALY burden of disease in the other PBCs is, in general, lower than the QALY burden of disease across those PBCs where outcome elasticities can be estimated (see Table 147).
Therefore, applying the same proportionate effects to a lower QALY burden generates a smaller health effect of a change in expenditure. p In essence, the difference between these estimates is that in column (1) of Table 149 the absolute effect on health associated with an absolute change in expenditure is extrapolated to the other PBCs, whereas in column (2) of Table 149 it is the relative effect on health of an absolute change in expenditure that is extrapolated. As we know that QALY burden differs between (and within) PBCs, and especially between the groups of PBCs with and without estimated outcome elasticities (see Table 147), it is the values based on QALY burden in column (2) of Table 149 that are regarded as most credible and represent our central or best estimate.
A detailed breakdown of changes in expenditure and changes in QALYs across all PBCs is shown in Table 150, where the analysis is based on QALY ratios and on QALY burden of disease. A comparison of these values suggests that QALY effects for the other PBCs are generally lower and therefore the cost per QALY for each of these PBCs are in general higher when based on a proportionate effect on QALY burden. Of course, we have not directly observed QoL effects in these PBCs but inferred them from the proportionate effects that we can observe. Insofar as investment and disinvestment opportunities in these PBCs might have been more valuable (offered greater improvement in QoL) than suggested by the implied PBC thresholds, then overall QALY effects will tend to be underestimated and the cost per QALY threshold overestimated.
For the reasons discussed in previous sections, we regard all the costs per QALY threshold reported in column (2) of Table 149 to be on balance conservative with respect to overall health effects of a change in expenditure. However, the estimate of £10,187 is based on an extrapolation of the proportionate effects to measures of burden on these PBCs, rather than observations of the direct impact of changes in expenditure on QoL in these types of disease. This is especially important in PBC 5, mental health disorders, which accounts for a large proportion of the change in overall expenditure (22%) and where a review of the evidence suggests that the investment and disinvestment opportunities in this PBC may have been more valuable than the implied PBC cost per QALY of £13,876 (see Addendum 3: characterisation of the investment and disinvestment decisions in mental health – depression and schizophrenia). The lower cost per QALY threshold for the 11 PBCs with outcome elasticities (£5128) might be regarded as more secure in this respect, but they only account for a proportion (38%) of any change in overall expenditure (see Table 155).
Table 151 reports how the estimated QALY effects based on measures of QALY burden for each PBC can be decomposed into that part associated with life-year effects adjusted for quality and that part associated with ‘pure’ QoL effects. These results are similar to those reported in Table 144 which were based on QALY ratios for the 11 PBCs with an estimated outcome elasticity. Those PBCs for which mortality is the major concern have a much greater share of total QALY effects associated with avoidance of premature death (e.g. PBCs 2 and 10) compared with those where QoL is the major concern (e.g. PBC 7). The differences tend to favour QALYs gained though avoidance of disability, which reflects the underestimation of the effects on ‘pure’ QoL when using QALY ratios based on estimates of YLL from GBD (see the discussion above). The exceptions are PBC 1 and PBC 18 + 19. The reason is that there are significant adjustments made based on differences in observed and recorded mortality (to adjust for differences in recording), as well as differences in YLL due to the GBD method of calculation (see Table 146).
PBC | (1) QALY change (total) | (2) QALY change (death) | % QALY gained | ||
---|---|---|---|---|---|
(3) For premature death | (4) For disability while alive | ||||
2 | Cancer | 2121 | 1968 | 93 | 7 |
10 | Circulatory | 8347 | 5727 | 69 | 31 |
11 | Respiratory | 28,072 | 1072 | 4 | 96 |
13 | Gastrointestinal | 3922 | 1446 | 37 | 63 |
1 | Infectious diseases | 74 | 13 | 18 | 82 |
4 | Endocrine | 6905 | 380 | 5 | 95 |
7 | Neurological | 1361 | 60 | 4 | 96 |
17 | Genitourinary | 34 | 8 | 22 | 78 |
16 | Trauma and injuries | 0 | 0 | N/A | N/A |
18 + 19 | Maternity and neonates | 14 | 10 | 69 | 31 |
3 | Disorders of blood | 1215 | 62 | 5 | 95 |
5 | Mental health | 10,878 | 949 | 9 | 91 |
6 | Learning disability | 207 | 41 | 20 | 80 |
8 | Problems of vision | 561 | 22 | 4 | 96 |
9 | Problems of hearing | 1168 | 9 | 1 | 99 |
12 | Dental problems | 578 | 1 | 0 | 100 |
14 | Skin | 103 | 38 | 37 | 63 |
15 | Musculoskeletal | 1005 | 50 | 5 | 95 |
20 | Poisoning and adverse events | 42 | 7 | 16 | 84 |
21 | Healthy individuals | 40 | 6 | 16 | 84 |
22 | Social care needs | 0 | 0 | N/A | N/A |
23 | Other | 0 | 0 | N/A | N/A |
The implied QALY per YLG and death averted are reported in Table 152. As expected, the implied QALY per PBC death averted across all 11 PBCs with outcome elasticities is higher (12.6 QALYs) than reported in Using ratios of quality-adjusted life-years to years of life lost (6.3 QALYs) because of the previous bias against QoL effects.
PBC | (1) QALY per YLG | (2) Implied QALY per excess death averted | (3) Implied QALY per PBC death averted | |
---|---|---|---|---|
2 | Cancer | 0.70 | 9.86 | 7.21 |
10 | Circulatory problems | 0.93 | 9.63 | 4.77 |
11 | Respiratory problems | 16.40 | 151.10 | 17.05 |
13 | Gastrointestinal problems | 1.78 | 26.66 | 16.78 |
All big four programmes | 2.66 | 30.02 | 10.82 | |
1 | Infectious diseases | 3.83 | 50.62 | 20.35 |
4 | Endocrine problems | 11.89 | 161.59 | 90.04 |
7 | Neurological problems | 14.86 | 202.05 | 90.92 |
17 | Genitourinary problems | 2.85 | 23.80 | 4.87 |
16 | Trauma and injuries | N/A | N/A | N/A |
18 + 19 | Maternity and neonates | 0.54 | 40.33 | 40.33 |
First 11 PBCs | 3.05 | 34.65 | 12.63 |
In Using ratios of quality-adjusted life-years to years of life lost, the ratios of QALYs to YLL due to disease in those PBCs where outcome elasticities could not be estimated could not be used to inform estimates of the threshold or indicate how any total health effects in these other PBCs are likely to be ‘shared’ between life-year effects adjusted for quality and that part associated with ‘pure’ QoL effects (see Table 144). By applying the observed proportionate effects of changes in expenditure to measures of QALY burden of disease in these other PBCs the likely share of any effects on QALYs between avoidance of premature mortality and avoidance of disability more closely reflect the nature of these types of diseases (see Table 151). As expected, a much greater proportion of QALY effects are associated with QoL during the disease compared with the 11 PBCs where mortality-based outcome elasticities could be estimated. The share of effects in particular PBCs are also much more credible. For example, in PBC 5 (mental health disorders) the overwhelming share of QALY effects are associated with QoL itself and for others, such as PBC 12 (dental problems), PBC 9 (problems of hearing) and PBC 8 (problems of vision), almost all effects are associated with QoL rather than mortality and life-years. For this, and the other reasons discussed above, the analysis based on measures of QALY burden are regarded as the best estimate of a cost per QALY ratio that reflects a more complete picture of the likely health effects of changes in overall expenditure.
Summary of the cost per quality-adjusted life-year threshold
The results of the three sequential steps of analysis described in this appendix are summarised in Table 153. In From mortality to life-years we explored ways in which the estimated effects on mortality from the econometrics work in Appendix 2 might be better translated into life-year effects by overcoming some of the limitations of mortality data available at PCT level and taking account of counterfactual deaths. The results of this analysis were reported in Table 122 and are repeated in column (1) of Table 153. These results can be interpreted as cost per QALY thresholds conditional on the assumption that all life-years are lived in full health and the QoL with disease is zero (equivalent to death).
PBC grouping | (1) Chapter 4, From mortality to life-years analysis | (2) Chapter 4, Adjusting life-years for quality-of-life analysis | (3) Chapter 4, Including quality-of-life effects during disease analysis |
---|---|---|---|
QoL associated with life extension | 1 | Norm | |
QoL during disease | 0 | 0 | Based on burden |
Best estimate | |||
Effect of expenditure on mortality | 1 year | 1 year | 1 year |
YLL per death averted | ∼ 4.1 | ∼ 4.1 | ∼ 4.1 |
QALYs per death averted | ∼ 4.1 | ∼ 3.5 | ∼ 14.9 |
(1) All big four programmes | £8080 | £9631 | £3036 |
(2) 11 PBCs (with mortality) | £15,628 | £18,622 | £5128 |
(3) All 23 PBCs | £17,663 | £21,047 | £10,187 |
Lower bound | |||
Effect of expenditure on mortality | Remainder of disease duration | Remainder of disease duration | Remainder of disease duration |
YLL per death averted | ∼ 4.1 | ∼ 4.1 | ∼ 4.1 |
QALYs per death averted | ∼ 4.1 | ∼ 3.5 | ∼ 14.9 |
(4) All big four programmes | £3846 | £4252 | £674 |
(5) 11 PBCs (with mortality) | £6106 | £6852 | £860 |
(6) All 23 PBCs | £6901 | £7744 | £1843 |
Upper bound | |||
Effect of expenditure on mortality | 1 year | 1 year | 1 year |
YLL per death averted | 2 | 2 | 2 |
QALYs per death averted | ∼ 2 | ∼ 1.9 | ∼ 7.2 |
(7) All big four programmes | £16,432 | £17,456 | £6292 |
(8) 11 PBCs (with mortality) | £32,387 | £34,492 | £10,626 |
(9) All 23 PBCs | £36,604 | £38,983 | £21,111 |
In Adjusting life-years for quality-of-life we considered how the estimated life-year effects might be adjusted for the QoL in which they are likely to be lived, taking account of the gender and the age at which life-years are gained or lost (see Table 135). The results of this analysis are repeated in Table 153, column (2). Finally, in the current section, we explored ways to also take account of the likely effects of changes in expenditure on QoL during disease as well as the effects associated with mortality and life-years [see Table 153, column (3)]. These estimates provide our central estimate of a cost per QALY threshold, because they make best use of available information while the assumptions required, which on balance are likely conservative with respect to health effects, appear more reasonable than the other alternatives available. q
The estimate of £5128 per QALY [see Table 153, line (2)] is restricted to the effects of changes in expenditure in the 11 PBCs where outcome elasticities can be estimated. Although this might be regarded as more secure, these PBCs only account for a proportion of the change in overall expenditure [approximately 38%, column (6) in Table 108]. The threshold of £10,187 uses the estimated proportionate effects of expenditure on the QALY burden of disease in these PBCs as a surrogate for proportionate effects in the others (i.e. assuming that the effects that can be observed will be similar to those that cannot). As discussed in Using estimates of the quality-adjusted life-year burden of disease, there are reasons to suspect that this may underestimate health effects in these PBCs which have most influence on the overall threshold. As in previous sections, no health effects are assigned to PBC 23 (GMS) on the basis that any health effects of this expenditure would be recorded in the other PBCs. r Therefore, the best or central estimate of cost per QALY threshold is £10,187 [see Table 153, column (3), line (3)].
This estimate reflects changes in undiscounted QALYs associated with changes in expenditure. Although all the health effects of a change in expenditure are restricted to 1 year (so no discounting is necessary) some of the QALY effects of a change in mortality in that year will occur in future years, so in principle should be discounted. However, discounting these life-year effects, even at the higher rate of 3.5% recommended by NICE, only increases the cost per QALY threshold to £10,333 (Table 154).
PBC scenario | Best estimate (£) | |
---|---|---|
(1) Undiscounted | (2) Discounteda | |
(1) All big four programmes | 3036 | 3097 |
(2) 11 PBCs (with mortality) | 5128 | 5218 |
(3) All 23 PBCsb | 10,187 | 10,333 |
As in previous sections of this chapter, the upper and lower bounds for the cost per QALY thresholds in Table 153, column (3) are based on making the necessary assumptions about duration of health effects and how long a death might be averted optimistic (providing the lower bound for the threshold) or conservative (an upper bound for the threshold). The lower bound [see Table 153, lines (4)–(6)] is based on assuming that health effects are not restricted to 1 year but apply to the whole of the remaining disease duration of the population at risk in PBCs during 1 year. Although this combines optimistic assumptions, it is possible that at least some part of a change in expenditure may prevent disease so will have an impact on populations that are incident to PBCs in the future. Such effects are not captured in any of the estimates presented in this chapter so all are conservative with respect to this type of health effects of expenditure. The upper bound [Table 153, lines (7)–(9)] is based on the combination of assuming that health effects are restricted to 1 year for the population currently at risk and that any death averted is only averted for 2 years (see Summary of cost per life-year estimates).
Which programme budget categories matter most?
Which PBCs have the greatest influence on the overall threshold depends, to a large extent, on how a change in overall expenditure is allocated to the different PBCs [Table 155, column (1)], i.e. those that account for a greater share of the change in expenditure will tend to have the greater influence. s
However, the overall threshold also depends on the proportionate effect of a change in PBC expenditure on the QALY burden associated with the PBCt and the scale of the QALY burden (for the population at risk) associated with the type of diseases that make up each PBC. u These determine the cost per QALY associated with each PBC [see Table 155, column (4)]. The share, attributable to each PBC, of the total health effects of a change in overall expenditure [see Table 155, column (2)] is the combined effect of all of these. The proportionate impact on the overall cost per QALY threshold of a 10% change in PBC health effects gives an indication of how sensitive the overall threshold is to the estimate of health effects associated with each PBC [see Table 155, column (3)]
PBC | (1) % share of change in overall expenditure | (2) % share of total health effects (QALY) | (3) Elasticity of the thresholda | (4) PBC cost per QALY (£) | |
---|---|---|---|---|---|
2 | Cancer | 3.99 | 3.18 | 0.32 | 12,772 |
10 | Circulatory | 6.92 | 12.52 | 1.25 | 5631 |
11 | Respiratory | 4.64 | 42.12 | 4.22 | 1123 |
13 | Gastrointestinal | 3.43 | 5.89 | 0.59 | 5944 |
1 | Infectious diseases | 1.74 | 0.11 | 0.01 | 158,349 |
4 | Endocrine | 3.67 | 10.36 | 1.04 | 3613 |
7 | Neurological | 3.58 | 2.04 | 0.20 | 17,844 |
17 | Genitourinary | 6.61 | 0.05 | 0.01 | 1,320,516 |
16 | Trauma and injuries | 2.15 | 0.00 | 0.00 | N/A |
18 + 19 | Maternity and neonates | 1.67 | 0.02 | < 0.01 | 813,578 |
3 | Disorders of blood | 1.22 | 1.82 | 0.18 | 6814 |
5 | Mental health | 22.23 | 16.32 | 1.63 | 13,876 |
6 | Learning disability | 3.35 | 0.31 | 0.03 | 109,806 |
8 | Problems of vision | 2.63 | 0.84 | 0.08 | 31,858 |
9 | Problems of hearing | 0.70 | 1.75 | 0.18 | 4047 |
12 | Dental problems | 2.56 | 0.87 | 0.09 | 30,030 |
14 | Skin | 1.14 | 0.16 | 0.02 | 75,158 |
15 | Musculoskeletal | 1.65 | 1.51 | 0.15 | 11,129 |
20 | Poisoning and adverse events | 0.48 | 0.06 | 0.01 | 76,909 |
21 | Healthy individuals | 2.00 | 0.06 | 0.01 | 336,325 |
22 | Social care needs | 7.36 | 0.00 | 0.00 | N/A |
23 | Other | 16.28 | 0.00 | 0.00 | N/A |
Although the 11 PBCs where outcome elasticities could be estimated only account for 38.4% of the change in overall expenditure, they account for 76.3% of the overall health effects. Within this group some PBCs contribute more than others. For example, PBC 11 (respiratory) accounts for a greater share of total health effects and has a higher elasticity (4.22%) than PBC 10 (circulatory), even though it accounts for a greater part of a change in overall expenditure. The reason is that the cost per QALY associated with changes in expenditure in PBC 11 is lower than PBC 10 and much lower than the overall threshold (so generates more health effects for the same, or even smaller, change in expenditure). The elasticities in Table 155, column (3) are instructive (e.g. the elasticity for PBC 11 suggests that even if the health effects of a change in expenditure in this PBC were overestimated by 30% the overall threshold would only increase by 12.7% to £11,477). All other PBCs have much less influence in this respect. Nonetheless, PBC 10 is important compared with others as it does contribute a large share of total health effects and has one of the highest elasticities (1.25%).
The other 12 PBCs, where outcome elasticities could not be estimated, account for the greater part of a change in overall expenditure (61.6%) but only 23.7% of the overall health effects (i.e. the cost per QALYs associated with a change in expenditure in these PBCs are, in general, higher). Of course, we have not directly observed QoL effects in these PBCs but inferred them from the proportionate effects that we can observe. Insofar as investment and disinvestment opportunities in these PBCs might have been more valuable (offered greater improvement in QoL) than suggested by the implied PBC thresholds in Table 155, column (4), the overall QALY effects will tend to be underestimated and the overall cost per QALY threshold will be overestimated.
Programme budget category 5 (mental health disorders) accounts for a large proportion of the change in overall expenditure (22.23%), contributes most to the overall health effects (16.32%) and has the highest elasticity (1.63%) compared with these other PBCs. The cost per QALY associated with this PBC (£13,876) is based on an extrapolation of estimated proportionate effects to a population-based measure of QALY burden in this PBC, rather than observations of the direct impact of changes in expenditure on QoL in the types of diseases that make up the PBC. Evidence that is available suggests that the investment and disinvestment opportunities in this PBC may have been more valuable than this implied cost per QALY (see Addendum 3: characterisation of the investment and disinvestment decisions in mental health – depression and schizophrenia). A search for evidence about interventions in those ICD-10 codes that contribute most to the PBC (based on prevalence or the contribution to the variance in PBC costs), suggests that pharmacological, psychological and social interventions for depression are all more cost-effective (in general much less than £10,000 per QALY) than the overall threshold, and significantly more valuable than the implied QALY threshold for this PBC. Based on the contribution that each ICD-10 code makes to variance in HES costs across PCTs, it is schizophrenia that contributes most. v Although interventions that may have been invested or disinvested in schizophrenia are, in general, less cost-effective (in general less than £24,000 per QALY) than those available for depression, they do not appear any less valuable than the implied cost per QALY of this PBC in Table 155. w
How uncertain are the estimates?
There are a number of sources of uncertainty which may contribute to an assessment of how uncertain a central or best estimate of the cost per QALY threshold might be. There are three reasons why uncertainty in the estimate of the threshold might be of policy interest: (i) the uncertainty in the parameters that determine the threshold might influence the mean or expected value of the threshold if they have a non-linear relationship to the threshold or when they have a multilinear relationship but are correlated with each other; (ii) the consequences of over- or underestimating the threshold differ so the uncertainty may have an influence on the extent to which a policy threshold (a single value that can be compared to the ICER of a new technology) should differ from the mean or expected value of the central or best estimate; and (iii) in conjunction with other methods of analysis it can indicate the potential value of gathering more information to improve these estimates in the future. Such analysis, known as value of information analysis, has firm foundations in statistical decision theory and has been applied to health-care decisions. A form of these analyses could be applied in subsequent research, ideally capturing some of the other sources of uncertainty. More recently, it has been applied to the decisions faced by NICE when considering if there is sufficient evidence to support the approval of a new technology. 95 Of course, hypothesis testing and the traditional rules of inference associated with it, such as statistical significance, p-values and confidence intervals, have no relevance when making unavoidable decisions about policy relevant quantities based on information currently available and the best use thereof. 96
An assessment of parameter uncertainty
Two sets of parameters are critical to the threshold, the expenditure elasticities estimated for each of the 23 PBCs, and the outcome elasticities estimated for 11 of these. These parameters are estimated with uncertainty, indicated by the standard errors on the relevant coefficients in the econometric analysis detailed in Appendix 2. As these statistical models estimate coefficients using normality on the relevant scale, normal distributions can be assigned to each of these estimated coefficients, each with a mean and standard deviation based on the results of the econometric analysis. These distributions represent the uncertainty in the mean estimate of each of the parameters and can be propagated through the various calculations required to estimate the overall cost per QALY threshold (i.e. through the sequence of analysis detailed in Adjusting life-years for quality-of-life, Including quality-of-life effects during disease and Which programme budget categories matter most?) using Monte Carlo simulation which randomly samples from the assigned distributions. The use of Monte Carlo simulation in this context is in essence Bayesian, where the standard errors from the frequentist econometric analysis are used to assign normal prior distributions with means equal to the point estimates and a standard deviation equal to the estimated standard errors. This is equivalent to a fully Bayesian analysis with initially uninformative priors which are updated through the analysis of expenditure and mortality data.
The results of each random sample from the Monte Carlo simulation represent one possible realisation of the overall threshold, given the uncertainty in estimates of the mean parameter values that determine it. By repeatedly sampling, a distribution of potential values that the overall threshold might take can be revealed. The results of this simulation are illustrated in Figure 51 showing a histogram of threshold values, and in Figure 52 showing the cumulative probability density function for a cost per QALY threshold based only on the 11 PBCs with estimated outcome elasticities and for all 23 PBCs. It represents the probability (on the y-axis) that the threshold lies below a particular value.
FIGURE 51.
Distribution of the cost per QALY threshold (all 23 PBCs).

FIGURE 52.
Cumulative probability density function for the cost per QALY threshold.
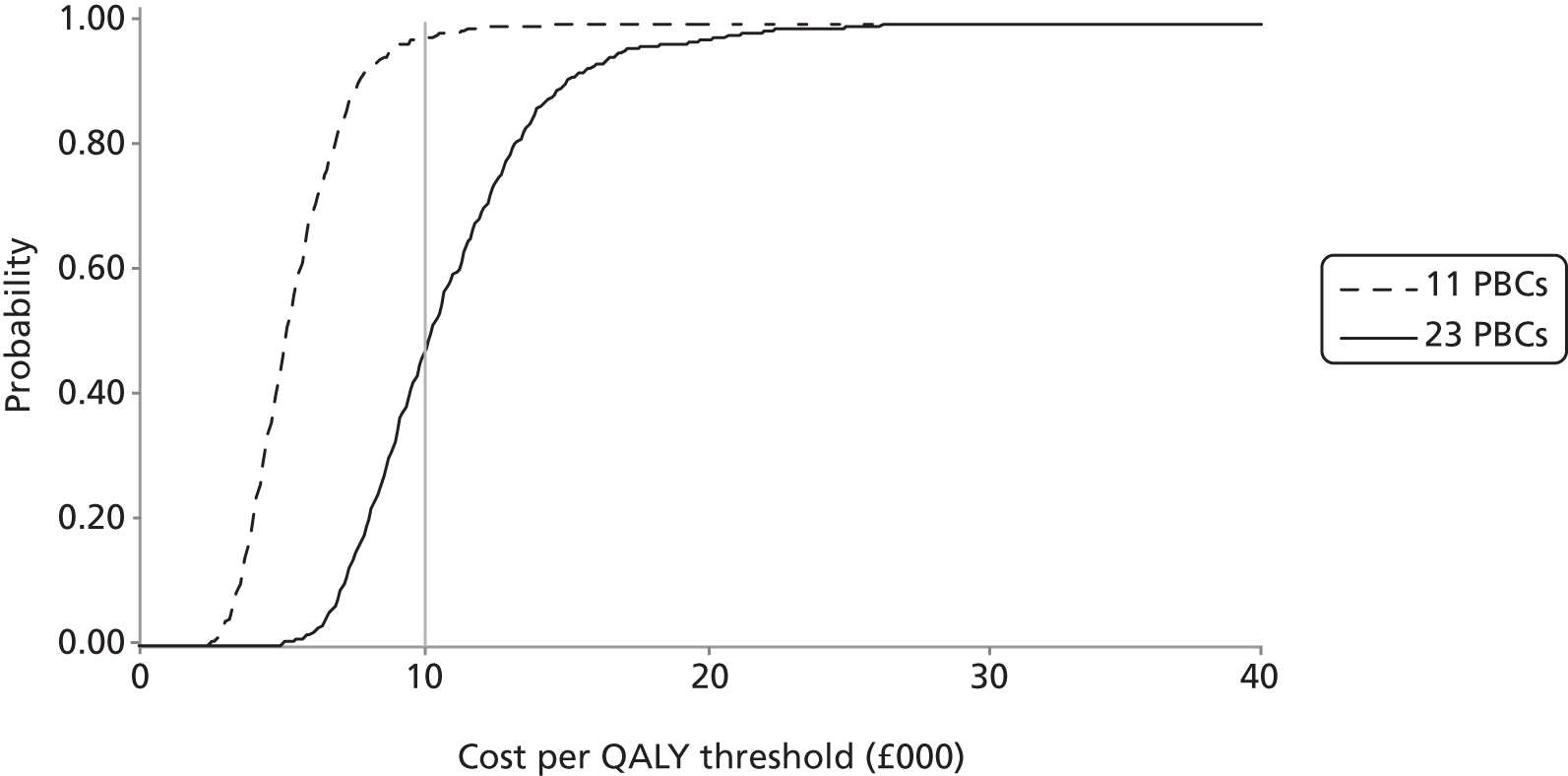
It has already been noted that restricting attention only to changes in expenditure in those 11 PBCs where an outcome elasticity can be estimated results in a much lower estimate of the threshold than considering all changes in expenditure across all PBCs. This lower estimate of £5144 per QALY is much less uncertain but these PBCs only account for 38% of a change in overall expenditure, so it is the higher estimate, for all 23 PBCs, that is of most relevance for policy (see Summary of the cost per quality-adjusted life-year threshold and Which programme budget categories matter most?). The fact that this estimate is more uncertain simply reflects the quality and quantity of data currently available. As useful analysis should endeavour to faithfully characterise uncertainty in policy relevant quantities, rather than select those quantities or questions for which precise estimates are possible, it is the more uncertain estimate for all 23 PBCs that should be of primary interest. The values that are used to generate Figure 52 are available in column (2) of Table 156. They indicate that the probability that the overall threshold is < £20,000 per QALY is 0.97 and the probability that is < £30,000 is 1.00.
Scenario | (1) 11 PBCs | (2) All 23 PBCs |
---|---|---|
Best estimate (deterministic) | £5128 | £10,187 |
Mean estimate (from the simulations) | £5114 | £10,092 |
Threshold value at the probability of (from the simulations) | ||
2.5% | £2956 | £6228 |
5.0% | £3237 | £6744 |
50.0% | £5250 | £10,378 |
95.0% | £8845 | £17,061 |
97.5% | £10,068 | £20,472 |
Probability (from the simulations) of the threshold being smaller than | ||
£3000 per QALY | 0.03 | 0.00 |
£4000 per QALY | 0.19 | 0.00 |
£5000 per QALY | 0.44 | 0.00 |
£6000 per QALY | 0.66 | 0.02 |
£7000 per QALY | 0.81 | 0.07 |
£8000 per QALY | 0.92 | 0.18 |
£9000 per QALY | 0.96 | 0.31 |
£10,000 per QALY | 0.97 | 0.45 |
£15,000 per QALY | 1.00 | 0.90 |
£20,000 per QALY | 1.00 | 0.97 |
£25,000 per QALY | 1.00 | 0.99 |
£30,000 per QALY | 1.00 | 1.00 |
Re-estimating the cost per quality-adjusted life-year threshold using 2008 expenditure data
The same methods of analysis can be applied to the econometric analysis of the 2008/9 expenditure and 2008–10 mortality data (see 2008/9 expenditure data and mortality data for 2008/9/10 in Chapter 3 and Analysis of programme budgeting expenditure for 2008/9 and mortality data for 2008/9/10 in Appendix 2). The differences between the 2006 analysis reported above and the analysis of expenditure in 2008 reported below (Table 157) are the (i) total PBC expenditure; (ii) estimated expenditure elasticities; (iii) estimated outcome elasticities; (iv) observed PBC deaths by age and gender; and (v) LE by age and gender. The other information about QoL norms (see Adjusting age-related quality-of-life for disease decrements), disease-related decrements (see Summary of the cost per quality-adjusted life-year threshold based only on mortality effects) and the information from GBD about incidence (by age and gender) and duration of disease (see Including quality-of-life effects during disease) remain unchanged between 2006 and 2008.
It should be noted that important improvements were made to the classification and collection of PBC expenditure data that took place after the 2006 data were collected. Therefore, the differences in threshold estimates for 2006 and 2008 partly reflect this (see Chapter 3, Comparing the cost of life-year estimates associated with different data sets and Comparing the cost of life-year estimates associated with different data sets in Appendix 2) so should not be overinterpreted. The results of the analysis of 2007 and 2008 expenditure are comparable in this respect, providing insights into how the threshold might change over time and with changes in the overall budget. For the purposes of this methodological research the 2008 expenditure and 2008–10 mortality data were the latest to be analysed.
PBC | (1) Total spend 2008/9 (£M) | Spend elasticities | Change in spend (£M) (% share) | (7) Outcome elasticitiesa | ||||
---|---|---|---|---|---|---|---|---|
(2) Unadjusted | (3) Analysis up to Chapter 4, Using ratios of quality-adjusted life-years to years of life lost (and Appendix 3) | (4) Analysis in Chapter 4, Using estimates of the quality-adjusted life-year burden of disease (and Appendix 3) | (5) Analysis up to Chapter 4, Using ratios of quality-adjusted life-years to years of life lost (and Appendix 3) | (6) Analysis in Chapter 4, Using estimates of the quality-adjusted life-year burden of disease (and Appendix 3) | ||||
2 | Cancer | 4843 | 0.525 | 0.525 | 0.724 | 25 (3.2) | 35 (4.5) | 0.307 |
10 | Circulatory | 6655 | 0.648 | 0.648 | 0.894 | 43 (5.5) | 59 (7.6) | 1.319 |
11 | Respiratory | 3994 | 0.652 | 0.652 | 0.900 | 26 (3.3) | 36 (4.6) | 1.808 |
13 | Gastrointestinal | 3989 | 0.456 | 0.456 | 0.629 | 18 (2.3) | 25 (3.2) | 1.364 |
All big four programmes | 19,481 | 113 (14.4) | 156 (19.8) | |||||
1 | Infectious diseases | 1201 | 1.545 | 1.545 | 2.132 | 19 (2.4) | 26 (3.3) | 0.504 |
4 | Endocrine | 2222 | 0.484 | 0.484 | 0.668 | 11 (1.4) | 15 (1.9) | 1.170 |
7 | Neurological | 3466 | 0.980 | 0.980 | 1.352 | 34 (4.3) | 47 (6) | 0.417 |
17 | Genitourinary | 3779 | 0.697 | 0.697 | 0.962 | 26 (3.4) | 36 (4.6) | 1.615 |
16 | Trauma and injuries | 3255 | 1.344 | 1.344 | 1.854 | 44 (5.6) | 60 (7.7) | – |
18 + 19 | Maternity and neonates | 3978 | 0.975 | 0.975 | 1.345 | 39 (4.9) | 54 (6.8) | 0.125 |
First 11 PBCs | 37,382 | 285 (36.3) | 393 (50.1) | |||||
3 | Disorders of blood | 998 | 1.171 | 2.291 | 1.616 | 23 (2.9) | 16 (2.1) | – |
5 | Mental health | 9794 | 1.036 | 2.027 | 1.429 | 198 (25.3) | 140 (17.9) | – |
6 | Learning disability | 2874 | 0.205 | 0.401 | 0.283 | 12 (1.5) | 8 (1) | – |
8 | Vision | 1688 | 0.654 | 1.279 | 0.902 | 22 (2.8) | 15 (1.9) | – |
9 | Hearing | 417 | 1.191 | 2.330 | 1.643 | 10 (1.2) | 7 (0.9) | – |
12 | Dental | 3198 | 0.513 | 1.003 | 0.708 | 32 (4.1) | 23 (2.9) | – |
14 | Skin | 1657 | 0.674 | 1.318 | 0.930 | 22 (2.8) | 15 (2) | – |
15 | Musculoskeletal | 4081 | 0.505 | 0.988 | 0.697 | 40 (5.1) | 28 (3.6) | – |
20 | Poisoning and adverse events | 938 | 0.562 | 1.099 | 0.775 | 10 (1.3) | 7 (0.9) | – |
21 | Healthy individuals | 1831 | 1.097 | 2.146 | 1.514 | 39 (5) | 28 (3.5) | – |
22 | Social care needs | 1874 | 0.911 | 1.782 | 1.257 | 33 (4.3) | 24 (3) | – |
23 | Other | 11,666 | 0.494 | 0.494 | 0.682 | 58 (7.4) | 80 (10.1) | – |
All 23 PBCs | 78,398 | 784 (100) | 784 (100) |
From mortality to life-years
In this section we summarise the calculation of net YLL, which take account of the fact that some of the observed deaths would have occurred anyway (had the same population not been at risk in the particular PBC) when estimating YLL (unobserved counterfactual deaths). In summary, to obtain net YLL, all observed deaths – both those that occur below and those that occur above LE (Table 158) – are taken into account. Those deaths occurring below LE generate YLL and those that occur at ages above LE generate YLG. By subtracting YLG from YLL to generate net YLL we take account of the fact that not all deaths below LE are excess deaths but some deaths above LE are.
PBC | 2008 | 2009 | 2010 | (7) Annual deaths < LE | (8) Annual deaths > LE | ||||
---|---|---|---|---|---|---|---|---|---|
(1) < LE | (2) > LE | (3) < LE | (4) > LE | (5) < LE | (6) > LE | ||||
1 | Infectious diseases | 3406 | 2586 | 3044 | 2190 | 2667 | 1894 | 3039 | 2223 |
2 | Cancer | 94,873 | 37,029 | 94,276 | 37,151 | 94,309 | 38,198 | 94,486 | 37,459 |
4 | Endocrine | 4033 | 2877 | 3834 | 2826 | 3816 | 2902 | 3894 | 2868 |
7 | Neurological | 9638 | 6859 | 9445 | 6939 | 9951 | 7480 | 9678 | 7093 |
10 | Circulatory | 80,894 | 76,292 | 76,048 | 73,342 | 74,035 | 73,719 | 76,992 | 74,451 |
11 | Respiratory | 32,083 | 35,180 | 29,912 | 33,304 | 29,691 | 33,176 | 30,562 | 33,887 |
13 | Gastrointestinal | 15,945 | 8259 | 15,361 | 8161 | 15,595 | 8372 | 15,633 | 8264 |
17 | Genitourinary | 4471 | 6667 | 4378 | 6900 | 4453 | 7166 | 4434 | 6911 |
18 + 19 | Maternity and neonates | 267 | 0 | 281 | 1 | 247 | 0 | 265 | 0 |
The estimates of net YLL calculated considering estimates of the LE for each PBC are detailed in Table 159.
PBC | (1) LE of males | (2) LE of females | Average 2006–8 | (7) Net YLL | ||||
---|---|---|---|---|---|---|---|---|
Deaths | (5) YLL | (6) YLG | ||||||
(3) < LE | (4) > LE | |||||||
1 | Infectious diseases | 79.6 | 83.6 | 2919 | 2344 | 53,926 | 15,132 | 38,794 |
2 | Cancer | 83.0 | 84.7 | 100,487 | 31,459 | 1,456,255 | 134,089 | 1,322,166 |
4 | Endocrine | 81.0 | 84.7 | 3945 | 2818 | 65,800 | 15,983 | 49,817 |
7 | Neurological | 79.6 | 83.3 | 9112 | 7659 | 137,791 | 47,722 | 90,069 |
10 | Circulatory | 83.0 | 86.5 | 89,434 | 62,009 | 1,049,459 | 278,421 | 771,038 |
11 | Respiratory | 80.3 | 84.0 | 29,828 | 34,621 | 306,838 | 229,403 | 77,434 |
13 | Gastrointestinal | 80.6 | 84.5 | 15,612 | 8286 | 271,395 | 46,141 | 225,254 |
17 | Genitourinary | 83.5 | 85.6 | 5058 | 6287 | 49,036 | 32,528 | 16,508 |
18 + 19 | Maternity and neonates | 78.7 | 83.1 | 265 | 0 | 19,783 | 1 | 19,781 |
The impact on the cost per life-year threshold is summarised in column (2) of Table 160, and a detailed breakdown is shown in Table 161.
PBC scenario | (1) 2006 | (2) 2008 |
---|---|---|
All big four programmes | £8080 | £10,220 |
11 PBCs (with mortality) | £15,628 | £23,360 |
All 23 PBCs (zero health effects for remaining 12 PBCs) | £57,497 | £64,275 |
All 23 PBCs (non-zero health effects for remaining 12 PBCs, except GMS)a | £17,663 | £25,214 |
PBC | (1) Change in spend (£M) | Using LE of the PBC population | |||
---|---|---|---|---|---|
(2) Net YLL | (3) Change in Net YLL | (4) Cost per YLG (£) | |||
2 | Cancer | 25 | 1,322,166 | 2131 | 11,931 |
10 | Circulatory problems | 43 | 771,038 | 6590 | 6544 |
11 | Respiratory problems | 26 | 77,434 | 913 | 28,528 |
13 | Gastrointestinal problems | 18 | 225,254 | 1401 | 12,983 |
All big four programmes | 10,220 | ||||
1 | Infectious diseases | 19 | 38,794 | 302 | 61,425 |
4 | Endocrine problems | 11 | 49,817 | 282 | 38,122 |
7 | Neurological problems | 34 | 90,069 | 368 | 92,282 |
17 | Genitourinary problems | 26 | 16,508 | 186 | 141,746 |
16 | Trauma and injuries | 44 | N/A | 0 | N/A |
18 + 19 | Maternity and neonates | 39 | 19,781 | 24 | 1,608,817 |
First 11 PBCs | 23,360 | ||||
3 | Disorders of blood | 23 | 979 | 23,360 | |
5 | Mental health disorders | 198 | 8496 | 23,360 | |
6 | Learning disability | 12 | 493 | 23,360 | |
8 | Problems of vision | 22 | 924 | 23,360 | |
9 | Problems of hearing | 10 | 416 | 23,360 | |
12 | Dental problems | 32 | 1374 | 23,360 | |
14 | Skin | 22 | 935 | 23,360 | |
15 | Musculoskeletal system | 40 | 1726 | 23,360 | |
20 | Poisoning and adverse events | 10 | 441 | 23,360 | |
21 | Healthy individuals | 39 | 1682 | 23,360 | |
22 | Social care needs | 33 | 1430 | 23,360 | |
23 | Other | 58 | 0 | N/A | |
All 23 PBCs | 25,214 |
The estimates of net YLL imply a number of excess deaths required to generate them in each PBC. The implied excess deaths associated with net YLL are reported in Table 162.
PBC | (1) Net YLL | (2) YLL per observed death | (3) Excess deaths | (4) Total deaths | (5) % excess deaths | |
---|---|---|---|---|---|---|
1 | Infectious diseases | 38,794 | 13.4 | 2934 | 5262 | 56 |
2 | Cancer | 1,322,166 | 14.1 | 93,917 | 131,945 | 71 |
4 | Endocrine | 49,817 | 13.7 | 3663 | 6762 | 54 |
7 | Neurological | 90,069 | 13.6 | 6642 | 16,771 | 40 |
10 | Circulatory | 771,038 | 10.5 | 74,217 | 151,443 | 49 |
11 | Respiratory | 77,434 | 9.2 | 8432 | 64,449 | 13 |
13 | Gastrointestinal | 225,254 | 15.2 | 15,049 | 23,897 | 63 |
17 | Genitourinary | 16,508 | 8.3 | 1978 | 11,345 | 17 |
18 + 19 | Maternity and neonates | 19,781 | 74.1 | 265 | 265 | 100 |
The cost per excess death and the cost per PBC death averted are reported in Table 163, and a detailed breakdown of changes in spend and excess or total deaths across PBCs is shown in Table 164. The cost per PBC death averted is, of course, significantly lower than the cost per excess death as excess deaths are only a proportion of total deaths (see Table 163).
PBC scenario | 2006–8 | 2008–10 | ||
---|---|---|---|---|
(1) Cost per excess death averted (£) | (2) Cost per PBC death averted (£) | (3) Cost per excess death averted (£) | (4) Cost per PBC death averted (£) | |
All big four programmes | 91,129 | 32,864 | 115,234 | 46,692 |
11 PBCs (with mortality) | 177,691 | 64,774 | 265,784 | 105,872 |
All 23 PBCs (zero health effects for remaining 12 PBCs) | 653,744 | 238,310 | 731,301 | 291,305 |
All 23 PBCs (non-zero health effects for remaining 12 PBCs, except GMS)a | 200,828 | 73,208 | 286,872 | 114,272 |
PBC | (1) Change in spend (£M) | PBC deaths | Excess deaths | |||||
---|---|---|---|---|---|---|---|---|
(2) Total PBC deaths | (3) Change in PBC deaths | (4) Cost per PBC death averted (£) | (5) Excess deaths | (6) Change in excess deaths | (7) Cost per excess death averted (£) | |||
2 | Cancer | 25 | 131,945 | 212.66 | 119,559 | 93,917 | 151.37 | 167,969 |
10 | Circulatory problems | 43 | 151,443 | 1294.40 | 33,316 | 74,217 | 634.34 | 67,983 |
11 | Respiratory problems | 26 | 64,449 | 759.74 | 34,276 | 8432 | 99.40 | 261,992 |
13 | Gastrointestinal problems | 18 | 23,897 | 148.64 | 122,379 | 15,049 | 93.60 | 194,332 |
All big four programmes | 46,692 | 0 | 115,234 | |||||
1 | Infectious diseases | 19 | 5262 | 40.97 | 452,858 | 2934 | 22.84 | 812,249 |
4 | Endocrine problems | 11 | 6762 | 38.29 | 280,856 | 3663 | 20.74 | 518,533 |
7 | Neurological problems | 34 | 16,771 | 68.54 | 495,603 | 6642 | 27.14 | 1,251,391 |
17 | Genitourinary problems | 26 | 11,345 | 127.71 | 206,253 | 1978 | 22.27 | 1,182,744 |
16 | Trauma and injuries | 44 | N/A | 0 | N/A | N/A | 0 | N/A |
18 + 19 | Maternity and neonates | 39 | 265 | 0.32 | 120,090,566 | 265 | 0.32 | 120,090,566 |
First 11 PBCs | 105,872 | 265,784 | ||||||
3 | Disorders of blood | 23 | 215.92 | 105,872 | 86.01 | 265,784 | ||
5 | Mental health disorders | 198 | 1874.69 | 105,872 | 746.76 | 265,784 | ||
6 | Learning disability | 12 | 108.86 | 105,872 | 43.36 | 265,784 | ||
8 | Problems of vision | 22 | 203.97 | 105,872 | 81.25 | 265,784 | ||
9 | Problems of hearing | 10 | 91.76 | 105,872 | 36.55 | 265,784 | ||
12 | Dental problems | 32 | 303.11 | 105,872 | 120.74 | 265,784 | ||
14 | Skin | 22 | 206.34 | 105,872 | 82.19 | 265,784 | ||
15 | Musculoskeletal system | 40 | 380.77 | 105,872 | 151.68 | 265,784 | ||
20 | Poisoning and adverse events | 10 | 97.40 | 105,872 | 38.80 | 265,784 | ||
21 | Healthy individuals | 39 | 371.11 | 105,872 | 147.83 | 265,784 | ||
22 | Social care needs | 33 | 315.43 | 105,872 | 125.65 | 265,784 | ||
23 | Other | 58 | 0 | N/A | 0 | N/A | ||
All 23 PBCs | 114,272 | 286,872 |
The number of YLGs associated with each excess death averted are reported for each PBC in Table 165 [see column (1)] and range from 74.6 years for PBC 18 + 19 to 8.3 years for PBC 17. On average, across all 11 PBCs each excess death averted is associated with 11.4 YLGs. The life-years associated with each observed death are reported for each PBC in Table 165, column (2) and range from 74.6 years in PBC 18 + 19 to 1.2 for PBC 11. On average, across all 11 PBCs each PBC death averted is associated with 4.5 YLGs.
PBC | (1) Implied YLL per excess death averted | (2) Implied YLL per PBC death averted | |
---|---|---|---|
2 | Cancer | 14.1 | 10.0 |
10 | Circulatory problems | 10.4 | 5.1 |
11 | Respiratory problems | 9.2 | 1.2 |
13 | Gastrointestinal problems | 15.0 | 9.4 |
All big four programmes | 11.3 | 4.6 | |
1 | Infectious diseases | 13.2 | 7.4 |
4 | Endocrine problems | 13.6 | 7.4 |
7 | Neurological problems | 13.6 | 5.4 |
17 | Genitourinary problems | 8.3 | 1.5 |
16 | Trauma and injuries | N/A | N/A |
18 + 19 | Maternity and neonates | 74.6 | 74.6 |
First 11 PBCs | 11.4 | 4.5 |
Summary of cost per life-year estimates
The cost per life-year threshold in lines (1)–(4) in Table 166 are regarded as the central or best estimates given the evidence available and the credibility of the alternative assumption that could be made. As explained in the Introduction, these are based on the conservative assumption that any health effects of changes in expenditure are restricted to 1 year, which, to some extent, may be offset by the more optimistic assumption that any death averted returns the individual to the mortality risk faced by the general population, matched for age and gender. See How uncertain are the estimates? for guidance in the interpretation of the upper and lower bound estimates.
PBC grouping | (1) 2006–8 | (2) 2008–10 |
---|---|---|
Best estimate | ||
Effect of expenditure on mortality | 1 year | 1 year |
YLL per PBC death averted | ∼ 4.1a | ∼ 4.5a |
(1) All big four programmes | £8080 | £10,220 |
(2) 11 PBCs (with mortality) | £15,628 | £23,360 |
(3) All 23 PBCs (zero health effects for remaining 12 PBCs) | £57,497 | £64,275 |
(4) All 23 PBCs (non-zero health effects for remaining 12 PBCs, except GMS)b | £17,663 | £25,214 |
Lower bound | ||
Effect of expenditure on mortality | Remainder of disease | Remainder of disease |
YLL per PBC death averted | ∼ 4.1a | ∼ 4.5a |
(5) All big four programmes | £3846 | £5083 |
(6) 11 PBCs (with mortality) | £6106 | £8579 |
(7) All 23 PBCs (zero health effects for remaining 12 PBCs) | £22,463 | £23,605 |
(8) All 23 PBCs (non-zero health effects for remaining 12 PBCs, except GMS)b | £6901 | £9260 |
Upper bound | ||
Effect of expenditure on mortality | 1 year | 1 year |
YLL per PBC death averted | 2 | 2 |
(9) All big four programmes | £16,432 | £23,346 |
(10) 11 PBCs (with mortality) | £32,387 | £52,936 |
(11) All 23 PBCs (zero health effects for remaining 12 PBCs) | £119,155 | £145,653 |
(12) All 23 PBCs (non-zero health effects for remaining 12 PBCs, except GMS)b | £36,604 | £57,136 |
Adjusting life-years for quality-of-life
The central or best estimates of the cost per life-year threshold, presented in Table 166 [see lines (2) and (4)] take no account of the health-related QoL in which years of life, expected to be gained or lost through changes in expenditure, are likely to be lived. In this section we examine the ways in which the life-years can be adjusted for quality, taking account of information that is available about (i) how QoL differs by age and gender, and (ii) how the quality-of-life-years associated with mortality changes might be affected by the types of diseases that make up each PBC.
Quality of life based on the general population
Quality-of-life norms (see Figure 49) can be applied to the YLL associated with all observed deaths in each PBC, taking account of gender and age at death. The results are reported in column (4)–(6) of Table 167.
PBC | Unadjusted life-years | QALYs | |||||
---|---|---|---|---|---|---|---|
(1) YLL | (2) YLG | (3) Net YLL | (4) YLG | (5) YLL | (6) Net YLG | ||
1 | Infectious diseases | 53,926 | 15,132 | 38,794 | 43,703 | 10,187 | 33,516 |
2 | Cancer | 1,456,255 | 134,089 | 1,322,166 | 1,129,191 | 89,231 | 1,039,960 |
4 | Endocrine | 65,800 | 15,983 | 49,817 | 52,465 | 10,598 | 41,867 |
7 | Neurological | 137,791 | 47,722 | 90,069 | 110,532 | 32,262 | 78,270 |
10 | Circulatory | 1,049,459 | 278,421 | 771,038 | 807,893 | 183,796 | 624,097 |
11 | Respiratory | 306,838 | 229,403 | 77,434 | 237,981 | 154,300 | 83,680 |
13 | Gastrointestinal | 271,395 | 46,141 | 225,254 | 214,756 | 30,811 | 183,945 |
17 | Genitourinary | 49,036 | 32,528 | 16,508 | 37,178 | 21,190 | 15,989 |
18 + 19 | Maternity and neonates | 19,783 | 1 | 19,781 | 17,176 | 1 | 17,175 |
The implications of the quality adjustment to a cost per QALY threshold that only accounts for the direct health effects of mortality are summarised in Table 168, and detailed in Table 169.
PBC scenario | 2006–8 | 2008–10 | ||
---|---|---|---|---|
(1) Cost per life-year threshold (£) | (2) Cost per QALY threshold (£) population norms | (3) Cost per life-year threshold (£) | (4) Cost per QALY threshold (£) population norms | |
All big four programmes | 8080 | 9631 | 10,220 | 12,338 |
11 PBCs | 15,628 | 18,622 | 23,360 | 28,045 |
All 23 PBCs | 17,663 | 21,047 | 25,214 | 30,270 |
PBC | (1) Change in spend (£M) | YLL using LE of PBC | ||
---|---|---|---|---|
(2) Change in QALY | (3) Cost per QALY gained (£) | |||
2 | Cancer | 25 | 1676 | 15,169 |
10 | Circulatory problems | 43 | 5334 | 8084 |
11 | Respiratory problems | 26 | 986 | 26,399 |
13 | Gastrointestinal problems | 18 | 1144 | 15,899 |
All big four programmes | 12,338 | |||
1 | Infectious diseases | 19 | 261 | 71,098 |
4 | Endocrine problems | 11 | 237 | 45,361 |
7 | Neurological problems | 34 | 320 | 106,193 |
17 | Genitourinary problems | 26 | 180 | 146,347 |
16 | Trauma and injuries | 44 | 0 | N/A |
18 + 19 | Maternity and neonates | 39 | 21 | 1,852,926 |
First 11 PBCs | 28,045 | |||
3 | Disorders of blood | 23 | 815 | 28,045 |
5 | Mental health disorders | 198 | 7077 | 28,045 |
6 | Learning disability | 12 | 411 | 28,045 |
8 | Problems of vision | 22 | 770 | 28,045 |
9 | Problems of hearing | 10 | 346 | 28,045 |
12 | Dental problems | 32 | 1144 | 28,045 |
14 | Skin | 22 | 779 | 28,045 |
15 | Musculoskeletal system | 40 | 1437 | 28,045 |
20 | Poisoning and adverse effects | 10 | 368 | 28,045 |
21 | Healthy individuals | 39 | 1401 | 28,045 |
22 | Social care needs | 33 | 1191 | 28,045 |
23 | Other | 58 | 0 | N/A |
All 23 PBCs | 30,270 |
Table 170 depicts the judgements over life-years, QoL weights and total QALYs implicit in calculations of the threshold cost per QALY in Table 166.
PBC | (1) Implied YLL per excess death averted | (2) Implied YLL per PBC death averted | (3) Implied QALYs gained per excess death averted | (4) Implied QALYs gained per PBC death averted | |
---|---|---|---|---|---|
2 | Cancer | 14.08 | 10.02 | 11.07 | 7.88 |
10 | Circulatory | 10.39 | 5.09 | 8.41 | 4.12 |
11 | Respiratory | 9.18 | 1.20 | 9.92 | 1.30 |
13 | Gastrointestinal | 14.97 | 9.43 | 12.22 | 7.70 |
All big four programmes | 11.28 | 4.57 | 9.34 | 3.78 | |
1 | Infectious diseases | 13.22 | 7.37 | 11.42 | 6.37 |
4 | Endocrine | 13.60 | 7.37 | 11.43 | 6.19 |
7 | Neurological | 13.56 | 5.37 | 11.78 | 4.67 |
17 | Genitourinary | 8.34 | 1.46 | 8.08 | 1.41 |
16 | Trauma and injuries | N/A | N/A | N/A | N/A |
18 + 19 | Maternity and neonates | 74.65 | 74.65 | 64.81 | 64.81 |
First 11 PBCs | 11.38 | 4.53 | 9.48 | 3.78 |
Adjusting age-related quality-of-life for disease decrements
By using age-related QoL disease decrements (exemplified in Figure 50) YLL can be adjusted for QoL of disease. The results are reported in columns (4)–(6) of Table 171.
PBC | Unadjusted life-years | QALYs | |||||
---|---|---|---|---|---|---|---|
(1) YLL | (2) YLG | (3) Net YLL | (4) YLG | (5) YLL | (6) Net YLG | ||
1 | Infectious diseases | 53,926 | 15,132 | 38,794 | 34,108 | 7524 | 26,584 |
2 | Cancer | 1,456,255 | 134,089 | 1,322,166 | 943,650 | 72,197 | 871,452 |
4 | Endocrine | 65,800 | 15,983 | 49,817 | 43,063 | 8334 | 34,729 |
7 | Neurological | 137,791 | 47,722 | 90,069 | 69,520 | 18,084 | 51,436 |
10 | Circulatory | 1,049,459 | 278,421 | 771,038 | 625,150 | 135,622 | 489,527 |
11 | Respiratory | 306,838 | 229,403 | 77,434 | 173,953 | 106,200 | 67,754 |
13 | Gastrointestinal | 271,395 | 46,141 | 225,254 | 162,441 | 22,060 | 140,380 |
17 | Genitourinary | 49,036 | 32,528 | 16,508 | 30,770 | 16,949 | 13,820 |
18 + 19 | Maternity and neonates | 19,783 | 1 | 19,781 | 16,100 | 1 | 16,099 |
The implications of the quality adjustment to a cost per QALY threshold that only accounts for the direct health effects of mortality are summarised in Table 172, and detailed in Table 173.
PBC scenario | 2006 | 2008 | ||
---|---|---|---|---|
(1) Cost per life-year threshold (£) | (2) Cost per QALY threshold (£) disease-related disutility | (3) Cost per life-year threshold (£) | (4) Cost per QALY threshold (£) disease-related disutility | |
All big four programmes | 8080 | 12,109 | 10,220 | 15,534 |
11 PBCs (with mortality) | 15,628 | 23,395 | 23,360 | 35,397 |
All 23 PBCs (zero health effects for remaining 12 PBCs) | 57,497 | 86,072 | 64,275 | 97,395 |
All 23 PBCs (non-zero health effects for remaining 12 PBCs, except GMS)a | 17,663 | 26,441 | 25,214 | 38,206 |
PBC | (1) Change in spend (£M) | YLL using LE of PBC | ||
---|---|---|---|---|
(2) Change in QALY | (3) Cost per QALY gained (£) | |||
2 | Cancer | 25 | 1405 | 18,102 |
10 | Circulatory problems | 43 | 4184 | 10,307 |
11 | Respiratory problems | 26 | 799 | 32,604 |
13 | Gastrointestinal problems | 18 | 873 | 20,833 |
All big four programmes | 15,534 | |||
1 | Infectious diseases | 19 | 207 | 89,638 |
4 | Endocrine problems | 11 | 197 | 54,685 |
7 | Neurological problems | 34 | 210 | 161,594 |
17 | Genitourinary problems | 26 | 156 | 169,315 |
16 | Trauma and injuries | 44 | 0 | N/A |
18 + 19 | Maternity and neonates | 39 | 20 | 1,976,769 |
First 11 PBCs | 35,397 | |||
3 | Disorders of blood | 23 | 646 | 35,397 |
5 | Mental health disorders | 198 | 5607 | 35,397 |
6 | Learning disability | 12 | 326 | 35,397 |
8 | Problems of vision | 22 | 610 | 35,397 |
9 | Problems of hearing | 10 | 274 | 35,397 |
12 | Dental problems | 32 | 907 | 35,397 |
14 | Skin | 22 | 617 | 35,397 |
15 | Musculoskeletal system | 40 | 1139 | 35,397 |
20 | Poisoning and adverse effects | 10 | 291 | 35,397 |
21 | Healthy individuals | 39 | 1110 | 35,397 |
22 | Social care needs | 33 | 943 | 35,397 |
23 | Other | 58 | 0 | N/A |
All 23 PBCs | 38,206 |
Table 174 depicts the judgements over life-years, QoL weights and total QALYs implicit in calculations of the threshold cost per QALY in Table 172.
PBC | (1) Implied YLL per excess death averted | (2) Implied YLL per PBC death averted | (3) Implied QALYs gained per excess death averted | (4) Implied QALYs gained per PBC death averted | |
---|---|---|---|---|---|
2 | Cancer | 14.08 | 10.02 | 9.28 | 6.60 |
10 | Circulatory | 10.39 | 5.09 | 6.60 | 3.23 |
11 | Respiratory | 9.18 | 1.20 | 8.04 | 1.05 |
13 | Gastrointestinal | 14.97 | 9.43 | 9.33 | 5.87 |
All big four programmes | 11.28 | 1.80 | 7.42 | 3.01 | |
1 | Infectious diseases | 13.22 | 7.37 | 9.06 | 5.05 |
4 | Endocrine | 13.60 | 7.37 | 9.48 | 5.14 |
7 | Neurological | 13.56 | 5.37 | 7.74 | 3.07 |
17 | Genitourinary | 8.34 | 1.46 | 6.99 | 1.22 |
16 | Trauma and injuries | N/A | N/A | N/A | N/A |
18 + 19 | Maternity and neonates | 74.65 | 74.65 | 60.75 | 60.75 |
First 11 PBCs | 11.38 | 4.53 | 6.77 | 2.99 |
Summary of the cost per quality-adjusted life-year threshold based only on mortality effects
The analysis to this point is summarised in Table 175. The three estimates of a cost per QALY threshold are based on assuming that each YLG is either lived in full health [see Table 175, column (1)]; lived in a QoL that reflects age and gender norms of the general population [see Table 175, column (2)]; or lived in a QoL that reflects the original disease state [see Table 175, column (3)].
PBC scenario | (1) QoL score = 1 | (2) QoL norm | (3) QoL diseased |
---|---|---|---|
Best estimate | |||
Effect of expenditure on mortality | 1 year | 1 year | 1 year |
YLL per death averteda | ∼ 4.5 | ∼ 4.5 | ∼ 4.5 |
QALYs per death averteda | ∼ 4.5 | ∼ 3.8 | ∼ 3.0 |
(1) All big four programmes | £10,220 | £12,338 | £15,534 |
(2) 11 PBCs | £23,360 | £28,045 | £35,397 |
(3) All 23 PBCs | £25,214 | £30,270 | £38,206 |
Lower bound | |||
Effect of expenditure on mortality | Remainder of disease | Remainder of disease | Remainder of disease |
YLL per death averteda | ∼ 4.5 | ∼ 4.5 | ∼ 4.5 |
QALYs per death averteda | ∼ 4.5 | ∼ 3.8 | ∼ 3.0 |
(4) All big four programmes | £5083 | £5811 | £7305 |
(5) 11 PBCs | £8579 | £9861 | £12,720 |
(6) All 23 PBCs | £9260 | £10,644 | £13,729 |
Upper bound | |||
Effect of expenditure on mortality | 1 year | 1 year | 1 year |
YLL per death averteda | 2 | 2 | 2 |
QALYs per death averteda | ∼ 2 | ∼ 1.8 | ∼ 1.4 |
(7) All big four programmes | £23,346 | £26,138 | £32,797 |
(8) 11 PBCs | £52,936 | £59,151 | £74,183 |
(9) All 23 PBCs | £57,136 | £63,844 | £80,069 |
Including quality-of-life effects during disease
In this section we explore how estimates of effects of expenditure that can be observed (i.e. on mortality) can be used to infer the likely effects on what cannot be directly observed (QoL), rather than making extreme assumptions that are not credible (e.g. assuming that changes in expenditure will have no effects on QoL outcomes). In Using estimates of the quality-adjusted life-year burden of disease, we described the use of ratios of QALYs lost to life-years lost due to disease and explored how the use of the QALY burden of disease is preferable to inform estimates of the threshold. Here we only present the results for the QALY burden of disease approach.
In Table 176, deaths and YLL from ONS (2008–10 mortality data) are compared with those from GBD. The factors used to adjust GBD information are reported in columns (4) and (7).
PBC | (1) Excess deaths ONS | Deaths | YLL | |||||
---|---|---|---|---|---|---|---|---|
(2) All deaths ONS | (3) All deaths GBD | (4) Adjustment factor (deaths) | (5) Net estimates ONS | (6) Total YLLGBD | (7) Adjustment factor (YLL) | |||
1 | Infectious diseases | 2934 | 5262 | 1408 | 3.737 | 38,794 | 25,142 | 1.543 |
2 | Cancer | 93,917 | 131,946 | 140,124 | 0.942 | 1,322,166 | 1,932,637 | 0.684 |
4 | Endocrine | 3663 | 6762 | 7509 | 0.901 | 49,817 | 95,401 | 0.522 |
7 | Neurological | 6642 | 16,771 | 12,854 | 1.305 | 90,069 | 164,796 | 0.547 |
10 | Circulatory | 74,217 | 151,443 | 178,454 | 0.849 | 771,038 | 1,750,608 | 0.440 |
11 | Respiratory | 8432 | 64,449 | 67,441 | 0.956 | 77,434 | 594,529 | 0.130 |
13 | Gastrointestinal | 15,049 | 23,897 | 28,329 | 0.844 | 225,254 | 396,829 | 0.568 |
17 | Genitourinary | 1978 | 11,345 | 8606 | 1.318 | 16,508 | 77,338 | 0.213 |
18 + 19 | Maternity and neonates | 265 | 265 | 2211 | 0.120 | 19,781 | 149,868 | 0.132 |
Total | 207,097 | 412,140 | 446,936 | 0.92 | 2,610,861 | 5,187,148 | 0.500 |
The threshold cost per QALY based on burden associated with 1 year of disease derived from GBD are summarised in Table 177, and detailed in Table 178.
PBC scenario | (1) 2006 | (2) 2008 |
---|---|---|
All big four programmes | £3036 | £4872 |
11 PBCs (with mortality) | £5128 | £8308 |
All 23 PBCs | £10,187 | £12,936 |
PBC | (1) Change in spend (£M) | QALY burden (HODaR and MEPS) | ||
---|---|---|---|---|
(2) Change in QALY | (3) Cost per QALY gained (£) | |||
2 | Cancer | 35 | 2064 | 16997 |
10 | Circulatory problems | 59 | 8453 | 7038 |
11 | Respiratory problems | 36 | 17,981 | 1998 |
13 | Gastrointestinal problems | 25 | 3441 | 7293 |
All big four programmes | 4872 | |||
1 | Infectious diseases | 26 | 1229 | 20,829 |
4 | Endocrine problems | 15 | 4749 | 3124 |
7 | Neurological problems | 47 | 8551 | 5480 |
17 | Genitourinary problems | 36 | 829 | 43,813 |
16 | Trauma and injuries | 60 | 0 | N/A |
18 + 19 | Maternity and neonates | 54 | 18 | 2,969,208 |
First 11 PBCs | 8308 | |||
3 | Disorders of blood | 16 | 1712 | 9419 |
5 | Mental health disorders | 140 | 7469 | 18,744 |
6 | Learning disability | 8 | 54 | 149,883 |
8 | Problems of vision | 15 | 333 | 45,788 |
9 | Problems of hearing | 7 | 1098 | 6239 |
12 | Dental problems | 23 | 533 | 42,472 |
14 | Skin | 15 | 152 | 101,042 |
15 | Musculoskeletal system | 28 | 1819 | 15,628 |
20 | Poisoning and adverse effects | 7 | 64 | 113,546 |
21 | Healthy individuals | 28 | 53 | 526,771 |
22 | Social care needs | 24 | N/A | |
23 | Other | 80 | N/A | |
All 23 PBCs | 12,936 |
Summary of the cost per quality-adjusted life-year threshold
The results of the three sequential steps of analysis are summarised in Table 179, for this year of analysis. They include (i) the cost per life-year [see Table 179, column (1)] based on the methods of analysis outlined in From mortality to life-years; (ii) the cost per life-year adjusted for QoL [see Table 179, column (2)] based on the methods of analysis outlined in Adjusting life-years for quality-of-life; and (iii) the cost per QALY [see Table 179, column (3)] based on the methods of analysis outlined in Including quality-of-life effects during disease. These estimates, in Table 179, column (3), take account of the likely effects of changes in expenditure on QoL during disease as well as the effects associated with mortality and life-years; making best use of available information, while the assumptions required appear more reasonable than the other alternatives available. For this reason these estimates remain our central or best estimates for all the waves of expenditure and mortality data.
PBC scenario | (1) Chapter 4, From mortality to life-years analysis | (2) Chapter 4, Adjusting life-years for quality of life analysis | (3) Chapter 4, Including quality-of-life effects during disease analysis |
---|---|---|---|
QoL associated with life extension | 1 | Norm | |
QoL during disease | 0 | 0 | Based on burden |
Best estimate | |||
Effect of expenditure on mortality | 1 year | 1 year | 1 year |
YLL per death averted | ∼ 4.5 | ∼ 4.5 | ∼ 4.5 |
QALYs per death averted | ∼ 4.5 | ∼ 3.8 | ∼ 15.0 |
(1) All big four programmes | £10,220 | £12,338 | £4872 |
(2) 11 PBCs (with mortality) | £23,360 | £28,045 | £8308 |
(3) All 23 PBCs | £25,214 | £30,270 | £12,936 |
Lower bound | |||
Effect of expenditure on mortality | Remainder of disease duration | Remainder of disease duration | Remainder of disease duration |
YLL per death averted | ∼ 4.5 | ∼ 4.5 | ∼ 4.5 |
QALYs per death averted | ∼ 4.5 | ∼ 3.8 | ∼ 15.0 |
(4) All big four programmes | £5083 | £5811 | £1194 |
(5) 11 PBCs (with mortality) | £8579 | £9861 | £1175 |
(6) All 23 PBCs | £9260 | £10,644 | £2018 |
Upper bound | |||
Effect of expenditure on mortality | 1 year | 1 year | 1 year |
YLL per death averted | 2 | 2 | 2 |
QALYs per death averted | ∼ 2 | ∼ 1.4 | ∼ 6.6 |
(7) All big four programmes | £23,346 | £26,138 | £11,040 |
(8) 11 PBCs (with mortality) | £52,936 | £59,151 | £18,827 |
(9) All 23 PBCs | £57,136 | £63,844 | £29,314 |
Which programme budget categories matter most?
The estimate of £8308 per QALY [see Table 179, line (2)] is restricted to the effects of changes in expenditure in the 11 PBCs where outcome elasticities can be estimated. However, these PBCs only account for a proportion of a change in overall expenditure (approximately 50%, Table 180). As was explained in Including quality-of-life effects during disease the QALY threshold of £12,936 [see Table 179, column (3), line (3)] uses the estimated proportionate effects of expenditure on the QALY burden of disease in the 11 PBCs as a surrogate for proportionate effects in the others (i.e. assuming that the effects that can be observed will be similar to those that cannot), and represents our central or best estimate. As in previous sections, no health effects are assigned to PBC 23 or 22 (GMS and social care) on the basis that any health effects of this expenditure would be recorded in the other PBCs. Although this estimate of £12,936 reflects changes in undiscounted QALYs associated with changes in expenditure, discounting the QALY effects only increases the cost per QALY threshold to £13,141 (Table 181). The effects of discounting are modest because (i) the health effects of a change in expenditure are restricted to 1 year (where no discounting is necessary); (ii) most of the total QALY effects occur in that year; (iii) it is only some of the life-year effects (adjusted for quality) of a change in mortality in that year that occur in future years that need to be discounted; and (iv) these need to be discounted only over 4.5 years on average.
PBC | (1) % share of change in overall expenditure | (2) % share of total health effects (QALY) | (3) Elasticity of the thresholda | (4) PBC cost per QALY (£) | |
---|---|---|---|---|---|
2 | Cancer | 4.47 | 3.41 | 0.34 | 16,997 |
10 | Circulatory | 7.59 | 13.95 | 1.40 | 7038 |
11 | Respiratory | 4.58 | 29.67 | 2.97 | 1998 |
13 | Gastrointestinal | 3.20 | 5.68 | 0.57 | 7293 |
1 | Infectious diseases | 3.27 | 2.03 | 0.20 | 20,829 |
4 | Endocrine | 1.89 | 7.84 | 0.78 | 3124 |
7 | Neurological | 5.98 | 14.11 | 1.41 | 5480 |
17 | Genitourinary | 4.64 | 1.37 | 0.14 | 43,813 |
16 | Trauma and injuries | 7.70 | 0.00 | 0.00 | N/A |
18 + 19 | Maternity and neonates | 6.83 | 0.03 | < 0.01 | 2,969,208 |
3 | Disorders of blood | 2.06 | 2.82 | 0.28 | 9419 |
5 | Mental health | 17.86 | 12.32 | 1.23 | 18,744 |
6 | Learning disability | 1.04 | 0.09 | 0.01 | 149,883 |
8 | Problems of vision | 1.94 | 0.55 | 0.05 | 45,788 |
9 | Problems of hearing | 0.87 | 1.81 | 0.18 | 6239 |
12 | Dental problems | 2.89 | 0.88 | 0.09 | 42,472 |
14 | Skin | 1.97 | 0.25 | 0.03 | 101,042 |
15 | Musculoskeletal | 3.63 | 3.00 | 0.30 | 15,628 |
20 | Poisoning and adverse events | 0.93 | 0.11 | 0.01 | 113,546 |
21 | Healthy individuals | 3.53 | 0.09 | 0.01 | 526,771 |
22 | Social care needs | 3.00 | 0.00 | 0.00 | N/A |
23 | Other | 10.14 | 0.00 | 0.00 | N/A |
PBC scenario | 2008–10 (£) discounted, best estimate |
---|---|
(1) All big four programmes | 4998 |
(2) 11 PBCs | 8467 |
(3) All 23 PBCs | 13,141 |
The upper and lower bounds for the cost per QALY thresholds in column (3) are based on making the necessary assumptions about duration of health effects and how long a death might be averted optimistic (providing the lower bound for the threshold) or conservative (an upper bound for the threshold). The lower bound [see Table 179, lines (4)–(6)] is based on assuming that health effects are not restricted to 1 year but apply to the whole of the remaining disease duration of the population at risk in PBCs during 1 year. Although this combines optimistic assumptions, it is possible that at least some part of a change in expenditure may prevent disease so will have an impact on populations that are incident to PBCs in the future. Such effects are not captured in any of the estimates presented in this report so all are conservative in this respect. The upper bound [see Table 179, lines (7)–(9)] is based on the combination of assuming that health effects are restricted to 1 year for the population currently at risk and that any death averted is only averted for 2 years (see Summary of cost per life-year estimates).
The estimated QALY effects associated with each PBC can be decomposed into that part due to life-year effects adjusted for quality and that part associated with effects on quality during disease (Table 182). Those PBCs for which mortality is the major concern have a much greater share of total QALY effects associated with avoidance of premature death (e.g. PBCs 2 and 10) compared with those where QoL is the major concern (e.g. PBC 7).
PBC | (1) QALY change (total) | (2) QALY change (death) | % QALY gained | ||
---|---|---|---|---|---|
(3) For premature death | (4) For disability while alive | ||||
2 | Cancer | 2064 | 1912 | 93 | 7 |
10 | Circulatory | 8453 | 5778 | 68 | 32 |
11 | Respiratory | 17,981 | 789 | 4 | 96 |
13 | Gastrointestinal | 3441 | 1268 | 3 | 63 |
1 | Infectious diseases | 1229 | 282 | 23 | 77 |
4 | Endocrine | 4749 | 254 | 5 | 95 |
7 | Neurological | 8551 | 335 | 4 | 96 |
17 | Genitourinary | 829 | 162 | 20 | 80 |
16 | Trauma and injuries | 0 | 0 | N/A | N/A |
18 + 19 | Maternity and neonates | 18 | 12 | 69 | 31 |
3 | Disorders of blood | 1712 | 88 | 5 | 95 |
5 | Mental health | 7469 | 652 | 9 | 91 |
6 | Learning disability | 54 | 11 | 20 | 80 |
8 | Problems of vision | 333 | 13 | 4 | 96 |
9 | Problems of hearing | 1098 | 8 | 1 | 99 |
12 | Dental problems | 533 | 1 | 0 | 100 |
14 | Skin | 152 | 56 | 37 | 63 |
15 | Musculoskeletal | 1819 | 90 | 5 | 95 |
20 | Poisoning and adverse events | 64 | 10 | 16 | 84 |
21 | Healthy individuals | 53 | 8 | 16 | 84 |
22 | Social care needs | 0 | 0 | N/A | N/A |
23 | Other | 0 | 0 | N/A | N/A |
How uncertain are the estimates?
In the earlier section How uncertain are the estimates? (pp. 432), the impact of uncertainty over the spend and outcome elasticities on estimates of the cost per QALY threshold has been illustrated and interpreted in detail using expenditure data from 2006/7. Here we repeat this analysis using expenditure data from 2008/9 and mortality data from 2008–10 (Table 183). Figure 53 shows the histogram of threshold values from the Monte Carlo simulation (where each random sample from the simulation represents one possible realisation of the overall threshold), and Figure 54 shows the cumulative probability density function for a cost per QALY threshold based on the 11 PBCs with estimated outcome elasticities and for all 23 PBCs.
FIGURE 53.
Histogram of simulation of undiscounted threshold (all 23 PBCs) (2008).
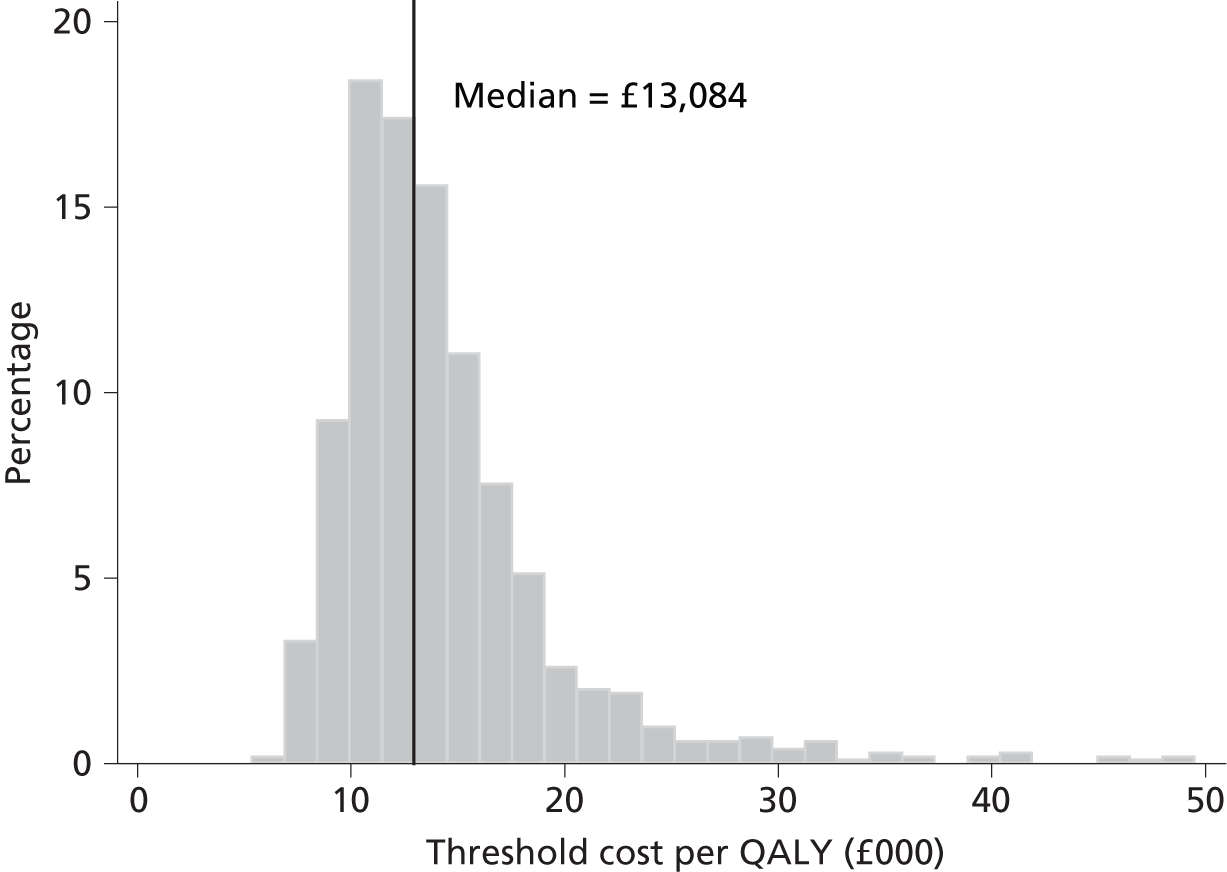
FIGURE 54.
Cumulative probability density function for the cost per QALY threshold (2008).

Scenario | (1) 11 PBCs | (2) All 23 PBCs |
---|---|---|
Best estimate (deterministic) | £8308 | £12,936 |
Mean estimate (from the simulations) | £8330 | £13,050 |
Threshold value at the probability of (from the simulations) | ||
2.5% | £5176 | £8141 |
5.0% | £5438 | £8760 |
50.0% | £8346 | £13,084 |
95.0% | £17,452 | £25,505 |
97.5% | £21,693 | £32,173 |
Probability (from the simulations) of the threshold being smaller than | ||
£5000 per QALY | 2% | 0% |
£6000 per QALY | 10% | 0% |
£7000 per QALY | 25% | 0% |
£8000 per QALY | 45% | 2% |
£9000 per QALY | 59% | 6% |
£10,000 per QALY | 71% | 13% |
£15,000 per QALY | 93% | 68% |
£20,000 per QALY | 97% | 89% |
£25,000 per QALY | 98% | 95% |
£30,000 per QALY | 99% | 97% |
£35,000 per QALY | 99% | 98% |
£40,000 per QALY | 99% | 99% |
£45,000 per QALY | 99% | 99% |
£50,000 per QALY | 99% | 99% |
Re-estimating the cost per quality-adjusted life-year threshold using 2007 expenditure data
The same methods of analysis were applied to the econometric analysis of the 2007/8 expenditure and 2007–9 mortality data (see Analysis of programme budgeting expenditure for 2007/8 and mortality data for 2007/8/9 in Appendix 2). Given the detailed reporting of the methods and interpretation of the analyses for other expenditure years (see Analysis of 2006/7 expenditure and 2006–8 mortality data and Re-estimating the cost per quality-adjusted life-year threshold using 2008 expenditure data), we will here only present the necessary tables of results (Tables 184–192).
PBC | (1) Total spend 2007/8 (£) | Spend elasticities | (4) Outcome elasticitiesa | ||
---|---|---|---|---|---|
(2) Unadjusted | (3) Adjusted | ||||
2 | Cancer | 4573 | 0.890 | 0.890 | 0.365 |
10 | Circulatory problems | 6325 | 0.293 | 0.293 | 1.277 |
11 | Respiratory problems | 3431 | 0.536 | 0.536 | 2.205 |
13 | Gastrointestinal problems | 3805 | 0.622 | 0.622 | 1.328 |
All big four programmes | 18,134 | ||||
1 | Infectious diseases | 1119 | 1.436 | 1.436 | 0.548 |
4 | Endocrine problems | 1997 | 0.264 | 0.264 | 0.566 |
7 | Neurological problems | 3165 | 1.035 | 1.035 | 0.339 |
17 | Genitourinary problems | 3439 | 1.004 | 1.004 | 1.855 |
16 | Trauma and injuries | 2918 | 1.686 | 1.686 | 0.369b |
18 + 19 | Maternity and neonates | 3662 | 0.514 | 0.514 | 0.110 |
First 11 PBCs | 34,434 | ||||
3 | Disorders of blood | 986 | 1.830 | 2.879 | – |
5 | Mental health disorders | 9171 | 1.145 | 1.801 | – |
6 | Learning disability | 2748 | 0.440 | 0.692 | – |
8 | Vision | 1556 | 1.170 | 1.841 | – |
9 | Hearing | 409 | 1.029 | 1.619 | – |
12 | Dental problems | 3014 | 0.424 | 0.667 | – |
14 | Problems of the skin | 1542 | 0.428 | 0.673 | – |
15 | Musculoskeletal system | 3848 | 0.806 | 1.268 | – |
20 | Poisoning and adverse events | 803 | 0.668 | 1.051 | – |
21 | Healthy individuals | 1594 | 0.986 | 1.551 | – |
22 | Social care needs | 1789 | 1.852 | 2.913 | – |
23 | Other | 11,763 | 0.563 | 0.563 | – |
All 23 PBCs | 73,656 |
PBC | 2007 | 2008 | 2009 | Annual deaths < LE | Annual deaths > LE | ||||
---|---|---|---|---|---|---|---|---|---|
< LE | > LE | < LE | > LE | < LE | > LE | ||||
1 | Infectious diseases | 3906 | 3731 | 3404 | 2588 | 3042 | 2192 | 3451 | 2837 |
2 | Cancer | 95,385 | 35,401 | 94,814 | 37,088 | 94,218 | 37,209 | 94,806 | 36,566 |
4 | Endocrine | 3970 | 2747 | 4031 | 2879 | 3832 | 2828 | 3944 | 2818 |
7 | Neurological | 8852 | 6494 | 9632 | 6865 | 9439 | 6945 | 9308 | 6768 |
10 | Circulatory | 80,687 | 78,404 | 80,834 | 76,352 | 75,993 | 73,397 | 79,172 | 76,051 |
11 | Respiratory | 29,571 | 35,029 | 32,059 | 35,204 | 29,890 | 33,326 | 30,507 | 34,520 |
13 | Gastrointestinal | 15,667 | 8367 | 15,937 | 8267 | 15,354 | 8168 | 15,653 | 8267 |
17 | Genitourinary | 4077 | 6553 | 4468 | 6670 | 4375 | 6903 | 4307 | 6709 |
18 + 19 | Maternity and neonates | 216 | 0 | 267 | 0 | 281 | 1 | 255 | 0 |
PBC | LE of males (years) | LE of females (years) | Average 2007–9 | Net YLL | ||||
---|---|---|---|---|---|---|---|---|
Deaths | YLL | YLG | ||||||
< LE | > LE | |||||||
1 | Infectious diseases | 79.6 | 83.6 | 3280 | 3008 | 57,715 | 19,085 | 38,629 |
2 | Cancer | 83.0 | 84.7 | 100,810 | 30,561 | 1,464,726 | 129,810 | 1,334,916 |
4 | Endocrine | 81.0 | 84.7 | 4004 | 2759 | 66,575 | 15,386 | 51,189 |
7 | Neurological | 79.6 | 83.3 | 8719 | 7357 | 135,760 | 44,925 | 90,835 |
10 | Circulatory | 83.0 | 86.5 | 92,729 | 62,494 | 1,069,632 | 276,368 | 793,264 |
11 | Respiratory | 80.3 | 84.0 | 29,668 | 35,359 | 304,168 | 230,245 | 73,922 |
13 | Gastrointestinal | 80.6 | 84.5 | 15,640 | 8280 | 271,092 | 45,500 | 225,593 |
17 | Genitourinary | 83.5 | 85.6 | 5008 | 6007 | 47,656 | 30,931 | 16,725 |
18 + 19 | Maternity and neonates | 78.7 | 83.1 | 255 | 0 | 18,844 | 1 | 18,843 |
PBC | Excess deaths ONS | Deaths | YLL | |||||
---|---|---|---|---|---|---|---|---|
All deaths ONS | All deaths GBD | Adjustment factor (deaths) | Net estimates ONS | Total YLL GBD | Adjustment factor (YLL) | |||
1 | Infectious diseases | 2925 | 6288 | 1408 | 4.47 | 38,629 | 25,142 | 1.54 |
2 | Cancer | 94,827 | 131,372 | 140,124 | 0.94 | 1,334,916 | 1,932,637 | 0.69 |
4 | Endocrine | 3765 | 6762 | 7509 | 0.90 | 51,189 | 95,401 | 0.54 |
7 | Neurological | 6692 | 16,076 | 12,854 | 1.25 | 90,835 | 164,796 | 0.55 |
10 | Circulatory | 76,322 | 155,223 | 178,454 | 0.87 | 793,264 | 1,750,608 | 0.45 |
11 | Respiratory | 8034 | 65,027 | 67,441 | 0.96 | 73,922 | 594,529 | 0.12 |
13 | Gastrointestinal | 15,064 | 23,920 | 28,329 | 0.84 | 225,593 | 396,829 | 0.57 |
17 | Genitourinary | 2005 | 11,016 | 8606 | 1.28 | 16,725 | 77,338 | 0.22 |
18 + 19 | Maternity and neonates | 255 | 255 | 2211 | 0.12 | 18,843 | 149,868 | 0.13 |
Total | 209,890 | 415,939 | 446,936 | 0.93 | 2,643,916 | 5,187,148 | 0.51 |
PBC scenario | (1) 2006 | (2) 2007 | (3) 2008 |
---|---|---|---|
All big four programmes | £3036 | £4549 | £4872 |
11 PBCs (with mortality) | £5128 | £8513 | £8308 |
All 23 PBCs | £10,187 | £13,554 | £12,936 |
PBC | (1) Change in spend (£M) | QALY burden (HODaR and MEPS) | ||
---|---|---|---|---|
(2) Change in QALY | (3) Cost per QALY gained (£) | |||
2 | Cancer | 51 | 3820 | 13,384 |
10 | Circulatory problems | 23 | 3462 | 6724 |
11 | Respiratory problems | 23 | 16,522 | 1398 |
13 | Gastrointestinal problems | 30 | 4166 | 7137 |
All big four programmes | 4549 | |||
1 | Infectious diseases | 20 | 1300 | 15,530 |
4 | Endocrine problems | 7 | 1143 | 5796 |
7 | Neurological problems | 41 | 6421 | 6409 |
17 | Genitourinary problems | 43 | 1224 | 35,449 |
16 | Trauma and injuries | 62 | ||
18 + 19 | Maternity and neonates | 24 | 7 | 3,250,386 |
First 11 PBCs | 8513 | |||
3 | Disorders of blood | 23 | 2695 | 8407 |
5 | Mental health disorders | 132 | 8316 | 15,863 |
6 | Learning disability | 15 | 117 | 129,512 |
8 | Problems of vision | 23 | 600 | 38,140 |
9 | Problems of hearing | 5 | 956 | 5534 |
12 | Dental problems | 16 | 444 | 36,177 |
14 | Skin | 8 | 98 | 84,977 |
15 | Musculoskeletal system | 39 | 2926 | 13,319 |
20 | Poisoning and adverse effects | 7 | 77 | 87,852 |
21 | Healthy individuals | 20 | 48 | 414,420 |
22 | Social care needs | 42 | N/A | |
23 | Other | 83 | N/A | |
All 23 PBCs | 13,554 |
PBC | (1) QALY change (total) | (2) QALY change (death) | % QALY gained | ||
---|---|---|---|---|---|
(3) For premature death | (4) For disability while alive | ||||
2 | Cancer | 3820 | 3542 | 93 | 7 |
10 | Circulatory | 3462 | 2369 | 68 | 32 |
11 | Respiratory | 16,522 | 687 | 4 | 96 |
13 | Gastrointestinal | 4166 | 1536 | 37 | 63 |
1 | Infectious diseases | 1300 | 258 | 20 | 80 |
4 | Endocrine | 1143 | 63 | 5 | 95 |
7 | Neurological | 6421 | 264 | 4 | 96 |
17 | Genitourinary | 1224 | 247 | 20 | 80 |
16 | Trauma and injuries | 0 | 0 | N/A | N/A |
18 + 19 | Maternity and neonates | 7 | 5 | 69 | 31 |
3 | Disorders of blood | 2695 | 139 | 5 | 95 |
5 | Mental health | 8316 | 726 | 9 | 91 |
6 | Learning disability | 117 | 23 | 20 | 80 |
8 | Problems of vision | 600 | 24 | 4 | 96 |
9 | Problems of hearing | 956 | 7 | 1 | 99 |
12 | Dental problems | 444 | 0 | 0 | 100 |
14 | Skin | 98 | 36 | 37 | 63 |
15 | Musculoskeletal | 2926 | 145 | 5 | 95 |
20 | Poisoning and adverse events | 77 | 12 | 16 | 84 |
21 | Healthy individuals | 48 | 7 | 16 | 84 |
22 | Social care needs | 0 | 0 | N/A | N/A |
23 | Other | 0 | 0 | N/A | N/A |
Summary of the cost per quality-adjusted life-year threshold
PBC scenario | Cost per QALY |
---|---|
QoL associated with life extension | Based on burden |
QoL during disease | Based on burden |
Best estimate | |
Effect of expenditure on mortality | 1 year |
YLL per death averted | ∼ 4.8 |
QALYs per death averted | ∼ 16.8 |
(1) All big four programmes | £4549 |
(2) 11 PBCs (with mortality) | £8513 |
(3) All 23 PBCs | £13,554 |
Lower bound | |
Effect of expenditure on mortality | Remainder of disease duration |
YLL per death averted | ∼ 4.8 |
QALYs per death averted | ∼ 16.8 |
(4) All big four programmes | £1116 |
(5) 11 PBCs (with mortality) | £1361 |
(6) All 23 PBCs | £2436 |
Upper bound | |
Effect of expenditure on mortality: | 1 year |
YLL per death averted: | 2 |
QALYs per death averted: | ∼ 7.0 |
(7) All big four programmes | £10,965 |
(8) 11 PBCs (with mortality) | £20,517 |
(9) All 23 PBCs | £32,670 |
PBC scenario | (1) 2007–9 |
---|---|
(1) All big four programmes | £4690 |
(2) 11 PBCs | £8718 |
(3) All 23 PBCs | £13,801 |
Addendum 1: data sources
General Practice Research Database
General Practice Research Database contains over 3 million active patient records drawn from approximately 400 primary care practices in the UK. The Medicines and Healthcare products Regulatory Agency manages the data set. The database has clinical and prescription data and can provide information to support pharmacovigilance (indication, utilisation and risk/benefit profiles of drugs) and formal pharmacoepidemiological studies, including information on demographics, medical symptoms, therapy (medicines, vaccines, devices) and treatment outcomes.
As of 29 March 2012 GPRD changed to the CPRD, an expanded data set that represents ‘The All England Data and Interventional Research Service’. CPRD was approached to provide information on the prevalence of disease by ICD-10 disease code. A sample set of data was analysed by researchers at Pharmatelligencex who were tasked with extracting data on prevalence of each disease state by ICD-10 code.
We were provided with access to data comprising of 22,313,086 rows/patient–ICD10 events (three digit)y representing 4,229,910 patients with data on new diagnosis of diseases observed between 1 January 2006 and 24 June 2011. Multiple events per patient are thus possible, and all patients are active in the data set (i.e. patients had at least one new diagnosis in the period of interest). Newly diagnosed (incident) events were defined using a wash-in period of 24 months (or from registration to index date if lower than 24 months). The sample contains 1873 unique ICD-10 codes in the data set. Seventy ICD-10 codes account for 50% of the total number of events, 166 for 75% and 306 for 90%.
Diagnoses are collected in CPRD using Read codes. These were mapped into three-character ICD-10 codes. Cross-mappings from Read V2 and Read V3 to ICD-10 were used in order to maximize the number of CPRD Read and ICD-10 codes included (33.2% of Read codes; 99.7% of ICD-10 codes). z
Unfortunately, due to the short collection period of CPRD it was not possible to directly observe prevalence only incidence over a period. Attempts were made to elicit a prevalence estimate through observed incidence data from CPRD coupled with clinical expertise on expected disease duration (provided by Dr Charlotte Haylock, York Teaching Hospital NHS Foundation Trust). Our approach classified expected duration for all ICD-10 diseases by three-digit code into one of five duration ‘buckets’. aa However, the limitations of the data were deemed too extensive to provide sufficient accuracy of estimates to represent a stronger estimate of prevalence than provided by GBD.
Global Burden of Disease
The WHO GBD project draws on a wide range of data sources to quantify global and regional effects of diseases, injuries and risk factors on population health. We were provided with access to the beta version of the WHO’s National Burden of Disease toolkit for the UK which represents a set of metrics on WHO prior estimates of mortality and burden of disease for WHO member states for 2004 (based on the GBD: 2004 update155). ab
The metrics of interest to our analysis included disease incidence, prevalence, duration and mortality. These metrics were provided by U-codes which were mapped to ICD-10 codes using direct WHO mapping algorithms. 157 In addition, in many cases each U-code was subdivided by disease sequelae which represent disease subcategories of each U-code. 157 As an individual may be represented in multiple sequelae in a single U-code to avoid double counting in the event of multiple sequelae in a given U-code our analysis uses prevalence estimates based on the sequelae with the largest prevalent population.
Our analysis uses two forms of prevalence data, ‘point prevalence’ and ‘annual prevalence’. ‘Point prevalence’ represents the instantaneous prevalence of a disease whereas ‘annual prevalence’ represents the extent of the prevalence population over a given year. To calculate ‘annual prevalence’ incidence of a disease was multiplied by expected disease duration rounded up to the nearest year.
All data was provided by age, given for both genders in fixed age buckets (either 8 or 19 buckets depending on the data of interest), as a result it was necessary to assume the relevant population could be represented by the mid-point of that bucket for the relevant metric.
Health Survey for England
The HSE comprises a series of annual surveys beginning in 1991. This survey is now commissioned and published by The NHS IC. It is designed to provide regular information on various aspects of the nation’s health. All surveys have covered the adult population aged 16 years and over living in private households in England. ac
In order to define the QoL norms for the population of the UK required for the analysis detailed in Chapter 4, Quality of life based on the general population, data from six Health Surveys for England (1996, 2003, 2004, 2005, 2006 and 2008) were pooled. Self-reported health status and EQ-5D data were extracted and used to generate mean health state utility values for the ‘normal’ population.
Surveys are not completed for people age under 16 years; as a result we have assumed that all persons aged 0–15 years have the same QoL norms as a person aged 16 years. In addition the number of surveys recorded for persons over 91 years of age is relatively small; as a result all persons aged over 91 years are assumed to have the same QoL norm as a person aged 91 years. The QoL norms for each age and gender are shown in Figure 3 in Quality of life based on the general population.
Health Outcomes Data Repository
Health Outcomes Data Repository represents a supplement of routine clinically coded data from the Cardiff and Vale NHS Hospitals Trust, UK, with survey data covering sociodemographic characteristics, QoL, utility and resource use information. 93 HODaR data were collected for subjects treated at Cardiff and Vale NHS Hospital from 2002 to 2004. Inpatients were surveyed 6 weeks post-discharge while outpatients are handed a survey package when they attend. More than 30,000 observations (aged ≥ 18 years) are available relating to approximately 2000 diagnoses of disease by ICD-10 code.
We used HODaR to estimate health-related QoL by ICD-10 diagnoses codes and age using EQ-5D. If data on a patient were provided with multiple diagnoses the primary condition was used.
Medical Expenditure Panel Survey
MEPS is a national representative survey of the US civilian non-institutionalised population, collecting information on health-care utilisation which began in 1996. 94 EQ-5D was employed to measure health-related QoL of the population in years 2000–2. There are about 38,000 adults (aged ≥ 18 years) completing EQ-5D relating to 700 ICD-10 code diagnoses. MEPS consists of a household component and an insurance component, both aimed at identifying the medical usage of individuals as well as how they are funded, their cost, and the scope and breadth of health insurance held and available.
As with HODaR, MEPS allowed us to estimate the health-related QoL by ICD-10 code and age. If data on a patient was provided with multiple conditions the primary condition was used.
Hospital Episode Statistics
Hospital Episode Statistics represents a collection of data with details on all admissions to the NHS hospital in England. It contains admitted patient care data from 1989 onwards, with more than 12 million new records added each year, and outpatient attendance data from 2003 onwards, with more than 40 million new records added each year.
Expenditure by ICD-10 codes and PCT was used to estimate the contribution to variance of each PBC. This was done by calculating the contribution of that ICD-10 code to the variance in expenditure between PCTs within a PBC (total costs allocated to individual ICD-10 codes were divided by the number of patients using services in the PCT). For our analysis we make use of HES data on the year 2007/8.
Patient-reported outcome measures
Introduced in 2009, the English NHS PROMs programme routinely collects self-reported health status of patients receiving surgery for four elective procedures: knee and hip replacement, groin hernia repair, and varicose vein surgery. Patients are invited to complete a questionnaire prior to surgery, and again 6 (or 3) months after surgery. 159 Differences in their self-reported health status are used to explore differences between provider performance in improving patient health. 160 The data that are collected include both condition-specific questions (the Oxford Hip Score, Oxford Knee Score and the Aberdeen Varicose Vein score; no condition-specific instrument is available for hernia) as well as the generic instrument, the EQ-5D (both the EQ-5D profile and the patient’s global assessment of their health, the EQ-VAS161). All NHS patients receiving these surgical procedures are invited to complete the PROMs questionnaires – in practice, for a variety of reasons, some patients do not participate, or complete only the pre-surgery, or the post-surgery, questionnaire – so the data do not cover 100% of patients. However, good coverage rates have been achieved (e.g. the response rate from hip surgery patients to April 2012 was 78% for the pre-surgery questionnaire, and 81% for the post-surgery questionnaire). 162
Patient-level data from the PROMs programme are freely available to download in anonymised form. Those data can also be linked to further information in the HES database, via requests to the NHS IC. Standardised reports on the PROMs data, including the average (case-mix adjusted) performance of providers, is regularly published by the NHS IC, currently on a quarterly basis.
There are plans to extend the PROMs programme in the future, in keeping with the Government’s NHS Outcomes Framework, and a number of pilot studies have been commissioned by the Department of Health in order to inform the roll-out to other NHS services. There is currently work under way around the potential use of PROMs in a wide range of long-term conditions; primary care; cancer survivorship; cardiovascular services; musculoskeletal; and cosmetic surgery.
Figures of prevalence distribution within programme budget categories
FIGURE 55.
Distribution of PBC 1 prevalence by age, gender and contributing ICD-10 code alongside proportion of patients and contribution to variance of each ICD-10 code. F00, female aged 0–4 years; F05, female aged 5–9 years; F15, female aged 10–14 years; F30, female aged 15–29 years; F45, female aged 30–44 years; F60: female aged 45–59 years; F70, female aged 60–69 years; F80, female aged ≥ 70 years; M00, male aged 0–4 years; M05, male aged 5–9 years; M15, male aged 10–14 years; M30, male aged 15–29 years; M45, male aged 30–44 years; M60: male aged 45–59 years; M70, male aged 60–69 years; M80, male aged ≥ 70 years.
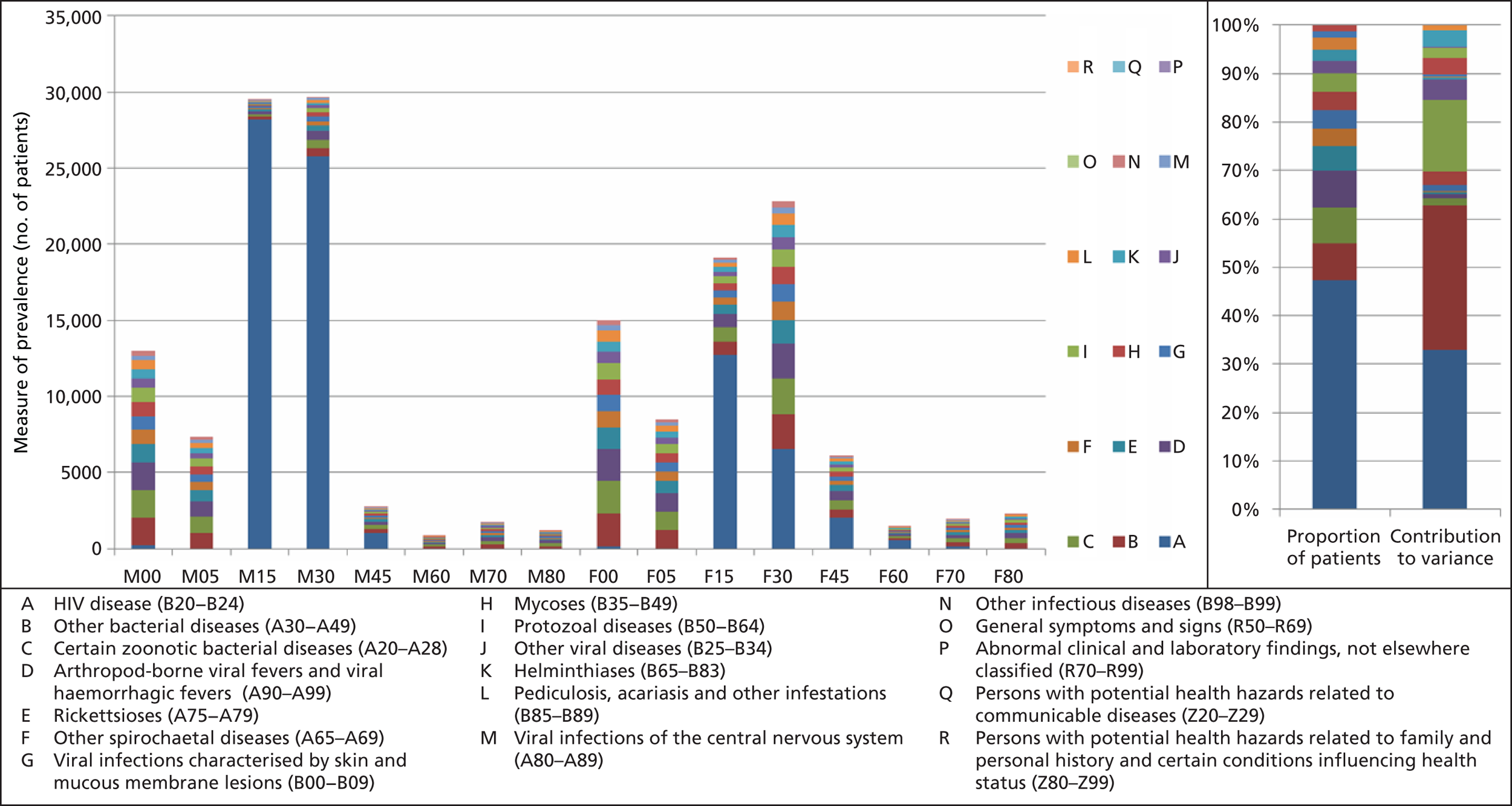
FIGURE 56.
Distribution of PBC 2 prevalence by age, gender and contributing ICD-10 code alongside proportion of patients and contribution to variance of each ICD-10 code. F00, female aged 0–4 years; F05, female aged 5–9 years; F15, female aged 10–14 years; F30, female aged 15–29 years; F45, female aged 30–44 years; F60: female aged 45–59 years; F70, female aged 60–69 years; F80, female aged ≥ 70 years; M00, male aged 0–4 years; M05, male aged 5–9 years; M15, male aged 10–14 years; M30, male aged 15–29 years; M45, male aged 30–44 years; M60: male aged 45–59 years; M70, male aged 60–69 years; M80, male aged ≥ 70 years.
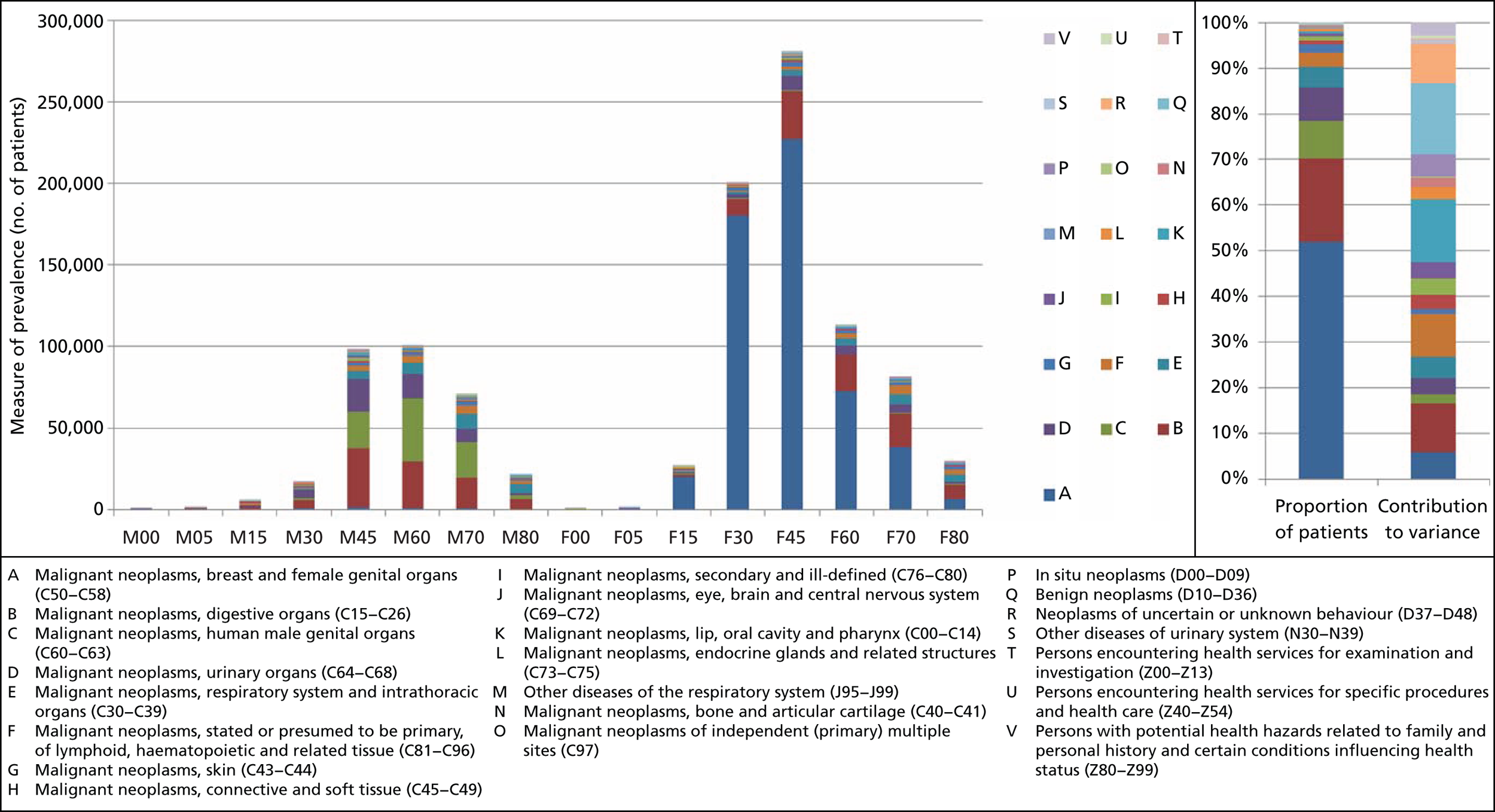
FIGURE 57.
Distribution of PBC 3 prevalence by age, gender and contributing ICD-10 code alongside proportion of patients and contribution to variance of each ICD-10 code. F00, female aged 0–4 years; F05, female aged 5–9 years; F15, female aged 10–14 years; F30, female aged 15–29 years; F45, female aged 30–44 years; F60: female aged 45–59 years; F70, female aged 60–69 years; F80, female aged ≥ 70 years; M00, male aged 0–4 years; M05, male aged 5–9 years; M15, male aged 10–14 years; M30, male aged 15–29 years; M45, male aged 30–44 years; M60: male aged 45–59 years; M70, male aged 60–69 years; M80, male aged ≥ 70 years.

FIGURE 58.
Distribution of PBC 4 prevalence by age, gender and contributing ICD-10 code alongside proportion of patients and contribution to variance of each ICD-10 code. ACTH, adrenocorticotrophic hormone; ADH, antidiuretic hormone; FSH, follicle-stimulating hormone; GH, growth hormone; LH, luteinising hormone; PTH, parathyroid hormone; TSH, thyroid-stimulating hormone. F00, female aged 0–4 years; F05, female aged 5–9 years; F15, female aged 10–14 years; F30, female aged 15–29 years; F45, female aged 30–44 years; F60: female aged 45–59 years; F70, female aged 60–69 years; F80, female aged ≥ 70 years; M00, male aged 0–4 years; M05, male aged 5–9 years; M15, male aged 10–14 years; M30, male aged 15–29 years; M45, male aged 30–44 years; M60: male aged 45–59 years; M70, male aged 60–69 years; M80, male aged ≥ 70 years.

FIGURE 59.
Distribution of PBC 5 prevalence by age, gender and contributing ICD-10 code alongside proportion of patients and contribution to variance of each ICD-10 code. F00, female aged 0–4 years; F05, female aged 5–9 years; F15, female aged 10–14 years; F30, female aged 15–29 years; F45, female aged 30–44 years; F60: female aged 45–59 years; F70, female aged 60–69 years; F80, female aged ≥ 70 years; M00, male aged 0–4 years; M05, male aged 5–9 years; M15, male aged 10–14 years; M30, male aged 15–29 years; M45, male aged 30–44 years; M60: male aged 45–59 years; M70, male aged 60–69 years; M80, male aged ≥ 70 years.

FIGURE 60.
Distribution of PBC 6 prevalence by age, gender and contributing ICD-10 code alongside proportion of patients and contribution to variance of each ICD-10 code. F00, female aged 0–4 years; F05, female aged 5–9 years; F15, female aged 10–14 years; F30, female aged 15–29 years; F45, female aged 30–44 years; F60: female aged 45–59 years; F70, female aged 60–69 years; F80, female aged ≥ 70 years; M00, male aged 0–4 years; M05, male aged 5–9 years; M15, male aged 10–14 years; M30, male aged 15–29 years; M45, male aged 30–44 years; M60: male aged 45–59 years; M70, male aged 60–69 years; M80, male aged ≥ 70 years.

FIGURE 61.
Distribution of PBC 7 prevalence by age, gender and contributing ICD-10 code alongside proportion of patients and contribution to variance of each ICD-10 code. F00, female aged 0–4 years; F05, female aged 5–9 years; F15, female aged 10–14 years; F30, female aged 15–29 years; F45, female aged 30–44 years; F60: female aged 45–59 years; F70, female aged 60–69 years; F80, female aged ≥ 70 years; M00, male aged 0–4 years; M05, male aged 5–9 years; M15, male aged 10–14 years; M30, male aged 15–29 years; M45, male aged 30–44 years; M60: male aged 45–59 years; M70, male aged 60–69 years; M80, male aged ≥ 70 years.
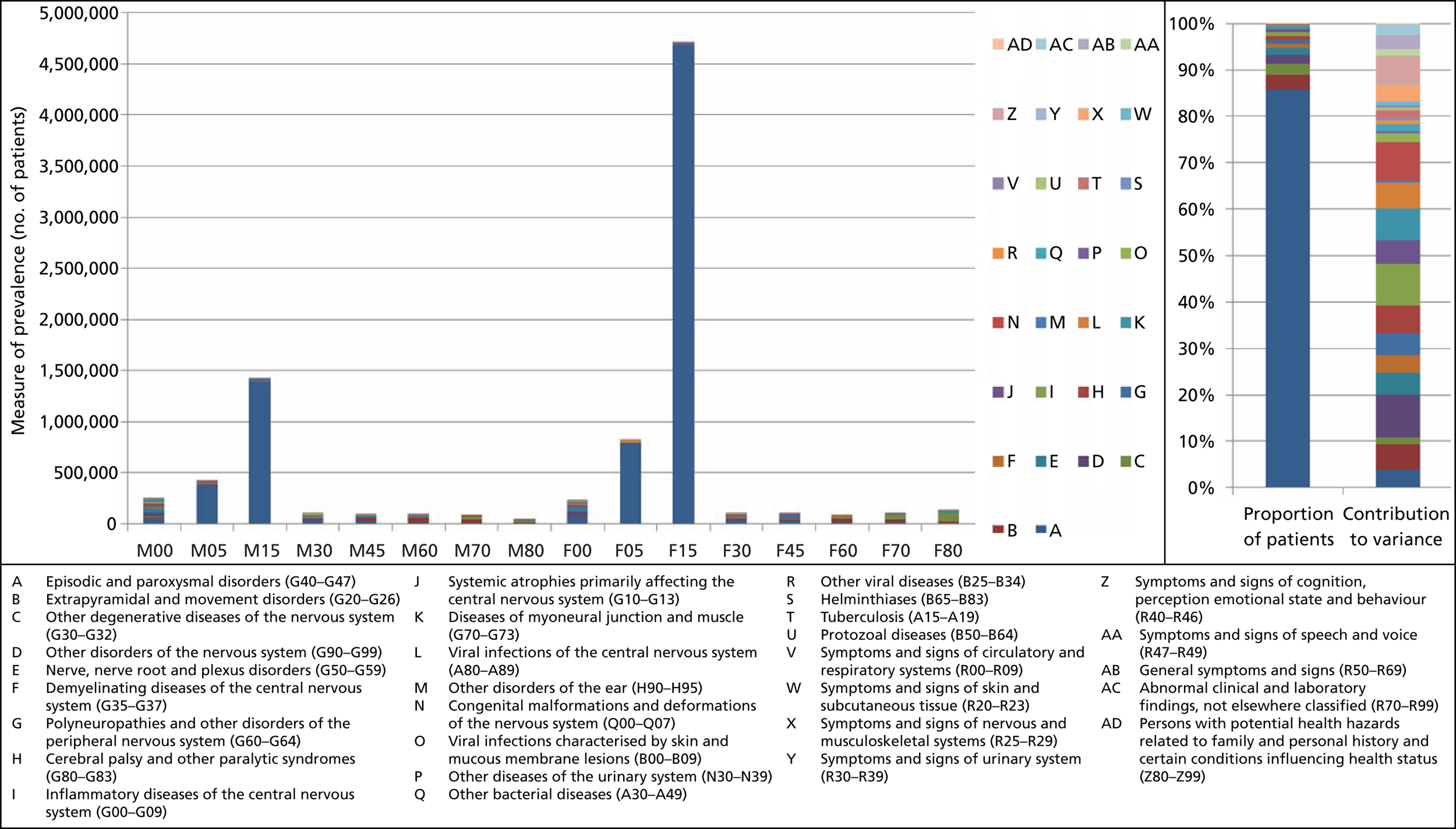
FIGURE 62.
Distribution of PBC 8 prevalence by age, gender and contributing ICD-10 code alongside proportion of patients and contribution to variance of each ICD-10 code. F00, female aged 0–4 years; F05, female aged 5–9 years; F15, female aged 10–14 years; F30, female aged 15–29 years; F45, female aged 30–44 years; F60: female aged 45–59 years; F70, female aged 60–69 years; F80, female aged ≥ 70 years; M00, male aged 0–4 years; M05, male aged 5–9 years; M15, male aged 10–14 years; M30, male aged 15–29 years; M45, male aged 30–44 years; M60: male aged 45–59 years; M70, male aged 60–69 years; M80, male aged ≥ 70 years.

FIGURE 63.
Distribution of PBC 9 prevalence by age, gender and contributing ICD-10 code alongside proportion of patients and contribution to variance of each ICD-10 code. F00, female aged 0–4 years; F05, female aged 5–9 years; F15, female aged 10–14 years; F30, female aged 15–29 years; F45, female aged 30–44 years; F60: female aged 45–59 years; F70, female aged 60–69 years; F80, female aged ≥ 70 years; M00, male aged 0–4 years; M05, male aged 5–9 years; M15, male aged 10–14 years; M30, male aged 15–29 years; M45, male aged 30–44 years; M60: male aged 45–59 years; M70, male aged 60–69 years; M80, male aged ≥ 70 years.

FIGURE 64.
Distribution of PBC 10 prevalence by age, gender and contributing ICD-10 code alongside proportion of patients and contribution to variance of each ICD-10 code. F00, female aged 0–4 years; F05, female aged 5–9 years; F15, female aged 10–14 years; F30, female aged 15–29 years; F45, female aged 30–44 years; F60: female aged 45–59 years; F70, female aged 60–69 years; F80, female aged ≥ 70 years; M00, male aged 0–4 years; M05, male aged 5–9 years; M15, male aged 10–14 years; M30, male aged 15–29 years; M45, male aged 30–44 years; M60: male aged 45–59 years; M70, male aged 60–69 years; M80, male aged ≥ 70 years.
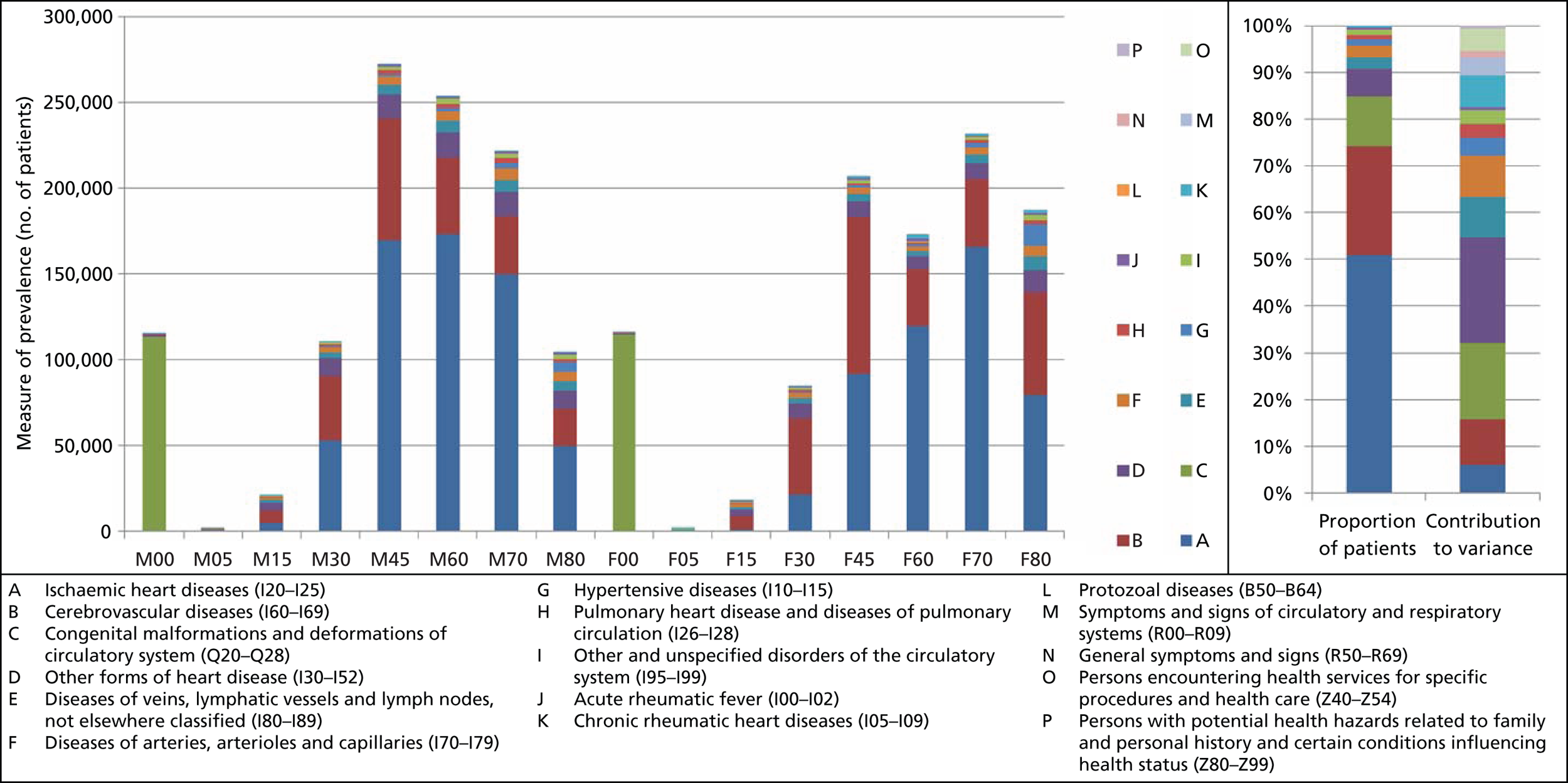
FIGURE 65.
Distribution of PBC 11 prevalence by age, gender and contributing ICD-10 code alongside proportion of patients and contribution to variance of each ICD-10 code. F00, female aged 0–4 years; F05, female aged 5–9 years; F15, female aged 10–14 years; F30, female aged 15–29 years; F45, female aged 30–44 years; F60: female aged 45–59 years; F70, female aged 60–69 years; F80, female aged ≥ 70 years; M00, male aged 0–4 years; M05, male aged 5–9 years; M15, male aged 10–14 years; M30, male aged 15–29 years; M45, male aged 30–44 years; M60: male aged 45–59 years; M70, male aged 60–69 years; M80, male aged ≥ 70 years.
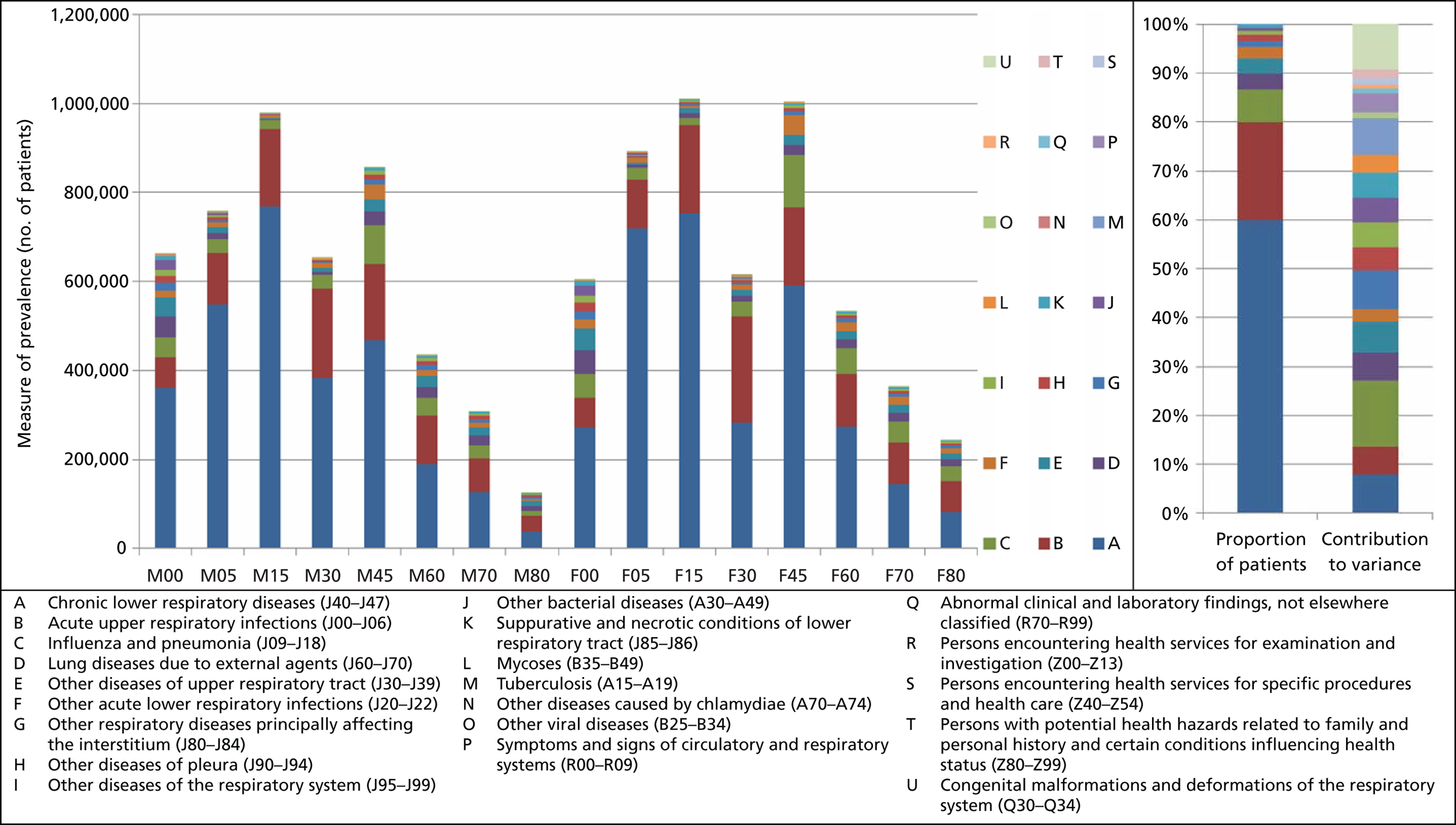
FIGURE 66.
Distribution of PBC 12 prevalence by age, gender and contributing ICD-10 code alongside proportion of patients and contribution to variance of each ICD-10 code. F00, female aged 0–4 years; F05, female aged 5–9 years; F15, female aged 10–14 years; F30, female aged 15–29 years; F45, female aged 30–44 years; F60: female aged 45–59 years; F70, female aged 60–69 years; F80, female aged ≥ 70 years; M00, male aged 0–4 years; M05, male aged 5–9 years; M15, male aged 10–14 years; M30, male aged 15–29 years; M45, male aged 30–44 years; M60: male aged 45–59 years; M70, male aged 60–69 years; M80, male aged ≥ 70 years.
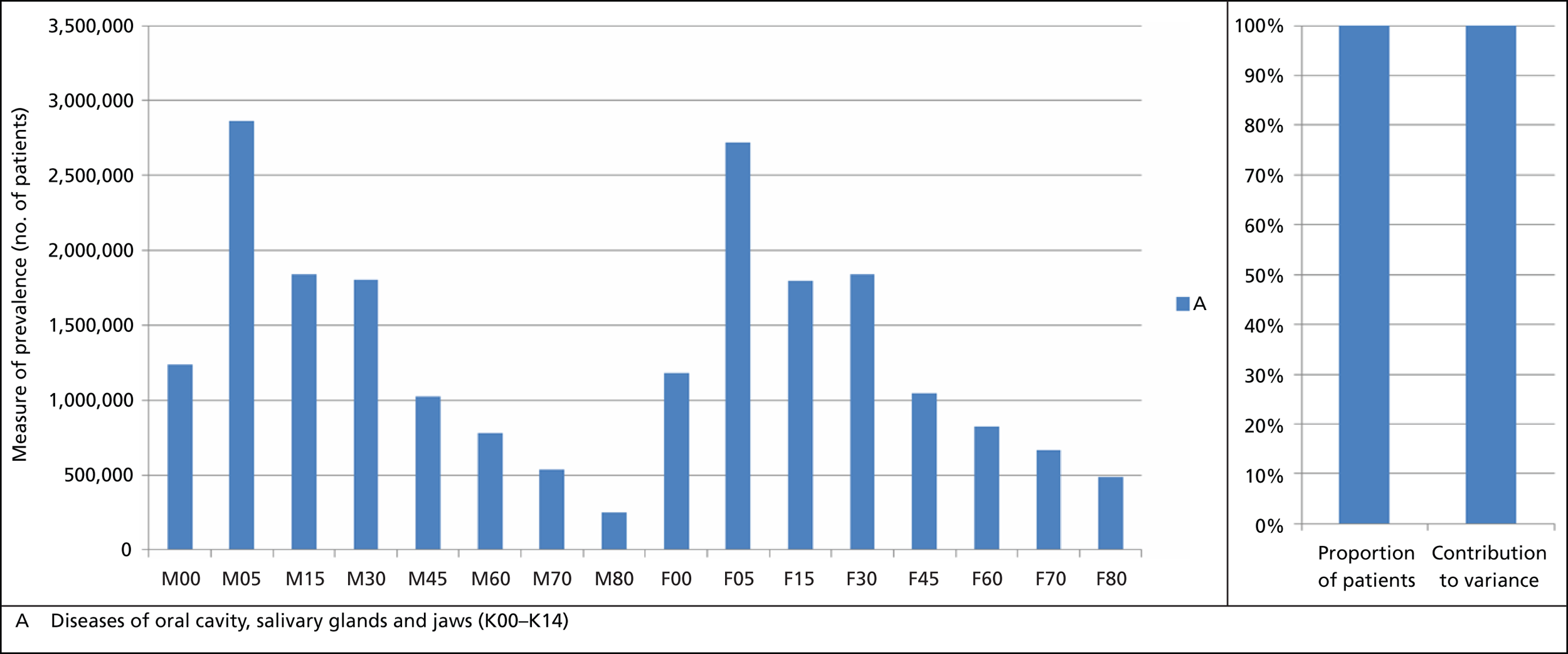
FIGURE 67.
Distribution of PBC 13 prevalence by age, gender and contributing ICD-10 code alongside proportion of patients and contribution to variance of each ICD-10 code. F00, female aged 0–4 years; F05, female aged 5–9 years; F15, female aged 10–14 years; F30, female aged 15–29 years; F45, female aged 30–44 years; F60: female aged 45–59 years; F70, female aged 60–69 years; F80, female aged ≥ 70 years; M00, male aged 0–4 years; M05, male aged 5–9 years; M15, male aged 10–14 years; M30, male aged 15–29 years; M45, male aged 30–44 years; M60: male aged 45–59 years; M70, male aged 60–69 years; M80, male aged ≥ 70 years.
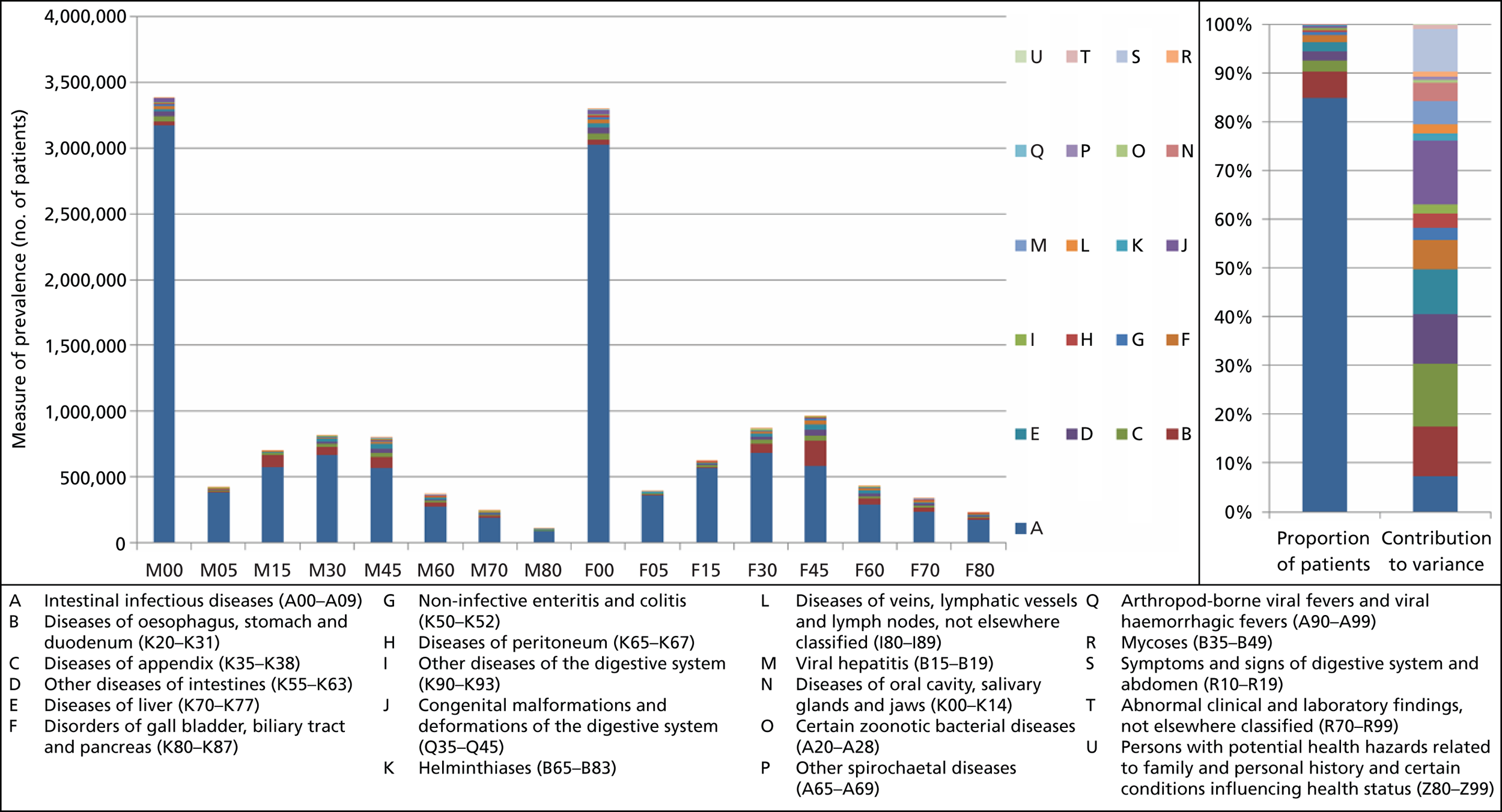
FIGURE 68.
Distribution of PBC 14 prevalence by age, gender and contributing ICD-10 code alongside proportion of patients and contribution to variance of each ICD-10 code. F00, female aged 0–4 years; F05, female aged 5–9 years; F15, female aged 10–14 years; F30, female aged 15–29 years; F45, female aged 30–44 years; F60: female aged 45–59 years; F70, female aged 60–69 years; F80, female aged ≥ 70 years; M00, male aged 0–4 years; M05, male aged 5–9 years; M15, male aged 10–14 years; M30, male aged 15–29 years; M45, male aged 30–44 years; M60: male aged 45–59 years; M70, male aged 60–69 years; M80, male aged ≥ 70 years.
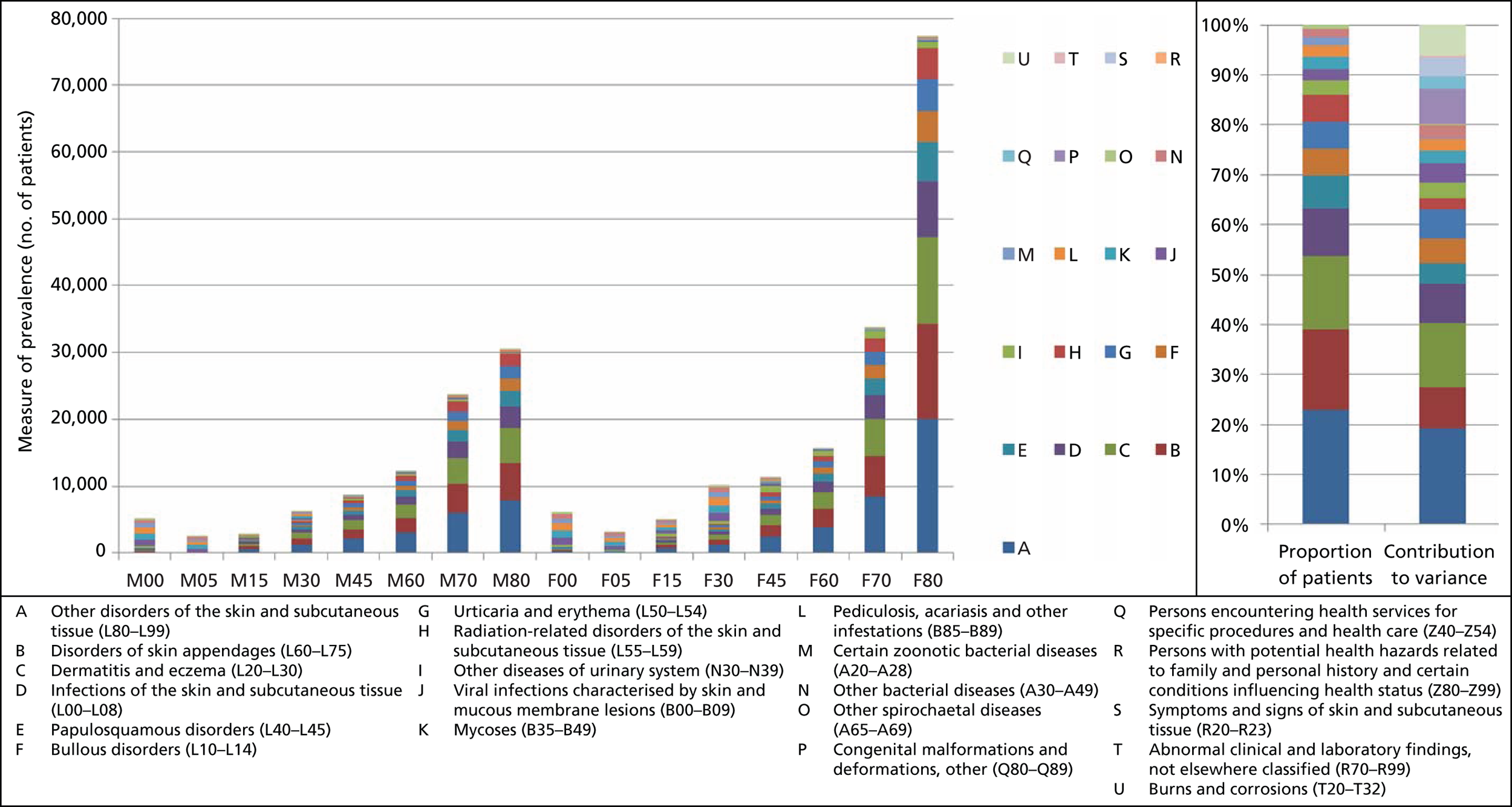
FIGURE 69.
Distribution of PBC 15 prevalence by age, gender and contributing ICD-10 code alongside proportion of patients and contribution to variance of each ICD-10 code. F00, female aged 0–4 years; F05, female aged 5–9 years; F15, female aged 10–14 years; F30, female aged 15–29 years; F45, female aged 30–44 years; F60: female aged 45–59 years; F70, female aged 60–69 years; F80, female aged ≥ 70 years; M00, male aged 0–4 years; M05, male aged 5–9 years; M15, male aged 10–14 years; M30, male aged 15–29 years; M45, male aged 30–44 years; M60: male aged 45–59 years; M70, male aged 60–69 years; M80, male aged ≥ 70 years.

FIGURE 70.
Distribution of PBC 17 prevalence by age, gender and contributing ICD-10 code alongside proportion of patients and contribution to variance of each ICD-10 code. F00, female aged 0–4 years; F05, female aged 5–9 years; F15, female aged 10–14 years; F30, female aged 15–29 years; F45, female aged 30–44 years; F60: female aged 45–59 years; F70, female aged 60–69 years; F80, female aged ≥ 70 years; M00, male aged 0–4 years; M05, male aged 5–9 years; M15, male aged 10–14 years; M30, male aged 15–29 years; M45, male aged 30–44 years; M60: male aged 45–59 years; M70, male aged 60–69 years; M80, male aged ≥ 70 years.

FIGURE 71.
Distribution of PBC 18 + 19 prevalence by age, gender and contributing ICD-10 code alongside proportion of patients and contribution to variance of each ICD-10 code. F00, female aged 0–4 years; F05, female aged 5–9 years; F15, female aged 10–14 years; F30, female aged 15–29 years; F45, female aged 30–44 years; F60: female aged 45–59 years; F70, female aged 60–69 years; F80, female aged ≥ 70 years; M00, male aged 0–4 years; M05, male aged 5–9 years; M15, male aged 10–14 years; M30, male aged 15–29 years; M45, male aged 30–44 years; M60: male aged 45–59 years; M70, male aged 60–69 years; M80, male aged ≥ 70 years.

FIGURE 72.
Distribution of PBC 20 prevalence by age, gender and contributing ICD-10 code alongside proportion of patients and contribution to variance of each ICD-10 code. F00, female aged 0–4 years; F05, female aged 5–9 years; F15, female aged 10–14 years; F30, female aged 15–29 years; F45, female aged 30–44 years; F60: female aged 45–59 years; F70, female aged 60–69 years; F80, female aged ≥ 70 years; M00, male aged 0–4 years; M05, male aged 5–9 years; M15, male aged 10–14 years; M30, male aged 15–29 years; M45, male aged 30–44 years; M60: male aged 45–59 years; M70, male aged 60–69 years; M80, male aged ≥ 70 years.

FIGURE 73.
Distribution of PBC 21 prevalence by age, gender and contributing ICD-10 code alongside proportion of patients and contribution to variance of each ICD-10 code. F00, female aged 0–4 years; F05, female aged 5–9 years; F15, female aged 10–14 years; F30, female aged 15–29 years; F45, female aged 30–44 years; F60: female aged 45–59 years; F70, female aged 60–69 years; F80, female aged ≥ 70 years; M00, male aged 0–4 years; M05, male aged 5–9 years; M15, male aged 10–14 years; M30, male aged 15–29 years; M45, male aged 30–44 years; M60: male aged 45–59 years; M70, male aged 60–69 years; M80, male aged ≥ 70 years.

Addendum 2: the role of data on local NHS decisions
The role of local data in this study
The aim of this research project, noted in Chapter 1, is to develop and demonstrate methods for threshold estimation that make best use of routinely available NHS data. The principal focus of that methodological development, as reflected in the main body of this report, has been the use of econometric methods to exploit observed variations in spending and health outcomes between PCTs, at the programme budget level of aggregation.
However, we also aimed to investigate, as a complementary element of the project, the extent to which there may be other, more disaggregated sources of evidence on investment and disinvestment decisions made by local NHS organisations which might inform our analysis.
Specifically, we set out to (a) identify and evaluate what data might be routinely available from local NHS organisations with respect to their decisions to increase or decrease spending on specific services, and (b) consider if and how such evidence might contribute directly toward the quantitative estimates of the threshold (e.g. by providing more granular, contextual information on spending decisions that might assist in the interpretation of model estimates). For example, we wished to explore whether or not there were any routinely collected data from local NHS organisations that could tell us something about which ICD-10 codes within a given PBC might be the focus of investment and disinvestment.
The work, which was undertaken, was therefore focused on the potential use of local data alongside the econometric analysis, rather than their potential use as an alternative means of identifying the marginal cost of a QALY in the NHS. 76
Sources of publicly available data on primary care trust investment and disinvestment
To help us identify possible sources of data on NHS spending decisions, we began by consulting a number of experts within the NHS, identified for us by our collaborator, Professor David Parkin (Chief Economist at NHS South East Coast). These included directors of finance, commissioning and public health. Those discussions helped direct us to a number of initiatives which involved the development of tools or evidence to inform resource allocation decisions, and helped to identify types and sources of documents published by PCTs that potentially contained relevant information on spending decisions. We then undertook a search for publicly available documents, in each case identifying what was available, and assessing its potential relevance for the purposes of this work outlined above. In evaluating each data source, the key considerations were:
-
Whether or not the data were routinely collected: routinely-collected data are preferred, as our overarching aim is to develop a set of methods to estimate the threshold, which can be readily updated from data routinely generated by the NHS.
-
Whether or not the data were in the public domain: published data are preferred to data that can only be obtained on request, because this would increase the cost and effort required in obtaining data from all relevant organisations.
-
Whether or not the data were collected and reported in a systematic and consistent manner that would facilitate comparisons between PCTs, and with sufficient detail to enable us to link spending decisions to specific programme budgets or ICD-10 codes. This aspect of the work was undertaken during 2010.
The following were identified as potential sources of data.
Programme budgeting tools: quadrant analysis – Spend and Outcome Tool
Data are available for 3 years, 2006/7, 2007/8 and 2008/9 under the Spend and Outcome Tool which is available to download (from Public Health England163). Expenditure data are organised by PBC only, with no lower level of disaggregation. The data show, for each PCT, the spend per head this year, the z-score of that spend, and the PCTs national ranking based on their z-score.
Outcomes data have also been captured, with different outcome measures within each PBC. Again, for each outcome there is a related z-score and the PCTs national ranking based on that z-score.
The tool enables users to see graphically how one PCT compares with others nationally, by SHA and by those PCTs similar to it by cluster (e.g. other PCTs in manufacturing towns). The quadrant analysis tool has the origin as the mean PCT for that PBC, with z-score for both expenditure and outcome equal to zero. The x-axis shows outcome, and the y-axis expenditure, both by z-score.
Although a useful tool, this source added little to the data already used in the econometric analysis, as it does not provide any additional information on the allocation of resources within PBCs.
Lists of interventions not normally funded
Most PCTs provide information about interventions not normally funded. However, these were of limited usefulness because most of the procedures listed are those that might be expected (cosmetic surgery, tattoo removal, etc.), and are not particularly informative about the marginal cost per QALY in the NHS. We did not find any information regarding whether or not any previously funded treatments had been added to these lists.
Special therapeutic and cancer committees
These are regionally based (not PCT or SHA) specialised committees that make decisions regarding spending on new cancer medicines and other special therapeutic areas. Although such decisions would be potentially of direct relevance, we were unable to find any public documentation on their processes or decision outcomes.
Quality, Innovation, Productivity and Prevention published data on efficiency savings in the NHS
Introduced in 2009, Quality, Innovation, Productivity and Prevention (QIPP) addresses the quality and productivity challenge faced by the NHS. Developed by NICE, the Cochrane Quality and Productivity topics identify areas where resources could be significantly reduced or stopped completely without reducing the quality of NHS care, releasing cash and/or resources to other areas in the NHS. Each Cochrane topic has been established from systematic reviews undertaken by reviewers at the Cochrane Collaboration.
Every month the Cochrane Collaboration informs NICE as to new or existing Cochrane reviews where they have found that the existing treatment option(s) is(are) harmful or ineffective and should not be used, or where evidence is unavailable or insufficient to support widespread use of that treatment in the NHS. NICE then completes an assessment of a Cochrane topic, to evaluate the efficiency savings that are likely against the QIPP criteria of likely ease of implementation, impact on productivity, and on the quality of care.
Savings per 100,000 patients are calculated, and then efficiency gains per PCT can be calculated. Once a topic has been accepted as best practise, users (PCTs) are encourage to submit their experience of implementing the changes, and the users achieving the best efficiency gains become QIPP examples of best practise.
The data show which procedures are considered an inefficient use of resources. However, to the extent these are based on the means of achieving the same or improved outcomes but with lower resources, they will not be revealing the marginal cost of producing a QALY in the NHS. Furthermore, there is incomplete information about the extent to which PCTs actually implement these recommendations.
NHS Right Care
This website (available at Public Health England164), has a section on the NHS Atlas of Variation in Health care, which seeks to reduce unwarranted variations in health care, defined as ‘. . . variation in the utilisation of health care services that cannot be explained by variation in patients or patients preferences’, to increase value and improve quality.
It also provides an Annual Population Value Review (currently third edition) which uses PB and marginal analysis to deliver QIPP. This provides, among other things, a 10-step, structured approach for PCTs to follow to establish where investment and disinvestment decisions could be made.
Further, it provides a tool for NHS foundation trusts to improve efficiency via service line management.
Although these tools may be being used by PCTs and foundation trusts, it was not clear to what extent that was the case, and there is no routine data on their use by NHS organisations or the decisions that resulted from that.
Health Investment Network: case studies of programme budgeting and marginal analysis
The NHS network, Health Investment Network, was established to provide the access to the latest knowledge and tools to help commissioners optimise their investment and disinvestment decisions. It provides case studies of PCTs which have used PB and marginal analysis to identify efficiency gains. This includes examples of ‘spend to save’ decisions, for example where an initial investment (e.g. in vascular checks for men in deprived areas) could be more than outweighed by savings. Such initiatives, although important, are not useful in identifying the marginal cost per QALY in the NHS. Other case studies identify ‘wish lists’ (areas which PCTs prioritise for additional spending, should budgets expand) and ‘hit lists’ (services that might be reduced, to free up resources for more cost-effective services). These case studies provide useful selected examples, but do not provide a routine or systematic reporting of such decisions across all PCTs.
Annual operating plans and strategic commissioning plans
Primary care trusts are required to publish, each year, operating plans and strategic commissioning plans detailing their planning for the coming year, including information on the way that PCTs have made decisions concerning resource allocation. As these reports are published annually, we considered that they constituted the most promising source of data, as they are produced routinely, and cover all PCTs.
Contact details and websites were identified for all 142 PCTs. Strategic commissioning plans were obtained for an initial 70 of these. These were used to identify any information provided about programmes of care or specific services where spending was planned to be increased or decreased. Those data were extracted and recorded into a spreadsheet, along with any relevant contextual information (e.g. relating to the process by which the decision had been made).
Our review of the data from the first 70 of these showed that there was considerable variation between the documents in terms of the level of detail and specificity about the services which were the subject of changes in spending. In many cases, the services were described in terms of broad initiatives which might have related to multiple programme budgets and ICD-10 codes. There was also variation in, and occasionally a lack of clarity about, the way in which spending changes were described. For example, in some cases changes were described in terms of absolute changes in spending, in others as net changes (once estimates of offsetting savings elsewhere had been taken into account), and in others it was not stated.
Given those concerns, the data were considered unlikely to be useful to complement the econometric analysis, and the research team decided not to proceed with further data extraction for the remaining PCTs.
Conclusions
The context within which this element of the work took place may be relevant to note. Although the NHS was not subject to the budget cuts imposed on other areas of government activity in response to the financial crisis, the NHS was required to make substantial productivity improvements within its existing budgets. This gave rise to a number of initiatives in response to the ‘productivity challenge’ and, generally, heightened interest in the identification of ways to improve efficiency; potential areas for disinvestment; and areas for investment which were motivated by ‘spend to save’. This may have made it more likely that we would observe disinvestment decisions. The NHS was also, during the course of this project, undergoing a period of restructuring. The transition from PCTs to CCGs, and the disestablishment of SHAs, may have had an effect on the availability of data and information relating to decision-making. It may also have broader implications for the availability of data in the future, given the change in administrative units.
Our review of local data sources suggested that there is very little routinely collected data on investment and disinvestment by local NHS organisations beyond the high-level aggregate data on spending by PBC which are used in the econometric analysis. More disaggregated data on spending decisions about specific services could, of course, be obtained by other means, for example by surveying PCTs, or by requesting such information from them using a freedom of information request. However, that would impose data collection costs and would need to be designed carefully to ensure that such efforts yielded complete and consistent information.
Addendum 3: characterisation of the investment and disinvestment decisions in mental health – depression and schizophrenia
Introduction
As has been highlighted in the main body of this project, it was not possible to produce an outcome equation for PBC 5, mental health problems, because no relevant mortality data was available from the NHS IC by PCT. Mental health represents a significant incidence and expenditure within the NHS. As a result, we investigated the direction of bias from the exclusion of mental health problems on our estimate of the cost-effectiveness threshold. To understand this bias we examined current investment decisions in mental health. Recent investments in treatments with ICERs above the estimated threshold would suggest that not including PBC 5 more directly in our calculation may underestimate the threshold, conversely if recent investment has ICERs below the estimated threshold it would suggest that its exclusion results in an overestimated threshold. We focussed on depression and schizophrenia because of their high prevalence and contribution to variance.
Method employed
To evaluate the direction of bias of the exclusion of PBC 5 we followed four steps to make the connection from the identification of the most significant ICD-10 codes of PBC 5 to considering the cost-effectiveness of the investment and disinvestment decisions made in the NHS around these disease areas. The strategy was as follows.
Step 1
-
Identify the mental health ICD-10 codes that are most influential and suitable on which to focus our analysis.
-
Done from number of patients and contribution to variance calculations using HES.
-
Step 2
-
Determine the medications or treatments used in the NHS to treat each of the significant ICD-10 codes.
-
There is likely to be a large crossover in the use of treatments for mental health areas, for example antipsychotics and cognitive–behavioural therapy (CBT) are both widely used.
-
We made use of the NHS Choices website coupled with clinical expertise for this identification.
-
Step 3
-
Identify the cost-effectiveness of the current treatments and medications used in the NHS.
-
This identification will be done from a range of sources including published HTAs, published guidance, Cost-Effectiveness Analysis Registry (or Tuft’s), NHS EED and MEDLINE searches.
-
This step relies heavily on the literature published; literature tends to cover historical activities many of which represent treatments of interest for this analysis. The case could be made that historical treatments that have not been evaluated have escaped evaluation due to their apparent cost-effectiveness, and are as such unlikely to be marginal activities.
-
Further difficulties arose in the identification of the relevant cost-effectiveness figure. Ideally, it would represent the cost per QALY relative to what would be performed if that activity was no longer available to the NHS.
-
Step 4
-
Connecting the available literature on the cost-effectiveness to recent investment and disinvestment decisions made in the NHS.
Results of analysis
Step 1: identification of relevant International Classification of Disease codes
We first rank ICD-10 codes by prevalence and contribution to variance. Prevalence is estimated from HES data. The contribution to variance is calculated as the variance in expenditure across PCTs for each ICD-10 code compared with the total variance in expenditure across PCTs for all ICD-10 codes within PBC 5. The most prevalent ICD-10 code was for mental and behavioural disorders due to use of alcohol (F10) at 7.48% of all ICD-10 codes within PBC 5 (Table 193). The ICD-10 code with the greatest contribution to variance was for schizophrenia (F20) with 45.16% (Table 194).
ICD-10 code | Description | % of mental health prevalence | Contribution to variance |
---|---|---|---|
F10 | Mental and behavioural disorders due to use of alcohol | 27.84 | 9.70 |
F20 | Schizophrenia | 10.01 | 45.16 |
F32 | Depressive episode | 9.96 | 6.91 |
F31 | Bipolar affective disorder | 6.19 | 6.38 |
F41 | Other anxiety disorders | 4.92 | 0.26 |
F60 | Specific personality disorders | 4.33 | 14.11 |
F03 | Unspecified dementia | 3.93 | 3.29 |
F01 | Vascular dementia | 3.32 | 1.58 |
G30 | Alzheimer‘s disease | 3.30 | 0.84 |
F33 | Recurrent depressive disorder | 2.83 | 3.68 |
ICD-10 code | Description | % of mental health prevalence | Contribution to variance |
---|---|---|---|
F20 | Schizophrenia | 10.01 | 45.16 |
F60 | Specific personality disorders | 4.33 | 14.11 |
F10 | Mental and behavioural disorders due to use of alcohol | 27.84 | 9.70 |
F32 | Depressive episode | 9.96 | 6.91 |
F31 | Bipolar affective disorder | 6.19 | 6.38 |
F33 | Recurrent depressive disorder | 2.83 | 3.68 |
F03 | Unspecified dementia | 3.93 | 3.29 |
F01 | Vascular dementia | 3.32 | 1.58 |
G30 | Alzheimer‘s disease | 3.30 | 0.84 |
F41 | Other anxiety disorders | 4.92 | 0.26 |
Depression (F32) and (F33) and schizophrenia (F20) have been chosen as the focus of our evaluation as they represent two of the largest mental health ICD-10 codes in terms of proportion of patients as well as proportion of variance in expenditure, as shown in Tables 193 and 194. In addition, they represent ICD-10 codes that involve interventions by the NHS that can be more clearly defined (in contrast to, for example, unspecified dementia and mental and behavioural disorders due to the use of alcohol). ad
Step 2: determination of treatment employed
Table 195 provided an overview of the main treatments for depression and schizophrenia. This list of treatments was identified using the NHS Choices website165 as well as discussion with our clinical representative for each of the respective illnesses. This list was used to inform a literature search of cost-effectiveness publications.
ICD-10 code | Disease | Treatment |
---|---|---|
F20 | Schizophrenia |
|
F32 and F33 | Depressive episode and recurrent depressive episode |
|
Step 3: evaluation of the relevant cost-effectiveness literature
Using the treatment categories identified in step 2 of this work, a systematic search was conducted to attempt to identify the range of literature on the cost-effectiveness of current NHS treatment of schizophrenia and depression. For both illnesses five online databases were searched: the Cost-Effectiveness Analysis Registry of the Tufts Medical Centre, NHS EED run by the Centre for Reviews and Dissemination at the University of York, MEDLINE, the NICE online database of Technical Appraisals and Clinical Guidelines, as well as NIHR’s Health Technology Assessments. All searches were conducted on the 19 October 2011.
The search strategies employed to search for relevant cost-effectiveness literature and details of the results can be found in the search strategy section at the end of this addendum. For schizophrenia, this approach identified 61 unique publications, five of which were deemed to be of broad relevance to this analysis. For depression, 65 publications were discovered, 10 of which were relevant. A paper of relevance to our analysis of mental health was deemed to be so if it presented cost-effectiveness results (in the form of a cost per QALY ICER) of a comparison of at least two of the treatments for either schizophrenia or depression identified in Analysis of 2006/7 expenditure and 2006/7/8 mortality data. These results could be from a de novo analysis or from a systematic review of the relevant literature.
Table 196 reports the cost-effectiveness results of antipsychotics for schizophrenia as first-line treatments. The NICE Clinical Guidelines for schizophrenia (CG82)166 demonstrate that the differences in costs and effects of the first- and second-generation treatments described are very similar with ICERs comparing each with no treatment ranging from £21,517 to £23,237 per QALY. Comparisons to active treatments result in ICERs of £5156 to £33,240 per QALY. 167,168
Study | Treatment | Comparator | Cost (£) | QALYs | ICER (£/QALY) |
---|---|---|---|---|---|
NICE CG82166 | Zotepine (Zoleptil®, Movianto) (second) | No treatment | 139,170 | 6.468 | 21,517 |
Paliperidone (Invega®, Johnson & Johnson) (second) | No treatment | 142,173 | 6.427 | 22,121 | |
Olanzapine (Zyprexa®, Eli Lilly) (second) | No treatment | 141,212 | 6.42 | 21,996 | |
Risperidone (Risperdal®, Johnson & Johnson) (second) | No treatment | 149,112 | 6.417 | 23,237 | |
Haloperidol (Haldol®, McNeil Laboratories) (first) | No treatment | 143,406 | 6.413 | 22,362 | |
Aripiprazole (Abilify®, Bristol-Myers Squibb) (second) | No treatment | 145,697 | 6.400 | 22,765 | |
Amisulpride (Arnival®, Sanofi-Aventis) (second) | No treatment | 147,920 | 6.392 | 23,141 | |
Knapp et al. 2008167 | Olanzapine (second) | Other antipsychotics | 5156 | ||
Davies et al. 2008168 | Clozapine (Clozaril®, Novartis) (second) | Other second-generation antipsychotics | 33,240 | ||
Aripiprazole then risperidone | Risperidone then olanzapine | 9440 |
The CG82 results are similar to the first-line treatment results from Bagnall et al. ,169 shown in Table 197. The cost-effectiveness of antipsychotics compared with no treatment as second, third or final therapy are < £20,000 per QALY.
Bagnall et al. 2003169 | ||||
---|---|---|---|---|
Antipsychotic | Line of treatment: ICER (£/QALY) | |||
First | Second | Third | Final | |
Chlorpromazine (Largactil®, Sanofi-Aventis) (first) | 21,989 | 15,185 | 15,419 | 15,303 |
Haloperidol (first) | 24,069 | 17,177 | 17,211 | 17,022 |
Clozapine (second) | 24,500 | 17,595 | 17,577 | 17,402 |
Olanzapine (second) | 25,719 | 18,869 | 18,808 | 18,865 |
Quetiapine (Seroquel®, AstraZeneca) (second) | 26,316 | 19,090 | 18,751 | 19,096 |
Zotepine (second) | 22,769 | 16,350 | 16,360 | 16,400 |
Risperidone (second) | 22,255 | 15,596 | 15,599 | 15,700 |
Ziprasidone (Geodon®, Pfizer) (second) | 21,935 | 15,192 | 15,191 | 15,224 |
Amisulpride (second) | 23,174 | 15,941 | 15,945 | 15,962 |
Sertindole (Serdolect®, Lundbeck) (atypical) | 23,181 | 16,297 | 16,308 | 16,354 |
Only one study reported the cost-effectiveness of a psychological or social intervention for schizophrenia. Barton et al. 170 conducted a randomised trial to estimate the clinical effectiveness and cost-effectiveness of social recovery orientated cognitive–behavioural therapy (SRCBT) against case management alone for people recently diagnosed with psychosis. SRCBT consisted of three stages of social recovery combined with CBT techniques including vocational case management. SRCBT was found to have an ICER of £18,844 per QALY compared with case management. However, it is not clear that all forms of CBT are well represented by this one study or that these results relate well to schizophrenia as this study was for the use of SRCBT for psychosis disorders in general.
Table 198 reports the cost-effectiveness results of publications identified in the systematic search of drug treatments for depression in the NHS. As was highlighted in Table 195, a range of drug treatments are available for depression, broadly falling into five categories: selective serotonin reuptake inhibitors (SSRIs), serotonin–norepinephrine reuptake inhibitors (SNRIs), tricyclic antidepressants (TCAs), monoamine oxidase inhibitors and lithium.
Study | Treatment | Comparator | Incremental cost | Incremental QALY (£) | ICER (£/QALY) |
---|---|---|---|---|---|
NICE CG90171 | Combined SSRI and CBT (severe depression) | SSRI alone | 5558 | ||
Duloxetine (Cymbalta®, Eli Lilly) (SNRI) | SSRI | 6300 | |||
Duloxetine (SNRI) | Mirtazapine (Avanza®, Organon) (TCA) | 2400 | |||
Duloxetine (SNRI) | Venlafaxine (Effexor®, Pfizer) (SNRI) | Dominates | |||
Escitalopram (Lexapro®, Forest Laboratories) (moderate depression) (SSRI) | Sertraline (Zoloft®, Pfizer) (SSRI) | 32,987 | |||
Escitalopram (severe depression) (SSRI) | Sertraline (SSRI) | 27,172 | |||
Lenox-Smith et al. 2009172 | Venlafaxine (major depression) (SNRI) | SSRI | 20,600 | ||
Fluoxetine (Prozac®, Eli Lilly) (SSRI) | Amitriptyline (Elavil®, Densa) (TCA) | Dominated | |||
Kendrick et al. 2006173 | SSRI | TCA | 2692 | ||
TCA | Lofepramine (Gamanil®, GlaxoSmithKline) (TCA) | Dominated | |||
SSRI | Lofepramine (TCA) | 5686 | |||
Hatziandreu et al. 1994174 | Sertraline (SSRI) | Dothiepin (TCA) | 2172 | ||
Peveler et al. 2005175 | SSRI | Lofepramine (TCA) | 0.035 | 199 | 5686 |
TCA | Lofepramine (TCA) | −0.004 | 93 | Dominated | |
SSRI | TCA | 0.039 | 105 | 2692 | |
Kendrick et al. 2009176 | SSRI + standard care | Standard care | 14,854 |
The NICE guideline CG90 tested the cost-effectiveness of numerous treatments for moderate and severe depression. It was found that across all the treatments tested the mean QALYs for moderate depression had a range of 0.053 and severe depression had a range of 0.065. The costs had a range of £408 for moderate and £396 for severe depression. The results suggest that mirtazapine has the lowest ICER for both moderate and severe depression. If mirtazapine is not a suitable treatment option than escitalopram or sertraline is preferred because escitalopram dominates venlafaxine and sertraline dominates the remaining antidepressants. The ICERs of escitalopram versus sertraline are £32,987 per QALY for moderate depression and £27,172 per QALY for severe. The authors thus suggest that according to these results escitalopram should be considered when mirtazapine and sertraline are not suitable. Other ICERs reported in CG90 can be found in Table 198. CG90 states that the economic evidence had limitations and these comparisons were considered insufficient to make specific recommendations for treatments.
Incremental cost-effectiveness ratios in other studies range from £2172 to £20,600 per QALY, with TCA alone being dominated by lofepramine (TCA) in two cases and fluoxetine (SSRI) being dominated by amitriptyline (TCA).
Table 199 provides the results of the combination therapies for moderate and severe depression presented in CG90171 and Simon et al. 177 These studies considered the impact of combined SSRI and CBT compared with SSRI alone. Both of these studies find combined CBT and antidepressant to have ICERs of < £8000 per QALY.
Study | Treatment | Comparator | Incremental cost (£) | Incremental QALY | ICER (£/QALY) |
---|---|---|---|---|---|
NICE CG90171 | Combined SSRI and CBT (moderate depression) | SSRI alone | 7052 | ||
Combined SSRI and CBT (severe depression) | SSRI alone | 5558 | |||
Simon et al. 2006177 | CBT + antidepressants in severe depression | Fluoxetine | 5777 | ||
CBT + antidepressants in moderate depression | Fluoxetine | 14,540 | |||
Kaltenthaler et al. 2002178 | BtB computerised CBT | TAU | 1209–7692 | ||
Kaltenthaler et al. 2006179 | BtB | TAU | 147 | 0.08 | 1801 |
Cope computerised CBT | TAU | 193 | 0.03 | 7139 | |
Overcoming depression computerised CBT | TAU | 64 | 0.01 | 5391 | |
FearFighter computerised CBT | Relaxation CBT | 138 | 0.058 | 2380 | |
Therapist lead CBT | Relaxation | 194 | 0.011 | 17,604 | |
Behavioural therapy steps computerised CBT | Relaxation | 360 | −0.01 | Dominated | |
Hollinghurst et al. 2010180 | Online computerised CBT | TAU | 17,173 |
In addition Table 199 provides results of analyses of computerised CBT compared with treatment as usual or relaxation. The results generally find CBT and computerised CBT to be highly cost-effective, with the exception of Behavioral Therapy Steps179 all ICERs are found to be < £18,000 per QALY.
Step 4: connection to investment and disinvestment decisions
In this section we discuss the investment and disinvestment decisions made considering the cost-effectiveness information in the previous section. If we believe that decision-makers will invest in treatments below their cost-effectiveness threshold and disinvest in treatments above this threshold then by considering the ICERs of treatments subject to investment and disinvestment we can create a range for their cost-effectiveness threshold. This approach and its role in the consideration of a cost-effectiveness threshold has been previously discussed by Appleby et al. 49 With a view of the cost-effectiveness threshold within PBC 5 we consider how its exclusion from our calculation of the threshold might influence our results.
To identify the broad areas of investment in the disease areas we make use of recent NICE guidance documents. Although NICE clinical guidance does not definitively represent observed shifts in practice and are often not well implemented in mental health trusts181 it can help to inform our evaluation. NICE guidance does not identify areas where disinvestment should occur within a disease; as a result, we have consulted experts in the respective fields to gain an understanding of any significant recent disinvestment decisions. For schizophrenia we were provided expert opinion by Professor Tim Kendall (Centre for Psychological Services Research, University of Sheffield) and for depression by Professor Simon Gilbody (Health Sciences, University of York).
For both schizophrenia and depression we will briefly discuss the areas of investment and disinvestment in two care categories: (i) drug treatments, and (ii) psychological and social interventions.
Results of step 4 for schizophrenia
Analysis of drug treatment
Antipsychotics used for the treatment of schizophrenia can be broadly identified as first or second generation (typical and atypical antipsychotics). To a certain extent there is still a debate over the relative strengths and weaknesses of each,182 and the significance of the adverse events associated with the second generation may still not be fully understood (such as the impact on new-onset type 2 diabetes183). However, our clinical experts indicated that clinicians were largely concerned with the adverse effects associated with the second-generation drugs, and the increasing evidence questioning the relative efficacy, such as Rosenheck et al. 182 who suggests that the first-generation drugs in many cases are just as effective. Recent NICE guidance leaves the choice of first- or second-generation drugs to the clinician to decide. 166
When considering the impact on our estimate of the threshold of the possible shift to first- from second-generation antipsychotics we must attempt to generalise about the relative cost-effectiveness of the two. Clearly this is difficult as each generation represents many different drugs. However, from CG82 the costs and benefits of the mainstream antipsychotics are broadly similar Table 196. This would suggest that a shift away from the second generation back towards the first would have little impact on the overall threshold as the costs and benefits associated with each are very similar.
Olanzapine came off patent in the third quarter of 2011. 184 Olanzapine and similar second-generation antipsychotics are associated with a cost of around £30M a year,ae clearly the introduction of generics to the market would significantly reduce this cost and thus increases the cost-effectiveness of these drugs. Although this shift does not fall within the years of our analysis, it will have a significant impact on the future value of the cost-effectiveness threshold.
The other significant area of debate, as identified by our clinician, is the role of the antipsychotic clozapine. It has often been viewed as the most effective antipsychotic drug for schizophrenia, however, the antipsychotic clozapine has been connected with some severe adverse events (such as myocarditis,185 agranulocytosis186 and central nervous system depression). This has led to the NICE guidelines advising clozapine only if an array of other antipsychotics has failed. 166 Although clozapine is highly clinically effective it is associated with a higher overall cost (a significant proportion due to the associated adverse events). As is shown in Table 196, Davies et al. 168 show clozapine to have an ICER of £33,240 when compared with other second-generation antipsychotics. Disinvestment of clozapine suggests that the threshold is < £33,240 per QALY. However, current investment in other first-line antipsychotics suggests that the threshold in mental health is > £23,237 per QALY.
Analysis of psychological and social intervention
In this section we discuss all non-drug-related interventions for schizophrenia. The NICE guidelines166 outline the provision of CBT, arts therapy and family interventions to treat schizophrenia; however, efficacy of these interventions is disputed187 and little is known about their cost-effectiveness. The systematic review only yielded one paper that was relevant to our analysis, as is shown in Table 200. The Barton et al. 170 study found that SRCBT had an ICER of < £20,000 per QALY relative to case management. However, as mentioned previously, this study may not represent all forms of CBT for schizophrenia.
Study | Treatment | Comparator | ICER (£/QALY) |
---|---|---|---|
Barton et al. 2009170 | SRCBT | Case management | 18,844 |
Our clinical advisors informed us that CBT provision varies significantly across PCTs and therefore represents an intervention likely to be subject to investment and disinvestment at the margin. The variation in CBT provision (and indeed other psychosocial/social interventions) is largely a result of the poor support for its efficacy and significant initial cost.
Other interventions of relevance to this investigation include art therapies and family interventions. As with CBT there is a significant variation in the provision of family interventions. No information on its cost-effectiveness was found from our search. Art therapies include music therapy; art therapy; and body movement or dance therapy. Our clinical advisors have highlighted increasing investigations into arts therapy, including the ‘Matisse trial’,188 publications around which have shown that art therapy as adjunctive therapy had little benefit over a comparator activity or treatment as usual. 187 No information on its cost-effectiveness was found from our search.
Early interventions in schizophrenia, which aims to identify and treat early symptoms associated with schizophrenia, have been a significant area of investment over recent years in the NHS. Although we were unable to identify any relevant cost-effectiveness literature around early interventions in schizophrenia it is generally expected that these represent cost-effective interventions over the long term. af
Although the lack of cost-effectiveness literature clearly limits the potential to directly associate these interventions with the wider cost-effectiveness threshold it is widely accepted that many social interventions for schizophrenia (specifically around CBT and family interventions) are cost-saving for the NHS,af as they reduce hospitalisation by reducing emergency hospital access and relapse rates that are high in schizophrenia representing the majority of related hospitalisations. 189
Investment in CBT with an expected ICER of £18,844 per QALY suggests that the threshold for mental health treatments is above this value.
Results of step 4 for depression
As Table 195 shows, depression is associated with a wider range of treatment than schizophrenia, specifically a wider range of drug treatments are available. As with the schizophrenia section of this addendum we will deal with the treatments under the two categories of drug treatments and psychological/social interventions. Electroconvulsive therapy, which is included in the treatment options available in the NHS as shown in Table 195, is excluded from this analysis based on expert opinion on the grounds of it being a very rarely used but extreme treatment that is not likely to be further subject to substantial investment or disinvestment, so is not relevant for our analysis.
Recent NICE clinical guidance171 highlights a range of key priorities for implementation. As with schizophrenia there is no guarantee that these are the areas of investment in depression care but it represents a suitable outline of the areas of interest. Several areas are highlighted:
-
early identification and diagnosis
-
low intensity psychological interventions (CBT, computerised CBT and group physical activity) for persistent subthreshold depressive symptoms or mild to moderate depression
-
reduced routine use of antidepressants for subthreshold depressive symptoms or mild depression
-
combination therapies (antidepressant and psychological) for moderate or severe depression
-
extension of therapy (antidepressant and psychological) beyond remission to reduce relapse
-
SSRIs are presented as the preferred type of antidepressant due to their equivalent efficacy and favourable risk–benefit ratio.
These are the areas of investment that our analysis will focus on.
Analysis of drug treatment
Our clinical advisors reported that the current area of activity in antidepressants is the creation of drugs such as escitalopram (a SSRI) and venlafaxine (a SNRI) that are relatively similar to generic treatments currently in the market. As these new drugs are covered by patents they are relatively expensive. Table 198 reports the results on the cost-effectiveness of these two drugs from NICE CG90171 as well as Lenox-Smith et al. 172 In both reports the drugs are compared with alternative SSRIs in moderate and severe depression. In both cases the newer SSRIs were approved by NICE with an ICER for escitalopram of £32,987 per QALY and for venlafaxine of £20,600 per QALY. If mirtazapine and sertraline are not suitable then the ICER of escitalopram for moderate depression is £5357 per QALY compared with citalopram. Although evidence was not available on whether or not clinicians were making use of these newer SSRIs, an investment in them away from alternative SSRIs may represent an increase in the cost-effectiveness threshold due to the relatively high ICERs reported in the two studies. However, the cost-effectiveness of each depends on what they displace and ICERs may be lower if the more cost-effective treatments have failed.
Investment decisions in the NHS for antidepressants are likely to represent changes in the type of antidepressant being prescribed rather than a shift from no treatment to treatment. The majority of trials discovered by systematic review given in Table 198 show that although the ICER of SSRIs compared with TCAs is very low,175 this is largely driven by very small gains in QALYs but for a similarly small increase in cost. As a result any observed investment in SSRIs away from TCAs is likely to lead to a small decrease in an observed threshold for the NHS.
Analysis of psychological and social intervention
The NICE guidelines reported in CG90 place a lot of focus on the provision of psychological interventions such as CBT (and computerised CBT) over antipsychotics wherever possible. Table 199 provides the results of the combination therapies for moderate and severe depression presented in CG90171 and Simon et al. 177 These considered the impact of combined SSRI and CBT compared with SSRI alone and concluded that combined therapies in both populations had ICERs of < £15,000 per QALY. According to our clinicians, this is an area that is likely to have had significant investment in recent years.
The two HTA reports in Table 199178,179 provide a good analysis of the cost-effectiveness of computerised CBT compared with treatment as usual. They show that the computerised CBTs investigated have ICER of < £8000 per QALY relative to TAU. Further analyses investigated different kinds of computerised CBT and found that compared with relaxation, CBT ICERs ranged from £2380 per QALY to dominated. Hollinghurst et al. 180 report that two CBT interventions compared to TAU had ICERs of £17,173.
As NICE guidelines encourage the use of CBT and our clinical experts believe this has been an area of increased investment, this review suggests that the threshold in mental health is > £17,173 per QALY.
Conclusion
There is very little accessible data on the investment and disinvestment decisions in specific areas of mental health and so we relied on the opinions of clinical experts. The NHS IC has some information on prescriptions of mental health treatments; however, it was not clear for which diseases these treatments were being used or for which line of therapy. As a result, these data were not included in our analysis as it was decided they may not represent the investment and disinvestment decisions that we were seeking to identify.
Most treatments reviewed had an ICER of < £24,000 per QALY. Two treatments had higher ICERs. Clozapine for the first-line treatment of schizophrenia was found to have an ICER of £33,240 per QALY compared to other second-generation antipsychotics. NICE’s recommendation to use clozapine only as a last-line treatment suggests that the threshold is < £33,240 per QALY. Escitalopram for moderate depression has been recommended by NICE and was reported to have an ICER of £32,987 per QALY compared with seratraline. Conclusions on the threshold from this finding are unclear. The cost-effectiveness of escitalopram in the NHS will depend on its use. If it is used rather than seratraline then the threshold may be over £32,987, but if it is used as third-line therapy than according to CG90 its use is less costly and more effective than the next best options.
How well the actual threshold reflects the ICERs reported above depends on how well clinical practice matches the clinical guidelines (i.e. whether or not the more cost-effective treatments are being used first).
Search strategies
Search strategy for schizophrenia
Cost-Effectiveness Analysis Registry search
Six keywords associated with the entire schizophrenia, schizotypal and delusional disorders. ICD-10 subchapters were searched for in the CEA Registry, these were: schizophrenia, schizotypal, delusional, psychotic, schizoaffective and psychosis. A search for any of these keywords in the registry yielded 18 different papers at the time of searching, with five of these being deemed suitable for our investigation (Barton et al. ,170 Davies et al. ,168 Jarbrink et al. ,190 Knapp et al. 167 and Davies et al. 191).
NHS Economic Evaluation Database search
A single relatively simple search strategy was defined to investigate NHS EED, this was as follows:
((Schizophrenia) AND (cost effectiveness):TI) and Economic evaluation:ZDT and Abstract:ZPS
This result strategy yielded 28 hits, only one of which was both relevant to our search and not discovered in the CEA Registry search (Rosenheck et al. ). 182
MEDLINE search
MEDLINE was searched using the strategy:
cost benefit analysis and (schizophrenia or schizotypal personality disorder or delusions) and Great britain(MeSH)
This strategy yielded 13 hits, none of which were both relevant and had not been previously identified through the CEA Registry or NHS EED searches.
National Institute for Health and Care Excellence technology appraisals and clinical guidelines
The NICE online database of published mental health-related technology appraisals and clinical guidelines (www.nice.org.uk/guidance/index.jsp?action=byTopic&o=7281) was searched for schizophrenia-related publications. Only one was found to fulfil our criteria for schizophrenia: CG82. 192
National Institute for Health Research’s Health Technology Assessments
Finally the NIHR’s database of published HTAs was searched. This activity discovered one additional relevant publication: HTA 00/20/01 – Bagnall et al. 169
Search strategy for depression
Cost-Effectiveness Analysis Registry search
Two keywords were searched on the CEA Registry, they were: depression and depressive. These keywords yielded 17 papers, five of which were deemed relevant for our purposes (Hollinghurst et al. ,180 Lenox-Smith et al. ,172 Kendrick et al. ,173 Simon et al. 177 and Hatzinandreu et al. 174).
NHS Economic Evaluation Database search
A search similar in structure to the search for schizophrenia papers was conducted in the NHS EED:
((depressive OR depression):TI AND (cost-effectiveness):TI) and Economic evaluation:ZDT and Abstract:ZPS) IN NHSEED
This yielded 43 hits, none of which were both relevant and previously undiscovered by the CEA registry search. Due to the complete nature of the CEA Registry and NHS EED searches, as well as time constraints on the systematic review, a MEDLINE search was not conducted as it was decided it would not provide sufficient added value.
National Institute for Health and Care Excellence technology appraisals and clinical guidelines
Searching the NICE database of technology appraisals and clinical guidelines yielded one publication deemed relevant to the analysis: CG90 – depression in adults. 193
National Institute for Health Research’s Health Technology Assessments
A search of the NIHR’s online database of published HTAs yielded four relevant publications:
HTA 01/23/01– Kaltenthaler et al. 178
HTA 04/01/01– Kaltenthaler et al. 179
HTA 96/61/11– Peveler et al. 175
HTA 01/70/05 – Kendrick et al. 176
Addendum 4: what type of health is forgone by the approval of a new technology?
The methods of analysis described in this work can identify not only how many QALYs are likely to be forgone across the NHS as a consequence of approving a technology which imposes incremental costs on the NHS, it can also indicate where those QALYs are likely to be forgone and how they are made up [i.e. the additional deaths, life-years lost (unadjusted and adjusted for QoL) and the QoL impacts on those with disease]. Based on the 2008 central estimate of the cost per QALY threshold, we will exemplify within this addendum the likely health displaced elsewhere in the NHS as a consequence of approving a new technology.
The example of ranibizumab for diabetic macular oedema
In 2011, NICE considered whether or not ranibizumab for the treatment of diabetic macular oedema in patients with central retinal thickness ≥ 400 micrometres should be approved for widespread use in the NHS (TA237105). Initially, this technology was rejected by NICE on the grounds that, at its current price, it would be unlikely to be cost-effective. In 2012, however, a rapid review of TA237106 approved ranibizumab if use was restricted to the most cost-effective subgroup (those with central retinal thickness ≥ 400 micrometres) and after a PAS for this subgroup of patients was offered (details of the PAS which provides a discount to the NHS is commercial in confidence). The committee concluded that the most plausible ICER for the subgroup of people with thicker retinas was likely to be higher than the manufacturer’s estimate (of £13,322 per QALY), but would be < £25,000 per QALY gained. 106
The appraisal and guidance documents106 provide the information required to estimate the additional NHS costs of treating this subgroup of patients each year. The original manufacturer submission presented an estimate of the numbers of patients in the NHS eligible to receive ranibizumab, based on its licensed indication. 107 These estimates are presented in Table 201. In the first year of implementation, up to 44,000 NHS patients would be eligible for treatment with ranibizumab based on its licensed indication. No consideration is made as to the size of the subpopulation approved for treatment; however, the RESTORE trial (that informs the submission) found approximately half of the participants in the study to be in this subpopulation [114 of 217 (52.5%)]. 105 The subgroup of patients where ranibizumab was ultimately approved is thus likely to be approximately 23,000 in the first year after approval.
Licensed indication | 2011 | 2012 | 2013 | 2014 | 2015 |
---|---|---|---|---|---|
Prevalent cases | 43,847 | 0 | 0 | 0 | 0 |
Incident cases | 0 | 5481 | 5481 | 5481 | 5481 |
Total eligible number of patients | 43,847 | 49,328 | 54,809 | 60,290 | 65,771 |
Subpopulation approved for treatment by NICE | |||||
Prevalent cases | 23,020 | 0 | 0 | 0 | 0 |
Incident cases | 0 | 2878 | 2878 | 2878 | 2878 |
Total eligible number of patients | 23,020 | 25,897 | 28,775 | 31,652 | 34,530 |
The incremental costs associated with the new treatment (compared with laser monotherapy) in the initial submission (TA237) were £3506 per patient (Table 202). 107 Given estimates reported in the rapid review are not available (commercial in confidence), we will use this estimate of incremental costs for the subpopulation of interest. These data suggest that the approval of ranibizumab in this subgroup at the original appraisal in 2011 (i.e. without a PAS) would impose just over £80M of additional NHS costs for treating the eligible population each year.
2011 | 2012 | 2013 | 2014 | 2015 | |
---|---|---|---|---|---|
Total eligible number of patients | 23,020 | 25,897 | 28,775 | 31,652 | 34,530 |
Total cost, without PAS (£) | 80,708,120 | 90,794,882 | 100,885,150 | 110,971,912 | 121,062,180 |
Total cost, 30% lower incremental costs (£) | 56,495,684 | 63,556,417 | 70,619,605 | 77,680,338 | 84,743,526 |
With introduction of the PAS, it is likely that a simple discount on the acquisition price of the new technology has been approved by the Department of Health. 106 Given the scale of the discount is not available (commercial in confidence), we assumed that this discount would reduce incremental costs by 30% (to £2454 per patient). After such a PAS, the approval of ranibizumab in this subgroup would impose just £56M (rather than £80M) of additional NHS costs for treating the eligible population in the first year.
What type of health is forgone by approval of a new technology?
Based on the 2008 central estimate of the cost per QALY threshold (£12,936 in Table 30), the approval of ranibizumab without a PAS would have been likely to displace 6184 QALYs elsewhere in the NHS. However, the analysis which underpins the threshold estimate can also be used to identify where the additional NHS cost of £80M are likely to impact and where and what type of health effects are likely to be forgone. This is illustrated in Table 203.
PBC | (1) Change in spend (£M) | (2) Additional deaths | (3) Life-years foregone | QALYs foregone | |||
---|---|---|---|---|---|---|---|
(4) Total QALYs forgone | (5) Due to premature death | (6) QoL effects | |||||
2 | Cancer | 3.58 | 30 | 300 | 211 | 195 | 16 |
10 | Circulatory problems | 6.07 | 182 | 928 | 863 | 590 | 273 |
11 | Respiratory problems | 3.67 | 107 | 129 | 1835 | 80 | 1754 |
13 | Gastrointestinal | 2.56 | 21 | 197 | 351 | 129 | 222 |
All big four programmes | 16 | 340 | 1554 | 3259 | 995 | 2265 | |
1 | Infectious diseases | 2.61 | 6 | 43 | 125 | 29 | 97 |
4 | Endocrine problems | 1.51 | 5 | 40 | 485 | 26 | 459 |
7 | Neurological problems | 4.78 | 10 | 52 | 873 | 34 | 838 |
17 | Genitourinary problems | 3.71 | 18 | 26 | 85 | 17 | 68 |
16 | Trauma and injuries | 6.16 | 0 | 0 | 0 | 0 | 0 |
18 + 19 | Maternity and neonates | 5.46 | 0 | 3 | 2 | 1 | 1 |
11 PBCs | 40 | 389 | 1717 | 4828 | 1101 | 3727 | |
3 | Disorders of blood | 1.65 | 3 | 13 | 175 | 9 | 166 |
5 | Mental health disorders | 14.29 | 23 | 103 | 762 | 67 | 696 |
6 | Learning disability | 0.83 | 0 | 2 | 6 | 1 | 4 |
8 | Problems of vision | 1.55 | 0 | 2 | 34 | 1 | 33 |
9 | Problems of hearing | 0.70 | 0 | 1 | 112 | 1 | 111 |
12 | Dental problems | 2.31 | 0 | 0 | 54 | 0 | 54 |
14 | Skin | 1.57 | 2 | 9 | 16 | 6 | 10 |
15 | Musculoskeletal system | 2.90 | 3 | 14 | 186 | 9 | 176 |
20 | Poisoning and adverse events | 0.74 | 0 | 2 | 7 | 1 | 5 |
21 | Healthy individuals | 2.83 | 0 | 1 | 5 | 1 | 5 |
22 | Social care needs | 2.40 | 0 | 0 | 0 | 0 | 0 |
23 | Other | 8.11 | 0 | 0 | 0 | 0 | 0 |
All 23 PBCs | 80 | 411 | 1864 | 6184 | 1197 | 4987 |
How the additional NHS cost of £80M will tend to affect spending in each of the 23 PBCs [see Table 203, column (1)] will be based on the estimated expenditure elasticities and total PBC expenditure (see Table 157). In calculations of the threshold, the inputs above (expenditure elasticities and total expenditure) allow predicting how a 1% change in total spend is distributed by PBC. The same rationale is used here to establish how the additional NHS cost of £80M will affect each PBC. Hence, changes in spend reported here will be proportional to changes in spend across PBCs evaluated in calculations of the threshold (as in Table 178).
The estimated outcome elasticities (see Table 157) allow the absolute changes in spend in each PBC described above to be translated into a change in deaths and life-year effects for the 11 PBCs where mortality effects could be estimated [see columns (2) and (3) of Table 145]. Applying the estimated proportional effect on the mortality burden of disease to measures of QALY (including the other PBCs) provides an estimate of the total QALY effect of the change in spend in each PBC [see Table 203, column (4)]. ag The QALY consequences of changing expenditure by £80M thus reflect PBC estimates of cost per QALY (e.g. for the cancer PBC, the predicted total health foregone of 211 QALYs was calculated from the change in spend of £3.58M and reflects the PBC-specific cost per QALY estimate of £16,997 reported for the threshold estimate in Table 178). In an analogous way, the comparison of life-year and total QALY effects allows the distinction to be made between QALY effects due the life-year effects of additional deaths and QALY effects due only to QoL [see Table 203, columns (5) and (6)].
The results reported in Table 203 suggests that approval is likely to result in 411 additional deaths [see Table 203, column (2)] and 1864 life-years [see Table 203, column (3)] forgone, most of which are likely to occur in circulatory, respiratory and cancer PBCs. However, the impact of approval of this technology on QALYs forgone due to premature death [see Table 203, column (5)] only accounts for a proportion of the total QALY effects [see Table 203, column (4)]. Most (4987) are associated with QoL forgone during disease [see Table 203, column (6)]. These QoL impacts are most likely to occur in respiratory, neurological and mental health PBCs. The PBC-level effects in Table 203 can also be examined at ICD-10 code level (Table 204) while recognising the caveats discussed in Chapter 4. ah For example, within the respiratory PBC, it appears to be chronic lower respiratory diseases (J40–J47) where most additional deaths, life-years and QoL would be forgone. In the mental health PBC the additional deaths appear to be associated with disorders due to psychoactive substance use (F10–F19) and mood (affective) disorders (F30–F39).
Total change in spend analysed = £80M | (1) Change in spend (£M) | (2) Life-years foregone | QALYs foregone | ||
---|---|---|---|---|---|
(3) Total QALYs forgone | (4) Due to premature death | (5) QoL effects | |||
Overall | 80 | 1864 | 6184 | 1197 | 4987 |
PBC specific | |||||
PBC 2 (cancer) | 3.58 | 300 | 211 | 195 | 16 |
Malignant neoplasms, digestive organs (C15–C26) | 81 | 56 | 53 | 3 | |
Malignant neoplasms, respiratory system and intrathoracic organs (C30–C39) | 69 | 46 | 45 | 1 | |
Malignant neoplasms, breast and female genital organs (C50–C58) | 55 | 43 | 35 | 8 | |
Malignant neoplasms, stated or presumed to be primary, of lymphoid, haematopoietic and related tissue (C81–C96) | 27 | 18 | 18 | 1 | |
Malignant neoplasms, human male genital organs (C60–C63) | 17 | 13 | 11 | 1 | |
Other ICD-10 codes in this PBC | 51 | 35 | 33 | ||
PBC 10 (circulatory) | 6.07 | 928 | 863 | 590 | 273 |
Ischaemic heart diseases (I20–I25) | 523 | 476 | 334 | 142 | |
Cerebrovascular diseases (I60–I69) | 190 | 183 | 117 | 66 | |
Other forms of heart disease (I30–I52) | 75 | 64 | 48 | 16 | |
Congenital malformations and deformations of the circulatory system (Q20–Q28) | 18 | 43 | 13 | 30 | |
Diseases of veins, lymphatic vessels and lymph nodes, not elsewhere classified (I80–I89) | 36 | 28 | 22 | 5 | |
Other ICD-10 codes in this PBC | 87 | 69 | 54 | 15 | |
PBC 11 (respiratory) | 3.67 | 129 | 1835 | 80 | 1754 |
Chronic lower respiratory diseases (J40–J47) | 57 | 1568 | 36 | 1532 | |
Lung diseases due to external agents (J60–J70) | 8 | 71 | 5 | 66 | |
Other diseases of upper respiratory tract (J30–J39) | 7 | 65 | 4 | 60 | |
Other respiratory diseases principally affecting the interstitium (J80–J84) | 3 | 26 | 2 | 24 | |
Other diseases of pleura (J90–J94) | 3 | 26 | 2 | 24 | |
Other ICD-10 codes in this PBC | 51 | 79 | 32 | 47 | |
PBC 7 (neurological) | 4.78 | 52 | 873 | 34 | 838 |
Episodic and paroxysmal disorders (G40–G47) | 10 | 640 | 7 | 633 | |
Extrapyramidal and movement disorders (G20–G26) | 10 | 72 | 6 | 66 | |
Other degenerative diseases of the nervous system (G30–G32) | 9 | 44 | 5 | 39 | |
Other disorders of the nervous system (G90–G99) | 2 | 22 | 1 | 21 | |
Nerve, nerve root and plexus disorders (G50–G59) | 2 | 19 | 1 | 18 | |
Other ICD-10 codes in this PBC | 19 | 75 | 13 | 62 | |
PBC 5 (mental health) | 14.29 | 103 | 762 | 67 | 696 |
Mental and behavioural disorders due to psychoactive substance use (F10–F19) | 39 | 311 | 27 | 283 | |
Mood (affective) disorders (F30–F39) | 2 | 129 | 1 | 128 | |
Organic, including symptomatic, mental disorders (F00–F09) | 34 | 89 | 20 | 69 | |
Neurotic, stress-related and somatoform disorders (F40–F48) | 1 | 72 | 1 | 71 | |
Behavioural syndromes associated with physiological disturbances and physical factors (F50–F59) | 3 | 44 | 2 | 42 | |
Other ICD-10 codes in this PBC | 24 | 118 | 15 | 103 |
The impact of a reduction in the price of this technology, either through value-based pricing or the PAS that was offered during the rapid review, can also be examined in the same way. The PAS was commercial in confidence, so here we will consider the hypothetical case that a 30% reduction in NHS costs (incremental costs) would make this technology cost-effective for this subgroup of patients. Such a discount would be expected to save 1855 QALYs including 126 deaths averted, 559 life-years (359 when adjusted for quality) and QoL effects during disease equivalent to 1496 QALYs, compared with approval of the technology at the original list price (Table 205).
PBC description | (1) Change in spend (£M) | (2) Additional deaths | (3) Life-years foregone | QALYs foregone | ||
---|---|---|---|---|---|---|
(4) Total QALYs forgone | (5) Due to premature death | (6) QoL effects | ||||
Before PAS | ||||||
All big four programmes | 16 | 340 | 1554 | 3259 | 995 | 2265 |
11 PBCs | 40 | 389 | 1717 | 4828 | 1101 | 3727 |
All 23 PBCs | 80 | 421 | 1864 | 6184 | 1197 | 4987 |
After PAS | ||||||
All big four programmes | 11 | 238 | 1088 | 2281 | 696 | 1585 |
11 PBCs | 28 | 272 | 1202 | 3380 | 771 | 2609 |
All 23 PBCs | 56 | 295 | 1305 | 4329 | 838 | 3491 |
Difference | ||||||
All big four programmes | –5 | –102 | –466 | –978 | –298 | –679 |
11 PBCs | –12 | –117 | –515 | –1448 | –330 | –1118 |
All 23 PBCs | –24 | –126 | –559 | –1855 | –359 | –1496 |
List of abbreviations
- 2SLS
- two-stage least squares
- A&E
- accident and emergency
- ACCA
- Association of Certified Accountants
- CARAN
- Combining Age-Related and Additional Needs
- CBT
- cognitive–behavioural therapy
- CCG
- Clinical Commissioning Group
- CEA
- cost-effectiveness analysis
- CPRD
- Clinical Practice Research Datalink
- DALY
- disability-adjusted life-year
- EED
- Economic Evaluation Database
- EQ-5D
- European Quality of Life-5 Dimensions
- EQ-VAS
- European Quality of Life-visual analogue scale
- EU
- European Union
- FCE
- finished consultant episode
- GBD
- Global Burden of Disease
- GDP
- gross domestic product
- GLS
- generalised least squared
- GMS
- general medical services
- GNP
- gross national product
- GP
- general practitioner
- GPRD
- General Practice Research Database
- HA
- housing association
- HCHS
- Hospital and Community Health Service
- HES
- Hospital Episode Statistics
- HIV
- human immunodeficiency virus
- HODaR
- Health Outcomes Data Repository
- HRG
- Healthcare Resource Group
- HSE
- Health Survey for England
- IAPT
- Improving Access to Psychological Therapies
- ICD-10
- International Classification of Diseases, Tenth Edition
- ICER
- incremental cost-effectiveness ratio
- IMD2007
- Index of Multiple Deprivation 2007 data set
- IV
- instrumental variable
- LA
- local association
- LE
- life expectancy
- LLT
- limiting long-term illness
- LM
- Lagrange multiplier
- LSOA
- lower super output area
- MEPS
- Medical Expenditure Panel Survey
- MeSH
- medical subject heading
- MFF
- market forces factor
- MRC
- Medical Research Council
- NAO
- National Audit Office
- NHB
- net health benefit
- NHS IC
- National Health Service Information Centre
- NICE
- National Institute for Health and Care Excellence
- NIHR
- National Institute for Health Research
- NSF
- National Service Framework
- OLS
- ordinary least squares
- ONS
- Office for National Statistics
- PAS
- Patient Access Scheme
- PB
- programme budgeting
- PBC
- programme budget category
- PBRA
- person-based resource allocation
- PCO
- primary care organisation
- PCT
- primary care trust
- PMS
- primary medical services
- PROM
- patient-reported outcome measure
- QALY
- quality-adjusted life-year
- QIPP
- Quality Innovation Productivity and Prevention
- QoL
- quality-of-life
- SHA
- Strategic Health Authority
- SMR
- standardised mortality rate
- SNRI
- serotonin-norepinephrine reuptake inhibitor
- SRCBT
- social recovery orientated cognitive–behavioural therapy
- SSRI
- selective serotonin reuptake inhibitor
- SYLLR
- standardised years of life lost rate
- TCA
- tricyclic antidepressant
- WHO
- World Health Organization
- WTP
- willingness to pay
- YLD
- years of life lived with disability
- YLG
- years of life gained
- YLL
- years of life lost