Notes
Article history
The research reported in this issue of the journal was funded by the HTA programme as project number 10/133/01. The contractual start date was in July 2012. The draft report began editorial review in November 2013 and was accepted for publication in September 2014. The authors have been wholly responsible for all data collection, analysis and interpretation, and for writing up their work. The HTA editors and publisher have tried to ensure the accuracy of the authors’ report and would like to thank the reviewers for their constructive comments on the draft document. However, they do not accept liability for damages or losses arising from material published in this report.
Declared competing interests of authors
Professor Kamlesh Khunti has acted as a consultant and speaker for Novartis Pharmaceuticals UK Ltd, Novo Nordisk, Sanofi-aventis, Eli Lilly and Company and Merck Sharp & Dohme Corp. He has received grants in support of investigator and investigator-initiated trials from Novartis Pharmaceuticals UK Ltd, Novo Nordisk, Sanofi-aventis, Eli Lilly and Company, Pfizer, Boehringer Ingelheim and Merck Sharp & Dohme Corp. Professor Kamlesh Khunti has also received funds for research and honoraria for speaking at meetings and has served on advisory boards for Eli Lilly and Company, Sanofi-aventis, Merck Sharp & Dohme Corp. and Novo Nordisk. Professor Melanie Davies has acted as consultant, advisory board member and speaker for Novo Nordisk, Sanofi-aventis, Eli Lilly and Company, Merck Sharp & Dohme Corp., Boehringer Ingelheim, Janssen and AstraZeneca. She has received grants in support of investigator and investigator-initiated trials from Novo Nordisk, Sanofi-aventis, Eli Lilly and Company, Boehringer Ingelheim, Merck Sharp & Dohme Corp. and GlaxoSmithKline.
Permissions
Copyright statement
© Queen’s Printer and Controller of HMSO 2015. This work was produced by Gillet et al. under the terms of a commissioning contract issued by the Secretary of State for Health. This issue may be freely reproduced for the purposes of private research and study and extracts (or indeed, the full report) may be included in professional journals provided that suitable acknowledgement is made and the reproduction is not associated with any form of advertising. Applications for commercial reproduction should be addressed to: NIHR Journals Library, National Institute for Health Research, Evaluation, Trials and Studies Coordinating Centre, Alpha House, University of Southampton Science Park, Southampton SO16 7NS, UK.
Chapter 1 Background
Rising prevalence and burden of diabetes
The number of cases of diagnosed diabetes in the UK increased to 3.2 million in 2013, with an estimated 850,000 people having the disease without knowing it, and as many as 7 million more at high risk of developing it. By 2025, if current trends continue, an estimated 5 million people will have diabetes. 1 Type 2 diabetes accounts for about 90% of cases of diabetes. 2 Of the total cost of diabetes, 75–80% is incurred in the treatment of complications associated with poor preventative care (e.g. poor glycaemic control) and the long duration of diabetes. 3 Identification of individuals at high risk of diabetes (HRD) can at least delay the onset of diabetes, and early intervention for diabetes can reduce or at least delay the onset of complications, which already account for around 10% of the total NHS budget. 4 This percentage is projected to rise to 17% over the next 20 years4 as a result of increasing rates of obesity and an ageing population.
Scope and context of the evaluation
This evaluation is concerned with which blood test to use when screening in order to identify cases of undiagnosed type 2 diabetes. Implicit in any evaluation of screening for diabetes is the concurrent opportunity to identify individuals at HRD and subsequently manage them to reduce their risk. The two main blood tests concerned are glycated haemoglobin (HbA1c) and fasting plasma glucose (FPG), these being the options recommended by the National Institute for Health and Care Excellence (NICE) for blood testing for diabetes and HRD. 5 A HbA1c test does not require an overnight fast and measures the amount of glucose that is being carried by the red blood cells in the body. The result indicates an individual’s average blood glucose levels over the previous 2–3 months. A FPG test directly measures glucose levels and is to be taken after an 8-hour overnight fast. A HbA1c or FPG measurement can be used both for the screening test and, where the first test is in the relevant diabetes range, for the confirmatory diagnostic test. The corresponding HbA1c and FPG definitions of diabetes are sufficient to make a diagnosis, the performance of HbA1c or FPG test no longer being assessed with reference to the oral glucose tolerance test (OGTT) as a gold standard test.
The starting context for the cost-effectiveness analysis is an individual attending an appointment at a general practice (GP) centre in England having been offered a NHS health check. From this point, a blood test may be offered either to all individuals or only to those exceeding a diabetes risk score threshold obtained from risk factor data.
The figure could be extended to include rescreening but we decided not to include this, as we have not modelled this as there was insufficient evidence on some key parameters that would be required. These issues are discussed in detail in Chapter 5, Secondary analyses of epidemiological studies.
There is the possibility that individuals offered a test will not accept the offer or not attend, as shown in Figure 1. NHS Health Checks is the Department of Health’s 5-year programme to reduce the vascular risk, especially for cardiovascular disease (CVD), of individuals between 40 and 74 years of age with elevated risk factors for these conditions. The evaluation, therefore, compares the cost-effectiveness of offering screening for diabetes and HRD using a HbA1c test versus a FPG test at the time of the health check appointment, as an addition to the other standard checks such as cholesterol and blood pressure levels.
FIGURE 1.
Scope of the evaluation. SBP, systolic blood pressure; TC, total cholesterol.

The main objectives of the modelling were as follows:
-
To assess which test is most cost-effective, following the 2012 NICE guidance on the identification of individuals with HRD as shown in Figure 2. Specifically, the recommended cut-off points for referral for preventative intervention (aimed at supporting diet and lifestyle changes) were adopted, that is 6.0% for HbA1c or 5.5 mmol/l for FPG.
-
To determine if the results and conclusions might be different in cohorts other than the Leicester Ethnic Atherosclerosis and Diabetes Risk (LEADER) cohort, in which the relative prevalence rates of diabetes and HRD differ markedly from those in LEADER. We also simultaneously examined the impact of alternative assumptions regarding uptake of HbA1c tests and FPG tests.
-
To assess which test is most cost-effective for screening strategies with lower HbA1c and FPG cut-off points than the NICE recommendations. These were included because some studies have suggested that intervention may be cost-effective at lower HbA1c cut-off points. 6
-
To determine if it is more cost-effective to use a random capillary glucose (RCG) test or the Leicester Practice Database Score (LPDS – see Chapter 2, Prescreening using the LPDS risk score) to prioritise who should receive the blood test (FPG or HbA1c).
FIGURE 2.
The NICE flow chart: identifying and managing risk of type 2 diabetes. BMI, body mass index. Figure reproduced with permission from NICE (2012) ‘PH 38 Preventing type 2 diabetes: risk identification and interventions for individuals at high risk’. London: NICE. 5 Available from http://guidance.nice.org.uk/PH38.

Absence of a single gold standard definition of diabetes
There no longer exists a single gold standard definition of diabetes. An individual can receive a diagnosis based on either HbA1c-based criteria or FPG-based criteria [or 2-hour plasma glucose (2hPG) criteria, but the associated test, the OGTT, is outside the scope of this assessment].
The cohorts identified by the alternative tests only partially overlap; this raises some issues to address around prognosis of individuals with differing diagnoses according to the two tests. Evidence on this is lacking, although we can estimate risk of diabetes and CVD through individuals’ risk factors.
It should be noted that diabetes risk assessment should be undertaken in all eligible 40- to 74-year-olds; it is not conditional on having a high CVD risk.
Chapter 2 Methods
Overview of approach
The model developed for these analyses is an adaptation of the model used to assess screening strategies as part of NICE’s Public Health Guidance Development work in 2012. 5
The modelling comprises two stages. The first stage entails a model to determine the individual screening outcomes from alternative strategies for screening and diagnosis. To populate this we used individual patient-level data from the Leicestershire-based LEADER study. We used these data to analyse the following questions:
-
What proportion of individuals would receive a blood test, taking account of two options to identify those in greatest need of a blood test? These options are the non-invasive LPDS risk score, which uses risk factors held in computer databases to calculate a score relating to an individual’s risk of developing diabetes (see Prescreening using the Leicester Practice Database Score), and a RCG test (see Prescreening using random capillary glucose).
-
Taking account of the different uptake rates (base case 20% higher for HbA1c than FPG), what proportion of individuals would be detected with diabetes or HRD (1) under HbA1c testing and (2) under FPG testing? HRD is defined as a HbA1c between 6.0% and 6.4%, or a FPG between 5.5 and 6.9 mmol/l.
The LEADER study did not include information on the RCG finger-prick test, so RCG values were incorporated into the LEADER data set using a mapping between HbA1c and RCG from the Anglo-Danish–Dutch Study of Intensive Treatment In People with Screen Detected Diabetes in Primary Care (ADDITION)-Cambridge study. This enabled us to answer the following question:
-
How do screening outcomes compare using the LPDS versus RCG testing as a prescreening tool?
The second stage entails using an adaptation of the Sheffield Type 2 Diabetes Model that was used to assess screening strategies as part of NICE’s Public Health Guidance Development work. The model is used to simulate the lifetime patient clinical pathways, incidence of complications and associated cost and health utility impacts. The assumptions for the modelling are described in detail later in the report, but the key ones are listed here:
-
Uptake rates of HbA1c tests (given that someone has attended a health check) were assumed to be 20% higher than for FPG tests (based on clinical advice), 95% and 75%, respectively, because of the need to fast for the FPG test.
-
The central estimates for the costs of screening tests (including staff time, transport and laboratory costs) were estimated to be £12.18 for a FPG test and £14.40 for a HbA1c test (see Units costs of prescreening and blood glucose tests for costing).
-
Cases of diabetes identified through screening are assumed to be treated at the point of diagnosis through routine care.
-
Cases identified as HRD are assumed to be offered a preventative intervention in the form of a less intensive group-based adaptation of the intervention used in the US Diabetes Prevention Programme.
-
Cases of undiagnosed diabetes and HRD missed by the screening process (because of either a risk score being below the threshold for blood testing or lack of uptake when offered a blood test) are modelled accordingly.
-
Economic modelling was used to estimate the lifetime discounted costs and quality-adjusted life-years (QALYs) and the net monetary benefit (NMB) associated with each screening strategy. The optimal strategy is the one with the highest NMB (assuming 1 QALY is valued at £20,000) among those with an incremental cost-effectiveness ratio (ICER) below £20,000/QALY compared with ‘no screening’ (this is not necessarily the one with the lowest ICER compared with no screening).
-
Uncertainty was analysed using one-way sensitivity analysis and probabilistic sensitivity analysis (PSA).
-
Evidence from studies other than LEADER, in particular from the University of East Anglia-Impaired Fasting Glucose (UEA-IFG) study, indicates that there may be significant regional variations in the relative prevalence of FPG versus HbA1c-defined diabetes and HRD. We therefore carried out some additional analyses based on alternative prevalence scenarios.
The model used for this evaluation naturally includes all of the inputs that would be included for an evaluation of screening for diabetes compared with no screening. Models of screening for diabetes necessitate inclusion of a large number of inputs and assumptions, as will become apparent during this section. For this test-specific screening evaluation, however, it was felt to be important that the key inputs are highlighted at the outset, namely those for which differential evidence exists for HbA1c versus FPG testing. The key inputs and methods are:
-
the prevalence of diabetes and HRD, for each of the HbA1c- and FPG-based definitions, in the LEADER cohort and other cohorts (see Prevalence of diabetes and high risk of diabetes)
-
the uptake rates when individuals are offered testing (see Uptake rates of blood tests)
-
the approach to mapping screening outcomes to subsequent glycaemic trajectories (see Discordance between the groups of individuals identified by glycated haemoglobin and those identified by fasting plasma glucose)
-
the approach to modelling the risks of diabetes and CVD in individuals with HRD, conditional on an individual’s HbA1c and FPG levels (see Rate of progression from high risk of diabetes and Fasting plasma glucose/glycated haemoglobin at baseline and risk of incident cardiovascular disease).
The costs of the tests are relatively small; therefore, they are not a key driver of relative long-term cost-effectiveness.
Prevalence of diabetes and high risk of diabetes
The Leicester Ethnic Atherosclerosis and Diabetes Risk cohort data set
We used the LEADER data set in all of the base case analyses to estimate the performance of the alternative screening strategies. The LEADER cohort is a combination of two systematic screening programmes conducted in Leicestershire, the ADDITION–Leicester study7 and the STAR (Screening Those At Risk) study. 8 These studies recruited from a population of over 950,000 in the relevant age range, approximately one-third of whom were resident in the City of Leicester. All individuals aged 40–75 years were invited to attend for screening and an OGTT was carried out according to World Health Organization (WHO) 1999 criteria. In addition, those aged 25–39 years and not of white European origin were invited for screening. Simultaneously, a HbA1c measurement was taken and measured on a correctly aligned assay analyser. The screening was conducted in a general practice centre, in a mobile screening unit or at one of the Leicester teaching hospitals, between February 2002 and August 2009. Those identified by the programme with HRD were offered an annual follow-up. In 2011, 9494 people who had been screened were included in the LEADER database. The data set includes HbA1c, FPG, 2hPG, family history of diabetes and routine demographics collected on all patients.
According to the 2001 census, 30% of this population classified themselves as belonging to Indian, Pakistani or Bangladeshi ethnic groups. Different strategies were used for participant recruitment in each study. The majority of participants (two-thirds) were screened regardless of risk of diabetes. For the remaining third, eligibility was subject to having a risk factor for diabetes, as recommended by Diabetes UK. Participants were recruited from 40 Leicestershire general practices from a range of deprivation levels.
Determining diagnostic outcome of individuals in the Leicester Ethnic Atherosclerosis and Diabetes Risk data set
The LEADER data set contains the LPDS risk score, results for a single FPG test (and, for some individuals, a second FPG result) and a single HbA1c test. In clinical practice, two test results are needed to diagnose diabetes but confirmatory test results were not available for all individuals in the LEADER data set. Where the LEADER test result was in the diabetes range, assumptions were necessary to determine whether or not an individual’s confirmatory test would result in a diagnosis of diabetes:
-
Not everyone with a first FPG ≥ 7 mmol/l in the LEADER data set had a second confirmatory FPG test. To populate the data set with sampled outcomes of confirmatory testing, we needed evidence on the proportion of confirmatory tests that confirm diabetes. We therefore undertook an analysis of the subset of individuals in the LEADER study in whom two FPG tests were undertaken. The results suggest that approximately 70% of repeat FPG tests confirm diabetes (i.e. FPG ≥ 7 mmol/l). We then sampled whether or not the second FPG result was confirmatory of diabetes, assuming that 70% would be confirmed.
-
For HbA1c testing, we assumed that the result of the first test would be replicated by the confirmatory test. Clinical experts advised that this was a reasonable assumption because HbA1c has much lower variation between consecutive test results than FPG. We have, however, included the cost of a second confirmatory HbA1c test where the initial test indicates diabetes, as the second test is required to make a formal diagnosis of diabetes.
For both HbA1c testing and FPG testing, where the result of the first test is below the cut-off for diabetes but in the range considered HRD, there is not a requirement to carry out a second confirmatory test.
Baseline characteristics and descriptive analyses
The baseline characteristics of the 8147 individuals from the LEADER data set aged 40–74 years for whom data were available for all data fields required for our analysis are shown in Table 1.
Characteristic | Mean | Standard deviation |
---|---|---|
Age (years) | 57.30 | 9.67 |
Systolic blood pressure (mmHg) | 137.01 | 19.68 |
HDL cholesterol (mmol/l) | 1.36 | 0.41 |
Total cholesterol (mmol/l) | 5.53 | 1.06 |
HbA1c (%) | 5.71 | 0.62 |
FPG (mmol/l) | 5.21 | 0.91 |
Number | Percentage | |
Male | 3874 | 47.6 |
White | 6199 | 76.1 |
Current smoker | 1480 | 18.2 |
Diabetes prevalence (HbA1c ≥ 6.5%) | 467 | 5.7 |
Diabetes prevalence (FPG ≥ 7.0 mmol/l)a | 150 | 1.8 |
Total diabetes prevalence (either test) | 513 | 6.3 |
Diabetes with both tests | 104 | 1.3 |
HRD prevalence (HbA1c 6.0–6.4%) | 1487 | 18.3 |
HRD prevalence (FPG 5.5–6.9 mmol/l) | 1936 | 23.8 |
Total HRD prevalence (either test) | 2823 | 34.7 |
HRD with both tests | 600 | 7.4 |
Total diabetes prevalence (based upon either HbA1c or FPG testing) is 6.6%, but only 1.3% test positive on both tests. HbA1c testing identifies more than three times as many individuals as FPG testing (5.7% compared with 1.8%).
The prevalence of HRD is similar with the two tests, but for the most part they identify different individuals, as, out of a total HRD prevalence of 34.7%, only 7.4% (fewer than one-quarter) are identified with both tests.
Although one of the two studies forming the LEADER cohort had a recruitment criterion of having at least one risk factor, this did not materially increase the risk of the LEADER cohort overall.
Table 2 shows how many individuals from the LEADER cohort belong to non-diabetic, HRD and diabetic subgroups based upon HbA1c or FPG values. The majority of people (62.8%) are not diagnosed as either diabetic or HRD with either of the two tests (italic text). Text in bold italics indicates percentages of individuals classified as at HRD (but not diabetic) for at least one of the criteria, whereas text in bold indicates percentages of individuals classified as diabetic for at least one of the criteria.
FPG subgroup (mmol/l) | HbA1c < 6.0% | HbA1c 6.0–6.4% | HbA1c ≥ 6.5% | Totals | ||||
---|---|---|---|---|---|---|---|---|
Number | Percentage | Number | Percentage | Number | Percentage | Number | Percentage | |
< 5.5 | 5114 | 62.8 | 853 | 10.5 | 94 | 1.2 | 6061 | 74.4 |
5.5–6.9 | 1067 | 13.1 | 600 | 7.4 | 269 | 3.3 | 1936 | 23.8 |
≥ 7.0 | 12 | 0.1 | 34 | 0.4 | 104 | 1.3 | 150 | 1.8 |
Totals | 6193 | 76.0 | 1487 | 18.3 | 467 | 5.7 | 8147 | 100 |
Figure 3 shows the distribution of HbA1c values in individuals aged between 40 and 74 years from the LEADER cohort. Individuals with HbA1c values between 6.0% and 6.4% are at HRD (green bars), whereas individuals with HbA1c values of 6.5% and above have diabetes (blue bars). Most of the population have HbA1c values under 6.0% (dark green bars).
FIGURE 3.
Histogram of the distribution of HbA1c values in people in the LEADER cohort.

Figure 4 shows the distribution of FPG values in individuals aged between 40 and 75 years from the LEADER cohort. Individuals with FPG values between 5.5 and 6.9 mmol/l are at HRD (green bars), whereas individuals with FPG values of 7.0 and over have diabetes (blue bars). Most of the population has FPG values under 5.5 (dark green bars). Comparison with Figure 3 shows clearly that, in the LEADER cohort, fewer individuals are diagnosed with diabetes using the FPG test than with the HbA1c test.
FIGURE 4.
Histogram of the distribution of FPG values (from single test) in people in the LEADER cohort.
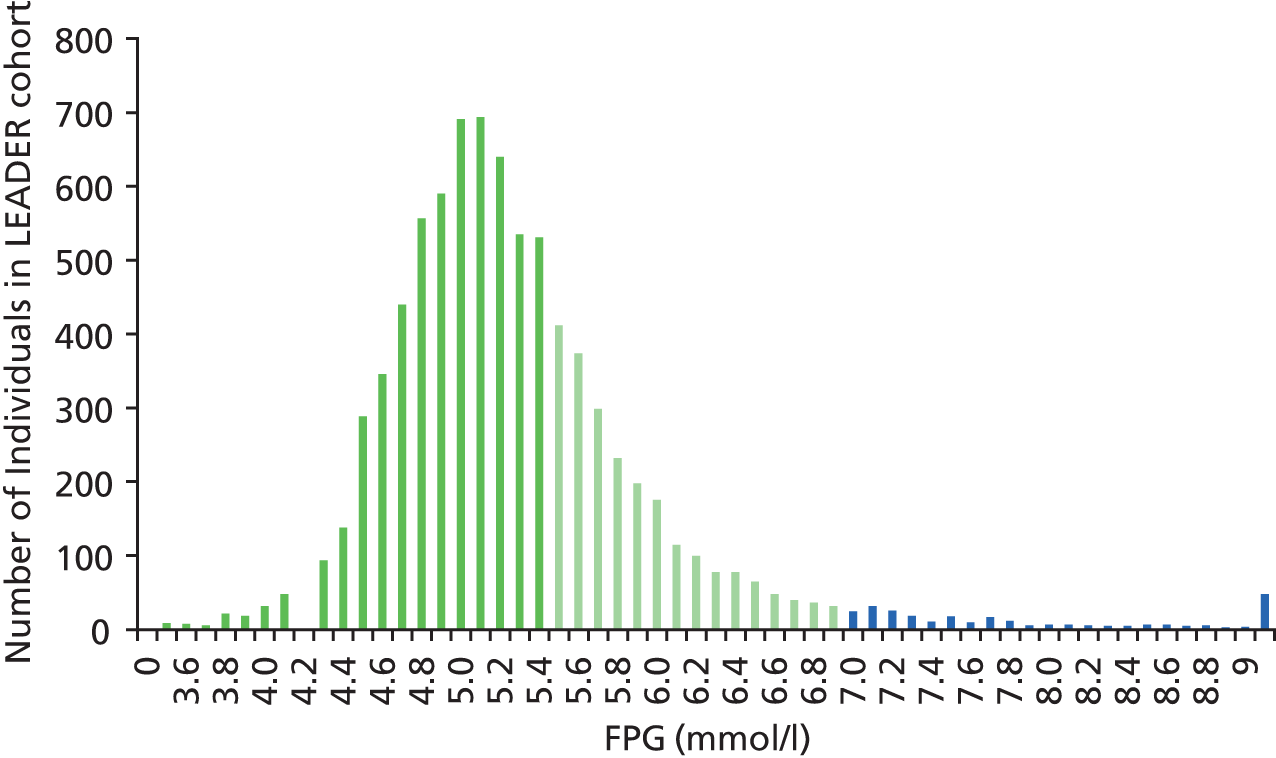
Figure 5 shows HbA1c values plotted against FPG values for each individual in the LEADER cohort aged between 40 and 75 years. The trend line (black dotted line) illustrates the positive correlation between FPG values and HbA1c values.
FIGURE 5.
Scatterplot of values of HbA1c against FPG for the LEADER cohort.

Figure 6 shows HbA1c values plotted against FPG values for individuals in the LEADER cohort who are diagnosed with diabetes according to either of the two tests. Individuals who are over the cut-off point for diabetes in both tests are represented with blue dots, whereas individuals who are over the cut-off point, in the HbA1c test or the repeated FPG test, are represented with black and dark green dots, respectively. Overall, the HbA1c test identifies more individuals with diabetes than does the FPG test.
FIGURE 6.
Scatterplot of values of FPG against HbA1c for individuals with diabetes (according to either the HbA1c or FPG test) in the LEADER cohort.
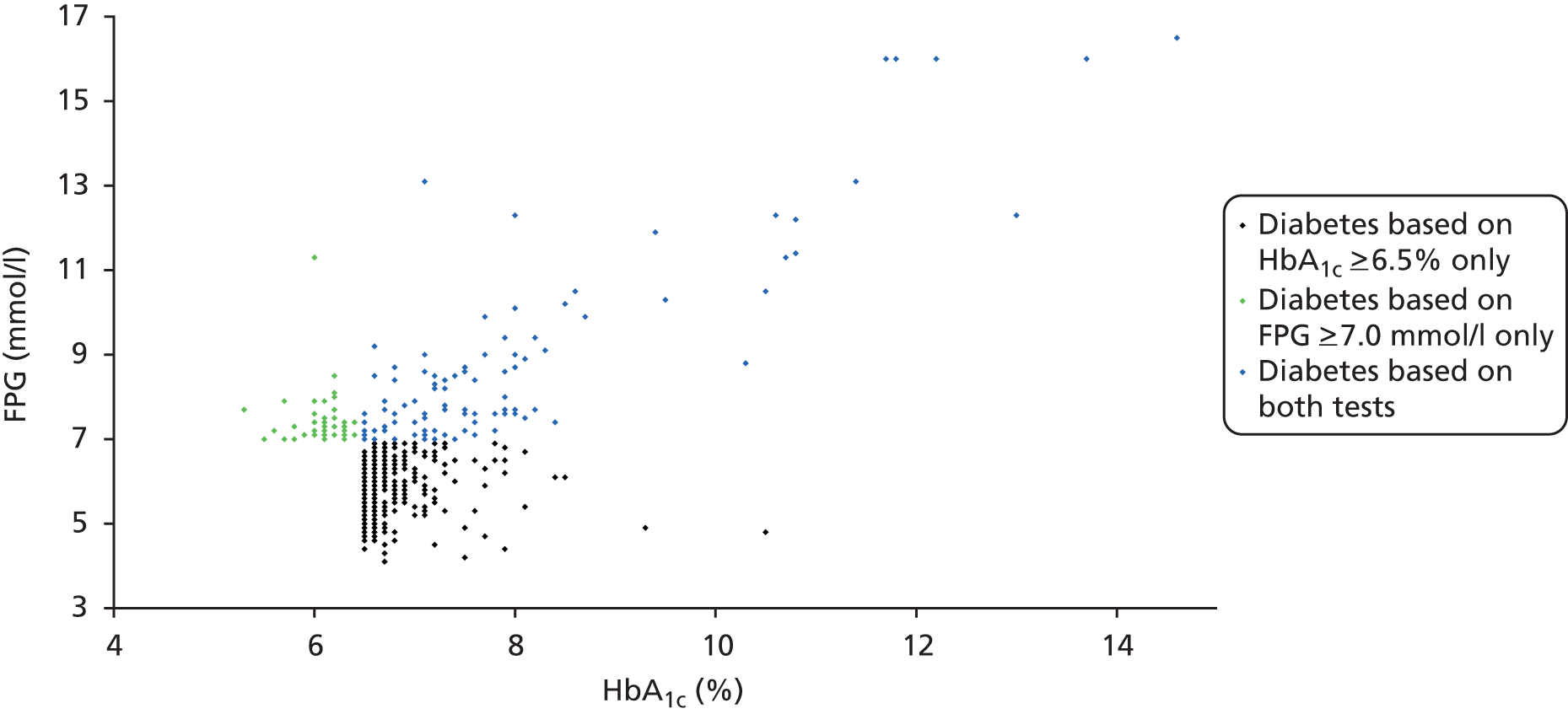
Figure 7 shows HbA1c values plotted against FPG values for individuals in the LEADER cohort who are at HRD according to either of the two tests. It clearly illustrates that the two tests predominantly identify different individuals, as only the green dots represent individuals who are below the cut-off points for HRD in both tests. Individuals who meet the criteria for HRD with just the HbA1c test or just the FPG test are represented with black and dark green dots, respectively. Some of the individuals diagnosed as being at HRD based on one of the two tests are diagnosed as having diabetes using the other test. Overall, the FPG test identifies more individuals with HRD than the HbA1c test. Note that most of these data are dependent only on results from a single FPG test, as individuals diagnosed with HRD using FPG testing will not be eligible for a second FPG test.
FIGURE 7.
Scatterplot of values of HbA1c against FPG for individuals defined as at high risk of diabetes (either by HbA1c or FPG) in the LEADER cohort. Fasting plasma glucose values are from a single test: either (i) FPG 5.5–6.9 mmol/l or (ii) FPG ≥ 7.0 mmol/l in first test but < 7.0 mmol/l on confirmatory testing (all values in mmol/l).

Prevalence of diabetes and high risk of diabetes among South Asians of 25–39 years of age in the Leicester Ethnic Atherosclerosis and Diabetes Risk cohort
Table 3 shows that, within the LEADER cohort, there are also significant differences in the relative prevalence of HbA1c to FPG-defined undiagnosed diabetes in South Asians under 40 years of age.
Diabetes | HRD | ||
---|---|---|---|
HbA1c (≥ 6.5%) | FPG (≥ 7.0 mmol/l) | HbA1c (6.0–6.4%) | FPG (5.5–6.9 mmol/l) |
3.3 | 0.5 | 10.4 | 11.2 |
Other UK cohorts providing estimates of prevalence of diabetes and high risk of diabetes using both glycated haemoglobin and fasting plasma glucose
During the later stages of the project, we became aware of some US studies that had reported higher prevalence of diabetes with FPG testing than with HbA1c testing. As a result of this, we contacted experts within the field with the aim of identifying any other UK-based screening (or prevention) cohorts that potentially had recorded both HbA1c and FPG measures at baseline. The purpose was to check if other cohorts had provided significantly different estimates of prevalence from those in the LEADER cohort. The studies identified are shown in Table 4. These were used to inform additional scenario analyses with alternative prevalence of diabetes and HRD, which are described in Scenario analyses – alternative prevalence and uptake rates.
Study (n) | Prevalence of diabetes | Prevalence of HRD | Source | |||
---|---|---|---|---|---|---|
HbA1c | FPG | HbA1c (≥ 6%) | HbA1c (≥ 5.7%) | FPG (≥ 5.5 mmol/l) | ||
LEADER (n = 8147) | 5.7 | 1.8 | 18.50 | 44.80 | 24.30 | Analysis of LEADER data set |
University of East Anglia Impaired Fasting Glucose (UEA-IFG) study (n = 3906) | 2.3 | 2.1 | 6.40 | 16.50 | 22.60 | From UEA-IFG study10 additional estimates (Max Bachmann, Professor of Health Services Research, Norwich Medical School, University of East Anglia, personal communication) |
Orkney (n = 1441) | 3.3 | 1.7 | 12.80 | − | − | Professor Sarah Wild, Centre for Population Health Sciences, University of Edinburgh, 2013, personal communication |
Fenland (n = 10,007) | 1.2 | 0.6 | 7.70 | 23.20 | 9.70 | Data from Fenland study provided by Professor N Wareham, MRC Epidemiology Unit, University of Cambridge |
1999–2006 National Health and Nutrition Examination Survey (n = 6890) | 2.3 | 3.6 | − | − | − | Carson et al. (2010)11 |
The study with the most different relative (HbA1c-defined to FPG-defined) prevalence of diabetes and HRD is the UEA-IFG study. This was the feasibility element prior to a large diabetes prevention programme in Norfolk, England,9 and screened 3906 participants aged between 45 and 70 years. Mean age was 59 years and mean body mass index (BMI) was 30 kg/m2. All participants had no previous diagnosis of diabetes and had at least one risk factor for glucose intolerance (a first-degree relative with type 2 diabetes, BMI > 25 kg/m2, waist circumference > 94 cm in men and > 80 cm in women, personal history of coronary heart disease (CHD) or gestational diabetes, or reported to have impaired fasting glucose (IFG) by their general practice or by themselves. All participants underwent a single FPG and HbA1c test between December 2009 and April 2010, and prevalence of HRD was 22.6% for FPG (5.5–6.9 mmol/l) compared with 6.4% for HbA1c (defined as 6.0–6.4%). We have no data on what proportion of the 22.6% would be filtered out if the ‘reported to have IFG’ eligibility criterion were removed. Nevertheless, the study provides a basis for specifying a scenario analysis with prevalence much higher for FPG testing (see Scenario analyses – alternative prevalence and uptake rates).
Other epidemiological evidence relevant to the model
A version of the Sheffield Diabetes Model (see The Sheffield Type 2 Diabetes Model) adapted for screening and prevention assessments was available at the start of the project. The model is described in a 2012 report for NICE. 12 During various prior projects, the model has drawn upon a wide array of evidence from published reviews (including the Waugh 200713 review of screening for diabetes), targeted searches and literature identified through topic experts. As a result, the vast majority of the evidence required for this assessment was already contained within the economic model. It was therefore neither necessary nor practicable to comprehensively search for, review and synthesise the vast volume of literature on all aspects of the epidemiology of diabetes within the scope of this project. We were also aware of an imminent review due to become available during the project, the 2013 update of evidence for screening for diabetes undertaken by Waugh and colleagues for the National Screening Committee. 14 The form, effectiveness and cost of preventative interventions in a real-world setting (see Form of intervention, Initial weight loss and Durability of reduction in risk) relied heavily on a clinical review undertaken for the NICE guidance. 15
The necessary endeavours to obtain and familiarise ourselves with the necessary data fields from the LEADER cohort had already been done during the NICE work. We were also aware of the recent publication of the final version of the LPDS risk score. 16
There were some areas where it was realised that new or updated evidence was required for the model. These include:
-
Revisiting rates of progression from HRD to diabetes, which was the subject of a recent meta-analysis that had become available (see Rate of progression from high risk of diabetes to diabetes)
-
Evidence on the multivariate risks for the incidence of diabetes and CVD according to both baseline HbA1c and FPG levels (see Adjusting an individual’s risk of diabetes to take account of both fasting plasma glucose and glycated haemoglobin and Fasting plasma glucose/glycated haemoglobin at baseline and risk of incident cardiovascular disease – evidence review). For this evidence, which typically necessitates a large epidemiological study to obtain adequate statistical power, it was decided that a systematic search for and synthesis of such epidemiological evidence would be both time-consuming and inefficient given the time available and existing sources of evidence at our disposal. We therefore identified studies from (1) literature already identified during previous work, (2) studies described in Section 3 of the 2013 update of evidence for screening14 and (3) evidence sources signposted by the clinical members of the team.
Economic evidence from other studies
A version of the Sheffield Diabetes Model (see The Sheffield Type 2 Diabetes Model), the economic model adapted for screening and prevention assessments, was available at the start of the project. Over various previous projects, this model has utilised evidence from previous literature reviews that include the economics of screening and prevention. These include a review of screening for diabetes (Waugh 200713) for the National Institute for Health Research Health Technology Assessment programme, and a review of key cost-effectiveness studies undertaken for the 2012 NICE guidance on risk identification. 5
The model already contained all of the economic parameters required for this assessment; therefore, it was considered unnecessary to undertake any new economic reviews or additional systematic reviews within this project.
Unit costs of tests (see Unit costs of prescreening and blood glucose tests) were drawn from the 2012 NICE economic modelling. 12
Chapter 6 of the 2013 update review of screening for diabetes14 included a review of economic studies since the 2007 review. 13 None of the studies, however, compared the long-term cost-effectiveness of alternative blood tests for diabetes; therefore, they were not of use for comparison with our results.
Defining the prescreening and blood glucose test strategies to be assessed
In this section, we describe the rationale by which we have defined the strategies to be assessed in our study. This covers prescreening methods examined, the blood glucose tests assessed and the thresholds for deeming an individual as at HRD. We then discuss the alternative combinations of these that were arrived at, forming a set of alternative overall screening strategies.
Laboratory blood tests: fasting plasma glucose and glycated haemoglobin
The options are:
-
Use HbA1c alone
-
The economic analysis is based on laboratory rather than point-of-care (POC) HbA1c testing. The possible impact of POC testing is considered further in Chapter 5, Point-of-care testing.
-
-
Use FPG alone
-
Similarly, the economic analysis is based on laboratory rather than POC FPG testing.
-
-
Combined HbA1c and FPG testing
-
As HbA1c and FPG identify only partially overlapping cohorts of individuals with HRD, undertaking both tests on individuals would identify a greater number of individuals at risk of diabetes than using either test alone (see Baseline characteristics and descriptive analyses), that is if an individual met the cut-off point for HRD on either test, then they would be classed as at HRD.
-
However, combined testing might be impractical from the viewpoint of resources, that is affordability and capacity within general practices and laboratories. It might also be confusing for doctors to explain to patients that they might have diabetes or a HRD according to one test but not the other. A UK expert statement has recommended against routine dual testing;17 therefore, we have not included any combination HbA1c/FPG testing options within any of our screening strategy options. The potential rationale for combination testing is considered further within Chapter 5, Discussion.
Reliability of glycated haemoglobin and fasting plasma glucose tests
There have historically been concerns about the reliability of both HbA1c testing and FPG testing. For HbA1c, there has been much effort to standardise assays over recent years, which resulted in WHO recommending that HbA1c can be used to diagnose diabetes and the UK expert group on HbA1c recently stating that UK laboratories now meet quality assurance requirements. 18 There are still some limitations, described within Appendix 1, but these are not believed to be of much consequence for the economic analysis.
Prescreening options
Prescreening using the Leicester Practice Database Score
The number of individuals undergoing blood tests can be reduced by filtering out a proportion with a low risk score for diabetes based on non-invasive measures available from electronic databases within primary care. The LPDS has been developed to help physicians assess the risk of an individual having diabetes from routinely available data in primary care systems. Since the economic analysis of risk identification undertaken for NICE5 which used the available draft version of the risk score at the time, the final version of the risk score was published in 2012,16 which includes the following risk factors:
-
age
-
gender
-
body mass index (BMI)
-
ethnicity [South Asian/other black and minority ethnic (BME)]
-
prescribed an anti-hypertensive
-
family history of diabetes.
Individuals in the LEADER cohort have an average LPDS of 5.31, with lower and upper interquartile ranges of 4.77 and 5.82, respectively.
Based on the LEADER data set, analysis shows that a screening strategy with a risk score cut-off point of 4.75 and HbA1c threshold for preventative intervention of 6.0% has a sensitivity of 94% for diabetes and 90% for HRD, while having to carry out blood tests on only 76% of individuals. In more affluent localities with populations with fewer or lower risk factors than in LEADER, a LPDS cut-off point of 4.75 would result in less than 76% of individuals being indicated for blood testing.
As the risk score includes ethnicity, it can be used in alternative populations with varying mixes of ethnicity.
Where routine primary care data are not available, particularly in settings outside a primary or secondary care setting such as a pharmacy, shopping centre, community or religious centre or the internet, the use of self-assessment using the Leicester Self-Assessment Score19 should not be precluded, the additional cost of the questionnaire compared with the LPDS having a negligible impact on the overall long-term cost-effectiveness.
Prescreening using random capillary glucose testing
Random capillary glucose testing is widely disregarded as a robust option for diagnostic screening because of its variability and poor test sensitivity at levels which give acceptable specificity. RCG testing is, however, another potential prescreening option to limit the number of people undertaking HbA1c or FPG blood tests. The device for undertaking RCG testing, often referred to as the ‘finger-prick test’, provides instant results that can be done within primary care and a decision made on how to interpret the results and what next steps to take (e.g. offer a HbA1c test) can be taken within the same consultation.
Variability and poor sensitivity are less of an issue if RCG is being considered as a means of identifying individuals most likely to have hyperglycaemia and in need of further diagnostic testing with a FPG or HbA1c test. In one study, the correlation between RCG and HbA1c was 0.62, a reasonably high correlation, suggesting that RCG could be useful as a prescreening tool. 18
As the LEADER study did not measure participants’ RCG levels, data on RCG were obtained from the ADDITION-Cambridge study. 20 This large study was conducted to evaluate the effectiveness and cost-effectiveness of intensive multifactorial treatment for people with screen-detected diabetes in primary care and used RCG and HbA1c tests as part of the initial screening protocol. The data supplied by the ADDITION team in Cambridge enabled us to construct a mapping between HbA1c and RCG values for individuals in the ADDITION study. For any individual’s HbA1c in the LEADER data set, an algorithm that we built was able to find the nearest HbA1c match in the ADDITION data. Then the algorithm could sample an RCG value from the subset of ADDITION RCG values corresponding to the matched HbA1c value. The sampled RCG values were then incorporated into the LEADER cohort data.
Formulation of set of screening strategies to evaluate
We use the term ‘screening strategy’ to refer to a permutation of any prescreen option with one of two blood test options.
Prescreen options:
-
no prescreen
-
use of the LPDS
-
use of a RCG test.
Blood test options:
-
FPG testing
-
HbA1c testing.
An example of a ‘simple strategy’ could be to use a FPG test on everybody (i.e. without a prior risk score or RCG). An example of a ‘stepped strategy’ would be to use the LPDS followed by a HbA1c test for those with a LPDS above a certain threshold. There is the additional ‘no screening’ option.
Each strategy includes a choice of one or two thresholds. For both LPDS and RCG, there is the choice of cut-offs for proceeding to a blood test. For individuals in the non-diabetic range of HbA1c or FPG, there is the choice of cut-off for labelling individuals as at HRD and thereby as eligible for referral for a preventative intervention.
Derivation of final set of screening strategies to model
The very large number of permutations of the components of a screening strategy means that there are lots of possible screening strategies that could be evaluated. The following seven steps describe the process and the rationale used to narrow down these options to a manageable set of strategies to be included in the modelling stage.
1. National Institute for Health and Care Excellence guidance for glycated haemoglobin
As a starting point, we include the strategy using HbA1c testing which was recommended by the 2012 NICE guidance,5 that is cut-off point of 6.5% or more for diabetes and of 6.0–6.4% for HRD.
2. National Institute for Health and Care Excellence guidance for fasting plasma glucose
Alongside the standard 7.0 mmol/l cut-off for diabetes, NICE recommended a cut-off of 5.5 mmol/l for the identification of individuals at HRD.
3. Choice of Leicester Practice Database Score threshold
Further modelling, carried out since the publication of the NICE guidance, undertook a thorough analysis of alternative LPDS prescreening thresholds and alternative HbA1c thresholds for intervening in individuals with HRD. This analysis indicated that it is likely to be more cost-effective to use a low LPDS threshold, for example 4.75, rather than a higher one such as 5.25. It also results in a reasonable proportion of individuals being offered a blood test (following discussions with clinician experts). A LPDS threshold of 4.75 was therefore chosen as most appropriate to use as a prescreening tool.
4. Scenario analyses: alternative glycated haemoglobin thresholds for defining high risk of diabetes
The further modelling described in step 3 also suggested that it may be cost-effective to intervene in individuals with HbA1c levels as low as 5.7% at least. For possible thresholds for labelling individuals as at HRD, we therefore chose to explore levels from 5.7% through to the current NICE recommendation of 6.0%.
5. Scenario analyses: alternative fasting plasma glucose thresholds for defining high risk of diabetes
We initially included a set of strategies with alternative FPG thresholds for HRD, ranging from a lower limit of 5.3 mmol/l (this was chosen as an ‘extreme’ lower case but was revisited during step 7) to an upper limit of 6.0 mmol/l [this was chosen as it is the higher of the two options recommended by WHO and the American Diabetes Association (ADA) for IFG]. 21
6. Random capillary glucose testing
The purpose of RCG testing is to reduce the number of individuals requiring a blood test. When evaluating RCG against the LPDS, the results might be dependent on an arbitrary choice of RCG cut-off point for being offered a subsequent blood test. To avoid this, we chose a RCG threshold that would reduce the number of blood tests required and result in the same proportion of individuals considered at HRD within the LEADER data set as equivalent strategies based on an LPDS cut-off point of 4.75 (see point 7 for more explanation of the need to ensure the same proportions identified as at HRD).
7. Adjusting thresholds to enable comparability between fasting plasma glucose and glycated haemoglobin strategies
Interim results indicated that it is possible to select a relatively low FPG cut-off point for defining HRD such that the FPG-based strategy is more cost-effective than the current NICE HbA1c-based strategy with a HbA1c cut-off for HRD of 6.0%. This is because the low FPG cut-off point results in a larger proportion of individuals being detected as at HRD and eligible for preventative intervention. Equally, a HbA1c cut-off point lower than 6.0% could then be found which would make HbA1c more cost-effective than FPG, and so on. To enable a ‘fair’, non-arbitrary comparison of HbA1c-based strategies versus FPG-based ones, it is necessary to take account of resource implications and the proportions labelled as at HRD. To do this, the two steps are:
-
Choose the proportion (or set of alternative proportions) of individuals identified as HRD on which the comparison is to be made. The proportion chosen and the corresponding HbA1c-based HRD thresholds are shown in Table 5.
-
For each given proportion of individuals identified as at HRD, identify a corresponding FPG threshold for HRD such that each pair of FPG and HbA1c thresholds results in the same proportion of individuals detected with HRD (and, therefore, offered the diet and exercise intervention) with each test – we refer to such pairwise thresholds as ‘ISO-resource’ thresholds. These thresholds, which take into account the base case assumed 20% higher uptake of offers of HbA1c testing compared with FPG testing (as discussed in Uptake rates of blood tests), are shown in Tables 6 and 7.
Screening strategy | Percentagea identified with HRD using the LEADER data set, adjusted for uptake of blood tests |
---|---|
NICE recommendations for high risk (with use of a risk score) | |
Assuming use LPDS cut-off of 4.75 to prescreen and HbA1c ≥ 6.0% | 16 |
Assuming use LPDS cut-off of 4.75 to prescreen and FPG ≥ 5.5 mmol/l | 16 |
NICE recommendations for high risk (without use of a risk score) | |
NICE recommendation for high risk, assuming no use of LPDS to prescreen and HbA1c ≥ 6.0% | 18 |
NICE recommendation for high risk, assuming no use of LPDS to prescreen and FPG ≥ 5.5 mmol/l | 23 |
Alternative thresholds for HRD/intervention | |
LPDS ≥ 4.75, HbA1c ≥ 5.8% | 27.5 |
HbA1c ≥ 5.8% | 33 |
LPDS ≥ 4.75, HbA1c ≥ 5.7% | 36 |
HbA1c ≥ 5.7% | 43 |
Strategy reference | Method used to prescreen who should be offered blood testing and prescreen threshold | Blood test used and threshold for offering intensive diet and exercise intervention to those with HRD detected by the threshold |
---|---|---|
No screening | ||
Screening for diabetes only | ||
LPDS 4.75/HbA1c 6.5 | LPDS ≥ 4.75 | HbA1c ≥ 6.5% (screening diabetes only – HbA1c) |
LPDS 4.75/FPG 7.0 | LPDS ≥ 4.75 | FPG ≥ 7.0 mmol/l (screening diabetes only – FPG) |
NICE-recommended strategies (diabetes and HRD): with use of risk score | ||
LPDS 4.75/HbA1c 6.0 | LPDS ≥ 4.75 | HbA1c ≥ 6.0% |
LPDS 4.75/FPG 5.5 | LPDS ≥ 4.75 | FPG ≥ 5.5 mmol/l |
Strategy reference | Method used to prescreen who should be offered blood testing and prescreen threshold | Blood test used and threshold for offering intensive diet and exercise intervention to those with HRD detected by the threshold |
---|---|---|
NICE-recommended strategies (diabetes and HRD): no use of risk score | ||
HbA1c 6.0 | – | HbA1c ≥ 6.0% |
FPG 5.5 | – | FPG ≥ 5.5 mmol/l |
NICE HbA1c threshold plus RCG (to be compared with LPDS 4.75/HbA1c 6.0) | ||
RCG 4.4/HbA1c 6.0 | RCG ≥ 4.4 | HbA1c ≥ 6.0% |
Other strategies (ISO resource as strategies HbA1c 6.0 and FPG 5.5) | ||
HbA1c 5.9 | – | HbA1c ≥ 5.9% |
FPG 5.6 | – | FPG ≥ 5.6 mmol/l |
Other strategies: ISO resource ≈27% HRD | ||
LPDS 4.75/HbA1c 5.8 | LPDS ≥ 4.75 | HbA1c ≥ 5.8% |
LPDS 4.75/FPG 5.2 | LPDS ≥ 4.75 | FPG ≥ 5.2 mmol/l |
Other strategies: ISO resource ≈32% HRD | ||
HbA1c 5.8 | – | Hba1c ≥ 5.8% |
FPG 5.2 | – | FPG ≥ 5.2 mmol/l |
Other strategies: ISO resource ≈36% HRD | ||
LPDS 4.75/HbA1c 5.7 | LPDS ≥ 4.75 | HbA1c ≥ 5.7% |
LPDS 4.75/FPG 5.0 | LPDS ≥ 4.75 | FPG ≥ 5.0 mmol/l |
RCG 4.2/HbA1c 5.7 | RCG ≥ 4.2 | HbA1c ≥ 5.7% |
Other strategies: ISO resource ≈43% HRD | ||
HbA1c 5.7 | – | HbA1c ≥ 5.7% |
FPG 5.0 | – | FPG ≥ 5.0 mmol/l |
Final set of National Institute for Health and Care Excellence guideline-based screening strategies
Table 6 shows the strategies that form the main analysis to be undertaken. Alternative strategies assessed as secondary analyses are shown in Table 7.
It should be emphasised that the NICE-based strategies are the ones that carry most weight, as they are based around current NICE guidance set out in 2012. 5 However, a modelling study suggested that it may be cost-effective to offer preventative interventions to individuals with HbA1c levels lower than 6%;6 therefore, it is possible that guidelines could change at some point in the future. We considered it useful to compare the cost-effectiveness of HbA1c versus FPG at lower cut-off points than the NICE recommended cut-off points for HRD.
Unit costs of prescreening and blood glucose tests
The full costs of tests include all costs associated with completing the test including nurse or health-care assistant time and laboratory costs. These are shown in Table 8. The costs for a HbA1c test and a FPG test are for laboratory tests (not POC tests).
Option | HCA/nurse time | Laboratory costs | Full cost | Year | Inflation uplift to 2013/14 rates | 2013/14 cost | Source |
---|---|---|---|---|---|---|---|
LPDS | – | – | – | – | 1.19 | £0.24 | Professor Kamlesh Khunti , University of Leicester, 2011, personal communication |
RCG | – | – | – | – | – | £3.34 | Estimate based on cost of an RCG relative to 2hPG in Chatterjee22 |
FPG | £4.13 | £6.10 | £10.23 | 2006 | 1.19 | £12.18 | Vascular Checks modelling Consultation (see table 3 in the Department of Health report23) |
HbA1c | – | – | – | – | – | £14.40 | Estimate based on difference in laboratory costs from FPG (Professor Kamlesh Khunti, University of Leicester, 2011, personal communication) |
There is no standard national source of unit costs for England for the blood tests for diabetes. The most recent costing in the UK is the one undertaken as part of the Vascular Checks modelling work,23 from which we obtained the cost of a FPG test. For this analysis, the most important issue is the difference in cost between a FPG test and a HbA1c test. The only difference in cost between a FPG test and a HbA1c test is the laboratory cost and we obtained this information from an estimate of these costs from Professor Kamlesh Khunti (University of Leicester, 2011, personal communication).
The cost for undertaking a RCG test was estimated based on a published study by Chatterjee. 22
Cost estimates were updated as appropriate for inflation. Annual inflation adjustments were obtained from the Hospital and Community Health Services Index reported in Unit Costs of Health and Social Care 2012. 24
Uptake rates of blood tests
Uptake rates for diabetes screening are often reported to be relatively low, with only 61% of patients taking up screening in the pilot diabetes screening programme in England. 25
We have not used this rate because the pilot programme was a research study in which participants were required to give consent, which some individuals may not wish to do, while some individuals may choose not to respond or attend for a variety of reasons.
For this economic evaluation, the appropriate rates need to reflect a setting where someone has already presented at the GP centre for the wider health check (i.e. for cholesterol and blood pressure). This makes the proportion accepting a blood test for HbA1c or FPG at the same time higher (if an individual is having cholesterol checked anyway, very few people would refuse to have the needle in a very short time longer to draw another sample for the HbA1c or FPG test).
There is currently no published evidence on rates of uptake of HbA1c testing and FPG testing within the NHS Health Checks programme, and we are not aware of any evidence from a similar setting elsewhere. Estimates were, therefore, based on discussions with clinical experts. Table 9 shows the estimates of uptake rates. These represent the proportion of people that have already presented at their GP centre for the health check who then agree to have the blood test for diabetes (or HRD).
Offer and uptake rates | Mean | SE | Distribution | Basis of distribution, where applicable | Sensitivity analysis |
---|---|---|---|---|---|
Offered health check | 100% | – | Fixed | – | – |
Proportion who take a RCG test when offered one as a prescreen at first health check attendance | 95% | 0.026 | Beta | Assumed 95% CI 100% to 90% | 85% |
Risk score data availability (LPDS) | 100% | – | Fixed | – | – |
Proportion who take screening HbA1c test when offered one during health check | 95% | 0.026 | Beta | Assumed 95% CI 100% to 90% | – |
Difference between uptake of FPG and HbA1c (FPG lower) when offered one during health check | 20% | 0.051 | Beta | Assumed 95% CI 10% to 30% | 10% |
Proportion who take confirmatory HbA1c at a repeat visit | For each sample run, set so that each sample value is 10% lower than sampled uptake for first HbA1c | – | – | ||
Proportion who take confirmatory FPG at a repeat visit | For each sample run, assumed to be the same as sample value for confirmatory HbA1c (see Uptake rates of blood tests for explanation) | – | – |
Two laboratory blood tests are required to make a diagnosis of diabetes. For the purpose of discussing uptake rates, we refer to the initial test as the ‘screening test’ and any second test to confirm if an individual has diabetes as the ‘confirmatory test’. The confirmatory test requires a second visit to the GP centre once the laboratory results for the first test have been sent to the GP centre.
The differential uptake between HbA1c and FPG testing is uncertain and was therefore explored within sensitivity and scenario analyses as discussed in Deterministic one-way sensitivity analyses and Scenario analyses – alternative prevalence and uptake rates, respectively). This is partly because there is variation across the country in instructions accompanying Health Checks invitations, in particular for practices that use FPG to test for diabetes. Some such practices request that individuals fast before their visit so that the FPG test can be taken at the same time as the blood test for cholesterol whereas others do not, in which case individuals indicated (after a prescreen if used) for a test for diabetes would need to return on a later date. The need for a separate visit would be expected to reduce the uptake of the test.
After discussion with clinical experts, it was considered to be conservative to assume no difference between the uptake of confirmatory HbA1c testing and uptake of confirmatory FPG testing. The rationale for this is that, given that an individual has been willing and able to attend for a first FPG, the reasons for lower uptake of FPG testing in general at the screening test (i.e. the inconvenience of fasting and/or visiting their GP in the morning) may not apply to that individual. In other words, having attended for a first FPG test, you may be as likely to return for a confirmatory FPG test (if needed) as an individual undergoing HbA1c testing would return for a confirmatory HbA1c test (if needed).
We assume 100% availability of data in GP databases to calculate the LPDS for each individual.
Monte Carlo sampling process for determining individual uptake and screening outcomes
For each individual in the model, random sampling was used to determine whether or not the individual accepts the offer of a blood test, based on the evidence and assumptions for uptake probabilities in Table 9.
If the stochastic screening outcome was HRD in the model, further random sampling was used to determine whether or not an individual would take up the offer of an intensive lifestyle intervention to reduce his or her risk of developing diabetes (see Referral for and uptake of preventative interventions in people with high-risk diabetes for evidence on uptake of prevention intervention). For any given individual in the model, the same sampled random numbers were used across the range of strategies to avoid introducing sampling bias.
Mapping individual screening outcomes to initial glycaemic trajectories
Discordance between the groups of individuals identified by glycated haemoglobin and those identified by fasting plasma glucose
As shown previously (see Baseline characteristics and descriptive analyses), there is limited concordance between the FPG test and the HbA1c test in terms of an individual’s screening outcome [classification as having diabetes, HRD or normal glucose tolerance (NGT)]. In other words, the subset of individuals classified as having diabetes with a HbA1c test only partially overlaps the subset classed as having diabetes with a FPG test. The same applies to the two subsets of individuals classified as having HRD and the two subsets classified as having NGT. For example, an individual may be diagnosed as having diabetes with a HbA1c test but as having a HRD with a FPG test, and vice versa. Similarly, an individual might be classed as having NGT with a FPG test but as having a HRD with a HbA1c test, and vice versa.
This lack of concordance is evidenced in published literature as well as being present in our analysis of the LEADER data set. According to one Dutch study,26 up to half of the subjects diagnosed at present using current glucose-defined criteria (fasting or post-glucose challenge) would not be diagnosed using HbA1c, and vice versa.
The economic model of screening that existed prior to this evaluation was designed to evaluate a single screening test. For a given screening outcome for an individual (diabetes, HRD or NGT), the prior model would apply an unambiguous corresponding natural history of future increases in glycaemia as follows:
-
Individuals with undiagnosed diabetes have a HbA1c trajectory (an increasing rate of HbA1c change) determined by their baseline HbA1c and a sampled HbA1c at clinical detection in the future [based on an average of 8% (see Time to clinical detection for cases of diabetes which are not screen detected)].
-
Individuals with undiagnosed HRD follow an individualised HbA1c trajectory determined according to a sampled outcome of whether they will progress to diabetes, and if so, when.
-
Individuals with NGT maintain their baseline HbA1c value.
However, when comparing two tests as in HbA1c versus FPG, for individuals with discordant screening outcomes between the two tests, the corresponding natural history in the model is ambiguous. The upshot of this is that, without some suitable modelling mechanism, the same individual might, for example, be assigned to an undiagnosed diabetes natural history when modelling HbA1c testing but undiagnosed HRD when modelling FPG testing.
This obviously would be an inappropriate departure from reality, as (in the absence of intervention) individuals follow the same trajectory in real life regardless of whether they have been screened with a FPG or HbA1c test. Because the modelled screening outcome (diabetes, HRD or NGT) determines the subsequent modelled glycaemic trajectory, such a departure would create spurious differences between the two strategies which could bias the modelled results. Within the economic model, we therefore derived a mechanism for ensuring that the underlying (untreated) glycaemic trajectory of an individual is the same regardless of whether a FPG test or HbA1c test has been used to screen them. Moreover, consistency is needed across all of the modelled screening strategies and not just on a pairwise basis.
Principle to ensure consistency
Individuals who meet the criterion for diabetes according to either the FPG or the HbA1c test (i.e. ≥ 7.0 mmol/l or ≥ 6.5%, respectively), for the purpose of modelling their glycaemic progression, are flagged in the model as having diabetes for all screening strategies. For any screening strategy that results in such an individual’s screening outcome being undetected diabetes, in the model, they follow the natural history of undiagnosed diabetes (while they remain undiagnosed; see Time to clinical detection for cases of diabetes which are not screen detected). This is considered a reasonable assumption because for most individuals, if the result of one of the tests (either FPG or HbA1c) would be in the diabetes range, say HbA1c, then it is likely that the pathophysiological defects in glucose metabolism would result in further glycaemic progression towards the diabetes threshold for the other measure (in this example FPG) within a few years. This assumption corroborates with a cross-sectional analysis that we undertook, using the LEADER cohort, which revealed that, among the subset of individuals with an initial FPG ≥ 7.5 mmol/l, 86% of individuals also had a HbA1c ≥ 6.5%.
Rate of progression from high risk of diabetes to diabetes
The landmark Finnish Diabetes Prevention Study (DPS) and American Diabetes Prevention Programme (DPP) trials were designed to assess the ability of intensive lifestyle interventions to reduce the risk of progression from impaired glucose tolerance (IGT) to diabetes. These trials reported incidence rates of diabetes of 23% over 4 years27 and 11% over 3 years,28 respectively. One review of progression rates has quoted higher annual rates of 5–10%. 29
Rates reported in some research studies may also be inflated to some extent by:
-
selective recruitment of individuals at particularly HRD, for example because of high average baseline BMI levels
-
the combination of the annual frequency of the OGTTs to test for diabetes during follow-up and the between-test variability of FPG and 2hPG measures that make up an OGTT.
The average progression rates in clinical practice may therefore be lower than in these trials, although there is likely to be significant variation regionally within England according to factors such as ethnicity, deprivation and other demographics.
It was decided that the best rates to use were those presented in a meta-analysis published in 2013. This reported progression rates approximately equivalent to 3.5% per year from a baseline HbA1c level of 6.0–6.4%. 30
Progression from fasting plasma glucose-identified high risk of diabetes to diabetes
We assume, based on advice from clinicians, that, where GP practices use FPG to identify individuals with HRD, they would continue to use a FPG to subsequently monitor them.
In the absence of knowledge of any evidence to the contrary, we also assume that the shape of the curves for the cumulative incidence of diabetes would be the same, regardless of whether or not annual monitoring of HRD is assessed by FPG or HbA1c testing.
The use of HbA1c as the sole glucose-related risk factor in some of the risk equations for complications of diabetes, and the fact that glucose control and switching in clinical practice is carried out with reference to HbA1c levels, necessitates that we identify the corresponding HbA1c level when individuals with FPG-identified HRD reach a FPG of 7.0 mmol/l (i.e. diabetes) – this HbA1c level is assumed to be 6.5%.
Adjusting an individual’s risk of diabetes to take account of both fasting plasma glucose and glycated haemoglobin
In this section, we describe the evidence review that we undertook to identify published literature reporting the independent contributions of FPG and HbA1c to the risks of developing diabetes.
As already discussed, a HbA1c test and a FPG test identify only partially overlapping groups of individuals with diabetes and at HRD. The average levels of HbA1c and FPG of individuals identified as at HRD with HbA1c testing may differ from the average levels under FPG testing. It cannot, therefore, be assumed that the average risk of diabetes for an individual identified at HRD with a HbA1c test is the same risk as that for an individual identified using a FPG test. It is, therefore, imperative that risks of developing diabetes take account of individual risk factors, in particular baseline FPG and HbA1c levels.
Additional literature was required to identify studies that had evaluated diabetes risk conditional on both baseline FPG and baseline HbA1c. In the model it was necessary to vary the risk of diabetes according to the individual’s FPG and HbA1c levels. Therefore, it was necessary to estimate the independent effects of these continuous measures on the probability of diabetes from published literature.
Given the time available for this particular topic, a new comprehensive literature search and review was not possible. However, we were able to rely on (1) studies identified in the 2013 evidence review update on screening for diabetes for the HTA,14 (2) studies which we had already identified as part of the work on the 2012 NICE risk assessment work5 and (3) additional studies identified from clinical experts. Studies were included in our review if they reported baseline measures for both FPG and HbA1c, and an analysis of the risk of diabetes.
We have identified 11 studies that have reported risk or incidence of diabetes by FPG and HbA1c score, as shown in Table 10. We aimed to identify a multivariate regression model that included FPG and HbA1c as continuous variables and that could be included within our individual-level simulation model. Table 10 summarises the population that was studied and the definition of diabetes used based on a HbA1c, FPG and/or 2hPG glucose.
Study | Population | Basis of diagnosis of diabetes | Baseline HbA1c (%) | Baseline FPG (mmol/l) | Baseline 2hPG (mmol/l) |
---|---|---|---|---|---|
Law et al. (2010)31 | Hong Kong Cardiovascular Risk Factor Prevalence Study, Hong Kong | ADA 2010, FPG ≥ 7.0 mmol/l, 2hPG ≥ 11.1 mmol/l and/or HbA1c ≥ 6.5% | N/A | N/A | N/A |
Valdes et al. (2011)32 | Asturias study, Spain | FPG ≥ 7.0 mmol/l, 2hPG ≥ 11 mmol/l and/or clinical diagnosis | Q1 3.4–4.8 | 5.0 | 5.3 |
Q2 4.9–5.1 | 5.2 | 5.7 | |||
Q3 5.2–5.4 | 5.3 | 5.8 | |||
Q4 5.5–6.9 | 5.6 | 6.6 | |||
Sato et al. (2010)33 | Kansai Healthcare Study, Japan | FPG ≥ 7.0 mmol/l or were taking an oral antidiabetic agent or insulin | N/A | N/A | N/A |
Ko et al. (2000)34 | The Diabetes and Endocrine Centre of the Prince of Wales Hospital, China | FPG ≥ 7.0 mmol/l | 5.78 | 5.36 | 7.55 |
Norberg et al. (2006)35 | Vasterbotten Intervention Programme, Sweden | FPG ≥ 7.0 mmol/l or 2hPG ≥ 12.2 mmol/l | M/D: 4.7 | M/D: 6.0 | M/D: 7.9 |
M/C: 4.3 | M/C: 5.3 | M/C: 6.2 | |||
W/D: 4.7 | W/D: 5.8 | W/D: 8.4 | |||
W/C: 4.3 | W/C: 5.2 | W/C: 7.2 | |||
Rasmussen et al. (2008)36 | ADDITION, Denmark | FPG ≥ 6.1 mmol/l or 2hPG ≥ 11.1 mmol/l | IFG: 5.6 | IFG: 5.8 | IFG: 6.2 |
IGT: 5.9 | IGT: 5.3 | IGT: 9.1 | |||
Selvin et al. (2011)37 | ARIC, USA | Definition 1: a single FPG value ≥ 7.0 mmol/l at baseline (visit 2). Definition 2: FPG values ≥ 7.0 mmol/l at two separate examinations | N/A | N/A | N/A |
Takahashi et al. (2010)38 | Tokyo, Japan | HbA1c ≥ 6.5% or self-reported, or commencement of glucose-lowering treatment | 5.4 | 5.5 | N/A |
Wang et al. (2011)39 | Indian tribes/communities in Arizona, North/South Dakota, and Oklahoma | HbA1c ≥ 6.5% or FPG ≥ 7.0 mmol/l or if on diabetes medications | N/A | N/A | N/A |
Law and colleagues31 describe the 8-year incidence of diabetes in a cohort of 530 non-diabetic Chinese individuals. 31 There were 47 diagnoses by 3 years and 81 at 8 years of follow-up. The authors report the hazard ratios from a multivariate Cox regression which includes covariates for HbA1c, FPG and 2hPG. The results suggest that HbA1c and FPG are independent predictors of a diagnosis of diabetes: the baseline hazard ratio for HbA1c was 3.74 [95% confidence interval (CI) 1.98 to 7.04] per 1% HbA1c and the hazard ratio for FPG was 1.76 (95% CI 1.13 to 2.74) per mmol/l. Unfortunately, the authors do not report mean baseline HbA1c or FPG in the article.
Valdes and colleagues32 report results from the Asturias study from northern Spain, in which the incidence of diabetes is reported for individuals with high FPG and/or high HbA1c. 32 The estimated cumulative incidence values and hazard ratios reported in that study are shown in Table 11.
Incidence/hazard ratio | FPG < 5.56 mmol/l | FPG ≥ 5.56 mmol/l | ||
---|---|---|---|---|
HbA1c < 5.5% | HbA1c ≥ 5.5% | HbA1c < 5.5% | HbA1c ≥ 5.5% | |
Cumulative incidence at 6 years (%) | 2 | 7 | 9 | 32 |
Hazard ratio vs. low risk | 1 | 3.5 | 4.5 | 16 |
Hazard ratio vs. high risk | 0.063 | 0.219 | 0.281 | 1 |
The Sato et al. 33 report results from a Japanese study in which study participants consisted of 9116 Japanese men aged 40–55 years with FPG less than 7.0 mmol/l who were not taking an oral antidiabetic agent or insulin at study entry. The study reports the results of a logistic regression which included categories for FPG and HbA1c. The estimated odds ratios for diabetes for each subgroup are shown in Table 12. The results suggest that both classifications are strong independent predictors of the diagnosis of diabetes.
FPG or HbA1c category | Odds ratio | 95% CI |
---|---|---|
FPG ≤ 5.5 mmol/l | 1.00 | − |
FPG 5.6–6.0 mmol/l | 3.28 | 2.57 to 4.18 |
FPG 6.1–7.0 mmol/l | 14.54 | 11.31 to 18.68 |
HbA1c ≤ 4.9% (5.3)a | 1.00 | − |
HbA1c 5.0–5.4% (5.4–5.7%)a | 1.71 | 1.26 to 2.31 |
HbA1c 5.5–5.9% (5.8–6.2%)a | 4.50 | 3.30 to 6.14 |
HbA1c 6.0–6.4% (6.3–6.7%)a | 11.04 | 7.23 to 16.87 |
HbA1c ≥ 6.5% (6.8)a | 33.58 | 18.88 to 66.78 |
Ko and colleagues34 categorised 208 subjects into groups based on their FPG (≥ 6.1, < 6.1 mmol/l) and HbA1c (≥ 6.1%, < 6.1%). The incidence of diabetes according to the OGTT test is reported after variable duration of follow-up. Since the OGTT is used to define diabetes at follow-up, the results have not been extracted here. This study was not used to estimate risk based on the diagnosis criteria and because it was measured in a Chinese population.
Norberg and colleagues35 report analyses of 468 participants in a Swedish study. They performed a logistic regression to predict the odds ratio of diagnosis of diabetes according to categories of HbA1c and whether or not the individual met the criteria for IFG at baseline (5.6–6.9 mmol/l). The results are reported in Table 13.
HbA1c/IFG | Odds ratio | 95% CI |
---|---|---|
HbA1c < 4.5% | 1.0 | – |
HbA1c 4.5–4.69% | 1.2 | 0.28 to 5.34 |
HbA1c ≥ 4.7% | 16 | 2.23 to 115.3 |
IFG | 18.8 | 2.88 to 123.4 |
Takahashi and colleagues38 report an odds ratio of 1.06 for FPG scores when added to HbA1c to predict diabetes. They report only cumulative incidence by categories of HbA1c.
Wang and colleagues39 describe analyses of 4549 American Indian men and women. They developed a logistic model for the risk of diabetes defined according to HbA1c ≥ 6.5% and FPG ≥ 7.0 mmol/l. The odds ratios for defined high-risk states according to these measures are reported in Table 14.
Risk group | FPG/HbA1c-defined diabetes | |
---|---|---|
Mean odds ratio | 95% CI | |
IFG | 2.34 | 1.81 to 3.03 |
6.0% ≤ HbA1c < 6.5% | 3.43 | 2.27 to 5.16 |
Two studies were identified as particularly useful for our applications:
Selvin and colleagues37 undertook analyses on a large population from the US Atherosclerosis in Communities (ARIC) study, which did not recruit based on glucose tests; therefore, representing a broad range of risk for diabetes. The ARIC study consisted of 12,485 individuals, after excluding individuals who identified their race as other than black or white, those with self-reported diabetes diagnosis, individuals with missing values for key variables, or individuals who were non-fasting. Maximum follow-up of participants was 15 years, and diabetes diagnosis was assessed by either glucose measurements or self-reported diagnosis.
The 10-year risks of FPG-defined diagnosed diabetes were stratified by categories of baseline FPG and HbA1c, as summarised in Table 15.
FPG category (mmol/l) | HbA1c < 5.7% | HbA1c ≥ 5.7% and < 6.5% | HbA1c ≥ 6.5% |
---|---|---|---|
< 5.56 | 2.65 | 9.69 | 20.00 |
≥ 5.56 and < 7.00 | 7.19 | 22.75 | 48.84 |
≥ 7.00 | 30.16 | 55.06 | 88.43 |
Rasmussen and colleagues,36 in the ADDITION-Denmark study, described a European population and analysed the data using continuous variables for FPG and HbA1c. The ADDITION-Denmark study was a population-based screening and intervention study for type 2 diabetes. This study included analysis of 607 individuals with IFG and 903 individuals with IGT identified as part of the screening programme. The definition of IFG corresponds to that of isolated IFG (5.6 mmol/l ≤ FPG < 6.1 mmol/l and 2hPG < 7.8 mmol/l), whereas IGT included isolated IGT and combined IFG and IGT (FPG < 6.1 mmol/l and 7.8 mmol/l ≤ 2hPG < 11.1 mmol/l). Incident diabetes was defined as one diabetic value of FPG (≥ 6.1 mmol/l) or 2hPG (≥ 11.1 mmol/l). The median follow-up for the groups was 2.5 and 2.1 years, respectively.
Rasmussen et al. 36 reported a statistical model for the hazard ratios for diabetes in those individuals who had IFG (FPG 5.6 mmol/l) at screening, adjusting for their HbA1c and FPG score in a multivariate model. They report a similar analysis in individuals who met the threshold for IGT at screening. Cumulative risks, progression rates and hazard ratios for progression to diabetes (≥ 6.1 mmol/l) were estimated with a regression model using interval censoring. The results of the regression model are reported in Table 16.
Blood glucose variable | Isolated IFG (5.6 mmol/l ≤ FBG < 6.1 mmol/l and 2hPG < 7.8 mmol/l) | IFG + IGT (7.8 mmol/l ≤ 2hBG < 11.1 mmol/l and FBG < 6.1 mmol/l) | ||
---|---|---|---|---|
Mean hazard ratio | 95% CI | Mean hazard ratio | 95% CI | |
HbA1c (per 1%) | 1.40 | 1.09 to 1.80 | 1.23 | 1.08 to 1.47 |
FBG (per mmol/l) | 3.19 | 2.33 to 4.37 | 1.65 | 1.43 to 1.92 |
2hPG (per mmol/l) | 1.10 | 1.00 to 1.21 | 1.26 | 1.18 to 1.35 |
These two studies from Selvin et al. 37 and Rasmussen et al. 36 provide useful information about the independent effects of FPG and HbA1c on diabetes risk. However, individually, the studies provide incomplete data on the independent effects of FPG and HbA1c on diabetes risk and could not be used directly in the cost-effectiveness model. Selvin et al. 37 described the absolute risk of diabetes for a cohort from the USA. These data may not be generalisable to a UK population in which the incidence of diabetes may be different. Rasmussen et al. 36 report the hazard ratios of HbA1c and FPG test scores on diabetes risk in a Danish population with either IFG or IGT. In order for these estimates of the hazard ratios to be applied to UK diabetes incidence rates, it would need to be established if they could be extrapolated to individuals who do not meet the criteria for either IFG or IGT. As a consequence of the limitations in both studies, we used the data to estimate parameters for the cost-effectiveness model that were based on the evidence provided by both studies.
We developed a simple simulation model to predict 10-year incidence of diabetes among individuals with baseline FPG and HbA1c test results. The simulation included parameters to adjust individuals’ risk according to their FPG and HbA1c test result using alternative hazard ratios. The simulated diabetes incidence rates for subgroups defined by Selvin et al. 37 are conditional on FPG and HbA1c to enable comparison of the simulated and observed diabetes incidence by subgroup.
The simple simulation model used data from the LEADER cohort to describe individual test results from HbA1c, FPG and 2hPG. Counts of the number of individuals in the subgroups defined by Selvin et al. 37 are detailed in Table 17. We do not have diabetes incidence data for the LEADER cohort; therefore, the baseline 7-year cumulative incidence of diabetes from the Finnish DPS was used to estimate the annual incidence of diabetes for individuals with the average glycaemic tests scores observed in the Finnish DPS control group. From this baseline risk, we adjusted an individual’s annual risk of developing diabetes according to his or her HbA1c and FPG test levels and hazard ratio parameters to estimate 10-year risk of diabetes. Hazard ratios parameters were taken from Rasmussen et al. ,36 but we included an additional analysis in which the simulation was calibrated to fit the Selvin et al. 37 data.
FPG (mmol/l) | HbA1c < 5.7% | HbA1c 5.7–6.5% | HbA1c ≥ 6.5% |
---|---|---|---|
< 5.56 | 4236 | 3029 | 128 |
5.56–7.0 | 421 | 1032 | 240 |
> 7.0 | 8 | 68 | 169 |
Analyses comparing the predicted cumulative incidence of diabetes in subjects from the LEADER cohort, using the risk equations described in Rasmussen et al. ,36 were conducted. Long-term survival estimates were extracted from the Finnish DPS along with estimates of mean baseline HbA1c, FPG and 2hPG. The mean estimates were used to estimate deviance from the mean in the LEADER cohort. Four predictive models were tested:
-
hazard ratio for HbA1c (1.23) and FPG (1.65) from the IGT subgroup
-
hazard ratio for HbA1c (1.23), FPG (1.65) and 2hPG (1.26) from the IGT subgroup
-
hazard ratio for HbA1c (1.40), FPG (3.19) and 2hPG (1.10) from the IFG subgroup
-
a modified analysis in which the baseline cumulative incidence from the Finnish DPS was increased to 0.82 to reduce incidence in the LEADER cohort and the hazard ratios HbA1c (1.4), FPG (1.65) and 2hPG (1.26) applied to investigate what magnitude of parameters were necessary to fit the Selvin et al. 37 study.
The predicted 10-year risks of diabetes according to the subgroups defined in Selvin et al. 37 are illustrated in Figure 8.
FIGURE 8.
Results of four predictive models for development of diabetes. (a) IGT model with only FPG and HbA1c used to modify risk; (b) IGT model with FPG, HbA1c and 2hPG to modify risk; (c) IFG model with FPG, HbA1c and 2hPG to modify risk; and (d) a calibrated model to match with Selvin et al. 37



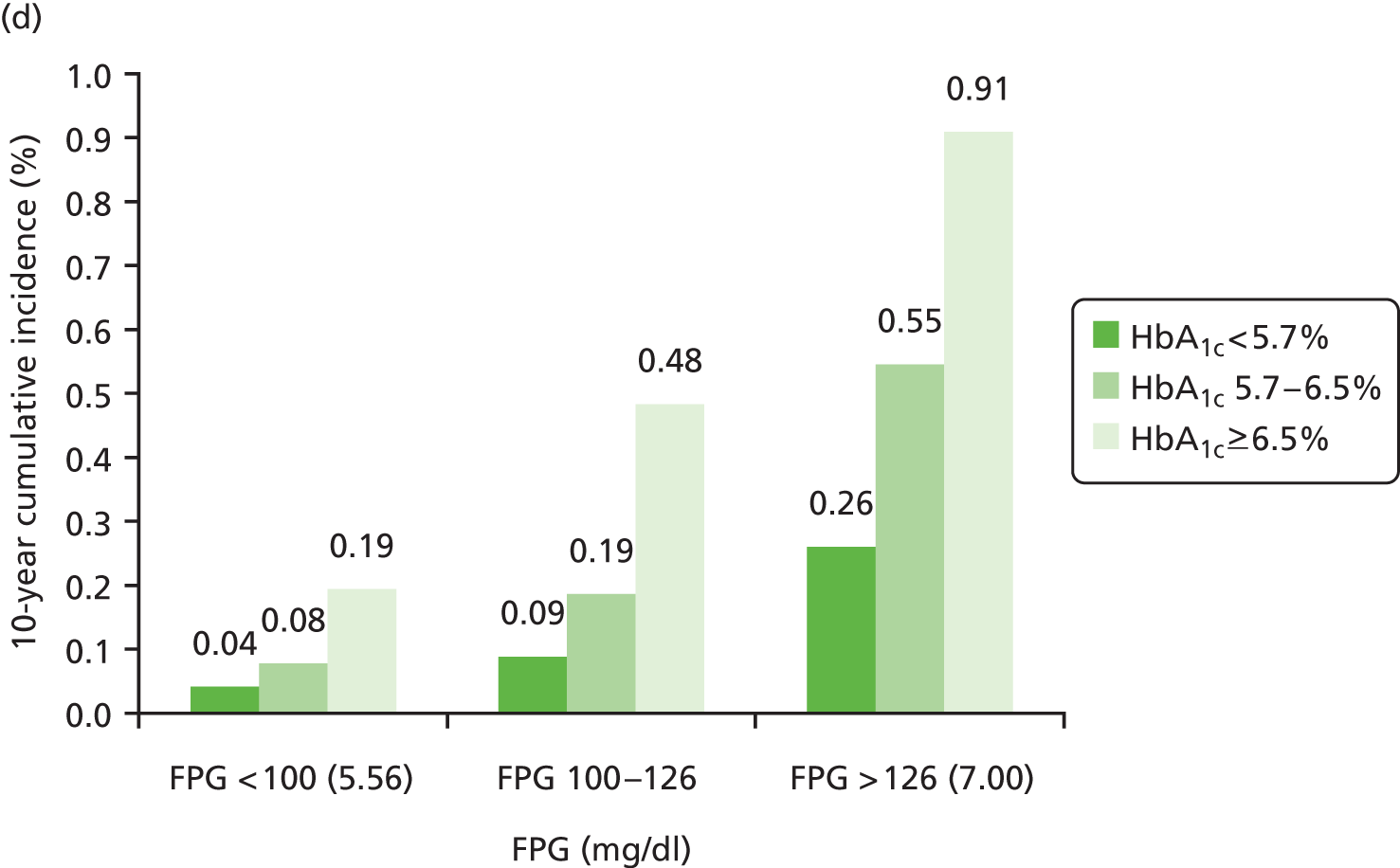
In all analyses, 10-year diabetes incidence increases for individuals with higher test scores on FPG and HbA1c. However, the difference in risk between the subgroups varies according to the assumed values for the hazard ratios. The simulated output in Figure 8a illustrates that the Rasmussen IGT model, with only FPG and HbA1c scores to adjust risk, does not generate sufficient risk differentiation between FPG and HbA1c subgroups. Greater differentiation in risk is achieved if 2hPG is included from the IGT model, as illustrated in Figure 8b. Estimates from the Rasmussen IFG model demonstrate differentiation of risk between FPG subgroups that more closely reflects estimates from Selvin et al. 37 reported in Table 15. However, this output overestimated risk in individuals with FPG ≥ 7.0 mmol/l. In the calibrated model, changing the HbA1c hazard ratio from that in Figure 8b and adjusting the baseline risk produced results more similar to Selvin et al. 37 (see Table 15) than found in the other analyses.
Fasting plasma glucose/glycated haemoglobin at baseline and risk of incident diabetes – evidence incorporated into the model
We chose to use the parameter estimates used to generate simulation output Figure 8d in the cost-effectiveness analysis. The final hazard ratios for FPG, HbA1c and 2hPG are reported in Table 18. These hazard ratio parameters were applied to the baseline risk of diabetes to generate individualised risk of diabetes estimates conditional on FPG, 2hPG and HbA1c.
Blood glucose variable | Mean hazard ratio | 95% CI |
---|---|---|
HbA1c (per 1%) | 1.40 | 1.09 to 1.80 |
FPG (per mmol/l) | 1.65 | 1.43 to 1.92 |
2hPG (per mmol/l) | 1.26 | 1.18 to 1.35 |
Fasting plasma glucose/glycated haemoglobin at baseline and risk of incident cardiovascular disease: evidence review
This section describes additional literature that was used to adjust risks of CVD to take account of both HbA1c and FPG. While HbA1c, systolic blood pressure (SBP), total cholesterol and high-density lipoprotein (HDL)-cholesterol are included within the published UK Prospective Diabetes Study (UKPDS) risk equations for CHD40 and stroke risk,1 FPG is not included.
The purpose of the following review was to identify studies that had evaluated CVD risk conditional on both baseline FPG and baseline HbA1c. In the model it was necessary to adjust individuals’ risk of diabetes according to their FPG and HbA1c. Therefore, it was necessary to estimate the independent effects of these continuous measures on the probability of CVD from published literature. Studies were identified from a previous literature review and were included if they reported baseline measures for FPG and HbA1c and an analysis of the risk of CVD.
We identified two articles that discussed multivariate risk factors for CVD events including both HbA1c and FPG in the regression model.
The first useful study is from a conference presentation in 200542 reporting the results of a multivariate regression of FPG and HbA1c scores to the risk of macrovascular complications. The study used data from 3538 participants in the UKPDS to determine if HbA1c and FPG are associated independently with incident macrovascular complications in type 2 diabetes. The data comprised 766 macrovascular events including fatal or non-fatal myocardial infarction, fatal or non-fatal stroke, non-fatal ischaemic heart disease and sudden death. Cox models were fitted to mean duration of follow-up of 9.7 years and adjusted for post-dietary run-in values for age, sex, ethnicity, HDL and low-density lipoprotein cholesterol, triglycerides, SBP and albuminuria (urine albumin ≥ 50 mg/l) and smoking status at time of diagnosis. FPG and HbA1c were used in the first analysis as baseline values and in the second analysis as time-dependent variables. Table 19 reports the results of a multivariate analysis of baseline and mean updated HbA1c. The updated mean HbA1c and FPG hazard ratios demonstrate the independent contribution of elevated HbA1c and FPG to the risk of developing macrovascular complications.
Variables | Baseline FPG, HbA1c | Updated mean FPG, HbA1c | ||||
---|---|---|---|---|---|---|
Hazard ratio | 95% CI | p-value | Hazard ratio | 95% CI | p-value | |
FPG (per mmol/l) | 1.06 | 1.02 to 1.10 | 0.0035 | 1.04 | 0.99 to 1.09 | 0.15 |
HbA1c | 0.97 | 0.91 to 1.03 | 0.32 | 1.12 | 1.03 to 1.21 | 0.006 |
The hazard ratios in Table 19 are applied in the model to estimate the probability of macrovascular events given an individual’s FPG and HbA1c score in the simulation.
The second study is a more recent study from Selvin and colleagues in 2010,43 which analysed the ARIC cohort to observe the risk of diabetes and CVD outcomes by HbA1c level. We have not directly used evidence from this study within the model, but present it here to show some comparison with the UKPDS. Hazard ratios for the progression to diabetes and CVD outcomes by categories of HbA1c (< 5%, 5–5.5%, 5.5–6%, 6–6.5%, > 6.5%) were presented. The results show that higher HbA1c is associated with a higher risk of diabetes and CVD outcomes. The analysis includes an adjusted model for FPG. Although the FPG estimates are not reported, the increased risk of diabetes and CVD outcomes remains significant, after adjusting for HbA1c.
Table 20 reports the hazard ratios for HbA1c categories and a continuous risk score per 1% increase in HbA1c reported in Selvin et al. 43 The results report greater sensitivity to HbA1c in a prediabetes population than that reported in the diagnosed UKPDS cohort. For example, baseline HbA1c is not a significant risk factor for CVD disease after adjustment for FPG in the UKPDS, whereas a high HbA1c at baseline in the ARIC cohort is a large and significant risk factor for coronary heart disease (hazard ratio 1.50) and stroke (hazard ratio 1.55). However, the difference may also be a result of geographical variation. For this reason, and because hazard ratios for FPG have not been reported, we have not used these estimates in the final model.
HbA1c category | Diagnosed diabetes | Coronary heart disease | Stroke | Death from any cause | ||||
---|---|---|---|---|---|---|---|---|
Hazard ratio | 95% CI | Hazard ratio | 95% CI | Hazard ratio | 95% CI | Hazard ratio | 95% CI | |
< 5% | 0.53 | 0.40 to 0.69 | 0.95 | 0.73 to 1.22 | 1.09 | 0.68 to 1.77 | 1.48 | 1.21 to 1.81 |
5–5.5% | 1.00 | – | 1.00 | – | 1.00 | – | 1.00 | – |
5.5–6% | 1.80 | 1.61 to 2.01 | 1.25 | 1.09 to 1.44 | 1.16 | 0.89 to 1.53 | 1.19 | 1.05 to 1.35 |
6–6.5% | 4.03 | 3.52 to 4.61 | 1.88 | 1.55 to 2.28 | 2.19 | 1.58 to 3.05 | 1.61 | 1.35 to 1.91 |
> 6.5% | 10.40 | 8.80 to 12.28 | 2.46 | 1.84 to 3.28 | 2.96 | 1.87 to 4.67 | 1.71 | 1.30 to 2.25 |
HbA1c 1% increase | 1.44 | 1.35 to 1.55 | 1.50 | 1.33 to 1.68 | 1.55 | 1.28 to 1.88 | 1.18 | 1.05 to 1.32 |
Fasting plasma glucose/glycated haemoglobin at baseline and risk of incident cardiovascular disease – evidence incorporated into the economic model
The impact of any difference in risk factors such as SBP, age and cholesterol identified by the two tests will be captured within the UKPDS risk equations which include these risk factors. 41,40 The UKPDS CHD risk equation also includes HbA1c but not FPG.
At any given HbA1c level, the FPG level for an individual would be expected to be higher for someone identified by a FPG test than by a HbA1c test (given that the former has implicitly met a FPG threshold criterion). It is therefore necessary to make some adjustment to the risk calculated using the UKPDS.
The most appropriate values to be used in the model were obtained from those in Kim et al. 42 and are shown in Table 21 (see Parameter values and distributions).
Parameter | Mean | SE (or 95% CI where stated) | Source | Distribution |
---|---|---|---|---|
Incidence of diabetes | ||||
Cohort baseline rate of progression from HRD to diabetes | 3.56% | 0.77% | Morris (2013)30 | Beta |
Glucose-related hazard ratios for risk of diabetes | ||||
Hazard ratio (per 0.5% HbA1c) for individualising risk | 1.23 | 95% CI 1.08 to 1.47 | Rasmussen (2008)36 | Log-normal |
Hazard ratio (per 0.5 mmol/l FPG) for individualising risk | 1.65 | 95% CI 1.43 to 1.92 | Rasmussen (2008)36 | Log-normal |
Hazard ratio (per 0.5 mmol/l 2hPG) for individualising risk | 1.26 | 95% CI 1.18 to 1.35 | Rasmussen (2008)36 | Log-normal |
Glucose-related hazard ratios for risk of CVD | ||||
Hazard ratio for HbA1c (per 1% HbA1c) | 1.12 | 95% CI 1.03 to 1.21 | Kim (2005)42 | Log-normal |
Hazard ratio for FPG (per 1 mmol/l FPG) | 1.04 | 95% CI 1.09 to 0.99 | Kim (2005)42 | Log-normal |
Hazard ratio for 2hPG (per 1 mmol/l) | 1.00 | − | Kim (2005)42 | Fixed |
Rate of HbA1c change per annum during preclinical period (undiagnosed diabetes) | ||||
HbA1c trajectories (rate of HbA1c change per annum) during preclinical period (undiagnosed diabetes) | ||||
Rate of change at 6.5% | 0.3% | 0.051% | Estimated based on assumed preclinical period of 4–5 years (see Time to clinical detection for cases of diabetes which are not screen detected) | Normal |
Rate of change at 9.0% | 0.6% | 0.102% |
Specification of the intervention included in the model to prevent diabetes in people at high risk of diabetes
Form of intervention
In the Finnish DPS, the intervention included an intensive first year during which detailed advice on how to achieve lifestyle goals relating to weight, intake of saturated fat, fibre intake and moderate exercise was provided. This included seven sessions with a nutritionist and supervised, individually tailored, circuit-type resistance training sessions. Following the initial intervention, there was a maintenance period, with a total duration of intervention of up to 6 years, depending on the time of recruitment into the study. 27 During the maintenance period, participants were offered ongoing support, including a visit to a nutritionist every 3 months, to help to sustain lifestyle improvements. Longer intervention (lasting for 5–6 years) did not seem to be more effective than shorter intervention (1–4 years). 44
In the real-life setting of current NHS care, clinical experts were clear that intensive active intervention that lasts for many years is not feasible. We have, therefore, continued with assumptions adopted for the modelling supporting the recent NICE guidance on prevention of diabetes. 12 This entailed group-based maintenance sessions every 4 months for years 2 to 4, each session costing £20 per person.
Initial weight loss
In line with modelling for the NICE guidance, we assumed a modestly intensive intervention with 11 contact hours per person for the initial course (i.e. excluding maintenance sessions), costing £150 per person and resulting in an average weight loss of 3.5 kg.
Durability of reduction in risk
In the Finnish DPS45 and US DPP,28 there was a tendency towards at least a partial regain of weight once maintenance intervention ceased. This regain in weight is likely to lead to some loss of the reduction in risk of diabetes.
The latest evidence from the Finnish DPS demonstrates parallel glucose trends among intervention and control study groups. 44 The authors conclude that the observed risk reduction in the DPS is likely to reflect a postponement of the disease rather than prevent it altogether.
In the economic model, after the initial weight loss during the first year of the intervention, weight was assumed to be regained at an even rate such that the weight change from baseline (compared with no intervention) was nil by the end of year 8 following the start of the intensive intervention. This assumption was based on clinical experts’ advice during the development of the 2012 NICE recommendations on risk assessment for diabetes. 5
Referral for and uptake of preventative interventions in people at high risk of diabetes
Based on advice from clinicians, it is assumed that 85% of individuals identified as at HRD are offered referral to an intervention programme. This is less than 100% because intervention is not appropriate for some individuals with existing co-morbidities. We then assume that 65% of individuals offered referral to a preventative intervention accept the offer, again based on advice from clinicians. This gives an overall rate of 55% of individuals identified as at HRD actually taking up preventative intervention.
Time to clinical detection for cases of diabetes which are not screen detected
Our economic model previously assumed that the lead-time between the point of potential screen detection and clinical detection is around 6–7 years13 based on the available evidence at the time.
However, additional evidence was published in 2012 from the Ely study. 46 This study compared the duration of clinically recognised diabetes in individuals who had been offered screening versus those had not been offered screening. The results suggested a lead-time of just 3 years. There are some caveats around this finding, in particular some dilution of the effect of screening through ad hoc opportunistic screening for diabetes and improved detection of risk factors for CVD (including diabetes) within primary care.
We have assumed a mean lead time of 4–5 years, that is between the two ‘extremes’ (which we have assumed to represent the 95% CIs for the true value when undertaking PSA).
Given the HbA1c level at which an individual is identified symptomatically with diabetes within clinical practice and the lead time before clinical detection, we can plot the (non-linear) upwards HbA1c trajectory for the individual. We have assumed clinical detection occurs on average at a HbA1c level of 8%, as per those recruited into the DESMOND (Diabetes Education and Self Management for Ongoing and Newly Diagnosed) study. 47
Benefits of early detection of diabetes
In this section, we summarise the assumptions made regarding the effect on treatment of a diagnosis of diabetes and thereby the benefits of earlier detection.
Tighter blood pressure control
In its guidance, NICE recommends a target of < 140 mmHg for SBP for individuals without diabetes but with treated hypertension. 48 Given the NHS Health Checks programme context of this evaluation, individuals are assumed to be managed to this level at baseline prior to assessment of risk of diabetes. For individuals with diabetes, the same target applies while an individual remains free of end-organ damage but, once damage has occurred (either microvascular or macrovascular complications), the target in NICE guidance is < 130 mmHg. 49
However, it may be difficult to achieve this target because control of hypertension, particularly SBP, is more difficult in patients with diabetes.
In the ADDITION-Europe study, SBP at 5 years was reduced by 2.86 mmHg more in the intensive treatment arm than in the routine care arm, both arms achieving substantial reductions of over 10 mmHg. 50 In the ADDITION-Leicester study, after 1 year, the blood pressure reduction was much larger in the intensive group (by 8 mmHg). 51
In view of this evidence, we assume that the reduction in SBP achieved in practice is 5 mmHg.
The actual difference in UK clinical practice today depends partly how tightly SBP is now being managed within the ongoing NHS Health Checks programme prior to identification of diabetes and the assessment of need for more aggressive antihypertensive therapy.
It is assumed that a 5 mmHg reduction could be achieved with one extra drug [at the average cost of a diuretic (bendrofluazide, 2.5 mg daily) and a beta-blocker (atenolol, 50 mg daily)].
Economic modelling
The majority of the analyses and uncertainty analysis described in this section are based around the multiethnic LEADER cohort. (For additional scenario-based analyses covering alternative prevalence and blood test uptake rates see Scenario analyses – alternative prevalence and uptake rates.)
Leicester Ethnic Atherosclerosis and Diabetes Risk cohort characteristics: inputs into the Sheffield Type 2 Diabetes Model
The LEADER cohort data set contained 8147 individuals for whom complete data were available for the required data fields (i.e. including HbA1c, FPG and risk factors for the LPDS risk score). As part of the PSA (see Uncertainty around Leicester Ethnic Atherosclerosis and Diabetes Risk-based analyses), bootstrapping ‘with replacement’ was carried out to obtain a new set of patients for each PSA sample run. The bootstrap procedure creates a new set of n sampled individuals by randomly sampling an individual from the full set of 8147 individuals. This process is then repeated until the set of n individuals (in this case 8147 – see Uncertainty around Leicester Ethnic Atherosclerosis and Diabetes Risk-based analyses) has been created for the next sample run of the PSA. The bootstrap procedure is carried out ‘with replacement’, which means that, after an individual has been sampled (for inclusion in the new sample set), he or she is replaced in the overall set before the next individual is sampled, thereby introducing uncertainty to capture the degree of uncertainty around the prevalence of diabetes and HRD in the LEADER cohort. 52
Analyses to be undertaken
Figure 9 is a summary of the various analyses undertaken, in particular which base case, scenario and sensitivity analyses are based on prevalence in the LEADER cohort and which are based on alternative prevalence scenarios.
FIGURE 9.
Schematic of analyses undertaken.

The model developed for these analyses is a screening and prevention adaptation of the Sheffield Type 2 Diabetes Model that was used to assess screening strategies as part of NICE’s Public Health Guidance on risk assessment for diabetes. 5 A fully detailed report on that work is available on the NICE website. 12
Briefly, the Sheffield Diabetes Model is an integrated health state simulation model of the natural history of diabetes and the lifetime cost-effectiveness of different treatments for type 2 diabetes. The model replicates patients’ risk of progression through five comorbidities: retinopathy, nephropathy, neuropathy, CHD and cerebrovascular disease. Patients can experience three of the major complications associated with diabetes: neuropathy, nephropathy and retinopathy. The time spent by patients in each state for each co-morbidity is recorded, for example years spent on dialysis, severe vision loss, together with transitions between states.
Total costs are obtained by adding the costs of therapy, the costs of one-off treatments (e.g. cost of amputation) and ongoing treatment of complications (e.g. treatment following stroke). The health benefit, the incremental QALYs, is obtained by applying quality of life measures (such as preference scores from the Harvard web-based database) to the time spent in the various diabetic health states. Cost-effectiveness estimates for potential interventions are obtained by dividing the total costs by the incremental QALYs.
Parameter values and distributions
Parameter values for screening and prevention-related parameters are shown in Tables 21 and 22, respectively.
Parameter | Mean | 95% CI | Source | Distribution |
---|---|---|---|---|
Modifiers of effectiveness of preventative intervention (for diabetes) | ||||
Specific adjustment (multiplier) to effectiveness of preventative interventions in phenotype identified by tests other than IGT-orientated OGTT | 0.70 | Assumed 0.5 to 1.0 | Assumption | Log-normal |
Assumed initial uptake of preventative interventions | 55% | Assumed 45% to 65% | Advice from clinical authors (Khunti, Davies) | Beta |
RRR per kg lost | 16% | 13% to 19% | Hamman (2006)53 | Log-normal |
The health state utilities in the model are shown in Table 23 and unit costs are shown in Table 24.
Health state utility parameter | Mean (SE) | Source | Distribution |
---|---|---|---|
Utility for diabetes with no complications | 0.785 (0.0530) | UKPDS 6256 | Beta |
Decrements for complications | |||
CHD | –0.055 (0.0064) | UKPDS 6256 | Gamma |
CHF | –0.108 (0.0309) | UKPDS 6256 | Gamma |
Stroke | –0.164 (0.0298) | UKPDS 6256 | Gamma |
Microalbuminuria | –0.011 (0.009) | Coffey & Associates57 | Gamma |
Macroalbuminuria | –0.011 (0.009) | Coffey & Associates57 | Gamma |
Dialysis | –0.078 (0.026) | Coffey & Associates57 | Gamma |
Post renal transplant | –0.052 (0.0133) | Mount Hood 4 Conference data | Gamma |
Neuropathy | –0.065 (0.008) | Coffey & Associates57 | Gamma |
Amputation | –0.280 (0.0559) | UKPDS 6256 | Gamma |
Proliferative retinopathy | –0.020 (0.0051) | Mount Hood 4 Conference data | Gamma |
Macular oedema | –0.020 (0.0051) | Mount Hood 4 Conference data | Gamma |
Severe vision loss | –0.074 (0.0255) | Mount Hood 4 Conference data | Gamma |
Weight (per kg) | –0.0025 (0.0011) | Weighted average of published studies58 | Gamma |
Unit costs | Mean | Distribution assumptions | Source | Distribution |
---|---|---|---|---|
Acute cost of MI – non-fatal MIa | £6153 | Mean –20%, +25% | UKPDS 6559 | Log-normal |
Acute cost of MI – fatal MIa | £1742 | Mean –20%, +25% | UKPDS 6559 | Log-normal |
Annual cost following MI | £702 | Mean –20%, +25% | UKPDS 6559 | Log-normal |
Acute cost of strokeb | ||||
Acute cost of stroke – non-fatal strokeb | £3579 | Mean –20%, +25% | UKPDS 6559 | Log-normal |
Acute cost of stroke – fatal strokeb | £5115 | Mean –20%, +25% | UKPDS 6559 | Log-normal |
Annual cost following stroke | £5892 | Mean –20%, +25% | Chambers et al.60 | Log-normal |
CHF incidence | £3594 | Mean – 20%, +25% | UKPDS 6559 | Log-normal |
CHF state cost | £909 | Mean –20%, +25% | UKPDS 6559 | Log-normal |
Haemodialysis per annum | £36,419 | Mean –20%, +25% | UK Transplant61 | Log-normal |
Peritoneal dialysis per annum | £18,210 | Mean –20%, +25% | UK Transplant61 | Log-normal |
Transplant – first year | £17,689 | Mean –20%, +25% | UK Transplant61 | Log-normal |
Cost of immunosuppression per annum | £5203 | Mean –20%, +25% | UK Transplant61 | Log-normal |
Annual cost of neuropathy | £214 | Mean –20%, +25% | Gordois et al.62 | Log-normal |
Amputation | £12,789 | Mean –20%, +25% | UKPDS 6559 | Log-normal |
Post-amputation costs per annum | £454 | Mean –20%, +25% | Palmer et al.63 | Log-normal |
Major hypoglycaemic episode | £659 | Mean –20%, +25% | Heaton et al.64 | Log-normal |
Retinal photocoagulation | £1073 | Mean –20%, +25% | UK National Screening Committee65 | Log-normal |
Severe vision loss per annum | £425 | Mean –20%, +25% | UKPDS 6559 | Log-normal |
Cost of management/monitoring – clinic visits, glucose tests, and proteinuria and eye screening | £269 | Mean –20%, +25% | Calculation | Log-normal |
Heart failure (temporary adverse event) | £3426 | Mean –20%, +25% | UKPDS 6559 | Log-normal |
Oedema | £42 | Mean –20%, + 5% | UKPDS 6559 | Log-normal |
Details of coefficients used within the CHD,40 stroke,41 CHD/stroke case fatality54 and congestive heart failure (CHF)55 risk equations used are shown in Tables 25–28.
Interpretation | Estimate (SE) | Distribution |
---|---|---|
Intercept | 0.0112 (0.001) | Normal |
Risk ratio for 1 year of age at diagnosis of diabetes | 1.059 (0.005) | Normal |
Risk ratio for female sex | 0.525 (0.054) | Normal |
Risk ratio for Afro-Caribbean ethnicity | 0.390 (0.102) | Normal |
Risk ratio for smoking | 1.350 (0.122) | Normal |
Risk ratio for 1% increase in HbA1c | 1.183 (0.036) | Normal |
Risk ratio for 10-mmHg increase in systolic blood pressure | 1.088 (0.026) | Normal |
Risk ratio for unit increase in logarithm of lipid ratio | 3.845 (0.640) | Normal |
Risk ratio for each year in duration of diagnosed diabetes | 1.078 (0.015) | Normal |
Parameter | Interpretation | Estimate | SE | Distribution |
---|---|---|---|---|
q0 | Intercept | 0.00186 | 0.0004 | Normal |
β1 | Risk ratio for 1 year of age at diagnosis of diabetes | 1.092 | 0.013 | Normal |
β2 | Risk ratio for female sex | 0.700 | 0.109 | Normal |
β3 | Risk ratio for smoking | 1.547 | 0.237 | Normal |
β4 | Risk ratio for atrial fibrillation | 8.554 | 2.963 | Normal |
β5 | Risk ratio for 10-mmHg increase in systolic blood pressure | 1.122 | 0.042 | Normal |
β6 | Risk ratio for unit increase in lipid ratio | 1.138 | 0.053 | Normal |
d | Risk ratio for each year in duration of diagnosed diabetes | 1.145 | 0.026 | Normal |
Parameter | Estimate | SE | Distribution |
---|---|---|---|
CHD | |||
Age | 0.048 | 0.024 | Normal |
HbA1c (per 1%) | 0.177 | 0.012 | Normal |
SBP | 0.140 | 0.061 | Normal |
Time to event, from diabetes diagnosis | 0.104 | 0.042 | Normal |
Stroke | |||
SBP | 0.246 | 0.078 | Normal |
Previous stroke | 2.21 | 0.545 | Normal |
Parameter | Estimate | SE | Distribution |
---|---|---|---|
Age | 0.093 | 0.016 | Normal |
HbA1c | 0.157 | 0.057 | Normal |
SBP | 0.114 | 0.056 | Normal |
λ | –8.018 | 0.408 | Normal |
γ | 1.711 | 0.158 | Normal |
Uncertainty around Leicester Ethnic Atherosclerosis and Diabetes Risk-based analyses
Probabilistic sensitivity analysis
This section describes how the existing functionality of the suite of models and associated input files to the Sheffield Type 2 Diabetes Model was developed further beyond its existing set-up for deterministic modelling, in order to incorporate uncertainty around modelled parameters from prevalence of diabetes and HRD right through to costs and health-related quality-of-life impact of diabetes complications. This enabled us to undertake PSA across all the alternative screening strategies.
Method to decide on how many probabilistic sensitivity analysis runs and how many individuals to simulate
It was realised that a large number of runs might be required to obtain stable results owing to the low prevalence of diabetes and uncertainty around a large number of model parameters. This could impose a considerable demand on computational time. In order to optimise this, we explored the trade-off between the number of parameter samples and the number of patients per sample. This is done by comparing the variances between separate batches of individuals with the variance across alternative sets of parameters. This entailed:
-
Running the same set of patients through the model 100 times to explore the variability due to parameter uncertainty. To do this we selected a batch of subjects with a ‘representative’ prevalence of diabetes and HRD.
-
Running alternative sampled cohorts of 500 individuals through the model, each run with the same sampled parameter values (all set at their mean) and then combining sets of results to explore the impact of cohort size on the variance between runs.
These issues have been explored previously,66 but the situation is complicated in screening models because the prevalence of diabetes (a key uncertain parameter) is inherently contained within the patient characteristics. Given this, and the fact that the exploratory analyses suggested that a much larger bootstrap size is needed for screening than for a diabetes treatment model, we decided to run each set of sampled parameters with the same number of patients as there are in the full LEADER cohort, that is with the 8147 individuals for which complete data were available for the required data fields. Bootstrapping with replacement was carried out to obtain the 8147 patients.
Interim analyses suggested that a relatively small number of PSA samples was sufficient for this analysis. This is because for screening, instead of the main driver being drug effectiveness, which often has significant uncertainty around it, effectiveness in this case is driven by a relatively certain prevalence of diabetes and HRD because of the large number of patients in the LEADER study. The final simulations were therefore undertaken with 60 PSA sample runs, each containing 8147 patients (i.e. nearly 490,000 patients in total).
Deterministic one-way sensitivity analyses
As uncertainty around prevalence is examined within scenario analyses (see Scenario analyses – alternative prevalence and uptake rates), this section concerns uncertainty around parameters unrelated to prevalence.
There are many uncertain parameters involved in models of the cost-effectiveness of screening for, and prevention of, type 2 diabetes. The emphasis of this evaluation concerns the relative cost-effectiveness of screening with alternative tests rather than the cost-effectiveness of screening for diabetes versus no screening. It is therefore important to prioritise sensitivity analyses for parameters for which there is differential evidence for HbA1c and FPG. The sensitivity analyses (see Table 29) were arrived at through detailed analysis of results, and sensitivity analyses undertaken during previous published economic evaluations of screening and prevention. The sensitivity analyses also reflect the base case conclusion that HbA1c-based testing appears to be more cost-effective than FPG, that is by testing uncertain parameters with alternative assumptions that would be more favourable to FPG.
Number | Uncertain parameter and sensitivity assumption | Value |
---|---|---|
SA1 | Mean HbA1c level at which previously undiagnosed diabetes is clinically/opportunistically detected Through a mix of rescreening and opportunistic screening for diabetes/HRD in routine care, many individuals with undiagnosed diabetes may not experience a rise in HbA1c to as high as the base case average of 8% before being diagnosed with diabetes |
Changed to 7.5% from 8.0% |
SA2 | Lead time between screen-detected and clinically detected diabetes The rate of HbA1c change during the ‘preclinical period’ (between onset of diabetes and clinical detection) was increased so that the average lead time between the point of screen detection and clinical detection is lower |
Reduced to 3 years from 4–5 years |
SA3 | Differential uptake of first FPG test (vs. HbA1c) We assumed the differential between uptake of first HbA1c tests and first FPG tests is lower, while maintaining no difference for a confirmatory test |
Reduced by 10% to 10% from base case of 20% |
SA4 | Discount rates A sensitivity analysis was undertaken using the discount rates applied to technology assessments outside Public Health |
Changed to 3.5% for both costs and QALYs (from 1.5% for both in base case) |
SA5 | Improvements in the effectiveness of the management of diagnosed diabetes | HbA1c annual rate of increase reduced from 0.2% per annum to 0.15% per annum for glucose-lowering drugs HbA1c annual rate of increase reduced from 0.1% to 0.075% per annum for insulin |
SA6 | Greater sustained prevention/delay of diabetes More optimistic scenario for the number of years for which weight loss is sustained (beyond year 1) per £50 per annum invested in maintenance intervention sessions during years 2 to 4 |
Changed from 2 to 3 years |
SA7 | Greater uptake of preventative intervention | Increased from 55% to 75% |
SA8 | Higher difference between the cost of a HbA1c test and a FPG test | Increased from £2.22 to £6 |
SA9 | Different natural history for FPG vs. HbA1c-identified diabetes
|
|
SA10 | Effectiveness of early intervention for type 2 diabetes – rate of HbA1c change (while HbA1c < 7.0%) | Increased from 0.15% per annum to 0.2% per annum |
SA11 | Cost of preventative intervention | Increased by 33% |
SA12 | Uptake of preventative intervention | Increased from 55% to 70% |
Sensitivity analyses SA1–8 were undertaken for the four NICE-related screening strategies (two with a risk score and two without): (1) LPDS 4.75/HbA1c 6.0, (2) HbA1c 4.75/FPG 5.5, (3) HbA1c 6.0 and (4) FPG 5.5. Sensitivity analyses SA 9–12 were additional ones run only for NICE-based strategies with a risk score, that is (1) LPDS 4.75/HbA1c 6.0 and (2) LPDS 4.75/FPG 5.5.
Scenario analyses – alternative prevalence and uptake rates
The most important additional analyses are ones reflecting alternative distributions of HbA1c and FPG, and thereby alternative prevalence of diabetes and HRD. All of the analyses described so far are based on the multiethnic LEADER cohort from Leicestershire (see The Leicester Ethnic Atherosclerosis and Diabetes Risk cohort data set). There are, however, variations in glucose distributions across the country, and lower average HbA1c levels have been reported in some other UK studies, for example 5.1% in the Whitehall II Study. 67
In the LEADER cohort, the prevalence of FPG-defined diabetes was relatively low, only 1.8%, compared with 5.7% for HbA1c-defined diabetes. Other regional subpopulations may have quite different relative prevalence of HbA1c-defined versus FPG-defined diabetes and HRD (see Other UK cohorts providing estimates of prevalence of diabetes and high risk of diabetes using both glycated haemoglobin and fasting plasma glucose). The UEA-IFG study is the study that differs most from LEADER in terms of the relative prevalence. 10
To test how sensitive the results and conclusions are to alternative glucose distributions, we repeated the analysis with four cohorts with alternative glucose distributions, such that the prevalence of HbA1c-defined diabetes is closer to that of FPG-defined diabetes. The scenarios concerned are labelled P_Sc1 to P_Sc4 in Table 30.
Alternative scenario | Diabetes (%) | HRD ≥ 6.0, < 6.5 (%) | Rationale |
---|---|---|---|
P_Sc1 | 2.1 | 6.9 | Low-case diabetes, low-case HRD (∼UEA-IFG study) |
P_Sc2 | 3.8 | 13.8 | Mid-case diabetes, mid-case HRD |
P_Sc3 | 3.8 | 20.2 | Mid-case diabetes, base case HRD |
P_Sc4 | 1.8 | 13.8 | Low-case diabetes, mid-case HRD |
The four alternative prevalence scenarios were obtained by adjusting the HbA1c distribution in the LEADER data set to result in prevalence rates as shown in Table 30 (although we were unable to obtain data for other cohorts on characteristics other than FPG and HbA1c). Alternative scenario P_Sc1 closely mirrors the prevalence according to the UEA-IFG study which, given the prevalence patterns across alternative studies shown in Table 4, is considered an ‘extreme’ opposite to the prevalence in the LEADER cohort. Scenarios P_Sc2, P_Sc3 and P_Sc4 can be seen as mid-range scenarios, that is in between the prevalence of the LEADER and UEA-IFG cohorts.
Owing to the complexity of adjusting the distributions of both HbA1c and FPG values in the LEADER data set so that the prevalence of diabetes and HRD matches the desired rates in Table 30, it was decided to keep the FPG levels unchanged and to just adjust HbA1c levels in order to obtain the desired differential prevalence between HbA1c-based and FPG-based definitions. The FPG-based prevalence for all of these scenarios is, therefore, 1.8% for diabetes and 23.8% for HRD (defined as 5.5–6.9 mmol/l).
In parallel with varying the prevalence, four alternative uptake scenarios were adopted for the uptake rates for first HbA1c and first FPG tests as shown in Table 31. Uptake was explored more extensively in the context of the prevalence scenarios P_Sc1 to P_Sc4 than within the LEADER-based analyses, as greater sensitivity to uptake rates was expected in this context.
Alternative scenario | Uptake for HbA1c (%) | Uptake for FPG (%), [difference from HbA1c (%)] |
---|---|---|
U_Sc1 | 95 | 85 (–10) |
U_Sc2 | 95 | 75 (–20) |
U_Sc3 | 95 | 65 (–30) |
U_Sc4 | 95 | 55 (–40) |
For each of the four prevalence scenarios, the scenarios were modelled separately in combination with each of the four uptake scenarios, thereby creating a set of 16 scenario analyses.
Perspective, horizon and discount rates
In line with the recently revised NICE recommendations for prevention interventions and consistent with NHS Health Checks now being the remit of local authorities, the base case discount rates used are 1.5% for both costs and QALYs. 68 We undertook sensitivity analyses using the alternative rate of 3.5% recommended by NICE for the evaluation of drugs.
The model time horizon was 80 years, which effectively allows modelling of an individual’s entire lifetime.
We adopted a public sector perspective, as is usual for evaluation of public health interventions.
Chapter 3 Results: intermediate screening outcomes
Comparison of pathways and outcomes of the screening process
Table 32 shows the screening yield and resource implications of each screening strategy evaluated.
Screening strategy | Risk score and glucose cut-off points | Percentage indicated and invited for laboratory blood test | Sensitivity of strategy to diabetes (assuming 100% uptake) | Sensitivity of strategy to HRDa (assuming 100% uptake) | Percentage of attendees undertaking all required steps of screeningb | Screening outcomes: proportion detected (taking account of uptake) compared with prevalence of undiagnosed cases (%) | |
---|---|---|---|---|---|---|---|
Diabetes: detected (prevalence) | HRD: detected (prevalence) | ||||||
Screening for diabetes only (for information) | |||||||
LPDS 4.75/HbA1c 6.5 | LPDS ≥ 4.75, HbA1c ≥ 6.5% | 76.0 | 94.2 | N/A | 94.3 | 4.4 (5.7) | 0 (0) |
LPDS 4.75/FPG 7.0 | LPDS ≥ 4.75, FPG ≥ 7.0 mmol/l | 76.0 | 96.0 | N/A | 74.7 | 1.2 (1.8) | 0 (0) |
Base case: NICE-recommended strategies (diabetes and HRD): with use of risk score | |||||||
LPDS 4.75/HbA1c 6.0 | LPDS ≥ 4.75, HbA1c ≥ 6.0% | 76.0 | 94.2 | 84.0 | 94.3 | 4.4 (5.7) | 15.8 (18.3) |
LPDS 4.75/FPG 5.5 | LPDS ≥ 4.75, FPG ≥ 5.5 mmol/l | 76.0 | 96.0 | 81.5 | 74.7 | 1.2 (1.8) | 15.9 (23.8) |
NICE-recommended strategies (diabetes and HRD): without use of risk score | |||||||
HbA1c 6.0 | HbA1c ≥ 6.0% | 100.0 | 100.0 | 100.0 | 94.2 | 4.6 (5.7) | 17.6 (18.3) |
FPG 5.5 | FPG ≥ 5.5 mmol/l | 100.0 | 100.0 | 100.0 | 74.7 | 1.5 (1.8) | 23.1 (23.8) |
NICE HbA1c threshold together with RCG for prescreening | |||||||
RCG 4.4/HbA1c 6.0 | RCG ≥ 4.4, HbA1c ≥ 6.0% | 94.4 | 98.1 | 95.3 | 89.5 | 4.4 (5.7) | 15.9 (18.3) |
Remaining strategies: potential future ones with alternative cut-off points for HRD | |||||||
Other strategies (ISO resource as strategies HbA1c 6.0 and FPG 5.5) | |||||||
HbA1c 5.9 | HbA1c ≥ 5.9% | 100.0 | 100.0 | 100.0 | 94.2 | 4.6 (5.7) | 24.7 (26) |
FPG 5.6 | FPG ≥ 5.6 mmol/l | 100.0 | 100.0 | 100.0 | 74.7 | 1.5 (1.8) | 18.6 (19.6) |
Other strategies: ISO resource ≈27% HRD | |||||||
LPDS 4.75/HbA1c 5.8 | LPDS ≥ 4.75, HbA1c ≥ 5.8% | 76.0 | 94.2 | 87.2 | 94.3 | 4.4 (5.7) | 28.7 (35.1) |
LPDS 4.75/FPG 5.2 | LPDS ≥ 4.75, FPG ≥ 5.2 mmol/l | 76.0 | 96.0 | 84.0 | 74.7 | 1.2 (1.8) | 26.6 (42.3) |
Other strategies: ISO resource ≈32% HRD | |||||||
HbA1c 5.8 | HbA1c ≥ 5.8% | 100.0 | 100.0 | 100.0 | 94.2 | 4.6 (5.7) | 33.3 (35.1) |
FPG 5.2 | FPG ≥ 5.2 mmol/l | 100.0 | 100.0 | 100.0 | 74.7 | 1.2 (1.8) | 31.7 (42.3) |
Other strategies: ISO resource ≈36% HRD | |||||||
LPDS 4.75/HbA1c 5.7 | LPDS ≥ 4.75, HbA1c ≥ 5.7% | 76.0 | 94.2 | 90.1 | 94.3 | 4.4 (5.7) | 35.9 (44.8) |
LPDS 4.75/FPG 5.0 | LPDS ≥ 4.75, FPG ≥ 5.0 mmol/l | 76.0 | 96.0 | 81.5 | 74.7 | 1.2 (1.8) | 35.8 (58.5) |
RCG 4.2/HbA1c 5.7 | RCG ≥ 4.2, HbA1c ≥ 5.7% | 88.1 | 95.7 | 90.1 | 89.5 | 4.3 (5.7) | 36.4 (35.1) |
Other strategies: ISO resource ≈43% HRD | |||||||
HbA1c 5.7 | HbA1c ≥ 5.7% | 100.0 | 100.0 | 100.0 | 94.2 | 4.6 (5.7) | 42.6 (44.8) |
FPG 5.0 | FPG ≥ 5.0 mmol/l | 100.0 | 100.0 | 100.0 | 74.7 | 1.2 (1.8) | 43.9 (58.5) |
The third column of Table 32 shows the percentage of 40- to 70-year-olds attending health checks who would be offered a blood test, taking account of those indicated as at low risk for diabetes at the prescreening stage and, therefore, not offered a blood test.
The fourth column shows the proportion of cases of undiagnosed diabetes that would be detected given any prescreening and assuming 100% uptake of blood tests.
The fifth column shows the proportion of cases of undiagnosed HRD that would be detected given any prescreening and assuming 100% uptake of blood tests.
The sixth column shows the proportion of health check attendees whose diabetes risk score is calculated and who, where applicable, take up any offers of an initial and confirmatory blood test.
The seventh column shows, for each screening strategy:
-
upper figure (within the bracket): the proportion of 40- to 74-year-olds eligible for risk assessment that would receive a diagnosis of diabetes, taking account of rates of uptake of blood tests
-
lower figure: the prevalence of undiagnosed diabetes among 40- to 74-year-olds eligible for risk assessment.
The eighth column shows:
-
upper figure: the proportion of 40- to 74-year-olds eligible for risk assessment that would receive a diagnosis of HRD, taking account of rates of uptake of blood tests
-
lower figure: the prevalence of undiagnosed HRD among 40- to 74-year-olds eligible for risk assessment.
Clearly, one of the main results is that the numbers of people detected with diabetes is strongly influenced by the glucose test, as the prevalence of HbA1c-defined diabetes is 5.7%, compared with a prevalence of 1.8% for FPG-defined diabetes. The sensitivities of the testing strategies are broadly similar when comparing HbA1c and FPG. For example, for the two strategies LPDS 4.75/HbA1c 6.5 and LPDS 4.75/FPG 7.0, the sensitivity for detecting diabetes is 94.2% and 96.0%, respectively, while sensitivity for HRD is 84.0% and 81.5%, respectively (all assuming 100% uptake of tests).
It is the proportion of cases detected with HRD that differs most across the strategies. The two NICE-recommended strategies which use a risk score (‘LPDS 4.75/HbA1c 6.0’ and ‘LPDS 4.75/FPG 5.5’) are estimated to detect 15.8% and 15.9%, respectively, of the total 40–74 years eligible population as at HRD. For the two NICE-recommended strategies that do not use a risk score, strategy ‘HbA1c 6.0%’ would detect HRD in slightly more people, at 17.6%, while strategy ‘FPG 5.5’ would detect HRD in considerably more, at 23.1%. These levels of detection are from an underlying prevalence of HRD of 18.3% for HbA1c 6.0–6.4% and 23.8% for FPG 5.5–6.9 mmol/l. Other potential future strategies, with lower thresholds than recommended by NICE for defining HRD, would detect even more cases of HRD, for example ‘LPDS 4.75/HbA1c 5.8’ would detect 28.7%, ‘LPDS 4.75/FPG 5.2’ would detect 26.6%, and ‘LPDS 4.75/HbA1c 5.9% or FPG 5.4’ would detect 25.7%.
Screening cost per case detected results
The screening cost per case of detected diabetes or HRD is shown in Table 33. This includes only the costs incurred up to the point of obtaining a definitive diagnosis, that is the cost of prescreening, blood tests for diabetes/HRD and associated staff and laboratory costs.
Test | Cost per case of diabetes detecteda | Cost per case of diabetes and HRD detecteda | Cost per person attending NHS Health Checksa | |||
---|---|---|---|---|---|---|
No risk score | With risk score | No risk score | With risk score | No risk score | With risk score | |
HbA1c | £309 | £257 | £65 | £56 | £14.35 | £11.23 |
FPG | £774 | £634 | £39 | £43 | £9.38 | £7.37 |
The cost per person of diabetes detected is lower when screening with a HbA1c test than with a FPG test because of the higher prevalence of undiagnosed diabetes (5.7% vs. 1.8%). When cases of HRD are included, the cost per case detected is lower for FPG because the test is cheaper (and, when no risk score is used, a greater number of cases of HRD are identified). The cost per person eligible for screening is lower for a FPG test because of its lower test cost.
This measure is of limited usefulness to decision makers as an indicator of value for money because:
-
It depends on the arbitrary definition of HRD. For example, progressive lowering of the cut-off point for defining HRD will inevitably reduce the cost per case detected because it results in identification of more cases. However, these additional people are at progressively lower risk of diabetes; therefore, they will, on average, have less capacity to benefit from the prevention intervention.
-
When cases of HRD are included in addition to diabetes, the cost per person attending health checks becomes very small, as shown in Table 33. As a result, any difference in the short-term screening cost of HbA1c testing versus FPG testing is unlikely to be a key driver of overall long-term cost-effectiveness, which takes account of many other elements that are important.
Chapter 4 Results: long-term cost-effectiveness modelling
Figure 10 summarises the various analyses, sensitivity analyses and scenario analyses, undertaken, in particular showing which cohorts they are based on. All analyses compare HbA1c testing with FPG testing except the analysis of LPDS versus RCG. The references in italics in the boxes of Figure 10 refer to the specific parts of Chapter 4 where the results are presented.
FIGURE 10.
Schematic of analyses undertaken.

The section starts by reporting the mean long-term cost-effectiveness results for HbA1c testing versus FPG testing for the NICE-recommended strategies that include use of a risk score for prescreening, first based on the LEADER cohort and then examining alternative prevalence scenarios. We then report results from one-way sensitivity analyses around non-prevalence related assumptions, before presenting results if no prescreening were undertaken and everyone was offered blood glucose testing. We move on to report some exploratory analyses of strategies with alternative cut-off points for preventative intervention to the NICE recommendations but which are ‘ISO-resource’ (as discussed earlier in Chapter 2, Derivation of final set of screening strategies to model). Finally, we compare use of the LPDS versus an RCG for prescreening.
Results for strategies recommended in National Institute for Health and Care Excellence guidance, based around the Leicester Ethnic Atherosclerosis and Diabetes Risk cohort
Figure 10 is a recap of the analyses undertaken.
Table 34 shows results comparing HbA1c testing with FPG testing assuming that any prescreening is undertaken with the LPDS. Results for the comparison of whether or not LPDS or RCG is the best tool for prescreening are shown later in Use of an RCG test or the Leicester Practice Database Score to prioritise who should receive the blood test.
Strategy | Glucose test and cut-off point for HRD | Total costs (including screening cost) | Total QALYs | Incremental costs vs. ‘no screening’ (discounted) | Incremental QALYs vs. ‘no screening’ (discounted) | ICER vs. ‘no screening’ | Net monetary benefit | Incremental net monetary benefit vs. ‘no screening’ | Probability most cost-effective of strategies within same NICE/ISO band (%)a |
---|---|---|---|---|---|---|---|---|---|
Per person attending an NHS Health Check | |||||||||
NICE-recommended strategies (diabetes and HRD): with use of risk score | |||||||||
LPDS 4.75/HbA1c 6.0 | LPDS ≥ 4.75, HbA1c ≥ 6.0% | £13,831 | 13.6390 | £66 | 0.0513 | £1289 | £258,950 | £960 | 98 |
LPDS 4.75/FPG 5.5 | LPDS ≥ 4.75, FPG ≥ 5.5 mmol/l | £13,843 | 13.6170 | £78 | 0.0293 | £2655 | £258,497 | £507 | 2 |
No screening and screening for diabetes only: for information only | |||||||||
Base case | No screening | £13,765 | 13.5877 | – | – | – | £257,990 | – | 0 |
LPDS 4.75/HbA1c 6.5 | LPDS ≥ 4.75, HbA1c ≥ 6.5% | £13,728 | 13.6153 | –£37 | 0.0276 | Dominates | £258,578 | £589 | 98 |
LPDS 4.75/FPG 7.0 | LPDS ≥ 4.75, FPG ≥ 7.0 mmol/l | £13,762 | 13.5982 | –£3 | 0.0104 | Dominates | £258,201 | £211 | 2 |
Table 34 shows the total costs and QALYs of each strategy in the third and fourth columns. The next two columns show the incremental costs and QALYs of the NICE-recommended strategies compared with the ‘no screening’ strategy. The seventh column shows the ICER of each strategy compared with ‘no screening’. The next column, ‘net monetary benefit’ of a strategy, reports the monetary value of the expected total QALYs less the total costs (including screening cost), valuing 1 QALY at £20,000. The NMB is a useful measure, as the most cost-effective strategy is easily identified by the highest NMB. The most cost-effective strategy is also the one with the highest incremental NMB versus ‘no screening’, shown in the ninth column. The final column shows the probability that a strategy is the most cost-effective out of the set of ‘comparable strategies’ (i.e. those within each subsection of the table, such as ‘NICE-recommended strategies (diabetes and HRD) – with use of risk score’.
The main findings from this analysis are as follows: if a LPDS cut-off point of 4.75 is used to determine which individuals receive a blood test, then screening everyone at the Health Check using a HbA1c test and an intervention cut-off point of 6.0% is more cost-effective than screening everyone at the Health Check using a FPG test with a cut-off point of 5.5 mmol/l. The incremental costs and QALYs of HbA1c testing compared with FPG testing are a saving of £12 (£66 – £78) and 0.0220 (0.0513–0.0293), respectively; therefore, HbA1c testing appears to marginally dominate FPG testing. PSA indicates a 98% probability that HbA1c 6.0% is more cost-effective than FPG 5.5 mmol/l.
While both of these strategies would identify around 16% of individuals as at HRD, more would be identified with diabetes in HbA1c testing than FPG testing (4.4% vs. 1.2%).
Probabilistic sensitivity analysis around the long-term cost-effectiveness for the National Institute for Health and Care Excellence-based strategies using the Leicester Ethnic Atherosclerosis and Diabetes Risk data set
Probabilistic sensitivity analysis process
Probabilistic sensitivity analysis was undertaken on each screening strategy with 60 PSA sample runs, each containing 8147 patients (i.e. nearly 490,000 patients in total).
To establish what number of PSA runs would be enough to ensure that results were stable, we investigated the stability of example model runs with pairs of strategies, checking whether or not the difference in net benefit between the strategies was stable enough to be meaningful. Figure 11 shows that the results become stable after a relatively small number of PSA sample runs (£20,000 cost/QALY threshold assumed).
FIGURE 11.
Cumulative mean difference and 95% confidence intervals for the mean difference in net benefit between strategies r4.75/HbA1c 6.0 and r4.75/FPG 5.5.

Cost-effectiveness acceptability curve for glycated haemoglobin versus fasting plasma glucose for National Institute for Health and Care Excellence-recommended strategies
The chart in Figure 12 shows the likelihood of each of the two NICE strategies (LPDS 4.75/HbA1c 6.0, LPDS 4.75/FPG 5.5) being the more cost-effective of the two strategies at alternative willingness-to-pay thresholds. It can be seen that, even at low willingness-to-pay thresholds, such as £6000 per QALY, HbA1c is 95% likely to be more cost-effective than FPG and remains extremely likely (97–98%) to be cost-effective at higher thresholds up to and beyond the usual NICE threshold of £20,000 per QALY.
FIGURE 12.
Likelihood of each of the two NICE strategies (with a risk score) being the more cost-effective at alternative acceptability thresholds.

Cost-effectiveness plane for glycated haemoglobin versus fasting plasma glucose for National Institute for Health and Care Excellence-recommended strategies
Figure 13 shows the cost-effectiveness plane for strategy LPDS 4.75/HbA1c 6.0 versus LPDS 4.75/FPG 5.5. Each dot represents the incremental cost and QALY results for each of the 60 sample runs of the PSA. The error bars show the 95% confidence intervals for the incremental costs and QALYs. The green ellipse indicates the range within which 95% of the data points are expected to lie (so there is 95% certainty that the true incremental costs and QALYs be within this range). The blue line is the £20,000 cost/QALY willingness-to-pay threshold; therefore, any dots below and to the right of the green line indicate that strategy LPDS 4.75/HbA1c 6.0 is more cost-effective than LPDS 4.75/FPG 5.5. As 98% of the dots lie below and to the right of the green line, this indicates that LPDS 4.75/HbA1c 6.0 is 98% likely to be the more cost-effective of the two strategies, as per Cost-effectiveness acceptability curve for glycated haemoglobin versus fasting plasma glucose for NICE-recommended strategies.
FIGURE 13.
Cost-effectiveness plane for strategy LPDS 4.75/HbA1c 6.0 vs. LPDS 4.75/FPG 5.5.

Drivers of benefits from diabetes prevention intervention
An interim model was modified and separate versions set up in such a way that the results from these separate models would facilitate identification of the relative contribution of diabetes prevention per se, blood pressure reduction and weight loss towards the benefits of the intensive lifestyle intervention to prevent diabetes. Analysis suggested that around half of the QALY gains are as a result of diabetes prevention per se, with one-third and one-sixth of gains a result of the weight loss and blood pressure-lowering effects of preventative intervention, respectively.
Scenario analyses to assess the effect of alternative evidence on prevalence and alternative assumptions for rates of uptake of blood tests
Scenario analyses were undertaken only around the NICE guideline-based strategies that include a risk score, that is LPDS 4.75/HbA1c 6.0 versus LPDS 4.75/FPG 5.5, because these strategies were more likely to be sensitive to alternative prevalence of diabetes and HRD than ISO-resource strategies (see Table 35).
Alternative prevalence scenario | Diabetes (HbA1c ≥ 6.5%) | HRD (HbA1c ≥ 6.0%, < 6.5%) | Uptake scenarios HbA1c/FPG | |||
---|---|---|---|---|---|---|
U_Sc1 95%/85% | U_Sc2 95%/75% | U_Sc3 95%/65% | U_Sc4 95%/55% | |||
P_Sc1 | 2.1% | 6.9% | −£165 (23%) | −£77 (38%) | −£41 (45%) | £86 (70%) |
P_Sc2 | 3.8% | 13.8% | £99 (70%) | £211 (85%) | £306 (93%) | £323 (85%) |
P_Sc3 | 3.8% | 20.2% | £224 (80%) | £298 (90%) | £368 (95%) | £461 (98%) |
P_Sc4 | 1.8% | 13.8% | −£101 (35%) | £33 (55%) | £121 (70%) | £146 (75%) |
Marginal net benefit figures greater than zero indicate that HbA1c is more cost-effective than FPG.
For the majority of scenario combinations of prevalence and uptake, HbA1c testing is very or highly likely to be more cost-effective than FPG testing.
The exceptions are where HbA1c-based prevalence of undiagnosed diabetes is much lower than LEADER and at a similar level to FPG-based prevalence, as in the UEA-IFG study, but it still depends on the relative prevalence of HRD and relative uptake rates as per Figure 14. These exceptions can be broken down into two cases:
-
If the prevalence of HbA1c-based HRD is very low compared with that for FPG (as in UEA-IFG), then FPG testing is more likely to be cost-effective than HbA1c testing, unless there is a very large difference in uptake of the tests (at least of the order 35%).
-
If the prevalence of HbA1c-based HRD is lower than that for FPG but higher than in UEA-IFG, then only if there is a small difference in uptake rates (less than 20%) is it likely that FPG testing is more cost-effective than HbA1c testing.
FIGURE 14.
Marginal net benefit for prevalence and uptake scenarios.

Deterministic, one-way (non-prevalence-related) sensitivity analysis results using Leicester Ethnic Atherosclerosis and Diabetes Risk data set
A set of eight deterministic sensitivity analyses were undertaken for the main comparison of HbA1c-based versus FPG-based testing.
Results are presented comparing the two NICE-recommended strategies that include a risk score and separately comparing the two corresponding strategies with no risk score. An additional four were carried out just in the context of the NICE-recommended strategies that include a risk score.
These analyses test how results change under different assumptions or parameter values in the model. The results shown in Tables 36 and 37 all indicate that HbA1c testing appears to be more cost-effective than FPG testing.
Number | Uncertain parameter and sensitivity assumption | Incremental costsa | Incremental QALYsa | Incremental net benefit at £20,000/QALY | Which is more cost-effective? |
---|---|---|---|---|---|
Base case (for reference) | –£12 | 0.0220 | £452 | HbA1c | |
SA1 | HbA1c at which clinically/opportunistically detected Through a mix of rescreening and opportunistic screening for HRD/diabetes, many individuals will not experience a rise in HbA1c to 8% before being diagnosed with diabetes. Alternative assumption used was 7.5% |
–£7 | 0.0188 | £383 | HbA1c |
SA2 | Lead time between screen-detected and clinically detected diabetes The rate of HbA1c change during the preclinical period was increased so that the average lead time between the point of screen detection and clinical detection is 3 years (instead of 4–5 years in the base case) |
–£12 | 0.0235 | £483 | HbA1c |
SA3 | Uptake of first FPG test We assumed the differential between a first HbA1c and FPG is only 10%, while maintaining no difference for a confirmatory test |
–£50 | 0.0153 | £356 | HbA1c |
SA4 | Discount rates A sensitivity analysis was undertaken using the discount rates applied to technology assessments outside of public health, namely 3.5% for both costs and QALYs |
£4 | 0.0157 | £309 | HbA1c |
SA5 | Improvements in the effectiveness of the management of diabetes | –£2 | 0.0238 | £478 | HbA1c |
SA6 | Greater sustained prevention/delay of diabetes More optimistic scenario with number of years for which weight loss sustained (beyond year 1) per £50 per annum years 2–4 maintenance, changed from 2 to 3 years |
£11 | 0.0206 | £402 | HbA1c |
SA7 | Greater uptake of preventative intervention Increased from 55% to 75% |
£7 | 0.0221 | £435 | HbA1c |
SA8 | Higher difference between cost of HbA1c and FPG Difference increased to £6 |
–£8 | 0.0220 | £448 | HbA1c |
SA9 | Different natural history for FPG vs. HbA1c-identified diabetes | ||||
Faster HbA1c progression for FPG vs. HbA1c | – £74 | 0.0198 | £470 | HbA1c | |
Slower HbA1c progression for FPG vs. HbA1c | £61 | 0.0146 | £230 | HbA1c | |
SA10 | Effectiveness of early intervention for type 2 diabetes Rate of HbA1c change (while HbA1c < 7.0%) increased from 0.15% per annum to 0.2% per annum |
£13 | 0.0219 | £424 | HbA1c |
SA11 | Increase cost of preventative intervention by 33% | –£4 | 0.0220 | £444 | HbA1c |
SA12 | Uptake of preventative intervention increased from 55% to 70% | £37 | 0.0222 | £409 | HbA1c |
No. | Uncertain parameter and sensitivity assumption | Incremental costsa | Incremental QALYsa | Incremental net benefit at £20,000/QALY | Which more cost-effective? |
---|---|---|---|---|---|
Base case (for reference) | –£30 | 0.0223 | £476 | HbA1c | |
SA1 | HbA1c at which clinically/opportunistically detected Through a mix of rescreening and opportunistic screening for diabetes/HRD, many individuals will not experience a rise in HbA1c to 8% before being diagnosed with diabetes. Alternative assumption used was 7.5% |
£2 | 0.0222 | £442 | HbA1c |
SA2 | Lead time between screen-detected and clinically detected diabetes The rate of HbA1c change during the preclinical period was increased so the average lead time between the point of screen detection and clinical detection is 3 years (instead of 4–5 years in the base case) |
–£10 | 0.0239 | £489 | HbA1c |
SA3 | Uptake of first FPG test We assumed the differential between a first HbA1c and FPG test is only 10%, whilst maintaining no difference for a confirmatory test |
–£53 | 0.0142 | £337 | HbA1c |
SA4 | Discount rates A sensitivity analysis was undertaken using the discount rates applied to technology assessments outside of public health, namely 3.5% for both costs and QALYs |
–£11 | 0.0158 | £327 | HbA1c |
SA5 | Improvements in the effectiveness of the management of diabetes | £9 | 0.0219 | £478 | HbA1c |
SA6 | Greater sustained prevention/delay of diabetes More optimistic scenario with number of years for which weight loss sustained (beyond year 1) per £50 per annum years 2–4 maintenance, changed from years 2–3 |
£0 | 0.0236 | £471 | HbA1c |
SA7 | Greater uptake of preventative intervention Increased from 55% to 75% |
£5 | 0.0216 | £428 | HbA1c |
SA8 | Higher difference between cost of HbA1c and FPG Difference increased to £6 |
–£26 | 0.0223 | £472 | HbA1c |
National Institute for Health and Care Excellence guidance strategies: results obtained without using a risk score
As per Table 38, if no risk score is used, the incremental costs and QALYs of using HbA1c 6.0% versus FPG 5.5 mmol/l are a saving of £30 (£75–£105) and 0.0224 (0.0566–0.0342), respectively; therefore, HbA1c testing appears to dominate FPG testing. PSA indicates that there is a 95% probability that HbA1c 6.0% is more cost-effective than FPG 5.5.
Strategy | Glucose test and cut-off point for HRD | Total costs (including screening cost) | Total QALYs | Incremental costs vs. ‘no screening’ (discounted) | Incremental QALYs vs. ‘no screening’ (discounted) | ICER vs. no screening | Net monetary benefit | Incremental net benefit vs. ‘no screening’ | Probability most cost-effective of strategies within same NICE/ISO band (%) |
---|---|---|---|---|---|---|---|---|---|
Per person eligible for diabetes risk assessment | |||||||||
NICE-recommended strategies without use of risk score (HbA1c 6.0 and FPG 5.5) plus FPG 5.6 (ISO resource as strategy HbA1c 6.0) | |||||||||
HbA1c 6.0 | HbA1c ≥ 6.0% | £13,841 | 13.6443 | £75 | 0.0566 | £1333 | £259,046 | £1056 | 97 |
FPG 5.5 | FPG ≥ 5.5 mmol/l | £13,870 | 13.6220 | £105 | 0.0342 | £3066 | £258,570 | £580 | 3 |
FPG 5.6 | FPG ≥ 5.6 mmol/l | £13,828 | 13.6193 | £63 | 0.0316 | £1990 | £258,559 | £569 | 0 |
With no risk score, more cases of HRD are identified with FPG screening, which partially offsets the benefits of HbA1c screening identifying more cases of undiagnosed diabetes (5.7% vs. 1.8%).
When comparing all four NICE-related strategies (i.e. with and without a risk score) together with no screening, screening everyone using HbA1c 6.0 provides the most QALYs, and when measured on the net benefit scale assuming a value for 1 QALY of £20,000, also has the highest expected net benefit. There are, however, disadvantages to not using a risk score (see Chapter 5, Statement of principal findings) even if there were the capacity to undertake blood tests on everyone.
Results for alternative cut-off points for preventative intervention to the National Institute for Health and Care Excellence recommendations (‘ISO-resource’ strategies)
Table 39 shows that, for any pair of ‘ISO-resource’ strategies (i.e. where the proportion of individuals identified as at HRD is the same for the HbA1c strategy as for the FPG strategy, as explained in Chapter 2, Derivation of final set of screening strategies to model), the HbA1c-based strategy is more cost-effective than the FPG-based strategy. This is clear from the fact that HbA1c has both a higher NMB and a higher incremental net benefit than ‘no screening’.
Strategy | Glucose test and cut-off point for HRD | Total costs (including screening cost) | Total QALYs | Incremental costs vs. ‘no screening’ (discounted) | Incremental QALYs vs. ‘no screening’ (discounted) | ICER vs. ‘no screening’ | Net monetary benefit | Incremental net benefit vs. ‘no screening’ | Probability most cost-effective of strategies within same NICE/ISO band (%) |
---|---|---|---|---|---|---|---|---|---|
Per person eligible for diabetes risk assessment | |||||||||
Other strategies: ISO resource ≈27% | |||||||||
LPDS 4.75/HbA1c 5.8 | LPDS ≥ 4.75, HbA1c ≥ 5.8% | £13,909 | 13.6556 | £144 | 0.0679 | £2124 | £259,203 | £1214 | 85 |
LPDS 4.75/FPG 5.2 | LPDS ≥ 4.75, FPG ≥ 5.2 mmol/l | £13,913 | 13.6303 | £148 | 0.0425 | £3478 | £258,693 | £703 | 2 |
Other strategies: ISO resource ≈32% | |||||||||
HbA1c 5.8 | HbA1c ≥ 5.8% | £13,941 | 13.6650 | £176 | 0.0773 | £2277 | £259,360 | £1370 | 92 |
FPG 5.2 | FPG ≥ 5.2 mmol/l | £13,942 | 13.6401 | £177 | 0.0523 | £3377 | £258,859 | £870 | 2 |
Other strategies: ISO resource ≈36% | |||||||||
LPDS 4.75/HbA1c 5.7 | LPDS ≥ 4.75, HbA1c ≥ 5.7% | £13,953 | 13.6634 | £188 | 0.0757 | £2482 | £259,316 | £1326 | 60 |
LPDS 4.75/FPG 5.0 | LPDS ≥ 4.75, FPG ≥ 5.0 mmol/l | £13,973 | 13.6417 | £208 | 0.0539 | £3848 | £258,861 | £871 | 2 |
Other strategies: ISO resource ≈43% | |||||||||
HbA1c 5.7 | HbA1c ≥ 5.7% | £14,003 | 13.6757 | £238 | 0.0880 | £2699 | £259,512 | £1522 | 100 |
FPG 5.0 | FPG ≥ 5.0 mmol/l | £14,037 | 13.6531 | £271 | 0.0653 | £4153 | £259,025 | £1035 | 0 |
Figure 15 aims to help to compare many strategies visually. It presents the results in the form of a cost-effectiveness plane, the x-axis showing incremental QALYs compared with no screening and the y-axis showing incremental cost compared with no screening. The bold dashed black diagonal line represents the acceptability threshold value of £20,000 per QALY gained, that is points below and to the right of this line would be considered cost-effective compared with ‘no screening’. This is equivalent to a strategy having a higher expected NMB than ‘no screening’.
FIGURE 15.
Incremental costs and QALYs vs. ‘no screening’ for each strategy.
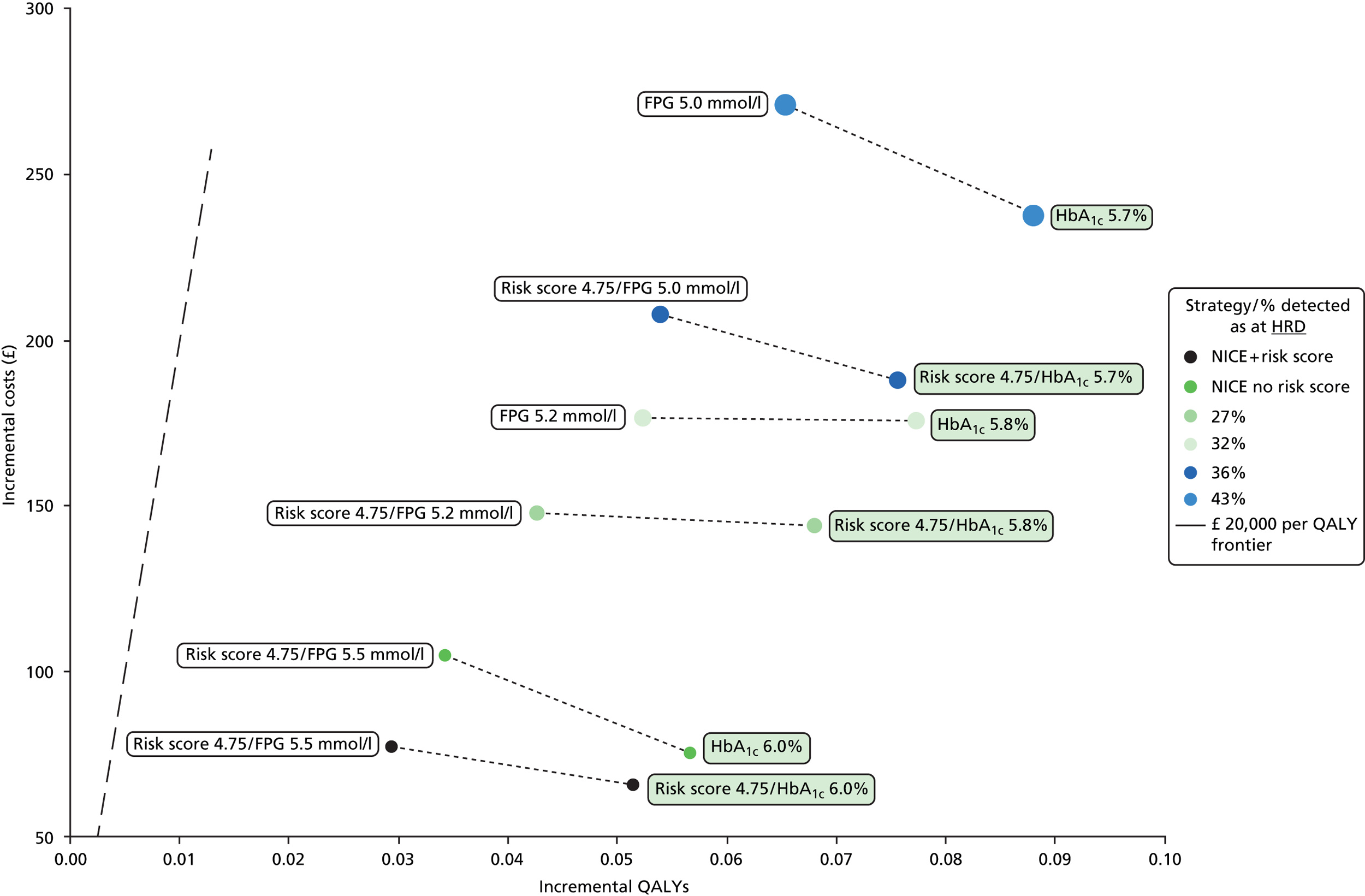
Visual presentation of all results based on Leicester Ethnic Atherosclerosis and Diabetes Risk cohort comparing glycated haemoglobin with fasting plasma glucose
The main purpose of Figure 15 is to show visually the results for HbA1c testing versus FPG, based on the LEADER cohort, for all analyses assessed. Comparable strategies are joined by dashed lines, whether these are the NICE-recommended strategies with a risk score, the NICE cut-off points for HRD but with no risk score, or potential alternative cut-off points. Corresponding strategies also have the same colour of dots. For the ISO-resource strategies, the size of the dot is in proportion to the percentage detected as at HRD.
When comparing any two strategies, dots further to the right of the origin provide more health benefits, that is QALYs. To determine which is the most cost-effective of a pair of strategies:
-
If Dot2 is further to the right and lower down on the y-axis than Dot1, then this is easy to interpret – strategy 2 provides more health benefit and incurs less cost and is therefore ‘dominant’ compared with strategy 1.
-
If Dot2 is further to the right but also higher up the y-axis, then we need to calculate the ICER and test to see if it is less than the £20,000 per QALY gained threshold. This can be easily done ‘by eye’ in the figure because the bold dashed sloping black line is a line of slope £20,000 per QALY gained, and so, if the slope from Dot1 to Dot2 is less steep than this dotted line, then its ICER will be lower than £20,000 per QALY and strategy 2 will be more cost-effective than strategy 1.
Two conclusions can be drawn from the comparisons:
-
For all comparisons, it turns out that the HbA1c-based strategies (box labels shaded in green) are further to the right and lower down than the corresponding FPG-based strategy (joined by a dashed line), so HbA1c is more cost-effective.
-
A secondary purpose of the figure is to show that the results indicate that the lower the threshold for preventative intervention, the more cost-effective the strategy. For example, if a line were joined from the LPDS 4.75/HbA1c 5.8 dot to the LPDS 4.75/HbA1c 5.7 dot, the slope would be less steep than the £20,000 per QALY gained line, so the results indicate that LPDS 4.75/HbA1c 5.7 is a more cost-effective strategy than LPDS 4.75/HbA1c 5.8.
Use of a random capillary glucose test or the Leicester Practice Database Score to prioritise who should receive the blood test
Once it had been established that HbA1c is more cost-effective than FPG, a separate analysis was undertaken to compare the cost-effectiveness of using an RCG test with using the LPDS risk score as a first step to prioritise individuals for further testing for diabetes or HRD using a HbA1c test (see Table 40).
Strategy | Total costs | Total QALYs | Net monetary benefit | Mean difference in net monetary benefit | 95% LCI for mean difference in net monetary benefit | Probability LPDS cost-effective vs. RCG (%) |
---|---|---|---|---|---|---|
6.0% HbA1c threshold for HRD | ||||||
LPDS 4.75/HbA1c 6.0 | £14,270 | 13.3816 | £253,361 | +£57 | +£39 | 88 |
RCG 4.4/HbA1c 6.0 | £14,271 | 13.3787 | £253,304 | – | – | – |
5.7% HbA1c threshold for HRD | ||||||
LPDS 4.75/HbA1c 5.7 | £14,410 | 13.4046 | £253,681 | +£9 | –£9 | 59 |
RCG 4.2/HbA1c 5.7 | £14,428 | 13.4050 | £253,672 | – | – | – |
The RCG and LPDS cut-off points for this comparison were chosen such that a similar proportion of individuals (with HbA1c ≥ 6.0%) would be identified. The comparisons were made in the context of (1) the NICE HbA1c threshold of 6.0% for HRD and (2) a lower HbA1c threshold of 5.7% for HRD.
If blood tests cannot be undertaken in all eligible individuals because of capacity or budget constraints, the LPDS is highly likely to be more cost-effective for prescreening than using an RCG test at a HbA1c cut-off point of 6.0% for HRD. At lower HbA1c thresholds for HRD, the choice appears to be much less certain.
There are a couple of factors that could not be taken account of within the modelling:
-
The LPDS might also identify individuals with slightly higher non-invasive risk factors for progression to diabetes. Unfortunately, there does not exist, to our knowledge, a risk equation that captures the variables included within the LPDS, plus HbA1c and FPG. Without this, it is not possible to account for these risk differences within the modelling.
-
An important part of the two-stage process of prescreening with a risk score, followed by a blood test if indicated, is that the risk score provides feedback to individuals on modifiable risk factors which may motivate them to make lifestyle changes to reduce their risk of developing diabetes. Such effects have not been quantified for use in the LPDS and therefore cannot be captured within the modelling.
Chapter 5 Discussion
Statement of principal findings
HbA1c testing is highly likely to be more cost-effective than FPG testing for screening for diabetes and HRD based on (1) the multiethnic LEADER cohort and (2) most alternative prevalence and uptake scenarios assessed. Although the absolute differences between tests in health gains per individual are small, the PSA shows that this conclusion is 95–98% certain in a region with similar relative (HbA1c- to FPG-defined) prevalence of undiagnosed diabetes and HRD as in the multiethnic Leicestershire-based LEADER cohort.
Scenario analyses indicate that, where the relative prevalence of HbA1c- to FPG-defined undiagnosed diabetes and HRD is lower than in the LEADER cohort, for example as in the UEA-IFG study, the relative cost-effectiveness depends on the size of the difference in uptake rates of screening for a HbA1c test versus a FPG test.
Given that only a few UK-based studies appear to exist that contain both baseline HbA1c and baseline FPG levels from a screening setting (or recruitment into a diabetes prevention programme), it is not possible to suggest to what extent the LEADER cohort is representative of the UK as a whole.
The prevalence patterns shown in Table 4 (see Chapter 2, Other UK cohorts providing estimates of prevalence of diabetes and high risk of diabetes using both glycated haemoglobin and fasting plasma glucose), together with the results from the scenario analyses (around alternative prevalence and uptake rates), would seem to suggest that HbA1c testing is likely to be more cost-effective than FPG testing in most localities; the exceptions are for combinations of prevalence and uptake that closely match one of the scenarios that reports a negative incremental net benefit in Table 35 in the results section.
Based on the LEADER cohort, sensitivity analyses around parameters unrelated to prevalence and uptake suggest that the conclusions are generally insensitive to a range of alternative assumptions around such parameters.
Lowering the thresholds for HRD and preventative intervention does not change the finding that HbA1c testing appears more cost-effective than FPG testing. This same finding was found for several ‘ISO-resource’ comparisons.
Owing to capacity or budget constraints, in most localities prescreening is likely to be necessary for the purpose of identifying which individuals should receive a blood test. In some areas with a very high diabetes risk profile (e.g. multiethnic localities), it may be more practical to carry out blood tests in everyone. In such cases, HbA1c testing remains highly likely to be more cost-effective than FPG testing. Note that this does not mean that the risk scoring should not be done at all (because drawing people’s attention to modifiable risk factors is likely to be beneficial).
Use of the LPDS risk score is likely to be more cost-effective than using an RCG test for prescreening. It should also be noted that a risk score may have additional effects not captured within the modelling – the calculation of the score may draw attention to high-risk factors for developing diabetes in the future (rather than just providing a normal/abnormal blood test result). This might prompt individuals to make lifestyle changes to reduce their risk, that is there may be an intervention effect even if their HbA1c or FPG is below the corresponding HRD threshold for intensive intervention.
Drivers of cost-effectiveness
In absolute terms, the expected differences in total long-term costs and QALYs between the two tests are small. This is because (1) the difference between the costs of a HbA1c test and a FPG test is small and (2) the prevalence of undiagnosed diabetes is relatively low (5.7% for HbA1c in the LEADER cohort) and cases undetected at screening will probably be diagnosed in clinical practice within around 5 years on average (or through rescreening, although we have not modelled this).
The key drivers of the cost-effectiveness are:
-
1. The relative prevalence of diabetes and HRD using HbA1c-based definitions versus FPG-based definitions. This depends very much on local variation.
-
In the LEADER cohort, both HbA1c testing and FPG testing would identify 16% of individuals as at HRD (if the LPDS is used to prescreen), but HbA1c testing identifies a larger number of people with undiagnosed diabetes (4.4% vs. 1.2%).
-
-
2. The higher uptake of HbA1c tests than FPG tests.
It is also worth mentioning uncertainty around the unit costs of the tests. As there is no single national reference source for data on such costs, and there is also variation between laboratories (depending particularly on volume of tests), there inevitably is some variation in quoted costs of the tests. Nevertheless, it is clear from sensitivity analysis that the cost-effectiveness of FPG versus HbA1c testing is likely to be very insensitive to this uncertainty given the absolute difference in screening cost per person in the model is only £2.22 (£14.40 vs. £12.18).
Strengths and limitations
This analysis represents, to our knowledge, the first economic analysis comparing the long-term cost-effectiveness of HbA1c testing with FPG testing to screen for diabetes and HRD. A particular strength is the allowance of an individual to have a different screening outcome (in terms of diabetes, HRD or NGT) according to the differing diagnostic definitions of HbA1c and FPG tests rather than based on the historical gold standard OGTT test.
Generalisability of the findings
The majority of our analysis was carried out based on the multiethnic LEADER cohort, with additional scenarios informed by prevalence of diabetes and HRD in other UK cohorts. Before HbA1c can be relied upon to be most cost-effective in a particular locality, given the variation in prevalence between cohorts, it is necessary to ascertain if the locality-specific prevalence is in line with those scenarios for which HbA1c testing is more cost-effective than FPG testing. The same clearly applies to non-UK populations.
Individuals of black and minority ethnic origin aged 25–39 years
The 2012 NICE guidance on risk assessment recommended that individuals of BME origin aged 25–39 years should also be assessed for risk of diabetes within the NHS Health Check programme because of their increased risk. 5
Taking the relative prevalence of FPG-defined and HbA1c-defined diabetes and HRD in the LEADER cohort, as shown in Table 3 (see Chapter 2, Prevalence of diabetes and high risk of diabetes among South Asians of 25–39 years of age in the Leicester Ethnic Atherosclerosis and Diabetes Risk cohort), together with the findings for the 40–74 years age group, it is very highly likely that HbA1c testing is more cost-effective than FPG testing among South Asians of age 25–39 years, and possibly for all BME ethnicities in this age range.
Rescreening
We have not extended the model structure to include rescreening because of important gaps in the evidence base, in particular:
-
The likelihood of attendance and completion of a subsequent diabetes reassessment given that an individual took up the offer of a blood test for diabetes at his or her initial health check.
-
The likelihood of attendance for, and completion of, risk assessment following a health check invite during a reassessment round, given that an individual either did not take up the initial offer of a health check or did not take up the offer of a blood test for diabetes.
-
The correlation between an individual’s HbA1c or FPG level at the initial health check and that at subsequent reassessment. This is a function of intertest variation, particularly for FPG testing, and the distribution of trajectories for the ‘true’ values of HbA1c or FPG between testing (e.g. some individuals identified with HRD at an initial health check will regress to NGT, some will remain with HRD and some will progress to diabetes).
This means that the model does not include either the costs or benefits of rescreening. The residual prevalence of diabetes in the LEADER cohort after a first round of offers/risk assessment was 2% for HbA1c and 1% for FPG. For HRD, prevalence was 7.1% for HbA1c and 10% for FPG. On the basis that the proportion of individuals with undetected diabetes or HRD after the initial assessment is likely to be proportional to the baseline prevalence of undiagnosed cases, there is a line of argument that inclusion of rescreening would be unlikely to materially influence the findings. However, the complexities involved in modelling rescreening are not straightforward and should evidence emerge to fill the gaps, it may be worth extending the analysis to confirm the findings in this report.
Uncertainties around the benefits of a diabetes screening programme
Risk profile of attendees at NHS Health Checks
To date, attendance for NHS Health Checks has, on average, been disappointing (49% in England between April 2012 and March 2013). 69 Moreover, current attendees may be over-represented by the ‘worried well’. The prevalence among these attendees may be lower than the eligible population prevalence. The consequence is that, for those that are successfully recruited for NHS Health Checks, the benefits of screening may be lower than our results suggest. However, this applies for both HbA1c and FPG testing; therefore, it is unlikely to influence the relative cost-effectiveness of the two tests.
The Anglo-Danish–Dutch Study of Intensive Treatment In People with Screen Detected Diabetes in Primary Care trial
The ADDITION trial was designed to assess the benefits of early intervention following screening for diabetes. This did not demonstrate the hoped for improvement in CVD outcomes from early intervention,50 leading to concerns about the effectiveness of screening for diabetes. However, there are caveats around the findings related to contamination between study arms, in particular around improvements in routine care over the course of the trial,14 such as increased prescription of statin therapy. Concerns about the effectiveness of screening would be greater if it were shown that earlier intervention had no beneficial effect on risk factors or if improvements in risk factors do not lead to reduced outcomes in the long run (e.g. over 10 years).
Storage of fasting plasma glucose samples and time to laboratory processing
The results assume similar storage methods and time to processing such that the decay in glucose values in clinical practice is similar to that in the LEADER study, which equally is assumed to be similar to those in epidemiological studies from which evidence for risk of diabetes and CVD are obtained.
The protocol used in LEADER, with samples stored at 4 °C for up to 2 hours, is likely to be more stringent than those typical in clinical practice. It is, therefore, likely that the diagnostic yield from screening in clinical practice using a FPG test may be lower than obtained in LEADER.
HbA1c levels are not subject to the same variation as glucose, namely according to the length of time between the blood sample being taken and the time of analysis.
Point-of-care testing
Our analysis has been based on laboratory testing rather than POC testing for both HbA1c and FPG. There have historically been concerns about standardisation of POC testing. 70 It is not a recommended option within the 2012 NICE guidance on risk assessment,5 although we understand that it is starting to be used in some localities. Some uncertainties about its validity in diabetes risk assessment remain, however. For example, there are uncertainties as to whether or not a POC test followed by a laboratory test is sufficient for a diagnosis of diabetes (for which two laboratory tests have historically been required).
Although we have not modelled POC testing, we consider that this would be unlikely to influence the conclusions because the number of visits to a GP centre is still likely to be lower for HbA1c than for FPG, and FPG testing will still require an overnight fast; therefore, the difference in uptake of the two tests is likely to remain. Any change to the difference in the unit costs of the POC HbA1c and FPG tests is likely to have an insignificant effect on cost-effectiveness.
Generalisability of evidence from studies of individuals with impaired glucose tolerance
Most of the existing evidence for the effectiveness of lifestyle interventions to prevention diabetes has come from studies of individuals with IGT; therefore, it may not be entirely applicable to our HbA1c- or FPG-identified cohorts. There is also uncertainty around whether or not the long-term rate of progression of diabetes differs depending on whether an individual was identified with a HbA1c or FPG test.
Individual choice of a glycated haemoglobin or a fasting plasma glucose test
Although there might be some instances across the country where practices might be willing to offer patients the choice of either a HbA1c test or a FPG test, we have been advised by clinical experts that it is unlikely that many would; therefore, this was considered a peripheral issue and has not been considered further.
Combined glycated haemoglobin and fasting plasma glucose testing
Some guidelines recommend against this on the grounds that it is confusing to patients. In addition, given the extra cost and time necessary for combined testing and existing resources within primary care, it seems highly unlikely that this could be routinely done for all individuals with the aim of identifying more individuals with HRD to offer intervention to (i.e. as a result of meeting either the HbA1c or the FPG criteria). A more realistic approach to such an aim might be to lower the cut-off point(s) for defining HRD below the existing NICE-recommended cut-off points, as discussed earlier.
However, combined testing might potentially have a role in aiming to identify a subset of individuals that meet both HbA1c and FPG criteria for HRD in the following contexts:
-
The Association of British Clinical Diabetologists position statement suggested that combined testing could be used,71 and that a potential role could be to identify high-risk individuals in whom both HbA1c and FPG levels fall just below the thresholds for HRD.
-
For further risk stratification of individuals who have been identified as at HRD having undergone one test (HbA1c or FPG). By taking the other test (FPG or HbA1c) as well, it would be possible to identify individuals in whom both cut-off points for HRD with HbA1c and FPG are exceeded, who are therefore at the highest risk of diabetes, and who may warrant a more intensive form of preventative intervention.
Comparison with related studies
It should be noted that the total and incremental cost and QALY figures reported in this document may be an order of magnitude different from previous work on screening and prevention, including the economic modelling for the 2012 NICE guidance on risk assessment. 12 This is because of different discount rates used as a result of the recent NICE recommendation to use 1.5% per annum for public health-related evaluations, rather than 3.5%. 68
Implications
Current implications for decision makers at national/local commissioner level
The economics of screening will vary at the local level according to the local diagnostic yield from screening, which itself will vary according to several factors.
Before HbA1c can be relied upon to be most cost-effective in a particular locality, given the variation in prevalence between cohorts, it may be desirable to ascertain if the locality-specific prevalence is in line with those scenarios for which our results indicate that HbA1c testing is likely to be more cost-effective than FPG testing.
Impact of ethnicity
Variations in ethnicity as well as deprivation are likely to be key determinants of variations between localities in prevalence of diabetes and HRD. Multiethnic populations tend to have a higher prevalence of diabetes and HRD.
Potential future implications for decision-makers at national/local commissioner level
The modelling suggests that a wide range of alternative risk assessment/intervention strategies would be cost-effective compared with no screening, and policy/commissioning decisions may therefore also be influenced by other criteria such as total cost to the NHS and capacity to deliver intensive interventions on a national scale.
The modelling suggests that the most cost-effective strategy would be to screen everybody using a HbA1c test and to offer those with HbA1c between 5.7% and 6.4% the intensive intervention. Based on the multiethnic LEADER data set, this HbA1c 5.7% strategy would identify 36% of those assessed (not of the whole population aged 40–74 years) as at ‘higher risk of diabetes’ (as well as identifying 5.7% with diabetes). This is more than double that under current NICE guidance, and delivery of an intensive lifestyle intervention to such a large number of people would be extremely unlikely to be manageable within current primary care capacity. In addition, from a budget perspective, assuming 70% of individuals are offered and attend a health check, between 2.3 and 2.4 million individuals would be identified as being at HRD (taking account of uptake of blood tests), which would cost around £350M for an intervention with 11 contact hours costing £150 per person (these costs exclude costs of ongoing maintenance intervention).
Giving consideration, at some point in the future, to intervening in individuals with both a high-risk score and a HbA1c level of at least 5.8% or 5.9% may be more realistic than intervening at HbA1c 5.7%.
Unanswered questions and recommendations for further research
Primary research/data collection
The recommendations considered in this section generally apply at the national level. However, if commissioners or practitioners are interested in undertaking research locally to aid their decision about which test to use, the two priorities for local data collection would be uptake rates and prevalence data (see Uptake rates for glycated haemoglobin testing and for fasting plasma glucose testing within NHS Health Checks and Prevalence data for those of 40–74 years of age).
Uptake rates for glycated haemoglobin testing and for fasting plasma glucose testing within NHS Health Checks
Reducing the uncertainty around the difference in uptake of the two tests would potentially be useful, as it would simplify the criteria for scenarios in which FPG testing might be more cost-effective than HbA1c testing.
Within follow-up beyond the initial 5-year programme of NHS Health Checks, it would be useful to collect data on uptake of subsequent offers of risk assessment conditional on taking up their original offer of a Health Check. This would inform modelling of rescreening.
Prevalence data for those of 40–74 years of age
If local data were collected and revealed a relative HbA1c-defined to FPG-defined prevalence of diabetes and HRD markedly different from the scenarios analysed here, it might be useful for the model be rerun to examine the difference this evidence would make to the cost-effectiveness.
If local data collection is problematic, it may be worth analysing the determinants of the large variation in prevalence between the LEADER cohort and the UEA-IFG cohort. If an explanatory model of HbA1c- and FPG-defined prevalence of diabetes and HRD could be constructed, this may be useful to local commissioners.
Prevalence may be worth analysing in future large epidemiological studies or prevention studies that screen individuals at the baseline.
Prevalence among those reaching 40 years of age
Each year, a new cohort of individuals will reach 40 years of age and be eligible for a Health Check (assuming a similar policy continues in the long run). The relative HbA1c- to FPG-defined prevalence of undiagnosed diabetes and HRD among such a cohort of 40-year-olds may differ from the current 40–74 years population. In particular, 40-year-olds are less likely to have undertaken opportunistic screening.
Unit costs of tests
Commissioners may wish to obtain local costs for tests, which will be influenced by local economies of scale and organisation of diagnostic services, in order to calculate the budgetary impact of a screening programme.
Secondary analyses of epidemiological studies
To inform a future assessment that includes rescreening, data sets may become available that provide evidence for the issues discussed in Rescreening.
Further evidence assessment
New evidence is likely to emerge over time about the natural history of FPG- versus HbA1c-defined HRD and diabetes. Any evidence that may strongly favour FPG testing might be considered grounds for re-examining the relative cost-effectiveness of HbA1c testing and FPG testing.
A recent paper by Faerch and colleagues,72 using data from the Whitehall II study, suggests the existence of alternative phenotypes among those who progress from HRD to diabetes. If and to what extent diabetes might be more progressive with one test versus another is unknown. Any difference would have some impact on the incidence of comorbidities and associated costs and QALYS.
To what extent ethnicity (and its associated influences on BMI) is the underlying determinant of the difference in the relative (HbA1c- to FPG-defined) prevalence of diabetes and HRD in the LEADER cohort versus the UEA-IFG cohort is unclear.
Converging prevalence of undiagnosed diabetes and high risk of diabetes
The relative prevalence of HbA1c- to FPG-defined undiagnosed diabetes and HRD will change over the coming years because HbA1c-based screening has only recently started and hence the current prevalence of undiagnosed HbA1c-defined diabetes and HRD is relatively high compared with that expected after a number of years of screening using HbA1c tests.
Looking beyond the horizon of the current NHS Health Checks programme, the main issue is how the relative prevalence of undiagnosed FPG-defined diabetes and HbA1c-defined diabetes may change. The current difference in prevalence (5.7% for HbA1c vs. 1.8% for FPG) is likely in part to be because of the history of opportunistic screening in the UK using OGTTs (which includes a FPG test). Over time, the difference in prevalence is likely to narrow to some extent, which is likely to reduce the superior cost-effectiveness of HbA1c testing. In addition, hypothetically, as the prevalences of undiagnosed HbA1c-defined diabetes and FPG-defined diabetes become closer, the cost-effectiveness of combined HbA1c and FPG screening may improve relative to using HbA1c alone.
Further modelling studies
Although there are several aspects of the evidence used for this assessment that ideally would be stronger, it is difficult to make firm recommendations for further research because the relative costs and benefits of undertaking further research are unclear (especially for primary data collection). Obtaining a quantified assessment of the likely value of further work using value-of-information techniques may be valuable further research in itself. However, reducing the uncertainty around some model parameters may have little impact on the relative cost-effectiveness.
Case for re-evaluation at some point within the next 3–10 years
There are several issues which, together, might justify further work and remodelling at some point:
-
Converging prevalence: if the prevalences of undiagnosed HbA1c- and FPG-defined diabetes and HRD converge as per Converging prevalence of undiagnosed high risk of diabetes and diabetes.
-
Rescreening: if evidence becomes available which meets the needs, discussed in Rescreening.
-
POC testing: if there is greater clarity around diagnostic pathways involving POC testing and if other evidence needed is identified (for example, the correlation between POC and laboratory FPG test results).
-
Completion of health checks: further analysis may be helpful to inform ongoing reassessment needs after the ongoing 5-year programme has been completed.
Cost-effectiveness among those of 40 years of age
As per Prevalence among those reaching 40 years of age, prevalence might differ among the subpopulation that turns 40 years of age each year and a separate evaluation may be justified for this.
Acknowledgements
The authors are grateful to:
The National Institute for Health Research Collaboration for Leadership in Applied Health Research and Care – East Midlands (NIHR CLAHRC – EM) and the NIHR Leicester–Loughborough Diet, Lifestyle and Physical Activity Biomedical Research Unit, which is a partnership between University Hospitals of Leicester NHS Trust, Loughborough University and the University of Leicester. These supported the contribution of the authors from the Leicester Diabetes Centre.
Professor Elizabeth Goyder, School of Health and Related Research (ScHARR), University of Sheffield, for providing advice on the implementation of the Department of Health’s NHS Health Checks programme in England.
Members of the MRC Epidemiology Unit at Cambridge University (Professor Simon Griffin and Dr Rebecca Simmons) for providing access to data from the ADDITION-Cambridge Study. Similarly, Professor Nick Wareham and Dr Nita Forouhi arranged and undertook analyses on data from the Fenland Study.
Professor Max Bachmann, Norwich Medical School, University of East Anglia, for providing results from additional analyses of HbA1c and FPG-based prevalence from the UEA-IFG study.
About the Sheffield Health Economics and Decision Science (HEDS) research group
The HEDS research group is part of the School of Health and Related Research (ScHARR), a multidisciplinary group within the Faculty of Medicine. Within the HEDS group, there is a range of specialties, including decision-analytic modelling and technology appraisal. The ScHARR Technology Assessment Group undertakes reviews of the clinical effectiveness and cost-effectiveness of health-care interventions for the NHS Research and Development (R&D) Health Technology Assessment (HTA) Programme on behalf of a range of policy-makers, including the NICE. Health economists and mathematical modellers work with systematic reviewers to undertake cost-effectiveness analyses using decision-analytic modelling for local and national health-care decision-making bodies. Members of HEDS also carry out and publish research papers on the role and methods of, and quality assurance in, modelling.
Contributions of authors
Mike Gillett, Research Fellow (Health Economics): principal investigator and conducted the economic modelling.
Alan Brennan, Professor of Health Economics and Decision Modelling: supervised the economic modelling.
Penny Watson, Research Associate (Health Economics): assisted with analysis of the LEADER data set and literature reviewing.
Kamlesh Khunti, Professor of Primary Care Diabetes and Vascular Medicine: provided advice on clinical and policy issues.
Melanie Davies, Professor of Diabetes Medicine and Honorary Consultant: provided advice on clinical and policy issues.
Samiul Mostafa, Clinical Research Fellow/SpR Diabetes Medicine: provided advice on clinical and policy issues.
Laura J Gray, Senior Lecturer of Population and Public Health Sciences: provided advice on LEADER data set.
The views expressed in this report do not necessarily reflect those of the NHS HTA programme or the UK Department of Health.
Disclaimers
This report presents independent research funded by the National Institute for Health Research (NIHR). The views and opinions expressed by authors in this publication are those of the authors and do not necessarily reflect those of the NHS, the NIHR, NETSCC, the HTA programme or the Department of Health. If there are verbatim quotations included in this publication the views and opinions expressed by the interviewees are those of the interviewees and do not necessarily reflect those of the authors, those of the NHS, the NIHR, NETSCC, the HTA programme or the Department of Health.
References
- The Press Association . Diabetes Total Soars to Top 3.2 Million. Health Service Journal 2014. http://www.hsj.co.uk/news/diabetes-total-soars-to-top-32-million/5067856.article#.UzRErM7KFGc (accessed February 2014).
- Holman N, Forouhi NG, Goyder E, Wild SH. The Association of Public Health Observatories (APHO) Diabetes Prevalence Model: estimates of total diabetes prevalence for England, 2010–2030. Diabet Med 2011;28:575-82. http://dx.doi.org/10.1111/j.1464-5491.2010.03216.x.
- NHS Evidence . Eyes on Evidence n.d.;29.
- The Cost of Diabetes Report. London: Diabetes UK; 2014.
- Preventing Type 2 Diabetes – Risk Identification and Interventions for Individuals at High Risk: Guidance. London: NICE; 2012.
- Zhuo X, Zhang P, Selvin E, Hoerger TJ, Ackermann RT, Li R, et al. Alternative HbA1c cutoffs to identify high-risk adults for diabetes prevention: a cost-effectiveness perspective. Am J Prev Med 2012;42:374-81. http://dx.doi.org/10.1016/j.amepre.2012.01.003.
- Webb DR, Khunti K, Srinivasan B, Gray LJ, Taub N, Campbell S, et al. Rationale and design of the ADDITION-Leicester study, a systematic screening programme and randomised controlled trial of multi-factorial cardiovascular risk intervention in people with type 2 diabetes mellitus detected by screening. Trials 2010;11. http://dx.doi.org/10.1186/1745-6215-11-16.
- Gray LJ, Tringham JR, Davies MJ, Webb DR, Jarvis J, Skinner TC, et al. Screening for type 2 diabetes in a multiethnic setting using known risk factors to identify those at high risk: a cross-sectional study. Vasc Health Risk Manag 2010;6:837-42. http://dx.doi.org/10.2147/VHRM.S12504.
- Irvine L, Barton GR, Gasper AV, Murray N, Clark A, Scarpello T, et al. Cost-effectiveness of a lifestyle intervention in preventing type 2 diabetes: economic evaluation alongside a clinical trial. Int J Technol Assess Health Care 2011;27:275-82. http://dx.doi.org/10.1017/S0266462311000365.
- Kumaravel B, Bachmann MO, Murray N, Dhatariya K, Fenech M, John WG, et al. Use of haemoglobin A1c to detect impaired fasting glucose or type 2 diabetes in a United Kingdom community based population. Diabetes Res Clin Pract 2012;96:211-16. http://dx.doi.org/10.1016/j.diabres.2011.12.004.
- Carson AP, Reynolds K, Fonseca VA, Muntner P. Comparison of A1C and fasting glucose criteria to diagnose diabetes among U.S. adults. Diabetes Care 2010;33:95-7. http://dx.doi.org/10.2337/dc09-1227.
- Gillett M, Chilcott J, Goyder E, Payne N, Thokala P, Freeman C, et al. Prevention of Type 2 Diabetes: Risk Identification and Interventions for Individuals at High Risk – Economic Review and Modelling 2012.
- Waugh N, Scotland G, Gillett M, Brennan A, Goyder E, Williams R, et al. Screening for type 2 diabetes: literature review and economic modelling. Health Technol Assess 2007;11. http://dx.doi.org/10.3310/hta11170.
- Waugh NR, Shyangdan D, Taylor-Phillips S, Suri G, Hall B. Screening for type 2 diabetes: a short report for the National Screening Committee. Health Technol Assess 2013;17. http://dx.doi.org/10.3310/hta17350.
- Johnson M, Jones R, Freeman C, Woods HB, Gillett M, Goyder E, et al. Can diabetes prevention programmes be translated effectively into real-world settings and still deliver improved outcomes? A synthesis of evidence. Diabet Med 2013;30:3-15. http://dx.doi.org/10.1111/dme.12018.
- Gray L, Davies M, Hiles S, Taub N, Webb D, Srinivasan B, et al. Detection of impaired glucose regulation and/or type 2 diabetes mellitus, using primary care electronic data, in a multiethnic UK community setting. Diabetologia 2012;55:959-66. http://dx.doi.org/10.1007/s00125-011-2432-x.
- John WG. on behalf of the UK Department of Health Advisory Committee on Diabetes . Use of HbA1c in the diagnosis of diabetes mellitus in the UK. The implementation of World Health Organization guidance 2011. Diabet Med 2012;29:1350-7. http://dx.doi.org/10.1111/j.1464-5491.2012.03762.x.
- John W, Hillson R, Alberti S. Use of haemoglobin A1c (HbA1c) in the diagnosis of diabetes mellitus. The implementation of World Health Organisation (WHO) guidance 2011. Pract Diabetes 2012;29:12-12a. http://dx.doi.org/10.1002/pdi.1648.
- Gray LJ, Taub NA, Khunti K, Gardiner E, Hiles S, Webb DR, et al. The Leicester risk assessment score for detecting undiagnosed type 2 diabetes and impaired glucose regulation for use in a multiethnic UK setting. Diabet Med 2010;27:887-95. http://dx.doi.org/10.1111/j.1464-5491.2010.03037.x.
- Echouffo-Tcheugui JB, Simmons RK, Williams KM, Barling RS, Prevost AT, Kinmonth AL, et al. The ADDITION-Cambridge trial protocol: a cluster – randomised controlled trial of screening for type 2 diabetes and intensive treatment for screen-detected patients. BMC Public Health 2009;9. http://dx.doi.org/10.1186/1471-2458-9-136.
- Definition and Diagnosis of Diabetes Mellitus and Intermediate Hyperglycaemia: Report of a WHO/IDF consultation. Geneva: World Health Organization; 2006.
- Chatterjee R, Narayan KM, Lipscomb J, Jackson SL, Long Q, Zhu M, et al. Screening for diabetes and prediabetes should be cost-saving in patients at high risk. Diabetes Care 2013;36:1981-7. http://dx.doi.org/10.2337/dc12-1752.
- Economic Modelling for Vascular Checks. London: Department of Health; 2008.
- Curtis L. Unit Costs of Health and Social Care 2012. Canterbury: Personal Social Services Research Unit (PSSRU), University of Kent; 2012.
- Goyder E, Wild S, Fischbacher C, Carlisle J, Peters J. Evaluating the impact of a national pilot screening programme for type 2 diabetes in deprived areas of England. Fam Pract 2008;25:370-5. http://dx.doi.org/10.1093/fampra/cmn054.
- van’t Riet E, Alssema M, Rijkelijkhuizen J. Relationship between A1c and glucose levels in the general Dutch population. Diabetes Care 2010;33:61-6. http://dx.doi.org/10.2337/dc09-0677.
- Tuomilehto J, Lindström J, Eriksson J, Valle T, Hämäläinen H, Ilanne-Parikka P, et al. Prevention of type 2 diabetes mellitus by changes in lifestyle among subjects with impaired glucose tolerance. N Engl J Med 2001;344:1343-50. http://dx.doi.org/10.1056/NEJM200105033441801.
- Knowler WC, Barrett-Connor E, Fowler SE, Hamman RF, Lachin JM, Walker EA, et al. Reduction in the incidence of type 2 diabetes with lifestyle intervention or metformin. N Engl J Med 2002;346:393-40. http://dx.doi.org/10.1056/NEJMoa012512.
- Unwin N, Shaw J, Zimmet P, Alberti K. Impaired glucose tolerance and impaired fasting glycaemia: the current status on definition and intervention. Diabet Med 2002;19:708-23. http://dx.doi.org/10.1046/j.1464-5491.2002.00835.x.
- Morris DH, Khunti K, Achana F, Srinivasan B, Gray LJ, Davies MJ, et al. Progression rates from HbA1c 6.0–6.4% and other prediabetes definitions to type 2 diabetes: a meta-analysis. Diabetologia 2013;56:1489-93. http://dx.doi.org/10.1007/s00125-013-2902-4.
- Law LS, Tso AW, Tam S, Wat NM, Cheung BM, Lam KS. Haemoglobin A1c is superior to fasting glucose in predicting the incidence of diabetes over 8 years among Chinese. Diabetes Res Clin Pract 2011;91:e53-6. http://dx.doi.org/10.1016/j.diabres.2010.11.014.
- Valdes S, Botas P, Delgado E, Alvarez F, Diaz-Cadorniga F. HbA(1c) in the prediction of type 2 diabetes compared with fasting and 2-h post-challenge plasma glucose: the Asturias study (1998–2005). Diabetes Metab 2011;37:27-32. http://dx.doi.org/10.1016/j.diabet.2010.07.002.
- Sato KK, Hayashi T, Harita N, Yoneda T, Nakamura Y, Endo G, et al. Combined measurement of fasting plasma glucose and A1C is effective for the prediction of type 2 diabetes: the Kansai Healthcare Study. Diabetes Care 2009;32:644-6. http://dx.doi.org/10.2337/dc08-1631.
- Ko GT, Chan JC, Tsang LW, Cockram CS. Combined use of fasting plasma glucose and HbA1c predicts the progression to diabetes in Chinese subjects. Diabetes Care 2000;23:1770-3. http://dx.doi.org/10.2337/diacare.23.12.1770.
- Norberg M, Eriksson JW, Lindahl B, Andersson C, Rolandsson O, Stenlund H, et al. A combination of HbA1c, fasting glucose and BMI is effective in screening for individuals at risk of future type 2 diabetes: OGTT is not needed. J Intern Med 2006;260:263-71. http://dx.doi.org/10.1111/j.1365-2796.2006.01689.x.
- Rasmussen SS, Glumer C, Sandbaek A, Lauritzen T, Borch-Johnsen K. Determinants of progression from impaired fasting glucose and impaired glucose tolerance to diabetes in a high-risk screened population: 3 year follow-up in the ADDITION study, Denmark. Diabetologia 2008;51:249-57. http://dx.doi.org/10.1007/s00125-007-0893-8.
- Selvin E, Steffes MW, Gregg E, Brancati FL, Coresh J. Performance of A1C for the classification and prediction of diabetes. Diabetes Care 2011;34:84-9. http://dx.doi.org/10.2337/dc10-1235.
- Takahashi O, Farmer AJ, Shimbo T, Fukui T, Glasziou PP. HbA1c to detect diabetes mellitus in healthy adults: when should we recheck?. Diabetes Care 2010;33:2016-17. http://dx.doi.org/10.2337/dc10-0588.
- Wang W, Lee ET, Howard BV, Fabsitz RR, Devereux RB, Welty TK. Fasting plasma glucose and hemoglobin A1c in identifying and predicting diabetes: the strong heart study. Diabetes Care 2011;34:363-8. http://dx.doi.org/10.2337/dc10-1680.
- Stevens RJ, Kothari V, Adler AI, Stratton IM, Holman RR. The UKPDS risk engine: a model for the risk of coronary heart disease in type II diabetes (UKPDS 56). Clin Sci 2001;101:671-9. http://dx.doi.org/10.1042/CS20000335.
- Kothari V, Stevens RJ, Adler AI, Stratton IM, Manley SE, Neil HA, et al. Risk of stroke in type 2 diabetes estimated by the UK Prospective Diabetes Study risk engine (UKPDS 60). Stroke 2002;33:1776-81. http://dx.doi.org/10.1161/01.STR.0000020091.07144.C7.
- Kim J, Stevens R, Holman R. FPG and HbA1c are independent risk factors for microvascular but not macrovascular complications in newly diagnosed type 2 diabetes. Diabetologia 2005;48.
- Selvin E, Steffes MW, Zhu H, Matsushita K, Wagenknecht L, Pankow J, et al. Glycated hemoglobin, diabetes, and cardiovascular risk in nondiabetic adults. N Engl J Med 2010;362:800-11. http://dx.doi.org/10.1056/NEJMoa0908359.
- Lindström J, Peltonen M, Eriksson JG, Ilanne-Parikka P, Aunola S, Keinanen-Kiukaanniemi S, et al. Improved lifestyle and decreased diabetes risk over 13 years: long-term follow-up of the randomised Finnish Diabetes Prevention Study (DPS). Diabetologia 2013;56:284-93. http://dx.doi.org/10.1007/s00125-012-2752-5.
- Lindström J, Ilanne-Parikka P, Peltonen M, Aunola S, Eriksson JG, Hemi K, et al. Sustained reduction in the incidence of type 2 diabetes by lifestyle intervention: follow-up of the Finnish Diabetes Prevention Study. Lancet 2006;368:1673-9. http://dx.doi.org/10.1016/S0140-6736(06)69701-8.
- Rahman M, Simmons RK, Hennings SH, Wareham NJ, Griffin SJ. How much does screening bring forward the diagnosis of type 2 diabetes and reduce complications? Twelve year follow-up of the Ely cohort. Diabetologia 2012;55:1651-9. http://dx.doi.org/10.1007/s00125-011-2441-9.
- Davies MJ, Heller S, Skinner TC, Campbell MJ, Carey ME, Cradock S, et al. Effectiveness of the diabetes education and self management for ongoing and newly diagnosed (DESMOND) programme for people with newly diagnosed type 2 diabetes: cluster randomised controlled trial. BMJ 2008;336:491-5. http://dx.doi.org/10.1136/bmj.39474.922025.BE.
- National Institute for Health and Care Excellence (NICE) . Hypertension. The Clinical Management of Primary Hypertension in Adults 2011.
- Type 2 Diabetes: National Clinical Guideline for Management in Primary and Secondary Care (Update). London: Royal College of Physicians; 2008.
- Griffin SJ, Borch-Johnsen K, Davies MJ, Khunti K, Rutten GE, Sandbæk A, et al. Effect of early intensive multifactorial therapy on 5-year cardiovascular outcomes in individuals with type 2 diabetes detected by screening (ADDITION-Europe): a cluster-randomised trial. Lancet 2012;378:156-67. http://dx.doi.org/10.1016/S0140-6736(11)60698-3.
- Webb DR, Khunti K, Gray LJ, Srinivasan BT, Farooqi A, Wareham N, et al. Intensive multifactorial intervention improves modelled coronary heart disease risk in screen-detected type 2 diabetes mellitus: a cluster randomized controlled trial. Diabet Med 2012;29:531-40. http://dx.doi.org/10.1111/j.1464-5491.2011.03441.x.
- The DECODE study group. European Diabetes Epidemiology Group. Diabetes epidemiology: collaborative analysis of diagnostic criteria in Europe . Glucose tolerance and mortality: comparison of WHO and American Diabetes Association diagnostic criteria. Lancet 1999;354:617-21. http://dx.doi.org/10.1016/S0140-6736(98)12131-1.
- Hamman RF, Wing RR, Edelstein SL, Lachin JM, Bray GA, Delahanty L, et al. Effect of weight loss with lifestyle intervention on risk of diabetes. Diabetes Care 2006;29:2102-7. http://dx.doi.org/10.2337/dc06-0560.
- Stevens RJ, Coleman RL, Adler AI, Stratton IM, Matthews DR, Holman RR. Risk factors for myocardial infarction case fatality and stroke case fatality in type 2 diabetes: UKPDS 66. Diabetes Care 2004;27:201-7. http://dx.doi.org/10.2337/diacare.27.1.201.
- Clarke PM, Gray AM, Briggs A, Farmer AJ, Fenn P, Stevens RJ, et al. A model to estimate the lifetime health outcomes of patients with type 2 diabetes: the United Kingdom Prospective Diabetes Study (UKPDS) Outcomes Model (UKPDS no. 68). Diabetologia 2004;47:1747-59. http://dx.doi.org/10.1007/s00125-004-1527-z.
- Clarke P, Gray A, Holman R. Estimating utility values for health states of type 2 diabetic patients using the EQ-5D (UKPDS 62). Med Decis Making 2002;22:340-9. http://dx.doi.org/10.1177/027298902400448902.
- Coffey JT, Brandle M, Zhou H, Marriott D, Burke R, Tabaei BP, et al. Valuing health-related quality of life in diabetes. Diabetes Care 2002;25:2238-43. http://dx.doi.org/10.2337/diacare.25.12.2238.
- Gillett M, Dallosso HM, Dixon S, Brennan A, Carey ME, Campbell MJ, et al. Delivering the diabetes education and self management for ongoing and newly diagnosed (DESMOND) programme for people with newly diagnosed type 2 diabetes: cost effectiveness analysis. BMJ 2010;341. http://dx.doi.org/10.1136/bmj.c4093.
- Clarke P, Gray A, Legood R, Briggs A, Holman R. The impact of diabetes-related complications on healthcare costs: results from the United Kingdom Prospective Diabetes Study (UKPDS Study No. 65). Diabet Med 2003;20:442-50. http://dx.doi.org/10.1046/j.1464-5491.2003.00972.x.
- Chambers M, Hutton J, Gladman J. Cost-effectiveness analysis of antiplatelet therapy in the prevention of recurrent stroke in the UK aspirin, dipyridamole and aspirin-dipyridamole. Pharmacoeconomics 1999;16:577-93. http://dx.doi.org/10.2165/00019053-199916050-00013.
- Cost-Effectiveness Of Transplantation. London: UK Transplant; 2007.
- Gordois A, Scuffham P, Shearer A, Oglesby A, Tobian JA. The health care costs of diabetic peripheral neuropathy in the US. Diabetes Care 2003;26:1790-5. http://dx.doi.org/10.2337/diacare.26.6.1790.
- Palmer AJ, Weiss C, Sendi PP, Neeser K, Brandt A, Singh G, et al. The cost-effectiveness of different management strategies for type I diabetes: a Swiss perspective. Diabetologia 2000;43:13-26. http://dx.doi.org/10.1007/s001250050003.
- Heaton A, Martin S, Brelje T. The economic effect of hypoglycemia in a health plan. Manag Care Interface 2003;16:23-7.
- Recommendations for Developing a Cost-Effective National Screening Strategy for Diabetic Retinopathy in the United Kingdom. London: UK National Screening Committee; 2013.
- O’Hagan A, Stevenson M, Madan J. Monte Carlo probabilistic sensitivity analysis for patient level simulation models: efficient estimation of mean and variance using ANOVA. Health Econ 2007;16:1009-23. http://dx.doi.org/10.1002/hec.1199.
- Christensen DL, Witte DR, Kaduka L, Jørgensen ME, Borch-Johnsen K, Mohan V, et al. Moving to an A1C-based diagnosis of diabetes has a different impact on prevalence in different ethnic groups. Diabetes Care 2010;33. http://dx.doi.org/10.2337/dc09-1843.
- Methods for the Development of NICE Public Health Guidance (Third Edition). London: NICE; 2012.
- NHS Health Checks Data: Number of Eligible People that Have Been Offered and Received NHS Health Checks. London: NHS England; 2013.
- Schindhelm R, Lenters-Westra E, Slingerland R. Glycated haemoglobin A(1c) (HbA(1c)) in the diagnosis of diabetes mellitus: don’t forget the performance of the HbA(1c) assay. Diabet Med 2010;27:1214-15. http://dx.doi.org/10.1111/j.1464-5491.2010.03088.x.
- Kilpatrick E, Winocour P. ABCD position statement on haemoglobin A1c for the diagnosis of diabetes. Pract Diabetes Int 2010;27:306-10. http://dx.doi.org/10.1002/pdi.1508.
- Faerch K, Witte DR, Tabak A, Perreault L, Herder C, Brunner EJ, et al. Trajectories of cardiometabolic risk factors before diagnosis of three subtypes of type 2 diabetes: a post-hoc analysis of the longitudinal Whitehall II cohort study. Lancet Diabetes Endocrinol 2013;1:43-51. http://dx.doi.org/10.1016/S2213-8587(13)70008-1.
- Bennett CM, Guo M, Dharmage SC. HbA(1c) as a screening tool for detection of type 2 diabetes: a systematic review. Diabet Med 2007;24:333-43. http://dx.doi.org/10.1111/j.1464-5491.2007.02106.x.
- Sacks DB. A1C versus glucose testing: a comparison. Diabetes Care 2011;34:518-23. http://dx.doi.org/10.2337/dc10-1546.
Appendix 1 Residual concerns about reliability of glycated haemoglobin and fasting plasma glucose tests
Glycated haemoglobin: limitations and practical use
The standardisation of HbA1c testing has much improved over recent years. Even so, recent data from an external Quality Assessment sample found a 4.3% coefficient of variation between laboratories, with results varying from 40 to 60 mmol/mol, and that the analytical variation for HbA1c, though reducing, was still greater than for FPG. 17
The principal remaining concerns are around:14
-
other conditions, such as haemoglobinopathy, that affect HbA1c levels
-
a growing body of evidence that some ethnic groups have naturally higher HbA1c levels (although the clinical significance of this is not known)
-
the effect of ageing on HbA1c levels. 73
Fasting plasma glucose: limitations and practical use
Glucose testing using a FPG test is currently still subject to less analytical (laboratory) variation than HbA1c testing. However, FPG testing suffers from much greater non-laboratory variation (biological variation and preanalytical variation). 74
Intra-individual biological variation has been reported to be between 5.7% and 8.3%, with inter-individual biological variation up to 12.5%. 74
As stated in Chapter 2, Determining diagnostic outcome of individuals in the Leicester Ethnic Atherosclerosis and Diabetes Risk data set, where the result of the first blood test is below the cut-off for diabetes but in the range considered HRD, there is not a requirement to carry out a second test.
For FPG in particular, this raises some concern about reliability of a HRD label in such cases, especially given the high biological variation and problems with stability of glucose levels within clinical practice. It is often argued that, even if such individuals test ‘false-positive’ based on a single FPG test, they will still obtain a degree of benefit from lifestyle intervention. This argument may not be economically sound, as the benefits of an intensive lifestyle intervention in lower risk patients may not justify the cost.
Ideal methods to avoid error before testing by stabilising glucose, such as placing tubes in ice water immediately after collection and/or separating cells from plasma within minutes, are impractical in a clinical setting. Across the UK, practices probably range from samples being kept in a fridge to being left on a shelf until collected and transported to a laboratory around 1pm. As glucose levels decrease in test tubes by 5–7% per hour because of glycolysis,74 a sample with a true blood glucose value of 126 mg/dl would fall to 111 mg/dl and 98 mg/dl after 2 hours and 4 hours, respectively, at room temperature. This is shown in Figure 16, alongside an alternative scenario where decay occurs at half the rate, that is 3% per hour.
FIGURE 16.
Decline in FPG over time under alternative decay assumptions.

The duration and implications of this overall lead time between a FPG sample being drawn and being processed at a laboratory are unclear. Qualitatively at least, we understand that fairly similar practices probably occurred in epidemiological and intervention studies from which the evidence for the modelling is sourced, although whether or not this was to the same extent is not known to us. It does, however, suggest that, where the lead time is long, some individuals may not be treated for diabetes when they should be.
Consistency of measurement of fasting plasma glucose between screening and prognostic research studies and UK clinical practice
For the modelling, calculation of the risks of CVD and other complications requires risk equations that include non-glycaemic risk factors and HbA1c. For FPG-based screening, this dictates that we use individuals’ corresponding HbA1c levels for the equations. Ideally, there would be consistency between the relationship between FPG and HbA1c in the screening outcome studies, the prognostic studies and what typically would be observed across clinical practice in the UK.
Screening outcome research studies of relevance to modelling
In the LEADER study, plasma glucose samples were kept at 4–8 °C for up to 2 hours before processing.
Glossary
- Glucose tolerance status
- This term is used to refer to the classification of an individual as having diabetes, high-risk of diabetes or normal glucose tolerance.
- High risk of diabetes
- Screening for diabetes also identifies individuals at ‘high risk of diabetes’ who need to form part of the economic evaluation of the two tests. This term, together with the use elsewhere of the terms ‘prediabetes’, ‘impaired glucose regulation’ and ‘non-diabetic hyperglycaemia’, largely describes similar groups of individuals, that is those with raised glucose levels above the normal range but below the threshold for diabetes. All of these terms rely on somewhat arbitrary levels of glucose or HbA1c. The terms ‘prediabetes’, ‘impaired glucose regulation’ and ‘non-diabetic hyperglycaemia’ label people as having a condition, which may be helpful in promoting lifestyle changes in order to reduce an individual’s risk of developing type 2 diabetes. For the purpose of this document, the term ‘high risk of diabetes’ has been chosen and abbreviated to ‘HRD’ as repeated use of the full term would make for clumsy reading. We do not use the other three terms except in reference to any previous studies that used them.
List of abbreviations
- 2hPG
- 2-hour plasma glucose
- ADA
- American Diabetes Association
- ADDITION
- Anglo-Danish–Dutch Study of Intensive Treatment In People with Screen Detected Diabetes in Primary Care
- ARIC
- Atherosclerosis in Communities
- BME
- black and minority ethnic
- BMI
- body mass index
- CHD
- coronary heart disease
- CHF
- congestive heart failure
- CI
- confidence interval
- CVD
- cardiovascular disease
- DPP
- Diabetes Prevention Programme
- DPS
- Diabetes Prevention Study
- FPG
- fasting plasma glucose
- GP
- general practice
- HbA1c
- glycated haemoglobin
- HDL
- high-density lipoprotein
- HRD
- high risk of diabetes
- ICER
- incremental cost-effectiveness ratio
- IFG
- impaired fasting glucose
- IGT
- impaired glucose tolerance
- LEADER
- Leicester Ethnic Atherosclerosis and Diabetes Risk
- LPDS
- Leicester Practice Database Score
- NGT
- normal glucose tolerance
- NICE
- National Institute for Health and Care Excellence
- NMB
- net monetary benefit
- OGTT
- oral glucose tolerance test
- POC
- point of care
- PSA
- probabilistic sensitivity analysis
- QALY
- quality-adjusted life-year
- RCG
- random capillary glucose
- SBP
- systolic blood pressure
- UEA-IFG
- University of East Anglia-Impaired Fasting Glucose
- UKPDS
- UK Prospective Diabetes Study
- WHO
- World Health Organization