Notes
Article history
The research reported in this issue of the journal was funded by the HTA programme as project number 11/46/23. The contractual start date was in January 2013. The report detailing the set up phase and initial outcomes began editorial review in June 2015 and was accepted for publication in July 2015. The authors have been wholly responsible for all data collection, analysis and interpretation, and for writing up their work. The HTA editors and production house have tried to ensure the accuracy of the authors’ report and would like to thank the reviewers for their constructive comments on the final report document. However, they do not accept liability for damages or losses arising from material published in this report. Should the study progress further, the full report will be published in the HTA journal.
Declared competing interests of authors
none
Permissions
Copyright statement
© Queen’s Printer and Controller of HMSO 2015. This work was produced by Simpson et al. under the terms of a commissioning contract issued by the Secretary of State for Health. This issue may be freely reproduced for the purposes of private research and study and extracts (or indeed, the full report) may be included in professional journals provided that suitable acknowledgement is made and the reproduction is not associated with any form of advertising. Applications for commercial reproduction should be addressed to: NIHR Journals Library, National Institute for Health Research, Evaluation, Trials and Studies Coordinating Centre, Alpha House, University of Southampton Science Park, Southampton SO16 7NS, UK.
Chapter 1 Background
Description of the health problem
During the 20th and 21st centuries, there have been four pandemics (global epidemics) of influenza (1918–19, 1957–8, 1968–9, 2009–10) producing very large numbers of clinical cases and, in the case of the first three, large numbers of deaths (with an estimated 20–40 million, 1 million, 1 million and 1.25 million deaths, respectively). This was owing to the population having little immunity to the novel influenza viruses involved (H1N1, H2N2, H3N2 and H1N1, respectively). The lack of herd immunity to the novel influenza viruses implicated (i.e. H1N1, H2N2 and H3N2) is believed to have been a key factor contributing to these very high numbers of deaths. 1 The influenza A subtype, H1N1 virus, which emerged in Mexico in March 2009, also produced a very large number of clinical cases, with one-third of the population being found to be infected by early 2010. 2 Previous vaccination to seasonal influenza vaccine conferred little or no cross-reactivity antibody responses. 3 Furthermore, persons aged < 30 years were found to have little evidence of cross-reactivity to the pandemic virus, with a proportion of older adults having pre-existing antibodies.
After the introduction of any new pandemic influenza, as well as front-line health-care workers, any new vaccination will be targeted at those who are considered to be at increased risk of serious illness or death from influenza infection, for example (1) those with any underlying medical conditions (e.g. chronic renal disease, immunosuppression resulting from disease or treatment, chronic heart disease, chronic respiratory disease); (2) those who may lack herd immunity (cross-reactivity) from exposure to previous pandemics or vaccinations; and (3) novel risk groups that are uniquely at risk because of a tropism exhibited by the virus. These national vaccination strategies have been shown to represent a potentially important approach to reduce both influenza-related illness and death, hence the considerable investment in this approach in many parts of the world.
Existing evidence
In the 2009 H1N1 pandemic, the earliest estimate of vaccine effectiveness (VE) in the UK was published in July 2010,4 4 months after the last case of influenza in that season (18 March 2010 in Scotland). 5 Further VE estimates were published in 2011. 6–8 Serology and data gathered in Scotland during 2009–10 also found that rates of immunisation for those most susceptible to serious influenza-like illness (ILI) were less than optimal. For instance, just one in four patients aged 5–50 years with serious underlying illnesses received monovalent A/H1N1 vaccine and an estimated 10,000 patients aged > 75 years with no underlying disease were issued the A/H1N1 vaccine in Scotland. There are multiple factors that might have led to poorer uptake among younger people in at-risk groups and these include having fewer comorbidities and concerns over VE or safety,9 and poorly targeted patient reminder/recall systems. 10 Furthermore, after the first round of vaccinations, the most socioeconomically deprived patients in Scotland were also found to be more susceptible and were no more likely to receive the vaccine than the most affluent people. 2 This increased susceptibility, however, was not explained by differences in local population density or between urban and rural areas or by differences in ethnicity.
Chapter 2 Research questions
Building on prior work,4,6 we aimed to enhance a previously used data linkage approach, which allowed the effectiveness of the 2009–10 H1N1 influenza pandemic vaccine to be determined. We wished to create a pandemic influenza reporting platform (Figure 1). The platform will allow rapid extraction and linkage of general practice, clinical, prescribing and vaccination data from sentinel general practices to virology information [real-time polymerase chain reaction (RT-PCR) swab and serology] using NHS Scotland’s Community Health Index (CHI) number, a unique patient identifier.
FIGURE 1.
Flow diagram for the Early estimation of pandemic influenza Antiviral and Vaccine Effectiveness project. HPS, Health Protection Scotland; ISD, Information Services Division.

We anticipate that the platform will help to rapidly determine, once a new pandemic is under way, the:
-
uptake and effectiveness of any new pandemic vaccine (if) available
-
analysis of any protective effect conferred by antivirals
-
clinical attack rate of pandemic influenza in general practice,
and, with stored serological information, determine:
-
the existence of any protective effect provided by previous exposure to and vaccination from A/H1N1 pandemic or seasonal influenza/identification of susceptible groups.
Chapter 3 Methods
This study involves a quantitative evaluation of aspects of any future vaccination or antiviral programme (implemented through general practice) to prevent pandemic influenza. Data extraction and linkage systems, and database analyses will help estimate VE and antiviral effectiveness. Data generated will also allow for common reports to be developed for UK and European colleagues (e.g. similar to Royal College of General Practitioners’ ‘flu spotter’ practices). 11
Pre-pandemic preparedness phase
This pre-pandemic phase of this project aimed to set up and test the data extraction systems, and create a repository of serology samples from patients registered with practices (see Figure 1).
Primary care data set
The Practice Team Information (PTI) network of general practices covers a 6% representative sample of the Scottish population (n = 300,000). 12 These general practices are given financial incentives by NHS National Services Scotland to record and code additional data electronically, over and above that routinely recorded for clinical care or as part of the PTI project (e.g. age, comorbidity, out-of-hours contacts and socioeconomic status), including influenza vaccination status. 13 Data from general practices within Scotland are of high quality and useful for epidemiological research. 14 The completeness of capture of contacts and accuracy of clinical event coding in general practice (using Read codes) has been found to be > 91%. 15 Furthermore, when influenza vaccination is provided as part of the general medical services contract, any variation between expected and reported vaccination uptake by practices is likely to be assessed by Health Board quality assurance and appraisal teams. 16
At the time of this report, 41 of the PTI practices had expressed an interest in participating, and 40 had completed all of the necessary University of Edinburgh contractual paperwork to participate in the study. All 41 practices had installed the necessary software for secure extract and transfer of data (Figure 2). This secure extraction system is supported by a Trusted Third Party, Albasoft Ltd, Inverness, UK. From these general practice data a database of information is generated (Table 1). These data have been tested for the presence of the necessary data fields for linkage and analysis.
FIGURE 2.
Secure data transfer from general practice. HPS, Health Protection Scotland; ID, identifier; ISD, Information Services Division; N3, the NHS national broadband network linking hospitals, medical centres and GPs; SMR01, Scottish Morbidity Record (hospital/mortality).

Variable | Data items | Data source |
---|---|---|
Age, sex | SMR01, general practice | |
Scottish Index of Multiple Deprivation/rural urbana | General practice | |
Hospital admission type Emergency/routine admission Date of first admission Length of stay |
SMR01 | |
Clinical condition codingb | Influenza vaccination | General practice |
Clinical condition codingb | Pneumococcal vaccination | General practice |
Prescription | Antiviral prescriptions | General practice |
Prescription | Asthma and COPD-related prescriptions | General practice |
Clinical condition codingb | Clinical at-risk groups (chronic respiratory disease, chronic heart disease, chronic kidney disease, chronic liver disease, chronic neurological disease, immunosuppression, diabetes, pregnancy) | General practice |
Diagnosis fields | Charlson comorbidities | SMR01 |
Palliative care field | General practice | |
Prescription, clinical attendance | Number of previous general practice consultations, prescribed drugs | General practice |
Clinical condition codingb | Smoking,a exercise statusa | General practice |
Number of previous hospital admissions | SMR01 | |
Clinical condition codingb | Pregnancy | General practice |
QOF exception reported (patient unsuitable, etc.) | General practice | |
Clinical condition codingb | Home oxygen | General practice |
Clinical condition coding,b diagnosis | Trauma | General practice, SMR01 |
Clinical condition coding,b diagnosis | ILI | General practice, SMR01, death records |
Clinical condition coding,b diagnosis | Asthma, and COPD symptoms and exacerbations | General practice, SMR01 |
Clinical condition coding,b diagnosis | Appendicitis | General practice, SMR01 |
Clinical condition coding,b diagnosis | Hernia | General practice, SMR01 |
RT-PCR swab results | ECOSS virology database |
The identifier CHI from general practice data was one-way encrypted (the encryption cannot be reversed) using pseudonymisation software (OpenPseudonymiser, version 0.9.7, University of Nottingham, Nottingham, UK). Once all practices have completed the necessary paperwork, the CHI will be used deterministically to link general practice data to other data sources, which will also have used the pseudonymisation software to encrypt CHI. The CHI data set lists everyone who is registered with a general practitioner or who is eligible for NHS screening services, and forms a unique identifier for NHS use. Since 2006 it has been mandated for use in all NHS Scotland communications and contacts, obviating the use of other personal identifiers, such as names and addresses. CHI is available on almost all data fields used in this project (> 97%). 17 Deterministic linkage using CHI has been carried out previously on data from 2000 to 2008 (without any issues),18 and is now routinely used by Health Protection Scotland (HPS) for data set linkages required for seasonal influenza monitoring.
Real-time polymerase chain reaction test and serology data set
General practitioners participating in this study are also involved in the Scottish Immunisation Programme Pandemic Influenza Primary care Reporting scheme. 19 Practices are encouraged to obtain nasal/throat swabs from patients of all ages who have symptoms that are suggestive of influenza. Each general practice is requested to submit five swab samples per week to the West of Scotland Specialist Virology Centre (WoSSVC), Glasgow, UK, for RT-PCR testing for a range of respiratory pathogens on any patient presenting for consultation in the practice with influenza-like symptoms. Sampling is carried out on all age groups independent of whether the patient has or has not been vaccinated. These data, along with results from swabbing carried out in primary and secondary care for routine diagnostic purposes in symptomatic patients outside the sentinel scheme, are entered into the Electronic Communication of Surveillance in Scotland database held by HPS. 20 These data are linked to general practice data to determine vaccination status, patient characteristics and ILIs that present to general practice (see Figure 2).
Secondary care and mortality data set
The Scottish Morbidity Record (SMR) database has information on all inpatient hospitalisations within Scotland (as well as information on death certification linked from National Records Scotland21) from 1981. Hospital data are reliable, with completeness and accuracy rates exceeding 90%. 22 Using the data extracted from participating general practices, a deterministic linkage can carried out using the CHI number (see Figure 2).
Serology data set
As part of the Early estimation of pandemic influenza Antiviral and Vaccine Effectiveness (EAVE) project, 2000 biochemistry samples are to be collected and stored in readiness for a future pandemic. Biochemistry laboratories serving participating general practices will set-aside serology samples for transfer to the WoSSVC laboratory for storage. Four biochemistry laboratories serving NHS Lothian, and NHS Greater Glasgow and Clyde Health Board areas [i.e. Glasgow Royal Infirmary, Western Infirmary, Southern General Hospital and the Regional Virus Laboratory Specialist Virology Centre (Edinburgh)] have been recruited by the University of Edinburgh, HPS and the WoSSVC laboratory. Serology samples from participating general practices were to be set-aside manually by laboratory staff and sent to the WoSSVC laboratory. During this project, however, new automated systems for sample handling were introduced at biochemistry laboratories, meaning straightforward manual set-aside by the laboratory staff was no longer feasible. We therefore allocated resource to create a Medical Laboratory Assistant (MLA) post within the WoSSVC laboratory, devoted to the manual collection and cataloguing of serology samples by visiting the four biochemistry laboratories and seeking out samples from EAVE practices. To ensure delivery of the EAVE project objectives, a special provision was entered into a service-level agreement between HPS (NHS National Services Scotland) and the WoSSVC (NHS Greater Glasgow and Clyde).
Secure data transfer systems have been implemented to allow linkage of patient vaccination status and other characteristics (e.g. age band, sex, comorbidity, socioeconomic status) to serology samples using deterministic data linkage (Figure 3). This is necessary to ensure that samples collected have representation across age, sex and comorbidity groups. Once the serology samples have been collated, patient vaccination status and other characteristic data will be transferred to, and securely held by, HPS. These data will represent the vaccination history and patient characteristics at, or near the time of, serology sample storage.
FIGURE 3.
Secure data/serology transfer from general practices and biochemistry units to WoSSVC for storage. ID, identifier.
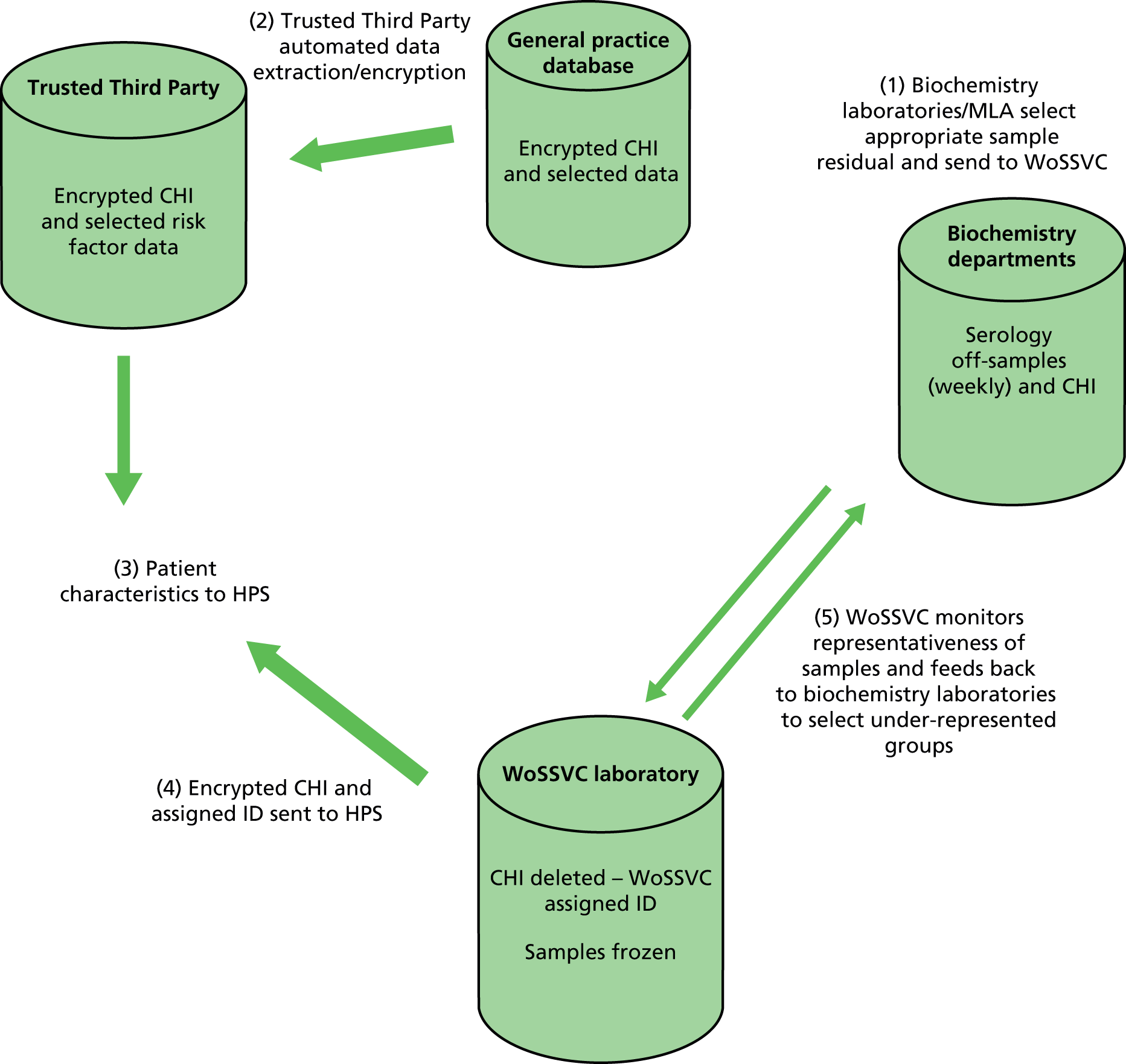
Once sampling has reached the total required, replacement of patients’ samples who have died or deregistered from participating practices will be carried out. The secure data transfer system for the general practice data required for linkage to serology has been successful tested, that is data from primary care practices have been transferred to HPS using NHS secure file transfer protocols.
Power calculations
A power calculation was carried out to determine the numbers needed to detect a difference in the relative risk of death between the vaccinated and unvaccinated groups. The power calculations are based on (unpublished) data available at HPS from a subset of the practices recruited. With 41 practices, a combined list size of approximately 300,000 patients is anticipated: 6% (n = 18,000) of patients will be aged 0–4 years, 18% (n = 54,000) aged > 65 years, and 17% of those aged < 65 years in an at-risk category on the basis of existing illnesses (n = 43,000). During the 2009–10 influenza pandemic, 15% of the population were vaccinated against A/H1N1 influenza and this figure is used throughout the proposed study; larger values to 20–25% will be associated with an increased power or smaller VEs detectable, and smaller values with a reduced power or the ability to detect only large VEs.
In a normal influenza season of 4 months, we report about 0.4–0.6% of the HPS surveillance cohort with a virological swab during the influenza season. This would translate to around 1300–1900 swabs in the EAVE cohort. During a pandemic we anticipate a greater frequency of swabbing, possibly double, and so base our calculations on a conservative availability of 1500 swabs. Assuming that 20% of those unvaccinated test positive for influenza (based on seasonal influenza figures from HPS) then we anticipate a 90% power to detect a VE of at least 43% (see Table 2). In a pandemic we anticipate a higher percentage positivity among the unvaccinated, and if this was 40% then VEs in excess of 28% would be detected. With increased swabbing in a pandemic, say at 3000 swabs, then VEs in excess of 20% would be detected with a 90% power.
Consultation rates for ILIs were around 30–50 per 100,000 per day during the peak of the pandemic. In the best-case scenario, the vaccine is available before the peak of the pandemic, and, in this case, over a 90-day observation period there is at least a 90% power to detect a 10% vaccine effect in the time to the first ILIs consultation from the beginning of the pandemic period (Table 2). If vaccination is available only during the pandemic period then the power will be lower. Assuming a 40% attack rate in the pandemic period then consultation rates will be lower (as some patients have already been exposed) and the average follow-up period lower. There will be at least an 80% power to detect vaccine effects of ≥ 20%.
Outcome | Exposure of interest | Statistical method useda | Effect size for influenza vaccine detectable at 90% power | Effect size for antiviral detectable at 90% power |
---|---|---|---|---|
RT-PCR | Influenza vaccination | Logistic regression | 20–43% | 25–39% |
Primary care, ILI | Influenza vaccination, antivirals | Cox proportional hazards | 10% | 9% |
Hospitalisation, IP | 55% | 48% | ||
Hospitalisation, IPC | 33% | 29% | ||
Hospitalisation, CVD | 36% | 32% | ||
Hospitalisation, all | 11% | 10% | ||
Deaths, IPb | – | – | ||
Deaths, IPCb | – | – | ||
Deaths, IPC plus CVD | 51% | 45% | ||
Deaths, all | 33% | 29% | ||
Hospitalisation, trauma | 26% | 23% | ||
Hospitalisation, appendicitis and herniab | – | – |
The rate of hospitalisations for influenza or pneumonia was 1 per 100,000 per day6 and there will be a 90% power to detect a vaccine effect of 55% in the time to first admission under the best-case scenario. For the combined influenza, pneumonia or chronic obstructive pulmonary disease (COPD) the rate was 2.8 per 100,000 per day;6 under the best-case scenario there is 90% power to detect a VE of 33%, and 66% if the vaccine is available during the pandemic. With an emergency hospitalisation rate of 2.3 per 100,000 per day for cerebrovascular and cardiovascular disease (CVD),6 VEs in excess of 36% can be detected with 90% power under the best-case scenario, whereas only those in excess of 75% can be detected if the vaccine becomes available during the pandemic. The rate for any emergency hospitalisations are much greater at 27.8 per 100,000 per day and the detectable VEs much lower. Under the best-case scenario we calculate a power of 90% to detect a VE in excess of 11%, and this rises to 21% if the vaccine becomes available only during the pandemic. Trauma admissions are used as negative controls and, with a rate of 4.5 per 100,000 per day, there is a power of 90% to detect VEs in excess of 26% under the best-case scenario, and 52% if the vaccine becomes available only during the pandemic.
For investigation of mortality, the rates of most of the end points are too low to be able to detect VEs of any reasonable magnitude. Based on data in the previous pandemic, the mortality rates of death from pneumonia are 0.11, COPD 0.24, CVD 0.78 and all cause 2.72 – all per 100,000 per day. 6 The principal analysis will be based on the combined end point of influenza, pneumonia, COPD or CVD, which has a rate of 1.14 per 100,000 per day; there is 90% power to detect a VE of 51% under the best-case scenario. For all-cause mortality the detectable VE is 33% at 90% power.
For the virological outcome of confirmed influenza, vaccine effects in excess of 50% would be anticipated for a well-matched vaccine and so this cohort should have sufficient power. For the less-specific outcomes of ILI consultations, vaccine effects around 20% are anticipated and, again, the study is adequately powered, even under the second scenario of the vaccine not being available until midway through the pandemic. For the hospitalisation outcomes, a VE of 32% was reported for the 2009 pandemic for hospitalisations for CVD5 and the current study has power to detect this and, also at the same level, for the combined influenza, pneumonia or COPD emergency hospitalisations. There is limited power to detect a reasonable vaccine effect on influenza or pneumonia hospitalisations or death. Detectable VEs are of the order of 33–50% and these are too high for such non-specific outcomes.
The power analysis for antiviral effectiveness is similar to that for vaccine exposure, although is much more speculative in view of the potential uncertainties surrounding the use of antivirals: some will receive antivirals for treatment of symptoms, and others will receive them for prophylactic treatment to prevent symptoms or reduce the symptoms. This should mean that individuals who receive antivirals prior to the event are expected to have fewer adverse outcomes of ILI consultations, hospitalisation and death than those who did not. For the same level of population exposure for the antivirals as vaccine (15%) then the detectable differences will be the same. During the 2009 pandemic the stockpile of antivirals was sufficient for 50% of the population, so we calculate powers assuming that 20% of the population are exposed. The detectable VEs assuming a best-case scenario are presented in Table 2. The detectable VEs will be lower if the use of antivirals is > 20%.
Statistical analysis plan
Vaccine uptake will be modelled through a logistic regression. For the virological test outcome of influenza positivity compared with no influenza among patients who have ILI symptoms, the primary analysis will be through a logistic regression generalised additive model, in which the time during the season is modelled as a spline function. For the consultation, hospitalisation and death outcomes the analysis will be based on a Poisson regression model or equivalent time-dependent Cox model.
Statistical scripts have been created, in R version 2.14.1 (The R Foundation for Statistical Computing, Vienna, Austria), which can calculate odds ratios (ORs: adjusted for age, sex, clinical risk group and deprivation) for differences in proportion of vaccine uptake between different groups of patients (sex, age, Scottish Index of Multiple Deprivation categories and at-risk groups) and for investigating trends in vaccine uptake. Vaccine uptake, and 95% confidence intervals (CIs) based on the Wilson method, will be reported for these broad categories at specific times during the pandemic.
For VE using information from linked virological swab data (a binary event), the logistic regression model will adjust for the effects of sex, age, socioeconomic status (Scottish Index of Multiple Deprivation23) and being in an at-risk morbidity group (the test-negative study design). A spline function for time during the pandemic season will be used to model the background rate of influenza and correct for any potential bias associated with the proportions of test-negative and test-positive patients at different periods during the pandemic. VE will be measured by comparing the results from swabs taken after vaccination among those vaccinated to swabs taken among those unvaccinated at the time the swab is collected. A delay of 14 days after vaccination will be used to establish the protective effect of the influenza vaccine. The adjusted estimate of VE = (1 – OR) × 100, where the OR is derived from the coefficient of vaccine status in the logistic model.
The rate of ILI, acute respiratory disease and other adverse outcomes – including hospitalisations and death – between vaccinated and unvaccinated cases will be ascertained. CIs for the rate ratio (RR) and tests of the differences between two rates will be carried out using the ‘midp method’ in the ‘rateratio’ function and ‘rate2by2.test’ function, respectively, using the ‘epitools’ package in R. 24 For small samples, CIs for the RR will be estimated using the Microsoft Excel® (version 14.5.1) workbook (Microsoft Corporation, Redmond, WA, USA). 25 Illness RRs, that is the ratio of the rate of admission to hospital or general practice consultation in the vaccinated compared with the rate of first admission to hospital or general practice consultation among those who did not receive the vaccine, will be calculated in both the ‘at-risk’ populations, age groups and the general population. This is a direct measure of VE. The unadjusted estimate of VE = (1 – RR) × 100.
Adjusted RRs of VE for prevention of hospitalisation/death/general practice consultation will be derived from time-dependent Cox models or the equivalent Poisson regression models, taking into account the time at risk and the possibility of multiple events (not for death). These models will adjust for sex, age, deprivation and clinical risk group, with vaccination group representing a time-dependent covariate. Individuals will be in the unvaccinated group (and will accumulate time at risk) until 14 days after the receipt of the vaccine and then they will switch to the vaccinated group. Within the Poisson regression models, the calendar time through the season will be modelled using spline functions.
In all models used to estimate the VE, propensity variables associated with the receipt of a vaccination and effect modifiers – such as vaccinations, consultations and hospitalisation in the previous influenza season, urban/rural status, smoking status, Charlson Score and pregnancy – will be used to control for the healthy vaccine effect. 26 This is in addition to the demographic variables, which will always be used.
Binomial analysis and logistic regression will be used to determine differences in seropositivity proportions owing to age, sex, comorbidity and vaccination status. Results will be expressed as the proportion seropositive rate with 95% CIs.
The analysis of antiviral use and the protective effect of antivirals will be analysed using similar approaches. The study cohort will provide information on the prescription of antivirals by general practice, although if there are other major routes of administration of antivirals then this information will not be captured, limiting the analyses that can be carried out here. Our initial plan is to include a binary variable of ever/never exposure to antivirals as an explanatory variable in the VE analyses. For the consultation, hospitalisation and death rates analysis, this antiviral exposure will be a second time-dependent exposure. If the data permit, we will consider using a measure of the volume of exposure, such as the length of the prescription. In models in which the primary aim is to estimate the effectiveness of vaccine, antiviral use will be included as a covariate. In models in which the principal aim is to investigate the effect of antivirals, exposure to the vaccine will be included, if appropriate. For example, antiviral effect may be estimated from a period before the vaccine becomes available and, in such instances, no adjustment needs to be made.
Post pandemic
With the emergence of any new pandemic influenza, the following will be calculated to evaluate any pandemic influenza vaccination programme:
-
From general practice data The vaccination uptake in the relevant ‘at-risk’ populations (patients aged < 65 years with ‘at-risk’ comorbidities and those aged > 65 years) and the general population recorded by general practices.
-
From linkage of general practice and Electronic Communication of Surveillance in Scotland data Influenza positivity from virological swab data in vaccinated and unvaccinated patients stratified by ‘at-risk’ populations, age, sex and socioeconomic status.
-
By combining general practice and SMR01 data Consultation for influenza-related morbidity (e.g. influenza, pneumonia, COPD and cardiac-related consultations) and issue of antiviral therapy from general practice data in vaccinated and unvaccinated patients stratified by ‘at-risk’ populations, age, sex and socioeconomic status. Mortality and influenza-related serious morbidity (e.g. influenza, pneumonia, COPD, and cardiac-related death and hospitalisation) in vaccinated and unvaccinated patients stratified by ‘at-risk’ populations, age, sex and socioeconomic status.
-
Data from general practice and virology data will permit the estimation of the clinical attack rate of pandemic influenza.
-
For patients with stored serology [linked to general practice data held by HPS (see Figure 3) and once a validated haemagglutination inhibition assay has been developed and prior to the introduction of any vaccination], cross-reactivity with previous exposure to A/H1N1 or A/H1N1 vaccination, other pandemic influenza or other seasonal influenza vaccination or exposure will be measured. A seropositive result will be determined using the universally accepted cut-off point of a 1 : 40 dilution for a panel of influenza strains. This will be undertaken by the WoSSVC in Glasgow.
Chapter 4 Ethical and governance processes, and pandemic study site set-up
All necessary approvals have been established in advance of any influenza pandemic. Permissions were obtained from the Privacy Advisory Committee (National Services NHS Scotland) (reference number 25/13), the Multicentre Research Ethics Committee (reference number 12/SS/0201), the National Caldicott Guardian (reference number 25/11/2013), 12 NHS Health Boards and the participating general practices. A poster to be displayed at the participating general practices regarding the collection of serology samples can be found in Appendix 1. Ethics and governance processes took 1 year. On the request of the National Caldicott Guardian, we sought accreditation by Health Improvement Scotland of the EAVE serology collection for storage as a human tissue bank. The necessary approvals were granted (GN13ID441), but this delayed serology collection by 12 months.
An Independent Steering Group was convened to oversee this work and comprised Neil Kelly (Chairperson, General Practitioner), Jonathan Van Tam (Professor of Health Protection), Punam Mangtani (Senior Lecturer in Epidemiology) and Elisabeth Ehrlich (Public and Patient Involvement representative). The Independent Steering Group met once during the study.
Chapter 5 Discussion
We have developed a new sentinel system that is capable of rapidly determining the estimated incidence of pandemic influenza and pandemic influenza vaccine and antiviral uptake and effectiveness in preventing influenza and influenza-related clinical outcomes.
We have all of the required regulatory approvals to allow rapid activation of the sentinel systems in the event of a pandemic. Of the 41 practices expressing an interest in participating, 40 have completed all the necessary paperwork to take part in the reporting platform. The data extraction tool has been installed in these practices. Data extraction and deterministic linkage systems have been tested. Four biochemistry laboratories have been recruited, and systems for serology collection and linkage of samples to general practice data have been put in place.
The process of obtaining research governance, and privacy approvals and other project-related paperwork required substantial project resource and time, and led to significant project delays. At the time of writing this report, serology collection had not begun and six practices had still to complete final paperwork. Serology delays were because of unanticipated regulatory approvals, usually applied for storage of human tissue being requested for our serology samples (serology being considered as not relevant material under the Human Tissue Act). 27 We have also had significant delays caused by biochemistry laboratory automation of serology handling. This required the creation of a new MLA post to help manually collect and catalogue the serology samples. It is anticipated that although these significant delays have ensued, all samples will be collected and linked to general practice data by the end of 2015. Practice recruitment was resource intensive. In particular, regular communication with the practices to ensure completion of the required paperwork (contract with the University of Edinburgh and invoicing) was required.
Similar to other sentinel systems,28 the sentinel system in use in Scotland uses clinician discretion based on specified criteria (and patient permission) in the decision to swab and test for influenza, rather than have a centrally managed process to ensure random sampling of patients for swabbing. This ensures that all available resources are used to maximise the number of eligible patients swabbed by clinicians at sentinel practices. In Scotland, reimbursement is available to practices for five swabs per day, and we therefore acknowledge that some eligible patients may not be swabbed. Any bias from eligible patient not being swabbed is limited by:
-
practices being required to swab the first two symptomatic patients per day, and at least the first two vaccinated patients (to receive reimbursement from HPS) so that not only vaccinated patients with ILI are swabbed
-
the case–control status of the patient not being known to the clinician, and therefore the clinician not being able to systematically swab vaccinated influenza-negative patients (as confirmation of influenza diagnosis can be determined only from the swab submitted for RT-PCR testing)
-
the measurement of incidence of non-influenza acute respiratory infection in vaccinated and unvaccinated groups (to ensure that they are similar in vaccinated and unvaccinated groups)
-
swabbing patterns being analysed to ascertain if systematic swabbing of only certain groups of patients has taken place (e.g. only particular age groups, the vaccinated or influenza-positive patients).
For swabs from symptomatic patients that take place outside the systematically collected sentinel subset (in primary and secondary care settings), we can identify these swabs and carry out subset analyses. In previous seasonal influenza VE analyses, no major bias has been introduced by including these non-sentinel swabs. 18
Maintenance of the project and activation in the event of a pandemic
Once the preparation phase of this project is completed, the project will be put into hibernation until a pandemic occurs. Overall responsibility for the EAVE project will remain with the principal investigator and co-investigators (as long as they remain in post).
Routine maintenance of the pandemic reporting platform will be carried out with the following elements: under a Trusted Third Party Agreement with the University of Edinburgh, Albasoft Ltd will carry out the requisite practice data extractions that are necessary in the event of a pandemic. This will require that the general practice data extraction and transfer (see Figures 2 and 3) will be routinely checked by the Trusted Third Party administering these systems, Albasoft Ltd and HPS. Iterations of data extractions may be required in the case of changes in coding or unforeseen developments in analysis methods.
Serology will be kept in storage until 2017, with a small turnover of samples (n = 200) in seasons 2015–16 and 2016–17. Beyond 2017, the serology storage will require further resources to maintain the freezing of the serology store and a regular refresh to ensure inclusion of serology samples containing antigenically drifted seasonal influenza and corresponding vaccine antibodies. However, under a service-level agreement, HPS and the WoSSVC have responsibility for maintaining the serology store.
The governance and privacy framework for undertaking this research, particularly in relation to secondary uses of data will require triennial review.
Recommendations for further research
The non-randomised nature of observational studies such as this are limited by the extent to which there may be dissimilarities between vaccinated and non-vaccinated persons, in both their likelihood of receiving vaccination, and in their subsequent care and follow-up, leading to overestimated benefits. 26 A suitable instrumental variable for these data should be constructed, validated and then included in the model to remove any effects of hidden bias. Further research should be focused on the development of a valid instrumental variable, for example census location data on influenza vaccine coverage similar to that used by Wong et al. 29
A National Pandemic Flu Service was not used in Scotland for the provision of antivirals (i.e. patients were assessed in primary care, identified as requiring an antiviral and used the normal process of receiving a prescription). Therefore, in the event of a more severe pandemic there may be a need to invoke randomisation as the fairest means to allocate a scare treatment resource, or to spread the risks associated with receiving an intervention that has not been as fully tested as would be the case outwith a pandemic. A coding option to differentiate and account (methodologically) patients who were randomised to intervention or control could be added to the statistical syntax in preparation for this.
Although the relatively modest size of our cohort makes it feasible to evaluate the pandemic influenza vaccine or antiviral in a whole population, analysis of subgroups (in particular older age groups or people with specific comorbidities, e.g. asthma) is likely to result in poorer precision and wide CIs. Work should therefore aim to address this by ensuring increased power to test VE in these subgroups of patients while minimising the effect of bias, such as health-seeking behaviour. This will require an increase in the number of practices participating in the EAVE reporting system. Consideration should also be given to link to other data sources, in particular to test for maternal outcomes in pregnant patients.
Conclusions
The EAVE sentinel surveillance system, which will provide a rapid evaluation of any available influenza vaccination or antiviral and the clinical attack rate, has been set up and is ready to be activated in the event of pandemic. The EAVE serology sample storage linked to general practice data, which aims to predict the attack rate of any new pandemic of influenza, will be completed by the end of 2015.
Acknowledgements
The authors would like to thank staff at Albasoft Ltd, HPS, WoSSVC and the Information Services Division, the general practices and biochemistry laboratories contributing data or serology to the study, and members of the Independent Steering Committee overseeing this work.
Contribution of authors
Dr Colin R Simpson (Reader in Population Health Sciences and Informatics) was the principal investigator and led the writing of this report.
Dr Nazir Lone (Clinical Fellow, Intensive Care), Dr Jim McMenamin (Consultant Epidemiologist), Dr Rory Gunson, Professor Chris Robertson (Professor of Statistics), Professor Lewis D Ritchie (Professor of Primary Care) and Professor Aziz Sheikh (Professor of Primary Care Research and Development) helped to design the study and commented on drafts of the report.
Professor Chris Robertson (Professor of Statistics) wrote the paper.
Data sharing statement
We have permission to create and analyse the EAVE linked data set from the Scottish National Caldicott Guardian, Privacy Advisory Committee, NHS research and development and general practices. Any additional analyses and researcher access can only be done so with the approval of the Public Benefits and Privacy Panel for Health and Social Care.
Disclaimers
This report presents independent research funded by the National Institute for Health Research (NIHR). The views and opinions expressed by authors in this publication are those of the authors and do not necessarily reflect those of the NHS, the NIHR, NETSCC, the HTA programme or the Department of Health. If there are verbatim quotations included in this publication the views and opinions expressed by the interviewees are those of the interviewees and do not necessarily reflect those of the authors, those of the NHS, the NIHR, NETSCC, the HTA programme or the Department of Health.
References
- Kilbourne ED. Influenza pandemics of the 20th Century. Emerg Infect Dis 2006;12:9-14. http://dx.doi.org/10.3201/eid1201.051254.
- McLeish NJ, Simmonds P, Robertson C, Handel I, McGilchrist M, Singh BK, et al. Sero-prevalence and incidence of A/H1N1 2009 influenza infection in Scotland in winter 2009–2010. PLOS ONE 2011;6. http://dx.doi.org/10.1371/journal.pone.0020358.
- Hancock K, Veguilla V, Lu X, Zhong W, Butler EN, Sun H, et al. Cross-reactive antibody responses to the 2009 pandemic H1N1 influenza virus. N Engl J Med 2009;361:1945-52. http://dx.doi.org/10.1056/NEJMoa0906453.
- Simpson CR, Ritchie LD, Robertson C, Sheikh A, McMenamin J. Vaccine effectiveness in pandemic influenza – primary care reporting (VIPER): an observational study to assess the effectiveness of the pandemic influenza A(H1N1)v vaccine. Health Technol Assess 2010;14. http://dx.doi.org/10.3310/hta14340-05.
- The Pandemic of Influenza A(H1N1) Infection in Scotland 2009–2010: Report on the Health Protection Response. Glasgow: HPS; 2010.
- Simpson CR, Ritchie LD, Robertson C, Sheikh A, McMenamin J. Effectiveness of H1N1 vaccine for the prevention of pandemic influenza in Scotland, UK: a retrospective observational cohort study. Lancet Infect Dis 2012;12:696-702. http://dx.doi.org/10.1016/S1473-3099(12)70133-0.
- Skowronski DM, Janjua NZ, De Serres G, Hottes TS, Dickinson JA, Crowcroft N, et al. Effectiveness of AS03 adjuvanted pandemic H1N1 vaccine: case-control evaluation based on sentinel surveillance system in Canada, autumn 2009. BMJ 2011;342. http://dx.doi.org/10.1136/bmj.c7297.
- Valenciano M, Kissling E, Cohen JM, Oroszi B, Barret AS, Rizzo C, et al. Estimates of pandemic influenza vaccine effectiveness in Europe, 2009–2010: results of Influenza Monitoring Vaccine Effectiveness in Europe (I-MOVE) multicentre case-control study. PLOS Med 2011;8. http://dx.doi.org/10.1371/journal.pmed.1000388.
- Coupland C, Harcourt S, Vinogradova Y, Smith G, Joseph C, Pringle M, et al. Inequalities in uptake of influenza vaccine by deprivation and risk group: time trends analysis. Vaccine 2007;25:7363-71. http://dx.doi.org/10.1016/j.vaccine.2007.08.032.
- Herrett E, van Staa T, Free C, Smeeth L. Text messaging reminders for influenza vaccine in primary care: protocol for a cluster randomised controlled trial (TXT4FLUJAB). BMJ Open 2014;4. http://dx.doi.org/10.1136/bmjopen-2013-004633.
- Hardelid P, Fleming DM, McMenamin J, Andrews N, Robertson C, Sebastian Pillai P, et al. Effectiveness of pandemic and seasonal influenza vaccine in preventing pandemic influenza A(H1N1) 2009 infection in England and Scotland 2009–2010. Euro Surveill 2011;16.
- Information Services Division Scotland . Continuous Morbidity Recording n.d. www.ndc.scot.nhs.uk/Dictionary-A-Z/Definitions/index.asp?Search=C&ID=152&Title=Continuous%20Morbidity%20Recording%20(CMR)&Title2 = CMR%20(Continuous%20Morbidity%20Recording) (accessed 17 July 2015).
- Information Services Division Scotland . General Practice – Practice Team Information n.d. www.isdscotland.org/pti (accessed 1 November 2009).
- Ekins-Daukes S, Helms PJ, Taylor MW, Simpson CR, McLay JS. Utility of routinely acquired primary care data for epidemiology and pharmacoepidemiology. Br J Clin Pharmacol 2005;59:684-90. http://dx.doi.org/10.1111/j.1365-2125.2005.02404.x.
- Whitelaw FG, Nevin SL, Milne RM, Taylor RJ, Taylor MW, Watt AH. Completeness and accuracy of morbidity and repeat prescribing records held on general practice computers in Scotland. Br J Gen Pract 1996;46:181-6.
- British Medical Association (BMA) . The Quality and Outcomes Framework Guidance n.d. http://webarchive.nationalarchives.gov.uk/20130107105354/http://www.dh.gov.uk/prod_consum_dh/groups/dh_digitalassets/@dh/@en/documents/digitalasset/dh_4088693.pdf (accessed 17 July 2015).
- The Scottish Government . Scottish Government EHealth Directorate CHI Programme End Programme Report n.d. www.ehealth.scot.nhs.uk/wp-content/documents/CHI-Programme-Report.pdf (accessed 17 July 2015).
- Simpson CR, Lone N, Kavanagh K, Ritchie LD, Robertson C, Sheikh A, et al. Trivalent inactivated seasonal influenza vaccine effectiveness for the prevention of laboratory confirmed influenza in a Scottish population 2000–2009. Euro Surveill 2015;20. http://dx.doi.org/10.2807/1560-7917.es2015.20.8.21043.
- Health Protection Scotland (HPS) . Influenza Surveillance Systems n.d. www.hps.scot.nhs.uk/resp/influenzasurveillancesystems.aspx (accessed 10 May 2015).
- Health Protection Scotland (HPS) . Electronic Communication of Surveillance in Scotland (ECOSS) n.d. www.hps.scot.nhs.uk/surveillance/SystemsDetail.aspx?id = 248 (accessed 10 May 2015).
- General Register Office for Scotland (GROS) . General Register Office for Scotland Statistics Library (Population Estimates) n.d. www.gro-scotland.gov.uk/statistics-and-data/statistics/statistics-by-theme/population/population-estimates/mid-year-population-estimates (accessed 12 September 2015).
- Harley K, Jones C. Quality of Scottish morbidity record (SMR) data. Health Bull 1996;54:410-17.
- Scottish Index of Multiple Deprivation. 2009 General Report. Edinburgh: Scottish Government National Statistics Publications; 2009.
- Graham PL, Mengersen K, Morton AP. Confidence limits for the ratio of two rates based on likelihood scores: non-iterative method. Stat Med 2003;22:2071-83. http://dx.doi.org/10.1002/sim.1405.
- Newcombe R. Confidence Intervals for Proportions and Related Quantities n.d. www.cardiff.ac.uk/medic/aboutus/departments/primarycareandpublichealth/ourresearch/resources/index.html (accessed 21 January 2010).
- Jackson LA, Jackson ML, Nelson JC, Neuzil KM, Weiss NS. Evidence of bias in estimates of influenza vaccine effectiveness in seniors. Int J Epidemiol 2006;35:337-44. http://dx.doi.org/10.1093/ije/dyi274.
- Human Tissue Authority . List of Materials Considered to Be ‘Relevant Material’ Under the Human Tissue Act 2004 n.d. www.hta.gov.uk/policies/list-materials-considered-be-’relevant-material’-under-human-tissue-act-2004 (accessed 27 May 2014).
- Skowronski DM, Masaro C, Kwindt TL, Mak A, Petric M, Li Y, et al. Estimating vaccine effectiveness against laboratory-confirmed influenza using a sentinel physician network: results from the 2005–2006 season of dual A and B vaccine mismatch in Canada. Vaccine 2007;25:2842-51. http://dx.doi.org/10.1016/j.vaccine.2006.10.002.
- Wong K, Campitelli MA, Stukel TA, Kwong JC. Estimating influenza vaccine effectiveness in community-dwelling elderly patients using the instrumental variable analysis method. Arch Intern Med 2012;172:484-91. http://dx.doi.org/10.1001/archinternmed.2011.2038.
Appendix 1 General practice poster for serology use
Appendix 2 Project protocol
Project title
Early estimation of pandemic influenza Antiviral and Vaccine Effectiveness (EAVE) – use of a unique community and laboratory national linked dataset (11\46\23).
Planned investigation
Research objectives
Building on prior work,1–3 we aim to enhance an approach used to determine effectiveness of the 2009 H1N1 influenza pandemic vaccine. 2 By linking primary care clinical, prescribing and vaccination data from 40 sentinel general practices to laboratory serology and swab information using a unique patient identifier the Community Health Index (CHI) number [HTA refs: 09/84/90] we will be able to determine once a new pandemic is underway:
-
the uptake and effectiveness of any new pandemic vaccine once available;
and using already collected serological information and swab data:
-
the existence of any protective effect provided by previous exposure to and vaccination from A/H1N1 pandemic or seasonal influenza/identification of susceptible groups
-
the attack rate of pandemic influenza
-
the analysis of any protective effect conferred by antivirals.
Existing research
During the 20th and 21st centuries, there have been four pandemics (global epidemics) of influenza (1918–1919, 1957–58, 1968–1969, 2009) producing very large numbers of clinical cases and in the case of the first three, large numbers of deaths (with an estimated 20–40 million, 1 million and 1 million deaths respectively). This was due to the population having little immunity to the novel influenza viruses involved (H1N1, H2N2, H3N2 respectively). 2 The lack of herd immunity to the novel influenza viruses implicated (i.e. H1N1, H2N2 and H3N2) is believed to have been a key factor contributing to these very high numbers of deaths in the 20th century. 1 The influenza A subtype H1N1 virus, which emerged in Mexico in March 2009,2 also produced a very large number of clinical cases with a third of the population being found to be infected by early 2010. 4 Previous vaccination to seasonal influenza vaccine conferred little or no cross-reactivity antibody responses. 5 Furthermore, persons under the age of 30 years were found to have little evidence of cross-reactivity to the pandemic virus, with a proportion of older adults having pre-existing antibodies.
After the introduction of any new pandemic influenza, as well as frontline healthcare workers, any new vaccination will be targeted at those who are considered to be at increased risk of serious illness or death from influenza-like illness, i.e. those with any underlying medical conditions: e.g. chronic renal disease; immunosuppression resulting from disease or treatment; chronic heart disease, etc. and also those that may lack herd immunity (cross-reactivity) from exposure to previous pandemics or vaccinations. These national vaccination strategies have been shown to represent a potentially important approach to reduce both influenza-related illness and death, hence the considerable investment in this approach in many parts of the world. However, in the 2009 H1N1 pandemic the earliest estimate of Vaccine Effectiveness (VE) in the UK was published in July 20102 four months after the last case of influenza in that season (18 March 2010 in Scotland). 6 Further VE estimates were published in 2011. 7–8 Serology and data gathered in Scotland during 2009/10 also found that rates of immunisation for those most susceptible to serious influenza-like illness were less than optimal. For instance, just one in four patients aged five to 50 years with serious underlying illnesses received monovalent H1N1/A vaccine and an estimated 10,000 patients aged > 75 years with no underlying disease were issued the H1N1/A vaccine in Scotland. Furthermore, the most socio-economically deprived patients in Scotland were also found, after the first round of vaccinations, to be more susceptible and were no more likely to receive the vaccine than the most affluent people. 4
Research methods
Design
Once the study commences, during a twenty-three month preparatory period, we will instigate a data and serology collection linkage system whereby, once a new pandemic occurs, we will be able to undertake a timely analysis of a large national retrospective observational cohort of patients using a unique community, hospital and laboratory linked dataset.
Setting
The Practice Team Information (PTI) network of 40 general practices currently covers a six per cent representative sample of the Scottish population (n = 300,000). These primary care practices are given financial incentives by NHS National Services Scotland to record and code additional data electronically, over and above that routinely recorded for clinical care or as part of the PTI project (e.g. age, co-morbidity, out of hours contacts and socio-economic status), including influenza vaccination status. 9 Data from general practices within Scotland have shown to be of high quality and useful for epidemiological research. 10 The completeness of capture of contacts and accuracy of clinical event coding in primary care (using Read codes) has been found to be above 91%. 11 The NHS in Scotland is free at the point of care. Access to secondary and tertiary care is usually provided through primary care with almost all of the population being registered with a general practitioner.
Once the pandemic is underway and using the unique Community Health Index (CHI) number, general practice patient level data will be extracted and linked to the Scottish Morbidity Record (SMR) catalogue which has information on all in-patient hospitalisations within Scotland (as well as information on death certification linked from the General Register Office for Scotland [GROS] now known as NRS12) from 1981. Hospital data are reliable, with completeness and accuracy rates exceeding 90%. 11
In a sub-study, data on vaccination and other patient characteristics will be linked to serological information collected from patients with influenza-like illness by practices. Scottish laboratories (West of Scotland Specialist Virology Laboratory & Regional Virus Laboratory Specialist Virology Centre [Edinburgh]) will store 2,000 biochemistry samples from a subset of participating practices. Once 2,000 specimens have been collected we will explore the replacement of patients’ samples who have died or deregistered from participating practices using deterministic data linkage between the laboratory and primary care. Using this technique we will also explore whether patient descriptions can be elucidated (e.g. age band, sex, comorbidity, socio-economic status) and stored in readiness for a pandemic. Once the pandemic begins, we will undertake a data linkage to determine other fields such as vaccination status (and other data including antiviral prescribing and influenza/influenza-like illness) of these patients. Their serum will then be tested to determine exposure to previous influenza viruses/vaccine (by the presence of antibodies) once a suitable validated test has been developed. Swabs submitted to test for new pandemic influenza will be compared to determine if previous vaccine or exposure confers protection.
Target population
300,000 people of all ages registered with participating practices throughout Scotland.
Recruitment
The forty general practices and two laboratories will be recruited by the University of Edinburgh and Health Protection Scotland (HPS).
Planned inclusion/exclusion criteria
All registered patients will be studied so there are no exclusion criteria.
Planned interventions
This study will involve a quantitative evaluation of aspects of any future vaccination programme (implemented through general practice) to prevent pandemic influenza. Vaccine effectiveness will be estimated by linking serology information generated from patients presenting with influenza-like illness (via swabs on a convenience sampling basis 10 per week per practice) to clinical information generated from primary care and hospitalisation and mortality data. Similar information on antivirals (if a well-matched antiviral is available) will also be extracted.
In a sub-study, stored residual biochemistry samples from practices will be used to elucidate important serological information on antibody cross-reactivity. This information will be used to inform policy-makers, clinicians and the public of the relative benefits of the vaccination programme and help target any vaccine at groups susceptible to infection.
Ethical and privacy arrangements
Permission to link the primary care, SMR, and laboratory databases for research purposes will be obtained from the Privacy Advisory Group. Further permissions will be sought from the appropriate Research Ethics Committee. Permissions from the general practices that contribute data to this project will be required.
Risks and anticipated benefits for trial participants and society, including how benefits justify risks
As this study will assess VE using routinely collected and de-identified data, this work will not add any known additional risks or benefits for the individual patients present in the databases. Informing patients as to the potential risks and benefits of vaccination will remain at the discretion of the appropriate primary care clinician. There are however potentially large societal benefits from targeting vaccination programmes to groups vulnerable to infection and assessing the effectiveness and impact of any vaccine for Scotland and the UK as a whole, but also for the international scientific community.
Statistical analysis
Odds and risk ratios (adjusted for age, sex and deprivation) will be calculated for differences in vaccine uptake rates between different groups of patients (sex, age, Scottish Index of Multiple Deprivation categories and at-risk groups) and for investigating trends in vaccine uptake. For VE using information from linked virological swab data and for serology data (both of which are binary events), a logistic regression model will be fitted adjusting for the effects of sex, age, socio-economic status and being in an at-risk morbidity group. VE will be measured by comparing swabs taken after vaccination with swabs taken before vaccination for all vaccinated individuals, and secondly by comparing swabs taken after vaccination among those vaccinated to swabs taken among those never vaccinated. A delay of fourteen days after vaccination will be used to establish the protective effect of the influenza vaccine. Propensity scores, such as vaccinations, consultations and hospitalisation in the previous flu season, and the effect modifiers will be used to control for the healthy vaccine effect. 13 In addition, using the cohort method the proportion of influenza-like illness, acute respiratory disease, and other adverse outcomes between vaccinated and unvaccinated cases will be ascertained. Confidence intervals for the rate ratio and tests of the differences between two rates will be carried out using the ‘midp method’ in the ‘rate ratio’ (RR) function and rate2by2.test function respectively using the ‘epitools’ package in R. 14 For small samples, confidence intervals for the RR will be estimated using the Excel workbook. 15
Illness RRs, i.e. the ratio of the rate of first admission to hospital or general practice (GP) consultation in the vaccinated compared to the rate of first admission to hospital or GP consultation among those who did not receive the vaccine will be calculated in both the ‘at-risk’ populations and the general population. This is a direct measure of VE. The unadjusted estimate of VE = (1 – RR) * 100. Adjusted RRs of VE for prevention of first hospitalisation/GP consultation will be derived from Poisson regression models, adjusting for sex, age, deprivation and clinical risk group.
Proposed outcome measures
With the emergence of any new pandemic influenza, we will calculate:
-
The vaccination uptake in the relevant ‘at risk’ populations (patients < 65 with ‘at-risk’ co-morbidities and those > 65 years) and the general population recorded by general practices;
-
Influenza positivity from virological swab data in vaccinated and unvaccinated patients stratified by ‘at-risk’ populations, age, sex and socio-economic status.
-
Influenza positivity from serology data in vaccinated and unvaccinated patients stratified by ‘at-risk’ populations, age, sex and socio-economic status, which will permit the estimation of incidence of influenza.
-
Consultation for influenza-related morbidity (e.g. influenza, pneumonia, COPD and cardiac-related consultations) and issue of antiviral therapy from general practice data in vaccinated and unvaccinated patients stratified by ‘at-risk’ populations, age, sex and socio-economic status;
-
Mortality and influenza-related serious morbidity (e.g. influenza, pneumonia, COPD and cardiac-related death and hospitalisation from SMR01 records) in vaccinated and unvaccinated patients stratified by ‘at-risk’ populations, age, sex and socio-economic status; and
-
For patients with stored serology (and prior to the introduction of any vaccination) whether cross-reactivity occurs due to previous exposure to A/H1N1 or A/H1N1 vaccination, other pandemic influenza or other seasonal influenza vaccination or exposure.
Project timetable and key milestones
Time point | Milestones |
---|---|
1st September 2012 | Study commencement |
1st September–1st July 2013 | Practice recruitment, regulatory approvals (Research Ethics and Privacy and Advisory Committees) and setting up of laboratory collection and storage and automated practice data collection operating procedures |
1st July 2013–30th June 2014 | Begin collection and storage of serology samples |
Test pilot data extraction and linkage. Development of statistical script | |
1st July 2014 (and every year hence until pandemic) | Assessment of serology samples to determine numbers collected. Pilot data practice data extraction and linkage (using CHI) |
1st August 2014 | Submission of analysis plan for publication |
Pandemic commencement | |
Month 1–2 | Serology samples tested for A/H1N1 pandemic/seasonal influenza seropositivity. Practice data extraction and linkage to virology SMR01 data |
Month 2 | Processed statistical dataset undergoes statistical analysis by Statistics and Modelling Group (Strathclyde University) |
Month 3 | Initial results published online (once validated) |
Month 6 | Final report |
Key references
1. Mooney JD, Weir A, McMenamin J, Ritchie LD, Macfarlane TV, Simpson CR, et al. The impact of pneumococcal vaccination in Scotland for those aged 65 and over during winter 2003/2004. BMC Infect Dis 2008;8:53.
2. Simpson CR, Ritchie LD, Robertson C, Sheikh A, McMenamin J. Vaccine effectiveness in pandemic influenza – primary care reporting (VIPER): an observational study to assess the effectiveness of the pandemic influenza A (H1N1)v vaccine. Health Technol Assess 2010;14:313–46.
3. Thomson WW, Shay DK, Weintraub E, Brammer L, Cox N, Anderson LJ, et al. Mortality Associated With Influenza and Respiratory Syncytial Virus in the United States JAMA 2003;289:179–86.
4. McLeish NJ, Simmonds P, Robertson C, Handel I, McGilchrist M, Singh BK, et al. Sero-prevalence and incidence of A/H1N1 2009 influenza infection in Scotland in winter 2009–2010. PLOS One 2011;6:e20358.
5. Hancock K, Veguilla V, Lu X, Zhong W, Butler EN, Sun H, et al. Cross-reactive antibody responses to the 2009 pandemic H1N1 influenza virus. N Engl J Med 2009;361:1945–52.
6. Health Protection Scotland. The Pandemic of Influenza A(H1N1) Infection in Scotland 2009–2010: Report on the Health Protection Response. Glasgow: Health Protection Scotland; 2010.
7. Skowronski DM, Janjua NZ, De Serres G, Hottes TS, Dickinson JA, Crowcroft N, et al. Effectiveness of AS03 adjuvanted pandemic H1N1 vaccine: case-control evaluation based on sentinel surveillance system in Canada, autumn 2009. BMJ 2011;342:c7297.
8. Valenciano M, Kissling E, Cohen JM, Oroszi B, Barret AS, Rizzo C, et al. Estimates of pandemic influenza vaccine effectiveness in Europe, 2009–2010: results of Influenza Monitoring Vaccine Effectiveness in Europe (I-MOVE) multicentre case-control study. PLOS Med 2011;8:e1000388.
9. Whitelaw FG, Nevin SL, Milne RM, Taylor RJ, Taylor MW, Watt AH: Completeness and accuracy of morbidity and repeat prescribing records held on general practice computers in Scotland. Br J Gen Pract 1996;46:181–6.
10. Ekins-Daukes S, Helms PJ, Taylor MW, Simpson CR, McLay JS. Utility of routinely acquired primary care data for epidemiology and pharmacoepidemiology. Br J Clin Pharmacol 2005;59:684–90.
11. Information Services Division Scotland. General Practice – Practice Team Information. URL: www.isdscotland.org/pti (accessed November 2009).
12. GRO Scotland: General Register Office for Scotland Statistics Library (population estimates). URL: www.gro-scotland.gov.uk/statistics/publications-and-data/populationestimates/index.html (accessed July 2005).
13. Jackson LA, Jackson ML, Nelson JC, Neuzil KM, Weiss NS. Evidence of bias in estimates of influenza vaccine effectiveness in seniors. Int J Epidemiol 2006;35:337–344.
14. Graham PL, Mengersen K & Morton AP. Confidence limits for the ratio of two rates based on likelihood scores: non-iterative method. Stat Med 2003;22:2071–83.
15. Newcombe R. Confidence Intervals for Proportions and Related Quantities. URL: www.cardiff.ac.uk/medic/aboutus/departments/primarycareandpublichealth/ourresearch/resources/index.html (accessed January 2010).
16. Hardelid P, Fleming DM, McMenamin J, Andrews N, Robertson C, Sebastian Pillai P, et al. Effectiveness of pandemic and seasonal influenza vaccine in preventing pandemic influenza A(H1N1) 2009 infection in England and Scotland 2009–2010. Euro Surveill 2011;16:19763.
List of abbreviations
- CHI
- Community Health Index
- CI
- confidence interval
- COPD
- chronic obstructive pulmonary disease
- CVD
- cerebrovascular and cardiovascular disease
- EAVE
- Early estimation of pandemic influenza Antiviral and Vaccine Effectiveness
- HPS
- Health Protection Scotland
- ILI
- influenza-like illness
- MLA
- medical laboratory assistant
- OR
- odds ratio
- PTI
- Practice Team Information
- RR
- rate ratio
- RT-PCR
- real-time polymerase chain reaction
- SMR
- Scottish Morbidity Record
- VE
- vaccine effectiveness
- WoSSVC
- West of Scotland Specialist Virology Centre