Notes
Article history
The research reported in this issue of the journal was funded by the HTA programme as project number 11/46/22. The contractual start date was in January 2013. The draft report began editorial review in June 2015 and was accepted for publication in August 2015. The authors have been wholly responsible for all data collection, analysis and interpretation, and for writing up their work. The HTA editors and publisher have tried to ensure the accuracy of the authors’ report and would like to thank the reviewers for their constructive comments on the draft document. However, they do not accept liability for damages or losses arising from material published in this report.Should the study progress further, the full report will be published in the HTA journal.
Declared competing interests of authors
SV, PRM, GMcC, AAK, MH, RB, EB, AB, TPvS, JJK, JSNVT, JSW and MGS were in receipt of grants from the National Institute for Health Research (NIHR), both for the conduct of this study and others. In addition, PRM reports grants from F. Hoffman La Roche outside the submitted work, and TPvS has participated in expert meetings with GlaxoSmithKline and Boehringer (not related to flu) and has provided methodological advice to Laser (including an observational study on the incidence of flu). The Clinical Practice Research Datalink is a joint venture between the UK Medicines and Healthcare products Regulatory Agency and NIHR.
Permissions
Copyright statement
© Queen’s Printer and Controller of HMSO 2015. This work was produced by Venkatesan et al. under the terms of a commissioning contract issued by the Secretary of State for Health. This issue may be freely reproduced for the purposes of private research and study and extracts (or indeed, the full report) may be included in professional journals provided that suitable acknowledgement is made and the reproduction is not associated with any form of advertising. Applications for commercial reproduction should be addressed to: NIHR Journals Library, National Institute for Health Research, Evaluation, Trials and Studies Coordinating Centre, Alpha House, University of Southampton Science Park, Southampton SO16 7NS, UK.
Chapter 1 Introduction
A surge in health-care demand can exceed capacity to provide normal standards of care during pandemics of severe influenza and other emerging infections. Surge is recognised in historic reports of influenza pandemics and current government guidance. 1,2 In such exceptional circumstances triage tools may aid decisions in identifying people who are most likely to benefit from higher levels of care. Triage tools have value only if they can reliably identify individuals who benefit from higher levels of care, thus maximising outcome for a limited health-care resource and allowing other essential health-care activity to continue. Practitioners and policy-makers require confidence in the evidence behind triage criteria, and reassurance that a triage tool is valid for use in a particular situation. However, it is, by definition, impossible to establish the relationships between the presenting clinical characteristics and outcome for a novel pathogen causing disease in a heterogeneous population of varying age and comorbidities without conducting rapid research during the early phase of that outbreak. Validation of a triage tool for use in a novel disease requires rapid research during the early phase of that outbreak. Rapid research should allow refinement and validation of triage tools so that in the event of surge a valid tool is available.
In 2009, during the early phase of the A/H1N1pdm2009 pandemic, health-seeking behaviour due to perceived risk of influenza increased quite out of proportion to influenza-like illness (ILI) activity in the community. 3 This placed exceptional pressure on primary health-care services and interfered with capacity to deliver both routine and emergency care to previously accepted standards. In England, the National Pandemic Flu Service, which relied upon clinical algorithms, was introduced in mid-July 2009 in order to relieve pressure on primary care services. 4 Later in the same outbreak, ILI in some regions exceeded the capacity of secondary care to continue some routine and specialist services.
In either situation, workload pressures may limit the time available for clinical decision-making, and health-care worker absence due to personal sickness or caring for dependants may limit the skill mix. Access to imaging and laboratory services may also be restricted. Health-care workers who are unfamiliar with clinical assessment and admission decision-making may be asked to fulfil ‘gatekeeper’ roles. 5 Together, these factors place increased reliance and emphasis on core clinical history-taking and examination skills for triage decisions, which may be devolved to less-experienced staff.
Triage tools for influenza
Provisional guidance6 suggested the use of the CURB-65 pneumonia score7 and the Pandemic Medical Early Warning Score (PMEWS)8 for hospital triage of adults in the UK. Importantly, neither score was ever intended for use in children. Most children can benefit from access to adult critical care facilities and general intensive care units when there is no paediatric intensive care unit capacity. Recognising this gap, a ‘toolkit’ of national guidance was developed in 2008 in the UK, which included newly developed Community Assessment Tools (CATs) for both children and adults in primary and secondary care, and matched hospital care pathways. 2 None of the recommended triage tools was validated in the context of a novel influenza outbreak at the onset of the A/H1N1pdm2009 outbreak.
CURB-65
‘CURB-65’ is a validated predictor of mortality from community-acquired pneumonia in adults but it was never intended for use in children. 7,9 CURB-65 was not designed to predict mortality from non-pneumonic presentations. 8–10
Pandemic Medical Early Warning Score
Challen et al. 11 proposed the PMEWS as a clinical triage tool to aid hospital admission decisions for adults in a pandemic situation. The PMEWS score attributes an ordinal value to ranges of physiological measurements (respiratory rate, oxygen saturation, heart rate, systolic blood pressure, temperature and neurological assessment) and patient characteristics (age, social factors, chronic disease and performance status) to generate a score of between 0 and 20 (Table 1). They validated PMEWS in adults presenting to hospital with community-acquired pneumonia and found that it was better than CURB-65 for predicting the need for admission and higher levels of care, but had limited ability to predict mortality. CURB-65 and PMEWS pose problems for use in primary care: CURB-65 is reliant on a contemporaneous serum urea value, PMEWS could be computationally complicated for some, and both are designed for use only with adults. Many people presenting with ILI are children. Other severity scoring tools exist but these are not suitable for use in primary care because of a greater dependence upon laboratory or radiological investigations.
Ring 1 value for each factor | |||||||
---|---|---|---|---|---|---|---|
Physiological measurement | Score | ||||||
3 | 2 | 1 | 0 | 1 | 2 | 3 | |
Respiratory rate (breaths per minute) | ≤ 8 | 9–18 | 19–25 | 26–29 | ≥ 30 | ||
Oxygen saturation (%) | < 89 | 90–93 | 94–96 | > 96 | |||
Heart rate (beats per minute) | ≤ 40 | 41–50 | 51–100 | 101–110 | 111–129 | ≥ 130 | |
Systolic blood pressure | ≤ 70 | 71–90 | 91–100 | > 100 | |||
Temperature (°C) | ≤ 35.0 | 35.1–36 | 36.1–37.9 | 38–38.9 | ≥ 39 | ||
Neurological assessment | Alert | Confused, agitated | Voice | Pain, unconscious | |||
PLUS | |||||||
Score 1 for each factor | |||||||
|
|||||||
Total P MEWS = |
Department of Health Community Assessment Tools
In 2009, the Department of Health in England published a package of care that included paediatric and adult CATs and patient pathways for use by the national health services of the UK nations in a severe pandemic event, in primary and secondary care, and matched hospital care pathways. 12 CATs were developed to help non-specialist front-line staff identify, when resources are limited, which sick children and adults are most likely to benefit from interventions and levels of care that are available only in hospitals. CATs were developed by paediatric and adult expert clinical development groups drawing on evidence that supports the recognition of severe influenza and severe pneumonia in the community in adults and children in resource-limited settings, severe chronic obstructive pulmonary disease in adults, potentially serious feverish illness in children and severe bronchiolitis in infants. 5,13–22 Clinicians were warned not to use the CATs and the pathways unless the local situation precluded normal admission and discharge processes.
CATs use six objective criteria and one subjective criterion based on simple (binary) clinical assessment (Figures 1 and 2). Meeting any CATs criterion warrants referral and admission to hospital. Criteria are (1) severe respiratory distress; (2) increased respiratory rate; (3) oxygen saturation of ≤ 92% on pulse oximetry breathing air or oxygen; (4) respiratory exhaustion; (5) severe dehydration or shock; (6) altered consciousness level; and (7) other clinical concern. Although criteria fields are common to paediatric and adult CATs, the abnormal physiological thresholds and clinical signs are age appropriate. Like PMEWS, there is no requirement for laboratory investigation to complete the assessment. However, CATs were intended for use only ‘during severe and exceptional circumstances when surge demand for health-care services leads to a need for strict triage’12 and, as such, were not deployed during the 2009–10 pandemic.
FIGURE 1.
Paediatric Community Assessment Tool for children aged < 16 years. © Crown Copyright, Department of Health, 2009, reproduced under Open Government Licence for public sector information.
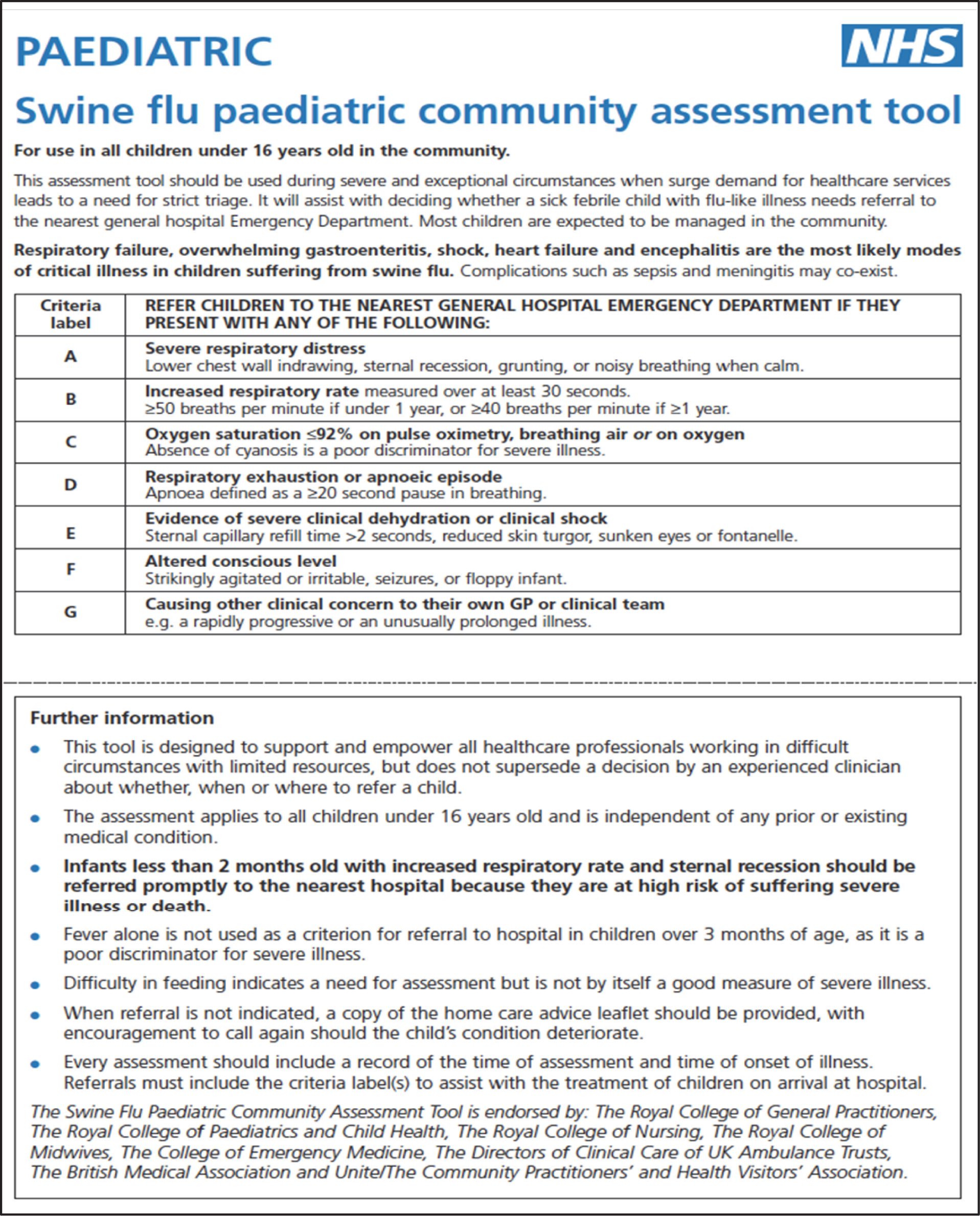
FIGURE 2.
Adult Community Assessment Tool for adults aged ≥ 16 years. © Crown Copyright, Department of Health, 2009, reproduced under Open Government Licence for public sector information.

Work underpinning this study
Goodacre et al. 23 conducted an evaluation of the discriminatory value of the CURB-65 score, PMEWS and CATs for predicting severe illness or mortality in 481 patients (346 aged < 16 years) presenting to hospital with suspected pandemic influenza. Initially they were unable to draw any conclusions regarding their clinical utility in a pandemic situation because of insufficient numbers of adults and a low incidence of severe outcome. In a later analysis of the same data, sensitivity, specificity and area under receiver operating characteristic (AUROC) values were reported for adults using these three triage tools with caveats regarding the power of the study. 24
In another study, PMEWS scores were calculated from a retrospective data enquiry of 300 adult patients with suspected pandemic influenza, who were assessed in the community by Ambulance Service emergency-care practitioners. AUROC curves suggest that PMEWS scores discriminate between decision to ‘treat and leave’ and ‘transfer for hospital assessment’. 25
The UK Pandemic Influenza Clinical Information Network (FLU-CIN) characterised polymerase chain reaction-confirmed pandemic influenza disease in a cohort of 1520 people [1040 adults, 480 children (aged < 16 years)] admitted to hospital. 26 FLU-CIN compared the clinical validity and utility of CATs, PMEWS and CURB-65 as predictors for interventions that are normally available only in hospital, higher levels of care and death using AUROC curve comparisons with 95% confidence intervals (CIs). 27 CATs showed the best predictive performance for level 2/3 admissions in both adults [AUROC: CATs 0.77 (95% CI 0.73 to 0.80); CURB-65 0.68 (95% CI 0.64 to 0.72); PMEWS 0.68 (95% CI 0.64 to 0.73), comparison of AUROCs; p < 0.001] and children [AUROC: CATs 0.74 (95% CI 0.68 to 0.80); CURB-65 0.52 (95% CI 0.46 to 0.59); PMEWS 0.69 (95% CI 0.62 to 0.75); p < 0.001].
Although the FLU-CIN cohort is limited to patients admitted to hospital with severe influenza and its complications, the data show that these triage tools are capable of predicting higher levels or care and/or death in children and adults. However, the FLU-CIN analysis did not include assessment of triage tools in primary care.
Appropriate use of such triage tools in the community could expedite referral to hospital and, when scores are high, immediate admission to level 2/3 care. Prompt admission and allocation of higher levels of care may be associated with improved patient outcomes. Another study26 by FLU-CIN found that delayed admission to hospital (≥ 4 days after symptom onset) was significantly associated with increased likelihood of admission to critical care and death.
Morbidity and mortality rates were low during the influenza A/H1N1pdm2009 pandemic compared with some previous influenza epidemics, such as that in 1989–90. 28 The use of antiviral therapy was generally low in the FLU-CIN cohort despite it being widely available at the time. A more severe pandemic may be associated with a greater acceptance of antiviral therapy, and this may impact upon need for higher levels of care and death. Consequently, criteria threshold values may need to be adjusted to optimise the receiver operating characteristic curve for each criterion and the AUROC curve for the various triage tools.
The validity and utility of using triage tools in the community to aid management decisions during a pandemic remains untested. Both PMEWS and CATs were developed with this purpose in mind and so we aimed to capture the criteria that would allow validation of these tools in this study.
Justification of this study
The validity and utility of triage tools need to be assessed, in a large community-based prospective study of patients presenting with ILI, to give confidence to general practitioner (GPs), who may be asked to use such tools in the event of surge, and policy-makers who may need to recommend their use to GPs. The routine use of electronic health records (EHRs) by GPs and existing permissions to access anonymous data for research purposes presents an opportunity to study GP assessments, management decisions and patient outcomes. Anonymous linkage of this GP-derived data to Hospital Episode Statistics (HES) data for hospital admissions and Office for National Statistics (ONS) data for causes of death permits a validation of the GP-derived data. Together, these processes should allow for the assessment and comparison of the validity and utility of triage tools in the community in relation to patient relevant outcomes (hospital admission, length of stay, higher levels of care and death).
The Health Protection Agency timeline for the UK 2009 pandemic showed only 12 weeks between identification of person-to-person transmission in the UK (first week of May) and peak influenza activity (last week of July) in the first pandemic wave. Prospective data collection with near real-time iterative and cumulative analysis is the only method for validating triage criteria and tools against a novel pathogen in such a short time.
As pandemics are unpredictable and infrequent, limited but potentially useful information will be gained from prospective feasibility and pilot work conducted in primary care during seasonal influenza while A/H1N1pdm2009 is still circulating. It would not be possible to conduct such a study properly during a pandemic without prior permissions, preparation of processes, feasibility and testing performance with a pilot. The UK National Institute for Health Research (NIHR) recognised the need to fund and support the rapid set-up of relevant research studies and ensure that these studies are successfully conducted so that their findings can inform the ongoing care of patients during an outbreak. This will require some changes to the usual processes undertaken by the Clinical Research Network, as well as the reprioritisation of both national and local resources in what may well be a challenging environment in terms of increased demands for patient care and falling staff numbers because of illness. Consequently, the Clinical Research Network has an urgent public health plan in place to ensure that urgent public health studies can be set up and delivered quickly and effectively. The Clinical Research Network’s urgent public health risk process will be activated at the request of the Department of Health. The FLU-CATs Study is one of the portfolio of studies that have been identified and granted the relevant research approvals in advance of an outbreak. 29
Conducting this study in real-time during the early stages of a pandemic, when the characteristics of the novel virus are not fully understood, is important as it allows refinement and validation of triage tools against the novel pathogen in preparation for possible surge. This cannot be done until a novel virus emerges. Dame Deirdre Hine has recommended that population-based studies be established that, in the early stages of a future pandemic, can measure the severity of the pandemic and support decision-making. 30
If the behaviour of the virus is markedly different in terms of severity between the first and subsequent waves, or evolves to cause severe disease in a particular organ system (as happened in the 1918–19 pandemic) then triage criteria may need to be adapted to reflect the consequent changes in health-care demand and clinical presentation.
The objective of this study was to establish processes now, in advance of a future pandemic, to validate the community triage tools capable of assisting hospital referral decisions for people of all ages for use if health-care demands exceed health-care capacity (surge).
The development of a triage tool has three distinct phases (set-up, pilot and implementation). It is expected that a triage tool would be implemented only in the exceptional circumstance of surge during an influenza pandemic or substantial outbreak of a novel emerging respiratory pathogen of public health interest. This study reports the set-up and pilot phases of this plan of work.
Chapter 2 Methods
The study was conducted in primary care settings in the UK. These are community settings in which medical care is provided by GPs. The study was restricted to those GPs whose practices participate in the Clinical Practice Research Datalink (CPRD).
The study is made possible by consistent routine use by GPs of EHRs, an autonomous software agent that sits beside that system, and anonymised patient-specific linkage to external data sources. Figure 3 outlines the source and flow of information. In principle, any of the electronic patient management systems in use could be used. We chose the Vision® version 3.01 (In Practice Systems Ltd, London, UK) platform because of the established linkage with CPRD. CPRD is the governmental centre, jointly funded by NIHR and the Medicines and Healthcare products Regulatory Agency (MHRA), which aims to provide anonymised health-care records for data services, interventional and observational research. 31 CPRD has ethical and regulatory approval to use anonymised patient data collected in over 650 participating GP practices and for linked–anonymised data access to individual patient data in the NHS England HES and mortality data in the ONS data set for approved purposes.
FIGURE 3.
Architecture of the real-time surveillance system.
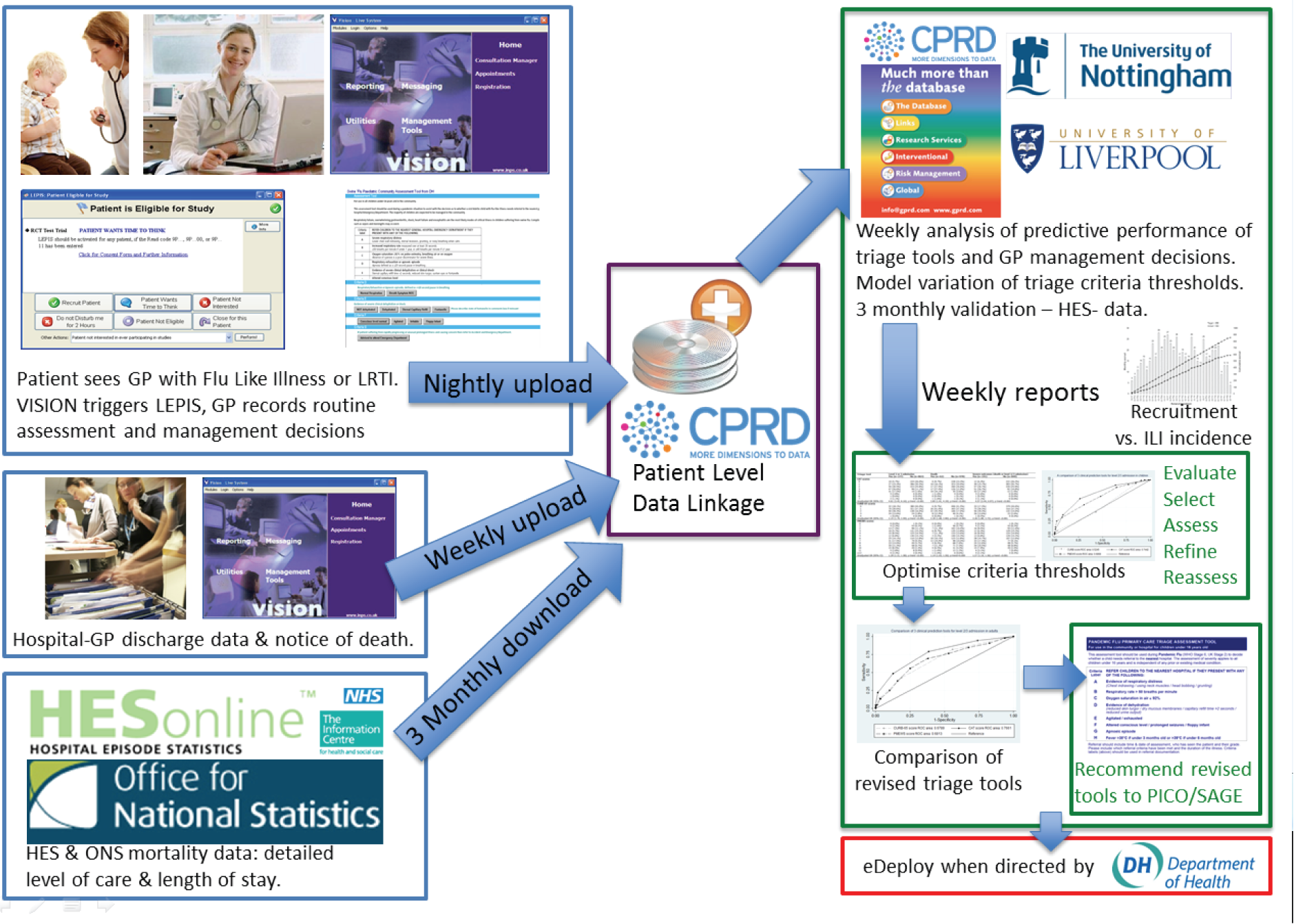
Eligible patients for the study were identified using Local Eligibility Patient Identification Software (LEPIS). LEPIS was developed for use with the electronic patient records software Vision® by researchers at Kings College London. LEPIS is an autonomous software agent that sits in the software background during a GP–patient consultation. It is remotely programmable by secure Ethernet to identify eligible patients using any combination of demographic features, Read codes and medications. LEPIS silently monitors the GP’s data entry for all consultations. When an eligible patient is identified, a pop-up window appears on the screen offering the opportunity to recruit that patient into a study. If recruited, LEPIS then ‘pipes’ the patient data to study specific processes. Further details of LEPIS are provided elsewhere. 32
Phase 1: set-up of the study, development of the technology and feasibility
This phase involved development of systems and feasibility testing. The information technology (IT) solution was deployed over 6 months between March and September 2013: in the UK this is not an endemic influenza season, and it occurred during an inter-pandemic period. Processes included set-up and validation of processes; optimisation of the clinical record entry screen; GP acceptability testing; establishing data-return format; check completeness of data returns; develop data clean-up algorithms; development of definitions, evaluation of completeness and validation of study outcomes using retrospective CPRD, HES and ONS data; and testing of LEPIS.
Developing the real-time surveillance system
Eligible patients for the study were identified during GP consultations using LEPIS loaded on participating GPs’ computers. LEPIS monitors the consultation record for entry of any eligible diagnostic Read code (see Appendix 1). When a participating GP enters a relevant diagnostic Read code, a pop-up window appears prompting them to consider recruiting the patient; not recruiting; or suspending further recruitment prompts for a period of time (Figure 4). If recruited, a form appears for structured entry of data related to the consultation (i.e. signs and symptoms, physiological measurements and decisions relating to treatment or hospital referral). The linkage between the LEPIS and Vision® systems enabled automatic triggering of tailor-made data entry forms based on age and sex for men, women and children (age < 16 years), with additional conditional questions relating to pregnancy status if female and age > 12 years. These forms are the electronic case report forms (eCRFs) for the study. The eCRFs were designed to encourage both positive and negative reporting. The eCRFs underwent revision after the first winter season to record if the GP actively decided not to make an assessment because the GP considered the feature to be grossly normal or abnormal. This important feature avoids bias in the analysis of data that might otherwise be considered missing at random, when, in reality, the data are missing because the GP considered the feature to be grossly normal or abnormal. This addresses one challenge of collecting data from routine consultation records, when many clinicians record only a limited number of important negative or positive features based on their personal practice.
FIGURE 4.
Screenshot of LEPIS pop-up window indicating an eligible patient.

Age- and gender-specific eCRFs were developed. Patients aged < 16 years are classified as ‘children’ and patients aged ≥ 16 years are classified as ‘adults’. Data collection forms for female patients > 12 years include a query on pregnancy status. A screenshot of a sample female adult LEPIS form is provided (Figure 5). The data collection form for children is identical to that for adults, except for three questions that are absent in the children’s form – blood pressure measurement, social isolation status and premorbid performance status – as these criteria are used only in the adult PMEWS triage tool.
FIGURE 5.
Sample data collection form for adult female.



Sociodemographic and relevant medical history data were extracted by a background process from the routine EHR to minimise GP workload.
The flagging system and web-based data collection form was user-tested by GPs at five practices. Sample web-based forms can be viewed at www.cprd.com/flucats/ (accessed 5 August 2015); these will be maintained for the duration of the study.
Data entry checks were incorporated in the eCRF so that measurement values could be entered only if they were within physiologically plausible ranges. To further minimise duplication of tasks, after the completion of each form, GPs are presented with a summary of their assessment along with any decisions made (to treat with antivirals and/or antibiotics, and referral to hospital). This can be cut and pasted, or downloaded in text or portable document format (PDF) for entry into the patient’s EHR. A screenshot of a sample GP summary generated following the submission of the data collection form is shown in Figure 6.
FIGURE 6.
Sample GP summary screen.

Eligibility criteria
All people (any age, any sex) presenting with ILI (regardless of date at onset of disease and prior medical history) to GPs participating in CPRD are eligible for this study. The challenge was to identify a set of Read codes that LEPIS would monitor and pop up to prompt GPs to recruit people with ILI, while not overburdening the GPs with irrelevant prompts.
Eligible Read codes were identified by systematic analysis of the CPRD database using a ‘reverse engineering’ process. First, we identified patients of any age admitted to hospital in the winter of 2010–11, who had a diagnosis of ‘influenza’, ‘an influenza-related condition’ or ‘complication of influenza’ in the HES data set, and extracted their CPRD/HES linked data. To identify these patients, we searched for terms such as ‘influenza’, ‘influenza-like illness’ and ‘acute respiratory infection’ (see Appendix 1 for initial list of eligible Read codes) in the Read-coded clinical terms dictionary. Next, the output was restricted to patients who had visited their GP in the 7 days prior to admission. Then we queried the CPRD data to list all Read codes used by GPs in these consultations that preceded the hospital admission. A total of 831 different Read codes were recorded for these GP consultations. Many of these consultations were unrelated to ILI. These Read codes were reviewed for relevance by PM and MGS, without sight of their frequency, to yield a shortlist of 39 codes. These codes were then listed by frequency and reviewed by the study steering group (see Appendix 2). We decided to use all of these codes at least initially as a previous consultation with GPs suggested that GPs prefer syndromic codes for ILI to reflect the diagnostic uncertainty in the absence of laboratory confirmation of influenza. It is our intention to refine the LEPIS trigger list at an interim analysis during the final phase of the study, that is, during a pandemic. 33
Recruitment of general practitioners
General practitioners from practices that were already contributing to the CPRD were invited to participate in the study by the CPRD team initially by post and followed up electronically. Further recruitment of CPRD GPs was conducted via the NIHR Primary Care Research Networks (PCRNs), and by CPRD and investigator activities at regional and national meetings of GP research groups. GP practices were required to install the LEPIS software on their practice computer system and were given remote IT support. GPs were briefed on the eligible Read codes so that they had the option of proactively triggering the LEPIS pop-up window. No monetary incentives were provided to GPs for participation in the study. Following correspondence with the Royal College of General Practitioners (RCGP), they were sent certificates to include in their continuing professional development portfolios.
Data linkages, validation of primary outcome measures, influenza surveillance data and expansion of study to other electronic health record systems
The study protocol included plans to validate the primary and secondary outcome events using HES data for ‘hospital admission within 24 hours of the consultation’ and ONS data for the outcome ‘death (all causes) within 30 days of the consultation’. In addition, at the request of NIHR reviewers, the feasibility study scope was expanded to explore linkages with Public Health England (PHE) data sets for data on microbiology and virology. The feasibility study also explored options for expansion of the study-specific technological infrastructure to all CPRD and non-CPRD GP practices in the event of a pandemic.
Automation of data extraction, analysis and reporting
Algorithms or ‘do files’ were developed and tested using Stata version 13 (StataCorp LP, College Station, TX, USA) to automate data linkage, extraction, analysis tasks and reporting to facilitate timely production of weekly and monthly reports on the incidence, progression and outcomes of pandemic influenza or a similar pandemic caused by a respiratory virus manifesting as ILI (see Appendix 3 for ‘do files’).
Evaluating user experience
Feedback on the ease of use of the study flagging and data collection system was obtained from participating GPs via e-mail consultation and one-to-one telephone interviews. Interviewees were provided a financial incentive for participation. A semistructured interview guide was used for the telephone interviews (see Appendix 4). Interviews were audio-recorded and transcribed verbatim for thematic analysis. Data were analysed using a thematic analysis approach that enables the researcher to identify, analyse and report themes or patterns in the data collected. The analysis followed the steps outlined by Braun and Clarke,34 beginning with familiarisation of the data through listening to the recording of the interviews, transcribing the data then reading and re-reading each interview, making initial codes in the margins.
Coding was conducted systematically, taking each interview initially in turn and then subsequently moving back and forth across the data set. In order to establish consistency in coding, and thereby enhance dependability and credibility, the transcripts were independently double-coded by two of the authors (SV and PRM). Initial themes were reviewed and refined further, and a revised thematic table of candidate themes was produced.
Phase 2: pilot study, data collection, extraction and statistical analysis
This phase involved data collection, extraction and statistical analysis from cases of ILI presenting to GPs. This pilot phase has run over two consecutive winter periods: 2013–14 and 2014–15 during an inter-pandemic period. We developed an automated weekly statistical evaluation of performance of triage criteria and tools. This phase is expected to continue to run in subsequent winter periods in a small number of practices as a maintenance process to ensure viability of processes as core systems continue to evolve and as EHR systems change.
Aim
To investigate the predictive performance of the triage tools CATs and the PMEWS for hospital admission and death in patients presenting with ILI to primary care, using a novel near real-time data collection, collation, linkage and analysis process.
Population and recruitment
Patients of all ages presenting to study GPs during the reporting period 19 March 2013 to 31 March 2015 (data upload 13 April 2015) with ILI were eligible for inclusion in the study and no exclusion criteria were applied. LEPIS was activated. Patient recruitment was determined by individual GP convenience rather than a specific sampling protocol. We estimated a minimum sample size of 1000 patients, assuming an event rate of 5% (based on the UK mortality and need for interventions in hospitalised influenza patients during the 2009 A/H1N1pdm2009 pandemic26) to test the hypothesis that the triage tools being tested would have an AUROC value of > 0.05, demonstrating the ability of the tools to discriminate between patients who experienced an outcome of interest and those who did not. 35,36
Design
Open cohort study involving follow-up for 30 days after the initial consultation for ILI.
Predictor variables
These include criteria from both the adult and child CATs (see Figures 1 and 2) and the adult PMEWS (see Table 1). Covariates that were extracted from the routine EHRs included patient sociodemographic characteristics (age, sex), comorbidities associated with an increased influenza risk (cardiovascular disease, chronic liver disease, neurological conditions, chronic renal disease, chronic respiratory disease, diabetes, immunosuppressive conditions), previous prescriptions of statins, antibiotics, influenza-specific antivirals, inhaled and oral corticosteroids, history of seasonal influenza vaccination, pneumococcal vaccination and Haemophilus influenzae type B vaccination. The detailed code list for disease covariates is provided in Appendix 5.
Outcome variables
There were two primary outcome variables to be collected from the GP EHR: hospital admission within 24 hours of GP assessment (binary categorical variable, coded as yes/no) and death (from all causes) within 30 days of GP assessment (binary categorical variable, coded as yes/no). Secondary outcome variables included ‘any need for augmented level of care (admission to high-dependency units or intensive care units coded as a binary categorical variable)’, length of hospital stay [three binary categorical variables were created using different thresholds of stay, < 48 hours (yes/no), ≥ 6 days (yes/no), ≥ 12 days (yes/no)], GP decision to refer to hospital (binary categorical variable, coded as yes/no), GP decision to prescribe antibiotics (binary categorical variable, coded as yes/no) and GP decision to prescribe influenza-specific antivirals (binary categorical variable, coded as yes/no).
Statistical analysis (planned and actual)
A descriptive analysis of patient sociodemographic and clinical characteristics was conducted. The planned analyses included an investigation of the association between each CATs criterion and the various outcome measures. Each was investigated, in turn, using univariate logistic regression analysis. In addition, a multivariable model was planned to identify which of the CATs criteria were significant independent predictors of outcome when included in the same model. A separate investigation of the association between other covariates (as listed above) and outcomes using a similar approach was also planned. The analysis plan also considered predictive performance of a combined CATs score (both non-weighted and weighted, which would incorporate all CATs criteria), as well as the effect of varying individual criteria and combined score thresholds (e.g. respiratory rate of > 30, > 35, > 40 breaths per minute or a combined CATs score of > 3, > 4, > 5). Finally, a comparison of predictive performance with the PMEWS score was planned for adults only. Predictive performance would be assessed using measures including sensitivity, specificity, positive predictive value, negative predictive value and AUROC curve values. Separate analyses were run for paediatric and adult patients. All statistical analyses were conducted using Stata.
Chapter 3 Ethics and consent
The CPRD has been granted generic ethics approval for observational studies that make use of only anonymised data and linked anonymised NHS health-care data (Multiple Research Ethics Committee ref. 05/MRE04/87). All CPRD studies require scientific approval from the MHRA Independent Scientific Advisory Committee (ISAC). The ISAC was established by the Secretary of State for Health in February 2006 to review the scientific merit of proposals for research using data from the CPRD as well as the Yellow Card Scheme database.
The clinical assessment data collection tool (or eCRF) was structured to capture evidence-based criteria recognised in national guidance. The study processes did not require the practitioner to make any change in their normal assessment; only to record the assessment in a structured manner. ISAC deemed that this was a non-interventional study and was exempt from the requirement for patient consent as (1) it involved the development and testing of an IT infrastructure for structured recording of the routine patient consultation rather than any departures from routine patient care and (2) made use of only linked anonymised data for analysis. This study protocol was approved by ISAC on 30 May 2012 (ISAC CPRD Protocol 12_043).
Chapter 4 Results
Phase 1: set-up of the study, development of the technology and feasibility
The general architecture of the real-time surveillance system is given in Figure 2. Data relating to each captured ILI GP consultation were uploaded on the CPRD database every night. The CPRD team then collated these data and sent weekly data to researchers based at the Universities of Nottingham and Liverpool. Additionally, on a monthly basis, the CPRD team sent background data (comorbidities, prescriptions, death, etc.) sourced from the routine electronic primary care record for all patients with captured ILI consultations. Each subsequent data instalment comprised the cumulative data acquired since the initiation of the study. The weekly and monthly data sets are described in detail in the FLU-CATs Data Handbook (see Appendix 6).
The Health and Social Care Information Centre (HSCIC) has responsibility for processing NHS England HES and linked ONS data since 1 April 2013. The study had permission to obtain 3-monthly HES and ONS linked data (as described in Data linkage) through existing agreements with HSCIC. HSCIC announced a delay in issue of HES data on 21 January 2014 for technical reasons and later imposed a moratorium on release of linked–anonymised data. As of 1 August 2015, CPRD has not received linked–anonymised HES data from HSCIC despite CPRD and the study investigators satisfying UK permissions. The retention of HES data by HSCIC has impacted on many research studies including FLU-CATs. It is anticipated that these validation analyses will be carried out as soon as the HES and ONS data are released to the researchers prior to the final phase of the study during a pandemic.
Development of real-time analysis and reporting
Three distinct stages of work were required:
-
Data cleaning and management Some data management of the weekly data as provided by CPRD was necessary to enable analyses. Most of the consultation-specific data collected via the web-based forms were provided as string variables, and data management mainly involved converting them to numeric variables or date variables where appropriate. These tasks have been automated and tested for accuracy in Stata 13 to enable an analyst to complete these usually labour- and time-intensive tasks in < 10 minutes once the data are received.
-
Analyses After cleaning, the following analyses were planned: descriptive analysis involving tabulation and summarising of the data; logistic regression analyses (unadjusted and adjusted) to investigate the association between each of the triage criterions and the primary outcomes; and an assessment of the predictive performance of different triage tools using AUROC curve comparisons. All analytical tasks have been automated using Stata 13 and can be completed in < 1 hour by an analyst.
-
Reporting Automated analyses and reporting mechanisms have been set up within Stata to report key findings from the weekly and monthly data sets. Findings currently being reported include frequency tables and charts, and results of unadjusted and adjusted logistic regression analyses. Weekly data set findings are reported in a Microsoft Excel® version 2010 (Microsoft Corporation, Redmond, WA, USA) spreadsheet and monthly findings in a Microsoft Word® version 2010 (Microsoft Corporation, Redmond, WA, USA) rich text format (RTF) document. This task has been semi-automated in Stata 13 to facilitate the production of weekly and monthly reports in Word and PDF format for policy-makers.
In addition, a Stata ‘do file’ was developed to extract background data from the CPRD database on comorbidities and select medication for all patients who underwent a FLU-CATs Study consultation. This has been tested by the CPRD team and has now been automated to provide the monthly data extracts. Detailed descriptions of each of the above three steps, along with instructions on how to use the Stata ‘do files’ are contained in the FLU-CATs Handbook (see Appendix 6). All ‘do files’ have been tested and validated with each of the weekly and monthly data uploads since the first weekly data tranche was received on 14 July 2014. The April 2015 data set (13 April 2015 tranche of weekly data) was the last tranche of data that was analysed at the time of preparation of this report. All findings presented in this report relate to the FLU-CATs data as of April 2015. Detailed results are provided below (see Phase 2: pilot study, data collection, extraction and statistical analysis).
Continuous weekly testing of these three processes has allowed us to reduce data management, analytical and reporting times to 1 day. By adapting the CPRD EHR structure we have also eliminated a separate electronic data entry step and streamlined data collation while maintaining data security.
We currently do not have sufficient data to report results from the AUROC analyses, even although we have recruited 863 eligible patients so far because influenza activity has been low and very few of our patients have progressed to the outcomes of interest.
Data linkage
A ‘do file’ was written to extract relevant variables (hospital admission, need for augmented level of care, length of stay and death) from HES and ONS data. This ‘do file’ has been tested by the CPRD team using historic HES and ONS data sets. CPRD have confirmed that the ‘do file’ is automated to periodically extract HES data for patients with FLU-CATs GP consultations. However, as of April 2015, no HES or ONS linked data have been received from HSCIC since January 2014. The HES/ONS data received in January 2014 related to the HES period up to the third quarter of 2013 and preceded the first winter influenza season that this study included. Therefore, validation of the few observed outcome events using the FLU-CATs pilot study data has not been possible.
Linkage to PHE data sets was explored and abandoned because of inability to anonymously link patients in CPRD with PHE records with confidence, mostly due to virology requests from primary care not including sufficient unique patient identifiers. As an alternative solution, linkage is being explored at a practice and patient level in collaboration with the RCGP Research and Surveillance Centre.
General practitioner participation and user experience
As of April 2015, a total of 30 GP practices participated in the FLU-CATs Study, although at any one time only 25 GP practices were actively recruiting. There were a total of 704 adult FLU-CATs consultations (702 single consultations and two repeat consultations) and 159 single consultations for children.
All 30 participating GPs were invited to be interviewed to evaluate the user experience. A total of six GPs agreed to be interviewed (Table 2). The interviews are summarised below.
Year qualified | Single-/multi-partner practice | Location group | Location type |
---|---|---|---|
1986 | Unknown | Urban | Urban – less sparse |
1985 | Six partners | Urban | Urban – less sparse |
1984 | Two partners | Urban | Urban – sparse |
1979 | Two partners | Urban | Urban – less sparse |
1986 | Senior partner, three other partners | Urban | Urban – less sparse |
1982 | Nine partners | Rural | Town and fringe – less sparse |
The purpose of conducting these interviews was to explore GPs’ experiences and views of being involved in a real-time influenza surveillance research project in order to help inform future consultations. GPs who participated in the FLU-CATs Study were sent invitation letters by CPRD: 11 GPs returned expressions of interest, of which six were interviewed over telephone. Each interview lasted between 10 and 20 minutes. A payment of £40 (based on the PCRN recommended rate of £80 per hour) was made to the GPs for their participation in the qualitative interviews.
The main finding that emerged from these interviews was that the LEPIS trigger pop-up and FLU-CATs data collection forms were easy to use. The simplicity of the eCRFs encouraged GPs to participate in the study despite there not being any financial incentive for participation. However, the setting up of the LEPIS system to enable data collection for the study was fraught with technical difficulties. Although the support from CPRD in resolving any technical issues surrounding LEPIS installation was appreciated by the GPs, technical difficulties persisted for some and this reduced patient recruitment. The FLU-CATs process was quite easy to conduct and did not interfere with the routine GP consultation. All interviewed GPs agreed that the FLU-CATs system, with a few modifications, was ready to be used in a pandemic scenario.
-
Introduction to, and involvement with, the FLU-CATs Study:
-
Of the six GPs interviewed, three were introduced to the FLU-CATs Study through the research networks (NIHR PCRN and other local research networks), one through a research coordinator and one directly by CPRD. The remaining GP did not remember exactly how he/she was introduced to the study but thought that it might have been through an e-mail.
-
-
Lack of financial incentive as a barrier to participation:
-
One GP said that the lack of financial incentive for the GPs’ participation in the FLU-CATs Study was ‘inevitably, a barrier’, whereas another GP said that it was not. One GP thought it might have been a barrier if the FLU-CATs Study was the first ever CPRD study in which the GP was participating but, as he/she had participated in a few before it was not a barrier for him/her. The consensus among the interviewed GPs, however, appeared to be that as the FLU-CATs Study was relatively straightforward and not ‘too onerous’, as one GP put it, the lack of a financial incentive was not a barrier to their participation. Interestingly, one GP said ‘My partners were a bit reluctant because there was no remuneration at all, but we thought it was relevant because it was relevant to the influenza season and I just thought it would focus our minds on how to manage respiratory tract infections’. One GP said the following about the lack of incentives for participation in the study: ‘I think the only difficulty, as I was saying at the beginning, with that is it’s a bit difficult to say why would I recommend doing it and my argument is because it makes me feel like a better person because I do, but does that make somebody else feel that it makes them feel like a better person if they do. I think the other thing is if you are thinking about incentives, I don’t think in general practice, the incentive needs to be particularly large, it just needs to be an appreciation of the fact that there are costs involved in doing things and the amount of money we got for [another study name] and [another study name], I would say was well in excess of what was necessary to reimburse us for the amount of work that we had done. And, I think if you were pitching something like FLU-CAT, looking for a price, then I think you’d be talking something like a couple of pounds a form not a couple of hundred pounds a form. Again, I think for the future of CPRD, I don’t think they would have to have terribly large incentives to make it a reason why people would want to do it. It’s perhaps where there has been some difficulty about it, it’s just the total lack of an incentive. And, basically, in general practice, if somebody isn’t paying you anything it’s costing you’.
-
-
LEPIS system installation:
-
LEPIS pop-up window According to one GP, this issue was serious enough to affect patient recruitment significantly: ‘I don’t think it pops up with consultations, so I don’t think we’ve hardly recruited anybody. So, it’s a real shame because it was easy when it worked, it was dead easy’.
-
Two GPs reported issues with the clinical IT system Vision while using the LEPIS system. According to one of them, ‘It was very difficult because we are on EMIS-WEB now but we were on Vision and it took a long time to get your software to work. Fortunately, we had a medical student who worked in our office temporarily who was sort of, you know, he was doing a Masters, who was very IT aware and he spent a lot of time talking to Vision and talking to you and trying to get it working and eventually he got it to work, but that was a real pain. And, now we’re on EMIS-WEB we can’t do it all because apparently it doesn’t work with EMIS anyway’. Another issue reported was that the LEPIS system would ‘hang’ while entering the FLU-CATs form and the GP did not use the system for a while because she did not want the screen to hang during a consultation. (EMIS® is an alternative EHR system.)
-
One recommendation to improve the system was to change the point at which LEPIS was triggered: ‘The biggest problem though I think with it is the fact that what triggers LEPIS is the receiving of the code not the selecting of the code and I don’t know, I mean I have said this before, that we use DXS which is the decisions support system and as soon as you select a code it activates DXS but if you select, let’s say flu-like illness, and then you type to the end of your clinical note, you then save it and LEPIS triggers, you’re less likely to want to interact with LEPIS than you would be if as soon as you’ve selected the code flu-like illness, it triggered it. And, that I think is the biggest obstacle to FLU-CAT being done, where it triggers, because you see by the time it triggers you’ve finished the consultation, you’ve finished the recording of the consultation and you either decide am I going to bother going back and doing it. . .I think that’s an inherent problem of the way that LEPIS works at the moment, that you really want to as soon as you put in that somebody’s got I don’t know, haematuria, you want it to bring up your haematuria study. You don’t want it to let you write the whole of your clinical history down and then trigger it’.
-
Another GP had reported technical difficulties with the LEPIS system, and had stopped recruiting for the study and intended to resume once the issue was resolved. The GP remarked that he/she would have found it useful to have received an e-mail or some communication indicating that the issue was resolved, as he/she had realised the system was fixed only on the morning of the telephone interview when checked to see if worked.
-
Despite having experienced difficulties with the LEPIS system, all interviewed GPs acknowledged the support from the CPRD team in helping resolve their technical glitches.
-
According to the CPRD team member responsible for the web development: ‘Yes, I mean it’s a mixed bag really, because you find some practices that are computer literate and some of them not computer literate and so we try to say this is how it works and some of them don’t actually grasp what’s required for the study, so they would say well it’s not working but they haven’t actually done the preliminary installation or for example like, if we take the example of the FLU-CATs, because although there is a wide range of Read-codes, perhaps they put a Read-code which is not in the list, so therefore obviously it would not pop out to say this patient is eligible . . . Yes, I mean you would know more than me when you describe the flu, everyone describes it differently, so that’s why we have included more and more Read-codes because if you. . .what we had done for flu example we say, oh this is only the ten Read-codes, but perhaps another doctor will describe it differently and it is another Read-code, although it means the same thing, but it is another Read-code, so we have worked towards including more Read-codes in order to encompass everything, or we try to educate . . . a big word educate, you can’t educate a doctor. . .but tell them that basically if they want to recruit for this study, if you want to take part, this is the list of the Read-codes. So, therefore there is that communication when you go’.
-
-
Nature of the FLU-CATs consultations:
-
All six GPs agreed that the FLU-CATs consultations were very similar to routine consultations and that the FLU-CATs eCRF was quite easy to complete. One GP said that he/she would fill out the FLU-CATs form after the consultation with the patient and remarked, ‘I supposed I had to make note of the blood pressure, which I might not have done had it been a normal viral illness, you know I wouldn’t necessarily have done that, yes’. Two GPs said they filled out the FLU-CATs forms during the consultation while the patient was still present. According to one of the GPs, ‘One of the main people that did our FLU-CATs study returns has been our nurse practitioner and she quite likes it, it’s quite helpful to her and I mean, I find it’s not at all unhelpful to me, provided I get it triggered at the right time’.
-
-
Readiness of the current FLU-CATs system for use by all GPs in a potential pandemic situation:
-
All six GPs thought that the FLU-CATs system would be ready for use in a pandemic situation with minor modifications. Two GPs expressed the need for the data entry to be better integrated within their clinical system; according to one of them, ‘I mean it doesn’t take that long. It [FLU-CATs form] was a couple of minutes extra I guess to do it compared to doing the consultation without it. So, in the middle of a pandemic that sort of translates to quite a lot of extra work I suppose. If you could capture straight into the notes without having to sort of. . .it’s a bit faffy at the end, sort of copying and pasting and it wasn’t the way the data was dropped into the notes would have been fine but it was pretty much impossible for anyone else to use really. But, otherwise it was okay’.
-
Another GP thought that the lack of pulse oximeters in every consulting room would need to be addressed before the FLU-CATs system is recommended for use in a pandemic situation.
-
All six interviewed GPs said that they would be very interested in reading any outputs that result from the FLU-CATs Study.
-
Phase 2: pilot study data collection, extraction and statistical analysis
To test the existing processes, three outcome measures were studied in the pilot: decision to (1) treat with influenza-specific antiviral drugs; (2) treat with antibiotics; and (3) refer to hospital. The relatively small number of deaths reported (which are well captured in primary care data) meant that we did not have sufficient numbers to look at death as an outcome. The non-availability of ONS data meant that we were not able to explore the causes of the few cases of death that may be unrelated to influenza. Likewise we could not assess the secondary outcome measures (such as admission to critical care or length of hospital stay) expected to be derived from HES data owing to the unavailability of HES data during the pilot phase.
There were 863 unique FLU-CATs observations in total: 704 adult consultations (702 single and two repeated) and 159 child consultations. There were 13 (1.8%) deaths in adults and two (1.3%) in children. GPs decided to refer 11 (1.6%) adults and six (3.8%) children to hospital. In the absence of linked HES and ONS data it is not possible to validate these results or explore the causes of death.
An important finding from the pilot study was that clinical measures are not consistently measured in all patients during consultations. In adult patients, temperature was not measured in 32%, respiratory rate was not measured in 60% and blood pressure was not measured in 74%. Children had a lower proportion of unmeasured values for temperature, at 14%, and respiratory rate, at 43%. Non-measurement was not the same as ‘non-assessment’ by the attending clinician; the ‘not measured’ category in clinical measurements, such as temperature, respiratory rate and heart rate, were further subdivided into ‘not measured as grossly normal’, ‘not measured as grossly abnormal’ and ‘not measured at all’.
In total, five adults, and no children, were prescribed antiviral drugs. A slightly higher proportion of adults (73.0%) were recommended treatment with antibiotics compared with children (69.0%). However, a greater proportion of children (3.8%) were referred to hospital compared with adults (1.6%). Completeness of data entry and a binary description of clinical variables are given in Table 3.
Data item | Adults (n = 704), n (%) | Children (n = 159), n (%) |
---|---|---|
Temperature | ||
Measured | 479 (68.04) | 136 (85.53) |
Not measured | 225 (31.96) | 23 (14.47) |
Not measured | 133 (18.89) | 16 (10.06) |
Grossly normal | 82 (11.65) | 5 (3.14) |
Grossly abnormal | 10 (1.42) | 2 (1.26) |
Respiratory ratea | ||
Measured | 280 (39.77) | 90 (56.60) |
Not measured | 424 (60.23) | 69 (43.40) |
Not measured | 217 (30.82) | 31 (19.50) |
Grossly normal | 201 (28.55) | 38 (23.90) |
Grossly abnormal | 6 (0.85) | 90 (56.60) |
Peripheral oxygen saturationb | ||
Measured | 417 (59.23) | 57 (35.85) |
Not measured | 287 (40.77) | 102 (64.15) |
Heart rate | ||
Measured | 478 (67.9) | 94 (59.12) |
Not measured | 226 (32.1) | 65 (40.88) |
Not measured | 138 (19.60) | 45 (28.30) |
Grossly normal | 86 (12.22) | 20 (12.58) |
Grossly abnormal | 2 (0.28) | 0 (0) |
Blood pressure | ||
Measured | 182 (25.85) | N/A |
Not measured | 522 (74.15) | |
Severe respiratory distress | ||
Yes | 34 (4.83) | 11 (6.92) |
No | 670 (95.17) | 148 (93.08) |
Respiratory exhaustion | ||
Yes | 10 (1.42) | 3 (1.89) |
No | 694 (98.58) | 156 (98.11) |
Severe dehydration | ||
Yes | 0 | 0 (0) |
No | 704 (100) | 100 (100) |
Sternal capillary refill time | ||
Normal | 443 (62.93) | 102 (64.15) |
> 2 seconds | 261 (37.07) | 57 (35.85) |
Patient on oxygen | ||
Yes | 1 (0.14) | 0 (0) |
No | 703 (99.86) | 159 (100) |
Patient on new oxygen | ||
Yes | 0 | 0 |
No | 1 (0.14) | 0 |
N/A | 703 (99.86) | 100 (100) |
New altered consciousness level | ||
No, patient alert | 702 (99.72) | 157 (98.74) |
Confused/agitated | 1 (0.14) | 2 (1.26) |
Responsive to pain only/unconscious (voice for children) | 1 (0.14) | 0 (0) |
Social isolation | ||
Yes | 62 (8.81) | N/A |
No | 609 (86.51) | |
Unknown | 33 (4.69) | |
Activity and ability to self-care | ||
Normal activity, ability to care for self | 531 (75.43) | N/A |
Limited activity, can care for self | 104 (14.77) | |
Housebound, can care for self | 11 (1.56) | |
Housebound, limited self-care | 6 (0.85) | |
Confined, no self-care | 5 (0.71) | |
Not assessed | 47 (6.68) | |
Decision to treat with antivirals | ||
Yes | 5 (0.71) | 0 (0) |
No | 699 (99.29) | 159 (100) |
Decision to treat with antibiotics | ||
Yes | 517 (73.44) | 110 (69.18) |
No | 187 (26.56) | 49 (30.82) |
Decision to refer to hospital | ||
Yes | 11 (1.56) | 6 (3.77) |
No | 693 (98.44) | 153 (96.23) |
Descriptive statistics for children and adults are presented in Table 4. As expected, children were observed to have higher mean and median temperature, respiratory rate and heart rate compared with adults.
Clinical measurements | Adults (n = 704) | Children (n = 159) |
---|---|---|
Temperature (°C) | ||
Mean (SD) | 36.82 (0.71) | 37.23 (1.03) |
Median (IQR) | 36.7 (36.3–37.2) | 37.05 (36.45–37.85) |
Respiratory rate (breaths per minute) | ||
Mean (SD) | 19.69 (5.63) | 24.5 (10.52) |
Median (IQR) | 18 (16–20) | 20 (18–28) |
Peripheral oxygen value (%) | ||
Mean (SD) | 96.94 (2.10) | N/A |
Median (IQR) | 98 (96–98) | |
Heart rate (beats per minute) | ||
Mean (SD) | 83.20 (13.21) | 102.02 (22.73) |
Median (IQR) | 82 (75–90) | 100 (80–120) |
Systolic blood pressure (mmHg) | ||
Mean (SD) | 132.83 (19.32) | N/A |
Median (IQR) | 131.5 (120–143) | |
Diastolic blood pressure (mmHg) | ||
Mean (SD) | 77.11 (10.27) | N/A |
Median (IQR) | 78 (70–84) |
The CATs criterion B (increased respiratory rate) could not be determined in 60.2% of adults and 43.4% of children owing to non-measurement of respiratory rate in these patients. The remaining six CATs criteria were estimated in all patients. Of these, CATs criterion G (other clinical concern) had the highest percentage of patients in both adults (21.0%) and in children (22.0%). CATs criterion F (new altered consciousness) was the least commonly seen criteria, observed in only two adult patients (0.3%) and two children (1.3%). Descriptive analyses for each of the CATs criteria are presented in Table 5, and key clinical concerns relating to CATs criterion G are presented in Table 6 (adults) and Table 7 (children).
Clinical measurements: CATs triage criterion | Adults (n = 704), n (%) | Children (n = 159), n (%) |
---|---|---|
A (severe respiratory distress) | ||
Yes | 34 (4.83) | 11 (6.92) |
No | 670 (95.17) | 148 (93.08) |
B (increased respiratory rate) | ||
Yes | 4 (0.57) | 12 (7.55) |
No | 276 (39.2) | 78 (49.06) |
Not measureda | 424 (60.23) | 69 (43.4) |
C (oxygen saturation of ≤ 92%) | ||
Yes | 15 (2.13) | 7 (4.40) |
No | 689 (97.87) | 152 (95.6) |
D (respiratory exhaustion) | ||
Yes | 10 (1.42) | 3 (1.89) |
No | 694 (98.58) | 156 (98.11) |
E (severe clinical dehydration/shock) | ||
Yes | 10 (1.42) | 5 (3.14) |
No | 694 (98.58) | 154 (96.86) |
F (new altered consciousness) | ||
Yes | 2 (0.28) | 2 (1.26) |
No | 702 (99.72) | 157 (98.74) |
G (other clinical concern) | ||
Yes | 148 (21.02) | 35 (22.01) |
No | 556 (78.98) | 124 (77.99) |
Theme | Frequency (n) |
---|---|
Other (diabetes, heart disease) | 44 |
Clinical signs suggestive of pneumonia (basal crepitations, rhonchi, crackles, decreased air entry and consolidation) | 39 |
Chronic lung disease (particularly asthma and chronic obstructive pulmonary disease) | 29 |
Chest pain (pleuritic chest wall pain) | 11 |
Sputum | 7 |
Wheezing | 6 |
Immunosuppression (long-term oral steroid use, other immunosuppressive treatment and conditions, such as sarcoidosis, which affect the immune system) | 6 |
Prolonged cough (> 4 weeks) | 3 |
Deterioration of symptoms | 1 |
Malignancy | 1 |
Theme | Frequency (n) |
---|---|
Clinical signs suggestive of pneumonia | 18 |
Other | 9 |
Chest related | 4 |
Cough | 2 |
Asthma | 1 |
Immunocompromised | 1 |
The association between Community Assessment Tools criteria and outcomes of interest
The distribution of events across various criteria and covariates resulted in a low number of events in some categories despite the pilot study reaching nearly 86% of the a priori sample size estimates, and so running a fully adjusted logistic regression model (adjusting for all confounders) was not possible. Therefore, an unadjusted and a multivariable model including all of the seven CATs criteria, adjusted for each other, was run. Generally, because the data were sparse, wide 95% CIs and variable omissions (from the analyses) were seen. Results from the children’s analyses are presented in Table 8 and results from the adults’ analyses are presented in Table 9.
Outcome | Unadjusted | Adjusteda | |||
---|---|---|---|---|---|
OR (95% CI) | p-value | OR (95% CI) | p-value | ||
1. Decision to treat with antivirals | |||||
CATs criterion | A | n/a | n/a | n/a | n/a |
B | n/a | n/a | n/a | n/a | |
C | n/a | n/a | n/a | n/a | |
D | n/a | n/a | n/a | n/a | |
E | n/a | n/a | n/a | n/a | |
F | n/a | n/a | n/a | n/a | |
G | n/a | n/a | n/a | n/a | |
2. Decision to treat with antibiotics | |||||
CATs criterion | A | 0.51 (0.15 to 1.75) | 0.2832 | 2.96 (0.12 to 70.38) | 0.5021 |
B | 0.30 (0.09 to 1.05) | 0.0587 | 0.14 (0.03 to 0.81) | 0.0282 | |
C | 2.77 (0.32 to 23.64) | 0.3519 | n/a | n/a | |
D | 0.89 (0.08 to 10.04) | 0.9241 | 0.13 (0.002 to 8.35) | 0.3392 | |
E | n/a | n/a | n/a | n/a | |
F | n/a | n/a | n/a | n/a | |
G | 10.07 (2.31 to 43.92) | 0.0021 | 7.54 (1.12 to 50.92) | 0.0382 | |
3. Decision to refer to hospital | |||||
CATs criterion | A | 8.00 (1.29 to 49.68) | 0.0256 | 2.89 (0.02 to 397.44) | 0.6731 |
B | 15.40 (1.28 to 185.61) | 0.0313 | 5.19 (0.12 to 234.07) | 0.3966 | |
C | 14.80 (2.18 to 100.66) | 0.0059 | 39.12 (0.55 to 2759.05) | 0.0913 | |
D | 15.10 (1.67 to 195.43) | 0.0377 | 19.77 (0.07 to 5498.53) | 0.2986 | |
E | 7.45 (0.70 to 79.35) | 0.0961 | n/a | n/a | |
F | 30.40 (1.65 to 558.88) | 0.0215 | n/a | n/a | |
G | 3.78 (0.73 to 19.63) | 0.1135 | 1.36 (0.03 to 72.39) | 0.8808 |
Outcome | Unadjusted | Adjusteda | |||
---|---|---|---|---|---|
OR (95% CI) | p-value | OR (95% CI) | p-value | ||
1. Decision to treat with antivirals | |||||
CATs criterion | A | 5.05 (0.55 to 46.41) | 0.1528 | 5.64 (0.53 to 59.97) | 0.1513 |
B | n/a | n/a | 0.99 (0.79 to 1.24) | 0.915 | |
C | n/a | n/a | n/a | n/a | |
D | n/a | n/a | n/a | n/a | |
E | n/a | n/a | n/a | n/a | |
F | 174.5 (9.22 to 3303.91) | < 0.001 | n/a | n/a | |
G | 15.42 (1.71 to 139.00) | 0.0148 | 9.88 (0.96 to 101.49) | 0.0539 | |
2. Decision to treat with antibiotics | |||||
CATs criterion | A | 1.73 (0.70 to 4.24) | 0.2329 | 1.19 (0.44 to 3.22) | 0.7269 |
B | 0.89 (0.86 to 0.93) | < 0.001 | 0.90 (0.87 to 0.94) | < 0.001 | |
C | 1.46 (0.41 to 5.22) | 0.5630 | 0.96 (0.22 to 4.15) | 0.9567 | |
D | n/a | n/a | n/a | n/a | |
E | 0.54 (0.15 to 1.92) | 0.3310 | 0.49 (0.12 to 1.99) | 0.3178 | |
F | 0.36 (0.02 to 5.79) | 0.4714 | 0.09 (0.004 to 1.87) | 0.1201 | |
G | 6.44 (3.31 to 12.55) | < 0.001 | 6.42 (3.17 to 12.99) | < 0.001 | |
3. Decision to refer to hospital | |||||
CATs criterion | A | 28.5 (8.20 to 99.04) | < 0.001 | 26.84 (5.62 to 128.05) | < 0.001 |
B | 0.90 (0.78 to 1.03) | 0.1303 | 0.98 (0.82 to 1.17) | 0.8272 | |
C | 35.43 (9.04 to 138.77) | < 0.001 | 11.67 (1.68 to 81.16) | 0.0130 | |
D | n/a | n/a | n/a | n/a | |
E | 36.75 (8.03 to 168.29) | < 0.001 | 48.99 (6.06 to 396.32) | < 0.001 | |
F | 69.20 (4.04 to 1185.94) | 0.0034 | 5.86 (0.003 to 11240.73) | 0.6467 | |
G | 3.21 (0.96 to 10.65) | 0.0573 | 2.51 (0.55 to 11.46) | 0.2350 |
Outcome 1: decision to treat with influenza-specific antiviral drugs
Given that only five adult patients were prescribed influenza-specific antiviral drugs, odds ratios (ORs) and 95% CIs could be obtained for only three criteria in the unadjusted and adjusted analyses. CATs criteria F and G showed strong positive associations (OR 174.5, 95% CI 9.2 to 3303.9 and OR 15.4, 95% CI 1.7 to 139.0, respectively) in the adjusted analyses, but none of the associations was statistically significant in the adjusted analyses.
None of the participating GPs decided to treat any of the recruited children with influenza-specific antiviral drugs, so no further analysis for this outcome was possible for this group.
Outcome 2: decision to treat with antibiotics
In adults, CATs criteria B and G were statistically significantly associated with this outcome in the unadjusted analyses, and remained statistically significant with consistent estimates in adjusted analyses. After adjusting for other CATs criteria, adults presenting with CATs criterion A showed a 10% decreased odds (95% CI 0.9 to 0.9) of being treated with antibiotics and those presenting with CATs criterion G were associated with an increased odds of being treated with antibiotics (OR 6.4, 95% CI 3.2 to 13.0).
In children, although CATs criterion G showed a 10-fold increase in odds of treatment with antibiotics (OR 10.1, 95% CI 2.3 to 43.9) in the unadjusted analyses, this was not seen to be statistically significant after adjustment for other CATs criteria.
Outcome 3: referral to hospital
In adults, CATs criteria A, C, E and F were positively and statistically significantly associated with referral to hospital in the unadjusted analyses. CATs criteria C and E remained statistically significant in the adjusted analyses, with increase in odds of hospital referral by 11.7 times (95% CI 1.7 to 81.2 times) and 49.0 times (95% CI 6.1 to 396.3 times), respectively.
In children, CATs criteria A, D and F were significantly associated with the outcome, although statistical significance was lost after adjustment for other CATs criteria.
Monthly data findings
Background variables including comorbidities and select treatments/vaccinations were extracted from the CPRD database for patients with FLU-CATs consultations (coded as ever having had a record of the comorbidity/treatment/vaccination before the FLU-CATs consultation date). In the 861 total of FLU-CATs patients, respiratory disease (25.9%) was the most commonly seen comorbidity, followed by diabetes (8.6%), renal disease (6.6%), cardiovascular disease (5.1%), neurological disease (2.2%), liver disease (0.4%) and immunosuppression (0.1%). Distribution of comorbidities in adults and children is presented in Table 10.
Comorbidity | Adult (N = 702a), n (%) | Children (N = 159), n (%) |
---|---|---|
Cardiovascular | 41 (4.58) | 3 (1.89) |
Liver | 3 (0.43) | 0 (0) |
Neurological | 17 (2.42) | 2 (1.26) |
Renal | 44 (6.27) | 13 (8.18) |
Respiratory | 209 (29.77) | 14 (8.81) |
Diabetes | 73 (10.40) | 1 (0.63) |
Immunosuppression | 1 (0.14) | 0 (0) |
Overall, 29.9% of all patients had been prescribed antibiotics, whereas only 0.9% had been prescribed influenza-specific antiviral drugs at some point before the FLU-CATs consultation. A total of 15.9% of all patients had past records of seasonal influenza vaccinations. Distribution of treatments and vaccinations in adults and children is presented in Table 11.
Drug/vaccination | Adult (N = 702a), n (%) | Children (N = 159), n (%) |
---|---|---|
Statin | 198 (28.21) | N/A |
Antibiotic | 245 (34.90) | 12 (7.55) |
Antiviral | 7 (1.00) | 1 (0.63) |
Inhaled steroids | 146 (79.20) | 9 (5.66) |
Oral steroids | 248 (35.33) | 32 (20.13) |
Seasonal influenza vaccine | 122 (17.38) | 15 (9.43) |
Hib vaccine | 2 (0.28) | 0 (0) |
Pneumococcal vaccine | 6 (0.85) | 0 (0) |
Comparison of Pandemic Medical Early Warning Score with Community Assessment Tools (adults only)
Given the large number of unmeasured/missing clinical measurements, PMEWS scores could only be calculated for a small proportion of adult patients in whom all necessary measurements were recorded.
Table 12 shows a comparison of the PMEWS score with the CATs score for the outcomes ‘decision to refer to hospital’ and ‘death’. Data in this pilot study are too sparse for inferential statistics.
Scores | Refer to hospital (N = 11), n (%) | Death (N = 13), n (%) | ||
---|---|---|---|---|
Yes | No | Yes | No | |
PMEWS | ||||
1 | 0 (0) | 10 (1.44) | 0 (0) | 10 (1.45) |
2 | 1 (9.09) | 14 (2.02) | 0 (0) | 15 (2.17) |
3 | 0 (0) | 15 (2.17) | 0 (0) | 15 (2.17) |
4 | 0 (0) | 7 (1.01) | 1 (7.69) | 6 (0.87) |
5 | 0 (0) | 8 (1.16) | 0 (0) | 8 (1.16) |
6 | 0 (0) | 2 (0.29) | 1 (7.69) | 1 (0.14) |
7 | 0 (0) | 1 (0.14) | 0 (0) | 1 (0.14) |
9 | 1 (9.09) | 1 (0.14) | 0 (0) | 2 (0.29) |
14 | 1 (9.09) | 0 (0) | 1 (7.69) | 0 (0) |
Missing | 8 (72.73) | 635 (91.63) | 10 (76.93) | 633 (91.61) |
CATs | ||||
0 | 2 (18.18) | 171 (24.68) | 4 (30.78) | 169 (24.46) |
1 | 0 (0) | 86 (12.41) | 0 (0) | 86 (12.45) |
2 | 3 (27.27) | 12 (1.73) | 2 (15.38) | 13 (1.88) |
3 | 0 (0) | 4 (0.58) | 0 (0) | 4 (0.58) |
4 | 2 (18.18) | 0 (0) | 1 (7.69) | 1 (0.14) |
Missing | 4 (36.37) | 420 (60.60) | 6 (46.15) | 418 (60.49) |
Automated weekly and monthly report generation
Stata ‘do files’ have been written to automate the logistic regression analyses and reporting of the results. The weekly and monthly ‘do files’ are described in detail in the FLU-CATs Handbook (see Appendix 6).
The weekly results are saved into an Excel spreadsheet with three worksheets – CATs criteria, clinical data and regression results. Sample screenshots of the weekly report are provided in Figure 7.
Monthly reports are saved as a RTF Word document containing plots and tables with frequencies of background CPRD data on comorbidities and treatments. A screenshot of a sample monthly report document in presented in Figure 8.
FIGURE 7.
Sample screenshots of the weekly automated spreadsheet.
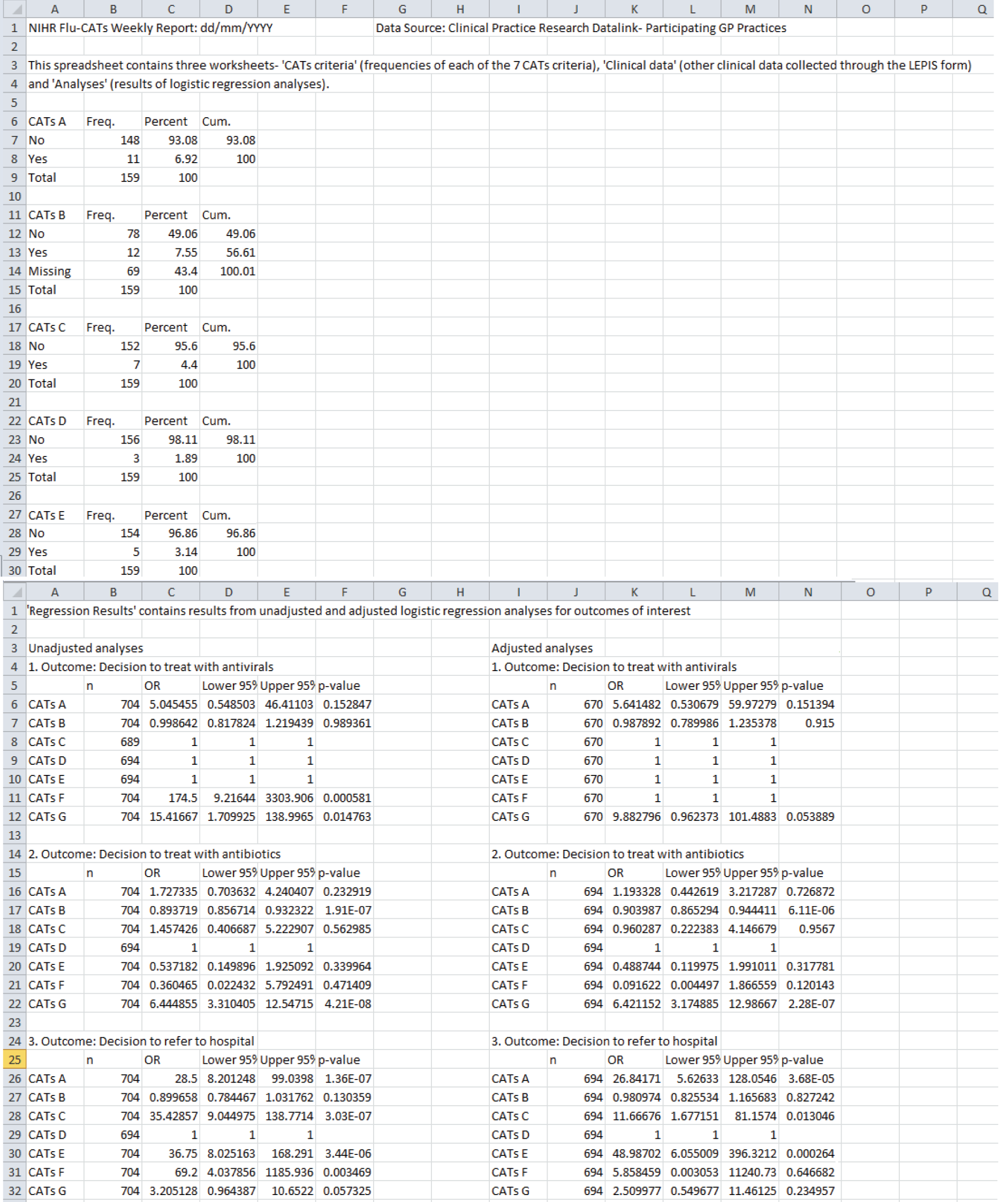
FIGURE 8.
Sample screenshots of the monthly automated report document.

Chapter 5 Discussion
Lessons learnt
Phase 1
The CPRD FLU-CATs team identified a number of technical and practical issues during the course of the feasibility study. These were captured via one-to-one interviews with the study team members and are listed below:
-
Monetary incentives would be necessary to increase GP participation unless there was a statutory requirement to systematically collect data on all possible influenza cases during a pandemic.
-
Funding for system maintenance and updating between study phases was not requested in the original grant. There is a cost associated with keeping the system updated and compliant with the evolving technological infrastructure, and to facilitate rapid re-activation in case of a pandemic.
-
External issues: bureaucratic processes, which are expected to be resolved in time, stalled provision by the HSCIC of contemporary HES/ONS linked data. Access to virology data from PHE is unlikely to be resolved because of lack of adherence to data standards by those requesting virology swabs tests in the community. Collaboration with the RCGP Research and Surveillance Centre may provide linked–anonymised virology surveillance data at a practice level and possibly at patient level.
-
Briefing of GPs on the study and LEPIS software installation: installing the LEPIS software was generally seen as being quite difficult and time intensive for practice staff. Many practices required regular technical advice from CPRD on this matter. Dedicated IT support is required to aid GPs at set-up.
-
LEPIS did not install at some of the practices, so these GPs were not able to participate in the study.
-
The reorganisation of primary health care from Primary Health Care Trusts to Clinical Commissioning Groups was associated with preferences for which EHRs are used across Clinical Commissioning Groups. The popularity of Vision appears to be declining in favour of EMIS. It is estimated that 50% of GPs now use EMIS. LEPIS does not work on EMIS and so an alternative solution will need to be developed. Thus, most importantly, to conduct the study in a pandemic situation we would need a flagging system that could work across several clinical IT systems.
-
Any decision aid based on the validated criteria would better be delivered separately on an open web-based platform or mobile phone ‘app’.
Phase 2
The two main lessons learnt regarding data analyses relate to missing data, clinical data not being measured and the delays in obtaining HES and ONS linkage data.
-
Missing clinical measurements Up to 74% of some clinical measurements (blood pressure in adults in this instance), were not recorded as part of GPs’ routine assessment of an adult person presenting with ILI. We would question the utility and adoption of triage tools that depend upon a clinical measurement that is not used in the routine assessment of ILI for use in a time-pressured pandemic situation. During the early phases of the pilot study, the low recording of clinical measurements prompted the researchers to modify the form to include two further categories: ‘not measured – grossly normal’ and ‘not measured – grossly abnormal’. This was based on informal feedback that the clinical examination did consider factors such as fever, respiratory rate, blood pressure, etc., but that formal measurements were triggered only if they appeared to be grossly abnormal. Our study was not an interventional study and therefore tried to approximate the ‘routine’ clinical consultation. Although failure of exact measurements resulted in poor recording of PMEWS criteria, we were able to derive the CATs criteria for the majority of patients. Therefore, it is reasonable to assume that CATs offers a more user-friendly and efficient option for GPs over a more data-intensive triage tool such as PMEWS.
-
Linkage with HES and ONS In the absence of contemporary linked data from HES and ONS, it was not possible to prospectively validate the primary outcomes (hospital admission and death) or study secondary outcomes (e.g. admission to intensive care, length of hospital stay) or examine cause of death. However, it is important to note that the CPRD primary care data routinely records death data and hospital admissions, so while there may be a slight underestimation of these data, it would still be possible to study these outcomes using primary care data alone (although possibly with a 1-month lag without active follow-up of flagged patients).
The LEPIS software and accompanying web-based data collection form have generally been regarded as being quick and easy to use after the initial set-up, taking an experienced user about a minute to complete. However, the research team has identified a number of limitations. One is that the LEPIS software is specific to GP practices that use the Vision system and incompatible with GP practices using EMIS, SystmOne® (Horsforth, Leeds, UK) or other EHR systems. Although the popularity of Vision is reducing, there are still about 650 practices using this system and contributing to CPRD. If all of these GPs could be deployed in the event of a pandemic, there could be a population-based surveillance system providing near real-time reports of the course of the pandemic. There were technical problems with compatibility of the LEPIS software with some configurations of the GP computer systems, which need to be resolved. Moreover, although being very easy to use, LEPIS was seen as being quite difficult to set up, and technical assistance was necessary to help GPs to install the system. Both of these points are important limitations that would prevent a rapid national level rollout using the existing LEPIS software to trigger data collection. A decision aid based on the validated criteria would better be delivered separately on an open web-based platform or mobile phone ‘app’.
A lack of any financial incentive was cited by several practices as a reason for not participating. Several GPs felt that even a minimal payment of a few pounds would be sufficient remuneration for the research activity. We will add a small payment for each case submitted in future seasons.
This study is one of the UK NIHR portfolio of pandemic preparedness studies. The investigators are funded to establish capacity and processes during an inter-pandemic period in readiness to run the study during the early stages of a future outbreak of pandemic influenza or other outbreak of severe acute respiratory infection of public health concern. Our results show that a web-based triage tool, which uses simple clinical assessments, can be used with ease during routine consultations, and that linkage to the patient’s EHR with automated background processes can be used to define the performance of triage criteria triage tool against outcome events. Using the processes that we have developed, the demonstration of the proof of concept indicates that the study will be of value during the early phases of a novel pandemic and capable of providing a valid triage tool in readiness for surge. If surge occurs, the web-based process could be switched from data collation to provide a decision aid. This would best be provided on common web-based platforms or mobile phone ‘apps’.
To scale up the study during a pandemic period will be challenging, as we recognise that about half of willing CPRD practices in the pilot had difficulties installing the LEPIS software, and half of all practices in England and Wales use a different EHR system. That said, the EMIS system also has capability to monitor consultations and trigger to structured data entry forms. The investigators are in negotiation with EMIS to develop a solution for that system.
This study adheres to the five principles of Dynamic Risk Assessment, as applied to the management of emergency situations by UK Government agencies (Evaluate, Select, Assess, Refine, Reassess). 37 That said, the proposed use of triage tools by the NHS in the UK during pandemic surge has been criticised, in particular because of lack of evidence of engagement with the general population and with local ethics committees. 38
The data collection period of the study to date covers two calendar years, including the set-up and pilot in sequential winter seasons. At most, only 25 GPs were recruiting cases. PHE described influenza activity in the UK as low in 2013–14 and moderate in 2014–15. 39 Perhaps as a result of these factors, and the low-to-moderate influenza activity, only a few of the 863 patients had primary outcomes of interest (death n = 15 and referral to hospital for admission n = 17).
It is possible that GPs chose to ignore the LEPIS prompts when patients with severe illness presented, thus introducing bias to the study. However, the hospital referral rate observed for this study of 19.7 per 1000 consultations is within the ranges quoted for several larger studies that examine variation in GP referral rates in the UK. 40
A potential limitation of studies such as this relates to possible misclassification of criteria as a result of clinicians’ preference to record only certain important negative or positive features. We avoided misclassification of data seen in our previous studies by use of required data entry fields with exclusive options.
This was a non-interventional study. Although the clinical assessment data collection tool (eCRF) was structured to capture evidence-based criteria, recognised in national guidance, it did not require the practitioner to make any change in his/her normal assessment – only to record the assessment. We thought that the presentation of a structured assessment form may result in personal reflection on the nature of the GP’s own assessment and so alter the completeness of future assessments. We were therefore surprised to find that some clinical measures are not consistently taken with many patients during consultations. Three-quarters of adults did not have their blood pressure taken, and 60% of adults and 43% of children did not have their respiratory rate measured. Our data collection system did allow GPs to record some clinical assessments, such as respiratory rate, as ‘not measured as grossly normal’ and ‘not measured as grossly abnormal’, which reflects pragmatic or usual practice. This finding is important, as it strongly suggests that criteria that rely upon a measurement such as blood pressure, and which is not being used consistently in routine assessment of ILI, should not be included in a triage tool that is designed for use during surge, when time and resources are in even shorter supply.
Denial of access to linked HES and ONS data by the HSCIC has meant that, at the time of writing, it has not been possible to validate the primary outcomes (admission to hospital and death) or secondary outcomes (admission to intensive care and length of hospital stay). A retrospective analysis of the existing data will be possible when the linked data are made available.
Chapter 6 Conclusions
The use of EHRs linked to background analytical processes allows near real-time analysis of GP assessments, management decisions and patients’ outcomes. The processes are dynamic and should allow refinement of triage criteria in the early stages of a future outbreak.
To be prepared, the study is continuing in pilot phase, as it is essential to maintain processes in an environment where EHRs and database processes are continually evolving. Future work will include exploring if minimal remuneration will improve recruitment, development of processes to include other EHR systems; and attempting to link to data on influenza surveillance at a practice and patient level.
Acknowledgements
We wish to acknowledge the following GPs for their cooperation in the project: Dr Jitendra Patel, Kings Langley Surgery, Kings Langley; Dr Hanif Rahim, St Bartholomew’s Medical Centre, Oxford; Dr Iain Jarvis, London Medical Practice, Gloucester; Dr Robert Moore, Greenford Road Medical Centre, Greenford; Dr John Edwards, Wolstanton Medical Centre, Newcastle Staffs; Dr Boghossian Tighe & Dr John Pittard, Staines Thameside Medical, Staines; Dr Peter Martin, Stifford Clays Health Centre, Grays; Dr Michael Heber, Cobtree Medical Practice, Maidstone; Dr Elizabeth Alborough, Barton Surgery, Dawlish; Dr Kevin Douglas, Claremont Medical Practice, Exmouth; Dr Gina Johnson, Kingfisher Practice, Luton; Dr Lindsey Compson, The Mauve Practice, Perth; Dr Tommy Hunter, Barns Medical Practice, Ayr; Dr Robin Fox, The Health Centre, Bicester; Dr James O’Donnell, Park House Surgery, St Helens; Dr Jonathan Unwin, Rosebank Surgery, Gloucester; Dr Andrew Freeman, Dartmouth Medical Practice, Dartmouth; Dr Marc Jacobs, Edzell Health Centre, Edzell; and Dr Monique King, Cherrymead Surgery, Loudwater. We also wish to acknowledge the contribution of 11 other GPs who contributed to the study.
Contribution of authors
Malcolm G Semple, Puja R Myles and Jonathan S Nguyen Van Tam conceived the study.
Jamie J Kirkham advised on the protocol and statistical analysis plan.
Malcolm G Semple with Tjeerd Pieter van Staa, and later Malcolm G Semple with Timothy J Williams, managed the project.
Sudhir Venkatesan and Puja R Myles wrote the automated statistical analysis scripts and conducted data analysis.
Malcolm G Semple, Sudhir Venkatesan and Puja R Myles drafted the report with input from all other authors.
Data sharing statement
Access to the CPRD data is available subject to a licence agreement containing details, terms and conditions of use and charges. Data Governance arrangements around access to data are approved and reviewed annually by the Confidentiality Advisory Group of the Health Research Authority. Among the key principles are that provision of data is for use for public-benefiting medical research only; attempts to identify patients, practices or clinicians is specifically prohibited and an ISAC protocol is required for all research that is, in any way, to be communicated to others. Please contact the CPRD Knowledge Centre for more information (kc@cprd.com).
Disclaimers
This report presents independent research funded by the National Institute for Health Research (NIHR). The views and opinions expressed by authors in this publication are those of the authors and do not necessarily reflect those of the NHS, the NIHR, NETSCC, the HTA programme or the Department of Health. If there are verbatim quotations included in this publication the views and opinions expressed by the interviewees are those of the interviewees and do not necessarily reflect those of the authors, those of the NHS, the NIHR, NETSCC, the HTA programme or the Department of Health.
References
- Report on the Pandemic of Influenza, 1918–19. London: His Majesty’s Stationery Office; 1920.
- Pandemic Flu: Management of Demand and Capacity in Healthcare Organisations. London: Her Majesty’s Stationery Office; 2009.
- Keramarou M, Cottrell S, Evans M, Moore C, Stiff R, Elliott C, et al. Two waves of pandemic influenza A (H1N1) 2009 in Wales: the possible impact of media coverage on consultation rates, April–December 2009. Euro Surveill 2011;16.
- Department of Health . Launch of the National Pandemic Flu Service 2009. http://webarchive.nationalarchives.gov.uk/+/www.dh.gov.uk/en/Publichealth/Flu/Swineflu/DH_102909 (accessed 11 August 2015).
- Lim WS. Pandemic flu: clinical management of patients with an influenza-like illness during an influenza pandemic. Thorax 2007;62:1-46. http://dx.doi.org/10.1136/thx.2006.073080.
- Department of Health . NHS Emergency Planning Guidance 2005: Underpinning Materials – Critical Care Contingency Planning in the Event of an Emergency Where the Numbers of Patients Substantially Exceeds Normal Critical Care Capacity 2007. www.dh.gov.uk/en/Publicationsandstatistics/Publications/PublicationsPolicyAndGuidance/DH_081282 (accessed 11 August 2015).
- Lim WS, van der Eerden MM, Laing R, Boersma WG, Karalus N, Town GI, et al. Defining community acquired pneumonia severity on presentation to hospital: an international derivation and validation study. Thorax 2003;58:377-82. http://dx.doi.org/10.1136/thorax.58.5.377.
- Challen K, Bentley A, Bright J, Walter D. Clinical review: mass casualty triage – pandemic influenza and critical care. Crit Care 2007;11. http://dx.doi.org/10.1186/cc5732.
- Lim WS, Baudouin SV, George RC, Hill AT, Jamieson C, Le Jeune I, et al. BTS Guidelines for the management of community acquired pneumonia in adults: update 2009. Thorax 2009;64:iii1-iii55. http://dx.doi.org/10.1136/thx.2009.121434.
- Mulrennan S, Tempone SS, Ling ITW, Williams SH, Gan G-C, Murray RJ, et al. Pandemic influenza (H1N1) 2009 pneumonia: CURB-65 score for predicting severity and nasopharyngeal sampling for diagnosis are unreliable. PLOS ONE 2010;5. http://dx.doi.org/10.1371/journal.pone.0012849.
- Challen K, Bright J, Bentley A, Walter D. Physiological-social score (PMEWS) vs. CURB-65 to triage pandemic influenza: a comparative validation study using community-acquired pneumonia as a proxy. BMC Health Serv Res 2007;7. http://dx.doi.org/10.1186/1472-6963-7-33.
- Department of Health and NHS . Swine Flu Clinical Package for Use When There Are Exceptional Demands on Healthcare Services n.d. http://webarchive.nationalarchives.gov.uk/20130107105354/http://www.dh.gov.uk/en/Publicationsandstatistics/Publications/PublicationsPolicyAndGuidance/DH_106495 (accessed 11 August 2015).
- British Thoracic Society . BTS guidelines for the management of community acquired pneumonia in childhood. Thorax 2002;57:i1-24. http://dx.doi.org/10.1136/thx.57.suppl_1.i1.
- BTS Guidelines for the Management of Community Acquired Pneumonia (CAP) in Adults. London: BTS; 2004.
- Chronic Obstructive Pulmonary Disease: Management of Chronic Obstructive Pulmonary Disease in Adults in Primary and Secondary Care. London: Royal College of Physicians of London; 2004.
- O’Driscoll BR, Howard LS, Davison AG. BTS guideline for emergency oxygen use in adult patients. Thorax 2008;63:vi1-68. http://dx.doi.org/10.1136/thx.2008.102947.
- Technical Bases for the Management of Pneumonia in Children at First-level Health Facilities. Geneva: WHO; 1991.
- Management of the Child with Cough or Difficult Breathing. WHO/ARI/9431. Geneva: WHO; 1994.
- Child and Adolescent Health and Development: IMCI Technical Seminars. WHO/FCH/CAH/0110. Geneva: WHO; 2001.
- Evidence Base for the Community Management of Pneumonia. Geneva: WHO; 2002.
- Bronchiolitis in Children. SIGN Guideline 91. Edinburgh: SIGN; 2006.
- Welsh A. Feverish Illness in Children: Assessment and Initial Management in Children Younger than 5 Years. London: RCOG Press; 2007.
- Goodacre S, Challen K, Wilson R, Campbell M. Evaluation of triage methods used to select patients with suspected pandemic influenza for hospital admission: cohort study. Health Technol Assess 2010;14. http://dx.doi.org/10.3310/hta14460-03.
- Challen K, Goodacre SW, Wilson R, Bentley A, Campbell M, Fitzsimmons C, et al. Evaluation of triage methods used to select patients with suspected pandemic influenza for hospital admission. Emerg Med J 2012;29:383-8. http://dx.doi.org/10.1136/emj.2010.104380.
- Gray JT, Challen K, Oughton L. Does the pandemic medical early warning score system correlate with disposition decisions made at patient contact by emergency care practitioners?. Emerg Med J 2010;27:943-7. http://dx.doi.org/10.1136/emj.2009.072959.
- Myles PR, Semple MG, Lim WS, Openshaw PJM, Gadd EM, Read RC, et al. Predictors of clinical outcome in a national hospitalised cohort across both waves of the influenza A/H1N1 pandemic 2009–2010 in the UK. Thorax 2012;67:709-17. http://dx.doi.org/10.1136/thoraxjnl-2011-200266.
- Myles PR, Nguyen-Van-Tam JS, Lim WS, Nicholson KG, Brett SJ, Enstone JE, et al. Comparison of CATs, CURB-65 and PMEWS as triage tools in pandemic influenza admissions to UK hospitals: case control analysis using retrospective data. PLOS ONE 2012;7. http://dx.doi.org/10.1371/journal.pone.0034428.
- Viboud C, Miller M, Olson DR, Osterholm M, Simonsen L. Preliminary estimates of mortality and years of life lost associated with the 2009 A/H1N1 pandemic in the US and comparison with past influenza seasons. PLOS Curr 2010;2. http://dx.doi.org/10.1371/currents.rrn1153.
- NIHR Clinical Research Network Urgent Public Health Risk Planning. London: NIHR; 2014.
- Hine D. The 2009 Influenza Pandemic: An Independent Review of the UK Response to the 2009 Influenza Pandemic. London: Cabinet Office, Her Majesty’s Government; 2010.
- Kousoulis AA, Rafi I, de Lusignan S. The CPRD and the RCGP: building on research success by enhancing benefits for patients and practices. Br J Gen Pract 2015;65:54-5. http://dx.doi.org/10.3399/bjgp15X683353.
- Van Staa T-P, Dyson L, McCann G, Padmanabhan S, Belatri R, Goldacre B, et al. The opportunities and challenges of pragmatic point-of-care randomised trials using routinely collected electronic records: evaluations of two exemplar trials. Health Technol Assess 2014;18. http://dx.doi.org/10.3310/hta18430.
- Prasad V, Atkinson O, Knox R, Myles PR, Nakafero G. Consultation with General Practitioners on Coding Preferences in Electronic Primary Care Patient Records. Nottingham: University of Nottingham; 2011.
- Braun V, Clarke V. Using thematic analysis in psychology. Qualitative Research in Psychology 2006;3:77-101. http://dx.doi.org/10.1191/1478088706qp063oa.
- Obuchowski NA, Lieber ML, Wians FH. ROC curves in clinical chemistry: uses, misuses, and possible solutions. Clin Chem 2004;50:1118-25. http://dx.doi.org/10.1373/clinchem.2004.031823.
- Obuchowski NA. Sample size tables for receiver operating characteristic studies. Am J Roentgenol 2000;175:603-8. http://dx.doi.org/10.2214/ajr.175.3.1750603.
- Generic Risk Assessments. London: The Stationery Office; 2009.
- Tillyard ARJ, Slowthe A. Pandemic triage: does the public know what this means?. BMJ 2009;339. www.bmj.com/rapid-response/2011/11/02/pandemic-triage-does-public-know-what-means (accessed 11 August 2015).
- Surveillance of Influenza and Other Respiratory Viruses in the United Kingdom: Winter 2013/14. London: PHE Publications; 2014.
- O’Donnell CA. Variation in GP referral rates: what can we learn from the literature?. Fam Pract 2000;17:462-71. http://dx.doi.org/ 10.1093/fampra/17.6.462.
Appendix 1 List of Read codes used to identify potential influenza cases in Hospital Episode Statistics data
H2z..00 Pneumonia or influenza NOS |
H2..00 Pneumonia and Influenza |
16L..00 Influenza-like symptoms |
H2y..00 Other specified pneumonia or influenza |
H2A..11 Influenza A(H1N1) swine flu |
H2A..00 Influenza due to Influenza A virus subtype H1N1 |
4JU0.00 Influenza H1 virus detected |
H051. Acute upper respiratory tract infection |
H05z. Upper respiratory tract infection (URTI) |
1W0..00 Possible Influenza A virus H1N1 subtype |
1J72.11 Suspected swine influenza |
1J72.00 Suspected Influenza A virus subtype H1N1 infection |
Hyu0700 [X] Influenza + other manifestations, virus not identified |
Hyu0600 [X] Influenza + other respiratory manifestations, virus not identified |
43k2.00 Influenza A antigen level |
43dF.00 Influenza A antibody level |
G520300 Acute myocarditis – influenza |
4JU4.00 Influenza A virus, other or untyped strain detected |
H29..00 Avian influenza |
Hyu0500 [X] Influenza + other manifestations, influenza virus identified |
H27.. Influenza |
H27z.12.Influenza like illness (ILL) |
H27y100 Influenza with gastrointestinal tract involvement |
H271z00 Influenza with Respiratory manifestations |
H270000 Influenza with bronchopneumonia |
H271000 Influenza with laryngitis |
H271100 Influenza with pharyngitits |
H270.00 Influenza with pneumonia |
H270z00 Influenza with pneumonia NOS |
H270.11 Chest infection – influenza with pneumonia |
H271.00 Influenza with other respiratory manifestation |
H27y.00 Influenza with other manifestations |
H27yz00 Influenza with other manifestations NOS |
H27y000 Influenza with encephalopathy |
4JU5.00 Influenza B virus detected |
43k3.00 Influenza B antigen level |
H270100 Influenza with pneumonia, influenza virus identified |
4JU2.00 Influenza H3 virus detected |
4JU3.00 Influenza H5 virus detected |
4J3M.00 Influenza A virus H1N1 subtype not detected |
Appendix 2 Influenza-related Read codes used by general practitioners for patient assessment prior to an admission to hospital with a discharge diagnosis of influenza and chosen to trigger Local Eligibility Patient Identification Software
Rank | Read code | Read code descriptor | Patients, n = 471 | Frequency (%) |
---|---|---|---|---|
1 | H27z.11 | Flu-like illness | 72 | 15.5 |
2 | H27..00 | Influenza | 70 | 15.0 |
3 | H06z011 | Chest infection | 60 | 12.9 |
4 | H2A..11 | Influenza A (H1N1) swine flu | 52 | 11.2 |
5 | H06z000 | Chest infection NOS | 41 | 8.8 |
6 | H26..00 | Pneumonia due to unspecified organism | 27 | 5.8 |
7 | H061.00 | Acute bronchiolitis | 19 | 4.1 |
8 | H06z100 | Lower resp tract infection | 14 | 3.0 |
9 | H2y..00 | Other specified pneumonia or influenza | 12 | 2.6 |
10 | H2z..00 | Pneumonia or influenza NOS | 11 | 2.4 |
11 | 16L..00 | Influenza-like symptoms | 9 | 1.9 |
12 | H06z111 | Respiratory tract infection | 8 | 1.7 |
13 | 1J72.11 | Suspected swine influenza | 7 | 1.5 |
14 | H2...00 | Pneumonia and influenza | 7 | 1.5 |
15 | H2A..00 | Influenza due to Influenza A virus subtype H1N1 | 7 | 1.5 |
16 | H062.00 | Acute lower respiratory tract infection | 6 | 1.3 |
17 | H21..00 | Lobar (pneumococcal) pneumonia | 6 | 1.3 |
18 | H27z.00 | Influenza NOS | 5 | 1.1 |
19 | H25..00 | Bronchopneumonia due to unspecified organism | 4 | 0.9 |
20 | 1W0..00 | Possible influenza A virus H1N1 subtype | 3 | 0.6 |
21 | H0...00 | Acute respiratory infections | 3 | 0.6 |
22 | H060.00 | Acute bronchitis | 3 | 0.6 |
23 | H20..00 | Viral pneumonia | 3 | 0.6 |
24 | H260.00 | Lobar pneumonia due to unspecified organism | 2 | 0.4 |
25 | H260000 | Lung consolidation | 2 | 0.4 |
26 | H261.00 | Basal pneumonia due to unspecified organism | 2 | 0.4 |
27 | H27z.12 | Influenza like illness | 2 | 0.4 |
28 | H28..00 | Atypical pneumonia | 2 | 0.4 |
29 | H5yy.11 | Respiratory infection NOS | 2 | 0.4 |
30 | 1J72.00 | Suspected influenza A virus subtype H1N1 infection | 1 | 0.2 |
31 | H20z.00 | Viral pneumonia NOS | 1 | 0.2 |
32 | H22..00 | Other bacterial pneumonia | 1 | 0.2 |
33 | H22z.00 | Bacterial pneumonia NOS | 1 | 0.2 |
34 | H23..00 | Pneumonia due to other specified organisms | 1 | 0.2 |
35 | H270000 | Influenza with bronchopneumonia | 1 | 0.2 |
36 | H270100 | Influenza with pneumonia, influenza virus identified | 1 | 0.2 |
37 | H27y.00 | Influenza with other manifestations | 1 | 0.2 |
38 | H30..00 | Bronchitis unspecified | 1 | 0.2 |
39 | H301.00 | Laryngotracheobronchitis | 1 | 0.2 |
Appendix 3 Stata algorithms for data management and analysis ‘do files’
Appendix 4 Semistructured interview guide used to evaluate general practitioner user experience of study technological interface
Introduction |
|
Decision to participate in the Flu-CATs |
|
Setting up the LEPIS system |
|
The Flu-CATs consultation |
|
Thoughts/reflections |
|
Appendix 5 Covariate code lists
Read code | Read term |
---|---|
History of cardiovascular disease at any point previous to the study consultation | |
G3...00 | Ischaemic heart disease |
G580.00 | Congestive heart failure |
G3...13 | IHD - Ischaemic heart disease |
G58..00 | Heart failure |
G3z..00 | Ischaemic heart disease |
G58z.00 | Heart failure NOS |
P6z..00 | Congenital heart anomaly NOS |
G580100 | Chronic congestive heart failure |
G580.12 | Right heart failure |
G3y..00 | Other specified ischaemic heart disease |
G34..00 | Other chronic ischaemic heart disease |
P6...00 | Other congenital heart anomalies |
G34z.00 | Other chronic ischaemic heart disease NOS |
P6z3.00 | Cyanotic congenital heart disease NOS |
G342.00 | Atherosclerotic cardiovascular disease |
P6y5.00 | Congenital heart block |
P5...12 | Congenital heart disease, septal and bulbar anomalies |
P6zz.00 | Congenital heart anomaly NOS |
P68..00 | Congenital heart disease |
G34y.00 | Other specified chronic ischaemic heart disease |
G583.00 | Heart failure with normal ejection fraction |
G34yz00 | Other specified chronic ischaemic heart disease NOS |
P6z2.00 | Acyanotic congenital heart disease NOS |
G580400 | Congestive heart failure due to valvular disease |
G583.11 | HFNEF - heart failure with normal ejection fraction |
Gyu3300 | [X]Other forms of chronic ischaemic heart disease |
P6y5000 | Congenital heart block, unspecified |
P6y5z00 | Congenital heart block NOS |
Gyu5g00 | [X]Cardiovascular disease, unspecified |
History of chronic liver disease at any point previous to the study consultation | |
J61..00 | Cirrhosis and chronic liver disease |
J614.00 | Chronic hepatitis |
J61z.00 | Chronic liver disease NOS |
J614z00 | Chronic hepatitis NOS |
J62..00 | Liver abscess and sequelae of chronic liver disease |
PB61.00 | Biliary atresia |
J61y.00 | Other non-alcoholic chronic liver disease |
J62y.00 | Other sequelae of chronic liver disease |
J61yz00 | Other non-alcoholic chronic liver disease NOS |
J614y00 | Chronic hepatitis unspecified |
PB61z00 | Biliary atresia NOS |
History of chronic neurological disease at any point previous to the study consultation | |
G66..00 | Stroke and cerebrovascular accident unspecified |
G65..12 | Transient ischaemic attack |
F20..00 | Multiple sclerosis |
F23..00 | Congenital cerebral palsy |
G66..12 | Stroke unspecified |
G64..13 | Stroke due to cerebral arterial occlusion |
G61..12 | Stroke due to intracerebral haemorrhage |
G664.00 | Cerebellar stroke syndrome |
G663.00 | Brain stem stroke syndrome |
F230100 | Cerebral palsy with spastic diplegia |
F23..11 | Congenital spastic cerebral palsy |
F137000 | Athetoid cerebral palsy |
F23z.00 | Congenital cerebral palsy NOS |
F23..12 | Infantile cerebral palsy |
G669.00 | Cerebral palsy, not congenital or infantile, acute |
F207.00 | Relapsing and remitting multiple sclerosis |
F23y200 | Spastic cerebral palsy |
F208.00 | Secondary progressive multiple sclerosis |
F23y400 | Ataxic diplegic cerebral palsy |
F23y000 | Ataxic infantile cerebral palsy |
F23yz00 | Other infantile cerebral palsy NOS |
F202.00 | Generalised multiple sclerosis |
F206.00 | Primary progressive multiple sclerosis |
F23y300 | Dyskinetic cerebral palsy |
F23y.00 | Other congenital cerebral palsy |
F200.00 | Multiple sclerosis of the brain stem |
Fyu9.00 | [X]Cerebral palsy and other paralytic syndromes |
F201.00 | Multiple sclerosis of the spinal cord |
Fyu9000 | [X]Other infantile cerebral palsy |
F137.11 | Athetoid cerebral palsy |
F29y100 | Postpolio syndrome |
F23y100 | Flaccid infantile cerebral palsy |
History of chronic renal disease at any point previous to the study consultation | |
1Z12.00 | Chronic kidney disease stage 3 |
K05..00 | Chronic renal failure |
1Z13.00 | Chronic kidney disease stage 4 |
K01..00 | Nephrotic syndrome |
1Z15.00 | Chronic kidney disease stage 3A |
1Z1C.00 | Chronic kidney disease stage 3 without proteinuria |
1Z1E.00 | Chronic kidney disease stage 3A without proteinuria |
1Z14.00 | Chronic kidney disease stage 5 |
1Z16.00 | Chronic kidney disease stage 3B |
K0Z..00 | Nephritis, nephrosis and nephrotic syndrome NOS |
1Z1G.00 | Chronic kidney disease stage 3B without proteinuria |
1Z1B.00 | Chronic kidney disease stage 3 with proteinuria |
ZV42000 | [V]Kidney transplanted |
K0…00 | Nephritis, nephrosis and nephrotic syndrome |
1Z1J.00 | Chronic kidney disease stage 4 without proteinuria |
1Z1D.00 | Chronic kidney disease stage 3A with proteinuria |
1Z1H.00 | Chronic kidney disease stage 4 with proteinuria |
1Z1F.00 | Chronic kidney disease stage 3B with proteinuria |
K011.00 | Nephrotic syndrome with membranous glomerulonephritis |
K01z.00 | Nephrotic syndrome NOS |
1Z1K.00 | Chronic kidney disease stage 5 with proteinuria |
K01x100 | Nephrotic syndrome in diabetes mellitus |
TB00111 | Renal transplant with complication, without blame |
K013.00 | Nephrotic syndrome with minimal change glomerulonephritis |
K015.00 | Nephrotic syndrome, focal and segmental glomerular lesions |
K016.00 | Nephrotic syndrome, diffuse membranous glomerulonephritis |
1Z1L.00 | Chronic kidney disease stage 5 without proteinuria |
K01x000 | Nephrotic syndrome in amyloidosis |
K014.00 | Nephrotic syndrome, minor glomerular abnormality |
K013.12 | Steroid sensitive nephrotic syndrome |
K01x400 | Nephrotic syndrome in systemic lupus erythematosus |
K01B.00 | Nephrotic syndrome, diffuse crescentic glomerulonephritis |
TB00100 | Kidney transplant with complication, without blame |
K0y..00 | Other specified nephritis, nephrosis or nephrotic syndrome |
K01w.00 | Congenital nephrotic syndrome |
K010.00 | Nephrotic syndrome with proliferative glomerulonephritis |
K01A.00 | Nephrotic syndrome, dense deposit disease |
Kyu2100 | [X]Other chronic renal failure |
K01x300 | Nephrotic syndrome in polyarteritis nodosa |
K01y.00 | Nephrotic syndrome with other pathological kidney lesions |
K012.00 | Nephrotic syndrome+membranoproliferative glomerulonephritis |
History of chronic respiratory disease at any point previous to the study consultation | |
H33..00 | Asthma |
H3...00 | Chronic obstructive pulmonary disease |
H333.00 | Acute exacerbation of asthma |
H33zz00 | Asthma NOS |
H33z100 | Asthma attack |
H34..00 | Bronchiectasis |
H36..00 | Mild chronic obstructive pulmonary disease |
H33..11 | Bronchial asthma |
H32..00 | Emphysema |
H33z011 | Severe asthma attack |
H31..00 | Chronic bronchitis |
H38..00 | Severe chronic obstructive pulmonary disease |
H330.00 | Extrinsic (atopic) asthma |
H330.12 | Childhood asthma |
663V100 | Mild asthma |
C370.00 | Cystic fibrosis |
H330.11 | Allergic asthma |
663V200 | Moderate asthma |
H331.00 | Intrinsic asthma |
1O2..00 | Asthma confirmed |
H330011 | Hay fever with asthma |
H330.13 | Hay fever with asthma |
H331.11 | Late onset asthma |
H31z.00 | Chronic bronchitis NOS |
H33z000 | Status asthmaticus NOS |
H58y300 | Interstitial lung disease NEC |
H330.14 | Pollen asthma |
H330111 | Extrinsic asthma with asthma attack |
H330000 | Extrinsic asthma without status asthmaticus |
H33z200 | Late-onset asthma |
663V300 | Severe asthma |
H33z111 | Asthma attack NOS |
H310000 | Chronic catarrhal bronchitis |
H312000 | Chronic asthmatic bronchitis |
H34z.00 | Bronchiectasis NOS |
H312100 | Emphysematous bronchitis |
H39..00 | Very severe chronic obstructive pulmonary disease |
H45..00 | Pneumoconiosis NOS |
H32z.00 | Emphysema NOS |
H330z00 | Extrinsic asthma NOS |
H3z..11 | Chronic obstructive pulmonary disease NOS |
Q317000 | Perinatal bronchopulmonary dysplasia |
H334.00 | Brittle asthma |
H312011 | Chronic wheezy bronchitis |
H43z.00 | Pneumoconiosis due to inorganic dust NOS |
H320.00 | Chronic bullous emphysema |
H331000 | Intrinsic asthma without status asthmaticus |
H331z00 | Intrinsic asthma NOS |
H340.00 | Recurrent bronchiectasis |
H311.00 | Mucopurulent chronic bronchitis |
C370z00 | Cystic fibrosis NOS |
66Yi.00 | Multiple COPD emergency hospital admissions |
H330100 | Extrinsic asthma with status asthmaticus |
A115.00 | Tuberculous bronchiectasis |
H40..00 | Coal workers’ pneumoconiosis |
C370200 | Cystic fibrosis with pulmonary manifestations |
H322.00 | Centrilobular emphysema |
SK07.00 | Subcutaneous emphysema |
H581.00 | Interstitial emphysema |
H331111 | Intrinsic asthma with asthma attack |
H33zz12 | Allergic asthma NEC |
Q317.00 | Perinatal chronic respiratory disease |
H591.00 | Chronic respiratory failure |
H320z00 | Chronic bullous emphysema NOS |
H341.00 | Post-infective bronchiectasis |
H331100 | Intrinsic asthma with status asthmaticus |
H42z.00 | Silica pneumoconiosis NOS |
H31y100 | Chronic tracheobronchitis |
H32yz00 | Other emphysema NOS |
Hyu3100 | [X]Other specified chronic obstructive pulmonary disease |
H311000 | Purulent chronic bronchitis |
H42..00 | Silica and silicate pneumoconiosis |
H32y.00 | Other emphysema |
H310z00 | Simple chronic bronchitis NOS |
H313.00 | Mixed simple and mucopurulent chronic bronchitis |
H32y200 | MacLeod’s unilateral emphysema |
H464.00 | Chronic respiratory conditions due to chemical fumes |
Q317z00 | Perinatal chronic respiratory disease NOS |
P861.00 | Congenital bronchiectasis |
H321.00 | Panlobular emphysema |
H320200 | Giant bullous emphysema |
H31y.00 | Other chronic bronchitis |
H31yz00 | Other chronic bronchitis NOS |
H43..00 | Pneumoconiosis due to other inorganic dust |
Q312.00 | Perinatal interstitial emphysema and related conditions |
H320000 | Segmental bullous emphysema |
H320100 | Zonal bullous emphysema |
C370100 | Cystic fibrosis with meconium ileus |
H582.00 | Compensatory emphysema |
C370y00 | Cystic fibrosis with other manifestations |
C370300 | Cystic fibrosis with intestinal manifestations |
Hyu3000 | [X]Other emphysema |
H320300 | Bullous emphysema with collapse |
H4y2100 | Chronic drug-induced interstitial lung disorders |
Q317y00 | Other specified perinatal chronic respiratory disease |
Q312111 | Perinatal mediastinal emphysema |
H464000 | Chronic emphysema due to chemical fumes |
H3y..11 | Other specified chronic obstructive pulmonary disease |
C370500 | Cystic fibrosis with distal intestinal obstruction syndrome |
H450.00 | Pneumoconiosis associated with tuberculosis |
H311100 | Fetid chronic bronchitis |
H420.00 | Talc pneumoconiosis |
H32y100 | Atrophic (senile) emphysema |
C370400 | Arthropathy in cystic fibrosis |
H32y111 | Acute interstitial emphysema |
C370000 | Cystic fibrosis with no meconium ileus |
H464z00 | Chronic respiratory conditions due to chemical fumes NOS |
H32y000 | Acute vesicular emphysema |
C370800 | Cystic fibrosis related cirrhosis |
History of diabetes at any point previous to the study consultation | |
C10..00 | Diabetes mellitus |
C10F.00 | Type 2 diabetes mellitus |
C100112 | Non-insulin dependent diabetes mellitus |
C109.00 | Non-insulin dependent diabetes mellitus |
C10E.00 | Type 1 diabetes mellitus |
C109.12 | Type 2 diabetes mellitus |
C100011 | Insulin dependent diabetes mellitus |
C100111 | Maturity onset diabetes |
C100100 | Diabetes mellitus, adult onset, no mention of complication |
C101.00 | Diabetes mellitus with ketoacidosis |
C10FJ00 | Insulin treated Type 2 diabetes mellitus |
C106.00 | Diabetes mellitus with neurological manifestation |
C106.12 | Diabetes mellitus with neuropathy |
C108.11 | IDDM-Insulin dependent diabetes mellitus |
C10FM00 | Type 2 diabetes mellitus with persistent microalbuminuria |
C108.12 | Type 1 diabetes mellitus |
C109.11 | NIDDM - Non-insulin dependent diabetes mellitus |
C109.13 | Type II diabetes mellitus |
C10EM00 | Type 1 diabetes mellitus with ketoacidosis |
C109J00 | Insulin treated Type 2 diabetes mellitus |
C10FL00 | Type 2 diabetes mellitus with persistent proteinuria |
C10F.11 | Type II diabetes mellitus |
C10FC00 | Type 2 diabetes mellitus with nephropathy |
C10F600 | Type 2 diabetes mellitus with retinopathy |
C107.00 | Diabetes mellitus with peripheral circulatory disorder |
C105.00 | Diabetes mellitus with ophthalmic manifestation |
C104.00 | Diabetes mellitus with renal manifestation |
66AJ.11 | Unstable diabetes |
C108.13 | Type I diabetes mellitus |
C10F900 | Type 2 diabetes mellitus without complication |
C10E.12 | Insulin dependent diabetes mellitus |
C10FN00 | Type 2 diabetes mellitus with ketoacidosis |
C100z00 | Diabetes mellitus NOS with no mention of complication |
C108900 | Insulin dependent diabetes maturity onset |
C10EK00 | Type 1 diabetes mellitus with persistent proteinuria |
C10ED00 | Type 1 diabetes mellitus with nephropathy |
C10E700 | Type 1 diabetes mellitus with retinopathy |
C10EL00 | Type 1 diabetes mellitus with persistent microalbuminuria |
C10FK00 | Hyperosmolar non-ketotic state in type 2 diabetes mellitus |
C101z00 | Diabetes mellitus NOS with ketoacidosis |
C104z00 | Diabetes mellitus with nephropathy NOS |
C11y000 | Steroid induced diabetes |
C10D.00 | Diabetes mellitus autosomal dominant type 2 |
C107.11 | Diabetes mellitus with gangrene |
C106z00 | Diabetes mellitus NOS with neurological manifestation |
C108700 | Insulin dependent diabetes mellitus with retinopathy |
C109900 | Non-insulin-dependent diabetes mellitus without complication |
C109400 | Non-insulin dependent diabetes mellitus with ulcer |
K01x100 | Nephrotic syndrome in diabetes mellitus |
C108500 | Insulin dependent diabetes mellitus with ulcer |
C10E.11 | Type I diabetes mellitus |
C106100 | Diabetes mellitus, adult onset, + neurological manifestation |
C10EN00 | Type 1 diabetes mellitus with ketoacidotic coma |
C10G.00 | Secondary pancreatic diabetes mellitus |
C10FB00 | Type 2 diabetes mellitus with polyneuropathy |
C105z00 | Diabetes mellitus NOS with ophthalmic manifestation |
C10F911 | Type II diabetes mellitus without complication |
C10F000 | Type 2 diabetes mellitus with renal complications |
C10FQ00 | Type 2 diabetes mellitus with exudative maculopathy |
C10C.11 | Maturity onset diabetes in youth |
C10FH00 | Type 2 diabetes mellitus with neuropathic arthropathy |
C10F200 | Type 2 diabetes mellitus with neurological complications |
C10EQ00 | Type 1 diabetes mellitus with gastroparesis |
C10FE00 | Type 2 diabetes mellitus with diabetic cataract |
C109600 | Non-insulin-dependent diabetes mellitus with retinopathy |
C10B000 | Steroid induced diabetes mellitus without complication |
C10F400 | Type 2 diabetes mellitus with ulcer |
C107.12 | Diabetes with gangrene |
Cyu2.00 | [X]Diabetes mellitus |
C105100 | Diabetes mellitus, adult onset, + ophthalmic manifestation |
C10FR00 | Type 2 diabetes mellitus with gastroparesis |
C10zz00 | Diabetes mellitus NOS with unspecified complication |
C10FJ11 | Insulin treated Type II diabetes mellitus |
C101000 | Diabetes mellitus, juvenile type, with ketoacidosis |
C10EE00 | Type 1 diabetes mellitus with hypoglycaemic coma |
C106000 | Diabetes mellitus, juvenile, + neurological manifestation |
C107z00 | Diabetes mellitus NOS with peripheral circulatory disorder |
C10FD00 | Type 2 diabetes mellitus with hypoglycaemic coma |
C106.13 | Diabetes mellitus with polyneuropathy |
C108400 | Unstable insulin dependent diabetes mellitus |
C10z.00 | Diabetes mellitus with unspecified complication |
C10y.00 | Diabetes mellitus with other specified manifestation |
C10FA00 | Type 2 diabetes mellitus with mononeuropathy |
C10FL11 | Type II diabetes mellitus with persistent proteinuria |
C10F100 | Type 2 diabetes mellitus with ophthalmic complications |
C10EP00 | Type 1 diabetes mellitus with exudative maculopathy |
C109C00 | Non-insulin dependent diabetes mellitus with nephropathy |
C10N100 | Cystic fibrosis related diabetes mellitus |
C10ER00 | Latent autoimmune diabetes mellitus in adult |
C10z100 | Diabetes mellitus, adult onset, + unspecified complication |
C108E00 | Insulin dependent diabetes mellitus with hypoglycaemic coma |
C10EJ00 | Type 1 diabetes mellitus with neuropathic arthropathy |
C10EA00 | Type 1 diabetes mellitus without complication |
C104100 | Diabetes mellitus, adult onset, with renal manifestation |
L180600 | Pre-existing diabetes mellitus, non-insulin-dependent |
C10F500 | Type 2 diabetes mellitus with gangrene |
C10E500 | Type 1 diabetes mellitus with ulcer |
C10E900 | Type 1 diabetes mellitus maturity onset |
C107200 | Diabetes mellitus, adult with gangrene |
C10E400 | Unstable type 1 diabetes mellitus |
C10FF00 | Type 2 diabetes mellitus with peripheral angiopathy |
C10E000 | Type 1 diabetes mellitus with renal complications |
C101100 | Diabetes mellitus, adult onset, with ketoacidosis |
C109J12 | Insulin treated Type II diabetes mellitus |
C107100 | Diabetes mellitus, adult, + peripheral circulatory disorder |
C10E300 | Type 1 diabetes mellitus with multiple complications |
C10F611 | Type II diabetes mellitus with retinopathy |
C108000 | Insulin-dependent diabetes mellitus with renal complications |
C10FG00 | Type 2 diabetes mellitus with arthropathy |
C10F300 | Type 2 diabetes mellitus with multiple complications |
C102100 | Diabetes mellitus, adult onset, with hyperosmolar coma |
C10FP00 | Type 2 diabetes mellitus with ketoacidotic coma |
C10D.11 | Maturity onset diabetes in youth type 2 |
C10EM11 | Type I diabetes mellitus with ketoacidosis |
C10E200 | Type 1 diabetes mellitus with neurological complications |
C101y00 | Other specified diabetes mellitus with ketoacidosis |
C109411 | Type II diabetes mellitus with ulcer |
C10EC00 | Type 1 diabetes mellitus with polyneuropathy |
C109J11 | Insulin treated non-insulin dependent diabetes mellitus |
C10EF00 | Type 1 diabetes mellitus with diabetic cataract |
C108A00 | Insulin-dependent diabetes without complication |
C106y00 | Other specified diabetes mellitus with neurological comps |
C109100 | Non-insulin-dependent diabetes mellitus with ophthalm comps |
C109000 | Non-insulin-dependent diabetes mellitus with renal comps |
C108512 | Type 1 diabetes mellitus with ulcer |
C108E11 | Type I diabetes mellitus with hypoglycaemic coma |
C109612 | Type 2 diabetes mellitus with retinopathy |
C109200 | Non-insulin-dependent diabetes mellitus with neuro comps |
C108D00 | Insulin dependent diabetes mellitus with nephropathy |
C109212 | Type 2 diabetes mellitus with neurological complications |
C10E100 | Type 1 diabetes mellitus with ophthalmic complications |
C108100 | Insulin-dependent diabetes mellitus with ophthalmic comps |
C109412 | Type 2 diabetes mellitus with ulcer |
C109E00 | Non-insulin depend diabetes mellitus with diabetic cataract |
C104y00 | Other specified diabetes mellitus with renal complications |
C108C00 | Insulin dependent diabetes mellitus with polyneuropathy |
C10E312 | Insulin dependent diabetes mellitus with multiple complicat |
C109B00 | Non-insulin dependent diabetes mellitus with polyneuropathy |
C108300 | Insulin dependent diabetes mellitus with multiple complicatn |
C10yz00 | Diabetes mellitus NOS with other specified manifestation |
C109C12 | Type 2 diabetes mellitus with nephropathy |
C108711 | Type I diabetes mellitus with retinopathy |
C109E11 | Type II diabetes mellitus with diabetic cataract |
C108511 | Type I diabetes mellitus with ulcer |
C108600 | Insulin dependent diabetes mellitus with gangrene |
C10zy00 | Other specified diabetes mellitus with unspecified comps |
C109H12 | Type 2 diabetes mellitus with neuropathic arthropathy |
C10FA11 | Type II diabetes mellitus with mononeuropathy |
C10EH00 | Type 1 diabetes mellitus with arthropathy |
C109500 | Non-insulin dependent diabetes mellitus with gangrene |
C108712 | Type 1 diabetes mellitus with retinopathy |
C10F311 | Type II diabetes mellitus with multiple complications |
C109D00 | Non-insulin dependent diabetes mellitus with hypoglyca coma |
C109011 | Type II diabetes mellitus with renal complications |
C109300 | Non-insulin-dependent diabetes mellitus with multiple comps |
C10yy00 | Other specified diabetes mellitus with other spec comps |
C108y00 | Other specified diabetes mellitus with multiple comps |
C108200 | Insulin-dependent diabetes mellitus with neurological comps |
C10E600 | Type 1 diabetes mellitus with gangrene |
C10FE11 | Type II diabetes mellitus with diabetic cataract |
C109012 | Type 2 diabetes mellitus with renal complications |
C102000 | Diabetes mellitus, juvenile type, with hyperosmolar coma |
C108F00 | Insulin dependent diabetes mellitus with diabetic cataract |
C109E12 | Type 2 diabetes mellitus with diabetic cataract |
C109H11 | Type II diabetes mellitus with neuropathic arthropathy |
C109511 | Type II diabetes mellitus with gangrene |
C10y100 | Diabetes mellitus, adult, + other specified manifestation |
C10z000 | Diabetes mellitus, juvenile type, + unspecified complication |
C102z00 | Diabetes mellitus NOS with hyperosmolar coma |
C103000 | Diabetes mellitus, juvenile type, with ketoacidotic coma |
C109D12 | Type 2 diabetes mellitus with hypoglycaemic coma |
C103z00 | Diabetes mellitus NOS with ketoacidotic coma |
C10EB00 | Type 1 diabetes mellitus with mononeuropathy |
C105000 | Diabetes mellitus, juvenile type, + ophthalmic manifestation |
C10E511 | Type I diabetes mellitus with ulcer |
C10E911 | Type I diabetes mellitus maturity onset |
C10E912 | Insulin dependent diabetes maturity onset |
C10FD11 | Type II diabetes mellitus with hypoglycaemic coma |
C10FC11 | Type II diabetes mellitus with nephropathy |
C108012 | Type 1 diabetes mellitus with renal complications |
C109G00 | Non-insulin dependent diabetes mellitus with arthropathy |
C109512 | Type 2 diabetes mellitus with gangrene |
C105y00 | Other specified diabetes mellitus with ophthalmic complicatn |
C10E411 | Unstable type I diabetes mellitus |
C108011 | Type I diabetes mellitus with renal complications |
C109C11 | Type II diabetes mellitus with nephropathy |
C10F411 | Type II diabetes mellitus with ulcer |
C10C.12 | Maturity onset diabetes in youth type 1 |
C10E611 | Type I diabetes mellitus with gangrene |
C108F11 | Type I diabetes mellitus with diabetic cataract |
C10FB11 | Type II diabetes mellitus with polyneuropathy |
C10E412 | Unstable insulin dependent diabetes mellitus |
C109F11 | Type II diabetes mellitus with peripheral angiopathy |
C10F011 | Type II diabetes mellitus with renal complications |
C108212 | Type 1 diabetes mellitus with neurological complications |
C108D11 | Type I diabetes mellitus with nephropathy |
C10FM11 | Type II diabetes mellitus with persistent microalbuminuria |
C10E712 | Insulin dependent diabetes mellitus with retinopathy |
C10E711 | Type I diabetes mellitus with retinopathy |
C108J12 | Type 1 diabetes mellitus with neuropathic arthropathy |
C109B11 | Type II diabetes mellitus with polyneuropathy |
C108411 | Unstable type I diabetes mellitus |
C108J11 | Type I diabetes mellitus with neuropathic arthropathy |
C108H00 | Insulin dependent diabetes mellitus with arthropathy |
C109211 | Type II diabetes mellitus with neurological complications |
C10E311 | Type I diabetes mellitus with multiple complications |
C10F111 | Type II diabetes mellitus with ophthalmic complications |
C108812 | Type 1 diabetes mellitus - poor control |
C109D11 | Type II diabetes mellitus with hypoglycaemic coma |
C109111 | Type II diabetes mellitus with ophthalmic complications |
C109F12 | Type 2 diabetes mellitus with peripheral angiopathy |
C10EA11 | Type I diabetes mellitus without complication |
C108z00 | Unspecified diabetes mellitus with multiple complications |
C103100 | Diabetes mellitus, adult onset, with ketoacidotic coma |
C107000 | Diabetes mellitus, juvenile +peripheral circulatory disorder |
C108E12 | Type 1 diabetes mellitus with hypoglycaemic coma |
C109A00 | Non-insulin dependent diabetes mellitus with mononeuropathy |
C10EG00 | Type 1 diabetes mellitus with peripheral angiopathy |
C108912 | Type 1 diabetes mellitus maturity onset |
C108412 | Unstable type 1 diabetes mellitus |
C10F211 | Type II diabetes mellitus with neurological complications |
C10EA12 | Insulin-dependent diabetes without complication |
Cyu2300 | [X]Unspecified diabetes mellitus with renal complications |
C10E012 | Insulin-dependent diabetes mellitus with renal complications |
C108B00 | Insulin dependent diabetes mellitus with mononeuropathy |
C108211 | Type I diabetes mellitus with neurological complications |
C109G12 | Type 2 diabetes mellitus with arthropathy |
C109A11 | Type II diabetes mellitus with mononeuropathy |
C108H11 | Type I diabetes mellitus with arthropathy |
C108911 | Type I diabetes mellitus maturity onset |
C10EN11 | Type I diabetes mellitus with ketoacidotic coma |
C109112 | Type 2 diabetes mellitus with ophthalmic complications |
C10EC11 | Type I diabetes mellitus with polyneuropathy |
C104000 | Diabetes mellitus, juvenile type, with renal manifestation |
C108A11 | Type I diabetes mellitus without complication |
C10EP11 | Type I diabetes mellitus with exudative maculopathy |
C10E112 | Insulin-dependent diabetes mellitus with ophthalmic comps |
C10E512 | Insulin dependent diabetes mellitus with ulcer |
C108B11 | Type I diabetes mellitus with mononeuropathy |
C10E111 | Type I diabetes mellitus with ophthalmic complications |
Kyu0300 | [X]Glomerular disorders in diabetes mellitus |
C10EE12 | Insulin dependent diabetes mellitus with hypoglycaemic coma |
C10EF12 | Insulin dependent diabetes mellitus with diabetic cataract |
C10EC12 | Insulin dependent diabetes mellitus with polyneuropathy |
C10E212 | Insulin-dependent diabetes mellitus with neurological comps |
C10ED12 | Insulin dependent diabetes mellitus with nephropathy |
C10EL11 | Type I diabetes mellitus with persistent microalbuminuria |
C108112 | Type 1 diabetes mellitus with ophthalmic complications |
C10FF11 | Type II diabetes mellitus with peripheral angiopathy |
C10F511 | Type II diabetes mellitus with gangrene |
History of immunosuppressive conditions at any point previous to the study consultation | |
43C3.11 | HIV positive |
A788.11 | Human immunodeficiency virus infection |
A788.00 | Acquired immune deficiency syndrome |
7840.00 | Total excision of spleen |
PK01.11 | Asplenia |
D415700 | Splenic infarction |
A788z00 | Acquired human immunodeficiency virus infection syndrome NOS |
PK0..00 | Anomalies of spleen |
PK01.00 | Absent spleen |
D415.00 | Other diseases of spleen |
D415z00 | Disease of spleen NOS |
A788000 | Acute human immunodeficiency virus infection |
A789000 | HIV disease resulting in mycobacterial infection |
8C31.00 | Transplant immunosuppression |
7840z00 | Total excision of spleen NOS |
A789400 | HIV disease resulting in multiple infections |
B905300 | Neoplasm of uncertain behaviour of spleen |
A789A00 | HIV disease resulting in wasting syndrome |
A789600 | HIV disease resulting in Burkitt’s lymphoma |
7841.00 | Other excision of spleen |
PK0z.00 | Anomalies of spleen NOS |
D415400 | Splenic atrophy |
A788y00 | Human immunodeficiency virus with other clinical findings |
A789700 | HIV dis resulting in both types of non-Hodgkin’s lymphoma |
A789900 | HIV disease resulting in lymphoid interstitial pneumonitis |
A788W00 | HIV disease resulting in unspecified malignant neoplasm |
A788200 | HIV infection with persistent generalised lymphadenopathy |
A789X00 | HIV dis reslt/oth mal neopl/lymph,h’matopoetc+reltd tissu |
A789100 | HIV disease resulting in cytomegaloviral disease |
A788500 | Human immunodeficiency virus with secondary infection |
A788400 | Human immunodeficiency virus with neurological disease |
A788300 | Human immunodeficiency virus with constitutional disease |
A788U00 | HIV disease result/haematological+immunologic abnorms,NEC |
A788X00 | HIV disease resulting/unspcf infectious+parasitic disease |
D415600 | Splenic fibrosis |
AyuC600 | [X]HIV disease resulting in other non-Hodgkin’s lymphoma |
AyuCB00 | [X]HIV disease result/haematological+immunologic abnorms,NEC |
AyuC300 | [X]HIV disease resulting in multiple infections |
AyuCC00 | [X]HIV disease resulting in other specified conditions |
AyuC400 | [X]HIV disease resulting/other infectious+parasitic diseases |
A789800 | HIV disease resulting in multiple malignant neoplasms |
AyuCD00 | [X]Unspecified human immunodeficiency virus [HIV] disease |
A788600 | Human immunodeficiency virus with secondary cancers |
B62y700 | Malignant lymphoma NOS of spleen |
B1z1.00 | Malignant neoplasm of spleen NEC |
History of immunosuppressive therapy in the year previous to the study consultation | |
8BAD.00 | Chemotherapy |
8BA5.00 | Oral chemotherapy |
7L16100 | Intravenous chemotherapy |
8BAD000 | Cancer chemotherapy |
7L18200 | Intramuscular chemotherapy |
7L1d.00 | Delivery of chemotherapy for neoplasm |
8BAL.00 | Combined pre-operative chemotherapy and radiotherapy |
7L1dz00 | Delivery of chemotherapy for neoplasm NOS |
7L1e.00 | Delivery of oral chemotherapy for neoplasm |
7L1ez00 | Delivery of oral chemotherapy for neoplasm NOS |
7L1dy00 | Other specified delivery of chemotherapy for neoplasm |
Appendix 6 FLU-CATs Data Handbook
Data sets
Data for the Flu-CATs Study are collected and uploaded on a weekly and on a monthly basis on to Dropbox by Emma Boyle from CPRD. Each week, two data sets are saved on to Dropbox: one each for children and adults. The following naming convention is followed for the weekly data sets: adults_YYYYMMDD and child_YYYYMMDD, with the date of the Monday for each week, for adult and children data sets, respectively.
Each monthly upload comprises four data sets: one data set each with LEPIS form data for adults and children (named form_adults_YYYY_MM and form_child_YYYY_MM); one ‘master file’ (named masterfile_YYYY_MM) containing data on comorbidities, selected treatments and vaccinations; and one ‘patient file’ (named patient_YYYY_MM) containing data that includes dates of death and transfer-out dates.
The weekly data sets are uploaded as tab-delimited text files on to Dropbox and the monthly data sets are saved as Stata files. Detailed data dictionaries for each of the weekly and monthly data sets are provided in the appendix.
‘Do files’
Data management Some data management is necessary for the weekly data sets before they can be analysed. The weekly data sets are saved as text files. They will first need to be downloaded off Dropbox and then ‘insheeted’ into Stata so as to convert them into Stata format. Once this is done, the relevant data management ‘do file‘ will need to be run.
Separate data management ‘do files’ have been prepared for adults and children. Each of the ‘do files’ is to be opened in Stata after the weekly FLU-CATs file has been ‘insheeted’ into Stata. Once ‘insheeted’, the ‘do file‘ may be executed and this should result in a complete run of the ‘do file’ with no errors. The resulting ‘clean’ file may then be saved as a Stata file. The ‘clean’ weekly data files will contain clinical measurements and other data collected through the LEPIS form in addition to each of the seven CATs criteria.
The monthly Flu-CATs data uploads require some minimal data management, which is included in the automation do file. The monthly data uploads do not require any additional data management. The automation and analysis ‘do files’ may be run directly on the files downloaded off Dropbox.
PMEWS and CATs A separate ‘do file’ has been written to calculate PMEWS scores in adults. Although the physiological data (MEWS) component can be carried out, using the weekly data downloads, to score the patient data, merging with the monthly data downloads from CPRD will be required as comorbidity data and patient age data are needed. The PMEWS ‘do file’ performs the necessary data management and generates cumulative PMEWS and CATs scores for the data set.
Data reports
‘Do files’ have been written to generate weekly and monthly FLU-CATs data reports. Separate ‘do files’ have been written for adult data and children data, although they are both identical except for the three variables that are present only in the adult data set (blood pressure, social isolation and performance status).
Weekly reports
For weekly reports, the ‘clean’ weekly Stata file is to be used. The input and output directories will need to be checked and modified if necessary. Once this has been done, the ‘do file’ may be executed. This should result in a complete run with no errors and a new Excel spreadsheet (titled ‘Results.xls’) with the weekly report should be created in the specified output folder. It is recommended that the results Excel spreadsheet is copied and re-saved in a different directory before running the next set of analyses, as these the ‘do files’ are programmed to overwrite the existing results spreadsheet.
At the time of writing this handbook, the latest tranche of weekly data for children (uploaded on 27 April 2015) does not have sufficient observations for outcome #1– ‘decision to treat with antivirals’ to obtain ORs, unadjusted or adjusted, for association with each of the CATs criteria. Because of this, the automated weekly report ‘do file’ terminates at this point. Until sufficient numbers for this outcome are obtained, the following is recommended:
-
Run the ‘do file’ until the error message is seen (until Outcome #1).
-
At this point, select portions of the ‘do file’ from Outcome #2 onwards and execute.
Monthly reports
From the monthly tranche of FLU-CATs data, the ‘master file’ and the ‘patient file’ will be used to generate a word document summarising findings for each month. As with the weekly data report ‘do files’, the directories with have to be checked and modified if necessary. Once this has been done, the ‘do file’ may be executed. This should result in a complete run with no errors and a new Word document (titled ‘mydoc1.rtf’) with the monthly report should be created in the specified output folder.
Notes
-
The monthly report ‘do file’ is best run in new Stata program window. If any changes are made to the ‘do file’ midway through executing the ‘do file’, save the changes (as a separate ‘do file’, if needed), close Stata and reopen the ‘do file’ in a new Stata window.
-
The monthly report document (opens in Word) will need to have all of the plots (saved as ‘myplot1.eps’, ‘myplot2.eps’, and so on) presented within the document to be saved in the same folder as the monthly report document itself. This must be kept in mind while e-mailing or sharing monthly reports– all plot files will have to be shared along with the report itself.
Data set descriptions
Weekly data set: adult (as provided by Clinical Practice Research Datalink)
Variable name | Type | Description |
---|---|---|
patid | long | Unique patient identifier |
pracid | int | Unique practice identifier |
formid | int | Unique LEPIS form identifier |
submit_date | long | Date of submission of FLU-CATs form (assumed to be the date of FLU-CATs consultation) |
cat | str5 | Category: adults/children (all adults in this data set) |
gender | byte | Gender: male or female |
birthyear | int | Patient’s year of birth |
mob | byte | Patient’s month of birth (for those aged under 16). 0 indicates no month set |
frddate | str10 | Date the patient first registered with the practice |
regdate | str10 | |
utsdate | str10 | Date at which the practice data is deemed to be of research quality |
todate | str10 | Date the patient transferred out of the practice, if relevant. Empty for patients who have not transferred out |
toreason | byte | Reason the patient transferred out of the practice |
deathdate | str10 | Date of death of patient |
lcdate | str10 | Date of the last collection for the practice |
accept | byte | Flag to indicate whether the patient has met certain quality standards: 1 = acceptable, 0 = unacceptable |
temperature | str32 | Body temperature: measured/not measured |
temperaturevalue | str4 | Body temperature value in °C |
severerespiratorydistress | str3 | Severe respiratory distress– yes/no |
respiratoryexhaustion | str3 | Respiratory exhaustion: yes/no |
respiratoryrate | str32 | Respiratory rate: measured/not measured |
respiratoryratevalue | str2 | Respiratory rate value in breaths per minute |
patientonoxygen | str3 | Is the patient on oxygen? yes/no |
patientonoxygenvalue | str2 | Is this oxygen need new and related to this episode? yes/no |
peripheraloxygensaturation | str30 | Peripheral oxygen saturation: measured/not measured |
peripheraloxygensaturationvalue | str3 | Peripheral oxygen saturation value (%) |
heartrate | str32 | Heart rate: measured/not measured |
heartratevalue | str3 | Numerical heart rate value |
bloodpressure | str12 | Blood pressure: measured/not measured |
bloodpressuresystolic | str3 | Systolic blood pressure value (mmHg) |
bloodpressurediastolic | str3 | Diastolic blood pressure value (mmHg) |
capillaryrefilltime | str40 | Capillary refill time: measured/not measured |
severedehydration | str2 | Signs of severe dehydration: yes/no |
newalteredconsciouslevel | str21 | New altered consciousness level: alert/confused or agitated/response to voice only/response to pain only |
socialisolation | str7 | Lives alone or no fixed abode: yes/no/unknown |
performancestatus | str48 | Patient’s usual activity and ability to self-care prior to this acute illness |
causingotherclinicalconcern | str3 | Causing other clinical concern? yes/no |
causingotherclinicalconcernvalue | str150 | Description of the clinical concern (≤ 150 characters) |
treatwithantivirals | str3 | Decision to treat with antivirals: yes/no |
treatwithantibiotics | str3 | Decision to treat with antibiotics: yes/no |
refertohospital | str3 | Decision to refer to hospital: yes/no |
Weekly data set: children (as provided by Clinical Practice Research Datalink)
Variable name | Type | Description |
---|---|---|
patid | long | Unique patient identifier |
pracid | int | Unique practice identifier |
formid | int | Unique LEPIS form identifier |
submit_date | long | Date of submission of Flu-CATs form (assumed to be the date of Flu-CATs consultation) |
cat | str5 | Category: adults/children (all children in this data set) |
gender | byte | Gender: male of female |
birthyear | int | Patient’s year of birth |
mob | byte | Patient’s month of birth (for those aged under 16). 0 indicates no month set |
frddate | str10 | Date the patient first registered with the practice |
regdate | str10 | |
utsdate | str10 | Date at which the practice data is deemed to be of research quality |
todate | str10 | Date the patient transferred out of the practice, if relevant. Empty for patients who have not transferred out |
toreason | byte | Reason the patient transferred out of the practice |
deathdate | str10 | Date of death of patient |
lcdate | str10 | Date of the last collection for the practice |
accept | byte | Flag to indicate whether the patient has met certain quality standards: 1 = acceptable, 0 = unacceptable |
temperature | str32 | Body temperature: measured/not measured |
temperaturevalue | str4 | Body temperature value in °C |
severerespiratorydistress | str3 | Severe respiratory distress: yes/no |
respiratoryexhaustion | str3 | Respiratory exhaustion: yes/no |
respiratoryrate | str32 | Respiratory rate: measured/not measured |
respiratoryratevalue | str2 | Respiratory rate value in breaths per minute |
patientonoxygen | str3 | Is the patient on oxygen? yes/no |
patientonoxygenvalue | str2 | Is this oxygen need new and related to this episode? yes/no |
peripheraloxygensaturation | str30 | Peripheral oxygen saturation: measured/not measured |
peripheraloxygensaturationvalue | str3 | Peripheral oxygen saturation value (%) |
heartrate | str32 | Heart rate: measured/not measured |
heartratevalue | str3 | Numerical heart rate value |
capillaryrefilltime | str40 | Capillary refill time: measured/not measured |
severedehydration | str2 | Signs of severe dehydration: yes/no |
newalteredconsciouslevel | str21 | New altered consciousness level: alert/confused or agitated/response to voice only/response to pain only |
causingotherclinicalconcern | str3 | Causing other clinical concern? yes/no |
causingotherclinicalconcernvalue | str150 | Description of the clinical concern (≤ 150 characters) |
treatwithantivirals | str3 | Decision to treat with antivirals: yes/no |
treatwithantibiotics | str3 | Decision to treat with antibiotics: yes/no |
refertohospital | str3 | Decision to refer to hospital: yes/no |
Monthly data sets
Four data sets are uploaded on to Dropbox by CPRD each month: two form data sets (one each for adults and children), one master file and 1 patient file. The two form files contain cumulative data from 4 weeks in one ‘monthly’ data set each for adults and children. The variables in the form files are identical to the variables in the respective weekly data extracts. Descriptions for the master file and the patient file are given below.
Master file
Variable name | Type | Description |
---|---|---|
patid | long | Unique patient identifier |
cat | str5 | Category: Adults/Children |
cardiovascular | float | Presence of cardiovascular disease: yes/no |
liver | float | Presence of liver disease: yes/no |
neurological | float | Presence of neurological disease: yes/no |
renal | float | Presence of renal disease: yes/no |
respiratory | float | Presence of respiratory disease: yes/no |
diabetes | float | Presence of diabetes: yes/no |
immune_supression | float | Presence of immunosuppression: yes/no |
statin | float | Prescription of statins: yes/no |
antibiotic | float | Prescription of antibiotics: yes/no |
antiviral | float | Prescription of antivirals: yes/no |
flu_vaccination | float | Seasonal influenza vaccination: yes/no |
hib | float | Haemophilus influenza type B vaccination: yes/no |
inhaled_steroids | float | Prescription of steroids (inhaled): yes/no |
oral_steroids | float | Prescription of steroids (oral): yes/no |
pneumococcal_vaccine | float | Pneumococcal vaccination: yes/no |
Patient file
Variable name | Type | Description |
---|---|---|
patid | long | Unique patient identifier |
pracid | int | Unique practice identifier |
cat | str5 | Category: adults/children |
formid | int | Unique LEPIS form identifier |
submit_date | float | Date of submission of FLU-CATs form (assumed to be the date of FLU-CATs consultation) |
gender | byte | Gender: male of female |
yob | int | Patient’s year of birth (Stata date format) |
mob | byte | Patient’s month of birth |
toreason | byte | Reason the patient transferred out of the practice |
year | float | Patient’s year of birth (YYYY format) |
regdate | float | |
todate | float | Date the patient transferred out of the practice, if relevant. Empty for patients who have not transferred out |
death | float | Date of death if the patient died |
Appendix 7 FLU-CATs protocol
NIHR Second Pandemic Influenza Themed Call: Detailed Project Description
Long Title: Real time refinement and validation of criteria and tools used in primary care to aid hospital referral decisions for patients of all ages in the event of surge during an influenza pandemic.
Short Title: Evaluation and refinement of pandemic influenza community assessment tools (the FLU-CATs study)
MG Semple, JS Nguyen Van Tam, PR Myles, JJ Kirkham, TJ Williams, TP van Staa
Summary
Design: A prospective analysis, linking criteria in a GP’s assessment of patients presenting with influenza like illness, to immediate management decisions and patient outcomes.
Objective: Assessment, refinement and validation of triage tools to guide GP referral of patients with influenza like illness during a pandemic in readiness for use should widespread illness exceed health care capacity (surge).
Method: GPs participating in the General Practice Research Database (GPRD) will record their assessment and management of patients with influenza like illness in LEPIS (Local Eligibility Patient Identification Service) an application linked to their routine in-practice software (Vision®). This is an established information technology based method for conducting large research studies in primary care.
High level hospital discharge data are routinely uploaded from secondary care to Vision® including: admission date, discharge date, main disease code, main procedure code and if death in hospital date of that death.
There is automated nightly linkage from Vision® and LEPIS to GPRD. This is collated by the GPRD research operations team and made available to external researchers on a weekly basis.
GPRD is linked to detailed Hospital Episode Summary data every three months. Data linked includes multiple disease codes, multiple procedure codes, length of admission, levels of augmented care (0 to 3), mortality, and pharmacoeconomics.
Analysis: Weekly cumulative analyses are planned using GPRD data. Three monthly analyses are planned using linked HES data as a gold standard.
Univariate and multivariate analyses using unconditional logistic regression will be used to investigate the association between triage criteria threshold values and primary outcomes (hospital admission, and death) and secondary outcomes (length of stay and augmented levels of care [high dependency/intensive care]). The threshold values of triage criteria will be refined by comparing the receiver operator characteristics at various thresholds of abnormality (e.g. respiratory rate of > 30, > 35, > 40).
The discriminatory value of existing and refined triage tools will be compared using logistic regression. Triage tools will be compared for their ability to predict outcomes using Area Under the Receiver Operating Characteristic Curve (AUROC) comparisons. The sensitivity, specificity, positive predictive value (PPV) and negative predictive value (NPV) will be calculated for triage tools using different score thresholds.
The virus, human behaviour, and models of health care provision in the community may change in time. Analysis will be reset between pandemic waves and appropriate comparisons made between pandemic waves.
Protocol amendment
The Award Board asked the investigators to consider add an extra resource to include virological diagnosis in a sub sample of patients.
We will explore linking GPRD to the HPA supra-regional microbiology network database to provide linked-anonymised results on microbiological investigations for respiratory pathogens. This will still require NIGB approval and Caldicott compliance. Preliminary enquiries have discovered that a supra-regional database is used by several of the nine HPA regions. This includes patient linked data on communicable diseases including the HPA/RCGP influenza virus swab results. GPRD is now linked to ten NHS datasets (including Hospital Episode Statistics, death certificates, cancer registry data, data from the Myocardial Infraction National Audit Program [MINAP]). These linkages are done on a regular basis using a Trusted Third Party and are all subject to NIGB approval. The project submitted involves already established links between GPRD, HES and Death Certifications. Establishing linkage to the HPA data-set would be a significant additional task for the study but one that would bring great benefit and avoid need for additional swabs to be taken.
Further details of protocol amendments including revised costs to resource linkage to the HPA are given at the end of this document.
Timeline
Phase one: (inter-pandemic – development / feasibility testing), duration six months during a summer season: set-up and validation of processes; optimisation of the clinical record entry screen; GP acceptability testing; establish data-return format; check completeness of data returns; develop data clean-up algorithms; development of definitions, evaluation of completeness and validation of study outcomes using historical GPRD and HES data; test of LEPIS study link. This will involve 3 to 5 practices experienced in developing GPRD/LEPIS research protocols.
Phase two: (inter-pandemic – pilot), duration six months in the consecutive winter season in 50 practices. Using data from cases of influenza like illness: test data-cleaning algorithms and the automated weekly evaluation of performance of triage criteria and tools. Rehearse report preparation to match expected ‘battle rhythm’ of key pandemic policy advisory bodies (SAGE and PICO). The project will be mothballed at end of phase two.
Phase three: will be activated in the event of a pandemic. The study link to LEPIS will be embedded in the Vision® software at all GPRD practices (currently 629) by the routine update process. GPs will be will prompted to complete assessment and outcome fields for cases presenting with influenza like illness. The GPRD research group will provide weekly data returns for these patients and three monthly linked HES data. We will run a weekly cumulative analysis against primary outcomes, allowing frequent refinement of criteria and tools against the novel pandemic strain, three monthly validation against HES data and plan adaptation of tools in readiness of surge.
An outline of the study as it would run in phases two and three is presented on page 20.
Has the project changed since the Expression of Interest was submitted? Yes
The title has changed to reflect that the aim of the study is to prospectively refine and validate the triage tools in real-time ready for use in the event of surge.
We have added Dr Jamie Kirkham, Centre for Medical Statistics and Health Evaluation, University of Liverpool, as a co-investigator to provide methodological and statistical support to the study at 3% WTE.
We have added a full time pre-doctoral research assistant based at the University of Nottingham to conduct statistical scripting and run analysis in year one. In lieu of this substantial change and additional salary costs, University of Nottingham have agreed to make a 100% institutional contribution to their estates and indirect costs.
Introduction
The potential for health care demand to exceed clinical capacity (surge) is recognised in historic reports of influenza pandemics and current government guidance.[1,2] Clinical triage tools capable of identifying the need for higher levels of care and risk of severe outcome have an important role in pandemic situations where secondary care capacity may be insufficient to meet demand; the time available for clinical decision making may be limited by workload pressures; and healthcare workers unfamiliar with clinical assessment and admission decision making may be asked to fulfil ‘gatekeeper’ roles.[3]
CURB-65 is a validated predictor of mortality from community acquired pneumonia in adults but was never intended for use in children.[4,5 CURB-65 does not perform as well in predicting higher levels of care and was not designed to predict mortality from non-pneumonic presentations.[6,7] Challen et al. proposed the Pandemic Medical Early Warning Score (PMEWS) as a clinical triage tool to aid hospital admission decisions for adults in a pandemic situation.[8] They validated PMEWS in adults presenting to hospital with community acquired pneumonia and found that it was better than CURB-65 for predicting the need for admission and higher levels of care but had limited ability to predict mortality. CURB-65 and PMEWS pose problems for use in primary care, the first being in-part reliant upon a contemporaneous serum urea value and the second is computationally complicated. Other severity scoring tools exist but these are not suitable for use in primary care due to a greater dependence upon laboratory or radiological investigations.
In 2009, the Department of Health England published a package of care that included Paediatric and Adult Community Assessment Tools (CATs) and patient pathways for use by the NHS in a severe pandemic event.[9] CATS were developed to help non-specialist front-line staff identify which sick children and adults are most likely to benefit from interventions and levels of care only available in hospitals when resources are limited. CATs were developed by paediatric and adult expert clinical development groups drawing on evidence that supports the recognition of severe influenza and severe pneumonia in the community in adults and children in resource limited settings; severe chronic obstructive pulmonary disease in adults; potentially serious feverish illness in children; and severe bronchiolitis in infants.[3,10,11,12,13,14,15,16,17,18,19] Clinicians were warned not to use the CATs and the pathways unless the local situation precluded normal admission and discharge processes.
CATs use six objective and one subjective criteria based on simple clinical assessment (figure 1). Meeting any CAT criterion warrants referral and admission to hospital. Criteria are: A) severe respiratory distress, B) increased respiratory rate, C) oxygen saturation > 2% on pulse oximetry breathing air or oxygen, D) respiratory exhaustion, E) severe dehydration or shock, F) altered consciousness level and G) other clinical concern. While criteria fields are common to adult and paediatric CATs, the abnormal physiological thresholds and clinical signs are age appropriate. Like PMEWS, there is no requirement for laboratory investigation to complete the assessment. However CATs were only intended for use ‘during severe and exceptional circumstances when surge demand for healthcare services leads to a need for strict triage”; and as such, were not deployed during the 2009/10 pandemic.
Work underpinning this study
Goodacre and colleagues (2010) conducted an evaluation of the discriminatory value of the CURB-65 score, PMEWS and CATs for predicting severe illness or mortality in patients with suspected pandemic influenza, but were unable to draw any conclusions regarding their clinical utility in a pandemic situation due to insufficient case numbers especially of adults, and a low incidence of severe outcome.[20]
Semple, Myles, Van-Tam and other members of the UK Pandemic Influenza Clinical Information Network (FLU-CIN) characterised PCR-confirmed pandemic influenza disease in a much larger cohort of 1520 people (1040 adults, 480 children (age < 16 years)) admitted to hospital.[21] (Thorax submitted) FLU-CIN compared the clinical validity and utility of CATs, PMEWS and CURB-65 as predictors for interventions normally only available in hospital, higher levels of care, and death using area under the Receiver Operating Characteristic (ROC) curve (AUROC) comparisons with 95% confidence intervals (paper submitted).[22] CATs showed the best predictive performance for level 2/3 admissions in both adults [AUROC: CAT 0.77 (0.73, 0.80); CURB-65 0.68 (0.64, 0.72); PMEWS 0.68 (0.64, 0.73), comparison of AUROCs p<0.001] and children [AUROC: CAT 0.74 (0.68, 0.80); CURB-65 0.52 (0.46, 0.59); PMEWS 0.69 (0.62, 0.75), p < 0.001].
While the FLU-CIN cohort is limited to patients admitted to hospital with severe influenza and its complications, the data show that triage tools are capable of predicting higher levels or care and or death in children and adults. However the FLU-CIN analysis does not include assessment of triage tools in primary care.
Appropriate use of such triage tools in the community could expedite referral to hospital and where scores are high, immediate admission to level 2/3 care. Prompt admission and allocation of higher levels of care may be associated with improved patient outcomes. Another study by FLU-CIN found that delayed admission to hospital (≥ 4 days after symptom onset) was significantly associated with increased likelihood of admission to critical care and death.[23]
The validity and utility of using triage tools in the community remains untested. Morbidity and mortality rates were low during the H1N1(2009) event when compared to some previous influenza epidemics such as the one in 1989/90 [24] and the use of anti-viral therapy was generally low in the FLU-CIN cohort despite it being widely available at the time. A more severe pandemic may be associated with a greater acceptance of anti-viral therapy and this may impact upon need for higher levels of care and death. Consequently criteria threshold values may need to be adjusted to optimize the ROC for each criterion and the AUROCs for the various triage tools.
Justification of this study
The validity and utility of the various triage tools needs to be assessed in a large community-based prospective study of patients presenting with influenza like illness to General Practitioners (GPs), to give confidence to clinicians who make be asked to use such tools in the event of surge, and policy makers who may need to recommend their use to GPs.
Linking GP assessment and management decisions to hospital episode data enables assessment and comparison of the validity and utility of triage in the community in relation to patient relevant outcomes (hospital admission, length of stay, higher levels of care and death).
It would not be possible to conduct this study during a pandemic without prior development of processes, feasibility and pilot studies.
The Health Protection Agency timeline for the UK 2009 pandemic shows only 12 weeks between identification of person-to-person transmission in the UK (first week May) and peak influenza activity (last week July) in the first pandemic wave. Prospective data collection with near real-time iterative and cumulative analysis is the only method for validating triage criteria and tools against a novel pathogen in such a short time.
Since pandemics are unpredictable and infrequent, limited but potentially useful information will be gained from prospective feasibility and pilot work conducted in primary care during seasonal influenza while H1N1v is still circulating.
Conducting this study in real-time during the early stages of a pandemic, when the characteristics of the novel virus are not fully understood, is important as it allows refinement and validation of triage tools against the novel pathogen in preparation for possible surge. This cannot be done until a novel virus emerges. Dame Diedre Hine has recommended that population based studies be established that, in the early stages of a future pandemic, can measure the severity of the pandemic and support decision making.[25]
The study method adheres to the five principles of Dynamic Risk Assessment and as applied to the management of emergency situations by UK Government agencies (Evaluate, Select, Assess, Refine, Reassess) (Home Office Guide to Operational Risk Assessment - Generic Risk Assessment 3.2. [Version 2 September 2008]).
If, as in the 1918/19 epidemic, the behaviour of the virus is markedly different in terms of severity between the first and subsequent waves or evolves to cause severe disease in a particular organ system; then triage criteria may need to be adapted to reflect the consequent changes in health care demand and clinical presentation.
Research objectives
Aim
To establish processes now, that can be used in the early stages of a future pandemic event to provide valid community triage tools capable of assisting hospital referral decisions for people of all ages for use if health care demands exceed health care capacity (surge).
Primary objective
From the start of a future pandemic event, to describe on a weekly cumulative basis the association between various triage tools and pandemic influenza outcomes. This will be supplemented as appropriate, with receiver operator characteristic (ROC) curves analysis and predictive values (positive and negative) of various community triage tools (Adult and Paediatric CATs, PMEWS) to predict outcomes (hospital admission and or death) in people of all ages presenting with pandemic influenza like illness to a large number of general practices in the UK; and to feed this information back to policy makers via the Pandemic Influenza Clinical and Operational Advisory Group’s Clinical Sub-group (PICO) and medical members of the Scientific Advisory Group for Emergencies (SAGE).
Secondary objectives
-
To provide reassurance that it is safe for people who do meet triage criteria and so would not be referred for hospital admission to continue to be managed in the community with advice.
-
To describe the associations between specific triage criteria and outcomes (hospital admission, length of stay, need for higher levels of care and or death).
-
Using sensitivity analysis to refine threshold values for specific triage criteria and outcomes (hospital admission, length of stay, need for higher levels of care and or death).
-
To describe demographics and clinical features of patients that meet threshold criteria and are referred for admission by GPs but are declined admission on assessment at hospital.
-
To describe demographics and clinical features of patients that present with influenza like illness to GPs and after assessment are not considered to need referral to hospital, yet die in the community within 30 days.
-
To describe demographics and clinical features of patients that present with influenza like illness to GPs and after assessment are not considered to need referral to hospital, yet die in hospital within 30 days.
We will analyse:
-
separately by age group: adults and children (< 16 years)
-
cumulatively for primary objectives.
-
weekly for primary objectives from initiation of study.
-
three monthly for secondary objectives.
Policy related
To provide evidence and reassurance to clinicians, policy makers and Ministers that any triage tools recommend for use in the event of surge are validated against the novel pathogen, are safe to use and clinically justified.
Plan of study
The study will run in three phases:
-
Phase one (inter-pandemic – development and feasibility). Proposed start date 01.04.2012. Duration six months during a summer season. At the start of this project, the researchers will evaluate in detail the validity and completeness of recording of the outcome measures in GPRD (analysing the data as collected up to the start of the study). Code lists will be developed. The frequency of recording of various data fields will be evaluated. In addition, comparisons will be made with HES in order to assess the completeness of recording of hospital admissions. These analyses will provide important information about the quality of GP recording of the outcomes. Based on the large number of published validation studies with GPRD, it is expected that major clinical outcomes are generally recorded well by GPs but that clinical details (such as length of hospital stay) may be less complete.
We will set-up and test the technology processes. This will involve 3 to 5 GP practices experienced in developing GPRD research protocols GPs will be involved in optimising the study record entry screen in LEPIS and do user acceptability testing. We will establish the data-return formats; check completeness of data recording by GPs in LEPIS; develop data clean-up algorithms; development of definitions, evaluation of completeness and validation of study outcomes using historical GPRD and HES data; test LEPIS study link.
There will be meetings with the oversight committee prior to commencement, at mid point and end of phase one.
-
Phase two (inter-pandemic – pilot), Proposed start date 01.10.2012. Duration six months in the consecutive winter season in 50 practices. Using data from cases of influenza like illness: test data-cleaning algorithms and the automated weekly evaluation of performance of triage criteria and tools. We will rehearse report preparation to match expected “battle rhythm” of key pandemic advisory bodies (SAGE and PICO). There will be meetings with the oversight committee prior to commencement, at mid point and end of phase two. The project will be mothballed at end of phase two.
-
Phase three will be activated in the event of a pandemic. The link to the web-based electronic record (LEPIS) will be embedded in the Vision® software at all GPRD practices (currently 629) by the routine update process. GPs will be will prompted to complete assessment and management fields for all cases presenting with influenza like illness. The GPRD research group will provide weekly data returns for these patients and three monthly linked hospital episode summary data. We will run a weekly cumulative analysis against outcomes, allowing frequent refinement of criteria and tools against the novel pandemic strain, and plan adaptation for use in readiness of surge.
In the event of imminent surge, and at the direction of policy makers at devolved Departments of Health, it will be possible to embed an algorithm behind the clinical record page in Vision®/LEPIS that will indicate that triage criteria have been met and so prompt the GP to consider hospital referral. Regional variations of Vision® software are provided to practices in devolved UK administrations, which will allow for variations in regional policy. A decision by one administration not to use triage tools during surge would be respected by not deploying the algorithm in that region. Because the GPRD is conducted in collaboration with the providers of the Vision® in-practice software, it would be possible to link all users of Vision® software to the algorithm, not just those participating in GPRD. We will explore the capability to provide and apply the algorithm to other providers of GP software. The study will continue during surge. The study will continue during surge. The triage algorithm will be removed when surge has ceased, and the study will continue.
Existing research
There have been two head to head comparisons of the triage tools proposed by DH guidance, both in cohort of patients already admitted to hospital.[20,22]
The validity and utility of using PMEWs, CATs (both triage tools) and CURB-65 (a validated predictor of mortality from community acquired pneumonia) to predict augmented levels of care and or death has been studied by the FLU-CIN in 1520 patients admitted to hospital with confirmed pandemic influenza A(H1N1)2009[22].
The ROC curves and AUROC values comparing the predictive value of the three clinical triage tools are described in figure 2. CATs showed the best predictive performance for augmented higher levels of care (effectively HDU and ITU admissions) in both adults [AUROC: CAT 0.77 (0.73, 0.80); CURB-65 0.68 (0.64, 0.72); PMEWS 0.68 (0.64, 0.73), comparison of AUROCs p < 0.001] and children [AUROC: CAT 0.74 (0.68, 0.80); CURB-65 0.52 (0.46, 0.59); PMEWS 0.69 (0.62, 0.75), p < 0.001]. CURB-65 and CAT had similar performance in predicting mortality in adults [AUROC: CAT 0.70 (0.63, 0.77); CURB-65 0.71 (0.65, 0.77); PME 5WS 0.60 (0.52, 0.67), p = 0.009] but CAT performed best as a predictor of mortality in children [AUROC: CAT 0.76 (0.66, 0.86); CURB-65 0.51 (0.39, 0.63); PMEWS 0.69 (0.55, 0.83), p = 0.002].
The receiver operator characteristic, sensitivity, specificity, positive predictive value (PPV) and negative predictive value (NPV) were calculated for each of the tools using various score thresholds (table 1). In adults, a CAT score ≥ 3 was the best predictor of level 2/3 admissions, death and combined severe outcome when compared to various cut-off scores for either CURB-65 or PMEWS. In children, a CAT score ≥ 3 was the best predictor of level 2/3 admission and combined severe outcome; performing marginally better than a PMEWS score > 9, both significantly better than CURB-65. In children, a PMEWS score > 9 was the best predictor of mortality in children; performing marginally better than a CAT score > 3, both significantly better than CURB-65.
Anonymised electronic general practice data has been used extensively across a wide spectrum of disease areas including seasonal and pandemic influenza. Watkins et al. used GPRD to determine the burden of seasonal influenza in childhood.[26] Hansell et al. (1999) investigated the epidemiology of respiratory disorders adopting similar methods.[27] Mangtani et al. (2004) investigated the effectiveness of influenza vaccination in the elderly.[28] Time series analysis of a similar nature to this proposed study was undertaken by Hajat et al. (1999) to determine the relationship between pollution and consultations for asthma and lower respiratory disorders.[29][30]
Research methods
Study design
Prospective observational maximum capture study using anonymised electronic general practice data linked to extended hospital episode summary data.
It is important to note that there will be no specific interventions made by the study.
None of the clinical features that constitute triage criteria are novel.
All clinical feature of interest are examined albeit with variations of adherence in current community practise.
Recruitment population
Any person presenting to a GPRD participating practice for consultation with a General Practitioner or Primary Care Nurse Practitioner regardless of date of onset of disease and prior medical history.
GPs participating in GPRD are committed to improving the health of their communities by providing anonymised data for a number of research and quality of service audit purposes.
Data collection templates with reminder flags are regularly uploaded to LEPIS and linked to Vision® in-practice software for such purposes.
Inclusion criteria
Any person presenting to a GPRD participating practice with influenza like illness regardless of date of onset of disease and prior medical history.
Exclusion criteria
People who present with any condition other than influenza like illness.
Proposed sample size
A formal sample size calculation is not possible and will depend to a great extent upon the clinical attack rate and hospitalisation rate (severity) of the pandemic strain. The clinical attack rate and hospitalisation rate of a future pandemic strain cannot be guessed, however the recent ‘mild’ pandemic does provide useful information.
In the first wave of the 2009 pandemic the clinical attack rate varied considerably between regions from 446 cases per 100,000 in the rural South-West to 1344 cases per 100,000 in London.[31] Most people experienced a mild, typical influenza like illness and the overall rate of hospitalisation ranged from 1.3% to 2.5% of those affected.
In the event of a pandemic and activation of phase 3 of the study, the study will recruit from all 629 practices participating in the GPRD currently actively caring for approximately 5.14 million people. Based on the limits of the clinical attack rates during the 2009 event, between 23,000 and 69,000 of these people would be expected to present in the first wave. Approximately 10,000 to 30,000 of these patients will present in the first 3 months before peak activity; forming the study cohort available for assessing, refining and validating triage tools in preparation for surge. Based on the limits of the hospitalisation rates previous described, 130 to 750 of these people would be admitted to hospital forming the group with a positive primary outcome measure in this study. In comparison, the FLU-CIN study was able to validate triage tools using similar method of analyses in a cohort of 1520 patients admitted to hospital where the positive primary outcomes groups comprised 250 (16.5%) people who required higher levels of care (level 2 HDU or 3 ITU) and 80 (5.3%) who died. Thus we are confident that this study will be sufficiently powered to assess, refine and validate the triage tools in the timetable described.
Data Sources: The General Practise Research Database (GPRD) and linked Hospital Episode data
The GPRD research group are named co-investigators in this application.
The General Practice Research Database (GPRD) is the world’s largest database of anonymised longitudinal medical records from primary care. The Secretary of State for Heath has owned the database since 1994. The GPRD is managed by Medicines and Healthcare products Regulatory Agency (MHRA). The database comprises near real-time comprehensive observational data from over 1000 GPs working in 629 general practices. It is a valuable tool for academic research in a broad range of areas including clinical epidemiology, disease patterns, disease management, outcomes research, and drug utilisation. The quality and reputation of the GPRD data make it an invaluable resource for researchers. There have been over 890 research papers published in peer-reviewed journals using data from this database. In July 2011 data was being collected on 5.14 million active patients of research standard or 8.3% of the UK population attending. The GPRD closely reflects the age, gender, and geographic distribution of the UK.[32] The quality of GPRD data has recently been reported in a systematic review. [33] While some acute musculoskeletal and metabolic conditions are not well recorded in GPRD, the majority of diagnoses were reliably coded and there is good agreement between more common diseases and other datasets. Importantly fro this study, influenza incidence rates derived from GPRD agree closely with data from national influenza surveillance systems.[30]
Detailed Hospital Episode Summary (HES) data is linked to GPRD every three months. HES data includes: detailed disease codes (ICD-10), procedure codes, levels of augmented care, length of stay and death. The ability of GPRD to conduct research across linked databases using this detailed clinical record data has been proven in a population-based study on incidence, risk factors, clinical complications and drug utilisation associated with seasonal influenza.[34]
GP clinical assessments and immediate management decisions are recorded on GPRD/LEPIS at time of consultation. This data is downloaded nightly and collated for access by researchers on a weekly basis. Data on hospital admission and death is typically entered on GPRD with in 48 hours of receipt of notification. Recently Jick et al. (2011) described the ability of GPRD to provide near real-time analysis of the epidemiology of pandemic influenza 2009.[35]
GPRD research group has gained ethical and National Information Governance Board (NIGB) approval to provide anonymised data and other healthcare data linked via the patient’s NHS number, sex, date of birth and post code.
GPs who contribute to the database use Vision® (In Practice Systems Ltd); a computer software package designed by an established user-group of experienced GPs, to make contemporaneous records of their routine consultations and enter study specific data in LEPIS; a linked application maintained by the GPRD research team.
General Practitioners participating in the GPRD will be prompted on screen to record their assessment and management of patients presenting with influenza like illness. A flag will appear during consultation requesting the GP to complete their assessment on the LEPIS study website. This is the limit of any variation from routine practise; which is to record assessment and key positive and negative rule-out features in in free text fields in Vision®. It is important to note that GP are not expected to diverge from their routine clinical assessment of patients and that GP management decisions are already routinely entered in Vision®.
Hospitals are required to transmit discharge summaries to GPs within 48 hours or suffer financial penalties. These contain high-level data: primary diagnosis, date of admission, date of discharge or death. This data is manually input to Vision®, usually within 48 hours of receipt.
Linked anonymised Vision® and LEPIS data is downloaded nightly in a silent background application to the GPRD research group. Weekly extracts of the data are provided to the wider research community as the GPRD.
Linkage to Hospital Episode Summary (HES) data is done by an external NHS group in a way that the GPRD research team does not see any identifying details. HES data includes: method of admission, disease coding (ICD-10), length of stay, level of augmented care, procedural coding (OPCS classification of interventions and procedures, v4.6), cause of death as certified, prescribing and pharmaco-economics. Levels of augmented care are defined by the Department of Health and Intensive Care Society Standard (2009). These high-level data in HES have been mandated for return from all NHS hospitals in England for all patients admitted to since April 2006 to support payment by results. HES data is linked to GPRD at three monthly intervals and are provided to the wider research community.
The Office for National Statistics (ONS) collects information on causes of deaths from death certificates. ONS data is linked to HES monthly and to GPRD every three months.
Notification of death is made by hospitals to GPs via standard operating procedures that ensure this data is handled as a priority to prevent inappropriate communications and facilitate appropriate support to bereaved relatives. Thus death as a status is entered on Vision® with little delay. Notice of ‘hospital admission’ is normally available within 48 hours of admission. The primary outcome measures of hospital admission and death are therefore available for use by GPRD following a nightly download. It is recognised that there is typically a delay of 48 hours after discharge before high-level hospital admission data is available from faxed hospital discharge letters. At the time of writing this proposal there is a 3-month interval in linkage of GPRD to HES and ONS certified cause of death data.
Part of our work in phases 1 and 2 will be study feasibility, conducting necessary validation and if necessary, initiate improved systems of data collection in the case of a pandemic.
Data management
The GPRD research group will undertake data collation and secure data warehousing in line with NIGB requirements. The GPRD research group will provide direct access to the GPRD warehouse for the statistician and epidemiologist. The researchers will have no access to patient identifiable data. All data analysis and will be maintained on secured computer servers within the University of Nottingham and University of Liverpool, only accessible to the research team and appropriately protected in accordance with Caldicott principles.
Definition of influenza like illness
Influenza like illness (ILI) will be defined per the World Health Organization criteria: ‘any person with sudden onset of fever (> 38 °C) and cough or sore throat in the absence of other diagnoses’. This closely matches the Centres for Disease Control (CDC) USA definition used for community incidence studies: ‘fever or recent history of fever (≥ 37.8 °C) plus cough and or sore throat in the absence of a known cause other than influenza’. This definition will include those people meeting the British Infection Society/British Thoracic Society/Health Protection Agency/Department of Health (England) guidance criteria (2007): ‘presence or recent history of fever (T > 38 °C) plus new cough in the context of influenza circulating in the community’. We have chosen not to adopt the Royal College of General Practitioners’ Syndromic Surveillance System algorithm as it depends on self-reporting of muscle ache and or headache, which are poorly reported by young children.
It is accepted that the definition of ILI may be revised in the event of a future pandemic, either on the basis of on-going surveillance information or in the event of emergence of a novel pneumonic pathogen. This is not a concern. This study’s aim is to establish automated processes capable of assessing and validating community triage tools in near-real time, which can be applied regardless of any variations of the case-defined entry criteria.
Predictor variables
Clinical features of patients presenting with influenza like illness as recorded by GPs on LEPIS during their routine consultation which include the criteria used by various triage tools:
-
Respiratory Rate (value)
-
Oxygen saturation (value)
-
Any sign of Severe Respiratory Distress (yes/no). Prompt ‘any of: lower chest wall indrawing, sternal recession, grunting, noisy breathing when calm, use of accessory muscles, supra-clavicular recession, tracheal tug, unable to complete sentences in one breath or feeling of suffocation’.
-
Respiratory Exhaustion (yes/no) or Apnoea reported (yes/no), apnoea defined as a ≥ 20-second pause in breathing
-
Blood pressure (systolic and diastolic values)
-
Sternal Capillary Refill Time (normal/> 2 secs)
-
Severe dehydration (yes/no). Prompt: ‘any of: reduced skin turgor, sunken eyes or fontanelle’.
-
Adults only: New altered conscious level (yes/no). Prompt ‘any of: new confusion or disorientation in person, place or time; AVCPU score < A; or ‘Mini (abbreviated) mental test score < 8’.
-
Children only: New altered conscious level (yes/no). Prompt ‘any of: new confusion or disorientation in person, place or time; AVPU score < A; or Mini mental test score < 8/10’.
-
Strikingly agitated (yes/no)
-
New seizures (yes/no)
-
Floppy infant (yes/no)
-
Social isolation (yes/no). Prompt: ‘any of: lives alone; no fixed abode’.
-
Chronic disease (yes/no). Prompt: ‘any of respiratory, cardiac, renal, metabolic, immune suppressed or sickle disease’.
-
Causing other clinical concern to their GP (yes/no). Prompt with ‘For example a rapidly progressive or an unusually prolonged illness’ (yes/no).
Demographic data to be collected silently by background application
-
Practice location.
-
Socioeconomic data at lower super output level (index of multiple deprivations).
-
Ethnicity.
-
Sex.
-
Age.
-
In cases of death, Office of National Statistics cause of death.
Outcome measures
Primary outcome measures
-
Hospital admission within 24 hours of GP assessment.
-
Death within 30 days of GP assessment (all causes). Complications leading to death may occur after some weeks (e.g. if prolonged admission to hospital). This outcome definition may miss cases that die as a direct consequence of their illness after 30 days, but based on 2009/10 experiences these are expected to be few.
Secondary outcome measures
-
Any need for augmented level of care during hospital admission i.e. level 2 – High Dependency and level 3 – Intensive/Critical Care, accepting that there are minor differences between paediatric and adult definitions of levels of care.
-
Length of hospital stay (stratified > 48 hours, ≥ 6 days and ≥ 12 days)
-
GP’s decision to refer for hospital admission.
Statistical analysis
Separate analyses will be made for paediatric and adult patients and will use age appropriate triage tools where available.
The datasets will be analysed using STATA statistical software.
Weekly cumulative analysis is planned using real-time data from GPRD followed by 3-monthly validation using HES and ONS linked GPRD data. A rolling analysis is required because the virus, human behaviour, and models of health care provision in the community may change in time. The analyses will be reset in keeping with key policy changes in order to assess the impact of any changes. For example the introduction of that automated National Pandemic Flu Service (NPFS) in July 2009 substantially reduced the numbers and altered the case mix of people presenting to GPs.[31]
Univariate and multivariate analysis (using unconditional logistic regression) will be used to investigate the association between triage criteria threshold values and primary outcomes (hospital admission and or death). The threshold values of triage criteria will be refined by describing the receiver operator characteristics of different thresholds of abnormality (e.g. respiratory rate > 30, > 35, > 40) to predict patient outcomes.
It is likely that in the first couple of months of the pandemic, when there are fewer cases, the analyses will be largely descriptive and focus on percentages and cross tabulations. However, as soon as it is feasible, the sensitivity, specificity, positive predictive value (PPV) and negative predictive value (NPV) will be calculated for each of the triage tools using different triage tool score thresholds. An example of analysis output is given in table 1. The discriminatory value of existing and refined triage tools will be compared using logistic regression. The performance of different triage tools will be compared on their ability to predict outcome using area under the Receiver Operating Characteristic curve (AUROC) comparisons. An example of analysis output is given in figure 2.
Cumulative analysis will be reset between pandemic waves and appropriate comparisons made between pandemic waves. Analyses can be reset if there is a substantial change to the model of pandemic influenza health care provision such as introduction of an automated National Pandemic Influenza Service which would be likely to alter the case mix presenting to GPs.
Risks/benefits to patients
For the individual patient there are no readily identifiable risks that result from the project. All data is anonymised to allow analysis without compromising patient confidentiality. It is possible that participating GPs will adapt their consultation practise to conduct clinical assessment in a structured format. This may benefit patients.
Once the study processes are developed and piloted, the methods can be activated to determine the appropriate management of other novel non-influenza severe acute respiratory pathogens, e.g. SARS coronavirus.
Risks/benefits to study
We have identified two system specific risks for the proposed study:
-
That despite prompts in the clinical software and other processes put in place to encourage data entry by GPs (such as GPRD newsletters and emails), that GPs will not enter their assessment in LEPIS and instead use other free-text fields in Vision®. To reduce this possibility, the software will be adapted to flag a reminder if an ILI diagnostic code is entered in Vision® and the LEPIS study page has not been completed.
-
It is accepted that there may be up to a week’s delay in availability of data from hospital discharge faxes that is dependent upon manual input to Vision® by GPs clerks (e.g. length of stay). There is potential for failure, reduced quality and increased delay of production of discharge faxes at some hospitals due to local pandemic influenza pressures on staff and financial penalties may be lifted. This will be mitigated by the very large size of the study recruiting from practices spread across the UK. This risk is not expected to affect data return for the primary outcome (hospital admission within 24 hours of GP assessment) as this data is returned automatically or notice of death which is handled as a priority to be communicated directly to GPs by clinical staff. Standard operating procedures are in place ensure rapid disseminating of notice of death in primary care systems to prevent inappropriate contacts and distress to bereaved relatives.
Consent
Patient consent is not required as all patient data is anonymised and no intervention is taking place. Patients and parents of patients at participating practices have the opportunity to opt out of the GPRD scheme at registration and any point thereafter. However after a patient has been assessed and their data transmitted in an anonymised format there is not capability to redact that data. Anonymised patient data in HES is subject to an NIGB section 251 approval for approved use without consent.
Ethics
GPRD research group has generic ethical approval for studies that only make use of anonymised data and linked anonymised Hospital Episode Statistics. All studies require scientific approval from the GPRD Independent Scientific Advisory Committee (ISAC). This study protocol will be submitted to ISAC for review. In the event that ethical review is required by ISAC, a submission will be made to a Multi-Centre Research Ethics Committee via the Integrated Research Application System.
The National Information Governance Board for Health and Social Care has authorised the Information Centre for Health and Social Care to provide anonymised Hospital Episode Statistics as the official statistical database on NHS hospital activity in England under a section 251 approval.
Outputs from the study
In Phase one and two, progress reports to the oversight group will be made every three months, with a final report being submitted at the end of phase two to NETSCC and Department of Health Pandemic Influenza Planning Team.
Reports will detail:
-
In Phase one
-
GP involvement in developing data capture screens.
-
User acceptability (GP) testing of data capture screens.
-
Completeness of data from GPRD based on retrospective analysis using HES-linked GPRD data as a gold standard for data completeness.
-
-
In Phase two
-
Weekly statistical analysis of cases of seasonal influenza to demonstrate capability of processes and retrospective validation against HES data.
-
An accrual graph of patients studied by week number plotted above the HPA Weekly National Influenza Report of GP reported ILI consulting rates in the UK.
-
During phase three (pandemic), summary results of statistical analysis and recommendations regarding the triage tools will be reported to the oversight committee on a fortnightly basis and shared with the Pandemic Influenza Clinical Operation Clinical Subgroup (PICO) and the Scientific Advisory Group for Emergencies (SAGE). The reporting cycle will be flexible and can be adapted to marry with the battle rhythm of these key sources of advice to policy makers. The research team have a deep understanding of the need to communicate their findings successfully.
Reports will detail:
-
An accrual graph of patients studied by week number plotted above the HPA Weekly National Influenza Report of GP reported ILI consulting rates in the UK.
-
Results of statistical analysis detailing ROC, Sensitivity, Specificity, PPV, NPV for triage criteria and AUROCs for triage tools against the primary outcomes: hospital admission, higher levels of care (2 – HDU, 3 –ITU) and death.
-
Recommendations regarding which criteria and tools to use in the event of surge.
In the post pandemic phase a final report will be produced and manuscripts prepared for publication.
Two of the investigators will present findings at an international conference.
Research governance
The University of Liverpool will act as sponsor for the study.
The proposed study will be undertaken in accordance with the University of Liverpool’s research governance procedures.
Dr MG Semple (Liverpool) and Prof Jonathan Van-Tam (Nottingham) will be joint guarantors for analysis and reports.
Study oversight committee
A formal steering committee is impractical. A small independent oversight group has already been convened which includes a research active principal in primary care with a particular interest in identification of severe illness in children and a consultant in infectious diseases who is an expert in translating evidence to policy and policy to practice. Both have already made recommendations to the protocol that have been accepted.
Dr Anthony Harnden, Principle in General Practice and Director of Primary Care Research Network Clinical (PCRN) Clinical Trials Unit Oxford. Dr Harnden is a member of PICO; appointed as an independent expert clinical advisor in Primary Care. He has published on identifying factors that identify children with serious infection in primary care, avoidable factors associated with chid deaths and the evidence base for interventions delivered to children in primary care.
Dr Barbara Bannister, Consultant in Infectious Diseases, and Consultant Clinical Advisor to Department of Health. Dr Bannister is a member of PICO; appointed as an expert clinical advisor in infectious diseases and as a representative of the DH Pandemic Influenza Programme. Dr Bannister supported the development and communication of operational guidance for medical professionals, including the mass use of antiviral and antibiotic therapy, clinical management guidelines, NPFS algorithms, triage and hospital pathways.
A NIHR NETSCC monitor or representative will be invited to join the group.
In phase one and two the investigators plan to meet with the overseers at least at commencement and at three monthly intervals.
In phase three, weekly reports will be presented to the overseers for ratification before being presented to SAGE, PICO and policy makers in DH.
Timetable and milestones
01/04/2012 – 30/09/2010 Phase one (inter-pandemic – development / feasibility), duration six months during a summer season: set-up and validation of processes, optimisation of the clinical record entry screen in LEPIS, GP acceptability testing, completeness of data, validation of linkage between Vision®, LEPIS, HES and GPRD, data-return format and development of data clean-up algorithms. Progress reports and meeting at 3 and 6 months with overseers.
01/10/2012 – 31/03/2013 Phase two (inter-pandemic – pilot), duration six months in a winter season in 50 practices. Using data from cases of influenza like illness: test data-cleaning algorithms and the automated weekly evaluation of performance of triage criteria and tools. Rehearsal of reporting procedures and reporting rhythm to PICO and SAGE. Progress reports and meeting at 3 and 6 months with overseers. A manuscript for publication will be produced at the completion of phase 2.
Phase three will be activated in the event of a pandemic, duration 12 months. We will run a weekly cumulative analysis, allowing refinement of criteria and tools against the novel pandemic strain in readiness for surge. Monthly summary reports to overseers during pandemic phase. When ready, refined and validated tools will be presented to overseers, seeking approval to reporting to policy makers. Meeting at least at 3, 6, and 9 months to overseers. A manuscript for publication will be produced in the post pandemic period and before month 12.
Expertise
The co-applicants at University of Liverpool (MGS) and Nottingham (JVT and PM) are experienced in conducting pragmatic research during outbreaks and collaborated during the 2009 pandemic on the FLU-CIN. Both groups have institutional support to reorganise work and time commitments in order to conduct this research project as they did in 2009/10.
Dr MG (Calum) Semple (principal investigator) is a Senior Lecturer in Child Health at the University of Liverpool and Consultant in Paediatric Respiratory Medicine at Alder Hey Children’s Hospital. His research interests include investigating the clinical and immunological factors associated with severe respiratory viral infections caused by human respiratory syncytial virus, human metapneumovirus and influenza virus. He was seconded to the Department of Health Pandemic Influenza Programme as a clinical advisor from 2008 to 2010 and supported the development of clinical guidance, community triage tools and hospital pathways in preparation for a future pandemic. At the onset of the 2009 A(H1N1) pandemic he joined the Pandemic Influenza Clinical Operational Group (PICO) as an independent expert advisor and with Professor Jonathan van Tam established the UK Pandemic Influenza Clinical Information Network (FLU-CIN).
Professor Jonathan Van Tam, University of Nottingham has a special interest in influenza: epidemiology; transmission; vaccinology; and pandemic preparedness, which now spans more than 20 years. He is an internationally renowned expert on influenza and senior editor of the textbook: Introduction to Pandemic Influenza. He has been a consultant to the World Health Organization since 2004 and sits on the UK Scientific Pandemic Influenza Committee (SPI), and its Pandemic Influenza Clinical and Operational Group (PICO). He was a core member of the UK Scientific Advisory Group for Emergencies (SAGE) during the 2009-10 pandemic crisis and simultaneously led the national FLU-CIN surveillance project for hospitalised pandemic flu cases; and two further ‘emergency’ pandemic research projects funded by NIHR. His unit is an official WHO Collaborating Centre for pandemic influenza and research, a Faculty of Public Health “national treasure” training location and an HPA Field Epidemiology training centre. He will provide this specialist expertise to the study, particularly around study development and design and policy-related analyses.
Dr Tjeerd-Pieter van Staa is head of GPRD Research. He is a physician with a MSc in Pharmaco-epidemiology from McGill University and PhD from Utrecht University. He has more than 15 years of significant research and industry experience in pharmaco-vigilance, epidemiology and pharmaco-economics. He has been the European Qualified Person for Pharmacovigilance. His current research activities concern the combination of prospective research methods with healthcare databases (including individual and cluster randomised clinical trials within the database and pharmaco-genetic research). He has implemented collaborative research of multiple health-data linkages, including cancer registries, MINAP, ALSPAC, air pollution and bowel screening. Development of pharmaco-epidemiological methods, including multidatabase analyses and visualisation and evaluation of data quality, is another research interest.
Dr Tim Williams is the GPRD research services manager. Tim Williams obtained a PhD from the University of Leeds in 1995 before working within the Post Graduate Medical School at the University of Surrey as a Research Fellow where he undertook technical and research aspects of UK primary care database pharmacoepidemiology. In 2001 he moved to his current position, as an epidemiologist within the General Practice Research Database division within the Medicines and Healthcare products Regulatory Agency. In 2003 he completed his MSc in Epidemiology from the London School of Hygiene and Tropical Medicine, and he is also an Honorary Research Fellow with Brighton and Sussex Medical School.
Dr Puja Myles is associate professor of health protection at the University of Nottingham. Her PhD thesis used a very large GP dataset to investigate risk factors for severe community acquired pneumonia. She is the analyst on the FLU-CIN. She will provide the data processing, analytical and statistical expertise to the study.
Dr Jamie Kirkham is a lecturer in Medical Statistics within the Department of Biostatistics, University of Liverpool. He has experience in statistical consultancy aimed specifically at improving the design and analysis of research projects. He also has experience in developing and validating clinical assessment tools (NIHR funded, Adverse Drug Reaction in Children project). He will provide methodological support to the project and assist with the statistical analysis.
Service use input
The NIHR Primary Care Research Network (PCRN) Clinical Study Group have reviewed the study outline.
A principle in primary care (AH member of oversight committee) has reviewed to full protocol.
The GPRD research group and development practices include physicians and principles in General Practice who have an appropriate vested interest in optimising the methods for data entry and developing appropriate triage tools for us in the communities they care for.
Research staff requirements/justification of resources
The use of data derived from a very large data set is a complex undertaking and requires specific skills to produce meaningful results. These include expertise in managing and manipulating large general practice datasets, ‘cleaning’ the data, extracting the relevant information, statistical processing and interpreting the findings appropriately. These need to be matched with relevant influenza clinical and public health skills, including in primary and acute care, health protection and respiratory epidemiology. The research team has therefore been drawn together to reflect these needs and includes expertise in handling large general practice datasets and the relevant clinical skills.
Phases 1 and 2: PM will lead in drafting the application for submission to the GPRD ISAC. These forms require detailed methodological description and would be difficult for someone without prior experience of primary care database studies. She will oversee the development of data cleaning and analysis algorithms and weekly report templates in preparation for Phase 3 as well as the validation of primary outcomes so that definitions can be revised if necessary. She will provide supervision to the research assistant for retrospective validation of real-time GPRD data using HES-linked GPRD data (which will be considered the ‘gold standard’ in terms of data completeness).
FT pre-doctoral research assistant: PM will be supported in this work by a full-time pre-doctoral research assistant. In order to keep costs down, we have opted for a pre-doctoral RA but this will then require the necessary input from PM as outlined previously.
Phase 3: PM: Ideally, data processing, analysis and production of weekly reports should be performed by a full-time research assistant under PM’s supervision. However, it is our experience that, due to the unpredictability of the timing of the pandemic and the need for an immediate start once the pandemic begins, it will not be possible to go through usual recruitment procedures for employing an RA. Moreover, even a very competent post-doctoral research assistant would need some level of training up in the project specific role. In a pandemic situation there will be no scope for delays which is why PM will take full responsibility of the data processing, analysis and production of weekly reports. This is usually a full-time responsibility but we anticipate that the preparation carried out in Phases 1 and 2 will enable PM to carry out these tasks at 60% whole time equivalent.
There are distinct differences in the time commitments required for the pre-pandemic phases (1 and 2) and the pandemic phase 3 which will require increased activity. Research staff time has been adjusted accordingly and to provide timely results to the overseers and policy makers.
Staff and role | Time commitment (WTE) for FEC | |
---|---|---|
Year 1 (phases 1 and 2), pre-pandemic | Year 2 (phase 3), pandemic | |
MGS (PI and developer of triage tools) | 10% | 20% |
PM (Very large database analyst) | 20% | 20% |
Pre-doctoral Research Assistant | 100% | nil |
JVT (Influenza Epidemiology) | 3% | 5% |
JK (Methodology and statistical support) | 3% | 3% |
Appendix 1 details costs for the technology solution planned by the research group, broken down by phases of study and years of study.
Duration of the proposed project
-
Phase one (inter-pandemic – development and feasibility). Proposed start date 01.04.2012. Duration six months during a summer season: set-up and validation of processes, optimisation of the clinical record entry screen, GP acceptability testing, validation of linkage to hospital episode data, data-return format and development of data clean-up algorithms.
-
Phase two (inter-pandemic – pilot). Proposed start date 01.10.2012. Duration six months in 10 to 20 practices during a winter season. Using data from people presenting with of influenza like illness: test data-cleaning algorithms and the automated weekly evaluation of performance of triage criteria and tools. The project will be mothballed at end of phase two.
-
Phase three will be activated in the event of a pandemic. The duration of a pandemic is hard to estimate. Based on the 2009 event, time from detection of person-to-person spread in the UK to peak activity was three months in the first wave with return to base line activity in a further one month. The second wave had a broader base and longer tail lasting approximately 5 months. The modified GP electronic record will be disseminated to all 629 GPRD practices by the routine update process. Weekly analysis and outputs as described will require increased commitment from all investigators. Costing phase three of the study for one year would allow checks on validity of tools to made between waves; would allow for variations in health seeking behaviour and GP clinical practise between waves, plus a three month wash up period for final analysis and reporting.
Ancillary costs
Weekly teleconferencing with all collaborators 1 hour, 5 lines, £40 per week = £4160.
Eight meetings (three monthly) of all collaborators £130 × 6 × 8 = £6240.
Eight meetings (three monthly) of PI + 1 (travel costs) with oversight committee (2). This will be conducted jointly with a NETSCC Monitor from or nominated by NETSCC £130 × 4 × 8 = £4160.
NETSCC will fund a maximum of two people to attend one international conference or one person to attend two conferences (later is preferred option) = £4500.
Costs of two open-access publications (based on BMJ group rates at 01.09.2011) 2 × £1700 = £3400.
Appendix 1
Detailed cost breakdown for research group data acquisition, processing and reporting
Activity | Phase 1, 6 months | Phase 2, 6 months | Phase 3 , 2 months |
---|---|---|---|
Project administration practice liaisona | |||
LEPIS software development / modification workb | |||
Web based data acquisitionc | |||
LEPIS running costsd | |||
GPRD data processing modificationse | |||
Develop data collation and cleaning algorithmsf | |||
Development of automatic reporting scripts and embedding analysis codeg | |||
Scientific input (TVS and TW)h | |||
System documentationi | |||
GPRD data processing system modification and testingj | |||
GPRD data costsk | |||
Weekly download of data for study patientsl | |||
Weekly execution of data extraction routinem | |||
Produce and send weekly reportn | |||
Subtotal |
Year one, Pre-pandemic (phases 1 and 2) | |
Year two, Pandemic (phase 3) | |
Total GRPD costs |
Notes
|
References
1. Chief Medical Officer, Great Britain Ministry of Health (1920) Reports on public health and medical subjects. No 4. Report on the pandemic of influenza, 1918–19. His Majesty’s Stationery Office, London.
2. Department of Health (2009) Pandemic Flu: Management of Demand and Capacity in Healthcare Organisations. Her Majesty’s Stationery Office, London.
3. Lim WS (2007) Pandemic flu: clinical management of patients with an influenza-like illness during an influenza pandemic. Provisional guidelines from the British Infection Society, British Thoracic Society, and Health Protection Agency in collaboration with the Department of Health. Thorax 62 Suppl 1: 1–46.
4. BTS (2009) BTS guidelines for the management of community acquired pneumonia in adults: update 2009. Thorax 64.
5. Lim W, van der Eerden M, Laing R, Boersma W, Karalus N, et al. (2003) Defining community acquired pneumonia severity on presentation to hospital: an international derivation and validation study. Thorax 58: 377–382.
6. Challen K, Bentley A, Bright J, Walter D (2007) Clinical review: Mass casualty triage- pandemic influenza and critical care. Critical Care 11.
7. Mulrennan S, Tempone SS, Ling IT, Williams SH, Gan GC, et al. (2010) Pandemic influenza (H1N1) 2009 pneumonia: CURB-65 score for predicting severity and nasopharyngeal sampling for diagnosis are unreliable. PLoS ONE 5: e12849.
8. Challen K, Bright J, Bentley A, Walter D (2007) Physiological-social score (PMEWS) vs. CURB-65 to triage pandemic influenza: a comparative validation study using community-acquired pneumonia as a proxy. BMC Health Services Research 7.
9. Department of Health & NHS (2009) Swine flu clinical package for use when there are exceptional demands on healthcare services. COI for Department of Health Gateway ref 12368 http://www.dh.gov.uk/en/Publicationsandstatistics/Publications/PublicationsPolicyAndGuidance/DH_106495.
10. British Thoracic Society (2004) Guidelines for the management of Community Acquired Pneumonia (CAP) in Adults. London: BTS.
11. British Thoracic Society (2002) Guidelines for the Management of Community Acquired Pneumonia in Childhood. Thorax 57(S1).
12. O’Driscoll BR, Howard LS, Davison AG (2008) BTS guideline for emergency oxygen use in adult patients. Thorax 63 Suppl 6: vi1–68.
13. World Health Organization (1991) Technical bases for the management of pneumonia in children at first-level health facilities. WHO/ARI/91.20.
14. World Health Organization (1994) Management of the child with cough or difficult breathing. WHO/ARI/94.31.
15. World Health Organization (2001) Child and adolescent health and development: IMCI technical seminars. WHO/FCH/CAH/01.10.
16. World Health Organization (2002) Evidence base for the community management of pneumonia. WHO/FCH/CAH/02.23.
17. National Collaborating Centre for Chronic Conditions (2004) Chronic obstructive pulmonary disease – Management of chronic obstructive pulmonary disease in adults in primary and secondary care. NICE Clinical Guideline 12. London: Royal College of Physicians of London.
18. Scottish Intercollegiate Guidelines Network (2006) Bronchiolitis in children. SIGN Guideline 91. Edinburgh: SIGN.
19. National Collaborating Centre for Women’s and Children’s Health (2007) Feverish illness in children. NICE Clinical Guideline 47. Welsh A, editor. London: RCOG Press.
20. Goodacre S, Challen K, Wilson R, Campbell M (2010) Evaluation of triage methods used to select patients with suspected pandemic influenza for hospital admission: a cohort study. Health Technology Assessment 14: 173–236.
21. Nguyen-Van-Tam J, Openshaw P, Hashim A (2010) Risk factors for hospitalisation and poor outcome with pandemic A/H1N1 influenza: United Kingdom first wave (May-September 2009). Thorax 65: 645–651.
22. P Myles, J Nguyen-Van-Tam, W-S Lim, K Nicholson, S Brett, et al. (September 2011) A comparison of three triage tools for 2009 pandemic influenza a(H1N1). European Scientific Working group on Influenza (ESWI) Fourth Conference. Malta. pp. A123P.
23. Myles P, Semple M, Lim W, Openshaw P, Gadd E, et al. (submitted) Predictors of clinical outcome in a national UK hospitalised cohort across both waves of the Influenza A/H1N1 pandemic 2009–2010.
24. Viboud C, Miller M, Olson D, Osterholm M, Simonsen L (2010) Preliminary Estimates of Mortality and Years of Life Lost Associated with the 2009 A/H1N1 Pandemic in the US and Comparison with Past Influenza Seasons. PLoS currents: RRN1153.
25. Hine D (2010) The 2009 Influenza Pandemic: An independent review of the UK response to the 2009 influenza pandemic. London: Cabinet Office HMG.
26. Watkins J (2004) Influenza: burden of disease in childhood. International Congress Series 1263: 263–266.
27. Hansell A, Hollowell J, Nichols T, McNiece R, Strachan D (1999) Use of the General Practice Research Database (GPRD) for respiratory epidemiology: a comparison with the 4th Morbidity Survey in General Practice (MSGP4). Thorax 54: 413–419.
28. Mangtani P, Cumberland P, Hodgson CR, Roberts JA, Cutts FT, et al. (2004) A cohort study of the effectiveness of influenza vaccine in older people, performed using the United Kingdom general practice research database. The Journal of Infectious Diseases 190: 1–10.
29. Hajat S, Haines A, Goubet SA, Atkinson RW, Anderson HR (1999) Association of air pollution with daily GP consultations for asthma and other lower respiratory conditions in London. Thorax 54: 597–605.
30. Jick H, Jick SS, Derby LE (1991) Validation of information recorded on general practitioner based computerised data resource in the United Kingdom. BMJ 302: 766–768.
31. Health Protection Agency (2009) Pandemic (H1N1) 2009 in England: an overview of initial epidemiological findings and implications for the second wave.
32. Hollowell J (1994) General practice research database (GPRD): scope and quality of data. Office of Population Censuses and Statistics (OPCS): 1–20.
33. Khan NF, Harrison SE, Rose PW (2010) Validity of diagnostic coding within the General Practice Research Database: a systematic review. The British Journal of General Practice: the journal of the Royal College of General Practitioners 60: e128–136.
34. Meier CR, Napalkov PN, Wegmuller Y, Jefferson T, Jick H (2000) Population-based study on incidence, risk factors, clinical complications and drug utilisation associated with influenza in the United Kingdom. European journal of clinical microbiology & infectious diseases : official publication of the European Society of Clinical Microbiology 19: 834–842.
35. Jick H, MacLaughlin D, Egger P, Wiggins P (2011) The United Kingdom 2009 Swine Flu Outbreak As Recorded in Real Time by General Practitioners. Epidemiology Research International 2011.
FIGURE 1.
Paediatric and adult DH/NHS Community Assessment Tools.
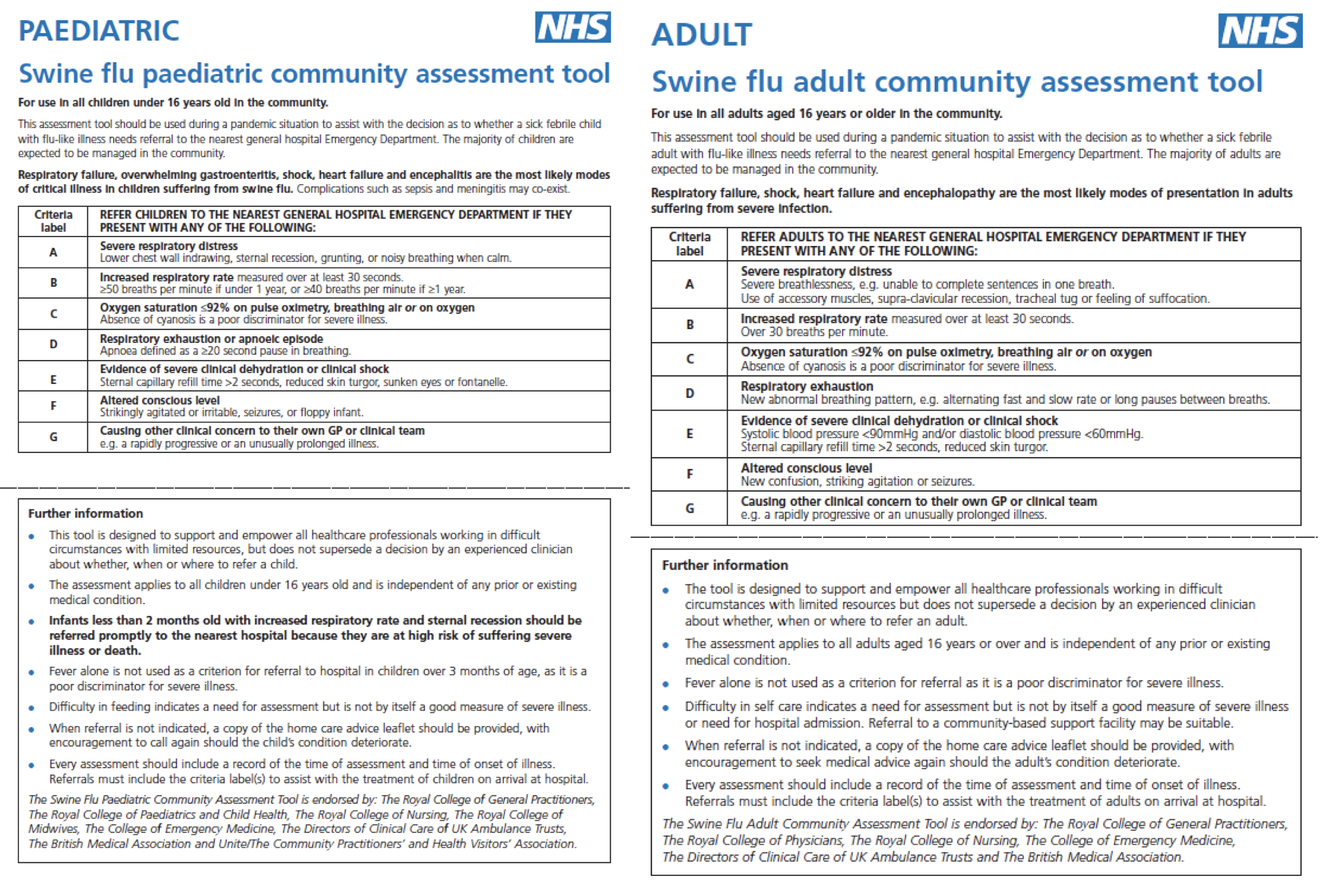
FIGURE 2.
ROC curves comparing the predictive value of CAT scores (solid lines), CURB-65 (grey dashed lines) and PMEWS (black dashed lines) in relation to admission to level 2 high dependency care and level 3 intensive care (upper) and mortality (lower) in adults (left panels) and children ≤ 16 years (right panels).
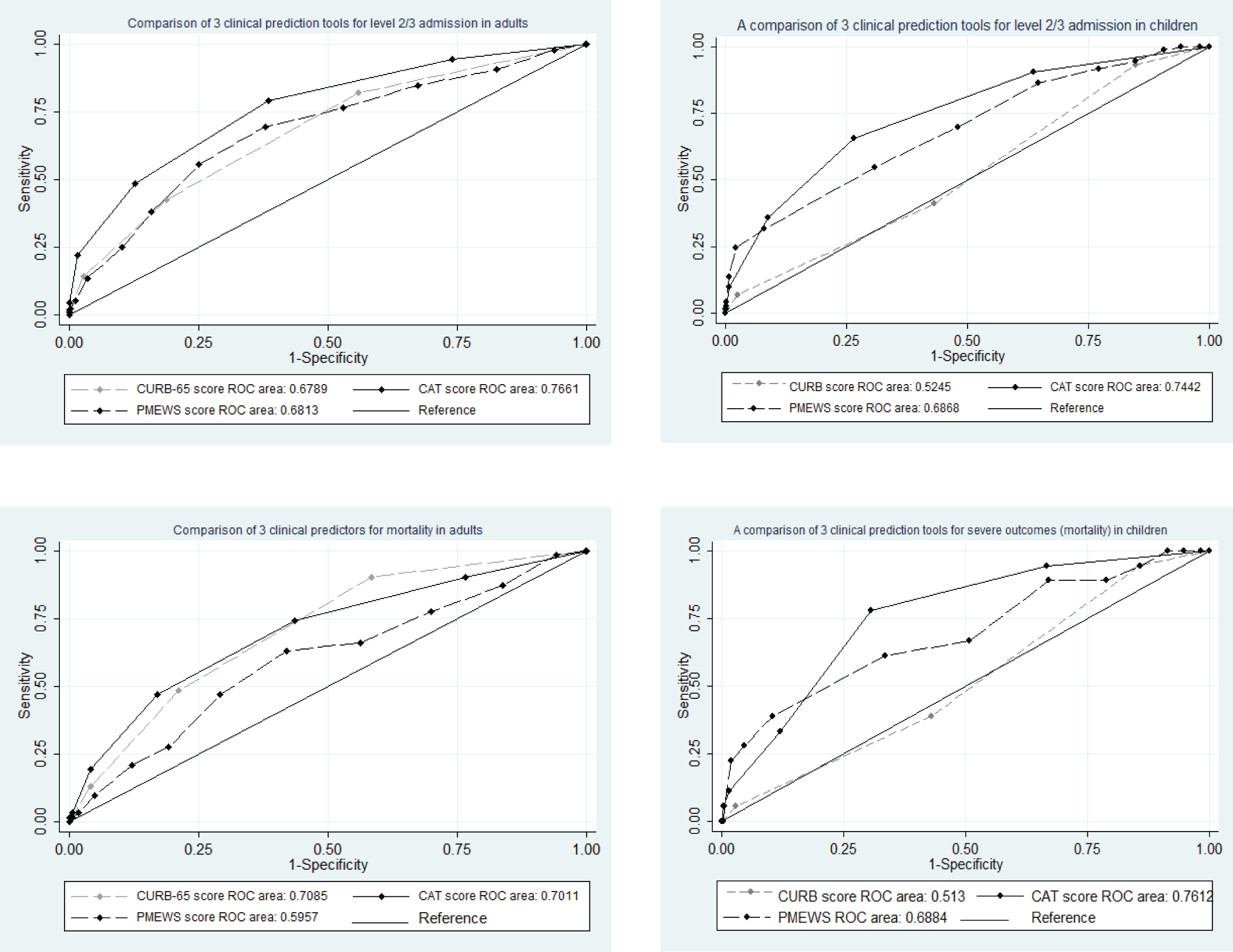
Outcome | Score | ROC area (95% CI) | Sensitivity, % (95% CI) | Specificity, % (95% CI) | PPV, % (95% CI) | NPV, % (95% CI) | |
---|---|---|---|---|---|---|---|
Level 2/3 admission | CURB-65 | ≥ 2 | 0.62 (0.58, 0.66) | 42.4 (35.0, 50.0) | 81.2 (78.5, 83.8) | 31.6 (25.8, 38.0) | 87.3 (84.8, 89.5) |
≥ 3 | 0.56 (0.53, 0.58) | 14.1 (9.4, 20.1) | 97.2 (95.9, 98.2) | 51.0 (36.3, 65.6) | 84.7 (82.3, 86.9) | ||
PMEWS | > 1 | 0.52 (0.51, 0.53) | 97.7 (94.3, 99.4) | 6.3 (4.7, 8.1) | 17.6 (15.3, 20.1) | 93.1 (83.3, 98.1) | |
> 2 | 0.54 (0.51, 0.56) | 90.4 (85.1, 94.3) | 17.4 (14.9, 20.1) | 18.3 (15.8, 21.1) | 89.8 (84.2, 94.0) | ||
> 3 | 0.54 (0.51, 0.56) | 90.4 (85.1, 94.3) | 17.4 (14.9, 20.1) | 18.3 (15.8, 21.1) | 89.8 (84.2, 94.0) | ||
> 4 | 0.59 (0.56, 0.62) | 84.7 (78.6, 89.7) | 32.6 (29.4, 35.8) | 20.5 (17.6, 23.6) | 91.2 (87.5, 94.1) | ||
> 5 | 0.62 (0.58, 0.65) | 76.3 (69.3, 82.3) | 47.0 (43.7, 50.4) | 22.8 (19.5, 26.4) | 90.6 (87.5, 93.2) | ||
> 7 | 0.65 (0.61, 0.69) | 55.4 (47.7, 62.8) | 74.9 (71.8, 77.7) | 31.1 (26.0, 36.5) | 89.1 (86.6, 91.3) | ||
> 9 | 0.57 (0.54, 0.61) | 24.9 (18.7, 31.9) | 89.7 (87.5, 91.6) | 33.1 (25.2, 41.8) | 85.3 (82.9, 87.6) | ||
> 11 | 0.52 (0.50, 0.54) | 5.1 (2.4, 9.4) | 98.7 (97.7, 99.4) | 45.0 (23.1, 68.5) | 83.5 (81.1, 85.8) | ||
CATs | ≥ 3 | 0.68 (0.64, 0.72) | 48.6 (41.0, 56.2) | 87.3 (84.8, 89.4) | 43.9 (36.8, 51.1) | 89.2 (86.9, 91.2) | |
≥ 4 | 0.60 (0.57, 0.63) | 22.0 (16.2, 28.9) | 98.4 (97.3, 99.1) | 73.6 (59.7, 84.7) | 86.0 (83.7, 88.1) | ||
≥ 5 | 0.52 (0.51, 0.54) | 4.5 (2.0, 8.7) | 100.0 (99.6, 100.0) | 100.0 (63.1, 100.0) | 83.6 (81.2, 85.8) | ||
Death | CURB-65 | ≥ 2 | 0.64 (0.57, 0.70) | 48.4 (35.5, 61.4) | 78.8 (76.1, 81.4) | 12.7 (8.7, 17.6) | 96.0 (94.4, 97.3) |
≥ 3 | 0.54 (0.50, 0.59) | 12.9 (5.7, 23.9) | 95.8 (94.4, 97.0) | 16.3 (7.3, 29.7) | 94.6 (92.9, 95.9) | ||
PMEWS | > 1 | 0.52 (0.50, 0.54) | 98.4 (91.3, 100.0) | 5.8 (4.4, 7.5) | 6.2 (4.8, 7.9) | 98.3 (90.8, 100.0) | |
> 2 | 0.52 (0.47, 0.56) | 87.1 (76.1, 94.3) | 16.3 (14.0, 18.7) | 6.2 (4.7, 8.0) | 95.2 (90.8, 97.9) | ||
> 3 | 0.52 (0.47, 0.56) | 87.1 (76.1, 94.3) | 16.3 (14.0, 18.7) | 6.2 (4.7, 8.0) | 95.2 (90.8, 97.9) | ||
> 4 | 0.54 (0.48, 0.59) | 77.4 (65.0, 87.1) | 30.1 (27.2, 33.0) | 6.6 (4.9, 8.6) | 95.5 (92.5, 97.5) | ||
> 5 | 0.55 (0.49, 0.61) | 66.1 (53.0, 77.7) | 43.7 (40.5, 46.8) | 6.9 (5.0, 9.3) | 95.3 (92.9, 97.1) | ||
> 7 | 0.59 (0.52, 0.65) | 46.8 (34.0, 59.9) | 70.8 (67.8, 73.6) | 9.2 (6.3, 13.0) | 95.4 (93.7, 96.8) | ||
> 9 | 0.54 (0.49, 0.60) | 21.0 (11.7, 33.2) | 87.7 (85.5, 89.7) | 9.8 (5.3, 16.1) | 94.6 (92.9, 96.0) | ||
> 11 | 0.51 (0.48, 0.53) | 3.2 (0.4, 11.2) | 98.2 (97.1, 98.9) | 10.0 (1.2, 31.7) | 94.1 (92.5, 95.5) | ||
CATs | ≥ 3 | 0.65 (0.58, 0.71) | 46.8 (34.0, 59.9) | 82.9 (80.4, 85.2) | 14.8 (10.1, 20.6) | 96.1 (94.6, 97.3) | |
≥ 4 | 0.58 (0.53, 0.63) | 19.4 (10.4, 31.4) | 95.8 (94.4, 97.0) | 22.6 (12.3, 36.2) | 94.9 (93.4, 96.2) | ||
≥ 5 | 0.51 (0.49, 0.54) | 3.2 (0.4, 11.2) | 99.4 (98.7, 99.8) | 25.0 (3.2, 65.1) | 94.2 (92.6, 95.5) | ||
Combined severe outcome (level 2/3 admission or death) | CURB | ≥ 2 | 0.63 (0.59, 0.66) | 43.5 (36.3, 50.8) | 81.9 (79.1, 84.4) | 35.0 (29.0, 41.5) | 86.6 (84.0, 88.8) |
≥ 3 | 0.56 (0.53, 0.58) | 14.1 (9.5, 19.9) | 97.4 (96.1, 98.4) | 55.1 (40.2, 69.3) | 83.5 (81.0, 85.7) | ||
PMEWS | > 1 | 0.52 (0.51, 0.53) | 97.9 (94.7, 99.4) | 6.4 (4.8, 8.2) | 19.0 (16.6, 21.6) | 93.1 (83.3, 98.1) | |
> 2 | 0.53 (0.51, 0.56) | 89.5 (84.3, 93.5) | 17.3 (14.8, 20.0) | 19.6 (17.0, 22.4) | 88.0 (82.1, 92.5) | ||
> 3 | 0.53 (0.51, 0.56) | 89.5 (84.3, 93.5) | 17.3 (14.8, 20.0) | 19.6 (17.0, 22.4) | 88.0 (82.1, 92.5) | ||
> 4 | 0.58 (0.55, 0.61) | 83.2 (77.2, 88.2) | 32.5 (29.4, 35.8) | 21.7 (18.8, 24.9) | 89.6 (85.7, 92.8) | ||
> 5 | 0.61 (0.57, 0.65) | 74.9 (68.1, 80.9) | 47.1 (43.7, 50.5) | 24.2 (20.8, 27.8) | 89.3 (86.0, 92.0) | ||
> 7 | 0.64 (0.60, 0.68) | 53.4 (46.1, 60.6) | 74.9 (71.9, 77.8) | 32.4 (27.2, 37.9) | 87.7 (85.1, 90.0) | ||
> 9 | 0.57 (0.53, 0.60) | 23.6 (17.7, 30.2) | 89.6 (87.4, 91.6) | 33.8 (25.9, 42.5) | 83.9 (81.3, 86.2) | ||
> 11 | 0.52 (0.50, 0.54) | 5.2 (2.5, 9.4) | 98.8 (97.8, 99.4) | 50.0 (27.2, 72.8) | 82.3 (79.8, 84.6) | ||
CATs | ≥ 3 | 0.68 (0.64, 0.72) | 48.2 (40.9, 55.5) | 87.8 (85.4, 89.9) | 46.9 (39.8, 54.2) | 88.3 (85.9, 90.4) | |
≥ 4 | 0.60 (0.57, 0.63) | 21.5 (15.9, 28.0) | 98.6 (97.5, 99.3) | 77.4 (63.8, 87.7) | 84.8 (82.4, 87.0) | ||
≥ 5 | 0.52 (0.51, 0.54) | 4.2 (1.8, 8.1) | 100.0 (99.6, 100.0) | 100.0 (63.1, 100.0) | 82.3 (79.8, 84.6) |
List of abbreviations
- AUROC
- area under receiver operator characteristic
- CAT
- Community Assessment Tool
- CI
- confidence interval
- CPRD
- Clinical Practice Research Datalink
- eCRF
- electronic case report form
- EHR
- electronic health record
- FLU-CIN
- Pandemic Influenza Clinical Information Network
- GP
- general practitioner
- HES
- Hospital Episode Statistics
- HSCIC
- Health and Social Care Information Centre
- ILI
- influenza-like illness
- ISAC
- Independent Scientific Advisory Committee
- IT
- information technology
- LEPIS
- Local Eligibility Patient Identification Software
- MHRA
- Medicines and Healthcare products Regulatory Agency
- NIHR
- National Institute for Health Research
- ONS
- Office for National Statistics
- OR
- odds ratio
- PCRN
- Primary Care Research Network
- portable document format
- PHE
- Public Health England
- PMEWS
- Pandemic Medical Early Warning Score
- RCGP
- Royal College of General Practitioners
- RTF
- rich text format