Notes
Article history
The research reported in this issue of the journal was funded by the HTA programme as project number 09/61/01. The contractual start date was in April 2011. The draft report began editorial review in April 2015 and was accepted for publication in December 2015. The authors have been wholly responsible for all data collection, analysis and interpretation, and for writing up their work. The HTA editors and publisher have tried to ensure the accuracy of the authors’ report and would like to thank the reviewers for their constructive comments on the draft document. However, they do not accept liability for damages or losses arising from material published in this report.
Declared competing interests of authors
Tim Eisen has declared share ownership and corporate sponsored research from AstraZeneca. He is on the advisory boards of AVEO, Astellas, Bristol-Myers Squibb (BMS), Bayer, Boehringer Ingelheim (BI), GlaxoSmithKline (GSK), Novartis, Pfizer and Roche. He has declared corporate-sponsored research from Bayer, GSK and Pfizer. He has received honoraria from AVEO, Astellas, BMS, Bayer, BI, GSK, Novartis, Pfizer and Roche. Tim Eisen is on part-time leave of absense from the University of Cambridge to work in AstraZeneca as Vice President, Head of Clinical Discovery Unit.
Permissions
Copyright statement
© Queen’s Printer and Controller of HMSO 2016. This work was produced by Field et al. under the terms of a commissioning contract issued by the Secretary of State for Health. This issue may be freely reproduced for the purposes of private research and study and extracts (or indeed, the full report) may be included in professional journals provided that suitable acknowledgement is made and the reproduction is not associated with any form of advertising. Applications for commercial reproduction should be addressed to: NIHR Journals Library, National Institute for Health Research, Evaluation, Trials and Studies Coordinating Centre, Alpha House, University of Southampton Science Park, Southampton SO16 7NS, UK.
Chapter 1 Introduction
Lung cancer: the clinical problem
Lung cancer kills more people than any other cancer, both in the UK and worldwide. It accounts for 13% of all malignant diagnoses in the UK, but > 20% of all UK deaths from malignancy (and 6% of total deaths). In the UK, in 2011, there were over 43,000 lung cancer diagnoses and nearly 35,000 lung cancer deaths. 1 Although improving, the 5-year survival rate from lung cancer in the UK, for all stages, is < 10% for both men and women – among the lowest for all cancer types1 (www.roycastle.org). Irrespective of age, about three-quarters of patients with lung cancer present at a late stage at which treatment is palliative and survival poor.
Based on the large piece of work undertaken by the International Association for the Study of Lung Cancer Lung Cancer Staging project,2 overall survival is best predicted by the stage of disease. Individuals with pT1aN0 have a 5-year survival rate of 77%, compared with 22% for pT4Nx disease, and as low as 2% in pT4-malignant effusion and nodules. The poor survival rates in the UK are mainly a result of most lung cancers being identified at a late stage; thus, there is a clear rationale for seriously considering a national screening programme.
The estimated cost of lung cancer to the UK NHS is £9071 per patient per year (compared with £2776 per patient per year for all cancer types),3 and the total cost of lung cancer to the UK economy is £2.4B, more than any other cancer. 3 This reflects the relatively high incidence of lung cancer, compounded by poor survival and high mortality. Although age-standardised lung cancer deaths have declined over the past few years, because of lower tobacco use and possibly greater public awareness, many ex-smokers remain at high risk of developing lung cancer. Their absolute risk is dependent on their age, smoking duration prior to tobacco cessation, interval since quitting and other factors, such as exposure to asbestos. This group of individuals now outnumbers current smokers both in the USA and in Europe, and will continue to do so over the next 2–3 decades. There is therefore a significant population at high risk of developing lung cancer, who could potentially benefit from screening and early detection.
Risk factors for lung cancer
Tobacco use is the major aetiological factor underlying lung cancer. The population attributable fraction for tobacco smoke (i.e. the percentage of lung cancer cases that are caused by tobacco) is 86%, representing around 34,600 cases in the UK (2010 figures). 4 Approximately 15% of lung cancer cases in never-smokers are most likely due to a range of environmental exposures and genetic factors, as well as second-hand smoke. 4 Other factors listed by the International Agency for Research on Cancer as having a causative role in lung cancer include ionising radiation (e.g. from X-rays, radon gas or previous radiotherapy) and occupational exposures, such as asbestos and silica. 5 Lung cancer is more common in people with a family history of the disease, particularly in siblings,6 and in those with a previous history of respiratory diseases [e.g. chronic obstructive pulmonary disease (COPD), chronic bronchitis, emphysema, tuberculosis and pneumonia]. 7,8 Lung cancer is more common in people from deprived socioeconomic groups:9 people from the most deprived socioeconomic quintile in England are 2.5 times as likely to develop lung cancer as those from the highest quintile (after adjusting for age; 2000–4 figures). 10 Furthermore, this trend has been consistent since the mid-1990s. 10,11 Recent work has supported the case that air pollution is independently associated with lung cancer. 12 Lung cancer incidence increases with age, with around 75% of new UK cases arising in individuals aged ≥ 65 years (2008–10 data). 1 Lung cancer is more common in males, with lifetime risks in the UK of around 7.1% for men and 5.6% for women. 1
Risk models for lung cancer prediction
Based on the known risk factors for lung cancer, a number of algorithmic risk models have been developed in order to predict a person’s likelihood of developing the disease. 13 Use of such models provides the potential to target screening or resources towards those at highest risk. 14 The majority of these risk models are based predominantly upon age and smoking, including those of Bach et al.,15 Spitz et al. ,16 Tammemagi et al. 17 and, more recently, that of Kovalchik et al. 18 However, the predictive accuracy of lung cancer risk models may be further improved by the addition of other epidemiological risk factors. 19 In the UK, the Liverpool Lung Project (LLP) risk model has been developed from a large case–control study of the same name. 20,21 The LLP risk prediction model incorporates age, gender, family history of lung cancer, smoking duration, personal history of other cancers and non-malignant respiratory diseases, and occupational exposure to asbestos. 20,21 The LLP model is a robust algorithm that has been validated in two international case–control populations (Harvard22 and European Union Early Lung Cancer) and one independent cohort (LLP 7500). 23 The LLP risk model has distinctive strengths. First, the predictor variables are all explicitly defined and can be readily assessed at the time of patient presentation, and, second, patients can be assigned to their appropriate risk class on the basis of information from the initial history alone. The utilisation of risk models, specifically the LLP risk model, has recently been highlighted by the National Cancer Institute (NCI). 24
Screening for lung cancer
Worldwide, a number of cohort studies and randomised controlled trials (RCTs) of screening for early detection of lung cancer have been, or are being, conducted. 25 These have used both chest X-ray and low-dose computed tomography (LDCT) screening; the latter is the superior method. 25
Chest radiography and sputum cytology lung cancer screening
The earliest lung screening trial was undertaken in London in the 1960s: > 55,000 individuals were randomised to either chest radiography every 6 months for 3 years, or chest radiography at the beginning and end of the 3-year period. 26 No mortality difference was found between the two groups. Three major trials in the USA, and one in Czechoslovakia, were developed in the 1970s; these trialled chest radiography with or without sputum cytology, but none showed any reduction in lung cancer mortality. A more recent trial is the lung component of the NCI Prostate, Lung, Colorectal and Ovarian Screening Trial (PLCO) of 150,000 individuals. In this trial, those in the intervention arm were offered chest radiography at baseline and then annually for 3 subsequent years (smokers) or 2 subsequent years (never-smokers); those in the control arm were given ‘usual care’ only. No mortality benefit was observed for the chest X-ray arm. 27,28
Low-dose computed tomography lung cancer screening
Low-dose computed tomography, which was introduced in the late 1990s, offers a major advance in imaging technology. 29 LDCT is more sensitive than chest radiography, and has enabled detection of lung tumours that are < 1 cm. Early studies using this technology include the USA-based Early Lung Cancer Action Project (ELCAP)30 in 1000 high-risk smokers; the Mayo Clinic project in 1520 individuals, including annual sputum cytology,31 the Milan study32 and a 3-year mass screening programme using a mobile computed tomography (CT) unit in Japan. 33 The ELCAP (observational) study was later expanded to an international collaboration including 30,000 subjects. The European Union–US Spiral CT Collaboration was initiated in 2001 in Liverpool. Subsequent meetings throughout Europe resulted in the development of collaborative protocols to provide a mechanism for different trial groups to work together, and for outcome data to be comparable between studies. The ultimate aim – pooling of results – was formalised in The Liverpool Statement 2005. 34
National Lung Cancer Screening trial
The first major RCT for lung cancer LDCT screening was the USA National Lung Cancer Screening Trial (NLST),35 which is a combination of two trials, one set up by the US NCI and the other by the American College of Radiology Imaging Network. The NLST started in 2002 and completed enrolment in 2004. The NLST recruited > 53,000 people aged 55–74 years, with a 30 pack-year smoking history, who had smoked within 15 years. These subjects were randomised to LDCT or chest X-ray, with lung cancer mortality as the outcome. The X-ray control arm of the study comprised data from those individuals who had been screened in the PLCO27 trial. The NLST reported a 20% relative reduction in lung cancer mortality in the LDCT arm. 36 Furthermore, all-cause mortality was reduced by 6.7% in the LDCT group compared with the chest radiography group.
European lung cancer screening trials
In Europe, the Dutch–Belgian randomised LDCT lung cancer screening trial (NELSON)37 was launched in 2003 and is currently under way. In planning the study, attention was focused on selecting a high-risk population to reduce the cost but retain the power of the study. Potential study participants were approached by letter with a questionnaire on their smoking exposure and asking whether or not they wished to be included in the trial. The questionnaire was initially sent to 335,441 men and women aged 50–75 years. Based on this data set, the selection criteria were developed, depending on duration of smoking, time since smoking cessation in ex-smokers, number of cigarettes smoked per day and the mean estimated expected lung cancer mortality rate. In this trial,37 LDCT screening takes place in years 1, 2 and 4, with 10 years of follow-up. The trial37 has 15,422 individuals, randomised in equal numbers to LDCT or ‘usual care’, with lung cancer mortality as the outcome. A number of small trials have been initiated, in anticipation of combination with partner studies, or a future meta-analysis. These include the Danish Lung Cancer Screening Trial (DLCST), Multi-centric Italian Lung Detection Trial (MILD),38,39 Italian Lung cancer Computed Tomography screening trial (ITALUNG),40,41 Detection and screening of early lung cancer by Novel imaging Technology and molecular assays trials in Italy42 and the French randomised pilot study, Depiscan. 43 ITALUNG,41 MILD38 and DLCST44 have all reported on their mortality data, which showed no difference in the CT-screened arm; however, none of the studies was powered to provide such a result. 39,41,44 A summary of the European randomised lung cancer screening trials is shown in Table 1.
Trial parameters | Trial name | |||||
---|---|---|---|---|---|---|
NELSON37 | DLCST45 | LUSI15 | DANTE42 | ITALUNG41 | MILD38 | |
Number of rounds | 4 | 5 | 5 | 5 | 4 | 5 |
Number of screening sites | 4 | 1 | 1 | 3 | 5 | 3 |
Vendor CT scanner | Siemens and Philips | Philips | Toshiba and Siemens | Philips | Siemens and General Electric | Siemens and Philips |
Number of rows | 16 | 16 | 16 and 128 | 1 and 16 | 1 and 16 | 6–16 |
Volumetric analysis | Yes | Yes | Yes | No | No | Yes |
Screen interval, years | 1, 2 and 2.5 | 1 | 1 | 1 | 1 | Randomisation to 1 or 2 |
Screen arm (n) | 7515 | 2052 | 2029 | 1276 | 1613 | 1185 and 1182 |
Control arm (n) | 7907 | 2052 | 2023 | 1196 | 1593 | 1630 |
Mean age at randomisation years (± SD) | 59 (6) | 57 (5) | 58 (5) | 65 (5) | 61 (4) | 59 (6) |
Current smokers at randomisation, % | 55 | 76 | 61 | 55 | 65 | 63 |
Mean pack-years (± SD) | 42 (19) | 36 (13) | 36 (18) | 47 (25) | 43 (18) | 43 (15) |
Females, % | 16 | 45 | 34 | 0 | 35 | 32 |
Follow-up since randomisation, years | 6 | 5 | 3 | 6 | 6 | 5 |
Person-years of follow-upa | 90,655 | 23,248 | 4073 | 13,541 | 14,453 | 15,589 |
Recruitment completed | Yes | Yes | Yes | Yes | Yes | No |
Screening completed | No | Yes | No | Yes | Yes | No |
Detection rate baseline, % | 0.9 | 0.8 | 1.1 | 2.2 | 1.5 | 0.8 |
Detection rate second round, % | 0.5 | 0.6 | 0.65 | 0.5 | 0.4 | 0.5 |
An overview of the European randomised lung cancer CT screening trials was prepared by the seven contributing principal investigators, and this paper also discussed the implementation of CT screening in Europe, post NLST. 47 The overview was based on the epidemiological, radiological and nodule management aspects of their trials at August 2010. 47,48 Including the data from the UK Lung Cancer Screening Trial (UKLS), 36,000 individuals will be available for a final mortality data testing after the NELSON37 trial has been reported.
Lung cancer screening in clinical practice in the UK
In order to make a case for the provision of screening as a service, we need a reliable estimate of the effect of LDCT screening on mortality in comparison with usual care. To date, only one trial (NLST35) has demonstrated a reduction in mortality from CT screening,49 but even when the results of NLST are combined with the three European trials that have reported on mortality (ITALUNG,41 MILD,38 DLCST45) there is still a 19% reduction. 46 If we assume that LDCT screening does reduce mortality, it is then necessary to demonstrate that this can be achieved in a cost-effective manner. This, in turn, requires information about benefits and harms, as well as the optimum way to select and recruit individuals at risk. The final results of NELSON37 and the pooled European RCTs will provide valuable information on mortality and cost-effectiveness data. Additionally, it is now apparent that some of the NLST35 participants had little chance of benefiting from the trial, as they were at very low risk of developing lung cancer; although this makes the reduction in mortality in the NLST more impressive, it also highlights the need for a more cost-effective approach and the minimisation of harms in those who are unlikely to benefit. In comparison, the NELSON37 study has adequate power to demonstrate a substantial benefit in a high-risk group.
The UK National Screening Committee50 has determined 22 criteria for the viability, effectiveness and appropriateness of a screening programme, 20 of which are relevant to LDCT lung cancer screening. Black et al. 51 have undertaken a systematic review of the literature in order to ascertain whether or not there was evidence for any clinical effectiveness utilising LDCT for lung cancer screening. This review was undertaken at a time when there was a paucity of real data and thus the conclusions were drawn from two small trials with very variable results. Not surprisingly, their conclusion stated that there was insufficient evidence at the time to support LDCT screening.
As part of feasibility studies prior to the start of the UKLS, a cost-effectiveness model was developed by Professor David Whynes, utilising the most conservative costings within the UK and NHS tariffs. This estimated the incremental cost-effectiveness ratio (ICER) of a single screen among a high-risk male population to be around £14,000 per quality-adjusted life-year (QALY) gained. 52
The UK Lung Cancer Screening trial
To begin to address some of these questions, the National Institute for Health Research Health Technology Assessment programme provided funding for a pilot study of LDCT screening in the UK population. Successful and cost-effective screening for lung cancer relies first upon identifying and targeting high-risk individuals:14 the US and European trials have used a combination of age and smoking history as trial eligibility criteria. However, as noted above, a number of more formal lung cancer risk prediction algorithms have been developed,13 and these may have utility in selecting those people who are suitable for screening. 14 In the UK, the risk model of choice is the LLP algorithm,20,21 and this model has been used as the basis for risk assessment in the UKLS.
The UKLS, the pilot phase of which is the subject of this report, is a multicentre RCT of LDCT for the early detection of lung cancer in high-risk subjects (≥ 5% risk over 5 years, based on the LLP model) aged 50–75 years. During the pilot phase of the UKLS, 4061 high-risk subjects were consented between August 2011 and February 2013.
The design of the main UKLS follows the Wald Single-Screen Design, which will enable early observation of the rate at which lung cancer incidence and mortality return to control levels, and in turn inform policy-makers on the appropriate screening frequency. 53
However, as only a pilot UKLS was undertaken, the data for mortality assessment can be calculated only when pooled with the other European Union screening trials. The UKLS incorporates a number of other elements that are specific to the area of lung cancer screening RCTs. First, UKLS is a population-based study, which randomly approached 250,000 individuals aged 50–75 years through local primary care trust (PCT) records. Second, UKLS utilises a validated lung cancer risk prediction model at an individual level23 to identify high-risk subjects, thus targeting resources to those who are most likely to benefit from screening. Third, UKLS is unique in having a formal care pathway plan, based on categorisation (size, volume and growth) of lung nodules. 53 Fourth, UKLS uses state-of-the-art volumetric analysis of lung nodules identified on LDCT scan: this has been used consistently by only one other trial – NELSON. 37
The UKLS pilot focused on two geographical areas, covering six PCTs around Liverpool and Cambridge. These areas were selected as they both have a specialist thoracic hospital [Liverpool Heart and Chest Hospital (LHCH), Merseyside and Papworth Hospital, Cambridgeshire]. Additionally, they cover very different populations in disparate areas of England, encompassing a broad range of socioeconomic groups that are representative of the UK as a whole. Liverpool is a large coastal port with an industrial heritage, and has the highest incidence of lung cancer in England, together with a very high-risk community within the region. Papworth is located in a rural area (individuals with lower risk of lung cancer but greater distances to travel for screening) but has adjacent cities whose populations are at significant risk of developing lung cancer, albeit lower than that of Liverpool.
Rationale for UK Lung Cancer Screening Trial pilot trial
By combining results of the UKLS pilot with those from NELSON37 and other European studies, it will be possible to model any likely mortality benefit of LDCT screening and treatment of early lesions. The pilot trial will also enable assessment of screening uptake, and factors that are unique to the UK population and the NHS. This will include testing the intervention against the criteria outlined by the UK National Screening Committee, especially some of those criteria concerning cost-effectiveness. The potential cost-effectiveness of UKLS has been maximised by the trial design (i.e. selection of a population at sufficiently high risk to yield a substantial number of tumours in return for the screening activity). 14 However, as the pilot trial is insufficiently powered to demonstrate a reduction in mortality, and a decision has been made not to fund the full UKLS, cost-effectiveness will have to be modelled.
Overall aim of the UK Lung Cancer Screening trial
The overall aim of the UKLS pilot is to contribute to the data required for an informed decision to be made regarding the introduction of population screening for lung cancer. This involves determining the best recruitment and screening strategies, introducing a screening protocol for the management of CT-detected nodules, establishing that the individuals selected for screening are at a sufficiently high risk for the benefits of the screening to outweigh the harms, and assessing the physical and psychological consequences and the health-economic implications of screening. Further objectives are to create a resource for future improvements to screening strategies and provide data for the European Union lung cancer pooling to ascertain European mortality.
Chapter 2 Methods
Objectives
The primary objective of this study was to carry out a pilot RCT examining the use of 16-channel (or higher) multidetector computed tomography in the screened group to identify early lung cancer. As UKLS is a pilot study, the main objectives are to provide data to facilitate decision-making by funders and policy-makers regarding the possibility of future introduction of lung cancer screening into the NHS. At this stage, the trial does not have sufficient power to draw any definitive conclusions regarding clinical or mortality benefits, or to provide categorical health-economic impact figures. However, it is possible to use the pilot data in algorithms to model the likely clinical and economic impact of a larger-scale screening project. The pilot study has also been designed to monitor the performance of all aspects of the clinical care pathway and the research evaluation technicalities, including a bespoke web-based UKLS database.
Trial design
The UKLS is a RCT of LDCT compared with usual care, for the early detection of lung cancer. The methods for the UKLS pilot study were derived from an initial feasibility study and follow the Wald Single-Screen Design. 53 Other screening trials have used this design, including the UK Flexisig Trial, the UK Aortic Aneurysm Screening Trial and the Singapore Breast Screening Trial. 54–56 UKLS is similar in methodology to NELSON,37 and this will allow a combined analysis of results to increase the statistical power of both trials.
Two main components were used in UKLS: an initial questionnaire-based screen to identify high-risk individuals from the population, followed by a RCT with intervention (LDCT) and control arms. Participants were allocated randomly to trial arms in a 1 : 1 ratio. Figure 1 illustrates the trial recruitment process. The UKLS design, patient information booklet and questionnaires were discussed with our patient representatives at each stage of the development of the trial.
FIGURE 1.
The UKLS recruitment and implementation process. DVD, digital versatile disc; GP, general practitioner; HES, Hospital Episode Statistics; MDT, multidisciplinary team; ONS, Office for National Statistics.

Ethical approval and research governance
The study received approval from the National Information Governance Board. Ethical approval for the study was given by Liverpool Central Research Ethics Committee in December 2010 (reference number 10/H1005/74). Site-specific assessments were obtained from the PCTs covering Liverpool, Sefton and Knowsley in the north, and Bedfordshire, Cambridgeshire and Peterborough in the south. The trial was registered with the International Standard Randomised Controlled Trial Register under the reference number 78513845.
A summary of the changes made to the original protocol is given in Appendix 1. Additionally, as fewer people than anticipated were at high risk of lung cancer (and therefore eligible for the trial), two alterations were made to the original plan. First, even though the response rate to the initial UKLS questionnaire was at the expected level, the anticipated proportion of responders with a risk of ≥ 5% over a 5-year period was far lower than expected. This observation may indicate that lower-risk people are more health conscious or simply more willing to participate in trials. Thus, in order to achieve the target of 4000 high-risk individuals, the number of population approaches was increased from 82,000 to 250,000 (this is expanded further below; see Sample size). Second, the LLP risk assessment model (used to determine eligibility for the trial) was adapted to include in the risk calculation a history of respiratory diseases (COPD, tuberculosis, bronchitis and emphysema) as well as pneumonia. Additionally, for the purposes of calculating lung cancer risk, a history of pipe or cigar smoking was classified in the same way as cigarette smoking. The refined model is known as the Liverpool Lung Project lung cancer risk prediction algorithm, version 2 (LLPv2; see Appendix 2).
Participants
Eligibility criteria
The eligibility criteria for trial participants are shown below.
Inclusion criteria
-
Five-year lung cancer risk ≥ 5%, based on the LLPv2 risk prediction model [includes age, gender, smoking duration (cigarettes, pipe and cigars), previous history of respiratory diseases (COPD, emphysema, bronchitis, pneumonia, tuberculosis), history of previous cancer, family history (early/late onset), exposure to asbestos].
-
Males and females aged between 50 and 75 years old.
-
Fully informed written consent given.
Exclusion criteria
-
Unable to give consent.
-
Comorbidity, which would unequivocally contraindicate either screening or treatment if lung cancer were detected.
-
A CT scan of the chest performed within the year preceding the invitation to be screened.
-
Any condition precluding written informed consent.
-
Inability to lie flat.
Setting and population approaches
As UKLS is a population study, its starting point was NHS PCT records covering regions in the vicinity of two thoracic hospitals specialising in lung cancer imaging, pathology and surgery: the LHCH and Papworth Hospital. Records relating to 249,988 individuals, aged 50–75 years, residing in specific PCT areas (Liverpool, Knowsley, Sefton, Cambridgeshire, Peterborough and Bedfordshire) were provided by the PCTs to a third-party data management company (DMC: Radar). Following exclusions by the PCTs, 247,354 of these individuals were approached by post by Radar. Approaches took place over two time periods: August 2011 to March 2012, and May to August 2012.
The initial contact from the DMC took the form of an invitation letter (on the respective PCT-headed notepaper), a UKLS participant information sheet and questionnaire 1 (see Appendix 3). This questionnaire covered smoking history and duration, personal history of non-malignant lung diseases (e.g. pneumonia, COPD, emphysema, bronchitis and tuberculosis) and previous malignancy, exposure to asbestos, and family history of lung and other cancers; in addition, it enquired whether or not the individual would be interested in participating in a screening study. For those who were unwilling to complete the entire questionnaire, and who were not interested in participating further, there was a shorter non-participation questionnaire (covering smoking status, lung cancer prior experience and concern, and educational level), which they were asked to return instead (see Appendix 3).
Response categorisation
Approached subjects were categorised as follows, based on their response to the first invitation: positive responders, individuals who returned questionnaire 1 and agreed to participate in UKLS; negative responders, individuals who declined to participate in UKLS but supplied some basic information by completing the shorter, non-participation questionnaire; and non-responders, individuals who did not respond to the first invitation.
Risk assessment/eligibility for trial
Completed questionnaire 1s (from positive responders) were returned to the DMC, scanned, and the data analysed automatically in order to identify individuals who were at high risk of developing lung cancer over the next 5 years (LLP risk score of ≥ 5). 20 A modified version of the LLP risk algorithm (LLPv2) was utilised for risk calculations (see Appendix 2). This incorporated additional respiratory parameters (i.e. COPD, emphysema, bronchitis and tuberculosis), as well as pneumonia, and also included both pipe and cigar usage within the smoking criteria. (Risk assessment was not carried out for negative responders, as this was not possible from the limited information that they supplied.) High-risk positive responders were contacted with a further questionnaire (questionnaire 2; see Appendix 3) to establish eligibility for the RCT (i.e. no exclusion criteria met)53 and sent a detailed patient information booklet: these people were also asked to consent to release their personal information to the UKLS research team. Non-responders to this second invitation were sent a reminder letter (see Appendix 3).
UK Lung Cancer Screening research clinics
Individuals responding to, and eligible on the basis of, the second questionnaire were invited to one of the recruitment centres (LHCH or Papworth Hospital). They were shown a digital versatile disc outlining the UKLS study57 and provided with an opportunity to ask any questions in a discussion group (n = 6–8) with the recruitment clinic administrator or on a one-to-one basis with a research nurse. Following informed consent, subjects underwent spirometry (forced expiratory volume in 1 second/forced vital capacity ratio), and provided blood, buccal swab, nasal brushings and sputum specimens. Recruits also completed a touchscreen questionnaire; this consisted of follow-up epidemiological and clinical questions, and psychosocial and quality-of-life questions, including the Hospital Anxiety and Depression Scale (HADS)58 and the Cancer Worry Scale-Revised (CWS-R)59,60 (adapted for lung cancer). All smokers (both CT screened and non-screened) were offered smoking cessation advice sheets and a list of local NHS stop smoking services.
Collection, storage and archiving of clinical samples
Up to 24 ml of blood were taken from each participant at the research clinic visit. Blood samples, buccal swabs, nasal brushings and sputum samples were labelled as detailed in the respective UKLS standard operating procedure and packaged. The packages were collected on a daily basis by courier for delivery to the University of Liverpool Experimental Cancer Medicine Centre Good Clinical Laboratory Practice laboratory, where they were processed and entered into their Laboratory Information Management system. A quality control check of these samples has been conducted (see Appendix 6).
Use of UK Lung Cancer Screening samples
A UKLS access committee has been established with external members of UKLS and also a patient representative. This access committee will oversee the release of UKLS data and specimens. The principle underlying release will be based on externally funded projects requesting the use of data/material, which will add considerable information and quality to the UKLS data.
Randomisation and blinding
Following attendance at a research clinic, recruits were randomised by computer into the intervention arm (LDCT scan, screen group) or the control arm (usual care, non-screen group) at a ratio of 1 : 1. Individuals were informed which group they were in within 2 weeks of randomisation. Owing to the nature of the intervention, a blinded trial design was not feasible.
Sequence generation, allocation concealment mechanism and implementation
The randomisation method was developed by the UKLS database designers (Artex, The Netherlands). Upon upload of subjects to the database, each subject was given a randomly generated unique code, consisting of eight characters (0–9; A–Z). All subjects who had given fully informed signed consent were available for randomisation; a minimum of two subjects from the same site (Liverpool or Papworth) were required in order for randomisation to be implemented. Each time randomisation took place, the computer generated a random shift number so that the order of characters in each participant’s unique code was shifted by this number. The rearranged codes were then ordered alphanumerically, and split on a 1 : 1 basis into A (intervention) or B (control) groups. The outcome was thus dependent both on the unique codes of the participants available for randomisation and the random shift number. Randomisation was carried out once per week by the trial manager or data manager, using a button function within the database. As the process was fully automated, the operator was unable to influence the process. Once participants had been randomised, it was not possible for their allocation to change. A more detailed example of the randomisation sequence is given in Appendix 4.
Interventions
Subjects in the intervention group received a thoracic LDCT scan several weeks post randomisation, either at LHCH, or Papworth Hospital. Full details are given in Chapter 4 (see Computed tomography acquisition).
Follow-up
Any lesions identified on LDCT screening were treated as per the planned UKLS care pathway in the study protocol53 [e.g. follow-up scan or referral to multidisciplinary team (MDT)] (see Figure 12). Health and mortality outcomes of UKLS participants in both study arms will be followed for 10 years, via the Office for National Statistics (ONS), the Hospital Episode Statistics database and the National Cancer Registration Service. The full protocol for the UKLS study is available online. 61
UK Lung Cancer Screening trial working with primary care
Although the UKLS did not use primary care-based recruitment of participants, it included strategies to engage effectively with the general practitioners (GPs) of participating patients. This took the form of updating the GPs in the PCT areas of the progress of the UKLS, with specific information on their own patients. The aim was to inform the GPs as to when their patients had been approached and recruited, and to provide them with CT scan results and any significant outcomes. GPs were also given information about how they could contact the study team with any queries or concerns.
Initial contact with general practitioners
The UKLS Project Management team wrote to the lead GP and the practice manager in the GP practice to inform them that individuals in their practice were being approached regarding the UKLS. They were provided with a copy of the 14-page UKLS patient information booklet and a poster to place in their surgery to inform patients that the UKLS was recruiting in their area. This enabled the GPs to respond to questions that their patients may have concerning the trial.
Spirometry result
Once a participant had been consented at the pilot site recruitment centre and the lung function (forced expiratory volume in 1 second/forced vital capacity) had been measured as part of the recruitment process, a letter was sent to the GP, with a copy of the lung function report, to enable them to act on lung function readings that indicated COPD.
Computed tomography scan results letter
Copies of letters sent to the UKLS recruits regarding CT scan results were also sent to their GPs, with details on the follow-up procedures.
Referral to multidisciplinary team
Details of participants referred to the MDTs with category 4 nodules, or participants reported to have a significant other finding (SOF), were reported to the GPs.
General practitioner questionnaire on significant other findings
A follow-up letter with a short questionnaire was sent to all the GPs at the end of the pilot, requesting the outcome of the SOFs.
General practitioner queries
The UKLS developed a mechanism for dealing with concerns and feedback from primary care. Like all studies of this kind, patients will often discuss their participation with their GPs; furthermore, GPs were informed about the involvement of their patients in the trial. As a consequence, although the trial was conducted largely independently of primary care (in terms of recruitment of patients and follow-up of patients with ‘positive’ screening results), GPs were made aware of the study and were able to contact the study team with any concerns.
We received a small number of written communications from GPs. They were read first by the ‘core’ study team (led by John Field) and a draft response was formulated. Then the UKLS primary care lead (David Weller) was sent the draft response for further input. There were no complaints of adverse clinical outcomes from the screening process within the correspondence received. On the whole, complaints related to (1) misunderstandings about who was responsible for follow-up investigations (although the study materials indicated that all such follow-up would be co-ordinated by the study team, in a couple of instances the GP thought he/she was responsible for ordering the investigations) and (2) concerns over patient anxiety that was generated through involvement in the study (again, through a misunderstanding of written materials and communications).
In all cases, the issues appeared to be addressed and resolved through our letters back to GPs – we did not hear further from the GPs concerned – and there are no ongoing unresolved issues.
Outcomes
Primary outcomes
-
Population-based recruitment based on risk stratification Compliance rate percentage (response to initial questionnaire); percentage with LLPv2 risk ≥ 5%; percentage response to questionnaire 2 ‘wishing to participate’.
-
Management of trial through web-based database The UKLS recruitment, CT images and clinical pathway were successfully managed through the bespoke web-based UKLS database.
-
Optimal characteristics of CT scan readers (radiologists vs. radiographers) Radiologists and radiographers were assessed at each pilot site and also at the second read site.
-
Characterisation of CT-detected nodules utilising volumetric analysis The proportions of the CT-screened individuals receiving a repeat CT scan (at 3, 6, 9 or 12 months) are reported.
-
Prevalence of lung cancer at baseline The baseline for the UKLS was found to be 1.7%. In addition, details are provided for those individuals who were diagnosed at the 3-month repeat scan).
Secondary outcomes
-
Sociodemographic factors affecting participation.
-
Psychosocial measures (cancer distress, anxiety, depression and decision satisfaction): likely psychosocial impact of participating in a lung cancer screening trial (HADS; CWS-R; decision satisfaction); preliminary data on reasons for non-participation/barriers to participation.
-
Cost-effectiveness modelling: modelling of health economics data regarding the likely consequences of undertaking a larger trial or population screening (using European Quality of Life-5 Dimensions questionnaire).
Tertiary outcome
Future pooling of pertinent data with similarly designed European lung cancer screening trials, such as NELSON. 37 Data to be pooled are those relating to lung cancer detection, incidence and mortality. (The pooling of the data, and subsequent interpretation, lies outside the remit of this report.)
Sample size
The original protocol for the pilot UKLS study allowed for a cohort of approximately 82,000 individuals to be approached, with the aim of identifying and randomising 4000 high-risk individuals from this cohort. Initially, 88,897 individuals were approached and, although the response rate was as expected, the number of responders at high risk of lung cancer was much lower than anticipated. (Data presented later in this report suggest that this may partly reflect a response bias.) The protocol was amended to enable a total of 250,000 individuals to be approached in order to provide at least 4000 high-risk individuals. The actual number approached (including the original cohort of 88,897) was 247,354; this yielded 4061 high-risk individuals who were enrolled into the RCT. However, a total of 8729 high-risk individuals were identified from the 250,000 approaches.
Data storage and analysis
Data were input to and stored on the bespoke UKLS system builder database, built by Artex, with data security approved by the NIGB. All contacts with participants, letters, CT findings, etc., were recorded in the database. Statistical and data analysis was undertaken using Stata version 12 (StataCorp LP, College Station, TX, USA).
Socioeconomic data analysis
Index of Multiple Deprivation (IMD) rank62 was obtained from postcode data in an anonymised form for all 249,988 individuals whose details were provided by the PCTs (including non-responders). UK IMD data were analysed and reported as ranks within quintiles based on England-wide population data: quintile 1 (Q1; most deprived) = IMD ranks 1–6496; quintile 2 (Q2; above average deprivation) = 6497–12,993; quintile 3 (Q3; average) = 12,994–19,489; quintile 4 (Q4; below average deprivation) = 1490–25,986; quintile 5 (Q5; least deprived) = 25,987–32,482.
The English Indices of Deprivation 201062 constitute the official measure of deprivation in England. The most commonly used of these 10 indices is the IMD, which is calculated from 38 separate indicators, based on weighted data from the following seven domains: income deprivation (22.5%); employment deprivation (22.5%); health deprivation and disability (13.5%); education, skills and training deprivation (13.5%); barriers to housing and services (9.3%); crime (9.3%); and living environment deprivation (9.3%). For the purposes of measuring deprivation, England is divided into 32,482 ‘lower layer super output areas’, with each area being carefully defined by its local geography and containing in the region of 1500 residents (minimum 1000 residents). IMD ranks, which provide a relative measure of deprivation in small areas across England, range from 1 (most deprived lower layer super output area) to 32,482 (least deprived lower layer super output area).
UK Lung Cancer Screening pilot trial statistical analysis plan
As this is a pilot trial, not powered for the end point of lung cancer mortality, analysis of the end point features is secondary in this plan, as the main UKLS was not funded. However, at the appropriate time, lung cancer mortality data will be analysed and pooled with the NELSON37 trial data. Risk scores were analysed based on a modification of the LLP risk model as published20,23 (see Appendix 2). The modifications took account of other smoking materials with the same projected risk as cigarette smoking and other respiratory diseases with the same projected risk as pneumonia.
Primary analyses
-
Descriptive tables of numbers in the pathway to recruitment; number approached, numbers and percentages not responding, responding negatively, responding positively, positive responders deemed eligible, recruited and randomised to each group. The same figures stratified by age, gender and region.
-
Descriptive tables of recruits to both arms, in terms of demographic variables, individual risk factors, and combined LLPv2 risk score. These will not be accompanied by formal inference, as the randomisation implies that any differences between the two arms will be by chance. 63
-
Diagnostic cascade in intervention arm. Number recruited, numbers and percentages screened, given further investigation, diagnoses of lung cancer.
-
Numbers (%) receiving specific further investigations: further imaging bronchoscopy, biopsy, surgical resections.
-
Clinical and tumour, nodes, metastases staging of cancers diagnosed as a result of the CT screen in the intervention arm.
-
Estimate associations between recommendation of further investigation and demographic variables, individual risk factors and the LLP risk score, using logistic regression. 64
-
Estimate associations between screen-detected cancer in the intervention arm and demographic variables, individual risk factors and LLP risk score, using logistic regression.
-
Compare lung cancer incidence over 2 years and stage distributions of lung cancers diagnosed between intervention and control arms, using Poisson regression.
-
Descriptive tables to illustrate results of (f–h).
Secondary analyses
-
Detailed analysis of demographic and risk factors by response and recruitment status, using logistic regression – first with response as end point and second with recruitment as end point.
-
Long-term follow-up of both arms for incidence by stage of lung cancer, to be analysed by proportional hazards regression. 65
-
Long-term follow-up of both arms for all-cause mortality, to be analysed by proportional hazards regression.
-
Estimate association of lung cancer incidence in follow-up with baseline findings and procedures in the intervention group, using proportional hazards regression.
-
Descriptive tables to illustrate results of (a–d).
-
Radiology and pathology reviews will be the subject of separate protocols.
Chapter 3 Recruitment of participants
Introduction and rationale
Successful introduction of any screening programme requires knowledge of the likely response and uptake, which can be affected, potentially, by a number of sociodemographic factors. From both an ethical and a cost-effectiveness point of view, it is imperative to target screening towards individuals who are at sufficiently high risk to warrant undergoing a screening intervention. It is also important to identify ‘hard-to-reach’ groups within the population so that there is the potential to target these groups specifically. This chapter therefore focuses on the response to the screening invitation, and the sociodemographic factors affecting risk of lung cancer and decision to participate in the trial.
Objectives and outcomes
To document overall response rate to the initial invitation to participate in UKLS, percentage of positive responders at high risk of lung cancer, and trial participation rate among high-risk responders. To assess the impact of specific sociodemographic factors (age, gender, socioeconomic status, region and smoking status) on trial uptake. To use these data to suggest particular demographic groups to be targeted to maximise screening uptake in any future lung cancer screening programme.
Methods
The methods for this chapter are described in detail in Chapter 2.
Results
Overall response rate and risk
Figure 2 and Appendix 5 show the overall response rate, numbers at high risk and clinic attendance. Details of 249,988 individuals in the 50- to 75-year age range were provided by the PCTs to the DMC. The PCTs sent additional information requesting that 2634 of these not be contacted (e.g. because they were recently deceased). The remaining 247,354 individuals were therefore approached by the DMC. Out of these, 148,608 (60.1%) were non-responders (no questionnaire returned), 22,788 (9.2%) were negative responders (non-participation questionnaire returned) and 75,958 (30.7%) were positive responders (questionnaire 1 returned; willing to participate). Of the positive responders, 8729 (11.5%) were classified by the LLPv2 risk prediction model to be at high risk (≥ 5% over the next 5 years) of developing lung cancer (mean LLPv2 risk score 8.77% vs. 1.00% for the low-risk group). Of the high-risk responders, 5967/8729 (68.4%) returned the second questionnaire and agreed to participate in the RCT. A total of 1291 individuals were subsequently excluded because they (1) did not meet the inclusion criteria (e.g. because they had had a previous CT scan of the chest); (2) had not completed the eligibility questionnaire correctly; (3) replied after maximum trial recruitment numbers had been reached; or (4) were unable to give fully informed consent. A total of 582 individuals either changed their mind or failed to attend clinic, and a further 33 attended the recruitment clinic but declined to consent. In total, therefore, 4061 individuals (5.3% of all positive responders and 46.5% of all high-risk positive responders) consented and were recruited into the RCT (see Figure 2).
FIGURE 2.
A Consolidated Standards of Reporting Trials diagram showing participant flow from initial contact to CT screening. DNA, did not attend; OFS, off study; Q, questionnaire.
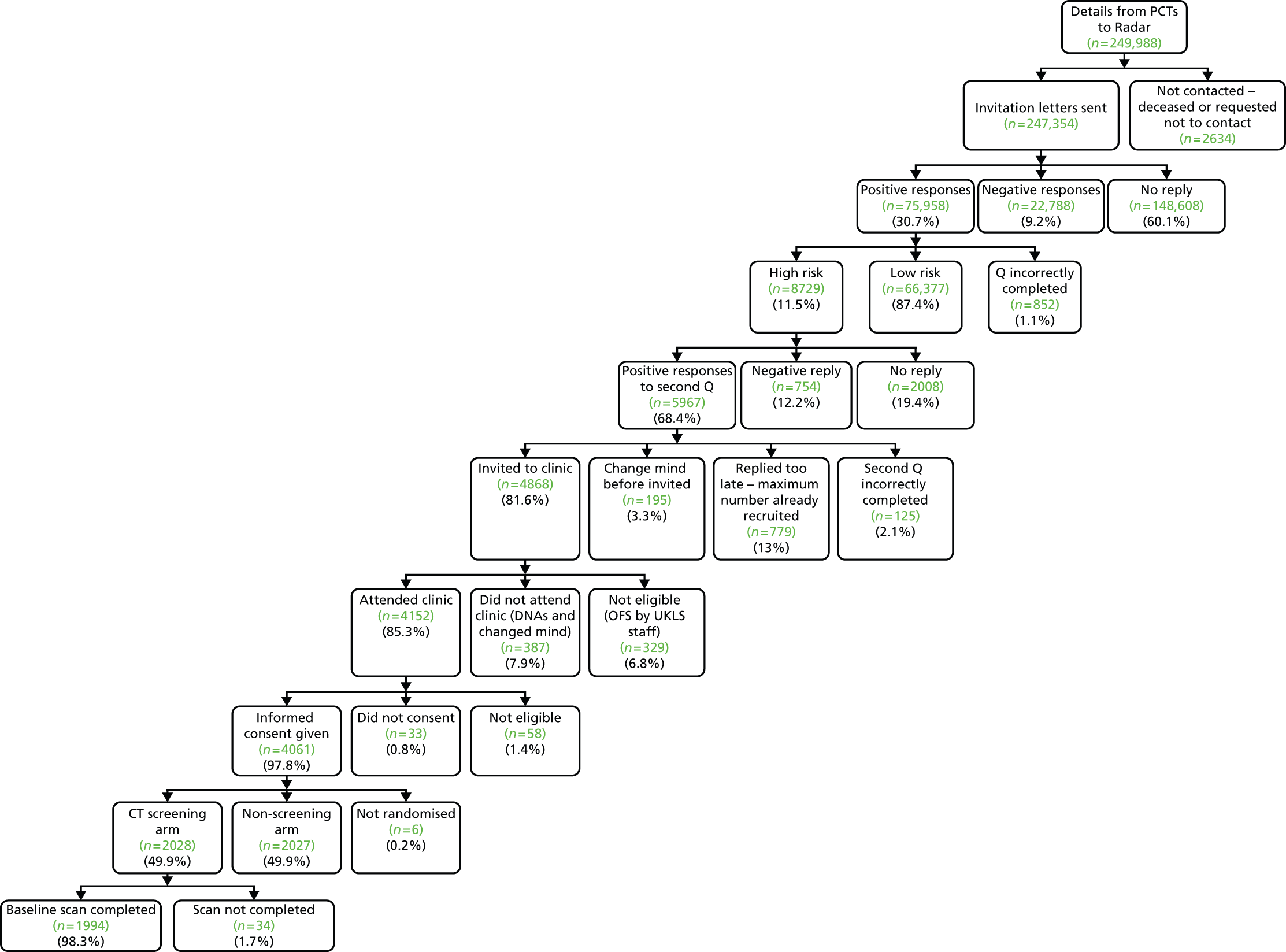
Factors influencing response rate and risk
Gender, age, socioeconomic status, region (north vs. south) and smoking status were analysed with respect to (1) response to the initial invitation letter; (2) risk status; and (3) participation of high-risk people in the UKLS screening trial. The actual numbers involved are tabulated in Appendix 5; trends are discussed in this chapter.
Gender
Men and women were approached in approximately equal numbers (n = 123,748 and n = 123,606, respectively), but females were slightly more likely to respond positively to the first questionnaire (30.3% of males and 31.1% of females were positive responders). However, men were 2.4 times more likely to be classified as at high LLP risk: 2016 (16.4%) of male and 832 (6.7%) of female positive responders had a high LLP risk score. The frequency of non-responders was greater for men than for women (62.0% of males; 58.2% of females) and the converse was true of negative responders (7.7% of males; 10.7% of females). Having been identified as high risk, and invited to participate in the trial, females were more likely than males to opt out of UKLS [45.5% vs. 35.8%; chi-squared (χ2) = 44.987; p < 0.0001].
Age
Positive response rate ranged from 26.6% in the 50–55 years age group to 35.0% in the 61–65 years age group, before dropping to 27.6% in the eldest (71–75 years) age group (Figure 3). Age is a major component of the LLP risk model, so unsurprisingly had a major impact upon risk classification: only 82 out of 16,273 positive responders (0.5%) in the 50–55 years age group were classified as high risk compared with 2046 (24.8%) in the 71–75 years group. In addition to the youngest (50–55 years) age group having the lowest positive response rate and the least likelihood of being at high risk, high-risk individuals in this age group were also less likely than those in any other age group to consent to participate in the RCT: only 29 out of 82 (35%) consented. Thus, overall in the 50–55 years age group, just 29 of 61,168 individuals originally approached (0.05%) were recruited to the RCT (Figure 4). The different age profiles of the original approached group compared with high-risk clinic attendees are shown in Figure 4. A total of 94.2% of high-risk clinic attendees were aged 61–75 years (compared with 58.1% of the 247,354 subjects originally approached).
FIGURE 3.
Impact of age on initial response rate, LLP risk and trial consent rate.

FIGURE 4.
Age distribution of the original approached population sample, positive responders, high-risk responders and recruits to the RCT. In the last two categories, percentages for the youngest age group are shown to the left of the y-axis. Nearly 95% of trial recruits were aged ≥ 61 years.

When lung cancer risk was considered by individual year of age, there was a steady increase in the percentage of people at high risk, with a progressive increase starting from age 59 years (6.1% at high risk; Figure 5).
FIGURE 5.
Percentage of UKLS positive responders (n = 75,958) with a LLP risk of ≥ 5%, by individual year of age. (There are eight individuals aged 76 years; these are included with the 75-year-old group.) See Appendix 5 for primary data.

Socioeconomic status
The English IMD rank62 was available on 247,328 individuals. Analysis of IMD data showed that the social demographic characteristics of the two recruitment areas (Liverpool and Cambridgeshire) were markedly different. Almost 45% of the Liverpool area residents approached for UKLS fell into the most deprived quintile of the English population, compared with < 10% in Cambridgeshire. However, as planned in the study design, the total UKLS approached sample was similar in socioeconomic distribution to the entire English population (Figure 6).
FIGURE 6.
Social demographics of UKLS sample. Distribution of individuals within IMD national quintiles, by area. Much more deprivation is seen in the Liverpool areas (Liverpool, Knowsley and Sefton) than in the Cambridgeshire areas (Cambridgeshire, Peterborough and Bedfordshire). However, the overall socioeconomic distribution of the UKLS sample is similar to that of the entire English population: this was an inherent feature of the study design. Q, quintile.
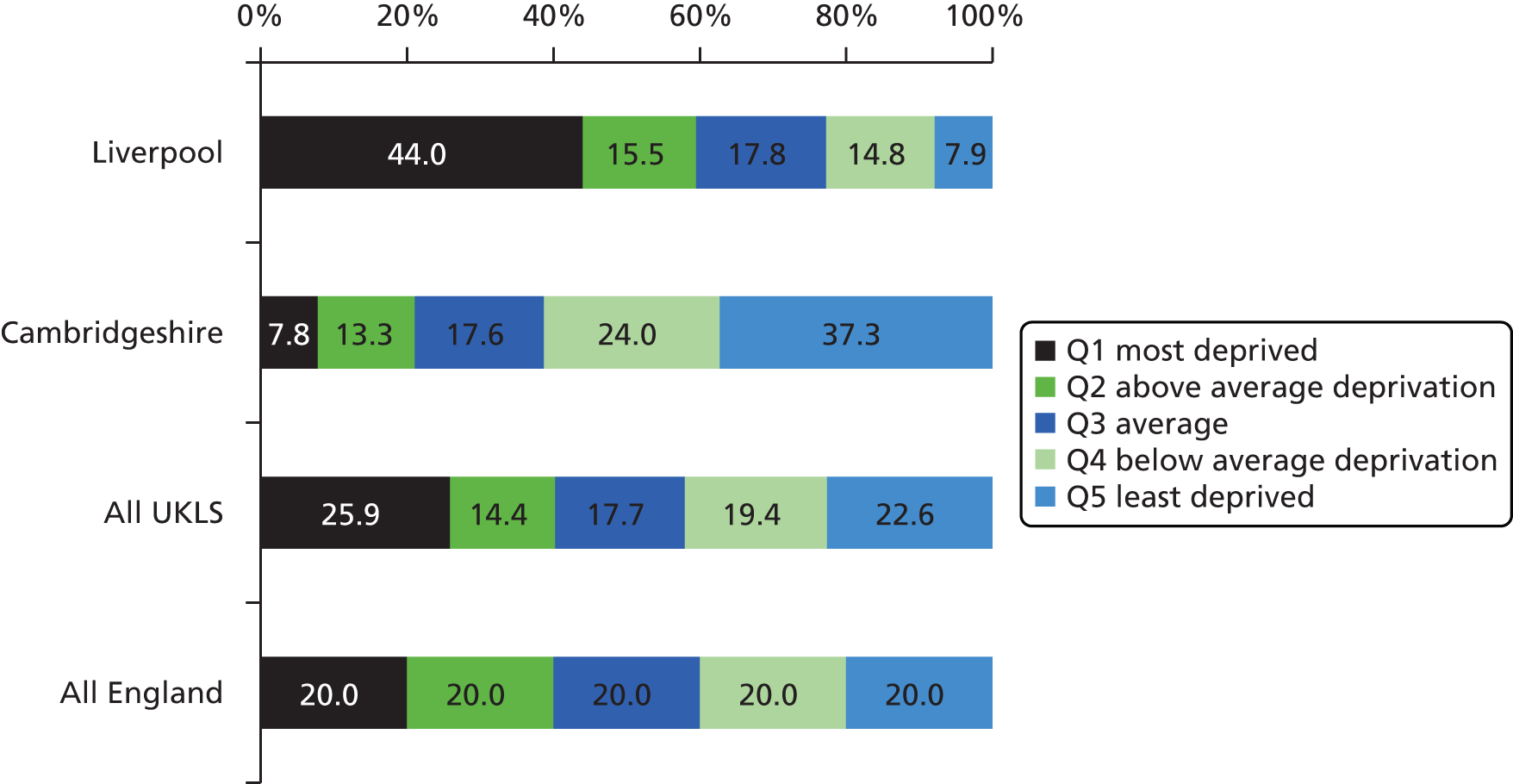
The positive response rate increased steadily with higher socioeconomic status: 21.7% of individuals in the lowest (most deprived) IMD quintile gave a positive response compared with 39.7% in the highest quintile (p < 0.001) (Figure 7). The proportion of individuals with a high LLP risk score decreased with higher socioeconomic status, ranging from 18.2% in the most deprived quintile to 8.3% in the least deprived quintile (p < 0.001) (Figure 8). As the social gradient of response and the social gradient of LLP risk were offset by each other, the sociodemographic spectrum of the individuals attending clinic was in proportion to that of the original approached sample (see Figure 8). People attending clinic therefore spanned all IMD quintiles in roughly equal numbers, including a representative proportion from more deprived postcodes (see Figure 8). However, of the high-risk individuals invited for screening, there was a trend towards individuals of higher socioeconomic status being more likely to consent to participate in the trial (see Figures 7 and 8).
FIGURE 7.
Impact of socioeconomic status on initial response rate (lower line), LLP risk (bars) and trial consent rate (upper line). Q, quintile.

FIGURE 8.
Socioeconomic distribution of the original approached population sample, positive responders, high-risk responders and recruits to the RCT. The social demographic spectrum of the high-risk individuals participating in the trial is roughly in proportion to that of the original approached sample. Q, quintile.

Region
There was a higher response rate from individuals in the southern region (Cambridgeshire, Peterborough and Bedfordshire) compared with the northern region (Liverpool, Knowsley and Sefton) (35.0% vs. 26.4%). Much, but not all, of this difference could be accounted for by the different social demographic spectrum between the two regions. When stratified by IMD quintile, there was still a tendency for a better response rate from the southern region than the northern region (Figure 9). Within each IMD quintile, individuals from the north were slightly more likely than those from the south to be at high risk of lung cancer (see Appendix 5). In the southern region, the likelihood of high-risk people consenting to the RCT increased with IMD quintile (from 18% to 60%), whereas in the northern region the likelihood of consent was static (around 45%) for the lowest four IMD quintiles, but dropped to 35% in the highest IMD quintile.
FIGURE 9.
Positive response rate to initial approach, stratified by both region (north/Liverpool and south/Cambridgeshire) and IMD quintile. A slightly better response rate was observed in the southern region in each IMD quintile. Q, quintile.
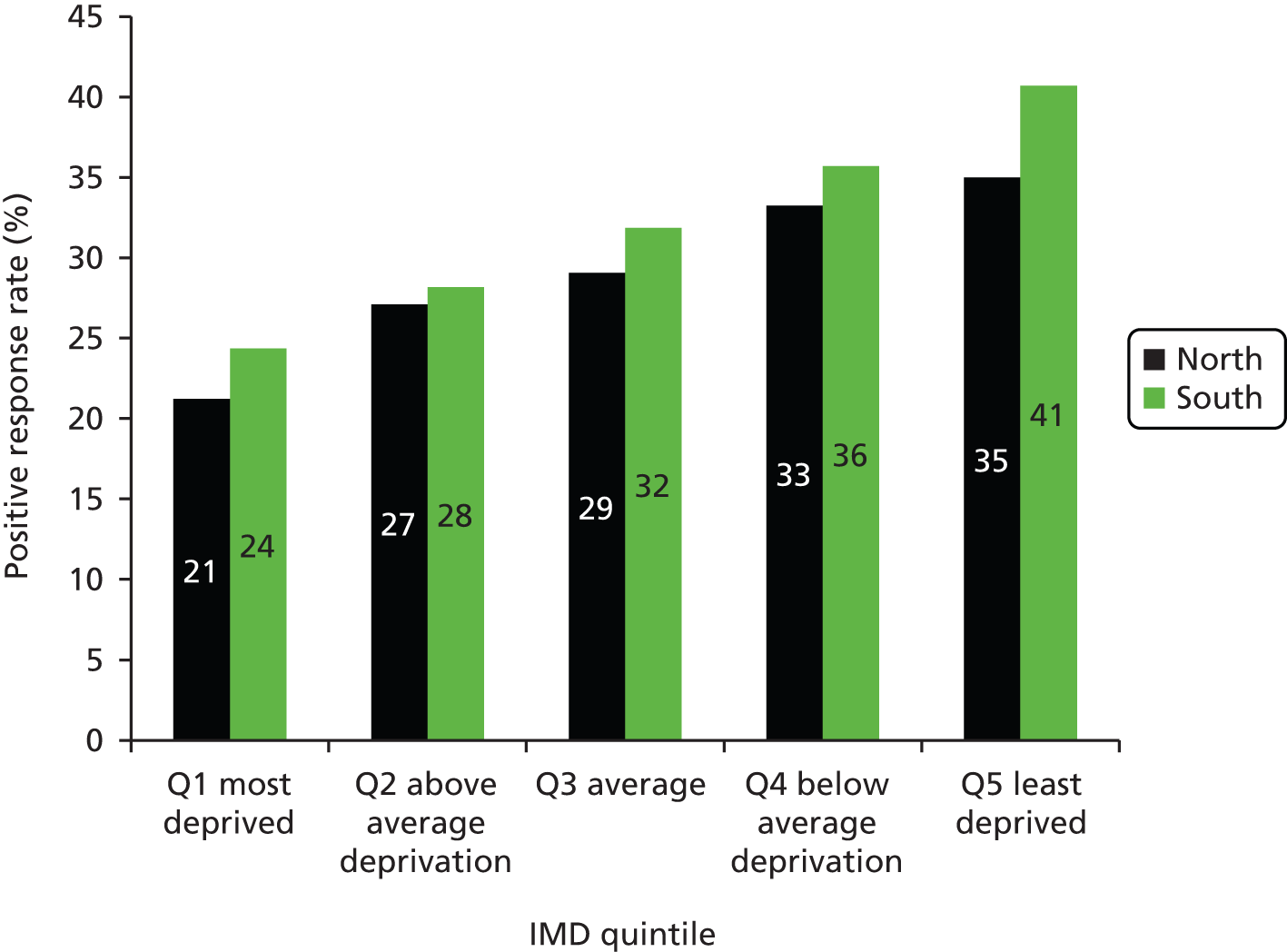
Smoking
Of the 75,958 positive responders, 73,934 (97.3%) gave information about their smoking habits. A total of 43.4% were never-smokers, 14.7% current smokers and 39.3% ex-smokers (Figure 10). Of the 22,788 negative responders, 22,024 (96.6%) provided information about their smoking habits. Never-smokers totalled 51.3%, 9.1% were current smokers and 36.2% ex-smokers (see Figure 10). National smoking data (from the ONS) for the 50+ years age group give an expected distribution of 49.5% never-smokers, 17.5% current smokers and 33% ex-smokers. 66 Thus, the observed values in our sample of both positive and negative responders are significantly different from population values (chi-squared test; p < 0.0001). The data suggest that ex-smokers are more likely to respond positively to a screening invitation, with current and never-smokers being less likely to respond positively. Negative responders are enriched with never-smokers, but comparatively few current smokers respond negatively. From expected population figures (and accounting for known figures from responders) it was possible to estimate the smoking status of the non-responders, of whom approximately 20% are probably current smokers (see Figure 10).
FIGURE 10.
Smoking status among UKLS positive and negative responders, and likely smoking status among non-responders, based on expected values from age 50+ years population figures less known figures from responders. In this case, ‘non-responders’ includes 2024 positive responders and 764 negative responders who did not provide their smoking status.

Unsurprisingly, smokers and ex-smokers were much more likely than never-smokers to have a LLP risk of ≥ 5%. Of the 75,958 positive responders, 3724 (33.5% of 11,130) current smokers and 4995 (16.7% of 29,855) ex-smokers were designated as high risk. However, only 10 (0.03% of 32,949) never-smokers had a high LLP risk score, and only two of these consented to participate in the RCT. In total, therefore, 21.3% of current and ex-smoking positive responders were high risk, and 9.9% participated in the RCT. Of the high-risk individuals, ex-smokers were more likely than current smokers to consent to participate in the RCT (49.9% vs. 42.1%, χ2 = 27.744; p < 0.0001).
Interaction between smoking and socioeconomic status
As people in more deprived socioeconomic groups are more likely to smoke, it is important to control for any possible confounding effects. Data were therefore stratified by both IMD quintile and smoking status. For all IMD national quintiles, the proportion of ex-smokers among positive responders was around 40%. Of the positive responders in the most deprived quintile, 27.6% were current smokers, decreasing to 9.1% in the least deprived quintile (see Appendix 5). Comparing positive and negative responders, there were proportionally more current smokers among positive responders, and proportionally more never-smokers among negative responders, across all IMD quintiles. There were proportionally more ex-smokers in positive responders in all but the most deprived IMD quintile (see Appendix 5).
Based on known smoking prevalence rates for 50- to 75-year-olds in each IMD quintile,67 it was possible to calculate the expected number of smokers in each IMD quintile for the 247,354 approached subjects (see Appendix 5). By subtracting the known number of smokers among positive and negative responders, this allowed an estimation of smoking status for non-responders in each IMD quintile. It was then possible to estimate the percentage of current smokers, ex-smokers and never-smokers who respond positively to the first screening invitation (Figure 11). The calculated response rate is generally lower among current smokers than the overall response rate for the relevant quintile (i.e. there is a smaller proportion of smokers among responders than would be expected from quintile-matched population figures). However, in the most and least deprived quintiles it appears that the response rate among smokers is similar to, or greater than, the overall response rate for that quintile (see Figure 11). For ex-smokers and never-smokers, positive response rate increases with socioeconomic status across all IMD quintiles, whereas the positive response rate among current smokers only rises in the upper two quintiles of IMD. The group least likely to respond positively are never-smokers in the most deprived IMD quintile (calculated as a 17.2% positive response rate), followed by current smokers in the three most deprived quintiles (calculated as 21.0–22.2% positive response rate). The highest positive response rate is seen in current smokers from the least deprived IMD quintile (calculated as a 45.2% positive response rate). The general trend across all IMD quintiles is consistent with the overall trend discussed above: ex-smokers are the most likely group to accept an invitation to be screened for lung cancer.
FIGURE 11.
Interaction between smoking status and socioeconomic status in determining initial response to the UKLS invitation. Calculated positive response rate, per cent, by smoking status and IMD quintile. The line (and percentages) denotes the known positive response rate of all individuals within that IMD quintile. Calculations were based on Health Survey for England population smoking figures for each IMD quintile, for an age group of 50–75 years (see Appendix 5). Q, quintile.

Discussion
The overall response rate from the 247,354 individuals approached from the population was 30.7%, which is consistent with the response rate anticipated when planning the trial, and the response rate from similar studies. A number of demographic factors were found to influence recruitment, participation and risk status. The main findings are discussed below.
Gender
Although women are slightly more likely than men to respond positively to an invitation to take part in UKLS, more men than women are designated as being at high LLP risk (6141 males vs. 2588 females, giving a gender ratio of 2.4 : 1). However, national lung cancer incidence figures for the 50–74 years age group in the UK give a much smaller male–female ratio of 1.34 : 1. 1 In addition, as high-risk women are significantly more likely than high-risk men to opt out of the screening trial, it is possible that women are under-represented in the trial. It will be interesting to consider the longer-term gender-related outcomes in the UKLS participants in future follow-up, as data become available through the ONS, Cancer Registry and Hospital Episode Statistics.
Age
Response rate was highest in the middle age group (61–65 years) and lowest in the youngest group (50–55 years). There was a fall-off in response in the oldest age group (71–75 years), who conceivably are less concerned about their risk of lung cancer or who anticipate more practical difficulties with participating in the study. Nevertheless, as the oldest age group has the greatest percentage at high LLP risk (24.8% of positive responders aged 71–75 years were high risk) this age group was reasonably well represented in terms of trial participation (22.4%, of clinic attendees were aged 71–75 years compared with 12.1% of the originally approached population). However, as around one-third of lung cancers in the UKLS age range occur in individuals of 71–75 years,1 it could be argued that it would be desirable to screen more people from this oldest group. The best represented age group in the RCT itself was the 66–70 years group, which accounted for 39.8% of trial participants.
Only 234 (5.8%) clinic attendees were aged ≤ 60 years, of whom only 29 (0.7%) were in the 50–55 years age group. Thus the youngest (50–55 years) age group yielded a trial participation rate of just 0.05% of the 61,168 who were originally invited to participate in UKLS. This has implications for cost-effectiveness, and, under the LLP model of risk prediction, suggests that it would not be prudent to include this age group in any future population-based lung screening studies or programmes.
In order to determine more accurately when response rate and risk increase to a point where screening is viable from a detection and cost-effectiveness point of view, we analysed LLP risk by individual year of age. The results suggest a fairly sharp increase at 59 years of age in the percentage of positive responders at high LLP risk, from around 3.1% at age 58 years to 6.1% at age 59 years, and 7.5% at age 60 years. This suggests that setting the lower cut-off point for eligibility at 60 years old would be a reasonable strategy for future studies. By considering data only from individuals aged between 60 and 75 years, 8339 out of the 49,468 positive responders in this age group (16.9%) are at high LLP risk (compared with just 1.5% for the 50–59 years group).
Socioeconomic group
There was a strong positive correlation between higher socioeconomic group (less deprived quintile of IMD) and positive response to the screening invitation. Similar trends have also been observed in other screening studies, and the lower uptake is considered to relate to barriers including fear and fatalistic beliefs about cancer68,69 and poorer self-rated health in people from lower socioeconomic groups. 70 Unlike with other cancers (e.g. breast cancer) there are marked sociodemographic differences in lung cancer risk, with individuals from lower socioeconomic groups being at greater risk of developing the disease: this largely relates to disparate tobacco use in different socioeconomic groups. It is therefore not ideal that those who are at highest risk are the least likely to take up the offer of screening. Our data suggest that this works at two levels: individuals at highest risk of lung cancer (i.e. from the lower socioeconomic groups) are less likely to respond to the initial screening invitation and also less likely to attend clinic after having been identified as at high risk. Consideration will have to be given to addressing this in any future screening programme. Within each socioeconomic quintile, although there is a significant difference in screening uptake between Liverpool and Cambridgeshire, the difference is not large. Most of the difference in uptake between the two regions therefore relates to the different social demographics of the two areas.
Smoking status
Analysis suggested that ex-smokers are the most responsive to a screening invitation, and the most likely to participate in the trial once identified as high risk. It could be argued that consciously deciding to stop smoking and being motivated to participate in screening are related decisions, perhaps made by individuals who are more health aware, or perceive greater risks from smoking. The initial response rate from current smokers was lower than would be expected based on age-matched population figures, possibly suggesting that current smokers are less likely to want to consider their cancer risk, or feel more threatened by the prospect of lung cancer screening. However, it was observed that, if smokers take the trouble to respond, they are more likely in percentage terms to be positive rather than negative responders. The converse is true for never-smokers, who perhaps (correctly) view their own risk as low and hence are over-represented among negative responders. Having been identified as at high risk, current smokers were significantly more likely than ex-smokers to opt out of the trial; conceivably, this could also be related to their perception of high risk or threat. Only two never-smokers participated in the RCT; it is clear that this is not a group to be targeted in a population-based screening approach.
Interaction between smoking and Index of Multiple Deprivation
The likelihood of a positive response to a CT screening invitation is lower both in more deprived socioeconomic groups and in current smokers. As smoking status and socioeconomic status are closely related, it is important to establish whether both independently affect response rate or if there is any confound. Predicted population smoking figures stratified by IMD quintile and age adjusted to the UKLS sample67 were therefore compared with the smoking prevalence seen among UKLS positive responders in each IMD quintile. This suggests that, in general, socioeconomic deprivation and current smoking status both act independently to lower the positive response rate. However, in the highest and lowest IMD quintiles, the impact of smoking status on response rate is much less marked. In the highest IMD quintile (least deprived), the calculated positive response rate among smokers is higher than the overall positive response rate. In the case of the results from the lowest (most deprived) IMD quintile, the minimal impact of smoking status partially offsets the trend for high-risk individuals being less likely to respond. As a general trend across all socioeconomic groups, ex-smokers are the most likely to respond positively (except in the least deprived quintile, in which a greater proportion of current smokers respond positively). It is clear that smoking status and socioeconomic status interact when predicting response rate, so in a possible future UK National lung Cancer CT screening programme, strategies may therefore need to be devised to target both current smokers and individuals from lower socioeconomic groups.
Conclusions
The demographic data from the population approached for the UKLS pilot provide a unique insight into the likely response to a lung cancer population screening trial in the UK. This will enable specific recommendations to be made regarding the implementation of any future UK-wide lung LDCT screening programme, such as initiating screening at age 60 years. Such a programme would need to give due consideration to ways to target those most at risk who may be least likely to take up offers of screening (i.e. the most deprived, current smokers and the those > 70 years) and women, who may perceive themselves to be at lower risk. Possible methods would be to incorporate concurrent advertising and/or utilise modified invitation materials.
Chapter 4 Radiological interventions and outcomes
Introduction
This chapter details the radiological methods used in the trial, including CT scan acquisition, the training and testing of CT scan readers, the CT scan reading process and the nodule management strategies used.
Rationale
Accurate and efficient CT scan reading is crucial to lung cancer screening. In this regard, one of the aims of the UKLS pilot study was to investigate the reliability of various methods of CT scan reading and CT scan reading strategies. The method of reading CT scans has potential implications not only for successful detection of lung cancers in a screening programme, but also for the cost-effectiveness of such a programme.
Objectives
To investigate the performance of:
-
UKLS thoracic radiologists in nodule detection and interpretation (i.e. nodule reporting), having undergone initial training
-
UKLS radiographers in nodule reporting, having undergone initial training, and the comparison of radiographer performance with that of radiologists both in the trial and in the wider literature
-
radiologists in nodule reporting when using radiographers as part of a ‘concurrent’ reading strategy.
Methods
Computed tomography acquisition, storage and transfer
Computed tomography scans were acquired using a Siemens Definition Flash 128 slice scanner (Siemens, Erlangen, Germany) at Papworth Hospital and LHCH. In the initial phase of the study, from November 2011 to December 2011, a Philips (Best, The Netherlands) Brilliance 65 scanner was utilised at LHCH.
Thoracic CT images were obtained (craniocaudally from lung apices to bases) during suspended maximal inspiration, in a single breath hold and without the administration of intravenous contrast. The field of view selected was the smallest diameter as measured from the widest point of the outer rib to outer rib (usually not > 35 cm). Thin detector collimation (0.5–0.625 mm) was used with a pitch of 0.9–1.1. Exposure factors were tailored to patient height and weight, with the aim of ensuring that CT dose index was kept at < 4 milliGray (mGy), with the effective radiation dose of < 2 milliSieverts. For a 70-kg patient, a peak kilovoltage of 120 was used and the milliampere-second was tailored to achieve a CT dose index of 1.6 mGy. Images were reconstructed at 1-mm thickness with 0.7 increment, using a moderate spatial frequency kernel.
Thin-section images were transmitted to the following locations: (1) a dedicated local Syngo Siemens (Forcheim, Germany) workstation (so that nodule volumetry could be performed); (2) local picture archiving and communication system servers (so that images could be stored and subsequently retrieved to the workstation); and (3) a central site [Royal Brompton Hospital, London) via the NHS Image Exchange Portal (Burnbank Systems, Ipswich, England) for the purposes of double reading.
Quality assurance
All local sites were required to have in place daily quality assurance practices for the CT scanner, using a water and body phantom. All doses were recorded at the time of acquisition on the UKLS database by radiographers.
During the process of CT scan reading, any reader (radiologist or radiographer) could highlight defects in the acquisition quality of any CT scan, such as image degradation due to breathing artefact. All such cases were reviewed and discussed by the local and central radiologist, with respect to diagnostic quality and a decision was made as to whether or not a repeat CT scan was required.
Additionally, every month for the first 6 months, and then quarterly, 10 randomly selected cases from both sites were reviewed at the central site by two radiographers. CT scans were assessed with respect to adequacy of craniocaudal coverage, adequacy of field of view, satisfactory degree of inspiration, correct reconstruction algorithms, presence of motion artefacts and the recorded radiation dose. Outliers were highlighted to the central site radiologist and feedback was provided to local sites if any of the acquisition parameters were deemed to be out of range.
Reading methods
To optimise sensitivity and specificity, all baseline CT scans were read by two thoracic radiologists at a local (LHCH or Papworth Hospital) and central (Royal Brompton Hospital) site (see Reading selection, training and testing). All discrepancies were reviewed by a third thoracic radiologist at the central site, who was the final arbiter. Once consensus had been achieved for all nodules, a letter was sent to the participant and his/her GP, outlining the results of the scan. The letter also detailed whether or not further follow-up CT scans within the UKLS were required. No computer aided detection software was used.
Nodule classification and management
Nodules were classified into one of four categories as per the UKLS nodule management protocol53 (Figure 12).
Category 1 Benign nodules fulfilling one of the following criteria: a benign pattern of calcification, fat, measuring < 3 mm in diameter or volume of < 15 mm3; or intrapulmonary lymph nodes fulfilling the following criteria: they lie within 5 mm of the pleura, are < 8 mm in diameter, smooth bordered and ovoid, and have at least one interlobular septum radiating from their surface.
Category 2 If solid and intraparenchymal, a maximum diameter of 3.1–4.9 mm or a volume of 15–49 mm3. If solid and pleural or juxtapleural, a maximum diameter of 3.1–4.9 mm. If non-solid or part solid, a maximum diameter of 3.1–4.9 mm. The solid component has a diameter of < 3 mm and/or volume of < 15 mm3. All non-solid/ground glass opacities, independent of diameter.
Category 3 If solid and intraparenchymal, a volume of 50–500 mm3. If solid and pleural or juxtapleural, a diameter 5–9.9 mm. If non-solid or part solid, a diameter of the ground-glass component of > 5 mm. If part solid, the solid component has a volume of 15–500 mm3 or a maximum diameter of 3.0–9.9 mm.
Category 4 If solid and intraparenchymal, a volume of > 500 mm3. If solid and pleural or juxtapleural, a diameter of ≥ 10 mm. If part solid, the solid component has a diameter of ≥ 10 mm or has a volume of > 500 mm3.
Nodules were managed as follows (see Figure 12):
No nodules or Category 1 nodules No further action required.
Category 2 nodules Follow-up CT scan at 12 months.
Category 3 nodules Follow-up CT scan at 3 months.
Category 4 nodules Referral to MDT.
When follow-up scans (at 3 or 12 months) were performed, the volume doubling time (VDT) of the nodule was calculated. VDTs were designated as < 400 days or ≥ 400 days.
Incidental findings
Incidental findings not related to thoracic malignancy, but regarded by both the central and local reader as likely to be clinically significant, were also highlighted to the participant’s GP. In such cases, a formal report with recommendations for further management was sent from the local site to the GP.
Nodule volumetry and transfer of nodule data to UK Lung Cancer Screening database
All CT scans were read using the ‘LungCARE’ application (LungCARE, version Somaris/5 VB 10A, Siemens Medical Solutions) on the Syngo Siemens workstation, which provides a value for nodule size, based on volume.
Studies loaded into LungCARE were presented in the following manner: 2 × 2 viewing partition with a default window setting level –500 Hounsfield units, width 1500 Hounsfield units, default display of transverse maximum intensity projections at 10 mm thickness in cine mode, 1-mm-collimation transverse images, 0.7-mm-collimation coronal images, and a panel for display of semiautomatic volumetric segmentation analysis if performed. Readers were free to alter maximum intensity projection thickness and window settings.
The reader was required to identify and mark all nodules of > 3-mm diameter or 15 mm3. All marked nodules were evaluated for size and recorded in the UKLS database using a non-commercial database electronic soft-copy entry pro forma (Artex Nodule Input for UKLS version 4.4, Logiton, The Netherlands). Options for nodule categorisation and segment location were available from drop-down menus, whereas the slice position of the nodule was entered using free text. Once a reading for a particular nodule had been completed, the information from the pro forma was copied and pasted into a structured Digital Imaging and Communications (DICOM) report in an extensible markup language (XML) format. The XML file was then transferred to a local network drive, so that the file could be uploaded to the patient’s record on the UKLS database via a computer with web access. The XML file contained information regarding a nodule’s size, table position location, lung and segment location, category and volume.
Reading of follow-up computed tomography scans
A key part of the UKLS protocol was the assessment of VDTs to identify significant nodule growth with a follow-up CT scan. If nodules were deemed to have been reliably segmented at baseline and follow-up then VDTs were automatically calculated in the UKLS database. For this to happen, CT scan readers were required to match the data of follow-up nodules with baseline nodules, by ‘dragging and dropping’ the data into the same row as the baseline nodule in the UKLS database (Figure 13). From October 2013, the UKLS protocol was changed such that follow-up CT scans were read by a single reader. This was done because the reading of follow-up CT scans largely consisted of the task of matching nodules on the follow-up scan to existing nodules that had already been identified. (This was in contrast with all baseline scans, for which double reading was used because of the theoretical benefits of using two readers to improve lung nodule detection and interpretation.)
FIGURE 13.
Screenshot from UKLS database. Nodules at baseline, 3-month and 12-month CT scans are matched in a row. The second figure in each box refers to the slice number and the third figure represents nodule volume. By hovering over the nodule, a figure for VDT is generated automatically. All nodules with a significant VDT of < 400 days are highlighted in purple (not shown in this example).
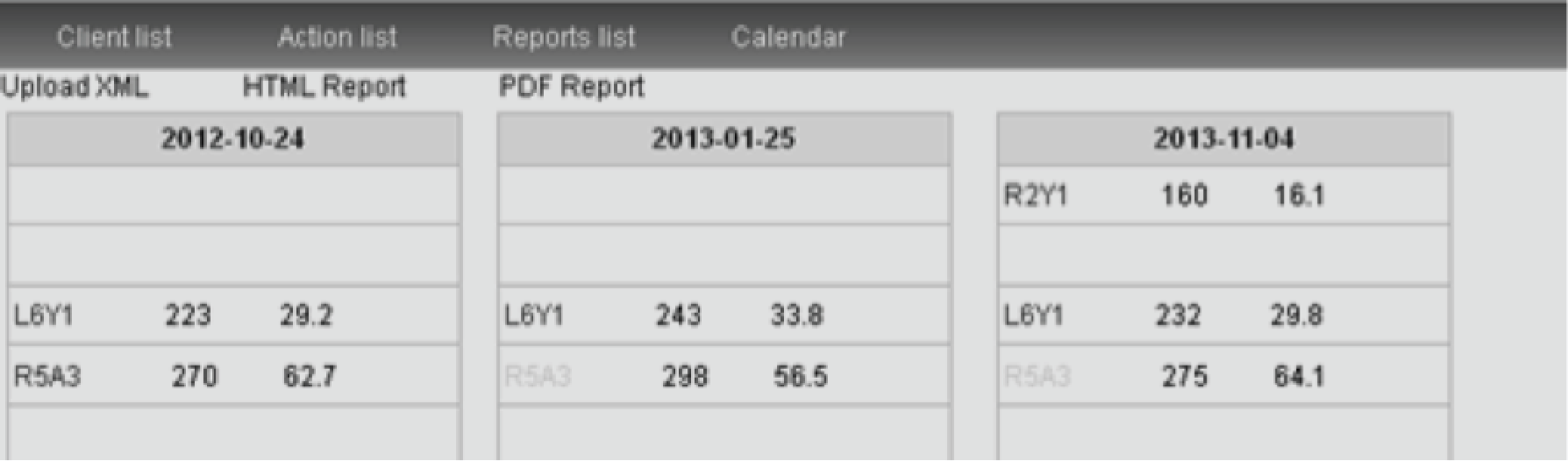
Reader selection, training and testing
Training and testing data set
Following written agreement from the NELSON37 board of investigators, 263 consecutive pre-anonymised LDCT screening studies were procured from this study. These scans had been performed at Utrecht Hospital, The Netherlands. This initial data set was enriched by a further 30 cases from the NELSON37 study containing ground-glass nodules and large category 4 nodules.
The selected NELSON37 cases were all pre-anonymised: each study had been given a unique reference number, and no patient identifiable data were visible. Volumetric data sets containing contiguous images were transferred via encrypted hard disk, in DICOM format, to the internal hard drive of the Syngo workstation (Siemens Medical Solutions, Forcheim, Germany) that was used at the three participating trial sites (Royal Brompton Hospital, London; LHCH, Liverpool; and Papworth Hospital, Cambridge).
The findings of the NELSON37 radiologists for each of the training CT scans were provided in a Microsoft Excel® (2007 version, Microsoft Corporation, Redmond, WA, USA) spreadsheet, and confirmed by two UKLS radiologists (AD and AN) from the Royal Brompton Hospital. Discrepancies between UKLS and NELSON37 radiologists’ findings were resolved by consensus opinion or by discussion with a third radiologist from the Royal Brompton Hospital (DMH). In addition, as the NELSON37 study did not specify intrapulmonary lymph nodes, these were also recorded by the UKLS radiologists (AD and AN).
Radiologists
All radiologist readers were experienced thoracic radiologists at cardiothoracic centres (LHCH, Papworth Hospital or Royal Brompton Hospital), with between 6 and 25 years’ experience, who regularly reported thoracic CT as part of daily clinical practice.
Twenty CT scans from the NELSON37 training data set, containing examples of all categories of nodules, were selected by the central site radiologist (AD). These cases, and the consensus findings, were shown to local radiologists by AD, and a tutorial on LungCARE was provided if local readers did not have prior experience of using this application. AD received UKLS database training from the database designer (Artex, The Netherlands) and, in turn, AD provided additional tutorials to other readers on uploading nodule data.
Prior to commencing formal reading as part of UKLS, all radiologists read a test set of cases (not part of the training set) containing at least 25 nodules, and were required to achieve a detection rate of at least 80% compared with the NELSON radiologists.
Radiographers
All radiographers were from cardiothoracic centres (LHCH, Papworth Hospital and Royal Brompton Hospital) and had experience in thoracic CT scan acquisition. None had previously reported CT scans. The radiographers were first given a PC-based presentation (prepared by AD) covering the basic principles of thoracic CT anatomy. They were then shown 20 CT scans containing examples of different types of lung nodules, as well as focal opacities such as pleural thickening and atelectasis that can mimic lung nodules. They were provided with the UKLS definitions of lung nodules. The radiographers were also tutored in the use of the LungCARE software on the Syngo workstation and the UKLS database.
Local site radiographers were trained on a further 80–100 CT scans, using a combination of direct supervision by the local radiologist on at least 20 CT scans and self-directed learning via the nodule data spreadsheet in the remainder. Radiographers at the Royal Brompton Hospital underwent training using a different method. Each radiographer was required to read and record their findings on 100 CT scans from the training set, and feedback was provided by a radiologist after every batch of 10 cases. The feedback included confirmation of nodules that were correctly identified, but highlighting missed nodules and overcalls.
Finally, prior to commencing reading of UKLS studies, all radiographers read test CT scans containing approximately 50 nodules, and were required to achieve an 80% detection rate compared with the NELSON37 radiologists.
Reading strategies tested
Reading performance of radiologists compared with radiographers of UK Lung Cancer Screening Trial scans (November 2011 to April 2012)
Each CT scan examination was read by a single radiologist at each of the two participating sites (radiologist A at local site 1 and radiologist B at local site 2). The CT scans were then transmitted to the central reading site for a second independent reading (by radiologist C).
The access rights to the UKLS database of the two reading radiologists at the participating sites were configured such that they were not able to view the recordings of other readers, but the central radiologist (radiologist C) could access these readings because radiologist C’s role was to identify any discrepant findings that required arbitration. The central radiologist viewed the readings of the local site radiologists only once he had completed his own reading.
Four radiographers who were able to commit at least 4 hours per week over the study period, and who had been trained using the methods described earlier, were selected as readers. Radiographer 1 read CT scans at local site 1, and radiographer 2 read CT scans at local site 2. Two radiographers (radiographers 3 and 4) read CT scans at the central site.
Sensitivity and false-positives per case were compared using McNemar’s test for nodules identified by radiographers and radiologists for the same CT scans. A subanalysis was conducted to examine the impact of time on radiographers’ and radiologists’ performance (i.e. to examine if there was a learning effect). A further subanalysis was conducted to identify whether or not radiographers’ and radiologists’ performance was changed by increasing the size threshold for nodules.
After the second reading, the radiologist at the central site (radiologist C) performed weekly reviews of all nodule candidates identified by radiologists for each subject to identify any discrepancies. Arbitration on discrepancies was provided at the central site by a thoracic radiologist with > 20 years’ experience (DMH), and the final consensus view was recorded on the database.
Reader performance of radiologists reading independently compared with radiologists using radiographers as concurrent readers (June 2012 to October 2012)
The same radiographers and radiologists were used for this study. An additional radiologist (radiologist D) also participated in reading at the central site. Radiologists either read CT scans independently as single readers (as described above) or concurrently with radiographers. The workflow in concurrent reading was as follows.
-
Each CT scan was first read by a radiographer on the LungCARE workstation, who uploaded his/her report to the UKLS database. The radiographer’s stored nodule recordings (in the form of a DICOM structured report) were then made available to the reading radiologist. For each recording, the radiologist had one of three options: accept a particular finding if he/she agreed with it; reject a finding if he/she disagreed with it; or amend the recording if he/she agreed that the finding represented a nodule but disagreed with its categorisation. Then, after reviewing the radiographer’s recordings, the radiologist performed an independent search to identify any additional nodules missed by the radiographer. The radiologist then saved his recordings as a new DICOM structured report, and uploaded this report to the UKLS database. Reading of CT scans between central and local sites was co-ordinated such that either the local site performed concurrent reading and the central site single reading, or vice versa. This ensured that a comparison between radiologists’ performance using single or concurrent reading could be made for the same CT scans.
-
All radiologists were asked to record on the UKLS database the time taken to read each CT scan and upload the data to the database, to the nearest minute.
-
After both local and central readings had been performed, the radiologists at the central site (radiologist C or D) reviewed all identified nodules to identify any discrepancies between readers. Arbitration on discrepancies was provided at the central site by a thoracic radiologist with > 20 years of experience (DMH), and the final consensus answer was recorded on the database. All agreed category 2–4 nodules and intrapulmonary lymph nodes were considered positive in the reference standard.
-
Sensitivity, the absolute number of false-positive detections and average false-positives per case [expressed as mean and standard deviation (SD)] were separately determined for the cohorts of CT scans read independently and concurrently per radiologist. The sensitivity of each radiologist was calculated by dividing the number of true-positive nodules detected by the total number of nodules in the reference standard for the cases read by that radiologist. The average number of false-positives per case was calculated by dividing the total number of false-positives by the total number of cases read by that radiologist. Differences in proportions were compared using the chi-squared test or the Fisher’s exact test in the case of smaller sample sizes, as appropriate. Differences in reading times between concurrent and independent reading for each reader were compared using the independent samples t-test. A post hoc analysis to determine the correlation between numbers of nodules and reading time was subsequently performed using Spearman’s rank correlation, and differences in correlation coefficients were analysed for statistical significance.
Results
Computed tomography acquisition
From 29 November 2011 to 8 June 2013, baseline CT scans were performed on 1994 participants. Seven of these 1994 scans (0.4%) required repeating (six because of breathing or motion artefact and one because of inadequate craniocaudal coverage).
The range of doses for baseline CT scans was 0.54–3.93 mGy, with a median dose of 1.62 mGy.
Overall performance of radiographers and radiologists
Radiographers 1–4 had sensitivities of 67.6%, 77.8%, 79.4% and 61.6%, respectively (mean sensitivity 71.6 ± 8.5%). Radiologists A–C had sensitivities of 88.9%, 87.0% and 74.0%, respectively (mean sensitivity 83.3 ± 8.1%).
The average numbers of false-positives per case for radiographers 1–4 were 1.2 (SD 2.1), 2.9 (SD 2.8), 0.6 (SD 1.0) and 1.1 (SD 1.3), respectively, whereas those of radiologists A–C were 0.5 (SD 0.8), 0.7 (SD 1.0) and 0.2 (SD 0.5), respectively.
Direct comparison of radiographer and radiologist performance
The sensitivities of each radiographer compared with those of the corresponding radiologists within a particular radiographer–radiologist combination are illustrated in Table 2.
Radiographer–radiologist combination | Number of CT scans read | Sensitivity | Difference | p-value | |
---|---|---|---|---|---|
Radiographer | Radiologist | ||||
1-A | 130 | 67.6 | 88.0 | 20.4 | 0.0008 |
1-C | 130 | 67.6 | 74.5 | 6.9 | 0.30 |
2-B | 139 | 77.8 | 87.4 | 9.6 | < 0.0001 |
2-C | 139 | 77.8 | 74.5 | –3.3 | 0.20 |
3-A | 68 | 81.0 | 92.2 | 11.2 | 0.01 |
3-B | 87 | 78.5 | 88.2 | 9.7 | 0.0087 |
3-C | 155 | 79.4 | 76.2 | –3.2 | 0.32 |
4-A | 64 | 53.8 | 86.6 | 32.8 | < 0.0001 |
4-B | 49 | 68.7 | 85.5 | 16.8 | 0.0051 |
4-C | 113 | 61.6 | 72.0 | 10.4 | 0.0119 |
Radiographer sensitivity was significantly lower than radiologist sensitivity in 7 of 10 radiographer–radiologist combinations (range of difference 9.7–32.8%; p < 0.05), and not significantly different in 3 of 10 combinations. Radiographers had significantly higher average false-positives per case than radiologists in 8 of 10 combinations (range of difference 0.4–2.6; p < 0.05), and there was no significant difference in the remaining two combinations (Table 3).
Radiographer–radiologist combination | False-positive rate | Difference | p-value | |
---|---|---|---|---|
Radiographer | Radiologist | |||
1-A | 1.1 ± 1.3 | 0.5 ± 0.8 | –0.6 | < 0.0001 |
1-C | 1.1 ± 1.3 | 0.1 ± 0.5 | –1.0 | < 0.0001 |
2-B | 2.8 ± 2.8 | 0.7 ± 1.1 | –2.1 | < 0.0001 |
2-C | 2.8 ± 2.8 | 0.2 ± 0.5 | –2.6 | < 0.0001 |
3-A | 0.9 ± 1.4 | 0.5 ± 0.8 | –0.4 | 0.0176 |
3-B | 1.4 ± 2.5 | 0.6 ± 1.0 | –0.8 | 0.0015 |
3-C | 1.2 ± 2.1 | 0.1 ± 0.5 | –1.1 | < 0.0001 |
4-A | 0.4 ± 0.8 | 0.5 ± 0.8 | 0.1 | 0.2009 |
4-B | 0.8 ± 1.2 | 0.8 ± 1.3 | 0 | 0.71 |
4-C | 0.6 ± 1.0 | 0.2 ± 0.5 | –0.4 | 0.0001 |
Reader performance in the first 10 weeks (period 1) compared with second 10 weeks (period 2)
The two radiographers with the lowest overall sensitivity (radiographers 1 and 4) showed a significant improvement in sensitivity between the first and second 10-week period (sensitivity 50.0% in period 1 vs. 74.1% in period 2 for radiographer 1; 41.8% in period 1 vs. 67.2% in period 2 for radiographer 4; p < 0.005), but their sensitivity in period 2 still did not reach the level of radiographers 2 and 3, who showed no significant difference in their sensitivity between the two periods.
Radiologists’ sensitivity did not significantly differ between the two periods. No radiographer or radiologist demonstrated a significant difference in average false-positives per case between the two periods.
Comparisons of sensitivity using alternate diameter thresholds
There were 236 reference standard nodules that were ≥ 5 mm in diameter. When considering only these nodules, the number of radiographer–radiologist combinations with significantly lower radiographer sensitivity decreased to 4 of 10 combinations (range of difference 17.2–37.3%; p < 0.05). No significant difference was seen in the remaining 6 of 10 combinations.
Comparison of radiologists’ performance when reporting alone and concurrently with radiographers
The overall sensitivity of each radiologist for the different reading methods is detailed in Table 4. The mean sensitivity for radiologists reading independently was 77.5 ± 11.2%, increasing to 90.8 ± 5.6% with the use of concurrent reading. For all but one radiologist (radiologist D), statistically significant higher sensitivity was achieved with concurrent reading compared with independent reading.
Reading method | Radiologist | |||
---|---|---|---|---|
A | B | C | D | |
Independent | 78.9 | 79.8 | 62.2 | 89.2 |
Concurrent | 90.4 | 98.2 | 84.5 | 90.1 |
Difference | 11.5 | 18.4 | 22.3 | 0.9 |
p-value | 0.01 | < 0.0001 | < 0.0001 | 0.97 |
There was a wide variation in the average false-positives per case. Although the overall mean of average false-positives per case increased from 0.33 ± 0.20 with independent reading to 0.60 ± 0.53 with concurrent reading, average false-positives per case ranged between 0.06 and 1.38, increasing with concurrent reading for radiologists A–C (and statistically significant for radiologists B and C) but decreasing for radiologist D (Table 5).
Reading method | Radiologist | |||
---|---|---|---|---|
A | B | C | D | |
Independent | 0.31 ± 0.75 | 0.47 ± 1.10 | 0.06 ± 0.25 | 0.48 ± 0.96 |
Concurrent | 0.37 ± 0.65 | 1.38 ± 1.46 | 0.21 ± 0.61 | 0.42 ± 0.76 |
Difference | 0.06 | 0.91 | 0.15 | –0.06 |
p-value | 0.56 | < 0.001 | 0.03 | 0.69 |
Reading times using independent and concurrent reading methods
The mean reading times per case for concurrent reading ranged from 6.2 to 8.6 minutes compared with 7.0–12.4 minutes for independent reading (Table 6). Concurrent reading was faster than independent reading for all radiologists, but this increase in reading speed was not statistically significant for radiologist C. Furthermore, the maximum decrease in mean reading time was just < 4 minutes (radiologist A).
Reading method | Radiologist | |||
---|---|---|---|---|
A | B | C | D | |
Independent | 12.4 (11.1 to 13.5) | 8.8 (7.8 to 9.8) | 7.0 (6.5 to 7.5) | 8.3 (7.4 to 9.3) |
Concurrent | 8.6 (7.9 to 9.3) | 6.2 (5.5 to 7.0) | 6.9 (6.4 to 7.4) | 7.0 (6.2 to 7.8) |
Difference | –3.8 | –2.6 | –0.1 | –1.3 |
p-value | < 0.0001 | 0.0001 | 0.65 | 0.03 |
Discussion
Nodule detection for lung cancer screening requires working in a systematic and uninterrupted fashion, with less emphasis on medical/radiological expertise. Systematic CT scan reading is time-consuming; subsequently entering information into the UKLS database takes more time. We investigated whether or not radiographers might be more appropriate readers than highly trained radiologists to carry out these tasks.
The results of our study show that in 7 of 10 pairings, radiographers were less sensitive than radiologists for the detection of lung nodules. Radiographers also generated significantly more false-positives than radiologists in 8 of 10 combinations. However, the mean (72%) and range (62–79%) of sensitivities of radiographers in this study group compares favourably to figures reported for radiologists in the literature (Table 7). Reassuringly, radiographers in general did not exceed three average false-positives per case, and are thus comparable with computer-aided detection (CAD) systems, where average false-positives per case range between 0.3 and 15 per case. 78 Therefore, we believe that these results show that the radiographers in this study (having initially undergone training and testing) may not yet be suitable to act as first and only readers in lung cancer screening. However, their performance compared reasonably well with reported results for radiologists and CAD systems in the literature, and therefore these results certainly do not disqualify radiographers from CT scan reading in lung cancer screening.
Authors | Year | Number and type of readers | Mean detection sensitivity | Range |
---|---|---|---|---|
Marten et al.71 | 2004 | Four radiologists | 40 | 21–57 |
Brochu et al.72 | 2007 | Three radiologists | 54 | 38–70 |
Rubin et al.73 | 2005 | Three radiologists | 50 | 41–60 |
Roos et al.74 | 2010 | Three radiologists | 53 | 44–59 |
Wormanns et al.75 | 2005 | Three radiologists | 64 | NR |
Beigelman-Aubry et al.76 | 2007 | Two radiologists | 52 | 46–58 |
Fraioli et al.77 | 2006 | Three radiologists | 57 | 46–68 |
Current study | 2012 | Three radiologists, four radiographers | 83 72 |
74–89 62–79 |
Instead, radiographers may be well placed to act as a form of reading aid to radiologists in lung cancer screening. We explored this by examining the performance of radiologists when reading alone compared with when reading alongside radiographers (concurrent reading). We found that the sensitivity of radiologists improved for the majority of radiologists (all apart from the most sensitive radiologist) when reading as part of a concurrent reading strategy. This improvement came at the expense of an increase in false-positive rates. Such a phenomenon is somewhat difficult to explain but it may be because once an opacity has been highlighted by a radiographer, there is a tendency for radiologists to ‘overcall’ such nodules and a difficulty in dismissing them.
Another potential benefit of using radiographers as concurrent readers is in the time saving for radiologists, although this was variable with a time saving of nearly 4 minutes for radiologist A, and barely any change for radiologist C. Perhaps more importantly, we showed that concurrent reading did not increase reading times. This is relevant, because another (less tangible) benefit of concurrent reading is that it may improve workflow for the radiologist and could potentially reduce reader fatigue. Tasks such as nodule marking, nodule segmentation and data entry are all steps that could be performed by radiographers to assist radiologists, as part of a concurrent reading strategy. An important question, for which the answer is currently unknown in the UK, is whether or not thoracic radiologists will volunteer to participate in lung cancer screening CT scan reading. Recruitment could be assisted if it is demonstrated that the reading process can be made efficient by the use of concurrent readers.
There are a number of variables that could influence the success of radiographer or concurrent reading strategies.
-
In UKLS, all nodules of > 3 mm or 15 mm3 were recorded. 74 There is emerging evidence to suggest that the risk of lung cancer in these small nodules (e.g. between 3 and 5 mm) is very low. 35,79 If the threshold for nodule size were to increase in a future lung cancer screening programme such that the total number of nodules recorded per CT scan reduced, this could have a substantial impact on reading times and performance. In fact, we showed that radiographer performance improved when very small nodules were disregarded from the analysis. Similarly, there is some recent evidence to suggest that a limit may be appropriate to the maximum number of nodules recorded in a single CT scan because patients with multiple nodules may actually be at lower risk of lung cancer. 80 In UKLS the maximum number of nodules recorded per CT scan was 20, but reading could be made more efficient if a lower limit was identified.
-
Another important consideration is whether or not future lung cancer screening programmes use volumetric analysis of nodule size. The benefits (e.g. increased reproducibility of nodule size) and the limitations (e.g. the inability to accurately segment ground glass or perivascular nodules) of currently commercially available volumetry applications are well described. 81 However, one advantage that is perhaps less well described is that it allows for a more automated evaluation of nodule size and growth, because VDTs can be readily and reliably calculated and growth can be more easily identified. This is important, because we have not shown (and there is no reason to believe) that radiographers would be satisfactorily proficient at identifying growth using visual subjective analysis. Incidentally, this can be a challenge for even experienced radiologists and volumetry also has the potential to assist radiologists in this regard (especially if they are reviewing a large number of nodules as part of a lung cancer screening programme).
-
Future studies need to be completed examining the role of radiographers compared with CAD as concurrent readers. At present, commercially available CAD systems are substantially limited by the number of false-positives that they generate (typically far greater than the results for radiographers in this study). 82 However, if future CAD systems addressed this issue, it may be that CAD software could be used instead of, or as well as, radiographers.
-
We have shown that a substantial proportion of screening CT scans identify potentially clinically significant incidental findings (see Chapter 5). Further work needs to be performed to evaluate whether or not radiographers can successfully recognise incidental findings, especially pneumonia and mediastinal lymphadenopathy, such that the reading process for radiologists could be further streamlined. Whether or not this is possible may well depend on the types of incidental findings that are deemed sufficiently significant to be highlighted in a lung cancer screening setting.
Chapter 5 Computed tomography scan findings and the early outcome of patients referred to the multidisciplinary team
Introduction
This chapter describes the outcomes of the baseline and follow-up CT scans, and documents any lung cancers that were identified. As all trial participants will be followed up over 10 years for health and mortality outcomes, it is possible here to give only an early indication of the outcomes for trial participants. This chapter should therefore not be regarded as giving complete information on the trial participants – only that information available to autumn 2014. It is therefore not possible to draw any definitive conclusions from the current data regarding the long-term effectiveness of lung screening.
Outcomes
The outcomes were the number of baseline and follow-up scans performed, categorisation of any lung nodules identified on scan, number of lung cancers diagnosed (including their staging and treatment) and any significant other findings. Mortality is not reported, as data are not mature.
Methods
Detailed methods regarding technical aspects of CT scanning/reading are given in the previous chapter. In brief, the trial participants who had been randomised to the intervention arm were given an appointment to attend for a single thoracic CT scan at either LHCH or Papworth Hospital. Scans were double-read by a radiologist at the local site (LHCH or Papworth) and a radiologist at the central site (Royal Brompton Hospital). Where necessary, arbitration was performed by an expert radiologist at the central site.
Any lung nodules identified on the CT scan were classified into four categories. Full details of these categories and the formal care pathway are shown in the previous chapter (see Figure 12). In summary, nodule categorisation and subsequent care was as follows:
-
No nodules or category 1 (benign) nodules No further action required.
-
Category 2 (small, probably benign) nodules Follow-up CT scan at 12 months.
-
Category 3 (larger, potentially malignant) nodules Follow-up CT scan at 3 months and 12 months.
-
Category 4 (higher chance of malignancy) nodules Immediate referral to MDT.
For category 2 or 3 nodules, the VDT of the nodule was calculated from the follow-up scan (at 3 or 12 months). The VDT was used to assess the likelihood of malignancy.
The UKLS protocol specified clear criteria for referral to the local lung cancer MDT. These were designed to select those at sufficiently high risk of lung cancer to justify further investigation. The subjects referred included those with a high risk of malignancy at baseline CT scan (category 4 nodules regarded as suspicious for lung cancer) and those of categories 2 and 3 with nodules that displayed a VDT of < 400 days. In addition, the protocol specified action for SOFs. These included abnormalities detected that required further action, either urgent, such as the finding of a cancer from another primary site, or less urgent, such as a likely pneumonia, requiring further imaging follow-up or treatment.
Computed tomography scan readers were required to make recommendations as per protocol wherever possible. However, radiologists were also at liberty to vary their recommendations on clinical grounds, if, in the opinion of both the local and central readers and local MDT chest physician, the CT scan appearances were not adequately covered by the protocol.
Details of subjects requiring follow-up for any of the criteria set out in the protocol were recorded in the UKLS database by the local investigators and from the GPs’ records. For lung cancer, stage, pathology and treatment offered were recorded.
Quality control for pathology of resected nodules
Quality control for histopathological examination of resected involved exchange between the reference thoracic pathologists at Liverpool (Professor John Gosney) and Papworth (Dr Doris Rassl) of a representative haematoxylin and eosin-stained section from all cases. This was accompanied, where necessary, by any immunolabelled sections used in diagnosis and/or classification of the lesion. Sections were reviewed ‘blind’ and responses were exchanged, with appropriate discussion, in cases of discordance.
Statistical analysis
Logistic regression was utilised in order to determine which factors were associated with requirement for further scans or investigations. Stata version 12 was used for analysis. The following variables (data obtained from questionnaire 1) were used in both univariate and multivariate analyses. For analyses including smoking status, the two never-smokers in the CT screening arm of the trial were removed from the analysis.
Variables used in logistic regression
Region (north or south), gender, age group (5-year bands), socioeconomic status (IMD), smoking status and duration, history of respiratory disease, history of mesothelioma, history of previous lung cancer, history of asbestos exposure and family history of cancer.
Results
A total of 4061 high-risk individuals consented to take part in the RCT. Six of these were not randomised because they had undergone a recent thoracic CT scan (so were not eligible; n = 3), changed their mind about participating (n = 1) or had no randomisation partner at the same hospital (n = 2). The remaining 4055 high-risk individuals were randomised into two groups. Following randomisation, 2028 individuals were assigned to the screening arm, and were offered a thoracic CT scan. Thirty-four of these individuals were not scanned. The most common reasons for this were that the individual withdrew following consent or a scan was felt to be inappropriate as a result of a change in his/her health. A total of 1994 individuals therefore underwent a baseline CT scan (see Figure 2). Details of individuals in both arms of the trial are shown in Table 8; the characteristics of people in both trial arms were very similar with respect to all factors considered.
Demographics | Total n = 4055, as n = 6 not randomised | |
---|---|---|
Screen arm (n = 2028) | Control arm (n = 2027) | |
Male–female ratio | 1529 : 499 (3.06 : 1) | 1507 : 520 (2.90 : 1) |
North–south ratio | 1023 : 1005 (1.02 : 1) | 1023 : 1004 (1.02 : 1) |
Age (years), mean (SD) | 67.1 (4.1) | 66.9 (4.1) |
Median age, years | 67 | 67 |
Median IMD rank | 17,374 | 17,704 |
Mean (SD) LLPv2 score | 8.87 (5.12) | 8.83 (4.71) |
Median LLPv2 score | 7.11 | 7.35 |
Never-smokers, n (%) | 2 (0.1) | 0 (0) |
Current smokers, n (%) | 777 (38.3) | 791 (39.0) |
Ex-smokers, n (%) | 1249 (61.6) | 1236 (61.0) |
Smoking duration 10–19 years, n (%)a | 117 (5.8) | 116 (5.7) |
Smoking duration 20+ years, n (%)a | 1895 (93.4) | 1907 (94.1) |
Smoking duration unknown, n (%)a | 14 (0.7) | 4 (0.2) |
Asbestos exposed, n (%) | 763 (37.6) | 763 (37.6) |
History of respiratory disease, n (%)b | 1056 (52.1) | 1023 (50.5) |
History of blood cancer, n (%)c | 26 (1.28) | 31 (1.53) |
History of solid tumour, n (%)d | 378 (18.6) | 396 (19.5) |
Family history of lung cancer, at any age, n (%) | 498 (24.6) | 554 (27.3) |
Family history of lung cancer < 60 years, n (%) | 215 (10.6) | 215 (10.6) |
Family history of lung cancer > 60 years, n (%) | 283 (14.0) | 339 (16.7) |
Family history of other cancer (not lung), n (%)d | 1026 (50.6) | 1019 (50.3) |
Figure 14 shows the outcome of the initial LDCT in a total of 1994 subjects. There were 979 (49.1%) subjects with either clear scans or category 1 nodules that were considered benign or who had a probability of malignancy that was too low to justify further investigation and follow-up, as per protocol.
FIGURE 14.
Diagnostic tree showing outcomes of 1994 baseline scans. a, One of these individuals had a third 3-month follow-up scan before referral to MDT; b, one person discharged from category 4 MDT at baseline also had several category 3 nodules – so was followed up as category 3, with 3-month and 12-month repeat scans, before final discharge. This person is not included in the category 3 figures. EOS, end of screening.
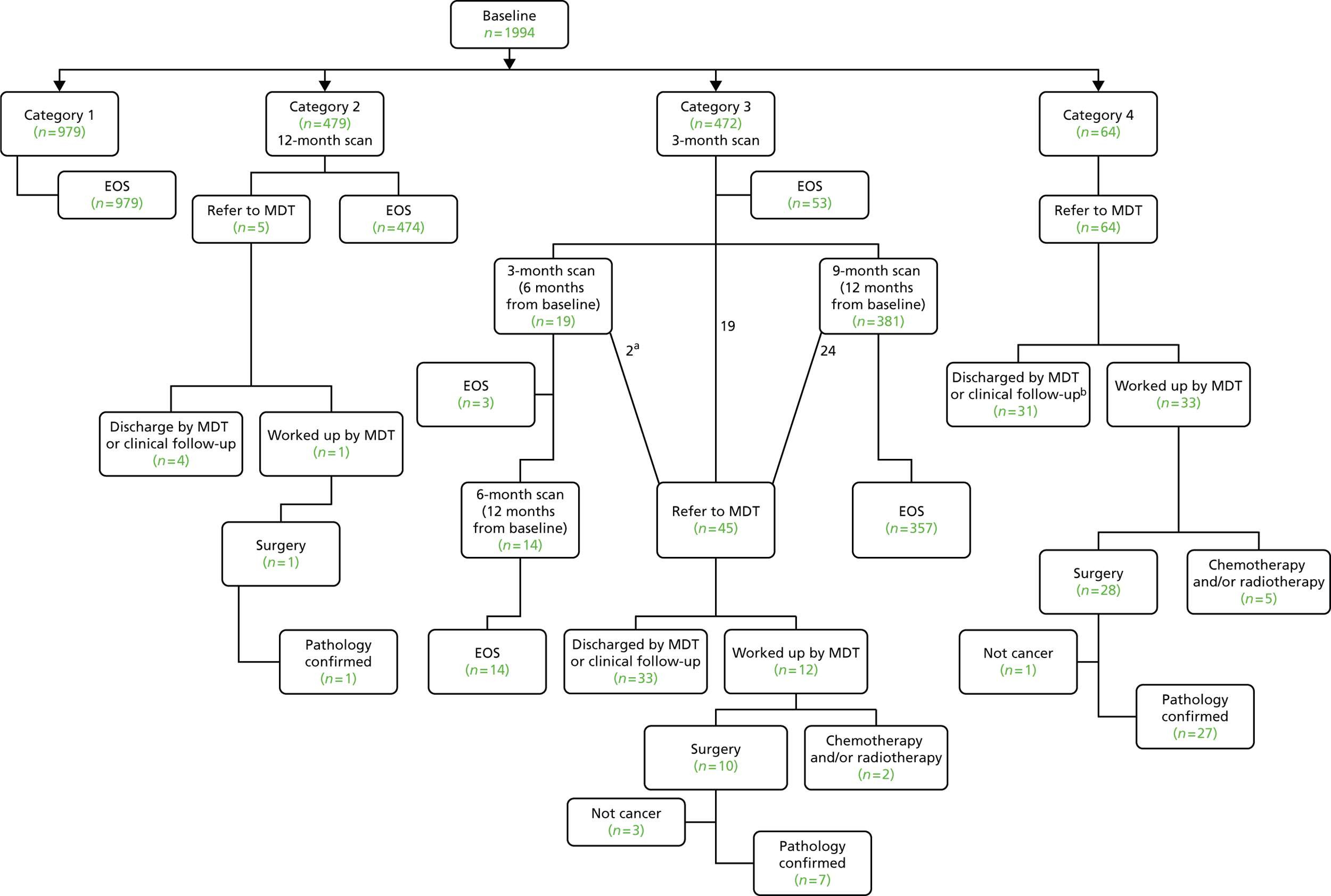
A total of 1015 (50.9%) participants had category 2–4 nodules. There were 479 (24%) subjects who had category 2 nodules, and who were therefore recommended for a 12-month repeat scan (see Figure 14). Of these, 474 subjects’ repeat CT scans were clear (no significant growth; VDT > 400 days), and the remaining five subjects (0.8%) were referred to the MDT. One of these subjects was diagnosed with cancer (which was resected) and four were discharged or are under follow-up with a presumed likely benign lesion.
There were 472 subjects (23.6%) who had category 3 nodules and therefore underwent a 3-month interval CT scan. Following the 3-month repeat scan, 53 (11.2%) subjects were discharged and 19 (4.0%) were referred to the MDT (two of these had cancer). Nineteen subjects underwent a further 3-month interval CT scan. This was part of a protocol amendment that was introduced to account for new nodules appearing on the 3-month interval CT scan; the concern was that potentially fast-growing cancers might be missed. Following the second 3-month interval CT scan, three subjects were discharged, one subject was referred to the MDT, and one subject underwent a further 3-month repeat scan before referral to the MDT; neither of these had cancer. The remainder comprised 14 subjects who underwent a 6-month CT scan (to complete a 12-month interval from the first CT scan). All of these CT scans have been completed with no malignancy detected. Following the first 3-month interval CT scan, 381 (80.7%) subjects were protocolled for a further 9-month interval CT scan; this resulted in 357 being discharged with no malignancy detected and 24 being referred to the MDT, five of whom had cancer. Thus, a total of 45 individuals initially classed as category 3 (9.5%) were referred to the MDT. Of these, 11 were thought to have cancer and 34 were discharged or are under surveillance for a likely benign diagnosis. One subject (with metastatic cancer) had chemotherapy and 10 had surgery. Altogether, 10 of 50 (20%) subjects referred to the MDT (who had category 2 or 3 nodules at baseline) had confirmed lung cancer.
Sixty-four subjects were referred to the MDT immediately following the baseline scan (see Figure 14). Thirty-one of these were discharged or are under MDT surveillance for nodules with a presumed benign diagnosis, and 33 were thought to have cancer and underwent further investigations. Five subjects had chemoradiotherapy and 28 had surgery, of whom 27 had histopathologically confirmed cancer. Thus 32 of 64 subjects (50%) referred to the MDT with category 4 findings at baseline had confirmed lung cancer.
Table 9 summarises the number of screened individuals assigned to each category, and the numbers referred to the MDT and identified cancers in each category.
Nodule category (management) | Category | Total | |||
---|---|---|---|---|---|
1 (discharged) | 2 (repeat scan at 12 months) | 3 (repeat scan at 3 months then 12 months) | 4 (immediate MDT referral) | ||
Number in category | 979 | 479 | 472 | 64 | 1994 |
Number referred to MDT | 0 (N/A) | 5 | 45 | 64 | 114 |
Number of confirmed lung cancers | 0 (N/A) | 1 | 9 | 32 | 42 |
Thus, 1952 of 1994 (97.9%) participants completed screening in the trial with no cancer found. Forty-two (2.1%) individuals were diagnosed with lung cancer.
Diagnostic work-up and false-positives
In the UKLS, we defined false-positives or rate of recall as those requiring further diagnostic investigation more immediately than a repeat annual screen, but who transpired on such investigation not to have lung cancer.
Overall, 951 of 1994 (47.7%) of subjects underwent at least one further CT scan after the initial screen. This comprised 479 subjects in category 2 who underwent a 12-month interval CT scan and 472 in category 3 (see Table 10). In addition, at the time of reporting, a further 414 CT scans have been carried out as per protocol (see Figure 14).
It should be noted that a repeat CT scan at 3 months for category 3 nodules was mandated by the protocol. There were a total of 536 subjects (i.e. 472 category 3, 64 category 4) with nodules requiring a repeat scan. Of the 64 category 4 individuals, 41 were found to have lung cancer.
Owing to our failsafe policy reflecting the single-screen design (i.e. follow-up of category 2 individuals), there were a further 479 individuals for whom a repeat screen was recommended at 12 months, only one of whom was found to have a confirmed cancer.
The referral rate to the MDT was low and there were relatively few people with benign disease who had invasive tests. One hundred and fourteen (5.7%) were referred to the MDT of whom 72 (3.6% of the total; 72/1994) did not have cancer.
For complete clarity, the proportion of false-positive tests is now provided in two ways, which allows an appreciation, in a patient-centred approach, of the variable impact on the subject in a trial or the patient in a programme. A ‘false-positive’ that mandates referral to the lung cancer MDT will usually be associated with significant psychological distress, and additional more or less invasive investigations with, in some cases, definitive treatment. An individual with a false-positive so defined is thus more likely to suffer harm than one defined in a different way; that is, those subjects who are recalled solely for further CT imaging to clarify the nature of a nodule. The latter is best termed ‘interval imaging rate’ and may, in screening programmes, merely mean continuing in the programme rather than referral to the MDT. For this reason, all category 3 lesions without cancer are reported separately as false-positives warranting interval imaging. Category 2 findings are not classified as false-positives warranting recall, as the cancer rate was found to be so low in this study that interval imaging would not be recommended.
Thus, on examining the number of UKLS participants referred to the MDT clinic, the false-positive rate is 3.6% (114 – 42/1994 = 3.6), whereas the interval imaging rate for the category 3 nodules is 23.2% (472 – 9/1994).
In total, 114 of 1994 (5.7%) participants were referred to the MDT, of whom 42 (2.1% of all screened) had lung cancer.
Details of cancer diagnoses
Of the 42 cancers with a confirmed diagnosis, 27 were diagnosed at LHCH, and 15 at Papworth Hospital. Twenty-five diagnoses were adenocarcinoma, 12 were squamous cell carcinoma, three were small cell carcinoma, one was a typical carcinoid and one was of unknown type (this patient was treated with palliation alone).
Pathological staging of cancers was completed for 35 of 42 cancers: 17 were T1aN0 (one Nx), six were T1bN0, two were T2aN0, two were T2bN0, two were T1aN1, three were T2aN1, two were T1–2N2 and one was T3N0. Clinical staging was recorded for a further seven cancers (Table 10). Thus, 67% of the screen-detected cancers that have so far been staged (28/42) were identified at stage 1. Details of all individuals referred to the MDT with a cancer diagnoses are shown in Table 10.
UKLS case number | Baseline nodule categorya | Gender | Age (years) | TNM | Final stage | Diagnosis | Treatment |
---|---|---|---|---|---|---|---|
1 | 4 | M | 59 | pT1a pN0 | IA | Adenocarcinoma | Surgery |
2 | 4 | M | 66 | pT1a pN0 | IA | Adenocarcinoma | Surgery |
3 | 4 | M | 66 | pT1a pN0 | IA | Adenocarcinoma | Surgery |
4 | 4 | M | 55 | pT1b pN0 | IA | Adenocarcinoma | Surgery |
5 | 4 | M | 63 | pT1a pN0 | IA | Adenocarcinoma | Surgery |
6 | 4 | F | 64 | pT1a pN0 | IA | Adenocarcinoma | Surgery |
7 | 4 | M | 67 | pT1b pN0 | IA | Small cell carcinoma | Surgery/chemotherapy |
8 | 4 | M | 62 | pT1a pN0 | IA | Squamous cell carcinoma | Surgery |
9 | 4 | M | 68 | pT1b pN0 | IA | Squamous cell carcinoma | Surgery |
10 | 4 | M | 67 | pT1a pN0 | IA | Squamous cell carcinoma | Surgery |
11 | 4 | M | 73 | pT1b pN0 | IA | Squamous cell carcinoma | Surgery |
12 | 4 | M | 71 | pT1a pN0 | IA | Adenocarcinoma | Surgery |
13 | 4 | M | 72 | cT1b cN0 cM0 | IA | Adenocarcinoma | Radiotherapy |
14 | 4 | M | 64 | pT1b pN0 | IA | Adenocarcinoma | Surgery |
15 | 4 | M | 68 | pT1a pN0 | IA | Adenocarcinoma | Surgery |
16 | 4 | M | 74 | cT1a cN0 cM0 | IA | Bronchogenic carcinoma | Palliative |
17 | 4 | M | 69 | pT1a pN0 | IA | Squamous cell carcinoma | Surgery |
18 | 4 | M | 70 | pT2a pN0 | IB | Adenocarcinoma | Surgery |
19 | 4 | M | 67 | pT2a pN0 | IB | Adenocarcinoma | Surgery |
20 | 4 | M | 68 | pT2a pN1 | IIA | Squamous cell carcinoma | Surgery/chemotherapy |
21 | 4 | F | 67 | pT1a pN1 | IIA | Squamous cell carcinoma | Surgery/chemotherapy |
22 | 4 | F | 64 | pT2b pN0 | IIA | Adenocarcinoma | Surgery/chemotherapy |
23 | 4 | F | 73 | pT2a pN1 | IIA | Small cell carcinoma | Surgery/chemotherapy |
24 | 4 | M | 63 | pT1a pN1 | IIA | Adenocarcinoma | Surgery/chemotherapy |
25 | 4 | M | 75 | pT2a pN1 | IIA | Squamous cell carcinoma | Surgery/chemotherapy |
26 | 4 | M | 64 | pT2b pN0 | IIA | Carcinoid | Surgery |
27 | 4 | M | 68 | pT1a pN2 | IIIA | Adenocarcinoma | Surgery |
28 | 4 | F | 69 | pT1b pN2 | IIIA | Adenocarcinoma | Surgery/chemotherapy |
29 | 4 | M | 63 | cT1a cN2 cM0 | IIIA | Small cell carcinoma | Chemotherapy |
30 | 4 | F | 60 | pT3 pN0 | IIB | Squamous cell carcinoma | Surgery/radiotherapy |
31 | 4 | M | 66 | cT4 cN3 cM1b | IV | Adenocarcinoma | Chemotherapy/radiotherapy |
32 | 4 | M | 64 | cT3 cN2 cM1b | IV | Squamous cell carcinoma | Palliative |
33 | 3 | M | 68 | pT1a pN0 | IA | Adenocarcinoma (two primaries) | Surgery |
34 | 3 | M | 69 | pT1a pN0 | IA | Adenocarcinoma | Surgery |
35 | 3 | M | 61 | pT1a pN0 | IA | Squamous cell carcinoma | Surgery |
36 | 3 | M | 70 | pT1a pN0 | IA | Adenocarcinoma | Surgery |
37 | 3 | F | 70 | pT1aNxb | IA | Adenocarcinoma | Surgery |
38 | 3 | M | 66 | pT1b pN0 | IA | Squamous cell carcinoma | Surgery |
39 | 3 | F | 69 | cT1a cN0 cM0 | IA | Adenocarcinoma | Radiotherapy |
40 | 3 | M | 71 | pT1a pN0 | IA | Adenocarcinoma | Surgery |
41 | 3 | F | 75 | cT4 cN2 cM1b | IV | Adenocarcinoma | Surgery (non-pulmonary) Radiotherapy/chemotherapy |
42 | 2 | F | 66 | pT1a pN0 | IA | Adenocarcinoma | Surgery |
Demographics of people diagnosed with lung cancer
Of the 42 individuals with a screen-detected lung cancer, 32 were men and 10 were women (i.e. 1.8% of all individuals screened, for both genders). The mean age at trial recruitment for the cancer patients was 66.9 years (median 67 years; range 55–75 years). This was similar to the mean age of all those screened (67.1 years). Seventeen of 42 (40.4%) people with a confirmed cancer were from the most deprived IMD quintile (Q1), five (11.9%) were from Q2, nine (21.43%) from Q3, four (9.5%) from Q4, and seven (16.7%) from the least deprived IMD quintile (Q5).
Predictors of subjects requiring further investigations
Variables from questionnaire 1 (see Appendix 3) were used in both univariate and multivariate logistic regression analyses to establish which factors were associated with participants requiring follow-up scans or investigations (i.e. category 2–4 individuals; n = 1015) compared with individuals with clear scans (category 1; n = 979). In the univariate analysis, both female gender [odds ratio (OR) 1.25, 95% confidence interval (CI) 1.02 to 1.53; p < 0.032] and a previous history of respiratory disease (OR 1.25, 95% CI 1.05 to 1.49; p < 0.012) were associated with requiring further scans. In the multivariate analysis, a previous history of respiratory disease (OR 1.28, 95% CI 1.06 to 1.54; p < 0.009) was associated with requiring further scans.
Further work-up by multidisciplinary team
Table 11 shows the further investigations and procedures that have so far been completed for subjects referred to the MDT. Only 8.6% of subjects who did not have confirmed cancer had needle biopsies and 4.3% had surgery. These are the two procedures most associated with complications. Overall, of 114 subjects referred to the MDT, 11 subjects (15.3%) without confirmed cancer had either a biopsy or surgery and nobody had both. This includes one subject with likely lung cancer. Thus, only 0.55% of subjects screened underwent semi-invasive or invasive tests for benign disease.
No cancer confirmed, N = 72 | Lung cancer confirmed, N = 42 | |||
---|---|---|---|---|
Investigation | n | % | n | % |
Further CT | 61 | 84.7 | 42 | 100.0 |
Needle biopsy | 7 | 9.7 | 15 | 35.7 |
EBUS | 1 | 1.4 | 3 | 8.3 |
PET | 13 | 18.05 | 37 | 88.9 |
Oncology referral | 1 | 1.4 | 13 | 36.1 |
Surgical referral | 4 | 5.5 | 35 | 83.3 |
Discharged | 3 | 4.3 | 21 | 58.3 |
Other outcome | 2 | 2.9 | 4 | 11.1 |
Outpatient follow-up | 21 | 30.0 | 21 | 58.3 |
Not suitable for MDT | 0 | 0.0 | 0 | 0 |
Treatment
Table 10 shows the treatment received by subjects with lung cancer. Reflecting the early stage, 35 of 42 subjects (83.3%) had surgery as their primary treatment. Of these, eight also had chemotherapy and one had radiotherapy. Chemotherapy was the primary treatment in three patients and radiotherapy was offered to two subjects who declined surgery. Treatment was palliative (supportive care) in two subjects.
Incidental findings
To date, over the course of the UKLS screening trial, 128 SOFs have been identified on the CT scans by the trial radiologists. Most of these findings related to thoracic disease, but 17 extrathoracic SOFs were also identified. Individuals with a SOF on scan were referred for further investigations and treatment to a relevant specialist or MDT, or their own GP. Details of the thoracic and extrathoracic SOFs are shown in Tables 12 and 13, respectively.
CT finding | n |
---|---|
Aortic dilatation | 4 |
Severe aortic valve calcification | 5 |
Mediastinal mass | 4 |
Mediastinal or hilar lymphadenopathy | 6 |
Pneumonia | 41 |
Bronchiectasis | 5 |
Pleural thickening | 8 |
Smoking related interstitial lung disease | 7 |
Severe emphysema | 9 |
Interstitial fibrosing lung disease (unspecified) | 6 |
Non-specific interstitial pneumonia | 2 |
Usual interstitial pneumonia | 12 |
Sarcoidosis | 1 |
Oesophageal thickening or dilatation | 2 |
Breast mass | 1 |
Lobar collapse | 2 |
Total | 115 |
CT finding | n |
---|---|
Biliary dilatation | 1 |
Adrenal mass | 3 |
Cirrhosis | 1 |
Hydronephrosis | 1 |
Liver mass | 1 |
Pancreatic cysts | 1 |
Renal mass | 3 |
Splenomegaly | 1 |
Thyroid mass | 1 |
Total | 13 |
Significant other findings were managed locally in Liverpool or Papworth. For any incidental findings that were identified before 9 December 2013, the participants’ GPs were contacted by UKLS and asked to provide follow-up and outcome data. The results from both Liverpool and Papworth are detailed below. Follow-up information for incidental findings arising after 9 December 2013 has not yet been requested, hence the figures below do not include all of the incidental findings detailed above (see Tables 12 and 13).
In the Liverpool cohort, 55 SOFs were identified, and the patients’ primary care physician informed. Seventeen different conditions were identified, including eight abdominal conditions. Thoracic conditions included pneumonia (n = 18), pulmonary fibrosis (n = 8), pleural thickening (n = 5), bronchiectasis (n = 3), lymphadenopathy (n = 3), severe emphysema (n = 3), lobar collapse (n = 2), oesophageal thickening (n = 2), mediastinal mass (n = 2), thyroid mass (n = 1) and thoracic aortic aneurysm (n = 1). Abdominal abnormalities included adrenal lesion (n = 2), abdominal aortic aneurysm (n = 2), cirrhosis of the liver (n = 1), splenomegaly (n = 1), renal mass (n = 1) and pancreatic cyst (n = 1). Further information on these patients was identified from secondary care records and 31 responses from primary care for follow-up data. Although the vast majority of pneumonias were managed in primary care, at least 22 of the others were referred to secondary and tertiary care for further investigation and management.
There were 48 SOFs in the Papworth cohort. The GPs of patients with SOFs were contacted by letter and asked to take appropriate action. For five cases with a potentially life-threatening condition the letter was followed up with a telephone call a few days later to ensure that action had been taken. Thoracic conditions included interstitial lung disease (n = 10), severe emphysema (n = 2), pneumonia (n = 16), atypical pneumonia (n = 4), lymphadenopathy (n = 3), posterior mediastinal mass (duplication cyst) (n = 1), thymoma (n = 1) and aortic calcification (n = 4). Abdominal conditions included renal mass (n = 2), adrenal mass (n = 1), hepatic mass (n = 1), abdominal aortic aneurysm (n = 1), biliary dilatation (n = 1) and hydronephrosis (n = 1). In 29 cases, outcomes are known from secondary care records or via the primary care follow-up questionnaire. Of these, the UKLS identified a diagnosis that was already known to the GP in three cases and no further action was taken. In 12 cases the GP treated the patient and in 14 cases the patient was referred to secondary or tertiary care.
Overall, about 5% of patients had a SOF identified. Nearly all of these were previously undiagnosed conditions. Their identification empowered primary care physicians to manage or appropriately refer on, for the patient’s benefit.
Discussion
These preliminary findings from the single screen UKLS study have confirmed several suppositions and hypotheses.
The lung cancer detection rate is 2.1%. This means that the LLP risk assessment model has performed as expected. All subjects had to have a 5-year risk of lung cancer of at least 5%. A > 1% risk on prevalence screening would therefore be expected. Unlike other CT screening studies, LLP avoids the problem of screening people at very low risk, for whom the harms of screening may outweigh the benefit.
The low threshold for imaging follow-up of abnormal findings resulted in 47.7% of subjects needing a follow-up CT scan at 3 months and/or 1 year. This was to be expected, as the UKLS protocol indicated repeat scans for people with category 2 nodules, which accounted for around 50% of nodules. However, only one lung cancer was detected from this category. Nine lung cancers have been confirmed from the group of category 3 nodules. For both of these categories the probability of finding lung cancer in the nodules is below the baseline risk of the population as a whole, and for category 3 (although numbers are too small to draw any real conclusion) it is 1.9%. Two lung cancers were identified by the 3-month interval CT scan, so it could be argued that this step is necessary. However, we are not able to comment on the effect on prognosis of detection of these lung cancers after 3 months rather than after a 12-month interval. Whether or not an annual screen is the best strategy for managing both category 2 and 3 nodules should be determined by reference to other studies with larger numbers and by monitoring outcomes of future screening programmes.
The immediate work-up strategy for the category 4 findings resulted in the greatest number of confirmed lung cancers; 50% of category 4 subjects referred to the MDT had lung cancer. Overall, only 5.7% of subjects were referred to the MDT, which demonstrates the utility of the imaging recall protocol. Furthermore, those subjects who underwent further investigation mostly underwent further imaging with few having minimally invasive or invasive procedures for benign disease. This rate of investigation of benign disease was similar to that found in NELSON37 and the NLST. Active treatment rates were high; 83% of subjects with confirmed lung cancer had surgery, reflecting the high proportion of patients with stage 1 or 2 disease. Of those patients who had surgery for later stage 3a disease, this was detected only at surgery, a feature thought to be associated with a more favourable prognosis.
A variety of significant other findings were detected. These included the detection of other cancers including malignant melanoma and renal carcinoma as well as important benign conditions such as severe emphysema. Not all of these benign diagnoses were known to the subject or their doctor. The detection of these other conditions (especially cancer) may clearly benefit subjects, although it is not possible to measure the effect in this study.
It is important to note how many more cancers appeared to be detected in the lower socioeconomic groups. Although numbers are relatively small there was a marked difference between the socioeconomic profile of the subjects screened (which was similar to the population as a whole) and that of those who had lung cancer. This raises a number of issues that may be important if screening programmes are to begin. As the risk of lung cancer is most strongly related to age and smoking, it is likely that the main reason for the observed rates in the lower socioeconomic groups is that the subjects smoked more. This would be in keeping with the way the LLPv2 selects individuals at a risk of at least 5% over 5 years. There will be a proportion of people who are at much greater risk by virtue of their smoking habit. This observation also has implications for the recruitment of people into programmes. At selection, we observed that the lower socioeconomic groups were more at risk yet were less likely to participate, and we now know that the same group, when recruited, does indeed have a greater prevalence of lung cancer. This serves to emphasise the importance of effective methods of recruitment of these relatively disadvantaged people into screening programmes if the effectiveness of such programmes is to be maximised.
Chapter 6 Psychosocial outcomes
Background
The importance of assessing psychosocial impact of lung cancer screening
Alongside clinical evidence, an evaluation of psychosocial harms and benefits is essential when considering the introduction of a new screening programme. Studies of cancer screening in the general population have highlighted adverse psychosocial effects, in particular those associated with abnormal, false-positive or inconclusive test results. 83,84 The psychosocial impact of LDCT screening for lung cancer has been examined in controlled trials outside the UK, in terms of effects of both trial allocation and screening results. 85–91 Overall, evidence from these trials suggests that LDCT screening does not produce long-term anxiety or other adverse effects that could deter high-risk individuals from further help-seeking or future participation in a lung screening programme. However, it is important to examine psychosocial effects within the UKLS context to ensure that any future lung screening programme is tailored to the specific needs of the UK high-risk population.
Barriers to uptake of lung cancer screening
Previous studies have highlighted some of the demographic and psychosocial barriers to lung screening uptake. Silvestri et al. 92 reported that smokers perceived fewer benefits and were less likely to consider lung cancer screening than non-smokers. Non-participants in NELSON88 were more likely to be female and former smokers, and to have a lower perceived risk of lung cancer and less-positive beliefs about the benefits of lung screening than participants. 93 In the first 88,897 individuals approached to take part in UKLS, participation was more likely in ex-smokers and those in higher socioeconomic groups. 94 Other barriers may include fears about lung screening tests and radiation exposure, and fatalistic beliefs about lung cancer. 95,96
Psychosocial impact of lung screening trial allocation
The psychosocial impact of trial group assignment has been examined in both the NELSON trial88 and the DLCST. 85 In NELSON,88 the intervention and control groups were equivalent in a range of psychosocial outcomes, both general and cancer-specific. Similarly, the DLCST found no differential effects of trial allocation on psychosocial outcomes at 1-year follow-up. 85 Although those in the control group reported slightly worse psychosocial outcomes on some measures at follow-up, the difference between groups was not significant when examining the mean increase in scores on each scale. The NCI’s PLCO87 reported poorer health-related quality of life (HRQL) in the intervention groups on one measure (mental component of the Short Form questionnaire-12 items), but no difference on any other psychosocial measure.
Psychosocial impact of lung screening results
Recent trials that have examined the psychosocial effects of lung screening results indicate that the negative impact of receiving an unfavourable result, especially in relation to cancer-specific distress, tends to diminish over time. The NELSON trial88 reported poorer quality of life, increased general anxiety and clinically elevated levels of cancer-specific distress at 2 months follow-up in high-risk individuals receiving an indeterminate CT scan result than in those receiving a negative (normal) result. However, these effects did not persist at 6 months’ follow-up (i.e. after the second screening round). 88,89
A similar pattern was observed in the Pittsburgh Lung Screening Study86 with a temporary increase in stated anxiety in those with an indeterminate screening result, although cancer-related fear did not return to baseline levels at 1-year follow-up. Participants in the PLCO who received at least one abnormal result across any of the cancers being screened, reported higher cancer-specific distress in the short term than in those who had received all normal screening results. 87 No significant differences were found on the mental or physical components of the Short Form questionnaire-12 items or were there differences found on any of the measures at 9 months’ follow-up, suggesting that negative psychosocial consequences of lung screening results are not long lasting.
Aim
To assess the psychosocial consequences of lung cancer screening.
Objectives
Specific objectives were to examine:
-
factors associated with trial non-uptake in high-risk individuals
-
short- and long-term impact of trial allocation on lung cancer-specific distress (primary outcome), and anxiety, depression and decision satisfaction (secondary outcomes)
-
impact of baseline CT scan result on lung cancer-specific distress, anxiety, depression and decision satisfaction
-
long-term impact of screening outcome on lung cancer-specific distress, anxiety, depression and decision satisfaction
-
short- and long-term impact of trial allocation on the primary outcome, adjusting for effect modifiers.
Hypotheses
-
Intervention arm participants will report higher short-term cancer distress than those in the control arm. In particular, those intervention arm participants who receive a positive result of their baseline CT scan will report higher short-term cancer distress than those with negative (normal) results.
-
There will be no difference in long-term cancer distress between trial arms or between screening outcome groups.
Methods
Participants
A random sample of 247,354 individuals, aged between 50 and 75 years, from six PCTs (three from the Liverpool area and three from the Cambridge area) were initially invited to take part in the UKLS by completing a risk assessment questionnaire based on the LLPv2. Individuals who were identified as being at high risk of lung cancer were then sent further information about the trial and invited to participate. Following completion of a further screening questionnaire to identify trial eligibility, people who met the criteria were invited to attend the recruitment centre. High-risk individuals who attended the recruitment centre were confirmed as eligible, and who consented to take part in the trial were included in the psychosocial outcomes component of the study. Individuals who actively declined to take part by completing an optional non-participation questionnaire are referred to as ‘negative responders’.
Procedure
At the UKLS recruitment centre, participants completed a baseline touchscreen questionnaire, which included a number of psychosocial measures (referred to as T0). Participants were sent a follow-up psychosocial questionnaire (T1) approximately 2 weeks after receiving either (1) the letter detailing that they had been assigned to the control arm of the trial (control group) or (2) the baseline CT scan result letter (intervention group). A freepost envelope was included with the questionnaire pack for the participant to use to return his/her completed questionnaire to the DMC. Between September 2012 and January 2013, a subset of just over 600 participants who did not return their T1 questionnaire received a reminder. A further follow-up questionnaire (T2) and freepost envelope were sent in January 2014 (10–27 months after attending the recruitment centre) to all participants who were still alive and not ‘off study’.
Measures
Lung cancer-specific distress
The revised six-item CWS-R59,60 was adapted to measure lung cancer-specific distress. The scale includes six items, each measured on a 4-point Likert scale (score range 6–24). Participants were asked to complete the scale, thinking about how they felt in the past week. The scale was found to have good internal consistency (T0 α = 0.81, T1 α = 0.85, T2 α = 0.85). A score of > 12.5 corresponds to a clinically significant threshold score on the General Health Questionnaire-28. 97 One question from the CWS-R was included in the non-participation questionnaire (‘How concerned are you, if at all, about the possibility of getting lung cancer some day?’). For comparisons between trial participants and negative responders who completed the non-participation questionnaire, responses to this question were recoded into three categories of affective risk perception: none (‘not at all’ concerned), lower (‘somewhat’ concerned) and higher (‘moderately’ or ‘very’ concerned).
General anxiety and depression
The HADS58 was used to measure general levels of anxiety and depression. Anxiety and depression subscales each included seven items on a 0–3 scale, anchored to how participants felt in the last week (score range 0–21). Good internal consistency was found both anxiety (T0 α = 0.81, T1 α = 0.87, T2 α = 0.87) and depression (T0 α = 0.73, T1 α = 0.84, T2 α = 0.84). Scores of 0–7 were classified as ‘normal’, 8–10 as ‘mild’ anxiety or depression, and 11–14 and 15–21 as ‘moderate’ and ‘severe’, respectively. 58
Decision satisfaction
Satisfaction with the decision to participate in the trial was measured using the Satisfaction with Decision Scale,98 a six-item scale with five response categories ranging from ‘strongly disagree’ to ‘strongly agree’. Scale scores were summed and then averaged so that possible scores ranged from 1 to 5 (T0 α = 0.94, T1 α = 0.95, T2 α = 0.96). Owing to a bimodal distribution in the current study, a binary variable was created to reflect lower decision satisfaction (score of < 5, ‘not very satisfied’) and higher decision satisfaction (score = 5, ‘very satisfied’).
Demographic variables
Educational attainment, marital status and ethnic group were included in the T0 questionnaire. Age (around the time of risk calculation) and gender were provided by the PCTs (via the DMC). For analyses examining barriers to trial uptake, age was categorised into three groups: younger age (≤ 65 years), recently retired (66–70 years) and older age (≥ 71 years). IMD scores and ranks were established by the DMC using participants’ postcodes. IMD ranks were analysed using standard quintiles based on England-wide population data: quintile 1, 1–6496; quintile 2, 6497–12,993; quintile 3, 12,994–19,489; quintile 4, 19,490–25,986; and quintile 5, 25,987–32,482. Quintile 1 reflects those most deprived and quintile 5 those least deprived.
Smoking status
Smoking status was collected in the UKLS questionnaire 1. Participants were identified as current smokers, ex-smokers or never-smokers. Very few high-risk participants had never smoked, hence this category was excluded during analyses.
Experience of lung cancer
Participants were asked in the T0 questionnaire whether they had a friend or close family member who had been diagnosed with lung cancer. Five responses were available and were categorised into two groups: ‘yes’ (included responses ‘yes, self’, ‘yes, someone close’, ‘yes, self and someone close’, ‘yes, prefer not to say who’) and ‘no’ (response ‘no’).
Computed tomography scan result
Participants in the intervention group were categorised according to their CT scan result both at baseline and longer-term follow-up (result of their most recent scan).
-
Negative Those who received a negative (normal) result.
-
Negative with incidental finding Those who received a significant incidental finding but no lung abnormality.
-
Positive for repeat scan Those who were recommended to attend for a repeat scan in 3 or 12 months.
-
Positive for MDT referral Those referred to the MDT with a major lung abnormality.
Long-term screening outcome
Screening outcome has been defined based on participants’ screening history and the outcome of their final CT scan (where relevant) at the time of T2 questionnaire completion.
-
True negative Those who received a negative (normal) result and no lung cancer diagnosed.
-
False positive Those who were recommended to attend for a repeat scan in 3 or 12 months, but then had a final negative (normal) result and no lung cancer diagnosed.
-
True positive Those who were recommended to attend for a repeat scan in 3 or 12 months or referred to the MDT with a major lung abnormality, and lung cancer was diagnosed.
-
Incidental finding Those who received a significant incidental finding (either at baseline scan or repeat scan) but no lung cancer diagnosed.
Participants who had a MDT referral but no lung cancer diagnosed were excluded from long-term outcome analyses, as were those still awaiting their final scan or result at the time of completing T2. Those whose results were not classified were also excluded, as they did not follow the standard care pathway and/or their psychosocial situation did not reflect their categorisation in the main trial, for example patients with mesothelioma were classified as category 1 (end of screening).
Statistical analyses
Analyses were conducted using SPSS version 20 (IBM Corporation, Armonk, NY, USA). A mean replacement strategy was used when participants were missing data on the psychosocial variables. Scale scores were assessed for normality and log-transformed in cases of non-normal distribution (cancer distress, anxiety and depression). Statistical associations between individual characteristics and trial participation were examined using the univariable and multivariable regression modelling. Attrition bias was assessed using the chi-squared test and independent t-tests.
Primary analyses
At each follow-up end point, psychosocial outcomes of trial allocation and screening results were assessed using (analyses of covariance (ANCOVAs; adjusted for baseline psychosocial score) and chi-squared tests, as appropriate.
The assumptions of ANCOVA, including homogeneity of regression slopes and linearity, were tested. Regression slopes for lung cancer distress scores were heterogeneous at short-term follow-up, therefore subgroup analyses were performed separately for high and low cancer distress thresholds (> 12.5 or < 12.5). Sensitivity analyses were performed to assess any confounding effects of data timing issues. At short-term assessment, participants were identified as protocol deviations and excluded from analyses if they completed their T1 questionnaire at < 10 days after their baseline scan result letter or control arm allocation letter was sent. At long-term assessment, participants who received a final scan or scan result within the 2 weeks prior to, or succeeding, T2 completion were identified as protocol deviations and were excluded from the data set.
To account for multiple testing, a conservative p-value of 0.01 was used to indicate statistical significance. Effect size was calculated using the η2 statistic (where a value of 0.01 represents a weak effect, 0.06 represents a moderate effect and 0.14 represents a strong effect). Following significant ANCOVA results, post hoc pairwise comparisons (using the Sidak correction and p-value of 0.05 to indicate statistical significance) were carried out to identify group differences.
Secondary analyses
Linear mixed-effects modelling was performed to account for both short- and long-term cancer distress. The model evaluated the impact of trial allocation after adjustment for baseline cancer distress, with a random subject intercept and slope separately for each outcome. Sensitivity analyses were conducted (separately for participants with low or high baseline cancer distress scores), each with different cancer distress effect modifiers included:
-
Univariable analysis evaluating the impact of trial allocation adjusting for baseline cancer distress only and each additional effect modifier separately.
-
Preconceived scientifically justified multivariable analysis evaluating the impact of trial allocation after adjustment for baseline cancer distress and other scientifically important effect modifiers: age group, smoking, deprivation quintile and time since attended recruitment centre.
-
Preconceived scientifically justified multivariable analysis evaluating the impact of trial allocation after adjustment for baseline cancer distress, and all other scientifically and statistically important effect modifiers. This involved a forward stepped model fitting process, assuming analysis (2) as the base model using the maximum likelihood approach for handling degrees of freedom and nested models. Effect modifiers were included if a likelihood ratio was significant at p ≤ 0.01 and were sequentially introduced in order of statistical importance. Covariates considered for inclusion as effect modifiers were trial site, gender, ethnicity, marital group and experience of lung cancer.
All trial group by main effect interactions were then assessed for statistical importance. After the optimal and parsimonious statistical model was found, parameter estimates, standard errors and p-values were estimated using the residual maximum likelihood approach to handling degrees of freedom.
For all the above, effect modifiers are summarised, interpreted and compared between nested analyses.
Results
Trial participation
A flow diagram of participation in the trial is shown in Figure 15. In total, 4061 individuals (5.3% of 75,958 positive responders; 46.5% of all high-risk positive responders) attended the recruitment clinic and were consented into the trial. Of these, 4039 trial participants completed baseline T0 psychosocial questionnaires: 4037 were randomised (n = 2018 intervention, n = 2019 control) and 3232 of the randomised participants completed T1 psychosocial questionnaires [n = 1653 (84%) intervention, n = 1579 (78%) control]. At T2, 2855 participants completed psychosocial questionnaires [n = 1553 (82%) intervention, n = 1302 (65%) control].
FIGURE 15.
Trial participation flow diagram.

Barriers to trial uptake
Of 8729 high-risk individuals, the current analysis compared 4061 individuals who attended the recruitment clinic and consented to participate with 2762 individuals who declined participation (i.e. 754 negative responders, 2008 non-responders). Of the negative responders, five were subsequently excluded because of reported gender discrepancies and one was reported as deceased.
Age, gender, smoking and socioeconomic group were statistically significantly associated with trial uptake (Table 14). Women were less likely to take part than men (OR 0.64; p < 0.001), and current smokers were less likely to take part than ex-smokers (OR 0.70; p < 0.001). Older individuals were less likely to participate in the trial than younger individuals aged ≤ 65 years (OR 0.73; p < 0.001) and those recently retired (OR 0.76; p < 0.001), but the difference in uptake between younger individuals and those recently retired was not statistically significant. Individuals in the highest socioeconomic group (quintile 5) were most likely to participate. Individuals in the lowest quintile were almost twice as likely to decline trial participation as those in the highest quintile (OR 0.56; p < 0.001).
Demographics | Non-participants (N = 2756),a n (%) | Trial participants (N = 4061),a n (%) | Univariable OR (95% CI) | p-value | Multivariable OR (95% CI)b | p-value |
---|---|---|---|---|---|---|
Gender | ||||||
Male | 1770 (64) | 3041 (75) | 1.00 (reference) | |||
Female | 986 (36) | 1020 (25) | 0.60 (0.54 to 0.67) | < 0.001*** | 0.64 (0.58 to 0.71) | < 0.001*** |
Age range | ||||||
Younger age (≤ 65 years) | 838 (30) | 1249 (31) | 1.00 (reference) | |||
Recently retired (66–70 years) | 1087 (39) | 1742 (43) | 1.08 (0.96 to 1.21) | 0.22 | 1.05 (0.93 to 1.18) | 0.47 |
Older population (≥ 71 years) | 831 (30) | 1070 (26) | 0.86 (0.76 to 0.98) | 0.02 | 0.73 (0.64 to 0.80) | < 0.001*** |
Older compared with recently retired | 0.80 (0.71 to 0.90) | < 0.001*** | 0.70 (0.62 to 0.79) | < 0.001*** | ||
Smoking | ||||||
Current smoker | 1334 (48) | 1568 (39) | 0.67 (0.61 to 0.74) | < 0.001*** | 0.70 (0.63 to 0.78) | < 0.001*** |
Ex-smoker | 1418 (51) | 2591 (61) | 1.00 (reference) | |||
Never smokedc | 4 (< 1) | 2 (< 1) | (–) | |||
Socioeconomic group | ||||||
Quintile 1 (most deprived) | 924 (34) | 1090 (27) | 0.52 (0.45 to 0.60) | < 0.001*** | 0.56 (0.49 to 0.65) | < 0.001*** |
Quintile 2 | 448 (16) | 487 (12) | 0.48 (0.40 to 0.57) | < 0.001*** | 0.49 (0.42 to 0.59) | < 0.001*** |
Quintile 3 | 483 (18) | 723 (18) | 0.66 (0.56 to 0.77) | < 0.001*** | 0.68 (0.58 to 0.80) | < 0.001*** |
Quintile 4 | 447 (16) | 732 (18) | 0.72 (0.61 to 0.85) | < 0.001*** | 0.73 (0.62 to 0.86) | < 0.001*** |
Quintile 5 (least deprived) | 453 (16) | 1029 (25) | 1.00 (reference) |
Individuals with a higher affective risk perception were less likely to take part in the trial compared with individuals reporting no or lower levels of affective risk perception (OR 0.52; p < 0.001 and OR 0.64; p < 0.001, respectively, after adjusting for age, gender, smoking and socioeconomic group). There was no statistically significant difference between ‘none’ and lower levels of affective risk perception (Table 15). There were no significant interactions between the included main effects (e.g. the interaction between gender and affective risk was not statistically important; p = 0.81).
Affective risk perception | Negative responders (N = 748), n (%) | Trial participants (N = 4061), n (%) | Univariable OR (95% CI) | p-value | Multivariable OR (95% CI)a | p-value |
---|---|---|---|---|---|---|
None | 129 (19) | 1054 (26) | 1.00 (reference) | |||
Lower | 213 (32) | 1493 (37) | 0.86 (0.69 to 1.09) | 0.22 | 0.82 (0.65 to 1.04) | 0.09 |
Higher | 329 (49) | 1478 (37) | 0.55 (0.45 to 0.69) | < 0.001*** | 0.52 (0.42 to 0.65) | < 0.001*** |
Higher compared with lower | 0.64 (0.53 to 0.77) | < 0.001*** | 0.64 (0.53 to 0.77) | < 0.001*** |
Attrition bias
Baseline touchscreen questionnaire completion
Descriptive statistics for those who did (n = 4039) and did not (n = 22) complete T0 questionnaires at the recruitment centre are shown in Table 33 (see Appendix 7). Statistical comparisons were not conducted because of the small number of non-completers.
Baseline computed tomography scan attendance
Of 2018 participants in the intervention arm, 31 did not attend their baseline CT scan. Descriptive statistics for scan attendees and non-attendees are provided in Table 34 (see Appendix 7). A trend towards lower decision satisfaction was observed in non-attendees, but this should be interpreted with caution because of the small sample size, which precluded formal statistical testing.
Follow-up questionnaire completion
Statistical comparisons between those who did and did not complete short- and long-term follow-up psychosocial questionnaires are displayed in Tables 16 and 17, respectively.
Demographics | Completers (N = 3232)a | Non-completers (N = 749)a | Test statistic (p-value) |
---|---|---|---|
Trial allocation, n (%) | |||
Intervention | 1653 (51) | 312 (42) | χ2(1) = 21.9 (< 0.001) |
Control | 1579 (49) | 437 (58) | |
Site, n (%) | |||
Liverpool | 1585 (49) | 420 (56) | χ2(1) = 12.0 (0.001) |
Papworth | 1647 (51) | 329 (44) | |
Age, mean (SD) | 67.73 (3.98) | 67.28 (4.46) | t(1041.7) = –2.5 (0.01) |
Gender, n (%) | |||
Male | 2446 (76) | 533 (71) | χ2(1) = 6.6 (0.01) |
Female | 786 (24) | 216 (29) | |
Education, n (%)b | |||
Up to GCSE/O-level or equivalent | 1007 (43) | 334 (57) | χ2(1) = 35.4 (< 0.001) |
Beyond GCSE/O-level or equivalent | 1310 (57) | 250 (43) | |
Ethnicity, n (%) | |||
White | 3190 (99) | 739 (99) | χ2(1) = 1.9 (0.17) |
Non-white | 26 (1) | 10 (1) | |
Marital status, n (%) | |||
Married/cohabiting | 2410 (75) | 508 (68) | χ2(1) = 14.6 (< 0.001) |
Not married/cohabitingc | 814 (25) | 240 (32) | |
IMD, n (%) | |||
Quintile 1 | 843 (26) | 222 (30) | χ2(4) = 23.6 (< 0.001) |
Quintile 2 | 367 (11) | 109 (15) | |
Quintile 3 | 574 (18) | 135 (18) | |
Quintile 4 | 578 (18) | 141 (19) | |
Quintile 5 | 870 (27) | 142 (19) | |
Smoking status (UKLS questionnaire 1), n (%) | |||
Current smoker | 1194 (37) | 338 (45) | χ2(1) = 17.4 (< 0.001) |
Ex-smoker | 2037 (63) | 410 (55) | |
Never-smoker | 1 (< 1)d | 1 (< 1)d | N/A |
Experience of lung cancer (T0), n (%) | |||
No | 1870 (58) | 396 (53) | χ2(1) = 6.3 (0.01) |
Yes | 1355 (42) | 352 (47) | |
Cancer distress (T0), mean (SD)e | 2.16 (0.28) 8.65 | 2.21 (0.31) 9.16 | t(3968) = 4.9 (< 0.001) |
Anxiety (T0), mean (SD)e | 1.52 (0.71) 3.59 | 1.65 (0.71) 4.20 | t(3973) = 4.3 (< 0.001) |
Depression (T0), mean (SD)e | 1.27 (0.67) 2.56 | 1.37 (0.66) 2.94 | t(3971) = 3.7 (< 0.001) |
Decision satisfaction (T0), n (%) | |||
Not very satisfied | 1916 (59) | 428 (57) | χ2(1) = 1.0 (0.32) |
Very satisfied | 1308 (41) | 317 (43) |
Demographics | Test statistic (p-value) | Completers (N = 2855)a | Non-completers (N = 1026)a |
---|---|---|---|
Trial allocation, n (%) | |||
Intervention | 1553 (54) | 335 (33) | χ2(1) = 142.9 (< 0.001) |
Control | 1302 (46) | 691 (67) | |
Site, n (%) | |||
Liverpool | 1336 (47) | 622 (61) | χ2(1) = 57.7 (< 0.001) |
Papworth | 1519 (53) | 404 (39) | |
Age, mean (SD) | 67.79 (3.98) | 67.19 (4.31) | t(1691.2) = –4.1 (< 0.001) |
Gender, n (%) | |||
Male | 2166 (76) | 736 (72) | χ2(1) = 6.8 (0.009) |
Female | 689 (24) | 290 (28) | |
Education, n (%)b | |||
Up to GCSE/O-level or equivalent | 940 (44) | 367 (53) | χ2(1) = 15.8 (< 0.001) |
Beyond GCSE/O-level or equivalent | 1196 (56) | 330 (47) | |
Ethnicity, n (%) | |||
White | 2825 (99) | 1004 (99) | χ2(1) = 4.4 (0.04) |
Non-white | 21 (1) | 15 (1) | |
Marital status, n (%) | |||
Married/cohabiting | 2174 (76) | 672 (66) | χ2(1) = 44.1 (< 0.001) |
Not married/cohabitingc | 675 (24) | 352 (34) | |
IMD, n (%) | |||
Quintile 1 | 668 (23) | 373 (36) | χ2(4) = 73.1 (< 0.001) |
Quintile 2 | 338 (12) | 126 (12) | |
Quintile 3 | 523 (18) | 170 (17) | |
Quintile 4 | 545 (19) | 157 (15) | |
Quintile 5 | 781 (27) | 200 (19) | |
Smoking status (UKLS questionnaire 1), n (%) | |||
Current smoker | 1009 (35) | 490 (48) | χ2(1) = 50.0 (< 0.001) |
Ex-smoker | 1845 (65) | 536 (52) | |
Never-smoker | 1 (< 1)d | 0 (0)d | N/A |
Experience of lung cancer (T0), n (%) | |||
No | 1683 (59) | 522 (51) | χ2(1) = 20.4 (< 0.001) |
Yes | 1166 (41) | 503 (49) | |
Cancer distress (T0), mean (SD)e | 2.16 (0.28) 8.64 | 2.20 (0.32) 9.06 | t(1615.1) = 4.5 (< 0.001) |
Anxiety (T0), mean (SD)e | 1.51 (0.71) 3.52 | 1.64 (0.71) 4.17 | t(3873) = 5.2 (< 0.001) |
Depression (T0), mean (SD)e | 1.25 (0.66) 2.51 | 1.38 (0.68) 2.96 | t(3871) = 5.0 (< 0.001) |
Decision satisfaction (T0), n (%) | |||
Not very satisfied | 1668 (59) | 612 (60) | χ2(1) = 0.52 (0.47) |
Very satisfied | 1179 (41) | 410 (40) |
At both time points, questionnaire completion was associated with older age (p ≤ 0.01), being male (p ≤ 0.01), being married or cohabiting (p < 0.001), higher educational attainment (p < 0.001), being an ex-smoker (compared with being a current smoker; p < 0.001) and taking part in the Papworth site (p ≤ 0.001). Note that a large number of data on educational level were missing or non-informative, therefore the results for this variable should be interpreted with caution. Questionnaire completion was also associated with deprivation (p < 0.001), with a greater proportion of completers in the highest IMD quintile at T1 and T2 and a greater proportion of non-completers in the lowest quintile at T2. Differences between completers and non-completers in ethnicity were not statistically significant at either follow-up.
A greater proportion of non-completers were from the control arm at each time point (both at p < 0.01). Although this suggests differential dropout, it should be noted that protocol exclusions were more frequent in the screening arm. Those who completed follow-up questionnaires reported significantly lower baseline scores on all psychosocial measures compared with non-completers (all at p < 0.001). However, the absolute differences were very small and scores were in the low range for both groups. Lung cancer experience was significantly associated with questionnaire completion, with a great proportion of non-completers having had experience of lung cancer (p ≤ 0.01). Differences between completers and non-completers in decision satisfaction were not statistically significant at either follow-up.
Baseline sample characteristics
Comparisons were made between the intervention and control arm, including only those who completed T0 questionnaires and were therefore in the psychosocial sample. As indicated in Table 18, demographic characteristics and baseline psychosocial scores were similar for the trial arms because of the adequate randomisation process, hence test statistics were not warranted.
Demographics | Intervention (N = 2018)a | Control (N = 2019)a |
---|---|---|
Site, n (%) | ||
Liverpool | 1003 (50) | 1016 (50) |
Papworth | 1002 (50) | 1016 (50) |
Age, mean (SD) | 67.72 (4.04) | 67.59 (4.13) |
Gender, n (%) | ||
Male | 1520 (75) | 1500 (74) |
Female | 498 (25) | 519 (26) |
Education, n (%)b | ||
Up to GCSE/O-level or equivalent | 678 (46) | 683 (46) |
Beyond GCSE/O-level or equivalent | 788 (54) | 791 (54) |
Ethnicity, n (%) | ||
White | 1992 (99) | 1992 (99) |
Non-white | 18 (1) | 19 (1) |
Marital status, n (%) | ||
Married/cohabiting | 1483 (74) | 1471 (73) |
Not married/cohabitingc | 528 (26) | 545 (27) |
IMD, n (%) | ||
Quintile 1 | 545 (27) | 533 (26) |
Quintile 2 | 243 (12) | 242 (12) |
Quintile 3 | 358 (18) | 361 (18) |
Quintile 4 | 353 (18) | 376 (19) |
Quintile 5 | 519 (26) | 507 (25) |
Smoking status (UKLS questionnaire 1), n (%) | ||
Current smoker | 772 (38) | 787 (39) |
Ex-smoker | 1244 (62) | 1232 (61) |
Never-smoker | 2 (< 1) | 0 (0) |
Experience of lung cancer (T0), n (%) | ||
No | 1168 (58) | 1126 (56) |
Yes | 846 (42) | 889 (44) |
Cancer distress (T0), mean (SD)d | 2.17 (0.29) 8.75 | 2.17 (0.29) 8.74 |
Anxiety (T0), mean (SD)d | 1.55 (0.71) 3.72 | 1.54 (0.71) 3.67 |
Depression (T0), mean (SD)d | 1.30 (0.68) 2.66 | 1.28 (0.67) 2.61 |
Decision satisfaction (T0), n (%) | ||
Not very satisfied | 1228 (61) | 1158 (58) |
Very satisfied | 786 (39) | 853 (42) |
Short-term outcomes analysis
The final sample for short-term psychosocial outcomes analysis (i.e. trial participants who had completed both T0 and T1 psychosocial questionnaires) consisted of 3232 individuals (see Figure 1). The mean age of participants was 67.7 years (see Table 16). The majority of participants were male (76%), married or cohabiting (75%), and of white ethnic origin (99%). One-third (31%) of the sample were educated up to and including General Certificate of Secondary Education, Ordinary level or equivalent, and 40% were educated beyond (data on educational level were missing or non-informative for 28% of the sample). Most of the sample comprised ex-smokers (63%). There was a spread of different levels of deprivation within the sample, with just over one-quarter being in the highest quintile and just over one-quarter being in the lowest quintile. Low mean levels of cancer distress, anxiety and depression were observed at baseline (see Tables 16 and 19). When categorised, 10% of the sample showed high levels of cancer distress at baseline, 8% showed moderate to severe levels of anxiety and 3% showed moderate to severe levels of depression. Just over 40% were very satisfied with their decision to take part in UKLS. Comparisons between trial allocation groups showed no significant differences in baseline characteristics (Table 19).
Demographics | Intervention (N = 1653)a | Control (N = 1579)a | Test statistic (p-value) |
---|---|---|---|
Site, n (%) | |||
Liverpool | 819 (50) | 766 (49) | χ2(1) = 0.35 (0.56) |
Papworth | 834 (51) | 813 (52) | |
Age, mean (SD) | 67.71 (3.94) | 67.75 (4.02) | t(3230) = 0.30 (0.77) |
Gender, n (%) | |||
Male | 1253 (76) | 1193 (76) | χ2(1) = 0.03 (0.87) |
Female | 400 (24) | 386 (24) | |
Education, n (%)b | |||
Up to GCSE/O-level or equivalent | 516 (44) | 491 (43) | χ2(1) = 0.40 (0.53) |
Beyond GCSE/O-level or equivalent | 654 (56) | 656 (57) | |
Ethnicity, n (%) | |||
White | 1632 (99) | 1558 (99) | χ2(1) = 0.01 (0.91) |
Non-white | 13 (1) | 13 (1) | |
Marital status, n (%) | |||
Married/cohabiting | 1239 (75) | 1171 (74) | χ2(1) = 0.33 (0.57) |
Not married/cohabitingc | 409 (25) | 405 (26) | |
IMD, n (%) | |||
Quintile 1 | 447 (27) | 396 (25) | χ2(4) = 3.28 (0.51) |
Quintile 2 | 193 (12) | 174 (11) | |
Quintile 3 | 292 (18) | 282 (18) | |
Quintile 4 | 280 (17) | 298 (19) | |
Quintile 5 | 441 (27) | 429 (27) | |
Smoking status (UKLS questionnaire 1), n (%) | |||
Current smoker | 621 (38) | 573 (36) | χ2(1) = 0.59 (0.44) |
Ex-smoker | 1031 (62) | 1006 (64) | |
Never-smoker | 1 (< 1) | 0 (0) | N/A |
Experience of lung cancer (T0), n (%) | |||
No | 974 (59) | 896 (57) | χ2(1) = 1.62 (0.20) |
Yes | 675 (41) | 680 (43) | |
Cancer distress (T0), mean (SD)d | 2.16 (0.28) 8.71 | 2.15 (0.28) 8.60 | t(3223) = –1.26 (0.21) |
Anxiety (T0), mean (SD)d | 1.53 (0.71) 3.63 | 1.51 (0.71) 3.55 | t(3225) = –0.74 (0.46) |
Depression (T0), mean (SD)d | 1.28 (0.68) 2.58 | 1.26 (0.67) 2.54 | t(3224) = –0.52 (0.60) |
Decision satisfaction (T0), mean (SD) | |||
Not very satisfied | 1000 (61) | 916 (58) | χ2(1) = 1.82 (0.18) |
Very satisfied | 651 (39) | 657 (42) |
Hypothesis 1: intervention arm participants will report higher short-term cancer distress than those in the control arm
As shown in Table 20, different effects of trial allocation on T1 lung cancer distress were found for participants who scored above and below the distress threshold (12.5) at baseline. For those with low baseline distress, T1 distress scores were significantly higher in the intervention group, although not to clinical levels, and with a very small effect size. For those with high baseline distress (10%, 326/3225), the effect of trial allocation on T1 cancer distress was not significant: mean levels of cancer distress remained high and bordered on clinical levels regardless of trial allocation (47%, 153/326). The effect of trial allocation on T1 general anxiety was not statistically significant. Significantly higher log-transformed depression scores were found in the control group, but the absolute difference was very small. When converted back to the original scale (0–21 range), depression scores were low and within the normal range for both control and intervention groups.
Outcome (T1) | Intervention, mean (95% CI)a | Control, mean (95% CI)a | Test statistic, p-value, effect size |
---|---|---|---|
Cancer distressb in low scorers at T0 (n = 2896) | 2.14 (2.13 to 2.16) | 2.11 (2.10 to 2.12) | F(1,2893) = 14.92, < 0.001, η2 = 0.005 |
8.54 (8.44 to 8.64) | 8.26 (8.16 to 8.36) | ||
Cancer distressb in high scorers at T0 (n = 326) | 2.50 (2.46 to 2.53) | 2.53 (2.50 to 2.57) | F(1,323) = 2.26, 0.13, η2 = 0.007 |
12.14 (11.73 to 12.55) | 12.61 (12.15 to 13.09) | ||
Anxietyb (n = 3209) | 1.54 (1.51 to 1.57) | 1.56 (1.53 to 1.59) | F(1,3206) = 1.23, 0.27, η2 < 0.001 |
3.67 (3.54 to 3.80) | 3.78 (3.64 to 3.92) | ||
Depressionb (n = 3208) | 1.26 (1.23 to 1.29) | 1.34 (1.31 to 1.37) | F(1,3205) = 14.36, < 0.001, η2 = 0.004 |
2.53 (2.42 to 2.63) | 2.81 (2.70 to 2.92) |
A significantly greater proportion of control arm participants were not very satisfied with their decision to take part (66%, 953/1451) than those in the intervention arm (58%, 875/1499) (χ2(1) = 16.7; p < 0.001).
Sensitivity analyses showed small changes in F-values but not in statistical significance levels, therefore further exclusions were not made.
Hypothesis 2: intervention arm participants with a positive baseline computed tomography scan result will report higher short-term cancer distress than those with negative results
As shown in Figure 15, 1653 participants who completed a T1 questionnaire were eligible for inclusion in the impact of baseline scan result analyses. Thirteen participants were excluded because of discrepancies in classification of their test result.
Preliminary analyses were conducted to test for potential covariates and are shown in Appendix 7, Table 35. Baseline differences between the screening result groups were not significant, hence only the baseline scores of each dependent variable were included as covariates.
Table 21 shows a statistically significant difference in T1 lung cancer distress among the different result groups. Participants who were positive for MDT referral (major lung abnormality) were significantly more distressed than each of the other result groups (negative mean difference 0.36; p < 0.001; negative with incidental finding mean difference 0.33; p < 0.001; positive for repeat scan mean difference 0.24; p < 0.001). Lung cancer distress scores for the MDT group approached clinical thresholds, with upper CIs crossing into clinically significant levels. Participants who were positive for a repeat scan also reported significantly greater T1 cancer distress than those receiving a negative (normal) result (mean difference 0.12; p < 0.001).
Outcome (T1) | Negative (N = 763),a,b mean (95% CI) | Negative with incidental finding (N = 41),a,b mean (95% CI) | Positive for repeat scan (N = 788),a,b mean (95% CI) | Positive for MDT referral (N = 48),a,b mean (95% CI) | Test statistic, p-value, effect size |
---|---|---|---|---|---|
Cancer distress (n = 1634) | 2.12 (2.10 to 2.13) | 2.15 (2.08 to 2.22) | 2.23 (2.22 to 2.25) | 2.47 (2.41 to 2.54) | F(3,1629) = 55.94, < 0.001, η2 = 0.093 |
8.32 (8.18 to 8.45) | 8.56 (7.97 to 9.19) | 9.34 (9.19 to 9.49)c | 11.88 (11.10 to 12.72)c, d, e | ||
Anxiety (n = 1630) | 1.51 (1.47 to 1.55) | 1.50 (1.32 to 1.68) | 1.56 (1.52 to 1.60) | 1.87 (1.70 to 2.04) | F(3,1625) = 5.79, 0.001, η2 = 0.011 |
3.54 (3.35 to 3.73) | 3.49 (2.75 to 4.39) | 3.76 (3.57 to 3.96) | 5.49 (4.48 to 6.67)c, d, e, f | ||
Depression (n = 1630) | 1.27 (1.23 to 1.31) | 1.20 (1.02 to 1.38) | 1.26 (1.22 to 1.30) | 1.40 (1.24 to 1.56) | F(3,1625) = 1.10, 0.35, η2 = 0.002 |
2.55 (2.41 to 2.70) | 2.31 (1.76 to 2.97) | 2.51 (2.37 to 2.66) | 3.05 (2.44 to 3.78) |
Differences in T1 general anxiety were found between groups. Those referred to MDT reported significantly greater anxiety than those receiving any other result (negative mean difference 0.36; p < 0.001; incidental finding mean difference 0.37; p = 0.022; positive for repeat scan mean difference 0.31; p = 0.003), although their scores were in the low/normal range. The difference in anxiety between the MDT referral group and the incidental finding group was not significant when the sensitivity analysis accounted for test timing issues. When participants who had completed their T1 questionnaires at more than a month after they had been sent their result were excluded (n = 174), the difference in anxiety for these groups was no longer significant (mean difference 0.28; p = 0.18). Differences in depression scores were not statistically significant for any of the screening result groups.
There was a significant association between screening result group and decision satisfaction (Table 22). A greater proportion of screening participants who were positive for MDT referral were very satisfied with their decision compared with the other three groups. Those who were positive for repeat scan had the greatest proportion not very satisfied with their decision.
Decision satisfaction (T1) | Negative (n = 702), n (%) | Negative with incidental finding (n = 39), n (%) | Positive for repeat scan (n = 705), n (%) | Positive for MDT referral (n = 42), n (%) | Test statistic (p-value) |
---|---|---|---|---|---|
Not very satisfied | 378 (54) | 22 (56) | 450 (64) | 18 (43) | χ2(3) = 18.8 (< 0.001) |
Very satisfied | 324 (46) | 17 (44) | 255 (36) | 24 (57) |
Long-term outcomes analysis
Data were available for 2855 trial participants for the long-term outcomes analysis (see Figure 15). Demographic and psychosocial characteristics of the sample at T2 were very similar to those of the sample included in T1 outcome analyses (see Table 19 and Appendix 7, Table 36).
Hypothesis 3: there will be no difference in long-term cancer distress between trial arms
Analyses of T2 cancer distress scores were split by high and low baseline scores because of differential short-term effects of trial allocation on these groups. These effects were not evident at long-term follow-up: in both T0 low- and high-scoring participants, the difference between trial arms in T2 cancer distress levels was not statistically significant (Table 23). Control group participants had significantly higher T2 anxiety and depression scores than those receiving the intervention; however, the absolute differences between trial arms were minimal and not of clinical significance. When converted to raw scores, all three measures for both trial arms were within the normal range.
Outcome (T2) | Intervention, mean (95% CI)a | Control, mean (95% CI)a | Test statistic,b p-value, effect size |
---|---|---|---|
Cancer distressc in low scorers at T0 (n = 2574) | 2.10 (2.09 to 2.11) | 2.09 (2.08 to 2.10) | F(1,2571) = 0.51, 0.48, η2 < 0.001 |
8.15 (8.05 to 8.25) | 8.10 (7.99 to 8.25) | ||
Cancer distressc in high scorers at T0 (n = 268) | 2.44 (2.39 to 2.48) | 2.46 (2.41 to 2.51) | F(1,265) = 0.43, 0.51, η2 = 0.002 |
11.43 (10.93 to 11.95) | 11.69 (11.11 to 12.30) | ||
Anxietyc (n = 2836) | 1.54 (1.51 to 1.57) | 1.61 (1.58 to 1.65) | F(1,2833) = 11.50, 0.001, η2 = 0.004 |
3.66 (3.52 to 3.80) | 4.02 (3.86 to 4.19 | ||
Depressionc (n = 2834) | 1.33 (1.30 to 1.36) | 1.39 (1.36 to 1.42) | F(1,2831) = 8.65, 0.003, η2 = 0.003 |
2.77 (2.67 to 2.89) | 3.01 (2.89 to 3.14) |
A significantly greater proportion of those in the control arm (74%, 883/1189) were not very satisfied with their decision to take part in screening compared with the intervention arm (60%, 855/1422, χ2(1) = 58.2; p < 0.001).
Hypothesis 4: there will be no difference in long-term cancer distress between screening outcome groups
The impact of screening outcome was assessed at long-term follow-up. Participants were excluded if they had no baseline scan, had a MDT referral but no lung cancer diagnosed, were awaiting their scan or results, or had results that were not classified (n = 267). Figure 16 indicates the final number of participants in each T2 screening outcome group: 23 true-positive (i.e. lung cancer diagnosed), 445 false-positive, 740 true-negative and 78 incidental screening results.
FIGURE 16.
Screening outcome groups at T2. Note: participants were categorised into screening outcome groups based on screening history and outcome of the final CT scan (where relevant) at the time of T2 questionnaire completion.

As shown in Appendix 7, Table 37, differences between T2 screening outcome groups on baseline measures were not observed.
Differences between screening outcome groups in T2 cancer distress, anxiety and depression were not statistically significant (Table 24). The raw scores of all psychosocial variables in all groups were observed to be within the normal range and not clinically relevant. Similarly, no differences were found in satisfaction with decision to take part in screening between the four groups (Table 25).
Outcome (T2) | True negative (N = 740),a,b mean (95% CI) | Incidental finding (N = 78),a,b mean (95% CI) | False positive (N = 445),a,b mean (95% CI) | True positive (N = 23),a,b mean (95% CI) | Test statistic,c p-value, effect size |
---|---|---|---|---|---|
Cancer distressd (n = 1279) | 2.11 (2.09 to 2.12) | 2.14 (2.09 to 2.19) | 2.14 (2.12 to 2.16) | 2.20 (2.10 to 2.30) | F(3,1274) = 2.88, 0.04, η2 = 0.007 |
8.22 (8.09 to 8.36) | 8.48 (8.06 to 8.93) | 8.51 (8.33 to 8.70) | 9.01 (8.16 to 9.96) | ||
Anxietyd (n = 1280) | 1.57 (1.53 to 1.62) | 1.45 (1.32 to 1.59) | 1.52 (1.47 to 1.58) | 1.37 (1.13 to 1.62) | F(3,1275) = 1.94, 0.12, η2 = 0.005 |
3.82 (3.61 to 4.03) | 3.28 (2.74 to 3.89) | 3.59 (3.34 to 3.85) | 2.94 (2.08 to 4.03) | ||
Depressiond (n = 1278) | 1.34 (1.30 to 1.39) | 1.22 (1.09 to 1.35) | 1.38 (1.33 to 1.44) | 1.26 (1.02 to 1.49) | F(3,1273) = 2.04, 0.11, η2 = 0.005 |
2.84 (2.68 to 3.00) | 2.38 (1.97 to 2.85) | 2.98 (2.78 to 3.20) | 2.52 (1.79 to 3.44) |
Decision satisfaction (T2) | True negative (N = 678),a n (%) | Incidental finding (N = 71),a n (%) | False positive (N = 408),a n (%) | True positive (N = 21),a n (%) | Test statistic (p-value) |
---|---|---|---|---|---|
Not very satisfied | 411 (61) | 39 (55) | 239 (59) | 6 (29) | χ2(3) = 9.29 (0.03) |
Very satisfied | 267 (39) | 32 (45) | 169 (41) | 15 (71) |
Secondary analysis: linear mixed-effects modelling
Impact of trial allocation
As an extension to ANCOVA, a linear mixed-effects multivariable regression model was fitted to examine the impact of trial allocation on cancer distress at both T1 and T2. The fixed effects included baseline cancer distress and trial allocation, and the random effects included a random intercept and slope. After adjustment for baseline cancer distress, the difference in logn mean distress between the intervention and control arm was 0.02 (95% CI –0.13 to 0.17; p = 0.42) (Table 26). When back-transformed to scores on the original distress scale, this equated to a relative increase of 2% for those in the intervention arm (95% CI –12% to 19%).
Effect modifiers | Logn difference in cancer distress (intervention – control) | |||
---|---|---|---|---|
Estimate | SE | p-value | 95% CI | |
Base model (baseline cancer distress and trial allocation) | 0.02 | 0.012 | 0.42 | –0.13 to 0.17 |
and gender | 0.02 | 0.011 | 0.40 | –0.13 to 0.16 |
and age groupa | 0.02 | 0.012 | 0.43 | –0.14 to 0.17 |
and smoking status | 0.01 | 0.012 | 0.46 | –0.14 to 0.17 |
and site | 0.02 | 0.011 | 0.43 | –0.14 to 0.16 |
and IMD | 0.01 | 0.012 | 0.46 | –0.14 to 0.16 |
and education groupb | 0.02 | 0.014 | 0.34 | –0.16 to 0.21 |
and ethnic group | 0.02 | 0.012 | 0.43 | –0.14 to 0.17 |
and time since recruitment centrec | 0.02 | 0.013 | 0.41 | –0.12 to 0.15 |
and result group | Not estimable | |||
and marital statusd | 0.02 | 0.013 | 0.43 | –0.14 to 0.18 |
and experience of lung cancer | 0.02 | 0.012 | 0.40 | –0.14 to 0.17 |
Univariable analysis
Low baseline cancer distress (< 12.5)
The overall pattern of cancer distress across trial allocation groups was examined, adjusting for baseline score and independently for additional effect modifiers. No statistical difference was observed between the intervention and control arms in cancer distress after adjustment for any additional effect modifier (see Table 26). For example, after adjusting for gender, the logn mean difference in cancer distress between intervention and control was 0.02 (95% CI –0.13 to 0.16; p = 0.40). This equated to a relative increase in original cancer distress scores of 2% in the intervention group (95% CI –12% to 17%) compared with the control group.
The differences in cancer distress between the category levels of each modifier are shown in Table 27. The following effect modifiers explained cancer distress (p < 0.001): age group, smoking status, trial site, socioeconomic group, marital status, gender, lung cancer experience and screening outcome group. Younger participants (≤ 65 years) were more distressed than those aged > 70 years, equating to a back-transformed increase of 6% in raw cancer distress scores for those up to age 65 years (95% CI 5% to 8%). Those aged 66–70 years were also more distressed than those aged > 70 years. Higher cancer distress scores were found in current smokers than in ex-smokers, participants who were recruited from the Liverpool area, those who were more deprived (quintiles 1–3 compared with quintile 5), those who were single or divorced (compared with married) individuals, females and those who had experience of lung cancer.
Baseline effect modifier | Logn difference in cancer distress | |||
---|---|---|---|---|
Estimate | SE | 95% CI | p-value | |
Age group, years | ||||
≤ 65 to > 70 | 0.06 | 0.008 | 0.05 to 0.08 | < 0.001 |
66–70 to > 70 | 0.04 | 0.007 | 0.02 to 0.05 | < 0.001 |
Smoking status | ||||
Ex-smoker – current smoker | –0.08 | 0.006 | –0.06 to –0.09 | < 0.001 |
Never smokeda – current smoker | 0.00 | 0.165 | 0.32 to –0.33 | 0.99 |
Site | ||||
Liverpool – Papworth | 0.07 | 0.006 | 0.06 to 0.08 | < 0.001 |
IMD | ||||
Most deprived (Q1) – most affluent (Q5) | 0.09 | 0.008 | 0.07 to 0.10 | < 0.001 |
Q2 – Q5 | 0.05 | 0.011 | 0.03 to 0.07 | < 0.001 |
Median affluent (Q3) – Q5 | 0.03 | 0.009 | 0.01 to 0.04 | 0.004 |
Q4 – Q5 | 0.02 | 0.009 | 0.00 to 0.04 | 0.029 |
Educationb | ||||
Up to GCSE – beyond GCSE | 0.01 | 0.007 | 0.00 to 0.03 | 0.05 |
Ethnic group | ||||
White – non-white | 0.01 | 0.035 | –0.06 to 0.08 | 0.79 |
Marital status | ||||
Married – single | –0.04 | 0.013 | –0.07 to –0.02 | 0.001 |
Married – living together | 0.01 | 0.013 | –0.02 to 0.04 | 0.41 |
Married – widowed | –0.03 | 0.011 | –0.05 to 0.00 | 0.03 |
Married – divorced | –0.05 | 0.010 | –0.07 to –0.03 | < 0.001 |
Gender | ||||
Female – male | 0.04 | 0.007 | 0.03 to 0.06 | < 0.001 |
Time since attended recruitment centre | ||||
3–6 months to < 3 months | 0.00 | 0.013 | –0.03 to 0.03 | 1.00 |
6–12 months to < 3 months | –0.05 | 0.016 | –0.09 to –0.01 | 0.03 |
> 12 months to < 3 months | –0.03 | 0.013 | –0.12 to 0.06 | 0.19 |
Result groupc | ||||
Negative with incidental finding – negative | 0.02 | 0.022 | –0.02 to 0.07 | 0.33 |
Positive for a repeat scan – negative | 0.09 | 0.009 | 0.07 to 0.11 | < 0.001 |
Positive for MDT referral – negative | 0.20 | 0.023 | 0.15 to 0.24 | < 0.001 |
Experience of lung cancer | ||||
Yes – no experience | 0.05 | 0.006 | 0.03 to 0.06 | < 0.001 |
As only intervention group participants received a CT scan, trial allocation group was fully nested with the result group effect modifier. Those who needed a repeat scan or MDT referral reported higher cancer distress than those who received a negative result.
High baseline cancer distress (≥ 12.5)
When the previous analyses were repeated in those with high baseline cancer distress scores, a similar pattern was observed to that found for low baseline cancer distress scorers (data not shown).
Impact of trial allocation adjusting for baseline cancer distress and other scientifically important effect modifiers
Low baseline cancer distress (< 12.5)
Having fit the scientifically justified and preconceived effect modifiers, the impact of trial allocation was not statistically significant. The logn mean difference was 0.03 (95% CI –0.14 to 0.19; p = 0.33) (Table 28, see left panel). Of the other effect modifiers included in the model, deprivation was significantly associated with distress, with those in the two most deprived groups being more distressed than those in the least deprived group (p < 0.01). Age group (p ≤ 0.01) and smoking status (p < 0.001) also influenced cancer distress, with participants up to the age of 70 years and current smokers (compared with ex-smokers) reporting higher cancer distress. Time since recruitment centre attendance was not significantly associated with distress.
Demographics | Multivariable analysis adjusted for scientifically important effect modifiers only (secondary analysis 2)a | Multivariable analysis adjusted for scientifically and statistically important effect modifiers (secondary analysis 3)b | |||||||
---|---|---|---|---|---|---|---|---|---|
Estimate | SE | 95% CI | p-value | Estimate | SE | 95% CI | p-value | ||
Allocation group (intervention – control) | 0.03 | 0.017 | –0.14 to 0.19 | 0.36 | 0.03 | 0.018 | –0.20 to 0.26 | 0.39 | |
Deprivation quintiles | |||||||||
Most deprived (Q1) – most affluent (Q5) | 0.07 | 0.010 | 0.05 to 0.09 | < 0.001 | 0.02 | 0.012 | –0.01 to 0.04 | 0.13 | |
Q2 – Q5 | 0.03 | 0.012 | 0.01 to 0.06 | 0.005 | 0.01 | 0.012 | –0.02 to 0.03 | 0.66 | |
Median deprived (Q3) – Q5 | 0.02 | 0.010 | 0.00 to 0.04 | 0.02 | 0.00 | 0.010 | –0.02 to 0.02 | 0.89 | |
Q4 – Q5 | 0.02 | 0.010 | 0.00 to 0.04 | 0.05 | 0.01 | 0.010 | –0.01 to 0.03 | 0.59 | |
Smoking status | |||||||||
Never smoked – current smoker | –0.04 | 0.158 | –0.35 to 0.27 | 0.79 | –0.03 | 0.157 | –0.34 to 0.27 | 0.83 | |
Ex-smoker – current smoker | –0.06 | 0.007 | –0.07 to –0.04 | < 0.001 | –0.06 | 0.007 | –0.08 to –0.05 | < 0.001 | |
Age group, years | |||||||||
Up to 65 to > 70 | 0.05 | 0.009 | 0.03 to 0.07 | < 0.001 | 0.05 | 0.009 | 0.03 to 0.07 | < 0.001 | |
66–70 to > 70 | 0.02 | 0.009 | 0.01 to 0.04 | 0.01 | 0.02 | 0.008 | 0.02 to 0.04 | 0.02 | |
Time since attended recruitment centre | |||||||||
3–6 months – < 3 months | –0.02 | 0.015 | –0.05 to 0.01 | 0.23 | –0.03 | 0.015 | –0.05 to 0.00 | 0.09 | |
6–12 months – < 3 months | –0.05 | 0.020 | –0.11 to –0.00 | 0.05 | –0.06 | 0.026 | –0.11 to –0.01 | 0.03 | |
> 12 months – < 3 months | –0.04 | 0.016 | –0.14 to 0.06 | 0.14 | –0.05 | 0.025 | –0.10 to 0.00 | 0.05 | |
Ln baseline cancer distress | 0.521 | 0.016 | 0.49 to 0.59 | < 0.001 | 0.555 | 0.013 | 0.53 to 0.58 | < 0.001 | |
Statistically important additional cancer distress effect modifiers | |||||||||
Site: Liverpool and Papworth | N/A | 0.06 | 0.008 | 0.04 to 0.07 | < 0.001 | ||||
Experience of lung cancer: yes – no | N/A | 0.03 | 0.007 | 0.02 to 0.05 | < 0.001 | ||||
Gender: female – male | N/A | 0.02 | 0.008 | 0.01 to 0.04 | 0.004 |
High baseline cancer distress (≥ 12.5)
The impact of trial allocation was not statistically significant in participants with high baseline cancer distress [logn(difference) –0.04, 95% CI –0.09 to 0.02; p = 0.20] (Table 29, left panel). Only ‘age group’ was found to influence cancer distress, with those aged < 70 years reporting more distress than those aged > 70 years (p < 0.001).
Demographics | Multivariable analysis adjusted for scientifically important effect modifiers only (secondary analysis 2)a | Multivariable analysis adjusted for scientifically and statistically important effect modifiers (secondary analysis 3)b | ||||||
---|---|---|---|---|---|---|---|---|
Estimate | SE | 95% CI | p-value | Estimate | SE | 95% CI | p-value | |
Allocation group (intervention – control) | –0.04 | 0.027 | –0.09 to 0.02 | 0.20 | –0.03 | 0.027 | –0.08 to 0.02 | 0.26 |
Deprivation quintiles | ||||||||
Most deprived (Q1) – most affluent (Q5) | 0.01 | 0.040 | –0.10 to 0.10 | 0.87 | –0.08 | 0.05 | –0.17 to 0.02 | 0.14 |
Q2 – Q5 | 0.00 | 0.054 | –0.10 to 0.11 | 0.95 | –0.06 | 0.058 | –0.17 to 0.06 | 0.34 |
Median deprived (Q3) – Q5 | 0.07 | 0.045 | –0.02 to 0.16 | 0.13 | 0.02 | 0.048 | –0.07 to 0.11 | 0.68 |
Q4 – Q5 | 0.06 | 0.042 | –0.02 to 0.14 | 0.16 | 0.03 | 0.043 | –0.06 to 0.11 | 0.49 |
Smoking status | ||||||||
Never smoked – current smoker | N/A | N/A | ||||||
Ex-smoker – current smoker | –0.04 | 0.027 | –0.09 to 0.02 | 0.18 | –0.04 | 0.027 | –0.09 to 0.02 | 0.17 |
Age (years) | ||||||||
≤ 65 to > 70 | 0.16 | 0.040 | 0.08 to 0.24 | < 0.001 | 0.16 | 0.041 | 0.08 to 0.24 | < 0.001 |
66–70 to > 70 | 0.18 | 0.038 | 0.10 to 0.25 | < 0.001 | 0.17 | 0.038 | 0.10 to 0.25 | < 0.001 |
Time since attended recruitment centre | ||||||||
3–6 months – < 3 months | 0.00 | 0.054 | –0.10 to 0.11 | 0.94 | –0.02 | 0.054 | –0.12 to 0.09 | 0.77 |
6–12 months – < 3 months | –0.12 | 0.066 | –0.25 to 0.01 | 0.07 | –0.11 | 0.065 | –0.24 to 0.02 | 0.08 |
> 12 months – < 3 months | –0.05 | 0.028 | –0.11 to 0.00 | 0.07 | –0.05 | 0.028 | –0.11 to 0.00 | 0.06 |
Ln baseline cancer distress | 0.669 | 0.119 | 0.43 to 0.90 | < 0.001 | 0.624 | 0.119 | 0.389 to 0.860 | < 001 |
Statistically important additional cancer distress effect modifiers | ||||||||
Site: Liverpool and Papworth | N/A | 0.09 | 0.036 | 0.02 to –0.16 | 0.02 | |||
Lung cancer experience: yes – no | N/A | 0.03 | 0.027 | –0.03 to 0.08 | 0.31 | |||
Gender: female – male | N/A | 0.03 | 0.028 | –0.02 to 0.09 | 0.22 |
Impact of trial allocation adjusting for baseline cancer distress and all other scientifically and statistically important effect modifiers
Low baseline cancer distress (< 12.5)
After fitting and adjusting for all terms in the model, the impact of trial allocation on cancer distress was not statistically significant [logn(difference) 0.03, 95% CI –0.20 to 0.26; p = 0.39]. A forward stepping model-fitting approach to nested models showed the following to be statistically important effect modifiers associated with higher cancer distress: Liverpool recruitment site (p < 0.001), female gender (p = 0.006) and having prior experience of lung cancer (p < 0.001) (see Table 28, right panel). After adjustment for the other effect modifiers, neither marital status (p = 0.24) nor ethnicity (p = 0.90) were found to exhibit a statistically significant effect on cancer distress.
Consistent with secondary analysis (2), the following scientifically justified effect modifiers were significant: age and smoking status. Current smokers reported higher distress than ex-smokers (p < 0.001) and participants aged up to 65 years reported higher distress than those aged > 70 years (p < 0.001).
Including recruitment site in the model removed the effect of deprivation quintile on cancer distress. From this, it can be surmised that the initial association between cancer distress and deprivation was likely to be confounded by recruitment site. Appendix 7 (see Table 38) shows that although half (50%) of those recruited from Liverpool were in the most deprived group and the rest distributed throughout the other four quintiles, almost half (46%) of those recruited from the Papworth site were in the least deprived group and the rest distributed throughout the other four quintiles.
High baseline cancer distress (≥ 12.5)
The same model that was found from the forward stepping process was fitted on the participants with high baseline cancer distress. There was no impact of trial allocation on cancer distress, after adjustment for the other explanatory variables (see Table 29, see right panel). However, it should be noted that the study was not powered to detect any differences in this particular subgroup, which is likely to be underpowered because of the relatively small sample size and effect size. The only significant influence on cancer distress was age group, with those aged ≤ 70 years reporting higher cancer distress than those aged > 70 years.
As with the analyses for low baseline cancer distress scorers, the inclusion of the recruitment site showed a trend (p = 0.02) towards higher cancer distress in participants from Liverpool than those at Papworth.
Discussion
The purpose of this chapter was to examine the psychosocial impact of participation in UKLS lung screening, in order to inform the implementation of a potential lung cancer screening programme. The present results should be interpreted alongside evidence of feasibility and cost-effectiveness when weighing up the benefit to harm ratio of LDCT lung screening for high-risk individuals in the UK.
Impact of trial allocation
Short-term negative consequences were observed in individuals allocated to receive LDCT screening, with higher cancer distress in the intervention arm at 2–4 weeks’ follow-up. However, this was a temporary effect that did not persist at long-term follow-up. Linear mixed-effects modelling confirmed that, despite the notional increase in cancer distress for those in the intervention arm in the short term (but not in the long term), there was little evidence of a meaningful difference between trial allocation groups once effect modifiers had been taken into account. Similarly, both the NELSON88 and PLCO87 trials found minimal long-term psychosocial effects of allocation to LDCT screening or no screening control. Although UKLS participants who were assigned to the control group reported slightly higher long-term anxiety and depression, the absolute differences were again small and not clinically significant. The latter trend is likely to reflect the control group’s disappointment and frustration at having been identified as high risk but denied the intervention, supported by the finding that a greater proportion were less satisfied with their decision to participate compared to the intervention arm. This finding mirrors the DLCST, in which control arm participants reported more negative consequences,85 perhaps perceiving a missed opportunity to gain reassurance from screening.
Overall, scores on measures of distress, anxiety and depression in the present trial were within the normal range in each arm and at each time point. For the minority of participants with pre-existing high cancer distress, short-term distress levels were high, regardless of trial allocation. These individuals could be identified for additional psychosocial support during routine lung screening. 97 Individual difference variables that adversely influenced levels of cancer distress over time, regardless of trial allocation, included female gender, younger age (< 65 years), smoking, deprivation and having prior experience of lung cancer. In addition, psychosocial outcomes were poorer in those recruited at the Liverpool site, over and above the effect of deprivation, suggesting that supportive interventions to improve the quality of care and minimise distress should be implemented alongside routine CT lung screening.
Impact of computed tomography screening results
The initial impact of trial allocation largely reflects transient adverse effects of positive screening results, which disappeared at up to 2 years’ follow-up. Within the intervention arm, there was a significant graded effect of screening result on short-term lung cancer distress. Unsurprisingly, participants who were referred to MDT because of a suspected major lung abnormality on their baseline scan reported higher cancer distress than any other screening result group, with levels close to threshold scores. Participants who required a repeat scan reported higher distress than those receiving an immediate ‘all-clear’ result. Differences in general anxiety (but not depression) were observed, with higher levels of short-term anxiety in participants who were referred to MDT. However, MDT group scores were within the normal range and they were also more at ease with their decision to take part in UKLS than any other screening outcome group. The latter finding has been reported in other screening evaluation studies, reflecting decision consolidation and the perception that further diagnostic tests have been carried out thoroughly and for personal benefit. 99
Adverse effects of screening result were not seen in the long term, when the final outcome of the screening episode was examined, although type II error cannot be discounted because of the relatively small number of participants (within the psychosocial sample) who eventually received a lung cancer diagnosis. Similarly, the absence of observed psychosocial effects in the small group of participants with incidental findings may reflect the heterogeneity of clinical diagnoses in this group. A number of participants were excluded from screening outcomes analyses, for example those referred to MDT but in whom lung cancer was not diagnosed; longer-term effects in these individuals are therefore unknown. The potential for distress caused by unfavourable or unexpected findings of CT lung screening should not be ruled out, and people who receive such results may benefit from further psychosocial support. On balance, however, the pattern of findings supports those of previous studies,87–89 which indicate long-term resolution of adverse screening effects.
Trial non-uptake
Although the overall trend towards minimal psychosocial consequences of the UKLS is encouraging, it is important to acknowledge the possibility of sample selection bias, which reduces the trial’s external validity and may mean that adverse effects were underestimated. A profile of potential risk factors for non-uptake of lung screening was revealed: high-risk individuals who were older (> 70 years of age), female, smokers, from a lower socioeconomic group or with a higher affective risk perception were less willing to participate in the trial. These findings are consistent with barriers to uptake reported in previous lung screening studies, including female gender,100 perceived threat associated with lung cancer and lung screening tests,95,96 and low perceived benefit among smokers. 92 Individuals from more affluent backgrounds may have a better understanding of the benefits of screening and face fewer barriers than those from poorer backgrounds. 101 Furthermore, these groups continued to be under-represented as the trial progressed, with greater attrition seen in women, smokers, less affluent individuals, those from the Liverpool area and those with higher baseline psychosocial scores. The same groups were also vulnerable to experiencing higher cancer distress when they did take part in the trial.
Summary and recommendations
The UKLS psychosocial evaluation indicates that short-term negative consequences of lung screening were modest and temporary. This pattern of psychosocial outcomes is likely to reassure clinicians and policy-makers who are weighing up the benefits and harms of routine lung cancer screening for high-risk groups in the UK. However, the present findings must be interpreted alongside evidence of feasibility, cost-effectiveness and reach. The psychosocial evaluation highlighted important subgroups who were under-represented in the trial and in whom lung cancer risk is known to be higher. In the case of a national lung cancer screening programme, efforts to improve uptake must include strategies for engaging women and targeting high-risk, hard-to-reach groups, including the those aged > 70 years, smokers and those from the poorest areas. This may involve targeted initiatives designed to raise awareness of lung cancer, such as the Be Clear on Cancer campaign102 and adapted screening invitation materials to prepare people for informed participation. Increasing uptake and providing support for underserved groups will be key to implementing an equitable lung cancer screening programme in the UK.
Chapter 7 Health economics
Introduction
This economic evaluation compares health technologies in terms of relative costs and relative outcomes, with a view to identifying which is superior. In general, a health technology is superior to another if it produces (1) the same or better outcomes at a lower cost; (2) better outcomes at the same cost; or (3) better outcomes at a higher cost, assuming that society believes that the enjoyment of better outcomes justifies the additional expenditure; from the economist’s perspective, it would be rational to replace an existing technology with a novel technology if the third listed item was found to meet any one of these criteria of cost-effectiveness.
The novel/existing technologies being compared here are population screening set against symptomatic presentation, in relation to the detection and subsequent treatment of lung cancers. The economic rationale for such a comparison is as follows. 103 Cancer is a progressive disease, evolving through a sequence of stages. Treatment is initiated once the disease is detected and, for any particular treatment, patient prognosis is a negative function of the stage of progression at detection. Thus, the later the stage at which the disease is diagnosed, the worse is the patient’s prognosis. The likelihood of detection from symptomatic presentation is a positive function of progression (i.e. the earlier the stage, the less likely are symptoms to present). Assuming that a testing procedure exists that can detect disease in an asymptomatic population, it follows that the administration of screening tests to such a population will identify tumours at earlier stages than would occur otherwise. Compared with unscreened patients, those with screen-detected cancers can receive earlier treatments and will achieve superior health outcomes (more years of life). However, these outcomes would be obtained only at the additional expense of both screening the population at risk and treating immediately all of those cases identified by the test results.
Demonstrating that cancer screening would be cost-effective by means of a RCT requires the estimation of (1) the net costs of screening over detection via symptomatic presentation among trial subjects; (2) net benefits, in terms of additional life expectancy on the part of screened subjects; and (3) the ratio of net benefits to net costs incurred. For acceptability, this ratio must be consistent with society’s criterion of acceptable value for money in securing health gains. The prevailing convention for acceptability in the UK’s public health-care system is £20,000–30,000 per QALY gained. 104
If informed by observation alone, the complete economic evaluation of any cancer screening trial is necessarily long term. Control subjects must be followed to identify the costs associated with the symptomatic presentation and treatment of disease corresponding with those cases detected and treated immediately as a result of screening. The necessary observation time will be the lead time of the cancer in question (i.e. by how many years screening advances diagnosis). A sufficient period of follow-up must elapse thereafter before a Kaplan–Meier-type estimation of survival gains for screened subjects compared with control subjects can be undertaken. To observe a mortality benefit, the observation period must allow for the control group tumours corresponding to screen-detected cancers to advance to a symptomatic state (lead time) and thence to death (survival time). Longer periods of follow-up improve the accuracy of such estimates and may in any case be required to ensure significance if the survival effects prove to be small. By way of example, the Nottingham colorectal cancer screening trial screened patients over 8 years and reported relative survival gains and cost-effectiveness over an average of 16 years of follow-up. 105 It was able to report differences in all-cause survival at 28 years from first recruitment. 106
UK Lung Cancer Screening evaluation model
The UKLS was very much smaller and very much briefer than the cited colorectal trial. Although the original intention had been to recruit more than 30,000 subjects and to follow them for at least 5 years,46 UKLS did not proceed beyond its pilot phase, whereby approximately 2000 subjects were screened and observed over 12–15 months. This short follow-up period precludes adopting the conventional approach to trial evaluation, namely, the measurement of long-term costs and outcomes in both the test and the control arms, and the comparison thereof. Of necessity, the observational element of the economic evaluation was restricted to those events and findings that occurred within the active trial period. Observable costs that accrued in the active period were the costs of (1) screening the target population; (2) rescreening or investigating patients with suspicious nodules according to the trial protocol;53 and (3) diagnostic work-up and treatment for the detected cancers. In that which follows, all costs are expressed in UK pounds sterling at 2011–12 prices. Where costs originally denominated in currencies other than sterling are reported, values have been converted to 2011–12 pounds sterling at purchasing power parity exchange rates.
Although the numbers and type of abnormality detected as a result of screening were also observable, the possible consequences of detection were not. Any survival benefits would accrue far beyond the current (observation) period. Being vital to the evaluation, these benefits were therefore modelled on the basis of observational data from other contexts. We presumed that the survival benefits of screening would be confined to those patients in whom cancers had been detected, and that such benefits would constitute the only source of health gain in the screening programme. For each of the cancers detected, the patient’s health gain would equal the life-years to be expected following screen detection and treatment less the life-years that the patient might expect as a result of treatment following eventual symptomatic presentation, assuming the patient had survived that long. In addition to future benefits, we modelled the future costs of the diagnostic work-up and treatment of the same cancers which would present eventually, had they not been screen detected. These were to be offset against the current period costs of the screening programme.
The UKLS recorded incidental findings, principally cases of pneumonia, bronchiectasis, interstitial lung disease and severe emphysema. Such findings are regularly reported in CT screening studies. 107 As the collection of cost and outcome data for individual non-cancer cases lay outside the trial protocol, the consequences of incidental findings have not been included in the UKLS economic evaluation. We note that only the effect of including costs and consequences of incidental findings would be indeterminate. Although it is probable that incidental findings would generate additional work-up and imaging costs (studies in both Canada108 and Italy109 have reported costs equivalent to around £9 per participant), it is equally likely that the earlier diagnosis of these non-cancer conditions could potentially generate compensating outcome gains.
Current costs
Costs were estimated from the NHS perspective. Cost-incurring events associated with the initial screens, and with ensuing work-up consequent upon the findings, were observed within the trial period. As per protocol, the number and frequency of scanning events depended upon test results, and these were as follows. (The figures presented here are those from an interim analysis, in November 2013; there are, therefore, small differences between these figures and the more recent ones reported in Chapter 5.) A total of 979 CT scans revealed no nodules of concern (category 1) and these subjects thereafter exited the trial; 479 CT scans revealed category 2 nodules, which required repeat scans at 12 months; 472 CT scans revealed category 3 nodules, which required repeat scans at 3 months – of these subjects, 19 required an additional scan at around 6 months; 398 of these subjects were rescanned at 12 months; and, finally, 64 CT scans yielded results of concern that was sufficient for subjects to be referred directly to the MDT (category 4). It follows that the execution of the UKLS protocol necessitated 3363 CT scans in total. The vast majority of these were, of course, negative for lung cancer, either at the initial scan or at subsequent scans, which served to eliminate false positives nominally detected in the earlier scans.
As a result of the CT findings, a total of 114 cases were referred to the MDT for work-up (i.e. further investigation and management decisions). Between them, the MDTs thereafter initiated 122 further CT scans with contrast, 20 guided needle biopsies, 50 positron emission tomography scans and four endobronchial ultrasound biopsies. With respect to treatment of the 42 cancers detected, we recorded 35 cases of surgery (primarily lobectomy and video-assisted thoracoscopic surgery) either alone or in combination with radiotherapy (n = 5) and/or chemotherapy (n = 11). Four patients had surgical biopsies or resections for benign disease and two patients were referred for palliative care.
The gross cost of events within the trial was estimated by combining the resource-use audit above with unit costs of each event type. For most events, the unit costs of procedures were 2011–12 National Health Service tariffs or reference costs110 as classified according to the appropriate Healthcare Resource Group coding. These unit costs derive from the financial returns from all relevant providers. Table 30 lists the unit costs of the events noted above, as a mean for the health-care system and as the extremes of the interquartile range across producers. As costs that are specific to individual types of surgery are unavailable, all surgery cases were considered as ‘complex thoracic procedures, with critical care as necessary’. Likewise, the surgical biopsies were classified as ‘major thoracic procedures’. Three procedures that played only a minor role in the UKLS event audit had no assigned Healthcare Resource Group per se (see Table 30). For these, we used updated unit costs that had been constructed by earlier researchers contributing to practice guidelines. 111
Procedure | HRG code | Mean unit cost (£) | Lower quartile (£) | Upper quartile (£) |
---|---|---|---|---|
CT scan (single area, no contrast) | RA08A | 84 | 63 | 99 |
MDT | CMDT_Oth | 104 | 48 | 118 |
CT scan with contrast (up to three areas) | RA13Z | 135 | 103 | 160 |
Guided needle biopsy | DZ03B | 863 | 530 | 1119 |
Bronchoscopy | DZ07 | 607 | 402 | 691 |
PET presurgery | RA39Z | 425 | 295 | 525 |
Surgery | DZ02 | 7502 | 6178 | 8338 |
Surgery: biopsy | DZ03B | 4295 | 1651 | 5853 |
Palliative care | SD01A | 340 | 159 | 459 |
Radiotherapy | 3039 | |||
Chemotherapy | } See text | 3883 | ||
EBUS | 1461 |
The mean gross cost of the UKLS protocol, as implemented, equals ΣCiNi across all events, where Ci = unit cost of event i, and Ni = number of occurrences of event i. Given UKLS events and unit costs, the mean gross current cost amounted to £687,617. This total comprised: (1) £282,490 for CT scans; (2) £72,592 for work-up via the MDTs; and (3) £332,534 for treatments of the detected cancers. To construct CIs, we assumed normality in all unit costs and converted the interquartile ranges for the eight principal event types (see Table 30) into SDs. We then re-estimated the gross cost calculation by simulation, using distributions governed by SDs about the mean unit costs [Palisade @RISK (version 4.5; Palisade Corporation, New York) with 20,000 iterations]. The simulation produced a 95% CI of £479,173 to £899,794.
Invitation and selection
Our calculations thus far have excluded the costs of selecting and inviting screening subjects. Obviously, subjects were invited to the UKLS, but the sequential invitation procedure was devised to target and recruit particular participants to a clinical trial, and to obtain both clinical and sociodemographic data. An invitation protocol addressing the needs of research does not provide a template for a pure screening protocol; including the actual recruitment costs for UKLS would therefore prove misleading when judging the likely cost-effectiveness of a screening programme. Were CT screening for lung cancer to be implemented in the UK, it seems probable that it would be organised in a fashion similar to other national cancer screening programmes (i.e. via a centralised, computerised call system). We constructed, accordingly, a hypothetical recruitment process to represent the invitation of the UKLS subjects who were actually screened.
We took our lead from UK colorectal screening, for which the current cost of inviting subjects to flexible sigmoidoscopy screening has been estimated at around £6 per invitation. 112 A programme of CT colonography in the Netherlands has been the subject of a very detailed audit,113 and the combined costs of invitation, reminders and distribution of results convert to a similar figure, around £5.50 per person invited. An additional requirement for lung cancer would be the restriction of potential subjects to a defined high-risk category, as cost-effectiveness is positively associated with disease prevalence. 52 Risk profiling following individual person-to-person contact is likely to prove expensive, but the presence of many propriety risk calculators freely available via the internet suggests the possibility of developing a cheaper alternative to judge eligibility. In our model we assumed that pure invitation and selection costs would have amounted to £10 per person invited. We assumed that, of those invited and selected, only 30% would agree thereafter to be screened. In other words, nearly 7000 individuals would have to be approached in an actual programme to achieve the numbers screened in the trial. This proportion is similar to that of those expressing an interest in screening following receipt of the initial UKLS questionnaire. It should be noted, however, that real-world cancer screening typically achieves a higher uptake rate when implemented as a formal programme. The uptake rate for the initial phases of the national colorectal screening programme, for example, was of the order of 50%. 114 Factoring these invitation/selection costs into the total caused gross current costs to increase by £67,260, or 10%, to £754,877 (95% CI £544,824 to £966,304).
Future benefits
To predict life-year gains consequent upon screen detection, we adapted an existing survival model based on life tables. 52 In brief, the model functions as follows. Life tables display general population mortality rates and survival rates, calculated for all causes of death. The mortality rate increases with age and life table data can be displayed as a survival curve, which maps the expected number of individuals, from a starting cohort, who survive at any given age. To estimate an individual’s life expectancy at any age, T, we calculate the number of ‘years alive’ in the cohort at every age. Summing these ‘years alive’ from T to the oldest possible age equals the total number of years lived by individuals from age T. In effect, this is the area under the survival curve. The expectation of a single life at age T is obtained by dividing the total number of years lived by the number alive at age T.
On to the survival curve for the general population we superimposed two cancer-related survival curves. First, a cohort, the members of which are destined to develop lung cancer, and will, prior to presentation, decline in numbers at the same rate as in the general population cohort. Following symptomatic presentation, the majority will die within a few years following treatment, whereas the mortality rates for survivors will revert to those of the general cohort. Second, screening an identical cohort would lead to the treatment of the cancers at an earlier age. Unless earlier intervention entails no mortality risk, the relative decline in numbers in this cohort will be initially greater than the relative decline of those in either a general population or a presymptomatic cancer cohort. However, assuming earlier detection offers improved longer-term survival; the relative decline will be lower than for the symptomatic cohort at higher ages. Each of these cancer-related survival curves yields a life expectancy at age T, and difference between the two expectancies is the gain from screening.
Modelling survival in a population screened at a particular age, TCT, therefore requires the specification of parameters. First, we require cancer-specific mortality rates following screen detection in order to modify population mortality rates. The observed pattern of initially high mortality following diagnosis, quickly tapering off, suggests a negative exponential formulation. Mortality rate from age TCT equals A × (T – TCT)–B, where (T–TCT) = 1, 2, 3 . . . and A and B are parameters. The specified rates are applied at ages beyond T, up to the age at which the population mortality rate exceeds the specified rate; thereafter, the rate following detection defaults to the population rate. Second, we require an estimate of lead time (TCT – TS) (i.e. the years elapsing between a cancer being screen-detected at age TCT and the same cancer, assuming it had been undetected, presenting symptomatically at age TS). Third, we require cancer-specific mortality rates following symptomatic detection in order to modify population mortality rates at ages beyond TS. We utilise an equivalent formulation for mortality rate adjustment from age TS [i.e. C × (T – TS)–D, where (T – TS) = 1, 2, 3 . . . and C and D are parameters].
Age-, gender- and stage-specific survival
The original, general, model52 failed to distinguish different cancer stages or different ages at screen detection. The detailed findings of UKLS, however, permit a more rigorous specification and enable us to simulate screen-detecting each of those cancers that are actually detected. We solved the survival model for each cancer individually, using life tables specific to the patient’s gender and his/her age at screening, assuming post-detection survival rates specific to the cancer stage at detection. As noted above, this required the assumption of lead times and two sets of mortality rate adjustments (screen detection vs. symptomatic presentation) for each individual cancer detected.
The UKLS detected 10 cancers among women and 32 among men, at ages ranging from 56 to 77 years. The numbers by stage were 28, 8, 3 and 3 for stages 1–4, respectively. Survival rates by stage and gender were assigned by assumption, informed by the literature, as follows.
The data available on which to build our survival assumptions were limited, because only the earliest of the clinical studies have accumulated sufficient follow-up time. With respect to post-treatment survival of screen-detected cancers, the principal source remains the US ELCAP, which reported 92% 10-year survival for patients in which operable stage 1 tumours were identified, and 80% survival for all cancers detected and treated. 115 A Japanese programme116 reported 90% 5-year survival for all screen-detected cancers, with 97% for those detected at stage 1. An earlier mobile programme in Japan117 reported 10-year survival at 88% for stage 1 and 50% for cancers at more advanced stages. Survival projections from ongoing trials have suggested 90% 2-year survival for cancers detected at early stages (stages 1 and 2) and 47% for cancers at advanced stages. 118 Although not trial based, audit studies from Alabama, USA,119 and from Taipei, Taiwan,120 indicated 5-year survival of > 70% for those treated for stage 1 cancers. As screening trials tend to focus on detecting early-stage disease, relatively little attention has been paid to the impact of screening on the survival of late-stage cancers. A recent review121 suggested that survival following treatable stage 3 cancer may be similar to that of early-stage disease and, with appropriate therapy, incurable stage 3 cancer could produce up to 23% 5-year survival. Although there is evidence that early chemotherapy improves survival for stage 4 cancers, the expected life-year gains are modest. 122,123
We used recent stage distributions and survival estimates for the UK124,125 as the basis for modelling post-presentation survival of the trial’s cancers, assuming that they had not been screen detected. We assumed that, were the patient to survive, a cancer which had been screen detected at stage S would have eventually presented at stage S or later (i.e. cancers would possibly progress but would never regress). For example, a stage 1 cancer could eventually present at any of the four stages according to the UK distribution, but a stage 3 cancer could present only at stages 3 or 4. Table 31 displays the survival rates assumed, based on the literature cited, and the implied values for the parameters for the life table modifications described above. It should be noted that survival rates for women in the absence of screening are superior to those of men, although the absence of gender-specific data required us to assume the same screen detection survival rates for both genders.
Stage at diagnosis | Males | Females | ||||||
---|---|---|---|---|---|---|---|---|
Survival, % | Parameters | Survival, % | Parameters | |||||
1 year | 5 year | A | B | 1 year | 5 year | C | D | |
Symptomatic presentation | ||||||||
Stage 1 or later | 31 | 10 | 0.69 | 0.9 | 35 | 13 | 0.65 | 0.95 |
Stage 2 or later | 25 | 8 | 0.75 | 1 | 28 | 10 | 0.72 | 1 |
Stage 3 or later | 22 | 6 | 0.78 | 0.9 | 25 | 8 | 0.75 | 1 |
Screen detection | ||||||||
Stage 1 or later | 90 | 70 | 0.1 | 0.4 | 90 | 70 | 0.1 | 0.4 |
Stage 2 or later | 75 | 40 | 0.25 | 0.47 | 75 | 40 | 0.25 | 0.47 |
Stage 3 or later | 55 | 22 | 0.45 | 0.7 | 55 | 22 | 0.45 | 0.7 |
Lead time
The final assumption required for outcome estimation is the lead time of screening. Although some modellers have presumed that lung cancer develops sufficiently rapidly to produce lead times of around 2 years,126–128 those actually engaged in screening have been more circumspect. Development times of 6 and 8 years between stages 1 (if screen detected) and four (if presenting) have been suggested for Japan129 and ELCAP,130 respectively, and these figures set the upper limits on lead times. A Danish CT trial has posted lead times of 4–5 years for early-stage cancers. 44 Analysis of the US NLST data suggests that the majority of, but by no means all, cancers would present within 5 years. 131
A possible approach to estimating lead time in the case of UKLS subjects would be to compare the mean subject ages at detection of the cancers by stage and the ages of symptomatic presentation currently observed in the UK. 124 The integer age differences amount to 3 years, 2 years and 1 year, for stages 1–3, respectively. In other words, the stage 1 cancers in UKLS were detected, on average, at an age 3 years lower than cancers of stage 1 or later, which present symptomatically. Although it is reasonable to expect lead times to fall by stage at detection, shorter lead times per se raise expected gains from screening in the survival model. To err on the side of caution, therefore, we assumed, first, the detection of stage 4 cancers would secure no survival advantage: stage 4 lead times, as it were, would correspond to the modest gains in earlier initiation of treatment. Second, lead times for stages 1–3 would be 6 years, 4 years and 2 years, respectively. Doubling our previous estimate ensured consistency with the more conservative published opinions. We note, in passing, that incorporating lead time in a life table model corrects for overdiagnosis, as it allows subjects to die of other causes before lead time elapses.
As the survival gain for each individual cancer depends on stage, age and gender, each gain accrues at a different time. For example, the model calculates that a 70-year-old male with stage 1 cancer would have died at 78.1 years in the absence of screening but would, as a result of early detection, gain 4.1 further years of life beyond that age. A 70-year-old male with stage 3 cancer would gain an extra 0.6 years of life beyond the age at which he would have died without screening, in 4.3 years’ time. To enable summation of survival gains accruing at different times we discounted future life-year gains to present values at 3.5% annually.
Predicted outcomes
The model predicted total life-year gains of 137.2 (discounted 89.4) from detecting and treating the 42 cancers. This translated to an average gain of 3.3 (95% CI 2.6 to 3.9) life-years per cancer, undiscounted, and 2.1 (95% CI 1.7 to 2.5) life-years, discounted. The average gain per person screened was 0.07 (discounted 0.05) life-years [i.e. 25.1 (16.4) life-days]. Most of the total life-year gains from screening (86% discounted) accrued as a result of the early detection and treatment of stage 1 cancers. It follows that model outcomes are especially sensitive to the parameters that are associated with stage 1 survival. Were we to assume a 7-year lead time for stage 1 cancers instead of a 6-year lead time, total gains would be 111.8 (discounted 73.3) life-years and the average gain per cancer would be 2.7 (95% CI 2.1 to 3.2) life-years per cancer, undiscounted, and 1.7 (95% CI 1.4 to 2.1) life-years, discounted. The assumption of a 5-year lead time increases total gains to 151.8 (discounted 98.7) life-years, and average gains to 3.6 (95% CI 2.8 to 4.4) life-years per cancer, undiscounted, and 2.4 (95% CI 1.9 to 2.8) life-years, discounted.
These predicted survival gains appear similar to that of a US state-transition simulation model,132 which used national epidemiological and clinical data. 133 The estimated survival gain from a single screen was 0.01–0.04 life-years per person screened, depending upon the assumptions made. 134 A more recent synthesis of five independent US models, again founded on the most recent clinical evidence, yielded predicted gains of between 0.02 and 0.09 life-years per person screened, depending on assumptions relating to screening frequency and subjects’ smoking history. 135
Future costs avoided
The eventual presentation of the UKLS cancers, had they not been detected and treated by screening, would have entailed costs of investigation and treatment following symptomatic presentation. The act of screening allowed these costs to be avoided, and it is appropriate to offset any such cost savings against the current screening-related costs of detection and treatment. We assumed that the treatment patterns following presentation of the potential cancers would have followed current practice. According to the National Lung Cancer Audit for 2012,136 20% of all lung cancers presented symptomatically at stages 1 and 2, with the remainder at later stages. Of those presenting, 95% were managed through a MDT and received work-up. Thereafter, 15% of patients were treated surgically, 30% received radiotherapy and 57% received chemotherapy, although many patients actually received no treatment with curative intent. 136
Gross costs of management in this scenario were calculated using the same unit costs (see Table 30). We assumed that the work-up for cancers presenting symptomatically would be as for the screen-detected cancers, although positron emission tomography-CT scans would be ordered only for those undergoing surgery. Following the assumed lead times, the costs of the early- and late-stage cancer presenting symptomatically would be incurred 6 years and 2 years in the future, respectively. From the life tables, we calculated that 12% (4%) of patients with early- (late-) stage cancers would have died before presentation and treatment, thereby obviating the need for any expenditure on management. The gross cost avoided (i.e. the cost of managing the cancers, which would have presented symptomatically) amounted to £213,658. This is 28% of the costs of management following screen detection and is equivalent, with discounting, to £189,379 in current values (95% CI £152,740 to £230,643).
Cost-effectiveness
The ICER of screen detection, based on UKLS and relative to no screening, is the additional costs per expected life-year gained. The mean net current cost of screen detection is the mean gross current cost (£754,877) minus the mean discounted costs avoided (£189,379), which amounts to £565,498 (95% CI £361,102 to £767,672). Given a predicted gain in discounted life-years of 89.4, the mean ICER amounts to £6325 per life-year gained. CIs were constructed by simulation, using the estimated cost distribution for the ICER’s numerator. Given the uncertainty over lead time, outcomes were assumed to vary uniformly between 73.0 and 98.7 life-years, the outcomes estimated assuming either 7- or 5-year lead times, respectively. The simulation produced 95% CIs for the ICER of £4109 to £9430.
Quality-adjusted life-years
Although the HRQL of UKLS subjects was measured using the European Quality of Life-5 Dimensions instrument prior to screening, an equivalent assessment post screening was precluded by the time constraint. In order to translate life-year gains into the more commonly quoted QALY gains, we followed the practice of other recent economic evaluations (see below). Most of the gains from a screening programme accrue to those treated successfully for early-stage cancers, with the result that the HRQL pre and post screening must be essentially similar to population norms. For the UK, HRQL norms for the genders/ages at which simulated deaths from cancer would have occurred lie in the range 0.71–0.78 relative to perfect health. 137 Adjusting life-year gains for each cancer detected by the HRQL coefficient for the patient’s expected age at death transforms the predicted total gain of 89.4 life-years into 66.8 QALYs, or 0.03 QALYs per person screened. The mean discounted gain per cancer detected was 1.6 QALYs. At 2.2 QALYs, expected mean gains for stage 1 cancers were considerably higher than QALY gains from detecting stage 2 cancers (0.6) and cancers at more advanced stages (0.2). With outcomes defined in terms of QALYs as opposed to life-years, the ICER equalled £8466 per QALY gained (95% CI £5516 to £12,634).
A modified protocol
The vast majority of cancers detected during the UKLS came via the category 3 or category 4 nodule management routes. Around 24% of trial subjects followed the category 2 route after their initial CT scan, yet that route yielded only one cancer. The question arises whether or not including the category 2 classification was, in itself, cost-effective. Our calculations thus far enable us to infer what would have happened had (1) only category 3 and 4 subjects proceeded to further investigation and treatment, and (2) both category 1 and 2 subjects immediately exited the trial. Under this revised scenario, the UKLS protocol would have been less costly, at the expense of one cancer remaining undetected.
Not rescreening the subjects classified as category 2, as the original protocol had required, would have saved £49,816 in CT scan costs. One fewer cancer detected would have avoided a MDT-directed work-up and treatment. Pro rata savings would amount to £1728 for work-up and £7917 for treatment. The single cancer would have presented symptomatically, at an expected future cost of £4508, discounted. Therefore, the net savings from excluding the category 2 arm would have been £54,953, making the net cost of the modified protocol £510,545. We make the conservative assumption that the cancer not detected would have been at stage 1. We have already estimated the expected gain from detecting a stage 1 cancer at 2.2 QALYs, discounted, making the outcome of the modified protocol 64.6 QALYs. It follows that the ICER of the modified protocol would be £7903 per QALY gained.
This revised ICER is marginally lower than the original baseline value, indicating that, as a result of the change in protocol, cost savings outweigh the reduction in outcome. The significance of this is best understood in reverse, by taking the modified protocol, rather than the original, as the reference case. The move from modified (no repeat CT scans for category 2 nodules) to original would entail the addition of £54,953 in costs for an outcome gain of 2.2 QALYs, implying an ICER of £24,978.
Comparison with other estimates
Until around 10 years ago, cost-effectiveness evaluations of CT screening consisted of technically sophisticated mathematical models, usually of the Markov stage transitions type. These models were based almost entirely on unsubstantiated assumptions, as was perhaps inevitable, given the paucity of clinical evidence at the time. The models were typically opaque, thereby frustrating attempts to discern reasons for differences in performance. It is probable, however, that differing assumptions (e.g. about sojourn time, lead time, interstage transition probabilities, the effect of screening on a subject’s cigarette smoking behaviour and the probabilities of harm owing to repeated screening) accounted for the unhelpfully wide range of ICERs predicted. 138
The publication of experimental data has enabled recent modellers to build on more robust foundations, yet the range of reported ICERs remains substantial. Consider just three examples. First, a US simulation;139 based on the state-transition model informed by national epidemiological and Mayo Clinic CT trial data132 estimated QALY gains from a single screen at 0.01–0.02 per person, producing an ICER in excess of £106,000 per QALY. The ICER for the annual screening of ever-smokers was at least £85,000 per QALY gained. Second, a US stage-shift model,140 using ELCAP protocols and outcomes data, Medicare tariffs and national survival rates by stage, produced baseline ICER estimates in the range £7900–17,560 (close to the UKLS estimate cited above). The model was re-estimated for annual screening and yielded baseline estimates of £19,060 or £31,800, depending on whether the model’s cancer stage predictions derived from the ELCAP- or the NLST-reported stage shift. 141 Finally, a study in Israel128 reported QALY gains of around 0.06 per person screened, along with an ICER of only £1005 per QALY gained.
Incremental cost-effectiveness ratios calculated from real data tend to be more transparent than those derived from mathematical models. Transparency facilitates comparison, and comparison reveals several likely explanations for variability in cost-effectiveness. To begin with, unit costs differ significantly by health-care system. Differences in unit cost enable the corresponding ICERs to differ, even when the same quantity of resources is being used to produce the same outcomes. Unit costs are substantially higher in the USA than they are in the UK and, for that matter, in virtually any other country. By way of example, our UKLS model included full investigation and surgical costs at < £10,000 per cancer (see Table 30), yet the US models included a cost of around £19,000 in the month of surgery142 or around £55,000 for diagnosing and treating localised cancer. 141 Although smaller than US costs, UK unit costs were significantly higher than those reported in the Israel study. For example, Table 30 costs for bronchoscopy and for lobectomy were, respectively, four and three times greater than their counterparts in Israel. Other things remaining equal, interventions appear the least (most) cost-effective in countries where the unit costs are highest (lowest).
The cost-effectiveness of screening is a function of disease prevalence. With a higher prevalence in the target population, more cases will be detected and more health gains will accrue for the same cost of screening. 52 In screening programmes and models, prevalence impacts in two ways. First, prevalence will be progressively raised by the gradual restriction of targets to individuals with higher and higher risk statuses. All things remaining equal, therefore, any programme involving a more stringent risk criterion for selection will record a greater yield of significant findings per person screened, and a lower ICER, than one with less strict criteria. Beyond the initial screen, costs and outcomes of screening depend on the positive predictive value of subject selection, and the different risk models used to identify targets in different studies have been shown to possess different positive predictive values. 143 Furthermore, it has been shown18 that a more accurate targeting of subjects in the NLST could have reduced considerably the number needed to be screened to prevent a cancer death, suggesting that the trial’s protocol was less efficient (and, implicitly, less cost-effective) than it might otherwise have been.
Second, the UKLS engaged a single screen, although other programmes, and their evaluations, have involved multiple screens. Unless disease incidence is especially high, the prevalence of disease in a population falls between the initial screen and any rescreen. A succession of screens will progressively increase total yield, although the increase at the margin would be expected to be lower than the increase in the costs of additional screening. This proposition can be illustrated by a simple simulation. In one of the US models,141 the proportion of positive results on rescreening was judged to be one-third of positives detected at the initial screen. Were we to assume similarly that a rescreen of UKLS subjects using the UKLS protocol would yield one-third of the outcomes detected in the actual screen, cancer management costs would be correspondingly lower, but screening costs would be the same as for the first screen. The ICER for the rescreen would be, at £19,096 per QALY gained, more than double the ICER for the single screen. Were the yield to be one-quarter of the original outcomes, the ICER would be even higher, at £24,173.
The USA’s long-running NLST has recently published an estimate of US$81,000 per QALY as its mean ICER. 144 This figure is nearly seven times greater than the corresponding estimate for the UKLS. Fortunately, the transparency of reporting makes cost-effectiveness comparisons straightforward, and Table 32 lists the principal similarities and differences between the two trials’ economic calculations. As is evident, all of the factors noted earlier (local unit costs, intensity of resource use, disease prevalence in the target population, multiple screens) contribute to an explanation of the difference in the ICERs.
Summary
-
Owing to the brief duration of the UKLS, observations pertinent to economic evaluation were limited to cost-incurring events associated with screening and the initial management of screen-detected cancers. Expected outcomes of the cancers detected were simulated on the basis of life tables and published survival data from other studies.
-
The baseline estimate for the ICER of once-only CT screening, under the UKLS protocol and with conservative assumptions, was £8466 per QALY gained (95% CI £5516 to £12,634). Health-economic analysis suggests that the intervention could be cost-effective.
-
A fully powered trial in the UK would provide these data but may now not be necessary following publication of the NLST and imminent results from NELSON. The key to establishing a cost-effective intervention is to design programmes that maximise efficiency, along the lines of methods used in UKLS.
-
The ICER estimated for UKLS is broadly consistent with the ICERs of other recent studies, once allowance is made for differences in efficiency between screening protocols. In this context, screening can be deemed to be more efficient if (1) costs are lower for given outcomes; (2) true-positive results among the target population are more probable; or (3) the additional outcomes of rescreening outweigh the additional costs incurred. Other things remaining equal, therefore, ICERs will be higher in programmes in which (1) unit costs of detection and management are higher; (2) lower-risk subjects are invited to be screened; and (3) screens are repeated at frequent intervals.
Number | NLST | UKLS | Inference |
---|---|---|---|
1 | Yield over three screening rounds = 2.0% of persons screened | Yield from a single prevalence screen = 2.1% of persons screened | UKLS had similar yield over fewer screening rounds |
2 | Screening and work-up costs per person screened = US$1965 | Screening and work-up costs per person screened = £212 (US$303 at current exchange rates) | Significantly higher detection costs in NLST (more intensive use of more costly resources) |
3 | Net treatment costs per person (screen-detected vs. no screening) = US$175 | Net treatment costs per person (screen-detected vs. no screening) = £60 (US$86) | Higher cost of treatment in NLST |
4 | Costs of patient time and travel to appointments included in total cost (evaluation adopts a social perspective, as recommended by the US Panel on Cost-Effectiveness in Health and Medicine) | Costs of patient time and travel to appointments not included in total costs (evaluation adopts a NHS perspective, as recommended by NICE) | Inclusion of patient costs makes NLST screening and management appear to be more expensive (and less cost-effective) |
5 | Outcome calculations based on US life table survival estimates | Outcome calculations based on UK life table survival estimates | Similar methods for outcome estimation were used |
6 | QALYs gained per person screened = 0.02 overall (0.03 in the 60–69 years age range) | QALYs gained per person screened = 0.03 | Expected gains per person screened were essentially similar |
7 | Mean ICER = US$81,000 overall, but far lower (US$52,000 and US$32,000) for the two highest-risk quintiles | Mean ICER = £8466 (US$12,106) | Screening lower-risk individuals inflated NLST’s ICER |
Chapter 8 Conclusions
Overview of the main findings from the UK Lung Cancer Screening trial
The pilot UKLS has been successfully completed with 4055 individuals randomised into either a CT screen arm or a non-screen arm, utilising the Wald Single Screen Design. 53 The specific successes include the demonstration that a population-based lung cancer screening trial can be undertaken in the UK, using a risk-based prediction model to select participants. The UKLS protocol-driven radiology, utilising volumetric analysis, and the UKLS care pathways have ensured the highest level of CT imaging assessment and management of participants as they progressed through the trial. The most significant clinical finding was the 1.7% prevalence of lung cancers at baseline, and that 75% of these were stage 1, thus considered to have a good 5-year clinical outcome. Almost half of the subjects with confirmed lung cancer were in the most deprived socioeconomic quintile. The baseline prevalence rate is considerably higher than that reported by the NLST35 or NELSON37 trials.
The harms and benefits were assessed through a detailed analysis of the psychosocial questionnaires and demonstrated that even though participation in the UKLS did cause some distress and anxiety in some of the participants, this was not found to be clinically significant. The cost-effectiveness of the UKLS was modelled on currently available data, and was shown to be beneath the National Institute for Health and Care Excellence (NICE) threshold for funding new technologies; the ICER of screen detection was £8466 per QALY gained (95% CI £5516 to £12,634) and could be considered to offer value for money within a NHS screening programme. To date, no cost-effectiveness data have been published for any of the international CT screening trials, thus we await the analysis of the pooled European screening trials data.
The UKLS web-based database was central to the organisational success of the trial, enabling continuous monitoring of the participants as they moved through the different stages of the process. Linkage of the UKLS database to the Siemens LungCARE volumetric analysis software provided an excellent methodology for following suspicious CT-detected nodules through the repeat scans. Indeed, linkage of the various questionnaires, as well as all of the participant letters, radiology and pathology reports within the database, provided a model management system for such screening trials.
The major limitation of the pilot UKLS is that it is not in a position to provide useful mortality data at this time; however, it is planned to pool the UKLS data with the largest of the European screening trials, the NELSON,37 and others in 2016. At this point, the mortality data will be released for both arms of the UKLS, and combined with the European data sets, to provide both mortality and cost-effectiveness data for Europe.
Implications of UK Lung Cancer Screening trial for lung cancer screening implementation
The UKLS pilot has provided, or will be able to provide, new information about the implementation of a UK lung cancer screening programme in the following key areas: (1) improving the diagnostic work-up and management of lung nodules; (2) determining the frequency of screening and its duration; and (3) appropriately selecting high-risk individuals.
Work-up of computed tomography-detected nodules
The UKLS incorporated nodule volumetric software as part of its protocol; this provides a more accurate and reliable measurement of lung nodule size and growth than diameter measurements. Despite this, however, a major implication of the findings from the UKLS is the need to lessen the burden of diagnostic work-up of indeterminate nodules. There were a total of 536 subjects (i.e. categories 3 and 4), with nodules requiring a repeat scan. Of the 64 category 4 nodules, 41 were found to have lung cancer. On examining the number of UKLS participants referred to the MDT clinic, the false-positive rate was 3.6% (114 – 42/1994 = 3.6), whereas the interval imaging rate for the category 3 nodules was 23.2% (472 – 9/1994).
As this work was undertaken within the context of a trial, we erred on the side of ‘over-reporting’ by incorporating category 2 nodules in the care pathway, and this has provided valuable data. However, in a UK national screening programme, one would consider a higher cut-off point for the classification of indeterminate nodules, most likely 100 mm3. The nodule management algorithms are likely to adopt a similar approach to that recently recommended by the British Thoracic Society for incidental detected nodules, although there may be a slight difference for a screening population. 145 Work-up of screen positives was meticulous, and was delivered according to strict protocol. However, sensitivity of the screen can be reliably estimated only on follow-up of screen negatives for subsequent cancer. This will be the subject of future research in the UKLS cohort.
Figure 17 shows an example of a possible pathway for management of nodules detected by LDCT in lung cancer screening; it is based on the successful NELSON37 and UKLS model, with modifications from more recent publications on size threshold and risk of malignancy.
FIGURE 17.
Pathway for management of indeterminate and positive findings detected by LDCT. GGN, ground glass nodules; PET, positron emission tomography. Notes: Volume measurements should be used in preference to diameter. Part solid module has both ground glass and solid components. GGNs have no solid component.
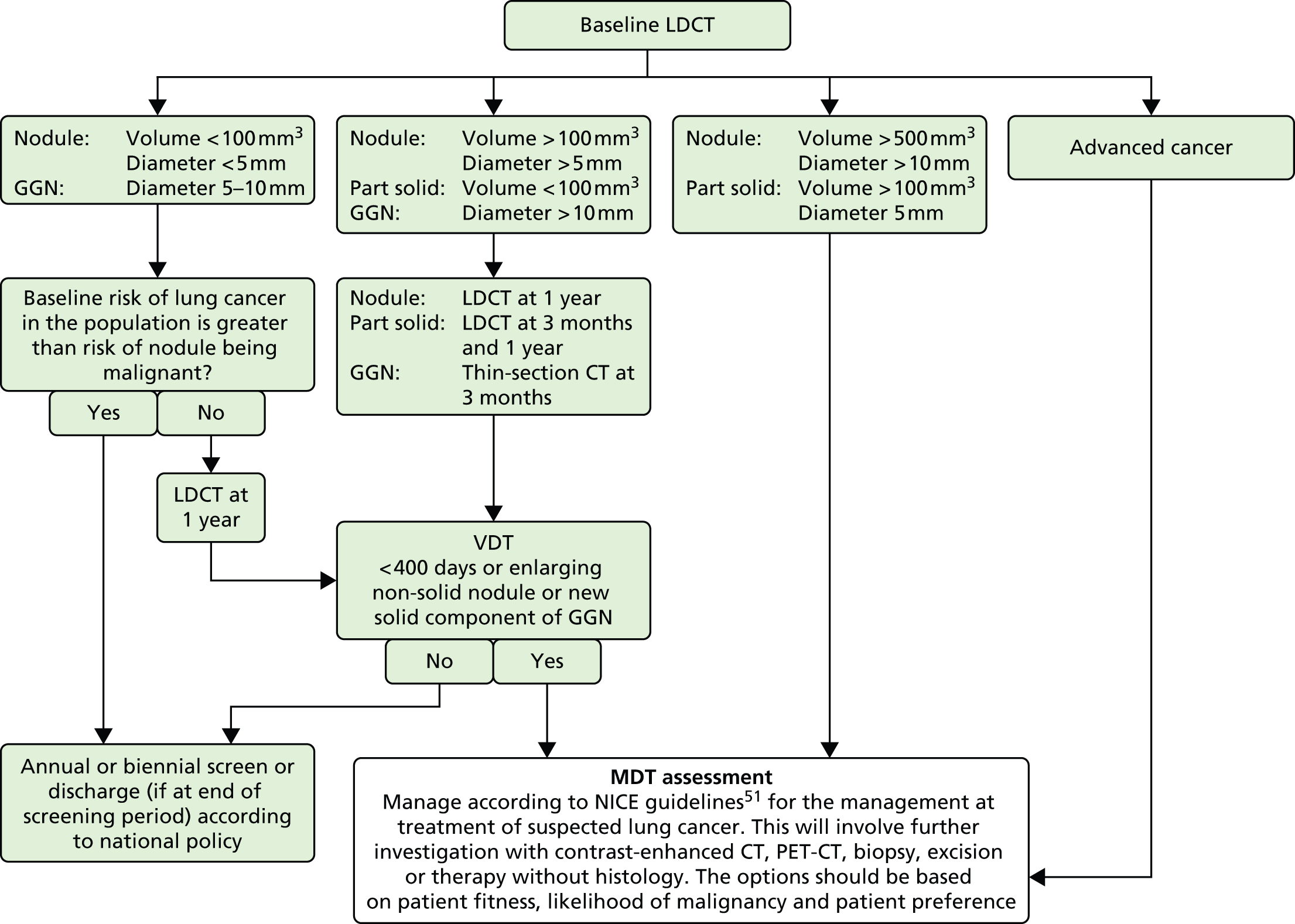
To date, the international lung cancer screening trials, including the UKLS, have not stipulated in their protocols the clinical work-up or the treatment interventions of CT-detected nodules that were referred to the MDT. The UKLS pilot was based at thoracic centres of excellence, where all of the clinical specialties were represented; multidisciplinary management of lung cancer is firmly established in the UK. The policies and pathways for managing suspected or proven lung cancer are summarised in the updated NICE146 and British Thoracic Society147 guidelines and the most recent British Thoracic Society guidelines on lung nodule management. 145 Subjects who enter a CT screening programme and have a possible cancer detected would have access to standard management based on the MDT.
Computed tomography reading for lung nodules
Issues around staffing of lung cancer screening, especially radiological reporting, will need to be addressed, especially when volumetric software analysis is incorporated. The UKLS study has convincingly shown that radiographers can also be used as assistant CT scan readers to aid radiologists; however, it will be necessary to set up the required training and accreditation systems prior to implementation of a national lung cancer screening programme. The UKLS CT data could contribute to a validated data set for training and testing in the future.
Screening age range/duration
The US Preventive Services Task Force has recently recommended screening yearly from 55 to 79 years of age, based on their microsimulation modelling, for people with a smoking history of at least 30 pack-years who had smoked within 15 years. However, the trials did use this age range, and the trial evidence necessarily pertains to shorter periods of intervention. The approach taken in UKLS was to use an individual risk score to assess eligibility. The results of UKLS suggest that screening might reasonably be offered from ages 60 to 75 years. As so few people aged < 60 years were at high risk of lung cancer, the recruitment activity below this age range was not productive. Screening at 70–75 years might be expected to prevent deaths up to the age of 79 years.
Screen interval
A far more difficult problem will be deciding on the screen interval. An annual screen was used in the NLST but the cost will be higher than a biennial or longer interval. It is possible to calculate the increase in mortality as a consequence of moving from an annual to a biennial screen. This has been modelled in a recent publication from the UKLS group. Various estimates under different potential scenarios suggest that 20–40% more lives might be saved with annual screening rather than biennial (Figure 18). 148 Follow-up of the UKLS population, to assess the time elapsed until the lung cancer incidence and mortality in the intervention and control groups converge, will give valuable information in terms of a safe interval. We suggest a strictly applied protocol for screen interval, based on our cost-effectiveness modelling given above.
FIGURE 18.
Screening flow chart.

Selection of high-risk individuals
There is clearly an imperative to offer screening specifically to those individuals who are most likely to benefit from the intervention. Therefore, a robust risk score should be used to select people for screening and this can be done at any point of recruitment, provided a validated score is available. Some scores select on smoking and age at a population level (as used in the NLST),36 whereas others consider a combination of factors (such as previous respiratory disease, previous cancer, family history of lung cancer and exposure to asbestos) in addition to smoking duration and age (as used in the UKLS). 23,94 A number of these risk parameters are readily obtainable in a general practice setting. 149 The utilisation of a risk prediction model to refine the selection process can also be successfully undertaken by an agency such as Radar, who worked very successfully with the UKLS.
The UKLS data also provide an opportunity to develop a risk prediction model that is based on the patient’s clinical data, integrated with imaging characteristics and, in the future, validated biomarkers.
Acceptability of screening
It is important for future policy to identify whether or not a screening intervention is acceptable, and to whom it is acceptable. There is a clinical need to ensure the acceptability of CT lung screening to those at higher risk, such as long-term smokers, and a public health imperative to ensure we put measures in place to include the ‘hard to reach’ groups that have the highest rates of lung cancer, and to understand the barriers to participating in lung cancer screening. The UKLS team have previously reported that participation was more likely in ex-smokers and those with a higher socioeconomic status94 in the first 88,897 individuals who were approached to take part in the UKLS.
Lung cancer screening and integrated smoking cessation
The financial burden of lung cancer is considerable: the estimated cost to the UK economy is £2.4B each year, £9071 per patient annually. This is far higher than the cost of any other cancer despite survival rates being among the lowest. More curative treatment and prevention resulting from integrated screening and smoking cessation programmes have the potential to reduce these costs. Thus the implementation of a UK national lung cancer screening programme should make use of the ‘teachable moment’ for smoking cessation, which would cascade into major beneficial health effects for all smoking-related diseases.
Strengths and limitations of the UK Lung Cancer Screening pilot trial
The UKLS is a true population-based study, which approached a specific age group via local PCT records. No other lung cancer screening study has adopted this approach. This has yielded important information about uptake from the general population, and highlighted the need for a more targeted approach. The UKLS is also the only trial to use a validated lung cancer risk prediction model, and shows that this approach can ensure that resources are devoted to those who are most likely to benefit from screening. The UKLS has shown that a protocolled management pathway, including advanced radiological techniques that effectively manage the detected lung cancer with minimal psychological and physical harm to those without cancer.
A limitation of the pilot UKLS is that, as a trial, it might be expected to recruit fewer people than a screening programme. It would not be fair to extrapolate from other screening programmes because of differences in the sociodemographic profile, but what can be said is that significant further work is the need to address recruitment from the hard-to-reach community. The UKLS was a pilot trial and was not powered to detect a mortality benefit. The planned full trial was not funded but would have addressed this and would have provided cost-effectiveness data, based on this. Instead, we had to use the (albeit very robust) NLST outcome data to develop a model to estimate cost-effectiveness. Although we have been conservative in our model, this is a weakness of the study. The UKLS was also unable to provide information on the value of subsequent yearly screens, in terms of either cancer detection rate or uptake. These data have been provided by other studies including the NLST.
Prioritised research recommendations
-
Recruitment Investigate methods to engage the hard to reach in future lung cancer screening and smoking cessation programmes.
-
Risk prediction modelling Access differing LLP risk levels (including COPD lung function), which should be utilised in a future programme, together with cost-effectiveness of screening.
-
CT nodule care pathway Reassess the cut-off point for indeterminate nodules, based on volume and VDT – as outlined in the recent NELSON89 publications. Also investigate the use of CAD in CT screening.
-
Work-up of CT-detected nodules Assess whether or not national methodologies for the work-up of CT screen-detected nodules are appropriate within a screening programme. Investigate significant other findings and outcomes. Develop methodologies to incorporate coronary artery score in future lung CT scan reports.
-
Training and accreditation Radiologists, as well as considering utilising radiographers in a future CT screening programme.
-
Availability of CT scanners for a future CT screening programme Needs to be assessed.
-
Psychosocial assessment Assess CT-screened individuals over a 15-year lifetime for harms and benefits.
-
Integrated smoking cessation programme with future CT screening programmes Combine both of these modalities for a synergetic effect.
-
Screen interval Assess if annual or biennial screening after two negative scans is appropriate for a future programme.
-
Screening age limit Assess whether or not 75 years is the appropriate cut-off point for lung cancer screening.
-
Pooling the UKLS data with the NELSON data The main UKLS was not funded and the time has now passed to undertake a full trial in the UK; however, we need to collaborate with the NELSON triallists to pool the UKLS data and examine the combined mortality and cost-effectiveness data. This will be the opportunity to follow up on the outcomes of the UKLS control arm.
-
Planning for a future lung cancer CT screening programme Start now (i.e. recruitment methodologies, service provision and screening programme protocol development).
Future prospects
In summary, the UKLS data reported in this document have made a very worthwhile contribution to the international data sets on lung cancer screening. Taking the UKLS pilot data in consort with the NLST mortality data, it is clear that the intervention can save lives from lung cancer, and may be practicable in the UK health setting. This should be in conjunction with promotion of, and support for, smoking cessation. There are no alternative strategies for significant reductions in lung cancer death rates that can be recommended now or in the next few years.
Although our results suggest that screening with UKLS eligibility and recruitment policies could be cost-effective, there is some uncertainty around this. There is a need for further research into methods of identification of the appropriate population and delivery of the service to that population. Recruitment into screening programmes based on risk assessment has to be done in the most effective way, and uptake of screening needs to be monitored. Further research on the interscreening interval is indicated. Special consideration should also be given to recruiting those in potentially hard-to-reach sections of society, including those with high levels of socioeconomic deprivation.
Any screening programme will need ongoing monitoring and evaluation, with risks and benefits regularly measured. Such risks and benefits should be conveyed to those offered screening to facilitate informed choice. There is a need to develop both information materials and protocols for regular audit and evaluation of a lung cancer screening programme, so that the National Screening Committee can deliberate on the subject with full knowledge of the implications.
Acknowledgements
We would like to thank the external members of the Trial Steering Committee for their advice and support for the project: Professor Ian Jacobs (University of Manchester), Professor Peter Armstrong (Radiologist, retired), Professor Deborah Ashby (Imperial College London), Dr Sanjay Popat (Royal Marsden) and Mr David Ardron as our service user representative.
Our thanks go also to the Data Monitoring and Ethics Committee comprising Professor Allan Hackshaw (University College London), Dr Catherine Hill (Institut Gustave Roussy, France) and Dr Robert Smith (American Cancer Society).
We are grateful to the participants and GPs who supported the study, giving so generously of their time and sharing their experiences with us, likewise the practice managers and administrative staff at all of the collaborating practices and PCTs who provided valuable assistance to us throughout the study.
We would like to thank a number of people who helped towards the successful completion of the study:
Christopher Hands managed the project for a period of time at inception. Gary Jeffers, Chantelle Murphy and Julie-Ann Bevan contributed to collecting data for the study and were responsible for sending all scan results letters to participants and their GPs. Dr Victoria Hughes was instrumental in setting up and running the trial within Papworth Hospital. Dr Margarita Perez-Casal gave invaluable support and advice in LHCH.
Dr Sadia Anwar, Dr Helen Roberts, Miss Susan Allen, Miss Emily Reason, Miss Clare Darlington, Mrs Susan Maycock (all from Nottingham University Hospitals), the patient volunteers and Mrs Lynn Faulds-Wood gave invaluable help with the production of the UKLS recruitment film.
The individuals at Papworth Hospital who ensured the smooth running of the trial and recruitment of participants: Lucie Garner, Sarah Lewis, Anne Joy, Geri Barrett, David Kirby, Helen Holcombe, SallyAnne Meakins, Leigh Clements, Katie Rintoul, Adama Fofana, Angela Reed and Richard Rowlands.
Liverpool Heart and Chest Hospital: Diane Jones, Colin Monaghan, Pauline Schofield, Lindsey Hodgson, Nicola Hoy, Peris Widdows, Sarah Feeney, James Chetter, Sheila Whyte, Claire McVey, Frances Johnston and Sharon Steele.
Royal Brompton & Harefield NHS Trust (RBHT): Christine Peacock, RBHT Radiology Services Manager, for releasing radiographers for CT scan reading, and the radiographers themselves, Natalie Gartland and Bruce Barton.
Individuals at Cardiff University for their help with statistical analysis: Ben Carter (statistical advice for the psychosocial analysis) and Noor Ali (contribution to the analysis of non-participation data).
The picture archiving and communication system managers at the local and central sites for their support and advice in the storage and transfer of CT images: David McCreavy (LHCH), Tom Wright (Papworth Hospital) and Adam Bell (RBHT).
In addition, thank you to the Liverpool Cancer Trials Unit for its support and guidance throughout the inception, delivery and reporting of the UKLS.
Finally, a special mention for Dr Joan Austoker, who was heavily involved in the psychosocial elements at the inception of the UKLS pilot but who sadly passed away suddenly before the trial started.
Contributions of authors
Professor John K Field (Clinical Professor of Molecular Oncology): chief investigator of the UKLS, developed the trial protocol, oversaw the management of trial and analysis; undertook management of staff and funding, preparation and writing of the final report, and editing of the manuscript.
Professor Stephen W Duffy (Professor of Screening): lead UKLS statistician; member of the UKLS team; developed the trial protocol, preparation of the analysis plan; undertook preparation and writing of the final report, and editing of the manuscript.
Professor David R Baldwin (Consultant Respiratory Physician and Honorary Professor of Medicine): respiratory lead for the UKLS; member of UKLS team; chaired the UKLS respiratory review group; assisted in developing the trial protocol and external evaluation of clinical data; undertook preparation and writing of the final report, and editing of the manuscript.
Dr Kate E Brain (Reader): UKLS psychosocial lead; member of the UKLS team; developed the questionnaires and evaluation of data; undertook preparation and contribution to the final report, and editing of the manuscript.
Dr Anand Devaraj (Consultant Radiologist): UKLS radiology second reader and signed off on all trial radiology reports; undertook preparation of data for report preparation and contribution to the final report, and editing of the manuscript.
Professor Tim Eisen (Consultant Medical Oncologist): member of UKLS team; provided advice for the development of the UKLS, and undertook editing of the final report.
Mrs Beverley A Green (UKLS Project Manager): oversaw trial management; supervised staff; reported to ethics and Health Technology Assessment (HTA); undertook preparation of data for, and contribution to, the final report, and editing of the manuscript.
Dr John A Holemans (Consultant Radiologist): UKLS radiologist; undertook all CT reporting at the LHCH, preparation of reports, and editing of the manuscript.
Mr Terry Kavanagh (Lung Cancer Patient Representative): member of UKLS team; provided advice on the UKLS application, all of the patient letters and the information sheets. Attended trial meetings and gave the patient’s perspective.
Dr Keith M Kerr (Consultant Pathologist): member of the UKLS team; provided advice on the UKLS pathology protocol and undertook editing of the manuscript.
Dr Martin Ledson (Consultant Respiratory Physician): UKLS respiratory physician; undertook the management of all of the patients who were referred through LHCH MDT, reporting at the LHCH; undertook preparation of the reports and editing of the manuscript.
Dr Kate J Lifford (Research Fellow): undertook the detailed analysis of the psychosocial questionnaires and prepared reports.
Dr Fiona E McRonald (Research Fellow in Molecular Oncology): contributed to the analysis of trial recruitment data, collated data with statisticians and took the lead in the preparation of the final report.
Dr Arjun Nair (Clinical Research Fellow in Radiology); undertook the analysis of the CT reporting systems of the radiologists and radiographers involved in the UKLS, and preparation of the reports.
Dr Richard D Page (Consultant Surgeon): member of UKLS team; provided advice on surgery and management of individuals with CT-detected nodules protocol, and undertook editing of the manuscript.
Professor Mahesh KB Parmar (Professor of Medical Statistics); member of the UKLS team; provided advice on the development of the UKLS protocol and running of the trial; undertook editing of the manuscript.
Dr Robert C Rintoul (Consultant Respiratory Physician) supported, in part, by the Cambridge Biomedical Research Centre and the Cambridge Cancer Centre: UKLS respiratory physician; assisted in setting up the Papworth UKLS recruitment clinics and oversaw the staff; undertook the management of all patients who were referred through the Papworth Hospital MDT, as well as preparation of reports and editing of the manuscript.
Dr Nicholas Screaton (Consultant Radiologist): UKLS radiologist; undertook all of the CT reporting at Papworth Hospital, as well as preparation of reports and editing of the manuscript.
Professor Nicholas J Wald (Professor of Epidemiology): UKLS advisor on trial design; developed the Wald Single Screen Design for the UKLS; member of the UKLS team; provided advice on the development of the UKLS protocol and running of the trial; undertook editing of the manuscript.
Professor David Weller (Director of Centre for Population Health Sciences): member of UKLS team; provided advice on the UKLS protocol and recruitment; undertook interaction with GP practices and editing of the manuscript.
Professor David K Whynes (Professor of Health Economics): UKLS Health Economist; member of the UKLS team; undertook the cost-effectiveness modelling for UKLS, as well as preparation of the report and editing of the manuscript.
Professor Paula R Williamson (Professor of Medical Statistics): member of the UKLS team; provided clinical trial support in Liverpool; provided advice on the development of the UKLS protocol and running the trial; undertook editing of the manuscript.
Dr Ghasem Yadegarfar (Research Fellow Statistician): UKLS statistician working within the Liverpool Cancer Trials Unit; undertook the downloading of data from the UKLS database, data cleaning and analysis on Stata, as well as preparation of the statistical reports.
Professor David M Hansell (Consultant Radiologist and the recipient of a National Institutes of Health Research Senior Investigator Award): lead UKLS radiologist; member of the UKLS team; chaired the UKLS radiology review group; assisted in developing trial protocol; undertook preparation and writing of the final report, and editing of the manuscript.
Publications
Baldwin DR, Duffy SW, Wald NJ, Page R, Hansell DM, Field JK. UK Lung Screen (UKLS) nodule management protocol: modelling of a single screen randomised controlled trial of low-dose CT screening for lung cancer. Thorax 2011;66:308–13.
Field JK, Baldwin D, Brain K, Devaraj A, Eisen T, Duffy SW, et al. CT screening for lung cancer in the UK: position statement by UKLS investigators following the NLST report. Thorax 2011;66:736–7.
Nair A, Baldwin DR, Field JK, Hansell DM, Devaraj A. Measurement methods and algorithms for the management of solid nodules. J Thorac Imaging 2012;27:230–9.
Field JK, Hansell DM, Duffy SW, Baldwin DR. CT screening for lung cancer: countdown to implementation. Lancet Oncol 2013;14:e591–600.
Field JK, Oudkerk M, Pedersen JH, Duffy SW. Prospects for population screening and diagnosis of lung cancer. Lancet 2013;382:732–41.
Baldwin DR, Hansell DM, Duffy SW, Field JK. Lung cancer screening with low dose computed tomography. BMJ 2014;348:g1970.
Duffy SW, Field JK, Allgood PC, Seigneurin A. Translation of research results to simple estimates of the likely effect of a lung cancer screening programme in the UK. Br J Cancer 2014;110:1834–40.
Field JK. Perspective: the screening imperative. Nature 2014;513:S7.
McRonald FE, Yadegarfar G, Baldwin DR, Devaraj A, Brain KE, Eisen T, et al. The UK Lung Screen (UKLS): demographic profile of first 88,897 approaches provides recommendations for population screening. Cancer Prev Res (Phila) 2014;7:362–71.
Ali N, Lifford KJ, Carter B, McRonald F, Yadegarfar G, Baldwin DR, et al. Barriers to uptake among high-risk individuals declining participation in lung cancer screening: a mixed methods analysis of the UK Lung Cancer Screening (UKLS) trial. BMJ Open 2015;5:e008254.
Field JK, Duffy SW, Baldwin DR, Whynes DK, Devaraj A, Brain KE, et al. UK Lung Cancer RCT Pilot Screening Trial: baseline findings from the screening arm provide evidence for the potential implementation of lung cancer screening. Thorax 2016;71:161–70.
Field JK, van Klaveren R, Pedersen JH, Pastorino U, Paci E, Becker N. European randomized lung cancer screening trials: Post NLST. J Surg Oncol 2013;108:280–6.
Nair A, Baldwin DR, Field JK, Hansell DM, Devaraj A. Measurement methods and algorithms for the management of solid nodules. J Thorac Imaging 2012;27:230–9.
Marcus MW, Raji OY, Field JK. Lung cancer screening: identifying the high risk cohort. J Thorac Dis 2015;7(Suppl. 2):156–2.
Field JK, Baldwin D, Brain K, Devaraj A, Eisen T, Duffy SW, et al. CT screening for lung cancer in the UK: position statement by UKLS investigators following the NLST report. Thorax 2011;66:736–7.
Data sharing statement
We are committed, in principle, to data sharing with fellow researchers, and are currently drawing up operating procedures for this. We anticipate that the data will be stored securely in Liverpool and reasonable requests for data for further research will be accommodated, subject to compliance with regulations, maintaining the integrity of information governance, and ensuring no loss of confidentiality on the part of the participants of the study. Requests that require considerable data manipulation and management on our part will need to be resourced by those requesting data.
Disclaimers
This report presents independent research funded by the National Institute for Health Research (NIHR). The views and opinions expressed by authors in this publication are those of the authors and do not necessarily reflect those of the NHS, the NIHR, NETSCC, the HTA programme or the Department of Health. If there are verbatim quotations included in this publication the views and opinions expressed by the interviewees are those of the interviewees and do not necessarily reflect those of the authors, those of the NHS, the NIHR, NETSCC, the HTA programme or the Department of Health.
References
- Cancer Research UK . Cancer Statistics for the UK 2012. www.cancerresearchuk.org/health-professional/cancer-statistics (accessed May 2016).
- Rami-Porta R, Ball D, Crowley J, Giroux DJ, Jett J, Travis WD, et al. The IASLC Lung Cancer Staging Project: proposals for the revision of the T descriptors in the forthcoming (seventh) edition of the TNM classification for lung cancer. J Thorac Oncol 2007;2:593-602. http://dx.doi.org/10.1097/JTO.0b013e31807a2f81.
- National Cancer Research Institute . Lung Cancer UK Price Tag Eclipses the Cost of Any Other Cancer 2012. www.cancerresearchuk.org/about-us/cancer-news/press-release/lung-cancer-uk-price-tag-eclipses-the-cost-of-any-other-cancer (accessed June 2012).
- Parkin DM. 2. Tobacco-attributable cancer burden in the UK in 2010. Br J Cancer 2011;105:6-13. http://dx.doi.org/10.1038/bjc.2011.475.
- Cogliano VJ, Baan R, Straif K, Grosse Y, Lauby-Secretan B, El Ghissassi F, et al. Preventable exposures associated with human cancers. J Natl Cancer Inst 2011;103:1827-39. http://dx.doi.org/10.1093/jnci/djr483.
- Cote ML, Liu M, Bonassi S, Neri M, Schwartz AG, Christiani DC, et al. Increased risk of lung cancer in individuals with a family history of the disease: a pooled analysis from the International Lung Cancer Consortium. Eur J Cancer 2012;48:1957-68. http://dx.doi.org/10.1016/j.ejca.2012.01.038.
- Brenner DR, McLaughlin JR, Hung RJ. Previous lung diseases and lung cancer risk: a systematic review and meta-analysis. PLOS ONE 2011;6. http://dx.doi.org/10.1371/journal.pone.0017479.
- Brenner DR, Boffetta P, Duell EJ, Bickeboller H, Rosenberger A, McCormack V, et al. Previous lung diseases and lung cancer risk: a pooled analysis from the International Lung Cancer Consortium. Am J Epidemiol 2012;176:573-85. http://dx.doi.org/10.1093/aje/kws151.
- Shack L, Jordan C, Thomson CS, Mak V, Moller H. Variation in incidence of breast, lung and cervical cancer and malignant melanoma of skin by socioeconomic group in England. BMC Cancer 2008;8. http://dx.doi.org/10.1186/1471-2407-8-271.
- Forman D. Cancer Incidence by Deprivation England, 1995–2004. London: National Cancer Intelligence Network; 2008.
- Rowan S. Trends in cancer incidence by deprivation, England and Wales, 1990–2002. Health Stat Q 2007;36:24-35.
- Raaschou-Nielsen O, Andersen ZJ, Beelen R, Samoli E, Stafoggia M, Weinmayr G, et al. Air pollution and lung cancer incidence in 17 European cohorts: prospective analyses from the European Study of Cohorts for Air Pollution Effects (ESCAPE). Lancet Oncol 2013;14:813-22. http://dx.doi.org/10.1016/S1470-2045(13)70279-1.
- Field JK, Chen Y, Marcus MW, McRonald FE, Raji OY, Duffy SW. The contribution of risk prediction models to early detection of lung cancer. J Surg Oncol 2013;108:304-11. http://dx.doi.org/10.1002/jso.23384.
- Van Klaveren RJ, de Koning HJ, Mulshine J, Hirsch FR. Lung cancer screening by spiral CT. What is the optimal target population for screening trials?. Lung Cancer 2002;38:243-52. http://dx.doi.org/10.1016/S0169-5002(02)00222-2.
- Bach PB, Kattan MW, Thornquist MD, Kris MG, Tate RC, Barnett MJ, et al. Variations in lung cancer risk among smokers. J Natl Cancer Inst 2003;95:470-8. http://dx.doi.org/10.1093/jnci/95.6.470.
- Spitz MR, Etzel CJ, Dong Q, Amos CI, Wei Q, Wu X, et al. An expanded risk prediction model for lung cancer. Cancer Prev Res (Phila) 2008;1:250-4. http://dx.doi.org/10.1158/1940-6207.CAPR-08-0060.
- Tammemagi MC, Katki HA, Hocking WG, Church TR, Caporaso N, Kvale PA, et al. Selection criteria for lung-cancer screening. N Engl J Med 2013;368:728-36. http://dx.doi.org/10.1056/NEJMoa1211776.
- Kovalchik SA, Tammemagi M, Berg CD, Caporaso NE, Riley TL, Korch M, et al. Targeting of low-dose CT screening according to the risk of lung-cancer death. N Engl J Med 2013;369:245-54. http://dx.doi.org/10.1056/NEJMoa1301851.
- Spitz MR, Hong WK, Amos CI, Wu X, Schabath MB, Dong Q, et al. A risk model for prediction of lung cancer. J Natl Cancer Inst 2007;99:715-26. http://dx.doi.org/10.1093/jnci/djk153.
- Cassidy A, Myles JP, van Tongeren M, Page RD, Liloglou T, Duffy SW, et al. The LLP risk model: an individual risk prediction model for lung cancer. Br J Cancer 2008;98:270-6. http://dx.doi.org/10.1038/sj.bjc.6604158.
- Cassidy A, Myles JP, Liloglou T, Duffy SW, Field JK. Defining high-risk individuals in a population-based molecular-epidemiological study of lung cancer. Int J Oncol 2006;28:1295-301. http://dx.doi.org/10.3892/ijo.28.5.1295.
- Garcia-Closas M, Kelsey KT, Wiencke JK, Xu X, Wain JC, Christiani DC. A case–control study of cytochrome P450 1A1, glutathione S-transferase M1, cigarette smoking and lung cancer susceptibility (Massachusetts, United States). Cancer Causes Control 1997;8:544-53. http://dx.doi.org/10.1023/A:1018481910663.
- Raji OY, Duffy SW, Agbaje OF, Baker SG, Christiani DC, Cassidy A, et al. Predictive accuracy of the Liverpool lung project risk model for stratifying patients for computed tomography screening for lung cancer: a case–control and cohort validation study. Ann Intern Med 2012;157:242-50. http://dx.doi.org/10.7326/0003-4819-157-4-201208210-00004.
- Peres J. Lung cancer screening gets risk-specific. J Natl Cancer Inst 2013;105:1-2. http://dx.doi.org/10.1093/jnci/djs631.
- Field JK, Duffy SW. Lung cancer screening: the way forward. Br J Cancer 2008;99:557-62. http://dx.doi.org/10.1038/sj.bjc.6604509.
- Brett GZ. Earlier diagnosis and survival in lung cancer. Br Med J 1969;4:260-2. http://dx.doi.org/10.1136/bmj.4.5678.260.
- Oken MM, Hocking WG, Kvale PA, Andriole GL, Buys SS, Church TR, et al. Screening by chest radiograph and lung cancer mortality: the Prostate, Lung, Colorectal, and Ovarian (PLCO) randomized trial. JAMA 2011;306:1865-73. http://dx.doi.org/10.1001/jama.2011.1591.
- Khan AF. Overview of the Prostate, Lung, Colorectal and Ovarian (PLCO) Cancer Screening Trial 2012. http://benchmarks.cancer.gov/2012/05/overview-of-the-prostate-lung-colorectal-and-ovarian-plco-cancer-screening-trial/ (accessed June 2012).
- Reeves AP, Kostis WJ. Computer-aided diagnosis for lung cancer. Radiol Clin North Am 2000;38:497-509. http://dx.doi.org/10.1016/S0033-8389(05)70180-9.
- Yankelevitz DF, Reeves AP, Kostis WJ, Zhao B, Henschke CI. Small pulmonary nodules: volumetrically determined growth rates based on CT evaluation. Radiology 2000;217:251-6. http://dx.doi.org/10.1148/radiology.217.1.r00oc33251.
- Swensen SJ, Viggiano RW, Midthun DE, Muller NL, Sherrick A, Yamashita K, et al. Lung nodule enhancement at CT: multicenter study. Radiology 2000;214:73-80. http://dx.doi.org/10.1148/radiology.214.1.r00ja1473.
- Pastorino U, Bellomi M, Landoni C, De Fiori E, Arnaldi P, Picchio M, et al. Early lung-cancer detection with spiral CT and positron emission tomography in heavy smokers: 2-year results. Lancet 2003;362:593-7. http://dx.doi.org/10.1016/S0140-6736(03)14188-8.
- Sone S, Takashima S, Li F, Yang Z, Honda T, Maruyama Y, et al. Mass screening for lung cancer with mobile spiral computed tomography scanner. Lancet 1998;351:1242-5. http://dx.doi.org/10.1016/S0140-6736(97)08229-9.
- Field JK, Smith RA, Duffy SW, Berg CD, van Klaveren R, Henschke CI, et al. The Liverpool Statement 2005: priorities for the European Union/United States spiral computed tomography collaborative group. J Thorac Oncol 2006;1:497-8. http://dx.doi.org/10.1016/S1556-0864(15)31618-X.
- Aberle DR, DeMello S, Berg CD, Black WC, Brewer B, Church TR, et al. Results of the two incidence screenings in the National Lung Screening Trial. N Engl J Med 2013;369:920-31. http://dx.doi.org/10.1056/NEJMoa1208962.
- Aberle DR, Adams AM, Berg CD, Black WC, Clapp JD, . National Lung Screening Trial Research Team . Reduced lung-cancer mortality with low-dose computed tomographic screening. N Engl J Med 2011;365:395-409.
- Van Klaveren RJ, Oudkerk M, Prokop M, Scholten ET, Nackaerts K, Vernhout R, et al. Management of lung nodules detected by volume CT scanning. N Engl J Med 2009;361:2221-9. http://dx.doi.org/10.1056/NEJMoa0906085.
- Pastorino U. Early detection of lung cancer. Respiration 2006;73:5-13. http://dx.doi.org/10.1159/000090990.
- Pastorino U, Rossi M, Rosato V, Marchiano A, Sverzellati N, Morosi C, et al. Annual or biennial CT screening versus observation in heavy smokers: 5-year results of the MILD trial. Eur J Cancer Prev 2012;21:308-15. http://dx.doi.org/10.1097/CEJ.0b013e328351e1b6.
- Picozzi G, Paci E, Lopez Pegna A, Bartolucci M, Roselli G, De Francisci A, et al. Screening of lung cancer with low dose spiral CT: results of a three year pilot study and design of the randomised controlled trial ‘Italung-CT’. Radiol Med 2005;109:17-26.
- Lopes Pegna A, Picozzi G, Mascalchi M, Carozzi FM, Carrozzi L, Comin C, et al. Design, recruitment and baseline results of the ITALUNG trial for lung cancer screening with low-dose CT. Lung Cancer 2009;64:34-40. http://dx.doi.org/10.1016/j.lungcan.2008.07.003.
- Infante M, Lutman FR, Cavuto S, Brambilla G, Chiesa G, Passera E, et al. Lung cancer screening with spiral CT baseline results of the randomized DANTE trial. Lung Cancer 2008;59:355-63. http://dx.doi.org/10.1016/j.lungcan.2007.08.040.
- Blanchon T, Brechot JM, Grenier PA, Ferretti GR, Lemarie E, Milleron B, et al. Baseline results of the Depiscan study: a French randomized pilot trial of lung cancer screening comparing low dose CT scan (LDCT) and chest X-ray (CXR). Lung Cancer 2007;58:50-8. http://dx.doi.org/10.1016/j.lungcan.2007.05.009.
- Saghir Z, Dirksen A, Ashraf H, Bach KS, Brodersen J, Clementsen PF, et al. CT screening for lung cancer brings forward early disease. The randomised Danish Lung Cancer Screening Trial: status after five annual screening rounds with low-dose CT. Thorax 2012;67:296-301. http://dx.doi.org/10.1136/thoraxjnl-2011-200736.
- Pedersen J, Ashraf H, Dirksen A, Bach K, Hansen H, Thoennesen P, et al. The Danish randomized lung cancer CT screening trial: overall design and results of the prevalence round. J Thorac Oncol 2009;5:608-14. http://dx.doi.org/10.1097/JTO.0b013e3181a0d98f.
- Field JK, Hansell DM, Duffy SW, Baldwin DR. CT screening for lung cancer: countdown to implementation. Lancet Oncol 2013;14:e591-600. http://dx.doi.org/10.1016/S1470-2045(13)70293-6.
- Field JK, van Klaveren R, Pedersen JH, Pastorino U, Paci E, Becker N, et al. European randomized lung cancer screening trials: post NLST. J Surg Oncol 2013;108:280-6. http://dx.doi.org/10.1002/jso.23383.
- Field JK, Oudkerk M, Pedersen JH, Duffy SW. Prospects for population screening and diagnosis of lung cancer. Lancet 2013;382:732-41. http://dx.doi.org/10.1016/S0140-6736(13)61614-1.
- Aberle DR, Berg CD, Black WC, Church TR, Fagerstrom RM, Galen B, et al. The National Lung Screening Trial: overview and study design. Radiology 2011;258:243-53. http://dx.doi.org/10.1148/radiol.10091808.
- Criteria for Appraising the Viability, Effectiveness and Appropriateness of a Screening Programme. London: Public Health England; 2014.
- Black C, de Verteuil R, Walker S, Ayres J, Boland A, Bagust A, et al. Population screening for lung cancer using computed tomography, is there evidence of clinical effectiveness? A systematic review of the literature. Thorax 2007;62:131-8. http://dx.doi.org/10.1136/thx.2006.064659.
- Whynes DK. Could CT screening for lung cancer ever be cost effective in the United Kingdom?. Cost Eff Resour Alloc 2008;6. http://dx.doi.org/10.1186/1478-7547-6-5.
- Baldwin DR, Duffy SW, Wald NJ, Page R, Hansell DM, Field JK. UK Lung Screen (UKLS) nodule management protocol: modelling of a single screen randomised controlled trial of low-dose CT screening for lung cancer. Thorax 2011;66:308-13. http://dx.doi.org/10.1136/thx.2010.152066.
- Atkin WS, Edwards R, Kralj-Hans I, Wooldrage K, Hart AR, Northover JM, et al. Once-only flexible sigmoidoscopy screening in prevention of colorectal cancer: a multicentre randomised controlled trial. Lancet 2010;375:1624-33. http://dx.doi.org/10.1016/S0140-6736(10)60551-X.
- Ashton HA, Buxton MJ, Day NE, Kim LG, Marteau TM, Scott RA, et al. The multicentre aneurysm screening study (MASS) into the effect of abdominal aortic aneurysm screening on mortality in men: a randomised controlled trial. Lancet 2002;360:1531-9. http://dx.doi.org/10.1016/S0140-6736(02)11522-4.
- Jakes RW, Duffy SW, Ng FC, Gao F, Ng EH. Mammographic parenchymal patterns and risk of breast cancer at and after a prevalence screen in Singaporean women. Int J Epidemiol 2000;29:11-9. http://dx.doi.org/10.1093/ije/29.1.11.
- UK Lung Cancer Screening Trial n.d. www.ukls.org (accessed November 2014).
- Zigmond AS, Snaith RP. The Hospital Anxiety and Depression Scale. Acta Psychiatr Scand 1983;67:361-70. http://dx.doi.org/10.1111/j.1600-0447.1983.tb09716.x.
- Lerman C, Daly M, Sands C, Balshem A, Lustbader E, Heggan T, et al. Mammography adherence and psychological distress among women at risk for breast cancer. J Natl Cancer Inst 1993;85:1074-80. http://dx.doi.org/10.1093/jnci/85.13.1074.
- Watson M, Lloyd S, Davidson J, Meyer L, Eeles R, Ebbs S, et al. The impact of genetic counselling on risk perception and mental health in women with a family history of breast cancer. Br J Cancer 1999;79:868-74. http://dx.doi.org/10.1038/sj.bjc.6690139.
- Field J. HTA Protocol n.d. www.hta.ac.uk/project/2382.asp (accessed June 2012).
- English Indices of Deprivation 2010. London: Department for Communities and Local Government; 2011.
- Senn S. Statistical Issues in Drug Development. Hoboken, NJ: Wiley; 2007.
- Clayton D, Hills M. Statistical Models in Epidemiology. Oxford: Oxford University Press; 1993.
- Collett D. Modelling Survival Data in Medical Research. London: Chapman and Hall; 2003.
- Robinson SH, Harris H. Smoking and Drinking Among Adults, 2009. London: Office for National Statistics; 2009.
- Health Survey for England, 2010. Colchester: UK Data Archive; 2015.
- Wardle J, Sutton S, Williamson S, Taylor T, McCaffery K, Cuzick J, et al. Psychosocial influences on older adults’ interest in participating in bowel cancer screening. Prev Med 2000;31:323-34. http://dx.doi.org/10.1006/pmed.2000.0725.
- Espinosa de Los Monteros K, Gallo LC. The relevance of fatalism in the study of Latinas’ cancer screening behavior: a systematic review of the literature. Int J Behav Med 2011;18:310-18. http://dx.doi.org/10.1007/s12529-010-9119-4.
- Miles A, Rainbow S, von Wagner C. Cancer fatalism and poor self-rated health mediate the association between socioeconomic status and uptake of colorectal cancer screening in England. Cancer Epidemiol Biomarkers Prev 2011;20:2132-40. http://dx.doi.org/10.1158/1055-9965.EPI-11-0453.
- Marten K, Seyfarth T, Auer F, Wiener E, Grillhosl A, Obenauer S, et al. Computer-assisted detection of pulmonary nodules: performance evaluation of an expert knowledge-based detection system in consensus reading with experienced and inexperienced chest radiologists. Eur Radiol 2004;14:1930-8. http://dx.doi.org/10.1007/s00330-004-2389-y.
- Brochu B, Beigelman-Aubry C, Goldmard JL, Raffy P, Grenier PA, Lucidarme O. Computer-aided detection of lung nodules on thin collimation MDCT: impact on radiologists’ performance. J Radiol 2007;88:573-8. http://dx.doi.org/10.1016/S0221-0363(07)89857-X.
- Rubin GD, Lyo JK, Paik DS, Sherbondy AJ, Chow LC, Leung AN, et al. Pulmonary nodules on multi-detector row CT scans: performance comparison of radiologists and computer-aided detection. Radiology 2005;234:274-83. http://dx.doi.org/10.1148/radiol.2341040589.
- Roos JE, Paik D, Olsen D, Liu EG, Chow LC, Leung AN, et al. Computer-aided detection (CAD) of lung nodules in CT scans: radiologist performance and reading time with incremental CAD assistance. Eur Radiol 2010;20:549-57. http://dx.doi.org/10.1007/s00330-009-1596-y.
- Wormanns D, Ludwig K, Beyer F, Heindel W, Diederich S. Detection of pulmonary nodules at multirow-detector CT: effectiveness of double reading to improve sensitivity at standard-dose and low-dose chest CT. Eur Radiol 2005;15:14-22. http://dx.doi.org/10.1007/s00330-004-2527-6.
- Beigelman-Aubry C, Raffy P, Yang W, Castellino RA, Grenier PA. Computer-aided detection of solid lung nodules on follow-up MDCT screening: evaluation of detection, tracking, and reading time. AJR Am J Roentgenol 2007;189:948-55. http://dx.doi.org/10.2214/AJR.07.2302.
- Fraioli F, Bertoletti L, Napoli A, Calabrese FA, Masciangelo R, Cortesi E, et al. Volumetric evaluation of therapy response in patients with lung metastases. Preliminary results with a computer system (CAD) and comparison with unidimensional measurements. Radiol Med 2006;111:365-75. http://dx.doi.org/10.1007/s11547-006-0035-2.
- Li Q. Recent progress in computer-aided diagnosis of lung nodules on thin-section CT. Comput Med Imaging Graph 2007;31:248-57. http://dx.doi.org/10.1016/j.compmedimag.2007.02.005.
- Henschke CI, Yip R, Yankelevitz DF, Smith JP. Definition of a positive test result in computed tomography screening for lung cancer: a cohort study. Ann Intern Med 2013;158:246-52. http://dx.doi.org/10.7326/0003-4819-158-4-201302190-00004.
- McWilliams A, Tammemagi MC, Mayo JR, Roberts H, Liu G, Soghrati K, et al. Probability of cancer in pulmonary nodules detected on first screening CT. N Engl J Med 2013;369:910-19. http://dx.doi.org/10.1056/NEJMoa1214726.
- Nair A, Baldwin DR, Field JK, Hansell DM, Devaraj A. Measurement methods and algorithms for the management of solid nodules. J Thorac Imaging 2012;27:230-9. http://dx.doi.org/10.1097/RTI.0b013e31824f83e1.
- Goo JM. A computer-aided diagnosis for evaluating lung nodules on chest CT: the current status and perspective. Korean J Radiol 2011;12:145-55. http://dx.doi.org/10.3348/kjr.2011.12.2.145.
- McCaffery K, Waller J, Forrest S, Cadman L, Szarewski A, Wardle J. Testing positive for human papillomavirus in routine cervical screening: examination of psychosocial impact. BJOG 2004;111:1437-43. http://dx.doi.org/10.1111/j.1471-0528.2004.00279.x.
- Brett J, Bankhead C, Henderson B, Watson E, Austoker J. The psychological impact of mammographic screening. A systematic review. Psychooncology 2005;14:917-38. http://dx.doi.org/10.1002/pon.904.
- Aggestrup LM, Hestbech MS, Siersma V, Pedersen JH, Brodersen J. Psychosocial consequences of allocation to lung cancer screening: a randomised controlled trial. BMJ Open 2012;2. http://dx.doi.org/10.1136/bmjopen-2011-000663.
- Byrne MM, Weissfeld J, Roberts MS. Anxiety, fear of cancer, and perceived risk of cancer following lung cancer screening. Med Decis Making 2008;28:917-25. http://dx.doi.org/10.1177/0272989X08322013.
- Taylor KL, Shelby R, Gelmann E, McGuire C. Quality of life and trial adherence among participants in the prostate, lung, colorectal, and ovarian cancer screening trial. J Natl Cancer Inst 2004;96:1083-94. http://dx.doi.org/10.1093/jnci/djh194.
- Van den Bergh KA, Essink-Bot ML, Borsboom GJ, Scholten ET, van Klaveren RJ, de Koning HJ. Long-term effects of lung cancer computed tomography screening on health-related quality of life: the NELSON trial. Eur Respir J 2011;38:154-61. http://dx.doi.org/10.1183/09031936.00123410.
- Van den Bergh KA, Essink-Bot ML, Borsboom GJ, Th Scholten E, Prokop M, de Koning HJ, et al. Short-term health-related quality of life consequences in a lung cancer CT screening trial (NELSON). Br J Cancer 2010;102:27-34. http://dx.doi.org/10.1038/sj.bjc.6605459.
- Van der Aalst CM, van den Bergh KA, Willemsen MC, de Koning HJ, van Klaveren RJ. Lung cancer screening and smoking abstinence: 2 year follow-up data from the Dutch-Belgian randomised controlled lung cancer screening trial. Thorax 2010;65:600-5. http://dx.doi.org/10.1136/thx.2009.133751.
- Van der Aalst CM, van Klaveren RJ, van den Bergh KA, Willemsen MC, de Koning HJ. The impact of a lung cancer computed tomography screening result on smoking abstinence. Eur Respir J 2011;37:1466-73. http://dx.doi.org/10.1183/09031936.00035410.
- Silvestri GA, Nietert PJ, Zoller J, Carter C, Bradford D. Attitudes towards screening for lung cancer among smokers and their non-smoking counterparts. Thorax 2007;62:126-30. http://dx.doi.org/10.1136/thx.2005.056036.
- Van den Bergh KA, Essink-Bot ML, van Klaveren RJ, de Koning HJ. Informed participation in a randomised controlled trial of computed tomography screening for lung cancer. Eur Respir J 2009;34:711-20. http://dx.doi.org/10.1183/09031936.00098908.
- McRonald FE, Yadegarfar G, Baldwin DR, Devaraj A, Brain KE, Eisen T, et al. The UK Lung Screen (UKLS): demographic profile of first 88897 approaches provides recommendations for population screening. Cancer Prev Res (Phila) 2014;7:362-71. http://dx.doi.org/10.1158/1940-6207.CAPR-13-0206.
- Jonnalagadda S, Bergamo C, Lin JJ, Lurslurchachai L, Diefenbach M, Smith C, et al. Beliefs and attitudes about lung cancer screening among smokers. Lung Cancer 2012;77:526-31. http://dx.doi.org/10.1016/j.lungcan.2012.05.095.
- Patel D, Akporobaro A, Chinyanganya N, Hackshaw A, Seale C, Spiro SG, et al. Attitudes to participation in a lung cancer screening trial: a qualitative study. Thorax 2012;67:418-25. http://dx.doi.org/10.1136/thoraxjnl-2011-200055.
- Brain K, Parsons E, Bennett P, Cannings-John R, Hood K. The evolution of worry after breast cancer risk assessment: 6-year follow-up of the TRACE study cohort. Psychooncology 2011;20:984-91.
- Holmes-Rovner M, Kroll J, Schmitt N, Rovner DR, Breer ML, Rothert ML, et al. Patient satisfaction with health care decisions: the satisfaction with decision scale. Med Decis Making 1996;16:58-64. http://dx.doi.org/10.1177/0272989X9601600114.
- Clements A, Henderson BJ, Tyndel S, Evans G, Brain K, Austoker J, et al. Diagnosed with breast cancer while on a family history screening programme: an exploratory qualitative study. Eur J Cancer Care (Engl) 2008;17:245-52. http://dx.doi.org/10.1111/j.1365-2354.2007.00837.x.
- Murthy VH, Krumholz HM, Gross CP. Participation in cancer clinical trials: race-, sex-, and age-based disparities. JAMA 2004;291:2720-6. http://dx.doi.org/10.1001/jama.291.22.2720.
- Von Wagner C. Inequalities in participation in an organized national colorectal cancer screening programme: results from the first 2.6 million invitations in England. Int J Epidemiol 2011;40:712-8. http://dx.doi.org/10.1093/ije/dyr008.
- Ironmonger L, Ohuma E, Ormiston-Smith N, Gildea C, Thomson CS, Peake MD. An evaluation of the impact of large-scale interventions to raise public awareness of a lung cancer symptom. Br J Cancer 2015;112:207-16. http://dx.doi.org/10.1038/bjc.2014.596.
- Whynes DK. Economics of cancer screening programmes. Expert Rev Pharmacoecon Outcomes Res 2004;4:61-9. http://dx.doi.org/10.1586/14737167.4.1.61.
- Guide to the Methods of Technology Appraisal. London: NICE; 2008.
- Whynes DK. Nottingham FOB Screening Trial . Cost-effectiveness of screening for colorectal cancer: evidence from the Nottingham faecal occult blood trial. J Med Screen 2004;11:11-5. http://dx.doi.org/10.1258/096914104772950691.
- Whynes DK, Mangham CM, Balfour TW, Scholefield JH. Analysis of deaths occurring within the Nottingham trial of faecal occult blood screening for colorectal cancer. Gut 2010;59:1088-93. http://dx.doi.org/10.1136/gut.2009.192971.
- Humphrey LL, Deffebach M, Pappas M, Baumann C, Artis K, Mitchell JP, et al. Screening for lung cancer with low-dose computed tomography: a systematic review to update the U.S. Preventive Services Task Force recommendation. Ann Intern Med 2013;159:411-20. http://dx.doi.org/10.7326/0003-4819-159-6-201309170-00690.
- Kucharczyk MJ, Menezes RJ, McGregor A, Paul NS, Roberts HC. Assessing the impact of incidental findings in a lung cancer screening study by using low-dose computed tomography. Can Assoc Radiol J 2011;62:141-5. http://dx.doi.org/10.1016/j.carj.2010.02.008.
- Priola AM, Priola SM, Giaj-Levra M, Basso E, Veltri A, Fava C, et al. Clinical implications and added costs of incidental findings in an early detection study of lung cancer by using low-dose spiral computed tomography. Clin Lung Cancer 2013;14:139-48. http://dx.doi.org/10.1016/j.cllc.2012.05.005.
- Department of Health. Reference Costs 2011–12 2012. www.gov.uk/government/uploads/system/uploads/attachment_data/file/213060/2011-12-reference-costs-publication.pdf (accessed May 2016).
- The Diagnosis and Treatment of Lung Cancer (Update of NICE Clinical Guideline 24). Cardiff: NCCC; 2011.
- Whyte S, Chilcott J, Cooper K, Essat M, Stevens J, Wong R, et al. Re-appraisal of the Options for Colorectal Cancer Screening: Report for the NHS Bowel Cancer Screening Programme. Sheffield: School of Health and Related Research; 2011.
- De Haan MC, Thomeer M, Stoker J, Dekker E, Kuipers EJ, van Ballegooijen M. Unit costs in population-based colorectal cancer screening using CT colonography performed in university hospitals in The Netherlands. Eur Radiol 2013;23:897-90. http://dx.doi.org/10.1007/s00330-012-2689-6.
- Logan RFA, Patnick J, Nickerson C, Coleman L, Rutter MD, von Wagner C, et al. Outcomes of the bowel cancer screening programme (BCSP) in England after the first 1 million tests. Gut 2012;61:1439-46. http://dx.doi.org/10.1136/gutjnl-2011-300843.
- International Early Lung Cancer Action Program Investigators . Survival of patients with stage 1 lung cancer detected on CT screening. N Engl J Med 2006;355:1763-71. http://dx.doi.org/10.1056/NEJMoa060476.
- Nawa T, Nakagawa T, Mizoue T, Kusano S, Chonan T, Fukai S, et al. Long-term prognosis of patients with lung cancer detected on low-dose chest computed tomography screening. Lung Cancer 2012;75:197-202. http://dx.doi.org/10.1016/j.lungcan.2011.07.002.
- Sone S, Nakayama T, Honda T, Tsushima K, Li F, Haniuda M, et al. Long-term follow-up study of a population-based 1996–1998 mass screening programme for lung cancer using mobile low-dose spiral computed tomography. Lung Cancer 2007;58:329-41. http://dx.doi.org/10.1016/j.lungcan.2007.06.022.
- Bach PB, Jett JR, Pastroino U, Tockman MS, Swensen SJ, Begg CB. Computed tomography screening and lung cancer outcomes. JAMA 2007;297:953-61. http://dx.doi.org/10.1001/jama.297.9.953.
- Cerfolio RJ, Bryant AS. Survival of patients with true pathologic stage 1 non-small cell lung cancer. Ann Thorac Surg 2009;88:917-23. http://dx.doi.org/10.1016/j.athoracsur.2009.05.040.
- Hung JJ, Jeng WJ, Hsu WH, Huang BS, Wu YC. Time trends of overall survival and survival after recurrence in completely resected stage I non-small cell lung cancer. J Thorac Oncol 2012;7:397-405. http://dx.doi.org/10.1097/JTO.0b013e31823b564a.
- Provencio M, Isla D, Sánchez A, Cantos B. Inoperable stage III non-small cell lung cancer: current treatment and role of vinorelbine. J Thorac Dis 2011;3:197-204.
- Cullen MH, Billingham LJ, Woodroffe CM, Chetiyawardana AD, Gower NH, Joshi R, et al. Mitomycin, ifosfamide, and cisplatin in unresectable non-small-cell lung cancer: effects on survival and quality of life. J Clin Oncol 1999;17:3188-94.
- Ternel JS, Greer JA, Muzikansky A, Gallagher ER, Admane S, Jackson VA, et al. Early palliative care for patients with metastatic non-small-cell lung cancer. N Engl J Med 2010;363:733-42. http://dx.doi.org/10.1056/NEJMoa1000678.
- Walters S, Maringe C, Coleman MP, Peake MD, Butler J, Young N, et al. Lung cancer survival and stage at diagnosis in Australia, Canada, Denmark, Norway, Sweden and the UK: a population-based study, 2004–2007. Thorax 2013;68:551-64. http://dx.doi.org/10.1136/thoraxjnl-2012-202297.
- Solomon T, Rachet B, Whitehead S, Coleman MP. Cancer Survival in England: Patients Diagnosed 2007–2011 and Followed up to 2012. London: Office for National Statistics; 2013.
- Goldberg SW, Mulshine JL, Hagstrom D, Pyenson BS. An actuarial approach to comparing early stage and late stage lung cancer mortality and survival. Popul Health Manag 2010;13:33-46. http://dx.doi.org/10.1089/pop.2009.0010.
- Wu D, Erwin D, Rosner GL. Sojourn time and lead time projection in lung cancer screening. Lung Cancer 2011;72:322-6. http://dx.doi.org/10.1016/j.lungcan.2010.10.010.
- Shmueli A, Fraifeld S, Peretz T, Gutfeld O, Gips M, Sosna J, et al. Cost-effectiveness of baseline low-dose computed tomography screening for lung cancer: the Israeli experience. Value Health 2013;16:922-31. http://dx.doi.org/10.1016/j.jval.2013.05.007.
- Soda H, Oka M, Tomita H, Nagashima S, Soda M, Kohno S. Length and lead time biases in radiologic screening for lung cancer. Respiration 1999;66:511-17. http://dx.doi.org/10.1159/000029450.
- Henschke CI, Yankelevitz DF, Kostis WJ. CT Screening for Lung Cancer. Semin Ultrasound CT MRI 2003;24:23-32. http://dx.doi.org/10.1016/S0887-2171(03)90022-9.
- Patz EF, Pinsky P, Gatsonis C, Sicks JD, Kramer BS, Tammemägi MC, et al. Overdiagnosis in low-dose computed tomography screening for lung cancer. JAMA 2014;174:269-74. http://dx.doi.org/10.1001/jamainternmed.2013.12738.
- McMahon PM, Kong CY, Johnson BE, Weinstein MC, Weeks JC, Kuntz KM, et al. Estimating long-term effectiveness of lung cancer screening in the Mayo CT screening study. Radiology 2008;248:278-87. http://dx.doi.org/10.1148/radiol.2481071446.
- Swensen SJ, Jett JR, Hartman TE, Midthun DE, Mandrekar SJ, Hillman SL, et al. CT screening for lung cancer: five-year prospective experience. Radiology 2005;235:259-65. http://dx.doi.org/10.1148/radiol.2351041662.
- McMahon PM, Kong CY, Weinstein MC, Tramontano AC, Cipriano LE, Johnson BE, et al. Adopting helical CT screening for lung cancer. Cancer 2008;113:3440-9. http://dx.doi.org/10.1002/cncr.23962.
- De Koning HJ, Meza R, Plevritis SK, Ten Haaf K, Munshi VN, Jeon J, et al. Benefits and harms of computed tomography lung cancer screening strategies: a comparative modeling study for the U.S. Preventive Services Task Force. Ann Intern Med 2014;160:311-20. http://dx.doi.org/10.7326/M13-2316.
- National Lung Cancer Audit Report 2013. Leeds: Health and Social Care Information Centre; 2013.
- Kind P, Hardman G, Macran S. UK Population Norms for EQ-5D (Discussion Paper 172). York: Centre for Health Economics, University of York; 1999.
- Black C, Bagust A, Boland A, Walker S, McLeod C, De Verteuil R, et al. The clinical effectiveness and cost-effectiveness of computed tomography screening for lung cancer: systematic reviews. Health Technol Assess 2006;10. http://dx.doi.org/10.3310/hta10030.
- McMahon PM, Kong CY, Bouzan C, Weinstein MC, Cipriano L, Tramontano AC, et al. Cost-effectiveness of CT screening for lung cancer in the US. J Thorac Oncol 2011;6:1841-8. http://dx.doi.org/10.1097/JTO.0b013e31822e59b3.
- Pyenson BS, Sander MS, Jiang Y, Kahn H, Mulshine JL. An actuarial analysis shows that offering lung cancer screening as an insurance benefit would save lives at relatively low cost. Health Aff (Millwood) 2012;31:770-9. http://dx.doi.org/10.1377/hlthaff.2011.0814.
- Villanti AC, Jiang Y, Abrams DB, Pyenson BS. A cost-utility analysis of lung cancer screening and the additional benefits of incorporating smoking cessation interventions. PLOS ONE 2013;8. http://dx.doi.org/10.1371/journal.pone.0071379.
- Cipriano LE, Romanus D, Earle CC, Neville BA, Halpern EF, Gazelle GS, et al. Lung cancer treatment costs, including patient responsibility, by disease stage and treatment modality, 1992 to 2003. Value Health 2011;14:41-52. http://dx.doi.org/10.1016/j.jval.2010.10.006.
- D’Amelio AM, Cassidy A, Asomaning K, Raji OY, Duffy SW, Field JK, et al. Comparison of discriminatory power and accuracy of three lung cancer risk models. Br J Cancer 2010;103:423-9. http://dx.doi.org/10.1038/sj.bjc.6605759.
- Black WC, Gareen IF, Soneji SS, Sicks JD, Keeler EB, Aberle DR, et al. Cost-effectiveness of CT screening in the National Lung Screening Trial. N Engl J Med 2014;371:1793-802. http://dx.doi.org/10.1056/NEJMoa1312547.
- Callister MEJ, Baldwin DR, Akram AR, Barnard S, Cane P, Draffan J, et al. British Society Guidelines for the investigation and management of pulmonary nodules. On behalf of the BTS Standards of Care Committe. Thorax 2015;70:ii1-54. http://dx.doi.org/10.1136/thoraxjnl-2015-207168.
- The Diagnosis and Treatment of Lung Cancer (Update). Cardiff: National Collaborating Centre for Cancer; 2011.
- Lim E, Baldwin D, Beckles M, Duffy J, Entwisle J, Faivre-Finn C, et al. Guidelines on the radical management of patients with lung cancer. Thorax 2010;65:1-27. http://dx.doi.org/10.1136/thx.2010.145938.
- Duffy SW, Field JK, Allgood PC, Seigneurin A. Translation of research results to simple estimates of the likely effect of a lung cancer screening programme in the United Kingdom. Br J Cancer 2014;110:1834-40. http://dx.doi.org/10.1038/bjc.2014.63.
- Iyen-Omofoman B, Tata LJ, Baldwin DR, Smith CJ, Hubbard RB. Using socio-demographic and early clinical features in general practice to identify people with lung cancer earlier. Thorax 2013;68:451-9. http://dx.doi.org/10.1136/thoraxjnl-2012-202348.
Appendix 1 Details of protocol amendments
Details of protocol change | Version: date |
---|---|
Minor administrative changes were made to this version of the protocol. Appendices were also amended to reflect the changes made to other related trial documentation | 2: 10 May 2011 |
An increase to the volume of blood collected from participants (from 20 to 24 ml) was documented here | 3: 20 September 2011 |
A clarification to the exclusion criteria was made here. It was made clear that previous chest CT scans, rather than previous CT scans within the last year, preclude participants from joining the trial | 4: 16 November 2011 |
The increase to the number of participants approached by UKLS from 82,000 to 250,000 was documented within this amendment | 5: 27 February 2012 |
The protocol was amended to reflect nodule management for new nodules identified at the 3-month repeat scan | 6: 4 April 2012 |
Changes were made in protocol version 7 to detail elements of psychosocial analysis. Changes were also made to the nodule care pathway to clarify detail of how nodule growth is measured, and to allow radiological and clinical decisions to be made in order to facilitate appropriate referrals to the MDT | 7: 18 May 2012 |
A change to the frequency of blood collected from participants referred to the MDT was included in this amendment. In addition, the location where tumour tissue is to be stored was changed. Clarification was made to the frequency of psychosocial questionnaires that are sent to participants | 8: 17 July 2012 |
The requirement for follow-up scans to have a second read by the radiologist in RBHT was removed. It was agreed that a 10% random sample would take place each month and the radiologist in RBHT would also assist with queries from the radiologists at the sites | 9: 28 March 2013 |
There was a change to the protocol to allow a further psychosocial questionnaire to be sent to participants 2 weeks after receiving the result of their follow-up scan | 10: 13 June 2013 |
We send out psychosocial questionnaires to individuals post randomisation to the non-screen arm. Individuals in the screen arm receive a psychosocial questionnaire 2 weeks after they receive each CT scan results letter. We will also be randomly selecting a subset of individuals from each arm to receive a psychosocial questionnaire at various time points over the 10-year follow-up period. The protocol was amended to reflect the fact that we will send all active participants a psychosocial questionnaire at certain time points, the first being before the end of 2013 The protocol was amended to reflect the fact that we will be requesting Hospital Episode Statistics data to provide follow-up data alongside the ONS and Cancer Registry data that we currently receive The protocol was amended to reflect the fact that we will write to GPs to request information on the treatment given to participants referred with significant incidental findings The protocol now includes clarification on the analysis that will be carried out on participants’ specimens |
11: 27 September 2013 |
This UKLS amendment is based on a retrospective change to the Liverpool Lung Project risk model, to reflect correctly that the LLPv2 risk model was utilised in the calculation of risk for all of the UKLS participants (see Appendix 2) | 12: 21 January 2014 |
Appendix 2 Details of the Liverpool Lung Project risk model (version 2)
Individuals were selected as eligible to take part in the trial on the basis that their risk of developing lung cancer over the next 5 years was ≥ 5%, based on the LLP risk algorithm. In practice, a lower cut-off point of 4.5% was used in order to correct for a potential underestimation of risk caused by grouping the ‘start smoking’ and ‘stop smoking’ ages into 10-year bands.
In addition, risks for the UKLS were calculated using LLPv2, a modified version of the published LLP model, which incorporates other potential respiratory risk factors (bronchitis, emphysema, tuberculosis and COPD) in addition to pneumonia, and treats cigar and pipe smoking as conferring an identical risk to cigarette smoking. This LLPv2 model was under development as an alternative method of calculating risk, and the circumstances leading to its use in the trial are described below.
A protocol deviation within the UKLS arose as a result of a computing error, whereby the prototype LLPv2 was used for risk calculation instead of the validated basic LLP model. The protocol deviation was confirmed to the UKLS Project Management Team on 17 August 2013. The impact of the computing error was that a proportion of individuals who had been recruited into the UKLS did not reach the high-risk criterion as defined by the basic LLP risk model (956 subjects: 469 in the non-screen arm and 487 in the screen arm).
A comparison of lung cancer prevalence was performed for individuals whose original (published LLP) risk score was > 5% and < 5% risk over 5 years. This indicated lung cancer prevalences of 1.65% and 1.64%, respectively. It was of note that 93% of the 956 ineligible trial participants had a > 3% risk of lung cancer, as defined by the original LLP model; this would be considered sufficiently high risk for inclusion in other lung cancer screening trials.
The protocol deviation was reported to both the Trial Steering Committee and the Data Monitoring Committee. The opinion of the Chairs of both committees was that this was an unfortunate event based on a computing error, but that all of the participants should continue to be included in the overall analysis, particularly as those erroneously included had the same prevalence of lung cancer as those recruited according to protocol. On 1 October 2013, the Ethics Committee indicated that they also agreed with this course of action. In addition, the sponsor and HTA were informed; they too agreed with the action taken.
The prevalence of lung cancer in the UKLS-screened participants who were accidentally included because of other respiratory illnesses (e.g. COPD, bronchitis, emphysema and tuberculosis), or smoking of cigars or pipes, was similar to the prevalence in those correctly included (1.64% compared with 1.65% at the time of analysis). This observation was so striking that, if the UKLS were to continue recruitment, the Project Management Team would submit a protocol amendment to include these subjects in future (i.e. if the main UKLS were funded).
The reporting of the methodology, risk calculation and selection criteria used in the pilot UKLS therefore reflects the actual use of LLPv2. Additional respiratory diseases (i.e. COPD, emphysema, bronchitis and tuberculosis) were added to the model alongside pneumonia. Pipes and cigars were also added into the model alongside cigarettes. This was not validated in other data sets but confirmed within the UKLS population. This is the case in the current report and in associated publications.
Appendix 3 UK Lung Cancer Screening trial documentation
Appendix 4 Trial randomisation method (outlined in Chapter 2)
The same randomisation process had previously been used in the NELSON89 lung cancer screening trial; it had therefore been tested and proven fit for purpose, and capable of achieving balance between the screening and control arms.
At the beginning of the study, the maximum number of subjects was decided (i.e. 250,000 subjects for UKLS). More than 250,000 random personal ID (PID) codes were then generated, each one consisting of eight characters (letters and/or digits), and a unique code was allocated to each subject in the UKLS database (positive and negative responders from the first stage). Individuals who had attended the recruitment clinics, and provided written informed consent, were flagged for randomisation. The PID codes corresponding to these people were used in the randomisation process.
As an example, consider the randomisation of 11 people who had signed consent forms:
-
Simply by sorting the PID codes corresponding to these people, there is already a random division (i.e. the first goes into the screening arm, the second into the control arm, third into the screening arm. . ., etc.). It is therefore pure chance as to which participants are allocated to which group.
-
To eliminate the possibility of staff influencing the process by knowledge of the PID codes, the randomisation programme draws a number between 1 and 7, and rotates the PID code by the same number of positions. The resulting shifted PID codes are sorted alphanumerically, thus the splitting of the group into screening and control arms is now performed on this new order. Addition or removal of one person in a group of PID codes to be randomised will impact upon the order of codes and therefore trial arm allocation. This adds a further random element to the process.
An example is shown below.
Original PID code | Original order | Random number shift of PID by: | |
---|---|---|---|
Three positions (original order rank) | Six positions (original order rank) | ||
ABDDJ3V1 | 1 | ADXP1DA0 (3) | 0WHG0ASC (6) |
CA0AFCKT | 2 | AFCKTCA0 (2) | 3FTGAMBK (8) |
DA0ADXP1 | 3 | AMS6HVYG (10) | 6HVYGAMS(10) |
GA0AW3MZ | 4 | ASC0WHG0 (6) | KTCA0AFC (2) |
GA1FDNSA | 5 | AW3MZGA0 (4) | MQZQ4JGA (11) |
HG0ASC0W | 6 | DJ3V1ABD (1) | MXTU8PGA (9) |
KA1FBXQM | 7 | FBXQMKA1 (7) | MZGA0AW3 (4) |
TGAMBK3F | 8 | FDNSAGA1 (5) | P1DA0ADX (3) |
TU8PGAMX | 9 | JGAMQZQ4 (11) | QMKA1FBX (7) |
VYGAMS6H | 10 | MBK3FTGA (8) | SAGA1FDN (5) |
ZQ4JGAMQ | 11 | PGAMXTU8 (9) | V1ABDDJ3 (1) |
Appendix 5 Data tables showing demographics of response and recruitment
This appendix relates to the trial recruitment procedure described in Chapter 3. Gender, age, socioeconomic status; region (north vs. south) and smoking status were analysed with respect to (1) response to the initial invitation letter; (2) risk status; and (3) participation of high-risk people in the UKLS trial. The numbers involved are tabulated in this appendix; the data are arranged in sets of tables, grouped according to which sociodemographic factor is being considered, as per the layout in Chapter 3. Further tables are included for when a more detailed analysis took place or when two sociodemographic factors were considered together. For the overall figures (on this page), the sections of the table showing the pathway to the RCT are shaded. For further clarification as to how the different analysis categories were defined, refer to the CONSORT diagram (see Figure 2).
Overall figures
Response to initial invitation letter
Positive response | Negative response | No response | Total | |
---|---|---|---|---|
Total | 75,958 (30.7%) | 22,788 (9.2%) | 148,608 (60.1%) | 247,354 (100.0%) |
Risk status
High risk | Low risk | Unknown risk | Total | |
---|---|---|---|---|
Total | 8729 (11.5%) | 66,377 (87.4%) | 852 (1.1%) | 75,958 (100.0%) |
Participation of high-risk people in the screening trial
Consented | Opted out | Not eligible | Total | |
---|---|---|---|---|
Total | 4061 (46.5%) | 3377 (38.7%) | 1291 (14.8%) | 8729 (100.0%) |
Gender
Gender | Positive response, n (%) | Negative response, n (%) | No response, n (%) | Total |
---|---|---|---|---|
Male | 37,525 (30.3) | 9557 (7.7) | 76,666 (62.0) | 123,748 |
Female | 38,433 (31.1) | 13,231 (10.7) | 71,942 (58.2) | 123,606 |
Total | 75,958 | 22,788 | 148,608 | 247,354 |
Gender | High risk, n (%) | Low risk, n (%) | Unknown risk, n (%) | Total |
---|---|---|---|---|
Male | 6141 (16.4) | 30,794 (82.1) | 590 (1.6) | 37,525 |
Female | 2588 (6.7) | 35,583 (92.6) | 262 (0.7) | 38,433 |
Total | 8729 | 66,377 | 852 | 75,958 |
Gender | Consented, n (%) | Opted out, n (%) | Not eligible, n (%) | Total |
---|---|---|---|---|
Male | 3041 (49.5) | 2198 (35.8) | 902 (14.7) | 6141 |
Female | 1020 (39.4) | 1179 (45.5) | 389 (15.0) | 2588 |
Total | 4061 | 3377 | 1291 | 8729 |
Age
Age group (years) | Positive response, n (%) | Negative response, n (%) | No response, n (%) | Total |
---|---|---|---|---|
50–55 | 16,273 (26.6) | 3502 (5.7) | 41,393 (67.7) | 61,168 |
56–60 | 12,892 (30.3) | 3177 (7.5) | 26,476 (62.2) | 42,545 |
61–65 | 21,226 (35.0) | 5573 (9.2) | 33,845 (55.8) | 60,644 |
66–70 | 17,302 (32.6) | 5817 (11.0) | 29,936 (56.4) | 53,055 |
71–75 | 8265 (27.6) | 4719 (15.8) | 16958 (56.6) | 29942 |
Total | 75,958 | 22,788 | 148,608 | 247,354 |
Age group (years) | High risk, n (%) | Low risk, n (%) | Unknown risk, n (%) | Total |
---|---|---|---|---|
50–55 | 82 (0.5) | 16,170 (99.4) | 21 (0.1) | 16,273 |
56–60 | 507 (3.9) | 12,331 (95.6) | 54 (0.4) | 12,892 |
61–65 | 2726 (12.8) | 18,255 (86.0) | 245 (1.2) | 21,226 |
66–70 | 3368 (19.5) | 13,617 (78.7) | 317 (1.8) | 17,302 |
71–75 | 2046 (24.8) | 6004 (72.6) | 215 (2.6) | 8265 |
Total | 8729 | 66,377 | 852 | 75,958 |
Age group (years) | Consented, n (%) | Opted out, n (%) | Not eligible, n (%) | Total |
---|---|---|---|---|
50–55 | 29 (35.4) | 43 (52.4) | 10 (12.2) | 82 |
56–60 | 205 (40.4) | 196 (38.7) | 106 (20.9) | 507 |
61–65 | 1298 (47.6) | 1001 (36.7) | 427 (15.7) | 2726 |
66–70 | 1618 (48.0) | 1277 (37.9) | 473 (14.0) | 3368 |
71–75 | 911 (44.5) | 860 (42.0) | 275 (13.4) | 2046 |
Total | 4061 | 3377 | 1291 | 8729 |
Risk status by individual year of age
Age, years | High risk | Low risk | Unknown risk | Total | % at high risk |
---|---|---|---|---|---|
50 | 1 | 2041 | 0 | 2042 | 0.0 |
51 | 0 | 1780 | 0 | 1780 | 0.0 |
52 | 1 | 1916 | 0 | 1917 | 0.1 |
53 | 1 | 2368 | 2 | 2371 | 0.0 |
54 | 28 | 3981 | 4 | 4013 | 0.7 |
55 | 51 | 4084 | 15 | 4150 | 1.2 |
56 | 53 | 3503 | 3 | 3559 | 1.5 |
57 | 52 | 2301 | 8 | 2361 | 2.2 |
58 | 63 | 1977 | 5 | 2045 | 3.1 |
59 | 138 | 2097 | 9 | 2244 | 6.1 |
60 | 201 | 2453 | 29 | 2683 | 7.5 |
61 | 285 | 3001 | 28 | 3314 | 8.6 |
62 | 343 | 3273 | 29 | 3645 | 9.4 |
63 | 421 | 3704 | 49 | 4174 | 10.1 |
64 | 773 | 4360 | 58 | 5191 | 14.9 |
65 | 904 | 3917 | 81 | 4902 | 18.4 |
66 | 658 | 3144 | 61 | 3863 | 17.0 |
67 | 755 | 3229 | 67 | 4051 | 18.6 |
68 | 727 | 2808 | 62 | 3597 | 20.2 |
69 | 654 | 2468 | 67 | 3189 | 20.5 |
70 | 574 | 1968 | 60 | 2602 | 22.1 |
71 | 667 | 1937 | 90 | 2694 | 24.8 |
72 | 446 | 1417 | 37 | 1900 | 23.5 |
73 | 377 | 1062 | 31 | 1470 | 25.6 |
74 | 354 | 943 | 30 | 1327 | 26.7 |
75a | 202 | 645 | 27 | 874 | 23.1 |
Socioeconomic status
IMD; all | Positive response, n (%) | Negative response, n (%) | No response, n (%) | Total |
---|---|---|---|---|
Q1 most deprived | 13,893 (21.7) | 4265 (6.7) | 45,882 (71.6) | 64,040 |
Q2 | 9813 (27.6) | 3300 (9.3) | 22,453 (63.1) | 35,566 |
Q3 | 13,337 (30.5) | 4375 (10.0) | 26,060 (59.5) | 43,772 |
Q4 | 16,707 (34.8) | 4933 (10.3) | 26,407 (55.0) | 48,047 |
Q5 least deprived | 22,198 (39.7) | 5913 (10.6) | 27,792 (49.7) | 55,903 |
Missing | 10 (0.0) | 2 (0.0) | 14 (0.0) | 26 |
Total | 75,958 | 22,788 | 148,608 | 247,354 |
IMD; all | High risk, n (%) | Low risk, n (%) | Unknown risk, n (%) | Total |
---|---|---|---|---|
Q1 most deprived | 2530 (18.2) | 11,102 (79.9) | 261 (1.9) | 13,893 |
Q2 | 1259 (12.8) | 8425 (85.9) | 129 (1.3) | 9813 |
Q3 | 1558 (11.7) | 11,625 (87.2) | 154 (1.2) | 13,337 |
Q4 | 1541 (9.2) | 15,033 (90.0) | 133 (0.8) | 16707 |
Q5 least deprived | 1840 (8.3) | 20,183 (90.9) | 175 (0.8) | 22,198 |
Missing | 1 (0.0) | 9 (0.0) | (0.0) | 10 |
Total | 8729 | 66,377 | 852 | 75,958 |
IMD; all | Consented, n (%) | Opted out, n (%) | Not eligible, n (%) | Total |
---|---|---|---|---|
Q1 most deprived | 1090 (43.1) | 1110 (43.9) | 330 (13.0) | 2530 |
Q2 | 487 (38.7) | 533 (42.3) | 239 (19.0) | 1259 |
Q3 | 723 (46.4) | 589 (37.8) | 246 (15.8) | 1558 |
Q4 | 732 (47.5) | 537 (34.8) | 272 (17.7) | 1541 |
Q5 least deprived | 1029 (55.9) | 607 (33.0) | 204 (11.1) | 1840 |
Missing | (0.0) | 1 (0.0) | (0.0) | 1 |
Total | 4061 | 3377 | 1291 | 8729 |
Region
Region | Positive response, n (%) | Negative response, n (%) | No response, n (%) | Total |
---|---|---|---|---|
North | 32,609 (26.4) | 9873 (8.0) | 81,049 (65.6) | 123,531 |
South | 43,349 (35.0) | 12,915 (10.4) | 67,559 (54.6) | 123,823 |
Total | 75,958 | 22,788 | 148,608 | 247,354 |
Region | High risk, n (%) | Low risk, n (%) | Unknown risk, n (%) | Total |
---|---|---|---|---|
North | 4496 (13.8) | 27674 (84.9) | 439 (1.3) | 32,609 |
South | 4233 (9.8) | 38703 (89.3) | 413 (1.0) | 43,349 |
Total | 8729 | 66377 | 852 | 75,958 |
Region | Consented, n (%) | Opted out, n (%) | Not eligible, n (%) | Total |
---|---|---|---|---|
North | 2050 (45.6) | 1785 (39.7) | 661 (14.7) | 4496 |
South | 2011 (47.5) | 1592 (37.6) | 630 (14.9) | 4233 |
Total | 4061 | 3377 | 1291 | 8729 |
Region and Index of Multiple Deprivation: north
IMD; north | Positive response, n (%) | Negative response, n (%) | No response, n (%) | Total |
---|---|---|---|---|
Q1 most deprived | 11,542 (21.2) | 3403 (6.3) | 39,448 (72.5) | 54,393 |
Q2 | 5178 (27.1) | 1602 (8.4) | 12,338 (64.5) | 19,118 |
Q3 | 6380 (29.1) | 1997 (9.1) | 13,567 (61.8) | 21,944 |
Q4 | 6095 (33.3) | 1761 (9.6) | 10,467 (57.1) | 18,323 |
Q5 least deprived | 3413 (35.0) | 1108 (11.4) | 5226 (53.6) | 9747 |
Missing | 1 (0.0) | 2 (0.0) | 3 (0.0) | 6 |
Total | 32,609 | 9873 | 81,049 | 123,531 |
IMD; north | High risk, n (%) | Low risk, n (%) | Unknown risk, n (%) | Total |
---|---|---|---|---|
Q1 most deprived | 2152 (18.6) | 9169 (79.4) | 221 (1.9) | 11542 |
Q2 | 702 (13.6) | 4412 (85.2) | 64 (1.2) | 5178 |
Q3 | 762 (11.9) | 5538 (86.8) | 80 (1.3) | 6380 |
Q4 | 576 (9.5) | 5472 (89.8) | 47 (0.8) | 6095 |
Q5 least deprived | 303 (8.9) | 3083 (90.3) | 27 (0.8) | 3413 |
Missing | 1 (0.0) | (0.0) | (0.0) | 1 |
Total | 4496 | 27,674 | 439 | 32,609 |
IMD, north | Consented, n (%) | Opted out, n (%) | Not eligible, n (%) | Total |
---|---|---|---|---|
Q1 most deprived | 1022 (47.5) | 902 (41.9) | 228 (10.6) | 2152 |
Q2 | 306 (43.6) | 277 (39.5) | 119 (17.0) | 702 |
Q3 | 363 (47.6) | 283 (37.1) | 116 (15.2) | 762 |
Q4 | 252 (43.8) | 200 (34.7) | 124 (21.5) | 576 |
Q5 least deprived | 107 (35.3) | 122 (40.3) | 74 (24.4) | 303 |
Missing | (0.0) | 1 (0.0) | (0.0) | 1 |
Total | 2050 | 1785 | 661 | 4496 |
Region and Index of Multiple Deprivation: south
IMD; south | Positive response, n (%) | Negative response, n (%) | No response, n (%) | Total |
---|---|---|---|---|
Q1 most deprived | 2351 (24.4) | 862 (8.9) | 6434 (66.7) | 9647 |
Q2 | 4635 (28.2) | 1698 (10.3) | 10,115 (61.5) | 16,448 |
Q3 | 6957 (31.9) | 2378 (10.9) | 12,493 (57.2) | 21,828 |
Q4 | 10612 (35.7) | 3172 (10.7) | 15,940 (53.6) | 29,724 |
Q5 least deprived | 18,785 (40.7) | 4805 (10.4) | 22,566 (48.9) | 46,156 |
Missing | 9 (0.0) | (0.0) | 11 (0.0) | 20 |
Total | 43,349 | 12,915 | 67,559 | 123,823 |
IMD; south | High risk, n (%) | Low risk, n (%) | Unknown risk, n (%) | Total |
---|---|---|---|---|
Q1 most deprived | 378 (16.1) | 1933 (82.2) | 40 (1.7) | 2351 |
Q2 | 557 (12.0) | 4013 (86.6) | 65 (1.4) | 4635 |
Q3 | 796 (11.4) | 6087 (87.5) | 74 (1.1) | 6957 |
Q4 | 965 (9.1) | 9561 (90.1) | 86 (0.8) | 10,612 |
Q5 least deprived | 1537 (8.2) | 17,100 (91.0) | 148 (0.8) | 18,785 |
Missing | (0.0) | 9 (0.0) | (0.0) | 9 |
Total | 4233 | 38,703 | 413 | 43,349 |
IMD, south | Consented, n (%) | Opted out, n (%) | Not eligible, n (%) | Total |
---|---|---|---|---|
Q1 most deprived | 68 (18.0) | 208 (55.0) | 102 (27.0) | 378 |
Q2 | 181 (32.5) | 256 (46.0) | 120 (21.5) | 557 |
Q3 | 360 (45.2) | 306 (38.4) | 130 (16.3) | 796 |
Q4 | 480 (49.7) | 337 (34.9) | 148 (15.3) | 965 |
Q5 least deprived | 922 (60.0) | 485 (31.6) | 130 (8.5) | 1537 |
Missing | (0.0) | (0.0) | (0.0) | 0 |
Total | 2011 | 1592 | 630 | 4233 |
Smoking status
Smoking | Positive response, n (%) | Negative response, n (%) | Total |
---|---|---|---|
Current smoker | 11,130 (14.7) | 2078 (9.1) | 13,208 |
Ex-smoker | 29,855 (39.3) | 8260 (36.2) | 38,115 |
Never-smoker | 32,949 (43.4) | 11,686 (51.3) | 44,635 |
Missing | 2024 (2.7) | 764 (3.4) | 2788 |
Total | 75,958 | 22,788 | 98,746 |
Smoking | High risk, n (%) | Low risk, n (%) | Unknown risk, n (%) | Total |
---|---|---|---|---|
Current smoker | 3724 (33.5) | 7080 (63.6) | 326 (2.9) | 11,130 |
Ex-smoker | 4995 (16.7) | 24,335 (81.5) | 525 (1.8) | 29,855 |
Never-smoker | 10 (0.0) | 32,938 (100.0) | 1 (0.0) | 32,949 |
Missing | (0.0) | 2024 (100.0) | (0.0) | 2024 |
Total | 8729 | 66,377 | 852 | 75,958 |
Smoking | Consented, n (%) | Opted out, n (%) | Not eligible, n (%) | Total |
---|---|---|---|---|
Current smoker | 1568 (42.1) | 1620 (43.5) | 536 (14.4) | 3724 |
Ex-smoker | 2491 (49.9) | 1753 (35.1) | 751 (15.0) | 4995 |
Never-smoker | 2 (20.0) | 4 (40.0) | 4 (40.0) | 10 |
Total | 4061 | 3377 | 1291 | 8729 |
Smoking, Index of Multiple Deprivation and response status
Positive responders
IMD vs. smoking; positive responders | Current smoker, n (%) | Ex-smoker, n (%) | Never-smoker, n (%) | Missing, n (%) | Total |
---|---|---|---|---|---|
Q1 most deprived | 3832 (27.6) | 5362 (38.6) | 4142 (29.8) | 557 (4.0) | 13,893 |
Q2 | 1659 (16.9) | 3962 (40.4) | 3890 (39.6) | 302 (3.1) | 9813 |
Q3 | 1796 (13.5) | 5410 (40.6) | 5766 (43.2) | 365 (2.7) | 13,337 |
Q4 | 1818 (10.9) | 6539 (39.1) | 7960 (47.6) | 390 (2.3) | 16,707 |
Q5 least deprived | 2024 (9.1) | 8578 (38.6) | 11,186 (50.4) | 410 (1.8) | 22,198 |
Missing | 1 (10.0) | 4 (40.0) | 5 (50.0) | (0.0) | 10 |
Total | 11,130 (14.7) | 29,855 (39.3) | 32,949 (43.4) | 2024 (2.7) | 75,958 (100.0) |
Negative responders
IMD vs. smoking; negative responders | Current smoker, n (%) | Ex-smoker, n (%) | Never-smoker, n (%) | Missing, n (%) | Total |
---|---|---|---|---|---|
Q1 most deprived | 672 (15.8) | 1771 (41.5) | 1677 (39.3) | 145 (3.4) | 4265 |
Q2 | 359 (10.9) | 1265 (38.3) | 1558 (47.2) | 118 (3.6) | 3300 |
Q3 | 380 (8.7) | 1565 (35.8) | 2270 (51.9) | 160 (3.7) | 4375 |
Q4 | 335 (6.8) | 1754 (35.6) | 2677 (54.3) | 167 (3.4) | 4933 |
Q5 least deprived | 332 (5.6) | 1904 (32.2) | 3503 (59.2) | 174 (2.9) | 5913 |
Missing | (0.0) | 1 (50.0) | 1 (50.0) | (0.0) | 2 |
Total | 2078 (9.1) | 8260 (36.2) | 11,686 (51.3) | 764 (3.4) | 22,788 (100.0) |
Population smoking figures by Index of Multiple Deprivation quintile
These figures are derived from the Health Survey for England 2010 (HSE), available via the UK Data Service (www.ukdataservice.ac.uk). Figures relate to the per-IMD quintile percentages for cigarette smoking in 3246 individuals aged 50–75 years. The age distribution within this sample from the HSE 2010 data did not differ significantly from that of the UKLS sample, so a direct comparison was possible.
IMD | Current smoker (%) | Ex-smoker (%) | Never-smoker (%) |
---|---|---|---|
Q1 most deprived | 28.2 | 34.3 | 37.5 |
Q2 | 22.2 | 35.5 | 42.4 |
Q3 | 18.5 | 38.1 | 43.4 |
Q4 | 12.8 | 34.9 | 52.4 |
Q5 least deprived | 8 | 35.9 | 56 |
Appendix 6 Summary report of UK Lung Cancer Screening sample quality control
The UKLS samples were randomly selected (within groups chosen to represent each recruitment centre) and subjected to standard biomolecule extraction, quantitation and quality control procedures. In some cases extractions were performed alongside samples from the LLP Biobank to provide a benchmark.
Number | Sample type | Extraction | Quality control |
---|---|---|---|
1 | EDTA Separator remnants | DNA by Qiagen kit | Spectrophotometry, agarose gel electrophoresis |
2 | Blood cells | DNA by Qiagen kit (QI Aamp® Blood Mini Kit, Qiagen, Manchester, UK) | Spectrophotometry, agarose gel electrophoresis |
3 | Blood cells | DNA by Source Bioscience (Nottingham, UK) | Spectrophotometry, agarose gel electrophoresis |
4 | Buccal scrape | DNA by Qiagen kit (Gentra® Puregene® Buccal Cell Kit, Qiagen, Manchester, UK) | Spectrophotometry, agarose gel electrophoresis |
5 | Nasal scrape | DNA by Qiagen kit | Spectrophotometry, agarose gel electrophoresis |
6 | Buccal scrape | RNA by Qiagen kit | Spectrophotometry, Qubit (Qubit™ fluorimeter, Life Technologies, Paisley, UK), Agilent Bioanalyzer (Agilent 2100 Bioanalyzer, Agilent Technologies UK Ltd, Stockport, UK) |
7 | Nasal scrape | RNA by Qiagen kit | Spectrophotometry, Qubit, Agilent Bioanalyzer |
8 | Plasma | miRNA by Norgen kit (Norgen Biotek Corp., Genflow, Lichfield, UK) | Quantitative RT-PCR (10 miRNA + RNU48) |
9 | Sputum | Shandon Cytospin® 3 (Thermo-Shandon, Runcorn, UK) | Sputum adequacy |
Deoxyribonucleic acid (DNA) was extracted for 10 of each of the approximately 4-ml remnants of 8-ml EDTA Separator tubes (Vacuette® K2 EDTA tubes, Greiner Bio-One Ltd, Stonehouse UK) after removal of plasma (see 1 in table) or from single aliquots of cell pellets from approximately 1.5 ml of ethylenediaminetetraacetic acid (EDTA) blood (2). Yields were better for cells than for plasma gel-tube remnants, equating to approximately 45 µg of DNA per 9-ml blood tube (in keeping with expectations) and 3.2 µg of DNA per 8-ml plasma gel tube. DNA quality, as assessed by spectrophotometry (A260-nm quantitation plus A260 nm : A280 nm and A260 nm : A230 nm ratios for DNA quality) was similar for all samples tested, but, again, gel tubes gave slightly poorer quality DNA (as often associated with lower yields). DNA integrity, assessed by agarose gel electrophoresis, was equivalent across all samples.
Having established a baseline for DNA yield in-house, blood cell samples from 80 individuals were commercially extracted by Source Bioscience (3). Quantitation and quality control were performed by Source Bioscience using a spectrophotometric plate reader. Results were analysed in respect to study site (Liverpool or Papworth), study nurse and date of collection. The majority of DNA yields were between 10 and 40 µg, in keeping with what was expected. There was some evidence of greater yields from Liverpool samples, but there was no difference in DNA quality and no obvious trend for any effect study nurse and date of collection.
Buccal (4) and nasal (5) DNA samples were prepared in parallel from samples stored in Saccomanno’s cytology fixative. In keeping with previous experience of these sampling techniques, there was considerable variation in DNA yield between samples. Although 90% of buccal samples gave > 20 ng of DNA (compared with 70% of nasal samples), the mean yield of DNA was greater for nasal samples (2.5 µg compared with 0.12 µg), with a concomitant increase in DNA quality. Therefore, although the majority of samples would be suitable for polymerase chain reaction (PCR)-based analysis, nasal samples might be better suited to more DNA-hungry discovery-phase investigations.
For ribonucleic acid (RNA), later samples of buccal (6) and nasal (7) scrapes, the nasal samples were superior in all measurements. Whereas 6 of 10 nasal samples gave Qubit concentrations of > 10 ng/µl (range 15–120 ng/µl) and RNA integrity number (RIN) values of 6.1–7.8, only 4 of 10 buccal samples gave similar amounts (range 15–69 ng/µl) but with lower RIN values of 0–5.9. The proportion of samples with very low yield is similar to those for DNA, indicating that sampling technique, rather than storage or extraction, may be a factor. The generally poor results for buccal RNA reflect the harsher environment in the buccal cavity for RNA (which is known to be more susceptible that DNA). Some improvement in yield might be achieved with new extraction techniques, but current results favour the use of nasal samples for RNA analysis. RNAlater can also be used for DNA extraction, so this may be a sensible alternative use for the buccal RNAlater samples.
Plasma (8) microribonucleic acid (microRNA) was subject to multiplex real-time and quantitative PCR for a panel consisting of RNU48 and 10 plasma miRNA previously reported to be expressed in plasma. Mean raw Ct (Cycle threshold) values for UKLS samples were significantly lower (Student’s t-test) and distributions significantly different (Mann–Whitney) for several assays, indicating that UKLS samples gave similar, or better, yields of microRNA than LLP samples collected over a similar time frame.
Sputum samples (9) have been prepared for cytological evaluation by standard means and are awaiting analysis by an independent cytologist.
Appendix 7 Psychosocial tables
Demographics | T0 completers (N = 4039)a | T0 non-completers (N = 22)a |
---|---|---|
Trial arm, n (%) | ||
Intervention | 2018 (50) | 10 (46) |
Control | 2019 (50) | 8 (36) |
Not randomised | 2 (< 1) | 4 (18) |
Site, n (%) | ||
Liverpool | 2033 (50) | 17 (77) |
Papworth | 2006 (50) | 5 (23) |
Age, mean (SD) | 67.66 (4.09) | 66.32 (4.10) |
Gender, n (%) | ||
Male | 3021 (75) | 20 (91) |
Female | 1018 (25) | 2 (9) |
Ethnicity, n (%) | ||
White | 3985 (99) | 8 (89) |
Non-white | 38 (1) | 1 (11) |
Marital status, n (%) | ||
Married/cohabiting | 2955 (73) | 8 (80) |
Not married/cohabitingb | 1073 (27) | 2 (20) |
IMD, n (%) | ||
Quintile 1 | 1079 (27) | 11 (50) |
Quintile 2 | 486 (12) | 1 (5) |
Quintile 3 | 719 (18) | 4 (18) |
Quintile 4 | 729 (18) | 3 (14) |
Quintile 5 | 1026 (25) | 3 (14) |
Smoking status (UKLS questionnaire 1), n (%) | ||
Current smoker | 1559 (39) | 9 (41) |
Ex-smoker | 2478 (61) | 13 (59) |
Never-smoker | 2 (< 1) | 0 (0) |
Demographics | Attended CT scan (N = 1987)a,b | Did not attend CT scan (N = 31)a |
---|---|---|
Site, n (%) | ||
Liverpool | 1001 (50) | 15 (48) |
Papworth | 986 (50) | 16 (52) |
Age, mean (SD) | 67.71 (4.03) | 68.42 (4.85) |
Gender, n (%) | ||
Male | 1498 (75) | 22 (71) |
Female | 489 (25) | 9 (29) |
Education, n (%)c | ||
Up to GCSE/O-level or equivalent | 667 (46) | 11 (55) |
Beyond GCSE/O-level or equivalent | 779 (54) | 9 (45) |
Ethnicity, n (%) | ||
White | 1961 (99) | 31 (100) |
Non-white | 18 (1) | 0 (0) |
Marital status, n (%) | ||
Married/cohabiting | 1462 (74) | 21 (70) |
Not married/cohabitingd | 519 (26) | 9 (30) |
IMD, n (%) | ||
Quintile 1 | 537 (27) | 8 (26) |
Quintile 2 | 239 (12) | 4 (13) |
Quintile 3 | 354 (18) | 4 (13) |
Quintile 4 | 347 (18) | 6 (19) |
Quintile 5 | 510 (26) | 9 (29) |
Smoking status (UKLS questionnaire 1), n (%) | ||
Current smoker | 759 (38) | 13 (42) |
Ex-smoker | 1226 (62) | 18 (58) |
Never-smoker | 2 (< 1) | 0 (0) |
Experience of lung cancer (T0), n (%) | ||
No | 1153 (58) | 15 (48) |
Yes | 830 (42) | 16 (52) |
Cancer distress (T0), mean (SD)e | 2.17 (0.29) | 2.22 (0.31) |
Anxiety (T0), mean (SD)e | 1.55 (0.71) | 1.63 (0.60) |
Depression (T0), mean (SD)e | 1.30 (0.68) | 1.43 (0.69) |
Decision satisfaction (T0), n (%) | ||
Not very satisfied | 1202 (61) | 26 (84) |
Very satisfied | 781 (39) | 5 (16) |
Demographics | Negative (N = 763)a | Negative with incidental finding (N = 41)a | Positive for repeat scan (N = 788)a | Positive for MDT referral (N = 48)a | Test statistic (p-value) |
---|---|---|---|---|---|
Site, n (%) | |||||
Liverpool | 376 (49) | 25 (61) | 385 (49) | 27 (56) | χ2(3) = 3.2 (0.37) |
Papworth | 387 (51) | 16 (39) | 403 (51) | 21 (44) | |
Age, mean (SD) | 67.63 (3.94) | 67.88 (3.69) | 67.76 (3.94) | 67.67 (3.95) | F(3,1636) = 0.2 (0.91) |
Gender, n (%) | |||||
Male | 594 (78) | 30 (73) | 581 (74) | 37 (77) | χ2(3) = 3.8 (0.29) |
Female | 169 (22) | 11 (27) | 207 (26) | 11 (23) | |
Education, n (%)b | |||||
Up to GCSE/O-level or equivalent | 228 (42) | 18 (60) | 256 (46) | 9 (29) | χ2(3) = 7.6 (0.06) |
Beyond GCSE/O-level or equivalent | 315 (58) | 12 (40) | 303 (54) | 22 (71) | |
Ethnicity, n (%) | |||||
White | 755 (99) | 40 (98) | 777 (> 99) | 47 (98) | N/A |
Non-white | 7 (1) | 1 (2) | 4 (1) | 1 (2) | |
Marital status, n (%) | |||||
Married/cohabiting | 585 (77) | 25 (61) | 583 (74) | 37 (77) | χ2(3) = 5.8 (0.12) |
Not married/cohabitingc | 177 (23) | 16 (39) | 201 (26) | 11 (23) | |
IMD, n (%) | |||||
Quintile 1 | 204 (27) | 11 (27) | 208 (26) | 19 (40) | N/A |
Quintile 2 | 94 (12) | 5 (12) | 90 (11) | 2 (4) | |
Quintile 3 | 135 (18) | 7 (17) | 139 (18) | 9 (19) | |
Quintile 4 | 121 (16) | 7 (17) | 144 (18) | 8 (17) | |
Quintile 5 | 209 (27) | 11 (27) | 207 (26) | 10 (21) | |
Smoking status (UKLS questionnaire 1), n (%) | |||||
Current smoker | 275 (36) | 12 (29) | 305 (39) | 23 (48) | χ2(3) = 4.6 (0.20) |
Ex-smoker | 488 (64) | 29 (71) | 482 (61) | 25 (52) | |
Never-smoker | 0 (0) | 0 (0) | 1 (< 1) | 0 (0) | N/A |
Experience of lung cancer (T0), n (%) | |||||
No | 462 (61) | 24 (59) | 453 (58) | 26 (54) | χ2(3) = 2.0 (0.57) |
Yes | 299 (39) | 17 (42) | 333 (42) | 22 (46) | |
Cancer distress (T0), mean (SD)d | 2.17 (0.28) 8.73 | 2.19 (0.29) 8.93 | 2.16 (0.29) 8.66 | 2.17 (0.32) 8.80 | F(3,1632) = 0.2 (0.88) |
Anxiety (T0), mean (SD)d | 1.55 (0.68) 3.69 | 1.43 (0.70) 3.19 | 1.53 (0.73) 3.61 | 1.49 (0.72) 3.44 | F(3,1634) = 0.4 (0.73) |
Depression (T0), mean (SD)d | 1.29 (0.66) 2.63 | 1. 19 (0.65) 2.28 | 1.28 (0.70) 2.59 | 1.10 (0.70) 2.01 | F(3,1634) = 1.3 (0.26) |
Decision satisfaction (T0), n (%) | |||||
Not very satisfied | 445 (58) | 26 (63) | 489 (62) | 31 (65) | χ2(3) = 2.8 (0.43) |
Very satisfied | 317 (42) | 15 (37) | 298 (38) | 17 (35) |
Demographics | Intervention (N = 1553)a | Control (N = 1302)a | Test statistic (p-value) |
---|---|---|---|
Site, n (%) | |||
Liverpool | 735 (47) | 601 (46) | χ2(1) = 0.39 (0.53) |
Papworth | 818 (53) | 701 (54) | |
Age, mean (SD) | 67.77 (3.98) | 67.82 (3.98) | t(2853) = 0.04 (0.77) |
Gender, n (%) | |||
Male | 1189 (77) | 977 (75) | χ2(1) = 0.90 (0.34) |
Female | 364 (23) | 325 (25) | |
Education, n (%)b | |||
Up to GCSE/O-level or equivalent | 509 (44) | 431 (44) | χ2(1) = 0.09 (0.77) |
Beyond GCSE/O-level or equivalent | 640 (56) | 556 (56) | |
Ethnicity, n (%) | |||
White | 1536 (99) | 1289 (99) | χ2(1) = 0.03 (0.86) |
Non-white | 11 (1) | 10 (1) | |
Marital status, n (%) | |||
Married/cohabiting | 1171 (76) | 1003 (77) | χ2(1) = 0.82 (0.37) |
Not married/cohabitingc | 377 (24) | 298 (23) | |
IMD, n (%) | |||
Quintile 1 | 378 (24) | 290 (22) | χ2(4) = 2.61 (0.63) |
Quintile 2 | 186 (12) | 152 (12) | |
Quintile 3 | 287 (19) | 236 (18) | |
Quintile 4 | 286 (18) | 259 (20) | |
Quintile 5 | 416 (27) | 365 (28) | |
Smoking status (UKLS questionnaire 1), n (%) | |||
Current smoker | 568 (37) | 441 (34) | χ2(1) = 2.30 (0.13) |
Ex-smoker | 984 (63) | 861 (66) | |
Never-smoker | 1 (< 1) | 0 (0) | N/A |
Experience of lung cancer (T0), n (%) | |||
No | 916 (59) | 767 (59) | χ2(1) = 0.001 (0.98) |
Yes | 634 (41) | 532 (41) | |
Cancer distress (T0), mean (SD)d | 2.16 (0.28) 8.69 | 2.15 (0.28) 8.58 | t(2845) = –1.24 (0.22) |
Anxiety (T0), mean (SD)d | 1.52 (0.71) 3.56 | 1.50 (0.71) 3.47 | t(2848) = –0.74 (0.46) |
Depression (T0), mean (SD)d | 1.26 (0.67) 2.54 | 1.25 (0.66) 2.47 | t(2845) = –0.71 (0.48) |
Decision satisfaction (T0), n (%) | |||
Not very satisfied | 932 (60) | 736 (57) | χ2(1) = 3.33 (0.07) |
Very satisfied | 618 (40) | 561 (43) |
Demographics | True negative (N = 740)a | Incidental finding (N = 78)a | False positive (N = 445)a | True positive (N = 23)a | Test statistic (p-value) |
---|---|---|---|---|---|
Site, n (%) | |||||
Liverpool | 348 (47) | 43 (55) | 218 (49) | 14 (61) | χ2(3) = 3.5 (0.33) |
Papworth | 392 (53) | 35 (45) | 227 (51) | 9 (39) | |
Age, mean (SD) | 67.69 (3.95) | 67.83 (3.95) | 67.88 (4.04) | 67.30 (4.63) | F(3,1282) = 0.3 (0.82) |
Gender, n (%) | |||||
Male | 584 (79) | 54 (69) | 336 (76) | 19 (83) | χχ2(3) = 5.2 (0.16) |
Female | 156 (21) | 24 (31) | 109 (25) | 4 (17) | |
Education, n (%)b | |||||
Up to GCSE/O-level or equivalent | 229 (41) | 33 (55) | 176 (51) | 8 (47) | χχ2(3) = 9.7 (0.02) |
Beyond GCSE/O-level or equivalent | 324 (59) | 27 (45) | 171 (49) | 9 (53) | |
Ethnicity, n (%) | |||||
White | 734 (99) | 77 (99) | 436 (99) | 23 (100) | N/A |
Non-white | 5 (1) | 1 (1) | 5 (1) | 0 (0) | |
Marital status, n (%) | |||||
Married/cohabiting | 563 (76) | 53 (68) | 336 (76) | 22 (96) | χ2(3) = 7.6 (0.06) |
Not married/cohabitingc | 177 (24) | 25 (32) | 106 (24) | 1 (4) | |
IMD, n (%) | |||||
Quintile 1 | 175 (24) | 24 (31) | 112 (25) | 8 (35) | N/A |
Quintile 2 | 94 (13) | 7 (9) | 57 (13) | 3 (13) | |
Quintile 3 | 140 (19) | 17 (22) | 69 (16) | 6 (26) | |
Quintile 4 | 127 (17) | 15 (19) | 86 (19) | 3 (13) | |
Quintile 5 | 204 (28) | 15 (19) | 121 (27) | 3 (13) | |
Smoking status (UKLS questionnaire 1), n (%) | |||||
Current smoker | 268 (36) | 30 (39) | 160 (36) | 11 (48) | χ2(3) = 1.5 (0.69) |
Ex-smoker | 472 (64) | 48 (62) | 285 (64) | 12 (52) | |
Never-smoker | 0 (0) | 0 (0) | 0 (0) | 0 (0) | N/A |
Experience of lung cancer (T0), n (%) | |||||
No | 445 (60) | 52 (67) | 250 (56) | 13 (57) | χ2(3) = 3.8 (0.29) |
Yes | 293 (40) | 26 (33) | 194 (44) | 10 (44) | |
Cancer distress (T0), mean (SD)d | 2.16 (0.27) 8.65 | 2.20 (0.29) 8.99 | 2.16 (0.29) 8.63 | 2.22 (0.31) 9.18 | F(3,1277) = 0.9 (0.46) |
Anxiety (T0), mean (SD)d | 1.52 (0.69) 3.59 | 1.38 (0.71) 2.97 | 1.55 (0.72) 3.73 | 1.55 (0.79) 3.70 | F(3,1280) = 1.4 (0.24) |
Depression (T0), mean (SD)d | 1.27 (0.65) 2.55 | 1.31 (0.65) 2.71 | 1. 26 (0.67) 2.53 | 1.27 (0.60) 2.55 | F(3,1278) = 0.1 (0.94) |
Decision satisfaction (T0), n (%) | |||||
Not very satisfied | 435 (59) | 48 (62) | 253 (57) | 13 (57) | χ2(3) = 0.7 (0.87) |
Very satisfied | 304 (41) | 30 (39) | 190 (43) | 10 (44) |
IMD | Liverpool: N = 2030 | Papworth: N = 1995 | ||
---|---|---|---|---|
Mean (SD) | n | Mean (SD) | n | |
Quintile 1 | 9.46 (3.32) | 1009 | 9.19 (2.47) | 68 |
Quintile 2 | 9.15 (2.82) | 305 | 9.29 (2.53) | 180 |
Quintile 3 | 9.07 (2.76) | 360 | 9.02 (2.59) | 356 |
Quintile 4 | 8.90 (2.63) | 249 | 9.26 (2.69) | 477 |
Quintile 5 | 8.94 (2.51) | 107 | 8.14 (2.37) | 914 |
List of abbreviations
- ANCOVA
- analysis of covariance
- CAD
- computer-aided detection
- CI
- confidence interval
- COPD
- chronic obstructive pulmonary disease
- CT
- computed tomography
- CWS-R
- Cancer Worry Scale-Revised
- DICOM
- Digital Imaging and Communications
- DLCST
- Danish Lung Cancer Screening Trial
- DMC
- data management company
- ELCAP
- Early Lung Cancer Action Project
- GP
- general practitioner
- HADS
- Hospital Anxiety and Depression Scale
- ICER
- incremental cost-effectiveness ratio
- IMD
- Index of Multiple Deprivation
- ITALUNG
- Italian Lung cancer Computed Tomography screening trial
- LDCT
- low-dose computed tomography
- LHCH
- Liverpool Heart and Chest Hospital
- LLP
- Liverpool Lung Project
- LLPv2
- Liverpool Lung Project lung cancer risk prediction algorithm, version 2
- MDT
- multidisciplinary team
- MILD
- Multi-centric Italian Lung Detection Trial
- NCI
- National Cancer Institute
- NELSON
- Nederlands Leuvens Longkanker Screenings Onderzoek (Dutch-Belgian Randomised Lung Cancer Screening Trial)
- NICE
- National Institute for Health and Care Excellence
- NLST
- National Lung Screening Trial
- ONS
- Office for National Statistics
- OR
- odds ratio
- PCT
- primary care trust
- PLCO
- Prostate, Lung, Colorectal and Ovarian Screening Trial
- QALY
- quality-adjusted life-year
- RCT
- randomised controlled trial
- SD
- standard deviation
- SOF
- significant other finding
- UKLS
- UK Lung Cancer Screening Trial
- VDT
- volume doubling time
- XML
- extensible markup language