Notes
Article history
The research reported in this issue of the journal was funded by the HTA programme as project number 08/116/300. The contractual start date was in July 2010. The draft report began editorial review in August 2014 and was accepted for publication in December 2015. The authors have been wholly responsible for all data collection, analysis and interpretation, and for writing up their work. The HTA editors and publisher have tried to ensure the accuracy of the authors’ report and would like to thank the reviewers for their constructive comments on the draft document. However, they do not accept liability for damages or losses arising from material published in this report.
Declared competing interests of authors
Torsten Lauritzen reports unrestricted grants from Novo Nordisk, AstraZeneca, Pfizer, GlaxoSmithKline, Servier and HemoCue during the conduct of the study. Torsten Lauritzen also owns stock/shares in Novo Nordisk. Guy EHM Ruttem reports grants from Novo Nordisk, GlaxoSmithKline and Merck during the conduct of the study and personal fees from Novo Nordisk and AstraZeneca outside the submitted work. Melanie J Davies has acted as a consultant, advisory board member and speaker for Novartis, Novo Nordisk, Sanofi-Aventis, Eli Lilly and Company, Merck Sharp & Dohme, Boehringer Ingelheim and Roche. Melanie J Davies has also received grants in support of investigator and investigator-initiated trials from Novartis, Novo Nordisk, Sanofi-Aventis, Eli Lilly and Company, Pfizer, Merck Sharp & Dohme and GlaxoSmithKline. Kamlesh Khunti has acted as a consultant and speaker for Novartis, Novo Nordisk, Sanofi-Aventis, Eli Lilly and Company and Merck Sharp & Dohme and has received grants in support of investigator and investigator-initiated trials from Novartis, Novo Nordisk, Sanofi-Aventis, Eli Lilly and Company, Pfizer, Boehringer Ingelheim and Merck Sharp & Dohme. Simon J Griffin reports grants from the Wellcome Trust, the Medical Research Council, NHS Research and Development, the National Institute for Health Research and the University of Aarhus (Denmark) and non-financial support from Bio-Rad Laboratories during the conduct of the study. Simon J Griffin has also received personal fees from Eli Lilly and Company, the Royal College of General Practitioners and Astra Zeneca outside the submitted work.
Permissions
Copyright statement
© Queen’s Printer and Controller of HMSO 2016. This work was produced by Simmons et al. under the terms of a commissioning contract issued by the Secretary of State for Health. This issue may be freely reproduced for the purposes of private research and study and extracts (or indeed, the full report) may be included in professional journals provided that suitable acknowledgement is made and the reproduction is not associated with any form of advertising. Applications for commercial reproduction should be addressed to: NIHR Journals Library, National Institute for Health Research, Evaluation, Trials and Studies Coordinating Centre, Alpha House, University of Southampton Science Park, Southampton SO16 7NS, UK.
Chapter 1 Introduction
Burden of diabetes
Type 2 diabetes is a common chronic disease, which affected 382 million people worldwide in 2013. 1 It is estimated that there will be 592 million individuals living with diabetes by 2035. Diabetes is a major cause of premature death. Global mortality attributable to known diabetes in adults aged 20–79 years in the year 2013 is estimated at 5.1 million deaths, which is 8.4% of total world mortality. 1 Diabetes is ranked among the leading causes of blindness, renal failure and lower limb amputation in virtually every developed society. People with diabetes have an increased risk of cardiovascular, cerebrovascular and peripheral vascular disease (PVD) and have a reduced risk of survival after suffering from silent ischaemia and myocardial infarction (MI) (compared with those without diabetes). Around 65% of individuals with type 2 diabetes die of cardiovascular disease (CVD). Diabetes is a very expensive chronic condition. It imposes a huge burden on national economies and health-care systems, as well as costs for individuals with diabetes and their families. Expenditure related to diabetes in 2010 was estimated to be approximately 10% of total health-care budgets in the UK and is projected to rise to 17% in 2035. 2
Preventing diabetes
The high social and economic cost of diabetes makes a compelling case for prevention of the disease. But where should governments and health services focus their attention? Most research has been completed on the tertiary prevention of diabetes, that is, the treatment of people with established disease. There have been significant improvements in the treatment of individuals with diabetes3 and there is good evidence that the development of long-term complications of diabetes can be significantly decreased by intensive treatment (IT) (see Screening and early treatment for early diabetes). We also have long-term evidence that diabetes can be prevented among those at high risk of developing the disease (primary prevention). 4 Intensive lifestyle and pharmacological interventions reduce the rate of progression of type 2 diabetes among people with impaired glucose tolerance (IGT). In a meta-analysis of published diabetes prevention trials, Gillies et al. 4 reported pooled hazard ratios of 0.51 [95% confidence interval (CI) 0.44 to 0.60] for lifestyle interventions compared with standard advice and 0.70 (95% CI 0.62 to 0.79) for oral diabetes drugs compared with the control. Longer-term follow-up of these trials provides evidence of the sustained prevention of diabetes. 5–7 Delaying or preventing diabetes in this way also reduces the risk of microvascular complications and reduces cardiovascular and all-cause mortality. 8,9
Secondary prevention strategies (i.e. earlier detection, e.g. by screening) have received little attention in the past. The current evidence base for the recommendation of screening and early treatment for diabetes is limited.
Screening and early treatment for diabetes
Type 2 diabetes meets many of the formal criteria for a disease for which screening is justified. 10 The condition is an important health problem associated with a substantial burden of suffering and health service cost. The natural history of the disease is well characterised. 11,12 The condition is frequently asymptomatic,13 with the true onset occurring several years before diagnosis. 11,14 Although detection of the condition may be improving in some parts of the world,15 nearly half of all people with diabetes remain undiagnosed. 1 When patients are diagnosed, many already have complications, such as CVD, chronic kidney disease and heart failure, retinopathy and neuropathy. 16–18 This suggests a potential window for earlier detection and treatment. Furthermore, there are a number of screening tests that are simple, safe and validated and that perform reasonably well when evaluated against recommended diagnostic criteria. 19 Modelling studies suggest that a programme of screening for diabetes would reduce both all-cause and diabetes-related mortality. 20–22 However, these estimates depend on a number of key assumptions. The only published trial of screening to date did not show an effect of population-based screening on mortality over 10 years of follow-up. 23
The National Screening Committee states that there should be an effective treatment for individuals identified through early detection, with evidence of early treatment leading to better outcomes than late treatment. 10 A screening programme for diabetes is most likely to seek to prevent CVD, the leading cause of premature death and disability among patients with diabetes. There is good evidence that the development of long-term complications of diabetes can be significantly decreased by IT. Results from the UK Prospective Diabetes Study (UKPDS) and the Kumamato trials have demonstrated the benefits of tight glycaemic24,25 and blood pressure control,26 whereas several trials, including the Collaborative Atorvastatin Diabetes Study27 and the Heart Protection Study (HPS),28 have confirmed the benefits of lipid-lowering drugs. The Steno study is one of the few trials to compare the benefits of targeted intensive multifactorial treatment with those of routine care (RC) for risk factors for CVD in individuals with established diabetes. 29 After 13 years of follow-up, patients receiving the IT had a 59% (95% CI 0.25% to 0.67%) lower risk of a CVD event and a 46% (95% CI 0.32% to 0.89%) lower risk of all-cause mortality than those receiving RC. When we started our research in 2010, there was no trial evidence that intensive multifactorial treatment improved CVD outcomes when commenced in the lead time between detection by screening and diagnosis in routine clinical practice.
In terms of preventing microvascular complications, treatment of individual risk factors such as blood pressure and glucose level reduces the risk of microvascular complications among clinically diagnosed patients. 24,30–32 IT of multiple risk factors in the Steno study was associated with a 61% (95% CI 13% to 83%) lower risk of nephropathy, a 58% (95% CI 14% to 79%) lower risk of retinopathy and a 63% (95% CI 21% to 82%) lower risk of autonomic neuropathy. 33 However, the effects on microvascular outcomes of starting multifactorial treatment earlier in the course of the disease are uncertain. Data from trials of IT of hyperglycaemia suggest that beneficial effects can be seen for microvascular outcomes in the short term, whereas cardiovascular benefits are evident only with longer follow-up. However, there remains some uncertainty about the merits of tight glycaemic control. 34,35
Little research has been completed on the potential effects of intensive multifactorial treatment on patient-reported outcome measures (PROMs) early in the course of the disease. For largely asymptomatic patients, such a treatment regime might be burdensome. Health practitioners might be reluctant to offer IT including the prescription of several medications and recommendations to change several lifestyle behaviours; this might lead to psychosocial stress and reduced satisfaction with treatment. 36 When assessing the effectiveness of early treatment, PROMs are a valuable complement to hard outcomes such as mortality and cardiovascular events. They are also increasingly being used as key performance indicators in chronic illness. PROMs reflect a patient’s assessment of his or her own health and well-being and involve questions about physical and social functioning and mental well-being. They may include both generic and disease-specific questions. The reliability of PROMs is similar to that of clinical measures such as blood pressure or blood glucose monitoring. 37 The use of PROMs has been recommended in the evaluation of health-care services and in regulatory decision-making. 38 Their use provides an opportunity to help drive changes in how health care is organised and delivered. 37
Evidence from the UKPDS study suggests that IT of blood pressure and blood glucose among newly diagnosed type 2 diabetes patients is not associated with adverse effects on quality of life. 39 The Action to Control Cardiovascular Risk in Diabetes (ACCORD) trial of intensive glycaemic control in US patients with long-standing type 2 diabetes was associated with modest improvements in satisfaction with diabetes treatment and did not lead to an increase in health-related quality of life. 40 The effects of intensive multifactorial treatment on PROMs among people with type 2 diabetes detected by screening are not known.
In addition to a lack of information on the effects of intensive multifactorial intervention on macrovascular, microvascular and PROMs early in the diabetes disease trajectory, little is known about the cost-effectiveness of such treatment. It is expected that increasing numbers of new patients will be identified as governments introduce national assessment programmes, such as the NHS Health Checks programme. 41 The balance of benefits, harms and costs of IT may not be the same for screen-detected individuals as for those with clinically diagnosed and long-standing diabetes.
The ADDITION-Europe trial
In 2001 a group of colleagues from Cambridge in the UK, Utrecht in the Netherlands and Copenhagen and Aarhus in Denmark came together to answer some of the outstanding questions about screening and early treatment for diabetes. Colleagues in Leicester were later invited to join the collaboration. The main aim of the Anglo–Danish–Dutch Study of Intensive Treatment in People with Screen-Detected Diabetes in Primary Care (ADDITION-Europe) was to investigate whether or not intensive multifactorial treatment improves outcomes compared with RC when commenced in the lead time between detection by screening and clinical diagnosis. This was a two-phase study consisting of a screening phase and a pragmatic, cluster-randomised, parallel-group trial. Results from the screening phase of the study have previously been reported. 42–46 This report concerns the results from the 5-year follow-up of the trial, which was funded in the UK by the National Institute for Health Research (NIHR) Health Technology Assessment programme.
Aims
To examine the effect of intensive multifactorial treatment compared with RC on (1) cardiovascular outcomes, (2) microvascular outcomes and (3) self-reported health status, well-being, diabetes-specific quality of life and treatment satisfaction after 5 years’ follow-up. A further aim was to assess the cost-effectiveness of intensive multifactorial treatment compared with RC in the UK setting.
Chapter 2 Trial design and methods
Design
The ADDITION-Europe trial was set up to evaluate the effectiveness of intensive multifactorial treatment with regard to macrovascular, microvascular and PROMs among individuals with screen-detected diabetes. It consisted of two phases – a screening phase and a pragmatic cluster-randomised parallel-group trial – in four centres (Denmark, Cambridge, UK, the Netherlands and Leicester, UK). This report concerns the results of the treatment trial (see Chapter 3). The main trial was supplemented with an economic evaluation to consider the cost-effectiveness of the intervention in the UK (see Chapter 4). A description of the trial protocol has already been published. 47
Ethical approval and research governance
The study was approved by local ethics committees in each centre and participants provided informed consent.
Randomisation, concealment and blinding
Of 1312 general practices invited to participate, 379 (29%) agreed and 343 clusters (26%) were randomised. Practices were randomly assigned by statisticians independent of the measurement teams to screening plus routine diabetes care or screening followed by intensive multifactorial treatment in a 1 : 1 ratio. Randomisation included stratification by county and number of full-time family physicians in Denmark and by single-handed or group status in the Netherlands. In Cambridge, randomisation included minimisation for the local district hospital and the number of patients per practice with diabetes. In Leicester, randomisation included minimisation for practice demographics, deprivation status and prevalence of type 2 diabetes.
Population-based stepwise screening took place between April 2001 and December 2006 among people aged 40–69 years (50–69 years in the Netherlands) without known diabetes, as previously described. 43,48–50 Screening programmes, which varied by centre (Table 1), consisted of a risk score51 (Cambridge) or self-completion questionnaires (Denmark52 and the Netherlands54) followed by capillary glucose testing or an invitation to attend an oral glucose tolerance test without prior risk assessment (Leicester). Individuals were diagnosed with diabetes according to the World Health Organization (WHO)’s 1999 criteria,56 including the requirement for confirmatory tests on separate occasions.
Centre | Screening programme | Intervention delivery | Outcome ascertainment |
---|---|---|---|
Cambridge, UK |
|
|
|
Denmark |
|
|
|
Leicester, UK |
|
|
|
The Netherlands |
|
|
|
Inclusion and exclusion criteria
All patients newly diagnosed with type 2 diabetes were eligible to participate in the treatment study unless their family physician indicated that they had contraindications to the proposed study medication, an illness with a life expectancy of < 12 months or psychological or psychiatric problems that were likely to invalidate informed consent. Overall, 3057 eligible participants with screen-detected diabetes agreed to take part (Denmark, n = 1533; Cambridge, n = 867; the Netherlands, n = 498; Leicester, n = 159).
Intervention
The characteristics of the interventions to promote IT in each centre have been described previously47–49,57 and are summarised in Table 1. We aimed to educate and support general practitioners (GPs) and practice nurses in target-driven management (using medication and promotion of a healthy lifestyle) of hyperglycaemia, blood pressure and cholesterol, based on the stepwise regimen used in the Steno-2 study. 29 Treatment targets and algorithms (Table 2) based on trial data in people with type 2 diabetes24,26,29,58,59 were the same for the IT groups in all centres. GPs were advised to consider prescribing an angiotensin-converting enzyme (ACE) inhibitor for patients with blood pressure ≥ 120/80 mmHg or a previous cardiovascular event58 and 75 mg of aspirin daily to patients without specific contraindications. Although treatment targets were specified and classes of medication recommended, prescribing decisions, including choice of individual drugs, were made by practitioners and patients. Following publication of the results of the HPS,28 the treatment algorithm included a recommendation to prescribe a statin to all patients with a cholesterol level of ≥ 3.5 mmol/l.
Treatment target | Treatment threshold | Baseline | Review 1 | Review 2 | Review 3 | |
---|---|---|---|---|---|---|
HbA1c | < 7.0% | > 6.5% | Diet | If HbA1c > 6.5%, prescribe metformin | If HbA1c > 6.5%, increase metformin dose/add a second medication (PGR or SU or TZD) | If HbA1c > 6.5%, add a third medication (PGR or SU or TZD) and consider adding insulin |
BP | ≤ 135/85 mmHga | ≥ 120/80 mmHg | If BP > 120/80 mmHg or CVD+, prescribe an ACE inhibitor titrated to the maximum dose | If BP > 135/85 mmHg, add a thiazide diuretic or calcium antagonist | If BP > 135/85 mmHg, add a thiazide diuretic or calcium antagonist | If BP > 135/85 mmHg, add a beta-blocker or alpha-blocker |
Cholesterol IHD– | < 5.0 mmol/l | ≥ 3.5 mmol/l | If TC ≥ 3.5 mmol/l, prescribe a statin | If TC > 5.0 mmol/l, increase statin dose up to the maximum | If TC > 5.0 mmol/l, increase statin dose up to the maximum | Consider adding a fibrate if TC > 5.0 mmol/l |
Cholesterol IHD+ | < 4.5 mmol/l | ≥ 3.5 mmol/l | If TC ≥ 3.5 mmol/, prescribe a statin | If TC > 4.5 mmol/l, increase statin dose up to the maximum | If TC > 4.5 mmol/l, increase statin dose up to the maximum | Consider adding a fibrate if TC > 4.5 mmol/l |
Aspirin | 75/80 mg of aspirin daily to all patients treated with antihypertensive medication and without specific contraindications |
Intensive treatment was promoted through the addition of several features to existing diabetes care. Small group or practice-based educational meetings were arranged with GPs and nurses to discuss the treatment targets and algorithms and lifestyle advice, including supporting evidence. Audit and feedback were included in follow-up meetings up to twice per year (the total number of practice meetings ranged from 2 to 10) or co-ordinated by post. In the Netherlands patients were seen in general practice by diabetes nurses who were authorised to prescribe medication and adjust doses under GP supervision. In Denmark and Cambridge practice staff were provided with educational materials for patients. In Denmark and the Netherlands patients were sent reminders if annual measures were overdue. In all centres practices received additional funding to support the delivery of care (up to the equivalent of three 10-minute consultations with a GP and three 15-minute consultations with a nurse per patient per year for 3 years). Leicester patients were referred to the Diabetes Education and Self-Management for Ongoing and Newly Diagnosed (DESMOND) structured education programme53 and were offered 2-monthly appointments with a diabetes nurse or physician in a community peripatetic clinic for 1 year and 4-monthly appointments thereafter. Clinic staff were prompted to contact patients defaulting from appointments.
In the RC group, GPs were provided only with the diagnostic test results. Patients with screen-detected diabetes received the standard pattern of diabetes care according to the recommendations applicable in each centre. 60–63
Data collection
Centrally trained staff undertook health assessments at baseline and after 5 years, including biochemical and anthropometric measures, and administered questionnaires, following standard operating procedures. Staff were unaware of study group allocation. Follow-up examinations took place from September 2008 until the end of December 2009. The mean [standard deviation (SD)] follow-up period was 5.3 (1.6) years. All biochemical measures were analysed in five regional laboratories at baseline and follow-up. Standardised self-report questionnaires were used to collect information on education, employment, ethnicity, lifestyle habits (smoking status, alcohol consumption), prescribed medication and health status. Questionnaires were completed at the same health assessment visit as the anthropometric and biochemical measurements. If participants did not complete follow-up questionnaires or measurements then the most recent values were obtained from general practice records along with information on prescribed medication.
Primary end point
The primary end point was a composite of first cardiovascular event, including cardiovascular mortality, cardiovascular morbidity (non-fatal MI and non-fatal stroke), revascularisation and non-traumatic amputation. All-cause mortality and each of the individual components of the primary end point were secondary outcomes. In each centre participants’ medical records or national registers were searched for potential end points by staff unaware of group allocation. For each possible end point, packs containing relevant clinical information (such as a death certificate, post-mortem report, medical records, hospital discharge summary, electrocardiographs and laboratory results) were prepared and sent to two members of the expert committees, who were unaware of group allocation, for independent adjudication according to an agreed protocol using standardised case report forms. Committee members met to reach consensus over discrepancies. The date of completion of follow-up for the primary end point was deemed to be the date of the first primary end point, the date of remeasurement at 5 years if no end point occurred or the date that the end-point search was undertaken if the participant did not experience an event or attend follow-up.
Secondary end points
Microvascular outcomes
Prespecified secondary outcomes included measures of kidney function, retinopathy and peripheral neuropathy.
Nephropathy was assessed by the urinary albumin–creatinine ratio (ACR) and the estimated glomerular filtration rate (eGFR). The urinary ACR was measured on spot urine and analysed at Aarhus Hospital (Aarhus, Denmark) and Steno Diabetes Centre (Gentofte, Denmark) using a Hitachi 912 Chemistry Analyzer (Tokyo, Japan); at Addenbrooke’s Hospital (Cambridge, UK) and Leicester Royal Infirmary (Leicester, UK) using an Olympus AU400 Chemistry Analyzer (Tokyo, Japan); and at the SHL Centre for Diagnostic Support in Primary Care (Etten-Leur, the Netherlands) using a Roche Hitachi Modular P Chemistry Analyzer (Basel, Switzerland). Repeated analyses of standardised trial control samples for urine creatinine during follow-up confirmed the reliability and precision of the laboratory methods with coefficients of variation (CVs) < 3.4% in all laboratories. Analyses of trial and external quality control samples of urine albumin revealed CVs between 2.0% and 9.8% in the Etten-Leur, Leicester and Gentofte laboratories and CVs of 4.9% for low concentrations and 3.4% for high concentrations in the Addenbrooke’s laboratory in Cambridge during the period of trial testing. Microalbuminuria was defined as an ACR of ≥ 2.5 mg/mmol for men and ≥ 3.5 mg/mmol for women and macroalbuminuria was defined as an ACR of ≥ 25 mg/mmol. Nephropathy was defined as the presence of either microalbuminuria or macroalbuminuria. The eGFR was calculated using data on serum creatinine, age, sex and ethnicity for each individual using the Modification of Diet in Renal Disease formula64 at baseline and follow-up. Change between the two time points was analysed as a continuous variable. Plasma creatinine was analysed with kinetic colourimetric methods at all laboratories at baseline and follow-up except in the Netherlands where an enzymatic method was used at follow-up. Repeated analyses of standardised control samples for creatinine during follow-up the confirmed reliability and precision of the laboratory methods, with CVs between 1.3% and 6.4%.
Retinopathy was assessed using gradable digital images taken using a retinal camera (two from each eye, one with the fovea in the centre and one with the macula in the centre). In the Netherlands and Leicester all retinal images were taken as part of the follow-up examination. In Denmark 81% of the images were taken as part of the study follow-up, with the remainder being obtained from routine health service records. All retinal images in Cambridge were retrieved from routine medical records. Only images taken in the 2 years preceding the follow-up visit were included in this analysis. Information on retinal photography devices used at the four centres is available on the study website [see www.addition.au.dk/ (accessed 30 June 2014)]. Retinal images were graded by three certified graders, who were unaware of the participants’ study group allocation, using a quantitative grading system and subsequently categorised according to the Early Treatment Diabetic Retinopathy Study (ETDRS) semiquantitative scale. 65 Two binary end points were then defined: (1) any retinopathy compared with no retinopathy and (2) severe or proliferative retinopathy compared with no, mild or moderate retinopathy.
Peripheral neuropathy was assessed using the self-administered Michigan Neuropathy Screening Instrument,66 which includes 13 questions about neuropathic symptoms. Responses to the questions are summed to calculate the total score. Responses of ‘yes’ to items 1–3, 5, 6, 8, 9, 11, 12, 14 and 15 each score 1 point and ‘no’ responses on items 7 and 13 each score 1 point. Item 10 is not included in the calculation of a score for neuropathy. Participants were defined as having peripheral neuropathy if they had a score of ≥ 7. The peripheral neuropathy scores were considered missing if categorisation was not possible because of unanswered items. 67
Patient-reported outcomes
As the participants were screen detected, no diabetes-specific measures were obtained at baseline. At follow-up, questionnaires were used to cover both generic and diabetes-specific measures.
Health status was assessed using the European Quality of Life-5 Dimensions (EQ-5D), a generic health-related quality of life questionnaire consisting of a classification system (EQ-5D profile) and a visual analogue scale [European Quality of Life visual analogue scale (EQ-VAS)]. The EQ-5D profile was completed by participants at baseline and follow-up; the EQ-VAS was completed at follow-up only. The EQ-5D profile covers five domains of health (mobility, self-care, usual activities, pain/discomfort and anxiety/depression), each with three levels of functioning: level 1, no problems; level 2, some problems; level 3, severe problems. This results in 243 health states, which are converted to a single index (‘utility’) using a population-specific algorithm. The standard UK value set gives utilities ranging from –0.594 to +1.00 (full health). 68 A value of 0 represents death; negative values imply a health state worse than death. The EQ-VAS is a graded, vertical line, anchored at 0 (worst imaginable health state) and 100 (best imaginable health state). Patients were asked to mark a point on the EQ-VAS that best reflected their actual health state. 69
The Short Form questionnaire-36 items (SF-36)70 generates a profile of scores on eight dimensions of health: (1) physical functioning; (2) role limitations because of physical problems; (3) social functioning; (4) bodily pain; (5) general mental health; (6) role limitations because of emotional problems; (7) vitality; and (8) general health perceptions. Two summary scales can be calculated: the physical component summary score and the mental component summary score. For all dimensions an average score can be calculated, with a range from 0 (least favourable health state) to 100 (most favourable health state). The SF-36 was completed at follow-up.
General well-being was assessed using the previously validated 12-item short form of the Well-Being Questionnaire (W-BQ12),71 which measures different aspects of the well-being of individuals, including diabetes patients. It can be scored as three subscales: negative well-being (a higher score means more negative well-being), energy (a higher score means more energy) and positive well-being (a higher score means more positive well-being). Each subscale consists of four items with a score range of 0–12. Furthermore, a score for general well-being can be calculated;72 this has a score range of 0–36 and a higher score indicates better well-being. The W-BQ12 was completed at follow-up.
Diabetes-specific quality of life was assessed using the previously validated Audit of Diabetes-Dependent Quality of Life (ADDQoL),73 a measure of patients’ perceived importance of diabetes and its treatment and its impact on quality of life. We used the ADDQoL 19, which includes 19 diabetes-specific items. For each item patients are asked how things would be without diabetes, with scores ranging from –3 (a great deal better) to 1 (worse), and to rate each item, with scores ranging from 3 (very important) to 0 (not at all important). A weighted rating per item can be calculated by multiplying the unweighted rating by the importance rating. The total ADDQoL score is the mean of all weighted ratings of applicable domains and ranges from –9 (maximum negative impact of diabetes) to 3 (maximum positive impact of diabetes). The ADDQoL was completed at follow-up.
Satisfaction with diabetes treatment was assessed using the previously validated Diabetes Treatment Satisfaction Questionnaire (DTSQ). 74 It consists of a six-item scale assessing treatment satisfaction and two items assessing the perceived frequency of hyperglycaemia and hypoglycaemia. The treatment satisfaction score ranges from 0 (very dissatisfied) to 36 (very satisfied). The DTSQ was completed at follow-up.
Sample size
An individually randomised trial would have required a total of 2700 individuals (1350 per group) to detect a 30% reduction in the cumulative risk of the primary end point at a 5% significance level and with 90% power, allowing for 10% loss to follow-up and assuming an event rate in the RC group of 3% per year (based on results from the UKPDS24). We expected a minimal effect of clustering within general practices, with an estimated intracluster correlation coefficient of 0.01; assuming an average of 10 participants per general practice, the design effect was 1.09 and so we inflated the estimated sample size for this cluster trial to 3000.
Statistical analysis
The analysis and reporting of this trial were undertaken in accordance with the Consolidated Standards of Reporting Trials (CONSORT) guidelines [see www.consort-statement.org/ (accessed 27 January 2016)]. Statistical analysis was undertaken in Stata 11.1 (StataCorp LP, College Station, TX, USA), SAS v9.2 (SAS Institute Inc., Cary, NC, USA) and Review Manager v5.1 (The Cochrane Collaboration, The Nordic Cochrane Centre, Copenhagen, Denmark), following a predefined analysis plan, which was finalised before preparation of the end-point data set, agreed with the Trial Steering Committee and deposited on the study website (see www.addition.au.dk/). The primary comparative analyses between the randomised groups were conducted on an intention-to-treat (ITT) basis without imputation of missing data.
Preliminary analyses
We summarised the baseline characteristics of individuals and general practices within each randomised group, by centre and overall.
Changes in mean values or percentages of other clinical and medication variables from baseline to follow-up were summarised in each randomised group and intervention effects and 95% CIs for these changes were estimated using the methods described in the primary and secondary analysis sections. We also calculated 10-year modelled CVD risk using the UKPDS model (version 3)75 at 5 years post diagnosis. This is a diabetes-specific risk assessment tool that estimates the absolute risk of fatal or non-fatal CVD within a defined time frame. The variables include age, sex, ethnicity, smoking status, glycated haemoglobin (HbA1c) level, systolic blood pressure, total to high-density lipoprotein (HDL) cholesterol ratio, atrial fibrillation (AF), previous MI or stroke, macroalbuminuria (ACR ≥ 30 mg/mmol), microalbuminuria (ACR ≥ 2.5 mg/mmol in men or ≥ 3.5 mg/mmol in women), duration of diagnosed diabetes and body mass index. We did not have data on AF in ADDITION-Europe participants and so all individuals were coded as zero (no AF). Centre-specific estimates of the difference in modelled CVD risk between treatment groups were combined using fixed-effects meta-analysis. Odds ratios (ORs) of meeting each of three treatment targets [HbA1c < 53 mmol/l (7.0%) if HbA1c > 6.5%; blood pressure ≤ 135/85 mmHg if ≥ 120/80 mm Hg; cholesterol < 5 mmol/l without ischaemic heart disease or < 4.5 mmol/l with ischaemic heart disease) comparing IT with RC were also estimated.
Primary analyses: cardiovascular outcomes
We plotted the cumulative probability of the primary end point. To assess intervention effects we used Cox regression to estimate a hazard ratio and 95% CI within each centre. As there were few participants and hence events in the Leicester centre, data for the primary end point and its components were grouped with data from Cambridge, the other UK centre. Because randomisation was at the practice level, robust standard errors (SEs) were calculated that take into account the two-level structure of the data (individuals clustered within practices) and any potential correlation between individuals within practices. We calculated the intracluster correlation coefficient for the primary end point. We combined centre-specific log-hazard ratios and SEs using fixed-effects meta-analysis and calculated the I2-statistic, which represents the proportion of variability (in log-hazard ratios) between centres that is due to heterogeneity. We tested the proportional hazards assumption by including a parameter for treatment × time interaction in each centre-specific Cox regression model. We analysed continuous intermediate end points within each centre using normal errors regression, with adjustment for the baseline value of the end point, excluding individuals who died or who were lost to follow-up. We combined the estimated differences in mean change from baseline across centres using fixed-effects meta-analysis. In the regression models we included individuals with a missing value of the outcome at baseline using the missing indicator method;76 variables with a skewed distribution were log-transformed. We estimated the effect of the intervention on prescribing end points using logistic regression within each centre and combined the estimated ORs across centres using fixed-effects meta-analysis. We undertook sensitivity analyses by excluding follow-up clinical data obtained from general practice records and prespecified subgroup analyses for the primary end point by including interaction terms between the intervention group and patient age and self-reported history of CVD, which were then pooled across centres. The cumulative incidence of the composite cardiovascular end point was calculated using the method for competing risks described by Gooley et al. ,77 with the competing events here being the primary end point and death from non-cardiovascular causes.
Secondary analyses: all-cause mortality
All-cause mortality data were analysed using the method described in the previous section for the composite CVD end point. Kaplan–Meier estimates of cumulative incidence were calculated.
Secondary analyses: microvascular outcomes
Binary end points (any albuminuria and any retinopathy) were analysed using a logistic regression model estimating the OR and 95% CI for the comparison between IT and RC separately within each centre. The continuous end points (ACR, eGFR) were analysed using a normal errors regression model with adjustment for baseline.
In both logistic and normal errors regression models, given the cluster-randomised design, SEs were adjusted using the cluster option in Stata to allow for correlation between patients within practices. The estimated ORs and differences in means from the four centres were pooled using fixed-effects meta-analysis and a forest plot was used to display the results. The I2-statistic, representing the proportion of variability between centres that is due to heterogeneity, was calculated. Intracluster correlation coefficient values were estimated for all of the microvascular outcomes.
Prespecified analyses of potential interactions between randomised groups and subgroups defined by the following baseline variables were undertaken: age (< 60 and ≥ 60 years), sex, HbA1c [< 6.6% (< 49 mmol/mol) and ≥ 6.6% (≥ 49 mmol/mol), which was the median value] and the presence of albuminuria. For ACR and eGFR, those participants with a missing baseline value of the variable were included in the analysis using the missing indicator method. 76 A sensitivity analysis using multiple imputation was used to investigate the impact of missing data on the retinopathy and peripheral neuropathy end points.
Secondary analyses: patient-reported outcomes
We presented mean scores and SDs for all PROMs at follow-up by centre and by randomised group. We used linear mixed-effects regression models to estimate the difference in each PROM at follow-up and 95% CI, comparing the IT group with the RC group. A random effect per general practice was included to account for intracluster correlation. All PROMs were left skewed. As an alternative to transformation, we wished to control for baseline levels of PROMs. These were not available for all measures so we included baseline EQ-5D score as a proxy for baseline quality of life; correction for baseline EQ-5D score greatly improved the normality of the residuals. The estimated differences in means from the four centres were then pooled using random-effects meta-analysis and a forest plot was used to display the estimated mean differences and 95% CIs for each centre and overall. We calculated the I2-statistic to represent the proportion of variability between centres attributable to heterogeneity.
Individuals who were lost to follow-up or who did not complete both the baseline and the follow-up questionnaires were excluded. Patients with missing data may be those who experienced more serious illness and greater disability and therefore these missing data are unlikely to be ‘missing completely at random’ but will rather be ‘missing at random’. Simply excluding these patients may lead to selection bias and therefore we used multiple imputation78 to perform a sensitivity analysis, imputing five data sets using patient characteristics at baseline and at 5 years’ follow-up and including all patients who were alive at follow-up.
Patient and public involvement
In the development of the ADDITION-Cambridge study, patients were involved in two pilot studies. The first was to assess the feasibility and uptake of the diabetes screening programme and to examine the effects of invitation to diabetes screening on anxiety, self-rated health and illness perceptions. 79 The second was a qualitative study of patients’ experiences of being screened for diabetes. 80 Further qualitative work was undertaken exploring practitioners’ experiences of taking part in the main ADDITION-Cambridge study. 81 In terms of patient involvement during the 5-year follow-up phase of the trial, we sent a newsletter to all ADDITION-Cambridge participants in 2008 outlining the results to date and informing them of our intention to invite them back for the 5-year follow-up assessment. We said to participants: ‘[a]ny ideas that you may have on the conduct and planning of the 5-year follow-up health check would be welcomed – please get in touch if you have any comments’. Responses fed into our 5-year planning. We also sent participants a newsletter in May 2012 with the 5-year study results and invited them and a guest to attend a local meeting during June/July 2012 where they had the chance to meet with ADDITION staff and hear about the results of the study. The five meetings were very well received and gave this special group of patients a chance to ask questions about the study and their diabetes treatment. Comments ranged from ‘very informative’ and ‘the content was useful and excellent’ to ‘the results of the study will motivate me to work harder at controlling my diabetes’. There was very strong support for being involved with a 10-year follow-up study. Similar feedback to study participants took place in the Netherlands and Denmark.
In ADDITION-Leicester, the intervention model was particularly suited to patient and public involvement, with ongoing contact with the clinical care team facilitating continuity and dialogue. IT participants were consulted and offered continued post-trial care delivered in a setting of their choice (primary or secondary care). A number of participants have become members of the local patient and public involvement group and are actively involved in steering local research. A newsletter describing the final results and thanking participants for their involvement was sent following completion of the 5-year outcome ascertainment for the entire cohort (July 2014). A significant number of participants were identified as being at high risk of diabetes during the ADDITION-Leicester study and have subsequently been involved with other studies aimed at the prevention of diabetes. We have also developed a black and minority ethnic panel that has contributed more widely to diabetes research in hard-to-reach groups.
Chapter 3 Trial results
Of the 343 general practices that were randomised, 318 (RC, n = 157; IT, n = 161) completed screening and included eligible participants. Participating practices in the Netherlands and the UK have been described previously. 43,48,49 All four centres had a diabetes prevalence of 3.5% and a nationally representative mean patient list of ≈7000 individuals (n = 7378 RC group, n = 7160 IT group). Baseline sociodemographic, biochemical, clinical and treatment characteristics of individuals in the two randomised groups were well matched overall (see Table 3). However, more patients were identified in IT than in RC practices in Denmark (n = 910 and 623, respectively) and more IT than RC participants had a previous history of ischaemic heart disease [International Statistical Classification of Diseases and Related Health Problems, Tenth Edition (ICD-10) codes I20–25: 11.2% vs. 8.5%, respectively] or other cardiac diagnoses (ICD-10 codes I30–I52: 8.4% vs. 4.5%, respectively). The mean age of ADDITION-Europe participants was 60 years and 58% were male, 94% were Caucasian and 41% were employed. Levels of CVD risk factors among participants at diagnosis were high and many participants were not receiving treatment for these risk factors. 50 Participant and practice flows are shown in Figure 1 (CONSORT diagram).
Characteristic | RC | IT | Between-group difference in change from baseline to follow-up | |||||||||||
---|---|---|---|---|---|---|---|---|---|---|---|---|---|---|
Baseline | Follow-up | Baseline | Follow-up | |||||||||||
% missing | n | % | % missing | n | % | % missing | n | % | % missing | n | % | Beta/OR | 95% CI | |
Demographic variables | ||||||||||||||
Male sex | 0 | 790 | 57.3 | – | – | – | 0 | 981 | 58.5 | – | – | – | – | – |
Age at diagnosis (years), mean (SD) | 0 | 60.2 | 6.8 | – | – | – | 0 | 60.3 | 6.9 | – | – | – | – | – |
White ethnicity | 3 | 1246 | 93.4 | – | – | – | 4 | 1539 | 95.8 | – | – | – | – | – |
Employed | 27 | 425 | 42.0 | – | – | – | 29 | 482 | 40.3 | – | – | – | – | – |
Clinical variables | ||||||||||||||
History of MI | 7 | 79 | 6.1 | – | – | – | 5 | 109 | 6.8 | – | – | – | – | – |
History of stroke | 8 | 24 | 1.9 | – | – | – | 7 | 45 | 2.9 | – | – | – | – | – |
Current smokers | 2 | 375 | 27.8 | 21 | 187 | 18.4 | 2 | 444 | 26.9 | 18 | 261 | 20.2 | 1.06 | 0.77 to 1.45 |
Units of alcohol per week, median (IQR) | 14 | 4.0 | 1 to 13 | 22 | 3.0 | 0 to 10 | 11 | 4.0 | 1 to 13 | 19 | 3.0 | 0 to 10 | –0.24 | –0.77 to 0.29 |
BMI (kg/m2), mean (SD) | 3 | 31.6 | 5.6 | 14 | 31.0 | 5.6 | 4 | 31.6 | 5.6 | 11 | 31.1 | 5.7 | 0.03 | –0.17 to 0.22 |
Weight (kg), mean (SD) | 3 | 90.3 | 17.6 | 7 | 88.4 | 17.8 | 4 | 90.9 | 17.5 | 5 | 89.1 | 18.2 | –0.02 | –0.58 to 0.55 |
Waist circumference (cm), mean (SD) | 2 | 106.8 | 13.5 | 17 | 105.3 | 13.6 | 4 | 107.1 | 13.5 | 13 | 105.4 | 13.6 | –0.30 | –1.01 to 0.42 |
HbA1c (%), median (IQR) | 6 | 6.6 | 6.1 to 7.3 | 5 | 6.5 | 6.1 to 7.1 | 5 | 6.5 | 6.1 to 7.3 | 4 | 6.4 | 6.0 to 6.9 | –0.08 | –0.14 to –0.02 |
HbA1c (%), mean (SD) | 6 | 7.0 | 1.5 | 5 | 6.7 | 0.95 | 5 | 7.0 | 1.6 | 4 | 6.6 | 0.95 | –0.08 | –0.14 to –0.02 |
Systolic blood pressure (mmHg), mean (SD) | 2 | 149.8 | 21.3 | 6 | 138.1 | 17.6 | 4 | 148.5 | 22.1 | 4 | 134.8 | 16.8 | –2.86 | –4.51 to –1.20 |
Diastolic blood pressure (mmHg), mean (SD) | 2 | 86.5 | 11.3 | 7 | 80.7 | 10.8 | 4 | 86.1 | 11.1 | 4 | 79.5 | 10.7 | –1.44 | –2.30 to –0.58 |
Total cholesterol (mmol/l), mean (SD) | 6 | 5.6 | 1.2 | 5 | 4.4 | 0.9 | 5 | 5.5 | 1.1 | 3 | 4.2 | 0.9 | –0.27 | –0.34 to –0.19 |
HDL cholesterol (mmol/l), median (IQR) | 7 | 1.2 | 1.0 to 1.5 | 7 | 1.3 | 1.1 to 1.6 | 7 | 1.2 | 1.0 to 1.5 | 4 | 1.2 | 1.0 to 1.5 | 0.00 | –0.03 to 0.02 |
LDL cholesterol (mmol/l), mean (SD) | 10 | 3.5 | 1.0 | 9 | 2.3 | 0.8 | 10 | 3.4 | 1.0 | 6 | 2.1 | 0.8 | –0.20 | –0.26 to –0.13 |
Triglycerides (mmol/l), median (IQR) | 6 | 1.7 | 1.2 to 2.4 | 6 | 1.6 | 1.1 to 2.3 | 6 | 1.6 | 1.2 to 2.3 | 4 | 1.5 | 1.0 to 2.1 | –0.05 | –0.12 to 0.01 |
Creatinine (µmol/l), mean (SD) | 8 | 84.9 | 18.6 | 6 | 79.8 | 29.9 | 7 | 83.4 | 17.1 | 5 | 81.0 | 30.5 | 1.81 | 0.10 to 3.53 |
Self-reported medication | ||||||||||||||
Any glucose-lowering drug | 3 | 7 | 0.5 | 6 | 681 | 56.4 | 4 | 8 | 0.5 | 3 | 990 | 65.0 | 1.53 | 1.25 to 1.89 |
Number of glucose-lowering drugs, median (IQR) | 3 | 0 | 0 to 0 | 6 | 1 | 0 to 1 | 4 | 0 | 0 to 0 | 3 | 1 | 0 to 1 | – | – |
Metformin | 3 | 5 | 0.4 | 6 | 583 | 48.3 | 4 | 6 | 0.4 | 3 | 835 | 54.8 | – | – |
Sulphonylurea | 3 | 2 | 0.1 | 6 | 215 | 17.8 | 4 | 2 | 0.1 | 3 | 291 | 19.1 | – | – |
Thiazolidinedione | 3 | 0 | 0 | 6 | 50 | 4.1 | 4 | 0 | 0 | 3 | 69 | 4.5 | – | – |
Insulin | 3 | 0 | 0 | 6 | 43 | 3.6 | 4 | 0 | 0 | 3 | 96 | 6.3 | – | – |
Other glucose-lowering drugs | 3 | 0 | 0 | 6 | 31 | 2.6 | 4 | 0 | 0 | 3 | 81 | 5.3 | – | – |
Any antihypertensive drugs | 3 | 585 | 43.7 | 6 | 911 | 75.4 | 4 | 752 | 46.7 | 3 | 1274 | 83.6 | 1.61 | 1.27 to 2.04 |
Number of antihypertensive drugs, median (IQR) | 3 | 0 | 0 to 1 | 6 | 2 | 1 to 3 | 4 | 0 | 0 to 2 | 3 | 2 | 1 to 3 | ||
ACE inhibitor or ARB | 3 | 248 | 18.5 | 6 | 721 | 59.7 | 4 | 345 | 21.4 | 3 | 1126 | 73.9 | 1.84 | 1.52 to 2.22 |
Beta-blocker | 3 | 252 | 18.8 | 6 | 285 | 23.6 | 4 | 366 | 22.7 | 3 | 462 | 30.3 | – | – |
Calcium antagonist | 3 | 166 | 12.4 | 6 | 326 | 27.0 | 4 | 202 | 12.6 | 3 | 446 | 29.3 | – | – |
Diuretic | 3 | 330 | 24.6 | 6 | 529 | 43.8 | 4 | 415 | 25.8 | 3 | 767 | 50.3 | – | – |
Other antihypertensive drugs | 3 | 23 | 1.7 | 6 | 49 | 4.1 | 4 | 32 | 2.0 | 3 | 70 | 4.6 | – | – |
Any cholesterol-lowering drugs | 3 | 206 | 15.4 | 6 | 889 | 73.6 | 4 | 274 | 17.0 | 3 | 1241 | 81.4 | – | – |
Statins | 3 | 200 | 14.9 | 6 | 864 | 71.5 | 4 | 271 | 16.8 | 3 | 1217 | 79.9 | 1.46 | 1.20 to 1.78 |
Aspirin | 3 | 169 | 12.6 | 6 | 504 | 41.7 | 4 | 249 | 15.5 | 3 | 1078 | 70.7 | – | – |
FIGURE 1.
Practice and participant flows in the ADDITION-Europe trial. Source: reproduced open access from Griffin et al. 55
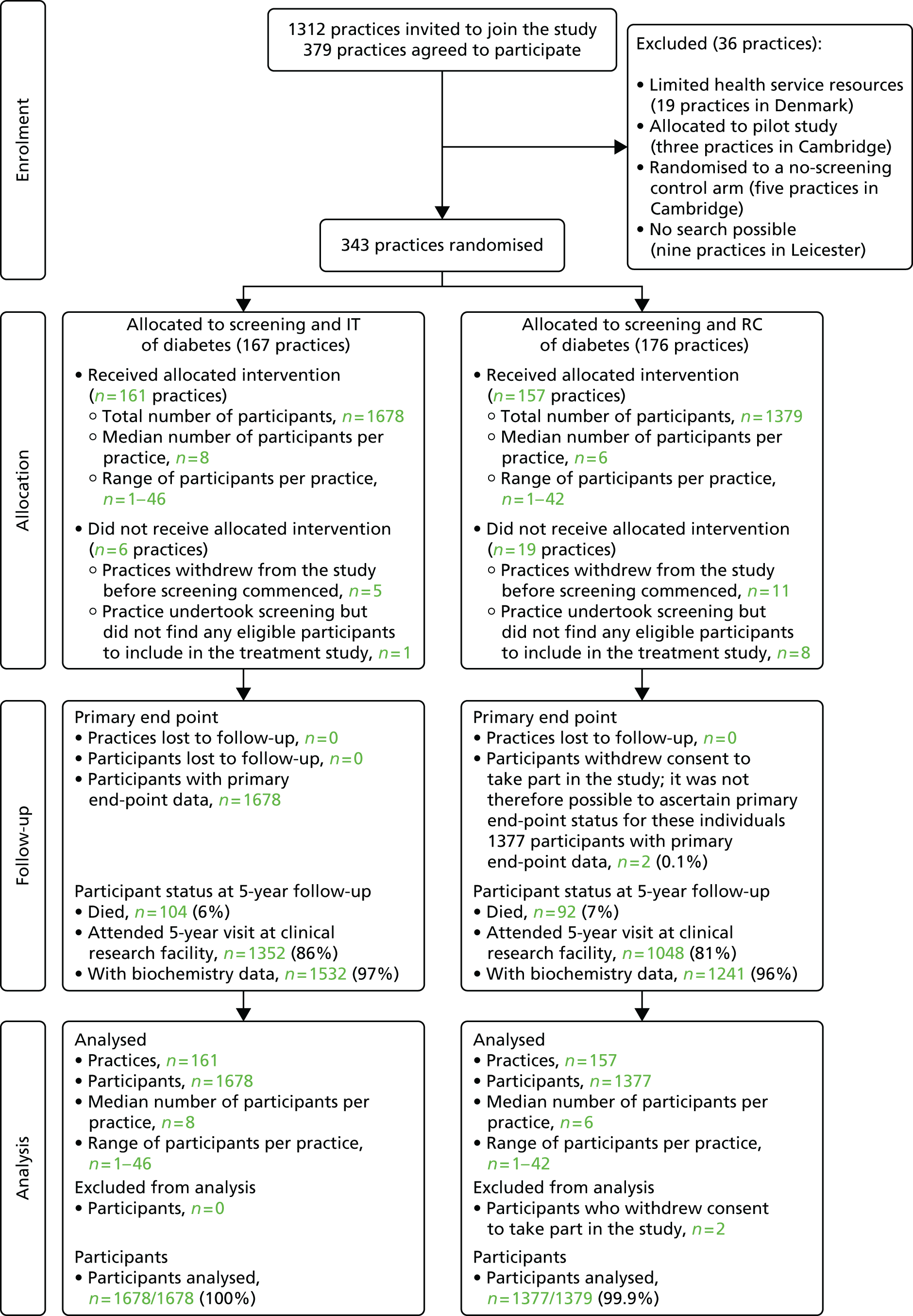
Of those who were still alive in 2009, 2400 (84%) individuals returned to a clinical research facility for a 5-year follow-up health assessment. We obtained biochemical and clinical data from GP records for a further 328 (11.5%) participants. Compared with those with follow-up data, participants with missing data were more likely to be from an ethnic minority group (10.2% vs. 5.7%; p = 0.04) and to have higher cholesterol (5.9 mmol/l vs. 5.6 mmol/l; p = 0.004) and low-density lipoprotein (LDL) cholesterol values (3.7 mmol/l vs. 3.4 mmol/l; p = 0.009) at baseline.
Changes in biochemical, clinical and treatment variables in the two trial groups are shown in Table 3. Prescription of antihypertensive, glucose-lowering and lipid-lowering medication increased in both groups. At follow-up, compared with the RC group, approximately 10% more participants were prescribed glucose-lowering, antihypertensive and lipid-lowering medication in the IT group. In addition, 15% more IT patients were prescribed ACE inhibitors or angiotensin receptor blockers (ARBs) and 30% more IT patients were prescribed aspirin at follow-up. Cardiovascular risk factors in both groups improved over 5 years of follow-up. The modest but statistically significant between-group differences in change from baseline for total and LDL cholesterol, systolic and diastolic blood pressure and HbA1c favoured the IT group.
The 10-year modelled CVD risk was 27.3% (SD 13.9%) at baseline in the whole trial cohort and 21.3% (SD 13.8%) at 5 years. Across all four centres there was a reduction in modelled CVD risk from baseline to 5 years in both the RC group (–5.0%, SD 12.2%) and the IT group (–6.9%, SD 9.0%). Figure 2 shows the distribution of CVD risk at baseline and follow-up by treatment group; the distribution of modelled CVD risk shifted to the left for both groups.
FIGURE 2.
Distribution of the UKPDS (version 3) modelled CVD risk score at baseline and 5 years’ follow-up in the ADDITION-Europe trial cohort by treatment group. (a) RC; and (b) IT. Source: reproduced open access from Black et al. 82


Within all four centres the modelled CVD risk was lower in the IT group than in the RC group at 5 years (Figure 3). The difference between the groups varied from –0.9% (95% CI –3.6% to 1.7%) in Cambridge to –4.8% (95% CI –8.4% to –1.3%) in the Netherlands. There was moderate variation between centres (I2 = 53.6%). For all centres combined, the 10-year modelled CVD risk was significantly lower (–2.0%, 95% CI –3.1% to –0.9%) in the IT group after adjustment for baseline cardiovascular risk and clustering by general practice.
FIGURE 3.
Difference in the UKPDS (version 3) modelled CVD risk score between treatment groups at 5 years’ follow-up in the ADDITION-Europe trial cohort, adjusted for baseline risk and accounting for clustering by general practice. Source: reproduced open access from Black et al. 82

Figure 4 shows the percentage of participants meeting treatment targets for cholesterol, HbA1c and blood pressure in each group at baseline and follow-up. More patients met treatment targets at follow-up than at baseline in both groups. The proportion meeting the targets was higher in the IT group than in the RC group. There was no difference between groups in the percentage of those reporting hypoglycaemia (χ2 = 4.44; p = 0.62). 74 The results were the same when excluding participants with follow-up clinical data obtained from GP records.
FIGURE 4.
Percentage of participants in each group of the ADDITION-Europe trial (±1 SE) for whom the treatment targets were met at baseline and follow-up at a mean of 5.3 years. BP, blood pressure. Source: reproduced open access from Griffin et al. 55
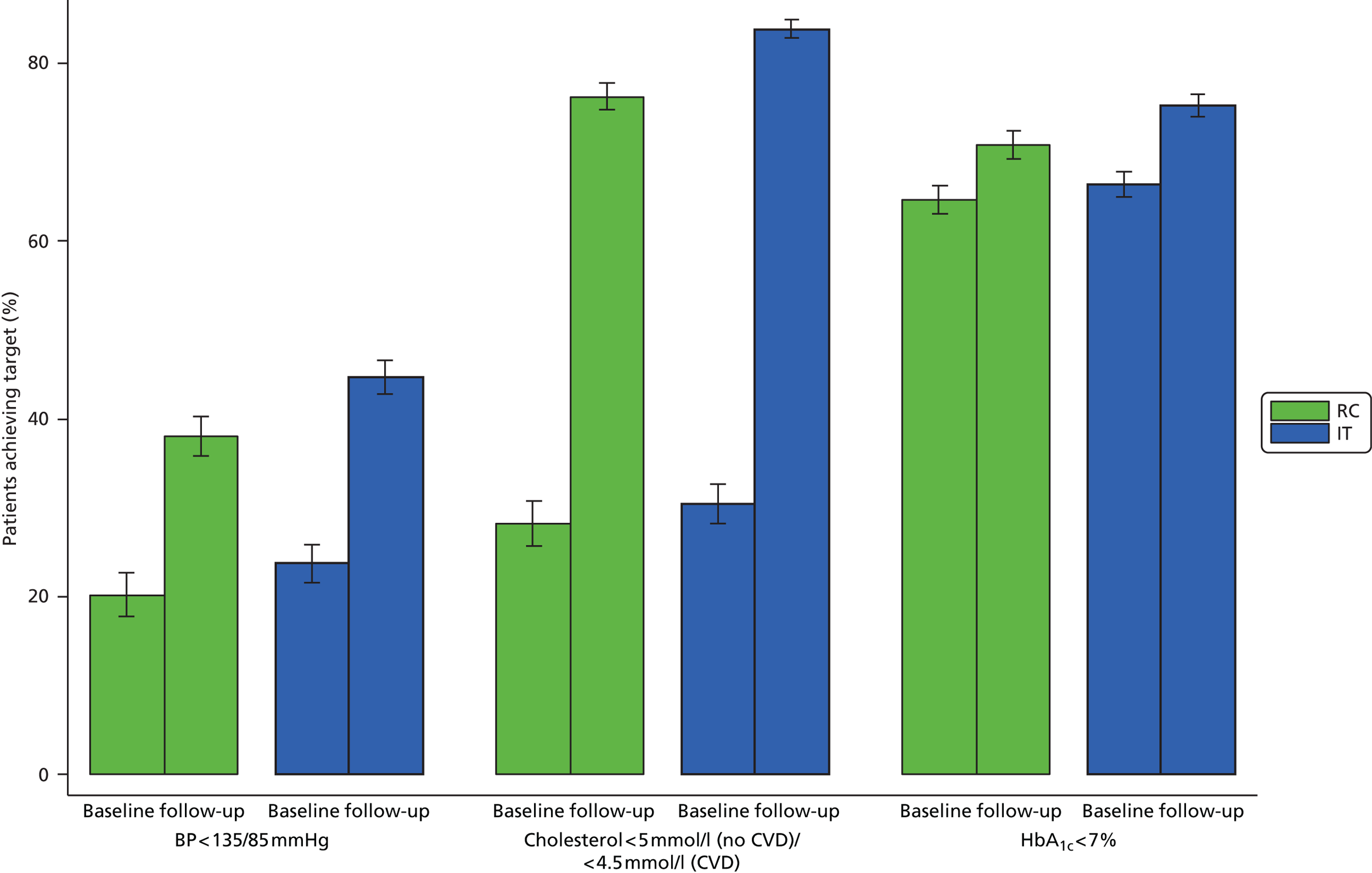
Primary analyses: cardiovascular outcomes
Primary end-point data were available for 99.9% (3055/3057) of participants. In total, 238 first cardiovascular events occurred during a mean (SD) follow-up period of 5.3 (1.6) years (Table 4). There were 121 events among 1678 participants (7.2%) in the IT group (incidence 13.5, 95% CI 11.3 to 16.1 per 1000 person-years) and 117 events among 1377 participants (8.5%) in the RC group (incidence 15.9, 95% CI 13.3 to 19.1 per 1000 person-years). The hazard ratio for the IT group compared with the RC group was 0.83 (95% CI 0.65 to 1.05; p = 0.12). The cumulative probability for the primary CVD end point appeared to diverge after 4 years of follow-up (Figure 5). In predefined subgroup analyses there were no interactions between the intervention and age or previous cardiovascular event (p > 0.1). The p-value was calculated using Cox regression and fixed-effects meta-analysis.
End point | RC (n = 1377), n (%) | IT (n = 1678), n (%) | IT vs. RC | |||
---|---|---|---|---|---|---|
Hazard ratioa | 95% CI | I2 (%)b | p-valuec | |||
Primary end point: composite cardiovascular eventsd | 117 (8.5) | 121 (7.2) | 0.83 | 0.65 to 1.05 | 0 | 0.12 |
Components of primary end point | ||||||
CVD death | 22 (1.6) | 26 (1.5) | 0.88 | 0.51 to 1.51 | 52 | |
MI | 32 (2.3) | 29 (1.7) | 0.70 | 0.41 to 1.21 | 0 | |
Stroke | 19 (1.4) | 22 (1.3) | 0.98 | 0.57 to 1.71 | 0 | |
Revascularisation | 44 (3.2) | 44 (2.6) | 0.79 | 0.53 to 1.18 | 0 | |
Amputation | 0 | 0 | – | – | – | |
Total mortality | 92 (6.7) | 104 (6.2) | 0.91 | 0.69 to 1.21 | 55 |
FIGURE 5.
Cumulative incidence of the composite cardiovascular end point in the RC and IT groups of the ADDITION-Europe trial (p = 0.12). Source: reproduced open access from Griffin et al. 55

However, estimated hazard ratios were 0.70 (95% CI 0.52 to 0.95) in those aged ≥ 60 years and 1.12 (95% CI 0.70 to 1.79) in those aged < 60 years. Hazard ratios for individual components of the composite end point all favoured the IT group (see Table 4), although none achieved statistical significance (Figure 6). There were no amputations as first events. The intracluster correlation coefficient for the primary end point was 0.002 (Denmark 0.014, UK 0.0000016, the Netherlands 0.025). This suggests that the cluster design had little effect on study power.
FIGURE 6.
The relative risk of the development of cardiovascular death, non-fatal MI, non-fatal stroke and revascularisation as a first event and the composite cardiovascular end point by country and overall in the IT group compared with the RC group. Source: reproduced open access from Griffin et al. 55

In total, there were 196 deaths (n = 60 cardiovascular, n = 97 cancer and n = 39 other), 104 (6.2%) in the IT group (mortality rate 11.6, 95% CI 9.6 to 14.0 per 1000 person-years) and 92 (6.7%) in the RC group (mortality rate 12.5, 95% CI 10.2 to 15.3 per 1000 person-years) (see Table 4). The combined mortality hazard ratio for the IT group compared with the RC group was 0.91 (95% CI 0.69 to 1.21). A Kaplan–Meier plot is shown in Figure 7 and country-specific hazard ratios in Figure 8. The heterogeneity of results between countries was not statistically significant. In the UK there were significantly fewer deaths in the IT group, whereas in Denmark there was a non-significant reduction in cumulative risk in the RC group.
FIGURE 7.
Kaplan–Meier estimates of all-cause mortality in the RC and IT groups in the ADDITION-Europe trial. Source: reproduced open access from Griffin et al. 55

FIGURE 8.
The relative risk of all-cause mortality by country and overall in the IT group compared with the RC group in the ADDITION-Europe trial. Source: reproduced open access from Griffin et al. 55

Secondary analyses: microvascular outcomes
Of the 2861 patients still alive at 5 years, 2493 (87.1%), 2710 (94.7%) and 2312 (80.8%) had data for urinary ACR, eGFR and peripheral neuropathy, respectively. Retinal photographs were retrieved for 2190 (76.5%) participants.
At 5 years’ follow-up any albuminuria was present in 316 (22.7%) participants in the IT group and 269 (24.4%) participants in the RC group. Macroalbuminuria was present in 56 (4.0%) and 37 (3.4%) participants in the IT and RC groups, respectively. Centre-specific ORs for any albuminuria favoured the IT group, but the pooled OR was not statistically significant (0.88, 95% CI 0.72 to 1.07; Figure 9). The pooled OR for macroalbuminuria was 1.15 (95% CI 0.76 to 1.74). In both groups the urinary ACR increased between baseline and follow-up. In the IT group the mean (SD) increase was 1.45 (SD 0.60) mg/mmol and in the RC group the mean (SD) increase was 1.30 (0.66) mg/mmol. The overall difference in means was –0.02 (95% CI –0.96 to 0.91) mg/mmol. There were no significant interactions between study group and any of the subgroups.
FIGURE 9.
Odds ratios and frequencies of any retinopathy, any peripheral neuropathy and any albuminuria at follow-up by study group in the ADDITION-Europe trial. Source: reproduced from Sandbæk et al. ,83 under the Creative Commons Attribution-NonCommercial-NoDerivs 3.0 licence (CC BY-NC-ND 3.0).

The mean (SD) eGFR increased between baseline and follow-up in both the IT group and the RC group [IT 4.31 (0.49) ml/minute, RC 6.44 (0.90) ml/minute; difference in means –1.39 (95% CI –2.97 to 0.19) ml/minute]. There were no significant interactions between treatment group and any of the subgroups concerning eGFR. The number of missing data was equally distributed between the two groups.
Retinopathy was present in 125 (10.1%) participants in the IT group and 116 (12.1%) patients in the RC group. Centre-specific ORs favoured the IT group but the pooled OR was not statistically significant (0.84, 95% CI 0.64 to 1.10) (see Figure 9). Imputation of missing values did not affect the estimates.
Individuals without retinal images at follow-up had significantly higher baseline mean HbA1c levels than individuals with retinal data [7.18% (55 mmol/mol) vs. 6.99% (53 mmol/mol), respectively; p = 0.044]. However, there was no difference between randomised groups (interaction p-value = 0.78). There was a significant interaction between retinopathy, randomised group and baseline HbA1c level (p = 0.007). IT appeared to be more effective among individuals with HbA1c ≥ 6.6% (≥ 49 mmol/mol) at baseline (OR 0.65, 95% CI 0.45 to 0.93) than among individuals with HbA1c < 6.6% (< 49 mmol/mol) at baseline (OR 1.17, 95% CI 0.75 to 1.82). There was no evidence of an interaction with either age or sex. Severe retinopathy was present in one participant in the IT group and in seven participants in the RC group.
Peripheral neuropathy was present in 63 (4.9%) participants in the IT group and 60 (5.9%) participants in the RC group (pooled OR 0.95, 95% CI 0.68 to 1.34) (see Figure 9). Non-responders to the neuropathy questionnaire had a higher body mass index (p = 0.055) and were more likely to be from a ethnic minority group (p = 0.004) than responders. Imputation of missing values did not affect the estimates.
The overall intracluster correlation coefficient values were as follows: retinopathy 0.014 (95% CI 0.00017 to 0.55), albuminuria 0.025 (95% CI 0.0066 to 0.091) and neuropathy 0.011 (95% CI 4.6 × 10–7 to 1). These results indicate that the impact of clustering on study power was small.
Secondary analyses: patient-reported outcomes
Data from 2861 participants were included in the multiple imputation analyses. Patients who completed questionnaires at baseline and follow-up (n = 2217) were more likely than those who did not complete questionnaires (n = 644) to be male (59.5% vs. 50.8%), of white ethnicity (95.2% vs. 89.9%) and employed (44.8% vs. 32.7%). Questionnaire completers were also less likely to smoke (24.3% vs. 34.0%), had higher levels of alcohol consumption (median 5 units per week vs. 3 units per week) and had higher EQ-5D scores (median 0.85 vs. 0.81) at baseline. They also had lower systolic blood pressure levels (148.5 mmHg vs. 151.4 mmHg). Other characteristics were comparable between treatment groups (data not shown).
Table 5 shows the PROM scores at follow-up, separately for each centre and by randomised group. EQ-5D values did not change between diagnosis and follow-up: the median (interquartile range) score was 0.85 (0.73 to 1.00) at baseline and 0.85 (0.73 to 1.00) at 5 years’ follow-up.
Measure | Centre | |||||||||||||||
---|---|---|---|---|---|---|---|---|---|---|---|---|---|---|---|---|
Denmark | Cambridge | Leicester | The Netherlands | |||||||||||||
IT | RC | IT | RC | IT | RC | IT | RC | |||||||||
n | Mean (SD) | n | Mean (SD) | n | Mean (SD) | n | Mean (SD) | n | Mean (SD) | n | Mean (SD) | n | Mean (SD) | n | Mean (SD) | |
SF-36 | ||||||||||||||||
PCS score | 665 | 46.7 (10.0) | 428 | 46.7 (9.6) | 350 | 43.9 (11.6) | 310 | 44.6 (11.3) | 59 | 44.3 (11.4) | 84 | 43.4 (10.5) | 177 | 46.8 (10.4) | 144 | 47.0 (10.5) |
MCS score | 665 | 55.3 (9,1) | 428 | 54.9 (8.5) | 350 | 53.4 (9.0) | 310 | 54.6 (8.4) | 59 | 50.9 (10.1) | 84 | 52.2 (9.8) | 177 | 54.3 (8.2) | 144 | 53.7 (7.4) |
EQ-5D score | 695 | 0.85 (0.21) | 463 | 0.84 (0.22) | 351 | 0.81 (0.23) | 312 | 0.83 (0.22) | 60 | 0.75 (0.31) | 85 | 0.79 (0.23) | 176 | 0.86 (0.18) | 144 | 0.82 (0.26) |
EQ-VAS | 691 | 76.9 (16.9) | 462 | 76.4 (18.5) | 355 | 76.1 (18.0) | 316 | 78.4 (16.4) | 60 | 78.3 (16.3) | 88 | 74.8 (18.4) | 175 | 76.5 (13.7) | 144 | 75.3 (15.6) |
W-BQ12 score | ||||||||||||||||
General | 680 | 28.5 (5.9) | 447 | 28.1 (6.3) | 346 | 25.5 (6.5) | 310 | 26.4 (5.9) | 58 | 25.3 (6.7) | 78 | 25.0 (6.3) | 171 | 27.6 (6.3) | 141 | 27.4 (5.7) |
Negative | 689 | 1.1 (2.0) | 458 | 1.1 (1.8) | 351 | 1.7 (2.4) | 314 | 1.4 (2.1) | 59 | 1.9 (2.5) | 86 | 2.1 (2.5) | 175 | 1.1 (1.9) | 143 | 1.1 (1.8) |
Energy | 691 | 8.1 (2.7) | 453 | 8.0 (2.8) | 352 | 7.0 (2.7) | 315 | 7.3 (2.6) | 58 | 7.1 (2.7) | 82 | 7.1 (2.3) | 174 | 8.5 (2.6) | 142 | 8.5 (2.3) |
Positive | 692 | 9.4 (2.5) | 456 | 9.2 (2.8) | 352 | 8.2 (2.8) | 315 | 8.4 (2.7) | 59 | 8.2 (2.6) | 85 | 8.0 (2.9) | 177 | 8.0 (3.1) | 144 | 8.1 (2.6) |
ADDQoL score | 552 | –0.73 (1.15) | 348 | –0.69 (1.07) | 315 | –0.84 (1.29) | 271 | –0.87 (1.30) | 50 | –1.20 (1.78) | 76 | –2.39 (2.52) | 169 | –0.55 (0.86) | 135 | –0.55 (0.92) |
DTSQ score | 648 | 30.9 (6.2) | 405 | 30.1 (6.7) | 344 | 31.5 (4.9) | 305 | 31.2 (5.4) | 60 | 33.0 (3.8) | 85 | 29.1 (7.3) | 174 | 31.2 (5.6) | 140 | 31.0 (5.6) |
The mean differences in PROMs comparing the IT group with the RC group are shown in Figures 10–13. There were no statistically significant differences in health status (see Figure 10), well-being (see Figure 11), diabetes-specific quality of life (see Figure 12) and satisfaction with diabetes treatment (see Figure 13) between the two groups. There was some heterogeneity between centres. The I2-statistic varied between 41% (W-BQ-positive) and 73% (EQ-VAS).
FIGURE 10.
Mean difference in health status between the IT group and the RC group by centre (boxes) after 5 years’ follow-up in the ADDITION-Europe trial and pooled estimates (diamonds) calculated by random-effects meta-analysis. Horizontal bars and diamond widths denote 95% CIs and box sizes indicate relative weight in the analysis. MCS, mental component summary; PCS, physical component summary. Source: reproduced from van den Donk et al. ,84 under the Creative Commons Attribution Noncommercial licence.

FIGURE 11.
Mean difference in W-BQ12 scores between the IT group and the RC group by centre (boxes) after 5 years’ follow-up in the ADDITION-Europe trial and pooled estimates (diamonds) calculated by random-effects meta-analysis. Horizontal bars and diamond widths denote 95% CIs and box sizes indicate relative weight in the analysis. Source: reproduced from van den Donk et al. ,84 under the Creative Commons Attribution Noncommercial licence.
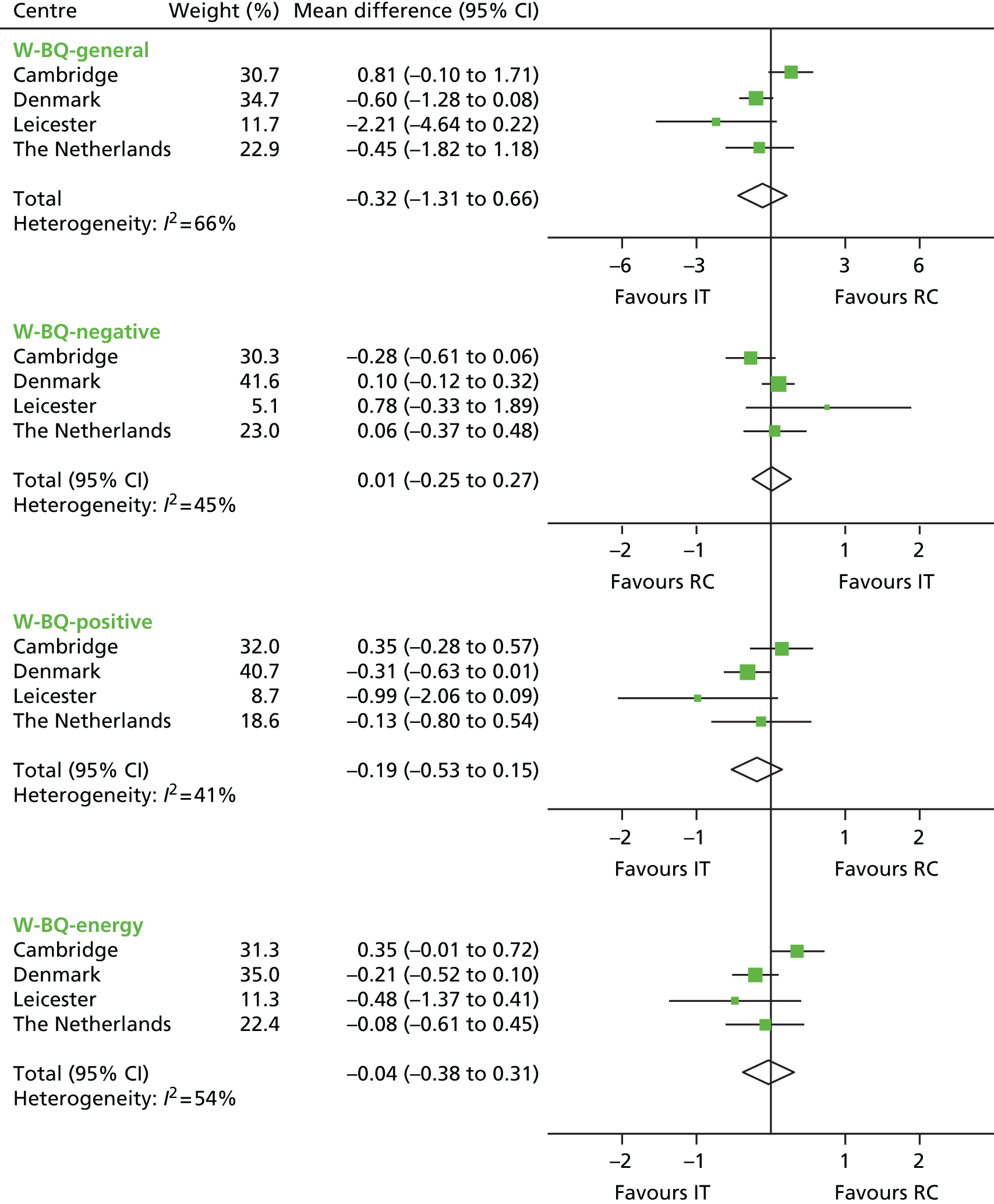
FIGURE 12.
Mean difference in ADDQoL scores between the IT group and the RC group by centre (boxes) after 5 years’ follow-up in the ADDITION-Europe trial and pooled estimates (diamonds) calculated by random-effects meta-analysis. Horizontal bars and diamond widths denote 95% CIs and box sizes indicate relative weight in the analysis. Source: reproduced from van den Donk et al. ,84 under the Creative Commons Attribution Noncommercial licence.
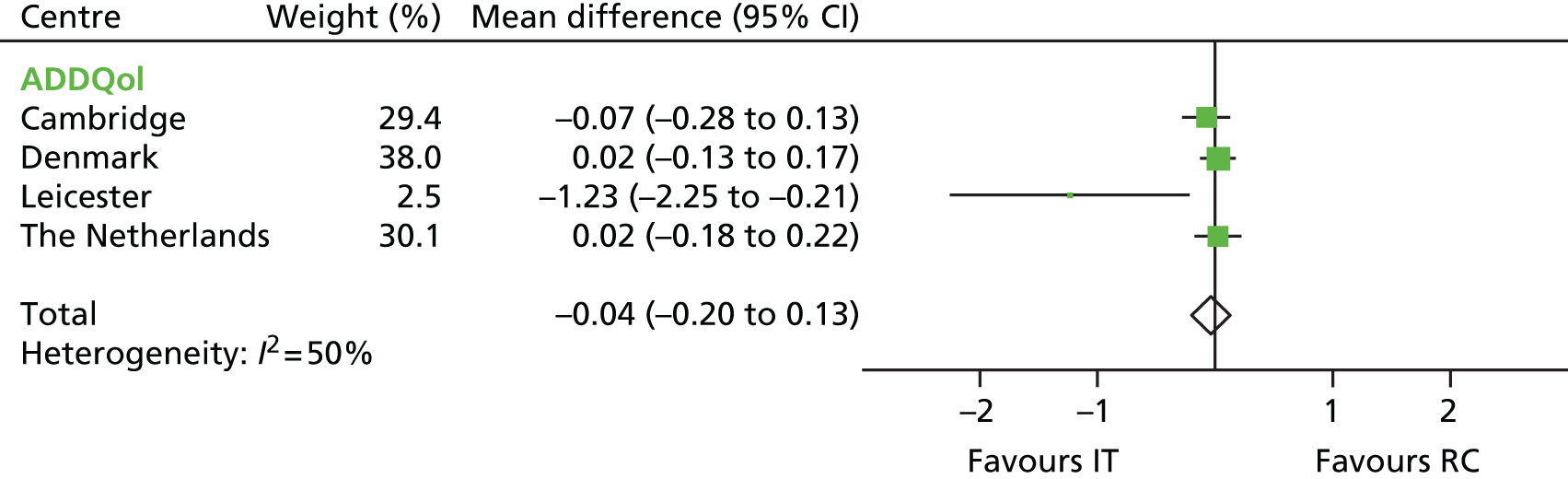
FIGURE 13.
Mean difference in DTSQ scores between the IT group and the RC group by centre (boxes) after 5 years’ follow-up in the ADDITION-Europe trial and pooled estimates (diamonds) calculated by random-effects meta-analysis. Horizontal bars and diamond widths denote 95% CIs and box sizes indicate relative weight in the analysis. Source: reproduced from van den Donk et al. ,84 under the Creative Commons Attribution Noncommercial licence.
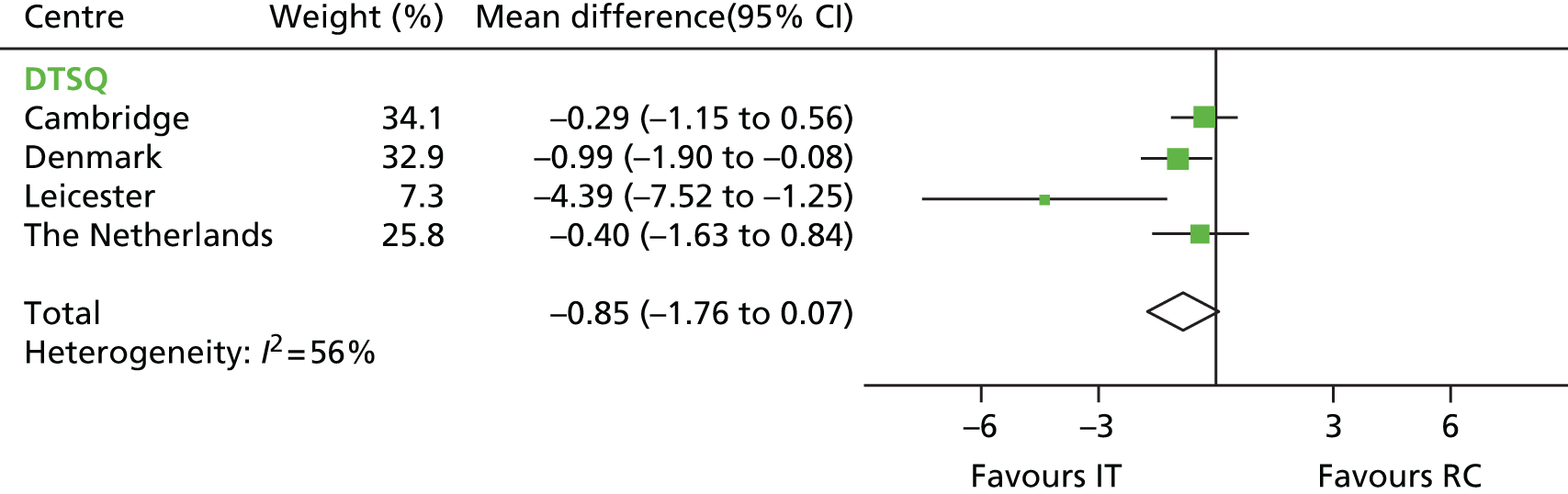
Multiple imputation analyses resulted in slightly different point estimates. However, the overall patterns remained the same and there were no statistically significant differences in any of the PROMs (results not shown).
Chapter 4 Economic evaluation
This chapter reports two analyses related to the cost-effectiveness of early IT of type 2 diabetes. First, we assessed the performance of the UKPDS outcomes model [UKPDS-OM, version 1.3; © Isis Innovation Ltd 2010; see www.dtu.ox.ac.uk/outcomesmodel (accessed 27 January 2016)]85 in predicting CVD risk in the ADDITION-Europe population; we wanted to investigate its suitability for modelling longer-term outcomes and costs for the ADDITION-Europe cohort. The second analysis concerns the short-term (1–6 years within-trial analysis) and long-term (10–30 years based on decision modelling) cost-effectiveness of the ADDITION intervention in the UK from a UK payer (NHS) perspective.
Validating the UK Prospective Diabetes Study outcomes model
Accurate prediction of cardiovascular risk among individuals with diabetes is important for providing prognostic information and targeting treatment to those at highest risk. It is also a key component of economic evaluations of interventions aimed at reducing the disease burden of diabetes and improving the quality and length of life for those with this chronic condition.
The UKPDS-OM is a cost-effectiveness analysis tool. It was derived from the UKPDS, a multicentre randomised controlled trial among 5102 individuals with newly diagnosed type 2 diabetes. 85 Participants were recruited from 23 UK centres. 86 The UKPDS-OM has been validated for the prediction of CVD and other complications in internal and external cohorts of people with clinically diagnosed diabetes. The observed and predicted cumulative incidence of CVD complications from diagnosis to 12 years’ follow-up were very similar when using an internal cohort. 85 Results varied from poor to moderate in terms of discrimination, calibration and absolute risk for external cohorts,87,88 with the UKPDS-OM tending to overestimate CVD risk. 89
The UKPDS-OM was developed using data from individuals recruited between 1977 and 1997. The treatment and costs of diabetes and related complications have changed since this time. The variables assessed by the model are also likely to vary over time and between countries. As such, we aimed to evaluate the performance of the UKPDS-OM in the ADDITION-Europe study, which included a multinational contemporary cohort of individuals with screen-detected diabetes.
Methods
The 5-year accumulated absolute risks of MI and stroke were estimated for each participant in the ADDITION-Europe study using the UKPDS-OM. 85 The model includes information on age at diagnosis, sex, ethnicity, duration of diabetes, weight, height, smoking status, presence or absence of AF, PVD, systolic blood pressure, HbA1c, total cholesterol, HDL cholesterol and years since pre-existing CVD events. Values for smoking status, systolic blood pressure, HbA1c, total cholesterol and HDL cholesterol were included at both baseline and 5 years’ follow-up.
As information on AF and PVD were not collected at baseline in the ADDITION-Europe study, and given that all patients had newly diagnosed diabetes, these variables were set to 0. The number of years since pre-existing ischaemic heart disease, congestive heart failure, amputation, blindness and renal failure were also set to 0 as this information was also not collected at baseline. Data on the number of years since any previous MI or stroke were collected in the ADDITION-Europe study and entered as appropriate into the model. As revascularisation is not a component of the UKPDS-OM and only a single case of amputation was reported during follow-up in the ADDITION-Europe study, only MI and stroke events were examined in this analysis.
Statistical analysis
We calculated the observed and predicted risk of non-fatal MI and non-fatal stroke by country and trial group using the UKPDS-OM. We employed multiple imputation to deal with missing data90 using the Markov chain Monte Carlo method and assuming an arbitrary missing pattern. A multivariate normal distribution was used to impute missing values for age, sex, weight, height, smoking status, total cholesterol, HDL cholesterol, systolic blood pressure and HbA1c. It has been previously demonstrated that multivariate normal imputation is less biased than complete-case analysis91 and produces similar results to other approaches despite the presence of binary and ordinal variables that do not follow a normal distribution. If the imputed HDL cholesterol value was greater than the total cholesterol value (five cases at baseline; one case at final follow-up), we assumed that HDL cholesterol (mmol/l) = total cholesterol – 0.1. For each patient with missing data we undertook five imputations and computed the average of the five risk estimates. 92
In the UKPDS-OM the categories of the variable ethnicity were white-Caucasian, Afro-Caribbean, and Asian-Indian. In the ADDITION-Europe cohort there were some unknown or unclassifiable values, for example mixed white + African, mixed white + Asian or others. These cases were unavoidably excluded from the analysis as ethnicity is improper for multiple imputation (156 cases).
We examined the discrimination of the UKPDS-OM by computing the area under the receiver operating characteristic (aROC) curve and assessed goodness of fit using the Hosmer–Lemeshow chi-square test. The same methods have been employed in previous studies for the validation of the UKPDS and Framingham risk engines. 87,88,93
In the UKPDS-OM, both MI and stroke are assigned a particular Weibull regression equation with age, sex, HbA1c and other variables as covariates. 85 To examine which distribution was the best fit for baseline risk of MI and stroke, we performed a number of survival regression analyses including exponential, log-normal, log-logistic, Weibull and generalised gamma. We computed minimal Bayesian information criterion (BIC) and Akaike information criterion (AIC) values to assess global model fit. 94 We then analysed the covariates with the best fit distribution to determine if they were significantly associated with MI and stroke. A p-value of < 0.10 was defined as statistically significant. 95 As risk factor values were not available for every year of follow-up in the ADDITION-Europe trial cohort, the average of the baseline and final follow-up values was used.
We also conducted a complete-case sensitivity analysis to examine whether or not the results were replicated after excluding patients for whom there were missing data (n = 781/2899; 26.9%). Statistical analyses were performed with SAS v9.3.
Results
Of the 3055 ADDITION-Europe participants, 156 were excluded as a result of unclassifiable or unknown ethnicity. Baseline values for age, sex, treatment group and HbA1c did not differ significantly between the included and excluded patients.
Observed and predicted risk of non-fatal myocardial infarction and stroke
The non-fatal MI and stroke rates for the ADDITION-Europe participants were 0.0228 and 0.0152, respectively. The observed risks of MI and stroke were lower than the predicted risks using the UKPDS-OM. When the data were broken down by country, overestimation was greater in the Dutch population than in the Danish and UK populations for both non-fatal MI and non-fatal stroke (Table 6).
Event | Centre | Intervention | Total n | Number of events | Observed risk (95% CI) (%) | Average predicted risk (%) |
---|---|---|---|---|---|---|
Non-fatal MI | All | RC | 1314 | 39 | 2.97 (2.12 to 4.04) | 6.86 |
IT | 1585 | 27 | 1.70 (1.13 to 2.47) | 6.77 | ||
Denmark | RC | 601 | 25 | 4.16 (2.71 to 6.08) | 6.46 | |
IT | 851 | 14 | 1.65 (0.90 to 2.74) | 6.16 | ||
The Netherlands | RC | 212 | 2 | 0.94 (0.11 to 3.37) | 8.45 | |
IT | 236 | 2 | 0.85 (0.10 to 3.03) | 8.74 | ||
UK | RC | 501 | 12 | 2.40 (1.24 to 4.15) | 6.67 | |
IT | 498 | 11 | 2.21 (1.12 to 3.92) | 6.87 | ||
Non-fatal stroke | All | RC | 1314 | 19 | 1.45 (0.87 to 2.25) | 2.30 |
IT | 1585 | 25 | 1.58 (1.02 to 2.32) | 2.34 | ||
Denmark | RC | 601 | 8 | 1.33 (0.58 to 2.61) | 2.24 | |
IT | 851 | 12 | 1.41 (0.73 to 2.45) | 2.05 | ||
The Netherlands | RC | 212 | 2 | 0.94 (0.11 to 3.37) | 3.00 | |
IT | 236 | 2 | 0.85 (0.10 to 3.03) | 3.63 | ||
UK | RC | 501 | 9 | 1.80 (0.82 to 3.38) | 3.08 | |
IT | 498 | 11 | 2.21 (1.11 to 3.92) | 2.21 |
Examination of the absolute event rates between intervention groups represents the impact of IT compared with RC. For non-fatal MI, the difference between intervention groups was higher than that predicted by the UKPDS-OM, suggesting that the UKPDS-OM underestimated the effect of IT. For non-fatal stroke, the difference was much smaller between the observed and predicted data, indicating only a slight overestimation (see Table 6).
Discrimination analysis
The aROC curve was 0.72 (95% CI 0.66 to 0.79) for non-fatal MI and 0.70 (95% CI 0.64 to 0.77) for non-fatal stroke, suggesting that the UKPDS-OM had moderate discriminatory ability. The Netherlands had the lowest aROC curve for MI (0.69) and the highest for stroke (0.79). Results from the Hosmer–Lemeshow test were non-significant in the overall trial cohort and in each country, suggesting that the goodness of fit was acceptable (Table 7).
Event | Centre | aROC curve (95% CI) | Chi-square value from the Hosmer–Lemeshow test | p-value from the Hosmer–Lemeshow test |
---|---|---|---|---|
MI | All centres | 0.72 (0.66 to 0.79) | 9.79 | 0.28 |
Denmark | 0.76 (0.66 to 0.85) | 7.61 | 0.47 | |
The Netherlands | 0.69 (0.45 to 0.94) | 7.11 | 0.53 | |
UK | 0.70 (0.59 to 0.81) | 8.01 | 0.43 | |
Stroke | All centres | 0.70 (0.64 to 0.77) | 9.10 | 0.33 |
Denmark | 0.76 (0.68 to 0.84) | 7.59 | 0.47 | |
The Netherlands | 0.79 (0.55 to 1.00) | 7.17 | 0.52 | |
UK | 0.65 (0.54 to 0.76) | 6.07 | 0.64 |
Survival regression analysis
Following survival analysis, an exponential distribution was the best for baseline risk for both MI and stroke based on BIC values. For AIC values, log-normal and exponential distributions provided the best fit for MI and stroke, respectively. As such, we used the exponential distribution in survival regression analysis with a selection of different covariates: age, sex, treatment group, smoking status, body mass index, HbA1c level, systolic blood pressure and ln(total cholesterol/HDL) for MI or total cholesterol/HDL for stroke. Age, sex and HbA1c level were significantly associated with non-fatal MI; age and sex were significantly associated with non-fatal stroke (Table 8).
Event | Distribution | BIC | AIC | Covariates significant (p > 0.10) from the exponential distribution |
---|---|---|---|---|
MI | Gamma | 656.12 | 585.50 | Age, sex, HbA1c |
Exponential | 641.45 | 583.43 | ||
Log-logistic | 648.95 | 585.13 | ||
Log-normal | 646.98 | 583.16 | ||
Weibull | 649.24 | 585.43 | ||
Stroke | Gamma | 434.02 | 364.41 | Age, sex |
Exponential | 418.54 | 360.50 | ||
Log-logistic | 426.21 | 362.39 | ||
Log-normal | 426.27 | 362.45 | ||
Weibull | 426.21 | 362.40 |
Complete-case analysis
In total, 2118 (out of 2899) participants were included in the complete-case analysis. Compared with participants excluded because of missing data, the included participants had a slightly lower mean age (60.09 vs. 60.84 years) and a higher mean HbA1c value (7.06% vs. 6.96%); there was also a higher proportion of men (59.0% vs. 55.3%) among the included participants. The results were generally similar to the results of the main imputed analysis in terms of observed and predicted risk and best fit distribution. The one exception was for the survival analysis, for which sex no longer remained significantly associated with stroke and HbA1c became significant.
Cost-effectiveness analysis
In our second analysis we aimed to examine the short- and long-term cost-effectiveness of IT compared with RC among people with screen-detected type 2 diabetes.
Methods
This analysis used data from the two UK centres (Cambridge and Leicester) in the ADDITION-Europe trial.
Costs
These included the cost of delivering the intervention and the routine cost to the NHS of treating diabetes and diabetes-related events observed during trial follow-up. All costs were calculated in UK pounds and monetary values were transformed to the 2009/10 UK national level using the Hospital and Community Health Services Pay and Prices Index,97,98 which coincided with the census date for outcome assessment.
Cost of delivering the ADDITION intervention
The cost of delivering the intervention included the costs of (1) the design of the materials, and consultation meetings with health professionals regarding development and production; (2) practitioner and patient meetings, which included the costs of delivering the meetings, the time of consultants and educators and the time of doctors and nurses; and (3) extra patient consultations and treatment (including prescription of cardioprotective medication and glucometers with strips) (Table 9). The unit costs for the time of doctors, nurses and other health professionals were obtained from standard UK unit cost references. 97,98 The volume of resources used was obtained from the ADDITION study protocol47 and relevant trial documents. Some costs were estimated from internal accounting during the trial. The cost for extra prescriptions in the IT group (compared with the RC group) was established in treatment algorithms in 2001 at the beginning of the ADDITION study and was transformed to 2009/10 prices. Intervention costs were different in Cambridge and Leicester and were averaged for the purposes of the cost-effectiveness analysis. For the long-term analysis we assumed that the additional prescription costs in the intervention arm would continue to be incurred each year.
Category | Category | Category | Personnel/item | Time | Other | Unit cost (£) | Cost (£) | Remark |
---|---|---|---|---|---|---|---|---|
Delivery | Materials | Design | 2 associated health professionals | 8 hours | – | 44a | 704 | |
Practitioner folders | 118 doctors and 52 nurses | – | – | 8.2 | 1394 | Internal accounting | ||
Patient folders | 513 patients (IT group) | – | – | 5.8 | 2975 | Internal accounting | ||
Handouts | – | – | – | – | 446 | Internal accounting | ||
Consultation meeting | 1 associated health professional (and 6 patients) | 2 hours | £5 for travel | 44a | 93 | Patient cost excluded | ||
Focus group meeting | 3 consultants | 3 hours | £5 for travel | 121b | 1104 | |||
3 nurses | 3 hours | £5 for travel | 44a | 411 | ||||
Preparatory meetings | Cambridge (in general practices) | 2 consultants | 3 hours × 100 | 121b | 72,600 | |||
4.5 doctors | 1.5 hours × 100 | 121b | 81,675 | |||||
2 nurses | 1.5 hours × 100 | 30c | 9000 | |||||
Leicester (in hospitals) | 2 educators | 6 hours × 6 | £54.1 for logistics × 6 | 44a | 3493 | |||
Subtotal | 173,895 | |||||||
Extra consultations | Cambridge (n = 452) | 10-minute GP visit | 3 visits × 3 years | 185d | 121,091 | Annual discount rate 3.5% | ||
10-minute nurse visit | 3 visits × 3 years | 30c | 19,637 | |||||
Leicester (n = 61) | GP initial visit + GP extra visits + annual review visit | 60 minutes + 20 minutes × 4 + 30 minutes | 165e,f | 28,534 | ||||
2 visits + annual review visit | (20 minutes + 30 minutes) × 4 | 165e,f | 20,748 | |||||
Subtotal | 190,010 | |||||||
Extra treatments | Cambridge (n = 452) | Extra prescription of SUs, ACE inhibitors and statins | Total cost = £262.5 per patient | 262.5 | 118,650 | Internal accounting | ||
Leicester (n = 61) | Extra prescription of SUs, ACE inhibitors and statins | Cost = £262.5 per patient | 262.5 | 16,013 | Internal accounting | |||
95% of participants issued with a glucometer and box of 50 strips at diagnosis | Glucometer cost = £1438; strips cost = £2968 | 4406 | 4406 | Internal accounting | ||||
Subtotal | 139,069 | |||||||
Total | 502,974 | |||||||
Cost per person | 980 |
Cost of the treatment of diabetes and diabetes-related complications
Unit treatment costs were obtained from published literature (Table 10). We collected the annual treatment cost of type 2 diabetes without complications and type 2 diabetes-related complications in the year of the event and in subsequent years. We extracted both inpatient costs (costs of admissions to hospital either as a day case or as an inpatient for ≥ 1 night) and non-inpatient costs (costs of all home, clinic and telephone contacts with GPs, nurses, podiatrists, opticians and dieticians and with eye and other hospital outpatient clinics) from the UKPDS,100 from where the majority of treatment costs used in this study were taken. In the short-term within-trial cost-effectiveness analysis and the long-term modelling analysis we used an additive method to sum the annual costs of multiple complications. 85
Event | Cost, year of event (£) | Cost, subsequent years (£) | Source | Utility decrement | Source | |
---|---|---|---|---|---|---|
Fatal | Non-fatal | |||||
Type 2 diabetes | – | 494.5 | 494.5 | Clarke et al.100 | –0.220 | Schwarz et al.101 |
Ischaemic heart disease | – | 3558.4 | 1175.2 | Clarke et al.100 | –0.090 | Clarke et al.102 |
MI | 2295.6 | 6861.8 | 1129.8 | Clarke et al.100 | –0.055 | Clarke et al.102 |
Heart failure | 3968.4 | 3968.4 | 1391.1 | Clarke et al.100 | –0.108 | Clarke et al.102 |
Stroke | 5786.8 | 4196.9 | 793.4 | Clarke et al.100 | –0.164 | Clarke et al.102 |
Revascularisation | – | 4943.1 | 316.3 | Valentine et al.103 | –0.059 | Valentine et al.103 |
Amputation | 13,664.2 | 13,664.2 | 788.7 | Clarke et al.100 | –0.280 | Clarke et al.102 |
Blindness | – | 1791.7 | 758.9 | Clarke et al.100 | –0.074 | Clarke et al.102 |
Renal failure | 30,599.2 | 30,599.2 | 30,599.2 | Schwarz et al.101 | –0.263 | Clarke et al.102 |
CVD death | 3724.3 | – | – | Valentine et al.103 | – |
Utility decrement
We collected published utility decrement data to calculate quality-adjusted life-years (QALYs) as the health outcome measurement for diabetes without complications and diabetes with complications, including ischaemic heart disease, MI, heart failure, stroke, revascularisation, amputation, blindness and renal failure (see Table 10). 102 The majority of these utility data were taken from the UKPDS study based on EQ-5D measurement. 104 The same value was assigned to the year of the event and to subsequent years. For patients with multiple events the additive method was used in which we summed utility decrements for each event. Thus, the health state of patients diagnosed with diabetes with no complications was assigned a utility of 1 – 0.22 = 0.78. Those with diabetes and a history of MI were assigned a value of 1 – 0.22 – 0.055 = 0.725. Utility decrements were applied until death; thus, a patient experiencing a MI would experience a 0.055 decrement to their utility in the year of the infarction and for every subsequent year.
Short-term cost-effectiveness analysis
We calculated the accumulated costs and QALYs for every year from diabetes diagnosis based on observed events (MI, stroke, revascularisation and amputation) in the ADDITION trial. Both costs and QALYs incurred after the first year were discounted at 3.5% per annum in line with current UK guidelines. 105 To adjust for baseline imbalances we used ordinary least squares regression analyses to calculate costs and QALYs as a function of intervention group (RC/IT), treatment centre (Cambridge/Leicester), age at diagnosis, sex and HbA1c level at baseline. Adjusted incremental treatment costs and QALYs were reported as means and 95% CIs.
Long-term modelling analysis
We used the UKPDS-OM (version 1.3) to perform long-term modelling analysis85 as it is derived from a UK population and focuses on cardiovascular complications. Our validation analysis described earlier96 concluded that the model provided a reasonable prediction of the incremental event rate although it tended to overestimate the absolute event rates.
Information from ADDITION trial participants at baseline was entered into the UKPDS-OM, including age at diagnosis, sex, ethnicity, duration of diabetes, weight, height, smoking status, systolic blood pressure, HbA1c, total cholesterol, HDL cholesterol and years since pre-existing CVD events. Values for smoking status, systolic blood pressure, HbA1c, total cholesterol and HDL cholesterol were also included from measurements taken at 1 and 5 years’ follow-up. For the years in between (2, 3 and 4 years) and for future years, the risk factor values were simulated by the UKPDS outcomes risk equations, that is, left to propagate through the long-term model. Data on AF, PVD, ischaemic heart disease, congestive heart failure, amputation, blindness and renal failure at diagnosis were not collected in the ADDITION study. Given that all participants were newly diagnosed, all values were set to zero for these variables.
To deal with missing data, multiple imputation was applied using the Markov chain Monte Carlo method assuming an arbitrary missing pattern. 90,106 A multivariate normal distribution was used to impute missing values of weight, height, smoking status, cholesterol, HDL cholesterol, systolic blood pressure and HbA1c. In the UKPDS-OM the required ethnicity categories were white Caucasian, Afro-Caribbean and Asian-Indian. There were some unknown or unclassifiable values, for example mixed white + African, mixed white + Asian, etc., in the ADDITION-UK trial. It was not suitable to replace these using multiple imputation and so we excluded these participants from the analysis (n = 25). After imputation, if the imputed HDL cholesterol value was higher than the cholesterol value (which was logically impossible), we assumed that HDL cholesterol (mmol/l) = cholesterol – 0.1 (five cases at baseline; one case at final follow-up). Five imputations were taken for each participant and we combined the results with Rubin’s rules. 90,92
Using the UKPDS-OM we performed a patient-level modelling analysis on time horizons of 10, 20 and 30 years with a discount rate of 3.5%. We report the 30-year simulation as the main result. For each time horizon, 1000 inner loops and 100 bootstraps were conducted. 107 Means and CIs at the patient level were used to conduct a further bootstrap analysis, adjusting for centre, age at diagnosis, sex and HbA1c at baseline as per the short-term analysis. Incremental cost-effectiveness ratios (ICERs) for the 10-, 20- and 30-year simulations are reported.
Analysis of uncertainty
Decision uncertainty is illustrated with a scatterplot of incremental cost–QALY pairs and the cost-effectiveness acceptability curve. 108 One-way sensitivity analyses were performed on treatment costs for events (±10%), utility decrements (±10%) and the discount rate (0%, 5%) using the 30-year simulation data with the results shown as a tornado diagram. 109 We also explored two scenarios with intervention costs of £750 (three-quarters of the cost) and £500 (half of the cost) to represent lower set-up costs (e.g. making use of previously designed materials).
Statistical analyses of within-trial data were performed using SAS v9.3. Analysis of long-term modelled scenarios was conducted using the UKPDS model and Microsoft Excel® 2013 (Microsoft Corporation, Redmond, WA, USA).
Results
Of 1026 participants in the ADDITION-UK study, two withdrew from the study and 25 were excluded from the modelling analysis because of unknown or unclassifiable ethnicity. Thus, 1024 participants were included in the short-term cost-effectiveness analysis and 999 were included in the long-term modelling analysis.
The total cost of delivering the IT intervention in ADDITION-UK was £502,974. This equates to £981 per person (£339 for materials and preparatory meetings, £370 for extra patient consultations and £272 for extra treatments) (see Table 9). The cost by centre was £90,379 (£1482 per person) in Leicester and £412,595 (£913 per person) in Cambridge.
Short-term cost-effectiveness analysis
There were no differences in cumulative QALYs over any time horizon from 1 to 5 years (Table 11). The cumulative incremental cost to the NHS (intervention cost and other expenditure incurred as a result of cardiovascular complications) ranged from £285 over a 1-year time horizon to £935 over 5 years (discounted at 3.5%). As IT was more costly and led to virtually no incremental health gains compared with RC over the first 5 years, we estimate that IT of people with screen-detected diabetes is, on average, not cost-effective in the short term.
Time horizon | RC | IT | Adjusted incremental cost (95% CI) (£) | Adjusted incremental QALYs (95% CI) | ICER (£) | P(ICER < £30,000) (%)b | ||||
---|---|---|---|---|---|---|---|---|---|---|
n | Cost, mean (SE) (£) | QALYs, mean (SE) | n | Cost, mean (SE) (£) | QALYs, mean (SE) | |||||
1 | 511 | 536.6 (0.8) | 0.779 (0.000) | 513 | 826.4 (1.7) | 0.778 (0.000) | 285.3 (199.3 to 371.3) | 0.0000 (–0.002 to 0.002) | ICER was infinite or IT group was dominated at all years | |
2 | 511 | 1138.7 (2.0) | 1.531 (0.000) | 513 | 1426.0 (2.0) | 1.530 (0.000) | 278.8 (151.4 to 406.2) | 0.0000 (–0.004 to 0.004) | ||
3 | 511 | 1705.8 (2.9) | 2.256 (0.000) | 513 | 2298.7 (3.0) | 2.254 (0.000) | 578.3 (388.6 to 768) | 0.0000 (–0.006 to 0.006) | ||
4 | 501 | 2239.3 (3.4) | 2.955 (0.000) | 509 | 3014.9 (3.6) | 2.954 (0.000) | 754.2 (531.5 to 976.9) | –0.0012 (–0.009 to 0.007) | ||
5 | 451 | 2804.4 (4.7) | 3.631 (0.000) | 455 | 3772.7 (4.7) | 3.627 (0.000) | 934.9 (653.6 to 1216.2) | –0.0040 (–0.016 to 0.008) | ||
10 | 501 | 6156.52 (20.02) | 6.450 (0.138) | 498 | 7435.86 (11.83) | 6.400 (0.140) | 1189.80 (1126.26 to 1244.96) | 0.0140 (–0.0013 to 0.0291) | 82,251.60 | 1.0 |
20 | 501 | 11,174.53 (31.27) | 9.324 (0.243) | 498 | 12,683.64 (20.70) | 9.157 (0.243) | 1495.66 (1368.31 to 1624.84) | 0.0428 (0.0013 to 0.0846) | 34,934.23 | 36.9 |
30 | 501 | 13,181.37 (22.38) | 10.076 (0.293) | 498 | 14,768.63 (29.19) | 9.818 (0.290) | 1745.31 (1564.44 to 1929.12) | 0.0465 (0.0014 to 0.0929) | 37,503.41 | 31.4 |
Long-term cost-effectiveness analysis
Intensive treatment was associated with positive incremental QALYs in the long term (0.0465 by 30 years, statistically significant at 20 years and beyond). The incremental cost of IT compared with RC at 10, 20 and 30 years also increased over time to £1745 at 30 years, yielding a point estimate ICER of £82,250 at 10 years, which fell to £35,000 at 20 years and rose slightly to £37,500 at 30 years (see Table 11). The unadjusted results suggest a lower point estimate QALY gain in the IT arm, which is reversed once adjustment is made for baseline differences. The result suggests that, although cost-effectiveness improves over time, it is still above commonly accepted thresholds in the UK (defined as reaching an ICER of £30,000 per QALY gained),105 even over a 30-year time horizon (Figure 14).
FIGURE 14.
Broken line chart showing the simulated ICERs at 10, 20 and 30 years for the ADDITION-Europe intervention. Source: reproduced from Tao et al. ,99 under the Creative Commons Attribution 4.0 International licence (CC BY 4.0).
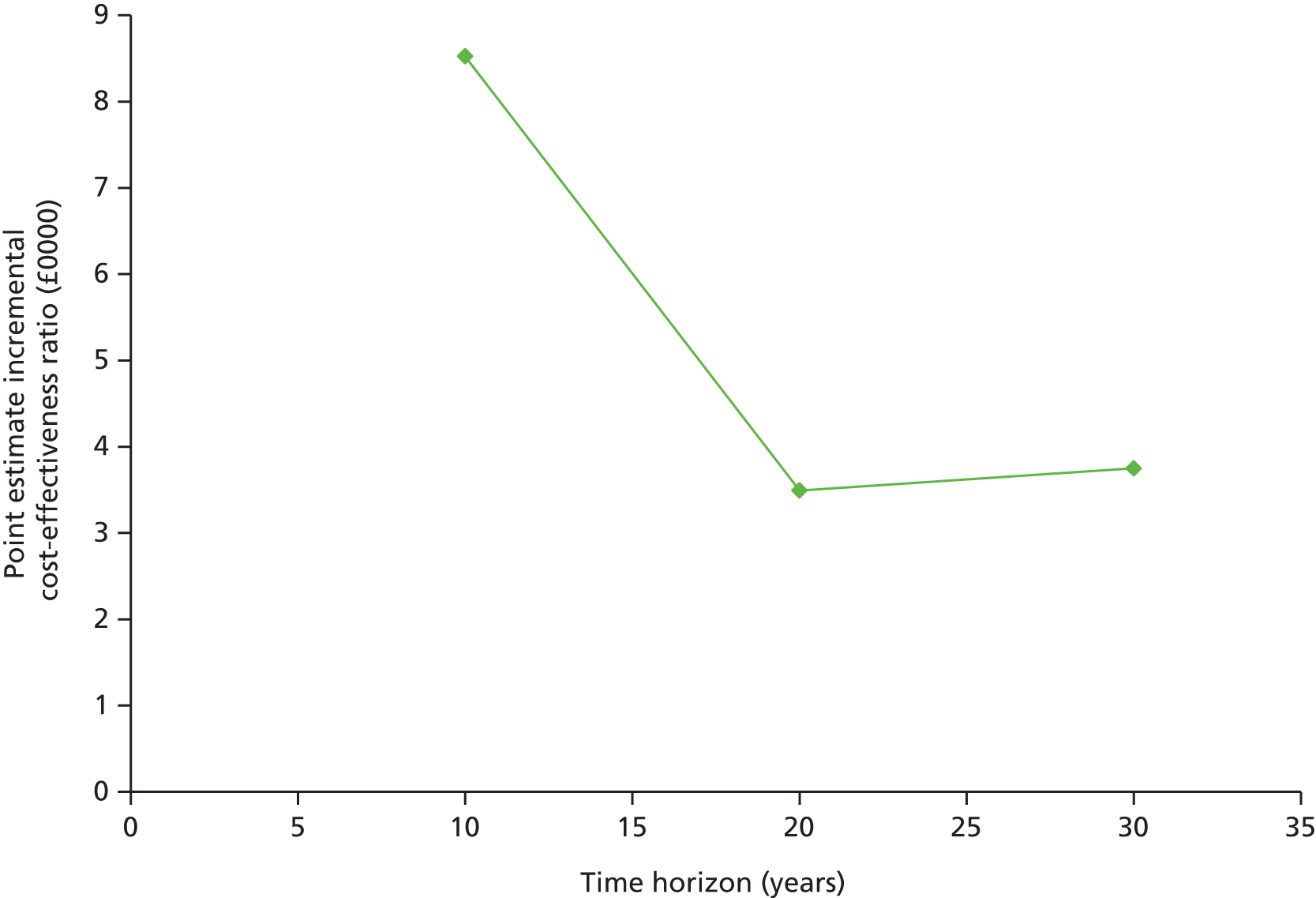
Analysis of uncertainty
Uncertainty and sensitivity analyses were performed on the predicted 30-year results. The cost-effectiveness plane based on bootstrap sampling shows the scatterplot of cost and QALY pairs under the base case (intervention cost of £981 per person) and two alternative scenarios with lower IT intervention costs (Figure 15) (£750 and £500 per person). Under these scenarios, 30-year point estimate ICERs are £37,503, £32,550 and £27,178. The cost at which the ICER falls to £30,000 is £631. If the intervention could be delivered ≤ £631 per patient, it may be considered cost-effective.
FIGURE 15.
Cost-effectiveness plane showing pairs of incremental costs and QALYs from bootstrap samples using three different costs of delivering the IT intervention. The points to the right of the lines are cost-effective. Source: reproduced from Tao et al. ,99 under the Creative Commons Attribution 4.0 International licence (CC BY 4.0).

Under all three scenarios, the majority of points are in the north-east quadrant, suggesting that IT is nearly always both more expensive and more effective (generates more QALYs) than RC, although the proportion of the probability mass in the north-west quadrant suggests that there is greater uncertainty around whether or not incremental QALYs are positive. The probability of cost-effectiveness according to the three different treatment costs (£981, £750 and £500) is 51.1%, 60.9% and 70.4% at a £20,000 per QALY gained threshold and 65.1%, 71.1% and 77.0% at a £30,000 per QALY gained threshold, respectively (Figure 16).
FIGURE 16.
Cost-effectiveness acceptability curves showing the probability of IT being more cost-effective than RC based on net benefit values from bootstrap samples using three different costs of delivering IT. The two dotted lines show the cost-effectiveness acceptability thresholds of £20,000 and £30,000 per QALY. Source: reproduced from Tao et al. ,99 under the Creative Commons Attribution 4.0 International licence (CC BY 4.0).

One-way sensitivity analyses varying unit treatment costs, utility decrements and the discount rate showed that the discount rate had the biggest impact on the ICER, with the impact of variations in utility decrements and treatment costs being minimal (Figure 17).
FIGURE 17.
Tornado diagram showing the influence of changing different parameters that contribute to the ICER in the long-term cost-effectiveness modelling analysis. The choice of discount rate has the greatest impact on the ICER (lower discount rate, unit costs and utility decrements all associated with a higher point estimate ICER). Source: reproduced from Tao et al. ,99 under the Creative Commons Attribution 4.0 International licence (CC BY 4.0).

Chapter 5 Discussion and conclusions
Change in treatment and risk factors
Results from the ADDITION-Europe trial show that screening for type 2 diabetes and early intensive multifactorial treatment of individuals found to have screen-detected diabetes is feasible in primary care. Screening identifies people with high levels of modifiable cardiovascular risk factors. The proportion of participants prescribed one or more blood pressure-, lipid- and glucose-lowering drugs and aspirin increased significantly from baseline to 5 years’ follow-up in both study groups. There were small differences in treatment between groups at 5 years favouring the IT group.
In both the IT group and the RC group, intermediate outcomes such as blood pressure and cholesterol improved considerably in the 5 years following diagnosis by screening; weight and glycaemia levels did not increase. In terms of change from baseline, and absolute levels of risk factors at 5 years, our results compare well with those of other trials in which patients were recruited at diabetes diagnosis and followed for 6 years. 110,111 However, the between-group differences observed at 1 year,49 which were clinically significant, were not maintained at 5 years and differences were smaller than those achieved in similar studies. 29,110 It is possible that adherence to treatment targets were suboptimal in this pragmatic trial. In three of the centres, the screening algorithm included the use of risk scores encompassing treatment for high blood pressure as one of the variables to predict diabetes risk. This limited the achievable differences in blood pressure levels between groups. Indeed, at baseline in the UKPDS, which began recruitment 20 years before the ADDITION-Europe study, 12% of patients were prescribed antihypertensive medication and 0.3% were prescribed lipid-lowering medication. In the ADDITION-Europe study, at baseline, 45% of patients were prescribed antihypertensive medication and 16% were prescribed lipid-lowering medication. Our trial was undertaken during a time of improvements in the delivery of diabetes care in general practice. This included the introduction of evidence-based guidelines in Denmark112 and the Netherlands113 and the Quality and Outcomes Framework for primary care in the UK. 114 Again, these guidelines and frameworks are likely to have reduced the achievable differences in treatment and risk factors between groups.
Despite increasing age and duration of diabetes there was a significant decline in 10-year modelled CVD risk in the 5 years following diagnosis in both the RC group [–5.0% (SD 12.2%)] and the IT group [–6.9% (SD 9.0%)]. Within all four centres the modelled CVD risk was lower in the IT group than in the RC group at 5 years. Comparable improvements in the CVD risk factors that drive modelled CVD risk have been reported among newly diagnosed patients enrolled in lifestyle interventions over 12 months. 54,115 Such changes were also observed among clinically diagnosed diabetes patients in the UKPDS trial at 6 years of follow-up. 110
Cardiovascular outcomes and all-cause mortality
The intervention to promote intensive target-driven management of individuals with screen-detected diabetes was associated with a non-statistically significant 17% relative reduction in the incidence of a composite cardiovascular event end point over 5 years. For all components of the primary end point, differences favoured the IT group. Differences were smallest for stroke and largest for MI. Cardiovascular event rates began to diverge after 4 years of follow-up. We found no evidence of an increased risk of adverse events including self-reported hypoglycaemia or mortality. The incidence of cardiovascular events in the ADDITION-Europe study (8.5% in the RC group) was lower than expected and less than event rates among newly diagnosed patients in the UKPDS (12.1%). 110
The combined mortality hazard ratio for the IT group compared with the RC group was 0.91 (95% CI 0.69 to 1.21). The heterogeneity in results between countries was not statistically significant. In the UK there were significantly fewer deaths in the IT group. In Denmark there was a non-significant reduction in cumulative risk in the RC group. The mortality rate (6.7% in the RC group) was also lower than expected. In the Hoorn study116 the mortality rate was 25% over 10 years in screen-detected patients and in newly diagnosed patients in Denmark111 the mortality rate was 33% over 7.4 years. The mortality rate in the ADDITION-Europe trial was similar to that seen in the Danish general population (without diabetes) of the same age between 1995 and 2006. 117 As before, this is most likely because of the high quality of care that was delivered to patients early in the course of the diabetes disease trajectory in both the RC group and the IT group.
Microvascular outcomes
Trial results for microvascular outcomes were similar to those for the cardiovascular outcomes. At 5 years, increases in prescribed treatment and improvements in cardiovascular risk factors were not associated with significant reductions in microvascular events. Differences between study groups favoured the IT group, with differences smallest for neuropathy and greatest for retinopathy. As for the CVD outcomes, the frequency of microvascular complications at 5 years’ follow-up was lower than expected. It was also lower than the reported frequencies among individuals with diabetes for a similar length of time. 32 We concluded that this was most likely because of the early detection of diabetes and the high-quality treatment that we observed in both study groups.
In terms of the nephropathy outcomes, 18.5% of ADDITION-Europe participants had any albuminuria at diagnosis. 50 This figure is not directly comparable to the estimate of 7.2% of patients with microalbuminuria at baseline in the UKPDS as a higher level of urinary albumin excretion was used to define microalbuminuria in the UKPDS. 32 The rate of progression of albuminuria in the ADDITION-Europe study, from 18.5% at baseline to 23.5% (1% per year), was half that observed in the UKPDS, despite ADDITION-Europe participants being older (mean age 60 years vs. 52 years in the UKPDS) and more likely to have high blood pressure at diagnosis. 32 Similarly, higher progression rates were reported in the VADT (Veterans Affairs Diabetes Trial),118 ACCORD119 and ADVANCE (Action in Diabetes and Vascular Disease: Preterax and Diamicron Modified Release Controlled Evaluation)120 trials. However, these studies recruited older people who had long-standing diabetes.
We observed a beneficial increase in eGFR in both the IT group and the RC group. This is probably linked to the treatment of multiple cardiovascular risk factors, including lipid-lowering treatment, glucose-lowering treatment and ACE or ARB treatment.
As with the nephropathy outcomes, retinopathy frequency was lower than expected. Just 12% of ADDITION-Europe RC patients developed retinopathy after 5 years of follow-up. In the UKPDS, 37% of patients had retinopathy at diagnosis and a further 22% developed the condition during the next 6 years. 31 Again, this is likely to reflect early treatment in the lead time between screen detection and clinical diagnosis.
A similar trend was observed for neuropathy, with 5% of the population reporting peripheral neuropathy at 5 years, with no difference between groups. This is markedly lower than the 11% reported in a population with an average diabetes duration of 16 years. 67 However, caution should be exercised in the interpretation of this result. We used the Michigan neuropathy questionnaire, which may not be sufficiently sensitive121 or reliable to detect early progress of neuropathy and/or differences between the IT group and the RC group. 122 There was heterogeneity in the effect size estimates for peripheral neuropathy across all centres, which supports this view.
Patient-reported outcomes
Compared with RC, IT was not associated with differences in any PROMs (health status, well-being, quality of life and treatment satisfaction) after 5 years of follow-up. Health status, as measured by the EQ-5D, did not change between diagnosis and follow-up. Again, we conclude that the time period within which our trial was undertaken, for example when targets for cholesterol and blood pressure became stricter for diabetes patients, resulted in smaller than expected differences between study groups.
Baseline health-related quality of life was high in our cohort (median EQ-5D score of 0.85). EQ-5D estimates remained stable over follow-up in spite of participants’ age increasing by 5 years. This is an important finding, particularly as the burden of treatment increased over this time. In a study among 1136 Dutch people with type 2 diabetes (mean age 64.9 years), the average EQ-5D score was 0.74 (SD 0.27). 123 Older patients, women and those with a longer duration of diabetes reported lower levels of health-related quality of life. In a cluster-randomised trial in 55 Dutch general practices, there was no change in diabetes-related health over 1 year between standard and intensive multifactorial therapy, although a negative effect on the SF-36 social functioning score could not be ruled out. 124 In the UKPDS, intensively treating blood pressure and blood glucose in newly diagnosed diabetes patients did not adversely affect health status. 39 In an ACCORD substudy, there was a difference in change in the SF-36 physical component score and the DTSQ scale over 4 years between the standard group and the intensive glucose-lowering group. 40 However, the absolute difference in the SF-36 score was only 0.5 units, considerably less than the general threshold of 3–5 points recognised as an important difference. ACCORD participants differed from ADDITION-Europe patients in that they were older, they had had clinically diagnosed diabetes for an average duration of 9–10 years, 35% were on insulin treatment and they had a different ethnic background. Overall, the findings from other studies are largely in line with the results from the ADDITION-Europe trial, that is, no significant differences in PROMs between treatment groups.
It is worth noting that, although pooled analyses from all centres showed no differences between the treatment groups, for some PROMs the results in Leicester clearly favoured the IT group. This might be linked to the nature of the intervention. This was delivered by an intermediate care team at a community-based diabetes specialist care facility. Patients also received weight management advice, a glucometer at diagnosis and the DESMOND structured education programme. 53 Other ADDITION-Europe centres relied on existing primary care teams to achieve the IT targets.
Validation of the UK Prospective Diabetes Study outcomes model
The UKPDS-OM had moderate discriminatory ability to predict non-fatal MI and stroke among ADDITION-Europe participants. However, the model overestimated absolute risk. As diabetes patients begin to be diagnosed earlier in the disease trajectory, and their CVD risk is therefore lower, the UKPDS-OM may need updating for CVD risk prediction in contemporary diabetes populations.
The accuracy of the UKPDS-OM (version 1.3) in estimating the risk of non-fatal MI and stroke varied between countries. It performed particularly poorly in the Netherlands compared with the UK and Denmark. This could be because of a difference in baseline characteristics between the ADDITION-Europe cohorts and the UKPDS cohort. The UKPDS population had some baseline characteristics associated with a lower risk of CVD, including lower mean age, mean body mass index and mean systolic blood pressure, and some characteristics associated with a higher risk, for example a higher percentage of men, a lower mean HDL cholesterol level and a slightly higher mean HbA1c level. The UKPDS also excluded people with previous CVD. However, the purpose of the UKPDS-OM is to provide coefficients for the inputted risk factors. As such, the accuracy of the predicted risk should be relatively insensitive to the baseline characteristics. We could reject a model on the basis of baseline characteristics only if the two populations were very different, for example predicting the risk of CVD based on a cohort of individuals aged 80 years and applying these risks to a cohort of individuals aged 20 years.
The most likely reason for the overestimation of risk is because of improvements in treatment between the time that the UKPDS data were collected (1977–97) and the time of ADDITION-Europe data collection (2002–6). The ADDITION-Europe intervention also included a much more comprehensive set of preventative treatments offered from an earlier stage in the disease. Our results are consistent with those of other studies examining the predictive ability of the UKPDS and Framingham models in populations with lower disease rates. 88,125
As AF and PVD data were not collected at baseline, these variables were set to zero in the UKPDS-OM. PVD was a risk factor only for amputation in the UKPDS-OM and so the omission of these data should not affect our results. AF is a risk factor for stroke in the UKPDS-OM;85 missing data for this variable are likely to underestimate stroke risk. However, in one study the prevalence of AF ranged from 1.2% to 2.8% among people aged 60 years126 and so missing data for this variable are unlikely to have a large effect on our results.
The UKPDS-OM performed differently in predicting the differences in CVD risk factors between the intervention groups. It underestimated the incremental risk of MI between groups and slightly overestimated differences for stroke. Assessment of the cost-effectiveness of health-care interventions necessitates knowledge of the increment between the comparator groups, rather than the difference in absolute levels of each group. It is ultimately a subjective decision whether or not these differences are sufficient to render the UKPDS-OM unsuitable for extrapolating the ADDITION-Europe data. The insight from our work shows that, if the UKPDS-OM is unadjusted, it will underestimate any reductions in MI risk attributable to IT and consequently potentially underestimate the cost-effectiveness of the intensive intervention (i.e. overestimate the ICER). The small disparity in the predicted compared with observed risk of stroke may be of limited consequence because of the effects of discounting.
As stated above, the UKPDS-OM had moderate discriminatory ability for predicting non-fatal MI and stroke. The aROC curve ranged from 0.65 to 0.79 and goodness of fit was acceptable. The UKPDS-OM is based on a set of Weibull survival regression equations. For the model to perform successfully, it is important to choose a suitable baseline distribution and relevant covariates. The best-fit model for the ADDITION-Europe data was an exponential distribution. Our analyses showed that age, sex and HbA1c level were important covariates and should be included in the UKPDS-OM.
Cost-effectiveness results
Cumulative costs and QALYs over a time horizon of 1–5 years indicated that IT was not cost-effective over the short term. This result may be linked to the improvements in cardiovascular risk factors that were observed in both trial groups between baseline and follow-up. 55 In the long-term modelling analysis, the 30-year simulated ICER was greater than the recommended UK National Institute for Health and Care Excellence threshold (£20,000–30,000 per QALY),105 suggesting that interventions to promote delivery of intensive multifactorial treatment in the ADDITION-UK study were not cost-effective compared with RC in the long term.
The intervention becomes cost-effective only if it can be delivered at a cost of < £631 per participant. The cost of delivering IT in our analysis (£981 per participant) was calculated based on the study protocol,47 which covered all aspects of the intervention. This figure may overestimate the cost of reproducing the intervention as trial materials would not require complete redevelopment (although some adaptation to local needs would be likely). Alternative approaches to influencing practitioner behaviour, such as point-of-care reminders and decision aids, might be cheaper than practice visits by specialists. Furthermore, treatment costs were based on payments made to GP practices according to predicted costs based on the study protocol rather than actual costs. These costs may have been overestimated as GPs prescribed less medication than expected. 55 Finally, as per our validation analysis, the UKPDS tends to overestimate the risks of cardiovascular events, which could have led to overestimation of the ICER.
Our finding of a general improvement in cost-effectiveness over the long term is exemplary of the nature of preventative treatments. As most of the costs of such interventions are borne ‘up front’, and chronic disease complications take a long time to develop, health and cost benefits are usually seen only over the longer term. We used a 30-year modelling horizon in our analyses as the average age of participants at baseline was 60 years. We therefore assumed that a 30-year time horizon would equate to a lifetime horizon for the majority of individuals. A key question for policy-makers is whether or not they are prepared to consider a lifetime horizon in their decision-making. The optimal time horizon for any economic evaluation is one that is sufficient to capture all impacts on incremental costs and outcomes. 127 In our case, this suggests a lifetime horizon. However, investing in an intervention now means spending the money from this year’s budget without seeing any benefit for many years. During this time personnel, governments and government policy may change. Introducing such polices is therefore a challenge given pressures (political or otherwise) to show the benefits from investment decisions over the short to medium term.
The DESMOND study examined the benefits of a structured education and self-management programme for people with newly diagnosed diabetes in the UK from 2004 to 2006. 53,128 The authors found that the intervention was likely to be cost-effective compared with usual care over a lifetime horizon (ICER £5387). One reason for the difference between our results and the results of the DESMOND study and other studies might be the early disease stage of participants in our study. Early detection might help prevent complications in the future by attenuating disease progression. However, intervening ‘too early’ might be less cost-effective. For example, intervening when an individual presents with diabetes-related symptoms may reduce the risk of an event that would otherwise have occurred in 5 years’ time. Intervening at an earlier stage, after detection of IGT, for example, may prevent an event that would not otherwise occur for 20 years. In general, individuals and society have a preference for benefits now rather than in the future. As such, they would value preventing an event in the next 5 years more highly than preventing the same event in 20 years’ time.
Strengths and limitations
The ADDITION-Europe study participants were drawn from representative population-based samples in three different European countries. Practices were recruited from a large geographical area in each country. Randomised practices (26% of those invited) were nationally representative in terms of key clinical and sociodemographic characteristics. 43,48,49 However, recruitment was non-random and the generalisability of our findings to other settings should be considered with caution. Furthermore, participants were predominantly white (93%), which might limit the extrapolation of our data to more ethnically diverse regions. Individuals were identified using a range of different screening programmes and were diagnosed according to 1999 WHO criteria. 56
The study intervention included algorithms and targets based on well-established trial data and we used a range of methods associated with changes in practitioner and patient behaviour. We randomised general practices rather than individuals (as the intervention was delivered mainly by practitioners) to minimise the risk of contamination. We achieved high levels of participant retention and independently adjudicated end-point ascertainment in both trial groups (99.9%). Trained staff were unaware of study group allocation. We assessed clinically important outcomes using standardised equipment and protocols. For the modelled CVD risk analysis, we used the latest version of the UKPDS risk score (version 3). This was derived from > 40,000 patient-years of data and 1115 CVD events. 75 For PROMs we used both generic and diabetes-specific measures.
There was variation between centres in the approaches to population-based screening for diabetes. However, all participants were diagnosed according to WHO guidelines. 56 Furthermore, the differences in screening programmes do not affect the internal validity of the study as they apply equally to both study groups in each centre. Rather, they increase the external validity or generalisability of the trial by including patients detected using a range of different screening programmes. There was also variation in the methods used to encourage the general practice teams to follow the treatment algorithms. However, there was one common set of treatment targets and algorithms for the IT group in all centres and, as stated above, this heterogeneity should increase generalisability without diminishing internal validity. We pooled centre-specific estimates using fixed-effects meta-analysis and formally tested for heterogeneity between centres using the I2-statistic. Although we acknowledge that this test has limited power, the primary outcome and all of its components did not exhibit significant between-centre heterogeneity and so we have not formally explored in this report explanations for any observed heterogeneity. Furthermore, there are a number of other centre-level covariates other than those previously mentioned relating to the different health systems in each country that may have contributed to variation in participant characteristics and response to the intervention. The different approaches taken in each centre to promote IT of multiple risk factors represent complex interventions. As the study was pragmatic, it is not possible to determine which aspects of the interventions might have been effective and which aspects might have been less so.
Cluster randomisation occurred before screening and patient recruitment. This design feature can introduce a difference between groups in participant characteristics. Although individuals in the IT and RC groups were well matched overall, in Denmark the intervention may have influenced the number and type of patients recruited to the IT group. Staff undertaking opportunistic screening may have conducted more tests in individuals at high CVD risk as they were aware of the potential benefits of early detection and treatment. This may have attenuated the effects of the intervention and might explain some of the differences in results between centres. Differences appeared to be present but might not have been statistically significant because of the limited power of the test of heterogeneity. Our intracluster correlation coefficients were small55 and did not adversely affect study power. Methods of screening, laboratory testing and outcome assessment were standardised across study groups in each centre in this pragmatic trial but did differ between centres. This may also have contributed to some of the observed heterogeneity. Measurement of biochemical outcomes differed slightly between baseline and follow-up in two centres but not between groups. Reassuringly, interlaboratory validation suggested that methods were consistent between centres. Primary end points were adjudicated using a standardised manual and case report form in each centre. Other potential sources of heterogeneity in our trial arise from variation between centres in participant characteristics. This might be because of differences in the screening programmes and underlying populations, different modes of delivering the intervention and other unmeasured factors.
For the CVD outcomes analyses there were few differences between those with and those without follow-up data. For the modelled CVD risk analysis, 27% of data were missing. Sensitivity analyses suggested that the primary analysis was likely to represent an accurate ITT analysis. We accounted for missing data at baseline using the missing indicator method and assumed that those lost to follow-up had a 10% higher risk than those with available data at 5 years. There was qualitatively no change to the final results and the difference in modelled CVD risk between groups remained significant. It is likely that we overestimated the level of modelled CVD risk in our contemporary trial cohort as the UKPDS model is based on a historical cohort diagnosed 20 years before the ADDITION-Europe study participants. However, this would not have affected the effect size estimate differentially by group. There was no difference between self-reported AF at 5 years between the RC group (13.3%) and the IT group (14.0%), suggesting that the exclusion of this variable from the UKPDS model was unlikely to affect our findings.
For the microvascular outcomes analysis there were differences in the proportions of individuals with data for different outcomes at 5 years. For example, 77% had retinopathy data and 95% had eGFR data. There was some evidence of healthy volunteer bias when examining the characteristics of participants with and without microvascular outcome data; however, absolute differences were small [e.g. 0.19% (2 mmol/mol) for HbA1c]. There were no differences between the IT group and the RC group in terms of the proportion with missing microvascular data. However, the prevalence of retinopathy and neuropathy might have been underestimated considering the adverse risk profile of individuals with missing data for these outcomes. Microvascular outcome assessment and laboratory testing were standardised across participants from the IT and RC groups in each centre but did differ between centres; for example, there was no standardised procedure for retinal photography. Some centres used routine secondary data sources, whereas others collected retinal data during remeasurement of participants at the 5-year follow-up examination. This variation might have led to differences in the precision of outcome assessment between centres but not between study groups. Furthermore, we assessed only gradable photos and they were coded using a standard scale (ETDRS65) by three experienced ophthalmologists who were unaware of study group allocation.
For the PROMs, patients who completed the questionnaires differed from those who did not. However, we believe that these differences are unlikely to have had an important effect on the outcomes as the differences were small, were not in one direction and were similar for both the IT group and the RC group. Our multiple imputation analysis confirmed the results of the main analysis, which supports our conjecture that selective dropout of patients did not introduce significant bias. As most of the PROMs were not measured at baseline, we were not able to examine changes in PROMs over time, nor adjust for baseline measurement of each PROM. This would not have been possible for diabetes-specific outcome measures such as the DTSQ and ADDQoL. We adjusted for a number of possible confounding variables, which were similar between the IT group and the RC group at baseline. We also adjusted all models for the baseline EQ-5D score as a proxy for baseline health status.
For the UKPDS-OM validation work we used multiple imputation, which allows the best use of the available data and takes into account the uncertainty in missing values. Both the imputed data set and the complete-case data set produced similar results, confirming the robustness of our results. Our validation work was limited by a lack of annual CVD risk factor measurement. In the UKPDS cohort, biochemical data were collected every year. We had to average baseline and follow-up values for the year of event in regression analyses as they were not available for every year. We did not have information on baseline AF, PVD, years since pre-existing CVD, blindness and renal failure. This might have introduced some bias in model prediction and influenced the efficiency of the model, most likely contributing to an underestimation of risk of CVD. We validated the UKPDS-OM using 5-year data and extrapolated the model to a longer time horizon in our cost-effectiveness work to estimate the 30-year incremental cost-effectiveness of the IT intervention. As follow-up continues more events will occur. We will revise our estimate of the cost-effectiveness of the intervention once longer-term data are available.
For the cost-effectiveness work we examined outcomes over both the short term and the long term and performed sensitivity analyses to test the robustness of our findings. We used data from the UKPDS, a UK study of individuals with diabetes and long-term CVD outcomes to calculate unit costs, utility decrements and modelled CVD risk. We adjusted for centre (Cambridge/Leicester) rather than practice as the intervention differed slightly between the centres; however, within the same centre practices shared standardised practitioner guidelines. Intraclass correlation coefficient values for GP practice for the primary outcome were very small in the main ADDITION-Europe trial, supporting our decision to adjust for centre rather than practice.
There were some limitations. First, we focused on only macrovascular outcomes (CVD and associated acute events). Microvascular outcomes such as retinopathy and nephropathy are also important in assessing the impact of type 2 diabetes on both quality of life and costs. Exclusion of these is likely to underestimate the benefit from any preventative intervention and thus underestimate cost-effectiveness (i.e. overestimate the ICER), particularly in the longer term as patients avoid CVD and live with diabetes long enough to develop microvascular complications.
Second, although the treatment protocol was identical, the lifestyle intervention was different in Leicester and Cambridge. To represent the cost of implementing an ‘ADDITION-like’ intervention that reflected a degree of diversity across the country we simply averaged the costs of the two interventions. We did, however, include centre in the adjusted analyses estimating incremental costs and QALYs. Results were similar when we adjusted for cluster (GP practice) and when running analyses separately by centre.
Third, we used an additive method to calculate treatment costs and utility decrements for individuals with multiple events. This is a commonly used method and was applied in the UKPDS-OM,100,129 but it is unclear if the cost and utility decrements of subsequent complications should be additive or multiplicative. A review of health state utilities in patients with type 2 diabetes published after completion of our analysis130 recommends the use of the Clarke data set for most comorbid events, or health state utilities very close to those used in our analysis, with one notable exception: we assigned a disutility of 0.263 for renal failure, whereas Beaudet et al. 130 recommend values between 0.204 and 0.164. Using a higher disutility will overestimate the health gain from averting renal failure. However, we estimated the difference in risk of renal failure over 30 years between the IT group and the RC group to be –0.05% (SE 0.06%). The impact of this on the incremental QALY gain per patient in each arm is therefore likely to be negligible.
Fourth, most of the equations in the UKPDS-OM predict future risk factors and events based on baseline values, time since diagnosis and the value of biometrics such as HbA1c level in the previous time period (year). It is an individual-level model in which patient data are calculated individually (rather than as mean values of a cohort). Thus, the model assumes that any trends present at 5 years will continue into the future, effectively assuming a continuation of the effect of the small differences in treatment generated by the intervention at 5 years. The 10-year follow-up of the UKPDS cohort found that, although between-group differences in HbA1c level and blood pressure were both lost within 12 months of the end of the active phase of the study, those who had previously achieved tighter control over HbA1c still had a lower event rate at 10 years than those who had not, while any benefit from lower blood pressure was not maintained. 131,132 Current guidelines and practice have changed since the initiation of the ADDITION-Europe trial, with patients recommended to receive at least as IT as the ‘intensive’ arm. Trial patients in the RC arm received a higher level of treatment than observed in ‘standard practice’, which may have diluted the observed incremental health gain. However, after 5 years of treatment, there was room for improvement in the prescription of cardioprotective treatment in both groups. Current evidence suggests that delays in treatment intensification in people with type 2 diabetes (clinical inertia) are still very common.
On the cost side, the official duration of the IT intervention was 3 years, for which practices were reimbursed for additional activity and prescriptions. We projected additional prescribed drug costs in the IT arm over the full 30 years under the assumption that patients in the RC arm would not increase their medication and patients in the IT arm would not decrease their medication over time. We may, therefore, have overestimated the cost of the IT arm. Whether this is true or not will be assessed once the 10-year follow-up data from the ADDITION-Europe cohort are available for analysis.
Finally, although we used the most appropriate CVD risk model available, as outlined earlier, the UKPDS-OM was derived using data from a historical cohort of people with clinically diagnosed diabetes. The ADDITION cohort included people with screen-detected diabetes. Other research125,133 has shown that the UKPDS-OM tends to overestimate absolute CVD risk, a finding replicated in our own investigation of the suitability of the UKPDS-OM to extrapolate ADDITION study data. 96 Furthermore, the utility decrements for MI and stroke derived from the ADDITION study were smaller than those in the UKPDS, a finding that is consistent with contemporary patients receiving better care and hence reporting higher quality of life. This underlines the importance of our sensitivity analyses, which showed that changes to these parameters did not substantially affect the ICER. Of the discount rate, intervention unit cost and utility decrement, variations in the discount rate had the biggest impact on the ICER.
Conclusion
Improvements in the quality of diabetes care in general practice during the duration of the ADDITION-Europe trial meant that treatment in the RC group was better than expected and not very different from the care provided in the IT group. 55 Cardiovascular risk factors (weight, blood pressure, cholesterol and modelled CVD risk82), including health-related behaviours (smoking), improved substantially following detection of diabetes by screening, even among those receiving RC. The small differences between groups in the intensity of treatment during the 5 years following diagnosis were associated with a non-significant 17% reduction in the primary composite cardiovascular end point in favour of the IT group. 55 There was no difference between the groups at 5 years in the prevalence of microvascular outcomes83 or in health status, well-being, quality of life or treatment satisfaction. 84 Recent trials of IT of glycaemia among patients with long-standing diabetes suggest that adverse effects occur early35,134 and that any benefit in terms of a reduction in cardiovascular risk takes longer than 5 years to be realised. 131 Whether or not the small difference in the intensity of treatment of multiple CVD risk factors early in the course of the disease in the ADDITION-Europe study will translate into a significant reduction in cardiovascular events and mortality over the longer term is uncertain.
We demonstrated that it was feasible to use the UKPDS-OM model to extrapolate the ADDITION-Europe data. 96 We took account of the possible underestimation of any reductions in MI and used the model in our cost-effectiveness analysis. Cost-effectiveness work showed that promotion of IT compared with RC was not cost-effective over a short- to medium-term time horizon for people with screen-detected diabetes in the UK. However, the intervention has the potential to be cost-effective if it can be delivered for approximately £630 per patient rather than £981. Such savings may be plausible through adaptation of predeveloped materials and economies of scale in delivery. Although there were few differences between screened and unscreened populations, the small proportion (2.9%) of individuals whose diabetes was detected by screening did appear to benefit. 23
Implications for clinical practice
The ADDITION-Europe randomised trial did not deliver conclusive evidence to justify implementation of a systematic screening and early treatment programme. Uncertainties remain, particularly concerning the overall cost-effectiveness of screening and early treatment for undiagnosed diabetes. However, the overall results of the ADDITION-Europe study suggest that earlier detection and treatment of diabetes is associated with net benefits. Although it remains uncertain whether or not IT is associated with a reduction in CVD or microvascular end points over the long term, there was no evidence that IT adversely affects PROMs early in the course of the disease. Furthermore, despite increases in age and diabetes duration, modelled CVD risk estimates decreased in the whole trial cohort from baseline to follow-up. Our research suggests that practitioners can safely treat multiple cardiovascular risk factors early and intensively in the diabetes disease trajectory when the rate of CVD risk progression may be slowed.
The observed absolute and relative risks of cardiovascular events and mortality among participants with an average HbA1c level of around 6.5% 5 years after diagnosis should reassure practitioners concerned about IT for hyperglycaemia. There was no interaction between IT and age or previous history of cardiovascular events, although there was a suggestion that benefits associated with IT were greatest among participants aged ≥ 60 years at diagnosis. Multifactorial treatment of screen-detected patients in both study groups was associated with improvements in cardiovascular risk factors and lower than expected rates of cardiovascular events and mortality.
In summary, primary care teams might consider the benefits of earlier detection (albeit not necessarily through population-based screening), lifestyle advice and IT for risk factors among those at high risk of diabetes and high risk of CVD. This might include opportunistic screening among those known to be at highest risk, based either on routinely available information such as age, body mass index and the presence of related conditions such as hypertension or on risk questionnaires/scores. Individuals identified in this way might be offered lifestyle interventions and preventative drug treatment appropriate to their level of risk.
Future research recommendations
Future research might focus on ways to increase the yield of programmes for the earlier detection of diabetes and assessment of cardiovascular risk. There is also a need to examine and implement effective and cost-effective care pathways for individuals who screen negative but who are at high risk of diabetes and CVD. The ADDITION-Europe study focused on the treatment of people with screen-detected diabetes, but the screening programme in each centre identified many more individuals without diabetes but who were at high risk of developing diabetes and CVD. In Denmark, for each person found with screen-detected diabetes, two people at high risk of developing diabetes and a further six people without diabetes but fulfilling guideline indications for preventative treatment of high risk factors for CVD were identified. Most of these people were not treated optimally according to current guidelines. 50,135 There is clearly an efficiency to combining strategies to identify individuals at high risk of both diabetes and CVD. Future research should also examine ways to intensify treatment and adherence. There was evidence of suboptimal treatment in this pragmatic trial. 55
Finally, future research should focus on whether or not the slowing of CVD risk progression observed in ADDITION-Europe trial participants in the first 5 years following diagnosis82 leads to a sustained reduction in CVD events over the long term. Outcomes tended to favour the IT group among ADDITION-Europe participants; however, the low frequency of events means that the 5-year duration of follow-up may have been insufficient to detect potentially clinically important differences between the RC group and the IT group. The apparent divergence of event rates from 4 years also suggests that further follow-up of the ADDITION-Europe trial is justified to examine whether early intensive multifactorial treatment reduces cardiovascular risk in the long term as seen in the UKPDS. 131 Longer-term follow-up of the ADDITION-Europe trial cohort alongside examination of microvascular, quality of life and cost data is therefore needed to establish the cost-effectiveness of IT among screen-detected patients.
Acknowledgements
We gratefully acknowledge the contribution of all participants, practice nurses and GPs in the ADDITION-Europe study. We are grateful to the independent end-point committees in Denmark (Professors Kristian Thygesen, Hans Ibsen, Ole Færgeman and Birger Thorsteinsson), the UK (Professor Jane Armitage and Dr Louise Bowman) and the Netherlands (Professors Cees Tack and Jaap Kappelle) and the Independent Data Monitoring and Safety Committee (Professor Christian Gluud, Professor Per Winkel and Professor Jørn Wetterslev). We thank Daniel Barnes for contributions to data cleaning, analysis and presentation.
The ADDITION-Denmark study was supported by the National Health Services in the counties of Copenhagen, Aarhus, Ringkøbing, Ribe and South Jutland in Denmark; the Danish Council for Strategic Research; the Danish Research Foundation for General Practice; the Novo Nordisk Foundation; the Danish Centre for Evaluation and Health Technology Assessment; the diabetes fund of the National Board of Health; the Danish Medical Research Council; and the Aarhus University Research Foundation. The trial has been given unrestricted grants from Novo Nordisk AS, Novo Nordisk Scandinavia AB, Novo Nordisk UK, Astra Denmark, Pfizer Denmark, GlaxoSmithKline Pharma Denmark, Servier Denmark A/S and HemoCue Denmark A/S. Parts of the grants from the Novo Nordisk Foundation, the Danish Council for Strategic Research and Novo Nordisk were transferred to the other centres.
Aside from the authors, the ADDITION-Denmark study group included Bendix Carstensen, Else-Marie Dalsgaard, Ynna Nielsen, Søren Bech-Morsing, Mette Vinther Skriver, Helle Terkildsen, Morten Charles, Merete Frandsen, Toke Bek and Henrik Andersen. We thank the Biochemistry Department at Steno Diabetes Centre for carrying out the biochemical assays and the following hospital departments for carrying out the clinical measurements: Steno Diabetes Centre Research Laboratory, Esbjerg Hospital Diabetes Clinic, Holstebro Hospital Research Department, Aabenraa Hospital Outpatient Clinic and Aarhus University Hospital Eye Department. We thank Toke Bek, Aarhus University Hospital Eye Department, and Henrik Lund Andersen, Glostrup Hospital Eye Department, for their contributions to the manual operating procedures for the retinal photography.
The ADDITION-Cambridge study was supported by the Wellcome Trust (grant reference number G061895), the Medical Research Council (grant reference number G0001164), the NIHR Health Technology Assessment programme (grant reference number 08/116/300), and NHS Research and Development support funding (including the Primary Care Research and Diabetes Research Networks). SJG received support from the Department of Health NIHR Programme Grants for Applied Research funding scheme (grant reference number RP-PG-0606-1259). NJW is a NIHR Senior Investigator. The views expressed in this publication are those of the authors and not necessarily those of the UK Department of Health. Bio-Rad provided equipment for HbA1c testing during the screening phase.
We are grateful to the ADDITION-Cambridge Independent Trial Steering Committee [Nigel Stott (chairperson), John Weinman, Richard Himsworth and Paul Little]. Aside from the authors, the ADDITION-Cambridge study team has included Rebecca Abbott, Amanda Adler, Judith Argles, Gisela Baker, Rebecca Bale, Roslyn Barling, Daniel Barnes, Mark Betts, Sue Boase, Sandra Bovan, Ryan Butler, Parinya Chamnan, Sean Dinneen, Pesheya Doubleday, Sue Emms, Mark Evans, Tom Fanshawe, Francis Finucane, Philippa Gash, Julie Grant, Wendy Hardeman, Robert Henderson, Susie Hennings, Muriel Hood, Garry King, Ann-Louise Kinmonth, Georgina Lewis, Christine May Hall, Joanna Mitchell, Richard Parker, Nicola Popplewell, A Toby Prevost, Emanuella De Lucia Rolfe, Lincoln Sergeant, Megan Smith, Stephen Sutton, Liz White, Fiona Whittle and Kate Williams. We thank the Cambridge University Hospitals NHS Foundation Trust Department of Clinical Biochemistry and the NIHR Cambridge Biomedical Research Centre Core Biochemical Assay Laboratory for carrying out the biochemical assays and the following people within the Medical Research Council Epidemiology Unit: Adam Dickinson (data management), Iain Morrison (information technology), Matt Sims (technical) and Paul Roberts, Kim Mwanza and James Sylvester (field epidemiology).
The ADDITION-Netherlands study was supported by unrestricted grants from Novo Nordisk, GlaxoSmithKline and Merck and by the Julius Center for Health Sciences and Primary Care, University Medical Center, Utrecht.
Aside from the authors, the ADDITION-Netherlands study team has included Kees Gorter, Paul Janssen, Lidian Izeboud, Jacqueline Berends, Marlies Blijleven, Bart Thoolen, Denise de Ridder, Jozien Bensing, Mehmet Akarsubasi, Paula Koekkoek, Carla Ruis, Geert Jan Biessels, Jaap Kappelle and Michiel van der Linden, all from Utrecht University. We thank the directors of the SHL Centre for Diagnostic Support in Primary Care, Etten-Leur (Wim Rutten and Paulien van Hessen), for their collaboration. Mardy Eckhardt, Erna Erdtsieck, Leandra Boonman and Annelies van der Smissen, all from the SHL, organised the screening and health assessments and carried out the biochemical assays. We thank the following people within the Julius Center: Nicole Boekema and Robert Veen (data management), Peter Zuithof (statistics) and Lizeth Vendrig and Annette Bak (staff recruitment). We thank Ronald P Stolk and Bruce HR Wolffenbuttel, University Medical Centre Groningen, University of Groningen, the Netherlands, for participation on the steering committee that designed the ADDITION-Netherlands study.
The ADDITION-Leicester was supported by the Department of Health and ad hoc Support Sciences, the NIHR Health Technology Assessment programme (grant reference number 08/116/300), NHS R&D support funding [including the Primary Care Research and Diabetes Research Networks and the Leicestershire, Northampton and Rutland (LNR) Collaboration for Leadership in Applied Health Research and Care (CLAHRC)] and the NIHR. MJD and KK receive support from the Department of Health NIHR Programme Grants for Applied Research funding scheme (grant reference number RP-PG-0606-1272).
Aside from the authors the ADDITION-Leicester study team has included Balasubramanian Thiagarajan Srinivasan, David Webb, Laura J Grey, Mary Quinn, Emma Wilmot, Samiul A Mostafa, Nitin Gholap, Hamid Mani, Winston Crasto, Steve Hiles, Joe Henson, Nick Taub, Janet Jarvis, Sukhjit Sehmi, Fiona Ablett, Champa Merry, Emma Healey, Julia Stockman, Sandra Campbell, Janette Barnett, Nil Radia, Mo Radia, Jo Howe, Lesley Bryan, Jane Brela, Jayne Hill, Helen Bray, Rachel Plummer, Zubeir Essat, Francis Pullen and Susan Enright. We thank the University Hospitals of Leicester NHS Trust Department of Clinical Biochemistry for carrying out biochemical assays.
Permission to use the DTSQ questionnaire was granted by Professor Clare Bradley, Health Psychology Research, Royal Holloway University of London, Surrey, UK.
We thank the Diabetes Trials Unit at Oxford University for the UKPDS-OM licence and the Health Economics Group at the University of East Anglia for methodological advice.
Contributions of authors
The following authors were involved in the conception of the original ADDITION-Europe study design: Knut Borch-Johnsen (Professor of Medicine), Melanie J Davies (Professor of Diabetes Medicine), Simon J Griffin (Professor of General Practice), Kamlesh Khunti (Professor of Primary Care Diabetes and Vascular Medicine), Torsten Lauritzen (Professor of General Practice), Guy EHM Rutten (Professor of General Practice), Annelli Sandbæk (Professor of General Practice) and Nicholas J Wareham (Professor of Epidemiology)
For Chapters 3 and 4, the following authors contributed to the design of the work; the acquisition, analysis and interpretation of data; drafting the work and revising it critically for important intellectual content; and preparing the results for publication: Knut Borch-Johnsen, Melanie J Davies, Simon J Griffin, Kamlesh Khunti, Torsten Lauritzen, Guy EHM Rutten, Annelli Sandbæk, Stephen J Sharp (Senior Statistician), Rebecca K Simmons (Senior Investigator Scientist, Diabetes Epidemiology), Maureen van den Donk (Scientific Staff Member, Epidemiology) and Nicholas J Wareham.
For the modelled CVD risk section of Chapter 3, James A Black (PhD student, Epidemiology) completed the data analysis, drafted the work and revised it critically for important intellectual content and prepared the results for publication.
For Chapter 4, the following authors contributed to the design of the work; conducted the economic analysis and interpretation of data, drafted the work and revised it critically for important intellectual content; and prepared the results for publication: Libo Tao (Research Fellow, Health Economics) and Edward CF Wilson (Senior Research Associate, Health Economics).
All authors gave final approval for this version of the work to be published. Professor Simon J Griffin agrees to be accountable for all aspects of the work in ensuring that questions related to the accuracy or integrity of any part of the work are appropriately investigated and resolved.
Publications
Lauritzen T, Griffin S, Borch-Johnsen K, Wareham NJ, Wolffenbuttel BH, Rutten G. The ADDITION study: proposed trial of the cost-effectiveness of an intensive multifactorial intervention on morbidity and mortality among people with Type 2 diabetes detected by screening. Int J Obes Relat Metab Disord 2000;24(Suppl 3):6–11.
Griffin SJ, Borch-Johnsen K, Davies M, Khunti K, Rutten GEHM, Sandbæk A, et al. Effect of early intensive multifactorial therapy on 5-year cardiovascular outcomes in individuals with type 2 diabetes detected by screening (ADDITION-Europe): a cluster-randomised trial. Lancet 2011;378:156–67.
van den Donk M, Sandbæk A, Borch-Johnsen K, Lauritzen T, Simmons RK, Wareham NJ, et al. Screening for type 2 diabetes. Lessons from the ADDITION-Europe study. Diabet Med 2011;28:1416–24.
Simmons RK, Echouffo-Tcheugui JB, Sharp SJ, Sargeant LA, Williams KM, Prevost AT, et al. Screening for type 2 diabetes and population mortality over 10 years (ADDITION-Cambridge): a cluster-randomised controlled trial. Lancet 2012;380:1741–8.
Simmons RK, Sharp SJ, Sandbæk A, Borch-Johnsen K, Davies MJ, Khunti K, et al. Does early intensive multifactorial treatment reduce total cardiovascular burden in individuals with screen-detected diabetes? Findings from the ADDITION-Europe cluster-randomized trial. Diabet Med 2012;29:e409–16.
Lauritzen T, Borch-Johnsen K, Davies MJ, Khunti K, Rutten GEHM, Sandbæk A, et al. Screening for diabetes. What do the results of the ADDITION trial mean for clinical practice? Diabetes Management 2013;3:367–78.
Tao L, Wilson ECF, Griffin SJ, Simmons RK. Performance of the UKPDS outcomes model for prediction of myocardial infarction and stroke in the ADDITION-Europe trial cohort. Value Health 2013;16:1074–80.
van den Donk M, Griffin SJ, Stellato RK, Simmons RK, Sandbæk A, Lauritzen T, et al. Effect of early intensive multifactorial therapy compared to routine care on self-reported health status, general wellbeing, diabetes specific quality of life and treatment satisfaction in screen-detected type 2 diabetes patients: the ADDITION-Europe study. Diabetologia 2013;56:2367–77.
Black JA, Sharp SJ, Wareham NJ, Sandbæk A, Rutten GE, Lauritzen T, et al. Change in cardiovascular risk factors following early diagnosis of type 2 diabetes: a cohort analysis of a cluster-randomised trial. Br J Gen Pract 2014;64:e208–16.
Black JA, Sharp SJ, Wareham NJ, Sandbæk A, Rutten GEHM, Lauritzen T, et al. Does early intensive multifactorial therapy reduce modelled cardiovascular risk in individuals with screen-detected diabetes? Results from the ADDITION-Europe cluster randomised trial. Diabet Med 2014;31:647–56.
Kuznetsov L, Griffin SJ, Davies MJ, Lauritzen T, Khunti K, Rutten GE, et al. Diabetes-specific quality of life but not health status is independently associated with glycaemic control among patients with type 2 diabetes: a cross-sectional analysis of the ADDITION-Europe trial cohort. Diabetes Res Clin Pract 2014;104:281–7.
Long GH, Cooper AJ, Wareham NJ, Griffin SJ, Simmons RK. Healthy behavior change and cardiovascular outcomes in newly diagnosed type 2 diabetic patients: a cohort analysis of the ADDITION-Cambridge study. Diabetes Care 2014;37:1712–20.
Sandbæk A, Griffin SJ, Sharp SJ, Simmons RK, Borch-Johnsen K, Rutten GEHM, et al. Effect of early multifactorial therapy compared with routine care on microvascular outcomes at 5 years in people with screen-detected diabetes. A Randomized Controlled Controlled Trial: the ADDITION-Europe study. Diabetes Care 2014;37:2015–23.
Black JA, Long GH, Sharp SJ, Kuznetsov L, Boothby CE, Griffin SJ, et al. Change in cardio-protective medication and health-related quality of life after diagnosis of screen-detected diabetes: results from the ADDITION-Cambridge cohort. Diabetes Res Clin Pract 2015;109:170–7.
Black JA, Simmons RK, Boothby CE, Davies MJ, Webb D, Khunti K, et al. Medication burden in the first 5 years following diagnosis of type 2 diabetes: findings from the ADDITION-UK trial cohort. BMJ Open Diabetes Res Care 2015;3:e000075.
Herman WH, Ye W, Griffin SJ, Simmons RK, Davies MJ, Khunti K, et al. Early detection and treatment of type 2 diabetes reduce cardiovascular morbidity and mortality: a simulation of the results of the Anglo-Danish-Dutch study of intensive treatment in people with screen-detected diabetes in primary care (ADDITION-Europe). Diabetes Care 2015;38:1449–55.
Kuznetsov L, Long GH, Griffin SJ, Simmons RK. Are changes in glycaemic control associated with diabetes-specific quality of life and health status in screen-detected type 2 diabetes patients? Four-year follow up of the ADDITION-Cambridge cohort. Diabetes Metab Res Rev 2015;31:69–75.
Tao L, Wilson EC, Wareham NJ, Sandbæk A, Rutten GE, Lauritzen T, et al. Cost-effectiveness of intensive multifactorial treatment compared with routine care for individuals with screen-detected type 2 diabetes: analysis of the ADDITION-UK cluster-randomized controlled trial. Diabet Med 2015;32:907–19.
All scientific papers arising from this report have been published as Open Access articles. As such, the papers are covered by a Creative Commons Attribution (CC BY 3.0) licence or the Creative Commons Attribution Non Commercial licence (CC BY-NC 3.0).
Data sharing statement
The ADDITION-Europe data are available to share via http://epi-meta.medschl.cam.ac.uk/ (accessed 28 January 2016).
Disclaimers
This report presents independent research funded by the National Institute for Health Research (NIHR). The views and opinions expressed by authors in this publication are those of the authors and do not necessarily reflect those of the NHS, the NIHR, NETSCC, the HTA programme or the Department of Health. If there are verbatim quotations included in this publication the views and opinions expressed by the interviewees are those of the interviewees and do not necessarily reflect those of the authors, those of the NHS, the NIHR, NETSCC, the HTA programme or the Department of Health.
References
- IDF Diabetes Atlas. Brussels: International Diabetes Federation; 2013.
- Hex N, Bartlett C, Wright D, Taylor M, Varley D. Estimating the current and future costs of type 1 and type 2 diabetes in the UK, including direct health costs and indirect societal and productivity costs. Diabet Med 2012;29:855-62. http://dx.doi.org/10.1111/j.1464-5491.2012.03698.x.
- Campbell SM, Roland MO, Middleton E, Reeves D. Improvements in quality of clinical care in English general practice 1998–2003: longitudinal observational study. BMJ 2005;331. http://dx.doi.org/10.1136/bmj.38632.611123.AE.
- Gillies CL, Abrams KR, Lambert PC, Cooper NJ, Sutton AJ, Hsu RT, et al. Pharmacological and lifestyle interventions to prevent or delay type 2 diabetes in people with impaired glucose tolerance: systematic review and meta-analysis. BMJ 2007;334. http://dx.doi.org/10.1136/bmj.39063.689375.55.
- Lindström J, Ilanne-Parikka P, Peltonen M, Aunola S, Eriksson JG, Hemiö K, et al. Sustained reduction in the incidence of type 2 diabetes by lifestyle intervention: follow-up of the Finnish Diabetes Prevention Study. Lancet 2006;368:1673-9. http://dx.doi.org/10.1016/S0140-6736(06)69701-8.
- Li G, Zhang P, Wang J, Gregg EW, Yang W, Gong Q, et al. The long-term effect of lifestyle interventions to prevent diabetes in the China Da Qing Diabetes Prevention Study: a 20-year follow-up study. Lancet 2008;371:1783-9. http://dx.doi.org/10.1016/S0140-6736(08)60766-7.
- Knowler WC, Fowler SE, Hamman RF, Christophi CA, Hoffman HJ, Brenneman AT, et al. 10-year follow-up of diabetes incidence and weight loss in the Diabetes Prevention Program Outcomes Study. Lancet 2009;374:1677-86. http://dx.doi.org/10.1016/S0140-6736(09)61457-4.
- Gong Q, Gregg EW, Wang J, An Y, Zhang P, Yang W, et al. Long-term effects of a randomised trial of a 6-year lifestyle intervention in impaired glucose tolerance on diabetes-related microvascular complications: the China Da Qing Diabetes Prevention Outcome Study. Diabetologia 2011;54:300-7. http://dx.doi.org/10.1007/s00125-010-1948-9.
- Li G, Zhang P, Wang J, An Y, Gong Q, Gregg EW, et al. Cardiovascular mortality, all-cause mortality, and diabetes incidence after lifestyle intervention for people with impaired glucose tolerance in the Da Qing Diabetes Prevention Study: a 23-year follow-up study. Lancet Diabet Endocrinol 2014;2:474-80. http://dx.doi.org/10.1016/S2213-8587(14)70057-9.
- National Screening Committee . Criteria for Appraisals the Viability, Effectiveness and Appropriateness of a Screening Programme 2010. www.screening.nhs.uk/criteria (accessed 19 March 2010).
- Harris MI, Klein R, Welborn TA, Knuiman MW. Onset of NIDDM occurs at least 4–7 yr before clinical diagnosis. Diabetes Care 1992;15:815-19. http://dx.doi.org/10.2337/diacare.15.7.815.
- Thompson TJ, Engelgau MM, Hegazy M, Ali MA, Sous ES, Badran A, et al. The onset of NIDDM and its relationship to clinical diagnosis in Egyptian adults. Diabet Med 1996;13:337-40. http://dx.doi.org/10.1002/(SICI)1096-9136(199604)13:4<337::AID-DIA71>3.0.CO;2-A 2.
- Lowe LP, Liu K, Greenland P, Metzger BE, Dyer AR, Stamler J. Diabetes, asymptomatic hyperglycemia, and 22-year mortality in black and white men. The Chicago Heart Association Detection Project in Industry Study. Diabetes Care 1997;20:163-9. http://dx.doi.org/10.2337/diacare.20.2.163.
- Rahman M, Simmons RK, Hennings SH, Wareham NJ, Griffin SJ. How much does screening bring forward the diagnosis of type 2 diabetes and reduce complications? Twelve year follow-up of the Ely cohort. Diabetologia 2012;55:1651-9. http://dx.doi.org/10.1007/s00125-011-2441-9.
- Goyder EC, McNally PG, Drucquer M, Spiers N, Botha JL. Shifting of care for diabetes from secondary to primary care, 1990–5: review of general practices. BMJ 1998;316:1505-6. http://dx.doi.org/10.1136/bmj.316.7143.1505.
- Plantinga LC, Crews DC, Coresh J, Miller ER, Saran R, Yee J, et al. Prevalence of chronic kidney disease in US adults with undiagnosed diabetes or prediabetes. Clin J Am Soc Nephrol 2010;5:673-82. http://dx.doi.org/10.2215/CJN.07891109.
- Flores-Le Roux JA, Comin J, Pedro-Botet J, Benaiges D, Puig-de Dou J, Chillarón JJ, et al. Seven-year mortality in heart failure patients with undiagnosed diabetes: an observational study. Cardiovasc Diabetol 2011;10. http://dx.doi.org/10.1186/1475-2840-10-39.
- Spijkerman AM, Dekker JM, Nijpels G, Adriaanse MC, Kostense PJ, Ruwaard D, et al. Microvascular complications at time of diagnosis of type 2 diabetes are similar among diabetic patients detected by targeted screening and patients newly diagnosed in general practice: the Hoorn screening study. Diabetes Care 2003;26:2604-8. http://dx.doi.org/10.2337/diacare.26.9.2604.
- Simmons RK, Echouffo-Tcheugui JB, Griffin SJ. Screening for type 2 diabetes: an update of the evidence. Diabetes Obes Metab 2010;12:838-44. http://dx.doi.org/10.1111/j.1463-1326.2010.01244.x.
- Kuo HS, Chang HJ, Chou P, Teng L, Chen TH. A Markov chain model to assess the efficacy of screening for non-insulin dependent diabetes mellitus (NIDDM). Int J Epidemiol 1999;28:233-40. http://dx.doi.org/10.1093/ije/28.2.233.
- Chang HJ, Kuo HS, Tung TH, Chou P, Chen TH. Evaluation of a population-based screening for type 2 diabetes: a community-based screening project in Puli, Taiwan. Prev Med 2000;31:396-402. http://dx.doi.org/10.1006/pmed.2000.0728.
- Kahn R, Alperin P, Eddy D, Borch-Johnsen K, Buse J, Feigelman J, et al. Age at initiation and frequency of screening to detect type 2 diabetes: a cost-effectiveness analysis. Lancet 2010;375:1365-74. http://dx.doi.org/10.1016/S0140-6736(09)62162-0.
- Simmons RK, Echouffo-Tcheugui JB, Sharp SJ, Sargeant LA, Williams KM, Prevost AT, et al. Screening for type 2 diabetes and population mortality over 10 years (ADDITION-Cambridge): a cluster-randomised controlled trial. Lancet 2012;380:1741-8. http://dx.doi.org/10.1016/S0140-6736(12)61422-6.
- UK Prospective Diabetes Study Group . Intensive blood-glucose control with sulphonylureas or insulin compared with conventional treatment and risk of complications in patients with type 2 diabetes (UKPDS 33). UK Prospective Diabetes Study (UKPDS) Group. Lancet 1998;352:837-53. http://dx.doi.org/10.1016/S0140-6736(98)07019-6.
- Ohkubo Y, Kishikawa H, Araki E, Miyata T, Isami S, Motoyoshi S, et al. Intensive insulin therapy prevents the progression of diabetic microvascular complications in Japanese patients with non-insulin-dependent diabetes mellitus: a randomized prospective 6-year study. Diabetes Res Clin Pract 1995;28:103-17. http://dx.doi.org/10.1016/0168-8227(95)01064-K.
- UK Prospective Diabetes Study Group . Tight blood pressure control and risk of macrovascular and microvascular complications in type 2 diabetes: UKPDS 38. UK Prospective Diabetes Study Group. BMJ 1998;317:703-13. http://dx.doi.org/10.1136/bmj.317.7160.703.
- Colhoun HM, Betteridge DJ, Durrington PN, Hitman GA, Neil HA, Livingstone SJ, et al. Primary prevention of cardiovascular disease with atorvastatin in type 2 diabetes in the Collaborative Atorvastatin Diabetes Study (CARDS): multicentre randomised placebo-controlled trial. Lancet 2004;364:685-96. http://dx.doi.org/10.1016/S0140-6736(04)16895-5.
- Collins R, Armitage J, Parish S, Sleigh P, Peto R. MRC/BHF Heart Protection Study of cholesterol-lowering with simvastatin in 5963 people with diabetes: a randomised placebo-controlled trial. Lancet 2003;361:2005-16. http://dx.doi.org/10.1016/S0140-6736(03)13636-7.
- Gaede P, Vedel P, Larsen N, Jensen GV, Parving HH, Pedersen O. Multifactorial intervention and cardiovascular disease in patients with type 2 diabetes. N Engl J Med 2003;348:383-93. http://dx.doi.org/10.1056/NEJMoa021778.
- Patel A, MacMahon S, Chalmers J, Neal B, Woodward M, . ADVANCE Collaborative Group . Effects of a fixed combination of perindopril and indapamide on macrovascular and microvascular outcomes in patients with type 2 diabetes mellitus (the ADVANCE trial): a randomised controlled trial. Lancet 2007;370:829-40. http://dx.doi.org/10.1016/S0140-6736(07)61303-8.
- Stratton IM, Kohner EM, Aldington SJ, Turner RC, Holman RR, Manley SE, et al. UKPDS 50: risk factors for incidence and progression of retinopathy in Type II diabetes over 6 years from diagnosis. Diabetologia 2001;44:156-63. http://dx.doi.org/10.1007/s001250051594.
- Adler AI, Stevens RJ, Manley SE, Bilous RW, Cull CA, Holman RR. UKPDS GROUP . Development and progression of nephropathy in type 2 diabetes: the United Kingdom Prospective Diabetes Study (UKPDS 64). Kidney Int 2003;63:225-32. http://dx.doi.org/10.1046/j.1523-1755.2003.00712.x.
- Gaede P, Lund-Andersen H, Parving HH, Pedersen O. Effect of a multifactorial intervention on mortality in type 2 diabetes. N Engl J Med 2008;358:580-91. http://dx.doi.org/10.1056/NEJMoa0706245.
- Ismail-Beigi F, Moghissi E, Tiktin M, Hirsch IB, Inzucchi SE, Genuth S. Individualizing glycemic targets in type 2 diabetes mellitus: implications of recent clinical trials. Ann Int Med 2011;154:554-9. http://dx.doi.org/10.7326/0003-4819-154-8-201104190-00007.
- Ray KK, Seshasai SR, Wijesuriya S, Sivakumaran R, Nethercott S, Preiss D, et al. Effect of intensive control of glucose on cardiovascular outcomes and death in patients with diabetes mellitus: a meta-analysis of randomised controlled trials. Lancet 2009;373:1765-72. http://dx.doi.org/10.1016/S0140-6736(09)60697-8.
- Peyrot M, Rubin RR, Lauritzen T, Snoek FJ, Matthews DR, Skovlund SE. Psychosocial problems and barriers to improved diabetes management: results of the Cross-National Diabetes Attitudes, Wishes and Needs (DAWN) Study. Diabetic Med 2005;22:1379-85. http://dx.doi.org/10.1111/j.1464-5491.2005.01644.x.
- Black N. Patient reported outcome measures could help transform healthcare. BMJ 2013;346. http://dx.doi.org/10.1136/bmj.f167.
- Darzi A. Quality and the NHS next stage review. Lancet 2008;371:1563-4. http://dx.doi.org/10.1016/S0140-6736(08)60672-8.
- UK Prospective Diabetes Study Group . Quality of life in type 2 diabetic patients is affected by complications but not by intensive policies to improve blood glucose or blood pressure control (UKPDS 37). U.K. Prospective Diabetes Study Group. Diabetes Care 1999;22:1125-36. http://dx.doi.org/10.2337/diacare.22.7.1125.
- Anderson RT, Narayan KM, Feeney P, Goff D, Ali MK, Simmons D, et al. Effect of intensive glycemic lowering on health-related quality of life in type 2 diabetes: ACCORD trial. Diabetes Care 2011;34:807-12. http://dx.doi.org/10.2337/dc10-1926.
- Putting Prevention First. Vascular Checks: Risk Assessment and Management. London: Department of Health; 2008.
- van den Donk M, Sandbæk A, Borch-Johnsen K, Lauritzen T, Simmons RK, Wareham NJ, et al. Screening for type 2 diabetes. Lessons from the ADDITION-Europe study. Diabetic Med 2011;28:1416-24. http://dx.doi.org/10.1111/j.1464-5491.2011.03365.x.
- Sargeant LA, Simmons RK, Barling RS, Butler R, Williams KM, Prevost AT, et al. Who attends a UK diabetes screening programme? Findings from the ADDITION-Cambridge study. Diabet Med 2010;27:995-1003. http://dx.doi.org/10.1111/j.1464-5491.2010.03056.x.
- Dalsgaard EM, Christensen JO, Skriver MV, Borch-Johnsen K, Lauritzen T, Sandbæk A. Comparison of different stepwise screening strategies for type 2 diabetes: finding from Danish general practice, Addition-DK. Primary Care Diabetes 2010;4:223-29. http://dx.doi.org/10.1016/j.pcd.2010.06.003.
- Dalsgaard EM, Lauritzen T, Christiansen T, Mai KS, Borch-Johnsen K, Sandbæk A. Socioeconomic factors related to attendance at a type 2 diabetes screening programme. Diabet Med 2009;26:518-25. http://dx.doi.org/10.1111/j.1464-5491.2009.02715.x.
- Janssen PG, Gorter KJ, Stolk RP, Rutten GE. Low yield of population-based screening for type 2 diabetes in the Netherlands: the ADDITION Netherlands study. Fam Pract 2007;24:555-61. http://dx.doi.org/10.1093/fampra/cmm052.
- Lauritzen T, Griffin S, Borch-Johnsen K, Wareham NJ, Wolffenbuttel BH, Rutten G. The ADDITION study: proposed trial of the cost-effectiveness of an intensive multifactorial intervention on morbidity and mortality among people with type 2 diabetes detected by screening. Int J Obes Relat Metab Disord 2000;24:6-11. http://dx.doi.org/10.1038/sj.ijo.0801420.
- Webb DR, Khunti K, Srinivasan B, Gray LJ, Taub N, Campbell S, et al. Rationale and design of the ADDITION-Leicester study, a systematic screening programme and randomised controlled trial of multi-factorial cardiovascular risk intervention in people with type 2 diabetes mellitus detected by screening. Trials 2010;11. http://dx.doi.org/10.1186/1745-6215-11-16.
- Janssen PG, Gorter KJ, Stolk RP, Rutten GE. Randomised controlled trial of intensive multifactorial treatment for cardiovascular risk in patients with screen-detected type 2 diabetes: 1-year data from the ADDITION Netherlands study. Br J Gen Pract 2009;59:43-8. http://dx.doi.org/10.3399/bjgp09X394851.
- Sandbæk A, Griffin SJ, Rutten G, Davies M, Stolk R, Khunti K, et al. Stepwise screening for diabetes identifies people with high but modifiable coronary heart disease risk. The ADDITION study. Diabetologia 2008;51:1127-34. http://dx.doi.org/10.1007/s00125-008-1013-0.
- Griffin SJ, Little PS, Hales CN, Kinmonth AL, Wareham NJ. Diabetes risk score: towards earlier detection of type 2 diabetes in general practice. Diabetes Metab Res 2000;16:164-71. http://dx.doi.org/10.1002/1520-7560(200005/06)16:3<164::AID-DMRR103>3.0.CO;2-R.
- Glumer C, Carstensen B, Sandbæk A, Lauritzen T, Jorgensen T, Borch-Johnsen K. A Danish diabetes risk score for targeted screening: the Inter99 study. Diabetes Care 2004;27:727-33. http://dx.doi.org/10.2337/diacare.27.3.727.
- Davies MJ, Heller S, Skinner TC, Campbell MJ, Carey ME, Cradock S, et al. Effectiveness of the diabetes education and self management for ongoing and newly diagnosed (DESMOND) programme for people with newly diagnosed type 2 diabetes: cluster randomised controlled trial. BMJ 2008;336:491-5. http://dx.doi.org/10.1136/bmj.39474.922025.BE.
- Ruige JB, de Neeling JN, Kostense PJ, Bouter LM, Heine RJ. Performance of an NIDDM screening questionnaire based on symptoms and risk factors. Diabetes Care 1997;20:491-6. http://dx.doi.org/10.2337/diacare.20.4.491.
- Griffin SJ, Borch-Johnsen K, Davies MJ, Khunti K, Rutten GE, Sandbæk A, et al. Effect of early intensive multifactorial therapy on 5-year cardiovascular outcomes in individuals with type 2 diabetes detected by screening (ADDITION-Europe): a cluster-randomised trial. Lancet 2011;378:156-67. http://dx.doi.org/10.1016/S0140-6736(11)60698-3.
- Definition, Diagnosis, and Classification of Diabetes Mellitus and its Complications: Report of a WHO Consultation. Geneva: WHO; 1999.
- Echouffo-Tcheugui JB, Simmons RK, Williams KM, Barling RS, Prevost AT, Kinmonth AL, et al. The ADDITION-Cambridge trial protocol: a cluster – randomised controlled trial of screening for type 2 diabetes and intensive treatment for screen-detected patients. BMC Public Health 2009;9. http://dx.doi.org/10.1186/1471-2458-9-136.
- Heart Outcomes Prevention Evaluation (HOPE) Study Investigators . Effects of ramipril on cardiovascular and microvascular outcomes in people with diabetes mellitus: results of the HOPE study and MICRO-HOPE substudy. Heart Outcomes Prevention Evaluation Study Investigators. Lancet 2000;355:253-9. http://dx.doi.org/10.1016/S0140-6736(99)12323-7.
- Pyorala K, Pedersen TR, Kjekshus J, Faergeman O, Olsson AG, Thorgeirsson G. Cholesterol lowering with simvastatin improves prognosis of diabetic patients with coronary heart disease. A subgroup analysis of the Scandinavian Simvastatin Survival Study (4S). Diabetes Care 1997;20:614-20. http://dx.doi.org/10.2337/diacare.20.4.614.
- Type 2 Diabetes in General Practice – Diagnosis and Treatment. Copenhagen: Royal College of General Practitioners; 1999.
- McIntosh A, Hutchinson A, Home PD, Brown F, Bruce A, Damerell A, et al. Clinical Guidelines and Evidence Review for Type 2 Diabetes: Management of Blood Glucose. Sheffield: ScHARR, University of Sheffield; 2001.
- Rutten GEHM, Verhoeven S, Heine RJ, De Grauw WJC, Cromme PVM, Reenders K. Dutch College of General Practitioners. Practice guideline diabetes mellitus type 2. Huisarts Wetensch 1999;42:67-84.
- Type 2 Diabetes: The Management of Type 2 Diabetes. London: NICE; 2009.
- Levey AS, Bosch JP, Lewis JB, Greene T, Rogers N, Roth D. A more accurate method to estimate glomerular filtration rate from serum creatinine: a new prediction equation. Modification of Diet in Renal Disease Study Group. Ann Intern Med 1999;130:461-70. http://dx.doi.org/10.7326/0003-4819-130-6-199903160-00002.
- Early Treatment Diabetic Retinopathy Study Research Group . Fundus photographic risk factors for progression of diabetic retinopathy. ETDRS report number 12. Ophthalmology 1991;98:823-33. http://dx.doi.org/10.1016/S0161-6420(13)38014-2.
- Feldman EL, Stevens MJ, Thomas PK, Brown MB, Canal N, Greene DA. A practical two-step quantitative clinical and electrophysiological assessment for the diagnosis and staging of diabetic neuropathy. Diabetes Care 1994;17:1281-9. http://dx.doi.org/10.2337/diacare.17.11.1281.
- Wu EQ, Borton J, Said G, Le TK, Monz B, Rosilio M, et al. Estimated prevalence of peripheral neuropathy and associated pain in adults with diabetes in France. Curr Med Res Opin 2007;23:2035-42. http://dx.doi.org/10.1185/030079907X210516.
- Dolan P. Modeling valuations for EuroQol health states. Med Care 1997;35:1095-108. http://dx.doi.org/10.1097/00005650-199711000-00002.
- Brooks R. EuroQol: the current state of play. Health Policy 1996;37:53-72. http://dx.doi.org/10.1016/0168-8510(96)00822-6.
- Ware JE, Snow KK, Kosinski M, Gandek B. SF-36 Health Survey. Manual and Interpretation Guide. Boston, MA: New England Medical Centre, the Health Institute; 1993.
- Pouwer F, van der Ploeg HM, Ader HJ, Heine RJ, Snoek FJ. The 12-item well-being questionnaire. An evaluation of its validity and reliability in Dutch people with diabetes. Diabetes Care 1999;22:2004-10. http://dx.doi.org/10.2337/diacare.22.12.2004.
- Pouwer F, Snoek FJ, van der Ploeg HM, Ader HJ, Heine RJ. The well-being questionnaire: evidence for a three-factor structure with 12 items (W-BQ12). Psychol Med 2000;30:455-62. http://dx.doi.org/10.1017/S0033291700001719.
- Bradley C, Todd C, Gorton T, Symonds E, Martin A, Plowright R. The development of an individualized questionnaire measure of perceived impact of diabetes on quality of life: the ADDQoL. Qual Life Res 1999;8:79-91. http://dx.doi.org/10.1023/A:1026485130100.
- Bradley C. Handbook of Psychology and Diabetes: a Guide to Psychological Measurements in Diabetes Research and Practice. Chur: Harwood Academic Press; 1994.
- Coleman R, Stevens R, Holman R. Updated UKPDS risk engine that estimates primary and secondary cardiovascular disease risk in people with recently-diagnosed or established type 2 diabetes. Diabetes 2012;61.
- White IR, Thompson SG. Adjusting for partially missing baseline measurements in randomized trials. Stat Med 2005;24:993-1007. http://dx.doi.org/10.1002/sim.1981.
- Gooley TA, Leisenring W, Crowley J, Storer BE. Estimation of failure probabilities in the presence of competing risks: new representations of old estimators. Stat Med 1999;18:695-706. http://dx.doi.org/10.1002/(SICI)1097-0258(19990330)18:6<695::AID-SIM60>3.0.CO;2-O.
- Donders AR, van der Heijden GJ, Stijnen T, Moons KG. Review: a gentle introduction to imputation of missing values. J Clin Epidemiol 2006;59:1087-91. http://dx.doi.org/10.1016/j.jclinepi.2006.01.014.
- Park P, Simmons RK, Prevost AT, Griffin SJ. Screening for type 2 diabetes is feasible, acceptable, but associated with increased short-term anxiety: a randomised controlled trial in British general practice. BMC Public Health 2008;8. http://dx.doi.org/10.1186/1471-2458-8-350.
- Eborall H, Davies R, Kinmonth AL, Griffin S, Lawton J. Patients’ experiences of screening for type 2 diabetes: prospective qualitative study embedded in the ADDITION (Cambridge) randomised controlled trial. BMJ 2007;335:490-3. http://dx.doi.org/10.1136/bmj.39308.392176.BE.
- Graffy J, Grant J, Williams K, Cohn S, Macbay S, Griffin S, et al. More than measurement: practice team experiences of screening for type 2 diabetes. Fam Pract 2010;27:386-94. http://dx.doi.org/10.1093/fampra/cmq022.
- Black JA, Sharp SJ, Wareham NJ, Sandbæk A, Rutten GE, Lauritzen T, et al. Does early intensive multifactorial therapy reduce modelled cardiovascular risk in individuals with screen-detected diabetes? Results from the ADDITION-Europe cluster randomized trial. Diabet Med 2014;31:647-56. http://dx.doi.org/10.1111/dme.12410.
- Sandbæk A, Griffin SJ, Sharp SJ, Simmons RK, Borch-Johnsen K, Rutten GE, et al. Effect of early multifactorial therapy compared with routine care on microvascular outcomes at 5 years in people with screen-detected diabetes: a randomised controlled trial: the ADDITION-Europe study. Diabetes Care 2014;37:2015-23. http://dx.doi.org/10.2337/dc13-1544.
- van den Donk M, Griffin SJ, Stellato RK, Simmons RK, Sandbæk A, Lauritzen T, et al. Effect of early intensive multifactorial therapy compared with routine care on self-reported health status, general well-being, diabetes-specific quality of life and treatment satisfaction in screen-detected type 2 diabetes mellitus patients (ADDITION-Europe): a cluster-randomised trial. Diabetologia 2013;56:2367-77. http://dx.doi.org/10.1007/s00125-013-3011-0.
- Clarke PM, Gray AM, Briggs A, Farmer AJ, Fenn P, Stevens RJ, et al. A model to estimate the lifetime health outcomes of patients with type 2 diabetes: the United Kingdom Prospective Diabetes Study (UKPDS) Outcomes Model (UKPDS no. 68). Diabetologia 2004;47:1747-59. http://dx.doi.org/10.1007/s00125-004-1527-z.
- UK Prospective Diabetes Study Group . UK Prospective Diabetes Study (UKPDS). VIII. Study design, progress and performance. Diabetologia 1991;34:877-90. http://dx.doi.org/10.1007/BF00400195.
- Guzder RN, Gatling W, Mullee MA, Mehta RL, Byrne CD. Prognostic value of the Framingham cardiovascular risk equation and the UKPDS risk engine for coronary heart disease in newly diagnosed type 2 diabetes: results from a United Kingdom study. Diabet Med 2005;22:554-62. http://dx.doi.org/10.1111/j.1464-5491.2005.01494.x.
- Simmons RK, Coleman RL, Price HC, Holman RR, Khaw KT, Wareham NJ, et al. Performance of the UK Prospective Diabetes Study risk engine and the Framingham risk equations in estimating cardiovascular disease in the EPIC-Norfolk cohort. Diabetes Care 2009;32:708-13. http://dx.doi.org/10.2337/dc08-1918.
- McEwan P, Bergenheim K, Currie C. External validation of the UKPDS outcomes model equations (UKPDS 68) and the UKPDS risk engine equations (UKPDS 56 and 60) in forecasting cardiovascular outcomes in people with type 2 diabetes. Value Health 2006;9:A37-8. http://dx.doi.org/10.1016/S1098-3015(10)64421-X.
- Rubin DB, Schenker N. Multiple imputation in health-care databases: an overview and some applications. Stat Med 1991;10:585-98. http://dx.doi.org/10.1002/sim.4780100410.
- Lee KJ, Carlin JB. Multiple imputation for missing data: fully conditional specification versus multivariate normal imputation. Am J Epidemiol 2010;171:624-32. http://dx.doi.org/10.1093/aje/kwp425.
- Rubin DB. Multiple Imputation for Nonresponse in Surveys. Hoboken, NJ: Wiley; 2004.
- van Dieren S, Peelen LM, Nöthlings U, van der Schouw YT, Rutten GE, Spijkerman AM, et al. External validation of the UK Prospective Diabetes Study (UKPDS) risk engine in patients with type 2 diabetes. Diabetologia 2011;54:264-70. http://dx.doi.org/10.1007/s00125-010-1960-0.
- Jones RH. Bayesian information criterion for longitudinal and clustered data. Stat Med 2011;30:3050-6. http://dx.doi.org/10.1002/sim.4323.
- Velentgas P, Dreyer NA, Nourjah P, Smith SR, Torchia MM. Developing a Protocol for Observational Comparative Effectiveness Research: A User’s Guide. Rockville, MD: Agency for Healthcare Research and Quality; 2013.
- Tao L, Wilson EC, Griffin SJ, Simmons RK. ADDITION-Europe study team . Performance of the UKPDS outcomes model for prediction of myocardial infarction and stroke in the ADDITION-Europe trial cohort. Value Health 2013;16:1074-80. http://dx.doi.org/10.1016/j.jval.2013.06.001.
- Curtis L. PSSRU Inflation Indices, Secondary PSSRU Inflation Indices. Canterbury: Personal Social Services Research Unit (PSSRU), University of Kent; 2004.
- Curtis L. Unit Costs of Health and Social Care 2010. Canterbury: Personal Social Services Research Unit (PSSRU), University of Kent; 2010.
- Tao L, Wilson EC, Wareham NJ, Sandbæk A, Rutten GE, Lauritzen T, et al. Cost-effectiveness of intensive multifactorial treatment compared with routine care for individuals with screen-detected type 2 diabetes: analysis of the ADDITION-UK cluster-randomized controlled trial. Diabet Med 2015;32:907-19. http://dx.doi.org/10.1111/dme.12711.
- Clarke P, Gray A, Legood R, Briggs A, Holman R. The impact of diabetes-related complications on healthcare costs: results from the United Kingdom Prospective Diabetes Study (UKPDS study no. 65). Diabet Med 2003;20:442-50. http://dx.doi.org/10.1046/j.1464-5491.2003.00972.x.
- Schwarz B, Gouveia M, Chen J, Nocea G, Jameson K, Cook J, et al. Cost-effectiveness of sitagliptin-based treatment regimens in European patients with type 2 diabetes and haemoglobin A1c above target on metformin monotherapy. Diabetes Obes Metab 2008;10:43-55. http://dx.doi.org/10.1111/j.1463-1326.2008.00886.x.
- Clarke P, Gray A, Holman R. Estimating utility values for health states of type 2 diabetic patients using the EQ-5D (UKPDS 62). Med Decis Making 2002;22:340-9. http://dx.doi.org/10.1177/027298902400448902.
- Valentine WJ, Bottomley JM, Palmer AJ, Brändle M, Foos V, Williams R, et al. PROactive 06: cost-effectiveness of pioglitazone in Type 2 diabetes in the UK. Diabet Med 2007;24:982-1002. http://dx.doi.org/10.1111/j.1464-5491.2007.02188.x.
- Szende A, Oppe M, Devlin N. EQ-5D Value Sets: Inventory, Comparative Review and User Guide. Dordrecht: Springer; 2007.
- Guide to the Methods of Technology Appraisal 2013. London: NICE; 2013.
- Yuan Y. Multiple imputation using SAS software. J Stat Softw 2011;45:1-25. http://dx.doi.org/10.18637/jss.v045.i06.
- Efron B. The bootstrap and Markov chain Monte Carlo. J Biopharm Stat 2011;21:1052-62. http://dx.doi.org/10.1080/10543406.2011.607736.
- Fenwick E, Byford S. A guide to cost-effectiveness acceptability curves. Br J Psychiatry 2005;187:106-8. http://dx.doi.org/10.1192/bjp.187.2.106.
- Eschenbach TG. Technical note: constructing tornado diagrams with spreadsheets. Eng Econ 2006;51:195-204. http://dx.doi.org/10.1080/00137910600695676.
- UK Prospective Diabetes Study Group . U.K. prospective diabetes study 16. Overview of 6 years’ therapy of type II diabetes: a progressive disease. U.K. Prospective Diabetes Study Group. Diabetes 1995;44:1249-58. http://dx.doi.org/10.2337/diab.44.11.1249.
- Olivarius NF, Beck-Nielsen H, Andreasen AH, Horder M, Pedersen PA. Randomised controlled trial of structured personal care of type 2 diabetes mellitus. BMJ 2001;323:970-5. http://dx.doi.org/10.1136/bmj.323.7319.970.
- Type 2 Diabetes in General Practice – An Evidence Based Guideline. Copenhagen: Royal College of General Practitioners in Denmark; 2004.
- Rutten GEHM, de Grauw WJC, Nijpels G, Goudswaard AN, Uitewaal PJM, van der Does FEE. Dutch guidelines on type 2 diabetes mellitus. Dutch College of General Practitioners. Huisarts Wetensch 2006;49:137-52.
- Doran T, Fullwood C, Gravelle H, Reeves D, Kontopantelis E, Hiroeh U, et al. Pay-for-performance programs in family practices in the United Kingdom. N Engl J Med 2006;355:375-84. http://dx.doi.org/10.1056/NEJMsa055505.
- Andrews RC, Cooper AR, Montgomery AA, Norcross AJ, Peters TJ, Sharp DJ, et al. Diet or diet plus physical activity versus usual care in patients with newly diagnosed type 2 diabetes: the Early ACTID randomised controlled trial. Lancet 2011;378:29-3. http://dx.doi.org/10.1016/s0140-6736(11)60442-x.
- Spijkerman AM, Dekker JM, Nijpels G, Jager A, Kostense PJ, van Hinsbergh VW, et al. Impact of diabetes duration and cardiovascular risk factors on mortality in type 2 diabetes: the Hoorn Study. Eur J Clin Invest 2002;32:924-30. http://dx.doi.org/10.1046/j.1365-2362.2002.01090.x.
- Carstensen B, Kristensen J, Ottosen P, Borch-Johnsen K. The Danish National Diabetes Register: trends in incidence, prevalence and mortality. Diabetologia 2008;51:2187-96. http://dx.doi.org/10.1007/s00125-008-1156-z.
- Duckworth W, Abraira C, Moritz T, Reda D, Emanuele N, Reaven PD, et al. Glucose control and vascular complications in veterans with type 2 diabetes. N Engl J Med 2009;360:129-39. http://dx.doi.org/10.1056/NEJMoa0808431.
- Gerstein HC, Miller ME, Byington RP, Goff DC, Bigger JT, Buse JB, et al. Effects of intensive glucose lowering in type 2 diabetes. N Engl J Med 2008;358:2545-59. http://dx.doi.org/10.1056/NEJMoa0802743.
- Patel A, MacMahon S, Chalmers J, Neal B, Billot L, Woodward M, et al. Intensive blood glucose control and vascular outcomes in patients with type 2 diabetes. N Engl J Med 2008;358:2560-72. http://dx.doi.org/10.1056/NEJMoa0802987.
- Herman WH, Pop-Busui R, Braffett BH, Martin CL, Cleary PA, Albers JW, et al. Use of the Michigan Neuropathy Screening Instrument as a measure of distal symmetrical peripheral neuropathy in type 1 diabetes: results from the Diabetes Control and Complications Trial/Epidemiology of Diabetes Interventions and Complications. Diabet Med 2012;29:937-44. http://dx.doi.org/10.1111/j.1464-5491.2012.03644.x.
- Rahman M, Griffin SJ, Rathmann W, Wareham NJ. How should peripheral neuropathy be assessed in people with diabetes in primary care? A population-based comparison of four measures. Diabet Med 2003;20:368-74. http://dx.doi.org/10.1046/j.1464-5491.2003.00931.x.
- Redekop WK, Koopmanschap MA, Stolk RP, Rutten GE, Wolffenbuttel BH, Niessen LW. Health-related quality of life and treatment satisfaction in Dutch patients with type 2 diabetes. Diabetes Care 2002;25:458-63. http://dx.doi.org/10.2337/diacare.25.3.458.
- Cleveringa FG, Minkman MH, Gorter KJ, van den Donk M, Rutten GE. Diabetes Care Protocol: effects on patient-important outcomes. A cluster randomized, non-inferiority trial in primary care. Diabet Med 2010;27:442-50. http://dx.doi.org/10.1111/j.1464-5491.2010.02968.x.
- Coleman RL, Stevens RJ, Retnakaran R, Holman RR. Framingham, SCORE, and DECODE risk equations do not provide reliable cardiovascular risk estimates in type 2 diabetes. Diabetes Care 2007;30:1292-3. http://dx.doi.org/10.2337/dc06-1358.
- Go AS, Hylek EM, Phillips KA, Chang Y, Henault LE, Selby JV, et al. Prevalence of diagnosed atrial fibrillation in adults: national implications for rhythm management and stroke prevention: the AnTicoagulation and Risk Factors in Atrial Fibrillation (ATRIA) Study. JAMA 2001;285:2370-5. http://dx.doi.org/10.1001/jama.285.18.2370.
- Drummond M, Sculpher M, Torrance G, O’Brien B, Stoddart G. Methods for the Economic Evaluation of Health Care Programmes. Oxford: Oxford University Press; 2005.
- Gillett M, Dallosso HM, Dixon S, Brennan A, Carey ME, Campbell MJ, et al. Delivering the diabetes education and self management for ongoing and newly diagnosed (DESMOND) programme for people with newly diagnosed type 2 diabetes: cost effectiveness analysis. BMJ 2010;341. http://dx.doi.org/10.1136/bmj.c4093.
- Brandle M, Zhou H, Smith BR, Marriott D, Burke R, Tabaei BP, et al. The direct medical cost of type 2 diabetes. Diabetes Care 2003;26:2300-4. http://dx.doi.org/10.2337/diacare.26.8.2300.
- Beaudet A, Clegg J, Thuresson PO, Lloyd A, McEwan P. Review of utility values for economic modeling in type 2 diabetes. Value Health 2014;17:462-70. http://dx.doi.org/10.1016/j.jval.2014.03.003.
- Holman RR, Paul SK, Bethel MA, Matthews DR, Neil HA. 10-year follow-up of intensive glucose control in type 2 diabetes. N Engl J Med 2008;359:1577-89. http://dx.doi.org/10.1056/NEJMoa0806470.
- Holman RR, Paul SK, Bethel MA, Neil HA, Matthews DR. Long-term follow-up after tight control of blood pressure in type 2 diabetes. N Engl J Med 2008;359:1565-76. http://dx.doi.org/10.1056/NEJMoa0806359.
- Kengne AP, Patel A, Colagiuri S, Heller S, Hamet P, Marre M, et al. The Framingham and UK Prospective Diabetes Study (UKPDS) risk equations do not reliably estimate the probability of cardiovascular events in a large ethnically diverse sample of patients with diabetes: the Action in Diabetes and Vascular Disease: Preterax and Diamicron-MR Controlled Evaluation (ADVANCE) Study. Diabetologia 2010;53:821-31. http://dx.doi.org/10.1007/s00125-010-1681-4.
- Hemmingsen B, Lund SS, Gluud C, Vaag A, Almdal T, Hemmingsen C, et al. Intensive glycaemic control for patients with type 2 diabetes: systematic review with meta-analysis and trial sequential analysis of randomised clinical trials. BMJ 2011;343. http://dx.doi.org/10.1136/bmj.d6898.
- Lauritzen T, Sandbæk A, Carlsen AH, Borch-Johnsen K. All-cause mortality and pharmacological treatment intensity following a high risk screening program for diabetes. A 6.6 year follow-up of the ADDITION study, Denmark. Prim Care Diabetes 2012;6:193-200. http://dx.doi.org/10.1016/j.pcd.2012.04.005.
Appendix 1 Further details of the intervention in each study centre
List of abbreviations
- ACCORD
- Action to Control Cardiovascular Risk in Diabetes
- ACE
- angiotensin-converting enzyme
- ACR
- albumin–creatinine ratio
- ADDITION-Europe
- Anglo–Danish–Dutch Study of Intensive Treatment in People with Screen-Detected Diabetes in Primary Care, a trial conducted in four centres: Denmark, the Netherlands, and Cambridge and Leicester (UK)
- ADDQoL
- Audit of Diabetes-Dependent Quality of Life
- AF
- atrial fibrillation
- AIC
- Akaike information criterion
- ARB
- angiotensin receptor blocker
- aROC
- area under the receiver operating characteristic
- BIC
- Bayesian information criterion
- CI
- confidence interval
- CONSORT
- Consolidated Standards of Reporting Trials
- CV
- coefficient of variation
- CVD
- cardiovascular disease
- DESMOND
- Diabetes Education and Self-Management for Ongoing and Newly Diagnosed
- DTSQ
- Diabetes Treatment Satisfaction Questionnaire
- eGFR
- estimated glomerular filtration rate
- EQ-5D
- European Quality of Life-5 Dimensions
- EQ-VAS
- European Quality of Life visual analogue scale
- ETDRS
- Early Treatment Diabetic Retinopathy Study
- GP
- general practitioner
- HbA1c
- glycated haemoglobin
- HDL
- high-density lipoprotein
- HPS
- Heart Protection Study
- ICD-10
- International Statistical Classification of Diseases and Related Health Problems, Tenth Edition
- ICER
- incremental cost-effectiveness ratio
- IGT
- impaired glucose tolerance
- IT
- intensive treatment
- ITT
- intention to treat
- LDL
- low-density lipoprotein
- MI
- myocardial infarction
- NIHR
- National Institute for Health Research
- OR
- odds ratio
- PROM
- patient-reported outcome measure
- PVD
- peripheral vascular disease
- QALY
- quality-adjusted life-year
- R&D
- Research and Development
- RC
- routine care
- SD
- standard deviation
- SE
- standard error
- SF-36
- Short Form questionnaire-36 items
- UKPDS
- UK Prospective Diabetes Study
- UKPDS-OM
- UK Prospective Diabetes Study outcomes model
- W-BQ12
- 12-item short form of the Well-Being Questionnaire
- WHO
- World Health Organization