Notes
Article history
The research reported in this issue of the journal was funded by the HTA programme as project number 07/37/69. The contractual start date was in June 2009. The draft report began editorial review in July 2015 and was accepted for publication in May 2016. The authors have been wholly responsible for all data collection, analysis and interpretation, and for writing up their work. The HTA editors and publisher have tried to ensure the accuracy of the authors’ report and would like to thank the reviewers for their constructive comments on the draft document. However, they do not accept liability for damages or losses arising from material published in this report.
Declared competing interests of authors
Simon Gates was a member of the National Institute for Health Research (NIHR) Efficacy and Mechanism Evaluation Board until February 2015 and the NIHR Standing Advisory Committee on Clinical Trials Units until December 2014 and is a member of the Medical Research Council Methodology Research Programme Panel. Sarah E Lamb is chairperson of the NIHR Health Technology Assessment Clinical Evaluation and Trials Board and member and chairperson of the NIHR Clinical Trials Unit Standing Advisory Committee. Gavin D Perkins is a member of the NIHR Health Services and Delivery Research Researcher-led Panel and is a NIHR Senior Investigator. Claire Hulme is a member of the HTA Commissioning Board.
Permissions
Copyright statement
© Queen’s Printer and Controller of HMSO 2017. This work was produced by Gates et al. under the terms of a commissioning contract issued by the Secretary of State for Health. This issue may be freely reproduced for the purposes of private research and study and extracts (or indeed, the full report) may be included in professional journals provided that suitable acknowledgement is made and the reproduction is not associated with any form of advertising. Applications for commercial reproduction should be addressed to: NIHR Journals Library, National Institute for Health Research, Evaluation, Trials and Studies Coordinating Centre, Alpha House, University of Southampton Science Park, Southampton SO16 7NS, UK.
Chapter 1 Introduction
Description of condition
Definition
Cardiac arrest is defined as the cessation of cardiac mechanical activity, as confirmed by the absence of signs of circulation. 1 The majority of cardiac arrests outside a hospital occur as a result of cardiac causes (e.g. ischaemic heart disease, myocardial infarction, arrhythmia). Other causes of cardiac arrest include trauma, submersion, drug overdose, asphyxia, exsanguination or other medical causes (e.g. stroke, pulmonary embolus). 1,2
There are three different mechanisms through which cardiac arrest occurs – the development of an arrhythmia that leads to loss of cardiac output [ventricular fibrillation (VF) or ventricular tachycardia (VT)], insufficient cardiac contraction to generate a cardiac output, pulseless electrical activity (PEA) and a failure of the electrical conduction system of the heart (asystole). 3
The manifestations of cardiac arrest are dramatic: within seconds of it occurring the blood supply to the brain and vital organs ceases. The victim loses consciousness and the process of cell death commences. There is a narrow window of opportunity (minutes) during which, if the heart can be restarted, the victim may be successfully resuscitated. The longer the victim remains in cardiac arrest, the worse the outcome and if attempts at restarting the heart are either delayed or unsuccessful then death will occur.
Chain of Survival
The Chain of Survival (Figure 1) describes a series of steps that need to be in place to optimise the chances of survival from out-of-hospital cardiac arrest (OHCA). 4
FIGURE 1.
The Chain of Survival. Reprinted from Jerry Nolan, Jasmeet Soar, Harald Eikeland. The Chain of Survival. Resuscitation (2006), 71;270–1 with permission from the Resuscitation Council (UK) and Laerdal Medical.
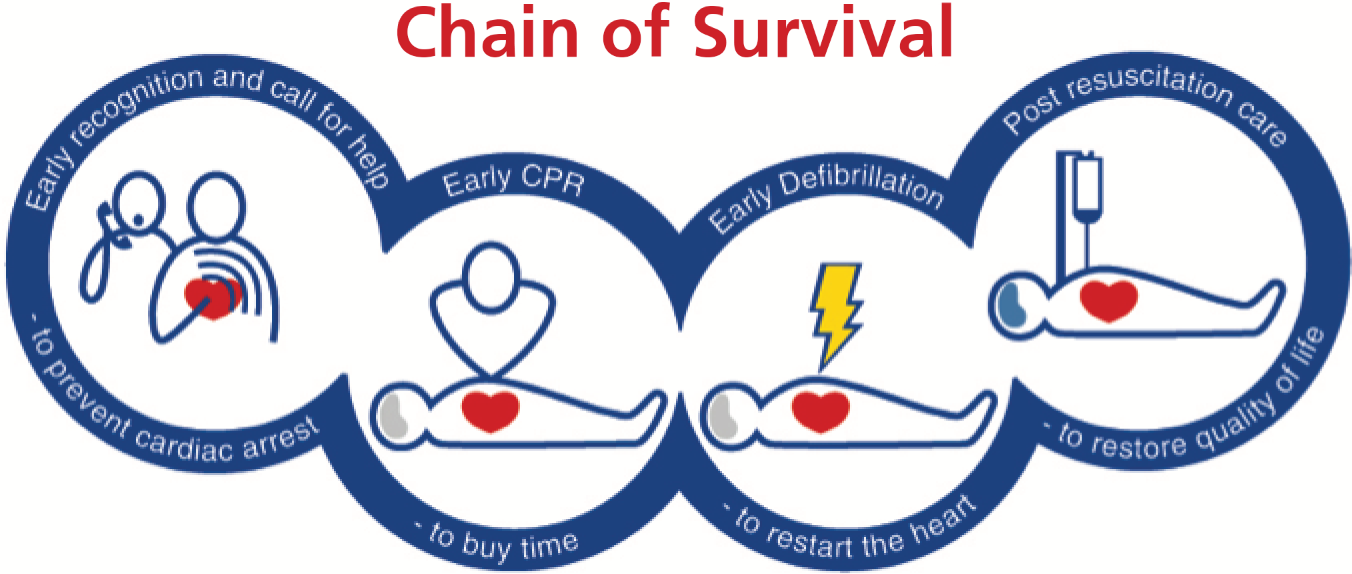
Early access
The first link in the chain is early access, which highlights the importance of identifying a patient at risk of cardiac arrest (e.g. someone suffering from an acute myocardial infarction) or someone who has sustained a cardiac arrest (identified by the loss of consciousness and absence of normal breathing) and getting a trained advanced life support (ALS) team to them as rapidly as possible.
High-quality cardiopulmonary resuscitation
The second link in the Chain of Survival is early cardiopulmonary resuscitation (CPR). CPR is the combination of chest compressions and ventilations and is optimally started by those who are initially at the scene of the collapse. This is known as bystander CPR. Bystander CPR increases the odds of survival by 1.23 [95% confidence interval (CI) 0.71 to 2.11] in the studies with the highest baseline survival rates and by 5.01 (95% CI 2.57 to 9.78) in the studies with the lowest baseline rates. 5 When the emergency services arrive on scene they will take over CPR. Current resuscitation guidelines highlight the importance of high-quality CPR for ensuring optimal outcomes from cardiac arrest. 6 High-quality CPR is defined as CPR that ensures that an adequate chest compression depth is achieved (5–6 cm), the compression rate is 100–120 per minute, interruptions are minimised and the chest is allowed to recoil between chest compressions.
Evidence supporting the importance of high-quality CPR is observational: there are no randomised trials evaluating different compression parameters. Nevertheless, high-quality CPR appears to be important to outcomes. 7 Experimental studies show a linear increase in cardiac output and coronary perfusion pressure with increasing compression depths. 8,9 Observational studies in humans found improved shock success10 and better return of spontaneous circulation (ROSC) rates and long-term survival with deeper chest compressions. 11,12 Faster chest compression rates (> 100 minutes) are associated with improved survival13–15 and ensuring that the chest is allowed to recoil between sequential chest compressions also appears to be important. 16
Interruptions in CPR are harmful. 17 A particularly critical time to minimise interruptions to CPR is around the time of attempted defibrillation. Prolonged pre-shock and peri-shock interruptions in CPR reduce the chances of shock success10 and survival. 18
Early defibrillation
Approximately one-quarter of OHCA in the UK occurs as a result of an arrhythmia: either VF or VT. These rhythms are referred to as shockable rhythms, as the arrhythmias may be terminated and cardiac function restored by the successful delivery of defibrillator shocks. The time from the onset of VF/VT to the delivery of a shock is critical to shock success and chances of survival. For every 60–90 seconds that a shock is delayed, the chance of survival falls by approximately 10%. 19
If a defibrillator is immediately available at the scene of a cardiac arrest, defibrillation should be attempted without delay. Where there is a delay in initiating CPR, there is a theoretical rationale that providing CPR before a shock improves coronary perfusion and thereby the chances of achieving sustained ROSC. 20 This concept was evaluated by the Resuscitation Outcomes Consortium in a cluster randomised trial that compared early analysis [30–60 seconds of emergency medical services (EMS)-administered CPR before initial rhythm analysis] with later analysis (180 seconds of CPR, before the initial electrocardiographic analysis). 21 The primary outcome was survival to hospital discharge with satisfactory functional status [a modified Rankin Scale (mRS) score of ≤ 3, on a scale of 0–6, with higher scores indicating greater disability]. The study21 enrolled 9933 patients (5290 to early analysis and 4643 to late analysis), but found no difference in outcomes (cluster-adjusted difference of –0.2%, 95% CI –1.1% to 0.7%).
Post hoc analyses found that for ambulance services with baseline VF survival of < 20%, ‘analyse late’ compared with ‘analyse early’ was associated with a lower chance of favourable functional survival (3.8% vs. 5.5%; OR 0.67, 95% CI 0.50 to 0.90). Conversely, in ambulance services with VF survival of > 20%, ‘analyse late’ was associated with a higher likelihood of favourable functional survival than ‘analyse early’ (7.5% vs. 6.1%; OR 1.22, 95% CI 0.98 to 1.52). 22
In the UK, the Joint Royal College Ambulance Liaison Committee (JRCALC) recommended that defibrillation should not be delayed to allow for a set period of predefibrillation CPR. In practical terms, this means that when an ambulance crew arrive at the scene of a cardiac arrest they will start CPR while the defibrillator/monitor is attached. Once attached, rhythm analysis and, if indicated, defibrillation should take place without further delay.
Post-resuscitation care
The return of a spontaneous circulation marks the start of the post-resuscitation care phase of treatment. 23 Unless the arrest has been relatively brief, most patients who achieve a ROSC will have an obtunded consciousness level, necessitating admission to intensive care. The focus of the post-resuscitation care phase of treatment is upon stabilising cardiac function to prevent a further arrest and minimising the consequences of the cardiac arrest on neurological outcome. This involves the use of targeted temperature management, avoidance of hyperglycaemia and cardiac reperfusion treatments. Most post-resuscitation care treatments are initiated following arrival in the emergency department and in the intensive care unit (ICU).
Incidence and burden of disease
Data from NHS England indicate that UK NHS ambulance services attend approximately 60,000 cardiac arrests each year; of those arrests attended, resuscitation is attempted in just less than half (28,000 cases). 24 Approximately 25% achieve an initial ROSC. However, only approximately one-third of those who achieve a ROSC survive to go home from hospital; thus, the overall survival to discharge rate is approximately 8%. The burden of disease is high, with an estimated 460,000 potential years of life lost, 270,000 of which are working years of life lost.
Functional survival after cardiac arrest is generally good, with the majority of those surviving doing so with a favourable neurological outcome. 25 Survivors may experience post-arrest problems, including anxiety, depression, post-traumatic stress and difficulties with cognitive function. 26
Despite the annual death toll exceeding that of dementia, stroke or lung cancer, there has been relatively little investment in research into this lethal condition. This has created a relatively weak evidence base compared with other diseases (e.g. there are 50-fold more trials per 10,000 deaths from myocardial infarction than deaths from cardiac arrest). A review of the National Institute for Health Research (NIHR) cardiovascular portfolio identified only 4 of 624 studies related to cardiac arrest. Until recently, the pattern was similar in the USA. 27
Existing evidence
At the time of initiating the PARAMEDIC (prehospital randomised assessment of a mechanical compression device in OHCA) trial, there were no large published randomised controlled trials (RCTs) evaluating the LUCAS-2 (Lund University Cardiopulmonary Assistance System-2; Jolife AB, Lund, Sweden) device. A systematic review of the literature in 201228 identified 16 studies investigating the LUCAS-2 device. Four of the studies were animal studies and 12 were human studies. Of the 12 human studies, one was a RCT and 11 were observational studies using either a cohort or before-or-after design (Figure 2).
FIGURE 2.
Forest plot from Gates et al. ’s28 systematic review of studies examining the LUCAS-2 device. M–H, Mantel–Haenszel; RR, risk ratio. Reproduced from Heart, Gates S, Smith JL, Ong GJ, Brace SJ, Perkins GD, 98, 908–13, 2012 with permission from BMJ Publishing Group Ltd.

The main finding of this review was that the existing evidence about the use of the LUCAS device is inconclusive. The animal studies tended to provide evidence that the LUCAS-2 device improved physiological end points, although the results were not consistent across studies.
Studies involving humans similarly lack consistency in the direction of benefit compared with harm. We chose not to perform any meta-analyses because of observed heterogeneity, varying study design and the high risk of bias in most of the included studies.
We concluded that the evidence base is insufficient for making any recommendations about the routine use of the LUCAS-2 device in clinical practice.
This conclusion is similar to the International Liaison Committee for Resuscitation Consensus on Science and Treatment’s recommendation,20 which advised that:
. . . there are insufficient data to support or refute the use of LUCAS-2 CPR instead of manual CPR. It may be reasonable to consider LUCAS-2 CPR to maintain continuous chest compression while undergoing computed tomography (CT) scan or similar diagnostic studies, when provision of manual CPR would be difficult.
Deakin et al. 20
Rationale for intervention
Because of the problems with manual chest compression, several mechanical devices have been proposed. These have potential advantages: they are able to provide compressions of a standard depth and frequency for long periods without interruption or fatigue and they free emergency medical personnel to attend to other tasks.
The LUCAS-2 is a mechanical device that provides automatic chest compressions. It delivers sternal compression at a constant rate, to a fixed depth, by a piston with the added feature of a suction cup that helps the chest return back to the normal position. It compresses 100 times per minute to a depth of 4–5 cm. It is easy to apply, stable in use, relatively light in weight (7.8 kg) and well adapted to use during patient movement on a stretcher and during ambulance transportation. The device is Conformité Européenne (CE) marked and has been on the market in Europe since 2002.
Detailed descriptions of the device and experimental data from animal studies showing increased cardiac output and cortical cerebral flow compared with manual standardised CPR have been published. 35,38
The LUCAS-2 device was introduced into a small number of ambulance services in the UK several years ago, despite the absence of evidence of its effectiveness from randomised trials. 39 It was subsequently withdrawn from routine use by several of the services because of lack of evidence about safety and efficacy and is now used only under restricted conditions. In the absence of evidence of clinical effectiveness or cost-effectiveness and the presence of some concerns regarding safety, the JRCALC, in discussion with the Department of Health, identified the need for large-scale clinical trials to evaluate the device. 40 Until such studies are completed, no further new purchases of the device are recommended by the JRCALC. A briefing note commissioned by the National Institute for Health and Care Excellence (NICE) concluded that ‘there is therefore an urgent need to evaluate this technology to discover whether it is effective and cost-effective in improving survival after cardiac arrest’. The need for a definitive trial is reinforced in the International Liaison Committee for Resuscitation’s analysis of knowledge gaps in resuscitation. 41
Chapter 2 Trial design and methods
Trial design
The PARAMEDIC trial was a pragmatic, cluster randomised trial, with ambulance service vehicles as the unit of randomisation, comparing mechanical chest compression using the LUCAS-2 device with standard manual chest compression, for patients in OHCA. The trial protocol has been published elsewhere. 42
The trial was undertaken in partnership with four NHS ambulance services (West Midlands, North East England, Welsh Ambulance Service and South Central). These organisations serve a total population of 13 million, spread over 62,160 km2. Vehicles were randomly allocated before the start of recruitment to carry the LUCAS-2 device (LUCAS-2 arm) or not (manual compression arm).
We chose to use a cluster randomised design because of costs and concerns that an individually randomised design would have a substantial risk of contamination among the manual compression arm. With individual randomisation, all vehicles taking part in the trial would have to carry a LUCAS-2 device and there would be a strong possibility that it would be used for patients who were allocated to manual compression, especially if the perception of paramedics was that the LUCAS-2 device made chest compression easier and allowed them to carry out other tasks more effectively.
Objectives
Primary objective
To evaluate the effect on mortality of using the LUCAS-2 device rather than manual chest compression during resuscitation by ambulance clinicians (paramedics, technicians, emergency care assistants, etc.) after OHCA at 30 days after the event.
Secondary objectives
To evaluate the effects of LUCAS-2 treatment on survival to 12 months, the cognitive and neurological outcomes of survivors and the cost-effectiveness of the LUCAS-2 device.
Selection of trial sites
The NHS organisations that delivered the trial were ambulance trusts. Initially (at the time of the funding application), we anticipated that three trusts would participate covering the West Midlands, Wales and Scotland. However, the Scottish Ambulance Service withdrew from the trial before the start of recruitment because its legal team was not happy with the research contract. The North East Ambulance Service and South Central Ambulance Service subsequently joined the trial.
Outcome measures
Primary outcome
Survival to 30 days post cardiac arrest.
Secondary outcomes
-
Survived event (sustained ROSC, with spontaneous circulation until admission and transfer of care to medical staff at the receiving hospital).
-
Survival to hospital discharge (the point at which the patient is discharged from the hospital acute care unit regardless of neurological status, outcome or destination).
-
Survival to 3 and 12 months.
-
Health-related quality of life (HRQL) at 3 and 12 months [Short Form questionnaire-12 items (SF-12)43 and EuroQol-5 Dimensions (EQ-5D)]. 44
-
Neurologically intact survival to 3 months [survival with a Cerebral Performance Category (CPC)45 score of 1 or 2].
-
Cognitive outcome at 12 months [as measured via the Mini Mental State Examination (MMSE)]. 46
-
Anxiety and depression at 12 months [as measured via the Hospital Anxiety and Depression Scale (HADS)]. 47
-
Post-traumatic stress at 12 months [as measured via the post-traumatic stress disorder (PTSD) Civilian Checklist (PCL-C)]. 48
-
Hospital length of stay.
-
Intensive care length of stay (see Appendix 1).
The outcomes defined by the Utstein convention1 for reporting outcomes from cardiac arrest are reported, as well as long-term follow-up at 12 months. We did not measure the incidence of injuries resulting from CPR, for three reasons: first, they are of little importance unless they result in differences in more substantive outcomes such as survival or duration of hospitalisation; second, they are difficult to measure and classify and may not be detected reliably; and, third, organising injury data collection from a large number of hospitals was felt to add significant organisational complexity to the trial, for little benefit.
The CPC score is a 5-point scale for describing the neurological outcome after cardiac arrest and is recommended by the Utstein guidelines. 1 There is a generally accepted split into good neurological outcome (CPC score of 1 or 2) and poor outcome (CPC score of 3–5). The definitions of the categories are:
-
CPC 1 Good cerebral performance: conscious, alert, able to work.
-
CPC 2 Moderate cerebral disability: conscious, sufficient cerebral function for independent activities of daily life. Able to work in sheltered environment.
-
CPC 3 Severe cerebral disability: conscious, dependent on others for daily support because of impaired brain function. Ranges from ambulatory state to severe dementia or paralysis.
-
CPC 4 Coma or vegetative state: any degree of coma without the presence of all of the brain death criteria.
-
CPC 5 Brain death.
However, recent studies have demonstrated that this score may be insensitive to some of the more subtle, but nevertheless important, longer-term neurocognitive and functional impairments that are experienced by survivors of cardiac arrest. 49,50 The spectrum of impairment of HRQL following cardiac arrest includes memory and cognitive dysfunction, affective disorders and PTSD. 48 The number of patients who are expected to survive to hospital discharge was anticipated to be in the region of 200–300, which allowed more intensive follow-up. We used four clinical outcome measures: the SF-12 is a standard quality-of-life measure that is short and easy to complete. The PTSD PCL-C48 is a 17-item questionnaire measuring the risk of developing PTSD and has been used in previous studies as a good surrogate for the clinical diagnosis of PTSD, which would require a face-to-face interview by a suitably trained professional. The HADS47 is a 14-item self-administered questionnaire, which has been previously used successfully to measure affective disorders in those surviving cardiac arrest. 51 The MMSE measures cognitive impairment. 52 In addition, the EQ-5D44 was used as a health utility measure for the health economic analysis.
Inclusion/exclusion criteria
Eligibility for clusters
All vehicles that were in service at each participating ambulance station, eligible to attend patients and could carry the device were included in the trial and randomised to one of the trial arms before the start of recruitment.
To maximise the efficiency of the trial, recruitment was concentrated predominantly in urban areas, where each vehicle would attend a higher number of cardiac arrests per year. This avoided the costs of supporting clusters in rural areas that were able to recruit very few patients, increased the size of clusters and increased the survival rate for the trial population by omitting patients who could not be reached quickly and had a very low chance of survival. These considerations should all have helped to improve power to detect a difference between the LUCAS-2 device and manual compression arms in the trial.
Eligibility for individual patients
Patients were eligible if they met all four of the criteria below:
-
Cardiac arrest in the out-of-hospital environment.
-
First ambulance resource was a trial vehicle.
-
Resuscitation attempt was initiated by the attending ambulance clinicians, in accordance with JRCALC guidelines. 53
-
The patient was known, or believed to be, aged ≥ 18 years.
The exclusion criteria were:
-
cardiac arrest caused by trauma
-
known or clinically apparent pregnancy.
All patients who fulfilled the eligibility criteria were included in the trial. The JRCALC Recognition of Life Extinction (ROLE) guidelines53 were applied to determine patients for whom a resuscitation attempt was inappropriate. This is the case when there is no chance of survival; when the resuscitation attempt would be futile and distressing for relatives, friends and health-care personnel; and when time and resources would be wasted undertaking such measures. If there was clear evidence that life was extinct (Box 1) or if the patient had a ‘do not attempt resuscitation’ order, ambulance staff were authorised to recognise death and withhold CPR.
Massive cranial and cerebral destruction.
Hemicorporectomy.
Massive truncal injury incompatible with life (including decapitation).
Decomposition/putrefaction.
Incineration.
Hypostasis.
Rigor mortis.
A valid ‘do not attempt resuscitation’ order or an advanced directive (living will) that states the wish of the patient not to undergo attempted resuscitation.
When the patient’s death is expected as a result of terminal illness.
Efforts would be futile, as defined by the combination of all three of the following being present:
-
more than 15 minutes since the onset of collapse.
-
no bystander CPR prior to arrival of the ambulance.
-
asystole (flat line) for > 30 seconds on the ECG monitor screen.
Exceptions are:
-
drowning
-
drug overdose/poisoning
-
trauma
-
submersion of adults for longer than 1 hour.
ECG, electrocardiography.
The LUCAS-2 device cannot be used if patients are too large or too small: the device fits patients with a sternum height of 17.0–30.3 cm and a chest width of < 45 cm. However, patient size was not an exclusion criterion because it would be impossible to apply correctly to the manual compression group, hence potentially introducing bias. Moreover, it was appropriate to include the small proportion of patients who were too large or too small for the LUCAS-2 device in the trial, in accordance with intention-to-treat (ITT) principles. The trial estimated the impact of the LUCAS-2 device on the survival rate among the whole cardiac arrest population. In one Swedish study,29 only 3 out of 159 patients (1.9%) were found to be too small or too large for the LUCAS device. We therefore anticipated that there would be only a small number of patients for whom the LUCAS-2 device could not be used, especially as the LUCAS-2 device accommodates larger patients than the LUCAS version 1 device that was used in the Swedish study.
Randomisation
Randomisation of trial vehicles to the LUCAS-2 and control arms was performed by the study statisticians before the devices were supplied to participating stations. As the number of LUCAS-2 devices was limited, it was inefficient to randomise vehicles in a 1 : 1 ratio. This would have entailed some vehicles at each station not contributing to the trial and, hence, non-inclusion of potentially eligible cardiac arrest patients. It would also be operationally more difficult for ambulance service staff, as procedures would be different between trial and non-trial vehicles. Because there was little cost associated with including additional standard care clusters, we included all eligible vehicles at each station in the trial, with the majority allocated to the control arm and a random selection (stratified by type of vehicle) allocated to the LUCAS-2 arm. This ensured that all eligible cardiac arrests were included in the trial. The number of LUCAS-2 devices allocated to each station was determined by the number of vehicles. In all of the stations, at least one device was allocated to a rapid response vehicle (RRV) and one to an ambulance (unless there were no vehicles of a type at a station). The number of vehicles available varied and it was not possible to ensure that allocation was in any precise ratio, but we aimed for the ratio of LUCAS-2 to standard care vehicles to be approximately 1 : 2. If new vehicles were brought into service at participating stations during the recruitment period, these were also randomised.
The randomisation sequence was computer generated, with stratification by station and type of vehicle. Once randomised, a vehicle’s allocation could not be changed and it remained in that group throughout the trial. Clusters were terminated if a vehicle left the trial permanently (e.g. was scrapped or withdrawn from front-line service).
Early in the trial it became apparent that ambulances and RRVs frequently moved between stations, and it was not possible to identify a set of vehicles that would be consistently present at a participating station. Hence, many of the vehicles initially randomised at a station moved elsewhere and were replaced by vehicles that had not been randomised. This resulted in a low proportion of potentially eligible cardiac arrests being attended by trial vehicles. We therefore tried as far as possible to randomise stations that were geographically close, so that most transfers of vehicles between stations would be to another station that was participating in the trial. This reduced the proportion of cardiac arrests that were attended by non-trial vehicles.
A slightly different method of randomisation was used by the North East Ambulance Service. This region used a different system of allocation of vehicles, in which vehicles did not have a base station, but were based at two main depots. From here, vehicles were allocated to stations as needed. This meant that there was a major problem with vehicle rotations; vehicles rarely stayed at the same station for a prolonged period, and there were no geographically close sets of stations around which vehicles tended to rotate. Because it was possible (for logistical reasons) to include only a limited number of stations in this region in the trial, the investigators and Trial Steering Committee (TSC) felt that it was appropriate to use a different system of randomisation for this region. This was intended to be equivalent to the usual system of randomisation by vehicle. The North East Ambulance Service randomisation system used ‘virtual vehicles’; at each station, each vehicle place was randomised to the LUCAS-2 or control arm, with stratification by type of vehicle. So, for example, if there were three ambulances at a station, number two might be randomised to the LUCAS-2 arm, so whichever ambulance filled that position on a particular day would carry the LUCAS-2 device and recruit patients to the LUCAS-2 arm. The disadvantage of this system was that the LUCAS-2 devices did not stay with the same vehicle, but had to be loaded on to the correct vehicle at the beginning of each shift and removed afterwards. However, the crews had done this for a previous trial, so were used to the process, and no problems of missing LUCAS-2 devices were encountered. This system ensured that cardiac arrests attended by ambulances from participating stations would be recruited to the trial. If we had used the same system in the North East Ambulance Service as elsewhere, then the recruitment rate would have been much lower.
Treatment allocation
A dispatch centre in each region co-ordinated the emergency response. The nearest available RRV or ambulance was dispatched to cases of suspected cardiac arrest. Back-up was provided by a second vehicle as soon as possible.
Treatment allocation of each individual participant was determined by the first trial vehicle to arrive on scene. If this was a LUCAS-2 device-containing vehicle, the patient was assigned to the LUCAS-2 arm, and if it was a non-LUCAS-2 device-containing vehicle (control), the patient was allocated to the manual compression arm. If the trial vehicle was not the first ambulance service vehicle to arrive on scene – that is, a double-manned ambulance or a single-manned RRV that was not part of the trial had already arrived and commenced resuscitation – the patient was not included in the trial. If the first response on scene was a community responder (volunteer members of the public trained in basic life support and defibrillation, despatched by ambulance control) or other responses (such as motorbike, helicopter or unmarked car) then the patient was included and their allocation was determined by the first trial vehicle to arrive, providing that continued resuscitation was indicated.
We aimed to include all eligible patients who were attended by a participating vehicle during the trial recruitment period. The attending ambulance clinicians determined whether or not a resuscitation attempt was appropriate, according to the JRCALC guidelines. 53 Patients were regarded as participating in the trial when a resuscitation attempt was initiated by the attending ambulance service personnel.
Consent
Ethical considerations
The occurrence of a cardiac arrest out of hospital is unpredictable. Within seconds of cardiac arrest, a person becomes unconscious and thus incapacitated. It was not therefore possible to obtain prospective consent directly from the research participant.
Treatment (in the form of CPR) must be started immediately in an attempt to save the person’s life. In this setting it was not practical to consult a carer or independent registered medical practitioner without placing the potential participant at risk of harm from delaying treatment.
Conducting research in emergency situations in which a patient lacks capacity is regulated by the Mental Capacity Act (2005)54 for England and Wales. The PARAMEDIC trial was approved in accordance with these requirements by the Coventry Research Ethics Committee (REC) (reference number 09/H1210/69). Ethics approval was also gained from the Scotland A REC, but the Scottish Ambulance Service subsequently withdrew from the study.
Approaching survivors
The nature of the condition meant that the majority (85–90%) of people in the study would not survive. Of those patients admitted to hospital alive, the majority (approximately 80%) would be comatose and admitted to an ICU (and thus remain incapacitated). Following admission to intensive care, approximately half of the people who initially survive die without regaining capacity (on average within 48 hours). The average duration of hospital stay for survivors is 18 days. 55
To avoid unnecessary distress to the relatives of the deceased, the timing of the approach was important and had to balance the need to inform at an early opportunity while determining – as accurately as possible – which patients had died. Pilot work for this trial established that it is not possible for ambulance services to determine with sufficient accuracy which patients have died, so the procedure was revised, based on the procedures of the ICON (Intensive Care Outcome Network) study. 56
The participating ambulance services conducted their own checks on patients’ survival using existing data systems, which differed between services. Where possible, ambulance services consulted the NHS Patient Demographics Service. Other checks carried out by either the ambulance service and/or the Warwick Clinical Trials Unit (WCTU), included contacts with hospitals, general practitioners (GPs), the Intensive Care National Audit and Research Centre (ICNARC) and local registrars of births and deaths (see Identification of cardiac arrests).
If a patient was transported to hospital, his/her clinical and contact details were sent to the study co-ordinating centre at the WCTU. Staff at the co-ordinating centre checked the status of each potential survivor with the Medical Research Information Service (MRIS) approximately 6 weeks after the patient’s cardiac arrest. This timing of the approach was selected to ensure that the majority of deaths had been included in the MRIS database. All of the survivors were flagged on the MRIS database and the trial co-ordinating centre was informed of any subsequent deaths.
After these checks, if someone was still believed to be alive then the co-ordinating centre contacted the person at his/her home address by letter to provide information about the study and the follow-up. If there was no response after 2 weeks, then the co-ordinating centre tried to contact the patient by telephone (if the telephone number was known) or by letter. If the patient wished to take part in the follow-up, then they could contact the WCTU by using the reply slip, telephone or e-mail. This gave the participants an opportunity to discuss the study and, if they were happy to proceed, a 3-month follow-up appointment was made. The consent form was either returned by post or signed at the 3-month follow-up visit. Patients who did not respond were approached again at 10 months post cardiac arrest in an attempt to invite them to participate in a 12-month visit.
In the event that the co-ordinating centre was notified (or had reason to believe) that a patient lacked capacity, an approach was made to his/her GP in order to establish if the patient had capacity to consent. In the event that a patient lacked capacity to consent, we sought the views of a personal consultee in order to establish the patient’s wishes. If a personal consultee could not be identified, a carer (unconnected with the study) determined if the patient would be likely to consent to follow-up.
Protection against bias
Cluster design
One of the major potential sources of bias in cluster randomised trials is selection bias, which can arise if different patients are selected for inclusion in the two trial arms. This can arise when clinicians or people selecting patients are aware of the allocation of the cluster and they may consciously or unconsciously apply inclusion criteria differently depending on the randomised intervention. There is greater scope for selection bias if a large proportion of potentially eligible patients are not included in the trial. In this trial, paramedics assessing patients for inclusion were aware of the allocations; however, we aimed to identify and include close to 100% of the eligible patients, using a combination of methods for identifying eligible patients (see Eligibility for individual patients). This should avoid most selection bias.
Threshold for resuscitation
As the ambulance clinicians who were delivering the interventions were not blinded, there was a possibility that bias could be introduced by different thresholds for resuscitation between the LUCAS-2 and standard care arms: if they believed strongly that the LUCAS-2 device was effective, some of them might have attempted resuscitation in the LUCAS-2 arm on patients who had no chance of survival, and for whom a resuscitation attempt was therefore inappropriate. This would have resulted in a group of patients with a very low probability of survival being recruited to the LUCAS-2 arm but not to the standard care arm, potentially masking any beneficial effect of the LUCAS-2 device. We used several strategies to prevent this bias from occurring, to detect if it was to happen and to correct it if necessary.
First, the criteria that were used to determine whether or not a resuscitation attempt was appropriate, and hence whether or not the patient was eligible, were as objective as possible. The JRCALC ROLE criteria53 were used by all of the participating ambulance services to determine when a resuscitation attempt is inappropriate, and this continued in the trial (see Eligibility for individual patients). Ambulance clinicians were therefore familiar with the application of these criteria and no change of practice was needed during the trial. However, there remained scope for differential application of the criteria to the two trial arms, so further strategies were devised.
Second, all ambulance clinicians in the trial were trained in the trial procedures57 to ensure that they understood the rationale for the trial and the importance of following the trial procedures. The training included a review of existing evidence so that participating ambulance clinicians understood the current position of equipoise regarding the effectiveness of the LUCAS-2 device and discussion of potential sources of bias in the trial and the importance of applying the inclusion/exclusion criteria rigorously to both arms. Training continued throughout the recruitment period to ensure that any new staff were trained before recruiting and that important messages were continually and correctly reinforced (see Appendix 2).
Third, we instituted a programme of regular monitoring of the characteristics of patients who were recruited to the two trial arms, the number of cardiac arrests in each arm when no resuscitation attempt was made and the proportion of cardiac arrests included in the trial in order to detect any imbalances that may be caused by different thresholds for resuscitation. We also monitored the presenting rhythm, proportion of witnessed and unwitnessed arrests, presence of bystander CPR and time from ‘999’ call to crew arrival (using ambulance computer log data). If a lower threshold for attempting resuscitation in the LUCAS-2 arm existed, then we would find a greater number of recruits and a greater proportion of cardiac arrests with resuscitation attempts, a greater proportion with unfavourable presenting rhythms, a lower proportion of witnessed arrests and with bystander CPR and longer times from ‘999’ call to start of resuscitation in the LUCAS-2 group.
Finally, if necessary, we corrected for any inclusion bias in the statistical analysis of the trial, by adjustment of the analysis to take account of imbalance in factors such as presenting rhythm, time since ‘999’ call and presence of bystander CPR. We expected any potential inclusion bias to affect only the group of patients who were least likely to survive and that it would not affect patients in whom a resuscitation attempt would always be made (e.g. those with presenting rhythms with the highest probability of survival) and therefore a comparison between the LUCAS-2 device and manual compression in the subgroups of patients in whom resuscitation was known to be appropriate would be unaffected.
Monitoring device usage
The LUCAS-2 devices continuously record data when switched on. Data on the date, time and duration of use are stored in the device’s internal memory and can be downloaded when the device is serviced. We intended to use these data to verify whether or not the LUCAS-2 device was used for all cardiac arrests in the LUCAS-2 group or any in the control group. However, in practice, obtaining access to the data was difficult, as they can be accessed only by the manufacturers at the time of device servicing and special software is required to interpret the data. Data were extracted from the devices during recruitment, and supplied to the trial team, but, through efforts to match up LUCAS-2 device usage with dates and times of resuscitations of recruited patients, it became apparent that it was possible to verify LUCAS-2 device use during resuscitation for only a small number of patients. The main reasons for this were, first, the lack of LUCAS-2 device clock synchronisation with the Universal Time clock; second, the difficulty of identifying the dates and times of LUCAS-2 device use for a resuscitation attempt, as opposed to device testing, demonstration or training; and, third, the lack of correspondence between the times of use recorded by the devices and those of recruitments recorded by ambulance services. Efforts to verify LUCAS-2 device use for resuscitation attempts based on the data recorded by the devices were discontinued.
Compliance was monitored by the direct report of ambulance service personnel on the patient report form (PRF). For each cardiac arrest, ambulance clinicians were asked to report whether or not the LUCAS-2 device had been used, and instances of non-compliance were followed up with the crews involved by the paramedic research fellows.
Monitoring quality of cardiopulmonary resuscitation
For interpretation of the trial’s results, it is helpful to understand the quality of CPR provided as standard care during the trial, as this may help to explain the observed differences. For example, if the CPR quality in the control group was extremely high, it would make it less likely that a treatment benefit would be seen in the LUCAS-2 group.
We originally planned to monitor CPR quality using data recorded by defibrillators during resuscitation attempts, from which we would calculate the compression fraction, that is, the percentage of time in which chest compressions are carried out (to ascertain pauses in the chest compressions). This would provide a measure of CPR quality in the control group and would allow verification of LUCAS-2 device use in the LUCAS-2 group. However, direct measurement of the quality of CPR in trial patients proved unachievable, for several reasons. First, different defibrillators were in use in the four ambulance services, which required different approaches to extract data. In two services, memory cards were required. These needed to be inserted before use, then removed and data extracted before the next use. This was operationally impractical. In the remaining two ambulance services, data were stored in the defibrillators, but extraction and analysis of them were challenging, and it proved to be extremely difficult to download the data reliably after resuscitation attempts. We were therefore forced to abandon attempts to collect data on CPR quality from trial patients.
Instead, we performed a study to estimate the ‘background’ CPR quality in each ambulance service. Approximately 20% of staff working in the trial areas were invited to take part in an evaluation of the quality of simulated CPR (using a manikin). Between February 2013 and June 2013 each staff member was asked to demonstrate ALS of an adult patient in VF, as they would do normally in the field. Data were recorded for around 5 minutes. The staff were able to work solo or as a double-person crew, whichever was their usual practice. We recorded compression depth, compression rate and compression fraction. Data were recorded anonymously and were not related back to staff performance.
Blinding
Because of the nature of the interventions, ambulance clinicians could not be blinded and were aware of treatment allocations. Control room personnel were blinded to the allocation of the ambulance service vehicles, to ensure that there was no bias in whether a LUCAS-2 device-containing or control vehicle was sent to an incident that was likely to be a cardiac arrest. Normally, the closest vehicle would be sent, which would not favour either LUCAS-2 or control arms of the trial. Ambulance service clinical staff were not blinded; vehicles randomly assigned to the LUCAS-2 arm were identified to them at the start of the shift during vehicle checks and through stickers in the cab and outside the vehicle.
Patients themselves were unconscious and therefore unaware of their treatment allocation at the time of the intervention, although 19 patients may have been subsequently unblinded by relatives or friends who were aware that the LUCAS-2 device was used. We sought to ensure blinding of outcome assessment as far as possible. Research nurses/paramedics assessing outcomes at 3 and 12 months’ follow-up were blinded to treatment group and endeavoured to maintain their blinding during the follow-up assessments. Mortality is an objective outcome, and its assessment was very unlikely to be influenced by knowledge of the treatment allocation.
Training
Paramedics seconded to work on the trial, along with clinical educator staff, trained all of the operational ambulance staff to use the LUCAS-2 device. Because of vehicle movements and staff rotations, staff-serviced vehicles were randomly assigned to both LUCAS-2 and manual groups; hence, all staff would potentially treat patients in the intervention arm. Training was carefully designed by the ambulance services on the basis of the manufacturer’s guidance. Because of the pragmatic design of this trial, training was developed in accordance with the process by which new technology would be introduced in routine practice into NHS ambulance services. This preparation included access to online training resources56 and included 1–2 hours of face-to-face training, updated annually. Training covered the study protocol and procedures, how to operate the LUCAS-2 device and the importance of high-quality CPR. Training included hands-on device deployment practice with a resuscitation manikin and emphasised the importance of rapid deployment with minimum interruptions in CPR. A competency checklist was completed before authorising staff to deploy the LUCAS-2 device correctly (see Appendix 2). Research paramedics reviewed all cases and provided feedback to individual staff as required. The rate of device use and reasons for non-use were fed back to participating services on a quarterly basis.
Clinical management of patients in the trial
The clinical management of patients in the trial was undertaken in accordance with the details given in the trial protocol.
The CPR that was delivered to all of the patients followed the International Liaison Committee for Resuscitation, European Resuscitation Council and Resuscitation Council (UK) guidelines that were in force during the study period. These guidelines are adopted for ambulance use by the JRCALC and the JRCALC’s guidelines for clinical practice form the basis for all of the resuscitation attempts that are delivered by ambulance crews. 53 At the commencement of the trial, the 2005 resuscitation guidelines were in place, but new resuscitation guidelines were published on 18 October 201058 and incorporated by all ambulance trusts that were involved in the trial during the following 12 months. All standard ALS interventions were provided, including drug administration, defibrillation and advanced airway management as required.
In both arms, if the patient did not respond despite full ALS intervention and remained asystolic for > 20 minutes, the resuscitation attempt could be discontinued. Unless these criteria were met, resuscitation was continued and the patient was transported to the nearest emergency department with ongoing CPR.
Intervention arm
The LUCAS-2 device used in the trial was the latest version of the LUCAS-2 device, manufactured by Jolife AB and distributed by Physio-Control UK, Watford, UK.
Patients who were allocated to the intervention arm (LUCAS-2 device) received mechanical chest compressions in place of standard manual chest compressions.
On arrival, after confirming cardiac arrest, manual CPR was commenced while the LUCAS-2 device was prepared and applied. Following this, the initial cardiac rhythm was assessed. If the patient was in VF or VT then a countershock was administered in accordance with JRCALC/ALS guidelines. Operational experience showed that the LUCAS-2 device could be deployed within 20–30 seconds of arrival at the patient’s location. Prior to intubation, compressions were provided using the 30 compressions/two ventilations mode. If the patient was intubated, asynchronous compressions and ventilations were provided, with a ventilation rate of 10 per minute.
Defibrillation was performed using the following sequence: pause LUCAS-2 device, analyse heart rhythm; if shock indicated, restart LUCAS-2 device, charge, deliver shock and continue CPR for 2 minutes. This minimised deleterious pre- and post-shock pauses in compressions. The LUCAS-2 device was used in place of standard chest compressions as long as continued resuscitation was indicated, including resuscitation in the field and during transport to hospital.
The trial intervention ceased after care was handed over to the medical team in the hospital or the patient was declared deceased according to the ROLE criteria. 53
Manual chest compression arm
On arrival, after confirming cardiac arrest, manual CPR was commenced and the initial cardiac rhythm was assessed. If the patient was in VF or pulseless VT, then a countershock was applied. Prior to intubation, compressions were provided using the 30 compressions/two ventilations mode. If the patient was intubated, asynchronous compressions and ventilations were provided, with a ventilation rate of 10 per minute.
Minimising interruptions in chest compressions is critical for optimising the chances that a shock is successful. However, it is currently considered unsafe to perform defibrillation during manual chest compression. Defibrillation was therefore performed using current UK recommendations, which are as follows: stop CPR; analyse heart rhythm, charge defibrillator, deliver shock, restart chest compressions and continue CPR for 2 minutes.
Serious adverse event reporting
Definitions
Adverse events
An adverse event (AE) is ‘Any untoward medical occurrence in a patient or clinical investigation participant taking part in health-care research, which does not necessarily have a causal relationship with the research’. 59
Serious adverse events
The definition of a serious adverse event (SAE) is an untoward and unexpected occurrence that:
-
results in death
-
is immediately life-threatening
-
requires hospitalisation or prolongation of existing hospitalisation
-
results in persistent or significant disability, or incapacity
-
consists of a congenital anomaly or birth defect (not relevant to this trial population). 59
Additional terms for device trials
For trials of devices, additional terms are used and are defined as follows.
Adverse device effect/event
Any unfavourable or unintended response to a medical device.
Serious adverse device effect/event
A serious adverse device effect/event (SADE) is an adverse device effect/event (ADE) that has resulted in any of the consequences of a SAE or might have led to those consequences if suitable action/intervention had not been taken.
Incident
Any malfunction or deterioration in the characteristics and/or performance of a device, as well as any inadequacy in the labelling or instructions for use, which, directly or indirectly, might lead or might have led, to the death of a patient or user, or other persons or to the serious deterioration in their state of health. 59
Events that should be reported
All AEs and SADEs and incidents were reported to the trial co-ordinating centre on the appropriate forms (see Appendix 3).
All of the patients in this trial were in an immediately life-threatening situation; many would not survive and all of those who did were hospitalised. These situations were, therefore, expected, and events leading to any of them were reported as SAE/SADEs only if their cause was clearly separate from the cardiac arrest. Events that were related to cardiac arrest and would be expected in patients undergoing attempted resuscitation (including death and hospitalisation) were not reported.
Therefore, events were reported as SAE/SADEs if they were:
-
serious
-
and were potentially related to trial participation (i.e. they may have resulted from study treatment such as use of the LUCAS-2 device)
-
and were unexpected (i.e. that the event was not an expected occurrence for patients who have had a cardiac arrest).
Examples of events that may be SAE/SADEs were the use of the LUCAS-2 device causing a new injury that endangered the patient, malfunction of the device causing injury to ambulance clinicians and malfunction of the device leading to inadequate chest compression.
Reporting serious adverse events
Events satisfying the criteria given above were reported to the study co-ordinating centre, using the event report form, as soon as they became apparent (see Appendix 3).
The SAE/SADE reports that were received by the co-ordinating centre were reviewed on receipt by the chief investigators, and those that were considered to satisfy the criteria for being related to the device and unexpected were notified to the main REC, the Medicines and Healthcare products Regulation Agency (MHRA) and manufacturer within 15 days of receipt.
The SAE reports were also reviewed by the Data Monitoring Committee (DMC) at its regular meetings. AEs that were not considered to be serious were logged and included in annual progress reports.
Data collection
Identification of cardiac arrests
Data were recorded on all of the cardiac arrests within the trial areas. This allowed assessment of the proportion of cardiac arrests that were enrolled into the trial and helped to ensure that no eligible cardiac arrests were missed. Data were collected by the attending ambulance clinicians, using the routinely completed PRF. Data from the PRFs were then transcribed on to the trial case report forms (CRFs) by the trial research fellows (see Appendix 4). The data collection was retrospective and each ambulance service had its own system, which meant that identifying cardiac arrest cases was challenging. The ambulance services, generally, had more than one system for identifying cardiac arrest cases to ensure that none was missed. One ambulance service had to go through all daily paper PRFs on station; others had electronic PRFs and were able to perform searches through a database. Other methods included linking in with national reporting of cardiac arrests to the Department of Health.
Data forms were collected in a central place at participating ambulance stations and collected by research paramedics on a weekly basis. For ineligible cardiac arrests (no resuscitation attempt, aged < 18 years, pregnant, traumatic aetiology, non-trial vehicle was first on scene), the ambulance service also sent the trial co-ordinating centre details of the arrests for monitoring purposes.
Hospitals did not undertake prospective data collection for trial participants because of the logistical difficulties that this would present. Hospitals were contacted, as necessary, to seek information about whether patients had been discharged or had died in hospital before contacting them for follow-up. Further details about length of stay in the ICU and hospital were also sought from hospitals, ICNARC and Hospital Episode Statistics (HES). Authority was granted by the Confidentiality Advisory Group to collect these data without seeking consent from the next of kin.
Deaths
Before admission
Deaths before admission to hospital were recorded by ambulance services and data for these patients were supplied to the trial database in anonymised form, as no personal identifiers were needed for follow-up. If a patient was transported to hospital, before transfer of identifiable data to the study co-ordinating centre, ambulance services conducted their own checks for survival. When access was granted locally, research fellows were able to search NHS Summary Care Records. They would also check with hospitals, where relevant.
To identify later deaths, all of the potential survivors had their status checked with MRIS approximately 6 weeks after their cardiac arrest. Therefore, the majority of deaths should have been included in the MRIS database. Deaths are normally included within 4 weeks of issue of a death certificate and we anticipated that the majority of certificates would be issued within a few days. All survivors were flagged on the MRIS database to ensure that the study was notified immediately if their death was registered. Issue of a death certificate may be delayed in some cases by referral to a coroner, but, in most cases, the coroner’s investigation will be concluded quickly and the delay to inclusion of the death on the MRIS database will be small. In addition, before writing to patients we also contacted their GP (if known) to check on survival.
At follow-up
Survivors were followed up approximately 90 days after their cardiac arrest, by a home visit or telephone contact from a study research nurse/paramedic. At this visit quality-of-life tools (SF-1243 and EQ-5D44) and an assessment of CPC score were completed.
If a patient was believed to be alive but had not responded to the invitation to take part in the follow-up or did not want to take part, we approached his/her GP, hospital or ambulance service for any repeat visits for information on the patient’s CPC score.
The second follow-up visit at 12 months included measurement of quality of life (SF-1241 and EQ-5D42), anxiety and depression (HADS47), post-traumatic stress (PCL-C48) and cognitive outcome (MMSE46). The NHS Demographics Batch Service was used to identify participants who had changed address since the last contact. Health service and social care resource use was reported in a patient self-completed questionnaire that was provided to participants at the 3- and 12-month follow-up visits (see Appendix 5).
Data management
All of the data collected during the trial were handled and stored in accordance with the Data Protection Act 1998. 60 Data were, as far as possible, anonymised, but this trial involved the use of identifiable personal data for follow-up. All transfer of data between ambulance services and the study co-ordinating centre used secure methods, such as encrypted e-mail. All of the study data were entered into a study-specific database, which was set up by the programming team at the WCTU at the start of the study. All specifications (i.e. database variables, validation checks, screens) were agreed between the programmer, statistician, chief investigators and trial co-ordinator. All trial documentation and data were archived after completion of the trial and are stored in accordance with the WCTU standard operating procedures61 (see Appendix 7).
Statistical methods
Power and sample size
Incidence of primary outcome
At the time of initiation of this trial, there were few data on the incidence of survival after cardiac arrest, and most of these referred to survival to hospital discharge rather than survival to 30 days. However, as most mortality will occur in the first days after cardiac arrest, we expected survival to hospital discharge and to 30 days to be similar. A systematic review, published in 2005,62 has summarised all European data. The overall incidence of survival to hospital discharge was 10.7%, with 21.2% survival to discharge for patients with an initial rhythm of VF. This review62 included eight studies from the UK, in which the mean survival to hospital discharge was 8.1% overall and 17.7% for patients with initial VF rhythm. Data on survival to discharge from audits of UK ambulance services were limited, because at the time of the study few ambulance services collected outcome data for patients beyond admission to hospital. Figures from the London Ambulance Service (2006–7) indicated a survival rate to discharge of 5.2% (95% CI 4.4% to 6.0%). 63 National audit data for England (2006) indicate that the proportion of patients for whom resuscitation is attempted (and who have ROSC at admission to hospital) varied between 10% and 26% for different ambulance services. 64 The overall national figure (2004–6) was 14–16%. Estimates of mortality in hospital vary from 50% to 70%; hence, the incidence of survival to discharge is expected to be between 4.5% and 8%. 65 A reasonable conservative estimate of survival to 30 days is 5% and we have used this value in the sample size calculations.
Intracluster correlation coefficient
No data currently exist from which a relevant intracluster correlation coefficient (ICC) for this trial can be calculated. We have therefore assumed a conservative value of 0.01 for the sample size calculation. We expected that, because the LUCAS-2 device and manual compression clusters recruited from the same geographical areas and hence the same populations, the ICC would be low. The value of the ICC was monitored at the interim analyses by the DMC.
Cluster size
Predicting the expected cluster size during the trial was difficult because of expected changes in the vehicles in service and the proportion of eligible cardiac arrests that they were likely to attend. Moreover, there was likely to be considerable variation in the number of cardiac arrests attended by each vehicle (i.e. variation in cluster size). Data from the West Midlands Ambulance Service suggested that each vehicle would attend around 10–20 cardiac arrests per year; allowing for non-resuscitations and periods off the road, a reasonable estimate of the cluster size over a 2-year recruitment period was 15.
The sample size was revised during recruitment in response to information that some of the parameters differed from the assumptions that were made at the start of the trial. The original sample size calculation is given, followed by the revised version.
Original sample size
The required sample size is sensitive to variation in several parameters that were not precisely known at the start of the trial, including the incidence of the primary outcome in the manual compression group and the ICC. We aimed to be able to detect, with 80% power, an increase in the incidence of survival to 30 days from 5% in the manual compression group to 7.5% in the LUCAS-2 group [a risk ratio (RR) of 1.5]. An increase in survival from 5% to 7.5% corresponds with a number needed to treat of 40 – or one extra life saved per 40 resuscitation attempts. This would translate into around 625 lives saved per year in the UK. In an individually randomised trial this would require 2942 participants. Allowing for clustering, assuming an ICC of 0.01 and a cluster size of 15, this would require 224 clusters if using a 1 : 1 randomisation ratio (112 LUCAS-2 group, 112 manual group; 3360 participants in total).
Because the number of LUCAS-2 devices that were available to the trial was limited, it was more efficient not to use a fixed 1 : 1 randomisation ratio (see Randomisations), but to randomise a number of LUCAS-2 devices among all of the vehicles at each ambulance station. This allowed inclusion in the trial of all cardiac arrests attended by vehicles from that station. The numbers of clusters required for 80% power to detect the difference specified above, with different randomisation ratios and cluster sizes, is shown in Table 1.
Cluster size | Control | Clusters required | Total number of participants | ||
---|---|---|---|---|---|
Total | LUCAS-2 | Control | |||
14 | 1 : 1 | 238 | 119 | 119 | 3332 |
1 : 2 | 260 | 87 | 173 | 3640 | |
15 | 1 : 1 | 224 | 112 | 112 | 3360 |
1 : 2 | 245 | 82 | 163 | 3675 | |
16 | 1 : 1 | 212 | 106 | 106 | 3392 |
1 : 2 | 231 | 77 | 154 | 3696 | |
18 | 1 : 1 | 192 | 96 | 96 | 3456 |
1 : 2 | 210 | 70 | 140 | 3780 | |
20 | 1 : 1 | 176 | 88 | 88 | 3520 |
1 : 2 | 192 | 64 | 128 | 3840 |
Our target was to randomise 82 LUCAS-2 clusters and 163 standard care clusters and a total sample size of 3675 participants. We expected to determine the primary outcome for close to 100% of trial participants, so no inflation of the sample size to allow for losses to follow-up of individual participants was proposed. With this sample size, the 95% CI around an estimated treatment effect of a RR of 1.50 would be 1.14 to 1.94, including adjustment for clustering.
Within this sample size we expected around 25% of patients to have an initial rhythm of VF (approximately 920 patients). This subgroup was expected to have significantly higher survival than the rest of the population, of around 15%. The number in this subgroup was sufficient to show an increase from 15% to 22.8% (RR 1.52) with 80% power, allowing for clustering.
The DMC monitored the values of all of the parameters of the sample size calculation at interim analyses and advised on any necessary modifications to the sample size.
Revision to sample size
The target sample size was reviewed in September 2012, after recruitment of 2469 patients, in response to an observed high level of non-compliance in the LUCAS-2 arm and to incorporate updated figures for the expected cluster size, ICC and ratio of control to LUCAS-2 clusters. The sample size re-estimation did not use any information from comparisons between the trial groups.
Because non-compliance would reduce the difference between the groups and potentially obscure a treatment effect due to the LUCAS-2 device, we used complier average causal effect (CACE) analysis66,67 as well as ITT analysis. This approach estimates the unadjusted odds ratio (OR) for the treatment effect among compliers, without introducing bias by ignoring the random assignment to groups. For re-estimating the sample size, we defined compliance in the LUCAS-2 group as use of the device, or non-use for legitimate reasons that would preclude its use in normal clinical practice (such as patients in whom the LUCAS-2 device was contraindicated or if space restriction meant that the LUCAS-2 device could not be deployed). Using this definition, we estimated that compliance would be around 70% at the end of the trial.
The expected average cluster size was calculated to be approximately nine and the control-to-LUCAS-2 ratio was 1.5 : 1. It was not possible to calculate an ICC from the interim trial data, but it was expected to be low and a lower value than was assumed in the original calculation (0.001 rather than 0.01) was used.
Using these figures, a sample size of 4344 would maintain the original power of the trial to detect an increase in survival from 5% to 7.5%, using CACE analysis rather than ITT analysis. The change to the sample size was approved by the DMC and TSC and the revised target of 4344 was adopted in December 2012.
Statistical analysis
We performed ITT analyses to estimate the treatment effect of the LUCAS-2 device and presented results as a point estimate (RR or mean difference), with uncertainty estimated by the 95% CI.
We also used CACE analyses to estimate the effect in cardiac arrests when the protocol was followed. 66 CACE estimates the treatment effect in people who were randomly assigned to the intervention and who actually received it, by comparing compliers in the intervention group with those participants in the control group who would have been compliers if they had been allocated to the intervention group. This analysis retains the advantages of randomisation and avoids introducing bias, hence CACE is preferred to per-protocol analysis. 67 CACE assumes that the probability of non-compliance with the LUCAS-2 device would be the same for people who were actually randomised to the control as for those who were randomised to the LUCAS-2 arm. If allocation is random, this assumption will hold. A second assumption is that outcomes are not affected just by being randomised to the LUCAS-2 or control groups; in other words, there is no systematic difference in outcomes between patients attended by LUCAS-2 device-containing vehicles and those attended by control vehicles, except that caused by the different treatments provided. CACE analysis enables us to estimate the unobserved proportion of the control group who would have been non-compliers if randomised to the LUCAS-2 group. We did two CACE analyses, defining compliers in different ways. In CACE 1, we treated as non-compliant those cases in which the LUCAS-2 device was not used for unknown or trial-related reasons that would not occur in real-life clinical practice (e.g. crew was not trained in trial procedures, crew misunderstood the trial protocol, the device was missing from the vehicle). This analysis omits trial-related non-use and should be a better estimate of the treatment effect in real-world clinical practice than an ITT analysis. In the CACE 2 analysis, we treated as compliant only those cases in which the LUCAS-2 device was actually used and this analysis therefore estimates efficacy, that is, the treatment effect in patients who received LUCAS-2 device treatment.
For ITT analyses, we used logistic regression models to obtain unadjusted and adjusted ORs and 95% CIs. The prespecified covariates used in the adjusted models were age, sex, response time, bystander CPR and initial rhythm. We attempted adjusting for the clustering design using multilevel logistic models using the Statistical Analysis Software (SAS) GLIMMIX procedure with logit link function based on the binomial distribution. Because of the extremely low survival rates in each cluster (vehicle), the multilevel models could not be fitted with the vehicle random effect, as this effect was not estimable. As a result, ordinary logistic regressions were fitted. We also undertook prespecified subgroup analyses by (1) initial rhythm (shockable vs. non-shockable); (2) cardiac arrest witnessed versus not witnessed; (3) type of vehicle (RRV vs. ambulance); (4) bystander CPR versus no bystander CPR; (5) region; (6) aetiology (presumed cardiac or non-cardiac); (7) age; and (8) response time. The analyses by region and type of vehicle were added during recruitment on the recommendation of the TSC. We fitted logistic regression models for the primary outcome measure with the inclusion of an interaction term to examine whether or not the treatment effect differed between the subgroups. Age and response times are continuous variables and we assessed these using multivariate fractional polynomials68 (see Appendix 8).
We did all analyses using SAS version 9.3 (SAS Institute, Marlow, UK). Interim analyses were conducted at least once per year during recruitment and supplied confidentially to the DMC. The DMC considered the results of the interim analysis and made recommendations to the TSC about continuation of recruitment or any modification to the trial that may have been necessary.
Approvals, registration and governance
The study was approved by the Coventry REC (reference number 09/H1210/69) and sponsored by the University of Warwick. It was conducted in accordance with the principles of good clinical practice and the Mental Capacity Act (2005). 54,69 The trial was registered on the International Standard Randomised Controlled Trial Number Register (ISRCTN08233942). Approval was given by the National Information Governance Board for Health and Social Care Ethics and Confidentiality Committee for access to personal data without consent (reference number ECC 2–02 (c)/2011). The manufacturers (Jolife AB) and distributors (Physio-Control UK) of the LUCAS-2 device had no role in the design, conduct, analysis or reporting of the trial. Their role was limited to supply and servicing of the LUCAS-2 devices and training of study co-ordinating centre personnel.
Several changes to the protocol and procedures were made during the trial (see Table 2). When these fulfilled the definition of ‘substantial amendments’ according to the UK Clinical Trials regulations, they were reviewed and approved by the ethics committee.
Staffing
The trial was co-ordinated by a team based at the WCTU. The exact personnel varied during the course of the trial but included a trial co-ordinator (who had overall management responsibility for the team), trial administrator, data manager and research nurse (who was responsible for co-ordinating follow-up) (Figure 3). The statistician, senior project manager and programmer were also based at the WCTU. Each ambulance service employed a number of research paramedics, who were seconded to the trial for its duration and had primary responsibility for delivering the trial in their area. They were based with the ambulance services, but worked closely with the central co-ordinating team. Their role was to deliver training, manage the devices and organise data collection. Some of the research paramedics additionally performed follow-up visits. These personnel were key to the successful delivery of the trial. As active paramedics, they had detailed knowledge of the procedures and challenges of their own ambulance service, and were able to design systems and procedures that would be successful in their area.
FIGURE 3.
Staff organogram. R&D, research and development.


Protocol amendments
There have been 11 substantial and non-substantial amendments to the trial documentation (Table 2).
Date of amendment | Amendment no. | Document(s) affected | Changes | New version number, date | Date approved by REC – Coventry | Date approved by lead R&D |
---|---|---|---|---|---|---|
23 April 2015 | 11 | N/A | MSc project: contact survivors enrolled in the PARAMEDIC trial to explore patient views and experiences of being Enrolled in an emergency care trial with a waiver of consent |
N/A | 5 May 2015 | |
17 February 2015 | 10 | N/A | Extension to end date of trial to end of June 2015 | N/A | 5 March 2015 | N/A |
1 May 2013 | 9 | Protocol, PIS | Clarifications to protocol, revised patient letters | Version 4.3, 1 April 2013 | 6 June 2013 | 8 July 2013 |
Version 5.1, 1 April 2013 | ||||||
4 March 2013 | 8 | Protocol | Revised sample size of 4344 | Version 4.2, 4 March 2013 | 25 March 2013 | 3 April 2013 |
8 November 2012 | 7 | N/A | MSc dissertation to go a full amendment | N/A | 21 November 2012 | 24 June 2013 |
12 June 2012 | 6 | PIS, ICF | Revise patient letter and add in another contact at 12 months | Version 5.0, 12 June 2012 | 3 July 2012 | 9 July 2012 |
5 April 2012 | 5 | N/A | Letter to inform REC of reward scheme (pilot) in Coventry area | N/A | 11 May 2012 | 9 July 2012 |
29 March 2012 | N/A | N/A | Letter to inform REC of Ian Jones’ dissertation | N/A | 13 July 2012 | |
22 December 2011 | 4 (minor amendment 2) | Information sheets | Minor changes to the invite letter and information sheets so that if we are doing only a 12-month visit the documents make more sense | Version 4.1, 15 December 2011 | 22 December 2011 | 6 January 2012 |
14 June 2011 | 3 (minor amendment 1) | N/A | Clarification that we will seek information from hospitals with regard to secondary outcomes | N/A | 20 June 2011 | 13 July 2011 |
21 March 2011 | 2 | Protocol | Sample size change Approaching survivors now we have NIGB approval Change from ‘paramedics’ to ‘ambulance clinicians’ throughout |
Version 4.1, 21 March 2011 | 4 May 2011 | 5 May 2011 |
21 March 2011 | 2 | Information sheets | Changes now we have NIGB approval Reply slip for patient representative to reply on behalf of patient |
Version 4.0, 16 December 2010 | 4 May 2011 | 5 May 2011 |
21 March 2011 | 2 | Consent form | Changes to consultee form – title changed from ‘Consent’ to ‘Agreement’. [Patient name] added instead of friend/relative, etc. | Version 4.0, 16 December 2010 | 4 May 2011 | 5 May 2011 |
12 August 2010 | 1 | Protocol | Several minor changes to text throughout, highlighted in yellow Change to primary outcome sections 2.2.1 and 2.3 Changes to sample size in section 2.4.4 Clarification of process if the first resource on scene is not a trial vehicle (section 2.5.2) Changes to section 2.10: Protection against bias: addition of subheadings Sections ‘7.3 Relationship with manufacturer’ and ‘8.4 Training’ added in Clarification that data will be collected for all of the cardiac arrest patients attended by trial vehicles, non-trial vehicles and those for which resuscitation attempts were not made for monitoring purposes. Only eligible cardiac arrests will be followed up and included in the analysis Addition of device related event capture to section 4 |
Version 3.0, 12 August 2010 | 16 September 2010 | 11 October 2010 (WMAS only) |
12 August 2010 | 1 | Information sheets 1 and 2 | Addition of trial ID to information sheet 1 Change of version and date to information sheets 1 and 2 |
Version 3.0, 12 August 2010 | 16 September 2010 | 11 October 2010 (WMAS only) |
12 August 2010 | 1 | N/A | Use of £5 vouchers for training paramedics Change to PIs: Scotland, B. Mason to G. Egan; and, West Midlands, R Cooke to G Bennett |
16 September 2010 | 11 October 2010 (WMAS only) |
Chapter 3 Trial results
Overview of recruitment
Recruitment of clusters
Four hundred and eighteen emergency vehicles (287 double-manned ambulances and 131 single-manned RRVs), at 86 ambulance stations, were included in the trial. One hundred and forty-seven vehicles (100 ambulances and 47 RRVs) were assigned to the LUCAS-2 group and 271 clusters (187 ambulances and 84 RRVs) were randomised to the control group (Table 3 and Figure 4). The overall LUCAS-2-to-control ratio was 1 : 1.8 (1 : 1.87 for ambulances and 1 : 1.79 for RRVs). Seventy-two clusters were terminated early during the recruitment period, for a variety of reasons, including the vehicle being taken out of front-line service, scrapped or transferred to a station that was out of the trial area.
Locality | Number of stations | Number of vehicles | ||||
---|---|---|---|---|---|---|
Ambulance | RRV | |||||
LUCAS-2 | Control | LUCAS-2 | Control | |||
West Midlands | ||||||
Birmingham | 24 | 27 | 49 | 12 | 24 | |
Black Country | 14 | 12 | 25 | 4 | 9 | |
Coventry & Warwickshire | 8 | 14 | 27 | 10 | 16 | |
South Central | ||||||
SCAS North | 3 | 9 | 13 | 3 | 8 | |
SCAS South | 2 | 6 | 18 | 2 | 3 | |
North East | ||||||
Durham | 2 | 1 | 2 | 1 | 1 | |
North Tyne | 6 | 1 | 5 | 1 | 0 | |
South Tyne | 7 | 3 | 6 | 2 | 4 | |
Teesside | 1 | 3 | 4 | 1 | 4 | |
Wales | ||||||
ABM East | 3 | 4 | 9 | 3 | 3 | |
ABM West | 4 | 5 | 7 | 1 | 4 | |
Cardiff | 2 | 5 | 8 | 1 | 3 | |
Llanelli | 1 | 2 | 2 | 2 | 2 | |
Newport | 2 | 4 | 6 | 2 | 2 | |
Vale of Glamorgan | 2 | 4 | 6 | 2 | 1 | |
Total | 100 | 187 | 47 | 84 |
FIGURE 4.
The Consolidated Standards of Reporting Trials (CONSORT) diagram for vehicles. a, A vehicle can be counted twice, as it can deliver/not deliver intervention on different occasions. Seventy-two (17%) of vehicles changed status. CA, cardiac arrest; CAT A, category A call.

Stations were opened for recruitment and vehicles were randomised between April 2010 and February 2013, so the duration of recruitment for each cluster varied between 4 and 38 months. The number of patients recruited by each vehicle was also very variable, ranging from 0 to 41 patients (Figure 5), with a mean cluster size of 13 patients in the LUCAS-2 arm and 11 in the control arm. Some of the zeros are accounted for by five stations with 17 vehicles that never commenced active recruitment. Individual ambulance staff attended on average 4.1 eligible cardiac arrests [standard deviation (SD) 3.6 eligible cardiac arrests] in the control group and 3.0 eligible cardiac arrests (SD 2.3 eligible cardiac arrests) in the LUCAS-2 group over the study period.
FIGURE 5.
Number of vehicles attending different numbers of cardiac arrests.
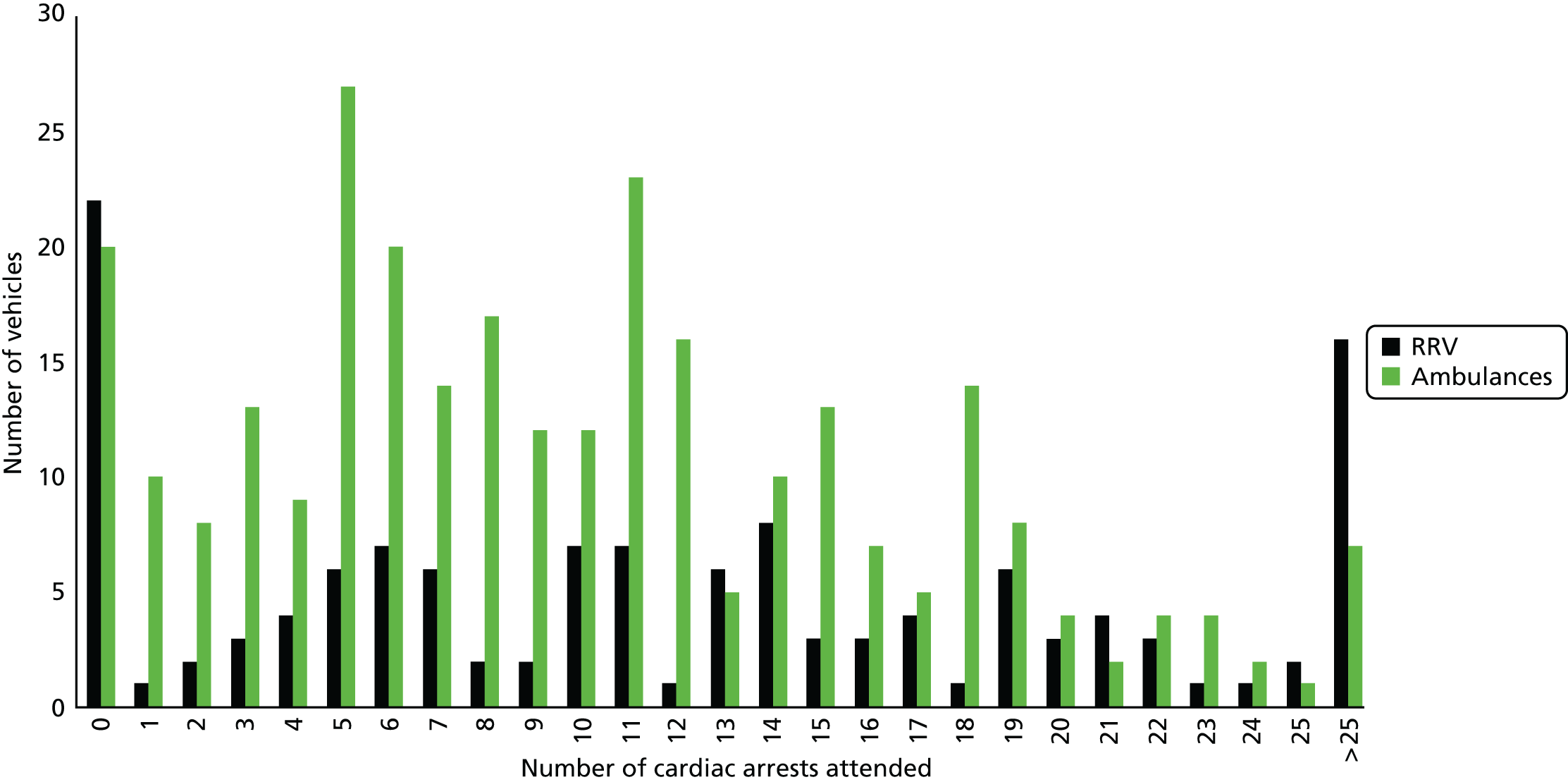
Recruitment of patients
Patients were recruited between 15 April 2010 and 10 June 2013. Recruitment began first in the West Midlands (start date 15 April 2010) and, subsequently, in the other regions (start dates: Wales, September 2011; South Central, October 2011; North East, May 2012). During the recruitment period there were 16.019 cardiac arrests attended by vehicles from participating stations and trial vehicles attended 11,171 emergency incidents. Cardiac arrest was confirmed and resuscitation was attempted in 4689 cases, of which 218 cases were ineligible and excluded. In total, 4471 patients were therefore enrolled in the study (Figure 6).
FIGURE 6.
Patient flow chart. a, Reasons that the LUCAS-2 device was not used: crew not trained, 78; crew error, 168; no device in vehicle, 26; unsuitable patient, 102 [patient too large, 58; patient too small, 22; other reasons (e.g. chest deformity), 22]; device issues, 14; not possible to use device, 140; and reason unknown, 110. Reasons for the LUCAS-2 device use in control arm were crew error.

In the LUCAS-2 arm, 638 cases received manual chest compression and in the control arm 11 cases received LUCAS-2 chest compression (see footnote a to Figure 6).
The majority of patients were recruited in the West Midlands (2723/4471, 60.9%), with smaller proportions in the other three regions. RRVs were the first vehicle in attendance for 1635 out of 4471 (36.6%) recruits, whereas ambulances were the first vehicle on scene for 2836 out of 4471 (63.4%) recruits (Table 4).
Region | Treatment arm | Total number of patients (percentage of total) | |||||
---|---|---|---|---|---|---|---|
LUCAS-2 | Control | ||||||
Ambulance | RRV | Total | Ambulance | RRV | Total | ||
North East | 80 | 106 | 186 | 226 | 131 | 357 | 543 (12.1%) |
South Central | 105 | 43 | 148 | 238 | 121 | 359 | 507 (11.3%) |
Wales | 230 | 116 | 346 | 231 | 121 | 352 | 698 (15.6%) |
West Midlands | 648 | 324 | 972 | 1078 | 673 | 1751 | 2723 (60.9%) |
Total (percentage of total) | 1063 (64.3%) | 589 (35.7%) | 1652 | 1773 (62.9%) | 1046 (37.1%) | 2819 | 4471 |
Differences in baseline characteristics between the groups were small (Table 5). Slightly more patients in the manual CPR group had cardiac arrest at home [2336/2819 (82.9%) vs. 1336/1652 (80.9%)] and witnessed arrest [1749/2819 (62.0%) vs. 1001/1652 (60.6%)].
Characteristic | Treatment arm | |
---|---|---|
LUCAS-2 (N = 1652) | Manual CPR (N = 2819) | |
Age (years) | ||
Mean (SD) | 71.0 (16.3) | 71.6 (16.1) |
Sex, n (%) | ||
Male | 1039 (63.0) | 1774 (62.9) |
Aetiology of cardiac arrest, n (%) | ||
Presumed cardiac | 1417 (85.8) | 2445 (86.7) |
Respiration | 125 (7.6) | 191 (6.8) |
Submersion | 5 (0.3) | 7 (0.3) |
Unknown | 48 (2.9) | 74 (2.6) |
Other (non-cardiac) | 57 (3.5) | 102 (3.6) |
Location, n (%) | ||
Home | 1336 (80.9) | 2336 (82.9) |
Public place | 225 (13.6) | 362 (12.8) |
Other | 91 (5.5) | 121 (4.3) |
Witnessed cardiac arrest, n (%) | 1001 (60.6) | 1749 (62.0) |
Bystander | 704 (42.6) | 1223 (43.4) |
EMS | 250 (15.1) | 449 (15.9) |
Non-EMS health care | 47 (2.8) | 75 (2.6) |
Not known | 0 | 2 (0.1) |
Bystander CPR before EMS arrival, n (%) | 716 (43.3) | 1238 (43.9) |
Not known | 90 (5.5) | 168 (6.0) |
Time (minutes) from emergency call to vehicle arrival, median (IQR) | 6.5 (4.8–9.1) | 6.3 (4.6–9.2) |
Initial rhythm, n (%) | ||
VF | 364 (22.0) | 597 (21.2) |
VT | 12 (0.7) | 18 (0.6) |
PEA | 398 (24.0) | 707 (25.0) |
Asystole | 824 (49.9) | 1384 (49.1) |
Not known | 54 (3.3) | 113 (4.0) |
Defibrillation before EMS arrival, n (%) | 19 (1.2) | 40 (1.9) |
Cumulative recruitment through time is shown in Figure 7.
FIGURE 7.
Cumulative recruitment: April 2010 to June 2013. Dotted line represents the original target recruitment; dashed line represents the revised target recruitment; and the green solid line represents actual recruitment.
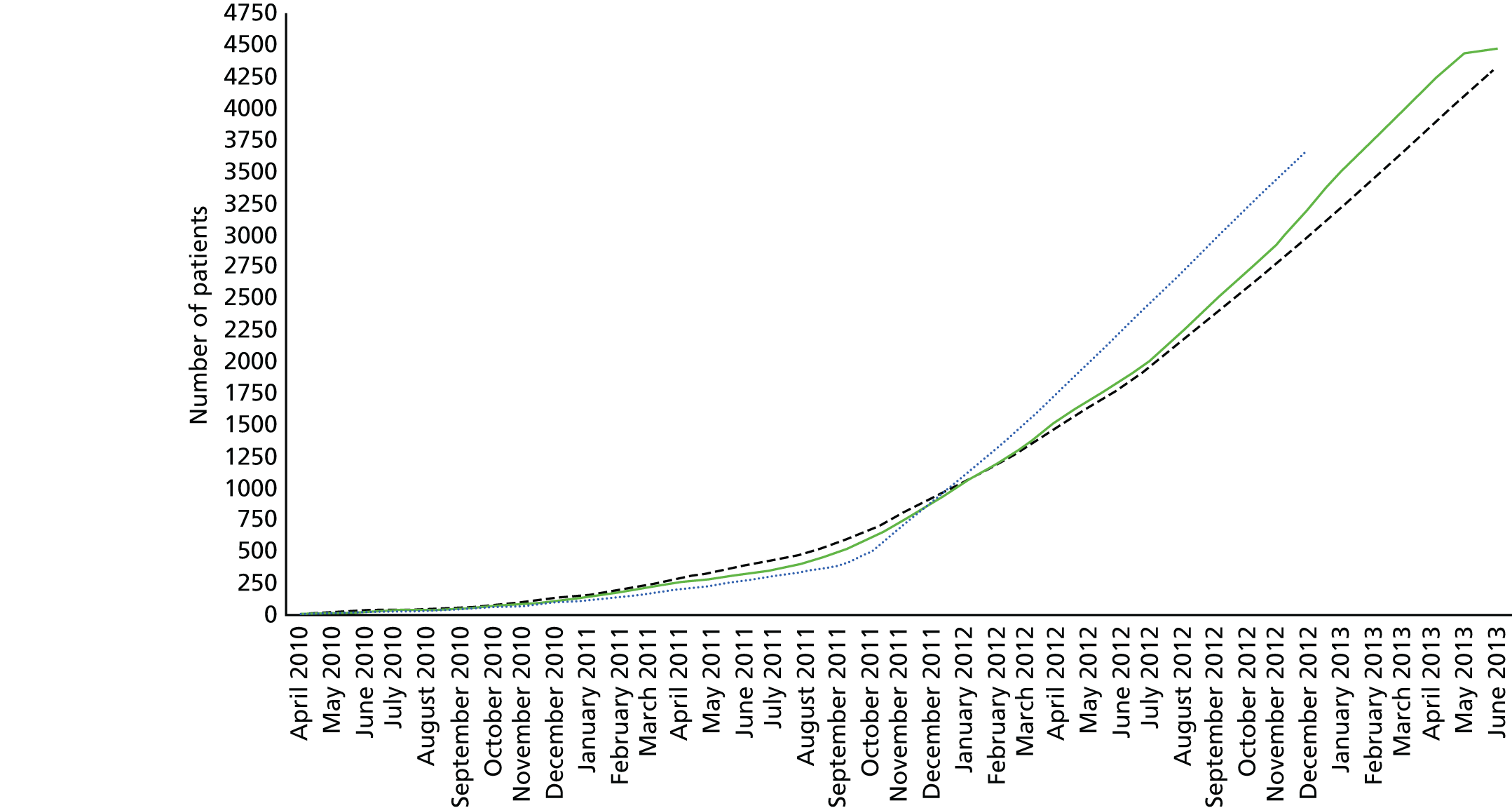
Treatment
A substantial proportion of the patients who were randomised to the LUCAS-2 group (638/1652, 38%) did not receive mechanical chest compressions. This was expected because in clinical practice there would be occasions in which the LUCAS-2 device would not be used and it is appropriate to include these cardiac arrests in a pragmatic trial. The LUCAS-2 device was used for 985 out of 1652 patients (60%) in the LUCAS-2 arm; in 29 out of 1652 (1.8%) cases the intervention used was unknown. The LUCAS-2 device was not used for trial-related reasons in 272 cases and 256 cases of non-use were classified as being for reasons that would occur in normal clinical practice. The reason for non-use was not known for 110 cases. In the control arm, the LUCAS-2 device was used in 11 out of 2819 cases (0.4%) as a result of crew error.
Treatments used were similar between the trial arms, although the proportion of patients receiving intravenous drugs was slightly higher in the LUCAS-2 group (1366/1652, 82.7%) than in the control group (2255/2819, 80.0%) (Table 6).
Treatment | Treatment arm, n (% of total) | |
---|---|---|
LUCAS-2 (N = 1652) | Manual CPR (N = 2819) | |
Intravenous drugs given | 1366 (82.7) | 2255 (80.0) |
Not known | 8 (0.5) | 14 (0.5) |
Intubated | 749 (45.3) | 1297 (46.0) |
Not known | 33 (2.0) | 48 (1.7) |
LMA/supraglottic airway device used | 435 (26.3) | 736 (26.1) |
Not known | 29 (1.9) | 47 (1.7) |
Transported to hospital | 1099 (66.5) | 1868 (66.2) |
Status at handover | ||
ROSC | 377 (22.8) | 658 (23.3) |
CPR in progress | 640 (38.7) | 1081 (38.4) |
Unknown | 82 (5.0) | 129 (4.6) |
Received allocated intervention | 985 (59.6) | 2808 (99.6) |
Did not receive allocated intervention | 638 (38.6) | 11 (0.4) |
Not known whether or not allocated intervention delivered | 29 (1.8) | 0 (0.0) |
Reasons for non-use of LUCAS-2 device | ||
Reason unknown | 110 | |
Not trained | 78 | |
Crew error | 168 | |
Not possible to use the LUCAS-2 device | 140 | |
No device in vehicle | 26 | |
Unsuitable patient | 102 | |
Device issues | 14 |
Follow-up
The number of patients followed up with visits at 3 and 12 months was limited by lack of response to the initial contact and patients declining participation in the follow-up (Table 7): 146 out of 278 survivors (52.5%) were followed up at 3 months and 143 out of 264 survivors (54.2%) at 12 months. The proportion of patients visited was slightly lower in the LUCAS-2 group than in the control group (49.0% vs. 54.1%), raising the possibility that there may have been differential losses between the trial arms (Table 8).
Follow-up | Treatment arm, n (% of total) | Total, n (% of total) (N = 4471) | |
---|---|---|---|
LUCAS-2 (N = 1652) | Control (N = 2819) | ||
From hospital discharge to 3-month follow-up | |||
Deceased prior to 3-month follow-up | 1556 (94.2) | 2636 (93.5) | 4192 (93.8) |
Death/alive status unknown | 0 (0.0) | 1 (0.0) | 1 (0.0) |
3-month follow-up | |||
Alive at 3-month follow-up | 96 (5.8) | 182 (6.5) | 278 (6.2) |
Follow-up complete (3-month visit done) | 47 (2.8) | 99 (3.5) | 146 (3.3) |
Patient declined (when contacted) | 19 (1.2) | 36 (1.3) | 55 (1.2) |
Lost to follow-up | 28 (1.7) | 47 (1.7) | 75 (1.7) |
Patient died (before visit could be done) | 2 (0.1) | 0 (0.0) | 2 (0.0) |
From hospital discharge to 12-month follow-up | |||
Deceased prior to 12-month follow-up | 1563 (94.6) | 2643 (93.8) | 4206 (94.1) |
Death/alive status unknown | 0 (0.0) | 1 (0.0) | 1 (0.0) |
12-month follow-up | |||
Alive at 12-month follow-up | 89 (5.4) | 175 (6.2) | 264 (5.9) |
Follow-up complete (12-month visit done) | 48 (2.9) | 95 (3.4) | 143 (3.2) |
Patient declined (when contacted) | 17 (1.0) | 33 (1.2) | 50 (1.1) |
Lost to follow-up | 23 (1.3) | 43 (1.5) | 66 (1.5) |
Withdrew | 1 (0.1) | 4 (0.1) | 5 (0.1) |
Patient died (before visit could be done) | 0 (0.0) | 0 (0.0) | 0 (0.0) |
Characteristic | Treatment arm | |
---|---|---|
LUCAS-2 (N = 47) | Control (N = 99) | |
Age (years) | ||
Mean (SD) | 60.6 (14.7) | 63.1 (14.1) |
Sex | ||
Male, n (%) | 35 (75) | 73 (74) |
Aetiology of cardiac arrest, n (%) | ||
Presumed cardiac | 42 (89.4) | 94 (95.0) |
Traumatic | 0 (0.0) | 0 (0.0) |
Respiration | 5 (10.6) | 3 (3.0) |
Submersion | 0 (0.0) | 0 (0.0) |
Unknown | 0 (0.0) | 0 (0.0) |
Other (non-cardiac) | 0 (0.0) | 2 (2.0) |
Location, n (%) | ||
Home | 26 (55.3) | 57 (57.6) |
Public place | 16 (34.0) | 34 (34.8) |
Other | 5 (10.6) | 8 (8.1) |
Not known | 0 (0.0) | 0 (0.0) |
Witnessed cardiac arrest, n (%) | 40 (85.1) | 90 (90.9) |
Bystander | 24 (60.0) | 52 (57.8) |
EMS | 15 (37.5) | 34 (37.8) |
Non-EMS health care | 1 (2.5) | 4 (4.4) |
Not known | 2 (4.2) | 3 (3.0) |
Bystander CPR before EMS arrival, n (%) | 18 (38.8) | 36 (36.4) |
Not known | 0 (0.0) | 5 (5.1) |
Time (minutes) from emergency call to vehicle arrival | ||
Median | 5.9 | 5.9 |
IQR | 4.2–7.0 | 4.3–8.2 |
Range | 2.5–56.8 | 2.1–30.4 |
Initial rhythm, n (%) | ||
VF | 37 (78.7) | 79 (79.8) |
VT | 3 (6.4) | 3 (3.0) |
PEA | 4 (8.5) | 10 (10.1) |
Asystole | 2 (4.3) | 2 (2.0) |
Not known | 1 (2.1) | 5 (5.1) |
Defibrillation before EMS arrival, n (%) | 1 (2.1) | 2 (2.0) |
Not known | 0 (0.0) | 1 (1.0) |
Outcomes: intention-to-treat analysis
Survival status could not be ascertained for one patient, who was from overseas and returned home < 30 days after the cardiac arrest, but it was known up to 12 months for all of the other participants. In the ITT analysis, 30-day survival was similar in the LUCAS-2 and control groups [LUCAS-2, 104/1652 (6.3%); control, 193/2818 (6.8%); adjusted OR 0.86, 95% CI 0.64 to 1.15].
The analyses did not show a clear advantage to the LUCAS-2 group for any outcome; there was very little effect of the LUCAS-2 device on ROSC (OR 0.99, 95% CI 0.86 to 1.14) and survived event (OR 0.97, 95% CI 0.82 to 1.14), but for survival at 30 days, 3 months and 12 months the point estimates favoured manual chest compression, although the 95% CIs included 1. The number of patients surviving with a favourable neurological outcome (i.e. a CPC score of 1 or 2) was lower in the LUCAS-2 group than in the control group (adjusted OR 0.72, 95% CI 0.52 to 0.99; Table 9).
Outcome | Treatment arm, n (% of total) | OR (95% CI) | ||
---|---|---|---|---|
LUCAS-2 (N = 1652) | Control (N = 2819) | |||
Unadjusted | Adjusted | |||
Survival to 30 days | 104 (6.3) | 193 (6.9) | 0.91 (0.71 to 1.17) | 0.86 (0.64 to 1.15) |
Not known | 0 (0.0) | 1 (0.04) | ||
ROSC | 522 (31.6) | 885 (31.4) | 1.02 (0.89 to 1.16) | 0.99 (0.86 to 1.14) |
Not known | 58 (3.5) | 82 (2.9) | ||
Survived event | 377 (22.8) | 658 (23.3) | 0.97 (0.83 to 1.14) | 0.97 (0.82 to 1.14) |
Not known | 82 (5.0) | 129 (4.6) | ||
Survival to 3 months | 96 (5.8) | 182 (6.5) | 0.89 (0.69 to 1.15) | 0.83 (0.61 to 1.12) |
Not known | 0 (0.0) | 1 (0.04) | ||
Survival to 12 months | 89 (5.4) | 175 (6.2) | 0.86 (0.60 to 1.12) | 0.83 (0.62 to 1.11) |
Survival with favourable neurological outcome (CPC score of 1 or 2) | 77 (4.7) | 168 (6.0) | 0.77 (0.59 to 1.02) | 0.72 (0.52 to 0.99) |
CPC (%) | ||||
1 | 67 (4.1) | 153 (5.4) | ||
2 | 10 (0.6) | 15 (0.5) | ||
3 | 14 (0.9) | 10 (0.4) | ||
4 | 2 (0.1) | 1 (0.0) | ||
5 | 1556 (94.2) | 2636 (93.5) | ||
Not known | 3 (0.2) | 4 (0.1) |
Complier average causal effect analyses
The CACE 1 analysis included cases for which the LUCAS-2 device was not used in situations when it would not be used in normal clinical practice. Its results were similar to the ITT analysis, which suggests that there is no advantage to the LUCAS-2 device when used as it would be in routine clinical practice. In the CACE 2 analysis, which included only cases for which the LUCAS-2 device was actually used in the intervention group, the effects of the LUCAS-2 device, again, were similar to the ITT analysis, although the estimate for survival with a CPC score of 1 or 2 was slightly more extreme. Therefore, there was not a substantial difference in the treatment effect of the LUCAS-2 device when it was actually used. A concern with the ITT analysis is that because it includes a large number of non-compliant cases, any benefit among patients for whom the device was actually used would be diluted by a lack of effect among cases that were non-compliant, leading to underestimation of the treatment effect. The CACE analyses demonstrate that the lack of advantage to the LUCAS-2 device in the ITT analysis is not due to dilution of the treatment effect (Table 10).
Survival | CACE analysis | |||||
---|---|---|---|---|---|---|
CACE 1 | CACE 2 | |||||
Treatment arm, n/N (% of total) | OR (95% CI) | Treatment arm, n/N (% of total) | OR (95% CI) | |||
LUCAS-2 | Control | LUCAS-2 | Control | |||
To 30 days | 81/1241 (6.5) | 153/2155 (7.1) | 0.92 (0.69 to 1.21) | 50/985 (5.1) | 99/1710 (5.8) | 0.87 (0.61 to 1.23) |
With a CPC score of 1 or 2 | 62/1238 (5.0) | 142/2151 (6.6) | 0.76 (0.56 to 1.03) | 38/983 (3.9) | 101/1701 (5.9) | 0.65 (0.45 to 0.96) |
Survived event | 297/779 (38.1) | 527/1378 (38.2) | 0.97 (0.84 to 1.18) | 232/632 (36.7) | 413/1077 (38.4) | 0.96 (0.79 to 1.16) |
Subgroup analyses
In the subgroup analyses there was no evidence of different treatment effects between the subgroups for 30-day survival according to whether or not the cardiac arrest was witnessed; the type of vehicle (ambulance or RRV); whether or not the patient received bystander CPR; aetiology; and region (Table 11). In the subgroup analysis by initial rhythm, there was a difference in treatment effect between patients with a shockable initial rhythm and those with PEA or asystole. Survival was lower in the LUCAS-2 group than in those with shockable initial rhythms (OR 0.71, 95% CI 0.52 to 0.98), but higher in the PEA/asystole group (OR 1.38, 95% CI 0.80 to 2.36).
Comparison | Subgroup | Treatment arm, n/N (% of total) | OR (95% CI) | Test of interaction (p-value) | |
---|---|---|---|---|---|
LUCAS-2 | Control | ||||
Initial rhythm | VF/VT | 69/376 (18.4) | 148/615 (24.1) | 0.71 (0.52 to 0.98) | 0.0390 |
PEA/asystole | 24/1222 (2.0) | 30/2091 (1.4) | 1.38 (0.80 to 2.36) | ||
Rhythm not known | 11/54 (20.4) | 15/113 (13.3) | – | ||
Witnessed status | Witnessed | 89/1001 (8.9) | 163/1749 (9.3) | 0.96 (0.73 to 1.25) | 0.6105 |
Not witnessed | 10/528 (1.9) | 21/864 (2.4) | 0.78 (0.36 to 1.66) | ||
Witnessed status not known | 123 (7.4) | 206 (7.3) | – | ||
Bystander CPR | Given | 42/716 (5.9) | 68/1238 (5.5) | 1.07 (0.72 to 1.59) | 0.3656 |
Not given | 59/846 (7.0) | 115/1413 (8.1) | 0.86 (0.61 to 1.17) | ||
Not known | 90 | 168 | – | ||
Type of vehicle | Ambulance | 60/1063 (5.6) | 127/1773 (7.7) | 0.78 (0.56 to 1.06) | 0.0928 |
Rapid response car | 44/589 (7.5) | 66/1045 (6.3) | 1.20 (0.81 to 1.78) | ||
Region | A | 16/186 (8.6) | 23/357 (6.4) | 1.37 (0.70 to 2.66) | 0.5217 |
B | 9/149 (6.1) | 33/359 (9.2) | 0.64 (0.30 to 1.37) | ||
C | 19/346 (5.5) | 22/352 (6.3) | 0.87 (0.46 to 1.64) | ||
D | 60/972 (6.2) | 115/1750 (6.6) | 0.94 (0.68 to 1.29) | ||
Aetiology | Presumed cardiac | 91/1417 (6.4) | 173/2445 (7.1) | 0.90 (0.69 to 1.17) | 0.1287 |
Other | 9/130 (6.9) | 7/198 (3.5) | 2.03 (0.74 to 5.59) |
The analyses of age and response time using fractional polynomial models did not show any interaction effects of these variables with the treatment effect of the LUCAS-2 device. Therefore, we did not find any evidence that the treatment effect of the LUCAS-2 device differs depending on the age of the patient or ambulance service response time.
Serious adverse events
Seven clinical AEs were reported in the LUCAS-2 group (three events of chest bruising, two of chest laceration and two of blood in mouth). No SAEs were reported. Fifteen device incidents occurred during operational use (four incidents in which alarms sounded, seven in which the device stopped working and four other device incidents). No adverse or SAEs were reported in the control group.
Follow-up at 3 months and 12 months
We considered using multiple imputation (MI) to attempt to correct for the effects of missing data, but we felt that the assumptions of any imputation procedure were unlikely to hold, given the large number of missing data and the high probability that missingness was related to outcome. Analyses therefore used available cases only, with no imputation.
At the 3-month follow-up (Table 12), SF-12 mental and physical scores and EQ-5D quality-of-life scores were slightly lower in the LUCAS-2 group than in the control group.
Outcome | Treatment arm | Difference (95% CI) | ||
---|---|---|---|---|
LUCAS-2 (N = 47) | Control (N = 99) | Unadjusted | Adjusted | |
SF-12 physical | ||||
Mean score (SD) | 38.9 (11.5) | 41.7 (10.9) | –2.8 (–6.7 to 1.1) | –3.0 (–7.0 to 1.1) |
Median score (IQR) | 40.8 (32.1–48.0) | 42.6 (34.7–49.4) | ||
Missing scores, n | 2 | 2 | ||
SF-12 mental | ||||
Mean score (SD) | 47.3 (13.4) | 48.9 (10.5) | –1.6 (–5.6 to 2.5) | –1.5 (–5.5 to 2.6) |
Median score (IQR) | 51.7 (38.2–57.3) | 50.2 (42.1–57.2) | ||
Missing scores, n | 2 | 2 | ||
EQ-5D | ||||
Mean score (SD) | 63.8 (23.5) | 72.0 (18.0) | –8.2 (–15.1 to –1.3) | –6.8 (–13.7 to –0.1) |
Median score (IQR) | 65.0 (50.0–80.0) | 75.0 (64.0–85.0) | ||
Missing scores, n | 0 | 0 |
In the 12-month follow-up (Table 13), all of the results were in the same direction, indicating worse outcomes in the LUCAS-2 group. For some of the outcomes, the 95% CIs excluded zero, whereas for others the data were compatible with a zero or small positive effect.
Outcome | Treatment arm | Difference (95% CI) | ||
---|---|---|---|---|
LUCAS-2 (N = 48) | Control (N = 95) | Unadjusted | Adjusted | |
SF-12 physical | ||||
Mean score (SD) | 40.1 (12.7) | 43.8 (10.7) | –3.8 (–7.8 to 0.2) | –3.5 (–7.4 to 0.4) |
Median score (IQR) | 40.7 (30.8–51.0) | 44.9 (36.1–52.5) | ||
Missing scores, n | 1 | 2 | ||
SF-12 mental | ||||
Mean score (SD) | 47.5 (11.5) | 49.4 (11.8) | –1.8 (–5.9 to 2.3) | –1.5 (–5.5 to 2.5) |
Median score (IQR) | 48.9 (42.9–55.3) | 50.8 (42.7–59.1) | ||
Missing scores, n | 1 | 2 | ||
EQ-5D | ||||
Mean score (SD) | 68.3 (22.0) | 75.0 (17.4) | –6.7 (–13.3 to –0.1) | –6.4 (–13.1 to 0.3) |
Median score (IQR) | 72.0 (54.5–87.5) | 80.0 (64.0–90.0) | ||
Missing scores, n | 0 | 0 | ||
MMSE | ||||
Mean score (SD) | 26.9 (3.7) | 28.0 (2.3) | –1.1 (–2.2 to –0.1) | –1.5 (–2.6 to –0.4) |
Median score (IQR) | 28.0 (27.0–29.0) | 29.0 (27.0–30.0) | ||
Missing scores, n | 1 | 1 | ||
HADS anxiety | ||||
Mean score (SD) | 6.7 (4.8) | 5.7 (4.2) | 0.9 (–0.6 to 2.5) | 0.6 (–0.9 to 2.1) |
Median score (IQR) | 6.0 (3.0–10.0) | 6.0 (2.0–8.0) | ||
Missing scores, n | 0 | 0 | ||
HADS depression | ||||
Mean score (SD) | 5.8 (4.4) | 4.4 (3.5) | 1.4 (1 to 2.8) | 1.1 (–0.2 to 2.5) |
Median score (IQR) | 5.0 (2.0–9.0) | 4.0 (1.0–7.0) | ||
Missing scores, n | 0 | 0 | ||
PCL-C | ||||
Mean score (SD) | 32.0 (12.9) | 30.2 (11.0) | 1.8 (–2.4 to 5.9) | 1.6 (–2.6 to 5.7) |
Median score (IQR) | 28.0 (22.0–36.0) | 28.0 (20.0–38.0) | ||
Missing scores, n | 3 | 2 |
All of the follow-up results should be interpreted cautiously because of the low percentage of patients known to be alive who were included in the follow-up. This was caused by refusal of consent and non-response to the invitation to participate in the follow-up and led to high numbers of missing data, with a consequent risk of bias. It is possible that those who declined to take part and those who did not respond may have had worse outcomes than those who responded. Slightly fewer people were followed up in the LUCAS-2 group, which could have been as a result of worse outcomes in that group.
The results of the ‘quality of CPR study’ are shown in Table 14. Data recording problems in one ambulance service meant that only compression depth was analysable from this service, so data for both compression rate and compression fraction were available from only three services.
Ambulance service | Number of personnel | Depth (mm), mean (SD) | Rate (compressions/minute), mean (SD) | Compression fraction, mean (SD) |
---|---|---|---|---|
A | 155 | 49.4 (8.0) | 131.8 (15.1) | 65.5 (9.0) |
B | 157 | 45.0 (6.8) | 119.4 (10.4) | 65.4 (11.4) |
C | 73 | 41.0 (4.8) | 117.6 (13.5) | 65.9 (9.1) |
D | 103 | 48.6 (8.4) | a | a |
Compression depth was fairly consistent across services, with the mean varying between 41 and 49.4 mm. Mean compression rate was substantially faster in one service than the other two that had data, and exceeded the guideline recommended rate of 120 compressions per minute. Compression fraction was very consistent across the three services that had data, at between 65% and 66%.
Chapter 4 Mechanical chest compression for out-of-hospital cardiac arrest: systematic review and meta-analysis
Introduction
At the time that the PARAMEDIC trial was initiated, we were aware of two other planned or ongoing trials of mechanical chest compression devices70,71 and two that had been published. 32,72 These trials used either the LUCAS device (original version of the battery-powered LUCAS-2) or the AutoPulse (ZOLL Medical Corporation, Chelmsford, MA, USA), which uses a load-distributing band system. A wide band fits around the chest, and its circumference is alternately shortened and lengthened, providing rhythmic chest compressions.
The two other large RCTs have recently been reported. 71,73 It is therefore useful to summarise the overall evidence for mechanical chest compression devices in OHCA in a systematic review and, where appropriate, meta-analysis.
Methods
Studies were eligible for inclusion if they were individually randomised or cluster randomised trials that compared the use of a mechanical chest compression device with standard manual chest compression in adult patients following OHCA. There was no restriction of eligibility based on language of publication. Quasi-randomised trials, for example those whose participants were randomised by birth date or days of the week, were excluded. Studies were not included in analyses if they reported insufficient information to allow assessment of their risk of bias. Screening, decisions about inclusion and data extraction were performed by one author and checked by a second author. The review protocol was not preregistered or published.
We searched electronic resources (MEDLINE, EMBASE and the Cochrane Central Register of Controlled Trials from 1990 to February 2015) and the reference lists of studies and review articles (last search February 2015). We based our search strategies on that published by the Cochrane review of mechanical chest compression devices,74 which used a combination of search terms to describe the condition (cardiac arrest), the intervention (mechanical compression devices) and the study design (RCTs) (see Appendix 9).
For each eligible study, we extracted information about the study’s population and methodology, and the following outcomes: ROSC; survived event (sustained ROSC until handover to a hospital emergency department); survival to hospital discharge or 30 days; and survival with good neurological outcome. Good neurological outcome was defined as either a CPC score of 1 or 2 or a mRS score of between 0 and 3. 75 When studies presented a treatment effect estimate that was adjusted for important covariates (e.g. clustering, initial rhythm, bystander CPR, EMS response time, age), we used this estimate in meta-analyses in preference to unadjusted results.
We used the Cochrane risk-of-bias tool to assess studies’ risk of bias. This assesses seven domains: (1) generation of random allocation sequence; (2) allocation concealment; (3) blinding of participants and study personnel; (4) blinding of outcome assessment; (5) incomplete outcome data; (6) selective reporting; and (7) other sources of bias. For each study, we assessed the methods that were used to address each potential source of bias and summarised them in tabular form. We did not produce an overall bias risk judgement or score, but assessed each domain separately (Table 15).
Study (trial acronym or first author) and year of study | Unit of randomisation | Study setting | Recruitment period | Intervention | Number of participants | Sequence generation | Allocation concealment | Blinding; patients and clinicians | Blinding: outcome assessment | Percentage of participants with missing data for each outcome | Selective reporting | Other sources of bias |
---|---|---|---|---|---|---|---|---|---|---|---|---|
ASPIRE, 200672 | Cluster – crossover at predetermined intervals | USA/Canada | 2004–5 | AutoPulse | 767; 51 clusters (EMS stations or groups of stations) | No information | Not concealed; ambulance staff aware of intervention | Not blinded | Unclear | Survival: 0% Survival with a CPC score of 1 or 2: 0.7%
|
No evidence | Participants with missing CPC scores were 8.3% of survivors |
Smekal, 201132 | Patient | Sweden | 2005–7 | LUCAS | 148 | No information | Sealed randomisation letter carried with device, opened at time of randomisation | Not blinded | Unclear | Survival: 0.7% ROSC: 1.4% Survived event: 0.7% |
No evidence | |
LINC, 201470 | Patient | Sweden, UK and the Netherlands | 2008–12 | LUCAS/LUCAS-2 | 2589 | No information | Sealed, opaque envelopes carried in ambulance and opened at the time of randomisation | Not blinded | Unclear | Survival: 1.1% ROSC: 0.1% Survived event: 0% Survival with a CPC score of 1 or 2: 1.1% |
No evidence | |
CIRC, 201471 | Patient | Austria, the Netherlands and the USA | 2009–11 | AutoPulse | 4231 | No information | Sealed randomisation cards opened when indication for CPR was found | Not blinded | ‘Not always blinded’ | Survival: 0.3% ROSC: 0% Survival with a mRS score of 0–3: 2.8%
|
No evidence | Participants with missing mRS scores were 27.7% of survivors |
PARAMEDIC, 201573 | Cluster | UK | 2010–13 | LUCAS-2 | 4471; 418 clusters (vehicles) | Computer generated Stratified by station and vehicle type |
Not concealed; ambulance staff aware of intervention | Not blinded | Survival from routine data Neurological status assessment blinded |
Survival: 0% ROSC: 3.1% Survived event: 4.7% Survival with a CPC score of 1 or 2: 0.2% |
No evidence | Participants with missing CPC score were 2.5% of survivors |
We combined studies using Review Manager software version 5.3 (RevMan, The Cochrane Collaboration, The Nordic Cochrane Centre, Copenhagen, Denmark). As there may be differences in treatment effect between trials, especially those using different devices, we used a random-effects model. We used the generic inverse variance method in RevMan to estimate the average treatment effect (OR) for each outcome, and the uncertainty around it, measured by the 95% CI. We also calculated 95% prediction intervals76 to estimate the range of plausible treatment effects. We quantified heterogeneity in each analysis by the τ2-statistic and I2-statistic. Studies were subgrouped by the type of mechanical compression device that was used, as different devices operate in different ways and hence could have different treatment effects. Our primary analysis compared mechanical compression with manual compression, and we performed a subgroup analysis by type of device to explore whether or not there was any evidence that treatment effects differed between devices.
Some of the included trials presented several results using different adjustments for covariates and design elements. We performed sensitivity analyses to explore the effects of using differently adjusted results for these trials. In addition, the PARAMEDIC trial71 presented CACE estimates, to estimate the treatment effect in the presence of non-compliance. 75,76 We performed additional sensitivity analyses to explore the effects of using these estimates.
Results
The search located five eligible studies55,67,69–71 (Figure 8).
FIGURE 8.
Flow chart of studies.
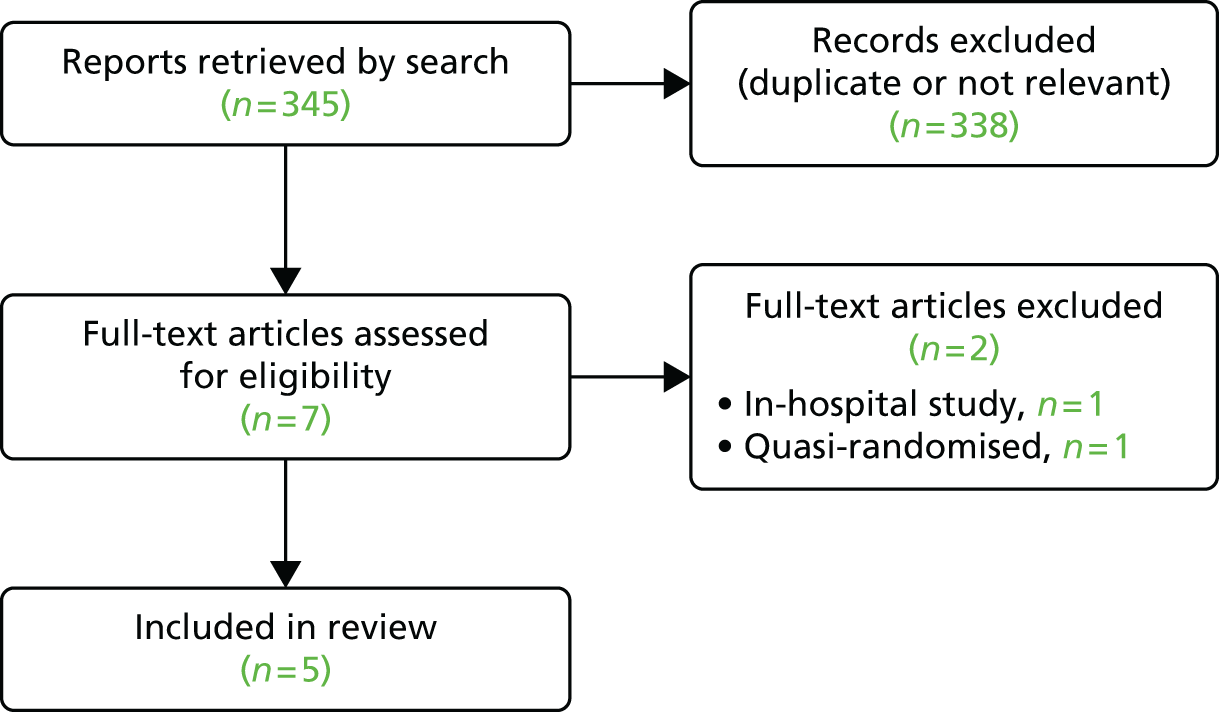
Two trials55,69 evaluated the AutoPulse device and three trials67,70,71 evaluated the LUCAS device. Two of the studies55,71 used a cluster randomised design: one study (PARAMEDIC)71 randomising by ambulance service vehicles and the other study [AutoPulse Assisted Prehospital International Resuscitation (ASPIRE)]55 using ambulance stations or groups of stations as the clusters; this study55 also incorporated crossovers at prespecified points between the intervention and control groups. The other three studies67,69,70 utilised individual randomisation, using sealed envelopes or cards carried with the device, which were accessed by the paramedic at the time of the resuscitation attempt.
There were a number of differences between the studies in addition to the chest compression device used, which may have caused differences in treatment effects and hence introduced heterogeneity into the meta-analyses. In two studies,67,70 the LUCAS-2 device was used as part of a modified treatment algorithm, whereas in the third LUCAS-2 study,71 mechanical chest compression was simply used to replace manual compression in the standard algorithm. One of the trials of AutoPulse conducted extensive training to optimise the quality of manual CPR that was provided to the control group;77 in contrast, other trials did not provide extra training, but the control group received CPR as it would be provided in standard clinical practice.
The randomisation methods of the studies appeared to be adequate, although four studies did not provide any information on the generation of the random allocation sequence. 55,67,69,70 One concern with individual randomisation was that it would be possible for ambulance staff to open randomisation envelopes early and subvert the randomisation scheme. No studies reported any problems with individual randomisation procedures, such as missing randomisation cards that could not be accounted for (which might indicate that crews had selected the intervention) or large numbers of eligible patients who were not recruited (which might suggest that the crew felt that the randomised allocation would not be good for the patient).
Blinding of clinicians providing care was clearly not possible and participants who survived may also have been aware of which allocation they had received. For example, use of the LUCAS-2 device may leave characteristic marks on the patient’s chest. The assessment of survival outcomes was unlikely to have been affected by whether or not the people assessing the outcome were blinded. One study71 stated that personnel assessing neurological status (via the CPC or mRS) were blinded; in other studies, this was unclear. It is conceivable that knowledge of treatment allocations could influence assessments of neurological status; if assessors had strong views on the effectiveness of the intervention being tested, they may have adjusted their threshold for allocating a patient to a mRS or CPC category. We cannot exclude this potential bias in studies for which outcome assessment was not blinded.
In all of the trials, the proportion of missing outcome data was low, when measured as a percentage of all of the study participants. However, in some trials, there was potentially bias because of missing data in the assessment of neurologically intact survival. This was because the missing data were concentrated among survivors; for example, in the Circulation Improving Resuscitation Care (CIRC) trial,71 although only 2.8% of participants had missing mRS data, they represented 27.7% of survivors. The populations included varied between trials. In the ASPIRE study,55 results were presented for a prespecified ‘primary’ population (patients who were in cardiac arrest at the time of EMS arrival and whose cardiac arrest was considered to be of cardiac origin). Patients who fulfilled exclusion criteria were treated according to trial allocation, but subsequently excluded (in order not to introduce delays to treatment); however, 304 ‘non-primary’ cases were also excluded from the main results. In the CIRC trial,71 there were also 522 post-randomisation exclusions of patients fulfilling exclusion criteria. However, this trial69 also excluded patients recruited in a prespecified run-in phase, an unspecified number of patients recruited early in the trial (after the run-in period) when compliance with AutoPulse was found to be poor as a result of battery issues and data from one site for a 3-month period when that site was non-compliant with the study protocol (number not stated).
The CIRC69 trial used a group sequential design, with predefined stopping boundaries for superiority, inferiority and equivalence (double triangular test). 77,78 The trial report presented treatment effect estimates that were adjusted for clinical covariates for all outcomes, but additionally adjusted the primary outcome (survival to hospital discharge) for the sequence of interim analyses. In this review we have used the results adjusted for covariates, but not for the interim analyses, because these are consistent and based on the data rather than the decision-making process. We explored the effect of the adjustment of the primary outcome for interim analyses with a sensitivity analysis.
The meta-analyses (see Figures 9–12) do not suggest an advantage to mechanical chest compression, using either device, for any of the outcomes. CIs and prediction intervals were wide, reflecting the low incidence of favourable outcomes after OHCA and consequent imprecision of treatment effect estimates.
For ROSC (Figure 9), although there was no evidence of an overall difference between mechanical and manual chest compression (average OR 0.96, 95% CI 0.85 to 1.10, 95% prediction interval 0.66 to 1.41), there was some evidence that the effects of the LUCAS-2 and AutoPulse devices were different (I2 = 78.5% for subgroup differences). There were data from only one AutoPulse trial,69 but that suggested a lower proportion achieving ROSC in the mechanical chest compression group.
FIGURE 9.
Return of spontaneous circulation. IV, inverse variance; SE, standard error.

Survival of event was reported only by trials that used the LUCAS-2 device (Figure 10). The results were consistent across trials and suggested no advantage to mechanical chest compression devices (OR 0.95, 95% CI 0.85 to1.07; 95% prediction interval 0.45 to 2.00).
FIGURE 10.
Survived event (i.e. sustained ROSC to handover to hospital emergency department). IV, inverse variance.

The analysis of survival to discharge or 30 days (Figure 11) again suggested no advantage to mechanical chest compression (OR 0.89, 95% CI 0.77 to 1.02; 95% prediction interval 0.71 to 1.12). The point estimate was in the direction of favouring manual chest compression and the upper 95% confidence limit was only just > 1. There was no evidence of heterogeneity of treatment effects. A sensitivity analysis using the estimate for the CIRC trial,71 adjusted for interim analyses as well as covariates, did not make a major difference to the overall average treatment effect (OR 0.94, 95% CI 0.79 to 1.11; 95% prediction interval 0.62 to 1.43). Similarly, sensitivity analyses using the CACE estimates for the PARAMEDIC trial71 did not make a substantial difference to the overall result.
FIGURE 11.
Survival to discharge from hospital or 30 days. IV, inverse variance.

Results for survival with good neurological outcome (Figure 12) were more heterogeneous than for other outcomes (I2 = 68%). This was not due to differences between the LUCAS-2 and AutoPulse devices, which were small (I2 = 11% for subgroup differences), but to inconsistency between the results of the two trials of each device. Reasons for the inconsistency were unclear. Overall, there was no evidence that the average treatment effect favoured mechanical chest compression, but the 95% prediction interval was very wide (OR 0.76, 95% CI 0.53 to 1.11; 95% prediction interval 0.17 to 3.49).
FIGURE 12.
Survival with CPC score of 1–2 or mRS score of 0–3. IV, inverse variance.
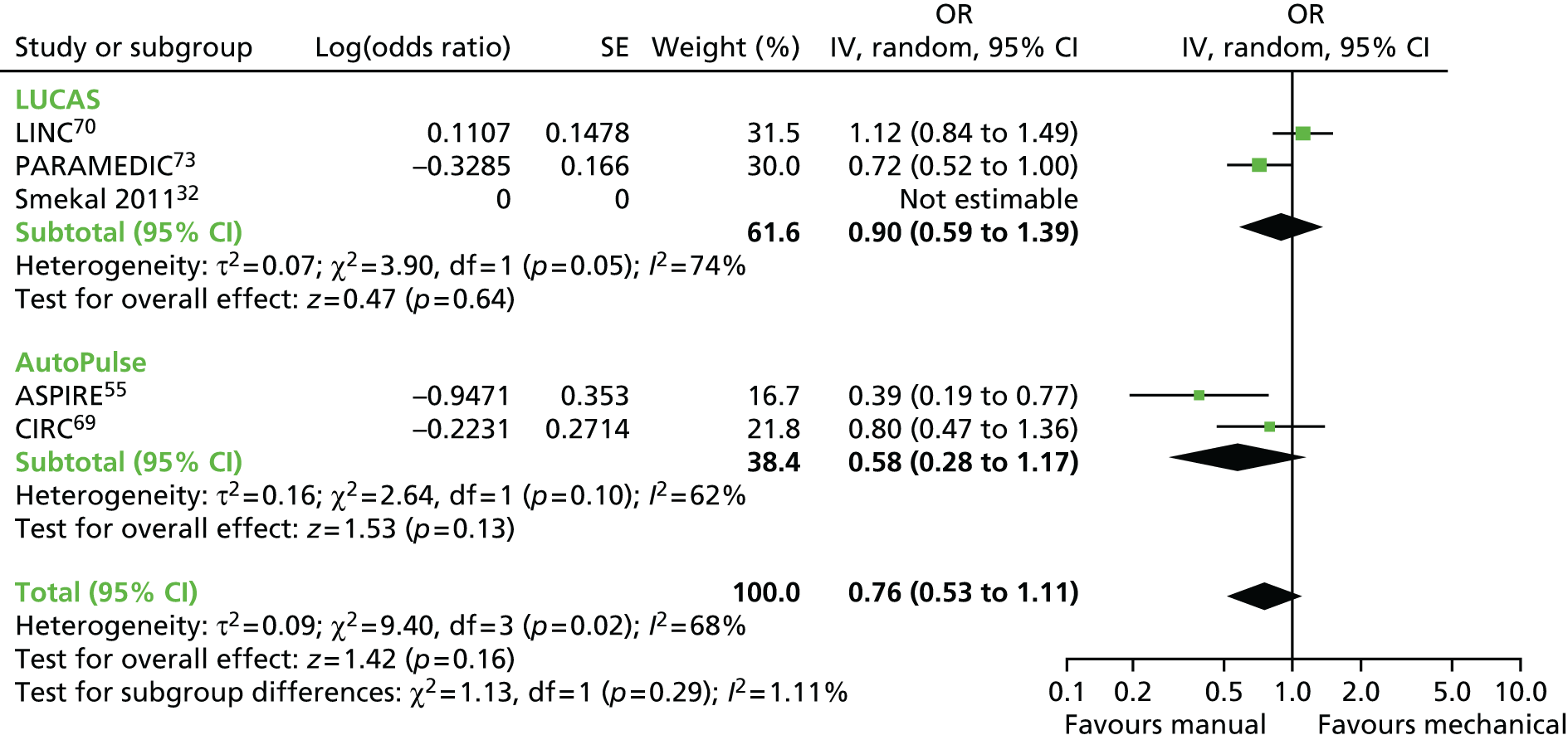
Discussion
The trials recruited unselected populations of patients, which were typical of clinical practice in the geographical areas in which they were conducted. Despite the large size of many of the trials included in the review, CIs around the combined treatment effect estimates were relatively wide because of the low survival rate from OHCA. The methodological quality of the included studies was generally good. Secure methods of randomisation were used and for most outcomes there were few missing data. Trials using a cluster randomised design were unable to conceal allocations in advance of assignment and ambulance crews would have been aware of the allocation. This could have led to inclusion bias, in two ways. First, patients might not be reported to the trial if it was felt that they were not receiving the best allocation. The PARAMEDIC trial71 guarded against this by including all eligible cardiac arrests that were attended by trial vehicles. It was not clear whether or not this was also the case in the ASPIRE study. 55 Second, the threshold for initiating a resuscitation attempt could have varied according to the intervention. For example, if a crew believed strongly that mechanical chest compression was better, they might initiate a resuscitation attempt in a situation in which they would not attempt resuscitation if manual chest compression was to be used. In the PARAMEDIC trial,71 the DMC reviewed evidence for differential thresholds for resuscitation but did not find evidence of any appreciable selection bias.
The use of the double triangular test design in the CIRC69 trial raises a number of issues. The adjustment of the final analysis to allow for the interim analyses had a large effect on the primary outcome (survival to hospital discharge), changing the point estimate of the OR from 0.89 to 1.06. The secondary analyses were not adjusted for the interim analyses, so the results for the primary and secondary outcomes were not directly comparable. In addition, the boundaries for equivalence in the double triangular test were very generous; if the ‘equivalence’ boundary was crossed, the 95% CI would be contained between log odds of –0.37 and 0.37 (i.e. an OR of 0.69 and 1.45). 69 This interval includes values that would represent substantial benefit and substantial harm, so the conclusion of ‘equivalence’ in this situation is questionable.
In some trials, data for neurologically intact survival were missing for a high proportion of survivors. This was most severe in the CIRC trial,71 in which data for this outcome were lacking for 27.7% of survivors. This reflects the difficulty of performing follow-up assessments on cardiac arrest survivors, but clearly has the potential to introduce bias. It is possible, or even likely, that there could be an association between missingness and neurological outcome. There are many plausible reasons why patients with poor outcomes may be more likely to be lost; for example, they may be harder to contact because they have moved to a residential care facility or they may be less willing or able to undertake follow-up assessments.
Chapter 5 Economic evaluation
Introduction
The economic evaluation was conducted to assess the cost-effectiveness of use of the LUCAS-2 device compared with manual chest compression (manual CPR) during resuscitation by ambulance staff after OHCA. The economic evaluation consisted of two distinct, but complementary, sets of analyses: a within-trial analysis over the 12-month trial period and a decision-analytic model that was constructed to extrapolate the results over the expected lifetime of the trial participants. The analyses were conducted from the NHS and Personal Social Services (PSS) perspective and they report cost per incremental quality-adjusted life-year (QALY) of the LUCAS-2 device compared with usual care (manual CPR). The analyses were conducted in line with best practice guidelines. 79
Methods
Within-trial analysis
The within-trial analysis aimed to determine the cost-effectiveness of the LUCAS-2 device compared with manual chest compression over the period of the trial (i.e. from cardiac arrest to 12 months’ follow-up). The analysis used QALYs as the main outcome and adopted the perspective of the NHS and PSS. Utility values were derived from patient questionnaires; resource use was obtained from a variety of sources, including the trial CRFs, large data sets (i.e. HES, ICNARC) and self-completed patient questionnaires. Neither costs nor QALYs were discounted given the 12-month time period. The results are reported as incremental cost-effectiveness ratios (ICERs).
Quality-adjusted life-years
Quality-adjusted life-years reflect both duration and quality of life and their estimation requires the production of utility weights for each health state observed in the trial population. HRQL was assessed using the EQ-5D,42 which has been validated for use in the critical care patient group. 80 Surviving patients completed the EQ-5D at 3 and 12 months post cardiac arrest. The EQ-5D responses were converted to health-state utility values using the UK tariff. 81 Utility values were combined with survival information to calculate QALYs for the trial period using an area under the curve (AUC) approach. As patients were unable to complete the measure at baseline, estimates had to be made of their baseline utility level. Following strategies previously used in studies that have dealt with this scenario,82–84 we assumed that patients who experienced a cardiac arrest had a baseline utility value of ‘0’ (which is equivalent to dead). We then assumed a linear transition from ‘0’ to the 3-month utility value and, similarly, from the 3- to the 12-month utility value. A utility weight of zero was assigned to patients who died within 3 months, which may underestimate total QALYs. We explored an alternative assumption in the sensitivity analysis, for which the survival days of these patients were assigned the average 3-month utility estimated in our sample.
Resource use and costs
The costs considered in this analysis included intervention costs (i.e. cost of the LUCAS-2 device and ambulance costs), costs of hospital inpatient stays, accident and emergency (A&E) admissions and outpatient visits and the use of primary care and community-based health and social care services (such as GP and social worker visits).
Resource use data were collected prospectively and retrospectively. Hospital resource utilisation was obtained through linkage with the HES data set. We extracted data from the HES for study participants from cardiac arrest to 12 months after randomisation. The data set records information on the total number of days in hospital, the number of in-person and telephone outpatient visits and the number of A&E admissions. To identify the number of hospital days spent in the ICU (Table 16) we used information from the ICNARC data set. Patients who stayed for < 24 hours in hospital were also identified. Some of these were regular admissions and, based on the profile of their procedural use, were assigned the cost of 1 day in ICU. Another group of patients who stayed for < 24 hours in hospital were recorded in HES as day case or regular day admissions. Hospital costs were obtained by multiplying the number of days or visits of each service by the corresponding unit cost derived from NHS reference costs databases. 85
Patient group | n | Missing | ICU length of stay (days) mean (SD) | Source |
---|---|---|---|---|
Survived 1–30 days | 588 | – | 3.15 (3.8) | ICNARC data and assumptiona |
Survived > 30 days | 211 | 87 | 7.10 (17.6) | ICNARC data |
Following hospital discharge, health-care resource use questionnaires were completed by surviving patients at 3 and 12 months post cardiac arrest. Patients were asked about their use of health and social services during the previous 3 months, including further inpatient and outpatient care and primary and community-based health and social services. For the 6-month period during which non-hospital resource use data were not collected (between 3 and 9 months post cardiac arrest), we used the average resource utilisation between the initial (0–3 months) and final (9–12 months) period. Patients who died within 3 months were assumed to have incurred no community-based health and social care costs. Health-care resource use was multiplied by the relevant unit costs extracted from the national reference costs (Table 17). 86
Resource item | Unit cost (£) | Source | Details/assumptions |
---|---|---|---|
LUCAS device cost per application | 232 | Own calculations: see Table 18 | A lifespan of 8 years was assumed |
Ambulance cost | 180 | NHS Reference Costs 2013–14 85 | ‘See and treat’ (if died on scene) |
231 | NHS Reference Costs 2013–14 85 | ‘See and treat and convey’ (if did not die on scene) | |
Hospital-based or residential care services | |||
ICU per day | 1382 | NHS Reference Costs 2013–14 85 | Non-specific general adult critical care patients predominate Average cost of 0–6 or more organs supported: SC, CCU01; CC, XC01Z-XC07Z |
Hospital inpatient stay per day | 275 | NHS Reference Costs 2013–14 85 | Non-elective inpatients; excess bed-days |
Hospital outpatient clinic appointment | 128 | NHS Reference Costs 2013–14 85 | Outpatient/consultant led |
Hospital A&E visit | 339 | NHS Reference Costs 2013–14 85 | Emergency medicine, category 3 investigation with category 4 treatment: SC, T01A; CC, VB02ZZ |
Nursing/residential home per day | 157 | Curtis, 201286 | Local authority residential care for older people Establishment cost per permanent resident week/7 days |
Primary care and community-based health and social services | |||
GP: surgery visit | 46 | Curtis, 201286 | Per-patient contact lasting 11.7 minutes, including direct care staff costs |
GP: home visit | 92 | Curtis, 201286 | (Per-patient contact lasting 11.7 minutes plus 12 minutes of travel time) × £3.90/minute cost of patient contact |
District nurse/health visitor visit | 45 | NHS Reference Costs 2013–14 85 | Average of district nurse, face to face: SC, NURS; CC, N02AF Average of health visitor, face to face: SC, HVM; CC, N03G |
Social worker visit | 79 | Curtis, 201286 | One-hour appointment |
Counsellor appointment | 50 | Curtis, 201286 | One-hour appointment |
Home help session | 24 | Curtis, 201286 | One-hour weekday session |
Speech and language therapist appointment | 84 | NHS Reference Costs 2013–14 85 | Speech and language therapist, adult, one to one: CC, A13A1 |
Psychologist appointment | 85 | NHS Reference Costs 2013–14 85 | Non-admitted non-face to face attendance, follow-up: SC, 656; CC, WF01C |
Day centre visit | 42 | Curtis, 201286 | Local authority day care for older people; per client session lasting 3.5 hours |
Lunch or social club session | 7 | Curtis, 201286 | Same cost as 1 hour of befriending older adults programme |
Meals on Wheels | 7 | Curtis, 201286 | Assuming one contact = one meal. Average cost of per ‘meal on wheels’ for the local authority |
Family Support session | 50 | Curtis, 201286 | Family support worker; per hour of client-related work |
A microcosting study was undertaken to establish the cost of the LUCAS-2 device and determine the relevant cost per application. This included (1) the cost of purchasing the device and accessories; (2) the cost of fitting the device to the ambulance; (3) maintenance costs; and (4) initial and ongoing staff training costs. The frequency of use observed in the trial was used to estimate the expected number of applications in order to calculate the expected cost per application (Table 18).
Cost item | Assumptions | Cost (£) for the trial period |
---|---|---|
Purchase cost (LUCAS-2 and accessories) | A one-off purchase cost, the LUCAS-2 device and necessary accessories were calculated using the purchase cost for the device itself, suction cups, battery, 12-V car cable and power supply for inside the ambulance. The cost of each these items was multiplied by the number of ambulances in the intervention trial arm. Battery chargers and a spare battery at each of the 90 stations with the LUCAS-2 device were costed. For spare LUCAS-2 parts it was assumed that one set of each spare would be required per 10 devices. Spare parts included a carry bag, stabilisation strap and patient straps | 148,504 |
Cost of fitting LUCAS-2 to vehicles | The total cost of fitting the device to ambulance vehicles required the cost of screws, chair strap, clips and net. One hour of labour was estimated to fit the strap per ambulance. The cost of fitting the device to one ambulance was then multiplied by the number of vehicles in the intervention arm | 783 |
Maintenance (assuming no repairs) | The planned preventative maintenance service was estimated to cost £250 for each LUCAS-2 device. This cost assumed no parts were needed and no repairs occurred | 35,750 |
Staff training (initial and ongoing) | Initial staff training: it was estimated that each regional ambulance trust had a mandatory training programme that paid paramedics 3 hours of overtime to attend. The per-paramedic cost was multiplied by the number of staff at each site who had been trained Ongoing staff training: one regional ambulance site reported a 30-minute training refresher for paramedics. It was assumed that paramedics in all sites would receive a similar 30-minute refresher course once per year. The cost per paramedic was multiplied by the total number of staff trained within the initial staff training |
46,450 |
Total costs | 231,488 | |
Number of applications | 996 | |
Cost per application | 232 |
Missing data
The primary analysis used MI to handle missing data using baseline characteristics (sex and age) to impute missing follow-up HRQL and resource use information. Unlike more simple imputation approaches, MI reflects both the structural uncertainty related to the parameters of the imputation model and the uncertainty arising from missing data. Practically, to obtain total costs and QALYs at 1 year for each patient, missing data on HRQL and resource use were addressed using chained equations. We used truncated models to reflect the specific distribution of HRQL and resource use and generated 10 data sets. Estimates from each imputed data set were combined following Rubin’s rule. 87 In the sensitivity analysis, we also report results from the complete-case analysis, which included only patients with non-missing utility values.
Cost-effectiveness analysis
The main cost-effectiveness outcome is the 1-year cost per QALY. ICERs were calculated where one intervention was more expensive and more effective or less effective and cheaper than the other. 88 The ICER is calculated by dividing the difference in mean cost between the two arms by the difference in mean QALYs between the two arms:
where Ci and Ei are the cost and effectiveness of the LUCAS-2 device and Cc and Ec are the cost and effectiveness of manual compression, and ΔC and ΔE are the incremental cost and effect of the intervention compared with the comparator. Thus, the ICER represents the cost per QALY gained. ICERs below the NICE willingness-to-pay threshold (λ) of £20,000 are considered to indicate cost-effectiveness. For the main analyses, as effects were observed within 12 months, no discounting of costs or effects was required. ITT analyses were conducted throughout.
Uncertainty and sensitivity analysis
Uncertainty was explored by conducting non-parametric bootstrapping via 1000 resampled analyses. Cost-effectiveness planes (scatterplots of the 1000 bootstrap replications) were created. 89,90 Sensitivity analyses were conducted to determine the impact of assumptions on the cost-effectiveness results. We compared results from complete-case analysis against MI and also carried out analyses using an average group cost for outliers with high costs. Owing to the large number of non-compliers, results of a per-protocol analysis were also reported. We also derived net monetary benefits (NMBs) for each patient using the following:
We then estimated linear regression models to identify predictors of NMB, including treatment arm.
Long-term decision-analytic model
To assess cost-effectiveness over the lifetime horizon, a decision tree combined with a Markov model was constructed91 (Figure 13). The model starts with a decision tree reflecting patients’ risk of death at different time points and patient CPC score at the end of the trial. The end points of the decision tree are the starting point of the lifetime Markov model. We chose to model the intervention impact from baseline application rather than simply extending outcomes and costs from 12 months onwards. The main motivation for this was to enable better capture of uncertainty during the trial period and allow propagation of this through the lifetime horizon. Beyond 1 year post cardiac arrest, costs, HRQL and survival were modelled in two subsets of patients: patients with (1) good neurological outcomes at 1 year (CPC score of 1 or 2) and (2) poor neurological outcomes at 1 year (CPC score of > 2). Relevant model parameters were extracted from the trial data and from the literature (Table 19). The parameters of interest included relative survival rates in these subgroups that were applied to UK reference mortality rates published by the Office for National Statistics (ONS) and annual cost and HRQL data for cardiac arrest survivors with/without good neurological outcomes (from trial data). Annual costs for patients with poor neurological outcomes were obtained from trial data (outpatient visits and community-based health and social care) and residential care costs were added, as these patients are likely to require daily support/institutionalisation. 86
FIGURE 13.
Structure of the decision-analytic model.

Parameters | Group/arm | Mean value | Distribution | Source |
---|---|---|---|---|
Decision tree | ||||
p (die on scene) | Manual CPR | 0.3274 | Beta | Trial data |
LUCAS-2 | 0.3290 | |||
p (die within 3 months | did not die on scene) | Manual CPR | 0.9029 | Beta | Trial data |
LUCAS-2 | 0.9134 | |||
p (die within 3–12 months | survived to 3 months) | Manual CPR | 0.0380 | Beta | Trial data |
LUCAS-2 | 0.0729 | |||
p (good neurological outcome | survive to 1 year) | Manual CPR | 0.8605 | Beta | Trial data |
LUCAS-2 | 0.7442 | |||
Markov model | ||||
Annual mortality rate | Fixed | ONS92 | ||
Excess mortality for patients with poor neurological outcomes | 1.67 | Log-normal | Phelps 201393 | |
Discount rate | 3.5% | Fixed | ||
Annual cost (£) | Good neurological outcome (CPC score of 1 or 2) | 3315 | Log-normal | Trial data |
Poor neurological outcome (CPC score of 3 or 4) | 43146 | Log-normal | Trial data and Curtis, 201286 | |
Utility | Good neurological outcome (CPC score of 1 or 2) | 0.75 | Beta | Trial data |
Poor neurological outcome (CPC score of 3 or 4) | 0.47 | Beta | Trial data |
A discount rate of 3.5% was applied to costs and effects in the Markov model (see Figure 13). The within-trial analysis was conducted using the statistical software Stata version 13.1 (StataCorp LP, College Station, TX, USA) and the decision-analytic model was built using Microsoft Excel® (Microsoft Corporation, Redmond, WA, USA).
Cost-effectiveness analysis
The ICER is calculated by dividing the difference in mean cost between the two arms by the difference in mean QALYs between the two arms:
where Ci and Ei are the expected cost and effectiveness of the LUCAS-2 device and are the expected cost and effectiveness of manual compression, and ΔC and ΔE are the incremental cost and effect of the intervention compared with the comparator. Thus, the ICER represents the cost per QALY gained. ICERs that are below the NICE willingness-to-pay threshold (λ) of £20,000 are considered to indicate cost-effectiveness. A discount rate of 3.5% was used to discount costs and benefits.
Uncertainty and sensitivity analysis
We first performed several one-way sensitivity analyses by adding and subtracting 20% of the main parameters of the model (i.e. costs, QALY and 1-year mortality) and assessed the subsequent impact on the ICERs. The value of 20% is arbitrary, but was considered likely to represent any uncertainty that might exist in parameter values. A probabilistic sensitivity analysis was then conducted for a more comprehensive account of uncertainty in the model parameters. Cost-effectiveness acceptability curves (CEACs) that show the probability of cost-effectiveness across a range of values for λ were created using the net benefit approach. 94 With this transformation, we avoid problems encountered with ICERs (same sign but opposite quadrants) and CEACs are much simpler to calculate. 95
Results
Among the 4771 patients who were enrolled in the study, 1652 were assigned to the intervention arm (LUCAS-2) and 2819 were assigned to the control group (manual CPR). During the trial, 985 (60%) patients in the intervention group received LUCAS-2 device treatment and 11 (< 1%) patients in the control group received mechanical CPR. 73 At 3 months, 96 (6%) patients survived in the LUCAS-2 group and 182 (6%) survived in the control group. At 12 months, 89 (5%) patients survived in the LUCAS-2 group and 177 (6%) survived in the manual CPR group (Table 20).
Follow-up | Treatment arm, n (%) | |
---|---|---|
Manual CPR | LUCAS-2 | |
3 months | ||
EQ-5D | 99 (54) | 47 (49) |
Resource use | 99 (54) | 46 (48) |
12 months | ||
EQ-5D | 95 (54) | 48 (54) |
Resource use | 93 (53) | 46 (52) |
One-year costs
Using the complete-case analysis, the average cost at 1 year in the LUCAS-2 group was higher than in the manual CPR group, with an incremental cost of £106.70, with hospital costs being the main cost driver (Table 21). Overall, the average cost in each category of costs is higher in the LUCAS-2 group than in the manual CPR group.
Arm | N | n missing | Mean (£) | Bootstrap 95% CI (£) | Minimum (£) | Maximum (£) |
---|---|---|---|---|---|---|
Complete-case data | ||||||
Costs to the NHS over 1 year | ||||||
Manual CPR | 2690 | 129 | 1294.00 | 1152.40 to 1435.70 | 0 | 41,945 |
LUCAS-2 | 1577 | 75 | 1400.70 | 1131.20 to 1670.30 | 0 | 123,660 |
ICU costs | ||||||
Manual CPR | 2762 | 57 | 959.20 | 826.80 to 1091.60 | 0 | 59,426 |
LUCAS-2 | 1622 | 30 | 1221.80 | 766.20 to 1677.50 | 0 | 317,860 |
Other hospital costs (A&E, outpatient, general ward) | ||||||
Manual CPR | 2772 | 47 | 521.50 | 428.60 to 614.30 | 0 | 75,767 |
LUCAS-2 | 1619 | 33 | 585.00 | 386.00 to 784.00 | 0 | 74,276 |
Hospital costs | ||||||
Manual CPR | 2732 | 87 | 1318.00 | 1136.30 to 1499.80 | 0 | 101,928 |
LUCAS-2 | 1599 | 53 | 1540.30 | 1083.40 to 1997.20 | 0 | 318,327 |
Community-based health and social care costs | ||||||
Manual CPR | 2716 | 103 | 31.90 | 23.10 to 40.60 | 0 | 8834 |
LUCAS-2 | 1593 | 59 | 91.10 | 21.20 to 160.90 | 0 | 50,138 |
Imputed data | ||||||
Costs to the NHS over 1 year | ||||||
Manual CPR | 2819 | – | 2021.30 | 1772.30 to 2270.20 | 0 | 41,945 |
LUCAS-2 | 1652 | – | 2831.00 | 2149.60 to 3512.30 | 0 | 123,660 |
ICU costs | ||||||
Manual CPR | 2819 | – | 1102.10 | 947.00 to 1257.20 | 0 | 59,426 |
LUCAS-2 | 1652 | – | 1447.30 | 883.40 to 2011.10 | 0 | 317,860 |
Other hospital costs (A&E, outpatients, general ward) | ||||||
Manual CPR | 2819 | – | 604.00 | 478.80 to 729.10 | 0 | 75,767 |
LUCAS-2 | 1652 | – | 724.60 | 507.50 to 941.70 | 0 | 74,276 |
Hospital costs | ||||||
Manual CPR | 2819 | – | 1706.10 | 1477.50 to 1934.60 | 0 | 101,928 |
LUCAS-2 | 1652 | – | 2171.80 | 1525.60 to 2818.00 | 0 | 318,327 |
Community-based health and social care costs | ||||||
Manual CPR | 2819 | – | 108.80 | 37.90 to 179.60 | 0 | 8834 |
LUCAS-2 | 1652 | – | 287.40 | 111.80 to 463.00 | 0 | 50,138 |
Total costs to the NHS divided by the number of 1-year survivors | ||||||
Manual CPR | 177 | – | 32,192 | 28,228 to 36,156 | ||
LUCAS-2 | 89 | – | 52,548 | 39,908 to 65,188 |
We also observed higher costs in the LUCAS-2 arm than in the manual CPR arm in all cost categories in analyses that followed MI. Using the imputed data sets, we computed the total cost incurred in each patient group (the sum of all costs across all patients) that we divided by the number of 1-year survivors in each group (i.e. 177 patients in the manual CPR arm and 89 patients in the LUCAS-2 arm). We obtained a costs per 1-year survivor of £32,192 in the manual CPR arm and of £52,548 in the LUCAS-2 arm.
Quality of life
The mean utility scores in each group were measured at 3 and 12 months (Table 22). At both 3 and 12 months, HRQL was higher in the manual CPR group than in the LUCAS-2 group. An independent-sample t-test indicated that these differences were statistically significant. Changes in HRQL between the 3- and 12-month assessments were not statistically significant. Table 22 also reports the average QALY over 1 year accrued by all patients in both groups based on the AUC calculations. The mean 1-year QALY is small as a result of the high 1-year mortality rate in the sample (> 95%). We observe a small difference in mean QALY over 1 year (0.007), with patients in the manual CPR group having a higher average QALY than patients in the LUCAS-2 group. HRQL of survivors at 12 months was also estimated by neurological outcome status (as measured via the CPC score). We found a significant difference in HRQL between patients with good neurological outcome (CPC score of 1 or 2; mean 0.75) and patients with poor neurological outcome (CPC score of 3 or 4; mean: 0.47).
Outcome | Treatment arm | |||||
---|---|---|---|---|---|---|
Manual CPR | LUCAS-2 | |||||
Number of participants with data | Mean | 95% CI | Number of participants with data | Mean | 95% CI | |
Utility score among survivors | ||||||
3 months | 99 | 0.780 | 0.732 to 0.828 | 47 | 0.647 | 0.555 to 0.738 |
12 months | 95 | 0.761 | 0.712 to 0.810 | 48 | 0.639 | 0.542 to 0.736 |
QALY over 12 months | ||||||
Complete case | 2741 | 0.026 | 0.021 to 0.031 | 1609 | 0.018 | 0.013 to 0.024 |
Imputed | 2818 | 0.042 | 0.036 to 0.048 | 1652 | 0.033 | 0.026 to 0.040 |
Cost-effectiveness at 1 year
Table 23 presents the cost-effectiveness results, showing the incremental costs and QALY for each arm of the trial, as well as the corresponding ICER. Results are shown for both the ITT and per-protocol analyses and, in each case, complete-case and MI results are presented. In addition, to obtain an approximation of the CACE, which was conducted for clinical outcomes, we inflate the ITT results using the proportion of compliers in the sample (i.e. 60%).
Analysis | Number of participants | Incremental | ICER | |
---|---|---|---|---|
Cost (£) | QALY | |||
MI (ITT) | 4771 | 809.60 | –0.0093 | Manual CPR dominates |
Complete case (ITT) | 4267 | 106.70 | –0.0072 | |
Complete case (ITT, average group cost for outliers) | 4267 | 39.20 | –0.0067 | |
MI (per protocol) | 3793 | 495.90 | –0.0142 | |
Complete case (per protocol) | 3391 | 296.40 | –0.0070 | |
Alternative QALY calculationa | 4771 | 809.60 | –0.0091 | |
QALY derived with SF-12 (complete case) | 4267 | 106.70 | –0.0046 | |
CACE (complete case) | 4267 | 177.80 | –0.012 |
At 1 year, we found an incremental QALY of –0.0072 and an incremental cost of £106.70, which indicates that the LUCAS-2 device is dominated by manual chest compression, that is, the LUCAS-2 device is more costly and less effective than manual chest compression. When a per-protocol analysis was conducted instead, manual compression still dominated and results from the MI led to the same conclusion. The conclusions remain unchanged when QALYs were derived using SF-12 instead of EQ-5D. Overall, the results suggest that manual chest compression dominated the LUCAS-2 device, with the LUCAS-2 device having higher costs and providing lower QALY benefits than manual CPR. Interpretation, however, should be tempered by the very small between-group differences observed in QALYs and the relatively small differences in costs.
In Figures 14 and 15, we present the results of the 1000 bootstrap replications in the cost-effectiveness plane for both the complete-case analysis and the analysis based on MI. In both cases, the 1000 estimates are spread mainly in the north-west quadrant of the cost-effectiveness plane, meaning that the LUCAS-2 device is more costly and less effective than manual chest compression; however, it is worth noting that QALY losses are minimal. In other words, these results confirm the finding that the LUCAS-2 device is dominated by manual CPR. None of the iterations is below conventional values of the threshold (£20,000 per QALY). It is worth noting that the iterations in the MI analysis are more concentrated in the north-west quadrant (i.e. only a small number of iterations correspond to a decrease in costs). This suggests that the complete-case analysis may underestimate the incremental costs of the LUCAS-2 device. A possible explanation is that data of more costly (e.g. older) patients are more likely to be missing.
FIGURE 14.
Cost-effectiveness plane for the LUCAS-2 device compared with manual chest compression. Complete-case analysis based on 1000 bootstrap replications.
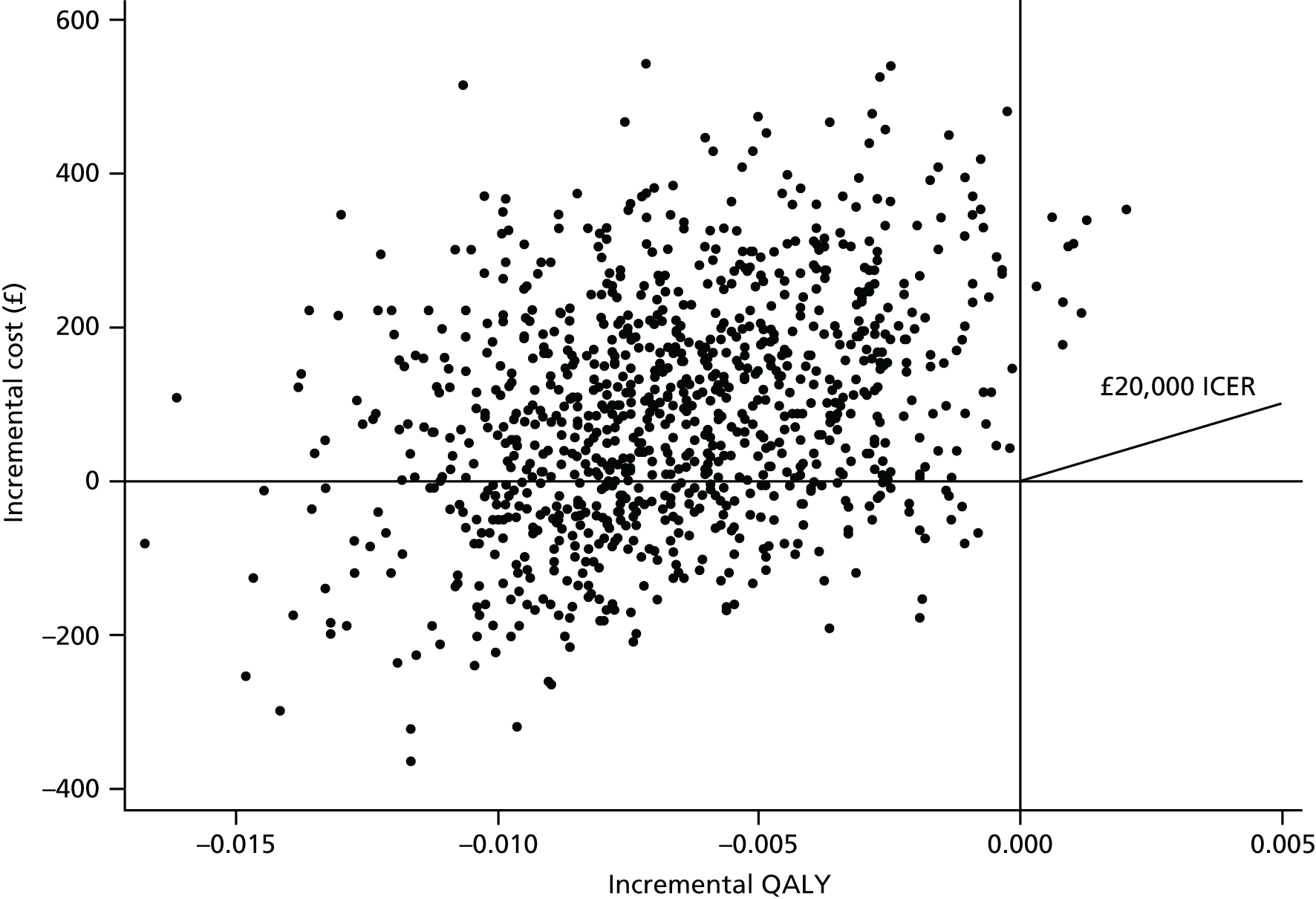
FIGURE 15.
Cost-effectiveness plane for the LUCAS-2 device compared with manual chest compression. MI analysis based on 1000 bootstrap replications.

Net monetary benefits
Linear regression models using age, sex and treatment allocation as covariates and independent variables were run to predict NMBs. Treatment allocation was found to be a significant predictor of NMB; NMB was significantly smaller (more negative) in the LUCAS-2 group.
Long-term cost-effectiveness (Markov model)
The cost-effectiveness estimates were extrapolated over a lifetime time horizon using the Markov model. The lifetime cost-effectiveness results obtained with the model are presented in Table 24. The base-case analysis is based on a cohort of patients aged 60 years, followed over 40 years, which corresponds to the average age of patients who survived at 1 year. Results suggest that the LUCAS-2 device is dominated by manual CPR, with an incremental cost of £2376.40 and an incremental QALY of –0.1286. This finding is robust to a range of sensitivity analyses as shown in Table 24.
Analysis | Incremental | ICER | |
---|---|---|---|
Cost (£) | QALY | ||
Base-case analysis (aged 60 years cohort) | 2376.40 | –0.1286 | Manual CPR dominates |
One-way sensitivity analyses | |||
Sensitivity to costs | |||
+20% of costs | 2851.60 | –0.1286 | Manual CPR dominates |
–20% of costs | 1901.10 | –0.1286 | Manual CPR dominates |
Sensitivity to QALY | |||
+20% of QALY | 2376.40 | –0.1543 | Manual CPR dominates |
–20% of QALY | 2376.40 | –0.1029 | Manual CPR dominates |
Sensitivity to 1-year mortality | |||
+20% 1-year mortality | –3987.50 | –0.0187 | £213,014 per QALY |
–20% 1-year mortality | 10,603.80 | –0.2401 | Manual CPR dominates |
Figure 16 shows the results from the probabilistic sensitivity analysis that takes parameter uncertainty into account. The CEAC indicates that the probability that the LUCAS-2 device is cost-effective is only around 20%, irrespective of the value of λ.
FIGURE 16.
Cost-effectiveness acceptability curves for the LUCAS-2 device compared with manual CPR.

Chapter 6 Discussion
Summary of main results
This pragmatic, cluster randomised trial found that the introduction of the LUCAS-2 device into routine ambulance service use did not improve outcomes after OHCA. There was no improvement in survival to 30 days or survival with a CPC score of 1 or 2. We did not identify any treatment-modifying variables, although there was a suggestion that the LUCAS-2 device might be more effective in patients with initial rhythms of PEA or asystole. In a systematic review, including the five existing trials of mechanical chest compression, there was no evidence of an advantage to mechanical chest compression for any outcomes, including survival and survival with good neurological function.
The findings of worse neurological outcomes and lower survival in patients presenting with an initially shockable rhythm was unexpected. Although these analyses were defined a priori, they were not the primary objective of the trial and should be interpreted with caution and deemed as hypothesis generating. One of these hypotheses is that interruptions in CPR during device deployment could cause reduced cardiac and cerebral perfusion. Alternatively, slightly more patients received adrenaline after randomisation in the LUCAS-2 group than in the control group, which might increase cardiac instability and impair cerebral microcirculation. Finally, deployment of the LUCAS-2 device before the first shock is likely to have led to a delay in the time to first shock, which might, in itself, reduce survival. 19
Strengths and limitations
This was a pragmatic study designed to assess the effectiveness of the LUCAS-2 device when implemented in a real-life setting. The intention of the trial was to test the LUCAS-2 device in an environment that was similar to the introduction of a new technology into the NHS.
We chose to use a cluster randomised design with vehicles as the unit of randomisation. The major advantages of this design were that it made implementation of the trial for participating ambulance service staff as simple as possible and it allowed inclusion of eligible cardiac arrests because recruitment was not dependent on a paramedic making a decision to randomise. This meant that one of the major potential drawbacks of cluster randomisation – selection bias – was avoided. Similarly, the design allowed recruitment to proceed quickly, and we successfully recruited nearly 4500 patients in 38 months. Selection bias could still be possible with this design, if there was a lower threshold for initiation of resuscitation if a LUCAS-2 device was present, although this potential bias could occur with any design and is not limited to cluster randomisation. The independent DMC monitored possible selection biases throughout the trial, by looking at the proportions of patients resuscitated when the LUCAS-2 device and control vehicles were first on scene and the characteristics of patients recruited to the two trial groups. There was no evidence of any substantial selection bias.
Our approach to training staff in use of the new technology was pragmatic and reflected the training that would be delivered when rolling out new technology across UK ambulance services. The training package was developed and delivered by experienced ambulance training staff. In the UK, the average ambulance paramedic encounters only one or two cardiac arrests per year and CPR update training is provided annually, so it is unlikely that, if the LUCAS-2 device was introduced into routine service, individuals would have extensive opportunities to practice with it and become expert in its use. The success of implementation is particularly important when considering the potential benefits and harms of mechanical chest compression, as interruptions in CPR and delays in device deployment are a major factor that can impact on outcomes.
Non-compliance in the LUCAS-2 arm was a major feature of this study: only 985 (60%) of 1652 patients randomly assigned to the LUCAS-2 group received mechanical chest compression. We expected that the LUCAS-2 device would not be used in approximately 15% of cases. These were situations in which use of the LUCAS-2 device would be impossible or inappropriate and would occur in clinical practice as often as they occurred in the trial. This includes patients who were too large or too small for the device, cases when the device malfunctioned, situations in which physical space restrictions or constraints made the use of the LUCAS-2 device impossible and cases in which a solo responder was first on scene and did not have the LUCAS-2 device. This usually occurred because the initial emergency call was not for cardiac arrest, so the LUCAS-2 device was not part of the equipment that would be initially taken to the patient. However, a significant proportion of non-uses of the LUCAS-2 device occurred for reasons that were related to the conduct of a research project and would not arise in normal clinical practice. These included the crew not having been trained in the trial procedures the LUCAS-2 device having been removed from the vehicle and errors by the crew about the study protocol.
The sample size was increased to maintain the power of the study to compensate for non-compliance in the LUCAS-2 arm. We also performed CACE analyses to investigate whether or not non-compliance affected the estimates of the treatment effect in the primary ITT analysis. The CACE analyses suggested that, if anything, outcomes were worse if the LUCAS-2 device was actually used and did not suggest that non-compliance had obscured a treatment benefit.
We attempted to measure the quality of CPR provided during the trial resuscitation attempts, in both the manual and LUCAS-2 arms. Unfortunately, this ultimately proved impossible. Our initial plan was to download electrocardiography (ECG) recordings from defibrillators, but this proved impossible because of the different models of defibrillator in use in the four ambulance services, their different data recording capabilities and the logistics of ensuring that the recordings were downloaded and stored at the appropriate time. We also attempted to use the data automatically recorded by the LUCAS-2 devices to verify their use during resuscitations. This was unsuccessful because of the difficulty of extracting data from the devices (it could be done only at the periodic servicing points) and the difficulty of reconciling the times and dates on the LUCAS-2 device recordings with the ambulance service records of the times and dates of resuscitation attempts. The data on performance of ambulance service personnel on the manikins may not be representative of the quality of CPR under field conditions.
The economic analysis was primarily based on data that were collected alongside the trial, which improves internal validity. In addition, linkage with large administrative data sets, including the HES data, was used to obtain resource use estimates that are more accurate than those obtained using retrospective surveys of patients. Of course, the long-term decision model relied on a number of assumptions and existing evidence was scarce for some parameters. We are confident, however, that it captured the most relevant relative costs and outcomes of the LUCAS-2 device compared with manual CPR. It is worth noting that a value of information analysis was not conducted, as the cost-effectiveness results were robust to a wide range of assumptions and the uncertainty around the dominance of manual CPR over the LUCAS-2 device was low (also, the key parameter of the model – the effectiveness of LUCAS-2 – was derived from the best possible source of evidence).
Other evidence
The systematic review of mechanical chest compression included five randomised trials, involving > 10,000 participants. The combined treatment effect did not suggest benefit to mechanical chest compression and no individual study found benefit. The results for survival with good neurological outcome were heterogeneous and both CIs and prediction intervals were wide and did not rule out benefit in some trials.
One important difference between the PARAMEDIC trial73 and other industry-sponsored trials70,71 was that they included elements that differed from routine practice. These included more intensive initial and re-training, a run-in period,71 and, in one study a threshold for quality of implementation, whereby patients were excluded if the threshold was not attained.
Another key difference between this and other recent trials was the absence of CPR feedback technology in the participating ambulance services. CPR feedback devices allow the measurement and adjustment of CPR quality. 96 Although international guidelines published in 2010 suggested that the devices could be considered as part of an overall strategy to improve CPR quality,3 their adoption into clinical practice has been variable. The scarcity of this technology limited our ability to report on the quality of CPR and monitor the performance of our implementation strategy. These findings serve to highlight the potential limitations of expecting the findings from efficacy trials to translate to real-life practice, for which the same degree of rigour, attention and assessment does not apply.
One earlier mechanical chest compression trial, ASPIRE,55 found unfavourable results for survival and neurological outcome, similar to the PARAMEDIC trial. 71 It was suggested that these effects were largely due to heterogeneity of treatment effects between sites and a re-analysis97 suggested that the unfavourable outcomes may have been due to protocol changes at one trial site (of five). However, the study investigators disagreed with this interpretation. 98 The finding of a similar result on survival with good CPC score in the PARAMEDIC trial71 gives some support to this finding.
Some of the other trials were successful in collecting at least some data on the quality of CPR provided in their manual compression arms. In the CIRC trial,71 CPR quality data were collected from 96% of participants and showed compression fractions in the first 5 minutes of 79.0% (SD 12.3%) in the manual group and 74.7% (SD 12.7%) in the mechanical arm. The target compression rates in the two arms of this trial were different: 100/minute in the manual arm and 80/minute in the mechanical arm. The target was achieved more often in the manual arm: median compression rate in the manual arm was 89.9 (IQR 79.3–100.3), but in the mechanical arm it was 65.9 (IQR 61.3–70.2). In LUCAS in Cardiac Arrest (LINC),70 compression fraction was recorded from 10% of patients and was 84% in the mechanical compression group and 78% in the manual group. The ASPIRE trial55 recorded compression fraction in the first 5 minutes from 45% of the manual compression group and 52% of the mechanical compression group; it was very similar, at 0.6 (SD 0.2) in the manual arm and 0.59 (SD 0.21) in the mechanical arm. The remaining two trials did not report any information on CPR quality. Recording of CPR quality in the context of a pragmatic trial is extremely challenging and may influence the trial’s interpretation. For example, although the CIRC trial71 did not demonstrate benefit to mechanical chest compression and the trial’s formal conclusion was equivalence (albeit with very wide boundaries for the definition of equivalence), the fact that manual compression was provided well allowed the claim that the mechanical device was as good as manual compression.
Chapter 7 Conclusions
This trial and a meta-analysis of the results from RCTs that enrolled > 10,000 patients do not suggest that mechanical chest compression devices are superior to manual chest compression when used routinely during resuscitation after OHCA. An economic evaluation showed that the LUCAS-2 device was unlikely to be cost-effective. The widespread deployment of devices based on clinical effectiveness does not seem justified. It is possible that mechanical chest compression devices will continue to play a role in resuscitation, as they can deliver chest compressions when manual CPR is difficult or impossible, such as during ambulance transport, and are likely to be the best treatment option in such situations. They may also have an important role in hospitals as a bridge to advanced treatments, such as extracorporeal membrane oxygenation.
Recommendations for research
Mechanical chest compression devices have not been evaluated for CPR during ambulance transport and further research is needed to establish whether or not there is any benefit in such situations.
The use of mechanical devices for in-hospital cardiac arrest has not been adequately evaluated in randomised trials. The results of prehospital studies may not extrapolate to the in-hospital setting because of differences in the patient population and response times, so further trials in this setting may be justified.
Acknowledgements
We would like to thank Gill Price, Regional Head of Clinical Evidence, Research and Development (R&D) Manager, West Midlands Ambulance Service; Sonia Byers, R&D Manager, North East Ambulance Service; Ed England, R&D Manager, South Central Ambulance Service; Ian Teague, Head of Training, South Central Ambulance Service; Susie Hennings, Project Manager, WCTU; Dr Sarah Duggan, Unit Manager, WCTU; Vikki Gordon, Research Nurse & Follow up Co-ordinator, WCTU; Claire Daffern, Quality & Audit Manager, WCTU; Bev Hoddell, Trial Co-ordinator for North East region, WCTU; Sonia Davis, Data Entry Clerk, WCTU; Sarah Rumble, Data Entry Clerk, WCTU; Charlotte Kaye, Trainee Trial Co-ordinator, WCTU; Charlotte Kelly, Health Economist, Academic Unit of Health Economics, Leeds Institute of Health Sciences, University of Leeds; and Thomas Mars, Report Co-ordinator/Editor, WCTU.
Contributions of authors
Simon Gates (Professor of Clinical Trials) was a member of the Trial Management Group, and was involved in the conception and design of the study, the interpretation of data and writing the report.
Ranjit Lall (Principal Research Fellow, Statistics) was a member of the Trial Management Group and was involved in data analysis and writing the report.
Tom Quinn (Professor of Nursing) was a member of the Trial Management Group and was involved in the conception and design of the study and writing the report.
Charles D Deakin (Medical Director) was a member of the Trial Management Group, was lead for the South Central Ambulance Service and was involved in writing the report.
Matthew W Cooke (Professor of Emergency Medicine) was a member of the Trial Management Group and was involved in the conception and design of the study and reviewing report.
Jessica Horton (Trial Co-ordinator) was a member of the Trial Management Group and was involved in study co-ordination and reviewing the report.
Sarah E Lamb (Director of Clinical Trials Unit) was a member of the Trial Management Group and was involved in the conception and design of the study and reviewing report.
Anne-Marie Slowther (Associate Professor, Clinical Ethics) provided specialist advice in ethics and was involved in the design of the study and reviewing report.
Malcolm Woollard (Professor of Pre-hospital Care) was a member of the Trial Management Group and was involved in the conception and design of the study.
Andy Carson (Medical Director) was involved in trial management and was lead for the West Midlands Ambulance Service.
Mike Smyth (Research Fellow, Paramedic) was involved in trial co-ordination, data collection and reviewing the report.
Kate Wilson (Research Fellow, Paramedic) was involved in trial co-ordination, data collection and reviewing the report.
Garry Parcell (Research Fellow, Paramedic) was involved in trial co-ordination, data collection and reviewing the report.
Andrew Rosser (Research Fellow, Paramedic) was involved in trial co-ordination, data collection and reviewing the report.
Richard Whitfield (Director of Research and Development) was involved in design of the study and reviewing the report and was the lead for the Welsh Ambulance Service.
Amanda Williams (Research Fellow, Paramedic) was involved in trial co-ordination, data collection and reviewing the report.
Rebecca Jones (Research Fellow, Paramedic) was involved in trial co-ordination, data collection and reviewing the report.
Helen Pocock (Research Fellow, Paramedic) was involved in trial co-ordination, data collection and reviewing the report.
Nicola Brock (Research Fellow, Paramedic) was involved in trial co-ordination, data collection and reviewing the report.
John JM Black (Medical Director) was involved in trial management and leading the trial in the South Central Ambulance Service.
John Wright (Consultant, Emergency Medicine) was involved in trial management in the North East Ambulance Service.
Kyee Han (Medical Director) was involved in trial management and was lead for the North East Ambulance Service.
Gary Shaw (Research Fellow, Paramedic) was involved in trial co-ordination, data collection and reviewing the report.
Laura Blair (Research Fellow, Paramedic) was involved in trial co-ordination, data collection and reviewing the report.
Joachim Marti (Lecturer, Health Economics) was involved in the health economic evaluation and writing and reviewing the report.
Claire Hulme (Professor of Health Economics) was involved in the design of the study, supervision of the health economics and writing and reviewing report.
Chris McCabe (Professor of Health Economics) was involved in the conception and design of the study and reviewing the report.
Silviya Nikolova (Lecturer, Health Economics) was involved in the health economic evaluation and writing and reviewing the report.
Zenia Ferreira (Research Assistant, Health Economics) was involved in the health economic evaluation and reviewing the report.
Gavin D Perkins (Professor of Critical Care) was a member of the Trial Management Group and was involved in the conception and design of the study and writing and reviewing the report.
Publications
Perkins GD, Lall R, Quinn T, Deakin CD, Cooke MW, Horton J, et al. Mechanical versus manual chest compression for out-of-hospital cardiac arrest (PARAMEDIC): a pragmatic, cluster randomised controlled trial. Lancet 2015;385:947–55.
Perkins GD, Quinn T, Deakin CD, Lall R, Gates S. Mechanical chest compression in the PARAMEDIC trial – authors’ reply. Lancet 2015;386:26–7.
Gates S, Quinn T, Deakin CD, Blair L, Couper K, Perkins GD. Mechanical chest compression for out-of-hospital cardiac arrest: systematic review and meta-analysis. Resuscitation 2015;94:91–7.
Rajagopal S, Kaye CR, Lall R, Deakin CD, Gates S, Pocock H, et al. Characteristics of patients who are not resuscitated in out of hospital cardiac arrests and opportunities to improve community response to cardiac arrest. Resuscitation 2016;109:110–15.
Pocock H, Deakin CD, Quinn T, Perkins GD, Horton J, Gates S. Human factors in prehospital research: lessons from the PARAMEDIC trial. Emerg Med J 2016;33:562–8.
Marti J, Hulme C, Ferreira Z, Nikolova S, Lall R, Kaye C, et al. The cost-effectiveness of a mechanical compression device in out hospital cardiac arrest. Resuscitation 2017; in press.
Data sharing statement
All of the trial data are held in electronic anonymised form by the WCTU. Requests for access to data should be directed to the WCTU manager.
Disclaimers
This report presents independent research funded by the National Institute for Health Research (NIHR). The views and opinions expressed by authors in this publication are those of the authors and do not necessarily reflect those of the NHS, the NIHR, NETSCC, the HTA programme or the Department of Health. If there are verbatim quotations included in this publication the views and opinions expressed by the interviewees are those of the interviewees and do not necessarily reflect those of the authors, those of the NHS, the NIHR, NETSCC, the HTA programme or the Department of Health.
References
- Jacobs I, Nadkarni V, Bahr J, Berg RA, Billi JE, Bossaert L, et al. Cardiac arrest and cardiopulmonary resuscitation outcome reports: update and simplification of the Utstein templates for resuscitation registries. A statement for healthcare professionals from a task force of the International Liaison Committee on resuscitation (American Heart Association, European Resuscitation Council, Australian Resuscitation Council, New Zealand Resuscitation Council, Heart and Stroke Foundation of Canada, InterAmerican Heart Foundation, Resuscitation Council of Southern Africa). Resuscitation 2004;63:233-49. https://doi.org/10.1016/j.resuscitation.2004.09.008.
- Nichol G, Thomas E, Callaway CW, Hedges J, Powell JL, Aufderheide TP, et al. Regional variation in out-of-hospital cardiac arrest incidence and outcome. JAMA 2008;300:1423-31. https://doi.org/10.1001/jama.300.12.1423.
- Deakin CD, Nolan JP, Soar J, Sunde K, Koster RW, Smith GB, et al. European Resuscitation Council Guidelines for Resuscitation 2010 Section 4. Adult advanced life support. Resuscitation 2010;81:1305-52. https://doi.org/10.1016/j.resuscitation.2010.08.017.
- Nolan J, Soar J, Eikeland H. The chain of survival. Resuscitation 2006;71:270-1. https://doi.org/10.1016/j.resuscitation.2006.09.001.
- Sasson C, Rogers MA, Dahl J, Kellermann AL. Predictors of survival from out-of-hospital cardiac arrest: a systematic review and meta-analysis. Circ Cardiovasc Qual Outcomes 2010;3:63-81. https://doi.org/10.1161/CIRCOUTCOMES.109.889576.
- Koster RW, Baubin MA, Bossaert LL, Caballero A, Cassan P, Castren M, et al. European Resuscitation Council Guidelines for Resuscitation 2010. Section 2. Adult basic life support and use of automated external defibrillators. Resuscitation 2010;81:1277-92. https://doi.org/10.1016/j.resuscitation.2010.08.009.
- Wik L, Steen PA, Bircher NG. Quality of bystander cardiopulmonary resuscitation influences outcome after prehospital cardiac arrest. Resuscitation 1994;28:195-203. https://doi.org/10.1016/0300-9572(94)90064-7.
- Babbs CF, Voorhees WD, Fitzgerald KR, Holmes HR, Geddes LA. Relationship of blood pressure and flow during CPR to chest compression amplitude: evidence for an effective compression threshold. Ann Emerg Med 1983;12:527-32. https://doi.org/10.1016/S0196-0644(83)80290-X.
- Ornato JP, Levine RL, Young DS, Racht EM, Garnett AR, Gonzalez ER. The effect of applied chest compression force on systemic arterial pressure and end-tidal carbon dioxide concentration during CPR in human beings. Ann Emerg Med 1989;18:732-7. https://doi.org/10.1016/S0196-0644(89)80005-8.
- Edelson DP, Abella BS, Kramer-Johansen J, Wik L, Myklebust H, Barry AM, et al. Effects of compression depth and pre-shock pauses predict defibrillation failure during cardiac arrest. Resuscitation 2006;71:137-45. https://doi.org/10.1016/j.resuscitation.2006.04.008.
- Stiell IG, Brown SP, Christenson J, Cheskes S, Nichol G, Powell J, et al. What is the role of chest compression depth during out-of-hospital cardiac arrest resuscitation?. Crit Care Med 2012;40:1192-8. https://doi.org/10.1097/CCM.0b013e31823bc8bb.
- Stiell IG, Brown SP, Nichol G, Cheskes S, Vaillancourt C, Callaway CW, et al. What is the optimal chest compression depth during out-of-hospital cardiac arrest resuscitation of adult patients?. Circulation 2014;130:1962-70. https://doi.org/10.1161/CIRCULATIONAHA.114.008671.
- Abella BS, Sandbo N, Vassilatos P, Alvarado JP, O’Hearn N, Wigder HN, et al. Chest compression rates during cardiopulmonary resuscitation are suboptimal: a prospective study during in-hospital cardiac arrest. Circulation 2005;111:428-34. https://doi.org/10.1161/01.CIR.0000153811.84257.59.
- Idris AH, Guffey D, Aufderheide TP, Brown S, Morrison LJ, Nichols P, et al. Relationship between chest compression rates and outcomes from cardiac arrest. Circulation 2012;125:3004-12. https://doi.org/10.1161/CIRCULATIONAHA.111.059535.
- Idris AH, Guffey D, Pepe PE, Brown SP, Brookes SC, Callaway CW, et al. Chest compression rates and survival following out-of-hospital cardiac arrest. Crit Care Med 2015;43:840-8. https://doi.org/10.1097/CCM.0000000000000824.
- Zuercher M, Hilwig RW, Ranger-Moore J, Nysaether J, Nadkarni VM, Berg MD, et al. Leaning during chest compressions impairs cardiac output and left ventricular myocardial blood flow in piglet cardiac arrest. Crit Care Med 2010;38:1141-6. https://doi.org/10.1097/CCM.0b013e3181ce1fe2.
- Vaillancourt C, Everson-Stewart S, Christenson J, Andrusiek D, Powell J, Nichol G, et al. The impact of increased chest compression fraction on return of spontaneous circulation for out-of-hospital cardiac arrest patients not in ventricular fibrillation. Resuscitation 2011;82:1501-7. https://doi.org/10.1016/j.resuscitation.2011.07.011.
- Cheskes S, Schmicker RH, Christenson J, Salcido DD, Rea T, Powell J, et al. Perishock pause: an independent predictor of survival from out-of-hospital shockable cardiac arrest. Circulation 2011;124:58-66. https://doi.org/10.1161/CIRCULATIONAHA.110.010736.
- Valenzuela TD, Roe DJ, Cretin S, Spaite DW, Larsen MP. Estimating effectiveness of cardiac arrest interventions: a logistic regression survival model. Circulation 1997;96:3308-13. https://doi.org/10.1161/01.CIR.96.10.3308.
- Deakin CD, Morrison LJ, Morley PT, Callaway CW, Kerber RE, Kronick SL, et al. Part 8: Advanced life support: 2010 International Consensus on Cardiopulmonary Resuscitation and Emergency Cardiovascular Care Science with Treatment Recommendations. Resuscitation 2010;81:e93-174. https://doi.org/10.1016/j.resuscitation.2010.08.027.
- Stiell IG, Nichol G, Leroux BG, Rea TD, Ornato JP, Powell J, et al. Early versus later rhythm analysis in patients with out-of-hospital cardiac arrest. N Engl J Med 2011;365:787-97. https://doi.org/10.1056/NEJMoa1010076.
- Rea T, Prince D, Morrison L, Callaway C, Aufderheide T, Daya M, et al. Association between survival and early versus later rhythm analysis in out-of-hospital cardiac arrest: do agency-level factors influence outcomes?. Ann Emerg Med 2014;64:1-8. https://doi.org/10.1016/j.annemergmed.2014.01.014.
- Nolan JP, Neumar RW, Adrie C, Aibiki M, Berg RA, Bottiger BW, et al. Post-cardiac arrest syndrome: epidemiology, pathophysiology, treatment, and prognostication. A Scientific Statement from the International Liaison Committee on Resuscitation; the American Heart Association Emergency Cardiovascular Care Committee; the Council on Cardiovascular Surgery and Anesthesia; the Council on Cardiopulmonary, Perioperative, and Critical Care; the Council on Clinical Cardiology; the Council on Stroke. Resuscitation 2008;79:350-79. https://doi.org/10.1016/j.resuscitation.2008.09.017.
- Perkins GD, Cooke MW. Variability in cardiac arrest survival: the NHS Ambulance Service Quality Indicators. Emerg Med J 2012;29:3-5. https://doi.org/10.1136/emermed-2011-200758.
- Soar J, Monsieurs KG, Ballance JH, Barelli A, Biarent D, Greif R, et al. European Resuscitation Council Guidelines for Resuscitation 2010. Section 9. Principles of Education in Resuscitation. Resuscitation 2010;81:1434-44. https://doi.org/10.1016/j.resuscitation.2010.08.014.
- Elliott VJ, Rodgers DL, Brett SJ. Systematic review of quality of life and other patient-centred outcomes after cardiac arrest survival. Resuscitation 2011;82:247-56. https://doi.org/10.1016/j.resuscitation.2010.10.030.
- Ornato JP, Becker LB, Weisfeldt ML, Wright BA. Cardiac arrest and resuscitation: an opportunity to align research prioritization and public health need. Circulation 2010;122:1876-9. https://doi.org/10.1161/CIRCULATIONAHA.110.963991.
- Gates S, Smith JL, Ong GJ, Brace SJ, Perkins GD. Effectiveness of the LUCAS device for mechanical chest compression after cardiac arrest: systematic review of experimental, observational and animal studies. Heart 2012;98:908-13. https://doi.org/10.1136/heartjnl-2011-301571.
- Axelsson C, Nestin J, Svensson L, Axelsson AB, Herlitz J. Clinical consequences of the introduction of mechanical chest compression in the EMS system for treatment of out-of-hospital cardiac arrest-a pilot study. Resuscitation 2006;71:47-55. https://doi.org/10.1016/j.resuscitation.2006.02.011.
- De Wilde R, van der Weijden P, de Haan M, Bosch J, de Nooij J, Harinck HIJ. ROSC at hospital admission in out of hospital cardiac arrest using LUCAS. Resuscitation 2008;77.
- Maule Y. Mechanical external chest compression: a new adjuvant technology in cardiopulmonary resuscitation. Urgences &Amp; Accueil 2007;7:4-7.
- Smekal D, Johansson J, Huzevka T, Rubertsson S. A pilot study of mechanical chest compressions with the LUCAS™ device in cardiopulmonary resuscitation. Resuscitation 2011;82:702-6. https://doi.org/10.1016/j.resuscitation.2011.01.032.
- Liao Q, Sjöberg T, Paskevicius A, Wolfnart B, Steen S. Manual versus mechanichal cardiopulmonary resuscitation. An experimental study in pigs. BMC Cardiovasc Disord 2010;10.
- Rubertsson S, Karlsten R. Increased cortical cerebral flow with LUCAS; a new device for mechanical chest compressions compared to standard external compressions during experimental cardiopulmonary resuscitation. Resuscitation 2005;65:357-63.
- Steen S, Liao Q, Pierre L, Paskevicius A, Sjöberg T. Evaluation of LUCAS, a new device for automatic mechanical compression and active decompression resuscitation. Resuscitation 2002;55:285-99. https://doi.org/10.1016/S0300-9572(02)00271-X.
- Olasveengen TM, Wik S, Steen PA. Quality of cardiopulmonary resuscitation before and during transport in out-of-hospital cardiac arrest. Resuscitation 2007;76:185-90.
- Truhlar A, Hejna P, Zabka L, Zatopkova L, Cerny V. Injuries caused by the autopulse and LUCAS II resuscitation systems compared to manual chest compressions. Resuscitation 2010;81.
- Steen S, Sjöberg T, Olsson P, Young M. Treatment of out-of-hospital cardiac arrest with LUCAS, a new device for automatic mechanical compression and active decompression resuscitation. Resuscitation 2005;67:25-30. https://doi.org/10.1016/j.resuscitation.2005.05.013.
- Woollard M, Mackway-Jones K. All that glistens is not gold. Emerg Med J 2008;25:251-2. https://doi.org/10.1136/emj.2008.057711.
- Joint Royal College Ambulance Liaison Committee (JRCALC) . Mechanical Compression: Request for Advice on Continued But Limited and Centrally Supervised Deployment by Ambulance Trusts That Are Currently Using LUCAS or AutoPulse Devices n.d. www.jrcalc.org.uk/wp-content/uploads/2013/11/LUCASForMedDirectors.pdf (accessed 20 January 2016).
- Gazmuri RJ, Nolan JP, Nadkarni VM, Arntz HR, Billi JE, Bossaert L, et al. Scientific knowledge gaps and clinical research priorities for cardiopulmonary resuscitation and emergency cardiovascular care identified during the 2005 International Consensus Conference on ECC and CPR Science with Treatment Recommendations. A consensus statement from the International Liaison Committee on Resuscitation; the American Heart Association Emergency Cardiovascular Care Committee; the Stroke Council; and the Cardiovascular Nursing Council. Resuscitation 2007;75:400-11. https://doi.org/10.1016/j.resuscitation.2007.09.008.
- Perkins GD, Woollard M, Cooke MW, Deakin C, Horton J, Lall R, et al. Prehospital randomised assessment of a mechanical compression device in cardiac arrest (PaRAMeDIC) trial protocol. Scand J Trauma Resusc Emerg Med 2010;18. https://doi.org/10.1186/1757-7241-18-58.
- Short Form 12 Health Survey, 1992–2002. Hanover, NH: Medical Outcomes Trust and Quality Metric Inc.; 2002.
- EuroQol Group . EuroQol: a new facility for the measurement of health-related quality of life. Health Policy 1990;16:199-208. https://doi.org/10.1016/0168-8510(90)90421-9.
- Safar P, Grenvik A, Safar P. Brain Failure and Resuscitation. New York, NY: Churchill Livingstone; 1981.
- Folstein MF, Folstein SE, McHugh PR. “Mini-mental State”. A practical method for grading the cognitive state of patients for the clinician. J Psychiatr Res 1975;12:189-98. https://doi.org/10.1016/0022-3956(75)90026-6.
- Zigmond AS, Snaith RP. The hospital anxiety and depression scale. Acta Psychiatr Scand 1983;67:361-70. https://doi.org/10.1111/j.1600-0447.1983.tb09716.x.
- Weathers F, Litz BT, Huska JA, Keane TM. PTSD Checklist Civilian Version. Boston, MA: National Center for PTSD Behavioral Science Division; 1994.
- Langhelle A, Nolan J, Herlitz J, Castren M, Wenzel V, Soreide E, et al. Recommended guidelines for reviewing, reporting, and conducting research on post-resuscitation care: the Utstein style. Resuscitation 2005;66:271-83. https://doi.org/10.1016/j.resuscitation.2005.06.005.
- Arawwawala D, Brett SJ. Clinical review: beyond immediate survival from resuscitation-long-term outcome considerations after cardiac arrest. Crit Care 2007;11. https://doi.org/10.1186/cc6139.
- O’Reilly SM, Grubb N, O’Carroll RE. Long-term emotional consequences of in-hospital cardiac arrest and myocardial infarction. Br J Clin Psychol 2004;43:83-95. https://doi.org/10.1348/014466504772812986.
- Sunnerhagen KS, Johansson O, Herlitz J, Grimby G. Life after cardiac arrest; a retrospective study. Resuscitation 1996;31:135-40. https://doi.org/10.1016/0300-9572(95)00903-5.
- Fisher JD, Brown SN, Cooke MW. UK Ambulance Service Clinical Practice Guidelines 2006. London: Joint Royal Colleges Ambulance Liaison Committee; 2006.
- Mental Capacity Act. London: HMSO; 2005.
- Nolan JP, Laver SR, Welch CA, Harrison DA, Gupta V, Rowan K. Outcome following admission to UK intensive care units after cardiac arrest: a secondary analysis of the ICNARC Case Mix Programme Database. Anaesthesia 2007;62:1207-16. https://doi.org/10.1111/j.1365-2044.2007.05232.x.
- Griffiths JA, Morgan K, Barber VS, Young JD. Study protocol: the Intensive Care Outcome Network (‘ICON’) study. BMC Health Serv Res 2008;8. https://doi.org/10.1186/1472-6963-8-132.
- PARAMEDIC – University of Warwick . PARAMEDIC Trial Procedures n.d. www.warwick.ac.uk/go/paramedic (accessed 20 January 2016).
- Resuscitation Council (UK) . Guidelines 2010 n.d. https://www.resus.org.uk/archive/guidelines-2010/ (accessed 20 January 2016).
- National Research Ethics Service (NRES) . Progress and Safety Reporting – Health Research Authority n.d. www.hra.nhs.uk/resources/during-and-after-your-study/progress-and-safety-reporting/ (accessed 20 January 2016).
- Data Protection Act. London: Stationery Office; 1998.
- Warwick Clinical Trials Unit (WCTU) . Standard Operating Procedures n.d. www2.warwick.ac.uk/fac/med/research/hscience/ctu/conducting/ (accessed 20 January 2016).
- Lafuente-Lafuente C, Melero-Bascones M. Active chest compression-decompression for cardiopulmonary resuscitation. Cochrane Database Syst Rev 2013;2. https://doi.org/10.1002/14651858.cd002751.pub3.
- Donohoe R, Mawson S. London Ambulance Service. Cardiac Arrest Annual Report 2006/2007. London: London Ambulance Service NHS Trust; 2007.
- Ambulance Service Association/Joint Royal Colleges Ambulance Liaison Committee (ASA/JRCALC) . National Out of Hospital CarDiac Arrest Project 2006.
- Pell JP, Sirel J, Marsden AK, Cobbe SM. Seasonal variations in out of hospital cardiopulmonary arrest. Heart 1999;82:680-3. https://doi.org/10.1136/hrt.82.6.680.
- Dunn G, Maracy M, Dowrick C, Ayuso-Mateos JL, Dalgard OS, Page H, et al. Estimating psychological treatment effects from a randomised controlled trial with both non-compliance and loss to follow-up. Br J Psychiatry 2003;183:323-31. https://doi.org/10.1192/bjp.183.4.323.
- Hewitt CE, Torgerson DJ, Miles JN. Is there another way to take account of noncompliance in randomized controlled trials?. CMAJ 2006;175. https://doi.org/10.1503/cmaj.051625.
- Royston P, Sauerbrei W. A new approach to modelling interactions between treatment and continuous covariates in clinical trials by using fractional polynomials. Stat Med 2004;23:2509-25. https://doi.org/10.1002/sim.1815.
- Research Governance Framework for Health and Social Care: Second Edition. London: Department of Health; 2005.
- Rubertsson S, Lindgren E, Smekal D, Ostlund O, Silfverstolpe J, Lichtveld RA, et al. Mechanical chest compressions and simultaneous defibrillation vs conventional cardiopulmonary resuscitation in out-of-hospital cardiac arrest: the LINC randomised trial. JAMA 2014;311:53-61. https://doi.org/10.1001/jama.2013.282538.
- Wik L, Olsen JA, Persse D, Sterz F, Lozano M, Brouwer MA, et al. Manual vs. integrated automatic load-distributing band CPR with equal survival after out of hospital cardiac arrest. The randomised CIRC trial. Resuscitation 2014;85:741-8. https://doi.org/10.1016/j.resuscitation.2014.03.005.
- Hallstrom A, Rea TD, Sayre MR, Christenson J, Anton AR, Mosesso VN, et al. Manual chest compression vs use of an automated chest compression device during resuscitation following out-of-hospital cardiac arrest: a randomized trial. JAMA 2006;295:2620-8. https://doi.org/10.1001/jama.295.22.2620.
- Perkins GD, Lall R, Quinn T, Deakin CD, Cooke MW, Horton J, et al. Mechanical versus manual chest compression for out-of-hospital cardiac arrest (PARAMEDIC): a pragmatic, cluster randomised controlled trial. Lancet 2015;385:947-55. https://doi.org/10.1016/S0140-6736(14)61886-9.
- Brooks SC, Hassan N, Bigham BL, Morrison LJ. Mechanical versus manual chest compressions for cardiac arrest. Cochrane Database Syst Rev 2014;2. https://doi.org/10.1002/14651858.cd007260.pub3.
- Perkins GD, Jacobs IG, Nadkarni VM, Berg RA, Bhanji F, Biarent D, et al. Cardiac arrest and cardiopulmonary resuscitation outcome reports: update of the Utstein Resuscitation Registry Templates for Out-of-Hospital Cardiac Arrest: a statement for healthcare professionals from a task force of the International Liaison Committee on Resuscitation (American Heart Association, European Resuscitation Council, Australian and New Zealand Council on Resuscitation, Heart and Stroke Foundation of Canada, InterAmerican Heart Foundation, Resuscitation Council of Southern Africa, Resuscitation Council of Asia); and the American Heart Association Emergency Cardiovascular Care Committee and the Council on Cardiopulmonary, Critical Care, Perioperative and Resuscitation. Resuscitation 2015;132:1286-300. https://doi.org/10.1161/cir.0000000000000144.
- Riley RD, Higgins JP, Deeks JJ. Interpretation of random effects meta-analyses. BMJ 2011;342. https://doi.org/10.1136/bmj.d549.
- Lerner EB, Persse D, Souders CM, Sterz F, Malzer R, Lozano M, et al. Design of the Circulation Improving Resuscitation Care (CIRC) trial: a new state of the art design for out-of-hospital cardiac arrest research. Resuscitation 2011;82:294-9. https://doi.org/10.1016/j.resuscitation.2010.11.013.
- Whitehead J, Brunier H. The double triangular test: a sequential test for the two-sided alternative with early stopping under the null hypothesis. Sequential Analysis 1990;9:117-36. https://doi.org/10.1080/07474949008836200.
- National Institute for Health and Care Excellence (NICE) . Guide to the Methods of Technology Appraisal 2013. https://publications.nice.org.uk/pmg9 (accessed 20 January 2016).
- Kaarlola A, Pettilä V, Kekki P. Performance of two measures of general health-related quality of life, the EQ-5D and the RAND-36 among critically ill patients. Intensive Care Med 2004;30:2245-52. https://doi.org/10.1007/s00134-004-2471-6.
- Dolan P. Modeling valuations for EuroQol health states. Med Care 1997;35:1095-108. https://doi.org/10.1097/00005650-199711000-00002.
- Thalanany MM, Mugford M, Hibbert C, Cooper NJ, Truesdale A, Robinson S, et al. Methods of data collection and analysis for the economic evaluation alongside a national, multi-centre trial in the UK: conventional ventilation or ECMO for Severe Adult Respiratory Failure (CESAR). BMC Health Serv Res 2008;8. https://doi.org/10.1186/1472-6963-8-94.
- Vainiola T, Roine RP, Pettilä V, Kantola T, Räsänen P, Sintonen H. Effect of health-related quality-of-life instrument and quality-adjusted life year calculation method on the number of life years gained in the critical care setting. Value Health 2011;14:1130-4. https://doi.org/10.1016/j.jval.2011.05.047.
- Ferguson ND, Scales DC, Pinto R, Wilcox ME, Cook DJ, Guyatt GH, et al. Integrating mortality and morbidity outcomes: using quality-adjusted life years in critical care trials. Am J Respir Crit Care Med 2013;187:256-61. https://doi.org/10.1164/rccm.201206-1057OC.
- Department of Health (DH) . NHS Reference Costs 2013–14 n.d. https://www.gov.uk/government/publications/nhs-reference-costs-2013-to-2014 (accessed 20 January 2016).
- Curtis L. Unit Costs of Health and Social Care 2012 2012. www.pssru.ac.uk/project-pages/unit-costs/2012/ (accessed 20 January 2016).
- Rubin DB. Multiple imputation after 18+ years. J Am Stat Assoc 1996;91:473-89. https://doi.org/10.1080/01621459.1996.10476908.
- Drummond MF, Sculpher MJ, Torrance GW, O’Brian BJ, Stoddart GL. Methods for the Economic Evaluation of Health Care Programmes. New York, NY: Oxford University Press; 2005.
- Van Hout BA, Al MJ, Gordon GS, Rutten FF. Costs, effects and C/E-ratios alongside a clinical trial. Health Econ 1994;3:309-19. https://doi.org/10.1002/hec.4730030505.
- Fenwick E, O’Brien BJ, Briggs A. Cost-effectiveness acceptability curves: facts, fallacies and frequently asked questions. Health Econ 2004;13:405-15. https://doi.org/10.1002/hec.903.
- Sonnenberg FA, Beck JR. Markov models in medical decision making: a practical guide. Med Decis Making 1993;13:322-38. https://doi.org/10.1177/0272989X9301300409.
- Office for National Statistics (ONS) . Historic and Projected Mortality Data (1951–2060) from the UK Life Tables, 2010-Based 2012. www.ons.gov.uk/ons/publications/re-reference-tables.html?edition=tcm%3A77-257453 (accessed 20 January 2016).
- Phelps R, Dumas F, Maynard C, Silver J, Rea T. Cerebral Performance Category and long-term prognosis following out-of-hospital cardiac arrest. Crit Care Med 2013;41:1252-7. https://doi.org/10.1097/CCM.0b013e31827ca975.
- Stinnet A, Mullahy J. Net health benefits: a new framework for the analysis of uncertainty in cost-effectiveness analysis. Med Decis Making 1998;18:S68-80. https://doi.org/10.1177/0272989X98018002S09.
- Ramsey S, Willke R, Briggs A, Brown R, Buxton M, Chawla A, et al. Good research practices for cost-effectiveness analysis alongside clinical trials: the ISPOR RCT-CEA Task Force report. Value Health 2005;8:521-33. https://doi.org/10.1111/j.1524-4733.2005.00045.x.
- Yeung J, Meeks R, Edelson D, Gao F, Soar J, Perkins GD. The use of CPR feedback/prompt devices during training and CPR performance: a systematic review. Resuscitation 2009;80:743-51. https://doi.org/10.1016/j.resuscitation.2009.04.012.
- Paradis NA, Young G, Lemeshow S, Brewer JE, Halperin HR. Inhomogeneity and temporal effects in AutoPulse Assisted Prehospital International Resuscitation: an exception from consent trial terminated early. Am J Emerg Med 2010;28:391-8. https://doi.org/10.1016/j.ajem.2010.02.002.
- Hallstrom A, Rea TD, Sayre MR, Christenson J, Cobb L, Mosesso VN, et al. The AutoPulse Assisted Prehospital International Resuscitation (ASPIRE) trial investigators respond to inhomogeneity and temporal effects assertions. Am J Emerg Med 2010;28:973-6. https://doi.org/10.1016/j.ajem.2010.07.001.
Appendix 1 In-hospital data fields
Length of stay | ||
Sedation status first 24 hours Lowest temperature first 24 hours (surrogate for therapeutic hypothermia) Number of days of basic cardiovascular, respiratory, organ support Number of days advanced cardiovascular, respiratory, renal, GI, neurological, dermatological, liver organ support provided Treatment withdrawn status Referral for organ donation Actual organ donation |
||
ICNARC CASE Mix – code | ||
Date of admission | DAH | |
Residence prior to admission to acute hospital | RESA | |
Age | DOB | |
Admission type | PA _V3 | |
Levels of care | Level 3 days | CCLOD |
Level 2 days | CCL2D | |
Level 1 days | CCL ID | |
Level 0 days | CCLOD | |
Date of ultimate discharge from ICU/HDU | DUDICU | |
Date of discharge from your hospital | DDH | |
Status at discharge from your hospital | HDIS | |
Destination post discharge from your hospital | DESTH V3 | |
Residence post discharge from hospital | RESD | |
Classification of surgery | To identify any procedures | CLASSNS |
Date of death | DOD | |
Date of ultimate discharge from hospital | (If transferred to another hospital) | DUDH |
Status at ultimate discharge from hospital | (If transferred to another hospital) | UHDIS |
Date of first critical care visit post discharge from your unit | DFCCD | |
Critical care visit post discharge from your unit | CCD |
Hospital Episode Statistics: PARAMEDIC – Data Extract Request [V1.0: October 2012 (CK) – updated March 2013 (CK)]
Data required for study participants for 12 months following randomisation.
Hospital Episode Statistics: inpatient
Data fields | Description |
---|---|
Admissions | |
Date of admission (admidate) | This field contains the date on which the patient was admitted to hospital at the start of a hospital spell; admidate is recorded on all episodes within a spell |
Method of admission (admimeth) | This field contains a code that identifies how the patient was admitted to hospital; admimeth is recorded on the first and also all subsequent episodes within the spell (i.e. where the spell is made up of more than one episode) |
Source of admission (admisorc) | This field contains a code that identifies where the patient was immediately prior to admission. Most patients are admitted from home, but there are some significant exceptions. In particular, this field differentiates between patients admitted from home and patients transferred from another hospital provider or institution |
Augmented/critical care period | |
ACP end date (acpend) | This field gives the end date of a period of augmented care |
ACP start date (acpstar) | This field states the start date of a period of augmented care |
High-dependency care level (depdays) | This field contains the number of days of high-dependency care in a period of augmented care |
Intensive care level days (intdays) | This field contains the number of days of intensive care in a period of augmented care |
Number of ACPs within episode (numacp) | This derived field gives the number of ACPs within episode |
Clinical | |
All diagnosis codes (diag_nn) | There are 20 fields (14 before April 2007 and seven before April 2002), diag_01 to diag_20, which contain information about a patient’s illness or condition. The field diag_01 contains the primary diagnosis. The other fields contain secondary/subsidiary diagnoses. The codes are defined in the ICD-10 |
All operative procedure codes (oper_nn) | There are 24 fields (12 before April 2007 and four prior to April 2002), oper_01 to oper_4, which contain information about a patient’s operations. The field oper_01 contains the main (i.e. most resource intensive) procedure. The other fields contain secondary procedures. The codes are defined in the tabular list of the Classification of Surgical Operations and Procedures; the current version is OPCS-4. Procedure codes start with a letter and are followed by two or three digits. The third digit identifies variations on a main procedure code containing two digits. The third digit is preceded by a full stop in OPCS4, but this is not stored in the field. A single operation may contain more than one procedure |
Patient classification (classpat) | This field identifies day cases, ordinary admissions, regular day and regular night attenders |
Discharges | |
Date of discharge (disdate) | This field contains the date on which the patient was discharged from hospital. It is present in the record only for the last episode of a spell |
Destination on discharge (disdest) | This field contains a code that identifies where the patient was due to go on leaving hospital. In most cases patients return home. For many patients the discharge destination is the same as source of admission (admisorc) |
Method of discharge (dismeth) | This field contains a code that defines the circumstances under which a patient left hospital. For the majority of patients this is when they are discharged by the consultant. This field is completed only for the last episode in a spell |
Episodes and spells | |
Date episode ended (epiend) | This field contains the date on which a patient left the care of a particular consultant |
Date episode started (epistart) | This field contains the date on which a patient was under the care of a particular consultant. If a patient has more than one episode in a spell, for each new episode there is a new value of epistart. However, the admission date that is copied to each new episode in a spell will remain unchanged and will be equal to the episode start date of the first episode in hospital |
Episode duration (epidur) | This field contains the difference in days between the episode start date (epistart) and the episode end date (epiend). If the episode is unfinished epidur is set as null |
Episode order (epiorder) | This field contains the number of the episode within the current spell. All spells start with an episode where epiorder is 01. Many spells finish with this episode, but if the patient moves to the care of another consultant then a new episode begins. Episode numbers increase by 1 for each new episode until the patient is discharged (this includes transfers to another NHS trust or PCT, i.e. the first episode in the new trust will have epiorder 01). If the same patient returns for a different spell in hospital, epiorder is again set to 01 |
Episode status (epistat) | This field tells you whether or not the episode had finished before the end of the HES data-year (i.e. whether the episode was still ‘live’ at midnight on 31 March). Because hospital providers are advised not to include clinical data (diagnosis and operation codes) in unfinished records, these are normally excluded from analyses. Also, if unfinished episodes are included in time series analyses – when data for more than 1 year are involved – there is a danger of counting the same episode twice |
HRGs | |
HES-generated HRG version (hrg_n.n) | This HES-derived field contains HRG values. HES adds the two most recent versions of HRG codes to records |
NHS-generated HRG code (hrgnhs) | The trust-derived HRG value as submitted to SUS takes into account the dominant grouping procedure (domproc) and may differ from the HES-derived HRG (HRG_n.n) |
Dominant procedure (domproc) | Contains the dominant procedure (operation) code assigned as part of the (NHS) HRG derivation process and submitted to SUS |
Patient | |
Age at start of episode (startage) | This derived field, calculated from episode start date (epistart) and date of birth (dob), contains the patient’s age in whole years [from 1 to 115 (1990–1 to 1994–5) and from 1 to 120 (1995–6 onwards)] |
Patient identifier – HES-generated (pseudo_hesid) | This field uniquely identifies a patient across all data-years. It is generated by matching records for the same patient using a combination of NHS number, local patient identifier, postcode, sex and date of birth |
Sex of patient (sex) | This field contains a code that defines the sex of the patient |
Socioeconomic | |
IMD decile group (md04_decile) | This field uses the IMD overall ranking to identify which 1 of 10 groups a super output area belongs to, from most deprived through to least deprived |
System | |
SUS record ID (susrecid) | SUS-generated record identifier |
Hospital Episode Statistics: outpatient
Data fields | Description |
---|---|
Appointments | |
Appointment date (apptdate) | The date when an appointment was scheduled |
First attendance (firstatt) | Indicates whether a patient is making a first attendance or follow-up attendance, and whether the consultation was face to face or via telephone |
Attendance type (atentype) | A field derived from ‘first appointment’ (firstatt) and ‘attended or did not attend’ (attended), used to identify if the attendance occurred and whether it was the first or subsequent |
Attended or did not attend (attended) | This indicates whether or not a patient attended for an appointment. If the patient did not attend it also indicates whether or not advanced warning was given |
Clinical | |
Diagnosis (diag_nn) | There are 12 fields (two before April 2007), diag_01 to diag_12, which contain information about a patient’s illness or condition. The field diag_01 contains the primary diagnosis. The other fields contain secondary/subsidiary diagnoses. The codes are defined in the International Statistical Classification of Diseases, Injuries and Causes of Death |
Main specialty (mainspef) | A code that defines the specialty under which the consultant is contracted. Compare with ‘treatment specialty’ (tretspef), the specialty under which the consultant worked |
Treatment specialty (tretspef) | This field contains a code that defines the specialty in which the consultant was working during the period of care. It can be compared with mainspef, the specialty under which the consultant is contracted. From April 2004, a new list of treatment specialties was introduced (see below). The new list describes the specialised service within which the patient was treated |
Medical staff type seeing patient (stafftyp) | Gives information about the type of care professional staff dealing with the patient during a consultant outpatient attendance, or nurse or midwife contact |
HRGs | |
NHS-generated HRG version no. (hrgnhsvn) | The version number for NHS-generated HRG code (hrgnhs) |
NHS-generated HRG code (hrgnhs) | The NHS-generated HRG code takes into account the dominant grouping procedure (domproc) and may differ from the HES-derived HRG (hrgorig) |
Patient | |
Patient identifier – HES-generated (pseudo_hesid) | This field uniquely identifies a patient across all data-years. It is generated by matching records for the same patient using a combination of NHS number, local patient identifier, postcode, sex and date of birth |
Sex of patient (sex) | This field contains a code which defines the sex of the patient |
Socioeconomic | |
IMD decile group (md04_decile) | This field uses the IMD overall ranking to identify which 1 of 10 groups a super output area belongs to, from most deprived to least deprived |
System | |
SUS record ID (susrecid) | SUS-generated record identifier |
Hospital Episode Statistics: accident and emergency
Data fields | Description |
---|---|
Attendances | |
Arrival mode (aearrivalmode) | The mode by which a patient arrived at an A&E department |
Arrival date (arrivaldate) | The arrival date of a patient in the A&E department |
Attendance category (aeattendcat) | An indication of whether a patient is making an initial or follow-up attendance within a particular A&E department:
|
Attendance disposal (aeattenddisp) | The way in which an A&E attendance might end |
Department type (aedepttype) | A classification of A&E department type according to the activity carried out |
Clinical | |
A&E diagnosis (diag n) | The A&E diagnosis code recorded for an A&E attendance. The CDS allows an unlimited number of diagnoses to be submitted; however, only the first 12 diagnoses are available within HES. The A&E diagnosis is a six-character code: diagnosis condition (n2), subanalysis (n1), anatomical area (n2) and anatomical side (anl) |
A&E investigation (invest n) | The A&E investigation recorded for an A&E attendance. The CDS allows an unlimited number of investigations to be submitted, however, only the first 12 investigations are available within HES. The A&E investigation is a six character code made up of investigation (n2) and local sub-analysis (up to an4). As the subanalysis is for local use, it cannot be classified |
A&E treatment (treat n) | The A&E treatment recorded for an A&E attendance. The CDS allows an unlimited number of treatments to be submitted; however, only the first 12 treatments are available within HES. The A&E treatment is a six-character code made up of treatment (n2), subanalysis (n1) and a local use section (up to an3). As the local use section is used for local codes, it cannot be classified |
HRGs | |
Dominant procedure (domproc) | The procedure that the HRG grouping algorithm has identified as having the greatest effect upon the resources consumed by a patient |
Trust HRG value (hrgnhs) | The trust-generated HRG code |
Trust HRG version (hrgnhsvn) | The version number for trust-generated HRG code (hrgnhs) |
Patient | |
Age on arrival (arrivalage) | This derived field, calculated from arrival date and date of birth |
Patient identifier – HES generated (pseudo_hesid) |
This field uniquely identifies a patient across all data-years. It is generated by matching records for the same patient using a combination of NHS number, local patient identifier, postcode, sex and date of birth |
Sex of patient (sex) | This field contains a code which defines the sex of the patient |
Residence | |
Patient’s PCT of residence (respct06) | This derived field contains the code for the PCT for the area in which the patient lived immediately before admission. It is derived from the patient’s postcode in the field homeadd |
Socioeconomic | |
IMD decile group (md04_decile) | This field uses the IMD overall ranking to identify which 1 of 10 groups a super output area belongs to, from most deprived to least deprived |
System | |
Record identifier | This is a record identifier that is created by the system. The eight digits store a decimal number |
SUS record ID (susrecid) | SUS-generated record identifier |
Treatment | |
Health authority of treatment (hatreat) | Health authority of treatment. This field is derived from the hospital provider code (procode). It indicates the health authority within which the treatment took place |
PCT of treatment (pcttreat) | PCT of treatment. It is derived from the main site postcode of the hospital provider code (procode), indicating the PCT area within which the organisation that was providing the treatment was located Note: (1) The PCT itself may be the provider of the treatment and (2) care provided at subsidiary sites will be attributed to the main trust location |
Appendix 2 Training documents
Appendix 3 Event reporting
Appendix 4 Case report forms
Appendix 5 Three-month follow-up questionnaires
SF-12 forms have been redacted for copyright reasons.
Appendix 6 Twelve-month follow-up questionnaires
SF-12 forms have been redacted for copyright reasons.
HADS forms have been redacted for copyright reasons.
MMSE forms have been redacted for copyright reasons.
Appendix 7 Data management plan
Appendix 8 Statistical analysis plan
Appendix 9 Systematic review search strategy
MEDLINE search strategy
-
exp Heart Arrest/
-
exp Death, Sudden/
-
cardiac arrest.tw.
-
heart arrest.tw.
-
cardiopulmonary arrest.tw.
-
sudden cardiac death$.tw.
-
sudden death$.tw.
-
or/1-7
-
exp Cardiopulmonary Resuscitation/
-
Heart Massage/
-
cpr.tw.
-
cardiopulmonary resuscitation.tw.
-
chest compression$.tw.
-
resuscitat$.tw.
-
or/9-14
-
Cardiopulmonary Resuscitation/is [Instrumentation]
-
autopulse.tw.
-
auto-pulse.tw.
-
thumper.tw.
-
lucas.tw.
-
hands-free.tw.
-
(pneumatic adj10 (pump or device)).tw.
-
(pneumatic adj10 compression$).tw.
-
(automat$ adj10 compression$).tw.
-
(device$ adj10 compression).tw.
-
(mechanical adj10 compression$).tw.
-
(machine$ adj10 compression$).tw.
-
piston$.tw.
-
load distributing.tw.
-
(vest adj10 compression).tw.
-
(mechanical adj10 cpr).tw.
-
(pneumatic adj10 cpr).tw.
-
(device adj10 cpr).tw.
-
(machine$ adj10 cpr).tw.
-
(vest adj10 cpr).tw.
-
or/16-35
-
8 and 15 and 36
-
randomised controlled trial.pt.
-
controlled clinical trial.pt.
-
randomized.ab.
-
placebo.ab.
-
drug therapy.fs.
-
randomly.ab.
-
trial.ab.
-
groups.ab.
-
38 or 39 or 40 or 41 or 42 or 43 or 44 or 45
-
exp animals/ not humans.sh.
-
46 not 47
-
37 and 48
Appendix 10 End-of-study information sheet
List of abbreviations
- A&E
- accident and emergency
- ADE
- adverse device effect/event
- AE
- adverse event
- ALS
- advanced life support
- ASPIRE
- AutoPulse Assisted Prehospital International Resuscitation
- AUC
- area under the curve
- CACE
- complier average causal effect
- CE
- Conformité Européenne
- CEAC
- cost-effectiveness acceptability curve
- CI
- confidence interval
- CIRC
- Circulation Improving Resuscitation Care
- CPC
- Cerebral Performance Category
- CPR
- cardiopulmonary resuscitation
- CRF
- case report form
- DMC
- Data Monitoring Committee
- ECG
- electrocardiography
- EMS
- emergency medical services
- EQ-5D
- EuroQol-5 Dimensions
- GP
- general practitioner
- HADS
- Hospital Anxiety and Depression Scale
- HES
- Hospital Episode Statistics
- HRQL
- health-related quality of life
- ICC
- intracluster correlation coefficient
- ICER
- incremental cost-effectiveness ratio
- ICNARC
- Intensive Care National Audit and Research Centre
- ICU
- intensive care unit
- ITT
- intention to treat
- JRCALC
- Joint Royal College Ambulance Liaison Committee
- LUCAS-2
- Lund University Cardiopulmonary Assistance System-2
- MHRA
- Medicines and Healthcare products Regulatory Agency
- MI
- multiple imputation
- MMSE
- Mini Mental State Examination
- MRIS
- Medical Research Information Service
- mRS
- modified Rankin Scale
- NICE
- National Institute for Health and Care Excellence
- NIHR
- National Institute for Health Research
- NMB
- net monetary benefit
- OHCA
- out-of-hospital cardiac arrest
- ONS
- Office for National Statistics
- OR
- odds ratio
- PARAMEDIC
- prehospital randomised assessment of a mechanical compression device in out-of-hospital cardiac arrest
- PCL-C
- Post-Traumatic Stress Disorder Civilian Checklist
- PEA
- pulseless electrical activity
- PRF
- patient report form
- PSS
- Personal Social Services
- PTSD
- post-traumatic stress disorder
- QALY
- quality-adjusted life-year
- RCT
- randomised controlled trial
- REC
- Research Ethics Committee
- ROLE
- Recognition of Life Extinction
- ROSC
- return of spontaneous circulation
- RR
- risk ratio
- RRV
- rapid response vehicle
- SADE
- serious adverse device effect/event
- SAE
- serious adverse event
- SD
- standard deviation
- SF-12
- Short Form questionnaire-12 items
- TSC
- Trial Steering Committee
- VF
- ventricular fibrillation
- VT
- ventricular tachycardia
- WCTU
- Warwick Clinical Trials Unit